- Privacy Policy

Home » Survey Research – Types, Methods, Examples
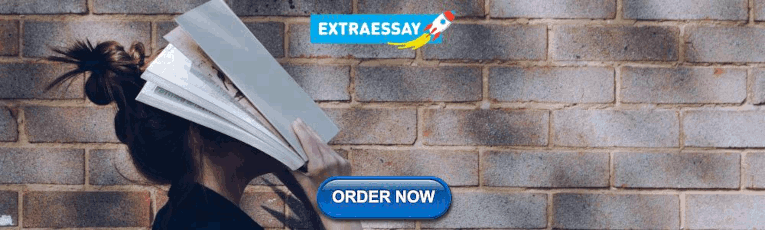
Survey Research – Types, Methods, Examples
Table of Contents

Survey Research
Definition:
Survey Research is a quantitative research method that involves collecting standardized data from a sample of individuals or groups through the use of structured questionnaires or interviews. The data collected is then analyzed statistically to identify patterns and relationships between variables, and to draw conclusions about the population being studied.
Survey research can be used to answer a variety of questions, including:
- What are people’s opinions about a certain topic?
- What are people’s experiences with a certain product or service?
- What are people’s beliefs about a certain issue?
Survey Research Methods
Survey Research Methods are as follows:
- Telephone surveys: A survey research method where questions are administered to respondents over the phone, often used in market research or political polling.
- Face-to-face surveys: A survey research method where questions are administered to respondents in person, often used in social or health research.
- Mail surveys: A survey research method where questionnaires are sent to respondents through mail, often used in customer satisfaction or opinion surveys.
- Online surveys: A survey research method where questions are administered to respondents through online platforms, often used in market research or customer feedback.
- Email surveys: A survey research method where questionnaires are sent to respondents through email, often used in customer satisfaction or opinion surveys.
- Mixed-mode surveys: A survey research method that combines two or more survey modes, often used to increase response rates or reach diverse populations.
- Computer-assisted surveys: A survey research method that uses computer technology to administer or collect survey data, often used in large-scale surveys or data collection.
- Interactive voice response surveys: A survey research method where respondents answer questions through a touch-tone telephone system, often used in automated customer satisfaction or opinion surveys.
- Mobile surveys: A survey research method where questions are administered to respondents through mobile devices, often used in market research or customer feedback.
- Group-administered surveys: A survey research method where questions are administered to a group of respondents simultaneously, often used in education or training evaluation.
- Web-intercept surveys: A survey research method where questions are administered to website visitors, often used in website or user experience research.
- In-app surveys: A survey research method where questions are administered to users of a mobile application, often used in mobile app or user experience research.
- Social media surveys: A survey research method where questions are administered to respondents through social media platforms, often used in social media or brand awareness research.
- SMS surveys: A survey research method where questions are administered to respondents through text messaging, often used in customer feedback or opinion surveys.
- IVR surveys: A survey research method where questions are administered to respondents through an interactive voice response system, often used in automated customer feedback or opinion surveys.
- Mixed-method surveys: A survey research method that combines both qualitative and quantitative data collection methods, often used in exploratory or mixed-method research.
- Drop-off surveys: A survey research method where respondents are provided with a survey questionnaire and asked to return it at a later time or through a designated drop-off location.
- Intercept surveys: A survey research method where respondents are approached in public places and asked to participate in a survey, often used in market research or customer feedback.
- Hybrid surveys: A survey research method that combines two or more survey modes, data sources, or research methods, often used in complex or multi-dimensional research questions.
Types of Survey Research
There are several types of survey research that can be used to collect data from a sample of individuals or groups. following are Types of Survey Research:
- Cross-sectional survey: A type of survey research that gathers data from a sample of individuals at a specific point in time, providing a snapshot of the population being studied.
- Longitudinal survey: A type of survey research that gathers data from the same sample of individuals over an extended period of time, allowing researchers to track changes or trends in the population being studied.
- Panel survey: A type of longitudinal survey research that tracks the same sample of individuals over time, typically collecting data at multiple points in time.
- Epidemiological survey: A type of survey research that studies the distribution and determinants of health and disease in a population, often used to identify risk factors and inform public health interventions.
- Observational survey: A type of survey research that collects data through direct observation of individuals or groups, often used in behavioral or social research.
- Correlational survey: A type of survey research that measures the degree of association or relationship between two or more variables, often used to identify patterns or trends in data.
- Experimental survey: A type of survey research that involves manipulating one or more variables to observe the effect on an outcome, often used to test causal hypotheses.
- Descriptive survey: A type of survey research that describes the characteristics or attributes of a population or phenomenon, often used in exploratory research or to summarize existing data.
- Diagnostic survey: A type of survey research that assesses the current state or condition of an individual or system, often used in health or organizational research.
- Explanatory survey: A type of survey research that seeks to explain or understand the causes or mechanisms behind a phenomenon, often used in social or psychological research.
- Process evaluation survey: A type of survey research that measures the implementation and outcomes of a program or intervention, often used in program evaluation or quality improvement.
- Impact evaluation survey: A type of survey research that assesses the effectiveness or impact of a program or intervention, often used to inform policy or decision-making.
- Customer satisfaction survey: A type of survey research that measures the satisfaction or dissatisfaction of customers with a product, service, or experience, often used in marketing or customer service research.
- Market research survey: A type of survey research that collects data on consumer preferences, behaviors, or attitudes, often used in market research or product development.
- Public opinion survey: A type of survey research that measures the attitudes, beliefs, or opinions of a population on a specific issue or topic, often used in political or social research.
- Behavioral survey: A type of survey research that measures actual behavior or actions of individuals, often used in health or social research.
- Attitude survey: A type of survey research that measures the attitudes, beliefs, or opinions of individuals, often used in social or psychological research.
- Opinion poll: A type of survey research that measures the opinions or preferences of a population on a specific issue or topic, often used in political or media research.
- Ad hoc survey: A type of survey research that is conducted for a specific purpose or research question, often used in exploratory research or to answer a specific research question.
Types Based on Methodology
Based on Methodology Survey are divided into two Types:
Quantitative Survey Research
Qualitative survey research.
Quantitative survey research is a method of collecting numerical data from a sample of participants through the use of standardized surveys or questionnaires. The purpose of quantitative survey research is to gather empirical evidence that can be analyzed statistically to draw conclusions about a particular population or phenomenon.
In quantitative survey research, the questions are structured and pre-determined, often utilizing closed-ended questions, where participants are given a limited set of response options to choose from. This approach allows for efficient data collection and analysis, as well as the ability to generalize the findings to a larger population.
Quantitative survey research is often used in market research, social sciences, public health, and other fields where numerical data is needed to make informed decisions and recommendations.
Qualitative survey research is a method of collecting non-numerical data from a sample of participants through the use of open-ended questions or semi-structured interviews. The purpose of qualitative survey research is to gain a deeper understanding of the experiences, perceptions, and attitudes of participants towards a particular phenomenon or topic.
In qualitative survey research, the questions are open-ended, allowing participants to share their thoughts and experiences in their own words. This approach allows for a rich and nuanced understanding of the topic being studied, and can provide insights that are difficult to capture through quantitative methods alone.
Qualitative survey research is often used in social sciences, education, psychology, and other fields where a deeper understanding of human experiences and perceptions is needed to inform policy, practice, or theory.
Data Analysis Methods
There are several Survey Research Data Analysis Methods that researchers may use, including:
- Descriptive statistics: This method is used to summarize and describe the basic features of the survey data, such as the mean, median, mode, and standard deviation. These statistics can help researchers understand the distribution of responses and identify any trends or patterns.
- Inferential statistics: This method is used to make inferences about the larger population based on the data collected in the survey. Common inferential statistical methods include hypothesis testing, regression analysis, and correlation analysis.
- Factor analysis: This method is used to identify underlying factors or dimensions in the survey data. This can help researchers simplify the data and identify patterns and relationships that may not be immediately apparent.
- Cluster analysis: This method is used to group similar respondents together based on their survey responses. This can help researchers identify subgroups within the larger population and understand how different groups may differ in their attitudes, behaviors, or preferences.
- Structural equation modeling: This method is used to test complex relationships between variables in the survey data. It can help researchers understand how different variables may be related to one another and how they may influence one another.
- Content analysis: This method is used to analyze open-ended responses in the survey data. Researchers may use software to identify themes or categories in the responses, or they may manually review and code the responses.
- Text mining: This method is used to analyze text-based survey data, such as responses to open-ended questions. Researchers may use software to identify patterns and themes in the text, or they may manually review and code the text.
Applications of Survey Research
Here are some common applications of survey research:
- Market Research: Companies use survey research to gather insights about customer needs, preferences, and behavior. These insights are used to create marketing strategies and develop new products.
- Public Opinion Research: Governments and political parties use survey research to understand public opinion on various issues. This information is used to develop policies and make decisions.
- Social Research: Survey research is used in social research to study social trends, attitudes, and behavior. Researchers use survey data to explore topics such as education, health, and social inequality.
- Academic Research: Survey research is used in academic research to study various phenomena. Researchers use survey data to test theories, explore relationships between variables, and draw conclusions.
- Customer Satisfaction Research: Companies use survey research to gather information about customer satisfaction with their products and services. This information is used to improve customer experience and retention.
- Employee Surveys: Employers use survey research to gather feedback from employees about their job satisfaction, working conditions, and organizational culture. This information is used to improve employee retention and productivity.
- Health Research: Survey research is used in health research to study topics such as disease prevalence, health behaviors, and healthcare access. Researchers use survey data to develop interventions and improve healthcare outcomes.
Examples of Survey Research
Here are some real-time examples of survey research:
- COVID-19 Pandemic Surveys: Since the outbreak of the COVID-19 pandemic, surveys have been conducted to gather information about public attitudes, behaviors, and perceptions related to the pandemic. Governments and healthcare organizations have used this data to develop public health strategies and messaging.
- Political Polls During Elections: During election seasons, surveys are used to measure public opinion on political candidates, policies, and issues in real-time. This information is used by political parties to develop campaign strategies and make decisions.
- Customer Feedback Surveys: Companies often use real-time customer feedback surveys to gather insights about customer experience and satisfaction. This information is used to improve products and services quickly.
- Event Surveys: Organizers of events such as conferences and trade shows often use surveys to gather feedback from attendees in real-time. This information can be used to improve future events and make adjustments during the current event.
- Website and App Surveys: Website and app owners use surveys to gather real-time feedback from users about the functionality, user experience, and overall satisfaction with their platforms. This feedback can be used to improve the user experience and retain customers.
- Employee Pulse Surveys: Employers use real-time pulse surveys to gather feedback from employees about their work experience and overall job satisfaction. This feedback is used to make changes in real-time to improve employee retention and productivity.
Survey Sample
Purpose of survey research.
The purpose of survey research is to gather data and insights from a representative sample of individuals. Survey research allows researchers to collect data quickly and efficiently from a large number of people, making it a valuable tool for understanding attitudes, behaviors, and preferences.
Here are some common purposes of survey research:
- Descriptive Research: Survey research is often used to describe characteristics of a population or a phenomenon. For example, a survey could be used to describe the characteristics of a particular demographic group, such as age, gender, or income.
- Exploratory Research: Survey research can be used to explore new topics or areas of research. Exploratory surveys are often used to generate hypotheses or identify potential relationships between variables.
- Explanatory Research: Survey research can be used to explain relationships between variables. For example, a survey could be used to determine whether there is a relationship between educational attainment and income.
- Evaluation Research: Survey research can be used to evaluate the effectiveness of a program or intervention. For example, a survey could be used to evaluate the impact of a health education program on behavior change.
- Monitoring Research: Survey research can be used to monitor trends or changes over time. For example, a survey could be used to monitor changes in attitudes towards climate change or political candidates over time.
When to use Survey Research
there are certain circumstances where survey research is particularly appropriate. Here are some situations where survey research may be useful:
- When the research question involves attitudes, beliefs, or opinions: Survey research is particularly useful for understanding attitudes, beliefs, and opinions on a particular topic. For example, a survey could be used to understand public opinion on a political issue.
- When the research question involves behaviors or experiences: Survey research can also be useful for understanding behaviors and experiences. For example, a survey could be used to understand the prevalence of a particular health behavior.
- When a large sample size is needed: Survey research allows researchers to collect data from a large number of people quickly and efficiently. This makes it a useful method when a large sample size is needed to ensure statistical validity.
- When the research question is time-sensitive: Survey research can be conducted quickly, which makes it a useful method when the research question is time-sensitive. For example, a survey could be used to understand public opinion on a breaking news story.
- When the research question involves a geographically dispersed population: Survey research can be conducted online, which makes it a useful method when the population of interest is geographically dispersed.
How to Conduct Survey Research
Conducting survey research involves several steps that need to be carefully planned and executed. Here is a general overview of the process:
- Define the research question: The first step in conducting survey research is to clearly define the research question. The research question should be specific, measurable, and relevant to the population of interest.
- Develop a survey instrument : The next step is to develop a survey instrument. This can be done using various methods, such as online survey tools or paper surveys. The survey instrument should be designed to elicit the information needed to answer the research question, and should be pre-tested with a small sample of individuals.
- Select a sample : The sample is the group of individuals who will be invited to participate in the survey. The sample should be representative of the population of interest, and the size of the sample should be sufficient to ensure statistical validity.
- Administer the survey: The survey can be administered in various ways, such as online, by mail, or in person. The method of administration should be chosen based on the population of interest and the research question.
- Analyze the data: Once the survey data is collected, it needs to be analyzed. This involves summarizing the data using statistical methods, such as frequency distributions or regression analysis.
- Draw conclusions: The final step is to draw conclusions based on the data analysis. This involves interpreting the results and answering the research question.
Advantages of Survey Research
There are several advantages to using survey research, including:
- Efficient data collection: Survey research allows researchers to collect data quickly and efficiently from a large number of people. This makes it a useful method for gathering information on a wide range of topics.
- Standardized data collection: Surveys are typically standardized, which means that all participants receive the same questions in the same order. This ensures that the data collected is consistent and reliable.
- Cost-effective: Surveys can be conducted online, by mail, or in person, which makes them a cost-effective method of data collection.
- Anonymity: Participants can remain anonymous when responding to a survey. This can encourage participants to be more honest and open in their responses.
- Easy comparison: Surveys allow for easy comparison of data between different groups or over time. This makes it possible to identify trends and patterns in the data.
- Versatility: Surveys can be used to collect data on a wide range of topics, including attitudes, beliefs, behaviors, and preferences.
Limitations of Survey Research
Here are some of the main limitations of survey research:
- Limited depth: Surveys are typically designed to collect quantitative data, which means that they do not provide much depth or detail about people’s experiences or opinions. This can limit the insights that can be gained from the data.
- Potential for bias: Surveys can be affected by various biases, including selection bias, response bias, and social desirability bias. These biases can distort the results and make them less accurate.
- L imited validity: Surveys are only as valid as the questions they ask. If the questions are poorly designed or ambiguous, the results may not accurately reflect the respondents’ attitudes or behaviors.
- Limited generalizability : Survey results are only generalizable to the population from which the sample was drawn. If the sample is not representative of the population, the results may not be generalizable to the larger population.
- Limited ability to capture context: Surveys typically do not capture the context in which attitudes or behaviors occur. This can make it difficult to understand the reasons behind the responses.
- Limited ability to capture complex phenomena: Surveys are not well-suited to capture complex phenomena, such as emotions or the dynamics of interpersonal relationships.
Following is an example of a Survey Sample:
Welcome to our Survey Research Page! We value your opinions and appreciate your participation in this survey. Please answer the questions below as honestly and thoroughly as possible.
1. What is your age?
- A) Under 18
- G) 65 or older
2. What is your highest level of education completed?
- A) Less than high school
- B) High school or equivalent
- C) Some college or technical school
- D) Bachelor’s degree
- E) Graduate or professional degree
3. What is your current employment status?
- A) Employed full-time
- B) Employed part-time
- C) Self-employed
- D) Unemployed
4. How often do you use the internet per day?
- A) Less than 1 hour
- B) 1-3 hours
- C) 3-5 hours
- D) 5-7 hours
- E) More than 7 hours
5. How often do you engage in social media per day?
6. Have you ever participated in a survey research study before?
7. If you have participated in a survey research study before, how was your experience?
- A) Excellent
- E) Very poor
8. What are some of the topics that you would be interested in participating in a survey research study about?
……………………………………………………………………………………………………………………………………………………………………………………………………………………………………………………………………….
9. How often would you be willing to participate in survey research studies?
- A) Once a week
- B) Once a month
- C) Once every 6 months
- D) Once a year
10. Any additional comments or suggestions?
Thank you for taking the time to complete this survey. Your feedback is important to us and will help us improve our survey research efforts.
About the author
Muhammad Hassan
Researcher, Academic Writer, Web developer
You may also like

Questionnaire – Definition, Types, and Examples

Case Study – Methods, Examples and Guide

Observational Research – Methods and Guide

Quantitative Research – Methods, Types and...

Qualitative Research Methods

Explanatory Research – Types, Methods, Guide
- Skip to main content
- Skip to primary sidebar
- Skip to footer
- QuestionPro

- Solutions Industries Gaming Automotive Sports and events Education Government Travel & Hospitality Financial Services Healthcare Cannabis Technology Use Case NPS+ Communities Audience Contactless surveys Mobile LivePolls Member Experience GDPR Positive People Science 360 Feedback Surveys
- Resources Blog eBooks Survey Templates Case Studies Training Help center

Home Market Research
Survey Research: Definition, Examples and Methods
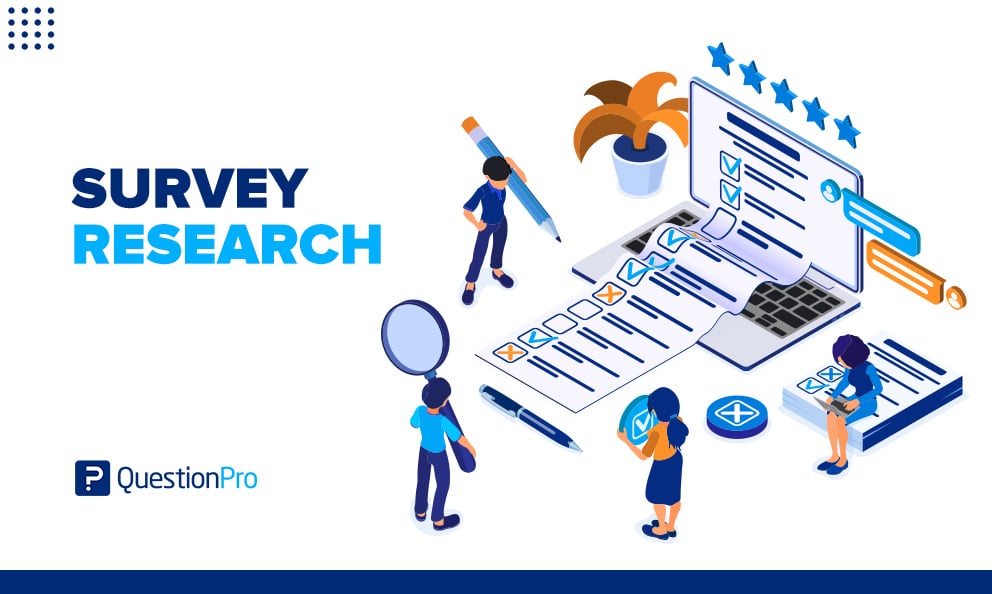
Survey Research is a quantitative research method used for collecting data from a set of respondents. It has been perhaps one of the most used methodologies in the industry for several years due to the multiple benefits and advantages that it has when collecting and analyzing data.
LEARN ABOUT: Behavioral Research
In this article, you will learn everything about survey research, such as types, methods, and examples.
Survey Research Definition
Survey Research is defined as the process of conducting research using surveys that researchers send to survey respondents. The data collected from surveys is then statistically analyzed to draw meaningful research conclusions. In the 21st century, every organization’s eager to understand what their customers think about their products or services and make better business decisions. Researchers can conduct research in multiple ways, but surveys are proven to be one of the most effective and trustworthy research methods. An online survey is a method for extracting information about a significant business matter from an individual or a group of individuals. It consists of structured survey questions that motivate the participants to respond. Creditable survey research can give these businesses access to a vast information bank. Organizations in media, other companies, and even governments rely on survey research to obtain accurate data.
The traditional definition of survey research is a quantitative method for collecting information from a pool of respondents by asking multiple survey questions. This research type includes the recruitment of individuals collection, and analysis of data. It’s useful for researchers who aim to communicate new features or trends to their respondents.
LEARN ABOUT: Level of Analysis Generally, it’s the primary step towards obtaining quick information about mainstream topics and conducting more rigorous and detailed quantitative research methods like surveys/polls or qualitative research methods like focus groups/on-call interviews can follow. There are many situations where researchers can conduct research using a blend of both qualitative and quantitative strategies.
LEARN ABOUT: Survey Sampling
Survey Research Methods
Survey research methods can be derived based on two critical factors: Survey research tool and time involved in conducting research. There are three main survey research methods, divided based on the medium of conducting survey research:
- Online/ Email: Online survey research is one of the most popular survey research methods today. The survey cost involved in online survey research is extremely minimal, and the responses gathered are highly accurate.
- Phone: Survey research conducted over the telephone ( CATI survey ) can be useful in collecting data from a more extensive section of the target population. There are chances that the money invested in phone surveys will be higher than other mediums, and the time required will be higher.
- Face-to-face: Researchers conduct face-to-face in-depth interviews in situations where there is a complicated problem to solve. The response rate for this method is the highest, but it can be costly.
Further, based on the time taken, survey research can be classified into two methods:
- Longitudinal survey research: Longitudinal survey research involves conducting survey research over a continuum of time and spread across years and decades. The data collected using this survey research method from one time period to another is qualitative or quantitative. Respondent behavior, preferences, and attitudes are continuously observed over time to analyze reasons for a change in behavior or preferences. For example, suppose a researcher intends to learn about the eating habits of teenagers. In that case, he/she will follow a sample of teenagers over a considerable period to ensure that the collected information is reliable. Often, cross-sectional survey research follows a longitudinal study .
- Cross-sectional survey research: Researchers conduct a cross-sectional survey to collect insights from a target audience at a particular time interval. This survey research method is implemented in various sectors such as retail, education, healthcare, SME businesses, etc. Cross-sectional studies can either be descriptive or analytical. It is quick and helps researchers collect information in a brief period. Researchers rely on the cross-sectional survey research method in situations where descriptive analysis of a subject is required.
Survey research also is bifurcated according to the sampling methods used to form samples for research: Probability and Non-probability sampling. Every individual in a population should be considered equally to be a part of the survey research sample. Probability sampling is a sampling method in which the researcher chooses the elements based on probability theory. The are various probability research methods, such as simple random sampling , systematic sampling, cluster sampling, stratified random sampling, etc. Non-probability sampling is a sampling method where the researcher uses his/her knowledge and experience to form samples.
LEARN ABOUT: Survey Sample Sizes
The various non-probability sampling techniques are :
- Convenience sampling
- Snowball sampling
- Consecutive sampling
- Judgemental sampling
- Quota sampling
Process of implementing survey research methods:
- Decide survey questions: Brainstorm and put together valid survey questions that are grammatically and logically appropriate. Understanding the objective and expected outcomes of the survey helps a lot. There are many surveys where details of responses are not as important as gaining insights about what customers prefer from the provided options. In such situations, a researcher can include multiple-choice questions or closed-ended questions . Whereas, if researchers need to obtain details about specific issues, they can consist of open-ended questions in the questionnaire. Ideally, the surveys should include a smart balance of open-ended and closed-ended questions. Use survey questions like Likert Scale , Semantic Scale, Net Promoter Score question, etc., to avoid fence-sitting.
LEARN ABOUT: System Usability Scale
- Finalize a target audience: Send out relevant surveys as per the target audience and filter out irrelevant questions as per the requirement. The survey research will be instrumental in case the target population decides on a sample. This way, results can be according to the desired market and be generalized to the entire population.
LEARN ABOUT: Testimonial Questions
- Send out surveys via decided mediums: Distribute the surveys to the target audience and patiently wait for the feedback and comments- this is the most crucial step of the survey research. The survey needs to be scheduled, keeping in mind the nature of the target audience and its regions. Surveys can be conducted via email, embedded in a website, shared via social media, etc., to gain maximum responses.
- Analyze survey results: Analyze the feedback in real-time and identify patterns in the responses which might lead to a much-needed breakthrough for your organization. GAP, TURF Analysis , Conjoint analysis, Cross tabulation, and many such survey feedback analysis methods can be used to spot and shed light on respondent behavior. Researchers can use the results to implement corrective measures to improve customer/employee satisfaction.
Reasons to conduct survey research
The most crucial and integral reason for conducting market research using surveys is that you can collect answers regarding specific, essential questions. You can ask these questions in multiple survey formats as per the target audience and the intent of the survey. Before designing a study, every organization must figure out the objective of carrying this out so that the study can be structured, planned, and executed to perfection.
LEARN ABOUT: Research Process Steps
Questions that need to be on your mind while designing a survey are:
- What is the primary aim of conducting the survey?
- How do you plan to utilize the collected survey data?
- What type of decisions do you plan to take based on the points mentioned above?
There are three critical reasons why an organization must conduct survey research.
- Understand respondent behavior to get solutions to your queries: If you’ve carefully curated a survey, the respondents will provide insights about what they like about your organization as well as suggestions for improvement. To motivate them to respond, you must be very vocal about how secure their responses will be and how you will utilize the answers. This will push them to be 100% honest about their feedback, opinions, and comments. Online surveys or mobile surveys have proved their privacy, and due to this, more and more respondents feel free to put forth their feedback through these mediums.
- Present a medium for discussion: A survey can be the perfect platform for respondents to provide criticism or applause for an organization. Important topics like product quality or quality of customer service etc., can be put on the table for discussion. A way you can do it is by including open-ended questions where the respondents can write their thoughts. This will make it easy for you to correlate your survey to what you intend to do with your product or service.
- Strategy for never-ending improvements: An organization can establish the target audience’s attributes from the pilot phase of survey research . Researchers can use the criticism and feedback received from this survey to improve the product/services. Once the company successfully makes the improvements, it can send out another survey to measure the change in feedback keeping the pilot phase the benchmark. By doing this activity, the organization can track what was effectively improved and what still needs improvement.
Survey Research Scales
There are four main scales for the measurement of variables:
- Nominal Scale: A nominal scale associates numbers with variables for mere naming or labeling, and the numbers usually have no other relevance. It is the most basic of the four levels of measurement.
- Ordinal Scale: The ordinal scale has an innate order within the variables along with labels. It establishes the rank between the variables of a scale but not the difference value between the variables.
- Interval Scale: The interval scale is a step ahead in comparison to the other two scales. Along with establishing a rank and name of variables, the scale also makes known the difference between the two variables. The only drawback is that there is no fixed start point of the scale, i.e., the actual zero value is absent.
- Ratio Scale: The ratio scale is the most advanced measurement scale, which has variables that are labeled in order and have a calculated difference between variables. In addition to what interval scale orders, this scale has a fixed starting point, i.e., the actual zero value is present.
Benefits of survey research
In case survey research is used for all the right purposes and is implemented properly, marketers can benefit by gaining useful, trustworthy data that they can use to better the ROI of the organization.
Other benefits of survey research are:
- Minimum investment: Mobile surveys and online surveys have minimal finance invested per respondent. Even with the gifts and other incentives provided to the people who participate in the study, online surveys are extremely economical compared to paper-based surveys.
- Versatile sources for response collection: You can conduct surveys via various mediums like online and mobile surveys. You can further classify them into qualitative mediums like focus groups , and interviews and quantitative mediums like customer-centric surveys. Due to the offline survey response collection option, researchers can conduct surveys in remote areas with limited internet connectivity. This can make data collection and analysis more convenient and extensive.
- Reliable for respondents: Surveys are extremely secure as the respondent details and responses are kept safeguarded. This anonymity makes respondents answer the survey questions candidly and with absolute honesty. An organization seeking to receive explicit responses for its survey research must mention that it will be confidential.
Survey research design
Researchers implement a survey research design in cases where there is a limited cost involved and there is a need to access details easily. This method is often used by small and large organizations to understand and analyze new trends, market demands, and opinions. Collecting information through tactfully designed survey research can be much more effective and productive than a casually conducted survey.
There are five stages of survey research design:
- Decide an aim of the research: There can be multiple reasons for a researcher to conduct a survey, but they need to decide a purpose for the research. This is the primary stage of survey research as it can mold the entire path of a survey, impacting its results.
- Filter the sample from target population: Who to target? is an essential question that a researcher should answer and keep in mind while conducting research. The precision of the results is driven by who the members of a sample are and how useful their opinions are. The quality of respondents in a sample is essential for the results received for research and not the quantity. If a researcher seeks to understand whether a product feature will work well with their target market, he/she can conduct survey research with a group of market experts for that product or technology.
- Zero-in on a survey method: Many qualitative and quantitative research methods can be discussed and decided. Focus groups, online interviews, surveys, polls, questionnaires, etc. can be carried out with a pre-decided sample of individuals.
- Design the questionnaire: What will the content of the survey be? A researcher is required to answer this question to be able to design it effectively. What will the content of the cover letter be? Or what are the survey questions of this questionnaire? Understand the target market thoroughly to create a questionnaire that targets a sample to gain insights about a survey research topic.
- Send out surveys and analyze results: Once the researcher decides on which questions to include in a study, they can send it across to the selected sample . Answers obtained from this survey can be analyzed to make product-related or marketing-related decisions.
Survey examples: 10 tips to design the perfect research survey
Picking the right survey design can be the key to gaining the information you need to make crucial decisions for all your research. It is essential to choose the right topic, choose the right question types, and pick a corresponding design. If this is your first time creating a survey, it can seem like an intimidating task. But with QuestionPro, each step of the process is made simple and easy.
Below are 10 Tips To Design The Perfect Research Survey:
- Set your SMART goals: Before conducting any market research or creating a particular plan, set your SMART Goals . What is that you want to achieve with the survey? How will you measure it promptly, and what are the results you are expecting?
- Choose the right questions: Designing a survey can be a tricky task. Asking the right questions may help you get the answers you are looking for and ease the task of analyzing. So, always choose those specific questions – relevant to your research.
- Begin your survey with a generalized question: Preferably, start your survey with a general question to understand whether the respondent uses the product or not. That also provides an excellent base and intro for your survey.
- Enhance your survey: Choose the best, most relevant, 15-20 questions. Frame each question as a different question type based on the kind of answer you would like to gather from each. Create a survey using different types of questions such as multiple-choice, rating scale, open-ended, etc. Look at more survey examples and four measurement scales every researcher should remember.
- Prepare yes/no questions: You may also want to use yes/no questions to separate people or branch them into groups of those who “have purchased” and those who “have not yet purchased” your products or services. Once you separate them, you can ask them different questions.
- Test all electronic devices: It becomes effortless to distribute your surveys if respondents can answer them on different electronic devices like mobiles, tablets, etc. Once you have created your survey, it’s time to TEST. You can also make any corrections if needed at this stage.
- Distribute your survey: Once your survey is ready, it is time to share and distribute it to the right audience. You can share handouts and share them via email, social media, and other industry-related offline/online communities.
- Collect and analyze responses: After distributing your survey, it is time to gather all responses. Make sure you store your results in a particular document or an Excel sheet with all the necessary categories mentioned so that you don’t lose your data. Remember, this is the most crucial stage. Segregate your responses based on demographics, psychographics, and behavior. This is because, as a researcher, you must know where your responses are coming from. It will help you to analyze, predict decisions, and help write the summary report.
- Prepare your summary report: Now is the time to share your analysis. At this stage, you should mention all the responses gathered from a survey in a fixed format. Also, the reader/customer must get clarity about your goal, which you were trying to gain from the study. Questions such as – whether the product or service has been used/preferred or not. Do respondents prefer some other product to another? Any recommendations?
Having a tool that helps you carry out all the necessary steps to carry out this type of study is a vital part of any project. At QuestionPro, we have helped more than 10,000 clients around the world to carry out data collection in a simple and effective way, in addition to offering a wide range of solutions to take advantage of this data in the best possible way.
From dashboards, advanced analysis tools, automation, and dedicated functions, in QuestionPro, you will find everything you need to execute your research projects effectively. Uncover insights that matter the most!
MORE LIKE THIS
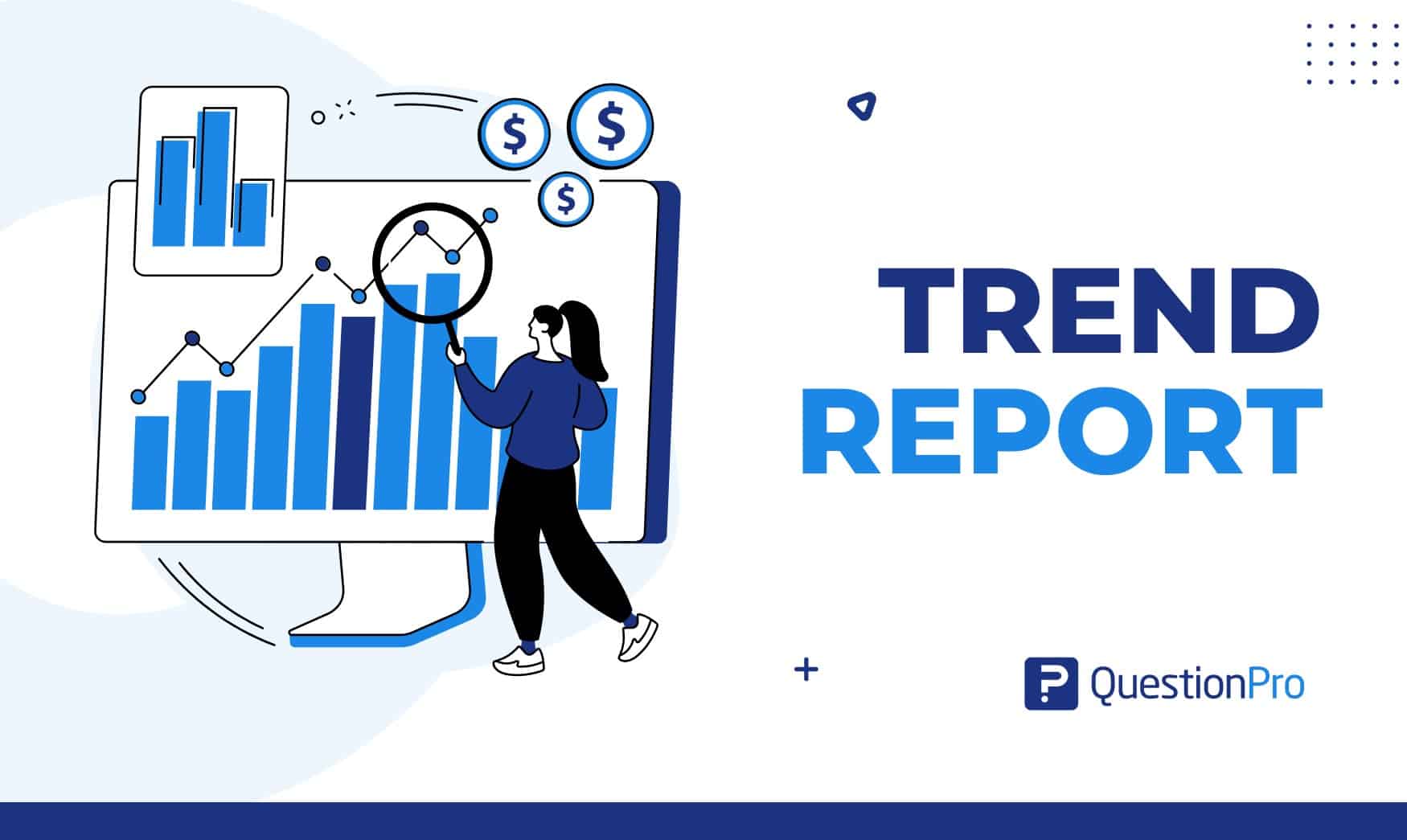
Trend Report: Guide for Market Dynamics & Strategic Analysis
May 29, 2024
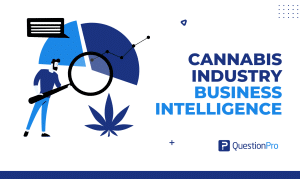
Cannabis Industry Business Intelligence: Impact on Research
May 28, 2024
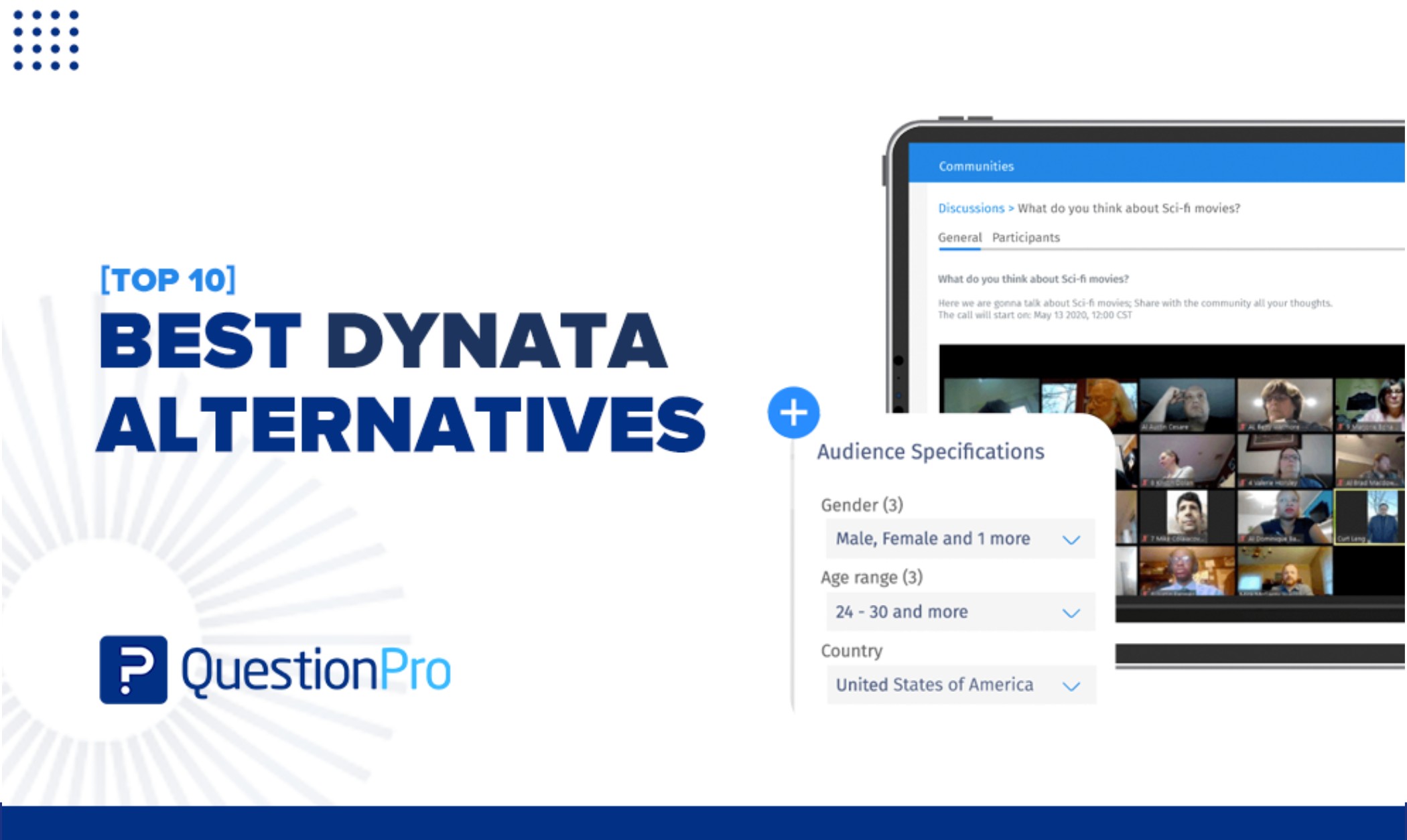
Top 10 Dynata Alternatives & Competitors
May 27, 2024
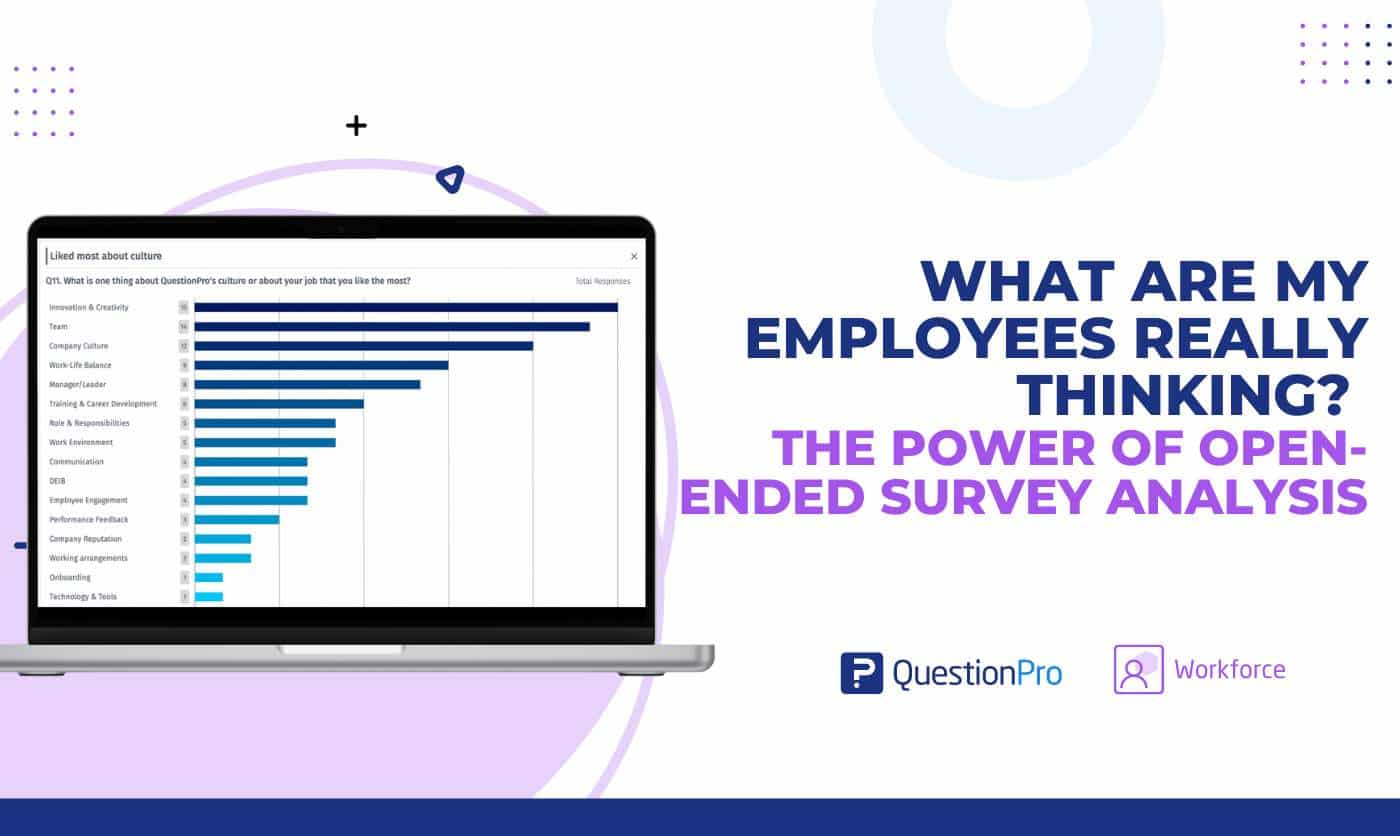
What Are My Employees Really Thinking? The Power of Open-ended Survey Analysis
May 24, 2024
Other categories
- Academic Research
- Artificial Intelligence
- Assessments
- Brand Awareness
- Case Studies
- Communities
- Consumer Insights
- Customer effort score
- Customer Engagement
- Customer Experience
- Customer Loyalty
- Customer Research
- Customer Satisfaction
- Employee Benefits
- Employee Engagement
- Employee Retention
- Friday Five
- General Data Protection Regulation
- Insights Hub
- Life@QuestionPro
- Market Research
- Mobile diaries
- Mobile Surveys
- New Features
- Online Communities
- Question Types
- Questionnaire
- QuestionPro Products
- Release Notes
- Research Tools and Apps
- Revenue at Risk
- Survey Templates
- Training Tips
- Uncategorized
- Video Learning Series
- What’s Coming Up
- Workforce Intelligence
Root out friction in every digital experience, super-charge conversion rates, and optimize digital self-service
Uncover insights from any interaction, deliver AI-powered agent coaching, and reduce cost to serve
Increase revenue and loyalty with real-time insights and recommendations delivered to teams on the ground
Know how your people feel and empower managers to improve employee engagement, productivity, and retention
Take action in the moments that matter most along the employee journey and drive bottom line growth
Whatever they’re are saying, wherever they’re saying it, know exactly what’s going on with your people
Get faster, richer insights with qual and quant tools that make powerful market research available to everyone
Run concept tests, pricing studies, prototyping + more with fast, powerful studies designed by UX research experts
Track your brand performance 24/7 and act quickly to respond to opportunities and challenges in your market
Explore the platform powering Experience Management
- Free Account
- For Digital
- For Customer Care
- For Human Resources
- For Researchers
- Financial Services
- All Industries
Popular Use Cases
- Customer Experience
- Employee Experience
- Net Promoter Score
- Voice of Customer
- Customer Success Hub
- Product Documentation
- Training & Certification
- XM Institute
- Popular Resources
- Customer Stories
- Artificial Intelligence
- Market Research
- Partnerships
- Marketplace
The annual gathering of the experience leaders at the world’s iconic brands building breakthrough business results, live in Salt Lake City.
- English/AU & NZ
- Español/Europa
- Español/América Latina
- Português Brasileiro
- REQUEST DEMO
- Experience Management
- What is a survey?
- Survey Research
Try Qualtrics for free
What is survey research.
15 min read Find out everything you need to know about survey research, from what it is and how it works to the different methods and tools you can use to ensure you’re successful.
Survey research is the process of collecting data from a predefined group (e.g. customers or potential customers) with the ultimate goal of uncovering insights about your products, services, or brand overall .
As a quantitative data collection method, survey research can provide you with a goldmine of information that can inform crucial business and product decisions. But survey research needs careful planning and execution to get the results you want.
So if you’re thinking about using surveys to carry out research, read on.
Get started with our free survey maker tool
Types of survey research
Calling these methods ‘survey research’ slightly underplays the complexity of this type of information gathering. From the expertise required to carry out each activity to the analysis of the data and its eventual application, a considerable amount of effort is required.
As for how you can carry out your research, there are several options to choose from — face-to-face interviews, telephone surveys, focus groups (though more interviews than surveys), online surveys , and panel surveys.
Typically, the survey method you choose will largely be guided by who you want to survey, the size of your sample , your budget, and the type of information you’re hoping to gather.
Here are a few of the most-used survey types:
Face-to-face interviews
Before technology made it possible to conduct research using online surveys, telephone, and mail were the most popular methods for survey research. However face-to-face interviews were considered the gold standard — the only reason they weren’t as popular was due to their highly prohibitive costs.
When it came to face-to-face interviews, organizations would use highly trained researchers who knew when to probe or follow up on vague or problematic answers. They also knew when to offer assistance to respondents when they seemed to be struggling. The result was that these interviewers could get sample members to participate and engage in surveys in the most effective way possible, leading to higher response rates and better quality data.
Telephone surveys
While phone surveys have been popular in the past, particularly for measuring general consumer behavior or beliefs, response rates have been declining since the 1990s .
Phone surveys are usually conducted using a random dialing system and software that a researcher can use to record responses.
This method is beneficial when you want to survey a large population but don’t have the resources to conduct face-to-face research surveys or run focus groups, or want to ask multiple-choice and open-ended questions .
The downsides are they can: take a long time to complete depending on the response rate, and you may have to do a lot of cold-calling to get the information you need.
You also run the risk of respondents not being completely honest . Instead, they’ll answer your survey questions quickly just to get off the phone.
Focus groups (interviews — not surveys)
Focus groups are a separate qualitative methodology rather than surveys — even though they’re often bunched together. They’re normally used for survey pretesting and designing , but they’re also a great way to generate opinions and data from a diverse range of people.
Focus groups involve putting a cohort of demographically or socially diverse people in a room with a moderator and engaging them in a discussion on a particular topic, such as your product, brand, or service.
They remain a highly popular method for market research , but they’re expensive and require a lot of administration to conduct and analyze the data properly.
You also run the risk of more dominant members of the group taking over the discussion and swaying the opinions of other people — potentially providing you with unreliable data.
Online surveys
Online surveys have become one of the most popular survey methods due to being cost-effective, enabling researchers to accurately survey a large population quickly.
Online surveys can essentially be used by anyone for any research purpose – we’ve all seen the increasing popularity of polls on social media (although these are not scientific).
Using an online survey allows you to ask a series of different question types and collect data instantly that’s easy to analyze with the right software.
There are also several methods for running and distributing online surveys that allow you to get your questionnaire in front of a large population at a fraction of the cost of face-to-face interviews or focus groups.
This is particularly true when it comes to mobile surveys as most people with a smartphone can access them online.
However, you have to be aware of the potential dangers of using online surveys, particularly when it comes to the survey respondents. The biggest risk is because online surveys require access to a computer or mobile device to complete, they could exclude elderly members of the population who don’t have access to the technology — or don’t know how to use it.
It could also exclude those from poorer socio-economic backgrounds who can’t afford a computer or consistent internet access. This could mean the data collected is more biased towards a certain group and can lead to less accurate data when you’re looking for a representative population sample.
When it comes to surveys, every voice matters.
Find out how to create more inclusive and representative surveys for your research.
Panel surveys
A panel survey involves recruiting respondents who have specifically signed up to answer questionnaires and who are put on a list by a research company. This could be a workforce of a small company or a major subset of a national population. Usually, these groups are carefully selected so that they represent a sample of your target population — giving you balance across criteria such as age, gender, background, and so on.
Panel surveys give you access to the respondents you need and are usually provided by the research company in question. As a result, it’s much easier to get access to the right audiences as you just need to tell the research company your criteria. They’ll then determine the right panels to use to answer your questionnaire.
However, there are downsides. The main one being that if the research company offers its panels incentives, e.g. discounts, coupons, money — respondents may answer a lot of questionnaires just for the benefits.
This might mean they rush through your survey without providing considered and truthful answers. As a consequence, this can damage the credibility of your data and potentially ruin your analyses.
What are the benefits of using survey research?
Depending on the research method you use, there are lots of benefits to conducting survey research for data collection. Here, we cover a few:
1. They’re relatively easy to do
Most research surveys are easy to set up, administer and analyze. As long as the planning and survey design is thorough and you target the right audience , the data collection is usually straightforward regardless of which survey type you use.
2. They can be cost effective
Survey research can be relatively cheap depending on the type of survey you use.
Generally, qualitative research methods that require access to people in person or over the phone are more expensive and require more administration.
Online surveys or mobile surveys are often more cost-effective for market research and can give you access to the global population for a fraction of the cost.
3. You can collect data from a large sample
Again, depending on the type of survey, you can obtain survey results from an entire population at a relatively low price. You can also administer a large variety of survey types to fit the project you’re running.
4. You can use survey software to analyze results immediately
Using survey software, you can use advanced statistical analysis techniques to gain insights into your responses immediately.
Analysis can be conducted using a variety of parameters to determine the validity and reliability of your survey data at scale.
5. Surveys can collect any type of data
While most people view surveys as a quantitative research method, they can just as easily be adapted to gain qualitative information by simply including open-ended questions or conducting interviews face to face.
How to measure concepts with survey questions
While surveys are a great way to obtain data, that data on its own is useless unless it can be analyzed and developed into actionable insights.
The easiest, and most effective way to measure survey results, is to use a dedicated research tool that puts all of your survey results into one place.
When it comes to survey measurement, there are four measurement types to be aware of that will determine how you treat your different survey results:
Nominal scale
With a nominal scale , you can only keep track of how many respondents chose each option from a question, and which response generated the most selections.
An example of this would be simply asking a responder to choose a product or brand from a list.
You could find out which brand was chosen the most but have no insight as to why.
Ordinal scale
Ordinal scales are used to judge an order of preference. They do provide some level of quantitative value because you’re asking responders to choose a preference of one option over another.
Ratio scale
Ratio scales can be used to judge the order and difference between responses. For example, asking respondents how much they spend on their weekly shopping on average.
Interval scale
In an interval scale, values are lined up in order with a meaningful difference between the two values — for example, measuring temperature or measuring a credit score between one value and another.
Step by step: How to conduct surveys and collect data
Conducting a survey and collecting data is relatively straightforward, but it does require some careful planning and design to ensure it results in reliable data.
Step 1 – Define your objectives
What do you want to learn from the survey? How is the data going to help you? Having a hypothesis or series of assumptions about survey responses will allow you to create the right questions to test them.
Step 2 – Create your survey questions
Once you’ve got your hypotheses or assumptions, write out the questions you need answering to test your theories or beliefs. Be wary about framing questions that could lead respondents or inadvertently create biased responses .
Step 3 – Choose your question types
Your survey should include a variety of question types and should aim to obtain quantitative data with some qualitative responses from open-ended questions. Using a mix of questions (simple Yes/ No, multiple-choice, rank in order, etc) not only increases the reliability of your data but also reduces survey fatigue and respondents simply answering questions quickly without thinking.
Find out how to create a survey that’s easy to engage with
Step 4 – Test your questions
Before sending your questionnaire out, you should test it (e.g. have a random internal group do the survey) and carry out A/B tests to ensure you’ll gain accurate responses.
Step 5 – Choose your target and send out the survey
Depending on your objectives, you might want to target the general population with your survey or a specific segment of the population. Once you’ve narrowed down who you want to target, it’s time to send out the survey.
After you’ve deployed the survey, keep an eye on the response rate to ensure you’re getting the number you expected. If your response rate is low, you might need to send the survey out to a second group to obtain a large enough sample — or do some troubleshooting to work out why your response rates are so low. This could be down to your questions, delivery method, selected sample, or otherwise.
Step 6 – Analyze results and draw conclusions
Once you’ve got your results back, it’s time for the fun part.
Break down your survey responses using the parameters you’ve set in your objectives and analyze the data to compare to your original assumptions. At this stage, a research tool or software can make the analysis a lot easier — and that’s somewhere Qualtrics can help.
Get reliable insights with survey software from Qualtrics
Gaining feedback from customers and leads is critical for any business, data gathered from surveys can prove invaluable for understanding your products and your market position, and with survey software from Qualtrics, it couldn’t be easier.
Used by more than 13,000 brands and supporting more than 1 billion surveys a year, Qualtrics empowers everyone in your organization to gather insights and take action. No coding required — and your data is housed in one system.
Get feedback from more than 125 sources on a single platform and view and measure your data in one place to create actionable insights and gain a deeper understanding of your target customers .
Automatically run complex text and statistical analysis to uncover exactly what your survey data is telling you, so you can react in real-time and make smarter decisions.
We can help you with survey management, too. From designing your survey and finding your target respondents to getting your survey in the field and reporting back on the results, we can help you every step of the way.
And for expert market researchers and survey designers, Qualtrics features custom programming to give you total flexibility over question types, survey design, embedded data, and other variables.
No matter what type of survey you want to run, what target audience you want to reach, or what assumptions you want to test or answers you want to uncover, we’ll help you design, deploy and analyze your survey with our team of experts.
Ready to find out more about Qualtrics CoreXM?
Get started with our free survey maker tool today
Related resources
Survey bias types 24 min read, post event survey questions 10 min read, best survey software 16 min read, close-ended questions 7 min read, survey vs questionnaire 12 min read, response bias 13 min read, double barreled question 11 min read, request demo.
Ready to learn more about Qualtrics?
Have a language expert improve your writing
Run a free plagiarism check in 10 minutes, automatically generate references for free.
- Knowledge Base
- Methodology
Research Design | Step-by-Step Guide with Examples
Published on 5 May 2022 by Shona McCombes . Revised on 20 March 2023.
A research design is a strategy for answering your research question using empirical data. Creating a research design means making decisions about:
- Your overall aims and approach
- The type of research design you’ll use
- Your sampling methods or criteria for selecting subjects
- Your data collection methods
- The procedures you’ll follow to collect data
- Your data analysis methods
A well-planned research design helps ensure that your methods match your research aims and that you use the right kind of analysis for your data.
Table of contents
Step 1: consider your aims and approach, step 2: choose a type of research design, step 3: identify your population and sampling method, step 4: choose your data collection methods, step 5: plan your data collection procedures, step 6: decide on your data analysis strategies, frequently asked questions.
- Introduction
Before you can start designing your research, you should already have a clear idea of the research question you want to investigate.
There are many different ways you could go about answering this question. Your research design choices should be driven by your aims and priorities – start by thinking carefully about what you want to achieve.
The first choice you need to make is whether you’ll take a qualitative or quantitative approach.
Qualitative research designs tend to be more flexible and inductive , allowing you to adjust your approach based on what you find throughout the research process.
Quantitative research designs tend to be more fixed and deductive , with variables and hypotheses clearly defined in advance of data collection.
It’s also possible to use a mixed methods design that integrates aspects of both approaches. By combining qualitative and quantitative insights, you can gain a more complete picture of the problem you’re studying and strengthen the credibility of your conclusions.
Practical and ethical considerations when designing research
As well as scientific considerations, you need to think practically when designing your research. If your research involves people or animals, you also need to consider research ethics .
- How much time do you have to collect data and write up the research?
- Will you be able to gain access to the data you need (e.g., by travelling to a specific location or contacting specific people)?
- Do you have the necessary research skills (e.g., statistical analysis or interview techniques)?
- Will you need ethical approval ?
At each stage of the research design process, make sure that your choices are practically feasible.
Prevent plagiarism, run a free check.
Within both qualitative and quantitative approaches, there are several types of research design to choose from. Each type provides a framework for the overall shape of your research.
Types of quantitative research designs
Quantitative designs can be split into four main types. Experimental and quasi-experimental designs allow you to test cause-and-effect relationships, while descriptive and correlational designs allow you to measure variables and describe relationships between them.
With descriptive and correlational designs, you can get a clear picture of characteristics, trends, and relationships as they exist in the real world. However, you can’t draw conclusions about cause and effect (because correlation doesn’t imply causation ).
Experiments are the strongest way to test cause-and-effect relationships without the risk of other variables influencing the results. However, their controlled conditions may not always reflect how things work in the real world. They’re often also more difficult and expensive to implement.
Types of qualitative research designs
Qualitative designs are less strictly defined. This approach is about gaining a rich, detailed understanding of a specific context or phenomenon, and you can often be more creative and flexible in designing your research.
The table below shows some common types of qualitative design. They often have similar approaches in terms of data collection, but focus on different aspects when analysing the data.
Your research design should clearly define who or what your research will focus on, and how you’ll go about choosing your participants or subjects.
In research, a population is the entire group that you want to draw conclusions about, while a sample is the smaller group of individuals you’ll actually collect data from.
Defining the population
A population can be made up of anything you want to study – plants, animals, organisations, texts, countries, etc. In the social sciences, it most often refers to a group of people.
For example, will you focus on people from a specific demographic, region, or background? Are you interested in people with a certain job or medical condition, or users of a particular product?
The more precisely you define your population, the easier it will be to gather a representative sample.
Sampling methods
Even with a narrowly defined population, it’s rarely possible to collect data from every individual. Instead, you’ll collect data from a sample.
To select a sample, there are two main approaches: probability sampling and non-probability sampling . The sampling method you use affects how confidently you can generalise your results to the population as a whole.
Probability sampling is the most statistically valid option, but it’s often difficult to achieve unless you’re dealing with a very small and accessible population.
For practical reasons, many studies use non-probability sampling, but it’s important to be aware of the limitations and carefully consider potential biases. You should always make an effort to gather a sample that’s as representative as possible of the population.
Case selection in qualitative research
In some types of qualitative designs, sampling may not be relevant.
For example, in an ethnography or a case study, your aim is to deeply understand a specific context, not to generalise to a population. Instead of sampling, you may simply aim to collect as much data as possible about the context you are studying.
In these types of design, you still have to carefully consider your choice of case or community. You should have a clear rationale for why this particular case is suitable for answering your research question.
For example, you might choose a case study that reveals an unusual or neglected aspect of your research problem, or you might choose several very similar or very different cases in order to compare them.
Data collection methods are ways of directly measuring variables and gathering information. They allow you to gain first-hand knowledge and original insights into your research problem.
You can choose just one data collection method, or use several methods in the same study.
Survey methods
Surveys allow you to collect data about opinions, behaviours, experiences, and characteristics by asking people directly. There are two main survey methods to choose from: questionnaires and interviews.
Observation methods
Observations allow you to collect data unobtrusively, observing characteristics, behaviours, or social interactions without relying on self-reporting.
Observations may be conducted in real time, taking notes as you observe, or you might make audiovisual recordings for later analysis. They can be qualitative or quantitative.
Other methods of data collection
There are many other ways you might collect data depending on your field and topic.
If you’re not sure which methods will work best for your research design, try reading some papers in your field to see what data collection methods they used.
Secondary data
If you don’t have the time or resources to collect data from the population you’re interested in, you can also choose to use secondary data that other researchers already collected – for example, datasets from government surveys or previous studies on your topic.
With this raw data, you can do your own analysis to answer new research questions that weren’t addressed by the original study.
Using secondary data can expand the scope of your research, as you may be able to access much larger and more varied samples than you could collect yourself.
However, it also means you don’t have any control over which variables to measure or how to measure them, so the conclusions you can draw may be limited.
As well as deciding on your methods, you need to plan exactly how you’ll use these methods to collect data that’s consistent, accurate, and unbiased.
Planning systematic procedures is especially important in quantitative research, where you need to precisely define your variables and ensure your measurements are reliable and valid.
Operationalisation
Some variables, like height or age, are easily measured. But often you’ll be dealing with more abstract concepts, like satisfaction, anxiety, or competence. Operationalisation means turning these fuzzy ideas into measurable indicators.
If you’re using observations , which events or actions will you count?
If you’re using surveys , which questions will you ask and what range of responses will be offered?
You may also choose to use or adapt existing materials designed to measure the concept you’re interested in – for example, questionnaires or inventories whose reliability and validity has already been established.
Reliability and validity
Reliability means your results can be consistently reproduced , while validity means that you’re actually measuring the concept you’re interested in.
For valid and reliable results, your measurement materials should be thoroughly researched and carefully designed. Plan your procedures to make sure you carry out the same steps in the same way for each participant.
If you’re developing a new questionnaire or other instrument to measure a specific concept, running a pilot study allows you to check its validity and reliability in advance.
Sampling procedures
As well as choosing an appropriate sampling method, you need a concrete plan for how you’ll actually contact and recruit your selected sample.
That means making decisions about things like:
- How many participants do you need for an adequate sample size?
- What inclusion and exclusion criteria will you use to identify eligible participants?
- How will you contact your sample – by mail, online, by phone, or in person?
If you’re using a probability sampling method, it’s important that everyone who is randomly selected actually participates in the study. How will you ensure a high response rate?
If you’re using a non-probability method, how will you avoid bias and ensure a representative sample?
Data management
It’s also important to create a data management plan for organising and storing your data.
Will you need to transcribe interviews or perform data entry for observations? You should anonymise and safeguard any sensitive data, and make sure it’s backed up regularly.
Keeping your data well organised will save time when it comes to analysing them. It can also help other researchers validate and add to your findings.
On their own, raw data can’t answer your research question. The last step of designing your research is planning how you’ll analyse the data.
Quantitative data analysis
In quantitative research, you’ll most likely use some form of statistical analysis . With statistics, you can summarise your sample data, make estimates, and test hypotheses.
Using descriptive statistics , you can summarise your sample data in terms of:
- The distribution of the data (e.g., the frequency of each score on a test)
- The central tendency of the data (e.g., the mean to describe the average score)
- The variability of the data (e.g., the standard deviation to describe how spread out the scores are)
The specific calculations you can do depend on the level of measurement of your variables.
Using inferential statistics , you can:
- Make estimates about the population based on your sample data.
- Test hypotheses about a relationship between variables.
Regression and correlation tests look for associations between two or more variables, while comparison tests (such as t tests and ANOVAs ) look for differences in the outcomes of different groups.
Your choice of statistical test depends on various aspects of your research design, including the types of variables you’re dealing with and the distribution of your data.
Qualitative data analysis
In qualitative research, your data will usually be very dense with information and ideas. Instead of summing it up in numbers, you’ll need to comb through the data in detail, interpret its meanings, identify patterns, and extract the parts that are most relevant to your research question.
Two of the most common approaches to doing this are thematic analysis and discourse analysis .
There are many other ways of analysing qualitative data depending on the aims of your research. To get a sense of potential approaches, try reading some qualitative research papers in your field.
A sample is a subset of individuals from a larger population. Sampling means selecting the group that you will actually collect data from in your research.
For example, if you are researching the opinions of students in your university, you could survey a sample of 100 students.
Statistical sampling allows you to test a hypothesis about the characteristics of a population. There are various sampling methods you can use to ensure that your sample is representative of the population as a whole.
Operationalisation means turning abstract conceptual ideas into measurable observations.
For example, the concept of social anxiety isn’t directly observable, but it can be operationally defined in terms of self-rating scores, behavioural avoidance of crowded places, or physical anxiety symptoms in social situations.
Before collecting data , it’s important to consider how you will operationalise the variables that you want to measure.
The research methods you use depend on the type of data you need to answer your research question .
- If you want to measure something or test a hypothesis , use quantitative methods . If you want to explore ideas, thoughts, and meanings, use qualitative methods .
- If you want to analyse a large amount of readily available data, use secondary data. If you want data specific to your purposes with control over how they are generated, collect primary data.
- If you want to establish cause-and-effect relationships between variables , use experimental methods. If you want to understand the characteristics of a research subject, use descriptive methods.
Cite this Scribbr article
If you want to cite this source, you can copy and paste the citation or click the ‘Cite this Scribbr article’ button to automatically add the citation to our free Reference Generator.
McCombes, S. (2023, March 20). Research Design | Step-by-Step Guide with Examples. Scribbr. Retrieved 27 May 2024, from https://www.scribbr.co.uk/research-methods/research-design/
Is this article helpful?
Shona McCombes

An official website of the United States government
The .gov means it’s official. Federal government websites often end in .gov or .mil. Before sharing sensitive information, make sure you’re on a federal government site.
The site is secure. The https:// ensures that you are connecting to the official website and that any information you provide is encrypted and transmitted securely.
- Publications
- Account settings
Preview improvements coming to the PMC website in October 2024. Learn More or Try it out now .
- Advanced Search
- Journal List
- J Korean Med Sci
- v.38(48); 2023 Dec 11
- PMC10713437

Designing, Conducting, and Reporting Survey Studies: A Primer for Researchers
Olena zimba.
1 Department of Clinical Rheumatology and Immunology, University Hospital in Krakow, Krakow, Poland.
2 National Institute of Geriatrics, Rheumatology and Rehabilitation, Warsaw, Poland.
3 Department of Internal Medicine N2, Danylo Halytsky Lviv National Medical University, Lviv, Ukraine.
Armen Yuri Gasparyan
4 Departments of Rheumatology and Research and Development, Dudley Group NHS Foundation Trust (Teaching Trust of the University of Birmingham, UK), Russells Hall Hospital, Dudley, UK.
Survey studies have become instrumental in contributing to the evidence accumulation in rapidly developing medical disciplines such as medical education, public health, and nursing. The global medical community has seen an upsurge of surveys covering the experience and perceptions of health specialists, patients, and public representatives in the peri-pandemic coronavirus disease 2019 period. Currently, surveys can play a central role in increasing research activities in non-mainstream science countries where limited research funding and other barriers hinder science growth. Planning surveys starts with overviewing related reviews and other publications which may help to design questionnaires with comprehensive coverage of all related points. The validity and reliability of questionnaires rely on input from experts and potential responders who may suggest pertinent revisions to prepare forms with attractive designs, easily understandable questions, and correctly ordered points that appeal to target respondents. Currently available numerous online platforms such as Google Forms and Survey Monkey enable moderating online surveys and collecting responses from a large number of responders. Online surveys benefit from disseminating questionnaires via social media and other online platforms which facilitate the survey internationalization and participation of large groups of responders. Survey reporting can be arranged in line with related recommendations and reporting standards all of which have their strengths and limitations. The current article overviews available recommendations and presents pointers on designing, conducting, and reporting surveys.
INTRODUCTION
Surveys are increasingly popular research studies that are aimed at collecting and analyzing opinions of diverse subject groups at certain periods. Initially and predominantly employed for applied social science research, 1 surveys have maintained their social dimension and transformed into indispensable tools for analyzing knowledge, perceptions, prevalence of clinical conditions, and practices in the medical sciences. 2 In rapidly developing disciplines with social dimensions such as medical education, public health, and nursing, online surveys have become essential for monitoring and auditing healthcare and education services 3 , 4 and generating new hypotheses and research questions. 5 In non-mainstream science countries with uninterrupted Internet access, online surveys have also been praised as useful studies for increasing research activities. 6
In 2016, the Medical Subject Headings (MeSH) vocabulary of the US National Library of Medicine introduced "surveys and questionnaires" as a structured keyword, defining survey studies as "collections of data obtained from voluntary subjects" ( https://www.ncbi.nlm.nih.gov/mesh/?term=surveys+and+questionnaires ). Such studies are instrumental in the absence of evidence from randomized controlled trials, systematic reviews, and cohort studies. Tagging survey reports with this MeSH term is advisable for increasing the retrieval of relevant documents while searching through Medline, Scopus, and other global databases.
Surveys are relatively easy to conduct by distributing web-based and non-web-based questionnaires to large groups of potential responders. The ease of conduct primarily depends on the way of approaching potential respondents. Face-to-face interviews, regular postmails, e-mails, phone calls, and social media posts can be employed to reach numerous potential respondents. Digitization and social media popularization have improved the distribution of questionnaires, expanded respondents' engagement, facilitated swift data processing, and globalization of survey studies. 7
SURVEY REPORTING GUIDANCE
Despite the ease of survey studies and their importance for maintaining research activities across academic disciplines, their methodological quality, reproducibility, and implications vary widely. The deficiencies in designing and reporting are the main reason for the inefficiency of some surveys. For instance, systematic analyses of survey methodologies in nephrology, transfusion medicine, and radiology have indicated that less than one-third of related reports provide valid and reliable data. 8 , 9 , 10 Additionally, no discussions of respondents' representativeness, reasons for nonresponse, and generalizability of the results have been pinpointed as drawbacks of some survey reports. The revealed deficiencies have justified the need for survey designing and data processing in line with reporting recommendations, including those listed on the EQUATOR Network website ( https://www.equator-network.org/ ).
Arguably, survey studies lack discipline-specific and globally-acceptable reporting guidance. The diversity of surveyed subjects and populations is perhaps the main confounder. Although most questionnaires contain socio-demographic questions, there are no reporting guidelines specifically tailored to comprehensively inquire specialists across different academic disciplines, patients, and public representatives.
The EQUATOR Network platform currently lists some widely promoted documents with statements on conducting and reporting web-based and non-web-based surveys ( Table 1 ). 11 , 12 , 13 , 14 The oldest published recommendation guides on postal, face-to-face, and telephone interviews. 1 One of its critical points highlights the need to formulate a clear and explicit question/objective to run a focused survey and to design questionnaires with respondent-friendly layout and content. 1 The Checklist for Reporting Results of Internet E-Surveys (CHERRIES) is the most-used document for reporting online surveys. 11 The CHERRIES checklist included points on ensuring the reliability of online surveys and avoiding manipulations with multiple entries by the same users. 11 A specific set of recommendations, listed by the EQUATOR Network, is available for specialists who plan web-based and non-web-based surveys of knowledge, attitude, and practice in clinical medicine. 12 These recommendations help design valid questionnaires, survey representative subjects with clinical knowledge, and complete transparent reporting of the obtained results. 12
COVID-19 = coronavirus disease 2019.
From January 2018 to December 2019, three rounds of surveying experts with interest in surveys and questionnaires allowed reaching consensus on a set of points for reporting web-based and non-web-based surveys. 13 The Consensus-Based Checklist for Reporting of Survey Studies included a rating of 19 items of survey reports, from titles to acknowledgments. 13 Finally, rapid recommendations on online surveys amid the coronavirus disease 2019 (COVID-19) pandemic were published to guide the authors on how to choose social media and other online platforms for disseminating questionnaires and targeting representative groups of respondents. 14
Adhering to a combination of these recommendations is advisable to minimize the limitations of each document and increase the transparency of survey reports. For cross-sectional analyses of large sample sizes, additionally consulting the STROBE standard of the EQUATOR Network may further improve the accuracy of reporting respondents' inclusion and exclusion criteria. In fact, there are examples of online survey reports adhering to both CHERRIES and STROBE recommendations. 15 , 16
ETHICS CONSIDERATIONS
Although health research authorities in some countries lack mandates for full ethics review of survey studies, obtaining formal review protocols or ethics waivers is advisable for most surveys involving respondents from more than one country. And following country-based regulations and ethical norms of research are therefore mandatory. 14 , 17
Full ethics review or exemption procedures are important steps for planning and conducting ethically sound surveys. Given the non-interventional origin and absence of immediate health risks for participants, ethics committees may approve survey protocols without a full ethics review. 18 A full ethics review is however required when the informational and psychological harms of surveys increase the risk. 18 Informational harms may result from unauthorized access to respondents' personal data and stigmatization of respondents with leaked information about social diseases. Psychological harms may include anxiety, depression, and exacerbation of underlying psychiatric diseases.
Survey questionnaires submitted for evaluation should indicate how informed consent is obtained from respondents. 13 Additionally, information about confidentiality, anonymity, questionnaire delivery modes, compensations, and mechanisms preventing unauthorized access to questionnaires should be provided. 13 , 14 Ethical considerations and validation are especially important in studies involving vulnerable and marginalized subjects with diminished autonomy and poor social status due to dementia, substance abuse, inappropriate sexual behavior, and certain infections. 18 , 19 , 20 Precautions should be taken to avoid confidentiality breaches and bot activities when surveying via insecure online platforms. 21
Monetary compensation helps attract respondents to fill out lengthy questionnaires. However, such incentives may create mechanisms deceiving the system by surveyees with a primary interest in compensation. 22 Ethics review protocols may include points on recording online responders' IP addresses and blocking duplicate submissions from the same Internet locations. 22 IP addresses are viewed as personal information in the EU, but not in the US. Notably, IP identification may deter some potential responders in the EU. 21
PATIENT KNOWLEDGE AND PERCEPTION SURVEYS
The design of patient knowledge and perception surveys is insufficiently defined and poorly explored. Although such surveys are aimed at consistently covering research questions on clinical presentation, prevention, and treatment, more emphasis is now placed on psychometric aspects of designing related questionnaires. 23 , 24 , 25 Targeting responsive patient groups to collect reliable answers is yet another challenge that can be addressed by distributing questionnaires to patients with good knowledge of their diseases, particularly those registering with university-affiliated clinics and representing patient associations. 26 , 27 , 28
The structure of questionnaires may differ for surveys of patient groups with various age-dependent health issues. Care should be taken when children are targeted since they often report a variety of modifiable conditions such as anxiety and depression, musculoskeletal problems, and pain, affecting their quality of life. 29 Likewise, gender and age differences should be considered in questionnaires addressing the quality of life in association with mental health and social status. 30 Questionnaires for older adults may benefit from including questions about social support and assistance in the context of caring for aging diseases. 31 Finally, addressing the needs of digital technologies and home-care applications may help to ensure the completeness of questionnaires for older adults with sedentary lifestyles and mobility disabilities. 32 , 33
SOCIAL MEDIA FOR QUESTIONNAIRE DISTRIBUTION
The widespread use of social media has made it easier to distribute questionnaires to a large number of potential responders. Employing popular platforms such as Twitter and Facebook has become particularly useful for conducting nationwide surveys on awareness and concerns about global health and pandemic issues. 34 , 35 When various social media platforms are simultaneously employed, participants' sociodemographic factors such as gender, age, and level of education may confound the study results. 36 Knowing targeted groups' preferred online networking and communication sites may better direct the questionnaire distribution. 37 , 38 , 39
Preliminary evidence suggests that distributing survey links via social-media accounts of individual users and organized e-groups with interest in specific health issues may increase their engagement and correctness of responses. 40 , 41
Since surveys employing social media are publicly accessible, related questionnaires should be professionally edited to easily inquire target populations, avoid sensitive and disturbing points, and ensure privacy and confidentiality. 42 , 43 Although counting e-post views is feasible, response rates of social-media distributed questionnaires are practically impossible to record. The latter is an inherent limitation of such surveys.
SURVEY SAMPLING
Establishing connections with target populations and diversifying questionnaire dissemination may increase the rigor of current surveys which are abundantly administered. 44 Sample sizes depend on various factors, including the chosen topic, aim, and sampling strategy (random or non-random). 12 Some topics such as COVID-19 and global health may easily attract the attention of large respondent groups motivated to answer a variety of questionnaire questions. In the beginning of the pandemic, most surveys employed non-random (non-probability) sampling strategies which resulted in analyses of numerous responses without response rate calculations. These qualitative research studies were mainly aimed to analyze opinions of specialists and patients exposed to COVID-19 to develop rapid guidelines and initiate clinical trials.
Outside the pandemic, and beyond hot topics, there is a growing trend of low response rates and inadequate representation of target populations. 45 Such a trend makes it difficult to design and conduct random (probability) surveys. Subsequently, hypotheses of current online surveys often omit points on randomization and sample size calculation, ending up with qualitative analyses and pilot studies. In fact, convenience (non-random or non-probability) sampling can be particularly suitable for previously unexplored and emerging topics when overviewing literature cannot help estimate optimal samples and entirely new questionnaires should be designed and tested. The limitations of convenience sampling minimize the generalizability of the conclusions since the sample representativeness is uncertain. 45
Researchers often employ 'snowball' sampling techniques with initial surveyees forwarding the questionnaires to other interested respondents, thereby maximizing the sample size. Another common technique for obtaining more responses relies on generating regular social media reminders and resending e-mails to interested individuals and groups. Such tactics can increase the study duration but cannot exclude the participation bias and non-response.
Purposive or targeted sampling is perhaps the most precise technique when knowing the target population size and respondents' readiness to correctly fill the questionnaires and ensure an exact estimate of response rate, close to 100%. 46
DESIGNING QUESTIONNAIRES
Correctness, confidentiality, privacy, and anonymity are critical points of inquiry in questionnaires. 47 Correctly worded and convincingly presented survey invitations with consenting options and reassurances of secure data processing may increase response rates and ensure the validity of responses. 47 Online surveys are believed to be more advantageous than offline inquiries for ensuring anonymity and privacy, particularly for targeting socially marginalized and stigmatized subjects. Online study design is indeed optimal for collecting more responses in surveys of sex- and gender-related and otherwise sensitive topics.
Performing comprehensive literature reviews, consultations with subject experts, and Delphi exercises may all help to specify survey objectives, identify questionnaire domains, and formulate pertinent questions. Literature searches are required for in-depth topic coverage and identification of previously published relevant surveys. By analyzing previous questionnaire characteristics, modifications can be made to designing new self-administered surveys. The justification of new studies should correctly acknowledge similar published reports to avoid redundancies.
The initial part of a questionnaire usually includes a short introduction/preamble/cover letter that specifies the objectives, target respondents, potential benefits and risks, and moderators' contact details for further inquiries. This part may motivate potential respondents to consent and answer questions. The specifics, volume, and format of other parts are dependent on revisions in response to pretesting and pilot testing. 48 The pretesting usually involves co-authors and other contributors, colleagues with the subject interest while the pilot testing usually involves 5-10 target respondents who are well familiar with the subject and can swiftly complete the questionnaires. The guidance obtained at the pretesting and pilot testing allows editing, shortening, or expanding questionnaire sections. Although guidance on questionnaire length and question numbers is scarce, some experts empirically consider 5 domains with 5 questions in each as optimal. 12 Lengthy questionnaires may be biased due to respondents' fatigue and inability to answer numerous and complicated questions. 46
Questionnaire revisions are aimed at ensuring the validity and consistency of questions, implying the appeal to relevant responders and accurate covering of all essential points. 45 Valid questionnaires enable reliable and reproducible survey studies that end up with the same responses to variably worded and located questions. 45
Various combinations of open-ended and close-ended questions are advisable to comprehensively cover all pertinent points and enable easy and quick completion of questionnaires. Open-ended questions are usually included in small numbers since these require more time to respond. 46 Also, the interpretation and analysis of responses to open-ended questions hardly contribute to generating robust qualitative data. 49 Close-ended questions with single and multiple-choice answers constitute the main part of a questionnaire, with single answers easier to analyze and report. Questions with single answers can be presented as 3 or more Likert scales (e.g., yes/no/do not know).
Avoiding too simplistic (yes/no) questions and replacing them with Likert-scale items may increase the robustness of questionnaire analyses. 50 Additionally, constructing easily understandable questions, excluding merged items with two or more points, and moving sophisticated questions to the beginning of a questionnaire may add to the quality and feasibility of the study. 50
Survey studies are increasingly conducted by health professionals to swiftly explore opinions on a wide range of topics by diverse groups of specialists, patients, and public representatives. Arguably, quality surveys with generalizable results can be instrumental for guiding health practitioners in times of crises such as the COVID-19 pandemic when clinical trials, systematic reviews, and other evidence-based reports are scarcely available or absent. Online surveys can be particularly valuable for collecting and analyzing specialist, patient, and other subjects' responses in non-mainstream science countries where top evidence-based studies are scarce commodities and research funding is limited. Accumulated expertise in drafting quality questionnaires and conducting robust surveys is valuable for producing new data and generating new hypotheses and research questions.
The main advantages of surveys are related to the ease of conducting such studies with limited or no research funding. The digitization and social media advances have further contributed to the ease of surveying and growing global interest toward surveys among health professionals. Some of the disadvantages of current surveys are perhaps those related to imperfections of digital platforms for disseminating questionnaires and analysing responses.
Although some survey reporting standards and recommendations are available, none of these comprehensively cover all items of questionnaires and steps in surveying. None of the survey reporting standards is based on summarizing guidance of a large number of contributors involved in related research projects. As such, presenting the current guidance with a list of items for survey reports ( Table 2 ) may help better design and publish related articles.
Disclosure: The authors have no potential conflicts of interest to disclose.
Author Contributions:
- Conceptualization: Zimba O.
- Formal analysis: Zimba O, Gasparyan AY.
- Writing - original draft: Zimba O.
- Writing - review & editing: Zimba O, Gasparyan AY.
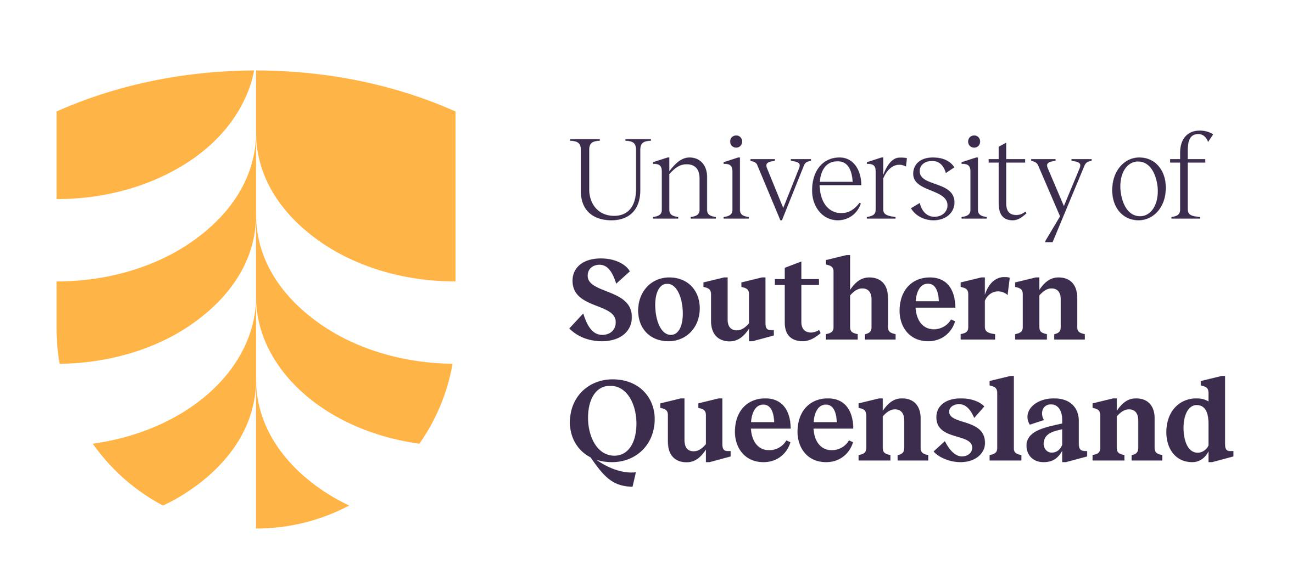
Want to create or adapt books like this? Learn more about how Pressbooks supports open publishing practices.
9 Survey research
Survey research is a research method involving the use of standardised questionnaires or interviews to collect data about people and their preferences, thoughts, and behaviours in a systematic manner. Although census surveys were conducted as early as Ancient Egypt, survey as a formal research method was pioneered in the 1930–40s by sociologist Paul Lazarsfeld to examine the effects of the radio on political opinion formation of the United States. This method has since become a very popular method for quantitative research in the social sciences.
The survey method can be used for descriptive, exploratory, or explanatory research. This method is best suited for studies that have individual people as the unit of analysis. Although other units of analysis, such as groups, organisations or dyads—pairs of organisations, such as buyers and sellers—are also studied using surveys, such studies often use a specific person from each unit as a ‘key informant’ or a ‘proxy’ for that unit. Consequently, such surveys may be subject to respondent bias if the chosen informant does not have adequate knowledge or has a biased opinion about the phenomenon of interest. For instance, Chief Executive Officers may not adequately know employees’ perceptions or teamwork in their own companies, and may therefore be the wrong informant for studies of team dynamics or employee self-esteem.
Survey research has several inherent strengths compared to other research methods. First, surveys are an excellent vehicle for measuring a wide variety of unobservable data, such as people’s preferences (e.g., political orientation), traits (e.g., self-esteem), attitudes (e.g., toward immigrants), beliefs (e.g., about a new law), behaviours (e.g., smoking or drinking habits), or factual information (e.g., income). Second, survey research is also ideally suited for remotely collecting data about a population that is too large to observe directly. A large area—such as an entire country—can be covered by postal, email, or telephone surveys using meticulous sampling to ensure that the population is adequately represented in a small sample. Third, due to their unobtrusive nature and the ability to respond at one’s convenience, questionnaire surveys are preferred by some respondents. Fourth, interviews may be the only way of reaching certain population groups such as the homeless or illegal immigrants for which there is no sampling frame available. Fifth, large sample surveys may allow detection of small effects even while analysing multiple variables, and depending on the survey design, may also allow comparative analysis of population subgroups (i.e., within-group and between-group analysis). Sixth, survey research is more economical in terms of researcher time, effort and cost than other methods such as experimental research and case research. At the same time, survey research also has some unique disadvantages. It is subject to a large number of biases such as non-response bias, sampling bias, social desirability bias, and recall bias, as discussed at the end of this chapter.
Depending on how the data is collected, survey research can be divided into two broad categories: questionnaire surveys (which may be postal, group-administered, or online surveys), and interview surveys (which may be personal, telephone, or focus group interviews). Questionnaires are instruments that are completed in writing by respondents, while interviews are completed by the interviewer based on verbal responses provided by respondents. As discussed below, each type has its own strengths and weaknesses in terms of their costs, coverage of the target population, and researcher’s flexibility in asking questions.
Questionnaire surveys
Invented by Sir Francis Galton, a questionnaire is a research instrument consisting of a set of questions (items) intended to capture responses from respondents in a standardised manner. Questions may be unstructured or structured. Unstructured questions ask respondents to provide a response in their own words, while structured questions ask respondents to select an answer from a given set of choices. Subjects’ responses to individual questions (items) on a structured questionnaire may be aggregated into a composite scale or index for statistical analysis. Questions should be designed in such a way that respondents are able to read, understand, and respond to them in a meaningful way, and hence the survey method may not be appropriate or practical for certain demographic groups such as children or the illiterate.
Most questionnaire surveys tend to be self-administered postal surveys , where the same questionnaire is posted to a large number of people, and willing respondents can complete the survey at their convenience and return it in prepaid envelopes. Postal surveys are advantageous in that they are unobtrusive and inexpensive to administer, since bulk postage is cheap in most countries. However, response rates from postal surveys tend to be quite low since most people ignore survey requests. There may also be long delays (several months) in respondents’ completing and returning the survey, or they may even simply lose it. Hence, the researcher must continuously monitor responses as they are being returned, track and send non-respondents repeated reminders (two or three reminders at intervals of one to one and a half months is ideal). Questionnaire surveys are also not well-suited for issues that require clarification on the part of the respondent or those that require detailed written responses. Longitudinal designs can be used to survey the same set of respondents at different times, but response rates tend to fall precipitously from one survey to the next.
A second type of survey is a group-administered questionnaire . A sample of respondents is brought together at a common place and time, and each respondent is asked to complete the survey questionnaire while in that room. Respondents enter their responses independently without interacting with one another. This format is convenient for the researcher, and a high response rate is assured. If respondents do not understand any specific question, they can ask for clarification. In many organisations, it is relatively easy to assemble a group of employees in a conference room or lunch room, especially if the survey is approved by corporate executives.
A more recent type of questionnaire survey is an online or web survey. These surveys are administered over the Internet using interactive forms. Respondents may receive an email request for participation in the survey with a link to a website where the survey may be completed. Alternatively, the survey may be embedded into an email, and can be completed and returned via email. These surveys are very inexpensive to administer, results are instantly recorded in an online database, and the survey can be easily modified if needed. However, if the survey website is not password-protected or designed to prevent multiple submissions, the responses can be easily compromised. Furthermore, sampling bias may be a significant issue since the survey cannot reach people who do not have computer or Internet access, such as many of the poor, senior, and minority groups, and the respondent sample is skewed toward a younger demographic who are online much of the time and have the time and ability to complete such surveys. Computing the response rate may be problematic if the survey link is posted on LISTSERVs or bulletin boards instead of being emailed directly to targeted respondents. For these reasons, many researchers prefer dual-media surveys (e.g., postal survey and online survey), allowing respondents to select their preferred method of response.
Constructing a survey questionnaire is an art. Numerous decisions must be made about the content of questions, their wording, format, and sequencing, all of which can have important consequences for the survey responses.
Response formats. Survey questions may be structured or unstructured. Responses to structured questions are captured using one of the following response formats:
Dichotomous response , where respondents are asked to select one of two possible choices, such as true/false, yes/no, or agree/disagree. An example of such a question is: Do you think that the death penalty is justified under some circumstances? (circle one): yes / no.
Nominal response , where respondents are presented with more than two unordered options, such as: What is your industry of employment?: manufacturing / consumer services / retail / education / healthcare / tourism and hospitality / other.
Ordinal response , where respondents have more than two ordered options, such as: What is your highest level of education?: high school / bachelor’s degree / postgraduate degree.
Interval-level response , where respondents are presented with a 5-point or 7-point Likert scale, semantic differential scale, or Guttman scale. Each of these scale types were discussed in a previous chapter.
Continuous response , where respondents enter a continuous (ratio-scaled) value with a meaningful zero point, such as their age or tenure in a firm. These responses generally tend to be of the fill-in-the blanks type.
Question content and wording. Responses obtained in survey research are very sensitive to the types of questions asked. Poorly framed or ambiguous questions will likely result in meaningless responses with very little value. Dillman (1978) [1] recommends several rules for creating good survey questions. Every single question in a survey should be carefully scrutinised for the following issues:
Is the question clear and understandable ?: Survey questions should be stated in very simple language, preferably in active voice, and without complicated words or jargon that may not be understood by a typical respondent. All questions in the questionnaire should be worded in a similar manner to make it easy for respondents to read and understand them. The only exception is if your survey is targeted at a specialised group of respondents, such as doctors, lawyers and researchers, who use such jargon in their everyday environment. Is the question worded in a negative manner ?: Negatively worded questions such as ‘Should your local government not raise taxes?’ tend to confuse many respondents and lead to inaccurate responses. Double-negatives should be avoided when designing survey questions.
Is the question ambiguous ?: Survey questions should not use words or expressions that may be interpreted differently by different respondents (e.g., words like ‘any’ or ‘just’). For instance, if you ask a respondent, ‘What is your annual income?’, it is unclear whether you are referring to salary/wages, or also dividend, rental, and other income, whether you are referring to personal income, family income (including spouse’s wages), or personal and business income. Different interpretation by different respondents will lead to incomparable responses that cannot be interpreted correctly.
Does the question have biased or value-laden words ?: Bias refers to any property of a question that encourages subjects to answer in a certain way. Kenneth Rasinky (1989) [2] examined several studies on people’s attitude toward government spending, and observed that respondents tend to indicate stronger support for ‘assistance to the poor’ and less for ‘welfare’, even though both terms had the same meaning. In this study, more support was also observed for ‘halting rising crime rate’ and less for ‘law enforcement’, more for ‘solving problems of big cities’ and less for ‘assistance to big cities’, and more for ‘dealing with drug addiction’ and less for ‘drug rehabilitation’. A biased language or tone tends to skew observed responses. It is often difficult to anticipate in advance the biasing wording, but to the greatest extent possible, survey questions should be carefully scrutinised to avoid biased language.
Is the question double-barrelled ?: Double-barrelled questions are those that can have multiple answers. For example, ‘Are you satisfied with the hardware and software provided for your work?’. In this example, how should a respondent answer if they are satisfied with the hardware, but not with the software, or vice versa? It is always advisable to separate double-barrelled questions into separate questions: ‘Are you satisfied with the hardware provided for your work?’, and ’Are you satisfied with the software provided for your work?’. Another example: ‘Does your family favour public television?’. Some people may favour public TV for themselves, but favour certain cable TV programs such as Sesame Street for their children.
Is the question too general ?: Sometimes, questions that are too general may not accurately convey respondents’ perceptions. If you asked someone how they liked a certain book and provided a response scale ranging from ‘not at all’ to ‘extremely well’, if that person selected ‘extremely well’, what do they mean? Instead, ask more specific behavioural questions, such as, ‘Will you recommend this book to others, or do you plan to read other books by the same author?’. Likewise, instead of asking, ‘How big is your firm?’ (which may be interpreted differently by respondents), ask, ‘How many people work for your firm?’, and/or ‘What is the annual revenue of your firm?’, which are both measures of firm size.
Is the question too detailed ?: Avoid unnecessarily detailed questions that serve no specific research purpose. For instance, do you need the age of each child in a household, or is just the number of children in the household acceptable? However, if unsure, it is better to err on the side of details than generality.
Is the question presumptuous ?: If you ask, ‘What do you see as the benefits of a tax cut?’, you are presuming that the respondent sees the tax cut as beneficial. Many people may not view tax cuts as being beneficial, because tax cuts generally lead to lesser funding for public schools, larger class sizes, and fewer public services such as police, ambulance, and fire services. Avoid questions with built-in presumptions.
Is the question imaginary ?: A popular question in many television game shows is, ‘If you win a million dollars on this show, how will you spend it?’. Most respondents have never been faced with such an amount of money before and have never thought about it—they may not even know that after taxes, they will get only about $640,000 or so in the United States, and in many cases, that amount is spread over a 20-year period—and so their answers tend to be quite random, such as take a tour around the world, buy a restaurant or bar, spend on education, save for retirement, help parents or children, or have a lavish wedding. Imaginary questions have imaginary answers, which cannot be used for making scientific inferences.
Do respondents have the information needed to correctly answer the question ?: Oftentimes, we assume that subjects have the necessary information to answer a question, when in reality, they do not. Even if a response is obtained, these responses tend to be inaccurate given the subjects’ lack of knowledge about the question being asked. For instance, we should not ask the CEO of a company about day-to-day operational details that they may not be aware of, or ask teachers about how much their students are learning, or ask high-schoolers, ‘Do you think the US Government acted appropriately in the Bay of Pigs crisis?’.
Question sequencing. In general, questions should flow logically from one to the next. To achieve the best response rates, questions should flow from the least sensitive to the most sensitive, from the factual and behavioural to the attitudinal, and from the more general to the more specific. Some general rules for question sequencing:
Start with easy non-threatening questions that can be easily recalled. Good options are demographics (age, gender, education level) for individual-level surveys and firmographics (employee count, annual revenues, industry) for firm-level surveys.
Never start with an open ended question.
If following a historical sequence of events, follow a chronological order from earliest to latest.
Ask about one topic at a time. When switching topics, use a transition, such as, ‘The next section examines your opinions about…’
Use filter or contingency questions as needed, such as, ‘If you answered “yes” to question 5, please proceed to Section 2. If you answered “no” go to Section 3′.
Other golden rules . Do unto your respondents what you would have them do unto you. Be attentive and appreciative of respondents’ time, attention, trust, and confidentiality of personal information. Always practice the following strategies for all survey research:
People’s time is valuable. Be respectful of their time. Keep your survey as short as possible and limit it to what is absolutely necessary. Respondents do not like spending more than 10-15 minutes on any survey, no matter how important it is. Longer surveys tend to dramatically lower response rates.
Always assure respondents about the confidentiality of their responses, and how you will use their data (e.g., for academic research) and how the results will be reported (usually, in the aggregate).
For organisational surveys, assure respondents that you will send them a copy of the final results, and make sure that you follow up with your promise.
Thank your respondents for their participation in your study.
Finally, always pretest your questionnaire, at least using a convenience sample, before administering it to respondents in a field setting. Such pretesting may uncover ambiguity, lack of clarity, or biases in question wording, which should be eliminated before administering to the intended sample.
Interview survey
Interviews are a more personalised data collection method than questionnaires, and are conducted by trained interviewers using the same research protocol as questionnaire surveys (i.e., a standardised set of questions). However, unlike a questionnaire, the interview script may contain special instructions for the interviewer that are not seen by respondents, and may include space for the interviewer to record personal observations and comments. In addition, unlike postal surveys, the interviewer has the opportunity to clarify any issues raised by the respondent or ask probing or follow-up questions. However, interviews are time-consuming and resource-intensive. Interviewers need special interviewing skills as they are considered to be part of the measurement instrument, and must proactively strive not to artificially bias the observed responses.
The most typical form of interview is a personal or face-to-face interview , where the interviewer works directly with the respondent to ask questions and record their responses. Personal interviews may be conducted at the respondent’s home or office location. This approach may even be favoured by some respondents, while others may feel uncomfortable allowing a stranger into their homes. However, skilled interviewers can persuade respondents to co-operate, dramatically improving response rates.
A variation of the personal interview is a group interview, also called a focus group . In this technique, a small group of respondents (usually 6–10 respondents) are interviewed together in a common location. The interviewer is essentially a facilitator whose job is to lead the discussion, and ensure that every person has an opportunity to respond. Focus groups allow deeper examination of complex issues than other forms of survey research, because when people hear others talk, it often triggers responses or ideas that they did not think about before. However, focus group discussion may be dominated by a dominant personality, and some individuals may be reluctant to voice their opinions in front of their peers or superiors, especially while dealing with a sensitive issue such as employee underperformance or office politics. Because of their small sample size, focus groups are usually used for exploratory research rather than descriptive or explanatory research.
A third type of interview survey is a telephone interview . In this technique, interviewers contact potential respondents over the phone, typically based on a random selection of people from a telephone directory, to ask a standard set of survey questions. A more recent and technologically advanced approach is computer-assisted telephone interviewing (CATI). This is increasing being used by academic, government, and commercial survey researchers. Here the interviewer is a telephone operator who is guided through the interview process by a computer program displaying instructions and questions to be asked. The system also selects respondents randomly using a random digit dialling technique, and records responses using voice capture technology. Once respondents are on the phone, higher response rates can be obtained. This technique is not ideal for rural areas where telephone density is low, and also cannot be used for communicating non-audio information such as graphics or product demonstrations.
Role of interviewer. The interviewer has a complex and multi-faceted role in the interview process, which includes the following tasks:
Prepare for the interview: Since the interviewer is in the forefront of the data collection effort, the quality of data collected depends heavily on how well the interviewer is trained to do the job. The interviewer must be trained in the interview process and the survey method, and also be familiar with the purpose of the study, how responses will be stored and used, and sources of interviewer bias. They should also rehearse and time the interview prior to the formal study.
Locate and enlist the co-operation of respondents: Particularly in personal, in-home surveys, the interviewer must locate specific addresses, and work around respondents’ schedules at sometimes undesirable times such as during weekends. They should also be like a salesperson, selling the idea of participating in the study.
Motivate respondents: Respondents often feed off the motivation of the interviewer. If the interviewer is disinterested or inattentive, respondents will not be motivated to provide useful or informative responses either. The interviewer must demonstrate enthusiasm about the study, communicate the importance of the research to respondents, and be attentive to respondents’ needs throughout the interview.
Clarify any confusion or concerns: Interviewers must be able to think on their feet and address unanticipated concerns or objections raised by respondents to the respondents’ satisfaction. Additionally, they should ask probing questions as necessary even if such questions are not in the script.
Observe quality of response: The interviewer is in the best position to judge the quality of information collected, and may supplement responses obtained using personal observations of gestures or body language as appropriate.
Conducting the interview. Before the interview, the interviewer should prepare a kit to carry to the interview session, consisting of a cover letter from the principal investigator or sponsor, adequate copies of the survey instrument, photo identification, and a telephone number for respondents to call to verify the interviewer’s authenticity. The interviewer should also try to call respondents ahead of time to set up an appointment if possible. To start the interview, they should speak in an imperative and confident tone, such as, ‘I’d like to take a few minutes of your time to interview you for a very important study’, instead of, ‘May I come in to do an interview?’. They should introduce themself, present personal credentials, explain the purpose of the study in one to two sentences, and assure respondents that their participation is voluntary, and their comments are confidential, all in less than a minute. No big words or jargon should be used, and no details should be provided unless specifically requested. If the interviewer wishes to record the interview, they should ask for respondents’ explicit permission before doing so. Even if the interview is recorded, the interviewer must take notes on key issues, probes, or verbatim phrases
During the interview, the interviewer should follow the questionnaire script and ask questions exactly as written, and not change the words to make the question sound friendlier. They should also not change the order of questions or skip any question that may have been answered earlier. Any issues with the questions should be discussed during rehearsal prior to the actual interview sessions. The interviewer should not finish the respondent’s sentences. If the respondent gives a brief cursory answer, the interviewer should probe the respondent to elicit a more thoughtful, thorough response. Some useful probing techniques are:
The silent probe: Just pausing and waiting without going into the next question may suggest to respondents that the interviewer is waiting for more detailed response.
Overt encouragement: An occasional ‘uh-huh’ or ‘okay’ may encourage the respondent to go into greater details. However, the interviewer must not express approval or disapproval of what the respondent says.
Ask for elaboration: Such as, ‘Can you elaborate on that?’ or ‘A minute ago, you were talking about an experience you had in high school. Can you tell me more about that?’.
Reflection: The interviewer can try the psychotherapist’s trick of repeating what the respondent said. For instance, ‘What I’m hearing is that you found that experience very traumatic’ and then pause and wait for the respondent to elaborate.
After the interview is completed, the interviewer should thank respondents for their time, tell them when to expect the results, and not leave hastily. Immediately after leaving, they should write down any notes or key observations that may help interpret the respondent’s comments better.
Biases in survey research
Despite all of its strengths and advantages, survey research is often tainted with systematic biases that may invalidate some of the inferences derived from such surveys. Five such biases are the non-response bias, sampling bias, social desirability bias, recall bias, and common method bias.
Non-response bias. Survey research is generally notorious for its low response rates. A response rate of 15-20 per cent is typical in a postal survey, even after two or three reminders. If the majority of the targeted respondents fail to respond to a survey, this may indicate a systematic reason for the low response rate, which may in turn raise questions about the validity of the study’s results. For instance, dissatisfied customers tend to be more vocal about their experience than satisfied customers, and are therefore more likely to respond to questionnaire surveys or interview requests than satisfied customers. Hence, any respondent sample is likely to have a higher proportion of dissatisfied customers than the underlying population from which it is drawn. In this instance, not only will the results lack generalisability, but the observed outcomes may also be an artefact of the biased sample. Several strategies may be employed to improve response rates:
Advance notification: Sending a short letter to the targeted respondents soliciting their participation in an upcoming survey can prepare them in advance and improve their propensity to respond. The letter should state the purpose and importance of the study, mode of data collection (e.g., via a phone call, a survey form in the mail, etc.), and appreciation for their co-operation. A variation of this technique may be to ask the respondent to return a prepaid postcard indicating whether or not they are willing to participate in the study.
Relevance of content: People are more likely to respond to surveys examining issues of relevance or importance to them.
Respondent-friendly questionnaire: Shorter survey questionnaires tend to elicit higher response rates than longer questionnaires. Furthermore, questions that are clear, non-offensive, and easy to respond tend to attract higher response rates.
Endorsement: For organisational surveys, it helps to gain endorsement from a senior executive attesting to the importance of the study to the organisation. Such endorsement can be in the form of a cover letter or a letter of introduction, which can improve the researcher’s credibility in the eyes of the respondents.
Follow-up requests: Multiple follow-up requests may coax some non-respondents to respond, even if their responses are late.
Interviewer training: Response rates for interviews can be improved with skilled interviewers trained in how to request interviews, use computerised dialling techniques to identify potential respondents, and schedule call-backs for respondents who could not be reached.
Incentives : Incentives in the form of cash or gift cards, giveaways such as pens or stress balls, entry into a lottery, draw or contest, discount coupons, promise of contribution to charity, and so forth may increase response rates.
Non-monetary incentives: Businesses, in particular, are more prone to respond to non-monetary incentives than financial incentives. An example of such a non-monetary incentive is a benchmarking report comparing the business’s individual response against the aggregate of all responses to a survey.
Confidentiality and privacy: Finally, assurances that respondents’ private data or responses will not fall into the hands of any third party may help improve response rates
Sampling bias. Telephone surveys conducted by calling a random sample of publicly available telephone numbers will systematically exclude people with unlisted telephone numbers, mobile phone numbers, and people who are unable to answer the phone when the survey is being conducted—for instance, if they are at work—and will include a disproportionate number of respondents who have landline telephone services with listed phone numbers and people who are home during the day, such as the unemployed, the disabled, and the elderly. Likewise, online surveys tend to include a disproportionate number of students and younger people who are constantly on the Internet, and systematically exclude people with limited or no access to computers or the Internet, such as the poor and the elderly. Similarly, questionnaire surveys tend to exclude children and the illiterate, who are unable to read, understand, or meaningfully respond to the questionnaire. A different kind of sampling bias relates to sampling the wrong population, such as asking teachers (or parents) about their students’ (or children’s) academic learning, or asking CEOs about operational details in their company. Such biases make the respondent sample unrepresentative of the intended population and hurt generalisability claims about inferences drawn from the biased sample.
Social desirability bias . Many respondents tend to avoid negative opinions or embarrassing comments about themselves, their employers, family, or friends. With negative questions such as, ‘Do you think that your project team is dysfunctional?’, ‘Is there a lot of office politics in your workplace?’, ‘Or have you ever illegally downloaded music files from the Internet?’, the researcher may not get truthful responses. This tendency among respondents to ‘spin the truth’ in order to portray themselves in a socially desirable manner is called the ‘social desirability bias’, which hurts the validity of responses obtained from survey research. There is practically no way of overcoming the social desirability bias in a questionnaire survey, but in an interview setting, an astute interviewer may be able to spot inconsistent answers and ask probing questions or use personal observations to supplement respondents’ comments.
Recall bias. Responses to survey questions often depend on subjects’ motivation, memory, and ability to respond. Particularly when dealing with events that happened in the distant past, respondents may not adequately remember their own motivations or behaviours, or perhaps their memory of such events may have evolved with time and no longer be retrievable. For instance, if a respondent is asked to describe his/her utilisation of computer technology one year ago, or even memorable childhood events like birthdays, their response may not be accurate due to difficulties with recall. One possible way of overcoming the recall bias is by anchoring the respondent’s memory in specific events as they happened, rather than asking them to recall their perceptions and motivations from memory.
Common method bias. Common method bias refers to the amount of spurious covariance shared between independent and dependent variables that are measured at the same point in time, such as in a cross-sectional survey, using the same instrument, such as a questionnaire. In such cases, the phenomenon under investigation may not be adequately separated from measurement artefacts. Standard statistical tests are available to test for common method bias, such as Harmon’s single-factor test (Podsakoff, MacKenzie, Lee & Podsakoff, 2003), [3] Lindell and Whitney’s (2001) [4] market variable technique, and so forth. This bias can potentially be avoided if the independent and dependent variables are measured at different points in time using a longitudinal survey design, or if these variables are measured using different methods, such as computerised recording of dependent variable versus questionnaire-based self-rating of independent variables.
- Dillman, D. (1978). Mail and telephone surveys: The total design method . New York: Wiley. ↵
- Rasikski, K. (1989). The effect of question wording on public support for government spending. Public Opinion Quarterly , 53(3), 388–394. ↵
- Podsakoff, P. M., MacKenzie, S. B., Lee, J.-Y., & Podsakoff, N. P. (2003). Common method biases in behavioral research: A critical review of the literature and recommended remedies. Journal of Applied Psychology , 88(5), 879–903. http://dx.doi.org/10.1037/0021-9010.88.5.879. ↵
- Lindell, M. K., & Whitney, D. J. (2001). Accounting for common method variance in cross-sectional research designs. Journal of Applied Psychology , 86(1), 114–121. ↵
Social Science Research: Principles, Methods and Practices (Revised edition) Copyright © 2019 by Anol Bhattacherjee is licensed under a Creative Commons Attribution-NonCommercial-ShareAlike 4.0 International License , except where otherwise noted.
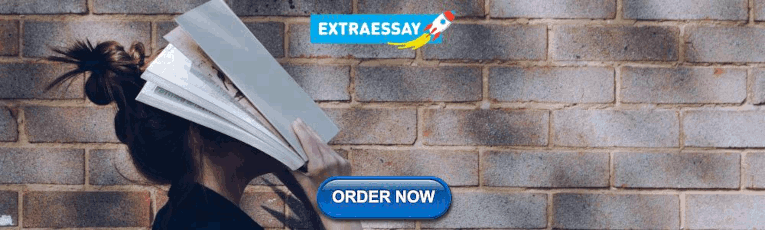
Share This Book
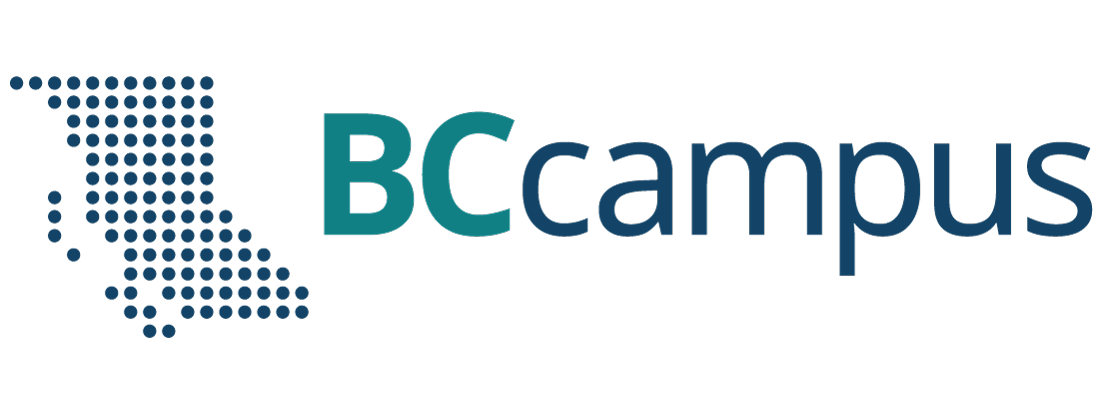
Want to create or adapt books like this? Learn more about how Pressbooks supports open publishing practices.
Chapter 9: Survey Research
Overview of Survey Research
Learning Objectives
- Define what survey research is, including its two important characteristics.
- Describe several different ways that survey research can be used and give some examples.
What Is Survey Research?
Survey research is a quantitative and qualitative method with two important characteristics. First, the variables of interest are measured using self-reports. In essence, survey researchers ask their participants (who are often called respondents in survey research) to report directly on their own thoughts, feelings, and behaviours. Second, considerable attention is paid to the issue of sampling. In particular, survey researchers have a strong preference for large random samples because they provide the most accurate estimates of what is true in the population. In fact, survey research may be the only approach in psychology in which random sampling is routinely used. Beyond these two characteristics, almost anything goes in survey research. Surveys can be long or short. They can be conducted in person, by telephone, through the mail, or over the Internet. They can be about voting intentions, consumer preferences, social attitudes, health, or anything else that it is possible to ask people about and receive meaningful answers. Although survey data are often analyzed using statistics, there are many questions that lend themselves to more qualitative analysis.
Most survey research is nonexperimental. It is used to describe single variables (e.g., the percentage of voters who prefer one presidential candidate or another, the prevalence of schizophrenia in the general population) and also to assess statistical relationships between variables (e.g., the relationship between income and health). But surveys can also be experimental. The study by Lerner and her colleagues is a good example. Their use of self-report measures and a large national sample identifies their work as survey research. But their manipulation of an independent variable (anger vs. fear) to assess its effect on a dependent variable (risk judgments) also identifies their work as experimental.
History and Uses of Survey Research
Survey research may have its roots in English and American “social surveys” conducted around the turn of the 20th century by researchers and reformers who wanted to document the extent of social problems such as poverty (Converse, 1987) [1] . By the 1930s, the US government was conducting surveys to document economic and social conditions in the country. The need to draw conclusions about the entire population helped spur advances in sampling procedures. At about the same time, several researchers who had already made a name for themselves in market research, studying consumer preferences for American businesses, turned their attention to election polling. A watershed event was the presidential election of 1936 between Alf Landon and Franklin Roosevelt. A magazine called Literary Digest conducted a survey by sending ballots (which were also subscription requests) to millions of Americans. Based on this “straw poll,” the editors predicted that Landon would win in a landslide. At the same time, the new pollsters were using scientific methods with much smaller samples to predict just the opposite—that Roosevelt would win in a landslide. In fact, one of them, George Gallup, publicly criticized the methods of Literary Digest before the election and all but guaranteed that his prediction would be correct. And of course it was. (We will consider the reasons that Gallup was right later in this chapter.) Interest in surveying around election times has led to several long-term projects, notably the Canadian Election Studies which has measured opinions of Canadian voters around federal elections since 1965. Anyone can access the data and read about the results of the experiments in these studies.
From market research and election polling, survey research made its way into several academic fields, including political science, sociology, and public health—where it continues to be one of the primary approaches to collecting new data. Beginning in the 1930s, psychologists made important advances in questionnaire design, including techniques that are still used today, such as the Likert scale. (See “What Is a Likert Scale?” in Section 9.2 “Constructing Survey Questionnaires” .) Survey research has a strong historical association with the social psychological study of attitudes, stereotypes, and prejudice. Early attitude researchers were also among the first psychologists to seek larger and more diverse samples than the convenience samples of university students that were routinely used in psychology (and still are).
Survey research continues to be important in psychology today. For example, survey data have been instrumental in estimating the prevalence of various mental disorders and identifying statistical relationships among those disorders and with various other factors. The National Comorbidity Survey is a large-scale mental health survey conducted in the United States . In just one part of this survey, nearly 10,000 adults were given a structured mental health interview in their homes in 2002 and 2003. Table 9.1 presents results on the lifetime prevalence of some anxiety, mood, and substance use disorders. (Lifetime prevalence is the percentage of the population that develops the problem sometime in their lifetime.) Obviously, this kind of information can be of great use both to basic researchers seeking to understand the causes and correlates of mental disorders as well as to clinicians and policymakers who need to understand exactly how common these disorders are.
And as the opening example makes clear, survey research can even be used to conduct experiments to test specific hypotheses about causal relationships between variables. Such studies, when conducted on large and diverse samples, can be a useful supplement to laboratory studies conducted on university students. Although this approach is not a typical use of survey research, it certainly illustrates the flexibility of this method.
Key Takeaways
- Survey research is a quantitative approach that features the use of self-report measures on carefully selected samples. It is a flexible approach that can be used to study a wide variety of basic and applied research questions.
- Survey research has its roots in applied social research, market research, and election polling. It has since become an important approach in many academic disciplines, including political science, sociology, public health, and, of course, psychology.
Discussion: Think of a question that each of the following professionals might try to answer using survey research.
- a social psychologist
- an educational researcher
- a market researcher who works for a supermarket chain
- the mayor of a large city
- the head of a university police force
- Converse, J. M. (1987). Survey research in the United States: Roots and emergence, 1890–1960 . Berkeley, CA: University of California Press. ↵
- The lifetime prevalence of a disorder is the percentage of people in the population that develop that disorder at any time in their lives. ↵
A quantitative approach in which variables are measured using self-reports from a sample of the population.
Participants of a survey.
Research Methods in Psychology - 2nd Canadian Edition Copyright © 2015 by Paul C. Price, Rajiv Jhangiani, & I-Chant A. Chiang is licensed under a Creative Commons Attribution-NonCommercial-ShareAlike 4.0 International License , except where otherwise noted.
Share This Book

Chapter 3 -- Survey Research Design and Quantitative Methods of Analysis for Cross-sectional Data
Almost everyone has had experience with surveys. Market surveys ask respondents whether they recognize products and their feelings about them. Political polls ask questions about candidates for political office or opinions related to political and social issues. Needs assessments use surveys that identify the needs of groups. Evaluations often use surveys to assess the extent to which programs achieve their goals. Survey research is a method of collecting information by asking questions. Sometimes interviews are done face-to-face with people at home, in school, or at work. Other times questions are sent in the mail for people to answer and mail back. Increasingly, surveys are conducted by telephone. SAMPLE SURVEYS Although we want to have information on all people, it is usually too expensive and time consuming to question everyone. So we select only some of these individuals and question them. It is important to select these people in ways that make it likely that they represent the larger group. The population is all the individuals in whom we are interested. (A population does not always consist of individuals. Sometimes, it may be geographical areas such as all cities with populations of 100,000 or more. Or we may be interested in all households in a particular area. In the data used in the exercises of this module the population consists of individuals who are California residents.) A sample is the subset of the population involved in a study. In other words, a sample is part of the population. The process of selecting the sample is called sampling . The idea of sampling is to select part of the population to represent the entire population. The United States Census is a good example of sampling. The census tries to enumerate all residents every ten years with a short questionnaire. Approximately every fifth household is given a longer questionnaire. Information from this sample (i.e., every fifth household) is used to make inferences about the population. Political polls also use samples. To find out how potential voters feel about a particular race, pollsters select a sample of potential voters. This module uses opinions from three samples of California residents age 18 and over. The data were collected during July, 1985, September, 1991, and February, 1995, by the Field Research Corporation (The Field Institute 1985, 1991, 1995). The Field Research Corporation is a widely-respected survey research firm and is used extensively by the media, politicians, and academic researchers. Since a survey can be no better than the quality of the sample, it is essential to understand the basic principles of sampling. There are two types of sampling-probability and nonprobability. A probability sample is one in which each individual in the population has a known, nonzero chance of being selected in the sample. The most basic type is the simple random sample . In a simple random sample, every individual (and every combination of individuals) has the same chance of being selected in the sample. This is the equivalent of writing each person's name on a piece of paper, putting them in plastic balls, putting all the balls in a big bowl, mixing the balls thoroughly, and selecting some predetermined number of balls from the bowl. This would produce a simple random sample. The simple random sample assumes that we can list all the individuals in the population, but often this is impossible. If our population were all the households or residents of California, there would be no list of the households or residents available, and it would be very expensive and time consuming to construct one. In this type of situation, a multistage cluster sample would be used. The idea is very simple. If we wanted to draw a sample of all residents of California, we might start by dividing California into large geographical areas such as counties and selecting a sample of these counties. Our sample of counties could then be divided into smaller geographical areas such as blocks and a sample of blocks would be selected. We could then construct a list of all households for only those blocks in the sample. Finally, we would go to these households and randomly select one member of each household for our sample. Once the household and the member of that household have been selected, substitution would not be allowed. This often means that we must call back several times, but this is the price we must pay for a good sample. The Field Poll used in this module is a telephone survey. It is a probability sample using a technique called random-digit dialing . With random-digit dialing, phone numbers are dialed randomly within working exchanges (i.e., the first three digits of the telephone number). Numbers are selected in such a way that all areas have the proper proportional chance of being selected in the sample. Random-digit dialing makes it possible to include numbers that are not listed in the telephone directory and households that have moved into an area so recently that they are not included in the current telephone directory. A nonprobability sample is one in which each individual in the population does not have a known chance of selection in the sample. There are several types of nonprobability samples. For example, magazines often include questionnaires for readers to fill out and return. This is a volunteer sample since respondents self-select themselves into the sample (i.e., they volunteer to be in the sample). Another type of nonprobability sample is a quota sample . Survey researchers may assign quotas to interviewers. For example, interviewers might be told that half of their respondents must be female and the other half male. This is a quota on sex. We could also have quotas on several variables (e.g., sex and race) simultaneously. Probability samples are preferable to nonprobability samples. First, they avoid the dangers of what survey researchers call "systematic selection biases" which are inherent in nonprobability samples. For example, in a volunteer sample, particular types of persons might be more likely to volunteer. Perhaps highly-educated individuals are more likely to volunteer to be in the sample and this would produce a systematic selection bias in favor of the highly educated. In a probability sample, the selection of the actual cases in the sample is left to chance. Second, in a probability sample we are able to estimate the amount of sampling error (our next concept to discuss). We would like our sample to give us a perfectly accurate picture of the population. However, this is unrealistic. Assume that the population is all employees of a large corporation, and we want to estimate the percent of employees in the population that is satisfied with their jobs. We select a simple random sample of 500 employees and ask the individuals in the sample how satisfied they are with their jobs. We discover that 75 percent of the employees in our sample are satisfied. Can we assume that 75 percent of the population is satisfied? That would be asking too much. Why would we expect one sample of 500 to give us a perfect representation of the population? We could take several different samples of 500 employees and the percent satisfied from each sample would vary from sample to sample. There will be a certain amount of error as a result of selecting a sample from the population. We refer to this as sampling error . Sampling error can be estimated in a probability sample, but not in a nonprobability sample. It would be wrong to assume that the only reason our sample estimate is different from the true population value is because of sampling error. There are many other sources of error called nonsampling error . Nonsampling error would include such things as the effects of biased questions, the tendency of respondents to systematically underestimate such things as age, the exclusion of certain types of people from the sample (e.g., those without phones, those without permanent addresses), or the tendency of some respondents to systematically agree to statements regardless of the content of the statements. In some studies, the amount of nonsampling error might be far greater than the amount of sampling error. Notice that sampling error is random in nature, while nonsampling error may be nonrandom producing systematic biases. We can estimate the amount of sampling error (assuming probability sampling), but it is much more difficult to estimate nonsampling error. We can never eliminate sampling error entirely, and it is unrealistic to expect that we could ever eliminate nonsampling error. It is good research practice to be diligent in seeking out sources of nonsampling error and trying to minimize them. DATA ANALYSIS Examining Variables One at a Time (Univariate Analysis) The rest of this chapter will deal with the analysis of survey data . Data analysis involves looking at variables or "things" that vary or change. A variable is a characteristic of the individual (assuming we are studying individuals). The answer to each question on the survey forms a variable. For example, sex is a variable-some individuals in the sample are male and some are female. Age is a variable; individuals vary in their ages. Looking at variables one at a time is called univariate analysis . This is the usual starting point in analyzing survey data. There are several reasons to look at variables one at a time. First, we want to describe the data. How many of our sample are men and how many are women? How many are black and how many are white? What is the distribution by age? How many say they are going to vote for Candidate A and how many for Candidate B? How many respondents agree and how many disagree with a statement describing a particular opinion? Another reason we might want to look at variables one at a time involves recoding. Recoding is the process of combining categories within a variable. Consider age, for example. In the data set used in this module, age varies from 18 to 89, but we would want to use fewer categories in our analysis, so we might combine age into age 18 to 29, 30 to 49, and 50 and over. We might want to combine African Americans with the other races to classify race into only two categories-white and nonwhite. Recoding is used to reduce the number of categories in the variable (e.g., age) or to combine categories so that you can make particular types of comparisons (e.g., white versus nonwhite). The frequency distribution is one of the basic tools for looking at variables one at a time. A frequency distribution is the set of categories and the number of cases in each category. Percent distributions show the percentage in each category. Table 3.1 shows frequency and percent distributions for two hypothetical variables-one for sex and one for willingness to vote for a woman candidate. Begin by looking at the frequency distribution for sex. There are three columns in this table. The first column specifies the categories-male and female. The second column tells us how many cases there are in each category, and the third column converts these frequencies into percents. Table 3.1 -- Frequency and Percent Distributions for Sex and Willingness to Vote for a Woman Candidate (Hypothetical Data) Sex Voting Preference Category Freq. Percent Category Freq. Percent Valid Percent Male 380 40.0 Willing to Vote for a Woman 460 48.4 51.1 Female 570 60.0 Not Willing to Vote for a Woman 440 46.3 48.9 Total 950 100.0 Refused 50 5.3 Missing Total 950 100.0 100.0 In this hypothetical example, there are 380 males and 570 females or 40 percent male and 60 percent female. There are a total of 950 cases. Since we know the sex for each case, there are no missing data (i.e., no cases where we do not know the proper category). Look at the frequency distribution for voting preference in Table 3.1. How many say they are willing to vote for a woman candidate and how many are unwilling? (Answer: 460 willing and 440 not willing) How many refused to answer the question? (Answer: 50) What percent say they are willing to vote for a woman, what percent are not, and what percent refused to answer? (Answer: 48.4 percent willing to vote for a woman, 46.3 percent not willing, and 5.3 percent refused to tell us.) The 50 respondents who didn't want to answer the question are called missing data because we don't know which category into which to place them, so we create a new category (i.e., refused) for them. Since we don't know where they should go, we might want a percentage distribution considering only the 900 respondents who answered the question. We can determine this easily by taking the 50 cases with missing information out of the base (i.e., the denominator of the fraction) and recomputing the percentages. The fourth column in the frequency distribution (labeled "valid percent") gives us this information. Approximately 51 percent of those who answered the question were willing to vote for a woman and approximately 49 percent were not. With these data we will use frequency distributions to describe variables one at a time. There are other ways to describe single variables. The mean, median, and mode are averages that may be used to describe the central tendency of a distribution. The range and standard deviation are measures of the amount of variability or dispersion of a distribution. (We will not be using measures of central tendency or variability in this module.) Exploring the Relationship Between Two Variables (Bivariate Analysis) Usually we want to do more than simply describe variables one at a time. We may want to analyze the relationship between variables. Morris Rosenberg (1968:2) suggests that there are three types of relationships: "(1) neither variable may influence one another .... (2) both variables may influence one another ... (3) one of the variables may influence the other." We will focus on the third of these types which Rosenberg calls "asymmetrical relationships." In this type of relationship, one of the variables (the independent variable ) is assumed to be the cause and the other variable (the dependent variable ) is assumed to be the effect. In other words, the independent variable is the factor that influences the dependent variable. For example, researchers think that smoking causes lung cancer. The statement that specifies the relationship between two variables is called a hypothesis (see Hoover 1992, for a more extended discussion of hypotheses). In this hypothesis, the independent variable is smoking (or more precisely, the amount one smokes) and the dependent variable is lung cancer. Consider another example. Political analysts think that income influences voting decisions, that rich people vote differently from poor people. In this hypothesis, income would be the independent variable and voting would be the dependent variable. In order to demonstrate that a causal relationship exists between two variables, we must meet three criteria: (1) there must be a statistical relationship between the two variables, (2) we must be able to demonstrate which one of the variables influences the other, and (3) we must be able to show that there is no other alternative explanation for the relationship. As you can imagine, it is impossible to show that there is no other alternative explanation for a relationship. For this reason, we can show that one variable does not influence another variable, but we cannot prove that it does. We can only show that it is more plausible or credible to believe that a causal relationship exists. In this section, we will focus on the first two criteria and leave this third criterion to the next section. In the previous section we looked at the frequency distributions for sex and voting preference. All we can say from these two distributions is that the sample is 40 percent men and 60 percent women and that slightly more than half of the respondents said they would be willing to vote for a woman, and slightly less than half are not willing to. We cannot say anything about the relationship between sex and voting preference. In order to determine if men or women are more likely to be willing to vote for a woman candidate, we must move from univariate to bivariate analysis. A crosstabulation (or contingency table ) is the basic tool used to explore the relationship between two variables. Table 3.2 is the crosstabulation of sex and voting preference. In the lower right-hand corner is the total number of cases in this table (900). Notice that this is not the number of cases in the sample. There were originally 950 cases in this sample, but any case that had missing information on either or both of the two variables in the table has been excluded from the table. Be sure to check how many cases have been excluded from your table and to indicate this figure in your report. Also be sure that you understand why these cases have been excluded. The figures in the lower margin and right-hand margin of the table are called the marginal distributions. They are simply the frequency distributions for the two variables in the whole table. Here, there are 360 males and 540 females (the marginal distribution for the column variable-sex) and 460 people who are willing to vote for a woman candidate and 440 who are not (the marginal distribution for the row variable-voting preference). The other figures in the table are the cell frequencies. Since there are two columns and two rows in this table (sometimes called a 2 x 2 table), there are four cells. The numbers in these cells tell us how many cases fall into each combination of categories of the two variables. This sounds complicated, but it isn't. For example, 158 males are willing to vote for a woman and 302 females are willing to vote for a woman. Table 3.2 -- Crosstabulation of Sex and Voting Preference (Frequencies) Sex Voting Preference Male Female Total Willing to Vote for a Woman 158 302 460 Not Willing to Vote for a Woman 202 238 440 Total 360 540 900 We could make comparisons rather easily if we had an equal number of women and men. Since these numbers are not equal, we must use percentages to help us make the comparisons. Since percentages convert everything to a common base of 100, the percent distribution shows us what the table would look like if there were an equal number of men and women. Before we percentage Table 3.2, we must decide which of these two variables is the independent and which is the dependent variable. Remember that the independent variable is the variable we think might be the influencing factor. The independent variable is hypothesized to be the cause, and the dependent variable is the effect. Another way to express this is to say that the dependent variable is the one we want to explain. Since we think that sex influences willingness to vote for a woman candidate, sex would be the independent variable. Once we have decided which is the independent variable, we are ready to percentage the table. Notice that percentages can be computed in different ways. In Table 3.3, the percentages have been computed so that they sum down to 100. These are called column percents . If they sum across to 100, they are called row percents . If the independent variable is the column variable, then we want the percents to sum down to 100 (i.e., we want the column percents). If the independent variable is the row variable, we want the percents to sum across to 100 (i.e., we want the row percents). This is a simple, but very important, rule to remember. We'll call this our rule for computing percents . Although we often see the independent variable as the column variable so the table sums down to 100 percent, it really doesn't matter whether the independent variable is the column or the row variable. In this module, we will put the independent variable as the column variable. Many others (but not everyone) use this convention. It would be helpful if you did this when you write your report. Table 3.3 -- Voting Preference by Sex (Percents) Voting Preference Male Female Total Willing to Vote for a Woman 43.9 55.9 51.1 Not Willing to Vote for a Woman 56.1 44.1 100.0 Total Percent 100.0 100.0 100.0 (Total Frequency) (360) (540) (900) Now we are ready to interpret this table. Interpreting a table means to explain what the table is saying about the relationship between the two variables. First, we can look at each category of the independent variable separately to describe the data and then we compare them to each other. Since the percents sum down to 100 percent, we describe down and compare across. The rule for interpreting percents is to compare in the direction opposite to the way the percents sum to 100. So, if the percents sum down to 100, we compare across, and if the percents sum across to 100, compare down. If the independent variable is the column variable, the percents will always sum down to 100. We can look at each category of the independent variable separately to describe the data and then compare them to each other-describe down and then compare across. In Table 3.3, row one shows the percent of males and the percent of females who are willing to vote for a woman candidate--43.9 percent of males are willing to vote for a woman, while 55.9 percent of the females are. This is a difference of 12 percentage points. Somewhat more females than males are willing to vote for a woman. The second row shows the percent of males and females who are not willing to vote for a woman. Since there are only two rows, the second row will be the complement (or the reverse) of the first row. It shows that males are somewhat more likely to be unwilling to vote for a woman candidate (a difference of 12 percentage points in the opposite direction). When we observe a difference, we must also decide whether it is significant. There are two different meanings for significance-statistical significance and substantive significance. Statistical significance considers whether the difference is great enough that it is probably not due to chance factors. Substantive significance considers whether a difference is large enough to be important. With a very large sample, a very small difference is often statistically significant, but that difference may be so small that we decide it isn't substantively significant (i.e., it's so small that we decide it doesn't mean very much). We're going to focus on statistical significance, but remember that even if a difference is statistically significant, you must also decide if it is substantively significant. Let's discuss this idea of statistical significance. If our population is all men and women of voting age in California, we want to know if there is a relationship between sex and voting preference in the population of all individuals of voting age in California. All we have is information about a sample from the population. We use the sample information to make an inference about the population. This is called statistical inference . We know that our sample is not a perfect representation of our population because of sampling error . Therefore, we would not expect the relationship we see in our sample to be exactly the same as the relationship in the population. Suppose we want to know whether there is a relationship between sex and voting preference in the population. It is impossible to prove this directly, so we have to demonstrate it indirectly. We set up a hypothesis (called the null hypothesis ) that says that sex and voting preference are not related to each other in the population. This basically says that any difference we see is likely to be the result of random variation. If the difference is large enough that it is not likely to be due to chance, we can reject this null hypothesis of only random differences. Then the hypothesis that they are related (called the alternative or research hypothesis ) will be more credible.
In the first column of Table 3.4, we have listed the four cell frequencies from the crosstabulation of sex and voting preference. We'll call these the observed frequencies (f o ) because they are what we observe from our table. In the second column, we have listed the frequencies we would expect if, in fact, there is no relationship between sex and voting preference in the population. These are called the expected frequencies (f e ). We'll briefly explain how these expected frequencies are obtained. Notice from Table 3.1 that 51.1 percent of the sample were willing to vote for a woman candidate, while 48.9 percent were not. If sex and voting preference are independent (i.e., not related), we should find the same percentages for males and females. In other words, 48.9 percent (or 176) of the males and 48.9 percent (or 264) of the females would be unwilling to vote for a woman candidate. (This explanation is adapted from Norusis 1997.) Now, we want to compare these two sets of frequencies to see if the observed frequencies are really like the expected frequencies. All we do is to subtract the expected from the observed frequencies (column three). We are interested in the sum of these differences for all cells in the table. Since they always sum to zero, we square the differences (column four) to get positive numbers. Finally, we divide this squared difference by the expected frequency (column five). (Don't worry about why we do this. The reasons are technical and don't add to your understanding.) The sum of column five (12.52) is called the chi square statistic . If the observed and the expected frequencies are identical (no difference), chi square will be zero. The greater the difference between the observed and expected frequencies, the larger the chi square. If we get a large chi square, we are willing to reject the null hypothesis. How large does the chi square have to be? We reject the null hypothesis of no relationship between the two variables when the probability of getting a chi square this large or larger by chance is so small that the null hypothesis is very unlikely to be true. That is, if a chi square this large would rarely occur by chance (usually less than once in a hundred or less than five times in a hundred). In this example, the probability of getting a chi square as large as 12.52 or larger by chance is less than one in a thousand. This is so unlikely that we reject the null hypothesis, and we conclude that the alternative hypothesis (i.e., there is a relationship between sex and voting preference) is credible (not that it is necessarily true, but that it is credible). There is always a small chance that the null hypothesis is true even when we decide to reject it. In other words, we can never be sure that it is false. We can only conclude that there is little chance that it is true. Just because we have concluded that there is a relationship between sex and voting preference does not mean that it is a strong relationship. It might be a moderate or even a weak relationship. There are many statistics that measure the strength of the relationship between two variables. Chi square is not a measure of the strength of the relationship. It just helps us decide if there is a basis for saying a relationship exists regardless of its strength. Measures of association estimate the strength of the relationship and are often used with chi square. (See Appendix D for a discussion of how to compute the two measures of association discussed below.) Cramer's V is a measure of association appropriate when one or both of the variables consists of unordered categories. For example, race (white, African American, other) or religion (Protestant, Catholic, Jewish, other, none) are variables with unordered categories. Cramer's V is a measure based on chi square. It ranges from zero to one. The closer to zero, the weaker the relationship; the closer to one, the stronger the relationship. Gamma (sometimes referred to as Goodman and Kruskal's Gamma) is a measure of association appropriate when both of the variables consist of ordered categories. For example, if respondents answer that they strongly agree, agree, disagree, or strongly disagree with a statement, their responses are ordered. Similarly, if we group age into categories such as under 30, 30 to 49, and 50 and over, these categories would be ordered. Ordered categories can logically be arranged in only two ways-low to high or high to low. Gamma ranges from zero to one, but can be positive or negative. For this module, the sign of Gamma would have no meaning, so ignore the sign and focus on the numerical value. Like V, the closer to zero, the weaker the relationship and the closer to one, the stronger the relationship. Choosing whether to use Cramer's V or Gamma depends on whether the categories of the variable are ordered or unordered. However, dichotomies (variables consisting of only two categories) may be treated as if they are ordered even if they are not. For example, sex is a dichotomy consisting of the categories male and female. There are only two possible ways to order sex-male, female and female, male. Or, race may be classified into two categories-white and nonwhite. We can treat dichotomies as if they consisted of ordered categories because they can be ordered in only two ways. In other words, when one of the variables is a dichotomy, treat this variable as if it were ordinal and use gamma. This is important when choosing an appropriate measure of association. In this chapter we have described how surveys are done and how we analyze the relationship between two variables. In the next chapter we will explore how to introduce additional variables into the analysis. REFERENCES AND SUGGESTED READING Methods of Social Research Riley, Matilda White. 1963. Sociological Research I: A Case Approach . New York: Harcourt, Brace and World. Hoover, Kenneth R. 1992. The Elements of Social Scientific Thinking (5 th Ed.). New York: St. Martin's. Interviewing Gorden, Raymond L. 1987. Interviewing: Strategy, Techniques and Tactics . Chicago: Dorsey. Survey Research and Sampling Babbie, Earl R. 1990. Survey Research Methods (2 nd Ed.). Belmont, CA: Wadsworth. Babbie, Earl R. 1997. The Practice of Social Research (8 th Ed). Belmont, CA: Wadsworth. Statistical Analysis Knoke, David, and George W. Bohrnstedt. 1991. Basic Social Statistics . Itesche, IL: Peacock. Riley, Matilda White. 1963. Sociological Research II Exercises and Manual . New York: Harcourt, Brace & World. Norusis, Marija J. 1997. SPSS 7.5 Guide to Data Analysis . Upper Saddle River, New Jersey: Prentice Hall. Data Sources The Field Institute. 1985. California Field Poll Study, July, 1985 . Machine-readable codebook. The Field Institute. 1991. California Field Poll Study, September, 1991 . Machine-readable codebook. The Field Institute. 1995. California Field Poll Study, February, 1995 . Machine-readable codebook.
Document Viewers
- Free PDF Viewer
- Free Word Viewer
- Free Excel Viewer
- Free PowerPoint Viewer

18 Different Types of Survey Methods + Pros & Cons
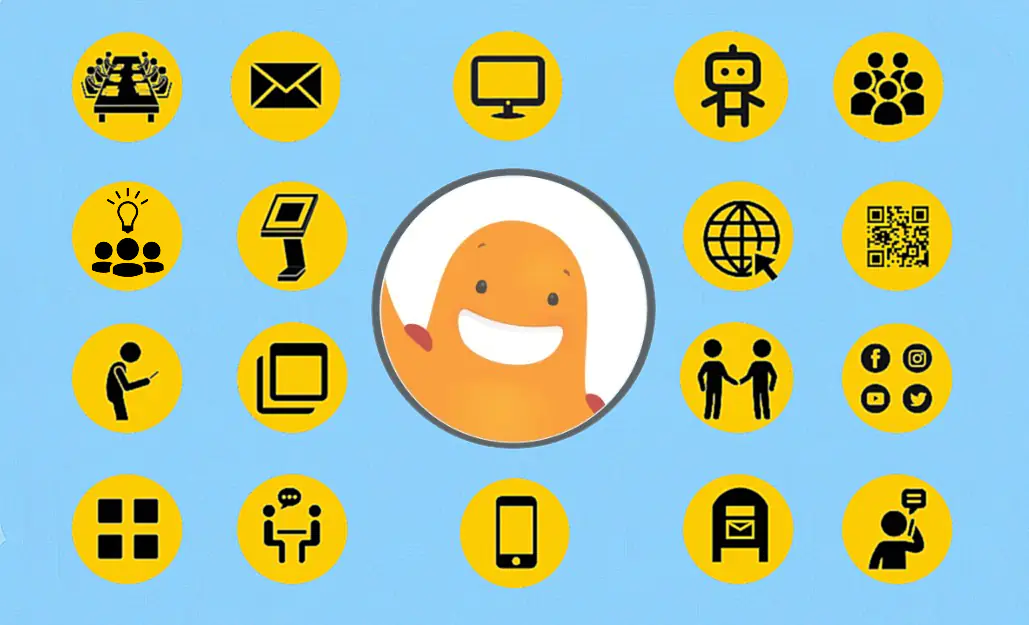
There are many reasons why surveys are important. Surveys help researchers find solutions, create discussions, and make decisions. They can also get to the bottom of the really important stuff, like, coffee or tea? Dogs or cats? Elvis or The Beatles? When it comes to finding the answers to these questions, there are 18 different types of survey methods to use.
Create your first survey, form, or poll now!
18 Different Types of Survey Methods
Different surveys serve different purposes, which is why there are a number of them to choose from. “What are the types of surveys I should use,” you ask? Here’s a look at the 18 types of survey methods researchers use today.
1. Interviews
Also known as in-person surveys or household surveys, this used to be one of the most popular types of survey to conduct. Researchers like them because they involve getting face-to-face with individuals. Of course, this method of surveying may seem antiquated when today we have online surveying at our fingertips. However, interviews still serve a purpose.
Researchers conduct interviews when they want to discuss something personal with people. For example, they may have questions that may require extensive probing to uncover the truth. Sure, some interviewees may be more comfortable answering questions confidentially behind a keyboard. However, a skilled interviewer is able to put them at ease and get genuine responses. They can often go deeper than you may be able to using other surveying methods.
Often, in-person interviews are recorded on camera. This way, an expert can review them afterward. They do this to determine if the answers given may be false based on an interviewee’s change in tone. A change in facial expressions and body movements may also be a signal they pick up on.
2. Intercept Surveys
While interviews tend to choose respondents and have controls in place, intercept surveys (or “man on the spot”) surveys are conducted at certain locations or events. This involves having an interviewer, or multiple interviewers, scoping out an area and asking people, generally at random, for their thoughts or viewpoints on a particular topic.
3. Focus Groups
These types of surveys are conducted in person as well. However, focus groups involve a number of people rather than just one individual. The group is generally small but demographically diverse and led by a moderator. The focus group may be sampling new products, or to have a discussion around a particular topic, often a hot-button one.
The purpose of a focus group survey is often to gauge people’s reaction to a product in a group setting or to get people talking, interacting—and yes, arguing—with the moderator taking notes on the group’s behavior and attitudes. This is often the most expensive survey method as a trained moderator must be paid. In addition, locations must be secured, often in various cities, and participants must be heavily incentivized to show up. Gift cards in the $75-100 range for each survey participant are the norm.
4. Panel Sampling
Recruiting survey-takers from a panel maintained by a research company is a surefire way to get respondents. Why? Because people have specifically signed up to take them. The benefit of these types of surveys for research, of course, is there you can be assured responses. In addition, you can filter respondents by a variety of criteria to be sure you’re speaking with your target audience.
The downside is data quality. These individuals get survey offers frequently. So, they may rush through them to get their inventive and move on to the next one. In addition, if you’re constantly tapping into the same people from the same panel, are you truly getting a representative sample?
5. Telephone Surveys
Most telephone survey research types are conducted through random digit dialing (RDD). RDD can reach both listed and unlisted numbers, improving sampling accuracy. Surveys are conducted by interviewers through computer-assisted telephone interviewing (CATI) software. CATI displays the questionnaire to the interviewer with a rotation of questions.
Telephone surveys started in the 1940s. In fact, in a recent blog , we recount how the predictions for the 1948 presidential election were completely wrong because of sampling bias in telephone surveys. Rising in popularity in the late 50s and early 60s when the telephone became common in most American households, telephone surveys are no longer a very popular method of conducting a survey. Why? Because many people refuse to take telephone surveys or simply are not answering calls from a number they don’t recognize.
6. Post-Call Surveys
If a telephone survey is going to be conducted, today it is usually a post-call survey. This is often accomplished through IVR, or interactive voice response. IVR means there is no interviewer involved. Instead, customers record answers to pre-recorded questions using numbers on their touch-tone keypads. If a question is open-ended, the interviewee can respond by speaking and the system records the answer. IVR surveys are often deployed to measure how a customer feels about a service they just received. For example, after calling your bank, you may be asked to stay on the line to answer a series of questions about your experience.
Most post-call surveys are either NPS surveys or customer satisfaction (CSAT) surveys. The former asks the customer “How likely are you to recommend our organization to a f riend or family based on your most recent interaction?” while the CSAT survey asks customers “How satisfied are you with the results of your most recent interaction?”. NPS survey results reflect how the customer feels about the brand, while CSAT surveys a re all about individual agent and contact center performance.
7. SMS Text Surveys
Many people rarely using their phone to talk anymore, and ignore calls from unknown numbers. This has given rise to the SMS (Short Messaging Service) text survey. SMS surveys are delivered via text to people who have opted in to receive notifications from the sender. This means that there is usually some level of engagement, improving response rates. The one downside is that questions typically need to be short, and answers are generally 1-2 words or simply numbers (this is why many NPS surveys, gauging customer satisfaction, are often conducted via SMS text). Be careful not to send too many text surveys, as a person can opt-out just as easily, usually by texting STOP.
8. Mail-in Surveys / Postal Surveys
These are delivered right to respondents’ doorsteps! Mail surveys were frequently used before the advent of the internet when respondents were spread out geographically and budgets were modest. After all, mail-in surveys didn’t require much cost other than the postage.
So are mail-in surveys going the way of the dinosaur? Not necessarily. They are still occasionally more valuable compared to different methods of surveying. Because they are going to a specific name and home address, they often feel more personalized. This personalization can prompt the recipient to complete the survey.
They’re also good for surveys of significant length. Most people have short attention spans, and won’t spend more than a few minutes on the phone or filling out an online survey. At least, not without an incentive! However, with a mail-in survey, the person can complete it at their leisure. They can fill out some of it, set it aside, and then come back to it later. This gives mail-in surveys a relatively high response rate.
9. Kiosk Surveys
These surveys happen on a computer screen at a physical location. You’ve probably seen them popping up in stores, hotel lobbies, hospitals, and office spaces. These days, they’re just about anywhere a researcher or marketer wants to collect data from customers or passers-by. Kiosk surveys provide immediate feedback following a purchase or an interaction. They collect responses while the experience is still fresh in the respondent’s mind. This makes their judgment more trustworthy. Below is an example of a SurveyLegend kiosk survey at McDonald’s. The kiosk survey collects information, thanks the respondent for their feedback, and then resets for the next customer. Read how to create your own kiosk survey here .
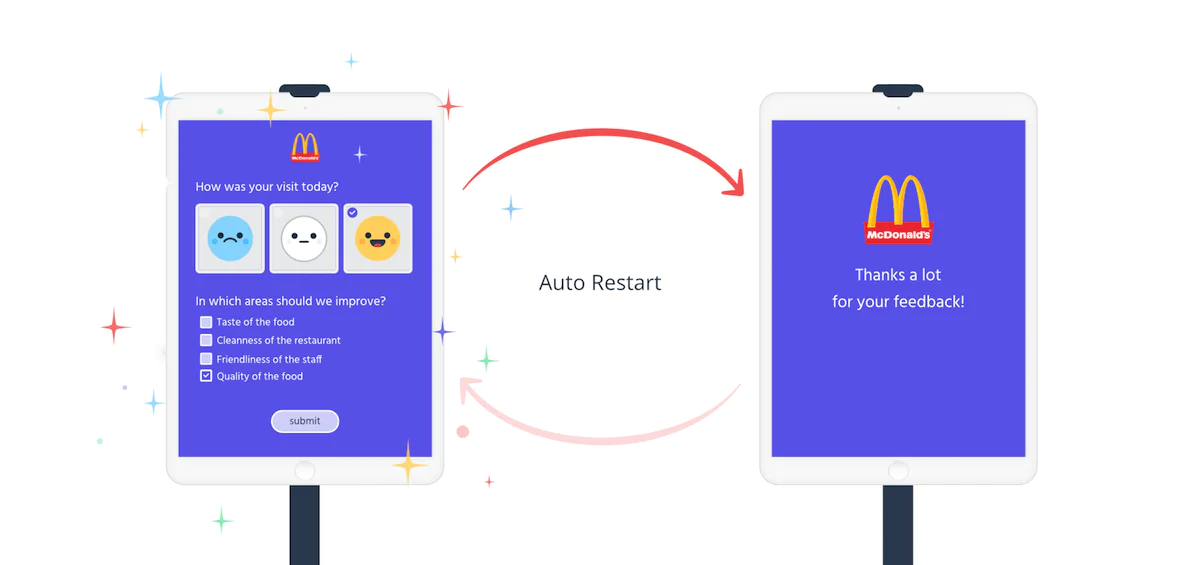
10. Email Surveys
Email surveys are one of the most effective surveying methods as they are delivered directly to your audience via their online account. They can be used by anyone for just about anything, and are easily customized for a particular audience. Another good thing about email surveys is you can easily see who did or did not open the survey and make improvements to it for a future send to increase response rates. You can also A/B test subject lines, imagery, and so on to see which is more effective. SurveyLegend offers dozens of different types of online survey questions, which we explore in our blog 12 Different Types of Survey Questions and When to Use Them (with Examples) .
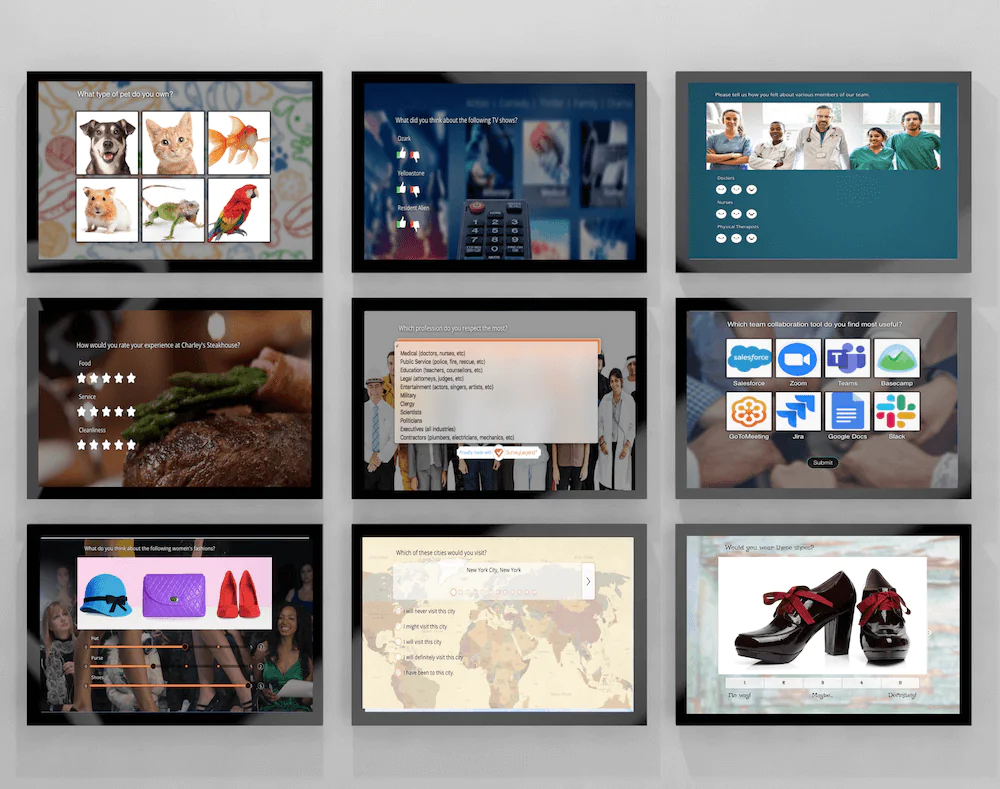
11. Pop-up Surveys
A pop-up survey is a feedback form that pops up on a website or app. Although the main window a person is reading on their screen remains visible, it is temporarily disabled until a user interacts with the pop-up, either agreeing to leave feedback or closing out of it. The survey itself is typically about the company whose site or app the user is currently visiting (as opposed to an intercept survey, which is an invitation to take a survey hosted on a different site).
A pop-up survey attempts to grab website visitors’ attention in a variety of ways, popping up in the middle of the screen, moving in from the side, or covering the entire screen. While they can be intrusive, they also have many benefits. Read about the benefits of pop-up surveys here .
12. Embedded Surveys
The opposite of pop-up surveys, these surveys live directly on your website or another website of your choice. Because the survey cannot be X’ed out of like a pop-up, it takes up valuable real estate on your site, or could be expensive to implement on someone else’s site. In addition, although the embedded survey is there at all times, it may not get the amount of attention a pop-up does since it’s not “in the respondent’s face.”
13. Social Media Surveys
There are more than 3.5 billion people are using social media worldwide, a number projected to increase to almost 4.5 billion in 2025. This makes social media extremely important to marketers and researchers. Using platforms such as Facebook, Twitter, Instagram, and the new Threads, many companies and organizations send out social media surveys regularly. Because people check their social media accounts quite regularly, it’s a good way to collect responses and monitor changes in satisfaction levels or popular opinion. Check out our blog on social media surveys for more benefits and valuable tips.
14. Mobile Surveys
Mobile traffic has now overtaken desktop computers as the most used device for accessing the internet, with more than 54% of the share. But don’t fret – you don’t have to create an entirely new survey to reach people on their phones or tablets. Online poll makers like SurveyLegend are responsive, so when you create a desktop version of a survey, it automatically becomes mobile-friendly. The survey renders, or displays, on any device or screen regardless of size, with elements on the page automatically rearranging themselves, shrinking, or expanding as necessary. Learn more about our responsive surveys .
15. Mobile App Surveys
Today, most companies have a mobile app. These can be an ideal way to conduct surveys as people have to willingly download your app; this means, they already have a level of engagement with your company or brand making them more likely to respond to your surveys.
16. QR Code Surveys
QR Code or QRC is an abbreviation of “Quick Response Code.” These two-dimensional encoded images, when scanned, deliver hidden information that’s stored on it. They’re different from barcodes because they can house a lot more information, including website URLs, phone numbers, or up to 4,000 characters of text. The recent QR code comeback provides a good opportunity for researchers to collect data. Place the QR code anywhere – on flyers, posters, billboards, commercials – and all someone had to do is scan it with the mobile device to have immediate access to a survey. Read more about the benefits of QR code surveys .
17. Delphi Surveys
A Delphi survey is a structured research method used to gather the collective opinions and insights of a panel of experts on a particular topic. The process involves several rounds of questionnaires or surveys. Each round is designed to narrow things down until a consensus or hypothyses can be formed. One of the key features of the Delphi survey research is that participants are unknown to each other, thereby eliminating influence.
18. AI Surveys
Artificial intelligence is the latest types of survey method. Using AI, researchers allow the technology to ask survey questions. These “Chatbots” can even ask follow-up questions on the spot based on a respondent’s answer. There can be drawbacks, however. If a person suspects survey questions are coming from AI, they may be less likely to respond (or may respond incorrectly to mess with the AI). Additionally, AI is not good with emotions, so asking sensitive questions in an emotionless manner could be off putting to people. Read more about AI Surveys .
Online Surveys: Ideal for Collecting Data and Feedback
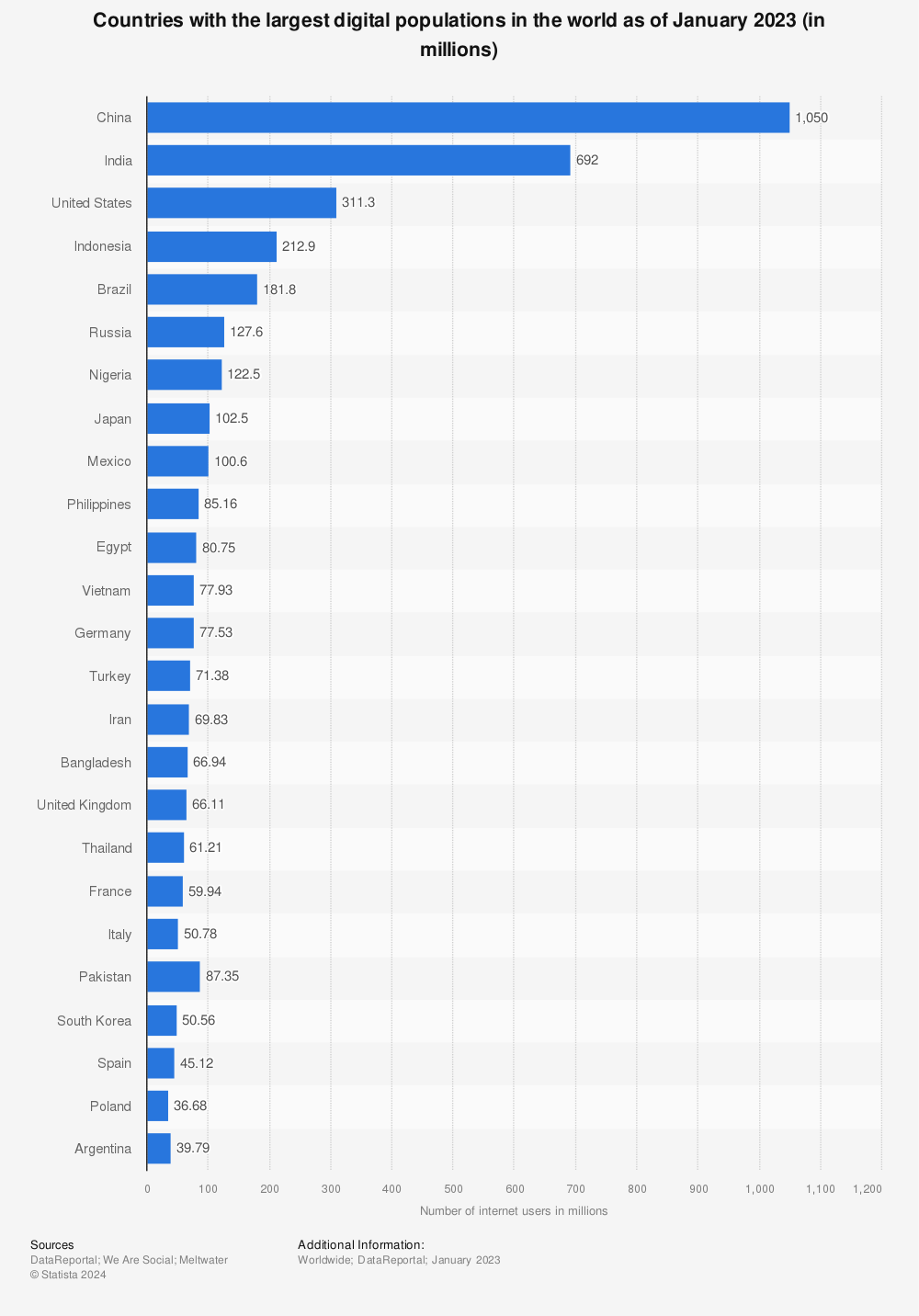
That’s not all. People can take online surveys just about anywhere thanks to mobile devices. The use of these devices across age groups is balancing out as well. Check out smartphone use by age group below.
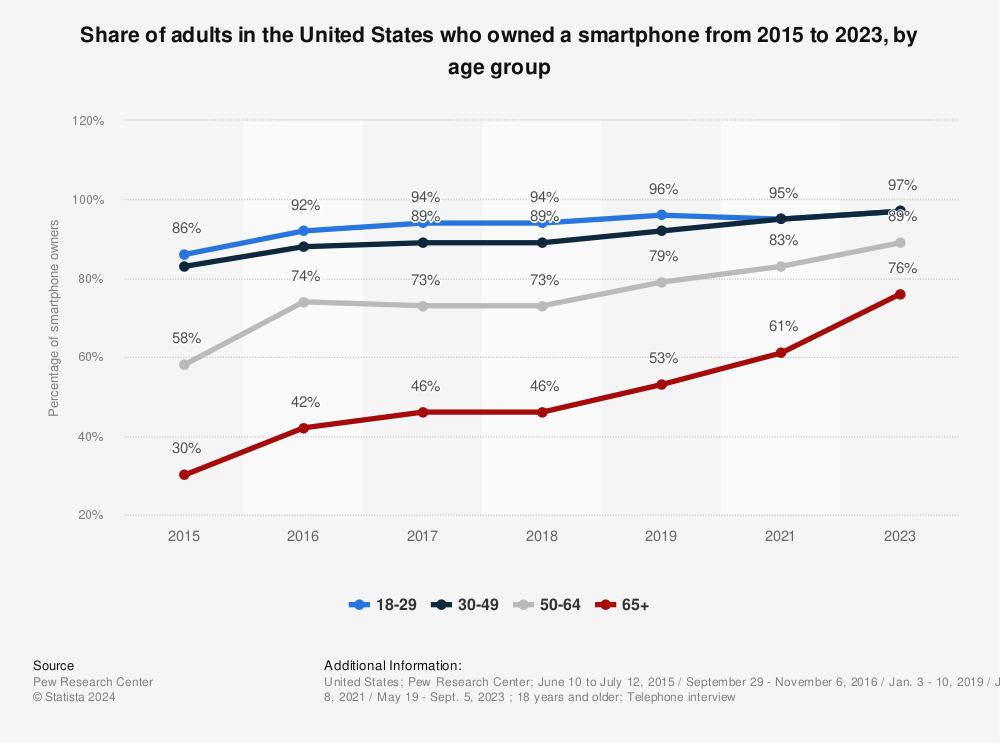
With more and more people accessing the internet through their mobile devices, now you can reach teens while they’re between classes and adults during their subway commute to work. Can’t say that for those other types of surveys !
Online surveys are also extremely cost-efficient. You don’t have to spend money on paper, printing, postage, or an interviewer. This significantly reduces set-up and administration costs. This also allows researchers and companies to send out a survey very expeditiously. Additionally, many online survey tools provide in-depth analysis of survey data. This saves you from having to spend money on further research once the survey is complete.
Researchers have their pick of options when it’s time to survey people. Which method you choose may depend upon cost, reach, and the types of questions.
Now, you may be wondering, “ Where can I make free surveys ?” You can get started with free online surveys using SurveyLegend! He re are a few things that make SurveyLegend the ideal choice for different types of surveys for research ( or for fun) .
- When it comes to surveys, brief is best to keep respondents attention. So, SurveyLegend automatically collects some data, such as the participant’s location, reducing the number of questions you have to ask.
- People like eye candy and many surveys are just plain dull. SurveyLegend offers beautifully rendered pre-designed surveys that will get your participant’s attention – and keep it through to completion!
- Today, most people take surveys on mobile devices. Often surveys desktop surveys don’t translate well, resulting in a high drop-off rate. SurveyLegend’s designs are responsive, automatically adjusting to any screen size.
What’s your favorite method of surveying people? (Hey… that’s a good topic for a survey!) Sound off in the comments!
Frequently Asked Questions (FAQs)
The 10 most common survey methods are online surveys, in-person interviews, focus groups, panel sampling, telephone surveys, post-call surveys, mail-in surveys, pop-up surveys, mobile surveys, and kiosk surveys.
Benefits of online surveys include their ability to reach a broad audience and that they are relatively inexpensive.
Kiosk surveys are surveys on a computer screen at the point of sale.
A focus group is an in-person interview or survey involving a group of people rather than just one individual. The group is generally small but demographically diverse, and led by a moderator.
Jasko Mahmutovic
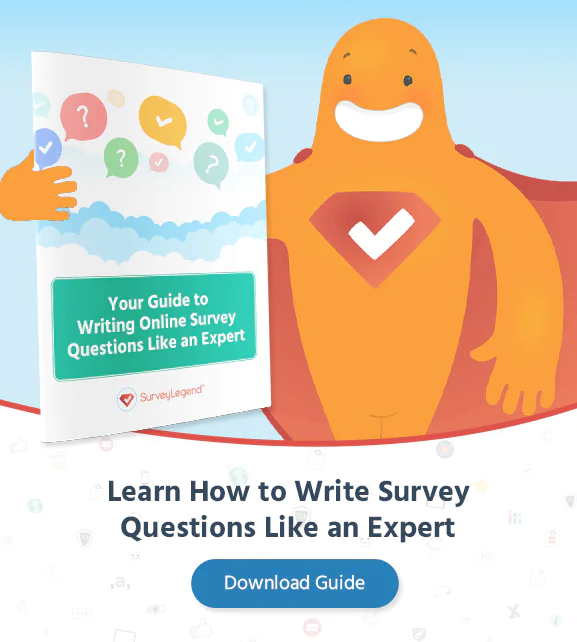
Related Articles You Might Like
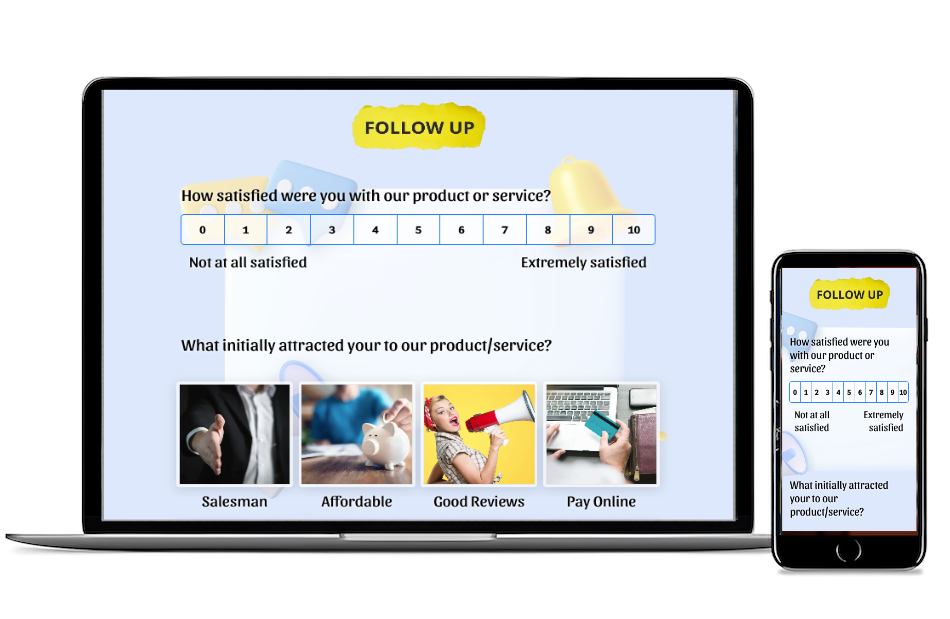
How To Create a Follow-up Survey & Questions To Ask
“The fortune is in the follow-up.” – Jim Rohn Rohn, an American entrepreneur, author, and motivational speaker who passed in 2009, understood the importance of follow-up. He would often...
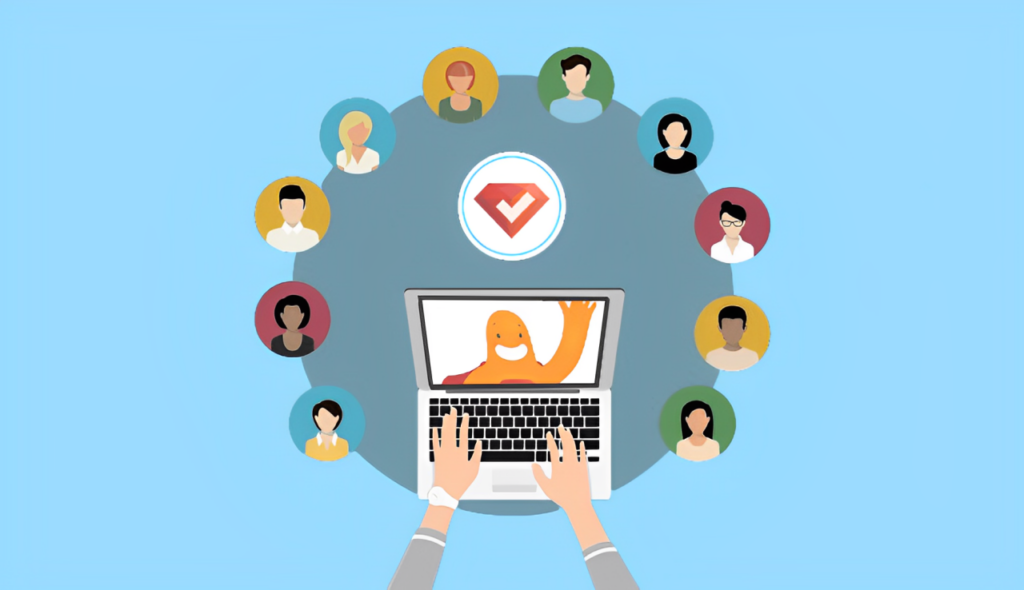
How To Create a Successful Webinar Survey & Questions To Ask
Webinars continue to fuel successful marketing initiatives and learning platforms. But not all webinars are created equal. If you’ve attended a virtual event in the past – and it’s 2024,...
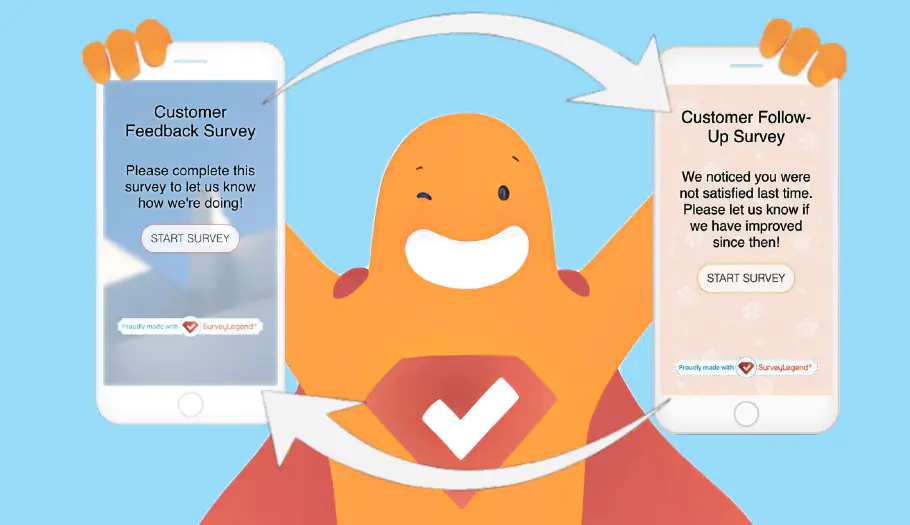
What Is A Closed-Loop Survey & Five Steps To Closing The Loop
When we talk about “closing the loop,” we’re not referring to that childhood method of tying shoelaces! In business, closing the loop refers to completing a cycle or ensuring...
Privacy Overview
- (855) 776-7763
All Products
BIGContacts CRM
Survey Maker
ProProfs.com
- Get Started Free
Want insights that improve experience & conversions?
Capture customer feedback to improve customer experience & grow conversions.
From Online to In-Person: Ultimate Guide to Survey Methods
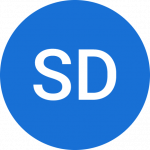
Author & Editor at ProProfs
Shivani Dubey specializes in crafting engaging narratives and exploring Customer Experience Management intricacies. She writes on vital topics like customer feedback, emerging UX and CX trends, and sentiment analysis.
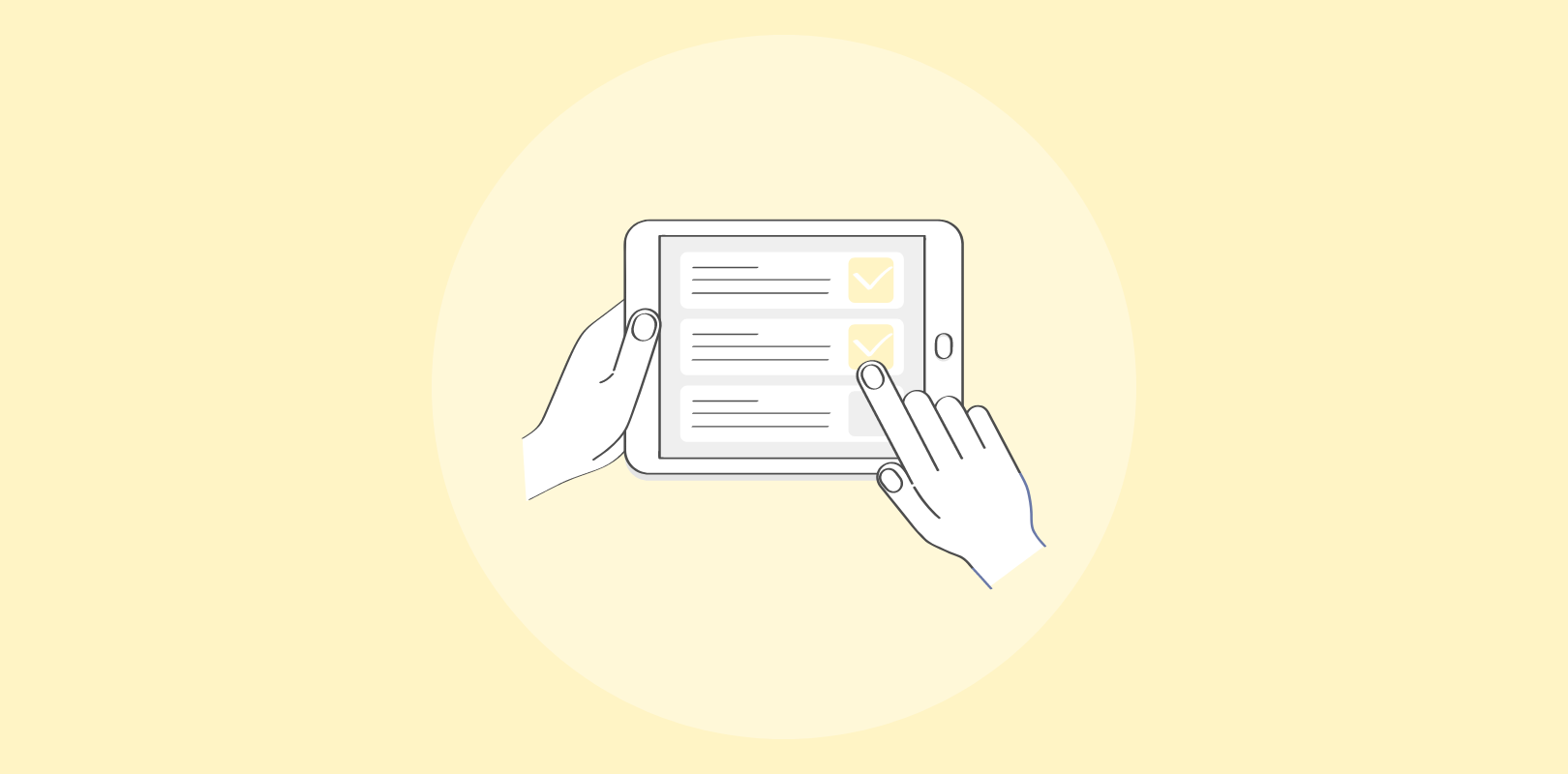
Ever filled out a quick survey on your phone or scribbled feedback on a napkin at your favorite café?
That’s the power of surveys in action—snappy tools that capture your thoughts at that moment.
Today, surveys have evolved far beyond the paper form, adapting to our digital lives. From a swift SMS post-purchase to an interactive kiosk at an event, each method offers a unique window into our experiences.
Let’s dive into the dynamic world of surveys, where every click, tap, and text helps shape better services and products.
What Are Survey Research Methods Anyway?
At its core, a survey method is a systematic approach to collecting data from a predefined group of respondents. This data is used to gain insights, make decisions, or measure opinions on various topics.
The beauty of surveys lies in their versatility—they can be adapted to online formats, face-to-face interactions, or even the good old paper-and-pencil approach.
Choosing the right survey method depends on a few key factors:
- who your target audience is,
- how much money you’re willing to spend,
- the timeframe you’re working within,
- and what you hope to achieve with the data.
Types of Survey Methods
In the realm of data collection, choosing the right survey method is akin to selecting the perfect tool for a job. Each method has its own set of strengths and weaknesses, tailored to specific research needs.
Let’s delve deeper into each type, armed with examples to highlight their practical applications.
Online Surveys: Digital Data Collection for Broad Reach and Efficiency
Online surveys have become the go-to method in the digital age, offering unparalleled convenience and reach. With free and easy-to-use tools like Qualaroo , you can embed surveys on your website , in your software product, or in a prototype as well.
It allows you to cast a wider net for the respondents and target a diverse audience for nuanced and inclusive insights.
Not only can you automate your research through timely surveys, but you can also set advanced triggers that target a specific customer segment based on factors like browsing source, purchase history, online behavior, and more.
With Qualaroo’s pop-up Nudges TM , you can collect contextual insights and check customers’ pulse, among many other customer satisfaction metrics.
For instance, an ecommerce company might use an online survey on its website to gauge consumer preferences for a new product line.
Mobile Surveys: Quick Feedback via Smartphones for Immediate Insights
The ubiquity of smartphones has given rise to mobile surveys, which are designed to be easily accessible on mobile devices.
You can embed in-app surveys on your mobile apps or use Qualaroo to create mobile web surveys to offer a seamless survey experience to the participants on their devices.
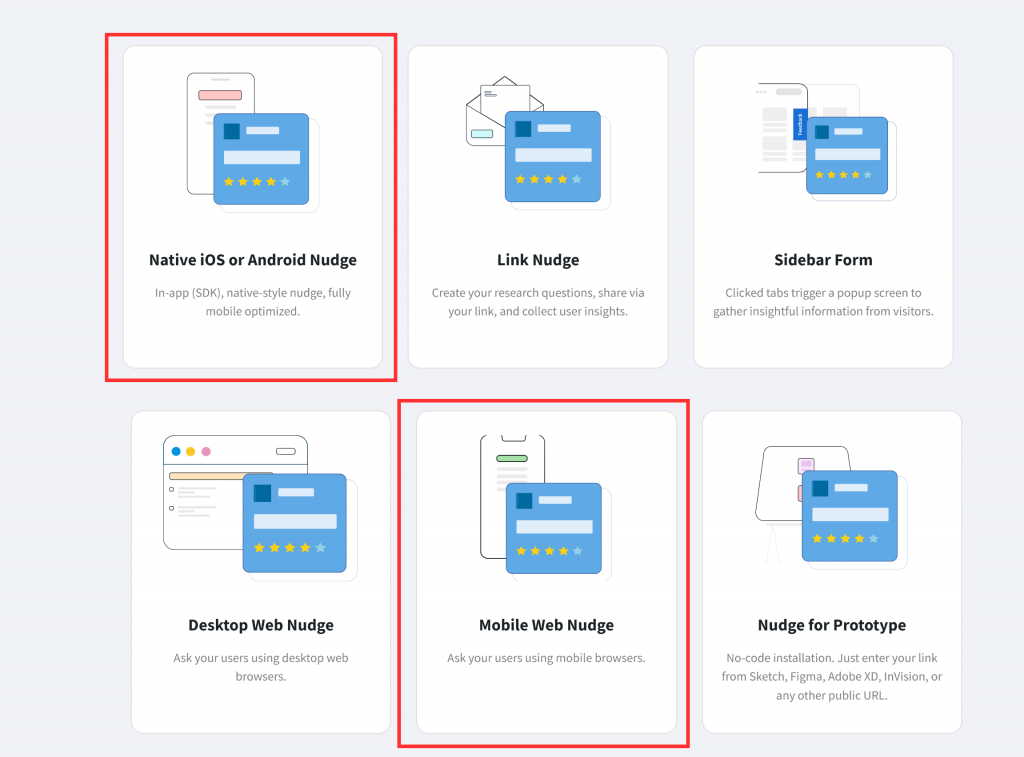
Retail companies, for example, might send a quick survey link via SMS to customers after a purchase to rate their shopping experience. Mobile surveys are excellent for capturing real-time feedback and are particularly effective in engaging younger demographics.
However, the design must be mobile-friendly, keeping questions short and straightforward to accommodate the smaller screen sizes and the typically shorter attention spans of users on the go.
Email Surveys: Personalized Questionnaires Delivered Directly to Inboxes
Email surveys are a widely used method where survey and survey invitations are sent directly to participants’ inboxes.
This method is highly cost-effective and allows for easy distribution to a large number of people in a short time. As a part of Qualaroo, ProProfs Survey Maker allows you to embed surveys into your emails, allowing participants to take the survey right when they open their email.
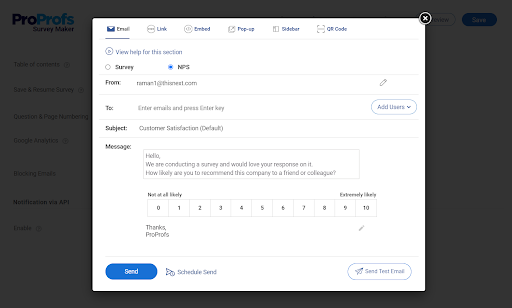
For example, a customer satisfaction survey might be emailed to customers after they’ve made a purchase, asking for feedback on their shopping experience.
SMS Surveys: Instant Surveys Through Text Messages for Concise Responses
SMS surveys involve sending short survey questions directly to participants’ mobile phones via text message.
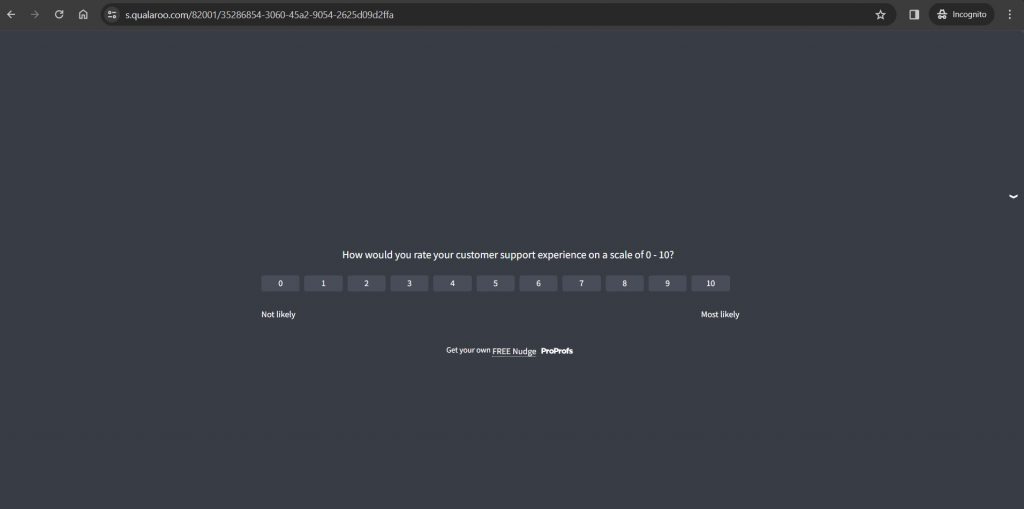
This method is excellent for reaching people on the go and is particularly effective for concise surveys with a limited number of questions.
An example might be a quick poll asking event attendees to rate their experience right after an event, maximizing the timeliness and relevance of the feedback.
However, the main limitations include the need to keep surveys extremely brief due to character limits and potential costs to respondents, depending on their mobile plans.
Telephone Surveys: Interactive Voice-Based Surveys for Detailed Feedback
Telephone surveys hark back to a time when the personal touch of a voice conversation was the primary mode of distance communication. Despite declining in popularity due to caller ID and privacy concerns, they’re still valuable in specific contexts.
For example, political polling organizations frequently use telephone surveys to gather opinions on candidates and issues, leveraging the ability to probe deeper into responses or clarify questions as needed.
The direct interaction can yield richer data, but researchers must contend with increasing refusal rates and the costs associated with staffing call centers.
Face-to-Face Interviews: Personal Interviews for Nuanced Understanding
Face-to-face interviews offer a depth of insight unmatched by other methods, allowing for the observation of non-verbal cues and the flexibility to adapt questions on the fly.
This method is particularly effective for sensitive topics or complex issues where understanding nuances is crucial.
An example would be a sociologist conducting in-depth interviews with participants to explore the impact of social media on mental health.
The major drawback is the logistical challenge and expense of conducting interviews, especially when the target population is geographically dispersed.
Cross-Sectional & Longitudinal Surveys: Snapshot Vs. Over-Time Analysis for Trend Observation
Cross-sectional surveys provide a snapshot of a particular moment, offering a broad overview of attitudes, opinions, or behaviors at a specific point in time.
For instance, a health organization might conduct a cross-sectional survey to assess smoking rates within a community. In contrast, longitudinal surveys track changes over time, offering insights into trends and causation.
An educational institution might use a longitudinal survey to follow a cohort of students throughout their academic career to study the impact of certain teaching methods on long-term success.
While cross-sectional surveys are relatively straightforward and cost-effective, longitudinal studies require a long-term commitment and can be more complex and costly due to their extended nature.
Panel Surveys: Consistent Feedback From the Same Group Over Various Topics
Panel surveys involve repeatedly surveying the same group of respondents over time, but unlike longitudinal surveys, they might focus on various topics at each wave.
This method is valuable for understanding changes within a population and how attitudes shift in response to events or interventions.
A media company, for instance, might maintain a panel of viewers to gauge reactions to different television programming over time. Panel surveys offer rich insights but require maintaining engagement with the panel members to minimize dropouts.
Kiosk Surveys: On-Location Digital Surveys for Immediate Feedback
Kiosk surveys are conducted on digital devices such as tablets or touch-screen stands located in physical locations where the target audience is present.
For instance, a retail store might set up a kiosk near the exit where customers can quickly rate their shopping experience or provide feedback on the service received.
One of the key advantages of kiosk surveys is the ability to capture real-time feedback while the experience is fresh in the respondents’ minds, leading to more accurate and actionable insights. Kiosk surveys can be highly engaging and visually appealing, encouraging participation.
However, challenges include the initial investment in hardware and software, potential issues with privacy and data security, and the need for regular maintenance of the physical setup.
Choosing the Right Path in Survey Research
Survey methods are essential tools in our quest for knowledge, offering a range of techniques to gather valuable insights. From quick SMS surveys to in-depth interviews, each method has its place, tailored to specific research needs and contexts.
As we conclude our exploration, remember that the strength of your findings lies in choosing the right survey approach.
So, whether you’re assessing customer satisfaction or exploring new trends, the right survey method and survey software can provide the clarity and direction needed to inform decisions and drive change
About the author
Shivani dubey.
Shivani has more than 3 years of experience in the modern creative content paradigm and technical writing verticals. She has been published in The Boss Magazine, Reseller Club, and HR Technologist. She is passionate about Artificial Intelligence and has a deep understanding of how organizations can leverage customer support technologies for maximum success. In her free time, she enjoys Nail art, playing with her guinea pigs, and chilling with a bowl of cheese fries.
Popular Posts in This Category
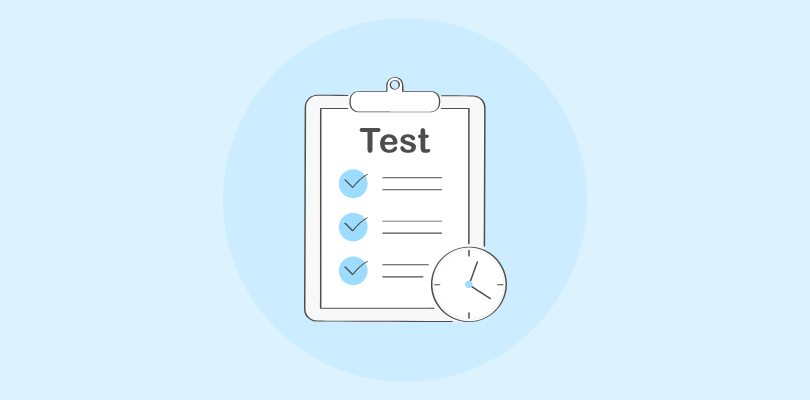
9 Best Employee Exit Survey Software To Streamline Offboarding
Your biggest growth opportunity might be right under your nose, what every sales lead needs to know about their growth lead and vice versa.
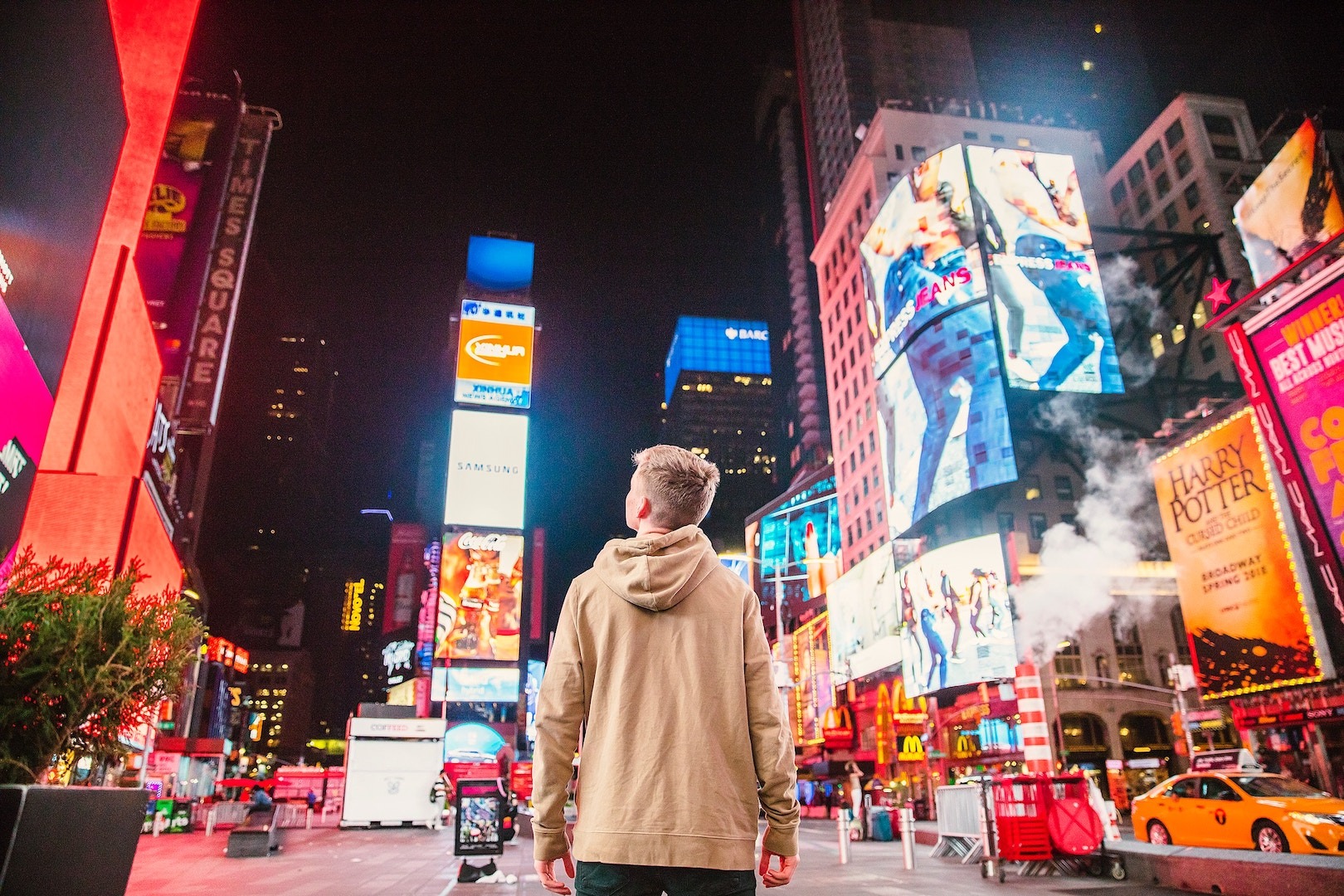
3 Simple Ways You Can Track ROI On Offline Marketing Efforts
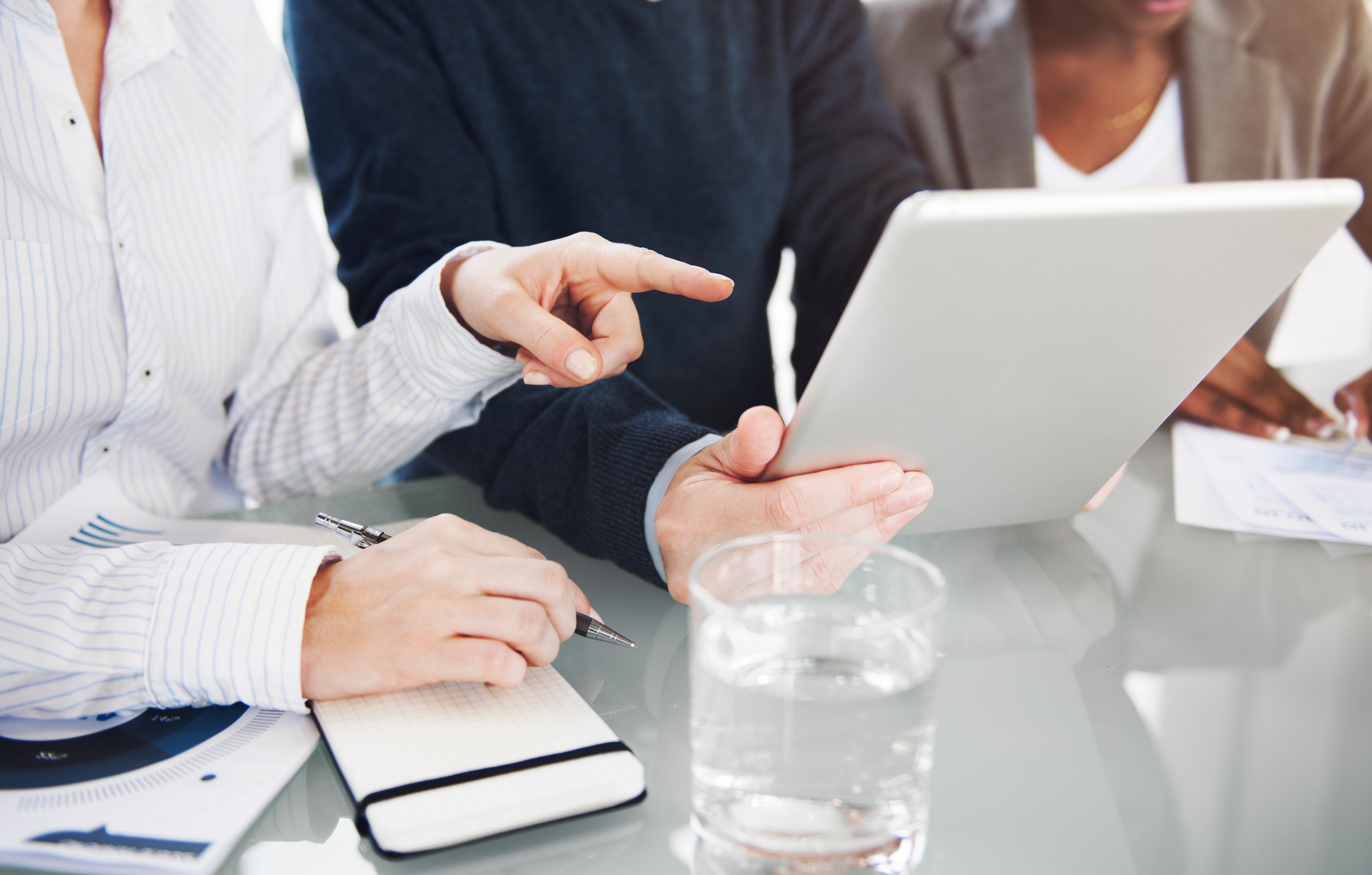
New Reporting Dashboard is Now in Beta!
3 ways to use the new qualaroo chat integrations.
This paper is in the following e-collection/theme issue:
Published on 27.5.2024 in Vol 8 (2024)
This is a member publication of University of Colorado Denver HARC
Lessons Learned From a Sequential Mixed-Mode Survey Design to Recruit and Collect Data From Case-Control Study Participants: Formative Evaluation
Authors of this article:

Original Paper
- Amanda D Tran 1 , MPH ;
- Alice E White 1 , MPH ;
- Michelle R Torok 1 , PhD ;
- Rachel H Jervis 2 , MPH ;
- Bernadette A Albanese 3 , MD, MPH ;
- Elaine J Scallan Walter 1 , MA, PhD
1 Department of Epidemiology, Colorado School of Public Health, University of Colorado, Aurora, CO, United States
2 Colorado Department of Public Health and Environment, Denver, CO, United States
3 Adams County Health Department, Brighton, CO, United States
Corresponding Author:
Elaine J Scallan Walter, MA, PhD
Department of Epidemiology
Colorado School of Public Health
University of Colorado
13001 East 17th Place
3rd Floor, Mail Stop B119
Aurora, CO, 80045
United States
Phone: 1 303 724 5162
Email: [email protected]
Background: Sequential mixed-mode surveys using both web-based surveys and telephone interviews are increasingly being used in observational studies and have been shown to have many benefits; however, the application of this survey design has not been evaluated in the context of epidemiological case-control studies.
Objective: In this paper, we discuss the challenges, benefits, and limitations of using a sequential mixed-mode survey design for a case-control study assessing risk factors during the COVID-19 pandemic.
Methods: Colorado adults testing positive for SARS-CoV-2 were randomly selected and matched to those with a negative SARS-CoV-2 test result from March to April 2021. Participants were first contacted by SMS text message to complete a self-administered web-based survey asking about community exposures and behaviors. Those who did not respond were contacted for a telephone interview. We evaluated the representativeness of survey participants to sample populations and compared sociodemographic characteristics, participant responses, and time and resource requirements by survey mode using descriptive statistics and logistic regression models.
Results: Of enrolled case and control participants, most were interviewed by telephone (308/537, 57.4% and 342/648, 52.8%, respectively), with overall enrollment more than doubling after interviewers called nonresponders. Participants identifying as female or White non-Hispanic, residing in urban areas, and not working outside the home were more likely to complete the web-based survey. Telephone participants were more likely than web-based participants to be aged 18-39 years or 60 years and older and reside in areas with lower levels of education, more linguistic isolation, lower income, and more people of color. While there were statistically significant sociodemographic differences noted between web-based and telephone case and control participants and their respective sample pools, participants were more similar to sample pools when web-based and telephone responses were combined. Web-based participants were less likely to report close contact with an individual with COVID-19 (odds ratio [OR] 0.70, 95% CI 0.53-0.94) but more likely to report community exposures, including visiting a grocery store or retail shop (OR 1.55, 95% CI 1.13-2.12), restaurant or cafe or coffee shop (OR 1.52, 95% CI 1.20-1.92), attending a gathering (OR 1.69, 95% CI 1.34-2.15), or sport or sporting event (OR 1.05, 95% CI 1.05-1.88). The web-based survey required an average of 0.03 (SD 0) person-hours per enrolled participant and US $920 in resources, whereas the telephone interview required an average of 5.11 person-hours per enrolled participant and US $70,000 in interviewer wages.
Conclusions: While we still encountered control recruitment challenges noted in other observational studies, the sequential mixed-mode design was an efficient method for recruiting a more representative group of participants for a case-control study with limited impact on data quality and should be considered during public health emergencies when timely and accurate exposure information is needed to inform control measures.
Introduction
Often used during disease outbreak investigations, case-control studies that retrospectively compare people who have a disease (case participants) with people who do not have the disease (control participants) are an efficient and relatively inexpensive method of identifying potential disease risk factors to guide control measures and interventions. Perhaps the most critical and challenging component of conducting a case-control study is the recruitment of appropriate control participants who are from the same source population as case participants [ 1 ]. Because control participants are not ill and may not be connected to the outbreak, they may be less motivated to complete a lengthy questionnaire that collects personal information and detailed exposure histories [ 2 - 4 ]. Moreover, with the increased use of mobile telephones and the routine use of caller ID, study participants contacted by traditional telephone-based survey methodologies may be less likely to answer the telephone [ 5 , 6 ], further reducing the opportunity for participant screening and recruitment.
Recruitment challenges are not unique to case-control studies, and other types of observational studies have shifted from traditional telephone interviews to web-based surveys with the goal of reaching larger groups of people more efficiently and at a lower cost [ 7 - 12 ]. While offering some advantages over traditional telephone interviews, web-based surveys often experience lower response rates and lower data quality [ 13 ], and some studies have found demographic differences between telephone and web-based survey participants, likely driven in part by disparities in internet connectivity and access [ 14 ]. For this reason, researchers have increasingly used both telephone interviews and web-based surveys in a sequential mixed-mode design, first contacting participants using a self-administered web-based survey, and then following up with nonresponders with an interviewer-administered telephone survey [ 15 ]. In other types of observational studies, this mixed-mode design has been shown to reduce selection bias, reduce costs, improve data quality, and result in higher response rates and faster participant recruitment [ 16 , 17 ], making it an appealing design choice for case-control studies.
In March 2020, the World Health Organization declared COVID-19 a global pandemic, and throughout many countries, public health or other governmental authorities implemented stay-at-home orders, travel restrictions, and other public health interventions to reduce disease transmission. In the absence of adequate data-driven evidence about community risk factors for COVID-19 transmission, we implemented a sequential mixed-mode case-control study design in Colorado to evaluate community exposures and behaviors associated with SARS-CoV-2 infection and inform public health control measures. While the benefits and limitations of sequential mixed-mode designs have been well-documented in other contexts [ 14 , 16 , 18 - 20 ], they have not been examined in the context of rapidly implemented epidemiological case-control studies. In this paper, we discuss the challenges, benefits, and limitations of using a sequential mixed-mode survey design using web-based surveys disseminated via SMS text message and telephone interviews for a case-control study assessing exposures during a public health emergency. Specific aims are (1) to compare the sociodemographic characteristics of web-based and telephone survey participants, (2) to evaluate the representativeness of survey participants to the sample population, (3) to assess the completeness of participant responses by survey mode, and (4) to estimate the time and resources required to recruit web-based and telephone survey participants.
Case-Control Study Design and Implementation
The case-control study was conducted among Colorado adults aged 18 years and older who had a positive (case) or negative (control) SARS-CoV-2 reverse transcription-polymerase chain reaction test result in Colorado’s electronic laboratory reporting (ELR) system with a specimen collection date from March 16 to April 29, 2021 [ 21 ]. Eligible individuals testing positive with a completed routine public health interview in Colorado’s COVID-19 surveillance system were randomly selected and individually matched on age (±10 years), zip code (urban areas) or region (rural and frontier areas), and specimen collection date (±3 days) with up to 20 individuals with a negative test, with the goal of enrolling 2 matched controls per enrolled case.
Self-administered (web-based) and interviewer-administered (telephone) case and control surveys were developed in Research Electronic Data Capture (REDCap; Vanderbilt University). REDCap is a secure, web-based platform designed to support data capture for research studies [ 22 ]. The surveys asked about contact with a person with confirmed or suspected COVID-19, travel history, employment, mask use, and community exposure settings (bar or club; church, religious, or spiritual gathering; gathering; grocery or retail shopping; gym or fitness center; health care setting; restaurant, cafe, or coffee shop; salon, spa, or barber; social event; or sports or sporting events) during the 14 days before illness onset or specimen submission. The full survey questionnaire is available in Multimedia Appendix 1 . Demographic data were obtained from Colorado’s COVID-19 case surveillance system and the control survey. Web-based surveys were offered in English and Spanish and included clarifying language, prompts, skip logic, text piping, and progress bars. Interviewers used computer-assisted telephone interviewing in REDCap with scripting and language line services when needed. Questions and response options were identically worded in the web-based and telephone surveys, with the exception of a “refused” option for questions in the telephone survey.
Using the Twilio integration in REDCap, selected individuals were sent an SMS text message to the telephone number provided at the time of testing (which may include both landlines and mobile phones) 3 to 7 days after their specimen collection date, inviting them to complete the web-based survey. A team of trained interviewers began contacting nonresponders for telephone interviews approximately 3 hours after the initial SMS text message was sent, making 1 contact attempt for individuals testing positive for SARS-CoV-2 and up to 2 contact attempts for those testing negative. Interviewers only contacted as many controls by telephone as needed to enroll 2 matched controls per enrolled case. The web-based survey link was resent via SMS text message or sent via email when requested. When possible, voicemail messages were left encouraging SMS text message recipients to complete the web-based survey. As the goal of the case-control study was to assess the risk of SARS-CoV-2 infection from community exposures, we only included surveys that had responses to all 15 community exposure questions. Partial surveys that did not have complete community exposure data were excluded from analyses. Individuals were also excluded if they reported living in an institution, close contact with a household member with confirmed or suspected COVID-19, receiving ≥1 dose of a COVID-19 vaccine (which was not universally available in Colorado at the time of the study), symptom onset date >7 days from specimen collection (case participants), a prior positive COVID-19 result (control participants), or providing personal identifying information in the web-based survey that was inconsistent with information from the ELR system (control participants).
Evaluation of a Sequential Mixed-Mode Survey Design
We evaluated the impact of conducting the COVID-19 case-control study using a sequential mixed-mode design by (1) comparing the sociodemographic characteristics of web-based and telephone survey participants, (2) evaluating the representativeness of study participants to the sample population, (3) assessing the completeness of participant responses by survey mode, and (4) estimating the time and resources required to recruit web-based and telephone survey participants. All analyses were performed using SAS (version 9.4; SAS Institute).
Comparison of Web-Based and Telephone Survey Participants
Case and control participants were eligible individuals who completed the web-based or telephone survey. We compared the demographic characteristics (age, gender, race and ethnicity, geographic location, working outside the home, and socioeconomic factors) of case and control participants completing the web-based and telephone survey to each other using 2-tailed t tests, Pearson χ 2 , or Fisher exact tests. Socioeconomic factors, which are not routinely asked in surveillance and therefore not included in the survey, were evaluated by aggregating mean scores for 4 Colorado EnviroScreen indicators (less than high school education, linguistic isolation, low income, and people of color) based on the participant’s county of residence. Colorado EnviroScreen (version 1.0; Colorado State University and the Colorado Department of Public Health and Environment) is a publicly available environmental justice mapping tool developed by the Colorado Department of Public Health and Environment and Colorado State University that evaluates 35 distinct environmental, health, economic, and demographic indicators. Colorado EnviroScreen scores range from 0 to 100, with the highest score representing the highest burden of health injustice.
Representativeness of Study Participants
We compared the demographic characteristics (as described earlier) of case and control participants completing the web-based and telephone surveys (separately and combined) to the sample pool of all randomly selected individuals testing positive (case sample pool) or negative (control sample pool) for SARS-CoV-2 using 2-tailed t tests, Pearson χ 2 , or Fisher exact tests.
Participant Responses
We evaluated data completeness and differential responses between web-based and telephone survey modes by comparing responses to exposure and behavior questions we deemed prone to social desirability bias (close contact with individuals with confirmed or suspected COVID-19, community exposures, travel, and mask use). Two bivariate logistic regression models, the first adjusting for case-control status and the second adjusting for case-control status and sociodemographic variables shown to be associated with mode effects (age, gender, race and ethnicity, and geographic location), examined the association between survey mode and participant response. Question nonresponse, where data were missing or refused, was evaluated for these questions as well as for other questions with free-text or multiple-choice response options (industry, occupation, reasons for COVID-19 testing, and mask type).
Time and Resource Needs
The time spent by study personnel contacting potential participants by SMS text message and telephone was obtained from self-recorded data in timesheets and used to calculate the person-hours required per enrolled participant. Total expenditures for the web-based and telephone surveys were calculated using staff wages and Twilio texting costs (an average of US $0.008 for a 160-character SMS text message).
Ethical Considerations
The case-control study was deemed by the Colorado Multiple Institutional Review Board to be public health surveillance and not human participant research and was therefore exempt from full approval and requirements for informed consent (protocol 21-2973).
Case and Control Participant Enrollment
The case sample pool included 1323 individuals. Of these, 318 (24%) responded to the web-based survey, and 331 (25%) were interviewed by telephone ( Figure 1 ). A total of 537 (40.6%) case participants were enrolled after excluding 78 (5.9%) partial and 34 (2.6%) ineligible survey responses. Of the 10,898 individuals in the control sample pool, 1072 (9.8%) responded to the web-based survey, and 1268 (11.6%) were interviewed by telephone. A total of 648 (5.9%) control participants were enrolled after excluding 1565 (14.4%) partial and 127 (1.2%) ineligible surveys. Of the enrolled case and control participants, most were interviewed by telephone (308/537, 57.4% and 342/648, 52.8%, respectively).

Case participants completing the web-based and telephone surveys were similar in age (mean 37, SD 13.21 and 14.69 years, respectively), whereas web-based control participants were slightly older than those completing the telephone survey (mean 38, SD 12.44 vs mean 36, SD 12.62 years, respectively; Table 1 ). For both case and control participants, those aged 40-59 years were more likely to complete the web-based survey, whereas participants aged 18-39 years and 60 years and older were more likely to complete the telephone survey. Web-based case and control participants were more likely to identify as female, White, non-Hispanic, reside in urban areas, and be less likely to work outside the home. Compared to web-based case and control participants, telephone participants had higher EnviroScreen scores for all socioeconomic indicators, indicating they resided in counties with larger populations of individuals with less than high school education, linguistic isolation, low income, and people of color.
a Individuals with a positive SARS-CoV-2 test result.
b Individuals with a negative SARS-CoV-2 test result.
c P <.05; control participant web-based versus telephone.
d P <.01; survey mode (web-based, telephone, and web-based and telephone combined) versus sample pool.
e P <.05; case participant web-based versus telephone.
f P <.05; survey mode (web-based, telephone, and web-based and telephone combined) versus sample pool.
g Information on sex and working outside the home were not available from Colorado’s electronic laboratory reporting system for control participants.
h Not available.
i Colorado EnviroScreen is an environmental justice mapping tool. Scores are assigned at the county level, with a higher score indicating that an area is more likely to be affected by the indicated health injustice.
There were statistically significant sociodemographic differences noted between web-based and telephone case and control participants and their respective sample pools ( Table 1 ). More web-based case participants identified as female (134/228, 58.8%) than those in the case sample pool (642/1318, 48.7%). More web-based control participants identified as White, non-Hispanic (205/267, 76.8%) than those in the control sample pool (4467/7812, 57.2%) and more often resided in urban areas (247/306, 80.7%) than those in the control sample pool (7841/10,898, 71.9%). Case and control participants were more similar to their respective sample pools when evaluated as a single group (total enrolled).
In the model adjusting for case or control status only, web-based participants were less likely to report close contact with an individual with COVID-19 when compared to telephone participants (odds ratio [OR] 0.70, 95% CI 0.53-0.94) but more likely to report community exposures including visiting a grocery store or retail shop (OR 1.55, 95% CI 1.13-2.12), visiting a restaurant or cafe or coffee shop (OR 1.52, 95% CI 1.20-1.92), attending a gathering outside the home (OR 1.69, 95% CI 1.34-2.15), or attending or participating in a sport or sporting event (OR 1.05, 95% CI 1.05-1.88) in 14 days before symptom onset or specimen collection ( Table 2 ). When adjusted for case or control status, age, gender, race and ethnicity, and geographic location, the only associations that remained statistically significant were close contact (adjusted OR 0.65, 95% CI 0.48-0.88) and gatherings (adjusted OR 1.44, 95% CI 1.12-1.85).
a Full survey questions are available in Multimedia Appendix 1 .
b Adjusted for case or control status.
c OR: odds ratio.
d Adjusted for case or control status, age, gender, race and ethnicity, and geographic location.
e N/A: not applicable.
Question nonresponse was low across both modalities, with similar ranges of missingness between the web-based survey (0/535, 0% to 22/535, 4.1%) and telephone survey (2/650, 0.3% to 34/650, 5.2%). Nonresponse to industry, occupation, and masking questions was higher in the telephone survey (9/650, 1.4% to 34/650, 5.2%) than the web-based survey (1/535, 0.2% to 22/535, 4.1%; Table 2 ).
Over the course of the study, staff spent a cumulative 15 hours randomly selecting and texting potential participants for the web-based survey, averaging 0.03 person-hours per enrolled participant (15 person-hours per 535 web-based participants) and US $500 in staff wages. Twilio texting costs were US $420, amounting to US $920 in total expenditures for the web-based survey. Comparatively, 3319 hours were spent by interviewers attempting to contact nonresponders by telephone, for an average of 5.11 person-hours per enrolled participant (3319 person-hours per 650 telephone participants) and US $70,000 in interviewer wages.
Principal Findings
While the web-based survey was more time- and cost-efficient than the telephone interview, participant enrollment was low, and there were statistically significant sociodemographic differences between the web-based case and control participants and their respective sample pools. Adding the follow-up telephone interview increased participant enrollment and the representativeness of both the case and control participants to sample pools. Participant responses to exposure and behavior questions and data completeness were similar between the 2 survey modalities.
Enrollment more than doubled for case and control participants after interviewers called individuals who did not respond to the web-based survey to complete the survey by telephone. Case participant enrollment for our mixed-mode study was higher than those for other COVID-19 case-control studies using telephone only (40.6% vs 3%-25% case participant enrollment in other studies), but control participant enrollment was lower (5.9% vs 9%-13% control participant enrollment in other studies) [ 23 - 25 ]. However, control participant enrollment in our sequential mixed-mode study may not be comparable to telephone-only COVID-19 case-control studies for 2 reasons. First, we texted up to 20 potential controls for every enrolled case participant in anticipation of lower response rates for the web-based survey, inflating the number of contacted controls in our response rate calculations. Second, we did not follow up with all potential controls by telephone once our quota of 2 controls per case was reached. In contrast, telephone-only studies only call as many controls as needed to enroll the desired number of matched control participants, which is typically less than 20.
We found sociodemographic differences between participants completing the survey on the web and by telephone. Web-based respondents were more likely to be female, identify as White, non-Hispanic, have higher levels of education, and reside in urban areas, which was consistent with other studies evaluating survey mode effects [ 12 , 26 , 27 ]. Contrary to other studies that found higher web-based response rates among those younger than 35 years of age [ 14 ], participants aged 18-39 years in our case-control study were more likely to respond to the telephone survey, as were participants aged 60 years and older, participants working outside the home, and participants residing in areas with a higher burden of health injustices. Some of these differences may be attributable to the timing of when potential participants were contacted. While potential participants were texted a link to complete the web-based survey only in the morning, telephone interviews were administered throughout the day, including in the late afternoon and evening when more people may be at home and not working. In addition, older participants and participants in lower socioeconomic settings may experience more barriers to completing a web-based survey, such as limited internet access or less comfort using mobile platforms [ 15 ], making them more likely to complete a telephone interview.
While there were sociodemographic differences between web-based and telephone participants and between web-based and telephone case and control participants and their respective sample pools, the sociodemographic characteristics of combined web-based and telephone survey participants were broadly representative of the sample pools. This indicates that the sequential mixed-mode design allowed for the recruitment of more representative case and control participant groups than if we had used a telephone or web-based survey alone, and the use of this survey design can help reduce selection bias in case-control studies.
Telephone surveys conducted by trained interviewers have several advantages over other modes of administration. Most importantly, trained interviewers can answer participants’ questions, add clarifying questions, and probe interviewees for more complete responses, leading to better data completeness and quality. While increasing data quality, telephone surveys can lead to social desirability bias as participants may alter answers to questions to seem more favorable or socially acceptable to an interviewer [ 19 , 20 ]. An advantage of using a web-based survey is that the absence of an interviewer may provide participants with the opportunity to answer questions more candidly, potentially reducing social desirability bias [ 19 , 20 ]. While we found that web-based participants were more likely to report certain community exposures, most of the differential responses between web-based and telephone participants were no longer statistically significant after adjusting for variables shown to be associated with mode effects (age, gender, race, ethnicity, and geographic location). This suggests that demographic differences between web-based and telephone participants may be confounding variables and should be considered when analyzing and interpreting data for case-control studies.
Limitations
This project was subject to several limitations. First, cases were randomly selected from persons reported in Colorado’s COVID-19 surveillance system who had already completed an interview with public health, which may impact study findings. For example, this method of case-participant selection may account for the high enrollment rates we had for our case-control study, and these individuals may systematically differ from those testing positive for SARS-CoV-2 who did not complete an initial interview with public health. Second, sample pool data were obtained from the ELR system for control participants, which had incomplete demographic data. The sample pool characteristics presented in this paper may not be accurate because of these missing data and, in turn, affect our evaluations of sample representativeness. Third, the socioeconomic characteristics of participants may be subject to ecological fallacy as we used county-level Colorado EnviroScreen scores as a proxy for individual socioeconomic status. Fourth, it is unclear whether the systematic differences noted between web-based and telephone participants were due to the survey mode itself or due to the additional contact attempts made to enroll telephone participants. Finally, this sequential mixed-mode case-control study was implemented during the COVID-19 pandemic, a period marked by various political and social factors that could have influenced who responded to our survey and their responses. As such, findings from this paper may not be generalizable to case-control studies evaluating other diseases or outbreaks.
Conclusions
Telephone interviews conducted as part of an outbreak investigation are time-consuming and costly [ 8 ]. Given the limited resources and staff at many public health agencies, it is critical to find methods to increase efficiency and reduce the costs of outbreak investigations. Web-based surveys are more time- and cost-efficient than telephone interviews, greatly reducing the workload for health departments. However, web-based surveys may appeal to specific demographics, have lower enrollment rates, and may require a larger sample pool or a longer time to enroll participants, which may not be feasible for small outbreaks or ideal for public health emergencies when timely data collection is crucial.
By using a sequential mixed-mode design, we were able to efficiently recruit participants for a case-control study with limited impact on data quality. Moreover, using the sequential mixed-mode approach allowed for maximal sample representativeness compared to a web-based or telephone interview alone. This is critical during public health emergencies, when timely and accurate exposure information is needed to inform control measures and policy. While the sequential mixed-mode design allowed us to reach more potential control participants with fewer resources, we still encountered the same challenges recruiting control participants noted in other studies.
Acknowledgments
The authors would like to thank the following people for their contributions to the conception, design, or management of the case-control study: Nisha Alden, Andrea Buchwald, Nicole Comstock, Lauren Gunn-Sandell, Tye Harlow, Breanna Kawasaki, Emma Schmoll, RX Schwartz, Ginger Stringer, and Rachel K Herlihy. This project was supported by a financial assistance award from the Centers for Disease Control and Prevention and awarded to the Colorado Department of Public Health and Environment. The Colorado Department of Public Health and Environment contracted with EJSW at the Colorado School of Public Health. The content is solely the responsibility of the authors and does not necessarily reflect the official views of the Centers for Disease Control and Prevention or the Colorado Department of Public Health and Environment.
Data Availability
The data sets generated and analyzed during this study are not publicly available due to Colorado state statutes and regulations, which limit data release based on maintaining confidentiality for potentially identifiable person-level data, but are available from the Colorado Department of Public Health and Environment upon reasonable request.
Authors' Contributions
ADT contributed to the study’s conception, reviewed current research, performed statistical analyses, interpreted results, and was the primary author of the manuscript. AEW, MRT, and EJSW made significant contributions to the study’s conception and interpretation of results and critically revised the manuscript. RHJ and BAA substantively reviewed and revised the manuscript for its content. All authors have read and agreed to the published version of the manuscript.
Conflicts of Interest
None declared.
Colorado COVID-19 case-control survey questionnaires.
- Schulz KF, Grimes DA. Case-control studies: research in reverse. Lancet. 2002;359(9304):431-434. [ CrossRef ] [ Medline ]
- Mook P, McCormick J, Kanagarajah S, Adak GK, Cleary P, Elson R, et al. Online market research panel members as controls in case-control studies to investigate gastrointestinal disease outbreaks: early experiences and lessons learnt from the UK. Epidemiol Infect. 2018;146(4):458-464. [ FREE Full text ] [ CrossRef ] [ Medline ]
- Waldram A, McKerr C, Gobin M, Adak G, Stuart JM, Cleary P. Control selection methods in recent case-control studies conducted as part of infectious disease outbreaks. Eur J Epidemiol. 2015;30(6):465-471. [ CrossRef ] [ Medline ]
- Aigner A, Grittner U, Becher H. Bias due to differential participation in case-control studies and review of available approaches for adjustment. PLoS One. 2018;13(1):e0191327. [ FREE Full text ] [ CrossRef ] [ Medline ]
- Czajka JL, Beyler A. Declining response rates in federal sureys: trends and implication. Mathematica Policy Research. 2016. URL: https://tinyurl.com/ycyte42a [accessed 2023-02-27]
- McClain C. Most Americans don't answer cellphone calls from unknown numbers. Pew Research Center. 2020. URL: https://tinyurl.com/3her7dc5 [accessed 2023-02-27]
- Yahata Y, Ohshima N, Odaira F, Nakamura N, Ichikawa H, Matsuno K, et al. Web survey-based selection of controls for epidemiological analyses of a multi-prefectural outbreak of enterohaemorrhagic Escherichia coli O157 in Japan associated with consumption of self-grilled beef hanging tender. Epidemiol Infect. 2018;146(4):450-457. [ FREE Full text ] [ CrossRef ] [ Medline ]
- Ghosh TS, Patnaik JL, Alden NB, Vogt RL. Internet- versus telephone-based local outbreak investigations. Emerg Infect Dis. 2008;14(6):975-977. [ FREE Full text ] [ CrossRef ] [ Medline ]
- Yasmin S, Pogreba-Brown K, Stewart J, Sunenshine R. Use of an online survey during an outbreak of Clostridium perfringens in a retirement community—Arizona, 2012. J Public Health Manag Pract. 2014;20(2):205-209. [ CrossRef ] [ Medline ]
- Srikantiah P, Bodager D, Toth B, Kass-Hout T, Hammond R, Stenzel S, et al. Web-based investigation of multistate salmonellosis outbreak. Emerg Infect Dis. 2005;11(4):610-612. [ FREE Full text ] [ CrossRef ] [ Medline ]
- Taylor M, Galanis E. Online population control surveys: a new method for investigating foodborne outbreaks. Epidemiol Infect. 2020;148:e93. [ FREE Full text ] [ CrossRef ] [ Medline ]
- Mauz E, Hoffmann R, Houben R, Krause L, Kamtsiuris P, Gößwald A. Mode equivalence of health indicators between data collection modes and mixed-mode survey designs in population-based health interview surveys for children and adolescents: methodological study. J Med Internet Res. 2018;20(3):e64. [ FREE Full text ] [ CrossRef ] [ Medline ]
- Fan W, Yan Z. Factors affecting response rates of the web survey: a systematic review. Comput Hum Behav. 2010;26(2):132-139. [ CrossRef ]
- Hollier LP, Pettigrew S, Slevin T, Strickland M, Minto C. Comparing online and telephone survey results in the context of a skin cancer prevention campaign evaluation. J Public Health (Oxf). 2017;39(1):193-201. [ FREE Full text ] [ CrossRef ] [ Medline ]
- de Leeuw ED. To mix or not to mix data collection modes in surveys. J Off Stat. 2005;21(2):233-255. [ FREE Full text ]
- Braekman E, Drieskens S, Charafeddine R, Demarest S, Berete F, Gisle L, et al. Mixing mixed-mode designs in a national health interview survey: a pilot study to assess the impact on the self-administered questionnaire non-response. BMC Med Res Methodol. 2019;19(1):212. [ FREE Full text ] [ CrossRef ] [ Medline ]
- Rivara FP, Koepsell TD, Wang J, Durbin D, Jaffe KM, Vavilala M, et al. Comparison of telephone with world wide web-based responses by parents and teens to a follow-up survey after injury. Health Serv Res. 2011;46(3):964-981. [ FREE Full text ] [ CrossRef ] [ Medline ]
- Bowling A. Mode of questionnaire administration can have serious effects on data quality. J Public Health (Oxf). 2005;27(3):281-291. [ FREE Full text ] [ CrossRef ] [ Medline ]
- Greene J, Speizer H, Wiitala W. Telephone and web: mixed-mode challenge. Health Serv Res. 2008;43(1 Pt 1):230-248. [ FREE Full text ] [ CrossRef ] [ Medline ]
- Jones MK, Calzavara L, Allman D, Worthington CA, Tyndall M, Iveniuk J. A comparison of web and telephone responses from a national HIV and AIDS survey. JMIR Public Health Surveill. 2016;2(2):e37. [ FREE Full text ] [ CrossRef ] [ Medline ]
- White AE, Tran AD, Torok MR, Jervis RH, Albanese BA, Buchwald AG, et al. Community exposures among Colorado adults who tested positive for SARS-CoV-2—a case-control study, March-December 2021. PLoS One. 2023;18(3):e0282422. [ FREE Full text ] [ CrossRef ] [ Medline ]
- Harris PA, Taylor R, Thielke R, Payne J, Gonzalez N, Conde JG. Research Electronic Data Capture (REDCap)—a metadata-driven methodology and workflow process for providing translational research informatics support. J Biomed Inform. 2009;42(2):377-381. [ FREE Full text ] [ CrossRef ] [ Medline ]
- Andrejko KL, Pry JM, Myers JF, Fukui N, DeGuzman JL, Openshaw J, et al. Effectiveness of face mask or respirator use in indoor public settings for prevention of SARS-CoV-2 infection—California, February-December 2021. MMWR Morb Mortal Wkly Rep. 2022;71(6):212-216. [ FREE Full text ] [ CrossRef ] [ Medline ]
- Andrejko KL, Pry J, Myers JF, Jewell NP, Openshaw J, Watt J, et al. Prevention of coronavirus disease 2019 (COVID-19) by mRNA-based vaccines within the general population of California. Clin Infect Dis. 2022;74(8):1382-1389. [ FREE Full text ] [ CrossRef ] [ Medline ]
- Fisher KA, Tenforde MW, Feldstein LR, Lindsell CJ, Shapiro NI, Files DC, et al. Community and close contact exposures associated with COVID-19 among symptomatic adults ≥18 years in 11 outpatient health care facilities—United States, July 2020. MMWR Morb Mortal Wkly Rep. 2020;69(36):1258-1264. [ FREE Full text ] [ CrossRef ] [ Medline ]
- Link MW, Mokdad AH. Alternative modes for health surveillance surveys: an experiment with web, mail, and telephone. Epidemiology. 2005;16(5):701-704. [ FREE Full text ] [ CrossRef ] [ Medline ]
- Roster CA, Rogers RD, Albaum G, Klein D. A comparison of response characteristics from web and telephone surveys. Int J Mark Res. 2018;46(3):359-373. [ CrossRef ]
Abbreviations
Edited by A Mavragani; submitted 19.01.24; peer-reviewed by M Couper, J Ziegenfuss; comments to author 13.02.24; revised version received 29.03.24; accepted 04.04.24; published 27.05.24.
©Amanda D Tran, Alice E White, Michelle R Torok, Rachel H Jervis, Bernadette A Albanese, Elaine J Scallan Walter. Originally published in JMIR Formative Research (https://formative.jmir.org), 27.05.2024.
This is an open-access article distributed under the terms of the Creative Commons Attribution License (https://creativecommons.org/licenses/by/4.0/), which permits unrestricted use, distribution, and reproduction in any medium, provided the original work, first published in JMIR Formative Research, is properly cited. The complete bibliographic information, a link to the original publication on https://formative.jmir.org, as well as this copyright and license information must be included.
- Open access
- Published: 27 May 2024
Youth not engaged in education, employment, or training: a discrete choice experiment of service preferences in Canada
- Meaghen Quinlan-Davidson 1 , 2 ,
- Mahalia Dixon 1 ,
- Gina Chinnery 3 ,
- Lisa D. Hawke 1 , 4 ,
- Srividya Iyer 5 , 6 ,
- Katherine Moxness 7 ,
- Matthew Prebeg 1 , 8 ,
- Lehana Thabane 9 , 10 , 11 &
- J. L. Henderson 1 , 4
BMC Public Health volume 24 , Article number: 1402 ( 2024 ) Cite this article
158 Accesses
1 Altmetric
Metrics details
Prior research has showed the importance of providing integrated support services to prevent and reduce youth not in education, employment, or training (NEET) related challenges. There is limited evidence on NEET youth’s perspectives and preferences for employment, education, and training services. The objective of this study was to identify employment, education and training service preferences of NEET youth. We acknowledge the deficit-based lens associated with the term NEET and use ‘upcoming youth’ to refer to this population group.
Canadian youth (14–29 years) who reported Upcoming status or at-risk of Upcoming status were recruited to the study. We used a discrete choice experiment (DCE) survey, which included ten attributes with three levels each indicating service characteristics. Sawtooth software was used to design and administer the DCE. Participants also provided demographic information and completed the Global Appraisal of Individual Needs–Short Screener. We analyzed the data using hierarchical Bayesian methods to determine service attribute importance and latent class analyses to identify groups of participants with similar service preferences.
A total of n =503 youth participated in the study. 51% of participants were 24–29 years of age; 18.7% identified as having Upcoming status; 41.1% were from rural areas; and 36.0% of youth stated that they met basic needs with a little left. Participants strongly preferred services that promoted life skills, mentorship, basic income, and securing a work or educational placement. Three latent classes were identified and included: (i) job and educational services (38.9%), or services that include career counseling and securing a work or educational placement; (ii) mental health and wellness services (34.9%), or services that offer support for mental health and wellness in the workplace and free mental health and substance use services; and (iii) holistic skills building services (26.1%), or services that endorsed skills for school and job success, and life skills.
Conclusions
This study identified employment, education, and training service preferences among Upcoming youth. The findings indicate a need to create a service model that supports holistic skills building, mental health and wellness, and long-term school and job opportunities.
Peer Review reports
Youth not in education, employment, or training (NEET) struggle to navigate school to work transitions and experience difficulties accessing jobs [ 1 ]. These youth are disconnected from school, have limited work experience [ 2 ], and experience a loss of economic, social, and human capital [ 3 ]. NEET status is associated with lower education, parental unemployment, low socioeconomic status, low self-confidence, more precarious housing, and young parenthood [ 4 , 5 , 6 , 7 , 8 ]. In Canada, the percentage of NEET youth (15–29 years) was estimated at 11% in 2022 [ 9 ]. Importantly, NEET status is not homogenous across the country, ranging from 36% in Nunavut, 20% in Northwest Territories, and 17% in Newfoundland and Labrador to 10% in Quebec, Prince Edward Island and British Columbia) [ 10 ]. Supporting and protecting these marginalized youth remains a challenge, particularly in light of the Coronavirus disease 2019 (COVID-19) pandemic, which adversely impacted the school to workforce transition for youth across the country [ 11 ]. Although the term NEET has been used to describe this population, it is considered stigmatizing and associated with a deficit-based lens[ 12 ]. As such, and in consultation with one of our youth team members, we refer to this population as ‘Upcoming youth’[ 13 ].
Upcoming status has gained attention across Canada in recent decades [ 14 ]. As an illustration of this focus, federal, provincial/territorial, and local programs exist to support Upcoming youth across the country [ 15 ]. Despite these efforts, evidence indicates program fragmentation, limited coordination across sectors and regions, and a lack of evaluation of these programs [ 16 ]. Further, these programs may be available to youth on a short-term basis and specific to youth who meet education, income, and age criteria [ 17 ]. There is a lack of knowledge of how to (re)engage Upcoming youth in general education and employment support services. Often the same limited outcomes are measured and reported (e.g., job attainment) with services focusing on these outcomes. At the same time, youth have not been asked what outcomes they prefer and accordingly what services they would like. Indeed, selective outcome reporting and lack of engagement of youth impairs the quality of evidence and contributes to research waste [ 18 ]. Given the heterogeneity of Upcoming status, this lack of evidence is particularly important for subgroups of youth (e.g., geographic location; socioeconomic status; mental health status) who face challenges in the school-to-work transition.
Prior global research has emphasized the importance of integrated, coordinated interventions that offer a range of support services (e.g., on-the-job, classroom-based, and social skills training) to prevent and reduce Upcoming status [ 19 , 20 , 21 , 22 , 23 ] [ 24 ]. Integrated youth service (IYS) models, which integrate education, employment, mental and physical health, substance use, peer support, and navigation in one, youth-friendly location have been established in Canada [ 25 ]. IYS deliver services that meet the needs, goals, and preferences of youth, and hold promise in serving vulnerable Upcoming youth through the provision of holistic services in a youth-friendly environment. Indeed, IYS models are investigating how to optimize employment, education, and training services as a critical component of supporting youth wellbeing and their successful transition to adulthood. This point is particularly important as Upcoming youth experience greater mental health and substance use (MHSU) concerns compared to youth who do not identify as Upcoming [ 26 , 27 ].
An essential component to designing and enhancing health and social services for Upcoming youth is understanding their perspectives [ 28 ]. Yet, there is a lack of evidence on Upcoming youth’s perspectives and preferences for employment, education, and training services within the Canadian context. For interventions to be relevant to the needs and experiences of youth—which will increase their chances of using the services and benefiting from them—it is important to understand what youth aim to achieve when participating in an intervention. Engaging youth in identifying service components and interventions will ensure that programs and services are relevant, feasible, and appropriate to this population group [ 29 ].
An approach that can be used to identify the demands and preferences of youth is the discrete choice experiment (DCE) [ 30 , 31 ]. The DCE is a quantitative method that requires participants to state their choice over sets of alternatives described in terms of several characteristics called attributes and the value placed on each attribute [ 30 , 31 ]. In this way, the DCE is able to identify the importance of attributes along which a variety of service options vary, as well as service preferences among subgroups. DCEs are one of the most popular methods for eliciting stated- preferences in health care [ 32 , 33 ]. They force participants to make trade-offs, identifying the importance of different service attributes [ 32 , 33 ]. Previous findings generated from DCE studies have been useful in informing service design and delivery, resource allocation, and policies, including the preferred design of IYS services [ 34 , 35 , 36 , 37 ].
Understanding service preferences from the perspective of Upcoming youth is critical for the development of interventions and policies that will help youth navigate the school-to-work transition. As such, the objective of the current study was to identify employment, education and training service preferences of Upcoming youth. As our approach to COVID-19-related impacts shifts, the need for this research is more urgent than ever, as a way to support vulnerable youth, reduce Upcoming status, prevent further exclusion, and help them on their path towards adulthood.
Discrete Choice Experiment (DCE)
A discrete choice experiment (DCE) methodology was used in this study, as described in the study protocol [ 13 ]. We followed the International Society for Pharmacoeconomics and Outcomes Research (ISPOR) guidelines on good research practices for conjoint analysis [ 38 ]. Attributes and levels were developed using the following methods. First, we reviewed the literature on relevant and preferred services for youth with Upcoming and at-risk Upcoming status [ 26 ]. An initial set of six attributes with three to four levels was developed from the literature review, highlighting components such as mental health, goals, and skills training. Second, focus groups were conducted among youth (16–29 years) with Upcoming and at-risk Upcoming status across Canada to obtain youth feedback on proposed service outcomes [ 39 ]. Thematic analysis [ 40 ] of the focus group data identified prominent attributes and levels, including skills training, mentorship, and networking. The project team included youth team members with lived/living experience of MHSU concerns and researchers; meetings were held with the team to refine the attributes and levels.
The list of attributes and levels were piloted among n = 9 youth (16–29 years) across Canada. Pilot participants completed the DCE with a member of the project team. The aim of the pilot was to obtain youth feedback on the proposed list of attributes and levels, as well as the design and functionality of the DCE. Based on pilot feedback, a final list of attributes and levels was developed. The final DCE list included ten attributes, each with three levels. The attributes included mentorship; skills for school and job success; technical skills; life skills; basic income; networking opportunities; securing a work or educational placement; career counselling; access to free mental health and substance use services; and support for mental health and wellness in the workplace. Using a 3 × 3 partial-profile design, we used Sawtooth software (version 9.14.2) [ 41 ] to administer the 14 randomized choice tasks. This design was chosen to optimize orthogonality, minimize participant burden, and ensure data robustness [ 42 ]. Table 1 shows a sample choice task; Additional File 1 contains the full list of attributes and levels in the study.
Participants and procedure
The study was approved by the Centre for Addiction and Mental Health’s (CAMH) Research Ethics Board in Toronto, Canada. This study consisted of n = 503 youth (14–29 years), recruited over a three-month period in late 2022 and early 2023. The sample size was based on a priori power calculations and exceeds the sample size of most DCE studies [ 13 ]. Study flyers with survey links were distributed through internal CAMH and external professional networks, as well as through social media (Facebook and Instagram).
Participants were eligible to complete the DCE if they were between the ages of 14 and 29 years; lived in Canada at the time of survey completion; and identified as Upcoming status or having ever been concerned of being at-risk of Upcoming status (self-identified). They were screened through an online survey sent via email, hosted on REDCap electronic software [ 43 ]. Participants gave informed consent and filled out anti-spam and eligibility questions. Those who were eligible were sent a link to complete the DCE through Sawtooth Software [ 41 ]. The survey was in English only. They also filled out self-report questionnaires on demographics, and mental health and substance use. Reminder emails to complete the survey were sent to participants once per week, with a maximum of three reminders sent. A total of n = 515 participants initiated the survey and n = 503 completed the survey, yielding a response rate of 97.7%. The median time to complete the DCE was 20.63 min. Participants received a $30 gift card as honorarium for survey completion.
Mental health and substance use measures
Participants completed the Global Appraisal of Individual Needs–Short Screener (GAIN-SS) (version 3) [ 44 ]. Internalizing disorders (depression, anxiety, somatic complaints, trauma etc.); externalizing disorders (hyperactivity, conduct problems, attention deficits, impulsivity etc.); and substance use disorders are domain subscales that are screened in the GAIN-SS [ 44 ]. The GAIN-SS also includes a crime/violence domain, however, low level of endorsement in this study precluded the inclusion of this subscale. Participants rated each administered symptom “never” to “within the past month”, indicating how recently they experienced symptom difficulties. Within each domain subscale, endorsed past month symptoms were counted and summed. Scores could range between 0–6, 0–7, and 0–5 for the Internalizing, Externalizing, and Substance Use Problems domain, respectively. Following previous literature, three or more items endorsed within the past month indicate a high likelihood of needing services and/or meeting threshold criteria for psychiatric diagnoses [ 44 , 45 ].
Demographic characteristics were collected. We included age (categorical measure), gender identity (man/boy [cis, trans]; woman/girl [cis, trans]; Gender diverse); ethnicity (White; Indigenous, Black, Asian, Mixed); region in Canada (Prairies, Western/Northern, Atlantic, Central); self-rated physical and mental health (good/very good/excellent; fair/poor) [ 46 ]; socioeconomic status (live comfortably; income meets needs with a little left; just meet basic expenses; don’t meet basic expenses); living arrangement (alone; with partner; with family; other); and area of residence (large city and suburbs of large city; small city, town, village or rural area).
Youth engagement
Following the McCain Model of Youth Engagement [ 47 ], and working with the Youth Engagement Initiative at the Margaret and Wallace McCain Centre for Child, Youth & Family Mental Health, we engaged youth throughout the study. To enhance study design, promote youth buy-in, and relevance of the study, youth were involved from project inception and implementation of study activities to interpretation of findings and manuscript development.
Statistical analysis
Statistical analyses were performed using Sawtooth Software version 9.14.2 [ 41 ] and Stata version 16.1 [ 48 ]. Descriptive statistics were calculated for all study variables overall and by latent-class grouping. Using hierarchical Bayesian methods within Sawtooth Software [ 41 ], utility estimates were calculated for each participant. Standardized zero-centered utilities were used and the average utility range of attribute levels was set to 100 (49) to calculate the estimates. Attributes with higher utility estimates indicated higher relative value compared with other attributes (Table 2 ).
To identify groups of participants with similar service preferences, we conducted latent class analyses [ 41 ]. To belong to a latent class, probabilities were assigned to each participant. Using different starting seeds, five replications for each latent class group was calculated, with log-likelihood decreases of 0.01 or less indicating convergence. Based on the analysis, we retained a three-class model. This model was determined by analyzing the Bayesian Information Criteria (BIC), Akaike Information Criteria (AIC), Consistent Akaike Information Criterion (CAIC), and Akaike’s Bayesian Information Criterion (ABIC); latent class sizes; and the interpretation of latent class groupings (Table 3. ). Team discussions with youth team members were held to review the importance scores and rankings and establish the names of the latent glass groupings (Table 4 ). Stata 16.1 [ 48 ] was used to compare latent classes on demographic characteristics and GAIN-SS scores using chi-square tests.
Table 5 presents participant demographic characteristics. The majority of participants were between 24 and 29 years of age; lived in urban areas; were engaged in employment and training only; and identified as White and girl/woman (Trans, cis). Almost two thirds of participants (65.79%) met threshold criteria for an internalizing disorder, followed by 36.62% with an externalizing disorder, and 8.65% with a substance use disorder.
Overall service preferences
The overall service preferences and importance scores of participants are presented in Table 2 . Participants positively endorsed services that promoted life skills, mentorship, basic income, and securing a work or educational placement. Participants were least likely to endorse technical skills. Within life skills services, youth positively endorsed services that included managing finances, taxes, and skills associated with self-care and cooking. The provision of a mentor who worked within the participant’s field of interest was preferred by all youth. Participants positively endorsed basic income until having secured employment that matched the basic income level. All youth preferred services that provided support to secure long-term job positions or school placements that aligned with their career interests or long-term goals.
Table 3. illustrates the fit indices of the latent class analysis. A three-class model was retained based on fit, size of latent class grouping, and interpretation of findings. Attribute importance scores and rankings are presented in Table 4 by latent class. There were some commonalities identified across the latent classes. All latent classes positively endorsed services that offered mentorship (mentors in their field of interest, with similar backgrounds, or peer mentors), basic income, and networking. Youth preferred the provision of a mentor with work experience in their field of interest. Participants also positively endorsed receiving basic income until 25 years of age (regardless of school or job status), or until they had found a job that matched the basic income level. In addition, all participants endorsed skills to network and opportunities to network in their area of interest.
Over 60% of participants from all of the latent classes reported fair/poor mental health. In addition, over 60% of participants in each latent class grouping met threshold criteria for an internalizing disorder, compared to the other GAIN-SS disorders. Furthermore, more participants identified as having lived in large cities/suburbs compared to small cities/towns in each latent class.
Latent Class 1: Job and educational services
The first latent class endorsed services that focused on education and long-term job services, focusing on a career trajectory ( n = 204, 38.9%). Attributes that drove these decisions included career counselling and securing a work or educational placement. Youth positively endorsed career counselling that helped to figure out career goals, create a resume, and complete job applications. Further, youth positively endorsed receiving long-term job positions or school placements that align with their career interests and long-term goals, as opposed to temporary or any job position. Participants from this latent class (Table 6 ) were more likely to be 24–29 years of age compared to other ages. Approximately 22.06% of youth in this latent class identified as Upcoming.
Latent Class 2: Mental health and wellness services
The second latent class endorsed mental health and wellness services ( n = 171, 34.9%). This latent class preferred that services offer support for mental health and wellness in the workplace and free mental health and substance use services. Specifically, participants positively endorsed the provision of on-site in-person, individual mental health and substance use services, as opposed to the provision of virtual or in-person group services. Further, youth positively endorsed ongoing access to a support worker to help in securing accommodations in the workplace, as opposed to learning how to advocate for oneself in workplace or support during job onboarding. Participants that endorsed this latent class (Table 5 ) tended to identify as Indigenous, Black, Asian, and Mixed; girl/woman (cis, trans); both student and employed; income met needs with a little left; and rated their physical health as good/excellent. Approximately 16.96% of youth in this latent class identified as Upcoming.
Latent Class 3: Holistic skills building services
Skills building was the focus of the third latent class ( n = 128, 26.1%). Participants positively endorsed skills for school and job success, as well as life skills. Specifically, youth were interested in learning about how to organize time; prioritize tasks; identify problems and solutions; as well as professionalism, communication and relationship building. Youth were also interested in life skills that focused on learning about how to manage finances and taxes, as well as self-care and cooking. Participants in this latent class (Table 5 ) tended to identify as White; employed only; living in an urban area; and income that just met basic expenses. Approximately 15.63% of youth in this latent class identified as Upcoming.
To our knowledge, this study was the first to identify employment, education, and training service preferences among Upcoming youth and those at-risk of Upcoming status using discrete choice experiment methods. The findings indicate that overall, youth value services that enhance their ability to deal effectively with life demands; receive advice and guidance by a mentor; and obtain financial support through basic income. In examining youth participants by latent class, the findings indicate a need to create a service model that supports long-term school and job opportunities, holistic skills building, and mental health and wellness. Job and educational services prioritized long-term job and school placements, with career counselling. Mental health and wellness services endorsed free, easily accessible and in-person support services. Meanwhile, holistic skills building focused on problem solving, communication, relationship building, and organization of time, as well as building skills to help youth manage daily life.
Participants highly endorsed services that promote life skills, mentorship, and basic income. For life skills, participants valued skills that included managing finances, taxes, and skills like self-care or cooking. Participants may have valued this service attribute because life skills empower youth. These skills are positive behaviours that give youth the knowledge, values, attitudes, and abilities necessary to effectively meet and deal with everyday challenges [ 50 , 51 ]. Prior research has shown how these skills strengthen psychosocial competencies, promote health and social relationships, and protect against risk-taking behaviours [ 51 ].
For mentorship, participants valued having a mentor who has work experience in the field they are interested in. Prior research has shown the negative associations between unemployment, exclusion, and economic hardship among Upcoming youth [ 52 , 53 ]. Participants may have chosen this service attribute as mentoring is a key component of career development. Career mentoring provides opportunities for career exploration and strengthening decision-making within this domain [ 54 , 55 , 56 , 57 ]. Research has shown the benefits of mentorship. Mentors are a positive resource, providing support and guiding youth as they navigate and succeed in their careers [ 54 , 58 , 59 ].
Youth also prioritized receiving basic income until they secured employment that matches the basic income level. Empirical evidence has shown associations between income and youth mental health outcomes [ 60 , 61 , 62 ]. Indeed, Johnson et al. [ 63 ] [ 63 ] posit that a universal basic income can positively affect health through behaviour, resources, and stress. Defined as income support to populations with minimal or no conditions [ 64 ], prior research has shown the benefits of a basic income plan in terms of poverty reduction, improvements in physical and mental health, economic growth, and human capital gains [ 65 , 66 , 67 , 68 , 69 ]. In a qualitative study in England [ 70 ], youth (14–24 years) reported that a universal basic income plan would improve their mental health through financial security, agency, greater equality, and improvements in relationships.
Differences in service preferences were observed among youth subgroups based on the identified latent classes. Youth who identify as Indigenous, Black, Asian, and Mixed prioritized mental health and wellness services compared to youth who identify as White. Previous literature has showed that Indigenous , Black, and racialized youth have experience longer wait times and poorer quality of mental health care compared to their White counterparts [ 71 , 72 , 73 ]. Prior literature has described how MHSU systems often do not consider or address the discrimination, systemic racism, economic marginalization, and intergenerational traumas that Indigenous, Black, and racialized populations experience within and outside of the service system [ 74 , 75 ]. These negative experiences adversely affect their access to and quality of MHSU care, leading to inequities in MHSU outcomes. To ensure that mental health and substance use services are culturally responsive, safe, effective, and available to Indigenous, Black, and racialized youth, services should incorporate their perspectives into service design and delivery. The finding that youth 24–29 years of age endorsed job and educational services focused on long term career planning could be attributed to being more advanced in thinking about their careers and a desire to find a career as opposed to a job [ 57 , 76 , 77 ]. It could also be due to older youth experiencing poorer labour market conditions [ 19 , 78 , 79 ]. To improve long-term job opportunities for youth, Canada’s labour standards need to be updated, ensuring protection and benefits to informal and non-standard youth workers (17).
A critical component for education, employment, and training services is raising awareness about these services and their benefits among youth. A 2019 survey among NEET youth (16–29 years) in Canada showed that 54% reported a hard time finding information on the labour market services, while 42% said the information available on these services was not easy to understand [ 80 ]. One way to address this issue is by delivering services to Upcoming youth at the local, community level. In fact, as IYS strengthen education, employment and training services, these community-based services can support youth by connecting them with local job opportunities. IYS can also work with other public, private and community organizations to change local, fragmented school and work policies [ 19 ]. Another way to address this issue would be to provide access to this information at an earlier age, as shown in a parliamentary enquiry in Victoria, Australia in which career management was recommended for incorporation into primary school curriculum[ 81 ].
All three latent classes preferred services that provided mentorship, basic income, and networking opportunities. Youth value mentorship opportunities from individuals with experience in their field of interest. Similarly, youth prioritized networking opportunities in their field of interest. Federal, provincial/territorial and local programs could harness this preference by creating mentorship and networking structures across public, private, and community organizations for youth [ 17 ]. Further, the provision of basic income would help support youth as they re-engage with school and the labour market [ 17 ]. Interestingly, technical skills were not endorsed by youth in this study. Although technical skills are endorsed as part of technical and vocational education and training programs [ 82 ], it may be that youth were not as concerned about enhancing technical skills as they were other services. Future research should investigate youth experiences of technical skill programs.
It is important to note that participants in all latent classes endorsed poor mental health, while a higher proportion of youth screened positive for internalizing disorders compared to other disorders. These findings are in line with prior literature, particularly in light of the COVID-19 pandemic [ 83 , 84 , 85 , 86 , 87 , 88 , 89 , 90 , 91 ]. It could also reflect the positionality of the researchers. The survey was administered by CAMH, a mental health teaching hospital, and could have been seen more among youth connected with mental health services compared to those not connected to CAMH. Prior research has showed that life skills training can promote positive development, mental wellbeing, and prevent risky behaviours [ 92 , 93 ]. The prioritization of long-term school and job placements among youth with mental health concerns indicates a need to strengthen these services for this cohort.
In fact, in 2020 the Individual Placement and Support (IPS) model [ 94 , 95 ], which provides mental health service users with personalized vocational support alongside mental health support to obtain employment, education, and training opportunities was launched in Alberta, British Columbia, Nova Scotia, Ontario and Quebec to strengthen existing IYS, including ACCESS Open Minds, Foundry, and Youth Wellness Hubs Ontario [ 96 ]. The program was implemented in 12 hubs across the country and is currently being evaluated. Despite the challenges that have arisen over the course of the pandemic, COVID-19 has highlighted an opportunity to improve the education, employment and training support systems that serve these youth. Some of the core principles of implementation of the IPS model align with findings from the current study and include integration of mental health treatment teams, employment specialists to support young people as they navigate the labour market, rapid job search approaches, and tailored job supports, among other principles [ 94 ].
Indeed, in building on the services endorsed in this study, it would be important to incorporate an evaluation framework such as the Consolidated Framework for Implementation Research [ 97 ] to evaluate the effectiveness and impact of these services. Determining potential outcomes that could be measured would also be important. Following the IPS model, for job and educational placements, services could implement the Youth Employment and Education Survey [ 94 , 95 ]. Potential outcomes could include status of school or employment, job permanency, educational placement duration, and satisfaction with the program, among others. For mental health and wellness support services, outcomes could focus on the number of in-person visits, satisfaction with the services, and self-reported mental health, among others. For holistic services, potential outcomes could focus on reporting and monitoring self-reported goals for problem-solving and communication, among others. It would be important to continuously assess and match services to Upcoming youth preferences.
We would like to acknowledge some limitations. This study includes a non-randomized sample of youth across Canada. Our study included less than 20% of youth who identified as Upcoming, which limits our ability to generalize the findings to this population group. Further research is needed among youth who identify as Upcoming to determine if these education, employment, and training services represent their preferences. Further, youth without stable and consistent internet access would also have been missed. We were unable to recruit large populations of youth from specific Indigenous and racialized backgrounds, although these did account for nearly half the sample. These groups may have different needs and preferences. Future research should investigate their perspectives on employment, education, and training services. In addition to the structure of the DCE survey, along with following a rigorous process in the development of the attributes and levels, there could have been some youth service priorities not assessed. Furthermore, as some of the attributes and levels built on each other, these commonalities could have influenced preference elicitation for specific service attributes. We tried to ensure that the survey was youth-friendly for all youth, however due to the cognitive capacity required to complete the survey, some youth with greater mental health and learning challenges may have been missed.
This study identified employment, education, and training service preferences among Upcoming youth and those at-risk of Upcoming status in Canada. The findings indicate a need at the federal, provincial/territorial, and local level to create a service model that supports school and job opportunities long-term; mental health and wellness; and building holistic skills. The model also requires community-based and youth-centred approaches in the design and delivery of these services. Our findings further support the need for widespread policy support for broader-spectrum IYS for Upcoming youth and those at-risk of Upcoming status.
Availability of data and materials
No datasets were generated or analysed during the current study.
Abbreviations
Akaike’s Bayesian Information Criterion
Akaike Information Criterion
Bayesian Information Criteria
Consistent Akaike Information Criterion
Centre for Addiction and Mental Health
Coronavirus disease 2019
Discrete Choice Experiment
Global Appraisal of Individual Needs Short Screener
Importance Scores
Individual Placement and Support model
International Society for Pharmacoeconomics and Outcomes Research
Integrated Youth Services
Mental health and substance use
Not in education, employment, or training
Standard Errors
Youth Wellness Hubs Ontario
Unit SE. Bridging the gap: New opportunities for 16–18 year olds not in education, employment or training. London: HMSO; 1999.
Google Scholar
Dea B, Glozier N, Purcell R, McGorry PD, Scott J, Feilds K-L, et al. A cross-sectional exploration of the clinical characteristics of disengaged (NEET) young people in primary mental healthcare. BMJ Open. 2014;4(12):e006378.
Article Google Scholar
Carcillo S. NEET youth in the aftermath of the crisis: Challenges and policies. Paris: OECD; 2015.
England PH. Local action on health inequalities: Reducing the number of young people not in employment, education or training. London: UCL Institute of Health Equity; 2014.
Dorsett R, Lucchino P. Explaining patterns in the school-to-work transition: an analysis using optimal matching. Adv Life Course Res. 2014;22:1–14.
Article PubMed Google Scholar
Henderson J, Hawke L, Chaim G. Not in employment, education or training: mental health, substance use, and disengagement in a multi-sectoral sample of service-seeking Canadian youth. Child Youth Serv Rev. 2017;75:138–45.
Benjet C, Hernández-Montoya D, Borges G, Méndez E, Medina-Mora ME, Aguilar-Gaxiola S. Youth who neither study nor work: mental health, education and employment. Salud Publica Mex. 2012;54(4):410–7.
Genda Y. Jobless youths and the NEET problem in Japan. SSJ. 2007;10:23–40.
Statistics Canada. 2023. Table 37–10–0196–01 Percentage of 15-to 29-year-olds in education and not in education by labour force status, highest level of education attained, age group and sex. https://doi.org/10.25318/3710019601-eng
Percentage of 15-to 29-year-olds in education and not in education by labour force status, highest level of education attained, age group and sex. Statistics Canada; 2023. Available from: https://www150.statcan.gc.ca/t1/tbl1/en/tv.action?pid=3710019601 .
Hanushek EA, Woessmann L. The economic impacts of learning losses. 2020.
Yates S, Payne M. Not so NEET? A critique of the use of ‘NEET’ in setting targets for interventions with young people. J Youth Stud. 2006;9(3):329–44.
Hawke LD, Hayes E, Iyer S, Killackey E, Chinnery G, Gariépy G, et al. Youth-oriented outcomes of education, employment and training interventions for upcoming youth: protocol for a discrete choice experiment. Early Interv Psychiatry. 2021;15(4):942–8.
Canada S. Education Indicators in Canada: an international perspective. Ottawa: Statistics Canada; 2009.
Mahboubi PHA. Lives put on hold: the impact of the COVID-19 pandemic on Canada’s Youth. Toronto: The C.D. Howe Institute; 2022.
Cukier W, Mo G, Karajovic S, Blanchette S, Hassannezhad Z, Elmi M, et al. Labour Market Implications for Racialized Youth. Toronto: The Diversity Institute & Future Skills Centre; 2023.
Government of Canada. 13 ways to modernize youth employment in Canada - strategies for a new world of work. Ottawa: Government of Canada; 2017.
Chalmers I, Glasziou P. Avoidable waste in the production and reporting of research evidence. Lancet. 2009;374(9683):86–9.
Paabort H, Flynn P, Beilmann M, Petrescu C. Policy responses to real world challenges associated with NEET youth: a scoping review. Front Sustain Cities. 2023;5:1154464.
Quintano C, Mazzocchi P, Rocca A. The determinants of Italian NEETs and the effects of the economic crisis. Genus. 2018;74(1):5.
Article PubMed PubMed Central Google Scholar
Ruesga S, Laxe F, Picatoste X. Sustainable development, poverty, and risk of exclusion for young people in the European Union: the case of NEETs. Sustainability. 2018;10:4708.
Robert S, Romanello L, Lesieur S, Kergoat V, Dutertre J, Ibanez G, et al. Effects of a systematically offered social and preventive medicine consultation on training and health attitudes of young people not in employment, education or training (NEETs): An interventional study in France. PLoS One. 2019;14(4):e0216226.
Article CAS PubMed PubMed Central Google Scholar
Haikkola L. Classed and gendered transitions in youth activation: the case of Finnish youth employment services. J Youth Stud. 2021;24(2):250–66. https://doi.org/10.1080/13676261.2020.1715358 .
Mawn L, Oliver EJ, Akhter N, Bambra CL, Torgerson C, Bridle C, et al. Are we failing young people not in employment, education or training (NEETs)? A systematic review and meta-analysis of re-engagement interventions. Syst Rev. 2017;6(1):16.
Research CIoH. IYS-Net aims to build a healthy future for Canada's youth. Ottawa: CIHR; 2023. Available from: https://cihr-irsc.gc.ca/e/53552.html .
Gariépy G, Danna SM, Hawke L, Henderson J, Iyer SN. The mental health of young people who are not in education, employment, or training: a systematic review and meta-analysis. Soc Psychiatry Psychiatr Epidemiol. 2022;57(6):1107–21.
Gariépy G, Iyer S. The mental health of young Canadians who are not working or in school. Can J Psychiatry. 2019;64(5):338–44.
Brownlie E, Chaim G, Heffernan O, Herzog T, Henderson J. Youth services system review: moving from knowledge gathering to implementation through collaboration, youth engagement, and exploring local community needs. Can J Commun Ment Health. 2017;36:1–17.
Darnay K, Hawke LD, Chaim G, Henderson J. INNOVATE Research: Youth Engagement Guidebook for Researchers. Toronto: Centre for Addiction and Mental Health; 2019.
Ryan M, Farrar S. Using conjoint analysis to elicit preferences for health care. BMJ. 2000;320(7248):1530–3.
Ryan M, Gerard K. Using discrete choice experiments to value health care programmes: current practice and future research reflections. Appl Health Econ Health Policy. 2003;2(1):55–64.
PubMed Google Scholar
Clark MD, Determann D, Petrou S, Moro D, de Bekker-Grob EW. Discrete choice experiments in health economics: a review of the literature. Pharmacoeconomics. 2014;32(9):883–902.
Janssen EM, Hauber AB, Bridges JFP. Conducting a discrete-choice experiment study following recommendations for good research practices: an application for eliciting patient preferences for diabetes treatments. Value Health. 2018;21(1):59–68.
Mangham LJ, Hanson K, McPake B. How to do (or not to do) … designing a discrete choice experiment for application in a low-income country. Health Policy Plan. 2009;24(2):151–8.
Hawke LD, Thabane L, Iyer SN, Jaouich A, Reaume-Zimmer P, Henderson J. Service providers endorse integrated services model for youth with mental health and substance use challenges: findings from a discrete choice experiment. BMC Health Serv Res. 2021;21(1):1035.
Hawke LD, Thabane L, Wilkins L, Mathias S, Iyer S, Henderson J. Don’t forget the caregivers! A discrete choice experiment examining caregiver views of integrated youth services. Patient. 2021;14(6):791–802.
Henderson J, Hawke LD, Iyer SN, Hayes E, Darnay K, Mathias S, et al. Youth perspectives on integrated youth services: a discrete choice conjoint experiment. Can J Psychiatry. 2022;67(7):524–33.
Bridges JF, Hauber AB, Marshall D, Lloyd A, Prosser LA, Regier DA, et al. Conjoint analysis applications in health–a checklist: a report of the ISPOR good research practices for conjoint analysis task force. Value Health. 2011;14(4):403–13.
Zhu N, Hawke LD, Prebeg M, Hayes E, Darnay K, Iyer SN, et al. Intervention outcome preferences for youth who are out of work and out of school: a qualitative study. BMC Psychol. 2022;10(1):180.
Braun V, Clarke V. Using thematic analysis in psychology. Qual Res Psychol. 2006;3(2):77–101.
Software S. Lighthouse Studio. Utah: Sawtooth Software; 2022.
Software S, The CBC. Advanced design module technical paper. Sequim: Sawtooth Software; 2008.
Harris PA, Taylor R, Thielke R, Payne J, Gonzalez N, Conde JG. Research electronic data capture (REDCap)–a metadata-driven methodology and workflow process for providing translational research informatics support. J Biomed Inform. 2009;42(2):377–81.
Dennis ML, Feeney T, Stevens LH. Global Appraisal of Individual Needs-Short Screener (GAIN-SS): Administration and Scoring Manual for the GAINSS Version 2.0.1. Bloomington: Chestnut Health Systems; 2006.
Dennis ML, Chan YF, Funk RR. Development and validation of the GAIN Short Screener (GSS) for internalizing, externalizing and substance use disorders and crime/violence problems among adolescents and adults. Am J Addict. 2006;15(Suppl 1):80–91.
PubMed PubMed Central Google Scholar
Canada S. Table 13–10–0763–01 Health characteristics of children and youth aged 1 to 17 years, Canadian Health Survey on Children and Youth 2019. 2019.
Heffernan OS, Herzog TM, Schiralli JE, Hawke LD, Chaim G, Henderson JL. Implementation of a youth-adult partnership model in youth mental health systems research: challenges and successes. Health Expect. 2017;20(6):1183–8.
StataCorp. Stata statistical software: release 16. 16th ed. TX: College Station: StataCorp LLC; 2019.
Bk O. Getting started with conjoint analysis: strategies for product design and pricing research. Madison: Research Publishers; 2006.
Taute F. Life skills training as part of employee assistance programs in South Africa. J Work Behav Health. 2008;22(4):97–106.
Comprehensive life skills framework. rights based and life cycle approach to building skills for empowerment. New York: UNICEF; 2012.
Egdell V, Beck V. A capability approach to understand the scarring effects of unemployment and job insecurity: developing the research agenda. Work Employ Soc. 2020;34(5):937–48.
Mojsoska-Blazevski N, Petreski M, Bojadziev MI. Youth survival in the labour market: employment scarring in three transition economies. The Economic and Labour Relations Review. 2017;28(2):312–31.
Bakshi AJ, Joshi J. The Interface between Positive Youth Development and Youth Career Development: New Avenues for Career Guidance Practice. In: Arulmani G, Bakshi AJ, Leong FTL, Watts AG, editors. Handbook of Career Development: International Perspectives. New York: Springer New York; 2014. p. 173–201.
Chapter Google Scholar
Hamilton SF, Hamilton MA. Development in youth enterprises. New Dir Youth Dev. 2012;2012(134):65–75, 9.
Mekinda MA. Support for career development in youth: program models and evaluations. New Dir Youth Dev. 2012;2012(134):45–54, 8.
Hamilton S, Hamilton MA. School, work, and emerging adulthood. In: Arnett J, Tanner JL, editors. Emerging adults in America: Coming of age in the 21st century. Washington, DC: American Psychological Association; 2006. p. 257–77.
Halpern R. Supporting vocationally oriented learning in the high school years: rationale, tasks, challenges. New Dir Youth Dev. 2012;2012(134):85–106 ( 10 ).
Bimrose J, Brown A. Mid-career progression and development: the role for career guidance and counseling. 2014. p. 203–22.
Pitchforth J, Fahy K, Ford T, Wolpert M, Viner RM, Hargreaves DS. Mental health and well-being trends among children and young people in the UK, 1995–2014: analysis of repeated cross-sectional national health surveys. Psychol Med. 2019;49(8):1275–85.
Haula T, Vaalavuo M. Mental health problems in youth and later receipt of social assistance: do parental resources matter? J Youth Stud. 2022;25(7):877–96.
Landstedt E, Coffey J, Nygren M. Mental health in young Australians: a longitudinal study. J Youth Stud. 2015;19:1–13.
Johnson MT, Johnson EA, Nettle D, Pickett KE. Designing trials of universal basic income for health impact: identifying interdisciplinary questions to address. J Public Health. 2021;44(2):408–16.
Wispelaere J, Stirton L. The many faces of universal basic income. The Political Quarterly. 2004;75:266–74.
Fromm E. The Psychological Aspects of the Guaranteed Income. 1966.
Garfinkel I, Huang CC. The Effects of a Basic Income Guarantee on Poverty and Income Distribution. In: Ackerman B, Alstott A, van Parijs P, editors. Redesigning Distribution: Basic income and stakeholder grants as cornerstones for real egalitarian capitalism (Real Utopias Project). New York: Verso Books; 2003. p143-174
Van Parijs P. The universal basic income: why Utopian Thinking matters, and how sociologists can contribute to It*. Polit Soc. 2013;41(2):171–82.
Forget E, Marando D, Surman T, Urban MC. Pilot lessons: how to design a basic income pilot project for Ontario. Mowat Publication. 2016;123:1-30.
Gibson M, Hearty W, Craig P. The public health effects of interventions similar to basic income: a scoping review. The Lancet Public Health. 2020;5:e165–76.
Johnson EA, Webster H, Morrison J, Thorold R, Mathers A, Nettle D, et al. What role do young people believe Universal Basic Income can play in supporting their mental health? J Youth Stud. 2023;1-20. https://doi.org/10.1080/13676261.2023.2256236
Fante-Coleman T, Jackson-Best F. Barriers and facilitators to accessing mental healthcare in Canada for Black youth: a scoping review. Adolescent Research Review. 2020;5(2):115–36.
Chiu M, Amartey A, Wang X, Kurdyak P. Ethnic differences in mental health status and service utilization: a population-based study in Ontario. Canada Can J Psychiatry. 2018;63(7):481–91.
Gajaria A, Guzder J, Rasasingham R. What’s race got to do with it? A proposed framework to address racism’s impacts on child and adolescent mental health in Canada. J Can Acad Child Adolesc Psychiatry. 2021;30(2):131–7.
Smye V, Browne AJ, Josewski V, Keith B, Mussell W. Social suffering: indigenous peoples’ experiences of accessing mental health and substance use services. Int J Environ Res Public Health. 2023;20(4):3288.
Cénat JM, Kogan C, Noorishad PG, Hajizadeh S, Dalexis RD, Ndengeyingoma A, et al. Prevalence and correlates of depression among Black individuals in Canada: the major role of everyday racial discrimination. Depress Anxiety. 2021;38(9):886–95.
Arnett J. Emerging adulthood: The winding road from the late teens through the twenties. New York: Oxford University Press; 2004.
Messersmith EE, Garrett JL, Davis-Kean PE, Malanchuk O, Eccles JS. Career development from adolescence through emerging adulthood insights from information technology occupations. J Adolesc Res. 2008;23(2):206–27.
Bradley SMG, Paniagua MN. Spatial variations and clustering in the rates of youth unemployment and NEET. Lancaster: Lancaster University Management School, Economics Department; 2019.
Caroleo F, Rocca A, Mazzocchi P, Quintano C. Being NEET in Europe before and after the economic crisis: an analysis of the micro and macro determinants. Soc Indic Res. 2020;149:1–34.
The Labour Make Information Council. Finding Their Path: What Youth Not In Employment, Education or Training (NEET) Want. Ottawa: The Labour Make Information Council; 2019.
Hooley T. Career education in Primary School. Education Services Australia: Melbourne; 2021.
UNESCO. Strategy for Technical and Vocational Education and Training. Paris: UNESCO; 2016.
McMahon G, Douglas A, Casey K, Ahern E. Disruption to well-being activities and depressive symptoms during the COVID-19 pandemic: the mediational role of social connectedness and rumination. J Affect Disord. 2022;309:274–81.
McNamara L. School recess and pandemic recovery efforts: ensuring a climate that supports positive social connection and meaningful play. FACETS. 2021;6:1814–30.
Cardenas MC, Bustos SS, Chakraborty R. A “parallel pandemic”: the psychosocial burden of COVID-19 in children and adolescents. Acta Paediatr. 2020;109(11):2187–8.
Almeida ILL, Rego JF, Teixeira ACG, Moreira MR. Social isolation and its impact on child and adolescent development: a systematic review. Rev Paul Pediatr. 2021;40:e2020385.
Saurabh K, Ranjan S. Compliance and psychological impact of quarantine in children and adolescents due to covid-19 pandemic. Indian J Pediatr. 2020;87(7):532–6.
Racine N, McArthur BA, Cooke JE, Eirich R, Zhu J, Madigan S. Global Prevalence of depressive and anxiety symptoms in children and adolescents during COVID-19: a meta-analysis. JAMA Pediatr. 2021;175(11):1142–50.
Blackwell CK, Mansolf M, Sherlock P, Ganiban J, Hofheimer JA, Barone CJ, et al. Youth well-being during the COVID-19 pandemic. Pediatrics. 2022;149(4):e2021054754.
Ezeoke OM, Kanaley MK, Brown DA, Negris OR, Das R, Lombard LS, et al. The impact of COVID-19 on adolescent wellness in Chicago. Child Care Health Dev. 2022;48(6):886–90.
Tombeau Cost K, Crosbie J, Anagnostou E, Charach A, Monga S, Kelley E, et al. Mostly worse, occasionally better: impact of COVID-19 pandemic on the mental health of Canadian children and adolescents. Eur Child Adolesc Psychiatry. 2022;31:1–14.
Foxcroft DR, Tsertsvadze A. Universal school-based prevention programs for alcohol misuse in young people. Cochrane Database Syst Rev. 2011;5:Cd009113.
Botvin GJ, Baker E, Dusenbury L, Botvin EM, Diaz T. Long-term follow-up results of a randomized drug abuse prevention trial in a white middle-class population. JAMA. 1995;273(14):1106–12.
Article CAS PubMed Google Scholar
Bond GR. Principles of the individual placement and support model: empirical support. Psychiatr Rehabil J. 1998;22(1):11–23.
Rinaldi M, Perkins R, Glynn E, Montibeller T, Clenaghan M, Rutherford J. Individual placement and support: from research to practice. Adv Psychiatr Treat. 2008;14(1):50–60.
Ontario YWH. CAMH, YWHO, ACCESS Open Minds and Foundry launch first-of-its kind initiative to help young people with mental health challenges find employment: YWHO; 2021. Available from: https://youthhubs.ca/en/camh-ywho-access-open-minds-and-foundry-launch-first-of-its-kind-initiative-to-help-young-people-with-mental-health-challenges-find-employment/ .
Powell BJ, Proctor EK, Glass JE. A systematic review of strategies for implementing empirically supported mental health interventions. Res Soc Work Pract. 2014;24(2):192–212.
Download references
Acknowledgements
We would like to thank the participants for their participation in this study. We would like to thank members of the Centre for Addiction and Mental Health’s Youth Engagement Initiative for their support of this study.
This research was funded by the Social Sciences and Humanities Research Council (SSHRC) (435–2019-0393).
Author information
Authors and affiliations.
Centre for Addiction and Mental Health, 80 Workman Way, Toronto, ON, M6J 1H4, Canada
Meaghen Quinlan-Davidson, Mahalia Dixon, Lisa D. Hawke, Matthew Prebeg & J. L. Henderson
Dalla Lana School of Public Health, University of Toronto, Toronto, ON, Canada
Meaghen Quinlan-Davidson
Orygen, Parkville, VIC, Australia
Gina Chinnery
Department of Psychiatry, University of Toronto, Toronto, ON, Canada
Lisa D. Hawke & J. L. Henderson
Department of Psychiatry, McGill University, Montreal, QC, Canada
Srividya Iyer
Douglas Research Centre, Montreal, QC, Canada
Batshaw Youth and Family Centres, Montreal, QC, Canada
Katherine Moxness
Department of Psychiatry, The Hospital for Sick Children, Toronto, ON, Canada
Matthew Prebeg
Department of Health Research Methods, Evidence, and Impact, McMaster University, Hamilton, ON, Canada
Lehana Thabane
St Joseph’s Healthcare Hamilton, Hamilton, ON, Canada
Faculty of Health Sciences, University of Johannesburg, Johannesburg, South Africa
You can also search for this author in PubMed Google Scholar
Contributions
MQD contributed to designing the research question and conducted the analysis, interpretation of the data, and drafted the manuscript. All authors read and approved the final manuscript. JLH contributed to designing the research, oversaw the conduct of the study, interpreted the data, reviewed the manuscript and provided study leadership; JLH is the overall guarantor of the work.
Corresponding author
Correspondence to J. L. Henderson .
Ethics declarations
Ethics approval and consent to participate.
The study was approved by the Research Ethics Board of the Centre for Addiction and Mental Health (124/2019). Informed consent was obtained from all participants in this study.
Consent for publication
Consent was obtained directly from participants.
Competing interests
The authors declare no competing interests.
Additional information
Publisher’s note.
Springer Nature remains neutral with regard to jurisdictional claims in published maps and institutional affiliations.
Supplementary Information
Supplementary material 1. final list of discrete choice experiment attributes and levels., rights and permissions.
Open Access This article is licensed under a Creative Commons Attribution 4.0 International License, which permits use, sharing, adaptation, distribution and reproduction in any medium or format, as long as you give appropriate credit to the original author(s) and the source, provide a link to the Creative Commons licence, and indicate if changes were made. The images or other third party material in this article are included in the article's Creative Commons licence, unless indicated otherwise in a credit line to the material. If material is not included in the article's Creative Commons licence and your intended use is not permitted by statutory regulation or exceeds the permitted use, you will need to obtain permission directly from the copyright holder. To view a copy of this licence, visit http://creativecommons.org/licenses/by/4.0/ . The Creative Commons Public Domain Dedication waiver ( http://creativecommons.org/publicdomain/zero/1.0/ ) applies to the data made available in this article, unless otherwise stated in a credit line to the data.
Reprints and permissions
About this article
Cite this article.
Quinlan-Davidson, M., Dixon, M., Chinnery, G. et al. Youth not engaged in education, employment, or training: a discrete choice experiment of service preferences in Canada. BMC Public Health 24 , 1402 (2024). https://doi.org/10.1186/s12889-024-18877-0
Download citation
Received : 29 November 2023
Accepted : 17 May 2024
Published : 27 May 2024
DOI : https://doi.org/10.1186/s12889-024-18877-0
Share this article
Anyone you share the following link with will be able to read this content:
Sorry, a shareable link is not currently available for this article.
Provided by the Springer Nature SharedIt content-sharing initiative
- Youth mental health and substance use
- Youth not in education
- Or training
- Service preferences
- Discrete choice experiment
BMC Public Health
ISSN: 1471-2458
- General enquiries: [email protected]
2024 Theses Doctoral
Artificial Intelligence vs. Human Coaches: A Mixed Methods Randomized Controlled Experiment on Client Experiences and Outcomes
Barger, Amber
The rise of artificial intelligence (AI) challenges us to explore whether human-to-human relationships can extend to AI, potentially reshaping the future of coaching. The purpose of this study was to examine client perceptions of being coached by a simulated AI coach, who was embodied as a vocally conversational live-motion avatar, compared to client perceptions of a human coach. It explored if and how client ratings of coaching process measures and outcome measures aligned between the two coach treatments. In this mixed methods randomized controlled trial (RCT), 81 graduate students enrolled in the study and identified a personally relevant goal to pursue. The study deployed an alternative-treatments between-subjects design, with one-third of participants receiving coaching from simulated AI coaches, another third engaging with seasoned human coaches, and the rest forming the control group. Both treatment groups had one 60-minute session guided by the CLEAR (contract, listen, explore, action, review) coaching model to support each person to gain clarity about their goal and identify specific behaviors that could help each make progress towards their goal. Quantitative data were captured through three surveys and qualitative input was captured through open-ended survey questions and 27 debrief interviews. The study utilized a Wizard of Oz technique from human-computer interaction research, ingeniously designed to sidestep the rapid obsolescence of technology by simulating an advanced AI coaching experience where participants unknowingly interacted with professional human coaches, enabling the assessment of responses to AI coaching in the absence of fully developed autonomous AI systems. The aim was to glean insights into client reactions to a future, fully autonomous AI with the expert capabilities of a human coach. Contrary to expectations from previous literature, participants did not rate professional human coaches higher than simulated AI coaches in terms of working alliance, session value, or outcomes, which included self-rated competence and goal achievement. In fact, both coached groups made significant progress compared to the control group, with participants convincingly engaging with their respective coaches, as confirmed by a novel believability index. The findings challenge prevailing assumptions about human uniqueness in relation to technology. The rapid advancement of AI suggests a revolutionary shift in coaching, where AI could take on a central and surprisingly effective role, redefining what we thought only human coaches could do and reshaping their role in the age of AI.
- Adult education
- Artificial intelligence--Educational applications
- Graduate students
- Educational technology--Evaluation
- Education, Higher--Technological innovations
- Education, Higher--Effect of technological innovations on
This item is currently under embargo. It will be available starting 2029-05-14.
More About This Work
- DOI Copy DOI to clipboard
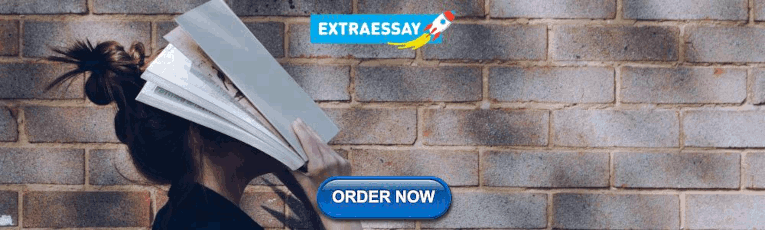
IMAGES
VIDEO
COMMENTS
Survey research means collecting information about a group of people by asking them questions and analyzing the results. To conduct an effective survey, follow these six steps: Determine who will participate in the survey. Decide the type of survey (mail, online, or in-person) Design the survey questions and layout.
Survey research is defined as "the collection of information from a sample of individuals through their responses to questions" ( Check & Schutt, 2012, p. 160 ). This type of research allows for a variety of methods to recruit participants, collect data, and utilize various methods of instrumentation. Survey research can use quantitative ...
Survey Research. Definition: Survey Research is a quantitative research method that involves collecting standardized data from a sample of individuals or groups through the use of structured questionnaires or interviews. The data collected is then analyzed statistically to identify patterns and relationships between variables, and to draw conclusions about the population being studied.
Survey research methods can be derived based on two critical factors: Survey research tool and time involved in conducting research. ... Survey research design. Researchers implement a survey research design in cases where there is a limited cost involved and there is a need to access details easily. This method is often used by small and large ...
Here, we cover a few: 1. They're relatively easy to do. Most research surveys are easy to set up, administer and analyze. As long as the planning and survey design is thorough and you target the right audience, the data collection is usually straightforward regardless of which survey type you use. 2.
Survey research means collecting information about a group of people by asking them questions and analysing the results. To conduct an effective survey, follow these six steps: Determine who will participate in the survey. Decide the type of survey (mail, online, or in-person) Design the survey questions and layout. Distribute the survey.
The survey is then constructed to test this model against observations of the phenomena. In contrast to survey research, a . survey. is simply a data collection tool for carrying out survey research. Pinsonneault and Kraemer (1993) defined a survey as a "means for gathering information about the characteristics, actions, or opinions of a ...
Step 2: Choose a type of research design. Step 3: Identify your population and sampling method. Step 4: Choose your data collection methods. Step 5: Plan your data collection procedures. Step 6: Decide on your data analysis strategies. Frequently asked questions. Introduction. Step 1. Step 2.
questionnaire design decisions can improve the quality of answers. 9.2. Open versus Closed Questions One of the first decisions a researcher must make when designing a survey question is whether to make it open (permitting respondents to answer in their own words) or closed (requiring respondents to select an answer from a set of choices ...
After settling on your research goal and beginning to design a questionnaire, the main considerations are the method of data collection, the survey instrument and the type of question you are going to ask. Methods of data collection include personal interviews, telephone, postal or electronic (Table 1).
Burns et al., 2008 12. A guide for the design and conduct of self-administered surveys of clinicians. This guide includes statements on designing, conducting, and reporting web- and non-web-based surveys of clinicians' knowledge, attitude, and practice. The statements are based on a literature review, but not the Delphi method.
Survey research is a research method involving the use of standardised questionnaires or interviews to collect data about people and their preferences, thoughts, and behaviours in a systematic manner. Although census surveys were conducted as early as Ancient Egypt, survey as a formal research method was pioneered in the 1930-40s by sociologist Paul Lazarsfeld to examine the effects of the ...
The survey research design is the use of a survey, administered either in written form or orally, to quan-tify, describe, or characterize an individual or a group. A survey is a series of questions or statements, called items, used in a questionnaire or an interview to mea-sure the self-reports or responses of respondents.
Descriptive research is the most common and conclusive form of survey research due to its quantitative nature. Unlike exploratory research methods, descriptive research utilizes pre-planned, structured surveys with closed-ended questions. It's also deductive, meaning that the survey structure and questions are determined beforehand based on existing theories or areas of inquiry.
The method of survey research is familiar and accessible, but this familiarity can obscure the careful decision-making required to design effective surveys. This guide identifies the key decisions involved with designing surveys for research purposes. Answering these questions will help to ensure that your survey collects the data you need.
Survey research is a quantitative and qualitative method with two important characteristics. First, the variables of interest are measured using self-reports. In essence, survey researchers ask their participants (who are often called respondents in survey research) to report directly on their own thoughts, feelings, and behaviours.
The experiment was conducted in the Detroit Metro Area Communities Study in 2021. We evaluated the adaptive design in five outcomes: 1) response rates, 2) demographic composition of respondents, 3) bias and variance of key survey estimates, 4) changes in significant results of regression models, and 5) costs.
Survey designs. Kerry Tanner, in Research Methods (Second Edition), 2018. Conclusion. Survey research designs remain pervasive in many fields. Surveys can appear deceptively simple and straightforward to implement. However valid results depend on the researcher having a clear understanding of the circumstances where their use is appropriate and the constraints on inference in interpreting and ...
Survey research design provides an unbiased representation of the population of interest (Owens, 2002). Survey research usually consists of methods of gathering data from usually a large number of ...
Chapter 3 -- Survey Research Design and Quantitative Methods of Analysis for Cross-sectional Data. ... Survey research is a method of collecting information by asking questions. Sometimes interviews are done face-to-face with people at home, in school, or at work. Other times questions are sent in the mail for people to answer and mail back.
While many books and articles guide various qualitative research methods and analyses, there is currently no concise resource that explains and differentiates among the most common qualitative approaches. We believe novice qualitative researchers, students planning the design of a qualitative study or taking an introductory qualitative research course, and faculty teaching such courses can ...
A Delphi survey is a structured research method used to gather the collective opinions and insights of a panel of experts on a particular topic. ... Born entrepreneur, passionate leader, motivator, great love for UI & UX design, strong believer in "less is more". Big advocate of bootstrapping. BS in Logistics Service Management. I don't ...
Types of Survey Methods. In the realm of data collection, choosing the right survey method is akin to selecting the perfect tool for a job. Each method has its own set of strengths and weaknesses, tailored to specific research needs. Let's delve deeper into each type, armed with examples to highlight their practical applications.
Background: Sequential mixed-mode surveys using both web-based surveys and telephone interviews are increasingly being used in observational studies and have been shown to have many benefits; however, the application of this survey design has not been evaluated in the context of epidemiological case-control studies. Objective: In this paper, we discuss the challenges, benefits, and limitations ...
A multi-method approach (i.e., multiple regression, relative importance analysis, and necessary condition analysis) was applied in a comparative contextualized research design (i.e., a three-study field survey research) involving 358 employees and 86 supervisors from an EU member country.
Prior research has showed the importance of providing integrated support services to prevent and reduce youth not in education, employment, or training (NEET) related challenges. There is limited evidence on NEET youth's perspectives and preferences for employment, education, and training services. The objective of this study was to identify employment, education and training service ...
In this mixed methods randomized controlled trial (RCT), 81 graduate students enrolled in the study and identified a personally relevant goal to pursue. The study deployed an alternative-treatments between-subjects design, with one-third of participants receiving coaching from simulated AI coaches, another third engaging with seasoned human ...
Regions densely populated with archaeological monuments pose significant challenges for construction investors and archaeologists during the planning stages of major construction projects. Recognising the archaeological potential of these areas is crucial for planning effective rescue excavations, which have become a standard procedure in construction. This study explores the utility of non ...