12.1 Mendel’s Experiments and the Laws of Probability
Learning objectives.
In this section, you will explore the following questions:
- Why was Mendel’s experimental work so successful?
- How do the sum and product rules of probability predict the outcomes of monohybrid crosses involving dominant and recessive alleles?
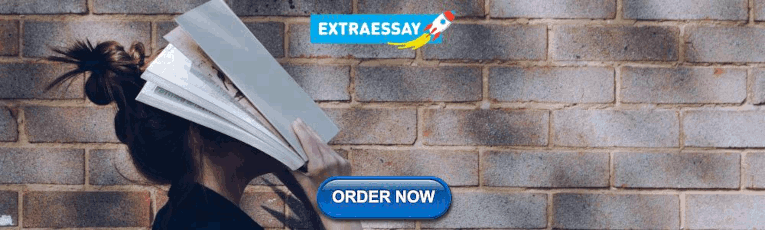
Connection for AP ® Courses
Genetics is the science of heredity. Austrian monk Gregor Mendel set the framework for genetics long before chromosomes or genes had been identified, at a time when meiosis was not well understood. Working with garden peas, Mendel found that crosses between true-breeding parents (P) that differed in one trait (e.g., color: green peas versus yellow peas) produced first generation (F1) offspring that all expressed the trait of one parent (e.g., all green or all yellow). Mendel used the term dominant to refer to the trait that was observed, and recessive to denote that non-expressed trait, or the trait that had “disappeared” in this first generation. When the F1 offspring were crossed with each other, the F2 offspring exhibited both traits in a 3:1 ratio. Other crosses (e.g., height: tall plants versus short plants) generated the same 3:1 ratio (in this example, tall to short) in the F2 offspring. By mathematically examining sample sizes, Mendel showed that genetic crosses behaved according to the laws of probability, and that the traits were inherited as independent events. In other words, Mendel used statistical methods to build his model of inheritance.
As you have likely noticed, the AP Biology course emphasizes the application of mathematics. Two rules of probability can be used to find the expected proportions of different traits in offspring from different crosses. To find the probability of two or more independent events (events where the outcome of one event has no influence on the outcome of the other event) occurring together, apply the product rule and multiply the probabilities of the individual events. To find the probability that one of two or more events occur, apply the sum rule and add their probabilities together.
The content presented in this section supports the learning objectives outlined in Big Idea 3 of the AP ® Biology Curriculum Framework. The AP ® learning objectives merge essential knowledge content with one or more of the seven science practices. These objectives provide a transparent foundation for the AP ® Biology course, along with inquiry-based laboratory experiences, instructional activities, and AP ® exam questions.
Teacher Support
Two rules of probability are used in solving genetics problems: the rule of multiplication and the rule of addition. The probability that independent events will occur simultaneously is the product of their individual probabilities. If two dices are tossed, what is the probability of landing two ones? A die has 6 faces, and assuming the die is not loaded, each face has the same probability of outcome. The probability of obtaining the number 1 is equal to the number on the die divided by the total number of sides: 1 6 1 6 . The probability of rolling two ones is equal to 1 6 × 1 6 = 1 36 1 6 × 1 6 = 1 36 .
The probability that any one of a set of mutually exclusive events will occur is the sum of their individual probabilities. The probability of rolling a 1 or a 2 is equal to 1 6 + 1 6 = 1 3 1 6 + 1 6 = 1 3 because the two outcomes are mutually exclusive. If we roll a 1, it cannot be a 2.
Tell students that Gregor Mendel was a monk who had received a solid scientific education and had excelled at mathematics. He brought this knowledge of science into his experiments with peas.
Engage students in describing what makes a good organism to study genetics. One approach is to ask the class if they would use elephants to study genetics. The disadvantages of using elephants actually highlight the advantages of using peas, corn, fruit flies, or mice for genetics studies: short life cycle, easy to maintain and handle, large number of offspring for statistical analysis, etc.
The concepts of statistics are not intuitive. Practice with dice and coins. Explain that the probability ratios are achieved with large numbers of trials.
Dominant traits are the ones expressed in a dominant/recessive situation. They do not usually repress the recessive trait. A dominant trait is not necessarily the most common trait in a population. For example, type O blood is a recessive trait, but it is the most frequent blood group in many ethnic groups. A dominant trait can be lethal. A dominant allele is not better than the recessive allele. Whether a trait is beneficial depends on the environment. Give the example of wing color in moths. Dark pigmentation is beneficial in a polluted environment where predators would not pick up the moths on dark tree barks. For example, the population peppered moths in 19th century London shifted so that their wing colors were darker to blend in with the soot of the Industrial Revolution. After pollution levels dropped, light pigmentation became more prevalent because it helped the moths to escape notice.
Johann Gregor Mendel (1822–1884) ( Figure 12.2 ) was a lifelong learner, teacher, scientist, and man of faith. As a young adult, he joined the Augustinian Abbey of St. Thomas in Brno in what is now the Czech Republic. Supported by the monastery, he taught physics, botany, and natural science courses at the secondary and university levels. In 1856, he began a decade-long research pursuit involving inheritance patterns in honeybees and plants, ultimately settling on pea plants as his primary model system (a system with convenient characteristics used to study a specific biological phenomenon to be applied to other systems). In 1865, Mendel presented the results of his experiments with nearly 30,000 pea plants to the local Natural History Society. He demonstrated that traits are transmitted faithfully from parents to offspring independently of other traits and in dominant and recessive patterns. In 1866, he published his work, Experiments in Plant Hybridization, 1 in the proceedings of the Natural History Society of Brünn.
Mendel’s work went virtually unnoticed by the scientific community that believed, incorrectly, that the process of inheritance involved a blending of parental traits that produced an intermediate physical appearance in offspring; this hypothetical process appeared to be correct because of what we know now as continuous variation. Continuous variation results from the action of many genes to determine a characteristic like human height. Offspring appear to be a “blend” of their parents’ traits when we look at characteristics that exhibit continuous variation. The blending theory of inheritance asserted that the original parental traits were lost or absorbed by the blending in the offspring, but we now know that this is not the case. Mendel was the first researcher to see it. Instead of continuous characteristics, Mendel worked with traits that were inherited in distinct classes (specifically, violet versus white flowers); this is referred to as discontinuous variation . Mendel’s choice of these kinds of traits allowed him to see experimentally that the traits were not blended in the offspring, nor were they absorbed, but rather that they kept their distinctness and could be passed on. In 1868, Mendel became abbot of the monastery and exchanged his scientific pursuits for his pastoral duties. He was not recognized for his extraordinary scientific contributions during his lifetime. In fact, it was not until 1900 that his work was rediscovered, reproduced, and revitalized by scientists on the brink of discovering the chromosomal basis of heredity.
Mendel’s Model System
Mendel’s seminal work was accomplished using the garden pea, Pisum sativum , to study inheritance. This species naturally self-fertilizes, such that pollen encounters ova within individual flowers. The flower petals remain sealed tightly until after pollination, preventing pollination from other plants. The result is highly inbred, or “true-breeding,” pea plants. These are plants that always produce offspring that look like the parent. By experimenting with true-breeding pea plants, Mendel avoided the appearance of unexpected traits in offspring that might occur if the plants were not true breeding. The garden pea also grows to maturity within one season, meaning that several generations could be evaluated over a relatively short time. Finally, large quantities of garden peas could be cultivated simultaneously, allowing Mendel to conclude that his results did not come about simply by chance.
Mendelian Crosses
Mendel performed hybridizations , which involve mating two true-breeding individuals that have different traits. In the pea, which is naturally self-pollinating, this is done by manually transferring pollen from the anther of a mature pea plant of one variety to the stigma of a separate mature pea plant of the second variety. In plants, pollen carries the male gametes (sperm) to the stigma, a sticky organ that traps pollen and allows the sperm to move down the pistil to the female gametes (ova) below. To prevent the pea plant that was receiving pollen from self-fertilizing and confounding his results, Mendel painstakingly removed all of the anthers from the plant’s flowers before they had a chance to mature.
Plants used in first-generation crosses were called P 0 , or parental generation one, plants ( Figure 12.3 ). Mendel collected the seeds belonging to the P 0 plants that resulted from each cross and grew them the following season. These offspring were called the F 1 , or the first filial ( filial = offspring, daughter or son), generation. Once Mendel examined the characteristics in the F 1 generation of plants, he allowed them to self-fertilize naturally. He then collected and grew the seeds from the F 1 plants to produce the F 2 , or second filial, generation. Mendel’s experiments extended beyond the F 2 generation to the F 3 and F 4 generations, and so on, but it was the ratio of characteristics in the P 0 −F 1 −F 2 generations that were the most intriguing and became the basis for Mendel’s postulates.
Garden Pea Characteristics Revealed the Basics of Heredity
In his 1865 publication, Mendel reported the results of his crosses involving seven different characteristics, each with two contrasting traits. A trait is defined as a variation in the physical appearance of a heritable characteristic. The characteristics included plant height, seed texture, seed color, flower color, pea pod size, pea pod color, and flower position. For the characteristic of flower color, for example, the two contrasting traits were white versus violet. To fully examine each characteristic, Mendel generated large numbers of F 1 and F 2 plants, reporting results from 19,959 F 2 plants alone. His findings were consistent.
What results did Mendel find in his crosses for flower color? First, Mendel confirmed that he had plants that bred true for white or violet flower color. Regardless of how many generations Mendel examined, all self-crossed offspring of parents with white flowers had white flowers, and all self-crossed offspring of parents with violet flowers had violet flowers. In addition, Mendel confirmed that, other than flower color, the pea plants were physically identical.
Once these validations were complete, Mendel applied the pollen from a plant with violet flowers to the stigma of a plant with white flowers. After gathering and sowing the seeds that resulted from this cross, Mendel found that 100 percent of the F 1 hybrid generation had violet flowers. Conventional wisdom at that time would have predicted the hybrid flowers to be pale violet or for hybrid plants to have equal numbers of white and violet flowers. In other words, the contrasting parental traits were expected to blend in the offspring. Instead, Mendel’s results demonstrated that the white flower trait in the F 1 generation had completely disappeared.
Importantly, Mendel did not stop his experimentation there. He allowed the F 1 plants to self-fertilize and found that, of F 2 -generation plants, 705 had violet flowers and 224 had white flowers. This was a ratio of 3.15 violet flowers per one white flower, or approximately 3:1. When Mendel transferred pollen from a plant with violet flowers to the stigma of a plant with white flowers and vice versa, he obtained about the same ratio regardless of which parent, male or female, contributed which trait. This is called a reciprocal cross —a paired cross in which the respective traits of the male and female in one cross become the respective traits of the female and male in the other cross. For the other six characteristics Mendel examined, the F 1 and F 2 generations behaved in the same way as they had for flower color. One of the two traits would disappear completely from the F 1 generation only to reappear in the F 2 generation at a ratio of approximately 3:1 ( Table 12.1 ).
Upon compiling his results for many thousands of plants, Mendel concluded that the characteristics could be divided into expressed and latent traits. He called these, respectively, dominant and recessive traits. Dominant traits are those that are inherited unchanged in a hybridization. Recessive traits become latent, or disappear, in the offspring of a hybridization. The recessive trait does, however, reappear in the progeny of the hybrid offspring. An example of a dominant trait is the violet-flower trait. For this same characteristic (flower color), white-colored flowers are a recessive trait. The fact that the recessive trait reappeared in the F 2 generation meant that the traits remained separate (not blended) in the plants of the F 1 generation. Mendel also proposed that plants possessed two copies of the trait for the flower-color characteristic, and that each parent transmitted one of its two copies to its offspring, where they came together. Moreover, the physical observation of a dominant trait could mean that the genetic composition of the organism included two dominant versions of the characteristic or that it included one dominant and one recessive version. Conversely, the observation of a recessive trait meant that the organism lacked any dominant versions of this characteristic.
So why did Mendel repeatedly obtain 3:1 ratios in his crosses? To understand how Mendel deduced the basic mechanisms of inheritance that lead to such ratios, we must first review the laws of probability.
Science Practice Connection for AP® Courses
Think about it.
Students are performing a cross involving seed color in garden pea plants. Yellow seed color is dominant to green seed color. What F1 offspring would be expected when cross true-breeding plants with green seeds with true-breading plants with yellow seeds? Express the answer(s) as percentage.
This question is an application of Learning Objectives 3.14 and Science Practice 2.2 because students are applying a mathematical routine (probability) to determine a Mendelian pattern of inheritance.
Possible answer:
Probability basics.
Probabilities are mathematical measures of likelihood. The empirical probability of an event is calculated by dividing the number of times the event occurs by the total number of opportunities for the event to occur. It is also possible to calculate theoretical probabilities by dividing the number of times that an event is expected to occur by the number of times that it could occur. Empirical probabilities come from observations, like those of Mendel. Theoretical probabilities come from knowing how the events are produced and assuming that the probabilities of individual outcomes are equal. A probability of one for some event indicates that it is guaranteed to occur, whereas a probability of zero indicates that it is guaranteed not to occur. An example of a genetic event is a round seed produced by a pea plant. In his experiment, Mendel demonstrated that the probability of the event “round seed” occurring was one in the F 1 offspring of true-breeding parents, one of which has round seeds and one of which has wrinkled seeds. When the F 1 plants were subsequently self-crossed, the probability of any given F 2 offspring having round seeds was now three out of four. In other words, in a large population of F 2 offspring chosen at random, 75 percent were expected to have round seeds, whereas 25 percent were expected to have wrinkled seeds. Using large numbers of crosses, Mendel was able to calculate probabilities and use these to predict the outcomes of other crosses.
The Product Rule and Sum Rule
Mendel demonstrated that the pea-plant characteristics he studied were transmitted as discrete units from parent to offspring. As will be discussed, Mendel also determined that different characteristics, like seed color and seed texture, were transmitted independently of one another and could be considered in separate probability analyses. For instance, performing a cross between a plant with green, wrinkled seeds and a plant with yellow, round seeds still produced offspring that had a 3:1 ratio of green:yellow seeds (ignoring seed texture) and a 3:1 ratio of round:wrinkled seeds (ignoring seed color). The characteristics of color and texture did not influence each other.
The product rule of probability can be applied to this phenomenon of the independent transmission of characteristics. The product rule states that the probability of two independent events occurring together can be calculated by multiplying the individual probabilities of each event occurring alone. To demonstrate the product rule, imagine that you are rolling a six-sided die (D) and flipping a penny (P) at the same time. The die may roll any number from 1–6 (D # ), whereas the penny may turn up heads (P H ) or tails (P T ). The outcome of rolling the die has no effect on the outcome of flipping the penny and vice versa. There are 12 possible outcomes of this action ( Table 12.2 ), and each event is expected to occur with equal probability.
Of the 12 possible outcomes, the die has a 2/12 (or 1/6) probability of rolling a two, and the penny has a 6/12 (or 1/2) probability of coming up heads. By the product rule, the probability that you will obtain the combined outcome 2 and heads is: (D 2 ) x (P H ) = (1/6) x (1/2) or 1/12 ( Table 12.3 ). Notice the word “and” in the description of the probability. The “and” is a signal to apply the product rule. For example, consider how the product rule is applied to the dihybrid cross: the probability of having both dominant traits (for example, yellow and round) in the F 2 progeny is the product of the probabilities of having the dominant trait for each characteristic, as shown here:
On the other hand, the sum rule of probability is applied when considering two mutually exclusive outcomes that can come about by more than one pathway. The sum rule states that the probability of the occurrence of one event or the other event, of two mutually exclusive events, is the sum of their individual probabilities. Notice the word “or” in the description of the probability. The “or” indicates that you should apply the sum rule. In this case, let’s imagine you are flipping a penny (P) and a quarter (Q). What is the probability of one coin coming up heads and one coin coming up tails? This outcome can be achieved by two cases: the penny may be heads (P H ) and the quarter may be tails (Q T ), or the quarter may be heads (Q H ) and the penny may be tails (P T ). Either case fulfills the outcome. By the sum rule, we calculate the probability of obtaining one head and one tail as [(P H ) × (Q T )] + [(Q H ) × (P T )] = [(1/2) × (1/2)] + [(1/2) × (1/2)] = 1/2 ( Table 12.3 ). You should also notice that we used the product rule to calculate the probability of P H and Q T , and also the probability of P T and Q H , before we summed them. Again, the sum rule can be applied to show the probability of having at least one dominant trait in the F 2 generation of a dihybrid cross:
To use probability laws in practice, it is necessary to work with large sample sizes because small sample sizes are prone to deviations caused by chance. The large quantities of pea plants that Mendel examined allowed him to calculate the probabilities of the traits appearing in his F 2 generation. As you will learn, this discovery meant that when parental traits were known, the offspring’s traits could be predicted accurately even before fertilization.
- 1 Johann Gregor Mendel, Versuche über Pflanzenhybriden Verhandlungen des naturforschenden Vereines in Brünn, Bd. IV für das Jahr , 1865 Abhandlungen, 3–47. [go here for the English translation here ]
As an Amazon Associate we earn from qualifying purchases.
This book may not be used in the training of large language models or otherwise be ingested into large language models or generative AI offerings without OpenStax's permission.
Want to cite, share, or modify this book? This book uses the Creative Commons Attribution License and you must attribute OpenStax.
Access for free at https://openstax.org/books/biology-ap-courses/pages/1-introduction
- Authors: Julianne Zedalis, John Eggebrecht
- Publisher/website: OpenStax
- Book title: Biology for AP® Courses
- Publication date: Mar 8, 2018
- Location: Houston, Texas
- Book URL: https://openstax.org/books/biology-ap-courses/pages/1-introduction
- Section URL: https://openstax.org/books/biology-ap-courses/pages/12-1-mendels-experiments-and-the-laws-of-probability
© Apr 26, 2024 OpenStax. Textbook content produced by OpenStax is licensed under a Creative Commons Attribution License . The OpenStax name, OpenStax logo, OpenStax book covers, OpenStax CNX name, and OpenStax CNX logo are not subject to the Creative Commons license and may not be reproduced without the prior and express written consent of Rice University.
Statistical Analysis of Genetic Data
Cite this chapter.
- Ton J. Cleophas MD, PhD, Associate-Professor 5 , 6 ,
- Aeilko H. Zwinderman Math D, PhD, Professor 7 &
- Toine F. Cleophas D Techn 8
In 1860, the benchmark experiments of the monk Gregor Mendel led him to propose the existence of genes. The results of Mendel’s pea data were astoundingly close to those predicted by his theory. When we recently looked into Mendel’s pea data and performed a chi-square test, we had to conclude the the chi-square value was too small not to reject the null-hypothesis. this would mean that Mendel’s reported data were so close to what he expected that we could only conclude that he had somewhat fudged the data (Table 1).
This is a preview of subscription content, log in via an institution to check access.
Access this chapter
- Available as PDF
- Read on any device
- Instant download
- Own it forever
Tax calculation will be finalised at checkout
Purchases are for personal use only
Institutional subscriptions
Unable to display preview. Download preview PDF.
Cornelisse CJ, Cornelis RS, Devilee P. Genes responsible for familial breast cancer. Pathol Res Pract 1996; 192: 684–693.
Article Google Scholar
Wijnen JT, Vasen HF, Khan PM, Zwinderman AH, van der Klift H, Mulder A, Tops C, Moller P, Fodde R. Clinical findings with implications for genetic testing in families with clustering of colorectal cancer. N Engl J Med 1998; 339: 511–518.
Jordan B (Ed.). DNA Microarrays: gene expression applications. Berlin: Springer-Verlag, 2001.
Google Scholar
Claverie JM. Computational methods for the identification of differential and coordinated gene expression. Hum Mol Genet 2001; 8: 1821–1832.
McLachlan G. Mixture.model clustering of microarray expression data. Aus Biometrics and New Zealand Stat Association Joint Conference, 2001, Christchurch, New Zealand.
Alizadeh AA, Duckers T, Van heeuwen R. Distinct types of diffuse large B-cell lymphoma identified by gene expression profiling. Nature 2000; 403: 503–511.
Eisen M. Cluster analysis and display of genome-wide expression patterns. Proc Natl Acad Sci USA, 1998; 95: 14863–14867.
Tavazoie S. Systematic determination of genetic network architecture. Nat Genet 1999; 22: 281–285.
Tamayo A. Interpreting patterns of gene-expression with self-organizing maps. Proc Natl Acad Sci USA, 1999; 96: 2907–2912.
Tibshirani R, Taylor J. Clustering methods for the analysis of DNA microarray data. Tech. rep. Stanford University, Dept of Statistics, Stanford.
Download references
Author information
Authors and affiliations.
European Interuniversity College of Pharmaceutical Medicine Lyon, France
Ton J. Cleophas MD, PhD, Associate-Professor ( President American College of Angiology, Co-Chair Module Statistics Applied to Clinical Trials, Internist-clinical pharmacologist )
Department Medicine, Albert Schweitzer Hospital, Dordrecht, The Netherlands
Department Biostatistics and Epidemiology, Academic Medical Center Amsterdam, The Netherlands
Aeilko H. Zwinderman Math D, PhD, Professor ( Co-Chair Module Statistics Applied to Clinical Trials, Professor of Statistics )
Technical University, Delft, The Netherlands
Toine F. Cleophas D Techn
You can also search for this author in PubMed Google Scholar
Rights and permissions
Reprints and permissions
Copyright information
© 2006 Springer Science+Business Media Dordrecht
About this chapter
Cleophas, T.J., Zwinderman, A.H., Cleophas, T.F. (2006). Statistical Analysis of Genetic Data. In: Statistics Applied to Clinical Trials. Springer, Dordrecht. https://doi.org/10.1007/978-1-4020-4650-6_23
Download citation
DOI : https://doi.org/10.1007/978-1-4020-4650-6_23
Publisher Name : Springer, Dordrecht
Print ISBN : 978-1-4020-4229-4
Online ISBN : 978-1-4020-4650-6
eBook Packages : Mathematics and Statistics Mathematics and Statistics (R0)
Share this chapter
Anyone you share the following link with will be able to read this content:
Sorry, a shareable link is not currently available for this article.
Provided by the Springer Nature SharedIt content-sharing initiative
- Publish with us
Policies and ethics
- Find a journal
- Track your research
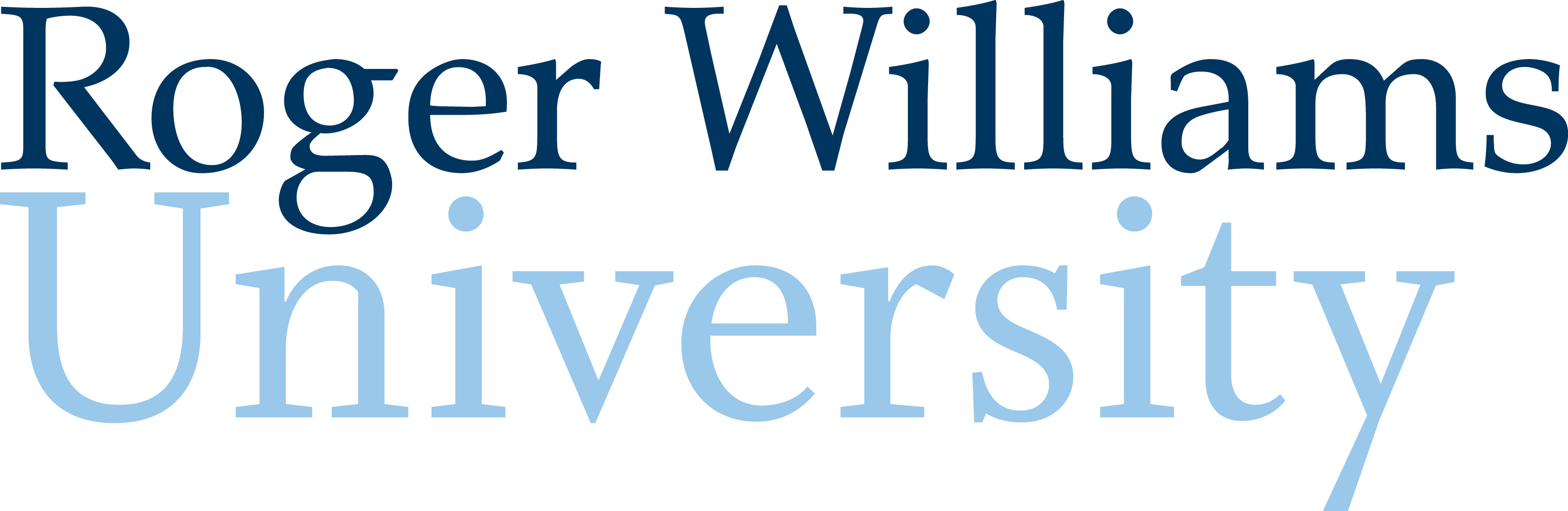
Want to create or adapt books like this? Learn more about how Pressbooks supports open publishing practices.
Chapter 18. Mendelian Genetics
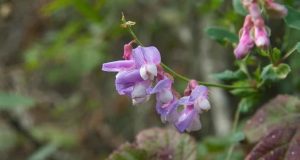
Chapter Outline
- 18.1 Mendel’s Experiments
- 18.2 Mendel’s Principles of Inheritance
- 18.3 Exceptions to Mendel’s Principles of Inheritance
Introduction
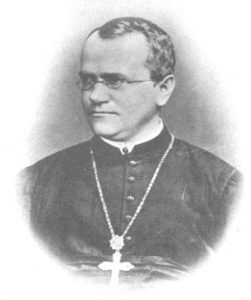
Genetics is the study of heredity. Johann Gregor Mendel (1822–1884) set the framework for genetics long before chromosomes or genes had been identified, at a time when meiosis was not well understood ( Figure 18.2 ). Mendel selected a simple biological system and conducted methodical, quantitative analyses using large sample sizes. Because of Mendel’s work, the fundamental principles of heredity were revealed. We now know that genes, carried on chromosomes, are the basic functional units of heredity with the capability to be replicated, expressed, or mutated. Today, the postulates put forth by Mendel form the basis of classical, or Mendelian, genetics. Not all genes are transmitted from parents to offspring according to Mendelian genetics, but Mendel’s experiments serve as an excellent starting point for thinking about inheritance.
18.1 | Mendel’s Experiments
Learning Objectives
By the end of this section, you will be able to:
- Describe the scientific reasons for the success of Mendel’s experimental work.
- Describe the expected outcomes of monohybrid crosses involving dominant and recessive alleles.
Johann Gregor Mendel (1822–1884) was a lifelong learner, teacher, scientist, and man of faith. As a young adult, he joined the Augustinian Abbey of St. Thomas in Brno in what is now the Czech Republic. Supported by the monastery, he taught physics, botany, and natural science courses at the secondary and university levels. In 1856, he began a decade-long research pursuit involving inheritance patterns in honeybees and plants, ultimately settling on pea plants as his primary model system . In 1865, Mendel presented the results of his experiments with nearly 30,000 pea plants to the local Natural History Society. He demonstrated that traits are transmitted faithfully from parents to offspring independently of other traits and in dominant and recessive patterns. In 1866, he published his work, Exp eriments in Plant Hybridization [1] in the proceedings of the Natural History Society of Brünn.
Mendel’s work went virtually unnoticed by the scientific community that believed, incorrectly, that the process of inheritance involved a blending of parental traits that produced an intermediate physical appearance in offspring; this hypothetical process appeared to be correct because of what we know now as continuous variation. Continuous variation results when many genes work together to determine a characteristic, such as human height or eye color. Offspring appear to be a “blend” of their parents’ traits when we look at characteristics that exhibit continuous variation.
Mendel worked with traits that were inherited in distinct classes, such as violet versus white flowers. These traits display discontinuous variation . Mendel’s choice of these kinds of traits allowed him to see that the traits were not blended in the offspring, nor were they absorbed, but rather that they kept their distinctness and could be passed on. In 1868, Mendel became abbot of the monastery and exchanged his scientific pursuits for his pastoral duties. He was not recognized for his extraordinary scientific contributions during his lifetime. In fact, it was not until 1900 that his work was rediscovered, reproduced, and revitalized by scientists on the brink of discovering the chromosomal basis of heredity.
18.1.1 Mendel’s Model System
Mendel’s seminal work was accomplished using the garden pea, Pisum sativum , to study inheritance. This species naturally self-fertilizes, such that pollen encounters ova within individual flowers. The flower petals remain sealed tightly until after pollination, preventing pollination from other plants. The result is highly inbred, or “true-breeding,” pea plants. These are plants that always produce offspring that look like the parent. By experimenting with true-breeding pea plants, Mendel avoided the appearance of unexpected traits in offspring that might occur if the plants were not true breeding. The garden pea also grows to maturity within one season, meaning that several generations could be evaluated over a relatively short time. Finally, large quantities of garden peas could be cultivated simultaneously, allowing Mendel to conclude that his results did not come about simply by chance.
18.1.2 Mendelian Crosses
Mendel performed hybridizations , which involve mating two true-breeding individuals that have different traits. In the pea, this is done by manually transferring pollen from one pea plant to the stigma of another pea plant. In plants, pollen carries the male gametes (sperm) to the stigma, a sticky organ that traps pollen and allows the sperm to move down the pistil to the female gametes (ova) below. To prevent the pea plant that was receiving pollen from self-fertilizing and confounding his results, Mendel painstakingly removed all of the pollen-producing anthers from the plant’s flowers before they had a chance to mature.
Plants used in first-generation crosses were called P , or parental generation, plants ( Figure 18.3 ). Mendel collected the seeds that resulted from each cross and grew them the following season. These offspring were called the F1 , or the first filial ( filial = offspring, daughter or son), generation. Once Mendel examined the characteristics in the F1 generation of plants, he allowed them to self-fertilize. He then collected and grew the seeds from the F1 plants to produce the F2 , or second filial, generation. Mendel’s experiments extended beyond the F2 generation to the F3 and F4 generations, and so on, but it was the ratio of characteristics in the P−F1−F2 generations that were the most intriguing and became the basis for Mendel’s principles.
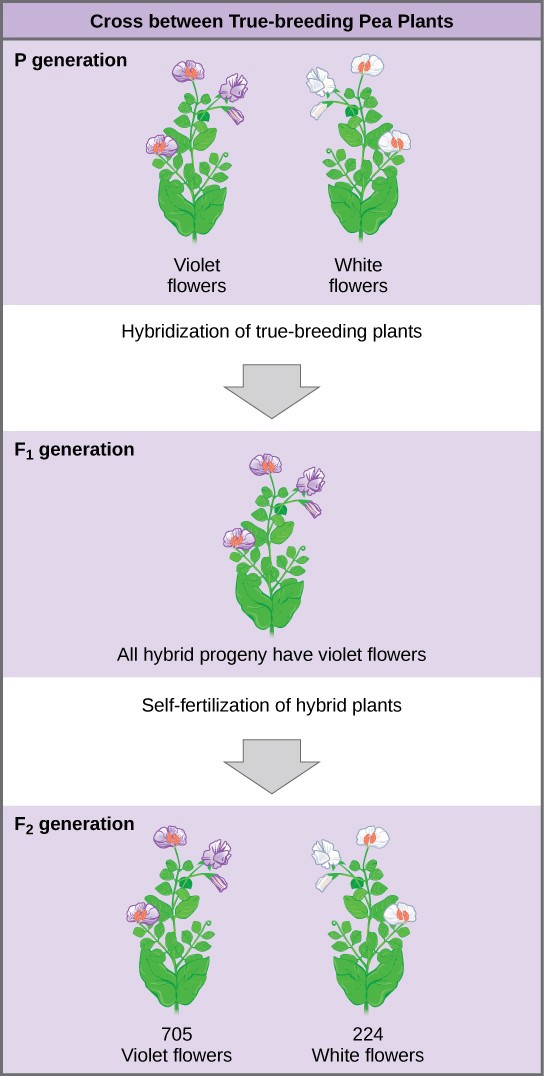
18.1.3 Garden Pea Characteristics Revealed the Basics of Heredity
In his 1865 publication, Mendel reported the results of his crosses involving seven different characteristics, each with two contrasting traits. A trait is defined as a variation in the physical appearance of a heritable characteristic. The characteristics include: tall vs. short plant height, wrinkled vs. round seeds, green vs. yellow seeds, violet vs. white flowers, etc. ( Table 18.1 ). To fully examine each characteristic, Mendel generated large numbers of F1 and F2 plants, reporting results from 19,959 F2 plants alone.
As an example, let us look at Mendel’s results for the flower color trait. First, Mendel confirmed that he had plants that bred true for white or violet flower color. Regardless of how many generations Mendel examined, all self-crossed offspring of parents with white flowers had white flowers, and all self-crossed offspring of parents with violet flowers had violet flowers. In addition, Mendel confirmed that, other than flower color, the pea plants were physically identical.
Once these validations were complete, Mendel applied pollen from a plant with violet flowers to the stigma of a plant with white flowers. After gathering and sowing the seeds that resulted from this cross, Mendel found that 100 percent of the F1 hybrid generation had violet flowers. Conventional wisdom at that time would have predicted the hybrid flowers to be pale violet or for hybrid plants to have equal numbers of white and violet flowers. In other words, the contrasting parental traits were expected to blend in the offspring. Instead, Mendel’s results demonstrated that the white flower trait in the F1 generation had completely disappeared.
Importantly, Mendel did not stop his experimentation there. He allowed the F1 plants to self-fertilize and found that, of F2- generation plants, 705 had violet flowers and 224 had white flowers. This was a ratio of 3.15 violet flowers per one white flower, or approximately 3:1. When Mendel transferred pollen from a plant with violet flowers to the stigma of a plant with white flowers and vice versa, he obtained about the same ratio regardless of which parent, male or female, contributed which trait. This is called a reciprocal cross —a paired cross in which the respective traits of the male and female in one cross become the respective traits of the female and male in the other cross. For the other six characteristics Mendel examined, the F1 and F2 generations behaved in the same way as they had for flower color. One of the two traits would disappear completely from the F1 generation only to reappear in the F2 generation at a ratio of approximately 3:1 ( Table 18.1 ).
Table 18.1 The Results of Mendel’s Garden Pea Hybridizations
18.2 | Mendel’s Principles of Inheritance
- Describe the three principles of inheritance.
- Explain the relationship between phenotype and genotype.
- Develop a Punnett square to calculate the expected proportions of genotypes and phenotypes in a monohybrid cross.
- Explain the purpose and methods of a test cross.
- Draw and interpret a pedigree.
Mendel generalized the results of his pea-plant experiments into three principles that describe the basis of inheritance in diploid organisms. They are: the principle of segregation, the principle of dominance, and the principle of independent assortment. Together, these principles summarize the basics of classical, or Mendelian, genetics.
18.2.1 The Principle of Segregation
Since the white flower trait reappeared in the F2 generation, Mendel saw that the traits remained separate (not blended) in the plants of the F1 generation. This led to the principle of segregation , which states that individuals have two copies of each trait, and that each parent transmits one of its two copies to its offspring.
We now know that the traits that are passed on are a result of genes that are inherited on chromosomes during meiosis and fertilization. The fact that the genetic factors proposed by Mendel were carried on chromosomes was proposed in 1902 by Walter and Sutton and Theodor Boveri ( Figure 18.4 ) as the Chromosomal Theory of Inheritance .
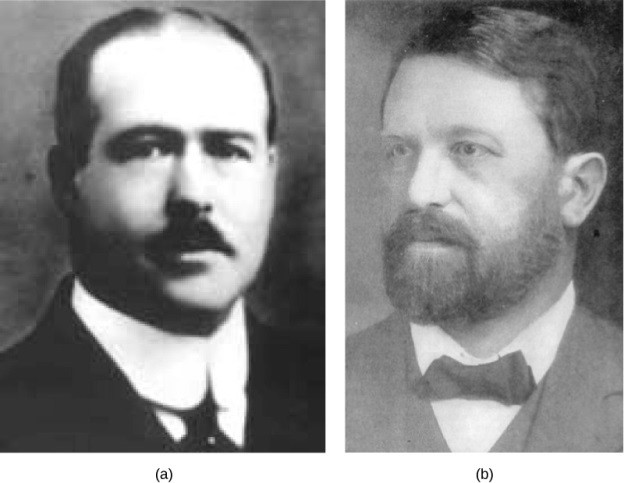
Different versions of genes are called alleles . Diploid organisms that have two identical alleles of a gene on their two homologous chromosomes are homozygous for that trait. Diploid organisms that have two different alleles of a gene on their two homologous chromosomes are heterozygous for that trait.
The physical basis of the principle of segregation is the first division of meiosis, in which the homologous chromosomes with their different versions of each gene are segregated into daughter nuclei. Since each gamete receives only one homolog of each chromosome, it follows that they receive only one allele for each trait. At fertilization, the zygote receives one of each homologous chromosome, and one of each allele, from each parent.
18.2.2 The Principle of Dominance
Upon compiling his results for many thousands of plants, Mendel concluded that the characteristics could be divided into dominant and recessive traits. Dominant traits are those that are expressed in a hybridization. Recessive traits become latent, or disappear, in the offspring of a hybridization but reappear in the progeny of the hybrid offspring. Thus, the violet-flower trait is dominant and the white-flower trait is recessive.
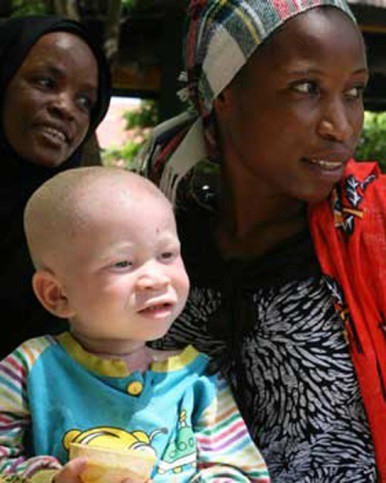
The principle of dominance states that in a heterozygote, only the dominant allele will be expressed. The recessive allele will remain “latent” but will be transmitted to offspring by the same manner in which the dominant allele is transmitted. The recessive trait will only be expressed by offspring that have two copies of this allele ( Figure 18. 5 ). Individuals with a dominant trait could have either two dominant versions of the trait or one dominant and one recessive version of the trait. Individuals with a recessive trait have two recessive alleles.
In Mendel’s experiments, the principle of dominance explains why the F1 heterozygous offspring were identical to one of the parents, rather than expressing both alleles. For a gene that is expressed in a dominant and recessive pattern, homozygous dominant and heterozygous organisms will look identical. The recessive allele will only be observed in homozygous recessive individuals. Some examples of human dominant and recessive traits are shown in Table 18.2 .
Table 18.2 Examples of dominant and recessive traits in humans.
The principles of segregation and dominance could be deduced by simple crosses that follow only one genetic trait. These crosses are called monohybrid crosses . Before we discuss the principle of independent assortment, let’s look at some tools and terminology used for monohybrid crosses.
18.2.3 Phenotypes and Genotypes
Several conventions exist for referring to genes and alleles. For the purposes of this chapter, we will abbreviate genes using the first letter of the gene’s corresponding dominant trait. For example, green is the dominant trait for pea pod color, so the pod-color gene would be abbreviated as G (note that it is customary to italicize gene designations). Furthermore, we will use uppercase and lowercase letters to represent dominant and recessive alleles, respectively. Therefore, we would refer to the genotype of a homozygous dominant pea plant with green pods as GG , a homozygous recessive pea plant with yellow pods as gg , and a heterozygous pea plant with green pods as Gg .
The two alleles for each given gene in a diploid organism may be expressed and interact to produce physical characteristics. The observable traits expressed by an organism are referred to as its phenotype . An organism’s underlying genetic makeup, which alleles it has, is called its genotype . Mendel’s hybridization experiments demonstrate the difference between phenotype and genotype. When true-breeding plants in which one parent had yellow pods and one had green pods were cross-fertilized, all of the F1 hybrid offspring had green pods. Although the hybrid offspring had the same phenotype as the true-breeding parent with green pods, we know that the genotype of the parent was homozygous dominant ( GG ), while the genotype of the F1 offspring was heterozygous ( Gg ). We know this since the yellow pod allele reappeared in some of the F2 offspring ( gg ).
18.2.4 Using Punnett Squares for Monohybrid Crosses
Punnett squares , devised by the British geneticist Reginald Punnett, can be used to predict the possible outcomes of a genetic cross or mating and their expected frequencies. To demonstrate a monohybrid cross, consider the case of true- breeding pea plants with yellow versus green pea seeds. The dominant seed color is yellow; therefore, the parental genotypes were YY for the plants with yellow seeds and yy for the plants with green seeds, respectively. To prepare a Punnett square, all possible combinations of the parental alleles are listed along the top (for one parent) and side (for the other parent) of a grid, representing their meiotic segregation into haploid gametes. Then the combinations of egg and sperm are made in the boxes in the table to show which alleles are combining. Each box then represents the diploid genotype of a zygote, or fertilized egg, that could result from this mating. Because each possibility is equally likely, genotypic ratios can be determined from a Punnett square. If the pattern of inheritance (dominant or recessive) is known, the phenotypic ratios can be inferred as well. For a monohybrid cross of two true-breeding parents, each parent contributes one type of allele. In this case, only one genotype is possible. All offspring are Yy and have yellow seeds ( Figure 18. 6 ).
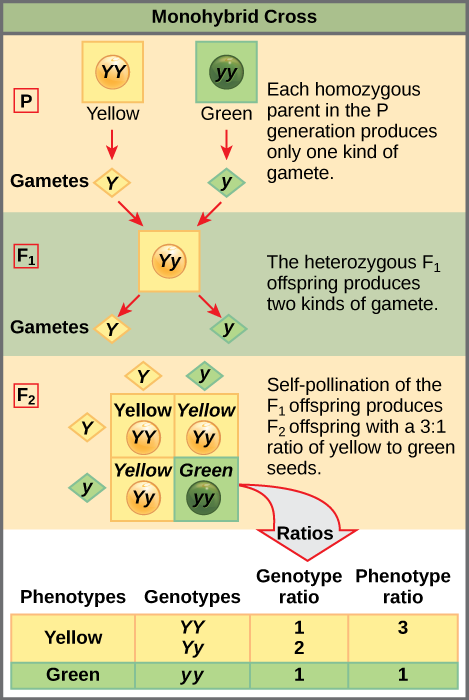
A self-cross of one of the Yy heterozygous offspring can be represented in a 2 × 2 Punnett square because each parent can donate one of two different alleles. Therefore, the offspring can potentially have one of four allele combinations: YY , Yy , yY , or yy ( Figure 18. 6 ). Notice that there are two ways to obtain the Yy genotype: a Y from the egg and a y from the sperm, or a y from the egg and a Y from the sperm. Both of these possibilities must be counted. Recall that Mendel’s pea- plant characteristics behaved in the same way in reciprocal crosses. Therefore, the two possible heterozygous combinations produce offspring that are genotypically and phenotypically identical despite their dominant and recessive alleles deriving from different parents.
Because fertilization is a random event, we expect each combination to be equally likely and for the offspring to exhibit a ratio of YY : Yy : yy genotypes of 1:2:1 ( Figure 18. 6 ). Furthermore, because the YY and Yy offspring have yellow seeds and are phenotypically identical, we expect the offspring to exhibit a phenotypic ratio of 3 yellow:1 green. Indeed, working with large sample sizes, Mendel observed approximately this ratio in every F2 generation resulting from crosses for individual traits.
Using a Test Cross to Determine Genotype
Beyond predicting the offspring of a cross between known homozygous or heterozygous parents, Mendel also developed a way to determine whether an organism that expressed a dominant trait was a heterozygote or a homozygote. Called the test cross , this technique is still used by plant and animal breeders. In a test cross, an organism with the dominant phenotype is crossed with an organism that is homozygous recessive for the same characteristic. If the dominant- expressing organism is a homozygote, then all F1 offspring will be heterozygotes expressing the dominant trait. Alternatively, if the dominant expressing organism is a heterozygote, the F1 offspring will exhibit a 1:1 ratio of heterozygotes and recessive homozygotes ( Figure 18. 7 ). The test cross further validates Mendel’s postulate that pairs of unit factors segregate equally.
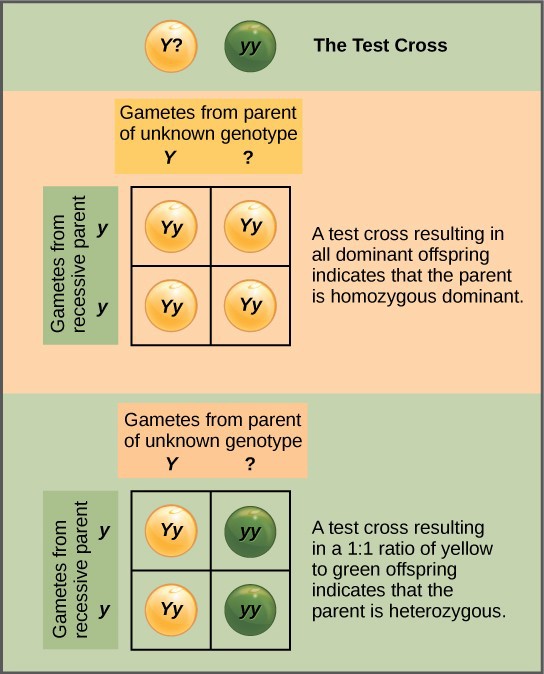
Concept Check
In pea plants, round peas (R) are dominant to wrinkled peas (r). You do a test cross between a pea plant with wrinkled peas (genotype rr) and a plant of unknown genotype that has round peas. You end up with three plants, all which have round peas.
- From this data, can you tell if the round pea parent plant is homozygous dominant or heterozygous?
- If the round pea parent plant is heterozygous, what is the probability that a random sample of 3 progeny peas will all be round?
18.2.5 Using Pedigrees to Study Inheritance Patterns
Many human diseases are inherited genetically. A healthy person in a family in which some members suffer from a recessive genetic disorder may want to know if he or she has the disease-causing gene and what risk exists of passing the disorder on to his or her offspring. Of course, doing a test cross in humans is unethical and impractical. Instead, geneticists use pedigree analysis to study the inheritance pattern of human genetic diseases.
Each row of a pedigree represents one generation of the family. Women are represented by circles; males by squares. People who had children together are connected with a horizontal line and their children are connected to this line with a vertical line. See Figure 18. 8 for an example of a pedigree for a human genetic disease.
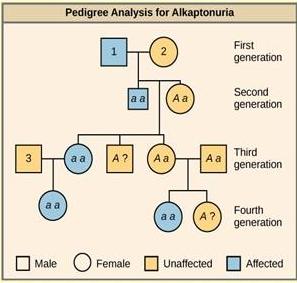
People with the recessive genetic disease alkaptonuria cannot properly metabolize two amino acids, phenylalanine and tyrosine. Affected individuals may have darkened skin and brown urine, and may suffer joint damage and other complications.
In this pedigree, individuals with the disorder are indicated in blue and have the genotype aa . Unaffected individuals are indicated in yellow and have the genotype AA or Aa . Note that it is often possible to determine a person’s genotype from the genotype of their offspring. For example, if neither parent has the disorder but their child does, both parents must be heterozygous. Two individuals on the pedigree have an unaffected phenotype but unknown genotype. Because they do not have the disorder, they must have at least one normal allele, so their genotype gets the “ A? ” designation.
What are the genotypes of the individuals labeled 1, 2, and 3?
18.2.6 Principle of Independent Assortment
Mendel’s principle of independent assortment states that genes do not influence each other with regard to the sorting of alleles into gametes, and every possible combination of alleles for every gene is equally likely to occur. The independent assortment of genes can be illustrated by a dihybrid cross, a cross between two true-breeding parents that express different traits for two characteristics. Consider the characteristics of seed color and seed texture for two pea plants, one that has green, wrinkled seeds ( yyrr ) and another that has yellow, round seeds ( YYRR ). Because each parent is homozygous, the principle of segregation indicates that the gametes for the green/wrinkled plant all are yr , and the gametes for the yellow/round plant are all YR . Therefore, the F1 generation of offspring all are YyRr ( Figure 18.9 ).
For the F2 generation, the principle of segregation requires that each gamete receive either an R allele or an r allele along with either a Y allele or a y allele. The principle of independent assortment states that a gamete into which an r allele sorted would be equally likely to contain either a Y allele or a y allele. Thus, there are four equally likely gametes that can be formed when the YyRr heterozygote is self-crossed, as follows: YR , Yr , yR , and yr . Arranging these gametes along the top and left of a 4 × 4 Punnett square gives us 16 equally likely genotypic combinations. From these genotypes, we infer a phenotypic ratio of 9 round/yellow:3 round/green:3 wrinkled/yellow:1 wrinkled/green ( Figure 18.9 ).
The physical basis for the principle of independent assortment also lies in meiosis I, in which the different homologous pairs line up in random orientations. Each gamete can contain any combination of paternal and maternal chromosomes (and therefore the genes on them) because the orientation of tetrads on the metaphase plane is random.
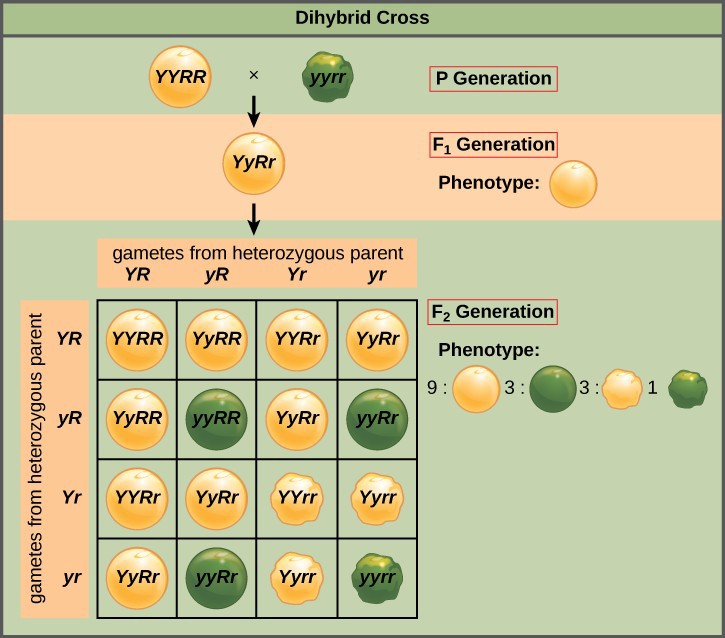
Testing the Hypothesis of Independent Assortment
To better appreciate the amount of labor and ingenuity that went into Mendel’s experiments, proceed through one of Mendel’s dihybrid crosses.
Question : What will be the offspring of a dihybrid cross?
Background : Consider that you have access to a large garden in which you can cultivate thousands of pea plants. There are several true-breeding plants with the following pairs of traits: tall plants with inflated pods, and dwarf plants with constricted pods. Before the plants have matured, you remove the pollen-producing organs from the tall/inflated plants in your crosses to prevent self-fertilization. When the plants mature, they are manually crossed by transferring pollen from the dwarf/constricted plants to the stigmata of the tall/inflated plants.
Hypothesis : Both trait pairs will sort independently according to Mendelian principles. When the true-breeding parents are crossed, all of the F1 offspring are tall and have inflated pods, which indicates that the tall (T ) and inflated (I) traits are dominant over the dwarf (t) and constricted (i) traits, respectively. A self-cross of the F1 heterozygotes results in 2,000 F2 progeny.
Test the hypothesis : You cross the dwarf and tall plants and then self-cross the offspring. For best results, this is repeated with hundreds or even thousands of pea plants. What special precautions should be taken in the crosses and in growing the plants?
If these traits sort independently, the ratios of tall:dwarf and inflated:constricted will each be 3:1. Each member of the F1 generation therefore has a genotype of TtIi . Figure 18.1 0 shows a cross between two TtIi individuals. There are 16 possible offspring genotypes. The offspring proportions: tall/inflated:tall/constricted:dwarf/inflated:dwarf/constricted show a 9:3:3:1 ratio. Notice from the grid that when considering the tall/dwarf and inflated/constricted trait pairs in isolation, they are each inherited in 3:1 ratios.
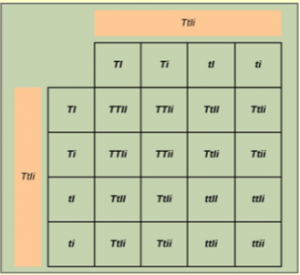
Analyze your data: You observe the following plant phenotypes in the F2 generation: 2706 tall/inflated, 930 tall/constricted, 888 dwarf/inflated, and 300 dwarf/constricted. Reduce these findings to a ratio and determine if they are consistent with Mendelian principles.
Form a conclusion: Were the results close to the expected 9:3:3:1 phenotypic ratio? Do the results support the prediction? What might be observed if far fewer plants were used, given that alleles segregate randomly into gametes? Try to imagine growing that many pea plants, and consider the potential for experimental error. For instance, what would happen if it was extremely windy one day?
18.3 | Exceptions to Mendel’s Principles of Inheritance
- Identify non-Mendelian inheritance patterns such as incomplete dominance, codominance, and sex linkage.
- Describe genetic linkage.
- Describe how chromosome maps are created.
- Explain the phenotypic outcomes of epistatic effects between genes.
Although Mendel’s principles still apply to some situations, many situations exist in which they do not apply. These “exceptions” to Mendelian genetics are discussed below.
18.3.1 Alternatives to Dominance and Recessiveness
Since Mendel’s experiments with pea plants, other researchers have found that the principle of dominance does not always hold true. Instead, several different patterns of inheritance have been found to exist.
Incomplete Dominance
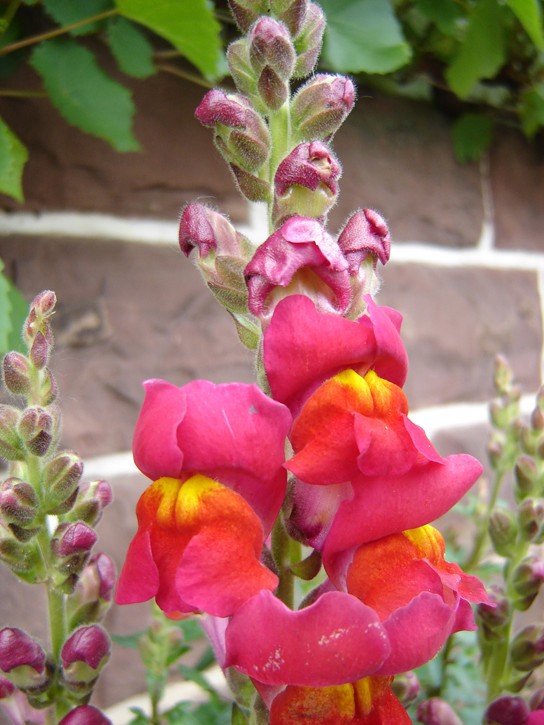
Mendel’s results, that traits are inherited as dominant and recessive pairs, contradicted the view at that time that offspring exhibited a blend of their parents’ traits. However, the heterozygote phenotype occasionally does appear to be intermediate between the two parents. For example, in the snapdragon, Antirrhinum majus ( Figure 18. 11 ), a cross between a homozygous parent with white flowers ( CWCW ) and a homozygous parent with red flowers ( CRCR ) will produce offspring with pink flowers ( CRCW ). (Note that different genotypic abbreviations are used for Mendelian extensions to distinguish these patterns from simple dominance and recessiveness.) This pattern of inheritance is described as incomplete dominance , denoting the expression of two contrasting alleles such that the individual displays an intermediate phenotype. The allele for red flowers is incompletely dominant over the allele for white flowers. However, the results of a heterozygote self-cross can still be predicted, just as with Mendelian dominant and recessive crosses. In this case, the genotypic ratio would be 1 CRCR :2 CRCW :1 CWCW , and the phenotypic ratio would be 1:2:1 for red:pink:white.
Codominance
A variation on incomplete dominance is codominance , in which both alleles for the same characteristic are simultaneously expressed in the heterozygote. An example of codominance is the MN blood groups of humans. The M and N alleles are expressed in the form of an M or N antigen present on the surface of red blood cells. Homozygotes ( LMLM and LNLN ) express either the M or the N allele, and heterozygotes ( LMLN ) express both alleles equally. In a self-cross between heterozygotes expressing a codominant trait, the three possible offspring genotypes are phenotypically distinct. However, the 1:2:1 genotypic ratio characteristic of a Mendelian monohybrid cross still applies.
Multiple Alleles
Mendel implied that only two alleles, one dominant and one recessive, could exist for a given gene. We now know that this is an oversimplification. Although individual humans (and all diploid organisms) can only have two alleles for a given gene, multiple alleles may exist at the population level such that many combinations of two alleles are observed. Note that when many alleles exist for the same gene, the convention is to denote the most common phenotype or genotype among wild animals as the wild type (often abbreviated “+”); this is considered the standard or norm. All other phenotypes or genotypes are considered variants of this standard, meaning that they deviate from the wild type. The variant may be recessive or dominant to the wild-type allele.
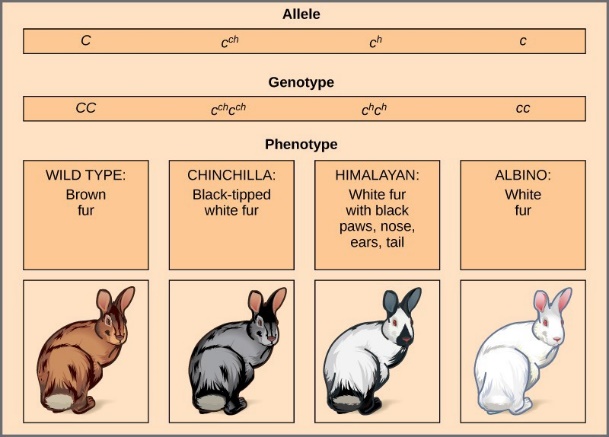
An example of multiple alleles is coat color in rabbits ( Figure 18. 12 ). Here, four alleles exist for the c gene. The wild-type version, C+C+ , is expressed as brown fur. The chinchilla phenotype, cchcch , is expressed as black-tipped white fur. The Himalayan phenotype, chch , has black fur on the extremities and white fur elsewhere. Finally, the albino, or “colorless” phenotype, cc , is expressed as white fur. In cases of multiple alleles, dominance hierarchies can exist. In this case, the wild- type allele is dominant over all the others, chinchilla is incompletely dominant over Himalayan and albino, and Himalayan is dominant over albino. This hierarchy, or allelic series, was revealed by observing the phenotypes of each possible heterozygote offspring.
An example of multiple allelism in humans pertains to ABO blood type. A person’s blood type (e.g., type A or type O) is caused by different combinations of three alleles: IA, IB, and IO. A person with type A blood could have either IAIA or IAIO genotype. A person with type B blood could have IBIB or IBIO genotype. A person with type O blood must have the IOIO genotype. Note that type AB blood is an example of codominance (IAIB).
The complete dominance of a wild-type phenotype over all other mutants often occurs as an effect of “dosage” of a specific gene product, such that the wild-type allele supplies the correct amount of gene product whereas the mutant alleles cannot. For rabbit fur color, the wild-type allele may supply a given dosage of fur pigment, whereas the mutants supply a lesser dosage or none at all.

Multiple Alleles Confer Drug Resistance in the Malaria Parasite
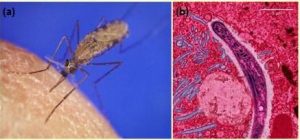
Malaria is a parasitic disease that is transmitted to humans by infected female Anopheles gambiae mosquitos ( Figure 18.13a ). It is characterized by cyclic high fevers, chills, flu-like symptoms, and severe anemia. Plasmodium falciparum is the most deadly causative agent of malaria ( Figure 18.13b ). When promptly and correctly treated, P. falciparum malaria has a mortality rate of 0.1%. However, in some parts of the world, the parasite has evolved resistance to commonly used malaria treatments, so the most effective malarial treatments can vary by geographic region.
In Southeast Asia, Africa, and South America, P. falciparum has developed resistance to the anti-malarial drugs chloroquine, mefloquine, and sulfadoxine-pyrimethamine. P. falciparum , which is haploid during the life stage in which it infects humans, has evolved multiple drug-resistant mutant alleles of the dhps gene. Varying degrees of sulfadoxine resistance are associated with each of these alleles. Being haploid, P. falciparum needs only one drug-resistant allele to express this trait.
Environmental Effects
Interestingly, the Himalayan phenotype in rabbits is the result of an allele that produces a temperature-sensitive gene product that only produces pigment in the cooler extremities of the rabbit’s body. In this case, the protein product of the gene does not fold correctly at high temperatures. A similar gene gives Siamese cats their distinctive coloration.
Temperature-sensitive proteins are also at work in arctic foxes and rabbits, which are white in the winter and darker colored during the summer. In these cases, the protein product of the gene does not fold correctly at colder temperatures. The mutation that caused this coloration was advantageous to these species, so they persisted in the populations.
18.3.2 X-Linked Traits are an Exception to the Principle of Segregation
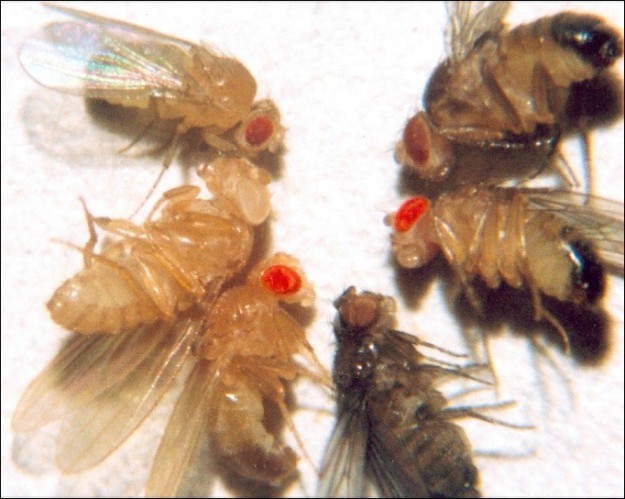
In humans, as well as in many other animals and some plants, the sex of the individual is determined by sex chromosomes. The sex chromosomes are one pair of non-homologous chromosomes. Until now, we have only considered inheritance patterns among non-sex chromosomes, or autosomes. In addition to 22 homologous pairs of autosomes, human females have a homologous pair of X chromosomes, whereas human males have an XY chromosome pair. Although the Y chromosome contains a small region of similarity to the X chromosome so that they can pair during meiosis, the Y chromosome is much shorter and contains many fewer genes. When a gene is present on the X chromosome, it is said to be X-linked .
Eye color in Drosophila was one of the first X-linked traits to be identified. Like humans, Drosophila males are XY and females are XX. In flies, the wild-type eye color is red (X W ) which is dominant to white eye color (X w ) ( Figure 18.1 4) . Females can be X W X W , X W X w or X w X w . However, Drosophila males lack a second allele copy on the Y chromosome, so their genotype can only be X W Y or X w Y. Males are said to be hemizygous , because they have only one allele for any X- linked characteristic. Hemizygosity makes the descriptions of dominance and recessiveness irrelevant for XY males.
In an X-linked cross, the genotypes of F1 and F2 offspring depend on whether the recessive trait was expressed by the male or the female in the P generation. When the P male expresses the white-eye phenotype and the female is homozygous red-eyed, all members of the F1 generation exhibit red eyes ( Figure 18.1 5 ). The F1 females are heterozygous (X W X w ), and the males are all X W Y, since they received their X chromosome from the homozygous dominant P female and their Y chromosome from the P male. A cross between a X W X w female and an X W Y male would produce only red-eyed females and both red- and white-eyed males. A cross between a homozygous white-eyed female and a male with red eyes would produce only heterozygous red-eyed females and only white-eyed males.
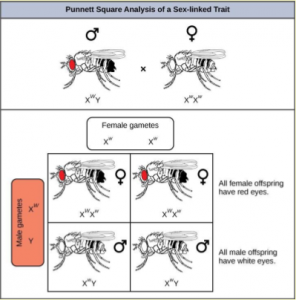
What ratio of offspring would result from a cross between a white-eyed male and a female that is heterozygous for red eye color?
In some groups of organisms with sex chromosomes, the gender with the non-homologous sex chromosomes is the female rather than the male. This is the case for all birds. In this case, sex-linked traits will be more likely to appear in the female, in which they are hemizygous.
Human Sex-linked Disorders
Sex-linkage studies in Morgan’s laboratory provided the fundamentals for understanding X-linked recessive disorders in humans, which included red-green color blindness, Types A and B hemophilia, and muscular dystrophy. Because human males need to inherit only one recessive mutant X allele to be affected, X-linked disorders are disproportionately observed in males. Females must inherit recessive X-linked alleles from both of their parents in order to express the trait. When they inherit one recessive X-linked mutant allele and one dominant X-linked wild-type allele, they are carriers of the trait and are typically unaffected. Carrier females can manifest mild forms of the trait due to the inactivation of the dominant allele located on one of the X chromosomes. However, female carriers can contribute the trait to their sons, resulting in the son exhibiting the trait, or they can contribute the recessive allele to their daughters, resulting in the daughters being carriers of the trait ( Figure 18.1 6 ). Although some Y-linked recessive disorders exist, typically they are associated with infertility in males and are therefore not transmitted to subsequent generations.
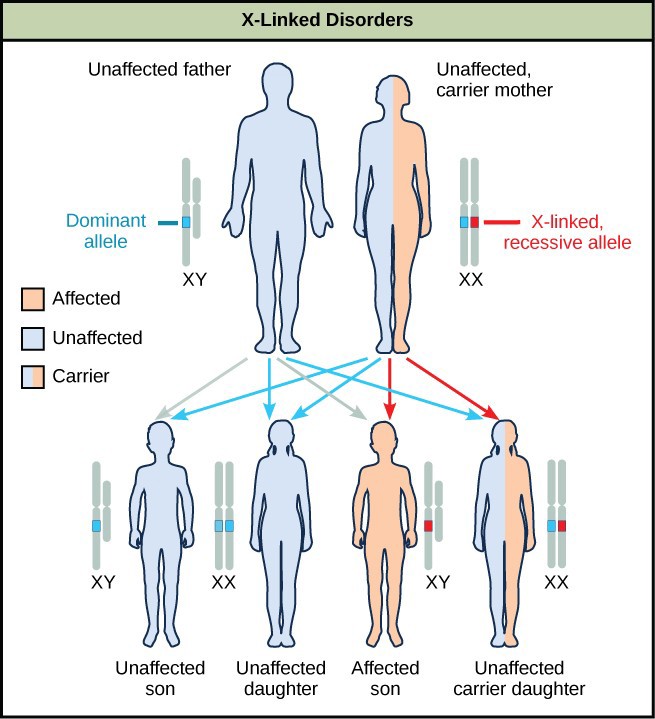
18.3.3 Lethal Alleles are Apparent Exceptions to the Principle of Segregation
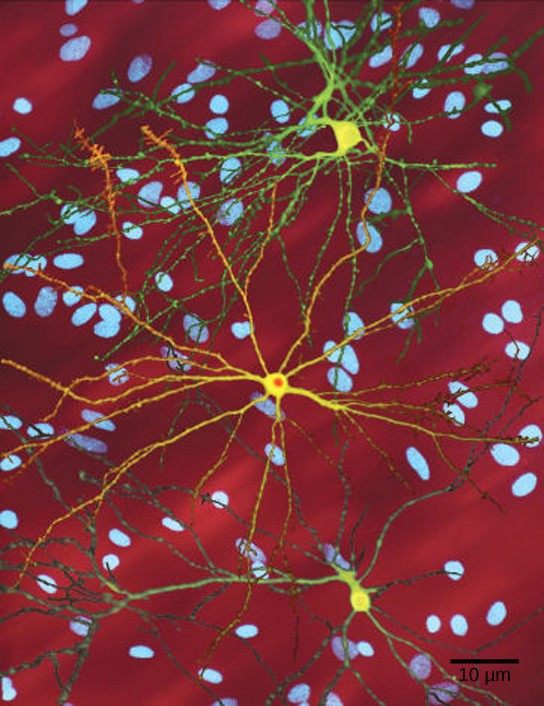
A large proportion of genes in an individual’s genome are essential for survival. Occasionally, a nonfunctional allele for an essential gene can arise by mutation and be transmitted in a population through heterozygous carriers. The wild-type allele functions at a capacity sufficient to sustain life and is therefore considered to be dominant over the nonfunctional allele. If two heterozygous parents mate, one quarter of their offspring will be homozygous recessive. Because the gene is essential, these individuals will die. This will cause the genotypic ratio among surviving offspring to be 2:1 rather than 3:1. This inheritance pattern is referred to as recessive lethal .
The dominant lethal inheritance pattern is one in which an allele is lethal both in the homozygote and the heterozygote. Dominant lethal alleles are very rare because, as you might expect, the allele only lasts one generation and is not transmitted. However, dominant lethal alleles might not be expressed until adulthood. The allele may be unknowingly passed on, resulting in a delayed death in both generations. An example of this in humans is Huntington disease, in which the nervous system gradually wastes away ( Figure 18.1 7 ). People who are heterozygous for the dominant Huntington allele ( Hh ) will inevitably develop the fatal disease. However, the onset of Huntington disease may not occur until age 40, at which point the afflicted persons may have already passed the allele to 50 percent of their offspring.
18.3.4 Linked Genes Violate the Principle of Independent Assortment
Although all of Mendel’s pea characteristics behaved according to the principle of independent assortment, we now know that some allele combinations are not inherited independently of each other. Genes that are located on different chromosomes will always sort independently. However, each chromosome contains hundreds or thousands of genes, organized linearly on chromosomes like beads on a string. Genes that are on the same chromosome are linked and are therefore likely to be inherited together. When homologs separate during meiosis I, entire chromosomes segregate into separate daughter cells, carrying all of their linked genes with them.
However, because of crossover, it is possible for two genes on the same chromosome to behave independently, or as if they are not linked. To understand this, let’s consider the biological basis of gene linkage and recombination.
Homologous chromosomes possess the same genes in the same order. However, since each homolog came from a different parent, the alleles may differ on homologous chromosome pairs. Prior to meiosis I, homologous chromosomes replicate and synapse so that genes on the homologs align with each other. At this stage, segments of homologous chromosomes cross over and exchange segments of genetic material ( Figure 18.1 8 ). Because the genes are aligned, the gene order is not altered. Instead, the result of recombination is that maternal and paternal alleles are combined onto the same chromosome. Across a given chromosome, several recombination events may occur, causing extensive shuffling of alleles.
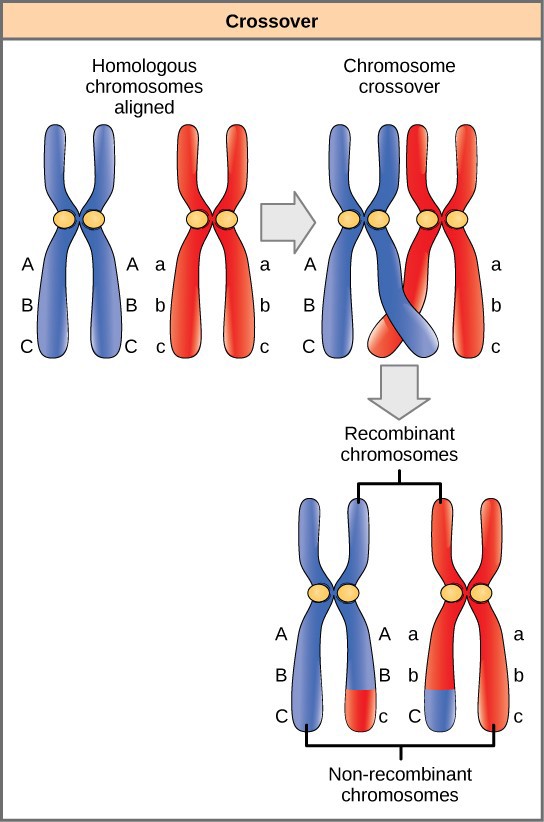
When two genes are located in close proximity on the same chromosome, their alleles are more likely to be transmitted through meiosis together. To exemplify this, imagine a dihybrid cross involving flower color and plant height in which the genes are next to each other on the chromosome. If the homologous chromosome from one parent has alleles for tall plants and red flowers, and the homolog from the other parent has alleles for short plants and yellow flowers, then when the gametes are formed, the tall and red alleles will go together into a gamete and the short and yellow alleles will go into other gametes. These are called the parental genotypes because they have been inherited intact from the parents of the individual producing gametes. Since the genes were close together on the same chromosomes, the chance of a crossover event happening between them is slim. Therefore, there will be no gametes with tall and yellow alleles and no gametes with short and red alleles. If you create the Punnett square with these gametes, you will see that the classical Mendelian prediction of a 9:3:3:1 outcome of a dihybrid cross would not apply
As the distance between two genes increases, the probability of crossovers between them increases, and the genes behave more as if they are on separate chromosomes. The further apart two linked genes are on a chromosome, the more progeny with nonparental genotypes will appear.
Genetic Linkage and Distances
Geneticists have used the proportion of nonparental gametes as a measure of how far apart genes are on a chromosome. Using this information, they have constructed elaborate maps of genes on chromosomes. Briefly, the more crossover that occurs between two linked genes, the further apart they are on the chromosome. The frequency of crossover is measured by counting the number of offspring that have nonparental genotypes. By using recombination frequency to predict genetic distance, the relative order of genes on chromosome 2 could be inferred.
18.3.5 Epistasis is an Exception to the Principle of Independent Assortment
Mendel’s studies in pea plants implied that every characteristic was distinctly and completely controlled by a single gene. In fact, single observable characteristics are almost always under the influence of multiple genes (each with two or more alleles) acting in unison. For example, at least eight genes contribute to eye color in humans.
Genes may function in complementary or synergistic fashions, such that two or more genes need to be expressed simultaneously to affect a phenotype. Genes may also oppose each other. In epistasis , the interaction between genes is antagonistic, such that one gene masks or interferes with the expression of another. Often the biochemical basis of epistasis is a gene pathway in which the expression of one gene is dependent on the function of a gene that precedes or follows it in the pathway.
An example of epistasis is pigmentation in mice. The wild-type coat color, agouti ( AA ), is dominant to solid-colored fur ( aa ). However, a separate gene ( C ) is necessary for pigment production. A mouse with a recessive c allele at this locus is unable to produce pigment and is albino regardless of the allele present at locus A . Therefore, the genotypes AAcc , Aacc , and aacc all produce an albino phenotype. A cross between heterozygotes for both genes ( AaCc x AaCc ) would generate offspring with a phenotypic ratio of 9 agouti:3 solid color:4 albino ( Figure 18.19 ). In this case, the C gene is epistatic to the A gene.
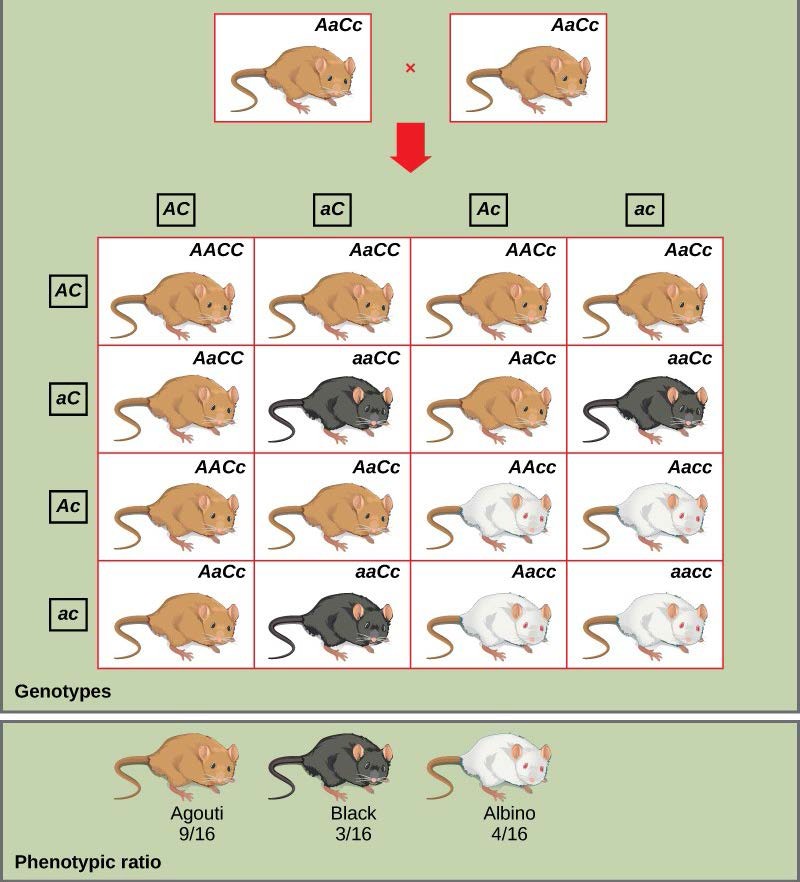
Epistasis can also occur when a dominant allele masks expression at a separate gene. Fruit color in summer squash is expressed in this way. Homozygous recessive expression of the W gene ( ww ) coupled with homozygous dominant or heterozygous expression of the Y gene ( YY or Yy ) generates yellow fruit, and the wwyy genotype produces green fruit. However, if a dominant copy of the W gene is present in the homozygous or heterozygous form, the summer squash will produce white fruit regardless of the Y alleles. A cross between white heterozygotes for both genes ( WwYy × WwYy ) would produce offspring with a phenotypic ratio of 12 white:3 yellow:1 green.
Finally, epistasis can be reciprocal such that either gene, when present in the dominant (or recessive) form, expresses the same phenotype. In the shepherd’s purse plant ( Capsella bursa-pastoris ), the characteristic of seed shape is controlled by two genes in a dominant epistatic relationship. When the genes A and B are both homozygous recessive ( aabb ), the seeds are ovoid. If the dominant allele for either of these genes is present, the result is triangular seeds. That is, every possible genotype other than aabb results in triangular seeds, and a cross between heterozygotes for both genes ( AaBb x AaBb ) would yield offspring with a phenotypic ratio of 15 triangular:1 ovoid.
As you work through genetics problems, keep in mind that any single characteristic that results in a phenotypic ratio that totals 16 is typical of a two-gene interaction. Recall the phenotypic inheritance pattern for Mendel’s dihybrid cross, which considered two non-interacting genes—9:3:3:1. Similarly, we would expect interacting gene pairs to also exhibit ratios expressed as 16 parts. Note that we are assuming the interacting genes are not linked; they are still assorting independently into gametes.
- Johann Gregor Mendel, Versuche über Pflanzenhybriden Verhandlungen des naturforschenden Vereines in Brünn, Bd. IV für das Jahr, 1865 Abhandlungen, 3–47. [for English translation see http://www.mendelweb.org/Mendel.plain.html] ↵
Introduction to Molecular and Cell Biology Copyright © 2020 by Katherine R. Mattaini is licensed under a Creative Commons Attribution-NonCommercial 4.0 International License , except where otherwise noted.
Share This Book

An official website of the United States government
The .gov means it’s official. Federal government websites often end in .gov or .mil. Before sharing sensitive information, make sure you’re on a federal government site.
The site is secure. The https:// ensures that you are connecting to the official website and that any information you provide is encrypted and transmitted securely.
- Publications
- Account settings
Preview improvements coming to the PMC website in October 2024. Learn More or Try it out now .
- Advanced Search
- Journal List
- Comput Struct Biotechnol J
Statistical methods for Mendelian randomization in genome-wide association studies: A review
Frederick j. boehm.
a Department of Biostatistics, University of Michigan, Ann Arbor, MI 48109, USA
b Center for Statistical Genetics, University of Michigan, Ann Arbor, MI 48109, USA
Graphical abstract

Genome-wide association studies have yielded thousands of associations for many common diseases and disease-related complex traits. The identified associations made it possible to identify the causal risk factors underlying diseases and investigate the causal relationships among complex traits through Mendelian randomization. Mendelian randomization is a form of instrumental variable analysis that uses SNP associations from genome-wide association studies as instruments to study and uncover causal relationships between complex traits. By leveraging SNP genotypes as instrumental variables, or proxies, for the exposure complex trait, investigators can tease out causal effects from observational data, provided that necessary assumptions are satisfied. We discuss below the development of Mendelian randomization methods in parallel with the growth of genome-wide association studies. We argue that the recent availability of GWAS summary statistics for diverse complex traits has motivated new Mendelian randomization methods with relaxed causality assumptions and that this area continues to offer opportunities for robust biological discoveries.
1. Introduction
Great interest has developed in inferring causal relationships between complex traits, i.e., traits that seemingly are not inherited in a Mendelian fashion, in observational human genetics studies. Discovery of such relationships is crucial to enhancing our understanding of the biology of health and disease. Inferring causal relationship in genetics is often carried out through Mendelian randomization (MR) analysis. MR analysis is a form of instrumental variable analysis in which genetic markers, typically single nucleotide polymorphisms (SNPs), serve as instruments, or proxies, for inferring causal effects of an exposure variable on an outcome variable [1] , [2] , [3] , [4] , [5] , [6] , [7] .
MR analysis is facilitated by the development of genome-wide association studies (GWAS), which present unique opportunities for discovery of causal relationships via MR [8] . A GWAS interrogates millions of single nucleotide polymorphisms (SNPs) to infer which are associated with the trait [9] , [10] . In the nearly 17 years since publication of early studies, researchers have reported thousands of novel SNP-trait associations from GWAS [10] , [11] , [12] , [13] . Ever larger sample sizes in GWAS have enhanced statistical power to detect associations and have refined our understanding of human health and disease. The association results from many large-scale GWAS are nowadays readily available, often in the form of summary statistics that include the marginal SNP p-values and/or their effect size estimates and standard errors [14] . The identified SNP associations are used as the main input for MR analysis and thus the wide availability of GWAS summary statistics clear the way for effective MR analysis in complex genetics studies.
Effective MR analyses are enabled by many MR methods developed in recent years. Methods for and uses of MR have appeared at a rapid and accelerating pace since the publication of the early GWAS ( Fig. 1 ). The overall trend of the methodology development is in the direction of increasingly sophisticated modeling of horizontal pleiotropy including both independent and correlated horizontal pleiotropy while attempting to maintain scalability and computational efficiency in the presence of multiple correlated SNPs.

Upward trend in article counts by year for Google Scholar keyword searches: 1. Mendelian randomization and 2. Genome-wide association study.
Here, we present a comprehensive review on 47 MR methods, primarily in the context of GWAS summary statistics, to help practitioners to choose which MR methods to use in applied data analysis. We discuss the basics form of MR analysis, the causality assumptions in MR, and how recent MR methods are developed to ensure robust results in the presence of assumption violations. We discuss in detail methods advances before briefly summarizing applications and directions for future research. Different from existing MR reviews [15] , [16] , [17] , we discuss in detail recent methods developments that enable modeling of horizontal pleiotropy and correlated horizontal pleiotropy and place these developments in the larger context of MR analysis with GWAS data. We present these recent methods developments for an audience of both statisticians and epidemiologists. We hope our review will facilitate the further advance of MR methods and their wide application on GWAS data.
2. Assumptions of Mendelian randomization
Mendelian randomization uses genetic markers in the form of SNP genotypes as the proxy (“instrument” or “instrumental variable”) for the “exposure,” a complex trait, and asks whether the “exposure” variable is causal for the “outcome” variable, which is typically a second complex trait. The exposure and outcome variables can be binary, a count, a time to event, or a continuous variable. For brevity and simplicity, we focus on continuous exposures and outcomes before considering other classes of outcome variables in a later section. Most MR studies use the “two-sample” MR design, in which one cohort of subjects has measurements for the exposure, while a second cohort has measurements for the outcome, with both cohorts sharing the same set of SNP instruments [18] . We focus on the two-sample MR design before considering the one-sample design and the partial two-sample design where samples are partially overlapped between the two cohorts.
MR aims to infer the causal effect of the exposure variable on the outcome variable in observational studies. The proper causal interpretation in an MR study requires the SNP instruments to satisfy three causality assumptions. The first assumption states that SNP instruments are associated with the exposure. If the first assumption, about the association between the SNP instruments and the exposure, holds, but this association is weak, then an amplification of biases, such as those due to violations of assumptions 2 and 3, may result [19] . Indeed, bias in causal estimates can increase with decreases in the strength of association between the SNP instrument and exposure [20] . The second assumption requires independence, conditional on the exposure and all measured and unmeasured confounders, between the SNP instruments and the outcome. The second MR assumption, often termed the exclusion restriction assumption, can also be stated as the need for the SNP instruments to affect the outcome only through the exposure. In a causal diagram, this means that the only path from the SNP instruments to the outcome is that containing the exposure. The last of the three assumptions states that the SNP instruments are independent of all (measured and unmeasured) confounders of the relationship between exposure and outcome. The third assumption ensures that the SNP instruments are independent of all confounders of the exposure-outcome relationship.
When the three MR assumptions hold, one may use instrumental variable statistical methods, with the genetic marker data, the exposure data, and the outcome data, to estimate and to test the causal effect of the exposure on the outcome [7] , [21] , [22] . In practice, careful evaluation of the assumptions is needed. Because assumptions 2 and 3, which involve absence of certain types of confounding, can’t be verified, practitioners must be cautious when planning MR analyses. Sensitivity analysis is highly recommended as a way to assess robustness of estimates in the presence of possible assumption violations [19] . We will discuss these sensitivity analyses in more detail in a later section and consider their evaluation in MR studies [19] .
The causal assumptions of MR also help one to understand the origin of the term “Mendelian randomization.” Recall Mendel’s inheritance laws [23] , under the assumption that alleles segregate randomly from parent to offspring, the offspring genotypes are unlikely to be associated with confounders of the exposure-outcome relationship. Additionally, reverse causation from the outcome or exposure to the genotypes is unanticipated since germ-line genotypes are fixed at conception and, thus, precede realization of other observed variables. Therefore, using SNP instruments in the instrumental variable analysis is often referred to as Mendelian randomization.
3. Statistical models and methods for MR with one instrument
We begin our discussion of Mendelian randomization in GWAS data by considering the simplest case, where there is a single SNP instrument and a single outcome variable. Approaches to MR with one SNP instrument can be classified into four categories: ratio of coefficients method, two-stage methods, likelihood-based methods, and semiparametric methods [15] . We discuss each of these before turning to methods that leverage multiple SNP instruments.
3.1. Ratio of coefficients method
The ratio of coefficients method, also known as the Wald method, estimates the causal effect of the exposure X on the outcome Y by using a single SNP instrument [24] . For a continuous outcome, the causal effect estimator, (1).
In Eq. (1), β ^ YZ and β ^ XZ are the slope estimates from the regressions of the outcome and exposure, respectively, on the SNP instrument. Since the Wald method requires only the regression coefficients, it can be used with summary data. However, the Wald method doesn’t accommodate multiple SNP instruments, which limits its direct use in the GWAS setting as will be discussed later.
3.2. Two-stage methods
A two-stage statistical model involves two regression models [15] , [25] . For a continuous outcome, one may perform “two-stage least squares,” which involves two linear regressions. First, the exposure variable is regressed on the instrument (i.e., the SNP genotypes) (Equation (2)). We denote each subject’s exposure variable value as x i in Equation (2), while α 0 denotes an intercept term and α 1 is the slope. z i denotes the SNP instrument genotype for subject i , while ∊ i , the error term, is assumed to be independent among subjects and normally distributed with a shared common variance and mean zero.
The resulting fitted values for the exposure variable from Eq. (2) are the independent variable in the second linear regression, where the outcome is the dependent variable. The causal effect estimate, then, is the coefficient β ^ 1 obtained from the second regression analysis (Eq. (3)). Note also that the random errors in Eq. (3) are assumed independent of those in Eq. (2). Like the ∊ i in Eq. (2), the τ i are assumed independent and identically distributed normal random errors.
Note that the uncertainty in the fitted values from the first regression is not considered when performing the second regression. For this reason, the variance of the estimator is incorrect in two-stage calculations [15] , [26] . This and other observations led researchers to develop likelihood-based MR methods.
3.3. Likelihood-based methods
Likelihood-based MR methods, unlike two-stage methods, provide maximum likelihood estimates with their many desirable properties [27] . Limited information maximum likelihood from econometrics is the earliest approach for likelihood-based inference in MR [28] , [29] . Limited information maximum likelihood with a single SNP instrument is modeled with two equations (Eqs. (4) and (5)), where the random errors follow a bivariate normal distribution [15] .
Limited information maximum likelihood is sometimes called the maximum likelihood counterpart of two-stage least squares, and it yields the same causal estimate as two-stage least squares and the ratio method when used with a single SNP instrument. Additionally, the limited information maximum likelihood framework can accommodate more than one SNP instrument by replacing α 1 z i 1 with the sum ∑ k = 1 K α k z ik .
One may also use Bayesian methods to obtain likelihood-based estimators [30] . Kleibergen [31] examined a model that is similar to that from the limited information maximum likelihood framework (Eq. (6)). The Bayesian model differs from the limited information maximum likelihood model in that the causal effect parameter, β 1 represents the effect between the true means for the exposure and the outcome. In the limited information maximum likelihood model, the causal effect is that of the measured effect on the measured outcome.
For each subject i , the exposure and outcome values come from a bivariate normal distribution. The mean of the exposure distribution is assumed linear in the SNP instrument, and the mean of the outcome distribution is linear in the mean exposure [15] , [32] . Kleibergen [31] demonstrated that the Bayesian model, with weak instruments, outperforms the limited information maximum likelihood model in terms of frequentist coverage levels.
3.4. Semiparametric methods
Semiparametric instrumental variable methods, which feature parametric and nonparametric components, typically assume a parametric model connecting the exposure and outcome, but don’t impose distributional assumptions on the errors [15] . Compared to fully parametric models, semiparametric models often are more robust to model misspecification [15] , [33] . We follow Burgess [15] by discussing three semiparametric strategies, generalized method of moments, continuous updating estimator, and G-estimation of structural mean models.
Generalized method of moments can be viewed as a more flexible form of two-stage least squares that handles heteroscedastic errors and nonlinearity in the two regressions [15] . Before specifying the model, we introduce notation. E Y ∨ do X = x is the conditional expectation of Y if we forced X to take value x for every subject [34] . The generalized method of moments equations, with a single SNP instrument, then, can be written as in Eq. (7).
The GMM estimate is the value of the vector β that satisfies Eq. (7), where f x i ; β = E Y ∨ do X = x . Pearl [35] developed numerical methods for obtaining estimates from Eq. (7).
Another semiparametric estimation method is G-estimation of a structural mean model [15] , [36] , [37] . We follow Burgess [15] by defining the potential outcome Y x as the outcome value that we would have observed had we set the exposure value X to x . For example, Y 0 denotes the observed outcome had we set the exposure to zero instead of its observed value x . The structural mean model for a continuous outcome is displayed in Eq. (8).
The causal effect parameter is β 1 . Burgess [15] derives the estimating equations for β 1 , after noting that the conditional expectation, E Y 0 | X = x , Z = z is independent of Z and reasoning that the causal effect is that value of β 1 that yields zero covariance between Z and E Y 0 ∨ X = x , Z = z (Eq. (9)).
Note that k indexes SNP instruments and ranges from 1 to K in Eq. (9).
3.5. Mendelian randomization with multiple independent SNP instruments
While using a single SNP instrument for MR analysis can be effective, Greenland [38] recognized that many genetic variants individually explain a small proportion of the variation in a trait, and, thus, sufficient statistical power of MR analysis with a single SNP instrument would require sample sizes in the tens of thousands [39] , [40] . Schatzkin [41] resolved this issue by proposing use of multiple SNP instruments in MR analysis. In particular, Palmer [42] reasoned that a single causal estimate derived from a collection of causal SNPs would have greater precision than an estimate derived from only one SNP [39] . Recognition of complex traits’ diverse genetic architectures has thus fueled development of two-sample MR methods with multiple SNP instruments [42] , [43] , [44] , [45] .
There are two important considerations for MR analysis with multiple SNP instruments. The first consideration is how to choose a subset of the available SNPs to serve as instruments. The solution to this task differs among published methods and majority of MR methods chose a set of independent SNPs as instruments [46] , [47] . The independent SNPs may be selected through linkage disequilibrium (LD) clumping [48] . The second consideration is how to make use of GWAS summary statistics for MR analysis, as sharing of GWAS summary statistics was encouraged and became a common practice [49] . [50] recognized that one could calculate the Wald ratio estimate of the causal effect from GWAS summary statistics, and [39] devised a strategy for determining a single causal effect from summary statistics for a collection of independent SNPs. Below, we discuss a number of MR methods that make use of multiple independent SNP instruments.
4. Statistical models and methods for MR with multiple instruments
4.1. methods with individual-level data.
One approach to integrating multiple SNP instruments into a MR framework is through calculation of allele scores [51] . Harbord [51] calculate an unweighted score as the number of exposure-increasing alleles in the subject’s genotypes. They also calculate a weighted allele score by using the exposure effects as weights. For example, a subject with g k copies of exposure-increasing alleles for SNP k has an unweighted allele score z = ∑ k = 1 K g k and a weighted allele score z = ∑ k = 1 K w k g k . Harbord [51] found that when weights are obtained from external data or from cross-validation or jackknife approaches applied to the analysis data, the allele score functions as a single instrumental variable and greatly diminishes the bias compared to that of the two-stage least squares estimator [15] , [52] .
Angrist [53] developed a LASSO-based method, sisVIVE, to identify invalid SNP instruments [54] . sisVIVE’s advantage over earlier methods is that it doesn’t require the analyst to know which SNP instruments are valid. Instead, it requires that at least 50% of the instruments be valid. sisVIVE outperforms two-stage least squares in many ways and performs similar to oracle two-stage least squares. Simulations and data analysis results reveal that sisVIVE is robust to possibly invalid instruments.
Tibshirani [55] , building on the research from [53] , implemented an adaptive LASSO-based estimator after recognizing that sisVIVE misclassifies valid SNP instruments as invalid when the invalid SNP instruments have strong effects on the exposure. Consistent selection of invalid SNP instruments, they found, depends on SNP instrument correlations. To address this issue, Tibshirani [55] proposed a median estimator with consistency that doesn’t depend on SNP instrument-exposure association strength or the SNP instrument correlation structure. They then applied methods from Windmeijer [56] to achieve a consistent estimator with the same asymptotic distribution as the oracle two-stages least squares. One important limitation of this thread of research is that both Angrist [53] and Tibshirani [55] require individual-level data.
Zou [57] , working with individual-level GWAS data, share naive and smoothed constrained instrumental variable methods. Central to their work is the proposal to create a new instrumental variable as a linear combination of genotype data from a collection of SNP instruments. They require that the new instrumental variable be standardized to have norm 1 and be orthogonal to potentially vertically pleiotropic traits. For a collection of SNPs, they choose the K -vector that meets these criteria and maximizes the correlation with the exposure. To obtain their second estimator, Zou [57] apply a l 0 penalty to constrain the number of SNP instruments that are given nonzero weights in the calculation of the new instrumental variable. They then compare their method with sisVIVE and allele score methods. Zou [57] find that both the smoothed version of their estimator and the allele score method are unbiased in their simulation settings.
Jiang [58] and Spiller [59] present a method, MR-GxE, that exploits gene by environment interactions to detect and to adjust for bias due to horizontal pleiotropy. Spiller [59] treat a SNP-covariate interaction as an instrumental variable, and, by so doing, they impose assumptions on that interaction. Together, these assumptions require that MR-GxE assume that horizontal pleiotropy effects are constant across the study cohort. The authors devise a three-step inferential procedure for two-sample MR with GWAS summary statistics. First, they estimate the SNP-exposure and SNP-outcome associations at a range of covariate values. Second, they regress the SNP-outcome estimated associations on the SNP-exposure estimated associations. Third, they treat the resulting slope as the causal effect estimate and the intercept as the mean horizontal pleiotropy effect.
4.2. Methods with summary data
The proliferation of publicly available GWAS data accelerates the development of MR methods with multiple SNP instruments that make use of GWAS summary statistics for model fitting. Our timeline highlights some of the many multi-instrument MR methods with GWAS summary statistics, with an increasing density of methods over time in the last three years ( Fig. 2 ). These methods include the inverse variance weighted MR [39] , MR-Egger [43] , weighted median estimation [60] , Bayesian weighted MR [61] , robust adjusted profile score [62] , MRMix [63] , CAUSE [64] , and MRAID [65] . These methods differ in their approaches to three considerations that include instrument selection (from among the available SNPs), modeling and controlling for horizontal pleiotropy, and statistical inference procedure. We summarize some of these MR methods that use independent or correlated SNP instruments below. We will cover the remaining MR methods that model horizontal pleiotropy in a later section.

Two-sample MR with GWAS summary statistics methods publications density increases over time.
Smith [39] termed their method “inverse variance weighting” (IVW) because each causal SNP’s effect is weighted by the inverse of the variance of the ratio estimator, and the overall causal effect is the sum of the weighted SNP causal effects. Specifically, Smith [39] combined causal effect ratio estimates from independent SNPs by using Eq. (10).
Spiller [60] , noting that consistency with IVW MR is not robust to invalid instrument use, developed a method for consistent MR analysis with weighted median-based estimators, building on research from [66] . Its breakdown point is 50%, meaning that up to 50% of SNP instruments can be invalid while maintaining consistency in estimation [67] . Specifically, let β ∼ j denote the j th ordered ratio estimate statistic, from least to greatest. If an odd number, say 2 K = 1 , of SNP instruments is used, then the simple median estimator is defined as the K + 1 ordered ratio estimate. For an even number, say 2 K , number of SNP instruments, choose the midpoint between the two middle-ranking ordered ratio estimates, β ∼ K + β ∼ K + 1 2 .
Due to the inefficiency of the simple median estimator, Spiller [60] define the weighted median estimator. To construct this estimator, order the ratio estimates from least to greatest, as with the simple median estimator. The weighted median estimator, then is the median of a distribution defined by having β ∼ j as the p j = 100 percentile, where w j is the weight assigned to the j th ordered ratio estimate. In this setting, the simple median estimator is seen to be the weighted median estimator when all weights are equal.
Spiller [60] also studied the penalized weighted median estimator. They defined the penalized weights, w i = w j ' × min 1 , 20 q j , where q j is the p-value resulting from the comparison of Q j = w j ' β ∼ j - β ^ IVW 2 to a χ 1 2 distribution [60] , [68] . The penalized weighted median estimator leaves most variants unaffected, but downweights those with outlying Wald ratio estimates. While the above MR methods all use independent SNP instruments, using independent instruments may not be ideal when there are multiple causal SNPs in linkage disequilibrium with each other. In this setting, discarding some of the causal SNPs may only capture a small proportion of trait variance explained by the exposure and lead to a power loss in MR [15] , [39] , [69] , [70] . Consequently, many MR methods have been recently developed to model multiple correlated instruments.
Cochran [69] developed methods for using correlated SNPs in IVW MR by inserting a covariance matrix into the standard IVW formulation. However, many MR methods use a set of pre-selected SNPs as instruments. Typically, these SNPs are selected to be statistically independent. This restriction to independent SNPs is needed for valid inference in methods like standard inverse variance weighting MR [39] .
4.3. Assumption violations and sensitivity analysis
It is worthwhile to consider ways in which the standard MR assumptions may be violated and strategies for identifying such violations. Let’s first consider the exclusion restriction assumption (assumption 2 above). It states that the SNP instrument must affect the outcome only through the exposure ( Fig. 3 B). The causal diagram in Fig. 3 A illustrates a scenario that violates this assumption [19] . Bias in causal effect estimation would result. However, if the intermediate is measured and treated as the exposure, then an unbiased estimate of causal effect is possible, since the intermediate captures the two pathways, intermediate -> outcome and intermediate -> exposure -> outcome, through which the SNP instrument affects the outcome Y [19] .

Causal diagrams for MR. A. Scenario where the genetic variant affects an intermediate variable on the pathway to the exposure. Because the intermediate affects the outcome through a pathway that doesn’t involve exposure, this scenario violates the exclusion restriction assumption. B. Scenario where the genetic variant affects an exposure, which in turn affects an outcome, possibly in the presence of unmeasured confounding. C. Correlated horizontal pleiotropy occurs when the genetic variant affects the exposure, which in turn affects the outcome, and the genetic variant affects the unobserved confounder, which in turn affects the exposure and the outcome independently.
Recognition of the many ways that the MR assumptions may be violated has inspired methods advances that enable assumption relaxations and has motivated use of sensitivity analysis to quantify the impact of possible assumption violations. Sensitivity analysis is recommended and widely used in MR studies because of the inability to verify the three MR assumptions with observational data. The goal of a sensitivity analysis is to gain insight into how the results might differ if the assumptions be violated. Because not all confounding variables are known or measured, assumptions 2 and 3 are not fully verifiable in MR studies. Recognition of this fact has inspired the development of sensitivity analysis tools. While we can’t assess whether a SNP instrument is associated with every confounder of the exposure-outcome association, it is possible to examine the associations of the SNP instrument with the measured covariates. While absence of such associations doesn’t guarantee satisfaction of the assumption, presence of SNP-covariate associations must be investigated carefully, as they may constitute assumption violations [71] .
Burgess [71] considered a collection of sensitivity analysis methods when working with GWAS summary statistics. Burgess [71] presented methods for both assessing the MR assumptions, to the extent possible, and performing robust analyses. For example, they used measured covariates to assess for possible associations with the SNP instruments. While they can’t rule out the possibility of unmeasured confounding, they can study the possibility of measured covariates serving as confounders.
To illustrate their approach, Burgess [71] shared a case study in which they examined the causal effect of C-reactive protein (CRP) levels on coronary artery disease risk with four genetic variants in the CRP gene region and 17 other genetic variants that affect coronary artery disease risk. They used measured covariates to probe for SNP-covariate associations, and followed it up with scatter plots and Cochran’s Q test on the causal estimates to inquire about whether the SNP instruments all identify the same causal parameter [72] , [73] , [74] . Additionally, Burgess [71] suggested using a funnel plot, like those in the meta-analysis literature, to visualize possible evidence of directional pleiotropy, where the average pleiotropic effects of the SNP instruments is nonzero [75] . Additionally, Egger regression can be useful in this setting [43] , [76] , [77] , [78] .
Spiller [59] point out that MR-GxE can be used as a sensitivity analysis tool to choose a set of valid SNP instruments from a collection of SNPs in settings where there may be a violation of the constant pleiotropy assumption.
MR-GENIUS is a framework for two-sample MR analysis when individual-level data are available [79] . Bowden [79] focused efforts on relaxing the exclusion restriction assumption and building on existing G-estimation methods to create an estimator that is robust to violations of the exclusion restriction and to additive unmeasured confounding [80] . They observe that their estimator, in some settings, reduces to that of [81] , which is widely used in econometrics, but not in the MR literature.
4.4. Explicit modeling of horizontal pleiotropy
Besides sensitivity analysis, several methods have been developed to directly model horizontal pleiotropy to ensure the validity of MR assumptions. Pleiotropy, where a single genetic variant affects multiple traits, has a long history of study in genetics and complex traits [82] , [83] , [84] . In MR setting, horizontal pleiotropy occurs when a SNP instrument affects the outcome through at least one pathway that bypasses the exposure variable [46] . The presence of horizontal pleiotropy constitutes a violation of the standard MR assumptions and can lead to biased causal effect estimates and diminished statistical power. Watanabe [46] documented widespread horizontal pleiotropy in GWAS. We discuss approaches to modeling and accounting for horizontal pleiotropy below.
In the short time since publication of [46] , researchers have recognized two types of horizontal pleiotropy [65] . The first occurs via exposure-independent paths. The resulting horizontal pleiotropic effects are independent of the SNP-exposure relationships. The second type of horizontal pleiotropy manifests in the presence of unobserved exposure-outcome confounding. It induces correlation between horizontal pleiotropic effects and SNP-exposure effects. Both types of horizontal pleiotropy violate standard MR modeling assumptions and can bias causal effect estimates and can increase false discoveries [65] . Early MR analyses avoided confounding from horizontal pleiotropy by discarding instrumental SNPs that might be associated with the outcome [46] , [47] . More recent methods have attempted to model horizontal pleiotropy [64] , [65] , [85] . CAUSE [64] and MRMix [63] both use a mixture of normal distributions to control for both types of horizontal pleiotropy. Modeling both types of horizontal pleiotropy is particularly challenging because the MR model likelihood often involves an integral that can’t be solved analytically. Because of this issue with the model likelihood, both MRMix and CAUSE use other, non-likelihood-based methods for inference.
Pierce [43] adapted Egger regression, originally developed to detect bias in meta-analyses, to detect bias from horizontal pleiotropy in two-sample MR studies with GWAS summary statistics or individual-level data [76] . Their approach is termed MR-Egger. They formulate their model as
where U designates confounders, X and Y denote the exposure and outcome, respectively, G is a SNP instrument, and ∊ X and ∊ Y are the zero-mean, normally distributed random errors.
Pierce [43] then write the outcome in terms of the SNP instrument j and the effects defined in Eq. (11) and define the new parameter Γ j = α j + β γ j (Eq. (12)).
In Eq. (12), α j = 0 when SNP j is a valid instrument.
MR-Egger fits the regression model in Eq. (13).
The notation in Eq. (13) could be a little misleading. The independent and dependent variables are γ ^ j and Γ ^ j , respectively, while the regression parameters are β 0 E and β E .
The slope coefficient β ^ E estimates the causal effect of the exposure on the outcome. Under the assumption that the SNP-exposure relationship is independent of the SNP’s pleiotropic effects, Egger’s test offers a valid test of the null causal hypothesis and consistently estimates the causal effect, even if all SNPs are invalid instruments [43] . In efforts to detect the presence of horizontal pleiotropy in two-sample MR studies with GWAS summary data, [72] developed the between-instrument heterogeneity test. In a meta-analysis of MR Wald ratio estimates, one per SNP instrument, they calculate Q in Eq. (14).
In Eq. (14), w k is the inverse variance of the Wald estimator, and μ F = ∑ k = 1 K w k β ^ XY k ∑ k = 1 K w k .
While the Q test tends to be conservative in small sample sizes, its power increases with increasing sample size and increasing degree of pleiotropy [72] .
Yuan [86] , working in the setting of two-sample MR with summary GWAS data, recognized that measurement error in outcome and exposure can give misleading results with the inferred causal arrow in the wrong direction. To work around this issue, they extended MR with a method that infers the causal direction between two traits. Specifically, Yuan [86] adapt Steiger’s Z test for a difference in correlations [87] to assess which of the two traits in an analysis has the stronger correlation with the SNP instrument. That with the stronger correlation is treated as the exposure. Yuan [86] applied their test in a study of DNA methylation and gene expression, where either direction of causality is plausible, and found that many methylation traits cause changes in gene expression.
Steiger [88] developed a weighted mode-based MR causal effect estimation method for two-sample MR analysis with GWAS summary data. They first calculate Wald ratio estimates for every instrumental SNP. They then apply smoothing to the empirical distribution of ratio estimates. The mode of this smoothed distribution is the simple mode-based estimate of the causal effect [88] . The inverse variance-weighted mode-based estimate is obtained by weighting the empirical distribution of ratio estimates by the inverse variance of each estimate. Steiger [88] compared their weighted and unweighted mode-based estimators with weighted and unweighted median-based estimators, MR Egger regression, and IVW. They found that their mode-based estimators demonstrated less bias and lower type I error rates than other estimators in some simulation settings. The mode-based estimators possessed lower statistical power to detect a causal effect compared to the IVW and weighted median-based methods, but their power exceeded that of MR Egger regression [88] . The mode-based estimators consistently estimated the causal effect when the mode across SNP instruments of the horizontal pleiotropy effects was zero [88] . In this manner, the mode-based estimators demonstrated a greater robustness to horizontal pleiotropy than did the other estimators.
Hartwig [89] approached the challenge of horizontal pleiotropy by developing three new methods for two-sample MR analysis with GWAS summary data: robust regression, penalized weights, and LASSO penalization. The first two can be viewed as modifications of MR-Egger and IVW methods. Together, they offer three strategies for downweighting or excluding variants with heterogeneous causal estimates. Recall that MR-Egger offers consistent causal effect estimates when there is no horizontal pleiotropy or when the horizontal pleiotropy effects adhere to the “Instrument Strength Independent of Direct Effect” (InSIDE) assumption [43] . The InSIDE assumption is satisfied when there is no correlation between the pleiotropic SNP instrument-outcome effects and the SNP instrument-exposure effects. Hartwig [89] uses MM estimation, which is one technique for robust linear regression [90] , to formulate an estimator with high breakdown point and high efficiency. The second estimation method, with penalized weights, is much like the penalized median estimator, from above, except that the second argument in the minimum function is, instead of the above. Finally the LASSO-based method fashions an estimator by applying a penalty to the objective function from MR-Egger to get Eq. (15) as the objective function.
Hartwig [89] studied two procedures for choosing λ : a heterogeneity stopping rule and a cross-validation rule. Taken together, these three methods offer additional contributions to a suite of sensitivity analysis tools that practitioners should consider.
Koller [91] introduced the contamination mixture method for robust and efficient MR analysis with hundreds of SNPs, some of which may be invalid instruments by violating one or more of the three core MR assumptions. Working in the setting of two-sample MR with GWAS data, they begin by considering a collection of candidate SNP instruments, which may contain some invalid SNP instruments. For each candidate SNP instrument, they calculate the Wald ratio estimate of the causal effect. With the collection of Wald ratio estimates, Koller [91] reason that the set of valid SNP instruments have Wald ratio estimates that arise from a normal distribution centered on the true causal parameter with variance equal to that of the Wald ratio estimator. The invalid instruments, however, are assumed to follow a normal distribution centered at zero with a variance greater than that of the Wald ratio estimator. They then work with profile log likelihood over a grid of causal parameter values to make inferences.
Zhao [62] presented MR-RAPS, a method that leverages a robust adjusted profile score to accomplish statistical inference in two-sample MR analyses with GWAS summary data. Dividing pleiotropic effects into systematic and idiosyncratic, they model the systematic pleiotropy with random effects. In so doing, no SNP satisfies the exclusion restriction assumption. Adjusting their profile likelihood estimator from the setting without pleiotropy, [62] obtain an estimator with consistency and asymptotic normality [92] . Idiosyncratic pleiotropy is addressed through robustification of the adjusted profile score [93] . They then demonstrate the properties of their estimator by analyzing simulated and real data [62] .
Huber [94] , working with a collection of SNP instruments, reasoned that if all three MR assumptions are satisfied, then the collection of Wald ratio estimates should be homogeneous; thus, any departures from homogeneity may signal a violation of one or more assumptions. They reported modified weights, in addition to studying weights derived from first order and second order approximations to the variance. Their modified weights lead to an estimator that is useful for quantifying heterogeneity and detecting outliers.
Bowden [95] developed the MR-TRYX framework for exploiting horizontal pleiotropy to identify alternative causal pathways. They did this by positing that, for a collection of putative SNP instruments, any heterogeneity in the Wald ratio estimates may be due to horizontal pleiotropy. Once they identify a SNP with outlying ratio estimates, they search GWAS results by querying the MR-Base database to identify complex traits (in other studies) that associate with the outlying SNP [96] . They follow up promising trait associations to account for their observations with multiple causal pathways.
Hemani [97] recognized that in some MR studies, the Wald ratio estimates for different SNP instruments may form clusters, and these clusters may represent distinct causal mechanisms and may identify the SNPs that are involved in them. They devised a method to detect these clusters in the collection of Wald ratio estimates and found SNP instruments that point to distinct causal pathways of biological importance [97] .
Fang [98] sought a probabilistic model that accounts for both linkage disequilibrium among SNP instruments and horizontal pleiotropy. They termed their method MR-LDP. Using an approach from [99] , [98] derived an approximate likelihood for their regression models of the exposure and outcome on the SNP instruments. Incorporation of a random effect into their models accounted for the variance in causal effect estimates due to horizontal pleiotropy. They situate inference within an empirical Bayesian framework and present a parameter-expanded variational Bayes expectation–maximization algorithm for estimation [100] . By jointly modeling the distribution of GWAS summary statistics and causal effects, [98] efficiently accommodates multiple SNP instruments in linkage disequilibrium. One limitation of MR-LDP is its reliance on the InSIDE assumption and resulting inability to accommodate correlated horizontal pleiotropy.
Liu [101] present IMRP, a method for causal effect estimation and horizontal pleiotropy detection. They describe a test to distinguish vertical pleiotropy from horizontal pleiotropy. By combining this test with two-sample MR analysis of GWAS summary statistics, they develop an iterative procedure for estimating the causal effect of exposure on outcome and test for presence of horizontal pleiotropy in the SNP instruments. The iterative procedure alternates between 1) updating the estimate of the causal effect and 2) testing for horizontal pleiotropy with the included SNP instruments. At each test for horizontal pleiotropy, some SNP instruments may be discarded if they demonstrate evidence of horizontal pleiotropic effects. The procedure ends when there remain only SNP instruments that don’t exhibit horizontal pleiotropy. In the authors’ simulations and data analysis sections, their new method performs about as well as GSMR, IVW, and MR-PRESSO in the settings with and without balanced pleiotropy and with and without satisfaction of the InSIDE assumption [101] . Finally, the authors note that IMRP is three orders of magnitude faster than simulations-based MR-PRESSO.
Zhu [102] build on research from Grant [103] to present a method that applies a l 1 penalty to coefficients of covariates in two-sample MR with GWAS summary statistics. Importantly, their method doesn’t penalize the SNP-exposure association. Instead, it shrinks towards zero those coefficients for covariates that demonstrate little pleiotropy. In so doing, they extend work from [104] , [105] to get a causal effect estimator that needs no valid SNP instruments and is robust to measured vertical pleiotropy and more statistically efficient than previous MR methods.
Burgess [106] consider two classes of horizontal pleiotropy with their method, MRCIP. They partition horizontal pleiotropic effects into correlated or idiosyncratic horizontal pleiotropy. Idiosyncratic horizontal pleiotropy is that with a large effect size, while correlated horizontal pleiotropy, like its definition elsewhere in our manuscript, refers to correlation between the effects of the SNP instruments on the exposure and the effects of the SNP instruments on the outcome. MRCIP relaxes both the exclusion restriction assumption and the InSIDE assumption by directly modeling the correlated horizontal pleiotropy with random effects. Furthermore, to accommodate the idiosyncratic pleiotropy, the authors downweight SNP instruments that demonstrate strong direct effects on the outcome. They find that their MRCIP provides valid causal inference even when there are no valid SNP instruments (i.e., when all SNP instruments are invalid) and when the InSIDE assumption is violated [106] . Their PRW-EM algorithm adds a reweighting step to the traditional expectation maximization algorithm to estimate the causal effect parameter in MR analysis [107] . Finally, they compare MRCIP to other MR methods with both simulated and real data sets [106] , like the developers of MR-RAPS, assume that the horizontal pleiotropy is balanced, i.e., that the mean horizontal pleiotropic effect is zero. One direction for future study is to augment MRCIP to permit unbalanced horizontal pleiotropy.
Dempster [108] build on other research using mixture models in the two-sample MR setting with GWAS summary statistics. Like MR-Clust, MR-PATH, as the method from [108] is called, works with the Wald ratio estimates from multiple SNP instruments. Coining the term “mechanistic heterogeneity” to refer to the distribution of Wald ratio estimates due to SNP involvement in distinct causal pathways, [108] specify a J -component normal mixture model. They use an expectation maximization algorithm to fit the model parameters (the component weights and the parameters for each of the J normal distributions) and suggest a Bayesian information criterion-based approach to choosing J [109] .
Schwarz [110] propose causal effect test statistics that are robust under weak instrument asymptotics [111] . Specifically, they extend three econometric methods to two-sample MR with GWAS summary statistics [112] , [113] , [114] and study the theoretical properties and the performance in applications. Schwarz [110] extend their methods to demonstrate conditions under which their estimators are equivalent to those in works from [62] , [55] , and [94] .
Moreira [115] developed BayesMR, a Bayesian framework for two-sample MR with individual-level data and discuss an approximation for use with GWAS summary statistics. Unlike many MR methods, BayesMR both aims to control for horizontal pleiotropy and to quantitatively assess the possibility of reverse causation, where the putative exposure is actually caused by the putative outcome. For independent SNP instruments, Moreira [115] permit each SNP instrument to have both a direct effect on the exposure and a horizontally pleiotropic direct effect on the outcome. Instrument effects and horizontally pleiotropic effects are assumed independent. Note that this is the Bayesian analog of the InSIDE assumption. After specifying the model, they present a nested sampling scheme [116] , [117] , [118] that simultaneously computes the model evidence for both causal directions (i.e., exposure causes outcome and outcome causes exposure) and acquires samples from the posterior distribution for Bayesian statistical inferences.
Handley [119] present BMRE, a Bayesian implementation of MR Egger. They apply weakly informative priors to the horizontal pleiotropy effect estimator in efforts to increase statistical power to detect a nonzero slope, i.e., the coefficient of the SNP instrument. They develop their method for two-sample MR with individual-level data. Simulations demonstrate that BMRE outperforms the MR Egger method while maintaining type I error rates in the examined scenarios. The authors emphasize a potential role for BMRE in MR sensitivity analysis.
Schmidt [120] found that multivariable MR, where multiple exposures jointly cause an outcome, consistently estimates the effect of the exposures on the outcome. They examined multivariable MR with both individual-level data and GWAS summary statistics. Schmidt [120] also develop a generalized version of Cochran’s Q test for quantifying SNP instrument strength and validity. However, this test requires knowledge of the covariances between the effects of the SNP instruments on the exposures. While these covariances could be estimated from individual-level data, such data are often unavailable in the GWAS setting. Importantly, the authors note that the estimands for multivariable MR and MR differ; multivariable MR estimates the direct causal effects of each exposure on the outcome, while MR estimates the total causal effect of an exposure on the outcome.
MR-link, reported by Sanderson [121] , aims to account for unobserved pleiotropy and linkage disequilibrium by using individual-level data on the outcome variable and summary statistics for the exposure. MR-link uses a three-step procedure to estimate causal effects.
- Use GCTA-COJO to obtain SNP instruments [122] .
- Prune all SNPs in linkage disequilibrium with the SNP instruments to obtain a set of “tag” SNPs with correlations less than 0.95.
- Solve for the causal effect estimate with ridge regression of the outcome on the matrix resulting from concatenation of the SNP instrument genotypes matrix and the tag SNPs genotypes matrix.
Note that in step 3, ridge regression is preferred to ordinary least squares due to the limited statistical power of ordinary least squares in this setting. Presumably, this is due to collinearity in the matrix of tag SNP genotypes.
4.5. Correlated horizontal pleiotropy
The second type of horizontal pleiotropy, from above, is sometimes called “correlated horizontal pleiotropy.” Correlated horizontal pleiotropy occurs when a SNP affects both the exposure and a variable that confounds the relationship between exposure and outcome ( Fig. 3 C). CAUSE and MRAID are two methods that aim to model correlated horizontal pleiotropy [64] , [65] . Their approaches to modeling correlated horizontal pleiotropy differ in important ways that ultimately affect performance. CAUSE allows for a proportion of SNPs to exhibit correlated pleiotropy, which [64] models as an effect on a shared, unobserved factor. The remaining SNPs are independent of this unobserved factor. Every SNP can have a nonzero pleiotropic effect on the outcome, and these pleiotropic effects are uncorrelated with the variant effects on the exposure. The model, then, is written as a mixture of biavariate normal distributions, and inference proceeds by borrowing ideas on adaptive shrinkage from [123] . Finally, [64] compares two models to determine whether the GWAS summary statistics are consistent with a causal effect of the exposure on the outcome. Specifically, they estimate the difference in the expected log pointwise posterior density for the model with causal effect fixed at zero and the model that permits a nonzero causal effect [124] .
Morrison [65] found that MRMix is not robust to misspecification of SNP effect sizes and often is biased. CAUSE, they found, yields overly conservative p-values [65] . These observations motivated [65] to develop a new method, MRAID. MRAID accommodates both individual-level data and GWAS summary statistics [65] . We focus on the approach that uses GWAS summary statistics. Two equations are central to MRAID modeling (Eqs. (16) and (17)).
The estimated marginal effects for the p SNP instruments on the exposure are denoted by β ^ X , while β ^ Y represents the estimated effects of the same SNPs on the outcome. The p by p SNP instrument correlation matrices for the exposure and outcome are written as Σ 1 and Σ 2 , respectively. Σ 1 and Σ 2 can be estimated with 1000 Genomes Project data, for example, by choosing a subset of 1000 Genomes Project subjects that have similar ancestry [125] . The p -vector error terms, e x and e y , follow multivariate normal distributions with mean zero and variances Σ 1 σ X 2 n 1 - 1 and Σ 2 σ Y 2 n 2 - 1 , respectively. Morrison [65] then constructs a Gibbs sampler to make likelihood-based inferences [126] . To facilitate computations, the investigators make several assumptions about the collection of SNPs:
- Relatively small proportion of SNPs have nonzero effects on the exposure
- A relatively small proportion of SNPs demonstrate horizontal pleiotropy.
- The chosen instrumental SNPs are more likely to display horizontal pleiotropy than are non-instrumental SNPs.
- Those SNPs that display horizontal pleiotropy are more likely to demonstrate uncorrelated horizontal pleiotropy than correlated horizontal pleiotropy.
By encoding these assumptions in prior distributions, Morrison [65] enables the inferential procedures to accommodate the observed data in the context of the assumptions on SNPs.
4.6. Binary, count, and time-to-event outcomes and exposures
While we’ve focused above on continuous outcomes and continuous exposures, some data are more naturally treated as binary, count, or time-to-event variables. MR methods for non-continuous outcomes and exposures is an active area of research. Kleibergen [31] and Geman [127] developed MR strategies for binary exposures and binary outcomes. Kleibergen [31] extended methods from Mendel [24] by fitting two logistic regressions, one for the outcome and one for the exposure. They then calculated the causal effect estimator as the ratio of the two logistic regression coefficient estimators. Geman [127] devised a new MR method for binary outcome and binary exposure by drawing on [128] and treating the exposure and outcome as correlated binary random variables. They implemented an iterative optimization algorithm to simultaneously infer the causal effect parameter and other parameters.
Researchers have also leveraged other generalized linear models for analysis in MR studies [129] . However, this area is relatively unexplored. Nelder et al. [130] treated hospitalizations as a count variable and modeled it with quasi-Poisson methods. Hazewinkel et al. [131] characterized statistical properties, including bias and power, with simulations of count random variables and binary random variables. With growing interest in modeling counts in biomedical data, where molecular phenotyping technologies now acquire RNA molecule counts and protein abundances, there may be opportunities for methods innovations in a generalized linear models framework for MR studies [132] , [133] , [134] , [135] .
Recently, multiple teams of investigators have analyzed time-to-event traits in MR studies [136] , [137] , [138] , [139] , [140] . He et al. [140] , for example, studied post-diagnosis survival time in women with breast cancer. They treated survival as a time-to-event outcome, which enabled them to model it with a proportional hazards model [141] in two-stage MR with individual-level data. Similarly, Tikkanen [139] , in a study of cardiovascular disease, applied proportional hazards regression when examining time to stroke as an outcome variable. In very recent work, Bowden [79] reported methods, MR GENIUS, that are robust to violations of the exclusion restriction assumption (under many assumed MR data generating processes). They considered binary, continuous, and time-to-event outcomes within their framework. We are unaware of MR studies that treat the exposure variable as a time-to-event, but it’s conceivable that future research will consider time-to-event traits as exposures in MR analyses.
5. Recent findings
5.1. omnigenic mr.
Recent research on the omnigenic hypothesis, which posits that every SNP’s effect on a trait is nonzero, has informed MR methods development [142] . Boyle [143] recognized the limitations of previous MR methods in the context of the omnigenic hypothesis. In response, Boyle [143] developed a MR method that uses all genome-wide SNPs as instruments. Using GWAS summary statistics as inputs, their method relies on a composite likelihood framework for scalable computation and allows for horizontal pleiotropy. Boyle [143] used extensive simulations, including those with model misspecifications, to characterize their method’s statistical power and robustness. Finally, they applied the new method to identify multiple complex traits that affect coronary artery disease and asthma. These causal relationships highlight the important roles of plasma lipids, blood pressure, and the immune system in CAD susceptibility and those of obesity and the immune system in asthma development.
5.2. Sample overlap in biobanks
The issue of overlapping samples in the two-sample MR with GWAS summary data has recently received attention in the published literature. Hemani [97] , working with data from the UK Biobank, found that SNP instruments derived from overlapping samples explained a higher proportion of the variance compared to those derived from non-overlapping samples. They argue that block jackknife resampling MR enables causal inference while avoiding bias due to overlapping samples. Wang [144] analyzed 2514 traits from the UK Biobank and evaluated the impact of winner’s curse on MR analysis. Winner’s curse, they found, amplifies weak instrument bias without inflating the false discovery rate. This finding led the authors to design a pseudoreplication process that reduces bias in MR studies. Sadreev [145] proposed a Bayesian approach for one-sample MR analysis where SNP instruments are permitted to exhibit pleiotropic effects on the outcome. Working in the setting of one-sample MR with individual-level data, they construct their model with a shrinkage prior, so that many SNP instruments have no horizontal pleiotropic effects. Their method relaxes the exclusion restriction assumption to permit horizontal pleiotropy (for some SNP instruments) and permits correlated SNP instruments, unlike many frequentist methods. Posterior inference proceeds by Markov chain Monte Carlo sampling. They also elaborate their model for a univariate exposure into that for a bivariate exposure to analyze the causal effects of body mass index and serum phenylalanine levels on blood pressure.
5.3. Bi-directional causal inference with MR to account for reverse causation
Reverse causation occurs when the outcome at an early time point has a causal effect on the exposure variable. While reverse causation is often assumed to not occur in MR study designs, [146] points out several scenarios where this assumption may not hold [147] . In recognition of this observation, [148] developed a framework, GRAPPLE, for performing two-sample MR with weak and strong instruments. Using GWAS summary statistics as inputs, GRAPPLE extends MR-RAPS and can detect presence of horizontally pleiotropic pathways, infer the causal direction, and perform multivariable MR. Central to the GRAPPLE framework is the observation that a horizontally pleiotropic pathway often gives rise to an additional mode in the profile likelihood. GRAPPLE uses the presence of multiple profile likelihood modes to diagnose horizontal pleiotropy with effects that are grouped by pathway. GRAPPLE facilitates identification of SNP instruments that contribute to the additional profile likelihood modes. A researcher using GRAPPLE may then recognize a confounding factor for each mode. With additional GWAS summary data with traits that reflect the confounding factors, GRAPPLE can fit multivariable MR models when the InSIDE assumption holds for the remaining horizontally pleiotropic effects [148] .
5.4. Practice recommendations
We now turn attention to the question of how to choose among the MR methods for a given analysis. We focus on the two-sample setting where only GWAS summary statistics are available. The large number of two-sample MR methods with summary statistics may challenge a practitioner seeking to develop an analysis plan. Early considerations include:
- assessing the plausibility of the MR assumptions
- determining which questions to address with sensitivity analysis
- assessing causal directionality between exposure and outcome variables
Detailed examples may be found in [149] , [150] , and [151] . In some settings, a practitioner may address these questions by a careful study of the relevant scientific literature. For example, there may be experimental studies that suggest a causal direction for the relationship between exposure and outcome. If both causal directions are plausible, then a bidirectional MR analysis may be needed. If the bidirectional MR analysis (and supporting scientific evidence) is consistent with a single causal direction, then a practitioner may proceed with unidirectional MR methods. Given the pervasive nature of horizontal pleiotropy and its potential impact on MR, we recommend using one or more MR methods explicitly model horizontal pleiotropy. Recent methods, such as CAUSE and MRAID, go a step further by modeling correlated horizontal pleiotropy [64] , [65] .
Other MR methods can be used in sensitivity analysis to assess robustness of findings to possible violations of MR assumptions. Methods like MR-clust have the potential to reveal additional relationships among genes that share a biological pathway [152] . The STROBE-MR statement has additional practice recommendations [153] .
6. Summary and outlook
Open research questions remain in the MR field. We have highlighted above three recent findings: omnigenic MR, methods for sample overlap in biobanks, and methods for bidirectional causal inference. While we discussed initial findings in these three areas, many opportunities for methods enhancements remain. For example, as biobank sizes grow in number of subjects and number of measured traits, there is increasing demand for MR methods that scale efficiently with large sample sizes. Development of methods for two-sample MR with overlapping samples opens opportunities to study rich biobank data, and we anticipate that this question - how to accommodate overlapping samples – will continue to be an active area of research.
In summary, we have presented a comprehensive review on 47 methods for MR analysis in GWAS ( Fig. 4 ). The overall trend of the methodology development for MR analysis is in the direction of increasingly sophisticated modeling of horizontal pleiotropy including both independent and correlated horizontal pleiotropy while attempting to maintain scalability and computational efficiency in the presence of multiple correlated SNPs. We hope our detailed review would benefit both methodology developers and applied analysts to further advance the development of MR methods and aid in their applications towards large, biobank-scale datasets, thus offering the possibility of discovering even more causal relationships among complex traits [154] .

Decision tree diagram for choosing among multiple-SNP MR methods.
CRediT authorship contribution statement
Frederick J. Boehm: Conceptualization, Investigation, Writing – original draft, Writing – review & editing, Visualization. Xiang Zhou: Conceptualization, Investigation, Writing – original draft, Writing – review & editing, Visualization, Supervision, Project administration.
Declaration of Competing Interest
The authors declare that they have no known competing financial interests or personal relationships that could have appeared to influence the work reported in this paper.
Acknowledgements
This study was supported by the National Institutes of Health (NIH) grant R01HG009124.
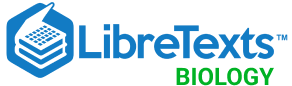
- school Campus Bookshelves
- menu_book Bookshelves
- perm_media Learning Objects
- login Login
- how_to_reg Request Instructor Account
- hub Instructor Commons
Margin Size
- Download Page (PDF)
- Download Full Book (PDF)
- Periodic Table
- Physics Constants
- Scientific Calculator
- Reference & Cite
- Tools expand_more
- Readability
selected template will load here
This action is not available.
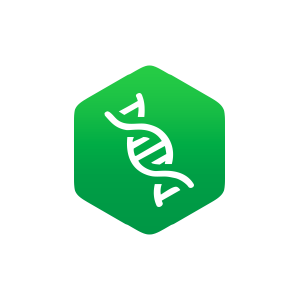
1.13: Introduction to Mendelian Genetics
- Last updated
- Save as PDF
- Page ID 73678
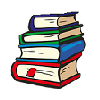
- Marjorie Hanneman, Walter Suza, Donald Lee, & Amy Kohmetscher
- Iowa State University via Iowa State University Digital Press
\( \newcommand{\vecs}[1]{\overset { \scriptstyle \rightharpoonup} {\mathbf{#1}} } \)
\( \newcommand{\vecd}[1]{\overset{-\!-\!\rightharpoonup}{\vphantom{a}\smash {#1}}} \)
\( \newcommand{\id}{\mathrm{id}}\) \( \newcommand{\Span}{\mathrm{span}}\)
( \newcommand{\kernel}{\mathrm{null}\,}\) \( \newcommand{\range}{\mathrm{range}\,}\)
\( \newcommand{\RealPart}{\mathrm{Re}}\) \( \newcommand{\ImaginaryPart}{\mathrm{Im}}\)
\( \newcommand{\Argument}{\mathrm{Arg}}\) \( \newcommand{\norm}[1]{\| #1 \|}\)
\( \newcommand{\inner}[2]{\langle #1, #2 \rangle}\)
\( \newcommand{\Span}{\mathrm{span}}\)
\( \newcommand{\id}{\mathrm{id}}\)
\( \newcommand{\kernel}{\mathrm{null}\,}\)
\( \newcommand{\range}{\mathrm{range}\,}\)
\( \newcommand{\RealPart}{\mathrm{Re}}\)
\( \newcommand{\ImaginaryPart}{\mathrm{Im}}\)
\( \newcommand{\Argument}{\mathrm{Arg}}\)
\( \newcommand{\norm}[1]{\| #1 \|}\)
\( \newcommand{\Span}{\mathrm{span}}\) \( \newcommand{\AA}{\unicode[.8,0]{x212B}}\)
\( \newcommand{\vectorA}[1]{\vec{#1}} % arrow\)
\( \newcommand{\vectorAt}[1]{\vec{\text{#1}}} % arrow\)
\( \newcommand{\vectorB}[1]{\overset { \scriptstyle \rightharpoonup} {\mathbf{#1}} } \)
\( \newcommand{\vectorC}[1]{\textbf{#1}} \)
\( \newcommand{\vectorD}[1]{\overrightarrow{#1}} \)
\( \newcommand{\vectorDt}[1]{\overrightarrow{\text{#1}}} \)
\( \newcommand{\vectE}[1]{\overset{-\!-\!\rightharpoonup}{\vphantom{a}\smash{\mathbf {#1}}}} \)
Learning Objectives
- Outline the experimental approach Mendel used to propose the idea that genes exist, control traits, and are inherited in predictable ways.
- Compare the methods used by Mendel and Punnett to predict trait inheritance.
Introduction
In plant and animal genetics research, the decisions a scientist will make are based on a high level of confidence in the predictable inheritance of the genes that control the trait being studied. This confidence comes from a past discovery by a biologist named Gregor Mendel, who explained the inheritance of trait variation using the idea of monogenic traits.
Monogenic characters are controlled by the following biological principles:
- Living things have genes in their cells that encode the information to control a single trait. These genes are stable and passed on from cell to cell without changing.
- The genes are in pairs in somatic cells. When these cells divide to form gametes, the pair of genes is divided. One gene from the pair goes into a gamete.
- Male gametes (pollen) combine with female gametes (eggs) in the wheat flower pistil and fuse to form the next generation (zygote). Gamete union is random.
- The zygote, again, has two copies of each gene. As the zygote grows into a multicellular seed and the seed grows into a plant, the same two gene copies are found in every cell.
Let’s take a short genetics history lesson to understand their confidence.
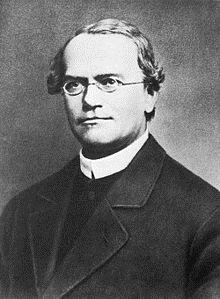
Mendel’s Peas
In the mid 1800’s, an Austrian monk named Gregor Mendel (Figure 1) decided he should try to understand how inherited traits are controlled. He needed a model organism he could work with in his research facility, a small garden in the monastery, and a research plan. His plan was designed to test a hypothesis for the inheritance of trait variation.
Since Mendel could obtain different varieties of peas that differed in easy to observe traits such as flower color, seed color and seed shape, and he could grow these peas in his garden, he chose peas as the model organism for conducting his inheritance control study. A model is easy to work with and often what you learn from the model you can apply to other organisms.
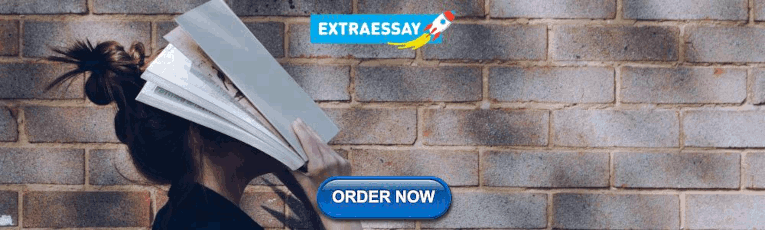
The Hypothesis
While many biologists were interested in trait inheritance, at the time Mendel conducted his experiments none of the biologists had published evidence that inheritance could be predicted. Mendel made this bold statement. His hypothesis was that he could observe “mathematical” regularities in the appearance of a trait that was passed on from parents to their offspring. Mendel had the idea that mathematical regularities could be observed and could be used to explain the biology of inheritance!
Mendel’s experimental plan was designed to test the hypothesis. He identified true breeding lines of peas by allowing them to self pollinate (which we will refer to as “selfing”) and examining their offspring. Pea plants have flowers that contain both male and female reproductive parts; if a pea flower is left undisturbed, the male and female gametes from the same flower will combine to produce seeds, the next generation. If the pea always made offspring like itself, Mendel had his true breeding line. He then made planned crosses between lines that differed by just one trait (monohybrid crosses). The controlled monohybrid cross was the first step in his experiment that allowed him to look for mathematical regularities in the data for three generations. Table 1 below shows the data from a series of these monohybrid cross experiments.
The Analysis
By summarizing his data in a single table, Mendel could look for those hypothesized math regularities. A regularity is a repeated observation.
Cross and Phenotypes
Round X Wrinkled
5474 Round 1850 Wrinkled
Cotyledon color
Yellow X Green
6022 Yellow 2001 Green
Seed coat color*
Gray X White
705 Gray 224 White
Inflated X Constricted
All inflated
882 Inflated 299 Constricted
Green X Yellow
428 Green 152 Yellow
Flower position
Axial X Terminal
651 Axial 207 Terminal
Stem length
Tall X Short
787 Tall 277 Short
*Gray seed coat also had purple flowers; White seed coat had white flowers.
Table 1 demonstrates that Mendel was serious about the math. He generated large numbers of offspring that allowed him to observe mathematical ratios. From his table of data, we can see mathematical patterns appear with every monohybrid cross he made.
- F 1 : All the plants had the same phenotype as one of the parents.
- F 2 : Both phenotypes are present, the phenotype that was not expressed in the F 1 appears again in the F 2 but is always the least frequently produced. The average ratio is about 3:1 for the two phenotypes.
What was striking to Mendel was that every character in his study exhibited the same kind of mathematical pattern. This suggested that the same fundamental processes inside the plant’s reproductive cells were at work controlling the inheritance of each trait.
Now Mendel had the task of providing a description of the fundamental biology process controlling each of these traits. He needed to come up with ideas that no one had yet proposed to explain biology.
New Idea #1:
The traits expressed in the pea plant were controlled by some kind of particle. These hereditary particles are stable and passed on intact from parent to offspring through the sex cells. (NOTE: Sex cells or gametes were not a new idea, Mendel was aware that biologists knew sexually reproducing plants and animals needed to make gametes.) We now call these particulate factors genes and will use that term in the rest of this reading.
New Idea #2:
Genes are stable, and genes can have alternative versions (alleles).
New Idea #3:
Genes are in pairs in somatic cells and these paired genes separate during gamete formation. Each gamete will have one gene from the pair of genes. The segregating of the paired genes from the somatic cells of the parent into gametes is random. Because segregation is random, a parent that has two different alleles for a gene pair will make two kinds of gametes and makes these gametes at equal frequencies.
From Mendel’s ideas, we can see that in a situation in which there was a normal version of a gene (we can call it the R gene) and an alternate version (r), the plant could produce gametes with just the R gene or just the r gene.
New Idea #4:
Plant flowers are designed to allow male gametes (pollen) to combine randomly with the female gametes (egg). When the gametes randomly come together, they bring the genes they carry to the same zygote. This means plants could have the genotype RR, Rr , or rr in families that have both the R and r alleles.
New Idea #5:
Mendel proposed that the genes controlling a trait not only paired in somatic cells, they also interacted in controlling the traits of the plants. For the traits in his experiment, he proposed that one allele interacted with the other in a dominant fashion. That means a plant that is the genotype RR would have the same phenotype as an Rr plant. The R allele is dominant to the r allele.
Ideas and Data advance science
Those were Mendel’s new ideas; he used them to make sense of his experiment data and observations. Let’s think like Mendel and apply those ideas.
All the F1 were the same
Mendel’s new ideas could explain this observation. Since his parents were true breeding, he was always making a cross between homozygous parents. Homo means the same, so the parents had two copies of the same version of the gene.
Crossing RR X rr plants to produce Rr
Since the R is dominant to r , then the Rr offspring (named the F 1 ) look the same (have the same phenotype) as the RR parent. Therefore, only one phenotype is observed in the F 1 . But the F 1 genotype is different from either parent. It is heterozygous (two different alleles).
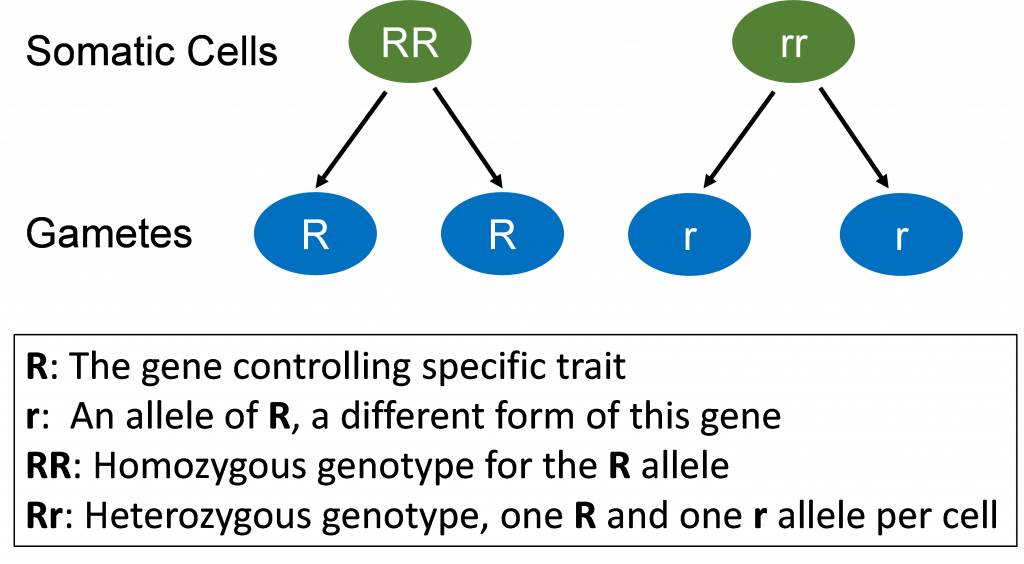
The F 2 : both traits appear in about a 3:1 ratio
Mendel could explain the reappearance of the recessive trait and the ratio by combining the idea of genes with the idea of random segregation. Mendel used simple algebra to explain this result.
First, he wrote out a mathematical expression to account for the gametes made in the male part of the F 1 flower or in the female part.
½ R + ½ r = all the gametes made (Figure 2).
Next, he reasoned that if pollen randomly united with the egg to combine the genes in the gametes, then algebra could be used to predict the result by multiplying the gamete expressions.
(½ R + ½ r) X (½ R + ½ r) = all the F 2 offspring made.
If we do the multiplication above, we get …
¼ RR + ¼ Rr + ¼ Rr + ¼ rr = ¼ RR + ½ Rr + ¼ rr = predicted fractions of F2 genotypes.
If this math is causing your brain to lose focus, you might be experiencing what Mendel’s contemporaries experienced when they read his published research paper. While many biologists were motivated to understand how the variation among animals and plants was controlled and inherited, it took biologists 30 years to recognize that Mendel’s new ideas to explain inheritance of traits in peas could be applied to inheritance of traits in other living organisms.
One possible explanation for this 30-year delay in appreciation is that it was difficult for biologists to understand how math could explain biology. One biologist that did understand what Mendel was describing was Punnett. Punnett decided to convert Mendel’s algebra into a more graphic representation of the process of gamete segregation and random union.
The Punnett Square
Math: (¼ RR + ½ Rr + ¼ rr).
Punnett designated the gametes made in the male and female parents with single letters (Figure 3). The diagram shows that when the gametes combine, the offspring (inside the squares) again have the genes in pairs in their cells. Accounting for the random union of gametes is accomplished with the four squares in the diagram. Two squares give the same Rr result, one the RR genotype and one rr . Both the algebra and diagram approaches provide the same prediction. Crossing an Rr with an Rr will produce three genotypes, RR, Rr and rr . They will be produced in a ratio based on the principle of segregation.
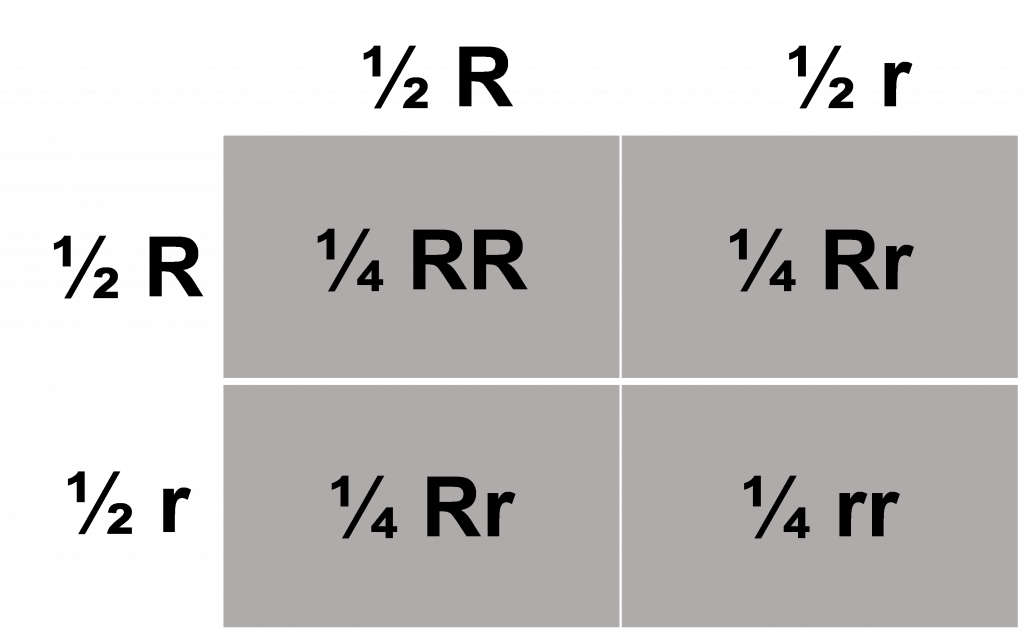
The genes controlling the monogenic traits behaved in predictable ways
Punnett’s diagram clarified for many biologists what Mendel was telling them in his published article. This was a challenging idea to understand because he was asking biologists to use something they could not see (genes) and explain something they could see (traits in peas or some other living organism).
Because Mendel recognized he was proposing a very different idea with the segregation principle, he was likely motivated to share the most convincing evidence possible. Mendel conducted additional experiments. One experiment was to test the hypothesis that there were two different kinds of F 2 which expressed the dominant trait, and these two types were being made by the F 1 in predictable fractions. How would Mendel show that F 2 which had the same phenotype did not always have the same genotype?
Mendel tested the breeding behavior of the F 2 . Mendel harvested all the selfed seed produced by his F 2 and grew progeny rows of F 3 . His segregation principle predicted that of the dominant F 2 , there should be two that are heterozygous for every one homozygote made (on average). The results of this experiment are summarized in Table 2 . Did Mendel’s data support the hypothesis?
Average ratio heterozygote F 2 to homozygote F 2 was 2.06 to 1.
The data show that, if we select a sample of F 2 with the dominant trait (Round seed or Yellow cotyledon), the principle of segregation predicts that there should be 2 heterozygotes for every 1 homozygotes.
Mendel’s data from rows of F 3 that all came from F 2 with the dominant trait supported his hypothesis. There were always two kinds of rows (true breeding and mixed) and the rows were in a 2:1 ratio. This fits with the principle of segregation .
By publishing these results in a scientific journal, Mendel allowed other scientists to learn from his work. This story reveals the real power of publishing research in the “permanent” scientific literature. The power of publication does not mean you were right with your science. The real power is that other scientists can find your paper, read it, think about your ideas, and then test them. In Mendel’s case, he was already dead when his fellow biologists discovered that his new ideas to explain the biology of peas were not only correct, but universal in their application.
Mendel’s Dihybrid Cross Experiments
Proper credit must be given to the idea of independent assortment. Gregor Mendel was the first to put this idea down on paper based on what he observed with his pea experiments. Furthermore, Mendel performed additional experiments to back up his ideas. Let’s examine his experiments with peas from the late 1800’s.
The outline below describes Mendel’s dihybrid cross experiments. The pattern observed in the results should look familiar!
The Experiment
- Parents: round seeds, yellow seeds (RRYY) x wrinkled seeds, green seeds (rryy).
- F 1 : All round and yellow seeds (RrYy).
- Selfing: F 1 (RrYy x RrYy):
Mendel explained his results as follows:
The F 1 plants have the genotype RrYy and can make four kinds of gametes RY, Ry, rY and ry.
Note that with both the Mendel algebra and Punnett square, the RRYY genotype occurs one time and the RrYy genotype occurs four times (Table 4). Mendel’s algebra and Punnett’s squares can be summarized to give the same results.
Selfing the F 2 to produce F 3
The easiest experiment to perform was to let the plants self-pollinate and then keep good records. After scoring his 556 F 2 seeds (Table 5) he took the 315 that were round and yellow and planted them in one part of his garden. The plants that grew were allowed to self-pollinate. Of the 315 round and yellow seeds planted, 301 plants matured and produced seed. The seed produced was the F 3 generation. At harvest, Mendel needed to exercise the utmost care. Each F 2 plant was handled separately. The seeds from the plant were harvested and Mendel then scored the F 3 seeds that came from the same F 2 plant. This can be referred to as F 2:3 data and the table below summarizes his complete experiment using all of the F 2 phenotypes.
Mendel’s F 2 data supported his principle of independent assortment. There were four different types of round yellow F 2 based on the kinds of progeny they could produce or their breeding behaviors. Based on the F 3 progeny produced, the F 2 genotype was deduced. For example, if a round, yellow seed gave all round progeny it must have the genotype RR . If it gave both round and wrinkled it was Rr .
Furthermore, the numbers of F 2 plants with each breeding behavior were in agreement with what was expected with independent assortment. There were four times as many round and yellow F 2 that gave all four phenotypes of F 3 seeds (138) compared to the round and yellow F 2 that were true breeding (38). Overall, there were nine types of breeding behaviors demonstrated in the F 2 demonstrating that there were nine F 2 genotypes. In all cases, the fractions observed in the F 2 agreed to the principle of independent assortment. Mendel’s well-planned experiment provided a convincing demonstration that genes behaved in this predictable manner.
The only thing better than performing an experiment that shows you were right about a new hypothesis is performing two experiments that show that you were right. That is what Gregor Mendel did! In his second experiment he crossed dihybrid F 1 plants with homozygous recessive plants in a test cross. This type of cross is named because the geneticist wants to perform a cross that will test or reveal the genotype of an organism. Therefore, a test cross is usually made between an organism with a dominant trait and a partner with a recessive version of this trait. Mendel performed the RrYy x rryy testcross and the expected progeny are shown in the Punnett square below:
RrYy gametes: RY, Ry, rY, ry
rryy gametes: all ry
The observed result closely matched the expected. The testcross experiment provides additional support for the principle of independent assortment.
Mendel established a rigorous precedent for using carefully planned multi-generation experiments to reveal the principles that governed trait inheritance. The beauty of Mendel’s accomplishments is that both the principles and his experimental approach can be applied to understanding the genetic control and inheritance of traits in many kinds of organisms still today.
Mendel’s principles of segregation and independent assortment are valid explanations for genetic variation observed in many organisms. Alleles of a gene pair may interact in a dominant vs. recessive manner or show a lack of dominance. Even so, these principles can be used to predict the future…at least the potential outcome of specific crosses.
Watch this video about Punnett Squares for more information

Snapsolve any problem by taking a picture. Try it in the Numerade app?
Concepts of Genetics
William s. klug, michael r. cummings, charlotte a. spencer, mendelian genetics - all with video answers.
Chapter Questions
In this chapter, we focused on the Mendelian postulates, probability, and pedigree analysis. We also considered some of the methods and reasoning by which these ideas, concepts, and techniques were developed. On the basis of these discussions, what answers would you propose to the following questions: (a) How was Mendel able to derive postulates concerning the behavior of "unit factors" during gamete formation, when he could not directly observe them? (b) How do we know whether an organism expressing a dominant trait is homozygous or heterozygous? (c) In analyzing genetic data, how do we know whether deviation from the expected ratio is due to chance rather than to another, independent factor? (d) since experimental crosses are not performed in humans, how do we know how traits are inherited?
Review the Chapter Concepts list on $\mathrm{p}$. 74 . The first five concepts provide a modern interpretation of Mendelian postulates. Based on these concepts, write a short essay that correlates Mendel's four postulates with what is now known about genes, alleles, and homologous chromosomes.
Albinism in humans is inherited as a simple recessive trait. For the following families, determine the genotypes of the parents and offspring. (When two alternative genotypes are possible, list both.) (a) Two normal parents have five children, four normal and one albino. (b) A normal male and an albino female have six children, all normal. (c) A normal male and an albino female have six children, three normal and three albino. (d) Construct a pedigree of the families in (b) and (c). Assume that one of the normal children in (b) and one of the albino children in (c) become the parents of eight children. Add these children to the pedigree, predicting their phenotypes (normal or albino).
Which of Mendel's postulates are illustrated by the pedigree that you constructed in Problem 3 ? List and define these postulates.
Discuss how Mendel's monohybrid results served as the basis for all but one of his postulates. Which postulate was not based on these results? Why?
What advantages were provided by Mendel's choice of the garden pea in his experiments?
Mendel crossed peas having round seeds and yellow cotyledons (seed leaves) with peas having wrinkled seeds and green cotyledons. All the $F_{1}$ plants had round seeds with yellow cotyledons. Diagram this cross through the $\mathrm{F}_{2}$ generation, using both the Punnett square and forked-line, or branch diagram, methods.
Based on the preceding cross, what is the probability that an organism in the $\mathrm{F}_{2}$ generation will have round seeds and green cotyledons and be true breeding?
Which of Mendel's postulates can only be demonstrated in crosses involving at least two pairs of traits? State the postulate.
Assume that you have a garden and some pea plants have solid leaves and others have striped leaves. You conduct a series of crosses $[(a) \text { through }(e)]$ and obtain the results given in the table. Define gene symbols and give the possible genotypes of the parents of each cross.
What is the basis for homology among chromosomes?
Two organisms, $A A B B C C D D E E$ and aabbccddee, are mated to produce an $\mathrm{F}_{1}$ that is self-fertilized. If the capital letters represent dominant, independently assorting alleles: (a) How many different genotypes will occur in the $\mathrm{F}_{2}$ ? (b) What proportion of the $\mathrm{F}_{2}$ genotypes will be recessive for all five loci? (c) Would you change your answers to (a) and/or (b) if the initial cross occurred between $A A b b C C$ddee$\times$aaBBccDDEE parents? (d) Would you change your answers to (a) and/or (b) if the initial cross occurred between $A A B B C C D D E E \times$ aabbccddEE parents?
Albinism, lack of pigmentation in humans, results from an autosomal recessive gene (a). Two parents with normal pigmentation have an albino child. (a) What is the probability that their next child will be albino? (b) What is the probability that their next child will be an albino girl? (c) What is the probability that their next three children will be albino?
Dentinogenesis imperfecta is a rare, autosomal, dominantly inherited disease of the teeth that occurs in about one in 8000 people (Witkop 1957 ). The teeth are somewhat brown in color, and the crowns wear down rapidly. Assume that a male with dentinogenesis imperfecta and no family history of the disease marries a woman with normal teeth. What is the probability that (a) their first child will have dentinogenesis imperfecta? (b) their first two children will have dentinogenesis imperfecta? (c) their first child will be a girl with dentinogenesis imperfecta?
In a study of black guinea pigs and white guinea pigs, 100 black animals were crossed with 100 white animals, and each cross was carried to an $\mathrm{F}_{2}$ generation. In 94 of the crosses, all the $\mathrm{F}_{1}$ offspring were black and an $\mathrm{F}_{2}$ ratio of 3 black: 1 white was obtained. In the other 6 cases, half of the $\mathrm{F}_{1}$ animals were black and the other half were white. Why? Predict the results of crossing the black and white $\mathrm{F}_{1}$ guinea pigs from the 6 exceptional cases.
Mendel crossed peas having round green seeds with peas having wrinkled yellow seeds. All $\mathrm{F}_{1}$ plants had seeds that were round and yellow. Predict the results of testcrossing these $\mathrm{F}_{1}$ plants.
Thalassemia is an inherited anemic disorder in humans. Affected individuals exhibit either a minor anemia or a major anemia. Assuming that only a single gene pair and two alleles are involved in the inheritance of these conditions, is thalassemia a dominant or recessive disorder?
A certain type of congenital deafness in humans is caused by a rare autosomal (not X-linked) dominant gene. (a) In a mating involving a deaf man and a deaf woman (both heterozygous), would you expect all the children to be deaf? Explain your answer. (b) In a mating involving a deaf man and a deaf woman (both heterozygous), could all the children have normal hearing? Explain your answer. (c) Another form of deafness is caused by a rare autosomal recessive gene. In a mating involving a deaf man and a deaf woman, could some of the children have normal hearing? Explain your answer.
In assessing data that fell into two phenotypic classes, a geneticist observed values of $250: 150 .$ She decided to perform a $\chi^{2}$ analysis by using the following two different null hypotheses: (a) the data fit a 3: 1 ratio, and (b) the data fit a 1: 1 ratio. Calculate the $\chi^{2}$ values for each hypothesis. What can be concluded about each hypothesis?
The basis for rejecting any null hypothesis is arbitrary. The researcher can set more or less stringent standards by deciding to raise or lower the $p$ value used to reject or not reject the hypothesis. In the case of the chi-square analysis of genetic crosses, would the use of a standard of $p=0.10$ be more or less stringent about not rejecting the null hypothesis? Explain.
Among dogs, short hair is dominant to long hair and dark coat color is dominant to white (albino) coat color. Assume that these two coat traits are caused by independently segregating gene pairs. For each of the crosses given below, write the most probable genotype (or genotypes if more than one answer is possible for the parents. It is important that you select a realistic symbol set and define each symbol below. Assume that for cross (d), you were interested in determining whether fur color follows a 3: 1 ratio. Set up (but do not complete the calculations) a Chi-square test for these data [fur color in cross $(\mathrm{d})]$.
Draw all possible conclusions concerning the mode of inheritance of the trait portrayed in each of the following limited pedigrees. (Each of the four cases is based on a different trait.) a. b. c. d.
In a family of eight children, what is the probability that (a) the third child is a girl? (b) six of the children are boys? (c) all the children are girls? (d) there are four boys and four girls? Assume that the probability of having a boy is equal to the probability of having a girl $(p=1 / 2)$.
In a family of six children, where one grandparent on either side has red hair, what mathematical expression predicts the probability that two of the children have red hair?
The autosomal (not X-linked) gene for brachydactyly, short fingers, is dominant to normal finger length. Assume that a female with brachydactyly in the heterozygous condition is married to a man with normal fingers. What is the probability that (a) their first child will have brachydactyly? (b) their first two children will have brachydactyly? (c) their first child will be a brachydactylous girl?
Galactosemia is a rare recessive disorder caused by the deficiency of galactose- 1 -phosphate uridylyltransferase, leading to the accumulation of toxic levels of galactitol in the blood. It leads to a $75 \%$ mortality rate in infants as infants cannot metabolize galactose from breast milk. In many countries, newborns are given a heel prick test to measure the levels of metabolic enzymes. As a genetic counselor, how would you explain to a couple whose baby has tested positive for galactosemia where the disease has come from?
Two true-breeding pea plants were crossed. One parent is round, terminal, violet, constricted, while the other expresses the respective contrasting phenotypes of wrinkled, axial, white, full. The four pairs of contrasting traits are controlled by four genes, each located on a separate chromosome. In the $\mathrm{F}_{1}$ only round, axial, violet, and full were expressed. In the $\mathrm{F}_{2},$ all possible combinations of these traits were expressed in ratios consistent with Mendelian inheritance. (a) What conclusion about the inheritance of the traits can be drawn based on the $\mathrm{F}_{1}$ results? (b) In the $\mathrm{F}_{2}$ results, which phenotype appeared most frequently? Write a mathematical expression that predicts the probability of occurrence of this phenotype. (c) Which $\mathrm{F}_{2}$ phenotype is expected to occur least frequently? Write a mathematical expression that predicts this probability. (d) In the $F_{2}$ generation, how often is either of the $P_{1}$ phenotypes likely to occur? (e) If the $F_{1}$ plants were testcrossed, how many different phenotypes would be produced? How does this number compare with the number of different phenotypes in the $\mathrm{F}_{2}$ generation just discussed?
Tay-Sachs disease (TSD) is an inborn error of metabolism that results in death, often by the age of $2 .$ You are a genetic counselor interviewing a phenotypically normal couple who tell you the male had a female first cousin (on his father's side) who died from TSD and the female had a maternal uncle with TSD. There are no other known cases in either of the families, and none of the matings have been between related individuals. Assume that this trait is very rare. (a) Draw a pedigree of the families of this couple, showing the relevant individuals. (b) Calculate the probability that both the male and female are carriers for TSD. (c) What is the probability that neither of them is a carrier? (d) What is the probability that one of them is a carrier and the other is not? [Hint: The $p$ values in (b), (c), and (d) should equal $1 .]$
Datura stramonium (the Jimsonweed) expresses flower colors of purple and white and pod textures of smooth and spiny. The results of two crosses in which the parents were not necessarily true breeding are shown at the top of the next column. (a) Based on these results, put forward a hypothesis for the inheritance of the purple/white and smooth/spiny traits. (b) Assuming that true-breeding strains of all combinations of traits are available, what single cross could you execute and carry to an $\mathrm{F}_{2}$ generation that will prove or disprove your hypothesis? Assuming your hypothesis is correct, what results of this cross will support it??
The wild-type (normal) fruit fly, Drosophila melanogaster, has straight wings and long bristles. Mutant strains have been isolated that have either curled wings or short bristles. The genes representing these two mutant traits are located on separate chromosomes. Carefully examine the data from the five crosses shown on the top of the following page (running across both columns). (a) Identify each mutation as either dominant or recessive. In each case, indicate which crosses support your answer. (b) Assign gene symbols and, for each cross, determine the genotypes of the parents.
An alternative to using the expanded binomial equation and Pascal's triangle in determining probabilities of phenotypes in a subsequent generation when the parents' genotypes are known is to use the following equation: $\frac{n !}{s ! t !} a^{s} b^{t}$ where $n$ is the total number of offspring, $s$ is the number of offspring in one phenotypic category, $t$ is the number of offspring in the other phenotypic category, $a$ is the probability of occurrence of the first phenotype, and $b$ is the probability of the second phenotype. Using this equation, determine the probability of a family of 5 offspring having exactly 2 children afflicted with sickle-cell anemia (an autosomal recessive disease $)$ when both parents are heterozygous for the sickle-cell allele.
To assess Mendel's law of segregation using tomatoes, a truebreeding tall variety (SS) is crossed with a true-breeding short variety $(s s) .$ The heterozygous $F_{1}$ tall plants $(S s)$ were crossed to produce two sets of $\mathrm{F}_{2}$ data, as follows. $\begin{array}{cc}\text { Set I } & \text { Set II } \\ 30 \text { tall } & 300 \text { tall } \\ 5 \text { short } & 50 \text { short }\end{array}$ (a) Using the $\chi^{2}$ test, analyze the results for both datasets. Calculate $\chi^{2}$ values and estimate the $p$ values in both cases. (b) From the above analysis, what can you conclude about the importance of generating large datasets in experimental conditions?
Albinism, caused by a mutational disruption in melanin (skin pigment production, has been observed in many species, including humans. In 1991 , the only documented observation of an albino humpback whale (named "Migaloo") was observed near New South Wales. Recently, Polanowski and coworkers (Polanowski, A., S. Robinson-Laverick, and D. Paton. 2012. Journal of Heredity $103: 130-133$ ) studied the genetics of humpback whales from the east coast of Australia, including Migaloo. (a) Do you think that Migaloo's albinism is more likely caused by a dominant or recessive mutation? Explain your reasoning. (b) What data would be helpful in determining the answer to part (a)?
(a) Assuming that Migaloo's albinism is caused by a rare recessive gene, what would be the likelihood of the establishment of a natural robust subpopulation of albino white humpback whales in this population? (b) Assuming that Migaloo's albinism is caused by a rare dominant gene, what would be the likelihood of the establishment of a natural robust subpopulation of albino white humpback whales in this population?
Assume that Migaloo's albinism is caused by a rare recessive gene. (a) In a mating of two heterozygous, normally pigmented whales, what is the probability that the first three offspring will all have normal pigmentation? (b) What is the probability that the first female offspring is normally pigmented? (c) What is the probability that the first offspring is a normally pigmented female?
Dentinogenesis imperfecta is a tooth disorder involving the production of dentin sialophosphoprotein, a bone-like component of the protective middle layer of teeth. The trait is inherited as an autosomal dominant allele located on chromosome 4 in humans and occurs in about 1 in 6000 to 8000 people. Assume that a man with dentinogenesis imperfecta, whose father had the disease but whose mother had normal teeth, married a woman with normal teeth. They have six children. What is the probability that their first child will be a male with dentinogenesis imperfecta? What is the probability that three of their six chil- dren will have the disease?
Thank you for visiting nature.com. You are using a browser version with limited support for CSS. To obtain the best experience, we recommend you use a more up to date browser (or turn off compatibility mode in Internet Explorer). In the meantime, to ensure continued support, we are displaying the site without styles and JavaScript.
- View all journals
- Explore content
- About the journal
- Publish with us
- Sign up for alerts
- Review Article
- Published: 20 February 2023
Mendelian inheritance revisited: dominance and recessiveness in medical genetics
- Johannes Zschocke ORCID: orcid.org/0000-0002-0046-8274 1 ,
- Peter H. Byers ORCID: orcid.org/0000-0001-7786-7030 2 , 3 &
- Andrew O. M. Wilkie ORCID: orcid.org/0000-0002-2972-5481 4
Nature Reviews Genetics volume 24 , pages 442–463 ( 2023 ) Cite this article
12 Citations
65 Altmetric
Metrics details
- Disease genetics
- Genetic variation
- Medical genetics
Understanding the consequences of genotype for phenotype (which ranges from molecule-level effects to whole-organism traits) is at the core of genetic diagnostics in medicine. Many measures of the deleteriousness of individual alleles exist, but these have limitations for predicting the clinical consequences. Various mechanisms can protect the organism from the adverse effects of functional variants, especially when the variant is paired with a wild type allele. Understanding why some alleles are harmful in the heterozygous state — representing dominant inheritance — but others only with the biallelic presence of pathogenic variants — representing recessive inheritance — is particularly important when faced with the deluge of rare genetic alterations identified by high throughput DNA sequencing. Both awareness of the specific quantitative and/or qualitative effects of individual variants and the elucidation of allelic and non-allelic interactions are essential to optimize genetic diagnosis and counselling.
This is a preview of subscription content, access via your institution
Access options
Access Nature and 54 other Nature Portfolio journals
Get Nature+, our best-value online-access subscription
24,99 € / 30 days
cancel any time
Subscribe to this journal
Receive 12 print issues and online access
176,64 € per year
only 14,72 € per issue
Buy this article
- Purchase on Springer Link
- Instant access to full article PDF
Prices may be subject to local taxes which are calculated during checkout
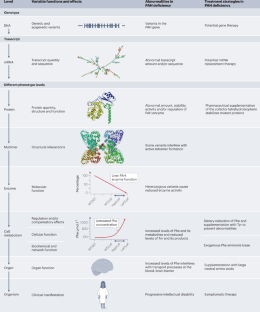
Similar content being viewed by others
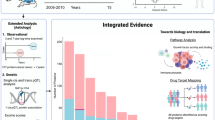
Identifying proteomic risk factors for cancer using prospective and exome analyses of 1463 circulating proteins and risk of 19 cancers in the UK Biobank
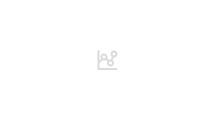
A deep catalogue of protein-coding variation in 983,578 individuals
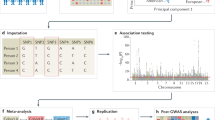
Genome-wide association studies
Mendel, G. Versuche über Pflanzenhybriden [German]. Verh. Naturforschenden Vereines Brünn. 4 , 3–47 (1866). English translation in Bateson, W. Mendel’s Principles of Heredity: A Defence. (Cambridge University Press, 1902).
Google Scholar
Zschocke, J., Byers, P. H. & Wilkie, A. O. M. Gregor Mendel and the concepts of dominance and recessiveness. Nat. Rev. Genet. 23 , 387–388 (2022).
Article CAS PubMed Google Scholar
Bateson, W. & Saunders, E. R. The facts of heredity in the light of Mendel’s discovery. Rep. Evol. Comm. R. Soc. 1 , 125–160 (1902).
Garrod, A. E. The incidence of alkaptonuria: a study in chemical individuality. Lancet 2 , 1616–1620 (1902).
Article CAS Google Scholar
McKusick, V. A. Mendelian Inheritance in Man (Johns Hopkins Univ. Press, 1966).
Nguengang Wakap, S. et al. Estimating cumulative point prevalence of rare diseases: analysis of the Orphanet database. Eur. J. Hum. Genet. 28 , 165–173 (2020).
Article PubMed Google Scholar
Van Hout, C. V. et al. Exome sequencing and characterization of 49,960 individuals in the UK Biobank. Nature 586 , 749–756 (2020). This article summarizes exome sequence data from almost 50,000 individuals in the UK biobank, including information on the prevalence of LoF variants and pathogenic variants of clinical importance per participant in the general population .
Article PubMed PubMed Central Google Scholar
Karczewski, K. J. et al. The mutational constraint spectrum quantified from variation in 141,456 humans. Nature 581 , 434–443 (2020). This study aggregates human sequence data from the Genome Aggregation Database (gnomAD), and describes the LOF observed/expected upper bound fraction score for estimating the likelihood of haplosufficiency versus haploinsufficiency for most protein-coding genes .
Article CAS PubMed PubMed Central Google Scholar
Collins, R. L. et al. A structural variation reference for medical and population genetics. Nature 581 , 444–451 (2020). This article describes a sequence-resolved analysis of all types of structural variants in almost 15,000 genomes across diverse global populations in gnomAD, and provides estimates of dosage sensitivity in the non-coding genome .
Muller, H. J. in Proc. Sixth Int. Congress of Genetics (ed. Jones, D. F.) 213–255 (Brooklyn Botanic Gardens, 1932).
Morgan, T. H., Bridges, C. B. & Sturtevant, A. H. The genetics of Drosophila . Bibliographica Genet. II , 1–262 (1925).
Orr, H. A. A test of Fisher’s theory of dominance. Proc. Natl Acad. Sci. USA 88 , 11413–11415 (1991).
Deutschbauer, A. M. et al. Mechanisms of haploinsufficiency revealed by genome-wide profiling in yeast. Genetics 169 , 1915–1925 (2005).
Lek, M. et al. Analysis of protein-coding genetic variation in 60,706 humans. Nature 536 , 285–291 (2016).
Minikel, E. V. et al. Evaluating drug targets through human loss-of-function genetic variation. Nature 581 , 459–464 (2020).
Balick, D. J., Jordan, D. M., Sunyaev, S. & Do, R. Overcoming constraints on the detection of recessive selection in human genes from population frequency data. Am. J. Hum. Genet. 109 , 33–49 (2022).
Backman, J. D. et al. Exome sequencing and analysis of 454,787 UK Biobank participants. Nature 599 , 628–634 (2021).
Cummings, B. B. et al. Transcript expression-aware annotation improves rare variant interpretation. Nature 581 , 452–458 (2020).
Cody, J. D. The consequences of abnormal gene dosage: lessons from chromosome 18. Trends Genet. 36 , 764–776 (2020).
Barton, A. R., Hujoel, M. L. A., Mukamel, R. E., Sherman, M. A. & Loh, P. R. A spectrum of recessiveness among Mendelian disease variants in UK Biobank. Am. J. Hum. Genet. 109 , 1298–1307 (2022).
Kingdom, R. et al. Rare genetic variants in genes and loci linked to dominant monogenic developmental disorders cause milder related phenotypes in the general population. Am. J. Hum. Genet. 109 , 1308–1316 (2022).
Fisher, R. A. The possible modification of the response of the wild type to recurrent mutations. Am. Nat. 62 , 679 (1928).
Article Google Scholar
Wright, S. Physiological and evolutionary theories of dominance. Am. Nat. 68 , 24–53 (1934).
Kacser, H. & Burns, J. A. The molecular basis of dominance. Genetics 97 , 639–666 (1981).
Omholt, S. W., Plahte, E., Oyehaug, L. & Xiang, K. Gene regulatory networks generating the phenomena of additivity, dominance and epistasis. Genetics 155 , 969–980 (2000).
Ishikawa, K., Makanae, K., Iwasaki, S., Ingolia, N. T. & Moriya, H. Post-translational dosage compensation buffers genetic perturbations to stoichiometry of protein complexes. PLoS Genet. 13 , e1006554 (2017).
Khan, Z. et al. Primate transcript and protein expression levels evolve under compensatory selection pressures. Science 342 , 1100–1104 (2013).
Kondrashov, F. A. & Koonin, E. V. A common framework for understanding the origin of genetic dominance and evolutionary fates of gene duplications. Trends Genet. 20 , 287–290 (2004).
Morrill, S. A. & Amon, A. Why haploinsufficiency persists. Proc. Natl Acad. Sci. USA 116 , 11866–11871 (2019). This paper analyses the functional characteristics of haploinsufficient genes, and proposes a dosage-stabilizing hypothesis of haploinsufficiency to explain its persistence over evolutionary time .
Ni, Z., Zhou, X. Y., Aslam, S. & Niu, D. K. Characterization of human dosage-sensitive transcription factor genes. Front. Genet. 10 , 1208 (2019).
Gilchrist, M. A. & Nijhout, H. F. Nonlinear developmental processes as sources of dominance. Genetics 159 , 423–432 (2001).
Veitia, R. A., Caburet, S. & Birchler, J. A. Mechanisms of Mendelian dominance. Clin. Genet. 93 , 419–428 (2018).
Johnson, A. F., Nguyen, H. T. & Veitia, R. A. Causes and effects of haploinsufficiency. Biol. Rev. Camb. Philos. Soc. 94 , 1774–1785 (2019).
Veitia, R. A. A generalized model of gene dosage and dominant negative effects in macromolecular complexes. FASEB J. 24 , 994–1002 (2010).
Thaxton, C. et al. Utilizing ClinGen gene–disease validity and dosage sensitivity curations to inform variant classification. Hum. Mutat. 43, 1031-1040 (2021).
Sopko, R. et al. Mapping pathways and phenotypes by systematic gene overexpression. Mol. Cell 21 , 319–330 (2006).
Antonarakis, S. E. Down syndrome and the complexity of genome dosage imbalance. Nat. Rev. Genet. 18 , 147–163 (2017).
Abel, H. J. et al. Mapping and characterization of structural variation in 17,795 human genomes. Nature 583 , 83–89 (2020).
Collins, R. L. et al. A cross-disorder dosage sensitivity map of the human genome. Cell 185 , 3041–3055.e25 (2022).
van Paassen, B. W. et al. PMP22 related neuropathies: Charcot–Marie–Tooth disease type 1A and hereditary neuropathy with liability to pressure palsies. Orphanet J. Rare Dis. 9 , 38 (2014).
Vavouri, T., Semple, J. I., Garcia-Verdugo, R. & Lehner, B. Intrinsic protein disorder and interaction promiscuity are widely associated with dosage sensitivity. Cell 138 , 198–208 (2009).
Stoebel, D. M., Dean, A. M. & Dykhuizen, D. E. The cost of expression of Escherichia coli lac operon proteins is in the process, not in the products. Genetics 178 , 1653–1660 (2008).
Makanae, K., Kintaka, R., Makino, T., Kitano, H. & Moriya, H. Identification of dosage-sensitive genes in Saccharomyces cerevisiae using the genetic tug-of-war method. Genome Res. 23 , 300–311 (2013).
Boyle, E. A., Li, Y. I. & Pritchard, J. K. An expanded view of complex traits: from polygenic to omnigenic. Cell 169 , 1177–1186 (2017).
Rice, A. M. & McLysaght, A. Dosage-sensitive genes in evolution and disease. BMC Biol. 15 , 78 (2017).
Rudnik-Schöneborn, S. et al. Andrological findings in infertile men with two (biallelic) CFTR mutations: results of a multicentre study in Germany and Austria comprising 71 patients. Hum. Reprod. 36 , 551–559 (2021).
Guldberg, P. et al. A European multicenter study of phenylalanine hydroxylase deficiency: classification of 105 mutations and a general system for genotype-based prediction of metabolic phenotype. Am. J. Hum. Genet. 63 , 71–79 (1998).
Ramsey, B. W. et al. A CFTR potentiator in patients with cystic fibrosis and the G551D mutation. N. Engl. J. Med. 365 , 1663–1672 (2011).
Carlock, G. et al. Developmental outcomes in duarte galactosemia. Pediatrics 143, e20182516 (2019).
Gouya, L. et al. The penetrance of dominant erythropoietic protoporphyria is modulated by expression of wildtype FECH. Nat. Genet. 30 , 27–28 (2002).
Rose, A. M. et al. Transcriptional regulation of PRPF31 gene expression by MSR1 repeat elements causes incomplete penetrance in retinitis pigmentosa. Sci. Rep. 6 , 19450 (2016).
Falkenberg, K. D. et al. Allelic expression imbalance promoting a mutant PEX6 allele causes Zellweger spectrum disorder. Am. J. Hum. Genet. 101 , 965–976 (2017).
Boussion, S. et al. TAR syndrome: clinical and molecular characterization of a cohort of 26 patients and description of novel noncoding variants of RBM8A. Hum. Mutat. 41 , 1220–1225 (2020).
Daum, H. et al. Smith–Lemli–Opitz syndrome: what is the actual risk for couples carriers of the DHCR7:c.964-1G>C variant? Eur. J. Hum. Genet. 28 , 938–942 (2020).
Plomin, R., Haworth, C. M. & Davis, O. S. Common disorders are quantitative traits. Nat. Rev. Genet. 10 , 872–878 (2009).
Deciphering Developmental Disorders Study. Prevalence and architecture of de novo mutations in developmental disorders. Nature 542 , 433–438 (2017). This study describes an analysis and meta-analysis of de novo pathogenic variants in more than 7,500 individuals with developmental disorders, estimation of their prevalence in the general population and the relative frequency of variants with quantitative and qualitative pathogenic effects, and identification of factors that influence the diagnostic yield .
Gröbner, R. et al. C1R mutations trigger constitutive complement 1 activation in periodontal Ehlers–Danlos syndrome. Front. Immunol. 10 , 2537 (2019).
Ilsley, M. D. et al. Corrupted DNA-binding specificity and ectopic transcription underpin dominant neomorphic mutations in KLF/SP transcription factors. BMC Genomics 20 , 417 (2019).
Otonkoski, T. et al. Physical exercise-induced hypoglycemia caused by failed silencing of monocarboxylate transporter 1 in pancreatic β cells. Am. J. Hum. Genet. 81 , 467–474 (2007).
Koyano-Nakagawa, N. et al. Etv2 regulates enhancer chromatin status to initiate Shh expression in the limb bud. Nat. Commun. 13 , 4221 (2022).
Oldridge, M. et al. De novo alu-element insertions in FGFR2 identify a distinct pathological basis for Apert syndrome. Am. J. Hum. Genet. 64 , 446–461 (1999).
Yu, K. & Ornitz, D. M. Uncoupling fibroblast growth factor receptor 2 ligand binding specificity leads to Apert syndrome-like phenotypes. Proc. Natl Acad. Sci. USA 98 , 3641–3643 (2001).
Tsuji, Y. et al. Systematic review of genotype–phenotype correlations in Frasier syndrome. Kidney Int. Rep. 6 , 2585–2593 (2021).
Swinnen, B., Robberecht, W. & Van Den Bosch, L. RNA toxicity in non-coding repeat expansion disorders. EMBO J. 39 , e101112 (2020).
Malik, I., Kelley, C. P., Wang, E. T. & Todd, P. K. Molecular mechanisms underlying nucleotide repeat expansion disorders. Nat. Rev. Mol. Cell Biol. 22 , 589–607 (2021).
Chiti, F. & Dobson, C. M. Protein misfolding, amyloid formation, and human disease: a summary of progress over the last decade. Annu. Rev. Biochem. 86 , 27–68 (2017).
Zlotogora, J. Dominance and homozygosity. Am. J. Med. Genet. 68 , 412–416 (1997).
Cubo, E. et al. Clinical manifestations of homozygote allele carriers in Huntington disease. Neurology 92 , e2101–e2108 (2019).
CAS PubMed Google Scholar
Tabrizi, S. J., Flower, M. D., Ross, C. A. & Wild, E. J. Huntington disease: new insights into molecular pathogenesis and therapeutic opportunities. Nat. Rev. Neurol. 16 , 529–546 (2020).
Jamilloux, Y. et al. Familial Mediterranean fever mutations are hypermorphic mutations that specifically decrease the activation threshold of the Pyrin inflammasome. Rheumatology 57 , 100–111 (2018).
Jeyakanthan, M. et al. Chemical basis for qualitative and quantitative differences between ABO blood groups and subgroups: implications for organ transplantation. Am. J. Transplant. 15 , 2602–2615 (2015).
Johnson, W. G. Metabolic interference and the +– heterozygote. a hypothetical form of simple inheritance which is neither dominant nor recessive. Am. J. Hum. Genet. 32 , 374–386 (1980).
CAS PubMed PubMed Central Google Scholar
Morissette, J. et al. Homozygotes carrying an autosomal dominant TIGR mutation do not manifest glaucoma. Nat. Genet. 19 , 319–321 (1998).
Huard, D. J. E. et al. Trifunctional high-throughput screen identifies promising scaffold to inhibit Grp94 and treat myocilin-associated glaucoma. ACS Chem. Biol. 13 , 933–941 (2018).
Brownlee, J. M., Heinz, B., Bates, J. & Moran, G. R. Product analysis and inhibition studies of a causative Asn to Ser variant of 4-hydroxyphenylpyruvate dioxygenase suggest a simple route to the treatment of Hawkinsinuria. Biochemistry 49 , 7218–7226 (2010).
Xu, D. et al. The evolving landscape of noncanonical functions of metabolic enzymes in cancer and other pathologies. Cell Metab. 33 , 33–50 (2021).
Pan, C., Li, B. & Simon, M. C. Moonlighting functions of metabolic enzymes and metabolites in cancer. Mol. Cell 81 , 3760–3774 (2021).
Baralle, F. E. & Giudice, J. Alternative splicing as a regulator of development and tissue identity. Nat. Rev. Mol. Cell Biol. 18 , 437–451 (2017).
Kim, H. K., Pham, M. H. C., Ko, K. S., Rhee, B. D. & Han, J. Alternative splicing isoforms in health and disease. Pflug. Arch. Eur. J. Physiol. 470 , 995–1016 (2018).
Gostyńska, K. B. et al. Mutation in exon 1a of PLEC, leading to disruption of plectin isoform 1a, causes autosomal-recessive skin-only epidermolysis bullosa simplex. Hum. Mol. Genet. 24 , 3155–3162 (2015).
Seo, A. et al. Mechanism for survival of homozygous nonsense mutations in the tumor suppressor gene BRCA1. Proc. Natl Acad. Sci. USA 115 , 5241–5246 (2018).
Mesman, R. L. S. et al. Alternative mRNA splicing can attenuate the pathogenicity of presumed loss-of-function variants in BRCA2. Genet. Med. 22 , 1355–1365 (2020).
Li, J. et al. Point mutations in exon 1B of APC reveal gastric adenocarcinoma and proximal polyposis of the stomach as a familial adenomatous polyposis variant. Am. J. Hum. Genet. 98 , 830–842 (2016).
Perenthaler, E. et al. Loss of UGP2 in brain leads to a severe epileptic encephalopathy, emphasizing that bi-allelic isoform-specific start-loss mutations of essential genes can cause genetic diseases. Acta Neuropathol. 139 , 415–442 (2020).
Dik, E., Naamati, A., Asraf, H., Lehming, N. & Pines, O. Human fumarate hydratase is dual localized by an alternative transcription initiation mechanism. Traffic 17 , 720–732 (2016).
Sternisha, S. M. & Miller, B. G. Molecular and cellular regulation of human glucokinase. Arch. Biochem. Biophys. 663 , 199–213 (2019).
Ferdinandusse, S. et al. An autosomal dominant neurological disorder caused by de novo variants in FAR1 resulting in uncontrolled synthesis of ether lipids. Genet. Med. 23 , 740–750 (2021).
Salvatore, D., Santoro, M. & Schlumberger, M. The importance of the RET gene in thyroid cancer and therapeutic implications. Nat. Rev. Endocrinol. 17 , 296–306 (2021).
Arighi, E. et al. Biological effects of the dual phenotypic Janus mutation of ret cosegregating with both multiple endocrine neoplasia type 2 and Hirschsprung’s disease. Mol. Endocrinol. 18 , 1004–1017 (2004).
Nicole, S. & Lory, P. New challenges resulting from the loss of function of Na(v)1.4 in neuromuscular diseases. Front. Pharmacol. 12 , 751095 (2021).
Liu, M., Yang, K. C. & Dudley, S. C. Jr Cardiac sodium channel mutations: why so many phenotypes? Nat. Rev. Cardiol. 11 , 607–615 (2014). This paper provides an exemplary review of the diverse functional effects of different pathogenic variants in the major cardiac sodium channel gene, based on the direct effect of the mutation on channel biophysics as well as age, sex, body temperature, cardiac regions and additional modifiers of channel behaviour .
Wiuf, C. Do ΔF508 heterozygotes have a selective advantage? Genet. Res. 78 , 41–47 (2001).
Allison, A. C. Polymorphism and natural selection in human populations. Cold Spring Harb. Symp. Quant. Biol. 29 , 137–149 (1964).
Krawczak, M. & Zschocke, J. A role for overdominant selection in phenylketonuria? Evidence from molecular data. Hum. Mutat. 21 , 394–397 (2003).
Miller, A. C. et al. Cystic fibrosis carriers are at increased risk for a wide range of cystic fibrosis-related conditions. Proc. Natl Acad. Sci. USA 117 , 1621–1627 (2020).
Penrose, L. S. The problem of anticipation in pedigrees of dystrophia myotonica. Ann. Eugen. 14 , 125–132 (1948).
Shay, J. W. & Wright, W. E. Telomeres and telomerase: three decades of progress. Nat. Rev. Genet. 20 , 299–309 (2019).
Vulliamy, T. et al. Disease anticipation is associated with progressive telomere shortening in families with dyskeratosis congenita due to mutations in TERC. Nat. Genet. 36 , 447–449 (2004).
Mangaonkar, A. A. & Patnaik, M. M. Short telomere syndromes in clinical practice: bridging bench and bedside. Mayo Clin. Proc. 93 , 904–916 (2018).
Monk, D., Mackay, D. J. G., Eggermann, T., Maher, E. R. & Riccio, A. Genomic imprinting disorders: lessons on how genome, epigenome and environment interact. Nat. Rev. Genet. 20 , 235–248 (2019).
Buiting, K., Williams, C. & Horsthemke, B. Angelman syndrome — insights into a rare neurogenetic disorder. Nat. Rev. Neurol. 12 , 584–593 (2016).
Cooper, D. N., Krawczak, M., Polychronakos, C., Tyler-Smith, C. & Kehrer-Sawatzki, H. Where genotype is not predictive of phenotype: towards an understanding of the molecular basis of reduced penetrance in human inherited disease. Hum. Genet. 132 , 1077–1130 (2013).
Martinelli, S. et al. Functional dysregulation of CDC42 causes diverse developmental phenotypes. Am. J. Hum. Genet. 102 , 309–320 (2018).
Cohen, A. S., Wilson, S. L., Trinh, J. & Ye, X. C. Detecting somatic mosaicism: considerations and clinical implications. Clin. Genet. 87 , 554–562 (2015).
Biesecker, L. G. & Spinner, N. B. A genomic view of mosaicism and human disease. Nat. Rev. Genet. 14 , 307–320 (2013).
Smedley, D. et al. A whole-genome analysis framework for effective identification of pathogenic regulatory variants in Mendelian disease. Am. J. Hum. Genet. 99 , 595–606 (2016).
Turner, H. & Jackson, L. Evidence for penetrance in patients without a family history of disease: a systematic review. Eur. J. Hum. Genet. 28 , 539–550 (2020).
Niemi, M. E. K. et al. Common genetic variants contribute to risk of rare severe neurodevelopmental disorders. Nature 562 , 268–271 (2018).
Goodrich, J. K. et al. Determinants of penetrance and variable expressivity in monogenic metabolic conditions across 77,184 exomes. Nat. Commun. 12 , 3505 (2021).
Fallerini, C. et al. Common, low-frequency, rare, and ultra-rare coding variants contribute to COVID-19 severity. Hum. Genet. 141 , 147–173 (2022).
Mars, N. et al. The role of polygenic risk and susceptibility genes in breast cancer over the course of life. Nat. Commun. 11 , 6383 (2020).
Schäffer, A. A. Digenic inheritance in medical genetics. J. Med. Genet. 50 , 641–652 (2013).
Deltas, C. Digenic inheritance and genetic modifiers. Clin. Genet. 93 , 429–438 (2018).
Jirsa, M. et al. Rotor syndrome in GeneReviews [Internet] (Univ. of Washington, 2019).
Bateson, W. Facts limiting the theory of heredity. Science 26 , 649–660 (1907).
Miko, I. Epistasis: gene interaction and phenotype effects. Nat. Educ. 1 , 197 (2008).
Phillips, P. C. Epistasis — the essential role of gene interactions in the structure and evolution of genetic systems. Nat. Rev. Genet. 9 , 855–867 (2008).
Kelly, R. J. et al. Molecular basis for H blood group deficiency in Bombay (Oh) and para-Bombay individuals. Proc. Natl Acad. Sci. USA 91 , 5843–5847 (1994).
Estiar, M. A. et al. Evidence for non-Mendelian inheritance in spastic paraplegia 7. Mov. Disord. 36 , 1664–1675 (2021).
Vockley, J. et al. Complex patterns of inheritance, including synergistic heterozygosity, in inborn errors of metabolism: implications for precision medicine driven diagnosis and treatment. Mol. Genet. Metab. 128 , 1–9 (2019).
Wei, W. H., Hemani, G. & Haley, C. S. Detecting epistasis in human complex traits. Nat. Rev. Genet. 15 , 722–733 (2014).
Hivert, V. et al. Estimation of non-additive genetic variance in human complex traits from a large sample of unrelated individuals. Am. J. Hum. Genet. 108 , 786–798 (2021).
Knudson, A. G. Jr Mutation and cancer: statistical study of retinoblastoma. Proc. Natl Acad. Sci. USA 68 , 820–823 (1971).
Turnbull, C., Sud, A. & Houlston, R. S. Cancer genetics, precision prevention and a call to action. Nat. Genet. 50 , 1212–1218 (2018).
Fernandez-Rozadilla, C. et al. Early colorectal cancers provide new evidence for a Lynch syndrome-to-CMMRD phenotypic continuum. Cancers 11, 1081 (2019).
Verkarre, V. et al. Paternal mutation of the sulfonylurea receptor ( SUR1 ) gene and maternal loss of 11p15 imprinted genes lead to persistent hyperinsulinism in focal adenomatous hyperplasia. J. Clin. Invest. 102 , 1286–1291 (1998).
Tukiainen, T. et al. Landscape of X chromosome inactivation across human tissues. Nature 550 , 244–248 (2017). This paper describes a comprehensive study of the pattern of X-chromosome inactivation in human female cells, including data on genes with variable ‘escape’ from inactivation and a discussion of the potential clinical relevance .
Balaton, B. P., Dixon-McDougall, T., Peeters, S. B. & Brown, C. J. The exceptional nature of the X chromosome. Hum. Mol. Genet. 27 , R242–R249 (2018).
Naqvi, S., Bellott, D. W., Lin, K. S. & Page, D. C. Conserved microRNA targeting reveals preexisting gene dosage sensitivities that shaped amniote sex chromosome evolution. Genome Res. 28 , 474–483 (2018).
Bellott, D. W. et al. Mammalian Y chromosomes retain widely expressed dosage-sensitive regulators. Nature 508 , 494–499 (2014).
Zhang, X. et al. Integrated functional genomic analyses of Klinefelter and Turner syndromes reveal global network effects of altered X chromosome dosage. Proc. Natl Acad. Sci. USA 117 , 4864–4873 (2020).
Di Stazio, M. et al. TBL1Y: a new gene involved in syndromic hearing loss. Eur. J. Hum. Genet. 27 , 466–474 (2019).
Dobyns, W. B. et al. Inheritance of most X-linked traits is not dominant or recessive, just X-linked. Am. J. Med. Genet. A 129A , 136–143 (2004).
Migeon, B. R. X-linked diseases: susceptible females. Genet. Med. 22 , 1156–1174 (2020).
Luo, S. et al. Biparental inheritance of mitochondrial DNA in humans. Proc. Natl Acad. Sci. USA 115 , 13039–13044 (2018).
Pagnamenta, A. T., Wei, W., Rahman, S. & Chinnery, P. F. Biparental inheritance of mitochondrial DNA revisited. Nat. Rev. Genet. 22 , 477–478 (2021).
Strande, N. T. et al. Evaluating the clinical validity of gene–disease associations: an evidence-based framework developed by the clinical genome resource. Am. J. Hum. Genet. 100 , 895–906 (2017).
Richards, S. et al. Standards and guidelines for the interpretation of sequence variants: a joint consensus recommendation of the American College of Medical Genetics and Genomics and the Association for Molecular Pathology. Genet. Med. 17 , 405–424 (2015). This paper reports the standard ACMG–AMP guidelines for classifying variants in molecular genetic diagnostics as (likely) pathogenic, (likely) benign or of uncertain significance, based on various criteria such as population data, computational data, functional data and segregation data .
Amendola, L. M. et al. Performance of ACMG–AMP variant-interpretation guidelines among nine laboratories in the clinical sequencing exploratory research consortium. Am. J. Hum. Genet. 98 , 1067–1076 (2016).
Harrison, S. M., Biesecker, L. G. & Rehm, H. L. Overview of specifications to the ACMG/AMP variant interpretation guidelines. Curr. Protoc. Hum. Genet. 103 , e93 (2019).
PubMed PubMed Central Google Scholar
Houge, G. et al. Stepwise ABC system for classification of any type of genetic variant. Eur. J. Hum. Genet. 30 , 150–159 (2022). This paper is an extension of the ACMG–AMG variant classification system, encompassing functional effect and clinical importance grades that are combined into a joint variant class to provide better estimates of variant significance in a clinical setting .
Zhang, S. et al. Base-specific mutational intolerance near splice sites clarifies the role of nonessential splice nucleotides. Genome Res. 28 , 968–974 (2018).
Scotti, M. M. & Swanson, M. S. RNA mis-splicing in disease. Nat. Rev. Genet. 17 , 19–32 (2016).
Manning, K. S. & Cooper, T. A. The roles of RNA processing in translating genotype to phenotype. Nat. Rev. Mol. Cell Biol. 18 , 102–114 (2017).
Sauna, Z. E. & Kimchi-Sarfaty, C. Understanding the contribution of synonymous mutations to human disease. Nat. Rev. Genet. 12 , 683–691 (2011). This paper comprehensively reviews the extent to which synonymous variants influence disease, the various molecular mechanisms that underlie these effects and the implications for future research and biomedical applications .
Spielmann, M. & Kircher, M. Computational and experimental methods for classifying variants of unknown clinical significance. Cold Spring Harb. Mol. Case Stud. 8, a006196 (2022).
Birolo, G. et al. Protein stability perturbation contributes to the loss of function in haploinsufficient genes. Front. Mol. Biosci. 8 , 620793 (2021).
Gerasimavicius, L., Liu, X. & Marsh, J. A. Identification of pathogenic missense mutations using protein stability predictors. Sci. Rep. 10 , 15387 (2020).
Coban-Akdemir, Z. et al. Identifying genes whose mutant transcripts cause dominant disease traits by potential gain-of-function alleles. Am. J. Hum. Genet. 103 , 171–187 (2018). This article describes an exemplary study of genes with premature termination codons that are predicted to escape nonsense-mediated decay and may be disease-causing through GoF effects .
Ferdinandusse, S. et al. A mutation creating an upstream translation initiation codon in SLC22A5 5′UTR is a frequent cause of primary carnitine deficiency. Hum. Mutat. 40 , 1899–1904 (2019).
Calvo, S. E., Pagliarini, D. J. & Mootha, V. K. Upstream open reading frames cause widespread reduction of protein expression and are polymorphic among humans. Proc. Natl Acad. Sci. USA 106 , 7507–7512 (2009).
Bhatia, S. et al. Disruption of autoregulatory feedback by a mutation in a remote, ultraconserved PAX6 enhancer causes aniridia. Am. J. Hum. Genet. 93 , 1126–1134 (2013).
Spielmann, M., Lupiáñez, D. G. & Mundlos, S. Structural variation in the 3D genome. Nat. Rev. Genet. 19 , 453–467 (2018). This article provides a comprehensive review of structural and quantitative chromosomal rearrangements that may affect the expression of distant genes through copy number alteration of regulatory elements or modification of the 3D genome by disrupting higher-order chromatin organization such as topologically associating domains .
Fuller, Z. L., Berg, J. J., Mostafavi, H., Sella, G. & Przeworski, M. Measuring intolerance to mutation in human genetics. Nat. Genet. 51 , 772–776 (2019).
Ziegler, A., Colin, E., Goudenège, D. & Bonneau, D. A snapshot of some pLI score pitfalls. Hum. Mutat. 40 , 839–841 (2019).
PubMed Google Scholar
Findlay, G. M. et al. Accurate classification of BRCA1 variants with saturation genome editing. Nature 562 , 217–222 (2018).
Przybyla, L. & Gilbert, L. A. A new era in functional genomics screens. Nat. Rev. Genet. 23 , 89–103 (2022).
Gussow, A. B., Petrovski, S., Wang, Q., Allen, A. S. & Goldstein, D. B. The intolerance to functional genetic variation of protein domains predicts the localization of pathogenic mutations within genes. Genome Biol. 17 , 9 (2016).
Petrovski, S., Wang, Q., Heinzen, E. L., Allen, A. S. & Goldstein, D. B. Genic intolerance to functional variation and the interpretation of personal genomes. PLoS Genet. 9 , e1003709 (2013).
Havrilla, J. M., Pedersen, B. S., Layer, R. M. & Quinlan, A. R. A map of constrained coding regions in the human genome. Nat. Genet. 51 , 88–95 (2019).
Li, G. C., Forster-Benson, E. T. C. & Sanders, C. R. Genetic intolerance analysis as a tool for protein science. Biochim. Biophys. Acta Biomembr. 1862 , 183058 (2020).
Hayeck, T. J. et al. Improved pathogenic variant localization via a hierarchical model of sub-regional intolerance. Am. J. Hum. Genet. 104 , 299–309 (2019).
Wiel, L. et al. MetaDome: pathogenicity analysis of genetic variants through aggregation of homologous human protein domains. Hum. Mutat. 40 , 1030–1038 (2019).
Silk, M. et al. MTR3D: identifying regions within protein tertiary structures under purifying selection. Nucleic Acids Res. 49 , W438–W445 (2021).
Qi, H. et al. MVP predicts the pathogenicity of missense variants by deep learning. Nat. Commun. 12 , 510 (2021).
Heyne, H. O. et al. Predicting functional effects of missense variants in voltage-gated sodium and calcium channels. Sci. Transl. Med. 12, eaay6848 (2020).
Bayrak, C. S. et al. Identification of discriminative gene-level and protein-level features associated with pathogenic gain-of-function and loss-of-function variants. Am. J. Hum. Genet. 108, 2301–2318 (2021).
Jumper, J. et al. Highly accurate protein structure prediction with AlphaFold. Nature 596 , 583–589 (2021).
Gerasimavicius, L., Livesey, B. J. & Marsh, J. A. Loss-of-function, gain-of-function and dominant-negative mutations have profoundly different effects on protein structure. Nat. Commun. 13 , 3895 (2022). This study investigates protein-level effects of pathogenic missense variants associated with different molecular mechanisms, showing that qualitative variant effects may be missed by current variant prioritization strategies, and highlighting ways to improve computational predictions through consideration of molecular disease mechanisms .
Epilepsy Genetics Initiative. De novo variants in the alternative exon 5 of SCN8A cause epileptic encephalopathy. Genet. Med. 20 , 275–281 (2018).
White, K. E. et al. Mutations that cause osteoglophonic dysplasia define novel roles for FGFR1 in bone elongation. Am. J. Hum. Genet. 76 , 361–367 (2005).
Bennett, J. T. et al. Mosaic activating mutations in FGFR1 cause encephalocraniocutaneous lipomatosis. Am. J. Hum. Genet. 98 , 579–587 (2016).
Ibrahimi, O. A., Zhang, F., Eliseenkova, A. V., Linhardt, R. J. & Mohammadi, M. Proline to arginine mutations in FGF receptors 1 and 3 result in Pfeiffer and Muenke craniosynostosis syndromes through enhancement of FGF binding affinity. Hum. Mol. Genet. 13 , 69–78 (2004).
Gruber, A. R., Lorenz, R., Bernhart, S. H., Neuböck, R. & Hofacker, I. L. The Vienna RNA websuite. Nucleic Acids Res. 36 , W70–W74 (2008).
Erlandsen, H. & Stevens, R. C. The structural basis of phenylketonuria. Mol. Genet. Metab. 68 , 103–125 (1999).
Higgs, D. R. et al. A review of the molecular genetics of the human α-globin gene cluster. Blood 73 , 1081–1104 (1989).
Magge, S. N. et al. Familial leucine-sensitive hypoglycemia of infancy due to a dominant mutation of the β-cell sulfonylurea receptor. J. Clin. Endocrinol. Metab. 89 , 4450–4456 (2004).
Marini, J. C. et al. Osteogenesis imperfecta. Nat. Rev. Dis. Prim. 3 , 17052 (2017).
Lee, J. & Hegele, R. A. Abetalipoproteinemia and homozygous hypobetalipoproteinemia: a framework for diagnosis and management. J. Inherit. Metab. Dis. 37 , 333–339 (2014).
Ramasamy, I. Update on the molecular biology of dyslipidemias. Clin. Chim. Acta 454 , 143–185 (2016).
Wohlfarter, Y. et al. Lost in promiscuity? An evolutionary and biochemical evaluation of HSD10 function in cardiolipin metabolism. Cell. Mol. Life Sci. 79 , 562 (2022).
Zschocke, J. et al. Progressive infantile neurodegeneration caused by 2-methyl-3-hydroxybutyryl-CoA dehydrogenase deficiency: a novel inborn error of branched-chain fatty acid and isoleucine metabolism. Pediatr. Res. 48 , 852–855 (2000).
Zschocke, J. HSD10 disease: clinical consequences of mutations in the HSD17B10 gene. J. Inherit. Metab. Dis. 35 , 81–89 (2012).
Rauschenberger, K. et al. A non-enzymatic function of 17β-hydroxysteroid dehydrogenase type 10 is required for mitochondrial integrity and cell survival. EMBO Mol. Med. 2 , 51–62 (2010).
Bhatta, A., Dienemann, C., Cramer, P. & Hillen, H. S. Structural basis of RNA processing by human mitochondrial RNase P. Nat. Struct. Mol. Biol. 28 , 713–723 (2021).
Plotnikov, A. N., Schlessinger, J., Hubbard, S. R. & Mohammadi, M. Structural basis for FGF receptor dimerization and activation. Cell 98 , 641–650 (1999).
Chung, W. C., Moyle, S. S. & Tsai, P. S. Fibroblast growth factor 8 signaling through fibroblast growth factor receptor 1 is required for the emergence of gonadotropin-releasing hormone neurons. Endocrinology 149 , 4997–5003 (2008).
Hong, S. et al. Dominant-negative kinase domain mutations in FGFR1 can explain the clinical severity of Hartsfield syndrome. Hum. Mol. Genet. 25 , 1912–1922 (2016).
Simonis, N. et al. FGFR1 mutations cause Hartsfield syndrome, the unique association of holoprosencephaly and ectrodactyly. J. Med. Genet. 50 , 585–592 (2013).
Cavenee, W. K. et al. Expression of recessive alleles by chromosomal mechanisms in retinoblastoma. Nature 305 , 779–784 (1983).
Stein, Y., Rotter, V. & Aloni-Grinstein, R. Gain-of-function mutant p53: all the roads lead to tumorigenesis. Int. J. Mol. Sci. 20 , 6197 (2019).
Kehrer-Sawatzki, H. et al. Phenotypic and genotypic overlap between mosaic NF2 and schwannomatosis in patients with multiple non-intradermal schwannomas. Hum. Genet. 137 , 543–552 (2018).
Nogué, C. et al. DGCR8 and the six hit, three-step model of schwannomatosis. Acta Neuropathol. 143 , 115–117 (2022).
Hoekstra, A. S. et al. Loss of maternal chromosome 11 is a signature event in SDHAF2, SDHD, and VHL-related paragangliomas, but less significant in SDHB-related paragangliomas. Oncotarget 8 , 14525–14536 (2017).
Burnichon, N. et al. Risk assessment of maternally inherited SDHD paraganglioma and phaeochromocytoma. J. Med. Genet. 54 , 125–133 (2017).
Twigg, S. R. et al. Cellular interference in craniofrontonasal syndrome: males mosaic for mutations in the X-linked EFNB1 gene are more severely affected than true hemizygotes. Hum. Mol. Genet. 22 , 1654–1662 (2013).
Mincheva-Tasheva, S., Nieto Guil, A. F., Homan, C. C., Gecz, J. & Thomas, P. Q. Disrupted excitatory synaptic contacts and altered neuronal network activity underpins the neurological phenotype in PCDH19-clustering epilepsy (PCDH19-CE). Mol. Neurobiol. 58 , 2005–2018 (2021).
Download references
Acknowledgements
The authors wish to thank U. Moog and S. Rudnik for helpful comments on earlier drafts of this manuscript, and J. Amberger for help with Online Mendelian Inheritance in Man (OMIM) statistics. A.O.M.W. acknowledges part-funding from NIHR Oxford Biomedical Research Centre.
Author information
Authors and affiliations.
Institute of Human Genetics, Medical University Innsbruck, Innsbruck, Austria
Johannes Zschocke
Department of Laboratory Medicine and Pathology, University of Washington, Seattle, WA, USA
Peter H. Byers
Department of Medicine (Medical Genetics), University of Washington, Seattle, WA, USA
MRC Weatherall Institute of Molecular Medicine, University of Oxford, Oxford, UK
Andrew O. M. Wilkie
You can also search for this author in PubMed Google Scholar
Contributions
All authors contributed to all aspects of the article; J.Z. developed the concept, wrote the initial draft of the manuscript and carried out major revisions.
Corresponding author
Correspondence to Johannes Zschocke .
Ethics declarations
Competing interests.
The authors declare no competing interests.
Peer review
Peer review information.
Nature Reviews Genetics thanks Alessandra Renieri, Zornitza Stark and Hans Waterham for their contribution to the peer review of this work.
Additional information
Publisher’s note Springer Nature remains neutral with regard to jurisdictional claims in published maps and institutional affiliations.
Related links
Matched Annotation from NCBI and EMBL-EBI (MANE): https://www.ncbi.nlm.nih.gov/refseq/MANE/
Online Mendelian Inheritance in Man: https://omim.org/statistics/geneMap
The earlier or more severe manifestation of a hereditary disease in the offspring than in the parent.
Different variants in the same gene often acting through different pathogenetic pathways cause a range of phenotypes that may be associated with different inheritance patterns.
A method to identify disease-causing variants in consanguineous families by focusing on autosomal regions with runs of homozygous genotypes inherited from a shared ancestor (that is, autozygous regions).
Different alleles of the same autosomal gene yield functionally distinct proteins with alternative phenotypes, both of which can be recognized in the (compound) heterozygous state.
Different pathogenic variants on the two alleles of the same autosomal gene, causing biallelic loss or modification of gene function.
A heterozygous variant codes for a structurally altered protein that interferes with the wild type (WT) protein.
The transcribed sequences of all protein-coding human genes.
The severity or extent of clinical manifestation in persons with a particular genotype. Variable expressivity indicates that individuals with the same genotype (for example, in the same family) may have quite different phenotypes.
The constellation of genetic variants in a particular gene or in the genome.
A genetic variant that causes inappropriate or novel protein functions such as uncontrolled activation or loss of regulation of the encoded protein, ectopic and/or illegitimate organ and/or cell-specific expression patterns, or novel (including toxic) protein or mRNA functions.
A gene or variant on one of two sex chromosomes, as opposed to autosomes.
Complete loss of one copy of a particular autosomal gene is usually asymptomatic. Haplosufficiency is a typical feature of recessive diseases and Mendelian wild type (WT) dominance.
Complete loss of one copy of a particular autosomal gene causes noticeable clinical effects; a single (haploid) normal allele does not suffice for normal development or homeostasis. Haploinsufficiency is the central pathogenetic mechanism in semi-dominant diseases caused by loss of function (LoF) variants.
Genetic variants that reduce but do not completely abolish the function of the encoded protein.
A genetic variant that causes complete loss of the encoded protein. Also known as a null variant.
A multifunctional protein performs two or more autonomous, independent and mechanistically different functions; specific impairment of one function by a pathogenic variant does not necessarily affect the other function(s).
The phenomenon whereby some heterozygous variants have advantageous clinical effects not observed in homozygous wild type (WT) or homozygous variant states.
The probability of clinical manifestation in persons with a particular genotype, which is frequently age-dependent. Penetrance may be complete (100%) or incomplete (reduced).
The measurable consequences of a genetic variant on the protein, cell, organ or clinical level.
A protein function is required in two or more different cellular processes or pathways; pathogenic variants in the respective gene cause different seemingly unrelated phenotypic traits.
The occurrence of an autosomal recessive (biallelic) disease in successive generations.
Describes the phenomenon whereby an autsomal genetic variant in its heterozygous state is associated with a less severe, intermediate phenotype than its homozygous state. Most quantitative variants are semi-dominant, but semi-dominance is also commonly observed for variants that have qualitative effects.
A protein has successive functions in cellular processes, such as sequential steps in an enzymatic reaction or transport processes, which are differently affected by genetic variants.
An additional copy of a particular autosomal gene (for example through a duplication that causes three instead of two gene copies) that has adverse clinical consequences.
A gene shows functional variability in expression or splicing, which is differently affected by genetic variants.
The phenomenon whereby some heterozygous variants cause a clinically adverse phenotype that is absent or less severe in wild type (WT) or variant homozygotes.
(WT). The genetic sequence or function defined as normal.
Rights and permissions
Springer Nature or its licensor (e.g. a society or other partner) holds exclusive rights to this article under a publishing agreement with the author(s) or other rightsholder(s); author self-archiving of the accepted manuscript version of this article is solely governed by the terms of such publishing agreement and applicable law.
Reprints and permissions
About this article
Cite this article.
Zschocke, J., Byers, P.H. & Wilkie, A.O.M. Mendelian inheritance revisited: dominance and recessiveness in medical genetics. Nat Rev Genet 24 , 442–463 (2023). https://doi.org/10.1038/s41576-023-00574-0
Download citation
Accepted : 14 December 2022
Published : 20 February 2023
Issue Date : July 2023
DOI : https://doi.org/10.1038/s41576-023-00574-0
Share this article
Anyone you share the following link with will be able to read this content:
Sorry, a shareable link is not currently available for this article.
Provided by the Springer Nature SharedIt content-sharing initiative
This article is cited by
Goldilocks principle and recessive disease.
- Alexandre Fabre
- Paul Guerry
European Journal of Human Genetics (2024)
Comparison of the ABC and ACMG systems for variant classification
- Gunnar Houge
- Eirik Bratland
- Andreas Laner
Mendel did not study common, naturally occurring phenotypes
- David Curtis
Journal of Genetics (2023)
Quick links
- Explore articles by subject
- Guide to authors
- Editorial policies
Sign up for the Nature Briefing newsletter — what matters in science, free to your inbox daily.

- Open access
- Published: 28 May 2024
Metabolome-wide Mendelian randomization for age at menarche and age at natural menopause
- Mojgan Yazdanpanah 1 ,
- Nahid Yazdanpanah 1 ,
- Isabel Gamache 1 ,
- Ken Ong 2 ,
- John R. B. Perry 2 , 3 &
- Despoina Manousaki ORCID: orcid.org/0000-0002-4133-0618 1 , 4
Genome Medicine volume 16 , Article number: 69 ( 2024 ) Cite this article
Metrics details
The role of metabolism in the variation of age at menarche (AAM) and age at natural menopause (ANM) in the female population is not entirely known. We aimed to investigate the causal role of circulating metabolites in AAM and ANM using Mendelian randomization (MR).
We combined MR with genetic colocalization to investigate potential causal associations between 658 metabolites and AAM and between 684 metabolites and ANM. We extracted genetic instruments for our exposures from four genome-wide association studies (GWAS) on circulating metabolites and queried the effects of these variants on the outcomes in two large GWAS from the ReproGen consortium. Additionally, we assessed the mediating role of the body mass index (BMI) in these associations, identified metabolic pathways implicated in AAM and ANM, and sought validation for selected metabolites in the Avon Longitudinal Study of Parents and Children (ALSPAC).
Our analysis identified 10 candidate metabolites for AAM, but none of them colocalized with AAM. For ANM, 76 metabolites were prioritized (FDR-adjusted MR P -value ≤ 0.05), with 17 colocalizing, primarily in the glycerophosphocholines class, including the omega-3 fatty acid and phosphatidylcholine (PC) categories. Pathway analyses and validation in ALSPAC mothers also highlighted the role of omega and polyunsaturated fatty acids levels in delaying age at menopause.
Conclusions
Our study suggests that metabolites from the glycerophosphocholine and fatty acid families play a causal role in the timing of both menarche and menopause. This underscores the significance of specific metabolic pathways in the biology of female reproductive longevity.
Female reproductive longevity, defined by the timing of menarche and menopause, exhibits significant variability driven by genetics, lifestyle, and environmental exposures [ 1 , 2 ], but the precise biological mechanisms underlying variations in reproductive aging are still not fully understood. However, the timing of both age at menarche (AAM) and age at natural menopause (ANM) appears to have significant effects on women’s health [ 3 ]. For example, the early onset of puberty has been linked to high risk-taking behaviors, reduced educational attainment [ 3 ], adult obesity, type 2 diabetes [ 4 ], cardiovascular diseases [ 5 ], susceptibility to cancers, and increased mortality rates [ 6 ]. Interestingly, women are more likely to experience an early natural menopause following either early or late menarche [ 7 ]. Therefore, identifying biomarkers that enhance our comprehension of the physiology of AAM and ANM variations, as well as their interconnectedness, is important. Moreover, these molecules may potentially serve as pharmacological targets to alter the duration of a woman’s reproductive lifespan.
Observational studies using large-scale metabolomics data have led to the discovery of a number of candidate biomarkers for various traits. Nevertheless, conducting case–control studies that simultaneously measure hundreds of circulating metabolites is cost-prohibitive but also susceptible to confounding and reverse causation, which restricts their ability to identify causal biomarkers. In recent years, large genome-wide association studies (GWAS) have identified genetic variants associated with the levels of numerous metabolites. Furthermore, large-scale GWAS datasets have become available for AAM and ANM, significantly advancing our knowledge of the genetic factors encompassing these traits. The availability of such GWAS data offers a valuable opportunity to investigate potential causal associations between circulating metabolites and AAM and ANM using Mendelian randomization (MR). MR is a well-established method in genetic epidemiology that explores whether a modifiable exposure is causally linked to a particular outcome [ 8 ]. Based on the use of genetic variants, randomly allocated at conception, to infer levels of these exposures, MR helps eliminate bias from confounding or reverse causation [ 9 ]. Two-sample MR uses data from separate GWAS for the exposure and outcome, enhancing statistical power for causal inference in complex health outcomes measured in large GWAS [ 10 ].
In this study, we conducted two-sample MR to investigate potential causal associations between hundreds of previously measured circulating metabolites and AAM or ANM using summary statistics from large GWAS [ 11 , 12 ]. We further explored the potential effects of body mass index (BMI) on the MR associations between the candidate metabolites and AAM and ANM. Colocalization analyses were conducted to differentiate between causal associations and genetic correlations due to variants in linkage disequilibrium (LD). Pathway and enrichment analyses were used to uncover potential biological processes influencing AAM and ANM. Finally, we sought validation for the causal associations with AAM and age at menopause for selected candidate metabolites directly measured in participants in the Avon Longitudinal Study of Parents and Children (ALSPAC).
Mendelian randomization assumptions
Univariable two-sample MR studies were performed to explore potential causal relationships between circulating metabolites and AAM and ANM. MR relies on three core assumptions: (1) The genetic instrument (IV) must have a strong association with the exposure (relevance assumption); (2) the genetic instrument should not be linked to confounding factors that connect the exposure to outcome (independence assumption); (3) the genetic instrument should affect the outcome only through the exposure (exclusion restriction assumption). Violation of this last assumption is known as horizontal pleiotropy.
Discovery datasets
For our MR analysis, we collected GWAS summary statistics for circulating metabolites on Europeans to use as sources for our exposures (Kettunen et al. [ 13 ], N = 24,925; Lotta et al. [ 14 ], N = 86,507; Long et al. [ 15 ], N = 1960; Shin et al. [ 16 ], N = 7824). The samples of the GWAS by Long et al. were derived from the TwinsUK cohort, while Shin et al. performed a GWAS meta-analysis of the TwinsUK and KORA cohorts. The GWAS by Lotta et al. was a meta-analysis of four cohorts (Fenland cohort, EPIC-Norfolk, INTERVAL) while Kettunen et al. meta-analyzed 14 GWAS including two GWAS from subsets of the FINRISK97 cohort. The methods used for metabolite measurements were liquid chromatography-mass spectrometry (LC–MS) (Long et al., Lotta et al., Shin et al.), and/or gas chromatography-mass spectrometry (GS-MS) (Shin et al.), and/or nuclear magnetic resonance spectrometry (NMR) (Kettunen et al. and Lotta et al.). All GWAS adjusted their metabolite measurements for age and sex of the participants, and additional covariates appear in Additional file 1 : Table S1. For the outcomes, we utilized summary statistics from the ReproGen consortium GWAS by Day et al. ( N total = 329,345, combining 40 studies with 23andMe and UK Biobank) [ 11 ] for AAM and from the largest-scale GWAS meta-analysis by Ruth et al. of four studies (1000 Genomes imputed studies, Breast Cancer Association Consortium, and UK Biobank, N total = 201,323) [ 12 ] for ANM. Units of measurement for the exposures (metabolite levels) were standard deviations (SD), while the outcomes were expressed in years in the respective GWAS. Additional file 1 : Table S1 provides additional details on each GWAS and Fig. 1 illustrates the overall study design.
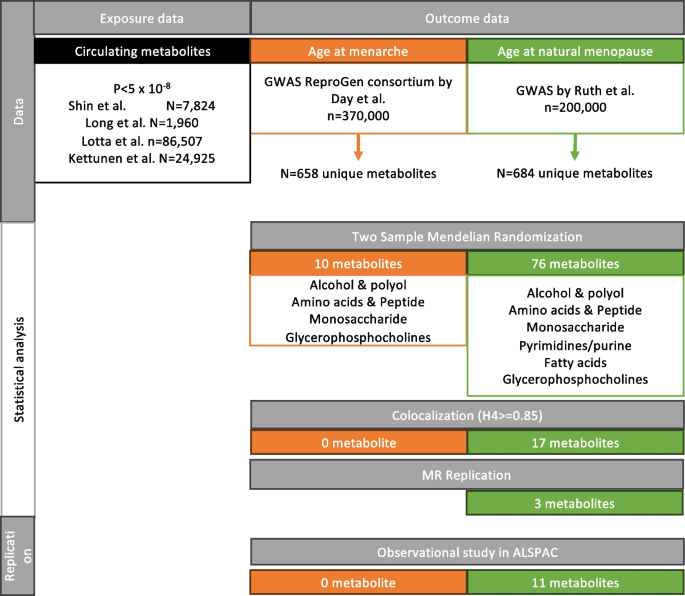
Flow chart of study design. Representation of the analytical steps and of the main results for both studied outcomes. Orange boxes refer to AAM, while green boxes refer to ANM
Instrumental variable selection
In order to satisfy the first MR assumption, we chose as IVs SNPs strongly associated with metabolite levels in the exposure GWAS ( P ≤ 5 × 10 −8 ). Among these, we selected independent SNPs (linkage disequilibrium (LD) r 2 < 0.001) within a 500-kb region using European ancestry reference data from the 1000 Genomes Project [ 17 ]. For SNPs that were not available in the outcome GWAS, we identified proxy SNPs in high LD ( r 2 > 0.8) using the SNIPA website ( https://snipa.helmholtz-muenchen.de/snipa3/ ). To further assess the first MR assumption, we filtered out metabolites for which the global F -statistic of the SNP-IVs was below 10, using the following formula: \(F=\frac{\frac{R2}{k}}{\frac{[1-R2]}{[n-k-1]}}\) , where n is the size of the cohort, k is the number of SNP-IVs, and R 2 is the proportion of the variance of each exposure explained by the SNP-IVs [ 18 ] (according to the formula \({R}^{2}\approx 2{\beta }^{2}f\times \left(1-f\right)\) where \(\beta\) and f denote the effect estimate and the effect allele frequency of the allele [ 19 ]). Summary statistics of the SNP-IVs used in our MR analysis can be found in Additional file 1 : Table S2.
Mendelian randomization analysis
We performed MR studies of the causal relationships between the exposures (metabolites) and outcomes (AAM and ANM) using the TwoSampleMR R package (v.0.5.5) [ 20 ]. We computed the MR Wald ratios for each genetic instrument of the exposures with the outcome, and when multiple SNP-IVs were available for a single metabolite, we meta-analyzed them using the inverse variance weighted (IVW) method [ 10 ]. Causal effects with type I error rate of less than 5% after correction for multiple testing using a false discovery rate (FDR) were considered significant.
Sensitivity analysis
To address potential violations of the third MR assumption, we conducted several sensitivity analyses to investigate the possibility of bias introduced by genetic instruments’ heterogeneity and pleiotropy. These analyses were performed on results that met the significance threshold and required the availability of multiple SNP-IVs. To assess pleiotropy, we employed both MR-Egger regression [ 21 ] and MR-PRESSO (Pleiotropy RESidual Sum and Outlier) [ 22 ] methods. MR-Egger, unlike the IVW method, does not constrain its intercept to zero, allowing for the detection of directional pleiotropy when the intercept significantly deviates from 0 ( p -value < 0.05). MR-Egger requires that the association of each variant with the exposure is not correlated with its pleiotropic effect, a condition known as the InSIDE assumption [ 21 ]. This is necessary to weaken the third assumption. The MR-PRESSO global test was also utilized to identify potential horizontal pleiotropy by estimating the presence of outlier SNP-IVs. As part of our sensitivity analyses, we applied Steiger filtering [ 23 ] to evaluate the directionality of the MR associations. This step ensured that the SNP-IVs were more strongly associated with the exposure (in this case, the metabolites) than with the outcomes (AAM and ANM). To assess heterogeneity, we implemented the Cochran Q heterogeneity test in both the IVW and MR-Egger analyses [ 24 ].
Multivariable MR analyses
To test the second MR assumption (“independence” assumption), we tested whether the association between the candidate metabolites and AAM or ANM, as determined by our MR analysis, could be influenced by body mass index (BMI), a possible confounder or mediator. Indeed, BMI is known to influence both AAM and ANM [ 2 , 25 , 26 , 27 ], and it also has an impact on certain metabolites [ 28 ]. In order to take this into account, we performed multivariable MR (MVMR) analysis. MVMR requires a larger number of genetic instruments for the exposures than the number of the exposures being tested in the model, which in this case are two: a metabolite and BMI. For these MVMR analyses, we used data from large available GWAS for childhood BMI ( n = 39,620) [ 29 ] and adult BMI ( n = ~ 700,000) [ 30 ].
Colocalization analyses
MR enables the detection of associations between two phenotypes; however, it is possible that the causal SNP for both phenotypes may not be the same. To explore this possibility, we performed a colocalization analysis to examine the potential influence of LD between the SNP-IVs for metabolites and the causal SNPs for AAM or ANM [ 31 ] on our causal MR associations. This analysis was performed using the coloc package in R [ 32 ], which computes posterior probabilities for four hypotheses: H0 (no association of the genomic locus with either trait), H1 (association with AAM or ANM but not with the metabolite level), H2 (association with the metabolite level but not with AAM or ANM), H3 (association with AAM or ANM and the metabolite level through two different causal SNPs in LD), and H4 (association with AAM or ANM and the metabolite level via one shared causal SNP). As parameters for prior probability, we used the default parameters, i.e., p 1 (prior probability of the exposure having a causal variant) = 1.0 × 10 −4 , p 2 (prior probability of the outcome having a causal variant) = 1.0 × 10 −4 , and p 12 (prior probability of the exposure and the outcome sharing the same causal variant) = 1.0 × 10 −5 . To estimate the posterior probability H4 for each genomic locus, which indicates the presence of a single causal variant for both the metabolites and AAM or ANM, we analyzed all SNPs with a minor allele frequency (MAF) > 0.01 within 1 MB of each metabolite SNP-IV. Colocalization analyses were performed for metabolites that showed evidence of MR association with AAM or ANM, using the available full summary-level results from the GWAS by Lotta et al. [ 14 ], Shin et al. [ 16 ], and Kettunen et al. [ 13 ] (full summary-level results from Long et al. are not available). If the posterior probabilities of H4 were greater than 0.8 for at least one of the SNP-IV associated with a candidate metabolite, this metabolite was considered colocalized with AAM or ANM.
Bidirectional MR
To test the directionality of our causal MR associations, in addition to the Steiger filtering, we performed reverse two-sample MR analyses, where AAM or ANM were the exposures and the colocalized metabolites were the outcomes. SNP-IVs for the two exposures (AAM or ANM) were extracted from the same ReproGen consortium GWAS and were strongly associated with the exposures at a GWAS p -value ≤ 5 × 10 −8 . The IVW method was used to evaluate the causal reverse associations, and we employed MR-Egger and two additional MR methods robust to pleiotropy, the weighted median [ 33 ], which assumes that at least half of the SNP-IVs are not pleiotropic, and the weighted mode [ 34 ], which assumes that the most common causal effect is consistent with the true causal effect.
Replication of our MR findings
We sought to replicate the findings for metabolites displaying significant associations in our main MR analysis by extracting IVs for these candidate metabolites from an independent cohort by Suhre et al. [ 35 ]. Since there was no available independent GWAS with available summary statistics for the outcome (ANM), we used the same GWAS meta-analysis by Ruth et al. [ 12 ]. We identified significant IVs associated with the metabolites in the Suhre et al. study and searched for proxies for missing SNPs in the ANM GWAS using the LDproxy function of LDlinkR ( r 2 > 0.8) [ 36 ]. Similar to our discovery MR, replication MR analyses were performed using the TwoSampleMR package [ 20 ].
Pathway and metabolite set enrichment analyses
To perform pathway and enrichment analyses based on the prioritized metabolites from our main MR and colocalization analyses, we first identified a single identifier per metabolite in the following databases: KEGG Compound [ 37 ], PubChem [ 38 ], BioCyc/HumanCyc [ 39 ], and Chemical Entities of Biological Interest (ChEBI) [ 40 ]. These databases provide the most frequently used and updated Human Metabolome Database (HMDB) identifiers in metabolomics [ 41 , 42 ]. Over-representation analysis (ORA) was implemented using the hypergeometric test to evaluate whether a particular metabolite set is represented more than expected by chance within the given compound list. Statistical significance was determined when FDR-corrected P -values were below 0.05. To perform ORA, we initially provided a list of compound names, which was then consolidated using conventional feature selection techniques to explore biologically significant patterns. This involved identifying whether a specific metabolite set was more prominently represented in the given compound list than would be expected by chance. After accounting for multiple testing, one-tailed P -values were calculated. We then used the Gene Multiple Association Network Integration Algorithm (GeneMANIA) tool ( http://www.genemania.org/ ) and Functional Mapping and Annotation of genetic associations (FUMA), a web-based tool ( https://fuma.ctglab.nl ), to construct a gene network to better characterize the functions of the main class of the MR-prioritized metabolites for AAM and ANM. Pathway analyses were performed using MetaboAnalyst [ 43 ], using “Enrichment Analysis” and “Joint-Pathway Analysis,” with the latter using the integration method of “Combine p values (pathway-level).” For the pathway and enrichment analyses, only metabolites which colocalized (H4 > 80%) with either AAM or ANM and who had identified metabolites (HMDB) were selected. These in silico follow-up analyses aimed to identify biologically meaningful pathways to which our candidate metabolites clustered, using quantitative metabolomic data.
Validation of selected candidate metabolites in the Avon Longitudinal Study of Parents and Children (ALSPAC) study
To validate our findings for selected candidate metabolites associated with AAM and ANM, we tested the association of directly measured levels of these metabolites with the two traits in ALSPAC. The ALSPAC is a population-based birth cohort study, which enrolled 14,541 pregnant women resident in Avon, UK, with expected delivery dates between 1 April 1991 and 31 December 1992 [ 44 , 45 ]. Of the initial pregnancies, there was a total of 13,988 children who were alive at 1 year of age. With additional phases of recruitment, the total sample size for analyses using any data collected after the age of seven is 15,447 pregnancies, resulting in 14,901 children being alive at 1 year of age. Overall, 8932 European children, among which n = 3919 girls, and their parents were closely monitored at regular intervals for 28 years using questionnaires and clinic-based assessments with full study details published elsewhere [ 46 , 47 ].
Age at onset of menarche was assessed based on a derived variable, combining repeated reports at different visits from age 8 years to age 17 years [ 48 ]. Age at menopause was assessed using questionnaires from 14,541 mothers in a recent follow-up visit in 2020 and was self-reported in a questionary (Variable number: C3b). Only mothers who had their menopause were kept for analysis [ 49 ]. Information was collected at two visits (Focus on Mothers 1 and 2 or FOM1 and FOM2). BMI measurements were calculated based on height and weight measurements of girls at clinical visits at ages 7, 8, and 11 years based on the formula weight (kg)/height (cm) 2 and were standardized to a mean of 0 and an SD of 1. Study data were collected and managed using REDCap electronic data capture tools hosted at the University of Bristol [ 50 ]. REDCap (Research Electronic Data Capture) is a secure, web-based software platform designed to support data capture for research studies. Missing BMI z -scores at age 8 years were imputed based on measurements at age 7 or 9 years. The maternal BMI was readily available as a derived variable, based on 2 clinic visits (FOM1 and FOM2).
Please note that the study website contains details of all the data that is available through a fully searchable data dictionary and variable search tool: http://www.bristol.ac.uk/alspac/researchers/our-data/
Metabolite measurements in ALSPAC
Nonfasted peripheral blood was collected from ALSPAC participants (children and mothers) at four different follow-up visits, at ages 7 (F7 visit), 15 (TF3 visit), 16 (TF4 visit), and 24 years (F24 visit) for child participants. Samples were processed within 4 h and stored at − 80 °C [ 51 ]. Fasting and post-prandial blood samples were also collected for a subset of ALSPAC participants at the Before Breakfast Study (BBS) at age 8 years. In mothers, metabolite levels were measured at a fasting state either at the FOM1 visit (average age 48 years, range 34–64 years) or the FOM2 visit (average age 51 years, range 39–66 years). Metabolomic profiling was done using the Nightingale NMR metabolomics platform (Helsinki, Finland), and 228 metabolic traits (and their ratios) were quantified in EDTA-plasma.
We assessed the associations between metabolites and age at menarche or menopause using linear regression. Subsequently, to assess the influence of BMI on these associations, we included childhood BMI at age 8 years as a covariate for AAM and mothers’ adult BMI at FOM1 or FOM2 as a covariate for age at menopause. We also conducted these models without including mothers who experienced early menopause, defined as before the age of 45 [ 49 ].
Causal relationships between metabolites and AAM or ANM
To evaluate the potential causal relationships between metabolites and AAM and ANM, we initially conducted univariate MR analyses, as outlined in the study design flowchart (Fig. 1 ). In total, we identified SNP-IVs for 658 metabolites for AAM and 684 for ANM (Additional file 1 : Table S3).
Our MR findings indicate causal relationships between ten circulating metabolites and AAM and 76 metabolites for ANM (at an FDR P -value ≤ 0.05) (Fig. 2 ). Among the identified metabolites for AAM, five metabolites belong to the glycerophosphocholine main class, two to the amino acids/peptides, and one to alcohols/polyols. All these metabolites, except X-11470, conferred an increase in AAM (Fig. 2 A, Additional file 1 : Table S3A), with effects ranging from 0.05 (mannose) to 0.25 (PC aa C32:3) years per 1 SD change in metabolite.
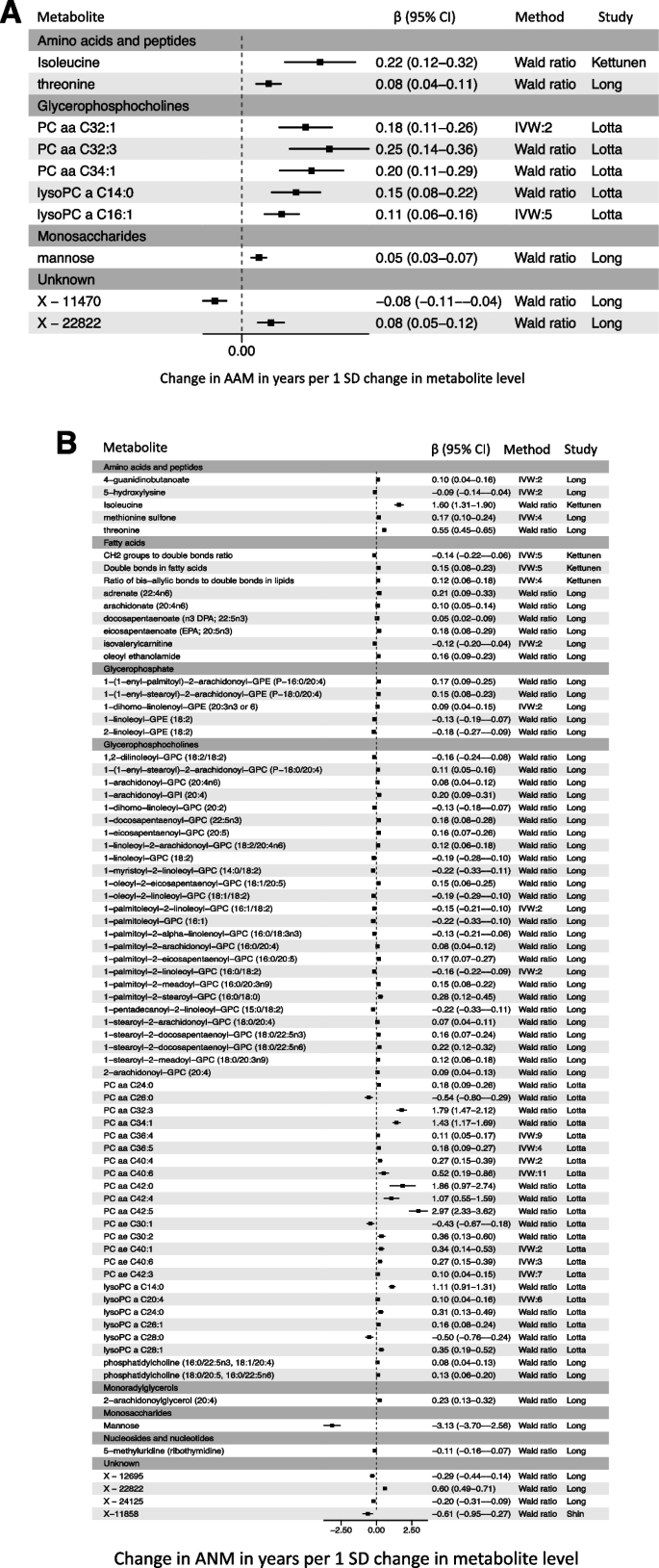
MR-prioritized metabolites for AAM ( A ) or ANM ( B ). Estimates (betas) express changes in years in AAM and ANM per SD increase in the circulating level of each metabolite. The results are grouped based on the main class of the metabolites
Contrarily, for ANM, metabolites within the same main class exhibited effects in different directions. The most prevalent main class was also the glycerophosphocholines, comprising of 50 of the 76 metabolites, followed by fatty acids with nine metabolites (Fig. 2 B, Additional file 1 : Table S3B). For ANM, we observed several metabolites, mostly phosphatidylcholine (PC) with absolute MR beta coefficients ranging between 0.05 (docosapentaenoate [n3 DPA; 22:5n3]) and 3.13 (mannose) years per SD increase in the metabolite level.
As statistical tests to evaluate pleiotropy, we performed MR-Egger, MR-PRESSO, and Cochran’s Q statistic. These tests did not suggest the presence of pleiotropy in the detected associations for metabolites with more than one SNP-IV (Additional file 1 : Tables S4Ai and S4Bi). Additionally, the results of Steiger filtering supported the presumed direction of the causal association, confirming that the candidate metabolites are likely responsible for the changes in AAM and ANM, rather than the inverse (Additional file 1 : Tables S4Aii and S4Bii).
Among the metabolites that met the significance threshold in our MR analyses, seven were common to both AAM and ANM, grouped into four major metabolic clusters: glycerophosphocholines [PC aa C32:3, PC aa C34:1, LysoPC a C14:0], amino acids and peptides [isoleucine, threonine], and monosaccharides [mannoses]. With the exception of mannose for ANM, increasing levels of all the other metabolites were consistently associated with later AAM and later ANM in our MR analyses (Fig. 3 and Additional file 1 : Table S5).
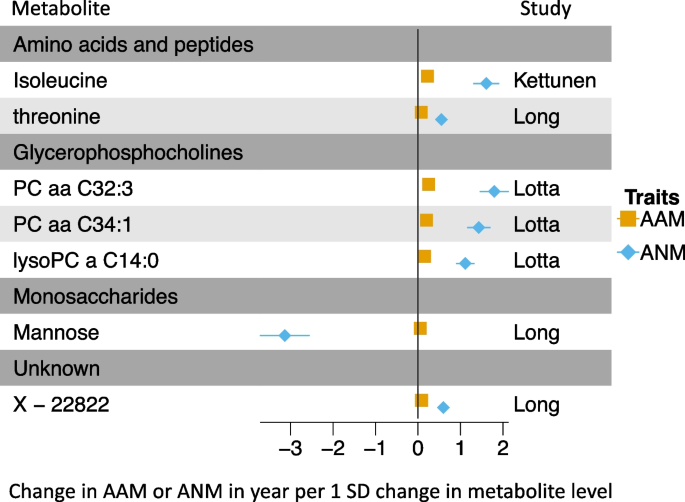
Shared MR-prioritized metabolites between AAM and ANM. Comparison of the effects of shared metabolites between AAM and ANM on the two outcomes. Estimates (betas) express changes in years in AAM and ANM per SD increase in the circulating level of each metabolite. The results are grouped based on the main class of the metabolites
Assessing the influence of BMI on the causal MR associations
To assess the influence of BMI on the causal relationships of the candidate metabolites with AAM or ANM, we conducted MVMR by including either childhood or adult BMI as second exposure in the MR model. These analyses were restricted to MR-prioritized metabolites with three or more SNP-IVs, resulting in one metabolite for AAM and nine for ANM. Among these metabolites, only lysoPC a C20:4 ( P -value = 0.015), PC aa C36:4 ( P -value = 0.047), PC aa C40:6 ( P -value = 0.031), and PC P-40:5 or PC O-40:6 ( P -value = 0.025) retained a suggestive ( P -value < 0.05) IVW MR estimate for causal association for ANM after adjusting for adult BMI (Additional file 1 : Table S6). The remaining causal associations of metabolites with AAM or ANM disappeared when child or adult BMI was taken into account, suggesting that BMI could potentially mediate or act as a confounder in these associations (Additional file 1 : Table S6).
Colocalization
In our colocalization analyses, we considered that the MR-prioritized metabolites colocalized with AAM or ANM if the posterior probabilities of the candidate metabolites and outcome sharing a single causal SNP (H4) for any of the SNP-IVs of each metabolite were greater than 0.8. We found evidence of colocalization with ANM for 17 MR-prioritized metabolites, mainly from the glycerophosphocholines class, but none for AAM (Additional file 1 : Table S7). The genes encompassing the SNP-IVs of the 17 colocalized metabolites were FADS1 , FADS2 , FEN1 , MYRF , and TMEM258 , suggesting the existence of shared pathways among the prioritized metabolites.
Bidirectional MR analysis
To further validate the directionality of the causal MR associations, we conducted reverse MR, which did not provide evidence for a causal effect of ANM on these metabolites (Additional file 1 : Table S8), confirming that the metabolites confer the changes in AAM or ANM, and not the opposite.
Replication MR analysis
We performed a replication MR analysis utilizing an independent metabolite cohort as a source of IVs for the MR-prioritized metabolites for ANM (Additional file 1 : Table S9). The results of the main MR association of omega-3 fatty acids with ANM replicated, with an increase of omega-3 fatty acids levels delaying the onset of ANM, and estimates consistently aligning across various MR methods.
Metabolic pathway and enrichment analysis
To uncover the biological mechanisms linking the 17 MR-identified and colocalized metabolites with ANM, we conducted a follow-up pathway analysis. Among these metabolites, we were able to identify 15 with Human Metabolome Database (HMDB) identifiers [ 52 ], which we used in our metabolite-based pathway and enrichment analysis (Additional file 1 : Table S10). Using the KEGG database, we identified a significant association between the glycerophosphocholines cluster and ANM (FDR P -value = 1.03 × 10 −9 ) (Additional file 1 : Table S11A). The pathways underlying this association encompass the metabolism of glycerophospholipids (FDR P -value = 2.13 × 10 −3 ), alpha-linolenic acid (FDR P -value = 2.13 × 10 −3 ), and linoleic acid (FDR P -value = 8.74 × 10 −3 ) (Additional file 1 : Table S11B).
The five genes where colocalization between metabolites and ANM occurred shared common networks and functions in our GeneMANIA and FUMA analyses (Fig. 4 , Additional file 1 : Table S12). Specifically, in our FUMA analysis, both FADS1 and FADS2 were linked to the metabolism of linoleic acid (adjusted P -value = 9.97 × 10 −4 ), alpha-linolenic omega-3, and linoleic omega-6 acids (adjusted P -value = 0.001), and to the biosynthesis of unsaturated fatty acids (adjusted P -value = 0.003) (Additional file 1 : Table S12C). This underlines the importance of the fatty acid pathway in the timing of ANM.
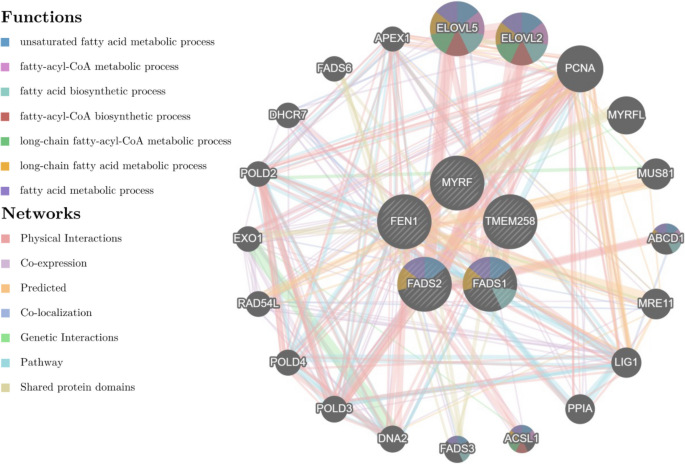
Pathway analysis of colocalized metabolites with ANM using GeneMANIA. Each circle represents a gene and its diverse interactions across the network. Pie-chart for each gene represents specific functions associated with lipid metabolism
Validation of selected candidate metabolites for AAM and ANM in ALSPAC
As a further step to validate the MR-prioritized metabolites for AAM and ANM, we conducted an observational study in an independent cohort, ALSPAC. In this cohort, the mean age at menarche was 12.21 ± 1.03 years ( N = 2456 girls across four visits), and the mean age at menopause was 49.03 ± 4.18 years ( N = 1626 post-menopausal mothers across the FOM1 and FOM2 visits). Regarding AAM, only two out of the 10 MR-prioritized metabolites were measured in this cohort. However, these two metabolites did not exhibit any association with AAM, with or without adjustment for childhood BMI (Additional file 1 : Table S13).
Nine out of the 17 colocalized metabolites for ANM were measured in ALSPAC. Six metabolites were found to be associated ( P -value < 0.05) with age at menopause in this cohort, all from the fatty acids class. Notably, omega-3 fatty acids displayed the largest effect, with a substantial delay in age at menopause ( β FOM2 = 4.12 ± 1.03 years per mmol/l increase in the metabolite level, P -value = 6.46 × 10 −5 , N = 863). With the exception of monounsaturated fatty acids, all remaining metabolites were consistently associated with a delay in age at menopause, with estimated effects ranging from 0.16 to 4.12 years per unit increase (mmol/l or percent) (Additional file 1 : Table S13). After adjusting for BMI, the results remained largely consistent with those from the unadjusted model, except for monounsaturated fatty acids. This discrepancy suggests that BMI may have mediating or confounding effects on the relationship of this metabolite with age at menopause. Furthermore, the majority of our results remained consistent even after excluding mothers with early menopause, defined as an age of menopause < 45 years [ 49 ] (Additional file 1 : Table S13). Overall, this analysis supports our finding that fatty acids, mostly those associated with omega-3 metabolism, appear to be important in the timing of age at menopause.
In this study, we employed Mendelian randomization (MR) and colocalization analyses to conduct a thorough investigation into the causal relationships between numerous circulating metabolites and the timing of menarche and menopause. We further validated our findings through an observational study in an independent cohort. Our results offer insights into the impact of metabolism, mostly that of the glycerophosphocholines and fatty acids, on female reproductive longevity, indicating that genetic predisposition to altered levels of circulating blood metabolites can be a risk factor for variations in AAM or ANM.
Our analysis highlights the role of many metabolites associated with the choline fraction, clustering within the phosphatidylcholine (PC) subclass, and of fatty acids, both essential nutriments from the diet [ 53 ], in the timing of age at menarche and menopause. Metabolism of both phosphatidylcholines and fatty acids involves the enzyme phosphatidylethanolamine N-methyltransferase (PEMT) [ 54 , 55 ], which is influenced by various factors, including sex hormone levels such as estrogens [ 56 ], suggesting links with the female fertility.
Phosphatidylcholine levels are also altered in physiological states, such as pregnancy and menopause [ 56 , 57 ], and pathological states of estrogen abundance or deficiency. More precisely, postmenopausal women are more susceptible to choline deficiency due to the decline in their estrogen levels, while pregnant women showed protection against its deficiency [ 56 , 57 ]. Furthermore, this metabolite subclass has been found to play a role in the regulation of menstrual cycle [ 58 ] and has shown protective effects on follicular development and oocyte maturation against an exogenous endocrine disruptor [ 59 ]. In our MR analysis, the majority of the metabolites associated with AAM or ANM and shared by both, belong to this subclass, with the majority conferring a delay of both outcomes. This underscores the significance of this class in the female reproductive system. Our results for AAM align with previous MR findings [ 60 ], while for ANM, further studies are needed to investigate the potential therapeutic effects of choline, or phosphocholine, supplementation on the timing of menopause.
During menopause, there is a shift in unsaturated fatty acid metabolism, and hormonal replacement therapy has been shown to restore different fatty acid levels in postmenopausal women [ 61 ] and in animal models [ 62 ]. Additionally, fatty acid levels have been found to impact menopausal symptoms [ 63 ]. Previous research supports the administration of omega-3 fatty acids to increase ovarian reserve [ 64 ], by potentially delaying the onset of menopause. Our main and replication MR analyses, pathway analysis, and observational study in ALSPAC converge to a delaying effect of omega-3, polyunsaturated, and monounsaturated fatty acids, on age at menopause, suggesting a protective role of polyunsaturated and omega-3 fatty acid supplementation in women at risk of premature menopause. A potential mechanism underlying these associations could be linked to the anti-inflammatory properties of omega-3 fatty acids, by reducing the production of proinflammatory cytokines [ 65 ]. Indeed, menopausal transition is linked to an increase in markers of inflammation, particularly in women with early menopause, which may suggest a detrimental effect of inflammation on ovarian longevity [ 66 , 67 , 68 ]. Thus, a lifetime exposure to higher levels of fatty acids with anti-inflammatory effects could potentially delay the onset of menopause, a hypothesis that merits to be tested in clinical trials.
The involvement of the FADS1 and FADS2 genes in ANM is an intriguing finding. These genes encode enzymes responsible for catalyzing the omega-3 and omega-6 lipid biosynthesis pathways [ 69 ]. The FADS locus, which is clustered on chromosome 11, exerts pleiotropic effects, mostly on lipid-associated metabolic traits, but recent GWAS evidence has linked it with female fertility [ 70 ]. Additionally, this locus has been targeted by natural selection multiple times in human history [ 53 , 69 , 71 ], including in populations with diets rich in meat and fish, which are significant sources of omega fatty acids and choline [ 53 ]. The selective pressure found in the European population was suggested to be caused by the diet transition across history [ 53 , 70 ]. However, it can also be possible that the selective pressure could also involve female fertility, potentially by delaying ANM and as such increasing the female reproductive longevity.
Overall, our findings provide new evidence on the role of lipid metabolism in female reproductive longevity, but the precise biological mechanisms behind our findings remain unclear and further studies need to be done to understand these associations.
Our study has multiple strengths. First, we used MR, a study design allowing for causal inference, by limiting confounding, reverse causation, and other biases common in observational epidemiology. The hypothesis-free design of our study offers a thorough screen for causal relationships between metabolites evaluated by non-targeted metabolomics and AAM or ANM. We conducted a number of sensitivity analyses and a replication MR study using instruments from an independent metabolite GWAS, which largely supported the main findings. Our colocalization analyses followed by pathway and enrichment studies provide further insight into the biological mechanisms underlying variations in ANM. Finally, our validation study, based on direct measurements of candidate metabolites in a cohort of women accurately reporting their AAM and ANM, further supports the role of selected MR-prioritized metabolites in these traits.
There are some considerable limitations in our study. Other than BMI, many factors influence the timing of menarche and menopause [ 1 , 2 ], among which lifestyle traits such as nutrition and physical activity [ 72 , 73 , 74 , 75 , 76 , 77 ], which could potentially confound the identified MR associations. However, information about lifestyle factors is not consistently available across cohorts and less GWAS are available of these traits, limiting our ability to adjust for them in multivariable MR. Also, higher BMI is correlated with lower socio-economic status and poor diet. The results of our observational study in ALSPAC girls can be hampered by the fact that the metabolite measurements were predominantly obtained under non-fasting conditions, which may have influenced our results toward the null [ 78 , 79 ]. Furthermore, the data collection in ALSPAC spanned nearly a decade, during which lifestyle elements may have changed, also potentially mitigating the effects of metabolites on the two outcomes. Analyzing a restricted time period could reduce this bias but might also lead to a loss of statistical power, emphasizing the need for replication in larger datasets with a shorter time frame. Moreover, the definition of age at menopause used in the ReproGen GWAS [ 12 ] differs from the definition of age at menopause used in the ALSPAC. While both definitions relied on self-reported age of menopause, which can be subject to memory bias, in the ReproGen GWAS, additional filtering was applied to isolate cases of natural menopause (see Additional file 1 : Table S1). Natural menopause is a term used to differentiate the spontaneous occurrence of menopause due to aging versus menopause induced by exogenous or pathological factors. These differences in definitions could potentially explain variations between our discovery and replication analyses. The inclusion of mothers with possible non-natural menopause and the fact that many mothers did not reach menopause during the most recent available follow-up visit in ALSPAC may have contributed to the lower-than-expected mean age at menopause. The memory bias limitation could be alleviated through replication analyses, even if the definitions of age at menopause differ between the discovery and replication phases. Additionally, metabolite levels were not necessarily measured by the same platforms across the metabolomic GWAS and ALSPAC. Also, there is a partial overlap of samples (from the UK Biobank) in the replication MR between the exposure and outcome GWAS cohorts. However, given the robustness of the association between the IVs and exposures ( F -statistic > 30), and the consistency in direction between the discovery MR and replication in ALSPAC, it is plausible to infer that bias may not necessarily be the driving force behind the observed association. This variation can also affect the results of our validation analysis in ALSPAC. Furthermore, our two-sample MR analyses can only test linear effects of the metabolite levels on AAM and ANM, and therefore, we cannot exclude non-linear effects (i.e., effects of extremely low or high metabolite levels) on these traits. Finally, our results are based on European GWAS data for both metabolites and AAM and ANM, and as such, they cannot be generalized to non-European populations.
Using complementary approaches leveraging human genomic and metabolomic data, we were able to identify circulating metabolites potentially influencing reproductive longevity. In keeping with previous research, our findings point to choline-containing phospholipids and fatty acids as molecules that affect the timing of both AAM and ANM. These results support the presence of differences in the metabolic profiles of women with altered pubertal or menopausal timing, while unraveling new biological pathways underpinning the female reproductive aging.
Availability of data and materials
The GWAS summary data of the five the metabolomic GWAS were obtained from GWAS catalog (accession date: 19 March 2024: Kettunen et al. GWAS: https://www.ebi.ac.uk/gwas/publications/27005778 [ 13 ]; Lotta et al. GWAS: https://www.ebi.ac.uk/gwas/publications/33414548 [ 14 ]; Shin et al. GWAS: https://www.ebi.ac.uk/gwas/publications/24816252 [ 16 ]). Full summary-level results from Long et al. and Suhre et al. are not available, but summary statistics for significant SNPs can be obtained from the GWAS publications (Long et al. GWAS: https://doi.org/ https://doi.org/10.1038/ng.3809 [ 15 ]; Suhre et al. GWAS: https://doi.org/ https://doi.org/10.1101/2022.06.12.22276286 [ 35 ]). The adult and child BMI summary-level GWAS data were also obtained from GWAS catalog (Yengo et al. GWAS: https://www.ebi.ac.uk/gwas/publications/30124842 [ 30 ]; Vogelezang et al. GWAS: https://www.ebi.ac.uk/gwas/publications/33045005 [ 29 ]). The GWAS data on the ages at menarche [ 11 ] and at natural menopause [ 12 ] were obtained from the REPROGEN Consortium site ( https://www.reprogen.org/data_download.html ). The ALSPAC data were accessed under the project number B4178. Additional file 1 (Additional_file_1.xlsx) includes the Tables S1 to S13. Additional file 2 (Additional_file_2.pdf) includes the MR-STROBE checklist.
Ceylan B, Özerdoğan N. Factors affecting age of onset of menopause and determination of quality of life in menopause. Turk J Obstet Gynecol. 2015;12(1):43–9.
Article PubMed PubMed Central Google Scholar
Yermachenko A, Dvornyk V. Nongenetic determinants of age at menarche: a systematic review. Biomed Res Int. 2014;2014: 371583.
Day FR, Elks CE, Murray A, Ong KK, Perry JR. Puberty timing associated with diabetes, cardiovascular disease and also diverse health outcomes in men and women: the UK Biobank study. Sci Rep. 2015;5:11208.
Elks CE, Ong KK, Scott RA, van der Schouw YT, Brand JS, Wark PA, et al. Age at menarche and type 2 diabetes risk: the EPIC-InterAct study. Diabetes Care. 2013;36(11):3526–34.
Prentice P, Viner RM. Pubertal timing and adult obesity and cardiometabolic risk in women and men: a systematic review and meta-analysis. Int J Obes (Lond). 2013;37(8):1036–43.
Article CAS PubMed Google Scholar
Charalampopoulos D, McLoughlin A, Elks CE, Ong KK. Age at menarche and risks of all-cause and cardiovascular death: a systematic review and meta-analysis. Am J Epidemiol. 2014;180(1):29–40.
Bjelland EK, Hofvind S, Byberg L, Eskild A. The relation of age at menarche with age at natural menopause: a population study of 336 788 women in Norway. Hum Reprod. 2018;33(6):1149–57.
Article CAS PubMed PubMed Central Google Scholar
Burgess S, Daniel RM, Butterworth AS, Thompson SG. Consortium EP-I Network Mendelian randomization: using genetic variants as instrumental variables to investigate mediation in causal pathways. Int J Epidemiol. 2015;44(2):484–95.
Article PubMed Google Scholar
Burgess S, Small DS, Thompson SG. A review of instrumental variable estimators for Mendelian randomization. Stat Methods Med Res. 2017;26(5):2333–55.
Burgess S, Foley CN, Zuber V. Inferring causal relationships between risk factors and outcomes from genome-wide association study data. Annu Rev Genomics Hum Genet. 2018;19:303–27.
Day FR, Thompson DJ, Helgason H, Chasman DI, Finucane H, Sulem P, et al. Genomic analyses identify hundreds of variants associated with age at menarche and support a role for puberty timing in cancer risk. Nat Genet. 2017;49(6):834–41.
Ruth KS, Day FR, Hussain J, Martinez-Marchal A, Aiken CE, Azad A, et al. Genetic insights into biological mechanisms governing human ovarian ageing. Nature. 2021;596(7872):393–7.
Kettunen J, Demirkan A, Wurtz P, Draisma HH, Haller T, Rawal R, et al. Genome-wide study for circulating metabolites identifies 62 loci and reveals novel systemic effects of LPA. Nat Commun. 2016;7:11122.
Lotta LA, Pietzner M, Stewart ID, Wittemans LBL, Li C, Bonelli R, et al. A cross-platform approach identifies genetic regulators of human metabolism and health. Nat Genet. 2021;53(1):54–64.
Long T, Hicks M, Yu HC, Biggs WH, Kirkness EF, Menni C, et al. Whole-genome sequencing identifies common-to-rare variants associated with human blood metabolites. Nat Genet. 2017;49(4):568–78.
Shin SY, Fauman EB, Petersen AK, Krumsiek J, Santos R, Huang J, et al. An atlas of genetic influences on human blood metabolites. Nat Genet. 2014;46(6):543–50.
The Genomes Project C, Auton A, Abecasis GR, Altshuler DM, Durbin RM, Abecasis GR, et al. A global reference for human genetic variation. Nature. 2015;526:68.
Palmer TM, Lawlor DA, Harbord RM, Sheehan NA, Tobias JH, Timpson NJ, et al. Using multiple genetic variants as instrumental variables for modifiable risk factors. Stat Methods Med Res. 2012;21(3):223–42.
Park JH, Wacholder S, Gail MH, Peters U, Jacobs KB, Chanock SJ, et al. Estimation of effect size distribution from genome-wide association studies and implications for future discoveries. Nat Genet. 2010;42(7):570–5.
Yavorska OO, Burgess S. MendelianRandomization: an R package for performing Mendelian randomization analyses using summarized data. Int J Epidemiol. 2017;46(6):1734–9.
Bowden J, Davey Smith G, Burgess S. Mendelian randomization with invalid instruments: effect estimation and bias detection through Egger regression. Int J Epidemiol. 2015;44(2):512–25.
Verbanck M, Chen CY, Neale B, Do R. Detection of widespread horizontal pleiotropy in causal relationships inferred from Mendelian randomization between complex traits and diseases. Nat Genet. 2018;50(5):693–8.
Hemani G, Tilling K, Davey SG. Orienting the causal relationship between imprecisely measured traits using GWAS summary data. PLoS Genet. 2017;13(11): e1007081.
Hemani G, Bowden J, Davey SG. Evaluating the potential role of pleiotropy in Mendelian randomization studies. Hum Mol Genet. 2018;27(R2):R195–208.
Farahmand M, Ramezani Tehrani F, Azizi F. Whether age of menarche is influenced by body mass index and lipoproteins profile? a retrospective study. Iran J Reprod Med. 2012;10(4):337–42.
PubMed PubMed Central Google Scholar
Al-Awadhi N, Al-Kandari N, Al-Hasan T, Almurjan D, Ali S, Al-Taiar A. Age at menarche and its relationship to body mass index among adolescent girls in Kuwait. BMC Public Health. 2013;13:29.
Zhu D, Chung HF, Pandeya N, Dobson AJ, Kuh D, Crawford SL, et al. Body mass index and age at natural menopause: an international pooled analysis of 11 prospective studies. Eur J Epidemiol. 2018;33(8):699–710.
Moore SC, Matthews CE, Sampson JN, Stolzenberg-Solomon RZ, Zheng W, Cai Q, et al. Human metabolic correlates of body mass index. Metabolomics. 2014;10(2):259–69.
Vogelezang S, Bradfield JP, Ahluwalia TS, Curtin JA, Lakka TA, Grarup N, et al. Novel loci for childhood body mass index and shared heritability with adult cardiometabolic traits. PLoS Genet. 2020;16(10): e1008718.
Yengo L, Sidorenko J, Kemper KE, Zheng Z, Wood AR, Weedon MN, et al. Meta-analysis of genome-wide association studies for height and body mass index in approximately 700000 individuals of European ancestry. Hum Mol Genet. 2018;27(20):3641–9.
Giambartolomei C, Vukcevic D, Schadt EE, Franke L, Hingorani AD, Wallace C, et al. Bayesian test for colocalisation between pairs of genetic association studies using summary statistics. PLoS Genet. 2014;10(5): e1004383.
Wallace C. Eliciting priors and relaxing the single causal variant assumption in colocalisation analyses. PLoS Genet. 2020;16(4): e1008720.
Bowden J, Davey Smith G, Haycock PC, Burgess S. Consistent estimation in Mendelian randomization with some invalid instruments using a weighted median estimator. Genet Epidemiol. 2016;40(4):304–14.
Hartwig FP, Davey Smith G, Bowden J. Robust inference in summary data Mendelian randomization via the zero modal pleiotropy assumption. Int J Epidemiol. 2017;46(6):1985–98.
Karsten S, Raghad A-I, Aziz B, Tanwir H, Anna H, Nisha S, et al. Lipoprotein profile and metabolic fine-mapping of genetic lipid risk loci. medRxiv. 2022:2022.06.12.22276286.
Myers TA, Chanock SJ, Machiela MJ. LDlinkR: An R Package for rapidly calculating linkage disequilibrium statistics in diverse populations. Front Genet. 2020;11:157. https://doi.org/10.3389/fgene.2020.00157 .
Kanehisa M, Goto S. KEGG: kyoto encyclopedia of genes and genomes. Nucleic Acids Res. 2000;28(1):27–30.
Kim S, Thiessen PA, Bolton EE, Chen J, Fu G, Gindulyte A, et al. PubChem substance and compound databases. Nucleic Acids Res. 2016;44(D1):D1202–13.
Romero P, Wagg J, Green ML, Kaiser D, Krummenacker M, Karp PD. Computational prediction of human metabolic pathways from the complete human genome. Genome Biol. 2005;6(1):R2.
Degtyarenko K, de Matos P, Ennis M, Hastings J, Zbinden M, McNaught A, et al. ChEBI: a database and ontology for chemical entities of biological interest. Nucleic Acids Res. 2008;36(Database issue):D344–50.
Booth SC, Weljie AM, Turner RJ. Computational tools for the secondary analysis of metabolomics experiments. Comput Struct Biotechnol J. 2013;4: e201301003.
Sas KM, Karnovsky A, Michailidis G, Pennathur S. Metabolomics and diabetes: analytical and computational approaches. Diabetes. 2015;64(3):718–32.
Pang Z, Chong J, Zhou G, de Lima Morais DA, L Chang, Barrette M, et al. MetaboAnalyst 5.0: narrowing the gap between raw spectra and functional insights. Nucleic Acids Research. 2021;49(W1):W388–96.
Smith D, Northstone K, Bowring C, Wells N, Crawford M, Pearson RM, et al. The Avon Longitudinal Study of Parents and Children - a resource for COVID-19 research: Generation 2 questionnaire data capture May-July 2020. Wellcome Open Res. 2020;5:278.
Northstone K, Lewcock M, Groom A, Boyd A, Macleod J, Timpson N, et al. The Avon Longitudinal Study of Parents and Children (ALSPAC): an update on the enrolled sample of index children in 2019. Wellcome Open Res. 2019;4:51.
Boyd A, Golding J, Macleod J, Lawlor DA, Fraser A, Henderson J, et al. Cohort Profile: the ‘children of the 90s’–the index offspring of the Avon Longitudinal Study of Parents and Children. Int J Epidemiol. 2013;42(1):111–27.
Fraser A, Macdonald-Wallis C, Tilling K, Boyd A, Golding J, Davey Smith G, et al. Cohort Profile: the Avon Longitudinal Study of Parents and Children: ALSPAC mothers cohort. Int J Epidemiol. 2013;42(1):97–110.
Roberts E, Fraser A, Gunnell D, Joinson C, Mars B. Timing of menarche and self-harm in adolescence and adulthood: a population-based cohort study. Psychol Med. 2020;50(12):2010–8.
Shuster LT, Rhodes DJ, Gostout BS, Grossardt BR, Rocca WA. Premature menopause or early menopause: Long-term health consequences. Maturitas. 2010;65(2):161–6.
Harris PA, Taylor R, Thielke R, Payne J, Gonzalez N, Conde JG. Research electronic data capture (REDCap)–a metadata-driven methodology and workflow process for providing translational research informatics support. J Biomed Inform. 2009;42(2):377–81.
Santos Ferreira DL, Williams DM, Kangas AJ, Soininen P, Ala-Korpela M, Smith GD, et al. Association of pre-pregnancy body mass index with offspring metabolic profile: Analyses of 3 European prospective birth cohorts. PLoS Med. 2017;14(8): e1002376.
Wishart DS, Tzur D, Knox C, Eisner R, Guo AC, Young N, et al. HMDB: the human metabolome database. Nucleic Acids Research. 2007;35(suppl_1):D521-D6.
Buckley MT, Racimo F, Allentoft ME, Jensen MK, Jonsson A, Huang H, et al. Selection in Europeans on fatty acid desaturases associated with dietary changes. Mol Biol Evol. 2017;34(6):1307–18.
Zeisel SH. Choline: critical role during fetal development and dietary requirements in adults. Annu Rev Nutr. 2006;26:229–50.
Resseguie M, Song J, Niculescu MD, da Costa KA, Randall TA, Zeisel SH. Phosphatidylethanolamine Nmethyltransferase (PEMT) gene expression is induced by estrogen in human and mouse primary hepatocytes. FASEB J. 2007;21(10):2622-32. https://doi.org/10.1096/fj.07-8227com .
Korsmo HW, Jiang X, Caudill MA. Choline: Exploring the growing science on its benefits for moms and babies. Nutrients. 2019;11(8):1823. https://doi.org/10.3390/nu11081823 .
Fischer LM, da Costa KA, Kwock L, Galanko J, Zeisel SH. Dietary choline requirements of women: effects of estrogen and genetic variation. Am J Clin Nutr. 2010;92(5):1113–9.
Draper CF, Duisters K, Weger B, Chakrabarti A, Harms AC, Brennan L, et al. Menstrual cycle rhythmicity: metabolic patterns in healthy women. Sci Rep. 2018;8(1):14568.
Lai FN, Liu XL, Li N, Zhang RQ, Zhao Y, Feng YZ, et al. Phosphatidylcholine could protect the defect of zearalenone exposure on follicular development and oocyte maturation. Aging (Albany NY). 2018;10(11):3486–506.
Cheng TS, Day FR, Perry JRB, Luan J, Langenberg C, Forouhi NG, Wareham NJ, Ong KK. Prepubertal dietary and plasma phospholipid fatty acids related to puberty timing: Longitudinal cohort and mendelian randomization analyses. Nutrients. 2021;13(6):1868. https://doi.org/10.3390/nu13061868 .
Cybulska AM, Skonieczna-Żydecka K, Drozd A, Rachubińska K, Pawlik J, Stachowska E, Jurczak A, Grochans E. Fatty acid profile of postmenopausal women receiving, and not receiving, hormone replacement therapy. Int J Environ Res Public Health. 2019;16(21):4273. https://doi.org/10.3390/ijerph16214273 .
Gortan Cappellari G, Losurdo P, Mazzucco S, Panizon E, Jevnicar M, Macaluso L, et al. Treatment with n-3 polyunsaturated fatty acids reverses endothelial dysfunction and oxidative stress in experimental menopause. J Nutr Biochem. 2013;24(1):371–9.
Abshirini M, Siassi F, Koohdani F, Qorbani M, Khosravi S, Aslani Z, et al. Higher intake of dietary n-3 PUFA and lower MUFA are associated with fewer menopausal symptoms. Climacteric. 2019;22(2):195–201.
Lipovac M, Aschauer J, Imhof H, Herrmann C, Sima M, Weiss P, et al. The effect of micronutrient supplementation on serum anti-Mullerian hormone levels: a retrospective pilot study. Gynecol Endocrinol. 2022;38(4):310–3.
Calder PC, Grimble RF. Polyunsaturated fatty acids, inflammation and immunity. Eur J Clin Nutr. 2002;56(Suppl 3):S14–9.
Ağaçayak E, Yaman Görük N, Küsen H, Yaman Tunç S, Başaranoğlu S, İçen MS, et al. Role of inflammation and oxidative stress in the etiology of primary ovarian insufficiency. Turk J Obstet Gynecol. 2016;13(3):109–15.
Yasui T, Maegawa M, Tomita J, Miyatani Y, Yamada M, Uemura H, et al. Changes in serum cytokine concentrations during the menopausal transition. Maturitas. 2007;56(4):396–403.
McCarthy M, Raval AP. The peri-menopause in a woman’s life: a systemic inflammatory phase that enables later neurodegenerative disease. J Neuroinflammation. 2020;17(1):317.
Ameur A, Enroth S, Johansson A, Zaboli G, Igl W, Johansson AC, et al. Genetic adaptation of fatty-acid metabolism: a human-specific haplotype increasing the biosynthesis of long-chain omega-3 and omega-6 fatty acids. Am J Hum Genet. 2012;90(5):809–20.
Mathieson I, Day FR, Barban N, Tropf FC, Brazel DM, e QC, et al. Genome-wide analysis identifies genetic effects on reproductive success and ongoing natural selection at the FADS locus. Nat Hum Behav. 2023.
Fumagalli M, Moltke I, Grarup N, Racimo F, Bjerregaard P, Jørgensen ME, et al. Greenlandic Inuit show genetic signatures of diet and climate adaptation. Science. 2015;349(6254):1343–7.
Nagata C, Wada K, Nakamura K, Tamai Y, Tsuji M, Shimizu H. Associations of physical activity and diet with the onset of menopause in Japanese women. Menopause. 2012;19(1):75–81.
Sapre S, Thakur R. Lifestyle and dietary factors determine age at natural menopause. J Midlife Health. 2014;5(1):3–5.
Nguyen NTK, Fan H-Y, Tsai M-C, Tung T-H, Huynh QTV, Huang S-Y, et al. Nutrient intake through childhood and early menarche onset in girls: systematic review and meta-analysis. Nutrients. 2020;12(9):2544.
Malina RM. Menarche in atheletes: a synthesis and hypothesis. Ann Hum Biol. 1983;10(1):1–24.
Chavarro J, Villamor E, Narváez J, Hoyos A. Socio-demographic predictors of age at menarche in a group of Colombian university women. Ann Hum Biol. 2004;31(2):245–57.
Cheng TS, Brage S, van Sluijs EMF, Ong KK. Pre-pubertal accelerometer-assessed physical activity and timing of puberty in British boys and girls: the Millennium Cohort Study. Int J Epidemiol. 2023;52(5):1316–27.
Kondoh H, Teruya T, Yanagida M. Metabolomics of human fasting: new insights about old questions. Open Biol. 2020;10(9):200176. https://doi.org/10.1098/rsob.200176 . Epub 2020 Sep 16.
Emwas AM, Al-Rifai N, Szczepski K, Alsuhaymi S, Rayyan S, Almahasheer H, et al. You are what you eat: application of metabolomics approaches to advance nutrition research. Foods. 2021;10(6):1249.
Download references
Acknowledgements
We are grateful to all the participants who contributed samples to the GWAS used in this analysis. We are extremely grateful to all the families who took part in the ALSPAC study, the midwives for their help in recruiting them, and the whole ALSPAC team, which includes interviewers, computer and laboratory technicians, clerical workers, research scientists, volunteers, managers, receptionists, and nurses.
DM is a Fonds de Recherche Quebec Santé (FRQS) Junior 1 scholar and has received a Career Development Award from ENRICH. The UK Medical Research Council and Wellcome (Grant ref: 217065/Z/19/Z) and the University of Bristol provide core support for ALSPAC. This publication is the work of the authors and DM will serve as guarantors for the contents of this paper.
Author information
Authors and affiliations.
Research Center of the Sainte-Justine University Hospital, Université de Montréal, 3175 Côte-Sainte-Catherine, Montréal, Québec, H3T 1C5, Canada
Mojgan Yazdanpanah, Nahid Yazdanpanah, Isabel Gamache & Despoina Manousaki
MRC Epidemiology Unit, School of Clinical Medicine, Wellcome-MRC Institute of Metabolic Science, University of Cambridge, Cambridge, CB2 0QQ, UK
Ken Ong & John R. B. Perry
Metabolic Research Laboratory, School of Clinical Medicine, Wellcome-MRC Institute of Metabolic Science, University of Cambridge, Cambridge, CB2 0QQ, UK
John R. B. Perry
Departments of Pediatrics, Biochemistry and Molecular Medicine, Université de Montréal, Montreal, Canada
Despoina Manousaki
You can also search for this author in PubMed Google Scholar
Contributions
D.M. conceived the study and supervised the analyses. N.Y., M.Y., and I.G. drafted the manuscript and performed the analyses. All authors contributed in the study design, reviewing and writing the manuscript. All authors read and approved the final manuscript.
Corresponding author
Correspondence to Despoina Manousaki .
Ethics declarations
Ethics approval and consent to participate.
Permission to access and analyze the data for the study was obtained from the ALSPAC Executive Committee ( http://www.bristol.ac.uk/alspac/researchers/access/ ). Ethics approval for this study was obtained by the Research Ethics Committee of the CHU Sainte-Justine University Hospital Center. This research conformed to the principles of the Helsinki.
Consent for publication
Informed participant consent from ALSPAC included the use of their data in research and publication of that research in journals, at conferences and on social media.
Competing interests
The authors declare that they have no competing interests.
Additional information
Publisher’s note.
Springer Nature remains neutral with regard to jurisdictional claims in published maps and institutional affiliations.
Supplementary Information
Additional file 1:.
Tables S1 to S13.
Additional file 2.
MR-STROBE checklist.
Rights and permissions
Open Access This article is licensed under a Creative Commons Attribution 4.0 International License, which permits use, sharing, adaptation, distribution and reproduction in any medium or format, as long as you give appropriate credit to the original author(s) and the source, provide a link to the Creative Commons licence, and indicate if changes were made. The images or other third party material in this article are included in the article's Creative Commons licence, unless indicated otherwise in a credit line to the material. If material is not included in the article's Creative Commons licence and your intended use is not permitted by statutory regulation or exceeds the permitted use, you will need to obtain permission directly from the copyright holder. To view a copy of this licence, visit http://creativecommons.org/licenses/by/4.0/ . The Creative Commons Public Domain Dedication waiver ( http://creativecommons.org/publicdomain/zero/1.0/ ) applies to the data made available in this article, unless otherwise stated in a credit line to the data.
Reprints and permissions
About this article
Cite this article.
Yazdanpanah, M., Yazdanpanah, N., Gamache, I. et al. Metabolome-wide Mendelian randomization for age at menarche and age at natural menopause. Genome Med 16 , 69 (2024). https://doi.org/10.1186/s13073-024-01322-7
Download citation
Received : 31 October 2023
Accepted : 22 March 2024
Published : 28 May 2024
DOI : https://doi.org/10.1186/s13073-024-01322-7
Share this article
Anyone you share the following link with will be able to read this content:
Sorry, a shareable link is not currently available for this article.
Provided by the Springer Nature SharedIt content-sharing initiative
- Metabolites
- Mendelian randomization
Genome Medicine
ISSN: 1756-994X
- Submission enquiries: [email protected]
- General enquiries: [email protected]
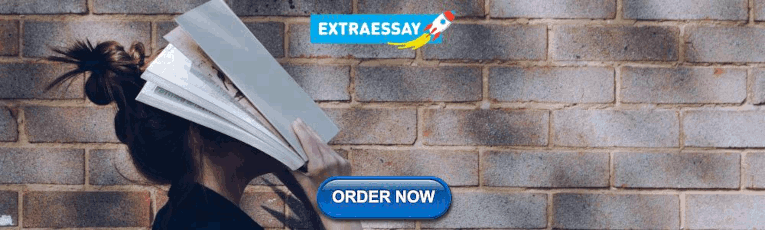
IMAGES
VIDEO
COMMENTS
If the X 2 value is greater than the value at a specific probability, then the null hypothesis has been rejected and a significant deviation from predicted values was observed. Using Mendel's laws, we can count phenotypes after a cross to compare against those predicted by probabilities (or a Punnett Square).
As a consequence, in a Mendelian genetic cross, the null hypothesis is usually an extrinsic hypothesis; in other words, the expected proportions can be predicted and calculated before the ...
The null hypothesis is usually an extrinsic hypothesis, where you knew the expected proportions before doing the experiment. Examples include a \(1:1\) sex ratio or a \(1:2:1\) ratio in a genetic cross. ... It uses the Mendel pea data from above. The "WEIGHT count" tells SAS that the "count" variable is the number of times each value of ...
certain conditions (for example, Mendelian inheritance of alleles) are true. A chi-squared test is used to determine how likely the observed data are if the null hypothesis is true. For example, in the coin flip example, the null hypothesis predicts that heads will appear 50% of the time and tails will appear 50% of the time.
An important question to answer in any genetic experiment is how can we decide if our data fits any of the Mendelian ratios we have discussed. A statistical test that can test out ratios is the Chi-Square or Goodness of Fit test. Let's test the following data to determine if it fits a 9:3:3:1 ratio. Enter the Chi-Square table at df = 3 and we ...
Null hypothesis—The pattern of inheritance follows Mendelian genetics and does not differ from a ratio of 3:1: $$ {\chi}^2=\varSigma \frac{{\left(O-E\right)}^2}{E} $$ E : If the flower colour inheritance followed Mendelian genetics, we would see that 3/4 of the total flowers would be violet and 1/4 would be white since violet is the dominant ...
License: CC BY: Attribution. Module 9: Mendelian Genetics is shared under a CC BY license and was authored, remixed, and/or curated by LibreTexts. Beginning students of biology always learn about Mendelian genetics. Inevitably, the study of inheritance always leads to additional questions. We now know that inheritance is much more complex, ….
Austrian monk Gregor Mendel set the framework for genetics long before chromosomes or genes had been identified, at a time when meiosis was not well understood. Working with garden peas, Mendel found that crosses between true-breeding parents (P) that differed in one trait (e.g., color: green peas versus yellow peas) produced first generation ...
the null- hypothesis of no difference with 1 degree of freedom if chi-square is larger than 3.84 or smaller than 0.004. In Mendel's data frequently very small chi-squares can be observed, as e.g., in the above example where it is as small as 0.0039. This means that the chi-square is too small not to reject the null-hypothesis. The results
1. Goodness of Fit: This is the chi-square application that is used in this. activity. It is used to compare collected data to an expected distribution. 2. 2 x 2 Contingency Table: This is used to compare the numerical responses between two independent groups. As an example, a 2 x 2 contingency table would be used to see if the use a particular ...
With the rapidly increasing availability of large genetic data sets in recent years, Mendelian Randomization (MR) has quickly gained popularity as a novel secondary analysis method. Leveraging ...
Mendelian randomization (MR) is a term that applies to the use of genetic variation to address causal questions about how modifiable exposures influence different outcomes. The principles of MR ...
An example of Mendelian genetics with two heterozygous parents, depicted at the left and top of each square. ... and indeed the LIGO team did not accept the null hypothesis. Fisher noted that Mendel could have derived his predictions from three simpler theoretical postulates, rather than from the data themselves. The evidential force of Mendel ...
Introduction. Figure 18.2 Johann Gregor Mendel is considered to be the father of genetics. Genetics is the study of heredity. Johann Gregor Mendel (1822-1884) set the framework for genetics long before chromosomes or genes had been identified, at a time when meiosis was not well understood ( Figure 18.2 ). Mendel selected a simple biological ...
If these differences alter the production, structure, or function of the protein, an observable or measurable change in the organism may occur. For example, Mendel identified two forms of a gene for seed color: one allele gave green seeds and the other gave yellow seeds. Figure 4.2.1 4.2. 1: Seven traits Mendel studied in peas.
Mendel's Experiment and the Reasoning Guiding Scientific Hypothesis Testing As you may recall, Mendel's theory proposed that dominant and recessive genes exist in pairs (e.g., ... fairness (i.e., to test the statistical null hypothesis that you have a fair coin), you could toss it 100 times. Suppose it lands heads 47 times and tails 53 ...
Mendelian randomization is a form of instrumental variable analysis that uses SNP associations from genome-wide association studies as instruments to study and uncover causal relationships between complex traits. By leveraging SNP genotypes as instrumental variables, or proxies, for the exposure complex trait, investigators can tease out causal ...
The ExSep model selection test is a method to analyze all genetic variables under the null hypothesis of no ExSep events. ... G. Mendelian randomization: genetic anchors for causal inference in ...
Introduction. In plant and animal genetics research, the decisions a scientist will make are based on a high level of confidence in the predictable inheritance of the genes that control the trait being studied. This confidence comes from a past discovery by a biologist named Gregor Mendel, who explained the inheritance of trait variation using ...
Mendelian Genetics; Concepts of Genetics William S. Klug, Michael R. Cummings, Charlotte A. Spencer. Chapter 3 Mendelian Genetics - all with Video Answers. Educators. Chapter Questions. 03:36. Problem 1 ... The basis for rejecting any null hypothesis is arbitrary. The researcher can set more or less stringent standards by deciding to raise or ...
composite null hypothesis [84] already implied the valid-ity of such extension. In brief, PLACO examines one gene at a time with two sets of Z-statistics as input and proceeds by dividing the composite null hypothesis of pleiotropy into three sub-null scenarios: (i) H 00: the gene is not associated neither of the two disorders. (ii) H 10:
Gregor Mendel's observation that some physical traits are inherited as discrete units that can completely disappear and reappear over successive generations 1 was a crucial step in the ...
The hypothesis-free design of our study offers a thorough screen for causal relationships between metabolites evaluated by non-targeted metabolomics and AAM or ANM. ... which may have influenced our results toward the null ... Consortium EP-I Network Mendelian randomization: using genetic variants as instrumental variables to investigate ...