7 Different Ways to Control for Confounding
Confounding can be controlled in the design phase of the study by using:
- Random assignment
- Restriction
Or in the data analysis phase by using:
- Stratification
- Inverse probability weighting
- Instrumental variable estimation
Here’s a quick summary of the similarities and differences between these methods:
In what follows, we will explain how each of these methods works, and discuss its advantages and limitations.
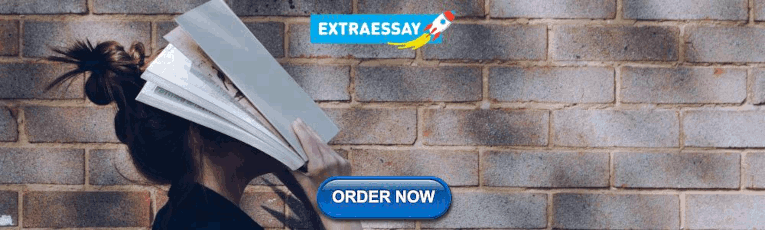
1. Random assignment
How it works.
Random assignment is a process by which participants are assigned, with the same chance, to either receive or not a certain exposure.
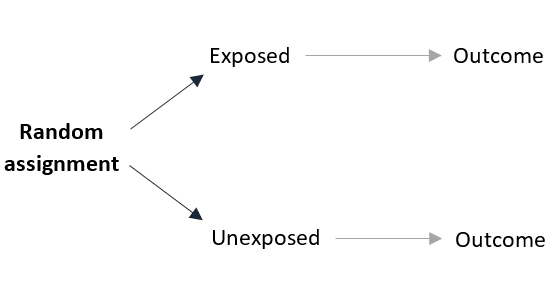
Randomizing the exposure adjusts for confounding by eliminating the influence of the confounder on the probability of receiving the exposure:
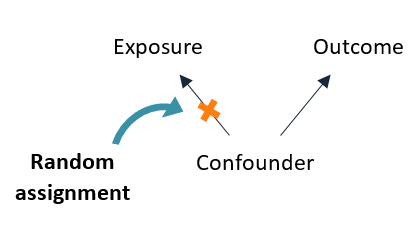
Advantage of random assignment:
Random assignment controls for confounding due to both measurable and unmeasurable causes. So it is especially useful when confounding variables are unknown or cannot be measured.
It also controls for time-varying confounders, that is when the exposure and the confounders are measured repeatedly in studies where participants are followed over time.
Limitation of random assignment:
Here are 3 reasons not to use random assignment:
- Ethical reason: Randomizing participants would be unethical when studying the effect of a harmful exposure, or on the contrary, when it is known for certain that the exposure is beneficial.
- Practical reason: Some exposures are very hard to randomize, like air pollution and education. Also, random assignment is not an option when we are analyzing observational data that we did not collect ourselves.
- Financial reason: Random assignment is a part of experimental designs where participants are followed over time, which turns out to be highly expensive in some cases.
Whenever the exposure cannot be randomly assigned to study participants, we will have to use an observational design and control for confounding by using another method from this list.
2. Restriction
Restriction refers to only including in the study participants of a certain confounder category, thereby eliminating its confounding effect.
For instance, if the relationship between smoking (the exposure) and heart disease (the outcome) is confounded by income, then restricting our study to only include participants of the same income category will eliminate its confounding effect:
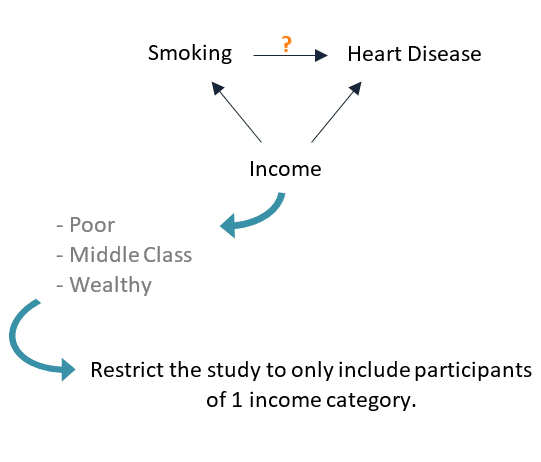
Advantage of restriction:
Unlike random assignment, restriction is easy to apply and also works for observational studies.
Limitation of restriction:
The biggest problem with restricting our study to 1 category of the confounder is that the results will not generalize well to the other categories. So restriction will limit the external validity of the study especially in cases where we have more than 1 confounder to control for.
3. Matching
Matching works by distributing the confounding variable evenly between the exposed and the unexposed groups.
The idea is to pair each exposed subject with an unexposed subject that shares the same characteristics regarding the variable that we want to control for. Then, by only analyzing participants for whom we found a match, we eliminate the confounding effect of that variable.
For example, suppose we want to control for income as a confounder of the relationship between smoking (the exposure) and heart disease (the outcome).
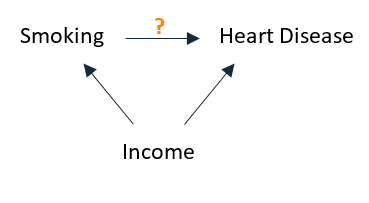
In this case, each smoker should be matched with a non-smoker of the same income category.
Here’s a step-by-step description of how this works:
Initially: The confounder is unequally distributed among the exposed and unexposed groups.
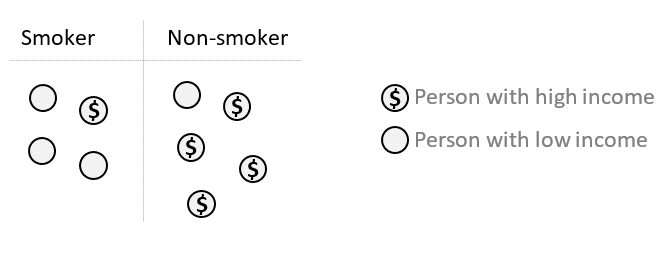
Step 1: Match each smoker with a non-smoker of the same income category.
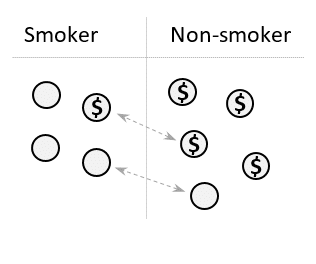
Step 2: Exclude all unmatched participants from the study.
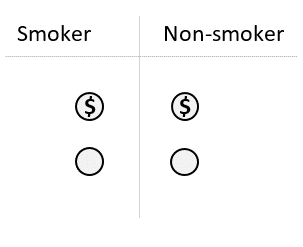
Result: The 2 groups will be balanced regarding the confounding variable.
Advantage of matching
Matching can be easy to apply in certain cases. For instance, matching on income in the example above can be done by selecting 1 smoker and 1 non-smoker from the same family, therefore having the same household income.
Limitation of matching
The more confounding variables we have to control for, the more difficult matching becomes, especially for continuous variables. The problem with matching on many characteristics is that a lot of participants will end up unmatched.
4. Stratification
Stratification controls for confounding by estimating the relationship between the exposure and the outcome within different subsets of the confounding variable, and then pooling these estimates.
Stratification works because, within each subset, the value of the confounder is the same for all participants and therefore cannot affect the estimated effect of the exposure on the outcome.
Here’s a step-by-step description of how to conduct a stratified analysis:
Step 1: Start by splitting the data into multiple subgroups (a.k.a. strata) according to the different categories of the confounding variable.
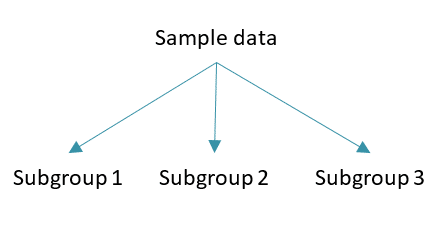
Step 2: Within each subgroup (or stratum), estimate the relationship between the exposure and the outcome.
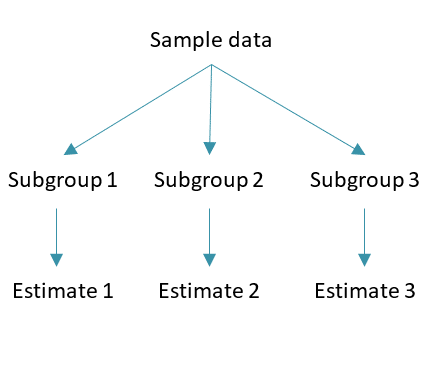
Step 3: Pool the obtained estimates:
- By averaging them.
- Or by weighting them by the size of each stratum — a method called standardization.
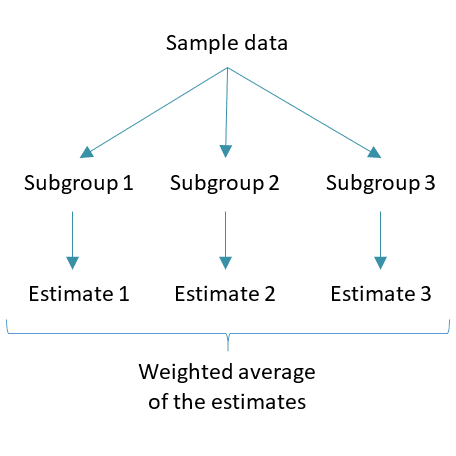
Result: The pooled estimate will be free of confounding.
Advantage of stratification
Stratified analysis is an old and intuitive method used to teach the logic of controlling for confounding. A more modern and practical approach would be regression analysis, which is next on our list.
Limitation of stratification
Stratification does not scale well, since controlling for multiple confounders simultaneously will lead to:
- Complex calculations.
- Subgroups that contain very few participants, and these will reflect the noise in the data more so than real effects.
5. Regression
Adjusting for confounding using regression simply means to include the confounding variable in the model used to estimate the influence of the exposure on the outcome.
A linear regression model, for example, will be of the form:
Where the coefficient β 1 will reflect the effect of the exposure on the outcome adjusted for the confounder.
Advantage of regression
Regression can easily control for multiple confounders simultaneously, as this simply means adding more variables to the model.
For more details on how to use it in practice, I wrote a separate article: An Example of Identifying and Adjusting for Confounding .
Limitation of regression
A regression model operates under certain assumptions that must be respected. For example, for linear regression these are:
- A linear relationship between the predictors (the exposure and the confounder) and the outcome.
- Independence, normality, and equal variance of the residuals.
6. Inverse probability weighting
Inverse probability weighting eliminates confounding by equalizing the frequency of the confounder between the exposed and the unexposed groups. This is done by counting each participant as many times as her inverse probability of being in a certain exposure category.
Here’s a step-by-step description of the process:
Suppose we want to control for income as a confounder of the relationship between smoking (the exposure) and heart disease (the outcome):
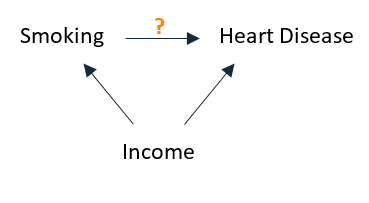
Initially: Since income and smoking are associated, participants of different income levels will have different probabilities of being smokers.
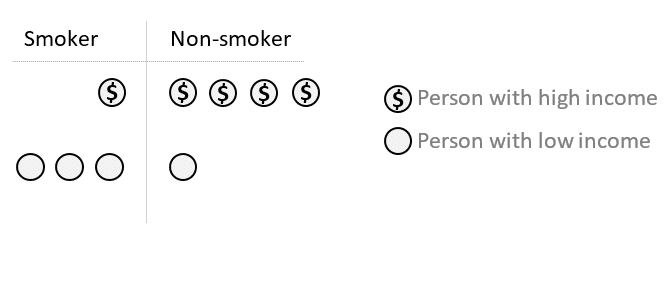
First, let’s focus on high income participants:
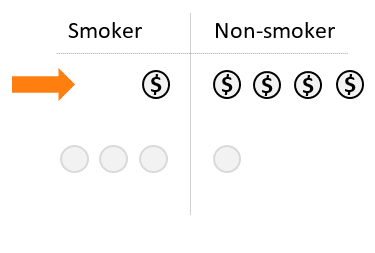
Step 1: Calculate the probability “P” that a person is a smoker.
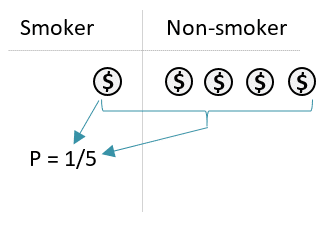
Step 2: Calculate the probability that a person is a non-smoker.
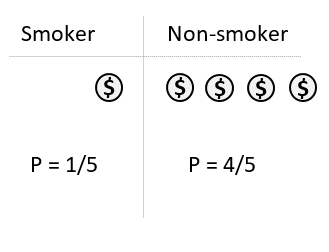
Step 3: Multiply each person by the inverse of their calculated probability. So each participant will no longer count as 1 person in the analysis. Instead, each will be counted as many times as their calculated inverse probability weight (i.e. 1 person will be 1/P persons).
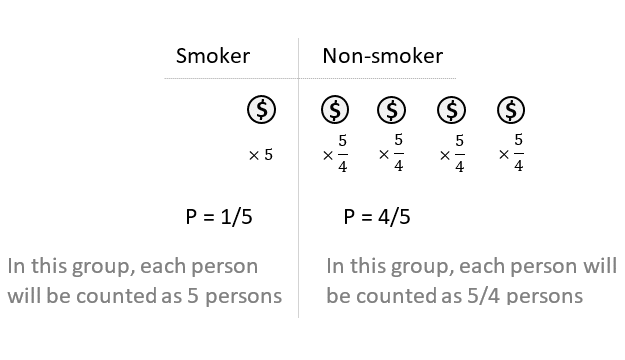
Now the smoking group has: 1 × 5 = 5 participants. And the non-smoking group also has: 4 × 5/4 = 5 participants
Finally, we have to repeat steps 1, 2, and 3 for participants in the low-income category.
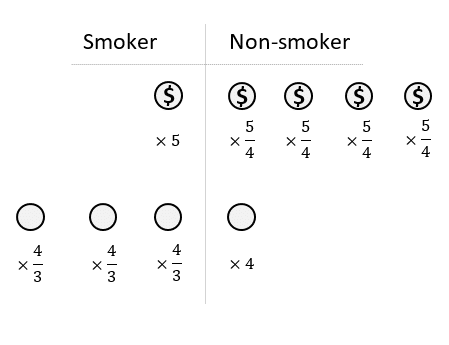
Result: The smoker and non-smoker groups are now balanced regarding income. So its confounding effect will be eliminated because it is no longer associated with the exposure.
Advantage of inverse probability weighting
This method is a type of what is referred to as G-methods that are used to control for time-varying confounders, that is when the exposure and the confounders are measured repeatedly in studies where participants are followed over time.
Limitation of inverse probability weighting
If some participants have very large weights (i.e. when their probability of being in a certain exposure category is very low), then each of these participants would be counted as a large number of people, which leads to instability in the estimation of the causal effect of the exposure on the outcome.
One solution would be to exclude from the study participants with very high or very low weights.
7. Instrumental variable estimation
The instrumental variable method estimates the unconfounded effect of the exposure on the outcome indirectly by using a variable — the instrumental variable — that represents the exposure but is not affected by confounding.
An instrumental variable satisfies 3 properties:
- It causes the exposure.
- It does not cause the outcome directly — it affects the outcome only through the exposure.
- Its association with the outcome is unconfounded.
Here’s a diagram that represents the relationship between the instrumental variable, the exposure, and the outcome:
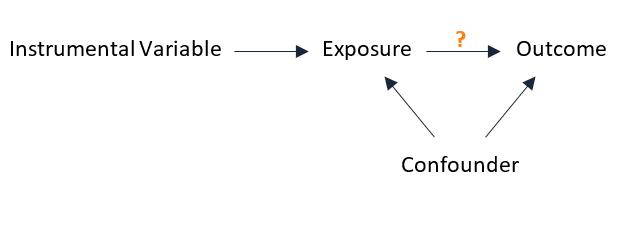
An instrumental variable is chosen so that nothing appears to cause it. So in a sense, it resembles the coin flip in a randomized experiment, because it appears to be randomly assigned.
How the instrumental variable can be used to study causality?
Looking at the data, if an association is found between the instrumental variable and the outcome then it must be causal, since according to property (3) above, their relationship is unconfounded. And because the instrumental variable affects the outcome only through the exposure, according to property (2), we can conclude that the exposure has a causal effect on the outcome.

So how to quantify this causal/unconfounded effect of the exposure on the outcome?
Let “α 1 ” denote the magnitude of the causal effect of the instrumental variable on the exposure, and “β 1 ” that of the exposure on the outcome.
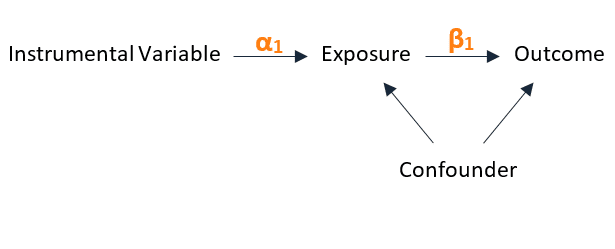
So our objective is to find β 1 .
Note that the simple regression model between the exposure and the outcome produces a confounded estimate of β 1 :
And therefore does not reflect the true β 1 that we are searching for.
So how to find this true unconfounded β 1 ?
Technically, if we think in terms of linear regression:
- α 1 is the change in the exposure CAUSED by a 1 unit change in the instrumental variable.
- β 1 is the change in the outcome CAUSED by a 1 unit change in the exposure.
It follows that a 1 unit change in the instrumental variable CAUSES an α 1 × β 1 change in the outcome (since the instrumental variable only affects the outcome through the exposure).
And as discussed above, any association between the instrumental variable and the outcome is causal. So, α 1 × β 1 can be estimated from the following regression model:
Where a 1 = α 1 × β 1
And because any association between the instrumental variable and the exposure is also causal (also unconfounded), the following model can be used to estimate α 1 :
Where b 1 = α 1
We end up with 2 equations:
- α 1 × β 1 = a 1
A simple calculation yields: β 1 = a 1 /b 1 which will be our estimated causal effect of the exposure on the outcome.
Advantage of instrumental variable estimation
Because the calculations that we just did are not dependent on any information about the confounder, we can use the instrumental variable approach to control for any measured, unmeasured, and unknown confounder.
This method is so powerful that it can be used in cases even where we do not know whether there is confounding or not between the exposure and the outcome, and which variables are suspect.
Limitation of instrumental variable estimation
In cases where the instrumental variable and the exposure are weakly correlated, the estimated effect of the exposure on the outcome will be biased.
The use of linear regression is also constrained by its assumptions, especially linearity and constant variance of the residuals.
As a rule of thumb, use the instrumental variable approach in cases where there are unmeasured confounders, otherwise, use other methods from this list since they will, in general, provide a better estimate of the causal relationship between the exposure and the outcome.
If you are interested, here are 3 Real-World Examples of Using Instrumental Variables .
- Hernán M, Robins JM. Causal Inference . Chapman & Hall/CRC; 2020.
- Roy J. A Crash Course in Causality: Inferring Causal Effects from Observational Data | Coursera.
- Pearl J, Mackenzie D. The Book of Why: The New Science of Cause and Effect . First edition. Basic Books; 2018.
Further reading
- Front-Door Criterion to Adjust for Unmeasured Confounding
- 4 Simple Ways to Identify Confounding
- 5 Real-World Examples of Confounding [With References]
- Why Confounding is Not a Type of Bias
- Using the 4 D-Separation Rules to Study a Causal Association
- List of All Biases in Research (Sorted by Popularity)
Random Assignment in Psychology: Definition & Examples
Julia Simkus
Editor at Simply Psychology
BA (Hons) Psychology, Princeton University
Julia Simkus is a graduate of Princeton University with a Bachelor of Arts in Psychology. She is currently studying for a Master's Degree in Counseling for Mental Health and Wellness in September 2023. Julia's research has been published in peer reviewed journals.
Learn about our Editorial Process
Saul Mcleod, PhD
Editor-in-Chief for Simply Psychology
BSc (Hons) Psychology, MRes, PhD, University of Manchester
Saul Mcleod, PhD., is a qualified psychology teacher with over 18 years of experience in further and higher education. He has been published in peer-reviewed journals, including the Journal of Clinical Psychology.
Olivia Guy-Evans, MSc
Associate Editor for Simply Psychology
BSc (Hons) Psychology, MSc Psychology of Education
Olivia Guy-Evans is a writer and associate editor for Simply Psychology. She has previously worked in healthcare and educational sectors.
In psychology, random assignment refers to the practice of allocating participants to different experimental groups in a study in a completely unbiased way, ensuring each participant has an equal chance of being assigned to any group.
In experimental research, random assignment, or random placement, organizes participants from your sample into different groups using randomization.
Random assignment uses chance procedures to ensure that each participant has an equal opportunity of being assigned to either a control or experimental group.
The control group does not receive the treatment in question, whereas the experimental group does receive the treatment.
When using random assignment, neither the researcher nor the participant can choose the group to which the participant is assigned. This ensures that any differences between and within the groups are not systematic at the onset of the study.
In a study to test the success of a weight-loss program, investigators randomly assigned a pool of participants to one of two groups.
Group A participants participated in the weight-loss program for 10 weeks and took a class where they learned about the benefits of healthy eating and exercise.
Group B participants read a 200-page book that explains the benefits of weight loss. The investigator randomly assigned participants to one of the two groups.
The researchers found that those who participated in the program and took the class were more likely to lose weight than those in the other group that received only the book.
Importance
Random assignment ensures that each group in the experiment is identical before applying the independent variable.
In experiments , researchers will manipulate an independent variable to assess its effect on a dependent variable, while controlling for other variables. Random assignment increases the likelihood that the treatment groups are the same at the onset of a study.
Thus, any changes that result from the independent variable can be assumed to be a result of the treatment of interest. This is particularly important for eliminating sources of bias and strengthening the internal validity of an experiment.
Random assignment is the best method for inferring a causal relationship between a treatment and an outcome.
Random Selection vs. Random Assignment
Random selection (also called probability sampling or random sampling) is a way of randomly selecting members of a population to be included in your study.
On the other hand, random assignment is a way of sorting the sample participants into control and treatment groups.
Random selection ensures that everyone in the population has an equal chance of being selected for the study. Once the pool of participants has been chosen, experimenters use random assignment to assign participants into groups.
Random assignment is only used in between-subjects experimental designs, while random selection can be used in a variety of study designs.
Random Assignment vs Random Sampling
Random sampling refers to selecting participants from a population so that each individual has an equal chance of being chosen. This method enhances the representativeness of the sample.
Random assignment, on the other hand, is used in experimental designs once participants are selected. It involves allocating these participants to different experimental groups or conditions randomly.
This helps ensure that any differences in results across groups are due to manipulating the independent variable, not preexisting differences among participants.
When to Use Random Assignment
Random assignment is used in experiments with a between-groups or independent measures design.
In these research designs, researchers will manipulate an independent variable to assess its effect on a dependent variable, while controlling for other variables.
There is usually a control group and one or more experimental groups. Random assignment helps ensure that the groups are comparable at the onset of the study.
How to Use Random Assignment
There are a variety of ways to assign participants into study groups randomly. Here are a handful of popular methods:
- Random Number Generator : Give each member of the sample a unique number; use a computer program to randomly generate a number from the list for each group.
- Lottery : Give each member of the sample a unique number. Place all numbers in a hat or bucket and draw numbers at random for each group.
- Flipping a Coin : Flip a coin for each participant to decide if they will be in the control group or experimental group (this method can only be used when you have just two groups)
- Roll a Die : For each number on the list, roll a dice to decide which of the groups they will be in. For example, assume that rolling 1, 2, or 3 places them in a control group and rolling 3, 4, 5 lands them in an experimental group.
When is Random Assignment not used?
- When it is not ethically permissible: Randomization is only ethical if the researcher has no evidence that one treatment is superior to the other or that one treatment might have harmful side effects.
- When answering non-causal questions : If the researcher is just interested in predicting the probability of an event, the causal relationship between the variables is not important and observational designs would be more suitable than random assignment.
- When studying the effect of variables that cannot be manipulated: Some risk factors cannot be manipulated and so it would not make any sense to study them in a randomized trial. For example, we cannot randomly assign participants into categories based on age, gender, or genetic factors.
Drawbacks of Random Assignment
While randomization assures an unbiased assignment of participants to groups, it does not guarantee the equality of these groups. There could still be extraneous variables that differ between groups or group differences that arise from chance. Additionally, there is still an element of luck with random assignments.
Thus, researchers can not produce perfectly equal groups for each specific study. Differences between the treatment group and control group might still exist, and the results of a randomized trial may sometimes be wrong, but this is absolutely okay.
Scientific evidence is a long and continuous process, and the groups will tend to be equal in the long run when data is aggregated in a meta-analysis.
Additionally, external validity (i.e., the extent to which the researcher can use the results of the study to generalize to the larger population) is compromised with random assignment.
Random assignment is challenging to implement outside of controlled laboratory conditions and might not represent what would happen in the real world at the population level.
Random assignment can also be more costly than simple observational studies, where an investigator is just observing events without intervening with the population.
Randomization also can be time-consuming and challenging, especially when participants refuse to receive the assigned treatment or do not adhere to recommendations.
What is the difference between random sampling and random assignment?
Random sampling refers to randomly selecting a sample of participants from a population. Random assignment refers to randomly assigning participants to treatment groups from the selected sample.
Does random assignment increase internal validity?
Yes, random assignment ensures that there are no systematic differences between the participants in each group, enhancing the study’s internal validity .
Does random assignment reduce sampling error?
Yes, with random assignment, participants have an equal chance of being assigned to either a control group or an experimental group, resulting in a sample that is, in theory, representative of the population.
Random assignment does not completely eliminate sampling error because a sample only approximates the population from which it is drawn. However, random sampling is a way to minimize sampling errors.
When is random assignment not possible?
Random assignment is not possible when the experimenters cannot control the treatment or independent variable.
For example, if you want to compare how men and women perform on a test, you cannot randomly assign subjects to these groups.
Participants are not randomly assigned to different groups in this study, but instead assigned based on their characteristics.
Does random assignment eliminate confounding variables?
Yes, random assignment eliminates the influence of any confounding variables on the treatment because it distributes them at random among the study groups. Randomization invalidates any relationship between a confounding variable and the treatment.
Why is random assignment of participants to treatment conditions in an experiment used?
Random assignment is used to ensure that all groups are comparable at the start of a study. This allows researchers to conclude that the outcomes of the study can be attributed to the intervention at hand and to rule out alternative explanations for study results.
Further Reading
- Bogomolnaia, A., & Moulin, H. (2001). A new solution to the random assignment problem . Journal of Economic theory , 100 (2), 295-328.
- Krause, M. S., & Howard, K. I. (2003). What random assignment does and does not do . Journal of Clinical Psychology , 59 (7), 751-766.

Related Articles
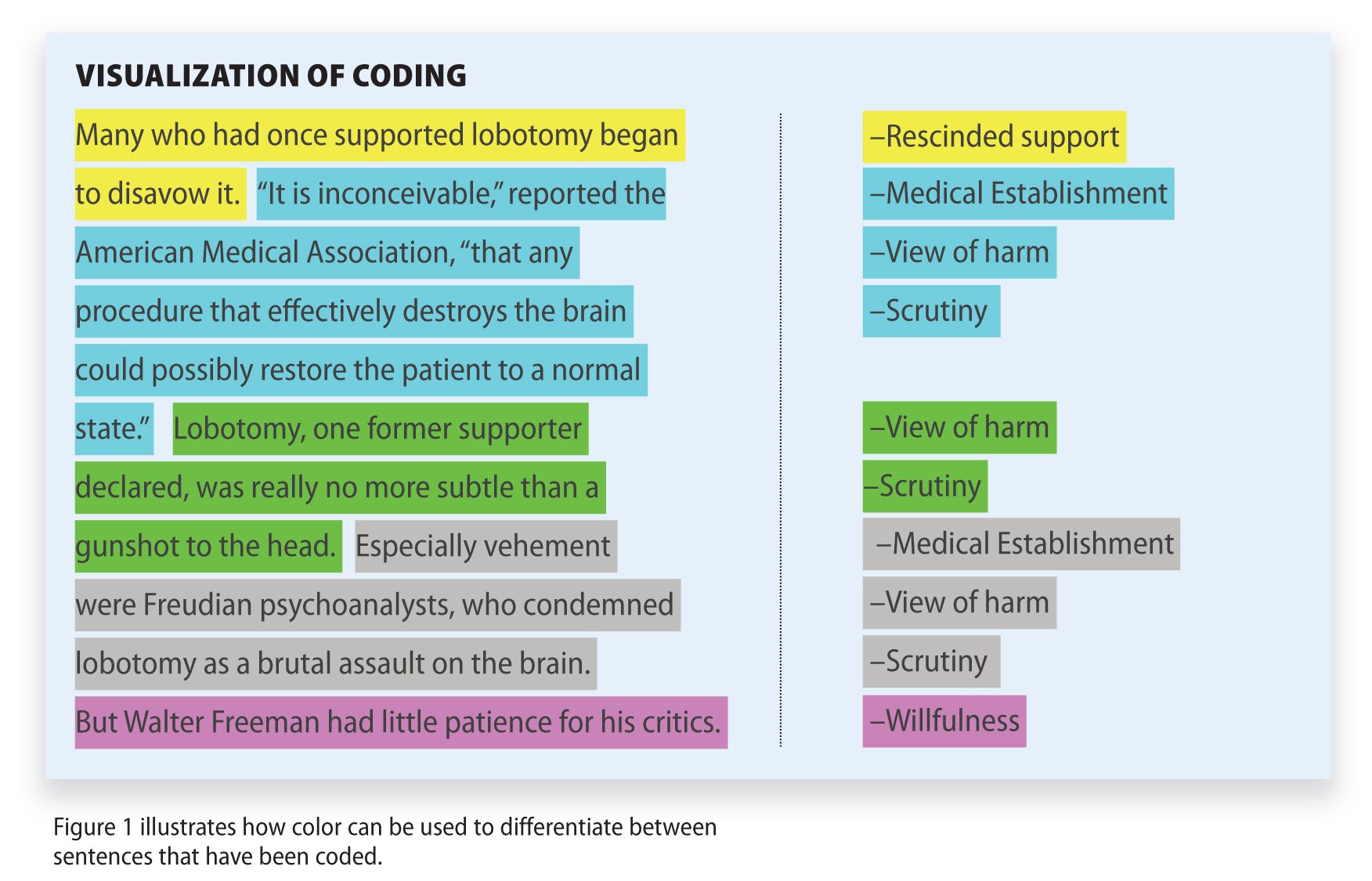
Research Methodology
Qualitative Data Coding
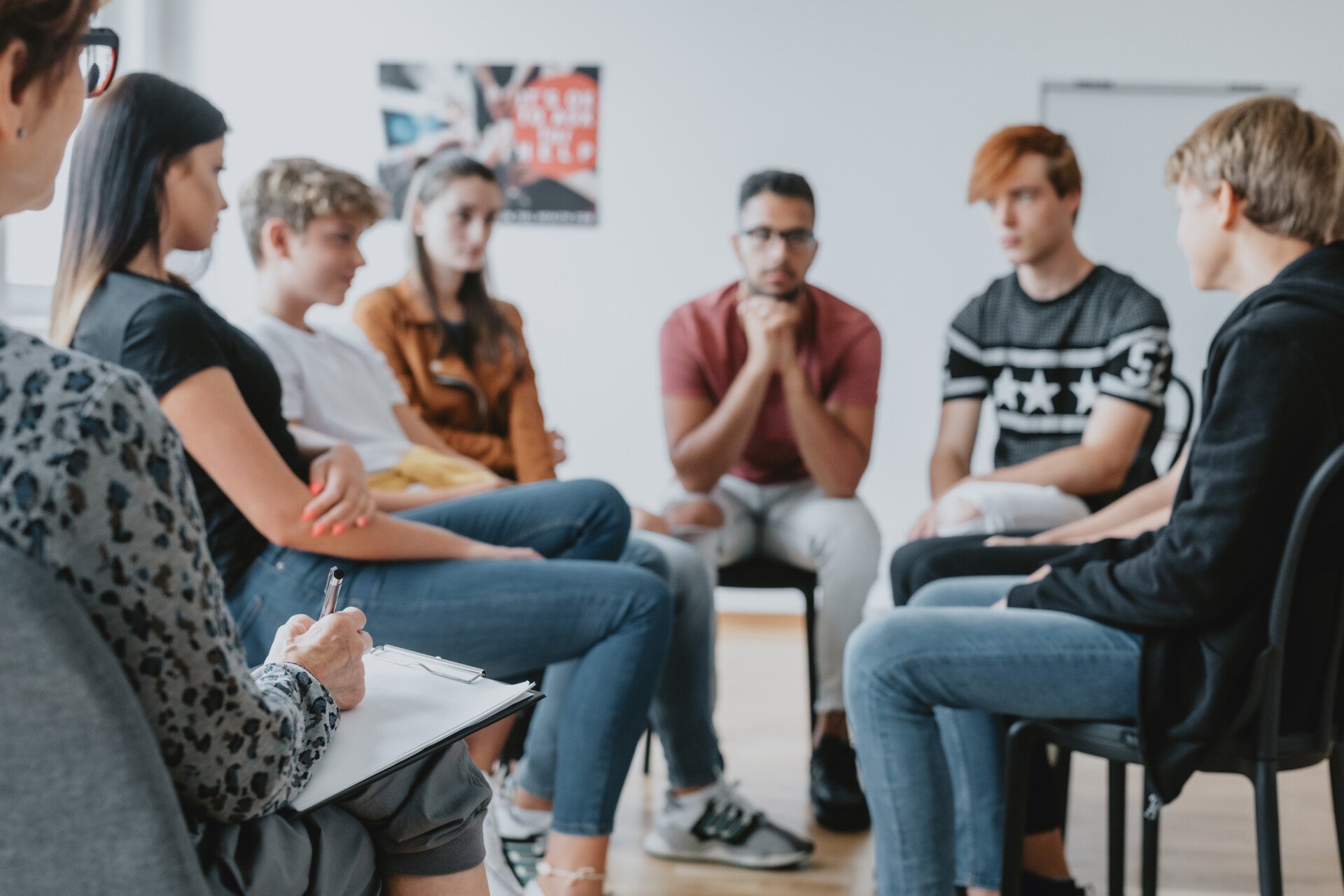
What Is a Focus Group?
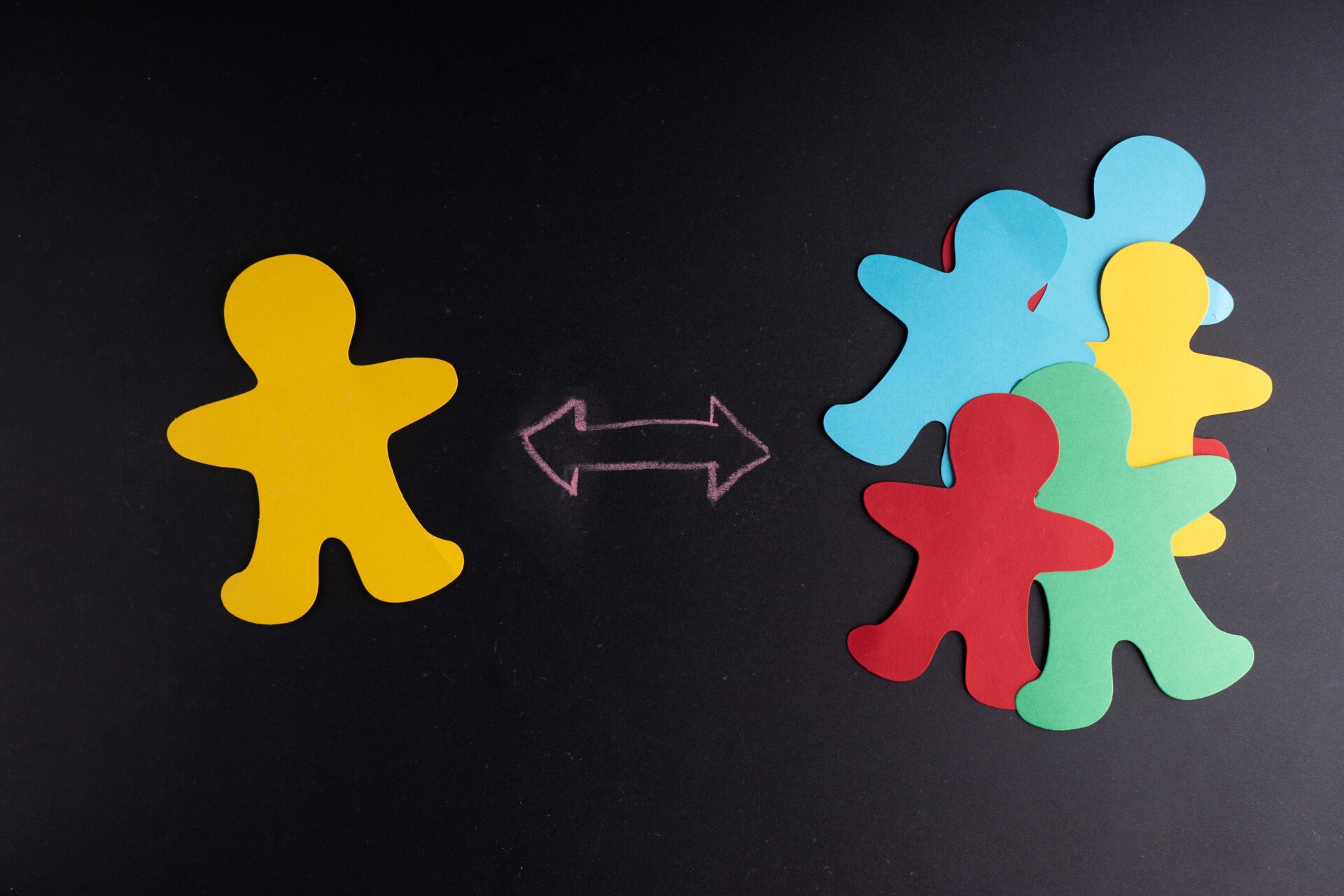
Cross-Cultural Research Methodology In Psychology
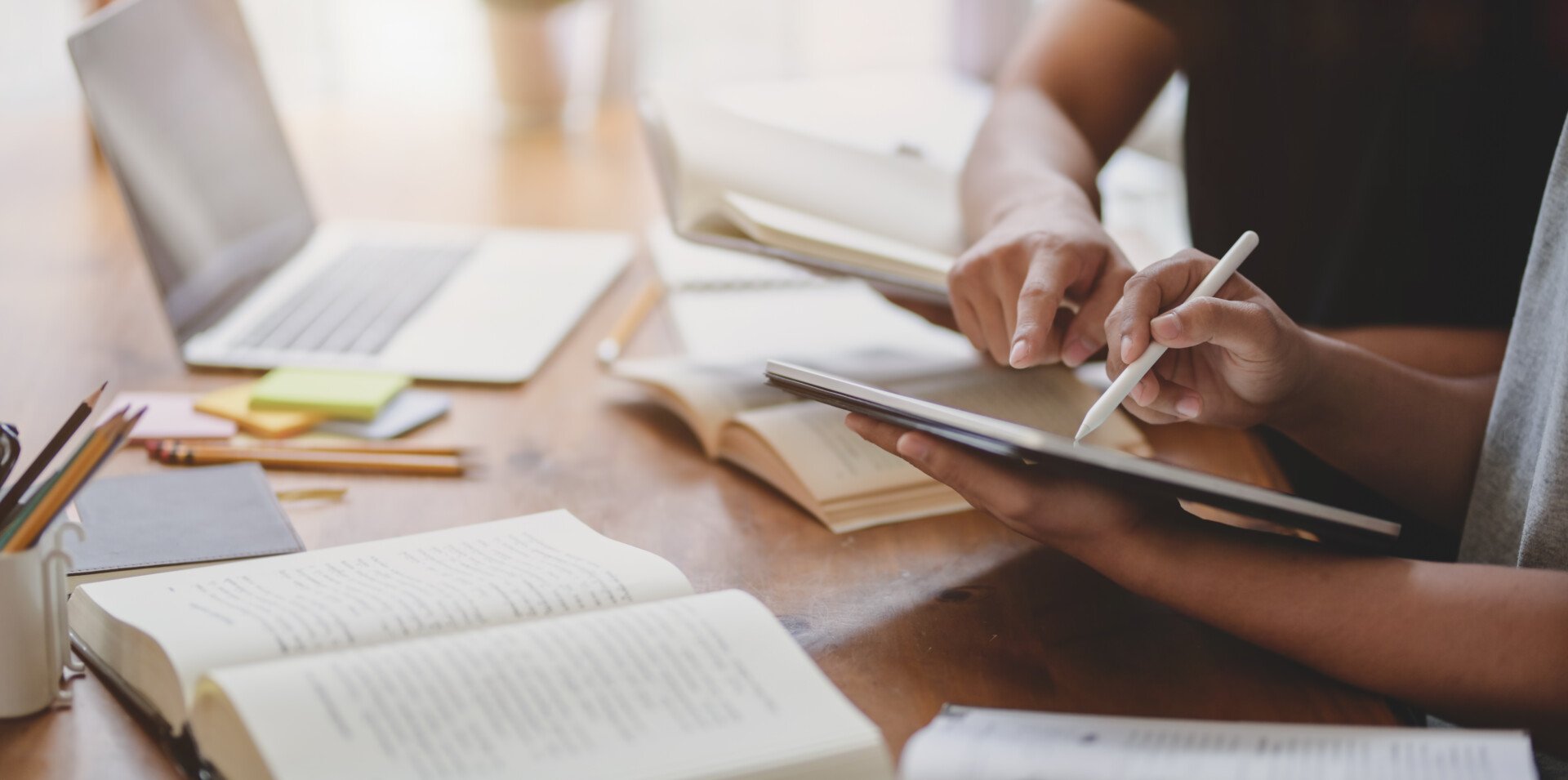
What Is Internal Validity In Research?
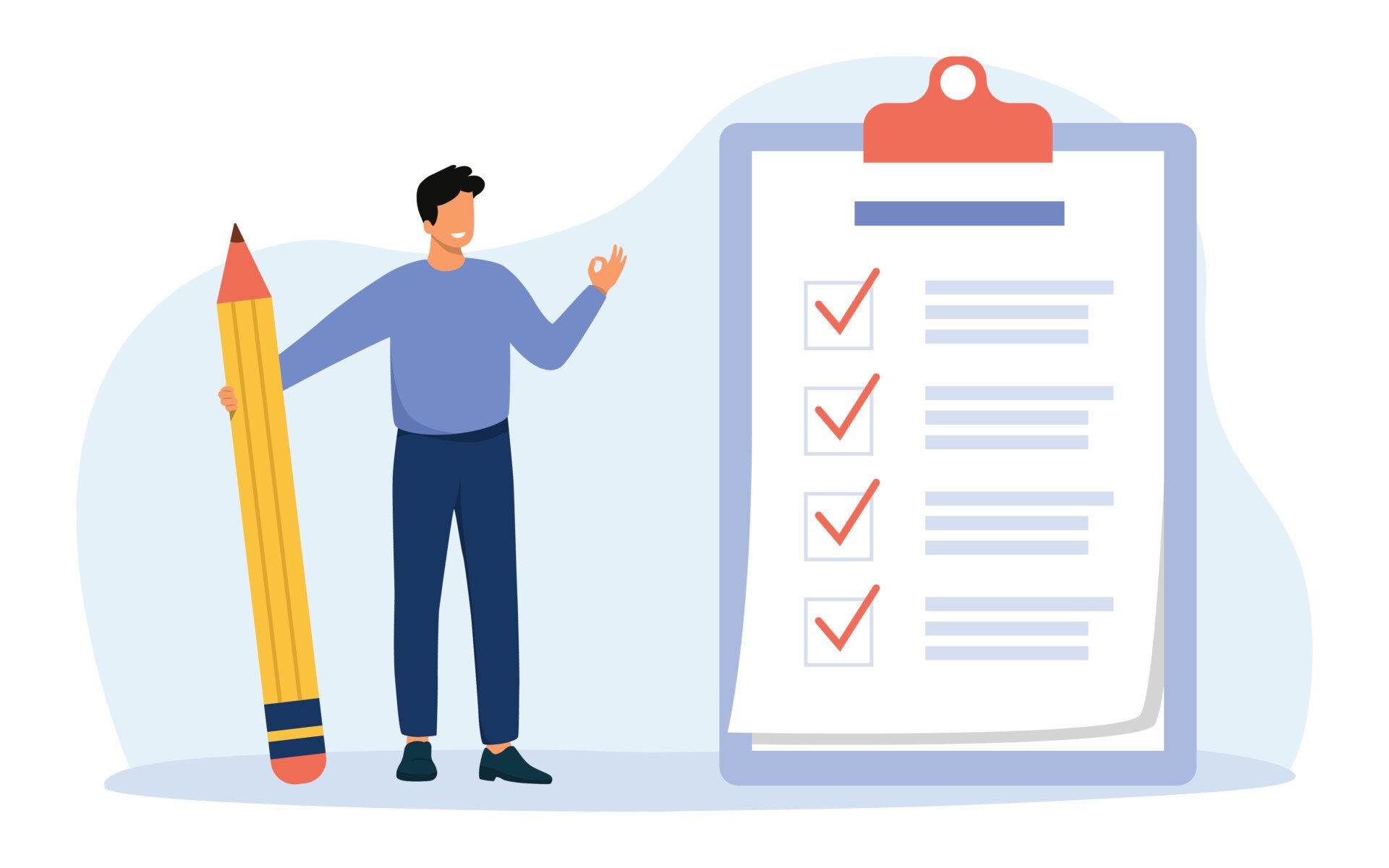
Research Methodology , Statistics
What Is Face Validity In Research? Importance & How To Measure
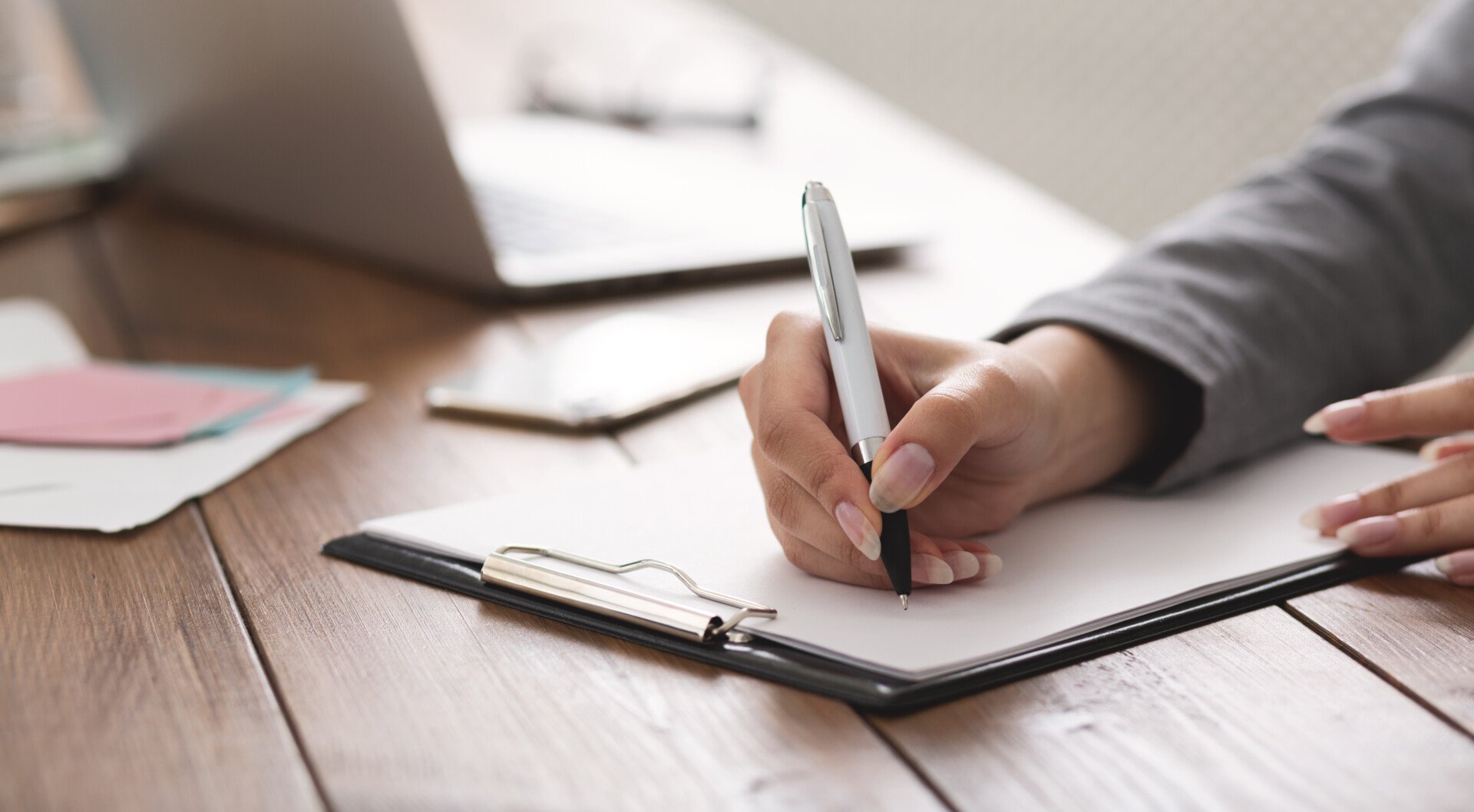
Criterion Validity: Definition & Examples
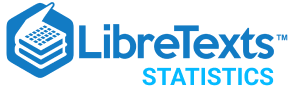
- school Campus Bookshelves
- menu_book Bookshelves
- perm_media Learning Objects
- login Login
- how_to_reg Request Instructor Account
- hub Instructor Commons
Margin Size
- Download Page (PDF)
- Download Full Book (PDF)
- Periodic Table
- Physics Constants
- Scientific Calculator
- Reference & Cite
- Tools expand_more
- Readability
selected template will load here
This action is not available.
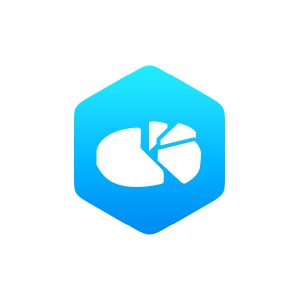
1.3: Threats to Internal Validity and Different Control Techniques
- Last updated
- Save as PDF
- Page ID 32915
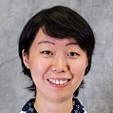
- Yang Lydia Yang
- Kansas State University
\( \newcommand{\vecs}[1]{\overset { \scriptstyle \rightharpoonup} {\mathbf{#1}} } \)
\( \newcommand{\vecd}[1]{\overset{-\!-\!\rightharpoonup}{\vphantom{a}\smash {#1}}} \)
\( \newcommand{\id}{\mathrm{id}}\) \( \newcommand{\Span}{\mathrm{span}}\)
( \newcommand{\kernel}{\mathrm{null}\,}\) \( \newcommand{\range}{\mathrm{range}\,}\)
\( \newcommand{\RealPart}{\mathrm{Re}}\) \( \newcommand{\ImaginaryPart}{\mathrm{Im}}\)
\( \newcommand{\Argument}{\mathrm{Arg}}\) \( \newcommand{\norm}[1]{\| #1 \|}\)
\( \newcommand{\inner}[2]{\langle #1, #2 \rangle}\)
\( \newcommand{\Span}{\mathrm{span}}\)
\( \newcommand{\id}{\mathrm{id}}\)
\( \newcommand{\kernel}{\mathrm{null}\,}\)
\( \newcommand{\range}{\mathrm{range}\,}\)
\( \newcommand{\RealPart}{\mathrm{Re}}\)
\( \newcommand{\ImaginaryPart}{\mathrm{Im}}\)
\( \newcommand{\Argument}{\mathrm{Arg}}\)
\( \newcommand{\norm}[1]{\| #1 \|}\)
\( \newcommand{\Span}{\mathrm{span}}\) \( \newcommand{\AA}{\unicode[.8,0]{x212B}}\)
\( \newcommand{\vectorA}[1]{\vec{#1}} % arrow\)
\( \newcommand{\vectorAt}[1]{\vec{\text{#1}}} % arrow\)
\( \newcommand{\vectorB}[1]{\overset { \scriptstyle \rightharpoonup} {\mathbf{#1}} } \)
\( \newcommand{\vectorC}[1]{\textbf{#1}} \)
\( \newcommand{\vectorD}[1]{\overrightarrow{#1}} \)
\( \newcommand{\vectorDt}[1]{\overrightarrow{\text{#1}}} \)
\( \newcommand{\vectE}[1]{\overset{-\!-\!\rightharpoonup}{\vphantom{a}\smash{\mathbf {#1}}}} \)
Internal validity is often the focus from a research design perspective. To understand the pros and cons of various designs and to be able to better judge specific designs, we identify specific threats to internal validity . Before we do so, it is important to note that the primary challenge to establishing internal validity in social sciences is the fact that most of the phenomena we care about have multiple causes and are often a result of some complex set of interactions. For example, X may be only a partial cause of Y or X may cause Y, but only when Z is present. Multiple causation and interactive effects make it very difficult to demonstrate causality. Turning now to more specific threats, Figure 1.3.1 below identifies common threats to internal validity.
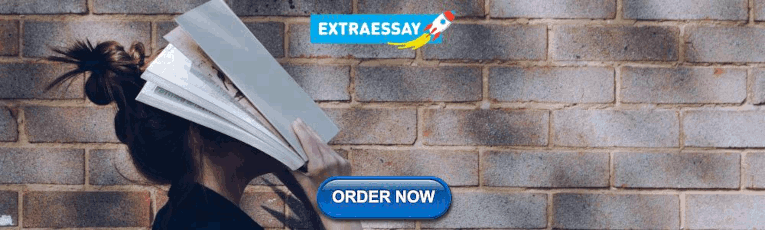
Different Control Techniques
All of the common threats mentioned above can introduce extraneous variables into your research design, which will potentially confound your research findings. In other words, we won't be able to tell whether it is the independent variable (i.e., the treatment we give participants), or the extraneous variable, that causes the changes in the dependent variable. Controlling for extraneous variables reduces its threats on the research design and gives us a better chance to claim the independent variable causes the changes in the dependent variable, i.e., internal validity. There are different techniques we can use to control for extraneous variables.
Random assignment
Random assignment is the single most powerful control technique we can use to minimize the potential threats of the confounding variables in research design. As we have seen in Dunn and her colleagues' study earlier, participants are not allowed to self select into either conditions (spend $20 on self or spend on others). Instead, they are randomly assigned into either group by the researcher(s). By doing so, the two groups are likely to be similar on all other factors except the independent variable itself. One confounding variable mentioned earlier is whether individuals had a happy childhood to begin with. Using random assignment, those who had a happy childhood will likely end up in each condition group. Similarly, those who didn't have a happy childhood will likely end up in each condition group too. As a consequence, we can expect the two condition groups to be very similar on this confounding variable. Applying the same logic, we can use random assignment to minimize all potential confounding variables (assuming your sample size is large enough!). With that, the only difference between the two groups is the condition participants are assigned to, which is the independent variable, then we are confident to infer that the independent variable actually causes the differences in the dependent variables.
It is critical to emphasize that random assignment is the only control technique to control for both known and unknown confounding variables. With all other control techniques mentioned below, we must first know what the confounding variable is before controlling it. Random assignment does not. With the simple act of randomly assigning participants into different conditions, we take care both the confounding variables we know of and the ones we don't even know that could threat the internal validity of our studies. As the saying goes, "what you don't know will hurt you." Random assignment take cares of it.
Matching is another technique we can use to control for extraneous variables. We must first identify the extraneous variable that can potentially confound the research design. Then we want to rank order the participants on this extraneous variable or list the participants in a ascending or descending order. Participants who are similar on the extraneous variable will be placed into different treatment groups. In other words, they are "matched" on the extraneous variable. Then we can carry out the intervention/treatment as usual. If different treatment groups do show differences on the dependent variable, we would know it is not the extraneous variables because participants are "matched" or equivalent on the extraneous variable. Rather it is more likely to the independent variable (i.e., the treatments) that causes the changes in the dependent variable. Use the example above (self-spending vs. others-spending on happiness) with the same extraneous variable of whether individuals had a happy childhood to begin with. Once we identify this extraneous variable, we do need to first collect some kind of data from the participants to measure how happy their childhood was. Or sometimes, data on the extraneous variables we plan to use may be already available (for example, you want to examine the effect of different types of tutoring on students' performance in Calculus I course and you plan to match them on this extraneous variable: college entrance test scores, which is already collected by the Admissions Office). In either case, getting the data on the identified extraneous variable is a typical step we need to do before matching. So going back to whether individuals had a happy childhood to begin with. Once we have data, we'd sort it in a certain order, for example, from the highest score (meaning participants reporting the happiest childhood) to the lowest score (meaning participants reporting the least happy childhood). We will then identify/match participants with the highest levels of childhood happiness and place them into different treatment groups. Then we go down the scale and match participants with relative high levels of childhood happiness and place them into different treatment groups. We repeat on the descending order until we match participants with the lowest levels of childhood happiness and place them into different treatment groups. By now, each treatment group will have participants with a full range of levels on childhood happiness (which is a strength...thinking about the variation, the representativeness of the sample). The two treatment groups will be similar or equivalent on this extraneous variable. If the treatments, self-spending vs. other-spending, eventually shows the differences on individual happiness, then we know it's not due to how happy their childhood was. We will be more confident it is due to the independent variable.
You may be thinking, but wait we have only taken care of one extraneous variable. What about other extraneous variables? Good thinking.That's exactly correct. We mentioned a few extraneous variables but have only matched them on one. This is the main limitation of matching. You can match participants on more than one extraneous variables, but it's cumbersome, if not impossible, to match them on 10 or 20 extraneous variables. More importantly, the more variables we try to match participants on, the less likely we will have a similar match. In other words, it may be easy to find/match participants on one particular extraneous variable (similar level of childhood happiness), but it's much harder to find/match participants to be similar on 10 different extraneous variables at once.
Holding Extraneous Variable Constant
Holding extraneous variable constant control technique is self-explanatory. We will use participants at one level of extraneous variable only, in other words, holding the extraneous variable constant. Using the same example above, for example we only want to study participants with the low level of childhood happiness. We do need to go through the same steps as in Matching: identifying the extraneous variable that can potentially confound the research design and getting the data on the identified extraneous variable. Once we have the data on childhood happiness scores, we will only include participants on the lower end of childhood happiness scores, then place them into different treatment groups and carry out the study as before. If the condition groups, self-spending vs. other-spending, eventually shows the differences on individual happiness, then we know it's not due to how happy their childhood was (since we already picked those on the lower end of childhood happiness only). We will be more confident it is due to the independent variable.
Similarly to Matching, we have to do this one extraneous variable at a time. As we increase the number of extraneous variables to be held constant, the more difficult it gets. The other limitation is by holding extraneous variable constant, we are excluding a big chunk of participants, in this case, anyone who are NOT low on childhood happiness. This is a major weakness, as we reduce the variability on the spectrum of childhood happiness levels, we decreases the representativeness of the sample and generalizabiliy suffers.
Building Extraneous Variables into Design
The last control technique building extraneous variables into research design is widely used. Like the name suggests, we would identify the extraneous variable that can potentially confound the research design, and include it into the research design by treating it as an independent variable. This control technique takes care of the limitation the previous control technique, holding extraneous variable constant, has. We don't need to excluding participants based on where they stand on the extraneous variable(s). Instead we can include participants with a wide range of levels on the extraneous variable(s). You can include multiple extraneous variables into the design at once. However, the more variables you include in the design, the large the sample size it requires for statistical analyses, which may be difficult to obtain due to limitations of time, staff, cost, access, etc.

Confounding and Effect Measure Modification
- 1
- | 2
- | 3
- | 4
- | 5
- | 6
- | 7
- | 8
- | 9

Control of Confounding in Study Design
Restriction, randomization in clinical trials.

All Modules

Module Topics
E pi_Tools.XLS
Class Slides
One of the conditions necessary for confounding to occur is that the confounding factor must be distributed unequally among the groups being compared. Consequently, one of the strategies employed for avoiding confounding is to restrict admission into the study to a group of subjects who have the same levels of the confounding factors. For example, in the hypothetical study looking at the association between physical activity and heart disease, suppose that age and gender were the only two confounders of concern. If so, confounding by these factors could have been avoided by making sure that all subjects were males between the ages of 40-50. This will ensure that the age distributions are similar in the groups being compared, so that confounding will be minimized.
This approach to controlling confounding is simple and effective, but it has several limitations:
- It reduces the number of subjects who are eligible (may cause sample size problem).
- Residual confounding can occur if you don't restrict narrowly enough. For example, in the study on exercise and heart disease, the investigators might have restricted the study to men aged 40-65. However, the age-related risk of heart disease still varies widely within this range as do levels of physical activity.
- You can't evaluate the effects of factors that have been restricted for. For example, if the study is limited to men aged 45-50, you can't use this study to examine the effects of gender or age (because these factors don't vary within your sample).
- Restriction limits generalizability. For example, if you restrict the study to men, you may not be able to generalize the findings to women.
Instead of restriction, one could also ensure that the study groups do not differ with respect to possible confounders such as age and gender by matching the two comparison groups. For example, for every active male between the ages of 40-50, we could find and enroll an inactiv e male between the ages of 40-50. In this way, the groups we are comparing can artificially be made similar with respect to these factors, so they cannot confound the relationship. This method actually requires the investigators to control confounding in both the design and analysis phases of the study, because the analysis of matched study groups differs from that of unmatched studies. Like restriction, this approach is straightforward, and it can be effective. However, it has the following disadvantages:
- It can be time-consuming and expensive.
- It limits sample size.
- You can't evaluate the effect of the factors you that you matched for.
Nevertheless, matching is useful in the following circumstances:
- When one needs to control for complex, multifaceted variables (e.g., heredity, environmental factors)
- When doing a case-control study in which there are many possible controls, but a smaller number of cases (e.g., 4:1 matching in the study examining the association between DES and vaginal cancer)
You previously studied randomization in the online module on Clinical Trials. Given the more detailed discussion in this current module of the conditions necessary for confounding to occur, it should be obvious why randomization is such a powerful method to control prevent confounding. If a large number of subjects are allocated to treatment groups by a random method that gives an equal chance of being in any treatment group, then it is likely that the groups will have similar distributions of age, gender, behaviors, and virtually all other known and as yet unknown possible confounding factors. Moreover, the investigators can get a sense of whether randomization has successfully created comparability among the groups by comparing their baseline characteristics.
return to top | previous page | next page
Content ©2016. All Rights Reserved. Date last modified: June 3, 2016. Wayne W. LaMorte, MD, PhD, MPH
Chapter 6: Experimental Research
6.2 experimental design, learning objectives.
- Explain the difference between between-subjects and within-subjects experiments, list some of the pros and cons of each approach, and decide which approach to use to answer a particular research question.
- Define random assignment, distinguish it from random sampling, explain its purpose in experimental research, and use some simple strategies to implement it.
- Define what a control condition is, explain its purpose in research on treatment effectiveness, and describe some alternative types of control conditions.
- Define several types of carryover effect, give examples of each, and explain how counterbalancing helps to deal with them.
In this section, we look at some different ways to design an experiment. The primary distinction we will make is between approaches in which each participant experiences one level of the independent variable and approaches in which each participant experiences all levels of the independent variable. The former are called between-subjects experiments and the latter are called within-subjects experiments.
Between-Subjects Experiments
In a between-subjects experiment , each participant is tested in only one condition. For example, a researcher with a sample of 100 college students might assign half of them to write about a traumatic event and the other half write about a neutral event. Or a researcher with a sample of 60 people with severe agoraphobia (fear of open spaces) might assign 20 of them to receive each of three different treatments for that disorder. It is essential in a between-subjects experiment that the researcher assign participants to conditions so that the different groups are, on average, highly similar to each other. Those in a trauma condition and a neutral condition, for example, should include a similar proportion of men and women, and they should have similar average intelligence quotients (IQs), similar average levels of motivation, similar average numbers of health problems, and so on. This is a matter of controlling these extraneous participant variables across conditions so that they do not become confounding variables.
Random Assignment
The primary way that researchers accomplish this kind of control of extraneous variables across conditions is called random assignment , which means using a random process to decide which participants are tested in which conditions. Do not confuse random assignment with random sampling. Random sampling is a method for selecting a sample from a population, and it is rarely used in psychological research. Random assignment is a method for assigning participants in a sample to the different conditions, and it is an important element of all experimental research in psychology and other fields too.
In its strictest sense, random assignment should meet two criteria. One is that each participant has an equal chance of being assigned to each condition (e.g., a 50% chance of being assigned to each of two conditions). The second is that each participant is assigned to a condition independently of other participants. Thus one way to assign participants to two conditions would be to flip a coin for each one. If the coin lands heads, the participant is assigned to Condition A, and if it lands tails, the participant is assigned to Condition B. For three conditions, one could use a computer to generate a random integer from 1 to 3 for each participant. If the integer is 1, the participant is assigned to Condition A; if it is 2, the participant is assigned to Condition B; and if it is 3, the participant is assigned to Condition C. In practice, a full sequence of conditions—one for each participant expected to be in the experiment—is usually created ahead of time, and each new participant is assigned to the next condition in the sequence as he or she is tested. When the procedure is computerized, the computer program often handles the random assignment.
One problem with coin flipping and other strict procedures for random assignment is that they are likely to result in unequal sample sizes in the different conditions. Unequal sample sizes are generally not a serious problem, and you should never throw away data you have already collected to achieve equal sample sizes. However, for a fixed number of participants, it is statistically most efficient to divide them into equal-sized groups. It is standard practice, therefore, to use a kind of modified random assignment that keeps the number of participants in each group as similar as possible. One approach is block randomization . In block randomization, all the conditions occur once in the sequence before any of them is repeated. Then they all occur again before any of them is repeated again. Within each of these “blocks,” the conditions occur in a random order. Again, the sequence of conditions is usually generated before any participants are tested, and each new participant is assigned to the next condition in the sequence. Table 6.2 “Block Randomization Sequence for Assigning Nine Participants to Three Conditions” shows such a sequence for assigning nine participants to three conditions. The Research Randomizer website ( http://www.randomizer.org ) will generate block randomization sequences for any number of participants and conditions. Again, when the procedure is computerized, the computer program often handles the block randomization.
Table 6.2 Block Randomization Sequence for Assigning Nine Participants to Three Conditions
Random assignment is not guaranteed to control all extraneous variables across conditions. It is always possible that just by chance, the participants in one condition might turn out to be substantially older, less tired, more motivated, or less depressed on average than the participants in another condition. However, there are some reasons that this is not a major concern. One is that random assignment works better than one might expect, especially for large samples. Another is that the inferential statistics that researchers use to decide whether a difference between groups reflects a difference in the population takes the “fallibility” of random assignment into account. Yet another reason is that even if random assignment does result in a confounding variable and therefore produces misleading results, this is likely to be detected when the experiment is replicated. The upshot is that random assignment to conditions—although not infallible in terms of controlling extraneous variables—is always considered a strength of a research design.
Treatment and Control Conditions
Between-subjects experiments are often used to determine whether a treatment works. In psychological research, a treatment is any intervention meant to change people’s behavior for the better. This includes psychotherapies and medical treatments for psychological disorders but also interventions designed to improve learning, promote conservation, reduce prejudice, and so on. To determine whether a treatment works, participants are randomly assigned to either a treatment condition , in which they receive the treatment, or a control condition , in which they do not receive the treatment. If participants in the treatment condition end up better off than participants in the control condition—for example, they are less depressed, learn faster, conserve more, express less prejudice—then the researcher can conclude that the treatment works. In research on the effectiveness of psychotherapies and medical treatments, this type of experiment is often called a randomized clinical trial .
There are different types of control conditions. In a no-treatment control condition , participants receive no treatment whatsoever. One problem with this approach, however, is the existence of placebo effects. A placebo is a simulated treatment that lacks any active ingredient or element that should make it effective, and a placebo effect is a positive effect of such a treatment. Many folk remedies that seem to work—such as eating chicken soup for a cold or placing soap under the bedsheets to stop nighttime leg cramps—are probably nothing more than placebos. Although placebo effects are not well understood, they are probably driven primarily by people’s expectations that they will improve. Having the expectation to improve can result in reduced stress, anxiety, and depression, which can alter perceptions and even improve immune system functioning (Price, Finniss, & Benedetti, 2008).
Placebo effects are interesting in their own right (see Note 6.28 “The Powerful Placebo” ), but they also pose a serious problem for researchers who want to determine whether a treatment works. Figure 6.2 “Hypothetical Results From a Study Including Treatment, No-Treatment, and Placebo Conditions” shows some hypothetical results in which participants in a treatment condition improved more on average than participants in a no-treatment control condition. If these conditions (the two leftmost bars in Figure 6.2 “Hypothetical Results From a Study Including Treatment, No-Treatment, and Placebo Conditions” ) were the only conditions in this experiment, however, one could not conclude that the treatment worked. It could be instead that participants in the treatment group improved more because they expected to improve, while those in the no-treatment control condition did not.
Figure 6.2 Hypothetical Results From a Study Including Treatment, No-Treatment, and Placebo Conditions
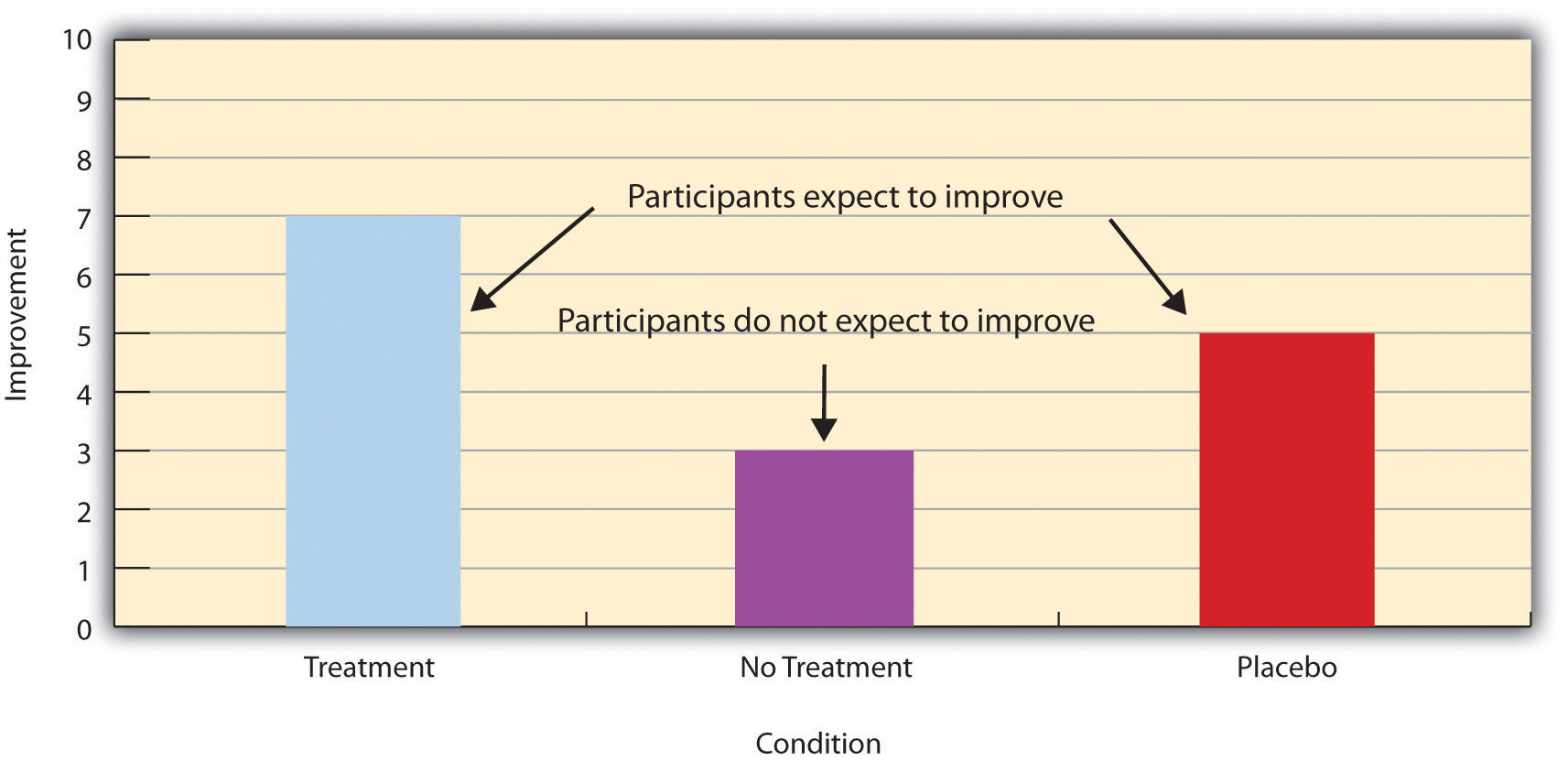
Fortunately, there are several solutions to this problem. One is to include a placebo control condition , in which participants receive a placebo that looks much like the treatment but lacks the active ingredient or element thought to be responsible for the treatment’s effectiveness. When participants in a treatment condition take a pill, for example, then those in a placebo control condition would take an identical-looking pill that lacks the active ingredient in the treatment (a “sugar pill”). In research on psychotherapy effectiveness, the placebo might involve going to a psychotherapist and talking in an unstructured way about one’s problems. The idea is that if participants in both the treatment and the placebo control groups expect to improve, then any improvement in the treatment group over and above that in the placebo control group must have been caused by the treatment and not by participants’ expectations. This is what is shown by a comparison of the two outer bars in Figure 6.2 “Hypothetical Results From a Study Including Treatment, No-Treatment, and Placebo Conditions” .
Of course, the principle of informed consent requires that participants be told that they will be assigned to either a treatment or a placebo control condition—even though they cannot be told which until the experiment ends. In many cases the participants who had been in the control condition are then offered an opportunity to have the real treatment. An alternative approach is to use a waitlist control condition , in which participants are told that they will receive the treatment but must wait until the participants in the treatment condition have already received it. This allows researchers to compare participants who have received the treatment with participants who are not currently receiving it but who still expect to improve (eventually). A final solution to the problem of placebo effects is to leave out the control condition completely and compare any new treatment with the best available alternative treatment. For example, a new treatment for simple phobia could be compared with standard exposure therapy. Because participants in both conditions receive a treatment, their expectations about improvement should be similar. This approach also makes sense because once there is an effective treatment, the interesting question about a new treatment is not simply “Does it work?” but “Does it work better than what is already available?”
The Powerful Placebo
Many people are not surprised that placebos can have a positive effect on disorders that seem fundamentally psychological, including depression, anxiety, and insomnia. However, placebos can also have a positive effect on disorders that most people think of as fundamentally physiological. These include asthma, ulcers, and warts (Shapiro & Shapiro, 1999). There is even evidence that placebo surgery—also called “sham surgery”—can be as effective as actual surgery.
Medical researcher J. Bruce Moseley and his colleagues conducted a study on the effectiveness of two arthroscopic surgery procedures for osteoarthritis of the knee (Moseley et al., 2002). The control participants in this study were prepped for surgery, received a tranquilizer, and even received three small incisions in their knees. But they did not receive the actual arthroscopic surgical procedure. The surprising result was that all participants improved in terms of both knee pain and function, and the sham surgery group improved just as much as the treatment groups. According to the researchers, “This study provides strong evidence that arthroscopic lavage with or without débridement [the surgical procedures used] is not better than and appears to be equivalent to a placebo procedure in improving knee pain and self-reported function” (p. 85).
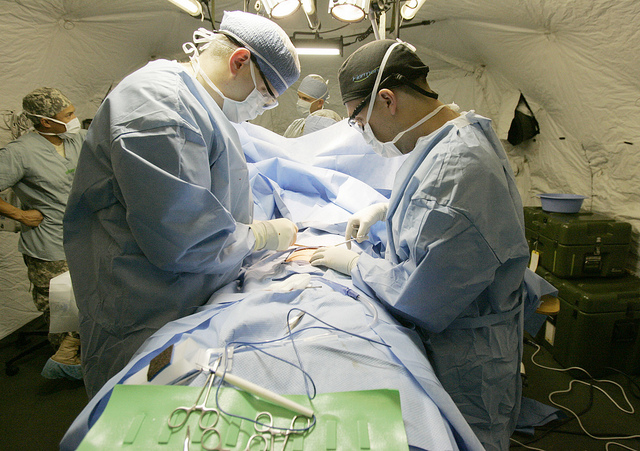
Research has shown that patients with osteoarthritis of the knee who receive a “sham surgery” experience reductions in pain and improvement in knee function similar to those of patients who receive a real surgery.
Army Medicine – Surgery – CC BY 2.0.
Within-Subjects Experiments
In a within-subjects experiment , each participant is tested under all conditions. Consider an experiment on the effect of a defendant’s physical attractiveness on judgments of his guilt. Again, in a between-subjects experiment, one group of participants would be shown an attractive defendant and asked to judge his guilt, and another group of participants would be shown an unattractive defendant and asked to judge his guilt. In a within-subjects experiment, however, the same group of participants would judge the guilt of both an attractive and an unattractive defendant.
The primary advantage of this approach is that it provides maximum control of extraneous participant variables. Participants in all conditions have the same mean IQ, same socioeconomic status, same number of siblings, and so on—because they are the very same people. Within-subjects experiments also make it possible to use statistical procedures that remove the effect of these extraneous participant variables on the dependent variable and therefore make the data less “noisy” and the effect of the independent variable easier to detect. We will look more closely at this idea later in the book.
Carryover Effects and Counterbalancing
The primary disadvantage of within-subjects designs is that they can result in carryover effects. A carryover effect is an effect of being tested in one condition on participants’ behavior in later conditions. One type of carryover effect is a practice effect , where participants perform a task better in later conditions because they have had a chance to practice it. Another type is a fatigue effect , where participants perform a task worse in later conditions because they become tired or bored. Being tested in one condition can also change how participants perceive stimuli or interpret their task in later conditions. This is called a context effect . For example, an average-looking defendant might be judged more harshly when participants have just judged an attractive defendant than when they have just judged an unattractive defendant. Within-subjects experiments also make it easier for participants to guess the hypothesis. For example, a participant who is asked to judge the guilt of an attractive defendant and then is asked to judge the guilt of an unattractive defendant is likely to guess that the hypothesis is that defendant attractiveness affects judgments of guilt. This could lead the participant to judge the unattractive defendant more harshly because he thinks this is what he is expected to do. Or it could make participants judge the two defendants similarly in an effort to be “fair.”
Carryover effects can be interesting in their own right. (Does the attractiveness of one person depend on the attractiveness of other people that we have seen recently?) But when they are not the focus of the research, carryover effects can be problematic. Imagine, for example, that participants judge the guilt of an attractive defendant and then judge the guilt of an unattractive defendant. If they judge the unattractive defendant more harshly, this might be because of his unattractiveness. But it could be instead that they judge him more harshly because they are becoming bored or tired. In other words, the order of the conditions is a confounding variable. The attractive condition is always the first condition and the unattractive condition the second. Thus any difference between the conditions in terms of the dependent variable could be caused by the order of the conditions and not the independent variable itself.
There is a solution to the problem of order effects, however, that can be used in many situations. It is counterbalancing , which means testing different participants in different orders. For example, some participants would be tested in the attractive defendant condition followed by the unattractive defendant condition, and others would be tested in the unattractive condition followed by the attractive condition. With three conditions, there would be six different orders (ABC, ACB, BAC, BCA, CAB, and CBA), so some participants would be tested in each of the six orders. With counterbalancing, participants are assigned to orders randomly, using the techniques we have already discussed. Thus random assignment plays an important role in within-subjects designs just as in between-subjects designs. Here, instead of randomly assigning to conditions, they are randomly assigned to different orders of conditions. In fact, it can safely be said that if a study does not involve random assignment in one form or another, it is not an experiment.
There are two ways to think about what counterbalancing accomplishes. One is that it controls the order of conditions so that it is no longer a confounding variable. Instead of the attractive condition always being first and the unattractive condition always being second, the attractive condition comes first for some participants and second for others. Likewise, the unattractive condition comes first for some participants and second for others. Thus any overall difference in the dependent variable between the two conditions cannot have been caused by the order of conditions. A second way to think about what counterbalancing accomplishes is that if there are carryover effects, it makes it possible to detect them. One can analyze the data separately for each order to see whether it had an effect.
When 9 Is “Larger” Than 221
Researcher Michael Birnbaum has argued that the lack of context provided by between-subjects designs is often a bigger problem than the context effects created by within-subjects designs. To demonstrate this, he asked one group of participants to rate how large the number 9 was on a 1-to-10 rating scale and another group to rate how large the number 221 was on the same 1-to-10 rating scale (Birnbaum, 1999). Participants in this between-subjects design gave the number 9 a mean rating of 5.13 and the number 221 a mean rating of 3.10. In other words, they rated 9 as larger than 221! According to Birnbaum, this is because participants spontaneously compared 9 with other one-digit numbers (in which case it is relatively large) and compared 221 with other three-digit numbers (in which case it is relatively small).
Simultaneous Within-Subjects Designs
So far, we have discussed an approach to within-subjects designs in which participants are tested in one condition at a time. There is another approach, however, that is often used when participants make multiple responses in each condition. Imagine, for example, that participants judge the guilt of 10 attractive defendants and 10 unattractive defendants. Instead of having people make judgments about all 10 defendants of one type followed by all 10 defendants of the other type, the researcher could present all 20 defendants in a sequence that mixed the two types. The researcher could then compute each participant’s mean rating for each type of defendant. Or imagine an experiment designed to see whether people with social anxiety disorder remember negative adjectives (e.g., “stupid,” “incompetent”) better than positive ones (e.g., “happy,” “productive”). The researcher could have participants study a single list that includes both kinds of words and then have them try to recall as many words as possible. The researcher could then count the number of each type of word that was recalled. There are many ways to determine the order in which the stimuli are presented, but one common way is to generate a different random order for each participant.
Between-Subjects or Within-Subjects?
Almost every experiment can be conducted using either a between-subjects design or a within-subjects design. This means that researchers must choose between the two approaches based on their relative merits for the particular situation.
Between-subjects experiments have the advantage of being conceptually simpler and requiring less testing time per participant. They also avoid carryover effects without the need for counterbalancing. Within-subjects experiments have the advantage of controlling extraneous participant variables, which generally reduces noise in the data and makes it easier to detect a relationship between the independent and dependent variables.
A good rule of thumb, then, is that if it is possible to conduct a within-subjects experiment (with proper counterbalancing) in the time that is available per participant—and you have no serious concerns about carryover effects—this is probably the best option. If a within-subjects design would be difficult or impossible to carry out, then you should consider a between-subjects design instead. For example, if you were testing participants in a doctor’s waiting room or shoppers in line at a grocery store, you might not have enough time to test each participant in all conditions and therefore would opt for a between-subjects design. Or imagine you were trying to reduce people’s level of prejudice by having them interact with someone of another race. A within-subjects design with counterbalancing would require testing some participants in the treatment condition first and then in a control condition. But if the treatment works and reduces people’s level of prejudice, then they would no longer be suitable for testing in the control condition. This is true for many designs that involve a treatment meant to produce long-term change in participants’ behavior (e.g., studies testing the effectiveness of psychotherapy). Clearly, a between-subjects design would be necessary here.
Remember also that using one type of design does not preclude using the other type in a different study. There is no reason that a researcher could not use both a between-subjects design and a within-subjects design to answer the same research question. In fact, professional researchers often do exactly this.
Key Takeaways
- Experiments can be conducted using either between-subjects or within-subjects designs. Deciding which to use in a particular situation requires careful consideration of the pros and cons of each approach.
- Random assignment to conditions in between-subjects experiments or to orders of conditions in within-subjects experiments is a fundamental element of experimental research. Its purpose is to control extraneous variables so that they do not become confounding variables.
- Experimental research on the effectiveness of a treatment requires both a treatment condition and a control condition, which can be a no-treatment control condition, a placebo control condition, or a waitlist control condition. Experimental treatments can also be compared with the best available alternative.
Discussion: For each of the following topics, list the pros and cons of a between-subjects and within-subjects design and decide which would be better.
- You want to test the relative effectiveness of two training programs for running a marathon.
- Using photographs of people as stimuli, you want to see if smiling people are perceived as more intelligent than people who are not smiling.
- In a field experiment, you want to see if the way a panhandler is dressed (neatly vs. sloppily) affects whether or not passersby give him any money.
- You want to see if concrete nouns (e.g., dog ) are recalled better than abstract nouns (e.g., truth ).
- Discussion: Imagine that an experiment shows that participants who receive psychodynamic therapy for a dog phobia improve more than participants in a no-treatment control group. Explain a fundamental problem with this research design and at least two ways that it might be corrected.
Birnbaum, M. H. (1999). How to show that 9 > 221: Collect judgments in a between-subjects design. Psychological Methods, 4 , 243–249.
Moseley, J. B., O’Malley, K., Petersen, N. J., Menke, T. J., Brody, B. A., Kuykendall, D. H., … Wray, N. P. (2002). A controlled trial of arthroscopic surgery for osteoarthritis of the knee. The New England Journal of Medicine, 347 , 81–88.
Price, D. D., Finniss, D. G., & Benedetti, F. (2008). A comprehensive review of the placebo effect: Recent advances and current thought. Annual Review of Psychology, 59 , 565–590.
Shapiro, A. K., & Shapiro, E. (1999). The powerful placebo: From ancient priest to modern physician . Baltimore, MD: Johns Hopkins University Press.
- Research Methods in Psychology. Provided by : University of Minnesota Libraries Publishing. Located at : http://open.lib.umn.edu/psychologyresearchmethods . License : CC BY-NC-SA: Attribution-NonCommercial-ShareAlike
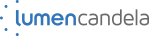
Privacy Policy
- Bipolar Disorder
- Therapy Center
- When To See a Therapist
- Types of Therapy
- Best Online Therapy
- Best Couples Therapy
- Best Family Therapy
- Managing Stress
- Sleep and Dreaming
- Understanding Emotions
- Self-Improvement
- Healthy Relationships
- Student Resources
- Personality Types
- Guided Meditations
- Verywell Mind Insights
- 2024 Verywell Mind 25
- Mental Health in the Classroom
- Editorial Process
- Meet Our Review Board
- Crisis Support
The Definition of Random Assignment According to Psychology
Kendra Cherry, MS, is a psychosocial rehabilitation specialist, psychology educator, and author of the "Everything Psychology Book."
:max_bytes(150000):strip_icc():format(webp)/IMG_9791-89504ab694d54b66bbd72cb84ffb860e.jpg)
Emily is a board-certified science editor who has worked with top digital publishing brands like Voices for Biodiversity, Study.com, GoodTherapy, Vox, and Verywell.
:max_bytes(150000):strip_icc():format(webp)/Emily-Swaim-1000-0f3197de18f74329aeffb690a177160c.jpg)
Materio / Getty Images
Random assignment refers to the use of chance procedures in psychology experiments to ensure that each participant has the same opportunity to be assigned to any given group in a study to eliminate any potential bias in the experiment at the outset. Participants are randomly assigned to different groups, such as the treatment group versus the control group. In clinical research, randomized clinical trials are known as the gold standard for meaningful results.
Simple random assignment techniques might involve tactics such as flipping a coin, drawing names out of a hat, rolling dice, or assigning random numbers to a list of participants. It is important to note that random assignment differs from random selection .
While random selection refers to how participants are randomly chosen from a target population as representatives of that population, random assignment refers to how those chosen participants are then assigned to experimental groups.
Random Assignment In Research
To determine if changes in one variable will cause changes in another variable, psychologists must perform an experiment. Random assignment is a critical part of the experimental design that helps ensure the reliability of the study outcomes.
Researchers often begin by forming a testable hypothesis predicting that one variable of interest will have some predictable impact on another variable.
The variable that the experimenters will manipulate in the experiment is known as the independent variable , while the variable that they will then measure for different outcomes is known as the dependent variable. While there are different ways to look at relationships between variables, an experiment is the best way to get a clear idea if there is a cause-and-effect relationship between two or more variables.
Once researchers have formulated a hypothesis, conducted background research, and chosen an experimental design, it is time to find participants for their experiment. How exactly do researchers decide who will be part of an experiment? As mentioned previously, this is often accomplished through something known as random selection.
Random Selection
In order to generalize the results of an experiment to a larger group, it is important to choose a sample that is representative of the qualities found in that population. For example, if the total population is 60% female and 40% male, then the sample should reflect those same percentages.
Choosing a representative sample is often accomplished by randomly picking people from the population to be participants in a study. Random selection means that everyone in the group stands an equal chance of being chosen to minimize any bias. Once a pool of participants has been selected, it is time to assign them to groups.
By randomly assigning the participants into groups, the experimenters can be fairly sure that each group will have the same characteristics before the independent variable is applied.
Participants might be randomly assigned to the control group , which does not receive the treatment in question. The control group may receive a placebo or receive the standard treatment. Participants may also be randomly assigned to the experimental group , which receives the treatment of interest. In larger studies, there can be multiple treatment groups for comparison.
There are simple methods of random assignment, like rolling the die. However, there are more complex techniques that involve random number generators to remove any human error.
There can also be random assignment to groups with pre-established rules or parameters. For example, if you want to have an equal number of men and women in each of your study groups, you might separate your sample into two groups (by sex) before randomly assigning each of those groups into the treatment group and control group.
Random assignment is essential because it increases the likelihood that the groups are the same at the outset. With all characteristics being equal between groups, other than the application of the independent variable, any differences found between group outcomes can be more confidently attributed to the effect of the intervention.
Example of Random Assignment
Imagine that a researcher is interested in learning whether or not drinking caffeinated beverages prior to an exam will improve test performance. After randomly selecting a pool of participants, each person is randomly assigned to either the control group or the experimental group.
The participants in the control group consume a placebo drink prior to the exam that does not contain any caffeine. Those in the experimental group, on the other hand, consume a caffeinated beverage before taking the test.
Participants in both groups then take the test, and the researcher compares the results to determine if the caffeinated beverage had any impact on test performance.
A Word From Verywell
Random assignment plays an important role in the psychology research process. Not only does this process help eliminate possible sources of bias, but it also makes it easier to generalize the results of a tested sample of participants to a larger population.
Random assignment helps ensure that members of each group in the experiment are the same, which means that the groups are also likely more representative of what is present in the larger population of interest. Through the use of this technique, psychology researchers are able to study complex phenomena and contribute to our understanding of the human mind and behavior.
Lin Y, Zhu M, Su Z. The pursuit of balance: An overview of covariate-adaptive randomization techniques in clinical trials . Contemp Clin Trials. 2015;45(Pt A):21-25. doi:10.1016/j.cct.2015.07.011
Sullivan L. Random assignment versus random selection . In: The SAGE Glossary of the Social and Behavioral Sciences. SAGE Publications, Inc.; 2009. doi:10.4135/9781412972024.n2108
Alferes VR. Methods of Randomization in Experimental Design . SAGE Publications, Inc.; 2012. doi:10.4135/9781452270012
Nestor PG, Schutt RK. Research Methods in Psychology: Investigating Human Behavior. (2nd Ed.). SAGE Publications, Inc.; 2015.
By Kendra Cherry, MSEd Kendra Cherry, MS, is a psychosocial rehabilitation specialist, psychology educator, and author of the "Everything Psychology Book."
Have a language expert improve your writing
Run a free plagiarism check in 10 minutes, automatically generate references for free.
- Knowledge Base
- Methodology
- Random Assignment in Experiments | Introduction & Examples
Random Assignment in Experiments | Introduction & Examples
Published on 6 May 2022 by Pritha Bhandari . Revised on 13 February 2023.
In experimental research, random assignment is a way of placing participants from your sample into different treatment groups using randomisation.
With simple random assignment, every member of the sample has a known or equal chance of being placed in a control group or an experimental group. Studies that use simple random assignment are also called completely randomised designs .
Random assignment is a key part of experimental design . It helps you ensure that all groups are comparable at the start of a study: any differences between them are due to random factors.
Table of contents
Why does random assignment matter, random sampling vs random assignment, how do you use random assignment, when is random assignment not used, frequently asked questions about random assignment.
Random assignment is an important part of control in experimental research, because it helps strengthen the internal validity of an experiment.
In experiments, researchers manipulate an independent variable to assess its effect on a dependent variable, while controlling for other variables. To do so, they often use different levels of an independent variable for different groups of participants.
This is called a between-groups or independent measures design.
You use three groups of participants that are each given a different level of the independent variable:
- A control group that’s given a placebo (no dosage)
- An experimental group that’s given a low dosage
- A second experimental group that’s given a high dosage
Random assignment to helps you make sure that the treatment groups don’t differ in systematic or biased ways at the start of the experiment.
If you don’t use random assignment, you may not be able to rule out alternative explanations for your results.
- Participants recruited from pubs are placed in the control group
- Participants recruited from local community centres are placed in the low-dosage experimental group
- Participants recruited from gyms are placed in the high-dosage group
With this type of assignment, it’s hard to tell whether the participant characteristics are the same across all groups at the start of the study. Gym users may tend to engage in more healthy behaviours than people who frequent pubs or community centres, and this would introduce a healthy user bias in your study.
Although random assignment helps even out baseline differences between groups, it doesn’t always make them completely equivalent. There may still be extraneous variables that differ between groups, and there will always be some group differences that arise from chance.
Most of the time, the random variation between groups is low, and, therefore, it’s acceptable for further analysis. This is especially true when you have a large sample. In general, you should always use random assignment in experiments when it is ethically possible and makes sense for your study topic.
Prevent plagiarism, run a free check.
Random sampling and random assignment are both important concepts in research, but it’s important to understand the difference between them.
Random sampling (also called probability sampling or random selection) is a way of selecting members of a population to be included in your study. In contrast, random assignment is a way of sorting the sample participants into control and experimental groups.
While random sampling is used in many types of studies, random assignment is only used in between-subjects experimental designs.
Some studies use both random sampling and random assignment, while others use only one or the other.
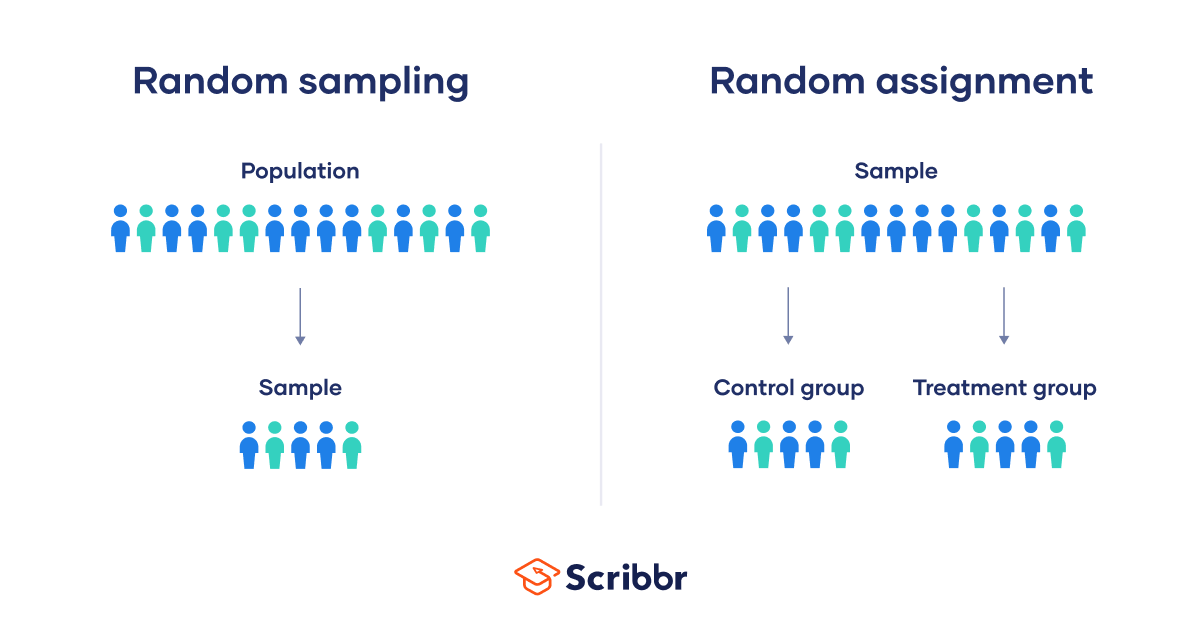
Random sampling enhances the external validity or generalisability of your results, because it helps to ensure that your sample is unbiased and representative of the whole population. This allows you to make stronger statistical inferences .
You use a simple random sample to collect data. Because you have access to the whole population (all employees), you can assign all 8,000 employees a number and use a random number generator to select 300 employees. These 300 employees are your full sample.
Random assignment enhances the internal validity of the study, because it ensures that there are no systematic differences between the participants in each group. This helps you conclude that the outcomes can be attributed to the independent variable .
- A control group that receives no intervention
- An experimental group that has a remote team-building intervention every week for a month
You use random assignment to place participants into the control or experimental group. To do so, you take your list of participants and assign each participant a number. Again, you use a random number generator to place each participant in one of the two groups.
To use simple random assignment, you start by giving every member of the sample a unique number. Then, you can use computer programs or manual methods to randomly assign each participant to a group.
- Random number generator: Use a computer program to generate random numbers from the list for each group.
- Lottery method: Place all numbers individually into a hat or a bucket, and draw numbers at random for each group.
- Flip a coin: When you only have two groups, for each number on the list, flip a coin to decide if they’ll be in the control or the experimental group.
- Use a dice: When you have three groups, for each number on the list, roll a die to decide which of the groups they will be in. For example, assume that rolling 1 or 2 lands them in a control group; 3 or 4 in an experimental group; and 5 or 6 in a second control or experimental group.
This type of random assignment is the most powerful method of placing participants in conditions, because each individual has an equal chance of being placed in any one of your treatment groups.
Random assignment in block designs
In more complicated experimental designs, random assignment is only used after participants are grouped into blocks based on some characteristic (e.g., test score or demographic variable). These groupings mean that you need a larger sample to achieve high statistical power .
For example, a randomised block design involves placing participants into blocks based on a shared characteristic (e.g., college students vs graduates), and then using random assignment within each block to assign participants to every treatment condition. This helps you assess whether the characteristic affects the outcomes of your treatment.
In an experimental matched design , you use blocking and then match up individual participants from each block based on specific characteristics. Within each matched pair or group, you randomly assign each participant to one of the conditions in the experiment and compare their outcomes.
Sometimes, it’s not relevant or ethical to use simple random assignment, so groups are assigned in a different way.
When comparing different groups
Sometimes, differences between participants are the main focus of a study, for example, when comparing children and adults or people with and without health conditions. Participants are not randomly assigned to different groups, but instead assigned based on their characteristics.
In this type of study, the characteristic of interest (e.g., gender) is an independent variable, and the groups differ based on the different levels (e.g., men, women). All participants are tested the same way, and then their group-level outcomes are compared.
When it’s not ethically permissible
When studying unhealthy or dangerous behaviours, it’s not possible to use random assignment. For example, if you’re studying heavy drinkers and social drinkers, it’s unethical to randomly assign participants to one of the two groups and ask them to drink large amounts of alcohol for your experiment.
When you can’t assign participants to groups, you can also conduct a quasi-experimental study . In a quasi-experiment, you study the outcomes of pre-existing groups who receive treatments that you may not have any control over (e.g., heavy drinkers and social drinkers).
These groups aren’t randomly assigned, but may be considered comparable when some other variables (e.g., age or socioeconomic status) are controlled for.
In experimental research, random assignment is a way of placing participants from your sample into different groups using randomisation. With this method, every member of the sample has a known or equal chance of being placed in a control group or an experimental group.
Random selection, or random sampling , is a way of selecting members of a population for your study’s sample.
In contrast, random assignment is a way of sorting the sample into control and experimental groups.
Random sampling enhances the external validity or generalisability of your results, while random assignment improves the internal validity of your study.
Random assignment is used in experiments with a between-groups or independent measures design. In this research design, there’s usually a control group and one or more experimental groups. Random assignment helps ensure that the groups are comparable.
In general, you should always use random assignment in this type of experimental design when it is ethically possible and makes sense for your study topic.
To implement random assignment , assign a unique number to every member of your study’s sample .
Then, you can use a random number generator or a lottery method to randomly assign each number to a control or experimental group. You can also do so manually, by flipping a coin or rolling a die to randomly assign participants to groups.
Cite this Scribbr article
If you want to cite this source, you can copy and paste the citation or click the ‘Cite this Scribbr article’ button to automatically add the citation to our free Reference Generator.
Bhandari, P. (2023, February 13). Random Assignment in Experiments | Introduction & Examples. Scribbr. Retrieved 21 May 2024, from https://www.scribbr.co.uk/research-methods/random-assignment-experiments/
Is this article helpful?
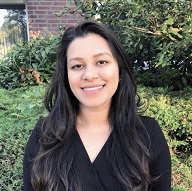
Pritha Bhandari
Other students also liked, a quick guide to experimental design | 5 steps & examples, controlled experiments | methods & examples of control, control groups and treatment groups | uses & examples.

An official website of the United States government
The .gov means it’s official. Federal government websites often end in .gov or .mil. Before sharing sensitive information, make sure you’re on a federal government site.
The site is secure. The https:// ensures that you are connecting to the official website and that any information you provide is encrypted and transmitted securely.
- Publications
- Account settings
Preview improvements coming to the PMC website in October 2024. Learn More or Try it out now .
- Advanced Search
- Journal List
- Evid Based Spine Care J
- v.3(1); 2012 Feb
Assessing bias: the importance of considering confounding
Andrea c. skelly.
1 Spectrum Research Inc, Tacoma, WA, USA
Joseph R. Dettori
Erika d. brodt, overview: why evaluate bias isn't statistical significance enough.
It is common to come across a study that reports that treatment A “provided significantly better pain relief” than treatment B.
When a difference in an outcome (eg, pain) between exposures (eg, treatment groups) is observed, one needs to consider whether the effect is truly because of exposure or if alternate explanations are possible. As we discussed in the previous issue of EBSJ, to evaluate the validity of a research study, factors that might distort the true association and/or influence its interpretation need to be carefully considered. This means evaluating the role of bias and considering the study's statistical precision. In the previous issue, we discussed aspects of statistical testing and precision to explore the question of whether an effect is real or due to chance. We also considered some caveats to concluding that a “statistically significant” result is clinically meaningful.
This article takes a look at the potential for bias and its impact.
Bias relates to systematic sources of error which need to be considered. Why? The internal validity of a study depends greatly on the extent to which biases have been accounted for and necessary steps taken to diminish their impact. In a poor-quality study, bias may be the primary reason the results are or are not “significant” statistically! Bias may preclude finding a true effect; it may lead to an inaccurate estimate (underestimate or overestimate) of the true association between exposure and an outcome. Significance testing in itself does not take into account factors which may bias study results.
Bias can be divided into three general categories: (1) selection bias; (2) information bias; and (3) confounding.
This article focuses on confounding. Recognizing it and controlling for its effects are important to a study's credibilty.
What is confounding?
Confounding is often referred to as a “mixing of effects” 1 , 2 wherein the effects of the exposure under study on a given outcome are mixed in with the effects of an additional factor (or set of factors) resulting in a distortion of the true relationship. In a clinical trial, this can happen when the distribution of a known prognostic factor differs between groups being compared.
Confounding factors may mask an actual association or, more commonly, falsely demonstrate an apparent association between the treatment and outcome when no real association between them exists.
The existence of confounding variables in studies make it difficult to establish a clear causal link between treatment and outcome unless appropriate methods are used to adjust for the effect of the confounders (more on this below). Confounding variables are those that may compete with the exposure of interest (eg, treatment) in explaining the outcome of a study. The amount of association “above and beyond” that which can be explained by confounding factors provides a more appropriate estimate of the true association which is due to the exposure.
General characteristics of confounders include the following:

A situation that contains both numbers 1 and 2 sets the stage for potential confounding ( Fig. 3 ).

How does confounding influence results? An example in spine surgery research:
Let's imagine that we wanted to know if treating index osteoporotic vertebral fractures with vertebroplasty increased the risk of subsequent vertebral fractures. We evaluate 400 patients with index vertebral fractures, 200 of whom received vertebroplasty and 200 did not. After 2 years, we identified 45 subsequent fractures with the following fictitious distribution:
At first glance, it looks like those who received vertebroplasty were at a much higher risk (two times the risk) compared with those who did not (this is called a “crude” estimate of the association). However, it is important to investigate whether other reasons could account for this difference. In particular, other variables that may influence the risk of subsequent vertebral fracture should be evaluated such as age, weight, and smoking status. The data below describes these variables at the time of the incident fracture. Note that age and weight are similar between groups. But, the difference in the proportion of patients who smoke is dramatically different in that 55% of the patients in the vertebroplasty group smoke compared with only 8% in the conservative care group, as outlined below.
If we stratify the results by smoking status, we note that the risk of subsequent fractures is similar between treatment groups in each stratum (smoking and nonsmoking) such that the relative risk (RR) is closer to 1 (no effect) compared with the overall results above where RR was 2.
Thus, smoking was a confounding factor distorting the true relationship between vertebroplasty and the risk of subsequent vertebral fractures.
Confounding by indication–a special and common case of confounding
With regard to the assessment of a technology or surgical procedure, confounding may take the form of an indication for use of that technology or procedure. 2 , 3 Such “confounding by indication” may be extremely important to consider in either studies of efficacy or of safety.
In a hypothetical study, let's suppose that all patients who received treatment A had more severe disease than those who received treatment B and that there was a statistically significant difference showing that treatment B resulted in better patient function. Is it valid to conclude that treatment B is truly better than treatment A? No! Given that the severity of the condition is likely associated with the outcome and that the severity is also associated with the treatment choice, the effects of the treatment cannot be separated from those of the severity.
To compare the effectiveness of two treatments, the only way to deal with this is to ensure that the study design requires patients with the same range of condition severity are included in both treatment groups and that choice of treatment is not based on condition severity.
Dealing with confounding
The potential for confounding should be considered in the design and implementation of the study. Factors which might be associated with the outcome other than the treatment need to be measured. To some extent, confounding can be accounted during analysis, assuming that such factors have been measured as part of the study.
Step 1: Measure and report all potential confounders
Patient characteristics are an often underreported or misreported set of measurements in spine care studies but are extremely important to quantify and report as they may be potential confounders. Diagnostic features, comorbidities, and any factor that might affect patient outcome needs to be measured and reported for each study group as well. Any and all of these characteristics, features, and factors may be potential confounders of the relationship between your “exposure of interest” (eg, a surgical treatment) and the outcome (eg, patient function). Planning for and measuring these attributes goes a long way toward dealing with the role of confounding.
Step 2: Routinely assess the role of confounding factors and adjust for them in analyses
There are a number of ways of assessing and adjusting for confounding, however a detailed discussion of this is beyond the scope of this article. Briefly, a few examples of how this could be accomplished include:
- During study planning, inclusion could be restricted by specific confounding variables, such as age.
- Several methods of “adjusting” the effect estimate as part of the analysis can be used. Stratification (as shown above) is one that can be relatively straightforward and involves looking at the association between the exposure and outcome for each factor category (or stratum) by calculating a stratum-specific estimate.
- Multivariate analysis, a set of statistical methods which allows for adjustment of multiple variables simultaneously via mathematical modeling, can also be used to “control” for confounding.
Basic concepts for these methods for control of confounding during analysis are the subject of future articles.
Step 3: Report adjusted and crude estimates of association and discuss limitations of the study that may be due to confounding and the magnitude of the influence
Regardless of the method used, an adjusted estimate should be obtained which reflects the degree of association between the exposure and disease that remains after the effects of the confounder have been “removed.” In general, if the adjusted estimate is different from the crude estimate by approximately 10% or more, the factor should be considered a confounder and the adjusted estimate used as a more reliable indicator of the effect of the exposure, ie, as an estimate of the effect “above and beyond” that is due to the confounder(s).
Failure to evaluate demographic and clinical factors as potential confounders can bias your study results and lead to erroneous conclusions. Study design must include the measurement and reporting of such factors. During analysis, the association between such factors and the outcome and your exposure of interest must be explored. A commonly overlooked type of confounding in the surgical literature is confounding by indication. This needs to be dealt with during study design to ensure that treatment groups include patients with the same range of condition severity and that treatment choice is not based on condition severity. In all likelihood, no matter how many variables one adjusts for, there will be residual confounding, possibly by factors that are unknown and cannot be measured.
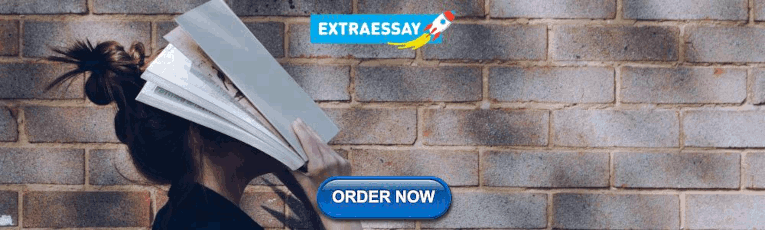
IMAGES
VIDEO
COMMENTS
Confounding variables (a.k.a. confounders or confounding factors) are a type of extraneous variable that are related to a study's independent and dependent variables. A variable must meet two conditions to be a confounder: It must be correlated with the independent variable. This may be a causal relationship, but it does not have to be.
Random assignment helps you separation causation from correlation and rule out confounding variables. As a critical component of the scientific method, experiments typically set up contrasts between a control group and one or more treatment groups. The idea is to determine whether the effect, which is the difference between a treatment group ...
Abstract. A Confounder is a variable whose presence affects the variables being studied so that the results do not reflect the actual relationship. There are various ways to exclude or control confounding variables including Randomization, Restriction and Matching. But all these methods are applicable at the time of study design.
In randomization the random assignment of study subjects to exposure categories to breaking any links between exposure and confounders. This reduces potential for confounding by generating groups that are fairly comparable with respect to known and unknown confounding variables. — How to control confounding effects by statistical analysis, 2012.
Random assignment controls for confounding due to both measurable and unmeasurable causes. So it is especially useful when confounding variables are unknown or cannot be measured. It also controls for time-varying confounders, that is when the exposure and the confounders are measured repeatedly in studies where participants are followed over ...
Random selection (also called probability sampling or random sampling) is a way of randomly selecting members of a population to be included in your study. On the other hand, random assignment is a way of sorting the sample participants into control and treatment groups. Random selection ensures that everyone in the population has an equal ...
Random assignment. Random assignment is the single most powerful control technique we can use to minimize the potential threats of the confounding variables in research design. As we have seen in Dunn and her colleagues' study earlier, participants are not allowed to self select into either conditions (spend $20 on self or spend on others).
These include transformations of variables, 16 shrinkage of parameter estimates 23 and random coefficient regression models. 24 Despite great flexibility when exploring associations between an exposure and an outcome while controlling for potential confounders, multivariable analysis does not directly identify whether a factor is a true confounder.
Restriction. One of the conditions necessary for confounding to occur is that the confounding factor must be distributed unequally among the groups being compared. Consequently, one of the strategies employed for avoiding confounding is to restrict admission into the study to a group of subjects who have the same levels of the confounding factors.
Objective: To review and describe randomization techniques used in clinical trials, including simple, block, stratified, and covariate adaptive techniques. Background: Clinical trials are required to establish treatment efficacy of many athletic training procedures. In the past, we have relied on evidence of questionable scientific merit to aid ...
Keywords. Confounding, sometimes referred to as confounding bias, is mostly described as a 'mixing' or 'blurring' of effects. 1 It occurs when an investigator tries to determine the effect of an exposure on the occurrence of a disease (or other outcome), but then actually measures the effect of another factor, a confounding variable.
Random assignment is a method for assigning participants in a sample to the different conditions, and it is an important element of all experimental research in psychology and other fields too. In its strictest sense, random assignment should meet two criteria. One is that each participant has an equal chance of being assigned to each condition ...
Random selection ensures that there will be no confounding variables, or factors that could influence the result of the experiment; confounding variables can render an experiment's findings ...
Random assignment refers to the use of chance procedures in psychology experiments to ensure that each participant has the same opportunity to be assigned to any given group in a study to eliminate any potential bias in the experiment at the outset. Participants are randomly assigned to different groups, such as the treatment group versus the ...
Instrumental variable. Instrumental variables to control confounding have been used in econometrics for decades but may also be useful in epidemiological studies to control confounding. 39 An instrumental variable is a factor that is associated with the exposure of interest (often a determinant of the exposure of interest), so that if we ...
Random assignment or random placement is an experimental technique for assigning human participants or animal subjects to different groups in an experiment (e.g., a treatment group versus a control group) using randomization, such as by a chance procedure (e.g., flipping a coin) or a random number generator. This ensures that each participant or subject has an equal chance of being placed in ...
Why does random assignment matter? Random assignment is an important part of control in experimental research, because it helps strengthen the internal validity of an experiment.. In experiments, researchers manipulate an independent variable to assess its effect on a dependent variable, while controlling for other variables. To do so, they often use different levels of an independent variable ...
Why does random assignment help control for confounding variables? a. Random assignment ensures that participants in a study are properly motivated to perform the experimental task that will be required of them. b. Random assignment eliminates individual differences by removing individuals with the same characteristics from the study and only ...
The bias can be while selecting the participant and the control (selection bias), owing to the confounding factors (confounding bias) and also while assessing the outcome (assessment bias). Randomization is the method adopted to eliminate the bias of selection and confounder. It has got two steps; generation of random number and concealment of ...
Study with Quizlet and memorize flashcards containing terms like A test that has low reliability cannot have good validity., Why does random assignment help control for confounding variables?, An article from the New York Times, published on April 24, 2007, summarized research conducted by of Dr. Vallortigara, a neuroscientist at the University of Trieste, Italy. In this study, Dr ...
Confounding is often referred to as a "mixing of effects" 1, 2 wherein the effects of the exposure under study on a given outcome are mixed in with the effects of an additional factor (or set of factors) resulting in a distortion of the true relationship. In a clinical trial, this can happen when the distribution of a known prognostic ...