A Systematic Literature Review on Malware Analysis
Ieee account.
- Change Username/Password
- Update Address
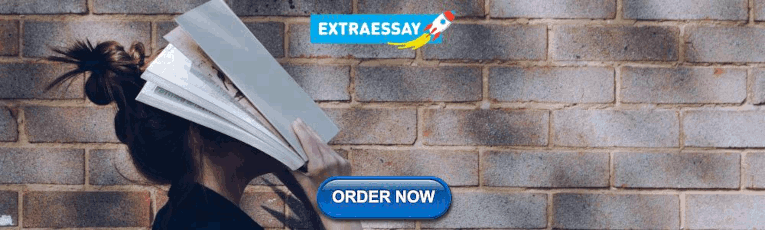
Purchase Details
- Payment Options
- Order History
- View Purchased Documents
Profile Information
- Communications Preferences
- Profession and Education
- Technical Interests
- US & Canada: +1 800 678 4333
- Worldwide: +1 732 981 0060
- Contact & Support
- About IEEE Xplore
- Accessibility
- Terms of Use
- Nondiscrimination Policy
- Privacy & Opting Out of Cookies
A not-for-profit organization, IEEE is the world's largest technical professional organization dedicated to advancing technology for the benefit of humanity. © Copyright 2024 IEEE - All rights reserved. Use of this web site signifies your agreement to the terms and conditions.
Click through the PLOS taxonomy to find articles in your field.
For more information about PLOS Subject Areas, click here .
Loading metrics
Open Access
Peer-reviewed
Research Article
Android malware analysis in a nutshell
Roles Conceptualization, Data curation, Formal analysis, Funding acquisition, Investigation, Methodology, Project administration, Resources, Supervision, Validation, Visualization, Writing – original draft, Writing – review & editing
* E-mail: [email protected] , [email protected]
Affiliations Security Engineering Lab, Computer Science Department, Prince Sultan University, Riyadh, KSA, Computer Science Department, King Abdullah II School of Information Technology, The University of Jordan, Amman, Jordan

Roles Conceptualization, Data curation, Formal analysis, Investigation, Methodology, Resources, Software, Validation, Visualization, Writing – original draft, Writing – review & editing
Affiliation Security Engineering Lab, Computer Science Department, Prince Sultan University, Riyadh, KSA
Affiliations Security Engineering Lab, Computer Science Department, Prince Sultan University, Riyadh, KSA, Electronics and Electrical Communication Engineering Department, Faculty of Electronic Engineering, Menoufia University, Menouf, Egypt
- Iman Almomani,
- Mohanned Ahmed,
- Walid El-Shafai
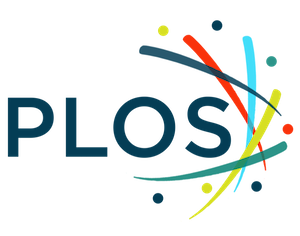
- Published: July 5, 2022
- https://doi.org/10.1371/journal.pone.0270647
- Reader Comments
This paper offers a comprehensive analysis model for android malware. The model presents the essential factors affecting the analysis results of android malware that are vision-based. Current android malware analysis and solutions might consider one or some of these factors while building their malware predictive systems. However, this paper comprehensively highlights these factors and their impacts through a deep empirical study. The study comprises 22 CNN (Convolutional Neural Network) algorithms, 21 of them are well-known, and one proposed algorithm. Additionally, several types of files are considered before converting them to images, and two benchmark android malware datasets are utilized. Finally, comprehensive evaluation metrics are measured to assess the produced predictive models from the security and complexity perspectives. Consequently, guiding researchers and developers to plan and build efficient malware analysis systems that meet their requirements and resources. The results reveal that some factors might significantly impact the performance of the malware analysis solution. For example, from a security perspective, the accuracy, F1-score, precision, and recall are improved by 131.29%, 236.44%, 192%, and 131.29%, respectively, when changing one factor and fixing all other factors under study. Similar results are observed in the case of complexity assessment, including testing time, CPU usage, storage size, and pre-processing speed, proving the importance of the proposed android malware analysis model.
Citation: Almomani I, Ahmed M, El-Shafai W (2022) Android malware analysis in a nutshell. PLoS ONE 17(7): e0270647. https://doi.org/10.1371/journal.pone.0270647
Editor: Sathishkumar V E, Hanyang University, KOREA, REPUBLIC OF
Received: April 30, 2022; Accepted: June 14, 2022; Published: July 5, 2022
Copyright: © 2022 Almomani et al. This is an open access article distributed under the terms of the Creative Commons Attribution License , which permits unrestricted use, distribution, and reproduction in any medium, provided the original author and source are credited.
Data Availability: The datasets that support the findings of this study are available online. These datasets were derived from the following resources available in the public domains: 1. https://www.sec.tu-bs.de/~danarp/drebin/download.html 2. https://www.impactcybertrust.org/dataset_view?idDataset=1275 .
Funding: This research was carried out without any financial support; however, the publication fee is sponsored by the Prince Sultan University, Saudi Arabia.
Competing interests: The authors have declared that no competing interests exist.
Introduction
Mal cious soft ware (Malware) is any software built for unauthorized purposes and mala fide aims. So, the malware affects the operating system performance and its running services due to its harmful behavior. Currently, android malware is one of the most critical threats that can encrypt or defect the operation of Android devices [ 1 ]. This is because Android malware applications (APKs) can steal or cipher sensitive data, show undesirable advertising, disrupt normal functions, or control the users’ devices without their knowledge [ 2 ].
There are a lot of groups and categories of Android malware APKs, such as worms, botnet, rootkits, ransomware, and Trojans [ 3 ]. These Android malware attacks can exploit metamorphic and polymorphic procedures to obfuscate traditional malware recognition and detection algorithms. Moreover, the Android malware developers have a tendency to modify small sections of the developed and implemented source codes to create other malware alternatives and threats that can evade the malware detection techniques [ 4 ]. Consequently, the identification process of Android malware attacks from the same malware family becomes tremendously challenging [ 5 ]. Therefore, efficient Android malware detection algorithms based on smart artificial intelligence (AI) tools need to be developed and implemented to identify and recognize the harmful effect of Android malware threats [ 6 , 7 ].
Android malware detection and identification algorithms are categorized into four main groups: static-based, dynamic-based, vision-based, or hybrid-based detection algorithms [ 8 – 12 ]. In static-based identification algorithms, the Android malware APKs are analyzed without executing them. So, these static-based algorithms depend on extracting some of the important features from the suspected source codes to identify and recognize the Android malware families. However, the main disadvantage of these static-based algorithms is that they are not robust to code obfuscation, and they need more computation steps during the process of extracting features [ 13 , 14 ]. In dynamic-based identification algorithms, the traces and features of the suspected source codes are examined and analyzed during their execution and running. The critical disadvantage of these algorithms is that they are more time-consuming and require additional storage resources [ 15 ].
On the other hand, in the hybrid-based identification algorithms, two or more types of identification categories are simultaneously employed to efficiently detect the Android malware attacks. But this malware identification category needs more sequential steps, high computational complexity, human intervention, and manual effort [ 16 ]. In vision-based malware identification algorithms, the Android malware APKs or their extracted features are converted to visual 2D digital images before the classification and detection process. Therefore, the main features of the Android malware APKs can be extracted and obtained by the unzipping or decompilation processes [ 17 , 18 ]. Then, the resulting 1D binary vectors of the extracted features (i.e., Android manifest, SMALI, and Classes.dex) are transformed to 2D vectors (grayscale images). In the last step, the resulting 2D grayscale images are forwarded to a well-developed malware classifier such as Convolutional Neural Networks (CNN)-based malware classifiers to detect and classify the category and family of the analyzed Android malware APKs.
Recently, Deep Learning (DL) and optimization algorithms are currently utilized and exploited in mitigating Android malware threats [ 19 – 22 ]. Thus, DL networks such as CNN algorithms are the most common AI and DL-based recognition & identification techniques used to detect malware attacks from the input malware visual images [ 23 – 25 ]. Furthermore, the CNN networks have the ability to efficiently distinguish various objects and aspects on the input visual images using well-tuned learning biases and weights based on utilizing optimization algorithms. Therefore, the CNN algorithms are the best choice for image classification challenges and applications, such as classifying malware images [ 26 – 29 ]. Consequently, efficient developed CNN algorithms can be used to automatically collect and obtain the rich and valuable features from Android malware visual images. Then, these obtained features are used to classify and identify the different families of Android malicious APKs.
Therefore, in our proposed work, without executing or running the Android APKs, we first converted their binary data into 2D images. After that, we employed a well-developed CNN-based Android malware detection algorithm to classify different categories of Android malware families from these 2D images. In addition, we tested and analyzed different 21 pre-trained CNN algorithms to check their detection performance in identifying and recognizing the Android malware classes from their visual images. Thus, the DL-based CNN algorithms differ from traditional Machine Learning (ML) algorithms that accomplish feature representation with specific parameter configuration or particular assumptions. Therefore, compared to conventional ML algorithms, the DL-based CNN algorithms can effectively discover complex patterns and obtain valuable features from multi-dimensional patterns like visual images.
In Android malware analysis and detection systems, many parameters and factors need to be considered that control the identification and recognition performance of the utilized malware classifiers. These parameters include (1) the analyzed Android dataset (balanced or imbalanced), (2) the utilized evaluation metrics (i.e., security or complexity metrics), (3) the type of malware analysis (static, dynamic, hybrid, or vision), and (4) the type of APK components selected to be analyzed in the detection process (i.e., Full APK file, android manifest file, SMALI file, or Classes.dex file).
So, this research is motivated by the importance of the area of android malware analysis and detection solutions due to the increased risk of such types of attacks. In addition, there are tremendous existing efforts utilizing vision-based algorithms to analyze and detect android malware with high accuracy. The most critical issue in the previous related works is that they only studied some parameters in their introduced malware detection systems. But, to achieve high detection accuracy and efficient malware analysis, many factors must be investigated that directly or indirectly affect the malware classification process.
As most current malware detection systems consider one or some factors while building their malware predictive systems, this motivates us to offer a comprehensive analysis model for Android malware. The model presents the essential factors affecting the analysis results of vision-based Android malware. Consequently, we comprehensively highlighted these factors and tested their impacts through a deep empirical study. The goal is to support researchers and developers by providing a clear guide on planning and building efficient Malware analysis systems that meet their requirements and available resources.
The significant contributions of our work are detailed as follows:
- Summarizing and comparing the most recent vision-based Android malware detection systems and the main factors studied by them.
- Proposing a nutshell vision-based model for efficiently detecting malware apps. This model considers a comprehensive set of factors that might impact the efficiency of malware analysis and detection solutions from the security and complexity perspectives. These factors include the nature of the malware datasets, APK conversion process & format, CNN algorithms used, and the evaluation metrics applied.
- Constructing a deep empirical study to implement all these factors and related parameters and analyze their impacts by running more than 450 experiments within the same environmen.
- Investigating the malware detection performance of different 22 CNN algorithms as part of the empirical study on the two most common imbalanced Android malware datasets (DREBIN and AMD). One of these CNN algorithms is developed from scratch for this research.
- Avoiding the need for static or dynamic analysis for classifying Android malware attacks by converting Android threats to visual images for easy and low-complex classification process using CNN algorithms. Thus, we achieved low computational complexity and, at the same time, obtained high detection accuracy.
- Studying the impact of different visual formats of Android malware APKs on the security and complexity performance of malware detection algorithms.
- Analyzing highly imbalanced Android malware datasets containing unbalanced malware classes to achieve proper detection performance.
- Report and analyze the experiments’ results whether the malware APKs were directly converted to images or rich extracted features from the Android APKs were converted to visual images.
- Performing a deep comparative analysis for the security and complexity metrics performance of all tested scenarios composed in the proposed comprehensive vision-based model.
The structure of this work is as follows. Section Related Work summarizes and compares the recent related studies. Section Proposed presents the proposed comprehensive android malware analysis model. Section Analysis illustrates the model evaluation and results discussions & analysis. Finally, Section Conclusions concludes the paper and offers some future directions.
Related work
This section summarizes and compares the previous work related to image-based malware detection algorithms and systems. Table 1 shows a summary and comparison in terms of type of image conversion (and if the process used involves unzipping, de-compilation, or both), used dataset(s), utilized CNN algorithms, performance evaluation measures considered such as model/prepossessing complexity and security measures.
- PPT PowerPoint slide
- PNG larger image
- TIFF original image
https://doi.org/10.1371/journal.pone.0270647.t001
Various algorithms were introduced in the literature that use unzipping, de-compilation, or both in the image conversion process. Regarding unzipping-related approaches [ 30 ], introduced a byte-level malware classification method by using Markov technique in classes.dex-to-image conversion and then using deep CNN for the classification. Moreover [ 31 ], proposed a system to classify malware by converting non-intuitive features into images to extract features using CNN and use the features in classical ML algorithms such as KNN to detect the malware family. [ 32 ] implemented and introduced a color visualization method on classes.dex and AndroidManifest.xml files in malware Android apps and classify the images using CNN-ResNet models. In [ 33 ] paper, classical machine algorithms such as Random Forest, K-nearest Neighbors, Decision Tree, Bagging, AdaBoost, and Gradient Boost were used for classification after constructing feature vectors from gray images, yielded from converting APK contents such as classes.dex to images. [ 34 ] proposed an approach to enhance blockchain user security by implementing RGB image visualization technique on three types of files in Android apps: classes.dex, AndroidManifest.xml, and Certificate. Then, train different classification models and apply a decision mechanism to detect malware versus benign. On the other hand, for de-compilation techniques [ 35 ], introduced a method called AdMat which treats Android apps as images by forming an adjacency matrix for each app and then feeding them to the CNN model to classify an app to malware or benign. Additionally [ 36 ], combined Opcodes, API packages, and API functions to construct RGB images and then use CNN for classification. [ 37 ] mapped permissions to severity levels [ 38 ]to create images to be fed to the CNN model for malware classification. Other methods such as [ 39 ] used network interactions as features to be converted to images to be input for CNN.
Different datasets were used in the previous papers to test the models and systems. The main ones were Drebin and AMD. Some of them used DREBIN alone, such as [ 31 , 36 ], and some of them used only AMD, such as [ 39 ]. However, most of them used a combination of both [ 32 , 33 , 35 , 37 ].
To evaluate the performance of the resulted predictive models, several metrics were used in the literature. Common metrics were accuracy, precision, recall, and F1-score [ 30 , 31 , 34 – 36 ]. Other metrics were used such as error rate, specificity, sensitivity, MSE, and FPR [ 31 , 32 , 36 , 37 ].
Even though different works have been introduced for malware detection analysis, none of them studied the approach comprehensively in terms of the used image conversion methods, datasets, CNN models, and evaluation metrics. This can be clearly observed in the comparison conducted among the related work and our proposed analysis model, as shown in Table 1 . For example, in terms of the employed CNN algorithms used, most of the related works examined a few models such as VGG16, ResNet, and customized CNN algorithms such as in [ 30 – 32 , 34 – 37 , 39 ]. Moreover, they did not take into consideration all different file formats of Android malware samples. For instance, the authors in [ 30 – 34 ] focused on the unzipping prepossessing without considering the impact of decompiling preprocessing for the Android malware APKs. On the other hand, the authors in [ 35 – 37 ] only considered the decompiling preprocessing. Additionally, few assessment metrics were used for performance evaluation and complexity & security analysis of the examined CNN algorithms. For example, some of the related studies used training time, prepossessing time, test time, APK file size, and RAM usage as complexity parameters, such as in [ 30 – 32 , 34 – 37 , 39 ]. However, none of these related works introduced a comprehensive analysis of all of these complexity metrics. Moreover, in terms of security measures used, the related works used various security metrics such as accuracy, precision, recall, and F1-score, such as in [ 30 – 32 , 34 – 37 , 39 ]. But many other assessment metrics must be considered and analyzed. For instance, the authors in [ 31 ] considered other metrics such as error rate and MSE, while the authors in [ 36 ] evaluated their suggested CNN algorithms using TPR and FPR. However, most related studies did not present deep and comprehensive security analyses such as the estimation of NPV, PPV, and FOR parameters that can provide more insights.
Therefore, in this paper, we introduce a comprehensive model that profoundly investigates the critical factors that might impact the performance of android malware analysis systems in terms of efficiency, complexity, and security perspectives. Our proposed work covers different APK file formats, and different scenarios of de-compilation & unzipping preprocessing to extract more features from the android APKs such as AM, DEX, de-compiled AM, and SMALI. Additionally, the proposed Android malware analysis model tests the performance of different 22 CNN algorithms in terms of comprehensive security and complexity metrics to deeply analyze their detection and computational efficiencies.
Proposed comprehensive Android malware analysis model
This section presents a nutshell model of building vision-based prediction models for Android malware detection systems. As shown in Fig 1 , primary factors should be considered as they will affect the Android malware analysis and detection processes. These factors include:
- Type of conversion : This defines how the Android malware apk is analyzed. One option is to keep it as is (compressed) and then convert it to an image. Another option is to decompile the apk file first using different tools such as apktool ( https://ibotpeaches.github.io/Apktool/ ). This tool decompiles the apk to generate smali files and the Android Manifest (AM) file. Then these files will be stacked and then converted to images. Additionally, the analysis system could consider only unzipping the apk file and then converting the resulting AM file and “classes.dex” (CD) files to images.
- Dataset Nature : The created or chosen Android malware dataset could severely impact the analysis model and the resulted predictive models. This includes the type of malware apps considered and their primary behavior, the number of families (classes), and whether the dataset is balanced or not.
- CNN Algorithms : The type of CNN algorithm that will be used to build the predictive model is vital to the performance of the malware detection systems. Therefore, this study has examined most of the well-known CNN algorithms (all currently implemented by Keras ( https://keras.io/ )) to provide a deep insight into the CNN algorithms’ impact on detecting Android malware applications.
- Evaluation : The way the Android malware analysis and predictive models are evaluated is critical to trade-off the system performance in terms of security and complexity. Therefore, the evaluation metrics must be carefully selected based on the system’s needs and available resources.
https://doi.org/10.1371/journal.pone.0270647.g001
The main flow of our proposed model is illustrated in Fig 2 . The first phase in the proposed nutshell model is the selection of the benchmarked android applications (apks) datasets that are heavily utilized in vision-based malware analysis systems. Therefore, both DREBIN [ 40 ] and AMD [ 41 ] have been selected. The reason behind choosing two different datasets is to show the impact of only changing the nature of the dataset that the system is analyzing and testing on the performance of the overall detection process. After that, the model processes these apks in different ways of conversions: (1) apk is kept compressed as is, (2) apk is decompiled using Apktool to produce android decompiled manifest file (DAM), and Smali files, and (3) apk is unzipped to generate android manifest (AM) file and Dex files. Then, the image conversion phase is started by converting all features resulting from the above files into images. These visual malware images are obtained by converting the extracted features’ binaries to 8-bit vectors and then converted to 2D grayscale images. For more details and explanations for the byte-to-image conversion process, it can be checked in [ 23 , 26 ].
https://doi.org/10.1371/journal.pone.0270647.g002
The final phase is applying 22 CNN models for training and testing the predictive models and then evaluating their performances using a comprehensive set of assessment metrics related to complexity such as time, CPU & storage utilization for both the per-processing and model execution phases. Additionally, 16 security-related metrics are also measured.
21 pre-trained CNN algorithms (VGG16, ResNet50, VGG19, DenseNet121, DenseNet169, DenseNet201, EfficientNetB0, EfficientNetB1, EfficientNetB2, EfficientNetB3, EfficientNetB4, EfficientNetB5, EfficientNetB6, EfficientNetB7, InceptionResNetV2, InceptionV3, MobileNet, MobileNetV2, MobileNetV3Large, MobileNetV3Small, and Xception) [ 42 – 44 ] are examined. These pretrained CNN algorithms are developed in Python and implemented in Keras and TensorFlow libraries [ 45 – 47 ]. Additionally, another CNN algorithms is developed from scratch in this research. This algorithm has different layers, as shown in Fig 3 .
https://doi.org/10.1371/journal.pone.0270647.g003
It consists of several sequential stages. The first stage is the processing of the input visual malware images through the input layer and the Batch-Normalization (BN) layer that normalizes the visual images by re-scaling and re-centering processes. The BN layer is also introduced in the proposed algorithm to stabilize the CNN network. Then, in the second stage, the superlative and furthermost effective features are extracted and accumulated through several 2D convolutional layers (Conv2D), containing the same padding and stride by one. The weights of each utilized Conv2D are initialized with an orthogonal matrix.
The number of employed filters in the Conv2D layers are 8, 16, 32, 64, 64, 256, respectively. Also, the Conv2D layers are interspersed with pooling layers called MaxPooling that selecting the most significant pixel values in a four-pixel space. So, the MaxPooling layers are characterized by reducing the computational burden of the proposed neural CNN network. After that, the GlobalAveragePooling2D is introduced to gather the most common features during the training process.
In the last stage, which is the decision-making, classification & detection stage, the spatial data is primarily converted to one-dimensional data by the flatten later. Next, three sequential fully connected layers (Dense) are utilized, each one of the first two Dense layers consists of 1024 nodes (neurons) whilst the last Dense layer consists of a number of nodes that equal the number of classified classes (eight malware classes in our proposed work). In addition, in the proposed CNN algorithm, we used the Dropout layer to prevent the overfitting problem. Furthermore, the Rectified Linear Unit (ReLU) is also utilized in all Conv2D and Dense layers as an activation function. But the ReLU is used in the last SoftMax layer to make the classification decision. Table 2 presents the specifications of all employed layers in the proposed CNN algorithm.
https://doi.org/10.1371/journal.pone.0270647.t002
Model evaluation and results analysis
This section describes and discusses the security and complexity analysis for the proposed comprehensive model. So, the in-detail analysis and testing of the employed 22 CNN algorithms are introduced in terms of different evaluation metrics. The simulation specifications of all examined CNN algorithms in the proposed comprehensive vision-based android malware detection model is summarized in Table 3 .
https://doi.org/10.1371/journal.pone.0270647.t003
Two imbalanced android datasets (DREBIN [ 40 ] and AMD [ 41 ]) are examined in the simulation analysis. Each one of these datasets contains eight android malware classes. The names and numbers of android malware APKs of the examined DREBIN and AMD datasets are presented in Table 4 .
https://doi.org/10.1371/journal.pone.0270647.t004
Assessment metrics
Security analysis
To assess the security of the proposed comprehensive model, we carried out extensive simulation experiments based on different vision-based scenarios. So, the examined 22 CNN algorithms, including the proposed one, are tested on five vision-based formats, which are: (1) direction conversion of an APK file to a visual image, (2) conversion of Android Manifest (AM) file extracted from the unzipping process to a visual image, (3) conversion of AM file extracted from the decompilation (DAM) process to a visual image, (4) conversion of Classes.dex (CD) file extracted from the unzipping process to a visual image, and (5) conversion of SMALI file extracted from the decompilation process to a visual image. All the above-mentioned security-related metrics are calculated. For simplicity in presenting and comparing the results, the accuracy, precision, recall, and F1-Score metrics are highlighted in each tested CNN algorithm for the five studied vision-based scenarios on two different android malware datasets (DREBIN & AMD), as shown in Tables 5 and 6 .
https://doi.org/10.1371/journal.pone.0270647.t005
https://doi.org/10.1371/journal.pone.0270647.t006
Tables 5 and 6 present the performance of all predictive models generated based on the DREBIN and AMD datasets from security perspectives. The results revealed that the proposed CNN algorithm achieves superior detection efficacy for the assessed security parameters compared to the other conventional CNN algorithms. Furthermore, it is demonstrated for the two examined android malware datasets that the DAM vision-based format introduces the best security performance for the proposed CNN algorithm and almost all tested CNN algorithms compared to other examined vision-based formats.
Moreover, Tables 5 and 6 show that the achievement of high detection efficacy depends on the proper selection of the CNN algorithm and the appropriate choice of utilized vision-based format. So, for example, in some tested cases, the DAM vision-based format is not the best vision-based scenario for some examined CNN algorithms. Therefore, based on the security target of the android malware analysis system, it can select the appropriate CNN model and vision-based format.
22 CNN models were implemented and applied on the two datasets. To simplify the presentation of the simulation results, we introduce only the confusion matrices and the accuracy & loss curves of the best-performed CNN model for the two investigated android malware datasets. Fig 4 presents the acquired confusion matrices of the proposed CNN algorithm for the two tested AMD and DREBIN android malware datasets for the best DAM image format. The security performance evaluation in terms of accuracy, recall, precision, and F1-Score can be estimated from these confusion matrices. It is demonstrated that the proposed CNN algorithm gives low false detection and low misclassification rate for the eight examined malware classes in both datasets. Fig 5 introduces the obtained accuracy & loss curves of the proposed CNN algorithm for the two tested AMD and DREBIN android malware datasets for the best DAM image format. The achieved results confirm that the proposed CNN algorithm provides the highest detection accuracy and the lowest detection loss compared to the other examined CNN algorithms, as also clarified in Tables 5 and 6 .
https://doi.org/10.1371/journal.pone.0270647.g004
https://doi.org/10.1371/journal.pone.0270647.g005
Table 7 shows the highest increase in the performance achieved among the different predictive models in terms of accuracy, F1-Score, precision, and recall. The comparison was conducted to show how various factors can affect the performance of the resulting predictive models in case of (a) only changing the type of conversion while keeping the same dataset and the applied CNN algorithm, (b) keeping the same conversion type and dataset while changing the applied CNN algorithm (c) keeping the type of conversion and applied CNN algorithm while changing the dataset itself. For example, the accuracy improvement reached 52.80% when CD type is used instead of the whole APK utilizing InceptionResNetV2 algorithm and AMD dataset. On the other hand, the accuracy improved by 107% when DAM type was used by our proposed algorithm (scratch) in comparison to the InceptionResNetV2 algorithm. The rest of the most significant F1-score, precision, and recall improvements have reached 95.16%, 71.44%, and 52.8%, respectively, when different conversion types were considered while applying the same CNN algorithm. Additionally, within the same conversion type, applying different CNN algorithms introduced 139.91% of F1-score improvement in the case of DAM type, 109.88% precision improvement in the case of SMALI type, and 107.04% in the case of DAM type.
https://doi.org/10.1371/journal.pone.0270647.t007
Similar behaviors were observed when DREBIN dataset was used. When applying the same CNN algorithm but considering different conversion types, the accuracy, F1-Score, precision, and recall have been improved by 77.55%, 149.71%, 126.16%, and 77.55%, respectively. However, the highest increase in the performance reached 131.29%, 236.44%, 192.00%, and 131.29% in terms of accuracy, F1-Score, precision and recall, respectively, when APK format was used and different CNN algorithms were applied.
The performance was also affected when the dataset itself was changed. For example, the amount of improvement in the accuracy, F1-Score, precision, and recall was higher when the DREBIN dataset was used, whether by changing the conversion type or the applied CNN algorithm, as also shown in Table 7 .
About the above comparisons and discussion, we can emphasize the impact of different factors on the performance of the android malware analysis systems. These factors need to be carefully addressed by the developers of the malware detection system to build predictive models that meet their needs.
In the following section, another way of assessing the malware analysis systems in terms of complexity. The developers can balance both the security and complexity measures when building their systems.
Complexity analysis
In addition to the security evaluation of the proposed comprehensive android malware analysis and predictive model, we have measured the complexity concerning the models’ execution and pre-processing phases. The models’ execution cost was calculated based on the model computational test time and CPU usage. Therefore, the complexity of all examined CNN algorithms, including our proposed algorithm, was measured when the two different android malware datasets were utilized, as shown in Tables 8 and 9 . The experiments’ outcomes reveal that (a) in the case of the DREBIN dataset, the test time was higher when APK as a whole was converted to an image, especially in our proposed CNN algorithm, VGG16, ResNet50, DenseNet121, DenseNet169, and EfficientNetB0, (b) there was variation among the models in regards to testing time even after using the same conversion type, (c) CPU usage in case of APK was less or close to the other types’ CPU usages in almost all CNN algorithms. In general, the CPU usage values were close in all algorithms for all conversion types, (d) overall, the test time was higher in DREBIN in comparison to AMD in all applied CNN algorithms, (e) fewer variations among the test time in the case of using AMD dataset in comparison to DREBIN with the highest value observed in EfficientNetB7, (f) CPU usage values were close for all conversion types and applied CNN algorithms in the case of using the AMD dataset, (g) our proposed CNN algorithm achieved lower testing time and CPU usage compared to other transfer learning CNN algorithms for the two tested datasets.
https://doi.org/10.1371/journal.pone.0270647.t008
https://doi.org/10.1371/journal.pone.0270647.t009
As discussed in the propose work section, the CNN algorithm is developed from scratch, and it is not a pre-trained CNN algorithm. Thus, as clarified in Table 2 (last row), our proposed CNN algorithm used a small number of trainable/non-trainable parameters compared to other pre-trained CNN algorithms. Therefore, it introduced a lower execution time.
Furthermore, the complexity of the pre-processing phases was measured in terms of (a) the speed of decompiling and unzipping processes for both the two tested android malware datasets, and (b) the size of the obtained visual images for all types of conversion considered in this research. Fig 6 demonstrates, in terms of histograms, the speed of decompiling and unzipping processes for the DREBIN and AMD datasets.
https://doi.org/10.1371/journal.pone.0270647.g006
The histogram distributions show the number of samples (y-axis) unzipped or decompiled as the time elapsed (x-axis). The general observation is that the unzipping process is faster than the decompiling for the two examined android malware datasets. This can be witnessed by counting the number of samples that can be unzipped by time. For example, in the case of the DREBIN dataset, more than 4000 apps took less than 0.005 seconds to be unzipped. In contrast, most apps (around 3000) took 2 to 3 seconds to be decompiled. AMD dataset apps took less time to unzip and decompile. For example, around 10000 apps took less than 0.01 seconds to be unzipped. In comparison, about 7000 apps took less than 3 seconds to be decompiled.
Similar outcomes are observed in the case of using the AMD dataset. However, overall, the unzipping and decompilation processes were faster in DREBIN than in the AMD dataset; this is due to the nature of the android apps included in this dataset.
Moreover, Figs 7 – 9 show the histogram distribution of the files size of the resulted images from the different types of conversations for the two datasets. Fig 7 presents the files size comparison between the DREBIN and AMD datasets of the produced APK images. It can be noticed that files size was much larger when the AMD dataset was used.
https://doi.org/10.1371/journal.pone.0270647.g007
https://doi.org/10.1371/journal.pone.0270647.g008
https://doi.org/10.1371/journal.pone.0270647.g009
The files size comparisons between the DREBIN and AMD datasets in the case of AM/DAM images and CD/SMALI images are shown in Figs 8 and 9 , respectively. The obtained results declare that the APK images have the largest files size compared to the other images resulting from other files types for both datasets. However, AM/DAM images are the smallest among them. Moreover, for all types of files and produced images, AMD was higher in size than DREBIN. Again this is due to the nature of the android apps included in this dataset.
Conclusions and future work
Android is the leading operating system worldwide, with around 70% market share. Consequently, attracting different security attackers to produce threatening malware apps that serve their bad intentions. On the other hand, security professionals are highly motivated to build efficient and smart android malware analysis and detection systems. These systems could be built based on vision-based approaches where the android apps or some of their components are converted to images. In this context, CNN algorithms are one of the best choices to generate vision-based predictive solutions.
The main shortcoming of the current related works is the focus on some factors when developing their malware analysis solutions, limiting the selection of best factors and practices that meet the target performance within the available resources.
Therefore, this study aims to provide a nutshell model for analyzing android malware apps that facilitates achieving high performance while respecting the system’s constraints. Furthermore, this research studied intensely the main factors that might significantly influence the performance of detecting android malware from security and complexity perspectives.
This study started by conducting a deep comparison among recent related works in the area of vision-based android malware analysis to check the primary factors considered by them and their ways of assessing them. Then we have built a comprehensive malware analysis model that captures essential aspects, processes, and practices that need to be considered to ensure the efficient building of malware detection systems. This model provides a thorough vision to developers on what to choose and why based on the systems’ needs and resources.
The primary factors that are included in our proposed model are: the type of conversions that decide on which features will be converted to images and how, dataset nature that depends on the kind of android malware apps included in the dataset, CNN algorithms that will be used to build the malware predictive solution, and most importantly the evaluation process that comprehensively assesses the performance of the malware analysis system in terms of complexity and security.
A deep empirical study has been conducted to evaluate the proposed model. The results reveal that the chosen factors and processes can significantly impact the performance of the analysis model, whether in terms of the security metrics such as accuracy, F1-score, precision, recall, or the complexity metrics such as test time, CPU usage, storage size, and pre-processing speed.
As a result, the proposed model will effectively direct the developers of malware analysis systems on which factors to adopt based on their requirements and the chosen factors’ impacts. Therefore, the researchers and developers can benefit from our model to trade off these factors to ensure building malware analysis systems that meet their goals.
For future work, other comprehensive models could be proposed for android malware analysis systems that are not vision-based. Additionally, we could introduce nutshell analysis models for different types of malware to other kinds of operating systems. Furthermore, we intend to study the effect of using variable byte sizes and different image sizes for the visual features of the Android malware applications. Moreover, a deep analysis of different misclassification and obfuscation classification scenarios can be investigated.
S1 and S2 Tables illustrate the security performance of different CNN algorithms utilizing DREBIN and AMD datasets, respectively. As mentioned before, these metrics were not included in the analysis section for simplicity in presenting the results and highlighting the main evaluation metrics in regards to the detection performance.
Supporting information
S1 table. security performance of models on drebin dataset based on other metrics..
https://doi.org/10.1371/journal.pone.0270647.s001
S2 Table. Security performance of models on AMD dataset based on other metrics.
https://doi.org/10.1371/journal.pone.0270647.s002
Acknowledgments
The authors would like to acknowledge the support of the Security Engineering Lab (SEL) at Prince Sultan University. Moreover, this research was done during the author Iman Almomani’s sabbatical year 2021/2022 from the University of Jordan, Amman–Jordan.
- View Article
- Google Scholar
- PubMed/NCBI
- 4. Naseer M, Rusdi JF, Shanono NM, Salam S, Muslim ZB, Abu NA, et al. Malware Detection: Issues and Challenges. In: Journal of Physics: Conference Series. vol. 1807. IOP Publishing; 2021. p. 012011.
- 9. Almomani I, Khayer A. Android applications scanning: The guide. In: 2019 International Conference on Computer and Information Sciences (ICCIS). IEEE; 2019. p. 1–5.
- 12. Acharya V, Ravi V, Mohammad N. EfficientNet-based Convolutional Neural Networks for Malware Classification. In: 2021 12th International Conference on Computing Communication and Networking Technologies (ICCCNT). IEEE; 2021. p. 1–6.
- 14. Al Khayer A, Almomani I, Elkawlak K. ASAF: Android static analysis framework. In: 2020 First International Conference of Smart Systems and Emerging Technologies (SMARTTECH). IEEE; 2020. p. 197–202.
- 23. Almomani I, Alkhayer A, El-Shafai W. An Automated Vision-Based Deep Learning Model for Efficient Detection of Android Malware Attacks. IEEE Access. 2022;.
- 24. Sriram S, Vinayakumar R, Sowmya V, Alazab M, Soman K. Multi-scale learning based malware variant detection using spatial pyramid pooling network. In: IEEE INFOCOM 2020-IEEE Conference on Computer Communications Workshops (INFOCOM WKSHPS). IEEE; 2020. p. 740–745.
- 25. Ganesan S, Ravi V, Krichen M, Sowmya V, Alroobaea R, Soman K. Robust malware detection using residual attention network. In: 2021 IEEE International Conference on Consumer Electronics (ICCE). IEEE; 2021. p. 1–6.
- 29. Yadav P, Menon N, Ravi V, Vishvanathan S, Pham TD. A two-stage deep learning framework for image-based android malware detection and variant classification. Computational Intelligence;.
- 32. Zhang H, Qin J, Zhang B, Yan H, Guo J, Gao F. A Multi-class Detection System for Android Malicious Apps Based on Color Image Features. In: International Conference on Security and Privacy in New Computing Environments. Springer; 2020. p. 186–206.
- 40. Arp D, Spreitzenbarth M, Hubner M, Gascon H, Rieck K, Siemens C. Drebin: Effective and explainable detection of android malware in your pocket. In: Ndss. vol. 14; 2014. p. 23–26.
- 41. Li Y, Jang J, Hu X, Ou X. Android malware clustering through malicious payload mining. In: International symposium on research in attacks, intrusions, and defenses. Springer; 2017. p. 192–214.
- 42. Brownlee J. Deep learning with Python: develop deep learning models on Theano and TensorFlow using Keras. 2016;.
- 43. Hodnett M, Wiley JF. R Deep Learning Essentials: A step-by-step guide to building deep learning models using TensorFlow, Keras, and MXNet. 2018;.
- 44. Vasilev I, Slater D, Spacagna G, Roelants P, Zocca V. Python Deep Learning: Exploring deep learning techniques and neural network architectures with Pytorch, Keras, and TensorFlow. 2019;.
- 45. Joseph FJJ, Nonsiri S, Monsakul A. Keras and TensorFlow: A hands-on experience. 2021; p. 85–111.
- 46. Géron A. Hands-on machine learning with Scikit-Learn, Keras, and TensorFlow: Concepts, tools, and techniques to build intelligent systems. 2019;.
- 47. Abadi M, Barham P, Chen J, Chen Z, Davis A, Dean J, et al. {TensorFlow}: A System for {Large-Scale} Machine Learning. In: 12th USENIX symposium on operating systems design and implementation (OSDI 16); 2016. p. 265–283.
Static Malware Analysis Using Machine Learning Methods
- Conference paper
- Cite this conference paper
- Hiran V. Nath 5 , 6 &
- Babu M. Mehtre 5
Part of the book series: Communications in Computer and Information Science ((CCIS,volume 420))
Included in the following conference series:
- International Conference on Security in Computer Networks and Distributed Systems
3301 Accesses
42 Citations
Malware analysis forms a critical component of cyber defense mechanism. In the last decade, lot of research has been done, using machine learning methods on both static as well as dynamic analysis. Since the aim and objective of malware developers have changed from just for fame to political espionage or financial gain, the malware is also getting evolved in its form, and infection methods. One of the latest form of malware is known as targeted malware, on which not much research has happened. Targeted malware, which is a superset of Advanced Persistent Threat (APT), is growing in its volume and complexity in recent years. Targeted Cyber attack (through targeted malware) plays an increasingly malicious role in disrupting the online social and financial systems. APTs are designed to steal corporate / national secrets and/or harm national/corporate interests. It is difficult to recognize targeted malware by antivirus, IDS, IPS and custom malware detection tools. Attackers leverage compelling social engineering techniques along with one or more zero day vulnerabilities for deploying APTs. Along with these, the recent introduction of Crypto locker and Ransom ware pose serious threats to organizations/nations as well as individuals. In this paper, we compare various machine-learning techniques used for analyzing malwares, focusing on static analysis.
This is a preview of subscription content, log in via an institution to check access.
Access this chapter
- Available as PDF
- Read on any device
- Instant download
- Own it forever
- Compact, lightweight edition
- Dispatched in 3 to 5 business days
- Free shipping worldwide - see info
Tax calculation will be finalised at checkout
Purchases are for personal use only
Institutional subscriptions
Unable to display preview. Download preview PDF.
The ‘ICEFOG’ APT: A tale of cloak and three daggers. Kaspersky Lab Global Research And Analysis Team(GREAT) (2013)
Google Scholar
Balduzzi, M., Ciangaglini, V., McArdle, R.: Targeted attacks detection with spunge. Trend Micro Research, EMEA (2013)
Becker, G.T., Regazzoni, F., Paar, C., Burleson, W.P.: Stealthy dopant-level hardware trojans (2013)
Bencsáth, B., Pék, G., Buttyán, L., Félegyházi, M.: The cousins of stuxnet: Duqu, flame, and gauss. Future Internet 4(4), 971–1003 (2012)
Article Google Scholar
Bilar, D.: Opcodes as predictor for malware. International Journal of Electronic Security and Digital Forensics 1(2), 156–168 (2007)
Blonce, A., Filiol, E., Frayssignes, L.: Portable document format (pdf) security analysis and malware threats. Tech. rep., Virology and Cryptology Laboratory, French Army Signals Academy (2008)
Cohen, W.W.: Fast effective rule induction. ICML 95, 115–123 (1995)
Desnos, A., Erra, R., Filiol, E.: Processor-dependent malware... and codes. arXiv preprint arXiv:1011.1638 (2010)
Dube, T., Raines, R., Peterson, G., Bauer, K., Grimaila, M., Rogers, S.: Malware type recognition and cyber situational awareness. In: Second International Conference on Social Computing (SocialCom), pp. 938–943. IEEE (2010)
Dube, T., Raines, R., Peterson, G., Bauer, K., Grimaila, M., Rogers, S.: Malware target recognition via static heuristics. Computers & Security 31(1), 137–147 (2012)
Dube, T.E.: A Novel Malware Target Recognition Architecture for Enhanced Cyberspace Situation Awareness. Ph.D Thesis, Air Force Institute of Technology, Wright-Patterson Air Force Base, Ohio (September 2011)
Dube, T.E., Raines, R.A., Grimaila, M.R., Bauer, K., Rogers, S.: Malware target recognition of unknown threats. IEEE Systems Journal 7(3) (September 2013)
Dube, T.E., Raines, R.A., Rogers, S.K.: Malware target recognition. US Patent 20, 120, 260, 342 (October 11, 2012)
Filiol, E.: Formalisation and implementation aspects of k-ary (malicious) codes. Journal in Computer Virology 3(2), 75–86 (2007)
Filiol, E.: Malicious cryptography techniques for unreversable (malicious or not) binaries. arXiv preprint arXiv:1009.4000 (2010)
Filiol, E., Helenius, M., Zanero, S.: Open problems in computer virology. Journal in Computer Virology 1(3-4), 55–66 (2006)
Kolter, J.Z., Maloof, M.A.: Learning to detect and classify malicious executables in the wild. The Journal of Machine Learning Research 7, 2721–2744 (2006)
MATH MathSciNet Google Scholar
Kolter, J.Z., Maloof, M.A.: Dynamic weighted majority: An ensemble method for drifting concepts. The Journal of Machine Learning Research 8, 2755–2790 (2007)
MATH Google Scholar
Kolter, J.Z., Maloof, M.A.: Learning to detect malicious executables in the wild. In: Proceedings of the Tenth ACM SIGKDD International Conference on Knowledge Discovery and Data Mining, pp. 470–478. ACM (2004)
Kolter, J.Z., Maloof, M.A.: Using additive expert ensembles to cope with concept drift. In: Proceedings of the 22nd International Conference on Machine Learning, pp. 449–456. ACM (2005)
Li, F., Lai, A., Ddl, D.: Evidence of advanced persistent threat: A case study of malware for political espionage. In: 6th International Conference on Malicious and Unwanted Software (Malware), pp. 102–109. IEEE (2011)
Lin, L., Kasper, M., Güneysu, T., Paar, C., Burleson, W.: Trojan side-channels: Lightweight hardware trojans through side-channel engineering. In: Clavier, C., Gaj, K. (eds.) CHES 2009. LNCS, vol. 5747, pp. 382–395. Springer, Heidelberg (2009)
Chapter Google Scholar
Liu, S.-T., Chen, Y.-M., Hung, H.-C.: N-victims: An approach to determine n-victims for apt investigations. In: Lee, D.H., Yung, M. (eds.) WISA 2012. LNCS, vol. 7690, pp. 226–240. Springer, Heidelberg (2012)
Lu, Y., Din, S., Zheng, C., Gao, B.: Using multi-feature and classifier ensembles to improve malware detection. Journal of CCIT 39(2), 57–72 (2010)
Lyda, R., Hamrock, J.: Using entropy analysis to find encrypted and packed malware. IEEE Security & Privacy 5(2), 40–45 (2007)
McDonald, G., Murchu, L.O., Doherty, S., Chien, E.: Stuxnet 0.5: The missing link. Symantec Security Response (online) 26 (2013)
Menn, J.: Key internet operator verisign hit by hackers. Reuters (February 2, 2012)
Muttik, I.: Zero-day malware. In: Virus Bulletin Conference (2010)
Prosecutors, Public: Messiah spyware infects middle east targets
Rafiq, N., Mao, Y.: Improving heuristics. In: Virus Bulletin Conference, pp. 9–12 (2008)
Raymond, D., Conti, G., Cross, T., Fanelli, R.: A control measure framework to limit collateral damage and propagation of cyber weapons. In: Fifth International Conference on Cyber Conflict (CyCon), pp. 1–16. IEEE (2013)
Santos, I., Brezo, F., Sanz, B., Laorden, C., Bringas, P.G.: Using opcode sequences in single-class learning to detect unknown malware. IET Information Security 5(4), 220–227 (2011)
Santos, I., Brezo, F., Ugarte-Pedrero, X., Bringas, P.G.: Opcode sequences as representation of executables for data-mining-based unknown malware detection. Information Sciences (2011)
Santos, I., Nieves, J., Bringas, P.G.: Semi-supervised learning for unknown malware detection. In: Abraham, A., Corchado, J.M., González, S.R., De Paz Santana, J.F. (eds.) International Symposium on DCAI. AISC, vol. 91, pp. 415–422. Springer, Heidelberg (2011)
Schultz, M.G., Eskin, E., Zadok, F., Stolfo, S.J.: Data mining methods for detection of new malicious executables. In: Proceedings of the 2001 IEEE Symposium on Security and Privacy, S&P 2001, pp. 38–49. IEEE (2001)
Shabtai, A., Moskovitch, R., Elovici, Y., Glezer, C.: Detection of malicious code by applying machine learning classifiers on static features: A state-of-the-art survey. Information Security Technical Report 14(1), 16–29 (2009)
Shafiq, M., Tabish, S., Farooq, M.: Pe-probe: leveraging packer detection and structural information to detect malicious portable executables. In: Proceedings of the Virus Bulletin Conference (VB), pp. 29–33 (2009)
Shafiq, M.Z., Tabish, S.M., Mirza, F., Farooq, M.: A framework for efficient mining of structural information to detect zero-day malicious portable executables. Tech. rep., TR-nexGINRC-2009-21 (January 2009), http://www.nexginrc.org/papers/tr21-zubair.pdf
Shafiq, M.Z., Tabish, S.M., Mirza, F., Farooq, M.: Pe-miner: mining structural information to detect malicious executables in realtime. In: Kirda, E., Jha, S., Balzarotti, D. (eds.) RAID 2009. LNCS, vol. 5758, pp. 121–141. Springer, Heidelberg (2009)
Sood, A., Enbody, R.: Targeted cyber attacks-a superset of advanced persistent threats. In: IEEE Computer and Reliability Societies, Michigan State University (2013)
Vasiliadis, G., Polychronakis, M., Ioannidis, S.: Gpu-assisted malware. In: 2010 5th International Conference on Malicious and Unwanted Software (MALWARE), pp. 1–6. IEEE (2010)
White, S.R.: Open problems in computer virus research. In: Virus Bulletin Conference (1998)
Zetter, K.: Google hack attack was ultra sophisticated, new details show. Wired Magazine 14 (2010)
Zhang, B., Yin, J., Hao, J., Zhang, D., Wang, S.: Malicious codes detection based on ensemble learning. In: Xiao, B., Yang, L.T., Ma, J., Muller-Schloer, C., Hua, Y. (eds.) ATC 2007. LNCS, vol. 4610, pp. 468–477. Springer, Heidelberg (2007)
Download references
Author information
Authors and affiliations.
Center for Information Assurance & Management, Institute for Development and Research in Banking Technology, India
Hiran V. Nath & Babu M. Mehtre
School of Computer and Information Sciences (SCIS), University of Hyderabad, India
Hiran V. Nath
You can also search for this author in PubMed Google Scholar
Editor information
Editors and affiliations.
Facultad de Informatica, Campus de Espinardo, s/n, 30., 100, Murcia, Spain
Gregorio Martínez Pérez
Indian Institute of Information Technology and Management, Technopark Campus, 695581, Trivandrum, Kerala, India
Sabu M. Thampi
Faculty of Computing and Mathematical Sciences, The University of Waikato, Waikato Mail Centre, Private Bag 3105, Hamilton, New Zealand
Guangdong University of Petrochemical Technology, P.R. China
Rights and permissions
Reprints and permissions
Copyright information
© 2014 Springer-Verlag Berlin Heidelberg
About this paper
Cite this paper.
Nath, H.V., Mehtre, B.M. (2014). Static Malware Analysis Using Machine Learning Methods. In: Martínez Pérez, G., Thampi, S.M., Ko, R., Shu, L. (eds) Recent Trends in Computer Networks and Distributed Systems Security. SNDS 2014. Communications in Computer and Information Science, vol 420. Springer, Berlin, Heidelberg. https://doi.org/10.1007/978-3-642-54525-2_39
Download citation
DOI : https://doi.org/10.1007/978-3-642-54525-2_39
Publisher Name : Springer, Berlin, Heidelberg
Print ISBN : 978-3-642-54524-5
Online ISBN : 978-3-642-54525-2
eBook Packages : Computer Science Computer Science (R0)
Share this paper
Anyone you share the following link with will be able to read this content:
Sorry, a shareable link is not currently available for this article.
Provided by the Springer Nature SharedIt content-sharing initiative
- Publish with us
Policies and ethics
- Find a journal
- Track your research
- Open access
- Published: 14 January 2020
An emerging threat Fileless malware: a survey and research challenges
- Sudhakar ORCID: orcid.org/0000-0001-7590-1995 1 , 2 &
- Sushil Kumar 3
Cybersecurity volume 3 , Article number: 1 ( 2020 ) Cite this article
31k Accesses
69 Citations
12 Altmetric
Metrics details
With the evolution of cybersecurity countermeasures, the threat landscape has also evolved, especially in malware from traditional file-based malware to sophisticated and multifarious fileless malware. Fileless malware does not use traditional executables to carry-out its activities. So, it does not use the file system, thereby evading signature-based detection system. The fileless malware attack is catastrophic for any enterprise because of its persistence, and power to evade any anti-virus solutions. The malware leverages the power of operating systems, trusted tools to accomplish its malicious intent. To analyze such malware, security professionals use forensic tools to trace the attacker, whereas the attacker might use anti-forensics tools to erase their traces. This survey makes a comprehensive analysis of fileless malware and their detection techniques that are available in the literature. We present a process model to handle fileless malware attacks in the incident response process. In the end, the specific research gaps present in the proposed process model are identified, and associated challenges are highlighted.
Introduction
Throughout the history of the malicious programs, there is one thing which had remained unchanged, the development of the malware program. Someone had to develop the code in such a manner so that no existing anti-virus (AV) software can detect its presence in the system. In 2002, the development of the malware industry had changed the entire threat landscape. This malware has the capability of residing in the system’s main memory undetected making least changes in the file system. This strategy has become the non-malware or fileless malware (Patten, 2017 ; Kumar et al., 2019a ).
When a system is detected as the compromised by some malicious program or malware, the very first thing a forensic expert will work to look for some malicious programs or software that should not be there. However, in this case, there is none because the fileless malware does not reside in the file system, it is a running program in the memory (Mansfield-Devine, 2017 ; Tian et al., 2019a ). The attacker has been using malware for its capabilities to control the compromised systems locally or remotely. Although, the operating systems itself providing several capabilities to the attacker.
The attackers are mostly involved in exploring vulnerabilities in the legitimate software that are already installed in the machine such as flash player, web-browser, PDF viewer and Microsoft office to exploit and load a script directly into the main memory without even touching the local file systems (Pontiroli & Martinez, 2015 ; Rani et al., 2019 ). In Windows Operating Systems, two most powerful tools and. NET framework are already installed which attacker can use to exploit the vulnerability, one is WMI (Windows Management Instrumentation) (Graeber, 2015 ) and second is PowerShell. WMI came into the limelight of the cybersecurity community when it was discovered that it is used maliciously as a component in the suite of exploits by Stuxnet (Falliere et al., 2011 ; Farwell & Rohozinski, 2011 ). Since then, WMI has been gaining popularity amongst the attackers, because it can perform system reconnaissance, AV/VM (Virtual Machine) detection, code execution, lateral movement, persistence, and data theft. Similarly, in the case of PowerShell, it is a highly flexible system shell and scripting platform for the attacker to provide all the features in the different stages of an intrusion. Since, it can also be used to bypass anti-virus detection, maintain persistence or infiltrate data. For example: In 2016, a hacker group infiltrates into the DNC (Democratic National Committee) with fileless malware. In this incident, the PowerShell and WMI were used as the attack vectors (Report, 2016 ).
In recent time, malware developers have adopted the high-level language in the development of the malicious codes that have changed the malware industry. After the release of Microsoft .Net framework, it became the center of attraction for all the windows software developers and unintentionally revolutionized the malware industry (Tian et al., 2019b ; Tian et al., 2019c ). It gives the malware writers a new and powerful arsenal equipped with all the features to make a malware undetected and stay ahead of the anti-virus software. With the use of this framework, malware creator may easily interact with the operating system and exploit vulnerabilities with the entire catalog of products with the help of the framework (Patten, 2017 ; Pontiroli & Martinez, 2015 ; Tian et al., 2019d ; Bhasin et al., 2018 ). The attacker uses a tool like PowerShell to coordinate attacks with the help of existing toolkits such as meterpreter (About the Metasploit Meterpreter, 2019 ), SET (Social Engineering Toolkit) (Pavković & Perkov, n.d. ), or the Metasploit Framework including an extensive list of modules that are already built-in and ready to use for the purpose of plotting additional attacks (Tian et al., 2018 ).
The fileless malware attacks in the organizations or targeted individuals are trending to compromise a targeted system avoids downloading malicious executable files usually to disk; instead, it uses the capability of web-exploits, macros, scripts, or trusted admin tools (Tan et al., 2018 ; Mansfield-Devine, 2018 ). Fileless malware can plot any attacks to the systems undetected like reconnaissance, execution, persistence, or data theft. There are not any limitations on what type of attacks can be possible with fileless malware. This survey includes infection mechanisms, legitimate system tools used in the process, analysis of major fileless malware, research challenges while handling such incidents in the process of incident and response. This type of study was not done in the literature, which includes the different perspectives of fileless malware. The main contributions of the paper are mentioned below.
The background of the malware with evasion and propagation techniques used to identify targets and stay undetected in the victim machines.
We analyze the behavior of all the fileless malware and discuss their persistent mechanisms in detail.
We analyze many solutions given by researchers to detect such malware by analyzing the malicious patterns in the process, registry, minor changes in file systems, and event logs.
We proposed a novel investigative model of incident handling and response, especially in fileless malware. The model includes all the phases with memory forensic, analysis and investigation of such incidents.
The rest of the paper is organized as shown in Table 1 .
Background of Fileless malware
Unlike traditional file-based malware attacks, instead of using real malicious executables, it leverages trusted, legitimate processes i.e. LOLBins (Living off the Land Binaries) (Living Off The Land Binaries And Scripts - (LOLBins and LOLScripts), 2019 ) and built-in tools of operating systems to attack and hide. The detailed comparisons between traditional file-based malware and fileless malware are mentioned in the Table 2 (Afianian et al., 2018 ). In this section, the formal definition of the fileless malware and execution techniques along with the system tools, is discussed. The section also elaborates on the infection technique used by such malware with attack vectors, as shown in Fig. 1 .
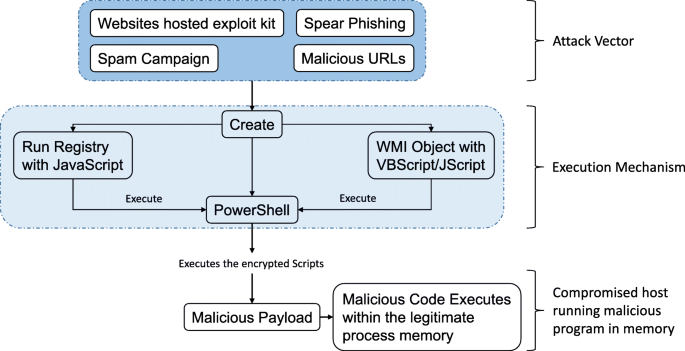
Infection flow of fileless malware
Fileless malware attacks do not download malicious files or write any content to the disk in order to compromise the systems. The attacker exploits merely the vulnerable application to inject malicious code directly into the main memory. The attacker can also leverage the trusted and widely used applications, i.e., Microsoft office or administration tools native to Windows OS like PowerShell and WMI to run scripts and load malicious code directly into volatile memory (Stop Fileless Attacksat Pre-execution, 2017 ; Zhang, 2018 ). The procedures and attack vectors are mentioned in Fig. 1 .
Execution of fileless malware
The malware authors have leveraged the advantage of two powerful legitimate windows applications Windows Management Instrumentation and PowerShell to execute their malicious binaries to make the attack undetected by AV solutions (Graeber, 2015 ).
The life cycle of the fileless malware works in three phases. First, attack vector, which has methods through which the attacker targets their victims. Second, the execution mechanism in this the initial malicious code could try to create a registry entry for its persistence or WMI object with VBScript/JScript to invoke an instance of PowerShell. Third, PowerShell can further able to execute the malicious program into the legitimate process memory directly without dropping any files to the file system making its target compromised by fileless malware; the infection life cycle is shown in Fig. 1 .
Windows management instrumentation
With the emergence of new technologies, many changes have occurred in the Windows Operating System over the period, but WMI remains powerful since Windows NT 4.0, and Windows 95. It has an excellent reputation in the security community to launch an attack across many phases of the attack life-cycle like reconnaissance, AV/VM detection, code execution, lateral movement, covert data storage, to persistence. Using these capabilities of WMI, an attacker can build a pure backdoor without even dropping the single file to the file system. In addition to this, it can be used to execute malicious JavaScript/VBScript directly in the memory to possibly evade the AV solutions (Graeber, 2015 ; O’Murchu & Gutierrez, 2015 ; Ruff, 2008 ).
PowerShell has a rich number of features to facilitate the attacker to even bypasses the detection capability of AV solutions to maintain persistence or spy on the system. Windows operating system has already whitelisted many modules for PowerShell. For example, evasive techniques (Bulazel & Yener, 2017 ) can be used to dynamically load PowerShell scripts into the memory without even writing anything on the file system (Pontiroli & Martinez, 2015 ; Case & Richard III, 2017 ).
Analysis of Fileless malware based on their persistent techniques
There are major three categories of fileless malware, which are described below by their persistence techniques and detailed classification of their attack vectors in Table 3 . The fileless malware can hide their location to make difficulties in the process of detection by traditional AV solutions and also for the security analyst (Demystifying Fileless Threats, 2019 ; Rivera & Inocencio, 2015 ).
Memory-resident malware
The malware that wholly resides in the main memory without touching the file systems. It uses only legitimate process or authentic windows files to execute and stays there until it triggered. The malware having such capabilities are described in this section:
Code Red (Zou et al., 2002 ; Danyliw & Householder, 2001 ; Rhodes, 2001 ): Code red infect Microsoft’s Internet Information Server (IIS) of version 4.0 and 5.0 having known buffer overflow vulnerability. The system is infected when server GET/default.ida request on TCP port 80 allowing the worm to run code on the server.
SQL Slammer (O’Murchu & Gutierrez, 2015 ; MS SQL Slammer/Sapphire Worm, 2003 ): SQL Slammer is a computer worm (SQL Slammer, 2019 ). It has the power to choke the bandwidth of the network resulting in a denial-of-service condition. The worm has used the method to propagate and infect by scanning the buffer-overflow vulnerability over the internet.
Lurk Trojan (Golovanov, 2012 ; Shulmin & Prokhorenko, 2016 ) : Lurk is a banking Trojan, infection could be possible either using command “regsrv32” and “netsh add helper dll” or via the ShellIconOverlayIdentifiers branch of the system registry. The Trojan uses its specific features to gain access to the users’ sensitive data, and with the help of it, an attacker can compromise their online banking services.
Poweliks (O’Murchu & Gutierrez, 2015 ; Team, 2017 ; Zeltser, 2017 ): Poweliks is fileless malware that is further being developed from file-based malware, known as Wowliks. The malware installs itself into the registry as well as use it to persist in the system, thus escape from AV solutions as it did not leave any files written on the disk. Also, the malware installs PowerShell in the background without alarming the defensive system if the system does not have it already. The system is penetrated by exploiting the Microsoft Office vulnerabilities and used the PowerShell along with JavaScript with shellcode to directly execute into legitimate memory. The attack vectors are compared between Wowliks and Poweliks in Table 4 .
Persistence mechanism - Poweliks uses system registries to achieve persistence, to stay undetected. It added two registries to the run key. First, in the form of JavaScript program encoded data written under (Default) value and other is the autorun entry that reads and decodes the encoded JavaScript data.
Windows registry malware
Registry is the database for storing low-level settings of the Windows operating system and some critical apps. In there, the malware authors managed to store complete malicious code into the registry in an encrypted manner, to make it undetected. To obtain the persistence, it can exploit some operating systems thumbnail cache using registry. However, the file is set to self-destruct once it carried out its malicious task (Wueest & Anand, 2017 ).
Kovter (Team, 2017 ; Fileless Malware - A Behavioural Analysis Of Kovter Persistence, 2016 ): Kovter can conceal itself in the registry and maintain persistence through the use of registry run key. The infection mechanism of Kovter has the feature to leave very few file traces of artifacts. It uses PowerShell for the execution of commands to achieve its malicious venture. Once the execution is completed PowerShell losses all the environment variables and it does not log the list of executed commands due to this, it leaves little chance to recover the script executed and the sample or information about the final payload (Zaharia, 2016 ).
Persistence mechanism - JavaScript code is added into the registry and is executed by a legitimate Windows file, mshta.exe, via WMI instead of mshtml.dll:
HKLM\path{\Software\Microsoft\Windows\Current Version\Run}.
Data: mshta javascript: {javascript code}.
Kovter decrypts the first stage JavaScript code, which resides in the registry leading to the second stage JavaScript containing a PowerShell script, which decodes the encoded shellcode and injects it to the legitimate windows process (regsvr32.exe) to execute, using a technique called Process Hollowing (Process Hollowing, 2019 ). The flow of injecting shellcode is mentioned in Fig. 2 .

Shellcode injection flow
PowerWare (Valdez & Sconzo, 2016 ): It is a fileless ransomware, which is mostly delivered via a macro-enabled Microsoft Word document. The malware uses the core utilities of windows operating the system such as PowerShell. By leveraging the capabilities of it, the ransomware entirely avoids writing any file on the disk and perform its malicious activities.
Rootkits fileless malware
An attacker can install this kind of malware after getting the administrator level privilege to hide the malicious code into the kernel of the Windows operating system. While this is not a 100% fileless infection either, it fits here.
Phase Bot (Zeltser, 2017 ; Phase Bot - A Fileless Rootkit (Part 1), 2014 ; Phase Bot - A Fileless Rootkit (Part 2), 2014 ): It is a type of bot, which can grab its’ victim information by applying form-grabbing approach (Sood et al., 2011 ) and stealing FTP data connection (Allman & Ostermann, 1999 ) with the ability to run without a file. Phase hides its relocatable code encrypted in the registry and uses PowerShell to read and execute this independent position code into memory. Both Phase Bot and Poweliks uses similar persistence mechanism.
Detection techniques for Fileless malware
In the case of fileless malware, PowerShell and WMI could be used to reconnaissance, establishing persistence, lateral movement, remote command execution, and file transfer, make it difficult to track evidence left behind during a compromise (Pontiroli & Martinez, 2015 ). In order to detect such malware infection, various techniques (Section 4.1–4.3) have been proposed by the researchers in their work. First two techniques (Section 4.1 and Section 4.2) are manual inspection techniques, need a security professional to look into the evidence prescribed by the researcher to successfully identify such attacks, whereas the third technique (Section 4.3) is only a concept yet to implement.
Detection by monitoring the behaviour of the system
In order to detect fileless malware, the system needs to consider two things. First, the processes which have elevated privileges after becoming live into the memory and Second, monitor the security events for the program execution by command-line console or PowerShell (Pontiroli & Martinez, 2015 ).
The attacker first aim is to gain the root access to its victim machine to take the full privilege of the PowerShell. To identify fileless infections, the system needs to monitor all the essential features that are accomplished by PowerShell capabilities, such as:
Remote command execution by the PowerShell.
Change of standard user privilege to administrative privilege to access WMI and .NET Framework base class library.
Programs, which are executing in the main memory, may be malicious.
It is essential to identify the principal sources of information such as network traffic, network connections, and suspicious modifications to particular Windows registry keys. In addition, the Windows event log, being on guard for clear indicators that may suggest the malicious activity has taken place. Some of the indispensable events need to be adequately monitored, such as:
Event ID 4688: The system needs to monitor all the newly created processes whose parent process is PowerShell.
Event ID 7040: If the service has been changing to auto-start from disabled/demand start of the Windows Remote Management (WS-Management).
Event ID 10148: This event is responsible for listening to the specific IP and Port for WS-Management related requests.
Detection by rule-based
Majority of malicious programs spread across the internet via targeted by the attacker or by the botnet to find the vulnerable victim is packed with Microsoft Office applications such as winword.exe, excel.exe, and powerpnt.exe. Furthermore, the detection of such programs, which trigger the cmd.exe or powershell.exe, could be malicious. Hence, the detection mechanism may work by the rule that can distinguish between benign process and malicious process (Valdez & Sconzo, 2016 ).
These similar rules can be implemented in the browsers to block such malicious apps from executing PowerShell and Command prompt. This should also help with other types of malware leveraging other Microsoft Office applications as mentioned in Fig. 3 .
CASE-1: ProcessName: cmd.exe AND (parentName: winword.exe OR parentName: excel.exe OR parentName: powerpnt.exe OR parentName: outlook.exe) AND chilprocessName: powershell.exe
CASE-2: ProcessName: cmd.exe AND (parentName: winword.exe OR parentName: excel.exe OR parentName: powerpnt.exe OR parentName: outlook.exe)
CASE-3: ProcessName: powershell.exe AND parentName: winword.exe
CASE-4: ProcessName: powershell.exe AND filemodCount: [1000 to *]
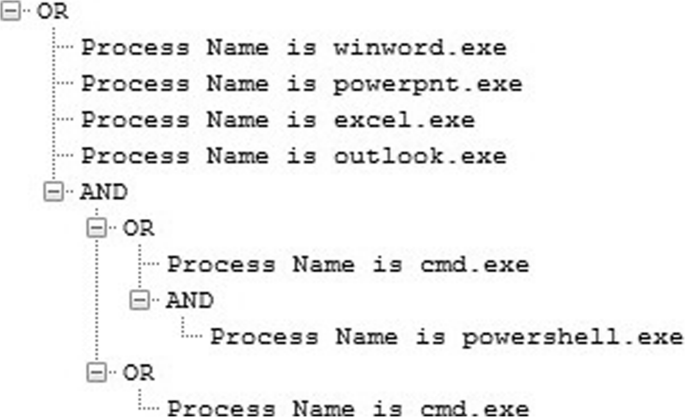
Malicious process flow
Detection by learning behavior of attack
A framework can be developed in the paradigm of client-server, wherein all the endpoints have a client deployed, and a server in the cloud. The framework is divided into three stages, like capturing events, tagging events, and learning from the events. In this system, the client can capture all the generated events by the host machine as mentioned in the Fig. 4 , to monitor the full stream of activity. In addition, the client also assigns a tag to each event appropriately to uncover the attacker’s progress. At last, in the server, many analysis engines working on the tagged-events supplied by the client to detect the malicious activity in the host machine. The tagged-events will be the raw data for learning algorithms and analyzing the behavior of the patterns to prevent or detect malicious activity through the co-relation amongst the event streams (Series, 2019 ).
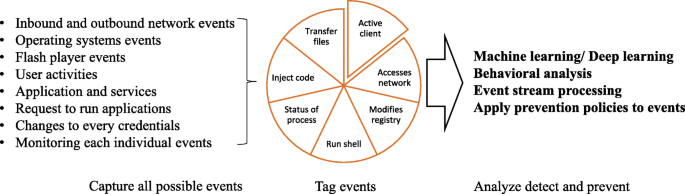
A framework to detect and prevent fileless malware attacks
Proposed process model for incident response
The proposed model is especially for malware related incidents both in real-time attacks and after attack scenarios. The process flow diagram and block diagram are thoroughly explained in Fig. 5 and Fig. 6 . The first five-phase (including incident response) is for the identification and investigation of the incident. Each organization should have an incident response team to handle cybersecurity incidents. The team can follow this process model and process flow to investigation the root cause of such attacks.
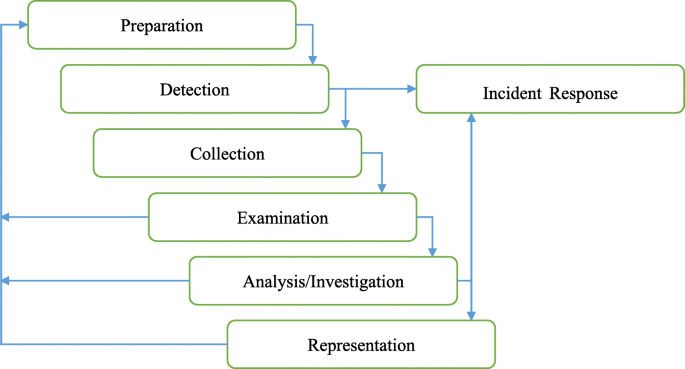
A process model for memory forensics
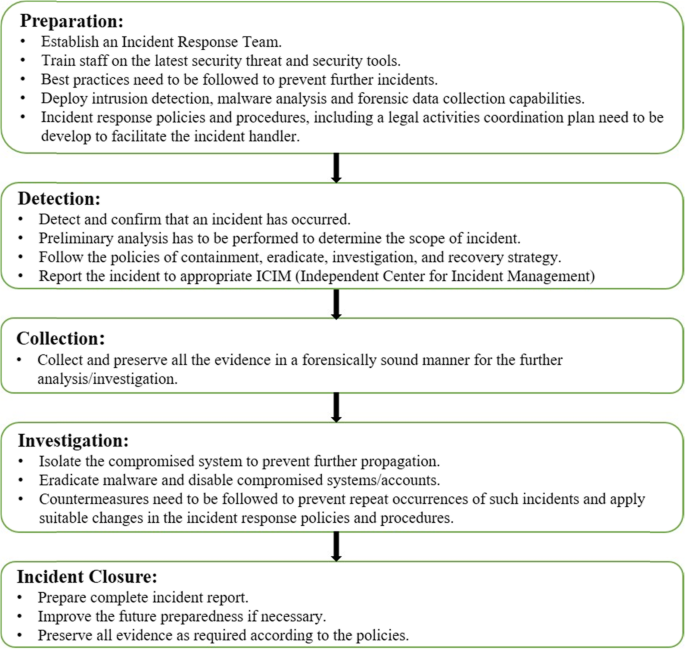
The process flow of cybersecurity incident handling and response
The analysis of evidence and artifacts are starts from the examination stage, where forensic examiner concludes whether the malware sample needs to investigate further. In this scenario, the attack pattern can be identified with the help of many techniques. Which are useful in detecting anomalies between the benign and malicious behavior of the system.
Preparation
The primary motive of this phase is continuous capability enhancement of the handling incident based on risk assessment and experiences of the incident handling team. In this context, regular training of the individual incident handler needs to be arranged to keep them ready for new security threats and related security tools for more sophisticated malicious programs like fileless malware, botnet, APT (Advanced Persistent Threat) and DDoS.
An incident handler needs to gather all available information about the incident. To facilitate the incident handler, forensic software and most advanced malware analysis tools are required. In case of occurrence of such incidents, an organization must have a policy in place containing reporting, information sharing, especially with outside organizations such as law enforcement (Khurana et al., 2009 ).
In the organization, security tools (anti-malware agents, intrusion detection/prevention systems, network sandboxes, and firewalls, etc.) must installed in their infrastructure to prevent and detect it from the security breaches and policy violations. If any alert are unauthorized events or anomalies triggers, then it requires a security analyst to examine logs and analyze the root cause of the unauthorized event (Shackleford, 2016 ). The malicious events could lead to the existence of malware, which could be determined by the various parameters, and quick validation can be done to confirm the attack. The validation is required to determine whether to investigate the suspected attack or ignore it. This phase can generate two branches – incident response and collection (Pilli et al., 2010 ).
- Incident response
The reactive and proactive response will be generated for intrusion detection according to the organization’s policy and guidelines. The damage already caused due to a cyberattack could be mitigated, and future action plans can be determined for such attacks. In addition, a similar response with more information obtained from the investigation can be initiated (Khurana et al., 2009 ; Pilli et al., 2010 ).
The evidence of the incident can be acquired from the sensors, which are in place in the network or from the compromised machines. The sensors can be honeypot/honeynet (Watson & Riden, 2008 ) to collect malicious samples or to capture the network behavior. All the collected evidence are preserved for further analysis and investigation purpose, as mentioned in Fig. 6 . All the analysis and investigation must be done on a copy of the original data, and the original evidence file is untouched to facilitate legal requirements (Khurana et al., 2009 ).
Examination
The traces obtained from various security sensors are integrated and fused to form one extensive data set on which analysis can be performed. The data set may contain redundant information, which needs to be removed and rectified efficiently. In this process, the crucial data should be intact (Khurana et al., 2009 ; Ren, 2004 ). The indicators of cybercrime can be searched from the extensive data set of evidence like malicious network traffic (Pilli et al., 2010 ; Tobergte & Curtis, 2013 ). The finding may be shared with the development team of security tools for improvement purposes (Case & Richard III, 2017 ; Cohen, 2017 ; Burdach, 2006 ).
Analysis & Investigation
While analysis of such incidents, an expert should consider the behavior of the systems such as registry entries, network communication, operating system fingerprinting, memory analysis, and process analysis to match for any malicious activities. Thorough investigations must be performed from a victim network or system through any intermediate nodes and communication pathway to pinpoint from where the attack originated.
The attacker can remove their footprints from the crime scene, such as securely delete the log files, payloads, registry entries, and cookies. IP spoofing and stepping stone attack strategies can also be used by the attacker to hide their IP address (Vacca, 2013 ). The investigation phase provides data for incident response and prosecution of the attacker (Khurana et al., 2009 ; Pilli et al., 2010 ).
Representation
All the findings from the different phases, such as examination and analysis/investigations, are collected and presented in a proper, understandable language for legal purposes with evidence. The detailed documentation can be presented in the form of a report with visualization so that they could be easily grasped (Khurana et al., 2009 ; Pilli et al., 2010 ).
Research challenges
Fileless malware already presents a significant problem, and it is gaining further popularity among attackers because it is undetectable by traditional file-based prevention and detection systems. Since, there are no files written on the disk, when the malware is persisting exclusively through process memory and registry files. The malicious process is not accessible without doing in-memory analysis because the source code of the process is not available. The fileless attacks can evade these AV tools without triggering alarms as deduce from the eq. 1.
In the process of prevention, detection, and collection of the malicious files or attack vectors in the infrastructure poses the various research challenges in incident handling and response, which are discussed in the following subsections. In Table 5 , the specific and common challenges are compared for both file-based and fileless malware.
Detection and collection
The first step to detect the fileless malware attacks is to identify the malicious pattern of the system and the malicious scripts, which are running in the memory. The malicious scripts are interpreted files such as JavaScript, Visual Basic, and PowerShell, which also need to consider as a parameter of detection engine. However, some points raise even more significant questions for the researcher (Gorelik & Moshailov, 2017 ):
Are we want to scan all text files, scripts, and XML files?
Are we to build a parser/interpreter for each type of interpreted files?
Are we to block any suspicious string, even if it is just a comment in the scripts?
Researchers are facing these questions when trying to balance false positive in early detection systems. The detection system must consider different attack vectors and source of evidence to block fileless attacks at the pre-execution stage. It must leverage the machine-learning algorithm to analyze command lines, scrutinize internet connections, monitor process behavior and protect the memory space of the running process to detect such attacks (Stop Fileless Attacksat Pre-execution, 2017 ). The system should collect all the relevant data from different sources to feed on the machine learning algorithm and get the best out of it (Tobergte & Curtis, 2013 ; Tian et al., 2019e ; Kumar et al., 2019b ). The data from the different sources may be quite large, so to handle the data, the system can use big data technologies (Sudhakar & S.K., 2018 ) with a machine learning algorithm for more efficient results.
The system is compromised with fileless malware having less possible to find traces of malicious activity. Although the evidence must be collected from different sources. Data fusion of all the evidence and logs collected from various security tools deployed in each host on the entire network is major challenges faced by the security researchers (Ren, 2004 ). The traces from the logs, attack vectors from various tools and reconnaissance of attributes from different hosts validate an attack. Characterization of anomalous network events, the malicious behavior of the system, and distinguishing attack traffic from legitimate traffic by searching for patterns of anomalies is a significant challenge (Stop Fileless Attacksat Pre-execution, 2017 ).
The large volume of data can be used to scrutinize to understand the relationship of attack and attacker intention, to do so the classification and clustering techniques can be used on the suspected data events to separate the benign events and malicious events (Aljaedi et al., 2011 ). Pattern recognition can be used to find anomalies in the malicious events. A malicious cluster can help to categorize the attack patterns and attack reconstruction to uncover the motivation and intention of the attacker can be a challenge (Almulhem, 2009 ).
All the collected evidence needs a thorough investigation to find the source IP of the attacker. Security researchers are facing problems to trace back the attacker IP address due to the advanced techniques of IP spoofing (Mitropoulos & Dimitrios Patsos, 2005 ). Identifying a mechanism to find the IP location mapping with attacker geolocation is itself a significant challenge (Nikkel, 2007 ).
In the whole process, the incident response is the phase where the result is reflected. The response should be quick and accurate so that the malicious activity should be mitigated and attacker unable to damage any further (Khurana et al., 2009 ; Aljaedi et al., 2011 ).
Security defenders should have a significant focus on detecting and preventing fileless malware attacks. The attackers use the legitimate application to fulfill their malicious motives. Since, the PowerShell and WMI can also be used to bypass signature-based detection systems, maintain persistence or ex-filtrate data makes it difficult to detect malicious activities. In this paper, we categorized most of the existing detection methods and types of fileless malware, which are targeting the real world. The proliferation of non-malware attacks has only accentuated this issue. Major global hacks against SWIFT (Society for Worldwide Interbank Financial Telecommunication) and the Ukraine power grid, among others, have served as clarion calls that critical infrastructure and worldwide financial systems will continue to be targeted by this type of sophisticated attacks.
Availability of data and materials
Not applicable.
About the Metasploit Meterpreter (2019). https://www.offensive-security.com/metasploit-unleashed/about-meterpreter/
Afianian A, Niksefat S, Sadeghiyan B, Baptiste D (2018) Malware dynamic analysis evasion techniques: A survey. arXiv preprint arXiv 1811:01190
Google Scholar
Aljaedi A, Lindskog D, Zavarsky P, Ruhl R, Almari F (2011) Comparative analysis of volatile memory forensics: Live response vs. memory imaging. In: 2011 IEEE Third International Conference on Privacy, Security, Risk and Trust and 2011 IEEE Third International Conference on Social Computing, pp 1253–1258. https://doi.org/10.1109/PASSAT/SocialCom.2011.68
Chapter Google Scholar
Allman, M., Ostermann, S.: FTP security considerations (1999). https://tools.ietf.org/html/rfc2577
Book Google Scholar
Almulhem, A.: Network forensics: Notions and challenges. In: 2009 IEEE International Symposium on Signal Processing and Information Technology (ISSPIT), pp. 463–466 (2009). doi: https://doi.org/10.1109/ISSPIT.2009.5407485 . IEEE
Bhasin V, Kumar S, Saxena P, Katti C (2018) Security architectures in wireless sensor network. Int J Inf Technol:1–12. https://doi.org/10.1007/s41870-018-0103-6
Bulazel A, Yener B (2017) A survey on automated dynamic malware analysis evasion and counter-evasion: pc, mobile, and web. In: Proceedings of the 1st reversing and offensive-oriented trends symposium, p 2 ACM
Burdach M (2006) Physical memory forensics. USA, Black Hat
Case A, Richard GG III (2017) Memory forensics: the path forward. Digit Investig 20:23–33
Article Google Scholar
Cohen M (2017) Scanning memory with Yara. Digit Investig 20:34–43. https://doi.org/10.1016/j.diin.2017.02.005
Danyliw R, Householder A (2001) CERT advisory CA-2001-19: "code red" worm exploiting buffer overflow in IIS indexing service DLL
Demystifying Fileless Threats (2019). https://www.mcafee.com/enterprise/en-in/lp/endpoint/fileless-attacks.htm
Falliere, N., Murchu, L.O., Chien, E.: W32. stuxnet dossier. White paper, Symantec Corp., Security Response 5(6), 29 (2011)
Farwell JP, Rohozinski R (2011) Stuxnet and the future of cyber war. Survival 53(1):23–40. https://doi.org/10.1080/00396338.2011.555586
Fileless Malware - A Behavioural Analysis Of Kovter Persistence (2016). https://airbus-cyber-security.com/fileless-malware-behavioural-analysis-kovter-persistence/
Golovanov, S.: A unique `bodiless' bot attacks news site visitors (2012). https://securelist.com/a-unique-bodiless-bot-attacks-news-site-visitors-3/32383/
Gorelik, M., Moshailov, R.: Fileless Malware: Attack Trend Exposed (2017). http://blog.morphisec.com/fileless-malware-attack-trend-exposed Accessed 2018-05-02
Graeber M (2015) Abusing windows management instrumentation (WMI) to build a persistent, asynchronous, and fileless backdoor. Black Hat, Las Vegas
Khurana H, Basney J, Bakht M, Freemon M, Welch V, Butler R (2009) Palantir: a framework for collaborative incident response and investigation. In: Proceedings of the 8th symposium on identity and trust on the internet, pp 38–51 ACM
Kumar S, Dohare U, Kumar K, Prasad Dora D, Naseer Qureshi K, Kharel R (2019a) Cybersecurity measures for geocasting in vehicular cyber physical system environments. IEEE Internet Things J 6(4):5916–5926. https://doi.org/10.1109/JIOT.2018.2872474
Kumar S, Singh K, Kumar S, Kaiwartya O, Cao Y, Zhou H (2019b) Delimitated anti jammer scheme for internet of vehicle: Machine learning based security approach. IEEE Access 7:113311–113323
Living Off The Land Binaries And Scripts - (LOLBins and LOLScripts) (2019). https://github.com/LOLBAS-Project/LOLBAS
Mansfield-Devine S (2017) Fileless attacks: compromising targets without malware. Netw Secur 2017(4):7–11
Mansfield-Devine, S.: The malware arms race. Computer Fraud & Security 2018(2), 15{20 (2018)
Mitropoulos S, Dimitrios Patsos CD (2005) Network forensics towards a classification of traceback mechanisms. In: Workshop of the 1st International Conference on Security and Privacy for Emerging Areas in Communication Networks, pp 9–16
MS SQL Slammer/Sapphire Worm (2003). https://www.giac.org/paper/gsec/3091/ms-sql-slammer-sapphire-worm/105136
Nikkel BJ (2007) An introduction to investigating IPv6 networks. Digit Investig 4(2):59–67. https://doi.org/10.1016/J.DIIN.2007.06.001
O’Murchu L, Gutierrez FP. The evolution of the fileless click-fraud malware poweliks. Symantec Corp. (2015)
Patten, D.: The evolution to fileless malware (2017). http://www.infosecwriters.com/Papers/DPatten Fileless.pdf
Pavković N, Perkov L Social engineering toolkit—a systematic approach to social engineering. In: 2011 proceedings of the 34th international convention MIPRO 2011 may 23 (pp. 1485-1489). IEEE
Phase Bot - A Fileless Rootkit (Part 1) (2014). https://www.malwaretech.com/2014/12/phase-bot-fileless-rootki.html
Phase Bot - A Fileless Rootkit (Part 2) (2014). https://www.malwaretech.com/2014/12/phase-bot-fileless-rootkit-part-2.html
Pilli ES, Joshi RC, Niyogi R (2010) Network forensic frameworks: survey and research challenges. Digit Investig 7(1–2):14–27. https://doi.org/10.1016/j.diin.2010.02.003.1112.6098
Pontiroli SM, Martinez FR (2015) The tao of .net and powershell malware analysis. In: Virus Bulletin Conference
Process Hollowing (2019). https://attack.mitre.org/techniques/T1093/
Rani R, Kumar S, Dohare U (2019) Trust evaluation for light weight security in sensor enabled internet of things: game theory oriented approach. IEEE Internet Things J 6(5):8421–8432. https://doi.org/10.1109/JIOT.2019.2917763
Ren, W.: On A Network Forensics Model For Information Security. ISTA, 229–234 (2004)
Carbon Black Threat Report: Non-malware attacks and Ransomware take center stage in 2016 (2016). https://www.carbonblack.com/2016/12/15/carbon-black-threat-report-non-malware-attacks-ransomware-takecenter-stage-2016/
Rhodes KA (2001) Code red, code red II, and SirCam attacks highlight need for proactive measures. GAO Testimony Before the Subcommittee on Government Efficiency
Rivera BS, Inocencio RU (2015) Doing more with less: a study of fileless infection attacks. VB 2015
Ruff N (2008) Windows memory forensics. J Comput Virol 4(2):83–100
Informational Series: What is Fileless malware? (2019). https://www.carbonblack.com/resources/definitions/what-is-fileless-malware/
Shackleford D (2016) Active breach detection: the next-generation security technology? SANS institute information security Reading room. In: SANS Institute Information Security Reading Room
Shulmin, A., Prokhorenko, M.: Lurk banker Trojan: exclusively for Russia (2016). https://securelist.com/lurk-banker-trojan-exclusively-for-russia/75040/
Sood, A.K., Enbody, R.J., Bansal, R.: The art of stealing banking information - form grabbing on fire (2011). https://www.virusbulletin.com/virusbulletin/2011/11/art-stealing-banking-information-form-grabbing-fire
SQL Slammer (2019). https://en.wikipedia.org/wiki/SQL Slammer
Stop Fileless Attacksat Pre-execution (2017). https://explore.bitdefender.com/solution-briefs/stop-fileless-attacks-pre-execution
Sudhakar P, S.K. (2018) An approach to improve load balancing in distributed storage systems for NoSQL databases: MongoDB. In: Pattnaik PK, Rautaray SS, Das H, Nayak J (eds) Progress in computing, analytics and networking. Springer, Singapore, pp 529–538
Tan Q, Gao Y, Shi J, Wang X, Fang B, Tian Z (2018) Toward a comprehensive insight into the eclipse attacks of tor hidden services. IEEE Internet Things J 6(2):1584–1593
Team CTG (2017) Threat spotlight: the truth about fileless malware [blog post]
Tian Z, Cui Y, An L, Su S, Yin X, Yin L, Cui X (2018) A real-time correlation of host-level events in cyber range service for smart campus. IEEE Access 6:35355–35364
Tian Z, Gao X, Su S, Qiu J, Du X, Guizani M (2019b) Evaluating reputation management schemes of internet of vehicles based on evolutionary game theory. IEEE Trans Veh Technol 68(6):5971–5980. https://doi.org/10.1109/TVT.2019.2910217
Tian Z, Li M, Qiu M, Sun Y, Su S (2019d) Block-def: a secure digital evidence framework using blockchain. Inf Sci 491:151–165
Tian Z, Luo C, Qiu J, Du X, Guizani M (2019e) A distributed deep learning system for web attack detection on edge devices. IEEE Transactions on Industrial Informatics
Tian Z, Shi W, Wang Y, Zhu C, Du X, Su S, Sun Y, Guizani N (2019a) Real-time lateral movement detection based on evidence reasoning network for edge computing environment. IEEE Trans Ind Inform 15(7):4285–4294. https://doi.org/10.1109/TII.2019.2907754
Tian Z, Su S, Shi W, Du X, Guizani M, Yu X (2019c) A data-driven method for future internet route decision modeling. Futur Gener Comput Syst 95:212–220
Tobergte DR, Curtis S (2013) The Art of Memory Forensics, vol 53, pp 1689–1699. https://doi.org/10.1017/CBO9781107415324.004 arXiv:1011.1669v3
Vacca, J.R.: Network Forensics. In: Computer and Information Security Handbook, 2nd edi edn., pp.649–660. Morgan Kaufmann Publishers is an imprint of Elsevier, United States (2013)
Valdez, R., Sconzo, M.: Threat alert: “PowerWare,” new Ransomware written in PowerShell, targets organizations via Microsoft word (2016). https://www.carbonblack.com/2016/03/25/threat-alert-powerwarenew-ransomware-written-in-powershell-targets-organizations-via-microsoft-word/
Watson D, Riden J (2008) The honeynet project: data collection tools, infrastructure, archives and analysis. Proceedings - WOMBAT Workshop on Information Security Threats Data Collection and Sharing, WISTDCS 2008:24–30. https://doi.org/10.1109/WISTDCS.2008.11
Wueest, C., Anand, H.: Living off the land and fileless attack techniques (2017). https://www.symantec.com/content/dam/symantec/docs/security-center/white-papers/istr-living-off-the-land-and-fileless-attack-techniques-en.pdf
Zaharia, A.: Understanding Fileless malware infections - the full guide (2016). https://heimdalsecurity.com/blog/fileless-malware-infections-guide/
Zeltser L (2017) The history of Fileless malware-looking beyond the buzzword
Zhang, E.: What is Fileless malware (or a non-malware attack)? Definition and best practices for Fileless malware protection (2018). https://digitalguardian.com/blog/what-fileless-malware-or-non-malware-attack-definition-and-best-practices-fileless-malware
Zou CC, Gong W, Towsley D (2002) Code red worm propagation modeling and analysis. In: Proceedings of the 9th ACM conference on computer and communications security, vol 147, p 138 ACM
Download references
Acknowledgements
Author information, authors and affiliations.
School of Computer & Systems Sciences, Jawaharlal Nehru University, 110067, New Delhi, India
Indian Computer Emergency Response Team, Ministry of Electronics & Information Technology, 110003, New Delhi, India
Sushil Kumar
You can also search for this author in PubMed Google Scholar
Contributions
The first author conceived the idea of the study and wrote the paper; all authors discussed and revised the final manuscript. All authors read and approved the final manuscript.
Corresponding author
Correspondence to Sudhakar .
Ethics declarations
Competing interests.
The authors declare that they have no competing interests.
Additional information
Publisher’s note.
Springer Nature remains neutral with regard to jurisdictional claims in published maps and institutional affiliations.
Rights and permissions
Open Access This article is distributed under the terms of the Creative Commons Attribution 4.0 International License ( http://creativecommons.org/licenses/by/4.0/ ), which permits unrestricted use, distribution, and reproduction in any medium, provided you give appropriate credit to the original author(s) and the source, provide a link to the Creative Commons license, and indicate if changes were made.
Reprints and permissions
About this article
Cite this article.
Sudhakar, Kumar, S. An emerging threat Fileless malware: a survey and research challenges. Cybersecur 3 , 1 (2020). https://doi.org/10.1186/s42400-019-0043-x
Download citation
Received : 29 July 2019
Accepted : 23 December 2019
Published : 14 January 2020
DOI : https://doi.org/10.1186/s42400-019-0043-x
Share this article
Anyone you share the following link with will be able to read this content:
Sorry, a shareable link is not currently available for this article.
Provided by the Springer Nature SharedIt content-sharing initiative
- Fileless malware
- Memory forensics
- Incident investigation
- Memory resident malware
Thank you for visiting nature.com. You are using a browser version with limited support for CSS. To obtain the best experience, we recommend you use a more up to date browser (or turn off compatibility mode in Internet Explorer). In the meantime, to ensure continued support, we are displaying the site without styles and JavaScript.
- View all journals
- My Account Login
- Explore content
- About the journal
- Publish with us
- Sign up for alerts
- Open access
- Published: 10 May 2024
PermDroid a framework developed using proposed feature selection approach and machine learning techniques for Android malware detection
- Arvind Mahindru 1 ,
- Himani Arora 2 ,
- Abhinav Kumar 3 ,
- Sachin Kumar Gupta 4 , 5 ,
- Shubham Mahajan 6 ,
- Seifedine Kadry 6 , 7 , 8 , 9 &
- Jungeun Kim 10
Scientific Reports volume 14 , Article number: 10724 ( 2024 ) Cite this article
131 Accesses
1 Altmetric
Metrics details
- Electrical and electronic engineering
- Engineering
The challenge of developing an Android malware detection framework that can identify malware in real-world apps is difficult for academicians and researchers. The vulnerability lies in the permission model of Android. Therefore, it has attracted the attention of various researchers to develop an Android malware detection model using permission or a set of permissions. Academicians and researchers have used all extracted features in previous studies, resulting in overburdening while creating malware detection models. But, the effectiveness of the machine learning model depends on the relevant features, which help in reducing the value of misclassification errors and have excellent discriminative power. A feature selection framework is proposed in this research paper that helps in selecting the relevant features. In the first stage of the proposed framework, t -test, and univariate logistic regression are implemented on our collected feature data set to classify their capacity for detecting malware. Multivariate linear regression stepwise forward selection and correlation analysis are implemented in the second stage to evaluate the correctness of the features selected in the first stage. Furthermore, the resulting features are used as input in the development of malware detection models using three ensemble methods and a neural network with six different machine-learning algorithms. The developed models’ performance is compared using two performance parameters: F-measure and Accuracy. The experiment is performed by using half a million different Android apps. The empirical findings reveal that malware detection model developed using features selected by implementing proposed feature selection framework achieved higher detection rate as compared to the model developed using all extracted features data set. Further, when compared to previously developed frameworks or methodologies, the experimental results indicates that model developed in this study achieved an accuracy of 98.8%.
Similar content being viewed by others
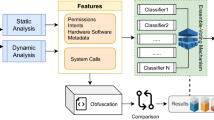
Evaluation and classification of obfuscated Android malware through deep learning using ensemble voting mechanism
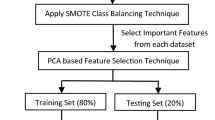
A study of dealing class imbalance problem with machine learning methods for code smell severity detection using PCA-based feature selection technique
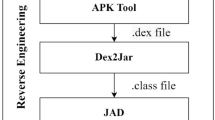
AndroMalPack: enhancing the ML-based malware classification by detection and removal of repacked apps for Android systems
Introduction.
Now-a-days, smartphones can do the same work as the computer has been doing. By the end of 2023, there will be around 6.64 billion smartphone users worldwide ( https://www.bankmycell.com/blog/how-many-phones-are-in-the-world ). According to the report ( https://www.statista.com/statistics/272307/market-share-forecast-for-smartphone-operating-systems/ ) at the end of 2023, Android operating systems captured 86.2% of the total segment. The main reason for its popularity is that its code is written in open source which attracts developers to develop Android apps on a daily basis. In addition to that it provides many valuable services such as process management, security configuration, and many more. The free apps that are provided in its official store are the second factor in its popularity. By the end of March 2023 data ( https://www.appbrain.com/stats/number-of-android-apps ), Android will have 2.6 billion apps in Google play store.
Nonetheless, the fame of the Android operating system has led to enormous security challenges. On the daily basis, cyber-criminals invent new malware apps and inject them into the Google Play store ( https://play.google.com/store?hl=en ) and third-party app stores. By using these malware-infected apps cyber-criminals steal sensitive information from the user’s phone and use that information for their own benefits. Google has developed the Google Bouncer ( https://krebsonsecurity.com/tag/google-bouncer/ ) and Google Play Protect ( https://www.android.com/play-protect/ ) for Android to deal with this unwanted malware, but both have failed to find out malware-infected apps 1 , 2 , 3 . According to the report published by Kaspersky Security Network, 6,463,414 mobile malware had been detected at the end of 2022 ( https://securelist.com/it-threat-evolution-in-q1-2022-mobile-statistics/106589/ ). Malware acts as a serious problem for the Android platform because it spreads through these apps. The challenging issue from the defender’s perspective is how to detect malware and enhance its performance. A traditional signature-based detection approach detects only the known malware whose definition is already known to it. Signature-based detection approaches are unable to detect unknown malware due to the limited amount of signatures present in its database. Hence, the solution is to develop a machine learning-based approach that dynamically learns the behavior of malware and helps humans in defending against malware attacks and enhancing mobile security.
Researchers and academicians have proposed different methods for analyzing and detecting malware from Android. Some of them have been proposed by using static analysis, for example, ANASTASIA 4 , DREBIN 5 , Droiddetector 6 and DroidDet 7 . On the other side, some researchers have proposed with the help of dynamic analysis, for example, IntelliDroid 8 , DroidScribe 9 , StormDroid 10 and MamaDroid 11 . But, the main constraints of these approaches are present in its implementation and time consumption because these models are developed with a number of features. On the other side, academicians and researchers 3 , 12 , 13 , 14 , 15 , 16 , 17 , 18 , 19 also proposed malware detection frameworks that are developed by using relevant features. But, they have restrictions too. They implemented only already proposed feature selection techniques in their work.
So, in this research paper, to overcome the hindrances a feature selection framework is proposed. This helps in the evaluation of appropriate feature sets with the goal of removing redundant features and enhances the effectiveness of the machine-learning trained model. Further, by selecting a significant features a framework named PermDroid is developed. The proposed framework is based on the principle of artificial neural network with six different machine learning techniques, i.e., Gradient descent with momentum (GDM), Gradient descent method with adaptive learning rate (GDA), Levenberg Marquardt (LM), Quasi-Newton (NM), Gradient descent (GD), and Deep Neural Network (DNN). These machine learning algorithms are considered on the basis of their performance in the literature 20 . In addition to this, three different ensemble techniques with three dissimilar combination rules are proposed in this research work to develop an effective malware detection framework. F-measure and Accuracy have been considered as performance parameters to evaluate the performance. From the literature review 21 , 22 , 23 , it is noticed that a number of authors have concentrated on bettering the functioning of the malware detection models. However, their study had a key flaw, they only used a small amount of data to develop and test the model. In order to address this issue, this study report takes into account 500,000 unique Android apps from various categories.

Steps are followed in developing Android malware detection framework.
The method for developing a reliable malware detection model is represented in Fig. 1 . The initial collection of Android application packages (.apk) comes from a variety of promised repositories (mentioned in “ Creation of experimental data set and extraction of features ” section). Anti-virus software is used to identify the class of .apk files at the next level (mentioned in “ Creation of experimental data set and extraction of features ” section). Then, features (such as API calls and permissions) are retrieved from the .apk file using various techniques described in the literature (mentioned in subsection 3.4). Additionally, a feature selection framework is applied to evaluate the extracted features (discussed in “ Proposed feature selection validation method ” section). Then, a model is developed using an artificial neural network using six different machine-learning techniques and three different ensemble models, employing the selected feature sets as input. Finally, F-measure and Accuracy are taken into consideration while evaluating the developed models. The following are the novel and distinctive contributions of this paper:
In this study, to develop efficient malware detection model half a million unique apps have been collected from different resources. Further, unique features are extracted by performing dynamic analysis in this study.
The methodology presented in this paper, is based on feature selection methodologies, which contributes in determining the significant features that are utilized to develop malware detection models.
In this study, we proposed three different ensemble techniques that are based on the principle of a heterogeneous approach.
Six different machine learning algorithms that are based on the principle of Artificial Neural Network (ANN) are trained by using relevant features.
When compared to previously developed frameworks and different anti-virus software in the market, the proposed Android malware detection framework can detect malware-infected apps in less time.
A cost-benefit analysis shows that the proposed Android malware detection framework is more effective in identifying malware-infected apps from the real world.
The remaining sections of this research paper are arranged as follows: “ Related work ” section presents the literature survey on Android malware detection as well as the creation of research questions. “ Research methodology ” section gives an overview of the research methodology used to create the Android malware detection framework. Different machine learning and ensemble techniques are addressed in “ Machine learning technique ” section. The proposed feature selection validation technique is discussed in “Proposed feature selection validation method” section. The experimental results are presented in “ Experimental setup and results ” section. Threats to validity are presented in “ Threats to validity ” section. Conclusion and the future scope are discussed in “ Conclusion and future work ” section.
Related work
The exploitation of the vulnerability is common these days to acquire higher privilege on Android platforms. Since 2008, cybercriminals have started targeting Android devices. An exploit app, from the perspective of Android security, can assist cyber-criminals in bypassing security mechanisms and gaining more access to users’ devices. Cybercriminals may exploit user data by selling their personal information for monetary gain if they took advantage of these privileges. The detection process, which has been used by researchers in the past and is based on Artificial Neural Networks (ANN) and feature selection techniques, is addressed in this subsection.
Androguard ( https://code.google.com/archive/p/androguard/ ) is a static analysis tool that detects malware on Android devices using the signature concept. Only malware that is already known to be present and whose definition is in the Androguard database is identified. It cannot, however, identify unidentified malware. Andromaly 23 , is developed on a dynamic analysis tool that uses a machine learning technique. It monitored CPU utilization, data transfer, the number of effective processes, and battery usage in real-time. The test was carried out on a few different types of simulated malware samples, but not on the applications that are present in the real-world. By using the semantics of the code in the form of code graphs collected from Android apps, Badhani et al. 24 developed malware detection methodology. Faruki et al. 21 introduced AndroSimilar, which is based on the principles of generated signatures that are developed from the extracted features, which are used to develop malware detection model.
Aurasium 25 takes control of an app’s execution by examining arbitrary security rules in real-time. It repackages Android apps with security policy codes and informs users of any privacy breaches. Aurasium has the problem of not being able to detect malicious behavior if an app’s signature changes. They performed dynamic analysis of Android apps and considered call-centric as a feature. The authors tested their method on over 2900 Android malware samples and found that it is effective at detecting malware activity. A web-based malware evaluation method has been proposed by Andrubis 26 , it operates on the premise that users can submit apps via a web service, and after examining their activity, it returns information on whether the app is benign or malicious. Ikram et al. 27 suggested an approach named as DaDiDroid based on weighted directed graphs of API calls to detect malware-infected apps. The experiment was carried out with 43,262 benign and 20,431 malware-infected apps, achieving a 91% accuracy rate. Shen et al. 28 developed an Android malware detection technique based on the information flow analysis principle. They implement N-gram analysis to determine common and unique behavioral patterns present in the complex flow. The experiment was carried out on 8,598 different Android apps with an accuracy of 82.0 percent. Yang et al. 29 proposed an approach named EnMobile that is based on the principle of entity characterization of the behavior of the Android app. The experiment was carried out on 6,614 different Android apps, and the empirical results show that their proposed approach outperformed four state-of-the-art approaches, namely Drebin, Apposcopy, AppContext, and MUDFLOW, in terms of recall and precision.
CrowDroid 34 , which is built using a behavior-based malware detection method, comprises of two components: a remote server and a crowdsourcing app that must both be installed on users’ mobile devices. CrowDroid uses a crowdsourcing app to send behavioral data to a remote server in the form of a log file. Further, they implemented 2-mean clustering approach to identify that the app belongs to malicious or benign class. But, the crowDroid app constantly depletes the device’s resources. Yuan et al. 52 proposed a machine learning approach named Droid-Sec that used 200 extracted static and dynamic features for developing the Android malware detection model. The empirical result suggests that the model built by using the deep learning technique achieved a 96% accuracy rate. TaintDroid 30 tracks privacy-sensitive data leakage in Android apps from third-party developers. Every time any sensitive data leaves the smartphone, TaintDroid records the label of the data, the app that linked with the data, as well as the data’s destination address.
Zhang et al. 53 proposed a malware detection technique based on the weighted contextual API dependency graph principle. An experiment was performed on 13500 benign samples and 2200 malware samples and achieved an acceptable false-positive rate of 5.15% for a vetting purpose.
AndroTaint 54 works on the principle of dynamic analysis. The features extracted were used to classify the Android app as dangerous, harmful, benign, or aggressive using a novel unsupervised and supervised anomaly detection method. Researchers have used numerous classification methods in the past, like Random forest 55 , J48 55 , Simple logistic 55 , Naïve Bayes 55 , Support Vector Machine 56 , 57 , K-star 55 , Decision tree 23 , Logistic regression 23 and k-means 23 to identify Android malware with a better percentage of accuracy. DroidDetector 6 , Droid-Sec 52 , and Deep4MalDroid 58 work on the convention of deep learning for identifying Android malware. Table 1 summarizes some of the existing malware detection frameworks for Android.
The artificial neural network (ANN) technique is used to identify malware on Android devices
Nix and Zhang 59 developed a deep learning algorithm by using a convolution neural network (CNN) and used API calls as a feature. They utilized the principle of Long Short-Term Memory (LSTM) and joined knowledge from its sequences. McLaughlin et al. 60 , implemented deep learning by using CNN and considered raw opcode as a feature to identify malware from real-world Android apps. Recently, researchers 6 , 58 used network parameters to identify malware-infected apps. Nauman et al. 61 , implemented connected, recurrent, and convolutional neural networks, and they also implemented DBN (Deep Belief Networks) to identify malware-infected apps from Android. Xiao et al. 62 , presented a technique that was based on the back-propagation of the neural networks on Markov chains and considered the system calls as a feature. They consider the system call sequence as a homogenous stationary Markov chain and employed a neural network to detect malware-infected apps. Martinelli et al. 63 , implemented a deep learning algorithm using CNN and consider the system call as a feature. They performed an experiment on a collection of 7100 real-world Android apps and identify that 3000 apps belong to distinct malware families. Xiao et al. 64 , suggested an approach that depends on the principle of LSTM (Long Short-Term Memory) and considers the system call sequence as a feature. They trained two LSTM models by the system call sequences for both the benign and malware apps and then compute the similarity score. Dimjas̈evic et al. 65 , evaluate several techniques for detecting malware apps at the repository level. The techniques worked on the tracking of system calls at the time the app is running in a sandbox environment. They performed an experiment on 12,000 apps and able to identify 96% malware-infected apps.
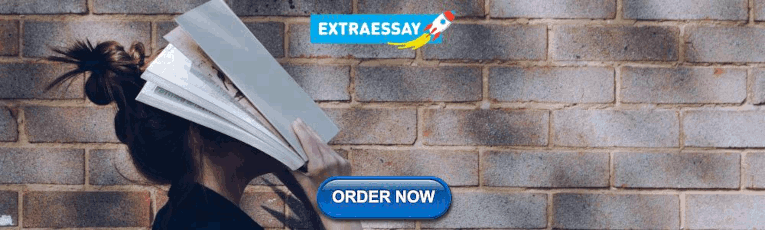
Using feature selection approaches, to detect Android malware
Table 2 shows the literature review for malware detection done by implementing feature selection techniques. Mas’ud et al. 66 proposed a functional solution to detect malware from the smartphone and can address the limitation of the environment of the mobile device. They implemented chi-square and information gain as feature selection techniques to select the best features from the extracted dataset. Further, with the help of selected best features, they employed K-Nearest Neighbour (KNN), Naïve Bayes (NB), Decision Tree (J48), Random Forest (RF), and Multi-Layer Perceptron (MLP) techniques to identify malware-infected apps. Mahindru and Sangal 3 developed a framework that works on the basis of feature selection approaches and used distinct semi-supervised, unsupervised, supervised, and ensemble techniques parallelly and identify 98.8% malware-infected apps. Yerima et al. 67 suggested an effective technique to detect malware from smartphones. They implemented mutual information as a feature selection approach to select the best features from the collected code and app characteristics that indicate the malicious activities of the app. To detect malware apps, from the wild, they trained selected features by using Bayesian classification and achieved an accuracy of 92.1%. Mahindru and Sangal 15 suggested a framework named as “PerbDroid” that is build by considering feature selection approaches and deep learning as a machine classifier. 2,00,000 Android apps in total were subjected to tests, with a detection rate of 97.8%. Andromaly 23 worked on the principle of the Host-based Malware Detection System that monitors features related to memory, hardware, and power events. After selecting the best features by implementing feature selection techniques, they employed distinct classification algorithms such as decision tree (J48), K-Means, Bayesian network, Histogram or Logistic Regression, Naïve Bayes (NB) to detect malware-infected apps. Authors 14 suggested a malware detection model based on semi-supervised machine learning approaches. They examined the proposed method on over 200,000 Android apps and found it to be 97.8% accurate. Narudin et al. 68 proposed a malware detection approach by considering network traffic as a feature. Further, they applied random forest, multi-layer perceptron, K-Nearest Neighbor (KNN), J48, and Bayes network machine learning classifiers out of which the K-Nearest Neighbor classifier attained an 84.57% true-positive rate for detection of the latest Android malware. Wang et al. 69 employed three different feature ranking techniques, i.e., t -test, mutual information, and correlation coefficient on 3,10,926, benign, and 4,868 malware apps using permission and detect 74.03% unknown malware. Previous researchers implement feature ranking approaches to select significant sets of features only. Authors 13 developed a framework named as “DeepDroid” based on deep learning algorithm. They use six different feature ranking algorithms on the extracted features dataset to select significant features. The tests involved 20,000 malware-infected apps and 100,000 benign ones. The detection rate of a framework proposed using Principal component analysis (PCA) was 94%. Researchers and Academicians 70 , 71 , 72 , 73 also implemented features selection techniques in the literature in different fields to select significant features for developing the models.
Research questions
To identify malware-infected apps and considering the gaps that are present in the literature following research questions are addressed in this research work:
RQ1 Does the filtering approach helps to identify that whether an app is a benign or malware-infected (first phase of the proposed feature selection framework)? To determine the statistical significance among malicious and benign apps, the t -test is used. After, determining significant features, a binary ULR investigation is applied to select more appropriate features. For analysis, all the thirty different feature data sets are assigned (shown in Table 5 ) as null hypotheses.
RQ2 Do already existing and presented work’s sets of features show an immense correlation with each other? To answer this question, both positive and negative correlations are examined to analyze the sets of features, which help in improving the detection rate.
RQ3 Can the identified features assist in determining whether the app is malware-infected or not? The primary objective of this question is to use the feature selection framework validation approach to determine the appropriate features. In this paper, four stages (i.e., ULR, t-test, Correlation analysis, and multivariate linear regression stepwise forward selection) are implemented to identify the appropriate features, that helps in identifying whether an app contains malicious behavior or not.
RQ4 Which classification algorithm among the implemented machine learning algorithms is most appropriate for identifying malware-infected apps? To answer to this question the efficiency of various machine learning approaches are evaluated. In this study, three different ensemble approaches and six different machine learning algorithms based on neural networks are considered.
RQ5 Is the feature collected (such as an app’s rating, API calls, permissions, and the number of people who have downloaded the app) sufficient for identifying a malicious app or not? This question helps in determining whether or not considering features can detect malware-infected apps in the real world. To answer this question, the performance of our suggested model is compared with previously published frameworks as well as several anti-virus scanners in the market.
Research methodology
Based on the research questions mentioned above, the methodology that is used in this research paper is mentioned in the following subsections. In order to improve the detection rate for malware, the obtained data set has been normalized, and dependent and independent variables have been selected.
Independent variables
In this study, the model is developed by applying the proposed feature selection approach, which helps in the detection of malware-infected apps. Additionally, as shown in Fig. 2 , five different strategies to select the best features are used. The best features are selected from other accessible features created on intermediate explore models at each level.
Dependent variables
The focus of this research is to find a link between Android apps and the features (such as app rating, API calls, permission, and the number of users who have downloaded an app) retrieved from the collected data set. The malware app characteristics are separated from the benign app features in the dependent variable of Android apps.
Creation of experimental data set and extraction of features
In this research paper, 70,000 .apk files from Google play store ( https://play.google.com/store?hl=en ), and more than 3 lacs .apk files from third-party app store i.e., Softonic ( https://en.softonic.com/android ), Android Authority ( https://www.androidauthority.com/apps/ ), CNET ( https://download.cnet.com/android/ ) belong to
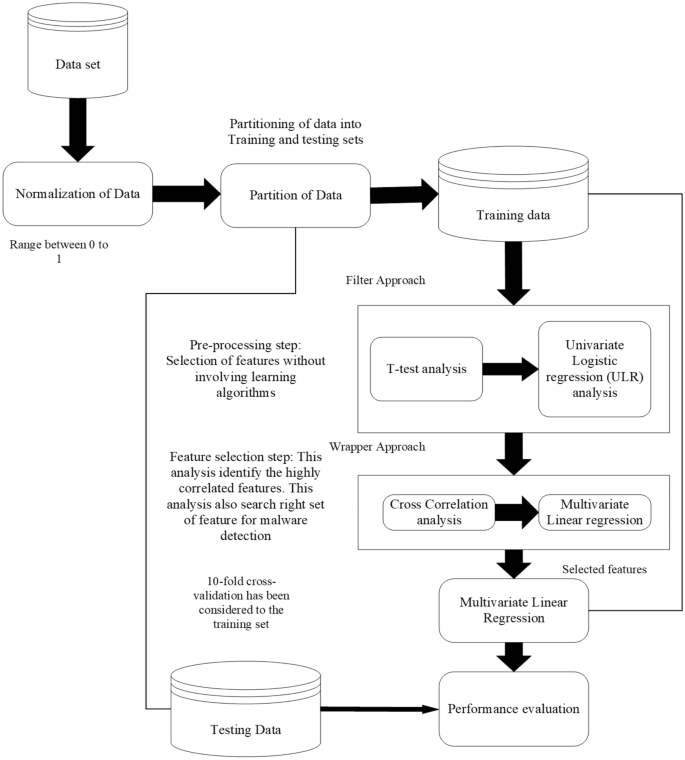
Proposed framework for feature selection and its validation.
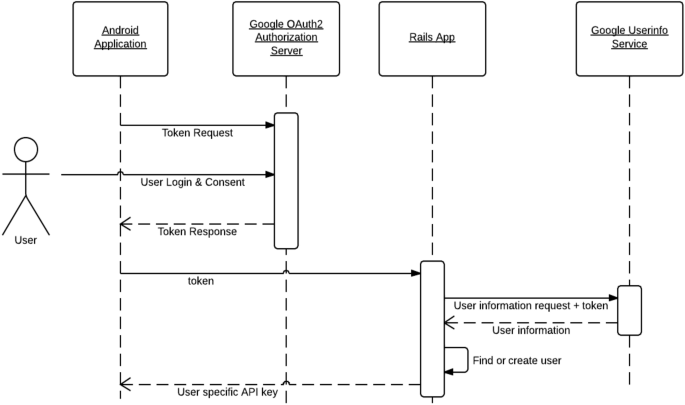
Sequence diagram showing reservation using Android app.
benign group and 70,000 malware-infected Android apps from 79 , 80 , 81 and Sanddroid ( http://sanddroid.xjtu.edu.cn:8080/ ) belongs to malicious group are collected to develop an effective malware detection framework. As seen in Table 3 , the .apk files we collected fall under thirty different categories. Collected malware-infected apps belong to ten different malware categories: AD (Adware), BA (Backdoor), HT (Hacker Tool), RA (Ransom), TR (Trojan), TB (Trojan-Banker), TC (Trojan-Clicker), TD (Trojan-Dropper), TS (Trojan-SMS) and TSY (Trojan-Spy). Classes are identified by using two distinct scanners i.e., VirusTotal ( https://www.virustotal.com/gui/ ) and Microsoft Windows Defender ( https://windows-defender.en.softonic.com/download ) and on the basis of its behavior defined in the study 82 .
To formulate an efficient malware detection framework, we extract 310 API calls and 1419 unique permissions ( https://github.com/ArvindMahindru66/Computer-and-security-dataset ), by implementing the procedure mentioned in the literature 3 , 13 , 15 , 83 . If an app requests the permission and API call during installation or runtime, we mark it as “1”; otherwise, we mark it as “0”. The following are some of the features of a certain app that have been extracted:
0,1,1,1,1,0,0,0,0,0,1,1,1,1,1,1,1,1,1,1,1,1,1,1,1,1,
0,0,0,0,0,0,0,0,0,0,1,1,1,1,1,1,1,1,1,1,1,1,1,0,0,0,
1,1,1,1,1,1,1,1,1,1,1,1,1,1,1,1,1,1,1,1,1,1,1,1,1,1,
1,1,1,1,1,1,1,1,1,1,0,0,0,0,0,0,0,0,0,0,0,0,0,0,0,0, and so on.
After extracting API calls and permissions from the collected data set from .apk files, it is divided into thirty different features data sets (Mahindru, Arvind (2024), “Android Benign and Malware Dataset”, Mendeley Data, V1, doi: 10.17632/rvjptkrc34.1). Table 4 illustrates the creation of various feature data sets as well as their explanations. These extracted features are divided into different sets on the basis of its behavior to which it belongs 3 , 13 , 15 , 83 . The main reasons to divide these extracted features into thirty different feature data sets are: to select significant features by using the proposed feature selection framework and to remove the complexity.
Figure 3 demonstrate the sequence diagram of an Android app by showing the example of an railway reservation app. How the process is started and how it is interact with other APIs and the permissions that are running in the background (Table 5 ).
Machine learning technique
ANN stands for artificial neural networks, and it is a computing system based on biological neural networks. These are able to perform certain tasks by utilizing certain examples, without using task-specific rules. Researchers are implementing ANN to solve different problems in malware detection, pattern recognition, classification, optimization, and associative memory 84 . In this paper, ANN is implemented to create a malware detection model. The structure of the ANN model is shown in Fig. 4 . ANN contains input nodes, hidden nodes, and output nodes.
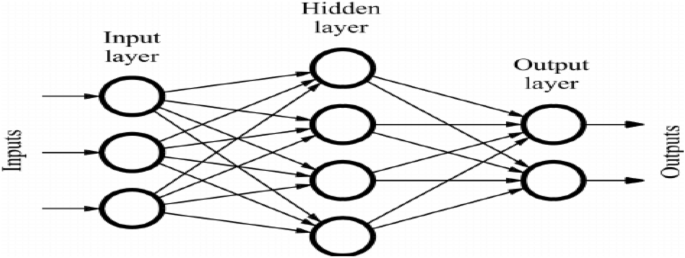
Artificial neural network.
The input layer employs a linear stimulation function, while the hidden and output layers employ squashed-S or sigmoidal functions. ANN can be presented as:
where B is the input vector, A is the weight vector and \(O^{'}\) denotes the desired output vector. In order to minimize the mean square error (MSE), the value of A is updated in each step. Mean square error can be calculated from the equation below:
Here, O is the actual output value, and \(O^{'}\) is the desired output value. Various methods were proposed by researchers 20 , 84 to train the neural network. In this research work, six different kinds of machine learning algorithms (namely, Gradient Descent approach, Quasi-Newton approach, Gradient Descent with Momentum approach, Levenber-Marquardt approach, Gradient Descent with Adaptive learning rate approach, and Deep neural network) are considered to develop malware detection model. These models are effective in the field of software fault prediction 20 , intrusion detection and desktop malware predictions 85 too.
Gradient descent with momentum approach
This approach accelerates the rate of convergence dramatically 20 , 84 . To obtain new weights, this approach combines the fraction diversity 20 , 84 , 86 . X is the updated weighed vector defined as:
where A denotes the momentum parameter value, \(X_k\) is the current weight vector and \(X_{k+1}\) is the update value of the weight vector and \((E_k)\) , used to identify the lower value in error space. Here, \(X_{k+1}\) relys on both the weight and the gradient. To determine the optimal value of A we implemented the cross-validation technique.
Gradient descent approach
This approach updates the weights to reduce the output error 20 , 84 , 86 . In Gradient descent (GD) approach, to identify the lower value in error space \((E_k)\) , the \(1^{st}\) - order derivative of the total error function is computed by considering, the following equation:
Redundancy weight vector X is modified by employing gradient vector G 20 , 84 , 86 . The up-dation of X is done through the following formula
where \(G_n\) is the gradient vector, \(O_{x+1}\) is the revised weight vector and \(\alpha\) is the gaining constant. To calculate the optimum value of \(\alpha\) , we implement cross-validation approach.
Gradient descent method with adaptive learning rate approach
In the GD approach, during training, the learning rate \((\alpha )\) remains stable. This approach is based on the concept that is quite perceptive to the approximation value of the learning rate. At the time of training, if the value of the learning rate is too high, the build model can be highly unstable and oscillate its value 20 . On the reverse of this, if the training value is too small, the procedure may take a long way to converge. Practically, it is not easy to find out the optimal value of \(\alpha\) before training. Actually, during the training process, the value of \(\alpha\) changes 20 . In each iteration, if the performance decline along with the required aim, the \(\alpha\) value is added by 1.05, and in reverse of this, if the performance increase by more than the factor of 1.04, then the \(\alpha\) value is incremented by 0.7 20 .
Levenberg Marquardt (LM) approach
The foundation of LM is an iterative technique that helps in locating the multivariate function’s minimal value. At the time of training, this value can be calculated as the sum of squares of real-valued with non-linera functions which helps in modifying the weights 20 , 87 . This method is quite stable and fast because it combines the Gauss Newton and the steepest descent approach. The iterative process for the same is given by
where \(X_{k+1}\) is the updated weight, \(X_k\) is the current weight, I is the identity matrix, \(\mu >0\) is named as combination coefficient and J is the Jacobian matrix. For a small value of \(\mu ,\) it becomes Gauss-Newton approach and for large, \(\mu ,\) it acts as GD approach. Representation of Jacobian matrix is : \(J=\) \(\begin{bmatrix} \frac{\partial E_{1,1}}{\partial X_1}&{} \frac{\partial E_{1,1}}{\partial X_2}&{}\cdots &{}\frac{\partial E_{1,1}}{\partial X_N}\\ \frac{\partial E_{1,2}}{\partial X_1}&{} \frac{\partial E_{1,2}}{\partial X_2}&{}\cdots &{}\frac{\partial E_{1,2}}{\partial X_N}\\ \vdots &{}\vdots &{}\vdots &{}\vdots \\ \frac{\partial E_{P,M}}{\partial X_1}&{} \frac{\partial E_{P,M}}{\partial X_2}&{}\cdots &{}\frac{\partial E_{P,M}}{\partial X_N}\\ \end{bmatrix}\) where P , N and M is the input patterns, weights and the output patterns.
Quasi-Newton approach
In order to compute the total error function, this approach requires the evaluation of the second order derivatives for each component of the gradient vector 20 , 84 . The iterative scheme for the Weight vector X is given as:
where \(X_k\) and \(X_{k+1}\) are the current and updated weight vectors, accordingly. H is the Hessian matrix given by \(H=\) \(\begin{bmatrix} \frac{\partial ^2E}{\partial X_1^2}&{} \frac{\partial ^2E}{\partial X_1X_2}&{}\cdots &{}\frac{\partial ^2E}{\partial X_1X_N}\\ \frac{\partial ^2E}{\partial X_1X_2}&{} \frac{\partial ^2E}{\partial X_2^2}&{}\cdots &{}\frac{\partial ^2E}{\partial X_2X_N}\\ \vdots &{}\vdots &{}\vdots &{}\vdots \\ \frac{\partial ^2E}{\partial X_1X_N}&{} \frac{\partial ^2E}{\partial X_2X_N}&{}\cdots &{}\frac{\partial ^2E}{\partial X_N^2} \end{bmatrix}\)
Deep learning neural network (DNN) approach
Convolutional Neural Networks (CNN) and Deep Belief Networks (DBN) are two deep architectures 88 that can be combined to create DNN. In this article, the DBN architecture to build our deep learning approach is implemented. The architecture of the deep learning method is demonstrated in Fig. 5 . The procedure is separated into two stages: supervised back-propagation and unsupervised pre-training. Restricted Boltzmann Machines (RBM) with a deep neural network is used to train the model with 100 epoches in the early stages of development. An iterative method is implemented to construct the model with unlabeled Android apps in the training step. Pre-trained DBN is fine-tuned with labeled Android apps in a supervised manner during the back-propagation step. In both stages of the training process, a model developed using deep learning methods uses an Android app.
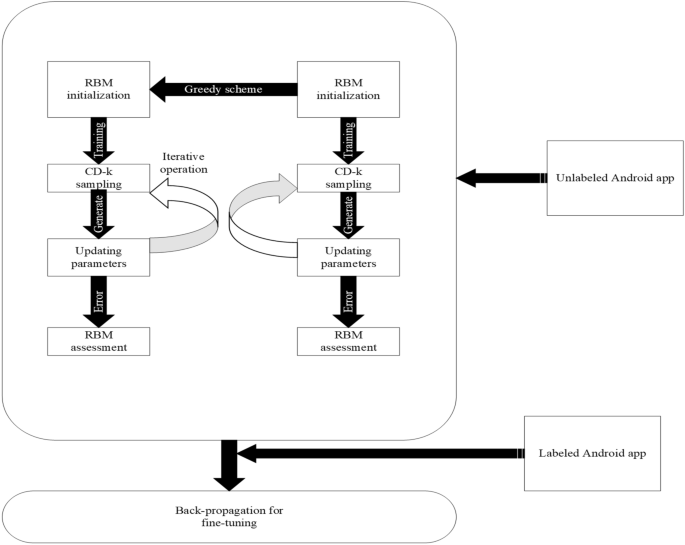
Deep learning neural network (DNN) method constructed with DBN.
Ensembles of classification models
In this study, three different ensemble models to detect malware from Android apps is also proposed. During development of the model, the outputs of all the classification models have been considered where the base machine learning algorithm allocated several priority levels and output is calculated by applying some combination rules. Ensemble approaches are divided into two types:
Homogenous ensemble approach: In this approach, all classification models, are of the same kinds, but the difference is in generating the training set.
Heterogenous ensemble approach: Here, all base classification approaches are of distinct types.
On the basis of combination rules, ensemble approaches are divided into two distinct categories:
Linear ensemble approach: While developing the model, with a linear ensemble approach an arbitrator combines the results that come from the base learners, i.e., selection of classification approach, average weighted, etc.
Nonlinear ensemble approach: While developing the model, with the nonlinear ensemble approach, it fed the result of the base classifier, which is a nonlinear malware detection model for example Decision tree (DT), Neural network (NN), etc.
In this work, a heterogenous ensemble approach having three distinct combination rules is adapted. The ensemble techniques are detailed in Table 6 .
BTE (best training ensemble) approach
The BTE technique is based on the observation that each classifier performs differently when the data set is partitioned 20 . Among the applied classifier, the best model is selected to train data set that are founded on the principles of certain performance parameters. In this research paper, accuracy is considered as a performance parameter. Algorithm 1 given below is considered to calculate the ensemble output \(E_{result}\) .
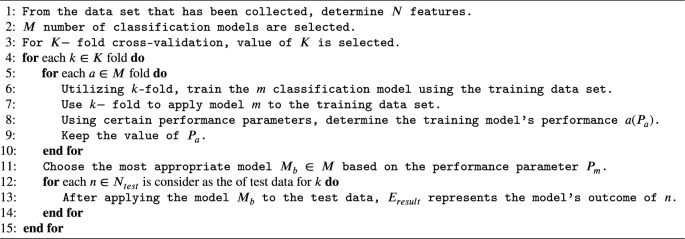
Best Training Ensemble (BTE) approach.
MVE (majority voting ensemble) approach
MVE approach, based on the principle to consider the output of the test data for each classifier, and the ensemble output \((E_{result})\) is concerned with the majority group differentiated by the base classifier 20 . Ensemble output \((E_{result})\) is calculated by implementing Algorithm 2.
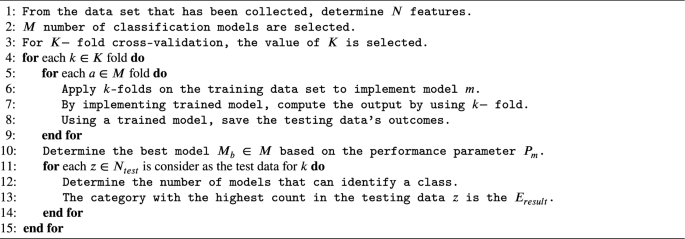
Majority Voting Ensemble (MVE) Approach.
NDTF (nonlinear ensemble decision tree forest) approach
In this study, to train the model with base leaner, is also considered. Further, the trained model is implemented the results on the corresponding testing data set to make the model for the final detection of malware apps. In this research paper, Decision tree forest (DTF) has been considered as a non-linear ensemble as a classifier which was suggested by Breiman in 2001. The developed model is based on the outcome of the collected results of the distinct decision trees. Algorithms 3 is used to calculate the result \((E_{result})\) .
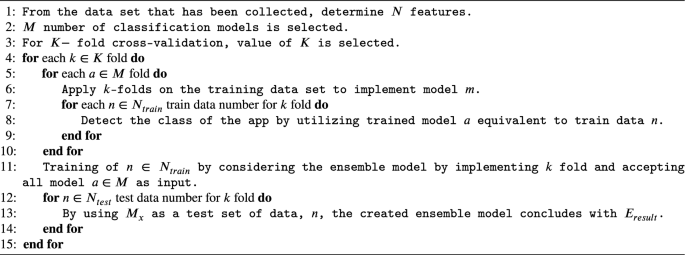
Nonlinear Ensemble Decision Tree Forest (NDTF) Approach.
Method for normalizing the data
In order to comply with the required diversity of input properties and prevent the saturation of the neurons, it is important to normalize the data prior to deploying a neural network spanning the range of 0 to 1 89 . The Min-max normalizing approach is used in this research study. This technique is work on the principle of a linear transformation, which brings each data point \(D_{q_i}\) of feature Q to a normalized value \(D_{q_i},\) that lies in between \(0-1.\)
To obtain the normalized value of \(D_{q_i}:\) , use the following equation:
The relative values of the relevance of the characteristic Q are min ( Q ) and max ( Q ).
Parameters considered for evaluation
This section provides definitions for the performance metrics needed to identify malicious apps. The confusion matrix is used to determine all of these characteristics. Actual and detected classification information is included in the confusion matrix, which was created using a detection approach. The constructed confusion matrix is shown in Table 7 . F-measure and accuracy are two performance parameters that are used to evaluate the performance of malware detection algorithms in this research. Formulas for evaluating the accuracy and F-measure are given below:
False positive (FP) A false positive occurs when the developed model identifies the positive class incorrectly.
False negative (FN) When the developed model successfully identifies the negative class, a false negative occurs.
True negative (TN) An accurate identification of the negative class by the developed model represents a true negative conclusion.
True positives (TP) An accurate identification of the positive class by the developed model represents a real positive conclusion.
Recall The data set’s positive classes that are made up of all other positive classes are identified.
where \(x= N_{Malware\rightarrow Malware},\) \(z= N_{Malware\rightarrow Benign}\)
Precision The accuracy measures the proportion of forecasts in the positive class that are indeed in the positive class.
where \(y= N_{Benign\rightarrow Malware}\)
Accuracy Accuracy is measured as 3 :
where \({N_{classes} = x+y+z+w}\) ,
\(w= N_{Benign\rightarrow Benign}\)
F-measure F-measure is measured as 3 :
Proposed feature selection validation method
The selection of relevant feature sets is an important challenge for data processing in various machine learning and data mining applications 90 , 91 , 92 . In the field of Android malware detection, a number of authors 13 , 14 , 15 , 69 , 93 , 94 applied only limited feature subset selection and feature ranking approaches i.e., Correlation, Goodman Kruskals, Information Gain, Chi-squared, Mutual Information, and t-test methods to detect malware. The first limitation of the previous studies is that they used a small data set (i.e., the number of malware or benign apps is less in number) to validate the proposed techniques. The additional significant disadvantage of the feature selection lies in the fact that after selecting the best features no comparison analyses were made among the classifiers model developed by reduced sets of features and by using all extracted feature sets. Mainly, the main reason for this is that the vast collection of features found in particular categories of the app (like books, entertainment, comics, game, etc.) makes it complex to produce a classifier by examining all the features as input. It is the best of our knowledge, that academicians and researchers were implemented these feature selection approaches individually; but no one selected features by combining all of these feature selection approaches. However, a framework for the feature selection approach has been given in this study, which helps in selecting the most appropriate features and enhance the effectiveness of the malware detection model. The suggested framework is applied to apps that have been gathered from the various repositories listed in section 2.4 and that fall under the thirty categories listed in Table 3 . Finally, we verified the framework by comparing the effectiveness of the models developed after implementing feature selection method with the efficiency of ones constructed using the whole data set initially formed.
Figure 2 demonstrates the phases of the proposed feature selection validation framework. Without using machine learning algorithms, this framework aims to determine whether the selected features are useful in detecting malicious apps. The wrapper strategy is used to pick the sets of features that are useful in identifying malware apps after all crucial components have been examined. It keeps track of the progress of the learning algorithm that was used to identify each feature subset. In this work, the selected features are investigated using linear discriminant analysis (LDA).
Data set Table 3 summarized the data set used in this research work. The considered data set belongs to 141 different malware families.
Normalization of data By using the Min-max normalizing approach, all features are normalized between the ranges of 0 and 1.
Partition of data We examined at the data set that wasn’t used for training in order to evaluate the proposed feature selection approach. Further, the data set is divided into two different parts one part is used for training, and the remaining is used for testing. The group ratios in the training and testing of the data sets are nearly identical.
Filter approach Pre-processing is the term that describes this technique because it eliminates extraneous features. In this step, the t -test and ULR analysis are implemented.
t-test analysis It examine the statistical significance of benign and malware apps using the t -test method. In a 2-class problem (malware apps and benign apps), analysis of the null hypothesis (H0) significant that the two populations are not equal, or it is seen that there is a noticeable variance among their mean values and features used by both of them are different 95 . Furthermore, it shows that the features affect the malware detection result. Hence, those features are considered, which have significant differences in their mean values, and others are excluded. Hence, it is essential to approve the null hypothesis (i.e., H0) and discard the alternative ones 95 . t -test is implemented on each of the attributes and then P value for each feature is calculated, which indicates how well it distinguishes the group of apps. According to research by 95 , features with an P value of < 0.05 show significant biases.
Univariate logistic regression (ULR) analysis After identifying features that make a significant difference between malware and benign apps, binary ULR analysis is implemented to test the correlation among features that helps in malware detection 95 . ULR analysis is implemented on each selected feature set, which helps in discovering whether the above-selected features were essential to detect the malware-infected apps or not. Only those features are considered, which are having P value < 0.05. From the results of the ULR analysis and t -test, the hypothesis are rejected and accepted mentioned in Table 5 .
Wrapper approach To determine optimum sets of the feature, cross-correlation analysis and multivariate linear regression stepwise forward selection is implemented in this stage.
Cross correlation analysis After finding the important features, the correlation analysis is implemented and then examination for both negative and positive correlation coefficients (i.e., r-value) between features is performed. If a feature has a value of r > = 0.7 or r-value < =0.7 with other features, i.e., have a higher correlation then the performance of these features is studied separately. Further, those features are selected, which perform better.
Multivariate linear regression stepwise forward selection It is not imply that, features that are achieved are relevant to develop malware detection framework. In this stage, ten-fold cross-validation technique is applied to determine the significant features.
Performance evaluation Further, to validate that proposed framework is able to identify malware-infected apps that were developed by implementing the steps mentioned above by using independent test data. Additionally, the efficiency of the essential feature sets used for malware detection is validated. On thirty different categories of Android apps, nine different machine learning classifiers were used to develop the investigation model. To evaluate the framework two separate performance parameters, are considered i.e., F-measure and Accuracy. The effectiveness of our detection model is then evaluated using the proposed malware detection methodology.
Evaluation of proposed framework
Three different approaches are used to evaluate our proposed framework:
Comparison with previously used classifiers Parameters like Accuracy and F-measure are compared with existing classifiers proposed by researchers in the literature to see if our suggested model is feasible or not.
Comparison with AV scanners To compare the effectiveness of our suggested work, ten different anti-virus scanners are considered and their performance is evaluated on the collected data set.
Detection of unknown and known malware families The proposed framework is also examined to see whether it can identify known and unknown malware families.
Experimental setup and results
The experimental setting used to develop the malware detection model is described in this portion of the paper. The model is developed using a Neural Network (NN) using six different types of machine learning algorithms, namely GD, NM, LM, GDA, GDM, DNN, and three ensemble techniques, including the best training, non-linear decision tree forest, and majority voting. These algorithms are applied on Android apps that were collected from different resources. Each category has a distinct number of benign and malicious apps (they are further separated into various families), which is sufficient for our analysis. Figure 6 presents PermDroid, our suggested framework.
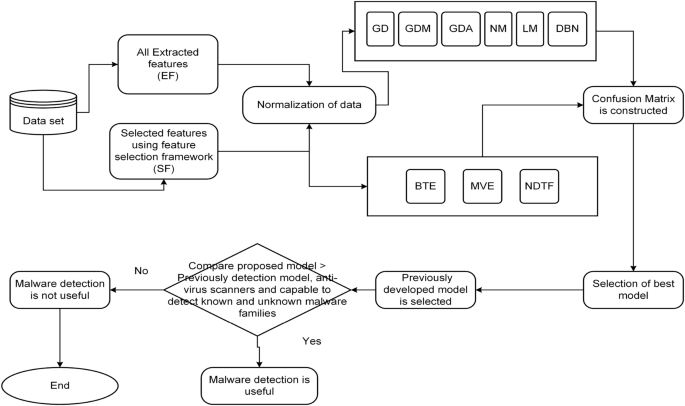
Proposed framework i.e., PermDroid.
Following are the phases that are pursued in this study, to develop an effective and efficient malware detection framework. The proposed feature selection framework is applied to all the extracted feature data sets, to select significant features. After that, six different machine learning algorithms based on the principle of neural network and three different ensemble algorithms are considered to develop a malware detection model. So, in this study, a total of 540 (30 different Android apps data sets * 9 different machine learning techniques * (one takes into account all extracted features, and another takes into account features identified using the suggested feature selection framework. )) different detection models are developed. The following are a detailed description of the model followed in this study:
Thirty different extracted feature data sets are used to implement the proposed feature selection framework.
The first stage, which involved identifying significant features, was employed as an input to train the model using various classification and ensemble machine learning approaches. In this research paper, ten-fold cross-validation technique is implemented to verify the develop model 16 . Further, outliers are eliminated, which effect the performance of the proposed framework. The performance of outliers is measured using the equation below:
The developed model using the aforementioned two processes is evaluated using the collected data set in order to determine whether or not the proposed framework is successful in identifying malicious apps.
Validation of the proposed feature selection framework
In this subsection, the selection of significant feature sets for malware detection is explained. Our analysis is started by using thirty different feature sets (mentioned in Table 4 ).
t-Test analysis
t -test analysis is used to determine the statistical significance of detecting the malware from Android apps. In this work, t -test is applied on extracted feature sets and calculated its P value. Further, in this study, the cut-off P value considered is 0.05, i.e., it denotes that feature sets that have P value < 0.05 has a strong prediction capability. Figure 7 illustrates the findings of a t -test performed on the thirty various categories of Android apps that comprise up our obtained data set. The P value is provided using two forms for simplicity of use (box with black circle \((\cdot)\) means P value < 0.05 and blank box \({}_ \Box\) means P value > than 0.05). The sets of features with emphasis P values of < 0.05 have a significant impact on identifying malicious or benign apps. Figure 7 shows how the S29, S27, S25, S23, S22, S21, S19, S18, S13, S10, S8, S5, S3, and S1 feature sets might help to detect malicious and benign apps in the Arcade and Action categories. As a result, in this study, we rule out the hypotheses H1, H3, H5, H8, H10, H13, H18, H19, H21, H22, H23, H25, H27, and H29, coming to the conclusion that these sets of features are capable of identifying apps in the Arcade and Action category that are malicious or benign.
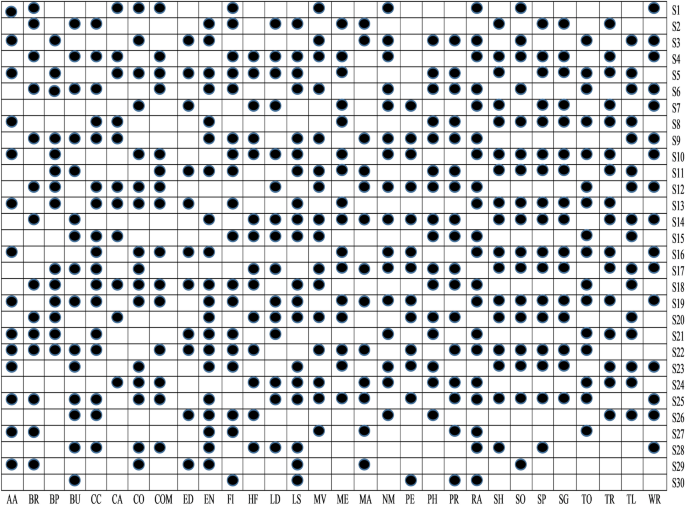
t -Test analysis.
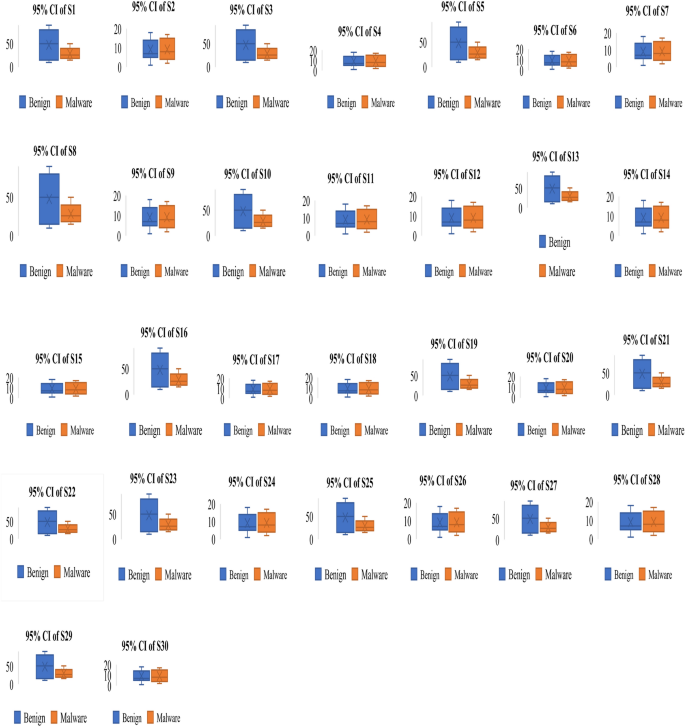
Error box-plots for all the set of permissions in Arcade and Action category apps.
To understand the relationship between malware and benign apps, we have drawn an error box-plot diagram. These box-plot diagrams verify the outcomes of the t -test analysis. If there is no overlapping in means and their confidence intervals (CI), then it means there will be a statistical difference between malware and benign apps else. There is no significant difference between them. An error box-plot of the 95% confidence intervals throughout the sets of features and the mean for Arcade and Action category apps is demonstrated in Fig. 8 . The outcomes of other categories of Android apps are of similar types. Based on Fig. 8 , we can observe that the boxes of S29, S27, S25, S23, S22, S21, S19, S18, S13, S10, S8, S5, S3, and S1 sets of feature do not overlap which means they are significantly different from each other. The mean value of the malware group is higher than the benign group apps. Based on error box-plots, we consider the hypotheses H1, H3, H5, H8, H10, H13, H18, H19, H21, H22, H23, H25, H27 and H29 concluding that these feature sets can able to identify the malware-infected apps for Arcade and Action category Android apps.
ULR analysis
To examine whether the selected sets of feature after implementing t -test analysis are significant to identify malware apps or not, in this study, ULR analysis is performed on selected sets of features. A set of features is considerably associated with malware detection if its P value is < 0.05. In every task, some sets of features are essential for the evolution of the malware detection model, while different sets of features do not seem to be appropriate for malware detection. The outcomes of the ULR approach are demonstrated in Fig. 9 . Equivalent to t-test analysis, the same representation is used as such in P values, i.e., blank box means P value > 0.05 and box having black square has P value \(\le\) to 0.05.
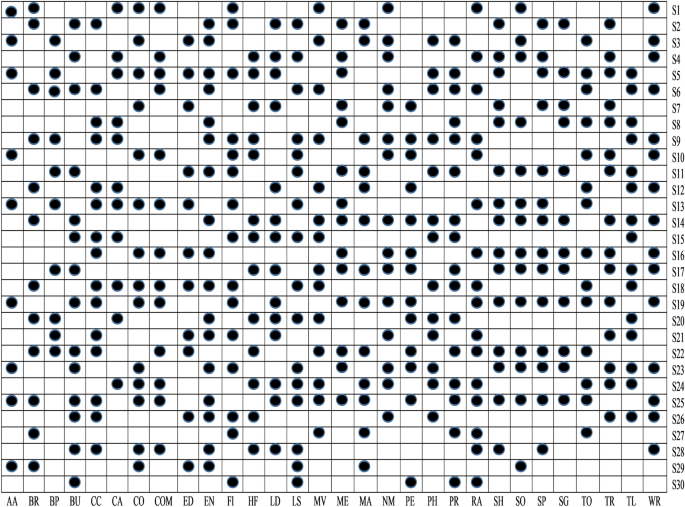
ULR analysis.
From Fig. 9 , it is clear that among thirty different categories of features, only S5, S3, S1, S13, S10, S23, S19, S29, and S25 sets of features are significant detectors of malware apps. As a result, we reject null hypotheses H1, H3, H5, H10, H13, H19, H23, H25, and H29 and conclude that these sets of features are directly related to the functioning of the apps. After implementing t -test and ULR analysis on our collected sets of features, rejection and acceptance of the hypotheses is done that is presented in the Table 5 . Figure 10 demonstrates the rejection and acceptance of the hypotheses for all of the thirty different categories of Android apps. The horizontal and vertical axes indicate the name of the hypothesis and the equivalent category of the Android app, accordingly. To represent the rejection and acceptance of the hypotheses, the cross symbol \((\times )\) and black circle \((\cdot )\) , are used respectively. Based on Fig. 10 , it is observed that only sixteen hypotheses out of thirty are accepted. Others are rejected for Arcade and Action category Android apps.
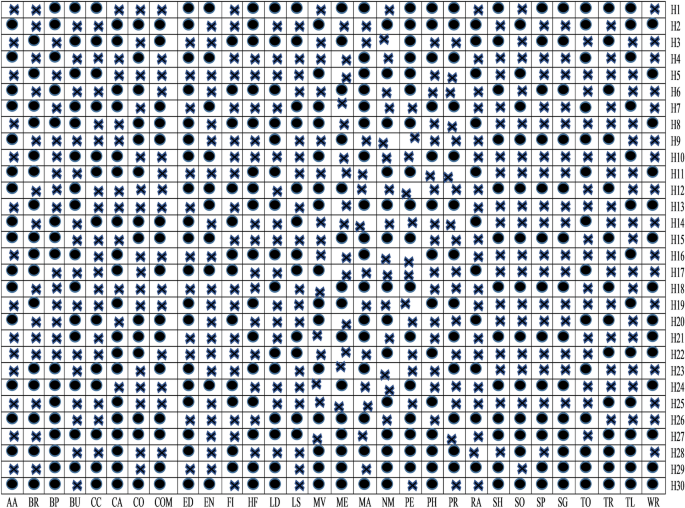
Hypothesis.
Cross correlation analysis
Figure 11 demonstrates the Pearson’s correlation between sets of features for all the categories of Android apps. The lower triangular (LT) and upper triangular (UT) matrices indicate the correlation in different sets of features for distinct Android app categories. The linear relation is evaluated by using the value of the correlation coefficient between distinct sets of extracted features from Android apps. In the present paper, Pearson’s correlation (r: Coefficient of correlation) is used to determine the linear relationship among distinct sets of features. The direction of the association is determined by whether the correlation coefficient, r , has a positive or negative sign. If the value of r is positive, it indicates that dependent and independent variables grow linearly or if the value of r is negative. Both the dependent and independent variables are inversely proportional to each other. Cross-correlation analysis is conducted only on the sets of features that were identified by implemented ULR and t -test analysis. If the relevant sets of features show a higher value of correlation (i.e., r -value \(\ge\) 0.7 or r -value \(\le -0.7\) ) with pertinent other sets of features, then the performance of these sets of feature separately and on the joint basis for malware detection is validated and consider those sets of feature which perform well. Figure 12 demonstrates the selected sets of the feature after implementing cross-correlation analysis. The selected sets of features are represented by utilizing a black circle \((\cdot)\) , demonstrating that equivalent sets of features are considered for this research paper.
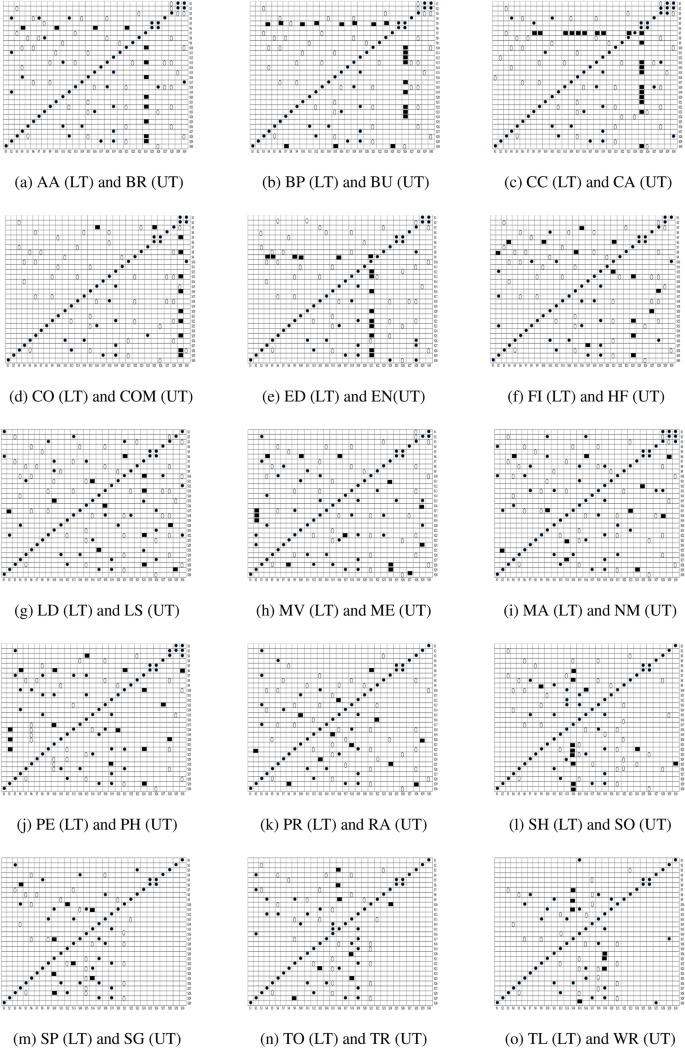
Correlation between set of features (here LT stands for lower triangle and UT stands for Upper triangle.
Stepwise forward selection for multivariate linear regression
After using cross-correlation analysis, the selected subset of features may or may not be important for creating the malware detection model. Further, a multivariate linear regression stepwise forward selection method is implemented in this study to discover the most important features for creating Android malware detection models. After applying multivariate linear regression stepwise on the retrieved feature data set, Fig. 13 shows a significant set of features. A set of features that were taken into account in this paper while building a malware detection model is represented by a black circle with the symbol \((\cdot )\) .
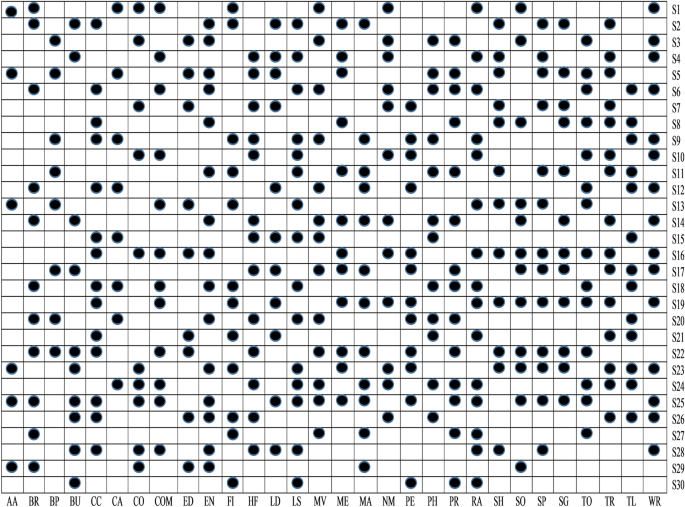
Features selected after implementing cross correlation analysis.
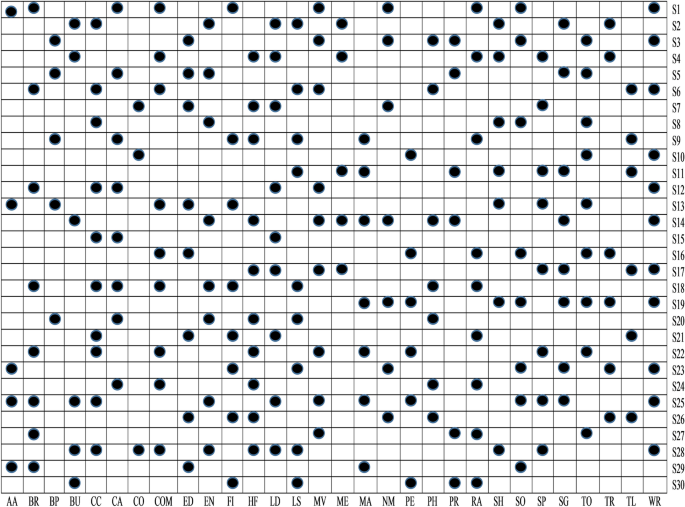
Features selected after implementing multivariate linear regression stepwise forward selection.
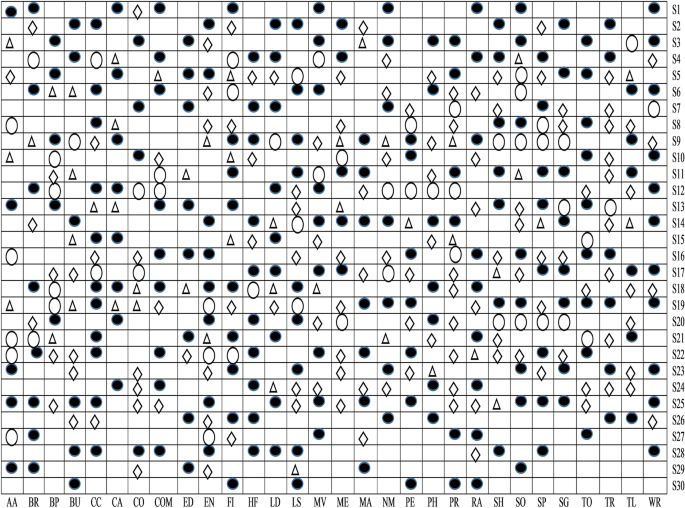
Selected sets of feature for malware detection.
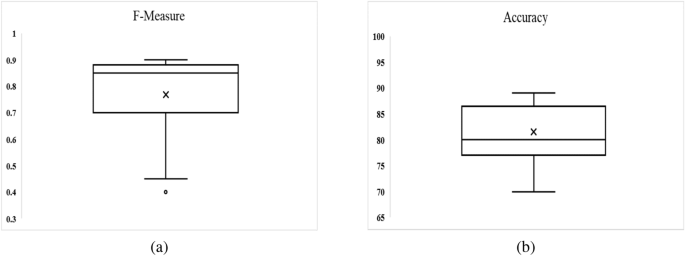
Results of testing data by considering performance parameters.
The overall outcome of the feature selection method
In this study, four distinct phases are used to identify relevant sets of features that will be taken into account while constructing the Android malware detection model. Some relevant sets of features are identified from the available sets of features in each stage based on the outcomes of the intermediate analysis. A selection of features from each of the thirty various categories of Android apps are shown in Fig. 14 . To make things easier, the selected feature sets are represented by four separate characters, as shown below:
Empty circle symbol: Features are relevant after implementing t -test analysis.
Triangle symbol: Features are relevant after implementing ULR analysis and t -test.
Diamond symbol: Features are relevant after applied cross-correlation analysis, ULR, and t -test.
Filled circle symbol: Features are relevant after implementing multivariate linear regression stepwise forward selection method, cross-correlation analysis, ULR, and t -test.
Evaluation on the basis of performance parameters
To examine set of features, a new data set is used that was not previously considered in this study. The model is originally built using ten-fold cross-validation, multivariate linear regression, and selected feature sets as input. Figure 15 illustrates the box-plot diagram for performance measures for all Android apps categories used in this study, including F-measure and Accuracy. It reveals that the outcome is computed as Accuracy of 82 percent and an average F-measure of 0.80.
Evaluation of the malware detection models developed using ANN
In this paper, we use a neural network to develop a model for malware detection using six different types of machine learning algorithms.
Two separate feature data sets are used as input to construct a model for identifying malware from Android apps (one comprises all extracted features (EF) and the other is used using the feature selection framework (SF). The following hardware was used to complete this task: a Core i7 processor with a 1 TB hard disc and 64 GB RAM. Each malware detection model’s performance is measured using two performance parameters: F-Measure and Accuracy. The outcomes of using a neural network with six different machine learning techniques to achieve performance metrics for various categories of Android apps are shown in Tables 8 and 9 . From Tables 8 and 9 , the following conclusions can be drawn:
The model developed by features selected using proposed framework (Model also developed by using distinct feature selection approaches are shown in Tables S1 to S14 in “Online Appendix A”) as an input produces better results when compared to a model constructed by taking into account all sets of features, presenting a significant value of F-measure and Accuracy for identifying malware.
In compared to the others, the neural network with Deep Neural Network (DNN) training method yields higher outcomes.
Figures 16 and 17 show the Accuracy and F-measure box-plot diagrams for each model built using classification methods. Each figure has two box plots, one containing all of the extracted features (EF) and the other containing only selected feature sets (SF).
The Box-plot diagram assists us in analyzing the performance of all the implemented approaches based on a single diagram. The line drawn in the middle of each box-plot diagram, i.e. the median, is used to determine its value. If a model’s median value is high, it’s regarded as the best model for detecting malware. It can be inferred from Figs. 16 and 17 that:
The models developed utilizing a significant set of features have high median values. The box-plot diagrams in Figs. 16 and 17 show that SF outperformed all extracted features in terms of detecting Android malware.
The DNN-based model yields the best results out of all the machine learning techniques for classification that have been used.
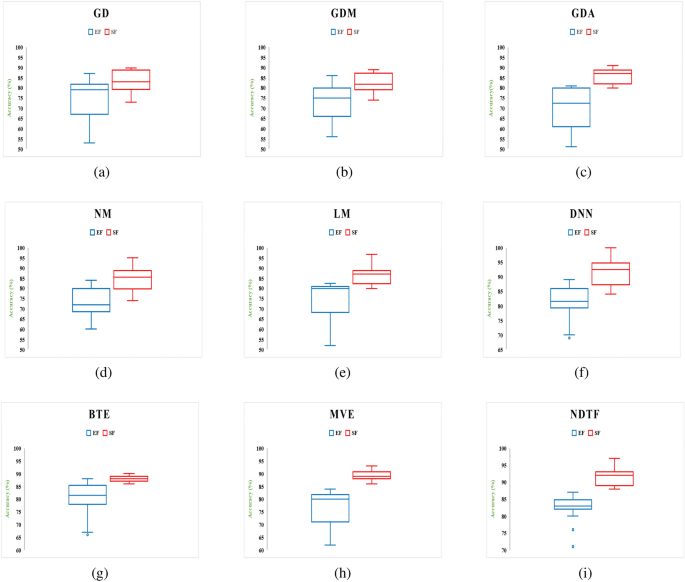
Box-plot diagram for measured performance parameter i.e., Accuracy.
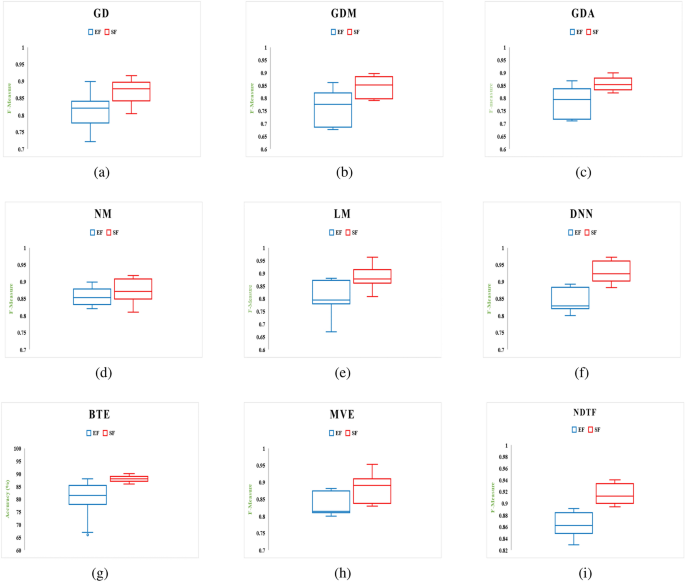
Box-plot diagram for measured performance parameter i.e., F-measure.
Evaluation of the malware detection models developed using ensemble techniques
In this study, three different heterogeneous ensemble approaches are considered for creating the Android malware detection model, each with a different combination rule (1 nonlinear and two linear). From Tables 8 and 9 and Figs. 16 and 17 , it can be revealed that the NDTF approach outperformed the BTE and MVE approaches. Further, it is also noticed that ensemble approaches detect more malware as compared to other implemented machine learning algorithms except DNN.
Comparison of the findings
In this study, paired Wilcoxon signed-rank tests to assess the relative performance of several feature sets and machine learning methods is employed. The Wilcoxon test with Bonferroni correction is used in this work for comparative review.
On the basis of detection approaches
To create a model that can determine whether an Android app is benign or malicious, nine different classification algorithms were evaluated. Two sets of features have been identified as inputs for developing malware detection models for thirty different categories of Android apps using two different performance parameters, namely F-Measure and Accuracy. One set of features takes into account all extracted features, and the other sets of selected features that are gained by implementing the framework of the feature selection method. Two sets of data are used for each strategy, each having 60 data points ((1 feature selection approach + 1 considering all retrieved features) * 30 Android app categories). The comparisons of pair-wise different machine learning techniques are shown in Table 10 .
There are two sections in Table 10 . The value of the significant difference between different pairings is shown in the second half of the table, and the calculated P value is shown in the first half. Using Bonferroni correction sets, the significant cutoff value is calculated. In this work, nine different machine learning algorithms were examined for creating malware detection models, resulting in a total of 36 potential pairs \({}^{9 techniques} C_2=36\) , with all results examined at a significance threshold of 0.05. We can rule out the null hypothesis if the P value is < 0.05/36 = 0.0013. According to the study, the null hypothesis for the test implies that no significant difference exists between the two procedures. Table 10 a shows that the P value is < 0.0013, indicating that there is a significant difference between the applied processes; out of 36 pairs of training techniques, 22 are offered as a significant outcome. By examining the mean difference value in Table 10 a, it can be seen that the DNN method outperformed the performance of other machine learning techniques. In addition, the value of the mean difference of ensemble techniques is better when compared to other models, with the exception of the model built using DNN.
On the basis of all selected sets of feature using proposed framework and extracted features
By taking into consideration each set of features, a total of 270 different data points ((3 ensemble techniques + neural network with six machine learning techniques) * 30 types of Android apps) are developed in this study (one for each performance measure). Wilcoxon signed-rank test performance was described in Table 10 b. It is seen from Table 10 b that there is a significant difference between the models developed because the P value is less than 0.05. Additionally, it is evident that the features taken into account employing the feature selection framework outperformed the model developed by using all extracted feature sets when comparing the mean difference values from Table 10 b to it.
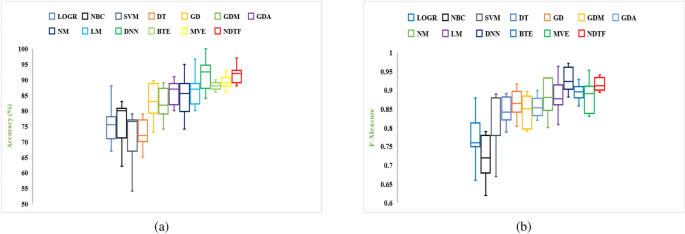
Measured performance parameters i.e., Accuracy and F-measure.
Proposed framework evaluation
Results comparison with previously employed classifiers.
In the present study, our newly developed malware detection model is also compared to the models developed using previously used classifiers such as decision tree analysis (DT), support vector machine (SVM), Naïve Bayes classifier (NBC), and logistic regression (LOGR). Two different sets of features (1 considering selected feature sets + 1 using all extracted features) are considered for 30 different categories of Android apps using two independent performance measures i.e., F-Measure and Accuracy. An aggregate of two sets i.e., 60 data points are employed for each classifier model are produced ((1 selected feature sets + 1 considering all extracted features)* 30 data sets). Figure 18 illustrates both the classifiers employed in this study and the most frequently used classifiers in the literature.
On the basis of Fig. 18 , it can be seen that the model produced using neural networks has a higher median value and achieves better results than the model developed using the literature’s used classifiers. Further, to decide that, which model produces better results, a pairwise Wilcoxon signed rank test is implemented. Table 11 summarizes the results of the Wilcoxon test with Bonferroni correction examination for accuracy outcomes. Further, the Table 11 is divided into two sections, the first of which indicates the P value and the second of which demonstrates the mean difference between different pairs of classifiers. We implemented thirteen different machine learning approaches in this research paper (4 previously applied classifier in the literature + 9 implemented classifier in this study); thus, an aggregate of seventy eight (78) individual pairs are possible \({}^{13techniques} C_2=78\) , and all classifier outcomes are examined at the 0.05 significance level. Only those null hypotheses with an P value is less than 0.05/78 = 0.000641 are rejected in this study. Table 11 shows that there is a significant difference between different implemented classifier approaches in a number of cases when the P value is less than 0.000641, i.e., 66 out of 78 pairs of classification approaches have significant outcomes. Table 11 demonstrates that the DNN approach outperforms other machine learning classifiers in terms of mean difference value.
Using cost-benefit analysis, comparison with previously employed classifiers
A cost-benefit analysis is used to evaluate the performance of developed model. Using the following equation, the cost-benefit analysis for each feature selection strategy is calculated:
In this case, \(Based_{cost}\) is determined by the correlation between the specified features set and the class error. The following equation can be used to compute \(Based_{cost}\) :
The multiple correlation coefficient between the error and the selected feature set is \(\rho _{SM.fault}\) , and the classification accuracy used to build a malware detection model using the selected feature set is \(Accuracy \ (SM)\) . The proposed model has a greater accuracy and a larger \(Based_{cost}\) since it has a higher multiple correlation coefficient. After adopting feature selection procedures, NAM stands for feature sets, while NSM stands for the number of selected features. The following equation can be used to determine \(Based_{cost}\) :
Instead of using the feature selection validation method, we use six other feature ranking approaches to evaluate PermDroid’s performance in this study. The naming standards used for the experiment are listed in Table 12 . The most important feature selection technique, as suggested in 96 , is the one that achieves a better value of cost-benefit. The cost-benefit analysis of different feature selection procedures is shown in Fig. 19 a,b. It is discovered that sets of features were selected after applying multivariate linear regression stepwise forward selection technique, cross-correlation analysis, ULR, and t -test to achieve a higher median Cost-benefit measure when compared to other feature selection techniques used by researchers in the literature.
In the literature academicians and researchers implemented different feature ranking and feature subset selection approaches i.e., Chi-squared test, Gain-ratio, Information-gain, Principal Component Analysis and Filtered subset evaluation. To evaluate the performance of our proposed feature selection approach, an experiment was performed by using Drebin data set and accuracy is measured and represented in Table 13 . Out of implemented six different feature selection techniques our proposed feature selection approach achieved an higher accuracy when compared to others.
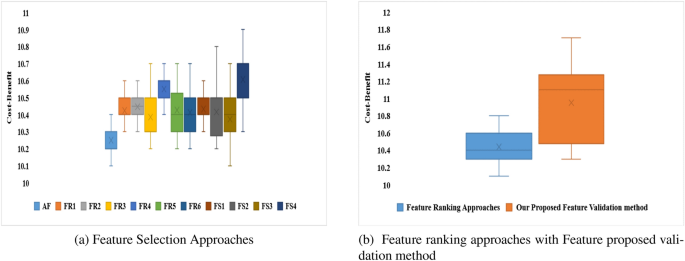
Calculated cost-benefit value.
Comparison of results based on the amount of time it takes to identify malware in real-world apps
In this section of the article, the performance of PermDroid is compared in terms of the time needed to identify malware in real-world apps. For this experiment, we download the data set from two different repositories Drebin ( https://www.sec.cs.tu-bs.de/~danarp/drebin/download.html ) and AMD ( http://amd.arguslab.org/ ) and experimented by implementing the individual frameworks. Table 14 shows that, when compared to the individual frameworks available in the literature, our suggested technique can identify malware in less time.
Comparison of the results on the basis of detection rate with different approaches or frameworks available in the literature
Furthermore, proposed malware detection model (i.e., PermDroid) is compared to previously developed techniques or frameworks present in the literature. The names, methodology, deployment, purpose, data collection, and detection rate of proposed methodologies or frameworks are listed in Table 15 . Empirical result revealed that our proposed framework produced a 3 percent greater detection rate. Experiment was performed by using Drebin data set ( https://www.sec.cs.tu-bs.de/~danarp/drebin/download.html ).
Comparison of results with different AV Scanners
Although PermDroid outperforms the classifiers used in the research, it should ultimately be similar to the results obtained using regular anti-virus software in the field for Android malware detection. For this study, ten different anti-virus softwares are selected from the market and used them on the data set that has been gathered in this study.
When compared to the various anti-viruses employed in the experiment, PermDroid performs significantly better. The results of the anti-virus scanner study are shown in Table 16 . The anti-virus scanners’ rates of virus detection vary widely. While the most effective scanners catch 97.1 percent of malware, some scanners only catch 82 percent of hazardous samples, which is probably a result of their inexperience with Android malware. PermDroid with DNN and NDTF outperform 1 out of 10 anti-virus scanners on the complete data set, with detection rates of 98.8% and 98.8%, respectively. Out of implemented different anti-virus scanners, it is discovered that at least two of them are capable of identifying every malware sample used in this study. As a result, it may conclude that PermDroid is more effective than many anti-virus scanners’ manually built signatures.
Identification of both well-known and new malware families
Detection of well-known malware families An experiment is also performed to identify whether or not our suggested framework, i.e., PermDroid, is capable of detecting malware from well-known families. The experiment is carried out on a sample of 20 families from each family (in our research paper, we collect 141 different malware families). According to empirical results, the suggested framework with DNN is capable of detecting an average of 98.8% of malware-infected apps, and the proposed framework with NDTF is likewise capable of doing the same. Table 17 lists the family names and the number of samples for each family, and Fig. 20 a,b show PermDroid’s detection performance for each family (Detection rates for some families are lower because of fewer samples in the data set).
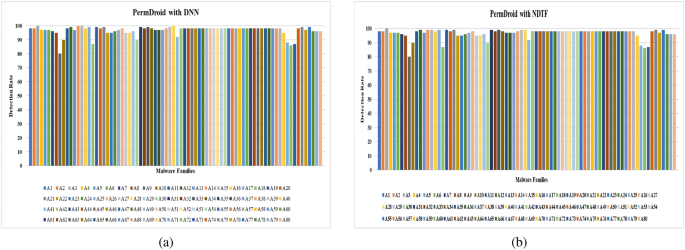
Detection rate of PermDroid with DNN and NDTF.
Detection of new malware families To examine if the suggested framework, is capable of identifying unknown malware families, PermDroid is trained with a random sample of 10 distinct families based on counting and then test is performed on the remaining families. Table 18 shows the outcomes in which PermDroid is trained with limited malware samples, which is required to generalize the characteristics of most malware families, and achieved a higher detection rate.
Experimental outcomes
The conclusions reached after conducting experimental work are presented in this section of the paper. The empirical work was done using a neural network and six different machine learning techniques, including GDA, NM, GD, GDM, LM, and DNN, as well as three ensemble approaches. The developed models outperform previously used classifiers in the literature (Table 11 ) and can detect malware from both known and unknown families (Table 18 , Fig. 20 ). Additionally, they increase the rate of detection by different Antivirus scanners (stated in Table 15 ). It is clear from Fig. 20 and Tables 14 , 15 , 16 , and 18 that:
PermDroid can detect 98.8% of Android malware, which is impossible for most AV scanners on the market.
With a detection rate of 98.8% for both known and unknown malware types, PermDroid is capable of finding malware.
The proposed framework is able to answer the research questions mentioned in “ Research questions ” section:
To verify the importance of the correlation between the feature sets and the malware detection model, the t -test and ULR analysis are used. It is discovered that there are several separate sets of features that are highly connected with the creation of malware detection models as a result of this research.
From Fig. 11 , it can be noticed that certain sets of features pass a high correlation with other sets of features (i.e., the case with a black square is having high negative correlation, and the case with a black circle is having a high positive correlation). It is essential to remove the collinearity among the features, for calculating the ability of each feature. In this manner, the models developed by selecting sets of the feature are capable to detect malware and do not suffer from the aspect of collinearity.
Forward stepwise selection process, ULR, correlation analysis, and t -test analysis are implemented to select features that are able to identify whether the app is malicious or not. The model built by applying the specified sets of features produces better outcomes when compared to the rest, according to t -test analysis.
Six various types of machine learning techniques based on neural network principles, such as NM, GD, LM, GDM, GDA, and DNN, as well as three ensemble approaches, are implemented in detecting whether an app is benign or malicious. From the Tables 8 and 9 , it is apparent that the model developed using an ANN and the Deep Neural Network (DNN) approach produces the best results when compared to other techniques.
Tables 8 and 9 and Figs. 18 , 19 and 20 show that our suggested model is effective in identifying malware from real-world apps when API calls, permissions, app rating, and the number of people that have downloaded the app are all considered features.
Threats to validity
In this section, threats to validity are discussed that are experienced while performing the experiment. Three different threats are mentioned below:
Construct validity The Android malware detection methodology in this research study is capable of detecting whether an app is benign or malicious, however it does not specify how many features are needed to find vulnerabilities in Android apps.
Internal validity The homogeneity of the data set employed in this research work is the second threat. Apps are collected from a variety of promised repositories. Any errors made while gathering data from these sources are not taken into account in this study. Although, it cannot promise that the data collected and retrieved for our analysis is 100 percent accurate, it can be believed that it assembled consistently.
External validity To train the Android malware detection algorithm, 141 different malware families are considered. Furthermore, the research can be extended to include other malware families in order to train the technique to identify malicious apps.
Conclusion and future work
This study suggests a framework for selecting small set of features that helps in detecting malware from Android apps. The following are our observations based on the basis of our proposed framework in this research paper:
Based on the feature selection method, it is discovered that there is a limited group of attributes that can detect malware or benign apps with greater accuracy and lower values of incorrectly classified errors.
Using our feature selection method sets S25, S28, S19, S14, S9, and S4 of features were discovered to be important malware detectors.
Based on the Wilcoxon signed-rank test, it is found that there is a significant difference between all extracted features and the selected feature sets. It is found that, after calculating the mean difference that the model developed with the input of the selected feature sets outperformed the model with the input of all extracted feature sets.
Different classification algorithms differ significantly, according to the Wilcoxon signed-rank test. By calculating the mean difference value, it is discovered that the model created by combining a neural network with the Deep-Learning machine-learning algorithm produced superior results than the other machine learning methods used in this study.
It may be inferred from the results of the experiments that the NDTF approach performed better than other ensemble methods.
Our used classifier outperformed the performance of the classifiers used in the literature, as shown in Fig. 20 and Tables 11 and 14 .
According to the results of the experiments (Tables 8 , 9 ), the malware detection model built was not significantly harmed after deleting 60% of the possible number of sets of features; in fact, in almost all cases, the results were better.
As shown in Table 18 and Fig. 20 , our proposed malware detection system can detect malware from both known and undiscovered malware families.
This study established that a malware detection method merely identifies whether an app is malicious or benign. Several avenues can be explored for future research. Firstly, a large amount of Android apps are required to develop the model, memorize and disclose information related to the data set. Second, it is also difficult to make a centralized system at the time of training and testing the model. Third, decentralized, privacy-preserving classifier model will be proposed for detecting Android malwares. Further, it is also be discovered how many permissions are necessary to evaluate whether an app is dangerous or not, more investigation may be done.
Data availibility
For materials should be addressed to corresponding authors.
Faruki, P. et al. Android security: A survey of issues, malware penetration, and defenses. IEEE Commun. Surv. Tutor. 17 (2), 998–1022 (2014).
Article Google Scholar
Gao, H., Cheng, S. & Zhang, W. Gdroid: Android malware detection and classification with graph convolutional network. Comput. Secur. 106 , 102264 (2021).
Mahindru, A. & Sangal, A. MLDroid—framework for android malware detection using machine learning techniques. Neural Comput. Appl. 33 , 1–58 (2020).
Google Scholar
Fereidooni, H., Conti, M., Yao, D. & Sperduti, A. Anastasia: Android malware detection using static analysis of applications. In 2016 8th IFIP International Conference on New Technologies, Mobility and Security (NTMS) , 1–5 (IEEE, 2016).
Arp, D. et al. Drebin: Effective and explainable detection of android malware in your pocket. Ndss 14 , 23–26 (2014).
Yuan, Z., Lu, Y. & Xue, Y. Droiddetector: Android malware characterization and detection using deep learning. Tsinghua Sci. Technol. 21 (1), 114–123 (2016).
Zhu, H. J. et al. Droiddet: Effective and robust detection of android malware using static analysis along with rotation forest model. Neurocomputing 272 , 638–646 (2018).
Wong, M. Y. & Lie, D. Intellidroid: A targeted input generator for the dynamic analysis of android malware. NDSS 16 , 21–24 (2016).
Dash, S. K., Suarez-Tangil, G., Khan, S., Tam, K., Ahmadi, M., Kinder, J. & Cavallaro, L. Droidscribe: Classifying android malware based on runtime behavior. In: 2016 IEEE Security and Privacy Workshops (SPW) , 252–261 (IEEE, 2016).
Chen, S., Xue, M., Tang, Z., Xu, L. & Zhu, H. Stormdroid: A streaminglized machine learning-based system for detecting android malware. In Proceedings of the 11th ACM on Asia Conference on Computer and Communications Security , 377–388 (2016).
Mariconti, E., Onwuzurike, L., Andriotis, P., Cristofaro, E. D., Ross, G. & Stringhini, G. Mamadroid: Detecting Android Malware by Building Markov Chains of Behavioral Models . arXiv:1612.04433 (2016)
Kabakus, A. T. DroidMalwareDetector: A novel android malware detection framework based on convolutional neural network. Expert Syst. Appl. 206 , 117833 (2022).
Mahindru, A. & Sangal, A. Deepdroid: Feature selection approach to detect android malware using deep learning. In: 2019 IEEE 10th International Conference on Software Engineering and Service Science (ICSESS) , 16–19 (IEEE, 2019).
Mahindru, A. & Sangal, A. Feature-based semi-supervised learning to detect malware from android. In Automated Software Engineering: A Deep Learning-Based Approach , 93–118 (Springer, 2020).
Mahindru, A. & Sangal, A. Perbdroid: Effective malware detection model developed using machine learning classification techniques. In A Journey Towards Bio-inspired Techniques in Software Engineering 103–139 (Springer, 2020).
Mahindru, A. & Sangal, A. Hybridroid: An empirical analysis on effective malware detection model developed using ensemble methods. J. Supercomput. 77 (8), 8209–8251 (2021).
Mahindru, A. & Sangal, A. Semidroid: A behavioral malware detector based on unsupervised machine learning techniques using feature selection approaches. Int. J. Mach. Learn. Cybern. 12 (5), 1369–1411 (2021).
Zhao, Y. et al. On the impact of sample duplication in machine-learning-based android malware detection. ACM Trans. Softw. Eng. Methodol. (TOSEM) 30 (3), 1–38 (2021).
Yumlembam, R., Issac, B., Jacob, S. M. & Yang L. IoT-based android malware detection using graph neural network with adversarial defense. IEEE Internet Things J. (2022).
Kumar, L., Misra, S. & Rath, S. K. An empirical analysis of the effectiveness of software metrics and fault prediction model for identifying faulty classes. Comput. Stand. Interfaces 53 , 1–32 (2017).
Faruki, P., Ganmoor, V., Laxmi, V., Gaur, M. S. & Bharmal, A. Androsimilar: Robust statistical feature signature for android malware detection. In Proceedings of the 6th International Conference on Security of Information and Networks , 152–159 (2013).
Milosevic, J., Malek, M. & Ferrante, A. Time, accuracy and power consumption tradeoff in mobile malware detection systems. Comput. Secur. 82 , 314–328 (2019).
Shabtai, A., Kanonov, U., Elovici, Y., Glezer, C. & Weiss, Y. Andromaly: A behavioral malware detection framework for android devices. J. Intell. Inf. Syst. 38 (1), 161–190 (2012).
Badhani, S. & Muttoo, S. K. Android malware detection using code graphs. In System Performance and Management Analytics , 203–215 (Springer, 2019).
Xu, R., Saïdi, H. & Anderson, R. Aurasium: Practical policy enforcement for android applications. In Presented as part of the 21st \(\{\) USENIX \(\}\) Security Symposium ( \(\{\) USENIX \(\}\) Security 12 ), 539–552 (2012).
Lindorfer, M., Neugschwandtner, M., Weichselbaum, L., Fratantonio, Y., Veen, V. V. D. & Platzer, C. (2014) Andrubis–1,000,000 apps later: A view on current android malware behaviors. In 2014 Third International Workshop on Building Analysis Datasets and Gathering Experience Returns for Security (BADGERS) , 3–17 (IEEE).
Ikram, M., Beaume, P. & Kâafar, M. A. Dadidroid: An Obfuscation Resilient Tool for Detecting Android Malware via Weighted Directed Call Graph Modelling . arXiv:1905.09136 (2019).
Shen, F., Vecchio, J. D., Mohaisen, A., Ko, S. Y. & Ziarek, L. Android malware detection using complex-flows. IEEE Trans. Mob. Comput. 18 (6), 1231–1245 (2018).
Yang, W., Prasad, M. R. & Xie, T. Enmobile: Entity-based characterization and analysis of mobile malware. In Proceedings of the 40th International Conference on Software Engineering , 384–394 (2018).
Enck, W. et al. Taintdroid: an information-flow tracking system for realtime privacy monitoring on smartphones. ACM Trans. Comput. Syst. (TOCS) 32 (2), 1–29 (2014).
Portokalidis, G., Homburg, P., Anagnostakis, K. & Bos, H. (2010) Paranoid android: Versatile protection for smartphones. In Proceedings of the 26th Annual Computer Security Applications Conference , 347–356.
Bläsing, T., Batyuk, L., Schmidt, A. D., Camtepe, S. A. & Albayrak, S. An android application sandbox system for suspicious software detection. In 2010 5th International Conference on Malicious and Unwanted Software , 55–62 (IEEE, 2010).
Aubery-Derrick, S. Detection of Smart Phone Malware . Unpublished Ph.D. Thesis, 1–211 (Electronic and Information Technology University, Berlin, 2011).
Burguera, I., Zurutuza, U. & Nadjm-Tehrani, S. Crowdroid: Behavior-based malware detection system for android. In Proceedings of the 1st ACM Workshop on Security and Privacy in Smartphones and Mobile Devices , 15–26 (2011).
Grace, M. C., Zhou, Y., Wang, Z. & Jiang, X. Systematic detection of capability leaks in stock android smartphones. In NDSS , vol 14, 19 (2012).
Grace, M., Zhou, Y., Zhang, Q., Zou, S. & Jiang, X. Riskranker: Scalable and accurate zero-day android malware detection. In Proceedings of the 10th International Conference on Mobile Systems, Applications, and Services , 281–294 (2012).
Zheng, C., Zhu, S., Dai, S., Gu, G., Gong, X., Han, X. & Zou, W. Smartdroid: An automatic system for revealing UI-based trigger conditions in android applications. In Proceedings of the Second ACM Workshop on Security and Privacy in Smartphones and Mobile Devices , 93–104 (2012).
Dini, G., Martinelli, F., Saracino, A. & Sgandurra, D. Madam: A multi-level anomaly detector for android malware. In International Conference on Mathematical Methods, Models, and Architectures for Computer Network Security , 240–253 (Springer, 2012).
Yan, L. K. & Yin, H. Droidscope: Seamlessly reconstructing the \(\{\) OS \(\}\) and Dalvik semantic views for dynamic android malware analysis. In Presented as part of the 21st \(\{\) USENIX \(\}\) Security Symposium ( \(\{\) USENIX \(\}\) Security 12 ), 569–584 (2012).
Backes, M., Gerling, S., Hammer, C., Maffei, M. & von Styp-Rekowsky, P. Appguard–enforcing user requirements on android apps. In International Conference on TOOLS and Algorithms for the Construction and Analysis of Systems , 543–548 (Springer, 2013).
Shahzad, F., Akbar, M., Khan, S. & Farooq, M. Tstructdroid: Realtime malware detection using in-execution dynamic analysis of kernel process control blocks on android . Tech Rep (National University of Computer and Emerging Sciences, Islamabad, 2013).
Rastogi, V., Chen, Y. & Enck, W. Appsplayground: Automatic security analysis of smartphone applications. In Proceedings of the third ACM Conference on Data and Application Security and Privacy , 209–220 (2013).
Rosen, S., Qian, Z. & Mao, Z. M. Appprofiler: A flexible method of exposing privacy-related behavior in android applications to end users. In Proceedings of the Third ACM Conference on Data and Application Security and Privacy , 221–232 (2013).
Desnos, A. et al . Androguard-reverse engineering, malware and goodware analysis of android applications. URL code google com/p/androguard 153 (2013).
Tam, K., Khan, S. J., Fattori, A. & Cavallaro, L. Copperdroid: Automatic reconstruction of android malware behaviors. In Ndss (2015).
Suarez-Tangil, G., Dash, S. K., Ahmadi, M., Kinder, J., Giacinto, G. & Cavallaro, L. Droidsieve: Fast and accurate classification of obfuscated android malware. In Proceedings of the Seventh ACM on Conference on Data and Application Security and Privacy , 309–320 (2017).
Idrees, F., Rajarajan, M., Conti, M., Chen, T. M. & Rahulamathavan, Y. Pindroid: A novel android malware detection system using ensemble learning methods. Comput. Secur. 68 , 36–46 (2017).
Martín, A., Menéndez, H. D. & Camacho, D. Mocdroid: Multi-objective evolutionary classifier for android malware detection. Soft. Comput. 21 (24), 7405–7415 (2017).
Karbab, E. B., Debbabi, M., Derhab, A. & Mouheb, D. Maldozer: Automatic framework for android malware detection using deep learning. Digit. Investig. 24 , S48–S59 (2018).
Lee, W. Y., Saxe, J. & Harang, R. Seqdroid: Obfuscated android malware detection using stacked convolutional and recurrent neural networks. In Deep Learning Applications for Cyber Security , 197–210 (Springer, 2019).
Alzaylaee, M. K., Yerima, S. Y. & Sezer, S. DL-Droid: Deep learning based android malware detection using real devices. Comput. Secur. 89 , 101663 (2020).
Yuan, Z., Lu, Y., Wang, Z. & Xue, Y. Droid-sec: Deep learning in android malware detection. In Proceedings of the 2014 ACM Conference on SIGCOMM , 371–372 (2014).
Zhang, M., Duan, Y., Yin, H. & Zhao, Z. Semantics-aware android malware classification using weighted contextual API dependency graphs. In Proceedings of the 2014 ACM SIGSAC Conference on Computer and Communications Security , 1105–1116 (2014).
Shankar, V. G., Somani, G., Gaur, M. S., Laxmi, V. & Conti, M. Androtaint: An efficient android malware detection framework using dynamic taint analysis. In 2017 ISEA Asia Security and Privacy (ISEASP) , 1–13 (IEEE, 2017).
Mahindru, A. & Singh, P. Dynamic permissions based android malware detection using machine learning techniques. In Proceedings of the 10th Innovations in Software Engineering Conference , 202–210 (2017).
Shi, B. et al. Prediction of recurrent spontaneous abortion using evolutionary machine learning with joint self-adaptive sime mould algorithm. Comput. Biol. Med. 148 , 105885 (2022).
Article PubMed Google Scholar
Zhang, Q., Wang, D. & Wang, Y. Convergence of decomposition methods for support vector machines. Neurocomputing 317 , 179–187 (2018).
Hou, S., Saas, A., Chen, L. & Ye, Y. Deep4maldroid: A deep learning framework for android malware detection based on linux kernel system call graphs. In 2016 IEEE/WIC/ACM International Conference on Web Intelligence Workshops (WIW) , 104–111 (IEEE, 2016).
Nix, R. & Zhang, J. Classification of android apps and malware using deep neural networks. In 2017 International Joint Conference on Neural Networks (IJCNN) , 1871–1878 (IEEE, 2017).
Zhang, X. A deep learning based framework for detecting and visualizing online malicious advertisement. Ph.D. Thesis, University of New Brunswick (2018)
Nauman, M., Tanveer, T. A., Khan, S. & Syed, T. A. Deep neural architectures for large scale android malware analysis. Clust. Comput. 21 (1), 569–588 (2018).
Xiao, X., Wang, Z., Li, Q., Xia, S. & Jiang, Y. Back-propagation neural network on Markov chains from system call sequences: a new approach for detecting android malware with system call sequences. IET Inf. Secur. 11 (1), 8–15 (2016).
Martinelli, F., Marulli, F. & Mercaldo, F. Evaluating convolutional neural network for effective mobile malware detection. Procedia Comput. Sci. 112 , 2372–2381 (2017).
Xiao, X., Zhang, S., Mercaldo, F., Hu, G. & Sangaiah, A. K. Android malware detection based on system call sequences and LSTM. Multim. Tools Appl. 78 (4), 3979–3999 (2019).
Dimjašević, M., Atzeni, S., Ugrina, I. & Rakamaric, Z. Evaluation of android malware detection based on system calls. In Proceedings of the 2016 ACM on International Workshop on Security and Privacy Analytics , 1–8 (2016).
Mas’ud, M. Z., Sahib, S., Abdollah, M. F., Selamat, S. R. & Yusof, R. Analysis of features selection and machine learning classifier in android malware detection. In 2014 International Conference on Information Science and Applications (ICISA) , 1–5 (IEEE, 2014).
Yerima, S. Y., Sezer, S., McWilliams, G. & Muttik, I. A new android malware detection approach using Bayesian classification. In 2013 IEEE 27th International Conference on Advanced Information Networking and Applications (AINA) , 121–128 (IEEE, 2013).
Narudin, F. A., Feizollah, A., Anuar, N. B. & Gani, A. Evaluation of machine learning classifiers for mobile malware detection. Soft. Comput. 20 (1), 343–357 (2016).
Wang, W. et al. Exploring permission-induced risk in android applications for malicious application detection. IEEE Trans. Inf. Forensics Secur. 9 (11), 1869–1882 (2014).
Ayar, M., Isazadeh, A., Gharehchopogh, F. S. & Seyedi, M. NSICA: Multi-objective imperialist competitive algorithm for feature selection in arrhythmia diagnosis. Comput. Biol. Med. 161 , 107025 (2023).
Article CAS PubMed Google Scholar
Hu, H. et al. Dynamic individual selection and crossover boosted forensic-based investigation algorithm for global optimization and feature selection. J. Bionic Eng. 20 , 1–27 (2023).
Zhong, C., Li, G., Meng, Z., Li, H. & He, W. A self-adaptive quantum equilibrium optimizer with artificial bee colony for feature selection. Comput. Biol. Med. 153 , 106520 (2023).
Zhou, P. et al. Unsupervised feature selection for balanced clustering. Knowl.-Based Syst. 193 , 105417 (2020).
Allix, K. et al. Empirical assessment of machine learning-based malware detectors for android. Empir. Softw. Eng. 21 (1), 183–211 (2016).
Narayanan, A., Chandramohan, M., Chen, L. & Liu, Y. A multi-view context-aware approach to android malware detection and malicious code localization. Empir. Softw. Eng. 23 (3), 1222–1274 (2018).
Azmoodeh, A., Dehghantanha, A. & Choo, K. K. R. Robust malware detection for internet of (battlefield) things devices using deep eigenspace learning. IEEE Trans. Sustain. Comput. 4 (1), 88–95 (2018).
Chen, K. Z., Johnson, N. M., D’Silva, V., Dai, S., MacNamara, K., Magrino, T. R., Wu, E. X., Rinard, M. & Song, D. X. Contextual policy enforcement in android applications with permission event graphs. In: NDSS , 234 (2013).
Yerima, S. Y., Sezer, S. & McWilliams, G. Analysis of Bayesian classification-based approaches for android malware detection. IET Inf. Secur. 8 (1), 25–36 (2014).
Gonzalez, H., Stakhanova, N. & Ghorbani, A. A. Droidkin: Lightweight detection of android apps similarity. In International Conference on Security and Privacy in Communication Networks , 436–453 (Springer, 2014) .
Kadir, A. F. A., Stakhanova, N. & Ghorbani, A. A. Android botnets: What urls are telling us. In International Conference on Network and System Security , 78–91 (Springer, 2015).
Zhou, Y. & Jiang, X. Android malware genome project. Disponibile a http://www.malgenomeproject.org (2012).
Garcia, J., Hammad, M. & Malek, S. Lightweight, obfuscation-resilient detection and family identification of android malware. ACM Trans. Softw. Eng. Methodol. (TOSEM) 26 (3), 1–29 (2018).
Mahindru, A. & Sangal, A. Parudroid: Validation of android malware detection dataset. J. Cybersecur. Inform. Manag. 3 (2), 42–52 (2020).
McCulloch, W. S. & Pitts, W. A logical calculus of the ideas immanent in nervous activity. Bull. Math. Biophys. 5 (4), 115–133 (1943).
Article MathSciNet Google Scholar
Faruk, M. J. H., Shahriar, H., Valero, M., Barsha, F. L., Sobhan, S., Khan, M. A., Whitman, M., Cuzzocrea, A., Lo, D., Rahman, A., et al . Malware detection and prevention using artificial intelligence techniques. In 2021 IEEE International Conference on Big Data (Big Data) , 5369–5377 (IEEE, 2021).
Battiti, R. First-and second-order methods for learning: Between steepest descent and newton’s method. Neural Comput. 4 (2), 141–166 (1992).
Levenberg, K. A method for the solution of certain non-linear problems in least squares. Q. Appl. Math. 2 (2), 164–168 (1944).
Bengio, Y. Learning deep architectures for AI. Found. Trends ® Mach. Learn. 2 (1), 1–127 (2009).
Kaur, J., Singh, S., Kahlon, K. S. & Bassi, P. Neural network-a novel technique for software effort estimation. Int. J. Comput. Theory Eng. 2 (1), 17 (2010).
Doraisamy, S., Golzari, S., Mohd, N., Sulaiman, M. N. & Udzir, N. I. A study on feature selection and classification techniques for automatic genre classification of traditional Malay music. In ISMIR , 331–336 (2008).
Forman, G. An extensive empirical study of feature selection metrics for text classification. J. Mach. Learn. Res. 3 (Mar), 1289–1305 (2003).
Furlanello, C., Serafini, M., Merler, S. & Jurman, G. Entropy-based gene ranking without selection bias for the predictive classification of microarray data. BMC Bioinform. 4 (1), 54 (2003).
Coronado-De-Alba, L. D., Rodríguez-Mota, A. & Escamilla-Ambrosio, P. J. Feature selection and ensemble of classifiers for android malware detection. In 2016 8th IEEE Latin-American Conference on Communications (LATINCOM) , 1–6 (IEEE, 2016).
Deepa, K., Radhamani, G. & Vinod, P. Investigation of feature selection methods for android malware analysis. Procedia Comput. Sci. 46 , 841–848 (2015).
Kothari, C. R. Research methodology: Methods and techniques. New Age International (2004).
Chaikla, N. & Qi, Y. Genetic algorithms in feature selection. In IEEE SMC’99 Conference Proceedings. 1999 IEEE International Conference on Systems, Man, and Cybernetics (Cat. No. 99CH37028) , vol 5, 538–540 (IEEE, 1999).
Onwuzurike, L. et al. Mamadroid: Detecting android malware by building Markov chains of behavioral models (extended version). ACM Trans. Privacy Secur. (TOPS) 22 (2), 1–34 (2019).
Hou, S., Ye, Y., Song, Y. & Abdulhayoglu, M. Hindroid: An intelligent android malware detection system based on structured heterogeneous information network. In Proceedings of the 23rd ACM SIGKDD International Conference on Knowledge Discovery and Data Mining , 1507–1515 (2017) .
Zhu, H. J. et al. HEMD: A highly efficient random forest-based malware detection framework for android. Neural Comput. Appl. 30 (11), 3353–3361 (2018).
Wang, W., Zhao, M. & Wang, J. Effective android malware detection with a hybrid model based on deep autoencoder and convolutional neural network. J. Ambient. Intell. Humaniz. Comput. 10 (8), 3035–3043 (2019).
Han, W., Xue, J., Wang, Y., Liu, Z. & Kong, Z. Malinsight: A systematic profiling based malware detection framework. J. Netw. Comput. Appl. 125 , 236–250 (2019).
Zou, D. et al. Intdroid: Android malware detection based on API intimacy analysis. ACM Trans. Softw. Eng. Methodol. (TOSEM) 30 (3), 1–32 (2021).
Mahindru, A. & Arora, H. Dnndroid: Android malware detection framework based on federated learning and edge computing. In International Conference on Advancements in Smart Computing and Information Security , 96–107 (Springer, 2022).
Mahindru, A. & Arora, H. Parudroid: Framework that enhances smartphone security using an ensemble learning approach. SN Comput. Sci. 4 (5), 630 (2023).
Mahindru, A., Sharma, S. K. & Mittal, M. Yarowskydroid: Semi-supervised based android malware detection using federation learning. In 2023 International Conference on Advancement in Computation & Computer Technologies (InCACCT) , 380–385 (IEEE, 2023).
Download references
Acknowlegment
This work was partly supported by the Technology Innovation Program funded by the Ministry of Trade, Industry & Energy (MOTIE) (No.20022899) and by the Technology Development Program of MSS (No.S3033853).
Author information
Authors and affiliations.
Department of Computer Science and applications, D.A.V. University, Sarmastpur, Jalandhar, 144012, India
Arvind Mahindru
Department of Mathematics, Guru Nanak Dev University, Amritsar, India
Himani Arora
Department of Nuclear and Renewable Energy, Ural Federal University Named after the First President of Russia Boris Yeltsin, Ekaterinburg, Russia, 620002
Abhinav Kumar
Department of Electronics and Communication Engineering, Central University of Jammu, Jammu, 181143, UT of J&K, India
Sachin Kumar Gupta
School of Electronics and Communication Engineering, Shri Mata Vaishno Devi University, Katra, 182320, UT of J&K, India
Department of Applied Data Science, Noroff University College, Kristiansand, Norway
Shubham Mahajan & Seifedine Kadry
Artificial Intelligence Research Center (AIRC), Ajman University, Ajman, 346, United Arab Emirates
Seifedine Kadry
MEU Research Unit, Middle East University, Amman 11831, Jordan
Applied Science Research Center, Applied Science Private University, Amman, Jordan
Department of Software, Department of Computer Science and Engineering, Kongju National University, Cheonan, 31080, Korea
Jungeun Kim
You can also search for this author in PubMed Google Scholar
Contributions
All the authors have contributed equally.
Corresponding authors
Correspondence to Arvind Mahindru , Sachin Kumar Gupta , Shubham Mahajan or Jungeun Kim .
Ethics declarations
Competing interests.
The authors declare no competing interests.
Additional information
Publisher's note.
Springer Nature remains neutral with regard to jurisdictional claims in published maps and institutional affiliations.
Supplementary Information
Supplementary information., rights and permissions.
Open Access This article is licensed under a Creative Commons Attribution 4.0 International License, which permits use, sharing, adaptation, distribution and reproduction in any medium or format, as long as you give appropriate credit to the original author(s) and the source, provide a link to the Creative Commons licence, and indicate if changes were made. The images or other third party material in this article are included in the article’s Creative Commons licence, unless indicated otherwise in a credit line to the material. If material is not included in the article’s Creative Commons licence and your intended use is not permitted by statutory regulation or exceeds the permitted use, you will need to obtain permission directly from the copyright holder. To view a copy of this licence, visit http://creativecommons.org/licenses/by/4.0/ .
Reprints and permissions
About this article
Cite this article.
Mahindru, A., Arora, H., Kumar, A. et al. PermDroid a framework developed using proposed feature selection approach and machine learning techniques for Android malware detection. Sci Rep 14 , 10724 (2024). https://doi.org/10.1038/s41598-024-60982-y
Download citation
Received : 14 October 2023
Accepted : 29 April 2024
Published : 10 May 2024
DOI : https://doi.org/10.1038/s41598-024-60982-y
Share this article
Anyone you share the following link with will be able to read this content:
Sorry, a shareable link is not currently available for this article.
Provided by the Springer Nature SharedIt content-sharing initiative
- Android apps
- Neural network
- Deep learning
- Feature selection
- Intrusion detection
- Permissions model
By submitting a comment you agree to abide by our Terms and Community Guidelines . If you find something abusive or that does not comply with our terms or guidelines please flag it as inappropriate.
Quick links
- Explore articles by subject
- Guide to authors
- Editorial policies
Sign up for the Nature Briefing newsletter — what matters in science, free to your inbox daily.

Help | Advanced Search
Computer Science > Cryptography and Security
Title: synthetic datasets for program similarity research.
Abstract: Program similarity has become an increasingly popular area of research with various security applications such as plagiarism detection, author identification, and malware analysis. However, program similarity research faces a few unique dataset quality problems in evaluating the effectiveness of novel approaches. First, few high-quality datasets for binary program similarity exist and are widely used in this domain. Second, there are potentially many different, disparate definitions of what makes one program similar to another and in many cases there is often a large semantic gap between the labels provided by a dataset and any useful notion of behavioral or semantic similarity. In this paper, we present HELIX - a framework for generating large, synthetic program similarity datasets. We also introduce Blind HELIX, a tool built on top of HELIX for extracting HELIX components from library code automatically using program slicing. We evaluate HELIX and Blind HELIX by comparing the performance of program similarity tools on a HELIX dataset to a hand-crafted dataset built from multiple, disparate notions of program similarity. Using Blind HELIX, we show that HELIX can generate realistic and useful datasets of virtually infinite size for program similarity research with ground truth labels that embody practical notions of program similarity. Finally, we discuss the results and reason about relative tool ranking.
Submission history
Access paper:.
- Other Formats

References & Citations
- Google Scholar
- Semantic Scholar
BibTeX formatted citation

Bibliographic and Citation Tools
Code, data and media associated with this article, recommenders and search tools.
- Institution
arXivLabs: experimental projects with community collaborators
arXivLabs is a framework that allows collaborators to develop and share new arXiv features directly on our website.
Both individuals and organizations that work with arXivLabs have embraced and accepted our values of openness, community, excellence, and user data privacy. arXiv is committed to these values and only works with partners that adhere to them.
Have an idea for a project that will add value for arXiv's community? Learn more about arXivLabs .
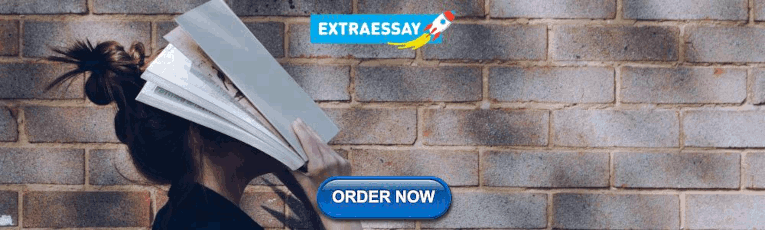
COMMENTS
This paper includes all the stuff Limitations of Static Malware Analysis and tools of Dynamic Malware Analysis and Deobfuscating malware. Discover the world's research 25+ million members
In this research paper, we present a protective mechanism that evaluates three ML algorithm approaches to malware detection and chooses the most appropriate one. According to statistics, the decision tree approach has the maximum detection accuracy (99.01%) and the lowest false positive rate (FPR; 0.021%) on a small dataset.
However, several pressing issues (e.g., unknown malware samples detection) still need to be addressed adequately. This article first presents a concise overview of malware along with anti-malware and then summarizes various research challenges. This is a theoretical and perspective article that is hoped to complement earlier articles and works.
In addition, Barriga and Yoo [38] survey the literature on malware evasion techniques and their impact on malware analysis techniques. This paper extends beyond that and includes recent AI-driven works used to overcome malware evasion techniques. 3. Taxonomy of malware classification. We present in this section the taxonomy of malware ...
In this survey, we review the key developments in the field of malware detection using AI and analyze core challenges. We systematically survey state-of-the-art methods across five critical aspects of building an accurate and robust AI-powered malware-detection model: malware sophistication, analysis techniques, malware repositories, feature selection, and machine learning vs. deep learning.
A Survey On Automated Dynamic Malware Analysis Evasion and Counter-Evasion: PC, Mobile, and Web. ROOTS: Proceedings of the 1st Reversing and Offensive-oriented Trends Symposium. Automated dynamic malware analysis systems are important in combating the proliferation of modern malware.
Table 2 summarizes notable papers that implemented machine learning to enhance malware analysis. For each paper, the lead author, year, and task given to the algorithm are listed. ... Y. Elovici, and L. Rokach, Malware Lab, Cyber Security Research Center, Ben-Gurion University of the Negev, Beer-Sheva, Israel; Department of Software and ...
Advanced malware poses a growing threat to the security of digital systems. This paper investigates the evolution of advanced malware, its stealthy characteristics, and the challenges it presents in contemporary cybersecurity. We analyse existing prevention strategies and propose an idea that leverages Python-based sandboxing, creating a virtual environment for malware analysis. This ...
daily 360,000 novel malware samples hit the scene [4]. As anti-malware becomes more avant-garde so as malwares in the wild, thereby escalating the arms race between malware guardians and writers. The quests for scalable and robust automated malware detection frameworks still have to go a long way. This article presents an overview of malwares
The paper is organized as follows: Section 2 describes the importance of moving from classical malware detection and analysis to smart and autonomous detection/analysis through the incorporation of advanced AI techniques. Moreover, we provide a classification of modern malware based on famous samples and high-profile cases.
Malware is a significant security danger on the Internet nowadays. Hostile to Virus organizations get a huge number of malwares tests each day. It is intended to harm PC frameworks without the information on the proprietor utilizing the framework and method headways are presenting enormous difficulties for scientists in both the scholarly world and the business. Malware tests are arranged and ...
2019. 2.1. Malware analysis. Malware analysis is a standard approach to understand the components and behaviour of malware, ransomware included. This analysis is useful to detect malware attacks and prevent similar attacks in the future. Malware analysis is broadly categorized into static and dynamic analysis.
This paper offers a comprehensive analysis model for android malware. The model presents the essential factors affecting the analysis results of android malware that are vision-based. Current android malware analysis and solutions might consider one or some of these factors while building their malware predictive systems. However, this paper comprehensively highlights these factors and their ...
Abstract. Malware analysis forms a critical component of cyber defense mechanism. In the last decade, lot of research has been done, using machine learning methods on both static as well as dynamic analysis. Since the aim and objective of malware developers have changed from just for fame to political espionage or financial gain, the malware is ...
Download a PDF of the paper titled Malware Analysis with Artificial Intelligence and a Particular Attention on Results Interpretability, by Benjamin Marais and 2 other authors. Download PDF Abstract: Malware detection and analysis are active research subjects in cybersecurity over the last years. Indeed, the development of obfuscation ...
This paper presents a systematic review of malware detection using Deep Learning techniques. On the basis of the evolution towards Deep Learning-based techniques, research taxonomy is proposed. Recent techniques for detecting malware on Android, iOS, IoT, Windows, APTs, and Ransomware are also explored and compared.
Enhancing Machine Learning Based Malware Detection Model by Reinforcement Learning. Malware detection is getting more and more attention due to the rapid growth of new malware. As a result, machine learning (ML) has become a popular way to detect malware variants. However, machine learning models can also be cheated.
Finally, Section 8 makes a conclusion of this paper. The following research questions have been brought out to help follow the process of systematic review conduction: ... For example, since malware analysis can be categorized into static/dynamic analysis according to the type of extracted features, "static/dynamic analysis" + "Android ...
This survey includes infection mechanisms, legitimate system tools used in the process, analysis of major fileless malware, research challenges while handling such incidents in the process of incident and response. This type of study was not done in the literature, which includes the different perspectives of fileless malware.
Extracting and synthesizing relevant information from selected high-quality research papers that were focused on malware detection in Windows desktop devices. ... Malware analysis plays a significant role in discovering patterns that can be used to detect and prevent future threats. Important information about the program or file is extracted ...
Further, the trained model is implemented the results on the corresponding testing data set to make the model for the final detection of malware apps. In this research paper, Decision tree forest ...
Our paper explores such adversarial vulnerabilities of neural network based malware classification system in the dynamic and online analysis environments. ... We offer recommendations for a balanced approach and a benchmark for much-needed future research into evasion attacks against malware classifiers, and develop more robust and trustworthy ...
Program similarity has become an increasingly popular area of research with various security applications such as plagiarism detection, author identification, and malware analysis. However, program similarity research faces a few unique dataset quality problems in evaluating the effectiveness of novel approaches. First, few high-quality datasets for binary program similarity exist and are ...