- Technical Support
- Find My Rep
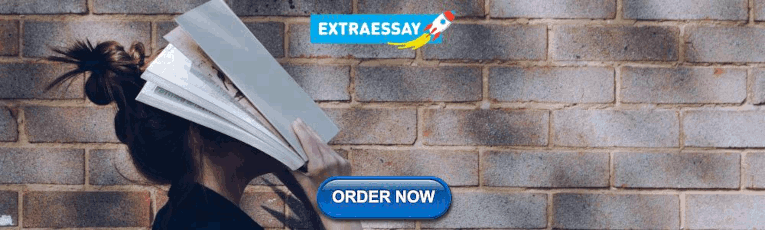
You are here
The SAGE Handbook of Quantitative Methodology for the Social Sciences
- David Kaplan - University of Wisconsin - Madison, USA
- Description
Click 'Additional Materials' for downloadable samples "The 24 chapters in this Handbook span a wide range of topics, presenting the latest quantitative developments in scaling theory, measurement, categorical data analysis, multilevel models, latent variable models, and foundational issues. Each chapter reviews the historical context for the topic and then describes current work, including illustrative examples where appropriate. The level of presentation throughout the book is detailed enough to convey genuine understanding without overwhelming the reader with technical material. Ample references are given for readers who wish to pursue topics in more detail. The book will appeal to both researchers who wish to update their knowledge of specific quantitative methods, and students who wish to have an integrated survey of state-of- the-art quantitative methods." —Roger E. Millsap, Arizona State University "This handbook discusses important methodological tools and topics in quantitative methodology in easy to understand language. It is an exhaustive review of past and recent advances in each topic combined with a detailed discussion of examples and graphical illustrations. It will be an essential reference for social science researchers as an introduction to methods and quantitative concepts of great use." —Irini Moustaki, London School of Economics, U.K.
"David Kaplan and SAGE Publications are to be congratulated on the development of a new handbook on quantitative methods for the social sciences. The Handbook is more than a set of methodologies, it is a journey. This methodological journey allows the reader to experience scaling, tests and measurement, and statistical methodologies applied to categorical, multilevel, and latent variables. The journey concludes with a number of philosophical issues of interest to researchers in the social sciences. The new Handbook is a must purchase." —Neil H. Timm, University of Pittsburgh The SAGE Handbook of Quantitative Methodology for the Social Sciences is the definitive reference for teachers, students, and researchers of quantitative methods in the social sciences, as it provides a comprehensive overview of the major techniques used in the field. The contributors, top methodologists and researchers, have written about their areas of expertise in ways that convey the utility of their respective techniques, but, where appropriate, they also offer a fair critique of these techniques. Relevance to real-world problems in the social sciences is an essential ingredient of each chapter and makes this an invaluable resource.
The handbook is divided into six sections:
• Scaling • Testing and Measurement • Models for Categorical Data • Models for Multilevel Data • Models for Latent Variables • Foundational Issues
These sections, comprising twenty-four chapters, address topics in scaling and measurement, advances in statistical modeling methodologies, and broad philosophical themes and foundational issues that transcend many of the quantitative methodologies covered in the book.
The Handbook is indispensable to the teaching, study, and research of quantitative methods and will enable readers to develop a level of understanding of statistical techniques commensurate with the most recent, state-of-the-art, theoretical developments in the field. It provides the foundations for quantitative research, with cutting-edge insights on the effectiveness of each method, depending on the data and distinct research situation.
See what’s new to this edition by selecting the Features tab on this page. Should you need additional information or have questions regarding the HEOA information provided for this title, including what is new to this edition, please email [email protected] . Please include your name, contact information, and the name of the title for which you would like more information. For information on the HEOA, please go to http://ed.gov/policy/highered/leg/hea08/index.html .
For assistance with your order: Please email us at [email protected] or connect with your SAGE representative.
SAGE 2455 Teller Road Thousand Oaks, CA 91320 www.sagepub.com
“The 24 chapters in this Handbook span a wide range of topics, presenting the latest quantitative developments in scaling theory, measurement, categorical data analysis, multilevel models, latent variable models, and foundational issues. Each chapter reviews the historical context for the topic and then describes current work, including illustrative examples where appropriate. The level of presentation throughout the book is detailed enough to convey genuine understanding without overwhelming the reader with technical material. Ample references are given for readers who wish to pursue topics in more detail. The book will appeal to both researchers who wish to update their knowledge of specific quantitative methods, and students who wish to have an integrated survey of state-of- the-art quantitative methods.”
“This handbook discusses important methodological tools and topics in quantitative methodology in easy to understand language. It is an exhaustive review of past and recent advances in each topic combined with a detailed discussion of examples and graphical illustrations. It will be an essential reference for social science researchers as an introduction to methods and quantitative concepts of great use.”
“David Kaplan and SAGE Publications are to be congratulated on the development of a new handbook on quantitative methods for the social sciences. The Handbook is more than a set of methodologies, it is a journey. This methodological journey allows the reader to experience scaling, tests and measurement, and statistical methodologies applied to categorical, multilevel, and latent variables. The journey concludes with a number of philosophical issues of interest to researchers in the social sciences. The new Handbook is a must purchase.”
"David Kaplan has convened a panel of top-notch methodologians, who take on the challenge in the writing of The SAGE Handbook of Quantitative Methodology for the Social Sciences (SHQM). The result is an engrossing collection of chapters that are sure to add screwdrivers, wrenches, and the occasional buzzsaw to your toolbox. A notable strength of the SHQM is the generally structure of each chapter. The chapters of the SHQM are a worthy accomplishment. The SHQM is both well conceived and well executed, providing the reader with numerous insights and a broader sense for the available tools of the quantitative methodological trade. It is most likely that few readers will have the opportunity to read this book from cover to cover, but should they feel so inspired, they will find the effort both rewarding and thought provoking."
"The Handbook provides an excellent introduction to broad range of state-of-the-art quantitative methods applicable to the social sciences. It shows the breadth and depth of advanced quantitative methods used by social scientists from numerous interrelated disciplines, it is rich with examples of real-world applications of these methods, and it provides suggestions for further readings and study in these areas. It is well worth reading cover-to-cover, and it is a very useful addition to the reference libraries of all quantitative social scientists, applied statisticians, and graduate students."
- Provides a comprehensive overview of the major techniques used in the field.
- Top methodologists and researchers have written about their areas of expertise
- Relevance to real-world problems in the social sciences is an essential ingredient of each chapter and makes this an invaluable resource.
- Indispensable to the teaching, study, and research of quantitative methods.
- Provides the foundations for quantitative research, with cutting-edge insights on the effectiveness of each method.
Sample Materials & Chapters
Chapter 1. Dual Scaling
Chapter 3. Principal Components Analysis with Nonlinear Optimal Scaling Transfo
Chapter 5. Test Modeling
Select a Purchasing Option

This title is also available on SAGE Knowledge , the ultimate social sciences online library. If your library doesn’t have access, ask your librarian to start a trial .

This title is also available on SAGE Research Methods , the ultimate digital methods library. If your library doesn’t have access, ask your librarian to start a trial .
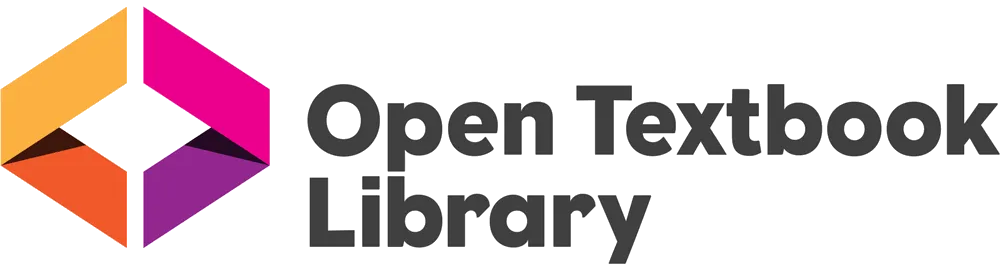
A Quick Guide to Quantitative Research in the Social Sciences
(12 reviews)

Christine Davies, Carmarthen, Wales
Copyright Year: 2020
Last Update: 2021
Publisher: University of Wales Trinity Saint David
Language: English
Formats Available
Conditions of use.

Learn more about reviews.

Reviewed by Jennifer Taylor, Assistant Professor, Texas A&M University-Corpus Christi on 4/18/24
This resource is a quick guide to quantitative research in the social sciences and not a comprehensive resource. It provides a VERY general overview of quantitative research but offers a good starting place for students new to research. It... read more
Comprehensiveness rating: 4 see less
This resource is a quick guide to quantitative research in the social sciences and not a comprehensive resource. It provides a VERY general overview of quantitative research but offers a good starting place for students new to research. It offers links and references to additional resources that are more comprehensive in nature.
Content Accuracy rating: 4
The content is relatively accurate. The measurement scale section is very sparse. Not all types of research designs or statistical methods are included, but it is a guide, so details are meant to be limited.
Relevance/Longevity rating: 4
The examples were interesting and appropriate. The content is up to date and will be useful for several years.
Clarity rating: 5
The text was clearly written. Tables and figures are not referenced in the text, which would have been nice.
Consistency rating: 5
The framework is consistent across chapters with terminology clearly highlighted and defined.
Modularity rating: 5
The chapters are subdivided into section that can be divided and assigned as reading in a course. Most chapters are brief and concise, unless elaboration is necessary, such as with the data analysis chapter. Again, this is a guide and not a comprehensive text, so sections are shorter and don't always include every subtopic that may be considered.
Organization/Structure/Flow rating: 5
The guide is well organized. I appreciate that the topics are presented in a logical and clear manner. The topics are provided in an order consistent with traditional research methods.
Interface rating: 5
The interface was easy to use and navigate. The images were clear and easy to read.
Grammatical Errors rating: 5
I did not notice any grammatical errors.
Cultural Relevance rating: 5
The materials are not culturally insensitive or offensive in any way.
I teach a Marketing Research course to undergraduates. I would consider using some of the chapters or topics included, especially the overview of the research designs and the analysis of data section.
Reviewed by Tiffany Kindratt, Assistant Professor, University of Texas at Arlington on 3/9/24
The text provides a brief overview of quantitative research topics that is geared towards research in the fields of education, sociology, business, and nursing. The author acknowledges that the textbook is not a comprehensive resource but offers... read more
Comprehensiveness rating: 3 see less
The text provides a brief overview of quantitative research topics that is geared towards research in the fields of education, sociology, business, and nursing. The author acknowledges that the textbook is not a comprehensive resource but offers references to other resources that can be used to deepen the knowledge. The text does not include a glossary or index. The references in the figures for each chapter are not included in the reference section. It would be helpful to include those.
Overall, the text is accurate. For example, Figure 1 on page 6 provides a clear overview of the research process. It includes general definitions of primary and secondary research. It would be helpful to include more details to explain some of the examples before they are presented. For instance, the example on page 5 was unclear how it pertains to the literature review section.
In general, the text is relevant and up-to-date. The text includes many inferences of moving from qualitative to quantitative analysis. This was surprising to me as a quantitative researcher. The author mentions that moving from a qualitative to quantitative approach should only be done when needed. As a predominantly quantitative researcher, I would not advice those interested in transitioning to using a qualitative approach that qualitative research would enhance their research—not something that should only be done if you have to.
Clarity rating: 4
The text is written in a clear manner. It would be helpful to the reader if there was a description of the tables and figures in the text before they are presented.
Consistency rating: 4
The framework for each chapter and terminology used are consistent.
Modularity rating: 4
The text is clearly divided into sections within each chapter. Overall, the chapters are a similar brief length except for the chapter on data analysis, which is much more comprehensive than others.
Organization/Structure/Flow rating: 4
The topics in the text are presented in a clear and logical order. The order of the text follows the conventional research methodology in social sciences.
I did not encounter any interface issues when reviewing this text. All links worked and there were no distortions of the images or charts that may confuse the reader.
Grammatical Errors rating: 3
There are some grammatical/typographical errors throughout. Of note, for Section 5 in the table of contents. “The” should be capitalized to start the title. In the title for Table 3, the “t” in typical should be capitalized.
Cultural Relevance rating: 4
The examples are culturally relevant. The text is geared towards learners in the UK, but examples are relevant for use in other countries (i.e., United States). I did not see any examples that may be considered culturally insensitive or offensive in any way.
I teach a course on research methods in a Bachelor of Science in Public Health program. I would consider using some of the text, particularly in the analysis chapter to supplement the current textbook in the future.
Reviewed by Finn Bell, Assistant Professor, University of Michigan, Dearborn on 1/3/24
For it being a quick guide and only 26 pages, it is very comprehensive, but it does not include an index or glossary. read more
For it being a quick guide and only 26 pages, it is very comprehensive, but it does not include an index or glossary.
Content Accuracy rating: 5
As far as I can tell, the text is accurate, error-free and unbiased.
Relevance/Longevity rating: 5
This text is up-to-date, and given the content, unlikely to become obsolete any time soon.
The text is very clear and accessible.
The text is internally consistent.
Given how short the text is, it seems unnecessary to divide it into smaller readings, nonetheless, it is clearly labelled such that an instructor could do so.
The text is well-organized and brings readers through basic quantitative methods in a logical, clear fashion.
Easy to navigate. Only one table that is split between pages, but not in a way that is confusing.
There were no noticeable grammatical errors.
The examples in this book don't give enough information to rate this effectively.
This text is truly a very quick guide at only 26 double-spaced pages. Nonetheless, Davies packs a lot of information on the basics of quantitative research methods into this text, in an engaging way with many examples of the concepts presented. This guide is more of a brief how-to that takes readers as far as how to select statistical tests. While it would be impossible to fully learn quantitative research from such a short text, of course, this resource provides a great introduction, overview, and refresher for program evaluation courses.
Reviewed by Shari Fedorowicz, Adjunct Professor, Bridgewater State University on 12/16/22
The text is indeed a quick guide for utilizing quantitative research. Appropriate and effective examples and diagrams were used throughout the text. The author clearly differentiates between use of quantitative and qualitative research providing... read more
Comprehensiveness rating: 5 see less
The text is indeed a quick guide for utilizing quantitative research. Appropriate and effective examples and diagrams were used throughout the text. The author clearly differentiates between use of quantitative and qualitative research providing the reader with the ability to distinguish two terms that frequently get confused. In addition, links and outside resources are provided to deepen the understanding as an option for the reader. The use of these links, coupled with diagrams and examples make this text comprehensive.
The content is mostly accurate. Given that it is a quick guide, the author chose a good selection of which types of research designs to include. However, some are not provided. For example, correlational or cross-correlational research is omitted and is not discussed in Section 3, but is used as a statistical example in the last section.
Examples utilized were appropriate and associated with terms adding value to the learning. The tables that included differentiation between types of statistical tests along with a parametric/nonparametric table were useful and relevant.
The purpose to the text and how to use this guide book is stated clearly and is established up front. The author is also very clear regarding the skill level of the user. Adding to the clarity are the tables with terms, definitions, and examples to help the reader unpack the concepts. The content related to the terms was succinct, direct, and clear. Many times examples or figures were used to supplement the narrative.
The text is consistent throughout from contents to references. Within each section of the text, the introductory paragraph under each section provides a clear understanding regarding what will be discussed in each section. The layout is consistent for each section and easy to follow.
The contents are visible and address each section of the text. A total of seven sections, including a reference section, is in the contents. Each section is outlined by what will be discussed in the contents. In addition, within each section, a heading is provided to direct the reader to the subtopic under each section.
The text is well-organized and segues appropriately. I would have liked to have seen an introductory section giving a narrative overview of what is in each section. This would provide the reader with the ability to get a preliminary glimpse into each upcoming sections and topics that are covered.
The book was easy to navigate and well-organized. Examples are presented in one color, links in another and last, figures and tables. The visuals supplemented the reading and placed appropriately. This provides an opportunity for the reader to unpack the reading by use of visuals and examples.
No significant grammatical errors.
The text is not offensive or culturally insensitive. Examples were inclusive of various races, ethnicities, and backgrounds.
This quick guide is a beneficial text to assist in unpacking the learning related to quantitative statistics. I would use this book to complement my instruction and lessons, or use this book as a main text with supplemental statistical problems and formulas. References to statistical programs were appropriate and were useful. The text did exactly what was stated up front in that it is a direct guide to quantitative statistics. It is well-written and to the point with content areas easy to locate by topic.
Reviewed by Sarah Capello, Assistant Professor, Radford University on 1/18/22
The text claims to provide "quick and simple advice on quantitative aspects of research in social sciences," which it does. There is no index or glossary, although vocabulary words are bolded and defined throughout the text. read more
The text claims to provide "quick and simple advice on quantitative aspects of research in social sciences," which it does. There is no index or glossary, although vocabulary words are bolded and defined throughout the text.
The content is mostly accurate. I would have preferred a few nuances to be hashed out a bit further to avoid potential reader confusion or misunderstanding of the concepts presented.
The content is current; however, some of the references cited in the text are outdated. Newer editions of those texts exist.
The text is very accessible and readable for a variety of audiences. Key terms are well-defined.
There are no content discrepancies within the text. The author even uses similarly shaped graphics for recurring purposes throughout the text (e.g., arrow call outs for further reading, rectangle call outs for examples).
The content is chunked nicely by topics and sections. If it were used for a course, it would be easy to assign different sections of the text for homework, etc. without confusing the reader if the instructor chose to present the content in a different order.
The author follows the structure of the research process. The organization of the text is easy to follow and comprehend.
All of the supplementary images (e.g., tables and figures) were beneficial to the reader and enhanced the text.
There are no significant grammatical errors.
I did not find any culturally offensive or insensitive references in the text.
This text does the difficult job of introducing the complicated concepts and processes of quantitative research in a quick and easy reference guide fairly well. I would not depend solely on this text to teach students about quantitative research, but it could be a good jumping off point for those who have no prior knowledge on this subject or those who need a gentle introduction before diving in to more advanced and complex readings of quantitative research methods.
Reviewed by J. Marlie Henry, Adjunct Faculty, University of Saint Francis on 12/9/21
Considering the length of this guide, this does a good job of addressing major areas that typically need to be addressed. There is a contents section. The guide does seem to be organized accordingly with appropriate alignment and logical flow of... read more
Considering the length of this guide, this does a good job of addressing major areas that typically need to be addressed. There is a contents section. The guide does seem to be organized accordingly with appropriate alignment and logical flow of thought. There is no glossary but, for a guide of this length, a glossary does not seem like it would enhance the guide significantly.
The content is relatively accurate. Expanding the content a bit more or explaining that the methods and designs presented are not entirely inclusive would help. As there are different schools of thought regarding what should/should not be included in terms of these designs and methods, simply bringing attention to that and explaining a bit more would help.
Relevance/Longevity rating: 3
This content needs to be updated. Most of the sources cited are seven or more years old. Even more, it would be helpful to see more currently relevant examples. Some of the source authors such as Andy Field provide very interesting and dynamic instruction in general, but they have much more current information available.
The language used is clear and appropriate. Unnecessary jargon is not used. The intent is clear- to communicate simply in a straightforward manner.
The guide seems to be internally consistent in terms of terminology and framework. There do not seem to be issues in this area. Terminology is internally consistent.
For a guide of this length, the author structured this logically into sections. This guide could be adopted in whole or by section with limited modifications. Courses with fewer than seven modules could also logically group some of the sections.
This guide does present with logical organization. The topics presented are conceptually sequenced in a manner that helps learners build logically on prior conceptualization. This also provides a simple conceptual framework for instructors to guide learners through the process.
Interface rating: 4
The visuals themselves are simple, but they are clear and understandable without distracting the learner. The purpose is clear- that of learning rather than visuals for the sake of visuals. Likewise, navigation is clear and without issues beyond a broken link (the last source noted in the references).
This guide seems to be free of grammatical errors.
It would be interesting to see more cultural integration in a guide of this nature, but the guide is not culturally insensitive or offensive in any way. The language used seems to be consistent with APA's guidelines for unbiased language.
Reviewed by Heng Yu-Ku, Professor, University of Northern Colorado on 5/13/21
The text covers all areas and ideas appropriately and provides practical tables, charts, and examples throughout the text. I would suggest the author also provides a complete research proposal at the end of Section 3 (page 10) and a comprehensive... read more
The text covers all areas and ideas appropriately and provides practical tables, charts, and examples throughout the text. I would suggest the author also provides a complete research proposal at the end of Section 3 (page 10) and a comprehensive research study as an Appendix after section 7 (page 26) to help readers comprehend information better.
For the most part, the content is accurate and unbiased. However, the author only includes four types of research designs used on the social sciences that contain quantitative elements: 1. Mixed method, 2) Case study, 3) Quasi-experiment, and 3) Action research. I wonder why the correlational research is not included as another type of quantitative research design as it has been introduced and emphasized in section 6 by the author.
I believe the content is up-to-date and that necessary updates will be relatively easy and straightforward to implement.
The text is easy to read and provides adequate context for any technical terminology used. However, the author could provide more detailed information about estimating the minimum sample size but not just refer the readers to use the online sample calculators at a different website.
The text is internally consistent in terms of terminology and framework. The author provides the right amount of information with additional information or resources for the readers.
The text includes seven sections. Therefore, it is easier for the instructor to allocate or divide the content into different weeks of instruction within the course.
Yes, the topics in the text are presented in a logical and clear fashion. The author provides clear and precise terminologies, summarizes important content in Table or Figure forms, and offers examples in each section for readers to check their understanding.
The interface of the book is consistent and clear, and all the images and charts provided in the book are appropriate. However, I did encounter some navigation problems as a couple of links are not working or requires permission to access those (pages 10 and 27).
No grammatical errors were found.
No culturally incentive or offensive in its language and the examples provided were found.
As the book title stated, this book provides “A Quick Guide to Quantitative Research in Social Science. It offers easy-to-read information and introduces the readers to the research process, such as research questions, research paradigms, research process, research designs, research methods, data collection, data analysis, and data discussion. However, some links are not working or need permissions to access them (pages 10 and 27).
Reviewed by Hsiao-Chin Kuo, Assistant Professor, Northeastern Illinois University on 4/26/21, updated 4/28/21
As a quick guide, it covers basic concepts related to quantitative research. It starts with WHY quantitative research with regard to asking research questions and considering research paradigms, then provides an overview of research design and... read more
As a quick guide, it covers basic concepts related to quantitative research. It starts with WHY quantitative research with regard to asking research questions and considering research paradigms, then provides an overview of research design and process, discusses methods, data collection and analysis, and ends with writing a research report. It also identifies its target readers/users as those begins to explore quantitative research. It would be helpful to include more examples for readers/users who are new to quantitative research.
Its content is mostly accurate and no bias given its nature as a quick guide. Yet, it is also quite simplified, such as its explanations of mixed methods, case study, quasi-experimental research, and action research. It provides resources for extended reading, yet more recent works will be helpful.
The book is relevant given its nature as a quick guide. It would be helpful to provide more recent works in its resources for extended reading, such as the section for Survey Research (p. 12). It would also be helpful to include more information to introduce common tools and software for statistical analysis.
The book is written with clear and understandable language. Important terms and concepts are presented with plain explanations and examples. Figures and tables are also presented to support its clarity. For example, Table 4 (p. 20) gives an easy-to-follow overview of different statistical tests.
The framework is very consistent with key points, further explanations, examples, and resources for extended reading. The sample studies are presented following the layout of the content, such as research questions, design and methods, and analysis. These examples help reinforce readers' understanding of these common research elements.
The book is divided into seven chapters. Each chapter clearly discusses an aspect of quantitative research. It can be easily divided into modules for a class or for a theme in a research method class. Chapters are short and provides additional resources for extended reading.
The topics in the chapters are presented in a logical and clear structure. It is easy to follow to a degree. Though, it would be also helpful to include the chapter number and title in the header next to its page number.
The text is easy to navigate. Most of the figures and tables are displayed clearly. Yet, there are several sections with empty space that is a bit confusing in the beginning. Again, it can be helpful to include the chapter number/title next to its page number.
Grammatical Errors rating: 4
No major grammatical errors were found.
There are no cultural insensitivities noted.
Given the nature and purpose of this book, as a quick guide, it provides readers a quick reference for important concepts and terms related to quantitative research. Because this book is quite short (27 pages), it can be used as an overview/preview about quantitative research. Teacher's facilitation/input and extended readings will be needed for a deeper learning and discussion about aspects of quantitative research.
Reviewed by Yang Cheng, Assistant Professor, North Carolina State University on 1/6/21
It covers the most important topics such as research progress, resources, measurement, and analysis of the data. read more
It covers the most important topics such as research progress, resources, measurement, and analysis of the data.
The book accurately describes the types of research methods such as mixed-method, quasi-experiment, and case study. It talks about the research proposal and key differences between statistical analyses as well.
The book pinpointed the significance of running a quantitative research method and its relevance to the field of social science.
The book clearly tells us the differences between types of quantitative methods and the steps of running quantitative research for students.
The book is consistent in terms of terminologies such as research methods or types of statistical analysis.
It addresses the headlines and subheadlines very well and each subheading should be necessary for readers.
The book was organized very well to illustrate the topic of quantitative methods in the field of social science.
The pictures within the book could be further developed to describe the key concepts vividly.
The textbook contains no grammatical errors.
It is not culturally offensive in any way.
Overall, this is a simple and quick guide for this important topic. It should be valuable for undergraduate students who would like to learn more about research methods.
Reviewed by Pierre Lu, Associate Professor, University of Texas Rio Grande Valley on 11/20/20
As a quick guide to quantitative research in social sciences, the text covers most ideas and areas. read more
As a quick guide to quantitative research in social sciences, the text covers most ideas and areas.
Mostly accurate content.
As a quick guide, content is highly relevant.
Succinct and clear.
Internally, the text is consistent in terms of terminology used.
The text is easily and readily divisible into smaller sections that can be used as assignments.
I like that there are examples throughout the book.
Easy to read. No interface/ navigation problems.
No grammatical errors detected.
I am not aware of the culturally insensitive description. After all, this is a methodology book.
I think the book has potential to be adopted as a foundation for quantitative research courses, or as a review in the first weeks in advanced quantitative course.
Reviewed by Sarah Fischer, Assistant Professor, Marymount University on 7/31/20
It is meant to be an overview, but it incredibly condensed and spends almost no time on key elements of statistics (such as what makes research generalizable, or what leads to research NOT being generalizable). read more
It is meant to be an overview, but it incredibly condensed and spends almost no time on key elements of statistics (such as what makes research generalizable, or what leads to research NOT being generalizable).
Content Accuracy rating: 1
Contains VERY significant errors, such as saying that one can "accept" a hypothesis. (One of the key aspect of hypothesis testing is that one either rejects or fails to reject a hypothesis, but NEVER accepts a hypothesis.)
Very relevant to those experiencing the research process for the first time. However, it is written by someone working in the natural sciences but is a text for social sciences. This does not explain the errors, but does explain why sometimes the author assumes things about the readers ("hail from more subjectivist territory") that are likely not true.
Clarity rating: 3
Some statistical terminology not explained clearly (or accurately), although the author has made attempts to do both.
Very consistently laid out.
Chapters are very short yet also point readers to outside texts for additional information. Easy to follow.
Generally logically organized.
Easy to navigate, images clear. The additional sources included need to linked to.
Minor grammatical and usage errors throughout the text.
Makes efforts to be inclusive.
The idea of this book is strong--short guides like this are needed. However, this book would likely be strengthened by a revision to reduce inaccuracies and improve the definitions and technical explanations of statistical concepts. Since the book is specifically aimed at the social sciences, it would also improve the text to have more examples that are based in the social sciences (rather than the health sciences or the arts).
Reviewed by Michelle Page, Assistant Professor, Worcester State University on 5/30/20
This text is exactly intended to be what it says: A quick guide. A basic outline of quantitative research processes, akin to cliff notes. The content provides only the essentials of a research process and contains key terms. A student or new... read more
This text is exactly intended to be what it says: A quick guide. A basic outline of quantitative research processes, akin to cliff notes. The content provides only the essentials of a research process and contains key terms. A student or new researcher would not be able to use this as a stand alone guide for quantitative pursuits without having a supplemental text that explains the steps in the process more comprehensively. The introduction does provide this caveat.
Content Accuracy rating: 3
There are no biases or errors that could be distinguished; however, it’s simplicity in content, although accurate for an outline of process, may lack a conveyance of the deeper meanings behind the specific processes explained about qualitative research.
The content is outlined in traditional format to highlight quantitative considerations for formatting research foundational pieces. The resources/references used to point the reader to literature sources can be easily updated with future editions.
The jargon in the text is simple to follow and provides adequate context for its purpose. It is simplified for its intention as a guide which is appropriate.
Each section of the text follows a consistent flow. Explanation of the research content or concept is defined and then a connection to literature is provided to expand the readers understanding of the section’s content. Terminology is consistent with the qualitative process.
As an “outline” and guide, this text can be used to quickly identify the critical parts of the quantitative process. Although each section does not provide deeper content for meaningful use as a stand alone text, it’s utility would be excellent as a reference for a course and can be used as an content guide for specific research courses.
The text’s outline and content are aligned and are in a logical flow in terms of the research considerations for quantitative research.
The only issue that the format was not able to provide was linkable articles. These would have to be cut and pasted into a browser. Functional clickable links in a text are very successful at leading the reader to the supplemental material.
No grammatical errors were noted.
This is a very good outline “guide” to help a new or student researcher to demystify the quantitative process. A successful outline of any process helps to guide work in a logical and systematic way. I think this simple guide is a great adjunct to more substantial research context.
Table of Contents
- Section 1: What will this resource do for you?
- Section 2: Why are you thinking about numbers? A discussion of the research question and paradigms.
- Section 3: An overview of the Research Process and Research Designs
- Section 4: Quantitative Research Methods
- Section 5: the data obtained from quantitative research
- Section 6: Analysis of data
- Section 7: Discussing your Results
Ancillary Material
About the book.
This resource is intended as an easy-to-use guide for anyone who needs some quick and simple advice on quantitative aspects of research in social sciences, covering subjects such as education, sociology, business, nursing. If you area qualitative researcher who needs to venture into the world of numbers, or a student instructed to undertake a quantitative research project despite a hatred for maths, then this booklet should be a real help.
The booklet was amended in 2022 to take into account previous review comments.
About the Contributors
Christine Davies , Ph.D
Contribute to this Page
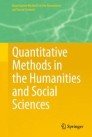
Book series
Quantitative Methods in the Humanities and Social Sciences
About this book series.
- Thomas DeFanti,
- Anthony Grafton,
- Thomas E. Levy,
- Lev Manovich,
- Alyn Rockwood
Book titles in this series
Humanities data in r.
Exploring Networks, Geospatial Data, Images, and Text
- Taylor Arnold
- Lauren Tilton
- Copyright: 2024
Available Renditions
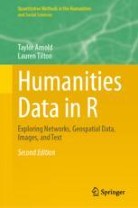
A Quantitative Portrait of Analytic Philosophy
Looking Through the Margins
- Eugenio Petrovich
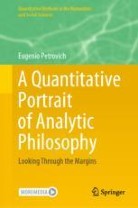
Database Computing for Scholarly Research
Case Studies Using the Online Cultural and Historical Research Environment
- Sandra R. Schloen
- Miller C. Prosser
- Copyright: 2023
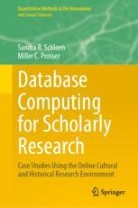
Who Wrote Citizen Kane?
Statistical Analysis of Disputed Co-Authorship
- Warren Buckland
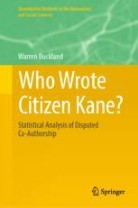
Capturing the Senses
Digital Methods for Sensory Archaeologies
- Giacomo Landeschi
- Eleanor Betts
- Open Access
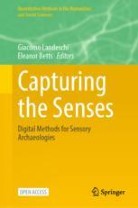
Publish with us
Advertisement
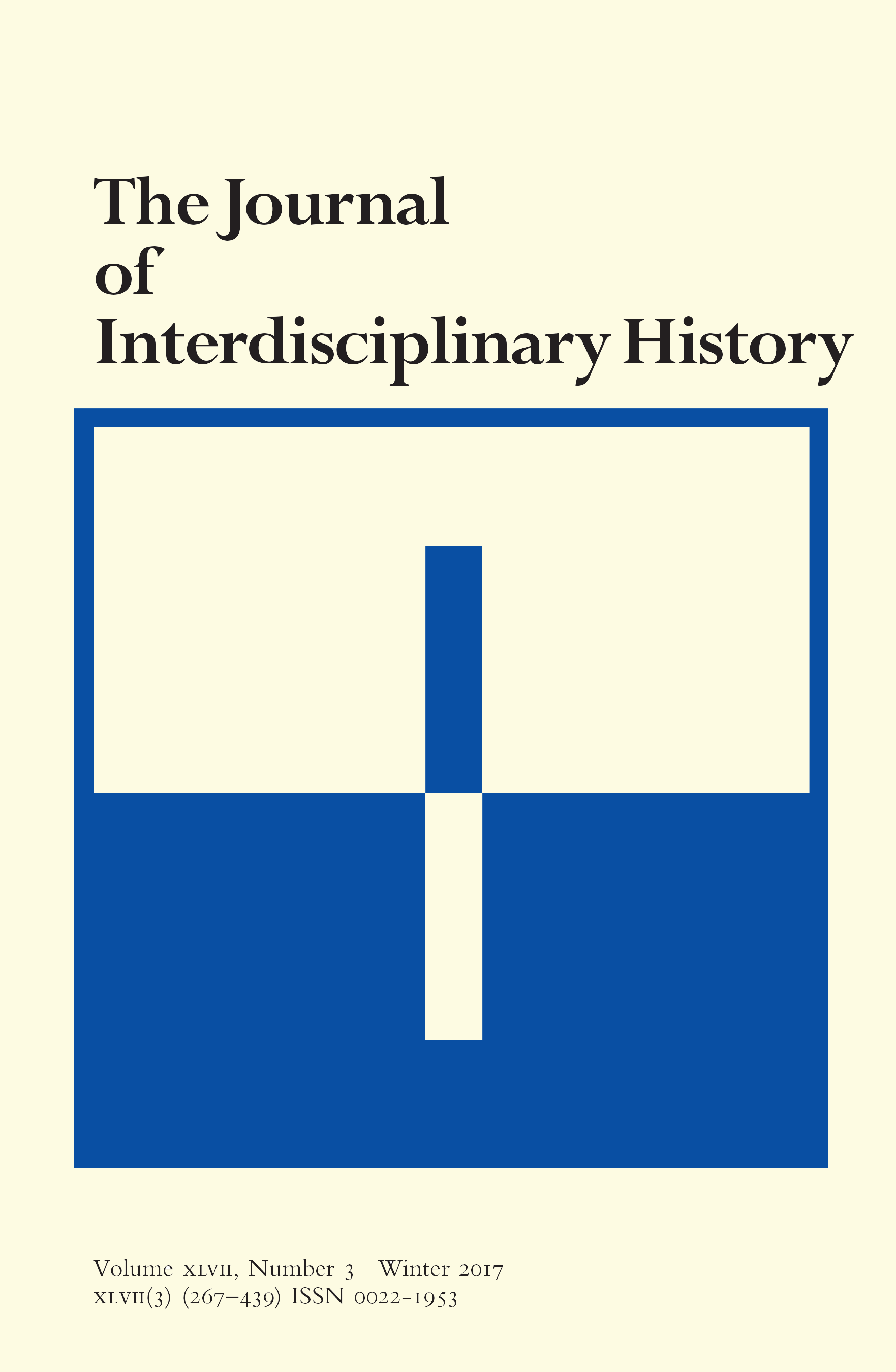
- Previous Article
- Next Article
Quantitative Methods in the Humanities: An Introduction
- Cite Icon Cite
- Permissions
- Article contents
- Figures & tables
- Supplementary Data
- Peer Review
- Search Site
A. E. C. M.; Quantitative Methods in the Humanities: An Introduction. The Journal of Interdisciplinary History 2020; 51 (1): 137–139. doi: https://doi.org/10.1162/jinh_r_01527
Download citation file:
- Ris (Zotero)
- Reference Manager
History is notoriously a “big tent” discipline. Because everything has a past, every subject has a history. The tools appropriate to ferret out those histories multiply just as easily as the topics, depending on the questions being asked and the nature of the evidence preserved (accidentally or otherwise) that might answer them. In what sense is History a coherent “discipline” at all? Is there more to hold it together than just a ferocious commitment to the past tense? Must historians adhere to a recognized and common methodology of practice, but of what might it consist, in the face of so much variety? These questions bedevil historians everywhere, especially when they are trying to figure out what their students should know and/or know how to do. Whatever the answers might be, these questions frame both the motivation for the book under review and its value for readers.
Written by two historians,...
Client Account
Sign in via your institution, email alerts, related articles, related book chapters, affiliations.
- Online ISSN 1530-9169
- Print ISSN 0022-1953
A product of The MIT Press
Mit press direct.
- About MIT Press Direct
Information
- Accessibility
- For Authors
- For Customers
- For Librarians
- Direct to Open
- Open Access
- Media Inquiries
- Rights and Permissions
- For Advertisers
- About the MIT Press
- The MIT Press Reader
- MIT Press Blog
- Seasonal Catalogs
- MIT Press Home
- Give to the MIT Press
- Direct Service Desk
- Terms of Use
- Privacy Statement
- Crossref Member
- COUNTER Member
- The MIT Press colophon is registered in the U.S. Patent and Trademark Office
This Feature Is Available To Subscribers Only
Sign In or Create an Account
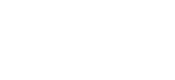
Quantitative Research: A Successful Investigation in Natural and Social Sciences
Mohajan, Haradhan (2020): Quantitative Research: A Successful Investigation in Natural and Social Sciences. Published in: Journal of Economic Development, Environment and People , Vol. 9, No. 4 (31 December 2020): pp. 52-79.
Research is the framework used for the planning, implementation, and analysis of a study. The proper choice of a suitable research methodology can provide an effective and successful original research. A researcher can reach his/her expected goal by following any kind of research methodology. Quantitative research methodology is preferred by many researchers. This article presents and analyzes the design of quantitative research. It also discusses the proper use and the components of quantitative research methodology. It is used to quantify attitudes, opinions, behaviors, and other defined variables and generalize results from a larger sample population by the way of generating numerical data. The purpose of this study is to provide some important fundamental concepts of quantitative research to the common readers for the development of their future projects, articles and/or theses. An attempt has been taken here to study the aspects of the quantitative research methodology in some detail.
All papers reproduced by permission. Reproduction and distribution subject to the approval of the copyright owners.
Contact us: [email protected]
This repository has been built using EPrints software .

Qualitative and quantitative research in the humanities and social sciences: how natural language processing (NLP) can help
- Published: 23 September 2021
- Volume 56 , pages 2751–2781, ( 2022 )
Cite this article
- Roberto Franzosi ORCID: orcid.org/0000-0001-8367-5190 1 ,
- Wenqin Dong 2 &
- Yilin Dong 2
1179 Accesses
2 Citations
4 Altmetric
Explore all metrics
The paper describes computational tools that can be of great help to both qualitative and quantitative scholars in the humanities and social sciences who deal with words as data. The Java and Python tools described provide computer-automated ways of performing useful tasks: 1. check the filenames well-formedness; 2. find user-defined characters in English language stories (e.g., social actors, i.e., individuals, groups, organizations; animals) (“find the character”) via WordNet; 3. aggregate words into higher-level aggregates (e.g., “talk,” “say,” “write” are all verbs of “communication”) (“find the ancestor”) via WordNet; 4. evaluate human-created summaries of events taken from multiple sources where key actors found in the sources may have been left out in the summaries (“find the missing character”) via Stanford CoreNLP POS and NER annotators; 5. list the documents in an event cluster where names or locations present close similarities (“check the character’s name tag”) using Levenshtein word/edit distance and Stanford CoreNLP NER annotator; 6. list documents categorized into the wrong event cluster (“find the intruder”) via Stanford CoreNLP POS and NER annotators; 7. classify loose documents into most-likely event clusters (“find the character’s home”) via Stanford CoreNLP POS and NER annotators or date matcher; 8. find similarities between documents (“find the plagiarist”) using Lucene. These tools of automatic data checking can be applied to ongoing projects or completed projects to check data reliability. The NLP tools are designed with “a fourth grader” in mind, a user with no computer science background. Some five thousand newspaper articles from a project on racial violence (Georgia 1875–1935) are used to show how the tools work. But the tools have much wider applicability to a variety of problems of interest to both qualitative and quantitative scholars who deal with text as data.
This is a preview of subscription content, log in via an institution to check access.
Access this article
Price includes VAT (Russian Federation)
Instant access to the full article PDF.
Rent this article via DeepDyve
Institutional subscriptions
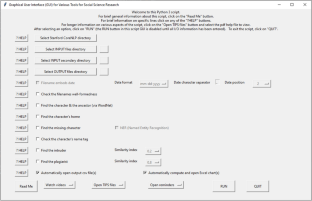
Similar content being viewed by others
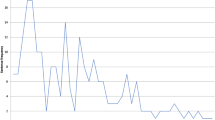
What’s in a text? Bridging the gap between quality and quantity in the digital era
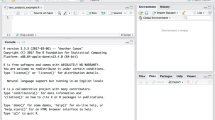
Beyond lexical frequencies: using R for text analysis in the digital humanities
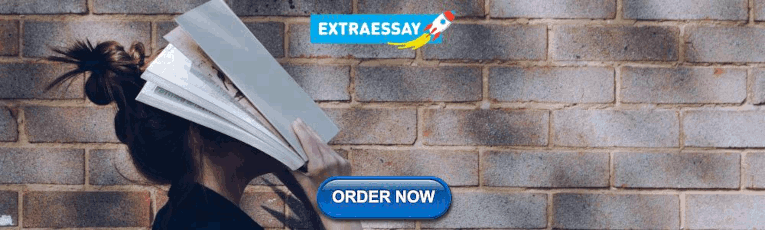
Text Mining and Big Textual Data: Relevant Statistical Models
On PEA see Koopmans and Rucht ( 2002 ) and (Hutter 2014 ); on PEA and its more rigorous methodological counterpart rooted in a linguistic theory of narrative and rhetoric, Quantitative Narrative Analysis (QNA), see Franzosi ( 2010 ).
See, for instance, Franzosi’s PC-ACE (Program for Computer-Assisted Coding of Events) at www.pc-ace.com (Franzosi 2010 ).
For recent surveys, see Evans and Aceves ( 2016 ), Edelmann et al. ( 2020 ).
The GitHub site will automatically install not only all the NLP Suite scripts but also Python and Anaconda required to run the scripts. It also provides extensive help on how to download and install a handful of external software required by some of the algorithms (e.g., Stanford CoreNLP, WordNet). The goal is to make it as easy as possible for non-technical users to take advantage of the tools with minimal investment.
We rely on the Python package openpyxl and ad hoc functions.
The newspaper collections found in Chronicling America of the Library of Congress ( http://chroniclingamerica.loc.gov/newspapers/ ), the Digital Library of Georgia ( http://dlg.galileo.usg.edu/MediaTypes/Newspapers.html?Welcome ), The Atlanta Constitution, Proquest, Readex.
Multiple cross-references are also possible, whereby a document deals with several different events.
Contrary to some protest event projects based on a single newspaper source (e.g., The New York Times in the “Dynamics of Collective Action, 1960–1995” project that involved several social scientists, notably, Doug McAdam, John McCarthy, Susan Olzak, Sarah Soule, and led to dozens of influential publications; see for all McAdam and Su 2002 ), the Georgia lynching project is based on multiple newspaper sources for each event.
Franzosi reports 1,600 distinct entries for subjects and objects and 7,000 for verbs for one of his projects (Franzosi 2010 : 93); similar figures are reported by Ericsson and Simon ( 1996 : 265–266) and Tilly ( 1995 : 414–415).
The most up-to-date numbers of terms are given in https://wordnet.princeton.edu/documentation/wnstats7wn .
A common critique of WordNet is that WordNet is better suited to account for concrete concepts than for abstract concepts. It is much easier to create hyponyms/hypernym relationships between “conifer” as a type of “tree”, a “tree” as a type of “plant”, and a “plant” as a type of “organism”. Not so easy to classify emotions like “fear” or “happiness” into hyponyms/hypernym relationships.
https://projects.csail.mit.edu/jwi/
The WordNet databases comprises both single words or combinations of two or more words that typically come together with a specific meaning (collocations, e.g., coming out, shut down, thumbs up, stand in line, customs duty). Over 80% of terms in the WordNet database are collocations, at least at the time of Miller et al.’s Introduction to WordNet manual (1993, p. 2). For the English language (but WordNet is available for some 200 languages) the database contains a very large set of terms. The most up-to-date numbers of terms are given in https://wordnet.princeton.edu/documentation/wnstats7wn .
Data aggregation is often referred to as “data reduction” in the social sciences and as “linguistic categorization” in linguistics (on linguistic categorization, see Taylor 2004 ; on verbs classification, Levin 1993 ; see also Franzosi 2010 : 61).
On the way up through the hierarchy, the script relies on the WordNet concepts of hypernym – the generic term used to designate a whole class of specific instances (Y is a hypernym of X if X is a (kind of) Y) – and holonym – the name of the whole of which the meronym names is a part. Y is a holonym of X if X is a part of Y.
Collocations are sets of two or more words that are usually together for a complete meaning, e.g., “coming out,” “sunny side up”. Over 80% of terms in the WordNet database are collocations, at least at the time of Miller et al.’s Introduction to WordNet manual (1993, p. 2). For the English language (but WordNet is available for some 200 languages) the database contains a very large set of terms. The most up-to-date numbers of terms in each category are given in https://wordnet.princeton.edu/documentation/wnstats7wn
The 25 top noun synsets are: act, animal, artifact, attribute, body, cognition, communication, event, feeling, food, group, location, motive, object, person, phenomenon, plant, possession, process, quantity, relation, shape, state, substance, time.
The 15 top verb synsets are: body, change, cognition, communication, competition, consumption, contact, creation, emotion, motion, perception, possession, social, stative, weather.
Unfortunately, there is no easy way to aggregate at levels lower than the top synsets. Wordnet is a linked graph where each node is a synset and synsets are interlinked by means of conceptual-semantic and lexical relations. In other words, it is not a simple tree structure: there is no way to tell at which level the synset is located at. For example, the synset “anger” can be traced from top level synset “feeling” and follows the path: feeling—> emotion—> anger. But it can also be traced from top level synset “state” and follows the path: state—> condition—> physiological condition—> arousal—> emotional arousal—> anger. In the first case, “anger” is at level 3 (assuming “feeling” and or other top synsets are level 1). In the second case, “anger” is at level 6. Programmatically, if one gives users more freedom to control the level of aggregating up, it is hard to build a user-friendly communication protocol. If the user wants to aggregate up to level 3 (two levels below the top synset), then should “anger” be considered as a level 3 synset? Does the user want “anger” to be considered as a level 3 synset? Since there is no clear definition of how far away a synset is from the root (top synsets), our algorithm aggregates all the way up to root.
Suppose that you wish to aggregate the verbs in your corpus under the label “violence.” WordNet top synsets for verbs do not include “violence” as a class. Verbs of violence may be listed under body, contact, social. You could use the Zoom IN/DOWN widget of Figure 24 to get a list of verbs in these top synsets, then manually go through the list to select only the verbs of violence of interest. That would mean go through manually the list of 956 verbs in the body class (e.g., to find there the verb “attack,” among others), the 2515 verbs of contact (e.g., to find there the verb “wrestle”), and the 1688 verbs of social (e.g., to find there the verb “abuse”). In total, 5159 distinct verbs. A restricted domain, for example newspaper articles of lynching, may have many fewer distinct verbs, indeed 2027, extracted using the lemma of the POS annotator for all the VB* tags. Whether using the WordNet dictionary (a better solution if the list of verbs of violence has to be used across different corpora) or the POS distinct verb tags, the dictionary list can then be used to annotate the documents in the corpus via the NLP Suite dictionary annotator GUI.
Current computational technology makes available a different approach to creating summaries: an automatic approach where summaries are generated automatically by a computer algorithm, rather than a human (Gambhir and Gupta 2017 ; Lloret and Palomar 2012 ; Nenkova and McKeown 2012 ).
We use the word “compilation”, rather than “summary”, since, by and large, we maintained the original newspaper language (e.g., the word “negro”, rather than “African American”) and original story line, however contrived the story may have appeared to be.
https://stanfordnlp.github.io/CoreNLP/ Manning et al. ( 2014 ).
More specifically, for locations, the NER tags used are: City, State_or_Province, Country. Several other NER values are also recognized and tagged (e.g., Numbers, Percentages, Money, Religion), but they are irrelevant in this context.
The column “List of Documents for Type of Error” may be split in several columns depending upon the number of documents found in error.
The algorithm can process all or selected NER values, comparing the associated word values either within a single event subdirectory or across all subdirectories (or all the files listed in a directory, for that matter).
We calculated the relativity index by using cosine similarity (Singhal 2001 ). We use the two list of NN, NNS, Location, Date, Person, and Organization from the j doc (L1) and from all other j-1 docs (L2) and compute cosine similarity between the two lists. We construct a vector from each list by mapping the word count onto each unique word. Then, relativity index is calculated as the cosine similarity between two vectors and n is the count of total unique words. For instance, L1 is {Alice: 2, doctor: 3, hospital: 1}, and L2 is {Bob:1, hospital: 2}. If we fix the order of all words as {Alice, doctor, hospital, Bob}, then the first vector (V1) is (2, 3, 1, 0), the second vector (V2) is (0, 0, 2, 1), and the length n of the vector is 4. The relativity is the dot product of two vectors divided by the product of two vector lengths. Documents with index of relativity significantly lower than the rest of the cluster are signalled as unlikely to belong to the cluster.
\({\text{relativity}}\;{\text{index}} = \frac{{\sum\nolimits_{i = 1}^{n} {\left( {V1_{i} V2_{i} } \right)} }}{{\sqrt {\sum\nolimits_{i = 1}^{n} {V1_{i}^{2} } } \sqrt {\sum\nolimits_{i = 1}^{n} {V2_{i}^{2} } } }}\)
The relativity index ranges from 0 to 1, where 0 means two documents are totally different, and 1 means two documents have exactly the same list of NN, NNS, Location, Date, Person, and Organization.
The bar chart displays the distribution of most frequent threshold index values as intervals, with most records in the 0.25 ~ 0.29 interval.
It should be noted that the use of the words plagiarism and plagiarist in this context should be taken with a grain of salt. First, the data do not tell us anything about who copied whom, but only that the two different newspapers shared content, wholly or in part; furthermore, the shared content may well have come from an unacknowledged wire service (on the development and spread of news wire services in the United States during the second half of the nineteenth century, see Brooker-Gross 1981 ; on computational tools for plagiarism and authorship attribution, see, for instance, Stein et al. 2011 ).
http://lucene.apache.org/core/downloads.html . For a summary of approaches to document similarities, see Forsyth and Sharoff ( 2014 ).
Other approaches are also available. After all, determining document similarity has been a major research area due to its wide application in information retrieval, document clustering, machine translation, etc. Existing approaches to determine document similarity can be grouped into two categories: knowledge-based similarity and content-based similarity (Benedetti et al., 2019 ).
Knowledge-based similarity approaches extract information from other sources to supplement the corpus, so as to draw from more document features to analyze. For example, Explicit Semantic Analysis (ESA) (Gabrilovich and Markovitch 2007 ) represents documents in high dimensional vectors based on the features extracted from both original articles and Wikipedia articles. Then, similarity of documents is calculated using vector space comparison algorithm. Since our main focus in this work is to detect plagiarism among texts in the same corpus, knowledge-based similarity approaches are not very fruitful.
Content-based similarity approaches focus on using only textual information contained in documents. Popular proposed techniques in this fields are Vector Space Models (Turney and Pantel 2010 ), probabilistic models such as Okapi BM-25 (Robertson and Zaragoza 2009 ). These methods all transform documents into some form of representations, and then either do a vector space comparison or query search match on the constructed representations.
document_duplicates.txt.
Users can specify different spans of temporal aggregation (e.g., year, quarter/year, month/year).
In this specific application, documents are newspapers where document name refers to the name of the paper (e.g., The New York Times) and document instance refers to a specific newspaper article (e.g., The New York Times_12-11-1912_1, referring to a The New York Times of December 11, 1912 on page 1). But the document name could refer to an ethnographic interview with document instance referring to an interviewer’s ID (by name or number), an interview’s location, time, or interviewee (by name or ID number).
The numbers in each row of the table add up to approximately the total number of newspaper articles in the corpus. This number of not exact due to the way the Lucene function “find top similar documents” computes similar documents with discrepancies numbering in the teens.
On the specific topic of lynching, see, for instance, the quantitative work by Beck and Tolnay ( 1990 ) or Franzosi et al. ( 2012 ) and the more qualitative work by Brundage ( 1993 ).
Aggarwal, C.C., Zhai, C.: A survey of text classification algorithms. In: Aggarwal, C.C., Zhai, C. (eds.) Mining Text Data, pp. 163–222. Springer, Boston (2012)
Chapter Google Scholar
Beck, E.M., Tolnay, S.: ‘The killing fields of the deep south: the market for cotton and the lynching of blacks, 1882–1930.’ Am. Sociol. Rev. 55 , 526–539 (1990)
Article Google Scholar
Beck, E.M., Tolnay, S.E.: Confirmed inventory of southern lynch victims, 1882–1930. Data file available from authors (2004).
Benedetti, F., Beneventano, D., Bergamaschi, S., Simonini, G.: Computing inter document similarity with Context Semantic Analysis. Inf. Syst. 80 , 136–147 (2019). https://doi.org/10.1016/j.is.2018.02.009
Białecki, A., Muir, R., & Ingersoll, G.: "Apache Lucene 4." SIGIR 2012 Workshop on Open Source Information Retrieval . August 16, 2012, Portland, OR, USA (2012).
Brundage, F.: Lynching in the New South: Georgia and Virginia, 1880–1930. University of Illinois Press, Urbana (1993)
Google Scholar
Johansson, J., Borg, M., Runeson, P., Mäntylä, M.V.:A replicated study on duplicate detection: using Apache Lucene to search among android defects. In: Proceedings of the 8th ACM/IEEE International Symposium on Empirical Software Engineering and Measurement, 8. ACM (2014)
Brooker-Gross, S.R.: News wire services in the nineteenth-century United States. J. Hist. Geogr. 7 (2), 167–179 (1981)
Cooper, J.W., Coden, A.R. Brown, E.W.: Detecting similar documents using salient terms. In: Proceedings of the Eleventh International Conference on Information and Knowledge Management , 245–251 (2002)
Edelmann, A., Wolff, T., Montagne, D., Bail, C.A.: Computational social science and sociology. Ann. Rev. Sociol. 46 , 61–81 (2020)
Ericsson, K.A., Herbert, S.: Protocol Analysis: Verbal Reports as Data, 2nd edn. MIT Press, Cambridge, MA (1996)
Evans, J.A., Aceves, P.: Machine translation: mining text for social theory. Ann. Rev. Sociol. 42 , 21–50 (2016)
Fellbaum, C. (ed.): WordNet. An Electronic Lexical Database. MIT Press, Cambridge, MA (1998)
Forsyth, R.S., Sharoff, S.: Document dissimilarity within and across languages: a benchmarking study. Liter. Linguistic Comput 29 (1), 6–22 (2014)
Franzosi, R.: Quantitative Narrative Analysis, vol. 162. Sage, Thousand Oaks, CA (2010)
Book Google Scholar
Franzosi, R., De Fazio, G., Vicari, S.: Ways of measuring agency: an application of quantitative narrative analysis to lynchings in Georgia (1875–1930). Sociol. Methodol. 42 (1), 1–42 (2012)
Gabrilovich, E., Markovitch, S.: Computing semantic relatedness using wikipedia-based explicit semantic analysis. IJcAI 7 , 1606–1611 (2007)
Gambhir, M., Gupta, V.: Recent automatic text summarization techniques: a survey. Artif. Intell. Rev. 47 , 1–66 (2017)
Grimm, J., Grimm, W.: [1812, 1857]. The original folk and fairy tales of the brothers Grimm: The Complete First Edition. [ Kinder- und Hausmärchen. Children’s and Household Tales ]. Translated and Edited by Jack Zipes. Princeton, NJ: Princeton University Press (2014)
Hutter, S.: Protest event analysis and its offspring. In: Donatella della Porta (ed.) Methodological Practices in Social Movement Research. Oxford: Oxford University Press, pp. 335–367 (2014)
Jacobs, J.: English fairy tales (Collected by Joseph Jacobs, Illustrated by John D. Batten) . London: David Nutt (1890)
Klandermans, B., Staggenborg, S. (eds.): Methods of Social Movement Research. University of Minnesota Press, Minneapolis (2002)
Koopmans, R., Rucht, D.: Protest event analysis. In: Klandermans, Bert, Staggenborg, Suzanne (eds.) Methods of Social Movement Research, pp. 231–59. University of Minnesota Press, Minneapolis (2002)
Kowsari, K., Meimandi, K.J., Heidarysafa, M., Mendu, S., Barnes, L., Brown, D.: Text classification algorithms: a survey. Information 2019 (10), 150 (2019)
Labov, W.: Language in the Inner City. University of Pennsylvania Press, Philadelphia (1972)
Lansdall‐Welfare, T., Sudhahar, S., Thompson, J., Lewis, J., FindMyPast Newspaper Team, and Cristianini, N.: Content analysis of 150 years of british periodicals. Proceedings of the National Academy of Sciences (PNAS), PNAS , Published online January 9, 2017 E457–E465 (2017)
Lansdall-Welfare, T., Cristianini, N.: History playground: a tool for discovering temporal trends in massive textual corpora. Digit. Scholar. Human. 35 (2), 328–341 (2020)
Levenshtein, V.I.: Binary codes capable of correcting deletions, insertions, and reversals. Doklady Akademii Nauk SSSR, 163(4):845–848, 1965 (Russian). English translation in Soviet Physics Doklady , 10(8):707–710, 1966. (Doklady is Russian for "Report". Sometimes transliterated in English as Doclady or Dokladi.) (1966)
Levin, B.: English Verb Classes and Alternations. The University of Chicago Press, Chicago (1993)
Lloret, E., Palomar, M.: Text summarisation in progress: a literature review. Artif. Intell. Rev. 37 , 1–41 (2012)
MacEachren, A.M., Roth, R.E., O'Brien, J., Li, B., Swingley, D., and Gahegan, M.: Visual semiotics & uncertainty visualization: an empirical study. IEEE Transactions on Visualization and Computer Graphics , Vol. 18, No. 12, December 2012 (2012)
Manning, C.D., Surdeanu, M., Bauer, J., Finkel, J., Bethard, S.J. and McClosky, D.: The stanford CoreNLP natural language processing toolkit. Proceedings of the 52nd Annual Meeting of the Association for Computational Linguistics: System Demonstrations , pp. 55–60 (2014)
McAdam, D., Yang, Su.: The war at home: antiwar protests and congressional voting, 1965–1973. Am. Sociol. Rev. 67 (5), 696–721 (2002)
McCandless, M., Hatcher, E., Gospodnetic, O.: Lucene in Action, Second Edition Covers Apache Lucene 3.0. Manning Publications Co, Greenwich, CT (2010)
Miller, G.A.: WordNet: a lexical database for english. Commun. ACM 38 (11), 39–41 (1995)
Miller, G.A., Beckwith, R., Fellbaum, C., Gross, D., Miller, K.J.: Introduction to WordNet: an on-line lexical database. Int. J. Lexicogr. 3 (4), 235–244 (1990)
Nenkova, A., McKeown, K.: A survey of text summarization techniques. In: Aggarwal, C.C., Cheng, X.Z. (eds.) Mining Text Data, pp. 43–76. Springer, Boston (2012)
Murchú, T.Ó., Lawless, S.: The problem of time and space: the difficulties in visualising spatiotemporal change in historical data. Proc. Dig. Human. 7 (8), 12 (2014)
Panitch, L.: Corporatism: a growth industry reaches the monopoly stage. Can. J. Polit. Sci. 21 (4), 813–818 (1988)
Robertson, S., Zaragoza, H.: The probabilistic relevance framework BM25 and beyond. Found. Trends® Inf Retr. 3 (4), 333–389 (2009).
Singhal, A.: Modern information retrieval: a brief overview. Bull. IEEE Comput. Soc. Tech. Comm. Data Eng. 24 (4), 35–43 (2001)
Stein, B., Lipka, N., Prettenhofer, P.: Plagiarism and authorship analysis. Lang. Resour. Eval. 45 (1), 63–82 (2011)
Taylor, J.R.: Linguistic Categorization. Oxford University Press, Oxford (2004)
Tilly, C.: Popular Contention in Great Britain, 1758–1834. Harvard University Press, Cambridge, MA (1995)
Turney, P.D., Pantel, P.: From frequency to meaning: vector space models of semantics. J. Artif. Int. Res. 37 (1), 141–188 (2010)
Zhang, H., Pan, J.: CASM: a deep-learning approach for identifying collective action events with text and image data from social media. Sociol. Methodol. 49 (1), 1–57 (2019)
Zhang, Y., Li, J.L.: Research and improvement of search engine based on Lucene. Int. Conf. Intell. Human-Mach. Syst. Cybern. 2 , 270–273 (2009)
Download references
Author information
Authors and affiliations.
Department of Sociology/Linguistics Program, Emory University, Atlanta, GA, USA
Roberto Franzosi
Carnegie Mellon University, Pittsburgh, PA, USA
Wenqin Dong & Yilin Dong
You can also search for this author in PubMed Google Scholar
Corresponding author
Correspondence to Roberto Franzosi .
Ethics declarations
Conflict of interest.
The authors have no relevant financial or non-financial interests to disclose.
Additional information
Publisher's note.
Springer Nature remains neutral with regard to jurisdictional claims in published maps and institutional affiliations.
See Figs. 23 , 24 and 25
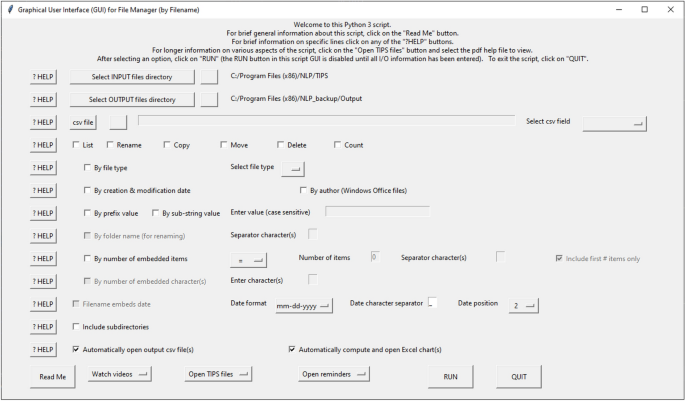
Screenshot of the Graphical User Interface (GUI) for the filename checker
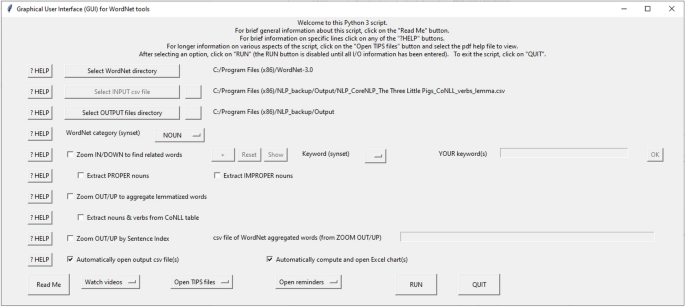
Graphical User Interface (GUI) for WordNet options
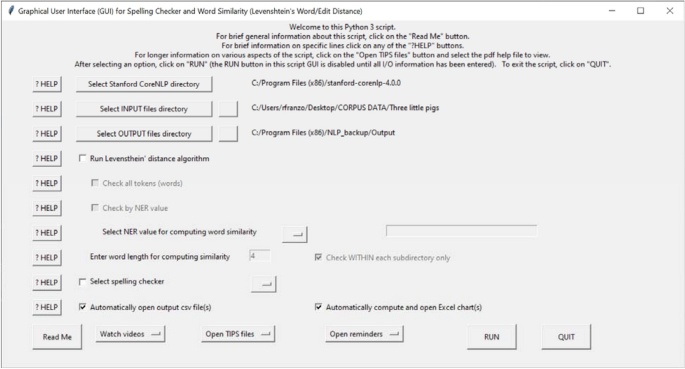
Graphical User Interface (GUI) for Word Similarities
Rights and permissions
Reprints and permissions
About this article
Franzosi, R., Dong, W. & Dong, Y. Qualitative and quantitative research in the humanities and social sciences: how natural language processing (NLP) can help. Qual Quant 56 , 2751–2781 (2022). https://doi.org/10.1007/s11135-021-01235-2
Download citation
Accepted : 02 September 2021
Published : 23 September 2021
Issue Date : August 2022
DOI : https://doi.org/10.1007/s11135-021-01235-2
Share this article
Anyone you share the following link with will be able to read this content:
Sorry, a shareable link is not currently available for this article.
Provided by the Springer Nature SharedIt content-sharing initiative
- Words as data
- Research in humanities and social sciences
- Social movements
- Natural language processing
- Computational linguistics
- Find a journal
- Publish with us
- Track your research
Thank you for visiting nature.com. You are using a browser version with limited support for CSS. To obtain the best experience, we recommend you use a more up to date browser (or turn off compatibility mode in Internet Explorer). In the meantime, to ensure continued support, we are displaying the site without styles and JavaScript.
- View all journals
- My Account Login
- Explore content
- About the journal
- Publish with us
- Sign up for alerts
- Open access
- Published: 24 May 2024
Beyond probability-impact matrices in project risk management: A quantitative methodology for risk prioritisation
- F. Acebes ORCID: orcid.org/0000-0002-4525-2610 1 ,
- J. M. González-Varona 2 ,
- A. López-Paredes 2 &
- J. Pajares 1
Humanities and Social Sciences Communications volume 11 , Article number: 670 ( 2024 ) Cite this article
Metrics details
- Business and management
The project managers who deal with risk management are often faced with the difficult task of determining the relative importance of the various sources of risk that affect the project. This prioritisation is crucial to direct management efforts to ensure higher project profitability. Risk matrices are widely recognised tools by academics and practitioners in various sectors to assess and rank risks according to their likelihood of occurrence and impact on project objectives. However, the existing literature highlights several limitations to use the risk matrix. In response to the weaknesses of its use, this paper proposes a novel approach for prioritising project risks. Monte Carlo Simulation (MCS) is used to perform a quantitative prioritisation of risks with the simulation software MCSimulRisk. Together with the definition of project activities, the simulation includes the identified risks by modelling their probability and impact on cost and duration. With this novel methodology, a quantitative assessment of the impact of each risk is provided, as measured by the effect that it would have on project duration and its total cost. This allows the differentiation of critical risks according to their impact on project duration, which may differ if cost is taken as a priority objective. This proposal is interesting for project managers because they will, on the one hand, know the absolute impact of each risk on their project duration and cost objectives and, on the other hand, be able to discriminate the impacts of each risk independently on the duration objective and the cost objective.
Introduction
The European Commission ( 2023 ) defines a project as a temporary organizational structure designed to produce a unique product or service according to specified constraints, such as time, cost, and quality. As projects are inherently complex, they involve risks that must be effectively managed (Naderpour et al. 2019 ). However, achieving project objectives can be challenging due to unexpected developments, which often disrupt plans and budgets during project execution and lead to significant additional costs. The Standish Group ( 2022 ) notes that managing project uncertainty is of paramount importance, which renders risk management an indispensable discipline. Its primary goal is to identify a project’s risk profile and communicate it by enabling informed decision making to mitigate the impact of risks on project objectives, including budget and schedule adherence (Creemers et al. 2014 ).
Several methodologies and standards include a specific project risk management process (Axelos, 2023 ; European Commission, 2023 ; Project Management Institute, 2017 ; International Project Management Association, 2015 ; Simon et al. 1997 ), and there are even specific standards and guidelines for it (Project Management Institute, 2019 , 2009 ; International Organization for Standardization, 2018 ). Despite the differences in naming each phase or process that forms part of the risk management process, they all integrate risk identification, risk assessment, planning a response to the risk, and implementing this response. Apart from all this, a risk monitoring and control process is included. The “Risk Assessment” process comprises, in turn, risk assessments by qualitative methods and quantitative risk assessments.
A prevalent issue in managing project risks is identifying the significance of different sources of risks to direct future risk management actions and to sustain the project’s cost-effectiveness. For many managers busy with problems all over the place, one of the most challenging tasks is to decide which issues to work on first (Ward, 1999 ) or, in other words, which risks need to be paid more attention to avoid deviations from project objectives.
Given the many sources of risk and the impossibility of comprehensively addressing them, it is natural to prioritise identified risks. This process can be challenging because determining in advance which ones are the most significant factors, and how many risks merit detailed monitoring on an individual basis, can be complicated. Any approach that facilitates this prioritisation task, especially if it is simple, will be welcomed by those willing to use it (Ward, 1999 ).
Risk matrices emerge as established familiar tools for assessing and ranking risks in many fields and industry sectors (Krisper, 2021 ; Qazi et al. 2021 ; Qazi and Simsekler, 2021 ; Monat and Doremus, 2020 ; Li et al. 2018 ). They are now so commonplace that everyone accepts and uses them without questioning them, along with their advantages and disadvantages. Risk matrices use the likelihood and potential impact of risks to inform decision making about prioritising identified risks (Proto et al. 2023 ). The methods that use the risk matrix confer higher priority to those risks in which the product of their likelihood and impact is the highest.
However, the probability-impact matrix has severe limitations (Goerlandt and Reniers, 2016 ; Duijm, 2015 ; Vatanpour et al. 2015 ; Ball and Watt, 2013 ; Levine, 2012 ; Cox, 2008 ; Cox et al. 2005 ). The main criticism levelled at this methodology is its failure to consider the complex interrelations between various risks and use precise estimates for probability and impact levels. Since then, increasingly more academics and practitioners are reluctant to resort to risk matrices (Qazi et al. 2021 ).
Motivated by the drawbacks of using risk matrices or probability-impact matrices, the following research question arises: Is it possible to find a methodology for project risk prioritisation that overcomes the limitations of the current probability-impact matrix?
To answer this question, this paper proposes a methodology based on Monte Carlo Simulation that avoids using the probability-impact matrix and allows us to prioritise project risks by evaluating them quantitatively, and by assessing the impact of risks on project duration and the cost objectives. With the help of the ‘MCSimulRisk’ simulation software (Acebes et al. 2024 ; Acebes et al. 2023 ), this paper determines the impact of each risk on project duration objectives (quantified in time units) and cost objectives (quantified in monetary units). In this way, with the impact of all the risks, it is possible to establish their prioritisation based on their absolute (and not relative) importance for project objectives. The methodology allows quantified results to be obtained for each risk by differentiating between the project duration objective and its cost objective.
With this methodology, it also confers the ‘Risk Assessment’ process cohesion and meaning. This process forms part of the general Risk Management process and is divided into two subprocesses: qualitative and quantitative risk analyses (Project Management Institute, 2017 ). Although Monte Carlo simulation is widely used in project risk assessments (Tong et al. 2018 ; Taroun, 2014 ), as far as we know, the literature still does not contain references that use the data obtained in a qualitative analysis (data related to the probability and impact of each identified risk) to perform a quantitative risk analysis integrated into the project model. Only one research line by A. Qazi (Qazi et al. 2021 ; Qazi and Dikmen, 2021 ; Qazi and Simsekler, 2021 ) appears, where the authors propose a risk indicator with which they determine the level of each identified risk that concerns the established threshold. Similarly, Krisper ( 2021 ) applies the qualitative data of risk factors to construct probability functions, but once again falls in the error of calculating the expected value of the risk for risk prioritisation. In contrast, the novelty proposed in this study incorporates into the project simulation model all the identified risks characterised by their probability and impact values, as well as the set of activities making up the project.
In summary, instead of the traditional risk prioritisation method to qualitatively estimate risk probabilities and impacts, we model probabilities and impacts (duration and cost) at the activity level as distribution functions. When comparing both methods (traditional vs. our proposal), the risk prioritisation results are entirely different and lead to a distinct ranking.
From this point, and to achieve our purpose, the article comes as follows. Literature review summarises the relevant literature related to the research. Methodology describes the suggested methodology. Case study presents the case study used to show how to apply the presented method before discussing the obtained results. Finally, Conclusions draws conclusions about the proposed methodology and identifies the research future lines that can be developed from it.
Literature review
This section presents the literature review on risk management processes and probability-impact matrices to explain where this study fits into existing research. This review allows us to establish the context where our proposal lies in integrated risk management processes. Furthermore, it is necessary to understand the reasons for seeking alternatives to the usual well-known risk matrices.
Risk management methodologies and standards
It is interesting to start with the definition of ‘Risk’ because it is a term that is not universally agreed on, even by different standards and norms. Thus, for example, the International Organization for Standardization ( 2018 ) defines it as “the effect of uncertainty on objectives”, while the Project Management Institute ( 2021 ) defines it as “an uncertain event or condition that, if it occurs, has a positive or negative effect on one or more project objectives”. This paper adopts the definition of risk proposed by Hillson ( 2014 ), who uses a particular concept: “risk is uncertainty that matters”. It matters because it affects project objectives and only the uncertainties that impact the project are considered a ‘risk’.
Other authors (Elms, 2004 ; Frank, 1999 ) identify two uncertainty categories: aleatoric, characterised by variability and the presence of a wide range of possible values; epistemic, which arises due to ambiguity or lack of complete knowledge. Hillson ( 2014 ) classifies uncertainties into four distinct types: aleatoric, due to the reliability of activities; stochastic, recognised as a risk event or a possible future event; epistemic, also due to ambiguity; ontological, that which we do not know (black swan). Except for ontological uncertainty, which cannot be modelled due to absolute ignorance of risk, the other identified uncertainties are incorporated into our project model. For this purpose, the probability and impact of each uncertainty are modelled as distribution functions to be incorporated into Monte Carlo simulation.
A risk management process involves analysing the opportunities and threats that can impact project objectives, followed by planning appropriate actions for each one. This process aims to maximise the likelihood of opportunities occurring and to minimise the likelihood of identified threats materialising.
Although it is true that different authors have proposed their particular way of understanding project risk management (Kerzner, 2022 ; Hillson and Simon, 2020 ; Chapman and Ward, 2003 ; Chapman, 1997 ), we wish to look at the principal methodologies, norms and standards in project management used by academics and practitioners to observe how they deal with risk (Axelos, 2023 ; European Commission, 2023 ; International Organization for Standardization, 2018 ; Project Management Institute, 2017 ; International Project Management Association, 2015 ) (Table 1 ).
Table 1 shows the main subprocesses making up the overall risk management process from the point of view of each different approach. All the aforementioned approaches contain a subprocess related to risk assessment. Some of these approaches develop the subprocess by dividing it into two parts: qualitative assessment and quantitative assessment. Individual project risks are ranked for further analyses or action with a qualitative assessment by evaluating the probability of their occurrence and potential impact. A quantitative assessment involves performing a numerical analysis of the joint effect of the identified individual risks and additional sources of uncertainty on the overall project objectives (Project Management Institute, 2017 ). In turn, all these approaches propose the probability-impact or risk matrix as a technique or tool for prioritising project risks.
Within this framework, a ranking of risks by a quantitative approach applies as opposed to the qualitative assessment provided by the risk matrix. To do so, we use estimates of the probability and impact associated with each identified risk. The project model includes these estimates to determine the absolute value of the impact of each risk on time and cost objectives.
Probability-impact matrix
The risk matrix, or probability-impact matrix, is a tool included in the qualitative analysis for risk management and used to analyse, visualise and prioritise risks to make decisions on the resources to be employed to combat them (Goerlandt and Reniers, 2016 ; Duijm, 2015 ). Its well-established use appears in different sectors, ranging from the construction industry (Qazi et al. 2021 ), oil and gas industries (Thomas et al. 2014 ), to the healthcare sector (Lemmens et al. 2022 ), engineering projects (Koulinas et al. 2021 ) and, of course, project management (International Organization for Standardization, 2019 ; Li et al. 2018 ).
In a table, the risk matrix represents the probability (usually on the vertical axis of the table) and impact (usually on the horizontal axis) categories (Ale et al. 2015 ). These axes are further divided into different levels so that risk matrices of 3×3 levels are found with three levels set for probability and three others to define impact, 5 × 5, or even more levels (Duijm, 2015 ; Levine, 2012 ; Cox, 2008 ). The matrix classifies risks into different risk categories, normally labelled with qualitative indicators of severity (often colours like “Red”, “Yellow” and “Green”). This classification combines each likelihood level with every impact level in the matrix (see an example of a probability-impact matrix in Fig. 1 ).
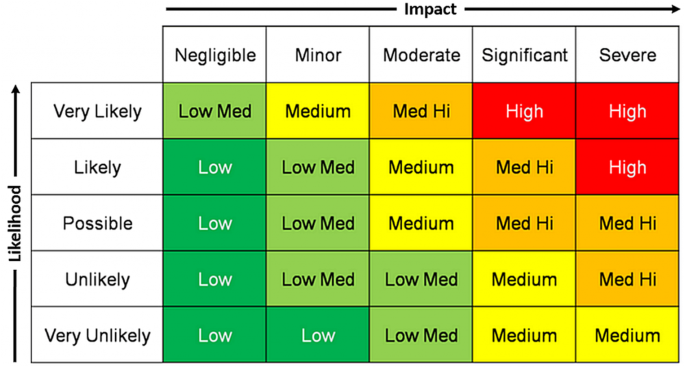
Probability – impact matrix. An example of use.
There are three different risk matrix typologies based on the categorisation of likelihood and impact: qualitative, semiquantitative, and quantitative. Qualitative risk matrices provide descriptive assessments of probability and consequence by establishing categories as “low,” “medium” or “high” (based on the matrix’s specific number of levels). In contrast, semiquantitative risk matrices represent the input categories by ascending scores, such as 1, 2, or 3 (in a 3×3 risk matrix), where higher scores indicate a stronger impact or more likelihood. Finally, in quantitative risk matrices, each category receives an assignment of numerical intervals corresponding to probability or impact estimates. For example, the “Low” probability level is associated with a probability interval [0.1 0.3] (Li et al. 2018 ).
Qualitative matrices classify risks according to their potential hazard, depending on where they fit into the matrix. The risk level is defined by the “colour” of the corresponding cell (in turn, this depends on the probability and impact level), with risks classified with “red” being the most important and the priority ones to pay attention to, but without distinguishing any risks in the different cells of the same colour. In contrast, quantitative risk matrices allow to classify risks according to their risk level (red, yellow, or green) and to prioritise each risk in the same colour by indicating which is the most important. Each cell is assigned a colour and a numerical value, and the product of the value is usually assigned to the probability level and the value assigned to the impact level (Risk = probability × impact).
Risk matrix use is frequent, partly due to its simple application and easy construction compared to alternative risk assessment methods (Levine, 2012 ). Risk matrices offer a well-defined structure for carrying out a methodical risk assessment, provide a practical justification for ranking and prioritising risks, visually and attractively inform stakeholders, among other reasons (Talbot, 2014 ; Ball and Watt, 2013 ).
However, many authors identify problems in using risk matrices (Monat and Doremus, 2020 ; Peace, 2017 ; Levine, 2012 ; Ni et al. 2010 ; Cox, 2008 ; Cox et al. 2005 ), and even the International Organization for Standardization ( 2019 ) indicates some drawbacks. The most critical problems identified in using risk matrices for strategic decision-making are that risk matrices can be inaccurate when comparing risks and they sometimes assign similar ratings to risks with significant quantitative differences. In addition, there is the risk of giving excessively high qualitative ratings to risks that are less serious from a quantitative perspective. This can lead to suboptimal decisions, especially when threats have negative correlations in frequency and severity terms. Such lack of precision can result in inefficient resource allocation because they cannot be based solely on the categories provided by risk matrices. Furthermore, the categorisation of the severity of consequences is subjective in uncertainty situations, and the assessment of probability, impact and risk ratings very much depends on subjective interpretations, which can lead to discrepancies between different users when assessing the same quantitative risks.
Given this background, several authors propose solutions to the posed problems. Goerlandt and Reniers ( 2016 ) review previous works that have attempted to respond to the problems identified with risk matrices. For example, Markowski and Mannan ( 2008 ) suggest using fuzzy sets to consider imprecision in describing ordinal linguistic scales. Subsequently, Ni et al. ( 2010 ) propose a methodology that employs probability and consequence ranks as independent score measures. Levine ( 2012 ) puts forward the use of logarithmic scales on probability and impact axes. Menge et al. (2018) recommend utilising untransformed values as scale labels due to experts’ misunderstanding of logarithmic scales. Ruan et al. ( 2015 ) suggest an approach that considers decision makers’ risk aversion by applying the utility theory.
Other authors, such as Duijm ( 2015 ), propose a continuous probability consequence diagram as an alternative to the risk matrix, and employing continuous scales instead of categories. They also propose utilising more comprehensive colour ranges in risk matrices whenever necessary to prioritise risks and to not simply accept them. In contrast, Monat and Doremus ( 2020 ) put forward a new risk prioritisation tool. Alternatively, Sutherland et al. ( 2022 ) suggest changing matrix size by accommodating cells’ size to the risk’s importance. Even Proto et al. ( 2023 ) recommend avoiding colour in risk matrices so that the provided information is unbiased due to the bias that arises when using coloured matrices.
By bearing in mind the difficulties presented by the results offered by risk matrices, we propose a quantitative method for risk prioritisation. We use qualitative risk analysis data by maintaining the estimate of the probability of each risk occurring and its potential impact. Nevertheless, instead of entering these data into the risk matrix, our project model contains them for Monte Carlo simulation. As a result, we obtain a quantified prioritisation of each risk that differentiates the importance of each risk according to the impact on cost and duration objectives.
Methodology
Figure 2 depicts the proposed method for prioritising project risks using quantitative techniques. At the end of the process, and with the prioritised risks indicating the absolute value of the impact of each risk on the project, the organisation can efficiently allocate resources to the risks identified as the most critical ones.
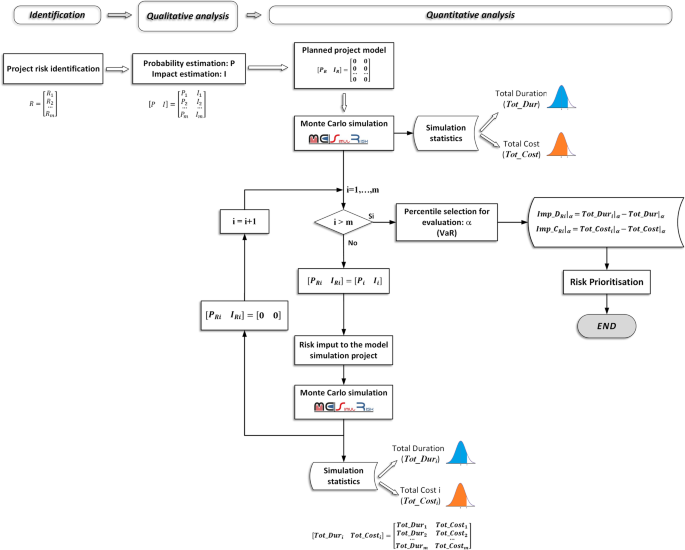
Quantitative Risk Assessment Flow Chart.
The top of the diagram indicates the risk phases that belong to the overall risk management process. Below them it reflects the steps of the proposed model that would apply in each phase.
The first step corresponds to the project’s “ risk identification ”. Using the techniques or tools established by the organisation (brainstorming, Delphi techniques, interviews, or others), we obtain a list of the risks ( R ) that could impact the project objectives (Eq. 1 ), where m is the number of risks identified in the project.
Next we move on to the “ risk estimation ” phase, in which a distribution function must be assigned to the probability that each identified risk will appear. We also assign the distribution function associated with the risk’s impact. Traditionally, the qualitative risk analysis defines semantic values (low, medium, high) to assign a level of probability and risk impact. These semantic values are used to evaluate the risk in the probability-impact matrix. Numerical scales apply in some cases, which help to assign a semantic level to a given risk (Fig. 3 ).
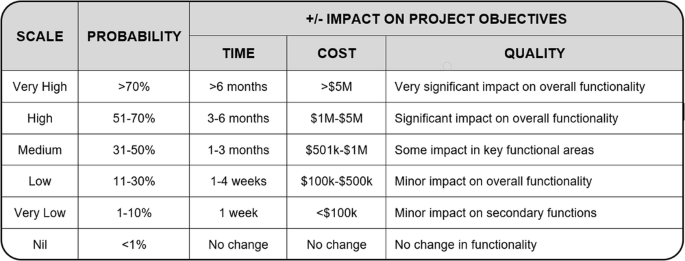
Source: Project Management Institute ( 2017 ).
Our proposed model includes the three uncertainty types put forward by Hillson ( 2014 ), namely aleatoric, stochastic and epistemic, to identify and assess different risks. Ontological uncertainty is not considered because it goes beyond the limits of human knowledge and cannot, therefore, be modelled (Alleman et al. 2018a ).
A risk can have aleatoric uncertainty as regards the probability of its occurrence, and mainly for its impact if its value can fluctuate over a set range due to its variability. This aleatoric risk uncertainty can be modelled using a probability distribution function (PDF), exactly as we do when modelling activity uncertainty (Acebes et al. 2015 , 2014 ). As the risk management team’s (or project management team’s) knowledge of the project increases, and as more information about the risk becomes available, the choice of the PDF (normal, triangular, beta, among others) and its parameters become more accurate.
A standard definition of risk is “an uncertain event that, if it occurs, may impact project objectives” (Project Management Institute, 2017 ). A risk, if defined according to the above statement, perfectly matches the stochastic uncertainty definition proposed by Hillson ( 2014 ). Moreover, one PDF that adequately models this type of uncertainty is a Bernoulli distribution function (Vose, 2008 ). Thus for deterministic risk probability estimates (the same as for risk impact), we model this risk (probability and impact) with a Bernoulli-type PDF that allows us to introduce this type of uncertainty into our simulation model.
Finally, epistemic uncertainties remain to be modelled, such as those for which we do not have absolute information about and that arise from a lack of knowledge (Damnjanovic and Reinschmidt, 2020 ; Alleman et al. 2018b ). In this case, risks (in likelihood and impact terms) are classified into different levels, and all these levels are assigned a numerical scale (as opposed to the methodology used in a qualitative risk analysis, where levels are classified with semantic values: “high”, “medium” and “low”).
“ Epistemic uncertainty is characterised by not precisely knowing the probability of occurrence or the magnitude of a potential impact. Traditionally, this type of risk has been identified with a qualitative term: “Very Low”, “Low”, “Medium”, “High” and “Very High” before using the probability-impact matrix. Each semantic category has been previously defined numerically by identifying every numerical range with a specific semantic value (Bae et al. 2004 ). For each established range, project managers usually know the limits (upper and lower) between which the risk (probability or impact) can occur. However, they do not certainly know the value it will take, not even the most probable value within that range. Therefore, we employ a uniform probability function to model epistemic uncertainty (i.e., by assuming that the probability of risk occurrence lies within an equiprobable range of values). Probabilistic representations of uncertainty have been successfully employed with uniform distributions to characterise uncertainty when knowledge is sparse or absent (Curto et al. 2022 ; Vanhoucke, 2018 ; Helton et al. 2006 ).
The choice of the number and range of each level should be subject to a thorough analysis and consideration by the risk management team. As each project is unique, there are ranges within which this type of uncertainty can be categorised. Different ranges apply to assess likelihood and impact. Furthermore for impact, further subdivision helps to distinguish between impact on project duration and impact on project costs. For example, when modelling probability, we can set five probability levels corresponding to intervals: [0 0.05], [0.05 0.2], [0.2 0.5], and so on. With the time impact, for example, on project duration, five levels as follows may apply: [0 1], [1 4], [4 12], …. (measured in weeks, for example).
Modelling this type of uncertainty requires the risk management team’s experience, the data stored on previous projects, and constant consultation with project stakeholders. The more project knowledge available, the more accurate the proposed model is for each uncertainty, regardless of it lying in the number of intervals, their magnitude or the type of probability function (PDF) chosen to model that risk.
Some authors propose using uniform distribution functions to model this type of epistemic uncertainty because it perfectly reflects lack of knowledge about the expected outcome (Eldosouky et al. 2014 ; Vose, 2008 ). On the contrary, others apply triangular functions, which require more risk knowledge (Hulett, 2012 ). Following the work by Curto et al. ( 2022 ), we employ uniform distribution functions.
As a result of this phase, we obtain the model and the parameters that model the distribution functions of the probability ( P ) and impact ( I ) of each identified risk in the previous phase (Eq. 2 ).
Once the risks identified in the project have been defined and their probabilities and impacts modelled, we move on to “ quantitative risk prioritisation ”. We start by performing MCS on the planned project model by considering only the aleatoric uncertainty of activities. In this way, we learn the project’s total duration and cost, which is commonly done in a Monte Carlo analysis. In Monte Carlo Methods (MCS), expert judgement and numerical methods are combined to generate a probabilistic result through simulation routine (Ammar et al. 2023 ). This mathematical approach is noted for its ability to analyse uncertain scenarios from a probabilistic perspective. MCS have been recognised as outperforming other methods due to their accessibility, ease of use and simplicity. MCS also allow the analysis of opportunities, uncertainties, and threats (Al-Duais and Al-Sharpi, 2023 ). This technique can be invaluable to risk managers and helpful for estimating project durations and costs (Ali Elfarra and Kaya, 2021 ).
As inputs to the simulation process, we include defining project activities (duration, cost, precedence relationship). We also consider the risks identified in the project, which are those we wish to prioritise and to obtain a list ordered by importance (according to their impact on not only duration, but also on project cost). The ‘MCSimulRisk’ software application (Acebes, Curto, et al. 2023 ; Acebes, De Antón, et al. 2023 ) allows us to perform MCS and to obtain the main statistics that result from simulation (including percentiles) that correspond to the total project duration ( Tot_Dur ) and to its total cost ( Tot_Cost ) (Eq. 3 ).
Next, we perform a new simulation by including the first of the identified risks ( R 1 ) in the project model, for which we know its probability ( P 1 ) and its Impact ( I 1 ). After MCS, we obtain the statistics corresponding to this simulation ([ Tot_Dur 1 Tot_Cost 1 ]). We repeat the same operation with each identified risk ( R i , i = 1, …, m ) and obtain the main statistics corresponding to each simulation (Eq. 4 ).
Once all simulations (the same number as risks) have been performed, we must choose a confidence percentile to calculate risk prioritisation (Rezaei et al. 2020 ; Sarykalin et al. 2008 ). Given that the total duration and cost results available to us, obtained by MCS, are stochastic and have variability (they are no longer constant or deterministic), we must choose a percentile (α) that conveys the risk appetite that we are willing to assume when calculating. Risk appetite is “ the amount and type of risk that an organisation is prepared to pursue, retain or take ” (International Organization for Standardization, 2018 ).
A frequently employed metric for assessing risk in finance is the Value at Risk (VaR) (Caron, 2013 ; Caron et al. 2007 ). In financial terms, it is traditional to choose a P95 percentile as risk appetite (Chen and Peng, 2018 ; Joukar and Nahmens, 2016 ; Gatti et al. 2007 ; Kuester et al. 2006 ; Giot and Laurent, 2003 ). However in project management, the P80 percentile is sometimes chosen as the most appropriate percentile to measure risk appetite (Kwon and Kang, 2019 ; Traynor and Mahmoodian, 2019 ; Lorance and Wendling, 2001 ).
Finally, after choosing the risk level we are willing to assume, we need to calculate how each risk impacts project duration ( Imp_D Ri ) and costs ( Imp_C Ri ). To do so, we subtract the original value of the total project expected duration and costs (excluding all risks) from the total duration and costs of the simulation in which we include the risk we wish to quantify (Eq. 5 ).
Finally, we present these results on two separate lists, one for the cost impact and one for the duration impact, by ranking them according to their magnitude.
In this section, we use a real-life project to illustrate how to apply the proposed method for quantitative risk prioritisation purposes. For this purpose, we choose an engineering, procurement and construction project undertaken in South America and used in the literature by Votto et al. ( 2020a , 2020b ).
Project description
The project used as an application example consists of the expansion of an industrial facility. It covers a wide spectrum of tasks, such as design and engineering work, procurement of machinery and its components, civil construction, installation of all machinery, as well as commissioning and starting up machines (Votto et al. 2020a , 2020b ).
Table 2 details the parameters that we use to define activities. The project comprises 32 activities, divided into three groups: engineering, procurement and construction (EPC). A fictitious initial activity ( Ai ) and a fictitious final activity ( Af ) are included. We employ triangular distribution functions, whose parameters are the minimum value ( Min ), the most probable value ( Mp ) and the maximum value ( Max ), to model the random duration of activities, expressed as days. We divide the cost of each activity in monetary units into a fixed cost ( FC ), independently of activity duration, and the variable cost ( VC ), which is directly proportional to project duration. As activity duration can vary, and the activity cost increases directly with its duration, the total project cost also exhibits random variations.
Under these conditions, the planned project duration is 300 days and has a planned cost of 30,000 (x1000) monetary units. Figure 4 shows the Planned Value Curve of the project.
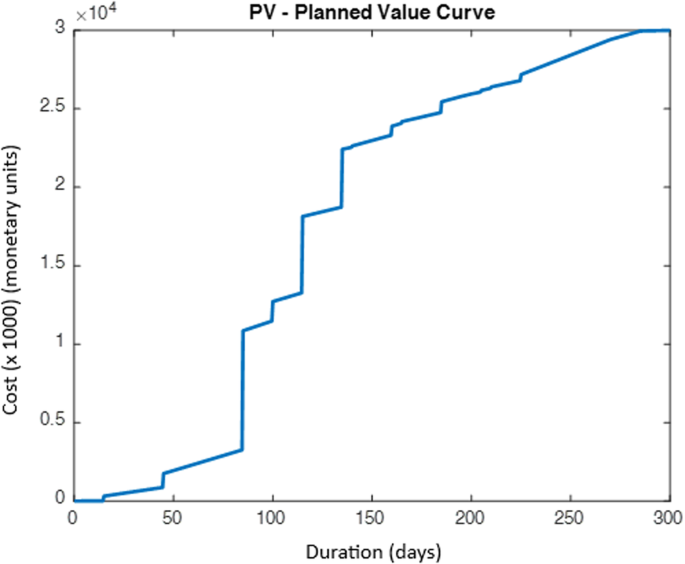
Planned value curve of the real-life project.
The next step in the methodology (Fig. 2 ) is to identify the project risks. To do this, the experts’ panel meets, analyses all the project documentation. Based on their personal experience with other similar projects and after consulting all the involved stakeholders, it provides a list of risks (see Table 3 ).
It identifies 11 risks, of which nine have the potential to directly impact the project duration objective (R1 to R9), while six may impact the cost objective (R10 to R15). The risks that might impact project duration and cost have two assigned codes. We identify the project phase and activity on which all the identified risks may have an impact (Table 3 ).
The next step is to estimate the likelihood and impact of the identified risks (qualitative analysis). Having analysed the project and consulted the involved stakeholders, the team determines the project’s different probability and impact levels (duration and cost). The estimation of these ranges depends on the project budget, the estimated project duration, and the team’s experience in assigning the different numerical values to each range. As a result, the project team is able to construct the probability-impact matrix shown in Fig. 5 .
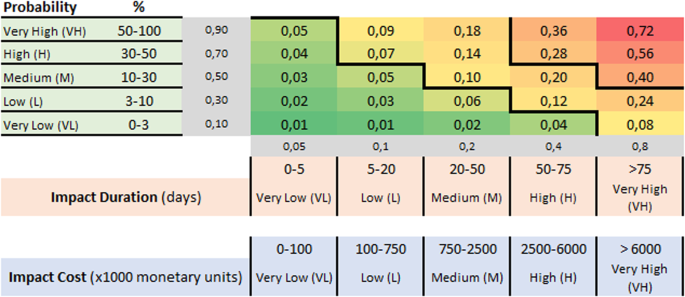
Estimation of the probability and impact ranges.
Each probability range for risk occurrence in this project is defined. Thus for a very low probability (VL), the assigned probability range is between 0 and 3% probability, for a low level (L), the assigned range lies between 3% and 10% probability of risk occurrence, and so on with the other established probability ranges (medium, high, very high).
The different impact ranges are also defined by differentiating between impacts in duration and cost terms. Thus a VL duration impact is between 0 and 5 days, while the same range (VL) in cost is between 0 and 100 (x1000) monetary units. Figure 5 shows the other ranges and their quantification in duration and cost terms.
The combination of each probability level and every impact level coincides in a cell of the risk matrix (Fig. 5 ) to indicate the risk level (“high”, “medium”, and “low”) according to the qualitative analysis. Each cell is assigned a numerical value by prioritising the risks at the same risk level. This work uses the matrix to compare the risk prioritisation results provided by this matrix to those provided by the proposed quantitative method.
A probability and impact value are assigned to each previously identified risk (Table 3 ). Thus, for example, for the risk called “Interruptions in the supply chain”, coded as R3 for impacting activity 13 duration, we estimate an L probability and a strong impact on duration (H). As this same risk might impact the activity 13 cost, it is also coded as R12, and its impact on cost is estimated as L (the probability is the same as in R3; Table 3 ).
Finally, to conclude the proposed methodology and to prioritise the identified risks, we use the “MCSimulRisk” software application by incorporating MCS (in this work, we employ 20,000 iterations in each simulation). Activities are modelled using triangular distribution functions to incorporate project information into the simulation application. Costs are modelled with fixed and variable costs depending on the duration of the corresponding activity. Furthermore, risks (probability and impact) are modelled by uniform distribution functions. Figure 6 depicts the project network and includes the identified risks that impact the corresponding activities.
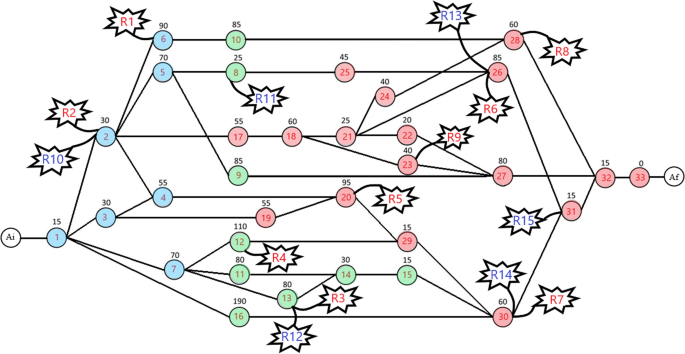
Network diagram of the project together with the identified risks.
Results and discussion
In order to obtain the results of prioritising the identified risks, we must specify a percentile that determines our risk aversion. This is the measure by which we quantify the risk. Figure 7 graphically justifies the choice of P95 as a risk measure, as opposed to a lower percentile, which corroborates the view in the literature and appears in Methodology . In Fig. 7 , we plot the probability distribution and cumulative distribution functions corresponding to the total project planned cost, together with the cost impact of one of the risks. The impact caused by the risk on the total cost corresponds to the set of iterations whose total cost is higher than that planned (bottom right of the histogram).
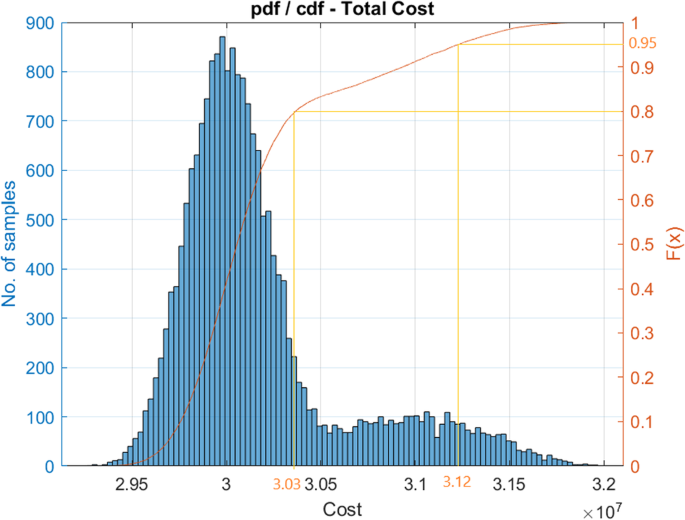
Source: MCSimulRisk.
By choosing P95 as VaR, we can consider the impact of a risk on the project in the measure. In this example, for P95 we obtain a total cost value of 3.12 × 10 7 monetary units. Choosing a lower percentile, e.g. P80, means that the value we can obtain with this choice can be considerably lower (3.03 × 10 7 monetary units), and might completely ignore the impact of the risk on the total project cost. However, project managers can choose the percentile that represents their risk aversion.
Once the percentile on which to quantify the risk is chosen, the “MCSimulRisk” application provides us with the desired results for prioritising project risks (Fig. 8 ). For the chosen percentile (P95), which represents our risk appetite for this project, the planned project duration is 323.43 days. In other words, with a 95% probability the planned project will be completed before 323.43 days. Similarly, the P95 corresponding to cost is 30,339 ×1000 monetary units. The application also provides us with the project duration in the first column of Fig. 8 after incorporating all the identified risks (corresponding to a P95 risk appetite) into the planned project. Column 2 of the same figure shows the project cost after incorporating the corresponding risk into the model.
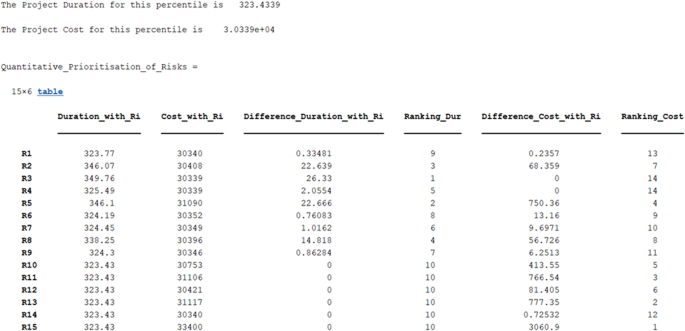
The first column corresponds to the risks identified. Columns Duration_with_Ri and Cost_with_Ri represent the simulation values, including the corresponding risk. Columns Difference_Duration_with_Ri and Difference_Cost_with_Ri represent the difference in duration and cost of each simulation concerning the value obtained for the chosen percentile. Finally, Ranking_Dur and Ranking_Cost represent the prioritisation of risks in duration and cost, respectively.
With the results in the first two columns (total project duration and cost after incorporating the corresponding risks), and by knowing the planned total project duration and cost (without considering risks) for a given percentile (P95), we calculate the values of the following columns in Fig. 8 . Thus column 3 represents the difference between the planned total project duration value (risk-free) and project duration by incorporating the corresponding risk that we wish to quantify. Column 4 prioritises the duration risks by ranking according to the duration that each risk contributes to the project. Column 5 represents the difference between the planned total project cost (risk-free) and the total project cost by incorporating the corresponding risk. Finally, Column 6 represents the ranking or prioritisation of the project risks according to their impact on cost.
To compare the results provided by this methodology in this paper we propose quantitative risk prioritisation, based on MCS. We draw up Table 4 with the results provided by the probability-impact matrix (Fig. 5 ).
The first set of columns in Table 4 corresponds to the implementation of the risk matrix (probability-impact matrix) for the identified risks. The second group of columns represents the prioritisation of risks according to their impact on duration (data obtained from Fig. 8 ). The third group corresponds to the risk prioritisation according to their impact on cost (data obtained from Fig. 8 ).
For the project proposed as an example, we find that risk R3 is the most important one if we wish to control the total duration because it corresponds to the risk that contributes the most duration to the project if it exists. We note that risks R10 to R15 do not impact project duration. If these risks materialise, their contribution to increase (or decrease, as the case may be) project duration is nil.
On the impact on project costs, we note that risk R15 is the most important. It is noteworthy that risk R5 is the fourth most important risk in terms of impact on the total project costs, even though it is initially identified as a risk that impacts project duration. Unlike cost risks (which do not impact the total project duration), the risks that can impact project duration also impact total costs.
We can see that the order of importance of the identified risks differs depending on our chosen method (risk matrix versus quantitative prioritisation). We independently quantify each risk’s impact on the cost and duration objectives. We know not only the order of importance of risks (R3, R5, etc.) but also the magnitude of their impact on the project (which is the absolute delay caused by a risk in duration terms or what is the absolute cost overrun generated by a risk in cost terms). It seems clear that one risk is more important than another, not only because of the estimation of its probability and impact but also because the activity on which it impacts may have a high criticality index or not (probability of belonging to the project’s critical path).
As expected, the contribution to the total duration of the identified risks that impact only cost is zero. The same is not valid for the risks identified to have an impact on duration because the latter also impacts the cost objective. We also see how the risks that initially impact a duration objective are more critical for their impact on cost than others that directly impact the project’s cost (e.g. R5).
Conclusions
The probability-impact matrix is used in project management to identify the risk to which the most attention should be paid during project execution. This paper studies how the risk matrix is adopted by a large majority of standards, norms and methodologies in project management and, at the same time, practitioners and academics recognise it as a fundamental tool in the qualitative risks analysis.
However, we also study how this risk matrix presents particular problems and offers erroneous and contradictory results. Some studies suggest alternatives to its use. Notwithstanding, it continues to be a widely employed tool in the literature by practitioners and academics. Along these lines, with this work we propose an alternative to the probability-impact matrix as a tool to know the most critical risk for a project that can prevent objectives from being fulfilled.
For this purpose, we propose a quantitative method based on MCS, which provides us with numerical results of the importance of risks and their impact on total duration and cost objectives. This proposed methodology offers significant advantages over other risk prioritisation methods and tools, especially the traditional risk matrix. The proposed case study reveals that risk prioritisation yields remarkably different results depending on the selected method, as our findings confirm.
In our case, we obtain numerical values for the impact of risks on total duration and cost objectives, and independently of one another. This result is interesting for project managers because they can focus decision-making on the priority order of risks and the dominant project objective (total duration or total cost) if they do not coincide.
From the obtained results, we find that the risks with an impact on the cost of activities do not influence the total duration result. The risks that impact project duration also impact the total cost target. This impact is more significant than that of a risk that impacts only the activity’s cost. This analysis leads us to believe that this quantitative prioritisation method has incredible potential for academics to extend their research on project risks and for practitioners to use it in the day-to-day implementation of their projects.
The proposed methodology will allow project managers to discover the most relevant project risks so they can focus their control efforts on managing those risks. Usually, implementing risk response strategies might be expensive (control efforts, insurance contracts, preventive actions, or others). Therefore, it is relevant to concentrate only on the most relevant risks. The proposed methodology allows project managers to select the most critical risks by overcoming the problems exhibited by previous methodologies like the probability-impact matrix.
In addition to the above, the risk prioritisation achieved by applying the proposed methodology is based on quantifying the impacts that risks may have on the duration and cost objectives of the project. Finally, we achieve an independent risk prioritisation in duration impact and project cost impact terms. This is important because the project manager can attach more importance to one risk or other risks depending on the priority objective that predominates in the project, the schedule or the total cost.
Undoubtedly, the reliability of the proposed method depends mainly on the accuracy of estimates, which starts by identifying risks and ends with modelling the probability and impact of each risk. The methodology we propose in this paper overcomes many of the problems of previous methodologies, but still has some limitations for future research to deal with. First of all, the results of simulations depend on the estimations of variables (probability distributions and their parameters, risk aversion parameters, etc.). Methodologies for improving estimations are beyond the scope of this research; we assume project teams are sufficient experts to make rational estimationsbased on experience and previous knowledge. Secondly, as risks are assumed to be independent, the contribution or effect of a particular risk can be estimated by including it in simulation and by computing its impact on project cost and duration. This is a reasonable assumption for most projects. In some very complex projects, however, risks can be related to one another. Further research should be done to face this situation.
As an additional research line, we plan to conduct a sensitivity study by simulating many different projects to analyse the robustness of the proposed method.
Finally, it is desirable to implement this methodology in real projects and see how it responds to the reality of a project in, for example, construction, industry, or any other sector that requires a precise and differentiated risk prioritisation.
Data availability
Data will be made available on request.
Acebes F, Curto D, De Antón J, Villafáñez, F (2024) Análisis cuantitativo de riesgos utilizando “MCSimulRisk” como herramienta didáctica. Dirección y Organización , 82(Abril 2024), 87–99. https://doi.org/10.37610/dyo.v0i82.662
Acebes F, De Antón J, Villafáñez F, Poza, D (2023) A Matlab-Based Educational Tool for Quantitative Risk Analysis. In IoT and Data Science in Engineering Management (Vol. 160). Springer International Publishing. https://doi.org/10.1007/978-3-031-27915-7_8
Acebes F, Pajares J, Galán JM, López-Paredes A (2014) A new approach for project control under uncertainty. Going back to the basics. Int J Proj Manag 32(3):423–434. https://doi.org/10.1016/j.ijproman.2013.08.003
Article Google Scholar
Acebes F, Pereda M, Poza D, Pajares J, Galán JM (2015) Stochastic earned value analysis using Monte Carlo simulation and statistical learning techniques. Int J Proj Manag 33(7):1597–1609. https://doi.org/10.1016/j.ijproman.2015.06.012
Al-Duais FS, Al-Sharpi RS (2023) A unique Markov chain Monte Carlo method for forecasting wind power utilizing time series model. Alex Eng J 74:51–63. https://doi.org/10.1016/j.aej.2023.05.019
Ale B, Burnap P, Slater D (2015) On the origin of PCDS - (Probability consequence diagrams). Saf Sci 72:229–239. https://doi.org/10.1016/j.ssci.2014.09.003
Ali Elfarra M, Kaya M (2021) Estimation of electricity cost of wind energy using Monte Carlo simulations based on nonparametric and parametric probability density functions. Alex Eng J 60(4):3631–3640. https://doi.org/10.1016/j.aej.2021.02.027
Alleman GB, Coonce TJ, Price RA (2018a) Increasing the probability of program succes with continuous risk management. Coll Perform Manag, Meas N. 4:27–46
Google Scholar
Alleman GB, Coonce TJ, Price RA (2018b) What is Risk? Meas N. 01(1):25–34
Ammar T, Abdel-Monem M, El-Dash K (2023) Appropriate budget contingency determination for construction projects: State-of-the-art. Alex Eng J 78:88–103. https://doi.org/10.1016/j.aej.2023.07.035
Axelos (2023) Managing Successful Projects with PRINCE2® 7th ed . (AXELOS Limited, Ed.; 7th Ed). TSO (The Stationery Office)
Bae HR, Grandhi RV, Canfield RA (2004) Epistemic uncertainty quantification techniques including evidence theory for large-scale structures. Comput Struct 82(13–14):1101–1112. https://doi.org/10.1016/j.compstruc.2004.03.014
Ball DJ, Watt J (2013) Further thoughts on the utility of risk matrices. Risk Anal 33(11):2068–2078. https://doi.org/10.1111/risa.12057
Article PubMed Google Scholar
Caron F (2013) Quantitative analysis of project risks. In Managing the Continuum: Certainty, Uncertainty, Unpredictability in Large Engineering Projects (Issue 9788847052437, pp. 75–80). Springer, Milano. https://doi.org/10.1007/978-88-470-5244-4_14
Caron F, Fumagalli M, Rigamonti A (2007) Engineering and contracting projects: A value at risk based approach to portfolio balancing. Int J Proj Manag 25(6):569–578. https://doi.org/10.1016/j.ijproman.2007.01.016
Chapman CB (1997) Project risk analysis and management– PRAM the generic process. Int J Proj Manag 15(5):273–281. https://doi.org/10.1016/S0263-7863(96)00079-8
Chapman CB, Ward S (2003) Project Risk Management: Processes, Techniques and Insights (John Wiley and Sons, Ed.; 2nd ed.). Chichester
Chen P-H, Peng T-T (2018) Value-at-risk model analysis of Taiwanese high-tech facility construction. J Manag Eng, 34 (2). https://doi.org/10.1061/(asce)me.1943-5479.0000585
Cox LA (2008) What’s wrong with risk matrices? Risk Anal 28(2):497–512. https://doi.org/10.1111/j.1539-6924.2008.01030.x
Cox LA, Babayev D, Huber W (2005) Some limitations of qualitative risk rating systems. Risk Anal 25(3):651–662. https://doi.org/10.1111/j.1539-6924.2005.00615.x
Creemers S, Demeulemeester E, Van de Vonder S (2014) A new approach for quantitative risk analysis. Ann Oper Res 213(1):27–65. https://doi.org/10.1007/s10479-013-1355-y
Article MathSciNet Google Scholar
Curto D, Acebes F, González-Varona JM, Poza D (2022) Impact of aleatoric, stochastic and epistemic uncertainties on project cost contingency reserves. Int J Prod Econ 253(Nov):108626. https://doi.org/10.1016/j.ijpe.2022.108626
Damnjanovic I, Reinschmidt KF (2020) Data Analytics for Engineering and Construction Project Risk Management . Springer International Publishing
Duijm NJ (2015) Recommendations on the use and design of risk matrices. Saf Sci 76:21–31. https://doi.org/10.1016/j.ssci.2015.02.014
Eldosouky IA, Ibrahim AH, Mohammed HED (2014) Management of construction cost contingency covering upside and downside risks. Alex Eng J 53(4):863–881. https://doi.org/10.1016/j.aej.2014.09.008
Elms DG (2004) Structural safety: Issues and progress. Prog Struct Eng Mater 6:116–126. https://doi.org/10.1002/pse.176
European Commission. (2023) Project Management Methodology. Guide 3.1 (European Union, Ed.). Publications Office of the European Union
Frank M (1999) Treatment of uncertainties in space nuclear risk assessment with examples from Cassini mission implications. Reliab Eng Syst Safe 66:203–221. https://doi.org/10.1016/S0951-8320(99)00002-2
Gatti S, Rigamonti A, Saita F, Senati M (2007) Measuring value-at-risk in project finance transactions. Eur Financ Manag 13(1):135–158. https://doi.org/10.1111/j.1468-036X.2006.00288.x
Giot P, Laurent S (2003) Market risk in commodity markets: a VaR approach. Energy Econ 25:435–457. https://doi.org/10.1016/S0140-9883(03)00052-5
Goerlandt F, Reniers G (2016) On the assessment of uncertainty in risk diagrams. Saf Sci 84:67–77. https://doi.org/10.1016/j.ssci.2015.12.001
Helton JC, Johnson JD, Oberkampf WL, Sallaberry CJ (2006) Sensitivity analysis in conjunction with evidence theory representations of epistemic uncertainty. Reliab Eng Syst Saf 91(10–11):1414–1434. https://doi.org/10.1016/j.ress.2005.11.055
Hillson D (2014) How to manage the risks you didn’t know you were taking. Paper presented at PMI® Global Congress 2014—North America, Phoenix, AZ. Newtown Square, PA: Project Management Institute
Hillson D, Simon P (2020) Practical Project Risk Management. THE ATOM METHODOLOGY (Third Edit, Issue 1). Berrett-Koehler Publishers, Inc
Hulett DT (2012) Acumen Risk For Schedule Risk Analysis - A User’s Perspective . White Paper. https://info.deltek.com/acumen-risk-for-schedule-risk-analysis
International Organization for Standardization. (2018). ISO 31000:2018 Risk management – Guidelines (Vol. 2)
International Organization for Standardization. (2019). ISO/IEC 31010:2019 Risk management - Risk assessment techniques
International Project Management Association. (2015). Individual Competence Baseline for Project, Programme & Portfolio Management. Version 4.0. In International Project Management Association (Vol. 4). https://doi.org/10.1002/ejoc.201200111
Joukar A, Nahmens I (2016) Estimation of the Escalation Factor in Construction Projects Using Value at Risk. Construction Research Congress , 2351–2359. https://doi.org/10.1061/9780784479827.234
Kerzner H (2022) Project Management. A Systems Approach to Planning, Scheduling, and Controlling (Inc. John Wiley & Sons, Ed.; 13th Editi)
Koulinas GK, Demesouka OE, Sidas KA, Koulouriotis DE (2021) A topsis—risk matrix and Monte Carlo expert system for risk assessment in engineering projects. Sustainability 13(20):1–14. https://doi.org/10.3390/su132011277
Krisper M (2021) Problems with Risk Matrices Using Ordinal Scales . https://doi.org/10.48550/arXiv.2103.05440
Kuester K, Mittnik S, Paolella MS (2006) Value-at-risk prediction: A comparison of alternative strategies. J Financ Econ 4(1):53–89. https://doi.org/10.1093/jjfinec/nbj002
Kwon H, Kang CW (2019) Improving project budget estimation accuracy and precision by analyzing reserves for both identified and unidentified risks. Proj Manag J 50(1):86–100. https://doi.org/10.1177/8756972818810963
Lemmens SMP, Lopes van Balen VA, Röselaers YCM, Scheepers HCJ, Spaanderman MEA (2022) The risk matrix approach: a helpful tool weighing probability and impact when deciding on preventive and diagnostic interventions. BMC Health Serv Res 22(1):1–11. https://doi.org/10.1186/s12913-022-07484-7
Levine ES (2012) Improving risk matrices: The advantages of logarithmically scaled axes. J Risk Res 15(2):209–222. https://doi.org/10.1080/13669877.2011.634514
Article ADS Google Scholar
Li J, Bao C, Wu D (2018) How to design rating schemes of risk matrices: a sequential updating approach. Risk Anal 38(1):99–117. https://doi.org/10.1111/risa.12810
Lorance RB, Wendling RV (2001) Basic techniques for analyzing and presentation of cost risk analysis. Cost Eng 43(6):25–31
Markowski AS, Mannan MS (2008) Fuzzy risk matrix. J Hazard Mater 159(1):152–157. https://doi.org/10.1016/j.jhazmat.2008.03.055
Article CAS PubMed Google Scholar
Menge DNL, MacPherson AC, Bytnerowicz TA et al. (2018) Logarithmic scales in ecological data presentation may cause misinterpretation. Nat Ecol Evol 2:1393–1402. https://doi.org/10.1038/s41559-018-0610-7
Monat JP, Doremus S (2020) An improved alternative to heat map risk matrices for project risk prioritization. J Mod Proj Manag 7(4):214–228. https://doi.org/10.19255/JMPM02210
Naderpour H, Kheyroddin A, Mortazavi S (2019) Risk assessment in bridge construction projects in Iran using Monte Carlo simulation technique. Pract Period Struct Des Constr 24(4):1–11. https://doi.org/10.1061/(asce)sc.1943-5576.0000450
Ni H, Chen A, Chen N (2010) Some extensions on risk matrix approach. Saf Sci 48(10):1269–1278. https://doi.org/10.1016/j.ssci.2010.04.005
Peace C (2017) The risk matrix: Uncertain results? Policy Pract Health Saf 15(2):131–144. https://doi.org/10.1080/14773996.2017.1348571
Project Management Institute. (2009) Practice Standard for Project Risk Management . Project Management Institute, Inc
Project Management Institute. (2017) A Guide to the Project Management Body of Knowledge: PMBoK(R) Guide. Sixth Edition (6th ed.). Project Management Institute Inc
Project Management Institute. (2019) The standard for Risk Management in Portfolios, Programs and Projects . Project Management Institute, Inc
Project Management Institute. (2021) A Guide to the Project Management Body of Knowledge: PMBoK(R) Guide. Seventh Edition (7th ed.). Project Management Institute, Inc
Proto R, Recchia G, Dryhurst S, Freeman ALJ (2023) Do colored cells in risk matrices affect decision-making and risk perception? Insights from randomized controlled studies. Risk Analysis , 1–15. https://doi.org/10.1111/risa.14091
Qazi A, Dikmen I (2021) From risk matrices to risk networks in construction projects. IEEE Trans Eng Manag 68(5):1449–1460. https://doi.org/10.1109/TEM.2019.2907787
Qazi A, Shamayleh A, El-Sayegh S, Formaneck S (2021) Prioritizing risks in sustainable construction projects using a risk matrix-based Monte Carlo Simulation approach. Sustain Cities Soc 65(Aug):102576. https://doi.org/10.1016/j.scs.2020.102576
Qazi A, Simsekler MCE (2021) Risk assessment of construction projects using Monte Carlo simulation. Int J Manag Proj Bus 14(5):1202–1218. https://doi.org/10.1108/IJMPB-03-2020-0097
Rehacek P (2017) Risk management standards for project management. Int J Adv Appl Sci 4(6):1–13. https://doi.org/10.21833/ijaas.2017.06.001
Rezaei F, Najafi AA, Ramezanian R (2020) Mean-conditional value at risk model for the stochastic project scheduling problem. Comput Ind Eng 142(Jul):106356. https://doi.org/10.1016/j.cie.2020.106356
Ruan X, Yin Z, Frangopol DM (2015) Risk Matrix integrating risk attitudes based on utility theory. Risk Anal 35(8):1437–1447. https://doi.org/10.1111/risa.12400
Sarykalin S, Serraino G, Uryasev S (2008) Value-at-risk vs. conditional value-at-risk in risk management and optimization. State-of-the-Art Decision-Making Tools in the Information-Intensive Age, October 2023 , 270–294. https://doi.org/10.1287/educ.1080.0052
Simon P, Hillson D, Newland K (1997) PRAM Project Risk Analysis and Management Guide (P. Simon, D. Hillson, & K. Newland, Eds.). Association for Project Management
Sutherland H, Recchia G, Dryhurst S, Freeman ALJ (2022) How people understand risk matrices, and how matrix design can improve their use: findings from randomized controlled studies. Risk Anal 42(5):1023–1041. https://doi.org/10.1111/risa.13822
Talbot, J (2014). What’s right with risk matrices? An great tool for risk managers… 31000risk. https://31000risk.wordpress.com/article/what-s-right-with-risk-matrices-3dksezemjiq54-4/
Taroun A (2014) Towards a better modelling and assessment of construction risk: Insights from a literature review. Int J Proj Manag 32(1):101–115. https://doi.org/10.1016/j.ijproman.2013.03.004
The Standish Group. (2022). Chaos report . https://standishgroup.myshopify.com/collections/all
Thomas P, Bratvold RB, Bickel JE (2014) The risk of using risk matrices. SPE Econ Manag 6(2):56–66. https://doi.org/10.2118/166269-pa
Tong R, Cheng M, Zhang L, Liu M, Yang X, Li X, Yin W (2018) The construction dust-induced occupational health risk using Monte-Carlo simulation. J Clean Prod 184:598–608. https://doi.org/10.1016/j.jclepro.2018.02.286
Traynor BA, Mahmoodian M (2019) Time and cost contingency management using Monte Carlo simulation. Aust J Civ Eng 17(1):11–18. https://doi.org/10.1080/14488353.2019.1606499
Vanhoucke, M (2018). The data-driven project manager: A statistical battle against project obstacles. In The Data-Driven Project Manager: A Statistical Battle Against Project Obstacles . https://doi.org/10.1007/978-1-4842-3498-3
Vatanpour S, Hrudey SE, Dinu I (2015) Can public health risk assessment using risk matrices be misleading? Int J Environ Res Public Health 12(8):9575–9588. https://doi.org/10.3390/ijerph120809575
Article CAS PubMed PubMed Central Google Scholar
Vose, D (2008). Risk Analysis: a Quantitative Guide (3rd ed.) . Wiley
Votto R, Lee Ho L, Berssaneti F (2020a) Applying and assessing performance of earned duration management control charts for EPC project duration monitoring. J Constr Eng Manag 146(3):1–13. https://doi.org/10.1061/(ASCE)CO.1943-7862.0001765
Votto R, Lee Ho L, Berssaneti F (2020b) Multivariate control charts using earned value and earned duration management observations to monitor project performance. Comput Ind Eng 148(Sept):106691. https://doi.org/10.1016/j.cie.2020.106691
Ward S (1999) Assessing and managing important risks. Int J Proj Manag 17(6):331–336. https://doi.org/10.1016/S0263-7863(98)00051-9
Download references
Acknowledgements
This research has been partially funded by the Regional Government of Castile and Leon (Spain) and the European Regional Development Fund (ERDF, FEDER) with grant VA180P20.
Author information
Authors and affiliations.
GIR INSISOC. Dpto. de Organización de Empresas y CIM. Escuela de Ingenierías Industriales, Universidad de Valladolid, Pº Prado de la Magdalena s/n, 47011, Valladolid, Spain
F. Acebes & J. Pajares
GIR INSISOC. Dpto. Economía y Administración de Empresas, Universidad de Málaga, Avda. Cervantes, 2, 29071, Málaga, Spain
J. M. González-Varona & A. López-Paredes
You can also search for this author in PubMed Google Scholar
Contributions
FA developed the conceptualisation and the methodology. JMG contributed to the literature review and interpretations of the results for the manuscript. FA and JP collected the experimental data and developed all the analyses and simulations. AL supervised the project. FA and JP wrote the original draft, while AL and JMG conducted the review and editing. All authors have read and agreed to the published version of the manuscript.
Corresponding author
Correspondence to F. Acebes .
Ethics declarations
Competing interests.
The authors declare no competing interests.
Ethical approval
Ethical approval was not required as the study did not involve human participants.
Informed consent
No human subjects are involved in this study.
Additional information
Publisher’s note Springer Nature remains neutral with regard to jurisdictional claims in published maps and institutional affiliations.
Supplementary information
Rights and permissions.
Open Access This article is licensed under a Creative Commons Attribution 4.0 International License, which permits use, sharing, adaptation, distribution and reproduction in any medium or format, as long as you give appropriate credit to the original author(s) and the source, provide a link to the Creative Commons licence, and indicate if changes were made. The images or other third party material in this article are included in the article’s Creative Commons licence, unless indicated otherwise in a credit line to the material. If material is not included in the article’s Creative Commons licence and your intended use is not permitted by statutory regulation or exceeds the permitted use, you will need to obtain permission directly from the copyright holder. To view a copy of this licence, visit http://creativecommons.org/licenses/by/4.0/ .
Reprints and permissions
About this article
Cite this article.
Acebes, F., González-Varona, J.M., López-Paredes, A. et al. Beyond probability-impact matrices in project risk management: A quantitative methodology for risk prioritisation. Humanit Soc Sci Commun 11 , 670 (2024). https://doi.org/10.1057/s41599-024-03180-5
Download citation
Received : 30 January 2024
Accepted : 13 May 2024
Published : 24 May 2024
DOI : https://doi.org/10.1057/s41599-024-03180-5
Share this article
Anyone you share the following link with will be able to read this content:
Sorry, a shareable link is not currently available for this article.
Provided by the Springer Nature SharedIt content-sharing initiative
Quick links
- Explore articles by subject
- Guide to authors
- Editorial policies

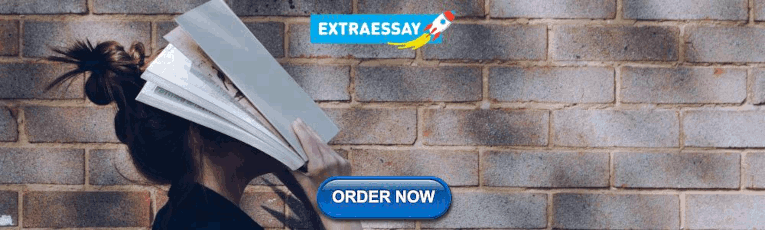
IMAGES
VIDEO
COMMENTS
Writing Research Proposals for Social Sciences and Humanities in a Higher Education Context
solid foundation in quantitative research methods in the social sciences. Moreover, we believe that this new edition can be a guide for quantitative researchers in the social sciences, specifically those who seek to work with R or transition from other programs to R.
Quantitative social science is an interdisciplinary field encompassing a large number of disciplines, including economics, education, political science, public policy, psy-chology, and sociology. In quantitative social science research, scholars analyze data to understand and solve problems about society and human behavior.
quantitative skills of careers in, for example, the financial sector as opposed to social scientific research. This programme does not set out to address such possible root causes. Rather, it starts from a recognition that, despite such influences and trends, there remain many centres of excellence in quantitative social science in Europe.
Mixed-methods research is a flexible approach, where the research design is determined by what we want to find out rather than by any predetermined epistemological position. In mixed-methods research, qualitative or quantitative components can predominate, or both can have equal status. 1.4. Units and variables.
Quantitative . Research Methods. T. his chapter focuses on research designs commonly used when conducting . quantitative research studies. The general purpose of quantitative research is to investigate a particular topic or activity through the measurement of variables in quantifiable terms. Quantitative approaches to conducting educational ...
The elephant in the room: Why should a social scientist read a book on "the humanities"? First, the title is somewhat misleading: The text focuses on historical and qualitative data that are often the subject of research in the humanities but are foundational to interpretive approaches in the social sciences as well.
Relevance to real-world problems in the social sciences is an essential ingredient of each chapter and makes this an invaluable resource. Indispensable to the teaching, study, and research of quantitative methods. Provides the foundations for quantitative research, with cutting-edge insights on the effectiveness of each method.
This book can be used to explain how quantitative methods can be part of the research instrumentation and the "toolbox" of scholars of Humanities and Social Sciences. The book contains numerous examples and a description of the main methods in use, with references to literature and available software.
This textbook offers an essential introduction to survey research and quantitative methods with clear instructions on how to conduct statistical tests with R. Building on the premise that we need to teach statistical methods in a holistic and practical format, the book guides students through the four main elements of survey research and quantitative analysis: (1) the importance of survey ...
The evolution of quantitative methods since the 1970s has been influenced by the growing interest in the social bond and phenomena of in-fluence and contagion, circulations and transfers, change, innovation, and life cycles. Special techniques have been developed to treat databases focused on relations and changes over time: network analysis ...
This resource is intended as an easy-to-use guide for anyone who needs some quick and simple advice on quantitative aspects of research in social sciences, covering subjects such as education, sociology, business, nursing. If you area qualitative researcher who needs to venture into the world of numbers, or a student instructed to undertake a quantitative research project despite a hatred for ...
About this book series. Quantitative Methods in the Humanities and Social Sciences is a book series designed to foster research-based conversation with all parts of the university campus - from buildings of ivy-covered stone to technologically savvy walls of glass. Scholarship from international researchers and the esteemed editorial board ...
Furthermore, it is grounded in the natural/physical sciences and adopts the ontological view that reality can be observed objectively, using the senses, quantified and measured, using numbers and ...
Quantitative methodologies: novel applications in the humanities and social sciences. At a time when data analysis is increasingly driving research and decision-making, computational analysis ...
History is notoriously a "big tent" discipline. Because everything has a past, every subject has a history. The tools appropriate to ferret out those histories multiply just as easily as the topics, depending on the questions being asked and the nature of the evidence preserved (accidentally or otherwise) that might answer them. In what sense is History a coherent "discipline" at all ...
behaviours. Social sciences can be classified into disciplines such as psychology (the science of human behaviours), sociology (the science of social groups), and economics (the science of firms, markets, and economies). The natural sciences are different from the social sciences in several respects. The natural
In this paper, I present an introduction to quantitative research methods in social sciences. The paper is intended for non-Economics undergraduate students, development researchers and ...
Quantitative research explains phenomena by collecting numerical unchanging d etailed data t hat. are analyzed using mathematically based methods, in particular statistics that pose questions of ...
Research is the framework used for the planning, implementation, and analysis of a study. The proper choice of a suitable research methodology can provide an effective and successful original research. A researcher can reach his/her expected goal by following any kind of research methodology. Quantitative research methodology is preferred by many researchers.
The paper describes computational tools that can be of great help to both qualitative and quantitative scholars in the humanities and social sciences who deal with words as data. The Java and Python tools described provide computer-automated ways of performing useful tasks: 1. check the filenames well-formedness; 2. find user-defined characters in English language stories (e.g., social actors ...
Research in the humanities, social sciences, and fine and creative arts has impact but defining, ... immediate as well as protracted. It includes the influence such research has upon 1 For example: ... that neither quantitative nor qualitative measures entirely suffice, so a combination of both, including ...
Keywords: critical review, quantitative research, qualitative research. 1. INTRODUCTION It is generally believed that qualitative and quantitative research methods are two frequently-used approaches utilised by researchers to collect effective data for their studies in a variety of disciplines, particularly in the filed of social science, such as
The project managers who deal with risk management are often faced with the difficult task of determining the relative importance of the various sources of risk that affect the project. This ...