
An official website of the United States government
The .gov means it’s official. Federal government websites often end in .gov or .mil. Before sharing sensitive information, make sure you’re on a federal government site.
The site is secure. The https:// ensures that you are connecting to the official website and that any information you provide is encrypted and transmitted securely.
- Publications
- Account settings
Preview improvements coming to the PMC website in October 2024. Learn More or Try it out now .
- Advanced Search
- Journal List
- Malays J Med Sci
- v.28(2); 2021 Apr
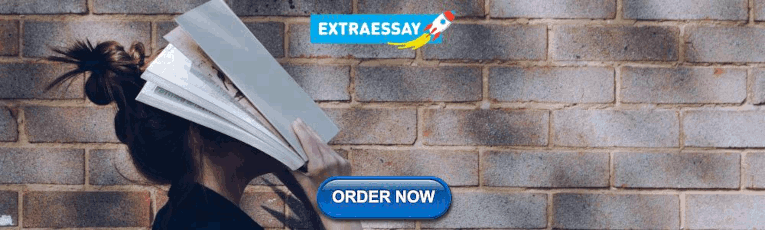
A Step-by-Step Process on Sample Size Determination for Medical Research
Determination of a minimum sample size required for a study is a major consideration which all researchers are confronted with at the early stage of developing a research protocol. This is because the researcher will need to have a sound prerequisite knowledge of inferential statistics in order to enable him/her to acquire a thorough understanding of the overall concept of a minimum sample size requirement and its estimation. Besides type I error and power of the study, some estimates for effect sizes will also need to be determined in the process to calculate or estimate the sample size. The appropriateness in calculating or estimating the sample size will enable the researchers to better plan their study especially pertaining to recruitment of subjects. To facilitate a researcher in estimating the appropriate sample size for their study, this article provides some recommendations for researchers on how to determine the appropriate sample size for their studies. In addition, several issues related to sample size determination were also discussed.
Introduction
Sample size calculation or estimation is an important consideration which necessitate all researchers to pay close attention to when planning a study, which has also become a compulsory consideration for all experimental studies ( 1 ). Moreover, nowadays, the selection of an appropriate sample size is also drawing much attention from researchers who are involved in observational studies when they are developing research proposals as this is now one of the factors that provides a valid justification for the application of a research grant ( 2 ). Sample size must be estimated before a study is conducted because the number of subjects to be recruited for a study will definitely have a bearing on the availability of vital resources such as manpower, time and financial allocation for the study. Nevertheless, a thorough understanding of the need to estimate or calculate an appropriate sample size for a study is crucial for a researcher to appreciate the effort expended in it.
Ideally, one can determine the parameter of a variable from a population through a census study. A census study recruits each and every subject in a population and an analysis is conducted to determine the parameter or in other words, the true value of a specific variable will be calculated in a targeted population. This approach of analysis is known as descriptive analysis. On the other hand, the estimate that is derived from a sample study is termed as a ‘statistic’ because it analyses sample data and subsequently makes inferences and conclusions from the results. This approach of analysis is known as inferential analysis, which is also the most preferred approach in research because drawing a conclusion from the sample data is much easier than performing a census study, due to various constraints especially in terms of cost, time and manpower.
In a census study, the accuracy of the parameters cannot be disputed because the parameters are derived from all subjects in the population. However, when statistics are derived from a sample, it is possible for readers to query to what extent these statistics are representative of the true values in the population. Thus, researchers will need to provide an additional piece of evidence besides the statistics, which is the P -value. The statistical significance or usually termed as ‘ P -value less than 0.05’, and it shall stand as an evidence or justification that the statistics derived from the sample can be inferred to the larger population. Some scholars may argue over the utility and versatility of P -value but it is nevertheless still applicable and acceptable until now ( 3 – 5 ).
Why It is Necessary to Perform a Sample Size Calculation or Estimation?
In order for the analysis to be conducted for addressing a specific objective of a study to be able to generate a statistically-significant result, a particular study must be conducted using a sufficiently large sample size that can detect the target effect sizes with an acceptable margin of error. In brief, a sample size is determined by three elements: i) type I error (alpha); ii) power of the study (1-type II error) and iii) effect size. A proper understanding of the concept of type I error and type II error will require a lengthy discussion. The prerequisite knowledge of statistical inference, probability and distribution function is also required to understand the overall concept ( 6 – 7 ). However, in sample size calculation, the values of both type I and type II errors are usually fixed. Type I error is usually fixed at 0.05 and sometimes 0.01 or 0.10, depending on the researcher. Meanwhile, power is usually set at 80% or 90% indicating 20% or 10% type II error, respectively. Hence, the only one factor that remains unspecified in the calculation of a sample size is the effect size of a study.
Effect size measures the ‘magnitude of effect’ of a test and it is independent of influences by the sample size ( 8 ). In other words, effect size measures the real effect of a test irrespective of its sample size. With reference to statistical tests, it is an expected parameter of a particular association (or correlation or relationship) with other tests in a targeted population. In a real setting, the parameter of a variable in a targeted population is usually unknown and therefore a study will be conducted to test and confirm these effect sizes. However, for the purpose of sample size calculation, it is still necessary to estimate the target effect sizes. By the same token, Cohen ( 9 ) presented in his article that a larger sample size is necessary to estimate small effect sizes and vice versa.
The main advantage of estimating the minimum sample size required is for planning purposes. For example, if the minimum sample size required for a particular study is estimated to be 300 subjects and a researcher already knows that he/she can only recruit 15 subjects in a month from a single centre. Thus, the researchers will need at least 20 months for data collection if there is only one study site. If the plan for data collection period is shorter than 20 months, then the researchers may consider to recruit subjects in more than one centre. In case where the researchers will not be able to recruit 300 subjects within the planned data collection period, the researchers may need to revisit the study objective or plan for a totally different study instead. If the researcher still wishes to pursue the study but is unable to meet the minimum required sample size; then it is likely that the study may not be able to reach a valid conclusion at the end, which will result in a waste of resources because it does not add any scientific contributions.
How to Calculate or Estimate Sample Size?
Sample size calculation serves two important functions. First, it aims to estimate a minimum sample size that can be sufficient for achieving a target level of accuracy in an estimate for a specific population parameter. In this instance, the researcher aims to produce an estimate that is expected to be equally accurate as an actual parameter in the target population. Second, it also aims to determine the level of statistical significance (i.e. P -value < 0.05) attained by these desired effect sizes. In other words, a researcher aims to infer the statistics derived from the sample to that of the larger population. In this case, a specific statistical test will be applied and the P -value will be calculated by using the statistical test (which will determine the level of statistical significance).
For univariate statistical test such as independent sample t -test or Pearson’s chi-square test, these sample size calculations can be done manually using a rather simple formula. However, the manual calculation can still be difficult for researchers who are non-statisticians. Various sample size software have now been introduced which make these sample size calculation easier. Nevertheless, a researcher may still experience some difficulty in using the software if he/she is not familiar with the concept of sample size calculation and the statistical tests. Therefore, various scholars have expended some effort to assist the researchers in the determination of sample sizes for various purposes by publishing sample size tables for various statistical tests ( 10 – 12 ). These sample sizes tables can be used to estimate the minimum sample size that is required for a study. Although such tables may have only a limited capacity for the selection of various effect sizes, and their corresponding sample size requirements; it is nonetheless much more practical and easier to use.
For some study objectives, it is often much easier to estimate the sample size based on a rule-of-thumb instead of manual calculation or sample size software. Taking an example of an objective of a study that needs to be answered using multivariate analysis, the estimation of an association between a set of predictors and an outcome can be very complicated if it involves many independent variables. In addition, the actual ‘effect size’ can range from low to high, which renders it even more difficult to be estimated. Therefore, it is recommended to adopt the conventional rule-of-thumb for estimating these sample sizes in these circumstances. Although some scholars have initially thought that the concept of rule-of-thumb may not be as scientifically robust when compared to actual calculations, it is still considered to be an acceptable approach ( 13 – 15 ). Table 1 illustrates some published articles for various sample size determinations for descriptive studies and statistical tests.
Summary of published articles related to sample size determination for various statistical tests
In brief, the present paper will be proposing five main steps for sample size determination as shown in Figure 1 . The following provides an initial description and then a discussion of each of these five steps:

Recommended steps in sample size determination
Step 1: To Understand the Objective of the Study
The objective of a study has to be measurable or in other words, can be determined by using statistical analysis. Sometimes, a single study may have several objectives. One of the common approaches to achieve this is to estimate the sample size required for every single objective and then the minimum required sample size for the study will be selected to be the highest number of all sample sizes calculated. However, this paper recommends that the minimum sample size be calculated only for the primary objective, which will remain valid as long as the primary objective is more important than all the other objectives. This also means that the calculation of minimum sample size for any other objectives (apart from the primary objective) will only be considered unless they are considered to be equally important as the primary objective. For the development of a research proposal, different institutions may apply different approaches for sample size determinations and hence, it is mandatory to adhere to their specific requirements for sample size determinations.
However, the estimation or calculation of sample size for every study objective can be further complicated by the fact that some of the secondary objectives may require a larger sample size than the primary objective. If the recruitment of a larger number of subjects is not an issue, then it will always be viable to obtain a larger sample size in order to accommodate the sample size requirements for each and every objective of the study. Otherwise, it may be advisable for a researcher to forgo some of the secondary objectives so that they will not be too burdensome for the him/her.
Step 2: To Select the Appropriate Statistical Analysis
Researchers have to decide the appropriate analysis or statistical test to be used to answer the study objective; regardless of whether the aim is to determine a single mean, or a prevalence, or correlation, or association, just to name a few. The formula that will be used to estimate or calculate the sample size will be the same as the formula for performing the statistical test that will be used to answer the objective of study. For example, if an independent sample t -test has to be used for analysis, then its sample size formula should be based on an independent sample t -test. Hence, there is no a single formula for sample size calculation or estimation which can apply universally to all situations and circumstances.
Step 3: To Calculate or Estimate the Sample Size
Estimating or calculating the sample size can be done either by using manual calculation, sample size software, sample size tables from scientific published articles, or by adopting various acceptable rule-of-thumbs. Since both the type I and type II errors are already pre-specified and fixed, hence only the effect size remains to be specified in order for the determination of an appropriate sample size. To illustrate this point, it will be easier to demonstrate by using a case scenario as an example. Say a researcher would like to study an effectiveness of a new diet programme to reduce weight. The researcher believes the new diet programme is better than the conventional diet programme. It was found that the conventional diet programme can reduce on average 1 kg in 1 month. How many subjects are required to prove that the new diet programme is better than the conventional diet programme?
Based on Step 1 and Step 2, a researcher has decided to apply the independent sample t -test to answer the objective of study. Next, the researcher will need to specify the effect size after having both type I error and power set at 0.05% and 80%, respectively (type II error = 20%). What margin of effect size will be appropriate? This shall depend on the condition itself or the underlying research rationale which can then be further classified into two categories. In the first category, the research rationale is to prove that the new diet programme (for reducing weight) is superior to the conventional diet programme. In this case, the researcher should aim for sizeably large effect size. In other words, the difference between means of the weight reduction (which constitutes part of the effect size for independent sample t -test) should be sufficiently large to demonstrate the superiority of the new diet programme over the conventional diet programme.
In the second category, the research rationale is to measure accurately the effectiveness of the new diet programme to reduce weight in comparison with conventional diet programme, irrespective of whether the difference between both programmes is large or small. In this situation, the difference does not matter since the researcher aims to measure an exact difference between them, which means that it can only tolerate a very low margin of difference. In this circumstance, the researcher will therefore only be able to accept the smaller effect sizes. The estimate of effect sizes in this instance can be reviewed either from literatures, pilot study, historical data and rarely by using an educated guess.
The acceptable or desirable effect size that can be found from the literature can vary over a wide range. Thus, one of the better options is to seek for the relevant information from published articles of recent studies (within 5 years) that applied almost similar research design such as used the same treatments and had reported about similar patient characteristics. If none of these published articles can provide a rough estimate of the desired effect size, then the researcher may have to consider conducting a pilot study to obtain a rough estimate of the closest approximation to the actual desired effect size. Besides, historical data or secondary data can also be used to estimate the desired effect size, provided that the researcher has access to the secondary data of the two diet programmes. However, it must be emphasised that deriving the effect size from secondary data may not always be feasible since the performance of the new intervention may still not yet have been assessed.
The last option is to estimate the desired effect size based on a scientifically or a clinically meaningful effect. This means the researcher, through his or her own knowledge and experience, is able to determine an expectation of the difference in effect, and then to set a target difference (namely, effect size) to be achieved. For example, a researcher makes an educated guess about the new diet programme, and requires it to achieve a minimum difference of 3 kg in weight reduction per month in order for it to demonstrate superiority over the conventional diet programme. Although it is always feasible to set a large effect size especially if the new diet programme has proven to be a more rigorous intervention and probably also costlier; however, there is also a risk for the study to might have possibly failed to report a statistically significant result if it has subsequently been found that the actual effect size is much smaller than that adopted by the study, after the analysis has been completed. Therefore, it is usually quite a challenging task to estimate an accurate effect size since the exact value of the effect size is not known until the study is completed. However, the researcher will still have to set the value of effect size for the purpose of sample size calculation or estimation.
Next is to calculate or estimate sample size either based on manual calculation, sample size software, sample size tables or by adopting a conventional rule of thumb. Referring to the example for illustration purposes, the sample size calculation was calculated by using the sample size software as follows; with a study setting of equal sample size for both groups, the mean reduction is set at only 1 kg with within group standard deviation estimated at 0.8 (derived from literature, pilot study or based on a reliable source), type I error at 0.05 and 80% power, a minimum sample size of 11 subjects are required for each group (both for new diet programme and conventional diet programme). The sample size was calculated using Power and Sample Size (PS) software (by William D Dupont and W Dale Plummer, Jr. is licensed under a Creative Commons Attribution-NonCommercial- NoDerivs 3.0 United States License).
Step 4: To Provide an Additional Allowance During Subject Recruitment to Cater for a Certain Proportion of Non-Response
After the minimum required sample size has been identified, it is necessary to provide additional allowances to cater for potential non-response subjects. A minimum required sample size simply means the minimum number of subjects a study must have after recruitment is completed. Thus, researchers must ideally be able to recruit subjects at least beyond the minimum required sample size. To avoid underestimation of sample size, researchers will need to anticipate the problem of non-response and then to make up for it by recruiting more subjects on top of the minimum sample size, usually by 20% to 30%. If, for example, the researcher is expecting a high non-response rate in a self-administered survey, then he/she should provide an allowance for it by adding more than 30% such as 40% to 50%. The occurrence of non-response could also happen in various other scenarios such as dropping out or loss to follow-up in a cohort study and experimental studies. Besides that, missing data or loss of records are also potential problems that can result in attrition in observational studies.
Referring to previous example as an illustration, by adding 20% of non-response rate in each group, 14 subjects are required in each group. The calculation should be done as follow:
Likewise, for a 30% non-response rate, the sample size required in each group will then be increased to 16 subjects (11/0.7 = 15.7 ≈ 16).
Step 5: To Write a Sample Size Statement
The sample size statement is important and it is usually included in the protocol or manuscript. In the existing research literatures, the sample size statement is written in various styles. This paper recommends for the sample size statement to start by reminding the readers or reviewers about the main objective of study. Hence, this paper recommends all the elements from Step 1 until Step 4 (study objective, appropriate statistical analysis, sample size estimation/calculation and non-response rate) should be fully stated in the sample size statement. Therefore, a proposed outline of this sample size statement of the previous example for two weight-losing diet programmes is as follows:
“This study hypothesised that the new diet programme is better than conventional diet programme in terms of weight reduction at a 1-month follow-up. Therefore, the sample size formula is derived from the independent sample t -test. Based on the results of a previous study (cite the appropriate reference), all the response within each subject group are assumed to be normally distributed with a within-group standard deviation (SD) of 0.80 kg. If the true mean difference of the new diet programme versus the conventional diet programme is estimated at 1.0 kg, the study will need to recruit 11 subjects in each group to be able to reject the null hypothesis that the population means of the new diet programme and conventional diet programme are found to be equal with a type I error of 0.05 and with at least 80% power of this study. By providing an additional allowance of 20% in sample recruitment due to possible non-response rate, the required sample size has been increased to 14 subjects in each group. The formula of sample size calculation is based on a study reported by Dupont and Plummer ( 31 ).”
Discussion on Effect Size Planning
Sample size is just an estimate indicating a minimum required number of sample data that is necessary to be collected to derive an accurate estimate for the target population or to obtain statistically significant results based on the desired effect sizes. In order to calculate or estimate sample size, researchers will need to provide some initial estimates for effect sizes. It is usually quite challenging to provide a reasonably accurate value of the effect size because the exact values of these effect sizes are usually not known and can only be derived from the study after the analysis is completed. Hence, the discrepancies of the effect sizes are commonly expected where the researchers will usually either overestimate or underestimate them.
A major problem often arises when the researchers overestimate the effect sizes during sample size estimation, which can lead to a failure of a study to detect a statistically significant result. To avoid such a problem, the researchers are encouraged to recruit more subjects than the minimum required sample size of the study. By referring to the same example previously (new diet programme versus conventional diet programme), if the required sample size is 11 subjects in each group, then researchers may consider recruiting more than 11 subjects such as 18 to 20 subjects in each group. This is possible if the researchers have the capability in terms of manpower and research grant to recruit more subjects and also if there are adequate number of subjects available to be recruited.
After the study is completed, if the difference in mean reduction was found not at least 1 kg after 1 month, then the result might not be statistically significant (depending on the actual value of the within-group SD) for a sample size of 11 subjects in each group. However, if the researchers had recruited 18 subjects in each group, the study will still obtain significant results even though the difference of mean reduction was 0.8 kg (if the within-group SD is estimated to be 0.8, and an equal sample size is planned for both groups, with type I error set at 0.05 and power of at least 80%). In this situation, researcher would still be able to draw a conclusion that the difference in mean reduction after one month was 0.8 kg, and this result was statistically significant. Such a conclusion is perhaps more meaningful than stating a non-significant result ( P > 0.05) for another study with only 11 subjects in each group.
However, it is necessary to always bear in mind that obtaining a larger sample size merely to show that P -value is less than 0.05 is not the right thing to do and it can also result in a waste of resources. Hence, the purpose of increasing the size of the sample from 11 to 18 per group is not merely for obtaining a P -value of less than 0.05; but more importantly, it is now able to draw a valid and clinically-significant conclusion from the smallest acceptable value of the effect size. In other words, the researcher is now able to tolerate a smaller effect size by stating that the difference in mean reduction of 0.8 kg is also considered to be a sizeable effect size because it is clinically significant. However, if the researcher insists that the difference in mean reduction should be at least 1.0 kg, then it will be necessary to maintain a minimum sample of only 11 subjects per group. It is now clear that such a subjective variation in the overall consideration of the magnitude of this effect size sometimes depends on the effectiveness and the cost of the new diet programme and hence, this will always require some degree of clinical judgement.
The concept of setting a desired value of the effect size is almost identical for all types of statistical test. The above example is only describing an analysis using the independent sample t -test. Since each statistical test may require a different effect size in its calculation or estimation of the sample size; thus, it is necessary for the researchers to be familiarised with each of these statistical tests in order to be able to set the desired values of the effect sizes for the study. In addition, further assistance may be sought from statisticians or biostatisticians for the determination of an adequate minimum sample size required for these studies.
Another Example of Sample Size Estimation Using General Rule of Thumb
Say a study aims to determine the association of factors with optimal HbA1c level as determined by its cut-off point of < 6.5% among patients with type 2 diabetes mellitus (T2DM). Previous study had already estimated that several significant factors were identified, and then included as three to four variables in the final model consisting of parameters that were selected from demographic profile of patients and clinical parameters (cite the appropriate reference). Now, the question is: How many T2DM patients should the study recruit in order to answer the study objective?
Step 1: To Understand the Objective of Study
The study aims to determine a set of independent variables that show a significant association with optimal HbA1c level (as determined by its cut-off point of < 6.5%) among T2DM patients.
Step 2: To Decide the Appropriate Statistical Analysis
In this example, the outcome variable is in the categorical and binary form, such as HbA1c level of < 6.5% versus ≥ 6.5%. On the other hand, there are about 3 to 4 independent variables, which can be expressed in both the categorical and numerical form. Therefore, an appropriate statistical analysis shall be logistic regression.
Step 3: To Estimate or Calculate the Sample Size Required
Since this study will require a multivariate regression analysis, thus it is recommended to estimate sample size based on the general rule of thumb. This is because the calculation of sample size for a multivariate regression analysis can be very complicated as the analysis will involve many variables and effect sizes. There are several general rules of thumb available for estimating the sample size for multivariate logistic regression. One of the latest rule of thumb is proposed by Bujang et al. ( 44 ). Two approaches are introduced here, namely: i) sample size estimation based on concept of event per variable (EPV) and ii) sample size estimation based on a simple formula.
- i) Sample size estimation based on a concept EPV 50
For EPV 50, the researcher will need to know the prevalence of the ‘good’ outcome category and the number of subjects in the ‘good’ outcome category to fit the rule of EPV 50 ( 14 , 44 ). Say, the prevalence of ‘good’ outcome category is reported at 70% (cite the appropriate reference). Then, with a total of four independent variables, the minimum sample size required in the ‘poor’ outcome category will be at least 200 subjects in order to fulfil the condition for EPV 50 (i.e. 200/4 = 50). On the other hand, by estimating the prevalence of ‘good’ outcome at 70.0%, this study will therefore need to recruit at least 290 subjects in order to ensure that a minimum 200 subjects will be obtained in the ‘poor’ outcome category (70/100 x 290 = 203, and 203 > 200).
- ii) Sample size estimation based on a formula of n = 100 + 50i (where i represents number of independent variable in the final model)
When using this formula, the researcher will first need to set the total number of independent variables in the final model ( 44 ). As stated in the example, the total number of independent variables were estimated to be about three to four (cite the appropriate reference). Then, with a total of four independent variables, the minimum required sample size will be 300 patients [(i.e. 100 + 50 ( 4 ) = 300].
Step 4: To Provide Additional Allowance for a Certain Proportion of Non-Response Rate
In order to make up for a rough estimate of 20.0% of non-response rate, the minimum sample size requirement is calculated to be 254 patients (i.e. 203/0.8) by estimating the sample size based on the EPV 50, and is calculated to be 375 patients (i.e. 300/0.8) by estimating the sample size based on the formula n = 100 + 50 i.
There were previously two approaches that were introduced to estimate sample size for logistic regression. Say, if the researcher chooses to apply the formula n = 100 + 50 i. Therefore, the sample size statement will be written as follows:
“The main objective of this study is to determine the association of factors with optimal HbA1c level as determined by its cut-off point of < 6.5% among patients with type 2 diabetes mellitus (T2DM). The sample size estimation is derived from the general rule of thumb for logistic regression proposed by Bujang et al. ( 44 ), which had established a simple guideline of sample size determination for logistic regression. In this study, Bujang et al. ( 44 ) suggested to calculate the sample size by basing on a formula n = 100 + 50 i. The estimated total number of independent variables was about three to four (cite the appropriate reference). Thus, with a total of four independent variables, the minimum required sample size will be 300 patients (i.e. 100 + 50 ( 4 ) = 300). By providing an additional allowance to cater for a possible dropout rate of 20%, this study will therefore need at least a sample size of 300/0.8 = 375 patients.”
Other Issues
Previously, there are four different approaches to estimate an effect size such as: i) by deriving it from the literature; ii) by using historical data or secondary data to estimate it; iii) by determining the clinical meaningful effect and last but not least and iv) by deriving it from the results of a pilot study. It is a controversial practice to estimate the effect size from a pilot study because it may not be accurate since the effect size has been derived from a small sample provided by a pilot study ( 52 – 55 ). In reality, many researchers often encounter great difficulties in the estimation of sample size either i) when the required effect size is not reported by the existing literature; or ii) if some new, innovative research proposals which may pose pioneering research questions that have never been addressed; or iii) if the research is examining a new intervention or exploring a new research area in where no similar studies have previously been conducted. Although there are many concerns about validity of using pilot studies for power calculation, further research is still being conducted in pilot studies in order to apply more scientifically robust approaches for using pilot studies in gathering preliminary support for subsequent research. For example, there are now many published studies regarding guidelines for estimating sample size requirements in pilot studies ( 54 – 61 ).
This article has sought to provide a brief but clear guidance on how to determine the minimum sample size requirements for all researchers. Sample size calculation can be a difficult task, especially for the junior researcher. However, the availability of sample size software, and sample size tables for sample size determination based on various statistical tests, and several recommended rules of thumb which can be helpful for guiding the researchers in the determination of an adequate sample size for their studies. For the sake of brevity and convenience, this paper hereby proposes a useful checklist that is presented in Table 2 , which aims to guide and assist all researchers to determine an adequate sample size for their studies.
A step-by-step guide for sample size determination
Acknowledgements
I would like to thank the Director General of Health, Ministry of Health Malaysia for his permission to publish this article. I would also thank Dr Ang Swee Hung and Mr Hoon Yon Khee for proofreading this article.
Conflict of Interest
- Technical Note
- Open access
- Published: 12 February 2016
When is enough, enough? Understanding and solving your sample size problems in health services research
- Victoria Pye 1 ,
- Natalie Taylor 1 ,
- Robyn Clay-Williams 1 &
- Jeffrey Braithwaite 1
BMC Research Notes volume 9 , Article number: 90 ( 2016 ) Cite this article
13 Citations
2 Altmetric
Metrics details
Health services researchers face two obstacles to sample size calculation: inaccessible, highly specialised or overly technical literature, and difficulty securing methodologists during the planning stages of research. The purpose of this article is to provide pragmatic sample size calculation guidance for researchers who are designing a health services study. We aimed to create a simplified and generalizable process for sample size calculation, by (1) summarising key factors and considerations in determining a sample size, (2) developing practical steps for researchers—illustrated by a case study and, (3) providing a list of resources to steer researchers to the next stage of their calculations. Health services researchers can use this guidance to improve their understanding of sample size calculation, and implement these steps in their research practice.
Sample size literature for randomized controlled trials and study designs in which there is a clear hypothesis, single outcome measure, and simple comparison groups is available in abundance. Unfortunately health services research does not always fit into these constraints. Rather, it is often cross-sectional, and observational (i.e., with no ‘experimental group’) with multiple outcomes measured simultaneously. It can also be difficult work with no a priori hypothesis. The aim of this paper is to guide researchers during the planning stages to adequately power their study and to avoid the situation described in Fig. 1 . By blending key pieces of methodological literature with a pragmatic approach, researchers will be equipped with valuable information to plan and conduct sufficiently powered research using appropriate methodological designs. A short case study is provided (Additional file 1 ) to illustrate how these methods can be applied in practice.
A statistician’s dilemma
The importance of an accurate sample size calculation when designing quantitative research is well documented [ 1 – 3 ]. Without a carefully considered calculation, results can be missed, biased or just plain incorrect. In addition to squandering precious research funds, the implications of a poor sample size calculation can render a study unethical, unpublishable, or both. For simple study designs undertaken in controlled settings, there is a wealth of evidence based guidance on sample size calculations for clinical trials, experimental studies, and various types of rigorous analyses (Table 1 ), which can help make this process relatively straightforward. Although experimental trials (e.g., testing new treatment methods) are undertaken within health care settings, research to further understand and improve the health service itself is often cross-sectional, involves no intervention, and is likely to be observing multiple associations [ 4 ]. For example, testing the association between leadership on hospital wards and patient re-admission, controlling for various factors such as ward speciality, size of team, and staff turnover, would likely involve collecting a variety of data (e.g., personal information, surveys, administrative data) at one time point, with no experimental group or single hypothesis. Multi-method study designs of this type create challenges, as inputs for an adequate sample size calculation are often not readily available. These inputs are typically: defined groups for comparison, a hypothesis about the difference in outcome between the groups (an effect size), an estimate of the distribution of the outcome (variance), and desired levels of significance and power to find these differences (Fig. 2 ).
Inputs for a sample size calculation
Even in large studies there is often an absence of funding for statistical support, or the funding is inadequate for the size of the project [ 5 ]. This is particularly evident in the planning phase, which is arguably when it is required the most [ 6 ]. A study by Altman et al. [ 7 ] of statistician involvement in 704 papers submitted to the British Medical Journal and Annals of Internal Medicine indicated that only 51 % of observational studies received input from trained biostatisticians and, even when accounting for contributions from epidemiologists and other methodologists, only 52 % of observational studies utilized statistical advice in the study planning phase [ 7 ]. The practice of health services researchers performing their own statistical analysis without appropriate training or consultation from trained statisticians is not considered ideal [ 5 ]. In the review decisions of journal editors, manuscripts describing studies requiring statistical expertise are more likely to be rejected prior to peer review if the contribution of a statistician or methodologist has not been declared [ 7 ].
Calculating an appropriate sample size is not only to be considered a means to an end in obtaining accurate results. It is an important part of planning research, which will shape the eventual study design and data collection processes. Attacking the problem of sample size is also a good way of testing the validity of the study, confirming the research questions and clarifying the research to be undertaken and the potential outcomes. After all it is unethical to conduct research that is knowingly either overpowered or underpowered [ 2 , 3 ]. A study using more participants then necessary is a waste of resources and the time and effort of participants. An underpowered study is of limited benefit to the scientific community and is similarly wasteful.
With this in mind, it is surprising that methodologists such as statisticians are not customarily included in the study design phase. Whilst a lack of funding is partially to blame, it might also be that because sample size calculation and study design seem relatively simple on the surface, it is deemed unnecessary to enlist statistical expertise, or that it is only needed during the analysis phase. However, literature on sample size normally revolves around a single well defined hypothesis, an expected effect size, two groups to compare, and a known variance—an unlikely situation in practice, and a situation that can only occur with good planning. A well thought out study and analysis plan, formed in a conjunction with a statistician, can be utilized effectively and independently by researchers with the help of available literature. However a poorly planned study cannot be corrected by a statistician after the fact. For this reason a methodologist should be consulted early when designing the study.
Yet there is help if a statistician or methodologist is not available. The following steps provide useful information to aid researchers in designing their study and calculating sample size. Additionally, a list of resources (Table 1 ) that broadly frame sample size calculation is provided to guide researchers toward further literature searches. Footnote 1
A place to begin
Merrifield and Smith [ 1 ], and Martinez-Mesa et al. [ 3 ] discuss simple sample size calculations and explain the key concepts (e.g., power, effect size and significance) in simple terms and from a general health research perspective. These are a useful reference for non-statisticians and a good place to start for researchers who need a quick reminder of the basics. Lenth [ 2 ] provides an excellent and detailed exposition of effect size, including what one should avoid in sample size calculation.
Despite the guidance provided by this literature, there are additional factors to consider when determining sample size in health services research. Sample size requires deliberation from the outset of the study. Figure 3 depicts how different aspects of research are related to sample size and how each should be considered as part of an iterative planning phase. The components of this process are detailed below.
Stages in sample size calculation
Study design and hypothesis
The study design and hypothesis of a research project are two sides of the same coin. When there is a single unifying hypothesis, clear comparison groups and an effect size, e.g., drug A will reduce blood pressure 10 % more than drug B, then the study design becomes clear and the sample size can be calculated with relative ease. In this situation all the inputs are available for the diagram in Fig. 2 .
However, in large scale or complex health services research the aim is often to further our understanding about the way the system works, and to inform the design of appropriate interventions for improvement. Data collected for this purpose is cross-sectional in nature, with multiple variables within health care (e.g., processes, perceptions, outputs, outcomes, costs) collected simultaneously to build an accurate picture of a complex system. It is unlikely that there is a single hypothesis that can be used for the sample size calculation, and in many cases much of the hypothesising may not be performed until after some initial descriptive analysis. So how does one move forward?
To begin, consider your hypothesis (one or multiple). What relationships do you want to find specifically? There are three reasons why you may not find the relationships you are looking for:
The relationship does not exist.
The study was not adequately powered to find the relationship.
The relationship was obscured by other relationships.
There is no way to avoid the first, avoiding the second involves a good understanding of power and effect size (see Lenth [ 2 ]), and avoiding the third requires an understanding of your data and your area of research. A sample size calculation needs to be well thought out so that the research can either find the relationship, or, if one is not found, to be clear why it wasn’t found. The problem remains that before an estimate of the effect size can be made, a single hypothesis, single outcome measure and study design is required. If there is more than one outcome measure, then each requires an independent sample size calculation as each outcome measure has a unique distribution. Even with an analysis approach confirmed (e.g., a multilevel model), it can be difficult to decide which effect size measure should be used if there is a lack of research evidence in the area, or a lack of consensus within the literature about which effect sizes are appropriate. For example, despite the fact that Lenth advises researchers to avoid using Cohen’s effect size measurements [ 2 ], these margins are regularly applied [ 8 ].
To overcome these challenges, the following processes are recommended:
Select a primary hypothesis. Although the study may aim to assess a large variety of outcomes and independent variables, it is useful to consider if there is one relationship that is of most importance. For example, for a study attempting to assess mortality, re-admissions and length of stay as outcomes, each outcome will require its own hypothesis. It may be that for this particular study, re-admission rates are most important, therefore the study should be powered first and foremost to address that hypothesis. Walker [ 9 ] describes why having a single hypothesis is easier to communicate and how the results for primary and secondary hypotheses should be reported.
Consider a set of important hypotheses and the ways in which you might have to answer each one. Each hypothesis will likely require different statistical tests and methods. Take the example of a study aiming to understand more about the factors associated with hospital outcomes through multiple tests for associations between outcomes such as length of stay, mortality, and readmission rates (dependent variables) and nurse experience, nurse-patient ratio and nurse satisfaction (independent variables). Each of these investigations may use a different type of analysis, a different statistical test, and have a unique sample size requirement. It would be possible to roughly calculate the requirements and select the largest one as the overall sample size for the study. This way, the tests that require smaller samples are sure to be adequately powered. This option requires more time and understanding than the first.
During the study planning phase, when a literature review is normally undertaken, it is important not only to assess the findings of previous research, but also the design and the analysis. During the literature review phase, it is useful to keep a record of the study designs, outcome measures, and sample sizes that have already been reported. Consider whether those studies were adequately powered by examining the standard errors of the results and note any reported variances of outcome variables that are likely to be measured.
One of the most difficult challenges is to establish an appropriate expected effect size. This is often not available in the literature and has to be a judgement call based on experience. However previous studies may provide insight into clinically significant differences and the distribution of outcome measures, which can be used to help determine the effect size. It is recommended that experts in the research area are consulted to inform the decision about the expected effect size [ 2 , 8 ].
Simulation and rules of thumb
For many study designs, simulation studies are available (Table 1 ). Simulation studies generally perform multiple simulated experiments on fictional data using different effect sizes, outcomes and sample sizes. From this, an estimation of the standard error and any bias can be identified for the different conditions of the experiments. These are great tools and provide ‘ball park’ figures for similar (although most likely not identical) study designs. As evident in Table 1 , simulation studies often accompany discussions of sample size calculations. Simulation studies also provide ‘rules of thumb’, or heuristics about certain study designs and the sample required for each one. For example, one rule of thumb dictates that more than five cases per variable are required for a regression analysis [ 10 ].
Before making a final decision on a hypothesis and study design, identify the range of sample sizes that will be required for your research under different conditions. Early identification of a sample size that is prohibitively large will prevent time being wasted designing a study destined to be underpowered. Importantly, heuristics should not be used as the main source of information for sample size calculation. Rules of thumb are rarely congruous with careful sample size calculation [ 10 ] and will likely lead to an underpowered study. They should only be used, along with the information gathered through the use of the other techniques recommended in this paper, as a guide to inform the hypothesis and study design.
Other considerations
Be mindful of multiple comparisons.
The nature of statistical significance is that one in every 20 hypotheses tested will give a (false) significant result. This should be kept in mind when running multiple tests on the collected data. The hypothesis and appropriate tests should be nominated before the data are collected and only those tests should be performed. There are ways to correct for multiple comparisons [ 9 ], however, many argue that this is unnecessary [ 11 ]. There is no definitive way to ‘fix’ the problem of multiple tests being performed on a single data set and statisticians continue to argue over the best methodology [ 12 , 13 ]. Despite its complexity, it is worth considering how multiple comparisons may affect the results, and if there would be a reasonable way to adjust for this. The decision made should be noted and explained in the submitted manuscript.
After reading some introductory literature around sample size calculation it should be possible to derive an estimate to meet the study requirements. If this sample is not feasible, all is not lost. If the study is novel, it may add to the literature regardless of sample size. It may be possible to use pilot data from this preliminary work to compute a sample size calculation for a future study, to incorporate a qualitative component (e.g., interviews, focus groups), for answering a research question, or to inform new research.
Post hoc power analysis
This involves calculating the power of the study retrospectively, by using the observed effect size in the data collected to add interpretation to an insignificant result [ 2 ]. Hoenig and Heisey [ 14 ] detail this concept at length, including the range of associated limitations of such an approach. The well-reported criticisms of post hoc power analysis should cultivate research practice that involves appropriate methodological planning prior to embarking on a project.
Health services research can be a difficult environment for sample size calculation. However, it is entirely possible that, provided that significance, power, effect size and study design have been appropriately considered, a logical, meaningful and defensible calculation can always be obtained, achieving the situation described in Fig. 4 .
A statistician’s dream
Literature summarising an aspect of sample size calculation is included in Table 1 , providing a comprehensive mix of different aspects. The list is not exhaustive, and is to be used as a starting point to allow researchers to perform a more targeted search once their sample size problems have become clear. A librarian was consulted to inform a search strategy, which was then refined by the lead author. The resulting literature was reviewed by the lead author to ascertain suitability for inclusion.
Merrifield A, Smith W. Sample size calculations for the design of health studies: a review of key concepts for non-statisticians. NSW Public Health Bull. 2012;23(8):142–7. doi: 10.1071/NB11017 .
Article Google Scholar
Lenth RV. Some practical guidelines for effective sample size determination. Am Stat. 2001;55(3):187–93. doi: 10.1198/000313001317098149 .
Martinez-Mesa J, Gonzalez-Chica DA, Bastos JL, Bonamigo RR, Duquia RP. Sample size: how many participants do i need in my research? An Bras Dermatol. 2014;89(4):609–15. doi: 10.1590/abd1806-4841.20143705 .
Article PubMed Central PubMed Google Scholar
Webb P, Bain C. Essential epidemiology: an introduction for students and health professionals. 2nd ed. Cambridge: Cambridge University Press; 2011.
Google Scholar
Omar RZ, McNally N, Ambler G, Pollock AM. Quality research in healthcare: are researchers getting enough statistical support? BMC Health Serv Res. 2006;6:2. doi: 10.1186/1472-6963-6-2 .
Maxwell SE, Kelley K, Rausch JR. Sample size planning for statistical power and accuracy in parameter estimation. Annu Rev Psychol. 2008;59:537–63. doi: 10.1146/annurev.psych.59.103006.093735 .
Article PubMed Google Scholar
Altman DG, Goodman SN, Schroter S. How statistical expertise is used in medical research. J Am Med Assoc. 2002;287(21):2817–20. doi: 10.1001/jama.287.21.2817 .
Sullivan GM, Feinn R. Using effect size—or why the P value is not enough. J Grad Med Educ. 2012;4(3):279–82. doi: 10.4300/JGME-D-12-00156.1 .
Walker AM. Reporting the results of epidemiologic studies. Am J Public Health. 1986;76(5):556–8.
Article PubMed Central CAS PubMed Google Scholar
Green SB. How many subjects does it take to do a regression analysis. Multivariate Behav Res. 1991;26(3):499–510. doi: 10.1207/s15327906mbr2603_7 .
Article CAS PubMed Google Scholar
Feise R. Do multiple outcome measures require p-value adjustment? BMC Med Res Methodol. 2002;2(1):8. doi: 10.1186/1471-2288-2-8 .
Savitz DA, Olshan AF. Describing data requires no adjustment for multiple comparisons: a reply from Savitz and Olshan. Am J Epidemiol. 1998;147(9):813–4. doi: 10.1093/oxfordjournals.aje.a009532 .
Savitz DA, Olshan AF. Multiple comparisons and related issues in the interpretation of epidemiologic data. Am J Epidemiol. 1995;142(9):904–8.
CAS PubMed Google Scholar
Hoenig JM, Heisey DM. The abuse of power: the pervasive fallacy of power calculations for data analysis. Am Stat. 2001;55(1):19–24. doi: 10.1198/000313001300339897 .
Noordzij M, Tripepi G, Dekker FW, Zoccali C, Tanck MW, Jager KJ. Sample size calculations: basic principles and common pitfalls. Nephrol Dial Transplant. 2010;25(5):1388–93. doi: 10.1093/ndt/gfp732 .
Vardeman SB, Morris MD. Statistics and ethics: some advice for young statisticians. Am Stat. 2003;57(1):21–6. doi: 10.1198/0003130031072 .
Dowd BE. Separated at birth: statisticians, social scientists, and causality in health services research. Health Serv Res. 2011;46(2):397–420. doi: 10.1111/j.1475-6773.2010.01203.x .
Dimick JB, Welch HG, Birkmeyer JD. Surgical mortality as an indicator of hospital quality: the problem with small sample size. J Am Med Assoc. 2004;292(7):847–51. doi: 10.1001/jama.292.7.847 .
Article CAS Google Scholar
Thomas DC, Siemiatycki J, Dewar R, Robins J, Goldberg M, Armstrong BG. The problem of multiple inference in studies designed to generate hypotheses. Am J Epidemiol. 1985;122(6):1080–95.
VanVoorhis CW, Morgan BL. Understanding power and rules of thumb for determining sample sizes. Tutor Quant Methods Psychol. 2007;3(2):43–50.
Van Belle G. Statistical rules of thumb. 2nd ed. New York: Wiley; 2011.
Serumaga-Zake PA, Arnab R, editors. A suggested statistical procedure for estimating the minimum sample size required for a complex cross-sectional study. The 7th international multi-conference on society, cybernetics and informatics: IMSCI, 2013 Orlando, Florida, USA; 2013.
Hsieh FY, Bloch DA, Larsen MD. A simple method of sample size calculation for linear and logistic regression. Stat Med. 1998;17(14):1623–34. doi: 10.1002/(SICI)1097-0258(19980730)17:14<1623:AID-SIM871>3.0.CO;2-S .
Alam MK, Rao MB, Cheng F-C. Sample size determination in logistic regression. Sankhya B. 2010;72(1):58–75. doi: 10.1007/s13571-010-0004-6 .
Peduzzi P, Concato J, Kemper E, Holford TR, Feinstein AR. A simulation study of the number of events per variable in logistic regression analysis. J Clin Epidemiol. 1996;49(12):1373–9. doi: 10.1016/S0895-4356(96)00236-3 .
Dupont WD, Plummer WD Jr. Power and sample size calculations for studies involving linear regression. Control Clin Trials. 1998;19(6):589–601. doi: 10.1016/S0197-2456(98)00037-3 .
Zhong B. How to calculate sample size in randomized controlled trial? J Thorac Dis. 2009;1(1):51–4.
PubMed Central PubMed Google Scholar
Maas CJM, Hox JJ. Sufficient sample sizes for multilevel modeling. Methodology. 2005;1(3):86–92. doi: 10.1027/1614-2241.1.3.86 .
Cohen MP. Sample size considerations for multilevel surveys. Int Stat Rev. 2005;73(3):279–87. doi: 10.1111/j.1751-5823.2005.tb00149.x .
Paccagnella O. Sample size and accuracy of estimates in multilevel models: new simulation results. Methodology. 2011;7(3):111–20. doi: 10.1027/1614-2241/A000029 .
Maas CJM, Hox JJ. Robustness issues in multilevel regression analysis. Stat Neerl. 2004;58(2):127–37. doi: 10.1046/j.0039-0402.2003.00252.x .
Download references
Authors’ contributions
VP drafted the paper, performed literature searches and tabulated the findings. NT made substantial contribution to the structure and contents of the article. RCW provided assistance with the figures and tables, as well as structure and contents of the article. Both RCW and NT aided in the analysis and interpretation of findings. JB provided input into the conception and design of the article and critically reviewed its contents. All authors read and approved the final manuscript.
Acknowledgements
We would like to acknowledge Emily Hogden for assistance with editing and submission. The funding source for this article is an Australian National Health and Medical Research Council (NHMRC) Program Grant, APP1054146.
Authors’ information
VP is a biostatistician with 7 years’ experience in health research settings. NT is a health psychologist with organizational behaviour change and implementation expertise. RCW is a health services researcher with expertise in human factors and systems thinking. JB is a professor of health services research and Foundation Director of the Australian Institute of Health Innovation.
Competing interests
The authors declare that they have no competing interests.
Author information
Authors and affiliations.
Australian Institute of Health Innovation, Faculty of Medicine and Health Sciences, Macquarie University, Level 6, 75 Talavera Road, Macquarie University, Sydney, NSW, 2109, Australia
Victoria Pye, Natalie Taylor, Robyn Clay-Williams & Jeffrey Braithwaite
You can also search for this author in PubMed Google Scholar
Corresponding author
Correspondence to Victoria Pye .
Additional file
Additional file 1. case study. this case study illustrates the steps of a sample size calculation., rights and permissions.
Open Access This article is distributed under the terms of the Creative Commons Attribution 4.0 International License ( http://creativecommons.org/licenses/by/4.0/ ), which permits unrestricted use, distribution, and reproduction in any medium, provided you give appropriate credit to the original author(s) and the source, provide a link to the Creative Commons license, and indicate if changes were made. The Creative Commons Public Domain Dedication waiver ( http://creativecommons.org/publicdomain/zero/1.0/ ) applies to the data made available in this article, unless otherwise stated.
Reprints and permissions
About this article
Cite this article.
Pye, V., Taylor, N., Clay-Williams, R. et al. When is enough, enough? Understanding and solving your sample size problems in health services research. BMC Res Notes 9 , 90 (2016). https://doi.org/10.1186/s13104-016-1893-x
Download citation
Received : 15 January 2016
Accepted : 28 January 2016
Published : 12 February 2016
DOI : https://doi.org/10.1186/s13104-016-1893-x
Share this article
Anyone you share the following link with will be able to read this content:
Sorry, a shareable link is not currently available for this article.
Provided by the Springer Nature SharedIt content-sharing initiative
- Sample size
- Effect size
- Health services research
- Methodologies
BMC Research Notes
ISSN: 1756-0500
- Submission enquiries: [email protected]
- General enquiries: [email protected]
- français
- español
- português
Related Links
Sample size determination in health studies : a practical manual / s. k. lwanga and s. lemeshow.

View Statistics
Other titles, description, collections.
- Publications
Show Statistical Information
- 1. Headquarters
Sample Size Calculator
Determines the minimum number of subjects for adequate study power, clincalc.com » statistics » sample size calculator, study group design.
Two independent study groups
One study group vs. population
Primary Endpoint
Dichotomous (yes/no)
Continuous (means)
Statistical Parameters
Dichotomous endpoint, two independent sample study, about this calculator.
This calculator uses a number of different equations to determine the minimum number of subjects that need to be enrolled in a study in order to have sufficient statistical power to detect a treatment effect. 1
Before a study is conducted, investigators need to determine how many subjects should be included. By enrolling too few subjects, a study may not have enough statistical power to detect a difference (type II error). Enrolling too many patients can be unnecessarily costly or time-consuming.
Generally speaking, statistical power is determined by the following variables:
- Baseline Incidence: If an outcome occurs infrequently, many more patients are needed in order to detect a difference.
- Population Variance: The higher the variance (standard deviation), the more patients are needed to demonstrate a difference.
- Treatment Effect Size: If the difference between two treatments is small, more patients will be required to detect a difference.
- Alpha: The probability of a type-I error -- finding a difference when a difference does not exist. Most medical literature uses an alpha cut-off of 5% (0.05) -- indicating a 5% chance that a significant difference is actually due to chance and is not a true difference.
- Beta: The probability of a type-II error -- not detecting a difference when one actually exists. Beta is directly related to study power (Power = 1 - β). Most medical literature uses a beta cut-off of 20% (0.2) -- indicating a 20% chance that a significant difference is missed.
Post-Hoc Power Analysis
To calculate the post-hoc statistical power of an existing trial, please visit the post-hoc power analysis calculator .
References and Additional Reading
- Rosner B. Fundamentals of Biostatistics . 7th ed. Boston, MA: Brooks/Cole; 2011.
Related Calculators
- Post-hoc Power Calculator
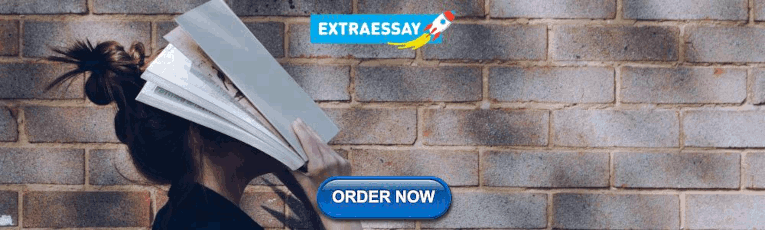
New and Popular
Cite this page.
Show AMA citation

We've filled out some of the form to show you this clinical calculator in action. Click here to start from scratch and enter your own patient data.
How to calculate sample size for different study designs in medical research?
Affiliation.
- 1 Department of Pharmacology, Govt. Medical College, Surat, Gujarat, India.
- PMID: 24049221
- PMCID: PMC3775042
- DOI: 10.4103/0253-7176.116232
Calculation of exact sample size is an important part of research design. It is very important to understand that different study design need different method of sample size calculation and one formula cannot be used in all designs. In this short review we tried to educate researcher regarding various method of sample size calculation available for different study designs. In this review sample size calculation for most frequently used study designs are mentioned. For genetic and microbiological studies readers are requested to read other sources.
Keywords: Medical research; sample size; study designs.
Publication types
Sample Size Calculators
For designing clinical research.

- Calculators
- CI for proportion
- CI for mean
- Means - effect size
- Means - sample size
- Proportions - effect size
- Proportions - sample size
- CI for proportion - sample size
- Survival analysis - sample size
- CI for risk ratio
- More calculators...
- Calculator finder
- About calculating sample size
If you are a clinical researcher trying to determine how many subjects to include in your study or you have another question related to sample size or power calculations, we developed this website for you. Our approach is based on Chapters 5 and 6 in the 4th edition of Designing Clinical Research (DCR-4), but the material and calculators provided here go well beyond an introductory textbook on clinical research methods.
This project was supported by the National Center for Advancing Translational Sciences, National Institutes of Health, through UCSF-CTSI Grant Numbers UL1 TR000004 and UL1 TR001872. Its contents are solely the responsibility of the authors and do not necessarily represent the official views of the NIH.
Please cite this site wherever used in published work:
Kohn MA, Senyak J. Sample Size Calculators [website]. UCSF CTSI. 11 January 2024. Available at https://www.sample-size.net/ [Accessed 28 May 2024]
This site was last updated on January 11, 2024.
Academia.edu no longer supports Internet Explorer.
To browse Academia.edu and the wider internet faster and more securely, please take a few seconds to upgrade your browser .
Enter the email address you signed up with and we'll email you a reset link.
- We're Hiring!
- Help Center
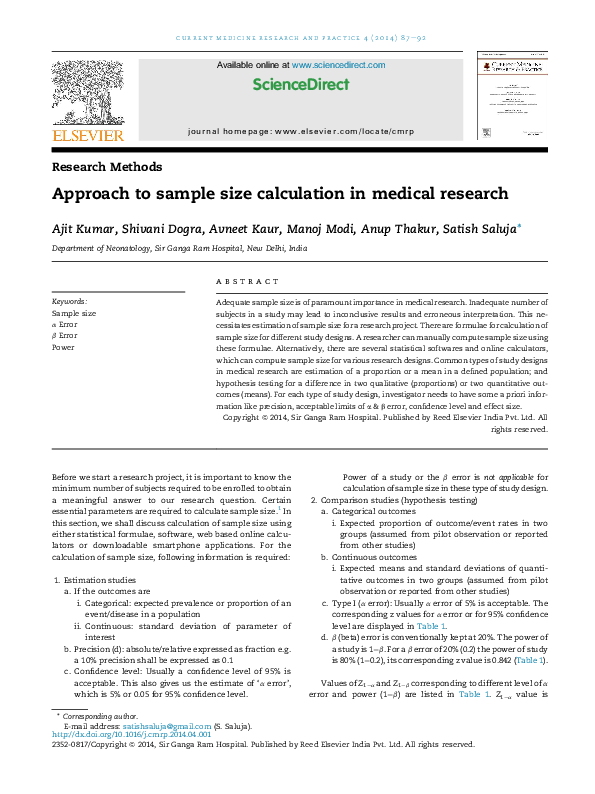
Approach to sample size calculation in medical research

2014, Current Medicine Research and Practice
Related Papers
Edwin Timothyus
Calculation of exact sample size is an important part of research design. It is very important to understand that different study design need different method of sample size calculation and one formula cannot be used in all designs. In this short review we tried to educate researcher regarding various method of sample size calculation available for different study designs. In this review sample size calculation for most frequently used study designs are mentioned. For genetic and microbiological studies readers are requested to read other sources.
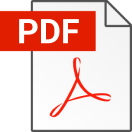
Indian Journal of Anaesthesia
Mohanchandra Mandal
Ayman Johargy
Journal of Ayub Medical College, Abbottabad : JAMC
One of frequently asked question by medical and dental students / researchers is how to determine the sample size. Sample size calculations is necessary for approval of research projects, clearance from ethical committees, approval of grant from funding bodies, publication requirement for journals and most important of all justify the authenticity of study results. Determining the sample size for a study is a crucial component. The goal is to include sufficient numbers of subjects so that statistically significant results can be detected. Using too few subjects' will result in wasted time, effort, money; animal lives etc. and may yield statistically inconclusive results. There are numerous situations in which sample size is determined that varies from study to study. This article will focus on the sample size determination for hypothesis testing that involves means, one sample t test, two independent sample t test, paired sample and one-way analysis of variance.
Journal of Investigative Dermatology
International journal of Ayurveda research
Supriya bhalerao
One of the pivotal aspects of planning a clinical study is the calculation of the sample size. It is naturally neither practical nor feasible to study the whole population in any study. Hence, a set of participants is selected from the population, which is less in number (size) but ...
BRIJESH SATHIAN
Dr. Padam Singh
An essential part of any medical research is to decide how many subjects need to be studied. A formal sample size calculation is a prerequisite to justify that the study with this size is capable of answering the research questions. This article highlights the statistical principles involved in sample size calculation along with formulae used in different situation illustrating with examples. The implications of deviations are also discussed.
Nephron Clinical Practice
Marlies Noordzij
The sample size is the number of patients or other experimental units that need to be included in a study to answer the research question. Pre-study calculation of the sample size is important; if a sample size is too small, one will not be able to detect an effect, while a sample that is too large may be a waste
RELATED PAPERS
Catina Feresin
David Miyamoto
Physical Review D
Marco Antônio Santos
Journal of Feminist Family Therapy
Samantha Marino
Journal of Mathematical Analysis and Applications
Faridah Fauzizah
Ezequiel Margutti
IEEE Access
Hongchuan Yu
Bone marrow transplantation
Natalia Creus
ASME 2011 Dynamic Systems and Control Conference and Bath/ASME Symposium on Fluid Power and Motion Control, Volume 1
Roberto Horowitz
Olle Inganäs
Journal of Urology
safwan jaradeh
monika yadav
Ailton Bueno Scorsoline
Journal of the College …
Irfan mirza
Proceedings of the 7th annual conference on Genetic and evolutionary computation
Tanasanee Phienthrakul
hukyytj jkthjfgr
Electronic Notes in Discrete Mathematics
Darya Kovalevskaya
Monthly Notices of the Royal Astronomical Society
Prof Richard G McMahon
SSRN Electronic Journal
Sean Corcoran
RELATED TOPICS
- We're Hiring!
- Help Center
- Find new research papers in:
- Health Sciences
- Earth Sciences
- Cognitive Science
- Mathematics
- Computer Science
- Academia ©2024
This paper is in the following e-collection/theme issue:
Published on 27.5.2024 in Vol 26 (2024)
Assessing the Content and Effect of Web-Based Decision Aids for Postmastectomy Breast Reconstruction: Systematic Review and Meta-Analysis of Randomized Controlled Trials
Authors of this article:

- Lin Yu 1 , MD ;
- Jianmei Gong 1 , PhD ;
- Xiaoting Sun 1 , MD ;
- Min Zang 1 , MD ;
- Lei Liu 1 * , PhD ;
- Shengmiao Yu 2 * , BS
1 School of Nursing, Liaoning University of Chinese Traditional Medicine, Shenyang, China
2 Outpatient Department, The Fourth Affiliated Hospital of China Medical University, Shenyang, China
*these authors contributed equally
Corresponding Author:
Lei Liu, PhD
School of Nursing, Liaoning University of Chinese Traditional Medicine
No.79 Chongshan Dong Road
Shenyang, 110000
Phone: 86 17824909908
Email: [email protected]
Background: Web-based decision aids have been shown to have a positive effect when used to improve the quality of decision-making for women facing postmastectomy breast reconstruction (PMBR). However, the existing findings regarding these interventions are still incongruent, and the overall effect is unclear.
Objective: We aimed to assess the content of web-based decision aids and its impact on decision-related outcomes (ie, decision conflict, decision regret, informed choice, and knowledge), psychological-related outcomes (ie, satisfaction and anxiety), and surgical decision-making in women facing PMBR.
Methods: This systematic review and meta-analysis followed the PRISMA (Preferred Reporting Items for Systematic Reviews and Meta-Analyses) guidelines. A total of 6 databases, PubMed, Embase, Cochrane Library, CINAHL, PsycINFO, and Web of Science Core Collection, were searched starting at the time of establishment of the databases to May 2023, and an updated search was conducted on April 1, 2024. MeSH (Medical Subject Headings) terms and text words were used. The Cochrane Risk of Bias Tool for randomized controlled trials was used to assess the risk of bias. The certainty of evidence was assessed using the Grading of Recommendations, Assessment, Development, and Evaluation approach.
Results: In total, 7 studies included 579 women and were published between 2008 and 2023, and the sample size in each study ranged from 26 to 222. The results showed that web-based decision aids used audio and video to present the pros and cons of PMBR versus no PMBR, implants versus flaps, and immediate versus delayed PMBR and the appearance and feel of the PMBR results and the expected recovery time with photographs of actual patients. Web-based decision aids help improve PMBR knowledge, decisional conflict (mean difference [MD]=–5.43, 95% CI –8.87 to –1.99; P =.002), and satisfaction (standardized MD=0.48, 95% CI 0.00 to 0.95; P =.05) but have no effect on informed choice (MD=–2.80, 95% CI –8.54 to 2.94; P =.34), decision regret (MD=–1.55, 95% CI –6.00 to 2.90 P =.49), or anxiety (standardized MD=0.04, 95% CI –0.50 to 0.58; P =.88). The overall Grading of Recommendations, Assessment, Development, and Evaluation quality of the evidence was low.
Conclusions: The findings suggest that the web-based decision aids provide a modern, low-cost, and high dissemination rate effective method to promote the improved quality of decision-making in women undergoing PMBR.
Trial Registration: PROSPERO CRD42023450496; https://www.crd.york.ac.uk/prospero/display_record.php?RecordID=450496
Introduction
Breast cancer (BC) is a major global health problem. In 2020, more than 2.3 million newly diagnosed cases and 685,000 deaths were associated with BC [ 1 ]. There has been a gradual increase in the incidence of BC globally over the past few decades, which has been attributed to lifestyle changes (eg, increase in BMI and decrease in birth rate), as well as an increase in screening detection as BC becomes more recognized [ 2 - 4 ]. Although BC has the highest incidence rate among all types of cancer, its mortality rate declined by 43% between 1989 and 2020, and it is concentrated in larger areas [ 2 , 5 ]. Advances in the early detection and treatment of BC have improved patient survival rates, which, in turn, have led to an increased focus on improving the quality of life of the survivors of BC. The surgical approach to BC is complex and usually involves the decision to undergo breast-conserving surgery and mastectomy. For women undergoing mastectomy, the change in appearance due to the missing breast can lead to various types of psychological problems including physical imagery discomfort, psychological distress, anxiety, and depression [ 6 ]. Postmastectomy breast reconstruction (PMBR) is now an option for women to restore their appearance [ 7 ].
However, when women face a PMBR decision, they must decide whether to use PMBR, and if they choose to do so, they should further decide on the timing and type of PMBR (ie, implant, autologous tissue, or combination) [ 8 , 9 ]. Delayed autologous PMBR results in a localized or regional recurrence rate similar to immediate PMBR [ 10 ]. A BC diagnosis can leave patients feeling anxious and uncertain, which is often exacerbated by presenting multiple, complex treatment options for women to choose from in a short period [ 11 ]. Most patients with BC who are considering PMBR immediately have clinically substantially decision conflict [ 12 , 13 ]. Patients experience postoperative complications leading to decision regret [ 14 ]. These issues can lead to poorer health outcomes, negative perceptions of the health care system, and lower quality of life [ 14 , 15 ]. Therefore, more preoperative patient education about possible complications includes the patient’s anatomy, which PMBR to choose, the associated pros and cons, and previous surgical and medication history. Women should be fully informed of their options and given the tools to weigh the pros and cons of each option, which may reduce the incidence of these adverse effects [ 16 ]. At the same time, personalized medicine is increasingly becoming the standard of care for patients with BC [ 17 ], and based on the current evidence, patients should have equal access to all eligible PMBR options [ 10 ]. In a sample of 126 patients who underwent mastectomy, a minority of patients made high-quality decisions about PMBR. Specifically, 43.3% of patients were adequately informed and accepted treatment decisions that were consistent with their preferences [ 11 ]. Therefore, patients and providers must work together through dialogue to optimize treatment options and engage in shared decision-making. However, it is not easy for inexperienced physicians to perform shared decision-making in an orderly and correct manner in a limited amount of time [ 18 ]. Decision aids may be helpful before a patient decides to undergo PMBR. Some studies [ 19 ] also suggest that decision aids may be helpful for some women even after undergoing a PMBR, as some women exhibit decision conflicts after the consultation. Decision aids are powerful tools to support patients in making informed choices based on their own values and are available via the internet, DVDs, and printed materials [ 20 ]. With the increasing popularity of the internet worldwide, web-based dissemination of information has been recognized as one of the most promising of all available formats (eg, leaflets, brochures, audio, and video) for delivering decision aids to patients. Web-based decision aids are characterized as being interactive, dynamic, and customizable [ 21 ]. On the one hand, web-based decision aids have a greater advantage in facilitating patient access than face-to-face interaction with physicians. On the other hand, decision aids on the internet can store and disseminate information over a longer period than traditional, static decision aids and can personalize the visit according to the patient’s values and preferences [ 21 - 23 ].
Prior Research
Paraskeva et al [ 24 ] conducted a systematic review exploring the effectiveness of interventions to assist women in making decisions about PMBR, which consisted of 6 studies with mixed results in terms of knowledge, decision-making, overall satisfaction, and quality of life. Berlin et al [ 25 ] assessed PMBR decision aids in a systematic review and meta-analysis, concluding that PMBR reduces decision conflict, improves information satisfaction, promotes participation in the decision-making process, and enhances the awareness of participation in the decision-making process. However, the authors included all types of trials (ie, quantitative and qualitative) and only meta-analyzed decision conflict. This review also did not include the effects of decision aids on outcome indicators such as psychologically relevant outcomes. Yang et al [ 26 ] conducted a meta-analysis exploring the effects of decision aids on decision-making in PMBR; however, the authors did not compare whether different forms of decision aids would have different effects. Zhao et al [ 27 ] conducted a scoping review with the aim of reviewing, comparing, and discussing the current incorporation of the adverse effects of BC treatments into decision aids and examined how web-based decision aids personalized BC treatment decision-making tools in patient–health care provider communication, clinician decision-making processes, and shared decision-making, as yet unassessed patient outcomes (eg, knowledge and anxiety). In summary, there is a lack of descriptions of the impact of web-based decision aids on the decision-making of women facing PMBR. Overall, existing systematic evaluations on related topics have produced mixed results, and more importantly, many primary trials [ 28 - 31 ], following these reviews, have produced conflicting results, which may provide new evidence. Therefore, there is a need for a new systematic evaluation to provide a comprehensive overview of the effectiveness of web-based decision aids on the quality of decision-making for women faced with PMBR, drawn from all available evidence from randomized controlled trials (RCTs) that meet high standards for evidence-based research.
The aim of this systematic review and meta-analysis was to assess the content of the web-based decision aids and evaluate their effectiveness on decision-related outcomes (ie, decision conflict, decision regret, informed choice, and knowledge), psychological-related outcomes (ie, satisfaction and anxiety), and surgical decision-making in women facing PMBR.
This is a systematic review and meta-analysis reported in accordance with PRISMA (Preferred Reporting Items for Systematic Reviews and Meta-Analyses; Multimedia Appendix 1 ) guidelines [ 32 ]. The protocol was registered in PROSPERO (CRD42023450496).
Eligibility and Exclusion Criteria
An overview of the inclusion and exclusion criteria can be found in Textbox 1 .
- The population included in the study was aged ≥18 years and women who had been diagnosed with breast cancer (BC) and were considering postmastectomy breast reconstruction (PMBR) but had not yet had the surgery and had internet access. If the patient, at the time of enrollment, had attempted PMBR; did not have BC (ie, were considering prophylactic mastectomy); and had an active psychiatric, cognitive, or visual impairment, they were not eligible.
Intervention
- Studies focusing on web-based decision aids (including websites and apps)
- Controls for usual care, counseling, health education pamphlets, and non–web-based decision aids
- The primary outcomes were decision-related outcomes (ie, informed choice, knowledge, decision conflict, and decision regret); psychological outcomes (ie, satisfaction and anxiety); and PMBR options and tool usability (ie, women’s feedback on use)
- Randomized controlled trials
Search Strategy
A systematic search of studies was carried out using English databases such as PubMed, Embase, Cochrane Library, CINAHL, PsycINFO, and Web of Science Core Collection from the date of inception of each database to May 2023, and an updated search was conducted on April 1, 2024, to cover new research. Medical Subject Headings terms and text words were used. The keywords used included “Mastectomy,” “mammaplasty,” “mastectomy,” “informed choice*,” “shared decision making,” “computer,” “web based,” and “Internet,” which are English search terms. These index terms and keywords were explored and modified according to the different grammatical rules of the database. Specific details of the search algorithm are available in Multimedia Appendix 2 . The reference lists of the included studies and relevant articles were hand-searched to identify other potentially eligible articles. The search was limited to articles in English and had no limitations with regard to publication year.
The results were input into EndNote X9, and duplicates were removed automatically. After removing duplicates, 2 reviewers independently screened the titles and abstracts of identified articles and removed irrelevant citations in accordance with the selection criteria. After the removal of irrelevant studies, the full texts of potentially relevant studies were retrieved. Next, both reviewers independently assessed the full texts. Any disagreements were settled by discussion with a third reviewer.
Data Extraction
Characteristics of the included RCTs (eg, author, year of publication, country, sample size, subject characteristics, form, content, development method and team, theoretical basis, duration of use, reading level, a brief description of the intervention in the control group, outcome measurements, follow-up, and results) were extracted into tables. We wrote to the authors to obtain more information about the results. Two reviewers compared the findings independently.
Risk of Bias Assessment
The quality of RCTs was evaluated using the Cochrane Handbook for RCTs [ 33 ]. The tool consists of 7 items: randomized sequence generation, allocation concealment, participant and personnel blinding, blinding for outcome assessment, incomplete outcome, data selective reporting, and other bias. The risk of bias for each domain was judged as low risk of bias, high risk of bias, or unclear risk of bias. The evaluation of study quality was performed independently by 2 reviewers, and a third reviewer was consulted if necessary.
Statistical Analysis
Statistical analysis was performed using Review Manager (version 5.3; Cochrane), illustrated using a forest plot when at least 2 studies were measured for the same outcomes for a PMBR decision at the longest follow-up time point [ 34 , 35 ]. We used mean differences (MDs) for continuous variables that were measured with the same instrument, standardized MDs (SMDs) when a similar outcome was assessed with different instruments, and relative risks for dichotomous variables. We calculated possible missing values such as SD and 95% CI [ 33 ]. In the study, heterogeneity was assessed via the Higgins I 2 statistic with I 2 values of ≤25%, 50%, and ≥75% deemed to represent low, medium, and high heterogeneity, respectively [ 33 ]. When there was no significant heterogeneity, the fixed effects model ( I 2 ≤50%) was used; otherwise, the random effects model was used, resulting in a more conservative summary effect estimate [ 33 ]. To identify potential sources of clinical heterogeneity, we also conducted a post hoc sensitivity analysis to determine the stability of the results by omitting each test [ 36 ].
Study Selection
Figure 1 shows the research selection process and results based on the PRISMA 2020 guidelines. A total of 844 studies were identified. A total of 129 of these studies were excluded because they were repetitive. After selecting titles and abstracts, 21 studies were included for the next stage. Consequently, 7 studies met the inclusion criteria.

Study Characteristics
The 7 studies included 579 women and were published between 2008 and 2023, and the sample size in each study ranged from 26 to 222. The average age of the women was approximately 50 years; they were in the early stages of BC and facing the PMBR decision. The studies were conducted in 3 countries; 6 studies were conducted in high-income countries—4 in the United States [ 30 , 31 , 37 , 38 ] and 2 in Australia [ 29 , 39 ]—and 1 in an upper–middle-income country, China [ 28 ]. Detailed characteristics of the included studies are shown in Table 1 .
a BC: breast cancer.
b DCS: Decision Conflict Scale.
c BR-DMPS: Breast Reconstruction-Decision-Making Process Scale.
d DRS: Decision Regret Scale.
e BIS: Body Image Scale.
f HADS: Hospital Anxiety and Depression Scale.
g DC: decisional conflict.
h DR: decisional regret.
i STAI: State-Trait Anxiety Inventory.
j BR: breast reconstruction.
k DQI: Decision Quality Index.
l DASS-21: Depression Anxiety Stress Scale.
m SSQ-6: Social Support Questionnaire.
Characteristics of the Interventions and Controls
The characteristics of the interventions and controls are shown in Multimedia Appendix 3 [ 28 - 31 , 37 - 39 ].
Characteristics of the Interventions
In total, 5 of the studies [ 28 - 30 , 38 , 39 ] explained that the web-based decision aids development team includes survivors of BC who have undergone mastectomy, plastic or reconstructive surgeons who perform PMBR, and software engineers. The methodology used to develop web-based decision aids includes qualitative research, evidence review and mentoring, and pilot study group meetings. The theoretical basis for the development of web-based decision aids is usually the International Patient Decision Aid Standards [ 29 , 30 , 39 ] or the Ottawa Decision Support Framework [ 28 ]. Except for 2 studies [ 28 , 37 ] that did not report the time of use, most web-based decision aids took between 20 and 74 minutes. Two web-based decision aids [ 29 , 30 , 39 ] were developed at a reading level written at a seventh- and eighth-grade reading level. The web-based decision aids content specifically includes the patient population and reconstruction options, including implant reconstruction (ie, tissue expanders and implant types), autologous flap reconstruction (ie, latissimus dorsi, rectus abdominis, and free flaps and deep epithelial perforator flaps in the lower abdomen), and skin-sparing and preserving mastectomies (ie, 1-phase and 2-phase procedures). There are also contraindications and general eligibility criteria. Timing of reconstruction includes immediate versus delayed reconstruction, as well as factors that influence the type and timing of reconstruction. It also includes information about the pros and cons of reconstruction versus no reconstruction, implants versus flaps, immediate versus delayed reconstruction, the look and feel of PMBR, and the expected recovery time. The probability of possible implant (eg, wrinkled breast appearance, periosteal contracture after radiation therapy, and possible need for implant replacement over time) and flap (eg, muscle weakness and flap failure) are clearly described in a balanced format with quotes of real patients’ opinions. The web-based decision aids show photographs, high-quality 3D animated images, pre- and postoperative photographs, audio, and video of actual patients of different skin colors and body types, A list of frequently asked questions from clinicians is also included. Elements in the tool include patient-tailored risk assessments, patient value clarification exercises, techniques for managing emotions, and strategies for communicating with family members about PMBR decisions. Women’s stories explaining their reasons for choosing particular methods and their impact on their lives are also included. Users enter their questions and the system prompts them to print a summary to use in a consultation with their physician. This customized printable page also helps patients discuss their concerns and options with their families.
Characteristics of the Controls
The control for the study by Politi et al [ 30 ] was the enhanced urgent care and American Society of Plastic Surgeons pamphlet on PMBR. Varelas et al [ 31 ] used traditional counseling. The control for the study by Fang et al [ 28 ] was the provider-provided urgent care+pamphlet, which describes the types of surgery, including mastectomy, implant-based PMBR, and autologous PMBR, as well as the advantages and disadvantages of the different types of surgeries. The control for the study by Manne et al [ 38 ] was the 56-page pamphlet available at no cost from the Cancer Support Community focusing on PMBR. For the study by Sherman et al [ 39 ], the control was the web-based access to excerpts of the public brochure, including basic information on breast surgery and reconstruction, but excluding content unique to the intervention group (ie, video interviews with patients or surgeons, and values clarification exercises). In the study by Mardinger et al [ 29 ], the control was the decision aids, which is unvalidated that contains 6 text-based pages that can be accessed in both interactive and noninteractive formats. The control for the study by Heller et al [ 37 ] was the group that received the standard patient education, including printed materials in books and pamphlets as well as personal instruction from the attending physician, physician-in-training, physician assistant, and nurse practitioner.
Outcome Measure
A total of 5 studies [ 28 , 29 , 31 , 38 , 39 ] measured decision conflict using the Decision Conflict Scale (DCS), and 1 study [ 30 ] measured decision conflict using the 4-item SURE scale. Three studies [ 28 , 29 , 39 ] measured decision regret using the Decision Regret Scale (DRS) and 2 studies [ 28 , 29 ] measured informed choice using the subdimension of the DCS—feeling informed. Knowledge was measured primarily by the percentage of correct answers to self-administered multiple-choice questions about specific plastic surgery procedures in 6 studies [ 28 - 31 , 37 , 38 ]. Satisfaction was measured using the Satisfaction with Decision Scale [ 29 ] and some scales adapted from those used in previous studies [ 28 , 37 - 39 ]. Anxiety was primarily measured using the Hospital Anxiety and Depression Scale [ 28 ] and the State-Trait Anxiety Inventory [ 31 , 38 ].
Decision-Related Outcomes
Decision conflict.
In total, 6 studies [ 28 - 31 , 38 , 39 ] investigated the impact of decision conflicts in PMBR. The 5 studies [ 28 , 29 , 31 , 38 , 39 ] that used DCS included in the meta-analysis showed a statistically significant positive impact of web-based decision aids interventions on decision conflict (MD=–5.43, 95% CI –8.87 to –1.99; P =.002). Heterogeneity experiments indicated that there was evidence of statistical heterogeneity in the expected summary results ( I 2 =63%; Figure 2 ). Politi et al [ 30 ] used the 4-item SURE DCS and reported that there was no difference between the 2 groups in terms of decisional conflict ( P >.05).

Decision Regret
In total, 3 studies [ 28 , 29 , 39 ] used DRS to investigate the impact of decision regret in PMBR. The meta-analysis showed that the difference in decision regret after the intervention was not statistically significant compared with the control group (MD=–1.55, 95% CI –6.00 to 2.90; P =.49). Heterogeneity experiments indicated that there was evidence of no statistical heterogeneity in the expected summary results ( I 2 =0%; Figure 2 ).
Informed Choice
In total, 2 studies [ 28 , 29 ] investigated the impact of informed choice by DCS in PMBR surgery. The meta-analysis showed that the difference in informed choice after the intervention was not statistically significant compared to the control group (MD=–2.80, 95% CI –8.54 to 2.94; P =.34). Heterogeneity experiments indicate that there was evidence of no statistical heterogeneity in the expected summary results ( I 2 =0%; Figure 2 ).
We did not conduct a meta-analysis of knowledge as an outcome because most of the instruments measuring knowledge were self-administered. The study by Heller et al [ 37 ] found significantly higher levels of knowledge in the web-based decision aids group, with a mean increase in correctly answered questions of 14% compared to 8% in the control group ( P =.02). Politi et al [ 30 ] found that participants using web-based decision aids had higher objective knowledge, answering an average of 85% (9.35/11) of the questions correctly compared to 58% (6.35/11) in the control group ( P <.001). Similarly, Varlas et al [ 31 ] showed improved knowledge assessment scores in both groups but significantly higher knowledge assessment scores in the intervention group (control=70.8%, SD 15.5%; intervention=83.1%, SD 13.8%; P =.02). However, Manne et al [ 38 ] reported similar effects of web-based decision aids on PMBR knowledge versus the booklet, and Fang et al [ 28 ] also reported no difference in the amount of PMBR-related medical information between web-based decision aids and the control group at 1 week after consultation ( P =.13), suggesting that women in both groups had a similar level of comprehension of medical information, whether using the booklet alone or in combination with the web-based decision aids. Mardinger et al [ 29 ] also reported that both groups had similar scores on the true or false PMBR knowledge questionnaire over time ( P >.05).
Psychological Outcomes
Satisfaction.
In total, 5 studies [ 28 , 29 , 31 , 38 , 39 ] used different scales to investigate the impact of satisfaction. The meta-analyses indicated that web-based decision aids may improve current form:satisfaction compared to controls, but the results were not statistically significant (SMD=0.48, 95% CI 0.00 to 0.95; P =.05). Heterogeneity experiments indicated that there was evidence of statistical heterogeneity in the expected summary results ( I 2 =79%; Figure 3 ). Similarly, Heller et al [ 37 ] reported a higher level of satisfaction with the way in which information about PMBR was obtained in the web-based decision aids group than in the control group ( P =.03).

A total of 3 studies used Hospital Anxiety and Depression Scale and State-Trait Anxiety Inventory [ 28 , 31 , 38 ] to investigate the impact of anxiety in PMBR. The meta-analysis showed that there was no statistically significant difference in the combination of SMD after intervention (SMD=0.04, 95% CI –0.50 to 0.58; P =.88). Heterogeneity experiments indicated that there was evidence of statistical heterogeneity in the expected summary results ( I 2 =61%; Figure 3 ). Heller et al [ 37 ] reported that in the web-based decision aids group, there was a trend toward lower levels of anxiety between the preoperative and postoperative visits, but the difference between the groups was not significant as determined by generalized estimating equation modeling.
Choice of Surgery
The surgical choices differed between the two groups in the study by Fang et al [ 28 ]: 56% (27/48) in the web-based decision aids group and 46% (22/48) in the control group opted for immediate PMBR ( P =.05). In addition, most patients chose implantable PMBR, with no difference between groups. Notably, the web-based decision aids group in the study by Mardinger et al [ 29 ] was unbalanced in terms of the choice of type of PMBR, with 10 (36%) women in the web-based decision aids group refusing PMBR compared with 6 (21%) women in the control group ( P =.20). The results of the study by Politi et al [ 30 ] showed that 95 (79.2%) women underwent reconstruction; among them, nearly all (92/95, 97%) underwent immediate PMBR, and there were no differences between groups in median preference scores for reconstruction, type, or time.
Evaluation of the Intervention
In total, 3 studies reported different benefits of web-based decision aids compared to controls. Heller et al [ 37 ] reported an upward trend in the number of patients in the web-based decision aids group who reported that they received all the necessary information and improved their ability to choose a PMBR plan, but the difference between the groups was not significant. Manne et al [ 38 ] reported that 81% of participants in the web-based decision aids found logging in and navigating easy and the length of time was rated as “just right,” and that the web-based decision aids were more helpful, interesting, and valuable than the brochures. Sherman et al [ 39 ] found that women in the intervention group found the web-based decision aids to be 2.94 (SD 0.76) informative, very useful, easy to use, contained enough information, and helped them to clarify their reconstruction ideas. However, Varelas et al [ 31 ] reported that surgeon satisfaction was also significantly higher in the intervention group than in the control group. Meanwhile, consultation time was shorter in the intervention group, but the difference was not statistically significant ( P =.46). Similarly, Politi et al [ 30 ] reported no difference between the web-based decision aids group and the control group in terms of mean counseling time after the intervention (29.7 vs 30.0 minutes; P >.05). Mardinger et al [ 29 ] showed that although women used both decision aids with comparable frequency, the total time spent counseling and the time spent per counseling session was significantly greater for women in the intervention than that for the control group ( P <.05). Women in the study by Fang et al [ 28 ] indicated no difference between the 2 groups in terms of perceived impact and utility of web-based decision aids on PMBR decisions.
Sensitivity Analysis
We conducted sensitivity analyses of decision conflict, satisfaction, and anxiety by removing each study. Sensitivity analysis showed that for decision conflict and satisfaction, after removing 1 study [ 31 ], contrary to the previous results, web-based decision aids did improve satisfaction (the I 2 range was 79%-12%) but did not improve decision conflict (the I 2 range was 63%-2%). We found that by removing the study by Manne et al [ 38 ], the stability of anxiety did not change but the heterogeneity was reduced from 62% to 0% ( Figure 4 ).

Risk of Bias
Figure 5 [ 28 - 31 , 37 - 39 ] presents the summary of the risk of deviation for the included studies. In 6 [ 28 - 31 , 37 , 39 ] of the 7 studies, the description of the method used in random assignment was clearly stated (ie, web-based automated randomization software and random number generator), and in the remaining study [ 38 ], the information obtained about random assignment was insufficient to make a definitive judgment. Of the 7 studies, 5 [ 30 , 31 , 37 - 39 ] were unable to make definitive judgments in this area because of underreporting, whereas in the remaining 2 trials [ 28 , 29 ] sufficient information was obtained about allocation concealment (individually sealed envelopes to conceal allocation). Furthermore, 6 studies [ 28 - 30 , 37 - 39 ] were judged to be at unclear risk of bias because the effect of unblinding was unknown, and 1 study [ 31 ] described the blinding of participants. Seven studies [ 28 - 31 , 37 - 39 ] achieved blinding of outcome evaluators (ie, clinic and surgical staff were blinded to condition assignment) or the blinding was unclear, but the outcome was objectively measured and not subjective to interpretation. Incomplete outcome data appeared to be adequately addressed in 7 studies [ 28 - 31 , 37 - 39 ] (ie, incomplete data were fairly evenly balanced across intervention groups or intention-to-treat analyses were reported). In addition, 3 studies [ 28 , 30 , 39 ] underwent clinical registration or reported relevant protocols, showing that outcomes were reported in full. The impact of selective reporting in the remaining 4 studies [ 29 , 31 , 37 , 38 ] was unclear, and this area was judged to be at unclear risk of bias. Information on other potential sources of bias was sufficient. Therefore, this area was judged to be at low risk of bias for all studies [ 28 - 31 , 37 - 39 ].

Certainty of Evidence
We assessed the certainty of evidence for the included RCTs using Grades of Recommendation Assessment, Development, and Evaluation (GRADE; Multimedia Appendix 4 ) except for decision regret, for which the certainty of evidence was low. The certainty of evidence was very low for the rest, that is, decision conflict, satisfaction, anxiety, and informed choice.
Principal Findings
Our systematic review and meta-analysis showed that the modules of web-based decision aids include basic information on PMBR, patient stories, risk assessment, value clarification, and emotion management and that patients can be directed to seek information and obtain personalized decision support based on their individual needs. Therefore, these web-based decision aids are helpful and recommended for women. Regarding the effectiveness of web-based decision aids, the results showed that they may improve PMBR knowledge, decision conflict, and satisfaction but have no effect on informed choice, decision regret, or anxiety. The overall GRADE quality of evidence for decision regret was low, and the overall GRADE quality of evidence for informed choice, decision conflict, and anxiety was very low.
The Content of Web-Based Decision Aids
First, regarding the content of web-based decision aids, few of the studies included in our systematic review and meta-analyses reported comprehensive development of their web-based decision aids. The types of decisions on which most web-based decision aids primarily focused were PMBR decision types and reconstruction times. In addition, some of the studies reported that the development of the tool was obtained through a decisional needs assessment. Research suggests that people tend to have decisional needs when confronted with known outcomes with multiple choices, uncertain outcomes, or valuing people differently and that unmet needs lead to poor quality decisions, which adversely affect health outcomes [ 40 ]. Research has shown that some patients have difficulty imagining plastic surgery without photos of women of different body types and skin colors when faced with a decision. Therefore, the use of 3D images during the counseling process is an acceptable web-based decision aid, and the results of our review suggest that web-based decision aids on PMBR decision-making show real photographs of patients by incorporating high-quality, 3D animated images and that viewing 3D images may increase presurgical preparation by giving patients a more realistic understanding of what is actually achievable after PMBR [ 41 ]. There are web-based decision aids that are designed with the goal of making patients more comfortable receiving information in a less-stressful environment outside of the hospital, and it also allows family members and friends who are members of the patient support group, but who may not necessarily be able to participate in the counseling, to receive specific information about the procedure and participate in the decision-making process. Women and their families are allowed to express their views about breast surgery because family members act as advocates and care coordinators in the decision-making process [ 42 ]. In this era of increasing emphasis on evidence-based medicine, the PMBR risk assessment calculator can help individualize and quantify risk to better inform surgical decisions and better manage patient expectations [ 43 ]. The purpose of the values clarification exercise is to help women assess, explore, and identify their personal values and to encourage them to think about how their values affect their decision-making. Using the values clarification exercise can help women increase their satisfaction with their appearance. Patient stories are also important to web-based decision aids, and research has shown that women express a need to learn about other women’s experiences to gain a deeper understanding of the impact of PMBR on their daily lives. Web-based decision aids have achieved this by telling the stories of patients who have had previous mastectomies, with or without PMBR. These stories illustrate the decision-making experiences of these patients and the impact of their decisions on their daily lives [ 44 ]. Another advantage of web-based decision aids is that they allow patients to absorb the information without being overwhelmed by other information or distracted by other issues. Research has shown that some people feel prepared and emotionally supported for PMBR decision-making, while others feel that the elements of supportive care are missing, making the inclusion of an emotion management module in web-based decision aids essential for women’s psychosocial support [ 45 ]. However, although the internet has become an easily accessible tool, there is still a persistent digital divide. Therefore, special attention should be given to the sociodemographic characteristics of the population, building more resources for health care infrastructure in underserved communities and providing free or discounted Wi-Fi connections and mobile devices in low-income areas [ 46 ]. These actions, combined with the popularity of smartphone users, are measures that may narrow the digital divide [ 21 ].
Effectiveness of Web-Based Decision Aids
In line with the results of a previous meta-analysis [ 26 ], web-based decision aids reduced decision conflict. Decision conflicts were as high as 45.68 (SD 23.40) among women who were newly diagnosed with early-stage BC in China [ 47 ]. Decision conflict was significantly higher among women who chose mastectomy with or without combined reconstruction compared to women who chose conservative breast surgery. Greater decision conflict is associated with less information, higher uncertainty in weighing choices based on personal values, and inadequate social support [ 40 ]. Women may second-guess their decisions after the fact, even if those decisions have already been made. Women who face PMBR decision-making need support in making this complex decision, especially those who do not have a strong preference for PMBR. Decision conflict can be reduced by addressing factors of uncertainty, such as providing information about the benefits and risks of each option and helping patients understand their own values [ 48 ]. Web-based decision aids can improve the quality of PMBR decision-making by enhancing patient knowledge and providing personalized risk assessments, reducing decision conflict [ 18 ].
Uncertainty about whether they are making the best decision can trigger emotional turmoil, and decision regret occurs when women compare the unfavorable outcome of a decision with alternative choices they may have [ 11 , 47 ]. The results of our meta-analysis showed that there was no effect of web-based decision aids on decision regret in the intervention group compared to the control. Women who choose decisions that result in unexpected clinical outcomes or lower-than-expected outcomes will inevitably experience decision regret, a very common but negative emotion, even though the patient’s preferences and needs are honored and considered in their treatment [ 49 ]. Decision regret can be used as an indicator of decision-making quality, which can contribute to performance improvement in the health care system. Other studies from a psychological perspective have shown that if a decision is regretted, the following “preference reversal” may cause patients to favor another unselected option, which may completely offset their health outcomes, with the degree of decision regret varying widely. However, Becerra Pérez et al [ 50 ] reported that most studies reported a low mean DRS, resulting in an overall mean score of 16.5 out of 100 across studies. It is important to note that there is no consensus on specific thresholds for clinically important decision regret based on DRS, and authors have rarely justified their choice of thresholds; therefore, minimum and maximum efficiency may limit our ability to perform statistical analyses [ 51 ].
Previous research has shown that women with BC who use decision aids receive more information that helps them make informed and values-based decisions [ 26 ]. Our results, in contrast, showed no effect of web-based decision aids on informed choice in the intervention group compared to the control group possibly because, compared to other forms, web-based decision aids require more effort. Therefore, some women in the web-based decision aids group may have been less inclined to seek more information and consider it carefully. This may explain why women in the web-based decision aids group did not feel less uninformed about their decisions [ 52 ]. The results of previous meta-analyses [ 25 , 26 ] suggest that web-based decision aids are promising interventions for improving knowledge related to PMBR decision-making and that web-based decision aids can help patients’ knowledge of PMBR and treatment options and can identify patients’ PMBR preferences and goals for quality decision-making with their health care providers; however, it is important to note that in this review, the impact of web-based decision aids on PMBR knowledge was mixed, which may be because most of the current instruments on PMBR decision-making knowledge measurement are self-administered scales. We found that web-based decision aids improved PMBR knowledge compared to a control group of some conventional education [ 37 ], traditional counseling [ 31 ], or conventional pamphlets [ 30 ]. When the control group was using pamphlets [ 19 , 28 ] or noninteractive decision aids [ 29 ] that contained similar information, web-based decision aids did not have a statistically significant effect on PMBR knowledge. Therefore, to elucidate the impact of web-based decision aids on knowledge, measurement studies using validated and sensitive instruments are needed.
Because the initial anxiety experienced by women may be related to the new diagnosis and anticipated surgery, this anxiety lessened once the surgery was over. There was no difference in the level of anxiety experienced after surgery between the 2 groups. Given the severity of a BC diagnosis, it is very reassuring that web-based decision aids did not exacerbate anxiety while providing benefits in terms of patient satisfaction and knowledge as well as surgeon satisfaction. Several studies have shown that patient satisfaction is higher when receiving PMBR information digitally [ 53 ]. Our study also suggests that web-based decision aids improve patient satisfaction with decision-making. Although most of the studies included in our systematic review reported that the use of web-based decision aids increased women’s satisfaction with PMBR, most of the measurement tools used to assess the outcomes used self-administered scales. Therefore, more high-quality evidence, including studies using validated and sensitive instruments, is needed to elucidate the impact of web-based decision aids on satisfaction [ 26 ].
Some of the outcome indicators in this review (ie, decision conflict, satisfaction, and anxiety) showed significant heterogeneity, which may be related to factors such as the fact that the measurement tools were different and the web-based decision aids were delivered in an inconsistent form and content. We conducted sensitivity analyses for decision conflict, satisfaction, and anxiety, and the adjusted total estimates of anxiety did not significantly change these results when studies were progressively omitted, excluding the study by Manne et al [ 38 ]. With respect to decision conflict and satisfaction, the adjusted total estimates changed significantly, a result that excludes the study by Varelas et al [ 31 ]. Contrary to the original results, the effect of web-based decision aids on improving satisfaction was statistically significant, and the effect of web-based decision aids on improving decision conflict was similar to the control group effect; therefore, the effect of web-based decision aids on decision conflict and satisfaction should be carefully interpreted. Regarding the heterogeneity of this meta-analysis, sensitivity analyses showed that the heterogeneity of all outcomes was also reduced by excluding 1 study.
Limitations
Some limitations of this review must be recognized. First, we did not perform an assessment of publication bias because only 7 studies were ultimately included in the analysis, which may cause publication bias. In addition, the included studies had no follow-up surveys and lacked evidence of the long-term impact of the interventions. Our findings serve as a reminder that even when statistical information is effectively communicated, participants may not make estimates of the same order of magnitude after a period. Finally, the number of included studies was small. Some studies had inconsistent outcome indicators and were therefore not included.
Conclusions
This review shows that web-based decision aids can increase knowledge and satisfaction, and reduce levels of decision conflict among women facing PMBR decision-making; however, there is no effect on informed choice, decision regret, or anxiety. Currently, web-based decision aids for women’s PMBR decision-making are relatively easy to implement in terms of content and form. Due to limitations in the number of included studies in our meta-analysis, well-designed studies, including multicenter RCTs using high-quality decision aids, are necessary in the future to further validate our conclusion that web-based decision aids play a role in the quality of decision-making for women facing PMBR.
Acknowledgments
The authors would like to thank the authors of studies in this review who took the time to become involved in guiding the development of this review.
Authors' Contributions
LY, LL, SY, JG, XS, and MZ made substantial contributions to the conception and design, acquisition of data, or analysis and interpretation of data and were involved in drafting the manuscript or revising it critically for important intellectual content. All authors provided final approval for the version to be published and agreed to be accountable for all aspects of the work in ensuring that questions related to the accuracy or integrity of any part of the work are appropriately investigated and resolved.
Conflicts of Interest
None declared.
PRISMA (Preferred Reporting Items for Systematic Reviews and Meta-Analyses) statement.
Search strategy.
Characteristics of the interventions and controls.
Certainty of evidence.
- Arnold M, Morgan E, Rumgay H, Mafra A, Singh D, Laversanne M, et al. Current and future burden of breast cancer: global statistics for 2020 and 2040. Breast. Dec 2022;66:15-23. [ FREE Full text ] [ CrossRef ] [ Medline ]
- Giaquinto AN, Sung H, Miller KD, Kramer JL, Newman LA, Minihan A, et al. Breast cancer statistics, 2022. CA Cancer J Clin. Nov 03, 2022;72(6):524-541. [ FREE Full text ] [ CrossRef ] [ Medline ]
- Pfeiffer RM, Webb-Vargas Y, Wheeler W, Gail MH. Proportion of U.S. trends in breast cancer incidence attributable to long-term changes in risk factor distributions. Cancer Epidemiol Biomarkers Prev. Oct 2018;27(10):1214-1222. [ FREE Full text ] [ CrossRef ] [ Medline ]
- Michaels E, Worthington RO, Rusiecki J. Breast cancer: risk assessment, screening, and primary prevention. Med Clin North Am. Mar 2023;107(2):271-284. [ CrossRef ] [ Medline ]
- Siegel RL, Miller KD, Fuchs HE, Jemal A. Cancer statistics, 2021. CA Cancer J Clin. Jan 12, 2021;71(1):7-33. [ FREE Full text ] [ CrossRef ] [ Medline ]
- Sun L, Ang E, Ang WH, Lopez V. Losing the breast: a meta-synthesis of the impact in women breast cancer survivors. Psychooncology. Feb 16, 2018;27(2):376-385. [ CrossRef ] [ Medline ]
- Kummerow KL, Du L, Penson DF, Shyr Y, Hooks MA. Nationwide trends in mastectomy for early-stage breast cancer. JAMA Surg. Jan 01, 2015;150(1):9-16. [ CrossRef ] [ Medline ]
- Li X, Meng M, Zhao J, Zhang X, Yang D, Fang J, et al. Shared decision-making in breast reconstruction for breast cancer patients: a scoping review. Patient Prefer Adherence. Dec 2021;15:2763-2781. [ FREE Full text ] [ CrossRef ] [ Medline ]
- Santosa KB, Qi J, Kim HM, Hamill JB, Wilkins EG, Pusic AL. Long-term patient-reported outcomes in postmastectomy breast reconstruction. JAMA Surg. Oct 01, 2018;153(10):891-899. [ FREE Full text ] [ CrossRef ] [ Medline ]
- Bargon CA, Young-Afat DA, Ikinci M, Braakenburg A, Rakhorst HA, Mureau MA, et al. Breast cancer recurrence after immediate and delayed postmastectomy breast reconstruction-A systematic review and meta-analysis. Cancer. Oct 01, 2022;128(19):3449-3469. [ FREE Full text ] [ CrossRef ] [ Medline ]
- Lee CN, Deal AM, Huh R, Ubel PA, Liu YJ, Blizard L, et al. Quality of patient decisions about breast reconstruction after mastectomy. JAMA Surg. Aug 01, 2017;152(8):741-748. [ FREE Full text ] [ CrossRef ] [ Medline ]
- Hoefel L, O’Connor AM, Lewis KB, Boland L, Sikora L, Hu J, et al. 20th anniversary update of the Ottawa decision support framework part 1: a systematic review of the decisional needs of people making health or social decisions. Med Decis Making. Jul 13, 2020;40(5):555-581. [ CrossRef ]
- Ter Stege JA, Oldenburg HS, Woerdeman LA, Witkamp AJ, Kieffer JM, van Huizum MA, et al. Decisional conflict in breast cancer patients considering immediate breast reconstruction. Breast. Feb 2021;55:91-97. [ FREE Full text ] [ CrossRef ] [ Medline ]
- Brehaut JC, O'Connor AM, Wood TJ, Hack TF, Siminoff L, Gordon E, et al. Validation of a decision regret scale. Med Decis Making. Jul 02, 2016;23(4):281-292. [ CrossRef ]
- Fortunato L, Loreti A, Cortese G, Spallone D, Toto V, Cavaliere F, et al. Regret and quality of life after mastectomy with or without reconstruction. Clin Breast Cancer. Jun 2021;21(3):162-169. [ CrossRef ] [ Medline ]
- Cai L, Momeni A. The impact of reconstructive modality and postoperative complications on decision regret and patient-reported outcomes following breast reconstruction. Aesthetic Plast Surg. Apr 29, 2022;46(2):655-660. [ CrossRef ] [ Medline ]
- Pinker K, Chin J, Melsaether AN, Morris EA, Moy L. Precision medicine and radiogenomics in breast cancer: new approaches toward diagnosis and treatment. Radiology. Jun 2018;287(3):732-747. [ CrossRef ] [ Medline ]
- Sowa Y, Inafuku N, Tsuge I, Yamanaka H, Katsube M, Sakamoto M, et al. Development and implementation of a decision aid for post-mastectomy breast reconstruction for Japanese women with breast cancer: a field-testing study. Breast Cancer. Jul 18, 2023;30(4):570-576. [ CrossRef ] [ Medline ]
- Manne SL, Topham N, Kirstein L, Virtue SM, Brill K, Devine KA, et al. Attitudes and decisional conflict regarding breast reconstruction among breast cancer patients. Cancer Nurs. 2016;39(6):427-436. [ FREE Full text ] [ CrossRef ] [ Medline ]
- Stacey D, Bennett CL, Barry MJ, Col NF, Eden KB, Holmes-Rovner M, et al. Decision aids for people facing health treatment or screening decisions. Cochrane Database Syst Rev. Oct 05, 2011;4(10):CD001431. [ CrossRef ] [ Medline ]
- Yu L, Li P, Yang S, Guo P, Zhang X, Liu N, et al. Web-based decision aids to support breast cancer screening decisions: systematic review and meta-analysis. J Comp Eff Res. Oct 2020;9(14):985-1002. [ FREE Full text ] [ CrossRef ] [ Medline ]
- Baptista S, Teles Sampaio E, Heleno B, Azevedo LF, Martins C. Web-based versus usual care and other formats of decision aids to support prostate cancer screening decisions: systematic review and meta-analysis. J Med Internet Res. Jun 26, 2018;20(6):e228. [ FREE Full text ] [ CrossRef ] [ Medline ]
- Tong G, Geng Q, Wang D, Liu T. Web-based decision aids for cancer clinical decisions: a systematic review and meta-analysis. Support Care Cancer. Nov 08, 2021;29(11):6929-6941. [ CrossRef ] [ Medline ]
- Paraskeva N, Guest E, Lewis-Smith H, Harcourt D. Assessing the effectiveness of interventions to support patient decision making about breast reconstruction: a systematic review. Breast. Aug 2018;40:97-105. [ FREE Full text ] [ CrossRef ] [ Medline ]
- Berlin NL, Tandon VJ, Hawley ST, Hamill JB, MacEachern MP, Lee CN, et al. Feasibility and efficacy of decision aids to improve decision making for postmastectomy breast reconstruction: a systematic review and meta-analysis. Med Decis Making. Dec 27, 2018;39(1):5-20. [ CrossRef ]
- Yang S, Yu L, Zhang C, Xu M, Tian Q, Cui X, et al. Effects of decision aids on breast reconstruction: a systematic review and meta-analysis of randomised controlled trials. J Clin Nurs. Apr 22, 2023;32(7-8):1025-1044. [ CrossRef ] [ Medline ]
- Zhao A, Larbi M, Miller K, O'Neill S, Jayasekera J. A scoping review of interactive and personalized web-based clinical tools to support treatment decision making in breast cancer. Breast. Feb 2022;61:43-57. [ FREE Full text ] [ CrossRef ] [ Medline ]
- Fang SY, Lin PJ, Kuo YL. Long-term effectiveness of a decision support app (Pink Journey) for women considering breast reconstruction surgery: pilot randomized controlled trial. JMIR Mhealth Uhealth. Dec 10, 2021;9(12):e31092. [ FREE Full text ] [ CrossRef ] [ Medline ]
- Mardinger C, Steve AK, Webb C, Sherman KA, Temple-Oberle C. Breast reconstruction decision aids decrease decisional conflict and improve decisional satisfaction: a randomized controlled trial. Plast Reconstr Surg. Feb 01, 2023;151(2):278-288. [ CrossRef ] [ Medline ]
- Politi MC, Lee CN, Philpott-Streiff SE, Foraker RE, Olsen MA, Merrill C, et al. A randomized controlled trial evaluating the BREASTChoice tool for personalized decision support about breast reconstruction after mastectomy. Ann Surg. Feb 2020;271(2):230-237. [ CrossRef ] [ Medline ]
- Varelas L, Egro FM, Evankovich N, Nguyen V. A randomized controlled trial to assess the use of a virtual decisional aid to improve knowledge and patient satisfaction in women considering breast reconstruction following mastectomy. Cureus. Dec 10, 2020;12(12):e12018. [ FREE Full text ] [ CrossRef ] [ Medline ]
- Page MJ, McKenzie JE, Bossuyt PM, Boutron I, Hoffmann TC, Mulrow CD, et al. The PRISMA 2020 statement: an updated guideline for reporting systematic reviews. J Clin Epidemiol. Jun 2021;134:178-189. [ FREE Full text ] [ CrossRef ] [ Medline ]
- Cumpston M, Li T, Page MJ, Chandler J, Welch VA, Higgins JP, et al. Updated guidance for trusted systematic reviews: a new edition of the Cochrane Handbook for Systematic Reviews of Interventions. Cochrane Database Syst Rev. Oct 03, 2019;10(10):ED000142. [ FREE Full text ] [ CrossRef ] [ Medline ]
- Lee AY, Wong AK, Hung TT, Yan J, Yang S. Nurse-led telehealth intervention for rehabilitation (telerehabilitation) among community-dwelling patients with chronic diseases: systematic review and meta-analysis. J Med Internet Res. Nov 02, 2022;24(11):e40364. [ FREE Full text ] [ CrossRef ] [ Medline ]
- Higgins JP, Green S. Cochrane Handbook for Systematic Reviews of Interventions. London, UK. The Cochrane Collaboration; 2008.
- Copas J, Shi JQ. Meta-analysis, funnel plots and sensitivity analysis. Biostatistics. Sep 2000;1(3):247-262. [ CrossRef ] [ Medline ]
- Heller L, Parker PA, Youssef A, Miller MJ. Interactive digital education aid in breast reconstruction. Plast Reconstr Surg. 2008;122(3):717-724. [ CrossRef ]
- Manne SL, Topham N, D'Agostino TA, Myers Virtue S, Kirstein L, Brill K, et al. Acceptability and pilot efficacy trial of a web-based breast reconstruction decision support aid for women considering mastectomy. Psychooncology. Dec 18, 2016;25(12):1424-1433. [ FREE Full text ] [ CrossRef ] [ Medline ]
- Sherman KA, Shaw LK, Winch CJ, Harcourt D, Boyages J, Cameron LD, et al. Reducing decisional conflict and enhancing satisfaction with information among women considering breast reconstruction following mastectomy: results from the BRECONDA randomized controlled trial. Plast Reconstr Surg. Oct 2016;138(4):592e-602e. [ CrossRef ] [ Medline ]
- O'Connor AM, Drake ER, Wells GA, Tugwell P, Laupacis A, Elmslie T. A survey of the decision-making needs of Canadians faced with complex health decisions. Health Expect. Jun 14, 2003;6(2):97-109. [ FREE Full text ] [ CrossRef ] [ Medline ]
- McCrorie AD, Begley AM, Chen JJ, McCorry NK, Paget G, McIntosh SA. Improving preparedness prior to reconstructive breast surgery via inclusion of 3D images during pre-operative counselling: a qualitative analysis. BMC Womens Health. Aug 31, 2021;21(1):323. [ FREE Full text ] [ CrossRef ] [ Medline ]
- Flitcroft K, Brennan M, Spillane A. Making decisions about breast reconstruction: a systematic review of patient-reported factors influencing choice. Qual Life Res. Sep 10, 2017;26(9):2287-2319. [ CrossRef ] [ Medline ]
- Kim JY, Mlodinow AS, Khavanin N, Hume KM, Simmons CJ, Weiss MJ, et al. Individualized risk of surgical complications: an application of the breast reconstruction risk assessment score. Plast Reconstr Surg Glob Open. 2015;3(5):e405. [ CrossRef ]
- Hung YT, Wu CF, Liang TH, Chou SS, Chen GL, Wu PN, et al. Developing a decision-aid website for breast cancer surgery: an action research approach. J Med Internet Res. Feb 04, 2019;21(2):e10404. [ FREE Full text ] [ CrossRef ] [ Medline ]
- Hansen ST, Willemoes Rasmussen LA. 'At least there is something in my bra': a qualitative study of women's experiences with oncoplastic breast surgery. J Adv Nurs. Oct 07, 2022;78(10):3304-3319. [ FREE Full text ] [ CrossRef ] [ Medline ]
- Sohn M, Yang J, Sohn J, Lee JH. Digital healthcare for dementia and cognitive impairment: a scoping review. Int J Nurs Stud. Apr 2023;140:104413. [ CrossRef ] [ Medline ]
- Zhuang HZ, Wang L, Yu XF, Chan SW, Gao YX, Li XQ, et al. Effects of decisional conflict, decision regret and self-stigma on quality of life for breast cancer survivors: a cross-sectional, multisite study in China. J Adv Nurs. Oct 2022;78(10):3261-3272. [ CrossRef ] [ Medline ]
- Subramanian L, Zhao J, Zee J, Knaus M, Fagerlin A, Perry E, et al. Use of a decision aid for patients considering peritoneal dialysis and in-center hemodialysis: a randomized controlled trial. Am J Kidney Dis. Sep 2019;74(3):351-360. [ FREE Full text ] [ CrossRef ] [ Medline ]
- Xu RH, Zhou LM, Wong EL, Wang D, Chang JH. Psychometric evaluation of the Chinese version of the decision regret scale. Front Psychol. Dec 3, 2020;11:583574. [ FREE Full text ] [ CrossRef ] [ Medline ]
- Becerra Pérez MM, Menear M, Brehaut JC, Légaré F. Extent and predictors of decision regret about health care decisions. Med Decis Making. Mar 14, 2016;36(6):777-790. [ CrossRef ]
- Bruce L, Khouri AN, Bolze A, Ibarra M, Richards B, Khalatbari S, et al. Long-term regret and satisfaction with decision following gender-affirming mastectomy. JAMA Surg. Oct 01, 2023;158(10):1070-1077. [ CrossRef ] [ Medline ]
- Osaka W, Nakayama K. Effect of a decision aid with patient narratives in reducing decisional conflict in choice for surgery among early-stage breast cancer patients: a three-arm randomized controlled trial. Patient Educ Couns. Mar 2017;100(3):550-562. [ CrossRef ] [ Medline ]
- Hoffman AS, Cantor SB, Fingeret MC, Housten AJ, Hanson SE, McGee JH, et al. Considering breast reconstruction after mastectomy: a patient decision aid video and workbook. Plast Reconstr Surg Glob Open. Nov 2019;7(11):e2500. [ FREE Full text ] [ CrossRef ] [ Medline ]
Abbreviations
Edited by S Ma, S Gardezi; submitted 22.10.23; peer-reviewed by A Trojan, T Lund-Jacobsen; comments to author 13.03.24; revised version received 16.04.24; accepted 16.04.24; published 27.05.24.
©Lin Yu, Jianmei Gong, Xiaoting Sun, Min Zang, Lei Liu, Shengmiao Yu. Originally published in the Journal of Medical Internet Research (https://www.jmir.org), 27.05.2024.
This is an open-access article distributed under the terms of the Creative Commons Attribution License (https://creativecommons.org/licenses/by/4.0/), which permits unrestricted use, distribution, and reproduction in any medium, provided the original work, first published in the Journal of Medical Internet Research, is properly cited. The complete bibliographic information, a link to the original publication on https://www.jmir.org/, as well as this copyright and license information must be included.
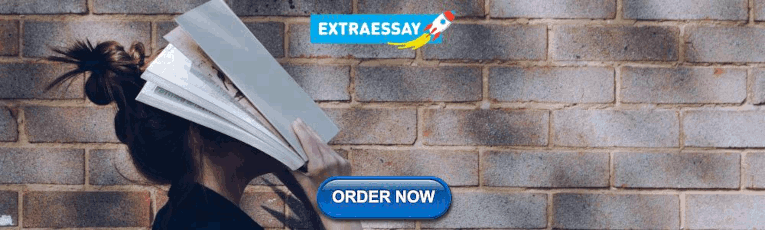
IMAGES
VIDEO
COMMENTS
Step 2: To Decide the Appropriate Statistical Analysis. In this example, the outcome variable is in the categorical and binary form, such as HbA1c level of < 6.5% versus ≥ 6.5%. On the other hand, there are about 3 to 4 independent variables, which can be expressed in both the categorical and numerical form.
Therefore, when estimating the sample size, population size is rarely important in medical research. 37 However, if the population is limited (e.g., in a study that evaluates an academic program, where the population is all students enrolled in the program), then the sample size equations can be adjusted for the population size. 37-39 The size ...
This article reviews basic statistical concepts in sample size estimation, discusses statistical considerations in the choice of a sample size for randomized controlled trials and observational studies, and provides strategies for reducing sample size when planning a study. ... Practical Statistics for Medical Research. CRC Press, Boca Raton ...
Sample Size Calculation in Medical Research Charan et al. 75 Annals of the National Academy of Medical Sciences (India)Vol. 57 No. 2/2021 2021. National Academy of Medical Sciences (India). Introduction Sample size is one of the key aspects of any research study. It is also one of the most overlooked part of clinical research.
Study design and hypothesis. The study design and hypothesis of a research project are two sides of the same coin. When there is a single unifying hypothesis, clear comparison groups and an effect size, e.g., drug A will reduce blood pressure 10 % more than drug B, then the study design becomes clear and the sample size can be calculated with relative ease.
1. Sample Size Calc ulation in Medical Rese arch: A Primer. Jaykaran Charan Rimplejeet Kaur Pankaj Bhardwaj Kuldeep S ingh Sneha R. Ambwani. Sanjeev Misra. Address for correspondence Rimplejeet ...
Sample Size Calculation in Medical Research: A Primer Go to citation Crossref Google Scholar Medication non‐adherence after allogeneic hematopoietic cell transplan...
any reviews on sample size estimation have focused more on specific study designs which often present technical equations and formula that are boring to statistically naïve health researchers. Therefore, this compendium reviews all the common sample size estimation formula in social science and health research with the aim of providing basic guidelines and principles to achieve valid sample ...
Presents the practical and statistical information needed to help investigators decide how large a sample to select from a population targeted for a health study or survey. Desgned to perform a cookbook function, the book uses explanatory text and abundant tabular calculations to vastly simplify the task of determining the minimum sample size ...
the sample size is low, the research outcome might not be reproduc - ible.1 Informal guidelines for sample size based on the experience ... International Medical Research Centre, Mail Code 6656, P.O. Box 9515, Jeddah 21423, Saudi Arabia. Email: [email protected] Abstract
Sample Size Calculator Determines the minimum number of subjects for adequate study power ClinCalc.com » Statistics » Sample ... (Power = 1 - β). Most medical literature uses a beta cut-off of 20% (0.2) -- indicating a 20% chance that a significant difference is missed. Post-Hoc Power Analysis. To calculate the post-hoc statistical power ...
This guide has sample size ready-reckoners for a number of common research designs. Each section is self-contained You need only read the section that applies to you. Examples There are examples in each section, aimed at helping you to describe your sample size calculation in a research proposal or ethics committee submission.
Therefore, when estimating the sample size, population size is rarely important in medical research. 37 However, if the population is limited (e.g., in a study that evaluates an academic program, where the population is all students enrolled in the program), then the sample size equations can be adjusted for the population size. 37 , 38 , 39 ...
Abstract. Calculation of exact sample size is an important part of research design. It is very important to understand that different study design need different method of sample size calculation and one formula cannot be used in all designs. In this short review we tried to educate researcher regarding various method of sample size calculation ...
Sample Size Calculators. If you are a clinical researcher trying to determine how many subjects to include in your study or you have another question related to sample size or power calculations, we developed this website for you. Our approach is based on Chapters 5 and 6 in the 4th edition of Designing Clinical Research (DCR-4), but the ...
Sample size computation for survey type of studies, observation studies and experimental studies based on means and proportions or rates, sensitivity - specificity tests for assessing the categorical outcome are presented in detail. Over the last decades, considerable interest has been focused on medical research designs and sample size ...
An essential part of any medical research is to decide how many subjects need to be studied. A formal sample size calculation is a prerequisite to justify that the study with this size is capable of answering the research questions. This article highlights the statistical principles involved in sample size calculation along with formulae used ...
Results: In total, 7 studies included 579 women and were published between 2008 and 2023, and the sample size in each study ranged from 26 to 222. The results showed that web-based decision aids used audio and video to present the pros and cons of PMBR versus no PMBR, implants versus flaps, and immediate versus delayed PMBR and the appearance ...