
Statistics Made Easy
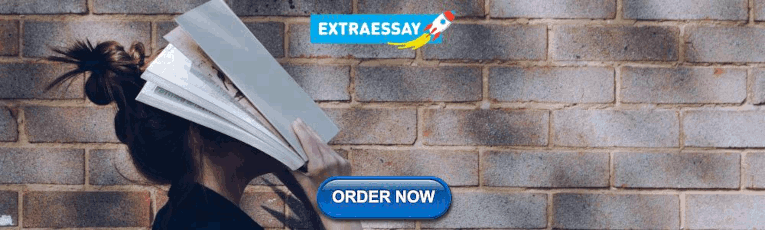
How to Write a Null Hypothesis (5 Examples)
A hypothesis test uses sample data to determine whether or not some claim about a population parameter is true.
Whenever we perform a hypothesis test, we always write a null hypothesis and an alternative hypothesis, which take the following forms:
H 0 (Null Hypothesis): Population parameter =, ≤, ≥ some value
H A (Alternative Hypothesis): Population parameter <, >, ≠ some value
Note that the null hypothesis always contains the equal sign .
We interpret the hypotheses as follows:
Null hypothesis: The sample data provides no evidence to support some claim being made by an individual.
Alternative hypothesis: The sample data does provide sufficient evidence to support the claim being made by an individual.
For example, suppose it’s assumed that the average height of a certain species of plant is 20 inches tall. However, one botanist claims the true average height is greater than 20 inches.
To test this claim, she may go out and collect a random sample of plants. She can then use this sample data to perform a hypothesis test using the following two hypotheses:
H 0 : μ ≤ 20 (the true mean height of plants is equal to or even less than 20 inches)
H A : μ > 20 (the true mean height of plants is greater than 20 inches)
If the sample data gathered by the botanist shows that the mean height of this species of plants is significantly greater than 20 inches, she can reject the null hypothesis and conclude that the mean height is greater than 20 inches.
Read through the following examples to gain a better understanding of how to write a null hypothesis in different situations.
Example 1: Weight of Turtles
A biologist wants to test whether or not the true mean weight of a certain species of turtles is 300 pounds. To test this, he goes out and measures the weight of a random sample of 40 turtles.
Here is how to write the null and alternative hypotheses for this scenario:
H 0 : μ = 300 (the true mean weight is equal to 300 pounds)
H A : μ ≠ 300 (the true mean weight is not equal to 300 pounds)
Example 2: Height of Males
It’s assumed that the mean height of males in a certain city is 68 inches. However, an independent researcher believes the true mean height is greater than 68 inches. To test this, he goes out and collects the height of 50 males in the city.
H 0 : μ ≤ 68 (the true mean height is equal to or even less than 68 inches)
H A : μ > 68 (the true mean height is greater than 68 inches)
Example 3: Graduation Rates
A university states that 80% of all students graduate on time. However, an independent researcher believes that less than 80% of all students graduate on time. To test this, she collects data on the proportion of students who graduated on time last year at the university.
H 0 : p ≥ 0.80 (the true proportion of students who graduate on time is 80% or higher)
H A : μ < 0.80 (the true proportion of students who graduate on time is less than 80%)
Example 4: Burger Weights
A food researcher wants to test whether or not the true mean weight of a burger at a certain restaurant is 7 ounces. To test this, he goes out and measures the weight of a random sample of 20 burgers from this restaurant.
H 0 : μ = 7 (the true mean weight is equal to 7 ounces)
H A : μ ≠ 7 (the true mean weight is not equal to 7 ounces)
Example 5: Citizen Support
A politician claims that less than 30% of citizens in a certain town support a certain law. To test this, he goes out and surveys 200 citizens on whether or not they support the law.
H 0 : p ≥ .30 (the true proportion of citizens who support the law is greater than or equal to 30%)
H A : μ < 0.30 (the true proportion of citizens who support the law is less than 30%)
Additional Resources
Introduction to Hypothesis Testing Introduction to Confidence Intervals An Explanation of P-Values and Statistical Significance
Featured Posts

Hey there. My name is Zach Bobbitt. I have a Masters of Science degree in Applied Statistics and I’ve worked on machine learning algorithms for professional businesses in both healthcare and retail. I’m passionate about statistics, machine learning, and data visualization and I created Statology to be a resource for both students and teachers alike. My goal with this site is to help you learn statistics through using simple terms, plenty of real-world examples, and helpful illustrations.
2 Replies to “How to Write a Null Hypothesis (5 Examples)”
you are amazing, thank you so much
Say I am a botanist hypothesizing the average height of daisies is 20 inches, or not? Does T = (ave – 20 inches) / √ variance / (80 / 4)? … This assumes 40 real measures + 40 fake = 80 n, but that seems questionable. Please advise.
Leave a Reply Cancel reply
Your email address will not be published. Required fields are marked *
Join the Statology Community
Sign up to receive Statology's exclusive study resource: 100 practice problems with step-by-step solutions. Plus, get our latest insights, tutorials, and data analysis tips straight to your inbox!
By subscribing you accept Statology's Privacy Policy.
Null Hypothesis Definition and Examples, How to State
What is the null hypothesis, how to state the null hypothesis, null hypothesis overview.

Why is it Called the “Null”?
The word “null” in this context means that it’s a commonly accepted fact that researchers work to nullify . It doesn’t mean that the statement is null (i.e. amounts to nothing) itself! (Perhaps the term should be called the “nullifiable hypothesis” as that might cause less confusion).
Why Do I need to Test it? Why not just prove an alternate one?
The short answer is, as a scientist, you are required to ; It’s part of the scientific process. Science uses a battery of processes to prove or disprove theories, making sure than any new hypothesis has no flaws. Including both a null and an alternate hypothesis is one safeguard to ensure your research isn’t flawed. Not including the null hypothesis in your research is considered very bad practice by the scientific community. If you set out to prove an alternate hypothesis without considering it, you are likely setting yourself up for failure. At a minimum, your experiment will likely not be taken seriously.
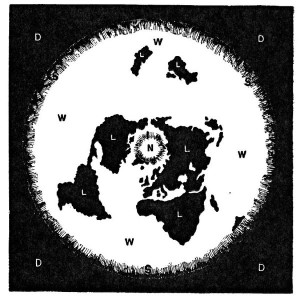
- Null hypothesis : H 0 : The world is flat.
- Alternate hypothesis: The world is round.
Several scientists, including Copernicus , set out to disprove the null hypothesis. This eventually led to the rejection of the null and the acceptance of the alternate. Most people accepted it — the ones that didn’t created the Flat Earth Society !. What would have happened if Copernicus had not disproved the it and merely proved the alternate? No one would have listened to him. In order to change people’s thinking, he first had to prove that their thinking was wrong .
How to State the Null Hypothesis from a Word Problem
You’ll be asked to convert a word problem into a hypothesis statement in statistics that will include a null hypothesis and an alternate hypothesis . Breaking your problem into a few small steps makes these problems much easier to handle.
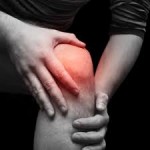
Step 2: Convert the hypothesis to math . Remember that the average is sometimes written as μ.
H 1 : μ > 8.2
Broken down into (somewhat) English, that’s H 1 (The hypothesis): μ (the average) > (is greater than) 8.2
Step 3: State what will happen if the hypothesis doesn’t come true. If the recovery time isn’t greater than 8.2 weeks, there are only two possibilities, that the recovery time is equal to 8.2 weeks or less than 8.2 weeks.
H 0 : μ ≤ 8.2
Broken down again into English, that’s H 0 (The null hypothesis): μ (the average) ≤ (is less than or equal to) 8.2
How to State the Null Hypothesis: Part Two
But what if the researcher doesn’t have any idea what will happen.
Example Problem: A researcher is studying the effects of radical exercise program on knee surgery patients. There is a good chance the therapy will improve recovery time, but there’s also the possibility it will make it worse. Average recovery times for knee surgery patients is 8.2 weeks.
Step 1: State what will happen if the experiment doesn’t make any difference. That’s the null hypothesis–that nothing will happen. In this experiment, if nothing happens, then the recovery time will stay at 8.2 weeks.
H 0 : μ = 8.2
Broken down into English, that’s H 0 (The null hypothesis): μ (the average) = (is equal to) 8.2
Step 2: Figure out the alternate hypothesis . The alternate hypothesis is the opposite of the null hypothesis. In other words, what happens if our experiment makes a difference?
H 1 : μ ≠ 8.2
In English again, that’s H 1 (The alternate hypothesis): μ (the average) ≠ (is not equal to) 8.2
That’s How to State the Null Hypothesis!
Check out our Youtube channel for more stats tips!
Gonick, L. (1993). The Cartoon Guide to Statistics . HarperPerennial. Kotz, S.; et al., eds. (2006), Encyclopedia of Statistical Sciences , Wiley.
If you're seeing this message, it means we're having trouble loading external resources on our website.
If you're behind a web filter, please make sure that the domains *.kastatic.org and *.kasandbox.org are unblocked.
To log in and use all the features of Khan Academy, please enable JavaScript in your browser.
AP®︎/College Statistics
Course: ap®︎/college statistics > unit 10.
- Idea behind hypothesis testing
Examples of null and alternative hypotheses
- Writing null and alternative hypotheses
- P-values and significance tests
- Comparing P-values to different significance levels
- Estimating a P-value from a simulation
- Estimating P-values from simulations
- Using P-values to make conclusions

Want to join the conversation?
- Upvote Button navigates to signup page
- Downvote Button navigates to signup page
- Flag Button navigates to signup page

Video transcript
9.1 Null and Alternative Hypotheses
The actual test begins by considering two hypotheses . They are called the null hypothesis and the alternative hypothesis . These hypotheses contain opposing viewpoints.
H 0 , the — null hypothesis: a statement of no difference between sample means or proportions or no difference between a sample mean or proportion and a population mean or proportion. In other words, the difference equals 0.
H a —, the alternative hypothesis: a claim about the population that is contradictory to H 0 and what we conclude when we reject H 0 .
Since the null and alternative hypotheses are contradictory, you must examine evidence to decide if you have enough evidence to reject the null hypothesis or not. The evidence is in the form of sample data.
After you have determined which hypothesis the sample supports, you make a decision. There are two options for a decision. They are reject H 0 if the sample information favors the alternative hypothesis or do not reject H 0 or decline to reject H 0 if the sample information is insufficient to reject the null hypothesis.
Mathematical Symbols Used in H 0 and H a :
equal (=) | not equal (≠) greater than (>) less than (<) |
greater than or equal to (≥) | less than (<) |
less than or equal to (≤) | more than (>) |
H 0 always has a symbol with an equal in it. H a never has a symbol with an equal in it. The choice of symbol depends on the wording of the hypothesis test. However, be aware that many researchers use = in the null hypothesis, even with > or < as the symbol in the alternative hypothesis. This practice is acceptable because we only make the decision to reject or not reject the null hypothesis.
Example 9.1
H 0 : No more than 30 percent of the registered voters in Santa Clara County voted in the primary election. p ≤ 30 H a : More than 30 percent of the registered voters in Santa Clara County voted in the primary election. p > 30
A medical trial is conducted to test whether or not a new medicine reduces cholesterol by 25 percent. State the null and alternative hypotheses.
Example 9.2
We want to test whether the mean GPA of students in American colleges is different from 2.0 (out of 4.0). The null and alternative hypotheses are the following: H 0 : μ = 2.0 H a : μ ≠ 2.0
We want to test whether the mean height of eighth graders is 66 inches. State the null and alternative hypotheses. Fill in the correct symbol (=, ≠, ≥, <, ≤, >) for the null and alternative hypotheses.
- H 0 : μ __ 66
- H a : μ __ 66
Example 9.3
We want to test if college students take fewer than five years to graduate from college, on the average. The null and alternative hypotheses are the following: H 0 : μ ≥ 5 H a : μ < 5
We want to test if it takes fewer than 45 minutes to teach a lesson plan. State the null and alternative hypotheses. Fill in the correct symbol ( =, ≠, ≥, <, ≤, >) for the null and alternative hypotheses.
- H 0 : μ __ 45
- H a : μ __ 45
Example 9.4
An article on school standards stated that about half of all students in France, Germany, and Israel take advanced placement exams and a third of the students pass. The same article stated that 6.6 percent of U.S. students take advanced placement exams and 4.4 percent pass. Test if the percentage of U.S. students who take advanced placement exams is more than 6.6 percent. State the null and alternative hypotheses. H 0 : p ≤ 0.066 H a : p > 0.066
On a state driver’s test, about 40 percent pass the test on the first try. We want to test if more than 40 percent pass on the first try. Fill in the correct symbol (=, ≠, ≥, <, ≤, >) for the null and alternative hypotheses.
- H 0 : p __ 0.40
- H a : p __ 0.40
Collaborative Exercise
Bring to class a newspaper, some news magazines, and some internet articles. In groups, find articles from which your group can write null and alternative hypotheses. Discuss your hypotheses with the rest of the class.
As an Amazon Associate we earn from qualifying purchases.
This book may not be used in the training of large language models or otherwise be ingested into large language models or generative AI offerings without OpenStax's permission.
Want to cite, share, or modify this book? This book uses the Creative Commons Attribution License and you must attribute Texas Education Agency (TEA). The original material is available at: https://www.texasgateway.org/book/tea-statistics . Changes were made to the original material, including updates to art, structure, and other content updates.
Access for free at https://openstax.org/books/statistics/pages/1-introduction
- Authors: Barbara Illowsky, Susan Dean
- Publisher/website: OpenStax
- Book title: Statistics
- Publication date: Mar 27, 2020
- Location: Houston, Texas
- Book URL: https://openstax.org/books/statistics/pages/1-introduction
- Section URL: https://openstax.org/books/statistics/pages/9-1-null-and-alternative-hypotheses
© Jan 23, 2024 Texas Education Agency (TEA). The OpenStax name, OpenStax logo, OpenStax book covers, OpenStax CNX name, and OpenStax CNX logo are not subject to the Creative Commons license and may not be reproduced without the prior and express written consent of Rice University.
Have a language expert improve your writing
Run a free plagiarism check in 10 minutes, generate accurate citations for free.
- Knowledge Base
Hypothesis Testing | A Step-by-Step Guide with Easy Examples
Published on November 8, 2019 by Rebecca Bevans . Revised on June 22, 2023.
Hypothesis testing is a formal procedure for investigating our ideas about the world using statistics . It is most often used by scientists to test specific predictions, called hypotheses, that arise from theories.
There are 5 main steps in hypothesis testing:
- State your research hypothesis as a null hypothesis and alternate hypothesis (H o ) and (H a or H 1 ).
- Collect data in a way designed to test the hypothesis.
- Perform an appropriate statistical test .
- Decide whether to reject or fail to reject your null hypothesis.
- Present the findings in your results and discussion section.
Though the specific details might vary, the procedure you will use when testing a hypothesis will always follow some version of these steps.
Table of contents
Step 1: state your null and alternate hypothesis, step 2: collect data, step 3: perform a statistical test, step 4: decide whether to reject or fail to reject your null hypothesis, step 5: present your findings, other interesting articles, frequently asked questions about hypothesis testing.
After developing your initial research hypothesis (the prediction that you want to investigate), it is important to restate it as a null (H o ) and alternate (H a ) hypothesis so that you can test it mathematically.
The alternate hypothesis is usually your initial hypothesis that predicts a relationship between variables. The null hypothesis is a prediction of no relationship between the variables you are interested in.
- H 0 : Men are, on average, not taller than women. H a : Men are, on average, taller than women.
Receive feedback on language, structure, and formatting
Professional editors proofread and edit your paper by focusing on:
- Academic style
- Vague sentences
- Style consistency
See an example
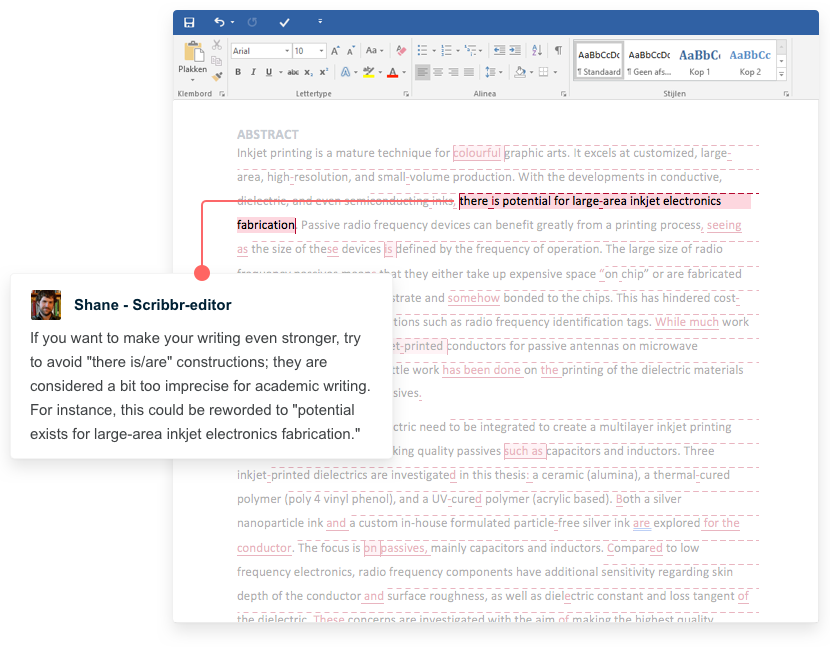
For a statistical test to be valid , it is important to perform sampling and collect data in a way that is designed to test your hypothesis. If your data are not representative, then you cannot make statistical inferences about the population you are interested in.
There are a variety of statistical tests available, but they are all based on the comparison of within-group variance (how spread out the data is within a category) versus between-group variance (how different the categories are from one another).
If the between-group variance is large enough that there is little or no overlap between groups, then your statistical test will reflect that by showing a low p -value . This means it is unlikely that the differences between these groups came about by chance.
Alternatively, if there is high within-group variance and low between-group variance, then your statistical test will reflect that with a high p -value. This means it is likely that any difference you measure between groups is due to chance.
Your choice of statistical test will be based on the type of variables and the level of measurement of your collected data .
- an estimate of the difference in average height between the two groups.
- a p -value showing how likely you are to see this difference if the null hypothesis of no difference is true.
Based on the outcome of your statistical test, you will have to decide whether to reject or fail to reject your null hypothesis.
In most cases you will use the p -value generated by your statistical test to guide your decision. And in most cases, your predetermined level of significance for rejecting the null hypothesis will be 0.05 – that is, when there is a less than 5% chance that you would see these results if the null hypothesis were true.
In some cases, researchers choose a more conservative level of significance, such as 0.01 (1%). This minimizes the risk of incorrectly rejecting the null hypothesis ( Type I error ).
The results of hypothesis testing will be presented in the results and discussion sections of your research paper , dissertation or thesis .
In the results section you should give a brief summary of the data and a summary of the results of your statistical test (for example, the estimated difference between group means and associated p -value). In the discussion , you can discuss whether your initial hypothesis was supported by your results or not.
In the formal language of hypothesis testing, we talk about rejecting or failing to reject the null hypothesis. You will probably be asked to do this in your statistics assignments.
However, when presenting research results in academic papers we rarely talk this way. Instead, we go back to our alternate hypothesis (in this case, the hypothesis that men are on average taller than women) and state whether the result of our test did or did not support the alternate hypothesis.
If your null hypothesis was rejected, this result is interpreted as “supported the alternate hypothesis.”
These are superficial differences; you can see that they mean the same thing.
You might notice that we don’t say that we reject or fail to reject the alternate hypothesis . This is because hypothesis testing is not designed to prove or disprove anything. It is only designed to test whether a pattern we measure could have arisen spuriously, or by chance.
If we reject the null hypothesis based on our research (i.e., we find that it is unlikely that the pattern arose by chance), then we can say our test lends support to our hypothesis . But if the pattern does not pass our decision rule, meaning that it could have arisen by chance, then we say the test is inconsistent with our hypothesis .
If you want to know more about statistics , methodology , or research bias , make sure to check out some of our other articles with explanations and examples.
- Normal distribution
- Descriptive statistics
- Measures of central tendency
- Correlation coefficient
Methodology
- Cluster sampling
- Stratified sampling
- Types of interviews
- Cohort study
- Thematic analysis
Research bias
- Implicit bias
- Cognitive bias
- Survivorship bias
- Availability heuristic
- Nonresponse bias
- Regression to the mean
Hypothesis testing is a formal procedure for investigating our ideas about the world using statistics. It is used by scientists to test specific predictions, called hypotheses , by calculating how likely it is that a pattern or relationship between variables could have arisen by chance.
A hypothesis states your predictions about what your research will find. It is a tentative answer to your research question that has not yet been tested. For some research projects, you might have to write several hypotheses that address different aspects of your research question.
A hypothesis is not just a guess — it should be based on existing theories and knowledge. It also has to be testable, which means you can support or refute it through scientific research methods (such as experiments, observations and statistical analysis of data).
Null and alternative hypotheses are used in statistical hypothesis testing . The null hypothesis of a test always predicts no effect or no relationship between variables, while the alternative hypothesis states your research prediction of an effect or relationship.
Cite this Scribbr article
If you want to cite this source, you can copy and paste the citation or click the “Cite this Scribbr article” button to automatically add the citation to our free Citation Generator.
Bevans, R. (2023, June 22). Hypothesis Testing | A Step-by-Step Guide with Easy Examples. Scribbr. Retrieved June 11, 2024, from https://www.scribbr.com/statistics/hypothesis-testing/
Is this article helpful?
Rebecca Bevans
Other students also liked, choosing the right statistical test | types & examples, understanding p values | definition and examples, what is your plagiarism score.
Null Hypothesis Examples
ThoughtCo / Hilary Allison
- Scientific Method
- Chemical Laws
- Periodic Table
- Projects & Experiments
- Biochemistry
- Physical Chemistry
- Medical Chemistry
- Chemistry In Everyday Life
- Famous Chemists
- Activities for Kids
- Abbreviations & Acronyms
- Weather & Climate
- Ph.D., Biomedical Sciences, University of Tennessee at Knoxville
- B.A., Physics and Mathematics, Hastings College
In statistical analysis, the null hypothesis assumes there is no meaningful relationship between two variables. Testing the null hypothesis can tell you whether your results are due to the effect of manipulating a dependent variable or due to chance. It's often used in conjunction with an alternative hypothesis, which assumes there is, in fact, a relationship between two variables.
The null hypothesis is among the easiest hypothesis to test using statistical analysis, making it perhaps the most valuable hypothesis for the scientific method. By evaluating a null hypothesis in addition to another hypothesis, researchers can support their conclusions with a higher level of confidence. Below are examples of how you might formulate a null hypothesis to fit certain questions.
What Is the Null Hypothesis?
The null hypothesis states there is no relationship between the measured phenomenon (the dependent variable ) and the independent variable , which is the variable an experimenter typically controls or changes. You do not need to believe that the null hypothesis is true to test it. On the contrary, you will likely suspect there is a relationship between a set of variables. One way to prove that this is the case is to reject the null hypothesis. Rejecting a hypothesis does not mean an experiment was "bad" or that it didn't produce results. In fact, it is often one of the first steps toward further inquiry.
To distinguish it from other hypotheses , the null hypothesis is written as H 0 (which is read as “H-nought,” "H-null," or "H-zero"). A significance test is used to determine the likelihood that the results supporting the null hypothesis are not due to chance. A confidence level of 95% or 99% is common. Keep in mind, even if the confidence level is high, there is still a small chance the null hypothesis is not true, perhaps because the experimenter did not account for a critical factor or because of chance. This is one reason why it's important to repeat experiments.
Examples of the Null Hypothesis
To write a null hypothesis, first start by asking a question. Rephrase that question in a form that assumes no relationship between the variables. In other words, assume a treatment has no effect. Write your hypothesis in a way that reflects this.
Are teens better at math than adults? | Age has no effect on mathematical ability. |
Does taking aspirin every day reduce the chance of having a heart attack? | Taking aspirin daily does not affect heart attack risk. |
Do teens use cell phones to access the internet more than adults? | Age has no effect on how cell phones are used for internet access. |
Do cats care about the color of their food? | Cats express no food preference based on color. |
Does chewing willow bark relieve pain? | There is no difference in pain relief after chewing willow bark versus taking a placebo. |
Other Types of Hypotheses
In addition to the null hypothesis, the alternative hypothesis is also a staple in traditional significance tests . It's essentially the opposite of the null hypothesis because it assumes the claim in question is true. For the first item in the table above, for example, an alternative hypothesis might be "Age does have an effect on mathematical ability."
Key Takeaways
- In hypothesis testing, the null hypothesis assumes no relationship between two variables, providing a baseline for statistical analysis.
- Rejecting the null hypothesis suggests there is evidence of a relationship between variables.
- By formulating a null hypothesis, researchers can systematically test assumptions and draw more reliable conclusions from their experiments.
- Difference Between Independent and Dependent Variables
- Examples of Independent and Dependent Variables
- What Is a Hypothesis? (Science)
- What 'Fail to Reject' Means in a Hypothesis Test
- Definition of a Hypothesis
- Null Hypothesis Definition and Examples
- Scientific Method Vocabulary Terms
- Null Hypothesis and Alternative Hypothesis
- Hypothesis Test for the Difference of Two Population Proportions
- How to Conduct a Hypothesis Test
- What Is a P-Value?
- What Are the Elements of a Good Hypothesis?
- Hypothesis Test Example
- What Is the Difference Between Alpha and P-Values?
- Understanding Path Analysis
- An Example of a Hypothesis Test
Null Hypothesis | Definition & Examples
Bridget is completing her M.S degree in Biology at Plymouth State University and received a B.S degree in Biology with a minor in Chemistry from Castleton University. She has experience teaching biology and botany for three years at Plymouth State University.
Karin has taught middle and high school Health and has a master's degree in social work.
Robert Ferdinand has taught university-level mathematics, statistics and computer science from freshmen to senior level. Robert has a PhD in Applied Mathematics.
Null Hypothesis: Questions for Additional Practice
1. The average life of a car battery of a certain brand is five years. This information is gathered using data obtained from people who have purchased and used this brand of battery over a period of several years. A researcher at the battery company develops a new type of car battery and claims that the average life of this battery is more than five years. To determine whether this claim is true, one would need to do some hypothesis testing. What would be the null hypothesis and the alternative hypothesis for this hypothesis test?
2. Past research data from a period of over several years states that the average life expectancy of whales is 100 years. A researcher at a laboratory wishes to test this hypothesis. To that end they procure a sample of life spans of several whales. What is the null hypothesis and the alternative hypothesis that this researcher will establish?
1. The researcher's null hypothesis will be: ''The average life of the new car battery is five years.''
Then their alternative hypothesis will read: ''The average life of the new car batter is more than five years.''
2. The null hypothesis for the researcher will state that, ''The average life expectancy of whales is exactly equal to 100 years.''
Their alternative hypothesis will read: ''The average life expectancy of whales is not equal to 100 years.''
What is an example of a null and alternative hypothesis?
A researcher conducts a scientific study to determine whether songbirds nest in forests with more canopy coverage. The null hypothesis would be that canopy cover has no effect on songbird nesting sites. The alternative hypothesis would be that songbirds nest in forest with increased canopy cover.
What is a null and alternative hypothesis?
The null hypothesis is a prediction that states there is no significant difference between variables or treatments, or the relationship is random. The alternative hypothesis is a prediction that states there is a significant difference between variables or treatments, the relationship is non-random.
Table of Contents
What is a null hypothesis, why test a null hypothesis, how to write a null hypothesis, lesson summary.
A hypothesis , in scientific studies, is defined as a proposed explanation for an observed phenomena that can be subject to further testing. A well formulated hypothesis must do two things: be able to be proven true or false, and the results that either prove or disprove the hypothesis must be reproducible.
The null hypothesis is defined as any observable differences in treatments or variables is likely due to chance. In other words, the null hypothesis states that there is no significant difference between two variables. In statistics, significant differences can be determined through statistical analysis and determine whether or not any differences are due to chance.
There are three common statistical tests that can be used to test for significant differences in data:
- Chi Square Test: mostly used for categorical data
- T-test: used to find differences among two groups
- ANOVA (Analysis of Variance): used to determine significant differences among more than two groups
The null hypothesis is important in research because it is usually tested against what the scientists or researchers are trying to prove or disprove. Testing the null hypothesis can ultimately validate or invalidate research.
Null vs. Alternative Hypothesis
If the null hypothesis in a scientific study states there are no significant differences between two variables, the alternative hypothesis provides an alternative explanation for such differences. Essentially, the alternative hypothesis is the opposite of the null hypothesis, and states observable differences between variables are non-random. The difference between the null hypothesis and the alternative hypothesis is that the null hypothesis assumes the results will be random and the alternative hypothesis assumes the results are non-random.
To unlock this lesson you must be a Study.com Member. Create your account

An error occurred trying to load this video.
Try refreshing the page, or contact customer support.
You must c C reate an account to continue watching
Register to view this lesson.
As a member, you'll also get unlimited access to over 88,000 lessons in math, English, science, history, and more. Plus, get practice tests, quizzes, and personalized coaching to help you succeed.
Get unlimited access to over 88,000 lessons.
Already registered? Log in here for access
Resources created by teachers for teachers.
I would definitely recommend Study.com to my colleagues. It’s like a teacher waved a magic wand and did the work for me. I feel like it’s a lifeline.
You're on a roll. Keep up the good work!
Just checking in. are you still watching.
- 0:00 What Is a Null Hypothesis?
- 1:08 What Is an Alternative…
- 1:55 Examples
- 3:01 Lesson Summary
At first glance, the null hypothesis looks like a statement suggesting the experiment has failed in some way. However, the null hypothesis is an essential part of the scientific method and the conclusions of an experimental study may be invalid without a null hypothesis. The following descriptions explain why the null hypothesis is necessary in scientific research.
- The null hypothesis is a required component of a well constructed experiment or study.
Formulating a scientific study using the scientific method first requires a question to be asked based on prior scientific knowledge or observed phenomena. The next step is to acquire as much background information on the subject you can. After that, you formulate your hypothesis. Essentially, the hypothesis is an educated prediction of an observed phenomena.
For example, plants growing in a north facing window appear to grow at a faster rate than plants growing in a south facing window. The formulated question may be something like, does the direction of plants influence the rate of growth? Then, further information is gathered based on north vs. south facing windows and plant growth, before formulating the hypothesis. After researching the topic, an alternative hypothesis can be formed based on the knowledge gleaned on the topic; for example, north facing plants grow at faster rate than south facing plants. The null hypothesis is that the direction of plants does not affect rate of growth. The rest of the scientific method is based on proving or disproving the hypotheses. This includes the methods, results, and conclusions. Without the null hypothesis, the remainder of the experiment can be considered invalid.
- The null hypothesis that is retained or rejected allows the researchers to redirect experimentation.
The null hypothesis is rarely accepted, instead it is either rejected or retained. In either case, it allows the researcher to explore other possible explanations and accurate predictions. If the null hypothesis is rejected, that means the data collected and analyzed shows that the relationship between the variables was significantly different and non-random. Using the example above, if data showed that the plants in the north facing window grew faster than plants in the south facing window, the null hypothesis is rejected. If data showed that both plants grew at the same rate, the null hypothesis would be retained, and further evidence would be needed to show whether this was due to some other variable, such as amount of sunlight, species, etc.
- The null hypothesis allows for less ambiguity in the data.
The null hypothesis provides a baseline for the data to be collected and analyzed. This is important in showing the results, specifically how the data was analyzed. If there were no significant differences in whether the plants growing in north or south facing windows grew at faster rates, the data analysis and visualizations (figures, graphs, or tables), would easily show that and be apparent to the reader.
An important difference in the null hypothesis and the alternative hypothesis is how it is written. The null hypothesis, essentially, is stating that any observable differences in variables is due to chance. The null hypothesis is written as H0 as a statement. The alternative hypothesis is written as H1 or HA also as a statement.
Null Hypothesis Examples
The following are examples of null hypotheses given different research questions or experimental designs .
- A researcher wants to determine whether sleeping 8 hours a day has an influence on stress levels.
- H0 : Sleeping eight hours a day has no influence on stress levels.
- H1 : Sleeping eight hours a day decreases stress levels.
- A researcher wants to determine whether adults over the age of 65 have a greater chance of heart disease.
- H0 : Adults over the age of 65 do not have a greater chance of heart disease.
- H1 : Adults over the age of 65 have a greater chance of heart disease.
A hypothesis is a proposed explanation for observed phenomena that can be tested. A null hypothesis states that there is no significant difference between treatments or variables. In statistical analysis, a significant difference determines whether a relationship between two variables is due to chance. An alternative hypothesis is the opposite of a null hypothesis and states the relationship between two variables is not due to chance. Null hypotheses in scientific research are essential because they provide a baseline for data, allows researchers to redirect experimentation, and makes the scientific method valid. The null hypothesis is written as H0 and the alternative hypothesis is written as H1 or HA . For example, a researcher wants to determine whether sugar consumption influences weight gain. The null hypothesis would state, sugar consumption does not influence weight gain. The alternative hypothesis would state, sugar consumption increases weight gain.
Video Transcript
A hypothesis is a speculation or theory based on insufficient evidence that lends itself to further testing and experimentation. With further testing, a hypothesis can usually be proven true or false. Let's look at an example. Little Susie speculates, or hypothesizes, that the flowers she waters with club soda will grow faster than flowers she waters with plain water. She waters each plant daily for a month (experiment) and proves her hypothesis true!
A null hypothesis is a hypothesis that says there is no statistical significance between the two variables in the hypothesis. It is the hypothesis that the researcher is trying to disprove. In the example, Susie's null hypothesis would be something like this: There is no statistically significant relationship between the type of water I feed the flowers and growth of the flowers. A researcher is challenged by the null hypothesis and usually wants to disprove it, to demonstrate that there is a statistically-significant relationship between the two variables in the hypothesis.
What Is an Alternative Hypothesis?
An alternative hypothesis simply is the inverse, or opposite, of the null hypothesis. So, if we continue with the above example, the alternative hypothesis would be that there IS indeed a statistically-significant relationship between what type of water the flower plant is fed and growth. More specifically, here would be the null and alternative hypotheses for Susie's study:
Null : If one plant is fed club soda for one month and another plant is fed plain water, there will be no difference in growth between the two plants.
Alternative : If one plant is fed club soda for one month and another plant is fed plain water, the plant that is fed club soda will grow better than the plant that is fed plain water.
Null Hypothesis: The Earth is flat.
Even before Christopher Columbus' time, Greek philosophers Pythagoras and Aristotle hypothesized the world was actually a sphere or circle. So according to these philosophers, their null hypothesis was that the world was flat. Their alternative hypothesis was that the earth was round. It wasn't until Christopher Columbus' voyage to the Americas that it was proven that Pythagoras and Aristotle's alternative hypotheses were correct.
Null Hypothesis: Drinking coffee in the morning will have no effect on level of alertness.
Even though it is scientifically proven that the caffeine in the coffee bean increases level of alertness, a researcher working for a big coffee company may want to disprove the null hypothesis that coffee does not have an effect on level of alertness. In this case, his alternative hypothesis would be that coffee does increase level of alertness.
Let's review. A hypothesis is a speculation or theory, based on insufficient evidence that lends itself to further testing and experimentation. With further testing, a hypothesis can usually be proven true or false. A null hypothesis is a hypothesis that says there is no statistical significance between the two variables. It is usually the hypothesis a researcher or experimenter will try to disprove or discredit. An alternative hypothesis is one that states there is a statistically significant relationship between two variables. It is usually the hypothesis a researcher or experimenter is trying to prove or has already proven.
Unlock Your Education
See for yourself why 30 million people use study.com, become a study.com member and start learning now..
Already a member? Log In
Recommended Lessons and Courses for You
Related lessons, related courses, recommended lessons for you.

Null Hypothesis | Definition & Examples Related Study Materials
- Related Topics
Browse by Courses
- Introduction to Psychology: Homework Help Resource
- CLEP Introductory Psychology Prep
- Research Methods in Psychology: Homework Help Resource
- UExcel Abnormal Psychology: Study Guide & Test Prep
- Research Methods in Psychology: Tutoring Solution
- Abnormal Psychology: Certificate Program
- Abnormal Psychology: Help and Review
- Abnormal Psychology: Tutoring Solution
- Research Methods in Psychology: Help and Review
- High School Psychology: Homeschool Curriculum
- High School Psychology: Credit Recovery
- High School Psychology Textbook
- Psychology 101: Intro to Psychology
- Psychology 102: Educational Psychology
- Psychology 103: Human Growth and Development
Browse by Lessons
- Formulation of Hypothesis & Examples
- Brown-Peterson Task | Overview, Procedure & Techniques
- Memory Consolidation | Definition & Examples
- Reactive Attachment Disorder: Symptoms & Treatment
- Social Cognition in Psychology | Definition & Examples
- Bilingualism and Multilingualism Across the Lifespan
- Conventional Personality in the Holland Code | Definition & Jobs
- Xenophilia | Definition & Examples
- Walker Evans | Biography, Photography & Works
- Bipolar 1 & 2 | Definition, Differences & Treatment
- Ironic Process Theory | Definition, Model & Examples
- Avoidant Personality Disorder: Symptoms & Treatment
- Source Amnesia | Causes, Prevention & Examples
- What is Suicidal Ideation? - Definition & Assessment
- What is Parental Alienation Syndrome?
Create an account to start this course today Used by over 30 million students worldwide Create an account
Explore our library of over 88,000 lessons
- Foreign Language
- Social Science
- See All College Courses
- Common Core
- High School
- See All High School Courses
- College & Career Guidance Courses
- College Placement Exams
- Entrance Exams
- General Test Prep
- K-8 Courses
- Skills Courses
- Teacher Certification Exams
- See All Other Courses
- Create a Goal
- Create custom courses
- Get your questions answered

Null Hypothesis – Simple Introduction
A null hypothesis is a precise statement about a population that we try to reject with sample data. We don't usually believe our null hypothesis (or H 0 ) to be true. However, we need some exact statement as a starting point for statistical significance testing.
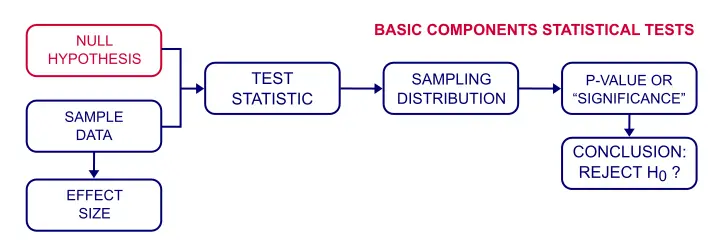
Null Hypothesis Examples
Often -but not always- the null hypothesis states there is no association or difference between variables or subpopulations. Like so, some typical null hypotheses are:
- the correlation between frustration and aggression is zero ( correlation analysis );
- the average income for men is similar to that for women ( independent samples t-test );
- Nationality is (perfectly) unrelated to music preference ( chi-square independence test );
- the average population income was equal over 2012 through 2016 ( repeated measures ANOVA ).
- Dutch, German, French and British people have identical average body weigths .the average body weight is equal for D
“Null” Does Not Mean “Zero”
A common misunderstanding is that “null” implies “zero”. This is often but not always the case. For example, a null hypothesis may also state that the correlation between frustration and aggression is 0.5. No zero involved here and -although somewhat unusual- perfectly valid. The “null” in “null hypothesis” derives from “nullify” 5 : the null hypothesis is the statement that we're trying to refute, regardless whether it does (not) specify a zero effect.
Null Hypothesis Testing -How Does It Work?
I want to know if happiness is related to wealth among Dutch people. One approach to find this out is to formulate a null hypothesis. Since “related to” is not precise, we choose the opposite statement as our null hypothesis: the correlation between wealth and happiness is zero among all Dutch people. We'll now try to refute this hypothesis in order to demonstrate that happiness and wealth are related all right. Now, we can't reasonably ask all 17,142,066 Dutch people how happy they generally feel.
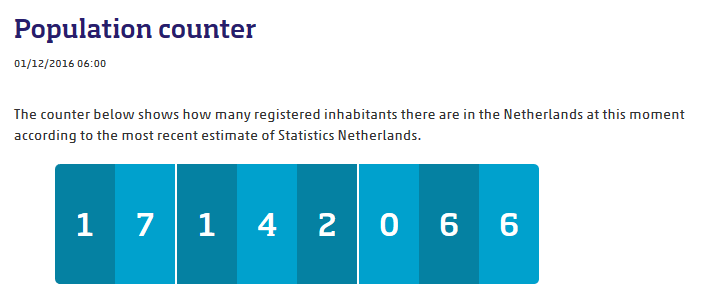
So we'll ask a sample (say, 100 people) about their wealth and their happiness. The correlation between happiness and wealth turns out to be 0.25 in our sample. Now we've one problem: sample outcomes tend to differ somewhat from population outcomes. So if the correlation really is zero in our population, we may find a non zero correlation in our sample. To illustrate this important point, take a look at the scatterplot below. It visualizes a zero correlation between happiness and wealth for an entire population of N = 200.
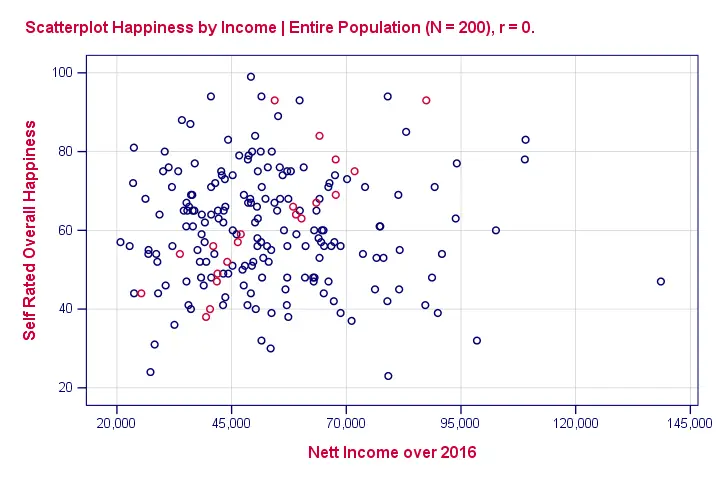
Now we draw a random sample of N = 20 from this population (the red dots in our previous scatterplot). Even though our population correlation is zero, we found a staggering 0.82 correlation in our sample . The figure below illustrates this by omitting all non sampled units from our previous scatterplot.
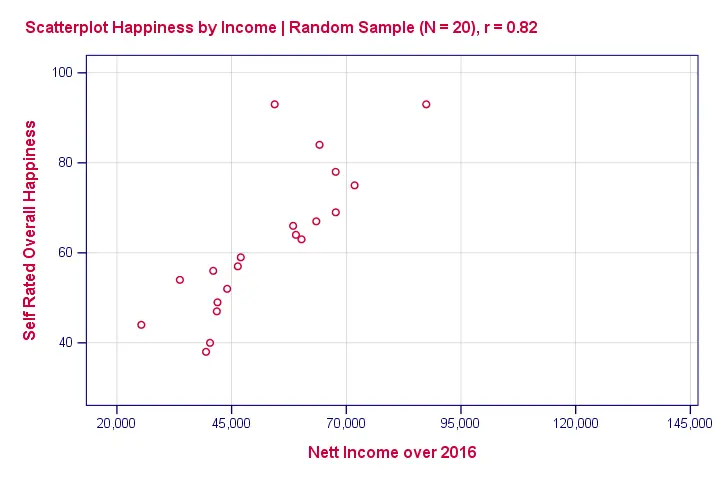
This raises the question how we can ever say anything about our population if we only have a tiny sample from it. The basic answer: we can rarely say anything with 100% certainty. However, we can say a lot with 99%, 95% or 90% certainty.
Probability
So how does that work? Well, basically, some sample outcomes are highly unlikely given our null hypothesis . Like so, the figure below shows the probabilities for different sample correlations (N = 100) if the population correlation really is zero.
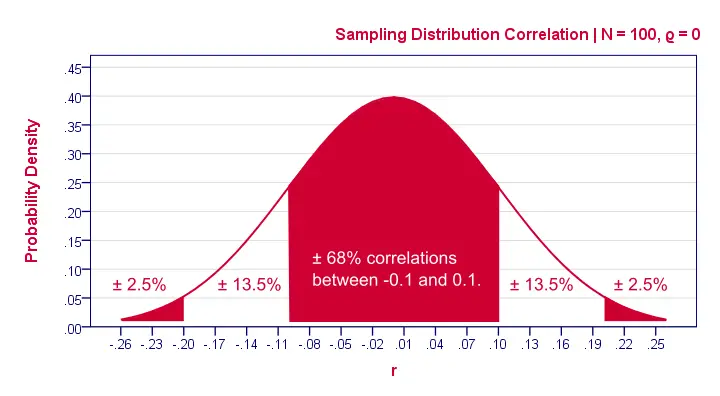
A computer will readily compute these probabilities. However, doing so requires a sample size (100 in our case) and a presumed population correlation ρ (0 in our case). So that's why we need a null hypothesis . If we look at this sampling distribution carefully, we see that sample correlations around 0 are most likely: there's a 0.68 probability of finding a correlation between -0.1 and 0.1. What does that mean? Well, remember that probabilities can be seen as relative frequencies. So imagine we'd draw 1,000 samples instead of the one we have. This would result in 1,000 correlation coefficients and some 680 of those -a relative frequency of 0.68- would be in the range -0.1 to 0.1. Likewise, there's a 0.95 (or 95%) probability of finding a sample correlation between -0.2 and 0.2.
We found a sample correlation of 0.25. How likely is that if the population correlation is zero? The answer is known as the p-value (short for probability value): A p-value is the probability of finding some sample outcome or a more extreme one if the null hypothesis is true. Given our 0.25 correlation, “more extreme” usually means larger than 0.25 or smaller than -0.25. We can't tell from our graph but the underlying table tells us that p ≈ 0.012 . If the null hypothesis is true, there's a 1.2% probability of finding our sample correlation.
Conclusion?
If our population correlation really is zero, then we can find a sample correlation of 0.25 in a sample of N = 100. The probability of this happening is only 0.012 so it's very unlikely . A reasonable conclusion is that our population correlation wasn't zero after all. Conclusion: we reject the null hypothesis . Given our sample outcome, we no longer believe that happiness and wealth are unrelated. However, we still can't state this with certainty.
Null Hypothesis - Limitations
Thus far, we only concluded that the population correlation is probably not zero . That's the only conclusion from our null hypothesis approach and it's not really that interesting. What we really want to know is the population correlation. Our sample correlation of 0.25 seems a reasonable estimate. We call such a single number a point estimate . Now, a new sample may come up with a different correlation. An interesting question is how much our sample correlations would fluctuate over samples if we'd draw many of them. The figure below shows precisely that, assuming our sample size of N = 100 and our (point) estimate of 0.25 for the population correlation.
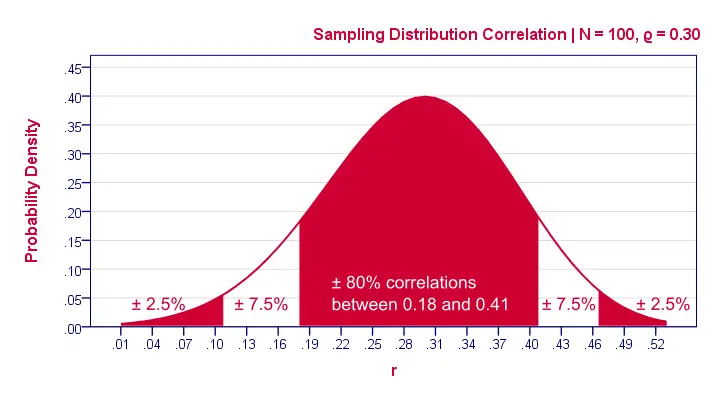
Confidence Intervals
Our sample outcome suggests that some 95% of many samples should come up with a correlation between 0.06 and 0.43. This range is known as a confidence interval . Although not precisely correct, it's most easily thought of as the bandwidth that's likely to enclose the population correlation . One thing to note is that the confidence interval is quite wide. It almost contains a zero correlation, exactly the null hypothesis we rejected earlier. Another thing to note is that our sampling distribution and confidence interval are slightly asymmetrical. They are symmetrical for most other statistics (such as means or beta coefficients ) but not correlations.
- Agresti, A. & Franklin, C. (2014). Statistics. The Art & Science of Learning from Data. Essex: Pearson Education Limited.
- Cohen, J (1988). Statistical Power Analysis for the Social Sciences (2nd. Edition) . Hillsdale, New Jersey, Lawrence Erlbaum Associates.
- Field, A. (2013). Discovering Statistics with IBM SPSS Newbury Park, CA: Sage.
- Howell, D.C. (2002). Statistical Methods for Psychology (5th ed.). Pacific Grove CA: Duxbury.
- Van den Brink, W.P. & Koele, P. (2002). Statistiek, deel 3 [Statistics, part 3]. Amsterdam: Boom.
Tell us what you think!
This tutorial has 17 comments:.
By John Xie on February 28th, 2023
“stop using the term ‘statistically significant’ entirely and moving to a world beyond ‘p < 0.05’”
“…, no p-value can reveal the plausibility, presence, truth, or importance of an association or effect.
Therefore, a label of statistical significance does not mean or imply that an association or effect is highly probable, real, true, or important. Nor does a label of statistical nonsignificance lead to the association or effect being improbable, absent, false, or unimportant.
Yet the dichotomization into ‘significant’ and ‘not significant’ is taken as an imprimatur of authority on these characteristics.” “To be clear, the problem is not that of having only two labels. Results should not be trichotomized, or indeed categorized into any number of groups, based on arbitrary p-value thresholds.
Similarly, we need to stop using confidence intervals as another means of dichotomizing (based, on whether a null value falls within the interval). And, to preclude a reappearance of this problem elsewhere, we must not begin arbitrarily categorizing other statistical measures (such as Bayes factors).”
Quotation from: Ronald L. Wasserstein, Allen L. Schirm & Nicole A. Lazar, Moving to a World Beyond “p<0.05”, The American Statistician(2019), Vol. 73, No. S1, 1-19: Editorial.
By Ruben Geert van den Berg on February 28th, 2023
Yes, partly agreed.
However, most students are still forced to apply null hypothesis testing so why not try to explain to them how it works?
An associated problem is that "significant" has a normal language meaning. Most people seem to confuse "statistically significant" with "real-world significant", which is unfortunate.
By the way, this same point applies to other terms such as "normally distributed". A normal distribution for dice rolls is not a normal but a uniform distribution ;-)
Keep up the good work!
SPSS tutorials
Privacy Overview
Cookie | Duration | Description |
---|---|---|
cookielawinfo-checkbox-analytics | 11 months | This cookie is set by GDPR Cookie Consent plugin. The cookie is used to store the user consent for the cookies in the category "Analytics". |
cookielawinfo-checkbox-functional | 11 months | The cookie is set by GDPR cookie consent to record the user consent for the cookies in the category "Functional". |
cookielawinfo-checkbox-necessary | 11 months | This cookie is set by GDPR Cookie Consent plugin. The cookies is used to store the user consent for the cookies in the category "Necessary". |
cookielawinfo-checkbox-others | 11 months | This cookie is set by GDPR Cookie Consent plugin. The cookie is used to store the user consent for the cookies in the category "Other. |
cookielawinfo-checkbox-performance | 11 months | This cookie is set by GDPR Cookie Consent plugin. The cookie is used to store the user consent for the cookies in the category "Performance". |
viewed_cookie_policy | 11 months | The cookie is set by the GDPR Cookie Consent plugin and is used to store whether or not user has consented to the use of cookies. It does not store any personal data. |

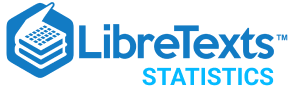
- school Campus Bookshelves
- menu_book Bookshelves
- perm_media Learning Objects
- login Login
- how_to_reg Request Instructor Account
- hub Instructor Commons
Margin Size
- Download Page (PDF)
- Download Full Book (PDF)
- Periodic Table
- Physics Constants
- Scientific Calculator
- Reference & Cite
- Tools expand_more
- Readability
selected template will load here
This action is not available.
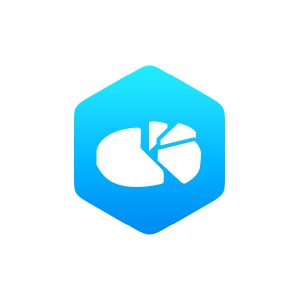
7.3: The Null Hypothesis
- Last updated
- Save as PDF
- Page ID 7115
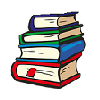
- Foster et al.
- University of Missouri-St. Louis, Rice University, & University of Houston, Downtown Campus via University of Missouri’s Affordable and Open Access Educational Resources Initiative
\( \newcommand{\vecs}[1]{\overset { \scriptstyle \rightharpoonup} {\mathbf{#1}} } \)
\( \newcommand{\vecd}[1]{\overset{-\!-\!\rightharpoonup}{\vphantom{a}\smash {#1}}} \)
\( \newcommand{\id}{\mathrm{id}}\) \( \newcommand{\Span}{\mathrm{span}}\)
( \newcommand{\kernel}{\mathrm{null}\,}\) \( \newcommand{\range}{\mathrm{range}\,}\)
\( \newcommand{\RealPart}{\mathrm{Re}}\) \( \newcommand{\ImaginaryPart}{\mathrm{Im}}\)
\( \newcommand{\Argument}{\mathrm{Arg}}\) \( \newcommand{\norm}[1]{\| #1 \|}\)
\( \newcommand{\inner}[2]{\langle #1, #2 \rangle}\)
\( \newcommand{\Span}{\mathrm{span}}\)
\( \newcommand{\id}{\mathrm{id}}\)
\( \newcommand{\kernel}{\mathrm{null}\,}\)
\( \newcommand{\range}{\mathrm{range}\,}\)
\( \newcommand{\RealPart}{\mathrm{Re}}\)
\( \newcommand{\ImaginaryPart}{\mathrm{Im}}\)
\( \newcommand{\Argument}{\mathrm{Arg}}\)
\( \newcommand{\norm}[1]{\| #1 \|}\)
\( \newcommand{\Span}{\mathrm{span}}\) \( \newcommand{\AA}{\unicode[.8,0]{x212B}}\)
\( \newcommand{\vectorA}[1]{\vec{#1}} % arrow\)
\( \newcommand{\vectorAt}[1]{\vec{\text{#1}}} % arrow\)
\( \newcommand{\vectorB}[1]{\overset { \scriptstyle \rightharpoonup} {\mathbf{#1}} } \)
\( \newcommand{\vectorC}[1]{\textbf{#1}} \)
\( \newcommand{\vectorD}[1]{\overrightarrow{#1}} \)
\( \newcommand{\vectorDt}[1]{\overrightarrow{\text{#1}}} \)
\( \newcommand{\vectE}[1]{\overset{-\!-\!\rightharpoonup}{\vphantom{a}\smash{\mathbf {#1}}}} \)
The hypothesis that an apparent effect is due to chance is called the null hypothesis, written \(H_0\) (“H-naught”). In the Physicians' Reactions example, the null hypothesis is that in the population of physicians, the mean time expected to be spent with obese patients is equal to the mean time expected to be spent with average-weight patients. This null hypothesis can be written as:
\[\mathrm{H}_{0}: \mu_{\mathrm{obese}}-\mu_{\mathrm{average}}=0 \]
The null hypothesis in a correlational study of the relationship between high school grades and college grades would typically be that the population correlation is 0. This can be written as
\[\mathrm{H}_{0}: \rho=0 \]
where \(ρ\) is the population correlation, which we will cover in chapter 12.
Although the null hypothesis is usually that the value of a parameter is 0, there are occasions in which the null hypothesis is a value other than 0. For example, if we are working with mothers in the U.S. whose children are at risk of low birth weight, we can use 7.47 pounds, the average birthweight in the US, as our null value and test for differences against that.
For now, we will focus on testing a value of a single mean against what we expect from the population. Using birthweight as an example, our null hypothesis takes the form:
\[\mathrm{H}_{0}: \mu=7.47 \nonumber \]
The number on the right hand side is our null hypothesis value that is informed by our research question. Notice that we are testing the value for \(μ\), the population parameter, NOT the sample statistic \(\overline{\mathrm{X}}\). This is for two reasons: 1) once we collect data, we know what the value of \(\overline{\mathrm{X}}\) is – it’s not a mystery or a question, it is observed and used for the second reason, which is 2) we are interested in understanding the population, not just our sample.
Keep in mind that the null hypothesis is typically the opposite of the researcher's hypothesis. In the Physicians' Reactions study, the researchers hypothesized that physicians would expect to spend less time with obese patients. The null hypothesis that the two types of patients are treated identically is put forward with the hope that it can be discredited and therefore rejected. If the null hypothesis were true, a difference as large or larger than the sample difference of 6.7 minutes would be very unlikely to occur. Therefore, the researchers rejected the null hypothesis of no difference and concluded that in the population, physicians intend to spend less time with obese patients.
In general, the null hypothesis is the idea that nothing is going on: there is no effect of our treatment, no relation between our variables, and no difference in our sample mean from what we expected about the population mean. This is always our baseline starting assumption, and it is what we seek to reject. If we are trying to treat depression, we want to find a difference in average symptoms between our treatment and control groups. If we are trying to predict job performance, we want to find a relation between conscientiousness and evaluation scores. However, until we have evidence against it, we must use the null hypothesis as our starting point.
Chi-Square (Χ²) Test & How To Calculate Formula Equation
Benjamin Frimodig
Science Expert
B.A., History and Science, Harvard University
Ben Frimodig is a 2021 graduate of Harvard College, where he studied the History of Science.
Learn about our Editorial Process
Saul Mcleod, PhD
Editor-in-Chief for Simply Psychology
BSc (Hons) Psychology, MRes, PhD, University of Manchester
Saul Mcleod, PhD., is a qualified psychology teacher with over 18 years of experience in further and higher education. He has been published in peer-reviewed journals, including the Journal of Clinical Psychology.
On This Page:
Chi-square (χ2) is used to test hypotheses about the distribution of observations into categories with no inherent ranking.
What Is a Chi-Square Statistic?
The Chi-square test (pronounced Kai) looks at the pattern of observations and will tell us if certain combinations of the categories occur more frequently than we would expect by chance, given the total number of times each category occurred.
It looks for an association between the variables. We cannot use a correlation coefficient to look for the patterns in this data because the categories often do not form a continuum.
There are three main types of Chi-square tests, tests of goodness of fit, the test of independence, and the test for homogeneity. All three tests rely on the same formula to compute a test statistic.
These tests function by deciphering relationships between observed sets of data and theoretical or “expected” sets of data that align with the null hypothesis.
What is a Contingency Table?
Contingency tables (also known as two-way tables) are grids in which Chi-square data is organized and displayed. They provide a basic picture of the interrelation between two variables and can help find interactions between them.
In contingency tables, one variable and each of its categories are listed vertically, and the other variable and each of its categories are listed horizontally.
Additionally, including column and row totals, also known as “marginal frequencies,” will help facilitate the Chi-square testing process.
In order for the Chi-square test to be considered trustworthy, each cell of your expected contingency table must have a value of at least five.
Each Chi-square test will have one contingency table representing observed counts (see Fig. 1) and one contingency table representing expected counts (see Fig. 2).
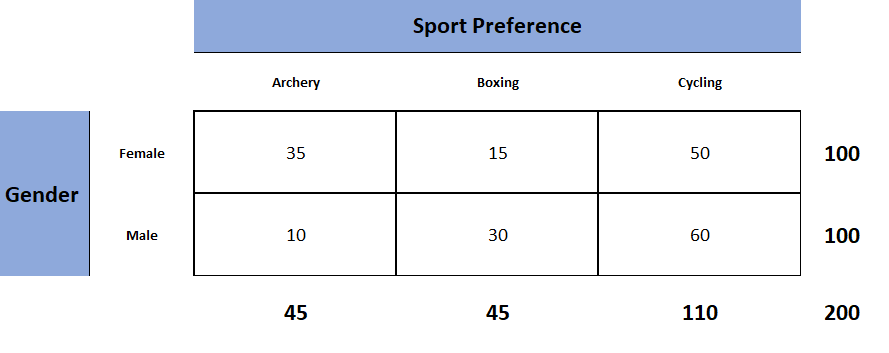
Figure 1. Observed table (which contains the observed counts).
To obtain the expected frequencies for any cell in any cross-tabulation in which the two variables are assumed independent, multiply the row and column totals for that cell and divide the product by the total number of cases in the table.
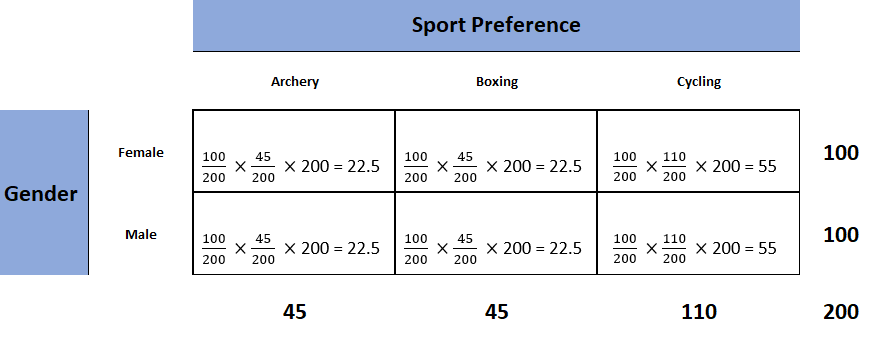
Figure 2. Expected table (what we expect the two-way table to look like if the two categorical variables are independent).
To decide if our calculated value for χ2 is significant, we also need to work out the degrees of freedom for our contingency table using the following formula: df= (rows – 1) x (columns – 1).
Formula Calculation
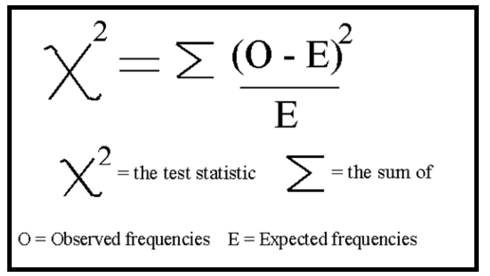
Calculate the chi-square statistic (χ2) by completing the following steps:
- Calculate the expected frequencies and the observed frequencies.
- For each observed number in the table, subtract the corresponding expected number (O — E).
- Square the difference (O —E)².
- Divide the squares obtained for each cell in the table by the expected number for that cell (O – E)² / E.
- Sum all the values for (O – E)² / E. This is the chi-square statistic.
- Calculate the degrees of freedom for the contingency table using the following formula; df= (rows – 1) x (columns – 1).
Once we have calculated the degrees of freedom (df) and the chi-squared value (χ2), we can use the χ2 table (often at the back of a statistics book) to check if our value for χ2 is higher than the critical value given in the table. If it is, then our result is significant at the level given.
Interpretation
The chi-square statistic tells you how much difference exists between the observed count in each table cell to the counts you would expect if there were no relationship at all in the population.
Small Chi-Square Statistic: If the chi-square statistic is small and the p-value is large (usually greater than 0.05), this often indicates that the observed frequencies in the sample are close to what would be expected under the null hypothesis.
The null hypothesis usually states no association between the variables being studied or that the observed distribution fits the expected distribution.
In theory, if the observed and expected values were equal (no difference), then the chi-square statistic would be zero — but this is unlikely to happen in real life.
Large Chi-Square Statistic : If the chi-square statistic is large and the p-value is small (usually less than 0.05), then the conclusion is often that the data does not fit the model well, i.e., the observed and expected values are significantly different. This often leads to the rejection of the null hypothesis.
How to Report
To report a chi-square output in an APA-style results section, always rely on the following template:
χ2 ( degrees of freedom , N = sample size ) = chi-square statistic value , p = p value .
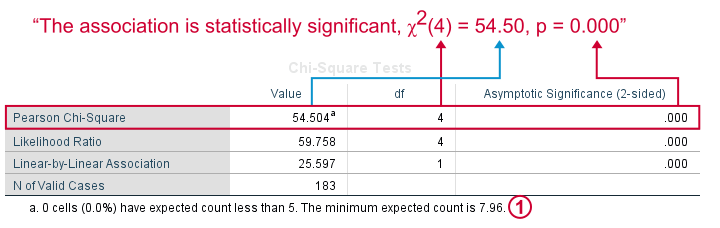
In the case of the above example, the results would be written as follows:
A chi-square test of independence showed that there was a significant association between gender and post-graduation education plans, χ2 (4, N = 101) = 54.50, p < .001.
APA Style Rules
- Do not use a zero before a decimal when the statistic cannot be greater than 1 (proportion, correlation, level of statistical significance).
- Report exact p values to two or three decimals (e.g., p = .006, p = .03).
- However, report p values less than .001 as “ p < .001.”
- Put a space before and after a mathematical operator (e.g., minus, plus, greater than, less than, equals sign).
- Do not repeat statistics in both the text and a table or figure.
p -value Interpretation
You test whether a given χ2 is statistically significant by testing it against a table of chi-square distributions , according to the number of degrees of freedom for your sample, which is the number of categories minus 1. The chi-square assumes that you have at least 5 observations per category.
If you are using SPSS then you will have an expected p -value.
For a chi-square test, a p-value that is less than or equal to the .05 significance level indicates that the observed values are different to the expected values.
Thus, low p-values (p< .05) indicate a likely difference between the theoretical population and the collected sample. You can conclude that a relationship exists between the categorical variables.
Remember that p -values do not indicate the odds that the null hypothesis is true but rather provide the probability that one would obtain the sample distribution observed (or a more extreme distribution) if the null hypothesis was true.
A level of confidence necessary to accept the null hypothesis can never be reached. Therefore, conclusions must choose to either fail to reject the null or accept the alternative hypothesis, depending on the calculated p-value.
The four steps below show you how to analyze your data using a chi-square goodness-of-fit test in SPSS (when you have hypothesized that you have equal expected proportions).
Step 1 : Analyze > Nonparametric Tests > Legacy Dialogs > Chi-square… on the top menu as shown below:
Step 2 : Move the variable indicating categories into the “Test Variable List:” box.
Step 3 : If you want to test the hypothesis that all categories are equally likely, click “OK.”
Step 4 : Specify the expected count for each category by first clicking the “Values” button under “Expected Values.”
Step 5 : Then, in the box to the right of “Values,” enter the expected count for category one and click the “Add” button. Now enter the expected count for category two and click “Add.” Continue in this way until all expected counts have been entered.
Step 6 : Then click “OK.”
The four steps below show you how to analyze your data using a chi-square test of independence in SPSS Statistics.
Step 1 : Open the Crosstabs dialog (Analyze > Descriptive Statistics > Crosstabs).
Step 2 : Select the variables you want to compare using the chi-square test. Click one variable in the left window and then click the arrow at the top to move the variable. Select the row variable and the column variable.
Step 3 : Click Statistics (a new pop-up window will appear). Check Chi-square, then click Continue.
Step 4 : (Optional) Check the box for Display clustered bar charts.
Step 5 : Click OK.
Goodness-of-Fit Test
The Chi-square goodness of fit test is used to compare a randomly collected sample containing a single, categorical variable to a larger population.
This test is most commonly used to compare a random sample to the population from which it was potentially collected.
The test begins with the creation of a null and alternative hypothesis. In this case, the hypotheses are as follows:
Null Hypothesis (Ho) : The null hypothesis (Ho) is that the observed frequencies are the same (except for chance variation) as the expected frequencies. The collected data is consistent with the population distribution.
Alternative Hypothesis (Ha) : The collected data is not consistent with the population distribution.
The next step is to create a contingency table that represents how the data would be distributed if the null hypothesis were exactly correct.
The sample’s overall deviation from this theoretical/expected data will allow us to draw a conclusion, with a more severe deviation resulting in smaller p-values.
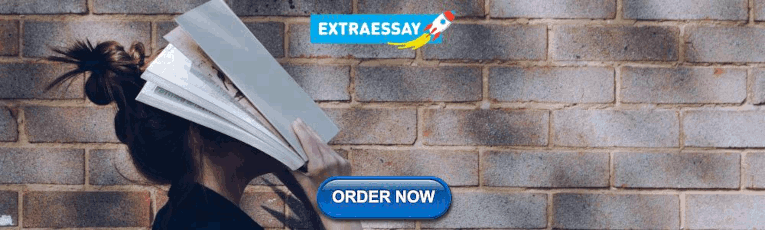
Test for Independence
The Chi-square test for independence looks for an association between two categorical variables within the same population.
Unlike the goodness of fit test, the test for independence does not compare a single observed variable to a theoretical population but rather two variables within a sample set to one another.
The hypotheses for a Chi-square test of independence are as follows:
Null Hypothesis (Ho) : There is no association between the two categorical variables in the population of interest.
Alternative Hypothesis (Ha) : There is no association between the two categorical variables in the population of interest.
The next step is to create a contingency table of expected values that reflects how a data set that perfectly aligns the null hypothesis would appear.
The simplest way to do this is to calculate the marginal frequencies of each row and column; the expected frequency of each cell is equal to the marginal frequency of the row and column that corresponds to a given cell in the observed contingency table divided by the total sample size.
Test for Homogeneity
The Chi-square test for homogeneity is organized and executed exactly the same as the test for independence.
The main difference to remember between the two is that the test for independence looks for an association between two categorical variables within the same population, while the test for homogeneity determines if the distribution of a variable is the same in each of several populations (thus allocating population itself as the second categorical variable).
Null Hypothesis (Ho) : There is no difference in the distribution of a categorical variable for several populations or treatments.
Alternative Hypothesis (Ha) : There is a difference in the distribution of a categorical variable for several populations or treatments.
The difference between these two tests can be a bit tricky to determine, especially in the practical applications of a Chi-square test. A reliable rule of thumb is to determine how the data was collected.
If the data consists of only one random sample with the observations classified according to two categorical variables, it is a test for independence. If the data consists of more than one independent random sample, it is a test for homogeneity.
What is the chi-square test?
The Chi-square test is a non-parametric statistical test used to determine if there’s a significant association between two or more categorical variables in a sample.
It works by comparing the observed frequencies in each category of a cross-tabulation with the frequencies expected under the null hypothesis, which assumes there is no relationship between the variables.
This test is often used in fields like biology, marketing, sociology, and psychology for hypothesis testing.
What does chi-square tell you?
The Chi-square test informs whether there is a significant association between two categorical variables. Suppose the calculated Chi-square value is above the critical value from the Chi-square distribution.
In that case, it suggests a significant relationship between the variables, rejecting the null hypothesis of no association.
How to calculate chi-square?
To calculate the Chi-square statistic, follow these steps:
1. Create a contingency table of observed frequencies for each category.
2. Calculate expected frequencies for each category under the null hypothesis.
3. Compute the Chi-square statistic using the formula: Χ² = Σ [ (O_i – E_i)² / E_i ], where O_i is the observed frequency and E_i is the expected frequency.
4. Compare the calculated statistic with the critical value from the Chi-square distribution to draw a conclusion.

Related Articles
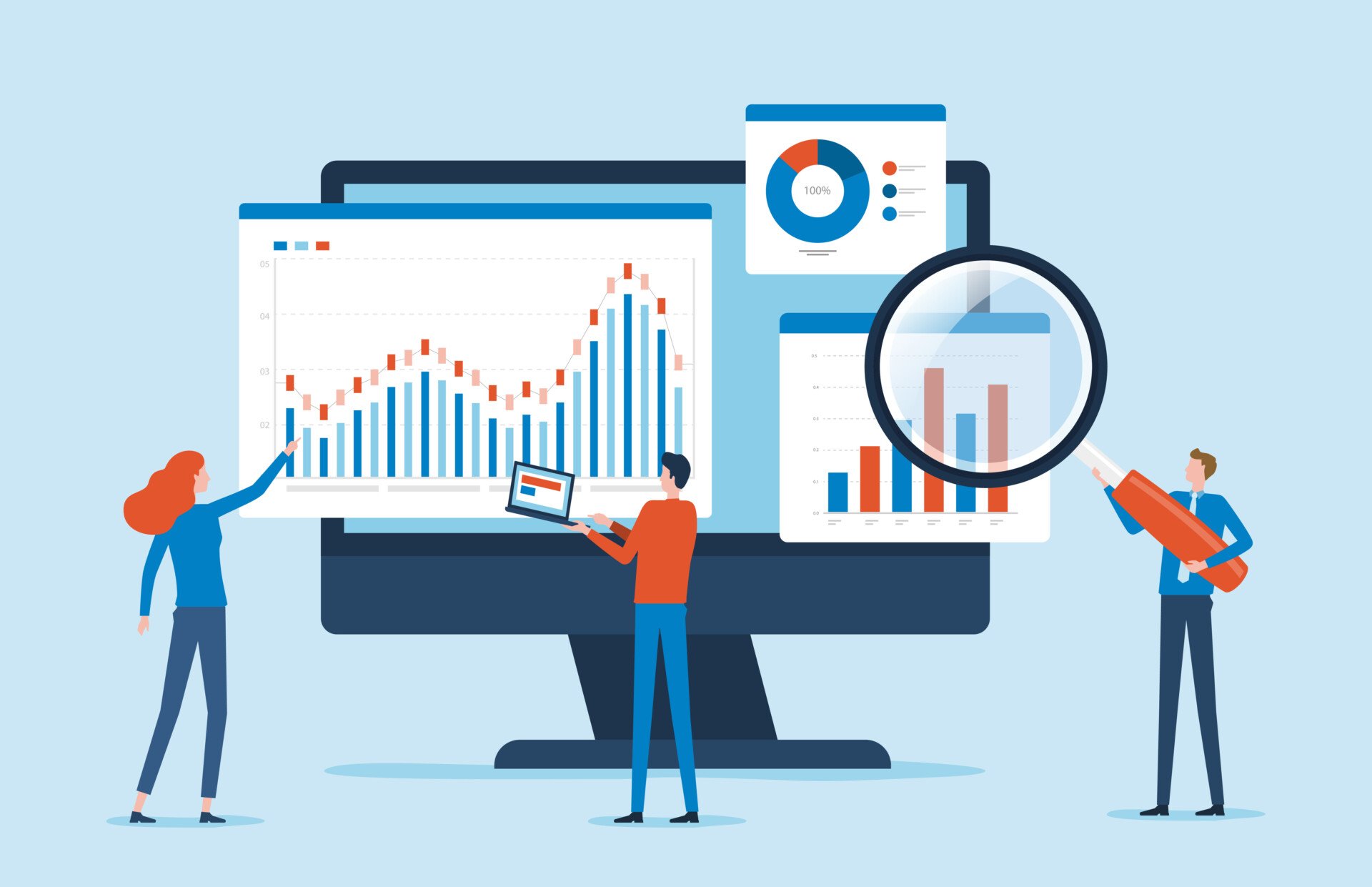
Exploratory Data Analysis
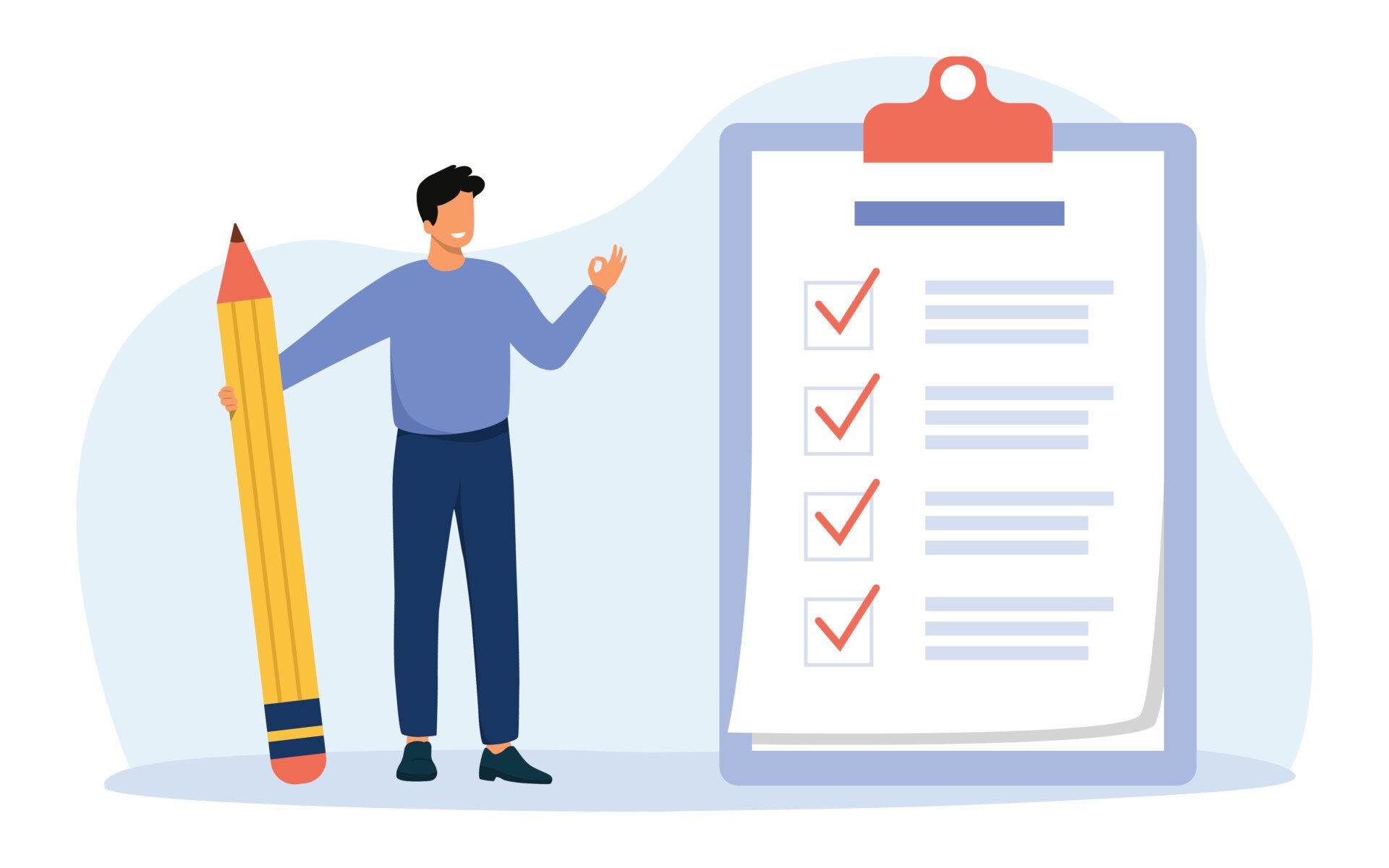
Research Methodology , Statistics
What Is Face Validity In Research? Importance & How To Measure
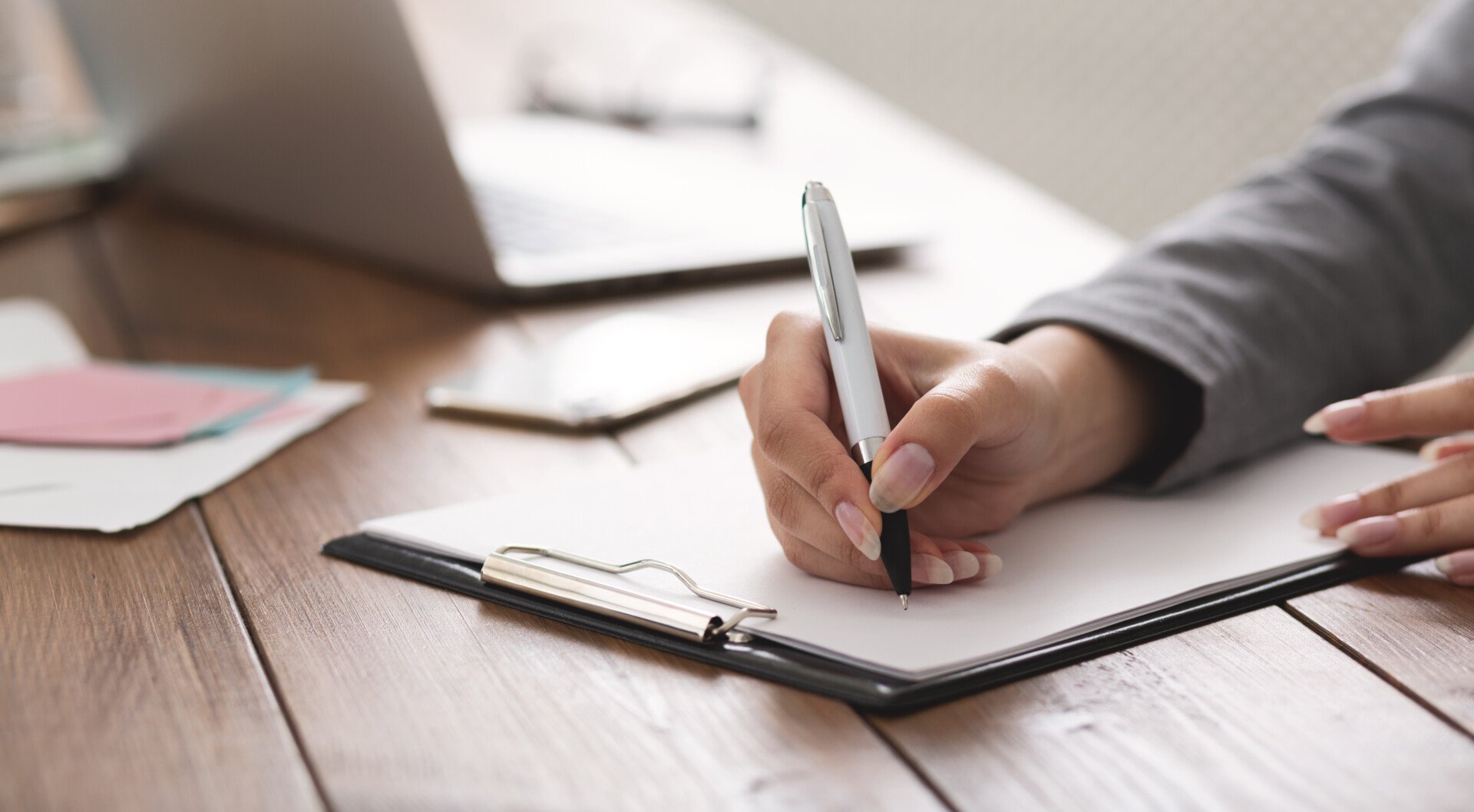
Criterion Validity: Definition & Examples
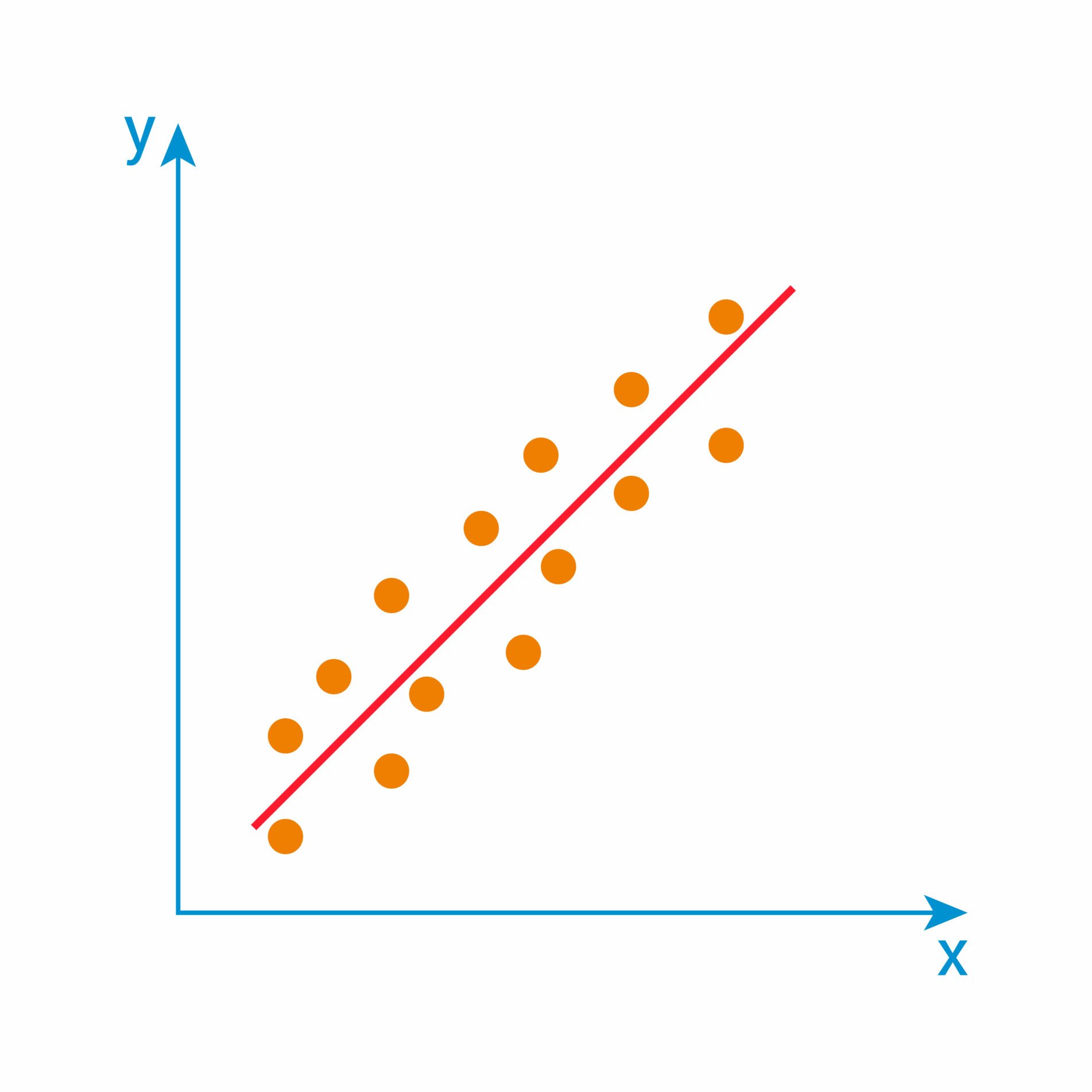
Convergent Validity: Definition and Examples
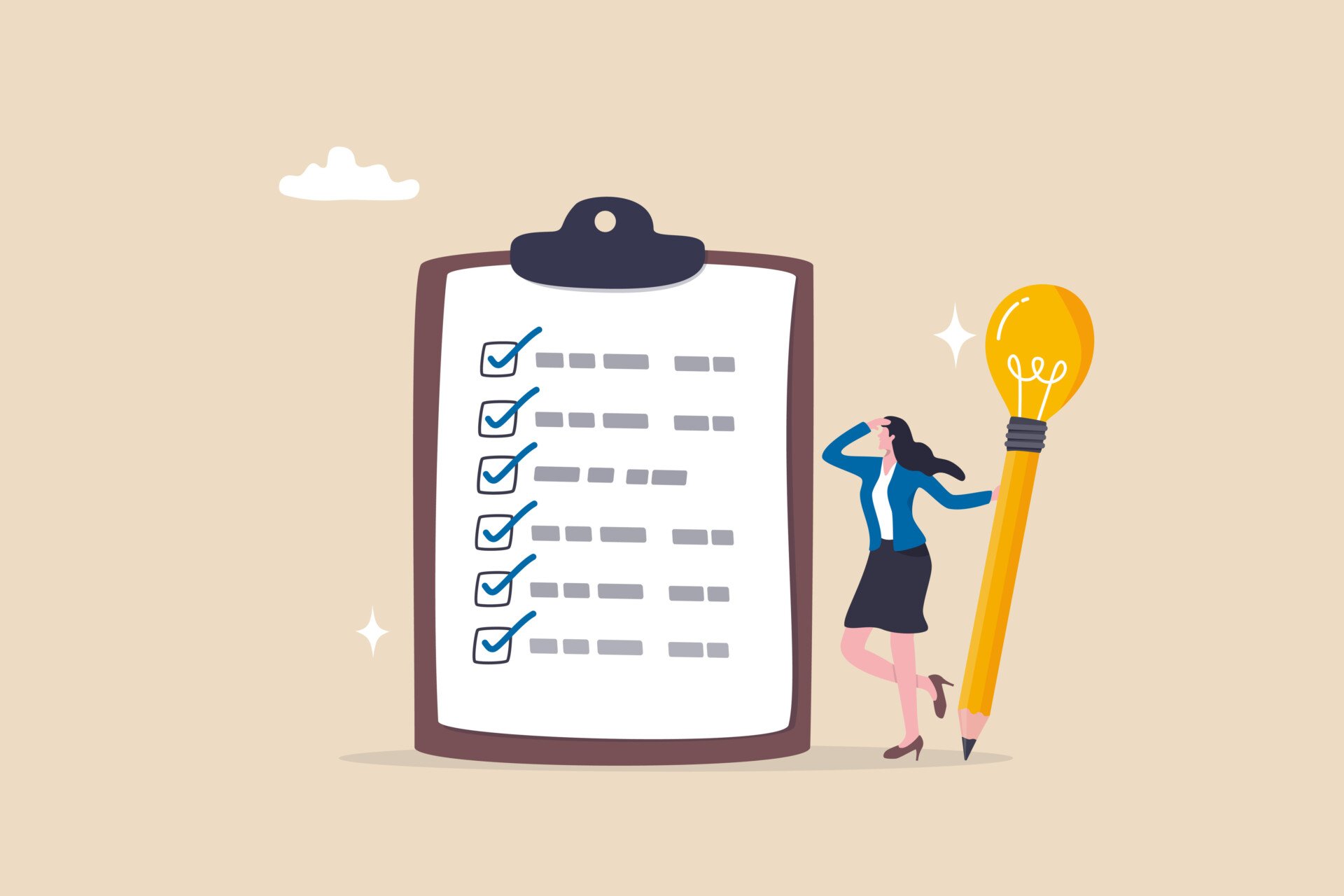
Content Validity in Research: Definition & Examples
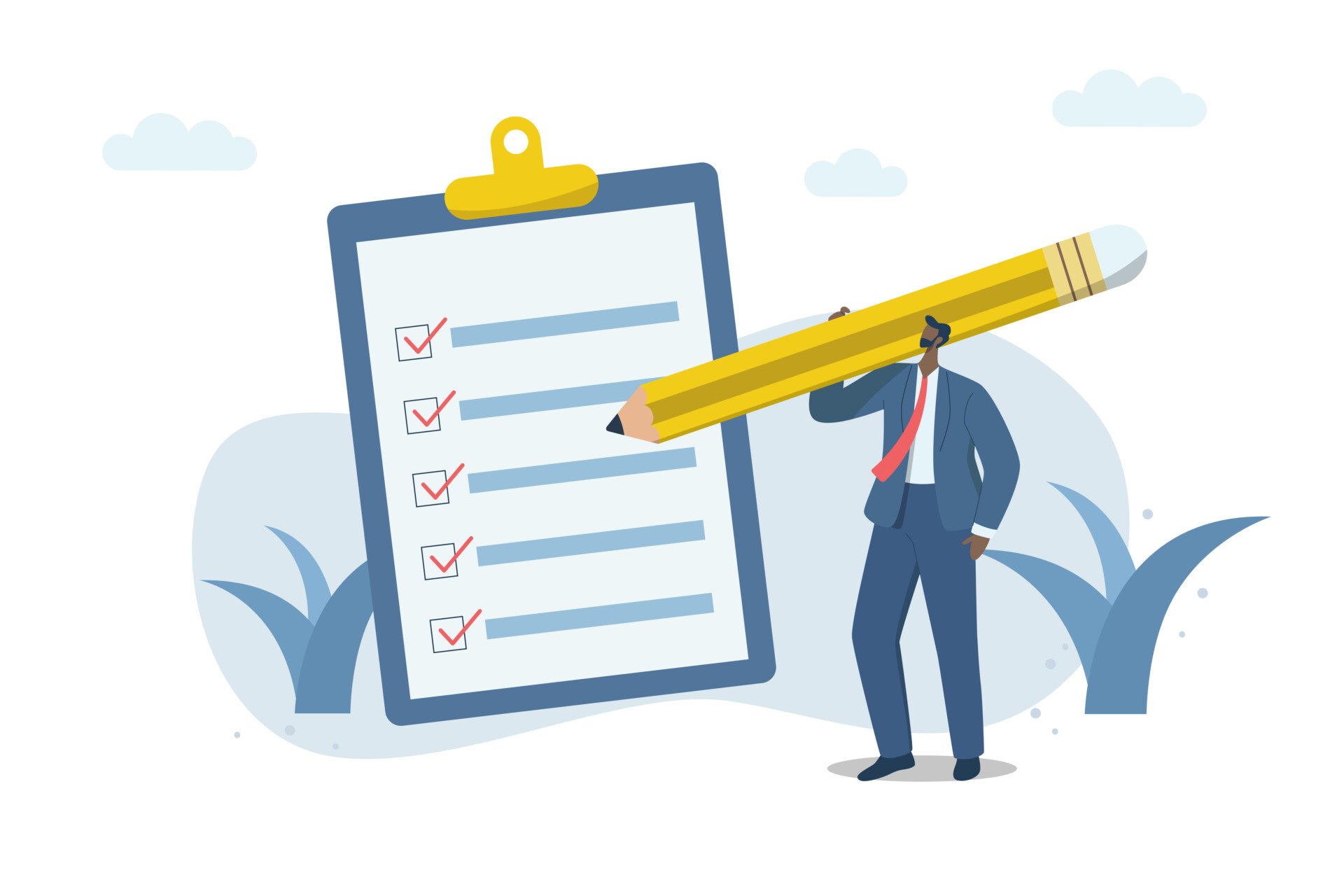
Construct Validity In Psychology Research
What is a scientific hypothesis?
It's the initial building block in the scientific method.
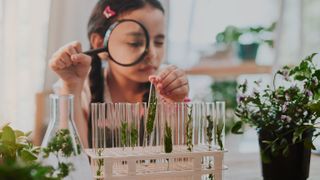
Hypothesis basics
What makes a hypothesis testable.
- Types of hypotheses
- Hypothesis versus theory
Additional resources
Bibliography.
A scientific hypothesis is a tentative, testable explanation for a phenomenon in the natural world. It's the initial building block in the scientific method . Many describe it as an "educated guess" based on prior knowledge and observation. While this is true, a hypothesis is more informed than a guess. While an "educated guess" suggests a random prediction based on a person's expertise, developing a hypothesis requires active observation and background research.
The basic idea of a hypothesis is that there is no predetermined outcome. For a solution to be termed a scientific hypothesis, it has to be an idea that can be supported or refuted through carefully crafted experimentation or observation. This concept, called falsifiability and testability, was advanced in the mid-20th century by Austrian-British philosopher Karl Popper in his famous book "The Logic of Scientific Discovery" (Routledge, 1959).
A key function of a hypothesis is to derive predictions about the results of future experiments and then perform those experiments to see whether they support the predictions.
A hypothesis is usually written in the form of an if-then statement, which gives a possibility (if) and explains what may happen because of the possibility (then). The statement could also include "may," according to California State University, Bakersfield .
Here are some examples of hypothesis statements:
- If garlic repels fleas, then a dog that is given garlic every day will not get fleas.
- If sugar causes cavities, then people who eat a lot of candy may be more prone to cavities.
- If ultraviolet light can damage the eyes, then maybe this light can cause blindness.
A useful hypothesis should be testable and falsifiable. That means that it should be possible to prove it wrong. A theory that can't be proved wrong is nonscientific, according to Karl Popper's 1963 book " Conjectures and Refutations ."
An example of an untestable statement is, "Dogs are better than cats." That's because the definition of "better" is vague and subjective. However, an untestable statement can be reworded to make it testable. For example, the previous statement could be changed to this: "Owning a dog is associated with higher levels of physical fitness than owning a cat." With this statement, the researcher can take measures of physical fitness from dog and cat owners and compare the two.
Types of scientific hypotheses
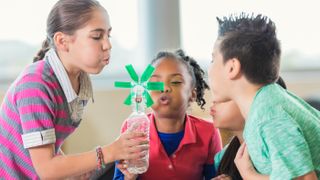
In an experiment, researchers generally state their hypotheses in two ways. The null hypothesis predicts that there will be no relationship between the variables tested, or no difference between the experimental groups. The alternative hypothesis predicts the opposite: that there will be a difference between the experimental groups. This is usually the hypothesis scientists are most interested in, according to the University of Miami .
For example, a null hypothesis might state, "There will be no difference in the rate of muscle growth between people who take a protein supplement and people who don't." The alternative hypothesis would state, "There will be a difference in the rate of muscle growth between people who take a protein supplement and people who don't."
If the results of the experiment show a relationship between the variables, then the null hypothesis has been rejected in favor of the alternative hypothesis, according to the book " Research Methods in Psychology " (BCcampus, 2015).
There are other ways to describe an alternative hypothesis. The alternative hypothesis above does not specify a direction of the effect, only that there will be a difference between the two groups. That type of prediction is called a two-tailed hypothesis. If a hypothesis specifies a certain direction — for example, that people who take a protein supplement will gain more muscle than people who don't — it is called a one-tailed hypothesis, according to William M. K. Trochim , a professor of Policy Analysis and Management at Cornell University.
Sometimes, errors take place during an experiment. These errors can happen in one of two ways. A type I error is when the null hypothesis is rejected when it is true. This is also known as a false positive. A type II error occurs when the null hypothesis is not rejected when it is false. This is also known as a false negative, according to the University of California, Berkeley .
A hypothesis can be rejected or modified, but it can never be proved correct 100% of the time. For example, a scientist can form a hypothesis stating that if a certain type of tomato has a gene for red pigment, that type of tomato will be red. During research, the scientist then finds that each tomato of this type is red. Though the findings confirm the hypothesis, there may be a tomato of that type somewhere in the world that isn't red. Thus, the hypothesis is true, but it may not be true 100% of the time.
Scientific theory vs. scientific hypothesis
The best hypotheses are simple. They deal with a relatively narrow set of phenomena. But theories are broader; they generally combine multiple hypotheses into a general explanation for a wide range of phenomena, according to the University of California, Berkeley . For example, a hypothesis might state, "If animals adapt to suit their environments, then birds that live on islands with lots of seeds to eat will have differently shaped beaks than birds that live on islands with lots of insects to eat." After testing many hypotheses like these, Charles Darwin formulated an overarching theory: the theory of evolution by natural selection.
"Theories are the ways that we make sense of what we observe in the natural world," Tanner said. "Theories are structures of ideas that explain and interpret facts."
- Read more about writing a hypothesis, from the American Medical Writers Association.
- Find out why a hypothesis isn't always necessary in science, from The American Biology Teacher.
- Learn about null and alternative hypotheses, from Prof. Essa on YouTube .
Encyclopedia Britannica. Scientific Hypothesis. Jan. 13, 2022. https://www.britannica.com/science/scientific-hypothesis
Karl Popper, "The Logic of Scientific Discovery," Routledge, 1959.
California State University, Bakersfield, "Formatting a testable hypothesis." https://www.csub.edu/~ddodenhoff/Bio100/Bio100sp04/formattingahypothesis.htm
Karl Popper, "Conjectures and Refutations," Routledge, 1963.
Price, P., Jhangiani, R., & Chiang, I., "Research Methods of Psychology — 2nd Canadian Edition," BCcampus, 2015.
University of Miami, "The Scientific Method" http://www.bio.miami.edu/dana/161/evolution/161app1_scimethod.pdf
William M.K. Trochim, "Research Methods Knowledge Base," https://conjointly.com/kb/hypotheses-explained/
University of California, Berkeley, "Multiple Hypothesis Testing and False Discovery Rate" https://www.stat.berkeley.edu/~hhuang/STAT141/Lecture-FDR.pdf
University of California, Berkeley, "Science at multiple levels" https://undsci.berkeley.edu/article/0_0_0/howscienceworks_19
Sign up for the Live Science daily newsletter now
Get the world’s most fascinating discoveries delivered straight to your inbox.
'The difference between alarming and catastrophic': Cascadia megafault has 1 especially deadly section, new map reveals
Arctic 'zombie fires' rising from the dead could unleash vicious cycle of warming
Epidurals may lower risk of complications after birth, study hints
Most Popular
- 2 100-foot 'walking tree' in New Zealand looks like an Ent from Lord of the Rings — and is the lone survivor of a lost forest
- 3 Save $400 on Unistellar's new smart binoculars during their early bird Kickstarter
- 4 10 'breathtaking' photos of our galaxy from the 2024 Milky Way Photographer of the Year contest
- 5 James Webb telescope finds carbon at the dawn of the universe, challenging our understanding of when life could have emerged
- 2 Neanderthals and humans interbred 47,000 years ago for nearly 7,000 years, research suggests
- 3 What is the 3-body problem, and is it really unsolvable?
- 4 Razor-thin silk 'dampens noise by 75%' — could be game-changer for sound-proofing homes and offices
- 5 Shigir Idol: World's oldest wood sculpture has mysterious carved faces and once stood 17 feet tall
Article Categories
Book categories, collections.
- Academics & The Arts Articles
- Math Articles
- Statistics Articles
How to Test a Null Hypothesis Based on One Population Proportion
Statistics for dummies.
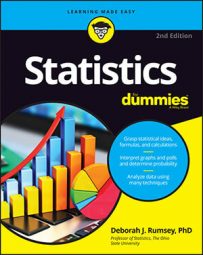
Sign up for the Dummies Beta Program to try Dummies' newest way to learn.
You can use a hypothesis test to test a statistical claim about a population proportion when the variable is categorical (for example, gender or support/oppose) and only one population or group is being studied (for example, all registered voters).
The test looks at the proportion ( p ) of individuals in the population who have a certain characteristic — for example, the proportion of people who carry cellphones. The null hypothesis is H 0 : p = p 0 , where p 0 is a certain claimed value of the population proportion, p . For example, if the claim is that 70% of people carry cellphones, p 0 is 0.70. The alternative hypothesis is one of the following:

The formula for the test statistic for a single proportion (under certain conditions) is:

and z is a value on the Z -distribution. To calculate the test statistic, do the following:
Calculate the sample proportion,

by taking the number of people in the sample who have the characteristic of interest (for example, the number of people in the sample carrying cellphones) and dividing that by n, the sample size.

where p o is the value in H o .
Calculate the standard error,

Divide your result from Step 2 by your result from Step 3.
To interpret the test statistic, look up your test statistic on the standard normal ( Z- ) distribution and calculate the p- value.
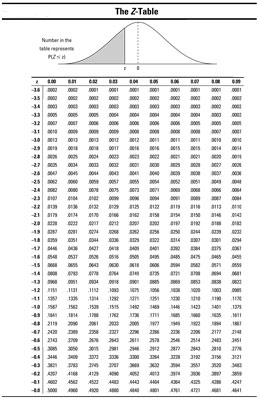
The conditions for using this test statistic are that

For example, suppose Cavifree claims that four out of five dentists recommend Cavifree toothpaste to their patients. In this case, the population is all dentists, and p is the proportion of all dentists who recommended Cavifree. The claim is that p is equal to “four out of five,” or p 0 is 4 divided by 5 = 0.80. You suspect that the proportion is actually less than 0.80. Your hypotheses are H 0 : p = 0.80 versus H a : p < 0.80.
Suppose that 151 out of your sample of 200 dental patients reported receiving a recommendation for Cavifree from their dentist. To find the test statistic for these results, follow these steps:
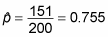
and n = 200.
Because p o = 0.80, take p(hat)-p 0 =0.755 – 0.80 = –0.045 as the numerator of the test statistic.
Next, the standard error equals
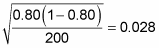
(the denominator of the test statistic).
The test statistic is
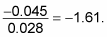
Because the resulting test statistic is negative, it means your sample results are –1.61 standard errors below (less than) the claimed value for the population. How often would you expect to get results like this if H 0 were true? The chance of being at or beyond (in this case less than) –1.61 is 0.0537. (Keep the negative with the number and look up –1.61 in the above Z -table.) This result is your p- value because H a is a less-than hypothesis.
Because the p- value is greater than 0.05 (albeit not by much), you don’t have quite enough evidence for rejecting H 0 . You conclude that the claim that 80% of dentists recommend Cavifree can’t be rejected, according to your data. However, it’s important to report the actual p -value too, so others can make their own decisions.
You might ask, “Hey, the sample proportion of 0.755 is way lower than the claimed proportion of 0.80. Why did the hypothesis test reject H 0 since 0.755 is less than 0.80?” Because in this case, 0.755 is not significantly less than 0.80. You also need to factor in variation using the standard error and the normal distribution to be able to say something about the entire population of dentists.
The letter p is used two different ways in this example: p -value and p . The letter p by itself indicates the population proportion, not the p -value. Don’t get confused. Whenever you report a p -value, be sure you add –value so it’s not confused with p, the population proportion .
About This Article
This article is from the book:.
- Statistics For Dummies ,
About the book author:
Deborah J. Rumsey , PhD, is an Auxiliary Professor and Statistics Education Specialist at The Ohio State University. She is the author of Statistics For Dummies, Statistics II For Dummies, Statistics Workbook For Dummies, and Probability For Dummies.
This article can be found in the category:
- Statistics ,
- Statistics For Dummies Cheat Sheet
- Checking Out Statistical Confidence Interval Critical Values
- Handling Statistical Hypothesis Tests
- Statistically Figuring Sample Size
- Surveying Statistical Confidence Intervals
- View All Articles From Book
- Skip to secondary menu
- Skip to main content
- Skip to primary sidebar
Statistics By Jim
Making statistics intuitive
Confidence Intervals: Interpreting, Finding & Formulas
By Jim Frost 10 Comments
What is a Confidence Interval?
A confidence interval (CI) is a range of values that is likely to contain the value of an unknown population parameter . These intervals represent a plausible domain for the parameter given the characteristics of your sample data. Confidence intervals are derived from sample statistics and are calculated using a specified confidence level.
Population parameters are typically unknown because it is usually impossible to measure entire populations. By using a sample, you can estimate these parameters. However, the estimates rarely equal the parameter precisely thanks to random sampling error . Fortunately, inferential statistics procedures can evaluate a sample and incorporate the uncertainty inherent when using samples. Confidence intervals place a margin of error around the point estimate to help us understand how wrong the estimate might be.
You’ll frequently use confidence intervals to bound the sample mean and standard deviation parameters. But you can also create them for regression coefficients , proportions, rates of occurrence (Poisson), and the differences between populations.
Related post : Populations, Parameters, and Samples in Inferential Statistics
What is the Confidence Level?
The confidence level is the long-run probability that a series of confidence intervals will contain the true value of the population parameter.
Different random samples drawn from the same population are likely to produce slightly different intervals. If you draw many random samples and calculate a confidence interval for each sample, a percentage of them will contain the parameter.
The confidence level is the percentage of the intervals that contain the parameter. For 95% confidence intervals, an average of 19 out of 20 include the population parameter, as shown below.

The image above shows a hypothetical series of 20 confidence intervals from a study that draws multiple random samples from the same population. The horizontal red dashed line is the population parameter, which is usually unknown. Each blue dot is a the sample’s point estimate for the population parameter. Green lines represent CIs that contain the parameter, while the red line is a CI that does not contain it. The graph illustrates how confidence intervals are not perfect but usually correct.
The CI procedure provides meaningful estimates because it produces ranges that usually contain the parameter. Hence, they present plausible values for the parameter.
Technically, you can create CIs using any confidence level between 0 and 100%. However, the most common confidence level is 95%. Analysts occasionally use 99% and 90%.
Related posts : Populations and Samples and Parameters vs. Statistics ,
How to Interpret Confidence Intervals
A confidence interval indicates where the population parameter is likely to reside. For example, a 95% confidence interval of the mean [9 11] suggests you can be 95% confident that the population mean is between 9 and 11.
Confidence intervals also help you navigate the uncertainty of how well a sample estimates a value for an entire population.
These intervals start with the point estimate for the sample and add a margin of error around it. The point estimate is the best guess for the parameter value. The margin of error accounts for the uncertainty involved when using a sample to estimate an entire population.
The width of the confidence interval around the point estimate reveals the precision. If the range is narrow, the margin of error is small, and there is only a tiny range of plausible values. That’s a precise estimate. However, if the interval is wide, the margin of error is large, and the actual parameter value is likely to fall somewhere within that more extensive range . That’s an imprecise estimate.
Ideally, you’d like a narrow confidence interval because you’ll have a much better idea of the actual population value!
For example, imagine we have two different samples with a sample mean of 10. It appears both estimates are the same. Now let’s assess the 95% confidence intervals. One interval is [5 15] while the other is [9 11]. The latter range is narrower, suggesting a more precise estimate.
That’s how CIs provide more information than the point estimate (e.g., sample mean) alone.
Related post : Precision vs. Accuracy
Confidence Intervals for Effect Sizes
Confidence intervals are similarly helpful for understanding an effect size. For example, if you assess a treatment and control group, the mean difference between these groups is the estimated effect size. A 2-sample t-test can construct a confidence interval for the mean difference.
In this scenario, consider both the size and precision of the estimated effect. Ideally, an estimated effect is both large enough to be meaningful and sufficiently precise for you to trust. CIs allow you to assess both of these considerations! Learn more about this distinction in my post about Practical vs. Statistical Significance .
Learn more about how confidence intervals and hypothesis tests are similar .
Related post : Effect Sizes in Statistics
Avoid a Common Misinterpretation of Confidence Intervals
A frequent misuse is applying confidence intervals to the distribution of sample values. Remember that these ranges apply only to population parameters, not the data values.
For example, a 95% confidence interval [10 15] indicates that we can be 95% confident that the parameter is within that range.
However, it does NOT indicate that 95% of the sample values occur in that range.
If you need to use your sample to find the proportion of data values likely to fall within a range, use a tolerance interval instead.
Related post : See how confidence intervals compare to prediction intervals and tolerance intervals .
What Affects the Widths of Confidence Intervals?
Ok, so you want narrower CIs for their greater precision. What conditions produce tighter ranges?
Sample size, variability, and the confidence level affect the widths of confidence intervals. The first two are characteristics of your sample, which I’ll cover first.
Sample Variability
Variability present in your data affects the precision of the estimate. Your confidence intervals will be broader when your sample standard deviation is high.
It makes sense when you think about it. When there is a lot of variability present in your sample, you’re going to be less sure about the estimates it produces. After all, a high standard deviation means your sample data are really bouncing around! That’s not conducive for finding precise estimates.
Unfortunately, you often don’t have much control over data variability. You can institute measurement and data collection procedures that reduce outside sources of variability, but after that, you’re at the mercy of the variability inherent in your subject area. But, if you can reduce external sources of variation, that’ll help you reduce the width of your confidence intervals.
Sample Size
Increasing your sample size is the primary way to reduce the widths of confidence intervals because, in most cases, you can control it more than the variability. If you don’t change anything else and only increase the sample size, the ranges tend to narrow. Need even tighter CIs? Just increase the sample size some more!
Theoretically, there is no limit, and you can dramatically increase the sample size to produce remarkably narrow ranges. However, logistics, time, and cost issues will constrain your maximum sample size in the real world.
In summary, larger sample sizes and lower variability reduce the margin of error around the point estimate and create narrower confidence intervals. I’ll point out these factors again when we get to the formula later in this post.
Related post : Sample Statistics Are Always Wrong (to Some Extent)!
Changing the Confidence Level
The confidence level also affects the confidence interval width. However, this factor is a methodology choice separate from your sample’s characteristics.
If you increase the confidence level (e.g., 95% to 99%) while holding the sample size and variability constant, the confidence interval widens. Conversely, decreasing the confidence level (e.g., 95% to 90%) narrows the range.
I’ve found that many students find the effect of changing the confidence level on the width of the range to be counterintuitive.
Imagine you take your knowledge of a subject area and indicate you’re 95% confident that the correct answer lies between 15 and 20. Then I ask you to give me your confidence for it falling between 17 and 18. The correct answer is less likely to fall within the narrower interval, so your confidence naturally decreases.
Conversely, I ask you about your confidence that it’s between 10 and 30. That’s a much wider range, and the correct value is more likely to be in it. Consequently, your confidence grows.
Confidence levels involve a tradeoff between confidence and the interval’s spread. To have more confidence that the parameter falls within the interval, you must widen the interval. Conversely, your confidence necessarily decreases if you use a narrower range.
Confidence Interval Formula
Confidence intervals account for sampling uncertainty by using critical values, sampling distributions, and standard errors. The precise formula depends on the type of parameter you’re evaluating. The most common type is for the mean, so I’ll stick with that.
You’ll use critical Z-values or t-values to calculate your confidence interval of the mean. T-values produce more accurate confidence intervals when you do not know the population standard deviation. That’s particularly true for sample sizes smaller than 30. For larger samples, the two methods produce similar results. In practice, you’d usually use a t-value.
Below are the confidence interval formulas for both Z and t. However, you’d only use one of them.

- x̄ = the sample mean, which is the point estimate.
- Z = the critical z-value
- t = the critical t-value
- s = the sample standard deviation
- s / √n = the standard error of the mean
The only difference between the two formulas is the critical value. If you’re using the critical z-value, you’ll always use 1.96 for 95% confidence intervals. However, for the t-value, you’ll need to know the degrees of freedom and then look up the critical value in a t-table or online calculator.
To calculate a confidence interval, take the critical value (Z or t) and multiply it by the standard error of the mean (SEM). This value is known as the margin of error (MOE) . Then add and subtract the MOE from the sample mean (x̄) to produce the upper and lower limits of the range.
Related posts : Critical Values , Standard Error of the Mean , and Sampling Distributions
Interval Widths Revisited
Think back to the discussion about the factors affecting the confidence interval widths. The formula helps you understand how that works. Recall that the critical value * SEM = MOE.
Smaller margins of error produce narrower confidence intervals. By looking at this equation, you can see that the following conditions create a smaller MOE:
- Smaller critical values, which you obtain by decreasing the confidence level.
- Smaller standard deviations, because they’re in the numerator of the SEM.
- Large samples sizes, because its square root is in the denominator of the SEM.
How to Find a Confidence Interval
Let’s move on to using these formulas to find a confidence interval! For this example, I’ll use a fuel cost dataset that I’ve used in other posts: FuelCosts . The dataset contains a random sample of 25 fuel costs. We want to calculate the 95% confidence interval of the mean.
However, imagine we have only the following summary information instead of the dataset.
- Sample mean: 330.6
- Standard deviation: 154.2
Fortunately, that’s all we need to calculate our 95% confidence interval of the mean.
We need to decide on using the critical Z or t-value. I’ll use a critical t-value because the sample size (25) is less than 30. However, if the summary didn’t provide the sample size, we could use the Z-value method for an approximation.
My next step is to look up the critical t-value using my t-table. In the table, I’ll choose the alpha that equals 1 – the confidence level (1 – 0.95 = 0.05) for a two-sided test. Below is a truncated version of the t-table. Click for the full t-distribution table .

In the table, I see that for a two-sided interval with 25 – 1 = 24 degrees of freedom and an alpha of 0.05, the critical value is 2.064.
Entering Values into the Confidence Interval Formula
Let’s enter all of this information into the formula.
First, I’ll calculate the margin of error:

Next, I’ll take the sample mean and add and subtract the margin of error from it:
- 330.6 + 63.6 = 394.2
- 330.6 – 63.6 = 267.0
The 95% confidence interval of the mean for fuel costs is 267.0 – 394.2. We can be 95% confident that the population mean falls within this range.
If you had used the critical z-value (1.96), you would enter that into the formula instead of the t-value (2.064) and obtain a slightly different confidence interval. However, t-values produce more accurate results, particularly for smaller samples like this one.
As an aside, the Z-value method always produces narrower confidence intervals than t-values when your sample size is less than infinity. So, basically always! However, that’s not good because Z-values underestimate the uncertainty when you’re using a sample estimate of the standard deviation rather than the actual population value. And you practically never know the population standard deviation.
Neyman, J. (1937). Outline of a Theory of Statistical Estimation Based on the Classical Theory of Probability . Philosophical Transactions of the Royal Society A . 236 (767): 333–380.
Share this:

Reader Interactions
April 23, 2024 at 8:37 am
February 24, 2024 at 8:29 am
Thank you so much
February 14, 2024 at 1:56 pm
If I take a sample and create a confidence interval for the mean, can I say that 95% of the mean of the other samples I will take can be found in this range?
February 23, 2024 at 8:40 pm
Unfortunately, that would be an invalid statement. The CI formula uses your sample to estimate the properties of the population to construct the CI. Your estimates are bound to be off by at least a little bit. If you knew the precise properties of the population, you could determine the range in which 95% of random samples from that population would fall. However, again, you don’t know the precise properties of the population. You just have estimates based on your sample.
September 29, 2023 at 6:55 pm
Hi Jim, My confusion is similar to one comment. What I cannot seem to understand is the concept of individual and many CIs and therefore statements such as X% of the CIs.
For a sampling distribution, which itself requires many samples to produce, we try to find a confidence interval. Then how come there are multiple CIs. More specifically “Different random samples drawn from the same population are likely to produce slightly different intervals. If you draw many random samples and calculate a confidence interval for each sample, a percentage of them will contain the parameter.” this is what confuses me. Is interval here represents the range of the samples drawn? If that is true, why is the term CI or interval used for sample range? If not, could you please explain what is mean by an individual CI or how are we calculating confidence interval for each sample? In the image depicting 19 out of 20 will have population parameter, is the green line the range of individual samples or the confidence interval?
Please try to sort this confusion out for me. I find your website really helpful for clearing my statistical concepts. Thank you in advance for helping out. Regards.
September 30, 2023 at 1:52 am
A key point to remember is that inferential statistics occur in the context of drawing many random samples from the same population. Of course, a single study typically draws a single sample. However, if that study were to draw another random sample, it would be somewhat different than the first sample. A third sample would be somewhat different as well. That produces the sampling distribution, which helps you calculate p-values and construct CIs. Inferential statistics procedures use the idea of many samples to incorporate random sampling error into the results.
For CIs, if you were to collect many random samples, a certain percentage of them will contain the population parameter. That percentage is the confidence interval. Again, a single study will only collect a single sample. However, picturing many CIs helps you understand the concept of the confidence level. In practice, a study generates one CI per parameter estimate. But the graph with multiple CIs is just to help you understand the concept of confidence level.
Alternatively, you can think of CIs as an object class. Suppose 100 disparate studies produce 95% CIs. You can assume that about 95 of those CIs actually contain the population parameter. Using statistical procedures, you can estimate the sampling distribution using the sample itself without collecting many samples.
I don’t know what you mean by “Interval here represents the range of samples drawn.” As I write in this article, the CI is an interval of values that likely contain the population parameter. Reread the section titled How to Interpret Confidence Intervals to understand what each one means.
Each CI is estimated from a single sample and a study generates one CI per parameter estimate. However, again, understanding the concept of the confidence level is easier when you picture multiple CIs. But if a single study were to collect multiple samples and produces multiple CIs, that graph is what you’d expect to see. Although, in the real world, you never know for sure whether a CI actually contains the parameter or not.
The green lines represent CIs that contain the population parameter. Red lines represent CIs that do not contain the population parameter. The graph illustrates how CIs are not perfect but they are usually correct. I’ve added text to the article to clarify that image.
I also show you how to calculate the CI for a mean in this article. I’m not sure what more you need to understand there? I’m happy to clarify any part of that.
I hope that helps!
July 6, 2023 at 10:14 am
Hi Jim, This was an excellent article, thank you! I have a question: when computing a CI in its single-sample t-test module, SPSS appears to use the difference between population and sample means as a starting point (so the formula would be (X-bar-mu) +/- tcv(SEM)). I’ve consulted multiple stats books, but none of them compute a CI that way for a single-sample t-test. Maybe I’m just missing something and this is a perfectly acceptable way of doing things (I mean, SPSS does it :-)), but it yields substantially different lower and upper bounds from a CI that uses the traditional X-bar as a starting point. Do you have any insights? Many thanks in advance! Stephen
July 7, 2023 at 2:56 am
Hi Stephen,
I’m not an SPSS user but that formula is confusing. They presented this formula as being for the CI of a sample mean?
I’m not sure why they’re subtracting Mu. For one thing, you almost never know what Mu is because you’d have to measure the entire population. And, if you knew Mu, you wouldn’t need to perform a t-test! Why would you use a sample mean (X-bar) if you knew the population mean? None of it makes sense to me. It must be an error of some kind even if just of documentation.
October 13, 2022 at 8:33 am
Are there strict distinctions between the terms “confident”, “likely”, and “probability”? I’ve seen a number of other sources exclaim that for a given calculated confidence interval, the frequentist interpretation of that is the parameter is either in or not in that interval. They say another frequent misinterpretation is that the parameter lies within a calculated interval with a 95% probability.
It’s very confusing to balance that notion with practical casual communication of data in non-research settings.
October 13, 2022 at 5:43 pm
It is a confusing issue.
In this strictest technical sense, the confidence level is probability that applies to the process but NOT an individual confidence interval. There are several reasons for that.
In the frequentist framework, the probability that an individual CI contains the parameter is either 100% or 0%. It’s either in it or out. The parameter is not a random variable. However, because you don’t know the parameter value, you don’t know which of those two conditions is correct. That’s the conceptual approach. And the mathematics behind the scenes are complementary to that. There’s just no way to calculate the probability that an individual CI contains the parameter.
On the other hand, the process behind creating the intervals will cause X% of the CIs at the Xth confidence level to include that parameter. So, for all 95% CIs, you’d expect 95% of them to contain the parameter value. The confidence level applies to the process, not the individual CIs. Statisticians intentionally used the term “confidence” to describe that as opposed to “probability” hoping to make that distinction.
So, the 95% confidence applies the process but not individual CIs.
However, if you’re thinking that if 95% of many CIs contain the parameter, then surely a single CI has a 95% probability. From a technical standpoint, that is NOT true. However, it sure sounds logical. Most statistics make intuitive sense to me, but I struggle with that one myself. I’ve asked other statisticians to get their take on it. The basic gist of their answers is that there might be other information available which can alter the actual probability. Not all CIs produced by the process have the same probability. For example, if an individual CI is a bit higher or lower than most other CIs for the same thing, the CIs with the unusual values will have lower probabilities for containing the parameters.
I think that makes sense. The only problem is that you often don’t know where your individual CI fits in. That means you don’t know the probability for it specifically. But you do know the overall probability for the process.
The answer for this question is never totally satisfying. Just remember that there is no mathematical way in the frequentist framework to calculate the probability that an individual CI contains the parameter. However, the overall process is designed such that all CIs using a particular confidence level will have the specified proportion containing the parameter. However, you can’t apply that overall proportion to your individual CI because on the technical side there’s no mathematical way to do that and conceptually, you don’t know where your individual CI fits in the entire distribution of CIs.
Comments and Questions Cancel reply
Analysis and visualization of interactions in R
OARC Statistical Methods and Data Analytics
Workshop outline
This workshop will teach you how to analyze and visualize interactions in regression models in R both using the emmeans package and with base R coding.
Topics discussed in the workshop:
- interpreting coefficients
- dummy variables for categorical predictors
- main effects models
- estimated marginal means
- estimating effects
- visualizing effects
- why we model interactions
- product terms
- general interpretation of coefficients in models with interactions
- interactions of two continuous variables
- interactions of two categorical variables
- interactions of a categorical variable and a continuous variable
- interactions in generalized linear models (logistic regression)
- Estimating simple effects through re-centering and changing reference groups
- Predictions
- Graphing predictions to visualize effects
Workshop packages
This workshop requires the emmeans and ggplot2 packages. This workshop is interactive, so if you wish to participate, please load both packages into R now
Workshop data set
The workshop data set contains data from an experiment of mice being fed 3 different diets.
- weight: weight in grams, the dependent variable
- age: age in weeks, from 2 to 16 weeks in increments of 2
- diet: diet type, one of high-“fat”, high-“starch”, or “control”
- sex: male or female
- litter: number of pups in litter, from 2 to 10
- hyper: 0=no hypertension, 1=hypertension
Note: the data are artificial. Do not make any inferences regarding mouse weights from these data.
Review of regression
Regression with a continuous predictor.
First, we review the interpretation of the coefficients of a regression model with a single continuous predictor.
In general, a regression coefficient for a predictor will be interpreted as the expected change in the dependent variable for a one-unit change in the predictor.
Below we model weight predicted by age:
\[{weight}_i = b_0 + b_{age}age_i + \epsilon_i\]
We use the lm() function to run a linear regression model.
Interpretation of coefficients:
- \(\hat{b}_0=19.86\) , the intercept is the expected outcome when all of the predictors equal zero. Thus, this is the expected weight for a mouse at week 0.
- \(\hat{b}_{age}=0.53\) , the slope of age. Mice are expected to gain .53 grams of weight per week.
Review of dummy variables
In regression models, categorical variables are typically represented by one or more dummy or indicator variables:
- 0 means not belonging to the category
- 1 means belonging to the category
A variable with \(k\) categories can be represented by \(k-1\) dummy variables.
For example, we can represent sex (2 categories) with a single dummy variable that equals 0 for males and equals 1 for females.
sex | female |
---|---|
male | 0 |
female | 1 |
female | 1 |
male | 0 |
For a 3-level categorical variable, we will need two dummies:
diet | fat | starch |
---|---|---|
fat | 1 | 0 |
starch | 0 | 1 |
starch | 0 | 1 |
control | 0 | 0 |
fat | 1 | 0 |
We do not need a third dummy variable for control, because being fat=0 and starch=0 implies membership to the control group.
The omitted category is often referred to as the reference group in regression models.
Factors in R
Factors can be used to represent categorical variables in R.
Essentially, factors are integer variables with labels for each integer value.
We use the factor() function to convert a character variable (or integer variable) to a factor .
To see the distinct categories of the factor in the order of their integer representation, use levels() on the factor variable.
The factor() function will use alphabetical ordering to order the categories by default.
If we want to specify the order, we use the levels= argument in factor() :
Although we will rarely need to use them, we can see the underlying integer values by converting the factor to a numeric vector:
If we are starting with an integer variable that we wish to convert to a factor , we use labels= in factor() to apply the text labels which will then become the levels themselves:
Important: In regression models, R will automatically create dummy variables for each level of factor except for the first, which will thus be the reference group.
Let’s make sex and diet into factor s.
Categorical variables in regression models
Categorical variables with \(k\) categories are represented in regression models by \(k-1\) dummy variables. We cannot add all \(k\) dummy variables to the model due to perfect collinearity.
The dummy variable omitted from the model represents the reference group, and the coefficients will represent differences from this reference group.
Let’s interpret the coefficients for a model where diet is predicting weight:
\[weight_i = b_0 + b_{fat}(diet_i=fat) + b_{sta}(diet_i=starch) + \epsilon_i\]
Coefficients:
- \(\hat{b}_0=22.51\) the expected weight for a mouse on the control diet (the reference group, i.e. when dummies fat=0 and starch=0 )
- \(\hat{b}_{fat}=4.01\) , mice on the high-fat diet are expected to weigh 4.01 grams more than mice on the control diet
- \(\hat{b}_{sta}=3.45\) , mice on the high-starch diet are expected to weigh 3.45 grams more than mice on the control diet
Main effects model with continuous and categorical
Let’s run a model where weight is the dependent variable, and diet, sex, and age are independent variables.
A model without interactions is sometimes called a main effects model, in which the variables’ effects do not depend on other variables.
Reference groups for our categorical variables (first level of factor):
- males for sex
- control for diet
\[weight_i = b_0 + b_{fem}(sex_i=female) + b_{fat}(diet_i=fat) + b_{sta}(diet_i=starch) + b_{age}age_i + \epsilon_i\]
Interpretation of regression coefficients:
- \(\hat{b}_0=18.18\) , the predicted weight for a male mouse on the control diet at age=0.
- \(\hat{b}_{fem}=-1.60\) , females are expected to weigh 1.6 grams less than males, holding diet and age constant
- \(\hat{b}_{fat}=4.00\) , mice on the high-fat diet are expected to weigh 4 grams more than mice on the control diet, holding sex and age constant
- \(\hat{b}_{sta}=3.45\) , mice on the high-starch diet are expected to weigh 3.45 grams more than mice on the control diet, holding sex and age constant
- \(\hat{b}_{age}=0.53\) , mice are expected to gain 0.53 grams per week, holding sex and diet constant
Load the auction data set from our website:
These data describe how much participants were willing to bid on items in a blind auction (all bidders make a single bid simultaneously), depending on characteristics of the item and how long the auction would last:
- bid: the amount of the bid in dollars
- hours: the number of hours before the auction ends
- quality: the quality of the item, as judged by the participant based on pictures and a description of the item; ranges from 0 (lowest) to 5 (highest)
- rarity: how hard to find the item is, one of “common”, “rare”, “unique”
- budget: the participants budget, categorized as “small” or “large”
- Currently, rarity and budget are character variables. Convert them both to factors, with the ordering of the levels for rarity and budget as (common, rare, unique) and (small, large), respectively.
- Run a main effects linear regression modeling bid (the outcome) predicted by hours and rarity.
- Interpret the coefficients
The emmeans package
Overview of the emmeans package.
The emmeans package can:
- compute estimated marginal means (EMMs) , which are predicted outcomes at specific values of predictors, possibly averaged over values of other predictors
- or calculate slopes (linear trends) for continuous variables
- generate graphs of model estimates and predictions, including interactions
The procedure for estimating effects (and contrasts) will differ depending on whether the variable whose effects we seek is categorical or continuous.
For effects of a categorical variable:
- use emmeans() to estimate EMMs across values of predictors (possibly involved in an interaction) and store these in an object of class emmGrid
- use contrast() on the emmGrid object (created by emmeans() ) to estimate effects and differences between effects
- use emmip() on the emmGrid object to graph the interaction
For effects (slopes) of a continuous variable we skip the emmeans() step and instead:
- use emtrends() on the object created by the regression function (e.g. lm object) to estimate slopes of the continuous variable
- use emmip() on the regression object to graph the interaction
One of the strengths of the emmeans package is that it supports regression models from many packages .
The emmeans() function
The emmeans() function calculates EMMs. The required arguments are:
- a regression model object (e.g. lm object) is the first argument
- for a factor variable, EMMs are calculated at each level of the factor
- for a numeric (continuous) variable, EMMs are calculated at the mean of the variable by default (we can specify values in at= )
- model variables not specified in specs= are averaged over
- when analyzing an interaction, we will generally specify the the variables involved in the interaction here
For example, below we use emmeans() to calculate EMMs across levels of diet based on our main effects model.
\[\begin{align}\widehat{weight}_{emm.con} &= b_0 + b_{fat}(0) + b_{sta}(0) + b_{fem}(0.5) + b_{age}(9) \\ &= 18.18 -1.6(0.5) + 0.53(9) \\ &= 22.2 \end{align}\]
\[\begin{align}\widehat{weight}_{emm.fat} &= b_0 + b_{fat}(1) + b_{sta}(0) + b_{fem}(0.5) + b_{age}(9) \\ &= 18.18 + 4(1) -1.6(0.5) + 0.53(9) \\ &= 26.2 \end{align}\]
We can add sex to specs= to get EMMs at each crossing of diet and sex (and at the mean age):
Once we have created an emmeans object ( class=emmGrid ), we next specify it in contrast() to estimate differences between the EMMs or in emmip() to graph the interaction.
A quick note about averaging over categorical covariates
Variables not specified in specs= are averaged over. For categorical variables, the default is to give equal weight to each category. This typically makes sense for experimental data where there is counterbalancing of categorical covariates.
In observational data, we instead often want the EMMs to reflect the proportional representation of the categories in the population. For example, races are not equally represented in the US population, and if we want our EMMs to reflect the proportional representation of race in the US, we can specify weights=proportional in emmeans :
Note: The method of averaging over covariates will typically only affect the estimation of EMMs themselves and should not affect estimation of simple effects and interaction contrasts (because those covariate effects are differenced out).
Pairwise comparisons among factor levels with emmeans::contrast()
Although we get estimates of differences between the high fat diet and control as well as high starch diet and control, we do not get a direct estimate of the difference between high fat and high starch.
We can easily obtain all pairwise comparisons among levels of factor by running contrast() on the emmGrid object.
Important arguments for contrast() :
- the first argument to contrast() is the emmGrid object produced by emmeans()
- the default is method="eff" , which requests contrasts of all levels with the average over all levels
- simple="diet" requests contrasts among all levels of diet
Multiple comparison adjustments for emmeans
In the output of contrast() estimating the effects of diet, a note at the bottom of the output indicates that p-values have been adjusted using Tukey’s method.
The emmeans package will by default apply adjustments that vary by the method= used.
An adjust= option can be added to many functions from the emmeans package to specify which method of adjustment to use.
For example, we can request no p-value adjustment with adjust="none"
See ?summary.emmGrid for a list of the many adjustments that are possible.
A discussion of multiple hypothesis adjustments is beyond the scope of this workshop, and henceforth we will not re-specify the adjustment.
Estimating slopes with emmeans::emtrends()
To estimate slopes of continuous variables, we skip the emmeans() step and use emtrends() , specifying:
- the first argument must be a fitted model object, not an emmGrid object
- e.g. if a factor variable is specified, slopes will be calculated at each level of the factor
- var= , the variable whose slopes we want to estimate
Below we request estimates of the slope of age for each diet group:
The slope estimates are the same because this is a main effects model, where the effects of variables are constrained to be the same across levels of other variables.
Graphing model predictions with emmeans::emmip()
The emmip() function is designed to create i nteraction p lots, but can be used to plot predictions from models without interactions.
Graphs of model predictions across values of the predictors are a common way to visualize predictor effects.
Important arguments for emmip() (see ?emmip for many more):
- the emmGrid object if we wish to visualize the effects of a categorical variable
- the regression model object if we wish to visualize the effects of a continuous variable
- Note: if an object created by emmeans() is used as the first argument (i.e. an emmGrid object), then only those variables specified in specs= in emmeans() can be specified in this formula
- CIs= , requests confidence intervals and is FALSE by default.
- xlab=, ylab=, tlab= , labels for the x-axis, y-axis, and moderator variable
Generally, the variable whose effect we wish to visualize should be mapped to the x-axis.
For example, let’s visualize the effects of diet for each sex (we will need to use the emm_me2 in which both sex and diet were specified in specs= ):
The graph above is actually a plot of the EMMs estimated for each combination of diet and sex in the object emm_me2 , and it allows us to visualize the differences between the expected weights for each diet and sex.
Graphing slopes with emmeans::emmip()
We can also visualize slopes with emmip() , using the following procedure:
- i.e., skip the emmeans() step
- specify the formula with the break variable on the left side of ~ and the slope variable on the right side
- use at= to specify the range of values to plot for the slope variable inside of list()
Here we visualize the slopes of age for each diet group:
… or each number between 2 and 16 in increments of 0.2:
Customizing emmip() plots with ggplot2
Conveniently, we can use ggplot2 functions and syntax to customize the plot:
If you’d like to build the plot from scratch using the emmeans estimates, you can save the data used to build the emmip() plot by specifying plotit=FALSE and saving the result to an object:
Then we can use ggplot2 functions to plot the data ourselves. Here we change the CI style from geom_pointrange to geom_ribbon :
Using the main effects model of bid regressed on hours and rarity (from the auction data) in Exercise 1:
- Estimate the slopes of hours for each rarity.
- Plot the slopes of hours for each rarity (from 2 to 9 hours).
Interactions and moderation models
Why model interactions.
An interaction allows the effect of a variable to depend on the value of another variable.
Main effects models assume the effect of a variable to be the same across values of another variable, whereas interactions relax this assumption.
If we enter the interaction of variable \(X\) and variable \(Z\) into a regression model, we can answer the following questions:
- The effects of \(X\) at specific levels of \(Z\) are often called simple effects or conditional effects
- How different are the effects of \(X\) at different values of \(Z\) ?
The same questions with the roles of \(X\) and \(Z\) reversed can also be answered.
The dependency of a variable’s effect on another variable is often called moderation or effect modification .
Product terms
An interaction between variables \(X\) and \(Z\) can be modeled with a product term (variable), \(XZ=X \times Z\) .
Although we can create these variables ourselves and add them to the regression model, R provides a convenient syntax for interactions in regression models that does not require the product term to be in the data set.
We don’t usually need to create these variables ourselves because R provides a convenient syntax for regression model formulas to model interactions using only the component variables:
- X:Z enters the product term \(XZ\) into the model
- X*Z enters both of the lower order terms, \(X\) and \(Z\) , as well as the product term \(XZ\) into the model
If either X or Z (or both) are factors, R will multiple each dummy variable representing that variable by the other variable.
The following two model specifications are equivalent.
Generally, we should include the lower order terms \(X\) and \(Z\) in a model with their interaction \(XZ\) , because omitting them constrains certain simple effects to zero, which can be result in a poorly fitting model.
General interpretation of coefficients in interaction models
For a model that includes \(X\) , \(Z\) , and their interaction \(XZ\) predicting an outcome \(Y\) :
\[Y_i = b_0 + b_XX_i + b_ZZ_i + b_{X \times Z}XZ_i + \epsilon_i\] the general interpretation of the coefficients will be:
- \(b_0\) , the intercept is again the expected outcome \(Y_i\) when all predictors equal zero, or \(X=0\) , \(Z=0\) , and \(XZ=0\)
- commonly misinterpreted as the main effect of \(X\)
- \(b_Z\) , the simple effect of \(Z\) when \(X=0\)
- interactions allow the effect of \(X\) to vary with \(Z\) , and the effect of \(Z\) to vary with \(X\)
The coefficient for the interaction is a difference between two simple effects. For example, \(b_{X \times Z}\) equals the difference in the simple effect of \(X\) when \(Z=1\) and the simple effect of \(X\) when \(Z=0\) .
- coefficients for lower order terms are interpreted as simple effects when the moderator is zero
- interaction coefficients will have 2 interpretations, reflecting that each variable in the interaction moderates the effect of the other
Interaction of two continuous variables
Regression model with an interaction of two continuous variables.
Let’s interpret the coefficients of a regression model that includes:
- the interaction of age and litter
\[weight_i = b_0 + b_{age}age_i + b_{lit}litter_i + b_{a \times l}(age_i)(litter_i) + b_{sex}(sex_i=female) + \epsilon_i\]
We include sex in the model to discuss how to handle other covariates when visualizing an interaction.
Interpretation:
- \(\hat{b}_0=20.52\) : a mouse at 0 weeks from a litter of 0 pups is expected to weight 20.52 grams
- \(\hat{b}_{age}=0.67\) : a mouse from a litter of 0 pups is expected to gain 0.67 grams per week
- \(\hat{b}_{lit}=0.024\) : at 0 weeks, mice are expected to weight 0.024 grams more per additional pup in the litter
- or, for each additional week, the expected change in weight for each additional pup (the litter effect) decreases by 0.024
As we can see above,
- the lower order terms are interpreted as simple effects of a variable when the interacting variable=0, (e.g. \(\hat{b}_{age}\) is the simple effect (slope) of the age when litter=0)
- interaction terms are interpreted as a difference between two simple effects, ( \(\hat{b}_{a \times l}\) is the difference between the simple effects of age for two litter values that differ by 1 (e.g. litter=1 vs litter=0))
With these coefficients, we can estimate the simple slope (effect) of age at different litter sizes (litter is the moderator here).
Simple effects not directly estimated by the regression will be calculated by adding the lower order term to the interaction term multiplied by the value of the moderator.
\[age.effect_{litter} = \hat{b}_{age} + \hat{b}_{a \times l}litter\] For example, the \(age\) effect when \(litter=0\) is:
\[\begin{align} age.effect_{0} &= \hat{b}_{age} + \hat{b}_{a \times l}0 \\ &= 0.67 - 0.024(0) \\ &= 0.67 \\ \end{align}\]
The \(age\) effect when \(litter=2\) is:
\[\begin{align} age.effect_{2} &= \hat{b}_{age} + \hat{b}_{a \times l}2 \\ &= 0.67 - 0.024(2) \\ &= 0.63 \end{align}\]
The \(age\) effect when \(litter=10\) is:
\[\begin{align} age.effect_{10} &= \hat{b}_{age} + \hat{b}_{a \times l}10 \\ &= 0.67 - 0.024(10) \\ &= 0.43 \end{align}\]
The age effect, the increase in weight per week, decreases as litter size increases. Litter appears to moderate the effect of age.
We can similarly estimate the effect of litter at various ages.
\[litter.effect_{age} = \hat{b}_{lit} + \hat{b}_{a \times l}age\] The \(litter\) effect when \(age=8\) is:
\[\begin{align} litter.effect_{8} &= \hat{b}_{lit} + \hat{b}_{a \times l}8 \\ &= 0.024 - 0.024(8) \\ &= -0.17 \end{align}\]
At 8 weeks, for each additional pup in the birth litter, a mouse is expected to weigh 0.17 grams less.
Using emmeans::emtrends() to estimate simple slopes
Because we wish to estimate the simple effects (slopes) of a continuous variable, we will use emtrends() .
Below, we will estimate the simple slopes of age at different values of litter. For this problem, litter is the moderator . When the moderator is continuous, we will have to choose values at which we wish to estimate simple effects/slopes of the other variable. Common choices are:
- substantively meaningful values, such as ages 18 and 21 for US citizens, or clinical cutoffs (e.g. for blood pressure and hypertension)
- minimum and maximum observed values
- mean, mean+sd, mean-sd
Let’s estimate the effects of age at litter sizes 2, 6, and 10 (min, mean, and max).
Here, we will use the following arguments:
- the first argument is the regression model object
- specs= , the name of the moderator, "litter"
- var= , the name of the variable whose simple effects we wish to estimate, "age"
- at= , a list() containing the values of the moderator (litter) at which to estimate the simple effects
We can see the decreasing slope of age as litter increases.
If you need p-values for the age slopes, put the result of emtrends() inside of test() :
Visualizing the interaction of two continuous variables with emmeans::emmip()
Now we will graph the slopes of age at specific values of litter using emmip() :
- to graph slopes, we use the regression model object as the first argument
- litter is the break variable, and age is the x-variable, so the formula is litter ~ age
- we specify values for both litter and age inside of a list() as an argument for at=
We see that mice from larger litters grow slower over time.
Using the auction dataset:
- Run a linear regression modeling bid predicted by hours, quality, and their interaction.
- Estimate the simple slopes of hours at each quality from 0 to 5.
- Graph the simple slopes of hours at each quality from 0 to 5.
Interaction of two categorical variables
Modeling the interaction of two categorical variables.
Now we will model the interaction of 2 categorical variables in a model that includes:
- interaction of diet and sex
The diet variable is represented by \(2\) dummy variables, while the sex variable is represented by \(1\) dummy variable, so the interaction will be represented by \(2 \times 1 = 2\) variables.
The regression model:
\[\begin{align}weight_i = &b_0 + b_{fat}(diet_i=fat) + b_{sta}(diet_i=starch) + b_{fem}(sex_i=female) + \\ &b_{f \times f}(diet_i=fat)(sex_i=female) + b_{s \times f}(diet_i=starch)(sex_i=female) + b_{age}age_i + \epsilon_i \end{align}\]
- \(\hat{b}_0 = 17.96\) : the intercept is the expected weight when all predictors equal 0, so a male on the control diet at week 0 is expected to weight 17.96 grams, \(\widehat{weight}_{con,mal,age=0}=17.96\)
- \(\hat{b}_{fat}=4.01\) : males on the high fat diet are expected to weigh 4.01 grams more than males on the control diet (i.e. the effect of the high fat diet when sex=0), holding age constant, \(\widehat{weight}_{fat,mal,age} - \widehat{weight}_{con,mal,age} = 4.01\)
- \(\hat{b}_{starch}=4.09\) : males on the high starch diet are expected to weigh 4.09 grams more than males on the control diet, holding age constant, \(\widehat{weight}_{sta,mal,age} - \widehat{weight}_{con,mal,age} = 4.09\)
- \(\hat{b}_{fem}=-1.17\) : females on the control diet are expected to weigh 1.17 grams less than males on the control diet (i.e. the sex effect when fat=0 and starch=0), holding age constant, \(\widehat{weight}_{con,fem,age} - \widehat{weight}_{con,mal,age} = -1.17\)
- \(\hat{b}_{age}=0.53\) : mice are expected to gain 0.53 grams per week, holding diet and sex constant, \(\widehat{weight}_{diet,sex,age} - \widehat{weight}_{diet,sex,age-1} = 0.53\)
- or, the difference between high fat females and high fat males is .004 grams less than the difference between control females and control males, holding age constant, \((\widehat{weight}_{fat,fem,age} - \widehat{weight}_{fat,mal,age}) - (\widehat{weight}_{con,fem,age} - \widehat{weight}_{con,mal,age})=-0.004\)
- or, the difference between high starch females and high starch males is 1.29 grams less than the difference between control females and control males, holding age constant
Questions not directly answered by the coefficients:
- how different are males on the high fat and high starch diets?
- what are the simple effects of diet for females?
- is (high fat - high starch) difference different between males and females?
These questions can be answered by functions in the emmeans pacakge (or by re-parameterizing the model).
Simple effects analysis with emmeans::contrast()
Two of the regression coefficients from the model with diet and sex interacted could be interpreted as the simple effects of diet for males.
We can estimate all of these simple effects easily with contrast() from emmeans .
The first step is creating an emmGrid object from the emmeans() function, and we should specify both variables involved in the interaction in specs= .
Then we specify the emmGrid object as the first argument to contrast , as well as:
- method="pairwise" for all pairwise comparisons of diet
- simple="diet" requests the simple effects of diet (for each sex)
Above we see the estimated simple effects of diet for each sex.
Although the control - fat contrast looks similar between the sexes, the other two contrasts do not:
- the interaction coefficient \(\hat{b}_{f \times f}=-0.004\) is the difference between the two control - fat contrasts ( \(-4.0117 - -4.0073 = -0.004\) )
- the interaction coefficient \(\hat{b}_{s \times f}=-1.29\) is the difference between the two control - strach contrasts
- the difference between the two fat - starch contrasts is not directly estimated in the model
For confidence intervals, we can put the contrast() inside of confint() :
If we are instead interested in simple sex effects for each diet, we simply specify simple="sex" instead:
Interaction contrasts using contrast()
One of the interaction contrasts (difference between simple effects) is not directly estimated in the regression model: the difference between ( fat - starch ) for males and ( fat - starch ) for females (or, equivalently, the difference between ( male - female ) for the fat diet vs ( male - female ) for the starch diet.)
We can easily get all interaction contrasts with contrast() , by specifying interaction=TRUE instead of simple= (but keeping method="pairwise" ):
The final contrast with estimate \(-1.28517\) is the one interaction not directly estimated by the regression model we ran.
Visualizing interactions of two categorical variables with emmeans::emmip()
To visualize the simple effects of diet across levels of sex (the moderator here), we specify in emmip() :
- the emmGrid object as the first argument
- sex is the break variable (moderators) and diet is the x.variable, so the formula is sex ~ diet
If we instead wish to visualize the simple effects of sex across diet, we reverse the formula:
Using the auction data set
- Run a linear regression modeling bid predicted by rarity, budget, and their interaction.
- Estimate the simple effects of rarity across budgets.
- Graph the simple effects of rarity across budgets.
Interaction of a categorical and continuous variable
Regression model with an interaction of a categorical and continuous variable.
We will discuss how to interpret and visualize the interaction of a categorical and continuous variable by modeling weight regressed on:
- the interaction of diet and age
\[\begin{align}weight_i = &b_0 + b_{fat}(diet_i=fat) + b_{sta}(diet_i=starch) + b_{age}age_i + \\ &b_{f \times a}(diet_i=fat)(age_i) + b_{s \times a}(diet_i=starch)(age_i) + b_{lit}litter_i\end{align}\]
- \(\hat{b}_0=20.66\) : mice on the control diet at 0 weeks from litters with 0 pups are expected to weigh 20.66 grams
- \(\hat{b}_{fat}=0.23\) : high-fat mice are expected to weight .23 grams more than control mice at 0 weeks (age=0), holding litter constant
- \(\hat{b}_{fat}=0.80\) : high-starch mice are expected to weight .23 grams more than control mice at 0 weeks, holding litter constant
- \(\hat{b}_{age}=0.29\) : mice on the control diet (the reference group, when fat=0 and starch=0) are expected to gain 0.29 grams per week (i.e. the simple slope of age), holding litter constant
- \(\hat{b}_{lit}=-0.19\) : mice are expected to weigh .19 grams less per additional pup in the litter, holding diet and age constant
- or, the difference between high-fat and control mice increases by .42 grams/week
- or, the difference between high-starch and control mice increases by .29 grams/week
Some quantities not directly estimated by this regression model:
- age slope for high-fat and high-starch mice
- difference between those 2 age slopes
Simple slopes analysis using emmeans::emtrends()
To estimate simple slopes, we again skip the step of estimating EMMs with emmeans() and use emtrends() directly, specifying:
- specs= , the moderator(s)
- var= , the variable whose simple slopes we want to estimate
We can see that mice on the control diet grow considerably slower than those on the other two diets.
For p-values, place the emtrends() within test() :
Simple effects analysis using emmeans::contrast()
We may also be interested in the simple effects of diets at specific ages.
We return to our procedure for estimating simple effects by first using emmeans() to obtain the EMMs.
- specify both interacting variables, diet and age, in specs=
- specify a list of ages (inside of list() ) at which to estimate the diet effects using at=
Then, to estimate the simple effects of diet, we use contrast() just as before, with method="pairwise" and simple="diet" :
Visualizing a categorical by continuous interaction using emmeans:emmip()
To graph the simple slopes of age by diet, we again skip the emmeans() step and proceed immediately to using emmip() .
- the first argument is the lm model object
- for the formula, we want age on the x-axis and separate lines/colors by diet, so we specify diet ~ age
- we must specify a list of ages to use with the at= argument, so we first specify the integers between 2 and 16
If instead we wish to plot simple effects of diet at specific ages we can use either the emmGrid object we created before (which has the ages specified)…
…or use the lm model object and specify the ages as above.
Using the auction dataset
- Run a linear regression modeling bid predicted by hours, rarity, and their interaction.
- Analyze the interaction both through estimation and graphing.
Interactions in generalized linear models
Considerations for interpreting interactions in generalized linear models.
The methods we have used thus far will continue to work for generalized linear models (GLMs), such as logistic and Poisson regression models.
GLMs use a link function to transform an outcome variable with limited range (e.g between 0 and 1) typically to a variable that can take on any value, to allow modeling linear relationships with the predictors.
- in logistic regression, the logit link function transforms probabilities (range \([0,1]\) ) to log-odds (range \((-\infty, \infty)\) )
- in Poisson regression, the log link function transforms counts (or rates) (range \([0,\infty]\) ) to log-counts (range \((-\infty, \infty)\) )
The predictors will have a linear relationship with the transformed outcome (e.g. the log-odds of the outcome), but a non-linear (i.e. curved) relationship with the untransformed outcome.
For example, here we show in a logistic regression that age has a linear relationship with the log-odds of hypertension.
…but a curved relationship with the probability of hypertension:
If we extend the range of age to beyond the observed data, we see the typical S-shape relationship between continuous predictors and probability of the outcome in logistic regression:
The advantage of plotting the predictor against the transformed outcome is that the non-parallelism associated with an interaction will be more obvious. On the other hand, most people will have a harder time interpreting on the transformed scale (i.e. how to interpret changes in log odds).
Additionally, effect estimates from GLMs are often expressed on two scales. In logistic regression:
- raw coefficients are intepreted as differences in log-odds of the outcome
- exponentiated coefficients are interpreted as odds ratios (multiplicative effects)
Thus, we can express simple effects in multiple ways.
We will use a logistic regression model to discuss analysis and visualization of interactions in GLMs.
Logistic regression model
Here we model the probability of hypertension (0/1) in a logistic regression that includes as predictors:
\[\begin{align}log\left(\frac{Pr(hyper_i=1)}{(1-Pr(hyper_i=1))}\right) = &b_0 + b_{fat}(diet_i=fat) + b_{sta}(diet_i=starch) + b_{age}age_i + \\ &b_{f \times a}(diet_i=fat)(age_i) + b_{s \times a}(diet_i=starch)(age_i) + b_{lit}litter_i\end{align}\]
- \(\hat{b}_0=-2.86\) , the expected log-odds of hypertension for control mouse at week 0 from a litter of 0 pups is -2.86
- \(\hat{b}_{fat}=-0.42\) , at week 0, high-fat mice are expected to have a decrease of 0.42 log odds of hypertension compared to control mice, holding litter constant
- \(\hat{b}_{sta}=-0.54\) , at week 0, high-starch mice are expected to have a decrease of -0.54 log odds of hypertension compared to control mice, holding litter constant
- \(\hat{b}_{age}=-0.033\) , control mice are expected have a decrease of -0.033 log odds of hypertension per week, holding litter constant
- \(\hat{b}_{lit}=-0.057\) , for each additional pup in the litter, the log odds of hypertension are expected to decrease by -0.057, holding diet and age constant
- or, the difference in log-odds between high-fat and control mice increases by 0.24 per week
- or, the difference in log-odds between high-starch and control mice increases by 0.18 per week
Odds Ratios
Coefficients in logistic regression are often exponentiated so that they be interpreted as odds ratios, except:
- the exponentiated intercept, which is interpreted as the expected odds when all predictors are zero
- exponentiated interaction coefficients, which are interpreted as ratios of odds ratios (see below)
- \(exp(\hat{b}_0)=0.057\) , the expected odds of hypertension for control mouse at week 0 from a litter of 0 pups is 0.057
- \(exp(\hat{b}_{fat})=0.66\) , at week 0, high-fat mice are expected to have a 34% decrease in the odds of hypertension compared to control mice, holding litter constant
- \(exp(\hat{b}_{sta})=0.58\) , at week 0, high-starch mice are expected to have a 42% decrease in the odds of hypertension compared to control mice, holding litter constant
- \(exp(\hat{b}_{age})=0.97\) , control mice are expected have a 3% decrease in the odds of hypertension per week, holding litter constant
- \(exp(\hat{b}_{lit})=0.94\) , for each additional pup in the litter, the odds of hypertension are expected to decrease 6%, holding diet and age constant
- or, the odds ratio comparing high-fat and control mice increases by 27% per week
- or, the odds ratio comparing high-starch and control mice increases by 19% per week
Exponentiated logistic regression interaction coefficients have a challenging interpretation – they are ratios of odds ratios. For example, if we compare the odds ratio representing the age effect (i.e. how much the odds of hypertension change when age increases by 1 week) between high fat and control mice, that ratio is \(exp(\hat{b}_{f \times a})=1.27\) . In other words, the increasing risk of hypertension with age is 26% larger for high fat mice than control mice.
Estimating logistic regression simple effects with contrast()
The procedure to estimate simple effects across levels of a moderator are the same as before.
For simple effects of a categorical variable, we begin by estimating EMMs with emmeans() . Here we estimate the simple effects of diet at ages 2, 9, and 16.
By default, these will be expressed in log-odds (logits).
If we instead want the EMMs expressed as probabilities, we can add type="response" :
Then, to estimate the simple effects we use contrast() . With the emmGrid object where EMMs are expressed in log-odds, the simple effects will be interpreted as differences in log-odds.
If we instead use contrast() on the emmGrid object where the EMMs are expressed as probabilities, the simple effects will be expressed as odds ratios:
Estimating logistic regression simple slopes with emtrends()
To estimate the simple slopes of age across diets, we use emtrends() , which by default will be expressed as changes in log-odds per week:
No option in emtrends() will transform these simple slopes to odds ratios, but we can do it manually:
Forest-style plots of odds ratios
Odds ratios are commonly displayed in plots that resemble Forest plots (often used for meta-analyses), where the odds ratio estimate is displayed with its confidence interval.
Using plot() on a contrast() object will produce a Forest-style plot of the simple effects.
Below we use the emmGrid object where the contrasts are expressed as odds ratios to plot odds ratios.
A reference line at x=1 is often added to signify “no effect”:
Interaction plots on the log-odds scale
Plotting the interaction on the log-odds scale will make the interaction more apparent (assuming it’s large enough), but log-odds units are hard to interpret.
Here we use emmip() to plot simple effects of diet at weeks 2, 9, and 16. We use the emmGrid object where EMMs are estimated in log odds.
We can also plot the simple slopes of age by diet using emmip() :
Interaction plots on the probability scale
Plotting the interaction on the log-odds scale will generally make the interaction less apparent because it is difficult to judge the parallelism of curves, but probability units are much more natural to interpret for most researchers.
To change the outcome to probabilities, we simply add type="response" to emmip() specifications we used before:
Do it yourself (DIY) analysis and visualization of interactions
Overview of diy analysis of interactions.
This section looks at methods for analyzing interactions with base R coding and visualizing interactions with the ggplot2 package.
One advantage of learning to analyze interactions without emmeans is that these methods will work for regression models and packages not supported by emmeans .
On the other hand, doing it yourself requires that you have a solid grasp of how to interpret regression coefficients.
DIY Estimating simple effects and slopes in R
As we have discussed throughout this workshop, the coefficient for the lower order term of \(X\) when it is interacted with \(Z\) represents effect of \(X\) when \(Z=0\) . We change the interpretation of this coefficient by changing the meaning of \(Z=0\) :
- for a continuous variable \(Z\) , we will recenter it to change the meaning of 0
- for a categorical variable \(Z\) , we will change the omitted, reference group
Re-centering a continuous moderator
Imagine we have a variable \(Z^* = Z - 5\) . If we model:
\[Y_i = b_0 + b_XX + b_{Z^*}Z^* + b_{X \times Z^*}XZ^* + \epsilon_i\]
\(b_X\) is interpreted as the effect of \(X\) when \(Z^*=0\) . However, when \(Z^*=0\) , \(Z=5\) , so we can also interpret \(b_X\) as the effect of \(X\) when \(Z=5\) .
Thus, we can estimate a coefficient that represents the effect of \(X\) at any value of \(Z\) by re-centering \(Z\) .
Let’s revisit our model with the interaction of diet and age:
The coefficients for diet above, \(b_{fat}\) and \(b_{sta}\) , are interpreted as the differences from the control diet when age=0.
If we instead want to estimate diet effects at other ages, we can re-center age, such as at ages 9 and 16 weeks.
Although we could add various re-centered versions a variable to a data set and enter them into regression models to estimate these simple effects…
… R provides a syntax shortcut that obviates the need for these variables in the data.
The I() function can be specified in regression formulas to allow operators like + , - , and ^ to be used as arithmetic operators.
Here, we estimate the effect of diet when age is 9 by specifying I(age-9) , which R interprets as “age variable minus 9”
Those lower order coefficients for diet, \(\hat{b}_{fat}=4.01\) and \(\hat{b}_{sta}=3.45\) are now interpreted as differences from the control group when age = 9.
They are the same as the diet simple effects at age=9 we estimated with contrast() from the emmeans package:
Let’s compare the coefficients from the model with uncentered age to the model with age centered at 9:
term | estimate | std.error | p.value | term | estimate | std.error | p.value |
---|---|---|---|---|---|---|---|
(Intercept) | 20.662 | 0.635 | 0.000 | (Intercept) | 23.296 | 0.398 | 0 |
dietfat | 0.234 | 0.785 | 0.766 | dietfat | 4.010 | 0.356 | 0 |
dietstarch | 0.803 | 0.785 | 0.307 | dietstarch | 3.447 | 0.356 | 0 |
age | 0.293 | 0.055 | 0.000 | I(age - 9) | 0.293 | 0.055 | 0 |
litter | -0.191 | 0.051 | 0.000 | litter | -0.191 | 0.051 | 0 |
dietfat:age | 0.420 | 0.078 | 0.000 | dietfat:I(age - 9) | 0.420 | 0.078 | 0 |
dietstarch:age | 0.294 | 0.078 | 0.000 | dietstarch:I(age - 9) | 0.294 | 0.078 | 0 |
Above we can see that only the intercept, \(\hat{b}_0\) , and the two diet simple effects, \(\hat{b}_{fat}\) and \(\hat{b}_{sta}\) have changed after re-centering.
Importantly, replacing a variable with a re-centered version of it will not change the overall fit of the model:
Mini-exercise : Recreate the estimates of the simple effects of diet at age=2 and age=16 shown in the output of contrast() above.
Re-centering a categorical moderator
Let’s revisit our model with the interaction of diet and uncentered age one more time:
The lower order coefficient for age, \(\hat{b}_{age}=0.29\) , is interpreted as the slope of age for the control group (i.e. fat=0 and starch=0).
We might be interested in the slope of age for the high-fat and high-starch groups. We can get those directly estimated by the model if we change the reference groups for diet.
Although we can create a new variable with a changed reference group using factor() , we can instead change reference groups of an existing factor variable temporarily for a regression model using relevel() , which has a ref= argument that specifies the reference group.
Here we change the reference group of diet to the high-fat group:
Several estimates have changed here, because all coefficients that involve diet are now interpreted as differences from the fat group (rather than differences from the control group as in our original model).
The \(\hat{b}_{age}=0.71\) coefficient is now interpreted as the slope of age for the high-fat group.
The final coefficient, \(\hat{b}_{s \times a}=-0.126\) is interpreted as the difference in age slopes between the high-starch mice and high-fat mice.
This estimate matches the slope of age for the high-fat group estimated by the emtrends() function:
The fit of the model with fat as the reference group is the same as the model with control as the reference group:
For confidence intervals for our new simple effects estimates, put the model object inside confint() :
Mini-exercise : Estimate the simple slope of age for the high-starch group by changing the reference group of diet.
DIY Prediction after regression
As we’ve seen, graphs of predictors vs predicted values are a common way to visualize regression effects.
The predict() function can be used on regression model objects to produce predictions for the current data or for new data.
Typically, to visualize an effect using model predictions, we will create a new data set of predictor values where:
- for the variable’s whose effects we wish to visualize, specify the range of values to appear on the x-axis
- for the moderator, specify values at which to evaluate and graph the simple effects
- the values of predictors whose effects we do not wish to visualize are fixed at constant values (e.g means or reference groups)
The function expand.grid() creates data frames where the values of the variables are fully crossed. Below, we create a dataset that crosses each of the 3 diet groups, with two ages, 2 and 16, and the mean of litter (litter=6), which should result in \(3 \times 2 \times 1 = 6\) rows of data.
Confidence intervals in graphs will generally be more accurate if we estimate more predictions, so we create a data set that includes all observed ages from 2 to 16 weeks:
Now, we can use predict() on the model object and add the predictions to the plotdata data set for plotting. Adding interval="confidence" requests confidence intervals as well.
As we see above, with interval="confidence" , predict() produces 3 new variables, fit , lwr , and upr , which are the predicted (mean) value, lower limit of the 95% CI for the predicted mean, and upper limit of the 95% CI, respectively.
We can use cbind() to add all 3 columns to plotdata.
We now have a dataset to plot the interaction.
DIY Graphing of predictions to visualize regression effects
We use the ggplot2 package to visualize our predictions.
Quick explanation of ggplot() syntax:
- the data set used for graphing
- which variables are mapped to aesthetics (visual aspects of the graph), inside of aes()
- e.g. geom_line() for line graphs, geom_point() for scatterplots
- geom functions inherit aesthetic mappings from the ggplot() function
- additional aesthetics specific to the geom can be specified in aes() inside the geom function
Below, we produce a graph of the plotdata data set with:
- age mapped to the x-axis
- fit, the predicted value mapped to the y-axis
- diet mapped to the color of the lines produced by geom_line()
We can add the 95% confidence intervals to our plot by mapping the lwr and upr columns in plotdata to ymin= and ymax= in geom_errorbar() .
Or in geom_pointrange() , which the emmeans package uses for confidence intervals…
…or using geom_ribbon() to produce confidence bands.
- fill= colors the insides of the band by a variable ( color= controls the border of the band).
- alpha= makes the bands transparent. Use a value between 0 and 1 (0 is completely transparent).
To graph the simple effects of diet at different ages, we need to subset plotdata to only those ages at which we wish to plot the diet effects:
Extending to three-way interactions
The methods we have discussed so far extend in a straightforward manner to interactions of 3 variables.
When using emmeans to analyze the interaction, generally we will need to specify one more variable in specs= than for an interaction of two variables.
Here we model a 3-way interaction of diet, sex, and age. Models with 3-way interactions can be difficult to interpret because of the number of coefficients estimated:
We can estimate the simple effects of diet for each sex and at ages 2, 9, and 16 using the same method as before:
To graph a three-way interaction with emmip() , we use a formula involving three variables: break.variable ~ x.variable | panel.variable , such that separate graph panels are created for each level of panel.variable :
As we see above, a three-way interaction implies that the interaction of two variables varies with a third variable. Here, we see that the interaction of sex and diet appears different across the 3 ages.
In the summary() output, the coefficients for dietfat:sexfemale and dietstarch:sexfemale represent the two-way interaction of the fat diet effect (vs control) and sex and the two-way interaction of the starch diet effect (vs control) and sex at age = 0, respectively. The three-way interaction coefficients allow these two-way interactions to vary by age. We can use contrast on the emm_3 object with arguments interaction = "pairwise" and by = "age" to estimate these two-way interactions at different ages.
We can also estimate simple slopes of age in the same way as before with emtrends() :
And graph the slopes with separate panels by the third variable (here sex):
Mini-exercise: try estimating and graphing the diet effects using DIY methods.
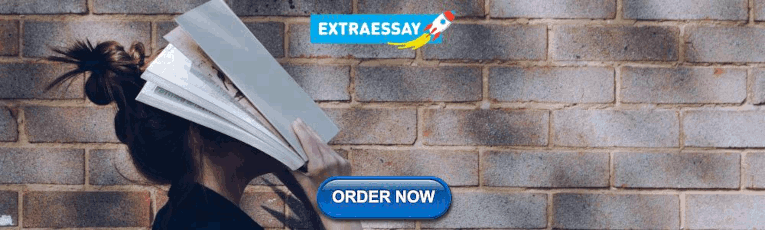
IMAGES
VIDEO
COMMENTS
The null and alternative hypotheses offer competing answers to your research question. When the research question asks "Does the independent variable affect the dependent variable?": The null hypothesis ( H0) answers "No, there's no effect in the population.". The alternative hypothesis ( Ha) answers "Yes, there is an effect in the ...
Null Hypothesis H 0: The correlation in the population is zero: ρ = 0. Alternative Hypothesis H A: The correlation in the population is not zero: ρ ≠ 0. For all these cases, the analysts define the hypotheses before the study. After collecting the data, they perform a hypothesis test to determine whether they can reject the null hypothesis.
Null Hypothesis Examples. "Hyperactivity is unrelated to eating sugar " is an example of a null hypothesis. If the hypothesis is tested and found to be false, using statistics, then a connection between hyperactivity and sugar ingestion may be indicated. A significance test is the most common statistical test used to establish confidence in a ...
Review. In a hypothesis test, sample data is evaluated in order to arrive at a decision about some type of claim.If certain conditions about the sample are satisfied, then the claim can be evaluated for a population. In a hypothesis test, we: Evaluate the null hypothesis, typically denoted with \(H_{0}\).The null is not rejected unless the hypothesis test shows otherwise.
Whenever we perform a hypothesis test, we always write a null hypothesis and an alternative hypothesis, which take the following forms: H0 (Null Hypothesis): Population parameter =, ≤, ≥ some value. HA (Alternative Hypothesis): Population parameter <, >, ≠ some value. Note that the null hypothesis always contains the equal sign.
A null hypothesis is rejected if the measured data is significantly unlikely to have occurred and a null hypothesis is accepted if the observed outcome is consistent with the position held by the null hypothesis. Rejecting the null hypothesis sets the stage for further experimentation to see if a relationship between two variables exists.
The first hypothesis is called the null hypothesis, denoted H 0. The null hypothesis always states that the population parameter is equal to the claimed value. For example, if the claim is that the average time to make a name-brand ready-mix pie is five minutes, the statistical shorthand notation for the null hypothesis in this case would be as ...
Step 1: Figure out the hypothesis from the problem. The hypothesis is usually hidden in a word problem, and is sometimes a statement of what you expect to happen in the experiment. The hypothesis in the above question is "I expect the average recovery period to be greater than 8.2 weeks.". Step 2: Convert the hypothesis to math.
It is the opposite of your research hypothesis. The alternative hypothesis--that is, the research hypothesis--is the idea, phenomenon, observation that you want to prove. If you suspect that girls take longer to get ready for school than boys, then: Alternative: girls time > boys time. Null: girls time <= boys time.
The actual test begins by considering two hypotheses.They are called the null hypothesis and the alternative hypothesis.These hypotheses contain opposing viewpoints. H 0, the —null hypothesis: a statement of no difference between sample means or proportions or no difference between a sample mean or proportion and a population mean or proportion. In other words, the difference equals 0.
A null hypothesis is a type of assumption used in statistics indicating that there is no significant difference between the samples from the underlying population. It is also known as the default hypothesis, represented by H 0. In contrast, there is a term "Alternative Hypothesis", represented by H 1. For every null hypothesis, there is an ...
Present the findings in your results and discussion section. Though the specific details might vary, the procedure you will use when testing a hypothesis will always follow some version of these steps. Table of contents. Step 1: State your null and alternate hypothesis. Step 2: Collect data. Step 3: Perform a statistical test.
One of the most basic concepts in statistics is hypothesis testing and something called The Null Hypothesis. This video breaks these concepts down into easy ...
Answer: H 0: p = 0.75. The null hypothesis is the prior claim that you want to test — in this case, that "75% of voters conclude the bond issue." The null and alternative hypotheses are always stated in terms of a population parameter ( p in this case). You decide to test the published claim that 75% of voters in your town favor a particular ...
Basic definitions. The null hypothesis and the alternative hypothesis are types of conjectures used in statistical tests to make statistical inferences, which are formal methods of reaching conclusions and separating scientific claims from statistical noise.. The statement being tested in a test of statistical significance is called the null hypothesis. . The test of significance is designed ...
We can break the process of null hypothesis testing down into a number of steps: Formulate a hypothesis that embodies our prediction ( before seeing the data) Collect some data relevant to the hypothesis. Specify null and alternative hypotheses. Fit a model to the data that represents the alternative hypothesis and compute a test statistic.
To distinguish it from other hypotheses, the null hypothesis is written as H 0 (which is read as "H-nought," "H-null," or "H-zero"). A significance test is used to determine the likelihood that the results supporting the null hypothesis are not due to chance. A confidence level of 95% or 99% is common. Keep in mind, even if the confidence level is high, there is still a small chance the ...
A hypothesis, in scientific studies, is defined as a proposed explanation for an observed phenomena that can be subject to further testing. A well formulated hypothesis must do two things: be able ...
You can use hypothesis tests to compare a population measure to a specified value, compare measures for two populations, determine whether a population follows a specified probability distribution, and so forth. Hypothesis testing is conducted as a six-step procedure: Null hypothesis. Alternative hypothesis. Level of significance. Test statistic.
A null hypothesis is a precise statement about a population that we try to reject with sample data. We don't usually believe our null hypothesis (or H 0) to be true. However, we need some exact statement as a starting point for statistical significance testing.
Hypothesis testing; T-test definition and formula explanation; Choosing the level of significance; T-distribution and p-value; Conclusion; Hypothesis testing. ... Null hypothesis (H₀) — the hypothesis that we have by default, or the accepted fact. Usually, it means the absence of an effect. By analogy with the trial process, it is ...
The null hypothesis in a correlational study of the relationship between high school grades and college grades would typically be that the population correlation is 0. This can be written as. H0: ρ = 0 (7.3.2) (7.3.2) H 0: ρ = 0. where ρ ρ is the population correlation, which we will cover in chapter 12. Although the null hypothesis is ...
Small Chi-Square Statistic: If the chi-square statistic is small and the p-value is large (usually greater than 0.05), this often indicates that the observed frequencies in the sample are close to what would be expected under the null hypothesis. The null hypothesis usually states no association between the variables being studied or that the ...
A scientific hypothesis is a tentative, testable explanation for a phenomenon in the natural world. It's the initial building block in the scientific method.Many describe it as an "educated guess ...
It is also known as the arithmetic mean, and it is the most common measure of central tendency. It is frequently called the "average." Learn how to find the mean and know when it is and is not a good statistic to use! How to Find the Mean. Finding the mean is very simple. Just add all the values and divide by the number of observations.
The null hypothesis is H 0: p = p 0, where p 0 is a certain claimed value of the population proportion, p. For example, if the claim is that 70% of people carry cellphones, p 0 is 0.70. The alternative hypothesis is one of the following: ... Dummies has always stood for taking on complex concepts and making them easy to understand. Dummies ...
We want to calculate the 95% confidence interval of the mean. However, imagine we have only the following summary information instead of the dataset. Sample mean: 330.6; Standard deviation: 154.2; N = 25; Fortunately, that's all we need to calculate our 95% confidence interval of the mean. We need to decide on using the critical Z or t-value.
mean, mean+sd, mean-sd; Let's estimate the effects of age at litter sizes 2, 6, and 10 (min, mean, and max). Here, we will use the following arguments: the first argument is the regression model object; specs=, the name of the moderator, "litter" var=, the name of the variable whose simple effects we wish to estimate, "age"