The application of deep learning in computer vision
Ieee account.
- Change Username/Password
- Update Address
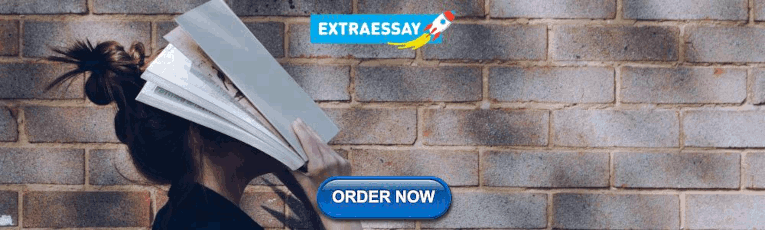
Purchase Details
- Payment Options
- Order History
- View Purchased Documents
Profile Information
- Communications Preferences
- Profession and Education
- Technical Interests
- US & Canada: +1 800 678 4333
- Worldwide: +1 732 981 0060
- Contact & Support
- About IEEE Xplore
- Accessibility
- Terms of Use
- Nondiscrimination Policy
- Privacy & Opting Out of Cookies
A not-for-profit organization, IEEE is the world's largest technical professional organization dedicated to advancing technology for the benefit of humanity. © Copyright 2024 IEEE - All rights reserved. Use of this web site signifies your agreement to the terms and conditions.
Editor’s Note: Special Issue on Robust Vision
- Editorial Notes
- Published: 24 May 2024
Cite this article
106 Accesses
Explore all metrics
Avoid common mistakes on your manuscript.
The International Journal of Computer Vision gratefully acknowledges the editorial work of the Guest Editors listed below. The special issue included an open call for papers on the topic, as well as extended papers invited from the associated workshop at ECCV 2022. The special issue features 32 papers which have been rigorously reviewed according to journal standards. The papers are available via the “Collections” link on the International Journal of Computer Vision homepage.
Oliver Zendel, Austrian Institute of Technology, Austria.
Adam Kortylewski , Max Planck Institute for Informatics, Germany.
Bernhard Egger, University of Erlangen-Nuremberg, Germany.
Additional information
Publisher's note.
Springer Nature remains neutral with regard to jurisdictional claims in published maps and institutional affiliations.
Rights and permissions
Reprints and permissions
About this article
Editor’s Note: Special Issue on Robust Vision. Int J Comput Vis (2024). https://doi.org/10.1007/s11263-024-02122-7
Download citation
Published : 24 May 2024
DOI : https://doi.org/10.1007/s11263-024-02122-7
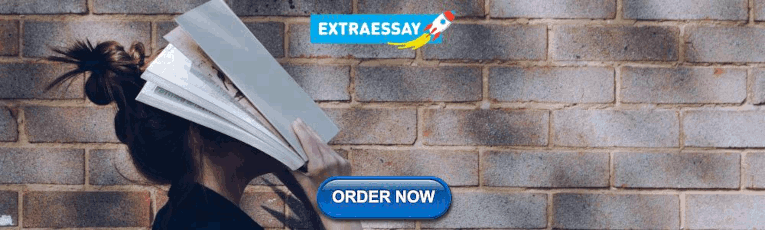
Share this article
Anyone you share the following link with will be able to read this content:
Sorry, a shareable link is not currently available for this article.
Provided by the Springer Nature SharedIt content-sharing initiative
- Find a journal
- Publish with us
- Track your research
Two big computer vision papers boost prospect of safer self-driving vehicles
New chip and camera technology bring closer potential of hands-free road time.
Like nuclear fusion and jet-packs, the self-driving car is a long-promised technology that has stalled for years - yet armed with research, boffins think they have created potential improvements.…
Citizens of Phoenix, San Francisco, and Los Angeles are able to take one of Waymo's self-driving taxis, first introduced to the public in December 2020. But they have not been without their glitches. Just last month in San Francisco, for example, one of the taxi service's autonomous vehicles drove down the wrong side of the street to pass a unicycle. In December last year, a Waymo vehicle hit a backwards-facing pickup truck, resulting in a report with the US National Highway Traffic Safety Administration (NHTSA) and a software update.
But this week, not one but two groups of researchers bidding to improve the performance of self-driving cars and other autonomous vehicles have published papers in the international science journal Nature.
A design for a new chip geared towards autonomous vehicles has arrived from China. Tsinghua University's Luping Shi and colleagues have taken inspiration from the human visual system by both combining low-accuracy, fast event-based detection with more accurate, but slower visualization of an image.
The researchers were able to show the chip — dubbed Tianmouc — could process pixel arrays quickly and robustly in an automotive driving perception system.
In a paper published today, the authors said: "We demonstrate the integration of a Tianmouc chip into an autonomous driving system, showcasing its abilities to enable accurate, fast and robust perception, even in challenging corner cases on open roads. The primitive-based complementary sensing paradigm helps in overcoming fundamental limitations in developing vision systems for diverse open-world applications."
In a separate paper, Davide Scaramuzza, University of Zurich robotics and perception professor, and his colleagues adopt a similar hybrid approach but apply it to camera technologies.
Youtube Video
Cameras for self-driving vehicles navigate a trade-off between bandwidth and latency. While high-res color cameras have good resolution, they require high bandwidth to detect rapid changes. Conversely, reducing the bandwidth increases latency, affecting the timely processing of data for potentially life-saving decision making.
To get out of this bind, the Swiss-based researchers developed a hybrid camera combining event processing with high-bandwidth image processing. Events cameras only record intensity changes, and report them as sparse measurements, meaning the system does not suffer from the bandwidth/latency trade-off.
The event camera is used to detect changes in the blind time between image frames using events. Event data converted into a graph, which changes over time and connects nearby points, is computed locally. The resulting hybrid object detector reduces the detection time in dangerous high-speed situations, according to an explanatory video.
In their paper, the authors say: "Our method exploits the high temporal resolution and sparsity of events and the rich but low temporal resolution information in standard images to generate efficient, high-rate object detections, reducing perceptual and computational latency."
They argue their use of a 20 frames per second RGB camera plus an event camera can achieve the same latency as a 5,000-fps camera with the bandwidth of a 45-fps camera without compromising accuracy.
"Our approach paves the way for efficient and robust perception in edge-case scenarios by uncovering the potential of event cameras," the authors write.
With a hybrid approach to both cameras and data processing in the offing, more widespread adoption of self-driving vehicles may be just around the corner. ®
Help | Advanced Search
Computer Science > Computer Vision and Pattern Recognition
Title: benchmarking and improving detail image caption.
Abstract: Image captioning has long been regarded as a fundamental task in visual understanding. Recently, however, few large vision-language model (LVLM) research discusses model's image captioning performance because of the outdated short-caption benchmarks and unreliable evaluation metrics. In this work, we propose to benchmark detail image caption task by curating high-quality evaluation datasets annotated by human experts, GPT-4V and Gemini-1.5-Pro. We also design a more reliable caption evaluation metric called CAPTURE (CAPtion evaluation by exTracting and coUpling coRE information). CAPTURE extracts visual elements, e.g., objects, attributes and relations from captions, and then matches these elements through three stages, achieving the highest consistency with expert judgements over other rule-based or model-based caption metrics. The proposed benchmark and metric provide reliable evaluation for LVLM's detailed image captioning ability. Guided by this evaluation, we further explore to unleash LVLM's detail caption capabilities by synthesizing high-quality data through a five-stage data construction pipeline. Our pipeline only uses a given LVLM itself and other open-source tools, without any human or GPT-4V annotation in the loop. Experiments show that the proposed data construction strategy significantly improves model-generated detail caption data quality for LVLMs with leading performance, and the data quality can be further improved in a self-looping paradigm. All code and dataset will be publicly available at this https URL .
Submission history
Access paper:.
- HTML (experimental)
- Other Formats

References & Citations
- Google Scholar
- Semantic Scholar
BibTeX formatted citation

Bibliographic and Citation Tools
Code, data and media associated with this article, recommenders and search tools.
- Institution
arXivLabs: experimental projects with community collaborators
arXivLabs is a framework that allows collaborators to develop and share new arXiv features directly on our website.
Both individuals and organizations that work with arXivLabs have embraced and accepted our values of openness, community, excellence, and user data privacy. arXiv is committed to these values and only works with partners that adhere to them.
Have an idea for a project that will add value for arXiv's community? Learn more about arXivLabs .
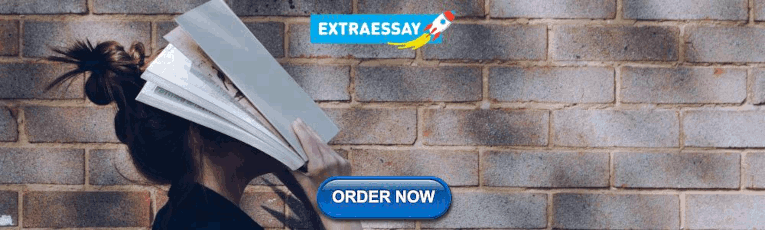
IMAGES
VIDEO
COMMENTS
This paper first reviews the main ideas of deep learning, and displays several related frequently-used algorithms for computer vision. Afterwards, the current research status of computer vision field is demonstrated in this paper, particularly the main applications of deep learning in the research field.
This study focuses on employing artificial intelligence and computer vision analysis to automatically identify aggregates quarries from satellite images within continental Spain.
This paper provide a survey of the recent technologies and theoretical concept explaining the development of computer vision especially related to image processing using different areas of...
Volume 16, Issue 1. Pages: 1-97. February 2022. Submit an article. View Calls for Papers. Journal Metrics. back. <em>IET Computer Vision</em> is an open access journal that introduces new horizons and sets the agenda for future avenues of research in a wide range of areas of computer vision.
We investigate algorithmic progress in image classification on ImageNet, perhaps the most well-known test bed for computer vision. We estimate a model, informed by work on neural scaling laws, and infer a decomposition of progress into the scaling of compute, data, and algorithms.
The International Journal of Computer Vision gratefully acknowledges the editorial work of the Guest Editors listed below. The special issue included an open call for papers on the topic, as well as extended papers invited from the associated workshop at ECCV 2022. The special issue features 32 papers which have been rigorously reviewed ...
Industry and Academic Research in Computer Vision. Iuliia Kotseruba, Manos Papagelis, John K. Tsotsos. yulia_k,papaggel,[email protected] York University Toronto, ON. ABSTRACT. This work aims to study the dynamic between research in the indus-try and academia in computer vision.
A Feature Paper should be a substantial original Article that involves several techniques or approaches, provides an outlook for future research directions and describes possible research applications. Feature papers are submitted upon individual invitation or recommendation by the scientific editors and must receive positive feedback from the ...
Cameras for self-driving vehicles navigate a trade-off between bandwidth and latency. While high-res color cameras have good resolution, they require high bandwidth to detect rapid changes ...
Image captioning has long been regarded as a fundamental task in visual understanding. Recently, however, few large vision-language model (LVLM) research discusses model's image captioning performance because of the outdated short-caption benchmarks and unreliable evaluation metrics. In this work, we propose to benchmark detail image caption task by curating high-quality evaluation datasets ...