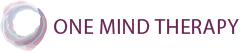
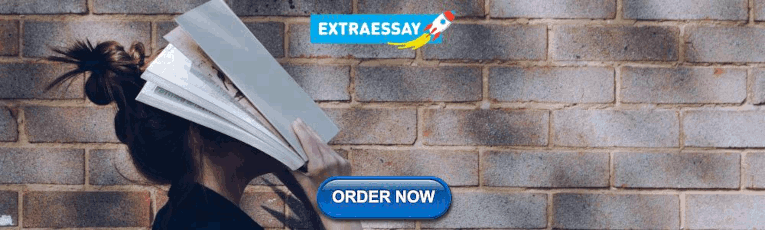
Operational Definition Psychology – Definition, Examples, and How to Write One
Elizabeth Research
Every good psychology study contains an operational definition for the variables in the research. An operational definition allows the researchers to describe in a specific way what they mean when they use a certain term. Generally, operational definitions are concrete and measurable. Defining variables in this way allows other people to see if the research has validity . Validity here refers to if the researchers are actually measuring what they intended to measure.
Definition: An operational definition is the statement of procedures the researcher is going to use in order to measure a specific variable.
We need operational definitions in psychology so that we know exactly what researchers are talking about when they refer to something. There might be different definitions of words depending on the context in which the word is used. Think about how words mean something different to people from different cultures. To avoid any confusion about definitions, in research we explain clearly what we mean when we use a certain term.
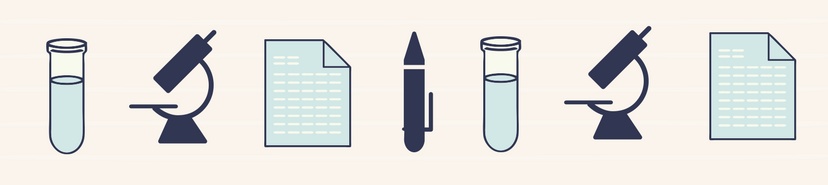
Operational Definition Examples
Example one:.
A researcher wants to measure if age is related to addiction. Perhaps their hypothesis is: the incidence of addiction will increase with age. Here we have two variables, age and addiction. In order to make the research as clear as possible, the researcher must define how they will measure these variables. Essentially, how do we measure someone’s age and how to we measure addiction?
Variable One: Age might seem straightforward. You might be wondering why we need to define age if we all know what age is. However, one researcher might decide to measure age in months in order to get someone’s precise age, while another researcher might just choose to measure age in years. In order to understand the results of the study, we will need to know how this researcher operationalized age. For the sake of this example lets say that age is defined as how old someone is in years.
Variable Two: The variable of addiction is slightly more complicated than age. In order to operationalize it the researcher has to decide exactly how they want to measure addiction. They might narrow down their definition and say that addiction is defined as going through withdrawal when the person stops using a substance. Or the researchers might decide that the definition of addiction is: if someone currently meets the DSM-5 diagnostic criteria for any substance use disorder. For the sake of this example, let’s say that the researcher chose the latter.
Final Definition: In this research study age is defined as participant’s age measured in years and the incidence of addiction is defined as whether or not the participant currently meets the DSM-5 diagnostic criteria for any substance use disorder.
Example Two
A researcher wants to measure if there is a correlation between hot weather and violent crime. Perhaps their guiding hypothesis is: as temperature increases so will violent crime. Here we have two variables, weather and violent crime. In order to make this research precise the researcher will have to operationalize the variables.
Variable One: The first variable is weather. The researcher needs to decide how to define weather. Researchers might chose to define weather as outside temperature in degrees Fahrenheit. But we need to get a little more specific because there is not one stable temperature throughout the day. So the researchers might say that weather is defined as the high recorded temperature for the day measured in degrees Fahrenheit.
Variable Two: The second variable is violent crime. Again, the researcher needs to define how violent crime is measured. Let’s say that for this study it they use the FBI’s definition of violent crime . This definition describes violent crime as “murder and nonnegligent manslaughter, forcible rape, robbery, and aggravated assault”.
However, how do we actually know how many violent crimes were committed on a given day? Researchers might include in the definition something like: the number of people arrested that day for violent crimes as recorded by the local police.
Final Definition: For this study temperature was defined as high recorded temperature for the day measured in degrees Fahrenheit. Violent crime was defined as the number of people arrested in a given day for murder, forcible rape, robbery, and aggravated assault as recorded by the local police.
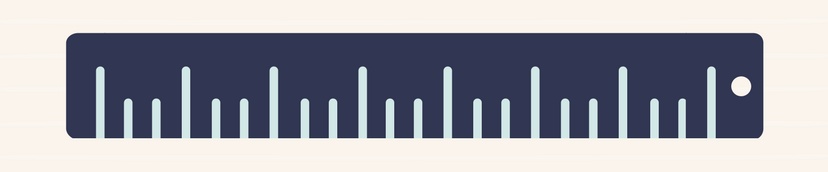
How to Write an Operational Definition
For the last example take the opportunity to see if you can write a clear operational definition for yourself. Imagine that you are creating a research study and you want to see if group therapy is helpful for treating social anxiety.
Variable One: How are you going to define group therapy? here are some things you might want to consider when creating your operational definition:
- What type of group therapy?
- Who is leading the therapy group?
- How long do people participate in the therapy group for?
- How can you “measure” group therapy?
There is no one way to write the operational definition for this variable. You could say something like group therapy was defined as a weekly cognitive behavioral therapy group led by a licensed MFT held over the course of ten weeks. Remember there are many ways to write an operational definition. You know you have written an effective one if another researcher could pick it up and create a very similar variable based on your definition.
Variable Two: The second variable you need to define is “effective treatment social anxiety”. Again, see if you can come up with an operational definition of this variable. This is a little tricky because you will need to be specific about what an effective treatment is as well as what social anxiety is. Here are some things to consider when writing your definition:
- How do you know a treatment is effective?
- How do you measure the effectiveness of treatment?
- Who provides a reliable definition of social anxiety?
- How can you measure social anxiety?
Again, there is no one right way to write this operational definition. If someone else could recreate the study using your definition it is probably an effective one. Here as one example of how you could operationalize the variable: social anxiety was defined as meeting the DSM-5 criteria for social anxiety and the effectiveness of treatment was defined as the reduction of social anxiety symptoms over the 10 week treatment period.
Final Definition: Take your definition for variable one and your definition for variable two and write them in a clear and succinct way. It is alright for your definition to be more than one sentence.
Why We Need Operational Definitions
There are a number of reasons why researchers need to have operational definitions including:
- Replicability
- Generalizability
- Dissemination
The first reason was mentioned earlier in the post when reading research others should be able to assess the validity of the research. That is, did the researchers measure what they intended to measure? If we don’t know how researchers measured something it is very hard to know if the study had validity.
The next reason it is important to have an operational definition is for the sake of replicability . Research should be designed so that if someone else wanted to replicate it they could. By replicating research and getting the same findings we validate the findings. It is impossible to recreate a study if we are unsure about how they defined or measured the variables.
Another reason we need operational definitions is so that we can understand how generalizable the findings are. In research, we want to know that the findings are true not just for a small sample of people. We hope to get findings that generalize to the whole population. If we do not have operational definitions it is hard to generalize the findings because we don’t know who they generalize to.
Finally, operational definitions are important for the dissemination of information. When a study is done it is generally published in a peer-reviewed journal and might be read by other psychologists, students, or journalists. Researchers want people to read their research and apply their findings. If the person reading the article doesn’t know what they are talking about because a variable is not clear it will be hard to them to actually apply this new knowledge.
Receive updates from my blog!

Operational Hypothesis
An Operational Hypothesis is a testable statement or prediction made in research that not only proposes a relationship between two or more variables but also clearly defines those variables in operational terms, meaning how they will be measured or manipulated within the study. It forms the basis of an experiment that seeks to prove or disprove the assumed relationship, thus helping to drive scientific research.
The Core Components of an Operational Hypothesis
Understanding an operational hypothesis involves identifying its key components and how they interact.
The Variables
An operational hypothesis must contain two or more variables — factors that can be manipulated, controlled, or measured in an experiment.
The Proposed Relationship
Beyond identifying the variables, an operational hypothesis specifies the type of relationship expected between them. This could be a correlation, a cause-and-effect relationship, or another type of association.
The Importance of Operationalizing Variables
Operationalizing variables — defining them in measurable terms — is a critical step in forming an operational hypothesis. This process ensures the variables are quantifiable, enhancing the reliability and validity of the research.
Constructing an Operational Hypothesis
Creating an operational hypothesis is a fundamental step in the scientific method and research process. It involves generating a precise, testable statement that predicts the outcome of a study based on the research question. An operational hypothesis must clearly identify and define the variables under study and describe the expected relationship between them. The process of creating an operational hypothesis involves several key steps:
Steps to Construct an Operational Hypothesis
- Define the Research Question : Start by clearly identifying the research question. This question should highlight the key aspect or phenomenon that the study aims to investigate.
- Identify the Variables : Next, identify the key variables in your study. Variables are elements that you will measure, control, or manipulate in your research. There are typically two types of variables in a hypothesis: the independent variable (the cause) and the dependent variable (the effect).
- Operationalize the Variables : Once you’ve identified the variables, you must operationalize them. This involves defining your variables in such a way that they can be easily measured, manipulated, or controlled during the experiment.
- Predict the Relationship : The final step involves predicting the relationship between the variables. This could be an increase, decrease, or any other type of correlation between the independent and dependent variables.
By following these steps, you will create an operational hypothesis that provides a clear direction for your research, ensuring that your study is grounded in a testable prediction.
Evaluating the Strength of an Operational Hypothesis
Not all operational hypotheses are created equal. The strength of an operational hypothesis can significantly influence the validity of a study. There are several key factors that contribute to the strength of an operational hypothesis:
- Clarity : A strong operational hypothesis is clear and unambiguous. It precisely defines all variables and the expected relationship between them.
- Testability : A key feature of an operational hypothesis is that it must be testable. That is, it should predict an outcome that can be observed and measured.
- Operationalization of Variables : The operationalization of variables contributes to the strength of an operational hypothesis. When variables are clearly defined in measurable terms, it enhances the reliability of the study.
- Alignment with Research : Finally, a strong operational hypothesis aligns closely with the research question and the overall goals of the study.
By carefully crafting and evaluating an operational hypothesis, researchers can ensure that their work provides valuable, valid, and actionable insights.
Examples of Operational Hypotheses
To illustrate the concept further, this section will provide examples of well-constructed operational hypotheses in various research fields.
The operational hypothesis is a fundamental component of scientific inquiry, guiding the research design and providing a clear framework for testing assumptions. By understanding how to construct and evaluate an operational hypothesis, we can ensure our research is both rigorous and meaningful.
Examples of Operational Hypothesis:
- In Education : An operational hypothesis in an educational study might be: “Students who receive tutoring (Independent Variable) will show a 20% improvement in standardized test scores (Dependent Variable) compared to students who did not receive tutoring.”
- In Psychology : In a psychological study, an operational hypothesis could be: “Individuals who meditate for 20 minutes each day (Independent Variable) will report a 15% decrease in self-reported stress levels (Dependent Variable) after eight weeks compared to those who do not meditate.”
- In Health Science : An operational hypothesis in a health science study might be: “Participants who drink eight glasses of water daily (Independent Variable) will show a 10% decrease in reported fatigue levels (Dependent Variable) after three weeks compared to those who drink four glasses of water daily.”
- In Environmental Science : In an environmental study, an operational hypothesis could be: “Cities that implement recycling programs (Independent Variable) will see a 25% reduction in landfill waste (Dependent Variable) after one year compared to cities without recycling programs.”
- Bipolar Disorder
- Therapy Center
- When To See a Therapist
- Types of Therapy
- Best Online Therapy
- Best Couples Therapy
- Best Family Therapy
- Managing Stress
- Sleep and Dreaming
- Understanding Emotions
- Self-Improvement
- Healthy Relationships
- Student Resources
- Personality Types
- Guided Meditations
- Verywell Mind Insights
- 2024 Verywell Mind 25
- Mental Health in the Classroom
- Editorial Process
- Meet Our Review Board
- Crisis Support
How to Write a Great Hypothesis
Hypothesis Definition, Format, Examples, and Tips
Kendra Cherry, MS, is a psychosocial rehabilitation specialist, psychology educator, and author of the "Everything Psychology Book."
:max_bytes(150000):strip_icc():format(webp)/IMG_9791-89504ab694d54b66bbd72cb84ffb860e.jpg)
Amy Morin, LCSW, is a psychotherapist and international bestselling author. Her books, including "13 Things Mentally Strong People Don't Do," have been translated into more than 40 languages. Her TEDx talk, "The Secret of Becoming Mentally Strong," is one of the most viewed talks of all time.
:max_bytes(150000):strip_icc():format(webp)/VW-MIND-Amy-2b338105f1ee493f94d7e333e410fa76.jpg)
Verywell / Alex Dos Diaz
- The Scientific Method
Hypothesis Format
Falsifiability of a hypothesis.
- Operationalization
Hypothesis Types
Hypotheses examples.
- Collecting Data
A hypothesis is a tentative statement about the relationship between two or more variables. It is a specific, testable prediction about what you expect to happen in a study. It is a preliminary answer to your question that helps guide the research process.
Consider a study designed to examine the relationship between sleep deprivation and test performance. The hypothesis might be: "This study is designed to assess the hypothesis that sleep-deprived people will perform worse on a test than individuals who are not sleep-deprived."
At a Glance
A hypothesis is crucial to scientific research because it offers a clear direction for what the researchers are looking to find. This allows them to design experiments to test their predictions and add to our scientific knowledge about the world. This article explores how a hypothesis is used in psychology research, how to write a good hypothesis, and the different types of hypotheses you might use.
The Hypothesis in the Scientific Method
In the scientific method , whether it involves research in psychology, biology, or some other area, a hypothesis represents what the researchers think will happen in an experiment. The scientific method involves the following steps:
- Forming a question
- Performing background research
- Creating a hypothesis
- Designing an experiment
- Collecting data
- Analyzing the results
- Drawing conclusions
- Communicating the results
The hypothesis is a prediction, but it involves more than a guess. Most of the time, the hypothesis begins with a question which is then explored through background research. At this point, researchers then begin to develop a testable hypothesis.
Unless you are creating an exploratory study, your hypothesis should always explain what you expect to happen.
In a study exploring the effects of a particular drug, the hypothesis might be that researchers expect the drug to have some type of effect on the symptoms of a specific illness. In psychology, the hypothesis might focus on how a certain aspect of the environment might influence a particular behavior.
Remember, a hypothesis does not have to be correct. While the hypothesis predicts what the researchers expect to see, the goal of the research is to determine whether this guess is right or wrong. When conducting an experiment, researchers might explore numerous factors to determine which ones might contribute to the ultimate outcome.
In many cases, researchers may find that the results of an experiment do not support the original hypothesis. When writing up these results, the researchers might suggest other options that should be explored in future studies.
In many cases, researchers might draw a hypothesis from a specific theory or build on previous research. For example, prior research has shown that stress can impact the immune system. So a researcher might hypothesize: "People with high-stress levels will be more likely to contract a common cold after being exposed to the virus than people who have low-stress levels."
In other instances, researchers might look at commonly held beliefs or folk wisdom. "Birds of a feather flock together" is one example of folk adage that a psychologist might try to investigate. The researcher might pose a specific hypothesis that "People tend to select romantic partners who are similar to them in interests and educational level."
Elements of a Good Hypothesis
So how do you write a good hypothesis? When trying to come up with a hypothesis for your research or experiments, ask yourself the following questions:
- Is your hypothesis based on your research on a topic?
- Can your hypothesis be tested?
- Does your hypothesis include independent and dependent variables?
Before you come up with a specific hypothesis, spend some time doing background research. Once you have completed a literature review, start thinking about potential questions you still have. Pay attention to the discussion section in the journal articles you read . Many authors will suggest questions that still need to be explored.
How to Formulate a Good Hypothesis
To form a hypothesis, you should take these steps:
- Collect as many observations about a topic or problem as you can.
- Evaluate these observations and look for possible causes of the problem.
- Create a list of possible explanations that you might want to explore.
- After you have developed some possible hypotheses, think of ways that you could confirm or disprove each hypothesis through experimentation. This is known as falsifiability.
In the scientific method , falsifiability is an important part of any valid hypothesis. In order to test a claim scientifically, it must be possible that the claim could be proven false.
Students sometimes confuse the idea of falsifiability with the idea that it means that something is false, which is not the case. What falsifiability means is that if something was false, then it is possible to demonstrate that it is false.
One of the hallmarks of pseudoscience is that it makes claims that cannot be refuted or proven false.
The Importance of Operational Definitions
A variable is a factor or element that can be changed and manipulated in ways that are observable and measurable. However, the researcher must also define how the variable will be manipulated and measured in the study.
Operational definitions are specific definitions for all relevant factors in a study. This process helps make vague or ambiguous concepts detailed and measurable.
For example, a researcher might operationally define the variable " test anxiety " as the results of a self-report measure of anxiety experienced during an exam. A "study habits" variable might be defined by the amount of studying that actually occurs as measured by time.
These precise descriptions are important because many things can be measured in various ways. Clearly defining these variables and how they are measured helps ensure that other researchers can replicate your results.
Replicability
One of the basic principles of any type of scientific research is that the results must be replicable.
Replication means repeating an experiment in the same way to produce the same results. By clearly detailing the specifics of how the variables were measured and manipulated, other researchers can better understand the results and repeat the study if needed.
Some variables are more difficult than others to define. For example, how would you operationally define a variable such as aggression ? For obvious ethical reasons, researchers cannot create a situation in which a person behaves aggressively toward others.
To measure this variable, the researcher must devise a measurement that assesses aggressive behavior without harming others. The researcher might utilize a simulated task to measure aggressiveness in this situation.
Hypothesis Checklist
- Does your hypothesis focus on something that you can actually test?
- Does your hypothesis include both an independent and dependent variable?
- Can you manipulate the variables?
- Can your hypothesis be tested without violating ethical standards?
The hypothesis you use will depend on what you are investigating and hoping to find. Some of the main types of hypotheses that you might use include:
- Simple hypothesis : This type of hypothesis suggests there is a relationship between one independent variable and one dependent variable.
- Complex hypothesis : This type suggests a relationship between three or more variables, such as two independent and dependent variables.
- Null hypothesis : This hypothesis suggests no relationship exists between two or more variables.
- Alternative hypothesis : This hypothesis states the opposite of the null hypothesis.
- Statistical hypothesis : This hypothesis uses statistical analysis to evaluate a representative population sample and then generalizes the findings to the larger group.
- Logical hypothesis : This hypothesis assumes a relationship between variables without collecting data or evidence.
A hypothesis often follows a basic format of "If {this happens} then {this will happen}." One way to structure your hypothesis is to describe what will happen to the dependent variable if you change the independent variable .
The basic format might be: "If {these changes are made to a certain independent variable}, then we will observe {a change in a specific dependent variable}."
A few examples of simple hypotheses:
- "Students who eat breakfast will perform better on a math exam than students who do not eat breakfast."
- "Students who experience test anxiety before an English exam will get lower scores than students who do not experience test anxiety."
- "Motorists who talk on the phone while driving will be more likely to make errors on a driving course than those who do not talk on the phone."
- "Children who receive a new reading intervention will have higher reading scores than students who do not receive the intervention."
Examples of a complex hypothesis include:
- "People with high-sugar diets and sedentary activity levels are more likely to develop depression."
- "Younger people who are regularly exposed to green, outdoor areas have better subjective well-being than older adults who have limited exposure to green spaces."
Examples of a null hypothesis include:
- "There is no difference in anxiety levels between people who take St. John's wort supplements and those who do not."
- "There is no difference in scores on a memory recall task between children and adults."
- "There is no difference in aggression levels between children who play first-person shooter games and those who do not."
Examples of an alternative hypothesis:
- "People who take St. John's wort supplements will have less anxiety than those who do not."
- "Adults will perform better on a memory task than children."
- "Children who play first-person shooter games will show higher levels of aggression than children who do not."
Collecting Data on Your Hypothesis
Once a researcher has formed a testable hypothesis, the next step is to select a research design and start collecting data. The research method depends largely on exactly what they are studying. There are two basic types of research methods: descriptive research and experimental research.
Descriptive Research Methods
Descriptive research such as case studies , naturalistic observations , and surveys are often used when conducting an experiment is difficult or impossible. These methods are best used to describe different aspects of a behavior or psychological phenomenon.
Once a researcher has collected data using descriptive methods, a correlational study can examine how the variables are related. This research method might be used to investigate a hypothesis that is difficult to test experimentally.
Experimental Research Methods
Experimental methods are used to demonstrate causal relationships between variables. In an experiment, the researcher systematically manipulates a variable of interest (known as the independent variable) and measures the effect on another variable (known as the dependent variable).
Unlike correlational studies, which can only be used to determine if there is a relationship between two variables, experimental methods can be used to determine the actual nature of the relationship—whether changes in one variable actually cause another to change.
The hypothesis is a critical part of any scientific exploration. It represents what researchers expect to find in a study or experiment. In situations where the hypothesis is unsupported by the research, the research still has value. Such research helps us better understand how different aspects of the natural world relate to one another. It also helps us develop new hypotheses that can then be tested in the future.
Thompson WH, Skau S. On the scope of scientific hypotheses . R Soc Open Sci . 2023;10(8):230607. doi:10.1098/rsos.230607
Taran S, Adhikari NKJ, Fan E. Falsifiability in medicine: what clinicians can learn from Karl Popper [published correction appears in Intensive Care Med. 2021 Jun 17;:]. Intensive Care Med . 2021;47(9):1054-1056. doi:10.1007/s00134-021-06432-z
Eyler AA. Research Methods for Public Health . 1st ed. Springer Publishing Company; 2020. doi:10.1891/9780826182067.0004
Nosek BA, Errington TM. What is replication ? PLoS Biol . 2020;18(3):e3000691. doi:10.1371/journal.pbio.3000691
Aggarwal R, Ranganathan P. Study designs: Part 2 - Descriptive studies . Perspect Clin Res . 2019;10(1):34-36. doi:10.4103/picr.PICR_154_18
Nevid J. Psychology: Concepts and Applications. Wadworth, 2013.
By Kendra Cherry, MSEd Kendra Cherry, MS, is a psychosocial rehabilitation specialist, psychology educator, and author of the "Everything Psychology Book."
Have a language expert improve your writing
Run a free plagiarism check in 10 minutes, automatically generate references for free.
- Knowledge Base
- Dissertation
- Operationalisation | A Guide with Examples, Pros & Cons
Operationalisation | A Guide with Examples, Pros & Cons
Published on 6 May 2022 by Pritha Bhandari . Revised on 10 October 2022.
Operationalisation means turning abstract concepts into measurable observations. Although some concepts, like height or age, are easily measured, others, like spirituality or anxiety, are not.
Through operationalisation, you can systematically collect data on processes and phenomena that aren’t directly observable.
- Self-rating scores on a social anxiety scale
- Number of recent behavioural incidents of avoidance of crowded places
- Intensity of physical anxiety symptoms in social situations
Instantly correct all language mistakes in your text
Be assured that you'll submit flawless writing. Upload your document to correct all your mistakes.
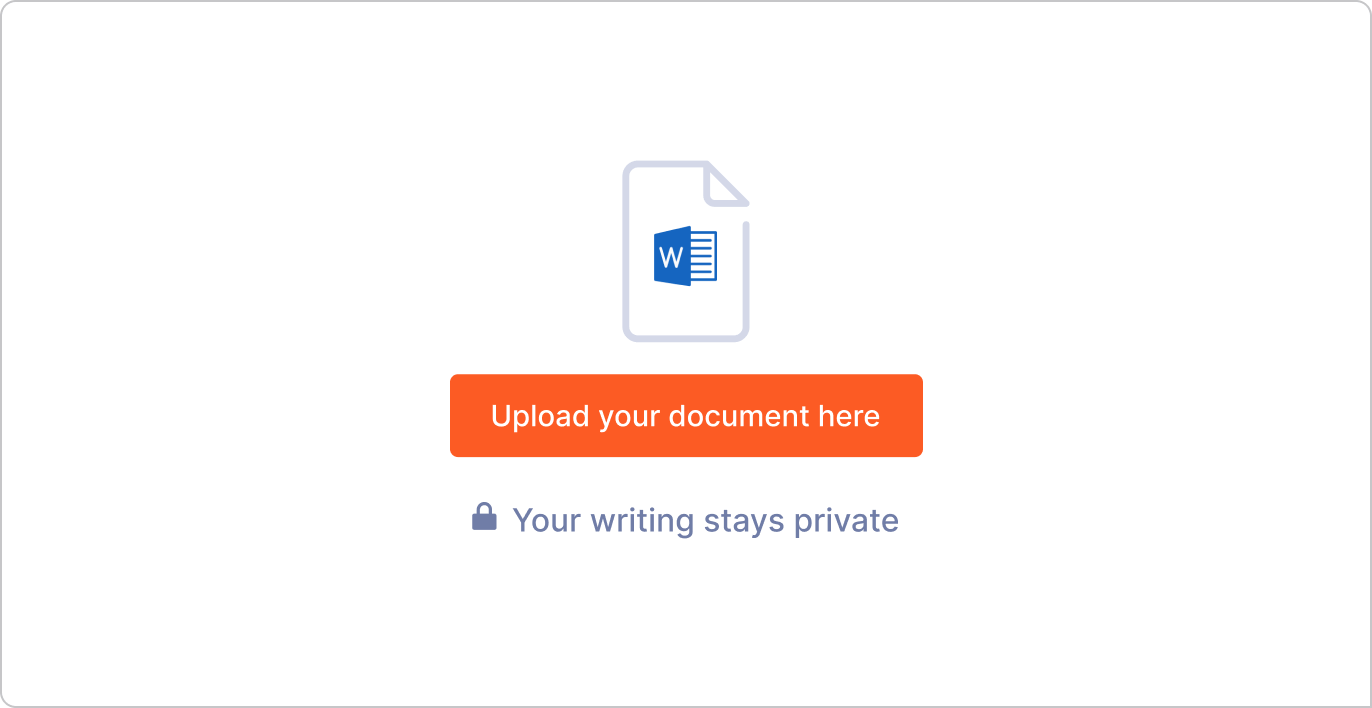
Table of contents
Why operationalisation matters, how to operationalise concepts, strengths of operationalisation, limitations of operationalisation, frequently asked questions about operationalisation.
In quantitative research , it’s important to precisely define the variables that you want to study.
Without transparent and specific operational definitions, researchers may measure irrelevant concepts or inconsistently apply methods. Operationalisation reduces subjectivity and increases the reliability of your study.
Your choice of operational definition can sometimes affect your results. For example, an experimental intervention for social anxiety may reduce self-rating anxiety scores but not behavioural avoidance of crowded places. This means that your results are context-specific and may not generalise to different real-life settings.
Generally, abstract concepts can be operationalised in many different ways. These differences mean that you may actually measure slightly different aspects of a concept, so it’s important to be specific about what you are measuring.
If you test a hypothesis using multiple operationalisations of a concept, you can check whether your results depend on the type of measure that you use. If your results don’t vary when you use different measures, then they are said to be ‘robust’.
Prevent plagiarism, run a free check.
There are three main steps for operationalisation:
- Identify the main concepts you are interested in studying.
- Choose a variable to represent each of the concepts.
- Select indicators for each of your variables.
Step 1: Identify the main concepts you are interested in studying
Based on your research interests and goals, define your topic and come up with an initial research question .
There are two main concepts in your research question:
- Social media behaviour
Step 2: Choose a variable to represent each of the concepts
Your main concepts may each have many variables , or properties, that you can measure.
For instance, are you going to measure the amount of sleep or the quality of sleep? And are you going to measure how often teenagers use social media, which social media they use, or when they use it?
- Alternate hypothesis: Lower quality of sleep is related to higher night-time social media use in teenagers.
- Null hypothesis: There is no relation between quality of sleep and night-time social media use in teenagers.
Step 3: Select indicators for each of your variables
To measure your variables, decide on indicators that can represent them numerically.
Sometimes these indicators will be obvious: for example, the amount of sleep is represented by the number of hours per night. But a variable like sleep quality is harder to measure.
You can come up with practical ideas for how to measure variables based on previously published studies. These may include established scales or questionnaires that you can distribute to your participants. If none are available that are appropriate for your sample, you can develop your own scales or questionnaires.
- To measure sleep quality, you give participants wristbands that track sleep phases.
- To measure night-time social media use, you create a questionnaire that asks participants to track how much time they spend using social media in bed.
After operationalising your concepts, it’s important to report your study variables and indicators when writing up your methodology section. You can evaluate how your choice of operationalisation may have affected your results or interpretations in the discussion section.
Operationalisation makes it possible to consistently measure variables across different contexts.
Scientific research is based on observable and measurable findings. Operational definitions break down intangible concepts into recordable characteristics.
Objectivity
A standardised approach for collecting data leaves little room for subjective or biased personal interpretations of observations.
Reliability
A good operationalisation can be used consistently by other researchers. If other people measure the same thing using your operational definition, they should all get the same results.
Operational definitions of concepts can sometimes be problematic.
Underdetermination
Many concepts vary across different time periods and social settings.
For example, poverty is a worldwide phenomenon, but the exact income level that determines poverty can differ significantly across countries.
Reductiveness
Operational definitions can easily miss meaningful and subjective perceptions of concepts by trying to reduce complex concepts to numbers.
For example, asking consumers to rate their satisfaction with a service on a 5-point scale will tell you nothing about why they felt that way.
Lack of universality
Context-specific operationalisations help preserve real-life experiences, but make it hard to compare studies if the measures differ significantly.
For example, corruption can be operationalised in a wide range of ways (e.g., perceptions of corrupt business practices, or frequency of bribe requests from public officials), but the measures may not consistently reflect the same concept.
Operationalisation means turning abstract conceptual ideas into measurable observations.
For example, the concept of social anxiety isn’t directly observable, but it can be operationally defined in terms of self-rating scores, behavioural avoidance of crowded places, or physical anxiety symptoms in social situations.
Before collecting data , it’s important to consider how you will operationalise the variables that you want to measure.
In scientific research, concepts are the abstract ideas or phenomena that are being studied (e.g., educational achievement). Variables are properties or characteristics of the concept (e.g., performance at school), while indicators are ways of measuring or quantifying variables (e.g., yearly grade reports).
The process of turning abstract concepts into measurable variables and indicators is called operationalisation .
Reliability and validity are both about how well a method measures something:
- Reliability refers to the consistency of a measure (whether the results can be reproduced under the same conditions).
- Validity refers to the accuracy of a measure (whether the results really do represent what they are supposed to measure).
If you are doing experimental research , you also have to consider the internal and external validity of your experiment.
Cite this Scribbr article
If you want to cite this source, you can copy and paste the citation or click the ‘Cite this Scribbr article’ button to automatically add the citation to our free Reference Generator.
Bhandari, P. (2022, October 10). Operationalisation | A Guide with Examples, Pros & Cons. Scribbr. Retrieved 7 June 2024, from https://www.scribbr.co.uk/thesis-dissertation/operationalisation/
Is this article helpful?
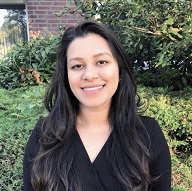
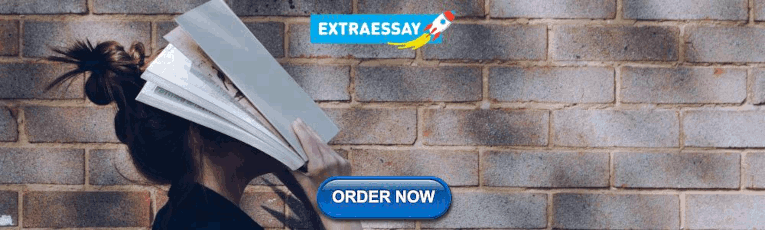
Pritha Bhandari
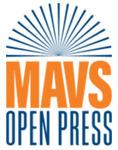
Want to create or adapt books like this? Learn more about how Pressbooks supports open publishing practices.
5.4 Operationalization
Learning objectives.
- Define and give an example of indicators for a variable
- Identify the three components of an operational definition
- Describe the purpose of multi-dimensional measures such as indexes, scales, and typologies and why they are used
Now that we have figured out how to define, or conceptualize, our terms we’ll need to think about operationalizing them. Operationalization is the process by which researchers conducting quantitative research spell out precisely how a concept will be measured. It involves identifying the specific research procedures we will use to gather data about our concepts. This of course requires that we know what research method(s) we will employ to learn about our concepts, and we’ll examine specific research methods later on in the text. For now, let’s take a broad look at how operationalization works. We can then revisit how this process works when we examine specific methods of data collection in later chapters. Remember, operationalization is only a process in quantitative research. Measurement in qualitative research will be discussed at the end of this section.
Operationalization works by identifying specific indicators that will be taken to represent the ideas we are interested in studying. If, for example, we are interested in studying masculinity, indicators for that concept might include some of the social roles prescribed to men in society such as breadwinning or fatherhood. Being a breadwinner or a father might therefore be considered indicators of a person’s masculinity. The extent to which a man fulfills either, or both, of these roles might be understood as clues (or indicators) about the extent to which he is viewed as masculine.
Let’s look at another example of indicators. Each day, Gallup researchers poll 1,000 randomly selected Americans to ask them about their well-being. To measure well-being, Gallup asks these people to respond to questions covering six broad areas: physical health, emotional health, work environment, life evaluation, healthy behaviors, and access to basic necessities. Gallup uses these six factors as indicators of the concept that they are really interested in, which is well-being .
Identifying indicators can be even simpler than the examples described thus far. What are the possible indicators of the concept of gender? Most of us would probably agree that “man” and “woman” are both reasonable indicators of gender, but you may want to include other options for people who identify as non-binary or other genders. Political party is another relatively easy concept for which to identify indicators. In the United States, likely indicators include Democrat and Republican and, depending on your research interest, you may include additional indicators such as Independent, Green, or Libertarian as well. Age and birthplace are additional examples of concepts for which identifying indicators is a relatively simple process. What concepts are of interest to you, and what are the possible indicators of those concepts?
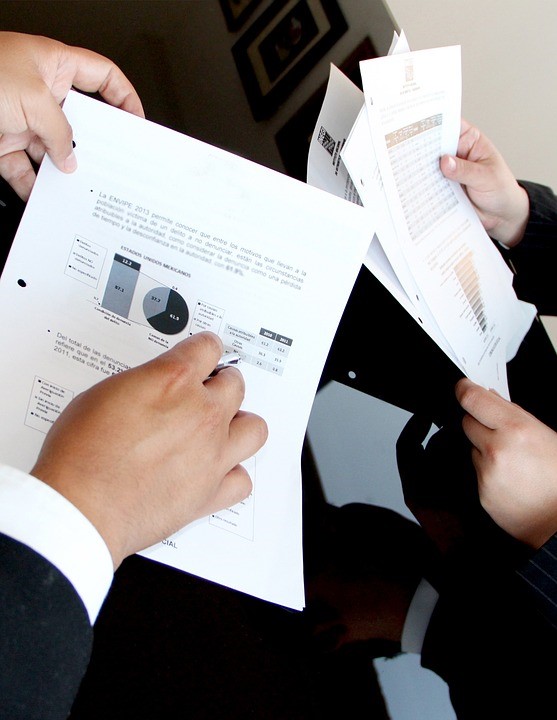
We have now considered a few examples of concepts and their indicators, but it is important we don’t make the process of coming up with indicators too arbitrary or casual. One way to avoid taking an overly casual approach in identifying indicators, as described previously, is to turn to prior theoretical and empirical work in your area. Theories will point you in the direction of relevant concepts and possible indicators; empirical work will give you some very specific examples of how the important concepts in an area have been measured in the past and what sorts of indicators have been used. Often, it makes sense to use the same indicators as researchers who have come before you. On the other hand, perhaps you notice some possible weaknesses in measures that have been used in the past that your own methodological approach will enable you to overcome.
Speaking of your methodological approach, another very important thing to think about when deciding on indicators and how you will measure your key concepts is the strategy you will use for data collection. A survey implies one way of measuring concepts, while focus groups imply a quite different way of measuring concepts. Your design choices will play an important role in shaping how you measure your concepts.
Operationalizing your variables
Moving from identifying concepts to conceptualizing them and then to operationalizing them is a matter of increasing specificity. You begin the research process with a general interest, identify a few concepts that are essential for studying that interest you, work to define those concepts, and then spell out precisely how you will measure those concepts. In quantitative research, that final stage is called operationalization.
An operational definition consists of the following components: (1) the variable being measured, (2) the measure you will use, (3) how you plan to interpret the results of that measure.
The first component, the variable, should be the easiest part. In much quantitative research, there is a research question that has at least one independent and at least one dependent variable. Remember that variables have to be able to vary. For example, the United States is not a variable. Country of birth is a variable, as is patriotism. Similarly, if your sample only includes men, gender is a constant in your study…not a variable.
Let’s pick a social work research question and walk through the process of operationalizing variables. Suppose we hypothesize that individuals on a residential psychiatric unit who are more depressed are less likely to be satisfied with care than those who are less depressed. Remember, this would be a negative relationship—as depression increases, satisfaction decreases. In this question, depression is the independent variable (the cause) and satisfaction with care is the dependent variable (the effect). We have our two variables—depression and satisfaction with care—so the first component is done. Now, we move onto the second component–the measure.
How do you measure depression or satisfaction? Many students begin by thinking that they could look at body language to see if a person were depressed. Maybe they would also verbally express feelings of sadness or hopelessness more often. A satisfied person might be happy around service providers and express gratitude more often. These may indicate depression, but they lack coherence. Unfortunately, what this “measure” is actually saying is that “I know depression and satisfaction when I see them.” While you are likely a decent judge of depression and satisfaction, you need to provide more information in a research study for how you plan to measure your variables. Your judgment is subjective, based on your own idiosyncratic experiences with depression and satisfaction. They couldn’t be replicated by another researcher. They also can’t be done consistently for a large group of people. Operationalization requires that you come up with a specific and rigorous measure for seeing who is depressed or satisfied.
Finding a good measure for your variable can take less than a minute. To measure a variable like age, you would probably put a question on a survey that asked, “How old are you?” To evaluate someone’s length of stay in a hospital, you might ask for access to their medical records and count the days from when they were admitted to when they were discharged. Measuring a variable like income might require some more thought, though. Are you interested in this person’s individual income or the income of their family unit? This might matter if your participant does not work or is dependent on other family members for income. Do you count income from social welfare programs? Are you interested in their income per month or per year? Measures must be specific and clear.
Depending on your research design, your measure may be something you put on a survey or pre/post-test that you give to your participants. For a variable like age or income, one well-worded question may suffice. Unfortunately, most variables in the social world are not so simple. Depression and satisfaction are multi-dimensional variables, as they each contain multiple elements. Asking someone “Are you depressed?” does not do justice to the complexity of depression, which includes issues with mood, sleeping, eating, relationships, and happiness. Asking someone “Are you satisfied with the services you received?” similarly omits multiple dimensions of satisfaction, such as timeliness, respect, meeting needs, and likelihood of recommending to a friend, among many others.
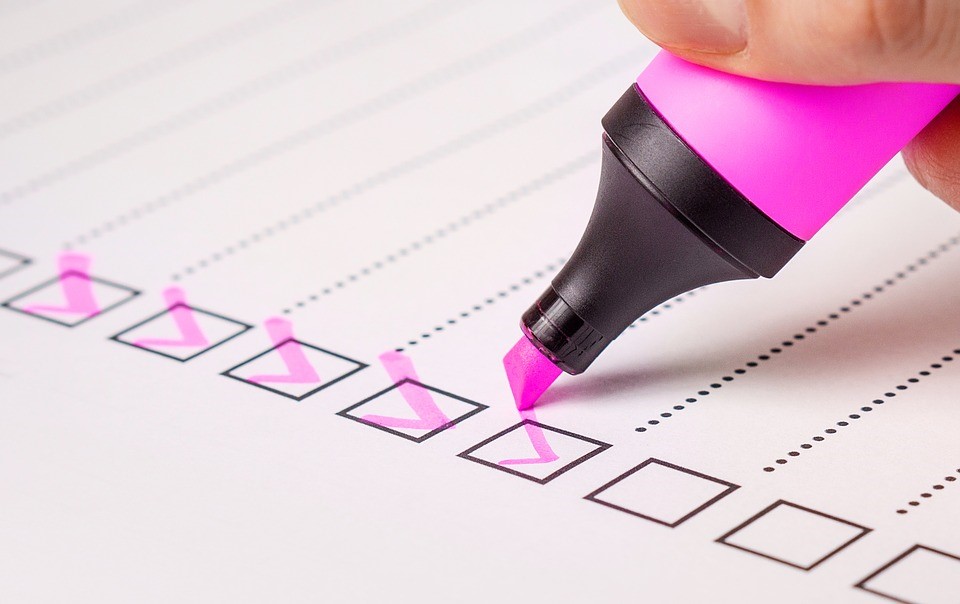
INDICES, SCALES, AND TYPOLOGIES
To account for a variable’s dimensions, a researcher might rely on an index, scale, or typology. An index is a type of measure that contains several indicators and is used to summarize some more general concept. An index of depression might ask if the person has experienced any of the following indicators in the past month: pervasive feelings of hopelessness, thoughts of suicide, over- or under-eating, and a lack of enjoyment in normal activities. On their own, some of these indicators like over- or under-eating might not be considered depression, but collectively, the answers to each of these indicators add up to an overall experience of depression. The index allows the researcher in this case to better understand what shape a respondent’s depression experience takes. If the researcher had only asked whether a respondent had ever experienced depression, she wouldn’t know what sorts of behaviors actually made up that respondent’s experience of depression.
Taking things one step further, if the researcher decides to rank order the various behaviors that make up depression, perhaps weighting suicidal thoughts more heavily than eating disturbances, then she will have created a scale rather than an index. Like an index, a scale is also a measure composed of multiple items or questions. But unlike indexes, scales are designed in a way that accounts for the possibility that different items may vary in intensity.
If creating your own scale sounds complicated, don’t worry! For most variables, this work has already been done by other researchers. You do not need to create a scale for depression because scales such as the Patient Health Questionnaire (PHQ-9) and the Center for Epidemiologic Studies Depression Scale (CES-D) and Beck’s Depression Inventory (BDI) have been developed and refined over dozens of years to measure variables like depression. Similarly, scales such as the Patient Satisfaction Questionnaire (PSQ-18) have been developed to measure satisfaction with medical care. As we will discuss in the next section, these scales have been shown to be reliable and valid. While you could create a new scale to measure depression or satisfaction, a study with rigor would pilot test and refine that scale over time to make sure it measures the concept accurately and consistently. This high level of rigor is often unachievable in student research projects, so using existing scales is recommended.
Another reason existing scales are preferable is that they can save time and effort. The Mental Measurements Yearbook provides a searchable database of measures for different variables. You can access this database from your library’s list of databases. At the University of Texas at Arlington, the Mental Measurements Yearbook can be searched directly or viewed online. If you can’t find anything in there, your next stop should be the methods section of the articles in your literature review. The methods section of each article will detail how the researchers measured their variables. In a quantitative study, researchers likely used a scale to measure key variables and will provide a brief description of that scale. A Google Scholar search such as “depression scale” or “satisfaction scale” should also provide some relevant results. As a last resort, a general web search may bring you to a scale for your variable.
Unfortunately, all of these approaches do not guarantee that you will be able to actually see the scale itself or get information on how it is interpreted. Many scales cost money to use and may require training to properly administer. You may also find scales that are related to your variable but would need to be slightly modified to match your study’s needs. Adapting a scale to fit your study is a possibility; however, you should remember that changing even small parts of a scale can influence its accuracy and consistency. Pilot testing is always recommended for adapted scales.
A final way of measuring multidimensional variables is a typology . A typology is a way of categorizing concepts according to particular themes. Probably the most familiar version of a typology is the micro, meso, macro framework. Students classify specific elements of the social world by their ecological relationship with the person. Let’s take the example of depression again. The lack of sleep associated with depression would be classified as a micro-level element while a severe economic recession would be classified as a macro-level element. Typologies require clearly stated rules on what data will get assigned to what categories, so carefully following the rules of the typology is important.
Once you have (1) your variable and (2) your measure, you will need to (3) describe how you plan to interpret your measure. Sometimes, interpreting a measure is incredibly easy. If you ask someone their age, you’ll probably interpret the results by noting the raw number (e.g., 22) someone provides. However, you could also re-code age into categories (e.g., under 25, 20-29-years-old, etc.). An index may also be simple to interpret. If there is a checklist of problem behaviors, one might simply add up the number of behaviors checked off–with a higher total indicating worse behavior. Sometimes an index will assign people to categories (e.g., normal, borderline, moderate, significant, severe) based on their total number of checkmarks. As long as the rules are clearly spelled out, you are welcome to interpret measures in a way that makes sense to you. Theory might guide you to use some categories or you might be influenced by the types of statistical tests you plan to run later on in data analysis.
For more complicated measures like scales, you should look at the information provided by the scale’s authors for how to interpret the scale. If you can’t find enough information from the scale’s creator, look at how the results of that scale are reported in the results section of research articles. For example, Beck’s Depression Inventory (BDI-II) uses 21 questions to measure depression. A person indicates on a scale of 0-3 how much they agree with a statement. The results for each question are added up, and the respondent is put into one of three categories: low levels of depression (1-16), moderate levels of depression (17-30), or severe levels of depression (31 and over).
In sum, operationalization specifies what measure you will be using to measure your variable and how you plan to interpret that measure. Operationalization is probably the trickiest component of basic research methods. Don’t get frustrated if it takes a few drafts and a lot of feedback to get to a workable definition.
Qualitative research and operationalization
As we discussed in the previous section, qualitative research takes a more open approach towards defining the concepts in your research question. The questions you choose to ask in your interview, focus group, or content analysis will determine what data you end up getting from your participants. For example, if you are researching depression qualitatively, you would not use a scale like the Beck’s Depression Inventory, which is a quantitative measure we described above. Instead, you would start off with a tentative definition of what depression means based on your literature review and use that definition to come up with questions for your participants. We will cover how those questions fit into qualitative research designs later on in the textbook. For now, remember that qualitative researchers use the questions they ask participants to measure their variables and that qualitative researchers can change their questions as they gather more information from participants. Ultimately, the concepts in a qualitative study will be defined by the researcher’s interpretation of what her participants say. Unlike in quantitative research in which definitions must be explicitly spelled out in advance, qualitative research allows the definitions of concepts to emerge during data analysis.
Spotlight on UTA School of Social Work
Are interactions with a social robot associated with changes in depression and loneliness.
Robust measurement is very important in research. Furthermore, providing a clear explanation of the measures used in a study helps others to understand the concepts being studied and interpret the findings as well as and helps other researchers to accurately replicate the study in different settings.
Dr. Noelle Fields and Ling Xu from the University of Texas at Arlington’s School of Social Work collaborated with Dr. Julienne Greer from the College of Liberal Arts on a pilot study that incorporated a participatory arts intervention with the social robot, NAO. The intervention took place with older adults living in an assisted living facility. The overall aim of this study was to help older adults improve their psychological well-being through participation in a theatre arts activity led by NAO.
The key outcome variables for this pilot study were psychological well-being measured by depression , loneliness , and engagement with the robot . Depression and loneliness were measured by two standardized scales: the 15-item Geriatric Depression Scale (Sheikh & Yesavage, 1986) and the revised 3-item UCLA loneliness scale (Hughes, Waite, Hawkley, & Cacioppo, 2004). However, engagement with the robot did not have a standardized measure. Thus, the research team utilized a measure to capture engagement with the robot based on previous research.
In this study, engagement with robot was defined as the degree of interaction or involvement with a robot. One way to measure engagement is for members of the research team (i.e., observers) to rate the level of participant engagement (see Table 1).
Table 1. Please circle 0-5 to indicate the participant’s engagement levels (definitions for each levels can be found in the example column).
This measurement was easy to apply in this study; however, it may lack the sensitivity to capture more detailed information about engagement, especially among older adult populations. Therefore, the researchers in this pilot study designed additional indicators to describe the participants’ reactions when interacting with a robot. More specifically, after watching a video of each participant interacting with NAO, each researcher gave an engagement score based on the following concepts: (1) attentiveness including focus on face of robot or gesture of robot, (2) smiling and/or laughter, (3) head nodding, and (4) facial/vocal expression that included eyes widening, eyebrows arching, and tonal changes in voice. Through video analysis, each of the concepts were counted and tabulated by independent researchers, and mean score among researchers on each concept was then calculated. Sum scores on total engagement were also adapted for analysis. See Table 2 for detailed information of this measurement.
Table 2: Each researcher should provide a score on each item below based on your observation of participants’ interaction with the robot.
The study found that participants reported improvements in mood, loneliness, and depression. The degree of difference/change was slightly greater in participants without dementia, perhaps suggesting social engagement and connection was a more profound attribute in cognitively intact older adults. Further research would be needed to confirm this hypothesis. Although the study is limited by its small scale and non-intervention control group, this exploratory pilot study supports the continuing development of participatory arts interventions with older adults using a social robotic platform. The benefits of performative participatory art between social robots and older adults is an emerging research area for human-robot social interactions and communications.
Key Takeaways
- Operationalization involves spelling out precisely how a concept will be measured.
- Operational definitions must include the variable, the measure, and how you plan to interpret the measure.
- Multi-dimensional concepts can be measured by an index, a scale, or a typology.
- It’s a good idea to look at how researchers have measured the concept in previous studies.
- Index- measure that contains several indicators and is used to summarize a more general concept
- Indicators- represent the concepts that we are interested in studying
- Operationalization- process by which researchers conducting quantitative research spell out precisely how a concept will be measured and how to interpret that measure
- Scale- composite measure designed in a way that accounts for the possibility that different items on an index may vary in intensity
- Typology- measure that categorizes concepts according to particular themes
Image attributions
Business charts by Pixabay CC-0
Checklist by TeroVesalainen CC-0
Foundations of Social Work Research Copyright © 2020 by Rebecca L. Mauldin is licensed under a Creative Commons Attribution-NonCommercial-ShareAlike 4.0 International License , except where otherwise noted.
Share This Book
- Skip to main content
- Skip to primary sidebar
IResearchNet
Operationalization
Operationalization definition.
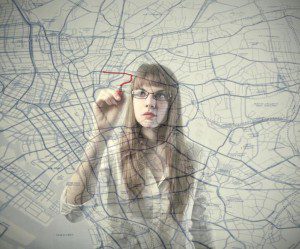
Examples of Operational Definitions
Imagine a researcher who is interested in helping curb aggression in schools by exploring if aggression is a response to frustration. To answer the question, the researcher must first define “aggression” and “frustration,” both conceptually and procedurally. In the example of frustration, the conceptual definition may be obstruction of goal-oriented behavior, but this definition is rarely specific enough for research. Therefore, an operational definition is needed that identifies how frustration and aggression will be measured or manipulated. In this example, frustration can be operationally defined in terms of responses to the question: How frustrated are you at this moment? The response options can be (a) not at all, (b) slightly, (c) moderately, and (d) very. The researcher could then classify people as frustrated if they answered “moderately” or “very” on the scale.
The researcher must also operationalize aggression in this particular study. However, one challenge of developing an operational definition is turning abstract concepts into observable (measurable) parts. For example, most people will agree that punching another person in the face with the goal of causing pain counts as an act of aggression, but people may differ on whether teasing counts as aggression. The ambiguity about the exact meaning of a concept is what makes operationalization essential for precise communication of methodological procedures within a study. In this particular example, aggression could be operational-ized as the number of times a student physically hits another person with intention to harm. Thus, having operationally defined the theoretical concepts, the relation between frustration and aggression can be investigated.
The Pros and Cons of Operationalization
Operationalization is an essential component in a theoretically centered science because it provides the means of specifying exactly how a concept is being measured or produced in a particular study. A precise operational definition helps ensure consistency in interpretation and collection of data, and thereby aids in replication and extension of the study. However, because most concepts can be operationally defined in many ways, researchers often disagree about the correspondence between the methods used in a particular study and the theoretical concept. In addition, when definitions become too specific, they are not always applicable or meaningful.
References:
- Emilio, R. (2003). What is defined in operational definitions? The case of operant psychology. Behavior and Philosophy, 31, 111-126.
- Underwood, B. J. (1957). Psychological research. New York: Appleton-Century-Crofts.
- Social Psychology Research Methods
- cognitive sophistication
- tolerance of diversity
- exposure to higher levels of math or science
- age (which is currently related to educational level in many countries)
- social class and other variables.
- For example, suppose you designed a treatment to help people stop smoking. Because you are really dedicated, you assigned the same individuals simultaneously to (1) a "stop smoking" nicotine patch; (2) a "quit buddy"; and (3) a discussion support group. Compared with a group in which no intervention at all occurred, your experimental group now smokes 10 fewer cigarettes per day.
- There is no relationship among two or more variables (EXAMPLE: the correlation between educational level and income is zero)
- Or that two or more populations or subpopulations are essentially the same (EXAMPLE: women and men have the same average science knowledge scores.)
- the difference between two and three children = one child.
- the difference between eight and nine children also = one child.
- the difference between completing ninth grade and tenth grade is one year of school
- the difference between completing junior and senior year of college is one year of school
- In addition to all the properties of nominal, ordinal, and interval variables, ratio variables also have a fixed/non-arbitrary zero point. Non arbitrary means that it is impossible to go below a score of zero for that variable. For example, any bottom score on IQ or aptitude tests is created by human beings and not nature. On the other hand, scientists believe they have isolated an "absolute zero." You can't get colder than that.
Research Hypothesis In Psychology: Types, & Examples
Saul Mcleod, PhD
Editor-in-Chief for Simply Psychology
BSc (Hons) Psychology, MRes, PhD, University of Manchester
Saul Mcleod, PhD., is a qualified psychology teacher with over 18 years of experience in further and higher education. He has been published in peer-reviewed journals, including the Journal of Clinical Psychology.
Learn about our Editorial Process
Olivia Guy-Evans, MSc
Associate Editor for Simply Psychology
BSc (Hons) Psychology, MSc Psychology of Education
Olivia Guy-Evans is a writer and associate editor for Simply Psychology. She has previously worked in healthcare and educational sectors.
On This Page:
A research hypothesis, in its plural form “hypotheses,” is a specific, testable prediction about the anticipated results of a study, established at its outset. It is a key component of the scientific method .
Hypotheses connect theory to data and guide the research process towards expanding scientific understanding
Some key points about hypotheses:
- A hypothesis expresses an expected pattern or relationship. It connects the variables under investigation.
- It is stated in clear, precise terms before any data collection or analysis occurs. This makes the hypothesis testable.
- A hypothesis must be falsifiable. It should be possible, even if unlikely in practice, to collect data that disconfirms rather than supports the hypothesis.
- Hypotheses guide research. Scientists design studies to explicitly evaluate hypotheses about how nature works.
- For a hypothesis to be valid, it must be testable against empirical evidence. The evidence can then confirm or disprove the testable predictions.
- Hypotheses are informed by background knowledge and observation, but go beyond what is already known to propose an explanation of how or why something occurs.
Predictions typically arise from a thorough knowledge of the research literature, curiosity about real-world problems or implications, and integrating this to advance theory. They build on existing literature while providing new insight.
Types of Research Hypotheses
Alternative hypothesis.
The research hypothesis is often called the alternative or experimental hypothesis in experimental research.
It typically suggests a potential relationship between two key variables: the independent variable, which the researcher manipulates, and the dependent variable, which is measured based on those changes.
The alternative hypothesis states a relationship exists between the two variables being studied (one variable affects the other).
A hypothesis is a testable statement or prediction about the relationship between two or more variables. It is a key component of the scientific method. Some key points about hypotheses:
- Important hypotheses lead to predictions that can be tested empirically. The evidence can then confirm or disprove the testable predictions.
In summary, a hypothesis is a precise, testable statement of what researchers expect to happen in a study and why. Hypotheses connect theory to data and guide the research process towards expanding scientific understanding.
An experimental hypothesis predicts what change(s) will occur in the dependent variable when the independent variable is manipulated.
It states that the results are not due to chance and are significant in supporting the theory being investigated.
The alternative hypothesis can be directional, indicating a specific direction of the effect, or non-directional, suggesting a difference without specifying its nature. It’s what researchers aim to support or demonstrate through their study.
Null Hypothesis
The null hypothesis states no relationship exists between the two variables being studied (one variable does not affect the other). There will be no changes in the dependent variable due to manipulating the independent variable.
It states results are due to chance and are not significant in supporting the idea being investigated.
The null hypothesis, positing no effect or relationship, is a foundational contrast to the research hypothesis in scientific inquiry. It establishes a baseline for statistical testing, promoting objectivity by initiating research from a neutral stance.
Many statistical methods are tailored to test the null hypothesis, determining the likelihood of observed results if no true effect exists.
This dual-hypothesis approach provides clarity, ensuring that research intentions are explicit, and fosters consistency across scientific studies, enhancing the standardization and interpretability of research outcomes.
Nondirectional Hypothesis
A non-directional hypothesis, also known as a two-tailed hypothesis, predicts that there is a difference or relationship between two variables but does not specify the direction of this relationship.
It merely indicates that a change or effect will occur without predicting which group will have higher or lower values.
For example, “There is a difference in performance between Group A and Group B” is a non-directional hypothesis.
Directional Hypothesis
A directional (one-tailed) hypothesis predicts the nature of the effect of the independent variable on the dependent variable. It predicts in which direction the change will take place. (i.e., greater, smaller, less, more)
It specifies whether one variable is greater, lesser, or different from another, rather than just indicating that there’s a difference without specifying its nature.
For example, “Exercise increases weight loss” is a directional hypothesis.
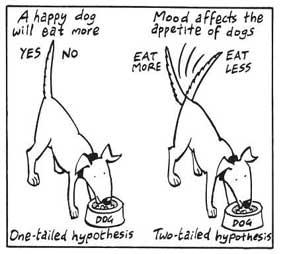
Falsifiability
The Falsification Principle, proposed by Karl Popper , is a way of demarcating science from non-science. It suggests that for a theory or hypothesis to be considered scientific, it must be testable and irrefutable.
Falsifiability emphasizes that scientific claims shouldn’t just be confirmable but should also have the potential to be proven wrong.
It means that there should exist some potential evidence or experiment that could prove the proposition false.
However many confirming instances exist for a theory, it only takes one counter observation to falsify it. For example, the hypothesis that “all swans are white,” can be falsified by observing a black swan.
For Popper, science should attempt to disprove a theory rather than attempt to continually provide evidence to support a research hypothesis.
Can a Hypothesis be Proven?
Hypotheses make probabilistic predictions. They state the expected outcome if a particular relationship exists. However, a study result supporting a hypothesis does not definitively prove it is true.
All studies have limitations. There may be unknown confounding factors or issues that limit the certainty of conclusions. Additional studies may yield different results.
In science, hypotheses can realistically only be supported with some degree of confidence, not proven. The process of science is to incrementally accumulate evidence for and against hypothesized relationships in an ongoing pursuit of better models and explanations that best fit the empirical data. But hypotheses remain open to revision and rejection if that is where the evidence leads.
- Disproving a hypothesis is definitive. Solid disconfirmatory evidence will falsify a hypothesis and require altering or discarding it based on the evidence.
- However, confirming evidence is always open to revision. Other explanations may account for the same results, and additional or contradictory evidence may emerge over time.
We can never 100% prove the alternative hypothesis. Instead, we see if we can disprove, or reject the null hypothesis.
If we reject the null hypothesis, this doesn’t mean that our alternative hypothesis is correct but does support the alternative/experimental hypothesis.
Upon analysis of the results, an alternative hypothesis can be rejected or supported, but it can never be proven to be correct. We must avoid any reference to results proving a theory as this implies 100% certainty, and there is always a chance that evidence may exist which could refute a theory.
How to Write a Hypothesis
- Identify variables . The researcher manipulates the independent variable and the dependent variable is the measured outcome.
- Operationalized the variables being investigated . Operationalization of a hypothesis refers to the process of making the variables physically measurable or testable, e.g. if you are about to study aggression, you might count the number of punches given by participants.
- Decide on a direction for your prediction . If there is evidence in the literature to support a specific effect of the independent variable on the dependent variable, write a directional (one-tailed) hypothesis. If there are limited or ambiguous findings in the literature regarding the effect of the independent variable on the dependent variable, write a non-directional (two-tailed) hypothesis.
- Make it Testable : Ensure your hypothesis can be tested through experimentation or observation. It should be possible to prove it false (principle of falsifiability).
- Clear & concise language . A strong hypothesis is concise (typically one to two sentences long), and formulated using clear and straightforward language, ensuring it’s easily understood and testable.
Consider a hypothesis many teachers might subscribe to: students work better on Monday morning than on Friday afternoon (IV=Day, DV= Standard of work).
Now, if we decide to study this by giving the same group of students a lesson on a Monday morning and a Friday afternoon and then measuring their immediate recall of the material covered in each session, we would end up with the following:
- The alternative hypothesis states that students will recall significantly more information on a Monday morning than on a Friday afternoon.
- The null hypothesis states that there will be no significant difference in the amount recalled on a Monday morning compared to a Friday afternoon. Any difference will be due to chance or confounding factors.
More Examples
- Memory : Participants exposed to classical music during study sessions will recall more items from a list than those who studied in silence.
- Social Psychology : Individuals who frequently engage in social media use will report higher levels of perceived social isolation compared to those who use it infrequently.
- Developmental Psychology : Children who engage in regular imaginative play have better problem-solving skills than those who don’t.
- Clinical Psychology : Cognitive-behavioral therapy will be more effective in reducing symptoms of anxiety over a 6-month period compared to traditional talk therapy.
- Cognitive Psychology : Individuals who multitask between various electronic devices will have shorter attention spans on focused tasks than those who single-task.
- Health Psychology : Patients who practice mindfulness meditation will experience lower levels of chronic pain compared to those who don’t meditate.
- Organizational Psychology : Employees in open-plan offices will report higher levels of stress than those in private offices.
- Behavioral Psychology : Rats rewarded with food after pressing a lever will press it more frequently than rats who receive no reward.

Related Articles
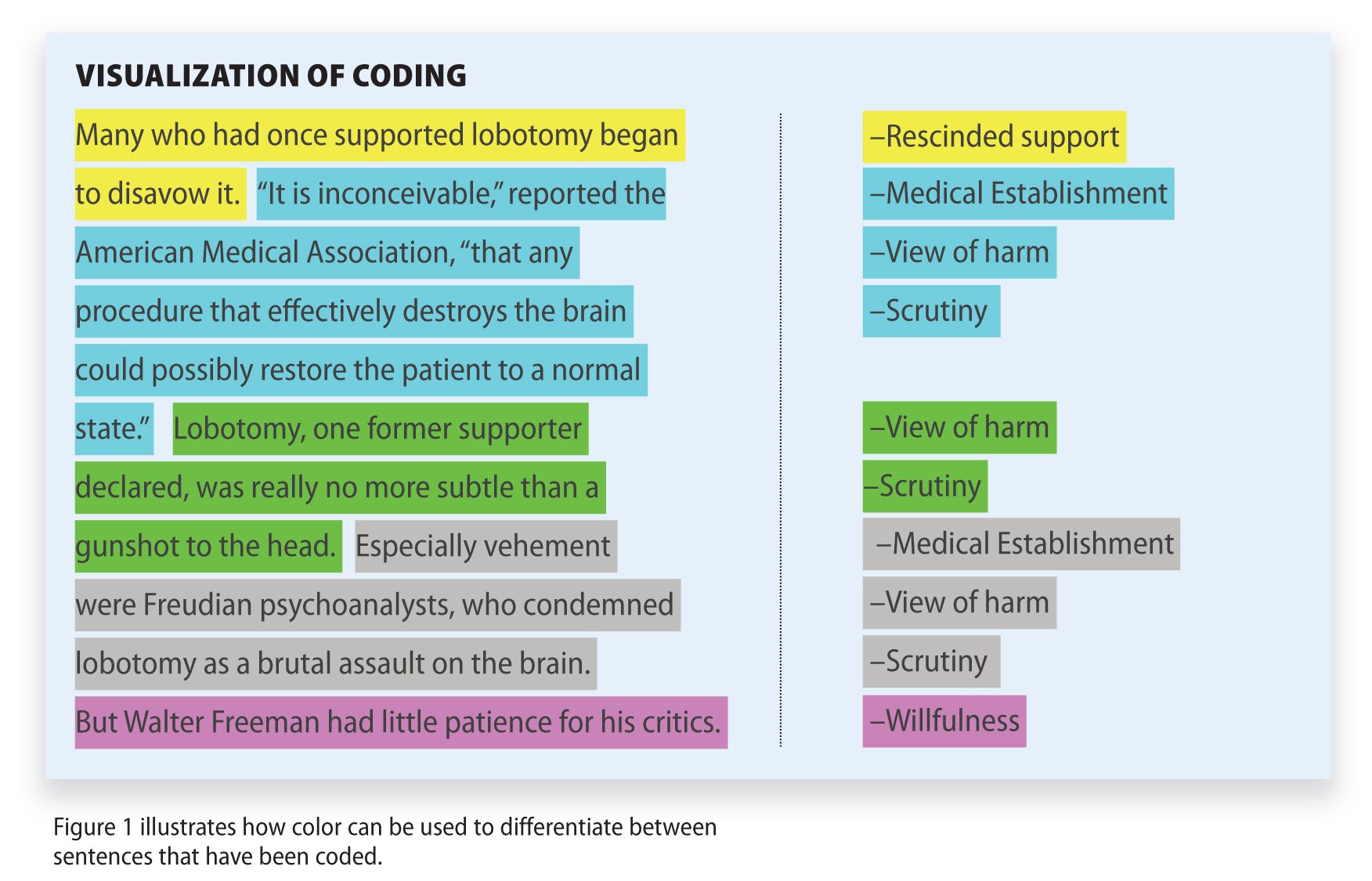
Research Methodology
Qualitative Data Coding
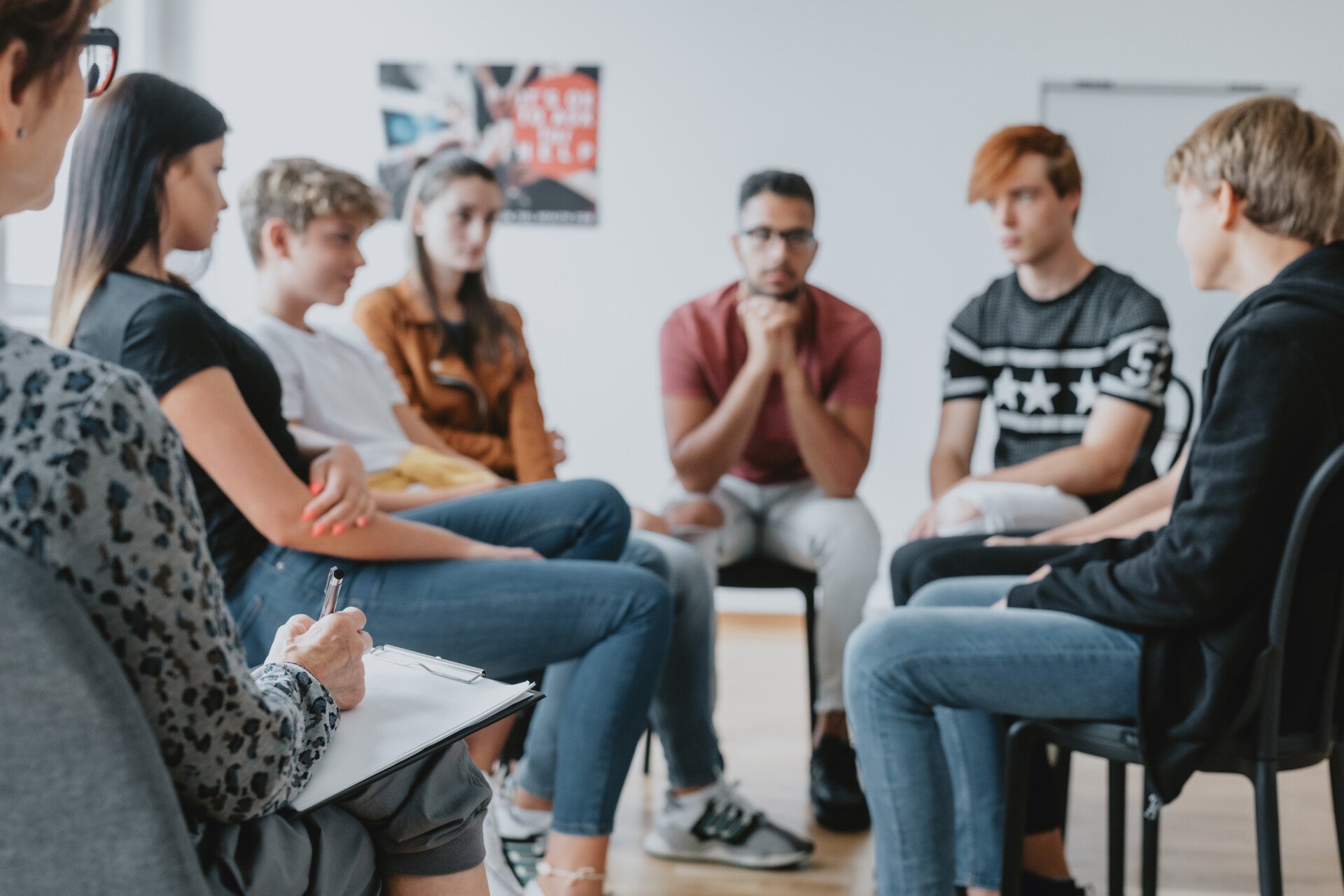
What Is a Focus Group?
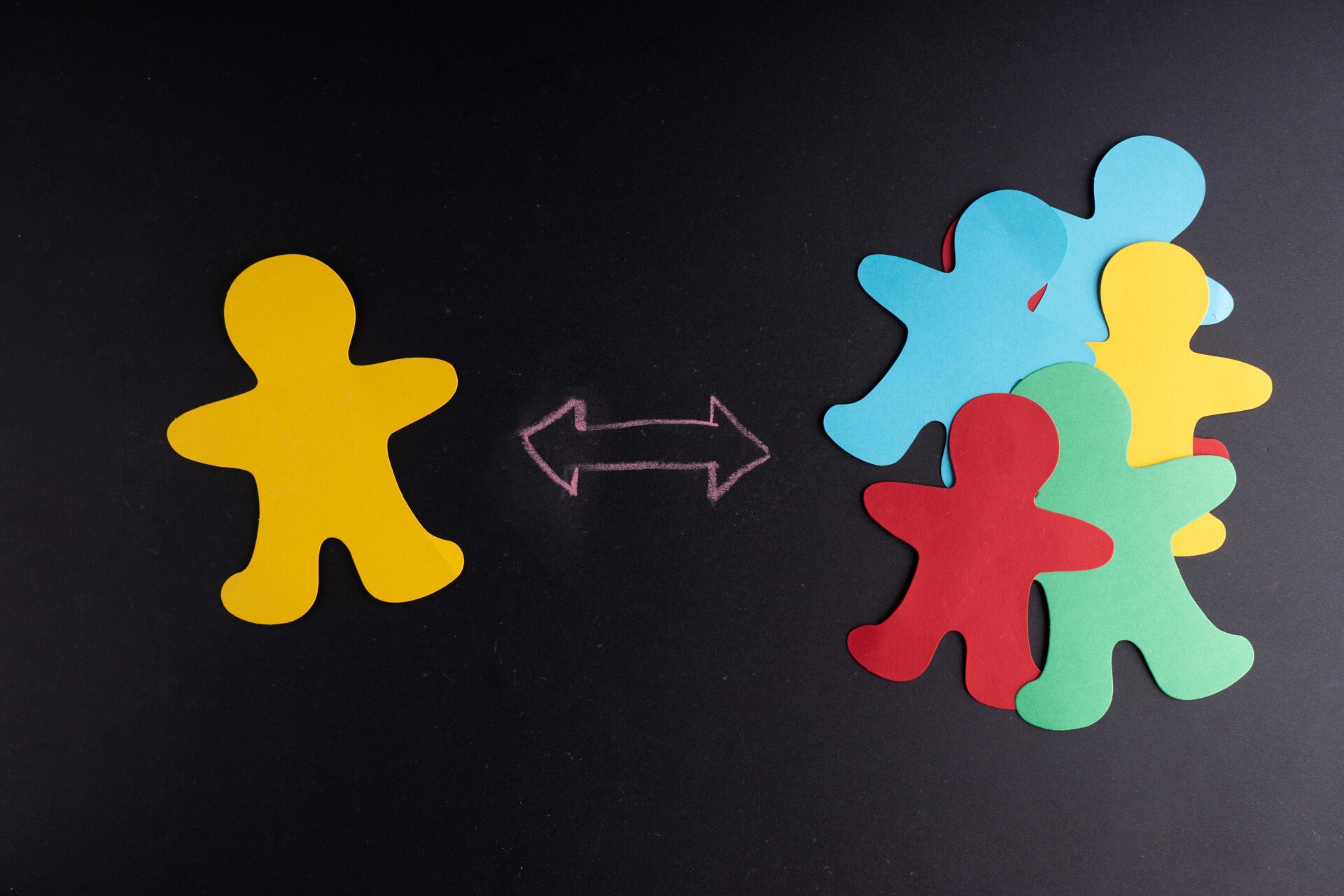
Cross-Cultural Research Methodology In Psychology
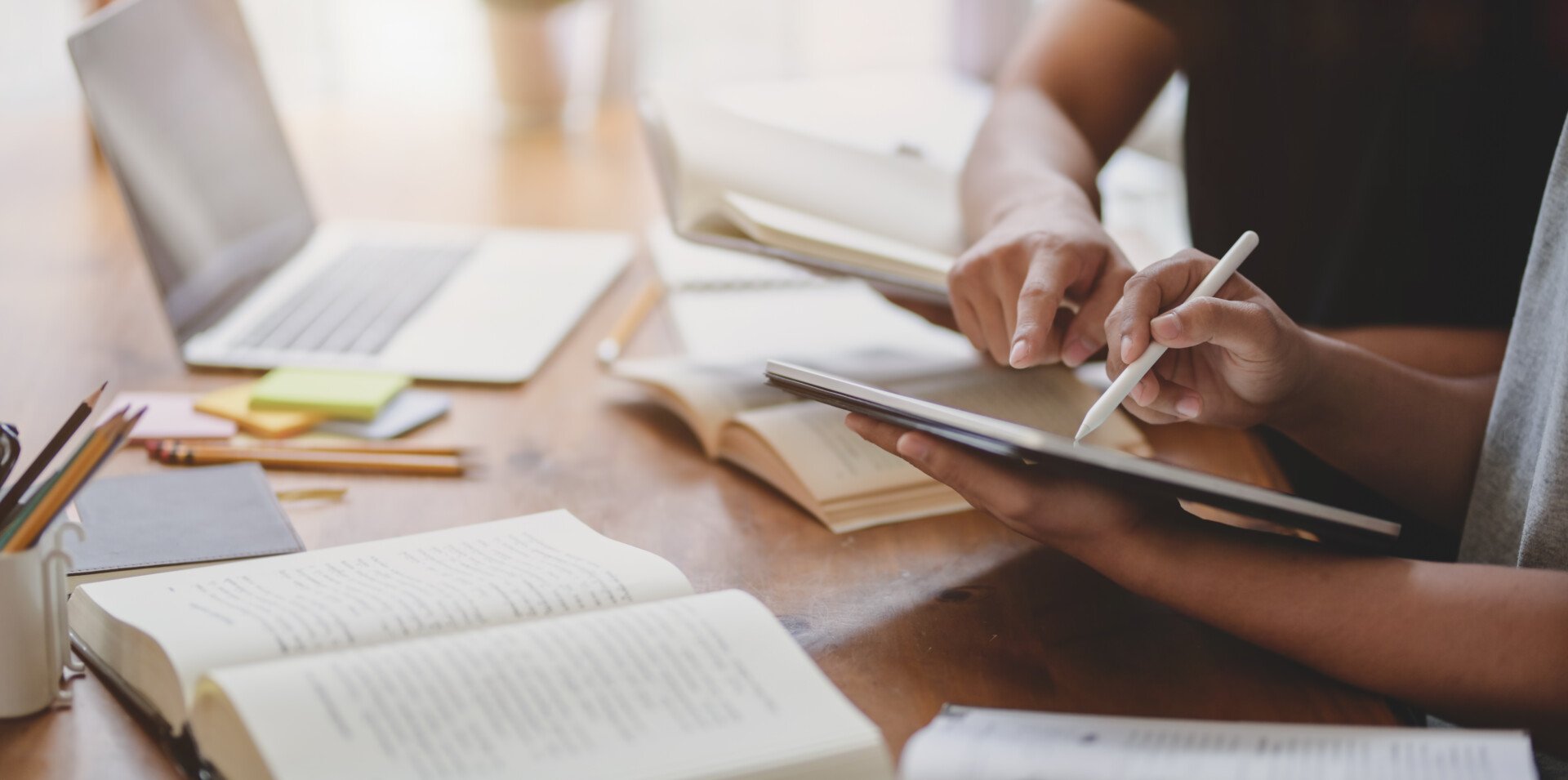
What Is Internal Validity In Research?
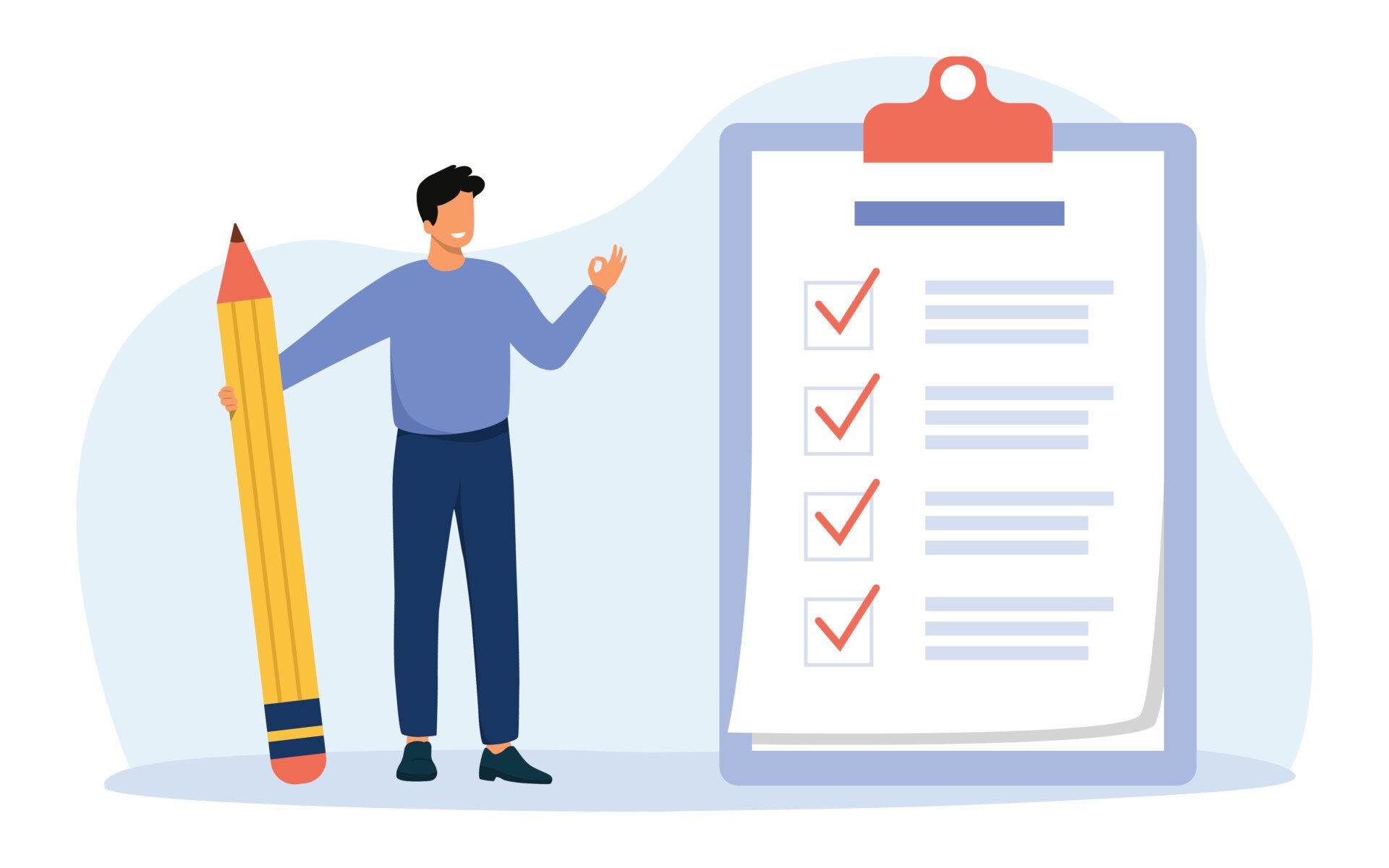
Research Methodology , Statistics
What Is Face Validity In Research? Importance & How To Measure
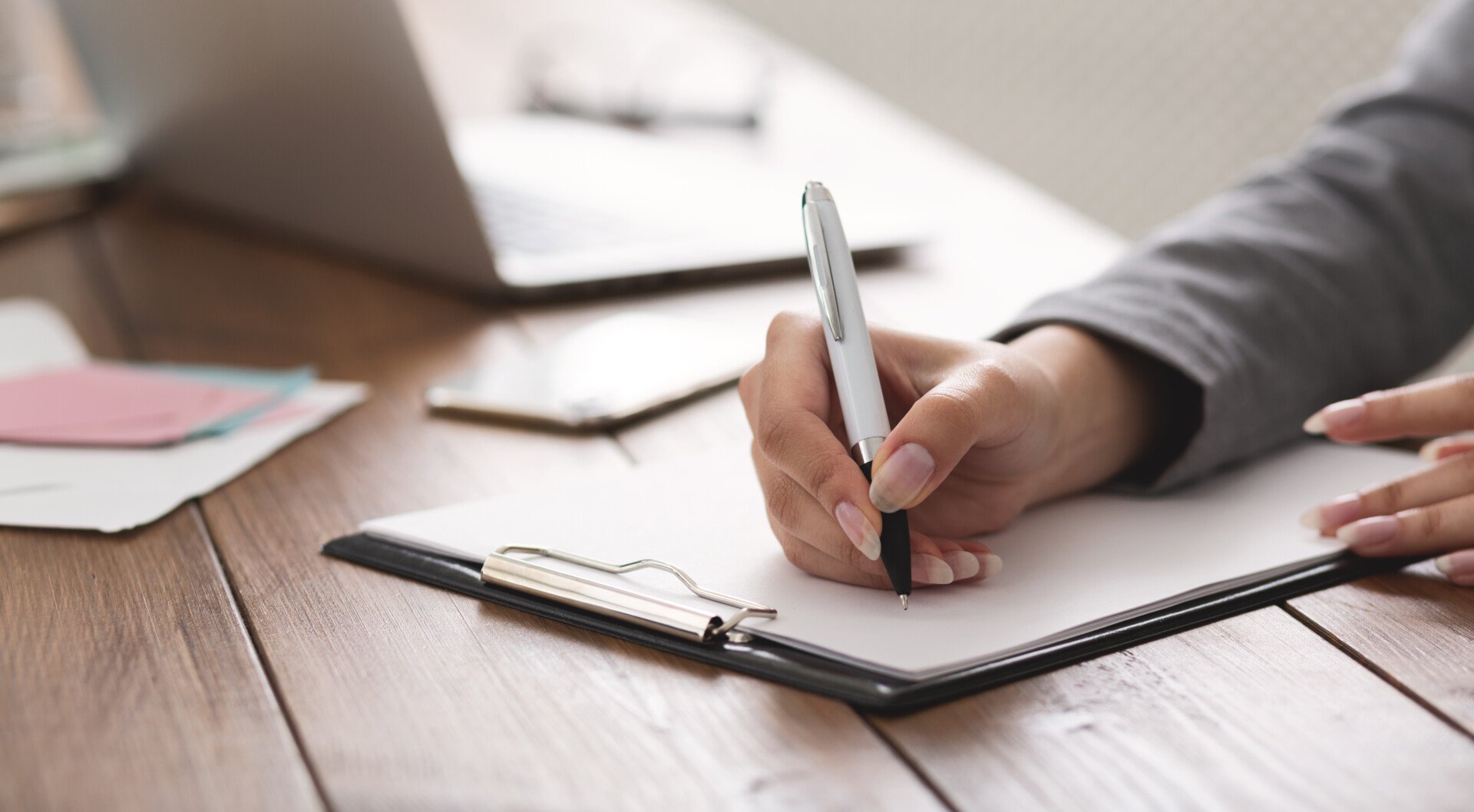
Criterion Validity: Definition & Examples
2.5 Designing a Research Study
Learning objectives.
- Define the concept of a variable, distinguish quantitative from categorical variables, and give examples of variables that might be of interest to psychologists.
- Explain the difference between a population and a sample.
- Distinguish between experimental and non-experimental research.
- Distinguish between lab studies, field studies, and field experiments.
Identifying and Defining the Variables and Population
Variables and operational definitions.
Part of generating a hypothesis involves identifying the variables that you want to study and operationally defining those variables so that they can be measured. Research questions in psychology are about variables. A variable is a quantity or quality that varies across people or situations. For example, the height of the students enrolled in a university course is a variable because it varies from student to student. The chosen major of the students is also a variable as long as not everyone in the class has declared the same major. Almost everything in our world varies and as such thinking of examples of constants (things that don’t vary) is far more difficult. A rare example of a constant is the speed of light. Variables can be either quantitative or categorical. A quantitative variable is a quantity, such as height, that is typically measured by assigning a number to each individual. Other examples of quantitative variables include people’s level of talkativeness, how depressed they are, and the number of siblings they have. A categorical variable is a quality, such as chosen major, and is typically measured by assigning a category label to each individual (e.g., Psychology, English, Nursing, etc.). Other examples include people’s nationality, their occupation, and whether they are receiving psychotherapy.
After the researcher generates his or her hypothesis and selects the variables he or she wants to manipulate and measure, the researcher needs to find ways to actually measure the variables of interest. This requires an operational definition —a definition of the variable in terms of precisely how it is to be measured. Most variables that researchers are interested in studying cannot be directly observed or measured and this poses a problem because empiricism (observation) is at the heart of the scientific method. Operationally defining a variable involves taking an abstract construct like depression that cannot be directly observed and transforming it into something that can be directly observed and measured. Most variables can be operationally defined in many different ways. For example, depression can be operationally defined as people’s scores on a paper-and-pencil depression scale such as the Beck Depression Inventory, the number of depressive symptoms they are experiencing, or whether they have been diagnosed with major depressive disorder. Researchers are wise to choose an operational definition that has been used extensively in the research literature.
Sampling and Measurement
In addition to identifying which variables to manipulate and measure, and operationally defining those variables, researchers need to identify the population of interest. Researchers in psychology are usually interested in drawing conclusions about some very large group of people. This is called the population . It could be all American teenagers, children with autism, professional athletes, or even just human beings—depending on the interests and goals of the researcher. But they usually study only a small subset or sample of the population. For example, a researcher might measure the talkativeness of a few hundred university students with the intention of drawing conclusions about the talkativeness of men and women in general. It is important, therefore, for researchers to use a representative sample—one that is similar to the population in important respects.
One method of obtaining a sample is simple random sampling , in which every member of the population has an equal chance of being selected for the sample. For example, a pollster could start with a list of all the registered voters in a city (the population), randomly select 100 of them from the list (the sample), and ask those 100 whom they intend to vote for. Unfortunately, random sampling is difficult or impossible in most psychological research because the populations are less clearly defined than the registered voters in a city. How could a researcher give all American teenagers or all children with autism an equal chance of being selected for a sample? The most common alternative to random sampling is convenience sampling , in which the sample consists of individuals who happen to be nearby and willing to participate (such as introductory psychology students). Of course, the obvious problem with convenience sampling is that the sample might not be representative of the population and therefore it may be less appropriate to generalize the results from the sample to that population.
Experimental vs. Non-Experimental Research
The next step a researcher must take is to decide which type of approach he or she will use to collect the data. As you will learn in your research methods course there are many different approaches to research that can be divided in many different ways. One of the most fundamental distinctions is between experimental and non-experimental research.
Experimental Research
Researchers who want to test hypotheses about causal relationships between variables (i.e., their goal is to explain) need to use an experimental method. This is because the experimental method is the only method that allows us to determine causal relationships. Using the experimental approach, researchers first manipulate one or more variables while attempting to control extraneous factors, and then they measure how the manipulated variables affect participants’ responses.
The terms independent variable and dependent variable are used in the context of experimental research. The independent variable is the variable the experimenter manipulates (it is the presumed cause) and the dependent variable is the variable the experimenter measures (it is the presumed effect).
Confounds are also a term that is rather specific to experimental research. A confound is an extraneous variable (so a variable other than the independent variable and dependent variable) that systematically varies along with the variables under investigation and therefore provides an alternative explanation for the results. When researchers design an experiment they need to ensure that they control for confounds; they need to ensure that extraneous variables don’t become confounding variables because in order to make a causal conclusion they need to make sure alternative explanations for the results have been ruled out.
As an example, if we manipulate the lighting in the room and examine the effects of that manipulation on workers’ productivity, then the lighting conditions (bright lights vs. dim lights) would be considered the independent variable and the workers’ productivity would be considered the dependent variable. If the bright lights are noisy then that noise would be a confound since the noise would be present whenever the lights are bright and the noise would be absent when the lights are dim. If noise is varying systematically with light then we wouldn’t know if a difference in worker productivity across the two lighting conditions is due to noise or light. So confounds are bad, they disrupt our ability to make causal conclusions about the nature of the relationship between variables. However, if there is noise in the room both when the lights are on and when the lights are off then noise is merely an extraneous variable (it is a variable other than the independent or dependent variable) and we don’t worry much about extraneous variables. This is because unless a variable varies systematically with the manipulated independent variable it cannot be a competing explanation for the results.
Non-Experimental Research
Researchers who are simply interested in describing characteristics of people, describing relationships between variables, and using those relationships to make predictions can use non-experimental or descriptive research. Using the non-experimental approach, the researcher simply measures variables as they naturally occur, but they do not manipulate them. For instance, if I just measured the number of traffic fatalities in America last year that involved the use of a cell phone but I did not actually manipulate cell phone use then this would be categorized as non-experimental research. Alternatively, if I stood at a busy intersection and recorded drivers’ genders and whether or not they were using a cell phone when they passed through the intersection to see whether men or women are more likely to use a cell phone when driving, then this would be non-experimental research. It is important to point out that non-experimental does not mean nonscientific. Non-experimental research is scientific in nature. It can be used to fulfill two of the three goals of science (to describe and to predict). However, unlike with experimental research, we cannot make causal conclusions using this method; we cannot say that one variable causes another variable using this method.
Laboratory vs. Field Research
The next major distinction between research methods is between laboratory and field studies. A laboratory study is a study that is conducted in the laboratory environment. In contrast, a field study is a study that is conducted in the real-world, in a natural environment.
Laboratory experiments typically have high internal validity . Internal validity refers to the degree to which we can confidently infer a causal relationship between variables. When we conduct an experimental study in a laboratory environment we have very high internal validity because we manipulate one variable while controlling all other outside extraneous variables. When we manipulate an independent variable and observe an effect on a dependent variable and we control for everything else so that the only difference between our experimental groups or conditions is the one manipulated variable then we can be quite confident that it is the independent variable that is causing the change in the dependent variable. In contrast, because field studies are conducted in the real-world, the experimenter typically has less control over the environment and potential extraneous variables, and this decreases internal validity, making it less appropriate to arrive at causal conclusions.
But there is typically a trade-off between internal and external validity . When internal validity is high, external validity tends to be low; and when internal validity is low, external validity tends to be high. External validity simply refers to the degree to which we can generalize the findings to other circumstances or settings, like the real-world environment. So laboratory studies are typically low in external validity, while field studies are typically high in external validity. Since field studies are conducted in the real-world environment it is far more appropriate to generalize the findings to that real-world environment than when the research is conducted in the more artificial sterile laboratory.
Finally, there are field studies which are nonexperimental in nature because nothing is manipulated. But there are also field experiments where an independent variable is manipulated in a natural setting and extraneous variables are controlled. Depending on their overall quality and the level of control of extraneous variables, such field experiments can have high external and high internal validity.

Share This Book
- Increase Font Size
Operational Definition in Research

In addition to careful planning, one of the keys to successful research is the use of operational definitions in measuring the concepts and variables we are studying or the terms we are using in our research documents.
The operational definition is the specific way a variable is measured in a particular study.
It is critical to operationally define a variable to lend credibility to the methodology and ensure the reproducibility of the study’s results. Another study may identify the same variable differently, making it difficult to compare the results of these two studies.
To begin with, the operational definition is different from the dictionary definition, which is often conceptual, descriptive, and consequently imprecise.
In contrast, an operational definition gives an obvious, precise, and communicable meaning to a concept used to ensure comprehensive knowledge of the idea by specifying how the idea is measured and applied within a particular set of circumstances.
This definition highlights two important things about an operational definition:
- It gives a precise meaning to the spoken or written word, forming a ‘common language between two or more people.
- It defines how a term, word, or phrase is used when it is applied in a specific context. This implies that a word may have different meanings when used in different situations.
An operational definition must be valid, which implies that it should measure what it is supposed to measure. It must also be reliable, meaning that the results should be the same even when done by different people or by one person at different times.
An operational definition ensures a succinct description of concepts and terms applied to a specific situation to facilitate the collection of meaningful and standardized data.
When collecting data, it is important to define every term very clearly to assure all those who collect and analyze the data have the same understanding.
Therefore, operational definitions should be very precise and framed to avoid variation and confusion in interpretation.
Suppose, for example, we want to know whether a professional journal may be considered as a ‘standard journal’ or not. Here is a possible operational definition of a standard journal.
We set in advance that a journal is considered standard if
- It contains an ISSN number.
- It is officially published from a public or private university or from an internationally recognized research organization;
- It is peer-reviewed;
- It has a recognized editorial /advisory board;
- It is published on a regular basis at least once a year,
- It has an impact factor.
Thus, the researcher knows exactly what to look for when determining whether a published journal is standard or not.
The operational definition of literacy rate as adopted by the Bangladesh Bureau of Statistics (BBS) in their Vital Registration System is as follows:
“Percentage of the population of 7 years and above who can write a letter among the total population.”
In sum, an operational definition serves four purposes:
- It establishes the rules and procedures the researcher uses to measure the variable.
- It provides unambiguous and consistent meaning to terms/variables that can be interpreted differently.
- It makes the collection of data and analysis more focused and efficient.
- It guides what type of data and information we are looking for.
By operationally defining a variable, a researcher can communicate a common methodology to another researcher.
Operational definitions lay down the ground rules and procedures that the investigator will use to observe and record behavior and write down facts without bias.
The sole purpose of defining the variables operationally is to keep them unambiguous, thereby reducing errors.
How to operationalize a variable?
There is no hard and first rule for operationally defining a variable. Operational definitions may vary depending on your purpose and how you measure them.
Neither are there any universally accepted definitions of all the variables. A researcher can logically choose a definition of a variable that will serve his or her purpose.
Whenever possible, operational definitions used by others in their work of good standing could be used to compare the results.
Suppose a study classifies students according to their grades: A, B, C, etc. But the task is not easy if you must determine which students fall in which grade since there is seldom any universal rule for grades.
To do this, you need an operational definition.
In the goiter prevalence survey of 2004a person was classified as iodine deficient for a urinary iodine excretion (IUE) <100 pg/L and severely iodine-deficient for a urinary iodine excretion (IUE) <20 pg/L. One may choose a different threshold, too, in defining the iodine deficiency.
As another example, suppose it is intended to assess mothers’ knowledge of family planning. A set of 20 questions has been designed such that for every correct answer, a score of 1 will be given to the respondents.
Suppose further that we want to make 4 categories of knowledge: ‘no knowledge,’ ‘low knowledge,’ ‘medium knowledge,’ and ‘high knowledge.’ We decide to define these knowledge levels as follows:
One might, however, choose a different range of scores to define the knowledge levels.
Based on the body mass index (BMI), for example, the international health risk classification is operationally defined as follows
For the classification of nutritional status, internationally accepted categories already exist, which are based on the so-called NCHS/WHO standard growth curves. For the indicator ‘weight-for-age’, for example, children are assessed to be
- Well-nourished (normal) if they are above 80% of the standard.
- Moderately malnourished (moderate underweight) if they are between 60% and 80% of the standard.
- Severely malnourished (severely under-weight) if they are below 60% of the standard.
The nutritional status can also be classified based on the weight-for-age Z-score (WAZ) values. The Z- score of cut-off values are:
A farmer may be classified as landless, medium, and big, depending on his possession of landholding size. One such classification is as follows:
Similarly, a business firm may be classified as large, medium, or small in terms of its investment, capital, and a number of employees or assets, which may vary widely by the type of business firm.
In demographic research, a person may be categorized as a child, those who are under five years of age, adolescents in the age range 12-19, adults aged 20-65, and old aged 65 and over.
Not only that, but variables also need operationally defined. The terms that indicate the relationship between variables need to be defined.
For example, in many stated hypotheses, we use such terms as ‘frequent,’ ‘greater than,’ ‘less than,’ ‘significant,’ ‘higher than,’ ‘favorable,’ ‘different,’ ‘efficient,’ and the like.
These terms must be clearly and unambiguously defined so that they make sense and allow the researcher to measure the variables in question.
Consider the following hypothesis.
- Visits of Family Welfare Assistants will motivate the women resulting in significantly higher use of contraceptives.
‘Visit’ is the independent variable to which we might associate numbers 0, 1, and 2, to mean the frequency of visits made during a stipulated period. The term ‘higher use’ may mean a higher rate (dependent variable) than before.
This can be measured as the difference between the present and the past rate or between a post-test and a pretest measurement:
Difference =.Average CPR (pretest) – Average CPR (posttest)
But how much ‘higher’ will be regarded as significant? Thus the term’ significant’ needs to be defined clearly. We may decide to statistically verify at a 5% level with a probability of at least 95% that the difference in usage level is significant.
Thus, the operational definition of terms tells us the meaning of their use and the way of measuring the difference and testing its statistical significance, thereby accepting or rejecting the hypothesis.
In a study on the comparison of the performance of nationalized commercial banks (NCB) and private commercial banks (PCB) by Hasan (1995), one of the hypotheses was of the following form:
- PCBs are more efficient than NCBs in private deposit collection.
The term ‘more efficient’ was assessed by testing the statistical significance of the differences in the mean deposits of the two banks in question at a 5% level.
The concept of operational definition also applies to other technical terms that are not universally defined.
Here are some examples of such terms with their operational definitions:
Operational Definition of Terms
What is the primary purpose of an operational definition in research?
The primary purpose of an operational definition is to provide a clear, precise, and communicable meaning to a concept, ensuring comprehensive understanding by specifying how the concept is measured and applied within a specific set of circumstances.
How does an operational definition differ from a dictionary definition?
While a dictionary definition is often conceptual, descriptive, and may be imprecise, an operational definition offers a specific, clear, and applicable meaning to a term or concept when used in a particular context.
Why is it essential to operationally define a variable in research?
Operationally defining a variable is crucial to lend credibility to the research methodology, ensure reproducibility of the study’s results, and provide a common methodology for communication between researchers.
What are the key characteristics of a good operational definition?
A good operational definition should be valid (measuring what it’s supposed to measure), reliable (providing consistent results across different instances or by different people), precise, and framed to avoid variation and confusion in interpretation.
Can operational definitions vary between studies or researchers?
Yes, operational definitions can vary depending on the study’s purpose and how variables are measured. There are no universally accepted definitions for all variables, allowing researchers to choose definitions that best serve their objectives.
Why is it important to define terms when collecting data?
Defining terms clearly ensures that everyone involved in collecting and analyzing the data has the same understanding, making the data collection process more focused and efficient and reducing potential errors or misinterpretations.
How can operational definitions help in comparing results across different studies?
By providing clear and specific criteria for measuring variables, operational definitions allow for a standardized approach. If multiple studies use the same or similar operational definitions, it becomes easier to compare and contrast their results.

Scientific Research and Methodology
2.2 conceptual and operational definitions.
Research studies usually include terms that must be carefully and precisely defined, so that others know exactly what has been done and there are no ambiguities. Two types of definitions can be given: conceptual definitions and operational definitions .
Loosely speaking, a conceptual definition explains what to measure or observe (what a word or a term means for your study), and an operational definitions defines exactly how to measure or observe it.
For example, in a study of stress in students during a university semester. A conceptual definition would describe what is meant by ‘stress.’ An operational definition would describe how the ‘stress’ would be measured.
Sometimes the definitions themselves aren’t important, provided a clear definition is given. Sometimes, commonly-accepted definitions exist, so should be used unless there is a good reason to use a different definition (for example, in criminal law, an ‘adult’ in Australia is someone aged 18 or over ).
Sometimes, a commonly-accepted definition does not exist, so the definition being used should be clearly articulated.
Example 2.2 (Operational and conceptual definitions) Players and fans have become more aware of concussions and head injuries in sport. A Conference on concussion in sport developed this conceptual definition ( McCrory et al. 2013 ) :
Concussion is a brain injury and is defined as a complex pathophysiological process affecting the brain, induced by biomechanical forces. Several common features that incorporate clinical, pathologic and biomechanical injury constructs that may be utilised in defining the nature of a concussive head injury include: Concussion may be caused either by a direct blow to the head, face, neck or elsewhere on the body with an “impulsive” force transmitted to the head. Concussion typically results in the rapid onset of short-lived impairment of neurological function that resolves spontaneously. However, in some cases, symptoms and signs may evolve over a number of minutes to hours. Concussion may result in neuropathological changes, but the acute clinical symptoms largely reflect a functional disturbance rather than a structural injury and, as such, no abnormality is seen on standard structural neuroimaging studies. Concussion results in a graded set of clinical symptoms that may or may not involve loss of consciousness. Resolution of the clinical and cognitive symptoms typically follows a sequential course. However, it is important to note that in some cases symptoms may be prolonged.
While this is all helpful… it does not explain how to identify a player with concussion during a game.
Rugby decided on this operational definition ( Raftery et al. 2016 ) :
… a concussion applies with any of the following: The presence, pitch side, of any Criteria Set 1 signs or symptoms (table 1)… [ Note : This table includes symptoms such as ‘convulsion,’ ‘clearly dazed,’ etc.]; An abnormal post game, same day assessment…; An abnormal 36–48 h assessment…; The presence of clinical suspicion by the treating doctor at any time…
Example 2.3 (Operational and conceptual definitions) Consider a study requiring water temperature to be measured.
An operational definition would explain how the temperature is measured: the thermometer type, how the thermometer was positioned, how long was it left in the water, and so on.

Example 2.4 (Operational definitions) Consider a study measuring stress in first-year university students.
Stress cannot be measured directly, but could be assessed using a survey (like the Perceived Stress Scale (PSS) ( Cohen et al. 1983 ) ).
The operational definition of stress is the score on the ten-question PSS. Other means of measuring stress are also possible (such as heart rate or blood pressure).
Meline ( 2006 ) discusses five studies about stuttering, each using a different operational definition:
- Study 1: As diagnosed by speech-language pathologist.
- Study 2: Within-word disfluences greater than 5 per 150 words.
- Study 3: Unnatural hesitation, interjections, restarted or incomplete phrases, etc.
- Study 4: More than 3 stuttered words per minute.
- Study 5: State guidelines for fluency disorders.
A study of snacking in Australia ( Fayet-Moore et al. 2017 ) used this operational definition of ‘snacking’:
…an eating occasion that occurred between meals based on time of day. — Fayet-Moore et al. ( 2017 ) (p. 3)
A study examined the possible relationship between the ‘pace of life’ and the incidence of heart disease ( Levine 1990 ) in 36 US cities. The researchers used four different operational definitions for ‘pace of life’ (remember the article was published in 1990!):
- The walking speed of randomly chosen pedestrians.
- The speed with which bank clerks gave ‘change for two $20 bills or [gave] two $20 bills for change.’
- The talking speed of postal clerks.
- The proportion of men and women wearing a wristwatch.
None of these perfectly measure ‘pace of life,’ of course. Nonetheless, the researchers found that, compared to people on the West Coast,
… people in the Northeast walk faster, make change faster, talk faster and are more likely to wear a watch… — Levine ( 1990 ) (p. 455)
Theory, hypothesis, and operationalization
Approach, theory, model.
First, you have to determine the general state of knowledge (or state of the art) as regards a certain objective. Are there already relevant attempts of explanation (models, theories, approaches, debates)? Many times there are theories already existing that provide a basis for discussing or looking at a certain problem.
When choosing a certain approach to explain complex circumstances, specific aspects of your problem area will be highlighted more prominently. Deciding on an approach means considering which questions can then be answered best. After choosing an approach it is necessary to use its related methods consequently.
Examples for approaches: «Education is an important prerequisite for a society's economic development» or «Earnings from tourism support national economy.»
Hypotheses and presumptions
Hypotheses are assumptions that could explain reality or - in other words - that could be the answer to your question. Such an assumption is based on the current state of research; it therefore delivers an answer that is theoretically possible («proposed solution») and applies at least to some extent to the question posed. When dealing with complex topics it is sometimes easier to develop a number of subordinate working hypotheses from just a few main hypotheses.
Example for a hypothesis: «Tourism offers children the possibility to earn money instead of going to school» or «The more tourists the fewer the children are going to school.»
Not all research projects are conducted by means of methods to test hypotheses. In social research, for example, there are reconstructive or interpretive methods as well. Here you try to explain and understand people's actions based on their interpretation of certain issues ( Bohnsack 2000: 12–13). However, also with such an approach researchers use hypotheses or presumptions to structure their work. The point is not to finally acknowledge or reject those hypotheses. You rather search for explanations that are plausible and comprehensible.
Example for a presumption: «In developing countries parents are skeptical about their children working for the tourism industry.»
However, most of the time one again acts on theses or presumptions. The point is not to finally acknowledge or reject those assumptions. One rather searches for explanations that are plausible and comprehensible.
Example for an explanation: «Parents don't worry about their children not going to school; they are afraid of losing their status when earning less than their children.»
Operationalization
It is necessary to operationalize the terms used in scientific research (that means particularly the central terms of a hypothesis). In order to guarantee the viability of a research method you have to define first which data will be collected by means of which methods. Research operations have to be specified to comprehend a subject matter in the first place ( Bopp 2000: 21). In order to turn the operationalized term into something manageable you determine its exact meaning during a research process.
Example for an operationalization: «When compared to other areas, tourist destinations are areas where children are less likely to go to school.»
Online Guidelines for Academic Research and Writing : The academic research process : Theory, hypothesis, and operationalization
Update: 28.10.2021 ( eLML ) - Contact - Print (PDF) - © OLwA 2011 (Creative Commons)
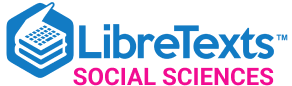
- school Campus Bookshelves
- menu_book Bookshelves
- perm_media Learning Objects
- login Login
- how_to_reg Request Instructor Account
- hub Instructor Commons
Margin Size
- Download Page (PDF)
- Download Full Book (PDF)
- Periodic Table
- Physics Constants
- Scientific Calculator
- Reference & Cite
- Tools expand_more
- Readability
selected template will load here
This action is not available.
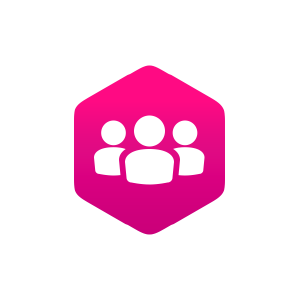
2.2: Concepts, Constructs, and Variables
- Last updated
- Save as PDF
- Page ID 26212
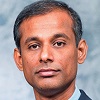
- Anol Bhattacherjee
- University of South Florida via Global Text Project
\( \newcommand{\vecs}[1]{\overset { \scriptstyle \rightharpoonup} {\mathbf{#1}} } \)
\( \newcommand{\vecd}[1]{\overset{-\!-\!\rightharpoonup}{\vphantom{a}\smash {#1}}} \)
\( \newcommand{\id}{\mathrm{id}}\) \( \newcommand{\Span}{\mathrm{span}}\)
( \newcommand{\kernel}{\mathrm{null}\,}\) \( \newcommand{\range}{\mathrm{range}\,}\)
\( \newcommand{\RealPart}{\mathrm{Re}}\) \( \newcommand{\ImaginaryPart}{\mathrm{Im}}\)
\( \newcommand{\Argument}{\mathrm{Arg}}\) \( \newcommand{\norm}[1]{\| #1 \|}\)
\( \newcommand{\inner}[2]{\langle #1, #2 \rangle}\)
\( \newcommand{\Span}{\mathrm{span}}\)
\( \newcommand{\id}{\mathrm{id}}\)
\( \newcommand{\kernel}{\mathrm{null}\,}\)
\( \newcommand{\range}{\mathrm{range}\,}\)
\( \newcommand{\RealPart}{\mathrm{Re}}\)
\( \newcommand{\ImaginaryPart}{\mathrm{Im}}\)
\( \newcommand{\Argument}{\mathrm{Arg}}\)
\( \newcommand{\norm}[1]{\| #1 \|}\)
\( \newcommand{\Span}{\mathrm{span}}\) \( \newcommand{\AA}{\unicode[.8,0]{x212B}}\)
\( \newcommand{\vectorA}[1]{\vec{#1}} % arrow\)
\( \newcommand{\vectorAt}[1]{\vec{\text{#1}}} % arrow\)
\( \newcommand{\vectorB}[1]{\overset { \scriptstyle \rightharpoonup} {\mathbf{#1}} } \)
\( \newcommand{\vectorC}[1]{\textbf{#1}} \)
\( \newcommand{\vectorD}[1]{\overrightarrow{#1}} \)
\( \newcommand{\vectorDt}[1]{\overrightarrow{\text{#1}}} \)
\( \newcommand{\vectE}[1]{\overset{-\!-\!\rightharpoonup}{\vphantom{a}\smash{\mathbf {#1}}}} \)
We discussed in Chapter 1 that although research can be exploratory, descriptive, or explanatory, most scientific research tend to be of the explanatory type in that they search for potential explanations of observed natural or social phenomena. Explanations require development of concepts or generalizable properties or characteristics associated with objects, events, or people. While objects such as a person, a firm, or a car are not concepts, their specific characteristics or behavior such as a person’s attitude toward immigrants, a firm’s capacity for innovation, and a car’s weight can be viewed as concepts.
Knowingly or unknowingly, we use different kinds of concepts in our everyday conversations. Some of these concepts have been developed over time through our shared language. Sometimes, we borrow concepts from other disciplines or languages to explain a phenomenon of interest. For instance, the idea of gravitation borrowed from physics can be used in business to describe why people tend to “gravitate” to their preferred shopping destinations. Likewise, the concept of distance can be used to explain the degree of social separation between two otherwise collocated individuals. Sometimes, we create our own concepts to describe a unique characteristic not described in prior research. For instance, technostress is a new concept referring to the mental stress one may face when asked to learn a new technology.
Concepts may also have progressive levels of abstraction. Some concepts such as a person’s weight are precise and objective, while other concepts such as a person’s personality may be more abstract and difficult to visualize. A construct is an abstract concept that is specifically chosen (or “created”) to explain a given phenomenon. A construct may be a simple concept, such as a person’s weight , or a combination of a set of related concepts such as a person’s communication skill , which may consist of several underlying concepts such as the person’s vocabulary , syntax , and spelling . The former instance (weight) is a unidimensional construct , while the latter (communication skill) is a multi-dimensional construct (i.e., it consists of multiple underlying concepts). The distinction between constructs and concepts are clearer in multi-dimensional constructs, where the higher order abstraction is called a construct and the lower order abstractions are called concepts. However, this distinction tends to blur in the case of unidimensional constructs.
Constructs used for scientific research must have precise and clear definitions that others can use to understand exactly what it means and what it does not mean. For instance, a seemingly simple construct such as income may refer to monthly or annual income, before-tax or after-tax income, and personal or family income, and is therefore neither precise nor clear. There are two types of definitions: dictionary definitions and operational definitions. In the more familiar dictionary definition, a construct is often defined in terms of a synonym. For instance, attitude may be defined as a disposition, a feeling, or an affect, and affect in turn is defined as an attitude. Such definitions of a circular nature are not particularly useful in scientific research for elaborating the meaning and content of that construct. Scientific research requires operational definitions that define constructs in terms of how they will be empirically measured. For instance, the operational definition of a construct such as temperature must specify whether we plan to measure temperature in Celsius, Fahrenheit, or Kelvin scale. A construct such as income should be defined in terms of whether we are interested in monthly or annual income, before-tax or after-tax income, and personal or family income. One can imagine that constructs such as learning , personality , and intelligence can be quite hard to define operationally.
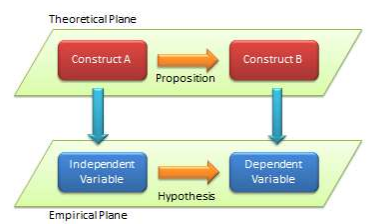
A term frequently associated with, and sometimes used interchangeably with, a construct is a variable. Etymologically speaking, a variable is a quantity that can vary (e.g., from low to high, negative to positive, etc.), in contrast to constants that do not vary (i.e., remain constant). However, in scientific research, a variable is a measurable representation of an abstract construct. As abstract entities, constructs are not directly measurable, and hence, we look for proxy measures called variables. For instance, a person’s intelligence is often measured as his or her IQ ( intelligence quotient ) score , which is an index generated from an analytical and pattern-matching test administered to people. In this case, intelligence is a construct, and IQ score is a variable that measures the intelligence construct. Whether IQ scores truly measures one’s intelligence is anyone’s guess (though many believe that they do), and depending on whether how well it measures intelligence, the IQ score may be a good or a poor measure of the intelligence construct. As shown in Figure 2.1, scientific research proceeds along two planes: a theoretical plane and an empirical plane. Constructs are conceptualized at the theoretical (abstract) plane, while variables are operationalized and measured at the empirical (observational) plane. Thinking like a researcher implies the ability to move back and forth between these two planes.
Depending on their intended use, variables may be classified as independent, dependent, moderating, mediating, or control variables. Variables that explain other variables are called independent variables , those that are explained by other variables are dependent variables , those that are explained by independent variables while also explaining dependent variables are mediating variables (or intermediate variables), and those that influence the relationship between independent and dependent variables are called moderating variables . As an example, if we state that higher intelligence causes improved learning among students, then intelligence is an independent variable and learning is a dependent variable. There may be other extraneous variables that are not pertinent to explaining a given dependent variable, but may have some impact on the dependent variable. These variables must be controlled for in a scientific study, and are therefore called control variables .
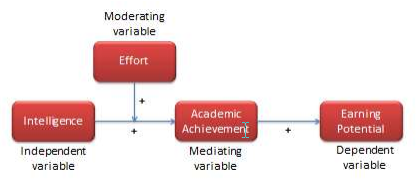
To understand the differences between these different variable types, consider the example shown in Figure 2.2. If we believe that intelligence influences (or explains) students’ academic achievement, then a measure of intelligence such as an IQ score is an independent variable, while a measure of academic success such as grade point average is a dependent variable. If we believe that the effect of intelligence on academic achievement also depends on the effort invested by the student in the learning process (i.e., between two equally intelligent students, the student who puts is more effort achieves higher academic achievement than one who puts in less effort), then effort becomes a moderating variable. Incidentally, one may also view effort as an independent variable and intelligence as a moderating variable. If academic achievement is viewed as an intermediate step to higher earning potential, then earning potential becomes the dependent variable for the independent variable academic achievement , and academic achievement becomes the mediating variable in the relationship between intelligence and earning potential. Hence, variable are defined as an independent, dependent, moderating, or mediating variable based on their nature of association with each other. The overall network of relationships between a set of related constructs is called a nomological network (see Figure 2.2). Thinking like a researcher requires not only being able to abstract constructs from observations, but also being able to mentally visualize a nomological network linking these abstract constructs.
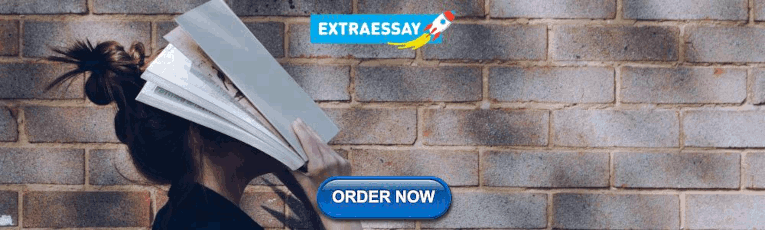
IMAGES
VIDEO
COMMENTS
Hypothesis example Based on your literature review, you choose to measure the variables quality of sleep and ... Operational definitions can easily miss meaningful and subjective perceptions of concepts by trying to reduce complex concepts to numbers. For example, asking consumers to rate their satisfaction with a service on a 5-point scale ...
An operational definition allows the researchers to describe in a specific way what they mean when they use a certain term. Generally, operational definitions are concrete and measurable. Defining variables in this way allows other people to see if the research has validity. Validity here refers to if the researchers are actually measuring what ...
Definition. An Operational Hypothesis is a testable statement or prediction made in research that not only proposes a relationship between two or more variables but also clearly defines those variables in operational terms, meaning how they will be measured or manipulated within the study. It forms the basis of an experiment that seeks to prove ...
A hypothesis is a tentative statement about the relationship between two or more variables. It is a specific, testable prediction about what you expect to happen in a study. It is a preliminary answer to your question that helps guide the research process. Consider a study designed to examine the relationship between sleep deprivation and test ...
for our operational definition of anxiety. As another example, consider the hypothesis that we proposed in the last chapter. We hypothesized that the effect of TV violence on older children's aggressive behavior at school will be less if the characters are not human. Although this appears to be a clear statement, more specific operational
Example: Hypothesis Based on your ... Operational definitions can easily miss meaningful and subjective perceptions of concepts by trying to reduce complex concepts to numbers. For example, asking consumers to rate their satisfaction with a service on a 5-point scale will tell you nothing about why they felt that way.
Define and give an example of indicators and attributes for a variable; Apply the three components of an operational definition to a variable; ... Remember, this would be an inverse relationship—as levels of depression increase, satisfaction decreases. In this hypothesis, level of depression is the independent (or predictor) variable and ...
(Some authors refer to this as the operational definition, ... for example, is more of a ... A hypothesis is a statement of the expected relationship between two or more variables. Like operationalizing a concept, constructing a hypothesis requires getting specific. A good hypothesis will not just predict that two (or more) variables are ...
In quantitative research, that final stage is called operationalization. An operational definition consists of the following components: (1) the variable being measured, (2) the measure you will use, (3) how you plan to interpret the results of that measure. The first component, the variable, should be the easiest part.
Examples of Operational Definitions. Imagine a researcher who is interested in helping curb aggression in schools by exploring if aggression is a response to frustration. To answer the question, the researcher must first define "aggression" and "frustration," both conceptually and procedurally. In the example of frustration, the ...
1 Chapter 5 Conceptualization, Operationalization, and Measurement Chapter Outline Measuring anything that exists Conceptions, concepts, and reality Conceptions as constructs Conceptualization Indicators and dimensions The interchangeability of indicators Real, nominal, and operational definitions Creating conceptual order Definitions in descriptive and explanatory studies
Examples include "achievement motivation" or "career choice" or "second language". You are describing a concept. On the other hand, OPERATIONAL VARIABLES (sometimes called "operational definitions") are how you actually measure this entity, or the concrete operations, measures, or procedures that you use to measure the concept in practice. If ...
Examples. A research hypothesis, in its plural form "hypotheses," is a specific, testable prediction about the anticipated results of a study, established at its outset. It is a key component of the scientific method. Hypotheses connect theory to data and guide the research process towards expanding scientific understanding.
Variables and Operational Definitions. Part of generating a hypothesis involves identifying the variables that you want to study and operationally defining those variables so that they can be measured. Research questions in psychology are about variables. A variable is a quantity or quality that varies across people or situations. For example ...
An example of operationally defining "personal space". In research design, especially in psychology, social sciences, life sciences and physics, operationalization or operationalisation is a process of defining the measurement of a phenomenon which is not directly measurable, though its existence is inferred from other phenomena.Operationalization thus defines a fuzzy concept so as to make it ...
Operational definitions play a crucial role in hypothesis testing by ensuring that variables are well-defined and measurable, allowing researchers to test their hypotheses effectively. Conclusion Operational definition Psychology is the backbone of empirical research in psychology.
The operational definition is the specific way a variable is measured in a particular study. It is critical to operationally define a variable to lend credibility to the methodology and ensure the reproducibility of the study's results. Another study may identify the same variable differently, making it difficult to compare the results of ...
Example 2.2 (Operational and conceptual definitions) Players and fans have become more aware of concussions and head injuries in sport. A Conference on concussion in sport developed this conceptual definition (McCrory et al. 2013):. Concussion is a brain injury and is defined as a complex pathophysiological process affecting the brain, induced by biomechanical forces.
It is necessary to operationalize the terms used in scientific research (that means particularly the central terms of a hypothesis). In order to guarantee the viability of a research method you have to define first which data will be collected by means of which methods. Research operations have to be specified to comprehend a subject matter in ...
As shown in Figure 2.1, scientific research proceeds along two planes: a theoretical plane and an empirical plane. Constructs are conceptualized at the theoretical (abstract) plane, while variables are operationalized and measured at the empirical (observational) plane. Thinking like a researcher implies the ability to move back and forth ...
The operational hypothesis should also define the relationship that is being measured and state how the measurement is occurring. It attempts to take an abstract idea and make it into a concrete, clearly defined method. It is used to inform readers how the experiment is going to measure the variables in a specific manner. An operational ...
An operational definition specifies concrete, replicable procedures designed to represent a construct. In the words of American psychologist S.S. Stevens (1935), "An operation is the performance which we execute in order to make known a concept." [1] [2] For example, an operational definition of "fear" (the construct) often includes measurable ...
20 terms. Ashley_102719. Preview. Exam 4 contemp. 111 terms. b3hrmad02. Preview. Study with Quizlet and memorize flashcards containing terms like The role of hypotheses, Example of hypothesis, The Research Hypothesis and more.