What is Holistic Coding in Qualitative Research? A Guide
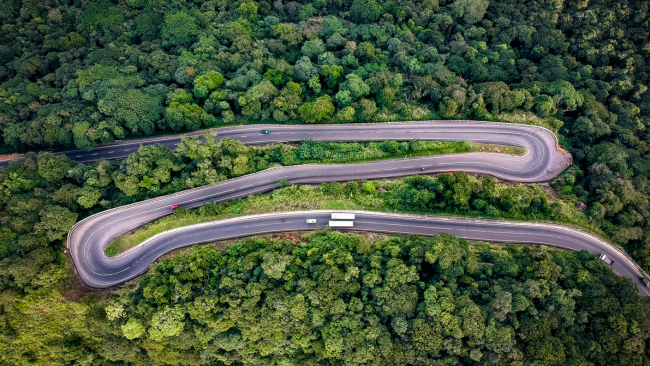
This is part of our Essential Guide to Coding Qualitative Data | Start a Free Trial of Delve | Take Our Free Online Qualitative Data Analysis Course
Holistic coding is a qualitative approach where you assign a single code to a data unit, such as a paragraph, transcript, or entire corpus, to capture its overall meaning. It allows researchers to get a 10,000-foot view of their data by identifying the key themes and concepts.
Saldaña (2012) coined the term, noting the time-saving benefits of capturing “the essence” of large quantitative datasets in the early stages of a study. This article offers an interpretation of Saldaña’s work on holistic coding and a practical guide for applying it to your research.
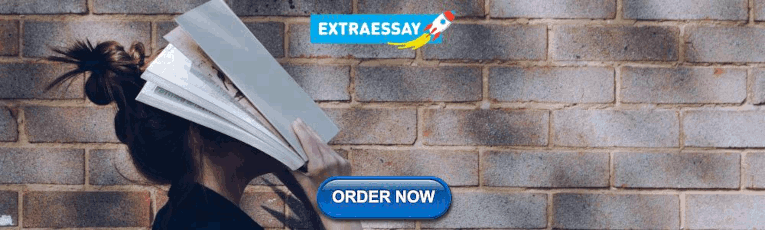
What is Holistic Coding?
Holistic coding is a first-cycle coding method, the initial stage of coding where the researcher identifies the main themes or concepts in the data, providing a high-level understanding of the data. It sets the stage for more organized second-cycle coding in later stages of research.
For reference, second-cycle coding methods like axial coding , elaborative coding , or descriptive coding are where researchers engage in a more analytical assessment and categorization process.
Holistic coding is also an inductive qualitative method where researchers assign meaning (and generate code) through iterative readings of the data. Holistic codes are like an initial discovery process that helps to unearth new themes or concepts from the data, instead of coding the data based on a preexisting study or theory.
By employing holistic coding, researchers gain a comprehensive understanding of the data they are working with. Like open coding , it provides a broad overview and aids in identifying potential categories or themes that help structure your research.
What Makes a Holistic Code?
Holistic codes can be single words, phrases, or short sentences. Saldaña adds that holistic codes are typically descriptive and do not usually include analytical or interpretive elements. Instead, they capture the “essence of the data unit.”
For example, a holistic code for a paragraph about a participant's experience of grief might be "Loss of a loved one." This code captures the overall meaning of the data unit (a paragraph) in a single phrase. It does not include the participant's thoughts or feelings about the loss.
You want a holistic code to be broad enough to capture various themes and concepts but specific enough to help identify overarching patterns and trends.
When to Use Holistic Coding?
Holistic coding is beneficial when you have a general idea of what to investigate within the data. From that broad starting point, you can familiarize yourself with your data before transitioning to more detailed, second-cycle coding methods (Saldaña, 2012).
Here are some instances where holistic coding can be particularly valuable:
Exploratory Research: Holistic coding is ideal for gaining a broad understanding and identifying potential themes or patterns in inductive, exploratory studies.
Preliminary Data Analysis: Holistic coding is a valuable tool in the early stages of qualitative research, providing an initial overview of the data and forming a foundation for further analysis.
Hypothesis Generation: Holistic coding helps researchers explore and identify potential categories or themes aligned with their research question or hypothesis.
Comparative Analysis: Holistic coding helps identify overarching patterns and themes when conducting comparative analysis across multiple cases or data sources.
Large-Scale Studies: Holistic coding is particularly useful in managing and navigating large amounts of data, such as extensive surveys or multiple interview transcripts.
Benefits and Limitations of Holistic Coding
While holistic coding is a helpful way to familiarize yourself with your data, it tends to lack nuance and detailed analysis. This broad-strokes approach in the first stages of your research sets the stage for more detailed and nuanced analysis in the subsequent steps.
Here are some benefits of using holistic coding:
It can help you to get a sense of the overall meaning of the data.
It can help you identify key themes and concepts in the data.
It can help you to develop a coding scheme for more detailed analysis.
Here are some limitations of using holistic coding:
It can be challenging to capture the nuances of the data with a single code.
It can be tough to compare different data units coded with the same holistic code.
It can be time-consuming to code large data units, even when applying just one code.
Overall, holistic coding provides a broad understanding of data but lacks subtlety.
Possible Applications of Holistic Coding
Holistic codes are helpful because they capture the essence of the data unit in a single code. Here are some examples of how to use holistic coding in qualitative research:
When studying the experience of grief, you could use “Loss” as a holistic coding to identify paragraphs where participants discuss the experience of losing a loved one.
Suppose you want to study the impact of a new educational program. Holistic coding lets you code complete interview transcripts to identify emerging themes and concepts. “Test scores” is a possible holistic code that helps organize your data.
A researcher studying the political views of a group of people might use holistic coding to code entire speeches. The goal is to identify top-level themes across participants. “Political ideology,” “Policy positions,” or “Values and beliefs” would be relevant holistic codes.
Applying Holistic Coding
To apply holistic coding effectively, here are the key steps:
1. Familiarize yourself with the data. Read the entire data set and upload it into a qualitative tool like Delve. Use the tool to record memos on the overall content and context. Consider the research question and how the data might help you to answer it as you read. Tools like Delve’s memo feature make recording, organizing, and storing your initial thoughts and ideas easy.
2. Determine the coding unit. The coding unit is the smallest unit of data that you will code. It can vary depending on the type of data and the research question.
For example, the coding unit could be a single paragraph or an entire transcript if you are coding interview transcripts. If you are coding text documents, the coding unit might be a single sentence, paragraph, or entire source. Smaller coding units require more time and effort upfront but give you a more granular view of the corpus.
💡 using Delve, you can determine the coding unit by highlighting the sentence, paragraph, or multiple paragraphs.
3. Create a holistic code. The code should capture the essence of one of the data's central themes. To do this, you can ask yourself the following questions:
What are the key concepts or themes that are present?
How much detail do I need to capture the meaning of the data?
What do I want to learn from using holistic coding?
How does the holistic code help answer my research question?
4. (Optional) Streamline your coding. Once you develop a holistic code, add the code to your qualitative data tool.
5. Note potential categories. After you’ve created codes, keep track of any potential categories or themes that emerge. You can use Delve to create themes and categories to organize your codes. Then you can also visualize how your codes aggregate , making it easier to categorize emergent patterns and themes in your data.
6. Iterate. Review your codes regularly to continuously refine your understanding of the data. This is an iterative process, so you may need to go back and forth between coding and revising your codes several times. Tools like Delve make it simple to edit, delete, and evolve codes as you go.
Record memos for new categories or emerging themes as you revise your codes.
It is important to be flexible and open to new ideas as you iterate.
This can be time-consuming but helps produce high-quality coding results.
7. Transition to second-cycle coding. Once you have completed the holistic coding phase, you can move on to more detailed coding methods in your second-cycle approach.
This approach gives you an initial sense of the data's overall contents and helps structure your codebook , paving the way for more detailed analysis and interpretation.
Holistic Coding With Delve
Delve’s intuitive, cloud-based coding software helps streamline the time-consuming and tedious parts of holistic coding.
Here are some of Delve’s specific features that streamline the holistic coding process:
Efficient coding : Delve's intuitive interface makes applying holistic codes to your data easy. You can simply drag and drop your data, select the text you want to code, and then assign a code. See how in this video: https://youtu.be/IhQmctWTbbo
Visualization : Delve's code visualization features make it easy to see how your codes aggregate. This can help you to identify patterns and relationships in your data.
Iteration : Delve makes it easy to iterate on your codes. The cloud-based coding tool makes it easy to organize, revise and refine your coding scheme as you learn more about your data.
Get started with Delve today!
Saldaña, J. (2012). The coding manual for qualitative researchers. 3rd ed. London, England: SAGE Publications.
Cite This Article
Delve, Ho, L., & Limpaecher, A. (2023c, July 16). What is Holistic Coding in Qualitative Analysis? A Guide https://delvetool.com/blog/holistic-coding
Academia.edu no longer supports Internet Explorer.
To browse Academia.edu and the wider internet faster and more securely, please take a few seconds to upgrade your browser .
Enter the email address you signed up with and we'll email you a reset link.
- We're Hiring!
- Help Center
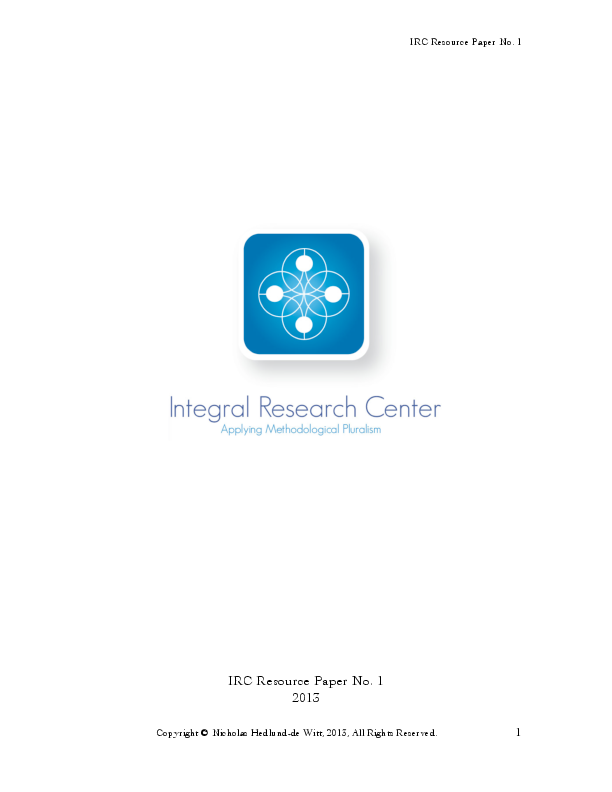
Coding: An Overview and Guide to Qualitative Data Analysis for Integral Researchers

RELATED PAPERS
Madalina Aur
Environmental Science & Technology
JOEL PEDERSEN
Call/Wa 0857 9154 2283|ukuran bak sortir lele pembesaran
bak sortir berkah
Journal of Pharmaceutical Negative Results
Norma Norma
Pascale Feghali
Said Rasool
Advances in Wavelet Theory and Their Applications in Engineering, Physics and Technology
NAJIB BEN AOUN
İlhan Tekeli
federica bianco
SN Comprehensive Clinical Medicine
Daniela Danieli
European journal of biochemistry
Dominique Pantaloni
ACS Catalysis
Osvaldo Cepeda Gutiérrez
Journal of School Psychology
Norris Haynes
Ghalib Jibara
Galician Medical Journal
Revista Sinalizar
MARGARIDA MARIA TELES
Edith Garneau
EUROPEAN RESEARCH STUDIES JOURNAL
Marek Goliński
Feminismo/s
Ana Garcia-Arroyo
RELATED TOPICS
- We're Hiring!
- Help Center
- Find new research papers in:
- Health Sciences
- Earth Sciences
- Cognitive Science
- Mathematics
- Computer Science
- Academia ©2024

Qualitative Data Coding 101
How to code qualitative data, the smart way (with examples).
By: Jenna Crosley (PhD) | Reviewed by:Dr Eunice Rautenbach | December 2020
As we’ve discussed previously , qualitative research makes use of non-numerical data – for example, words, phrases or even images and video. To analyse this kind of data, the first dragon you’ll need to slay is qualitative data coding (or just “coding” if you want to sound cool). But what exactly is coding and how do you do it?
Overview: Qualitative Data Coding
In this post, we’ll explain qualitative data coding in simple terms. Specifically, we’ll dig into:
- What exactly qualitative data coding is
- What different types of coding exist
- How to code qualitative data (the process)
- Moving from coding to qualitative analysis
- Tips and tricks for quality data coding
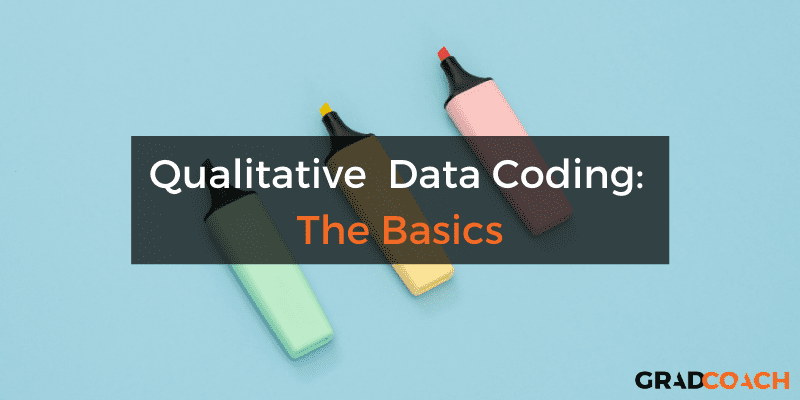
What is qualitative data coding?
Let’s start by understanding what a code is. At the simplest level, a code is a label that describes the content of a piece of text. For example, in the sentence:
“Pigeons attacked me and stole my sandwich.”
You could use “pigeons” as a code. This code simply describes that the sentence involves pigeons.
So, building onto this, qualitative data coding is the process of creating and assigning codes to categorise data extracts. You’ll then use these codes later down the road to derive themes and patterns for your qualitative analysis (for example, thematic analysis ). Coding and analysis can take place simultaneously, but it’s important to note that coding does not necessarily involve identifying themes (depending on which textbook you’re reading, of course). Instead, it generally refers to the process of labelling and grouping similar types of data to make generating themes and analysing the data more manageable.
Makes sense? Great. But why should you bother with coding at all? Why not just look for themes from the outset? Well, coding is a way of making sure your data is valid . In other words, it helps ensure that your analysis is undertaken systematically and that other researchers can review it (in the world of research, we call this transparency). In other words, good coding is the foundation of high-quality analysis.
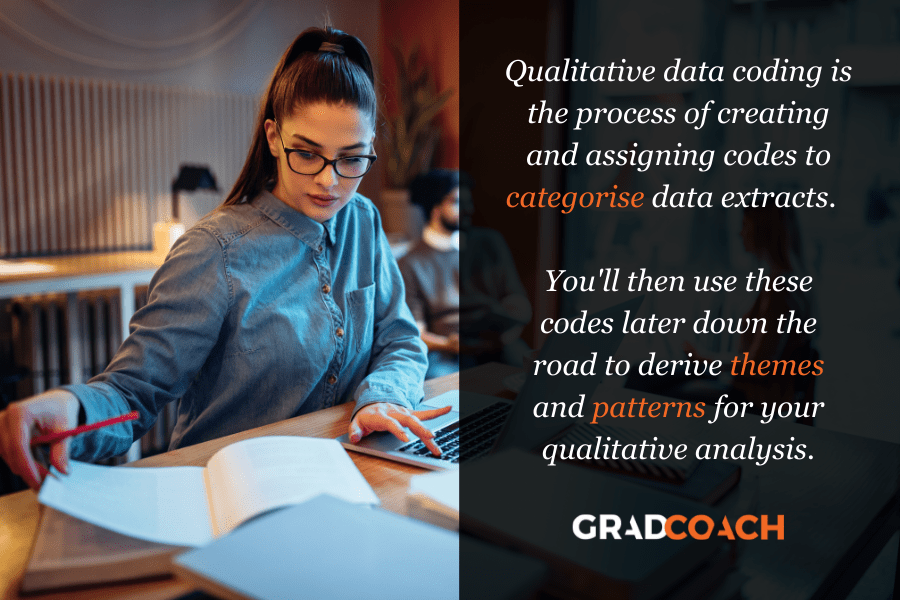
What are the different types of coding?
Now that we’ve got a plain-language definition of coding on the table, the next step is to understand what overarching types of coding exist – in other words, coding approaches . Let’s start with the two main approaches, inductive and deductive .
With deductive coding, you, as the researcher, begin with a set of pre-established codes and apply them to your data set (for example, a set of interview transcripts). Inductive coding on the other hand, works in reverse, as you create the set of codes based on the data itself – in other words, the codes emerge from the data. Let’s take a closer look at both.
Deductive coding 101
With deductive coding, we make use of pre-established codes, which are developed before you interact with the present data. This usually involves drawing up a set of codes based on a research question or previous research . You could also use a code set from the codebook of a previous study.
For example, if you were studying the eating habits of college students, you might have a research question along the lines of
“What foods do college students eat the most?”
As a result of this research question, you might develop a code set that includes codes such as “sushi”, “pizza”, and “burgers”.
Deductive coding allows you to approach your analysis with a very tightly focused lens and quickly identify relevant data . Of course, the downside is that you could miss out on some very valuable insights as a result of this tight, predetermined focus.
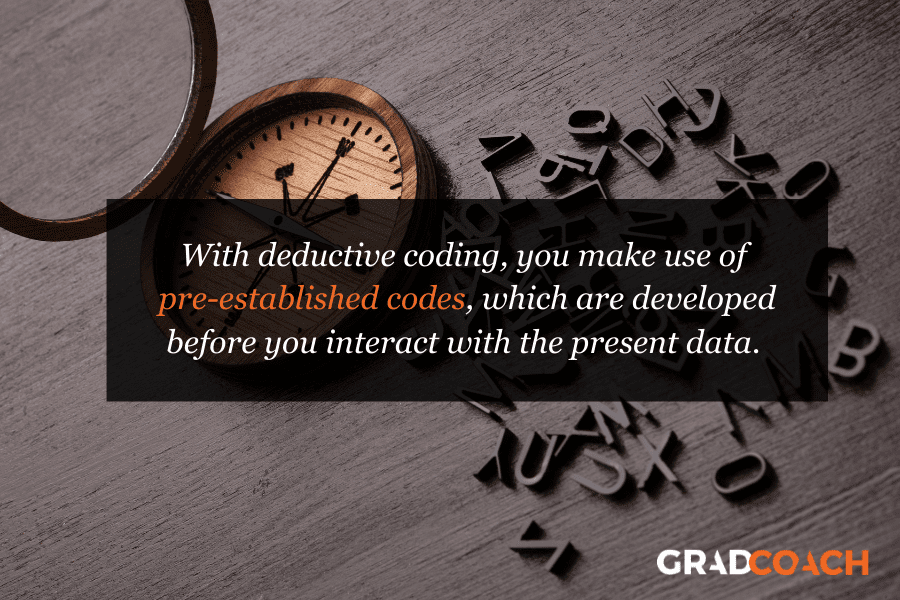
Inductive coding 101
But what about inductive coding? As we touched on earlier, this type of coding involves jumping right into the data and then developing the codes based on what you find within the data.
For example, if you were to analyse a set of open-ended interviews , you wouldn’t necessarily know which direction the conversation would flow. If a conversation begins with a discussion of cats, it may go on to include other animals too, and so you’d add these codes as you progress with your analysis. Simply put, with inductive coding, you “go with the flow” of the data.
Inductive coding is great when you’re researching something that isn’t yet well understood because the coding derived from the data helps you explore the subject. Therefore, this type of coding is usually used when researchers want to investigate new ideas or concepts , or when they want to create new theories.
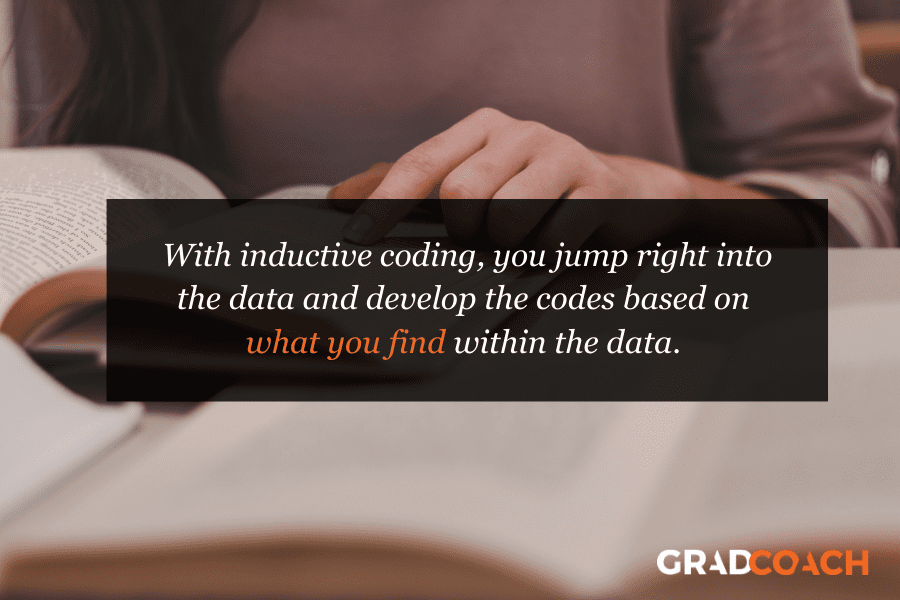
A little bit of both… hybrid coding approaches
If you’ve got a set of codes you’ve derived from a research topic, literature review or a previous study (i.e. a deductive approach), but you still don’t have a rich enough set to capture the depth of your qualitative data, you can combine deductive and inductive methods – this is called a hybrid coding approach.
To adopt a hybrid approach, you’ll begin your analysis with a set of a priori codes (deductive) and then add new codes (inductive) as you work your way through the data. Essentially, the hybrid coding approach provides the best of both worlds, which is why it’s pretty common to see this in research.
Need a helping hand?
How to code qualitative data
Now that we’ve looked at the main approaches to coding, the next question you’re probably asking is “how do I actually do it?”. Let’s take a look at the coding process , step by step.
Both inductive and deductive methods of coding typically occur in two stages: initial coding and line by line coding .
In the initial coding stage, the objective is to get a general overview of the data by reading through and understanding it. If you’re using an inductive approach, this is also where you’ll develop an initial set of codes. Then, in the second stage (line by line coding), you’ll delve deeper into the data and (re)organise it according to (potentially new) codes.
Step 1 – Initial coding
The first step of the coding process is to identify the essence of the text and code it accordingly. While there are various qualitative analysis software packages available, you can just as easily code textual data using Microsoft Word’s “comments” feature.
Let’s take a look at a practical example of coding. Assume you had the following interview data from two interviewees:
What pets do you have?
I have an alpaca and three dogs.
Only one alpaca? They can die of loneliness if they don’t have a friend.
I didn’t know that! I’ll just have to get five more.
I have twenty-three bunnies. I initially only had two, I’m not sure what happened.
In the initial stage of coding, you could assign the code of “pets” or “animals”. These are just initial, fairly broad codes that you can (and will) develop and refine later. In the initial stage, broad, rough codes are fine – they’re just a starting point which you will build onto in the second stage.
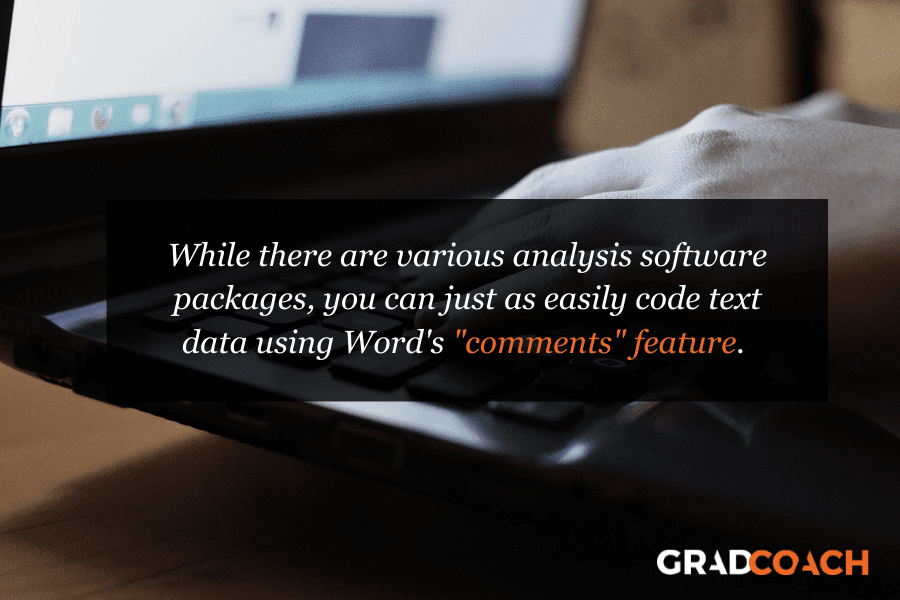
How to decide which codes to use
But how exactly do you decide what codes to use when there are many ways to read and interpret any given sentence? Well, there are a few different approaches you can adopt. The main approaches to initial coding include:
- In vivo coding
Process coding
- Open coding
Descriptive coding
Structural coding.
- Value coding
Let’s take a look at each of these:
In vivo coding
When you use in vivo coding , you make use of a participants’ own words , rather than your interpretation of the data. In other words, you use direct quotes from participants as your codes. By doing this, you’ll avoid trying to infer meaning, rather staying as close to the original phrases and words as possible.
In vivo coding is particularly useful when your data are derived from participants who speak different languages or come from different cultures. In these cases, it’s often difficult to accurately infer meaning due to linguistic or cultural differences.
For example, English speakers typically view the future as in front of them and the past as behind them. However, this isn’t the same in all cultures. Speakers of Aymara view the past as in front of them and the future as behind them. Why? Because the future is unknown, so it must be out of sight (or behind us). They know what happened in the past, so their perspective is that it’s positioned in front of them, where they can “see” it.
In a scenario like this one, it’s not possible to derive the reason for viewing the past as in front and the future as behind without knowing the Aymara culture’s perception of time. Therefore, in vivo coding is particularly useful, as it avoids interpretation errors.
Next up, there’s process coding , which makes use of action-based codes . Action-based codes are codes that indicate a movement or procedure. These actions are often indicated by gerunds (words ending in “-ing”) – for example, running, jumping or singing.
Process coding is useful as it allows you to code parts of data that aren’t necessarily spoken, but that are still imperative to understanding the meaning of the texts.
An example here would be if a participant were to say something like, “I have no idea where she is”. A sentence like this can be interpreted in many different ways depending on the context and movements of the participant. The participant could shrug their shoulders, which would indicate that they genuinely don’t know where the girl is; however, they could also wink, showing that they do actually know where the girl is.
Simply put, process coding is useful as it allows you to, in a concise manner, identify the main occurrences in a set of data and provide a dynamic account of events. For example, you may have action codes such as, “describing a panda”, “singing a song about bananas”, or “arguing with a relative”.
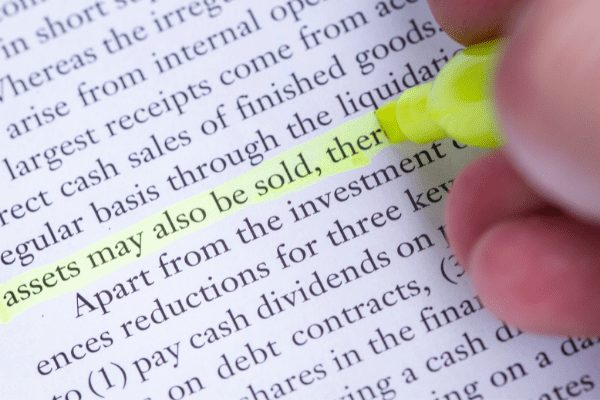
Descriptive coding aims to summarise extracts by using a single word or noun that encapsulates the general idea of the data. These words will typically describe the data in a highly condensed manner, which allows the researcher to quickly refer to the content.
Descriptive coding is very useful when dealing with data that appear in forms other than traditional text – i.e. video clips, sound recordings or images. For example, a descriptive code could be “food” when coding a video clip that involves a group of people discussing what they ate throughout the day, or “cooking” when coding an image showing the steps of a recipe.
Structural coding involves labelling and describing specific structural attributes of the data. Generally, it includes coding according to answers to the questions of “ who ”, “ what ”, “ where ”, and “ how ”, rather than the actual topics expressed in the data. This type of coding is useful when you want to access segments of data quickly, and it can help tremendously when you’re dealing with large data sets.
For example, if you were coding a collection of theses or dissertations (which would be quite a large data set), structural coding could be useful as you could code according to different sections within each of these documents – i.e. according to the standard dissertation structure . What-centric labels such as “hypothesis”, “literature review”, and “methodology” would help you to efficiently refer to sections and navigate without having to work through sections of data all over again.
Structural coding is also useful for data from open-ended surveys. This data may initially be difficult to code as they lack the set structure of other forms of data (such as an interview with a strict set of questions to be answered). In this case, it would useful to code sections of data that answer certain questions such as “who?”, “what?”, “where?” and “how?”.
Let’s take a look at a practical example. If we were to send out a survey asking people about their dogs, we may end up with a (highly condensed) response such as the following:
Bella is my best friend. When I’m at home I like to sit on the floor with her and roll her ball across the carpet for her to fetch and bring back to me. I love my dog.
In this set, we could code Bella as “who”, dog as “what”, home and floor as “where”, and roll her ball as “how”.
Values coding
Finally, values coding involves coding that relates to the participant’s worldviews . Typically, this type of coding focuses on excerpts that reflect the values, attitudes, and beliefs of the participants. Values coding is therefore very useful for research exploring cultural values and intrapersonal and experiences and actions.
To recap, the aim of initial coding is to understand and familiarise yourself with your data , to develop an initial code set (if you’re taking an inductive approach) and to take the first shot at coding your data . The coding approaches above allow you to arrange your data so that it’s easier to navigate during the next stage, line by line coding (we’ll get to this soon).
While these approaches can all be used individually, it’s important to remember that it’s possible, and potentially beneficial, to combine them . For example, when conducting initial coding with interviews, you could begin by using structural coding to indicate who speaks when. Then, as a next step, you could apply descriptive coding so that you can navigate to, and between, conversation topics easily.
Step 2 – Line by line coding
Once you’ve got an overall idea of our data, are comfortable navigating it and have applied some initial codes, you can move on to line by line coding. Line by line coding is pretty much exactly what it sounds like – reviewing your data, line by line, digging deeper and assigning additional codes to each line.
With line-by-line coding, the objective is to pay close attention to your data to add detail to your codes. For example, if you have a discussion of beverages and you previously just coded this as “beverages”, you could now go deeper and code more specifically, such as “coffee”, “tea”, and “orange juice”. The aim here is to scratch below the surface. This is the time to get detailed and specific so as to capture as much richness from the data as possible.
In the line-by-line coding process, it’s useful to code everything in your data, even if you don’t think you’re going to use it (you may just end up needing it!). As you go through this process, your coding will become more thorough and detailed, and you’ll have a much better understanding of your data as a result of this, which will be incredibly valuable in the analysis phase.
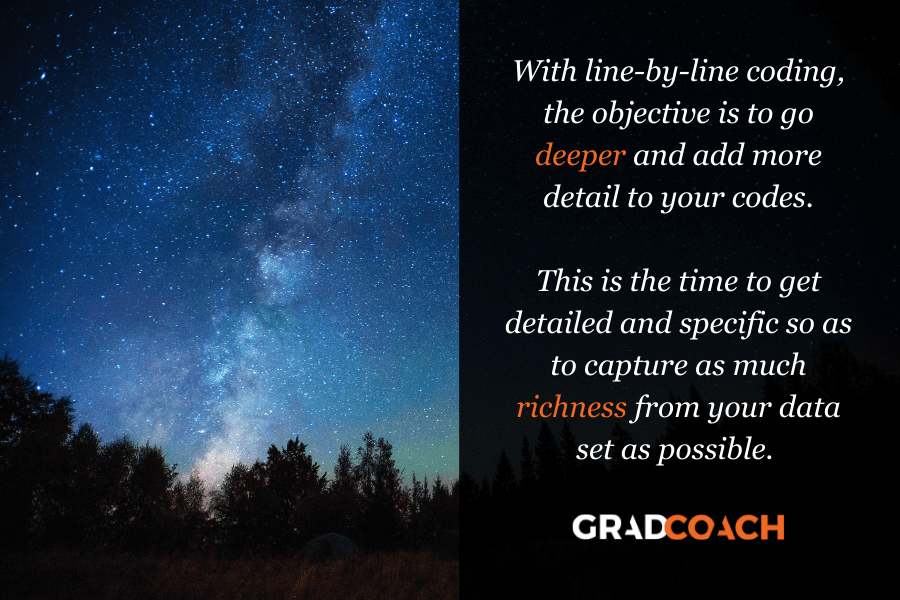
Moving from coding to analysis
Once you’ve completed your initial coding and line by line coding, the next step is to start your analysis . Of course, the coding process itself will get you in “analysis mode” and you’ll probably already have some insights and ideas as a result of it, so you should always keep notes of your thoughts as you work through the coding.
When it comes to qualitative data analysis, there are many different types of analyses (we discuss some of the most popular ones here ) and the type of analysis you adopt will depend heavily on your research aims, objectives and questions . Therefore, we’re not going to go down that rabbit hole here, but we’ll cover the important first steps that build the bridge from qualitative data coding to qualitative analysis.
When starting to think about your analysis, it’s useful to ask yourself the following questions to get the wheels turning:
- What actions are shown in the data?
- What are the aims of these interactions and excerpts? What are the participants potentially trying to achieve?
- How do participants interpret what is happening, and how do they speak about it? What does their language reveal?
- What are the assumptions made by the participants?
- What are the participants doing? What is going on?
- Why do I want to learn about this? What am I trying to find out?
- Why did I include this particular excerpt? What does it represent and how?
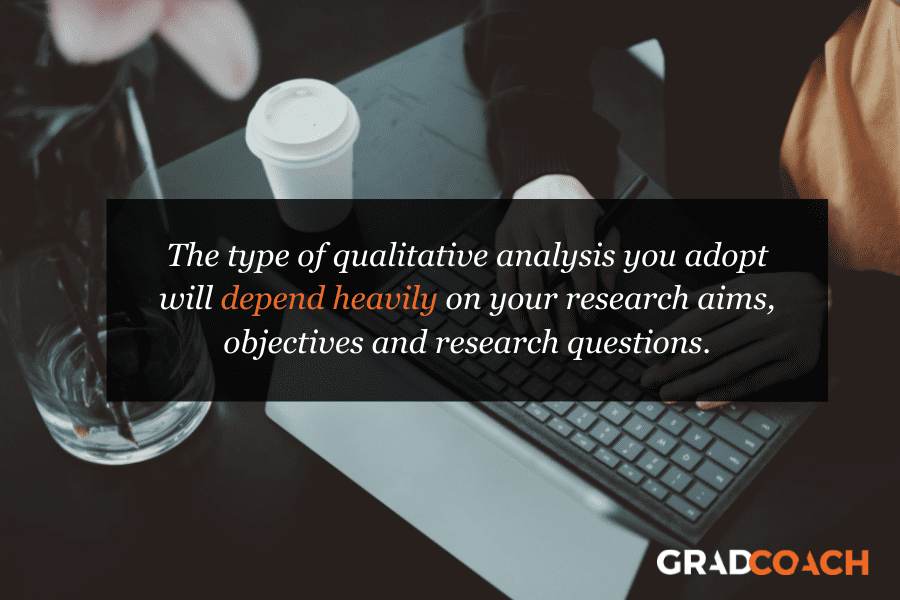
Code categorisation
Categorisation is simply the process of reviewing everything you’ve coded and then creating code categories that can be used to guide your future analysis. In other words, it’s about creating categories for your code set. Let’s take a look at a practical example.
If you were discussing different types of animals, your initial codes may be “dogs”, “llamas”, and “lions”. In the process of categorisation, you could label (categorise) these three animals as “mammals”, whereas you could categorise “flies”, “crickets”, and “beetles” as “insects”. By creating these code categories, you will be making your data more organised, as well as enriching it so that you can see new connections between different groups of codes.
Theme identification
From the coding and categorisation processes, you’ll naturally start noticing themes. Therefore, the logical next step is to identify and clearly articulate the themes in your data set. When you determine themes, you’ll take what you’ve learned from the coding and categorisation and group it all together to develop themes. This is the part of the coding process where you’ll try to draw meaning from your data, and start to produce a narrative . The nature of this narrative depends on your research aims and objectives, as well as your research questions (sounds familiar?) and the qualitative data analysis method you’ve chosen, so keep these factors front of mind as you scan for themes.
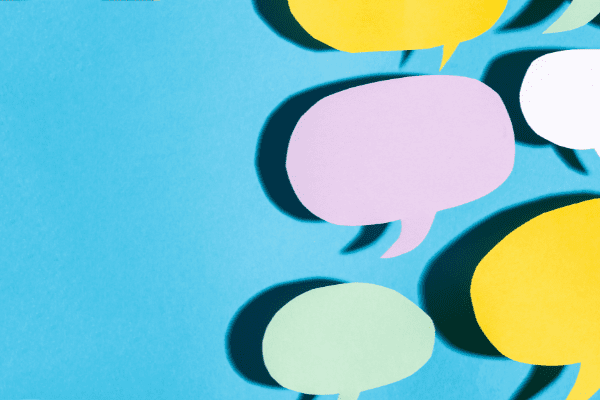
Tips & tricks for quality coding
Before we wrap up, let’s quickly look at some general advice, tips and suggestions to ensure your qualitative data coding is top-notch.
- Before you begin coding, plan out the steps you will take and the coding approach and technique(s) you will follow to avoid inconsistencies.
- When adopting deductive coding, it’s useful to use a codebook from the start of the coding process. This will keep your work organised and will ensure that you don’t forget any of your codes.
- Whether you’re adopting an inductive or deductive approach, keep track of the meanings of your codes and remember to revisit these as you go along.
- Avoid using synonyms for codes that are similar, if not the same. This will allow you to have a more uniform and accurate coded dataset and will also help you to not get overwhelmed by your data.
- While coding, make sure that you remind yourself of your aims and coding method. This will help you to avoid directional drift , which happens when coding is not kept consistent.
- If you are working in a team, make sure that everyone has been trained and understands how codes need to be assigned.
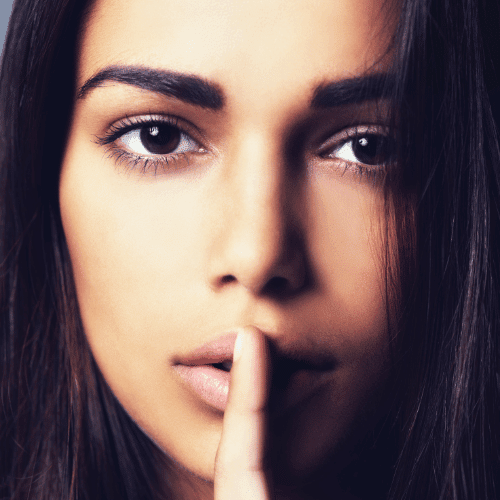
Psst... there’s more!
This post was based on one of our popular Research Bootcamps . If you're working on a research project, you'll definitely want to check this out ...
You Might Also Like:
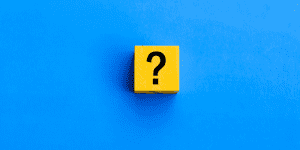
31 Comments
I appreciated the valuable information provided to accomplish the various stages of the inductive and inductive coding process. However, I would have been extremely satisfied to be appraised of the SPECIFIC STEPS to follow for: 1. Deductive coding related to the phenomenon and its features to generate the codes, categories, and themes. 2. Inductive coding related to using (a) Initial (b) Axial, and (c) Thematic procedures using transcribe data from the research questions
Thank you so much for this. Very clear and simplified discussion about qualitative data coding.
This is what I want and the way I wanted it. Thank you very much.
All of the information’s are valuable and helpful. Thank for you giving helpful information’s. Can do some article about alternative methods for continue researches during the pandemics. It is more beneficial for those struggling to continue their researchers.
Thank you for your information on coding qualitative data, this is a very important point to be known, really thank you very much.
Very useful article. Clear, articulate and easy to understand. Thanks
This is very useful. You have simplified it the way I wanted it to be! Thanks
Thank you so very much for explaining, this is quite helpful!
hello, great article! well written and easy to understand. Can you provide some of the sources in this article used for further reading purposes?
You guys are doing a great job out there . I will not realize how many students you help through your articles and post on a daily basis. I have benefited a lot from your work. this is remarkable.
Wonderful one thank you so much.
Hello, I am doing qualitative research, please assist with example of coding format.
This is an invaluable website! Thank you so very much!
Well explained and easy to follow the presentation. A big thumbs up to you. Greatly appreciate the effort 👏👏👏👏
Thank you for this clear article with examples
Thank you for the detailed explanation. I appreciate your great effort. Congrats!
Ahhhhhhhhhh! You just killed me with your explanation. Crystal clear. Two Cheers!
D0 you have primary references that was used when creating this? If so, can you share them?
Being a complete novice to the field of qualitative data analysis, your indepth analysis of the process of thematic analysis has given me better insight. Thank you so much.
Excellent summary
Thank you so much for your precise and very helpful information about coding in qualitative data.
Thanks a lot to this helpful information. You cleared the fog in my brain.
Glad to hear that!
This has been very helpful. I am excited and grateful.
I still don’t understand the coding and categorizing of qualitative research, please give an example on my research base on the state of government education infrastructure environment in PNG
Wahho, this is amazing and very educational to have come across this site.. from a little search to a wide discovery of knowledge.
Thanks I really appreciate this.
Thank you so much! Very grateful.
This was truly helpful. I have been so lost, and this simplified the process for me.
Just at the right time when I needed to distinguish between inductive and
deductive data analysis of my Focus group discussion results very helpful
Very useful across disciplines and at all levels. Thanks…
Submit a Comment Cancel reply
Your email address will not be published. Required fields are marked *
Save my name, email, and website in this browser for the next time I comment.
- Print Friendly
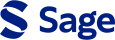
The Coding Manual for Qualitative Researchers
Student resources.
Welcome to the companion website for The Coding Manual for Qualitative Research , third edition, by Johnny Saldaña. This website offers a wealth of additional resources to support students and lecturers including:
CAQDAS links giving guidance and links to a variety of qualitative data analysis software.
Code lists including data extracted from the author’s study, “Lifelong Learning Impact: Adult Perceptions of Their High School Speech and/or Theatre Participation” (McCammon, Saldaña, Hines, & Omasta, 2012), which you can download and make your own practice manipulations to the data.
Coding examples from SAGE journals providing actual examples of coding at work, giving you insight into coding procedures.
Three sample interview transcripts that allow you to test your coding skills.
Group exercises for small and large groups encourage you to get to grips with basic principles of coding, partner development, categorization and qualitative data analysis
Flashcard glossary of terms enables you to test your knowledge of the terminology commonly used in qualitative research and coding.
About the book
Johnny Saldaña’s unique and invaluable manual demystifies the qualitative coding process with a comprehensive assessment of different coding types, examples and exercises. The ideal reference for students, teachers, and practitioners of qualitative inquiry, it is essential reading across the social sciences and neatly guides you through the multiple approaches available for coding qualitative data.
Its wide array of strategies, from the more straightforward to the more complex, is skilfully explained and carefully exemplified, providing a complete toolkit of codes and skills that can be applied to any research project. For each code Saldaña provides information about the method's origin, gives a detailed description of the method, demonstrates its practical applications, and sets out a clearly illustrated example with analytic follow up.
This international bestseller is an extremely usable, robust manual and is a must-have resource for qualitative researchers at all levels.
This website may contain links to both internal and external websites. All links included were active at the time the website was launched. SAGE does not operate these external websites and does not necessarily endorse the views expressed within them. SAGE cannot take responsibility for the changing content or nature of linked sites, as these sites are outside of our control and subject to change without our knowledge. If you do find an inactive link to an external website, please try to locate that website by using a search engine. SAGE will endeavour to update inactive or broken links when possible.
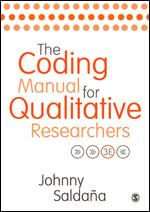
- Search Menu
- Browse content in Arts and Humanities
- Browse content in Archaeology
- Anglo-Saxon and Medieval Archaeology
- Archaeological Methodology and Techniques
- Archaeology by Region
- Archaeology of Religion
- Archaeology of Trade and Exchange
- Biblical Archaeology
- Contemporary and Public Archaeology
- Environmental Archaeology
- Historical Archaeology
- History and Theory of Archaeology
- Industrial Archaeology
- Landscape Archaeology
- Mortuary Archaeology
- Prehistoric Archaeology
- Underwater Archaeology
- Urban Archaeology
- Zooarchaeology
- Browse content in Architecture
- Architectural Structure and Design
- History of Architecture
- Residential and Domestic Buildings
- Theory of Architecture
- Browse content in Art
- Art Subjects and Themes
- History of Art
- Industrial and Commercial Art
- Theory of Art
- Biographical Studies
- Byzantine Studies
- Browse content in Classical Studies
- Classical History
- Classical Philosophy
- Classical Mythology
- Classical Literature
- Classical Reception
- Classical Art and Architecture
- Classical Oratory and Rhetoric
- Greek and Roman Papyrology
- Greek and Roman Epigraphy
- Greek and Roman Law
- Greek and Roman Archaeology
- Late Antiquity
- Religion in the Ancient World
- Digital Humanities
- Browse content in History
- Colonialism and Imperialism
- Diplomatic History
- Environmental History
- Genealogy, Heraldry, Names, and Honours
- Genocide and Ethnic Cleansing
- Historical Geography
- History by Period
- History of Emotions
- History of Agriculture
- History of Education
- History of Gender and Sexuality
- Industrial History
- Intellectual History
- International History
- Labour History
- Legal and Constitutional History
- Local and Family History
- Maritime History
- Military History
- National Liberation and Post-Colonialism
- Oral History
- Political History
- Public History
- Regional and National History
- Revolutions and Rebellions
- Slavery and Abolition of Slavery
- Social and Cultural History
- Theory, Methods, and Historiography
- Urban History
- World History
- Browse content in Language Teaching and Learning
- Language Learning (Specific Skills)
- Language Teaching Theory and Methods
- Browse content in Linguistics
- Applied Linguistics
- Cognitive Linguistics
- Computational Linguistics
- Forensic Linguistics
- Grammar, Syntax and Morphology
- Historical and Diachronic Linguistics
- History of English
- Language Evolution
- Language Reference
- Language Acquisition
- Language Variation
- Language Families
- Lexicography
- Linguistic Anthropology
- Linguistic Theories
- Linguistic Typology
- Phonetics and Phonology
- Psycholinguistics
- Sociolinguistics
- Translation and Interpretation
- Writing Systems
- Browse content in Literature
- Bibliography
- Children's Literature Studies
- Literary Studies (Romanticism)
- Literary Studies (American)
- Literary Studies (Asian)
- Literary Studies (European)
- Literary Studies (Eco-criticism)
- Literary Studies (Modernism)
- Literary Studies - World
- Literary Studies (1500 to 1800)
- Literary Studies (19th Century)
- Literary Studies (20th Century onwards)
- Literary Studies (African American Literature)
- Literary Studies (British and Irish)
- Literary Studies (Early and Medieval)
- Literary Studies (Fiction, Novelists, and Prose Writers)
- Literary Studies (Gender Studies)
- Literary Studies (Graphic Novels)
- Literary Studies (History of the Book)
- Literary Studies (Plays and Playwrights)
- Literary Studies (Poetry and Poets)
- Literary Studies (Postcolonial Literature)
- Literary Studies (Queer Studies)
- Literary Studies (Science Fiction)
- Literary Studies (Travel Literature)
- Literary Studies (War Literature)
- Literary Studies (Women's Writing)
- Literary Theory and Cultural Studies
- Mythology and Folklore
- Shakespeare Studies and Criticism
- Browse content in Media Studies
- Browse content in Music
- Applied Music
- Dance and Music
- Ethics in Music
- Ethnomusicology
- Gender and Sexuality in Music
- Medicine and Music
- Music Cultures
- Music and Media
- Music and Religion
- Music and Culture
- Music Education and Pedagogy
- Music Theory and Analysis
- Musical Scores, Lyrics, and Libretti
- Musical Structures, Styles, and Techniques
- Musicology and Music History
- Performance Practice and Studies
- Race and Ethnicity in Music
- Sound Studies
- Browse content in Performing Arts
- Browse content in Philosophy
- Aesthetics and Philosophy of Art
- Epistemology
- Feminist Philosophy
- History of Western Philosophy
- Metaphysics
- Moral Philosophy
- Non-Western Philosophy
- Philosophy of Language
- Philosophy of Mind
- Philosophy of Perception
- Philosophy of Science
- Philosophy of Action
- Philosophy of Law
- Philosophy of Religion
- Philosophy of Mathematics and Logic
- Practical Ethics
- Social and Political Philosophy
- Browse content in Religion
- Biblical Studies
- Christianity
- East Asian Religions
- History of Religion
- Judaism and Jewish Studies
- Qumran Studies
- Religion and Education
- Religion and Health
- Religion and Politics
- Religion and Science
- Religion and Law
- Religion and Art, Literature, and Music
- Religious Studies
- Browse content in Society and Culture
- Cookery, Food, and Drink
- Cultural Studies
- Customs and Traditions
- Ethical Issues and Debates
- Hobbies, Games, Arts and Crafts
- Lifestyle, Home, and Garden
- Natural world, Country Life, and Pets
- Popular Beliefs and Controversial Knowledge
- Sports and Outdoor Recreation
- Technology and Society
- Travel and Holiday
- Visual Culture
- Browse content in Law
- Arbitration
- Browse content in Company and Commercial Law
- Commercial Law
- Company Law
- Browse content in Comparative Law
- Systems of Law
- Competition Law
- Browse content in Constitutional and Administrative Law
- Government Powers
- Judicial Review
- Local Government Law
- Military and Defence Law
- Parliamentary and Legislative Practice
- Construction Law
- Contract Law
- Browse content in Criminal Law
- Criminal Procedure
- Criminal Evidence Law
- Sentencing and Punishment
- Employment and Labour Law
- Environment and Energy Law
- Browse content in Financial Law
- Banking Law
- Insolvency Law
- History of Law
- Human Rights and Immigration
- Intellectual Property Law
- Browse content in International Law
- Private International Law and Conflict of Laws
- Public International Law
- IT and Communications Law
- Jurisprudence and Philosophy of Law
- Law and Politics
- Law and Society
- Browse content in Legal System and Practice
- Courts and Procedure
- Legal Skills and Practice
- Primary Sources of Law
- Regulation of Legal Profession
- Medical and Healthcare Law
- Browse content in Policing
- Criminal Investigation and Detection
- Police and Security Services
- Police Procedure and Law
- Police Regional Planning
- Browse content in Property Law
- Personal Property Law
- Study and Revision
- Terrorism and National Security Law
- Browse content in Trusts Law
- Wills and Probate or Succession
- Browse content in Medicine and Health
- Browse content in Allied Health Professions
- Arts Therapies
- Clinical Science
- Dietetics and Nutrition
- Occupational Therapy
- Operating Department Practice
- Physiotherapy
- Radiography
- Speech and Language Therapy
- Browse content in Anaesthetics
- General Anaesthesia
- Neuroanaesthesia
- Clinical Neuroscience
- Browse content in Clinical Medicine
- Acute Medicine
- Cardiovascular Medicine
- Clinical Genetics
- Clinical Pharmacology and Therapeutics
- Dermatology
- Endocrinology and Diabetes
- Gastroenterology
- Genito-urinary Medicine
- Geriatric Medicine
- Infectious Diseases
- Medical Toxicology
- Medical Oncology
- Pain Medicine
- Palliative Medicine
- Rehabilitation Medicine
- Respiratory Medicine and Pulmonology
- Rheumatology
- Sleep Medicine
- Sports and Exercise Medicine
- Community Medical Services
- Critical Care
- Emergency Medicine
- Forensic Medicine
- Haematology
- History of Medicine
- Browse content in Medical Skills
- Clinical Skills
- Communication Skills
- Nursing Skills
- Surgical Skills
- Browse content in Medical Dentistry
- Oral and Maxillofacial Surgery
- Paediatric Dentistry
- Restorative Dentistry and Orthodontics
- Surgical Dentistry
- Medical Ethics
- Medical Statistics and Methodology
- Browse content in Neurology
- Clinical Neurophysiology
- Neuropathology
- Nursing Studies
- Browse content in Obstetrics and Gynaecology
- Gynaecology
- Occupational Medicine
- Ophthalmology
- Otolaryngology (ENT)
- Browse content in Paediatrics
- Neonatology
- Browse content in Pathology
- Chemical Pathology
- Clinical Cytogenetics and Molecular Genetics
- Histopathology
- Medical Microbiology and Virology
- Patient Education and Information
- Browse content in Pharmacology
- Psychopharmacology
- Browse content in Popular Health
- Caring for Others
- Complementary and Alternative Medicine
- Self-help and Personal Development
- Browse content in Preclinical Medicine
- Cell Biology
- Molecular Biology and Genetics
- Reproduction, Growth and Development
- Primary Care
- Professional Development in Medicine
- Browse content in Psychiatry
- Addiction Medicine
- Child and Adolescent Psychiatry
- Forensic Psychiatry
- Learning Disabilities
- Old Age Psychiatry
- Psychotherapy
- Browse content in Public Health and Epidemiology
- Epidemiology
- Public Health
- Browse content in Radiology
- Clinical Radiology
- Interventional Radiology
- Nuclear Medicine
- Radiation Oncology
- Reproductive Medicine
- Browse content in Surgery
- Cardiothoracic Surgery
- Gastro-intestinal and Colorectal Surgery
- General Surgery
- Neurosurgery
- Paediatric Surgery
- Peri-operative Care
- Plastic and Reconstructive Surgery
- Surgical Oncology
- Transplant Surgery
- Trauma and Orthopaedic Surgery
- Vascular Surgery
- Browse content in Science and Mathematics
- Browse content in Biological Sciences
- Aquatic Biology
- Biochemistry
- Bioinformatics and Computational Biology
- Developmental Biology
- Ecology and Conservation
- Evolutionary Biology
- Genetics and Genomics
- Microbiology
- Molecular and Cell Biology
- Natural History
- Plant Sciences and Forestry
- Research Methods in Life Sciences
- Structural Biology
- Systems Biology
- Zoology and Animal Sciences
- Browse content in Chemistry
- Analytical Chemistry
- Computational Chemistry
- Crystallography
- Environmental Chemistry
- Industrial Chemistry
- Inorganic Chemistry
- Materials Chemistry
- Medicinal Chemistry
- Mineralogy and Gems
- Organic Chemistry
- Physical Chemistry
- Polymer Chemistry
- Study and Communication Skills in Chemistry
- Theoretical Chemistry
- Browse content in Computer Science
- Artificial Intelligence
- Computer Architecture and Logic Design
- Game Studies
- Human-Computer Interaction
- Mathematical Theory of Computation
- Programming Languages
- Software Engineering
- Systems Analysis and Design
- Virtual Reality
- Browse content in Computing
- Business Applications
- Computer Security
- Computer Games
- Computer Networking and Communications
- Digital Lifestyle
- Graphical and Digital Media Applications
- Operating Systems
- Browse content in Earth Sciences and Geography
- Atmospheric Sciences
- Environmental Geography
- Geology and the Lithosphere
- Maps and Map-making
- Meteorology and Climatology
- Oceanography and Hydrology
- Palaeontology
- Physical Geography and Topography
- Regional Geography
- Soil Science
- Urban Geography
- Browse content in Engineering and Technology
- Agriculture and Farming
- Biological Engineering
- Civil Engineering, Surveying, and Building
- Electronics and Communications Engineering
- Energy Technology
- Engineering (General)
- Environmental Science, Engineering, and Technology
- History of Engineering and Technology
- Mechanical Engineering and Materials
- Technology of Industrial Chemistry
- Transport Technology and Trades
- Browse content in Environmental Science
- Applied Ecology (Environmental Science)
- Conservation of the Environment (Environmental Science)
- Environmental Sustainability
- Environmentalist Thought and Ideology (Environmental Science)
- Management of Land and Natural Resources (Environmental Science)
- Natural Disasters (Environmental Science)
- Nuclear Issues (Environmental Science)
- Pollution and Threats to the Environment (Environmental Science)
- Social Impact of Environmental Issues (Environmental Science)
- History of Science and Technology
- Browse content in Materials Science
- Ceramics and Glasses
- Composite Materials
- Metals, Alloying, and Corrosion
- Nanotechnology
- Browse content in Mathematics
- Applied Mathematics
- Biomathematics and Statistics
- History of Mathematics
- Mathematical Education
- Mathematical Finance
- Mathematical Analysis
- Numerical and Computational Mathematics
- Probability and Statistics
- Pure Mathematics
- Browse content in Neuroscience
- Cognition and Behavioural Neuroscience
- Development of the Nervous System
- Disorders of the Nervous System
- History of Neuroscience
- Invertebrate Neurobiology
- Molecular and Cellular Systems
- Neuroendocrinology and Autonomic Nervous System
- Neuroscientific Techniques
- Sensory and Motor Systems
- Browse content in Physics
- Astronomy and Astrophysics
- Atomic, Molecular, and Optical Physics
- Biological and Medical Physics
- Classical Mechanics
- Computational Physics
- Condensed Matter Physics
- Electromagnetism, Optics, and Acoustics
- History of Physics
- Mathematical and Statistical Physics
- Measurement Science
- Nuclear Physics
- Particles and Fields
- Plasma Physics
- Quantum Physics
- Relativity and Gravitation
- Semiconductor and Mesoscopic Physics
- Browse content in Psychology
- Affective Sciences
- Clinical Psychology
- Cognitive Psychology
- Cognitive Neuroscience
- Criminal and Forensic Psychology
- Developmental Psychology
- Educational Psychology
- Evolutionary Psychology
- Health Psychology
- History and Systems in Psychology
- Music Psychology
- Neuropsychology
- Organizational Psychology
- Psychological Assessment and Testing
- Psychology of Human-Technology Interaction
- Psychology Professional Development and Training
- Research Methods in Psychology
- Social Psychology
- Browse content in Social Sciences
- Browse content in Anthropology
- Anthropology of Religion
- Human Evolution
- Medical Anthropology
- Physical Anthropology
- Regional Anthropology
- Social and Cultural Anthropology
- Theory and Practice of Anthropology
- Browse content in Business and Management
- Business Ethics
- Business Strategy
- Business History
- Business and Technology
- Business and Government
- Business and the Environment
- Comparative Management
- Corporate Governance
- Corporate Social Responsibility
- Entrepreneurship
- Health Management
- Human Resource Management
- Industrial and Employment Relations
- Industry Studies
- Information and Communication Technologies
- International Business
- Knowledge Management
- Management and Management Techniques
- Operations Management
- Organizational Theory and Behaviour
- Pensions and Pension Management
- Public and Nonprofit Management
- Strategic Management
- Supply Chain Management
- Browse content in Criminology and Criminal Justice
- Criminal Justice
- Criminology
- Forms of Crime
- International and Comparative Criminology
- Youth Violence and Juvenile Justice
- Development Studies
- Browse content in Economics
- Agricultural, Environmental, and Natural Resource Economics
- Asian Economics
- Behavioural Finance
- Behavioural Economics and Neuroeconomics
- Econometrics and Mathematical Economics
- Economic History
- Economic Systems
- Economic Methodology
- Economic Development and Growth
- Financial Markets
- Financial Institutions and Services
- General Economics and Teaching
- Health, Education, and Welfare
- History of Economic Thought
- International Economics
- Labour and Demographic Economics
- Law and Economics
- Macroeconomics and Monetary Economics
- Microeconomics
- Public Economics
- Urban, Rural, and Regional Economics
- Welfare Economics
- Browse content in Education
- Adult Education and Continuous Learning
- Care and Counselling of Students
- Early Childhood and Elementary Education
- Educational Equipment and Technology
- Educational Strategies and Policy
- Higher and Further Education
- Organization and Management of Education
- Philosophy and Theory of Education
- Schools Studies
- Secondary Education
- Teaching of a Specific Subject
- Teaching of Specific Groups and Special Educational Needs
- Teaching Skills and Techniques
- Browse content in Environment
- Applied Ecology (Social Science)
- Climate Change
- Conservation of the Environment (Social Science)
- Environmentalist Thought and Ideology (Social Science)
- Natural Disasters (Environment)
- Social Impact of Environmental Issues (Social Science)
- Browse content in Human Geography
- Cultural Geography
- Economic Geography
- Political Geography
- Browse content in Interdisciplinary Studies
- Communication Studies
- Museums, Libraries, and Information Sciences
- Browse content in Politics
- African Politics
- Asian Politics
- Chinese Politics
- Comparative Politics
- Conflict Politics
- Elections and Electoral Studies
- Environmental Politics
- European Union
- Foreign Policy
- Gender and Politics
- Human Rights and Politics
- Indian Politics
- International Relations
- International Organization (Politics)
- International Political Economy
- Irish Politics
- Latin American Politics
- Middle Eastern Politics
- Political Behaviour
- Political Economy
- Political Institutions
- Political Methodology
- Political Communication
- Political Philosophy
- Political Sociology
- Political Theory
- Politics and Law
- Politics of Development
- Public Policy
- Public Administration
- Quantitative Political Methodology
- Regional Political Studies
- Russian Politics
- Security Studies
- State and Local Government
- UK Politics
- US Politics
- Browse content in Regional and Area Studies
- African Studies
- Asian Studies
- East Asian Studies
- Japanese Studies
- Latin American Studies
- Middle Eastern Studies
- Native American Studies
- Scottish Studies
- Browse content in Research and Information
- Research Methods
- Browse content in Social Work
- Addictions and Substance Misuse
- Adoption and Fostering
- Care of the Elderly
- Child and Adolescent Social Work
- Couple and Family Social Work
- Developmental and Physical Disabilities Social Work
- Direct Practice and Clinical Social Work
- Emergency Services
- Human Behaviour and the Social Environment
- International and Global Issues in Social Work
- Mental and Behavioural Health
- Social Justice and Human Rights
- Social Policy and Advocacy
- Social Work and Crime and Justice
- Social Work Macro Practice
- Social Work Practice Settings
- Social Work Research and Evidence-based Practice
- Welfare and Benefit Systems
- Browse content in Sociology
- Childhood Studies
- Community Development
- Comparative and Historical Sociology
- Economic Sociology
- Gender and Sexuality
- Gerontology and Ageing
- Health, Illness, and Medicine
- Marriage and the Family
- Migration Studies
- Occupations, Professions, and Work
- Organizations
- Population and Demography
- Race and Ethnicity
- Social Theory
- Social Movements and Social Change
- Social Research and Statistics
- Social Stratification, Inequality, and Mobility
- Sociology of Religion
- Sociology of Education
- Sport and Leisure
- Urban and Rural Studies
- Browse content in Warfare and Defence
- Defence Strategy, Planning, and Research
- Land Forces and Warfare
- Military Administration
- Military Life and Institutions
- Naval Forces and Warfare
- Other Warfare and Defence Issues
- Peace Studies and Conflict Resolution
- Weapons and Equipment

A newer edition of this book is available.
- < Previous chapter
- Next chapter >

28 Coding and Analysis Strategies
Johnny Saldaña, School of Theatre and Film, Arizona State University
- Published: 04 August 2014
- Cite Icon Cite
- Permissions Icon Permissions
This chapter provides an overview of selected qualitative data analytic strategies with a particular focus on codes and coding. Preparatory strategies for a qualitative research study and data management are first outlined. Six coding methods are then profiled using comparable interview data: process coding, in vivo coding, descriptive coding, values coding, dramaturgical coding, and versus coding. Strategies for constructing themes and assertions from the data follow. Analytic memo writing is woven throughout the preceding as a method for generating additional analytic insight. Next, display and arts-based strategies are provided, followed by recommended qualitative data analytic software programs and a discussion on verifying the researcher’s analytic findings.
Coding and Analysis Strategies
Anthropologist Clifford Geertz (1983) charmingly mused, “Life is just a bowl of strategies” (p. 25). Strategy , as I use it here, refers to a carefully considered plan or method to achieve a particular goal. The goal in this case is to develop a write-up of your analytic work with the qualitative data you have been given and collected as part of a study. The plans and methods you might employ to achieve that goal are what this article profiles.
Some may perceive strategy as an inappropriate if not colonizing word, suggesting formulaic or regimented approaches to inquiry. I assure you that that is not my intent. My use of strategy is actually dramaturgical in nature: strategies are actions that characters in plays take to overcome obstacles to achieve their objectives. Actors portraying these characters rely on action verbs to generate belief within themselves and to motivate them as they interpret the lines and move appropriately on stage. So what I offer is a qualitative researcher’s array of actions from which to draw to overcome the obstacles to thinking to achieve an analysis of your data. But unlike the pre-scripted text of a play in which the obstacles, strategies, and outcomes have been predetermined by the playwright, your work must be improvisational—acting, reacting, and interacting with data on a moment-by-moment basis to determine what obstacles stand in your way, and thus what strategies you should take to reach your goals.
Another intriguing quote to keep in mind comes from research methodologist Robert E. Stake (1995) who posits, “Good research is not about good methods as much as it is about good thinking” (p. 19). In other words, strategies can take you only so far. You can have a box full of tools, but if you do not know how to use them well or use them creatively, the collection seems rather purposeless. One of the best ways we learn is by doing . So pick up one or more of these strategies (in the form of verbs) and take analytic action with your data. Also keep in mind that these are discussed in the order in which they may typically occur, although humans think cyclically, iteratively, and reverberatively, and each particular research project has its own unique contexts and needs. So be prepared for your mind to jump purposefully and/or idiosyncratically from one strategy to another throughout the study.
QDA (Qualitative Data Analysis) Strategy: To Foresee
To foresee in QDA is to reflect beforehand on what forms of data you will most likely need and collect, which thus informs what types of data analytic strategies you anticipate using.
Analysis, in a way, begins even before you collect data. As you design your research study in your mind and on a word processor page, one strategy is to consider what types of data you may need to help inform and answer your central and related research questions. Interview transcripts, participant observation field notes, documents, artifacts, photographs, video recordings, and so on are not only forms of data but foundations for how you may plan to analyze them. A participant interview, for example, suggests that you will transcribe all or relevant portions of the recording, and use both the transcription and the recording itself as sources for data analysis. Any analytic memos (discussed later) or journal entries you make about your impressions of the interview also become data to analyze. Even the computing software you plan to employ will be relevant to data analysis as it may help or hinder your efforts.
As your research design formulates, compose one to two paragraphs that outline how your QDA may proceed. This will necessitate that you have some background knowledge of the vast array of methods available to you. Thus surveying the literature is vital preparatory work.
QDA Strategy: To Survey
To survey in QDA is to look for and consider the applicability of the QDA literature in your field that may provide useful guidance for your forthcoming data analytic work.
General sources in QDA will provide a good starting point for acquainting you with the data analytic strategies available for the variety of genres in qualitative inquiry (e.g., ethnography, phenomenology, case study, arts-based research, mixed methods). One of the most accessible is Graham R. Gibbs’ (2007) Analysing Qualitative Data , and one of the most richly detailed is Frederick J. Wertz et al.'s (2011) Five Ways of Doing Qualitative Analysis . The author’s core texts for this article came from The Coding Manual for Qualitative Researchers ( Saldaña, 2009 , 2013 ) and Fundamentals of Qualitative Research ( Saldaña, 2011 ).
If your study’s methodology or approach is grounded theory, for example, then a survey of methods works by such authors as Barney G. Glaser, Anselm L. Strauss, Juliet Corbin and, in particular, the prolific Kathy Charmaz (2006) may be expected. But there has been a recent outpouring of additional book publications in grounded theory by Birks & Mills (2011) , Bryant & Charmaz (2007) , Stern & Porr (2011) , plus the legacy of thousands of articles and chapters across many disciplines that have addressed grounded theory in their studies.
Particular fields such as education, psychology, social work, health care, and others also have their own QDA methods literature in the form of texts and journals, plus international conferences and workshops for members of the profession. Most important is to have had some university coursework and/or mentorship in qualitative research to suitably prepare you for the intricacies of QDA. Also acknowledge that the emergent nature of qualitative inquiry may require you to adopt different analytic strategies from what you originally planned.
QDA Strategy: To Collect
To collect in QDA is to receive the data given to you by participants and those data you actively gather to inform your study.
QDA is concurrent with data collection and management. As interviews are transcribed, field notes are fleshed out, and documents are filed, the researcher uses the opportunity to carefully read the corpus and make preliminary notations directly on the data documents by highlighting, bolding, italicizing, or noting in some way any particularly interesting or salient portions. As these data are initially reviewed, the researcher also composes supplemental analytic memos that include first impressions, reminders for follow-up, preliminary connections, and other thinking matters about the phenomena at work.
Some of the most common fieldwork tools you might use to collect data are notepads, pens and pencils, file folders for documents, a laptop or desktop with word processing software (Microsoft Word and Excel are most useful) and internet access, a digital camera, and a voice recorder. Some fieldworkers may even employ a digital video camera to record social action, as long as participant permissions have been secured. But everything originates from the researcher himself or herself. Your senses are immersed in the cultural milieu you study, taking in and holding on to relevant details or “significant trivia,” as I call them. You become a human camera, zooming out to capture the broad landscape of your field site one day, then zooming in on a particularly interesting individual or phenomenon the next. Your analysis is only as good as the data you collect.
Fieldwork can be an overwhelming experience because so many details of social life are happening in front of you. Take a holistic approach to your entree, but as you become more familiar with the setting and participants, actively focus on things that relate to your research topic and questions. Of course, keep yourself open to the intriguing, surprising, and disturbing ( Sunstein & Chiseri-Strater, 2012 , p. 115), for these facets enrich your study by making you aware of the unexpected.
QDA Strategy: To Feel
To feel in QDA is to gain deep emotional insight into the social worlds you study and what it means to be human.
Virtually everything we do has an accompanying emotion(s), and feelings are both reactions and stimuli for action. Others’ emotions clue you to their motives, attitudes, values, beliefs, worldviews, identities, and other subjective perceptions and interpretations. Acknowledge that emotional detachment is not possible in field research. Attunement to the emotional experiences of your participants plus sympathetic and empathetic responses to the actions around you are necessary in qualitative endeavors. Your own emotional responses during fieldwork are also data because they document the tacit and visceral. It is important during such analytic reflection to assess why your emotional reactions were as they were. But it is equally important not to let emotions alone steer the course of your study. A proper balance must be found between feelings and facts.
QDA Strategy: To Organize
To organize in QDA is to maintain an orderly repository of data for easy access and analysis.
Even in the smallest of qualitative studies, a large amount of data will be collected across time. Prepare both a hard drive and hard copy folders for digital data and paperwork, and back up all materials for security from loss. I recommend that each data “chunk” (e.g., one interview transcript, one document, one day’s worth of field notes) get its own file, with subfolders specifying the data forms and research study logistics (e.g., interviews, field notes, documents, Institutional Review Board correspondence, calendar).
For small-scale qualitative studies, I have found it quite useful to maintain one large master file with all participant and field site data copied and combined with the literature review and accompanying researcher analytic memos. This master file is used to cut and paste related passages together, deleting what seems unnecessary as the study proceeds, and eventually transforming the document into the final report itself. Cosmetic devices such as font style, font size, rich text (italicizing, bolding, underlining, etc.), and color can help you distinguish between different data forms and highlight significant passages. For example, descriptive, narrative passages of field notes are logged in regular font. “Quotations, things spoken by participants, are logged in bold font.” Observer’s comments, such as the researcher’s subjective impressions or analytic jottings, are set in italics.
QDA Strategy: To Jot
To jot in QDA is to write occasional, brief notes about your thinking or reminders for follow up.
A jot is a phrase or brief sentence that will literally fit on a standard size “sticky note.” As data are brought and documented together, take some initial time to review their contents and to jot some notes about preliminary patterns, participant quotes that seem quite vivid, anomalies in the data, and so forth.
As you work on a project, keep something to write with or to voice record with you at all times to capture your fleeting thoughts. You will most likely find yourself thinking about your research when you're not working exclusively on the project, and a “mental jot” may occur to you as you ruminate on logistical or analytic matters. Get the thought documented in some way for later retrieval and elaboration as an analytic memo.
QDA Strategy: To Prioritize
To prioritize in QDA is to determine which data are most significant in your corpus and which tasks are most necessary.
During fieldwork, massive amounts of data in various forms may be collected, and your mind can get easily overwhelmed from the magnitude of the quantity, its richness, and its management. Decisions will need to be made about the most pertinent of them because they help answer your research questions or emerge as salient pieces of evidence. As a sweeping generalization, approximately one half to two thirds of what you collect may become unnecessary as you proceed toward the more formal stages of QDA.
To prioritize in QDA is to also determine what matters most in your assembly of codes, categories, themes, assertions, and concepts. Return back to your research purpose and questions to keep you framed for what the focus should be.
QDA Strategy: To Analyze
To analyze in QDA is to observe and discern patterns within data and to construct meanings that seem to capture their essences and essentials.
Just as there are a variety of genres, elements, and styles of qualitative research, so too are there a variety of methods available for QDA. Analytic choices are most often based on what methods will harmonize with your genre selection and conceptual framework, what will generate the most sufficient answers to your research questions, and what will best represent and present the project’s findings.
Analysis can range from the factual to the conceptual to the interpretive. Analysis can also range from a straightforward descriptive account to an emergently constructed grounded theory to an evocatively composed short story. A qualitative research project’s outcomes may range from rigorously achieved, insightful answers to open-ended, evocative questions; from rich descriptive detail to a bullet-pointed list of themes; and from third-person, objective reportage to first-person, emotion-laden poetry. Just as there are multiple destinations in qualitative research, there are multiple pathways and journeys along the way.
Analysis is accelerated as you take cognitive ownership of your data. By reading and rereading the corpus, you gain intimate familiarity with its contents and begin to notice significant details as well as make new insights about their meanings. Patterns, categories, and their interrelationships become more evident the more you know the subtleties of the database.
Since qualitative research’s design, fieldwork, and data collection are most often provisional, emergent, and evolutionary processes, you reflect on and analyze the data as you gather them and proceed through the project. If preplanned methods are not working, you change them to secure the data you need. There is generally a post-fieldwork period when continued reflection and more systematic data analysis occur, concurrent with or followed by additional data collection, if needed, and the more formal write-up of the study, which is in itself an analytic act. Through field note writing, interview transcribing, analytic memo writing, and other documentation processes, you gain cognitive ownership of your data; and the intuitive, tacit, synthesizing capabilities of your brain begin sensing patterns, making connections, and seeing the bigger picture. The purpose and outcome of data analysis is to reveal to others through fresh insights what we have observed and discovered about the human condition. And fortunately, there are heuristics for reorganizing and reflecting on your qualitative data to help you achieve that goal.
QDA Strategy: To Pattern
To pattern in QDA is to detect similarities within and regularities among the data you have collected.
The natural world is filled with patterns because we, as humans, have constructed them as such. Stars in the night sky are not just a random assembly; our ancestors pieced them together to form constellations like the Big Dipper. A collection of flowers growing wild in a field has a pattern, as does an individual flower’s patterns of leaves and petals. Look at the physical objects humans have created and notice how pattern oriented we are in our construction, organization, and decoration. Look around you in your environment and notice how many patterns are evident on your clothing, in a room, and on most objects themselves. Even our sometimes mundane daily and long-term human actions are reproduced patterns in the form of roles, relationships, rules, routines, and rituals.
This human propensity for pattern making follows us into QDA. From the vast array of interview transcripts, field notes, documents, and other forms of data, there is this instinctive, hardwired need to bring order to the collection—not just to reorganize it but to look for and construct patterns out of it. The discernment of patterns is one of the first steps in the data analytic process, and the methods described next are recommended ways to construct them.
QDA Strategy: To Code
To code in QDA is to assign a truncated, symbolic meaning to each datum for purposes of qualitative analysis.
Coding is a heuristic—a method of discovery—to the meanings of individual sections of data. These codes function as a way of patterning, classifying, and later reorganizing them into emergent categories for further analysis. Different types of codes exist for different types of research genres and qualitative data analytic approaches, but this article will focus on only a few selected methods. First, a definition of a code:
A code in qualitative data analysis is most often a word or short phrase that symbolically assigns a summative, salient, essence-capturing, and/or evocative attribute for a portion of language-based or visual data. The data can consist of interview transcripts, participant observation fieldnotes, journals, documents, literature, artifacts, photographs, video, websites, e-mail correspondence, and so on. The portion of data to be coded can... range in magnitude from a single word to a full sentence to an entire page of text to a stream of moving images.... Just as a title represents and captures a book or film or poem’s primary content and essence, so does a code represent and capture a datum’s primary content and essence. [ Saldaña, 2009 , p. 3]
One helpful pre-coding task is to divide long selections of field note or interview transcript data into shorter stanzas . Stanza division “chunks” the corpus into more manageable paragraph-like units for coding assignments and analysis. The transcript sample that follows illustrates one possible way of inserting line breaks in-between self-standing passages of interview text for easier readability.
Process Coding
As a first coding example, the following interview excerpt about an employed, single, lower-middle-class adult male’s spending habits during the difficult economic times in the U.S. during 2008–2012 is coded in the right-hand margin in capital letters. The superscript numbers match the datum unit with its corresponding code. This particular method is called process coding, which uses gerunds (“-ing” words) exclusively to represent action suggested by the data. Processes can consist of observable human actions (e.g., BUYING BARGAINS), mental processes (e.g., THINKING TWICE), and more conceptual ideas (e.g., APPRECIATING WHAT YOU’VE GOT). Notice that the interviewer’s (I) portions are not coded, just the participant’s (P). A code is applied each time the subtopic of the interview shifts—even within a stanza—and the same codes can (and should) be used more than once if the subtopics are similar. The central research question driving this qualitative study is, “In what ways are middle-class Americans influenced and affected by the current [2008–2012] economic recession?”
Different researchers analyzing this same piece of data may develop completely different codes, depending on their lenses and filters. The previous codes are only one person’s interpretation of what is happening in the data, not the definitive list. The process codes have transformed the raw data units into new representations for analysis. A listing of them applied to this interview transcript, in the order they appear, reads:
BUYING BARGAINS
QUESTIONING A PURCHASE
THINKING TWICE
STOCKING UP
REFUSING SACRIFICE
PRIORITIZING
FINDING ALTERNATIVES
LIVING CHEAPLY
NOTICING CHANGES
STAYING INFORMED
MAINTAINING HEALTH
PICKING UP THE TAB
APPRECIATING WHAT YOU’VE GOT
Coding the data is the first step in this particular approach to QDA, and categorization is just one of the next possible steps.
QDA Strategy: To Categorize
To categorize in QDA is to cluster similar or comparable codes into groups for pattern construction and further analysis.
Humans categorize things in innumerable ways. Think of an average apartment or house’s layout. The rooms of a dwelling have been constructed or categorized by their builders and occupants according to function. A kitchen is designated as an area to store and prepare food and the cooking and dining materials such as pots, pans, and utensils. A bedroom is designated for sleeping, a closet for clothing storage, a bathroom for bodily functions and hygiene, and so on. Each room is like a category in which related and relevant patterns of human action occur. Of course, there are exceptions now and then, such as eating breakfast in bed rather than in a dining area or living in a small studio apartment in which most possessions are contained within one large room (but nonetheless are most often organized and clustered into subcategories according to function and optimal use of space).
The point here is that the patterns of social action we designate into particular categories during QDA are not perfectly bounded. Category construction is our best attempt to cluster the most seemingly alike things into the most seemingly appropriate groups. Categorizing is reorganizing and reordering the vast array of data from a study because it is from these smaller, larger, and meaning-rich units that we can better grasp the particular features of each one and the categories’ possible interrelationships with one another.
One analytic strategy with a list of codes is to classify them into similar clusters. Obviously, the same codes share the same category, but it is also possible that a single code can merit its own group if you feel it is unique enough. After the codes have been classified, a category label is applied to each grouping. Sometimes a code can also double as a category name if you feel it best summarizes the totality of the cluster. Like coding, categorizing is an interpretive act, for there can be different ways of separating and collecting codes that seem to belong together. The cut-and-paste functions of a word processor are most useful for exploring which codes share something in common.
Below is my categorization of the fifteen codes generated from the interview transcript presented earlier. Like the gerunds for process codes, the categories have also been labeled as “-ing” words to connote action. And there was no particular reason why fifteen codes resulted in three categories—there could have been less or even more, but this is how the array came together after my reflections on which codes seemed to belong together. The category labels are ways of answering “why” they belong together. For at-a-glance differentiation, I place codes in CAPITAL LETTERS and categories in upper and lower case Bold Font :
Category 1: Thinking Strategically
Category 2: Spending Strategically
Category 3: Living Strategically
APPRECIATING WHAT YOU'VE GOT
Notice that the three category labels share a common word: “strategically.” Where did this word come from? It came from analytic reflection on the original data, the codes, and the process of categorizing the codes and generating their category labels. It was the analyst’s choice based on the interpretation of what primary action was happening. Your categories generated from your coded data do not need to share a common word or phrase, but I find that this technique, when appropriate, helps build a sense of unity to the initial analytic scheme.
The three categories— Thinking Strategically , Spending Strategically , and Living Strategically —are then reflected upon for how they might interact and interplay. This is where the next major facet of data analysis, analytic memos, enters the scheme. But a necessary section on the basic principles of interrelationship and analytic reasoning must precede that discussion.
QDA Strategy: To Interrelate
To interrelate in QDA is to propose connections within, between, and among the constituent elements of analyzed data.
One task of QDA is to explore the ways our patterns and categories interact and interplay. I use these terms to suggest the qualitative equivalent of statistical correlation, but interaction and interplay are much more than a simple relationship. They imply interrelationship . Interaction refers to reverberative connections—for example, how one or more categories might influence and affect the others, how categories operate concurrently, or whether there is some kind of “domino” effect to them. Interplay refers to the structural and processual nature of categories—for example, whether some type of sequential order, hierarchy, or taxonomy exists; whether any overlaps occur; whether there is superordinate and subordinate arrangement; and what types of organizational frameworks or networks might exist among them. The positivist construct of “cause and effect” becomes influences and affects in QDA.
There can even be patterns of patterns and categories of categories if your mind thinks conceptually and abstractly enough. Our minds can intricately connect multiple phenomena but only if the data and their analyses support the constructions. We can speculate about interaction and interplay all we want, but it is only through a more systematic investigation of the data—in other words, good thinking—that we can plausibly establish any possible interrelationships.
QDA Strategy: To Reason
To reason in QDA is to think in ways that lead to causal probabilities, summative findings, and evaluative conclusions.
Unlike quantitative research, with its statistical formulas and established hypothesis-testing protocols, qualitative research has no standardized methods of data analysis. Rest assured, there are recommended guidelines from the field’s scholars and a legacy of analytic strategies from which to draw. But the primary heuristics (or methods of discovery) you apply during a study are deductive , inductive , abductive , and retroductive reasoning. Deduction is what we generally draw and conclude from established facts and evidence. Induction is what we experientially explore and infer to be transferable from the particular to the general, based on an examination of the evidence and an accumulation of knowledge. Abduction is surmising from the evidence that which is most likely, those explanatory hunches based on clues. “Whereas deductive inferences are certain (so long as their premises are true) and inductive inferences are probable, abductive inferences are merely plausible” ( Shank, 2008 , p. 1). Retroduction is historic reconstruction, working backwards to figure out how the current conditions came to exist.
It is not always necessary to know the names of these four ways of reasoning as you proceed through analysis. In fact, you will more than likely reverberate quickly from one to another depending on the task at hand. But what is important to remember about reasoning is:
to base your conclusions primarily on the participants’ experiences, not just your own
not to take the obvious for granted, as sometimes the expected won't always happen. Your hunches can be quite right and, at other times, quite wrong
to examine the evidence carefully and make reasonable inferences
to logically yet imaginatively think about what is going on and how it all comes together.
Futurists and inventors propose three questions when they think about creating new visions for the world: What is possible (induction)? What is plausible (abduction)? What is preferable (deduction)? These same three questions might be posed as you proceed through QDA and particularly through analytic memo writing, which is retroductive reflection on your analytic work thus far.
QDA Strategy: To Memo
To memo in QDA is to reflect in writing on the nuances, inferences, meanings, and transfer of coded and categorized data plus your analytic processes.
Like field note writing, perspectives vary among practitioners as to the methods for documenting the researcher’s analytic insights and subjective experiences. Some advise that such reflections should be included in field notes as relevant to the data. Others advise that a separate researcher’s journal should be maintained for recording these impressions. And still others advise that these thoughts be documented as separate analytic memos. I prescribe the latter as a method because it is generated by and directly connected to the data themselves.
An analytic memo is a “think piece” of reflexive free writing, a narrative that sets in words your interpretations of the data. Coding and categorizing are heuristics to detect some of the possible patterns and interrelationships at work within the corpus, and an analytic memo further articulates your deductive, inductive, abductive, and retroductive thinking processes on what things may mean. Though the metaphor is a bit flawed and limiting, think of codes and their consequent categories as separate jigsaw puzzle pieces, and their integration into an analytic memo as the trial assembly of the complete picture.
What follows is an example of an analytic memo based on the earlier process coded and categorized interview transcript. It is not intended as the final write-up for a publication but as an open-ended reflection on the phenomena and processes suggested by the data and their analysis thus far. As the study proceeds, however, initial and substantive analytic memos can be revisited and revised for eventual integration into the final report. Note how the memo is dated and given a title for future and further categorization, how participant quotes are occasionally included for evidentiary support, and how the category names are bolded and the codes kept in capital letters to show how they integrate or weave into the thinking:
March 18, 2012 EMERGENT CATEGORIES: A STRATEGIC AMALGAM There’s a popular saying now: “Smart is the new rich.” This participant is Thinking Strategically about his spending through such tactics as THINKING TWICE and QUESTIONING A PURCHASE before he decides to invest in a product. There’s a heightened awareness of both immediate trends and forthcoming economic bad news that positively affects his Spending Strategically . However, he seems unaware that there are even more ways of LIVING CHEAPLY by FINDING ALTERNATIVES. He dines at all-you-can-eat restaurants as a way of STOCKING UP on meals, but doesn’t state that he could bring lunch from home to work, possibly saving even more money. One of his “bad habits” is cigarettes, which he refuses to give up; but he doesn’t seem to realize that by quitting smoking he could save even more money, not to mention possible health care costs. He balks at the idea of paying $1.50 for a soft drink, but doesn’t mind paying $6.00–$7.00 for a pack of cigarettes. Penny-wise and pound-foolish. Addictions skew priorities. Living Strategically , for this participant during “scary times,” appears to be a combination of PRIORITIZING those things which cannot be helped, such as pet care and personal dental care; REFUSING SACRIFICE for maintaining personal creature-comforts; and FINDING ALTERNATIVES to high costs and excessive spending. Living Strategically is an amalgam of thinking and action-oriented strategies.
There are several recommended topics for analytic memo writing throughout the qualitative study. Memos are opportunities to reflect on and write about:
how you personally relate to the participants and/or the phenomenon
your study’s research questions
your code choices and their operational definitions
the emergent patterns, categories, themes, assertions, and concepts
the possible networks (links, connections, overlaps, flows) among the codes, patterns, categories, themes, assertions, and concepts
an emergent or related existent theory
any problems with the study
any personal or ethical dilemmas with the study
future directions for the study
the analytic memos generated thus far [labeled “metamemos”]
the final report for the study [adapted from Saldaña, 2013 , p. 49]
Since writing is analysis, analytic memos expand on the inferential meanings of the truncated codes and categories as a transitional stage into a more coherent narrative with hopefully rich social insight.
QDA Strategy: To Code—A Different Way
The first example of coding illustrated process coding, a way of exploring general social action among humans. But sometimes a researcher works with an individual case study whose language is unique, or with someone the researcher wishes to honor by maintaining the authenticity of his or her speech in the analysis. These reasons suggest that a more participant-centered form of coding may be more appropriate.
In Vivo Coding
A second frequently applied method of coding is called in vivo coding. The root meaning of “in vivo” is “in that which is alive” and refers to a code based on the actual language used by the participant ( Strauss, 1987 ). What words or phrases in the data record you select as codes are those that seem to stand out as significant or summative of what is being said.
Using the same transcript of the male participant living in difficult economic times, in vivo codes are listed in the right-hand column. I recommend that in vivo codes be placed in quotation marks as a way of designating that the code is extracted directly from the data record. Note that instead of fifteen codes generated from process coding, the total number of in vivo codes is thirty. This is not to suggest that there should be specific numbers or ranges of codes used for particular methods. In vivo codes, though, tend to be applied more frequently to data. Again, the interviewer’s questions and prompts are not coded, just the participant's responses:
The thirty in vivo codes are then extracted from the transcript and listed in the order they appear to prepare them for analytic action and reflection:
“SKYROCKETED”
“TWO-FOR-ONE”
“THE LITTLE THINGS”
“THINK TWICE”
“ALL-YOU-CAN-EAT”
“CHEAP AND FILLING”
“BAD HABITS”
“DON'T REALLY NEED”
“LIVED KIND OF CHEAP”
“NOT A BIG SPENDER”
“HAVEN'T CHANGED MY HABITS”
“NOT PUTTING AS MUCH INTO SAVINGS”
“SPENDING MORE”
“ANOTHER DING IN MY WALLET”
“HIGH MAINTENANCE”
“COUPLE OF THOUSAND”
“INSURANCE IS JUST WORTHLESS”
“PICK UP THE TAB”
“IT ALL ADDS UP”
“NOT AS BAD OFF”
“SCARY TIMES”
Even though no systematic reorganization or categorization has been conducted with the codes thus far, an analytic memo of first impressions can still be composed:
March 19, 2012 CODE CHOICES: THE EVERYDAY LANGUAGE OF ECONOMICS After eyeballing the in vivo codes list, I noticed that variants of “CHEAP” appear most often. I recall a running joke between me and a friend of mine when we were shopping for sales. We’d say, “We're not ‘cheap,’ we're frugal .” There’s no formal economic or business language is this transcript—no terms such as “recession” or “downsizing”—just the everyday language of one person trying to cope during “SCARY TIMES” with “ANOTHER DING IN MY WALLET.” The participant notes that he’s always “LIVED KIND OF CHEAP” and is “NOT A BIG SPENDER” and, due to his employment, “NOT AS BAD OFF” as others in the country. Yet even with his middle class status, he’s still feeling the monetary pinch, dining at inexpensive “ALL-YOU-CAN-EAT” restaurants and worried about the rising price of peanut butter, observing that he’s “NOT PUTTING AS MUCH INTO SAVINGS” as he used to. Of all the codes, “ANOTHER DING IN MY WALLET” stands out to me, particularly because on the audio recording he sounded bitter and frustrated. It seems that he’s so concerned about “THE LITTLE THINGS” because of high veterinary and dental charges. The only way to cope with a “COUPLE OF THOUSAND” dollars worth of medical expenses is to find ways of trimming the excess in everyday facets of living: “IT ALL ADDS UP.”
Like process coding, in vivo codes could be clustered into similar categories, but another simple data analytic strategy is also possible.
QDA Strategy: To Outline
To outline in QDA is to hierarchically, processually, and/or temporally assemble such things as codes, categories, themes, assertions, and concepts into a coherent, text-based display.
Traditional outlining formats and content provide not only templates for writing a report but templates for analytic organization. This principle can be found in several CAQDAS (Computer Assisted Qualitative Data Analysis Software) programs through their use of such functions as “hierarchies,” “trees,” and “nodes,” for example. Basic outlining is simply a way of arranging primary, secondary, and sub-secondary items into a patterned display. For example, an organized listing of things in a home might consist of:
Large appliances
Refrigerator
Stove-top oven
Microwave oven
Small appliances
Coffee maker
Dining room
In QDA, outlining may include descriptive nouns or topics but, depending on the study, it may also involve processes or phenomena in extended passages, such as in vivo codes or themes.
The complexity of what we learn in the field can be overwhelming, and outlining is a way of organizing and ordering that complexity so that it does not become complicated. The cut-and-paste and tab functions of a word processor page enable you to arrange and rearrange the salient items from your preliminary coded analytic work into a more streamlined flow. By no means do I suggest that the intricate messiness of life can always be organized into neatly formatted arrangements, but outlining is an analytic act that stimulates deep reflection on both the interconnectedness and interrelationships of what we study. As an example, here are the thirty in vivo codes generated from the initial transcript analysis, arranged in such a way as to construct five major categories:
“DON’T REALLY NEED”
“HAVEN’T CHANGED MY HABITS”
Now that the codes have been rearranged into an outline format, an analytic memo is composed to expand on the rationale and constructed meanings in progress:
March 19, 2012 NETWORKS: EMERGENT CATEGORIES The five major categories I constructed from the in vivo codes are: “SCARY TIMES,” “PRIORTY,” “ANOTHER DING IN MY WALLET,” “THE LITTLE THINGS,” and “LIVED KIND OF CHEAP.” One of the things that hit me today was that the reason he may be pinching pennies on smaller purchases is that he cannot control the larger ones he has to deal with. Perhaps the only way we can cope with or seem to have some sense of agency over major expenses is to cut back on the smaller ones that we can control. $1,000 for a dental bill? Skip lunch for a few days a week. Insulin medication to buy for a pet? Don’t buy a soft drink from a vending machine. Using this reasoning, let me try to interrelate and weave the categories together as they relate to this particular participant: During these scary economic times, he prioritizes his spending because there seems to be just one ding after another to his wallet. A general lifestyle of living cheaply and keeping an eye out for how to save money on the little things compensates for those major expenses beyond his control.
QDA Strategy: To Code—In Even More Ways
The process and in vivo coding examples thus far have demonstrated only two specific methods of thirty-two documented approaches ( Saldaña, 2013 ). Which one(s) you choose for your analysis depends on such factors as your conceptual framework, the genre of qualitative research for your project, the types of data you collect, and so on. The following sections present a few other approaches available for coding qualitative data that you may find useful as starting points.
Descriptive Coding
Descriptive codes are primarily nouns that simply summarize the topic of a datum. This coding approach is particularly useful when you have different types of data gathered for one study, such as interview transcripts, field notes, documents, and visual materials such as photographs. Descriptive codes not only help categorize but also index the data corpus’ basic contents for further analytic work. An example of an interview portion coded descriptively, taken from the participant living in tough economic times, follows to illustrate how the same data can be coded in multiple ways:
For initial analysis, descriptive codes are clustered into similar categories to detect such patterns as frequency (i.e., categories with the largest number of codes), interrelationship (i.e., categories that seem to connect in some way), and initial work for grounded theory development.
Values Coding
Values coding identifies the values, attitudes, and beliefs of a participant, as shared by the individual and/or interpreted by the analyst. This coding method infers the “heart and mind” of an individual or group’s worldview as to what is important, perceived as true, maintained as opinion, and felt strongly. The three constructs are coded separately but are part of a complex interconnected system.
Briefly, a value (V) is what we attribute as important, be it a person, thing, or idea. An attitude (A) is the evaluative way we think and feel about ourselves, others, things, or ideas. A belief (B) is what we think and feel as true or necessary, formed from our “personal knowledge, experiences, opinions, prejudices, morals, and other interpretive perceptions of the social world” ( Saldaña, 2009 , pp. 89–90). Values coding explores intrapersonal, interpersonal, and cultural constructs or ethos . It is an admittedly slippery task to code this way, for it is sometimes difficult to discern what is a value, attitude, or belief because they are intricately interrelated. But the depth you can potentially obtain is rich. An example of values coding follows:
For analysis, categorize the codes for each of the three different constructs together (i.e., all values in one group, attitudes in a second group, and beliefs in a third group). Analytic memo writing about the patterns and possible interrelationships may reveal a more detailed and intricate worldview of the participant.
Dramaturgical Coding
Dramaturgical coding perceives life as performance and its participants as characters in a social drama. Codes are assigned to the data (i.e., a “play script”) that analyze the characters in action, reaction, and interaction. Dramaturgical coding of participants examines their objectives (OBJ) or wants, needs, and motives; the conflicts (CON) or obstacles they face as they try to achieve their objectives; the tactics (TAC) or strategies they employ to reach their objectives; their attitudes (ATT) toward others and their given circumstances; the particular emotions (EMO) they experience throughout; and their subtexts (SUB) or underlying and unspoken thoughts. The following is an example of dramaturgically coded data:
Not included in this particular interview excerpt are the emotions the participant may have experienced or talked about. His later line, “that’s another ding in my wallet,” would have been coded EMO: BITTER. A reader may not have inferred that specific emotion from seeing the line in print. But the interviewer, present during the event and listening carefully to the audio recording during transcription, noted that feeling in his tone of voice.
For analysis, group similar codes together (e.g., all objectives in one group, all conflicts in another group, all tactics in a third group), or string together chains of how participants deal with their circumstances to overcome their obstacles through tactics (e.g., OBJ: SAVING MEAL MONEY > TAC: SKIPPING MEALS). Explore how the individuals or groups manage problem solving in their daily lives. Dramaturgical coding is particularly useful as preliminary work for narrative inquiry story development or arts-based research representations such as performance ethnography.
Versus Coding
Versus coding identifies the conflicts, struggles, and power issues observed in social action, reaction, and interaction as an X VS. Y code, such as: MEN VS. WOMEN, CONSERVATIVES VS. LIBERALS, FAITH VS. LOGIC, and so on. Conflicts are rarely this dichotomous. They are typically nuanced and much more complex. But humans tend to perceive these struggles with an US VS. THEM mindset. The codes can range from the observable to the conceptual and can be applied to data that show humans in tension with others, themselves, or ideologies.
What follows are examples of versus codes applied to the case study participant’s descriptions of his major medical expenses:
As an initial analytic tactic, group the versus codes into one of three categories: the Stakeholders , their Perceptions and/or Actions , and the Issues at stake. Examine how the three interrelate and identify the central ideological conflict at work as an X vs. Y category. Analytic memos and the final write-up can detail the nuances of the issues.
Remember that what has been profiled in this section is a broad brushstroke description of just a few basic coding processes, several of which can be compatibly “mixed and matched” within a single analysis (see Saldaña’s [2013] The Coding Manual for Qualitative Researchers for a complete discussion). Certainly with additional data, more in-depth analysis can occur, but coding is only one approach to extracting and constructing preliminary meanings from the data corpus. What now follows are additional methods for qualitative analysis.
QDA Strategy: To Theme
To theme in QDA is to construct summative, phenomenological meanings from data through extended passages of text.
Unlike codes, which are most often single words or short phrases that symbolically represent a datum, themes are extended phrases or sentences that summarize the manifest (apparent) and latent (underlying) meanings of data ( Auerbach & Silverstein, 2003 ; Boyatzis, 1998 ). Themes, intended to represent the essences and essentials of humans’ lived experiences, can also be categorized or listed in superordinate and subordinate outline formats as an analytic tactic.
Below is the interview transcript example used in the coding sections above. (Hopefully you are not too fatigued at this point with the transcript, but it’s important to know how inquiry with the same data set can be approached in several different ways.) During the investigation of the ways middle-class Americans are influenced and affected by the current (2008–2012) economic recession, the researcher noticed that participants’ stories exhibited facets of what he labeled “economic intelligence” or EI (based on the formerly developed theories of Howard Gardner’s multiple intelligences and Daniel Goleman’s emotional intelligence). Notice how themeing interprets what is happening through the use of two distinct phrases—ECONOMIC INTELLIGENCE IS (i.e., manifest or apparent meanings) and ECONOMIC INTELLIGENCE MEANS (i.e., latent or underlying meanings):
Unlike the fifteen process codes and thirty in vivo codes in the previous examples, there are now fourteen themes to work with. In the order they appear, they are:
EI IS TAKING ADVANTAGE OF UNEXPECTED OPPORTUNITY
EI MEANS THINKING BEFORE YOU ACT
EI IS BUYING CHEAP
EI MEANS SACRIFICE
EI IS SAVING A FEW DOLLARS NOW AND THEN
EI MEANS KNOWING YOUR FLAWS
EI IS SETTING PRIORITIES
EI IS FINDING CHEAPER FORMS OF ENTERTAINMENT
EI MEANS LIVING AN INEXPENSIVE LIFESTYLE
EI IS NOTICING PERSONAL AND NATIONAL ECONOMIC TRENDS
EI MEANS YOU CANNOT CONTROL EVERYTHING
EI IS TAKING CARE OF ONE’S OWN HEALTH
EI MEANS KNOWING YOUR LUCK
There are several ways to categorize the themes as preparation for analytic memo writing. The first is to arrange them in outline format with superordinate and subordinate levels, based on how the themes seem to take organizational shape and structure. Simply cutting and pasting the themes in multiple arrangements on a word processor page eventually develops a sense of order to them. For example:
A second approach is to categorize the themes into similar clusters and to develop different category labels or theoretical constructs . A theoretical construct is an abstraction that transforms the central phenomenon’s themes into broader applications but can still use “is” and “means” as prompts to capture the bigger picture at work:
Theoretical Construct 1: EI Means Knowing the Unfortunate Present
Supporting Themes:
Theoretical Construct 2: EI is Cultivating a Small Fortune
Theoretical Construct 3: EI Means a Fortunate Future
What follows is an analytic memo generated from the cut-and-paste arrangement of themes into an outline and into theoretical constructs:
March 19, 2012 EMERGENT THEMES: FORTUNE/FORTUNATELY/UNFORTUNATELY I first reorganized the themes by listing them in two groups: “is” and “means.” The “is” statements seemed to contain positive actions and constructive strategies for economic intelligence. The “means” statements held primarily a sense of caution and restriction with a touch of negativity thrown in. The first outline with two major themes, LIVING AN INEXPENSIVE LIFESTYLE and YOU CANNOT CONTROL EVERYTHING also had this same tone. This reminded me of the old children’s picture book, Fortunately/Unfortunately , and the themes of “fortune” as a motif for the three theoretical constructs came to mind. Knowing the Unfortunate Present means knowing what’s (most) important and what’s (mostly) uncontrollable in one’s personal economic life. Cultivating a Small Fortune consists of those small money-saving actions that, over time, become part of one's lifestyle. A Fortunate Future consists of heightened awareness of trends and opportunities at micro and macro levels, with the understanding that health matters can idiosyncratically affect one’s fortune. These three constructs comprise this particular individual’s EI—economic intelligence.
Again, keep in mind that the examples above for coding and themeing were from one small interview transcript excerpt. The number of codes and their categorization would obviously increase, given a longer interview and/or multiple interviews to analyze. But the same basic principles apply: codes and themes relegated into patterned and categorized forms are heuristics—stimuli for good thinking through the analytic memo-writing process on how everything plausibly interrelates. Methodologists vary in the number of recommended final categories that result from analysis, ranging anywhere from three to seven, with traditional grounded theorists prescribing one central or core category from coded work.
QDA Strategy: To Assert
To assert in QDA is to put forward statements that summarize particular fieldwork and analytic observations that the researcher believes credibly represent and transcend the experiences.
Educational anthropologist Frederick Erickson (1986) wrote a significant and influential chapter on qualitative methods that outlined heuristics for assertion development . Assertions are declarative statements of summative synthesis, supported by confirming evidence from the data, and revised when disconfirming evidence or discrepant cases require modification of the assertions. These summative statements are generated from an interpretive review of the data corpus and then supported and illustrated through narrative vignettes—reconstructed stories from field notes, interview transcripts, or other data sources that provide a vivid profile as part of the evidentiary warrant.
Coding or themeing data can certainly precede assertion development as a way of gaining intimate familiarity with the data, but Erickson’s methods are a more admittedly intuitive yet systematic heuristic for analysis. Erickson promotes analytic induction and exploration of and inferences about the data, based on an examination of the evidence and an accumulation of knowledge. The goal is not to look for “proof” to support the assertions but plausibility of inference-laden observations about the local and particular social world under investigation.
Assertion development is the writing of general statements, plus subordinate yet related ones called subassertions , and a major statement called a key assertion that represents the totality of the data. One also looks for key linkages between them, meaning that the key assertion links to its related assertions, which then link to their respective subassertions. Subassertions can include particulars about any discrepant related cases or specify components of their parent assertions.
Excerpts from the interview transcript of our case study will be used to illustrate assertion development at work. By now, you should be quite familiar with the contents, so I will proceed directly to the analytic example. First, there is a series of thematically related statements the participant makes:
“Buy one package of chicken, get the second one free. Now that was a bargain. And I got some.”
“With Sweet Tomatoes I get those coupons for a few bucks off for lunch, so that really helps.”
“I don’t go to movies anymore. I rent DVDs from Netflix or Redbox or watch movies online—so much cheaper than paying over ten or twelve bucks for a movie ticket.”
Assertions can be categorized into low-level and high-level inferences . Low-level inferences address and summarize “what is happening” within the particulars of the case or field site—the “micro.” High-level inferences extend beyond the particulars to speculate on “what it means” in the more general social scheme of things—the “meso” or “macro.” A reasonable low-level assertion about the three statements above collectively might read: The participant finds several small ways to save money during a difficult economic period . A high-level inference that transcends the case to the macro level might read: Selected businesses provide alternatives and opportunities to buy products and services at reduced rates during a recession to maintain consumer spending.
Assertions are instantiated (i.e., supported) by concrete instances of action or participant testimony, whose patterns lead to more general description outside the specific field site. The author’s interpretive commentary can be interspersed throughout the report, but the assertions should be supported with the evidentiary warrant . A few assertions and subassertions based on the case interview transcript might read (and notice how high-level assertions serve as the paragraphs’ topic sentences):
Selected businesses provide alternatives and opportunities to buy products and services at reduced rates during a recession to maintain consumer spending. Restaurants, for example, need to find ways during difficult economic periods when potential customers may be opting to eat inexpensively at home rather than spending more money by dining out. Special offers can motivate cash-strapped clientele to patronize restaurants more frequently. An adult male dealing with such major expenses as underinsured dental care offers: “With Sweet Tomatoes I get those coupons for a few bucks off for lunch, so that really helps.” The film and video industries also seem to be suffering from a double-whammy during the current recession: less consumer spending on higher-priced entertainment, resulting in a reduced rate of movie theatre attendance (currently 39 percent of the American population, according to CNN); coupled with a media technology and business revolution that provides consumers less costly alternatives through video rentals and internet viewing: “I don’t go to movies anymore. I rent DVDs from Netflix or Redbox or watch movies online—so much cheaper than paying over ten or twelve bucks for a movie ticket.”
“Particularizability”—the search for specific and unique dimensions of action at a site and/or the specific and unique perspectives of an individual participant—is not intended to filter out trivial excess but to magnify the salient characteristics of local meaning. Although generalizable knowledge serves little purpose in qualitative inquiry since each naturalistic setting will contain its own unique set of social and cultural conditions, there will be some aspects of social action that are plausibly universal or “generic” across settings and perhaps even across time. To work toward this, Erickson advocates that the interpretive researcher look for “concrete universals” by studying actions at a particular site in detail, then comparing those to other sites that have also been studied in detail. The exhibit or display of these generalizable features is to provide a synoptic representation, or a view of the whole. What the researcher attempts to uncover is what is both particular and general at the site of interest, preferably from the perspective of the participants. It is from the detailed analysis of actions at a specific site that these universals can be concretely discerned, rather than abstractly constructed as in grounded theory.
In sum, assertion development is a qualitative data analytic strategy that relies on the researcher’s intense review of interview transcripts, field notes, documents, and other data to inductively formulate composite statements that credibly summarize and interpret participant actions and meanings, and their possible representation of and transfer into broader social contexts and issues.
QDA Strategy: To Display
To display in QDA is to visually present the processes and dynamics of human or conceptual action represented in the data.
Qualitative researchers use not only language but illustrations to both analyze and display the phenomena and processes at work in the data. Tables, charts, matrices, flow diagrams, and other models help both you and your readers cognitively and conceptually grasp the essence and essentials of your findings. As you have seen thus far, even simple outlining of codes, categories, and themes is one visual tactic for organizing the scope of the data. Rich text, font, and format features such as italicizing, bolding, capitalizing, indenting, and bullet pointing provide simple emphasis to selected words and phrases within the longer narrative.
“Think display” was a phrase coined by methodologists Miles and Huberman (1994) to encourage the researcher to think visually as data were collected and analyzed. The magnitude of text can be essentialized into graphics for “at-a-glance” review. Bins in various shapes and lines of various thicknesses, along with arrows suggesting pathways and direction, render the study as a portrait of action. Bins can include the names of codes, categories, concepts, processes, key participants, and/or groups.
As a simple example, Figure 28.1 illustrates the three categories’ interrelationship derived from process coding. It displays what could be the apex of this interaction, LIVING STRATEGICALLY, and its connections to THINKING STRATEGICALLY, which influences and affects SPENDING STRATEGICALLY.
Figure 28.2 represents a slightly more complex (if not playful) model, based on the five major in vivo codes/categories generated from analysis. The graphic is used as a way of initially exploring the interrelationship and flow from one category to another. The use of different font styles, font sizes, and line and arrow thicknesses are intended to suggest the visual qualities of the participant’s language and his dilemmas—a way of heightening in vivo coding even further.
Accompanying graphics are not always necessary for a qualitative report. They can be very helpful for the researcher during the analytic stage as a heuristic for exploring how major ideas interrelate, but illustrations are generally included in published work when they will help supplement and clarify complex processes for readers. Photographs of the field setting or the participants (and only with their written permission) also provide evidentiary reality to the write-up and help your readers get a sense of being there.
QDA Strategy: To Narrate
To narrate in QDA is to create an evocative literary representation and presentation of the data in the form of creative nonfiction.
All research reports are stories of one kind or another. But there is yet another approach to QDA that intentionally documents the research experience as story, in its traditional literary sense. Narrative inquiry plots and story lines the participant’s experiences into what might be initially perceived as a fictional short story or novel. But the story is carefully crafted and creatively written to provide readers with an almost omniscient perspective about the participants’ worldview. The transformation of the corpus from database to creative nonfiction ranges from systematic transcript analysis to open ended literary composition. The narrative, though, should be solidly grounded in and emerge from the data as a plausible rendering of social life.
A simple illustration of category interrelationship.
An illustration with rich text and artistic features.
The following is a narrative vignette based on interview transcript selections from the participant living through tough economic times:
Jack stood in front of the soft drink vending machine at work and looked almost worriedly at the selections. With both hands in his pants pockets, his fingers jingled the few coins he had inside them as he contemplated whether he could afford the purchase. One dollar and fifty cents for a twenty-ounce bottle of Diet Coke. One dollar and fifty cents. “I can practically get a two-liter bottle for that same price at the grocery store,” he thought. Then Jack remembered the upcoming dental surgery he needed—that would cost one thousand dollars—and the bottle of insulin and syringes he needed to buy for his diabetic, “high maintenance” cat—about one hundred and twenty dollars. He sighed, took his hands out of his pockets, and walked away from the vending machine. He was skipping lunch that day anyway so he could stock up on dinner later at the cheap-but-filling-all-you-can-eat Chinese buffet. He could get his Diet Coke there.
Narrative inquiry representations, like literature, vary in tone, style, and point of view. The common goal, however, is to create an evocative portrait of participants through the aesthetic power of literary form. A story does not always have to have a moral explicitly stated by its author. The reader reflects on personal meanings derived from the piece and how the specific tale relates to one’s self and the social world.
QDA Strategy: To Poeticize
To poeticize in QDA is to create an evocative literary representation and presentation of the data in the form of poetry.
One form for analyzing or documenting analytic findings is to strategically truncate interview transcripts, field notes, and other pertinent data into poetic structures. Like coding, poetic constructions capture the essence and essentials of data in a creative, evocative way. The elegance of the format attests to the power of carefully chosen language to represent and convey complex human experience.
In vivo codes (codes based on the actual words used by participants themselves) can provide imagery, symbols, and metaphors for rich category, theme, concept, and assertion development, plus evocative content for arts-based interpretations of the data. Poetic inquiry takes note of what words and phrases seem to stand out from the data corpus as rich material for reinterpretation. Using some of the participant’s own language from the interview transcript illustrated above, a poetic reconstruction or “found poetry” might read:
Scary Times Scary times... spending more (another ding in my wallet) a couple of thousand (another ding in my wallet) insurance is just worthless (another ding in my wallet) pick up the tab (another ding in my wallet) not putting as much into savings (another ding in my wallet) It all adds up. Think twice: don't really need skip Think twice, think cheap: coupons bargains two-for-one free Think twice, think cheaper: stock up all-you-can-eat (cheap—and filling) It all adds up.
Anna Deavere Smith, a verbatim theatre performer, attests that people speak in forms of “organic poetry” in everyday life. Thus in vivo codes can provide core material for poetic representation and presentation of lived experiences, potentially transforming the routine and mundane into the epic. Some researchers also find the genre of poetry to be the most effective way to compose original work that reflects their own fieldwork experiences and autoethnographic stories.
QDA Strategy: To Compute
To compute in QDA is to employ specialized software programs for qualitative data management and analysis.
CAQDAS is an acronym for Computer Assisted Qualitative Data Analysis Software. There are diverse opinions among practitioners in the field about the utility of such specialized programs for qualitative data management and analysis. The software, unlike statistical computation, does not actually analyze data for you at higher conceptual levels. CAQDAS software packages serve primarily as a repository for your data (both textual and visual) that enable you to code them, and they can perform such functions as calculate the number of times a particular word or phrase appears in the data corpus (a particularly useful function for content analysis) and can display selected facets after coding, such as possible interrelationships. Certainly, basic word-processing software such as Microsoft Word, Excel, and Access provide utilities that can store and, with some pre-formatting and strategic entry, organize qualitative data to enable the researcher’s analytic review. The following internet addresses are listed to help in exploriong these CAQDAS packages and obtaining demonstration/trial software and tutorials:
AnSWR: www.cdc.gov/hiv/topics/surveillance/resources/software/answr
ATLAS.ti: www.atlasti.com
Coding Analysis Toolkit (CAT): cat.ucsur.pitt.edu/
Dedoose: www.dedoose.com
HyperRESEARCH: www.researchware.com
MAXQDA: www.maxqda.com
NVivo: www.qsrinternational.com
QDA Miner: www.provalisresearch.com
Qualrus: www.qualrus.com
Transana (for audio and video data materials): www.transana.org
Weft QDA: www.pressure.to/qda/
Some qualitative researchers attest that the software is indispensable for qualitative data management, especially for large-scale studies. Others feel that the learning curve of CAQDAS is too lengthy to be of pragmatic value, especially for small-scale studies. From my own experience, if you have an aptitude for picking up quickly on the scripts of software programs, explore one or more of the packages listed. If you are a novice to qualitative research, though, I recommend working manually or “by hand” for your first project so you can focus exclusively on the data and not on the software.
QDA Strategy: To Verify
To verify in QDA is to administer an audit of “quality control” to your analysis.
After your data analysis and the development of key findings, you may be thinking to yourself, “Did I get it right?” “Did I learn anything new?” Reliability and validity are terms and constructs of the positivist quantitative paradigm that refer to the replicability and accuracy of measures. But in the qualitative paradigm, other constructs are more appropriate.
Credibility and trustworthiness ( Lincoln & Guba, 1985 ) are two factors to consider when collecting and analyzing the data and presenting your findings. In our qualitative research projects, we need to present a convincing story to our audiences that we “got it right” methodologically. In other words, the amount of time we spent in the field, the number of participants we interviewed, the analytic methods we used, the thinking processes evident to reach our conclusions, and so on should be “just right” to persuade the reader that we have conducted our jobs soundly. But remember that we can never conclusively “prove” something; we can only, at best, convincingly suggest. Research is an act of persuasion.
Credibility in a qualitative research report can be established through several ways. First, citing the key writers of related works in your literature review is a must. Seasoned researchers will sometimes assess whether a novice has “done her homework” by reviewing the bibliography or references. You need not list everything that seminal writers have published about a topic, but their names should appear at least once as evidence that you know the field’s key figures and their work.
Credibility can also be established by specifying the particular data analytic methods you employed (e.g., “Interview transcripts were taken through two cycles of process coding, resulting in five primary categories”), through corroboration of data analysis with the participants themselves (e.g., “I asked my participants to read and respond to a draft of this report for their confirmation of accuracy and recommendations for revision”) or through your description of how data and findings were substantiated (e.g., “Data sources included interview transcripts, participant observation field notes, and participant response journals to gather multiple perspectives about the phenomenon”).
Creativity scholar Sir Ken Robinson is attributed with offering this cautionary advice about making a convincing argument: “Without data, you’re just another person with an opinion.” Thus researchers can also support their findings with relevant, specific evidence by quoting participants directly and/or including field note excerpts from the data corpus. These serve both as illustrative examples for readers and to present more credible testimony of what happened in the field.
Trustworthiness , or providing credibility to the writing, is when we inform the reader of our research processes. Some make the case by stating the duration of fieldwork (e.g., “Seventy-five clock hours were spent in the field”; “The study extended over a twenty-month period”). Others put forth the amounts of data they gathered (e.g., “Twenty-seven individuals were interviewed”; “My field notes totaled approximately 250 pages”). Sometimes trustworthiness is established when we are up front or confessional with the analytic or ethical dilemmas we encountered (e.g., “It was difficult to watch the participant’s teaching effectiveness erode during fieldwork”; “Analysis was stalled until I recoded the entire data corpus with a new perspective.”).
The bottom line is that credibility and trustworthiness are matters of researcher honesty and integrity . Anyone can write that he worked ethically, rigorously, and reflexively, but only the writer will ever know the truth. There is no shame if something goes wrong with your research. In fact, it is more than likely the rule, not the exception. Work and write transparently to achieve credibility and trustworthiness with your readers.
The length of this article does not enable me to expand on other qualitative data analytic strategies, such as to conceptualize, abstract, theorize, and write. Yet there are even more subtle thinking strategies to employ throughout the research enterprise, such as to synthesize, problematize, persevere, imagine, and create. Each researcher has his or her own ways of working, and deep reflection (another strategy) on your own methodology and methods as a qualitative inquirer throughout fieldwork and writing provides you with metacognitive awareness of data analytic processes and possibilities.
Data analysis is one of the most elusive processes in qualitative research, perhaps because it is a backstage, behind-the-scenes, in-your-head enterprise. It is not that there are no models to follow. It is just that each project is contextual and case specific. The unique data you collect from your unique research design must be approached with your unique analytic signature. It truly is a learning-by-doing process, so accept that and leave yourself open to discovery and insight as you carefully scrutinize the data corpus for patterns, categories, themes, concepts, assertions, and possibly new theories through strategic analysis.
Auerbach, C. F. , & Silverstein, L. B. ( 2003 ). Qualitative data: An introduction to coding and analysis . New York: New York University Press.
Google Scholar
Google Preview
Birks, M. , & Mills, J. ( 2011 ). Grounded theory: A practical guide . London: Sage.
Boyatzis, R. E. ( 1998 ). Transforming qualitative information: Thematic analysis and code development . Thousand Oaks, CA: Sage.
Bryant, A. , & Charmaz, K. (Eds.). ( 2007 ). The Sage handbook of grounded theory . London: Sage.
Charmaz, K. ( 2006 ). Constructing grounded theory: A practical guide through qualitative analysis . Thousand Oaks, CA: Sage.
Erickson, F. ( 1986 ). Qualitative methods in research on teaching. In M. C. Wittrock (Ed.), Handbook of research on teaching (3rd ed.) (pp. 119–161). New York: Macmillan.
Geertz, C. ( 1983 ). Local knowledge: Further essays in interpretive anthropology . New York: Basic Books.
Gibbs, G. R. ( 2007 ). Analysing qualitative data . London: Sage.
Lincoln, Y. S. , & Guba, E. G. ( 1985 ). Naturalistic inquiry . Newbury Park, CA: Sage.
Miles, M. B. , & Huberman, A. M. ( 1994 ). Qualitative data analysis (2nd ed.). Thousand Oaks, CA: Sage.
Saldaña, J. ( 2009 ). The coding manual for qualitative researchers . London: Sage.
Saldaña, J. ( 2011 ). Fundamentals of qualitative research . New York: Oxford University Press.
Saldaña, J. ( 2013 ). The coding manual for qualitative researchers (2nd ed.). London: Sage.
Shank, G. ( 2008 ). Abduction. In L. M. Given (Ed.), The Sage encyclopedia of qualitative research methods (pp. 1–2). Thousand Oaks, CA: Sage.
Stake, R. E. ( 1995 ). The art of case study research . Thousand Oaks, CA: Sage.
Stern, P. N. , & Porr, C. J. ( 2011 ). Essentials of accessible grounded theory . Walnut Creek, CA: Left Coast Press.
Strauss, A. L. ( 1987 ). Qualitative analysis for social scientists . Cambridge: Cambridge University Press.
Sunstein, B. S. , & Chiseri-Strater, E. ( 2012 ). FieldWorking: Reading and writing research (4th ed.). Boston: Bedford/St. Martin’s.
Wertz, F. J. , Charmaz, K. , McMullen, L. M. , Josselson, R. , Anderson, R. , & McSpadden, E. ( 2011 ). Fives ways of doing qualitative analysis: Phenomenological psychology, grounded theory, discourse analysis, narrative research, and intuitive inquiry . New York: Guilford.
- About Oxford Academic
- Publish journals with us
- University press partners
- What we publish
- New features
- Open access
- Institutional account management
- Rights and permissions
- Get help with access
- Accessibility
- Advertising
- Media enquiries
- Oxford University Press
- Oxford Languages
- University of Oxford
Oxford University Press is a department of the University of Oxford. It furthers the University's objective of excellence in research, scholarship, and education by publishing worldwide
- Copyright © 2024 Oxford University Press
- Cookie settings
- Cookie policy
- Privacy policy
- Legal notice
This Feature Is Available To Subscribers Only
Sign In or Create an Account
This PDF is available to Subscribers Only
For full access to this pdf, sign in to an existing account, or purchase an annual subscription.
Research, Digital, UX and a PhD.
A Guide to Coding Qualitative Data
Published September 18, 2014 by Salma Patel
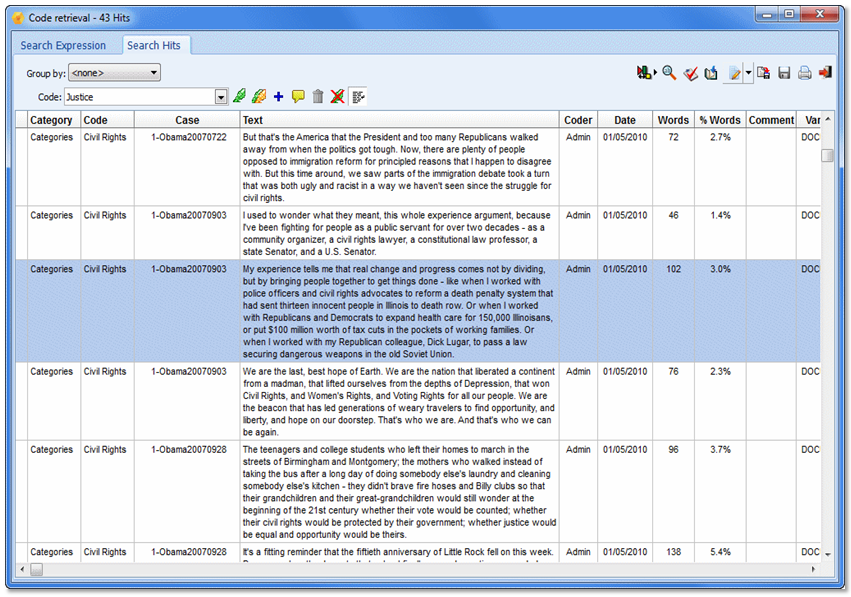
Coding qualitative data can be a daunting task, especially for the first timer. Below are my notes, which is a useful summary on coding qualitative data (please note, most of the text has been taken directly from The Coding Manual for Qualitative Researchers by Johnny Saldana ).
Background to Coding
A coding pattern can be characterised by:
- similarity (things happen the same way)
- difference (they happen in predictably different ways)
- frequency (they happen often or seldom)
- sequence (they happen in a certain order)
- correspondence (they happen in relation to other activities or events)
- causation (one appears to cause another)
A theme is an outcome of coding
Questions to consider when you are coding:
- what are people doing? What are they trying to accomplish?
- How, exactly, do they do this? What specific means and/or strategies do they use?
- How do members talk about, characterise, and understand what is going on?
- what assumptions are they making?
- what do I see going on here?
- what did I learn from these notes?
- why did I include them?
- what surprised me? (To track your assumptions)
- what intrigued me? (To track your positionality)
- what disturbed me? (To track the tensions within your value, attitude, and belief systems)
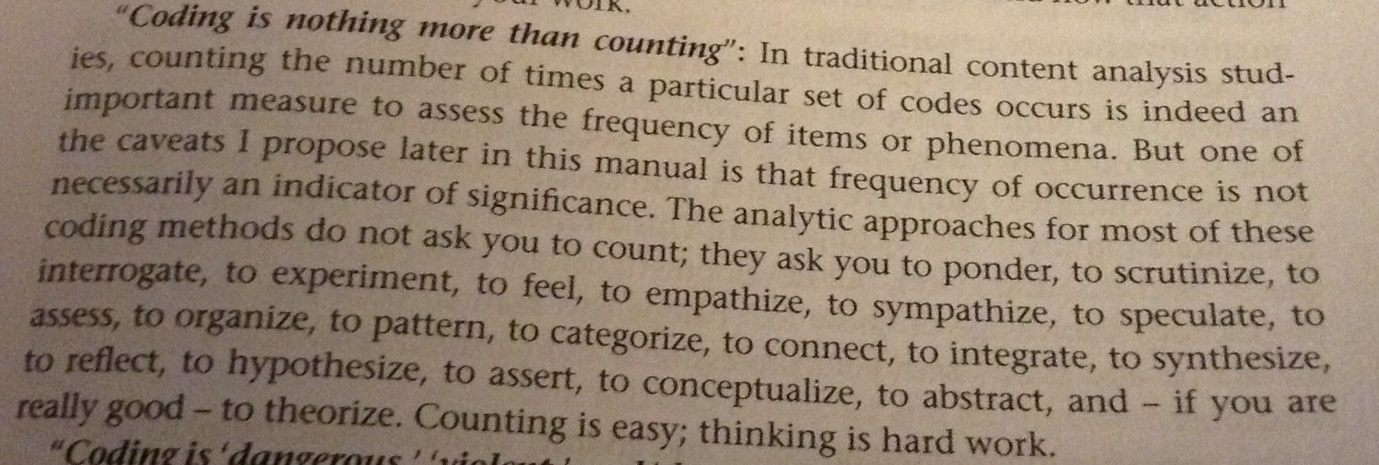
Writing Analytic Memos
Gordon-Finlayson (2010) emphasises that “coding is simply a structure on which reflection (via memo writing) happens. It is memo-writing that is the engine go grounded theory, not coding”. Glazer and Holton (2004) further clarify that “Memos present hypotheses about connections between categories and/or their properties and begin to integrate these connections with clusters of other categories to generate the theory”.
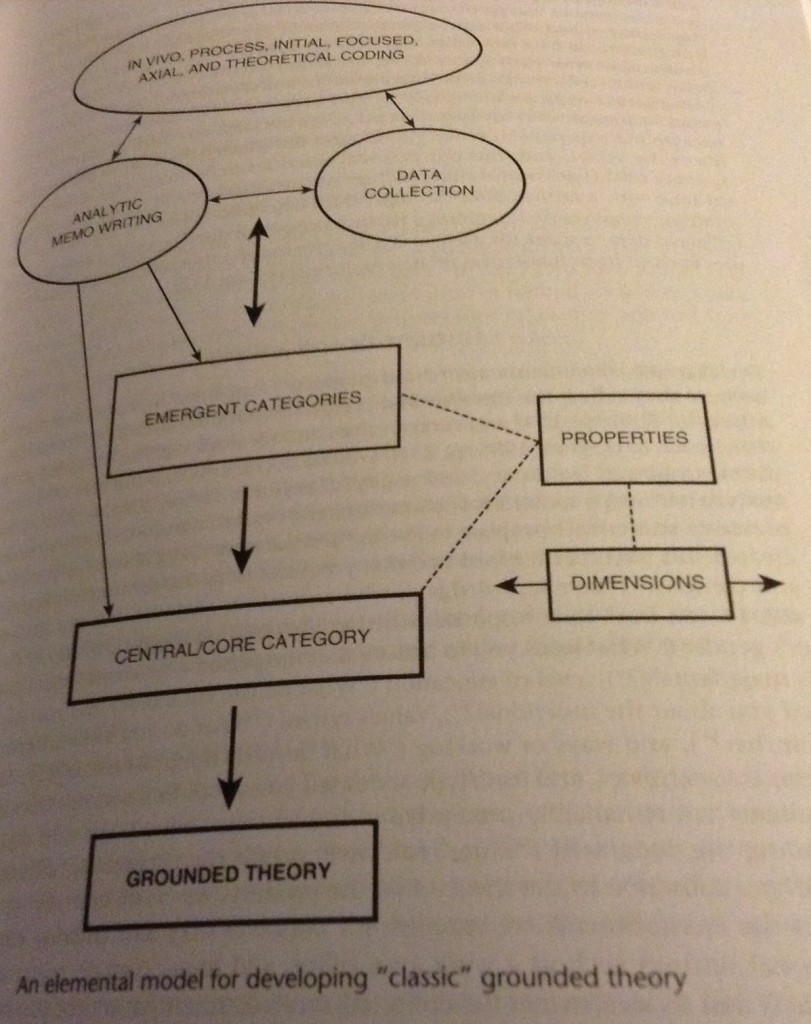
The coding cycles
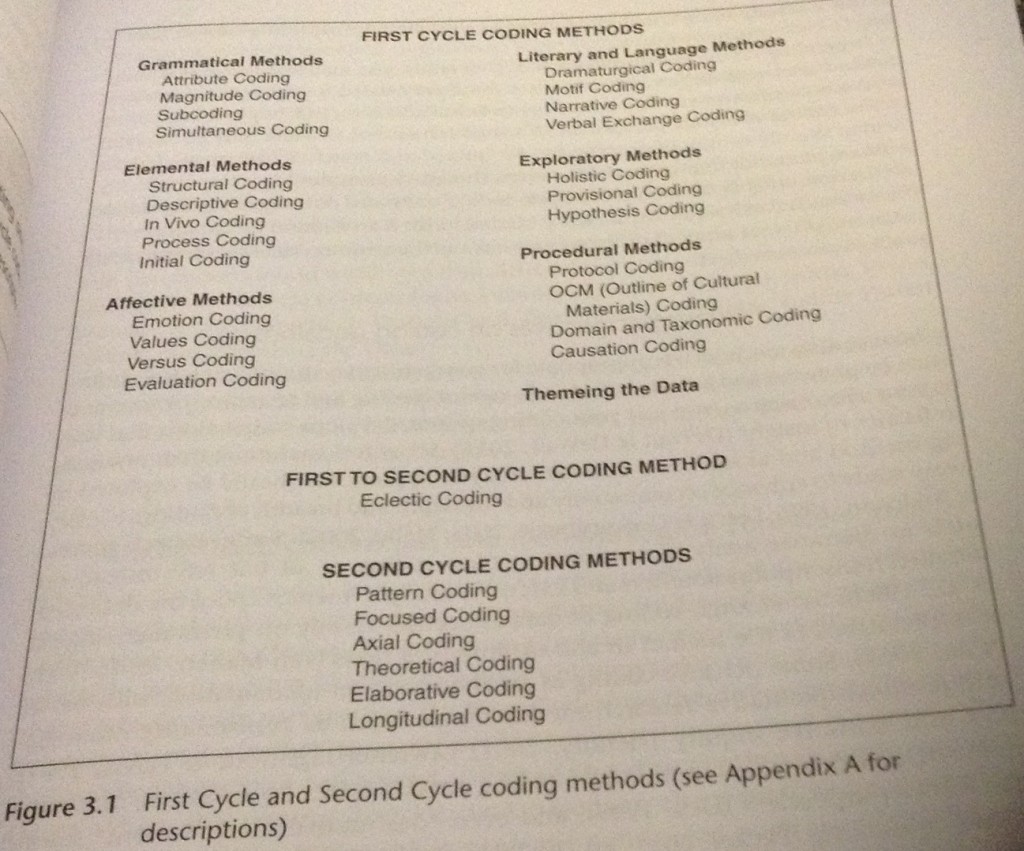
Depending on the qualitative coding method(s) you employ, the choice may have numerical conversion and transformation possibilities for basic descriptive statistics for mixed method studies.
First Cycle Coding
1. Grammatical Methods include
- attribute coding (essential information about the data and demographic characteristics of the participants for future management and reference)
- magnitude coding (applies alphanumeric or symbolic codes to data, to describe their variable characteristics such as intensity or frequency, example, Strongly (STR) Moderately (MOD) No opinions (NO). They can be qualitative, quantitative and/or nominal indicators to enhance description, and it’s a way of quantitizing and qualitizing data
- sub coding and simultaneous coding.
2. Elemental methods are primary approaches to data analysis. They include:
- structural coding is a question-based code that acts as a labelling and index device, allowing researchers to quickly access data likely to be relevant to a particular analysis from a larger data set. It’s used as a categorisation technique for further qualitative data analysis.
- descriptive coding summarises in a word or noun the basic topic of a passage of qualitative data.
- In Vivo Coding refers to coding with a word or short phrase from the actual language found in the qualitative data record.
- Process coding uses gerunds (“-ing” words) exclusively to connote action in the data.
- Initial Coding is breaking down qualitative data into discrete parts, closely examining them, and comparing them for similarities and differences.
3. Affective methods investigate subjective qualities of human experience (eg emotions, values, conflicts, judgements) by directly acknowledging and naming those experiences. They include:
- Emotion coding labels the emotion recalled or experienced
- Values coding assess a participant’s integrated value, attitude, and belief systems. (side note: Questionnaires and surveys such as Likert scales and semantic differentials, are designed to collect and measure a participant’s values, attitudes, and beliefs about selected subjects).
- Versus Coding acknowledges that humans are frequently in conflict, and the codes identify which individuals, groups, or systems are struggling for power.
- Evaluation Coding focuses on how we can analyse data that judge the merit and worth of programs and policies.
4. Literary and Language Methods are a contemporary approach to the analysis of Oral communication. They include Dramaturgical Coding, Motif Coding, Narrative coding and Verbal Exchange Coding, and all explore underlying sociological, psychological and cultural constructs.
5. Exploratory Methods are preliminary assignment of codes to the data, after which the researcher might proceed to more specific First Cycle or Second Cycle coding methods.
- Holistic Coding applies a single code to each large unit of data in the corpus to capture a sense of the overall contents and the possible categories that may develop.
- Provisional Coding begins with a “start list” of researcher- generated codes based on what preparatory investigation suggest might appear in the data before they are analysed.
- Hypothesis Coding applies researcher-developed “hunches” of what might occur in the data before or after they have been initially analysed.
6. Procedural Methods consist of pre- established systems or very specific ways of analysing qualitative data. They include:
- Protocol Coding is coding data according to a pre-established, recommended, standardised or prescribed system.
- OCM (Outline of Cultural Materials) Coding is a systematic coding system for ethnographic studies.
- Domain and Taxonomic Coding is an ethnographic method for discovering the cultural knowledge people use to organise their behaviours and interpret their experiences.
- Causation coding is to locate, extract, and/or infer causal beliefs from qualitative data.
Code Mapping and Landscaping
Code Mapping is categorising and organising the codes, and code landscaping is presenting these codes in a visual manner, for example by using a Wordle graphic.
Operational Model Diagramming can be used to map or diagram the emergent sequences or networks of your codes and categories related to your study in a sophisticated way.
Second Cycle Coding
Second cycle coding is reorganising and condensing the vast array of initial analytic details into a “main dish”. They include:
1. Pattern coding is a way of grouping summaries into a smaller number of sets, themes, or constructs.
2. Focused coding searches for the most frequent or significant codes. It categorises coded data based on thematic or conceptual similarity
3. Axial coding describes a category’s properties and dimensions and explores how the categories and subcategories relate to each other.
4. Theoretical coding progresses towards discovering the central or core category that identifies the primary theme of the research
5. Elaborative coding builds on a previous study’s codes, categories, and themes while a current and related study is underway. This method employs additional qualitative data to support or modify the researcher’s observations developed in an earlier project.
6. Longitudinal coding is the attribution of selected change processes to qualitative data collected and compared across time.
After Second Cycle Coding
Code weaving is the actual integration of key code words and phrases into narrative form to see how the puzzle pieces for together. Codeweave the primary codes, categories, themes, and/or concepts of your analysis into as few sentences as possible. Try writing several variations to investigate how the items might interrelate, suggest causation, indicate a process, or work holistically to create a broader theme. Search for evidence in the data that supports your summary statements, and/or disconfirming evidence that suggests revision of your statements.
From Coding to Theorising
A social science theory has three main characteristics: it predicts and controls action through an if-then logic; explains how and/or why something happens by stating it’s cause(s); and provides insights and guidance for improving social life.
The stage at which I seem to find a theory emerging in my mind is when I create categories of categories.
Use categories and analytic memos as sources of theory.
If I cannot develop a theory, then I will be satisfied with my construction of a key assertion, a summative and data supported statement about the particulars of a research study, rather than generalisable and transferable meanings of my findings to other settings and contexts.
Findings at a glance can be presented as follows:
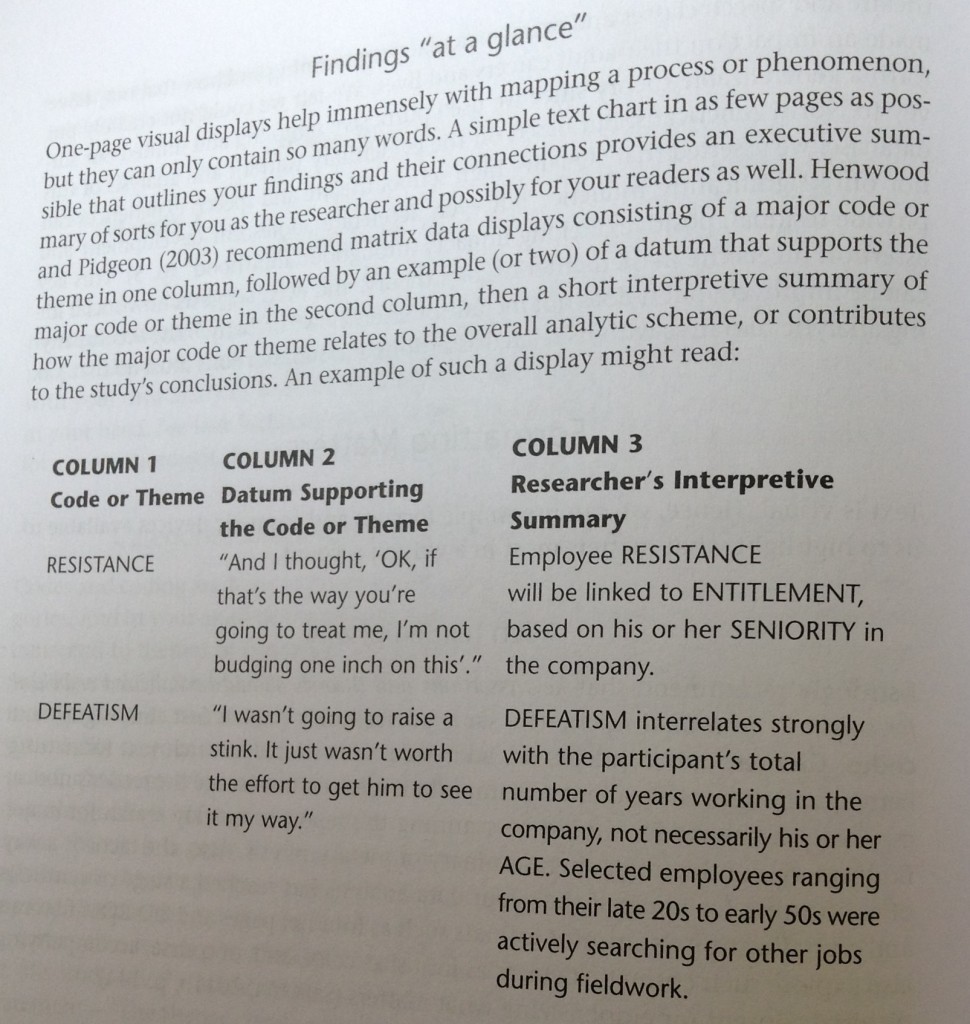
The coding journey should be noted in the analytical memos and discussed in your dissertation.
Related Posts:
- Thematic Analysis - how do you generate themes?
- Saturation in qualitative research samples
- Performance Analysis Workshop
- Notes from workshop (GDS): How to carry out basic…
- Forward Research Plan - Childminder service in public beta
Published in Headline Qualitative Research Research Methods
- coding data
- coding qualitative data
- qualitative research
- research design
10 Comments
Very helpful article. Can you please list the references you mentioned in the article? which book are you refering to explain the coding types?
Thanks. Which specific reference would you like? I can look it up in the book.
Same question. You show a number of books in the various images, none of which look familiar. Could you list/cite those resources? That would be most helpful
The reference is mentioned at the top of the article. It links to the book these images are all from. See here: https://www.amazon.co.uk/gp/product/1446247376 (Sadana, 2012)
Best wishes, Salma
I also got a a question regarding the second cycle coding. Is it possible to use multiple coding forms? For example, can I code my interviews by using pattern coding, focused coding and axial coding? Or would I have to decide on one?
Yes Lara, you can use multiple coding forms. Just keep a note of it for your write up.
Thank you so much, Salma Patel, for taking the time to lay this critical and complex process out with all the illustrations. It’s so helpful to me. May your work and life continue to flourish.
thank you so much Ms. Patel
great article. thanks a lot
Great article. Very helpful! Can you point us to any examples of how researchers have coded data using some of these techniques? It would be helpful to see this in action, especially in helping understand how these different aspects of coding sit side by side in the analytical process. Thanks again!
Leave a Reply Cancel reply
Your email address will not be published. Required fields are marked *
Save my name, email, and website in this browser for the next time I comment.
- AI & NLP
- Churn & Loyalty
- Customer Experience
- Customer Journeys
- Customer Metrics
- Feedback Analysis
- Product Experience
- Product Updates
- Sentiment Analysis
- Surveys & Feedback Collection
- Try Thematic
Welcome to the community
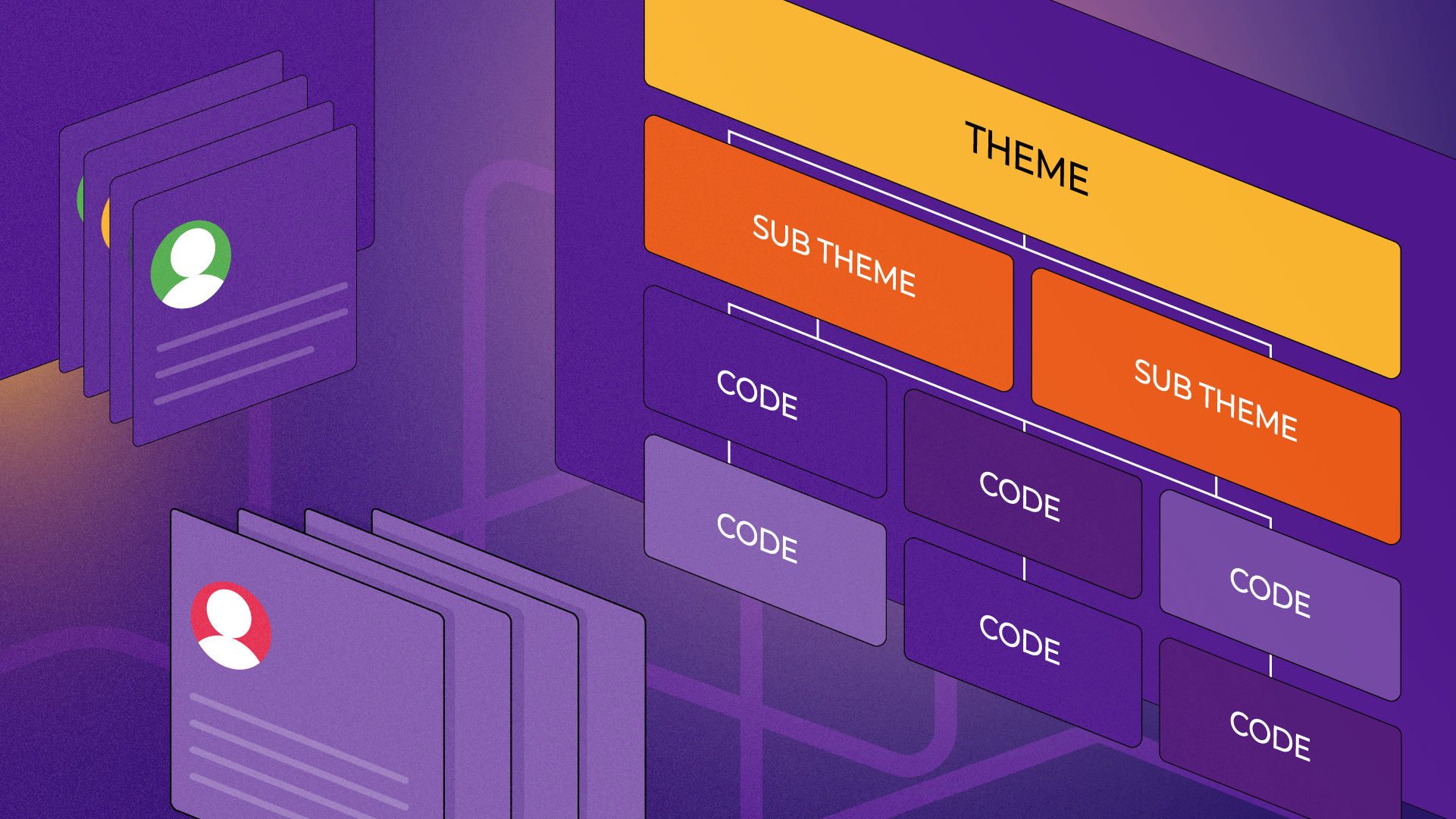
Coding Qualitative Data: How to Code Qualitative Research
How many hours have you spent sitting in front of Excel spreadsheets trying to find new insights from customer feedback?
You know that asking open-ended survey questions gives you more actionable insights than asking your customers for just a numerical Net Promoter Score (NPS) . But when you ask open-ended, free-text questions, you end up with hundreds (or even thousands) of free-text responses.
How can you turn all of that text into quantifiable, applicable information about your customers’ needs and expectations? By coding qualitative data.
Keep reading to learn:
- What coding qualitative data means (and why it’s important)
- Different methods of coding qualitative data
- How to manually code qualitative data to find significant themes in your data
What is coding in qualitative research?
Coding is the process of labeling and organizing your qualitative data to identify different themes and the relationships between them.
When coding customer feedback , you assign labels to words or phrases that represent important (and recurring) themes in each response. These labels can be words, phrases, or numbers; we recommend using words or short phrases, since they’re easier to remember, skim, and organize.
Coding qualitative research to find common themes and concepts is part of thematic analysis . Thematic analysis extracts themes from text by analyzing the word and sentence structure.
Within the context of customer feedback, it's important to understand the many different types of qualitative feedback a business can collect, such as open-ended surveys, social media comments, reviews & more.
What is qualitative data analysis?
Qualitative data analysis is the process of examining and interpreting qualitative data to understand what it represents.
Qualitative data is defined as any non-numerical and unstructured data; when looking at customer feedback, qualitative data usually refers to any verbatim or text-based feedback such as reviews, open-ended responses in surveys , complaints, chat messages, customer interviews, case notes or social media posts
For example, NPS metric can be strictly quantitative, but when you ask customers why they gave you a rating a score, you will need qualitative data analysis methods in place to understand the comments that customers leave alongside numerical responses.
Methods of qualitative data analysis
Thematic analysis.
This refers to the uncovering of themes, by analyzing the patterns and relationships in a set of qualitative data. A theme emerges or is built when related findings appear to be meaningful and there are multiple occurences. Thematic analysis can be used by anyone to transform and organize open-ended responses, online reviews and other qualitative data into significant themes.
Content analysis:
This refers to the categorization, tagging and thematic analysis of qualitative data. Essentially content analysis is a quantification of themes, by counting the occurrence of concepts, topics or themes. Content analysis can involve combining the categories in qualitative data with quantitative data, such as behavioral data or demographic data, for deeper insights.
Narrative analysis:
Some qualitative data, such as interviews or field notes may contain a story on how someone experienced something. For example, the process of choosing a product, using it, evaluating its quality and decision to buy or not buy this product next time. The goal of narrative analysis is to turn the individual narratives into data that can be coded. This is then analyzed to understand how events or experiences had an impact on the people involved.
Discourse analysis:
This refers to analysis of what people say in social and cultural context. The goal of discourse analysis is to understand user or customer behavior by uncovering their beliefs, interests and agendas. These are reflected in the way they express their opinions, preferences and experiences. It’s particularly useful when your focus is on building or strengthening a brand , by examining how they use metaphors and rhetorical devices.
Framework analysis:
When performing qualitative data analysis, it is useful to have a framework to organize the buckets of meaning. A taxonomy or code frame (a hierarchical set of themes used in coding qualitative data) is an example of the result. Don't fall into the trap of starting with a framework to make it faster to organize your data. You should look at how themes relate to each other by analyzing the data and consistently check that you can validate that themes are related to each other .
Grounded theory:
This method of analysis starts by formulating a theory around a single data case. Therefore the theory is “grounded’ in actual data. Then additional cases can be examined to see if they are relevant and can add to the original theory.
Why is it important to code qualitative data?
Coding qualitative data makes it easier to interpret customer feedback. Assigning codes to words and phrases in each response helps capture what the response is about which, in turn, helps you better analyze and summarize the results of the entire survey.
Researchers use coding and other qualitative data analysis processes to help them make data-driven decisions based on customer feedback. When you use coding to analyze your customer feedback, you can quantify the common themes in customer language. This makes it easier to accurately interpret and analyze customer satisfaction.
What is thematic coding?
Thematic coding, also called thematic analysis, is a type of qualitative data analysis that finds themes in text by analyzing the meaning of words and sentence structure.
When you use thematic coding to analyze customer feedback for example, you can learn which themes are most frequent in feedback. This helps you understand what drives customer satisfaction in an accurate, actionable way.
To learn more about how Thematic analysis software helps you automate the data coding process, check out this article .
Automated vs. Manual coding of qualitative data
Methods of coding qualitative data fall into three categories: automated coding and manual coding, and a blend of the two.
You can automate the coding of your qualitative data with thematic analysis software . Thematic analysis and qualitative data analysis software use machine learning, artificial intelligence (AI) , and natural language processing (NLP) to code your qualitative data and break text up into themes.
Thematic analysis software is autonomous , which means…
- You don’t need to set up themes or categories in advance.
- You don’t need to train the algorithm — it learns on its own.
- You can easily capture the “unknown unknowns” to identify themes you may not have spotted on your own.
…all of which will save you time (and lots of unnecessary headaches) when analyzing your customer feedback.
Businesses are also seeing the benefit of using thematic analysis software. The capacity to aggregate data sources into a single source of analysis helps to break down data silos, unifying the analysis and insights across departments . This is now being referred to as Omni channel analysis or Unified Data Analytics .
Use Thematic Analysis Software
Try Thematic today to discover why leading companies rely on the platform to automate the coding of qualitative customer feedback at scale. Whether you have tons of customer reviews, support chat or open-ended survey responses, Thematic brings every valuable insight to the surface, while saving you thousands of hours.
Advances in natural language processing & machine learning have made it possible to automate the analysis of qualitative data, in particular content and framework analysis. The most commonly used software for automated coding of qualitative data is text analytics software such as Thematic .
While manual human analysis is still popular due to its perceived high accuracy, automating most of the analysis is quickly becoming the preferred choice. Unlike manual analysis, which is prone to bias and doesn’t scale to the amount of qualitative data that is generated today, automating analysis is not only more consistent and therefore can be more accurate, but can also save a ton of time, and therefore money.
Our Theme Editor tool ensures you take a reflexive approach, an important step in thematic analysis. The drag-and-drop tool makes it easy to refine, validate, and rename themes as you get more data. By guiding the AI, you can ensure your results are always precise, easy to understand and perfectly aligned with your objectives.
Thematic is the best software to automate code qualitative feedback at scale.
Don't just take it from us. Here's what some of our customers have to say:
I'm a fan of Thematic's ability to save time and create heroes. It does an excellent job using a single view to break down the verbatims into themes displayed by volume, sentiment and impact on our beacon metric, often but not exclusively NPS.
It does a superlative job using GenAI in summarizing a theme or sub-theme down to a single paragraph making it clear what folks are trying to say. Peter K, Snr Research Manager.
Thematic is a very intuitive tool to use. It boasts a robust level of granularity, allowing the user to see the general breadth of verbatim themes, dig into the sub-themes, and further into the sentiment of the open text itself. Artem C, Sr Manager of Research. LinkedIn.
AI-powered software to transform qualitative data at scale through a thematic and content analysis.
How to manually code qualitative data
For the rest of this post, we’ll focus on manual coding. Different researchers have different processes, but manual coding usually looks something like this:
- Choose whether you’ll use deductive or inductive coding.
- Read through your data to get a sense of what it looks like. Assign your first set of codes.
- Go through your data line-by-line to code as much as possible. Your codes should become more detailed at this step.
- Categorize your codes and figure out how they fit into your coding frame.
- Identify which themes come up the most — and act on them.
Let’s break it down a little further…
Deductive coding vs. inductive coding
Before you start qualitative data coding, you need to decide which codes you’ll use.
What is Deductive Coding?
Deductive coding means you start with a predefined set of codes, then assign those codes to the new qualitative data. These codes might come from previous research, or you might already know what themes you’re interested in analyzing. Deductive coding is also called concept-driven coding.
For example, let’s say you’re conducting a survey on customer experience . You want to understand the problems that arise from long call wait times, so you choose to make “wait time” one of your codes before you start looking at the data.
The deductive approach can save time and help guarantee that your areas of interest are coded. But you also need to be careful of bias; when you start with predefined codes, you have a bias as to what the answers will be. Make sure you don’t miss other important themes by focusing too hard on proving your own hypothesis.
What is Inductive Coding?
Inductive coding , also called open coding, starts from scratch and creates codes based on the qualitative data itself. You don’t have a set codebook; all codes arise directly from the survey responses.
Here’s how inductive coding works:
- Break your qualitative dataset into smaller samples.
- Read a sample of the data.
- Create codes that will cover the sample.
- Reread the sample and apply the codes.
- Read a new sample of data, applying the codes you created for the first sample.
- Note where codes don’t match or where you need additional codes.
- Create new codes based on the second sample.
- Go back and recode all responses again.
- Repeat from step 5 until you’ve coded all of your data.
If you add a new code, split an existing code into two, or change the description of a code, make sure to review how this change will affect the coding of all responses. Otherwise, the same responses at different points in the survey could end up with different codes.
Sounds like a lot of work, right? Inductive coding is an iterative process, which means it takes longer and is more thorough than deductive coding. A major advantage is that it gives you a more complete, unbiased look at the themes throughout your data.
Combining inductive and deductive coding
In practice, most researchers use a blend of inductive and deductive approaches to coding.
For example, with Thematic, the AI inductively comes up with themes, while also framing the analysis so that it reflects how business decisions are made . At the end of the analysis, researchers use the Theme Editor to iterate or refine themes. Then, in the next wave of analysis, as new data comes in, the AI starts deductively with the theme taxonomy.
Categorize your codes with coding frames
Once you create your codes, you need to put them into a coding frame. A coding frame represents the organizational structure of the themes in your research. There are two types of coding frames: flat and hierarchical.
Flat Coding Frame
A flat coding frame assigns the same level of specificity and importance to each code. While this might feel like an easier and faster method for manual coding, it can be difficult to organize and navigate the themes and concepts as you create more and more codes. It also makes it hard to figure out which themes are most important, which can slow down decision making.
Hierarchical Coding Frame
Hierarchical frames help you organize codes based on how they relate to one another. For example, you can organize the codes based on your customers’ feelings on a certain topic:
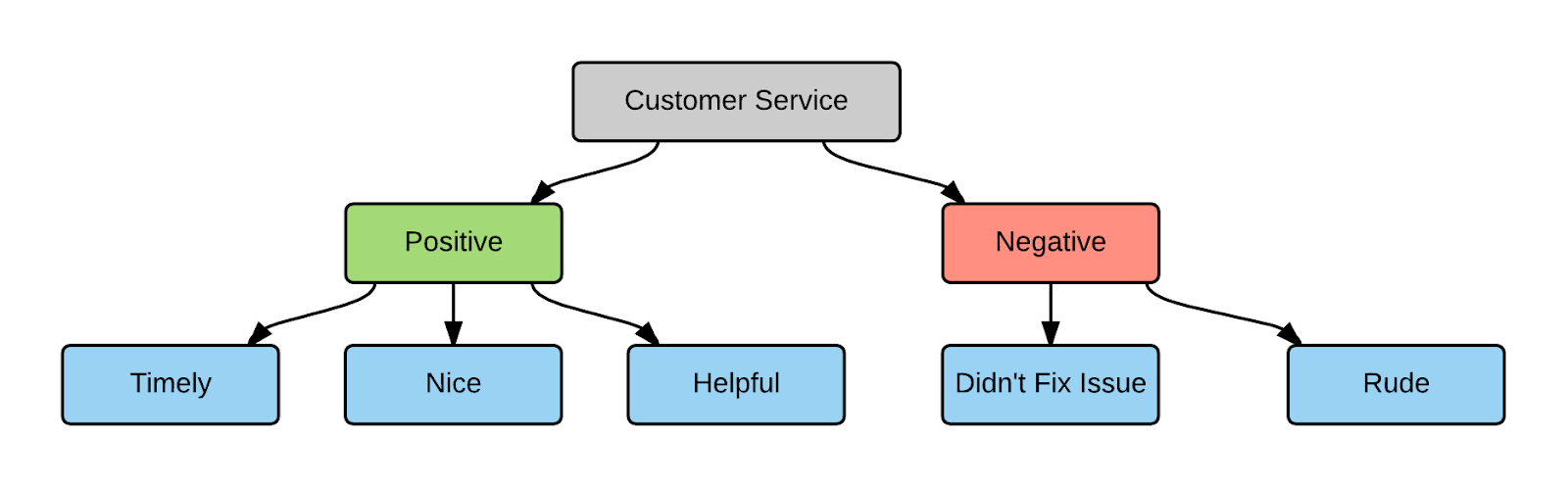
In this example:
- The top-level code describes the topic (customer service)
- The mid-level code specifies whether the sentiment is positive or negative
- The third level details the attribute or specific theme associated with the topic
Hierarchical framing supports a larger code frame and lets you organize codes based on organizational structure. It also allows for different levels of granularity in your coding.
Whether your code frames are hierarchical or flat, your code frames should be flexible. Manually analyzing survey data takes a lot of time and effort; make sure you can use your results in different contexts.
For example, if your survey asks customers about customer service, you might only use codes that capture answers about customer service. Then you realize that the same survey responses have a lot of comments about your company’s products. To learn more about what people say about your products, you may have to code all of the responses from scratch! A flexible coding frame covers different topics and insights, which lets you reuse the results later on.
Tips for manually coding qualitative data
Now that you know the basics of coding your qualitative data, here are some tips on making the most of your qualitative research.
Use a codebook to keep track of your codes
As you code more and more data, it can be hard to remember all of your codes off the top of your head. Tracking your codes in a codebook helps keep you organized throughout the data analysis process. Your codebook can be as simple as an Excel spreadsheet or word processor document. As you code new data, add new codes to your codebook and reorganize categories and themes as needed.
Make sure to track:
- The label used for each code
- A description of the concept or theme the code refers to
- Who originally coded it
- The date that it was originally coded or updated
- Any notes on how the code relates to other codes in your analysis
How to create high-quality codes - 4 tips
1. cover as many survey responses as possible..
The code should be generic enough to apply to multiple comments, but specific enough to be useful in your analysis. For example, “Product” is a broad code that will cover a variety of responses — but it’s also pretty vague. What about the product? On the other hand, “Product stops working after using it for 3 hours” is very specific and probably won’t apply to many responses. “Poor product quality” or “short product lifespan” might be a happy medium.
2. Avoid commonalities.
Having similar codes is okay as long as they serve different purposes. “Customer service” and “Product” are different enough from one another, while “Customer service” and “Customer support” may have subtle differences but should likely be combined into one code.
3. Capture the positive and the negative.
Try to create codes that contrast with each other to track both the positive and negative elements of a topic separately. For example, “Useful product features” and “Unnecessary product features” would be two different codes to capture two different themes.
4. Reduce data — to a point.
Let’s look at the two extremes: There are as many codes as there are responses, or each code applies to every single response. In both cases, the coding exercise is pointless; you don’t learn anything new about your data or your customers. To make your analysis as useful as possible, try to find a balance between having too many and too few codes.
Group responses based on themes, not words
Make sure to group responses with the same themes under the same code, even if they don’t use the same exact wording. For example, a code such as “cleanliness” could cover responses including words and phrases like:
- Looked like a dump
- Could eat off the floor
Having only a few codes and hierarchical framing makes it easier to group different words and phrases under one code. If you have too many codes, especially in a flat frame, your results can become ambiguous and themes can overlap. Manual coding also requires the coder to remember or be able to find all of the relevant codes; the more codes you have, the harder it is to find the ones you need, no matter how organized your codebook is.
Make accuracy a priority
Manually coding qualitative data means that the coder’s cognitive biases can influence the coding process. For each study, make sure you have coding guidelines and training in place to keep coding reliable, consistent, and accurate .
One thing to watch out for is definitional drift, which occurs when the data at the beginning of the data set is coded differently than the material coded later. Check for definitional drift across the entire dataset and keep notes with descriptions of how the codes vary across the results.
If you have multiple coders working on one team, have them check one another’s coding to help eliminate cognitive biases.
Conclusion: 6 main takeaways for coding qualitative data
Here are 6 final takeaways for manually coding your qualitative data:
- Coding is the process of labeling and organizing your qualitative data to identify themes. After you code your qualitative data, you can analyze it just like numerical data.
- Inductive coding (without a predefined code frame) is more difficult, but less prone to bias, than deductive coding.
- Code frames can be flat (easier and faster to use) or hierarchical (more powerful and organized).
- Your code frames need to be flexible enough that you can make the most of your results and use them in different contexts.
- When creating codes, make sure they cover several responses, contrast one another, and strike a balance between too much and too little information.
- Consistent coding = accuracy. Establish coding procedures and guidelines and keep an eye out for definitional drift in your qualitative data analysis.
Some more detail in our downloadable guide
If you’ve made it this far, you’ll likely be interested in our free guide: Best practices for analyzing open-ended questions.
The guide includes some of the topics covered in this article, and goes into some more niche details.
If your company is looking to automate your qualitative coding process, try Thematic !
If you're looking to trial multiple solutions, check out our free buyer's guide . It covers what to look for when trialing different feedback analytics solutions to ensure you get the depth of insights you need.
Happy coding!
Authored by Alyona Medelyan, PhD – Natural Language Processing & Machine Learning
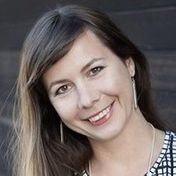
CEO and Co-Founder
Alyona has a PhD in NLP and Machine Learning. Her peer-reviewed articles have been cited by over 2600 academics. Her love of writing comes from years of PhD research.
We make it easy to discover the customer and product issues that matter.
Unlock the value of feedback at scale, in one platform. Try it for free now!
- Questions to ask your Feedback Analytics vendor
- How to end customer churn for good
- Scalable analysis of NPS verbatims
- 5 Text analytics approaches
- How to calculate the ROI of CX
Our experts will show you how Thematic works, how to discover pain points and track the ROI of decisions. To access your free trial, book a personal demo today.
Recent posts
When two major storms wreaked havoc on Auckland and Watercare’s infrastructurem the utility went through a CX crisis. With a massive influx of calls to their support center, Thematic helped them get inisghts from this data to forge a new approach to restore services and satisfaction levels.
Become a qualitative theming pro! Creating a perfect code frame is hard, but thematic analysis software makes the process much easier.
Qualtrics is one of the most well-known and powerful Customer Feedback Management platforms. But even so, it has limitations. We recently hosted a live panel where data analysts from two well-known brands shared their experiences with Qualtrics, and how they extended this platform’s capabilities. Below, we’ll share the

Holistic Thinking and Qualitative E-Research Design
by Janet Salmons, PhD Manager for Sage Research Methods Community Dr. Salmons is the author of a series of books, including a new edition of Doing Qualitative Research Online . She will be a research fellow at the Center for Advanced Internet Studies in Bochum, Germany in 2024. Dr. Salmons will be offering a special webinar from the Center for Advanced Internet Studies: “How to do research in a digital world.” Registration is now open for this free May 7 event. Find your time zone here . Use the code COMMUNIT24 for 25% off through December 31, 2024 when you purchase the book from Sage.
Qualitative research design is challenging. Technology adds new considerations.
All researchers are faced with a set of design decisions, and the challenge of creating a plan that shows how all the pieces fit together. Online researchers face additional challenges, because they must also consider the implications of the technologies used in the study. Technology tools and platforms are not neutral. The technology itself has an influence on the study, whether we are using online communications with stakeholders and participants, an app for a survey, or an online archive where we find extant data.
I created an E-Research Framework as a model researchers can use to evaluate their choices. It is presented as a circle to show that the design process is iterative and holistic, not linear.
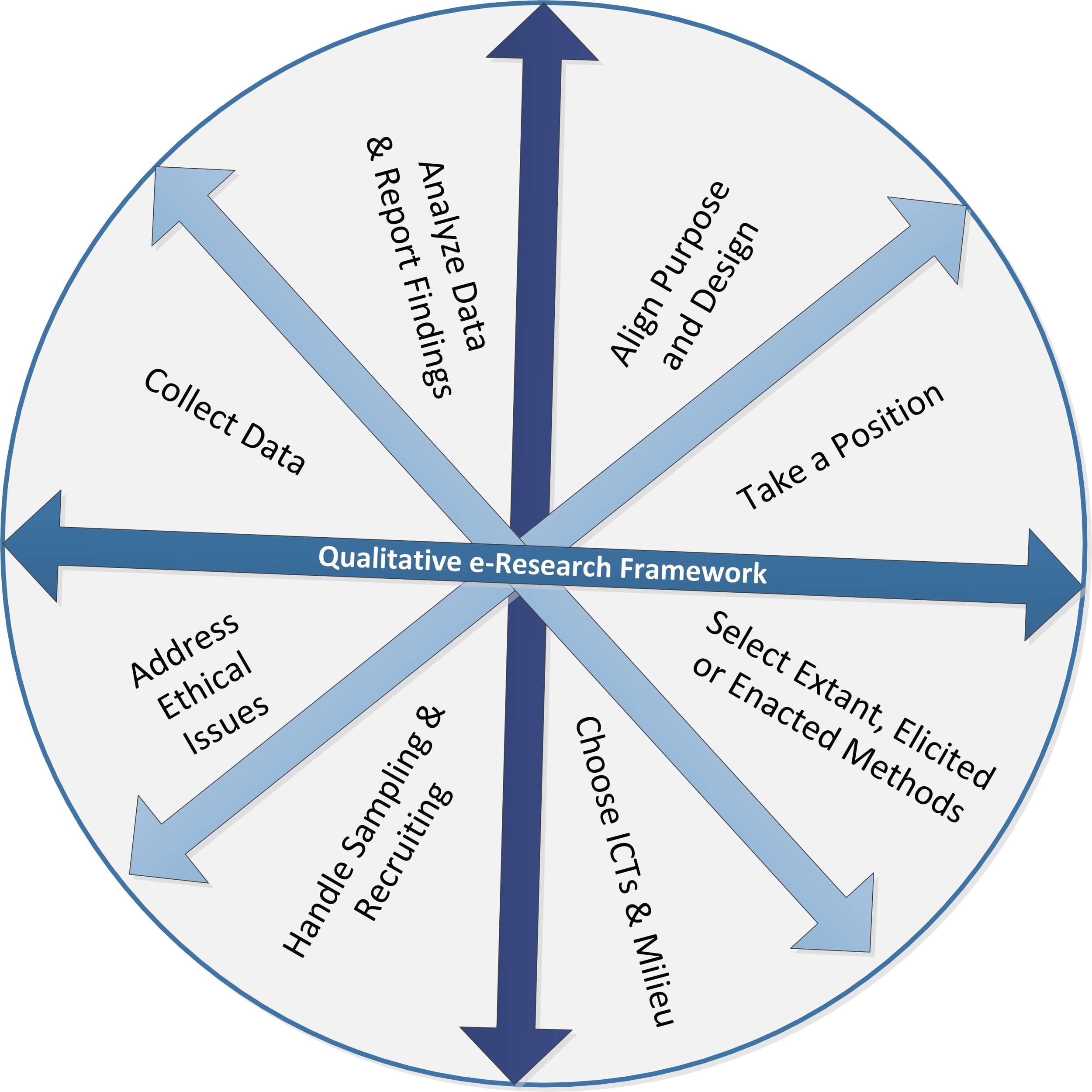
The Qualitative e-Research Framework (Salmons, 2012, 2016, 2022) c.vision2lead
Let’s look at key questions for each part of the framework.
Aligning purpose and design in qualitative e-research:
Are theories and epistemologies, methodologies, and methods appropriate for the study?
Have all elements of the design been fully addressed?
Taking a position as a researcher undertaking qualitative e-research:
Are you transparent about taking an insider or outsider position?
Do you explain implications related to your position, including any conflicts of interest or risks of researcher bias?
Selecting extant, elicited, or enacted methods for collecting data online:
Do data collection methods fit the purpose of the study, in the context of the research problem and population?
Are ICT functions, features, and/or limitations addressed with the selected approach(es)?
Are the specific methods (interviews, observations, document reviews, etc.) appropriate to the study?
Selecting information and communications technologies (ICTs) and the setting for qualitative e-research:
What ICT features will be used and why?
Will the study collect text-based, audio, and/or visual data?
Will the setting be in a public or private online milieu?
Handling sampling and recruiting in qualitative e-research:
Will the study engage human participants? If so, what sampling approaches are appropriate given the purpose of the study and target population?
Will participants be recruited online, if so, how?
In a study using extant data, how will archives or datasets be selected? What criteria will be used for selection of specific documents, posts or user-generated content?
Addressing ethical issues in qualitative e-research:
Have you taken appropriate steps to protect human subjects and, where appropriate, their avatars or online representations?
Have you obtained proper informed consent?
Do you have permission to access and use posts, documents, profiles, or images?
Have you committed to acting with integrity in all phases and contacts with others throughout the study?
Collecting the data online in qualitative e-research:
Are you experienced with all features of the selected technology?
For studies using extant methods, are you familiar with the setting, archive, databases, or other online environment where the data will be collected?
Are you familiar with programming or software tools needed to collect Big Data or to mine or trawl for data?
Do you have a plan for recording observations?
For studies using elicited or enacted methods, do you have a plan for conducting interviews, focus groups, or other interactions with participants with either prepared questions or a guide?
Analyzing the data and reporting on qualitative e-research:
Do you have a plan for preparing, organizing, and coding all types of data?
Do youthe proper permissions for using image, excerpts, or quotations in published reports?
For in-depth explanations and guidance, see Doing Qualitative Research Online . Use the code COMMUNIT24 for 25% off through December 31, 2024 when you purchase the book from Sage.
Learn more!
Find full text of the first edition of Doing Qualitative Research Online , as well as Qualitative Online Interviews and Cases in Online Interview Research, on SAGE Research Methods. If you don’t have access to this database through an academic library, you can explore it with a free trial .
More Sage Research Methods posts about online research methods
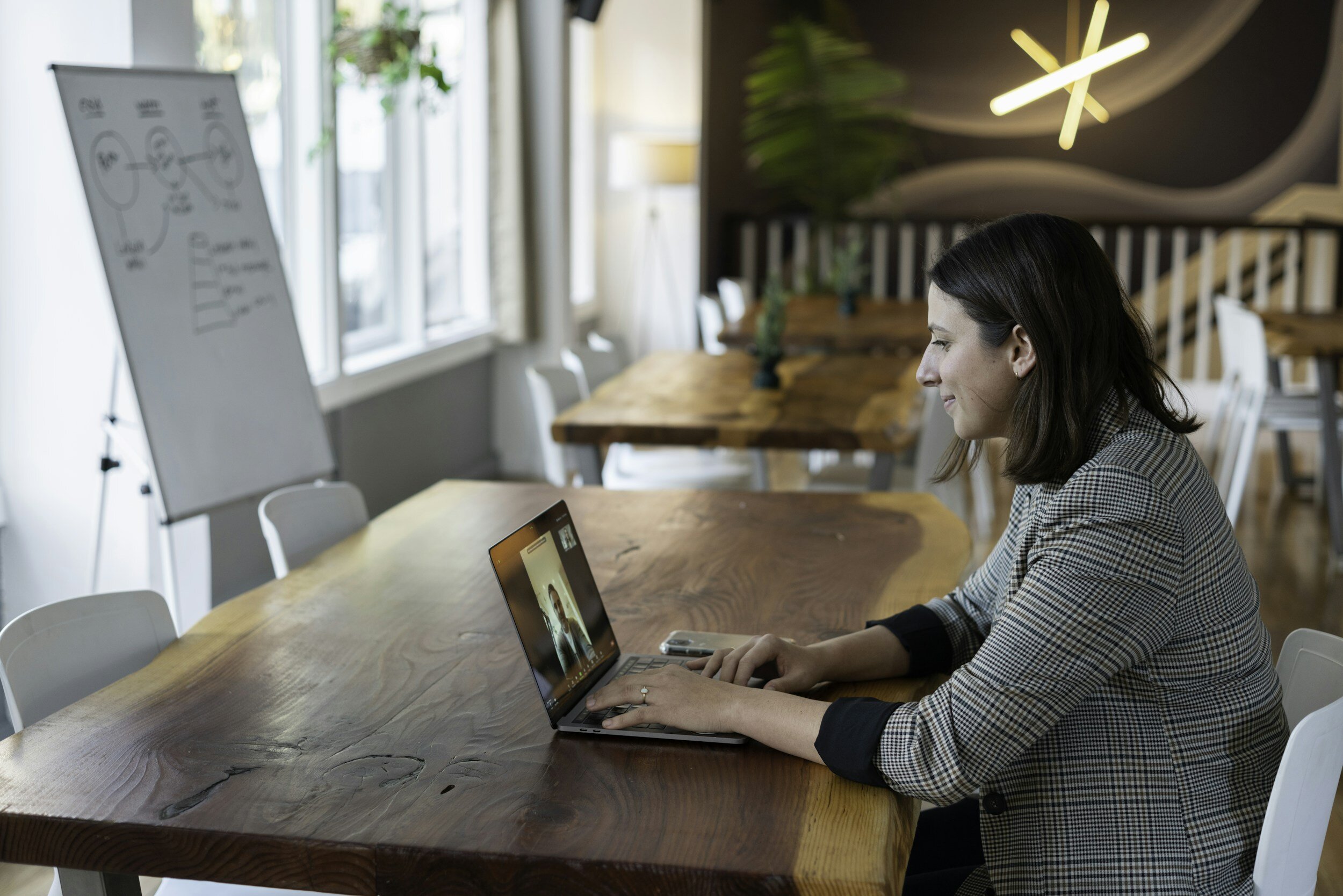
There are lots of questions to consider when using videoconference platforms for scholarly interviews.
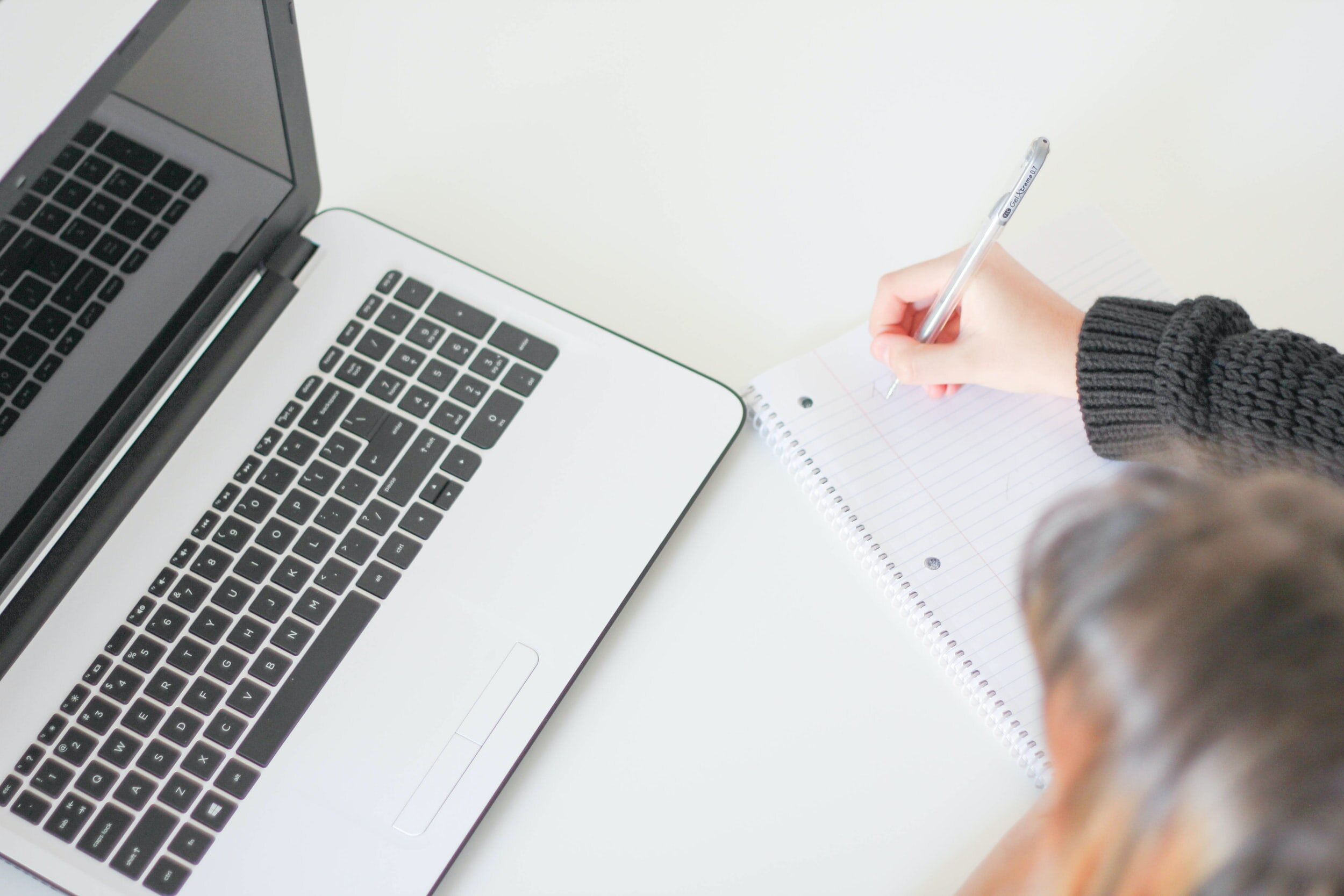
Suggestions and resources to help you collect data with online interviews.
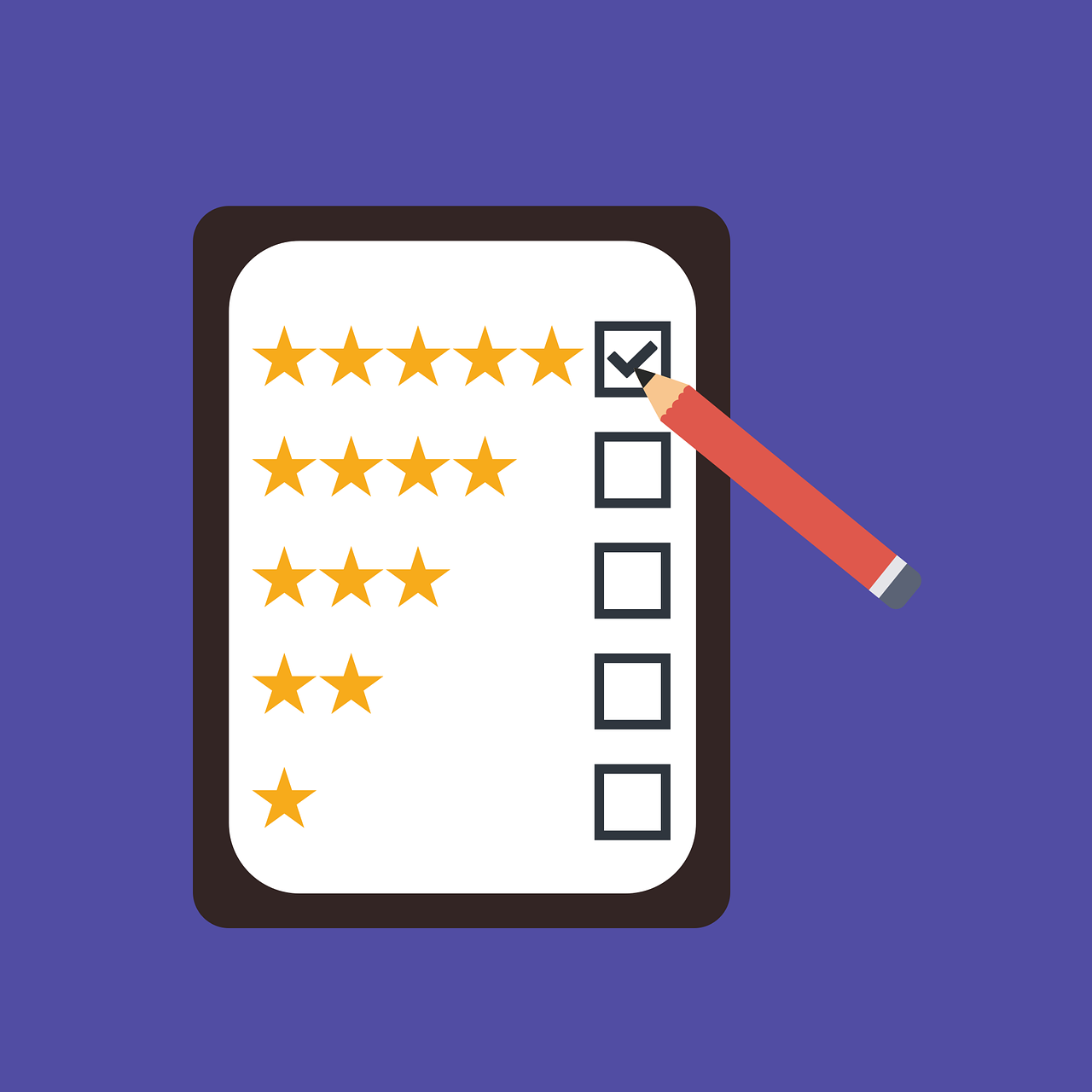
When survey tools were first available online, it seemed like the process of data collection would get much easier. Find open-access articles and author interviews.
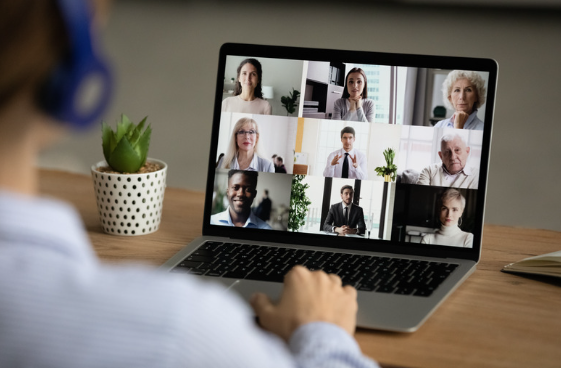
Learn about ethics and consent for online research from the perspective of an institutional review board member.
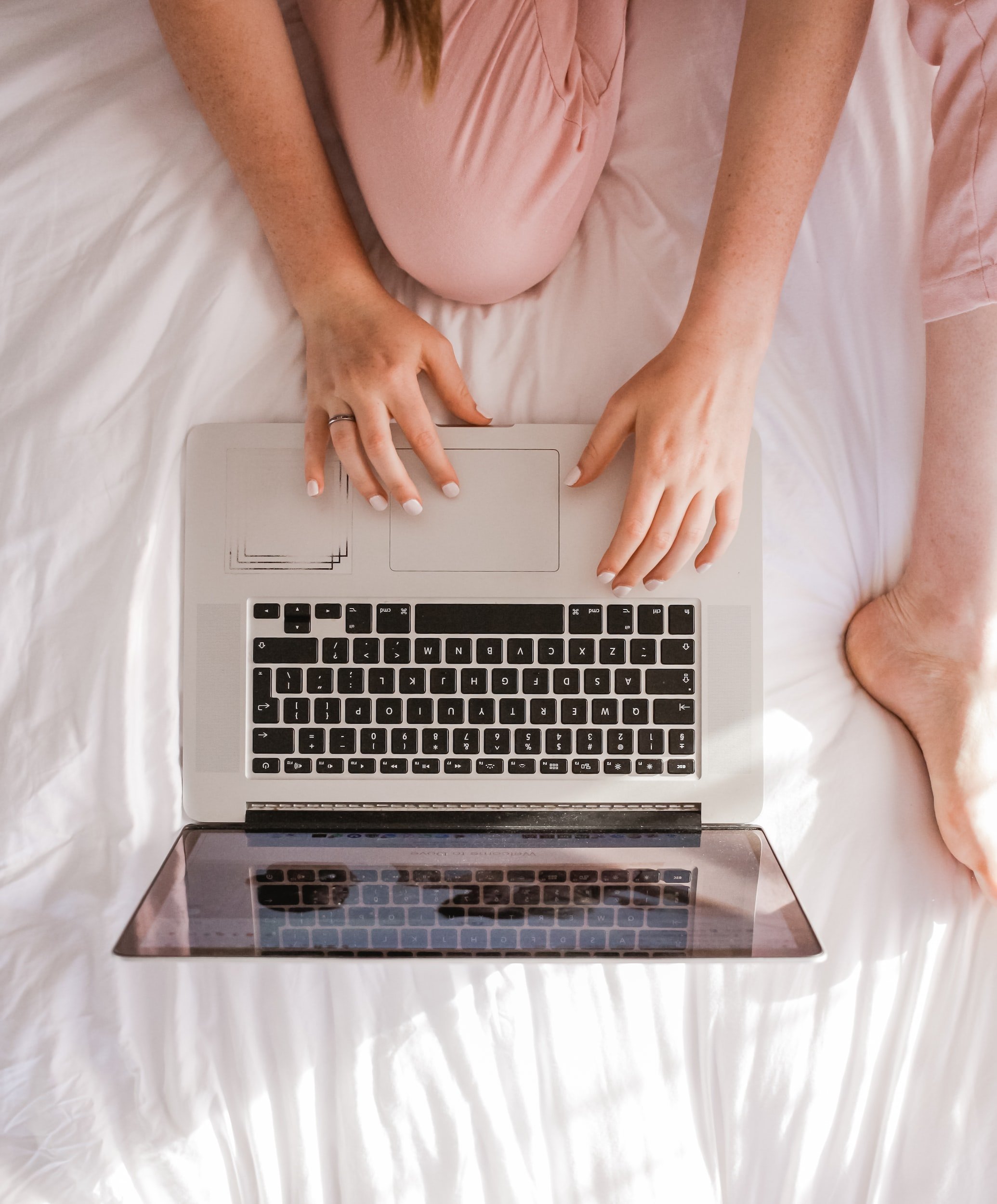
Thinking about designing a study using online surveys? Learn from these researchers’ experiences.
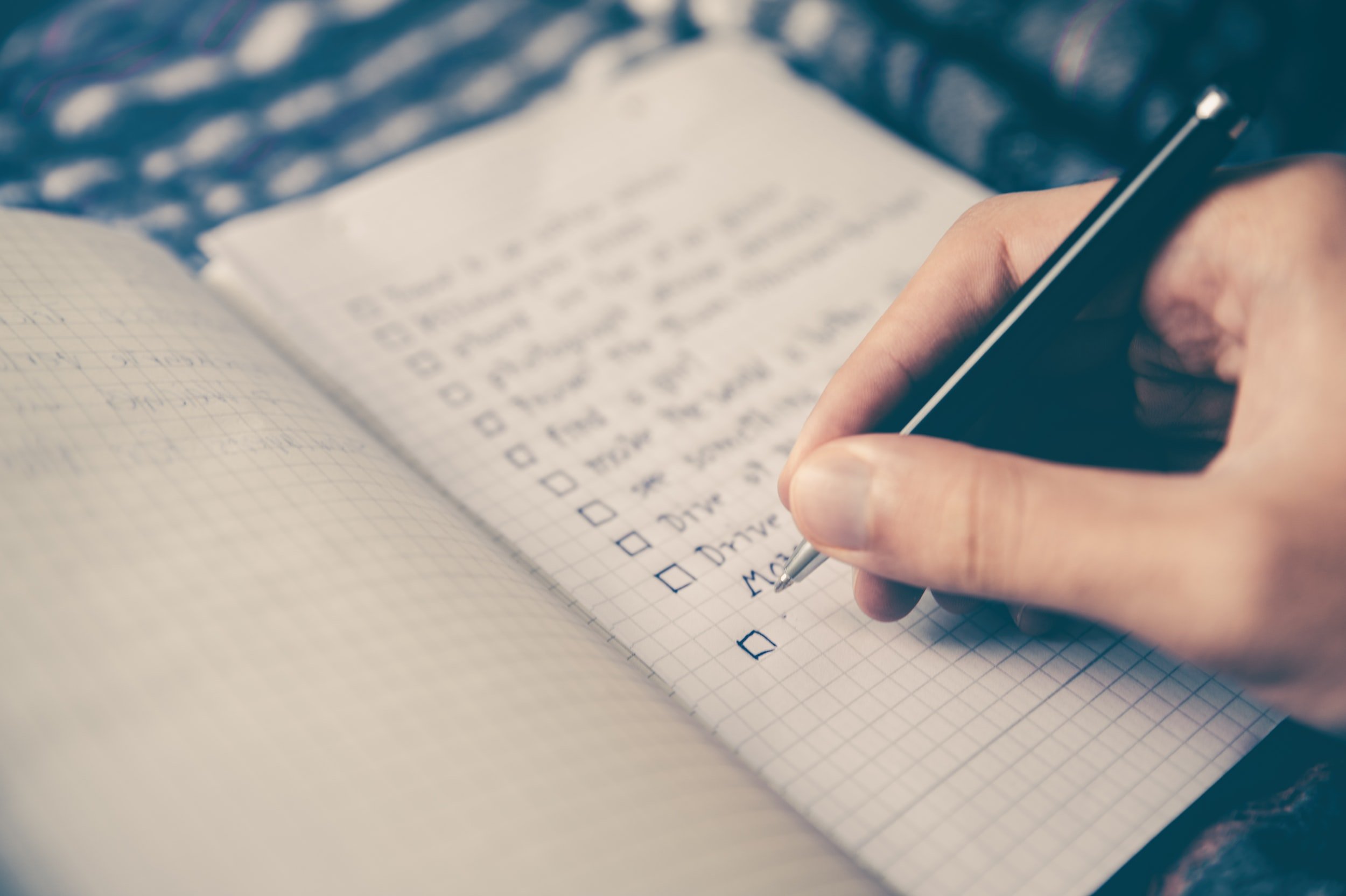
Ways researchers and participants relate can be collaborative, as Laura Wilson and Emma Dickinson discussed in this SAGE Methodspace interview.
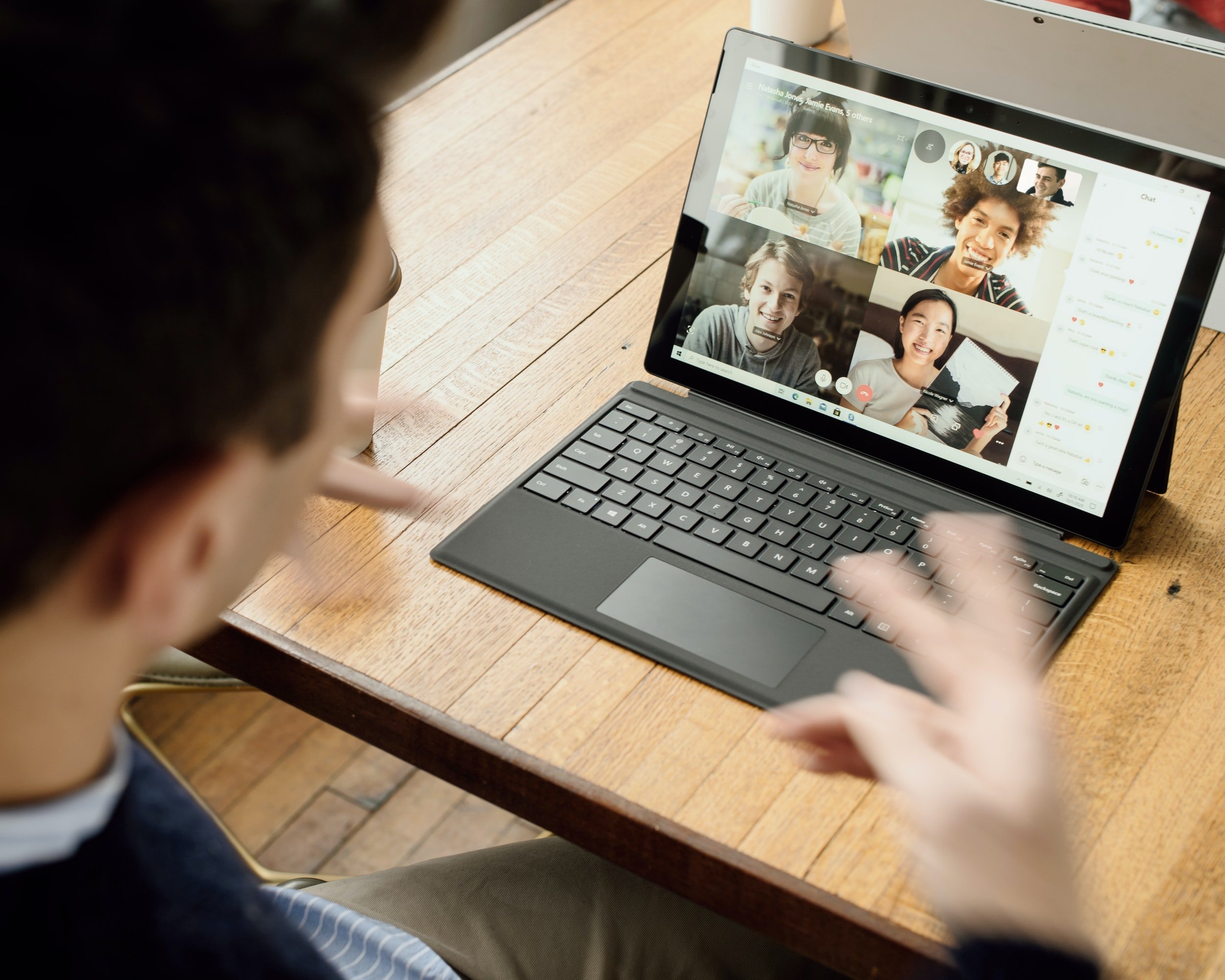
The steps you take to prepare for a videoconference presentation or meeting will make the difference between an engaging or a frustrating experience.
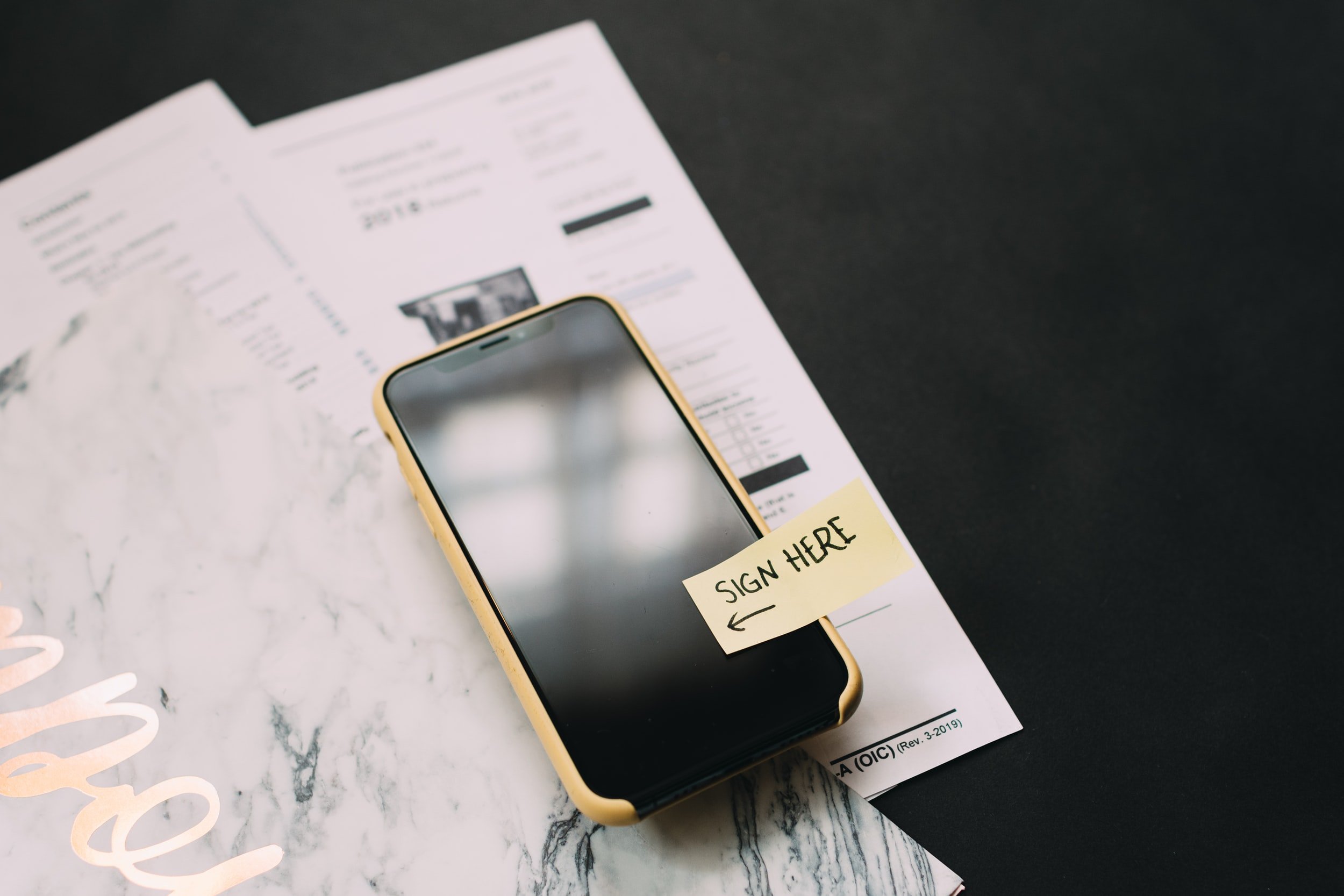
How can you reduce or eliminate fraudulent responses to online surveys? Jennifer Lawlor explains.
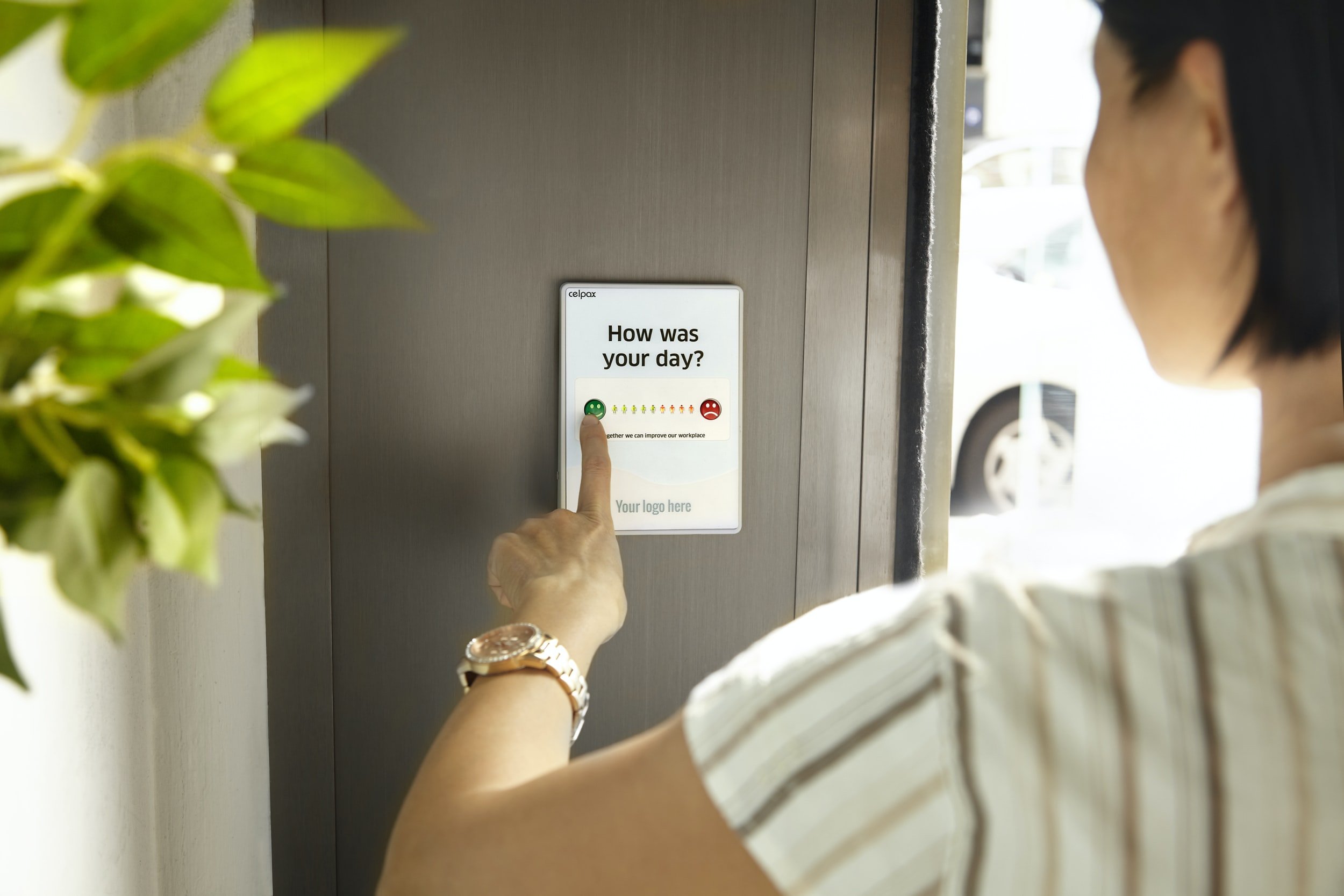
Dr. Jan Eichhorn offers tips for survey research.
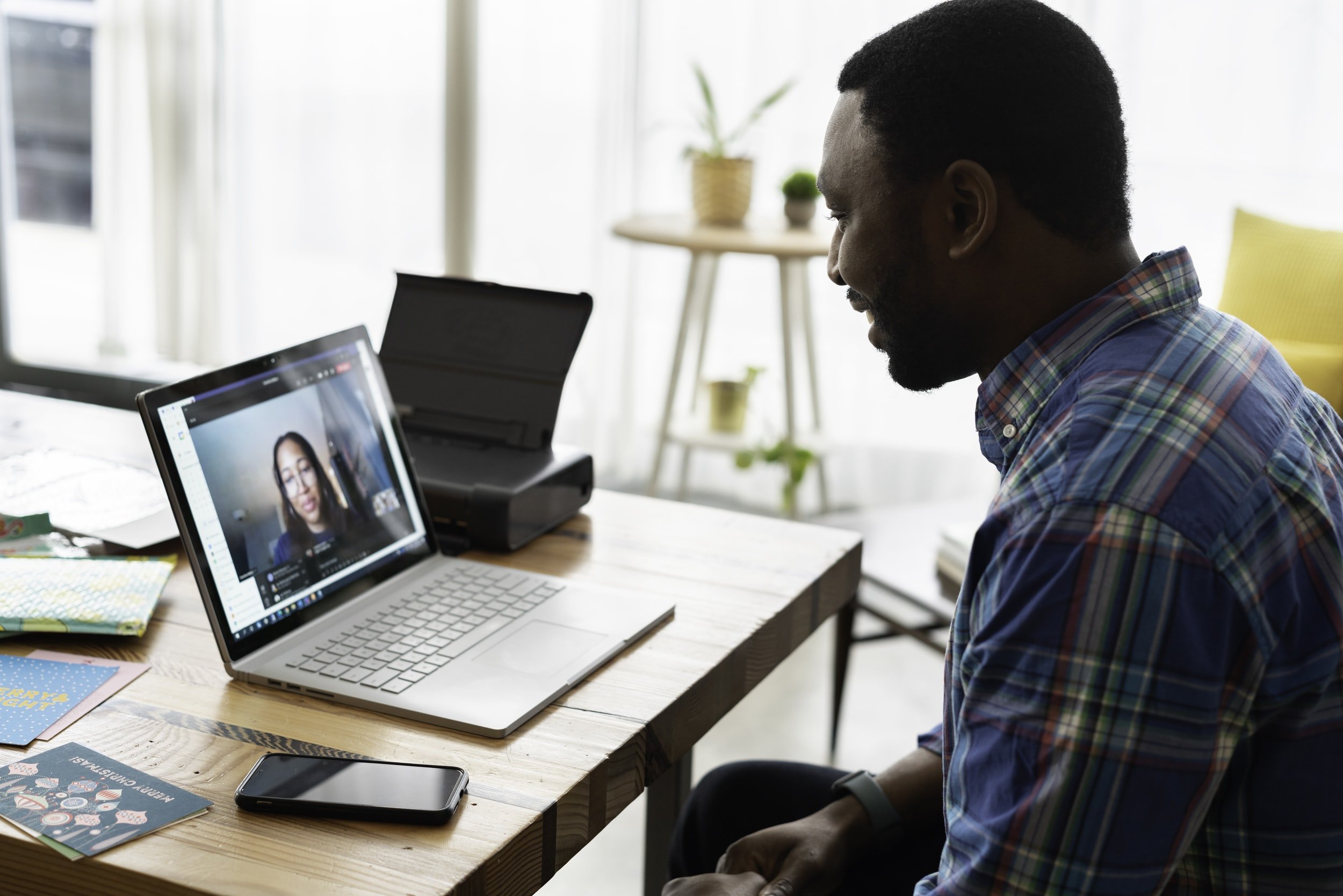
How can you interview participants online about sensitive topics? Tips from Janet Salmons, author of Doing Qualitative Research Online.
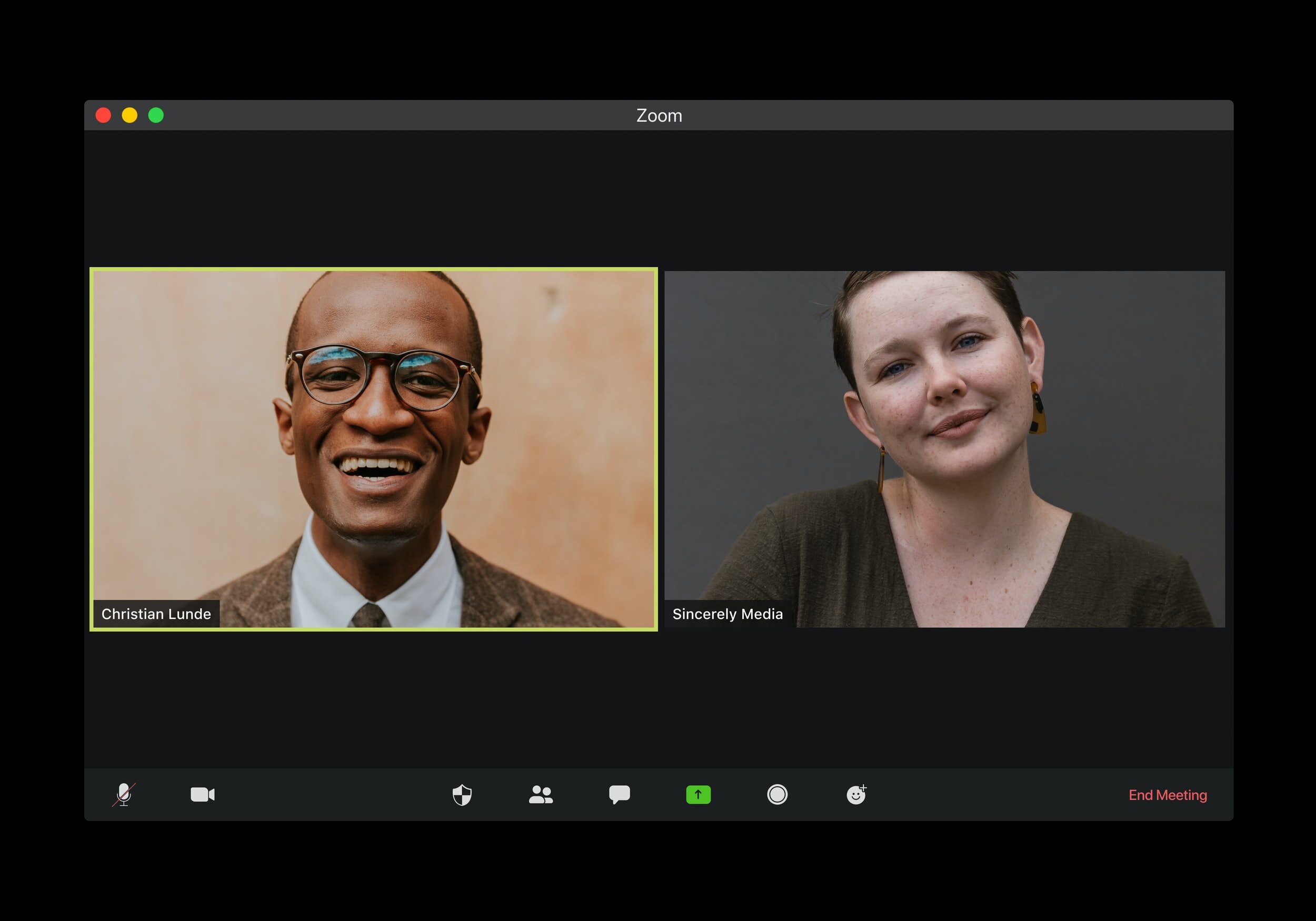
Find a new open access article with tips for online interviews.
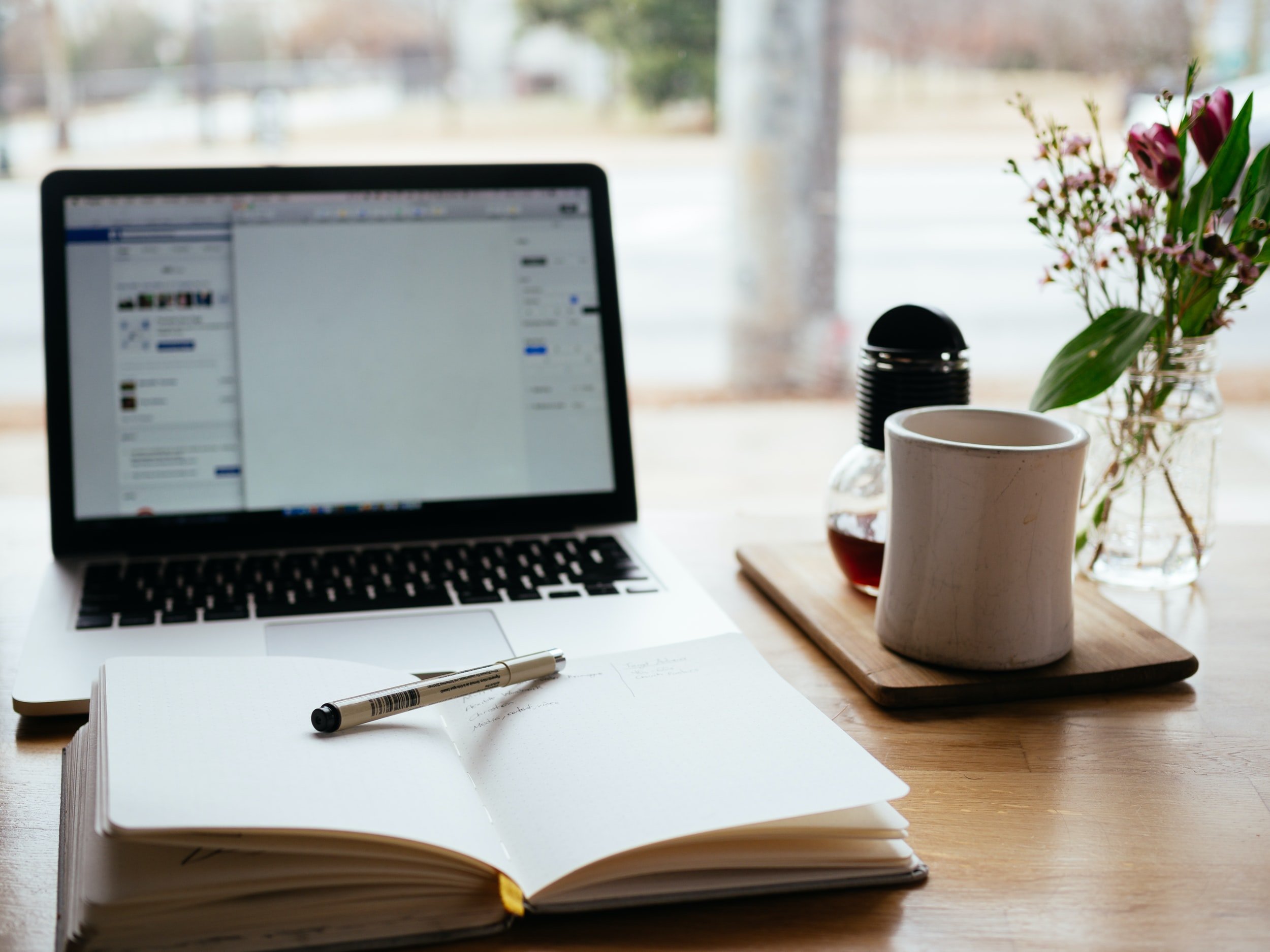
Interested in collecting data online from social media or participants? Join this webinar with Luke Sloan and Janet Salmons!
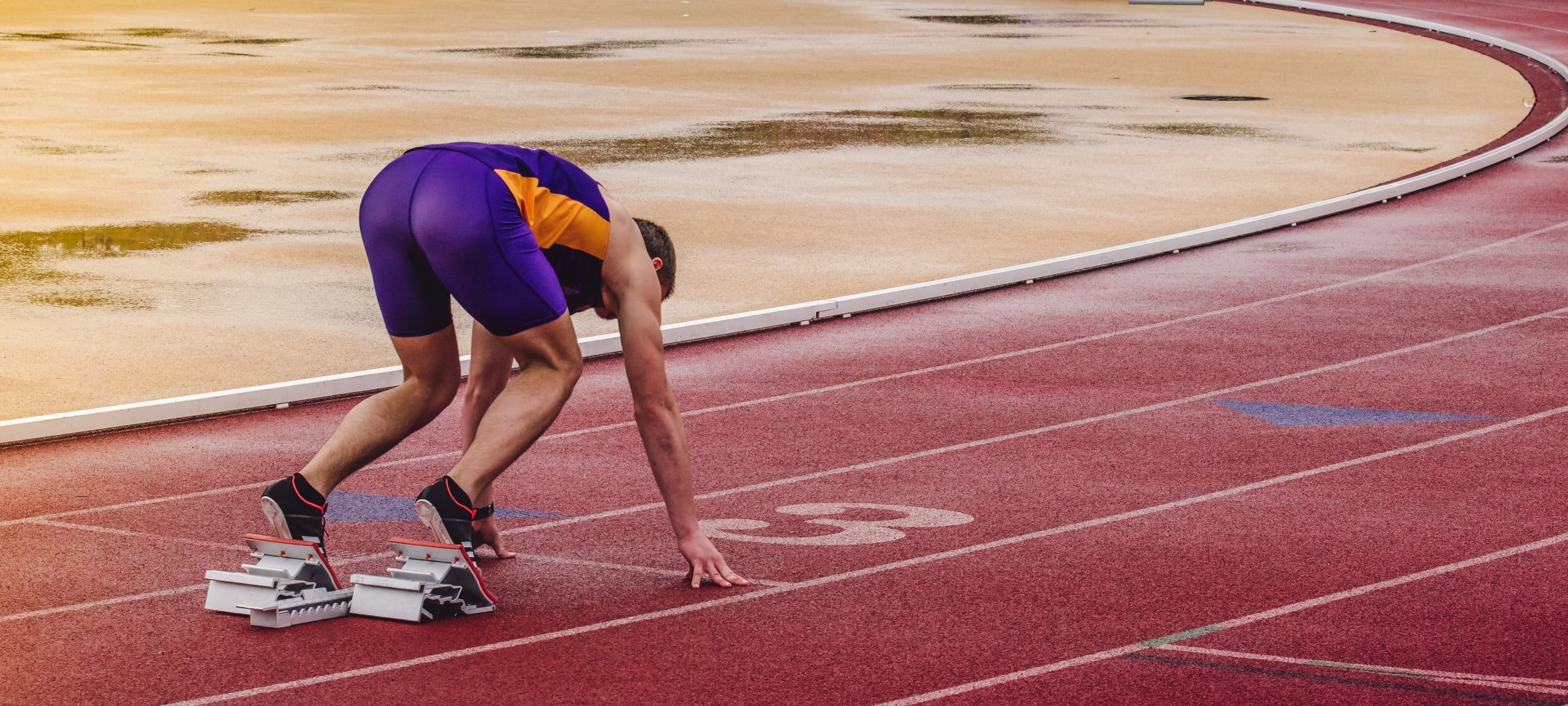
Want to prepare for this webinar? Register, then read our work and get your questions ready!
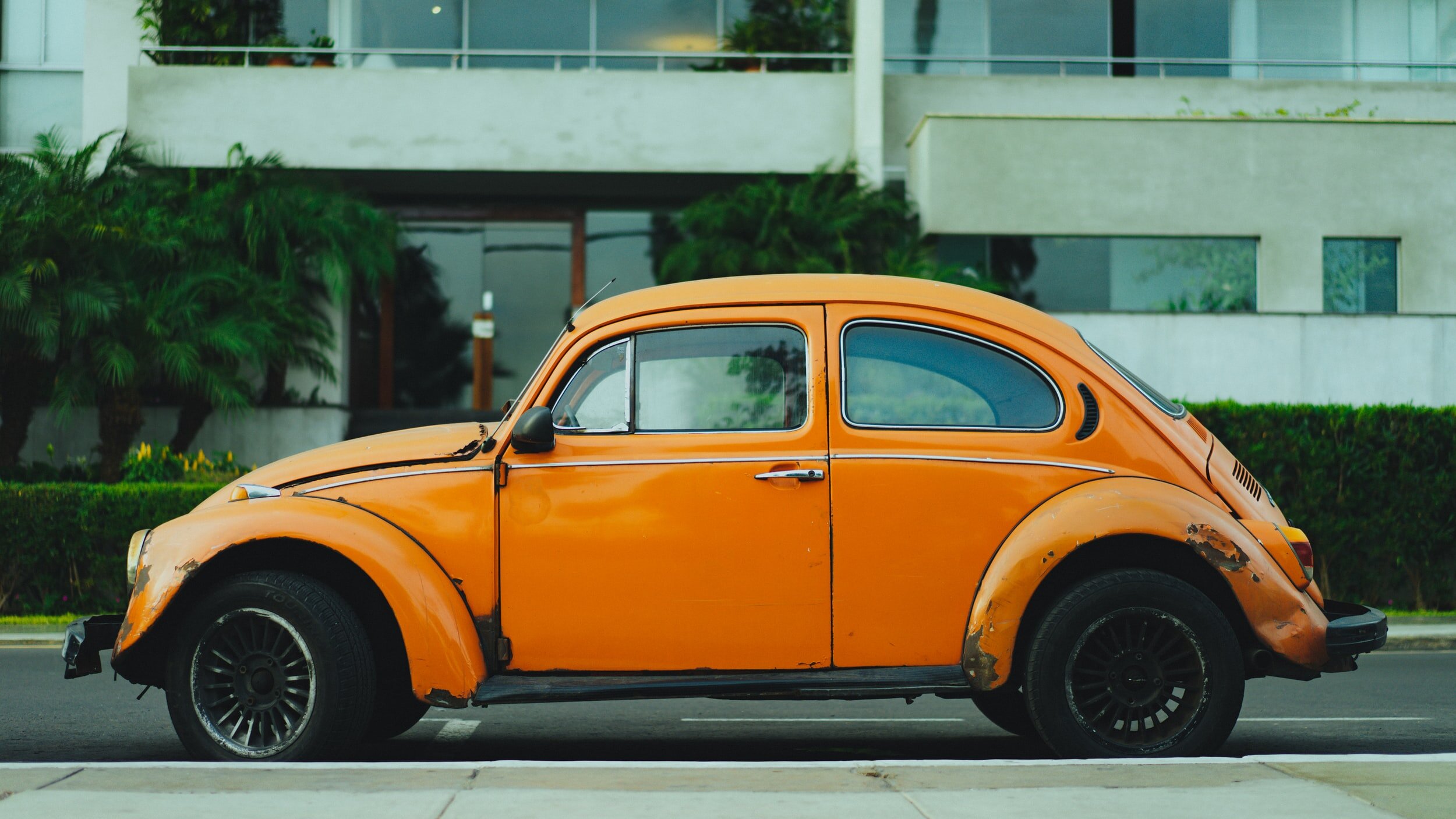
Join us for the Women in Social & Behavioral Science Wikipedia Edit-a-thon
Designing qualitative research with the total quality framework.
Research Design Review
A discussion of qualitative & quantitative research design, a holistic approach to qualitative analysis.

A holistic approach to analysis acknowledges that (1) these unique contributions to our research are central to why we conduct qualitative research and (2) importantly, qualitative researchers owe it to their participants — who have given so much of themselves for our purposes — to maintain the integrity of their lived experiences.
How does the researcher do this?
At the conclusion of each IDI or focus group discussion, the interviewer or moderator should reflect on their understanding of what was learned from the participant(s). To do this, the researcher will use their notes and the audio and/or video recording of the session. It is useful to use Excel or something similar to log the key takeaways associated with the research objectives. By doing this exercise after each IDI or group discussion, the researcher is absorbing a complete “picture” of each participant’s or group’s attitudes, behaviors, and experiences. From there, the researcher can look across participants or groups to contrast and compare.
Crucially, however, the researcher is not necessarily contrasting and comparing simply based on the use of terminology or other obvious, manifest content. Instead, the researcher considers the entirety of what they have learned about each individual or group of participants as revealed in a combination of obvious, subtle, and contextual interconnections within the data.
This holistic approach begins in the beginning — before transcripts and coding — and, with concerted effort, is maintained throughout the analysis process.
Share this:
- Click to share on Reddit (Opens in new window)
- Click to share on Twitter (Opens in new window)
- Click to share on LinkedIn (Opens in new window)
- Click to share on Facebook (Opens in new window)
- Click to share on Tumblr (Opens in new window)
- Click to email a link to a friend (Opens in new window)
- Click to print (Opens in new window)
Leave a comment Cancel reply
This site uses Akismet to reduce spam. Learn how your comment data is processed .

- Already have a WordPress.com account? Log in now.
- Subscribe Subscribed
- Copy shortlink
- Report this content
- View post in Reader
- Manage subscriptions
- Collapse this bar
Qualitative Data Coding
Saul Mcleod, PhD
Editor-in-Chief for Simply Psychology
BSc (Hons) Psychology, MRes, PhD, University of Manchester
Saul Mcleod, PhD., is a qualified psychology teacher with over 18 years of experience in further and higher education. He has been published in peer-reviewed journals, including the Journal of Clinical Psychology.
Learn about our Editorial Process
Olivia Guy-Evans, MSc
Associate Editor for Simply Psychology
BSc (Hons) Psychology, MSc Psychology of Education
Olivia Guy-Evans is a writer and associate editor for Simply Psychology. She has previously worked in healthcare and educational sectors.
Coding is the process of analyzing qualitative data (usually text) by assigning labels (codes) to chunks of data that capture their essence or meaning. It allows you to condense, organize and interpret your data.
A code is a word or brief phrase that captures the essence of why you think a particular bit of data may be useful. A good analogy is that a code describes data like a hashtag describes a tweet.
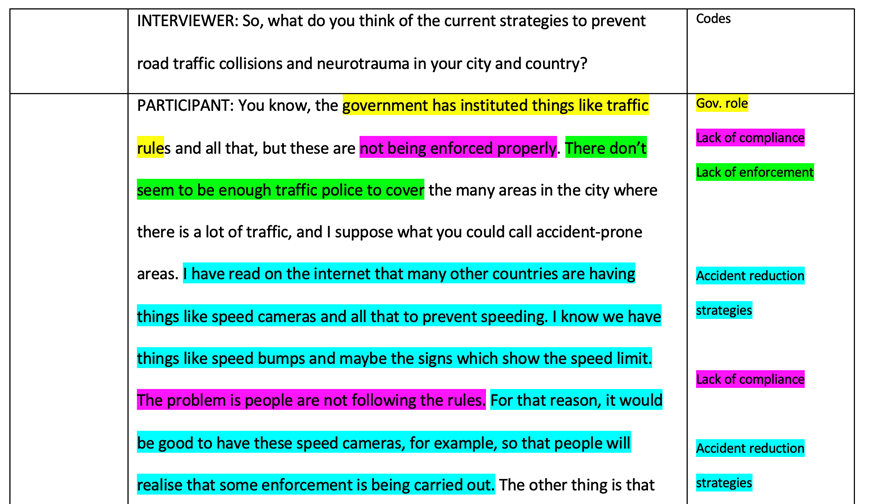
Coding is an iterative process, with researchers refining and revising their codes as their understanding of the data evolves.
The ultimate goal is to develop a coherent and meaningful coding scheme that captures the richness and complexity of the participants’ experiences and helps answer the research questions.
Step 1: Familiarize yourself with the data
- Read through your data (interview transcripts, field notes, documents, etc.) several times. This process is called immersion.
- Think and reflect on what may be important in the data before making any firm decisions about ideas, or potential patterns.
Step 2: Decide on your coding approach
- Will you use predefined deductive codes (based on theory or prior research), or let codes emerge from the data (inductive coding)?
- Will a piece of data have one code or multiple?
- Will you code everything or selectively? Broader research questions may warrant coding more comprehensively.
If you decide not to code everything, it’s crucial to:
- Have clear criteria for what you will and won’t code
- Be transparent about your selection process in research reports
- Remain open to revisiting uncoded data later in analysis
Step 3: Do a first round of coding
- Go through the data and assign initial codes to chunks that stand out
- Create a code name (a word or short phrase) that captures the essence of each chunk
- Keep a codebook – a list of your codes with descriptions or definitions
- Be open to adding, revising or combining codes as you go
Descriptive codes
- In vivo coding / Semantic coding : This method uses words or short phrases directly from the participant’s own language as codes. It deals with the surface-level content, labeling what participants directly say or describe. It identifies keywords, phrases, or sentences that capture the literal content. Participant : “I was just so overwhelmed with everything.” Code : “overwhelmed”
- Process coding : Uses gerunds (“-ing” words) to connote observable or conceptual action in the data. Participant : “I started by brainstorming ideas, then I narrowed them down.” Codes : “brainstorming ideas,” “narrowing down”
- Open coding : A form of initial coding where the researcher remains open to any possible theoretical directions indicated by the data. Participant : “I found the class really challenging, but I learned a lot.” Codes : “challenging class,” “learning experience”
- Descriptive coding : Summarizes the primary topic of a passage in a word or short phrase. Participant : “I usually study in the library because it’s quiet.” Code : “study environment”
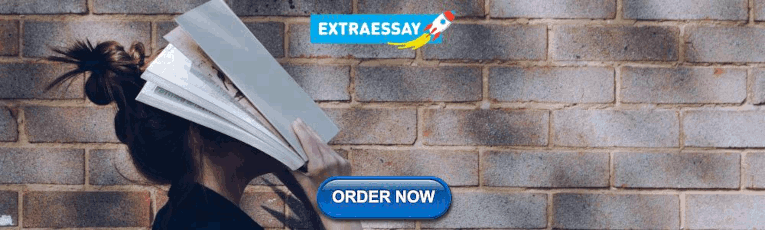
Step 4: Review and refine codes
- Look over your initial codes and see if any can be combined, split up, or revised
- Ensure your code names clearly convey the meaning of the data
- Check if your codes are applied consistently across the dataset
- Get a second opinion from a peer or advisor if possible
Interpretive codes
Interpretive codes go beyond simple description and reflect the researcher’s understanding of the underlying meanings, experiences, or processes captured in the data.
These codes require the researcher to interpret the participants’ words and actions in light of the research questions and theoretical framework.
For example, latent coding is a type of interpretive coding which goes beyond surface meaning in data. It digs for underlying emotions, motivations, or unspoken ideas the participant might not explicitly state
Latent coding looks for subtext, interprets the “why” behind what’s said, and considers the context (e.g. cultural influences, or unconscious biases).
- Example: A participant might say, “Whenever I see a spider, I feel like I’m going to pass out. It takes me back to a bad experience as a kid.” A latent code here could be “Feelings of Panic Triggered by Spiders” because it goes beyond the surface fear and explores the emotional response and potential cause.
It’s useful to ask yourself the following questions:
- What are the assumptions made by the participants?
- What emotions or feelings are expressed or implied in the data?
- How do participants relate to or interact with others in the data?
- How do the participants’ experiences or perspectives change over time?
- What is surprising, unexpected, or contradictory in the data?
- What is not being said or shown in the data? What are the silences or absences?
Theoretical codes
Theoretical codes are the most abstract and conceptual type of codes. They are used to link the data to existing theories or to develop new theoretical insights.
Theoretical codes often emerge later in the analysis process, as researchers begin to identify patterns and connections across the descriptive and interpretive codes.
- Structural coding : Applies a content-based phrase to a segment of data that relates to a specific research question. Research question : What motivates students to succeed? Participant : “I want to make my parents proud and be the first in my family to graduate college.” Interpretive Code : “family motivation” Theoretical code : “Social identity theory”
- Value coding : This method codes data according to the participants’ values, attitudes, and beliefs, representing their perspectives or worldviews. Participant : “I believe everyone deserves access to quality healthcare.” Interpretive Code : “healthcare access” (value) Theoretical code : “Distributive justice”
Pattern codes
Pattern coding is often used in the later stages of data analysis, after the researcher has thoroughly familiarized themselves with the data and identified initial descriptive and interpretive codes.
By identifying patterns and relationships across the data, pattern codes help to develop a more coherent and meaningful understanding of the phenomenon and can contribute to theory development or refinement.
For Example
Let’s say a researcher is studying the experiences of new mothers returning to work after maternity leave. They conduct interviews with several participants and initially use descriptive and interpretive codes to analyze the data. Some of these codes might include:
- “Guilt about leaving baby”
- “Struggle to balance work and family”
- “Support from colleagues”
- “Flexible work arrangements”
- “Breastfeeding challenges”
As the researcher reviews the coded data, they may notice that several of these codes relate to the broader theme of “work-family conflict.”
They might create a pattern code called “Navigating work-family conflict” that pulls together the various experiences and challenges described by the participants.
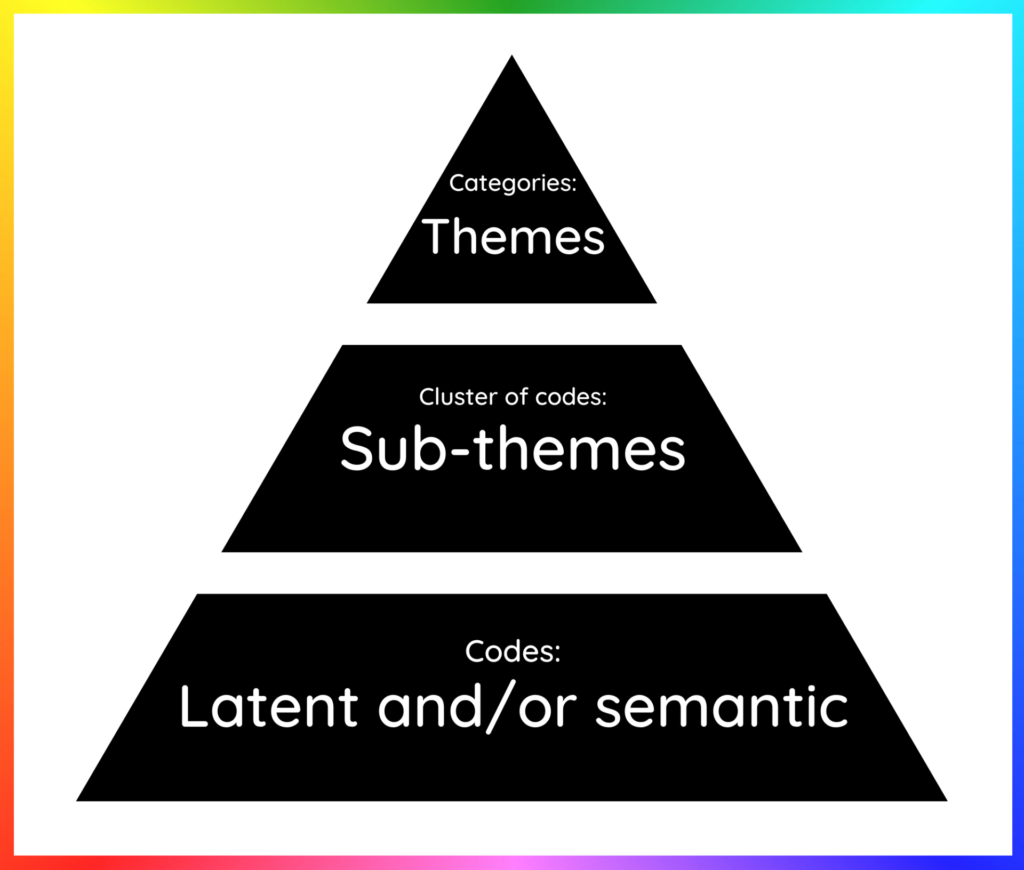
Related Articles
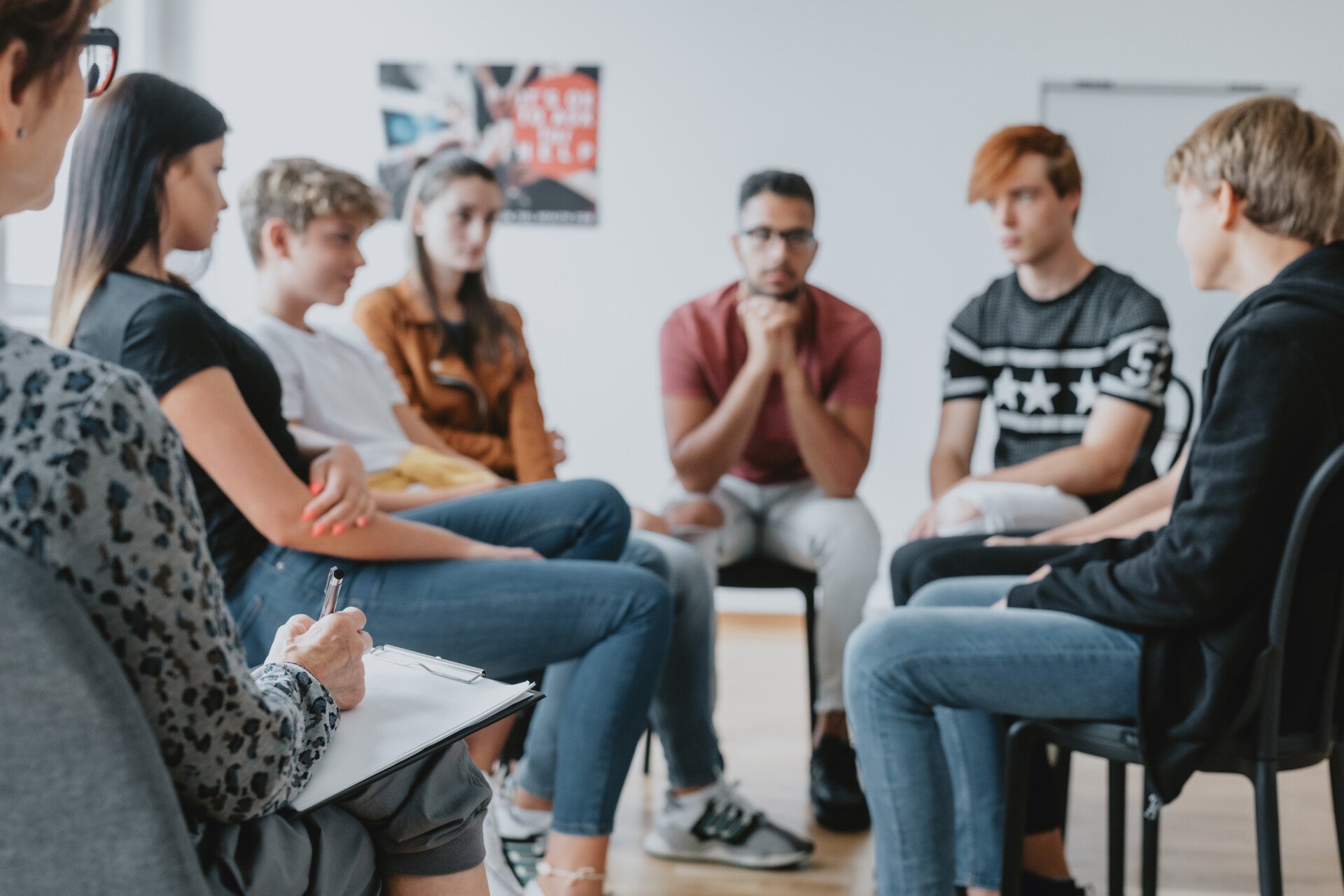
Research Methodology
What Is a Focus Group?
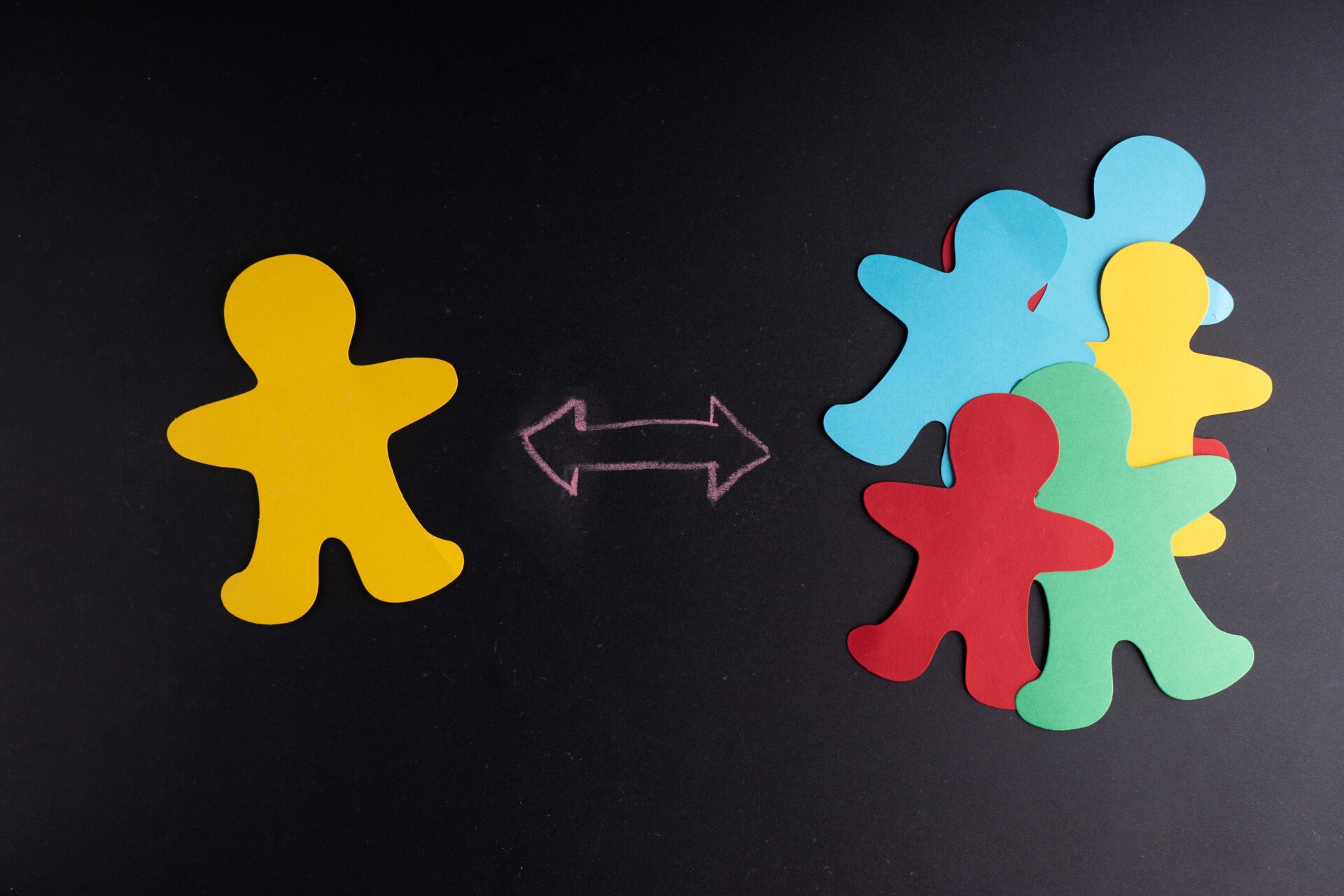
Cross-Cultural Research Methodology In Psychology
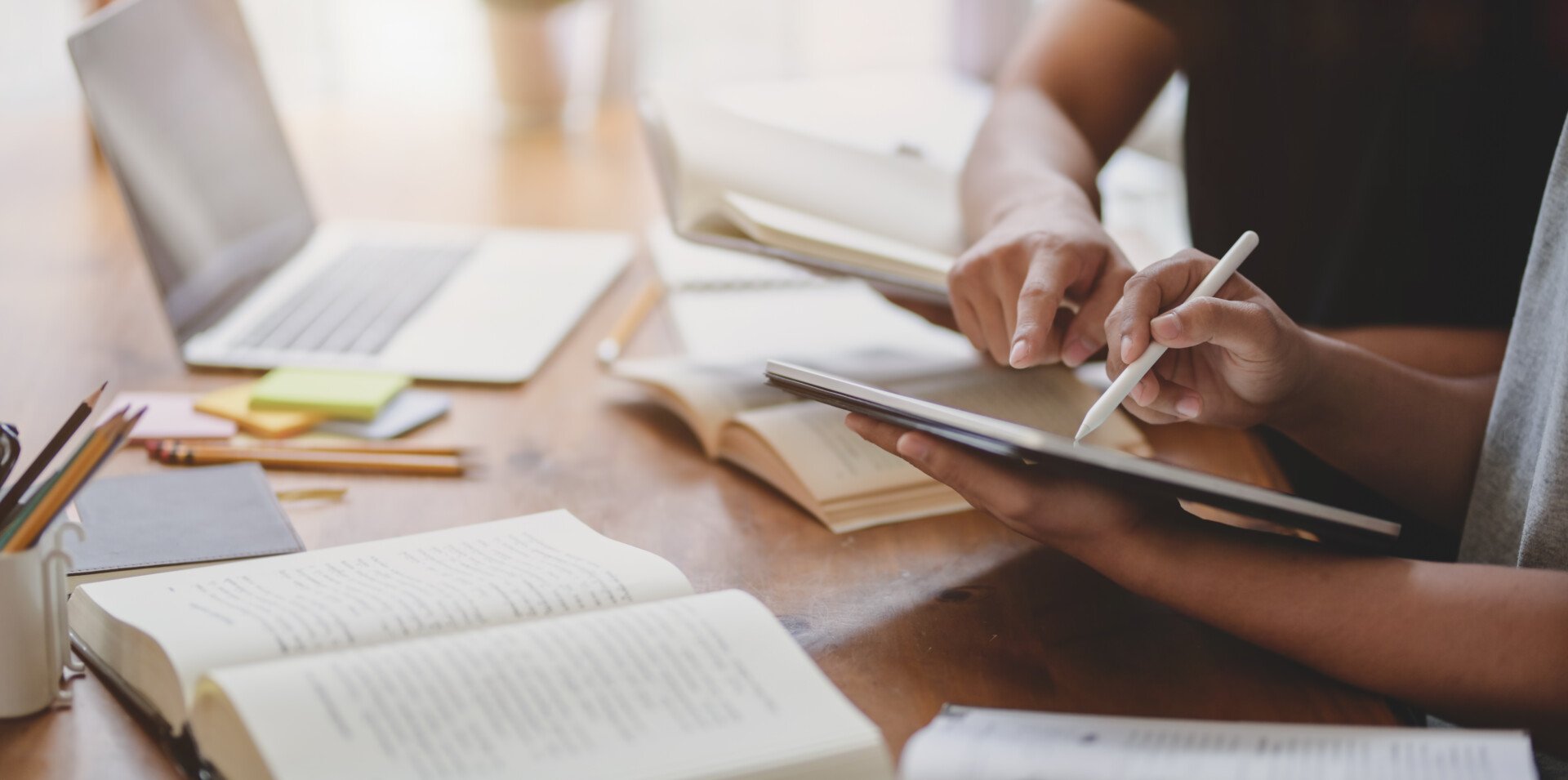
What Is Internal Validity In Research?
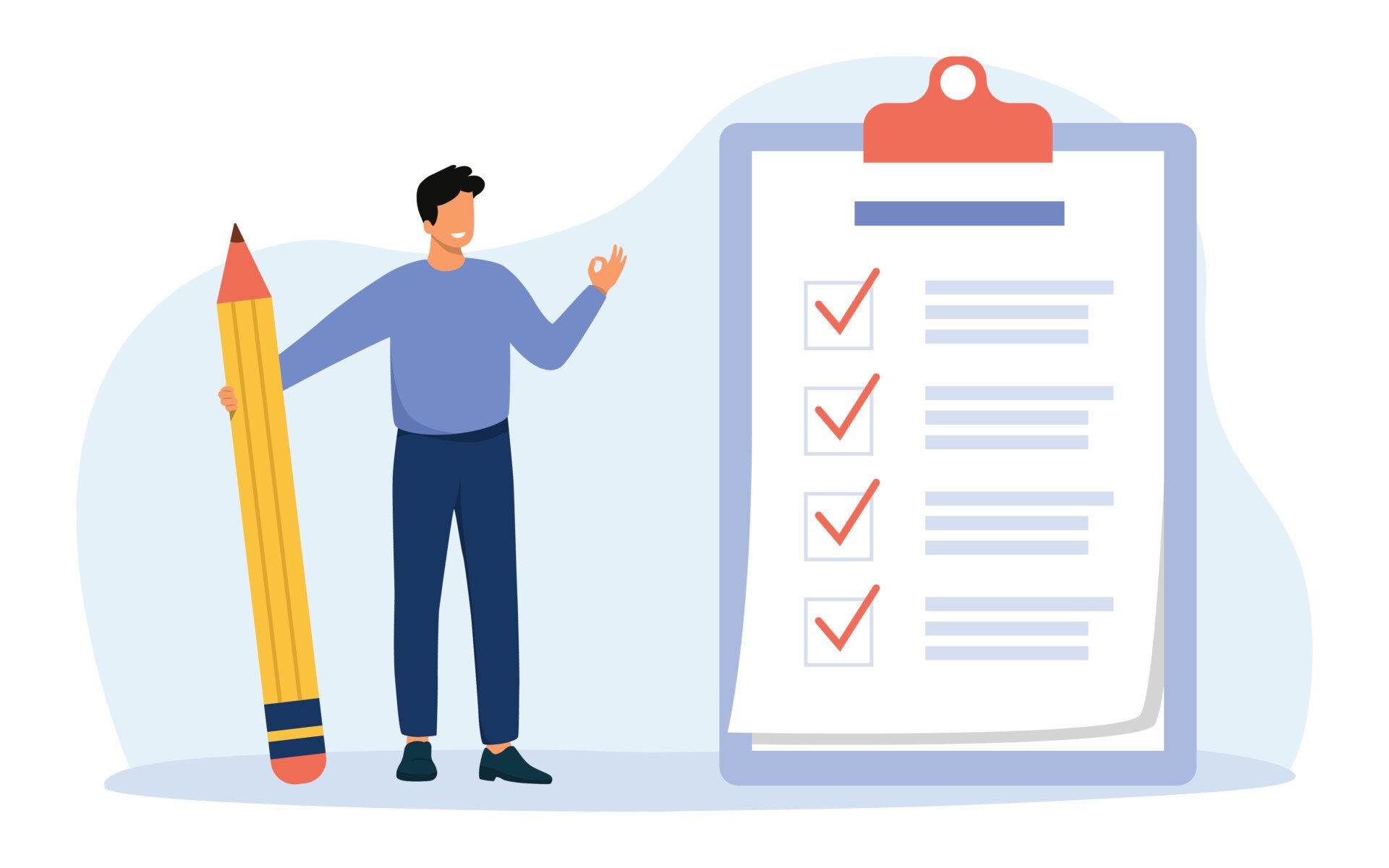
Research Methodology , Statistics
What Is Face Validity In Research? Importance & How To Measure
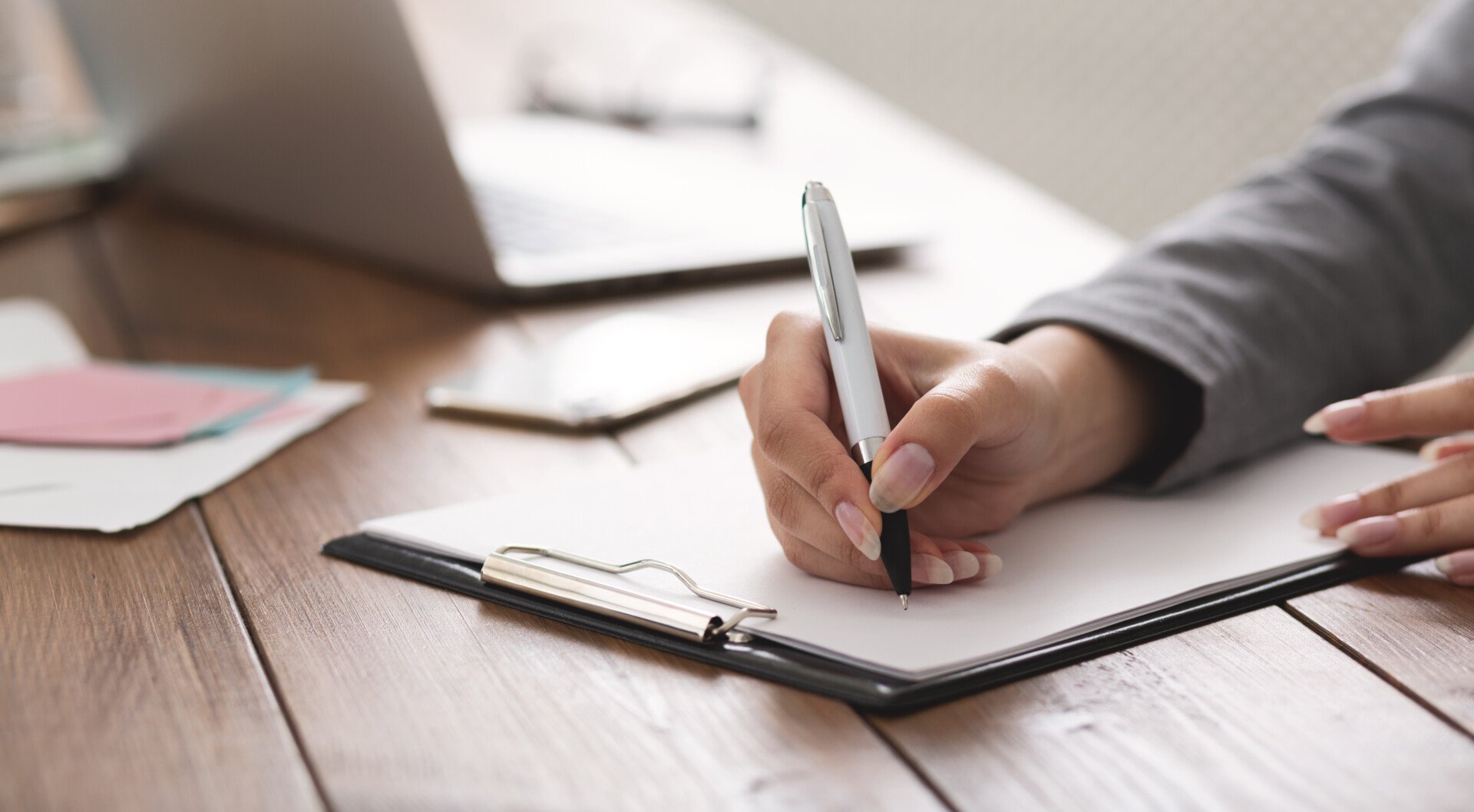
Criterion Validity: Definition & Examples
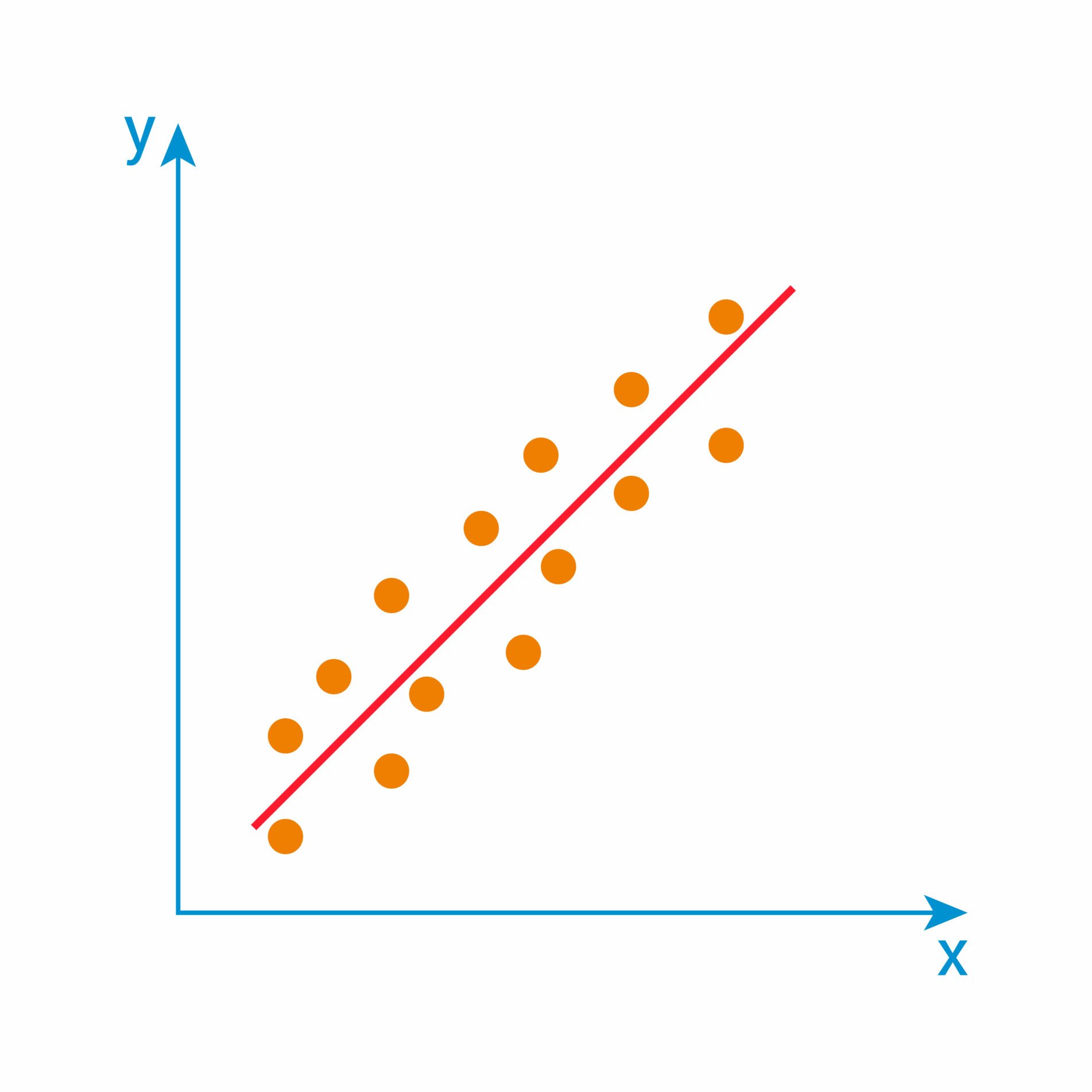
Convergent Validity: Definition and Examples

An official website of the United States government
The .gov means it’s official. Federal government websites often end in .gov or .mil. Before sharing sensitive information, make sure you’re on a federal government site.
The site is secure. The https:// ensures that you are connecting to the official website and that any information you provide is encrypted and transmitted securely.
- Publications
- Account settings
Preview improvements coming to the PMC website in October 2024. Learn More or Try it out now .
- Advanced Search
- Journal List
- Neurol Res Pract

How to use and assess qualitative research methods
Loraine busetto.
1 Department of Neurology, Heidelberg University Hospital, Im Neuenheimer Feld 400, 69120 Heidelberg, Germany
Wolfgang Wick
2 Clinical Cooperation Unit Neuro-Oncology, German Cancer Research Center, Heidelberg, Germany
Christoph Gumbinger
Associated data.
Not applicable.
This paper aims to provide an overview of the use and assessment of qualitative research methods in the health sciences. Qualitative research can be defined as the study of the nature of phenomena and is especially appropriate for answering questions of why something is (not) observed, assessing complex multi-component interventions, and focussing on intervention improvement. The most common methods of data collection are document study, (non-) participant observations, semi-structured interviews and focus groups. For data analysis, field-notes and audio-recordings are transcribed into protocols and transcripts, and coded using qualitative data management software. Criteria such as checklists, reflexivity, sampling strategies, piloting, co-coding, member-checking and stakeholder involvement can be used to enhance and assess the quality of the research conducted. Using qualitative in addition to quantitative designs will equip us with better tools to address a greater range of research problems, and to fill in blind spots in current neurological research and practice.
The aim of this paper is to provide an overview of qualitative research methods, including hands-on information on how they can be used, reported and assessed. This article is intended for beginning qualitative researchers in the health sciences as well as experienced quantitative researchers who wish to broaden their understanding of qualitative research.
What is qualitative research?
Qualitative research is defined as “the study of the nature of phenomena”, including “their quality, different manifestations, the context in which they appear or the perspectives from which they can be perceived” , but excluding “their range, frequency and place in an objectively determined chain of cause and effect” [ 1 ]. This formal definition can be complemented with a more pragmatic rule of thumb: qualitative research generally includes data in form of words rather than numbers [ 2 ].
Why conduct qualitative research?
Because some research questions cannot be answered using (only) quantitative methods. For example, one Australian study addressed the issue of why patients from Aboriginal communities often present late or not at all to specialist services offered by tertiary care hospitals. Using qualitative interviews with patients and staff, it found one of the most significant access barriers to be transportation problems, including some towns and communities simply not having a bus service to the hospital [ 3 ]. A quantitative study could have measured the number of patients over time or even looked at possible explanatory factors – but only those previously known or suspected to be of relevance. To discover reasons for observed patterns, especially the invisible or surprising ones, qualitative designs are needed.
While qualitative research is common in other fields, it is still relatively underrepresented in health services research. The latter field is more traditionally rooted in the evidence-based-medicine paradigm, as seen in " research that involves testing the effectiveness of various strategies to achieve changes in clinical practice, preferably applying randomised controlled trial study designs (...) " [ 4 ]. This focus on quantitative research and specifically randomised controlled trials (RCT) is visible in the idea of a hierarchy of research evidence which assumes that some research designs are objectively better than others, and that choosing a "lesser" design is only acceptable when the better ones are not practically or ethically feasible [ 5 , 6 ]. Others, however, argue that an objective hierarchy does not exist, and that, instead, the research design and methods should be chosen to fit the specific research question at hand – "questions before methods" [ 2 , 7 – 9 ]. This means that even when an RCT is possible, some research problems require a different design that is better suited to addressing them. Arguing in JAMA, Berwick uses the example of rapid response teams in hospitals, which he describes as " a complex, multicomponent intervention – essentially a process of social change" susceptible to a range of different context factors including leadership or organisation history. According to him, "[in] such complex terrain, the RCT is an impoverished way to learn. Critics who use it as a truth standard in this context are incorrect" [ 8 ] . Instead of limiting oneself to RCTs, Berwick recommends embracing a wider range of methods , including qualitative ones, which for "these specific applications, (...) are not compromises in learning how to improve; they are superior" [ 8 ].
Research problems that can be approached particularly well using qualitative methods include assessing complex multi-component interventions or systems (of change), addressing questions beyond “what works”, towards “what works for whom when, how and why”, and focussing on intervention improvement rather than accreditation [ 7 , 9 – 12 ]. Using qualitative methods can also help shed light on the “softer” side of medical treatment. For example, while quantitative trials can measure the costs and benefits of neuro-oncological treatment in terms of survival rates or adverse effects, qualitative research can help provide a better understanding of patient or caregiver stress, visibility of illness or out-of-pocket expenses.
How to conduct qualitative research?
Given that qualitative research is characterised by flexibility, openness and responsivity to context, the steps of data collection and analysis are not as separate and consecutive as they tend to be in quantitative research [ 13 , 14 ]. As Fossey puts it : “sampling, data collection, analysis and interpretation are related to each other in a cyclical (iterative) manner, rather than following one after another in a stepwise approach” [ 15 ]. The researcher can make educated decisions with regard to the choice of method, how they are implemented, and to which and how many units they are applied [ 13 ]. As shown in Fig. 1 , this can involve several back-and-forth steps between data collection and analysis where new insights and experiences can lead to adaption and expansion of the original plan. Some insights may also necessitate a revision of the research question and/or the research design as a whole. The process ends when saturation is achieved, i.e. when no relevant new information can be found (see also below: sampling and saturation). For reasons of transparency, it is essential for all decisions as well as the underlying reasoning to be well-documented.

Iterative research process
While it is not always explicitly addressed, qualitative methods reflect a different underlying research paradigm than quantitative research (e.g. constructivism or interpretivism as opposed to positivism). The choice of methods can be based on the respective underlying substantive theory or theoretical framework used by the researcher [ 2 ].
Data collection
The methods of qualitative data collection most commonly used in health research are document study, observations, semi-structured interviews and focus groups [ 1 , 14 , 16 , 17 ].
Document study
Document study (also called document analysis) refers to the review by the researcher of written materials [ 14 ]. These can include personal and non-personal documents such as archives, annual reports, guidelines, policy documents, diaries or letters.
Observations
Observations are particularly useful to gain insights into a certain setting and actual behaviour – as opposed to reported behaviour or opinions [ 13 ]. Qualitative observations can be either participant or non-participant in nature. In participant observations, the observer is part of the observed setting, for example a nurse working in an intensive care unit [ 18 ]. In non-participant observations, the observer is “on the outside looking in”, i.e. present in but not part of the situation, trying not to influence the setting by their presence. Observations can be planned (e.g. for 3 h during the day or night shift) or ad hoc (e.g. as soon as a stroke patient arrives at the emergency room). During the observation, the observer takes notes on everything or certain pre-determined parts of what is happening around them, for example focusing on physician-patient interactions or communication between different professional groups. Written notes can be taken during or after the observations, depending on feasibility (which is usually lower during participant observations) and acceptability (e.g. when the observer is perceived to be judging the observed). Afterwards, these field notes are transcribed into observation protocols. If more than one observer was involved, field notes are taken independently, but notes can be consolidated into one protocol after discussions. Advantages of conducting observations include minimising the distance between the researcher and the researched, the potential discovery of topics that the researcher did not realise were relevant and gaining deeper insights into the real-world dimensions of the research problem at hand [ 18 ].
Semi-structured interviews
Hijmans & Kuyper describe qualitative interviews as “an exchange with an informal character, a conversation with a goal” [ 19 ]. Interviews are used to gain insights into a person’s subjective experiences, opinions and motivations – as opposed to facts or behaviours [ 13 ]. Interviews can be distinguished by the degree to which they are structured (i.e. a questionnaire), open (e.g. free conversation or autobiographical interviews) or semi-structured [ 2 , 13 ]. Semi-structured interviews are characterized by open-ended questions and the use of an interview guide (or topic guide/list) in which the broad areas of interest, sometimes including sub-questions, are defined [ 19 ]. The pre-defined topics in the interview guide can be derived from the literature, previous research or a preliminary method of data collection, e.g. document study or observations. The topic list is usually adapted and improved at the start of the data collection process as the interviewer learns more about the field [ 20 ]. Across interviews the focus on the different (blocks of) questions may differ and some questions may be skipped altogether (e.g. if the interviewee is not able or willing to answer the questions or for concerns about the total length of the interview) [ 20 ]. Qualitative interviews are usually not conducted in written format as it impedes on the interactive component of the method [ 20 ]. In comparison to written surveys, qualitative interviews have the advantage of being interactive and allowing for unexpected topics to emerge and to be taken up by the researcher. This can also help overcome a provider or researcher-centred bias often found in written surveys, which by nature, can only measure what is already known or expected to be of relevance to the researcher. Interviews can be audio- or video-taped; but sometimes it is only feasible or acceptable for the interviewer to take written notes [ 14 , 16 , 20 ].
Focus groups
Focus groups are group interviews to explore participants’ expertise and experiences, including explorations of how and why people behave in certain ways [ 1 ]. Focus groups usually consist of 6–8 people and are led by an experienced moderator following a topic guide or “script” [ 21 ]. They can involve an observer who takes note of the non-verbal aspects of the situation, possibly using an observation guide [ 21 ]. Depending on researchers’ and participants’ preferences, the discussions can be audio- or video-taped and transcribed afterwards [ 21 ]. Focus groups are useful for bringing together homogeneous (to a lesser extent heterogeneous) groups of participants with relevant expertise and experience on a given topic on which they can share detailed information [ 21 ]. Focus groups are a relatively easy, fast and inexpensive method to gain access to information on interactions in a given group, i.e. “the sharing and comparing” among participants [ 21 ]. Disadvantages include less control over the process and a lesser extent to which each individual may participate. Moreover, focus group moderators need experience, as do those tasked with the analysis of the resulting data. Focus groups can be less appropriate for discussing sensitive topics that participants might be reluctant to disclose in a group setting [ 13 ]. Moreover, attention must be paid to the emergence of “groupthink” as well as possible power dynamics within the group, e.g. when patients are awed or intimidated by health professionals.
Choosing the “right” method
As explained above, the school of thought underlying qualitative research assumes no objective hierarchy of evidence and methods. This means that each choice of single or combined methods has to be based on the research question that needs to be answered and a critical assessment with regard to whether or to what extent the chosen method can accomplish this – i.e. the “fit” between question and method [ 14 ]. It is necessary for these decisions to be documented when they are being made, and to be critically discussed when reporting methods and results.
Let us assume that our research aim is to examine the (clinical) processes around acute endovascular treatment (EVT), from the patient’s arrival at the emergency room to recanalization, with the aim to identify possible causes for delay and/or other causes for sub-optimal treatment outcome. As a first step, we could conduct a document study of the relevant standard operating procedures (SOPs) for this phase of care – are they up-to-date and in line with current guidelines? Do they contain any mistakes, irregularities or uncertainties that could cause delays or other problems? Regardless of the answers to these questions, the results have to be interpreted based on what they are: a written outline of what care processes in this hospital should look like. If we want to know what they actually look like in practice, we can conduct observations of the processes described in the SOPs. These results can (and should) be analysed in themselves, but also in comparison to the results of the document analysis, especially as regards relevant discrepancies. Do the SOPs outline specific tests for which no equipment can be observed or tasks to be performed by specialized nurses who are not present during the observation? It might also be possible that the written SOP is outdated, but the actual care provided is in line with current best practice. In order to find out why these discrepancies exist, it can be useful to conduct interviews. Are the physicians simply not aware of the SOPs (because their existence is limited to the hospital’s intranet) or do they actively disagree with them or does the infrastructure make it impossible to provide the care as described? Another rationale for adding interviews is that some situations (or all of their possible variations for different patient groups or the day, night or weekend shift) cannot practically or ethically be observed. In this case, it is possible to ask those involved to report on their actions – being aware that this is not the same as the actual observation. A senior physician’s or hospital manager’s description of certain situations might differ from a nurse’s or junior physician’s one, maybe because they intentionally misrepresent facts or maybe because different aspects of the process are visible or important to them. In some cases, it can also be relevant to consider to whom the interviewee is disclosing this information – someone they trust, someone they are otherwise not connected to, or someone they suspect or are aware of being in a potentially “dangerous” power relationship to them. Lastly, a focus group could be conducted with representatives of the relevant professional groups to explore how and why exactly they provide care around EVT. The discussion might reveal discrepancies (between SOPs and actual care or between different physicians) and motivations to the researchers as well as to the focus group members that they might not have been aware of themselves. For the focus group to deliver relevant information, attention has to be paid to its composition and conduct, for example, to make sure that all participants feel safe to disclose sensitive or potentially problematic information or that the discussion is not dominated by (senior) physicians only. The resulting combination of data collection methods is shown in Fig. 2 .

Possible combination of data collection methods
Attributions for icons: “Book” by Serhii Smirnov, “Interview” by Adrien Coquet, FR, “Magnifying Glass” by anggun, ID, “Business communication” by Vectors Market; all from the Noun Project
The combination of multiple data source as described for this example can be referred to as “triangulation”, in which multiple measurements are carried out from different angles to achieve a more comprehensive understanding of the phenomenon under study [ 22 , 23 ].
Data analysis
To analyse the data collected through observations, interviews and focus groups these need to be transcribed into protocols and transcripts (see Fig. 3 ). Interviews and focus groups can be transcribed verbatim , with or without annotations for behaviour (e.g. laughing, crying, pausing) and with or without phonetic transcription of dialects and filler words, depending on what is expected or known to be relevant for the analysis. In the next step, the protocols and transcripts are coded , that is, marked (or tagged, labelled) with one or more short descriptors of the content of a sentence or paragraph [ 2 , 15 , 23 ]. Jansen describes coding as “connecting the raw data with “theoretical” terms” [ 20 ]. In a more practical sense, coding makes raw data sortable. This makes it possible to extract and examine all segments describing, say, a tele-neurology consultation from multiple data sources (e.g. SOPs, emergency room observations, staff and patient interview). In a process of synthesis and abstraction, the codes are then grouped, summarised and/or categorised [ 15 , 20 ]. The end product of the coding or analysis process is a descriptive theory of the behavioural pattern under investigation [ 20 ]. The coding process is performed using qualitative data management software, the most common ones being InVivo, MaxQDA and Atlas.ti. It should be noted that these are data management tools which support the analysis performed by the researcher(s) [ 14 ].

From data collection to data analysis
Attributions for icons: see Fig. Fig.2, 2 , also “Speech to text” by Trevor Dsouza, “Field Notes” by Mike O’Brien, US, “Voice Record” by ProSymbols, US, “Inspection” by Made, AU, and “Cloud” by Graphic Tigers; all from the Noun Project
How to report qualitative research?
Protocols of qualitative research can be published separately and in advance of the study results. However, the aim is not the same as in RCT protocols, i.e. to pre-define and set in stone the research questions and primary or secondary endpoints. Rather, it is a way to describe the research methods in detail, which might not be possible in the results paper given journals’ word limits. Qualitative research papers are usually longer than their quantitative counterparts to allow for deep understanding and so-called “thick description”. In the methods section, the focus is on transparency of the methods used, including why, how and by whom they were implemented in the specific study setting, so as to enable a discussion of whether and how this may have influenced data collection, analysis and interpretation. The results section usually starts with a paragraph outlining the main findings, followed by more detailed descriptions of, for example, the commonalities, discrepancies or exceptions per category [ 20 ]. Here it is important to support main findings by relevant quotations, which may add information, context, emphasis or real-life examples [ 20 , 23 ]. It is subject to debate in the field whether it is relevant to state the exact number or percentage of respondents supporting a certain statement (e.g. “Five interviewees expressed negative feelings towards XYZ”) [ 21 ].
How to combine qualitative with quantitative research?
Qualitative methods can be combined with other methods in multi- or mixed methods designs, which “[employ] two or more different methods [ …] within the same study or research program rather than confining the research to one single method” [ 24 ]. Reasons for combining methods can be diverse, including triangulation for corroboration of findings, complementarity for illustration and clarification of results, expansion to extend the breadth and range of the study, explanation of (unexpected) results generated with one method with the help of another, or offsetting the weakness of one method with the strength of another [ 1 , 17 , 24 – 26 ]. The resulting designs can be classified according to when, why and how the different quantitative and/or qualitative data strands are combined. The three most common types of mixed method designs are the convergent parallel design , the explanatory sequential design and the exploratory sequential design. The designs with examples are shown in Fig. 4 .

Three common mixed methods designs
In the convergent parallel design, a qualitative study is conducted in parallel to and independently of a quantitative study, and the results of both studies are compared and combined at the stage of interpretation of results. Using the above example of EVT provision, this could entail setting up a quantitative EVT registry to measure process times and patient outcomes in parallel to conducting the qualitative research outlined above, and then comparing results. Amongst other things, this would make it possible to assess whether interview respondents’ subjective impressions of patients receiving good care match modified Rankin Scores at follow-up, or whether observed delays in care provision are exceptions or the rule when compared to door-to-needle times as documented in the registry. In the explanatory sequential design, a quantitative study is carried out first, followed by a qualitative study to help explain the results from the quantitative study. This would be an appropriate design if the registry alone had revealed relevant delays in door-to-needle times and the qualitative study would be used to understand where and why these occurred, and how they could be improved. In the exploratory design, the qualitative study is carried out first and its results help informing and building the quantitative study in the next step [ 26 ]. If the qualitative study around EVT provision had shown a high level of dissatisfaction among the staff members involved, a quantitative questionnaire investigating staff satisfaction could be set up in the next step, informed by the qualitative study on which topics dissatisfaction had been expressed. Amongst other things, the questionnaire design would make it possible to widen the reach of the research to more respondents from different (types of) hospitals, regions, countries or settings, and to conduct sub-group analyses for different professional groups.
How to assess qualitative research?
A variety of assessment criteria and lists have been developed for qualitative research, ranging in their focus and comprehensiveness [ 14 , 17 , 27 ]. However, none of these has been elevated to the “gold standard” in the field. In the following, we therefore focus on a set of commonly used assessment criteria that, from a practical standpoint, a researcher can look for when assessing a qualitative research report or paper.
Assessors should check the authors’ use of and adherence to the relevant reporting checklists (e.g. Standards for Reporting Qualitative Research (SRQR)) to make sure all items that are relevant for this type of research are addressed [ 23 , 28 ]. Discussions of quantitative measures in addition to or instead of these qualitative measures can be a sign of lower quality of the research (paper). Providing and adhering to a checklist for qualitative research contributes to an important quality criterion for qualitative research, namely transparency [ 15 , 17 , 23 ].
Reflexivity
While methodological transparency and complete reporting is relevant for all types of research, some additional criteria must be taken into account for qualitative research. This includes what is called reflexivity, i.e. sensitivity to the relationship between the researcher and the researched, including how contact was established and maintained, or the background and experience of the researcher(s) involved in data collection and analysis. Depending on the research question and population to be researched this can be limited to professional experience, but it may also include gender, age or ethnicity [ 17 , 27 ]. These details are relevant because in qualitative research, as opposed to quantitative research, the researcher as a person cannot be isolated from the research process [ 23 ]. It may influence the conversation when an interviewed patient speaks to an interviewer who is a physician, or when an interviewee is asked to discuss a gynaecological procedure with a male interviewer, and therefore the reader must be made aware of these details [ 19 ].
Sampling and saturation
The aim of qualitative sampling is for all variants of the objects of observation that are deemed relevant for the study to be present in the sample “ to see the issue and its meanings from as many angles as possible” [ 1 , 16 , 19 , 20 , 27 ] , and to ensure “information-richness [ 15 ]. An iterative sampling approach is advised, in which data collection (e.g. five interviews) is followed by data analysis, followed by more data collection to find variants that are lacking in the current sample. This process continues until no new (relevant) information can be found and further sampling becomes redundant – which is called saturation [ 1 , 15 ] . In other words: qualitative data collection finds its end point not a priori , but when the research team determines that saturation has been reached [ 29 , 30 ].
This is also the reason why most qualitative studies use deliberate instead of random sampling strategies. This is generally referred to as “ purposive sampling” , in which researchers pre-define which types of participants or cases they need to include so as to cover all variations that are expected to be of relevance, based on the literature, previous experience or theory (i.e. theoretical sampling) [ 14 , 20 ]. Other types of purposive sampling include (but are not limited to) maximum variation sampling, critical case sampling or extreme or deviant case sampling [ 2 ]. In the above EVT example, a purposive sample could include all relevant professional groups and/or all relevant stakeholders (patients, relatives) and/or all relevant times of observation (day, night and weekend shift).
Assessors of qualitative research should check whether the considerations underlying the sampling strategy were sound and whether or how researchers tried to adapt and improve their strategies in stepwise or cyclical approaches between data collection and analysis to achieve saturation [ 14 ].
Good qualitative research is iterative in nature, i.e. it goes back and forth between data collection and analysis, revising and improving the approach where necessary. One example of this are pilot interviews, where different aspects of the interview (especially the interview guide, but also, for example, the site of the interview or whether the interview can be audio-recorded) are tested with a small number of respondents, evaluated and revised [ 19 ]. In doing so, the interviewer learns which wording or types of questions work best, or which is the best length of an interview with patients who have trouble concentrating for an extended time. Of course, the same reasoning applies to observations or focus groups which can also be piloted.
Ideally, coding should be performed by at least two researchers, especially at the beginning of the coding process when a common approach must be defined, including the establishment of a useful coding list (or tree), and when a common meaning of individual codes must be established [ 23 ]. An initial sub-set or all transcripts can be coded independently by the coders and then compared and consolidated after regular discussions in the research team. This is to make sure that codes are applied consistently to the research data.
Member checking
Member checking, also called respondent validation , refers to the practice of checking back with study respondents to see if the research is in line with their views [ 14 , 27 ]. This can happen after data collection or analysis or when first results are available [ 23 ]. For example, interviewees can be provided with (summaries of) their transcripts and asked whether they believe this to be a complete representation of their views or whether they would like to clarify or elaborate on their responses [ 17 ]. Respondents’ feedback on these issues then becomes part of the data collection and analysis [ 27 ].
Stakeholder involvement
In those niches where qualitative approaches have been able to evolve and grow, a new trend has seen the inclusion of patients and their representatives not only as study participants (i.e. “members”, see above) but as consultants to and active participants in the broader research process [ 31 – 33 ]. The underlying assumption is that patients and other stakeholders hold unique perspectives and experiences that add value beyond their own single story, making the research more relevant and beneficial to researchers, study participants and (future) patients alike [ 34 , 35 ]. Using the example of patients on or nearing dialysis, a recent scoping review found that 80% of clinical research did not address the top 10 research priorities identified by patients and caregivers [ 32 , 36 ]. In this sense, the involvement of the relevant stakeholders, especially patients and relatives, is increasingly being seen as a quality indicator in and of itself.
How not to assess qualitative research
The above overview does not include certain items that are routine in assessments of quantitative research. What follows is a non-exhaustive, non-representative, experience-based list of the quantitative criteria often applied to the assessment of qualitative research, as well as an explanation of the limited usefulness of these endeavours.
Protocol adherence
Given the openness and flexibility of qualitative research, it should not be assessed by how well it adheres to pre-determined and fixed strategies – in other words: its rigidity. Instead, the assessor should look for signs of adaptation and refinement based on lessons learned from earlier steps in the research process.
Sample size
For the reasons explained above, qualitative research does not require specific sample sizes, nor does it require that the sample size be determined a priori [ 1 , 14 , 27 , 37 – 39 ]. Sample size can only be a useful quality indicator when related to the research purpose, the chosen methodology and the composition of the sample, i.e. who was included and why.
Randomisation
While some authors argue that randomisation can be used in qualitative research, this is not commonly the case, as neither its feasibility nor its necessity or usefulness has been convincingly established for qualitative research [ 13 , 27 ]. Relevant disadvantages include the negative impact of a too large sample size as well as the possibility (or probability) of selecting “ quiet, uncooperative or inarticulate individuals ” [ 17 ]. Qualitative studies do not use control groups, either.
Interrater reliability, variability and other “objectivity checks”
The concept of “interrater reliability” is sometimes used in qualitative research to assess to which extent the coding approach overlaps between the two co-coders. However, it is not clear what this measure tells us about the quality of the analysis [ 23 ]. This means that these scores can be included in qualitative research reports, preferably with some additional information on what the score means for the analysis, but it is not a requirement. Relatedly, it is not relevant for the quality or “objectivity” of qualitative research to separate those who recruited the study participants and collected and analysed the data. Experiences even show that it might be better to have the same person or team perform all of these tasks [ 20 ]. First, when researchers introduce themselves during recruitment this can enhance trust when the interview takes place days or weeks later with the same researcher. Second, when the audio-recording is transcribed for analysis, the researcher conducting the interviews will usually remember the interviewee and the specific interview situation during data analysis. This might be helpful in providing additional context information for interpretation of data, e.g. on whether something might have been meant as a joke [ 18 ].
Not being quantitative research
Being qualitative research instead of quantitative research should not be used as an assessment criterion if it is used irrespectively of the research problem at hand. Similarly, qualitative research should not be required to be combined with quantitative research per se – unless mixed methods research is judged as inherently better than single-method research. In this case, the same criterion should be applied for quantitative studies without a qualitative component.
The main take-away points of this paper are summarised in Table Table1. 1 . We aimed to show that, if conducted well, qualitative research can answer specific research questions that cannot to be adequately answered using (only) quantitative designs. Seeing qualitative and quantitative methods as equal will help us become more aware and critical of the “fit” between the research problem and our chosen methods: I can conduct an RCT to determine the reasons for transportation delays of acute stroke patients – but should I? It also provides us with a greater range of tools to tackle a greater range of research problems more appropriately and successfully, filling in the blind spots on one half of the methodological spectrum to better address the whole complexity of neurological research and practice.
Take-away-points
Acknowledgements
Abbreviations, authors’ contributions.
LB drafted the manuscript; WW and CG revised the manuscript; all authors approved the final versions.
no external funding.
Availability of data and materials
Ethics approval and consent to participate, consent for publication, competing interests.
The authors declare no competing interests.
Publisher’s Note
Springer Nature remains neutral with regard to jurisdictional claims in published maps and institutional affiliations.
- Open access
- Published: 11 May 2024
Nursing students’ stressors and coping strategies during their first clinical training: a qualitative study in the United Arab Emirates
- Jacqueline Maria Dias 1 ,
- Muhammad Arsyad Subu 1 ,
- Nabeel Al-Yateem 1 ,
- Fatma Refaat Ahmed 1 ,
- Syed Azizur Rahman 1 , 2 ,
- Mini Sara Abraham 1 ,
- Sareh Mirza Forootan 1 ,
- Farzaneh Ahmad Sarkhosh 1 &
- Fatemeh Javanbakh 1
BMC Nursing volume 23 , Article number: 322 ( 2024 ) Cite this article
470 Accesses
Metrics details
Understanding the stressors and coping strategies of nursing students in their first clinical training is important for improving student performance, helping students develop a professional identity and problem-solving skills, and improving the clinical teaching aspects of the curriculum in nursing programmes. While previous research have examined nurses’ sources of stress and coping styles in the Arab region, there is limited understanding of these stressors and coping strategies of nursing students within the UAE context thereby, highlighting the novelty and significance of the study.
A qualitative study was conducted using semi-structured interviews. Overall 30 students who were undergoing their first clinical placement in Year 2 at the University of Sharjah between May and June 2022 were recruited. All interviews were recorded and transcribed verbatim and analyzed for themes.
During their first clinical training, nursing students are exposed to stress from different sources, including the clinical environment, unfriendly clinical tutors, feelings of disconnection, multiple expectations of clinical staff and patients, and gaps between the curriculum of theory classes and labatories skills and students’ clinical experiences. We extracted three main themes that described students’ stress and use of coping strategies during clinical training: (1) managing expectations; (2) theory-practice gap; and (3) learning to cope. Learning to cope, included two subthemes: positive coping strategies and negative coping strategies.
Conclusions
This qualitative study sheds light from the students viewpoint about the intricate interplay between managing expectations, theory practice gap and learning to cope. Therefore, it is imperative for nursing faculty, clinical agencies and curriculum planners to ensure maximum learning in the clinical by recognizing the significance of the stressors encountered and help students develop positive coping strategies to manage the clinical stressors encountered. Further research is required look at the perspective of clinical stressors from clinical tutors who supervise students during their first clinical practicum.
Peer Review reports
Nursing education programmes aim to provide students with high-quality clinical learning experiences to ensure that nurses can provide safe, direct care to patients [ 1 ]. The nursing baccalaureate programme at the University of Sharjah is a four year program with 137 credits. The programmes has both theoretical and clinical components withs nine clinical courses spread over the four years The first clinical practicum which forms the basis of the study takes place in year 2 semester 2.
Clinical practice experience is an indispensable component of nursing education and links what students learn in the classroom and in skills laboratories to real-life clinical settings [ 2 , 3 , 4 ]. However, a gap exists between theory and practice as the curriculum in the classroom differs from nursing students’ experiences in the clinical nursing practicum [ 5 ]. Clinical nursing training places (or practicums, as they are commonly referred to), provide students with the necessary experiences to ensure that they become proficient in the delivery of patient care [ 6 ]. The clinical practicum takes place in an environment that combines numerous structural, psychological, emotional and organizational elements that influence student learning [ 7 ] and may affect the development of professional nursing competencies, such as compassion, communication and professional identity [ 8 ]. While clinical training is a major component of nursing education curricula, stress related to clinical training is common among students [ 9 ]. Furthermore, the nursing literature indicates that the first exposure to clinical learning is one of the most stressful experiences during undergraduate studies [ 8 , 10 ]. Thus, the clinical component of nursing education is considered more stressful than the theoretical component. Students often view clinical learning, where most learning takes place, as an unsupportive environment [ 11 ]. In addition, they note strained relationships between themselves and clinical preceptors and perceive that the negative attitudes of clinical staff produce stress [ 12 ].
The effects of stress on nursing students often involve a sense of uncertainty, uneasiness, or anxiety. The literature is replete with evidence that nursing students experience a variety of stressors during their clinical practicum, beginning with the first clinical rotation. Nursing is a complex profession that requires continuous interaction with a variety of individuals in a high-stress environment. Stress during clinical learning can have multiple negative consequences, including low academic achievement, elevated levels of burnout, and diminished personal well-being [ 13 , 14 ]. In addition, both theoretical and practical research has demonstrated that increased, continual exposure to stress leads to cognitive deficits, inability to concentrate, lack of memory or recall, misinterpretation of speech, and decreased learning capacity [ 15 ]. Furthermore, stress has been identified as a cause of attrition among nursing students [ 16 ].
Most sources of stress have been categorized as academic, clinical or personal. Each person copes with stress differently [ 17 ], and utilizes deliberate, planned, and psychological efforts to manage stressful demands [ 18 ]. Coping mechanisms are commonly termed adaptation strategies or coping skills. Labrague et al. [ 19 ] noted that students used critical coping strategies to handle stress and suggested that problem solving was the most common coping or adaptation mechanism used by nursing students. Nursing students’ coping strategies affect their physical and psychological well-being and the quality of nursing care they offer. Therefore, identifying the coping strategies that students use to manage stressors is important for early intervention [ 20 ].
Studies on nursing students’ coping strategies have been conducted in various countries. For example, Israeli nursing students were found to adopt a range of coping mechanisms, including talking to friends, engaging in sports, avoiding stress and sadness/misery, and consuming alcohol [ 21 ]. Other studies have examined stress levels among medical students in the Arab region. Chaabane et al. [ 15 ], conducted a systematic review of sudies in Arab countries, including Saudi Arabia, Egypt, Jordan, Iraq, Pakistan, Oman, Palestine and Bahrain, and reported that stress during clinical practicums was prevalent, although it could not be determined whether this was limited to the initial clinical course or occurred throughout clinical training. Stressors highlighted during the clinical period in the systematic review included assignments and workload during clinical practice, a feeling that the requirements of clinical practice exceeded students’ physical and emotional endurance and that their involvement in patient care was limited due to lack of experience. Furthermore, stress can have a direct effect on clinical performance, leading to mental disorders. Tung et al. [ 22 ], reported that the prevalence of depression among nursing students in Arab countries is 28%, which is almost six times greater than the rest of the world [ 22 ]. On the other hand, Saifan et al. [ 5 ], explored the theory-practice gap in the United Arab Emirates and found that clinical stressors could be decreased by preparing students better for clinical education with qualified clinical faculty and supportive preceptors.
The purpose of this study was to identify the stressors experienced by undergraduate nursing students in the United Arab Emirates during their first clinical training and the basic adaptation approaches or coping strategies they used. Recognizing or understanding different coping processes can inform the implementation of corrective measures when students experience clinical stress. The findings of this study may provide valuable information for nursing programmes, nurse educators, and clinical administrators to establish adaptive strategies to reduce stress among students going clinical practicums, particularly stressors from their first clinical training in different healthcare settings.
A qualitative approach was adopted to understand clinical stressors and coping strategies from the perspective of nurses’ lived experience. Qualitative content analysis was employed to obtain rich and detailed information from our qualitative data. Qualitative approaches seek to understand the phenomenon under study from the perspectives of individuals with lived experience [ 23 ]. Qualitative content analysis is an interpretive technique that examines the similarities and differences between and within different areas of text while focusing on the subject [ 24 ]. It is used to examine communication patterns in a repeatable and systematic way [ 25 ] and yields rich and detailed information on the topic under investigation [ 23 ]. It is a method of systematically coding and categorizing information and comprises a process of comprehending, interpreting, and conceptualizing the key meanings from qualitative data [ 26 ].
Setting and participants
This study was conducted after the clinical rotations ended in April 2022, between May and June in the nursing programme at the College of Health Sciences, University of Sharjah, in the United Arab Emirates. The study population comprised undergraduate nursing students who were undergoing their first clinical training and were recruited using purposive sampling. The inclusion criteria for this study were second-year nursing students in the first semester of clinical training who could speak English, were willing to participate in this research, and had no previous clinical work experience. The final sample consisted of 30 students.
Research instrument
The research instrument was a semi structured interview guide. The interview questions were based on an in-depth review of related literature. An intensive search included key words in Google Scholar, PubMed like the terms “nursing clinical stressors”, “nursing students”, and “coping mechanisms”. Once the questions were created, they were validated by two other faculty members who had relevant experience in mental health. A pilot test was conducted with five students and based on their feedback the following research questions, which were addressed in the study.
How would you describe your clinical experiences during your first clinical rotations?
In what ways did you find the first clinical rotation to be stressful?
What factors hindered your clinical training?
How did you cope with the stressors you encountered in clinical training?
Which strategies helped you cope with the clinical stressors you encountered?
Data collection
Semi-structured interviews were chosen as the method for data collection. Semi structured interviews are a well-established approach for gathering data in qualitative research and allow participants to discuss their views, experiences, attitudes, and beliefs in a positive environment [ 27 ]. This approach allows for flexibility in questioning thereby ensuring that key topics related to clinical learning stressors and coping strategies would be explored. Participants were given the opportunity to express their views, experiences, attitudes, and beliefs in a positive environment, encouraging open communication. These semi structured interviews were conducted by one member of the research team (MAS) who had a mental health background, and another member of the research team who attended the interviews as an observer (JMD). Neither of these researchers were involved in teaching the students during their clinical practicum, which helped to minimize bias. The interviews took place at the University of Sharjah, specifically in building M23, providing a familiar and comfortable environment for the participant. Before the interviews were all students who agreed to participate were provided with an explanation of the study’s purpose. The time and location of each interview were arranged. Before the interviews were conducted, all students who provided consent to participate received an explanation of the purpose of the study, and the time and place of each interview were arranged to accommodate the participants’ schedules and preferences. The interviews were conducted after the clinical rotation had ended in April, and after the final grades had been submitted to the coordinator. The timings of the interviews included the month of May and June which ensured that participants have completed their practicum experience and could reflect on the stressors more comprehensively. The interviews were audio-recorded with the participants’ consent, and each interview lasted 25–40 min. The data were collected until saturation was reached for 30 students. Memos and field notes were also recorded as part of the data collection process. These additional data allowed for triangulation to improve the credibility of the interpretations of the data [ 28 ]. Memos included the interviewers’ thoughts and interpretations about the interviews, the research process (including questions and gaps), and the analytic progress used for the research. Field notes were used to record the interviewers’ observations and reflections on the data. These additional data collection methods were important to guide the researchers in the interpretation of the data on the participants’ feelings, perspectives, experiences, attitudes, and beliefs. Finally, member checking was performed to ensure conformability.
Data analysis
The study used the content analysis method proposed by Graneheim and Lundman [ 24 ]. According to Graneheim and Lundman [ 24 ], content analysis is an interpretive technique that examines the similarities and differences between distinct parts of a text. This method allows researchers to determine exact theoretical and operational definitions of words, phrases, and symbols by elucidating their constituent properties [ 29 ]. First, we read the interview transcripts several times to reach an overall understanding of the data. All verbatim transcripts were read several times and discussed among all authors. We merged and used line-by-line coding of words, sentences, and paragraphs relevant to each other in terms of both the content and context of stressors and coping mechanisms. Next, we used data reduction to assess the relationships among themes using tables and diagrams to indicate conceptual patterns. Content related to stress encountered by students was extracted from the transcripts. In a separate document, we integrated and categorized all words and sentences that were related to each other in terms of both content and context. We analyzed all codes and units of meaning and compared them for similarities and differences in the context of this study. Furthermore, the emerging findings were discussed with other members of the researcher team. The final abstractions of meaningful subthemes into themes were discussed and agreed upon by the entire research team. This process resulted in the extraction of three main themes in addition to two subthemes related to stress and coping strategies.
Ethical considerations
The University of Sharjah Research Ethics Committee provided approval to conduct this study (Reference Number: REC 19-12-03-01-S). Before each interview, the goal and study procedures were explained to each participant, and written informed consent was obtained. The participants were informed that participation in the study was voluntary and that they could withdraw from the study at any time. In the event they wanted to withdraw from the study, all information related to the participant would be removed. No participant withdrew from the study. Furthermore, they were informed that their clinical practicum grade would not be affected by their participation in this study. We chose interview locations in Building M23that were private and quiet to ensure that the participants felt at ease and confident in verbalizing their opinions. No participant was paid directly for involvement in this study. In addition, participants were assured that their data would remain anonymous and confidential. Confidentiality means that the information provided by participants was kept private with restrictions on how and when data can be shared with others. The participants were informed that their information would not be duplicated or disseminated without their permission. Anonymity refers to the act of keeping people anonymous with respect to their participation in a research endeavor. No personal identifiers were used in this study, and each participant was assigned a random alpha-numeric code (e.g., P1 for participant 1). All digitally recorded interviews were downloaded to a secure computer protected by the principal investigator with a password. The researchers were the only people with access to the interview material (recordings and transcripts). All sensitive information and materials were kept secure in the principal researcher’s office at the University of Sharjah. The data will be maintained for five years after the study is completed, after which the material will be destroyed (the transcripts will be shredded, and the tapes will be demagnetized).
In total, 30 nursing students who were enrolled in the nursing programme at the Department of Nursing, College of Health Sciences, University of Sharjah, and who were undergoing their first clinical practicum participated in the study. Demographically, 80% ( n = 24) were females and 20% ( n = 6) were male participants. The majority (83%) of study participants ranged in age from 18 to 22 years. 20% ( n = 6) were UAE nationals, 53% ( n = 16) were from Gulf Cooperation Council countries, while 20% ( n = 6) hailed from Africa and 7% ( n = 2) were of South Asian descent. 67% of the respondents lived with their families while 33% lived in the hostel. (Table 1 )
Following the content analysis, we identified three main themes: (1) managing expectations, (2) theory-practice gap and 3)learning to cope. Learning to cope had two subthemes: positive coping strategies and negative coping strategies. An account of each theme is presented along with supporting excerpts for the identified themes. The identified themes provide valuable insight into the stressors encountered by students during their first clinical practicum. These themes will lead to targeted interventions and supportive mechanisms that can be built into the clinical training curriculum to support students during clinical practice.
Theme 1: managing expectations
In our examination of the stressors experienced by nursing students during their first clinical practicum and the coping strategies they employed, we identified the first theme as managing expectations.
The students encountered expectations from various parties, such as clinical staff, patients and patients’ relatives which they had to navigate. They attempted to fulfil their expectations as they progressed through training, which presented a source of stress. The students noted that the hospital staff and patients expected them to know how to perform a variety of tasks upon request, which made the students feel stressed and out of place if they did not know how to perform these tasks. Some participants noted that other nurses in the clinical unit did not allow them to participate in nursing procedures, which was considered an enormous impediment to clinical learning, as noted in the excerpt below:
“…Sometimes the nurses… They will not allow us to do some procedures or things during clinical. And sometimes the patients themselves don’t allow us to do procedures” (P5).
Some of the students noted that they felt they did not belong and felt like foreigners in the clinical unit. Excerpts from the students are presented in the following quotes;
“The clinical environment is so stressful. I don’t feel like I belong. There is too little time to build a rapport with hospital staff or the patient” (P22).
“… you ask the hospital staff for some guidance or the location of equipment, and they tell us to ask our clinical tutor …but she is not around … what should I do? It appears like we do not belong, and the sooner the shift is over, the better” (P18).
“The staff are unfriendly and expect too much from us students… I feel like I don’t belong, or I am wasting their (the hospital staff’s) time. I want to ask questions, but they have loads to do” (P26).
Other students were concerned about potential failure when working with patients during clinical training, which impacted their confidence. They were particularly afraid of failure when performing any clinical procedures.
“At the beginning, I was afraid to do procedures. I thought that maybe the patient would be hurt and that I would not be successful in doing it. I have low self-confidence in doing procedures” (P13).
The call bell rings, and I am told to answer Room No. XXX. The patient wants help to go to the toilet, but she has two IV lines. I don’t know how to transport the patient… should I take her on the wheelchair? My eyes glance around the room for a wheelchair. I am so confused …I tell the patient I will inform the sister at the nursing station. The relative in the room glares at me angrily … “you better hurry up”…Oh, I feel like I don’t belong, as I am not able to help the patient… how will I face the same patient again?” (P12).
Another major stressor mentioned in the narratives was related to communication and interactions with patients who spoke another language, so it was difficult to communicate.
“There was a challenge with my communication with the patients. Sometimes I have communication barriers because they (the patients) are of other nationalities. I had an experience with a patient [who was] Indian, and he couldn’t speak my language. I did not understand his language” (P9).
Thus, a variety of expectations from patients, relatives, hospital staff, and preceptors acted as sources of stress for students during their clinical training.
Theme 2: theory-practice gap
Theory-practice gaps have been identified in previous studies. In our study, there was complete dissonance between theory and actual clinical practice. The clinical procedures or practices nursing students were expected to perform differed from the theory they had covered in their university classes and skills lab. This was described as a theory–practice gap and often resulted in stress and confusion.
“For example …the procedures in the hospital are different. They are different from what we learned or from theory on campus. Or… the preceptors have different techniques than what we learned on campus. So, I was stress[ed] and confused about it” (P11).
Furthermore, some students reported that they did not feel that they received adequate briefing before going to clinical training. A related source of stress was overload because of the volume of clinical coursework and assignments in addition to clinical expectations. Additionally, the students reported that a lack of time and time management were major sources of stress in their first clinical training and impacted their ability to complete the required paperwork and assignments:
“…There is not enough time…also, time management at the hospital…for example, we start at seven a.m., and the handover takes 1 hour to finish. They (the nurses at the hospital) are very slow…They start with bed making and morning care like at 9.45 a.m. Then, we must fill [out] our assessment tool and the NCP (nursing care plan) at 10 a.m. So, 15 only minutes before going to our break. We (the students) cannot manage this time. This condition makes me and my friends very stressed out. -I cannot do my paperwork or assignments; no time, right?” (P10).
“Stressful. There is a lot of work to do in clinical. My experiences are not really good with this course. We have a lot of things to do, so many assignments and clinical procedures to complete” (P16).
The participants noted that the amount of required coursework and number of assignments also presented a challenge during their first clinical training and especially affected their opportunity to learn.
“I need to read the file, know about my patient’s condition and pathophysiology and the rationale for the medications the patient is receiving…These are big stressors for my learning. I think about assignments often. Like, we are just focusing on so many assignments and papers. We need to submit assessments and care plans for clinical cases. We focus our time to complete and finish the papers rather than doing the real clinical procedures, so we lose [the] chance to learn” (P25).
Another participant commented in a similar vein that there was not enough time to perform tasks related to clinical requirements during clinical placement.
“…there is a challenge because we do not have enough time. Always no time for us to submit papers, to complete assessment tools, and some nurses, they don’t help us. I think we need more time to get more experiences and do more procedures, reduce the paperwork that we have to submit. These are challenges …” (P14).
There were expectations that the students should be able to carry out their nursing duties without becoming ill or adversely affected. In addition, many students reported that the clinical environment was completely different from the skills laboratory at the college. Exposure to the clinical setting added to the theory-practice gap, and in some instances, the students fell ill.
One student made the following comment:
“I was assisting a doctor with a dressing, and the sight and smell from the oozing wound was too much for me. I was nauseated. As soon as the dressing was done, I ran to the bathroom and threw up. I asked myself… how will I survive the next 3 years of nursing?” (P14).
Theme 3: learning to cope
The study participants indicated that they used coping mechanisms (both positive and negative) to adapt to and manage the stressors in their first clinical practicum. Important strategies that were reportedly used to cope with stress were time management, good preparation for clinical practice, and positive thinking as well as engaging in physical activity and self-motivation.
“Time management. Yes, it is important. I was encouraging myself. I used time management and prepared myself before going to the clinical site. Also, eating good food like cereal…it helps me very much in the clinic” (P28).
“Oh yeah, for sure positive thinking. In the hospital, I always think positively. Then, after coming home, I get [to] rest and think about positive things that I can do. So, I will think something good [about] these things, and then I will be relieved of stress” (P21).
Other strategies commonly reported by the participants were managing their breathing (e.g., taking deep breaths, breathing slowly), taking breaks to relax, and talking with friends about the problems they encountered.
“I prefer to take deep breaths and breathe slowly and to have a cup of coffee and to talk to my friends about the case or the clinical preceptor and what made me sad so I will feel more relaxed” (P16).
“Maybe I will take my break so I feel relaxed and feel better. After clinical training, I go directly home and take a long shower, going over the day. I will not think about anything bad that happened that day. I just try to think about good things so that I forget the stress” (P27).
“Yes, my first clinical training was not easy. It was difficult and made me stressed out…. I felt that it was a very difficult time for me. I thought about leaving nursing” (P7).
I was not able to offer my prayers. For me, this was distressing because as a Muslim, I pray regularly. Now, my prayer time is pushed to the end of the shift” (P11).
“When I feel stress, I talk to my friends about the case and what made me stressed. Then I will feel more relaxed” (P26).
Self-support or self-motivation through positive self-talk was also used by the students to cope with stress.
“Yes, it is difficult in the first clinical training. When I am stress[ed], I go to the bathroom and stand in the front of the mirror; I talk to myself, and I say, “You can do it,” “you are a great student.” I motivate myself: “You can do it”… Then, I just take breaths slowly several times. This is better than shouting or crying because it makes me tired” (P11).
Other participants used physical activity to manage their stress.
“How do I cope with my stress? Actually, when I get stressed, I will go for a walk on campus” (P4).
“At home, I will go to my room and close the door and start doing my exercises. After that, I feel the negative energy goes out, then I start to calm down… and begin my clinical assignments” (P21).
Both positive and negative coping strategies were utilized by the students. Some participants described using negative coping strategies when they encountered stress during their clinical practice. These negative coping strategies included becoming irritable and angry, eating too much food, drinking too much coffee, and smoking cigarettes.
“…Negative adaptation? Maybe coping. If I am stressed, I get so angry easily. I am irritable all day also…It is negative energy, right? Then, at home, I am also angry. After that, it is good to be alone to think about my problems” (P12).
“Yeah, if I…feel stress or depressed, I will eat a lot of food. Yeah, ineffective, like I will be eating a lot, drinking coffee. Like I said, effective, like I will prepare myself and do breathing, ineffective, I will eat a lot of snacks in between my free time. This is the bad side” (P16).
“…During the first clinical practice? Yes, it was a difficult experience for us…not only me. When stressed, during a break at the hospital, I will drink two or three cups of coffee… Also, I smoke cigarettes… A lot. I can drink six cups [of coffee] a day when I am stressed. After drinking coffee, I feel more relaxed, I finish everything (food) in the refrigerator or whatever I have in the pantry, like chocolates, chips, etc” (P23).
These supporting excerpts for each theme and the analysis offers valuable insights into the specific stressors faced by nursing students during their first clinical practicum. These insights will form the basis for the development of targeted interventions and supportive mechanisms within the clinical training curriculum to better support students’ adjustment and well-being during clinical practice.
Our study identified the stressors students encounter in their first clinical practicum and the coping strategies, both positive and negative, that they employed. Although this study emphasizes the importance of clinical training to prepare nursing students to practice as nurses, it also demonstrates the correlation between stressors and coping strategies.The content analysis of the first theme, managing expectations, paves the way for clinical agencies to realize that the students of today will be the nurses of tomorrow. It is important to provide a welcoming environment where students can develop their identities and learn effectively. Additionally, clinical staff should foster an environment of individualized learning while also assisting students in gaining confidence and competence in their repertoire of nursing skills, including critical thinking, problem solving and communication skills [ 8 , 15 , 19 , 30 ]. Another challenge encountered by the students in our study was that they were prevented from participating in clinical procedures by some nurses or patients. This finding is consistent with previous studies reporting that key challenges for students in clinical learning include a lack of clinical support and poor attitudes among clinical staff and instructors [ 31 ]. Clinical staff with positive attitudes have a positive impact on students’ learning in clinical settings [ 32 ]. The presence, supervision, and guidance of clinical instructors and the assistance of clinical staff are essential motivating components in the clinical learning process and offer positive reinforcement [ 30 , 33 , 34 ]. Conversely, an unsupportive learning environment combined with unwelcoming clinical staff and a lack of sense of belonging negatively impact students’ clinical learning [ 35 ].
The sources of stress identified in this study were consistent with common sources of stress in clinical training reported in previous studies, including the attitudes of some staff, students’ status in their clinical placement and educational factors. Nursing students’ inexperience in the clinical setting and lack of social and emotional experience also resulted in stress and psychological difficulties [ 36 ]. Bhurtun et al. [ 33 ] noted that nursing staff are a major source of stress for students because the students feel like they are constantly being watched and evaluated.
We also found that students were concerned about potential failure when working with patients during their clinical training. Their fear of failure when performing clinical procedures may be attributable to low self-confidence. Previous studies have noted that students were concerned about injuring patients, being blamed or chastised, and failing examinations [ 37 , 38 ]. This was described as feeling “powerless” in a previous study [ 7 , 12 ]. In addition, patients’ attitudes towards “rejecting” nursing students or patients’ refusal of their help were sources of stress among the students in our study and affected their self-confidence. Self-confidence and a sense of belonging are important for nurses’ personal and professional identity, and low self-confidence is a problem for nursing students in clinical learning [ 8 , 39 , 40 ]. Our findings are consistent with a previous study that reported that a lack of self-confidence was a primary source of worry and anxiety for nursing students and affected their communication and intention to leave nursing [ 41 ].
In the second theme, our study suggests that students encounter a theory-practice gap in clinical settings, which creates confusion and presents an additional stressors. Theoretical and clinical training are complementary elements of nursing education [ 40 ], and this combination enables students to gain the knowledge, skills, and attitudes necessary to provide nursing care. This is consistent with the findings of a previous study that reported that inconsistencies between theoretical knowledge and practical experience presented a primary obstacle to the learning process in the clinical context [ 42 ], causing students to lose confidence and become anxious [ 43 ]. Additionally, the second theme, the theory-practice gap, authenticates Safian et al.’s [ 5 ] study of the theory-practice gap that exists United Arab Emirates among nursing students as well as the need for more supportive clinical faculty and the extension of clinical hours. The need for better time availability and time management to complete clinical tasks were also reported by the students in the study. Students indicated that they had insufficient time to complete clinical activities because of the volume of coursework and assignments. Our findings support those of Chaabane et al. [ 15 ]. A study conducted in Saudi Arabia [ 44 ] found that assignments and workload were among the greatest sources of stress for students in clinical settings. Effective time management skills have been linked to academic achievement, stress reduction, increased creativity [ 45 ], and student satisfaction [ 46 ]. Our findings are also consistent with previous studies that reported that a common source of stress among first-year students was the increased classroom workload [ 19 , 47 ]. As clinical assignments and workloads are major stressors for nursing students, it is important to promote activities to help them manage these assignments [ 48 ].
Another major challenge reported by the participants was related to communicating and interacting with other nurses and patients. The UAE nursing workforce and population are largely expatriate and diverse and have different cultural and linguistic backgrounds. Therefore, student nurses encounter difficulty in communication [ 49 ]. This cultural diversity that students encounter in communication with patients during clinical training needs to be addressed by curriculum planners through the offering of language courses and courses on cultural diversity [ 50 ].
Regarding the third and final theme, nursing students in clinical training are unable to avoid stressors and must learn to cope with or adapt to them. Previous research has reported a link between stressors and the coping mechanisms used by nursing students [ 51 , 52 , 53 ]. In particular, the inability to manage stress influences nurses’ performance, physical and mental health, attitude, and role satisfaction [ 54 ]. One such study suggested that nursing students commonly use problem-focused (dealing with the problem), emotion-focused (regulating emotion), and dysfunctional (e.g., venting emotions) stress coping mechanisms to alleviate stress during clinical training [ 15 ]. Labrague et al. [ 51 ] highlighted that nursing students use both active and passive coping techniques to manage stress. The pattern of clinical stress has been observed in several countries worldwide. The current study found that first-year students experienced stress during their first clinical training [ 35 , 41 , 55 ]. The stressors they encountered impacted their overall health and disrupted their clinical learning. Chaabane et al. [ 15 ] reported moderate and high stress levels among nursing students in Bahrain, Egypt, Iraq, Jordan, Oman, Pakistan, Palestine, Saudi Arabia, and Sudan. Another study from Bahrain reported that all nursing students experienced moderate to severe stress in their first clinical placement [ 56 ]. Similarly, nursing students in Spain experienced a moderate level of stress, and this stress was significantly correlated with anxiety [ 30 ]. Therefore, it is imperative that pastoral systems at the university address students’ stress and mental health so that it does not affect their clinical performance. Faculty need to utilize evidence-based interventions to support students so that anxiety-producing situations and attrition are minimized.
In our study, students reported a variety of positive and negative coping mechanisms and strategies they used when they experienced stress during their clinical practice. Positive coping strategies included time management, positive thinking, self-support/motivation, breathing, taking breaks, talking with friends, and physical activity. These findings are consistent with those of a previous study in which healthy coping mechanisms used by students included effective time management, social support, positive reappraisal, and participation in leisure activities [ 57 ]. Our study found that relaxing and talking with friends were stress management strategies commonly used by students. Communication with friends to cope with stress may be considered social support. A previous study also reported that people seek social support to cope with stress [ 58 ]. Some students in our study used physical activity to cope with stress, consistent with the findings of previous research. Stretching exercises can be used to counteract the poor posture and positioning associated with stress and to assist in reducing physical tension. Promoting such exercise among nursing students may assist them in coping with stress in their clinical training [ 59 ].
Our study also showed that when students felt stressed, some adopted negative coping strategies, such as showing anger/irritability, engaging in unhealthy eating habits (e.g., consumption of too much food or coffee), or smoking cigarettes. Previous studies have reported that high levels of perceived stress affect eating habits [ 60 ] and are linked to poor diet quality, increased snacking, and low fruit intake [ 61 ]. Stress in clinical settings has also been linked to sleep problems, substance misuse, and high-risk behaviors’ and plays a major role in student’s decision to continue in their programme.
Implications of the study
The implications of the study results can be grouped at multiple levels including; clinical, educational, and organizational level. A comprehensive approach to addressing the stressors encountered by nursing students during their clinical practicum can be overcome by offering some practical strategies to address the stressors faced by nursing students during their clinical practicum. By integrating study findings into curriculum planning, mentorship programs, and organizational support structures, a supportive and nurturing environment that enhances students’ learning, resilience, and overall success can be envisioned.
Clinical level
Introducing simulation in the skills lab with standardized patients and the use of moulage to demonstrate wounds, ostomies, and purulent dressings enhances students’ practical skills and prepares them for real-world clinical scenarios. Organizing orientation days at clinical facilities helps familiarize students with the clinical environment, identify potential stressors, and introduce interventions to enhance professionalism, social skills, and coping abilities Furthermore, creating a WhatsApp group facilitates communication and collaboration among hospital staff, clinical tutors, nursing faculty, and students, enabling immediate support and problem-solving for clinical situations as they arise, Moreover, involving chief nursing officers of clinical facilities in the Nursing Advisory Group at the Department of Nursing promotes collaboration between academia and clinical practice, ensuring alignment between educational objectives and the needs of the clinical setting [ 62 ].
Educational level
Sharing study findings at conferences (we presented the results of this study at Sigma Theta Tau International in July 2023 in Abu Dhabi, UAE) and journal clubs disseminates knowledge and best practices among educators and clinicians, promoting awareness and implementation of measures to improve students’ learning experiences. Additionally we hold mentorship training sessions annually in January and so we shared with the clinical mentors and preceptors the findings of this study so that they proactively they are equipped with strategies to support students’ coping with stressors during clinical placements.
Organizational level
At the organizational we relooked at the available student support structures, including counseling, faculty advising, and career advice, throughout the nursing program emphasizing the importance of holistic support for students’ well-being and academic success as well as retention in the nursing program. Also, offering language courses as electives recognizes the value of communication skills in nursing practice and provides opportunities for personal and professional development.
For first-year nursing students, clinical stressors are inevitable and must be given proper attention. Recognizing nursing students’ perspectives on the challenges and stressors experienced in clinical training is the first step in overcoming these challenges. In nursing schools, providing an optimal clinical environment as well as increasing supervision and evaluation of students’ practices should be emphasized. Our findings demonstrate that first-year nursing students are exposed to a variety of different stressors. Identifying the stressors, pressures, and obstacles that first-year students encounter in the clinical setting can assist nursing educators in resolving these issues and can contribute to students’ professional development and survival to allow them to remain in the profession. To overcome stressors, students frequently employ problem-solving approaches or coping mechanisms. The majority of nursing students report stress at different levels and use a variety of positive and negative coping techniques to manage stress.
The present results may not be generalizable to other nursing institutions because this study used a purposive sample along with a qualitative approach and was limited to one university in the Middle East. Furthermore, the students self-reported their stress and its causes, which may have introduced reporting bias. The students may also have over or underreported stress or coping mechanisms because of fear of repercussions or personal reasons, even though the confidentiality of their data was ensured. Further studies are needed to evaluate student stressors and coping now that measures have been introduced to support students. Time will tell if these strategies are being used effectively by both students and clinical personnel or if they need to be readdressed. Finally, we need to explore the perceptions of clinical faculty towards supervising students in their first clinical practicum so that clinical stressors can be handled effectively.
Data availability
The data sets are available with the corresponding author upon reasonable request.
Almarwani AM. The effect of integrating a nursing licensure examination preparation course into a nursing program curriculum: a quasi-experimental study. Saudi J Health Sci. 2022;11:184–9.
Article Google Scholar
Horntvedt MT, Nordsteien A, Fermann T, Severinsson E. Strategies for teaching evidence-based practice in nursing education: a thematic literature review. BMC Med Educ. 2018;18:172.
Article PubMed PubMed Central Google Scholar
Larsson M, Sundler AJ, Blomberg K, Bisholt B. The clinical learning environment during clinical practice in postgraduate district nursing students’ education: a cross-sectional study. Nurs Open. 2023;10:879–88.
Article PubMed Google Scholar
Sellberg M, Palmgren PJ, Möller R. A cross-sectional study of clinical learning environments across four undergraduate programs using the undergraduate clinical education environment measure. BMC Med Educ. 2021;21:258.
Saifan A, Devadas B, Mekkawi M, Amoor H, Matizha P, James J, et al. Managing the theory-practice gap in nursing education and practice: hearing the voices of nursing students in the United Arab Emirates. J Nurs Manag. 2021;29:1869–79.
Flott EA, Linden L. The clinical learning environment in nursing education: a concept analysis. J Adv Nurs. 2016;72:501–13.
Kalyani MN, Jamshidi N, Molazem Z, Torabizadeh C, Sharif F. How do nursing students experience the clinical learning environment and respond to their experiences? A qualitative study. BMJ Open. 2019;9:e028052.
Mahasneh D, Shoqirat N, Alsaraireh A, Singh C, Thorpe L. From learning on mannequins to practicing on patients: nursing students’ first-time experience of clinical placement in Jordan. SAGE Open Nurs. 2021;7:23779608211004298.
PubMed PubMed Central Google Scholar
Stubin C. Clinical stress among undergraduate nursing students: perceptions of clinical nursing faculty. Int J Nurs Educ Scholarsh. 2020;17:20190111.
Ahmed WAM. Anxiety and related symptoms among critical care nurses in Albaha, Kingdom of Saudi Arabia. AIMS Med Sci. 2015;2:303–9.
Alhassan. Duke Phillips. 2024.
Ekstedt M, Lindblad M, Löfmark A. Nursing students’ perception of the clinical learning environment and supervision in relation to two different supervision models - a comparative cross-sectional study. BMC Nurs. 2019;18:49.
Bradshaw C, Murphy Tighe S, Doody O. Midwifery students’ experiences of their clinical internship: a qualitative descriptive study. Nurse Educ Today. 2018;68:213–7.
McCarthy B, Trace A, O’Donovan M, O’Regan P, Brady-Nevin C, O’Shea M, et al. Coping with stressful events: a pre-post-test of a psycho-educational intervention for undergraduate nursing and midwifery students. Nurse Educ Today. 2018;61:273–80.
Chaabane S, Chaabna K, Bhagat S, Abraham A, Doraiswamy S, Mamtani R, et al. Perceived stress, stressors, and coping strategies among nursing students in the Middle East and North Africa: an overview of systematic reviews. Syst Rev. 2021;10:136.
Pines EW, Rauschhuber ML, Norgan GH, Cook JD, Canchola L, Richardson C, et al. Stress resiliency, psychological empowerment and conflict management styles among baccalaureate nursing students. J Adv Nurs. 2012;68:1482–93.
Lazarus RS. Coping theory and research: past, present, and future. Psychosom Med. 1993;55:234–47.
Article CAS PubMed Google Scholar
Boyd MA. Essentials of psychiatric nursing. Philadelphia, PA: Wolters Kluwer; 2017.
Google Scholar
Labrague LJ, McEnroe-Petitte DM, Gloe D, Thomas L, Papathanasiou IV, Tsaras K. A literature review on stress and coping strategies in nursing students. J Ment Health. 2017;26:471–80.
Ni C, Lo D, Liu X, Ma J, Xu S, Li L. Chinese female nursing students’ coping strategies, self-esteem and related factors in different years of school. J Nurs Educ Pract. 2012;2:33–41.
Jan LK, Popescu L. Israel’s nursing students’ stress sources and coping strategies during their first clinical experience in hospital wards-a qualitative research. Soc Work Rev / Rev Asistenta Soc. 2014;13:163–88.
Tung YJ, Lo KKH, Ho RCM, Tam WSW. Prevalence of depression among nursing students: a systematic review and meta-analysis. Nurse Educ Today. 2018;63:119–29.
Speziale HS, Streubert HJ, Carpenter DR. Qualitative research in nursing: advancing the humanistic imperative. Philadelphia, PA: Lippincott Williams & Wilkins; 2011.
Graneheim UH, Lundman B. Qualitative content analysis in nursing research: concepts, procedures and measures to achieve trustworthiness. Nurse Educ Today. 2004;24:105–12.
Bryman A. Integrating quantitative and qualitative research: how is it done? Qual Res. 2006;6:97–113.
Holloway I, Wheeler S. Qualitative research in nursing and healthcare. New York, NY: Wiley; 2013.
Richards L, Morse J. A user’s guide to qualitative methods. London, UK: Sage; 2007.
Lincoln Y, Guba EG. The SAGE handbook of qualitative research. Newbury Park, CA: SAGE Publications Inc; 2017.
Park S, Park KS. Family stigma: a concept analysis. Asian Nurs Res. 2014;8:165–71.
Onieva-Zafra MD, Fernández-Muñoz JJ, Fernández-Martínez E, García-Sánchez FJ, Abreu-Sánchez A, Parra-Fernández ML. Anxiety, perceived stress and coping strategies in nursing students: a cross-sectional, correlational, descriptive study. BMC Med Educ. 2020;20:370.
Albloushi M, Ferguson L, Stamler L, Bassendowski S, Hellsten L, Kent-Wilkinson A. Saudi female nursing students experiences of sense of belonging in the clinical settings: a qualitative study. Nurse Educ Pract. 2019;35:69–74.
Arkan B, Ordin Y, Yılmaz D. Undergraduate nursing students’ experience related to their clinical learning environment and factors affecting to their clinical learning process. Nurse Educ Pract. 2018;29:127–32.
Bhurtun HD, Azimirad M, Saaranen T, Turunen H. Stress and coping among nursing students during clinical training: an integrative review. J Nurs Educ. 2019;58:266–72.
Jamshidi N, Molazem Z, Sharif F, Torabizadeh C, Kalyani MN. The challenges of nursing students in the clinical learning environment: a qualitative study. ScientificWorldJournal. 2016;2016:1846178.
Porter SL. First year nursing students’ perceptions of stress and resilience during their initial clinical placement and the introduction of a stress management app: a mixed methods approach. A thesis submitted in partial fulfilment of the requirements of Edinburgh Napier University, for the award of Doctor of Philosophy. 2019. https://www.napier.ac.uk/~/media/worktribe/output-2086663/first-year-nursing-students-perceptions-of-stress-and-resilience-during-their-initial.pdf
Panda S, Dash M, John J, Rath K, Debata A, Swain D, et al. Challenges faced by student nurses and midwives in clinical learning environment - A systematic review and meta-synthesis. Nurse Educ Today. 2021;101:104875.
Ahmadi G, Shahriari M, Keyvanara M, Kohan S. Midwifery students’ experiences of learning clinical skills in Iran: a qualitative study. Int J Med Educ. 2018;9:64–71.
Harrison-White K, Owens J. Nurse link lecturers’ perceptions of the challenges facing student nurses in clinical learning environments: a qualitative study. Nurse Educ Pract. 2018;32:78–83.
Grobecker PA. A sense of belonging and perceived stress among baccalaureate nursing students in clinical placements. Nurse Educ Today. 2016;36:178–83.
Msiska G, Kamanga M, Chilemba E, Msosa A, Munkhondya TE. Sources of stress among undergraduate nursing students during clinical practice: a Malawian perspective. Open J Nurs. 2019;9:1.
Joolaee S, Amiri SRJ, Farahani MA, Varaei S. Iranian nursing students’ preparedness for clinical training: a qualitative study. Nurse Educ Today. 2015;35:e13–7.
Günay U, Kılınç G. The transfer of theoretical knowledge to clinical practice by nursing students and the difficulties they experience: a qualitative study. Nurse Educ Today. 2018;65:81–6.
Farzi S, Shahriari M, Farzi S. Exploring the challenges of clinical education in nursing and strategies to improve it: a qualitative study. J Educ Health Promot. 2018;7:115.
Hamaideh SH, Al-Omari H, Al-Modallal H. Nursing students’ perceived stress and coping behaviors in clinical training in Saudi Arabia. J Ment Health. 2017;26:197–203.
Yaghoobi A, Mohagheghi H, Zade MY, Ganji K, Olfatii N. The effect of time management training on test anxiety and academic achievement motivation among high school students. J Sch Psychol. 2014;3:131–44.
Kebriaei A, Bidgoli MS, Saeedi A. Relationship between use of time management skills and satisfaction with spending time among students of Zahedan University of Medical Sciences. J Med Educ Dev. 2014;6:79–88.
Chen YW, Hung CH. Predictors of Taiwanese baccalaureate nursing students’ physio-psycho-social responses during clinical practicum. Nurse Educ Today. 2014;34:73–7.
Ab Latif R, Mat Nor MZ. Stressors and coping strategies during clinical practice among diploma nursing students. Malays J Med Sci. 2019;26:88–98.
Al-Yateem N, Almarzouqi A, Dias JM, Saifan A, Timmins F. Nursing in the United Arab Emirates: current challenges and opportunities. J Nurs Manag. 2021;29:109–12.
Baraz-Pordanjani S, Memarian R, Vanaki Z. Damaged professional identity as a barrier to Iranian nursing students’ clinical learning: a qualitative study. J Clin Nurs Midwifery. 2014;3:1–15.
Labrague LJ, McEnroe-Petitte DM, Papathanasiou IV, Edet OB, Tsaras K, Leocadio MC, et al. Stress and coping strategies among nursing students: an international study. J Ment Health. 2018;27:402–8.
Madian AAEM, Abdelaziz MM, Ahmed HAE. Level of stress and coping strategies among nursing students at Damanhour University, Egypt. Am J Nurs Res. 2019;7:684–96.
Wu CS, Rong JR, Huang MZ. Factors associated with perceived stress of clinical practice among associate degree nursing students in Taiwan. BMC Nurs. 2021;20:89.
Zhao FF, Lei XL, He W, Gu YH, Li DW. The study of perceived stress, coping strategy and self-efficacy of Chinese undergraduate nursing students in clinical practice. Int J Nurs Pract. 2015;21:401–9.
Bektaş H, Terkes N, Özer Z. Stress and ways of coping among first year nursing students: a Turkish perspective. J Hum Sci. 2018;15:319–30.
John B, Al-Sawad M. Perceived stress in clinical areas and emotional intelligence among baccalaureate nursing students. J Indian Acad Appl Psychol. 2015;41:76–85.
Mapfumo JS, Chitsiko N, Chireshe R. Teaching practice generated stressors and coping mechanisms among student teachers in Zimbabwe. S Afr J Educ. 2012;32:155–66.
Timmins F, Corroon AM, Byrne G, Mooney B. The challenge of contemporary nurse education programmes. Perceived stressors of nursing students: mental health and related lifestyle issues. J Psychiatr Ment Health Nurs. 2011;18:758–66.
Hegberg NJ, Tone EB. Physical activity and stress resilience: considering those at-risk for developing mental health problems. Ment Health Phys Act. 2015;8:1–7.
Shudifat RM, Al-Husban RY. Perceived sources of stress among first-year nursing students in Jordan. J Psychosoc Nurs Ment Health Serv. 2015;53:37–43.
El Ansari W, Adetunji H, Oskrochi R. Food and mental health: relationship between food and perceived stress and depressive symptoms among university students in the United Kingdom. Cent Eur J Public Health. 2014;22:90–7.
Dias JM, Aderibigbe SA, Abraham MS. Undergraduate nursing students’ mentoring experiences in the clinical practicum: the United Arab Emirates (UAE) perspective. J Nurs Manag. 2022;30:4304–13.
Download references
Acknowledgements
The authors are grateful to all second year nursing students who voluntarily participated in the study.
No funding was received. Not applicable.
Author information
Authors and affiliations.
Department of Nursing, College of Health Sciences, University of Sharjah, POBox, Sharjah, 272272, UAE
Jacqueline Maria Dias, Muhammad Arsyad Subu, Nabeel Al-Yateem, Fatma Refaat Ahmed, Syed Azizur Rahman, Mini Sara Abraham, Sareh Mirza Forootan, Farzaneh Ahmad Sarkhosh & Fatemeh Javanbakh
Health Care Management, College of Health Sciences, University of Sharjah, Sharjah, United Arab Emirates
Syed Azizur Rahman
You can also search for this author in PubMed Google Scholar
Contributions
JMD conceptualized the idea and designed the methodology, formal analysis, writing original draft and project supervision and mentoring. MAS prepared the methodology and conducted the qualitative interviews and analyzed the methodology and writing of original draft and project supervision. NY, FRA, SAR, MSA writing review and revising the draft. SMF, FAS, FJ worked with MAS on the formal analysis and prepared the first draft.All authors reviewed the final manuscipt of the article.
Corresponding author
Correspondence to Jacqueline Maria Dias .
Ethics declarations
Ethics approval and consent to participate.
The Research Ethics Committee (REC) under) the Office of the Vice Chancellor for Research and Graduate Studies UOS approved this study (REC 19-12-03-01-S). Additionally, a written consent was obtained from all participants and the process followed the recommended policies and guidelines of the Declaration of Helsinki.
Consent for publication
Not applicable.
Competing interests
Dr Fatma Refaat Ahmed is an editorial board member in BMC Nursing. Other authors do not have any conflict of interest
Additional information
Publisher’s note.
Springer Nature remains neutral with regard to jurisdictional claims in published maps and institutional affiliations.
Electronic supplementary material
Below is the link to the electronic supplementary material.
Supplementary Material 1
Rights and permissions.
Open Access This article is licensed under a Creative Commons Attribution 4.0 International License, which permits use, sharing, adaptation, distribution and reproduction in any medium or format, as long as you give appropriate credit to the original author(s) and the source, provide a link to the Creative Commons licence, and indicate if changes were made. The images or other third party material in this article are included in the article’s Creative Commons licence, unless indicated otherwise in a credit line to the material. If material is not included in the article’s Creative Commons licence and your intended use is not permitted by statutory regulation or exceeds the permitted use, you will need to obtain permission directly from the copyright holder. To view a copy of this licence, visit http://creativecommons.org/licenses/by/4.0/ . The Creative Commons Public Domain Dedication waiver ( http://creativecommons.org/publicdomain/zero/1.0/ ) applies to the data made available in this article, unless otherwise stated in a credit line to the data.
Reprints and permissions
About this article
Cite this article.
Dias, J.M., Subu, M.A., Al-Yateem, N. et al. Nursing students’ stressors and coping strategies during their first clinical training: a qualitative study in the United Arab Emirates. BMC Nurs 23 , 322 (2024). https://doi.org/10.1186/s12912-024-01962-5
Download citation
Received : 06 January 2024
Accepted : 22 April 2024
Published : 11 May 2024
DOI : https://doi.org/10.1186/s12912-024-01962-5
Share this article
Anyone you share the following link with will be able to read this content:
Sorry, a shareable link is not currently available for this article.
Provided by the Springer Nature SharedIt content-sharing initiative
- Clinical practicums
- Coping strategies
- Nursing students
BMC Nursing
ISSN: 1472-6955
- General enquiries: [email protected]
- Open access
- Published: 13 May 2024
Patient medication management, understanding and adherence during the transition from hospital to outpatient care - a qualitative longitudinal study in polymorbid patients with type 2 diabetes
- Léa Solh Dost ORCID: orcid.org/0000-0001-5767-1305 1 , 2 ,
- Giacomo Gastaldi ORCID: orcid.org/0000-0001-6327-7451 3 &
- Marie P. Schneider ORCID: orcid.org/0000-0002-7557-9278 1 , 2
BMC Health Services Research volume 24 , Article number: 620 ( 2024 ) Cite this article
274 Accesses
Metrics details
Continuity of care is under great pressure during the transition from hospital to outpatient care. Medication changes during hospitalization may be poorly communicated and understood, compromising patient safety during the transition from hospital to home. The main aims of this study were to investigate the perspectives of patients with type 2 diabetes and multimorbidities on their medications from hospital discharge to outpatient care, and their healthcare journey through the outpatient healthcare system. In this article, we present the results focusing on patients’ perspectives of their medications from hospital to two months after discharge.
Patients with type 2 diabetes, with at least two comorbidities and who returned home after discharge, were recruited during their hospitalization. A descriptive qualitative longitudinal research approach was adopted, with four in-depth semi-structured interviews per participant over a period of two months after discharge. Interviews were based on semi-structured guides, transcribed verbatim, and a thematic analysis was conducted.
Twenty-one participants were included from October 2020 to July 2021. Seventy-five interviews were conducted. Three main themes were identified: (A) Medication management, (B) Medication understanding, and (C) Medication adherence, during three periods: (1) Hospitalization, (2) Care transition, and (3) Outpatient care. Participants had varying levels of need for medication information and involvement in medication management during hospitalization and in outpatient care. The transition from hospital to autonomous medication management was difficult for most participants, who quickly returned to their routines with some participants experiencing difficulties in medication adherence.
Conclusions
The transition from hospital to outpatient care is a challenging process during which discharged patients are vulnerable and are willing to take steps to better manage, understand, and adhere to their medications. The resulting tension between patients’ difficulties with their medications and lack of standardized healthcare support calls for interprofessional guidelines to better address patients’ needs, increase their safety, and standardize physicians’, pharmacists’, and nurses’ roles and responsibilities.
Peer Review reports
Introduction
Continuity of patient care is characterized as the collaborative engagement between the patient and their physician-led care team in the ongoing management of healthcare, with the mutual objective of delivering high-quality and cost-effective medical care [ 1 ]. Continuity of care is under great pressure during the transition of care from hospital to outpatient care, with a risk of compromising patients’ safety [ 2 , 3 ]. The early post-discharge period is a high-risk and fragile transition: once discharged, one in five patients experience at least one adverse event during the first three weeks following discharge, and more than half of these adverse events are drug-related [ 4 , 5 ]. A retrospective study examining all discharged patients showed that adverse drug events (ADEs) account for up to 20% of 30-day hospital emergency readmissions [ 6 ]. During hospitalization, patients’ medications are generally modified, with an average of nearly four medication changes per patient [ 7 ]. Information regarding medications such as medication changes, the expected effect, side effects, and instructions for use are frequently poorly communicated to patients during hospitalization and at discharge [ 8 , 9 , 10 , 11 ]. Between 20 and 60% of discharged patients lack knowledge of their medications [ 12 , 13 ]. Consideration of patients’ needs and their active engagement in decision-making during hospitalization regarding their medications are often lacking [ 11 , 14 , 15 ]. This can lead to unsafe discharge and contribute to medication adherence difficulties, such as non-implementation of newly prescribed medications [ 16 , 17 ].
Patients with multiple comorbidities and polypharmacy are at higher risk of ADE [ 18 ]. Type 2 diabetes is one of the chronic health conditions most frequently associated with comorbidities and patients with type 2 diabetes often lack care continuum [ 19 , 20 , 21 ]. The prevalence of patients hospitalized with type 2 diabetes can exceed 40% [ 22 ] and these patients are at higher risk for readmission due to their comorbidities and their medications, such as insulin and oral hypoglycemic agents [ 23 , 24 , 25 ].
Interventions and strategies to improve patient care and safety at transition have shown mixed results worldwide in reducing cost, rehospitalization, ADE, and non-adherence [ 26 , 27 , 28 , 29 , 30 , 31 , 32 , 33 , 34 , 35 ]. However, interventions that are patient-centered, with a patient follow-up and led by interprofessional healthcare teams showed promising results [ 34 , 35 , 36 ]. Most of these interventions have not been implemented routinely due to the extensive time to translate research into practice and the lack of hybrid implementation studies [ 37 , 38 , 39 , 40 , 41 ]. In addition, patient-reported outcomes and perspectives have rarely been considered, yet patients’ involvement is essential for seamless and integrated care [ 42 , 43 ]. Interprofessional collaboration in which patients are full members of the interprofessional team, is still in its infancy in outpatient care [ 44 ]. Barriers and facilitators regarding medications at the transition of care have been explored in multiple qualitative studies at one given time in a given setting (e.g., at discharge, one-month post-discharge) [ 8 , 45 , 46 , 47 , 48 ]. However, few studies have adopted a holistic methodology from the hospital to the outpatient setting to explore changes in patients’ perspectives over time [ 49 , 50 , 51 ]. Finally, little is known about whether, how, and when patients return to their daily routine following hospitalization and the impact of hospitalization weeks after discharge.
In Switzerland, continuity of care after hospital discharge is still poorly documented, both in terms of contextual analysis and interventional studies, and is mainly conducted in the hospital setting [ 31 , 35 , 52 , 53 , 54 , 55 , 56 ]. The first step of an implementation science approach is to perform a contextual analysis to set up effective interventions adapted to patients’ needs and aligned to healthcare professionals’ activities in a specific context [ 41 , 57 ]. Therefore, the main aims of this study were to investigate the perspectives of patients with type 2 diabetes and multimorbidities on their medications from hospital discharge to outpatient care, and on their healthcare journey through the outpatient healthcare system. In this article, we present the results focusing on patients’ perspectives of their medications from hospital to two months after discharge.
Study design
This qualitative longitudinal study, conducted from October 2020 to July 2021, used a qualitative descriptive methodology through four consecutive in-depth semi-structured interviews per participant at three, 10-, 30- and 60-days post-discharge, as illustrated in Fig. 1 . Longitudinal qualitative research is characterized by qualitative data collection at different points in time and focuses on temporality, such as time and change [ 58 , 59 ]. Qualitative descriptive studies aim to explore and describe the depth and complexity of human experiences or phenomena [ 60 , 61 , 62 ]. We focused our qualitative study on the 60 first days after discharge as this period is considered highly vulnerable and because studies often use 30- or 60-days readmission as an outcome measure [ 5 , 63 ].
This qualitative study follows the Consolidated Criteria for Reporting Qualitative Research (COREQ). Ethics committee approval was sought and granted by the Cantonal Research Ethics Commission, Geneva (CCER) (2020 − 01779).
Recruitment took place during participants’ hospitalization in the general internal medicine divisions at the Geneva University Hospitals in the canton of Geneva (500 000 inhabitants), Switzerland. Interviews took place at participants’ homes, in a private office at the University of Geneva, by telephone or by secure video call, according to participants’ preference. Informal caregivers could also participate alongside the participants.
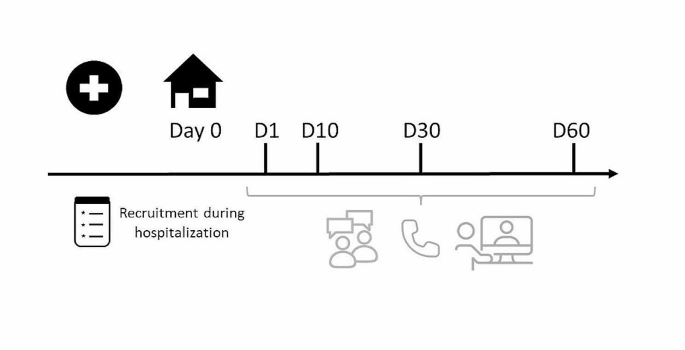
Study flowchart
Researcher characteristics
All the researchers were trained in qualitative studies. The diabetologist and researcher (GG) who enrolled the patients in the study was involved directly or indirectly (advice asked to the Geneva University Hospital diabetes team of which he was a part) for most participants’ care during hospitalization. LS (Ph.D. student and community pharmacist) was unknown to participants and presented herself during hospitalization as a “researcher” and not as a healthcare professional to avoid any risk of influencing participants’ answers. This study was not interventional, and the interviewer (LS) invited participants to contact a healthcare professional for any questions related to their medication or medical issues.
Population and sampling strategy
Patients with type 2 diabetes were chosen as an example population to describe polypharmacy patients as these patients usually have several health issues and polypharmacy [ 20 , 22 , 25 ]. Inclusions criteria for the study were: adult patients with type 2 diabetes, with at least two other comorbidities, hospitalized for at least three days in a general internal medicine ward, with a minimum of one medication change during hospital stay, and who self-managed their medications once discharged home. Exclusion criteria were patients not reachable by telephone following discharge, unable to give consent (patients with schizophrenia, dementia, brain damage, or drug/alcohol misuse), and who could not communicate in French. A purposive sampling methodology was applied aiming to include participants with different ages, genders, types, and numbers of health conditions by listing participants’ characteristics in a double-entry table, available in Supplementary Material 1 , until thematic saturation was reached. Thematic saturation was considered achieved when no new code or theme emerged and new data repeated previously coded information [ 64 ]. The participants were identified if they were hospitalized in the ward dedicated to diabetes care or when the diabetes team was contacted for advice. The senior ward physician (GG) screened eligible patients and the interviewer (LS) obtained written consent before hospital discharge.
Data collection and instruments
Sociodemographic (age, gender, educational level, living arrangement) and clinical characteristics (reason for hospitalization, date of admission, health conditions, diabetes diagnosis, medications before and during hospitalization) were collected by interviewing participants before their discharge and by extracting participants’ data from electronic hospital files by GG and LS. Participants’ pharmacies were contacted with the participant’s consent to obtain medication records from the last three months if information regarding medications before hospitalization was missing in the hospital files.
Semi-structured interview guides for each interview (at three, 10-, 30- and 60-days post-discharge) were developed based on different theories and components of health behavior and medication adherence: the World Health Organization’s (WHO) five dimensions for adherence, the Information-Motivation-Behavioral skills model and the Social Cognitive Theory [ 65 , 66 , 67 ]. Each interview explored participants’ itinerary in the healthcare system and their perspectives on their medications. Regarding medications, the following themes were mentioned at each interview: changes in medications, patients’ understanding and implication; information on their medications, self-management of their medications, and patients’ medication adherence. Other aspects were mentioned in specific interviews: patients’ hospitalization and experience on their return home (interview 1), motivation (interviews 2 and 4), and patient’s feedback on the past two months (interview 4). Interview guides translated from French are available in Supplementary Material 2 . The participants completed self-reported and self-administrated questionnaires at different interviews to obtain descriptive information on different factors that may affect medication management and adherence: self-report questionnaires on quality of life (EQ-5D-5 L) [ 68 ], literacy (Schooling-Opinion-Support questionnaire) [ 69 ], medication adherence (Adherence Visual Analogue Scale, A-VAS) [ 70 ] and Belief in Medication Questionnaire (BMQ) [ 71 ] were administered to each participant at the end of selected interviews to address the different factors that may affect medication management and adherence as well as to determine a trend of determinants over time. The BMQ contains two subscores: Specific-Necessity and Specific-Concerns, addressing respectively their perceived needs for their medications, and their concerns about adverse consequences associated with taking their medication [ 72 ].
Data management
Informed consent forms, including consent to obtain health data, were securely stored in a private office at the University of Geneva. The participants’ identification key was protected by a password known only by MS and LS. Confidentiality was guaranteed by pseudonymization of participants’ information and audio-recordings were destroyed once analyzed. Sociodemographic and clinical characteristics, medication changes, and answers to questionnaires were securely collected by electronic case report forms (eCRFs) on RedCap®. Interviews were double audio-recorded and field notes were taken during interviews. Recorded interviews were manually transcribed verbatim in MAXQDA® (2018.2) by research assistants and LS and transcripts were validated for accuracy by LS. A random sample of 20% of questionnaires was checked for accuracy for the transcription from the paper questionnaires to the eCRFs. Recorded sequences with no link to the discussed topics were not transcribed and this was noted in the transcripts.
Data analysis
A descriptive statistical analysis of sociodemographic, clinical characteristics and self-reported questionnaire data was carried out. A thematic analysis of transcripts was performed, as described by Braun and Clarke [ 73 ], by following six steps: raw data was read, text segments related to the study objectives were identified, text segments to create new categories were identified, similar or redundant categories were reduced and a model that integrated all significant categories was created. The analysis was conducted in parallel with patient enrolment to ensure data saturation. To ensure the validity of the coding method, transcripts were double coded independently and discussed by the research team until similar themes were obtained. The research group developed and validated an analysis grid, with which LS coded systematically the transcriptions and met regularly with the research team to discuss questions on data analysis and to ensure the quality of coding. The analysis was carried out in French, and the verbatims of interest cited in the manuscript were translated and validated by a native English-speaking researcher to preserve the meaning.
In this analysis, we used the term “healthcare professionals” when more than one profession could be involved in participants’ medication management. Otherwise, when a specific healthcare professional was involved, we used the designated profession (e.g. physicians, pharmacists).
Patient and public involvement
During the development phase of the study, interview guides and questionnaires were reviewed for clarity and validity and adapted by two patient partners, with multiple health conditions and who experienced previously a hospital discharge. They are part of the HUG Patients Partners + 3P platform for research and patient and public involvement.
Interviews and participants’ descriptions
A total of 75 interviews were conducted with 21 participants. In total, 31 patients were contacted, seven refused to participate (four at the project presentation and three at consent), two did not enter the selection criteria at discharge and one was unreachable after discharge. Among the 21 participants, 15 participated in all interviews, four in three interviews, one in two interviews, and one in one interview, due to scheduling constraints. Details regarding interviews and participants characteristics are presented in Tables 1 and 2 .
The median length of time between hospital discharge and interviews 1,2,3 and 4 was 5 (IQR: 4–7), 14 (13-20), 35 (22-38), and 63 days (61-68), respectively. On average, by comparing medications at hospital admission and discharge, a median of 7 medication changes (IQR: 6–9, range:2;17) occurred per participant during hospitalization and a median of 7 changes (5–12) during the two months following discharge. Details regarding participants’ medications are described in Table 3 .
Patient self-reported adherence over the past week for their three most challenging medications are available in Supplementary Material 3 .
Qualitative analysis
We defined care transition as the period from discharge until the first medical appointment post-discharge, and outpatient care as the period starting after the first medical appointment. Data was organized into three key themes (A. Medication management, B. Medication understanding, and C. Medication adherence) divided into subthemes at three time points (1. Hospitalization, 2. Care transition and 3. Outpatient care). Figure 2 summarizes and illustrates the themes and subthemes with their influencing factors as bullet points.
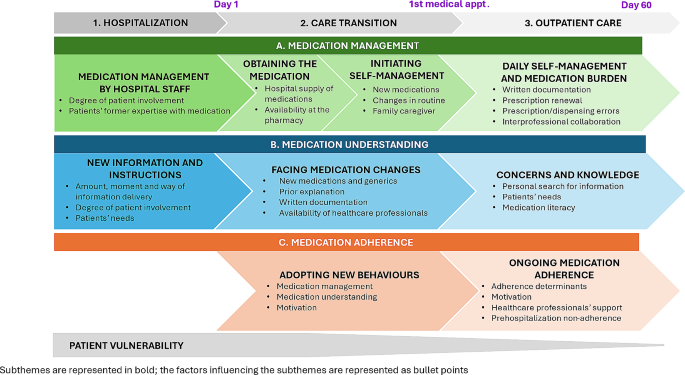
Participants’ medication management, understanding and adherence during hospitalization, care transition and outpatient care
A. Medication management
A.1 medication management during hospitalization: medication management by hospital staff.
Medications during hospitalization were mainly managed by hospital healthcare professionals (i.e. nurses and physicians) with varying degrees of patient involvement: “At the hospital, they prepared the medications for me. […] I didn’t even know what the packages looked like.” Participant 22; interview 1 (P22.1) Some participants reported having therapeutic education sessions with specialized nurses and physicians, such as the explanation and demonstration of insulin injection and glucose monitoring. A patient reported that he was given the choice of several treatments and was involved in shared decision-making. Other participants had an active role in managing and optimizing dosages, such as rapid insulin, due to prior knowledge and use of medications before hospitalization.
A.2 Medication management at transition: obtaining the medication and initiating self-management
Once discharged, some participants had difficulties obtaining their medications at the pharmacy because some medications were not stored and had to be ordered, delaying medication initiation. To counter this problem upstream, a few participants were provided a 24-to-48-hour supply of medications at discharge. It was sometimes requested by the patient or suggested by the healthcare professionals but was not systematic. The transition from medication management by hospital staff to self-management was exhausting for most participants who were faced with a large amount of new information and changes in their medications: “ When I was in the hospital, I didn’t even realize all the changes. When I came back home, I took away the old medication packages and got out the new ones. And then I thought : « my God, all this…I didn’t know I had all these changes » ” P2.1 Written documentation, such as the discharge prescription or dosage labels on medication packages, was helpful in managing their medication at home. Most participants used weekly pill organizers to manage their medications, which were either already used before hospitalization or were introduced post-discharge. The help of a family caregiver in managing and obtaining medications was reported as a facilitator.
A.3 Medication management in outpatient care: daily self-management and medication burden
A couple of days or weeks after discharge, most participants had acquired a routine so that medication management was less demanding, but the medication burden varied depending on the participants. For some, medication management became a simple action well implemented in their routine (“It has become automatic” , P23.4), while for others, the number of medications and the fact that the medications reminded them of the disease was a heavy burden to bear on a daily basis (“ During the first few days after getting out of the hospital, I thought I was going to do everything right. In the end, well [laughs] it’s complicated. I ended up not always taking the medication, not monitoring the blood sugar” P12.2) To support medication self-management, some participants had written documentation such as treatment plans, medication lists, and pictures of their medication packages on their phones. Some participants had difficulties obtaining medications weeks after discharge as discharge prescriptions were not renewable and participants did not see their physician in time. Others had to visit multiple physicians to have their prescriptions updated. A few participants were faced with prescription or dispensing errors, such as prescribing or dispensing the wrong dosage, which affected medication management and decreased trust in healthcare professionals. In most cases, according to participants, the pharmacy staff worked in an interprofessional collaboration with physicians to provide new and updated prescriptions.
B. Medication understanding
B.1 medication understanding during hospitalization: new information and instructions.
The amount of information received during hospitalization varied considerably among participants with some reporting having received too much, while others saying they received too little information regarding medication changes, the reason for changes, or for introducing new medications: “They told me I had to take this medication all my life, but they didn’t tell me what the effects were or why I was taking it.” P5.3
Hospitalization was seen by some participants as a vulnerable and tiring period during which they were less receptive to information. Information and explanations were generally given verbally, making it complicated for most participants to recall it. Some participants reported that hospital staff was attentive to their needs for information and used communication techniques such as teach-back (a way of checking understanding by asking participants to say in their own words what they need to know or do about their health or medications). Some participants were willing to be proactive in the understanding of their medications while others were more passive, had no specific needs for information, and did not see how they could be engaged more.
B.2 Medication understanding at transition: facing medication changes
At hospital discharge, the most challenging difficulty for participants was to understand the changes made regarding their medications. For newly diagnosed participants, the addition of new medications was more difficult to understand, whereas, for experienced participants, changes in known medications such as dosage modification, changes within a therapeutic class, and generic substitutions were the most difficult to understand. Not having been informed about changes caused confusion and misunderstanding. Therefore, medication reconciliation done by the patient was time-consuming, especially for participants with multiple medications: “ They didn’t tell me at all that they had changed my treatment completely. They just told me : « We’ve changed a few things. But it was the whole treatment ». ” P2.3 Written information, such as the discharge prescription, the discharge report (brief letter summarizing information about the hospitalization, given to the patient at discharge), or the label on the medication box (written by the pharmacist with instructions on dosage) helped them find or recall information about their medications and diagnoses. However, technical terms were used in hospital documentations and were not always understandable. For example, this participant said: “ On the prescription of valsartan, they wrote: ‘resume in the morning once profile…’[once hypertension profile allows]… I don’t know what that means.” P8.1 In addition, some documents were incomplete, as mentioned by a patient who did not have the insulin dosage mentioned on the hospital prescription. Some participants sought help from healthcare professionals, such as pharmacists, hospital physicians, or general practitioners a few days after discharge to review medications, answer questions, or obtain additional information.
B.3 Medication understanding in the outpatient care: concerns and knowledge
Weeks after discharge, most participants had concerns about the long-term use of their medications, their usefulness, and the possible risk of interactions or side effects. Some participants also reported having some lack of knowledge regarding indications, names, or how the medication worked: “I don’t even know what Brilique® [ticagrelor, antiplatelet agent] is for. It’s for blood pressure, isn’t it?. I don’t know.” P11.4 According to participants, the main reasons for the lack of understanding were the lack of information at the time of prescribing and the large number of medications, making it difficult to search for information and remember it. Participants sought information from different healthcare professionals or by themselves, on package inserts, through the internet, or from family and friends. Others reported having had all the information needed or were not interested in having more information. In addition, participants with low medication literacy, such as non-native speakers or elderly people, struggled more with medication understanding and sought help from family caregivers or healthcare professionals, even weeks after discharge: “ I don’t understand French very well […] [The doctor] explained it very quickly…[…] I didn’t understand everything he was saying” P16.2
C. Medication adherence
C.2 medication adherence at transition: adopting new behaviors.
Medication adherence was not mentioned as a concern during hospitalization and a few participants reported difficulties in medication initiation once back home: “I have an injection of Lantus® [insulin] in the morning, but obviously, the first day [after discharge], I forgot to do it because I was not used to it.” P23.1 Participants had to quickly adopt new behaviors in the first few days after discharge, especially for participants with few medications pre-hospitalization. The use of weekly pill organizers, alarms and specific storage space were reported as facilitators to support adherence. One patient did not initiate one of his medications because he did not understand the medication indication, and another patient took her old medications because she was used to them. Moreover, most participants experienced their hospitalization as a turning point, a time when they focused on their health, thought about the importance of their medications, and discussed any new lifestyle or dietary measures that might be implemented.
C.3 Medication adherence in outpatient care: ongoing medication adherence
More medication adherence difficulties appeared a few weeks after hospital discharge when most participants reported nonadherence behaviors, such as difficulties implementing the dosage regimen, or intentionally discontinuing the medication and modifying the medication regimen on their initiative. Determinants positively influencing medication adherence were the establishment of a routine; organizing medications in weekly pill-organizers; organizing pocket doses (medications for a short period that participants take with them when away from home); seeking support from family caregivers; using alarm clocks; and using specific storage places. Reasons for nonadherence were changes in daily routine; intake times that were not convenient for the patient; the large number of medications; and poor knowledge of the medication or side effects. Healthcare professionals’ assistance for medication management, such as the help of home nurses or pharmacists for the preparation of weekly pill-organizers, was requested by participants or offered by healthcare professionals to support medication adherence: “ I needed [a home nurse] to put my pills in the pillbox. […] I felt really weak […] and I was making mistakes. So, I’m very happy [the doctor] offered me [home care]. […] I have so many medications.” P22.3 Some participants who experienced prehospitalization non-adherence were more aware of their non-adherence and implemented strategies, such as modifying the timing of intake: “I said to my doctor : « I forget one time out of two […], can I take them in the morning? » We looked it up and yes, I can take it in the morning.” P11.2 In contrast, some participants were still struggling with adherence difficulties that they had before hospitalization. Motivations for taking medications two months after discharge were to improve health, avoid complications, reduce symptoms, reduce the number of medications in the future or out of obligation: “ I force myself to take them because I want to get to the end of my diabetes, I want to reduce the number of pills as much as possible.” P14.2 After a few weeks post-hospitalization, for some participants, health and illness were no longer the priority because of other life imperatives (e.g., family or financial situation).
This longitudinal study provided a multi-faceted representation of how patients manage, understand, and adhere to their medications from hospital discharge to two months after discharge. Our findings highlighted the varying degree of participants’ involvement in managing their medications during their hospitalization, the individualized needs for information during and after hospitalization, the complicated transition from hospital to autonomous medication management, the adaptation of daily routines around medication once back home, and the adherence difficulties that surfaced in the outpatient care, with nonadherence prior to hospitalization being an indicator of the behavior after discharge. Finally, our results confirmed the lack of continuity in care and showed the lack of patient care standardization experienced by the participants during the transition from hospital to outpatient care.
This in-depth analysis of patients’ experiences reinforces common challenges identified in the existing literature such as the lack of personalized information [ 9 , 10 , 11 ], loss of autonomy during hospitalization [ 14 , 74 , 75 ], difficulties in obtaining medication at discharge [ 11 , 45 , 76 ] and challenges in understanding treatment modifications and generics substitution [ 11 , 32 , 77 , 78 ]. Some of these studies were conducted during patients’ hospitalization [ 10 , 75 , 79 ] or up to 12 months after discharge [ 80 , 81 ], but most studies focused on the few days following hospital discharge [ 9 , 11 , 14 , 82 ]. Qualitative studies on medications at transition often focused on a specific topic, such as medication information, or a specific moment in time, and often included healthcare professionals, which muted patients’ voices [ 9 , 10 , 11 , 47 , 49 ]. Our qualitative longitudinal methodology was interested in capturing the temporal dynamics, in-depth narratives, and contextual nuances of patients’ medication experiences during transitions of care [ 59 , 83 ]. This approach provided a comprehensive understanding of how patients’ perspectives and behaviors evolved over time, offering insights into the complex interactions of medication management, understanding and adherence, and turning points within their medication journeys. A qualitative longitudinal design was used by Fylan et al. to underline patients’ resilience in medication management during and after discharge, by Brandberg et al. to show the dynamic process of self-management during the 4 weeks post-discharge and by Lawton et al. to examine how patients with type 2 diabetes perceived their care after discharge over a period of four years [ 49 , 50 , 51 ]. Our study focused on the first two months following hospitalization and future studies should focus on following discharged and at-risk patients over a longer period, as “transitions of care do not comprise linear trajectories of patients’ movements, with a starting and finishing point. Instead, they are endless loops of movements” [ 47 ].
Our results provide a particularly thorough description of how participants move from a state of total dependency during hospitalization regarding their medication management to a sudden and complete autonomy after hospital discharge impacting medication management, understanding, and adherence in the first days after discharge for some participants. Several qualitative studies have described the lack of shared decision-making and the loss of patient autonomy during hospitalization, which had an impact on self-management and created conflicts with healthcare professionals [ 75 , 81 , 84 ]. Our study also highlights nuanced patient experiences, including varying levels of patient needs, involvement, and proactivity during hospitalization and outpatient care, and our results contribute to capturing different perspectives that contrast with some literature that often portrays patients as more passive recipients of care [ 14 , 15 , 74 , 75 ]. Shared decision-making and proactive medication are key elements as they contribute to a smoother transition and better outcomes for patients post-discharge [ 85 , 86 , 87 ].
Consistent with the literature, the study identifies some challenges in medication initiation post-discharge [ 16 , 17 , 88 ] but our results also describe how daily routine rapidly takes over, either solidifying adherence behavior or generating barriers to medication adherence. Participants’ nonadherence prior to hospitalization was a factor influencing participants’ adherence post-hospitalization and this association should be further investigated, as literature showed that hospitalized patients have high scores of non-adherence [ 89 ]. Mortel et al. showed that more than 20% of discharged patients stopped their medications earlier than agreed with the physician and 25% adapted their medication intake [ 90 ]. Furthermore, patients who self-managed their medications had a lower perception of the necessity of their medication than patients who received help, which could negatively impact medication adherence [ 91 ]. Although participants in our study had high BMQ scores for necessity and lower scores for concerns, some participants expressed doubts about the need for their medications and a lack of motivation a few weeks after discharge. Targeted pharmacy interventions for newly prescribed medications have been shown to improve medication adherence, and hospital discharge is an opportune moment to implement this service [ 92 , 93 ].
Many medication changes were made during the transition of care (a median number of 7 changes during hospitalization and 7 changes during the two months after discharge), especially medication additions during hospitalization and interruptions after hospitalization. While medication changes during hospitalization are well described, the many changes following discharge are less discussed [ 7 , 94 ]. A Danish study showed that approximately 65% of changes made during hospitalization were accepted by primary healthcare professionals but only 43% of new medications initiated during hospitalization were continued after discharge [ 95 ]. The numerous changes after discharge may be caused by unnecessary intensification of medications during hospitalization, delayed discharge letters, lack of standardized procedures, miscommunication, patient self-management difficulties, or in response to an acute situation [ 96 , 97 , 98 ]. During the transition of care, in our study, both new and experienced participants were faced with difficulties in managing and understanding medication changes, either for newly prescribed medication or changes in previous medications. Such difficulties corroborate the findings of the literature [ 9 , 10 , 47 ] and our results showed that the lack of understanding during hospitalization led to participants having questions about their medications, even weeks after discharge. Particular attention should be given to patients’ understanding of medication changes jointly by physicians, nurses and pharmacists during the transition of care and in the months that follow as medications are likely to undergo as many changes as during hospitalization.
Implication for practice and future research
The patients’ perspectives in this study showed, at a system level, that there was a lack of standardization in healthcare professional practices regarding medication dispensing and follow-up. For now, in Switzerland, there are no official guidelines on medication prescription and dispensation during the transition of care although some international guidelines have been developed for outpatient healthcare professionals [ 3 , 99 , 100 , 101 , 102 ]. Here are some suggestions for improvement arising from our results. Patients should be included as partners and healthcare professionals should systematically assess (i) previous medication adherence, (ii) patients’ desired level of involvement and (iii) their needs for information during hospitalization. Hospital discharge processes should be routinely implemented to standardize hospital discharge preparation, medication prescribing, and dispensing. Discharge from the hospital should be planned with community pharmacies to ensure that all medications are available and, if necessary, doses of medications should be supplied by the hospital to bridge the gap. A partnership with outpatient healthcare professionals, such as general practitioners, community pharmacists, and homecare nurses, should be set up for effective asynchronous interprofessional collaboration to consolidate patients’ medication management, knowledge, and adherence, as well as to monitor signs of deterioration or adverse drug events.
Future research should consolidate our first attempt to develop a framework to better characterize medication at the transition of care, using Fig. 2 as a starting point. Contextualized interventions, co-designed by health professionals, patients and stakeholders, should be tested in a hybrid implementation study to test the implementation and effectiveness of the intervention for the health system [ 103 ].
Limitations
This study has some limitations. First, the transcripts were validated for accuracy by the interviewer but not by a third party, which could have increased the robustness of the transcription. Nevertheless, the interviewer followed all methodological recommendations for transcription. Second, patient inclusion took place during the COVID-19 pandemic, which may have had an impact on patient care and the availability of healthcare professionals. Third, we cannot guarantee the accuracy of some participants’ medication history before hospitalization, even though we contacted the participants’ main pharmacy, as participants could have gone to different pharmacies to obtain their medications. Fourth, our findings may not be generalizable to other populations and other healthcare systems because some issues may be specific to multimorbid patients with type 2 diabetes or to the Swiss healthcare setting. Nevertheless, issues encountered by our participants regarding their medications correlate with findings in the literature. Fifth, only 15 out of 21 participants took part in all the interviews, but most participants took part in at least three interviews and data saturation was reached. Lastly, by its qualitative and longitudinal design, it is possible that the discussion during interviews and participants’ reflections between interviews influenced participants’ management, knowledge, and adherence, even though this study was observational, and no advice or recommendations were given by the interviewer during interviews.
Discharged patients are willing to take steps to better manage, understand, and adhere to their medications, yet they are also faced with difficulties in the hospital and outpatient care. Furthermore, extensive changes in medications not only occur during hospitalization but also during the two months following hospital discharge, for which healthcare professionals should give particular attention. The different degrees of patients’ involvement, needs and resources should be carefully considered to enable them to better manage, understand and adhere to their medications. At a system level, patients’ experiences revealed a lack of standardization of medication practices during the transition of care. The healthcare system should provide the ecosystem needed for healthcare professionals responsible for or involved in the management of patients’ medications during the hospital stay, discharge, and outpatient care to standardize their practices while considering the patient as an active partner.
Data availability
The anonymized quantitative survey datasets and the qualitative codes are available in French from the corresponding author on reasonable request.
Abbreviations
adverse drug events
Adherence Visual Analogue Scale
Belief in Medication Questionnaire
Consolidated Criteria for Reporting Qualitative Research
case report form
standard deviation
World Health Organization
American Academy of Family Physician. Continuity of Care, Definition of 2020. Accessed 10 July 2022 https://www.aafp.org/about/policies/all/continuity-of-care-definition.html
Kripalani S, LeFevre F, Phillips CO, Williams MV, Basaviah P, Baker DW. Deficits in communication and information transfer between hospital-based and primary care physicians: implications for patient safety and continuity of care. JAMA. 2007;297(8):831–41.
Article CAS PubMed Google Scholar
World Health Organization (WHO). Medication Safety in Transitions of Care. 2019.
Forster AJ, Murff HJ, Peterson JF, Gandhi TK, Bates DW. The incidence and severity of adverse events affecting patients after discharge from the hospital. Ann Intern Med. 2003;138(3):161–7.
Article PubMed Google Scholar
Krumholz HM. Post-hospital syndrome–an acquired, transient condition of generalized risk. N Engl J Med. 2013;368(2):100–2.
Article CAS PubMed PubMed Central Google Scholar
Banholzer S, Dunkelmann L, Haschke M, Derungs A, Exadaktylos A, Krähenbühl S, et al. Retrospective analysis of adverse drug reactions leading to short-term emergency hospital readmission. Swiss Med Wkly. 2021;151:w20400.
Blozik E, Signorell A, Reich O. How does hospitalization affect continuity of drug therapy: an exploratory study. Ther Clin Risk Manag. 2016;12:1277–83.
Article PubMed PubMed Central Google Scholar
Allen J, Hutchinson AM, Brown R, Livingston PM. User experience and care for older people transitioning from hospital to home: patients’ and carers’ perspectives. Health Expect. 2018;21(2):518–27.
Daliri S, Bekker CL, Buurman BM, Scholte Op Reimer WJM, van den Bemt BJF, Karapinar-Çarkit F. Barriers and facilitators with medication use during the transition from hospital to home: a qualitative study among patients. BMC Health Serv Res. 2019;19(1):204.
Bekker CL, Mohsenian Naghani S, Natsch S, Wartenberg NS, van den Bemt BJF. Information needs and patient perceptions of the quality of medication information available in hospitals: a mixed method study. Int J Clin Pharm. 2020;42(6):1396–404.
Foulon V, Wuyts J, Desplenter F, Spinewine A, Lacour V, Paulus D, et al. Problems in continuity of medication management upon transition between primary and secondary care: patients’ and professionals’ experiences. Acta Clin Belgica: Int J Clin Lab Med. 2019;74(4):263–71.
Article Google Scholar
Micheli P, Kossovsky MP, Gerstel E, Louis-Simonet M, Sigaud P, Perneger TV, et al. Patients’ knowledge of drug treatments after hospitalisation: the key role of information. Swiss Med Wkly. 2007;137(43–44):614–20.
PubMed Google Scholar
Ziaeian B, Araujo KL, Van Ness PH, Horwitz LI. Medication reconciliation accuracy and patient understanding of intended medication changes on hospital discharge. J Gen Intern Med. 2012;27(11):1513–20.
Allen J, Hutchinson AM, Brown R, Livingston PM. User experience and care integration in Transitional Care for older people from hospital to home: a Meta-synthesis. Qual Health Res. 2016;27(1):24–36.
Mackridge AJ, Rodgers R, Lee D, Morecroft CW, Krska J. Cross-sectional survey of patients’ need for information and support with medicines after discharge from hospital. Int J Pharm Pract. 2018;26(5):433–41.
Mulhem E, Lick D, Varughese J, Barton E, Ripley T, Haveman J. Adherence to medications after hospital discharge in the elderly. Int J Family Med. 2013;2013:901845.
Fallis BA, Dhalla IA, Klemensberg J, Bell CM. Primary medication non-adherence after discharge from a general internal medicine service. PLoS ONE. 2013;8(5):e61735.
Zhou L, Rupa AP. Categorization and association analysis of risk factors for adverse drug events. Eur J Clin Pharmacol. 2018;74(4):389–404.
Moreau-Gruet F. La multimorbidité chez les personnes de 50 ans et plus. Résultats basés sur l’enqête SHARE (Survey of Health, Ageing and Retirement in Europe. Obsan Bulletin 4/2013. 2013(Neuchâtel: OBservatoire suisse de la santé).
Iglay K, Hannachi H, Joseph Howie P, Xu J, Li X, Engel SS, et al. Prevalence and co-prevalence of comorbidities among patients with type 2 diabetes mellitus. Curr Med Res Opin. 2016;32(7):1243–52.
Sibounheuang P, Olson PS, Kittiboonyakun P. Patients’ and healthcare providers’ perspectives on diabetes management: a systematic review of qualitative studies. Res Social Adm Pharm. 2020;16(7):854–74.
Müller-Wieland D, Merkel M, Hamann A, Siegel E, Ottillinger B, Woker R, et al. Survey to estimate the prevalence of type 2 diabetes mellitus in hospital patients in Germany by systematic HbA1c measurement upon admission. Int J Clin Pract. 2018;72(12):e13273.
Blanc AL, Fumeaux T, Stirnemann J, Dupuis Lozeron E, Ourhamoune A, Desmeules J, et al. Development of a predictive score for potentially avoidable hospital readmissions for general internal medicine patients. PLoS ONE. 2019;14(7):e0219348.
Hansen LO, Greenwald JL, Budnitz T, Howell E, Halasyamani L, Maynard G, et al. Project BOOST: effectiveness of a multihospital effort to reduce rehospitalization. J Hosp Med. 2013;8(8):421–7.
Khalid JM, Raluy-Callado M, Curtis BH, Boye KS, Maguire A, Reaney M. Rates and risk of hospitalisation among patients with type 2 diabetes: retrospective cohort study using the UK General Practice Research Database linked to English Hospital Episode statistics. Int J Clin Pract. 2014;68(1):40–8.
Lussier ME, Evans HJ, Wright EA, Gionfriddo MR. The impact of community pharmacist involvement on transitions of care: a systematic review and meta-analysis. J Am Pharm Assoc. 2020;60(1):153–.
van der Heijden A, de Bruijne MC, Nijpels G, Hugtenburg JG. Cost-effectiveness of a clinical medication review in vulnerable older patients at hospital discharge, a randomized controlled trial. Int J Clin Pharm. 2019;41(4):963–71.
Bingham J, Campbell P, Schussel K, Taylor AM, Boesen K, Harrington A, et al. The Discharge Companion Program: an interprofessional collaboration in Transitional Care Model Delivery. Pharm (Basel). 2019;7(2):68.
Google Scholar
Farris KB, Carter BL, Xu Y, Dawson JD, Shelsky C, Weetman DB, et al. Effect of a care transition intervention by pharmacists: an RCT. BMC Health Serv Res. 2014;14:406.
Meslot C, Gauchet A, Hagger MS, Chatzisarantis N, Lehmann A, Allenet B. A Randomised Controlled Trial to test the effectiveness of planning strategies to improve Medication Adherence in patients with Cardiovascular Disease. Appl Psychol Health Well Being. 2017;9(1):106–29.
Garnier A, Rouiller N, Gachoud D, Nachar C, Voirol P, Griesser AC, et al. Effectiveness of a transition plan at discharge of patients hospitalized with heart failure: a before-and-after study. ESC Heart Fail. 2018;5(4):657–67.
Daliri S, Bekker CL, Buurman BM, Scholte Op Reimer WJM, van den Bemt BJF, Karapinar-Çarkit F. Medication management during transitions from hospital to home: a focus group study with hospital and primary healthcare providers in the Netherlands. Int J Clin Pharm. 2020.
Hansen LO, Young RS, Hinami K, Leung A, Williams MV. Interventions to reduce 30-day rehospitalization: a systematic review. Ann Intern Med. 2011;155(8):520–8.
Leppin AL, Gionfriddo MR, Kessler M, Brito JP, Mair FS, Gallacher K, et al. Preventing 30-day hospital readmissions: a systematic review and meta-analysis of randomized trials. JAMA Intern Med. 2014;174(7):1095–107.
Donzé J, John G, Genné D, Mancinetti M, Gouveia A, Méan M et al. Effects of a Multimodal Transitional Care Intervention in patients at high risk of readmission: the TARGET-READ Randomized Clinical Trial. JAMA Intern Med. 2023.
Rodrigues CR, Harrington AR, Murdock N, Holmes JT, Borzadek EZ, Calabro K, et al. Effect of pharmacy-supported transition-of-care interventions on 30-Day readmissions: a systematic review and Meta-analysis. Ann Pharmacother. 2017;51(10):866–89.
Lam MYY, Dodds LJ, Corlett SA. Engaging patients to access the community pharmacy medicine review service after discharge from hospital: a cross-sectional study in England. Int J Clin Pharm. 2019;41(4):1110–7.
Hossain LN, Fernandez-Llimos F, Luckett T, Moullin JC, Durks D, Franco-Trigo L, et al. Qualitative meta-synthesis of barriers and facilitators that influence the implementation of community pharmacy services: perspectives of patients, nurses and general medical practitioners. BMJ Open. 2017;7(9):e015471.
En-Nasery-de Heer S, Uitvlugt EB, Bet PM, van den Bemt BJF, Alai A, van den Bemt P et al. Implementation of a pharmacist-led transitional pharmaceutical care programme: process evaluation of medication actions to reduce hospital admissions through a collaboration between Community and Hospital pharmacists (MARCH). J Clin Pharm Ther. 2022.
Morris ZS, Wooding S, Grant J. The answer is 17 years, what is the question: understanding time lags in translational research. J R Soc Med. 2011;104(12):510–20.
De Geest S, Zúñiga F, Brunkert T, Deschodt M, Zullig LL, Wyss K, et al. Powering Swiss health care for the future: implementation science to bridge the valley of death. Swiss Med Wkly. 2020;150:w20323.
Noonan VK, Lyddiatt A, Ware P, Jaglal SB, Riopelle RJ, Bingham CO 3, et al. Montreal Accord on patient-reported outcomes (PROs) use series - paper 3: patient-reported outcomes can facilitate shared decision-making and guide self-management. J Clin Epidemiol. 2017;89:125–35.
Hesselink G, Schoonhoven L, Barach P, Spijker A, Gademan P, Kalkman C, et al. Improving patient handovers from hospital to primary care: a systematic review. Ann Intern Med. 2012;157(6):417–28.
(OFSP) Interprofessionnalité dans le domaine de la santé Soins ambulatoire. Accessed 4 January 2024. https://www.bag.admin.ch/bag/fr/home/strategie-und-politik/nationale-gesundheitspolitik/foerderprogramme-der-fachkraefteinitiative-plus/foerderprogramme-interprofessionalitaet.html
Mitchell SE, Laurens V, Weigel GM, Hirschman KB, Scott AM, Nguyen HQ, et al. Care transitions from patient and caregiver perspectives. Ann Fam Med. 2018;16(3):225–31.
Davoody N, Koch S, Krakau I, Hägglund M. Post-discharge stroke patients’ information needs as input to proposing patient-centred eHealth services. BMC Med Inf Decis Mak. 2016;16:66.
Ozavci G, Bucknall T, Woodward-Kron R, Hughes C, Jorm C, Joseph K, et al. A systematic review of older patients’ experiences and perceptions of communication about managing medication across transitions of care. Res Social Adm Pharm. 2021;17(2):273–91.
Fylan B, Armitage G, Naylor D, Blenkinsopp A. A qualitative study of patient involvement in medicines management after hospital discharge: an under-recognised source of systems resilience. BMJ Qual Saf. 2018;27(7):539–46.
Fylan B, Marques I, Ismail H, Breen L, Gardner P, Armitage G, et al. Gaps, traps, bridges and props: a mixed-methods study of resilience in the medicines management system for patients with heart failure at hospital discharge. BMJ Open. 2019;9(2):e023440.
Brandberg C, Ekstedt M, Flink M. Self-management challenges following hospital discharge for patients with multimorbidity: a longitudinal qualitative study of a motivational interviewing intervention. BMJ Open. 2021;11(7):e046896.
Lawton J, Rankin D, Peel E, Douglas M. Patients’ perceptions and experiences of transitions in diabetes care: a longitudinal qualitative study. Health Expect. 2009;12(2):138–48.
Mabire C, Bachnick S, Ausserhofer D, Simon M. Patient readiness for hospital discharge and its relationship to discharge preparation and structural factors: a cross-sectional study. Int J Nurs Stud. 2019;90:13–20.
Meyers DC, Durlak JA, Wandersman A. The quality implementation framework: a synthesis of critical steps in the implementation process. Am J Community Psychol. 2012;50(3–4):462–80.
Meyer-Massetti C, Hofstetter V, Hedinger-Grogg B, Meier CR, Guglielmo BJ. Medication-related problems during transfer from hospital to home care: baseline data from Switzerland. Int J Clin Pharm. 2018;40(6):1614–20.
Neeman M, Dobrinas M, Maurer S, Tagan D, Sautebin A, Blanc AL, et al. Transition of care: a set of pharmaceutical interventions improves hospital discharge prescriptions from an internal medicine ward. Eur J Intern Med. 2017;38:30–7.
Geese F, Schmitt KU. Interprofessional Collaboration in Complex Patient Care Transition: a qualitative multi-perspective analysis. Healthc (Basel). 2023;11(3).
Craig P, Dieppe P, Macintyre S, Michie S, Nazareth I, Petticrew M. Developing and evaluating complex interventions: the new Medical Research Council guidance. Int J Nurs Stud. 2013;50(5):587–92.
Thomson R, Plumridge L, Holland J, Editorial. Int J Soc Res Methodol. 2003;6(3):185–7.
Audulv Å, Hall EOC, Kneck Å, Westergren T, Fegran L, Pedersen MK, et al. Qualitative longitudinal research in health research: a method study. BMC Med Res Methodol. 2022;22(1):255.
Kim H, Sefcik JS, Bradway C. Characteristics of qualitative descriptive studies: a systematic review. Res Nurs Health. 2017;40(1):23–42.
Sandelowski M. Whatever happened to qualitative description? Res Nurs Health. 2000;23(4):334–40.
Bradshaw C, Atkinson S, Doody O. Employing a qualitative description Approach in Health Care Research. Glob Qual Nurs Res. 2017;4:2333393617742282.
PubMed PubMed Central Google Scholar
Bellone JM, Barner JC, Lopez DA. Postdischarge interventions by pharmacists and impact on hospital readmission rates. J Am Pharm Assoc (2003). 2012;52(3):358–62.
Hennink MM, Kaiser BN, Marconi VC. Code saturation versus meaning saturation: how many interviews are Enough? Qual Health Res. 2016;27(4):591–608.
World Health Organization. Adherence to long-term therapies: evidence for action. 2003.
Fisher JD, Fisher WA, Amico KR, Harman JJ. An information-motivation-behavioral skills model of adherence to antiretroviral therapy. Health Psychol. 2006;25(4):462–73.
Bandura A. Health promotion from the perspective of social cognitive theory. Psychol Health. 1998;13(4):623–49.
ShiftEUROQOL Research FOndation EQ 5D Instruments. Accessed 30 July 2022 https://euroqol.org/eq-5d-instruments/sample-demo/
Jeppesen KM, Coyle JD, Miser WF. Screening questions to predict limited health literacy: a cross-sectional study of patients with diabetes mellitus. Ann Fam Med. 2009;7(1):24–31.
Giordano TP, Guzman D, Clark R, Charlebois ED, Bangsberg DR. Measuring adherence to antiretroviral therapy in a diverse population using a visual analogue scale. HIV Clin Trials. 2004;5(2):74–9.
Horne R, Weinman J, Hankins M. The beliefs about medicines questionnaire: the development and evaluation of a new method for assessing the cognitive representation of medication. Psychol Health. 1999;14(1):1–24.
Horne R, Chapman SC, Parham R, Freemantle N, Forbes A, Cooper V. Understanding patients’ adherence-related beliefs about medicines prescribed for long-term conditions: a meta-analytic review of the necessity-concerns Framework. PLoS ONE. 2013;8(12):e80633.
Braun V, Clarke V. Using thematic analysis in psychology. Qualitative Res Psychol. 2006;3(2):77–101.
Waibel S, Henao D, Aller M-B, Vargas I, Vázquez M-L. What do we know about patients’ perceptions of continuity of care? A meta-synthesis of qualitative studies. Int J Qual Health Care. 2011;24(1):39–48.
Rognan SE, Jørgensen MJ, Mathiesen L, Druedahl LC, Lie HB, Bengtsson K, et al. The way you talk, do I have a choice?’ Patient narratives of medication decision-making during hospitalization. Int J Qualitative Stud Health Well-being. 2023;18(1):2250084.
Michel B, Hemery M, Rybarczyk-Vigouret MC, Wehrle P, Beck M. Drug-dispensing problems community pharmacists face when patients are discharged from hospitals: a study about 537 prescriptions in Alsace. Int J Qual Health Care. 2016;28(6):779–84.
Bruhwiler LD, Hersberger KE, Lutters M. Hospital discharge: what are the problems, information needs and objectives of community pharmacists? A mixed method approach. Pharm Pract (Granada). 2017;15(3):1046.
Knight DA, Thompson D, Mathie E, Dickinson A. Seamless care? Just a list would have helped!’ Older people and their carer’s experiences of support with medication on discharge home from hospital. Health Expect. 2013;16(3):277–91.
Gualandi R, Masella C, Viglione D, Tartaglini D. Exploring the hospital patient journey: what does the patient experience? PLoS ONE. 2019;14(12):e0224899.
Norberg H, Håkansson Lindqvist M, Gustafsson M. Older individuals’ experiences of Medication Management and Care after Discharge from Hospital: an interview study. Patient Prefer Adherence. 2023;17:781–92.
Jones KC, Austad K, Silver S, Cordova-Ramos EG, Fantasia KL, Perez DC, et al. Patient perspectives of the hospital discharge process: a qualitative study. J Patient Exp. 2023;10:23743735231171564.
Hesselink G, Flink M, Olsson M, Barach P, Dudzik-Urbaniak E, Orrego C, et al. Are patients discharged with care? A qualitative study of perceptions and experiences of patients, family members and care providers. BMJ Qual Saf. 2012;21(Suppl 1):i39–49.
Murray SA, Kendall M, Carduff E, Worth A, Harris FM, Lloyd A, et al. Use of serial qualitative interviews to understand patients’ evolving experiences and needs. BMJ. 2009;339:b3702.
Berger ZD, Boss EF, Beach MC. Communication behaviors and patient autonomy in hospital care: a qualitative study. Patient Educ Couns. 2017;100(8):1473–81.
Davis RE, Jacklin R, Sevdalis N, Vincent CA. Patient involvement in patient safety: what factors influence patient participation and engagement? Health Expect. 2007;10(3):259–67.
Greene J, Hibbard JH. Why does patient activation matter? An examination of the relationships between patient activation and health-related outcomes. J Gen Intern Med. 2012;27(5):520–6.
Mitchell SE, Gardiner PM, Sadikova E, Martin JM, Jack BW, Hibbard JH, et al. Patient activation and 30-day post-discharge hospital utilization. J Gen Intern Med. 2014;29(2):349–55.
Weir DL, Motulsky A, Abrahamowicz M, Lee TC, Morgan S, Buckeridge DL, et al. Failure to follow medication changes made at hospital discharge is associated with adverse events in 30 days. Health Serv Res. 2020;55(4):512–23.
Kripalani S, Goggins K, Nwosu S, Schildcrout J, Mixon AS, McNaughton C, et al. Medication nonadherence before hospitalization for Acute Cardiac events. J Health Commun. 2015;20(Suppl 2):34–42.
Mortelmans L, De Baetselier E, Goossens E, Dilles T. What happens after Hospital Discharge? Deficiencies in Medication Management encountered by geriatric patients with polypharmacy. Int J Environ Res Public Health. 2021;18(13).
Mortelmans L, Goossens E, Dilles T. Beliefs about medication after hospital discharge in geriatric patients with polypharmacy. Geriatr Nurs. 2022;43:280–7.
Bandiera C, Ribaut J, Dima AL, Allemann SS, Molesworth K, Kalumiya K et al. Swiss Priority setting on implementing Medication Adherence interventions as Part of the European ENABLE COST action. Int J Public Health. 2022;67.
Elliott R, Boyd M, Nde S. at e. Supporting adherence for people starting a new medication for a long-term condition through community pharmacies: a pragmaticrandomised controlled trial of the New Medicine Service. 2015.
Grimmsmann T, Schwabe U, Himmel W. The influence of hospitalisation on drug prescription in primary care–a large-scale follow-up study. Eur J Clin Pharmacol. 2007;63(8):783–90.
Larsen MD, Rosholm JU, Hallas J. The influence of comprehensive geriatric assessment on drug therapy in elderly patients. Eur J Clin Pharmacol. 2014;70(2):233–9.
Viktil KK, Blix HS, Eek AK, Davies MN, Moger TA, Reikvam A. How are drug regimen changes during hospitalisation handled after discharge: a cohort study. BMJ Open. 2012;2(6):e001461.
Strehlau AG, Larsen MD, Søndergaard J, Almarsdóttir AB, Rosholm J-U. General practitioners’ continuation and acceptance of medication changes at sectorial transitions of geriatric patients - a qualitative interview study. BMC Fam Pract. 2018;19(1):168.
Anderson TS, Lee S, Jing B, Fung K, Ngo S, Silvestrini M, et al. Prevalence of diabetes medication intensifications in older adults discharged from US Veterans Health Administration Hospitals. JAMA Netw Open. 2020;3(3):e201511.
Royal Pharmaceutical Society. Keeping patients safewhen they transfer between care providers– getting the medicines right June 2012. Accessed 27 October 2023 https://www.rpharms.com/Portals/0/RPS%20document%20library/Open%20access/Publications/Keeping%20patients%20safe%20transfer%20of%20care%20report.pdf
International Pharmaceutical Federation (FIP). Medicines reconciliation: A toolkit for pharmacists. Accessed 23 September 2023 https://www.fip.org/file/4949
Californian Pharmacist Assiociation Transitions of Care Resource Guide. https://cdn.ymaws.com/www.cshp.org/resource/resmgr/Files/Practice-Policy/For_Pharmacists/transitions_of_care_final_10.pdf
Royal Collegue of Physicians. Medication safety at hospital discharge: Improvement guide and resource. Accessed 18 September 2023 https://www.rcplondon.ac.uk/file/33421/download
Douglas N, Campbell W, Hinckley J. Implementation science: buzzword or game changer. J Speech Lang Hear Res. 2015;58.
Download references
Acknowledgements
The authors would like to thank all the patients who took part in this study. We would also like to thank the Geneva University Hospitals Patients Partners + 3P platform as well as Mrs. Tourane Corbière and Mr. Joël Mermoud, patient partners, who reviewed interview guides for clarity and significance. We would like to thank Samuel Fabbi, Vitcoryavarman Koh, and Pierre Repiton for the transcriptions of the audio recordings.
This research did not receive any specific grant from funding agencies in the public, commercial, or not-for-profit sectors.
Open access funding provided by University of Geneva
Author information
Authors and affiliations.
School of Pharmaceutical Sciences, University of Geneva, Geneva, Switzerland
Léa Solh Dost & Marie P. Schneider
Institute of Pharmaceutical Sciences of Western Switzerland, University of Geneva, Geneva, Switzerland
Division of Endocrinology, Diabetes, Hypertension and Nutrition, Department of Medicine, Geneva University Hospitals, Geneva, Switzerland
Giacomo Gastaldi
You can also search for this author in PubMed Google Scholar
Contributions
LS, GG, and MS conceptualized and designed the study. LS and GG screened and recruited participants. LS conducted the interviews. LS, GG, and MS performed data analysis and interpretation. LS drafted the manuscript and LS and MS worked on the different versions. MS and GG approved the final manuscript.
Corresponding authors
Correspondence to Léa Solh Dost or Marie P. Schneider .
Ethics declarations
Ethics approval and consent to participate.
Ethics approval was sought and granted by the Cantonal Research Ethics Commission, Geneva (CCER) (2020 − 01779), and informed consent to participate was obtained from all participants.
Consent for publication
Informed consent for publication was obtained from all participants.
Competing interests
The authors declare no competing interests.
Additional information
Publisher’s note.
Springer Nature remains neutral with regard to jurisdictional claims in published maps and institutional affiliations.
Electronic supplementary material
Below is the link to the electronic supplementary material.
Supplementary Material 1
Supplementary material 2, supplementary material 3, rights and permissions.
Open Access This article is licensed under a Creative Commons Attribution 4.0 International License, which permits use, sharing, adaptation, distribution and reproduction in any medium or format, as long as you give appropriate credit to the original author(s) and the source, provide a link to the Creative Commons licence, and indicate if changes were made. The images or other third party material in this article are included in the article’s Creative Commons licence, unless indicated otherwise in a credit line to the material. If material is not included in the article’s Creative Commons licence and your intended use is not permitted by statutory regulation or exceeds the permitted use, you will need to obtain permission directly from the copyright holder. To view a copy of this licence, visit http://creativecommons.org/licenses/by/4.0/ . The Creative Commons Public Domain Dedication waiver ( http://creativecommons.org/publicdomain/zero/1.0/ ) applies to the data made available in this article, unless otherwise stated in a credit line to the data.
Reprints and permissions
About this article
Cite this article.
Solh Dost, L., Gastaldi, G. & Schneider, M. Patient medication management, understanding and adherence during the transition from hospital to outpatient care - a qualitative longitudinal study in polymorbid patients with type 2 diabetes. BMC Health Serv Res 24 , 620 (2024). https://doi.org/10.1186/s12913-024-10784-9
Download citation
Received : 28 June 2023
Accepted : 26 February 2024
Published : 13 May 2024
DOI : https://doi.org/10.1186/s12913-024-10784-9
Share this article
Anyone you share the following link with will be able to read this content:
Sorry, a shareable link is not currently available for this article.
Provided by the Springer Nature SharedIt content-sharing initiative
- Continuity of care
- Transition of care
- Patient discharge
- Medication management
- Medication adherence
- Qualitative research
- Longitudinal studies
- Patient-centered care
- Interprofessional collaboration
- Type 2 diabetes
BMC Health Services Research
ISSN: 1472-6963
- General enquiries: [email protected]
Qualitative Insights into Organizational Value Creation: Decoding Characteristics of Metaverse Platforms
- Open access
- Published: 15 May 2024
Cite this article
You have full access to this open access article
- Fabian Tingelhoff ORCID: orcid.org/0000-0002-7235-6001 1 ,
- Raphael Schultheiss 1 ,
- Sofia Marlena Schöbel 2 &
- Jan Marco Leimeister 1
141 Accesses
Explore all metrics
The significance of metaverse platforms is growing in both research and practical applications. To utilize the chances and opportunities metaverse platforms offer, research and practice must understand how these platforms create value, which has not been adequately explored. Our research explores the characteristics of metaverse platforms that facilitate value creation for organizations in both B2B and B2C sectors. Employing a qualitative inductive approach, we conducted 15 interviews with decision-makers from international corporations active in the metaverse. We identified 26 metaverse platform characteristics, which we categorized into six dimensions based on the DeLone and McLean Information Systems success model. Subsequently, we provide examples to illustrate the application of these identified characteristics within metaverse platforms. This study contributes to the academic discourse by uncovering the characteristics that shape the competitive landscape of emerging metaverse platforms. Leveraging these characteristics may offer metaverse providers a competitive edge in attracting complementary organizations to their platforms.
Similar content being viewed by others
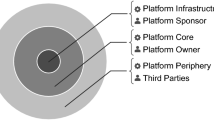
Three layers of abstraction: a conceptual framework for theorizing digital multi-sided platforms
Creating the Foundation for a Functioning Internal Platform
Tracing the Roots of the Organizational Benefits of IT Services
Avoid common mistakes on your manuscript.
1 Introduction
Recently, companies have begun to invest in metaverse platforms. McKinsey and J.P. Morgan project the metaverse as a trillion-dollar opportunity (McKinsey, 2022 ; Moy & Gadgil, 2022 ), while Gartner predicts 30% of companies will serve customers via metaverse platforms by 2027 (Gartner, 2022 ). This culminates in JP Morgan’s assessment, emphasizing that “metaverses will likely infiltrate every sector in some way in the coming years” (Moy & Gadgil, 2022 ). Indeed, with annual spending over $54 billion, expenditure on metaverses nearly doubled that of music purchases in 2021 (Moy & Gadgil, 2022 ). Researchers attribute this growth to metaverses revolutionizing virtual societal and economic interactions (Di Pietro & Cresci, 2021 ). Specifically, the metaverse benefit from network effects, becoming more appealing as user and organizational participation increases (Gawer & Cusumano, 2014 ; Tingelhoff et al., 2024 ). Accordingly, metaverse users benefit from the presence of more participating organizations, and reciprocally, having many users renders the metaverse more attractive for organizations (Kim et al., 2016 ).
While research deems metaverses only as viable once many users and complementors participate, about 500 companies already joined a metaverse platform, which only amounts to about 0.00015% of all companies (Brimco, 2024 ; Newzoo, 2022 ). A major contributing circumstance to the discrepancy of predicted and actual predicted and actual corporate engagement in metaverses is the inconsistencies of how different metaverse platforms influence organizational value creation. While past studies have examined how organizations mitigate challenges when creating value on metaverse platforms (e.g., Schöbel & Tingelhoff, 2023 ), organizations find it challenging to join metaverses due to the diverse ways in which various metaverses support value creation. Ultimately, it has not been fully explored how organizations can utilize characteristics of metaverse platforms (e.g., personalization and immersion) in their offerings to maximize their value proposition. When selecting a metaverse platform, organizations must prioritize its characteristics carefully to guarantee it fits their strategy. This study aims to uncover which metaverse platform features aid or hinder organizational value creation, leading to the following research questions:
RQ1 : What platform characteristics influence an organization’s ability to create value on a metaverse platform?
RQ2 : How have these characteristics already been configured in existing metaverse platforms?
This study seeks to contribute towards the research questions using qualitative data. We analyzed interviews with 15 decision-makers, all tasked with strategizing for corporations' engagements on metaverse platforms. We built on the DeLone and McLean Information Systems success model (D&M IS success model) (DeLone & McLean, 1992 , 2002 , 2003 ) to structure our data collection and analysis. From this, we identified 26 platform characteristics across six dimensions. Furthermore, we discovered these characteristics, such as accessibility and privacy, play different roles in metaverse platforms compared to traditional ones, a topic we expand on in our discussion. To substantiate our findings, we illustrate how these characteristics are divergently implemented in two existing metaverse platforms: Roblox and Decentraland.
Our study deepens the understanding of how organizations adopt metaverse platforms, laying groundwork for future research into corporate activities within these platforms. The study also broadens our knowledge of how emerging technologies' features support value-creation, beyond known platform mechanisms. Our results can help metaverse providers design more effective and appealing platforms. Moreover, by clarifying which platform features affect value creation, our study aids organizations in deciding how to offer their products or services on metaverse platforms. In summary, this study enables informed decisions about engaging with metaverse platforms for organizations.
This paper is structured as follows. The next section introduces foundational knowledge on metaverse platform ecosystems and outlines the structuring of their characteristics. Following that, we detail the methodology, then report and analyze the study's results. Subsequently, we exemplify our findings with case studies of two prominent metaverse platforms. In the sixth section, we explore the study's limitations and suggest directions for future research. The paper concludes with a summary of key insights.
2 Theoretical Background
2.1 the metaverse platform ecosystem and how it can create organizational value.
The metaverse is a multi-user virtual platform built on Web3 technology to revolutionize how people interact in any context (Di Pietro & Cresci, 2021 ). The malleable features of this emerging platform generate an immersive user experience, mimicking the real world sans physical constraints (Jaynes et al., 2003 ), thus enabling new and richer kinds of social and business interactions (e.g., through immersive 3D communication) (Bourlakis et al., 2009 ). By combining technologies like self-sovereign identity, virtual reality, and blockchain, these platforms evolve into parallel societies and economies. Though social media platforms (e.g., Facebook) or e-commerce platforms (e.g., Amazon) have already impacted human interactions and purchasing behaviors, this unique technology blend is unprecedented in virtual environments (Wang et al., 2021 ), enabling users to construct a parallel life with all its facets (i.e., work and leisure) in one platform for the first time.
The organizational structures around metaverse platforms classify them as an emergent platform ecosystem type (Schöbel & Leimeister, 2023 ). An ecosystem can be described as the underlying structure among partners, designed to enhance interactions and deliver a core value proposition (Adner, 2017 ). Generally, Typically, a platform is any product, service, or technology used by ecosystem actors to innovate and create complementary offerings (Gawer & Cusumano, 2014 ). It is typically integrated into ecosystems to help actors fulfill their value propositions (Gawer & Cusumano, 2014 ). For instance, the Apple App Store is integrated into the Apple ecosystem, including hardware and operating systems, enabling partners to create valuable apps and services.
Value is defined by an individual’s overall utility assessment based on their perception of input and output (Zeithaml, 1988 ). Two processual value components exist: use value and exchange value (Bowman & Ambrosini, 2000 ). Use value describes the recipient’s perception of a product or service based on their needs and values. Thus, use value stems from an organization's products and services, shaped by the recipient's personal and situational evaluation. Conversely, exchange value is what the recipient is willing to give in return for the use value. It can take other forms than monetary compensation, such as brand awareness or customer relationship, and is realized through the reciprocal transfer via the platform (Sanders & Simons, 2009 ).
Platform ecosystems comprise three major roles: platform leaders, complementors, and users. Platform leaders (or orchestrators) are the providers of the platform ecosystem (Gawer & Cusumano, 2002 ; Oliveira et al., 2019 ). They orchestrate the platform and are responsible for its regulation, maintenance, and adoption of standards within the ecosystem. Complementors provide products and services built for the platform, enhancing the ecosystem's core value. Lastly, users procure the complementors’ products and services via the platform. Usually, they access the platform and its complementary offering directly through a user interface. Consequently, in a metaverse platform ecosystem, value creation occurs through the reciprocal exchange of use and exchange values between complementors and users. Users receive products or services in the metaverse (use value) and are willing to compensate complementors with money and attention (exchange value). Accordingly, complementors are the source, users are the target, and the metaverse platform is the locus of value creation.
Some researchers contend that metaverse platform ecosystems challenge traditional role allocations (Schöbel & Leimeister, 2023 ). Notably, metaverse platforms represent early examples of decentralized governance models (Goldberg & Schär, 2023 ). Decentralized platforms are collectively owned by stakeholders rather than a single orchestrator. This collective ownership model is known as a Decentralized Autonomous Organization (DAO). On such a platform, users and complementors can impact decisions about platform governance, such as technical standards or the maximum amount of land created for users. This shifts the power balance among ecosystem participants, unlike in traditional platforms. In decentralized platforms, power is distributed; complementors and customers can propose and implement changes, not just the orchestrator (Goldberg & Schär, 2023 ). reducing the orchestrator's role also affects the direct relationships between customers and complementors (Yoo et al., 2023 ). While traditionally, both parties only interacted through the platform and, hence, the orchestrator, metaverses enable customers and complementors to interact directly. This is further emphasized by the fact that a metaverse does not require transaction intermediaries (e.g., banks). Specifically, the metaverse enables the direct exchanges of value items (Tapscott & Tapscott, 2017 ). This uniqueness to metaverse platforms potentially leads to simpler, more flexible, and efficient business processes by reducing communication complexities.
While many researchers contributed conceptualizing the metaverse, Schöbel et al. ( 2023 ) made the first effort to distinguish different types of metaverse platforms. Their taxonomy evaluates the technologies in a metaverse platform's infrastructure to highlight differences in value propositions. For example, they argue that a metaverse platform ecosystem like Decentraland focuses more on the creator economy and, hence, the creation and distribution of value items. In contrast, game-based platforms like Roblox focus on entertaining experiences that deepen user-brand relationships, alongside transactional value. This visualizes the paucity of platform types and business foci under the metaverse umbrella.
2.2 The DeLone and McLean IS Success Model
Identifying key success factors for organizational value creation is essential for insights that stay relevant in the rapidly changing metaverse platform ecosystems (Schöbel & Leimeister, 2023 ). In this context, past research employed value creation or value co-creation theory, predominantly focusing on aspects of value creation that are within an organization’s control. For instance, value creation theory typically centers on the consumer's perceived assessment, which organizations can influence by modifying their offerings or engaging in value co-creation with consumers. This aspect of how organizations can steer this process has been explored in existing literature, including in the context of the metaverse (e.g., Schöbel & Tingelhoff, 2023 ; Tingelhoff et al., 2024 ).
Conversely, our study examines platform characteristics that impact an organization's ability to create value. These external factors, often outside organizations' control, significantly influence value creation dynamics in technology-driven settings like metaverse platforms. In this context, the DeLone and McLean IS success model (D&M IS success model) (DeLone & McLean, 1992 , 2002 , 2003 ) has emerged as a cornerstone in the domain of Information Systems (Wang, 2008 ), enabling analysis of platform characteristics and their effects on organizational value creation. It provides a multifaceted perspective, deconstructing information systems into six key dimensions: information quality, system quality, service quality, usage intentions, user satisfaction, and net benefits.
Firstly, metaverse platforms are inherently complex digital ecosystems that thrive on exchanging information (Schöbel & Leimeister, 2023 ). The dimension of information quality is directly tied to the nature of data presented in metaverses, its accuracy, timeliness, and relevance. High-quality information will invariably influence user and organizational decision-making within a metaverse platform (Balica et al., 2022 ). System quality mirrors the technical prowess of a metaverse platform. As immersive environments, metaverse platforms demand high system performance, ease of navigation, and reliability. The better the system quality, the more seamless the user experience, thus attracting more complementors (Schöbel & Tingelhoff, 2023 ). Service quality in the metaverse context indicates the support structures in place. This could involve technical support, user guidelines, and assistance in content creation (Park & Kim, 2022 ). A high-quality service structure can significantly enhance the desirability of a metaverse platform for users and, in turn, organizations (Jo & Park, 2022 ).
The next dimensions, usage intentions and user satisfaction , arise from the interaction of the prior dimensions. Usage intentions resonate with how frequently users and organizations engage with the metaverse platform. A platform with a higher intent of usage becomes a hotspot for value creation and exchange, which is paramount in a networked environment like the metaverse (Ataman et al., 2023 ). Conversely, increased user satisfaction levels in a metaverse indicate that the platform effectively meets or surpasses the multifaceted expectations of users and organizations. When users are satisfied with their experiences, they are more likely to invest time, resources, and encourage peer participation, thus amplifying the platform's network effects and cementing its value-creation potential for organizations (Golf-Papez et al., 2022 ).
Lastly, the dimension of net benefits encapsulates the tangible and intangible outcomes that organizations derive from their engagement with an information system. When organizations can measure the positive impact of their involvement in a metaverse platform—whether in terms of revenue, brand recognition, skills acquisition, or social connections—it reinforces their commitment to the platform and ensures sustained engagement (Polyviou & Pappas, 2022 ). This sustained engagement, powered by recognized net benefits, can indicate the platform's long-term value-creation potential for organizations (Periyasami & Periyasamy, 2022 ).
While the D&M IS success model is tried and tested across various IS contexts, its inherent adaptability makes it particularly apt for metaverse platforms. The model’s validity at both individual and organizational levels (Petter et al., 2008 ) makes it a versatile tool for understanding an emergent and complex environment like the metaverse. Furthermore, previous validations of this model in diverse IS environments, from enterprise systems to e-commerce platforms (Wang, 2008 ), have shown its robustness and adaptability (Ahlan, 2014 ; Al-Kofahi et al., 2020 ). Applying it to the metaverse, an amalgamation of various IS types, seems like a logical progression.
The essence of the D&M IS success model is its capability to elucidate key dimensions that contribute to its capability to support complementors to create and deliver value through an information system (Wang, 2008 ). Yet, the interplay of these dimensions over time and the progressive stages of adoption need to be considered for a more comprehensive understanding. This aspect is vital as it bridges the D&M IS success model with real-world technological adoption behaviors and patterns, making it more contextually relevant, especially for evolving digital realms like the metaverse. By integrating process steps, one can understand not just what supports value creation (as indicated by the D&M IS success model) but also how and when these characteristics manifest and influence organizational value creation over the adoption lifecycle. To expand theories for a temporal interplay of characteristics, Ahlan ( 2014 ) proposed three process steps: system creation, system use, and system impact. This hierarchical order of influence characteristics can be applied to the D&M IS success model, where information, system, and service quality correspond to the system creation, usage intention and user satisfaction to system usage, and net benefits to system impact. The combined model is depicted in Fig. 1 .
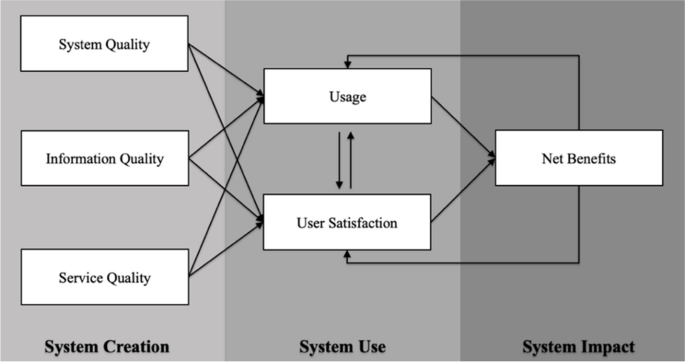
Research Model, based on DeLone and McLean ( 1992 , 2002 , 2003 ) and Ahlan ( 2014 )
The initial phase of any technological endeavor involves its conceptualization, development, and implementation (Weber et al., 2023 ). This phase is particularly crucial within the metaverse context as it lays the foundation for the user experience. Information, system, and service quality become essential metrics at this juncture. The quality and relevance of information guide the design and functionalities of the metaverse platform (Bayraktar et al., 2023 ). System quality ensures the platform’s technical soundness and scalability, which is vital to handling the dynamic nature of the metaverse’s ever-evolving virtual landscapes and foundational technologies (Peukert et al., 2022 ). Service quality, conversely, pertains to the support mechanisms, ensuring that complementors have a smooth onboarding process and immediate resolution to any technical hitches (Xi et al., 2023 ). A robust system creation phase, bolstered by these quality metrics, sets the tone for subsequent adoption and usage.
Once a system has been created and launched, its success is predominantly gauged by its acceptance and the extent of its use. Here, usage intention is a precursor, indicating initial interest and potential adoption rates (Jeong & Kim, 2023 ). However, for the system to embed itself into the daily routines of users and organizations, satisfaction becomes pivotal (Xi et al., 2023 ). As users engage with the metaverse platform, their experiences, which are shaped by immersive interactions, realistic representations, and the fulfillment of intended purposes, dictate their satisfaction levels. Satisfied users not only continue their engagement but also promote organic growth through positive word-of-mouth and peer recommendations (Mladenović et al., 2023 ).
For metaverse platforms, the system impact, as denoted by net benefits, encapsulates how effectively platform characteristics support organizational value creation (Polyviou & Pappas, 2022 ). This could manifest in diverse ways, from driving innovation in product or service offerings, facilitating unique customer engagement models, to fostering new revenue streams or enhancing brand visibility within the virtual realms (Hadi et al., 2023 ). Moreover, it might also encompass intangible benefits such as enhanced collaborative potentials, access to new market segments, or the ability to test and iterate offerings in risk-mitigated virtual scenarios (Yoo et al., 2023 ). Thus, system impact, in this context, underscores whether the metaverse platform meets the technical and experiential needs of its complementors and provides a conducive environment for organizations to harness its potential and realize tangible value.
In summary, the adapted D&M IS success model provides an exhaustive framework to unpack, understand, and measure how metaverse platform characteristics influence organizational value creation. By mapping its six dimensions to the specific characteristics of metaverse platforms along Ahlan ( 2014 )’s process steps, this study aims to clarify the characteristics that influence organizational value creation in this burgeoning digital frontier.
3 Methodology
3.1 ensuring the quality of qualitative data.
This study investigates how metaverse platform characteristics impact organizational value creation. The phenomenon of the metaverse is relatively new, and less is known about how organizations create value on metaverse platforms. To gain exploratory insights into organizational value creation, we adopted a qualitative research method (Draper, 2004 ).
To ensure our qualitative data's validity and reliability, we adhered to Lincoln and Guba ( 1985 )’s criteria: credibility, transferability, dependability, and confirmability. Credibility refers to the data's internal validity and its alignment with reality (Merriam & Grenier, 2019 ). We selected our interviewees based on three criteria. First, we considered whether interviewees had a holistic overview of organizational value creation. This includes individuals who are not only involved in strategic decision-making but also with a broad understanding of how various organizational units contribute to value creation. Second, as we explored metaverse platforms, our participants needed substantial experience in the metaverse sector. This ensures that our data comes from individuals who are not only familiar with the concept but are actively engaged in its application and development within their organizations. Third, we focused on participants whose organizations actively offer products or services on metaverse platforms (e.g., Roblox or Decentraland). This practical involvement ensures our insights are grounded in real-world experiences and challenges. To guarantee a similar level of abstraction and comparability of our findings, we selected interview partners based on similar levels of responsibility regarding the metaverse. Furthermore, to increase credibility and mitigate possible biases in the data collection and analysis (Valenzuela & Shrivastava, 2002 ), the first three authors of the paper coded the data independently. Specifically, we employed the qualitative inductive approach described by Gioia et al. ( 2013 ), which is designed to enhance qualitative rigor in conducting and presenting inductive research. This approach is particularly suitable for inductively developing grounded theory, offering rich and detailed theoretical descriptions, which, in our case, pertains to the value creation on metaverse platforms. In line with the method proposed by Gioia et al. ( 2013 ), we constructed first-order concepts, second-order themes, and aggregate dimensions, with the latter reflecting the theoretical constructs.
To ensure intercoder reliability, the coders critically discussed their initial coding results until the first-order codes were sufficiently reviewed, and a second iteration could start that led to a detailed description of our second-order constructs. During this process, the coders aimed for the codes to be on a comparable level of abstraction and still reflect the individual experiences of each informant. Our analysis was rooted in a hermeneutic approach, emphasizing the shared understanding of the nature of metaverse platforms. Following the insights of Paterson and Higgs ( 2005 ), we embraced hermeneutics for its dialogical nature, which in our research manifested through the interviews conducted between experts and the interviewer.
To improve the transferability—whether the results of one study can be transferred to different settings with different participants—of our results, we have provided sufficient contextual information about each informant and their interview in Appendix 1. Dependability describes the extent to which the findings of a study can be replicated (Merriam & Grenier, 2019 ). We noticed a high degree of concept/coding saturation (95% of codes surfaced) after the 11th interview, which aligns with previous studies on the method (Guest et al., 2006 ) and the topic at hand (Schöbel & Tingelhoff, 2023 ). Finally, confirmability mainly concerns objectivity and addressing potential research biases that can result, for instance, from inherent values and beliefs but also the mere presence or timing of follow-up questions (Valenzuela & Shrivastava, 2002 ). We addressed confirmability by independently executing tasks simultaneously and comparing results (e.g., for data coding and interpretation). Further, all the researchers were at least aware of their potential biases and influences, which we critically, openly, and proactively addressed during the planning and execution of this research.
3.2 Data Collection
In our qualitative study, we interviewed 15 metaverse decision-makers from multilateral organizations in both B2B and B2C markets. These participants represent the mission and vision of an organization; they have the highest responsibility for their organization’s metaverse strategy, were familiar with key metaverse platforms, and understood how different organizational units contribute to value creation. In other words, we interviewed CEOs and senior leaders who are deeply familiar with their organizations and can insightfully discuss how metaverse platforms align with their business models to create value. Interviewees explicitly consented to their interviews being recorded and their personal data being published, both before and after the interviews. This procedure adhered to ethical research standards, ensuring transparency and reliability in data collection.
We refer to individual participants using the abbreviation IP (interview partner) and their number (1–15). We used a structured interview guide to ensure interviewees fully understood the platform characteristics being studied. The guide featured open-ended, concise questions, supplemented with follow-up probes to resolve ambiguities. Additionally, we provided contextual information and examples where necessary to aid comprehension. We began by asking participants about their demographics. This was followed by general questions about the metaverse (how they defined it, for instance). We then focused on which platform characteristics they deemed crucial for strategic decisions regarding their metaverse offerings. We explicitly referenced the three process steps of system creation, system use, and system impact. In addition to discussing the impact on their organizations, we invited opinions on future metaverse platform developments and their relevance to the interviewee’s organization.
Interviews were held online (Lo Iacono et al., 2016 ) and conducted in the interviewees' native languages when possible (Harzing & Maznevski, 2002 ). To address the potential for translation-induced alterations, we employed a rigorous process. First, we conducted 66% of the interviews in the native languages of both interviewees and interviewers, reducing the necessity for translation. The remaining interviews were conducted in English. For interviews needing translation, a bilingual, topic-knowledgeable co-author handled the task. A second bilingual co-author reviewed and verified the transcripts against the audio recordings for translation accuracy. The interviews lasted between 26 and 56 min (mean: 34). In one interview (IP 5), technical difficulties obstructed the recording. The interviewer—in this case, the second author— immediately after the interview reconstructed the interview details from notes and memory.
We based our coding, for which we used the Atlas.ti22 software, on the transcripts (Gioia et al., 2013 ). The first three authors coded simultaneously and independently. According to Gioia et al. ( 2013 ), the first-order coding was an open coding mechanism that remained faithful to the words of each interviewee. Ending up with a high number of codes prompted us to summarize those codes where the informants addressed the same topic but with different terminology. Since the initial open coding was executed independently, ongoing researcher discussions shaped the final constructs. After identifying platform characteristics, we assessed their relative importance. To this end, we presented the ordered variables to our interview partners for an ordinal rating of relative strategic importance. To substantiate our findings, we showcased the highest-rated characteristics using two practical examples. Roblox and Decentraland serve as leading examples of early metaverse platforms (Schöbel et al., 2023 ). As current recipients of immense funding and publicity from practitioners (Kamin, 2021 ; Wang & Ho, 2022 ), they are ideal subjects for further investigation.
In line with our research goals, we identified 26 platform characteristics and categorized them according to the six dimensions of the D&M IS success model. Additionally, our metaverse experts ranked these characteristics based on their importance and relevance to organizational value creation in metaverse environments. Figure 2 reports the characteristics per dimension ranked by their average importance (with 1 indicating the highest importance). However, the rankings reflect the experts' average views. Though indicative of relative importance, they are not meant as precise statistical measures. Given this, we advise that small differences in rankings, particularly those within 0.1 or 0.2, may be negligible.
In subsequent sections, we detail our empirical findings and analyze each of the six success dimensions.
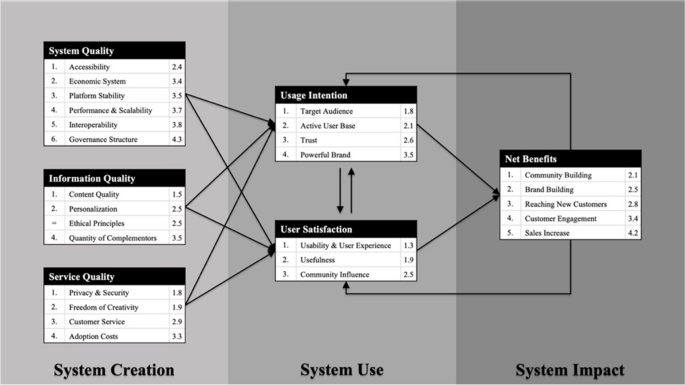
Adapted Research Model (including the average ranks by IPs)
4.1 System Creation: System Quality, Information Quality, and Service Quality
The system quality dimension encompassed the most platform characteristics. In this dimension, interview partners (IPs 1, 2, 4, 8, 9, 12, 13, 14, and 15) ranked accessibility —ease of access for complementors and users—as the most crucial. The interviewees specifically mentioned that platforms must be accessible through various devices, including tablets, smartphones, and desktop PCs (IPs 1, 2, and 9). IP 9 elaborates:
It is all about being available across the devices. The more devices you have available for the platform, the easier it is to enter. I mean, as we all know, traffic is coming from mobile and desktop. […] So, you know, if your platform is only compatible with PCs, you’re missing out on a big portion of the market. Footnote 1
However, accessibility encompasses not only device compatibility but also the ease of navigating the platform's functions. “One should be able to move around and execute actions without having to read a one-hour manual,” argued IP8 when asked about the necessary functionalities regarding the system quality of a metaverse platform. Similarly, IP 14 emphasized that operability is currently more important than functionality.
Interviewees (IPs 4, 12, 14, and 15) also deemed an integrated economic system essential. The interviewees highlighted the necessity of integrated payment systems (IP 12) and their ability to create new user experiences (IP 15). This includes a currency that offers voting rights on decentralized platforms. Further, economic systema enable new functionalities, such as play-to-earn, where users are rewarded with items or tokens of monetary value. In this way, a platform can ensure its most active users receive a higher voting share. Additionally, new business models—for instance, transferring goods from the virtual to the physical world and vice versa—require digital ownership structures that metaverse platforms must provide (IP 14).
Across all dimensions, platform stability emerged as the most frequently mentioned characteristic (IPs 2, 3, 4, 6, 7, 9, 11, 12, 14, and 15) . Interviewees expressed concerns that platform bugs, like a faulty login page, could harm their brand image (IPs 4, 6, and 9). While some worried that these “bugs lead to users exiting the metaverse altogether” (IP11), others cautioned against entry barriers: IP12 warned that “[t]he metaverse-guest’s fear about crossing the threshold into the virtual immersion must be addressed for them to use it at all.”
Information quality represents the second dimension in the D&M IS success model. Content quality emerged as the primary concern in the interviews (IPs 3, 4, 6, 7, 8, 10, 12, 13, 14, and 15). Organizations worry about losing potential customers if the platform's overall user experience and other complementors' offerings are unsatisfactory. “So, you know, if the content is not attractive,” IP 14 explained, “people will just hop onto it, take a look, and probably never come back. If you don’t engage your participants, that becomes an issue.” Specifically, IP14 worries that a bad experience on one platform could deter customers from using any metaverse platform. Compared to other platforms, this concern is particularly pertinent for metaverse platforms given their current stage of development. Additionally, companies consider the connotation of the content produced on a platform. For instance, companies may hesitate to join game-based metaverses due to concerns that users could associate the game's characteristics with the company. IP12 specified this concern: “We try hard to avoid becoming a Disneyland. This is not just a game and fun. It is totally okay if it is around fun; that is not wrong. […] Even though a platform might have entertainment aspects, we also want to be able to educate our customers in a serious manner.”
Personalization (IPs 1, 8, 11, and 14) is another integral metaverse platform characteristic. This encompasses two facets of customization. First, organizations aim to align their virtual presence with their brand identity: “Nobody wants to access a generic platform with this great template-world. No, everybody wants their own logos, their own trees, their own information channels” (IP 11). In addition to platform design flexibility, organizations also aim to offer personalized products to their customers. This introduces several technological challenges, as discussed by Duan et al. ( 2021 ). To provide personalized features effectively, orchestrators must balance individual customization with maintaining a cohesive design across the platform. This creates tension among stakeholders, as personalization challenges the standardization and regulations needed for a unified look. IP11 cited Meta’s AI builder as a notable example of addressing these challenges:
The amount that individualization and customization are wanted by the customers is extremely effortful. […] There is now this AI builder by Meta. It’s a prototype based on voice recognition. So, Mark Zuckerberg is standing there [on the metaverse platform] as an avatar and says: ‘Hey, I need an island,’ and then an island appears. And then he says, ‘I want trees,’ and a palm tree appears. […] And this is how you could build customized yet standardized objects for your customers in a few minutes.
Service quality marks the last dimension within the system creation phase. Here, aspects of privacy and security (IPs 1, 3, 6, 11, 12, 13, and 14) emerged as the focal platform characteristic. This focus stems from the unique types of data collected by metaverse platforms. With advancements enabling the collection of micro-movements and face-tracking data, organizations can now access unprecedented types of personal data. Aware of these risks, interviewees unanimously agreed that “if it [a metaverse platform] is not built safely, it won’t be successful” (IP 6). In other words, our interviewees require security and privacy concepts to develop a metaverse platform “into a safe space” (IP 13). Nevertheless, organizations often require sensitive data to effectively serve their customers. For instance, a hotel company testing its designs in the metaverse necessitates personal data on user movements within the platform. Consequently, organizations are concerned that customer reluctance to share data on metaverse platforms, coupled with their cautious approach to data collection, may restrict product sales (IPs 1 and 14). This creates tension between the need for privacy and safety versus the drive for product offerings and sales, often prioritizing the latter at the expense of the former. Therefore, striking the correct balance becomes crucial for complementors, given its significant influence on a platform's operational capabilities.
4.2 System Use: Usage Intention and User Satisfaction
Usage intention determines the target users of a platform and their manner of engagement. Naturally, organizations aim to reach their target audience upon entering a metaverse platform (IPs 1, 6, 10, 11, 12, and 14). IP 10 explains that large corporations might easily financially engage with any major platform, whereas small and medium enterprises (SMEs) must carefully choose platforms where their selected target audience is most active. For instance, while Roblox’s age demographic is age 13 to 25, the users of Meta’s Horizon are potentially older (IP 6). Additionally, complementors seek platforms with an active user base (IPs 3, 4, 6, 7, 8, 9, and 15). Platforms are appealing if “used by active users on a daily basis” (IP 4), as complementors aim to “engage and interact with users to enter a common dialogue and exchange” (IP 7). In other words, complementors view the metaverse as a venue for initiating interactions with current and potential customers. In this context, the metaverse acts as an additional channel for organizations to actively engage with their target audience (Hadi et al., 2023 ). Some companies aim to educate customers and create leads in the metaverse (IP 12), others focus on product-centric community creation to foster customer loyalty (IPs 3 and 6). This is consistent with the metaverse's focus on social interaction and immersion (Schöbel et al., 2023 ).
The user satisfaction dimension relates to the benefits users perceive from a platform. This perception shapes how users view the complementors on the platform. As a result, organizations favor platforms that cater to user needs. In this regard, the most critical platform characteristic concerns usability and user experience (IPs 1, 3, 4, 7, 8, 9, 10, 12, 13, and 14). This aspect is closely linked with system availability, a key part of the system quality dimension. It emphasizes a user-friendly experience throughout the application phase and the entire user journey (IP 7). IP 8 desires platforms that include “elements to positively surprise the user,” a point further elaborated by IP 4:
“Then another point would be the platform usage, this is all about how to access this platform and send the user out to discover the world and the many, many leads of the platform. How could we even make the consumer journey way easier to access the metaverse? […] How could we make this […] extend reality? How could we remove all the initial steps to make it like a natural, accessible environment?”
IP 4's concerns focus on how a metaverse platform's unique features are practically implemented. More specifically, metaverses are meant to extend reality by adding features for business and leisure (Bourlakis et al., 2009 ). For instance, making crypto wallet sign-up optional could increase platform accessibility. However, this could restrict the functionality of the platform's economic system, as seen in Decentraland's guest login feature. Therefore, platforms and complementors must find compromises to balance this tension.
Most interviewees agreed that a metaverse platform must offer added value to its users, essentially making it useful (IPs 1, 2, 3, 4, 6, 7, 8, 12, and 14). A metaverse platform “needs to provide an inherent added value to its users to convince [complementors] to have potential” (IP8). This implies that a metaverse platform must offer value to users even without the contributions of complementors. Here, value means any desirable outcome users perceive from using the platform. This challenges Bowman and Ambrosini ( 2000 )’ value theory that positions complementors as the exclusive source of use value in traditional ecosystems. Nonetheless, complementors prefer metaverse platforms that are already part of a functional, value-adding ecosystem (IPs 1, 4, 7, and 8).
The rationale behind these characteristics lies in the competitive dynamics of early-stage platform markets. IP 3 noted that platforms offering the highest perceived value will outlast others, becoming attractive investments for complementors. Although complementors are crucial to a digital platform's value proposition, they approach metaverse platforms with skepticism, demanding evidence of past performance. Complementors aim to minimize investment and brand reputation risks by insisting on metaverse platforms demonstrating success (i.e., adding user value) before participating. Such requirements pose significant challenges for emerging platforms. Larger orchestrators like Meta (formerly Facebook) and Microsoft benefit from established credibility, whereas smaller, entrepreneurial platforms face stricter scrutiny, complicating the development of strong complementor ecosystems.
4.3 System Impact: Net Benefits
Net benefits describe the positive outcomes expected by complementors from their participation in a platform. Unlike previous dimensions, which focus on the metaverse's structure and customer relations, this dimension concentrates on the direct interaction between complementors and the platform. Foremost, complementors value community building (IPs 3, 4, 6, and 14). Our experts view the metaverse as enhancing bidirectional relationships between brands and communities, beyond what is typical on social media. IP3 highlighted this advantage, noting it “allowing our fans to give feedback to us.” More specifically, companies can nurture their communities while profiting from their responses. Product development is a field where this potential is already visible (see customer engagement ). As an example, Starwood Hotels first constructed their properties in the metaverse world of “Second Life” (Gates ( n.d. )). Subsequently, they invited customers to experience the space in 3D and offer feedback. Only after several iterations did Starwood begin real-world construction. Moreover, complementors can benefit from community building in various other use cases. For instance, the energy drink brand Prime engages its audience through the metaverse and NFTs (see customer engagement ), fostering community feelings and boosting beverage consumption (see increased sales ). Thus, community building serves as a steppingstone, not just an end goal. This aligns with previous studies on community building and social media (e.g., Guo et al., 2016 ; Sledgianowski & Kulviwat, 2008 ).
Brand building (IPs 3, 6, 7, 10, and 14) involves marketing activities with enduring effects. IP 14, among others, highlighted marketing as the most viable current business model outcome in the metaverse. Interviewees see metaverses as crucial for educating customers about a brand (IPs 3, 6, 7, 14). As with every online marketing, the metaverse can be used to engage customers with the core principles of one’s brand (IP 14), to provide information and experiences about products (IPs 7 and 10), and to create strong emotional bonds, such as customer loyalty (IP 6). Yet, metaverses go beyond traditional functions by offering real-life brand experiences. For instance, in the metaverse, customers can test-drive a BMW (BMW, 2023 ), feel the difference of Nike shoes (Nike, 2023 ), or experience Givenchy's brand values at a virtual pool party (Smith, 2022 ). Immersing users to the point where fiction and reality blur (Lee et al., 2022 ) allows for deeper and more impactful brand experiences (Hadi et al., 2023 ). Therefore, interviewees stressed the importance of a metaverse platform's brand-building tools in choosing the right metaverse.
Although many interviewees (IPs 1, 2, 3, 6, 7, 8, 10, and 15) mentioned increased sales as a significant platform benefit, it received the lowest ranking across all dimensions, falling into the 80th percentile. This finding was surprising, given the portrayal of the metaverse as a prime venue for trading, evidenced by trends in NFTs and virtual real estate. However, our interviewees either did not see its revenue potential (yet) or appreciated its other unique opportunities, disregarding financial benefit. For instance, IP3 asserted that “there is no revenue potential out of this [metaverse],” contrasting with IP2's statement that “we just don’t want to burn money.” Ultimately, all interviewees agreed that sales increase was among the least important net benefits. However, metaverse usage becomes much more apparent when comparing the results of B2B companies to B2C companies. In our sample, all B2C interview partners used metaverse platforms for community or brand-building, indicating that consumer brands predominantly utilize these platforms to foster their existing assets (mainly customer base and brand equity). This emphasizes that, for these companies, the metaverse represents not a quick profit avenue but a long-term, sustainable investment. Conversely, B2B organizations show no clear tendencies. While five interviewees (IPs 6, 8, 11, 13, and 14) evaluated community and brand-building as the most important, two indicated reaching new customers and customer engagement as their highest priorities (IPs 3 and 2, respectively). However, none ranked increased sales first. This is in line with previous research on organizational value creation in metaverses. For instance, according to Schöbel and Tingelhoff ( 2023 ), organizations lack the foundational knowledge to understand a metaverse platform’s opportunities and challenges, thus hindering efficient decision-making in the B2B sector. Alternatively, the diversity in organizational needs within the B2B sector might explain the varied preferences. Nonetheless, the unanimous lack of priority for increased sales in both B2B and B2C groups highlights the metaverse's broader benefits, offering extensive marketing and customer interaction possibilities beyond mere e-commerce.
5 Discussion of Results: Illustrating How to Transfer Platform Characteristics
Building on earlier discussions about crucial metaverse platform characteristics for complementors, certain orchestrators are now incorporating features that align with these metaverse attributes. Decentraland and Roblox are two leading examples of early metaverse platforms. With 42 million daily active users globally, Roblox stands as one of the most popular metaverse platforms (Gollmer, 2022 ). Conversely, Decentraland has approximately 8,000 daily users, according to internal Decentraland Foundation data (Decentraland, 2022 ). Despite smaller user numbers, Decentraland remains a leading name among decentralized metaverse platforms (Brooke, 2022 ). Furthermore, Decentraland (IPs 2, 3, 4, 6, 7, 8, 9, and 15) and Roblox (IPs 3, 4, 6, and 13) were the most frequently mentioned metaverse platforms by our interviewees, referencing these platforms continuously as metaverse examples.
Studies indicate that both platforms possess the technological capabilities essential for metaverse classification, such as immersion or creator economy aspects (Schöbel et al., 2023 ). For instance, Decentraland encourages creativity by allowing users to own and develop land parcels. Each platform offers a comprehensive visualization of its virtual world. Collaborating with these platforms allows organizations to bypass the need for hiring game designers or creating immersive worlds from scratch. Thus, both platforms serve as accessible entry points into the metaverse. The key distinction lies in Roblox's centralized structure versus Decentraland's decentralized governance. The Roblox Corporation, a US-based public software company, controls significant updates to Roblox. In contrast, Decentraland operates as a DAO, where ownership and decision-making powers are distributed among users and complementors via the virtual currency, MANA. This model enables stakeholders to vote on governance issues and how the treasury is allocated (Brooke, 2022 ). We will use these two platforms as practical examples to illustrate our interview findings.
5.1 Illustration of System Creation
Both platforms’ user experiences are quite diverging. Roblox stands out for its accessibility , supporting web browsers, mobile devices, and Xbox consoles, aligning with our interviewees' preferences. Additionally, Roblox offers both 2D and immersive 3D experiences via virtual reality (VR) headsets. Despite its maturity, Roblox has yet to incorporate augmented reality (AR) technology (Shin, 2022 ; Yang et al., 2022a , b ). Metaverses aim to blend virtual and physical realities, enabling seamless information exchange between the two (Marabelli & Newell, 2022 ). AR offers significant advantages by overlaying virtual content onto the real world, intertwining both realities. Body sensors can further enhance user immersion by integrating physical movements into the virtual experience (Park & Kim, 2022 ). Despite our interviewees' emphasis on accessibility, metaverse platforms infrequently implement these immersive features (Park & Kim, 2022 ).
Although Roblox boasts an open and accessible design, it restricts users with a compulsory sign-in process that demands personal information, including date of birth. Additionally, signing in necessitates users' acceptance of Roblox’s terms of use and privacy policy. This requirement raises privacy and security concerns due to the submission of private data for platform access (IP 1, 3, 6, 11, 12, 13, and 14). Past research highlights the critical role of data security measures like privacy guidelines, stressing the need for user empowerment in decisions regarding data collection and storage (Guidi & Michienzi, 2022 ; Ning et al., 2021 ). Figure 3 shows Roblox’s access page.

Roblox’s Web Access
Decentraland features a distinct economic system, a priority reflected in its user account setup. Unlike other platforms, Decentraland registration requires a third-party crypto wallet, such as MetaMask, instead of a platform-specific account. This shows that Decentraland, unlike Roblox, is not interested in being the proprietor of its users’ data. A crypto wallet is essential for owning and trading virtual goods and currencies, a fundamental aspect of metaverse platforms (Di Pietro & Cresci, 2021 ; Oliver et al., 2010 ; Tayal et al., 2022 ; Vidal-Tomás, 2022 ). Using a crypto wallets as accounts, Decentraland’s users can execute peer-to-peer economic transactions without intermediaries. This aligns with our interview results, where interviewees highlighted the need for integrated payment systems (IP 12) as enabler for unique user experiences (IP 15).
User-generated content (UGC) is a defining characteristic of metaverse platforms. UGC empowers users to enrich the platform with their creations, ranging from services and products to various content. While some researchers argue that all content must be user-generated in a metaverse (Ayiter, 2012 ), others believe metaverses should primarily enable user creativity (Dionisio et al., 2013 ) to facilitate UGC (Oliver et al., 2010 ). For instance, Roblox incorporates gaming elements (Getchell et al., 2010 ) and actively supports users in crafting their games from the ground up (Metcalf, 2022 ). The Roblox engine allows users to seamlessly transition between diverse game genres, like puzzles and sports —this is, in part, related to the metaverse vision of persistency and interoperability between different platforms. Additionally, Roblox offers resources for users to learn programming, further empowering them to design their games. Roblox's open creation platform has led to the development of over 32 million unique virtual experiences, indicating its focus on freedom of creativity . Greater creative freedom for content creators fosters more innovation in the design of experiences (Orgaz et al., 2012 ).
Similarly, creativity extends to the creation and sale of digital goods (Boughzala et al., 2012 ; Kim, 2021 ). Roblox, along with its users and complementors, can create and sell these digital items. Roblox thus empowers its users to transact and monetize their content, a vital characteristic of metaverse platforms (Popescu et al., 2022 ; Tayal et al., 2022 ). Yet, Roblox conducts all transactions with its proprietary currency, Robux, which can be purchased via the platform using traditional payment methods, such as credit cards. Studies indicate that transactions in FIAT currency benefit users by eliminating currency conversion and lock-in mechanisms (Gadalla et al., 2013 ; Hwang & Lee, 2022 ; Papagiannidis et al., 2008 ; Vidal-Tomás, 2022 ; Yang et al., 2022a , b ). Despite the emphasized importance of interoperability by research (Di Pietro & Cresci, 2021 ; Kim, 2021 ) and our interviewees (IPs 1, 7, and 14), Roblox lacks this feature. Although purchases on Roblox are persistent (Braud et al., 2021 ; Falchuk et al., 2018 ), they are not transferable to other platforms (Wang et al., 2021 ). Figure 4 shows the Roblox Avatar shop.
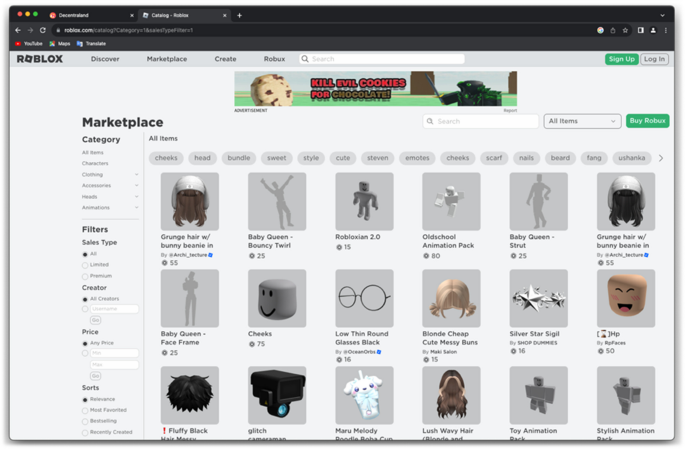
Roblox’s Avatar Shop
Decentraland also prioritizes personalization . For instance, the platform offers extensive character customization options available even to guest users. Beyond many free features, Decentraland also offers paid personalization options, such as skins or collectibles. This is an essential feature of metaverse platforms, as scholars agree on the importance of designing avatars resembling the actual appearance of the user (e.g., Dionisio et al., 2013 ; Gadalla et al., 2013 ; Hwang & Lee, 2022 ; Shin, 2022 ). However, some researchers describe real-time 3D scans as the pinnacle of avatar customization (Schöbel et al., 2023 ). Photorealistic depictions of users offer significant advantages. This approach mitigates safety concerns related to anonymity and potential irresponsible behavior in virtual spaces (Falchuk et al., 2018 ; Guidi & Michienzi, 2022 ; Sykownik et al., 2022 ). Yet, achieving photorealism introduces several technological challenges. Implementing face scans requires infrastructure like depth sensors, while photorealistic rendering demands high computing power and significant data storage, both of which are already current bottlenecks in expanding virtual worlds. Decentraland's approach to character customization, depicted in Fig. 5 , strikes a balance between photorealism and anonymized character appearances.
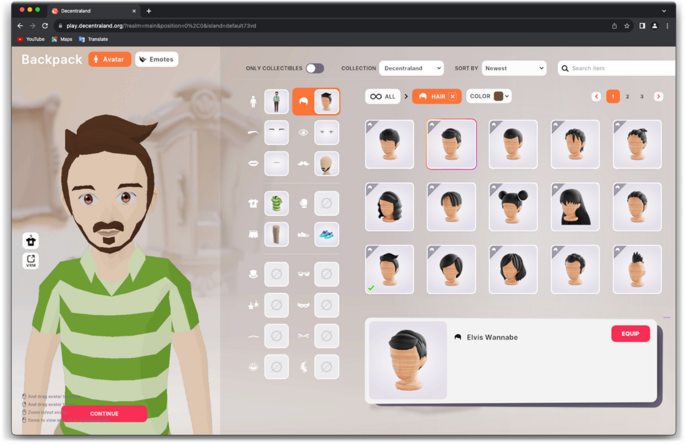
Decentraland’s Character Customization Page
Transitioning between locations on both metaverse platforms resulted in significant loading times. During our Decentraland test, specifically when creating the character, the platform crashed, erasing all progress (see Fig. 6 ). While platform stability was mentioned by almost every interviewee (IPs 2, 3, 4, 6, 7, 9, 11, 12, 14, and 15), it cannot always be guaranteed. These issues stem largely from the high demands for computational power and data transfer (Choi & Kim, 2017 ). The integration of technologies like VR, decentralized ledgers, photorealism, and platform interconnectivity means metaverses will eventually generate more data than current storage capacities can handle (Schöbel et al., 2023 ). Therefore, “hosting and handling a metaverse platform will require significantly more effort than organizing traditional two-sided market platforms” (Schöbel et al., 2023 , p. 8). These challenges can already be observed in the platforms’ governing decisions. To manage computational and data-sharing loads, both platforms cap the number of users per server, as computational power, rendering, and data traffic requirements increase exponentially with each additional user. Imposing these limitations, however, contradicts fundamental metaverse principles like unrestricted user movement (Jaynes et al., 2003 ; Owens et al., 2011 ) and independence (Davis et al., 2009 ; Khansulivong et al., 2022 ). Further, social interactions are a cornerstone of the metaverse concept (Davis et al., 2009 ; Wang et al., 2021 ). The present state of technology and governance in metaverse platforms indicates that a fully realized metaverse remains a distant goal (Peukert et al., 2022 ; Schöbel et al., 2023 ).
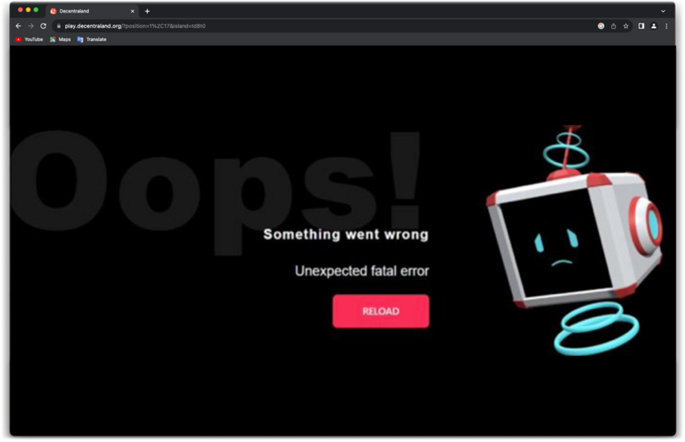
Error Message while using Decentraland
5.2 Illustration of System Usage
Past research has identified trust as a critical component for the functionality of an ecosystem (Lang et al., 2019 ; Tawaststjerna & Olander, 2021 ). Stakeholders rely on trustful interactions among ecosystem actors, particularly in e-commerce and social media platforms (Bonina & Eaton, 2020 ). E-commerce platforms serve as centers for financial transactions. Customers and complementors must trust these platforms to accurately and error-free conduct their transactions. Given that many transactions on these platforms are one-time events, complementors face challenges in building trust through rapport with customers. Hence, e-commerce platforms (such as Amazon) act as trusted intermediaries (Friedrich et al., 2019 ; Molla & Licker, 2001 ; Torkzadeh & Dhillon, 2002 ). Users prepay for products, trusting in timely delivery, whereas complementors trust the platform to compensate them for sales. Thus, trust among its actors is essential for an e-commerce platform's proper function (Friedrich et al., 2019 ). Similarly, social media platforms manage valuable data. Unlike e-commerce platforms, social media platforms facilitate the sharing of personal information, like preferences and opinions, rather than financial transactions. On social media, users express their identities by customizing profiles, engaging with content, and sharing their posts (Krasnova et al., 2017 ; Lin & Lu, 2011 ). The importance of trust in social media was underscored when Twitter sold verification checkmarks without vetting accounts. As users trusted the check would verify an individual’s or company’s authenticity, many took announcements from fake accounts as serious news. This led to global stock market turmoil, with some companies losing billions in market value due to misinformation (Mac et al., 2022 ). Experts concluded that Twitter, as an information mediator, “undermine[s] the original purpose […] – to help users know they can trust information being shared” (Duffy, 2022 ). This instance highlights the crucial need for trust within social media ecosystems (Sledgianowski & Kulviwat, 2008 ).
The metaverse stands out as a platform that integrates functionalities from e-commerce, social media, collaboration, and education into a single environment (Tingelhoff et al., 2024 ). Consequently, it is designed to support both financial transactions and the exchange of private information. Therefore, trust may play an even more critical role for ecosystem actors in the metaverse than in other digital environments (Badruddoja et al., 2022 ; Wang et al., 2022 ). Existing research consistently demonstrates how user experience influences trust in digital ecosystems (Seckler et al., 2015 ) and automated systems (Yang et al., 2017 ).
At its heart, the metaverse is focused on delivering a distinctive user experience (Tingelhoff et al., 2024 ). Immersion and automation technologies make user experience even more crucial in the metaverse compared to other digital platforms. Our interview partners mirrored this. For instance, IP4 emphasized the importance of removing obstacles to enhance the accessibility and enjoyment of the virtual world. Moreover, IP9 identified user experience as essential for the success of both the platform and its complementors. IP8 summarized: “The deciding factor is clearly customer experience, meaning that a user can develop a positive feeling on the metaverse platform and then leave it with a smile on their face.” This can be further supported by anecdotal evidence from our research team. When the previously described error message occurred while testing Decentraland (see Fig. 6 ), our first author exclaimed: “If the platform isn't stable enough to create an avatar, how can I trust it with my financial transactions?”.
5.3 Illustration of System Impact
Given the vast range of integration options Roblox and Decentraland provide for complementors, their business models and resulting net benefits can significantly vary. Even within a single platform, complementors often pursue varied objectives through their participation. For instance, Adidas launched an NFT collection to potentially attract new customers and boost sales (Bain, 2023 ),, whereas Nike concentrated on brand building . On Roblox, Nike created Nikeland, a virtual world where users engage in games themed around Nike shoes and interact with one another. Players navigate the map using features like sprinting or hoverboards, underscoring sportiness and innovation—qualities Nike aims to embody (Marr, 2022 ).
Nike additionally focuses on community building . Specifically, it nurtures a sense of community by blending collaborative and competitive dynamics. Competitive features are key in metaverses, engaging users, encouraging social interaction, and presenting challenges to tackle (Martins et al., 2022 ; Quintín et al., 2016 ). Nikeland features a leaderboard, allowing users to continuously compare achievements with peers. Users can also team up for challenges, promoting user collaboration (Martins et al., 2022 ). These game elements are proven to influence brand image within the metaverse (Oliver et al., 2010 ).
Beyond subconsciously building brand associations (Kim et al., 2022 ; Wagner et al., 2009 ), Nike actively educates customers on its brand values during gameplay. According to our interviewees (e.g., IP 12) and existing research (e.g., Tingelhoff et al., 2024 ), metaverse platforms deeply engage and educate customers through immersive content (Lee et al., 2022 ). Specifically, in Nikeland, the content is centered on Nike-related content. For instance, players interact with a guide named “Nike Coach.” Wearing a Nike shirt, the coach is presented as an authority on health and sports. The coach assigns quests and offers advice on increasing physical activity. Through this, Nike seeks to solidify its reputation as a sports authority. Additionally, the coach explicitly conveys Nike's values and goals, educating customers. Experts (Hazan et al., 2022 ) and researchers (Dwivedi et al., 2022 ) have underscored the metaverse's unique ability to blend gaming with e-commerce in that manner. Figure 7 depicts a screenshot of Nikeland with the previously discussed elements highlighted.
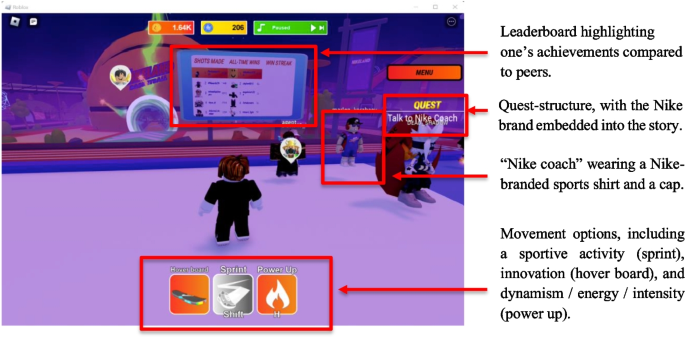
Playing games in Nikeland, Nike’s virtual world on the Roblox platform
We caution against assuming that the net benefits for complementors can be definitively assessed from an external perspective. Nevertheless, Decentraland and Roblox demonstrate consideration for several identified characteristics conducive to complementors' value creation. Both platforms also exhibit limitations within certain dimensions, which, from our observation, detract from their overall performance. This may stem from the platforms' evolving nature, underscoring the imperative for ongoing enhancement. Moreover, the presence of the variables from our analysis in both platforms is irrespective of their governance structure . This reinforces the view that the organizational structure of a metaverse platform is not the primary distinguishing factor for complementors. Our interview findings, illustrated in Fig. 2 , support this observation.
6 Contributions and Implications
Our study offers several contributions to researchers and practitioners. Theoretically, our study enriches understanding of ecosystem dynamics in metaverse platforms. Specifically, we explored the role and relationships of complementors within metaverse platforms. We applied the D&M IS success model, a well-established framework, to examine the platform-business model fit across different contexts. From its six dimensions, we pinpointed 26 characteristics specific to metaverse platforms. These characteristics significantly impact complementors' value creation in metaverse environments. They progress the conceptualization of the of metaverse’s capabilities and the tensions with which complementors must deal within the metaverse ecosystem. Consequently, our study encourages researchers to explore the metaverse's nature, meaning, and ecosystem players further.
From a practitioner’s perspective, our study guides both orchestrators and complementors. For metaverse decision-makers, our findings highlight essential platform characteristics vital for effective value creation. This understanding can lead to complementors aligning with platforms that resonate with their business models and, conversely, enable orchestrators to refine their platforms to support organizational value creation better. The implications are dual: improving the alignment between business models and platforms, and fostering platform evolution to better facilitate value creation. Ultimately, these insights could promote stronger business cases in metaverse platforms, accelerating adoption among businesses and consumers.
7 Limitations and Future Research
Our study’s limitations provide grounds for future research. First, a consensus on defining the metaverse remains elusive. While conceptual papers discuss the metaverse's nature (e.g., Hadi et al., 2023 ), others like Peukert et al. ( 2022 ) highlight its ongoing evolution, complicating its definition. While this study aims to contribute to the technological design choices during its development, we also want to highlight the need to replicate our findings in the future to determine their validity over time. Second, our sample spanned experts from the B2B and B2C contexts and several industries, resulting in a reasonably general framework. Future research could replicate this study with industry-specific experts to explore how metaverse applications can be customized to meet distinct industry needs. Third, most of our interviewees were from Europe, with only one from Hong Kong and another from the USA. Therefore, cultural differences might yield varied findings. Given the concentration of metaverse developments in North America and Asia, conducting studies in these regions could uncover additional insights. Finally, our interviewees worked at companies already involved in metaverses or actively considering them, which could have biased our results. Future research should engage experts holding critical views on the metaverse to contrast their perspectives with our findings.
8 Conclusion
This study aimed to highlight key characteristics of metaverse platforms crucial for complementors seeking to maximize their value creation. Our research draws on platform ecosystem and value creation theories to provide a foundational understanding of how complementors generate value on metaverse platforms. We interviewed 15 metaverse decision-makers across various organizations, identifying 26 characteristics of metaverse platforms that impact complementors' ability to create value. We structured these characteristics according to the six dimensions of the DeLone and McLean IS success model and exemplified them through Decentraland and Roblox.
The journey to fully comprehend metaverse platforms is ongoing. This study clarifies the design characteristics of metaverse platforms and introduces a framework to aid complementors in choosing suitable platforms. Additionally, it empowers researchers and practitioners to design new metaverse platforms purposefully. Ultimately, our research seeks to lay the groundwork for future inquiries in this domain.
Data Availability
All data supporting the findings of this study are available within the paper and its Appendix. Any further data are available from the corresponding author upon reasonable request.
All quotes were translated to English for this paper.
Adner, R. (2017). Ecosystem as structure: An actionable construct for strategy. Journal of Management, 43 (1), 39–58.
Article Google Scholar
Ahlan, A. R. (2014). Implementation of input-process-output model for measuring information system project success. TELKOMNIKA Indonesian Journal of Electrical Engineering, 12 (7), 5603–5612.
Google Scholar
Al-Kofahi, M. K., Hassan, H., Mohamad, R., Intan, T., & Com, M. (2020). Information systems success model: A review of literature. International Journal of Innovation, Creativity and Change, 12 (8)
Ataman, K., Myhr, N., & Nistor, C. (2023). Disruptive Innovation as a Network Dilemma: A Conceptual Model. Journal of Behavioral and Applied Management, 23 (2), 104–113.
Ayiter, E. (2012). Further dimensions: Text, typography and play in the Metaverse. International Conference on Cyberworlds
Badruddoja, S., Dantu, R., He, Y., Thompson, M., Salau, A., & Upadhyay, K. (2022). Trusted AI with Blockchain to Empower Metaverse. Fourth International Conference on Blockchain Computing and Applications (BCCA) .
Bain, M. (2023). How Web3 Is Shaking Up Digital Marketing. Business of Fashion. https://www.businessoffashion.com/articles/technology/the-state-of-fashion-2023-report-web3-digital-marketing-nfts/
Balica, R. -Ş, Majerová, J., & Cuţitoi, A.-C. (2022). Metaverse applications, technologies, and infrastructure: Predictive algorithms, real-time customer data analytics, and virtual navigation tools. Linguistic and Philosophical Investigations, 21 , 219–235.
Bayraktar, E., Tatoglu, E., Aydiner, A. S., & Delen, D. (2023). Business analytics adoption and technological intensity: An efficiency analysis. Information Systems Frontiers, 1–18
BMW. (2023). The iX1 Metaverse Experience. Retrieved February 2023 from https://www.bmw.com/en/events/dreams.html
Bonina, C., & Eaton, B. (2020). Cultivating open government data platform ecosystems through governance: Lessons from Buenos Aires, Mexico City and Montevideo. Government Information Quarterly, 37 (3), 101479.
Boughzala, I., de Vreede, G.-J., & Limayem, M. (2012). Team collaboration in virtual worlds: Editorial to the special issue. Journal of the Association for Information Systems, 13 (10), 715–734.
Bourlakis, M., Papagiannidis, S., & Li, F. (2009). Retail spatial evolution: Paving the way from traditional to metaverse retailing. Electronic Commerce Research, 9 (1), 135–148.
Bowman, C., & Ambrosini, V. (2000). Value creation versus value capture: Towards a coherent definition of value in strategy. British Journal of Management, 11 (1), 1–15.
Braud, A., Fromentoux, G., Radier, B., & Le Grand, O. (2021). The Road to European Digital Sovereignty with Gaia-X and IDSA. IEEE Network, 35 (2), 4–5.
Brimco. (2024). Business Statistics You Should Know in 2024. Retrieved March 2024 from https://www.brimco.io/business-statistics-to-know/#:~:text=There%20are%20an%20estimated%20334%20million%20companies%20worldwide&text=This%20is%20a%20marginal%20growth%20from%202021%20when%20there%20were%20314.21%20million .
Brooke, C. (2022). Best Metaverse Platforms to Play in 2022 . Business2Community. https://www.business2community.com/cryptocurrency/best-metaverse-platforms
Choi, H.-S., & Kim, S.-H. (2017). A content service deployment plan for metaverse museum exhibitions—Centering on the combination of beacons and HMDs. International Journal of Information Management, 37 (1), 1519–1527.
Davis, A., Murphy, J. D., Owens, D., Khazanchi, D., & Zigurs, I. (2009). Avatars, people, and virtual worlds: Foundations for research in metaverses. Journal of the Association for Information Systems, 10 (2), 90–117.
Decentraland. (2022). How Many DAU Does Decentraland Have? https://decentraland.org/blog/announcements/how-many-dau-does-decentraland-have/
DeLone, W. H., & McLean, E. R. (2002). Information systems success revisited. Proceedings of the 35th Annual Hawaii International Conference on System Sciences
DeLone, W. H., & McLean, E. R. (1992). Information systems success: The quest for the dependent variable. Information Systems Research, 3 (1), 60–95.
DeLone, W. H., & McLean, E. R. (2003). The DeLone and McLean model of information systems success: A ten-year update. Journal of Management Information Systems, 19 (4), 9–30.
Dionisio, J. D. N., III. (2013). 3D virtual worlds and the metaverse: Current status and future possibilities. ACM Computing Surveys (CSUR), 45 (3), 1–38.
Di Pietro, R., & Cresci, S. (2021). Metaverse: Security and Privacy Issues. IEEE International Conference on Trust, Privacy and Security in Intelligent Systems and Applications (TPS-ISA), 3 , 281–288
Draper, J. (2004). The relationship between research question and research design. In Research into Practice: Essential Skills for Reading and Applying Research in Nursing and Health Care (Vol. 2, pp. 69–84). Bailliere Tindall
Duan, H., Li, J., Fan, S., Lin, Z., Wu, X., & Cai, W. (2021, October). Metaverse for social good: A university campus prototype. In: Proceedings of the 29th ACM international conference on multimedia, pp 153–161. https://doi.org/10.1145/3474085.3479238
Duffy, C. (2022). Twitter relaunches option to pay for blue check marks. CNN Business . https://edition.cnn.com/2022/12/12/tech/twitter-verification-relaunch/index.html
Dwivedi, Y. K., Hughes, L., Wang, Y., Alalwan, A. A., Ahn, S. J., Balakrishnan, J., Barta, S., Belk, R., Buhalis, D., & Dutot, V. (2022). Metaverse marketing: How the metaverse will shape the future of consumer research and practice. Psychology & Marketing, 40 (4), 750–76.
Falchuk, B., Loeb, S., & Neff, R. (2018). The social metaverse: Battle for privacy. IEEE Technology and Society Magazine, 37 (2), 52–61.
Friedrich, T., Schlauderer, S., & Overhage, S. (2019). The impact of social commerce feature richness on website stickiness through cognitive and affective factors: An experimental study. Electronic Commerce Research and Applications, 36 , 100861.
Gadalla, E., Keeling, K., & Abosag, I. (2013). Metaverse-retail service quality: A future framework for retail service quality in the 3D internet. Journal of Marketing Management, 29 (13–14), 1493–1517.
Gartner. (2022). Estimated metaverse use case among consumers and businesses worldwide in 2026. Statista. Retrieved January 2022 from https://www.statista.com/statistics/1290160/projected-metaverse-use-reach-global-consumers-businesses/
Gawer, A., & Cusumano, M. A. (2002). Platform leadership: How Intel, Microsoft, and Cisco drive industry innovation (Vol. 5). Harvard Business School Press Boston, MA
Gawer, A., & Cusumano, M. A. (2014). Industry platforms and ecosystem innovation. Journal of Product Innovation Management, 31 (3), 417–433.
Getchell, K., Oliver, I., Miller, A., & Allison, C. (2010). Metaverses as a platform for game based learning. IEEE International Conference on Advanced Information Networking and Applications, 24 , 1195–1202.
Gioia, D. A., Corley, K. G., & Hamilton, A. L. (2013). Seeking qualitative rigor in inductive research: Notes on the Gioia methodology. Organizational Research Methods, 16 (1), 15–31.
Goldberg, M., & Schär, F. (2023). Metaverse governance: An empirical analysis of voting within Decentralized Autonomous Organizations. Journal of Business Research, 160 , 113764.
Golf-Papez, M., Heller, J., Hilken, T., Chylinski, M., de Ruyter, K., Keeling, D. I., & Mahr, D. (2022). Embracing falsity through the metaverse: The case of synthetic customer experiences. Business Horizons, 65 (6), 739–749.
Gollmer, P. (2022). Web3: Die Internet-Revolution, die aus der Abhängigkeit von Big Tech führen soll. Neue Züricher Zeitung. https://www.nzz.ch/technologie/ist-web3-die-zukunft-des-internets-oder-nur-ein-weiterer-hype-ld.1664275?reduced=true
Guest, G., Bunce, A., & Johnson, L. (2006). How many interviews are enough? An experiment with data saturation and variability. Field Methods, 18 (1), 59–82.
Guidi, B., & Michienzi, A. (2022). SocialFi: towards the new shape of social media. ACM SIGWEB Newsletter (Summer), 1–8
Guo, J., Liu, Z., & Liu, Y. (2016). Key success factors for the launch of government social media platform: Identifying the formation mechanism of continuance intention. Computers in Human Behavior, 55 , 750–763.
Hadi, R., Melumad, S., & Park, E. S. (2023). The Metaverse: A New Digital Frontier for Consumer Behavior. Journal of Consumer Psychology, AHEAD-OF-PRINT.
Harzing, A.-W., & Maznevski, M. (2002). The interaction between language and culture: A test of the cultural accommodation hypothesis in seven countries. Language and Intercultural Communication, 2 (2), 120–139.
Hazan, E., Kelly, G., Khan, H., Spillecke, D., & Yee, L. (2022). Marketing in the metaverse: An opportunity for innovation and experimentation.
Hwang, R., & Lee, M. (2022). The Influence of Music Content Marketing on User Satisfaction and Intention to Use in the Metaverse: A Focus on the SPICE Model. Businesses, 2 (2), 141–155.
Jaynes, C., Seales, W. B., Calvert, K., Fei, Z., & Griffioen, J. (2003). The Metaverse: a networked collection of inexpensive, self-configuring, immersive environments. Proceedings of the Workshop on Virtual Environments.
Jeong, S.-H., & Kim, H.-K. (2023). Effect of Trust in Metaverse on Usage Intention through Technology Readiness and Technology Acceptance Model. Tehnički Vjesnik, 30 (3), 837–845.
Jo, H., & Park, S. (2022). Roles of Information Security and Benefits in Forming User Satisfaction of Metaverse. International Conference on Computational Science and Computational Intelligence (CSCI)
Kamin, D. (2021). Investors Snap Up Metaverse Real Estate in a Virtual Land Boom. New York Times. https://www.nytimes.com/2021/11/30/business/metaverse-real-estate.html
Khansulivong, C., Wicha, S., & Temdee, P. (2022). Adaptive of New Technology for Agriculture Online Learning by Metaverse: A Case Study in Faculty of Agriculture, National University of Laos. Joint International Conference on Digital Arts, Media and Technology with ECTI Northern Section Conference on Electrical, Electronics, Computer and Telecommunications Engineering (ECTI DAMT & NCON) , 428–432.
Kim, J. (2021). Advertising in the Metaverse: Research Agenda. Journal of Interactive Advertising, 21 (3), 141–144.
Kim, H. J., Kim, I., & Lee, H. (2016). Third-party mobile app developers’ continued participation in platform-centric ecosystems: An empirical investigation of two different mechanisms. International Journal of Information Management, 36 (1), 44–59.
Kim, D. J., Salvacion, M., Salehan, M., & Kim, D. W. (2022). An empirical study of community cohesiveness, community attachment, and their roles in virtual community participation. European Journal of Information Systems, 1–28.
Krasnova, H., Veltri, N. F., Eling, N., & Buxmann, P. (2017). Why men and women continue to use social networking sites: The role of gender differences. The Journal of Strategic Information Systems, 26 (4), 261–284.
Lang, N., von Szczepanski, K., & Wurzer, C. (2019). The emerging art of ecosystem management. Boston Consulting Group, 1–20
Lee, H.-K., Park, S., & Lee, Y. (2022). A proposal of virtual museum metaverse content for the MZ generation. Digital Creativity, 33 (2), 1–17.
Lin, K.-Y., & Lu, H.-P. (2011). Why people use social networking sites: An empirical study integrating network externalities and motivation theory. Computers in Human Behavior, 27 (3), 1152–1161.
Lincoln, Y. S., & Guba, E. G. (1985). Naturalistic inquiry . Sage.
Book Google Scholar
Lo Iacono, V., Symonds, P., & Brown, D. H. (2016). Skype as a tool for qualitative research interviews. Sociological Research Online, 21 (2), 103–117.
Mac, R., Mullin, B., Conger, K., & Isaac, M. (2022). A Verifiable Mess: Twitter Users Create Havoc by Impersonating Brands. New York Times. https://www.nytimes.com/2022/11/11/technology/twitter-blue-fake-accounts.html
Marabelli, M., & Newell, S. (2022). Everything you Always Wanted to Know About the Metaverse* (*But Were Afraid to Ask). Academy of Management Annual Meeting, 1–40.
Marr, B. (2022). The Amazing Ways Nike Is Using The Metaverse, Web3 And NFTs. Forbes. https://www.forbes.com/sites/bernardmarr/2022/06/01/the-amazing-ways-nike-is-using-the-metaverse-web3-and-nfts/?sh=62e049e256e9
Martins, D., Oliveira, L., & Amaro, A. C. (2022). Life in Second Life through the eyes of the residents. Iberian Conference on Information Systems and Technologies (CISTI), 17 , 1–6.
McKinsey. (2022). Value creation in the metaverse: The real business of the virtual world. McKinsey Research. https://www.mckinsey.com/~/media/mckinsey/business%20functions/marketing%20and%20sales/our%20insights/value%20creation%20in%20the%20metaverse/Value-creation-in-the-metaverse.pdf
Merriam, S. B., & Grenier, R. S. (2019). Qualitative research in practice: Examples for Discussion and Analysis. John Wiley & Sons
Metcalf, M. (2022). How Creativity & Deep Audience Knowledge Made Roblox A Sensation. Latana. https://latana.com/post/roblox-deep-dive/
Mladenović, D., Ismagilova, E., Filieri, R., & Dwivedi, Y. K. (2023). MetaWOM–toward a sensory word-of-mouth (WOM) in the metaverse. International Journal of Contemporary Hospitality Management
Molla, A., & Licker, P. S. (2001). E-commerce systems success: An attempt to extend and respecify the Delone and MacLean model of IS success. Journal of Electronic Commerce Research, 2 (4), 131–141.
Moy, C., & Gadgil, A. (2022). Opportunities in the metaverse: How businesses can explore the metaverse and navigate the hype vs. reality . Onyx by J.P. Morgan. Retrieved March 2022 from https://www.jpmorgan.com/content/dam/jpm/treasury-services/documents/opportunities-in-the-metaverse.pdf
Newzoo. (2022). The Metaverse, Blockchain Gaming, and NFTs: Navigating the Internet’s Uncharted Waters . Retrieved March 2024 from https://resources.newzoo.com/hubfs/Reports/Metaverse/2022_Newzoo_Metaverse_Blockchain_NFT_Report.pdf?utm_campaign=Metaverse%20Report%202022&utm_medium=email&_hsmi=217738092&_hsenc=p2ANqtz--lsLjwfkn5vmoHZ3vlIlMt5jH5co1McK_yqbxz5Sjt76ruFm6Hc1lnkiXT1Iu_HKHixVUYNGAFyG0THr5G4wRIMUc0SZ4GcdoW--edcMxde4v1eq8&utm_content=217738092&utm_source=hs_automation
Nike. (2023). Nikeland on Roblox. https://www.nike.com/kids/nikeland-roblox
Ning, H., Wang, H., Lin, Y., Wang, W., Dhelim, S., Farha, F., Ding, J., & Daneshmand, M. (2021). A Survey on Metaverse: the State-of-the-art, Technologies, Applications, and Challenges. arXiv preprint , arXiv:2111.09673 , 1–34.
Oliver, I. A., Miller, A. H., & Allison, C. (2010). Virtual worlds, real traffic: interaction and adaptation. Proceedings of the Annual ACM SIGMM Conference on Multimedia Systems
Oliveira, S., Barros, M. I., Lima, G. D., Farias, L. B. (2019). Investigations into Data Ecosystems: A systematic mapping study. Knowledge and Information Systems, 61 (2), 589–630.
Orgaz, G. B., R-moreno, M. D., Camacho, D., & Barrero, D. F. (2012). Clustering avatars behaviours from virtual worlds interactions. Proceedings of the 4th International Workshop on Web Intelligence & Communities
Owens, D., Mitchell, A., Khazanchi, D., & Zigurs, I. (2011). An empirical investigation of virtual world projects and metaverse technology capabilities. ACM SIGMIS Database: The DATABASE for Advances in Information Systems, 42 (1), 74–101.
Papagiannidis, S., Bourlakis, M., & Li, F. (2008). Making real money in virtual worlds: MMORPGs and emerging business opportunities, challenges and ethical implications in metaverses. Technological Forecasting and Social Change, 75 (5), 610–622.
Park, S.-M., & Kim, Y.-G. (2022). A Metaverse: Taxonomy, components, applications, and open challenges. IEEE Access, 10 , 4209–4251.
Paterson, M., & Higgs, J. (2005). Using hermeneutics as a qualitative research approach in professional practice. Qualitative Report, 10 (2), 339–357.
Periyasami, S., & Periyasamy, A. P. (2022). Metaverse as future promising platform business model: Case study on fashion value chain. Businesses, 2 (4), 527–545.
Petter, S., DeLone, W., & McLean, E. (2008). Measuring information systems success: Models, dimensions, measures, and interrelationships. European Journal of Information Systems, 17 , 236–263.
Peukert, C., Weinhardt, C., Hinz, O., & van der Aalst, W. M. (2022). Metaverse: How to Approach Its Challenges from a BISE Perspective. Business & Information Systems Engineering, 64 , 401–406.
Polyviou, A., & Pappas, I. O. (2022). Chasing metaverses: Reflecting on existing literature to understand the business value of metaverses. Information Systems Frontiers, 25 , 2417–28.
Popescu, G. H., Valaskova, K., & Horak, J. (2022). Augmented Reality Shopping Experiences, Retail Business Analytics, and Machine Vision Algorithms in the Virtual Economy of the Metaverse. Journal of Self-Governance & Management Economics, 10 (2), 67–81.
Quintín, E., Sanz, C., & Zangara, A. (2016). The impact of role-playing games through Second Life on the oral practice of linguistic and discursive sub-competences in English. International Conference on Collaboration Technologies and Systems (CTS), 148–155
Sanders, L., & Simons, G. (2009). A social vision for value co-creation in design. Open Source Business Resource(December 2009).
Schöbel, S. M., & Leimeister, J. M. (2023). Metaverse platform ecosystems. Electronic Markets, 33 (1), 12.
Schöbel, S. M., & Tingelhoff, F. (2023). Overcoming Challenges to Enable the Potential of Metaverse Platforms — A Qualitative Approach to Understand Value Creation. AIS Transactions of Human-Computer Interaction, 15 (1), 1–23.
Schöbel, S. M., Karatas, J., Tingelhoff, F., & Leimeister, J. M. (2023). Not everything is a Metaverse?! A Practitioners Perspective on Characterizing Metaverse Platforms. Hawaii International Conference on System Science, 56 , 544–553.
Seckler, M., Heinz, S., Forde, S., Tuch, A. N., & Opwis, K. (2015). Trust and distrust on the web: User experiences and website characteristics. Computers in Human Behavior, 45 , 39–50.
Shin, D. (2022). The actualization of meta affordances: Conceptualizing affordance actualization in the metaverse games. Computers in Human Behavior, 133 , 1–13.
Sledgianowski, D., & Kulviwat, S. (2008). Social network sites: Antecedents of user adoption and usage
Smith, S. (2022). Givenchy Parfums enters Metaverse with Roblox. TheIndustry.Beauty. https://theindustry.beauty/givenchy-parfums-enters-metaverse-with-roblox/
Stephen Gates. (n.d.). Aloft Hotels in Second Life . Retrieved December 2022 from https://stephengates.com/portfolio/aloft-hotels-second-life/
Sykownik, P., Maloney, D., Freeman, G., & Masuch, M. (2022). Something Personal from the Metaverse: Goals, Topics, and Contextual Factors of Self-Disclosure in Commercial Social VR. CHI Conference on Human Factors in Computing Systems , 1–17.
Tapscott, D., & Tapscott, A. (2017). How blockchain will change organizations. MIT Sloan Management Review, 58 (2), 10–13.
Tawaststjerna, T., & Olander, H. (2021). Managing digital transformation in digital business ecosystems. International Journal of Innovation Management, 25 (10), 2140003.
Tayal, S., Rajagopal, K., & Mahajan, V. (2022). Virtual Reality based Metaverse of Gamification. International Conference on Computing Methodologies and Communication (ICCMC) , 1597–1604
Tingelhoff, F., Klug, S., & Elshan, E. (2024). The Diffusion of the Metaverse: How YouTube Influencers Shape Metaverse Mass Adoption and What This Means for Organizations. California Management Review , forthcoming.
Torkzadeh, G., & Dhillon, G. (2002). Measuring factors that influence the success of Internet commerce. Information Systems Research, 13 (2), 187–204.
Valenzuela, D., & Shrivastava, P. (2002). Interview as a method for qualitative research (Presentation). Southern Cross University and the Southern Cross Institute of Action Research (SCIAR) . https://d1wqtxts1xzle7.cloudfront.net/31820583/Interview_as_a_Method_for_QR-libre.pdf?1390950384=&response-content-disposition=inline%3B+filename%3DInterview_as_a_Method_for_QR.pdf&Expires=1698950691&Signature=HRcpfWOlmyQlAQWJesqeOUaT9WdxxfRUOJ-o~ADETWEgE-Gd7QQ2ILhPYEyXo8DGGTEBwfy4g4Fa4nyQoxiOTSybbmNAZ6Dvyfd19KsekeygVuJtJCJgQA2jtMD21L1d4m3Fqts8Vvee5uTwFQ5sUlMzQsEnhOzBW-0Kr7N-zFDO9nl4MA3treOlaaTiXScP84p~EN~lnnhFPUsPM1sNPkWAvFT17Nj8m0PGQE8Yx07XsstcnWDAHLbSdJIBChNoDpNsHoEHLgVEW4mEE-wn2YouqJMyf~gdzGreBlza-XFcD3AGgJzJwDTxPTF3p78MQGqXunb7UeBDP4fKvIhCNw__&Key-Pair-Id=APKAJLOHF5GGSLRBV4ZA
Vidal-Tomás, D. (2022). The new crypto niche: NFTs, play-to-earn, and metaverse tokens. Finance Research Letters, 47 , 1–13.
Wagner, C., Liu, L., Schneider, C., Prasarnphanich, P., & Chen, H. (2009). Creating a successful professional virtual community: A sustainable digital ecosystem for idea sharing. 3rd IEEE International Conference on Digital Ecosystems and Technologies.
Wang, Y. S. (2008). Assessing e-commerce systems success: A respecification and validation of the DeLone and McLean model of IS success. Information Systems Journal, 18 (5), 529–557.
Wang, M., & Ho, C. (2022). Metaverse Platforms — Roblox Overtakes Decentraland (MANA) and Sandbox (SAND). Bybit . https://blog.bybit.com/en-US/post/metaverse-platforms-roblox-overtakes-decentraland-mana-and-sandbox-sand--bltc3d581e1ae0fa13e/
Wang, D., Yan, X., & Zhou, Y. (2021). Research on Metaverse: Concept, development and standard system. International Conference on Electronics, Communications and Information Technology (CECIT) , 2 , 983–991
Wang, Y., Su, Z., Zhang, N., Xing, R., Liu, D., Luan, T. H., & Shen, X. (2022). A survey on metaverse: Fundamentals, security, and privacy. IEEE Communications Surveys & Tutorials 25
Weber, M., Engert, M., Schaffer, N., Weking, J., & Krcmar, H. (2023). Organizational Capabilities for AI Implementation—Coping with Inscrutability and Data Dependency in AI. Information Systems Frontiers, 25 (4), 1549–1569.
Xi, N., Chen, J., Gama, F., Riar, M., & Hamari, J. (2023). The challenges of entering the metaverse: An experiment on the effect of extended reality on workload. Information Systems Frontiers, 25 (2), 659–680.
Yang, D., Zhou, J., Chen, R., Song, Y., Song, Z., Zhang, X., Wang, Q., Wang, K., Zhou, C., & Sun, J. (2022a). Expert consensus on the metaverse in medicine. Clinical eHealth, 5 , 1–9.
Yang, Q., Zhao, Y., Huang, H., Xiong, Z., Kang, J., & Zheng, Z. (2022b). Fusing blockchain and AI with metaverse: A survey. IEEE Open Journal of the Computer Society, 3 , 122–136.
Yang, X. J., Unhelkar, V. V., Li, K., & Shah, J. A. (2017). Evaluating effects of user experience and system transparency on trust in automation. Proceedings of the 2017 ACM/IEEE international conference on human-robot interaction.
Yoo, K., Welden, R., Hewett, K., & Haenlein, M. (2023). The merchants of meta: A research agenda to understand the future of retailing in the metaverse. Journal of Retailing, 99 (2), 173–192.
Zeithaml, V. A. (1988). Consumer perceptions of price, quality, and value: A means-end model and synthesis of evidence. Journal of Marketing, 52 (3), 2–22.
Download references
Acknowledgements
For this research, Fabian Tingelhoff received funding from the Konrad-Adenauer-Foundation (KAS).
Open access funding provided by University of St.Gallen
Author information
Authors and affiliations.
Institute of Information Systems and Digital Business, University of St.Gallen, St. Gallen, Switzerland
Fabian Tingelhoff, Raphael Schultheiss & Jan Marco Leimeister
Information Systems, University of Osnabrück, Osnabrück, Germany
Sofia Marlena Schöbel
You can also search for this author in PubMed Google Scholar
Corresponding author
Correspondence to Fabian Tingelhoff .
Ethics declarations
Conflict of interest.
The authors declare that they had no conflict of interest when constructing this manuscript.
Additional information
Publisher's note.
Springer Nature remains neutral with regard to jurisdictional claims in published maps and institutional affiliations.
See Table 1
Rights and permissions
Open Access This article is licensed under a Creative Commons Attribution 4.0 International License, which permits use, sharing, adaptation, distribution and reproduction in any medium or format, as long as you give appropriate credit to the original author(s) and the source, provide a link to the Creative Commons licence, and indicate if changes were made. The images or other third party material in this article are included in the article's Creative Commons licence, unless indicated otherwise in a credit line to the material. If material is not included in the article's Creative Commons licence and your intended use is not permitted by statutory regulation or exceeds the permitted use, you will need to obtain permission directly from the copyright holder. To view a copy of this licence, visit http://creativecommons.org/licenses/by/4.0/ .
Reprints and permissions
About this article
Tingelhoff, F., Schultheiss, R., Schöbel, S.M. et al. Qualitative Insights into Organizational Value Creation: Decoding Characteristics of Metaverse Platforms. Inf Syst Front (2024). https://doi.org/10.1007/s10796-024-10494-x
Download citation
Accepted : 02 May 2024
Published : 15 May 2024
DOI : https://doi.org/10.1007/s10796-024-10494-x
Share this article
Anyone you share the following link with will be able to read this content:
Sorry, a shareable link is not currently available for this article.
Provided by the Springer Nature SharedIt content-sharing initiative
- Metaverse Platform Ecosystem
- Organizational Value Creation
- Platform Characteristics
- Complementors
- Find a journal
- Publish with us
- Track your research
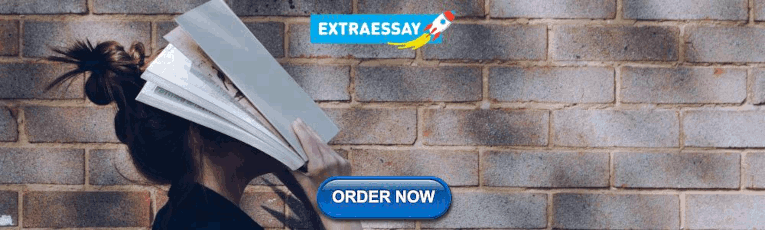
IMAGES
VIDEO
COMMENTS
Holistic codes are helpful because they capture the essence of the data unit in a single code. Here are some examples of how to use holistic coding in qualitative research: When studying the experience of grief, you could use "Loss" as a holistic coding to identify paragraphs where participants discuss the experience of losing a loved one.
coding, Focused coding, Axial coding, Theoretical coding, Elaborative coding, and Longitudinal coding. Finally, Theming the data, which includes eclectic coding. lies in between the first and second cycles. Table 2 presents a summary of how each of Saldaňa's (2012) 32 coding methods can be applied to analyzing and interpreting information that
Chapter Summary. This chapter first presents the purposes and goals of The Coding Manual for Qualitative Researchers. It then provides definitions and examples of codes and categories and their roles in qualitative data analysis. The procedures and mechanics of coding follow, along with discussions of analytic software and team collaboration.
Holistic Coding Provisional Coding. Hypothesis Coding Protocol Coding. Causation Coding ... In qualitative data analysis, a code is a researcher-generated construct that symbolizes ... Some research methodologists believe that coding is merely technical, preparatory work for higher level thinking about the study. But we believe that coding is ...
Provisional Coding is well-suited for studies that build on or attempt to corroborate previous research o Hypothesis Coding: is a deductive approach (as opposed to the inductive approach of grounded theory methods) based on the application of a researcher-generated, predetermined list of codes onto qualitative data specifically to assess or ...
Step 1 - Initial coding. The first step of the coding process is to identify the essence of the text and code it accordingly. While there are various qualitative analysis software packages available, you can just as easily code textual data using Microsoft Word's "comments" feature.
Welcome to the companion website for The Coding Manual for Qualitative Research, third edition, by Johnny Saldaña. This website offers a wealth of additional resources to support students and lecturers including: CAQDAS links giving guidance and links to a variety of qualitative data analysis software.. Code lists including data extracted from the author's study, "Lifelong Learning Impact ...
This chapter provides an overview of selected qualitative data analytic strategies with a particular focus on codes and coding. Preparatory strategies for a qualitative research study and data management are first outlined. Six coding methods are then profiled using comparable interview data: process coding, in vivo coding, descriptive coding ...
Arguably, a multimodal representation of data produces a more holistic analysis than textual analysis alone (Markle et al., 2011; Silver & Patashnick, 2011), enhancing rigor and lending credibility to the conclusions drawn from qualitative research . The key consideration, which this article addresses, is exploring the added value of a more ...
5. Exploratory Methods are preliminary assignment of codes to the data, after which the researcher might proceed to more specific First Cycle or Second Cycle coding methods. Holistic Coding applies a single code to each large unit of data in the corpus to capture a sense of the overall contents and the possible categories that may develop.
Transparency is once again a central issue of debate across types of qualitative research. Ethnographers focus on whether to name people, places, or to share data (Contreras 2019; Guenther 2009; Jerolmack and Murphy 2017; Reyes 2018b) and whether our data actually match the claims we make (e.g., Jerolmack and Khan 2014).Work on how to conduct qualitative data analysis, on the other hand, walks ...
Keywords: psychotherapy, qualitative, intervention, holistic, ... From this initial inductive coding scheme, a list of preliminary themes was generated based on the summaries and collective interpretation of the coded material. ... with a substantial body of qualitative research focusing on the perspective of clients (Timulak, 2010; Timulak and ...
that can help pave the way to the researcher's interpretive judgements and improve the ir quality. By using this paper, novice researchers will be able to reflect more carefully on the ...
Create new codes based on the second sample. Go back and recode all responses again. Repeat from step 5 until you've coded all of your data. If you add a new code, split an existing code into two, or change the description of a code, make sure to review how this change will affect the coding of all responses.
The Qualitative Report Volume 27 Number 11 Article 8 11-9-2022 Contextual Coding in Qualitative Research Involving Participants with Diverse Sociocultural Backgrounds Ahtisham Younas Memorial University of Newfoundland, Canada, [email protected] Angela Cuoco Tor Vergata University of Rome, Italy, [email protected] Ercole Vellone
Holistic Thinking and Qualitative E-Research Design. Identifying & Planning Research Research Design Research Ethics. Jan 31, 2023. by Janet Salmons, PhD Manager for Sage Research Methods Community. Dr. Salmons is the author of a series of books, including a new edition of Doing Qualitative Research Online. She will be a research fellow at the ...
Specifically, qualitative research differs from research models that focus on the creation of knowledge in a contained and event-oriented manner. As we will show, the holistic nature of the research process, the interplay between different phases of it, from topic selection to write-up, makes qualitative research a unique and important ...
The greatest strength of the qualitative research approach lies in the richness and depth of the healthcare exploration and description it makes. In health research, these methods are considered as the most humanistic and person-centered way of discovering and uncovering thoughts and actions of human beings. Table 1.
Qualitative research is a type of research that collects and analyzes non-numerical data to ... generating holistic and integrated descriptions of a wide range of structural and social determinants across ... qualitative coding software programs such as MAXQDA, Atlas.ti, and others are rapidly evolving and incorporating AI to ...
Coding until blue in the face, particularly in the beginning phase of analysis, has the potential effect of "slicing and dicing" participants' lived experiences thereby weakening their unique contributions to the research study. A holistic approach to analysis acknowledges that (1) these unique contributions to our research are central to ...
This article will first outline and describe grounded theory analysis. Charmaz (2006) version of grounded theory is adopted here as it most closely aligns with a design approach to research enquiry incorporating pragmatism, symbolic interactionism, and an interpretivist view of research. The focus in this article will be on the nature and depth of interaction with the data required and the ...
Coding is an iterative process, with researchers refining and revising their codes as their understanding of the data evolves. The ultimate goal is to develop a coherent and meaningful coding scheme that captures the richness and complexity of the participants' experiences and helps answer the research questions.
Abstract. This paper aims to provide an overview of the use and assessment of qualitative research methods in the health sciences. Qualitative research can be defined as the study of the nature of phenomena and is especially appropriate for answering questions of why something is (not) observed, assessing complex multi-component interventions ...
It is a method of systematically coding and categorizing information and comprises a ... throughout the nursing program emphasizing the importance of holistic support for students' well-being and academic success as well as retention in the nursing program. ... Streubert HJ, Carpenter DR. Qualitative research in nursing: advancing the ...
Study design. This qualitative longitudinal study, conducted from October 2020 to July 2021, used a qualitative descriptive methodology through four consecutive in-depth semi-structured interviews per participant at three, 10-, 30- and 60-days post-discharge, as illustrated in Fig. 1.Longitudinal qualitative research is characterized by qualitative data collection at different points in time ...
Data Analysis and Coding. For all four approaches, when conducting the data analysis, researchers need to be able to code, sort, and identify themes and relationships from the data. ... Debates to assure quality in qualitative research have been the point of conversations among scholars for decades (e.g. Denzin & Lincoln, 2011; ...
The significance of metaverse platforms is growing in both research and practical applications. To utilize the chances and opportunities metaverse platforms offer, research and practice must understand how these platforms create value, which has not been adequately explored. Our research explores the characteristics of metaverse platforms that facilitate value creation for organizations in ...
That is to say, a well-crafted research design ensures that the study is methodologically sound, enabling researchers to draw valid conclusions and contribute meaningfully to the body of knowledge in their field. This study was designed to triangulate qualitative and quantitative methods, as required in a mixed-methods approach.
The purpose of this article is to offer a critical discussion on resisting robotic qualitative analysis. We argue that health and social science researchers, as well as qualitative researchers from other disciplines must resist thin engagement with qualitative data analysis, so that they avoid the mechanical and lifeless production of research findings and rather move towards soulful ...