- En español – ExME
- Em português – EME
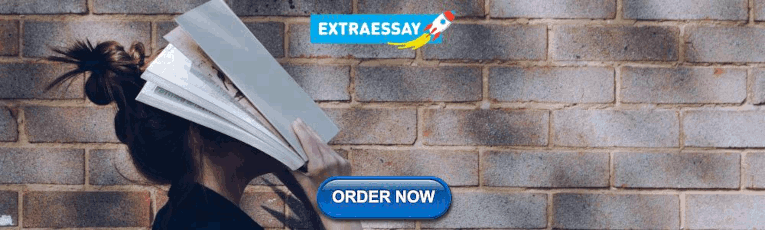
What are sampling methods and how do you choose the best one?
Posted on 18th November 2020 by Mohamed Khalifa
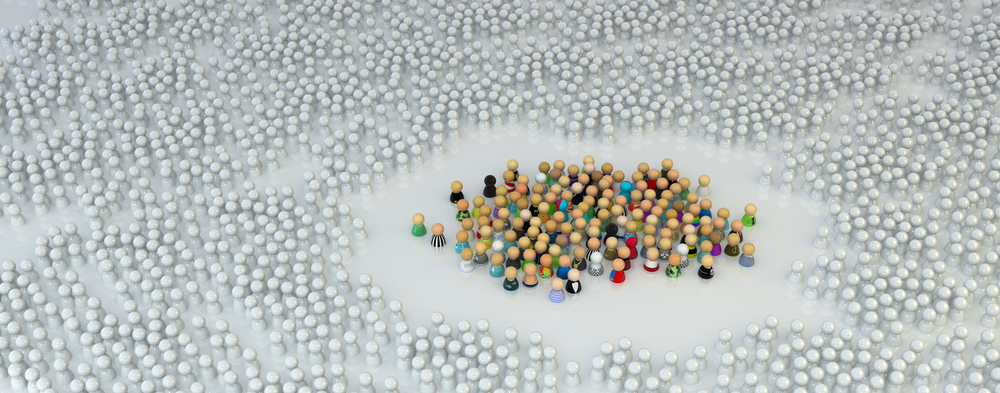
This tutorial will introduce sampling methods and potential sampling errors to avoid when conducting medical research.
Introduction to sampling methods
Examples of different sampling methods, choosing the best sampling method.
It is important to understand why we sample the population; for example, studies are built to investigate the relationships between risk factors and disease. In other words, we want to find out if this is a true association, while still aiming for the minimum risk for errors such as: chance, bias or confounding .
However, it would not be feasible to experiment on the whole population, we would need to take a good sample and aim to reduce the risk of having errors by proper sampling technique.
What is a sampling frame?
A sampling frame is a record of the target population containing all participants of interest. In other words, it is a list from which we can extract a sample.
What makes a good sample?
A good sample should be a representative subset of the population we are interested in studying, therefore, with each participant having equal chance of being randomly selected into the study.
We could choose a sampling method based on whether we want to account for sampling bias; a random sampling method is often preferred over a non-random method for this reason. Random sampling examples include: simple, systematic, stratified, and cluster sampling. Non-random sampling methods are liable to bias, and common examples include: convenience, purposive, snowballing, and quota sampling. For the purposes of this blog we will be focusing on random sampling methods .
Example: We want to conduct an experimental trial in a small population such as: employees in a company, or students in a college. We include everyone in a list and use a random number generator to select the participants
Advantages: Generalisable results possible, random sampling, the sampling frame is the whole population, every participant has an equal probability of being selected
Disadvantages: Less precise than stratified method, less representative than the systematic method
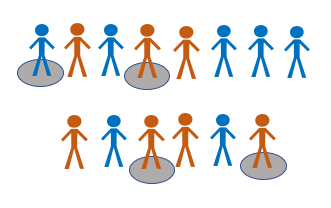
Example: Every nth patient entering the out-patient clinic is selected and included in our sample
Advantages: More feasible than simple or stratified methods, sampling frame is not always required
Disadvantages: Generalisability may decrease if baseline characteristics repeat across every nth participant
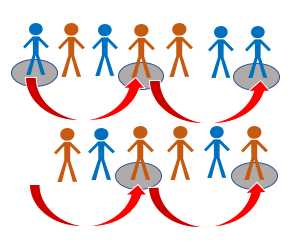
Example: We have a big population (a city) and we want to ensure representativeness of all groups with a pre-determined characteristic such as: age groups, ethnic origin, and gender
Advantages: Inclusive of strata (subgroups), reliable and generalisable results
Disadvantages: Does not work well with multiple variables
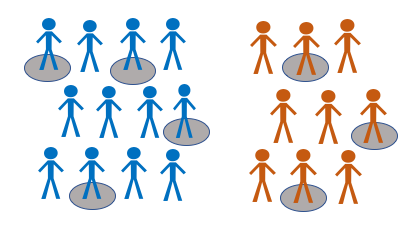
Example: 10 schools have the same number of students across the county. We can randomly select 3 out of 10 schools as our clusters
Advantages: Readily doable with most budgets, does not require a sampling frame
Disadvantages: Results may not be reliable nor generalisable
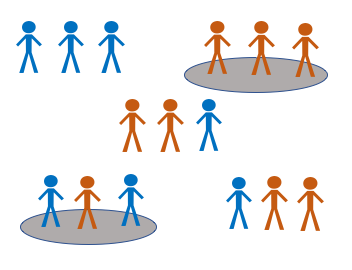
How can you identify sampling errors?
Non-random selection increases the probability of sampling (selection) bias if the sample does not represent the population we want to study. We could avoid this by random sampling and ensuring representativeness of our sample with regards to sample size.
An inadequate sample size decreases the confidence in our results as we may think there is no significant difference when actually there is. This type two error results from having a small sample size, or from participants dropping out of the sample.
In medical research of disease, if we select people with certain diseases while strictly excluding participants with other co-morbidities, we run the risk of diagnostic purity bias where important sub-groups of the population are not represented.
Furthermore, measurement bias may occur during re-collection of risk factors by participants (recall bias) or assessment of outcome where people who live longer are associated with treatment success, when in fact people who died were not included in the sample or data analysis (survivors bias).
By following the steps below we could choose the best sampling method for our study in an orderly fashion.
Research objectiveness
Firstly, a refined research question and goal would help us define our population of interest. If our calculated sample size is small then it would be easier to get a random sample. If, however, the sample size is large, then we should check if our budget and resources can handle a random sampling method.
Sampling frame availability
Secondly, we need to check for availability of a sampling frame (Simple), if not, could we make a list of our own (Stratified). If neither option is possible, we could still use other random sampling methods, for instance, systematic or cluster sampling.
Study design
Moreover, we could consider the prevalence of the topic (exposure or outcome) in the population, and what would be the suitable study design. In addition, checking if our target population is widely varied in its baseline characteristics. For example, a population with large ethnic subgroups could best be studied using a stratified sampling method.
Random sampling
Finally, the best sampling method is always the one that could best answer our research question while also allowing for others to make use of our results (generalisability of results). When we cannot afford a random sampling method, we can always choose from the non-random sampling methods.
To sum up, we now understand that choosing between random or non-random sampling methods is multifactorial. We might often be tempted to choose a convenience sample from the start, but that would not only decrease precision of our results, and would make us miss out on producing research that is more robust and reliable.
References (pdf)
Mohamed Khalifa
Leave a reply cancel reply.
Your email address will not be published. Required fields are marked *
Save my name, email, and website in this browser for the next time I comment.
No Comments on What are sampling methods and how do you choose the best one?
Thank you for this overview. A concise approach for research.
really helps! am an ecology student preparing to write my lab report for sampling.
I learned a lot to the given presentation.. It’s very comprehensive… Thanks for sharing…
Very informative and useful for my study. Thank you
Oversimplified info on sampling methods. Probabilistic of the sampling and sampling of samples by chance does rest solely on the random methods. Factors such as the random visits or presentation of the potential participants at clinics or sites could be sufficiently random in nature and should be used for the sake of efficiency and feasibility. Nevertheless, this approach has to be taken only after careful thoughts. Representativeness of the study samples have to be checked at the end or during reporting by comparing it to the published larger studies or register of some kind in/from the local population.
Thank you so much Mr.mohamed very useful and informative article
Subscribe to our newsletter
You will receive our monthly newsletter and free access to Trip Premium.
Related Articles
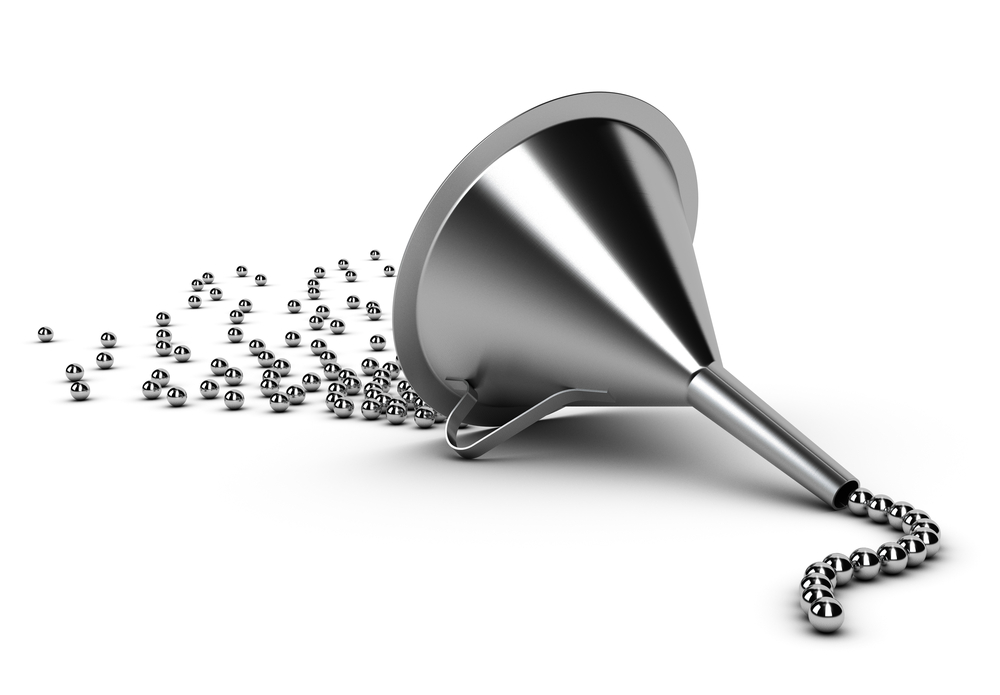
How to read a funnel plot
This blog introduces you to funnel plots, guiding you through how to read them and what may cause them to look asymmetrical.
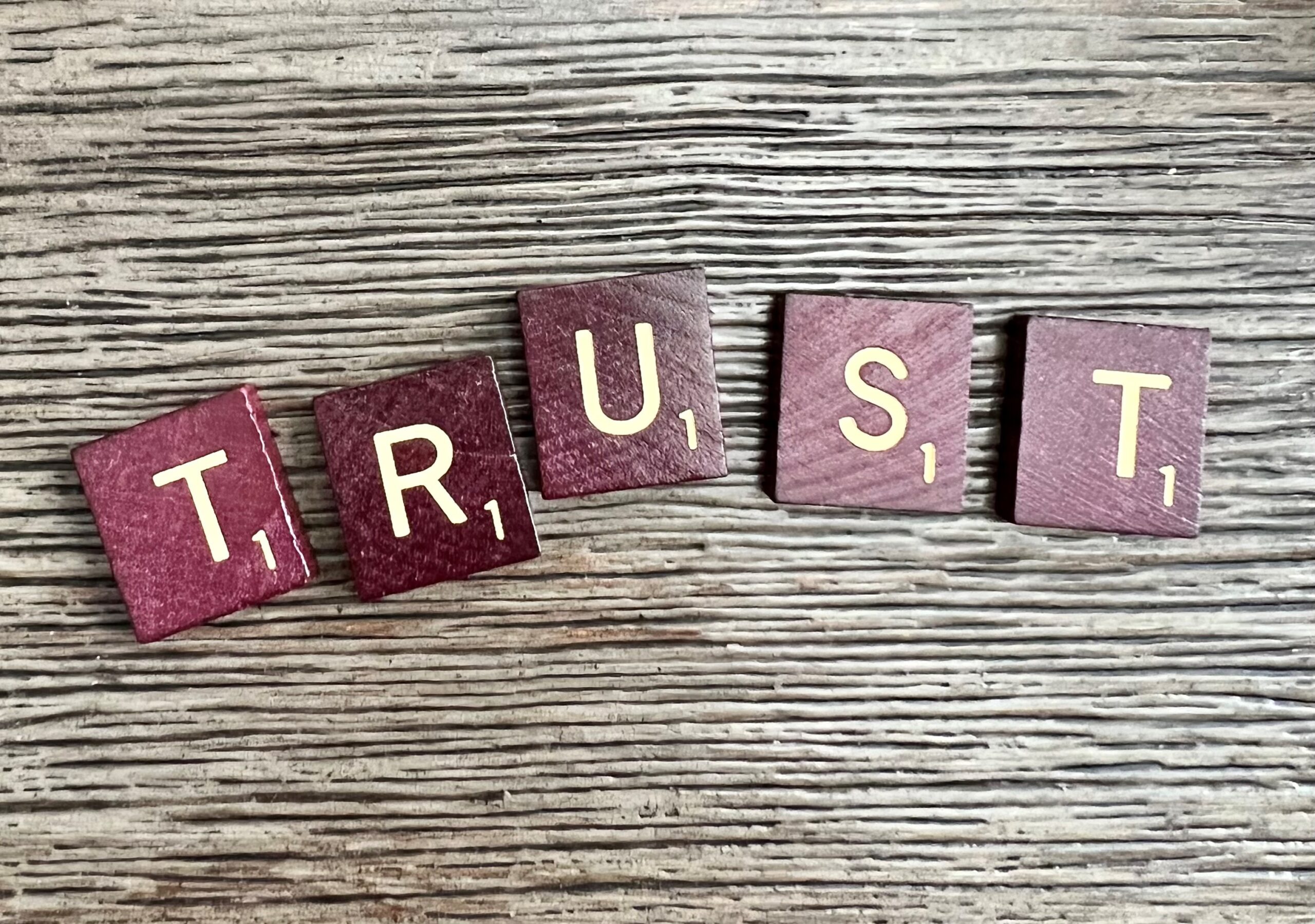
Internal and external validity: what are they and how do they differ?
Is this study valid? Can I trust this study’s methods and design? Can I apply the results of this study to other contexts? Learn more about internal and external validity in research to help you answer these questions when you next look at a paper.
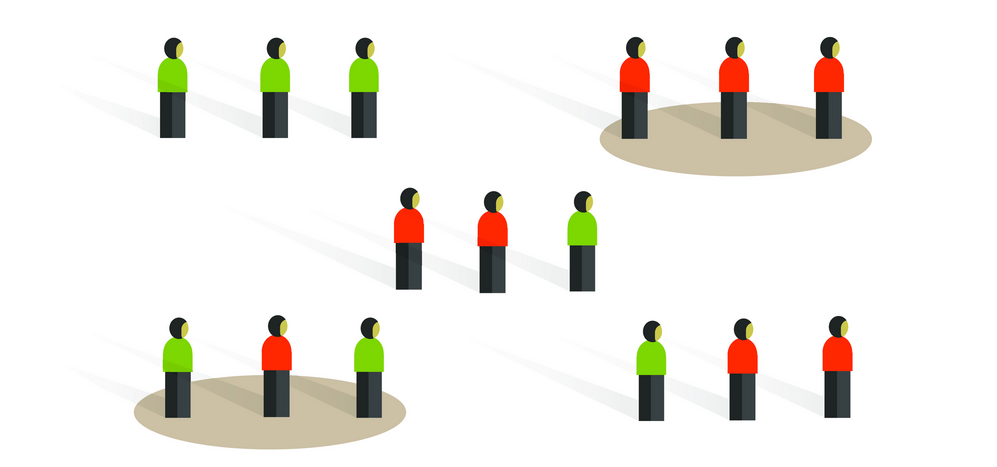
Cluster Randomized Trials: Concepts
This blog summarizes the concepts of cluster randomization, and the logistical and statistical considerations while designing a cluster randomized controlled trial.
- Utility Menu

GA4 Tracking Code

bok_logo_2-02_-_harvard_left.png
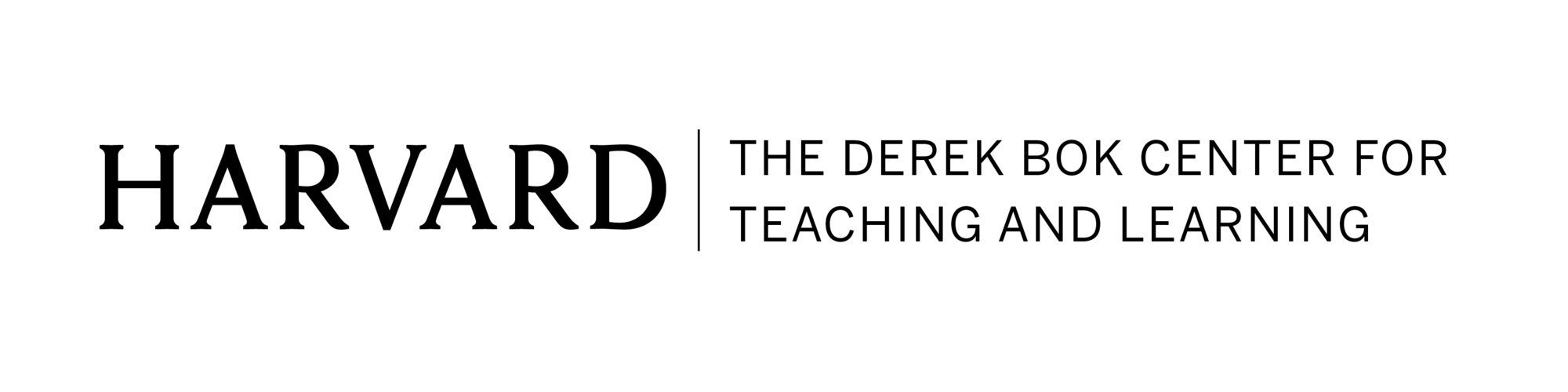
Statistical Sampling Case Study
To learn about sampling techniques in social science research, students practice tackling a real-world research problem through discussing a hypothetical case..
- To enable students to understand the benefits and drawbacks of various sampling techniques.
- To provide students with experience designing sampling methods to address a real-world research problem.
Class: Sociology 128: Models of Social Science Research
Introduction/Background: This course introduces sociology students to concepts and strategies in social science research. In week five, students learn about nonrandom and random sampling techniques (snowball sampling, simple random sampling, etc.). In discussion section later that week, students apply this knowledge to a hypothetical case study where a researcher aims to study the experiences of homeless people in the United States.
Before Class:
Students learned about the pros and cons of various sampling techniques in lecture.
During Class:
- In discussion section, students received a handout about various types of sampling techniques, as well as a hypothetical research scenario about researching a population of homeless people in New York City. The instructor and students reviewed the sampling techniques they had learned, including what types of social science research questions each technique would enable researchers to answer.
- Students were then broken up into groups of 2-3. Guided by four questions on the handout, students analyzed the research problem and discussed within their groups the pros and cons of various sampling techniques. The instructor moved between the groups and provided feedback as students were deciding how to answer each question.
- Once each group determined how they would approach the problem, they shared out their choice with the class. The class discussed the benefits and drawbacks to each group's choices.
Students left class with a deep understanding of a hypothetical research scenario and the various considerations they would have to take into account when deciding how to sample a population. They would later use this knowledge in developing their group projects at the end of the semester.
The research scenario prompt, attached
Submitted by Matthew Clair, Teaching Fellow, Harvard Department of Sociology
More activities like this
- Adaptive Scenario Exercise
- Complex Stats, Simple Tech
- Caminalcule Taxonomy Exercise
- The Minimal Group Paradigm
- How to Read a Sociology Article
Further Filter By
Activity type.
- Discussion (102) Apply Discussion filter
- Research (79) Apply Research filter
- Presentation (55) Apply Presentation filter
- Role Play (54) Apply Role Play filter
- Homework (44) Apply Homework filter
- Pair and Share (42) Apply Pair and Share filter
- Case Study (31) Apply Case Study filter
- Do Now (31) Apply Do Now filter
- Lab (30) Apply Lab filter
- Game (29) Apply Game filter
- Debate (24) Apply Debate filter
- Peer Instruction (23) Apply Peer Instruction filter
- Quick Write (23) Apply Quick Write filter
- Field Trip (21) Apply Field Trip filter
- Lecture (20) Apply Lecture filter
- Jigsaw (18) Apply Jigsaw filter
- Concept Map (14) Apply Concept Map filter
- Sequence Reconstruction (8) Apply Sequence Reconstruction filter
- Speed Dating (3) Apply Speed Dating filter
- Statement Correction (1) Apply Statement Correction filter
- General Education (44) Apply General Education filter
- Government (38) Apply Government filter
- Physics (20) Apply Physics filter
- English (13) Apply English filter
- Organismic and Evolutionary Biology (13) Apply Organismic and Evolutionary Biology filter
- Sociology (12) Apply Sociology filter
- Statistics (12) Apply Statistics filter
- Expository Writing (10) Apply Expository Writing filter
- Romance Languages and Literatures (10) Apply Romance Languages and Literatures filter
- Germanic Languages and Literatures (9) Apply Germanic Languages and Literatures filter
- History (9) Apply History filter
- Humanities (9) Apply Humanities filter
- Music (9) Apply Music filter
- Mathematics (8) Apply Mathematics filter
- Molecular and Cellular Biology (8) Apply Molecular and Cellular Biology filter
- Economics (7) Apply Economics filter
- Freshman Seminars (6) Apply Freshman Seminars filter
- Social Studies (6) Apply Social Studies filter
- Astronomy (5) Apply Astronomy filter
- Chemistry and Chemical Biology (5) Apply Chemistry and Chemical Biology filter
- Psychology (5) Apply Psychology filter
- Computer Science (4) Apply Computer Science filter
- East Asian Languages and Civilizations (4) Apply East Asian Languages and Civilizations filter
- History of Science (4) Apply History of Science filter
- Linguistics (4) Apply Linguistics filter
- Stem Cell and Regenerative Biology (4) Apply Stem Cell and Regenerative Biology filter
- Comparative Literature (3) Apply Comparative Literature filter
- History and Literature (3) Apply History and Literature filter
- African and African American Studies (2) Apply African and African American Studies filter
- Classics (2) Apply Classics filter
- Earth and Planetary Science (2) Apply Earth and Planetary Science filter
- Education (2) Apply Education filter
- History of Art and Architecture (2) Apply History of Art and Architecture filter
- Public Health (2) Apply Public Health filter
- Studies of Women, Gender, and Sexuality (2) Apply Studies of Women, Gender, and Sexuality filter
- Systems Biology (2) Apply Systems Biology filter
- Anthropology (1) Apply Anthropology filter
- Biomedical Engineering (1) Apply Biomedical Engineering filter
- Near Eastern Languages and Civilizations (1) Apply Near Eastern Languages and Civilizations filter
- Psychiatry (1) Apply Psychiatry filter
- Visual and Environmental Studies (1) Apply Visual and Environmental Studies filter
Learning Objective
- Collaborate (107) Apply Collaborate filter
- Develop Subject Specific Intuitions (101) Apply Develop Subject Specific Intuitions filter
- Make Real World Connections to Course Material (101) Apply Make Real World Connections to Course Material filter
- Interpret Primary Sources to Propose a Model or Argument (95) Apply Interpret Primary Sources to Propose a Model or Argument filter
- Learn Foundational Knowledge (82) Apply Learn Foundational Knowledge filter
- Develop Communication Skills (73) Apply Develop Communication Skills filter
- Defend a Position in a Model or Argument (55) Apply Defend a Position in a Model or Argument filter
- Evaluate and Critique a Model or Argument (45) Apply Evaluate and Critique a Model or Argument filter
- Reflect on the Learning Process (Metacognition) (44) Apply Reflect on the Learning Process (Metacognition) filter
- Compare the Strengths and Weaknesses of Different Methods (23) Apply Compare the Strengths and Weaknesses of Different Methods filter
Length of Activity
- Full Class (142) Apply Full Class filter
- 10 to 30 minutes (49) Apply 10 to 30 minutes filter
- Multiple Classes (31) Apply Multiple Classes filter
- up to 10 minutes (22) Apply up to 10 minutes filter
- Full Semester (19) Apply Full Semester filter

Sampling Methods & Strategies 101
Everything you need to know (including examples)
By: Derek Jansen (MBA) | Expert Reviewed By: Kerryn Warren (PhD) | January 2023
If you’re new to research, sooner or later you’re bound to wander into the intimidating world of sampling methods and strategies. If you find yourself on this page, chances are you’re feeling a little overwhelmed or confused. Fear not – in this post we’ll unpack sampling in straightforward language , along with loads of examples .
Overview: Sampling Methods & Strategies
- What is sampling in a research context?
- The two overarching approaches
Simple random sampling
Stratified random sampling, cluster sampling, systematic sampling, purposive sampling, convenience sampling, snowball sampling.
- How to choose the right sampling method
What (exactly) is sampling?
At the simplest level, sampling (within a research context) is the process of selecting a subset of participants from a larger group . For example, if your research involved assessing US consumers’ perceptions about a particular brand of laundry detergent, you wouldn’t be able to collect data from every single person that uses laundry detergent (good luck with that!) – but you could potentially collect data from a smaller subset of this group.
In technical terms, the larger group is referred to as the population , and the subset (the group you’ll actually engage with in your research) is called the sample . Put another way, you can look at the population as a full cake and the sample as a single slice of that cake. In an ideal world, you’d want your sample to be perfectly representative of the population, as that would allow you to generalise your findings to the entire population. In other words, you’d want to cut a perfect cross-sectional slice of cake, such that the slice reflects every layer of the cake in perfect proportion.
Achieving a truly representative sample is, unfortunately, a little trickier than slicing a cake, as there are many practical challenges and obstacles to achieving this in a real-world setting. Thankfully though, you don’t always need to have a perfectly representative sample – it all depends on the specific research aims of each study – so don’t stress yourself out about that just yet!
With the concept of sampling broadly defined, let’s look at the different approaches to sampling to get a better understanding of what it all looks like in practice.
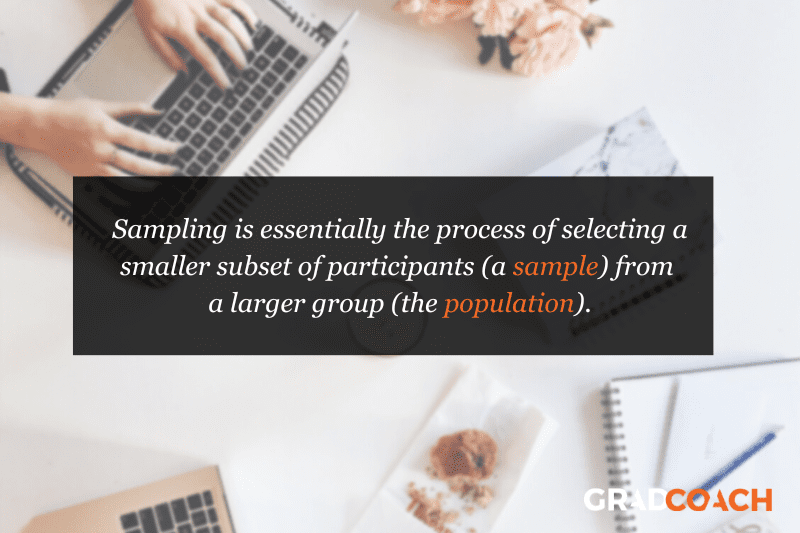
The two overarching sampling approaches
At the highest level, there are two approaches to sampling: probability sampling and non-probability sampling . Within each of these, there are a variety of sampling methods , which we’ll explore a little later.
Probability sampling involves selecting participants (or any unit of interest) on a statistically random basis , which is why it’s also called “random sampling”. In other words, the selection of each individual participant is based on a pre-determined process (not the discretion of the researcher). As a result, this approach achieves a random sample.
Probability-based sampling methods are most commonly used in quantitative research , especially when it’s important to achieve a representative sample that allows the researcher to generalise their findings.
Non-probability sampling , on the other hand, refers to sampling methods in which the selection of participants is not statistically random . In other words, the selection of individual participants is based on the discretion and judgment of the researcher, rather than on a pre-determined process.
Non-probability sampling methods are commonly used in qualitative research , where the richness and depth of the data are more important than the generalisability of the findings.
If that all sounds a little too conceptual and fluffy, don’t worry. Let’s take a look at some actual sampling methods to make it more tangible.
Need a helping hand?
Probability-based sampling methods
First, we’ll look at four common probability-based (random) sampling methods:
Importantly, this is not a comprehensive list of all the probability sampling methods – these are just four of the most common ones. So, if you’re interested in adopting a probability-based sampling approach, be sure to explore all the options.
Simple random sampling involves selecting participants in a completely random fashion , where each participant has an equal chance of being selected. Basically, this sampling method is the equivalent of pulling names out of a hat , except that you can do it digitally. For example, if you had a list of 500 people, you could use a random number generator to draw a list of 50 numbers (each number, reflecting a participant) and then use that dataset as your sample.
Thanks to its simplicity, simple random sampling is easy to implement , and as a consequence, is typically quite cheap and efficient . Given that the selection process is completely random, the results can be generalised fairly reliably. However, this also means it can hide the impact of large subgroups within the data, which can result in minority subgroups having little representation in the results – if any at all. To address this, one needs to take a slightly different approach, which we’ll look at next.
Stratified random sampling is similar to simple random sampling, but it kicks things up a notch. As the name suggests, stratified sampling involves selecting participants randomly , but from within certain pre-defined subgroups (i.e., strata) that share a common trait . For example, you might divide the population into strata based on gender, ethnicity, age range or level of education, and then select randomly from each group.
The benefit of this sampling method is that it gives you more control over the impact of large subgroups (strata) within the population. For example, if a population comprises 80% males and 20% females, you may want to “balance” this skew out by selecting a random sample from an equal number of males and females. This would, of course, reduce the representativeness of the sample, but it would allow you to identify differences between subgroups. So, depending on your research aims, the stratified approach could work well.
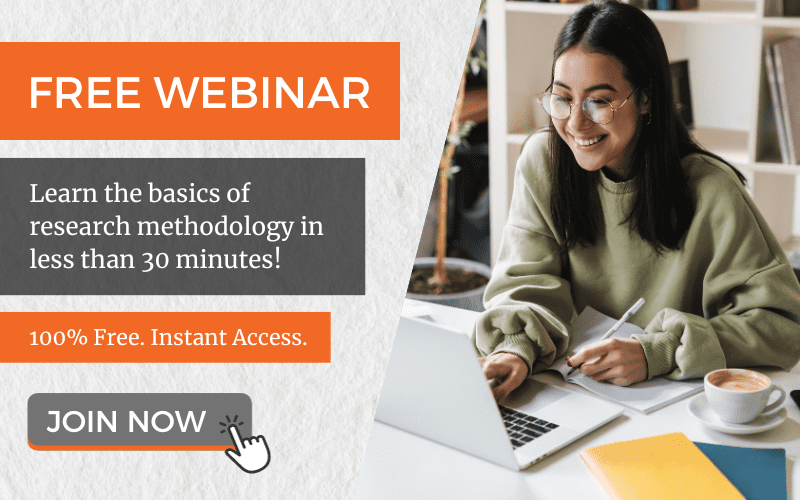
Next on the list is cluster sampling. As the name suggests, this sampling method involves sampling from naturally occurring, mutually exclusive clusters within a population – for example, area codes within a city or cities within a country. Once the clusters are defined, a set of clusters are randomly selected and then a set of participants are randomly selected from each cluster.
Now, you’re probably wondering, “how is cluster sampling different from stratified random sampling?”. Well, let’s look at the previous example where each cluster reflects an area code in a given city.
With cluster sampling, you would collect data from clusters of participants in a handful of area codes (let’s say 5 neighbourhoods). Conversely, with stratified random sampling, you would need to collect data from all over the city (i.e., many more neighbourhoods). You’d still achieve the same sample size either way (let’s say 200 people, for example), but with stratified sampling, you’d need to do a lot more running around, as participants would be scattered across a vast geographic area. As a result, cluster sampling is often the more practical and economical option.
If that all sounds a little mind-bending, you can use the following general rule of thumb. If a population is relatively homogeneous , cluster sampling will often be adequate. Conversely, if a population is quite heterogeneous (i.e., diverse), stratified sampling will generally be more appropriate.
The last probability sampling method we’ll look at is systematic sampling. This method simply involves selecting participants at a set interval , starting from a random point .
For example, if you have a list of students that reflects the population of a university, you could systematically sample that population by selecting participants at an interval of 8 . In other words, you would randomly select a starting point – let’s say student number 40 – followed by student 48, 56, 64, etc.
What’s important with systematic sampling is that the population list you select from needs to be randomly ordered . If there are underlying patterns in the list (for example, if the list is ordered by gender, IQ, age, etc.), this will result in a non-random sample, which would defeat the purpose of adopting this sampling method. Of course, you could safeguard against this by “shuffling” your population list using a random number generator or similar tool.
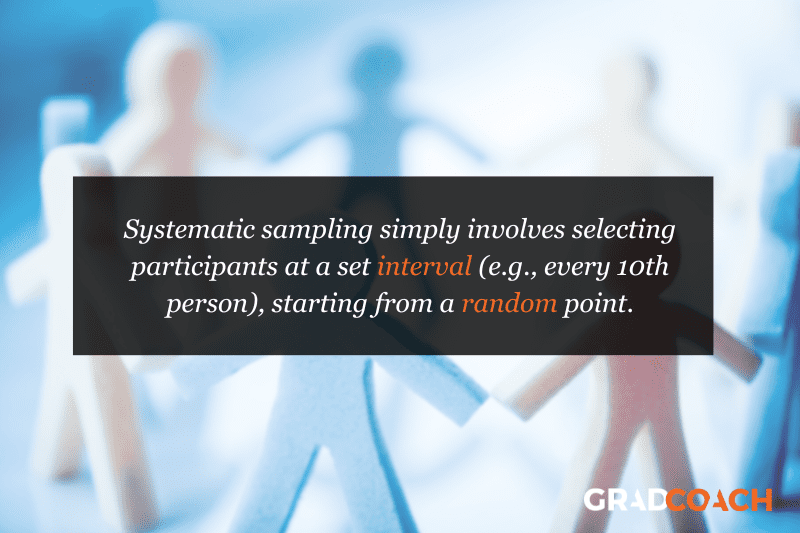
Non-probability-based sampling methods
Right, now that we’ve looked at a few probability-based sampling methods, let’s look at three non-probability methods :
Again, this is not an exhaustive list of all possible sampling methods, so be sure to explore further if you’re interested in adopting a non-probability sampling approach.
First up, we’ve got purposive sampling – also known as judgment , selective or subjective sampling. Again, the name provides some clues, as this method involves the researcher selecting participants using his or her own judgement , based on the purpose of the study (i.e., the research aims).
For example, suppose your research aims were to understand the perceptions of hyper-loyal customers of a particular retail store. In that case, you could use your judgement to engage with frequent shoppers, as well as rare or occasional shoppers, to understand what judgements drive the two behavioural extremes .
Purposive sampling is often used in studies where the aim is to gather information from a small population (especially rare or hard-to-find populations), as it allows the researcher to target specific individuals who have unique knowledge or experience . Naturally, this sampling method is quite prone to researcher bias and judgement error, and it’s unlikely to produce generalisable results, so it’s best suited to studies where the aim is to go deep rather than broad .
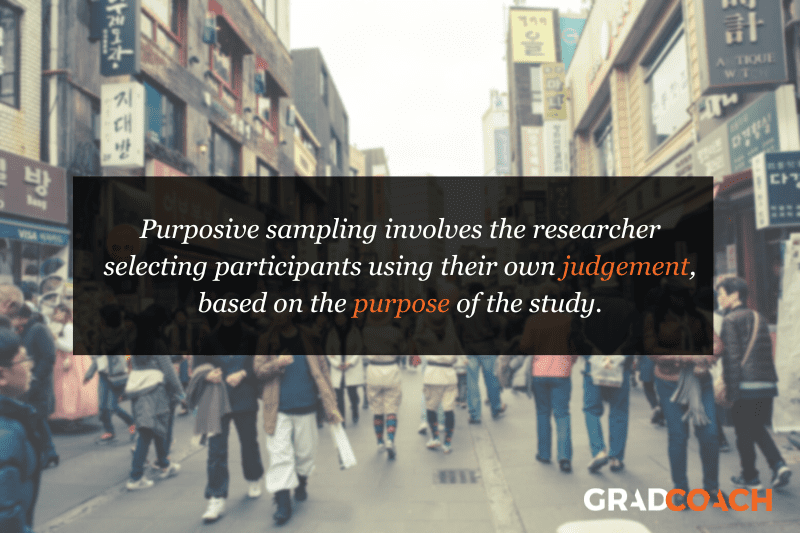
Next up, we have convenience sampling. As the name suggests, with this method, participants are selected based on their availability or accessibility . In other words, the sample is selected based on how convenient it is for the researcher to access it, as opposed to using a defined and objective process.
Naturally, convenience sampling provides a quick and easy way to gather data, as the sample is selected based on the individuals who are readily available or willing to participate. This makes it an attractive option if you’re particularly tight on resources and/or time. However, as you’d expect, this sampling method is unlikely to produce a representative sample and will of course be vulnerable to researcher bias , so it’s important to approach it with caution.
Last but not least, we have the snowball sampling method. This method relies on referrals from initial participants to recruit additional participants. In other words, the initial subjects form the first (small) snowball and each additional subject recruited through referral is added to the snowball, making it larger as it rolls along .
Snowball sampling is often used in research contexts where it’s difficult to identify and access a particular population. For example, people with a rare medical condition or members of an exclusive group. It can also be useful in cases where the research topic is sensitive or taboo and people are unlikely to open up unless they’re referred by someone they trust.
Simply put, snowball sampling is ideal for research that involves reaching hard-to-access populations . But, keep in mind that, once again, it’s a sampling method that’s highly prone to researcher bias and is unlikely to produce a representative sample. So, make sure that it aligns with your research aims and questions before adopting this method.
How to choose a sampling method
Now that we’ve looked at a few popular sampling methods (both probability and non-probability based), the obvious question is, “ how do I choose the right sampling method for my study?”. When selecting a sampling method for your research project, you’ll need to consider two important factors: your research aims and your resources .
As with all research design and methodology choices, your sampling approach needs to be guided by and aligned with your research aims, objectives and research questions – in other words, your golden thread. Specifically, you need to consider whether your research aims are primarily concerned with producing generalisable findings (in which case, you’ll likely opt for a probability-based sampling method) or with achieving rich , deep insights (in which case, a non-probability-based approach could be more practical). Typically, quantitative studies lean toward the former, while qualitative studies aim for the latter, so be sure to consider your broader methodology as well.
The second factor you need to consider is your resources and, more generally, the practical constraints at play. If, for example, you have easy, free access to a large sample at your workplace or university and a healthy budget to help you attract participants, that will open up multiple options in terms of sampling methods. Conversely, if you’re cash-strapped, short on time and don’t have unfettered access to your population of interest, you may be restricted to convenience or referral-based methods.
In short, be ready for trade-offs – you won’t always be able to utilise the “perfect” sampling method for your study, and that’s okay. Much like all the other methodological choices you’ll make as part of your study, you’ll often need to compromise and accept practical trade-offs when it comes to sampling. Don’t let this get you down though – as long as your sampling choice is well explained and justified, and the limitations of your approach are clearly articulated, you’ll be on the right track.
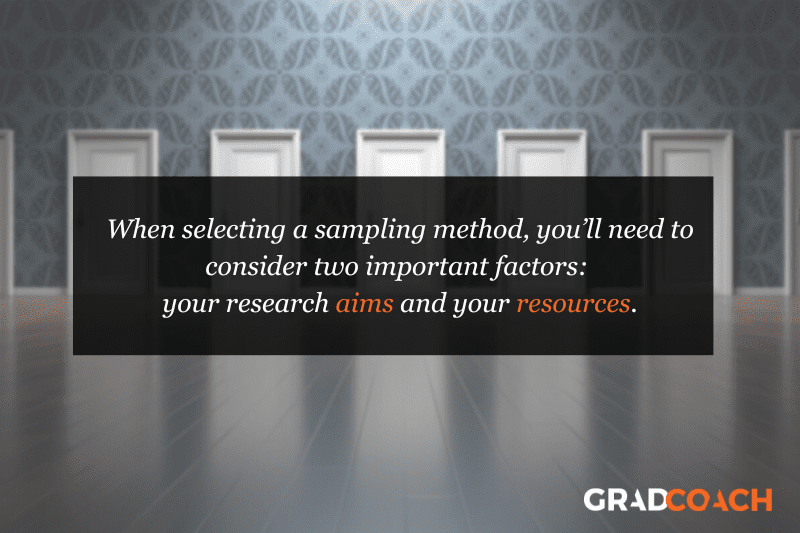
Let’s recap…
In this post, we’ve covered the basics of sampling within the context of a typical research project.
- Sampling refers to the process of defining a subgroup (sample) from the larger group of interest (population).
- The two overarching approaches to sampling are probability sampling (random) and non-probability sampling .
- Common probability-based sampling methods include simple random sampling, stratified random sampling, cluster sampling and systematic sampling.
- Common non-probability-based sampling methods include purposive sampling, convenience sampling and snowball sampling.
- When choosing a sampling method, you need to consider your research aims , objectives and questions, as well as your resources and other practical constraints .
If you’d like to see an example of a sampling strategy in action, be sure to check out our research methodology chapter sample .
Last but not least, if you need hands-on help with your sampling (or any other aspect of your research), take a look at our 1-on-1 coaching service , where we guide you through each step of the research process, at your own pace.
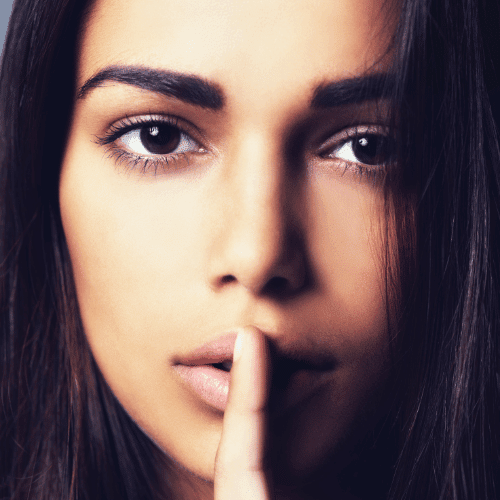
Psst... there’s more!
This post was based on one of our popular Research Bootcamps . If you're working on a research project, you'll definitely want to check this out ...
You Might Also Like:
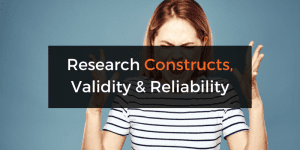
Excellent and helpful. Best site to get a full understanding of Research methodology. I’m nolonger as “clueless “..😉
Excellent and helpful for junior researcher!
Grad Coach tutorials are excellent – I recommend them to everyone doing research. I will be working with a sample of imprisoned women and now have a much clearer idea concerning sampling. Thank you to all at Grad Coach for generously sharing your expertise with students.
Submit a Comment Cancel reply
Your email address will not be published. Required fields are marked *
Save my name, email, and website in this browser for the next time I comment.
- Print Friendly

Research Writing and Analysis
- NVivo Group and Study Sessions
- SPSS This link opens in a new window
- Statistical Analysis Group sessions
- Using Qualtrics
- Dissertation and Data Analysis Group Sessions
- Defense Schedule - Commons Calendar This link opens in a new window
- Research Process Flow Chart
- Research Alignment Chapter 1 This link opens in a new window
- Step 1: Seek Out Evidence
- Step 2: Explain
- Step 3: The Big Picture
- Step 4: Own It
- Step 5: Illustrate
- Annotated Bibliography
- Literature Review This link opens in a new window
- Systematic Reviews & Meta-Analyses
- How to Synthesize and Analyze
- Synthesis and Analysis Practice
- Synthesis and Analysis Group Sessions
- Problem Statement
- Purpose Statement
- Conceptual Framework
- Theoretical Framework
- Quantitative Research Questions
- Qualitative Research Questions
- Trustworthiness of Qualitative Data
- Analysis and Coding Example- Qualitative Data
- Thematic Data Analysis in Qualitative Design
- Dissertation to Journal Article This link opens in a new window
- International Journal of Online Graduate Education (IJOGE) This link opens in a new window
- Journal of Research in Innovative Teaching & Learning (JRIT&L) This link opens in a new window
Writing a Case Study

What is a case study?

A Case study is:
- An in-depth research design that primarily uses a qualitative methodology but sometimes includes quantitative methodology.
- Used to examine an identifiable problem confirmed through research.
- Used to investigate an individual, group of people, organization, or event.
- Used to mostly answer "how" and "why" questions.
What are the different types of case studies?

Note: These are the primary case studies. As you continue to research and learn
about case studies you will begin to find a robust list of different types.
Who are your case study participants?

What is triangulation ?
Validity and credibility are an essential part of the case study. Therefore, the researcher should include triangulation to ensure trustworthiness while accurately reflecting what the researcher seeks to investigate.

How to write a Case Study?
When developing a case study, there are different ways you could present the information, but remember to include the five parts for your case study.

Was this resource helpful?
- << Previous: Thematic Data Analysis in Qualitative Design
- Next: Journal Article Reporting Standards (JARS) >>
- Last Updated: May 3, 2024 8:12 AM
- URL: https://resources.nu.edu/researchtools

- Privacy Policy

Home » Case Study – Methods, Examples and Guide
Case Study – Methods, Examples and Guide
Table of Contents

A case study is a research method that involves an in-depth examination and analysis of a particular phenomenon or case, such as an individual, organization, community, event, or situation.
It is a qualitative research approach that aims to provide a detailed and comprehensive understanding of the case being studied. Case studies typically involve multiple sources of data, including interviews, observations, documents, and artifacts, which are analyzed using various techniques, such as content analysis, thematic analysis, and grounded theory. The findings of a case study are often used to develop theories, inform policy or practice, or generate new research questions.
Types of Case Study
Types and Methods of Case Study are as follows:
Single-Case Study
A single-case study is an in-depth analysis of a single case. This type of case study is useful when the researcher wants to understand a specific phenomenon in detail.
For Example , A researcher might conduct a single-case study on a particular individual to understand their experiences with a particular health condition or a specific organization to explore their management practices. The researcher collects data from multiple sources, such as interviews, observations, and documents, and uses various techniques to analyze the data, such as content analysis or thematic analysis. The findings of a single-case study are often used to generate new research questions, develop theories, or inform policy or practice.
Multiple-Case Study
A multiple-case study involves the analysis of several cases that are similar in nature. This type of case study is useful when the researcher wants to identify similarities and differences between the cases.
For Example, a researcher might conduct a multiple-case study on several companies to explore the factors that contribute to their success or failure. The researcher collects data from each case, compares and contrasts the findings, and uses various techniques to analyze the data, such as comparative analysis or pattern-matching. The findings of a multiple-case study can be used to develop theories, inform policy or practice, or generate new research questions.
Exploratory Case Study
An exploratory case study is used to explore a new or understudied phenomenon. This type of case study is useful when the researcher wants to generate hypotheses or theories about the phenomenon.
For Example, a researcher might conduct an exploratory case study on a new technology to understand its potential impact on society. The researcher collects data from multiple sources, such as interviews, observations, and documents, and uses various techniques to analyze the data, such as grounded theory or content analysis. The findings of an exploratory case study can be used to generate new research questions, develop theories, or inform policy or practice.
Descriptive Case Study
A descriptive case study is used to describe a particular phenomenon in detail. This type of case study is useful when the researcher wants to provide a comprehensive account of the phenomenon.
For Example, a researcher might conduct a descriptive case study on a particular community to understand its social and economic characteristics. The researcher collects data from multiple sources, such as interviews, observations, and documents, and uses various techniques to analyze the data, such as content analysis or thematic analysis. The findings of a descriptive case study can be used to inform policy or practice or generate new research questions.
Instrumental Case Study
An instrumental case study is used to understand a particular phenomenon that is instrumental in achieving a particular goal. This type of case study is useful when the researcher wants to understand the role of the phenomenon in achieving the goal.
For Example, a researcher might conduct an instrumental case study on a particular policy to understand its impact on achieving a particular goal, such as reducing poverty. The researcher collects data from multiple sources, such as interviews, observations, and documents, and uses various techniques to analyze the data, such as content analysis or thematic analysis. The findings of an instrumental case study can be used to inform policy or practice or generate new research questions.
Case Study Data Collection Methods
Here are some common data collection methods for case studies:
Interviews involve asking questions to individuals who have knowledge or experience relevant to the case study. Interviews can be structured (where the same questions are asked to all participants) or unstructured (where the interviewer follows up on the responses with further questions). Interviews can be conducted in person, over the phone, or through video conferencing.
Observations
Observations involve watching and recording the behavior and activities of individuals or groups relevant to the case study. Observations can be participant (where the researcher actively participates in the activities) or non-participant (where the researcher observes from a distance). Observations can be recorded using notes, audio or video recordings, or photographs.
Documents can be used as a source of information for case studies. Documents can include reports, memos, emails, letters, and other written materials related to the case study. Documents can be collected from the case study participants or from public sources.
Surveys involve asking a set of questions to a sample of individuals relevant to the case study. Surveys can be administered in person, over the phone, through mail or email, or online. Surveys can be used to gather information on attitudes, opinions, or behaviors related to the case study.
Artifacts are physical objects relevant to the case study. Artifacts can include tools, equipment, products, or other objects that provide insights into the case study phenomenon.
How to conduct Case Study Research
Conducting a case study research involves several steps that need to be followed to ensure the quality and rigor of the study. Here are the steps to conduct case study research:
- Define the research questions: The first step in conducting a case study research is to define the research questions. The research questions should be specific, measurable, and relevant to the case study phenomenon under investigation.
- Select the case: The next step is to select the case or cases to be studied. The case should be relevant to the research questions and should provide rich and diverse data that can be used to answer the research questions.
- Collect data: Data can be collected using various methods, such as interviews, observations, documents, surveys, and artifacts. The data collection method should be selected based on the research questions and the nature of the case study phenomenon.
- Analyze the data: The data collected from the case study should be analyzed using various techniques, such as content analysis, thematic analysis, or grounded theory. The analysis should be guided by the research questions and should aim to provide insights and conclusions relevant to the research questions.
- Draw conclusions: The conclusions drawn from the case study should be based on the data analysis and should be relevant to the research questions. The conclusions should be supported by evidence and should be clearly stated.
- Validate the findings: The findings of the case study should be validated by reviewing the data and the analysis with participants or other experts in the field. This helps to ensure the validity and reliability of the findings.
- Write the report: The final step is to write the report of the case study research. The report should provide a clear description of the case study phenomenon, the research questions, the data collection methods, the data analysis, the findings, and the conclusions. The report should be written in a clear and concise manner and should follow the guidelines for academic writing.
Examples of Case Study
Here are some examples of case study research:
- The Hawthorne Studies : Conducted between 1924 and 1932, the Hawthorne Studies were a series of case studies conducted by Elton Mayo and his colleagues to examine the impact of work environment on employee productivity. The studies were conducted at the Hawthorne Works plant of the Western Electric Company in Chicago and included interviews, observations, and experiments.
- The Stanford Prison Experiment: Conducted in 1971, the Stanford Prison Experiment was a case study conducted by Philip Zimbardo to examine the psychological effects of power and authority. The study involved simulating a prison environment and assigning participants to the role of guards or prisoners. The study was controversial due to the ethical issues it raised.
- The Challenger Disaster: The Challenger Disaster was a case study conducted to examine the causes of the Space Shuttle Challenger explosion in 1986. The study included interviews, observations, and analysis of data to identify the technical, organizational, and cultural factors that contributed to the disaster.
- The Enron Scandal: The Enron Scandal was a case study conducted to examine the causes of the Enron Corporation’s bankruptcy in 2001. The study included interviews, analysis of financial data, and review of documents to identify the accounting practices, corporate culture, and ethical issues that led to the company’s downfall.
- The Fukushima Nuclear Disaster : The Fukushima Nuclear Disaster was a case study conducted to examine the causes of the nuclear accident that occurred at the Fukushima Daiichi Nuclear Power Plant in Japan in 2011. The study included interviews, analysis of data, and review of documents to identify the technical, organizational, and cultural factors that contributed to the disaster.
Application of Case Study
Case studies have a wide range of applications across various fields and industries. Here are some examples:
Business and Management
Case studies are widely used in business and management to examine real-life situations and develop problem-solving skills. Case studies can help students and professionals to develop a deep understanding of business concepts, theories, and best practices.
Case studies are used in healthcare to examine patient care, treatment options, and outcomes. Case studies can help healthcare professionals to develop critical thinking skills, diagnose complex medical conditions, and develop effective treatment plans.
Case studies are used in education to examine teaching and learning practices. Case studies can help educators to develop effective teaching strategies, evaluate student progress, and identify areas for improvement.
Social Sciences
Case studies are widely used in social sciences to examine human behavior, social phenomena, and cultural practices. Case studies can help researchers to develop theories, test hypotheses, and gain insights into complex social issues.
Law and Ethics
Case studies are used in law and ethics to examine legal and ethical dilemmas. Case studies can help lawyers, policymakers, and ethical professionals to develop critical thinking skills, analyze complex cases, and make informed decisions.
Purpose of Case Study
The purpose of a case study is to provide a detailed analysis of a specific phenomenon, issue, or problem in its real-life context. A case study is a qualitative research method that involves the in-depth exploration and analysis of a particular case, which can be an individual, group, organization, event, or community.
The primary purpose of a case study is to generate a comprehensive and nuanced understanding of the case, including its history, context, and dynamics. Case studies can help researchers to identify and examine the underlying factors, processes, and mechanisms that contribute to the case and its outcomes. This can help to develop a more accurate and detailed understanding of the case, which can inform future research, practice, or policy.
Case studies can also serve other purposes, including:
- Illustrating a theory or concept: Case studies can be used to illustrate and explain theoretical concepts and frameworks, providing concrete examples of how they can be applied in real-life situations.
- Developing hypotheses: Case studies can help to generate hypotheses about the causal relationships between different factors and outcomes, which can be tested through further research.
- Providing insight into complex issues: Case studies can provide insights into complex and multifaceted issues, which may be difficult to understand through other research methods.
- Informing practice or policy: Case studies can be used to inform practice or policy by identifying best practices, lessons learned, or areas for improvement.
Advantages of Case Study Research
There are several advantages of case study research, including:
- In-depth exploration: Case study research allows for a detailed exploration and analysis of a specific phenomenon, issue, or problem in its real-life context. This can provide a comprehensive understanding of the case and its dynamics, which may not be possible through other research methods.
- Rich data: Case study research can generate rich and detailed data, including qualitative data such as interviews, observations, and documents. This can provide a nuanced understanding of the case and its complexity.
- Holistic perspective: Case study research allows for a holistic perspective of the case, taking into account the various factors, processes, and mechanisms that contribute to the case and its outcomes. This can help to develop a more accurate and comprehensive understanding of the case.
- Theory development: Case study research can help to develop and refine theories and concepts by providing empirical evidence and concrete examples of how they can be applied in real-life situations.
- Practical application: Case study research can inform practice or policy by identifying best practices, lessons learned, or areas for improvement.
- Contextualization: Case study research takes into account the specific context in which the case is situated, which can help to understand how the case is influenced by the social, cultural, and historical factors of its environment.
Limitations of Case Study Research
There are several limitations of case study research, including:
- Limited generalizability : Case studies are typically focused on a single case or a small number of cases, which limits the generalizability of the findings. The unique characteristics of the case may not be applicable to other contexts or populations, which may limit the external validity of the research.
- Biased sampling: Case studies may rely on purposive or convenience sampling, which can introduce bias into the sample selection process. This may limit the representativeness of the sample and the generalizability of the findings.
- Subjectivity: Case studies rely on the interpretation of the researcher, which can introduce subjectivity into the analysis. The researcher’s own biases, assumptions, and perspectives may influence the findings, which may limit the objectivity of the research.
- Limited control: Case studies are typically conducted in naturalistic settings, which limits the control that the researcher has over the environment and the variables being studied. This may limit the ability to establish causal relationships between variables.
- Time-consuming: Case studies can be time-consuming to conduct, as they typically involve a detailed exploration and analysis of a specific case. This may limit the feasibility of conducting multiple case studies or conducting case studies in a timely manner.
- Resource-intensive: Case studies may require significant resources, including time, funding, and expertise. This may limit the ability of researchers to conduct case studies in resource-constrained settings.
About the author
Muhammad Hassan
Researcher, Academic Writer, Web developer
You may also like

Questionnaire – Definition, Types, and Examples

Observational Research – Methods and Guide

Quantitative Research – Methods, Types and...

Qualitative Research Methods

Explanatory Research – Types, Methods, Guide

Survey Research – Types, Methods, Examples
Case-Control Studies

Introduction
Cohort studies have an intuitive logic to them, but they can be very problematic when one is investigating outcomes that only occur in a small fraction of exposed and unexposed individuals. They can also be problematic when it is expensive or very difficult to obtain exposure information from a cohort. In these situations a case-control design offers an alternative that is much more efficient. The goal of a case-control study is the same as that of cohort studies, i.e., to estimate the magnitude of association between an exposure and an outcome. However, case-control studies employ a different sampling strategy that gives them greater efficiency.
Learning Objectives
After completing this module, the student will be able to:
- Define and explain the distinguishing features of a case-control study
- Describe and identify the types of epidemiologic questions that can be addressed by case-control studies
- Define what is meant by the term "source population"
- Describe the purpose of controls in a case-control study
- Describe differences between hospital-based and population-based case-control studies
- Describe the principles of valid control selection
- Explain the importance of using specific diagnostic criteria and explicit case definitions in case-control studies
- Estimate and interpret the odds ratio from a case-control study
- Identify the potential strengths and limitations of case-control studies
Overview of Case-Control Design
In the module entitled Overview of Analytic Studies it was noted that Rothman describes the case-control strategy as follows:
"Case-control studies are best understood by considering as the starting point a source population , which represents a hypothetical study population in which a cohort study might have been conducted. The source population is the population that gives rise to the cases included in the study. If a cohort study were undertaken, we would define the exposed and unexposed cohorts (or several cohorts) and from these populations obtain denominators for the incidence rates or risks that would be calculated for each cohort. We would then identify the number of cases occurring in each cohort and calculate the risk or incidence rate for each. In a case-control study the same cases are identified and classified as to whether they belong to the exposed or unexposed cohort. Instead of obtaining the denominators for the rates or risks, however, a control group is sampled from the entire source population that gives rise to the cases. Individuals in the control group are then classified into exposed and unexposed categories. The purpose of the control group is to determine the relative size of the exposed and unexposed components of the source population. Because the control group is used to estimate the distribution of exposure in the source population, the cardinal requirement of control selection is that the controls be sampled independently of exposure status."
To illustrate this consider the following hypothetical scenario in which the source population is the state of Massachusetts. Diseased individuals are red, and non-diseased individuals are blue. Exposed individuals are indicated by a whitish midsection. Note the following aspects of the depicted scenario:
- The disease is rare.
- There is a fairly large number of exposed individuals in the state, but most of these are not diseased.

If we somehow had exposure and outcome information on all of the subjects in the source population and looked at the association using a cohort design, we might find the data summarized in the contingency table below.
In this hypothetical example, we have data on all 6,000,000 people in the source population, and we could compute the probability of disease (i.e., the risk or incidence) in both the exposed group and the non-exposed group, because we have the denominators for both the exposed and non-exposed groups.
The table above summarizes all of the necessary information regarding exposure and outcome status for the population and enables us to compute a risk ratio as a measure of the strength of the association. Intuitively, we compute the probability of disease (the risk) in each exposure group and then compute the risk ratio as follows:
The problem , of course, is that we usually don't have the resources to get the data on all subjects in the population. If we took a random sample of even 5-10% of the population, we would have few diseased people in our sample, certainly not enough to produce a reasonably precise measure of association. Moreover, we would expend an inordinate amount of effort and money collecting exposure and outcome data on a large number of people who would not develop the outcome.
We need a method that allows us to retain all the people in the numerator of disease frequency (diseased people or "cases") but allows us to collect information from only a small proportion of the people that make up the denominator (population, or "controls"), most of whom do not have the disease of interest. The case-control design allows us to accomplish this. We identify and collect exposure information on all the cases, but identify and collect exposure information on only a sample of the population. Once we have the exposure information, we can assign subjects to the numerator and denominator of the exposed and unexposed groups. This is what Rothman means when he says,
"The purpose of the control group is to determine the relative size of the exposed and unexposed components of the source population."
In the above example, we would have identified all 1,300 cases, determined their exposure status, and ended up categorizing 700 as exposed and 600 as unexposed. We might have ransomly sampled 6,000 members of the population (instead of 6 million) in order to determine the exposure distribution in the total population. If our sampling method was random, we would expect that about 1,000 would be exposed and 5,000 unexposed (the same ratio as in the overall population). We calculate a similar measure as the risk ratio above, but substituting in the denominator a sample of the population ("controls") instead of the whole population:
Note that when we take a sample of the population, we no longer have a measure of disease frequency, because the denominator no longer represents the population. Therefore, we can no longer compute the probability or rate of disease incidence in each exposure group. We also can't calculate a risk or rate difference measure for the same reason. However, as we have seen, we can compute the relative probability of disease in the exposed vs. unexposed group. The term generally used for this measure is an odds ratio , described in more detail later in the module.
Consequently, when the outcome is uncommon, as in this case, the risk ratio can be estimated much more efficiently by using a case-control design. One would focus first on finding an adequate number of cases in order to determine the ratio of exposed to unexposed cases. Then, one only needs to take a sample of the population in order to estimate the relative size of the exposed and unexposed components of the source population. Note that if one can identify all of the cases that were reported to a registry or other database within a defined period of time, then it is possible to compute an estimate of the incidence of disease if the size of the population is known from census data. While this is conceptually possible, it is rarely done, and we will not discuss it further in this course.

A Nested Case-Control Study
Suppose a prospective cohort study were conducted among almost 90,000 women for the purpose of studying the determinants of cancer and cardiovascular disease. After enrollment, the women provide baseline information on a host of exposures, and they also provide baseline blood and urine samples that are frozen for possible future use. The women are then followed, and, after about eight years, the investigators want to test the hypothesis that past exposure to pesticides such as DDT is a risk factor for breast cancer. Eight years have passed since the beginning of the study, and 1.439 women in the cohort have developed breast cancer. Since they froze blood samples at baseline, they have the option of analyzing all of the blood samples in order to ascertain exposure to DDT at the beginning of the study before any cancers occurred. The problem is that there are almost 90,000 women and it would cost $20 to analyze each of the blood samples. If the investigators could have analyzed all 90,000 samples this is what they would have found the results in the table below.
Table of Breast Cancer Occurrence Among Women With or Without DDT Exposure
If they had been able to afford analyzing all of the baseline blood specimens in order to categorize the women as having had DDT exposure or not, they would have found a risk ratio = 1.87 (95% confidence interval: 1.66-2.10). The problem is that this would have cost almost $1.8 million, and the investigators did not have the funding to do this.
While 1,439 breast cancers is a disturbing number, it is only 1.6% of the entire cohort, so the outcome is relatively rare, and it is costing a lot of money to analyze the blood specimens obtained from all of the non-diseased women. There is, however, another more efficient alternative, i.e., to use a case-control sampling strategy. One could analyze all of the blood samples from women who had developed breast cancer, but only a sample of the whole cohort in order to estimate the exposure distribution in the population that produced the cases.
If one were to analyze the blood samples of 2,878 of the non-diseased women (twice as many as the number of cases), one would obtain results that would look something like those in the next table.
Odds of Exposure: 360/1079 in the cases versus 432/2,446 in the non-diseased controls.
Totals Samples analyzed = 1,438+2,878 = 4,316
Total Cost = 4,316 x $20 = $86,320
With this approach a similar estimate of risk was obtained after analyzing blood samples from only a small sample of the entire population at a fraction of the cost with hardly any loss in precision. In essence, a case-control strategy was used, but it was conducted within the context of a prospective cohort study. This is referred to as a case-control study "nested" within a cohort study.
Rothman states that one should look upon all case-control studies as being "nested" within a cohort. In other words the cohort represents the source population that gave rise to the cases. With a case-control sampling strategy one simply takes a sample of the population in order to obtain an estimate of the exposure distribution within the population that gave rise to the cases. Obviously, this is a much more efficient design.
It is important to note that, unlike cohort studies, case-control studies do not follow subjects through time. Cases are enrolled at the time they develop disease and controls are enrolled at the same time. The exposure status of each is determined, but they are not followed into the future for further development of disease.
As with cohort studies, case-control studies can be prospective or retrospective. At the start of the study, all cases might have already occurred and then this would be a retrospective case-control study. Alternatively, none of the cases might have already occurred, and new cases will be enrolled prospectively. Epidemiologists generally prefer the prospective approach because it has fewer biases, but it is more expensive and sometimes not possible. When conducted prospectively, or when nested in a prospective cohort study, it is straightforward to select controls from the population at risk. However, in retrospective case-control studies, it can be difficult to select from the population at risk, and controls are then selected from those in the population who didn't develop disease. Using only the non-diseased to select controls as opposed to the whole population means the denominator is not really a measure of disease frequency, but when the disease is rare , the odds ratio using the non-diseased will be very similar to the estimate obtained when the entire population is used to sample for controls. This phenomenon is known as the r are-disease assumption . When case-control studies were first developed, most were conducted retrospectively, and it is sometimes assumed that the rare-disease assumption applies to all case-control studies. However, it actually only applies to those case-control studies in which controls are sampled only from the non-diseased rather than the whole population.
The difference between sampling from the whole population and only the non-diseased is that the whole population contains people both with and without the disease of interest. This means that a sampling strategy that uses the whole population as its source must allow for the fact that people who develop the disease of interest can be selected as controls. Students often have a difficult time with this concept. It is helpful to remember that it seems natural that the population denominator includes people who develop the disease in a cohort study. If a case-control study is a more efficient way to obtain the information from a cohort study, then perhaps it is not so strange that the denominator in a case-control study also can include people who develop the disease. This topic is covered in more detail in EP813 Intermediate Epidemiology.
Retrospective and Prospective Case-Control Studies
Students usually think of case-control studies as being only retrospective, since the investigators enroll subjects who have developed the outcome of interest. However, case-control studies, like cohort studies, can be either retrospective or prospective. In a prospective case-control study, the investigator still enrolls based on outcome status, but the investigator must wait to the cases to occur.
When is a Case-Control Study Desirable?
Given the greater efficiency of case-control studies, they are particularly advantageous in the following situations:
- When the disease or outcome being studied is rare.
- When the disease or outcome has a long induction and latent period (i.e., a long time between exposure and the eventual causal manifestation of disease).
- When exposure data is difficult or expensive to obtain.
- When the study population is dynamic.
- When little is known about the risk factors for the disease, case-control studies provide a way of testing associations with multiple potential risk factors. (This isn't really a unique advantage to case-control studies, however, since cohort studies can also assess multiple exposures.)
Another advantage of their greater efficiency, of course, is that they are less time-consuming and much less costly than prospective cohort studies.
The DES Case-Control Study
A classic example of the efficiency of the case-control approach is the study (Herbst et al.: N. Engl. J. Med. Herbst et al. (1971;284:878-81) that linked in-utero exposure to diethylstilbesterol (DES) with subsequent development of vaginal cancer 15-22 years later. In the late 1960s, physicians at MGH identified a very unusual cancer cluster. Eight young woman between the ages of 15-22 were found to have cancer of the vagina, an uncommon cancer even in elderly women. The cluster of cases in young women was initially reported as a case series, but there were no strong hypotheses about the cause.
In retrospect, the cause was in-utero exposure to DES. After World War II, DES started being prescribed for women who were having troubles with a pregnancy -- if there were signs suggesting the possibility of a miscarriage, DES was frequently prescribed. It has been estimated that between 1945-1950 DES was prescribed for about 20% of all pregnancies in the Boston area. Thus, the unborn fetus was exposed to DES in utero, and in a very small percentage of cases this resulted in development of vaginal cancer when the child was 15-22 years old (a very long latent period). There were several reasons why a case-control study was the only feasible way to identify this association: the disease was extremely rare (even in subjects who had been exposed to DES), there was a very long latent period between exposure and development of disease, and initially they had no idea what was responsible, so there were many possible exposures to consider.
In this situation, a case-control study was the only reasonable approach to identify the causative agent. Given how uncommon the outcome was, even a large prospective study would have been unlikely to have more than one or two cases, even after 15-20 years of follow-up. Similarly, a retrospective cohort study might have been successful in enrolling a large number of subjects, but the outcome of interest was so uncommon that few, if any, subjects would have had it. In contrast, a case-control study was conducted in which eight known cases and 32 age-matched controls provided information on many potential exposures. This strategy ultimately allowed the investigators to identify a highly significant association between the mother's treatment with DES during pregnancy and the eventual development of adenocarcinoma of the vagina in their daughters (in-utero at the time of exposure) 15 to 22 years later.
For more information see the DES Fact Sheet from the National Cancer Institute.
An excellent summary of this landmark study and the long-range effects of DES can be found in a Perspective article in the New England Journal of Medicine. A cohort of both mothers who took DES and their children (daughters and sons) was later formed to look for more common outcomes. Members of the faculty at BUSPH are on the team of investigators that follow this cohort for a variety of outcomes, particularly reproductive consequences and other cancers.
Selecting & Defining Cases and Controls
The "case" definition.
Careful thought should be given to the case definition to be used. If the definition is too broad or vague, it is easier to capture people with the outcome of interest, but a loose case definition will also capture people who do not have the disease. On the other hand, an overly restrictive case definition is employed, fewer cases will be captured, and the sample size may be limited. Investigators frequently wrestle with this problem during outbreak investigations. Initially, they will often use a somewhat broad definition in order to identify potential cases. However, as an outbreak investigation progresses, there is a tendency to narrow the case definition to make it more precise and specific, for example by requiring confirmation of the diagnosis by laboratory testing. In general, investigators conducting case-control studies should thoughtfully construct a definition that is as clear and specific as possible without being overly restrictive.
Investigators studying chronic diseases generally prefer newly diagnosed cases, because they tend to be more motivated to participate, may remember relevant exposures more accurately, and because it avoids complicating factors related to selection of longer duration (i.e., prevalent) cases. However, it is sometimes impossible to have an adequate sample size if only recent cases are enrolled.
Sources of Cases
Typical sources for cases include:
- Patient rosters at medical facilities
- Death certificates
- Disease registries (e.g., cancer or birth defect registries; the SEER Program [Surveillance, Epidemiology and End Results] is a federally funded program that identifies newly diagnosed cases of cancer in population-based registries across the US )
- Cross-sectional surveys (e.g., NHANES, the National Health and Nutrition Examination Survey)
Selection of the Controls
As noted above, it is always useful to think of a case-control study as being nested within some sort of a cohort, i.e., a source population that produced the cases that were identified and enrolled. In view of this there are two key principles that should be followed in selecting controls:
- The comparison group ("controls") should be representative of the source population that produced the cases.
- The "controls" must be sampled in a way that is independent of the exposure, meaning that their selection should not be more (or less) likely if they have the exposure of interest.
If either of these principles are not adhered to, selection bias can result (as discussed in detail in the module on Bias).

Note that in the earlier example of a case-control study conducted in the Massachusetts population, we specified that our sampling method was random so that exposed and unexposed members of the population had an equal chance of being selected. Therefore, we would expect that about 1,000 would be exposed and 5,000 unexposed (the same ratio as in the whole population), and came up with an odds ratio that was same as the hypothetical risk ratio we would have had if we had collected exposure information from the whole population of six million:
What if we had instead been more likely to sample those who were exposed, so that we instead found 1,500 exposed and 4,500 unexposed among the 6,000 controls? Then the odds ratio would have been:
This odds ratio is biased because it differs from the true odds ratio. In this case, the bias stemmed from the fact that we violated the second principle in selection of controls. Depending on which category is over or under-sampled, this type of bias can result in either an underestimate or an overestimate of the true association.
A hypothetical case-control study was conducted to determine whether lower socioeconomic status (the exposure) is associated with a higher risk of cervical cancer (the outcome). The "cases" consisted of 250 women with cervical cancer who were referred to Massachusetts General Hospital for treatment for cervical cancer. They were referred from all over the state. The cases were asked a series of questions relating to socioeconomic status (household income, employment, education, etc.). The investigators identified control subjects by going door-to-door in the community around MGH from 9:00 AM to 5:00 PM. Many residents are not home, but they persist and eventually enroll enough controls. The problem is that the controls were selected by a different mechanism than the cases, AND the selection mechanism may have tended to select individuals of different socioeconomic status, since women who were at home may have been somewhat more likely to be unemployed. In other words, the controls were more likely to be enrolled (selected) if they had the exposure of interest (lower socioeconomic status).

Sources for "Controls"
Population controls:.
A population-based case-control study is one in which the cases come from a precisely defined population, such as a fixed geographic area, and the controls are sampled directly from the same population. In this situation cases might be identified from a state cancer registry, for example, and the comparison group would logically be selected at random from the same source population. Population controls can be identified from voter registration lists, tax rolls, drivers license lists, and telephone directories or by "random digit dialing". Population controls may also be more difficult to obtain, however, because of lack of interest in participating, and there may be recall bias, since population controls are generally healthy and may remember past exposures less accurately.
Example of a Population-based Case-Control Study: Rollison et al. reported on a "Population-based Case-Control Study of Diabetes and Breast Cancer Risk in Hispanic and Non-Hispanic White Women Living in US Southwestern States". (ALink to the article - Citation: Am J Epidemiol 2008;167:447–456).
"Briefly, a population-based case-control study of breast cancer was conducted in Colorado, New Mexico, Utah, and selected counties of Arizona. For investigation of differences in the breast cancer risk profiles of non-Hispanic Whites and Hispanics, sampling was stratified by race/ethnicity, and only women who self-reported their race as non-Hispanic White, Hispanic, or American Indian were eligible, with the exception of American Indian women living on reservations. Women diagnosed with histologically confirmed breast cancer between October 1999 and May 2004 (International Classification of Diseases for Oncology codes C50.0–C50.6 and C50.8–C50.9) were identified as cases through population-based cancer registries in each state."
"Population-based controls were frequency-matched to cases in 5-year age groups. In New Mexico and Utah, control participants under age 65 years were randomly selected from driver's license lists; in Arizona and Colorado, controls were randomly selected from commercial mailing lists, since driver's license lists were unavailable. In all states, women aged 65 years or older were randomly selected from the lists of the Centers for Medicare and Medicaid Services (Social Security lists). Of all women contacted, 68 percent of cases and 42 percent of controls participated in the study."
"Odds ratios and 95% confidence intervals were calculated using logistic regression, adjusting for age, body mass index at age 15 years, and parity. Having any type of diabetes was not associated with breast cancer overall (odds ratio = 0.94, 95% confidence interval: 0.78, 1.12). Type 2 diabetes was observed among 19% of Hispanics and 9% of non-Hispanic Whites but was not associated with breast cancer in either group."
In this example, it is clear that the controls were selected from the source population (principle 1), but less clear that they were enrolled independent of exposure status (principle 2), both because drivers' licenses were used for selection and because the participation rate among controls was low. These factors would only matter if they impacted on the estimate of the proportion of the population who had diabetes.
Hospital or Clinic Controls:

- They have diseases that are unrelated to the exposure being studied. For example, for a study examining the association between smoking and lung cancer, it would not be appropriate to include patients with cardiovascular disease as control, since smoking is a risk factor for cardiovascular disease. To include such patients as controls would result in an underestimate of the true association.
- Second, control patients in the comparison should have diseases with similar referral patterns as the cases, in order to minimize selection bias. For example, if the cases are women with cervical cancer who have been referred from all over the state, it would be inappropriate to use controls consisting of women with diabetes who had been referred primarily from local health centers in the immediate vicinity of the hospital. Similarly, it would be inappropriate to use patients from the emergency room, because the selection of a hospital for an emergency is different than for cancer, and this difference might be related to the exposure of interest.
The advantages of using controls who are patients from the same facility are:
- They are easier to identify
- They are more likely to participate than general population controls.
- They minimize selection bias because they generally come from the same source population (provided referral patterns are similar).
- Recall bias would be minimized, because they are sick, but with a different diagnosis.
Example: Several years ago the vascular surgeons at Boston Medical Center wanted to study risk factors for severe atherosclerosis of the lower extremities. The cases were patients who were referred to the hospital for elective surgery to bypass severe atherosclerotic blockages in the arteries to the legs. The controls consisted of patients who were admitted to the same hospital for elective joint replacement of the hip or knee. The patients undergoing joint replacement were similar in age and they also were following the same referral pathways. In other words, they met the "would" criterion: if one of the joint replacement surgery patients had developed severe atherosclerosis in their leg arteries, they would have been referred to the same hospital.
Friend, Neighbor, Spouse, and Relative Controls:
Occasionally investigators will ask cases to nominate controls who are in one of these categories, because they have similar characteristics, such as genotype, socioeconomic status, or environment, i.e., factors that can cause confounding, but are hard to measure and adjust for. By matching cases and controls on these factors, confounding by these factors will be controlled. However, one must be careful that the controls satisfy the two fundamental principles. Often, they do not.
How Many Controls?
Since case-control studies are often used for uncommon outcomes, investigators often have a limited number of cases but a plentiful supply of potential controls. In this situation the statistical power of the study can be increased somewhat by enrolling more controls than cases. However, the additional power that is achieved diminishes as the ratio of controls to cases increases, and ratios greater than 4:1 have little additional impact on power. Consequently, if it is time-consuming or expensive to collect data on controls, the ratio of controls to cases should be no more than 4:1. However, if the data on controls is easily obtained, there is no reason to limit the number of controls.
Methods of Control Sampling
There are three strategies for selecting controls that are best explained by considering the nested case-control study described on page 3 of this module:
- Survivor sampling: This is the most common method. Controls consist of individuals from the source population who do not have the outcome of interest.
- Case-base sampling (also known as "case-cohort" sampling): Controls are selected from the population at risk at the beginning of the follow-up period in the cohort study within which the case-control study was nested.
- Risk Set Sampling: In the nested case-control study a control would be selected from the population at risk at the point in time when a case was diagnosed.
The Rare Outcome Assumption
It is often said that an odds ratio provides a good estimate of the risk ratio only when the outcome of interest is rare, but this is only true when survivor sampling is used. With case-base sampling or risk set sampling, the odds ratio will provide a good estimate of the risk ratio regardless of the frequency of the outcome, because the controls will provide an accurate estimate of the distribution in the source population (i.e., not just in non-diseased people).
More on Selection Bias
Always consider the source population for case-control studies, i.e. the "population" that generated the cases. The cases are always identified and enrolled by some method or a set of procedures or circumstances. For example, cases with a certain disease might be referred to a particular tertiary hospital for specialized treatment. Alternatively, if there is a database or a disease registry for a geographic area, cases might be selected at random from the database. The key to avoiding selection bias is to select the controls by a similar, if not identical, mechanism in order to ensure that the controls provide an accurate representation of the exposure status of the source population.
Example 1: In the first example above, in which cases were randomly selected from a geographically defined database, the source population is also defined geographically, so it would make sense to select population controls by some random method. In contrast, if one enrolled controls from a particular hospital within the geographic area, one would have to at least consider whether the controls were inherently more or less likely to have the exposure of interest. If so, they would not provide an accurate estimate of the exposure distribution of the source population, and selection bias would result.
Example 2: In the second example above, the source population was defined by the patterns of referral to a particular hospital for a particular disease. In order for the controls to be representative of the "population" that produced those cases, the controls should be selected by a similar mechanism, e.g., by contacting the referring health care providers and asking them to provide the names of potential controls. By this mechanism, one can ensure that the controls are representative of the source population, because if they had had the disease of interest they would have been just as likely as the cases to have been included in the case group (thus fulfilling the "would" criterion).
Example 3: A food handler at a delicatessen who is infected with hepatitis A virus is responsible for an outbreak of hepatitis which is largely confined to the surrounding community from which most of the customers come. Many (but not all) of the infected cases are identified by passive and active surveillance. How should controls be selected? In this situation, one might guess that the likelihood of people going to the delicatessen would be heavily influenced by their proximity to it, and this would to a large extent define the source population. In a case-control study undertaken to identify the source, the delicatessen is one of the exposures being tested. Consequently, even if the cases were reported to the state-wide surveillance system, it would not be appropriate to randomly select controls from the state, the county, or even the town where the delicatessen is located. In other words, the "would" criterion doesn't work here, because anyone in the state with clinical hepatitis would end up in the surveillance system, but someone who lived far from the deli would have a much lower likelihood of having the exposure. A better approach would be to select controls who were matched to the cases by neighborhood, age, and gender. These controls would have similar access to go to the deli if they chose to, and they would therefore be more representative of the source population.
Analysis of Case-Control Studies
The computation and interpretation of the odds ratio in a case-control study has already been discussed in the modules on Overview of Analytic Studies and Measures of Association. Additionally, one can compute the confidence interval for the odds ratio, and statistical significance can also be evaluated by using a chi-square test (or a Fisher's Exact Test if the sample size is small) to compute a p-value. These calculations can be done using the Case-Control worksheet in the Excel file called EpiTools.XLS.

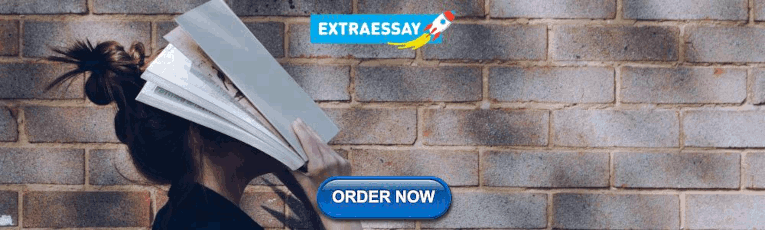
Advantages and Disadvantages of Case-Control Studies
Advantages:
- They are efficient for rare diseases or diseases with a long latency period between exposure and disease manifestation.
- They are less costly and less time-consuming; they are advantageous when exposure data is expensive or hard to obtain.
- They are advantageous when studying dynamic populations in which follow-up is difficult.
Disadvantages:
- They are subject to selection bias.
- They are inefficient for rare exposures.
- Information on exposure is subject to observation bias.
- They generally do not allow calculation of incidence (absolute risk).
Case-based risk analysis model for rainstorm inundation in metro systems based on a bayesian network
- ORIGINAL PAPER
- Published: 06 May 2024
Cite this article
- Chao Zhang ORCID: orcid.org/0000-0001-7519-4039 1 ,
- Tingxin Qin 1 ,
- Wan Wang 1 ,
- Fengjiao Xu 1 &
- Qian Zhou 1
10 Accesses
Explore all metrics
The intensities and frequencies of extreme rainstorms are increasing, which may result in severe inundation of urban metro systems. Although there is some risk assessment research on regional metro systems based on spatiotemporal data, the characteristics of specific metro stations and shortcomings in the emergency response process need more consideration. In this paper, a risk analysis model for rainstorm inundation in metro systems based on a Bayesian network and a practical case study are proposed. First, the risk factors are obtained by integrating general mechanism analysis and the case study. Second, an event evolution diagram is established to represent the comprehensive evolution process of a potential event. Third, the risk analysis model is established using a Bayesian network model considering the quantitative causal relationships between risk factors. This model is used to analyze the risk of supporting emergency management, including emergency preparation based on critical risk factor sensitivity identification, prewarning response strategy development based on risk analysis as rainstorms occur, and rescue strategy development based on risk analysis as rainstorm water flows into metro tunnels. Furthermore, this model can be flexibly improved as natural hazards and metro systems change and as new problems are exposed in practical cases.
This is a preview of subscription content, log in via an institution to check access.
Access this article
Price includes VAT (Russian Federation)
Instant access to the full article PDF.
Rent this article via DeepDyve
Institutional subscriptions
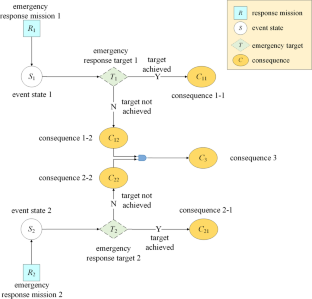
Similar content being viewed by others
Application of the hidden markov model in a dynamic risk assessment of rainstorms in dalian, china.
Multihazard Risk Assessment from Qualitative Methods to Bayesian Networks: Reviewing Recent Contributions and Exploring New Perspectives
Scenario-based risk framework selection and assessment model development for natural disasters: a case study of typhoon storm surges, data availability.
Not applicable.
Code availability
References .
Aoki Y, Yoshizawa A, Taminato T (2016) Anti-inundation measures for underground stations of Tokyo metro. Procedia Eng 165:2–10. https://doi.org/10.1016/j.proeng.2016.11.730
Article Google Scholar
Banuls VA, Turoff M, Hiltz SR (2013) Collaborative scenario modeling in emergency management through cross-impact. Technol Forecast Soc Change 80(9):1756–1774. https://doi.org/10.1016/j.techfore.2012.11.007
China Urban Rail Transit Association (2022) 2021 annual statistical and analysis report of urban rail transit. www.camet.org.cn/tjxx/9944 . Accessed 6 February 2023
Forero-Ortiz E, Martínez-Gomariz E, Porcuna MC, Locatelli L, Russo B (2020) Flood risk assessment in an underground railway system under the impact of climate change—a case study of the Barcelona metro. Sustainability 12:5291. https://doi.org/10.3390/su12135291
Gao L, Zhang L, Li X, Zhou S (2019) Evaluating metropolitan flood coping capabilities under heavy storms. J Hydrol Eng 24:05019011. https://doi.org/10.1061/(asce)he.1943-5584.0001793
Guan C, Dong D, Shen F, Gao X, Chen L (2022) Hierarchical structure model of safety risk factors in new coastal towns: a systematic analysis using the DEMATEL-ISM-SNA method. Int J Environ Res Public Health 19:10496. https://doi.org/10.3390/ijerph191710496
IEC (2019) IEC 31010: 2019 Risk management — Risk assessment techniques. IEC, Geneva, Switerland
ISO (2018) ISO 31000: 2018 Risk management — Guidelines. ISO, Geneva, Switzerland
Jiao L, Li D, Zhang Y, Zhu Y, Huo X, Wu Y (2021) Identification of the key influencing factors of urban rail transit station resilience against disasters caused by rainstorms. Land 10:1298. https://doi.org/10.3390/land10121298
Kang B, Chhipi-Shrestha G, Deng Y, Mori J, Hewage K, Sadiq R (2018) Development of a predictive model for Clostridium difficile infection incidence in hospitals using Gaussian mixture model and Dempster-Shafer theory. Stoch Environ Res Risk Assess 32:1743–1758. https://doi.org/10.1007/s00477-017-1459-z
Lai W-L, Wang H-R, Wang C, Zhang J, Zhao Y (2017) Waterlogging risk assessment based on self-organizing map (SOM) artificial neural networks: a case study of an urban storm in Beijing. J Mt Sci 14:898–905. https://doi.org/10.1007/s11629-016-4035-y
Lyu H-M, Wang G-F, Shen J, Lu L-H, Wang G-Q (2016) Analysis and GIS mapping of flooding hazards on 10 May 2016, Guangzhou. China Water 8:447. https://doi.org/10.3390/w8100447
Lyu H-M, Sun W-J, Shen S-L, Arulrajah A (2018) Flood risk assessment in metro systems of mega-cities using a GIS-based modeling approach. Sci Total Environ 626:1012–1025. https://doi.org/10.1016/j.scitotenv.2018.01.138
Article CAS Google Scholar
Lyu H-M, Shen S-L, Zhou A, Yang J (2019) Perspectives for flood risk assessment and management for mega-city metro system. Tunn Undergr Space Technol 84:31–44. https://doi.org/10.1016/j.tust.2018.10.019
Lyu H-M, Zhou W-H, Shen S-L, Zhou A-N (2020) Inundation risk assessment of metro system using AHP and TFN-AHP in Shenzhen. Sustain Cities Soc 56:102103. https://doi.org/10.1016/j.scs.2020.102103
Norsys Software Corp (2023) Netica’s help system. www.norsys.com/WebHelp/NETICA.htm . Accessed 6 February 2023
Pearl J (1986) Fusion, Propagation, and Structuring in Belief Networks. Artif Intell 29(3):241–288. https://doi.org/10.1016/0004-3702(86)90072-X
Quan R-S (2014) Rainstorm waterlogging risk assessment in central urban area of Shanghai based on multiple scenario simulation. Nat Hazards 73:1569–1585. https://doi.org/10.1007/s11069-014-1156-x
Quan R, Zhang L, Liu M, Lu M, Wang J, Niu H (2011) Risk assessment of rainstorm waterlogging on subway in central urban area of Shanghai, China based on scenario simulation. In: 2011 19th international conference on geoinformatics. IEEE, Shanghai, China, pp 1–6. https://doi.org/10.1109/GeoInformatics.2011.5981176
Sun Z, Zhang J, Zhang Q, Hu Y, Yan D, Wang C (2014) Integrated risk zoning of drought and waterlogging disasters based on fuzzy comprehensive evaluation in Anhui Province, China. Nat Hazards 71:1639–1657. https://doi.org/10.1007/s11069-013-0971-9
Sun S, Zhai J, Li Y, Huang D, Wang G (2020) Urban waterlogging risk assessment in well-developed region of Eastern China. Phys Chem Earth Parts a/b/c 115:102824. https://doi.org/10.1016/j.pce.2019.102824
Sun D, Wang H, Lall U, Huang J, Liu G (2022) Subway travel risk evaluation during flood events based on smart card data. Geomat Nat Hazards Risk 13:2796–2818. https://doi.org/10.1080/19475705.2022.2134056
The Central People’s Government of the People’s Republic of China (2022) Investigation report of rainstorm disaster in Zhengzhou, Henan Province. http://www.xunxian.gov.cn/eportal/fileDir/xunxian/resource/cms/article/3656823/4038345/%E6%B2%B3%E5%8D%97%E9%83%91%E5%B7%9E%E2%80%9C7%C2%B720%E2%80%9D%E7%89%B9%E5%A4%A7%E6%9A%B4%E9%9B%A8%E7%81%BE%E5%AE%B3%E8%B0%83%E6%9F%A5%E6%8A%A5%E5%91%8A.pdf . Accessed 20 July 2022
Wang Y, Liang Y, Sun H (2020) A regret theory-based decision-making method for urban rail transit in emergency response of rainstorm disaster. J Adv Transp 2020:1–12. https://doi.org/10.1155/2020/3235429
Wang J, Liu S, Song Y, Wang J, Wu H (2021) Environmental risk assessment of subway station construction to achieve sustainability using the intuitionistic fuzzy analytic hierarchy process and set pair analysis. Discrete Dyn Nat Soc 2021:1–17. https://doi.org/10.1155/2021/5541493
Wang G, Liu Y, Hu Z, Zhang G, Liu J, Lyu Y, Gu Y, Huang X, Zhang Q, Liu L (2021) Flood risk assessment of subway systems in metropolitan areas under land subsidence scenario: a case study of Beijing. Remote Sens 13:637. https://doi.org/10.3390/rs13040637
Wu H, Wang J (2020) Assessment of waterlogging risk in the deep foundation pit projects based on projection pursuit model. Adv Civ Eng 2020:1–11. https://doi.org/10.1155/2020/2569531
Wu H, Wang J (2021) A method for prediction of waterlogging economic losses in a subway station project. Mathematics 9:1421. https://doi.org/10.3390/math9121421
Wu J, Zhou R, Xu S, Wu Z (2017) Probabilistic analysis of natural gas pipeline network accident based on Bayesian network. J Loss Prev Process Ind 46:126–136. https://doi.org/10.1016/j.jlp.2017.01.025
Xu Q, Han L, Xu K (2022) Causal analysis and prevention measures for extreme heavy rainstorms in Zhengzhou to protect human health. Behav Sci 12:176. https://doi.org/10.3390/bs12060176
Yin Z, Yin J, Xu S, Wen J (2011) Community-based scenario modelling and disaster risk assessment of urban rainstorm waterlogging. J Geogr Sci 21:274–284. https://doi.org/10.1007/s11442-011-0844-7
Yu H, Liang C, Li P, Niu K, Du F, Shao J, Liu Y (2019) Evaluation of waterlogging risk in an urban subway station. Adv Civ Eng 2019:1–12. https://doi.org/10.1155/2019/5393171
Zhang C, Wu J, Hu X, Ni S (2018) A probabilistic analysis model of oil pipeline accidents based on an integrated Event-Evolution-Bayesian (EEB) model. Process Saf Environ Prot 117:694–703. https://doi.org/10.1016/j.psep.2018.06.017
Zhang C, Wang W, Xu F, Chen Y, Qin T (2022) A risk treatment strategy model for oil pipeline accidents based on a bayesian decision network model. Int J Environ Res Public Health 19:13053. https://doi.org/10.3390/ijerph192013053
Download references
Acknowledgements
This work was supported by the National Natural Science Foundation of China (Grant No. 72274181) and the Fundamental Research Funds for the Central Business Unit (Grant No. 512020Y-7502).
This work was funded by the National Natural Science Foundation of China (Grant No. 72274181) and the Fundamental Research Funds for the Central Business Unit (Grant No. 512020Y-7502).
Author information
Authors and affiliations.
Subinstitute for Public Safety Standardization, China National Institute of Standardization, Beijing, 100191, China
Chao Zhang, Tingxin Qin, Wan Wang, Fengjiao Xu & Qian Zhou
You can also search for this author in PubMed Google Scholar
Contributions
C.Z.: Conceptualization, methodology, writing—reviewing and editing, funding acquisition. W.W.: Writing—Original draft. F.X.: Investigation, formal analysis. Q.Z.: Formal analysis, visualization. T.Q.: Supervision, writing—reviewing and editing. All the authors have read and agreed to the published version of the manuscript.
Corresponding author
Correspondence to Chao Zhang .
Ethics declarations
Competing interests.
The authors have no competing interests to declare that they are relevant to the content of this article.
Additional information
Publisher's note.
Springer Nature remains neutral with regard to jurisdictional claims in published maps and institutional affiliations.
Rights and permissions
Springer Nature or its licensor (e.g. a society or other partner) holds exclusive rights to this article under a publishing agreement with the author(s) or other rightsholder(s); author self-archiving of the accepted manuscript version of this article is solely governed by the terms of such publishing agreement and applicable law.
Reprints and permissions
About this article
Zhang, C., Qin, T., Wang, W. et al. Case-based risk analysis model for rainstorm inundation in metro systems based on a bayesian network. Stoch Environ Res Risk Assess (2024). https://doi.org/10.1007/s00477-024-02737-9
Download citation
Accepted : 23 April 2024
Published : 06 May 2024
DOI : https://doi.org/10.1007/s00477-024-02737-9
Share this article
Anyone you share the following link with will be able to read this content:
Sorry, a shareable link is not currently available for this article.
Provided by the Springer Nature SharedIt content-sharing initiative
- Case-based risk analysis model
- Rainstorm inundation
- Metro system
- Bayesian network
- Find a journal
- Publish with us
- Track your research
- Open access
- Published: 09 May 2024
Examining the feasibility of assisted index case testing for HIV case-finding: a qualitative analysis of barriers and facilitators to implementation in Malawi
- Caroline J. Meek 1 , 2 ,
- Tiwonge E. Mbeya Munkhondya 3 ,
- Mtisunge Mphande 4 ,
- Tapiwa A. Tembo 4 ,
- Mike Chitani 4 ,
- Milenka Jean-Baptiste 2 ,
- Dhrutika Vansia 4 ,
- Caroline Kumbuyo 4 ,
- Jiayu Wang 2 ,
- Katherine R. Simon 4 ,
- Sarah E. Rutstein 5 ,
- Clare Barrington 2 ,
- Maria H. Kim 4 ,
- Vivian F. Go 2 &
- Nora E. Rosenberg 2
BMC Health Services Research volume 24 , Article number: 606 ( 2024 ) Cite this article
Metrics details
Assisted index case testing (ICT), in which health care workers take an active role in referring at-risk contacts of people living with HIV for HIV testing services, has been widely recognized as an evidence-based intervention with high potential to increase status awareness in people living with HIV. While the available evidence from eastern and southern Africa suggests that assisted ICT can be an effective, efficient, cost-effective, acceptable, and low-risk strategy to implement in the region, it reveals that feasibility barriers to implementation exist. This study aims to inform the design of implementation strategies to mitigate these feasibility barriers by examining “assisting” health care workers’ experiences of how barriers manifest throughout the assisted ICT process, as well as their perceptions of potential opportunities to facilitate feasibility.
In-depth interviews were conducted with 26 lay health care workers delivering assisted ICT in Malawian health facilities. Interviews explored health care workers’ experiences counseling index clients and tracing these clients’ contacts, aiming to inform development of a blended learning implementation package. Transcripts were inductively analyzed using Dedoose coding software to identify and describe key factors influencing feasibility of assisted ICT. Analysis included multiple rounds of coding and iteration with the data collection team.
Participants reported a variety of barriers to feasibility of assisted index case testing implementation, including sensitivities around discussing ICT with clients, privacy concerns, limited time for assisted index case testing amid high workloads, poor quality contact information, and logistical obstacles to tracing. Participants also reported several health care worker characteristics that facilitate feasibility (knowledge, interpersonal skills, non-stigmatizing attitudes and behaviors, and a sense of purpose), as well as identified process improvements with the potential to mitigate barriers.
Conclusions
Maximizing assisted ICT’s potential to increase status awareness in people living with HIV requires equipping health care workers with effective training and support to address and overcome the many feasibility barriers that they face in implementation. Findings demonstrate the need for, as well as inform the development of, implementation strategies to mitigate barriers and promote facilitators to feasibility of assisted ICT.
Trial registration
NCT05343390. Date of registration: April 25, 2022.
Peer Review reports
Introduction
To streamline progress towards its goal of ending AIDS as a public health threat by 2030, the Joint United Nations Programme on HIV/AIDS (UNAIDS) launched a set of HIV testing and treatment targets [ 1 ]. Adopted by United Nations member states in June 2021, the targets call for 95% of all people living with HIV (PLHIV) to know their HIV status, 95% of all PLHIV to be accessing sustained antiretroviral therapy (ART), and 95% of all people receiving ART to achieve viral suppression by 2025 [ 2 ]. Eastern and southern Africa has seen promising regional progress towards these targets in recent years, and the region is approaching the first target related to status awareness in PLHIV- in 2022, 92% of PLHIV in the region were aware of their status [ 3 ]. However, several countries in the region lag behind [ 4 ], and as 2025 approaches, it is critical to scale up adoption of evidence-based interventions to sustain and accelerate progress.
Index case testing (ICT), which targets provision of HIV testing services (HTS) for sexual partners, biological children, and other contacts of known PLHIV (“index clients”), is a widely recognized evidence-based intervention used to identify PLHIV by streamlining testing efforts to populations most at risk [ 5 , 6 , 7 ]. Traditional approaches to ICT rely on passive referral, in which index clients invite their contacts for testing [ 5 ]. However, the World Health Organization (WHO) and the President’s Emergency Plan for HIV/AIDS Relief (PEPFAR) have both recommended assisted approaches to ICT [ 6 , 8 , 9 , 10 ], in which health care workers (HCWs) take an active role in referral of at-risk contacts for testing, due to evidence of improved effectiveness in identifying PLHIV compared to passive approaches [ 10 , 11 , 12 , 13 , 14 ]. As a result, there have been several efforts to scale assisted ICT throughout eastern and southern Africa in recent years [ 15 , 16 , 17 , 18 , 19 , 20 ]. In addition to evidence indicating that assisted ICT can be effective in increasing HIV testing and case-finding [ 16 , 17 , 21 , 22 , 23 , 24 ], implementation evidence [ 25 ] from the region suggests that assisted ICT can be an efficient [ 14 ], acceptable [ 5 , 13 , 15 , 18 , 20 , 21 , 26 ], cost-effective [ 27 ], and low-risk [ 21 , 22 , 24 , 28 , 29 ] strategy to promote PLHIV status awareness. However, the few studies that focus on feasibility, or the extent to which HCWs can successfully carry out assisted ICT [ 25 ], suggest that barriers exist to feasibility of effective implementation [ 18 , 19 , 20 , 30 , 31 , 32 ]. Developing informed implementation strategies to mitigate these barriers requires more detailed examination of how these barriers manifest throughout the assisted ICT process, as well as of potential opportunities to facilitate feasibility, from the perspective of the HCWs who are doing the “assisting”.
This qualitative analysis addresses this need for further detail by exploring “assisting” HCWs’ perspectives of factors that influence the feasibility of assisted ICT, with a unique focus on informing development of effective implementation strategies to best support assisted ICT delivery in the context of an implementation science trial in Malawi.
This study was conducted in the Machinga and Balaka districts of Malawi. Malawi is a country in southeastern Africa in which 7.1% of the population lives with HIV and 94% of PLHIV know their status [ 4 ]. Machinga and Balaka are two relatively densely populated districts in the southern region of Malawi [ 33 ] with HIV prevalence rates similar to the national average [ 34 ]. We selected Machinga and Balaka because they are prototypical of districts in Malawi implementing Ministry of Health programs with support from an implementing partner.
Malawi has a long-established passive ICT program, and in 2019 the country also adopted an assisted component, known as voluntary assisted partner notification, as part of its national HIV testing policy [ 32 ]. In Malawi, ICT is conducted through the following four methods, voluntarily selected by the index client: 1) passive referral, in which HCWs encourage the index client to refer partners for voluntary HTS, 2) contract referral, in which HCWs establish an informal ‘contract’ with index clients that agrees upon a date that the HCW can contact the contact clients if they have not yet presented for HTS; 3) provider referral, in which HCWs contact and offer voluntary HTS to contact clients; and 3) dual referral, in which HCWs accompany and provide support to index clients in disclosing their status and offering HTS to their partners [ 8 ].
While Malawi has one of the lowest rates of qualified clinical HCWs globally (< 5 clinicians per 100,000 people) [ 35 ], the country has a strong track record of shifting HTS tasks to lay HCWs, who have been informally trained to perform certain health care delivery functions but do not have a formal professional/para-professional certification or tertiary education degree, in order to mitigate this limited medical workforce capacity [ 32 , 36 ]. In Malawi, lay HCW roles include HIV Diagnostic Assistants (who are primarily responsible for HIV testing and counseling, including index case counseling) and community health workers (who are responsible for a wider variety of tasks, including index case counseling and contact tracing) [ 32 ]. Non-governmental organization implementing partners, such as the Tingathe Program, play a critical role in harnessing Malawian lay HCW capacity to rapidly and efficiently scale up HTS, including assisted ICT [ 32 , 37 , 38 , 39 ].
Study design
Data for this analysis were collected as part of formative research for a two-arm cluster randomized control trial examining a blended learning implementation package as a strategy for building HCW capacity in assisted ICT [ 40 ]. Earlier work [ 32 ] established the theoretical basis for testing the blended learning implementation package, which combines individual asynchronous modules with synchronous small-group interactive sessions to enhance training and foster continuous quality improvement. The formative research presented in this paper aimed to further explore factors influencing feasibility of the assisted ICT from the perspective of HCWs in order to inform development of the blended learning implementation package.
Prior to the start of the trial (October-December 2021), the research team conducted 26 in-depth interviews (IDIs) with lay HCWs at 14 of the 34 facilities included in the parent trial. We purposively selected different types of facilities (hospitals, health centers, and dispensaries) in both districts and from both randomization arms, as this served as a qualitative baseline for a randomized trial. Within these facilities, we worked with facility supervisors to purposively select HCWs who were actively engaged in Malawi’s ICT program from the larger sample of HCWs eligible for the parent trial (had to be at least 18 years old, employed full-time at one of the health facilities included in the parent trial, and involved in counseling index clients and/or tracing their contacts). The parent trial enrolled 306 HCWs, who were primarily staff hired by Tingathe Program to support facilities implementing Malawi’s national HIV program.
Data collection
IDIs were conducted by three trained Malawian interviewers in a private setting using a semi-structured guide. IDIs were conducted over the phone when possible ( n = 18) or in-person at sites with limited phone service ( n = 8). The semi-structured guide was developed for this study through a series of rigorous, iterative discussions among the research team (Additional file 1 ). The questions used for this analysis were a subset of a larger interview. The interview guide questions for this analysis explored HCWs’ experiences with assisted ICT, including barriers and facilitators to implementation. Probing separately about the processes of counseling index clients and tracing their contacts, interviewers asked questions such as “What is the first thing that comes to mind when you think of counseling index clients/tracing contacts?”, “What aspects do you [like/not like] about…?” and “What do your colleagues say about…?”. When appropriate, interviewers probed further about how specific factors mentioned by the participant facilitate or impede the ICT implementation experience.
The IDIs lasted from 60–90 min and were conducted in Chichewa, a local language in Malawi. Eleven audio recordings were transcribed verbatim in Chichewa before being translated into English and 15 recordings were directly translated and transcribed into English. Interviewers summarized each IDI after it was completed, and these summaries were discussed with the research team routinely.
Data analysis
The research team first reviewed all of the interview summaries individually and then met multiple times to discuss initial observations, refining the research question and scope of analysis. A US-based analyst (CJM) with training in qualitative analysis used an inductive approach to develop a codebook, deriving broad codes from the implementation factors mentioned by participants throughout their interviews. Along with focused examination of the transcripts, she consulted team members who had conducted the IDIs with questions or clarifications. CJM regularly met with Malawian team members (TEMM, MM, TAT) who possess the contextual expertise necessary to verify and enhance meaning. She used the Dedoose (2019) web application to engage in multiple rounds of coding, starting with codes representing broad implementation factors and then further refining the codebook as needed to capture the nuanced manifestations of these barriers and facilitators. Throughout codebook development and refinement, the analyst engaged in memoing to track first impressions, thought processes, and coding decisions. The analyst presented the codebook and multiple rounds of draft results to the research team. All transcripts and applied codes were also reviewed in detail by additional team members (MJB, DV). Additional refinements to the codebook and results interpretations were iteratively made based on team feedback.
Ethical clearance
Ethical clearance was provided by UNC’s IRB, Malawi’s National Health Sciences Research Committee, and the Baylor College of Medicine IRB. Written informed consent was obtained from all participants in the main study and interviewers confirmed verbal consent before starting the IDIs.
Participant characteristics are described in Table 1 below.
Factors influencing feasibility of assisted ICT: barriers and facilitators
Participants described a variety of barriers and facilitators to feasibility of assisted ICT, manifesting across the index client counseling and contact client tracing phases of the implementation process. Identified barriers included sensitivities around discussing ICT with clients, privacy concerns, limited time for ICT amid high workloads, poor quality contact information, and logistical obstacles to tracing. In addition to these barriers, participants also described several HCW characteristics that facilitated feasibility: ICT knowledge, interpersonal skills, positive attitudes towards clients, and sense of purpose. Barriers and facilitators are mapped to the ICT process in Fig. 1 and described in greater detail in further sections.
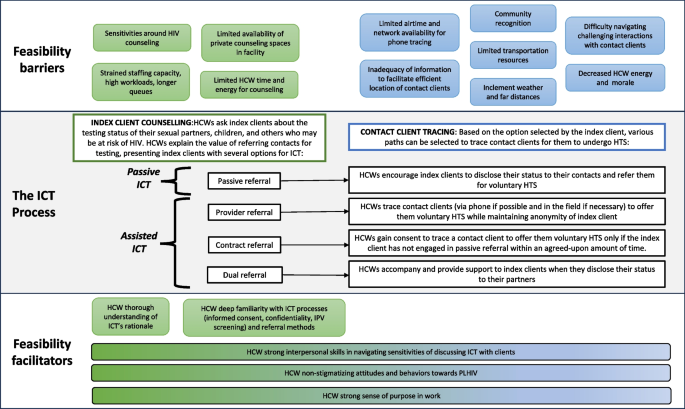
Conceptual diagram mapping feasibility barriers and facilitators to the ICT process
Feasibility barriers
Sensitivities around discussing ict with clients.
Participants described ICT as a highly sensitive topic to approach with clients. Many expressed a feeling of uncertainty around how open index clients will be to sharing information about their contacts, as well as how contacts will react when approached for HTS. When asked about difficult aspects of counseling index clients, many HCWs mentioned clients’ hesitance or declination to participate in assisted ICT and share their contacts. Further, several HCWs mentioned that some index clients would provide false contact information. These index client behaviors were often attributed to confidentiality concerns, fear of unwanted status disclosure, and fear of the resulting implications of status disclosure: “They behave that way because they think you will be telling other people about their status…they also think that since you know it means their life is done, you will be looking at them differently .” Populations commonly identified as particularly likely to hesitate, refuse, or provide false information included youth (described as “ shy ” “ thinking they know a lot ” and “ difficult to reveal their contacts ”) and newly diagnosed clients (“it may be hard for them to accept [their HIV diagnosis]” ). One participant suggested that efforts to pair index clients with same-sex HCWs could make them more comfortable to discuss their contacts.
When asked about the first things that come to mind when starting to trace contacts, many participants discussed wondering how they will be received by the contact and preparing themselves to approach the contact. When conducting provider or contract referral, HCWs described a variety of challenging reactions that can occur when they approach a contact for HTS- including delay or refusal of testing, excessive questioning about the identity of the index client who referred them for testing, and even anger or aggression. Particularly mentioned in the context of male clients, these kinds of reactions can lead to stress and uncertain next steps for HCWs: “I was very tensed up. I was wondering to myself what was going to happen…he was talking with anger.”
Participants also noted the unique sensitivities inherent in conducting dual referral and interacting with sexual partners of index clients, explaining that HIV disclosure can create acute conflict in couples due to perceived blame and assumptions of infidelity. They recounted these scenarios as particularly difficult to navigate, with high stakes that require high-quality counseling skills: “sometimes if you do not have good counseling the marriage happens to get to an end.” . Some participants discussed concern about index client risk of intimate partner violence (IPV) upon partner disclosure: “they think that if they go home and [disclose their HIV status], the marriage will end right there, or for some getting to a point of [being] beaten.”
Privacy concerns
Participants also reported that clients highly value privacy, which can be difficult to secure throughout the ICT process. In the facility, while participants largely indicated that counseling index clients was much more successful when conducted in a private area, many reported limited availability of private counseling space. One participant described this challenge: “ if I’m counseling an index client and people keep coming into the room…this compromises the whole thing because the client becomes uncomfortable in the end.” Some HCWs mentioned working around this issue through use of screens, “do-not-disturb” signs, outdoor spots, and tents.
Participants also noted maintaining privacy as a challenge when tracing contact clients in the field, as they sometimes find clients in a situation that is not conducive to private conversations. One participant described: “ we get to the house and find that there are 4, 5 people with our [contact client]…it doesn’t go well…That is a mission gone wrong. ” Participants also noted that HCWs are also often easily recognizable in the community due to their bikes and cars, which exacerbates the risk of compromising privacy. To address privacy challenges in the community, participants reported strategies to increase discretion, including dressing to blend in with the community, preparing an alternate reason to be looking for the client, and offering HTS to multiple people or households to avoid singling out one person.
Limited time for ICT amid high workloads
Some participants indicated that strained staffing capacity leads HCWs to have to perform multiple roles, expressing challenges in balancing their ICT work with their other tasks. As one participant described, “Sometimes it is found that you are assigned a task here at the hospital to screen anyone who comes for blood testing, but you are also supposed to follow up [with] the contacts the same day- so it becomes a problem…you fail to follow up [with] the contacts.” Some also described being the only, or one of few staff responsible for ICT: “You’re doing this work alone, so you can see that it is a big task to do it single-handedly.” The need to counsel each index client individually, as a result of confidentiality concerns, further increases workload for the limited staff assigned to this work. Further, HCWs often described contact tracing in the field as time-consuming and physically taxing, which leaves them less time and energy for counseling. Many HCWs noted the need to hire more staff dedicated to ICT work.
High workloads also resulted in shorter appointments and less time to counsel index clients, which participants reported limits the opportunity for rapport that facilitates openness or probes for detailed information about sexual partners. Participants emphasized the importance of having enough time to meaningfully engage with index clients: “For counseling you cannot have a limit to say, ‘I will talk to him for 5 min only.’ …That is not counseling then. You are supposed to stay up until…you feel that this [person] is fulfilled.” . In addition, high workload can reduce the capacity of HCWs to deliver quality counseling: “So you find that as you go along with the counseling, you can do better with the first three clients but the rest, you are tired and you do short cuts.”
High workloads also lead to longer queues, which may deter clients from coming into the clinic or cause them to leave before receiving services: “Sometimes because of shortage of staff, it happens that you have been assigned a certain task that you were supposed to do but at the same time there are clients who were supposed to be counseled. As a result, because you spent more time on the other task as a result you lose out some of the clients because you find that they have gone.” In response to long queues, several participants described ‘fast-tracking’ contact clients who come in for HTS in effort to maximize case-finding by prioritizing those who have been identified as at risk of HIV.
Poor quality contact information
Participants repeatedly discussed the importance of eliciting accurate information about a person’s sexual partners, including where, when, and how to best contact them. As one participant said, “ Once the index has given us the wrong information then everything cannot work, it becomes wrong…if he gives us full information [with] the right details then everything becomes successful and happens without a problem. ” Adequate information is a critical component of the ICT process, and incorrect or incomplete information delays or prevents communication with contact clients.
Inadequate information, which can include incorrect or incomplete names, phone numbers, physical addresses, and contextual details, can arise from a variety of scenarios. Most participants mentioned index clients providing incorrect information as a concern. This occurred either intentionally to avoid disclosure or unintentionally if information was not known. Poor quality contact information also results from insufficient probing and poor documentation, which is often exacerbated by aforementioned HCW time and energy constraints. In one participant’s words, “The person who has enlisted the contact…is the key person who can make sure that our tracing is made easy.” Participants noted the pivotal role of the original HCW who first interacts with the index client in not only eliciting correct locator information but also eliciting detailed contextual information. For example, details about a contact client’s profession are helpful to trace the client at a time when they will likely be at home. Other helpful information included nicknames, HIV testing history, and notes about confidentiality concerns.
Logistical obstacles to tracing
Some contact clients are reached by phone whereas others must be physically traced in the community. Some participants reported difficulty with tracing via phone, frequently citing network problems and lack of sufficient airtime allocated by the facility. Participants also reported that some clients were unreachable by phone, necessitating physical tracing. Physically tracing a contact client requires a larger investment of resources than phone tracing, especially when the client lives at a far distance from the clinic. Participants frequently discussed having to travel far distances to reach contact clients, an issue some saw as exacerbated by people who travel to clinics at far distances due to privacy concerns.
While most participants reported walking or biking to reach contact clients in the community, some mentioned using a motorcycle or Tingathe vehicle. However, access to vehicles is often limited and these transportation methods require additional expenses for fuel. Walking or biking was also reported to expose HCWs to inclement weather, including hot or rainy seasons, and potential safety risks such as violence.
Participants reported that traveling far distances can be physically taxing and time-consuming, sometimes rendering them too tired or busy to attend to other tasks. Frequent travel influenced HCW morale, particularly when a tracing effort did not result in successfully recruiting a contact client. Participants frequently described this perception of wasted time and energy as “ painful ”, with the level of distress often portrayed as increasing with the distance travelled. As one HCW said, “You [can] find out that he gave a false address. That is painful because it means you have done nothing for the person, you travelled for nothing.”
HCWs described multiple approaches used to strategically allocate limited resources for long distances. These approaches included waiting to physically trace until there are multiple clients in a particular area, reserving vehicle use for longer trips, and coordinating across HCWs to map out contact client locations. HCWs also mentioned provision of rain gear and sun protection to mitigate uncomfortable travel. Another approach involved allocating contact tracing to HCWs based in the same communities as the contact clients.
Feasibility facilitators
Hcw knowledge about ict.
Participants reported that HCWs with a thorough understanding of ICT’s rationale and purpose can facilitate client openness. Clients were more likely to engage with HCWs about assisted ICT if they understood the benefits to themselves and their loved ones. One HCW stated, “If the person understands why we need the information, they will give us accurate information.”
Participants also discussed the value of deep HCW familiarity with ICT procedures and processes, particularly regarding screening clients for IPV and choosing referral method. One participant described the importance of clearly explaining various referral methods to clients: “So…people come and choose the method they like…when you explain things clearly it is like the index client is free to choose a method which the contact can use for testing”. Thorough knowledge of available referral methods allows HCWs to actively engage with index clients to discuss strategies to refer contacts in a way that fits their unique confidentiality needs, which was framed as particularly important when IPV is identified as a concern. Multiple participants suggested the use of flipcharts or videos, saying these would save limited HCW time and energy, fill information gaps, and provide clients with a visual aid to supplement the counseling. Others suggested recurring opportunities for training, to continuously “refresh” their ICT knowledge in order to facilitate implementation.
HCW interpersonal skills
In addition, HCWs’ ability to navigate sensitive conversations about HIV was noted as a key facilitator of successful implementation. Interpersonal skills were mentioned as mitigating the role’s day-to-day uncertainty by preparing HCWs to engage with clients, especially newly diagnosed clients: “ I need to counsel them skillfully so that they understand what I mean regardless that they have just tested positive for HIV.”
When discussing strategies to build HCW skills in counseling index clients and tracing contact clients, participants suggested establishing regular opportunities to discuss challenges and share approaches to address these challenges: “ I think that there should be much effort on the [HCWs] doing [ICT]. For example, what do I mean, they should be having a meeting with the facility people to ask what challenges are you facing and how can we end them?”. Another participant further elaborated, saying “We should be able to share experiences with our [colleagues] so that we can all learn from one another. And also, there are other people who are really brilliant at their job. Those people ought to come visit us and see how we are doing. That is very motivating.”
HCW non-stigmatizing attitudes and behaviors
Participants also highlighted the role of empathy and non-judgement in building trust with clients: “ Put yourself in that other person’s shoes. In so doing, the counseling session goes well. Understanding that person, that what is happening to them can also happen to you. ”. Participants viewed trust-building as critical to facilitating client comfort and openness: “if they trust you enough, they will give you the right information.” Further, participants associated HCW assurance of confidentiality with promoting trust and greater information sharing: “ Also assuring them on the issue of confidentiality because confidentiality is a paramount. If there will not be confidentiality then the clients will not reveal.”
HCW sense of purpose
Lastly, several participants reported that a sense of purpose and desire to help people motivated them to overcome the challenges of delivering assisted ICT. One participant said, “ Some of these jobs are a ministry. Counseling is not easy. You just need to tell yourself that you are there to help that person. ” Many seemed to take comfort in the knowledge that their labors, however taxing, would ultimately allow people to know their status, take control of their health, and prevent the spread of HIV. Participants framed the sense of fulfillment from successful ICT implementation as a mitigating factor amidst challenges: “ If [the contact client] has accepted it then I feel that mostly I have achieved the aim of being in the health field…that is why it is appealing to me ”.
Participants described a variety of barriers to assisted ICT implementation, including sensitivities around discussing ICT with clients, privacy concerns, limited time for ICT amid high workloads, poor quality contact information, and logistical obstacles to tracing. These barriers manifested across each step of the process of counseling index clients and tracing contacts. However, participants also identified HCW characteristics and process improvements that can mitigate these barriers.
Further, participants’ descriptions of the assisted ICT process revealed the intimately interconnected nature of factors that influence feasibility of assisted ICT. Sensitivities around HIV, privacy limitations, time constraints, and HCW characteristics all contribute to the extent to which counseling index clients elicits adequate information to facilitate contact tracing. Information quality has implications for HCW capacity, as inadequate information can lead to wasted resources, including HCW time and energy, on contact tracing. The opportunity cost of wasted efforts, which increases as the distance from which the contact client lives from the clinic increases, depletes HCW morale. The resulting acceleration of burnout, which is already fueled by busy workloads and the inherent uncertainty of day-to-day ICT work, further impairs HCW capacity to effectively engage in quality counseling that elicits adequate information from index clients. This interconnectedness suggests that efforts to mitigate barriers at any step of the assisted ICT process may have the potential to ripple across the whole process.
Participants’ descriptions of client confidentiality and privacy concerns, as well as fear of consequences of disclosure, align with previous studies that emphasize stigma as a key barrier to assisted ICT [ 15 , 18 , 19 , 20 , 30 , 31 ] and the overall HIV testing and treatment cascade [ 41 ]. Our findings suggest that anticipated stigma, or the fear of discrimination upon disclosure [ 42 ], drives several key barriers to feasibility of assisted ICT implementation. Previous studies also highlight the key role of HCWs in mitigating barriers related to anticipated stigma; noting the key role of HCW ICT knowledge, interpersonal skills, and non-stigmatizing attitudes/behaviors in securing informed consent from clients for ICT, tailoring the referral strategy to minimize risk to client confidentiality and safety, building trust and rapport with the client, and eliciting accurate contact information from index clients to facilitate contact tracing [ 18 , 19 , 20 , 30 ].
Our findings also reflect previous evidence of logistical challenges related to limited time, space, and resources that can present barriers to feasibility for HCWs [ 18 , 19 , 20 , 30 , 31 ]. Participants in the current study described these logistical challenges as perpetuating HCW burnout, making it harder for them to engage in effective counseling. Cumulative evidence of barriers across different settings (further validated by this study) suggests that assisted ICT implementation may pose greater burden on HCWs than previously thought [ 7 ]. However, our findings also suggest that strategic investment in targeted implementation strategies has the potential to help overcome these feasibility barriers.
In our own work, these findings affirmed the rationale for and informed the development of the blended learning implementation package tested in our trial [ 40 , 43 ]. Findings indicated the need for evidence-based training and support to promote HCW capacity to foster facilitating characteristics. Participants discussed the value of "refresher" opportunities in building knowledge, as well as the value of learning from other’s experiences. The blended learning implementation package balances both needs by providing time for HCWs to master ICT knowledge and skills with a combination of asynchronous, digitally delivered content (which allows for continuous review as a "refresher") and in-person sessions (which allow for sharing, practicing, and feedback). Our findings also highlight the value of flexible referral methods that align with the client’s needs, so our training content includes a detailed description of each referral method process. Further, our training content emphasizes client-centered, non-judgmental counseling as our findings add to cumulative evidence of stigma as a key barrier to assisted ICT implementation [ 41 ].
In addition, participants frequently mentioned informal workarounds currently in use to mitigate barriers or offered up ideas for potential solutions to try. Our blended learning implementation package streamlines these problem-solving processes by offering monthly continuous quality improvement sessions at each facility in our enhanced arm. These sessions allow for structured time to discuss identified barriers, share ideas to mitigate barriers, and develop solutions for sustained process improvement tailored to their specific setting. Initial focus areas for continuous quality improvement discussions include use of space, staffing, allocation of airtime and vehicles, and documentation, which were identified as barriers to feasibility in the current study.
Our study provides a uniquely in-depth examination of HCWs’ experiences implementing assisted ICT, exploring how barriers can manifest and interact with each other at each step of the process to hinder successful implementation. Further, our study has a highly actionable focus on informing development of implementation strategies to support HCWs implementing assisted ICT. Our study also has limitations. Firstly, while our sole focus on HCWs allowed for deeper exploration of assisted ICT from the perspective of those actually implementing it on the ground, this meant that our analysis did not include perspectives of index or contact clients. In addition, we did not conduct sub-group analyses as interpretation of results would be limited by our small sample size.
Assisted ICT has been widely recognized as an evidence-based intervention with high promise to increase PLHIV status awareness [ 5 , 6 , 7 , 10 , 12 , 13 , 14 , 15 , 16 , 17 , 18 , 19 , 20 , 21 , 23 , 24 , 26 , 27 , 28 , 29 ], which is important as countries in eastern and southern Africa strive to reach global UNAIDS targets. Study findings support cumulative evidence that HCWs face a variety of feasibility barriers to assisted ICT implementation in the region; further, the study’s uniquely in-depth focus on the experiences of those doing the “assisting” enhances understanding of how these barriers manifest and informs the development of implementation strategies to mitigate these barriers. Maximizing assisted ICT’s potential to increase HIV testing requires equipping HCWs with effective training and support to address and overcome the many feasibility barriers they face in implementation. Findings demonstrate the need for, as well as inform the development of, implementation strategies to mitigate barriers and promote facilitators to feasibility of assisted ICT.
Availability of data and materials
Qualitative data on which this analysis is based, as well as data collection materials and codebooks, are available from the last author upon reasonable request. The interview guide is included as an additional file.
Abbreviations
Acquired Immunodeficiency Syndrome
Antiretroviral Therapy
Health Care Worker
Human Immunodeficiency Virus
HIV Testing Services
Index Case Testing
In-Depth Interview
Intimate Partner Violence
Institutional Review Board
President’s Emergency Plan for HIV/AIDS Relief
People Living With HIV
Joint United Nations Programme on HIV/AIDS
World Health Organization
UNAIDS. Prevailing against pandemics by putting people at the centre. Geneva: UNAIDS; 2020.
Google Scholar
Frescura L, Godfrey-Faussett P, Feizzadeh AA, El-Sadr W, Syarif O, Ghys PD, et al. Achieving the 95 95 95 targets for all: A pathway to ending AIDS. PLoS One. 2022;17(8):e0272405.
Article CAS PubMed PubMed Central Google Scholar
UNAIDS. UNAIDS global AIDS update 2023: The path that ends AIDS. New York: United Nations; 2023.
Book Google Scholar
UNAIDS. UNAIDS data 2023. Geneva: Joint United Nations Programme on HIV/AIDS; 2023.
Kahabuka C, Plotkin M, Christensen A, Brown C, Njozi M, Kisendi R, et al. Addressing the first 90: A highly effective partner notification approach reaches previously undiagnosed sexual partners in Tanzania. AIDS Behav. 2017;21(8):2551–60.
Article PubMed PubMed Central Google Scholar
Lasry A, Medley A, Behel S, Mujawar MI, Cain M, Diekman ST, et al. Scaling up testing for human immunodeficiency virus infection among contacts of index patients - 20 countries, 2016–2018. MMWR Morb Mortal Wkly Rep. 2019;68(21):474–7.
Onovo A, Kalaiwo A, Agweye A, Emmanuel G, Keiser O. Diagnosis and case finding according to key partner risk populations of people living with HIV in Nigeria: A retrospective analysis of community-led index partner testing services. EClinicalMedicine. 2022;43:101265.
World Health Organization (WHO). Guidelines on HIV self-testing and partner notification : supplement to Consolidated guidelines on HIV testing services. 2016. https://apps.who.int/iris/bitstream/handle/10665/251655/9789241549868-eng.pdf?sequence=1 . Accessed 19 Apr 2024.
Watts H. Why PEPFAR is going all in on partner notification services. 2019. https://programme.ias2019.org/PAGMaterial/PPT/1934_117/Why%20PEPFAR%20is%20all%20in%20for%20PNS%2007192019%20rev.pptx . Accessed 19 Apr 2024.
Dalal S, Johnson C, Fonner V, Kennedy CE, Siegfried N, Figueroa C, et al. Improving HIV test uptake and case finding with assisted partner notification services. AIDS. 2017;31(13):1867–76.
Article PubMed Google Scholar
Mathews C, Coetzee N, Zwarenstein M, Lombard C, Guttmacher S, Oxman A, et al. A systematic review of strategies for partner notification for sexually transmitted diseases, including HIV/AIDS. Int J STD AIDS. 2002;13(5):285–300.
Hogben M, McNally T, McPheeters M, Hutchinson AB. The effectiveness of HIV partner counseling and referral services in increasing identification of HIV-positive individuals a systematic review. Am J Prev Med. 2007;33(2 Suppl):S89-100.
Brown LB, Miller WC, Kamanga G, Nyirenda N, Mmodzi P, Pettifor A, et al. HIV partner notification is effective and feasible in sub-Saharan Africa: opportunities for HIV treatment and prevention. J Acquir Immune Defic Syndr. 2011;56(5):437–42.
Sharma M, Ying R, Tarr G, Barnabas R. Systematic review and meta-analysis of community and facility-based HIV testing to address linkage to care gaps in sub-Saharan Africa. Nature. 2015;528(7580):S77-85.
Edosa M, Merdassa E, Turi E. Acceptance of index case HIV testing and its associated factors among HIV/AIDS Clients on ART follow-up in West Ethiopia: A multi-centered facility-based cross-sectional study. HIV AIDS (Auckl). 2022;14:451–60.
PubMed Google Scholar
Williams D, MacKellar D, Dlamini M, Byrd J, Dube L, Mndzebele P, et al. HIV testing and ART initiation among partners, family members, and high-risk associates of index clients participating in the CommLink linkage case management program, Eswatini, 2016–2018. PLoS ONE. 2021;16(12):e0261605.
Remera E, Nsanzimana S, Chammartin F, Semakula M, Rwibasira GN, Malamba SS, et al. Brief report: Active HIV case finding in the city of Kigali, Rwanda: Assessment of voluntary assisted partner notification modalities to detect undiagnosed HIV infections. J Acquir Immune Defic Syndr. 2022;89(4):423–7.
Article CAS PubMed Google Scholar
Quinn C, Nakyanjo N, Ddaaki W, Burke VM, Hutchinson N, Kagaayi J, et al. HIV partner notification values and preferences among sex workers, fishermen, and mainland community members in Rakai, Uganda: A qualitative study. AIDS Behav. 2018;22(10):3407–16.
Monroe-Wise A, Maingi Mutiti P, Kimani H, Moraa H, Bukusi DE, Farquhar C. Assisted partner notification services for patients receiving HIV care and treatment in an HIV clinic in Nairobi, Kenya: a qualitative assessment of barriers and opportunities for scale-up. J Int AIDS Soc. 2019;22 Suppl 3(Suppl Suppl 3):e25315.
Liu W, Wamuti BM, Owuor M, Lagat H, Kariithi E, Obong’o C, et al. “It is a process” - a qualitative evaluation of provider acceptability of HIV assisted partner services in western Kenya: experiences, challenges, and facilitators. BMC Health Serv Res. 2022;22(1):616.
Myers RS, Feldacker C, Cesar F, Paredes Z, Augusto G, Muluana C, et al. Acceptability and effectiveness of assisted human immunodeficiency virus partner services in Mozambique: Results from a pilot program in a public. Urban Clinic Sex Transm Dis. 2016;43(11):690–5.
Rosenberg NE, Mtande TK, Saidi F, Stanley C, Jere E, Paile L, et al. Recruiting male partners for couple HIV testing and counselling in Malawi’s option B+ programme: an unblinded randomised controlled trial. Lancet HIV. 2015;2(11):e483–91.
Mahachi N, Muchedzi A, Tafuma TA, Mawora P, Kariuki L, Semo BW, et al. Sustained high HIV case-finding through index testing and partner notification services: experiences from three provinces in Zimbabwe. J Int AIDS Soc. 2019;22 Suppl 3(Suppl Suppl 3):e25321.
Cherutich P, Golden MR, Wamuti B, Richardson BA, Asbjornsdottir KH, Otieno FA, et al. Assisted partner services for HIV in Kenya: a cluster randomised controlled trial. Lancet HIV. 2017;4(2):e74–82.
Proctor E, Silmere H, Raghavan R, Hovmand P, Aarons G, Bunger A, et al. Outcomes for implementation research: conceptual distinctions, measurement challenges, and research agenda. Adm Policy Ment Health. 2011;38(2):65–76.
Kamanga G, Brown L, Jawati P, Chiwanda D, Nyirenda N. Maximizing HIV partner notification opportunities for index patients and their sexual partners in Malawi. Malawi Med J. 2015;27(4):140–4.
Rutstein SE, Brown LB, Biddle AK, Wheeler SB, Kamanga G, Mmodzi P, et al. Cost-effectiveness of provider-based HIV partner notification in urban Malawi. Health Policy Plan. 2014;29(1):115–26.
Wamuti BM, Welty T, Nambu W, Chimoun FT, Shields R, Golden MR, et al. Low risk of social harms in an HIV assisted partner services programme in Cameroon. J Int AIDS Soc. 2019;22 Suppl 3(Suppl Suppl 3):e25308.
Henley C, Forgwei G, Welty T, Golden M, Adimora A, Shields R, et al. Scale-up and case-finding effectiveness of an HIV partner services program in Cameroon: an innovative HIV prevention intervention for developing countries. Sex Transm Dis. 2013;40(12):909–14.
Klabbers RE, Muwonge TR, Ayikobua E, Izizinga D, Bassett IV, Kambugu A, et al. Health worker perspectives on barriers and facilitators of assisted partner notification for HIV for refugees and Ugandan nationals: A mixed methods study in West Nile Uganda. AIDS Behav. 2021;25(10):3206–22.
Mugisha N, Tirera F, Coulibaly-Kouyate N, Aguie W, He Y, Kemper K, et al. Implementation process and challenges of index testing in Cote d’Ivoire from healthcare workers’ perspectives. PLoS One. 2023;18(2):e0280623.
Rosenberg NE, Tembo TA, Simon KR, Mollan K, Rutstein SE, Mwapasa V, et al. Development of a Blended Learning Approach to Delivering HIV-Assisted Contact Tracing in Malawi: Applied Theory and Formative Research. JMIR Form Res. 2022;6(4):e32899.
Government of Malawi National Statistical Office. 2018 Malawi population and housing census : main report. 2019. https://malawi.unfpa.org/sites/default/files/resource-pdf/2018%20Malawi%20Population%20and%20Housing%20Census%20Main%20Report%20%281%29.pdf . Accessed 19 April 2024.
Wolock TM, Flaxman S, Chimpandule T, Mbiriyawanda S, Jahn A, Nyirenda R, et al. Subnational HIV incidence trends in Malawi: large, heterogeneous declines across space. medRxiv (PREPRINT). 2023. https://www.ncbi.nlm.nih.gov/pmc/articles/PMC9915821/ . Accessed 19 Apr 2024.
World Health Organization (WHO). Medical doctors (per 10,000). 2020. https://www.who.int/data/gho/data/indicators/indicator-details/GHO/medical-doctors-(per-10-000-population . Accessed 19 Apr 2024.
Flick RJ, Simon KR, Nyirenda R, Namachapa K, Hosseinipour MC, Schooley A, et al. The HIV diagnostic assistant: early findings from a novel HIV testing cadre in Malawi. AIDS. 2019;33(7):1215–24.
Kim MH, Ahmed S, Buck WC, Preidis GA, Hosseinipour MC, Bhalakia A, et al. The Tingathe programme: a pilot intervention using community health workers to create a continuum of care in the prevention of mother to child transmission of HIV (PMTCT) cascade of services in Malawi. J Int AIDS Soc. 2012;15(Suppl 2):17389.
Simon KR, Hartig M, Abrams EJ, Wetzel E, Ahmed S, Chester E, et al. The Tingathe Surge: a multi-strategy approach to accelerate HIV case finding in Malawi. Public Health Action. 2019;9(3):128–34.
Ahmed S, Kim MH, Dave AC, Sabelli R, Kanjelo K, Preidis GA, et al. Improved identification and enrolment into care of HIV-exposed and -infected infants and children following a community health worker intervention in Lilongwe, Malawi. J Int AIDS Soc. 2015;18(1):19305.
Tembo TA, Mollan K, Simon K, Rutstein S, Chitani MJ, Saha PT, et al. Does a blended learning implementation package enhance HIV index case testing in Malawi? A protocol for a cluster randomised controlled trial. BMJ Open. 2024;14(1):e077706.
Nyblade L, Mingkwan P, Stockton MA. Stigma reduction: an essential ingredient to ending AIDS by 2030. Lancet HIV. 2021;8(2):e106–13.
Nyblade L, Stockton M, Nyato D, Wamoyi J. Perceived, anticipated and experienced stigma: exploring manifestations and implications for young people’s sexual and reproductive health and access to care in North-Western Tanzania. Cult Health Sex. 2017;19(10):1092–107.
Tembo TA, Simon KR, Kim MH, Chikoti C, Huffstetler HE, Ahmed S, et al. Pilot-Testing a Blended Learning Package for Health Care Workers to Improve Index Testing Services in Southern Malawi: An Implementation Science Study. J Acquir Immune Defic Syndr. 2021;88(5):470–6.
Download references
Acknowledgements
We are grateful to the Malawian health care workers who shared their experiences through in-depth interviews, as well as to the study team members in Malawi and the United States for their contributions.
Research reported in this publication was funded by the National Institutes of Health (R01 MH124526) with support from the University of North Carolina at Chapel Hill Center for AIDS Research (P30 AI50410) and the Fogarty International Center of the National Institutes of Health (D43 TW010060 and R01 MH115793-04). The funders had no role in trial design, data collection and analysis, decision to publish or preparation of the manuscript.
Author information
Authors and affiliations.
RTI International, Research Triangle Park, NC, USA
Caroline J. Meek
Gillings School of Global Public Health, University of North Carolina at Chapel Hill, Chapel Hill, NC, USA
Caroline J. Meek, Milenka Jean-Baptiste, Jiayu Wang, Clare Barrington, Vivian F. Go & Nora E. Rosenberg
Kamuzu University of Health Sciences, Blantyre, Malawi
Tiwonge E. Mbeya Munkhondya
Baylor College of Medicine Children’s Foundation, Lilongwe, Malawi
Mtisunge Mphande, Tapiwa A. Tembo, Mike Chitani, Dhrutika Vansia, Caroline Kumbuyo, Katherine R. Simon & Maria H. Kim
Department of Medicine, Division of Infectious Diseases, University of North Carolina at Chapel Hill, Chapel Hill, NC, USA
Sarah E. Rutstein
You can also search for this author in PubMed Google Scholar
Contributions
TAT, KRS, SER, MHK, VFG, and NER contributed to overall study conceptualization, with CJM, CB, and NER leading conceptualization of the analysis presented in this study. Material preparation and data collection were performed by TEMM, MM, TAT, MC, and CK. Analysis was led by CJM with support from MJB and DV. The first draft of the manuscript was written by CJM with consultation from NER, TEMM, MM, TAT, MJB, and DV. JW provided quantitative analysis support for participant characteristics. All authors commented on previous versions of the manuscript. All authors read and approved the final manuscript.
Corresponding author
Correspondence to Caroline J. Meek .
Ethics declarations
Ethics approval and consent to participate.
Ethical clearance was provided by the Malawi National Health Science Research Committee (NHSRC; #20/06/2566), University of North Carolina Institution Review Board (UNC IRB; #20–1810) and the Baylor College of Medicine institutional review board (Baylor IRB; H-48800). The procedures used in this study adhere to the tenets of the Declaration of Helsinki. Written informed consent for participation was obtained from all study participants prior to enrollment in the parent study. Interviewers also engaged in informal verbal discussion of consent immediately ahead of in-depth interviews.
Consent for publication
Not applicable. No identifying information is included in the manuscript.
Competing interests
The authors declare no competing interests.
Additional information
Publisher’s note.
Springer Nature remains neutral with regard to jurisdictional claims in published maps and institutional affiliations.
Supplementary Information
Supplementary material 1., rights and permissions.
Open Access This article is licensed under a Creative Commons Attribution 4.0 International License, which permits use, sharing, adaptation, distribution and reproduction in any medium or format, as long as you give appropriate credit to the original author(s) and the source, provide a link to the Creative Commons licence, and indicate if changes were made. The images or other third party material in this article are included in the article's Creative Commons licence, unless indicated otherwise in a credit line to the material. If material is not included in the article's Creative Commons licence and your intended use is not permitted by statutory regulation or exceeds the permitted use, you will need to obtain permission directly from the copyright holder. To view a copy of this licence, visit http://creativecommons.org/licenses/by/4.0/ . The Creative Commons Public Domain Dedication waiver ( http://creativecommons.org/publicdomain/zero/1.0/ ) applies to the data made available in this article, unless otherwise stated in a credit line to the data.
Reprints and permissions
About this article
Cite this article.
Meek, C.J., Munkhondya, T.E.M., Mphande, M. et al. Examining the feasibility of assisted index case testing for HIV case-finding: a qualitative analysis of barriers and facilitators to implementation in Malawi. BMC Health Serv Res 24 , 606 (2024). https://doi.org/10.1186/s12913-024-10988-z
Download citation
Received : 31 August 2023
Accepted : 12 April 2024
Published : 09 May 2024
DOI : https://doi.org/10.1186/s12913-024-10988-z
Share this article
Anyone you share the following link with will be able to read this content:
Sorry, a shareable link is not currently available for this article.
Provided by the Springer Nature SharedIt content-sharing initiative
- HIV testing and counseling
- Index case testing
- Assisted partner notification services
- Implementation science
- Health care workers
BMC Health Services Research
ISSN: 1472-6963
- General enquiries: [email protected]
Thank you for visiting nature.com. You are using a browser version with limited support for CSS. To obtain the best experience, we recommend you use a more up to date browser (or turn off compatibility mode in Internet Explorer). In the meantime, to ensure continued support, we are displaying the site without styles and JavaScript.
- View all journals
- Explore content
- About the journal
- Publish with us
- Sign up for alerts
- Published: 08 May 2024
A meta-analysis on global change drivers and the risk of infectious disease
- Michael B. Mahon ORCID: orcid.org/0000-0002-9436-2998 1 , 2 na1 ,
- Alexandra Sack 1 , 3 na1 ,
- O. Alejandro Aleuy 1 ,
- Carly Barbera 1 ,
- Ethan Brown ORCID: orcid.org/0000-0003-0827-4906 1 ,
- Heather Buelow ORCID: orcid.org/0000-0003-3535-4151 1 ,
- David J. Civitello 4 ,
- Jeremy M. Cohen ORCID: orcid.org/0000-0001-9611-9150 5 ,
- Luz A. de Wit ORCID: orcid.org/0000-0002-3045-4017 1 ,
- Meghan Forstchen 1 , 3 ,
- Fletcher W. Halliday 6 ,
- Patrick Heffernan 1 ,
- Sarah A. Knutie 7 ,
- Alexis Korotasz 1 ,
- Joanna G. Larson ORCID: orcid.org/0000-0002-1401-7837 1 ,
- Samantha L. Rumschlag ORCID: orcid.org/0000-0003-3125-8402 1 , 2 ,
- Emily Selland ORCID: orcid.org/0000-0002-4527-297X 1 , 3 ,
- Alexander Shepack 1 ,
- Nitin Vincent ORCID: orcid.org/0000-0002-8593-1116 1 &
- Jason R. Rohr ORCID: orcid.org/0000-0001-8285-4912 1 , 2 , 3 na1
Nature ( 2024 ) Cite this article
192 Altmetric
Metrics details
- Infectious diseases
Anthropogenic change is contributing to the rise in emerging infectious diseases, which are significantly correlated with socioeconomic, environmental and ecological factors 1 . Studies have shown that infectious disease risk is modified by changes to biodiversity 2 , 3 , 4 , 5 , 6 , climate change 7 , 8 , 9 , 10 , 11 , chemical pollution 12 , 13 , 14 , landscape transformations 15 , 16 , 17 , 18 , 19 , 20 and species introductions 21 . However, it remains unclear which global change drivers most increase disease and under what contexts. Here we amassed a dataset from the literature that contains 2,938 observations of infectious disease responses to global change drivers across 1,497 host–parasite combinations, including plant, animal and human hosts. We found that biodiversity loss, chemical pollution, climate change and introduced species are associated with increases in disease-related end points or harm, whereas urbanization is associated with decreases in disease end points. Natural biodiversity gradients, deforestation and forest fragmentation are comparatively unimportant or idiosyncratic as drivers of disease. Overall, these results are consistent across human and non-human diseases. Nevertheless, context-dependent effects of the global change drivers on disease were found to be common. The findings uncovered by this meta-analysis should help target disease management and surveillance efforts towards global change drivers that increase disease. Specifically, reducing greenhouse gas emissions, managing ecosystem health, and preventing biological invasions and biodiversity loss could help to reduce the burden of plant, animal and human diseases, especially when coupled with improvements to social and economic determinants of health.
This is a preview of subscription content, access via your institution
Access options
Access Nature and 54 other Nature Portfolio journals
Get Nature+, our best-value online-access subscription
24,99 € / 30 days
cancel any time
Subscribe to this journal
Receive 51 print issues and online access
185,98 € per year
only 3,65 € per issue
Buy this article
- Purchase on Springer Link
- Instant access to full article PDF
Prices may be subject to local taxes which are calculated during checkout
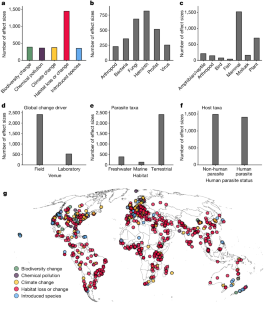
Similar content being viewed by others
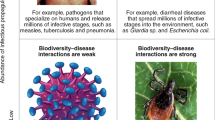
Towards common ground in the biodiversity–disease debate
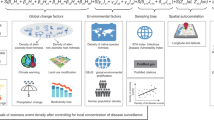
Biological invasions facilitate zoonotic disease emergences
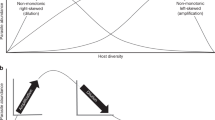
Measuring the shape of the biodiversity-disease relationship across systems reveals new findings and key gaps
Data availability.
All the data for this Article have been deposited at Zenodo ( https://doi.org/10.5281/zenodo.8169979 ) 52 and GitHub ( https://github.com/mahonmb/GCDofDisease ) 53 .
Code availability
All the code for this Article has been deposited at Zenodo ( https://doi.org/10.5281/zenodo.8169979 ) 52 and GitHub ( https://github.com/mahonmb/GCDofDisease ) 53 . R markdown is provided in Supplementary Data 1 .
Jones, K. E. et al. Global trends in emerging infectious diseases. Nature 451 , 990–994 (2008).
Article ADS CAS PubMed PubMed Central Google Scholar
Civitello, D. J. et al. Biodiversity inhibits parasites: broad evidence for the dilution effect. Proc. Natl Acad. Sci USA 112 , 8667–8671 (2015).
Halliday, F. W., Rohr, J. R. & Laine, A.-L. Biodiversity loss underlies the dilution effect of biodiversity. Ecol. Lett. 23 , 1611–1622 (2020).
Article PubMed PubMed Central Google Scholar
Rohr, J. R. et al. Towards common ground in the biodiversity–disease debate. Nat. Ecol. Evol. 4 , 24–33 (2020).
Article PubMed Google Scholar
Johnson, P. T. J., Ostfeld, R. S. & Keesing, F. Frontiers in research on biodiversity and disease. Ecol. Lett. 18 , 1119–1133 (2015).
Keesing, F. et al. Impacts of biodiversity on the emergence and transmission of infectious diseases. Nature 468 , 647–652 (2010).
Cohen, J. M., Sauer, E. L., Santiago, O., Spencer, S. & Rohr, J. R. Divergent impacts of warming weather on wildlife disease risk across climates. Science 370 , eabb1702 (2020).
Article CAS PubMed PubMed Central Google Scholar
Rohr, J. R. et al. Frontiers in climate change-disease research. Trends Ecol. Evol. 26 , 270–277 (2011).
Altizer, S., Ostfeld, R. S., Johnson, P. T. J., Kutz, S. & Harvell, C. D. Climate change and infectious diseases: from evidence to a predictive framework. Science 341 , 514–519 (2013).
Article ADS CAS PubMed Google Scholar
Rohr, J. R. & Cohen, J. M. Understanding how temperature shifts could impact infectious disease. PLoS Biol. 18 , e3000938 (2020).
Carlson, C. J. et al. Climate change increases cross-species viral transmission risk. Nature 607 , 555–562 (2022).
Halstead, N. T. et al. Agrochemicals increase risk of human schistosomiasis by supporting higher densities of intermediate hosts. Nat. Commun. 9 , 837 (2018).
Article ADS PubMed PubMed Central Google Scholar
Martin, L. B., Hopkins, W. A., Mydlarz, L. D. & Rohr, J. R. The effects of anthropogenic global changes on immune functions and disease resistance. Ann. N. Y. Acad. Sci. 1195 , 129–148 (2010).
Rumschlag, S. L. et al. Effects of pesticides on exposure and susceptibility to parasites can be generalised to pesticide class and type in aquatic communities. Ecol. Lett. 22 , 962–972 (2019).
Allan, B. F., Keesing, F. & Ostfeld, R. S. Effect of forest fragmentation on Lyme disease risk. Conserv. Biol. 17 , 267–272 (2003).
Article Google Scholar
Brearley, G. et al. Wildlife disease prevalence in human‐modified landscapes. Biol. Rev. 88 , 427–442 (2013).
Rohr, J. R. et al. Emerging human infectious diseases and the links to global food production. Nat. Sustain. 2 , 445–456 (2019).
Bradley, C. A. & Altizer, S. Urbanization and the ecology of wildlife diseases. Trends Ecol. Evol. 22 , 95–102 (2007).
Allen, T. et al. Global hotspots and correlates of emerging zoonotic diseases. Nat. Commun. 8 , 1124 (2017).
Sokolow, S. H. et al. Ecological and socioeconomic factors associated with the human burden of environmentally mediated pathogens: a global analysis. Lancet Planet. Health 6 , e870–e879 (2022).
Young, H. S., Parker, I. M., Gilbert, G. S., Guerra, A. S. & Nunn, C. L. Introduced species, disease ecology, and biodiversity–disease relationships. Trends Ecol. Evol. 32 , 41–54 (2017).
Barouki, R. et al. The COVID-19 pandemic and global environmental change: emerging research needs. Environ. Int. 146 , 106272 (2021).
Article CAS PubMed Google Scholar
Nova, N., Athni, T. S., Childs, M. L., Mandle, L. & Mordecai, E. A. Global change and emerging infectious diseases. Ann. Rev. Resour. Econ. 14 , 333–354 (2021).
Zhang, L. et al. Biological invasions facilitate zoonotic disease emergences. Nat. Commun. 13 , 1762 (2022).
Olival, K. J. et al. Host and viral traits predict zoonotic spillover from mammals. Nature 546 , 646–650 (2017).
Guth, S. et al. Bats host the most virulent—but not the most dangerous—zoonotic viruses. Proc. Natl Acad. Sci. USA 119 , e2113628119 (2022).
Nelson, G. C. et al. in Ecosystems and Human Well-Being (Millennium Ecosystem Assessment) Vol. 2 (eds Rola, A. et al) Ch. 7, 172–222 (Island Press, 2005).
Read, A. F., Graham, A. L. & Raberg, L. Animal defenses against infectious agents: is damage control more important than pathogen control? PLoS Biol. 6 , 2638–2641 (2008).
Article CAS Google Scholar
Medzhitov, R., Schneider, D. S. & Soares, M. P. Disease tolerance as a defense strategy. Science 335 , 936–941 (2012).
Torchin, M. E. & Mitchell, C. E. Parasites, pathogens, and invasions by plants and animals. Front. Ecol. Environ. 2 , 183–190 (2004).
Bellay, S., de Oliveira, E. F., Almeida-Neto, M. & Takemoto, R. M. Ectoparasites are more vulnerable to host extinction than co-occurring endoparasites: evidence from metazoan parasites of freshwater and marine fishes. Hydrobiologia 847 , 2873–2882 (2020).
Scheffer, M. Critical Transitions in Nature and Society Vol. 16 (Princeton Univ. Press, 2020).
Rohr, J. R. et al. A planetary health innovation for disease, food and water challenges in Africa. Nature 619 , 782–787 (2023).
Reaser, J. K., Witt, A., Tabor, G. M., Hudson, P. J. & Plowright, R. K. Ecological countermeasures for preventing zoonotic disease outbreaks: when ecological restoration is a human health imperative. Restor. Ecol. 29 , e13357 (2021).
Hopkins, S. R. et al. Evidence gaps and diversity among potential win–win solutions for conservation and human infectious disease control. Lancet Planet. Health 6 , e694–e705 (2022).
Mitchell, C. E. & Power, A. G. Release of invasive plants from fungal and viral pathogens. Nature 421 , 625–627 (2003).
Chamberlain, S. A. & Szöcs, E. taxize: taxonomic search and retrieval in R. F1000Research 2 , 191 (2013).
Newman, M. Fundamentals of Ecotoxicology (CRC Press/Taylor & Francis Group, 2010).
Rohatgi, A. WebPlotDigitizer v.4.5 (2021); automeris.io/WebPlotDigitizer .
Lüdecke, D. esc: effect size computation for meta analysis (version 0.5.1). Zenodo https://doi.org/10.5281/zenodo.1249218 (2019).
Lipsey, M. W. & Wilson, D. B. Practical Meta-Analysis (SAGE, 2001).
R Core Team. R: A Language and Environment for Statistical Computing Vol. 2022 (R Foundation for Statistical Computing, 2020); www.R-project.org/ .
Viechtbauer, W. Conducting meta-analyses in R with the metafor package. J. Stat. Softw. 36 , 1–48 (2010).
Pustejovsky, J. E. & Tipton, E. Meta-analysis with robust variance estimation: Expanding the range of working models. Prev. Sci. 23 , 425–438 (2022).
Lenth, R. emmeans: estimated marginal means, aka least-squares means. R package v.1.5.1 (2020).
Bartoń, K. MuMIn: multi-modal inference. Model selection and model averaging based on information criteria (AICc and alike) (2019).
Burnham, K. P. & Anderson, D. R. Multimodel inference: understanding AIC and BIC in model selection. Sociol. Methods Res. 33 , 261–304 (2004).
Article MathSciNet Google Scholar
Marks‐Anglin, A. & Chen, Y. A historical review of publication bias. Res. Synth. Methods 11 , 725–742 (2020).
Nakagawa, S. et al. Methods for testing publication bias in ecological and evolutionary meta‐analyses. Methods Ecol. Evol. 13 , 4–21 (2022).
Gurevitch, J., Koricheva, J., Nakagawa, S. & Stewart, G. Meta-analysis and the science of research synthesis. Nature 555 , 175–182 (2018).
Bates, D., Mächler, M., Bolker, B. & Walker, S. Fitting linear mixed-effects models using lme4. J. Stat. Softw. 67 , 1–48 (2015).
Mahon, M. B. et al. Data and code for ‘A meta-analysis on global change drivers and the risk of infectious disease’. Zenodo https://doi.org/10.5281/zenodo.8169979 (2024).
Mahon, M. B. et al. Data and code for ‘A meta-analysis on global change drivers and the risk of infectious disease’. GitHub github.com/mahonmb/GCDofDisease (2024).
Download references
Acknowledgements
We thank C. Mitchell for contributing data on enemy release; L. Albert and B. Shayhorn for assisting with data collection; J. Gurevitch, M. Lajeunesse and G. Stewart for providing comments on an earlier version of this manuscript; and C. Carlson and two anonymous reviewers for improving this paper. This research was supported by grants from the National Science Foundation (DEB-2109293, DEB-2017785, DEB-1518681, IOS-1754868), National Institutes of Health (R01TW010286) and US Department of Agriculture (2021-38420-34065) to J.R.R.; a US Geological Survey Powell grant to J.R.R. and S.L.R.; University of Connecticut Start-up funds to S.A.K.; grants from the National Science Foundation (IOS-1755002) and National Institutes of Health (R01 AI150774) to D.J.C.; and an Ambizione grant (PZ00P3_202027) from the Swiss National Science Foundation to F.W.H. The funders had no role in study design, data collection and analysis, decision to publish or preparation of the manuscript.
Author information
These authors contributed equally: Michael B. Mahon, Alexandra Sack, Jason R. Rohr
Authors and Affiliations
Department of Biological Sciences, University of Notre Dame, Notre Dame, IN, USA
Michael B. Mahon, Alexandra Sack, O. Alejandro Aleuy, Carly Barbera, Ethan Brown, Heather Buelow, Luz A. de Wit, Meghan Forstchen, Patrick Heffernan, Alexis Korotasz, Joanna G. Larson, Samantha L. Rumschlag, Emily Selland, Alexander Shepack, Nitin Vincent & Jason R. Rohr
Environmental Change Initiative, University of Notre Dame, Notre Dame, IN, USA
Michael B. Mahon, Samantha L. Rumschlag & Jason R. Rohr
Eck Institute of Global Health, University of Notre Dame, Notre Dame, IN, USA
Alexandra Sack, Meghan Forstchen, Emily Selland & Jason R. Rohr
Department of Biology, Emory University, Atlanta, GA, USA
David J. Civitello
Department of Ecology and Evolutionary Biology, Yale University, New Haven, CT, USA
Jeremy M. Cohen
Department of Botany and Plant Pathology, Oregon State University, Corvallis, OR, USA
Fletcher W. Halliday
Department of Ecology and Evolutionary Biology, Institute for Systems Genomics, University of Connecticut, Storrs, CT, USA
Sarah A. Knutie
You can also search for this author in PubMed Google Scholar
Contributions
J.R.R. conceptualized the study. All of the authors contributed to the methodology. All of the authors contributed to investigation. Visualization was performed by M.B.M. The initial study list and related information were compiled by D.J.C., J.M.C., F.W.H., S.A.K., S.L.R. and J.R.R. Data extraction was performed by M.B.M., A.S., O.A.A., C.B., E.B., H.B., L.A.d.W., M.F., P.H., A.K., J.G.L., E.S., A.S. and N.V. Data were checked for accuracy by M.B.M. and A.S. Analyses were performed by M.B.M. and J.R.R. Funding was acquired by D.J.C., J.R.R., S.A.K. and S.L.R. Project administration was done by J.R.R. J.R.R. supervised the study. J.R.R. and M.B.M. wrote the original draft. All of the authors reviewed and edited the manuscript. J.R.R. and M.B.M. responded to reviewers.
Corresponding author
Correspondence to Jason R. Rohr .
Ethics declarations
Competing interests.
The authors declare no competing interests.
Peer review
Peer review information.
Nature thanks Colin Carlson and the other, anonymous, reviewer(s) for their contribution to the peer review of this work. Peer reviewer reports are available.
Additional information
Publisher’s note Springer Nature remains neutral with regard to jurisdictional claims in published maps and institutional affiliations.
Extended data figures and tables
Extended data fig. 1 prisma flowchart..
The PRISMA flow diagram of the search and selection of studies included in this meta-analysis. Note that 77 studies came from the Halliday et al. 3 database on biodiversity change.
Extended Data Fig. 2 Summary of the number of studies (A-F) and parasite taxa (G-L) in the infectious disease database across ecological contexts.
The contexts are global change driver ( A , G ), parasite taxa ( B , H ), host taxa ( C , I ), experimental venue ( D , J ), study habitat ( E , K ), and human parasite status ( F , L ).
Extended Data Fig. 3 Summary of the number of effect sizes (A-I), studies (J-R), and parasite taxa (S-a) in the infectious disease database for various parasite and host contexts.
Shown are parasite type ( A , J , S ), host thermy ( B , K , T ), vector status ( C , L , U ), vector-borne status ( D , M , V ), parasite transmission ( E , N , W ), free living stages ( F , O , X ), host (e.g. disease, host growth, host survival) or parasite (e.g. parasite abundance, prevalence, fecundity) endpoint ( G , P , Y ), micro- vs macroparasite ( H , Q , Z ), and zoonotic status ( I , R , a ).
Extended Data Fig. 4 The effects of global change drivers and subsequent subcategories on disease responses with Log Response Ratio instead of Hedge’s g.
Here, Log Response Ratio shows similar trends to that of Hedge’s g presented in the main text. The displayed points represent the mean predicted values (with 95% confidence intervals) from a meta-analytical model with separate random intercepts for study. Points that do not share letters are significantly different from one another (p < 0.05) based on a two-sided Tukey’s posthoc multiple comparison test with adjustment for multiple comparisons. See Table S 3 for pairwise comparison results. Effects of the five common global change drivers ( A ) have the same directionality, similar magnitude, and significance as those presented in Fig. 2 . Global change driver effects are significant when confidence intervals do not overlap with zero and explicitly tested with two-tailed t-test (indicated by asterisks; t 80.62 = 2.16, p = 0.034 for CP; t 71.42 = 2.10, p = 0.039 for CC; t 131.79 = −3.52, p < 0.001 for HLC; t 61.9 = 2.10, p = 0.040 for IS). The subcategories ( B ) also show similar patterns as those presented in Fig. 3 . Subcategories are significant when confidence intervals do not overlap with zero and were explicitly tested with two-tailed one sample t-test (t 30.52 = 2.17, p = 0.038 for CO 2 ; t 40.03 = 4.64, p < 0.001 for Enemy Release; t 47.45 = 2.18, p = 0.034 for Mean Temperature; t 110.81 = −4.05, p < 0.001 for Urbanization); all other subcategories have p > 0.20. Note that effect size and study numbers are lower here than in Figs. 3 and 4 , because log response ratios cannot be calculated for studies that provide coefficients (e.g., odds ratio) rather than raw data; as such, all observations within BC did not have associated RR values. Despite strong differences in sample size, patterns are consistent across effect sizes, and therefore, we can be confident that the results presented in the main text are not biased because of effect size selection.
Extended Data Fig. 5 Average standard errors of the effect sizes (A) and sample sizes per effect size (B) for each of the five global change drivers.
The displayed points represent the mean predicted values (with 95% confidence intervals) from the generalized linear mixed effects models with separate random intercepts for study (Gaussian distribution for standard error model, A ; Poisson distribution for sample size model, B ). Points that do not share letters are significantly different from one another (p < 0.05) based on a two-sided Tukey’s posthoc multiple comparison test with adjustment for multiple comparisons. Sample sizes (number of studies, n, and effect sizes, k) for each driver are as follows: n = 77, k = 392 for BC; n = 124, k = 364 for CP; n = 202, k = 380 for CC; n = 517, k = 1449 for HLC; n = 96, k = 355 for IS.
Extended Data Fig. 6 Forest plots of effect sizes, associated variances, and relative weights (A), Funnel plots (B), and Egger’s Test plots (C) for each of the five global change drivers and leave-one-out publication bias analyses (D).
In panel A , points are the individual effect sizes (Hedge’s G), error bars are standard errors of the effect size, and size of the points is the relative weight of the observation in the model, with larger points representing observations with higher weight in the model. Sample sizes are provided for each effect size in the meta-analytic database. Effect sizes were plotted in a random order. Egger’s tests indicated significant asymmetries (p < 0.05) in Biodiversity Change (worst asymmetry – likely not bias, just real effect of positive relationship between diversity and disease), Climate Change – (weak asymmetry, again likely not bias, climate change generally increases disease), and Introduced Species (relatively weak asymmetry – unclear whether this is a bias, may be driven by some outliers). No significant asymmetries (p > 0.05) were found in Chemical Pollution and Habitat Loss/Change, suggesting negligible publication bias in reported disease responses across these global change drivers ( B , C ). Egger’s test included publication year as moderator but found no significant relationship between Hedge’s g and publication year (p > 0.05) implying no temporal bias in effect size magnitude or direction. In panel D , the horizontal red lines denote the grand mean and SE of Hedge’s g and (g = 0.1009, SE = 0.0338). Grey points and error bars indicate the Hedge’s g and SEs, respectively, using the leave-one-out method (grand mean is recalculated after a given study is removed from dataset). While the removal of certain studies resulted in values that differed from the grand mean, all estimated Hedge’s g values fell well within the standard error of the grand mean. This sensitivity analysis indicates that our results were robust to the iterative exclusion of individual studies.
Extended Data Fig. 7 The effects of habitat loss/change on disease depend on parasite taxa and land use conversion contexts.
A) Enemy type influences the magnitude of the effect of urbanization on disease: helminths, protists, and arthropods were all negatively associated with urbanization, whereas viruses were non-significantly positively associated with urbanization. B) Reference (control) land use type influences the magnitude of the effect of urbanization on disease: disease was reduced in urban settings compared to rural and peri-urban settings, whereas there were no differences in disease along urbanization gradients or between urban and natural settings. C) The effect of forest fragmentation depends on whether a large/continuous habitat patch is compared to a small patch or whether disease it is measured along an increasing fragmentation gradient (Z = −2.828, p = 0.005). Conversely, the effect of deforestation on disease does not depend on whether the habitat has been destroyed and allowed to regrow (e.g., clearcutting, second growth forests, etc.) or whether it has been replaced with agriculture (e.g., row crop, agroforestry, livestock grazing; Z = 1.809, p = 0.0705). The displayed points represent the mean predicted values (with 95% confidence intervals) from a metafor model where the response variable was a Hedge’s g (representing the effect on an infectious disease endpoint relative to control), study was treated as a random effect, and the independent variables included enemy type (A), reference land use type (B), or land use conversion type (C). Data for (A) and (B) were only those studies that were within the “urbanization” subcategory; data for (C) were only those studies that were within the “deforestation” and “forest fragmentation” subcategories. Sample sizes (number of studies, n, and effect sizes, k) in (A) for each enemy are n = 48, k = 98 for Virus; n = 193, k = 343 for Protist; n = 159, k = 490 for Helminth; n = 10, k = 24 for Fungi; n = 103, k = 223 for Bacteria; and n = 30, k = 73 for Arthropod. Sample sizes in (B) for each reference land use type are n = 391, k = 1073 for Rural; n = 29, k = 74 for Peri-urban; n = 33, k = 83 for Natural; and n = 24, k = 58 for Urban Gradient. Sample sizes in (C) for each land use conversion type are n = 7, k = 47 for Continuous Gradient; n = 16, k = 44 for High/Low Fragmentation; n = 11, k = 27 for Clearcut/Regrowth; and n = 21, k = 43 for Agriculture.
Extended Data Fig. 8 The effects of common global change drivers on mean infectious disease responses in the literature depends on whether the endpoint is the host or parasite; whether the parasite is a vector, is vector-borne, has a complex or direct life cycle, or is a macroparasite; whether the host is an ectotherm or endotherm; or the venue and habitat in which the study was conducted.
A ) Parasite endpoints. B ) Vector-borne status. C ) Parasite transmission route. D ) Parasite size. E ) Venue. F ) Habitat. G ) Host thermy. H ) Parasite type (ecto- or endoparasite). See Table S 2 for number of studies and effect sizes across ecological contexts and global change drivers. See Table S 3 for pairwise comparison results. The displayed points represent the mean predicted values (with 95% confidence intervals) from a metafor model where the response variable was a Hedge’s g (representing the effect on an infectious disease endpoint relative to control), study was treated as a random effect, and the independent variables included the main effects and an interaction between global change driver and the focal independent variable (whether the endpoint measured was a host or parasite, whether the parasite is vector-borne, has a complex or direct life cycle, is a macroparasite, whether the study was conducted in the field or lab, habitat, the host is ectothermic, or the parasite is an ectoparasite).
Extended Data Fig. 9 The effects of five common global change drivers on mean infectious disease responses in the literature only occasionally depend on location, host taxon, and parasite taxon.
A ) Continent in which the field study occurred. Lack of replication in chemical pollution precluded us from including South America, Australia, and Africa in this analysis. B ) Host taxa. C ) Enemy taxa. See Table S 2 for number of studies and effect sizes across ecological contexts and global change drivers. See Table S 3 for pairwise comparison results. The displayed points represent the mean predicted values (with 95% confidence intervals) from a metafor model where the response variable was a Hedge’s g (representing the effect on an infectious disease endpoint relative to control), study was treated as a random effect, and the independent variables included the main effects and an interaction between global change driver and continent, host taxon, and enemy taxon.
Extended Data Fig. 10 The effects of human vs. non-human endpoints for the zoonotic disease subset of database and wild vs. domesticated animal endpoints for the non-human animal subset of database are consistent across global change drivers.
(A) Zoonotic disease responses measured on human hosts responded less positively (closer to zero when positive, further from zero when negative) than those measured on non-human (animal) hosts (Z = 2.306, p = 0.021). Note, IS studies were removed because of missing cells. (B) Disease responses measured on domestic animal hosts responded less positively (closer to zero when positive, further from zero when negative) than those measured on wild animal hosts (Z = 2.636, p = 0.008). These results were consistent across global change drivers (i.e., no significant interaction between endpoint and global change driver). As many of the global change drivers increase zoonotic parasites in non-human animals and all parasites in wild animals, this may suggest that anthropogenic change might increase the occurrence of parasite spillover from animals to humans and thus also pandemic risk. The displayed points represent the mean predicted values (with 95% confidence intervals) from a metafor model where the response variable was a Hedge’s g (representing the effect on an infectious disease endpoint relative to control), study was treated as a random effect, and the independent variable of global change driver and human/non-human hosts. Data for (A) were only those diseases that are considered “zoonotic”; data for (B) were only those endpoints that were measured on non-human animals. Sample sizes in (A) for zoonotic disease measured on human endpoints across global change drivers are n = 3, k = 17 for BC; n = 2, k = 6 for CP; n = 25, k = 39 for CC; and n = 175, k = 331 for HLC. Sample sizes in (A) for zoonotic disease measured on non-human endpoints across global change drivers are n = 25, k = 52 for BC; n = 2, k = 3 for CP; n = 18, k = 29 for CC; n = 126, k = 289 for HLC. Sample sizes in (B) for wild animal endpoints across global change drivers are n = 28, k = 69 for BC; n = 21, k = 44 for CP; n = 50, k = 89 for CC; n = 121, k = 360 for HLC; and n = 29, k = 45 for IS. Sample sizes in (B) for domesticated animal endpoints across global change drivers are n = 2, k = 4 for BC; n = 4, k = 11 for CP; n = 7, k = 20 for CC; n = 78, k = 197 for HLC; and n = 1, k = 2 for IS.
Supplementary information
Supplementary information.
Supplementary Discussion, Supplementary References and Supplementary Tables 1–3.
Reporting Summary
Peer review file, supplementary data 1.
R markdown code and output associated with this paper.
Supplementary Table 4
EcoEvo PRISMA checklist.
Rights and permissions
Springer Nature or its licensor (e.g. a society or other partner) holds exclusive rights to this article under a publishing agreement with the author(s) or other rightsholder(s); author self-archiving of the accepted manuscript version of this article is solely governed by the terms of such publishing agreement and applicable law.
Reprints and permissions
About this article
Cite this article.
Mahon, M.B., Sack, A., Aleuy, O.A. et al. A meta-analysis on global change drivers and the risk of infectious disease. Nature (2024). https://doi.org/10.1038/s41586-024-07380-6
Download citation
Received : 02 August 2022
Accepted : 03 April 2024
Published : 08 May 2024
DOI : https://doi.org/10.1038/s41586-024-07380-6
Share this article
Anyone you share the following link with will be able to read this content:
Sorry, a shareable link is not currently available for this article.
Provided by the Springer Nature SharedIt content-sharing initiative
By submitting a comment you agree to abide by our Terms and Community Guidelines . If you find something abusive or that does not comply with our terms or guidelines please flag it as inappropriate.
Quick links
- Explore articles by subject
- Guide to authors
- Editorial policies
Sign up for the Nature Briefing: Anthropocene newsletter — what matters in anthropocene research, free to your inbox weekly.

- Share full article
Advertisement
Supported by
Study Suggests Genetics as a Cause, Not Just a Risk, for Some Alzheimer’s
People with two copies of the gene variant APOE4 are almost certain to get Alzheimer’s, say researchers, who proposed a framework under which such patients could be diagnosed years before symptoms.

By Pam Belluck
Scientists are proposing a new way of understanding the genetics of Alzheimer’s that would mean that up to a fifth of patients would be considered to have a genetically caused form of the disease.
Currently, the vast majority of Alzheimer’s cases do not have a clearly identified cause. The new designation, proposed in a study published Monday, could broaden the scope of efforts to develop treatments, including gene therapy, and affect the design of clinical trials.
It could also mean that hundreds of thousands of people in the United States alone could, if they chose, receive a diagnosis of Alzheimer’s before developing any symptoms of cognitive decline, although there currently are no treatments for people at that stage.
The new classification would make this type of Alzheimer’s one of the most common genetic disorders in the world, medical experts said.
“This reconceptualization that we’re proposing affects not a small minority of people,” said Dr. Juan Fortea, an author of the study and the director of the Sant Pau Memory Unit in Barcelona, Spain. “Sometimes we say that we don’t know the cause of Alzheimer’s disease,” but, he said, this would mean that about 15 to 20 percent of cases “can be tracked back to a cause, and the cause is in the genes.”
The idea involves a gene variant called APOE4. Scientists have long known that inheriting one copy of the variant increases the risk of developing Alzheimer’s, and that people with two copies, inherited from each parent, have vastly increased risk.
The new study , published in the journal Nature Medicine, analyzed data from over 500 people with two copies of APOE4, a significantly larger pool than in previous studies. The researchers found that almost all of those patients developed the biological pathology of Alzheimer’s, and the authors say that two copies of APOE4 should now be considered a cause of Alzheimer’s — not simply a risk factor.
The patients also developed Alzheimer’s pathology relatively young, the study found. By age 55, over 95 percent had biological markers associated with the disease. By 65, almost all had abnormal levels of a protein called amyloid that forms plaques in the brain, a hallmark of Alzheimer’s. And many started developing symptoms of cognitive decline at age 65, younger than most people without the APOE4 variant.
“The critical thing is that these individuals are often symptomatic 10 years earlier than other forms of Alzheimer’s disease,” said Dr. Reisa Sperling, a neurologist at Mass General Brigham in Boston and an author of the study.
She added, “By the time they are picked up and clinically diagnosed, because they’re often younger, they have more pathology.”
People with two copies, known as APOE4 homozygotes, make up 2 to 3 percent of the general population, but are an estimated 15 to 20 percent of people with Alzheimer’s dementia, experts said. People with one copy make up about 15 to 25 percent of the general population, and about 50 percent of Alzheimer’s dementia patients.
The most common variant is called APOE3, which seems to have a neutral effect on Alzheimer’s risk. About 75 percent of the general population has one copy of APOE3, and more than half of the general population has two copies.
Alzheimer’s experts not involved in the study said classifying the two-copy condition as genetically determined Alzheimer’s could have significant implications, including encouraging drug development beyond the field’s recent major focus on treatments that target and reduce amyloid.
Dr. Samuel Gandy, an Alzheimer’s researcher at Mount Sinai in New York, who was not involved in the study, said that patients with two copies of APOE4 faced much higher safety risks from anti-amyloid drugs.
When the Food and Drug Administration approved the anti-amyloid drug Leqembi last year, it required a black-box warning on the label saying that the medication can cause “serious and life-threatening events” such as swelling and bleeding in the brain, especially for people with two copies of APOE4. Some treatment centers decided not to offer Leqembi, an intravenous infusion, to such patients.
Dr. Gandy and other experts said that classifying these patients as having a distinct genetic form of Alzheimer’s would galvanize interest in developing drugs that are safe and effective for them and add urgency to current efforts to prevent cognitive decline in people who do not yet have symptoms.
“Rather than say we have nothing for you, let’s look for a trial,” Dr. Gandy said, adding that such patients should be included in trials at younger ages, given how early their pathology starts.
Besides trying to develop drugs, some researchers are exploring gene editing to transform APOE4 into a variant called APOE2, which appears to protect against Alzheimer’s. Another gene-therapy approach being studied involves injecting APOE2 into patients’ brains.
The new study had some limitations, including a lack of diversity that might make the findings less generalizable. Most patients in the study had European ancestry. While two copies of APOE4 also greatly increase Alzheimer’s risk in other ethnicities, the risk levels differ, said Dr. Michael Greicius, a neurologist at Stanford University School of Medicine who was not involved in the research.
“One important argument against their interpretation is that the risk of Alzheimer’s disease in APOE4 homozygotes varies substantially across different genetic ancestries,” said Dr. Greicius, who cowrote a study that found that white people with two copies of APOE4 had 13 times the risk of white people with two copies of APOE3, while Black people with two copies of APOE4 had 6.5 times the risk of Black people with two copies of APOE3.
“This has critical implications when counseling patients about their ancestry-informed genetic risk for Alzheimer’s disease,” he said, “and it also speaks to some yet-to-be-discovered genetics and biology that presumably drive this massive difference in risk.”
Under the current genetic understanding of Alzheimer’s, less than 2 percent of cases are considered genetically caused. Some of those patients inherited a mutation in one of three genes and can develop symptoms as early as their 30s or 40s. Others are people with Down syndrome, who have three copies of a chromosome containing a protein that often leads to what is called Down syndrome-associated Alzheimer’s disease .
Dr. Sperling said the genetic alterations in those cases are believed to fuel buildup of amyloid, while APOE4 is believed to interfere with clearing amyloid buildup.
Under the researchers’ proposal, having one copy of APOE4 would continue to be considered a risk factor, not enough to cause Alzheimer’s, Dr. Fortea said. It is unusual for diseases to follow that genetic pattern, called “semidominance,” with two copies of a variant causing the disease, but one copy only increasing risk, experts said.
The new recommendation will prompt questions about whether people should get tested to determine if they have the APOE4 variant.
Dr. Greicius said that until there were treatments for people with two copies of APOE4 or trials of therapies to prevent them from developing dementia, “My recommendation is if you don’t have symptoms, you should definitely not figure out your APOE status.”
He added, “It will only cause grief at this point.”
Finding ways to help these patients cannot come soon enough, Dr. Sperling said, adding, “These individuals are desperate, they’ve seen it in both of their parents often and really need therapies.”
Pam Belluck is a health and science reporter, covering a range of subjects, including reproductive health, long Covid, brain science, neurological disorders, mental health and genetics. More about Pam Belluck
The Fight Against Alzheimer’s Disease
Alzheimer’s is the most common form of dementia, but much remains unknown about this daunting disease..
How is Alzheimer’s diagnosed? What causes Alzheimer’s? We answered some common questions .
A study suggests that genetics can be a cause of Alzheimer’s , not just a risk, raising the prospect of diagnosis years before symptoms appear.
Determining whether someone has Alzheimer’s usually requires an extended diagnostic process . But new criteria could lead to a diagnosis on the basis of a simple blood test .
The F.D.A. has given full approval to the Alzheimer’s drug Leqembi. Here is what to know about i t.
Alzheimer’s can make communicating difficult. We asked experts for tips on how to talk to someone with the disease .
- Election 2024
- Entertainment
- Newsletters
- Photography
- Personal Finance
- AP Investigations
- AP Buyline Personal Finance
- AP Buyline Shopping
- Press Releases
- Israel-Hamas War
- Russia-Ukraine War
- Global elections
- Asia Pacific
- Latin America
- Middle East
- Election Results
- Delegate Tracker
- AP & Elections
- Auto Racing
- 2024 Paris Olympic Games
- Movie reviews
- Book reviews
- Personal finance
- Financial Markets
- Business Highlights
- Financial wellness
- Artificial Intelligence
- Social Media
Supreme Court sides with music producer in copyright case over sample in Flo Rida hit
FILE - Supreme Court is seen on Capitol Hill in Washington, Thursday, April 25, 2024. (AP Photo/J. Scott Applewhite)
FILE - The Supreme Court of the United States is seen in Washington, March 26, 2024. (AP Photo/Amanda Andrade-Rhoades, File)
- Copy Link copied
WASHINGTON (AP) — The Supreme Court sided with a music producer in a copyright case Thursday, allowing him to seek more than a decade’s worth of damages over a sample used in a hit Flo Rida song.
The 6-3 decision came in a case filed by Sherman Nealy, who was suing over music used in the 2008 song “In the Ayer,” by the rapper Flo Rida. It also was featured on TV shows like “So You Think You Can Dance.”
Nealy’s suit says he didn’t know his former collaborator had inked a deal with a record company while he was in prison that allowed the sampling of the song “Jam the Box.” He sued in 2018 for damages going back to the song’s release.
Copyright law says suits must be filed within three years of the violation, or the point when it’s discovered. The record company, Warner Chappell, argued that means Nealy would only be entitled to three years’ worth of royalties at most.
The question of how far back damages can go has split appeals courts, and it’s one that industry groups like the Recording Industry Association of America called on the Supreme Court to decide.
The opinion handed down Thursday was written by Justice Elena Kagan, and joined by her liberal colleagues Sonia Sotomayor and Ketanji Brown Jackson as well as conservative justices John Roberts, Brett Kavanaugh and Amy Coney Barrett.
“There is no time limit on monetary recovery. So a copyright owner possessing a timely claim is entitled to damages for infringement, no matter when the infringement occurred,” Kagan wrote.
An attorney for Nealy, Wes Earnhardt, said the opinion gives clarity on an important issue.
Three conservative justices dissented. Justice Neil Gorsuch wrote that the majority sidestepped the important question: Whether Nealy’s claim was valid to begin with, or whether copyright holders should have to show some kind of fraud in order to sue over older violations. The dissenters said the suit should have been dismissed.
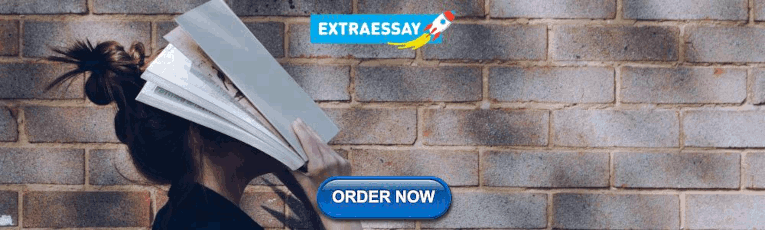
IMAGES
VIDEO
COMMENTS
This paper will examine the available methods in sampling participants for qualitative study. Specifically, the paper will discuss the sampling frame suitable for case study, such as single-case (holistic and embedded), multi-case, and a snowball or network sampling procedure. Discussion will also involve challenges anticipated for each ...
Presenting individual case studies has highlighted how purposive sampling can be integrated into varying contexts dependent on study design. The sampling strategies clearly situate each study in terms of trustworthiness for data collection and analysis. The selected approach to purposive sampling used in each case aligns to the research ...
Extreme case sampling: Purposeful selection of the most unusual cases. Typical case sampling: Selection of the most typical or average participants. ... Descriptive content analysis studies are not based on a specific qualitative tradition and are varied in their methods of analysis. The analysis of the content aims to identify themes, and ...
We could choose a sampling method based on whether we want to account for sampling bias; a random sampling method is often preferred over a non-random method for this reason. Random sampling examples include: simple, systematic, stratified, and cluster sampling. Non-random sampling methods are liable to bias, and common examples include ...
Presenting individual case studies has highlighted how purposive sampling can be integrated into varying contexts dependent on study design. The sampling strategies clearly situate each study in terms of trustworthiness for data collection and analysis. The selected approach to purposive sampling used in each case aligns to the research ...
Research design is the key that unlocks before the both the researcher and the audience all the primary elements of the research—the purpose of the research, the research questions, the type of case study research to be carried out, the sampling method to be adopted, the sample size, the techniques of data collection to be adopted and the ...
In week five, students learn about nonrandom and random sampling techniques (snowball sampling, simple random sampling, etc.). In discussion section later that week, students apply this knowledge to a hypothetical case study where a researcher aims to study the experiences of homeless people in the United States. Students learned about the pros ...
Sampling methods are crucial for conducting reliable research. In this article, you will learn about the types, techniques and examples of sampling methods, and how to choose the best one for your study. Scribbr also offers free tools and guides for other aspects of academic writing, such as citation, bibliography, and fallacy.
Sampling Strategies in Qualitative Research . TimRapley . Put simply, sampling really matters. It matters in relation to an array of issues, for the whole trajectory of ... The one-off case study, conceived and executed in magnificent isolation, has no place in modern social science and little more than anecdotal value to a policy
To learn about sampling techniques in social science research, students practice tackling a real-world research problem through discussing a hypothetical case. Goals: To enable students to understand the benefits and drawbacks of various sampling techniques. To provide students with experience designing sampling methods to address a real-world ...
The purpose of case study research is twofold: (1) to provide descriptive information and (2) to suggest theoretical relevance. Rich description enables an in-depth or sharpened understanding of the case. It is unique given one characteristic: case studies draw from more than one data source. Case studies are inherently multimodal or mixed ...
The essential topics related to the selection of participants for a health research are: 1) whether to work with samples or include the whole reference population in the study (census); 2) the sample basis; 3) the sampling process and 4) the potential effects nonrespondents might have on study results. We will refer to each of these aspects ...
Case study protocol is a formal document capturing the entire set of procedures involved in the collection of empirical material . It extends direction to researchers for gathering evidences, empirical material analysis, and case study reporting . This section includes a step-by-step guide that is used for the execution of the actual study.
Stratified random sampling. Stratified random sampling is similar to simple random sampling, but it kicks things up a notch. As the name suggests, stratified sampling involves selecting participants randomly, but from within certain pre-defined subgroups (i.e., strata) that share a common trait.For example, you might divide the population into strata based on gender, ethnicity, age range or ...
This paper will examine the available methods in sampling participants for qualitative study. Specifically, the paper will discuss the sampling frame suitable for case study, such as single-case (holistic and embedded), multi-case, and a snowball or network sampling procedure. Discussion will also involve challenges anticipated for each procedure.
Revised on November 20, 2023. A case study is a detailed study of a specific subject, such as a person, group, place, event, organization, or phenomenon. Case studies are commonly used in social, educational, clinical, and business research. A case study research design usually involves qualitative methods, but quantitative methods are ...
Purposive sampling is useful for. case study in three situations: (1) when a researcher wants to select unique cases that are especially informative, (2) when a researcher would like to select ...
A Case study is: An in-depth research design that primarily uses a qualitative methodology but sometimes includes quantitative methodology. Used to examine an identifiable problem confirmed through research. Used to investigate an individual, group of people, organization, or event. Used to mostly answer "how" and "why" questions.
Cluster sampling- she puts 50 into random groups of 5 so we get 10 groups then randomly selects 5 of them and interviews everyone in those groups --> 25 people are asked. 2. Stratified sampling- she puts 50 into categories: high achieving smart kids, decently achieving kids, mediumly achieving kids, lower poorer achieving kids and clueless ...
Defnition: A case study is a research method that involves an in-depth examination and analysis of a particular phenomenon or case, such as an individual, organization, community, event, or situation. It is a qualitative research approach that aims to provide a detailed and comprehensive understanding of the case being studied.
With a case-control sampling strategy one simply takes a sample of the population in order to obtain an estimate of the exposure distribution within the population that gave rise to the cases. Obviously, this is a much more efficient design. It is important to note that, unlike cohort studies, case-control studies do not follow subjects through ...
The intensities and frequencies of extreme rainstorms are increasing, which may result in severe inundation of urban metro systems. Although there is some risk assessment research on regional metro systems based on spatiotemporal data, the characteristics of specific metro stations and shortcomings in the emergency response process need more consideration. In this paper, a risk analysis model ...
Assisted index case testing (ICT), in which health care workers take an active role in referring at-risk contacts of people living with HIV for HIV testing services, has been widely recognized as an evidence-based intervention with high potential to increase status awareness in people living with HIV. While the available evidence from eastern and southern Africa suggests that assisted ICT can ...
The database resulting from our literature search includes 972 studies and 2,938 observations of global change drivers on disease or parasitism from 1,006 parasite taxa, 480 host taxa and 1,497 ...
A Case Study of Prioritizing Real Integration in School Mergers: Arts and Letters 305 United ... An analysis of DOE data for class sizes in each school suggests that even while expanding the number of classes to meet the caps, creating these two grade cohorts could work in the spaces available in each building.
The new study had some limitations, including a lack of diversity that might make the findings less generalizable. Most patients in the study had European ancestry. While two copies of APOE4 also ...
In fact, the study on risk factors for erysipelas (Pitché et al., 2015) was a prospective case case-control study. Thus, it is important to remember that the nature of the study (case-control or cohort) depends on the sampling method.
Radiant cooling floors combined with ventilation systems have been widely applied in large space buildings. However, there has been a lack of research on system control strategies for their adaptation to weather changes. This study aimed to find control strategies for radiant cooling floors combined with displacement ventilation systems used in large space buildings in order to achieve energy ...
However the range of participants was from 1-700. Regarding differences by methodology, he found case studies had a mean sample size of 188; ethnographies 128; grounded theory, 59; narrative inquiry, 18; and phenomenology, 21. (Guetterman, 2015: 10-13). So there was no uniform answer to the question and the ranges varied according to methodology.
WASHINGTON (AP) — The Supreme Court sided with a music producer in a copyright case Thursday, allowing him to seek more than a decade's worth of damages over a sample used in a hit Flo Rida song. The 6-3 decision came in a case filed by Sherman Nealy, who was suing over music used in the 2008 song "In the Ayer," by the rapper Flo Rida.