- Skip to Content
- Catalog Home
- Institution Home
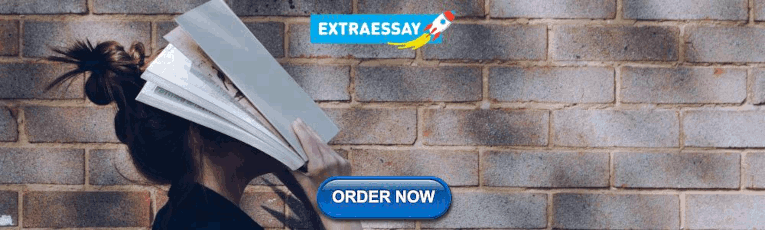
Graduate School of Arts and Sciences Programs and Policies 2023–2024
- Yale University Publications /
- Graduate School of Arts and Sciences /
- Degree-Granting Departments and Programs /
Statistics and Data Science
Current edition: graduate archive . click to change..
203.432.0666 http://statistics.yale.edu M.A., M.S., Ph.D.
Chair Joseph Chang
Directors of Graduate Studies Andrew Barron (24 Hlh, [email protected] ) John Emerson (24 Hlh, [email protected] )
Professors Donald Andrews ( Economics ), Andrew Barron, Jeffrey Brock ( Mathematics ), Joseph Chang, Katarzyna Chawarska ( Child Study Center ), Xiaohong Chen ( Economics ), Nicholas Christakis ( Sociology ), Ronald Coifman ( Mathematics ), James Duncan ( Radiology and Biomedical Imaging ), John Emerson ( Adjunct ), Alan Gerber ( Political Science ), Mark Gerstein ( Molecular Biophysics and Biochemistry ), Anna Gilbert, John Hartigan ( Emeritus ), Edward Kaplan ( School of Management/Operations Research ), Harlan Krumholz ( Internal Medicine ), John Lafferty, Zongming Ma, David Pollard ( Emeritus ), Nils Rudi ( School of Management ), Jasjeet Sekhon, Donna Spiegelman ( Biostatistics ), Daniel Spielman, Hemant Tagare ( Radiology and Biomedical Engineering ), Van Vu ( Mathematics ), Yihong Wu, Heping Zhang ( Biostatistics ), Hongyu Zhao ( Biostatistics ), Harrison Zhou, Steven Zucker ( Computer Science )
Associate Professors P.M. Aronow ( Political Science ), Forrest Crawford ( Biostatistics ), Amin Karbasi ( Electrical Engineering ), Vahideh Manshadi ( School of Management/Operations ), Ethan Meyers ( Visiting ), Sekhar Tatikonda
Assistant Professors Elisa Celis, Zhou Fan, Joshua Kalla ( Political Science ), Roy Lederman, Lu Lu, Fredrik Savje ( Political Science ), Dustin Scheinost ( Radiology and Biomedical Imaging ), Andre Wibisono ( Computer Science ), Zhuoran Yang, Ilker Yildirim ( Psychology ), Ilias Zadik
Fields of Study
Fields of study include the main areas of statistical theory (with emphasis on foundations, Bayes theory, decision theory, nonparametric statistics), probability theory (stochastic processes, asymptotics, weak conver g ence), information theory, bioinformatics and genetics, classification, data mining and machine learning, neural nets, network science, optimization, statistical computing, and graphical models and methods.
Special Requirements for the Ph.D. Degree in Statistics and Data Science
There is no foreign language requirement. Students take at least twelve courses, usually during the first two years. The department strongly recommends that students take S&DS 551 (Stochastic Processes), S&DS 600 (Advanced Probability), S&DS 610 (Statistical Inference), S&DS 612 (Linear Models), S&DS 625 (Statistical Case Studies), S&DS 631 (Optimization and Computation), S&DS 632 (Advanced Optimization Techniques), and S&DS 661 (Data Analysis), and requires that students take S&DS 626 (Practical Work). Substitutions are possible with the permission of the director of graduate studies (DGS); courses from other complementary departments such as Mathematics and Computer Science are encouraged. With the permission of the DGS and under special circumstances, appropriate courses may be taken at the undergraduate level in departments outside of Statistics and Data Science to fulfill these elective requirements.
The qualifying examination consists of three parts: a written report on an analysis of a data set, one or more written examination(s), and an oral examination. The examinations are taken as scheduled by the department. All parts of the qualifying examination must be completed before the beginning of the third year. A prospectus for the dissertation should be submitted no later than the first week of March in the third year. The prospectus must be accepted by the department before the end of the third year if the student is to register for a fourth year. Upon successful completion of the qualifying examination and the prospectus (and meeting of Graduate School requirements), the student is admitted to candidacy. Students are expected to attend weekly departmental seminars.
Students normally serve as teaching fellows for several terms to acquire professional training. All students are required to be teaching fellows for a minimum of two terms, regardless of the nature of their funding. The timing of this teaching is at the discretion of the DGS.
Combined Ph.D. Program
The Department of Statistics and Data Science also offers, in conjunction with the Department of Political Science, a combined Ph.D. in Statistics and Data Science and Political Science. For further details, see Political Science .
Master’s Degrees
M.A. in Statistics
Three different M.A. in Statistics are offered. All require completion of eight term courses approved by the DGS; of which one must be in probability, one must be in statistical theory, and one must be in data analysis. The remaining five elective courses may include courses from other departments and, with the permission of the DGS and under special circumstances, appropriate courses may be taken at the undergraduate level in departments outside of Statistics and Data Science.
M.A. in Statistics (en route to the Ph.D. in Statistics and Data Science) This degree requires an average grade of HP or higher, and two terms of residence.
M.A. in Statistics (en route to the Ph.D. in other areas of study) Pursuit of this degree requires an application process managed by the DGS of Statistics and Data Science followed by approval from the DGSs from both programs and the cognizant Graduate School dean. All eight courses for this degree must earn grades of HP or higher. Most of the courses for the M.A. in Statistics should be in addition to the requirements of the primary Ph.D. program. This degree also has an academic teaching fellow requirement, to be determined by the DGSs from both programs and the cognizant Graduate School dean.
Terminal M.A. in Statistics Students are also admitted directly to a terminal master of arts program in Statistics. Students must earn an average grade of HP or higher and receive at least one grade of Honors. Full-time students must take a minimum of four courses per term. Part-time students are also accepted into the program. All students are expected to complete two terms of full-time tuition and residence, or the equivalent, at Yale. See Degree Requirements: Terminal M.A./M.S. Degrees, under Policies and Regulations.
Terminal M.S. in Statistics and Data Science Students are also admitted directly to a terminal master of science program in Statistics and Data Science. To qualify for the M.S., the student must successfully complete an approved program of twelve term courses with an average grade of HP or higher and receive at least two grades of Honors, chosen in consultation with the DGS. With the permission of the DGS and under special circumstances, appropriate courses may be taken at the undergraduate level in departments outside of Statistics and Data Science to fulfill elective requirements. Full-time students must take a minimum of four courses per term. Part-time students are also accepted into the program. All students are expected to complete three terms of full-time tuition and residence, or the equivalent, at Yale. See Degree Requirements: Terminal M.A./M.S. Degrees, under Policies and Regulations.
Program information is available online at http://statistics.yale.edu .
S&DS 501a / E&EB 510a, Introduction to Statistics: Life Sciences Jonathan Reuning-Scherer
Statistical and probabilistic analysis of biological problems, presented with a unified foundation in basic statistical theory. Problems are drawn from genetics, ecology, epidemiology, and bioinformatics. TTh 1pm-2:15pm
S&DS 502a, Introduction to Statistics: Political Science Jonathan Reuning-Scherer
Statistical analysis of politics, elections, and political psychology. Problems presented with reference to a wide array of examples: public opinion, campaign finance, racially motivated crime, and public policy. Note: S&DS 501 – 506 offer a basic introduction to statistics, including numerical and graphical summaries of data, probability, hypothesis testing, confidence intervals, and regression. Each course focuses on applications to a particular field of study and is taught jointly by two instructors, one specializing in statistics and the other in the relevant area of application. The first seven weeks are attended by all students in S&DS 501 – 506 together as general concepts and methods of statistics are developed. The course separates for the last six and a half weeks, which develop the concepts with examples and applications. Computers are used for data analysis. These courses are alternatives; they do not form a sequence, and only one may be taken for credit. TTh 1pm-2:15pm
S&DS 503a, Introduction to Statistics: Social Sciences Jonathan Reuning-Scherer
Descriptive and inferential statistics applied to analysis of data from the social sciences. Introduction of concepts and skills for understanding and conducting quantitative research. Note: S&DS 501 – 506 offer a basic introduction to statistics, including numerical and graphical summaries of data, probability, hypothesis testing, confidence intervals, and regression. Each course focuses on applications to a particular field of study and is taught jointly by two instructors, one specializing in statistics and the other in the relevant area of application. The first seven weeks are attended by all students in S&DS 501 – 506 together as general concepts and methods of statistics are developed. The course separates for the last six and a half weeks, which develop the concepts with examples and applications. Computers are used for data analysis. These courses are alternatives; they do not form a sequence, and only one may be taken for credit. TTh 1pm-2:15pm
S&DS 505a, Introduction to Statistics: Medicine Jay Emerson and Jonathan Reuning-Scherer
Statistical methods relied upon in medicine and medical research. Practice in reading medical literature competently and critically, as well as practical experience performing statistical analysis of medical data. Note: S&DS 501 – 506 offer a basic introduction to statistics, including numerical and graphical summaries of data, probability, hypothesis testing, confidence intervals, and regression. Each course focuses on applications to a particular field of study and is taught jointly by two instructors, one specializing in statistics and the other in the relevant area of application. The first seven weeks are attended by all students in S&DS 501 – 506 together as general concepts and methods of statistics are developed. The course separates for the last six and a half weeks, which develop the concepts with examples and applications. Computers are used for data analysis. These courses are alternatives; they do not form a sequence, and only one may be taken for credit. TTh 1pm-2:15pm
S&DS 506a, Introduction to Statistics: Data Analysis Robert Wooster and Jonathan Reuning-Scherer
An introduction to probability and statistics with emphasis on data analysis. Note: S&DS 501 – 506 offer a basic introduction to statistics, including numerical and graphical summaries of data, probability, hypothesis testing, confidence intervals, and regression. Each course focuses on applications to a particular field of study and is taught jointly by two instructors, one specializing in statistics and the other in the relevant area of application. The first seven weeks are attended by all students in S&DS 501 – 506 together as general concepts and methods of statistics are developed. The course separates for the last six and a half weeks, which develop the concepts with examples and applications. Computers are used for data analysis. These courses are alternatives; they do not form a sequence, and only one may be taken for credit. TTh 1pm-2:15pm
S&DS 530a / PLSC 530a, Data Exploration and Analysis Ethan Meyers
Survey of statistical methods: plots, transformations, regression, analysis of variance, clustering, principal components, contingency tables, and time series analysis. The R computing language and web data sources are used. HTBA
S&DS 538a, Probability and Statistics Joseph Chang
Fundamental principles and techniques of probabilistic thinking, statistical modeling, and data analysis. Essentials of probability: conditional probability, random variables, distributions, law of large numbers, central limit theorem, Markov chains. Statistical inference with emphasis on the Bayesian approach: parameter estimation, likelihood, prior and posterior distributions, Bayesian inference using Markov chain Monte Carlo. Introduction to regression and linear models. Computers are used throughout for calculations, simulations, and analysis of data. Prerequisite: after or concurrently with MATH 118 or MATH 120 . TTh 1pm-2:15pm
S&DS 540a, An Introduction to Probability Theory Robert Wooster
Introduction to probability theory. Topics include probability spaces, random variables, expectations and probabilities, conditional probability, independence, discrete and continuous distributions, central limit theorem, Markov chains, and probabilistic modeling. This course may be appropriate for non-S&DS graduate students. Prerequisite: MATH 115 or equivalent. MW 2:30pm-3:45pm
S&DS 541a, Probability Theory Yihong Wu
A first course in probability theory: probability spaces, random variables, expectations and probabilities, conditional probability, independence, some discrete and continuous distributions, central limit theorem, Markov chains, probabilistic modeling. Prerequisite: calculus of functions of several variables. MW 9am-10:15am
S&DS 542a, Theory of Statistics Andrew Barron
Principles of statistical analysis: maximum likelihood, sampling distributions, estimation, confidence intervals, tests of significance, regression, analysis of variance, and the method of least squares. Prerequisite: S&DS 541 . HTBA
S&DS 565a, Introductory Machine Learning John Lafferty
This course covers the key ideas and techniques in machine learning without the use of advanced mathematics. Basic methodology and relevant concepts are presented in lectures, including the intuition behind the methods. Assignments give students hands-on experience with the methods on different types of data. Topics include linear regression and classification, tree-based methods, clustering, topic models, word embeddings, recurrent neural networks, dictionary learning, and deep learning. Examples come from a variety of sources including political speeches, archives of scientific articles, real estate listings, natural images, and others. Programming is central to the course and is based on the Python programming language. TTh 11:35am-12:50pm
S&DS 572a, YData: Data Science for Political Campaigns Joshua Kalla
Political campaigns have become increasingly data driven. Data science is used to inform where campaigns compete, which messages they use, how they deliver them, and among which voters. In this course, we explore how data science is being used to design winning campaigns. Students gain an understanding of what data is available to campaigns, how campaigns use this data to identify supporters, and the use of experiments in campaigns. The course provides students with an introduction to political campaigns, an introduction to data science tools necessary for studying politics, and opportunities to practice the data science skills presented in S&DS 523 . W 1:30pm-3:20pm
S&DS 580a, Neural Data Analysis Ethan Meyers
We discuss data analysis methods that are used in the neuroscience community. Methods include classical descriptive and inferential statistics, point process models, mutual information measures, machine learning (neural decoding) analyses, dimensionality reduction methods, and representational similarity analyses. Each week we read a research paper that uses one of these methods, and we replicate these analyses using the R or Python programming language. Emphasis is on analyzing neural spiking data, although we also discuss other imaging modalities such as magneto/electro-encephalography (EEG/MEG), two-photon imaging, and possibility functional magnetic resonance imaging data (fMRI). Data we analyze includes smaller datasets, such as single neuron recordings from songbird vocal motor system, as well as larger data sets, such as the Allen Brain observatory’s simultaneous recordings from the mouse visual system. TTh 2:30pm-3:45pm
S&DS 600a, Advanced Probability Sekhar Tatikonda
Measure theoretic probability, conditioning, laws of large numbers, convergence in distribution, characteristic functions, central limit theorems, martingales. Some knowledge of real analysis is assumed. TTh 2:30pm-3:45pm
S&DS 610a, Statistical Inference Harrison Zhou
A systematic development of the mathematical theory of statistical inference covering methods of estimation, hypothesis testing, and confidence intervals. An introduction to statistical decision theory. Knowledge of probability theory at the level of S&DS 541 is assumed. TTh 11:35am-12:50pm
S&DS 612a, Linear Models Zongming Ma
The geometry of least squares; distribution theory for normal errors; regression, analysis of variance, and designed experiments; numerical algorithms (with particular reference to the R statistical language); alternatives to least squares. Prerequisites: linear algebra and some acquaintance with statistics. MW 11:35am-12:50pm
S&DS 625a, Statistical Case Studies Brian Macdonald
Statistical analysis of a variety of statistical problems using real data. Emphasis on methods of choosing data, acquiring data, assessing data quality, and the issues posed by extremely large data sets. Extensive computations using R. Enrollment limited; requires permission of the instructor. HTBA
S&DS 627a, Statistical Consulting Jay Emerson
Statistical consulting and collaborative research projects often require statisticians to explore new topics outside their area of expertise. This course exposes students to real problems, requiring them to draw on their expertise in probability, statistics, and data analysis. Students complete the course with individual projects supervised jointly by faculty outside the department and by one of the instructors. Students enroll for both terms ( S&DS 627 and 628 ) and receive one credit at the end of the year. Enrollment limited; requires permission of the instructor. ½ Course cr F 2:30pm-4:30pm
S&DS 631a / AMTH 631a, Optimization and Computation Zhuoran Yang
An introduction to optimization and computation motivated by the needs of computational statistics, data analysis, and machine learning. This course provides foundations essential for research at the intersections of these areas, including the asymptotic analysis of algorithms, an understanding of condition numbers, conditions for optimality, convex optimization, gradient descent, linear and conic programming, and NP hardness. Model problems come from numerical linear algebra and constrained least squares problems. Other useful topics include data structures used to represent graphs and matrices, hashing, automatic differentiation, and randomized algorithms. Prerequisites: multivariate calculus, linear algebra, probability, and permission of the instructor. Enrollment is limited, with preference given to graduate students in Statistics and Data Science. TTh 1pm-2:15pm
S&DS 645b / CB&B 645b, Statistical Methods in Computational Biology Hongyu Zhao
Introduction to problems, algorithms, and data analysis approaches in computational biology and bioinformatics. We discuss statistical issues arising in analyzing population genetics data, gene expression microarray data, next-generation sequencing data, microbiome data, and network data. Statistical methods include maximum likelihood, EM, Bayesian inference, Markov chain Monte Carlo, and methods of classification and clustering; models include hidden Markov models, Bayesian networks, and graphical models. Offered every other year. Prerequisite: S&DS 538 , S&DS 542 , or S&DS 661 . Prior knowledge of biology is not required, but some interest in the subject and a willingness to carry out calculations using R is assumed. Th 10am-11:50am
S&DS 665a, Intermediate Machine Learning John Lafferty
S&DS 365 is a second course in machine learning at the advanced undergraduate or beginning graduate level. The course assumes familiarity with the basic ideas and techniques in machine learning, for example as covered in S&DS 265 . The course treats methods together with mathematical frameworks that provide intuition and justifications for how and when the methods work. Assignments give students hands-on experience with machine learning techniques, to build the skills needed to adapt approaches to new problems. Topics include nonparametric regression and classification, kernel methods, risk bounds, nonparametric Bayesian approaches, graphical models, attention and language models, generative models, sparsity and manifolds, and reinforcement learning. Programming is central to the course, and is based on the Python programming language and Jupyter notebooks. MW 1pm-2:15pm
S&DS 688a, Computational and Statistical Trade-offs in High Dimensional Statistics Ilias Zadik
Modern statistical tasks require the use of both computationally efficient and statistically accurate methods. But, can we always find a computationally efficient method that achieves the information-theoretic optimal statistical guarantees? If not, is this an artifact of our techniques, or a potentially fundamental source of computational hardness? This course surveys a new and growing research area studying such questions on the intersection of high dimensional statistics and theoretical computer science. We discuss various tools to explain the presence of such “computational-to-statistical gaps” for several high dimensional inference models. These tools include the “low-degree polynomials” method, statistical query lower bounds, and more. We also discuss connections with other fields such as statistical physics and cryptography. Prerequisites: maturity with probability theory (equivalent of 241/541) and linear algebra and a familiarity with basic algorithms and mathematical statistics. T 4pm-5:50pm
S&DS 690a, Independent Study Jay Emerson
By arrangement with faculty. Approval of DGS required. HTBA
S&DS 700a, Departmental Seminar Staff
Presentations of recent breakthroughs in statistics and data science. 0 Course cr M 4pm-5:30pm
Print Options
Send Page to Printer
Print this page.
Download Page (PDF)
The PDF will include all information unique to this page.
Download 2022-2023 Graduate PDF
Search form
Institute for foundations of data science debuts with interdisciplinary vision.

The future of data science at Yale will reside on one of the top floors of a re-imagined Kline Tower on Science Hill, starting in 2023 — but the university, its researchers, and the new Institute for Foundations of Data Science (FDS) aren’t waiting for next year.
Doctors, economists, seismologists, psychologists, engineers, computer scientists — faculty from dozens of disciplines — are already using powerful data science tools to infuse their research with next-generation insights. They’re making discoveries in cyber security, urbanization, autism, cardiology, and artificial intelligence.
Now they will be aided by Yale’s new Institute for Foundations of Data Science. Yale launched the new initiative on Oct. 14 with presentations from 20 faculty members currently taking research in bold new directions thanks to innovative mathematical, statistical, and algorithmic methods of working with data.
By integrating faculty from across campus the university will help scholars apply new methods of data science to their work and inspire advances in foundational research in a range of disciplines.

“ I can’t think of anything more important than using data-driven approaches to finding solutions,” said Yale President Peter Salovey, citing climate change, political polarization, health care, and medical discoveries as vital areas for data-driven research that “transcend” a single department or discipline.
“ This is a university-wide priority,” Salovey added. “It’s an exciting moment, and there’s a lot more to come.”
In recent years, the university has made major investments in faculty and infrastructure to elevate Yale’s data science capabilities. In 2018, the University Science Strategy Committee identified data science as a leading priority ; since then, Yale has hired 25 new ladder faculty members in the Departments of Computer Science, and Statistics and Data Science.

“ This is a day we’ve been working toward for a long time,” said Provost Scott Strobel, who led the University Science Strategy Committee, during the recent launch event. He noted that faculty members have told him that “the future of science is data science.”
FDS’s eventual home will be on an upper floor of Kline Tower, which is being renovated to house the Faculty of Arts and Sciences’ departments of Statistics and Data Science, Mathematics, and Astronomy. The renovation will be completed in 2023.
The institute combines the foundations of data science methodology with applications that can benefit society, said Daniel Spielman, the inaugural James A. Attwood Director of FDS, and a Sterling Professor of Computer Science, Statistics and Data Science, and Mathematics.
FDS does this by integrating faculty from departments and schools across the university.
“ Almost every major advance in data science started with a scholar in another field who had a problem they could not solve,” said Spielman, who recently won the Breakthrough Prize in Mathematics for his work in theoretical computer science and mathematics.

Some of the faculty members who outlined their research during the Oct. 14 launch event included:
- Karen Seto, the Frederick C. Hixon Professor of Geography and Urbanization Science at the School of the Environment, who applies machine learning techniques to a wealth of satellite imaging data, documenting and evaluating changes to the landscape due to urbanization.
- Rohan Khera, an assistant professor of medicine at Yale School of Medicine and assistant professor of biostatistics at Yale School of Public Health, who uses novel methods to detect heart disease signatures from data in wearable technology.
- James Duncan, the Ebenezer K. Hunt Professor of Biomedical Engineering, Electrical Engineering and Radiology, and Biomedical Imaging at the School of Engineering & Applied Science (SEAS), who is developing predictive models to gauge the effectiveness of autism therapy, based on data science techniques applied to brain imaging.
- Jeffrey Park, professor of Earth and Planetary Sciences in the Faculty of Arts and Sciences (FAS), who uses data science methods to make a wealth of interactive seismic wave information available to researchers in real time.
- Amin Karbasi, associate professor of electrical engineering, computer science, and statistics and data science at SEAS, who is exploring innovative ways to design the human labeling component of raw data that is used in artificial intelligence systems.
- Bryan Kelly, a professor of finance at the School of Management, who uses machine learning methods to make more effective predictions about key components in asset valuation.
- Forrest Crawford, associate professor of biostatistics, statistics and data science, ecology and evolutionary biology, and management, who uses advanced mathematics models to reveal hidden structures in epidemiology, such as mapping and tracking social distancing behavior during the COVID-19 pandemic.
- Elisa Celis, an assistant professor of statistics and data science at FAS, who analyzes the objectivity of data used in a variety of algorithms affecting everything from politics and policing to consumer behavior.
“ Together, we have an opportunity to make an incredible impact,” Celis said.
FDS is planning additional events around campus in the coming months to highlight ongoing data science-related research. The institute is also seeking applicants for multi-year postdoctoral positions for independent scholars working on the foundations of data science.
“ This is just a taste of what’s happening,” Spielman said. “We could easily hear from 80 faculty members — but we’d be here all day. That’s why we’re starting an institute.”
Campus & Community
Science & Technology
Social Sciences
Media Contact
Fred Mamoun: [email protected] , 203-436-2643

Mapping the seafloor sediment superhighway

Maurie McInnis returns to Yale in a new role: President-elect

Screening room: Yale Law clinic offers legal guidance for documentaries

To avoid infection spread, how long a quarantine is sufficient? It depends
- Show More Articles
DiscoverDataScience.org
PhD in Data Science – Your Guide to Choosing a Doctorate Degree Program
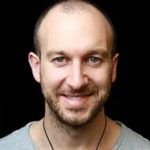
Created by aasif.faizal
Professional opportunities in data science are growing incredibly fast. That’s great news for students looking to pursue a career as a data scientist. But it also means that there are a lot more options out there to investigate and understand before developing the best educational path for you.
A PhD is the most advanced data science degree you can get, reflecting a depth of knowledge and technical expertise that will put you at the top of your field.
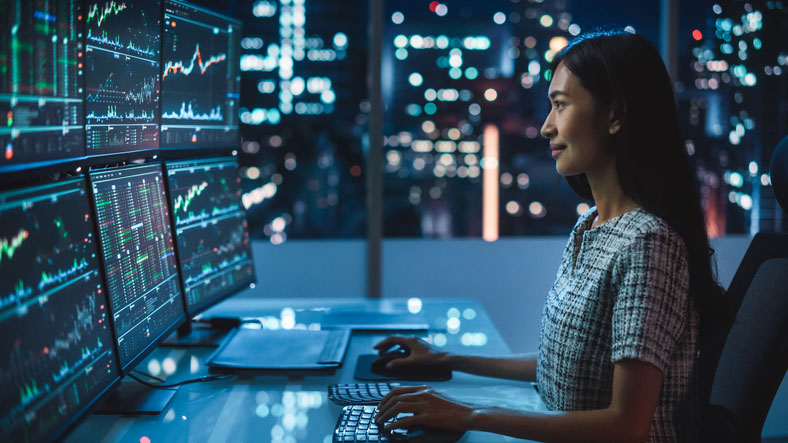
This means that PhD programs are the most time-intensive degree option out there, typically requiring that students complete dissertations involving rigorous research. This means that PhDs are not for everyone. Indeed, many who work in the world of big data hold master’s degrees rather than PhDs, which tend to involve the same coursework as PhD programs without a dissertation component. However, for the right candidate, a PhD program is the perfect choice to become a true expert on your area of focus.
If you’ve concluded that a data science PhD is the right path for you, this guide is intended to help you choose the best program to suit your needs. It will walk through some of the key considerations while picking graduate data science programs and some of the nuts and bolts (like course load and tuition costs) that are part of the data science PhD decision-making process.
Data Science PhD vs. Masters: Choosing the right option for you
If you’re considering pursuing a data science PhD, it’s worth knowing that such an advanced degree isn’t strictly necessary in order to get good work opportunities. Many who work in the field of big data only hold master’s degrees, which is the level of education expected to be a competitive candidate for data science positions.
So why pursue a data science PhD?
Simply put, a PhD in data science will leave you qualified to enter the big data industry at a high level from the outset.
You’ll be eligible for advanced positions within companies, holding greater responsibilities, keeping more direct communication with leadership, and having more influence on important data-driven decisions. You’re also likely to receive greater compensation to match your rank.
However, PhDs are not for everyone. Dissertations require a great deal of time and an interest in intensive research. If you are eager to jumpstart a career quickly, a master’s program will give you the preparation you need to hit the ground running. PhDs are appropriate for those who want to commit their time and effort to schooling as a long-term investment in their professional trajectory.
For more information on the difference between data science PhD’s and master’s programs, take a look at our guide here.
Topics include:
- Can I get an Online Ph.D in Data Science?
- Overview of Ph.d Coursework
Preparing for a Doctorate Program
Building a solid track record of professional experience, things to consider when choosing a school.
- What Does it Cost to Get a Ph.D in Data Science?
- School Listings
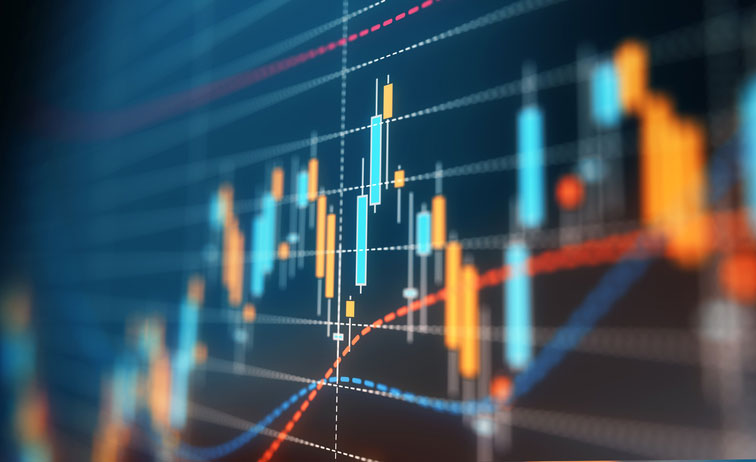
Data Science PhD Programs, Historically
Historically, data science PhD programs were one of the main avenues to get a good data-related position in academia or industry. But, PhD programs are heavily research oriented and require a somewhat long term investment of time, money, and energy to obtain. The issue that some data science PhD holders are reporting, especially in industry settings, is that that the state of the art is moving so quickly, and that the data science industry is evolving so rapidly, that an abundance of research oriented expertise is not always what’s heavily sought after.
Instead, many companies are looking for candidates who are up to date with the latest data science techniques and technologies, and are willing to pivot to match emerging trends and practices.
One recent development that is making the data science graduate school decisions more complex is the introduction of specialty master’s degrees, that focus on rigorous but compact, professional training. Both students and companies are realizing the value of an intensive, more industry-focused degree that can provide sufficient enough training to manage complex projects and that are more client oriented, opposed to research oriented.
However, not all prospective data science PhD students are looking for jobs in industry. There are some pretty amazing research opportunities opening up across a variety of academic fields that are making use of new data collection and analysis tools. Experts that understand how to leverage data systems including statistics and computer science to analyze trends and build models will be in high demand.
Can You Get a PhD in Data Science Online?
While it is not common to get a data science Ph.D. online, there are currently two options for those looking to take advantage of the flexibility of an online program.
Indiana University Bloomington and Northcentral University both offer online Ph.D. programs with either a minor or specialization in data science.
Given the trend for schools to continue increasing online offerings, expect to see additional schools adding this option in the near future.
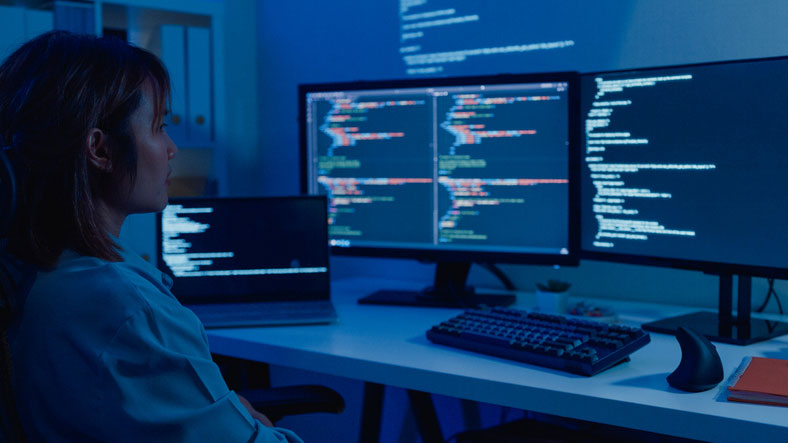
Overview of PhD Coursework
A PhD requires a lot of academic work, which generally requires between four and five years (sometimes longer) to complete.
Here are some of the high level factors to consider and evaluate when comparing data science graduate programs.
How many credits are required for a PhD in data science?
On average, it takes 71 credits to graduate with a PhD in data science — far longer (almost double) than traditional master’s degree programs. In addition to coursework, most PhD students also have research and teaching responsibilities that can be simultaneously demanding and really great career preparation.
What’s the core curriculum like?
In a data science doctoral program, you’ll be expected to learn many skills and also how to apply them across domains and disciplines. Core curriculums will vary from program to program, but almost all will have a core foundation of statistics.
All PhD candidates will have to take a qualifying exam. This can vary from university to university, but to give you some insight, it is broken up into three phases at Yale. They have a practical exam, a theory exam and an oral exam. The goal is to make sure doctoral students are developing the appropriate level of expertise.
Dissertation
One of the final steps of a PhD program involves presenting original research findings in a formal document called a dissertation. These will provide background and context, as well as findings and analysis, and can contribute to the understanding and evolution of data science. A dissertation idea most often provides the framework for how a PhD candidate’s graduate school experience will unfold, so it’s important to be thoughtful and deliberate while considering research opportunities.
Since data science is such a rapidly evolving field and because choosing the right PhD program is such an important factor in developing a successful career path, there are some steps that prospective doctoral students can take in advance to find the best-fitting opportunity.
Join professional associations
Even before being fully credentials, joining professional associations and organizations such as the Data Science Association and the American Association of Big Data Professionals is a good way to get exposure to the field. Many professional societies are welcoming to new members and even encourage student participation with things like discounted membership fees and awards and contest categories for student researchers. One of the biggest advantages to joining is that these professional associations bring together other data scientists for conference events, research-sharing opportunities, networking and continuing education opportunities.
Leverage your social network
Be on the lookout to make professional connections with professors, peers, and members of industry. There are a number of LinkedIn groups dedicated to data science. A well-maintained professional network is always useful to have when looking for advice or letters of recommendation while applying to graduate school and then later while applying for jobs and other career-related opportunities.
Kaggle competitions
Kaggle competitions provide the opportunity to solve real-world data science problems and win prizes. A list of data science problems can be found at Kaggle.com . Winning one of these competitions is a good way to demonstrate professional interest and experience.
Internships
Internships are a great way to get real-world experience in data science while also getting to work for top names in the world of business. For example, IBM offers a data science internship which would also help to stand out when applying for PhD programs, as well as in seeking employment in the future.
Demonstrating professional experience is not only important when looking for jobs, but it can also help while applying for graduate school. There are a number of ways for prospective students to gain exposure to the field and explore different facets of data science careers.
Get certified
There are a number of data-related certificate programs that are open to people with a variety of academic and professional experience. DeZyre has an excellent guide to different certifications, some of which might help provide good background for graduate school applications.
Conferences
Conferences are a great place to meet people presenting new and exciting research in the data science field and bounce ideas off of newfound connections. Like professional societies and organizations, discounted student rates are available to encourage student participation. In addition, some conferences will waive fees if you are presenting a poster or research at the conference, which is an extra incentive to present.
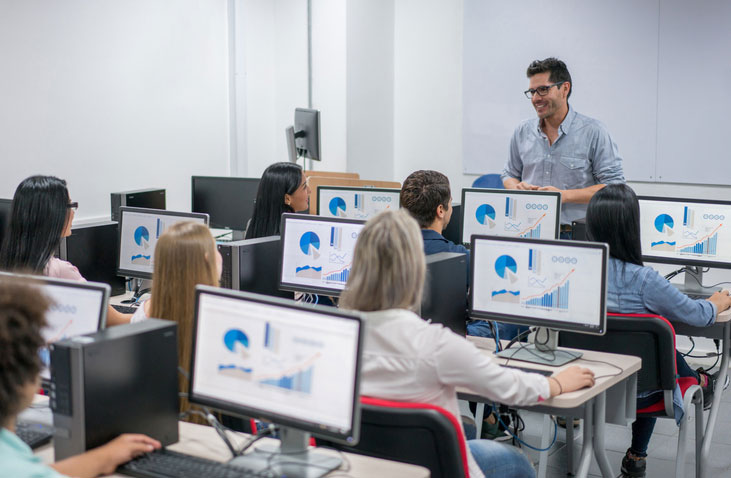
It can be hard to quantify what makes a good-fit when it comes to data science graduate school programs. There are easy to evaluate factors, such as cost and location, and then there are harder to evaluate criteria such as networking opportunities, accessibility to professors, and the up-to-dateness of the program’s curriculum.
Nevertheless, there are some key relevant considerations when applying to almost any data science graduate program.
What most schools will require when applying:
- All undergraduate and graduate transcripts
- A statement of intent for the program (reason for applying and future plans)
- Letters of reference
- Application fee
- Online application
- A curriculum vitae (outlining all of your academic and professional accomplishments)
What Does it Cost to Get a PhD in Data Science?
The great news is that many PhD data science programs are supported by fellowships and stipends. Some are completely funded, meaning the school will pay tuition and basic living expenses. Here are several examples of fully funded programs:
- University of Southern California
- University of Nevada, Reno
- Kennesaw State University
- Worcester Polytechnic Institute
- University of Maryland
For all other programs, the average range of tuition, depending on the school can range anywhere from $1,300 per credit hour to $2,000 amount per credit hour. Remember, typical PhD programs in data science are between 60 and 75 credit hours, meaning you could spend up to $150,000 over several years.
That’s why the financial aspects are so important to evaluate when assessing PhD programs, because some schools offer full stipends so that you are able to attend without having to find supplemental scholarships or tuition assistance.
Can I become a professor of data science with a PhD.? Yes! If you are interested in teaching at the college or graduate level, a PhD is the degree needed to establish the full expertise expected to be a professor. Some data scientists who hold PhDs start by entering the field of big data and pivot over to teaching after gaining a significant amount of work experience. If you’re driven to teach others or to pursue advanced research in data science, a PhD is the right degree for you.
Do I need a master’s in order to pursue a PhD.? No. Many who pursue PhDs in Data Science do not already hold advanced degrees, and many PhD programs include all the coursework of a master’s program in the first two years of school. For many students, this is the most time-effective option, allowing you to complete your education in a single pass rather than interrupting your studies after your master’s program.
Can I choose to pursue a PhD after already receiving my master’s? Yes. A master’s program can be an opportunity to get the lay of the land and determine the specific career path you’d like to forge in the world of big data. Some schools may allow you to simply extend your academic timeline after receiving your master’s degree, and it is also possible to return to school to receive a PhD if you have been working in the field for some time.
If a PhD. isn’t necessary, is it a waste of time? While not all students are candidates for PhDs, for the right students – who are keen on doing in-depth research, have the time to devote to many years of school, and potentially have an interest in continuing to work in academia – a PhD is a great choice. For more information on this question, take a look at our article Is a Data Science PhD. Worth It?
Complete List of Data Science PhD Programs
Below you will find the most comprehensive list of schools offering a doctorate in data science. Each school listing contains a link to the program specific page, GRE or a master’s degree requirements, and a link to a page with detailed course information.
Note that the listing only contains true data science programs. Other similar programs are often lumped together on other sites, but we have chosen to list programs such as data analytics and business intelligence on a separate section of the website.
Boise State University – Boise, Idaho PhD in Computing – Data Science Concentration
The Data Science emphasis focuses on the development of mathematical and statistical algorithms, software, and computing systems to extract knowledge or insights from data.
In 60 credits, students complete an Introduction to Graduate Studies, 12 credits of core courses, 6 credits of data science elective courses, 10 credits of other elective courses, a Doctoral Comprehensive Examination worth 1 credit, and a 30-credit dissertation.
Electives can be taken in focus areas such as Anthropology, Biometry, Ecology/Evolution and Behavior, Econometrics, Electrical Engineering, Earth Dynamics and Informatics, Geoscience, Geostatistics, Hydrology and Hydrogeology, Materials Science, and Transportation Science.
Delivery Method: Campus GRE: Required 2022-2023 Tuition: $7,236 total (Resident), $24,573 total (Non-resident)
View Course Offerings
Bowling Green State University – Bowling Green, Ohio Ph.D. in Data Science
Data Science students at Bowling Green intertwine knowledge of computer science with statistics.
Students learn techniques in analyzing structured, unstructured, and dynamic datasets.
Courses train students to understand the principles of analytic methods and articulating the strengths and limitations of analytical methods.
The program requires 60 credit hours in the studies of Computer Science (6 credit hours), Statistics (6 credit hours), Data Science Exploration and Communication, Ethical Issues, Advanced Data Mining, and Applied Data Science Experience.
Students must also complete 21 credit hours of elective courses, a qualifying exam, a preliminary exam, and a dissertation.
Delivery Method: Campus GRE: Required 2022-2023 Tuition: $8,418 (Resident), $14,410 (Non-resident)
Brown University – Providence, Rhode Island PhD in Computer Science – Concentration in Data Science
Brown University’s database group is a world leader in systems-oriented database research; they seek PhD candidates with strong system-building skills who are interested in researching TupleWare, MLbase, MDCC, Crowd DB, or PIQL.
In order to gain entrance, applicants should consider first doing a research internship at Brown with this group. Other ways to boost an application are to take and do well at massive open online courses, do an internship at a large company, and get involved in a large open-source software project.
Coding well in C++ is preferred.
Delivery Method: Campus GRE: Required 2022-2023 Tuition: $62,680 total
Chapman University – Irvine, California Doctorate in Computational and Data Sciences
Candidates for the doctorate in computational and data science at Chapman University begin by completing 13 core credits in basic methodologies and techniques of computational science.
Students complete 45 credits of electives, which are personalized to match the specific interests and research topics of the student.
Finally, students complete up to 12 credits in dissertation research.
Applicants must have completed courses in differential equations, data structures, and probability and statistics, or take specific foundation courses, before beginning coursework toward the PhD.
Delivery Method: Campus GRE: Required 2022-2023 Tuition: $37,538 per year
Clemson University / Medical University of South Carolina (MUSC) – Joint Program – Clemson, South Carolina & Charleston, South Carolina Doctor of Philosophy in Biomedical Data Science and Informatics – Clemson
The PhD in biomedical data science and informatics is a joint program co-authored by Clemson University and the Medical University of South Carolina (MUSC).
Students choose one of three tracks to pursue: precision medicine, population health, and clinical and translational informatics. Students complete 65-68 credit hours, and take courses in each of 5 areas: biomedical informatics foundations and applications; computing/math/statistics/engineering; population health, health systems, and policy; biomedical/medical domain; and lab rotations, seminars, and doctoral research.
Applicants must have a bachelor’s in health science, computing, mathematics, statistics, engineering, or a related field, and it is recommended to also have competency in a second of these areas.
Program requirements include a year of calculus and college biology, as well as experience in computer programming.
Delivery Method: Campus GRE: Required 2022-2023 Tuition: $10,858 total (South Carolina Resident), $22,566 total (Non-resident)
View Course Offerings – Clemson
George Mason University – Fairfax, Virginia Doctor of Philosophy in Computational Sciences and Informatics – Emphasis in Data Science
George Mason’s PhD in computational sciences and informatics requires a minimum of 72 credit hours, though this can be reduced if a student has already completed a master’s. 48 credits are toward graduate coursework, and an additional 24 are for dissertation research.
Students choose an area of emphasis—either computer modeling and simulation or data science—and completed 18 credits of the coursework in this area. Students are expected to completed the coursework in 4-5 years.
Applicants to this program must have a bachelor’s degree in a natural science, mathematics, engineering, or computer science, and must have knowledge and experience with differential equations and computer programming.
Delivery Method: Campus GRE: Required 2022-2023 Tuition: $13,426 total (Virginia Resident), $35,377 total (Non-resident)
Harrisburg University of Science and Technology – Harrisburg, Pennsylvania Doctor of Philosophy in Data Sciences
Harrisburg University’s PhD in data science is a 4-5 year program, the first 2 of which make up the Harrisburg master’s in analytics.
Beyond this, PhD candidates complete six milestones to obtain the degree, including 18 semester hours in doctoral-level courses, such as multivariate data analysis, graph theory, machine learning.
Following the completion of ANLY 760 Doctoral Research Seminar, students in the program complete their 12 hours of dissertation research bringing the total program hours to 36.
Delivery Method: Campus GRE: Required 2022-2023 Tuition: $14,940 total
Icahn School of Medicine at Mount Sinai – New York, New York Genetics and Data Science, PhD
As part of the Biomedical Science PhD program, the Genetics and Data Science multidisciplinary training offers research opportunities that expand on genetic research and modern genomics. The training also integrates several disciplines of biomedical sciences with machine learning, network modeling, and big data analysis.
Students in the Genetics and Data Science program complete a predetermined course schedule with a total of 64 credits and 3 years of study.
Additional course requirements and electives include laboratory rotations, a thesis proposal exam and thesis defense, Computer Systems, Intro to Algorithms, Machine Learning for Biomedical Data Science, Translational Genomics, and Practical Analysis of a Personal Genome.
Delivery Method: Campus GRE: Not Required 2022-2023 Tuition: $31,303 total
Indiana University-Purdue University Indianapolis – Indianapolis, Indiana PhD in Data Science PhD Minor in Applied Data Science
Doctoral candidates pursuing the PhD in data science at Indiana University-Purdue must display competency in research, data analytics, and at management and infrastructure to earn the degree.
The PhD is comprised of 24 credits of a data science core, 18 credits of methods courses, 18 credits of a specialization, written and oral qualifying exams, and 30 credits of dissertation research. All requirements must be completed within 7 years.
Applicants are generally expected to have a master’s in social science, health, data science, or computer science.
Currently a majority of the PhD students at IUPUI are funded by faculty grants and two are funded by the federal government. None of the students are self funded.
IUPUI also offers a PhD Minor in Applied Data Science that is 12-18 credits. The minor is open to students enrolled at IUPUI or IU Bloomington in a doctoral program other than Data Science.
Delivery Method: Campus GRE: Required 2022-2023 Tuition: $9,228 per year (Indiana Resident), $25,368 per year (Non-resident)
Jackson State University – Jackson, Mississippi PhD Computational and Data-Enabled Science and Engineering
Jackson State University offers a PhD in computational and data-enabled science and engineering with 5 concentration areas: computational biology and bioinformatics, computational science and engineering, computational physical science, computation public health, and computational mathematics and social science.
Students complete 12 credits of common core courses, 12 credits in the specialization, 24 credits of electives, and 24 credits in dissertation research.
Students may complete the doctoral program in as little as 5 years and no more than 8 years.
Delivery Method: Campus GRE: Required 2022-2023 Tuition: $8,270 total
Kennesaw State University – Kennesaw, Georgia PhD in Analytics and Data Science
Students pursuing a PhD in analytics and data science at Kennesaw State University must complete 78 credit hours: 48 course hours and 6 electives (spread over 4 years of study), a minimum 12 credit hours for dissertation research, and a minimum 12 credit-hour internship.
Prior to dissertation research, the comprehensive examination will cover material from the three areas of study: computer science, mathematics, and statistics.
Successful applicants will have a master’s degree in a computational field, calculus I and II, programming experience, modeling experience, and are encouraged to have a base SAS certification.
Delivery Method: Campus GRE: Required 2022-2023 Tuition: $5,328 total (Georgia Resident), $19,188 total (Non-resident)
New Jersey Institute of Technology – Newark, New Jersey PhD in Business Data Science
Students may enter the PhD program in business data science at the New Jersey Institute of Technology with either a relevant bachelor’s or master’s degree. Students with bachelor’s degrees begin with 36 credits of advanced courses, and those with master’s take 18 credits before moving on to credits in dissertation research.
Core courses include business research methods, data mining and analysis, data management system design, statistical computing with SAS and R, and regression analysis.
Students take qualifying examinations at the end of years 1 and 2, and must defend their dissertations successfully by the end of year 6.
Delivery Method: Campus GRE: Required 2022-2023 Tuition: $21,932 total (New Jersey Resident), $32,426 total (Non-resident)
New York University – New York, New York PhD in Data Science
Doctoral candidates in data science at New York University must complete 72 credit hours, pass a comprehensive and qualifying exam, and defend a dissertation with 10 years of entering the program.
Required courses include an introduction to data science, probability and statistics for data science, machine learning and computational statistics, big data, and inference and representation.
Applicants must have an undergraduate or master’s degree in fields such as mathematics, statistics, computer science, engineering, or other scientific disciplines. Experience with calculus, probability, statistics, and computer programming is also required.
Delivery Method: Campus GRE: Required 2022-2023 Tuition: $37,332 per year
View Course Offering
Northcentral University – San Diego, California PhD in Data Science-TIM
Northcentral University offers a PhD in technology and innovation management with a specialization in data science.
The program requires 60 credit hours, including 6-7 core courses, 3 in research, a PhD portfolio, and 4 dissertation courses.
The data science specialization requires 6 courses: data mining, knowledge management, quantitative methods for data analytics and business intelligence, data visualization, predicting the future, and big data integration.
Applicants must have a master’s already.
Delivery Method: Online GRE: Required 2022-2023 Tuition: $16,794 total
Stevens Institute of Technology – Hoboken, New Jersey Ph.D. in Data Science
Stevens Institute of Technology has developed a data science Ph.D. program geared to help graduates become innovators in the space.
The rigorous curriculum emphasizes mathematical and statistical modeling, machine learning, computational systems and data management.
The program is directed by Dr. Ted Stohr, a recognized thought leader in the information systems, operations and business process management arenas.
Delivery Method: Campus GRE: Required 2022-2023 Tuition: $39,408 per year
University at Buffalo – Buffalo, New York PhD Computational and Data-Enabled Science and Engineering
The curriculum for the University of Buffalo’s PhD in computational and data-enabled science and engineering centers around three areas: data science, applied mathematics and numerical methods, and high performance and data intensive computing. 9 credit course of courses must be completed in each of these three areas. Altogether, the program consists of 72 credit hours, and should be completed in 4-5 years. A master’s degree is required for admission; courses taken during the master’s may be able to count toward some of the core coursework requirements.
Delivery Method: Campus GRE: Required 2022-2023 Tuition: $11,310 per year (New York Resident), $23,100 per year (Non-resident)
University of Colorado Denver – Denver, Colorado PhD in Big Data Science and Engineering
The University of Colorado – Denver offers a unique program for those students who have already received admission to the computer science and information systems PhD program.
The Big Data Science and Engineering (BDSE) program is a PhD fellowship program that allows selected students to pursue research in the area of big data science and engineering. This new fellowship program was created to train more computer scientists in data science application fields such as health informatics, geosciences, precision and personalized medicine, business analytics, and smart cities and cybersecurity.
Students in the doctoral program must complete 30 credit hours of computer science classes beyond a master’s level, and 30 credit hours of dissertation research.
The BDSE fellowship requires students to have an advisor both in the core disciplines (either computer science or mathematics and statistics) as well as an advisor in the application discipline (medicine and public health, business, or geosciences).
In addition, the fellowship covers full stipend, tuition, and fees up to ~50k for BDSE fellows annually. Important eligibility requirements can be found here.
Delivery Method: Campus GRE: Required 2022-2023 Tuition: $55,260 total
University of Marylan d – College Park, Maryland PhD in Information Studies
Data science is a potential research area for doctoral candidates in information studies at the University of Maryland – College Park. This includes big data, data analytics, and data mining.
Applicants for the PhD must have taken the following courses in undergraduate studies: programming languages, data structures, design and analysis of computer algorithms, calculus I and II, and linear algebra.
Students must complete 6 qualifying courses, 2 elective graduate courses, and at least 12 credit hours of dissertation research.
Delivery Method: Campus GRE: Required 2022-2023 Tuition: $16,238 total (Maryland Resident), $35,388 total (Non-resident)
University of Massachusetts Boston – Boston, Massachusetts PhD in Business Administration – Information Systems for Data Science Track
The University of Massachusetts – Boston offers a PhD in information systems for data science. As this is a business degree, students must complete coursework in their first two years with a focus on data for business; for example, taking courses such as business in context: markets, technologies, and societies.
Students must take and pass qualifying exams at the end of year 1, comprehensive exams at the end of year 2, and defend their theses at the end of year 4.
Those with a degree in statistics, economics, math, computer science, management sciences, information systems, and other related fields are especially encouraged, though a quantitative degree is not necessary.
Students accepted by the program are ordinarily offered full tuition credits and a stipend ($25,000 per year) to cover educational expenses and help defray living costs for up to three years of study.
During the first two years of coursework, they are assigned to a faculty member as a research assistant; for the third year students will be engaged in instructional activities. Funding for the fourth year is merit-based from a limited pool of program funds
Delivery Method: Campus GRE: Required 2022-2023 Tuition: $18,894 total (in-state), $36,879 (out-of-state)
University of Nevada Reno – Reno, Nevada PhD in Statistics and Data Science
The University of Nevada – Reno’s doctoral program in statistics and data science is comprised of 72 credit hours to be completed over the course of 4-5 years. Coursework is all within the scope of statistics, with titles such as statistical theory, probability theory, linear models, multivariate analysis, statistical learning, statistical computing, time series analysis.
The completion of a Master’s degree in mathematics or statistics prior to enrollment in the doctoral program is strongly recommended, but not required.
Delivery Method: Campus GRE: Required 2022-2023 Tuition: $5,814 total (in-state), $22,356 (out-of-state)
University of Southern California – Los Angles, California PhD in Data Sciences & Operations
USC Marshall School of Business offers a PhD in data sciences and operations to be completed in 5 years.
Students can choose either a track in operations management or in statistics. Both tracks require 4 courses in fall and spring of the first 2 years, as well as a research paper and courses during the summers. Year 3 is devoted to dissertation preparation and year 4 and/or 5 to dissertation defense.
A bachelor’s degree is necessary for application, but no field or further experience is required.
Students should complete 60 units of coursework. If the students are admitted with Advanced Standing (e.g., Master’s Degree in appropriate field), this requirement may be reduced to 40 credits.
Delivery Method: Campus GRE: Required 2022-2023 Tuition: $63,468 total
University of Tennessee-Knoxville – Knoxville, Tennessee The Data Science and Engineering PhD
The data science and engineering PhD at the University of Tennessee – Knoxville requires 36 hours of coursework and 36 hours of dissertation research. For those entering with an MS degree, only 24 hours of course work is required.
The core curriculum includes work in statistics, machine learning, and scripting languages and is enhanced by 6 hours in courses that focus either on policy issues related to data, or technology entrepreneurship.
Students must also choose a knowledge specialization in one of these fields: health and biological sciences, advanced manufacturing, materials science, environmental and climate science, transportation science, national security, urban systems science, and advanced data science.
Applicants must have a bachelor’s or master’s degree in engineering or a scientific field.
All students that are admitted will be supported by a research fellowship and tuition will be included.
Many students will perform research with scientists from Oak Ridge national lab, which is located about 30 minutes drive from campus.
Delivery Method: Campus GRE: Required 2022-2023 Tuition: $11,468 total (Tennessee Resident), $29,656 total (Non-resident)
University of Vermont – Burlington, Vermont Complex Systems and Data Science (CSDS), PhD
Through the College of Engineering and Mathematical Sciences, the Complex Systems and Data Science (CSDS) PhD program is pan-disciplinary and provides computational and theoretical training. Students may customize the program depending on their chosen area of focus.
Students in this program work in research groups across campus.
Core courses include Data Science, Principles of Complex Systems and Modeling Complex Systems. Elective courses include Machine Learning, Complex Networks, Evolutionary Computation, Human/Computer Interaction, and Data Mining.
The program requires at least 75 credits to graduate with approval by the student graduate studies committee.
Delivery Method: Campus GRE: Not Required 2022-2023 Tuition: $12,204 total (Vermont Resident), $30,960 total (Non-resident)
University of Washington Seattle Campus – Seattle, Washington PhD in Big Data and Data Science
The University of Washington’s PhD program in data science has 2 key goals: training of new data scientists and cyberinfrastructure development, i.e., development of open-source tools and services that scientists around the world can use for big data analysis.
Students must take core courses in data management, machine learning, data visualization, and statistics.
Students are also required to complete at least one internship that covers practical work in big data.
Delivery Method: Campus GRE: Required 2022-2023 Tuition: $17,004 per year (Washington resident), $30,477 (non-resident)
University of Wisconsin-Madison – Madison, Wisconsin PhD in Biomedical Data Science
The PhD program in Biomedical Data Science offered by the Department of Biostatistics and Medical Informatics at UW-Madison is unique, in blending the best of statistics and computer science, biostatistics and biomedical informatics.
Students complete three year-long course sequences in biostatistics theory and methods, computer science/informatics, and a specialized sequence to fit their interests.
Students also complete three research rotations within their first two years in the program, to both expand their breadth of knowledge and assist in identifying a research advisor.
Delivery Method: Campus GRE: Required 2022-2023 Tuition: $10,728 total (in-state), $24,054 total (out-of-state)
Vanderbilt University – Nashville, Tennessee Data Science Track of the BMI PhD Program
The PhD in biomedical informatics at Vanderbilt has the option of a data science track.
Students complete courses in the areas of biomedical informatics (3 courses), computer science (4 courses), statistical methods (4 courses), and biomedical science (2 courses). Students are expected to complete core courses and defend their dissertations within 5 years of beginning the program.
Applicants must have a bachelor’s degree in computer science, engineering, biology, biochemistry, nursing, mathematics, statistics, physics, information management, or some other health-related field.
Delivery Method: Campus GRE: Required 2022-2023 Tuition: $53,160 per year
Washington University in St. Louis – St. Louis, Missouri Doctorate in Computational & Data Sciences
Washington University now offers an interdisciplinary Ph.D. in Computational & Data Sciences where students can choose from one of four tracks (Computational Methodologies, Political Science, Psychological & Brain Sciences, or Social Work & Public Health).
Students are fully funded and will receive a stipend for at least five years contingent on making sufficient progress in the program.
Delivery Method: Campus GRE: Required 2022-2023 Tuition: $59,420 total
Worcester Polytechnic Institute – Worcester, Massachusetts PhD in Data Science
The PhD in data science at Worcester Polytechnic Institute focuses on 5 areas: integrative data science, business intelligence and case studies, data access and management, data analytics and mining, and mathematical analysis.
Students first complete a master’s in data science, and then complete 60 credit hours beyond the master’s, including 30 credit hours of research.
Delivery Method: Campus GRE: Required 2022-2023 Tuition: $28,980 per year
Yale University – New Haven, Connecticut PhD Program – Department of Stats and Data Science
The PhD in statistics and data science at Yale University offers broad training in the areas of statistical theory, probability theory, stochastic processes, asymptotics, information theory, machine learning, data analysis, statistical computing, and graphical methods. Students complete 12 courses in the first year in these topics.
Students are required to teach one course each semester of their third and fourth years.
Most students complete and defend their dissertations in their fifth year.
Applicants should have an educational background in statistics, with an undergraduate major in statistics, mathematics, computer science, or similar field.
Delivery Method: Campus GRE: Required 2022-2023 Tuition: $46,900 total
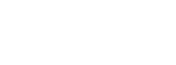
- Related Programs
- Yale-BI Joint Selection Committee (JSC) and Advisors
- Yale-BI Joint Research Committee (JRC) and Fellows
- Fellowship Gallery
- Publications
- Training & Collaboration
- News & Events
INFORMATION FOR
- Residents & Fellows
- Researchers
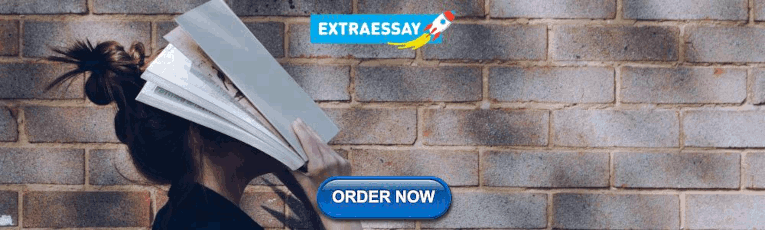
The Center for Biomedical Data Science
Cbds latest news, single-cell profiling reveals insights about immunity in idiopathic pulmonary fibrosis, next generation research uses real-world data to identify most effective hypertension drugs for patients, leeds receives va research award for ai, housing study, cheung receives nih grant to research water contaminants and human health, conversations with dr. naftali kaminski: behind the scenes of a new medical podcast, from the journals, center for biomedical data science events, yale nlp/llm interest group talks, bids rising star/research in progress seminar.
Large Scale Genomic Study of Addiction
New! Call for submissions to AI for Drug Discovery Workshop by May 6, 22nd International Conference on Artificial Intelligence in Medicine in Salt Lake City, Utah.
Organized by the Yale-Boehringer Ingelheim Biomedical Data Science Fellowship Program
- Public Health
Programs of study are offered in the areas of Biostatistics (PhD or MS), Chronic Disease Epidemiology (PhD or MS), Environmental Health Sciences (PhD), Epidemiology of Microbial Diseases (PhD), Epidemiology Infectious Disease (MS), Health Informatics (MS), Health Policy and Management (PhD), or Social and Behavioral Sciences (PhD).
- Programs of Study
- PhD - Doctor of Philosophy
- MS - Master of Science
Christian Tschudi
Director of Graduate Studies
Melanie Elliot
Administrative Director of Graduate Student Affairs
- [email protected]
- 203-785-6383
Katie Doucet
Student Affairs Advisor
- 203-785-4257
Admission Requirements
Standardized testing requirements.
GRE is required for:
Biostatistics (PhD and MS) Chronic Disease Epidemiology (PhD) Health Policy Management (PhD)
GRE is not accepted for:
Environmental Health Sciences (PhD) Epidemiology of Infectious Diseases (MS) Epidemiology of Microbial Diseases (PhD) Social and Behavioral Sciences (PhD) Health Informatics (MS)
Either GRE or MCAT is required for:
Chronic Disease Epidemiology (MS)
English Language Requirement
TOEFL iBT or IELTS Academic is required of most applicants whose native language is not English.
You may be exempt from this requirement if you have received (or will receive) an undergraduate degree from a college or university where English is the primary language of instruction, and if you have studied in residence at that institution for at least three years.
Admission Information
The Public Health program offers a PhD in the following subfields: Biostatistics , Chronic Disease Epidemiology , Environmental Health Sciences , Epidemiology of Microbial Diseases , Health Policy and Management , and Social and Behavioral Sciences . The Public Health MS program subfields are: Biostatistics , Chronic Disease Epidemiology , Epidemiology of Infectious Diseases , and Health Informatics . Within the MS Biostatistics program, there are three pathways: Standard, Data Science, and Implementation and Prevention Science Methods. Within the Epidemiology of Infectious Diseases program, there are two specializations: Quantitative and Clinical. The MS in Biostatistics and Health Informatics are 2-year programs. The MS In Chronic Disease Epidemiology and Epidemiology of Infectious Diseases are 1-year programs. Applicants should select “Public Health” on the application and then the program which best matches their research interests. MS Biostatistics and Epidemiology Infectious Diseases applicants should indicate their preferred pathway or specialization in the relevant section of the application. The Biostatistics pathway can be changed during the first semester of study.
At the Yale School of Public Health, you will join a community dedicated to nurturing your intellectual and professional success in the field of public health. You will learn from national and international leaders in science-based public policy, health interventions, and basic research so that you step into a career with impact. YSPH offers many opportunities for innovation and collaboration, and students explore the interdisciplinary nature of public health through coursework and collaboration across the University.
Academic Information
Program Advising Guidelines
GSAS Advising Guidelines
Academic Resources
Academic calendar.
The Graduate School's academic calendar lists important dates and deadlines related to coursework, registration, financial processes, and milestone events such as graduation.
Featured Resource
Registration Information and Dates
https://registration.yale.edu/
Students must register every term in which they are enrolled in the Graduate School. Registration for a given term takes place the semester prior, and so it's important to stay on top of your academic plan. The University Registrar's Office oversees the systems that students use to register. Instructions about how to use those systems and the dates during which registration occurs can be found on their registration website.
Financial Information
Phd stipend & funding.
PhD students at Yale are normally full-funded for a minimum of five years. During that time, our students receive a twelve-month stipend to cover living expenses and a fellowship that covers the full cost of tuition and student healthcare.
- PhD Student Funding Overview
- Graduate Financial Aid Office
- PhD Stipends
- Health Award
- Tuition and Fees
Master's Funding
While Master's programs are not generally funded, there are resources available to students to help navigate financial responsibilities during graduate school.
- Master's Student Funding Overview
- Yale Student Grants Database
- Student Employment
- Loans for US Citizens
- Loans for Non-US Citizens
Alumni Insights
Below you will find alumni placement data for our departments and programs.
- Mission, Facts and Figures
- Deans, Chairs and Staff
- Leadership Council
- Dean in the News
- Get Involved
- DEIB Mission
- Message from DEIB Associate Dean
- News and Media
- Reading Lists
- The Yale and Slavery Research Project
- Photo Gallery
- Winslow Medal
- Coat of Arms & Mace
- $50 Million Challenge
- For Pandemic Prevention and Global Health
- For Understanding the Health Impacts of Climate Change
- For Health Equity and Justice
- For Powering Health Solutions through Data Science
- For Future Leaders
- For Faculty Leaders
- For Transformational Efforts
Data, Leadership, and Collaboration at the School of Public Health
- An abiding love for Yale turns into a lasting gift – in 15 minutes
- Endowed Professorship Created at Critical Time for Yale School of Public Health
- Brotherly encouragement spurs gift to support students
- Prestipino creates opportunities for YSPH students, now and later
- Alumna gives back to the school that “opened doors” in male-dominated field
- For Public Health, a Broad Mission and a Way to Amplify Impact
- Couple Endows Scholarship to Put Dreams in Reach for YSPH Students
- A Match Made at YSPH
- A HAPPY Meeting of Public Health and the Arts
- Generous Gift Bolsters Diversity & Inclusion
- Alumni Donations Aid Record Number of YSPH Students
- YSPH’s Rapid Response Fund Needs Donations – Rapidly
- Podiatric Medicine and Orthopedics as Public Health Prevention
- Investing in Future Public Health Leaders
- Support for Veterans and Midcareer Students
- Donor Eases Burden for Policy Students
- A Personal Inspiration for Support of Cancer Research
- Reducing the Burden of Student Debt
- Learning About Global Health Through Global Travel
- A Meeting in Dubai, and a Donation to the School
- Rapid Response Fund
- Planned Giving
- Testimonials
- Faculty, Postdoc Jobs
- For the Media
- Issues List
- PDF Issues for Download
- Editorial Style Guide
- Social Media
- Shared Humanity Podcast
- Health & Veritas Podcast
- Accreditation
- Faculty Directory by Name
- Career Achievement Awards
- Annual Research Awards
- Teaching Spotlights
- Biostatistics
- Chronic Disease Epidemiology
- Climate Change and Health Concentration
- Environmental Health Sciences
- Epidemiology of Microbial Diseases
- Global Health
- Health Policy and Management
- Maternal and Child Health Promotion Track
- Public Health Modeling Concentration
- Regulatory Affairs Track
- Social & Behavioral Sciences
- U.S. Health Justice Concentration
- Why Public Health at Yale
- Events and Contact
- What Does it Take to be a Successful YSPH Student?
- How to Apply and FAQs
- Incoming Student Gateway
- Traveling to Yale
- Meet Students and Alumni
- Past Internship Spotlights
- Student-run Organizations
- MS and PhD Student Leaders
- Staff Spotlights
- Life in New Haven
- Libraries at Yale
- The MPH Internship Experience
- Practicum Course Offerings
- Summer Funding and Fellowships
- Downs Fellowship Committee
- Stolwijk Fellowship
- Climate Change and Health
- Career Management Center
- What You Can Do with a Yale MPH
- MPH Career Outcomes
- MS Career Outcomes
- PhD Career Outcomes
- Employer Recruiting
- Tuition and Expenses
- External Funding and Scholarships
- External Fellowships for PhD Candidates
- Alumni Spotlights
- Bulldog Perks
- Stay Involved
- Board of Directors
- Emerging Majority Affairs Committee
- Award Nomination Form
- Board Nomination Form
- Alumni Engagement Plus
- Mentorship Program
- The Mentoring Process
- For Mentors
- For Students
- Recent Graduate Program
- Transcript and Verification Requests
- Applied Practice and Student Research
- Competencies and Career Paths
- Applied Practice and Internships
- Student Research
- Seminar and Events
- Competencies and Career paths
- Why the YSPH Executive MPH
- Message from the Program Director
- Two-year Hybrid MPH Schedule
- The Faculty
- Student Profiles
- Newsletter Articles
- Approved Electives
- Physicians Associates Program
- Joint Degrees with International Partners
- MS in Biostatistics Standard Pathway
- MS Implementation and Prevention Science Methods Pathway
- MS Data Sciences Pathway
- Internships and Student Research
- Competencies
- Degree Requirements - Quantitative Specialization
- Degree Requirements - Clinical Specialization
- Degree Requirements- PhD Biostatistics Standard Pathway
- Degree Requirements- PhD Biostatistics Implementation and Prevention Science Methods Pathway
- Meet PhD Students in Biostatistics
- Meet PhD Students in CDE
- Degree Requirements and Timeline
- Meet PhD Students in EHS
- Meet PhD Students in EMD
- Meet PhD Students in HPM
- Degree Requirements - PhD in Social and Behavioral Sciences
- Degree Requirements - PhD SBS Program Maternal and Child Health Promotion
- Meet PhD Students in SBS
- Differences between MPH and MS degrees
- Academic Calendar
- Translational Alcohol Research Program
- Molecular Virology/Epidemiology Training Program (MoVE-Kaz)
- For Public Health Practitioners and Workforce Development
- Course Description
- Instructors
- Registration
- Coursera Offerings
- Non-degree Students
- International Initiatives & Partnerships
- NIH-funded Summer Research Experience in Environmental Health (SREEH)
- Summer International Program in Environmental Health Sciences (SIPEHS)
- 2022 Student Awards
- APHA Annual Meeting & Expo
- National Public Health Week (NPHW)
- Leaders in Public Health
- YSPH Dean's Lectures
- The Role of Data in Public Health Equity & Innovation Conference
- Innovating for the Public Good
- Practice- and community-based research and initiatives
- Practice and community-based research and initiatives
- Activist in Residence Program
- Publications
- Health Care Systems and Policy
- Heart Disease and Stroke
- Panels, Seminars and Workshops (Recordings)
- Rapid Response Fund Projects
- SalivaDirect™
- Emerging Infections Program - COVID-NET
- Public Health Modeling Unit Projects
- HIV-AIDS-TB
- The Lancet 2023 Series on Breastfeeding
- 'Omics
- News in Biostatistics
- Biostatistics Overview
- Seminars and Events
- Seminar Recordings
- Statistical Genetics/Genomics, Spatial Statistics and Modeling
- Causal Inference, Observational Studies and Implementation Science Methodology
- Health Informatics, Data Science and Reproducibility
- Clinical Trials and Outcomes
- Machine Learning and High Dimensional Data Analysis
- News in CDE
- Nutrition, Diabetes, Obesity
- Maternal and Child Health
- Outcomes Research
- Health Disparities
- Women's Health
- News in EHS
- EHS Seminar Recordings
- Climate change and energy impacts on health
- Developmental origins of health and disease
- Environmental justice and health disparities
- Enviromental related health outcomes
- Green chemistry solutions
- Novel approaches to assess environmental exposures and early markers of effect
- 1,4 Dioxane
- Reproducibility
- Tissue Imaging Mass Spectrometry
- Alcohol and Cancer
- Olive Oil and Health
- Lightning Talks
- News in EMD
- Antimicrobial Resistance
- Applied Public Health and Implementation Science
- Emerging Infections and Climate Change
- Global Health/Tropical Diseases
- HIV and Sexually Transmitted Infections
- Marginalized Population Health & Equity
- Pathogen Genomics, Diagnostics, and Molecular Epidemiology
- Vector-borne and Zoonotic Diseases
- Disease Areas
- EMD Research Day
- News in HPM
- Health Systems Reform
- Quality, Efficiency and Equity of Healthcare
- Substance Abuse and Mental Health
- Modeling: Policy, Operations and Disease
- Pharmaceuticals, Vaccines and Medical Devices
- Health and Wellbeing
- News in SBS
- Aging Health
- Community Engagement
- Health Equity
- Mental Health
- Reproductive Health
- Sexuality and Health
- Nutrition, Exercise
- Stigma Prevention
- Community Partners
- For Public Health Practitioners
- Reports and Publications
- Fellows Stipend Application
- Agency Application
- Past Fellows
- PHFP in the News
- Frequently Asked Questions
- International Activity
- Research Publications
- Grant Listings
- Modeling Analyses
- 3 Essential Questions Series
INFORMATION FOR
- Prospective Students
- Incoming Students
- myYSPH Members
Yale School of Public Health (YSPH) has long emphasized both data science and data-driven health leadership. Equitable data science makes transformational and impactful research possible, resulting in innovations that advance and improve public health, from identifying new cancer treatments to creating guidelines reducing pollution’s health impact.
A new two-part gift to YSPH builds on this fundamental focus on data, while supporting an ongoing, collaborative educational program with the Yale School of Management (SOM). The gift, from former PepsiCo CEO Indra Nooyi, a 1980 graduate of the Yale School of Management, and her husband Raj Nooyi, will advance the school’s efforts to train students as leaders in data-driven public health improvement of people’s wellbeing at local, national, and global scales. The Nooyis have previously supported YSPH with an endowed professorship.
“I am so grateful to Indra and Raj for their strategic, farsighted support of these two important programs,” said YSPH Dean Megan L. Ranney. “We can’t be great public health data science leaders without investment – or without partnerships.”
Leading the Way with Data
“The Yale School of Public Health has a long record of accomplishment in data-driven, impactful research. During the COVID-19 pandemic, its researchers provided policymakers in Connecticut and internationally with timely data-driven insights to help guide emergency response. The school also educates future leaders who use public health data science and data equity to enable transformational research in the face of quickly changing methods, technology, and societal shifts,” says Indra Nooyi. “That track record was a significant factor in our decision to support the school.”
The Nooyis’ gift creates an endowed data science resource fund that can be used to advance teaching and research at the school. For example, recent research showed that RSV vaccines would decrease illness and death if deployed like flu shots and was used to develop a dynamic new tool for evaluating the effectiveness of large-scale public health interventions. In April, the school held an international gathering to improve equity in accessing and using high-quality health data. But as sources of data explode and artificial intelligence becomes more powerful, YSPH must expand its efforts to remain a leader in the field.
The Nooyis’ gift has enabled YSPH to recruit one of the world’s most influential public health data scientists. The school recently appointed award-winning statistician Bhramar Mukherjee, PhD, as its inaugural Senior Associate Dean of Public Health Data Science and Data Equity. The deanship, made possible by the Nooyis’ gift, is an important new leadership position that reflects the school’s focus on data science as a critical pillar for the future of public health. She joins YSPH on Aug. 1, 2024.
“Dr. Bhramar Mukherjee works at the cutting edge of public health data collection and analysis, with an eye towards global data equity,” Ranney said. “Her recruitment advances our goal of leading the future of public health data science.”
A Collaborative Degree
A portion of the Nooyis’ gift supports the continued success of the Health Care Management Program, run by YSPH and the Yale School of Management. Students take classes at both schools, earning a Master of Public Health (MPH) degree in healthcare management, and can pursue an MBA. Graduates of the program have become leaders of hospitals, public health systems, and health startups.
Howard Forman, a professor at both schools and a diagnostic radiologist at Yale New Haven Hospital, directs the program. “Our program is the best in the country, and the Nooyis’ support will help us remain financially affordable to students,” notes Forman.
One of the marquee events of the Health Care Management Program is a yearly conference that brings over 500 health care professionals to Yale SOM, including many of the program’s current students and alumni. The event celebrated its twentieth year in 2024. “The conference draws on academic rigor but emphasizes practice,” says Forman. “It’s a place where alumni and students can learn from each other.”
Ranney notes that YSPH is Yale’s newest independent school, transitioning from being a department of the Yale School of Medicine. “That means gifts like these have an outsize impact. Indra and Raj’s gift allows our school to continue to grow as world leaders of public health.”
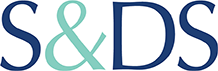
Department of Statistics and Data Science
Terminal ma/ms programs.
The Department offers a broad training program comprised of the main areas of statistical theory (with emphasis on foundations, Bayes theory, decision theory, nonparametric statistics), probability theory, stochastic processes, asymptotics, information theory, machine learning, data analysis, statistical computing, and graphical methods.
With this background, graduates of the program have found excellent positions in industry, and government. See the list of M.A. alumni for examples.
We now offer both the 8-course M.A. in Statistics and a new 12-course M.S. in Statistics & Data Science! Students may apply to either program but are also allowed to change between programs during their study. Petitions for such changes should be done at the start of a new semester using the Departmental Transfer Form .
Recent placements
Very recent graduates of the terminal Master’s program have continued their graduate education at Yale, the University of Michigan School of Business, the University British Columbia, Stanford University, and Purdue University. Other recent graduates have been employed by Oliver Wyman, Captrust, Deutsch Bank, RAAP, Progressive Leasing, Mathematica, McKinsey, 7 Eleven, and Facebook. These are just a sample of recent graduate activity, and we encourage all alumni to keep in touch and let us know of any updates!
Data Science
- Undergraduate Program
Introduction to the Major
Data science combines computational and inferential reasoning to draw conclusions based on data about different aspects of the real world. Data scientists come from all walks of life, all areas of study, and all backgrounds. They share an appreciation for the practical use of mathematical and scientific thinking and the power of computing to understand and solve problems for business, research, and societal impact.
The Division offers a major and minor in Data Science, as well as coursework such as Data 8: Foundations of Data Science , its connector courses , and Data Science modules that are broadly accessible to all students.
The Data Science Curriculum
Students will gain deep technical knowledge and an understanding of the social and human contexts and ethical implications of how data are collected, analyzed, and used. Students also gain expertise in applying knowledge in a chosen Domain Emphasis; a few examples include:
- Computational Biology
- Geospatial Information & Technology
- Human Behavior & Psychology
- Inequalities in Society
- Social Policy and Law
Amplify Your Major
- Help shape the Data Science program as part of a student team working on data analytics, curriculum development, communications, human resources, etc.
- Apply to be a data discovery consultant .
- Make an impact with cutting-edge research and gain hands-on skills with the Data Science Discovery Program .
- Join a student organization such as those in the Data Science Nexus .
I think that the broadest possible set of people in the world need to be owners of the data. Statistics Professor Ani Adhikari, Co-creator of Data 8
- Four-Year Student Timeline
Explore Your Major
- Review major and college requirements . Meet with a Data Science major advisor and your college advisor to create a 4-year schedule.
- Talk to Data Science peer advisors about life in the major.
- Join or form a study group.
- Take Data 8 .
Connect and Build Community
- Get 1:1 mentoring with Berkeley Connect .
- Find academic support at the Student Learning Center and other tutoring resources.
- Check out Data Scholars , a community of underrepresented and nontraditional students in Data Science.
Discover Your Passions
- Enroll in a connector course to see how Data 8 concepts apply in a variety of academic areas.
- Visit the Career Counseling Library to learn more about your academic and professional interests.
- Discover new interests in a Freshman Seminar , L&S 1 , or a student-run DeCal course .
Engage Locally and Globally
- Get involved in Data Scholars or other ways to engage in your classes from the start.
- Look into volunteer and student organization opportunities across campus.
- Explore study, internship, and research abroad options with Berkeley Study Abroad .
Reflect and Plan Your Future
- Sign up for Handshake and CareerMail .
- Visit Berkeley Career Engagement and the Career Counseling Library and develop a plan for getting career ready.
- Meet alumni at a Career Connections event.
- Explore Winter Break Externships with alums.
Second Year
- Read through the major Domain Emphasis options, noting those that most interest you.
- Complete lower division prerequisites and declare the major .
- Review the Data Science Piazza forum .
- Consider augmenting your major with a certificate or minor .
- Sign up for the Data Science email list and start attending department events .
- Engage in public service and leadership experiences by participating in a Public Service Center Program .
- Reflect on successes, setbacks, and lessons learned in your first year.
- Take advantage of the free interactive course textbooks for core Data Science courses.
- Enroll in a Big Ideas Course or Discovery Course .
- Learn about democratizing data science at Data & Tech for All Week .
- Look into student opportunities to get involved in the Data Science Education Program.
- Discuss and explore options to study abroad while making degree progress.
- Meet with a Career Educator to discuss your career or graduate school goals.
- Try some self-assessment activities to explore different industries within data science (e.g., social justice).
- Get to know faculty to develop rapport for letters of recommendation.
- Narrow down your Domain Emphasis preferences and start upper division coursework.
- Review degree progress with your advisors.
- Get involved with the Data Science Education Program to develop a portfolio and make an impact on campus.
- Help shape the Data Science program by joining a student team, serving as a data discovery consultant , or becoming a peer advisor .
- Join a working group to collaborate under the broad rubric of data-intensive social science.
- Become a Golden Bear Orientation Leader and welcome new students to UC Berkeley.
- Explore team-based, data research projects in the Data Science Discovery Program .
- Pursue independent projects with help from the Data Discovery Consultants or D-Lab workshops .
- Consider applying to the Data Science Honors Program or writing a senior thesis.
- Experience life outside of Berkeley via campus visitor and exchange programs , UCDC , Cal in the Capital , or the Cal-in-Sacramento Fellowship .
- Attend conferences or get involved in Data Science-related projects to supplement your curriculum.
- Attend an internship fair and apply for summer internships.
- Develop a portfolio that demonstrates your analytical and problem-solving skills.
- Conduct informational interviews .
- Develop your network. Update your resume and LinkedIn profile.
Fourth Year
- Do a degree check to ensure you are on track to graduate.
- Complete any “bucket list” courses.
- Finish remaining major, college, and campus requirements.
- Join a professional organization related to your interests.
- Volunteer and give back to the community.
- Attend a variety of performances, events, and activities to expand your mind.
- Connect with alumni groups and build your network as you prepare to graduate.
- Attend lectures and speaker series hosted by the Berkeley Institute for Data Science (BIDS) or other related research units .
- Take on a leadership role in a student organization.
- Find research and funding opportunities in the OURS database .
- Consider gap year opportunities prior to embarking on your next academic or career adventure.
- Explore service opportunities after graduation, such as Peace Corps , Teach for America , or U.S. Department of State .
- Attend Employer Info Sessions and On-Campus Recruiting .
- Join the Data Science alumni group on LinkedIn.
- Ask professors and graduate student instructors for recommendation letters.
- Take advantage of Alumni Services .
What Can I Do With My Major?
The Data Science BA positions students to help inform and develop solutions for a range of pressing challenges, from adapting industry to a new world of data, to amplifying learning in education, to helping communities recover from disaster. Our alumni are employed in a wide variety of roles and industries.
Jobs and Employers
- Analytics Intern, Major League Baseball
- Applications Engineer, Accenture
- Data Analyst, Political Campaign
- Data Engineer, Atlassian
- Data Scientist, Uber
- Machine Learning Engineer, IBM
- Product Analyst, Hive
- Product Manager, Zynga
- Site Reliability Engineer, Adobe
- Software Engineer, Salesforce
- Technical Staff, Oracle
Graduate Programs
- Computer Science, Masters
- Earth and Environmental Science, PhD
- Information and Data Science, Masters
Examples gathered from LinkedIn and an internal survey of recent Berkeley graduates.
Connect With Us
Attend department events with students, staff, and faculty. Visit data.berkeley.edu for news and updates. Also check out events hosted by the Berkeley Institute for Data Science (BIDS): events.berkeley.edu/BIDS .
Join our undergraduate email list-serve and view past issues of our student newsletter at data.berkeley.edu/academics/data-science-undergraduate-studies/advising/advising-resources .
Look out for information about annual events including:
- Data & Tech for All Week
- Cal Water Data Hackathon
- Women in Data Science (WIDS)
- Hack for Social Good
- Career Connections for Data Science
Visit data.berkeley.edu/academics/undergraduate-programs/data-science-advising for information about our advisors, advising hours and locations, and how to request an appointment.
For quick questions, email us at [email protected] or [email protected] .
How to Use this Map
Use this map to help plan and guide your experience at UC Berkeley, including academic, co-curricular, and discovery opportunities. Everyone’s Berkeley experience is different and activities in this map are suggestions. Always consult with your advisors whenever possible for new opportunities and updates.
- What Can I Do with My Major?

Download the PDF Print Version
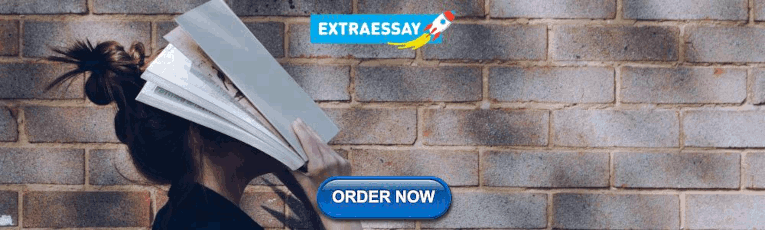
IMAGES
VIDEO
COMMENTS
Department of Statistics and Data Science. Yale University Kline Tower 219 Prospect Street New Haven, CT 06511. Mailing Address: PO Box 208290, New Haven, CT 06520-8290. Shipping Address (packages and Federal Express): 266 Whitney Avenue, New Haven, CT 06511. Department Phone: 203.432.0666
Department of Statistics and Data Science. Yale University Kline Tower 219 Prospect Street New Haven, CT 06511. Mailing Address: PO Box 208290, New Haven, CT 06520-8290. Shipping Address (packages and Federal Express): 266 Whitney Avenue, New Haven, CT 06511. Department Phone: 203.432.0666
Terminal M.S. in Statistics and Data Science Students are also admitted directly to a terminal master of science program in Statistics and Data Science. To qualify for the M.S., the student must successfully complete an approved program of twelve term courses with an average grade of HP or higher and receive at least two grades of Honors ...
1) Identify the program and degree you want. 2) Verify the application deadline for your program. 3) Determine what standardized tests you need to take. Register early. 4) Complete your application. Decide whether you will apply for a PhD or a terminal Master's (MA, MS) in one of the programs available at the Graduate School of Arts and Sciences.
The Graduate School of Arts and Sciences offers MA, MS, and PhD degrees, as well as non-degree programs, in more than 70 fields of study. ... Below you will find alumni placement data for our departments and programs. ... School offers a wide variety of resources to help you navigate your intellectual and professional growth while at Yale. Use ...
The future of data science at Yale will reside on one of the top floors of a re-imagined Kline Tower on Science Hill, starting in 2023 — but the university, its researchers, and the new Institute for Foundations of Data Science (FDS) aren't waiting for next year.. Doctors, economists, seismologists, psychologists, engineers, computer scientists — faculty from dozens of disciplines ...
The Graduate School of Arts and Sciences (GSAS) is one unit within a large, complex, and historic university. In addition to the Graduate School, Yale has an undergraduate school (Yale College) and 13 professional schools that award post-baccalaureate degrees in law, medicine, business, drama, art, architecture, music, nursing, etc.
Dr. Qingyu Chen is an incoming tenure-track assistant professor at Biomedical Informatics & Data Science, School of Medicine at Yale. He is setting up his lab in 2024. Dr. Chen has led the major milestone in AI and data science in healthcare from data generation to method development and further to practical applications.
The PhD in statistics and data science at Yale University offers broad training in the areas of statistical theory, probability theory, stochastic processes, asymptotics, information theory, machine learning, data analysis, statistical computing, and graphical methods. Students complete 12 courses in the first year in these topics.
Data Science. The Yale School of Public Health has a long history of contributing to the development of quantitative methodologies and tools for rigorous scientific research to address the most challenging problems in biology, medicine and public health. Our researchers are working to: Interpretation of findings. The school is a leader in:
The Center for Biomedical Data Science acts as a focus for research and education in biomedical data science at Yale. CBDS Latest News. Copy Link. May 21, 2024. Leeds Receives VA Research Award for AI, Housing Study. ... PhD. Yale NLP/LLM Interest Group Talks. Kamino: A Scalable Architecture to Support Medical AI Research Using Large Real-World ...
The Biostatistics data science pathway combines rigorous statistical training with the development of advanced computational skills to solve the public health challenges of tomorrow. Required courses cover epidemiology, regression models, databases, machine learning and more. Students will become familiar with data science programming tools (e ...
Statistics and Data Science* Optional; Mathematics Subject Test Optional: Translational Molecular Medicine, Pharmacology and Physiology (BBS) ... When you take this test, please specify Yale University Graduate School of Arts and Sciences (also known as the Office of Graduate Admissions) as a score recipient. To identify us in the IELTS system ...
At the Yale School of Public Health, you will join a community dedicated to nurturing your intellectual and professional success in the field of public health. You will learn from national and international leaders in science-based public policy, health interventions, and basic research so that you step into a career with impact.
Yale School of Public Health (YSPH) has long emphasized both data science and data-driven health leadership. Equitable data science makes transformational and impactful research possible, resulting in innovations that advance and improve public health, from identifying new cancer treatments to creating guidelines reducing pollution's health impact.
Department of Statistics and Data Science. Yale University Kline Tower 219 Prospect Street New Haven, CT 06511. Mailing Address: PO Box 208290, New Haven, CT 06520-8290. Shipping Address (packages and Federal Express): 266 Whitney Avenue, New Haven, CT 06511. Department Phone: 203.432.0666
Data science combines computational and inferential reasoning to draw conclusions based on data about different aspects of the real world. Data scientists come from all walks of life, all areas of study, and all backgrounds. ... Earth and Environmental Science, PhD; Information and Data Science, Masters; Examples gathered from LinkedIn and an ...