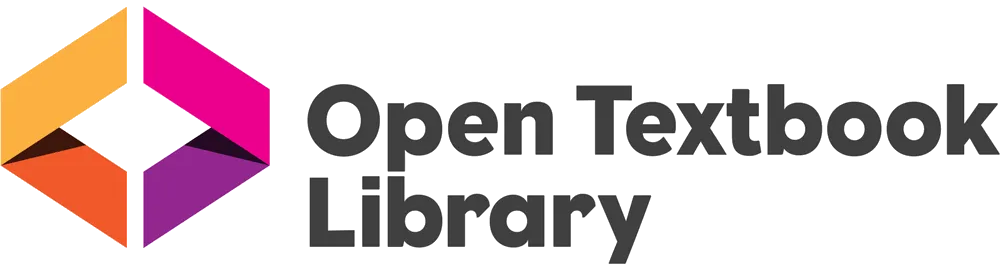
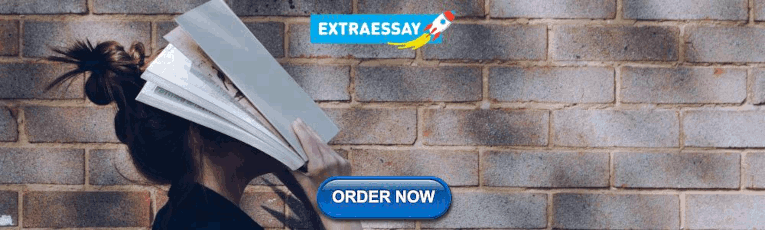
Social Data Analysis

Mikaila Mariel Lemonik Arthur, Rhode Island College
Roger Clark, Rhode Island College
Copyright Year: 2021
Last Update: 2023
Publisher: Rhode Island College Digital Publishing
Language: English
Formats Available
Conditions of use.

Learn more about reviews.
Reviewed by Alice Cheng, Associate Professor, North Carolina State University on 12/19/23
Social Data Analysis: A Comprehensive Guide" truly lives up to its title by offering a comprehensive exploration of both quantitative and qualitative data analysis in the realm of social research. The book provides an in-depth understanding of the... read more
Comprehensiveness rating: 4 see less
Social Data Analysis: A Comprehensive Guide" truly lives up to its title by offering a comprehensive exploration of both quantitative and qualitative data analysis in the realm of social research. The book provides an in-depth understanding of the subject matter, making it a valuable resource for readers seeking a thorough grasp of social data analysis.
The comprehensiveness of the book is evident in several key aspects:
Coverage of Quantitative and Qualitative Methods:
The book effectively covers both quantitative and qualitative data analysis, acknowledging the importance of a balanced approach in social research. Readers benefit from a holistic understanding of various analytical methods, allowing them to choose the most suitable approach for their research questions. Focus on SPSS for Quantitative Analysis:
The dedicated section on quantitative data analysis with SPSS demonstrates the book's commitment to providing practical guidance. Readers are taken through the nuances of using SPSS, from basic functions to more advanced analysis, enhancing their proficiency in a widely used statistical software. Real-World Application Using GSS Data:
The integration of data from the 2021 General Social Survey (GSS) and the modified GSS Codebook adds a practical dimension to the book. Readers have the opportunity to apply their learning to real-world scenarios, fostering a deeper understanding of social data analysis in action. Consideration of Ethical Practices:
The book's mention of survey weights and their exclusion from the learning dataset reflects a commitment to ethical data analysis practices. This attention to ethical considerations enhances the comprehensiveness of the book by addressing important aspects of responsible research. Supplementary Resources and Glossary:
The inclusion of a glossary ensures that readers, especially those new to the field, can easily grasp the terminology used. The availability of supplementary resources, such as a modified GSS Codebook, further supports readers in applying their knowledge beyond theoretical discussions. Recognition of Alternative Tools:
Acknowledging the existence of alternative tools, such as R, demonstrates the book's awareness of the diversity in data analysis approaches. While focusing on SPSS, the book encourages readers to explore other options, contributing to a more nuanced and well-rounded education in social data analysis. Overall, the book's comprehensiveness lies not only in its coverage of various data analysis methods but also in its commitment to providing practical, ethical, and diverse perspectives on social data analysis. It serves as an inclusive and accessible guide for readers at different levels of expertise.
Content Accuracy rating: 4
"Social Data Analysis: A Comprehensive Guide" maintains a commendable level of accuracy throughout its content. The authors demonstrate a meticulous approach to presenting information, ensuring that concepts are explained with precision and clarity. The accuracy is particularly notable in the sections covering quantitative data analysis with SPSS, where step-by-step instructions are provided for readers to follow, minimizing the risk of misinterpretation.
The use of real-world examples from the 2021 General Social Survey enhances the book's accuracy by grounding theoretical discussions in practical applications. The modified GSS Codebook is a thoughtful addition, contributing to the accuracy of the learning experience by providing a clear reference for variables used in the examples.
The authors' acknowledgment of the limitation regarding survey weights in the learning dataset reflects a commitment to transparency and ethical research practices. While the book focuses on a specific statistical software (SPSS), it accurately recognizes alternative tools like R, allowing readers to make informed decisions based on their preferences and requirements.
The glossary aids in maintaining accuracy by providing clear definitions of key terms, ensuring that readers have a precise understanding of the terminology used. Additionally, the reference to external resources, such as IBM's list of resellers and related guides from Kent State, contributes to the accuracy of the book by directing readers to authoritative sources for further information.
In conclusion, "Social Data Analysis: A Comprehensive Guide" upholds a high level of accuracy, presenting information in a manner that is both reliable and accessible. The book's attention to detail, reliance on real-world examples, and commitment to ethical considerations collectively contribute to its overall accuracy as a valuable resource for those engaging in social data analysis.
Relevance/Longevity rating: 4
"Social Data Analysis: A Comprehensive Guide" stands out for its relevance in the field of social research and data analysis. Several key aspects contribute to the book's contemporary and practical relevance:
Integration of Current Data:
The incorporation of data from the 2021 General Social Survey (GSS) ensures that the book's examples and applications are based on recent and relevant datasets. This contemporary approach allows readers to engage with real-world scenarios and analyze data reflective of current social trends. Focus on SPSS and Alternative Tools:
The book's emphasis on using SPSS for quantitative data analysis aligns with the software's widespread use in the social sciences. This focus enhances the book's relevance for readers in academic and professional settings where SPSS is commonly employed. Moreover, the acknowledgment of alternative tools, such as R, adds relevance by catering to a diverse audience with varying software preferences. Practical Applications:
The inclusion of practical examples, screenshots, and step-by-step instructions in the section on quantitative data analysis with SPSS enhances the book's relevance. Readers can directly apply the concepts learned, fostering a hands-on learning experience that is directly applicable to their research or academic pursuits. Ethical Considerations:
The discussion on ethical considerations, particularly the mention of survey weights and their exclusion from the learning dataset, adds relevance by addressing contemporary concerns in research methodology. This ethical awareness aligns with current discussions surrounding responsible and transparent research practices. Diversity of Analytical Approaches:
The book's acknowledgment of alternative methods, such as qualitative and mixed methods data analysis with Dedoose, contributes to its relevance by recognizing the diversity of approaches within the social sciences. This inclusivity allows readers to explore different analytical methods based on their research needs. Supplementary Resources:
The provision of supplementary resources, including the modified GSS Codebook and references to external guides, enhances the book's relevance. These resources offer readers additional tools and information to extend their learning beyond the book, ensuring that they stay updated on best practices and advancements in social data analysis. In summary, "Social Data Analysis: A Comprehensive Guide" remains relevant by incorporating current data, addressing ethical considerations, and catering to a diverse audience with practical examples and alternative tools. The book's contemporary approach aligns with the evolving landscape of social research and data analysis, making it a valuable and relevant resource for students, researchers, and practitioners alike.
Clarity rating: 4
"Social Data Analysis: A Comprehensive Guide" excels in clarity, offering readers a lucid and accessible journey through the intricate landscape of social data analysis. Several factors contribute to the clarity of the book:
Clear Explanations and Language:
The authors employ clear and concise language, making complex concepts in social data analysis accessible to a broad audience. Technical terms are explained in a straightforward manner, enhancing comprehension for readers regardless of their prior knowledge in the field. Step-by-Step Instructions:
The section on quantitative data analysis with SPSS stands out for its clarity due to the inclusion of step-by-step instructions. Readers are guided through processes, ensuring that they can follow and replicate actions easily. This approach fosters a practical understanding of how to apply the theoretical concepts discussed. Visual Aids and Examples:
The use of visual aids, such as screenshots and examples, enhances clarity by providing readers with visual cues to reinforce textual explanations. Real-world examples from the 2021 General Social Survey help readers connect theoretical concepts to practical applications, furthering their understanding. Logical Organization:
The book follows a logical and well-organized structure, moving from introducing social data analysis to specific tools and methods. This logical progression aids in the clarity of the learning journey, allowing readers to build on their understanding progressively. Glossary for Terminology:
The inclusion of a glossary ensures that readers can easily reference and understand key terminology. This contributes to overall clarity by preventing confusion about specialized terms used in the context of social data analysis. Consideration of Different Audiences:
The book is mindful of different audiences by providing options for both students and faculty. This consideration adds clarity by tailoring content to the specific needs and perspectives of these distinct reader groups. Transparency Regarding Limitations:
The book's transparency regarding limitations, such as the exclusion of survey weights from the learning dataset, contributes to clarity. Readers are made aware of the scope and purpose of the dataset, avoiding potential confusion about its applicability to real-world scenarios. In summary, "Social Data Analysis: A Comprehensive Guide" is characterized by its clarity, achieved through clear explanations, practical examples, logical organization, and thoughtful consideration of the diverse needs of its readership. The book effectively demystifies social data analysis, making it an approachable and enlightening resource for individuals at various levels of expertise.
Consistency rating: 4
"Social Data Analysis: A Comprehensive Guide" maintains a high level of consistency throughout its content, ensuring a cohesive and reliable learning experience. The consistency is evident in the uniform and clear language used across chapters, providing a seamless transition for readers as they navigate different sections of the book. The logical organization of topics and the structured approach to quantitative data analysis with SPSS contribute to a consistent learning curve, allowing readers to progressively build on their knowledge. Additionally, the inclusion of real-world examples and visual aids is consistently applied, enhancing the practicality of the book. The authors' commitment to ethical considerations, such as the transparency about the exclusion of survey weights in the learning dataset, reflects a consistent adherence to responsible research practices. Overall, the book's internal coherence, both in language and content, ensures that readers experience a consistent and reliable guide in their exploration of social data analysis.
Modularity rating: 3
"Social Data Analysis: A Comprehensive Guide" excels in modularity, providing a well-organized and modular structure that enhances the learning experience. The book is divided into distinct sections, each focusing on specific aspects of social data analysis. This modular approach allows readers to navigate the content efficiently, catering to different learning preferences and enabling targeted study.
The modularity is evident in the clear demarcation of chapters, from the introduction of social data analysis to the practical application of quantitative data analysis with SPSS and qualitative data analysis with Dedoose. Each section is designed as a standalone module, contributing to a structured and cohesive learning path.
Furthermore, within each module, the book maintains a modular design with sub-sections, ensuring that readers can easily locate and focus on specific topics of interest. The step-by-step instructions provided in the quantitative data analysis section exemplify this modular design, breaking down complex processes into manageable and easily digestible components.
The inclusion of supplementary resources, such as the modified GSS Codebook and glossary, adds to the modularity by offering readers standalone references that complement the main content. This modularity enhances the accessibility of the book, allowing readers to customize their learning experience based on their specific needs and interests.
In conclusion, the modularity of "Social Data Analysis: A Comprehensive Guide" contributes to the book's effectiveness as an educational resource. The well-structured and modular design facilitates a flexible and user-friendly learning experience, making it a valuable tool for readers seeking to navigate the complexities of social data analysis at their own pace.
Organization/Structure/Flow rating: 4
"Social Data Analysis: A Comprehensive Guide" is a well-structured and informative book that serves as an invaluable resource for students and faculty delving into the realm of social data analysis. The authors adeptly navigate readers through the intricacies of both quantitative and qualitative data analysis, placing a specific emphasis on the use of SPSS (Statistical Package for the Social Sciences) for quantitative analysis.
The book begins with a solid foundation, introducing readers to the concept of social data analysis. The initial sections provide a clear understanding of the importance and application of both quantitative and qualitative methods in social research. Notably, the authors strike a balance between theory and practical application, ensuring that readers can grasp the concepts and implement them effectively.
The heart of the book lies in its detailed exploration of quantitative data analysis with SPSS. The authors guide readers through the usage of this powerful statistical software, offering practical insights and step-by-step instructions. The inclusion of screenshots and examples using data from the 2021 General Social Survey enhances the book's accessibility, allowing readers to follow along seamlessly.
Furthermore, the book goes beyond theoretical discussions and provides a modified GSS Codebook for the data used in the text. This resource is invaluable for readers who wish to apply their knowledge to real-world scenarios. The authors' emphasis on the importance of survey weights and their exclusion from the learning dataset demonstrates a commitment to ethical and accurate data analysis practices.
The inclusion of a glossary enriches the learning experience by providing clear definitions of key terms. Additionally, the section on qualitative and mixed methods data analysis with Dedoose broadens the scope of the book, catering to readers interested in a diverse range of analytical approaches.
While the book excels in elucidating complex topics, it does not shy away from acknowledging alternative tools. The authors rightly introduce R as an open-source alternative, recognizing its significance and suggesting that R supplements to the book may be available in the future.
In conclusion, "Social Data Analysis: A Comprehensive Guide" stands out as a comprehensive and accessible resource for individuals venturing into the field of social data analysis. The authors' expertise, coupled with practical examples and supplementary resources, make this book a valuable companion for students, faculty, and anyone keen on mastering the art and science of social data analysis.
Interface rating: 4
The text is free of significant interface issues, including navigation problems, distortion of images/charts, and any other display features that may distract or confuse the reader.
Grammatical Errors rating: 5
The book contains no grammatical errors
Cultural Relevance rating: 5
The text is not culturally insensitive or offensive in any way.
Table of Contents
- Acknowledgements
- How to Use This Book
- Section I. Introducting Social Data Analysis
- Section II. Quantitative Data Analysis
- Section III. Qualitative Data Analysis
- Section IV. Quantitative Data Analysis with SPSS
- Section V. Qualitative and Mixed Methods Data Analysis with Dedoose
- Modified GSS Codebook for the Data Used in this Text
- Works Citied
- About the Authors
Ancillary Material
About the book.
Social data analysis enables you, as a researcher, to organize the facts you collect during your research. Your data may have come from a questionnaire survey, a set of interviews, or observations. They may be data that have been made available to you from some organization, national or international agency or other researchers. Whatever their source, social data can be daunting to put together in a way that makes sense to you and others.
This book is meant to help you in your initial attempts to analyze data. In doing so it will introduce you to ways that others have found useful in their attempts to organize data. You might think of it as like a recipe book, a resource that you can refer to as you prepare data for your own consumption and that of others. And, like a recipe book that teaches you to prepare simple dishes, you may find this one pretty exciting. Analyzing data in a revealing way is at least as rewarding, we’ve found, as it is to cook up a yummy cashew carrot paté or a steaming corn chowder. We’d like to share our pleasure with you.
About the Contributors
Mikaila Mariel Lemonik Arthur is Professor of Sociology at Rhode Island College, where she has taught a wide variety of courses including Social Research Methods, Social Data Analysis, Senior Seminar in Sociology, Professional Writing for Justice Services, Comparative Law and Justice, Law and Society, Comparative Perspectives on Higher Education, and Race and Justice. She has written a number of books and articles, including both those with a pedagogical focus (including Law and Justice Around the World, published by the University of California Press) and those focusing on her scholarly expertise in higher education (including Student Activism and Curricular Change in Higher Education, published by Routledge). She has expertise and experience in academic program review, translating research findings for policymakers, and disability accessibility in higher education, and has served as a department chair and as Vice President of the RIC/AFT, her faculty union. Outside of work, she enjoys reading speculative fiction, eating delicious vegan food, visiting the ocean, and spending time with amazing humans.
Roger Clark is Professor Emeritus of Sociology at Rhode Island College, where he continues to teach courses in Social Research Methods and Social Data Analysis and to coauthor empirical research articles with undergraduate students. He has coauthored two textbooks, An Invitation to Social Research (with Emily Stier Adler) and Gender Inequality in Our Changing World: A Comparative Approach (with Lori Kenschaft and Desirée Ciambrone). He has been ranked by the USTA in its New England 60- and 65-and-older divisions, shot four holes in one on genuine golf courses, and run multiple half and full marathons. Like the Energizer Bunny, he keeps on going and going, but, given his age, leaves it to your imagination where
Contribute to this Page
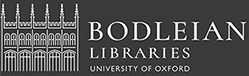
Data and Statistics for Social Sciences: Data analysis tools & training
- General & Macrodata
- Economics & Finance
- Politics & IR
- Art & culture
- Energy & Environment
- Health & Social care
- Science & Technology
- Media, IT and Communication
- Public Opinion
- Data Archives & Services
- Data analysis tools & training
- Introduction
- Definitions
To support social scientists and others who are required to gather and handle data, the SSL has created a Data Area providing access to PCs which have specialised and restricted-licence data software installed: NVivo and IBM SPSS Statistics . Any reader may use these PCs.
The SSL also provides safe access to secure research data via the SafePod that can be booked and used by researchers internal and external to the University. For general enquiries about the SafePod Network, contact 01334 463901 or email SafePod Network (SPN) .
Go to the SSL Data Services page for more information.
The process of systematically applying statistical and/or logical techniques to describe and illustrate, condense and recap, and evaluate data. There four types of data analysis: descriptive, diagnostic, predictive and prescriptive.
The representation of information in the form of a chart, diagram, picture, etc.
Involves collecting, combining, and visualising various types of geospatial data . It is used to model and represent how people, objects, and phenomena interact within space, as well as to make predictions based on trends in the relationships between places.
Involves the identification, examination, and interpretation of patterns and themes in textual data and determines how these patterns and themes help answer the research questions at hand.
Involves analysing number-based data, or data that can be easily “converted” into numbers without losing any meaning (which includes categorical and numerical data) using various statistical techniques.
Data analysis tools
- Quantitative
- Qualitative
- Visualisation tools
See below some data-driven tools used to understand complex concepts:
- Apache Spark A unified analytics engine for large-scale data processing built on data science; also popular for data pipelines and machine learning models development.
- Python An increasingly popular tool for data analysis . In recent years, a number of libraries have reached maturity, allowing R and Stata users to take advantage of the beauty, flexibility, and performance of Python without sacrificing the functionality these older programs have accumulated over the years.
- R A free software environment for statistical computing and graphics. It compiles and runs on a wide variety of UNIX platforms, Windows and MacOS. R provides a wide variety of statistical (linear and nonlinear modelling, classical statistical tests, time-series analysis, classification, clustering, etc.) and graphical techniques, and is highly extensible. R is freely available online.
- SPSS This data analysis software allows for the editing and analysis of all types of quantitative data, whether structured data or relational databases. It works with all common file formats and can be used for formulaic analysis or graphing data. more... less... More information is available on SOLO and on the SPSS website . Guides are available in the SSL Data Area or you can consult the SPSS Introductory Guide (PDF, 519 KB). IT services offer SPSS training as part of their course catalog and SPSS can be purchased for personal use through the University store .
- Stata A powerful and flexible general-purpose statistical software package used in research, among others in the fields of economics, sociology, political science. It's capabilities include data management, statistical analysis, graphics, simulations, regression, and custom programming. more... less... STATA is available to eligible students and staff in departments and centres in the Manor Road Building (MRB); to be eligible you must be nominated by your department/centre. Students can also purchase STATA at a reduced cost for their own devices from the supplier Timberlake .
Software packages comprised of tools designed to facilitate a qualitative approach to qualitative data, which include texts, graphics, audio or video. These packages (sometimes referred as CAQDAS - Computer Assisted/Aided Qualitative Data Analysis) may also enable the incorporation of quantitative (numeric) data and/or include tools for taking quantitative approaches to qualitative data. Here are some more popular packages -
- Atlas.ti Software for the qualitative analysis of large bodies of textual, graphical, audio and video data. It offers a variety of tools for accomplishing the tasks associated with any systematic approach to "soft" data, i.e. material which cannot be analysed by formal, statistical approaches in meaningful ways. more... less... You can download a trial version from the website, it is free and works without time limit. Free training webinars are offered on the website.
- MAXQDA An alternative to Nvivo handles a similar range of data types allowing organisation, colour coding and retrieval of data. Text, audio or video may equally be dealt with by this software package. A range of data visualisation tools are also included. more... less... Trial licences available from MAXQDA
- NVivo A qualitative data analysis (QDA) computer software package designed for qualitative researchers working with very rich text-based and/or multimedia information, where deep levels of analysis on small or large volumes of data are required. more... less... NVivo is installed on PCs in the SSL Data Area; also available from IT services shop.
- ArcGIS A geographic information system that can be used by anyone working with geospatial data or in fact any statistical information that includes geographical variables such as location, elevation, population density and so on. If the information being used features a geographical representation of the world as part of the mix then ArcGIS should be of interest. more... less... Use ArcGIS to: View maps/mapped information as part of analysis; Compile geographic data; Build and edit maps to help analysis or visualisation; Amend properties and fields in geospatial databases and generally manage such information; Develop projects that draw on the large user base and functionality this package has built up. It can be used with any geo-spatial data such as the Landscan population database.ArcGIS Desktop is available on library computers in the Social Science Library (can be found in the all programs menu).
- Atlas.ti Now fuelled by OpenAI, computer-assisted qualitative data analysis software that facilitates analysis of qualitative data for qualitative research, quantitative research, and mixed methods research. more... less... You can download a trial version from the website, it is free and works without time limit. Free training webinars are offered on the website.
- MapInfo A complete, desktop mapping solution for the geographic information system (GIS) analyst to visualize, analyze, edit, interpret, and output data — revealing relationships, patterns, and trends. more... less... Offered on a 30-day free trial.
- Geospatial Analysis online This free online resource introduces concepts, methods and tools, provides many examples using a variety of software tools such as ArcGIS, etc. to clarify the concepts discussed. It aims to be comprehensive (but not necessarily exhaustive) in terms of concepts and techniques, representative and independent in terms of software tools, and above all practical in terms of application and implementation.
- Blender This free and open source 3D creation suite supports the entirety of the 3D pipeline — modeling, rigging, animation, simulation, rendering, compositing and motion tracking, in the context of research data in particular. more... less... The suite is free to download from the website ITLC offers either a face-to-face , or online courses: go to LinkedIn Learning through Webauth using your single sign-on. An overview course on 3D modelling taught by ITLC uses SketchUp, Blender and image manipulation software.
- Datawrapper An online data-visualisation tool for making interactive charts which are responsive and embeddable in a website.
- QGIS A cross-platform, free and open-source desktop geographic information system (GIS) application. more... less... Online course through LinkedIn Learning.
- R A tool used for data analysis and visualisation.
- Shiny Using the free Shiny package, these analyses and visualisations can be published as interactive webpages just using R. more... less... R and Shiny are available as both face-to-face and online courses.
- Tableau Public An easy to use, free and powerful tool for creating interactive dashboards and data visualisations that can be shared publicly and embedded in your personal site. more... less... Check out a face-to-face course offered by the ITLC.
Online tools and services
- Subscription online tools and services
- Free/free trial online tools & services
- Google tools
- Bloomberg A global financial database, where you can find nearly any type of financial data. If a company is publicly traded, Bloomberg will have some information on it. Also included are profiles of over a million people. more... less... To book a time slot for the Bloomberg workstation in the Sainsbury Library please use this form: https://outlook.office365.com/owa/calendar/[email protected]/bookings/ Note: if you are a non-SBS student you MUST book during our normal staffed hours.
- Eikon A financial market intelligence database and a set of financial analysis tools that provide information on markets, indices, company and economic information and historical financial data. more... less... Limited remote access to Eikon. Current members of the University can request a temporary login to access Eikon remotely by emailing Sainsbury Library . Logins are issued for three to seven days, depending on demand. Currently loans are for 3 days.
- S&P Capital IQ Extra username and password are required for this resource. Please email [email protected] for an account. By requesting an account via [email protected] you are consenting to us sharing this data (your name, email address and University card expiry date). You can read the Oxford University student privacy policy at https://compliance.admin.ox.ac.uk/current-students-and-staff and the S&P Global privacy policy at https://www.spglobal.com/en/privacy/privacy-policy-english. Please let us know if you have any questions. more... less... S&P Capital IQ combines information on companies, markets, and people worldwide with tools for analysis, financial modelling, market analysis, screening, targeting, and relationship and workflow management.
- UKDS.Stat: International aggregate data Hosts economic and social datasets provided by the World Bank, OECD, International Monetary Fund, United Nations, and International Energy Agency. more... less... Key datasets include World Energy Balances, World Development Indicators, Balance of Payments Statistics, Direction of Trade Statistics, International Financial Statistics, World Economic Outlook, Main Economic Indicators, Quarterly National Accounts, and the Human Rights Atlas. Datasets also cover statistics on science, environment, education, health, and in depth regional statistics. You can find comprehensive platform and data guides on accessing, exploring and visualising data in UKDS.Stat.
- Exploratory Offers various tools to make data analysis more accessible and collaborative. For example, Exploratory’s Analytics View facilitates the use a wide range of advanced open source AI / Machine Learning algorithms to discover hidden patterns and trends in your data effectively; Data Wrangling allows to join/merge multiple data sets together with various options, or filter data by using other data sets; Visualisation tools give an easier way to spot patterns or trends by comparing them together side by side, or visualise the relationship between pairs of two categorical columns. Offered on a free trial basis.
- GESIS: MISSY (Microdata Information System) Part of the service infrastructure of the German Microdata Lab, MISSY is an online service platform that provides structured metadata for official statistics. It includes metadata at the study and variable level as well as reports and tools for data handling and analysis. more... less... All documentation in MISSY refers to EU and national (German microcensus) microdata available for scientific purposes.
- SeekTable Free web reporting tool, providing online pivot tables, charts & datagrids builder, simple data exploration with drill-down, search driven analytics (natural lang queries), export crosstabs to Excel, PDF, CSV, HTML, share and publish reports for public access.
- Social Data Science Lab An ESRC Data Investment, part of the Big Data Network for the social sciences brings together crime, social, computer, and statistical scientists to study the empirical, methodological, theoretical and technical dimensions of New and Emerging Forms of Data in social, policy and business contexts. This empirical social data science programme is complemented by a focus on ethics and the development of new methodological tools and technical solutions for the UK academic, public and private sectors. more... less... The Lab develops and supports the COSMOS Open Data Analytics software, that provides ethical access to social media data for social science researchers.
- Metabase (Stitch) Stitch can replicate data from all your sources to a central warehouse. From there, it's easy to use Metabase to perform the in-depth analysis you need. more... less... 2 weeks free trial
- Dataset Search Enables users to find data sets stored across the web through a simple keyword search. The tool surfaces information about data sets hosted in thousands of repositories across the web, making these data sets universally accessible and useful.
- Looker Studio A free tool that turns your data into informative, easy to read, easy to share, and fully customizable dashboards and reports. more... less... Looker studio help
- Public Data Explorer Makes large public-interest datasets easy to explore, visualise and communicate. As the charts and maps animate over time, the changes in the world become easier to understand. Navigate between different views, make your own comparisons, and share your findings.
- IT Training
- Training calendars
- Online training
- SAGE research help
- Bodleian iSkills Workshops in Information Discovery & Scholarly Communications The programme designed to help you to make effective use of scholarly materials. It covers, among other things, managing your research data, information discovery, keeping up to date with new research, responsible research metrics, understanding copyright and looking after your intellectual property, open Access publishing and complying with funder mandates for open access.
- IT Learning Centre ITLC offers both classroom-based and online video courses to University members. Lunchtime sessions, online resources and some courses are free; there is a charge for other courses. more... less... You can search classroom-based courses via online course booking system which allows you to book or cancel a taught course and to manage your notifications using your Single Sign-On. Having problems? See Help Centre Frequently Asked Questions .
- Q-Step The programme designed to promote a step-change in quantitative social science training. The Oxford Q-Step Centre (OQC) enables undergraduates across the Social Sciences to have access to enhanced training in Quantitative Methods, through lectures and data-labs. It is hosted by the Department of Politics and International Relations, in close co-operation with the Department of Sociology, and based in the Manor Road Building. See Courses and Resources.
Data training in the University
External data training & events.
Online training includes the University's subscription to LinkedIn Learning , a resource of online, video-based courses that University members can access at any time for free using their single sign-on credentials.
ITLC also offers self-service learning resources through its IT Learning Portfolio , a range of resources that you can download and use to develop your IT digital skills for study, research and work.
- LinkedIn Learning: Data Analysis Learn the latest quantitative and qualitative data analysis skills for effective business decision-making and explore the necessary tools, such as Microsoft Excel, Tableau, SQL, Python, R, and more.
You can also try
- edX Data Analysis courses Free access to college courses from leading universities.
For students embarking on their dissertation projects, or those supervising them, the UK Data Service offers dissertation resources, data and events to help with planning and completing a dissertation project.
UK Data Service student pages
The student web pages in the Learning Hub bring all student resources together in one place. On these pages, you can find information about the UK Data Service, its data collections and how you can find and access these.
If you’re unsure about why you might want to use existing data sources in your research project, then watch video Secondary analysis What and why?
You can also find out more about using survey data in a dissertation or finding and accessing data for your project .
Want to boost up your data skills? Try interactive data skills modules for users who want to get to grips with key aspects of survey, longitudinal or aggregate data.
Useful data
The UK Data Service holds a wealth of data on many different topics that students may wish to explore in their dissertation projects.
UKDS student pages provide guides on top surveys for dissertation projects. This includes the Quick start guide: British Social Attitudes (BSA) and Quick start guide: Health Survey for England (HSE) .
The Browse Data pages also allow you to search data by key themes or data types.
Free workshop
UKDS is also hosting the following free workshop for dissertation students. If you are interested but can’t attend UKDS' YouTube channel includes recordings of all webinars and workshops. You can also find video tutorials such as using SPSS playlist .
Dissertation Award
The UK Data Service Dissertation Award recognises students who use data from the UK Data Service in their undergraduate dissertations. Each year we give awards to the three best dissertations from the academic year.

- CASES A collection of case studies of real social research, specially commissioned and designed to help you understand abstract methodological concepts in practice.
- Datasets A collection of teaching datasets and instructional guides that give students a chance to learn data analysis by practicing themselves.
- Video Contains tutorials, case study videos, expert interviews, and more, covering the entire research methods and statistics curriculum.
- Methodspace An online network for the community of researchers engaged in research methods. more... less... The site is created for students and researchers to network and share research, resources and debates. Methodspace users have free access to selected journal articles, book chapters, etc which highlight emerging topics in the field.
- << Previous: Data Archives & Services
- Last Updated: May 8, 2024 10:41 AM
- URL: https://libguides.bodleian.ox.ac.uk/ssdata
Website feedback
Accessibility Statement - https://visit.bodleian.ox.ac.uk/accessibility
Google Analytics - Bodleian Libraries use Google Analytics cookies on this web site. Google Analytics anonymously tracks individual visitor behaviour on this web site so that we can see how LibGuides is being used. We only use this information for monitoring and improving our websites and content for the benefit of our users (you). You can opt out of Google Analytics cookies completely (from all websites) by visiting https://tools.google.com/dlpage/gaoptout
© Bodleian Libraries 2021. Licensed under a Creative Commons Attribution 4.0 International Licence

An official website of the United States government
The .gov means it's official. Federal government websites often end in .gov or .mil. Before sharing sensitive information, make sure you're on a federal government site.
The site is secure. The https:// ensures that you are connecting to the official website and that any information you provide is encrypted and transmitted securely.
- Publications
- Account settings
- Browse Titles
NCBI Bookshelf. A service of the National Library of Medicine, National Institutes of Health.
National Research Council; Division of Behavioral and Social Sciences and Education; Commission on Behavioral and Social Sciences and Education; Committee on Basic Research in the Behavioral and Social Sciences; Gerstein DR, Luce RD, Smelser NJ, et al., editors. The Behavioral and Social Sciences: Achievements and Opportunities. Washington (DC): National Academies Press (US); 1988.

The Behavioral and Social Sciences: Achievements and Opportunities.
- Hardcopy Version at National Academies Press
5 Methods of Data Collection, Representation, and Analysis
This chapter concerns research on collecting, representing, and analyzing the data that underlie behavioral and social sciences knowledge. Such research, methodological in character, includes ethnographic and historical approaches, scaling, axiomatic measurement, and statistics, with its important relatives, econometrics and psychometrics. The field can be described as including the self-conscious study of how scientists draw inferences and reach conclusions from observations. Since statistics is the largest and most prominent of methodological approaches and is used by researchers in virtually every discipline, statistical work draws the lion’s share of this chapter’s attention.
Problems of interpreting data arise whenever inherent variation or measurement fluctuations create challenges to understand data or to judge whether observed relationships are significant, durable, or general. Some examples: Is a sharp monthly (or yearly) increase in the rate of juvenile delinquency (or unemployment) in a particular area a matter for alarm, an ordinary periodic or random fluctuation, or the result of a change or quirk in reporting method? Do the temporal patterns seen in such repeated observations reflect a direct causal mechanism, a complex of indirect ones, or just imperfections in the data? Is a decrease in auto injuries an effect of a new seat-belt law? Are the disagreements among people describing some aspect of a subculture too great to draw valid inferences about that aspect of the culture?
Such issues of inference are often closely connected to substantive theory and specific data, and to some extent it is difficult and perhaps misleading to treat methods of data collection, representation, and analysis separately. This report does so, as do all sciences to some extent, because the methods developed often are far more general than the specific problems that originally gave rise to them. There is much transfer of new ideas from one substantive field to another—and to and from fields outside the behavioral and social sciences. Some of the classical methods of statistics arose in studies of astronomical observations, biological variability, and human diversity. The major growth of the classical methods occurred in the twentieth century, greatly stimulated by problems in agriculture and genetics. Some methods for uncovering geometric structures in data, such as multidimensional scaling and factor analysis, originated in research on psychological problems, but have been applied in many other sciences. Some time-series methods were developed originally to deal with economic data, but they are equally applicable to many other kinds of data.
- In economics: large-scale models of the U.S. economy; effects of taxation, money supply, and other government fiscal and monetary policies; theories of duopoly, oligopoly, and rational expectations; economic effects of slavery.
- In psychology: test calibration; the formation of subjective probabilities, their revision in the light of new information, and their use in decision making; psychiatric epidemiology and mental health program evaluation.
- In sociology and other fields: victimization and crime rates; effects of incarceration and sentencing policies; deployment of police and fire-fighting forces; discrimination, antitrust, and regulatory court cases; social networks; population growth and forecasting; and voting behavior.
Even such an abridged listing makes clear that improvements in methodology are valuable across the spectrum of empirical research in the behavioral and social sciences as well as in application to policy questions. Clearly, methodological research serves many different purposes, and there is a need to develop different approaches to serve those different purposes, including exploratory data analysis, scientific inference about hypotheses and population parameters, individual decision making, forecasting what will happen in the event or absence of intervention, and assessing causality from both randomized experiments and observational data.
This discussion of methodological research is divided into three areas: design, representation, and analysis. The efficient design of investigations must take place before data are collected because it involves how much, what kind of, and how data are to be collected. What type of study is feasible: experimental, sample survey, field observation, or other? What variables should be measured, controlled, and randomized? How extensive a subject pool or observational period is appropriate? How can study resources be allocated most effectively among various sites, instruments, and subsamples?
The construction of useful representations of the data involves deciding what kind of formal structure best expresses the underlying qualitative and quantitative concepts that are being used in a given study. For example, cost of living is a simple concept to quantify if it applies to a single individual with unchanging tastes in stable markets (that is, markets offering the same array of goods from year to year at varying prices), but as a national aggregate for millions of households and constantly changing consumer product markets, the cost of living is not easy to specify clearly or measure reliably. Statisticians, economists, sociologists, and other experts have long struggled to make the cost of living a precise yet practicable concept that is also efficient to measure, and they must continually modify it to reflect changing circumstances.
Data analysis covers the final step of characterizing and interpreting research findings: Can estimates of the relations between variables be made? Can some conclusion be drawn about correlation, cause and effect, or trends over time? How uncertain are the estimates and conclusions and can that uncertainty be reduced by analyzing the data in a different way? Can computers be used to display complex results graphically for quicker or better understanding or to suggest different ways of proceeding?
Advances in analysis, data representation, and research design feed into and reinforce one another in the course of actual scientific work. The intersections between methodological improvements and empirical advances are an important aspect of the multidisciplinary thrust of progress in the behavioral and social sciences.
- Designs for Data Collection
Four broad kinds of research designs are used in the behavioral and social sciences: experimental, survey, comparative, and ethnographic.
Experimental designs, in either the laboratory or field settings, systematically manipulate a few variables while others that may affect the outcome are held constant, randomized, or otherwise controlled. The purpose of randomized experiments is to ensure that only one or a few variables can systematically affect the results, so that causes can be attributed. Survey designs include the collection and analysis of data from censuses, sample surveys, and longitudinal studies and the examination of various relationships among the observed phenomena. Randomization plays a different role here than in experimental designs: it is used to select members of a sample so that the sample is as representative of the whole population as possible. Comparative designs involve the retrieval of evidence that is recorded in the flow of current or past events in different times or places and the interpretation and analysis of this evidence. Ethnographic designs, also known as participant-observation designs, involve a researcher in intensive and direct contact with a group, community, or population being studied, through participation, observation, and extended interviewing.
Experimental Designs
Laboratory experiments.
Laboratory experiments underlie most of the work reported in Chapter 1 , significant parts of Chapter 2 , and some of the newest lines of research in Chapter 3 . Laboratory experiments extend and adapt classical methods of design first developed, for the most part, in the physical and life sciences and agricultural research. Their main feature is the systematic and independent manipulation of a few variables and the strict control or randomization of all other variables that might affect the phenomenon under study. For example, some studies of animal motivation involve the systematic manipulation of amounts of food and feeding schedules while other factors that may also affect motivation, such as body weight, deprivation, and so on, are held constant. New designs are currently coming into play largely because of new analytic and computational methods (discussed below, in “Advances in Statistical Inference and Analysis”).
Two examples of empirically important issues that demonstrate the need for broadening classical experimental approaches are open-ended responses and lack of independence of successive experimental trials. The first concerns the design of research protocols that do not require the strict segregation of the events of an experiment into well-defined trials, but permit a subject to respond at will. These methods are needed when what is of interest is how the respondent chooses to allocate behavior in real time and across continuously available alternatives. Such empirical methods have long been used, but they can generate very subtle and difficult problems in experimental design and subsequent analysis. As theories of allocative behavior of all sorts become more sophisticated and precise, the experimental requirements become more demanding, so the need to better understand and solve this range of design issues is an outstanding challenge to methodological ingenuity.
The second issue arises in repeated-trial designs when the behavior on successive trials, even if it does not exhibit a secular trend (such as a learning curve), is markedly influenced by what has happened in the preceding trial or trials. The more naturalistic the experiment and the more sensitive the meas urements taken, the more likely it is that such effects will occur. But such sequential dependencies in observations cause a number of important conceptual and technical problems in summarizing the data and in testing analytical models, which are not yet completely understood. In the absence of clear solutions, such effects are sometimes ignored by investigators, simplifying the data analysis but leaving residues of skepticism about the reliability and significance of the experimental results. With continuing development of sensitive measures in repeated-trial designs, there is a growing need for more advanced concepts and methods for dealing with experimental results that may be influenced by sequential dependencies.
Randomized Field Experiments
The state of the art in randomized field experiments, in which different policies or procedures are tested in controlled trials under real conditions, has advanced dramatically over the past two decades. Problems that were once considered major methodological obstacles—such as implementing randomized field assignment to treatment and control groups and protecting the randomization procedure from corruption—have been largely overcome. While state-of-the-art standards are not achieved in every field experiment, the commitment to reaching them is rising steadily, not only among researchers but also among customer agencies and sponsors.
The health insurance experiment described in Chapter 2 is an example of a major randomized field experiment that has had and will continue to have important policy reverberations in the design of health care financing. Field experiments with the negative income tax (guaranteed minimum income) conducted in the 1970s were significant in policy debates, even before their completion, and provided the most solid evidence available on how tax-based income support programs and marginal tax rates can affect the work incentives and family structures of the poor. Important field experiments have also been carried out on alternative strategies for the prevention of delinquency and other criminal behavior, reform of court procedures, rehabilitative programs in mental health, family planning, and special educational programs, among other areas.
In planning field experiments, much hinges on the definition and design of the experimental cells, the particular combinations needed of treatment and control conditions for each set of demographic or other client sample characteristics, including specification of the minimum number of cases needed in each cell to test for the presence of effects. Considerations of statistical power, client availability, and the theoretical structure of the inquiry enter into such specifications. Current important methodological thresholds are to find better ways of predicting recruitment and attrition patterns in the sample, of designing experiments that will be statistically robust in the face of problematic sample recruitment or excessive attrition, and of ensuring appropriate acquisition and analysis of data on the attrition component of the sample.
Also of major significance are improvements in integrating detailed process and outcome measurements in field experiments. To conduct research on program effects under field conditions requires continual monitoring to determine exactly what is being done—the process—how it corresponds to what was projected at the outset. Relatively unintrusive, inexpensive, and effective implementation measures are of great interest. There is, in parallel, a growing emphasis on designing experiments to evaluate distinct program components in contrast to summary measures of net program effects.
Finally, there is an important opportunity now for further theoretical work to model organizational processes in social settings and to design and select outcome variables that, in the relatively short time of most field experiments, can predict longer-term effects: For example, in job-training programs, what are the effects on the community (role models, morale, referral networks) or on individual skills, motives, or knowledge levels that are likely to translate into sustained changes in career paths and income levels?
Survey Designs
Many people have opinions about how societal mores, economic conditions, and social programs shape lives and encourage or discourage various kinds of behavior. People generalize from their own cases, and from the groups to which they belong, about such matters as how much it costs to raise a child, the extent to which unemployment contributes to divorce, and so on. In fact, however, effects vary so much from one group to another that homespun generalizations are of little use. Fortunately, behavioral and social scientists have been able to bridge the gaps between personal perspectives and collective realities by means of survey research. In particular, governmental information systems include volumes of extremely valuable survey data, and the facility of modern computers to store, disseminate, and analyze such data has significantly improved empirical tests and led to new understandings of social processes.
Within this category of research designs, two major types are distinguished: repeated cross-sectional surveys and longitudinal panel surveys. In addition, and cross-cutting these types, there is a major effort under way to improve and refine the quality of survey data by investigating features of human memory and of question formation that affect survey response.
Repeated cross-sectional designs can either attempt to measure an entire population—as does the oldest U.S. example, the national decennial census—or they can rest on samples drawn from a population. The general principle is to take independent samples at two or more times, measuring the variables of interest, such as income levels, housing plans, or opinions about public affairs, in the same way. The General Social Survey, collected by the National Opinion Research Center with National Science Foundation support, is a repeated cross sectional data base that was begun in 1972. One methodological question of particular salience in such data is how to adjust for nonresponses and “don’t know” responses. Another is how to deal with self-selection bias. For example, to compare the earnings of women and men in the labor force, it would be mistaken to first assume that the two samples of labor-force participants are randomly selected from the larger populations of men and women; instead, one has to consider and incorporate in the analysis the factors that determine who is in the labor force.
In longitudinal panels, a sample is drawn at one point in time and the relevant variables are measured at this and subsequent times for the same people. In more complex versions, some fraction of each panel may be replaced or added to periodically, such as expanding the sample to include households formed by the children of the original sample. An example of panel data developed in this way is the Panel Study of Income Dynamics (PSID), conducted by the University of Michigan since 1968 (discussed in Chapter 3 ).
Comparing the fertility or income of different people in different circumstances at the same time to find correlations always leaves a large proportion of the variability unexplained, but common sense suggests that much of the unexplained variability is actually explicable. There are systematic reasons for individual outcomes in each person’s past achievements, in parental models, upbringing, and earlier sequences of experiences. Unfortunately, asking people about the past is not particularly helpful: people remake their views of the past to rationalize the present and so retrospective data are often of uncertain validity. In contrast, generation-long longitudinal data allow readings on the sequence of past circumstances uncolored by later outcomes. Such data are uniquely useful for studying the causes and consequences of naturally occurring decisions and transitions. Thus, as longitudinal studies continue, quantitative analysis is becoming feasible about such questions as: How are the decisions of individuals affected by parental experience? Which aspects of early decisions constrain later opportunities? And how does detailed background experience leave its imprint? Studies like the two-decade-long PSID are bringing within grasp a complete generational cycle of detailed data on fertility, work life, household structure, and income.
Advances in Longitudinal Designs
Large-scale longitudinal data collection projects are uniquely valuable as vehicles for testing and improving survey research methodology. In ways that lie beyond the scope of a cross-sectional survey, longitudinal studies can sometimes be designed—without significant detriment to their substantive interests—to facilitate the evaluation and upgrading of data quality; the analysis of relative costs and effectiveness of alternative techniques of inquiry; and the standardization or coordination of solutions to problems of method, concept, and measurement across different research domains.
Some areas of methodological improvement include discoveries about the impact of interview mode on response (mail, telephone, face-to-face); the effects of nonresponse on the representativeness of a sample (due to respondents’ refusal or interviewers’ failure to contact); the effects on behavior of continued participation over time in a sample survey; the value of alternative methods of adjusting for nonresponse and incomplete observations (such as imputation of missing data, variable case weighting); the impact on response of specifying different recall periods, varying the intervals between interviews, or changing the length of interviews; and the comparison and calibration of results obtained by longitudinal surveys, randomized field experiments, laboratory studies, onetime surveys, and administrative records.
It should be especially noted that incorporating improvements in methodology and data quality has been and will no doubt continue to be crucial to the growing success of longitudinal studies. Panel designs are intrinsically more vulnerable than other designs to statistical biases due to cumulative item non-response, sample attrition, time-in-sample effects, and error margins in repeated measures, all of which may produce exaggerated estimates of change. Over time, a panel that was initially representative may become much less representative of a population, not only because of attrition in the sample, but also because of changes in immigration patterns, age structure, and the like. Longitudinal studies are also subject to changes in scientific and societal contexts that may create uncontrolled drifts over time in the meaning of nominally stable questions or concepts as well as in the underlying behavior. Also, a natural tendency to expand over time the range of topics and thus the interview lengths, which increases the burdens on respondents, may lead to deterioration of data quality or relevance. Careful methodological research to understand and overcome these problems has been done, and continued work as a component of new longitudinal studies is certain to advance the overall state of the art.
Longitudinal studies are sometimes pressed for evidence they are not designed to produce: for example, in important public policy questions concerning the impact of government programs in such areas as health promotion, disease prevention, or criminal justice. By using research designs that combine field experiments (with randomized assignment to program and control conditions) and longitudinal surveys, one can capitalize on the strongest merits of each: the experimental component provides stronger evidence for casual statements that are critical for evaluating programs and for illuminating some fundamental theories; the longitudinal component helps in the estimation of long-term program effects and their attenuation. Coupling experiments to ongoing longitudinal studies is not often feasible, given the multiple constraints of not disrupting the survey, developing all the complicated arrangements that go into a large-scale field experiment, and having the populations of interest overlap in useful ways. Yet opportunities to join field experiments to surveys are of great importance. Coupled studies can produce vital knowledge about the empirical conditions under which the results of longitudinal surveys turn out to be similar to—or divergent from—those produced by randomized field experiments. A pattern of divergence and similarity has begun to emerge in coupled studies; additional cases are needed to understand why some naturally occurring social processes and longitudinal design features seem to approximate formal random allocation and others do not. The methodological implications of such new knowledge go well beyond program evaluation and survey research. These findings bear directly on the confidence scientists—and others—can have in conclusions from observational studies of complex behavioral and social processes, particularly ones that cannot be controlled or simulated within the confines of a laboratory environment.
Memory and the Framing of Questions
A very important opportunity to improve survey methods lies in the reduction of nonsampling error due to questionnaire context, phrasing of questions, and, generally, the semantic and social-psychological aspects of surveys. Survey data are particularly affected by the fallibility of human memory and the sensitivity of respondents to the framework in which a question is asked. This sensitivity is especially strong for certain types of attitudinal and opinion questions. Efforts are now being made to bring survey specialists into closer contact with researchers working on memory function, knowledge representation, and language in order to uncover and reduce this kind of error.
Memory for events is often inaccurate, biased toward what respondents believe to be true—or should be true—about the world. In many cases in which data are based on recollection, improvements can be achieved by shifting to techniques of structured interviewing and calibrated forms of memory elicitation, such as specifying recent, brief time periods (for example, in the last seven days) within which respondents recall certain types of events with acceptable accuracy.
- “Taking things altogether, how would you describe your marriage? Would you say that your marriage is very happy, pretty happy, or not too happy?”
- “Taken altogether how would you say things are these days—would you say you are very happy, pretty happy, or not too happy?”
Presenting this sequence in both directions on different forms showed that the order affected answers to the general happiness question but did not change the marital happiness question: responses to the specific issue swayed subsequent responses to the general one, but not vice versa. The explanations for and implications of such order effects on the many kinds of questions and sequences that can be used are not simple matters. Further experimentation on the design of survey instruments promises not only to improve the accuracy and reliability of survey research, but also to advance understanding of how people think about and evaluate their behavior from day to day.
Comparative Designs
Both experiments and surveys involve interventions or questions by the scientist, who then records and analyzes the responses. In contrast, many bodies of social and behavioral data of considerable value are originally derived from records or collections that have accumulated for various nonscientific reasons, quite often administrative in nature, in firms, churches, military organizations, and governments at all levels. Data of this kind can sometimes be subjected to careful scrutiny, summary, and inquiry by historians and social scientists, and statistical methods have increasingly been used to develop and evaluate inferences drawn from such data. Some of the main comparative approaches are cross-national aggregate comparisons, selective comparison of a limited number of cases, and historical case studies.
Among the more striking problems facing the scientist using such data are the vast differences in what has been recorded by different agencies whose behavior is being compared (this is especially true for parallel agencies in different nations), the highly unrepresentative or idiosyncratic sampling that can occur in the collection of such data, and the selective preservation and destruction of records. Means to overcome these problems form a substantial methodological research agenda in comparative research. An example of the method of cross-national aggregative comparisons is found in investigations by political scientists and sociologists of the factors that underlie differences in the vitality of institutions of political democracy in different societies. Some investigators have stressed the existence of a large middle class, others the level of education of a population, and still others the development of systems of mass communication. In cross-national aggregate comparisons, a large number of nations are arrayed according to some measures of political democracy and then attempts are made to ascertain the strength of correlations between these and the other variables. In this line of analysis it is possible to use a variety of statistical cluster and regression techniques to isolate and assess the possible impact of certain variables on the institutions under study. While this kind of research is cross-sectional in character, statements about historical processes are often invoked to explain the correlations.
More limited selective comparisons, applied by many of the classic theorists, involve asking similar kinds of questions but over a smaller range of societies. Why did democracy develop in such different ways in America, France, and England? Why did northeastern Europe develop rational bourgeois capitalism, in contrast to the Mediterranean and Asian nations? Modern scholars have turned their attention to explaining, for example, differences among types of fascism between the two World Wars, and similarities and differences among modern state welfare systems, using these comparisons to unravel the salient causes. The questions asked in these instances are inevitably historical ones.
Historical case studies involve only one nation or region, and so they may not be geographically comparative. However, insofar as they involve tracing the transformation of a society’s major institutions and the role of its main shaping events, they involve a comparison of different periods of a nation’s or a region’s history. The goal of such comparisons is to give a systematic account of the relevant differences. Sometimes, particularly with respect to the ancient societies, the historical record is very sparse, and the methods of history and archaeology mesh in the reconstruction of complex social arrangements and patterns of change on the basis of few fragments.
Like all research designs, comparative ones have distinctive vulnerabilities and advantages: One of the main advantages of using comparative designs is that they greatly expand the range of data, as well as the amount of variation in those data, for study. Consequently, they allow for more encompassing explanations and theories that can relate highly divergent outcomes to one another in the same framework. They also contribute to reducing any cultural biases or tendencies toward parochialism among scientists studying common human phenomena.
One main vulnerability in such designs arises from the problem of achieving comparability. Because comparative study involves studying societies and other units that are dissimilar from one another, the phenomena under study usually occur in very different contexts—so different that in some cases what is called an event in one society cannot really be regarded as the same type of event in another. For example, a vote in a Western democracy is different from a vote in an Eastern bloc country, and a voluntary vote in the United States means something different from a compulsory vote in Australia. These circumstances make for interpretive difficulties in comparing aggregate rates of voter turnout in different countries.
The problem of achieving comparability appears in historical analysis as well. For example, changes in laws and enforcement and recording procedures over time change the definition of what is and what is not a crime, and for that reason it is difficult to compare the crime rates over time. Comparative researchers struggle with this problem continually, working to fashion equivalent measures; some have suggested the use of different measures (voting, letters to the editor, street demonstration) in different societies for common variables (political participation), to try to take contextual factors into account and to achieve truer comparability.
A second vulnerability is controlling variation. Traditional experiments make conscious and elaborate efforts to control the variation of some factors and thereby assess the causal significance of others. In surveys as well as experiments, statistical methods are used to control sources of variation and assess suspected causal significance. In comparative and historical designs, this kind of control is often difficult to attain because the sources of variation are many and the number of cases few. Scientists have made efforts to approximate such control in these cases of “many variables, small N.” One is the method of paired comparisons. If an investigator isolates 15 American cities in which racial violence has been recurrent in the past 30 years, for example, it is helpful to match them with 15 cities of similar population size, geographical region, and size of minorities—such characteristics are controls—and then search for systematic differences between the two sets of cities. Another method is to select, for comparative purposes, a sample of societies that resemble one another in certain critical ways, such as size, common language, and common level of development, thus attempting to hold these factors roughly constant, and then seeking explanations among other factors in which the sampled societies differ from one another.
Ethnographic Designs
Traditionally identified with anthropology, ethnographic research designs are playing increasingly significant roles in most of the behavioral and social sciences. The core of this methodology is participant-observation, in which a researcher spends an extended period of time with the group under study, ideally mastering the local language, dialect, or special vocabulary, and participating in as many activities of the group as possible. This kind of participant-observation is normally coupled with extensive open-ended interviewing, in which people are asked to explain in depth the rules, norms, practices, and beliefs through which (from their point of view) they conduct their lives. A principal aim of ethnographic study is to discover the premises on which those rules, norms, practices, and beliefs are built.
The use of ethnographic designs by anthropologists has contributed significantly to the building of knowledge about social and cultural variation. And while these designs continue to center on certain long-standing features—extensive face-to-face experience in the community, linguistic competence, participation, and open-ended interviewing—there are newer trends in ethnographic work. One major trend concerns its scale. Ethnographic methods were originally developed largely for studying small-scale groupings known variously as village, folk, primitive, preliterate, or simple societies. Over the decades, these methods have increasingly been applied to the study of small groups and networks within modern (urban, industrial, complex) society, including the contemporary United States. The typical subjects of ethnographic study in modern society are small groups or relatively small social networks, such as outpatient clinics, medical schools, religious cults and churches, ethnically distinctive urban neighborhoods, corporate offices and factories, and government bureaus and legislatures.
As anthropologists moved into the study of modern societies, researchers in other disciplines—particularly sociology, psychology, and political science—began using ethnographic methods to enrich and focus their own insights and findings. At the same time, studies of large-scale structures and processes have been aided by the use of ethnographic methods, since most large-scale changes work their way into the fabric of community, neighborhood, and family, affecting the daily lives of people. Ethnographers have studied, for example, the impact of new industry and new forms of labor in “backward” regions; the impact of state-level birth control policies on ethnic groups; and the impact on residents in a region of building a dam or establishing a nuclear waste dump. Ethnographic methods have also been used to study a number of social processes that lend themselves to its particular techniques of observation and interview—processes such as the formation of class and racial identities, bureaucratic behavior, legislative coalitions and outcomes, and the formation and shifting of consumer tastes.
Advances in structured interviewing (see above) have proven especially powerful in the study of culture. Techniques for understanding kinship systems, concepts of disease, color terminologies, ethnobotany, and ethnozoology have been radically transformed and strengthened by coupling new interviewing methods with modem measurement and scaling techniques (see below). These techniques have made possible more precise comparisons among cultures and identification of the most competent and expert persons within a culture. The next step is to extend these methods to study the ways in which networks of propositions (such as boys like sports, girls like babies) are organized to form belief systems. Much evidence suggests that people typically represent the world around them by means of relatively complex cognitive models that involve interlocking propositions. The techniques of scaling have been used to develop models of how people categorize objects, and they have great potential for further development, to analyze data pertaining to cultural propositions.
Ideological Systems
Perhaps the most fruitful area for the application of ethnographic methods in recent years has been the systematic study of ideologies in modern society. Earlier studies of ideology were in small-scale societies that were rather homogeneous. In these studies researchers could report on a single culture, a uniform system of beliefs and values for the society as a whole. Modern societies are much more diverse both in origins and number of subcultures, related to different regions, communities, occupations, or ethnic groups. Yet these subcultures and ideologies share certain underlying assumptions or at least must find some accommodation with the dominant value and belief systems in the society.
The challenge is to incorporate this greater complexity of structure and process into systematic descriptions and interpretations. One line of work carried out by researchers has tried to track the ways in which ideologies are created, transmitted, and shared among large populations that have traditionally lacked the social mobility and communications technologies of the West. This work has concentrated on large-scale civilizations such as China, India, and Central America. Gradually, the focus has generalized into a concern with the relationship between the great traditions—the central lines of cosmopolitan Confucian, Hindu, or Mayan culture, including aesthetic standards, irrigation technologies, medical systems, cosmologies and calendars, legal codes, poetic genres, and religious doctrines and rites—and the little traditions, those identified with rural, peasant communities. How are the ideological doctrines and cultural values of the urban elites, the great traditions, transmitted to local communities? How are the little traditions, the ideas from the more isolated, less literate, and politically weaker groups in society, transmitted to the elites?
India and southern Asia have been fruitful areas for ethnographic research on these questions. The great Hindu tradition was present in virtually all local contexts through the presence of high-caste individuals in every community. It operated as a pervasive standard of value for all members of society, even in the face of strong little traditions. The situation is surprisingly akin to that of modern, industrialized societies. The central research questions are the degree and the nature of penetration of dominant ideology, even in groups that appear marginal and subordinate and have no strong interest in sharing the dominant value system. In this connection the lowest and poorest occupational caste—the untouchables—serves as an ultimate test of the power of ideology and cultural beliefs to unify complex hierarchical social systems.
Historical Reconstruction
Another current trend in ethnographic methods is its convergence with archival methods. One joining point is the application of descriptive and interpretative procedures used by ethnographers to reconstruct the cultures that created historical documents, diaries, and other records, to interview history, so to speak. For example, a revealing study showed how the Inquisition in the Italian countryside between the 1570s and 1640s gradually worked subtle changes in an ancient fertility cult in peasant communities; the peasant beliefs and rituals assimilated many elements of witchcraft after learning them from their persecutors. A good deal of social history—particularly that of the family—has drawn on discoveries made in the ethnographic study of primitive societies. As described in Chapter 4 , this particular line of inquiry rests on a marriage of ethnographic, archival, and demographic approaches.
Other lines of ethnographic work have focused on the historical dimensions of nonliterate societies. A strikingly successful example in this kind of effort is a study of head-hunting. By combining an interpretation of local oral tradition with the fragmentary observations that were made by outside observers (such as missionaries, traders, colonial officials), historical fluctuations in the rate and significance of head-hunting were shown to be partly in response to such international forces as the great depression and World War II. Researchers are also investigating the ways in which various groups in contemporary societies invent versions of traditions that may or may not reflect the actual history of the group. This process has been observed among elites seeking political and cultural legitimation and among hard-pressed minorities (for example, the Basque in Spain, the Welsh in Great Britain) seeking roots and political mobilization in a larger society.
Ethnography is a powerful method to record, describe, and interpret the system of meanings held by groups and to discover how those meanings affect the lives of group members. It is a method well adapted to the study of situations in which people interact with one another and the researcher can interact with them as well, so that information about meanings can be evoked and observed. Ethnography is especially suited to exploration and elucidation of unsuspected connections; ideally, it is used in combination with other methods—experimental, survey, or comparative—to establish with precision the relative strengths and weaknesses of such connections. By the same token, experimental, survey, and comparative methods frequently yield connections, the meaning of which is unknown; ethnographic methods are a valuable way to determine them.
- Models for Representing Phenomena
The objective of any science is to uncover the structure and dynamics of the phenomena that are its subject, as they are exhibited in the data. Scientists continuously try to describe possible structures and ask whether the data can, with allowance for errors of measurement, be described adequately in terms of them. Over a long time, various families of structures have recurred throughout many fields of science; these structures have become objects of study in their own right, principally by statisticians, other methodological specialists, applied mathematicians, and philosophers of logic and science. Methods have evolved to evaluate the adequacy of particular structures to account for particular types of data. In the interest of clarity we discuss these structures in this section and the analytical methods used for estimation and evaluation of them in the next section, although in practice they are closely intertwined.
A good deal of mathematical and statistical modeling attempts to describe the relations, both structural and dynamic, that hold among variables that are presumed to be representable by numbers. Such models are applicable in the behavioral and social sciences only to the extent that appropriate numerical measurement can be devised for the relevant variables. In many studies the phenomena in question and the raw data obtained are not intrinsically numerical, but qualitative, such as ethnic group identifications. The identifying numbers used to code such questionnaire categories for computers are no more than labels, which could just as well be letters or colors. One key question is whether there is some natural way to move from the qualitative aspects of such data to a structural representation that involves one of the well-understood numerical or geometric models or whether such an attempt would be inherently inappropriate for the data in question. The decision as to whether or not particular empirical data can be represented in particular numerical or more complex structures is seldom simple, and strong intuitive biases or a priori assumptions about what can and cannot be done may be misleading.
Recent decades have seen rapid and extensive development and application of analytical methods attuned to the nature and complexity of social science data. Examples of nonnumerical modeling are increasing. Moreover, the widespread availability of powerful computers is probably leading to a qualitative revolution, it is affecting not only the ability to compute numerical solutions to numerical models, but also to work out the consequences of all sorts of structures that do not involve numbers at all. The following discussion gives some indication of the richness of past progress and of future prospects although it is by necessity far from exhaustive.
In describing some of the areas of new and continuing research, we have organized this section on the basis of whether the representations are fundamentally probabilistic or not. A further useful distinction is between representations of data that are highly discrete or categorical in nature (such as whether a person is male or female) and those that are continuous in nature (such as a person’s height). Of course, there are intermediate cases involving both types of variables, such as color stimuli that are characterized by discrete hues (red, green) and a continuous luminance measure. Probabilistic models lead very naturally to questions of estimation and statistical evaluation of the correspondence between data and model. Those that are not probabilistic involve additional problems of dealing with and representing sources of variability that are not explicitly modeled. At the present time, scientists understand some aspects of structure, such as geometries, and some aspects of randomness, as embodied in probability models, but do not yet adequately understand how to put the two together in a single unified model. Table 5-1 outlines the way we have organized this discussion and shows where the examples in this section lie.
A Classification of Structural Models.
Probability Models
Some behavioral and social sciences variables appear to be more or less continuous, for example, utility of goods, loudness of sounds, or risk associated with uncertain alternatives. Many other variables, however, are inherently categorical, often with only two or a few values possible: for example, whether a person is in or out of school, employed or not employed, identifies with a major political party or political ideology. And some variables, such as moral attitudes, are typically measured in research with survey questions that allow only categorical responses. Much of the early probability theory was formulated only for continuous variables; its use with categorical variables was not really justified, and in some cases it may have been misleading. Recently, very significant advances have been made in how to deal explicitly with categorical variables. This section first describes several contemporary approaches to models involving categorical variables, followed by ones involving continuous representations.
Log-Linear Models for Categorical Variables
Many recent models for analyzing categorical data of the kind usually displayed as counts (cell frequencies) in multidimensional contingency tables are subsumed under the general heading of log-linear models, that is, linear models in the natural logarithms of the expected counts in each cell in the table. These recently developed forms of statistical analysis allow one to partition variability due to various sources in the distribution of categorical attributes, and to isolate the effects of particular variables or combinations of them.
Present log-linear models were first developed and used by statisticians and sociologists and then found extensive application in other social and behavioral sciences disciplines. When applied, for instance, to the analysis of social mobility, such models separate factors of occupational supply and demand from other factors that impede or propel movement up and down the social hierarchy. With such models, for example, researchers discovered the surprising fact that occupational mobility patterns are strikingly similar in many nations of the world (even among disparate nations like the United States and most of the Eastern European socialist countries), and from one time period to another, once allowance is made for differences in the distributions of occupations. The log-linear and related kinds of models have also made it possible to identify and analyze systematic differences in mobility among nations and across time. As another example of applications, psychologists and others have used log-linear models to analyze attitudes and their determinants and to link attitudes to behavior. These methods have also diffused to and been used extensively in the medical and biological sciences.
Regression Models for Categorical Variables
Models that permit one variable to be explained or predicted by means of others, called regression models, are the workhorses of much applied statistics; this is especially true when the dependent (explained) variable is continuous. For a two-valued dependent variable, such as alive or dead, models and approximate theory and computational methods for one explanatory variable were developed in biometry about 50 years ago. Computer programs able to handle many explanatory variables, continuous or categorical, are readily available today. Even now, however, the accuracy of the approximate theory on given data is an open question.
Using classical utility theory, economists have developed discrete choice models that turn out to be somewhat related to the log-linear and categorical regression models. Models for limited dependent variables, especially those that cannot take on values above or below a certain level (such as weeks unemployed, number of children, and years of schooling) have been used profitably in economics and in some other areas. For example, censored normal variables (called tobits in economics), in which observed values outside certain limits are simply counted, have been used in studying decisions to go on in school. It will require further research and development to incorporate information about limited ranges of variables fully into the main multivariate methodologies. In addition, with respect to the assumptions about distribution and functional form conventionally made in discrete response models, some new methods are now being developed that show promise of yielding reliable inferences without making unrealistic assumptions; further research in this area promises significant progress.
One problem arises from the fact that many of the categorical variables collected by the major data bases are ordered. For example, attitude surveys frequently use a 3-, 5-, or 7-point scale (from high to low) without specifying numerical intervals between levels. Social class and educational levels are often described by ordered categories. Ignoring order information, which many traditional statistical methods do, may be inefficient or inappropriate, but replacing the categories by successive integers or other arbitrary scores may distort the results. (For additional approaches to this question, see sections below on ordered structures.) Regression-like analysis of ordinal categorical variables is quite well developed, but their multivariate analysis needs further research. New log-bilinear models have been proposed, but to date they deal specifically with only two or three categorical variables. Additional research extending the new models, improving computational algorithms, and integrating the models with work on scaling promise to lead to valuable new knowledge.
Models for Event Histories
Event-history studies yield the sequence of events that respondents to a survey sample experience over a period of time; for example, the timing of marriage, childbearing, or labor force participation. Event-history data can be used to study educational progress, demographic processes (migration, fertility, and mortality), mergers of firms, labor market behavior, and even riots, strikes, and revolutions. As interest in such data has grown, many researchers have turned to models that pertain to changes in probabilities over time to describe when and how individuals move among a set of qualitative states.
Much of the progress in models for event-history data builds on recent developments in statistics and biostatistics for life-time, failure-time, and hazard models. Such models permit the analysis of qualitative transitions in a population whose members are undergoing partially random organic deterioration, mechanical wear, or other risks over time. With the increased complexity of event-history data that are now being collected, and the extension of event-history data bases over very long periods of time, new problems arise that cannot be effectively handled by older types of analysis. Among the problems are repeated transitions, such as between unemployment and employment or marriage and divorce; more than one time variable (such as biological age, calendar time, duration in a stage, and time exposed to some specified condition); latent variables (variables that are explicitly modeled even though not observed); gaps in the data; sample attrition that is not randomly distributed over the categories; and respondent difficulties in recalling the exact timing of events.
Models for Multiple-Item Measurement
For a variety of reasons, researchers typically use multiple measures (or multiple indicators) to represent theoretical concepts. Sociologists, for example, often rely on two or more variables (such as occupation and education) to measure an individual’s socioeconomic position; educational psychologists ordinarily measure a student’s ability with multiple test items. Despite the fact that the basic observations are categorical, in a number of applications this is interpreted as a partitioning of something continuous. For example, in test theory one thinks of the measures of both item difficulty and respondent ability as continuous variables, possibly multidimensional in character.
Classical test theory and newer item-response theories in psychometrics deal with the extraction of information from multiple measures. Testing, which is a major source of data in education and other areas, results in millions of test items stored in archives each year for purposes ranging from college admissions to job-training programs for industry. One goal of research on such test data is to be able to make comparisons among persons or groups even when different test items are used. Although the information collected from each respondent is intentionally incomplete in order to keep the tests short and simple, item-response techniques permit researchers to reconstitute the fragments into an accurate picture of overall group proficiencies. These new methods provide a better theoretical handle on individual differences, and they are expected to be extremely important in developing and using tests. For example, they have been used in attempts to equate different forms of a test given in successive waves during a year, a procedure made necessary in large-scale testing programs by legislation requiring disclosure of test-scoring keys at the time results are given.
An example of the use of item-response theory in a significant research effort is the National Assessment of Educational Progress (NAEP). The goal of this project is to provide accurate, nationally representative information on the average (rather than individual) proficiency of American children in a wide variety of academic subjects as they progress through elementary and secondary school. This approach is an improvement over the use of trend data on university entrance exams, because NAEP estimates of academic achievements (by broad characteristics such as age, grade, region, ethnic background, and so on) are not distorted by the self-selected character of those students who seek admission to college, graduate, and professional programs.
Item-response theory also forms the basis of many new psychometric instruments, known as computerized adaptive testing, currently being implemented by the U.S. military services and under additional development in many testing organizations. In adaptive tests, a computer program selects items for each examinee based upon the examinee’s success with previous items. Generally, each person gets a slightly different set of items and the equivalence of scale scores is established by using item-response theory. Adaptive testing can greatly reduce the number of items needed to achieve a given level of measurement accuracy.
Nonlinear, Nonadditive Models
Virtually all statistical models now in use impose a linearity or additivity assumption of some kind, sometimes after a nonlinear transformation of variables. Imposing these forms on relationships that do not, in fact, possess them may well result in false descriptions and spurious effects. Unwary users, especially of computer software packages, can easily be misled. But more realistic nonlinear and nonadditive multivariate models are becoming available. Extensive use with empirical data is likely to force many changes and enhancements in such models and stimulate quite different approaches to nonlinear multivariate analysis in the next decade.
Geometric and Algebraic Models
Geometric and algebraic models attempt to describe underlying structural relations among variables. In some cases they are part of a probabilistic approach, such as the algebraic models underlying regression or the geometric representations of correlations between items in a technique called factor analysis. In other cases, geometric and algebraic models are developed without explicitly modeling the element of randomness or uncertainty that is always present in the data. Although this latter approach to behavioral and social sciences problems has been less researched than the probabilistic one, there are some advantages in developing the structural aspects independent of the statistical ones. We begin the discussion with some inherently geometric representations and then turn to numerical representations for ordered data.
Although geometry is a huge mathematical topic, little of it seems directly applicable to the kinds of data encountered in the behavioral and social sciences. A major reason is that the primitive concepts normally used in geometry—points, lines, coincidence—do not correspond naturally to the kinds of qualitative observations usually obtained in behavioral and social sciences contexts. Nevertheless, since geometric representations are used to reduce bodies of data, there is a real need to develop a deeper understanding of when such representations of social or psychological data make sense. Moreover, there is a practical need to understand why geometric computer algorithms, such as those of multidimensional scaling, work as well as they apparently do. A better understanding of the algorithms will increase the efficiency and appropriateness of their use, which becomes increasingly important with the widespread availability of scaling programs for microcomputers.
Over the past 50 years several kinds of well-understood scaling techniques have been developed and widely used to assist in the search for appropriate geometric representations of empirical data. The whole field of scaling is now entering a critical juncture in terms of unifying and synthesizing what earlier appeared to be disparate contributions. Within the past few years it has become apparent that several major methods of analysis, including some that are based on probabilistic assumptions, can be unified under the rubric of a single generalized mathematical structure. For example, it has recently been demonstrated that such diverse approaches as nonmetric multidimensional scaling, principal-components analysis, factor analysis, correspondence analysis, and log-linear analysis have more in common in terms of underlying mathematical structure than had earlier been realized.
Nonmetric multidimensional scaling is a method that begins with data about the ordering established by subjective similarity (or nearness) between pairs of stimuli. The idea is to embed the stimuli into a metric space (that is, a geometry with a measure of distance between points) in such a way that distances between points corresponding to stimuli exhibit the same ordering as do the data. This method has been successfully applied to phenomena that, on other grounds, are known to be describable in terms of a specific geometric structure; such applications were used to validate the procedures. Such validation was done, for example, with respect to the perception of colors, which are known to be describable in terms of a particular three-dimensional structure known as the Euclidean color coordinates. Similar applications have been made with Morse code symbols and spoken phonemes. The technique is now used in some biological and engineering applications, as well as in some of the social sciences, as a method of data exploration and simplification.
One question of interest is how to develop an axiomatic basis for various geometries using as a primitive concept an observable such as the subject’s ordering of the relative similarity of one pair of stimuli to another, which is the typical starting point of such scaling. The general task is to discover properties of the qualitative data sufficient to ensure that a mapping into the geometric structure exists and, ideally, to discover an algorithm for finding it. Some work of this general type has been carried out: for example, there is an elegant set of axioms based on laws of color matching that yields the three-dimensional vectorial representation of color space. But the more general problem of understanding the conditions under which the multidimensional scaling algorithms are suitable remains unsolved. In addition, work is needed on understanding more general, non-Euclidean spatial models.
Ordered Factorial Systems
One type of structure common throughout the sciences arises when an ordered dependent variable is affected by two or more ordered independent variables. This is the situation to which regression and analysis-of-variance models are often applied; it is also the structure underlying the familiar physical identities, in which physical units are expressed as products of the powers of other units (for example, energy has the unit of mass times the square of the unit of distance divided by the square of the unit of time).
There are many examples of these types of structures in the behavioral and social sciences. One example is the ordering of preference of commodity bundles—collections of various amounts of commodities—which may be revealed directly by expressions of preference or indirectly by choices among alternative sets of bundles. A related example is preferences among alternative courses of action that involve various outcomes with differing degrees of uncertainty; this is one of the more thoroughly investigated problems because of its potential importance in decision making. A psychological example is the trade-off between delay and amount of reward, yielding those combinations that are equally reinforcing. In a common, applied kind of problem, a subject is given descriptions of people in terms of several factors, for example, intelligence, creativity, diligence, and honesty, and is asked to rate them according to a criterion such as suitability for a particular job.
In all these cases and a myriad of others like them the question is whether the regularities of the data permit a numerical representation. Initially, three types of representations were studied quite fully: the dependent variable as a sum, a product, or a weighted average of the measures associated with the independent variables. The first two representations underlie some psychological and economic investigations, as well as a considerable portion of physical measurement and modeling in classical statistics. The third representation, averaging, has proved most useful in understanding preferences among uncertain outcomes and the amalgamation of verbally described traits, as well as some physical variables.
For each of these three cases—adding, multiplying, and averaging—researchers know what properties or axioms of order the data must satisfy for such a numerical representation to be appropriate. On the assumption that one or another of these representations exists, and using numerical ratings by subjects instead of ordering, a scaling technique called functional measurement (referring to the function that describes how the dependent variable relates to the independent ones) has been developed and applied in a number of domains. What remains problematic is how to encompass at the ordinal level the fact that some random error intrudes into nearly all observations and then to show how that randomness is represented at the numerical level; this continues to be an unresolved and challenging research issue.
During the past few years considerable progress has been made in understanding certain representations inherently different from those just discussed. The work has involved three related thrusts. The first is a scheme of classifying structures according to how uniquely their representation is constrained. The three classical numerical representations are known as ordinal, interval, and ratio scale types. For systems with continuous numerical representations and of scale type at least as rich as the ratio one, it has been shown that only one additional type can exist. A second thrust is to accept structural assumptions, like factorial ones, and to derive for each scale the possible functional relations among the independent variables. And the third thrust is to develop axioms for the properties of an order relation that leads to the possible representations. Much is now known about the possible nonadditive representations of both the multifactor case and the one where stimuli can be combined, such as combining sound intensities.
Closely related to this classification of structures is the question: What statements, formulated in terms of the measures arising in such representations, can be viewed as meaningful in the sense of corresponding to something empirical? Statements here refer to any scientific assertions, including statistical ones, formulated in terms of the measures of the variables and logical and mathematical connectives. These are statements for which asserting truth or falsity makes sense. In particular, statements that remain invariant under certain symmetries of structure have played an important role in classical geometry, dimensional analysis in physics, and in relating measurement and statistical models applied to the same phenomenon. In addition, these ideas have been used to construct models in more formally developed areas of the behavioral and social sciences, such as psychophysics. Current research has emphasized the communality of these historically independent developments and is attempting both to uncover systematic, philosophically sound arguments as to why invariance under symmetries is as important as it appears to be and to understand what to do when structures lack symmetry, as, for example, when variables have an inherent upper bound.
Many subjects do not seem to be correctly represented in terms of distances in continuous geometric space. Rather, in some cases, such as the relations among meanings of words—which is of great interest in the study of memory representations—a description in terms of tree-like, hierarchial structures appears to be more illuminating. This kind of description appears appropriate both because of the categorical nature of the judgments and the hierarchial, rather than trade-off, nature of the structure. Individual items are represented as the terminal nodes of the tree, and groupings by different degrees of similarity are shown as intermediate nodes, with the more general groupings occurring nearer the root of the tree. Clustering techniques, requiring considerable computational power, have been and are being developed. Some successful applications exist, but much more refinement is anticipated.
Network Models
Several other lines of advanced modeling have progressed in recent years, opening new possibilities for empirical specification and testing of a variety of theories. In social network data, relationships among units, rather than the units themselves, are the primary objects of study: friendships among persons, trade ties among nations, cocitation clusters among research scientists, interlocking among corporate boards of directors. Special models for social network data have been developed in the past decade, and they give, among other things, precise new measures of the strengths of relational ties among units. A major challenge in social network data at present is to handle the statistical dependence that arises when the units sampled are related in complex ways.
- Statistical Inference and Analysis
As was noted earlier, questions of design, representation, and analysis are intimately intertwined. Some issues of inference and analysis have been discussed above as related to specific data collection and modeling approaches. This section discusses some more general issues of statistical inference and advances in several current approaches to them.
Causal Inference
Behavioral and social scientists use statistical methods primarily to infer the effects of treatments, interventions, or policy factors. Previous chapters included many instances of causal knowledge gained this way. As noted above, the large experimental study of alternative health care financing discussed in Chapter 2 relied heavily on statistical principles and techniques, including randomization, in the design of the experiment and the analysis of the resulting data. Sophisticated designs were necessary in order to answer a variety of questions in a single large study without confusing the effects of one program difference (such as prepayment or fee for service) with the effects of another (such as different levels of deductible costs), or with effects of unobserved variables (such as genetic differences). Statistical techniques were also used to ascertain which results applied across the whole enrolled population and which were confined to certain subgroups (such as individuals with high blood pressure) and to translate utilization rates across different programs and types of patients into comparable overall dollar costs and health outcomes for alternative financing options.
A classical experiment, with systematic but randomly assigned variation of the variables of interest (or some reasonable approach to this), is usually considered the most rigorous basis from which to draw such inferences. But random samples or randomized experimental manipulations are not always feasible or ethically acceptable. Then, causal inferences must be drawn from observational studies, which, however well designed, are less able to ensure that the observed (or inferred) relationships among variables provide clear evidence on the underlying mechanisms of cause and effect.
Certain recurrent challenges have been identified in studying causal inference. One challenge arises from the selection of background variables to be measured, such as the sex, nativity, or parental religion of individuals in a comparative study of how education affects occupational success. The adequacy of classical methods of matching groups in background variables and adjusting for covariates needs further investigation. Statistical adjustment of biases linked to measured background variables is possible, but it can become complicated. Current work in adjustment for selectivity bias is aimed at weakening implausible assumptions, such as normality, when carrying out these adjustments. Even after adjustment has been made for the measured background variables, other, unmeasured variables are almost always still affecting the results (such as family transfers of wealth or reading habits). Analyses of how the conclusions might change if such unmeasured variables could be taken into account is essential in attempting to make causal inferences from an observational study, and systematic work on useful statistical models for such sensitivity analyses is just beginning.
The third important issue arises from the necessity for distinguishing among competing hypotheses when the explanatory variables are measured with different degrees of precision. Both the estimated size and significance of an effect are diminished when it has large measurement error, and the coefficients of other correlated variables are affected even when the other variables are measured perfectly. Similar results arise from conceptual errors, when one measures only proxies for a theoretical construct (such as years of education to represent amount of learning). In some cases, there are procedures for simultaneously or iteratively estimating both the precision of complex measures and their effect on a particular criterion.
Although complex models are often necessary to infer causes, once their output is available, it should be translated into understandable displays for evaluation. Results that depend on the accuracy of a multivariate model and the associated software need to be subjected to appropriate checks, including the evaluation of graphical displays, group comparisons, and other analyses.
New Statistical Techniques
Internal resampling.
One of the great contributions of twentieth-century statistics was to demonstrate how a properly drawn sample of sufficient size, even if it is only a tiny fraction of the population of interest, can yield very good estimates of most population characteristics. When enough is known at the outset about the characteristic in question—for example, that its distribution is roughly normal—inference from the sample data to the population as a whole is straightforward, and one can easily compute measures of the certainty of inference, a common example being the 95 percent confidence interval around an estimate. But population shapes are sometimes unknown or uncertain, and so inference procedures cannot be so simple. Furthermore, more often than not, it is difficult to assess even the degree of uncertainty associated with complex data and with the statistics needed to unravel complex social and behavioral phenomena.
Internal resampling methods attempt to assess this uncertainty by generating a number of simulated data sets similar to the one actually observed. The definition of similar is crucial, and many methods that exploit different types of similarity have been devised. These methods provide researchers the freedom to choose scientifically appropriate procedures and to replace procedures that are valid under assumed distributional shapes with ones that are not so restricted. Flexible and imaginative computer simulation is the key to these methods. For a simple random sample, the “bootstrap” method repeatedly resamples the obtained data (with replacement) to generate a distribution of possible data sets. The distribution of any estimator can thereby be simulated and measures of the certainty of inference be derived. The “jackknife” method repeatedly omits a fraction of the data and in this way generates a distribution of possible data sets that can also be used to estimate variability. These methods can also be used to remove or reduce bias. For example, the ratio-estimator, a statistic that is commonly used in analyzing sample surveys and censuses, is known to be biased, and the jackknife method can usually remedy this defect. The methods have been extended to other situations and types of analysis, such as multiple regression.
There are indications that under relatively general conditions, these methods, and others related to them, allow more accurate estimates of the uncertainty of inferences than do the traditional ones that are based on assumed (usually, normal) distributions when that distributional assumption is unwarranted. For complex samples, such internal resampling or subsampling facilitates estimating the sampling variances of complex statistics.
An older and simpler, but equally important, idea is to use one independent subsample in searching the data to develop a model and at least one separate subsample for estimating and testing a selected model. Otherwise, it is next to impossible to make allowances for the excessively close fitting of the model that occurs as a result of the creative search for the exact characteristics of the sample data—characteristics that are to some degree random and will not predict well to other samples.
Robust Techniques
Many technical assumptions underlie the analysis of data. Some, like the assumption that each item in a sample is drawn independently of other items, can be weakened when the data are sufficiently structured to admit simple alternative models, such as serial correlation. Usually, these models require that a few parameters be estimated. Assumptions about shapes of distributions, normality being the most common, have proved to be particularly important, and considerable progress has been made in dealing with the consequences of different assumptions.
More recently, robust techniques have been designed that permit sharp, valid discriminations among possible values of parameters of central tendency for a wide variety of alternative distributions by reducing the weight given to occasional extreme deviations. It turns out that by giving up, say, 10 percent of the discrimination that could be provided under the rather unrealistic assumption of normality, one can greatly improve performance in more realistic situations, especially when unusually large deviations are relatively common.
These valuable modifications of classical statistical techniques have been extended to multiple regression, in which procedures of iterative reweighting can now offer relatively good performance for a variety of underlying distributional shapes. They should be extended to more general schemes of analysis.
In some contexts—notably the most classical uses of analysis of variance—the use of adequate robust techniques should help to bring conventional statistical practice closer to the best standards that experts can now achieve.
Many Interrelated Parameters
In trying to give a more accurate representation of the real world than is possible with simple models, researchers sometimes use models with many parameters, all of which must be estimated from the data. Classical principles of estimation, such as straightforward maximum-likelihood, do not yield reliable estimates unless either the number of observations is much larger than the number of parameters to be estimated or special designs are used in conjunction with strong assumptions. Bayesian methods do not draw a distinction between fixed and random parameters, and so may be especially appropriate for such problems.
A variety of statistical methods have recently been developed that can be interpreted as treating many of the parameters as or similar to random quantities, even if they are regarded as representing fixed quantities to be estimated. Theory and practice demonstrate that such methods can improve the simpler fixed-parameter methods from which they evolved, especially when the number of observations is not large relative to the number of parameters. Successful applications include college and graduate school admissions, where quality of previous school is treated as a random parameter when the data are insufficient to separately estimate it well. Efforts to create appropriate models using this general approach for small-area estimation and undercount adjustment in the census are important potential applications.
Missing Data
In data analysis, serious problems can arise when certain kinds of (quantitative or qualitative) information is partially or wholly missing. Various approaches to dealing with these problems have been or are being developed. One of the methods developed recently for dealing with certain aspects of missing data is called multiple imputation: each missing value in a data set is replaced by several values representing a range of possibilities, with statistical dependence among missing values reflected by linkage among their replacements. It is currently being used to handle a major problem of incompatibility between the 1980 and previous Bureau of Census public-use tapes with respect to occupation codes. The extension of these techniques to address such problems as nonresponse to income questions in the Current Population Survey has been examined in exploratory applications with great promise.
Computer Packages and Expert Systems
The development of high-speed computing and data handling has fundamentally changed statistical analysis. Methodologies for all kinds of situations are rapidly being developed and made available for use in computer packages that may be incorporated into interactive expert systems. This computing capability offers the hope that much data analyses will be more carefully and more effectively done than previously and that better strategies for data analysis will move from the practice of expert statisticians, some of whom may not have tried to articulate their own strategies, to both wide discussion and general use.
But powerful tools can be hazardous, as witnessed by occasional dire misuses of existing statistical packages. Until recently the only strategies available were to train more expert methodologists or to train substantive scientists in more methodology, but without the updating of their training it tends to become outmoded. Now there is the opportunity to capture in expert systems the current best methodological advice and practice. If that opportunity is exploited, standard methodological training of social scientists will shift to emphasizing strategies in using good expert systems—including understanding the nature and importance of the comments it provides—rather than in how to patch together something on one’s own. With expert systems, almost all behavioral and social scientists should become able to conduct any of the more common styles of data analysis more effectively and with more confidence than all but the most expert do today. However, the difficulties in developing expert systems that work as hoped for should not be underestimated. Human experts cannot readily explicate all of the complex cognitive network that constitutes an important part of their knowledge. As a result, the first attempts at expert systems were not especially successful (as discussed in Chapter 1 ). Additional work is expected to overcome these limitations, but it is not clear how long it will take.
Exploratory Analysis and Graphic Presentation
The formal focus of much statistics research in the middle half of the twentieth century was on procedures to confirm or reject precise, a priori hypotheses developed in advance of collecting data—that is, procedures to determine statistical significance. There was relatively little systematic work on realistically rich strategies for the applied researcher to use when attacking real-world problems with their multiplicity of objectives and sources of evidence. More recently, a species of quantitative detective work, called exploratory data analysis, has received increasing attention. In this approach, the researcher seeks out possible quantitative relations that may be present in the data. The techniques are flexible and include an important component of graphic representations. While current techniques have evolved for single responses in situations of modest complexity, extensions to multiple responses and to single responses in more complex situations are now possible.
Graphic and tabular presentation is a research domain in active renaissance, stemming in part from suggestions for new kinds of graphics made possible by computer capabilities, for example, hanging histograms and easily assimilated representations of numerical vectors. Research on data presentation has been carried out by statisticians, psychologists, cartographers, and other specialists, and attempts are now being made to incorporate findings and concepts from linguistics, industrial and publishing design, aesthetics, and classification studies in library science. Another influence has been the rapidly increasing availability of powerful computational hardware and software, now available even on desktop computers. These ideas and capabilities are leading to an increasing number of behavioral experiments with substantial statistical input. Nonetheless, criteria of good graphic and tabular practice are still too much matters of tradition and dogma, without adequate empirical evidence or theoretical coherence. To broaden the respective research outlooks and vigorously develop such evidence and coherence, extended collaborations between statistical and mathematical specialists and other scientists are needed, a major objective being to understand better the visual and cognitive processes (see Chapter 1 ) relevant to effective use of graphic or tabular approaches.
Combining Evidence
Combining evidence from separate sources is a recurrent scientific task, and formal statistical methods for doing so go back 30 years or more. These methods include the theory and practice of combining tests of individual hypotheses, sequential design and analysis of experiments, comparisons of laboratories, and Bayesian and likelihood paradigms.
There is now growing interest in more ambitious analytical syntheses, which are often called meta-analyses. One stimulus has been the appearance of syntheses explicitly combining all existing investigations in particular fields, such as prison parole policy, classroom size in primary schools, cooperative studies of therapeutic treatments for coronary heart disease, early childhood education interventions, and weather modification experiments. In such fields, a serious approach to even the simplest question—how to put together separate estimates of effect size from separate investigations—leads quickly to difficult and interesting issues. One issue involves the lack of independence among the available studies, due, for example, to the effect of influential teachers on the research projects of their students. Another issue is selection bias, because only some of the studies carried out, usually those with “significant” findings, are available and because the literature search may not find out all relevant studies that are available. In addition, experts agree, although informally, that the quality of studies from different laboratories and facilities differ appreciably and that such information probably should be taken into account. Inevitably, the studies to be included used different designs and concepts and controlled or measured different variables, making it difficult to know how to combine them.
Rich, informal syntheses, allowing for individual appraisal, may be better than catch-all formal modeling, but the literature on formal meta-analytic models is growing and may be an important area of discovery in the next decade, relevant both to statistical analysis per se and to improved syntheses in the behavioral and social and other sciences.
- Opportunities and Needs
This chapter has cited a number of methodological topics associated with behavioral and social sciences research that appear to be particularly active and promising at the present time. As throughout the report, they constitute illustrative examples of what the committee believes to be important areas of research in the coming decade. In this section we describe recommendations for an additional $16 million annually to facilitate both the development of methodologically oriented research and, equally important, its communication throughout the research community.
Methodological studies, including early computer implementations, have for the most part been carried out by individual investigators with small teams of colleagues or students. Occasionally, such research has been associated with quite large substantive projects, and some of the current developments of computer packages, graphics, and expert systems clearly require large, organized efforts, which often lie at the boundary between grant-supported work and commercial development. As such research is often a key to understanding complex bodies of behavioral and social sciences data, it is vital to the health of these sciences that research support continue on methods relevant to problems of modeling, statistical analysis, representation, and related aspects of behavioral and social sciences data. Researchers and funding agencies should also be especially sympathetic to the inclusion of such basic methodological work in large experimental and longitudinal studies. Additional funding for work in this area, both in terms of individual research grants on methodological issues and in terms of augmentation of large projects to include additional methodological aspects, should be provided largely in the form of investigator-initiated project grants.
Ethnographic and comparative studies also typically rely on project grants to individuals and small groups of investigators. While this type of support should continue, provision should also be made to facilitate the execution of studies using these methods by research teams and to provide appropriate methodological training through the mechanisms outlined below.
Overall, we recommend an increase of $4 million in the level of investigator-initiated grant support for methodological work. An additional $1 million should be devoted to a program of centers for methodological research.
Many of the new methods and models described in the chapter, if and when adopted to any large extent, will demand substantially greater amounts of research devoted to appropriate analysis and computer implementation. New user interfaces and numerical algorithms will need to be designed and new computer programs written. And even when generally available methods (such as maximum-likelihood) are applicable, model application still requires skillful development in particular contexts. Many of the familiar general methods that are applied in the statistical analysis of data are known to provide good approximations when sample sizes are sufficiently large, but their accuracy varies with the specific model and data used. To estimate the accuracy requires extensive numerical exploration. Investigating the sensitivity of results to the assumptions of the models is important and requires still more creative, thoughtful research. It takes substantial efforts of these kinds to bring any new model on line, and the need becomes increasingly important and difficult as statistical models move toward greater realism, usefulness, complexity, and availability in computer form. More complexity in turn will increase the demand for computational power. Although most of this demand can be satisfied by increasingly powerful desktop computers, some access to mainframe and even supercomputers will be needed in selected cases. We recommend an additional $4 million annually to cover the growth in computational demands for model development and testing.
Interaction and cooperation between the developers and the users of statistical and mathematical methods need continual stimulation—both ways. Efforts should be made to teach new methods to a wider variety of potential users than is now the case. Several ways appear effective for methodologists to communicate to empirical scientists: running summer training programs for graduate students, faculty, and other researchers; encouraging graduate students, perhaps through degree requirements, to make greater use of the statistical, mathematical, and methodological resources at their own or affiliated universities; associating statistical and mathematical research specialists with large-scale data collection projects; and developing statistical packages that incorporate expert systems in applying the methods.
Methodologists, in turn, need to become more familiar with the problems actually faced by empirical scientists in the laboratory and especially in the field. Several ways appear useful for communication in this direction: encouraging graduate students in methodological specialties, perhaps through degree requirements, to work directly on empirical research; creating postdoctoral fellowships aimed at integrating such specialists into ongoing data collection projects; and providing for large data collection projects to engage relevant methodological specialists. In addition, research on and development of statistical packages and expert systems should be encouraged to involve the multidisciplinary collaboration of experts with experience in statistical, computer, and cognitive sciences.
A final point has to do with the promise held out by bringing different research methods to bear on the same problems. As our discussions of research methods in this and other chapters have emphasized, different methods have different powers and limitations, and each is designed especially to elucidate one or more particular facets of a subject. An important type of interdisciplinary work is the collaboration of specialists in different research methodologies on a substantive issue, examples of which have been noted throughout this report. If more such research were conducted cooperatively, the power of each method pursued separately would be increased. To encourage such multidisciplinary work, we recommend increased support for fellowships, research workshops, and training institutes.
Funding for fellowships, both pre-and postdoctoral, should be aimed at giving methodologists experience with substantive problems and at upgrading the methodological capabilities of substantive scientists. Such targeted fellowship support should be increased by $4 million annually, of which $3 million should be for predoctoral fellowships emphasizing the enrichment of methodological concentrations. The new support needed for research workshops is estimated to be $1 million annually. And new support needed for various kinds of advanced training institutes aimed at rapidly diffusing new methodological findings among substantive scientists is estimated to be $2 million annually.
- Cite this Page National Research Council; Division of Behavioral and Social Sciences and Education; Commission on Behavioral and Social Sciences and Education; Committee on Basic Research in the Behavioral and Social Sciences; Gerstein DR, Luce RD, Smelser NJ, et al., editors. The Behavioral and Social Sciences: Achievements and Opportunities. Washington (DC): National Academies Press (US); 1988. 5, Methods of Data Collection, Representation, and Analysis.
- PDF version of this title (16M)
In this Page
Other titles in this collection.
- The National Academies Collection: Reports funded by National Institutes of Health
Recent Activity
- Methods of Data Collection, Representation, and Analysis - The Behavioral and So... Methods of Data Collection, Representation, and Analysis - The Behavioral and Social Sciences: Achievements and Opportunities
Your browsing activity is empty.
Activity recording is turned off.
Turn recording back on
Connect with NLM
National Library of Medicine 8600 Rockville Pike Bethesda, MD 20894
Web Policies FOIA HHS Vulnerability Disclosure
Help Accessibility Careers
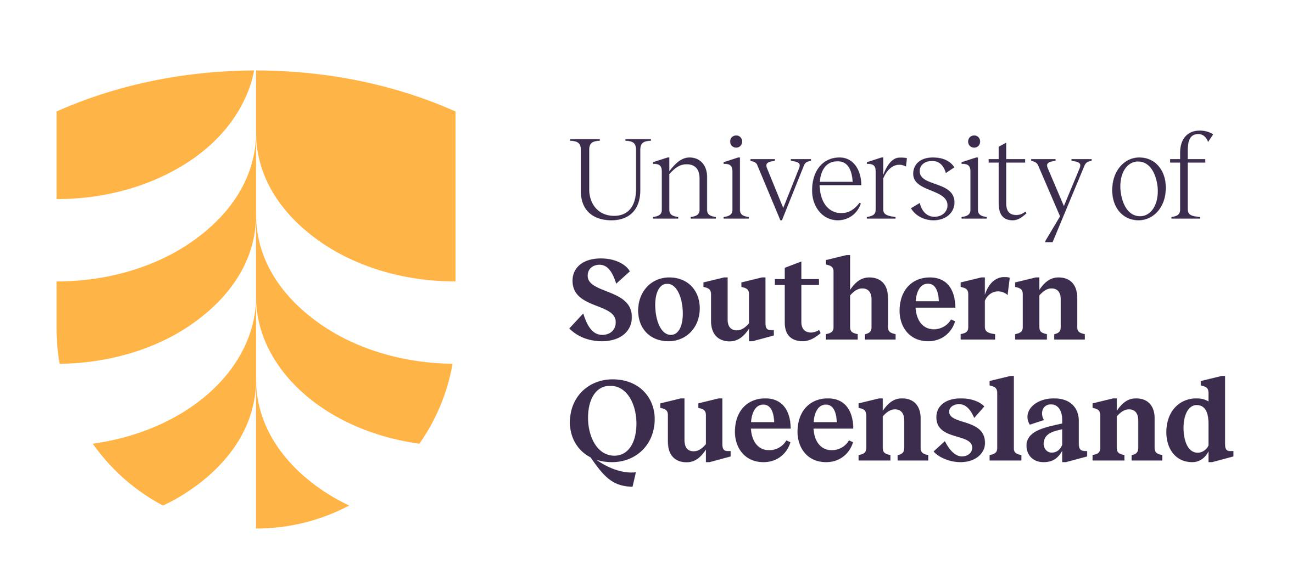
Want to create or adapt books like this? Learn more about how Pressbooks supports open publishing practices.
13 Qualitative analysis
Qualitative analysis is the analysis of qualitative data such as text data from interview transcripts. Unlike quantitative analysis, which is statistics driven and largely independent of the researcher, qualitative analysis is heavily dependent on the researcher’s analytic and integrative skills and personal knowledge of the social context where the data is collected. The emphasis in qualitative analysis is ‘sense making’ or understanding a phenomenon, rather than predicting or explaining. A creative and investigative mindset is needed for qualitative analysis, based on an ethically enlightened and participant-in-context attitude, and a set of analytic strategies. This chapter provides a brief overview of some of these qualitative analysis strategies. Interested readers are referred to more authoritative and detailed references such as Miles and Huberman’s (1984) [1] seminal book on this topic.
Grounded theory
How can you analyse a vast set of qualitative data acquired through participant observation, in-depth interviews, focus groups, narratives of audio/video recordings, or secondary documents? One of these techniques for analysing text data is grounded theory —an inductive technique of interpreting recorded data about a social phenomenon to build theories about that phenomenon. The technique was developed by Glaser and Strauss (1967) [2] in their method of constant comparative analysis of grounded theory research, and further refined by Strauss and Corbin (1990) [3] to further illustrate specific coding techniques—a process of classifying and categorising text data segments into a set of codes (concepts), categories (constructs), and relationships. The interpretations are ‘grounded in’ (or based on) observed empirical data, hence the name. To ensure that the theory is based solely on observed evidence, the grounded theory approach requires that researchers suspend any pre-existing theoretical expectations or biases before data analysis, and let the data dictate the formulation of the theory.
Strauss and Corbin (1998) describe three coding techniques for analysing text data: open, axial, and selective. Open coding is a process aimed at identifying concepts or key ideas that are hidden within textual data, which are potentially related to the phenomenon of interest. The researcher examines the raw textual data line by line to identify discrete events, incidents, ideas, actions, perceptions, and interactions of relevance that are coded as concepts (hence called in vivo codes ). Each concept is linked to specific portions of the text (coding unit) for later validation. Some concepts may be simple, clear, and unambiguous, while others may be complex, ambiguous, and viewed differently by different participants. The coding unit may vary with the concepts being extracted. Simple concepts such as ‘organisational size’ may include just a few words of text, while complex ones such as ‘organizational mission’ may span several pages. Concepts can be named using the researcher’s own naming convention, or standardised labels taken from the research literature. Once a basic set of concepts are identified, these concepts can then be used to code the remainder of the data, while simultaneously looking for new concepts and refining old concepts. While coding, it is important to identify the recognisable characteristics of each concept, such as its size, colour, or level—e.g., high or low—so that similar concepts can be grouped together later . This coding technique is called ‘open’ because the researcher is open to and actively seeking new concepts relevant to the phenomenon of interest.
Next, similar concepts are grouped into higher order categories . While concepts may be context-specific, categories tend to be broad and generalisable, and ultimately evolve into constructs in a grounded theory. Categories are needed to reduce the amount of concepts the researcher must work with and to build a ‘big picture’ of the issues salient to understanding a social phenomenon. Categorisation can be done in phases, by combining concepts into subcategories, and then subcategories into higher order categories. Constructs from the existing literature can be used to name these categories, particularly if the goal of the research is to extend current theories. However, caution must be taken while using existing constructs, as such constructs may bring with them commonly held beliefs and biases. For each category, its characteristics (or properties) and the dimensions of each characteristic should be identified. The dimension represents a value of a characteristic along a continuum. For example, a ‘communication media’ category may have a characteristic called ‘speed’, which can be dimensionalised as fast, medium, or slow . Such categorisation helps differentiate between different kinds of communication media, and enables researchers to identify patterns in the data, such as which communication media is used for which types of tasks.
The second phase of grounded theory is axial coding , where the categories and subcategories are assembled into causal relationships or hypotheses that can tentatively explain the phenomenon of interest. Although distinct from open coding, axial coding can be performed simultaneously with open coding. The relationships between categories may be clearly evident in the data, or may be more subtle and implicit. In the latter instance, researchers may use a coding scheme (often called a ‘coding paradigm’, but different from the paradigms discussed in Chapter 3) to understand which categories represent conditions (the circumstances in which the phenomenon is embedded), actions/interactions (the responses of individuals to events under these conditions), and consequences (the outcomes of actions/interactions). As conditions, actions/interactions, and consequences are identified, theoretical propositions start to emerge, and researchers can start explaining why a phenomenon occurs, under what conditions, and with what consequences.
The third and final phase of grounded theory is selective coding , which involves identifying a central category or a core variable, and systematically and logically relating this central category to other categories. The central category can evolve from existing categories or can be a higher order category that subsumes previously coded categories. New data is selectively sampled to validate the central category, and its relationships to other categories—i.e., the tentative theory. Selective coding limits the range of analysis, and makes it move fast. At the same time, the coder must watch out for other categories that may emerge from the new data that could be related to the phenomenon of interest (open coding), which may lead to further refinement of the initial theory. Hence, open, axial, and selective coding may proceed simultaneously. Coding of new data and theory refinement continues until theoretical saturation is reached—i.e., when additional data does not yield any marginal change in the core categories or the relationships.
The ‘constant comparison’ process implies continuous rearrangement, aggregation, and refinement of categories, relationships, and interpretations based on increasing depth of understanding, and an iterative interplay of four stages of activities: comparing incidents/texts assigned to each category to validate the category), integrating categories and their properties, delimiting the theory by focusing on the core concepts and ignoring less relevant concepts, and writing theory using techniques like memoing, storylining, and diagramming. Having a central category does not necessarily mean that all other categories can be integrated nicely around it. In order to identify key categories that are conditions, action/interactions, and consequences of the core category, Strauss and Corbin (1990) recommend several integration techniques, such as storylining, memoing, or concept mapping, which are discussed here. In storylining , categories and relationships are used to explicate and/or refine a story of the observed phenomenon. Memos are theorised write-ups of ideas about substantive concepts and their theoretically coded relationships as they evolve during ground theory analysis, and are important tools to keep track of and refine ideas that develop during the analysis. Memoing is the process of using these memos to discover patterns and relationships between categories using two-by-two tables, diagrams, or figures, or other illustrative displays. Concept mapping is a graphical representation of concepts and relationships between those concepts—e.g., using boxes and arrows. The major concepts are typically laid out on one or more sheets of paper, blackboards, or using graphical software programs, linked to each other using arrows, and readjusted to best fit the observed data.
After a grounded theory is generated, it must be refined for internal consistency and logic. Researchers must ensure that the central construct has the stated characteristics and dimensions, and if not, the data analysis may be repeated. Researcher must then ensure that the characteristics and dimensions of all categories show variation. For example, if behaviour frequency is one such category, then the data must provide evidence of both frequent performers and infrequent performers of the focal behaviour. Finally, the theory must be validated by comparing it with raw data. If the theory contradicts with observed evidence, the coding process may need to be repeated to reconcile such contradictions or unexplained variations.
Content analysis
Content analysis is the systematic analysis of the content of a text—e.g., who says what, to whom, why, and to what extent and with what effect—in a quantitative or qualitative manner. Content analysis is typically conducted as follows. First, when there are many texts to analyse—e.g., newspaper stories, financial reports, blog postings, online reviews, etc.—the researcher begins by sampling a selected set of texts from the population of texts for analysis. This process is not random, but instead, texts that have more pertinent content should be chosen selectively. Second, the researcher identifies and applies rules to divide each text into segments or ‘chunks’ that can be treated as separate units of analysis. This process is called unitising . For example, assumptions, effects, enablers, and barriers in texts may constitute such units. Third, the researcher constructs and applies one or more concepts to each unitised text segment in a process called coding . For coding purposes, a coding scheme is used based on the themes the researcher is searching for or uncovers as they classify the text. Finally, the coded data is analysed, often both quantitatively and qualitatively, to determine which themes occur most frequently, in what contexts, and how they are related to each other.
A simple type of content analysis is sentiment analysis —a technique used to capture people’s opinion or attitude toward an object, person, or phenomenon. Reading online messages about a political candidate posted on an online forum and classifying each message as positive, negative, or neutral is an example of such an analysis. In this case, each message represents one unit of analysis. This analysis will help identify whether the sample as a whole is positively or negatively disposed, or neutral towards that candidate. Examining the content of online reviews in a similar manner is another example. Though this analysis can be done manually, for very large datasets—e.g., millions of text records—natural language processing and text analytics based software programs are available to automate the coding process, and maintain a record of how people’s sentiments fluctuate with time.
A frequent criticism of content analysis is that it lacks a set of systematic procedures that would allow the analysis to be replicated by other researchers. Schilling (2006) [4] addressed this criticism by organising different content analytic procedures into a spiral model. This model consists of five levels or phases in interpreting text: convert recorded tapes into raw text data or transcripts for content analysis, convert raw data into condensed protocols, convert condensed protocols into a preliminary category system, use the preliminary category system to generate coded protocols, and analyse coded protocols to generate interpretations about the phenomenon of interest.
Content analysis has several limitations. First, the coding process is restricted to the information available in text form. For instance, if a researcher is interested in studying people’s views on capital punishment, but no such archive of text documents is available, then the analysis cannot be done. Second, sampling must be done carefully to avoid sampling bias. For instance, if your population is the published research literature on a given topic, then you have systematically omitted unpublished research or the most recent work that is yet to be published.
Hermeneutic analysis
Hermeneutic analysis is a special type of content analysis where the researcher tries to ‘interpret’ the subjective meaning of a given text within its sociohistoric context. Unlike grounded theory or content analysis—which ignores the context and meaning of text documents during the coding process—hermeneutic analysis is a truly interpretive technique for analysing qualitative data. This method assumes that written texts narrate an author’s experience within a sociohistoric context, and should be interpreted as such within that context. Therefore, the researcher continually iterates between singular interpretation of the text (the part) and a holistic understanding of the context (the whole) to develop a fuller understanding of the phenomenon in its situated context, which German philosopher Martin Heidegger called the hermeneutic circle . The word hermeneutic (singular) refers to one particular method or strand of interpretation.
More generally, hermeneutics is the study of interpretation and the theory and practice of interpretation. Derived from religious studies and linguistics, traditional hermeneutics—such as biblical hermeneutics —refers to the interpretation of written texts, especially in the areas of literature, religion and law—such as the Bible. In the twentieth century, Heidegger suggested that a more direct, non-mediated, and authentic way of understanding social reality is to experience it, rather than simply observe it, and proposed philosophical hermeneutics , where the focus shifted from interpretation to existential understanding. Heidegger argued that texts are the means by which readers can not only read about an author’s experience, but also relive the author’s experiences. Contemporary or modern hermeneutics, developed by Heidegger’s students such as Hans-Georg Gadamer, further examined the limits of written texts for communicating social experiences, and went on to propose a framework of the interpretive process, encompassing all forms of communication, including written, verbal, and non-verbal, and exploring issues that restrict the communicative ability of written texts, such as presuppositions, language structures (e.g., grammar, syntax, etc.), and semiotics—the study of written signs such as symbolism, metaphor, analogy, and sarcasm. The term hermeneutics is sometimes used interchangeably and inaccurately with exegesis , which refers to the interpretation or critical explanation of written text only, and especially religious texts.
Finally, standard software programs, such as ATLAS.ti.5, NVivo, and QDA Miner, can be used to automate coding processes in qualitative research methods. These programs can quickly and efficiently organise, search, sort, and process large volumes of text data using user-defined rules. To guide such automated analysis, a coding schema should be created, specifying the keywords or codes to search for in the text, based on an initial manual examination of sample text data. The schema can be arranged in a hierarchical manner to organise codes into higher-order codes or constructs. The coding schema should be validated using a different sample of texts for accuracy and adequacy. However, if the coding schema is biased or incorrect, the resulting analysis of the entire population of texts may be flawed and non-interpretable. However, software programs cannot decipher the meaning behind certain words or phrases or the context within which these words or phrases are used—such sarcasm or metaphors—which may lead to significant misinterpretation in large scale qualitative analysis.
- Miles, M. B., & Huberman, A. M. (1984). Qualitative data analysis: A sourcebook of new methods . Newbury Park, CA: Sage Publications. ↵
- Glaser, B., & Strauss, A. (1967). The discovery of grounded theory: Strategies for qualitative research . New York: Aldine Pub Co. ↵
- Strauss, A., & Corbin, J. (1990). Basics of qualitative research: Grounded theory procedures and techniques , Beverly Hills: Sage Publications. ↵
- Schiling, J. (2006). On the pragmatics of qualitative assessment: Designing the process for content analysis. European Journal of Psychological Assessment , 22(1), 28–37. ↵
Social Science Research: Principles, Methods and Practices (Revised edition) Copyright © 2019 by Anol Bhattacherjee is licensed under a Creative Commons Attribution-NonCommercial-ShareAlike 4.0 International License , except where otherwise noted.
Share This Book
- Survey Software The world’s leading omnichannel survey software
- Online Survey Tools Create sophisticated surveys with ease.
- Mobile Offline Conduct efficient field surveys.
- Text Analysis
- Close The Loop
- Automated Translations
- NPS Dashboard
- CATI Manage high volume phone surveys efficiently
- Cloud/On-premise Dialer TCPA compliant Cloud & on-premise dialer
- IVR Survey Software Boost productivity with automated call workflows.
- Analytics Analyze survey data with visual dashboards
- Panel Manager Nurture a loyal community of respondents.
- Survey Portal Best-in-class user friendly survey portal.
- Voxco Audience Conduct targeted sample research in hours.

Find the best survey software for you! (Along with a checklist to compare platforms)
Get Buyer’s Guide
- 100+ question types
- Drag-and-drop interface
- Skip logic and branching
- Multi-lingual survey
- Text piping
- Question library
- CSS customization
- White-label surveys
- Customizable ‘Thank You’ page
- Customizable survey theme
- Reminder send-outs
- Survey rewards
- Social media
- Website surveys
- Correlation analysis
- Cross-tabulation analysis
- Trend analysis
- Real-time dashboard
- Customizable report
- Email address validation
- Recaptcha validation
- SSL security
Take a peek at our powerful survey features to design surveys that scale discoveries.
Download feature sheet.
- Hospitality
- Financial Services
- Academic Research
- Customer Experience
- Employee Experience
- Product Experience
- Market Research
- Social Research
- Data Analysis
Explore Voxco
Need to map Voxco’s features & offerings? We can help!
Watch a Demo
Download Brochures
Get a Quote
- NPS Calculator
- CES Calculator
- A/B Testing Calculator
- Margin of Error Calculator
- Sample Size Calculator
- CX Strategy & Management Hub
- Market Research Hub
- Patient Experience Hub
- Employee Experience Hub
- Market Research Guide
- Customer Experience Guide
- The Voxco Guide to Customer Experience
- NPS Knowledge Hub
- Survey Research Guides
- Survey Template Library
- Webinars and Events
- Feature Sheets
- Try a sample survey
- Professional services
Find the best customer experience platform
Uncover customer pain points, analyze feedback and run successful CX programs with the best CX platform for your team.
Get the Guide Now

We’ve been avid users of the Voxco platform now for over 20 years. It gives us the flexibility to routinely enhance our survey toolkit and provides our clients with a more robust dataset and story to tell their clients.
VP Innovation & Strategic Partnerships, The Logit Group
- Client Stories
- Voxco Reviews
- Why Voxco Research?
- Careers at Voxco
- Vulnerabilities and Ethical Hacking
Explore Regional Offices
- Cloud/On-premise Dialer TCPA compliant Cloud on-premise dialer
- Predictive Analytics
- Customer 360
- Customer Loyalty
- Fraud & Risk Management
- AI/ML Enablement Services
- Credit Underwriting
Get Buyer’s Guide
- SMS surveys
- Banking & Financial Services
- Retail Solution
- Risk Management
- Customer Lifecycle Solutions
- Net Promoter Score
- Customer Behaviour Analytics
- Customer Segmentation
- Data Unification
Explore Voxco
Watch a Demo
Download Brochures
- CX Strategy & Management Hub
- Blogs & White papers
- Case Studies

VP Innovation & Strategic Partnerships, The Logit Group
- Why Voxco Intelligence?
- Our clients
- Client stories
- Featuresheets

Examining Data Analysis Techniques in Social Research: Qualitative vs. Quantitative
SHARE THE ARTICLE ON
Data analysis provides social researchers with the tool to unlock insights and understand complex social phenomena. You can interpret the data, and uncover relationships and patterns to address human behavior and social experiences.
Social research, as we all know, focuses on expanding our knowledge on social dynamics. Data analysis in social science research provides you with empirical evidence to dig deeper and explore human experience, attitudes, interactions, and social structures. Social data analysis enables you to assess the effectiveness of policies and programs, helping you make informed decisions and design effective interventions.
In this blog we focus on exploring quantitative and qualitative data analysis in social science research.
What is data analysis in research?
In research, data analysis refers to employing statistical and logical techniques to evaluate and synthesize the data collected. It allows researchers to extract meaningful insights from an unstructured mass of data.
Extracting insights and meaning from data gives us a better understanding of the world and different phenomena and empowers improved decision-making.
Different data will need to be analyzed using different techniques. Within this article, we will explore the different kinds of data in research and the different methods of data analysis used to analyze them.
Read how Voxco helped Siena College conduct more than one hundred polls and to 3M phone calls.
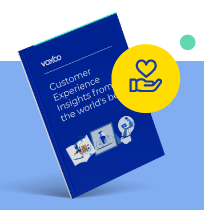
Types of Data in Research
There are three main types of data in research:
- Qualitative Data: Qualitative data is used to describe qualities or characteristics and generally refers to the descriptive findings collected through different methods of research. It refers to data that is non-numerical in nature and is, therefore, not quantifiable. Some examples of qualitative data are blood type, ethnic group, color , etc.
- Quantitative Data: The type of data whose value takes distinct figures or counts that are associated with a numerical value. It refers to quantifiable information that can be used to conduct statistical analysis and mathematical computations. Some examples of quantitative data are cost, age, and weight.
- Categorical Data: Categorical data refers to the types of data that can be divided into groups. Categorical variables can only take one of a limited and usually fixed number of possible values. Some examples of categorical data are race, gender, age group, etc.
Key objectives of data analysis in social research
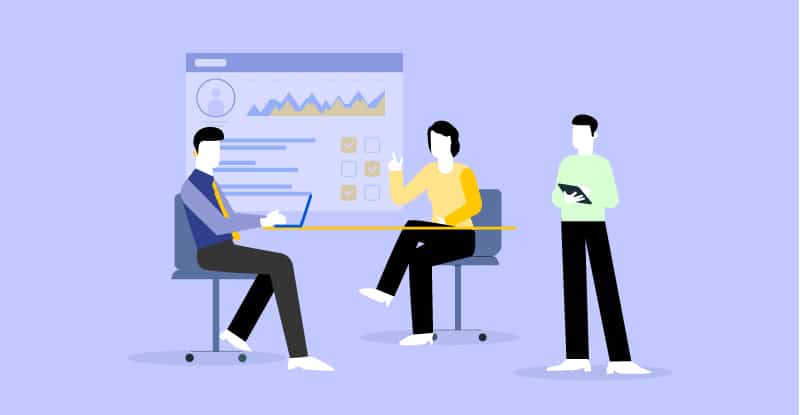
The followings are the primary objectives of data analysis in social research.
- Data analysis techniques help you describe and summarize the social phenomenon you are studying. It provides you with statistical values such as means, medians, frequencies, and standard deviation, giving you a snapshot of the collected data.
- It helps facilitate exploratory analysis allowing you to uncover previously unknown insights. The analysis provides a foundation for further research by identifying patterns and relationships for hypothesis generation.
- Data analysis in social science research enables you to make inferences and draw meaningful conclusions about the target population based on the research sample. By gathering empirical evidence, you can generalize the research results to the larger population, ensuring the external validity.
Related read: Importance of social research.
Challenges in social research data analysis
While data analysis is central to social research and offers multiple benefits, it is not without its challenges. Here are some common obstacles you may encounter when performing data analysis in social science research.
- Data quality – It is important to ensure that you remove any missing or inconsistent data to main data integrity and validity.
- Selecting the proper data analysis technique – You must have a good understanding of various analysis techniques to select the one that is appropriate for the research.
- Interpreting complex results – You need to communicate the findings effectively and provide a clear explanation of the implication of your research result.
Unlock the true potential of your survey data.
Explore how easy it is to conduct sophisticated statistical analysis and create one-click summaries, custom live dashboards, and in-depth reports with Voxco Analytics.
See Voxco survey software in action with a Free demo.
Data analysis in social research using a qualitative approach
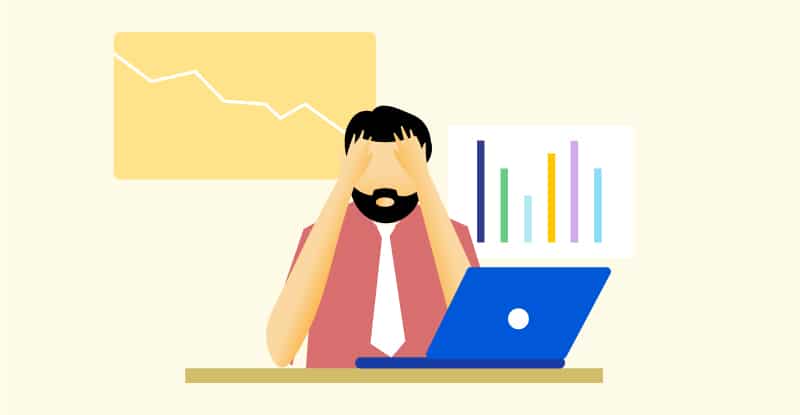
Let’s take a look at how data analysis is conducted in qualitative research and the different methods that are commonly used to do so.
Data preparation for qualitative data analysis –
Before you dive into analyzing your qualitative social research data, you need to prepare the data to make sense of the rich information.
Step 1: Data familiarization:
You need to start by getting familiar with the qualitative or textual data you have gathered. Take the time to read and re-read the interviews or feedback to gain a holistic understanding of the content.
Step 2: Coding and categorization:
This step involves assigning codes or labels to segments of data. Coding helps you identify themes, concepts, and patterns within your data. Organize your codes into categories (grouping related codes together) and themes (overarching ideas that arise from the data).
Step 3: Theme and pattern identification:
Once you have assigned codes, you can start identifying common themes. Look for recurring responses to questions, or identify shared experiences. You can now identify similarities and differences across the data and participants.
How do we identify patterns in qualitative data
When analysing and looking for patterns in textual information, there are many different methods that can be used, including:
- Word-based Method: The word-based method generally involves manually reading through the gathered data to find repetitive themes or commonly used words.
- Scrutiny-based Technique: The scrutiny-based technique is used to derive conclusions based solely on what is already known by the researcher. This is a popular method of text analysis for identifying correlations and patterns within textual information.
- Variable Partitioning : Variable partitioning, or dynamic partitioning, can be used to split variables so that more coherent descriptions and explanations can be extracted from vast vast data.
6 Data analysis methods in qualitative social research -
There are six main analysis methods in quantitative research that you can use in data analysis for social research. Let’s look at these six methods.
- Narrative analysis.
- Qualitative content analysis.
- Grounded theory.
- Discourse analysis.
- Thematic analysis.
- Interpretive phenomenological analysis.
Let’s explore these six qualitative data analysis methods.
1. Narrative Analysis:
Narrative analysis, or narrative inquiry, is a qualitative research method where researchers interpret texts or visual data in a storied form. There are different approaches to narrative analysis, including; functional, thematic, structural, and dialogic.
2. Qualitative Content Analysis:
This is a straightforward method of qualitative research where patterns within a piece of content are evaluated. It can be used with different forms of content, such as words, phrases, and/or images.
3. Grounded Theory:
This method of qualitative analysis is used to create new theories using the data collected by using a series of “tests” and “revisions”. Grounded theory (GT) follows a structured but flexible methodology focusing on social processes or actions.
4. Discourse Analysis:
This method is used to study written, vocal, sign language, or any significant semiotic event, in relation to its social context. It allows researchers to examine a language beyond just sentences and explains how these sentences function in a social context.
5. Thematic Analysis:
The thematic analysis involves looking for patterns by taking large bodies of data and grouping them based on shared themes or similarities to answer the research question being addressed. This method of qualitative data analysis is widely used in the field of psychology.
6. Interpretive Phenomenological Analysis (IPA):
It is an approach to psychological qualitative research and has an ideographic focus. It provides a detailed examination of a person and their lived experiences. The aim of IPA is to understand how participants make sense of their personal and social world.
Leverage online survey tools that enable you to perform text analysis and sentiment analysis to extract insights from your qualitative research data.
Looking for robust, agile, and powerful survey software?
Download our guide to see what features your platform must have.
In this guide, you’ll discover:
- The risks and benefits of adopting new survey software.
- What features to look out for when you’re making a purchase decision?
- A definitive checklist to compare platforms.
Data analysis in social research using a quantitative approach
Let’s now delve into how you can conduct data analysis in quantitative research and the different methods that are commonly used to do so.
Data preparation for quantitative data analysis
Before quantitative data can be analyzed, it must first be prepared using the following three steps:
Step 1: Data Validation:
Data validation refers to comparing the gathered data against defined rules to ensure that it is within the required quality parameters without any bias. It generally involves checking for the following; fraud, screening, procedure, and completeness.
Step 2: Data Editing:
Data editing refers to reviewing and adjusting after checking for missing, invalid, or inconsistent entries within the data records.
Step 3: Data Coding:
As the name suggests, data coding involves deriving codes from observed data. It refers to transforming and organizing gathered information into a set of meaningful and cohesive categories.
2 data analysis methods in quantitative social research
There are two main methods of data analysis used in quantitative research:
- Descriptive analysis.
- Inferential analysis.
1. Descriptive Statistics:
This quantitative method of data analysis is used to describe the basic features of data in a study and provides simple summaries about the measures and sample.
It helps researchers understand the details of a sample group and doesn’t aim to make assumptions or predictions about the entire population. Descriptive analysis generally includes the first set of statistics covered before moving on to inferential statistics.
Some common statistical tests used in descriptive statistics are mean, median, mode, skewness, and standard deviation.
2. Inferential Statistics:
Inferential statistics differs from descriptive statistics as it aims to make inferences about the population rather than about a specific data set or sample. It, therefore, allows researchers to make assumptions and predictions about an entire population.
There are two main kinds of predictions made using inferential statistics, including predictions about the differences between groups within a population and predictions about the relationships between variables relevant to a population.
Some common inferential methods used in quantitative data analysis are regression analysis, frequency tables, analysis of variance (ANOVA), cross-tabulation, and correlational research. Leverage a data analysis tool that streamlines the entire process of quantitative data analysis and automates any manual work.
Start collecting insights & make data-driven decisions.
Voxco is trusted by 500+ global brands & Top 50MR to gather, measure, uncover, and act on meaningful insights.
Data analysis encompasses both quantitative and qualitative methods. Quantitative methods in social science research allow objective insights with the help of statistical analysis. Qualitative methods in social science research provide exploratory insights with the help of textual analysis. Through data analysis in social science research, you uncover patterns, establish correlations, and gain a deeper understanding of social systems. You can contribute to the discipline with evidence-based insights and generate knowledge that informs decision-making, policies, and interventions advancing our understanding of human behavior and social phenomenon.
Explore all the survey question types possible on Voxco
Explore Voxco Survey Software
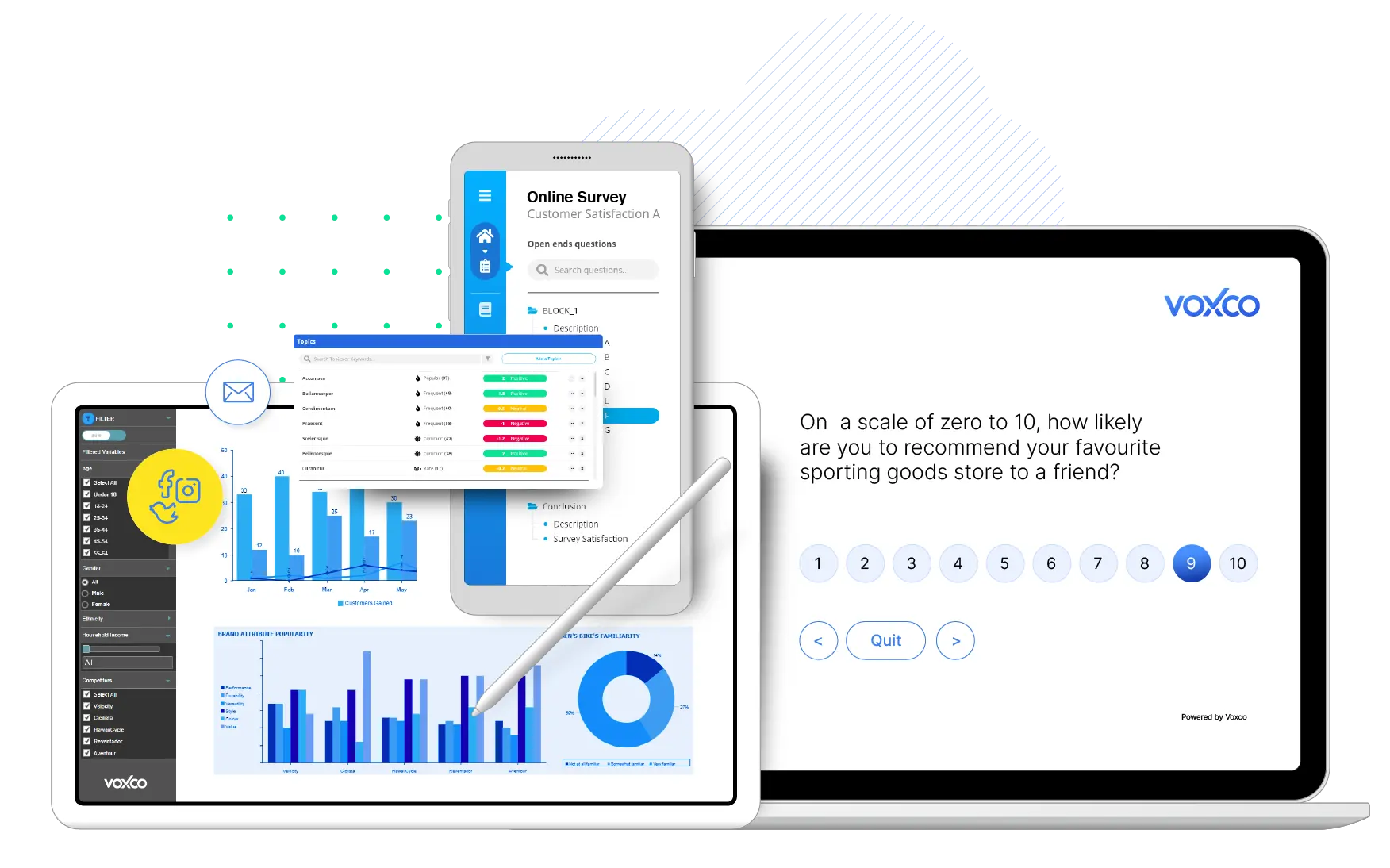
+ Omnichannel Survey Software
+ Online Survey Software
+ CATI Survey Software
+ IVR Survey Software
+ Market Research Tool
+ Customer Experience Tool
+ Product Experience Software
+ Enterprise Survey Software
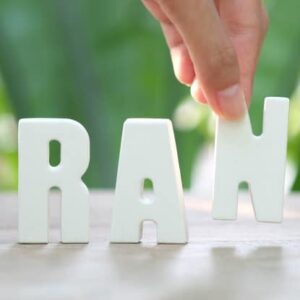
Unaided Brand Awareness
Unaided Brand Awareness SHARE THE ARTICLE ON Share on facebook Share on twitter Share on linkedin Table of Contents What is Unaided Brand Awareness? Brand
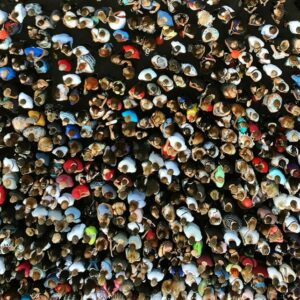
What is a cross-sectional study?
Cross Sectional Study: The Science Behind the Moment Try a free Voxco Online sample survey! Unlock your Sample Survey SHARE THE ARTICLE ON Table of
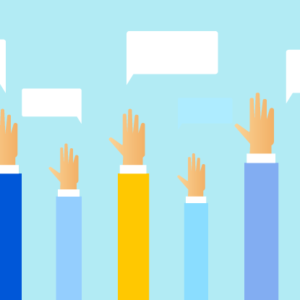
Public Opinion Polls
Public Opinion Polls SHARE THE ARTICLE ON Share on facebook Share on twitter Share on linkedin Table of Contents What is a public opinion poll?
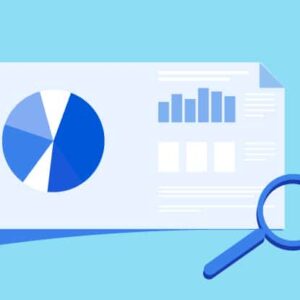
Association vs causation
Difference between Association and Causation SHARE THE ARTICLE ON Table of Contents What is Association? Association is a statistical relationship between two variables. This relationship
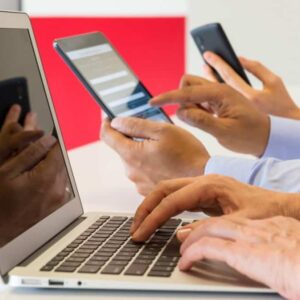
A Comprehensive Guide to Understanding kano analysis for Product Development
A Comprehensive Guide to Understanding kano analysis for Product Development SHARE THE ARTICLE ON Table of Contents In today’s highly competitive business landscape, understanding and

Emerging trends in Patient Experience and how to meet them
With the addition of more and more touchpoints for interacting with patients, the scope of what is considered the traditional patient experience has greatly expanded.
We use cookies in our website to give you the best browsing experience and to tailor advertising. By continuing to use our website, you give us consent to the use of cookies. Read More
- University Libraries
- Research Guides
- Topic Guides
- Data resources for social science
- Research datasets for secondary analysis
Data resources for social science: Research datasets for secondary analysis
- Aggregate data and tables
- State Resources
- National Resources
- International Resources
- Historical Maps and Aerial Photos
- Research data management guide
- Data analysis applications & data literacy/skills
- Searching, citing, and engaging literatures
- For grad students & faculty
- How...? (Vendors' help sites)
- Accessing library resources off campus
What's on this page
This part of the guide identifies key collections of data that can be exported and analyzed in analytical software. In general, you can select microdata based on narrative descriptions and data documentation. See the aggregate data tab for data in tables, which often can be exported and combined for analysis.
Resources on this page are grouped under these headings: Overview of issues in locating data; Major data repositories ; VT Library data services ; International demographic/economic data collections , US-centric data repositories ; Governance data collections ; International electoral data portals , US social & opinion data collections ; International social survey archives ; Miscellaneous data collections, and guidance on citing data you use.
Data can be hard to find and to work with . Statista is a large, reasonably easy to use tool for finding statistics from around the word. Sage Sage Data [formerly called Data Planet ] is even more comprehensive, but it helps to be familiar with the ways statistical agencies organize and describe their data if you want to use it effectively.
The VT library has experts to help you. The VT libraries has a team of informatics consultants to help you with methodology, interpretation, visualization, and management/curation of your research data. Some tabs in this guide are maintained by members of the library's data service group.
Demographic and economic datasets: international
Electoral data.
- Access to these data requires you to createa free, personal account, which then allows you to save customized datasets for future reference and to receive automatic updates to the data when they become available.
- Tools + Resources section lists open data sources recommended by MEDSL
- Research section offers "explainers" and academic papers
Cite the data you use
Temporary, trial access only -- use while you can.
The University Libraries at Virginia Tech regularly secure short-term, trial access to online resources in order to gauge their appropriateness to our university's teaching and research missions. These trials run in October, February, and sometimes April. Most trials run 30 days.
This box highlights some of these opportunities. All active trials are listed in a sidebar in the main Databases A-Z directory and as a tab atop this libguide.
Each entry includes a link to a user survey. I and other subject librarians invite you to email us your detailed assessments of resources. Responses from the Virginia Tech community are vital to the library's deliberations about whether and when to acquire or enhance databases and the like.
As appropriate I will list all currently active trials and user survey links in a resource trials tab in this and my other libguides. Entries for trials I may include in the body of my libguides will go away when the trial period ends.
Data portals and repositories around the world
If you want to start by searching for variables:
- Google Dataset Search Discovery tool for numerical and geospatial data, but (like VT Discovery Search) its reliability depends on how dataset providers comply with technical standards for describing data (ie, metadata).
If you prefer to start by browsing by topic or place:
- International Social Survey Programme The ISSP is a cross-national survey program conducting annual surveys in a broad group of countries. The survey asks questions on a variety of topics . You can download full datasets or analyze online through the GESIS Archive .
US (and mostly US) data collections
- Virginia Tech's institutional membership entitles members of the VT community both to download datasets and to deposit their research data for permanent curation and access; create a free ICPSR "My data" account and log in with it in order to download data.
- Some datasets have access/use restrictions that may require approval by VT's institutional review board (among other offices) and by ICPSR prior to access; in some cases you researchers are required to work only in secure "data enclaves." For highly sensitive data, such approvals can add months to the beginning of the research timeline. Restricted data at ICPSR are conspicuously marked. (These restrictions are to protect research respondents' identities in areas like drug use, sexuality, and criminal behavior.)
- Qualitative Data Repository Based at Syracuse University, QDR selects, ingests, curates, archives, manages, durably preserves, and provides access to digital data used in qualitative and multi-method social inquiry. The repository develops and publicizes common standards and methodologically informed practices for these activities, as well as for the reuse and citation of qualitative data. VT Libraries provides Tech's institutional membership in QDR. (In fact, Virginia Tech is the QDR's very first institutional member.)
- VTechData Virginia Tech’s institutional data repository is a platform for depositing and providing public access to datasets and related research products created by Virginia Tech faculty, staff, and students. Other research universities may offer similar repositories.
- U.S. Government Information: Stats/Data A handy point of departure, this libguide from UC San Diego identifies key data providers and major statistical publications from US federal agencies. (Also includes some databases restricted to UCSD.)
- See the Federal Committee on Statistical Methodology (FCSM) site for technical standards and guidelines behind the federal data.
- United States Census Bureau Data Repository The US Census Bureau Data Repository preserves and disseminates survey instruments, specifications, data dictionaries, codebooks, and other materials provided by the US Census Bureau. ICPSR, the host of this data repository, has also listed additional Census-related data collections from its larger holdings.
- ResearchDataGov.ORG: Application Portal for Restricted Data for Federal Statistics ResearchDataGov is a web portal and application system for discovering and requesting restricted-access microdata from various US federal statistical agencies . These data must be accessed and used only within a Federal Statistical Research Data Center -- Virginia Tech has an arrangement for VT faculty researchers to apply to use the FSRDC at Georgetown University. Access to these data will take several months, not moments: it requires application, then approval by the federal agency(ies) that generated the datasets -- as well as the Georgetown FSRDC administrator; Tech's Institute for Society, Culture, and Environment; and other campus offices . See VT application procedures at ISCE more... less... The data described in ResearchDataGov.org are owned by and accessed through the agencies and units of the federal statistical system. Data access is determined by the owning or distributing agency and is limited to specific physical or virtual data enclaves. Even though all data assets are listed in a single inventory, they are not necessarily available for use in the same location(s). Multiple data assets accessed in the same location may not be able to be used together due to disclosure risk and other requirements. Please note the access modality of the data in which you are interested and seek guidance from the owning agency about whether assets can be linked or otherwise used together. ICPSR developed ResearchDataGov with support and guidance from the Census Bureau, the Office of Management and Budget, and the Interagency Council on Statistical Policy.
- DataLumos Some government digital data were distributed on disk or tape and not posted online, and some data that were available have moved or taken down over the years. DataLumos is ICPSR's archive for valuable US government agencies' social data resources.
- Data/stats sources in other VT research guides You can search for data sources and statistics resources in other VT Libraries' research guides. Here is a basic starter list. Sort and filter it in various ways and use its search box as a point of departure for more.
Virginia and nearby state official data portals
- Virginia Open Data Portal
- Virginia Geographic Information Network
- Maryland 's Open Data Portal
- Maryland's Mapping and GIS Data Portal
- Open Data DC
- DC Map Data
- LINC: Log Into North Carolina
- Tennessee Open Data Portal
- T ransparent Tennessee OpenMaps
- TNMap Open Data Portal
- [Kentucky] KyGovMaps Open Data Portal
- Map West Virginia
- WV State GIS Data Clearinghouse
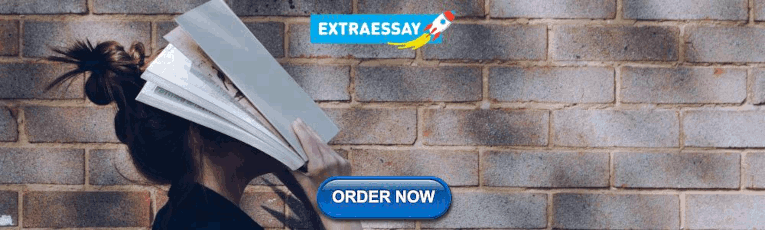
US social/opinion surveys
College librarian for social sciences & history.

Data support in VT Libraries
- Data & informatics consultants VT Libraries' in-house consultants for social and natural sciences, engineering, and arts/visualization
- Statistical consulting by SAIG Tech's Statistical Applications and Innovations Group offers walk-in consulting hours in the Newman Library Data Transformation Lab (room 3010) four afternoons a week to address your quick questions or to help with research projects requiring less than 30 minutes of assistance. Walk-in hours are available only when classes are in session.
- Data management & curation (VT Libraries) The Tech Libraries offer data management and curation support for researchers throughout the research lifecycle, from the planning stages through publishing and disseminating research.
- Virtual Computer Labs (TLOS remote access) Tech's Technology-enhanced Learning and Online Strategies (TLOS) office transitioned 250 computers in its campus labs to virtual-only access via the VT VPN as a Covid protection measure. This page tells you how. This list of applications on those TLOS lab machines shows which ones are available remotely. TLOS licenses for statistical software often expire every August, and updates may be delayed.
Ask a Librarian
Data about governance.
- Regional Authority Index . RAI tracks regional authority on an annual basis from 1950 to 2010 in 81 countries. Datasets include annual scores in for 231 regional governments/tiers and 81 countries for 1950-2010
- International Authority Index . MIA measures delegation and pooling of international authority for 76 international governmental organizations for 1950-2010. The MIA data are annual.
Miscellaneous data collections
Find library resources by their format.
- Audio books
- Book reviews
- Business, company & industry info
- Center for Research Libraries research collections
- Citation & style manuals
- Demographic visualizations (GIS)
- Engineering standards
- Foreign language learning materials
- Magazines, journals, other periodicals
- Manuscripts
- Movie reviews & criticisms
- Patents & trademarks
- Pleasure reading books
- Primary source databases
- Reserves (for Blacksburg classes)
- Speeches & transcripts
- Temporary, trial access
- Tests & measures
- Theses & dissertations
- Tutorial & educational resources
International social survey datasets
- WorldPublicOpinion.org WorldPublicOpinion.org presents articles summarize polling data and analyses from numerous sources with links to questionnaires and results. Full datasets can be downloaded from http://drum.lib.umd.edu/handle/1903/10117 . WorldPublicOpinion.org is an international collaborative project managed by the Program for Public Consultation at the University of Maryland.
- LatinoBarometer
- AsianBarometer
- AfroBarometer
- ArabBarometer
- EurasiaBarometer .
- Latin American Databank (Roper Center) LAD provides a portal for Latin American datasets acquired, processed and archived by the Roper Center for Public Opinion Research. This valuable collections includes data from public opinion surveys conducted by the survey research community in Latin America and the Caribbean, including universities, institutes, individual scholars, private polling and public opinion research firms.
- ESS - National Pages links to the European Social Survey in the participating countries in local language.
- Next: Aggregate data and tables >>
- Last Updated: May 8, 2024 4:27 PM
A big data analysis of the adoption of quoting encouragement policy on Twitter during the 2020 U.S. presidential election
- Research Article
- Open access
- Published: 19 May 2024
Cite this article
You have full access to this open access article
- Amirhosein Bodaghi ORCID: orcid.org/0000-0002-9284-474X 1 &
- Jonathan J. H. Zhu 2
42 Accesses
Explore all metrics
This research holds significance for the fields of social media and communication studies through its comprehensive evaluation of Twitter’s quoting encouragement policy enacted during the 2020 U.S. presidential election. In addressing a notable gap in the literature, this study introduces a framework that assesses both the quantitative and qualitative effects of specific platform-wide policy interventions, an aspect lacking in existing research. Employing a big data approach, the analysis includes 304 million tweets from a randomly sampled cohort of 86,334 users, using a systematic framework to examine pre-, within-, and post-intervals aligned with the policy timeline. Methodologically, SARIMAX models and linear regression are applied to the time series data on tweet types within each interval, offering an examination of temporal trends. Additionally, the study characterizes short-term and long-term adopters of the policy using text and sentiment analyses on quote tweets. Results show a significant retweeting decrease and modest quoting increase during the policy, followed by a swift retweeting resurgence and quoting decline post-policy. Users with fewer connections or higher activity levels adopt quoting more. Emerging quoters prefer shorter, positive quote texts. These findings hold implications for social media policymaking, providing evidence for refining existing policies and shaping effective interventions.
Avoid common mistakes on your manuscript.
Introduction
The introduction of the quote tweet feature by Twitter in April 2015 marked a significant development in the platform’s functionality. While a conventional retweet merely reproduces the original tweet, serving as a symbol of agreement and endorsement between users involved [ 1 ], the quote tweet feature allows users to include their own commentary when sharing a tweet. Consequently, this feature has given rise to various novel applications, including the expression of opinions, public replies, and content forwarding [ 2 ]. Notably, owing to the perennial significance of the US presidential elections [ 3 , 4 ], Twitter instituted a novel policy on October 9, 2020, advising users to abstain from mere retweeting and advocating instead for the utilization of quote tweets supplemented by individual perspectives. This policy remained in effect until December 16, 2020. Indeed, before the policy change, retweeting on Twitter was simple. With a single click, users could share a post with their followers. However, during the time policy was held, clicking the retweet button no longer automatically shared the post. Instead, Twitter prompted users to add their own thoughts or comments before sharing. This essentially created a “Quote Tweet.” This extra step was intended to encourage users to share more thoughtfully. Importantly, adding text to the quote tweet was optional. Users could still leave the comment section blank and share the post without any additional commentary. This option essentially replicated the old retweet functionality.
Significance of the research
This research holds significance in the realm of social media and communication studies, particularly in understanding the impact of policy interventions on user behavior. The significance can be delineated through various dimensions. First, the study provides a comprehensive evaluation of the effectiveness of Twitter’s quoting encouragement policy implemented during the 2020 U.S. presidential election. By employing a robust big data approach and sophisticated analytical methods, the research goes beyond anecdotal observations, offering a nuanced understanding of how such policies influence user engagement. This contribution is valuable for social media platforms seeking evidence-based insights into the outcomes of policy interventions, aiding in the refinement of existing policies and the formulation of new ones. Second, the findings offer actionable insights for social media policymakers and practitioners involved in the delicate task of shaping user behavior. Understanding the quantitative and qualitative effects of the policy shift allows for the optimization of future interventions, fostering more effective communication strategies on platforms like Twitter. Policymakers can leverage the identified user characteristics and behavioral patterns to tailor interventions that resonate with the diverse user base, thereby enhancing the impact of social media policies. Finally, the research enriches the theoretical landscape by applying the Motivation Crowding Theory, Theory of Planned Behavior (TPB), and Theory of Diffusion of Innovation (DOI) to the context of social media policy adoption. This interdisciplinary approach contributes to theoretical advancements, offering a framework that can be applied beyond the scope of this study. As theories from economics and psychology are employed to understand user behavior in the digital age, the research paves the way for cross-disciplinary collaborations and a more holistic comprehension of online interactions.
Research gap
Despite the existing body of literature on quoting behavior on Twitter, there is a conspicuous gap in addressing the unique policy implemented by Twitter from October 9 to December 16, 2020, encouraging users to quote instead of retweeting. Previous studies have explored the use of quotes in various contexts, such as political discourse and the spread of misinformation, but none have specifically examined the impact of a platform-wide policy shift promoting quoting behavior. In addition, while some studies have investigated user behaviors associated with quoting, retweeting, and other tweet types, there is a lack of a comprehensive framework that assesses the quantitative and qualitative effects of a specific policy intervention. The current study introduces a detailed evaluation framework, incorporating time series analysis, text analysis, and sentiment analysis, providing a nuanced understanding of the Twitter quoting encouragement policy’s impact on user engagement. Moreover, previous research has explored user characteristics in the context of social media engagement but has not specifically addressed how users' attributes may influence their response to a platform-wide policy change. The current study bridges this gap by investigating how factors like social network size and activity levels correlate with users’ adoption of the quoting encouragement policy. Finally, while some studies have assessed the immediate effects of policy interventions, there is a lack of research investigating the longitudinal impact after the withdrawal of such policies. The current study extends the temporal dimension by examining user behavior during the pre-, within-, and post-intervals, offering insights into the sustained effects and user adaptation following the cessation of the quoting encouragement policy. By addressing these research gaps, the current study seeks to provide a holistic examination of the quoting encouragement policy on Twitter, contributing valuable insights to the fields of social media studies, policy evaluation, and user behavior analysis.
Research objectives
This study aims to assess the effectiveness of the Twitter policy implemented from October 9 to December 16, 2020, which encouraged users to utilize the quote tweet feature instead of simple retweeting. Specifically, the research objectives are twofold: (1) to determine the adoption rate of this policy and evaluate its success, and (2) to identify user characteristics based on their reactions to this policy. The outcomes of this research contribute to both the evaluation of the Twitter policy and the development of more effective approaches to policymaking. Stier et al. [ 5 ] proposed a comprehensive framework comprising four phases for the policymaking process: agenda setting, policy formulation, policy implementation, and evaluation. According to this framework, the evaluation phase involves assessing the outcomes of the policy, considering the perspectives of stakeholders involved in the previous phases. In this context, the present research examines the Twitter quoting encouragement policy, which represents an intervention in the daily usage patterns of Twitter users, through both quantitative and qualitative analyses. The quantitative effects analysis, particularly the achievements observed, provide valuable insights for evaluating the efficacy of the quoting encouragement policy by Twitter. Additionally, the results obtained from the qualitative analyses facilitate policy implementation, which refers to the process of translating policies into practical action under the guidance of an authoritative body.
Quantitative effects
In this section, we present the hypotheses formulated to assess the quantitative effects of the Twitter quoting encouragement policy. The hypotheses are as follows:
H1: The intervention is expected to have a negative impact on users’ retweeting behavior. We hypothesize that the policy promoting the use of quote tweets instead of simple retweets will lead to a reduction in the frequency of retweeting among users.
H2: The intervention is unlikely to significantly affect other types of user behavior, such as posting original or reply tweets, as well as quotes. We anticipate that any observed changes in the rates of these tweet types would be of minimal magnitude and primarily influenced by factors unrelated to the intervention.
H3: The termination of the intervention is anticipated to have a positive effect on users' retweeting behavior. We hypothesize that the discontinuation of the policy encouraging quote tweets will result in an increase in users' retweeting activity.
H4: Similar to H2, the conclusion of the intervention is not expected to impact other tweet types (excluding quotes) in terms of posting behavior. This suggests the presence of a prevailing opinion inertia, where users tend to maintain their existing patterns and tendencies when posting original, reply, and non-quote tweets.
These hypotheses serve as a foundation for analyzing the quantitative effects of the Twitter quoting encouragement policy and investigating its influence on users’ tweet behaviors. Through rigorous analysis, we aim to shed light on the impact of the intervention and its implications for user engagement on the platform.
Qualitative effects
The qualitative effects can be examined from two distinct perspectives: User Characteristics and Text Characteristics. Moreover, the analysis encompasses three intervals, namely the Pre-Interval (prior to the policy implementation), Within Interval (during the policy implementation), and Post-Interval (after the policy withdrawal). The hypotheses for each perspective are as follows:
User characteristics
H5: Users with a larger social network (i.e., more friends) are expected to exhibit a lesser increase in their quoting rate during the Within Interval.
H6: Users who demonstrate a regular pattern of activity, characterized by a lower frequency of overall Twitter engagement (such as publishing at least one tweet type on more days), are more inclined to experience an elevation in their quoting rate during the Within Interval.
H7: Users who engage in a higher volume of retweeting activities during the Pre-Interval are more likely to observe an increase in their quoting rate during the Within Interval.
H8: The swifter users experience an increase in their quoting rate during the Within Interval, the sooner they are likely to discontinue quoting tweets upon entering the Post-Interval.
Text characteristics
H9: Short-term quoters tend to exhibit a comparatively smaller change in the length of their quote texts compared to long-term quoters. This is primarily due to the involuntary nature of the former, whereas the latter are more intentionally created.
H10: The sentiment of quote texts from short-term quoters is generally more likely to elicit a greater range of emotions compared to those from long-term quoters. This difference is attributable, at least in part, to the intervention's influence on short-term quoters.
H11: The quote texts of short-term quoters are generally more prone to receiving a higher number of retweets compared to those of long-term quoters. This can be attributed to factors such as longer text length, less deliberative content, and the presence of heightened emotional elements in the latter.
These hypotheses form the basis for analyzing the qualitative effects of the Twitter quoting encouragement policy, enabling a comprehensive understanding of user and text characteristics during different intervals. By examining these effects, we aim to shed light on the nuanced dynamics that underlie users’ quoting behavior and its implications on social interaction and engagement within the Twitter platform.
Theoretical framework
In alignment with the two main parts of this research, which examine the quantitative and qualitative effects of the recent Twitter policy, the theoretical framework is also divided into two contexts: one for quantitative analysis and the other for investigating qualitative effects. For the quantitative analyses, the motivation crowding theory has played a central role in shaping the corresponding hypotheses. This theory suggests that providing extrinsic incentives for specific behavior can sometimes undermine intrinsic motivation to engage in that behavior [ 6 ]. Although the motivation crowding theory originated in the realm of economics [ 7 ], this study aims to apply it to the adoption of policies within the context of Twitter. By treating the quoting encouragement policy as an incentive, this research seeks to quantify the impact of this incentive during its implementation and withdrawal. Hypotheses 1–4 have been formulated to guide these quantitative analyses and explore the potential influence of the undermining effect on the adoption rate after the policy withdrawal.
Regarding the qualitative analyses, the TPB and the DOI serve as foundational frameworks for developing hypotheses related to user and text characteristics. The TPB explains behavior based on individuals' beliefs through three key components: attitude, subjective norms, and perceived behavioral control, which collectively shape behavioral intentions. Drawing on the TPB, hypotheses 5–8 aim to characterize different users based on their behaviors and attitudes toward the new policy. The DOI provides a platform for distinguishing users based on the time of adoption. In line with this theory, hypotheses 9–11 have been formulated to address characteristics that facilitate early adoption based on the content of quote texts. Figure 1 illustrates the theoretical framework of this study, highlighting its key components.
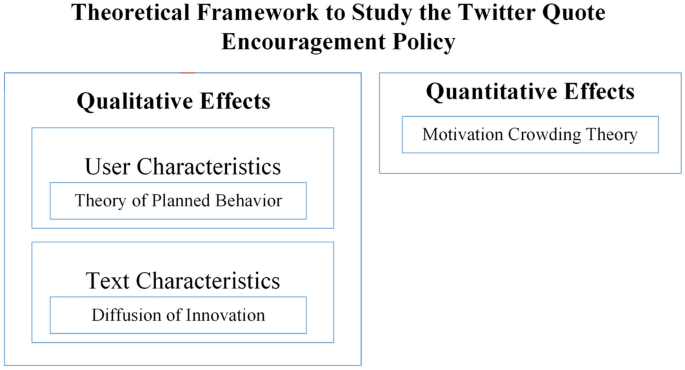
Uniqueness and generalizability
To the best of our knowledge, this research represents the first comprehensive study to investigate the impact of the quoting encouragement policy implemented by Twitter. In comparison to the limited existing studies that have examined Twitter policies in the past, this research distinguishes itself through both the scale of the dataset utilized and the breadth of the analyses conducted. These unique aspects contribute to the applicability of this study in two key areas: methodology and findings. In terms of methodology, the presented approach incorporates an interrupted time series analysis framework, coupled with text and sentiment analyses, to examine policy interventions on a large scale. This framework enables researchers to develop various approaches for analyzing interventions within the realm of social media and big data. With regards to the findings, the extraction of qualitative and quantitative patterns from such a vast dataset yields novel insights. Particularly noteworthy is the ability to juxtapose these macro and micro results, leading to a deeper understanding of the policy’s effects. The findings of this study hold potential value for practitioners and policymakers not only on Twitter but also on other global platforms like Instagram and YouTube. However, it is important to consider certain modifications, such as adapting the follower-to-following ratio, when applying these findings to undirected networks like Facebook, where mutual agreement is necessary for link creation. Moreover, the analysis of this policy, which was implemented during the presidential election, provides valuable insights into its potential impact on public attention. Public attention has recently been identified as a critical factor in the success of presidential candidates [ 8 ]. Therefore, understanding the effects of the quoting encouragement policy can contribute to a better understanding of the dynamics surrounding public attention during such critical periods. Indeed, the uniqueness of this research lies in its pioneering examination of the Twitter quoting encouragement policy, extensive dataset, and comprehensive analyses. These distinct features enhance the applicability of the research in terms of methodology and findings, with potential implications for other global platforms and the study of public attention in political contexts.
Literature review
Given the nature of this research, which focuses on a novel Twitter policy that promotes quoting instead of retweeting, the literature review examines three perspectives: (1) Quote, (2) Engagement, and (3) Hashtag Adoption. These perspectives encompass relevant aspects that align with the scope of this study.
Garimella et al. [ 2 ] conducted a study on the utilization of the newly introduced “quote RT” feature on Twitter, specifically examining its role in political discourse and the sharing of political opinions within the broader social network. Their findings indicated that users who were more socially connected and had a longer history on Twitter were more likely to employ quote RTs. Furthermore, they observed that quotes facilitated the dissemination of political discourse beyond its original source. In a different context, Jang et al. [ 9 ] employed the rate of quotes as a measure to identify and detect fake news on Twitter. Their research focused on leveraging quotes as a means of analyzing the spread of misinformation and distinguishing it from authentic news. Li et al. [ 10 ] tried to identify users with high dissemination capability under different topics. Additionally, Bodaghi et al. [ 11 ] investigated the characteristics of users involved in the propagation of fake news, considering quotes and their combined usage with other tweet types such as retweets and replies. Their analysis aimed to gain insights into the user behaviors associated with the dissemination of false information. South et al. [ 12 ] utilized the quoter model, which mimics the information generation process of social media accounts, to evaluate the reliability and resilience of information flow metrics within a news–network ecosystem. This study focused on assessing the validity of these metrics in capturing the dynamics between news outlets engaged in a similar information dissemination process. By reviewing these studies, we can identify their relevance to the understanding of quoting behavior and its implications within different contexts, such as political discourse and the spread of misinformation. However, it is important to note that these previous works primarily focused on the usage of quotes and their effects without specifically addressing the Twitter policy under investigation in this study.
The concept of engagement on social media platforms, particularly in relation to political communication and online interactions, has been extensively explored in previous studies. Boulianne et al. [ 13 ] conducted research on the engagement rate with candidates’ posts on social media and observed that attack posts tend to receive higher levels of engagement, while tagging is associated with a general trend of lower engagement. Lazarus et al. [ 14 ] focused on President Trump’s tweets and found that engagement levels vary depending on the substantive content of the tweet, with negatively toned tweets and tweets involving foreign policy receiving higher engagement compared to other types of tweets. Yue et al. [ 15 ] delved into how nonprofit executives in the U.S. engage with online audiences through various communication strategies and tactics. Ahmed et al. [ 16 ] examined Twitter political campaigns during the 2014 Indian general election. Bodaghi et al. [ 17 ] conducted a longitudinal analysis on Olympic gold medalists on Instagram, investigating their characteristics as well as the rate of engagement they receive from their followers. Hou et al. [ 18 ] studied the engagement differences between scholars and non-scholars on Twitter. Hoang et al. [ 19 ] aimed at predicting whether a post is going to be forwarded or not. Munoz et al. [ 20 ] proposed an index as a tool to measure engagement based on the tweet and follower approach.
The decision of an online social network user to join a discussion group is not solely influenced by the number of friends who are already members of the group. Backstrom et al. [ 21 ] discovered that factors such as the relationships between friends within the group and the level of activity in the group also play a significant role in the user’s decision. Hu et al. [ 22 ] performed an empirical study on Sina Weibo to understand the selectivity of retweeting behaviors. Moreover, Balestrucci et al. [ 23 ] studied how credulous users engage with social media content. Bodaghi et al. [ 24 ] explored the impact of dissenting opinions on the engagement rate during the process of information spreading on Twitter. Wells et al. [ 25 ] examined the interactions between candidate communications, social media, partisan media, and news media during the 2015–2016 American presidential primary elections. They found that social media activity, particularly in the form of retweets of candidate posts, significantly influenced news media coverage of specific candidates. Yang et al. [ 26 ] investigated the tweet features that trigger customer engagement and found a positive correlation between the rate of quoting and the number of positive quotes. Bodaghi et al. [ 27 ] studied the role of users’ position in Twitter graphs in their engagement with viral tweets. They demonstrated how different patterns of engagement can arise from various forms of graph structures, leading to the development of open-source software for characterizing spreaders [ 28 , 29 ].
Hashtag adoption
The adoption and usage of hashtags on Twitter have been investigated in several studies, shedding light on the factors influencing individual behavior and the role of social networks. Zhang et al. [ 30 ] explored the behavior of Twitter users in adopting hashtags and specifically focused on the effect of “structure diversity” on individual behavior. Their findings suggest that users' behavior in online social networks is not solely influenced by their friends but is also significantly affected by the number of groups to which these friends belong. Tian et al. [ 31 ] investigated the impact of preferred behaviors among a heterogeneous population on social propagation within multiplex-weighted networks. Their research shed light on the diverse adoption behaviors exhibited by individuals with varying personalities in real-world scenarios. Examining hashtag use on Twitter, Monster et al. [ 32 ] examined how social network size influences people's likelihood of adopting novel labels. They found that individuals who follow fewer users tend to use a larger number of unique hashtags to refer to events, indicating greater malleability and variability in hashtag use. Rathnayake [ 33 ] sought to conceptualize networking events from a platform-oriented view of media events, emphasizing the role of hashtags in bottom-up construction. Hashtags played a key role in this taxonomy, reflecting their significance in organizing and categorizing discussions around specific events. Furthermore, Bodaghi et al. [ 34 ] demonstrated that the size of a user's friend network also impacts broader aspects, such as their decision to participate in an information-spreading process. The characteristics and dynamics of an individual’s social network play a role in shaping their behavior and engagement with hashtags. These studies collectively contribute to our understanding of hashtag adoption and its relationship to social networks, providing insights into the factors that influence individuals’ decisions to adopt and use hashtags in online platforms like Twitter.
Method and analysis
Data collection.
For this study, a random sample of 86,334 users from the United States was selected. The data collection process involved crawling their tweets, specifically the last 3200 tweets if available, until October 2020. The crawling process continued for these users at seven additional time intervals until February 2021. This resulted in a total of eight waves of data, encompassing all the tweets from these 86,334 users starting from the 3200th tweet prior to the first crawling time in October 2020, up until their last tweet on February 2, 2021. The eight waves of crawled data were then merged into a final dataset, and any overlapping tweets were removed. The final dataset consists of a data frame containing 304,602,173 unique tweets from the 86,334 users. Each tweet in the dataset is associated with 23 features, resulting in a dataset size exceeding 31 GB. Additionally, another dataset was created by crawling the user characteristics of these 86,334 users, such as the number of followers, friends, and statuses. The dataset includes four types of tweets: Retweet, Quote, Reply, and Original. Each tweet in the dataset belongs to only one of these types (pure mode) or a combination of types (hybrid mode). The hybrid modes are represented in two forms: (1) a retweet of a quote and (2) a reply that contains a quote. To maintain consistency and focus on pure modes in the dataset, the former was considered solely as a retweet, and the latter was treated as a quote only. As a result, the approximate counts of the four tweet types (Retweet, Quote, Reply, and Original) in the dataset are 143 M, 23 M, 77 M, and 61 M, respectively. To ensure a more recent focus on activities, the analysis specifically considered data from October 9, 2019, onwards. This date, October 9, 2019, was chosen as it is one year prior to Twitter’s issuance of the quoting encouragement policy. By using this cutoff date, the analysis concentrates on the data relevant to the policy's implementation and subsequent effects.
Data exploration
This section explores three aspects of the data: (1) the average number of tweets per user in each tweet type, (2) the number of active users in each tweet type, and (3) the usage of hashtags. The analysis includes all 86,334 users in the dataset. The exploration is conducted across three intervals: (1) pre-interval (from October 9, 2019, to October 8, 2020), (2) within-interval (from October 9, 2020, to December 15, 2020), and (3) post-interval (from December 16, 2020, to February 2, 2021). The code used for these explorations is publicly available. Footnote 1 Figure 2 presents the results for the first two aspects. The plots on the left-hand side illustrate the average number of tweets published in each tweet type, namely Original, Quote, Reply, and Retweet. The plots on the right-hand side display the number of active users in each tweet type. Active users in a specific type on a given day are defined as users who have published at least one tweet in that type on that day.
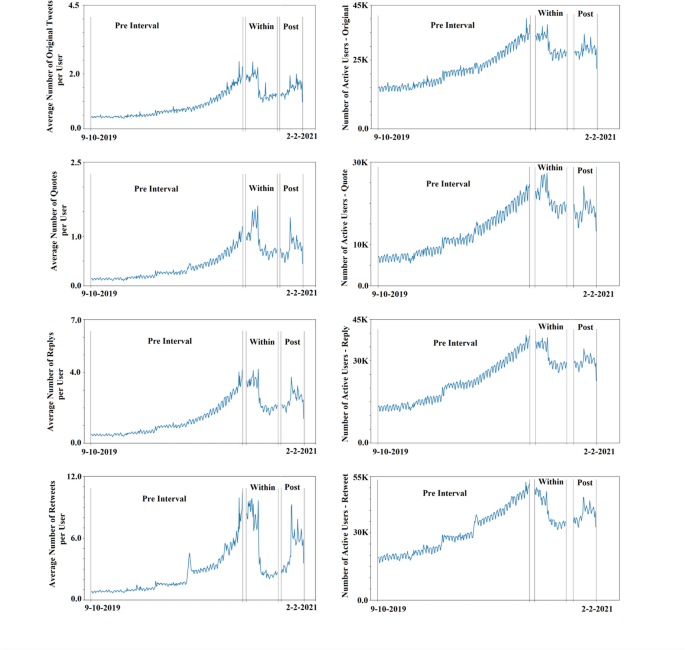
Daily rates of user activities during pre-, within-, and post-intervals
To analyze the usage of hashtags, the first step is to identify political hashtags. This involves extracting all the hashtags used in the dataset from September 1, 2020, to February 1, 2021, excluding the last day of the dataset (February 2, 2021) due to incomplete data collection. The following intervals are defined based on this period:
Pre-Interval: September 1, 2020, to October 8, 2020.
Within-Interval: October 9, 2020, to December 15, 2020.
Post-Interval: December 16, 2020, to February 1, 2021.
The extraction process yields a total of 1,126,587 hashtags. From this set, the 100 most frequently used hashtags are selected for further analysis. These selected hashtags are then reviewed and annotated by two referees, considering their political context. Through consensus between the referees, 32 hashtags out of the initial 100 are identified as political. The results of the usage analysis on these selected political hashtags are presented in Fig. 3 .
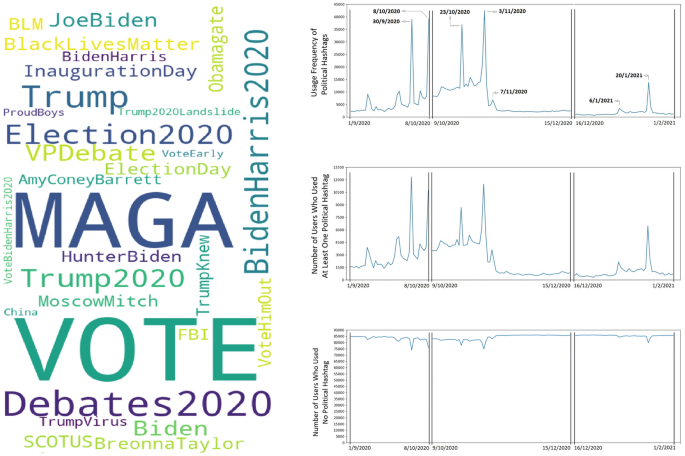
Usage of political hashtags. The left plot presents a word cloud depicting the 32 most frequently repeated political hashtags. The right plot displays the distribution of these political hashtags. The upper plot labels the significant dates associated with spikes in usage
Table 1 displays the key dates corresponding to the significant spikes observed in the plots depicted in Fig. 3 . These events directly influenced the patterns observed in the dataset.
Measurements of quantitative effects
To perform quantitative analysis, the data frame of each user was extracted by segregating all tweets associated with the same user ID. This process resulted in the creation of 86,334 individual data frames, each corresponding to a unique user. Subsequently, each user's data frame was divided into three distinct time intervals as follows:
Pre Interval [2019-10-09 to 2020-10-08]: This interval encompasses the year prior to the implementation of the new Twitter policy on 2020-10-09. Hence, the end of this interval is set as 2020-10-08.
Within Interval [2020-10-09 to 2020-12-15]: This interval spans from the policy’s inception on the first day, i.e., 2020-10-09, until its termination by Twitter on the last day, i.e., 2020-12-15.
Post Interval [2020-12-16 to 2021-02-02]: This interval commences on the day immediately following the removal of the policy, i.e., 2020-12-16, and continues until the last day on which a user published a tweet within the dataset. The dataset's coverage concludes on 2021-02-02, which represents the latest possible end date for this interval if a user had any tweet published on that date.
Impact analysis of the Twitter policy
The objective of this analysis is to assess the individual impact of the new Twitter policy, which promotes quoting instead of retweeting, on each user. Specifically, we aim to examine how the rate and quantity of published tweets per day have been altered following the implementation or removal of the new policy. Figure 4 illustrates the slopes and levels of a selected tweet type (quote) within each interval for a given user. Given the presence of four tweet types and three intervals, it is necessary to fit a total of 12 models for each user, corresponding to each tweet type within each interval.
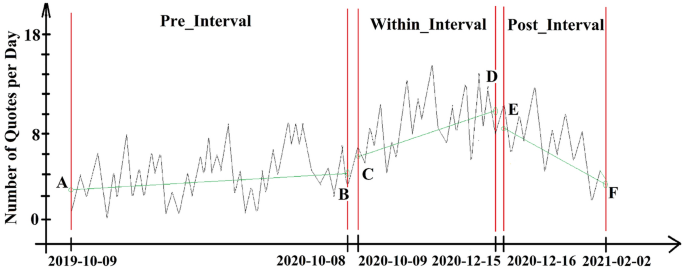
Slope and levels of number of quotes published by users during three intervals. This figure displays the slope and levels of the three intervals (pre-, within-, and post-intervals) for the number of quotes published by each user. The green lines depict the linear regression of the time series for each interval. The slope of pre-interval, within-interval, and post-interval corresponds to the slope of AB, CD, and EF lines, respectively. The start/end levels of pre-interval, within-interval, and post-interval are represented by A/B, C/D, and E/F, respectively
To analyze the impact of the new policy for each tweet type within a specific interval, we applied linear regression using the Ordinary Least Squares method (Eq. 1 ) in Python for users who had at least 7 data points with non-zero values.
where y is the number of tweets per day, x is the number of days, \(\alpha\) is the coefficient representing the slope, \(\varepsilon\) is the error, and \(\beta\) is the level. We then checked for the presence of autocorrelation in the residuals using the Durbin–Watson test (Eq. 2 ). If no autocorrelation was detected, we used linear regression to calculate the slopes and levels.
where d is the Durbin–Watson statistic, \({{\text{e}}}_{{\text{i}}}\) is the residual at observation i, n is the number of observations. The Durbin–Watson statistic ranges from 0 to 4. A value around 2 indicates no autocorrelation, while values significantly less than 2 suggest positive autocorrelation, and values significantly greater than 2 suggest negative autocorrelation. However, if autocorrelation was present, we employed linear regression with autoregressive errors (Eq. 3 ).
where \(\delta = {\widehat{{\varnothing }}}_{1}{\delta }_{i-1}+ {\widehat{{\varnothing }}}_{2}{\delta }_{i-2}+\dots + {\widehat{{\varnothing }}}_{p}{\delta }_{i-p}-{\widehat{\theta }}_{1}{e}_{i-1}- {\widehat{\theta }}_{2}{e}_{i-2}-\dots -{\widehat{\theta }}_{q}{e}_{i-q}+{\varepsilon }_{i}\)
In this equation, the errors are modelled using an ARIMA (p, d, q), where p and q represent the lags in the autoregressive (AR) and moving-average (MA) models, respectively, and d is the differencing value. We utilized the SARIMAX (p, d, q) model in Python to implement this regression, where the exogenous variable X (in Eq. 1 ) represents the number of days.
To determine the best values for the model's parameters, we conducted a grid search to generate a set of potential parameter combinations. We then evaluated the results for each combination based on the following criteria: (1) All achieved coefficients must be significant, (2) Akaike Information Criterion (AIC) based on Eq. ( 4 ) should be less than 5000, and (3) The Ljung–Box coefficient based on Eq. ( 5 ) should be significant (> 0.05).
where \(\mathcal{L}\) is the maximum log-likelihood of the model, k is the number of estimated parameters. A lower AIC value indicates a better estimation of the model orders.
where n is the sample size, \({\rho }_{k}\) is the sample autocorrelation at lag k. The test statistic follows a chi-squared distribution with degrees of freedom equal to the number of lags considered. The null hypothesis is that there is no autocorrelation up to the specified lag. A p-value greater than 0.05 suggests that there is no significant autocorrelation in the residuals, indicating an adequate fit. Finally, among the selected results, the model with the lowest sigma2 shown by Eq. ( 6 ), indicating less variance in the residuals, was chosen as the best-fit model.
where \({e}_{i}\) is the residual at observation i, \(\overline{e }\) is the mean of the residuals, and n is the number of observations. In the case of time series analysis, the residuals are the differences between the observed values and the values predicted by the ARIMA or SARIMAX model. The parameter values corresponding to this model were considered the optimal fit. The entire process for obtaining the slope and level findings is depicted in Fig. 5 , and the results are presented in Table 2 .
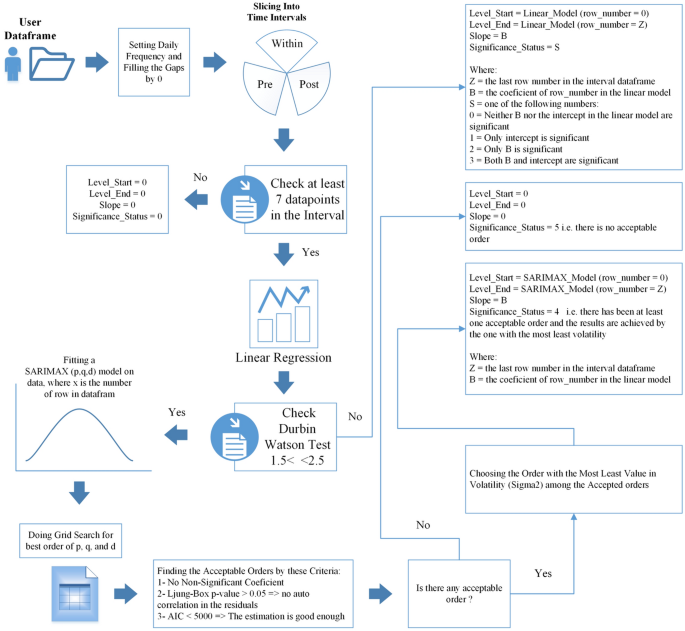
Flowchart illustrating the overall analysis procedure for slope and level assessments
Table 2 illustrates variations in the slope and level of tweeting between intervals. For instance, a level change of − 1.025 indicates a daily decrease of approximately 1.025 quotes from pre-interval to within-interval. Similarly, a slope change of 0.003 reflects an increase of around 0.003 quotes per day in the slope of quoting during the same transition. The table provides additional insights into slope and level changes for other tweet types across different intervals.
Analysis of qualitative effects
In this section, we aim to investigate the changes in user behavior towards the Twitter policy based on user characteristics such as the number of followers, number of friends, and number of statuses. To achieve this, we consider users whose obtained models are significant in both paired intervals (pre-within or within-post). We calculate the correlations between the values of these characteristics and the rate of change in the slope of each tweet type between the intervals. The results of this analysis are presented in Table 3 .
For instance based on Table 3 , investigating the relationships, a notable negative correlation of − 0.042 is observed between the number of friends a user has and the rate of slope change for quote publishing, specifically from the pre-interval to the within-interval. Additionally, a significant negative correlation of − 0.079 is evident between the number of quotes published in the post-interval and the number of retweets previously published in the within-interval. Further detailed explanations and implications are presented in the “ Results ” section.
The analysis of text characteristics focuses on examining the impact of the new policy on the length and sentiment of quote texts. Specifically, we are interested in understanding how the quote texts of two different user groups, namely “short-term quoters” and “long-term quoters,” have changed in terms of length and sentiment from the pre-interval to the within-interval. We define the two groups as follows:
Short-term Quoter: A user who did not engage in quoting during the pre-interval but started quoting in the within interval.
Long-term Quoter: A user who engaged in quoting during the pre-interval and continued to do so in the within interval. A quoter is defined as a user whose average number of quotes in all tweets exceeds a certain threshold.
For the analysis, we extract three characteristics from the quote text: (1) the number of characters (excluding spaces), (2) the sentiment score, and (3) the number of times the quote has been retweeted. We preprocess the text by performing tasks such as removing non-ASCII characters, emojis, mentions, and hashtags. To calculate the sentiment score, we utilize the sentiment analyzer from the Python NLTK package, which is based on VADER, Footnote 2 a lexicon, and rule-based sentiment analysis tool specifically designed for sentiments expressed in social media. The sentiment score calculated by VADER is a compound score that represents the overall sentiment of a text. The score is computed based on the valence (positivity or negativity) of individual words in the text (Eq. 7 ).
where \({S}_{compound}\) is the compound sentiment score, V is the valence score of word and is normalized to be between − 1 (most negative) and 1 (most positive), and I is the intensity of word. The weights are determined by the intensity of each word's sentiment. The rate of change in the average value of these characteristics from the pre to within intervals is then calculated for each user. Finally, we compute the average rates of change separately for short-term and long-term quoters, as presented in Table 4 .
As illustrated in Table 4 , 34,317 users in the dataset exhibited a quote-publishing rate exceeding 0.05 during the pre and within intervals, indicating more than 5 quotes per every 100 published tweets. These users observed a marginal increase (0.006) in the average sentiment of their tweets from pre-intervals to within-intervals. Conversely, 5900 users in the dataset, who had no quotes in the pre-interval but exceeded 0.01 of all their tweets as quotes during the pre and within intervals, experienced a decrease of 0.242 per day in their rate of retweeting from pre-intervals to within-intervals. Further detailed explanations and implications are presented in the “ Results ” section.
Impact of the Twitter policy
The findings of the impact analysis are presented in Table 2 , illustrating the changes in slopes for different tweet types. It is observed that the slope of each tweet type, except for quotes, decreased upon entering the within interval, while quotes experienced a slight increase (0.0031). Notably, prior to the implementation of the new policy, there was a substantial increase in the number of daily tweets across all types. Therefore, the decline in levels during the within intervals relative to the pre-intervals can be attributed to this initial surge in activity. Another significant result is the considerable decrease in the number of daily published quotes during the post-interval compared to the within-interval. Additionally, a significant decrease (− 2.785) and increase (11.587) are observed in the slope of retweets per day during the within and post intervals, respectively. These notable changes in both retweet and quote rates highlight the impact of Twitter's new policy. When examining these results from a broader perspective, two trends emerge: (1) during the transition from the pre-interval to the within interval, the slope of all tweet types, except for quotes, decreased, and (2) from the within-interval to the post-interval, the slope of all tweet types increased, except for quotes. These trends underscore the pronounced impact of the new policy implemented by Twitter. In conclusion, it can be inferred that the policy has achieved some progress. However, determining the true success of the policy requires considering Twitter's overarching goals within a broader context, encompassing both short-term and long-term consequences.
The correlations between user characteristics and slope changes in each tweet type during different intervals are presented in Table 3 . The results, particularly the correlations between slope changes in quoting and retweeting and other user characteristics, can be examined from three perspectives: the pre-within transition, within-post transition, and a comparison of pre-within to post-within.
Pre-within transitions
Regarding the pre-within transition, several noteworthy relationships can be observed. Firstly, there is an inverse relationship between the number of friends a user has and the slope change for the quote type. This suggests that users with a larger number of friends exhibit less improvement in their quoting rate during the within-interval (following the implementation of the Twitter policy). Similarly, the number of statuses published by users also demonstrates a negative correlation with the slope change for quotes. In other words, users who tend to publish a higher number of statuses show less inclination to increase their quoting rate during the within interval. Additionally, significant relationships emerge between the slope change in quoting during the pre-within interval and both retweet counts and the number of data points. This indicates that users who have engaged in more retweets are more likely to exhibit a propensity for quoting during the within-interval. Similar relationships can be observed between the slope change in quoting during the pre-within interval and other tweet types, suggesting that more active users are more influenced by the changes in the quoting behavior.
Within-post transitions
Analyzing the within-post transitions, several significant relationships can be observed. Firstly, the slope change in retweeting during the within-post interval exhibits a significant relationship with the number of quotes and original tweets during the within interval. This implies that users who have a higher number of quotes and original tweets in their activity would experience a greater increase in the retweeting rate after the policy cancellation (post-interval). However, the slope change in retweeting during the within-post interval does not show a significant relationship with the slope change in any other tweet type, except for an inverse relationship with original tweets. In other words, users who engage more in original tweets during the within-interval are likely to exhibit a lower increase in the rate of retweeting during the post-interval. Regarding the slope change in quoting during the within-post interval, a significant negative relationship is observed with the number of retweets during the within interval. This indicates that users who have a higher number of retweets during the within-interval are likely to experience a lower increase in the quoting rate during the post-interval. This relationship holds true for users who have quoted more during the within interval as well.
Pre-within to within-post comparison
Comparing the slope change in quoting and retweeting between the pre-within and within-post transitions, it can be observed that users who experienced an increase in their quoting or retweeting rate during the pre-within transition tend to exhibit a higher inclination to decrease it during the within-post transition. Additionally, a significant inverse relationship is evident between the slope change in quoting during the pre-within interval and the slope change in retweeting during the within-post interval. This implies that users who witnessed a greater increase in their quoting rate during the pre-within transition are likely to experience a larger decrease in their retweeting rate during the within-post transition.
The results of the text analysis, specifically length, sentiment, and the number of retweets, are presented in Table 4 . Examining the results reveals several key findings. Firstly, the quote texts of long-term quoters have undergone a reduction in length during the within interval compared to the pre-interval, across all threshold levels. However, for short-term quoters, this reduction in quote length only occurs at threshold levels equal to or above 0.05. Furthermore, among those whose quote texts have been shortened (at threshold levels of 0.05 and 0.075), short-term quoters experience a greater reduction in length compared to long-term quoters. Regarding sentiment analysis, the results indicate an overall increase in the sentiment score of quote texts from the pre-interval to the within interval. However, this increase is more pronounced for short-term quoters compared to long-term quoters.
Additionally, for both categories and across all threshold levels, the number of retweets received by quotes has decreased from the pre-interval to the within-interval. This decrease is particularly significant for long-term quoters, except at threshold level 0 for short-term quoters. This observation aligns with expectations since short-term quoters did not have any quotes during the pre-interval, resulting in their sentiment score being subtracted from the sentiment scores of quotes during the within interval. Notably, the decrease in the number of retweets is more substantial for long-term quoters, except at a threshold level of 0.075, where it is slightly higher for short-term quoters. In summary, by considering a threshold of 0.075 as an indicator, we can conclude that the Twitter policy has influenced quote texts in the following ways: (1) There is a greater reduction in the number of characters for short-term quoters compared to long-term quoters, and (2) The increase in sentiment score is more significant for short-term quoters relative to long-term quoters.
The findings pertaining to the hypotheses are outlined in Table 5 .
Quantitative findings
The quantitative analysis, based on hypotheses H1–4, reveals that the intervention has a negative impact on users’ retweeting behavior, while other tweet types remain relatively unaffected. However, the cessation of the intervention leads to an increase in the retweeting rate and a decrease in the quoting rate. When considering only the period when the policy was in effect, namely the within-interval, it can be concluded that the policy was partially successful. Despite a minor increase in the quoting rate, the significant decline in retweeting indicates a positive outcome. However, when examining the long-term effects after discontinuation of the policy, i.e., the post-interval, the policy can be regarded as a failure, as the retweeting rate experienced a dramatic increase while the quoting rate decreased substantially. Although Twitter did not enforce users to quote instead of retweeting nor provide any explicit promotion or reward for quoting, the quoting encouragement policy may have influenced users' perceptions and served as a virtual external incentive for initiating quoting behavior. This phenomenon can be explained by the adaptive nature of the brain in perceiving rewards based on recent levels and ranges of rewards, fictive outcomes, social comparisons, and other relevant factors [ 35 , 36 ]. The motivation crowding theory offers a framework for discussing this observation. When an extrinsic reward is removed, the level of intrinsic motivation diminishes compared to a scenario where no additional reward was initially provided [ 37 ]. In the case of Twitter's policy, users may have perceived the extrinsic incentive of adding a few extra characters to a retweet as rewarding and complied accordingly. However, once this external incentive was eliminated, the residual intrinsic motivation decreased below its initial level. This explains the subsequent decline in the quoting rate during the post-interval, accompanied by a surge in retweeting activity.
Qualitative findings
The qualitative analysis, focusing on hypotheses H5–11, reveals several noteworthy patterns. Users with a smaller number of friends and higher levels of overall tweet activity are more inclined to align with the policy and increase their quoting rate during the within interval. Furthermore, users who experienced an increase in their quoting rate during the within interval are more likely to decrease their quoting rate following the policy withdrawal in the post interval. Additionally, users who adopted quoting behavior as a result of the policy during the within interval demonstrated a tendency to publish quotes with shorter text length and more positive emotions. Two observed patterns can be explained respectively by the TPB and the DOI. The TPB posits that an individual’s behavioral intentions are influenced by three components, with subjective norms playing a significant role [ 38 ]. The impact of subjective norms is contingent upon the connections an individual has with others. Users with a smaller number of friends have fewer channels through which subjective norms can exert pressure. Consequently, these users are less influenced by societal norms that have not yet accommodated the new policy. Hence, users with fewer friends are more likely to be early adopters of the policy. Moreover, recent research [ 39 ] suggests that TPB, along with the theory of the Spiral of Silence, can potentially explain the avoidance of adoption, particularly when adoption involves expressing individual beliefs. Furthermore, the DOI provides insights into the adoption process, suggesting that adopters can be categorized into distinct groups based on the timing of their adoption [ 40 ]. Through this categorization, shared characteristics in terms of personality, socioeconomic status, and communication behaviors emerge. Early adopters, characterized by a greater tolerance for uncertainty and change, often exhibit higher levels of upward mobility within their social and socioeconomic contexts, as well as enhanced self-efficacy [ 41 ]. These characteristics are reflected in the more positive emotions expressed in their quote posts.
Implications
This study carries implications from both practical and theoretical perspectives. From a practical standpoint, the findings provide valuable guidance for practitioners in developing a multistage model that captures users’ behavior towards a new social media policy at an aggregate level. Such a model is crucial for designing efficient strategies aimed at expediting the adoption process among the majority of users. Leveraging the quantitative analysis method employed in this study, practitioners can first evaluate the impact of the policy, and then, using the qualitative analysis method, identify users who are more inclined to adopt or reject the policy based on their characteristics and text behavior. Gaining insights into user tendencies towards policy adoption or rejection in advance can inform a series of initiatives, including targeted user categorization to introduce or withhold the policy during its initial stages. An illustrative study by Xu et al. [ 42 ] explored public opinion on Twitter during Hurricane Irma across different stages, analyzing over 3.5 million tweets related to the disaster to discern distinct thematic patterns that emerged during each stage. Their findings assist practitioners in utilizing Twitter data to devise more effective strategies for crisis management. From a theoretical perspective, the findings contribute to the advancement of theories such as the TPB and the DOI in the realm of cyberspace. According to TPB, subjective norms play a significant role in shaping human behavior. This study revealed that users with a smaller number of friends are more inclined to accept the new policy. This suggests that users who have fewer connections are more likely to deviate from the prevailing norm in which the adoption of the new policy has not yet gained traction. Furthermore, the higher rates of positivity observed in the quote texts of short-term quoters, relative to their long-term counterparts, contribute to the extension of the Innovation Diffusion Theory regarding policy adoption and expand our understanding of the possible manifestations of early adopters' characteristics in the context of social media.
For a more nuanced understanding, it is noteworthy to explore the impact of events on user behavior. While events like debates can undeniably influence user activity levels, this impact is likely experienced across all types of users, such as quoters and retweeters. Our analysis, examining individual users across multiple time intervals that encompass these events, allows us to observe user-specific behavioral evolution. The extracted patterns thus represent dominant shifts in spreading behavior observed in the majority, irrespective of their original preference (retweeting or quoting). This observed consistency suggests that the policy's influence may extend beyond just event-driven fluctuations. The consistent shift in information-sharing behavior throughout the study period points towards the possible contribution of additional factors beyond isolated events.
Conclusion and future works
This research employed a big data approach to analyze the Twitter quoting encouragement policy, examining both its quantitative and qualitative effects. The research timeline was divided into three distinct intervals: pre, within, and post-intervals. Time series analysis was then utilized to identify changes in the rates of different tweet types across these intervals. Additionally, text and sentiment analysis, along with correlation methods, were applied to explore the relationships between user characteristics and their responses to the policy. The results revealed a short-term success followed by a long-term failure of the policy. Moreover, a set of user characteristics was identified, shedding light on their adherence to the policy and their quoting tendencies during the policy’s implementation. These findings have significant implications for the development and evaluation of new policies in the realm of social media, offering valuable insights for the design of more effective strategies.
The study of policy adoption on social media is still in its early stages, particularly in the realm of data analytics and behavioral research [ 43 ]. Future studies can build upon this research and explore additional factors and techniques to deepen our understanding. For example, the impact of aggregations, such as crowd emotional contagion, convergence behavior, and adaptive acceptance, can be modelled as exogenous factors in the analysis [ 44 , 45 ]. Additionally, incorporating new techniques for sentiment analysis, as highlighted in studies by Zhao et al. [ 46 ], and Erkantarci et al. [ 47 ], as well as semantic techniques [ 48 ], can further enhance computational analyses. Moreover, future research can consider factors related to the continuance of use [ 49 ] to examine the reasons behind policy rejection by users who initially adopted it. The inclusion of census data, search logs of users [ 50 ], user demographics [ 51 ], and the analysis of interconnections within a graph [ 52 ] would be valuable additions to the analysis. These additional data sources can provide a more comprehensive understanding of user behaviors and interactions. Furthermore, it is important to consider bot filtering techniques to ensure the accuracy and reliability of the findings. This step is particularly crucial for extending the research beyond Twitter and examining policy adoption in non-cyber spaces. By exploring these avenues of research, future studies can advance our knowledge of policy adoption on social media, providing valuable insights into user behaviors, motivations, and the effectiveness of policy interventions. Finally, this study’s data collection and storage methods share similarities with those employed in prior efforts [ 53 ]. However, there remains significant potential for innovation in this area.
Data availability
The datasets analyzed during the current study are available from the corresponding author on reasonable request.
Code availability
Codes are publicly available by this link: https://github.com/AmirhoseinBodaghi/TwitterPolicyProject .
https://github.com/AmirhoseinBodaghi/TwitterPolicyProject .
Valence Aware Dictionary and sEntiment Reasoner.
Weber, I., Garimella, V. R. K., & Batayneh, A. (2013). Secular vs. Islamist polarization in Egypt on twitter . ASONAM.
Book Google Scholar
Garimella, K., Weber, I., & Choudhury, M.D. (2016). Quote RTs on Twitter: Usage of the new feature for political discourse. WebSci’ 16 Germany.
Gallego, M., & Schofield, N. (2017). Modeling the effect of campaign advertising on US presidential elections when differences across states matter. Mathematical Social Sciences, 90 , 160–181.
Article Google Scholar
Jones, M. A., McCune, D., & Wilson, J. M. (2020). New quota-based apportionment methods: The allocation of delegates in the Republican Presidential Primary. Mathematical Social Sciences., 108 , 122–137.
Stier, S., Schünemann, W. J., & Steiger, S. (2018). Of activists and gatekeepers: Temporal and structural properties of policy networks on Twitter. New Media and Society, 20 (5), 1910–1930.
Frey, B. S., & Jegen, R. (2001). Motivation crowding theory. Journal of Economic Survey, 15 , 589–611.
Kreps, D. (1997). Intrinsic motivation and extrinsic incentives. American Economic Review, 87 , 359–364.
Google Scholar
Stiles, E. A., Swearingen, C. D., & Seiter, L. M. (2022). Life of the party: Social networks, public attention, and the importance of shocks in the presidential nomination process. Social Science Computer Review . https://doi.org/10.1177/08944393221074599
Jang, Y., Park, C. H., & Seo, Y. S. (2019). Fake news analysis modeling using quote retweet. Electronics, 8 (12), 1377.
Li, K., Zhu, H., Zhang, Y., & Wei, J. (2022). Dynamic evaluation method on dissemination capability of microblog users based on topic segmentation. Physica A: Statistical Mechanics and its Applications, 608 , 128264. https://doi.org/10.1016/j.physa.2022.128264
Bodaghi, A., & Oliveira, J. (2020). The characteristics of rumor spreaders on Twitter: A quantitative analysis on real data. Computer Communications, 160 , 674–687.
South, T., Smart, B., Roughan, M., & Mitchell, L. (2022). Information flow estimation: A study of news on Twitter. Online Social Networks and Media, 31 , 100231. https://doi.org/10.1016/j.osnem.2022.100231
Boulianne, S., & Larsson, A. O. (2021). Engagement with candidate posts on Twitter, Instagram, and Facebook during the 2019 election. New Media and Society, 1–22.
Lazarus, J., & Thornton, J. R. (2021). Bully pulpit? Twitter users’ engagement with president trump’s tweets. Social Science Computer Review., 39 (5), 961–980.
Yue, C. A., Qin, Y. S., Vielledent, M., Men, L. R., & Zhou, A. (2021). Leadership going social: How U.S. nonprofit executives engage publics on Twitter. Telematics and Informatics, 65 , 101710. https://doi.org/10.1016/j.tele.2021.101710
Ahmed, S., Jaidka, K., & Cho, J. (2021). The 2014 Indian elections on Twitter: A comparison of campaign strategies of political parties. Telematics and Informatics, 33 (4), 1071–1087.
Bodaghi, A., & Oliveira, J. (2022). A longitudinal analysis on Instagram characteristics of Olympic champions. Social Network Analysis and Mining, 12 , 3.
Hou, J., Wang, Y., Zhang, Y., & Wang, D. (2022). How do scholars and non-scholars participate in dataset dissemination on Twitter. Journal of Informetrics., 16 (1), 101223. https://doi.org/10.1016/j.joi.2021.101223
Hoang, T. B. N., & Mothe, J. (2018). Predicting information diffusion on Twitter—Analysis of predictive features. Journal of Computational Science, 28 , 257–264. https://doi.org/10.1016/j.jocs.2017.10.010
Munoz, M. M., Rojas-de-Gracia, M.-M., & Navas-Sarasola, C. (2022). Measuring engagement on Twitter using a composite index: An application to social media influencers. Journal of Informetrics, 16 (4), 101323. https://doi.org/10.1016/j.joi.2022.101323
Backstrom, L., Huttenlocher, D., Kleinberg, J., & Lan, X. (2006). Group formation in large social networks: membership, growth, and evolution. Proceedings of the 12th ACM SIGKDD international conference on Knowledge discovery and data mining (KDD ’06) (pp. 44–54). Association for Computing Machinery.
Chapter Google Scholar
Hu, J., Luo, Y., & Yu, J. (2018). An empirical study on selectiviey of retweeting behaviors under multiple exposures in social networks. Journal of Computational Science, 28 , 228–235. https://doi.org/10.1016/j.jocs.2017.11.004
Balestrucci, A., De Nicola, R., Petrocchi, M., & Trubiani, C. (2021). A behavioural analysis of credulous Twitter users. Online Social Networks and Media., 23 , 100133. https://doi.org/10.1016/j.osnem.2021.100133
Bodaghi, A., & Goliaei, S. (2018). A novel model for rumor spreading on social networks with considering the influence of dissenting opinions. Advances in Complex Systems, 21 , 1850011.
Wells, C., Shah, D., Lukito, J., Pelled, A., Pevehouse, J. C., & Yang, J. (2020). Trump, Twitter, and news media responsiveness: A media systems approach. New Media and Society, 22 (4), 659–682.
Yang, D., & Fujimura, S. (2019). What Will Influence customer's engagement the strategies and goals of tweet. IEEE international conference on industrial engineering and engineering management ( IEEM ), pp. 364–368.
Bodaghi, A., & Oliveira, J. (2022). The theater of fake news spreading, who plays which role? A study on real graphs of spreading on Twitter. Expert Systems with Applications, 189 , 116110.
Bodaghi, A., Oliveira, J., & Zhu, J. J. H. (2021). The fake news graph analyzer: An open-source software for characterizing spreaders in large diffusion graphs. Software Impacts. 100182.
Bodaghi, A., Oliveira, J., & Zhu, J. J. H. (2022). The Rumor Categorizer: An open-source software for analyzing rumor posts on Twitter. Software Impacts. 100232.
Zhang, A., Zheng, M., & Pang, B. (2018). Structural diversity effect on hashtag adoption in Twitter. Physica A: Statistical Mechanics and its Applications., 493 , 267–275.
Tian, Y., Tian, H., Cui, Y., Zhu, X., & Cui, Q. (2023). Influence of behavioral adoption preference based on heterogeneous population on multiple weighted networks. Applied Mathematics and Computation, 446 , 127880. https://doi.org/10.1016/j.amc.2023.127880
Monster, I., & Lev-Ari, S. (2018). The effect of social network size on hashtag adoption on Twitter. Cognitive Science, 42 (8), 3149–3158.
Rathnayake, C. (2021). Uptake, polymorphism, and the construction of networked events on Twitter. Telematics and Informatics, 57 , 101518.
Bodaghi, A., Goliaei, S., & Salehi, M. (2019). The number of followings as an influential factor in rumor spreading. Applied Mathematics and Computation, 357 , 167–184.
Seymour, B., & McClure, S. M. (2008). Anchors, scales and the relative coding of value in the brain. Current Opinion in Neurobiology, 18 , 173–178.
Murayama, K., Matsumoto, M., Izuma, K., & Matsumoto, K. (2010). Neural basis of the undermining effect of monetary reward on intrinsic motivation. Proceedings of the National Academy of Sciences of USA, 107 , 20911–20916.
Camerer, C. (2010). Removing financial incentives demotivates the brain. Proceedings of the National Academy of Sciences, 107 (49), 20849–20850.
Ajzen, I. (1991). The theory of planned behavior. Organizational Behavior and Human Decision Processes, 50 (2), 179–211.
Wu, T. Y., Xu, X., & Atkin, D. (2020). The alternatives to being silent: Exploring opinion expression avoidance strategies for discussing politics on Facebook. Internet Research, 30 (6), 1709–1729.
Everett, R. (2003). Diffusion of innovations (5th ed.). Simon and Schuster. ISBN 978-0-7432-5823-4.
Straub, E. T. (2009). Understanding technology adoption: Theory and future directions for informal learning. Review of Educational Research, 79 (2), 625–649.
Xu, Z., Lachlan, K., Ellis, L., & Rainear, A. M. (2020). Understanding public opinion in different disaster stages: A case study of Hurricane Irma. Internet Research, 30 (2), 695–709.
Motiwalla, L., Deokar, A. V., Sarnikar, S., & Dimoka, A. (2019). Leveraging data analytics for behavioral research. Information Systems Frontiers, 21 , 735–742.
Mirbabaie, M., Bunker, D., Stieglitz, S., & Deubel, A. (2020). Who sets the tone? Determining the impact of convergence behaviour archetypes in social media crisis communication. Information System Frontiers, 22 , 339–351. https://doi.org/10.1007/s10796-019-09917-x
Iannacci, F., Fearon, C., & Pole, K. (2021). From acceptance to adaptive acceptance of social media policy change: A set-theoretic analysis of B2B SMEs. Information Systems Frontiers, 23 , 663–680.
Zhao, X., & Wong, C. W. (2023). Automated measures of sentiment via transformer- and lexicon-based sentiment analysis (TLSA). Journal of Computational Social Science . https://doi.org/10.1007/s42001-023-00233-8
Erkantarci, B., & Bakal, G. (2023). An empirical study of sentiment analysis utilizing machine learning and deep learning algorithms. Journal of Computational Social Science . https://doi.org/10.1007/s42001-023-00236-5
Bodaghi, A., & Oliveira, J. (2024). A financial anomaly prediction approach using semantic space of news flow on twitter. Decision Analytics Journal, 10 , 100422. https://doi.org/10.1016/j.dajour.2024.100422
Franque, F. B., Oliveira, T., Tam, C., & Santini, F. O. (2020). A meta-analysis of the quantitative studies in continuance intention to use an information system. Internet Research, 31 (1), 123–158.
Feng, Y., & Shah, C. (2022). Unifying telescope and microscope: A multi-lens framework with open data for modeling emerging events. Information Processing and Management, 59 (2), 102811.
Brandt, J., Buckingham, K., Buntain, C., Anderson, W., Ray, S., Pool, J. R., & Ferrari, N. (2020). Identifying social media user demographics and topic diversity with computational social science: A case study of a major international policy forum. Journal of Computational Social Science, 3 , 167–188.
Antonakaki, D., Fragopoulou, P., & Ioannidis, S. (2021). A survey of Twitter research: Data model, graph structure, sentiment analysis and attacks. Expert Systems with Applications, 164 , 114006.
Bodaghi, A. (2019). Newly emerged rumors in Twitter. Zenodo. https://doi.org/10.5281/zenodo.2563864
Download references
Acknowledgements
The study was funded by City University of Hong Kong Centre for Communication Research (No. 9360120) and Hong Kong Institute of Data Science (No. 9360163). We would also like to express our sincere appreciation to Pastor David Senaratne and his team at Haggai Tourist Bungalow in Colombo, Sri Lanka, for their generous hospitality. Their support provided a conducive environment for the corresponding author to complete parts of this manuscript.
Author information
Authors and affiliations.
School of Computing, Ulster University, Belfast, Northern Ireland, UK
Amirhosein Bodaghi
Department of Media and Communication, City University of Hong Kong, Kowloon, Hong Kong
Jonathan J. H. Zhu
You can also search for this author in PubMed Google Scholar
Corresponding author
Correspondence to Amirhosein Bodaghi .
Ethics declarations
Conflict of interest.
On behalf of all authors, the corresponding author states that there is no conflict of interest.
Additional information
Publisher's note.
Springer Nature remains neutral with regard to jurisdictional claims in published maps and institutional affiliations.
Rights and permissions
Open Access This article is licensed under a Creative Commons Attribution 4.0 International License, which permits use, sharing, adaptation, distribution and reproduction in any medium or format, as long as you give appropriate credit to the original author(s) and the source, provide a link to the Creative Commons licence, and indicate if changes were made. The images or other third party material in this article are included in the article's Creative Commons licence, unless indicated otherwise in a credit line to the material. If material is not included in the article's Creative Commons licence and your intended use is not permitted by statutory regulation or exceeds the permitted use, you will need to obtain permission directly from the copyright holder. To view a copy of this licence, visit http://creativecommons.org/licenses/by/4.0/ .
Reprints and permissions
About this article
Bodaghi, A., Zhu, J.J.H. A big data analysis of the adoption of quoting encouragement policy on Twitter during the 2020 U.S. presidential election. J Comput Soc Sc (2024). https://doi.org/10.1007/s42001-024-00291-6
Download citation
Received : 06 January 2024
Accepted : 07 May 2024
Published : 19 May 2024
DOI : https://doi.org/10.1007/s42001-024-00291-6
Share this article
Anyone you share the following link with will be able to read this content:
Sorry, a shareable link is not currently available for this article.
Provided by the Springer Nature SharedIt content-sharing initiative
- Quote retweets
- Social media
- Time series analysis
- Text analysis
- Policy intervention
- Find a journal
- Publish with us
- Track your research
- Technical Support
- Find My Rep
You are here
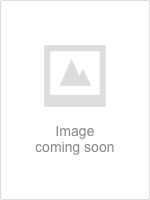
An Introduction to Political and Social Data Analysis Using R
- Thomas M. Holbrook - Professor Emeritus, University of Wisconsin
- Description
See what’s new to this edition by selecting the Features tab on this page. Should you need additional information or have questions regarding the HEOA information provided for this title, including what is new to this edition, please email [email protected] . Please include your name, contact information, and the name of the title for which you would like more information. For information on the HEOA, please go to http://ed.gov/policy/highered/leg/hea08/index.html .
For assistance with your order: Please email us at [email protected] or connect with your SAGE representative.
SAGE 2455 Teller Road Thousand Oaks, CA 91320 www.sagepub.com
Supplements
Clarity in communication is absolutely essential in introductory methodology and data science courses. Holbrook's way with words makes complicated statistical and computational language easy to understand and instills confidence in students.
The book introduces many useful concepts without getting too bogged down in any individual concept. Students get a huge, wide exposure to content. This will help facilitate class conversations on day one , which is a real leverage.
Holbrook introduces complex and technically challenging concepts in a way that, for those new to the world of R, is approachable and easy to understand. Fantastic introductory text for undergraduate study.
Chapter 1 provides a strong overview of the research process. While it talks a lot about data, it does so in a non-technical way that I think most undergraduates would be able to get through reasonably well . The examples provided are relevant and broadly interesting.
This text is structured well for taking students through Data Analysis for political science. Students are walked through both the meaning of the statistics examined and how the computer can be made to generate them. Each section builds on the preceding sections in a clear manner. The only problem with this book is that I wish I had written it. I can't wait to use it .
Professor Holbrook's book provides an accessible entry-point for students of all levels to use data for politica l and social research, while offering clear and easy-to-understand guidance to the use of the R software .
This is a highly engaging and practical introduction to social research using R. All the essentials are here with little to no distracting material that might confuse already anxious students. I highly recommend this text .
- Practical data analysis approaches in this text focus on using statistics to understand data and research, rather that focusing on learning statistics for its own sake.
- Just enough R code in this text helps students use this programming environment to get results with a minimum of coding and no loading complex data analysis packages.
- Simple political and social science examples throughout ensure students see the context of the data analysis they are doing.
- Posit.cloud instructions in Chapter 2 help instructors make using R straightforward for students.
- R code accompanies most graphs and tables so students can run the code themselves and follow along.
- Concepts and Calculation Exercises focus on concepts first with simple calculations so students can confirm their knowledge.
- R Problems ask students to use the R commands from earlier in the chapter to analyze data and interpret the results.
For instructors
Select a purchasing option, related products.

Thank you for visiting nature.com. You are using a browser version with limited support for CSS. To obtain the best experience, we recommend you use a more up to date browser (or turn off compatibility mode in Internet Explorer). In the meantime, to ensure continued support, we are displaying the site without styles and JavaScript.
- View all journals
- My Account Login
- Explore content
- About the journal
- Publish with us
- Sign up for alerts
- Open access
- Published: 15 May 2024
Supply chain transformational leadership and resilience: the mediating role of ambidextrous business model
- Taiwen Feng 1 na1 ,
- Zhihui Si 1 na1 ,
- Wenbo Jiang 2 &
- Jianyu Tan 3
Humanities and Social Sciences Communications volume 11 , Article number: 628 ( 2024 ) Cite this article
260 Accesses
1 Altmetric
Metrics details
- Business and management
The global prevalence of COVID-19 has caused many supply chain disruptions, which calls for firms to build resilient supply chains. Prior research primarily examined the effects of firm resources or capabilities on supply chain resilience (SCR), with limited attention given to the critical role of supply chain transformational leadership (SCTL). Based on social learning theory, we explore how SCTL impacts SCR via an ambidextrous business model and the moderating role of paradox cognition. We employ hierarchical regression analysis to verify the hypotheses with data from 317 Chinese firms. The results show that SCTL has a positive impact on proactive and reactive SCR, and the ambidextrous business model mediates this relationship. Furthermore, paradox cognition strengthens the effect of SCTL on the ambidextrous business model. This study contributes to literature and practices in the field of transformational leadership and SCR by providing unique insights into how to improve SCR from a leadership perspective.
Similar content being viewed by others
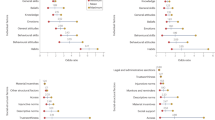
Determinants of behaviour and their efficacy as targets of behavioural change interventions
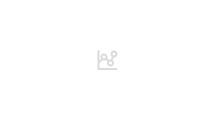
The impact of artificial intelligence on employment: the role of virtual agglomeration
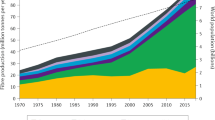
The environmental price of fast fashion
Introduction.
Affected by the global prevalence of COVID-19, frequent supply chain disruptions have occurred (Nikolopoulos et al., 2021 ; Jiang et al., 2023 ; Shen and Sun, 2023 ). Since supply chains are increasingly complex, firms are more likely to suffer from supply chain disruptions (Lechler et al., 2019 ; Spieske and Birkel, 2021 ; Xi et al., 2024 ). It will be difficult for a firm lacking resilient supply chains to survive and compete within a dynamic and uncertain condition. Supply chain resilience (SCR) reflects the capability of a system to maintain desirable functions before/during disruptions and/or timely recover to its normal functions after disruptive events (Gu et al., 2021 ). Understanding the enablers of SCR would help the firm better respond to potential risks caused by supply chain disruptions (Vanpoucke and Ellis, 2020 ).
Firm leaders could play critical roles in reducing disruption risk in supply chains and building a more resilient supply chain (Khunwishit et al., 2018 ). However, little research has checked the effect of transformational leadership within the supply chain context. We define supply chain transformational leadership (SCTL) as a continual influence that the focal firm demonstrates modeling values and reformative behaviors, which motivates its supply chain partners to act similarly with inspiration and close relationships.
While previous studies mainly focused on the roles of specific resources or capabilities on SCR, such as agility, redundancy, and collaboration (Al Naimi et al., 2021 ; Tukamuhabwa et al., 2015 ), the strategic role of SCTL has largely been ignored. Previous studies suggest that transformational leadership encourages employees’ reaction to changes in a firm (Peng et al., 2021 ) and increases team resilience (Dimas et al., 2018 ). Hence, high levels of SCTL could operate as role-modeling behaviors for the focal firm’s partners and foster a more resilient supply chain. According to social learning theory (Bandura, 1977 ; Brown et al., 2005 ), the focal firm with high levels of SCTL acts as a reliable role model whom its followers trust and attempt to emulate. That is to say, supply chain partners can learn transformative behaviors by observing the focal firm. As a result, the focal firm with high levels of SCTL acts as a benchmark for its supply chain followers to build a resilient supply chain. Therefore, we propose that SCTL may strengthen SCR.
Firms throughout supply chains often face conflicting objectives while implementing organizational learning to improve SCR (Lee and Rha, 2016 ). That is, they must balance different types of learning strategies, such as exploring potential opportunities to transform supply chains while also exploiting current resources to optimize supply chains. The tension of balancing exploitation and exploration is termed organizational ambidexterity (Kristal et al., 2010 ). According to an ambidexterity perspective (Aslam et al., 2022 ; Eng et al., 2023 ), the focal firm with high levels of SCTL prefers to deal with supply chain disruptions through both exploring external opportunities and exploiting internal resources. However, little is known about how SCTL affects SCR via organizational ambidexterity.
Our research devotes to filling this research gap through clarifying the mediating effect of an ambidextrous business model that encompasses both novelty and efficiency within the SCTL–SCR link. We define an ambidextrous business model as a firm’s boundary-spanning transaction mode developed to create and capture value by both balancing activities of redesigning a novel business model and reorganizing elements of an existing one. Specifically, a novelty-centered business model could help firms explore a new value proposition to meet changing demands in disruptions, whereas an efficiency-centered business model improves inter-organizational transaction efficiency by facilitating supply chain visibility and reducing transaction cost (Wei et al., 2017 ; Zott and Amit, 2008 ). Drawing on social learning theory (Ojha et al., 2018 ), the focal firm with high levels of SCTL may demonstratively build an ambidextrous business model by fostering a supportive organizational context. Then, the ambidextrous business model in the focal firm motivates other supply chain partners to emulate and actively take a similar business model, improving SCR through shared supply chain ambidexterity. In this manner, an ambidextrous business model may mediate the SCTL–SCR relationship.
Furthermore, the focal firm with paradoxical thinking and cognition could also influence its learning strategies (Brusoni and Rosenkranz, 2014 ). That is, paradoxical thinking and cognition would affect the focal firm’s attitude and identification towards tensions (explore or exploit) arising from its contrasting strategic agendas (Smith and Lewis, 2011 ). When the focal firm possesses high levels of paradox cognition, it is more likely to recognize and embrace tensions, making well-balanced strategic decisions through developing transformational leadership. Hence, we propose that paradox cognition enhances the impact of SCTL on an ambidextrous business model.
In sum, this study explores three questions to uncover the impact of SCTL on SCR. First, whether SCTL is positively related to SCR? Second, does ambidextrous business model mediate the SCTL–SCR relationship? Third, does paradox cognition strengthen the role of SCTL on ambidextrous business model? By answering the above questions, this study makes a contribution to research and practices in the field of transformational leadership and SCR.
Literature review and hypotheses development
Supply chain resilience.
Resilience, a multidisciplinary construct originating from engineering, ecology, and psychology (Holling, 1973 ; Novak et al., 2021 ). Although most scholars have viewed resilience as an ability to resist and/or rebound from disruptive events (El Baz and Ruel, 2021 ; Namdar et al., 2018 ), there still lacks a normative definition widely accepted. Later, resilience is extended and applied to the field of social sciences, such as supply chain management and operational management. Due to the prevalence of COVID-19, resilience is particularly valued in global supply chains as supply chains become increasingly complex (Spieske and Birkel, 2021 ).
The major divergences of SCR concentrate on two aspects: influencing scope and attributive level. With regard to the influencing scope, some authors only treat SCR as a reactive capability (Brandon-Jones et al., 2014 ; El Baz and Ruel, 2021 ), while others propose that both reactive and proactive components are indispensable (Gu et al., 2021 ). With regard to the attributive level, SCR is often viewed as a firm’s capability (Ambulkar et al., 2015 ); however, it is more acceptable to belong to a whole supply chain system (Scholten et al., 2020 ). Hence, we define SCR as the capability of a system to maintain its expected functions before disruptions and timely recover to its normal functions during facing interruptions.
SCR has been segmented into various dimensions corresponding to different nodes, disruptive phases, or sub-capabilities. For example, Pournader et al. ( 2016 ) argue that SCR could be divided by the organizational boundary into supplier, internal, and customer resilience. Han et al. ( 2020 ) suggest that SCR could be classified into stages of readiness, response, and recovery. Jüttner and Maklan ( 2011 ) propose that flexibility, velocity, visibility, and collaboration are essential sub-capabilities comprising SCR. Following Cheng and Lu’s study ( 2017 ), we divide SCR into two dimensions: proactive and reactive SCR. Proactive SCR is the capability of a supply chain system to mitigate shocks and keep its normal state before/during possible disruptions. Reactive SCR means the capability of a supply chain system to quickly respond and return to its normal state after experiencing disruptions.
Although previous research has revealed diverse factors in formulating SCR (Razak et al., 2023 ; Scholten and Schilder, 2015 ), transformational leadership is rarely discussed. Prior studies mainly examine the roles of four groups of resources and capabilities in building SCR, including reengineering, collaboration, agility, and risk management culture (Belhadi et al., 2022 ). First, supply chain reengineering is positively related to SCR. Resources and capabilities, such as network structure, security, redundancy, efficiency, innovation, contingency planning, and market position, usually contribute to the realignment of structures and processes within supply chains (Han et al., 2020 ; Tukamuhabwa et al., 2017 ), which could help firms deal with new changes. Second, supply chain collaboration is valuable to build SCR. By developing information sharing, risk and revenue sharing, trust, communication, coordination, and integration, the cooperation among different supply chain partners becomes mutually high-quality (Ali et al., 2017 ; Dubey et al., 2021 ; Zhu et al., 2024 ). Third, supply chain agility facilitates the construction of SCR. Flexibility, velocity, visibility, ambidexterity, market sensitiveness, and disruption mitigation (El Baz and Ruel, 2021 ; Gu et al., 2021 ; Jain et al., 2017 ; Kochan and Nowicki, 2018 ) can increase the responsiveness of a supply chain system when facing dynamic business environment. Fourth, supply chain risk management culture, which involves risk awareness, knowledge management, and training and development of a risk management team, can create a proper culture atmosphere in favor of SCR (Belhadi et al., 2022 ).
Beyond four fostering factors, some research has also identified the interactive effects of mixed resources or capabilities on SCR, like industry 4.0 technologies, social capital, leadership, and business model (Belhadi et al., 2024 ; Gölgeci and Kuivalainen, 2020 ; Shashi et al., 2020 ; Shin and Park, 2021 ). However, we still lack knowledge about the strategic role of transformational leadership in fostering SCR. Antecedents of SCR in existing literature are shown in Table 1 .
Supply chain transformational leadership and supply chain resilience
Transformational leadership refers to leaders’ suitable behaviors that drive their followers’ reformative behaviors through continuous motivation and partnership (Bass, 1985 , 1999 ). Existing literature demonstrates that transformational leadership could affect employee attitude (Peng et al., 2021 ) and team resilience in a firm (Dimas et al., 2018 ), while the strategic role of transformational leadership across an entire supply chain system needs more explanation. According to social learning theory (Brown et al., 2005 ), we regard the focal firm with high levels of SCTL as a credible role model whom other supply chain partners respect, trust, and emulate. In this manner, other supply chain partners are likely to learn transformative behaviors by observing the focal firm.
We view the development of SCTL as a role modeling-learning process. That is, the focal firm with high levels of SCTL has an exemplary influence on other supply chain partners via observing and learning from benchmarks. Specifically, SCTL includes three elements: inspiration, intellectual stimulation, and individualized consideration (Defee et al., 2010 ). Inspiration implies that the focal firm with high levels of SCTL often articulates a compelling vision about a desirable future for the supply chain system. The focal firm, with intellectual stimulation, tends to stimulate other supply chain partners to solve issues by adopting creative and innovative methods. Individualized consideration helps the focal firm understand differentiated demands of supply chain followers, and assists them respectively. Based on social learning theory (Bommer et al., 2005 ), the focal firm’s transformative behaviors benefit its followers by the conveyance of competence. Before/during disruptive events, the focal firm clarifies a reliable vision and motivates followers to observe what it does to improve firm resilience. Targeted support makes it easier for other supply chain partners to master and emulate the focal firm’s resilient actions. In addition, coordination and trust among firms are developed in the social learning process (Mostafa, 2019 ), constructing closer supply chain relationships. Therefore, SCTL could enhance the proactive dimension of SCR.
The focal firm with high levels of SCTL would not only strengthen the proactive dimension of SCR, but also contribute to the reactive dimension of SCR. Drawing on social learning theory (Bommer et al., 2005 ), the focal firm’s transformative behaviors increase the self-efficacy of other supply chain partners. After supply chain disruptions, the focal firm demonstrates its response and encourages followers to achieve quick recovery through their differentially new insights. Besides, as firms in the supply chain are closely connected, all members’ resilient actions would transform into SCR when there are common goals and effective interactions (Gölgeci and Kuivalainen, 2020 ). In this manner, SCTL contributes to the reactive aspect of SCR. Hence, we hypothesize:
H1: SCTL has a positive influence on (a) proactive dimension and (b) reactive dimension of SCR.
Supply chain transformational leadership and ambidextrous business model
Ambidexterity is a special dynamic ability balancing exploration and exploitation simultaneously (Kristal et al., 2010 ; Lee and Rha, 2016 ). Previous literature has identified that different leadership styles, such as transformational leadership, could foster ambidexterity in firms (Jansen et al., 2008 ; Tarba et al., 2020 ). Ambidextrous business model means a firm’s boundary-spanning transaction mode developed to create and catch business value by balancing activities of redesigning novel governance, content, and structure and reorganizing elements of an existing business model. Miller ( 1996 ) identifies that novelty and efficiency are classic themes of designing business models. Specifically, a novelty-centered business model aims to create value and catch potential opportunities by redesigning a new business model, while an efficiency-centered business model devotes to increasing efficiency and decreasing operational cost by reconstructing the current business model (Feng et al., 2022 ; Wei et al., 2017 ; Zott and Amit, 2008 ). Under the context of plurality, change, and scarcity, leaders in firms have more intentions to make decisions from an ambidexterity perspective (Smith and Lewis, 2011 ). According to social learning theory (Wang and Feng, 2023 ), leaders in the focal firm with high levels of SCTL tend to express a committed attitude and take exemplary actions to maintain balancing operations. In other words, employees would be guided to conduct certain transformative behaviors, raising a flexible organizational culture with their leaders’ values.
SCTL, which is viewed as a role model-building process, includes three components: inspiration, intellectual stimulation, and individualized consideration (Defee et al., 2010 ). First, the focal firm with high levels of SCTL often articulates a compelling vision and sets high-quality standards. Inspiration by the focal firm’s leaders shows necessary confidence in their subordinates’ abilities and encourages employees to recognize the importance of individual effort in creating and capturing value through exploring and exploiting business opportunities. Additionally, the focal firm’s leaders promote collective goal-setting and collaboration among employees based on a shared vision, creating a supportive organizational context characterized by discipline, stretch, and trust (Ojha et al., 2018 ; Xi et al., 2023 ). Second, the focal firm with high levels of SCTL pays much attention to meeting emerging challenges. Intellectual stimulation by the focal firm’s leaders demonstrates transformative ideas and stimulates their employees to provide new insights under a challenging but supportive atmosphere, increasing organizational creativity and contributing to a stretch context (Elkins and Keller, 2003 ). Third, the focal firm with high levels of SCTL actively understands and helps its internal members. Individualized consideration by the focal firm’s leaders offers differentiated support via one-to-one knowledge exchange and creates a heartwarming condition that promotes more assistance among employees, fostering a culture of support and trust (Bommer et al., 2005 ). While a supportive organizational context is developed (Pan et al., 2021 ), a firm with high levels of SCTL prefers to design an ambidextrous business model. Thus, we hypothesize:
H2: SCTL has a positive influence on an ambidextrous business model.
Ambidextrous business model and supply chain resilience
The development of an ambidextrous business model could be recognized as a role model-engaging process. According to social learning theory (Wang and Feng, 2023 ), the focal firm with high levels of ambidextrous business model would serve as an example that provides a flexible business model for its followers. Then, supply chain followers are likely to trust and attempt to emulate the focal firm’s business model when sensing or experiencing frequent supply chain disruptions.
In detail, the focal firm with a high level of ambidextrous business model shows its supply chain partners how to maintain agility before/during disruptions through a proper organization arrangement. A novelty-centered business model could help other firms realize that they must create and capture value through designing new activities of governance, content, and structure to predict/respond to changing environments before/during disruptions. An efficiency-centered business model guides followers to continuously change the current supply chain into a more robust system (Wei et al., 2017 ; Zott and Amit, 2008 ). Besides, when all firms with high levels of ambidextrous business models tend to balance novelty and efficiency simultaneously, they would contribute to a more robust supply chain by preventive supply chain ambidexterity. Therefore, the ambidextrous business model enhances the proactive dimension of SCR.
The focal firm with high levels of the ambidextrous business model provides other supply chain members a valuable frame to quickly react after disruptions as well. Specifically, a novelty-centered business model stimulates other firms to adopt new ideas and norms in solving issues after disruptive events, improving their adaptability and responsiveness. An efficiency-centered business model helps followers achieve greater transaction efficiency and lower transaction costs, facilitating the adjustment of actions and strategies to rapidly respond to disruptions. In addition, firms with high levels of ambidextrous business models jointly balance novelty and efficiency, establishing a more resilient supply chain through responsive supply chain ambidexterity. SCTL contributes to the reactive dimension of SCR. Hence, we hypothesize:
H3: Ambidextrous business model has a positive influence on (a) proactive dimension and (b) reactive dimension of SCR.
In sum, the ambidextrous business model serves as a proper mediator within the role modeling-learning process. Drawing on social learning theory, the focal firm with high levels of SCTL demonstrates an ambidextrous business model through fostering a supportive organizational context. And then other supply chain partners would actively learn and emulate the focal firm’s typical business model based on their trust and common values, improving SCR by supply chain ambidexterity. An ambidextrous business model could transform SCTL into proactive and reactive dimensions of SCR. Thus, we hypothesize:
H4: Ambidextrous business model mediates the relationship between SCTL and (a) proactive dimension and (b) reactive dimension of SCR.
The moderating role of paradox cognition
Paradox cognition refers to an epistemic framework and process recognizing and juxtaposing contradictory demands, which could make latent tensions within organizations more explicit (Smith and Tushman, 2005 ). The focal firm with paradoxical thinking and cognition could influence learning strategies (Brusoni and Rosenkranz, 2014 ; Sheng et al., 2023 ). That is, paradox cognition may affect the focal firm’s attitude and identification towards tensions (explore or exploit) arising from its contrasting strategic agendas (Smith and Lewis, 2011 ). Based on social learning theory (Bandura, 1977 ), when the focal firm possesses high levels of paradox cognition, it is more likely to recognize the importance of ambidexterity. In this manner, leaders’ transformative behaviors in the focal firm with high levels of SCTL would be more easily accepted and emulated by employees to balance both explorative and exploitive learning activities (Han et al., 2022 ), which may help build an ambidextrous business model. By contrast, when the focal firm has low levels of paradox cognition, it tends to choose either novelty or efficiency in designing a business model. The SCTL-ambidextrous business model relationship becomes less important because contradictions in the focal firm are latent. Hence, we hypothesize:
H5: Paradox cognition enhances the impact of SCTL on an ambidextrous business model.
Combining the hypotheses above, we build a conceptual model to check the influence of SCTL on SCR (including proactive and reactive SCR), the mediating role of the ambidextrous business model within the SCTL–SCR relationship, and the moderating effect of paradox cognition. The conceptual model is illustrated in Fig. 1 .
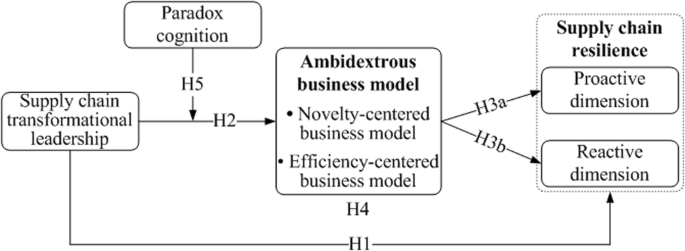
This figure represents the hypothetical relationships among constructs.
Research design
Procedures and data collection.
We gathered data from Chinese manufacturers. Affected by the COVID-19 pandemic, manufacturing firms in China suffered from many supply chain disruptions, prompting leaders to realize the necessity of keeping a resilient supply chain (Lin et al., 2021 ; Shen and Sun, 2023 ). It is a challenging objective for manufacturing firms in China as they account for a large share of total exports in the global supply chains. Thus, China provided an appropriate context to explore the antecedents of SCR.
Due to the regional imbalanced characteristic of the Chinese economic force and transportation network (Feng et al., 2019 ; Hosseini et al., 2019 ), we selected sampling firms in five typical provinces: Guangdong, Jiangsu, Shandong, Henan, and Inner Mongolia. Guangdong, Jiangsu, and Shandong, in the eastern coastal areas of China, had relatively high levels of economic force and transportation networks. Henan, in the middle area of China, had average levels of economic force and transportation network. By contrast, Inner Mongolia, in the north and west of China, had relatively low levels of economic force and transportation network.
We adopted three steps to design a questionnaire. First, 12 firm executives, including the chief executive officer, general manager, or vice president, were interviewed to confirm the content validity of our study issue. All these individuals were required to be knowledgeable about their firms’ internal operations as well as external partnerships. Second, an initial questionnaire was developed through literature and expert review, translation, and back-translation. Third, a pre-test with another 20 executives was conducted to provide useful suggestions for modification, forming the formal questionnaire.
We randomly chose 200 firms in each province above and sought cooperation via a cover letter introducing the research intention. All participants were ensured confidentiality. Invitations were sent through emails or telephones, and 435 firms agreed to join our survey total. To mitigate common method bias (CMB), we split each questionnaire into two parts (including parts A and B) and invited different respondents in each firm to complete one part respectively. Part A featured demographic characteristics, competitive intensity, SCTL, novelty-centered business model, and SCR, whereas part B included paradox cognition and efficiency-centered business model.
We distributed and received back the questionnaires through emails from May 2020 to December 2020. 317 valid questionnaires were gathered, with an effective response rate of 72.9%. The final sample included 72 firms in Guangdong, 62 firms in Jiangsu, 67 firms in Shandong, 56 firms in Henan, and 60 firms in Inner Mongolia. The average working experience of 634 respondents was 7.19 years. 64.8% of our respondents held the posts of chief executive officer, general manager, or vice president, and 35.2% were operations directors. The detailed features of sampled firms are presented in Table 2 .
We utilized two steps to verify non-response bias (Armstrong and Overton, 1977 ). First, firm size and ownership were compared for the nonresponding and responding firms. Second, differences in firm size, firm age, industry, and ownership between the early and late responses were also examined. These results of the independent t -test suggested that non-response bias in this study was not a serious issue.
We selected the seven-point Likert scale adopted or adapted from previous studies to measure all constructs in the questionnaire (1 = strongly disagree, 7 = strongly agree).
Supply chain transformational leadership
A refined seven-item scale from Defee et al. ( 2010 ) was applied to measure SCTL. SCTL was operationalized as respondents’ perceptions of their firms’ influences, which are often the outcome of behavioral factors, including inspiration, intellectual stimulation, and individualized consideration.
Paradox cognition
A seven-item scale from Smith and Lewis ( 2011 ) was used to measure paradox cognition. Respondents were requested to evaluate the degree of their own firms’ dual awareness when making strategic decisions in the last three years.
Ambidextrous business model
A ten-item scale and a nine-item scale were adjusted by Zott and Amit ( 2007 ) to measure the novelty-centered business model and efficiency-centered business model in turn. Additionally, the average value of these two variables was calculated to measure the ambidextrous business model. This approach not only kept convenience to reserve and made logical interpretations for the useful information from both parts but reflected the nature of ambidexterity–seemingly contradictory yet coexisting tensions (Lubatkin et al., 2006 ; Zhang et al., 2015 ).
Following Cheng and Lu ( 2017 ), SCR was divided into two dimensions: proactive and reactive SCR Two altered four-item scales were adopted for proactive and reactive SCR separately (Ambulkar et al., 2015 ; Brandon-Jones et al., 2014 ; Wieland and Wallenburg, 2013 ).
Control variables
To mitigate the roles of other factors on analytical results as much as possible, we controlled five demographic characteristics, including firm size, firm age, industry, ownership, and competitive intensity (Ambulkar et al., 2015 ; Gölgeci and Ponomarov, 2015 ). Firm age and firm size were measured by the natural logarithm of the number of years since foundation and the natural logarithm of the number of employees, respectively (Li et al., 2008 ). One dummy variable was to control industry (1 = high-tech firm, 0 = otherwise), and two dummy variables (including state-owned and collective firms and private firms) were to control ownership. A four-item scale was adjusted by Jaworski and Kohli ( 1993 ) to measure competitive intensity.
Reliability and validity
First, we did a reliability test and explorative factor analysis (EFA). All constructs revealed high reliability with a Cronbach’s alpha value of more than 0.7 (Flynn et al., 1990 ). Seven principal components were extracted, which was consistent with constructs in the scales (Table 3 ). Second, we made a confirmatory factor analysis (CFA) by AMOS 24.0 to ensure validity. The results indicated that the measurement model had good fit indices: χ ²/d f = 2.034; RMSEA = 0.057; CFI = 0.928; NNFI = 0.923; SRMR = 0.038. All constructs’ composite reliability (CR) was more than 0.7, with item loadings varying from 0.760 to 0.939, and all average variance extracted (AVE) values were more than 0.5 (Table 3 ). Thus, the results indicated sufficient convergent validity. Besides, the comparison between shared variances of constructs and the square root of AVE demonstrated that all correlations were less than the corresponding square roots of AVEs (Table 4 ), identifying acceptable discriminant validity. Tables 3 and 4 reported the measure items, reliability, and validity assessment.
Common method bias
We utilized three means to test CMB. First, Harman’s single-factor test was conducted, revealing that there were seven principal components (Table 3 ), and no single factor accounted for most variances in these measures. Second, the fit indices of CFA between the actual seven-factor model and the one-factor model were compared, indicating that the one-factor model got significantly worse fit indices. Third, another common method factor was supplemented to the seven-factor CFA model above and it discovered that the fit indices did not change significantly. As a result, there was no serious CMB.
We adopted hierarchical regression analysis and the bootstrapping method by SPSS 23.0 to examine the research hypotheses. First, the effect of SCTL on SCR was examined. Then, the influence of SCTL on the ambidextrous business model, the effect of the ambidextrous business model on SCR, and the mediating impact of the ambidextrous business model within the SCTL–SCR link were tested. Finally, the moderating effect of paradox cognition in the SCTL–ambidextrous business model relationship was examined. Table 5 reports the results of the hierarchical regression model.
To minimize possible multicollinearity, we generated an interaction with mean-centering of both the independent variable and the moderating variable (Aiken and West, 1991 ). The maximal value of the variance inflation factor (VIF) is 1.739, which is much less than the recommended cut-off of 10. Thus, the multicollinearity is not serious.
H1a and H1b predict the positive impact of SCTL on both dimensions of SCR. Models 5 and 9 in Table 5 show that SCTL has a significantly positive effect on the proactive dimension ( β = 0.122, p < 0.05) and reactive dimension ( β = 0.166, p < 0.01). Therefore, H1a and H1b are supported.
H2 predicts the positive influence of SCTL on the ambidextrous business model. Model 2 in Table 5 indicates that SCTL has a significantly positive impact on ambidextrous business models ( β = 0.140, p < 0.05). Hence, H2 is supported.
H3a and H3b predict the positive role of the ambidextrous business model on both dimensions of SCR. Models 6 and 10 in Table 5 suggest that the ambidextrous business model has a positive effect on the proactive dimension ( β = 0.241, p < 0.001) and reactive dimension ( β = 0.256, p < 0.001). Therefore, H3a and H3b are supported.
H4a and H4b hypothesize that the ambidextrous business model mediates the relationships between SCTL and two dimensions of SCR. According to Baron and Kenny ( 1986 ), Models 2, 5, and 7 in Table 5 jointly demonstrate that the ambidextrous business model ( β = 0.228, p < 0.001) fully mediates the relationship between SCTL ( β = 0.090, p > 0.1) and proactive dimension, which supports H4a. Similarly, Models 2, 9, and 11 in Table 5 collectively exhibit that the ambidextrous business model ( β = 0.237, p < 0.001) partially mediates the relationship between SCTL ( β = 0.133, p < 0.05) and reactive dimension, which supports H4b.
To ensure the robustness of the results, we further used the PROCESS macro to conduct a bootstrapped mediation analysis. As depicted in Table 6 , the results keep consistency with the corresponding results in Table 5 , ensuring the effectiveness of analytical findings before.
H5 hypothesizes that paradox cognition strengthens the impact of SCTL on the ambidextrous business model. Model 3 in Table 5 presents that the interaction of SCTL and paradox cognition is significantly positive ( β = 0.094, p < 0.1), which supports H5. Moreover, we applied a simple slope analysis to verify the moderating effect of paradox cognition so that a clearer explanation could be given. As illustrated in Fig. 2 , when levels of paradox cognition are higher, the role of SCTL in the ambidextrous business model becomes stronger. Hence, the result further supports a strengthened effect of paradox cognition in the SCTL–ambidextrous business model relationship.
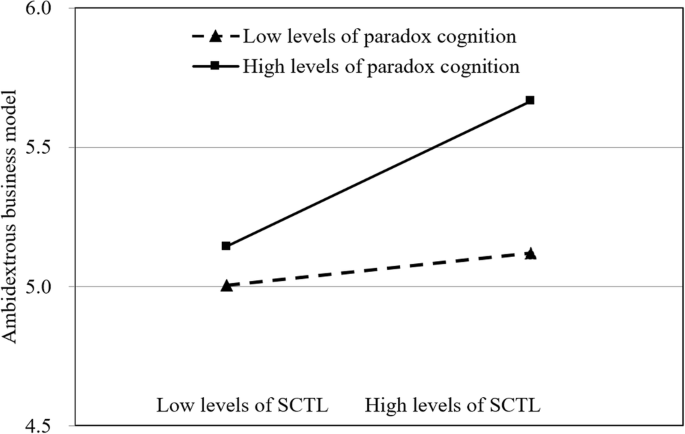
This figure reflects the moderating effect of paradox cognition on the relationship between supply chain transformational leadership and ambidextrous business model.
Discussions and implications
Discussions.
This study intends to verify the impact of SCTL on both dimensions of SCR (including proactive and reactive SCR) through the ambidextrous business model and the moderating role of paradox cognition. Our results exhibit that SCTL has a positive influence on proactive and reactive SCR. This finding is similar to studies that explore the effect of leader–member exchange on network resilience performance in the supply chain context (Shin and Park, 2021 ) or the effect of transformational supply chain leadership on operational performance (Defee et al., 2010 ). However, these studies only emphasize the necessity of inter-organizational relationships and capabilities within the influential process of supply chain leadership on supply chain performance. Our results show that SCTL contributes to proactive and reactive SCR in a social learning process where both firm resilience and supply chain collaboration are indispensable parts.
Our results demonstrate that an ambidextrous business model mediates the impact of SCTL on SCR. This finding is inconsistent with existing studies about the antecedents or consequences of business models (Schoemaker et al., 2018 ; Shashi et al., 2020 ). One possible explanation is that the ambidextrous business model aims at designing new business models to capture and create value while also reconfiguring new combinations to improve transaction efficiency. Our results also indicate that the ambidextrous business model fully mediates the relationship between SCTL and proactive SCR while partially mediates the relationship between SCTL and reactive SCR. That is, the ambidextrous business model occupies a more important position in the SCTL-proactive dimension link. A possible reason could be that compared with intellectual stimulation, the influence of inspiration and individualized consideration is more dispersive within a longer time, improving the necessity of an ambidextrous business model. These results provide new insights to realize how SCTL enhances SCR.
In addition, we identify that paradox cognition strengthens the effect of SCTL on an ambidextrous business model. When the focal firm has high levels of paradox cognition, it tends to recognize the importance of ambidexterity. In this manner, the focal firm’s transformative behaviors would be more easily accepted and emulated by employees to balance both explorative and exploitive learning activities (Han et al., 2022 ), building an ambidextrous business model. This outcome verifies our research hypothesis, indicating the importance of paradox cognition in the SCTL–ambidextrous business model link.
Theoretical contributions
This study contributes to managerial research in three aspects. First, we enrich the antecedents of SCR by confirming the role of SCTL. Existing studies emphasize the impacts of specific resources or capabilities on SCR, such as agility, redundancy, and collaboration (Al Naimi et al., 2021 ; Tukamuhabwa et al., 2015 ), while the strategic effect of SCTL is rarely discussed. Previous literature has identified that transformational leadership could improve employee attitude (Peng et al., 2021 ) and team resilience (Dimas et al., 2018 ) at the firm level. Our research extends the concept of transformational leadership to the whole supply chain system and proposes that the focal firm with high levels of SCTL can improve proactive and reactive SCR. Hence, we contribute to the field of SCTL and SCR.
Second, we reveal the ‘black box’ of how SCTL impacts SCR by examining the mediating role of the ambidextrous business model. Existing studies reveal the influence of transformational leadership on organizational ambidexterity (Eng et al., 2023 ) and the impact of organizational ambidexterity on SCR (Aslam et al., 2022 ), while we still lack understanding of how SCTL affects SCR. Previous literature has demonstrated that redesigning a supply chain with high levels of concentration plays a significant role in protecting firm performance when suffering from disruptions (Liu et al., 2023 ). Hence, we contribute to the SCTL and SCR literature by showing a partial mediating effect of the ambidextrous business model in the SCTL–proactive SCR relationship and a fully mediating effect of ambidextrous business model in the SCTL–reactive SCR relationship.
Third, we clarify the boundary condition for the SCTL–ambidextrous business model relationship by examining the moderating effect of paradox cognition. Existing studies show that the efficiency of the learning process would be influenced by external stakeholders (Song et al., 2020 ; Wang and Feng, 2023 ), while the interactive role of internal factors is largely ignored. Previous literature has argued that organizational learning may be influenced by paradoxical thinking and cognition (Brusoni and Rosenkranz, 2014 ). Our findings suggest that paradox cognition would affect the focal firm’s attitude and identification towards tensions (explore or exploit) arising from its contrasting strategic agendas. Under high levels of paradox cognition, the focal firm is more likely to recognize and embrace tensions, making well-balanced decisions. Thus, the efficiency of social learning from SCTL to ambidextrous business model improves, which further emphasizes the necessity of developing paradox cognition within the learning process.
Managerial implications
This study offers three suggestions for managerial practice. First, managers should undertake leading roles and encourage member firms within the supply chain to improve SCR. In a dynamic and uncertain context, the focal firm with high levels of SCTL is effective to motivate its supply chain partners’ transformative behaviors. Managers should develop a reliable role model whom their followers trust and attempt to emulate. They should also develop two types of SCR, including proactive and reactive SCR. Additionally, they should articulate a compelling vision for all supply chain members, providing individualized training to meet the differentiated needs of firms and stimulating supply chain partners to create new insights with a supportive and challenging atmosphere.
Second, managers should establish an ambidextrous business model in firms. The focal firm with high levels of SCTL often demonstrates an ambidextrous business model by fostering a supportive organizational context. Managers should design an ambidextrous business model balancing both novelty and efficiency. Furthermore, they are suggested to motivate other supply chain followers to learn and emulate the focal firm’s transformative behaviors through a shared system vision, promoting communication and coordination among supply chain members.
Third, managers should foster a paradox cognition framework within their firms. Under high levels of paradox cognition, the focal firm is more likely to recognize the importance of ambidexterity and solve tensions from an ambidexterity perspective. Transformative behaviors of the focal firm would be more easily accepted and emulated by its employees. Managers should provide a proper organizational context for employees to improve their paradoxical thinking and cognition to quickly respond to disruptions.
Conclusion and limitations
Drawing on social learning theory, this study clarifies the impact of SCTL on SCR. Our findings reveal that SCTL has a positive influence on both proactive and reactive SCR. In addition, the ambidextrous business model fully mediates the relationship between SCTL and proactive SCR while also partially mediating the relationship between SCTL and reactive SCR. Paradox cognition strengthens the effect of SCTL on the ambidextrous business model.
This study has a few limitations, of course. First, we must demonstrate the effect of SCTL on SCR. Future research could try investigating the roles of other factors, such as transactional leadership to enrich antecedents of SCR. Second, this study only explores the mediating role of the ambidextrous business model between SCTL and SCR. In the future, other possible realization paths from the configurational perspective should be verified (Feng and Sheng, 2023 ). Third, we must identify the moderating impact of paradox cognition within the SCTL–ambidextrous business model relationship. Scholars are suggested to discover more possible boundary conditions like dynamic environment, and build a moderated mediation model to further explore the roles of potential moderators.
Data availability
All data generated and analyzed during the current study are included in this article and a supplementary Excel spreadsheet called ‘Dataset’ which contains all items’ values from questionnaires and other control variables’ values.
Aiken LS, West SG (1991) Multiple regression: testing and interpreting interactions. Sage, Newbury Park, CA
Google Scholar
Al Naimi M, Faisal MN, Sobh R, Uddin SMF (2021) Antecedents and consequences of supply chain resilience and reconfiguration: an empirical study in an emerging economy. J Enterp Inf Manag 34(6):1722–1745
Article Google Scholar
Ali A, Mahfouz A, Arisha A (2017) Analysing supply chain resilience: integrating the constructs in a concept mapping framework via a systematic literature review. Supply Chain Manag: Int J 22(1):16–39
Ambulkar S, Blackhurst J, Grawe S (2015) Firm’s resilience to supply chain disruptions: scale development and empirical examination. J Oper Manag 33-34(1):111–122
Armstrong JS, Overton TS (1977) Estimating nonresponse bias in mail surveys. J Mark Res 14(3):396–402
Aslam H, Syed TA, Blome C, Ramish A, Ayaz K (2022) The multifaceted role of social capital for achieving organizational ambidexterity and supply chain resilience. IEEE Trans Eng Manag https://doi.org/10.1109/TEM.2022.3174069
Bandura A (1977) Social learning theory. General Learning Press, New York
Baron RM, Kenny DA (1986) The moderator-mediator variable distinction in social psychological research: conceptual, strategic, and statistical considerations. J Personal Soc Psychol 51(6):1173–1182
Article CAS Google Scholar
Bass BM (1985) Leadership and performance beyond expectations. Free Press, New York
Bass BM (1999) Two decades of research and development in transformational leadership. Eur J Work Organ Psychol 8(1):9–32
Belhadi A, Kamble S, Fosso Wamba S, Queiroz MM (2022) Building supply-chain resilience: an artificial intelligence-based technique and decision-making framework. Int J Prod Res 60(14):4487–4507
Belhadi A, Mani V, Kamble SS, Khan SAR, Verma S (2024) Artificial intelligence-driven innovation for enhancing supply chain resilience and performance under the effect of supply chain dynamism: an empirical investigation. Ann Oper Res 333:627–652
Bommer WH, Rich GA, Rubin RS (2005) Changing attitudes about change: longitudinal effects of transformational leader behavior on employee cynicism about organizational change. J Organ Behav 26(7):733–753
Brandon-Jones E, Squire B, Autry CW, Petersen KJ (2014) A contingent resource-based perspective of supply chain resilience and robustness. J Supply Chain Manag 50(3):55–73
Brown ME, Treviño LK, Harrison DA (2005) Ethical leadership: a social learning perspective for construct development and testing. Organ Behav Hum Decis Process 97(2):117–134
Brusoni S, Rosenkranz NA (2014) Reading between the lines: learning as a process between organizational context and individuals’ proclivities. Eur Manag J. 32(1):147–154
Cheng JH, Lu KL (2017) Enhancing effects of supply chain resilience: Insights from trajectory and resource-based perspectives. Supply Chain Manag: Int J 22(4):329–340
Chowdhury MMH, Quaddus M (2017) Supply chain resilience: conceptualization and scale development using dynamic capability theory. Int J Prod Econ 188:185–204
Defee CC, Stank TPT, Esper T (2010) Performance implications of transformational supply chain leadership and followership. Int J Phys Distrib Logist Manag 40(10):763–791
Dimas ID, Rebelo T, Lourenço PR, Pessoa CIP (2018) Bouncing back from setbacks: on the mediating role of team resilience in the relationship between transformational leadership and team effectiveness. J Psychol 152(6):358–372
Article PubMed Google Scholar
Dubey R, Gunasekaran A, Childe SJ, Fosso Wamba S, Roubaud D, Foropon C (2021) Empirical investigation of data analytics capability and organizational flexibility as complements to supply chain resilience. Int J Prod Res 59(1):110–128
El Baz J, Ruel S (2021) Can supply chain risk management practices mitigate the disruption impacts on supply chains’ resilience and robustness? Evidence from an empirical survey in a COVID-19 outbreak era. Int J Prod Econ 233:107972
Elkins T, Keller RT (2003) Leadership in research and development organizations: a literature review and conceptual framework. Leadersh Q 14(4-5):587–606
Eng TY, Mohsen K, Wu LC (2023) Wireless information technology competency and transformational leadership in supply chain management: implications for innovative capability. Inf Technol People 36(3):969–995
Feng T, Sheng H (2023) Identifying the equifinal configurations of prompting green supply chain integration and subsequent performance outcome. Bus Strateg Environ 32(8):5234–5251
Feng T, Wang D, Lawton A, Luo BN (2019) Customer orientation and firm performance: the joint moderating effects of ethical leadership and competitive intensity. J Bus Res 100:111–121
Feng T, Yang S, Sheng H (2022) Supply chain integration and novelty-centered business model design: an organizational learning perspective. Eur Manag J https://doi.org/10.1016/j.emj.2022.12.002
Flynn BB, Sakakibara S, Schroeder RG, Bates KA, Flynn EJ (1990) Empirical research methods in operations management. J Oper Manag 9(2):250–284
Gölgeci I, Ponomarov SY (2015) How does firm innovativeness enable supply chain resilience? The moderating role of supply uncertainty and interdependence. Technol Anal Strat Manag 27(3):267–282
Gölgeci I, Kuivalainen O (2020) Does social capital matter for supply chain resilience? The role of absorptive capacity and marketing-supply chain management alignment. Ind Mark Manag 84:63–74
Gu M, Yang L, Huo B (2021) The impact of information technology usage on supply chain resilience and performance: an ambidextrous view. Int J Prod Econ 232:107956
Han G, Bai Y, Peng G (2022) Creating team ambidexterity: the effects of leader dialectical thinking and collective team identification. Eur Manag J 40(2):175–181
Han Y, Chong WK, Li D (2020) A systematic literature review of the capabilities and performance metrics of supply chain resilience. Int J Prod Res 58(15):4541–4566
Holling CS (1973) Resilience and stability of ecological systems. Annu Rev Ecol Syst 4(1):1–23
Hosseini S, Ivanov D, Dolgui A (2019) Review of quantitative methods for supply chain resilience analysis. Transp Res Part E 125:285–307
Jain V, Kumar S, Soni U, Chandra C (2017) Supply chain resilience: model development and empirical analysis. Int J Prod Res 55(22):6779–6800
Jansen JJ, George G, Van den Bosch FA, Volberda HW (2008) Senior team attributes and organizational ambidexterity: the moderating role of transformational leadership. J Manag Stud 45(5):982–1007
Jaworski BJ, Kohli AK (1993) Market orientation: antecedents and consequences. J Mark 57(3):53–70
Jiang Y, Feng T, Huang Y (2024) Antecedent configurations toward supply chain resilience: the joint impact of supply chain integration and big data analytics capability. J Oper Manag 70(2):257–284
Jüttner U, Maklan S (2011) Supply chain resilience in the global financial crisis: an empirical study. Supply Chain Manag: Int J 16(4):246–259
Khunwishit S, Choosuk C, Webb G (2018) Flood resilience building in Thailand: assessing progress and the effect of leadership. Int J Disaster Risk Sci 9(1):44–54
Kochan CG, Nowicki DR (2018) Supply chain resilience: a systematic literature review and typological framework. Int J Phys Distrib Logist Manag 48(8):842–865
Kristal MM, Huang X, Roth AV (2010) The effect of an ambidextrous supply chain strategy on combinative competitive capabilities and business performance. J Oper Manag 28(5):415–429
Lechler S, Canzaniello A, Rossmann B, von der Gracht HA, Hartmann E (2019) Real-time data processing in supply chain management: revealing the uncertainty dilemma. Int J Phys Distrib Logist Manag 49(10):1003–1019
Lee SM, Rha JS (2016) Ambidextrous supply chain as a dynamic capability: building a resilient supply chain. Manag Decis 54(1):2–23
Li JJ, Poppo L, Zhou KZ (2008) Do managerial ties in China always produce value? Competition, uncertainty, and domestic vs. foreign firms. Strat Manag J 29(4):383–400
Lin Y, Fan D, Shi X, Fu M (2021) The effects of supply chain diversification during the COVID-19 crisis: evidence from Chinese manufacturers. Transp Res Part E: Logist Transp Rev 155:102493
Liu F, Liu C, Wang X, Park K, Fang M (2023) Keep concentrated and carry on: redesigning supply chain concentration in the face of COVID-19. Int J Logist Res Appl https://doi.org/10.1080/13675567.2023.2175803
Lubatkin MH, Simsek Z, Ling Y, Veiga JF (2006) Ambidexterity and performance in small-to medium-sized firms: the pivotal role of top management team behavioral integration. J Manag 32(5):646–672
Miller D (1996) Configurations revisited. Strat Manag J 17(7):505–512
Mostafa AMS (2019) Transformational leadership and restaurant employees customer-oriented behaviours: the mediating role of organizational social capital and work engagement. Int J Contemp Hosp Manag 31(3):1166–1182
Namdar J, Li X, Sawhney R, Pradhan N (2018) Supply chain resilience for single and multiple sourcing in the presence of disruption risks. Int J Prod Res 56(6):2339–2360
Nikolopoulos K, Punia S, Schäfers A, Tsinopoulos C, Vasilakis C (2021) Forecasting and planning during a pandemic: COVID-19 growth rates, supply chain disruptions, and governmental decisions. Eur J Oper Res 290(1):99–115
Article MathSciNet PubMed Google Scholar
Novak DC, Wu Z, Dooley KJ (2021) Whose resilience matters? Addressing issues of scale in supply chain resilience. J Bus Logist 42(3):323–335
Ojha D, Acharya C, Cooper D (2018) Transformational leadership and supply chain ambidexterity: mediating role of supply chain organizational learning and moderating role of uncertainty. Int J Prod Econ 197:215–231
Pan Y, Verbeke A, Yuan W (2021) CEO transformational leadership and corporate entrepreneurship in China. Manag Organ Rev 17(1):45–76
Peng J, Li M, Wang Z, Lin Y (2021) Transformational leadership and employees’ reactions to organizational change: evidence from a meta-analysis. J Appl Behav Sci 57(3):369–397
Pournader M, Rotaru K, Kach AP, Razavi Hajiagha SH (2016) An analytical model for system-wide and tier-specific assessment of resilience to supply chain risks. Supply Chain Manag: Int J 21(5):589–609
Razak GM, Hendry LC, Stevenson M (2023) Supply chain traceability: a review of the benefits and its relationship with supply chain resilience. Prod Plan Control 34(11):1114–1134
Schoemaker PJ, Heaton S, Teece D (2018) Innovation, dynamic capabilities, and leadership. Calif Manag Rev 61(1):15–42
Scholten K, Schilder S (2015) The role of collaboration in supply chain resilience. Supply Chain Manag: Int J 20(4):471–484
Scholten K, Stevenson M, van Donk DP (2020) Dealing with the unpredictable: supply chain resilience. Int J Oper Prod Manag 40(1):1–10
Shashi, Centobelli P, Cerchione R, Ertz M (2020) Managing supply chain resilience to pursue business and environmental strategies. Bus Strategy Environ 29(3):1215–1246
Shen ZM, Sun Y (2023) Strengthening supply chain resilience during COVID-19: a case study of JD.com. J Oper Manag 69(3):359–383
Sheng H, Feng T, Liu L (2023) The influence of digital transformation on low-carbon operations management practices and performance: does CEO ambivalence matter? Int J Prod Res 61(18):6215–6229
Shin N, Park S (2021) Supply chain leadership driven strategic resilience capabilities management: a leader-member exchange perspective. J Bus Res 122:1–13
Smith WK, Tushman ML (2005) Managing strategic contradictions: a top management model for managing innovation streams. Organ Sci 16(5):522–536
Smith WK, Lewis MW (2011) Toward a theory of paradox: a dynamic equilibrium model of organizing. Acad Manag Rev 36(2):381–403
Song M, Yang MX, Zeng KJ, Feng W (2020) Green knowledge sharing, stakeholder pressure, absorptive capacity, and green innovation: evidence from Chinese manufacturing firms. Bus Strategy Environ 29(3):1517–1531
Spieske A, Birkel H (2021) Improving supply chain resilience through industry 4.0: a systematic literature review under the impressions of the COVID-19 pandemic. Comput Ind Eng 158:107452
Article PubMed PubMed Central Google Scholar
Tarba SY, Jansen JJ, Mom TJ, Raisch S, Lawton TC (2020) A microfoundational perspective of organizational ambidexterity: critical review and research directions. Long Range Plan 53(6):102048
Tukamuhabwa B, Stevenson M, Busby J (2017) Supply chain resilience in a developing country context: a case study on the interconnectedness of threats, strategies and outcomes. Supply Chain Manag: Int J 22(6):486–505
Tukamuhabwa BR, Stevenson M, Busby J, Zorzini M (2015) Supply chain resilience: definition, review and theoretical foundations for further study. Int J Prod Res 53(18):5592–5623
Vanpoucke E, Ellis SC (2020) Building supply-side resilience-a behavioural view. Int J Oper Prod Manag 40(1):11–33
Wang J, Feng T (2023) Supply chain ethical leadership and green supply chain integration: a moderated mediation analysis. Int J Logist Res Appl 26(9):1145–1171
Article MathSciNet Google Scholar
Wei Z, Song X, Wang D (2017) Manufacturing flexibility, business model design, and firm performance. Int J Prod Econ 193:87–97
Wieland A, Wallenburg CM (2013) The influence of relational competencies on supply chain resilience: a relational view. Int J Phys Distrib Logist Manag 43(4):300–320
Xi M, Fang W, Feng T (2023) Green intellectual capital and green supply chain integration: the mediating role of supply chain transformational leadership. J Intellect Cap 24(4):877–899
Xi M, Liu Y, Fang W, Feng T (2024) Intelligent manufacturing for strengthening operational resilience during the COVID-19 pandemic: a dynamic capability theory perspective. Int J Prod Econ 267:109078
Zhang Y, Waldman DA, Han YL, Li XB (2015) Paradoxical leader behaviors in people management: antecedents and consequences. Acad Manag J 58(2):538–566
Zhu J, Feng T, Lu Y, Jiang W (2024) Using blockchain or not? A focal firm’s blockchain strategy in the context of carbon emission reduction technology innovation. Bus Strategy Environ 33(4):3505–3531
Zott C, Amit R (2007) Business model design and the performance of entrepreneurial firms. Organ Sci 18(2):181–199
Zott C, Amit R (2008) The fit between product market strategy and business model: implications for firm performance. Strateg Manag J 29(1):1–26
Download references
Acknowledgements
This work was partially supported by the National Natural Science Foundation of China (72172040), the Fundamental Research Funds for the Central Universities (HIT.HSS.ESD202333), and the Taishan Scholar Project of Shandong Province (tsqn201909154).
Author information
These authors contributed equally: Taiwen Feng, Zhihui Si.
Authors and Affiliations
School of Economics and Management, Harbin Institute of Technology (Weihai), Weihai, China
Taiwen Feng & Zhihui Si
School of Economics and Management, Dalian University of Technology, Dalian, China
Wenbo Jiang
College of New Energy, Harbin Institute of Technology (Weihai), Weihai, China
You can also search for this author in PubMed Google Scholar
Contributions
Taiwen Feng: Conceptualization, investigation, data curation, funding acquisition, supervision, writing-review and editing. Zhihui Si: Methodology, data curation, formal analysis, writing-original draft, and editing. Wenbo Jiang: Investigation, data curation, writing-review, and editing. Jianyu Tan: Data curation, writing-review, and editing.
Corresponding author
Correspondence to Wenbo Jiang .
Ethics declarations
Competing interests.
The authors declare no competing interests.
Ethical approval
The survey process and procedures used in this study adhere to the tenets of the Declaration of Helsinki. Ethics approval was obtained from the Professor Committee at the School of Economics and Management of Harbin Institute of Technology (Weihai), China. The ethical approval protocol number 2020-01.
Informed consent
The data collection process was conducted with strict adherence to ethical considerations. Informed consent was given to all respondents, and respondents were assured that data would be treated confidentially and used only for research purposes. They were also informed that all private information, including their names and companies’ names, would be anonymized in the study results.
Additional information
Publisher’s note Springer Nature remains neutral with regard to jurisdictional claims in published maps and institutional affiliations.
Supplementary information
Rights and permissions.
Open Access This article is licensed under a Creative Commons Attribution 4.0 International License, which permits use, sharing, adaptation, distribution and reproduction in any medium or format, as long as you give appropriate credit to the original author(s) and the source, provide a link to the Creative Commons licence, and indicate if changes were made. The images or other third party material in this article are included in the article’s Creative Commons licence, unless indicated otherwise in a credit line to the material. If material is not included in the article’s Creative Commons licence and your intended use is not permitted by statutory regulation or exceeds the permitted use, you will need to obtain permission directly from the copyright holder. To view a copy of this licence, visit http://creativecommons.org/licenses/by/4.0/ .
Reprints and permissions
About this article
Cite this article.
Feng, T., Si, Z., Jiang, W. et al. Supply chain transformational leadership and resilience: the mediating role of ambidextrous business model. Humanit Soc Sci Commun 11 , 628 (2024). https://doi.org/10.1057/s41599-024-03099-x
Download citation
Received : 21 November 2023
Accepted : 23 April 2024
Published : 15 May 2024
DOI : https://doi.org/10.1057/s41599-024-03099-x
Share this article
Anyone you share the following link with will be able to read this content:
Sorry, a shareable link is not currently available for this article.
Provided by the Springer Nature SharedIt content-sharing initiative
Quick links
- Explore articles by subject
- Guide to authors
- Editorial policies


A systematic analysis of the contribution of genetics to multimorbidity and comparisons with primary care data
- Find this author on Google Scholar
- Find this author on PubMed
- Search for this author on this site
- ORCID record for Louise M Allan
- ORCID record for Frank Dudbridge
- For correspondence: [email protected] [email protected]
- ORCID record for Luke C Pilling
- ORCID record for João Delgado
- Info/History
- Supplementary material
- Preview PDF
Background Multimorbidity, the presence of two or more conditions in one person, is increasingly prevalent. Yet shared biological mechanisms of specific pairs of conditions often remain poorly understood. We address this gap by integrating large-scale primary care and genetic data to elucidate potential causes of multimorbidity.
Methods We defined chronic, common, and heritable conditions in individuals aged ≥65 years, using two large representative healthcare databases [CPRD (UK) N=2,425,014 and SIDIAP (Spain) N=1,053,640], and estimated heritability using the same definitions in UK Biobank (N=451,197). We used logistic regression models to estimate the co-occurrence of pairs of conditions in the primary care data.
Linkage disequilibrium score regression was used to estimate genetic similarity between pairs of conditions. Meta-analyses were conducted across healthcare databases, and up to three sources of genetic data, for each condition pair. We classified pairs of conditions as across or within-domain based on the international classification of disease.
Findings We identified N=72 chronic conditions, with 43·6% of 2546 pairs showing higher co-occurrence than expected and evidence of shared genetics. Notably, across-domain pairs like iron deficiency anaemia and peripheral arterial disease exhibited substantial shared genetics (genetic correlation R g =0·45[95% Confidence Intervals 0·27:0·64]). N=33 pairs displayed negative genetic correlations, such as skin cancer and rheumatoid arthritis ( R g =-0·14[-0·21:-0·06]), indicating potential protective mechanisms. Discordance between genetic and primary care data was also observed, e.g., abdominal aortic aneurysm and bladder cancer co-occurred but were not genetically correlated (Odds-Ratio=2·23[2·09:2·37], R g =0·04[-0·20:0·28]) and schizophrenia and fibromyalgia were less likely to co-occur but were positively genetically correlated (OR=0·84[0·75:0·94], R g =0·20[0·11:0·29]).
Interpretation Most pairs of chronic conditions show evidence of shared genetics and co-occurrence in primary care, suggesting shared mechanisms. The identified shared mechanisms, negative correlations and discordance between genetic and observational data provide a foundation for future research on prevention and treatment of multimorbidity.
Funding UK Medical Research Council [MR/W014548/1].
Competing Interest Statement
ARL is now an employee of AstraZeneca and has interests in the company. The work undertaken here was prior to his appointment. SK's group has received funding support from Amgen BioPharma outside of this work. JB is a part time employee of Novo Nordisk Research Centre Oxford, limited, unrelated to this work. TF has consulted for several pharmaceutical companies. All other authors have no disclosures to declare.
Funding Statement
This work was supported by the UK Medical Research Council [grant number MR/W014548/1]. This study was supported by the National Institute for Health and Care Research (NIHR) Exeter Biomedical Research Centre (BRC), the NIHR Leicester BRC, the NIHR Oxford BRC, the NIHR Peninsula Applied Research Collaboration, and the NIHR HealthTech Research Centre. KB is partly funded by the NIHR Applied Research Collaboration South-West Peninsula. JM is funded by an NIHR Advanced Fellowship (NIHR302270). The views expressed are those of the authors and not necessarily those of the NIHR or the Department of Health and Social Care. CV acknowledges research funding by a "Contratos para la intensificacion de la actividad investigadora en el Sistema Nacional de Salud" contract (INT23/00040) from the Spanish Ministry of Science and Innovation.
Author Declarations
I confirm all relevant ethical guidelines have been followed, and any necessary IRB and/or ethics committee approvals have been obtained.
The details of the IRB/oversight body that provided approval or exemption for the research described are given below:
This study was approved by the relevant ethics committees: SIDIAP Scientific and Ethical Committees (19/518-P) on 18/12/2019. The SIDIAP database is based on opt-out presumed consent. If a patient decides to opt out, their routine data would be excluded of the database. CPRD ISAC committee protocol number 23_003109. The Northwest Multi-Centre Research Ethics Committee approved the collection and use of UK Biobank data for health-related research (Research Ethics Committee reference 11/NW/0382). UKB was granted under Application Number 9072.
I confirm that all necessary patient/participant consent has been obtained and the appropriate institutional forms have been archived, and that any patient/participant/sample identifiers included were not known to anyone (e.g., hospital staff, patients or participants themselves) outside the research group so cannot be used to identify individuals.
I understand that all clinical trials and any other prospective interventional studies must be registered with an ICMJE-approved registry, such as ClinicalTrials.gov. I confirm that any such study reported in the manuscript has been registered and the trial registration ID is provided (note: if posting a prospective study registered retrospectively, please provide a statement in the trial ID field explaining why the study was not registered in advance).
I have followed all appropriate research reporting guidelines, such as any relevant EQUATOR Network research reporting checklist(s) and other pertinent material, if applicable.
↵ * = joint first authors
↵ # = joint senior authors
Data Availability
We cannot make individual-level data available. Researchers can apply to UK Biobank ( https://www.ukbiobank.ac.uk/enable-your-research/ ), CPRD ( https://www.cprd.com/research-applications ), and SIDIAP ( https://www.sidiap.org/index.php/en/solicituds-en ). We have made our diagnostic code lists, code and results available on our GitHub ( https://github.com/GEMINI-multimorbidity/ ) site and Shiny website ( https://gemini-multimorbidity.shinyapps.io/atlas/ ). GWAS summary statistics will be available following acceptance at the GWAS Catalog ( https://www.ebi.ac.uk/gwas/home ).
View the discussion thread.
Supplementary Material
Thank you for your interest in spreading the word about medRxiv.
NOTE: Your email address is requested solely to identify you as the sender of this article.

Citation Manager Formats
- EndNote (tagged)
- EndNote 8 (xml)
- RefWorks Tagged
- Ref Manager
- Tweet Widget
- Facebook Like
- Google Plus One
Subject Area
- Genetic and Genomic Medicine
- Addiction Medicine (324)
- Allergy and Immunology (629)
- Anesthesia (166)
- Cardiovascular Medicine (2388)
- Dentistry and Oral Medicine (289)
- Dermatology (207)
- Emergency Medicine (380)
- Endocrinology (including Diabetes Mellitus and Metabolic Disease) (840)
- Epidemiology (11783)
- Forensic Medicine (10)
- Gastroenterology (703)
- Genetic and Genomic Medicine (3758)
- Geriatric Medicine (350)
- Health Economics (636)
- Health Informatics (2402)
- Health Policy (935)
- Health Systems and Quality Improvement (902)
- Hematology (341)
- HIV/AIDS (782)
- Infectious Diseases (except HIV/AIDS) (13328)
- Intensive Care and Critical Care Medicine (769)
- Medical Education (366)
- Medical Ethics (105)
- Nephrology (400)
- Neurology (3515)
- Nursing (199)
- Nutrition (528)
- Obstetrics and Gynecology (676)
- Occupational and Environmental Health (665)
- Oncology (1827)
- Ophthalmology (538)
- Orthopedics (219)
- Otolaryngology (287)
- Pain Medicine (234)
- Palliative Medicine (66)
- Pathology (447)
- Pediatrics (1035)
- Pharmacology and Therapeutics (426)
- Primary Care Research (423)
- Psychiatry and Clinical Psychology (3185)
- Public and Global Health (6156)
- Radiology and Imaging (1283)
- Rehabilitation Medicine and Physical Therapy (750)
- Respiratory Medicine (830)
- Rheumatology (379)
- Sexual and Reproductive Health (372)
- Sports Medicine (324)
- Surgery (402)
- Toxicology (50)
- Transplantation (172)
- Urology (146)
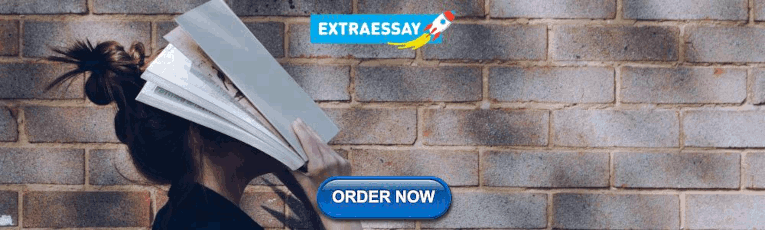
IMAGES
VIDEO
COMMENTS
Data Analysis for Social Scientists. Learn methods for harnessing and analyzing data to answer questions of cultural, social, economic, and policy interest. The course is free to audit. Learners can take a proctored exam and earn a course certificate by paying a fee, which varies by ability to pay. Please scroll down for more information on the ...
The social science data seeks researchers with training or a demonstrable aptitude for social science work and programming to refine and extend their skills through the generation, analysis, and ...
For many researchers unfamiliar with qualitative research, determining how to conduct qualitative analyses is often quite challenging. Part of this challenge is due to the seemingly limitless approaches that a qualitative researcher might leverage, as well as simply learning to think like a qualitative researcher when analyzing data. From framework analysis (Ritchie & Spencer, 1994) to content ...
"Data Analysis for Social Science is a great textbook for any undergraduate research methods course. I especially like that it teaches point estimates and uncertainty separately. In the past, when I taught these concepts together, I found students were overwhelmed. Breaking them up makes the statistics easier to understand.
Resources. Data Analysis for Social Science provides a friendly introduction to the statistical concepts and programming skills needed to conduct and evaluate social scientific studies. Assuming no prior knowledge of statistics and coding and only minimal knowledge of math, the book teaches the fundamentals of survey research, predictive models ...
Dr. Florian G. Hartmann is a research associate at the Chair of Social Science Methodology at the University of the Federal Armed Forces in Munich. Dr. Johannes Kopp is Professor of Sociology at the University of Trier. Dr. Daniel Lois holds the professorship for Social Science Methodology at the University of the Federal Armed Forces Munich.
Social data analysis enables you, as a researcher, to organize the facts you collect during your research. Your data may have come from a questionnaire survey, a set of interviews, or observations. They may be data that have been made available to you from some organization, national or international agency or other researchers. Whatever their source, social data can be daunting to put ...
The programme designed to promote a step-change in quantitative social science training. The Oxford Q-Step Centre (OQC) enables undergraduates across the Social Sciences to have access to enhanced training in Quantitative Methods, through lectures and data-labs. It is hosted by the Department of Politics and International Relations, in close co ...
Preview. Accessible, engaging, and informative, this text will help any social science student approach statistics with confidence. With a well-paced and well-judged integrated approach rather than a simple linear trajectory, this book progresses at a realistic speed that matches the pace at which statistics novices actually learn.
Meta-analysis methodology has improved dramatically over the last few years, leading the charge towards a credibility revolution in the social sciences and beyond. Recent advances include solutions to: p-hacking, model uncertainty, collinearity, and to the lack of robustness in earlier approaches to publication bias correction. Yet few applied ...
Qualitative research can prevent some of these problems. Such methods can help to understand data quality, inform design and analysis decisions and guide interpretation of results. The ...
In this course you will be introduced to the basic ideas behind the qualitative research in social science. You will learn about data collection, description, analysis and interpretation in qualitative research. Qualitative research often involves an iterative process. We will focus on the ingredients required for this process: data collection ...
The text starts with an introduction to computerized data analysis and the social research process, then walks users through univariate, bivariate, and multivariate analysis using SPSS. The book contains applications from across the social sciences—sociology, political science, social work, criminal justice, health—so it can be used in ...
This chapter concerns research on collecting, representing, and analyzing the data that underlie behavioral and social sciences knowledge. Such research, methodological in character, includes ethnographic and historical approaches, scaling, axiomatic measurement, and statistics, with its important relatives, econometrics and psychometrics. The field can be described as including the self ...
Data Analysis Using Regression and Multilevel/Hierarchical Models, first published in 2007, is a comprehensive manual for the applied researcher who wants to perform data analysis using linear and nonlinear regression and multilevel models. ... The substantive focus of many social science research problems leads directly to the consideration of ...
Jan Schwalbach is a postdoctoral researcher at the Department Data Services for the Social Sciences at GESIS - Leibniz Institute for the Social Sciences. His research revolves around digital behavioral data and legislative politics, including computational text analysis, survey experiments, as well as the provision and harmonization of large ...
The master's program in Social Data Analytics is a terminal degree program designed to be completed in two semesters. The program requires eight courses including an optional intensive Research Internship that is attached to a faculty Directed Research Practicum. Brown undergraduates who enter the program as fifth-year Master's students are ...
Content analysis is the systematic analysis of the content of a text—e.g., who says what, to whom, why, and to what extent and with what effect—in a quantitative or qualitative manner. Content analysis is typically conducted as follows. First, when there are many texts to analyse—e.g., newspaper stories, financial reports, blog postings ...
The researcher used the Statistical Package for Social Science (SPSS) version 25.0 software to gather and analyse data since it is among the fastest at performing activities such as statistical ...
Qualitative methods in social science research provide exploratory insights with the help of textual analysis. The goal of data analysis in social science is to interpret and summarise findings.
Qualitative methods in social science research provide exploratory insights with the help of textual analysis. Through data analysis in social science research, you uncover patterns, establish correlations, and gain a deeper understanding of social systems. You can contribute to the discipline with evidence-based insights and generate knowledge ...
Second Edition. Apply statistics to your everyday life. Statistics and Data Analysis for Social Science helps students to build a strong foundational understanding of statistics by providing clarity around when and why statistics useful. Rather than focusing on the "how to" of statistics, author Eric J. Krieg simplifies the complexity of ...
Here you will find data compiled by federal agencies, tools, and resources to conduct research, develop web and mobile applications, design data visualizations, and more.Browse by topic from the landing page or access the searchable data catalog from the Data menu at the top of the page. Files may be in TXT, HTML, XLS, CSV. or other formats.
This research holds significance for the fields of social media and communication studies through its comprehensive evaluation of Twitter's quoting encouragement policy enacted during the 2020 U.S. presidential election. In addressing a notable gap in the literature, this study introduces a framework that assesses both the quantitative and qualitative effects of specific platform-wide policy ...
By. Eve Glasberg. May 20, 2024. Until the middle of the 20th century, few thought of science as a social system, instead seeing scientific discovery as the work of individual geniuses. Columbia's Department of Sociology played a pivotal role in advancing the social study of science. Researchers from the Columbia program analyzed how science ...
Practical data analysis approaches in this text focus on using statistics to understand data and research, rather that focusing on learning statistics for its own sake.; Just enough R code in this text helps students use this programming environment to get results with a minimum of coding and no loading complex data analysis packages.; Simple political and social science examples throughout ...
We employ hierarchical regression analysis to verify the hypotheses with data from 317 Chinese firms. ... to the field of social sciences, such as supply chain management and operational ...
Water Resources Research is an AGU hydrology journal publishing original research articles and commentaries on hydrology, water resources, and the social sciences of water. Abstract River algal blooms have become a global environmental problem due to their large impact range and environmental hazards. ... 2.3 Data Collection and Analysis.
Background Multimorbidity, the presence of two or more conditions in one person, is increasingly prevalent. Yet shared biological mechanisms of specific pairs of conditions often remain poorly understood. We address this gap by integrating large-scale primary care and genetic data to elucidate potential causes of multimorbidity. Methods We defined chronic, common, and heritable conditions in ...