Research Hypothesis In Psychology: Types, & Examples
Saul Mcleod, PhD
Editor-in-Chief for Simply Psychology
BSc (Hons) Psychology, MRes, PhD, University of Manchester
Saul Mcleod, PhD., is a qualified psychology teacher with over 18 years of experience in further and higher education. He has been published in peer-reviewed journals, including the Journal of Clinical Psychology.
Learn about our Editorial Process
Olivia Guy-Evans, MSc
Associate Editor for Simply Psychology
BSc (Hons) Psychology, MSc Psychology of Education
Olivia Guy-Evans is a writer and associate editor for Simply Psychology. She has previously worked in healthcare and educational sectors.
On This Page:
A research hypothesis, in its plural form “hypotheses,” is a specific, testable prediction about the anticipated results of a study, established at its outset. It is a key component of the scientific method .
Hypotheses connect theory to data and guide the research process towards expanding scientific understanding
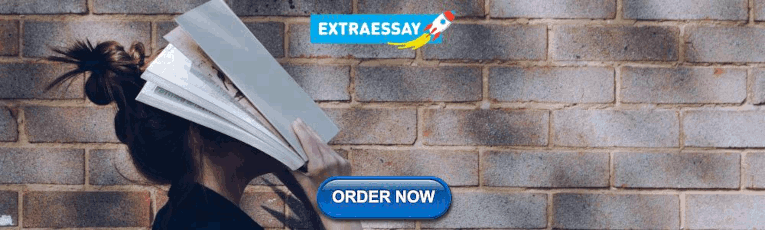
Some key points about hypotheses:
- A hypothesis expresses an expected pattern or relationship. It connects the variables under investigation.
- It is stated in clear, precise terms before any data collection or analysis occurs. This makes the hypothesis testable.
- A hypothesis must be falsifiable. It should be possible, even if unlikely in practice, to collect data that disconfirms rather than supports the hypothesis.
- Hypotheses guide research. Scientists design studies to explicitly evaluate hypotheses about how nature works.
- For a hypothesis to be valid, it must be testable against empirical evidence. The evidence can then confirm or disprove the testable predictions.
- Hypotheses are informed by background knowledge and observation, but go beyond what is already known to propose an explanation of how or why something occurs.
Predictions typically arise from a thorough knowledge of the research literature, curiosity about real-world problems or implications, and integrating this to advance theory. They build on existing literature while providing new insight.
Types of Research Hypotheses
Alternative hypothesis.
The research hypothesis is often called the alternative or experimental hypothesis in experimental research.
It typically suggests a potential relationship between two key variables: the independent variable, which the researcher manipulates, and the dependent variable, which is measured based on those changes.
The alternative hypothesis states a relationship exists between the two variables being studied (one variable affects the other).
A hypothesis is a testable statement or prediction about the relationship between two or more variables. It is a key component of the scientific method. Some key points about hypotheses:
- Important hypotheses lead to predictions that can be tested empirically. The evidence can then confirm or disprove the testable predictions.
In summary, a hypothesis is a precise, testable statement of what researchers expect to happen in a study and why. Hypotheses connect theory to data and guide the research process towards expanding scientific understanding.
An experimental hypothesis predicts what change(s) will occur in the dependent variable when the independent variable is manipulated.
It states that the results are not due to chance and are significant in supporting the theory being investigated.
The alternative hypothesis can be directional, indicating a specific direction of the effect, or non-directional, suggesting a difference without specifying its nature. It’s what researchers aim to support or demonstrate through their study.
Null Hypothesis
The null hypothesis states no relationship exists between the two variables being studied (one variable does not affect the other). There will be no changes in the dependent variable due to manipulating the independent variable.
It states results are due to chance and are not significant in supporting the idea being investigated.
The null hypothesis, positing no effect or relationship, is a foundational contrast to the research hypothesis in scientific inquiry. It establishes a baseline for statistical testing, promoting objectivity by initiating research from a neutral stance.
Many statistical methods are tailored to test the null hypothesis, determining the likelihood of observed results if no true effect exists.
This dual-hypothesis approach provides clarity, ensuring that research intentions are explicit, and fosters consistency across scientific studies, enhancing the standardization and interpretability of research outcomes.
Nondirectional Hypothesis
A non-directional hypothesis, also known as a two-tailed hypothesis, predicts that there is a difference or relationship between two variables but does not specify the direction of this relationship.
It merely indicates that a change or effect will occur without predicting which group will have higher or lower values.
For example, “There is a difference in performance between Group A and Group B” is a non-directional hypothesis.
Directional Hypothesis
A directional (one-tailed) hypothesis predicts the nature of the effect of the independent variable on the dependent variable. It predicts in which direction the change will take place. (i.e., greater, smaller, less, more)
It specifies whether one variable is greater, lesser, or different from another, rather than just indicating that there’s a difference without specifying its nature.
For example, “Exercise increases weight loss” is a directional hypothesis.
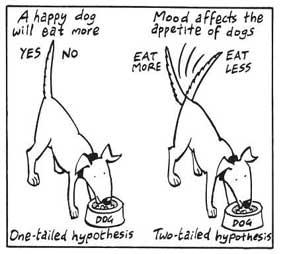
Falsifiability
The Falsification Principle, proposed by Karl Popper , is a way of demarcating science from non-science. It suggests that for a theory or hypothesis to be considered scientific, it must be testable and irrefutable.
Falsifiability emphasizes that scientific claims shouldn’t just be confirmable but should also have the potential to be proven wrong.
It means that there should exist some potential evidence or experiment that could prove the proposition false.
However many confirming instances exist for a theory, it only takes one counter observation to falsify it. For example, the hypothesis that “all swans are white,” can be falsified by observing a black swan.
For Popper, science should attempt to disprove a theory rather than attempt to continually provide evidence to support a research hypothesis.
Can a Hypothesis be Proven?
Hypotheses make probabilistic predictions. They state the expected outcome if a particular relationship exists. However, a study result supporting a hypothesis does not definitively prove it is true.
All studies have limitations. There may be unknown confounding factors or issues that limit the certainty of conclusions. Additional studies may yield different results.
In science, hypotheses can realistically only be supported with some degree of confidence, not proven. The process of science is to incrementally accumulate evidence for and against hypothesized relationships in an ongoing pursuit of better models and explanations that best fit the empirical data. But hypotheses remain open to revision and rejection if that is where the evidence leads.
- Disproving a hypothesis is definitive. Solid disconfirmatory evidence will falsify a hypothesis and require altering or discarding it based on the evidence.
- However, confirming evidence is always open to revision. Other explanations may account for the same results, and additional or contradictory evidence may emerge over time.
We can never 100% prove the alternative hypothesis. Instead, we see if we can disprove, or reject the null hypothesis.
If we reject the null hypothesis, this doesn’t mean that our alternative hypothesis is correct but does support the alternative/experimental hypothesis.
Upon analysis of the results, an alternative hypothesis can be rejected or supported, but it can never be proven to be correct. We must avoid any reference to results proving a theory as this implies 100% certainty, and there is always a chance that evidence may exist which could refute a theory.
How to Write a Hypothesis
- Identify variables . The researcher manipulates the independent variable and the dependent variable is the measured outcome.
- Operationalized the variables being investigated . Operationalization of a hypothesis refers to the process of making the variables physically measurable or testable, e.g. if you are about to study aggression, you might count the number of punches given by participants.
- Decide on a direction for your prediction . If there is evidence in the literature to support a specific effect of the independent variable on the dependent variable, write a directional (one-tailed) hypothesis. If there are limited or ambiguous findings in the literature regarding the effect of the independent variable on the dependent variable, write a non-directional (two-tailed) hypothesis.
- Make it Testable : Ensure your hypothesis can be tested through experimentation or observation. It should be possible to prove it false (principle of falsifiability).
- Clear & concise language . A strong hypothesis is concise (typically one to two sentences long), and formulated using clear and straightforward language, ensuring it’s easily understood and testable.
Consider a hypothesis many teachers might subscribe to: students work better on Monday morning than on Friday afternoon (IV=Day, DV= Standard of work).
Now, if we decide to study this by giving the same group of students a lesson on a Monday morning and a Friday afternoon and then measuring their immediate recall of the material covered in each session, we would end up with the following:
- The alternative hypothesis states that students will recall significantly more information on a Monday morning than on a Friday afternoon.
- The null hypothesis states that there will be no significant difference in the amount recalled on a Monday morning compared to a Friday afternoon. Any difference will be due to chance or confounding factors.
More Examples
- Memory : Participants exposed to classical music during study sessions will recall more items from a list than those who studied in silence.
- Social Psychology : Individuals who frequently engage in social media use will report higher levels of perceived social isolation compared to those who use it infrequently.
- Developmental Psychology : Children who engage in regular imaginative play have better problem-solving skills than those who don’t.
- Clinical Psychology : Cognitive-behavioral therapy will be more effective in reducing symptoms of anxiety over a 6-month period compared to traditional talk therapy.
- Cognitive Psychology : Individuals who multitask between various electronic devices will have shorter attention spans on focused tasks than those who single-task.
- Health Psychology : Patients who practice mindfulness meditation will experience lower levels of chronic pain compared to those who don’t meditate.
- Organizational Psychology : Employees in open-plan offices will report higher levels of stress than those in private offices.
- Behavioral Psychology : Rats rewarded with food after pressing a lever will press it more frequently than rats who receive no reward.

Related Articles
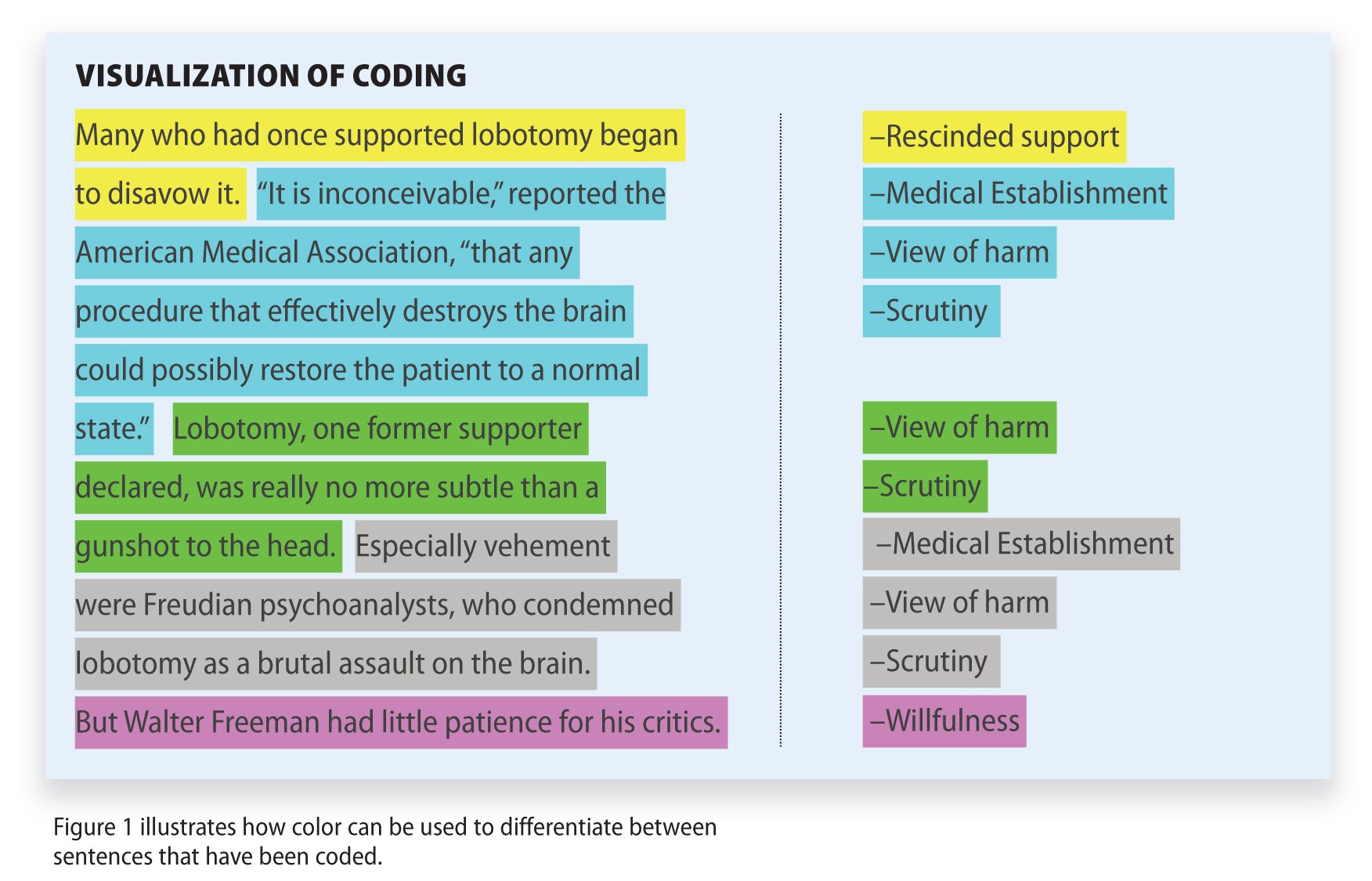
Research Methodology
Qualitative Data Coding
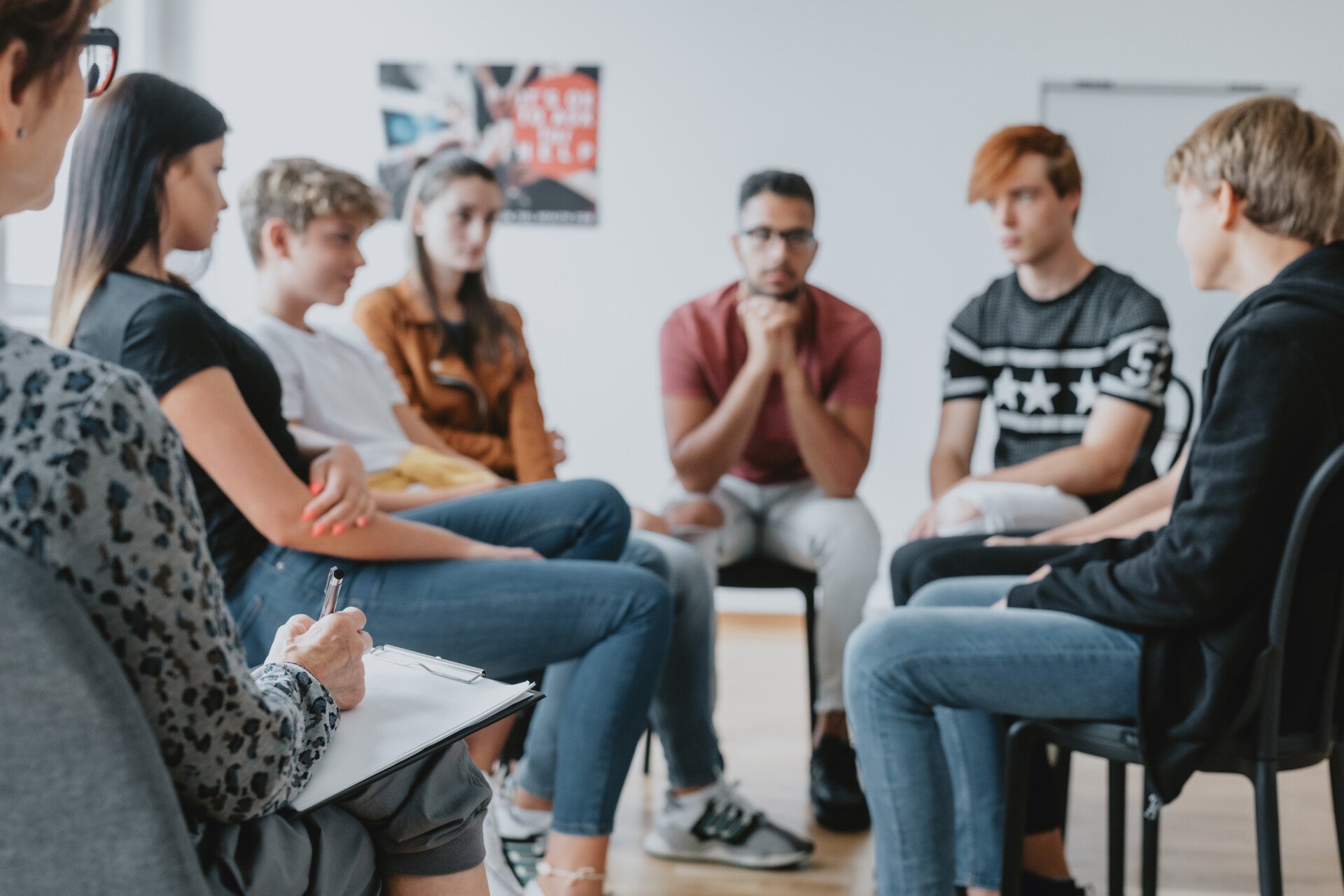
What Is a Focus Group?
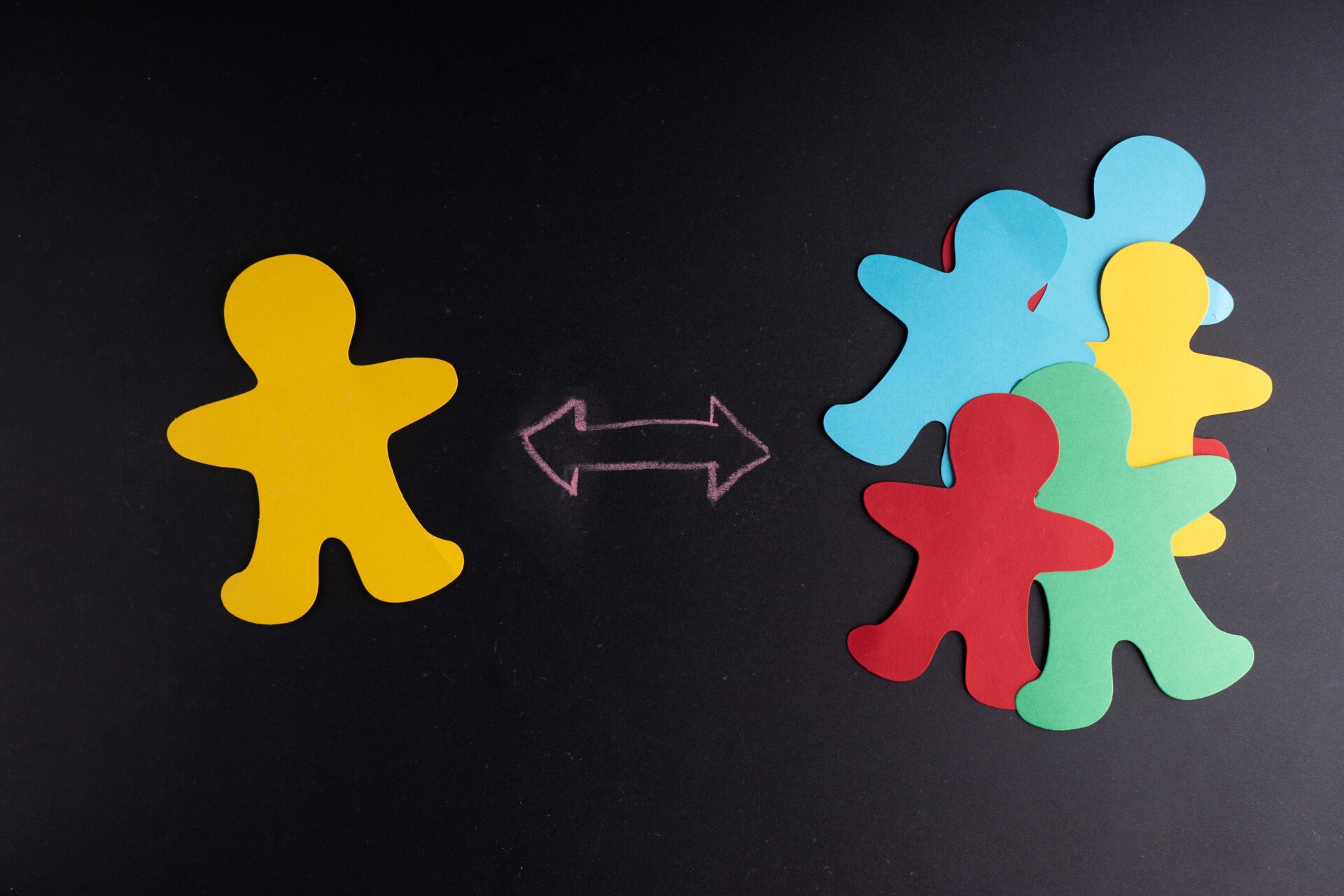
Cross-Cultural Research Methodology In Psychology
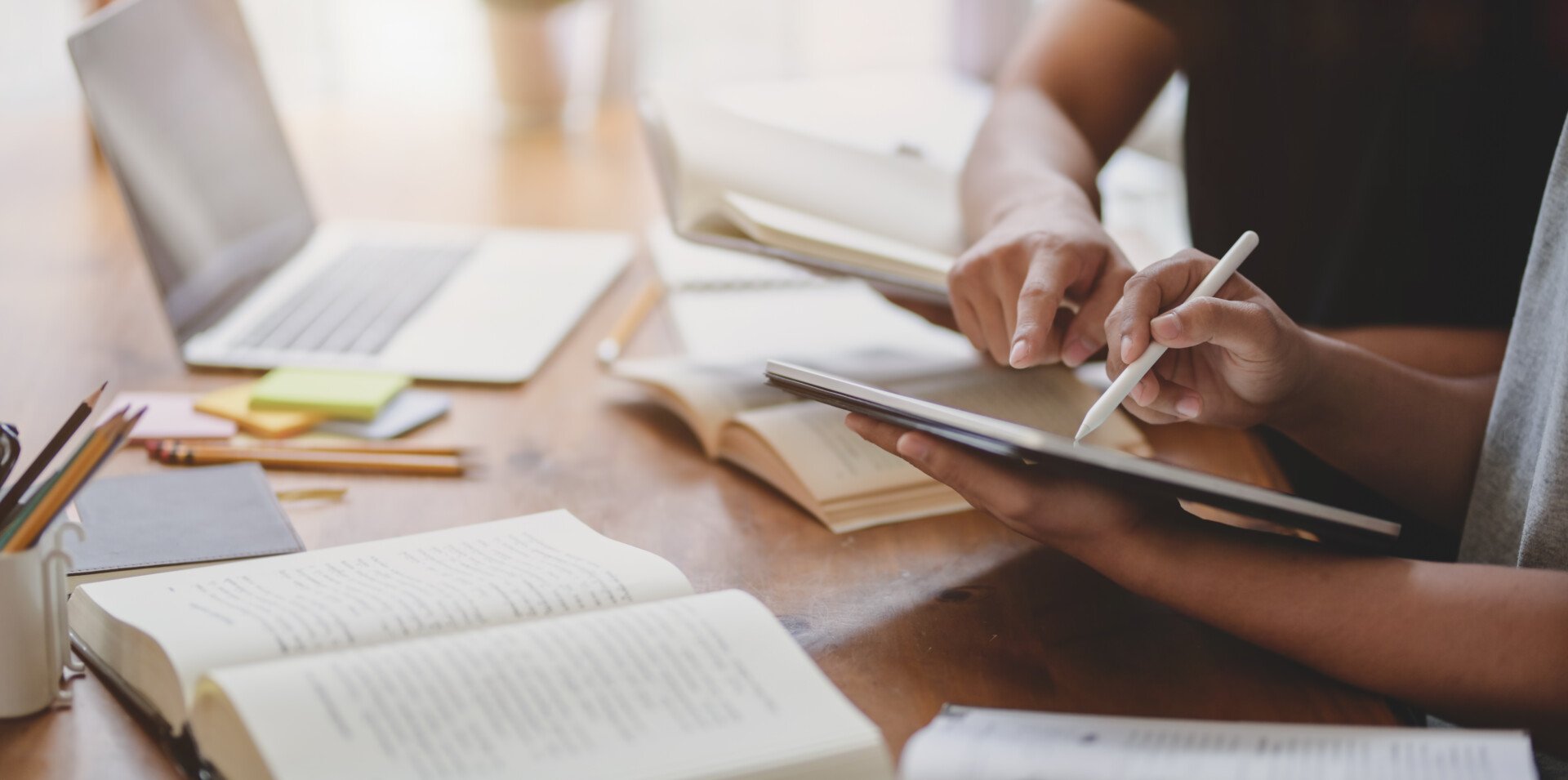
What Is Internal Validity In Research?
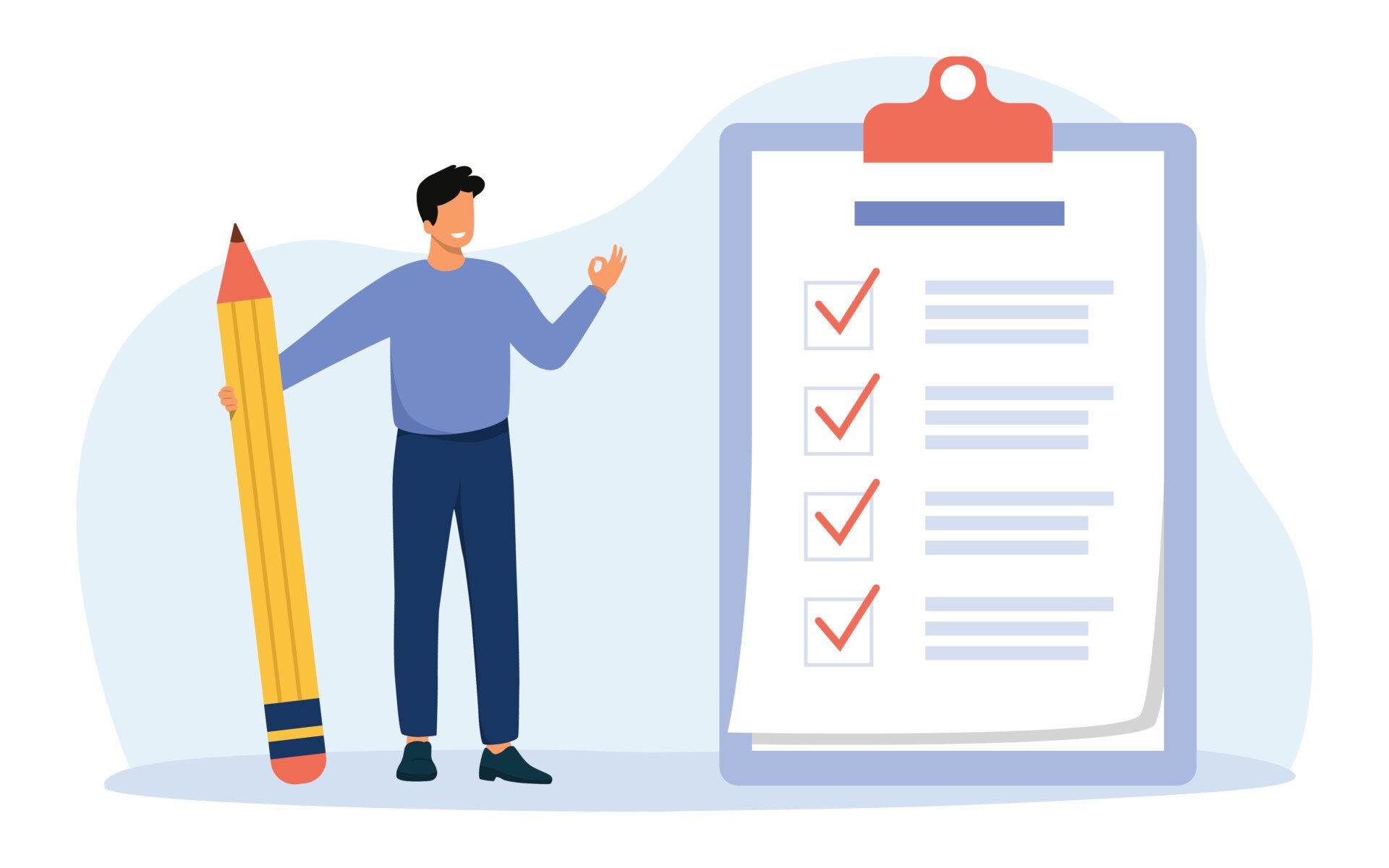
Research Methodology , Statistics
What Is Face Validity In Research? Importance & How To Measure
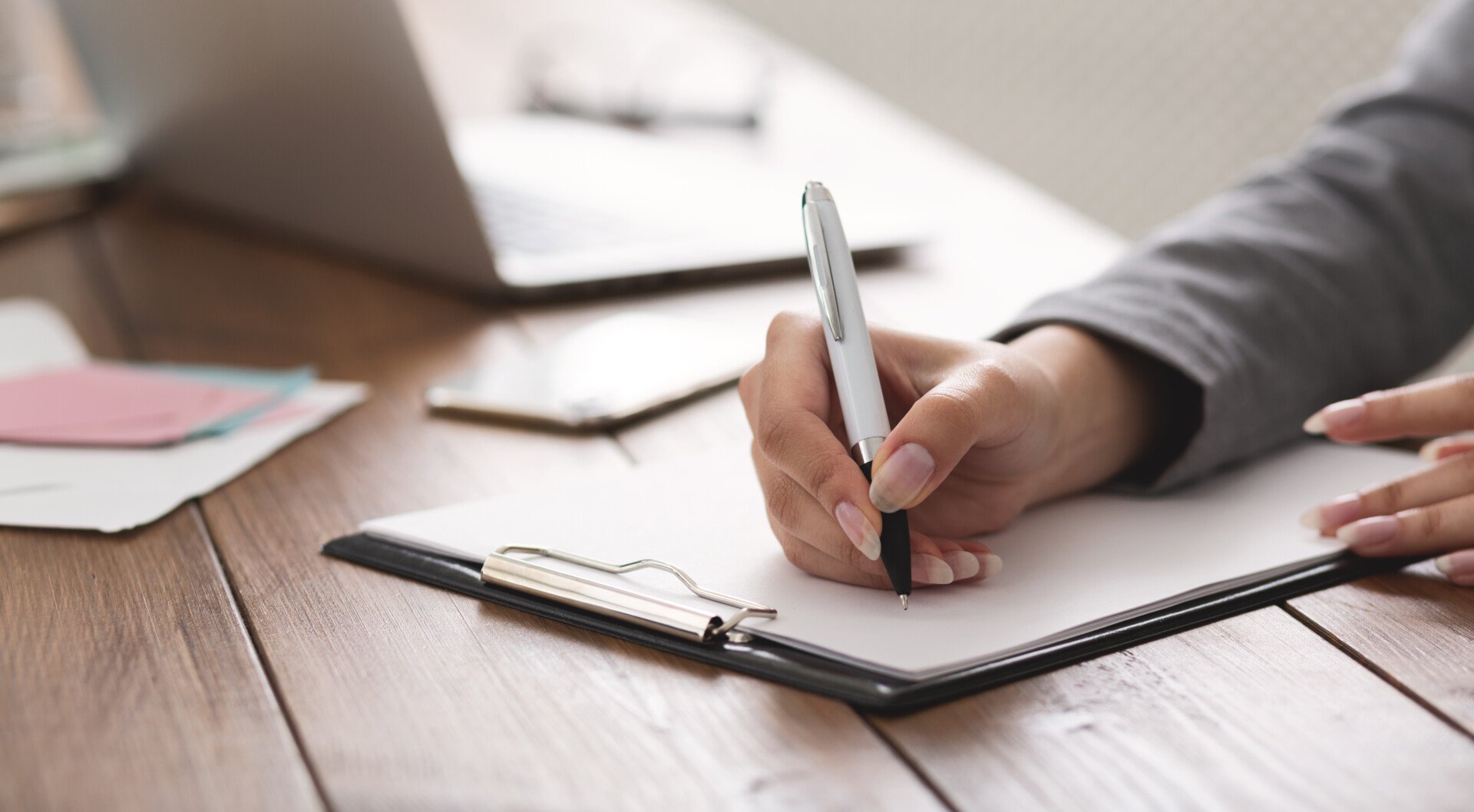
Criterion Validity: Definition & Examples
- Bipolar Disorder
- Therapy Center
- When To See a Therapist
- Types of Therapy
- Best Online Therapy
- Best Couples Therapy
- Best Family Therapy
- Managing Stress
- Sleep and Dreaming
- Understanding Emotions
- Self-Improvement
- Healthy Relationships
- Student Resources
- Personality Types
- Guided Meditations
- Verywell Mind Insights
- 2024 Verywell Mind 25
- Mental Health in the Classroom
- Editorial Process
- Meet Our Review Board
- Crisis Support
How to Write a Great Hypothesis
Hypothesis Definition, Format, Examples, and Tips
Kendra Cherry, MS, is a psychosocial rehabilitation specialist, psychology educator, and author of the "Everything Psychology Book."
:max_bytes(150000):strip_icc():format(webp)/IMG_9791-89504ab694d54b66bbd72cb84ffb860e.jpg)
Amy Morin, LCSW, is a psychotherapist and international bestselling author. Her books, including "13 Things Mentally Strong People Don't Do," have been translated into more than 40 languages. Her TEDx talk, "The Secret of Becoming Mentally Strong," is one of the most viewed talks of all time.
:max_bytes(150000):strip_icc():format(webp)/VW-MIND-Amy-2b338105f1ee493f94d7e333e410fa76.jpg)
Verywell / Alex Dos Diaz
- The Scientific Method
Hypothesis Format
Falsifiability of a hypothesis.
- Operationalization
Hypothesis Types
Hypotheses examples.
- Collecting Data
A hypothesis is a tentative statement about the relationship between two or more variables. It is a specific, testable prediction about what you expect to happen in a study. It is a preliminary answer to your question that helps guide the research process.
Consider a study designed to examine the relationship between sleep deprivation and test performance. The hypothesis might be: "This study is designed to assess the hypothesis that sleep-deprived people will perform worse on a test than individuals who are not sleep-deprived."
At a Glance
A hypothesis is crucial to scientific research because it offers a clear direction for what the researchers are looking to find. This allows them to design experiments to test their predictions and add to our scientific knowledge about the world. This article explores how a hypothesis is used in psychology research, how to write a good hypothesis, and the different types of hypotheses you might use.
The Hypothesis in the Scientific Method
In the scientific method , whether it involves research in psychology, biology, or some other area, a hypothesis represents what the researchers think will happen in an experiment. The scientific method involves the following steps:
- Forming a question
- Performing background research
- Creating a hypothesis
- Designing an experiment
- Collecting data
- Analyzing the results
- Drawing conclusions
- Communicating the results
The hypothesis is a prediction, but it involves more than a guess. Most of the time, the hypothesis begins with a question which is then explored through background research. At this point, researchers then begin to develop a testable hypothesis.
Unless you are creating an exploratory study, your hypothesis should always explain what you expect to happen.
In a study exploring the effects of a particular drug, the hypothesis might be that researchers expect the drug to have some type of effect on the symptoms of a specific illness. In psychology, the hypothesis might focus on how a certain aspect of the environment might influence a particular behavior.
Remember, a hypothesis does not have to be correct. While the hypothesis predicts what the researchers expect to see, the goal of the research is to determine whether this guess is right or wrong. When conducting an experiment, researchers might explore numerous factors to determine which ones might contribute to the ultimate outcome.
In many cases, researchers may find that the results of an experiment do not support the original hypothesis. When writing up these results, the researchers might suggest other options that should be explored in future studies.
In many cases, researchers might draw a hypothesis from a specific theory or build on previous research. For example, prior research has shown that stress can impact the immune system. So a researcher might hypothesize: "People with high-stress levels will be more likely to contract a common cold after being exposed to the virus than people who have low-stress levels."
In other instances, researchers might look at commonly held beliefs or folk wisdom. "Birds of a feather flock together" is one example of folk adage that a psychologist might try to investigate. The researcher might pose a specific hypothesis that "People tend to select romantic partners who are similar to them in interests and educational level."
Elements of a Good Hypothesis
So how do you write a good hypothesis? When trying to come up with a hypothesis for your research or experiments, ask yourself the following questions:
- Is your hypothesis based on your research on a topic?
- Can your hypothesis be tested?
- Does your hypothesis include independent and dependent variables?
Before you come up with a specific hypothesis, spend some time doing background research. Once you have completed a literature review, start thinking about potential questions you still have. Pay attention to the discussion section in the journal articles you read . Many authors will suggest questions that still need to be explored.
How to Formulate a Good Hypothesis
To form a hypothesis, you should take these steps:
- Collect as many observations about a topic or problem as you can.
- Evaluate these observations and look for possible causes of the problem.
- Create a list of possible explanations that you might want to explore.
- After you have developed some possible hypotheses, think of ways that you could confirm or disprove each hypothesis through experimentation. This is known as falsifiability.
In the scientific method , falsifiability is an important part of any valid hypothesis. In order to test a claim scientifically, it must be possible that the claim could be proven false.
Students sometimes confuse the idea of falsifiability with the idea that it means that something is false, which is not the case. What falsifiability means is that if something was false, then it is possible to demonstrate that it is false.
One of the hallmarks of pseudoscience is that it makes claims that cannot be refuted or proven false.
The Importance of Operational Definitions
A variable is a factor or element that can be changed and manipulated in ways that are observable and measurable. However, the researcher must also define how the variable will be manipulated and measured in the study.
Operational definitions are specific definitions for all relevant factors in a study. This process helps make vague or ambiguous concepts detailed and measurable.
For example, a researcher might operationally define the variable " test anxiety " as the results of a self-report measure of anxiety experienced during an exam. A "study habits" variable might be defined by the amount of studying that actually occurs as measured by time.
These precise descriptions are important because many things can be measured in various ways. Clearly defining these variables and how they are measured helps ensure that other researchers can replicate your results.
Replicability
One of the basic principles of any type of scientific research is that the results must be replicable.
Replication means repeating an experiment in the same way to produce the same results. By clearly detailing the specifics of how the variables were measured and manipulated, other researchers can better understand the results and repeat the study if needed.
Some variables are more difficult than others to define. For example, how would you operationally define a variable such as aggression ? For obvious ethical reasons, researchers cannot create a situation in which a person behaves aggressively toward others.
To measure this variable, the researcher must devise a measurement that assesses aggressive behavior without harming others. The researcher might utilize a simulated task to measure aggressiveness in this situation.
Hypothesis Checklist
- Does your hypothesis focus on something that you can actually test?
- Does your hypothesis include both an independent and dependent variable?
- Can you manipulate the variables?
- Can your hypothesis be tested without violating ethical standards?
The hypothesis you use will depend on what you are investigating and hoping to find. Some of the main types of hypotheses that you might use include:
- Simple hypothesis : This type of hypothesis suggests there is a relationship between one independent variable and one dependent variable.
- Complex hypothesis : This type suggests a relationship between three or more variables, such as two independent and dependent variables.
- Null hypothesis : This hypothesis suggests no relationship exists between two or more variables.
- Alternative hypothesis : This hypothesis states the opposite of the null hypothesis.
- Statistical hypothesis : This hypothesis uses statistical analysis to evaluate a representative population sample and then generalizes the findings to the larger group.
- Logical hypothesis : This hypothesis assumes a relationship between variables without collecting data or evidence.
A hypothesis often follows a basic format of "If {this happens} then {this will happen}." One way to structure your hypothesis is to describe what will happen to the dependent variable if you change the independent variable .
The basic format might be: "If {these changes are made to a certain independent variable}, then we will observe {a change in a specific dependent variable}."
A few examples of simple hypotheses:
- "Students who eat breakfast will perform better on a math exam than students who do not eat breakfast."
- "Students who experience test anxiety before an English exam will get lower scores than students who do not experience test anxiety."
- "Motorists who talk on the phone while driving will be more likely to make errors on a driving course than those who do not talk on the phone."
- "Children who receive a new reading intervention will have higher reading scores than students who do not receive the intervention."
Examples of a complex hypothesis include:
- "People with high-sugar diets and sedentary activity levels are more likely to develop depression."
- "Younger people who are regularly exposed to green, outdoor areas have better subjective well-being than older adults who have limited exposure to green spaces."
Examples of a null hypothesis include:
- "There is no difference in anxiety levels between people who take St. John's wort supplements and those who do not."
- "There is no difference in scores on a memory recall task between children and adults."
- "There is no difference in aggression levels between children who play first-person shooter games and those who do not."
Examples of an alternative hypothesis:
- "People who take St. John's wort supplements will have less anxiety than those who do not."
- "Adults will perform better on a memory task than children."
- "Children who play first-person shooter games will show higher levels of aggression than children who do not."
Collecting Data on Your Hypothesis
Once a researcher has formed a testable hypothesis, the next step is to select a research design and start collecting data. The research method depends largely on exactly what they are studying. There are two basic types of research methods: descriptive research and experimental research.
Descriptive Research Methods
Descriptive research such as case studies , naturalistic observations , and surveys are often used when conducting an experiment is difficult or impossible. These methods are best used to describe different aspects of a behavior or psychological phenomenon.
Once a researcher has collected data using descriptive methods, a correlational study can examine how the variables are related. This research method might be used to investigate a hypothesis that is difficult to test experimentally.
Experimental Research Methods
Experimental methods are used to demonstrate causal relationships between variables. In an experiment, the researcher systematically manipulates a variable of interest (known as the independent variable) and measures the effect on another variable (known as the dependent variable).
Unlike correlational studies, which can only be used to determine if there is a relationship between two variables, experimental methods can be used to determine the actual nature of the relationship—whether changes in one variable actually cause another to change.
The hypothesis is a critical part of any scientific exploration. It represents what researchers expect to find in a study or experiment. In situations where the hypothesis is unsupported by the research, the research still has value. Such research helps us better understand how different aspects of the natural world relate to one another. It also helps us develop new hypotheses that can then be tested in the future.
Thompson WH, Skau S. On the scope of scientific hypotheses . R Soc Open Sci . 2023;10(8):230607. doi:10.1098/rsos.230607
Taran S, Adhikari NKJ, Fan E. Falsifiability in medicine: what clinicians can learn from Karl Popper [published correction appears in Intensive Care Med. 2021 Jun 17;:]. Intensive Care Med . 2021;47(9):1054-1056. doi:10.1007/s00134-021-06432-z
Eyler AA. Research Methods for Public Health . 1st ed. Springer Publishing Company; 2020. doi:10.1891/9780826182067.0004
Nosek BA, Errington TM. What is replication ? PLoS Biol . 2020;18(3):e3000691. doi:10.1371/journal.pbio.3000691
Aggarwal R, Ranganathan P. Study designs: Part 2 - Descriptive studies . Perspect Clin Res . 2019;10(1):34-36. doi:10.4103/picr.PICR_154_18
Nevid J. Psychology: Concepts and Applications. Wadworth, 2013.
By Kendra Cherry, MSEd Kendra Cherry, MS, is a psychosocial rehabilitation specialist, psychology educator, and author of the "Everything Psychology Book."
- Skip to main content
- Skip to primary sidebar
- Skip to footer
- QuestionPro

- Solutions Industries Gaming Automotive Sports and events Education Government Travel & Hospitality Financial Services Healthcare Cannabis Technology Use Case NPS+ Communities Audience Contactless surveys Mobile LivePolls Member Experience GDPR Positive People Science 360 Feedback Surveys
- Resources Blog eBooks Survey Templates Case Studies Training Help center

Home Market Research
Research Hypothesis: What It Is, Types + How to Develop?
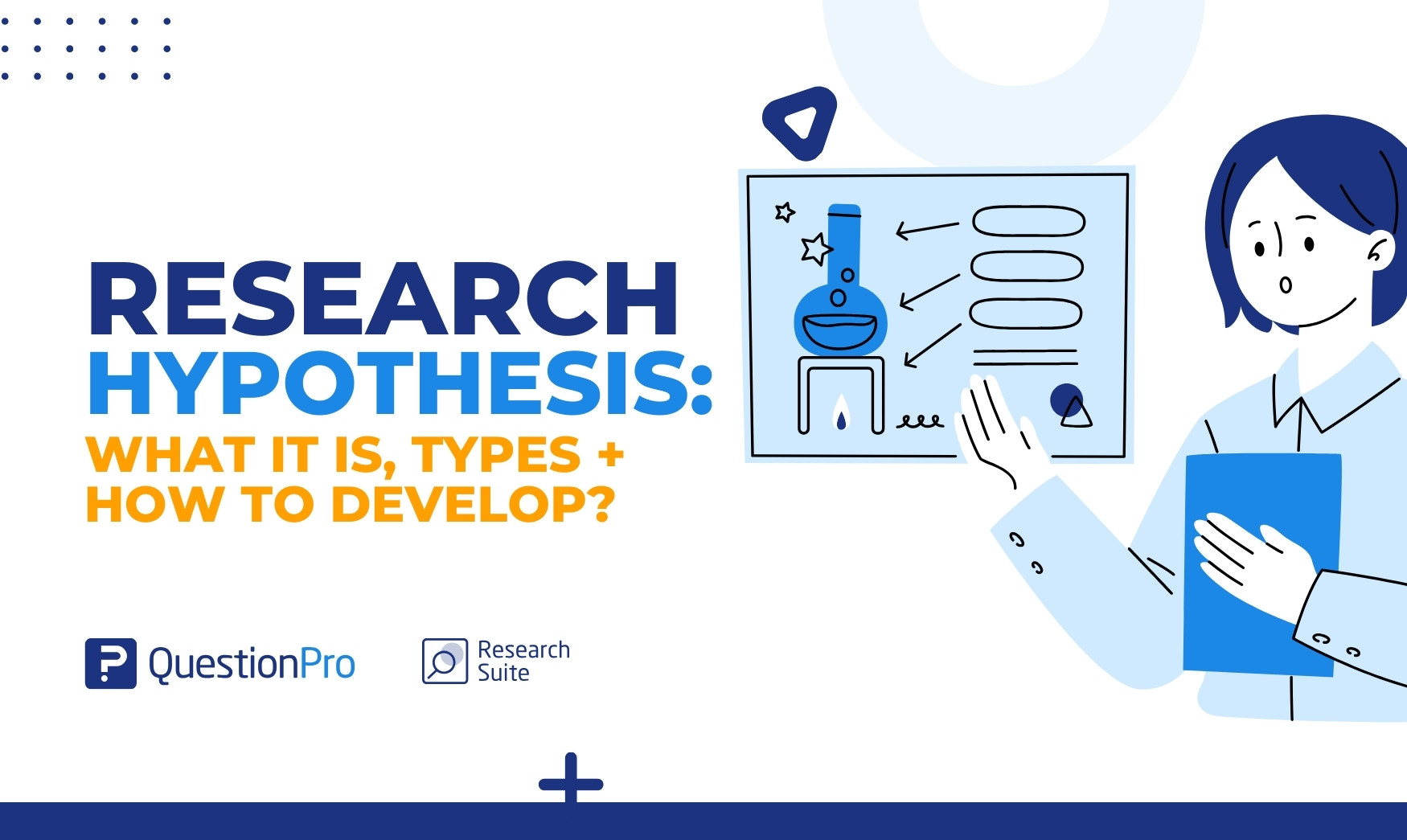
A research study starts with a question. Researchers worldwide ask questions and create research hypotheses. The effectiveness of research relies on developing a good research hypothesis. Examples of research hypotheses can guide researchers in writing effective ones.
In this blog, we’ll learn what a research hypothesis is, why it’s important in research, and the different types used in science. We’ll also guide you through creating your research hypothesis and discussing ways to test and evaluate it.
What is a Research Hypothesis?
A hypothesis is like a guess or idea that you suggest to check if it’s true. A research hypothesis is a statement that brings up a question and predicts what might happen.
It’s really important in the scientific method and is used in experiments to figure things out. Essentially, it’s an educated guess about how things are connected in the research.
A research hypothesis usually includes pointing out the independent variable (the thing they’re changing or studying) and the dependent variable (the result they’re measuring or watching). It helps plan how to gather and analyze data to see if there’s evidence to support or deny the expected connection between these variables.
Importance of Hypothesis in Research
Hypotheses are really important in research. They help design studies, allow for practical testing, and add to our scientific knowledge. Their main role is to organize research projects, making them purposeful, focused, and valuable to the scientific community. Let’s look at some key reasons why they matter:
- A research hypothesis helps test theories.
A hypothesis plays a pivotal role in the scientific method by providing a basis for testing existing theories. For example, a hypothesis might test the predictive power of a psychological theory on human behavior.
- It serves as a great platform for investigation activities.
It serves as a launching pad for investigation activities, which offers researchers a clear starting point. A research hypothesis can explore the relationship between exercise and stress reduction.
- Hypothesis guides the research work or study.
A well-formulated hypothesis guides the entire research process. It ensures that the study remains focused and purposeful. For instance, a hypothesis about the impact of social media on interpersonal relationships provides clear guidance for a study.
- Hypothesis sometimes suggests theories.
In some cases, a hypothesis can suggest new theories or modifications to existing ones. For example, a hypothesis testing the effectiveness of a new drug might prompt a reconsideration of current medical theories.
- It helps in knowing the data needs.
A hypothesis clarifies the data requirements for a study, ensuring that researchers collect the necessary information—a hypothesis guiding the collection of demographic data to analyze the influence of age on a particular phenomenon.
- The hypothesis explains social phenomena.
Hypotheses are instrumental in explaining complex social phenomena. For instance, a hypothesis might explore the relationship between economic factors and crime rates in a given community.
- Hypothesis provides a relationship between phenomena for empirical Testing.
Hypotheses establish clear relationships between phenomena, paving the way for empirical testing. An example could be a hypothesis exploring the correlation between sleep patterns and academic performance.
- It helps in knowing the most suitable analysis technique.
A hypothesis guides researchers in selecting the most appropriate analysis techniques for their data. For example, a hypothesis focusing on the effectiveness of a teaching method may lead to the choice of statistical analyses best suited for educational research.
Characteristics of a Good Research Hypothesis
A hypothesis is a specific idea that you can test in a study. It often comes from looking at past research and theories. A good hypothesis usually starts with a research question that you can explore through background research. For it to be effective, consider these key characteristics:
- Clear and Focused Language: A good hypothesis uses clear and focused language to avoid confusion and ensure everyone understands it.
- Related to the Research Topic: The hypothesis should directly relate to the research topic, acting as a bridge between the specific question and the broader study.
- Testable: An effective hypothesis can be tested, meaning its prediction can be checked with real data to support or challenge the proposed relationship.
- Potential for Exploration: A good hypothesis often comes from a research question that invites further exploration. Doing background research helps find gaps and potential areas to investigate.
- Includes Variables: The hypothesis should clearly state both the independent and dependent variables, specifying the factors being studied and the expected outcomes.
- Ethical Considerations: Check if variables can be manipulated without breaking ethical standards. It’s crucial to maintain ethical research practices.
- Predicts Outcomes: The hypothesis should predict the expected relationship and outcome, acting as a roadmap for the study and guiding data collection and analysis.
- Simple and Concise: A good hypothesis avoids unnecessary complexity and is simple and concise, expressing the essence of the proposed relationship clearly.
- Clear and Assumption-Free: The hypothesis should be clear and free from assumptions about the reader’s prior knowledge, ensuring universal understanding.
- Observable and Testable Results: A strong hypothesis implies research that produces observable and testable results, making sure the study’s outcomes can be effectively measured and analyzed.
When you use these characteristics as a checklist, it can help you create a good research hypothesis. It’ll guide improving and strengthening the hypothesis, identifying any weaknesses, and making necessary changes. Crafting a hypothesis with these features helps you conduct a thorough and insightful research study.
Types of Research Hypotheses
The research hypothesis comes in various types, each serving a specific purpose in guiding the scientific investigation. Knowing the differences will make it easier for you to create your own hypothesis. Here’s an overview of the common types:
01. Null Hypothesis
The null hypothesis states that there is no connection between two considered variables or that two groups are unrelated. As discussed earlier, a hypothesis is an unproven assumption lacking sufficient supporting data. It serves as the statement researchers aim to disprove. It is testable, verifiable, and can be rejected.
For example, if you’re studying the relationship between Project A and Project B, assuming both projects are of equal standard is your null hypothesis. It needs to be specific for your study.
02. Alternative Hypothesis
The alternative hypothesis is basically another option to the null hypothesis. It involves looking for a significant change or alternative that could lead you to reject the null hypothesis. It’s a different idea compared to the null hypothesis.
When you create a null hypothesis, you’re making an educated guess about whether something is true or if there’s a connection between that thing and another variable. If the null view suggests something is correct, the alternative hypothesis says it’s incorrect.
For instance, if your null hypothesis is “I’m going to be $1000 richer,” the alternative hypothesis would be “I’m not going to get $1000 or be richer.”
03. Directional Hypothesis
The directional hypothesis predicts the direction of the relationship between independent and dependent variables. They specify whether the effect will be positive or negative.
If you increase your study hours, you will experience a positive association with your exam scores. This hypothesis suggests that as you increase the independent variable (study hours), there will also be an increase in the dependent variable (exam scores).
04. Non-directional Hypothesis
The non-directional hypothesis predicts the existence of a relationship between variables but does not specify the direction of the effect. It suggests that there will be a significant difference or relationship, but it does not predict the nature of that difference.
For example, you will find no notable difference in test scores between students who receive the educational intervention and those who do not. However, once you compare the test scores of the two groups, you will notice an important difference.
05. Simple Hypothesis
A simple hypothesis predicts a relationship between one dependent variable and one independent variable without specifying the nature of that relationship. It’s simple and usually used when we don’t know much about how the two things are connected.
For example, if you adopt effective study habits, you will achieve higher exam scores than those with poor study habits.
06. Complex Hypothesis
A complex hypothesis is an idea that specifies a relationship between multiple independent and dependent variables. It is a more detailed idea than a simple hypothesis.
While a simple view suggests a straightforward cause-and-effect relationship between two things, a complex hypothesis involves many factors and how they’re connected to each other.
For example, when you increase your study time, you tend to achieve higher exam scores. The connection between your study time and exam performance is affected by various factors, including the quality of your sleep, your motivation levels, and the effectiveness of your study techniques.
If you sleep well, stay highly motivated, and use effective study strategies, you may observe a more robust positive correlation between the time you spend studying and your exam scores, unlike those who may lack these factors.
07. Associative Hypothesis
An associative hypothesis proposes a connection between two things without saying that one causes the other. Basically, it suggests that when one thing changes, the other changes too, but it doesn’t claim that one thing is causing the change in the other.
For example, you will likely notice higher exam scores when you increase your study time. You can recognize an association between your study time and exam scores in this scenario.
Your hypothesis acknowledges a relationship between the two variables—your study time and exam scores—without asserting that increased study time directly causes higher exam scores. You need to consider that other factors, like motivation or learning style, could affect the observed association.
08. Causal Hypothesis
A causal hypothesis proposes a cause-and-effect relationship between two variables. It suggests that changes in one variable directly cause changes in another variable.
For example, when you increase your study time, you experience higher exam scores. This hypothesis suggests a direct cause-and-effect relationship, indicating that the more time you spend studying, the higher your exam scores. It assumes that changes in your study time directly influence changes in your exam performance.
09. Empirical Hypothesis
An empirical hypothesis is a statement based on things we can see and measure. It comes from direct observation or experiments and can be tested with real-world evidence. If an experiment proves a theory, it supports the idea and shows it’s not just a guess. This makes the statement more reliable than a wild guess.
For example, if you increase the dosage of a certain medication, you might observe a quicker recovery time for patients. Imagine you’re in charge of a clinical trial. In this trial, patients are given varying dosages of the medication, and you measure and compare their recovery times. This allows you to directly see the effects of different dosages on how fast patients recover.
This way, you can create a research hypothesis: “Increasing the dosage of a certain medication will lead to a faster recovery time for patients.”
10. Statistical Hypothesis
A statistical hypothesis is a statement or assumption about a population parameter that is the subject of an investigation. It serves as the basis for statistical analysis and testing. It is often tested using statistical methods to draw inferences about the larger population.
In a hypothesis test, statistical evidence is collected to either reject the null hypothesis in favor of the alternative hypothesis or fail to reject the null hypothesis due to insufficient evidence.
For example, let’s say you’re testing a new medicine. Your hypothesis could be that the medicine doesn’t really help patients get better. So, you collect data and use statistics to see if your guess is right or if the medicine actually makes a difference.
If the data strongly shows that the medicine does help, you say your guess was wrong, and the medicine does make a difference. But if the proof isn’t strong enough, you can stick with your original guess because you didn’t get enough evidence to change your mind.
How to Develop a Research Hypotheses?
Step 1: identify your research problem or topic..
Define the area of interest or the problem you want to investigate. Make sure it’s clear and well-defined.
Start by asking a question about your chosen topic. Consider the limitations of your research and create a straightforward problem related to your topic. Once you’ve done that, you can develop and test a hypothesis with evidence.
Step 2: Conduct a literature review
Review existing literature related to your research problem. This will help you understand the current state of knowledge in the field, identify gaps, and build a foundation for your hypothesis. Consider the following questions:
- What existing research has been conducted on your chosen topic?
- Are there any gaps or unanswered questions in the current literature?
- How will the existing literature contribute to the foundation of your research?
Step 3: Formulate your research question
Based on your literature review, create a specific and concise research question that addresses your identified problem. Your research question should be clear, focused, and relevant to your field of study.
Step 4: Identify variables
Determine the key variables involved in your research question. Variables are the factors or phenomena that you will study and manipulate to test your hypothesis.
- Independent Variable: The variable you manipulate or control.
- Dependent Variable: The variable you measure to observe the effect of the independent variable.
Step 5: State the Null hypothesis
The null hypothesis is a statement that there is no significant difference or effect. It serves as a baseline for comparison with the alternative hypothesis.
Step 6: Select appropriate methods for testing the hypothesis
Choose research methods that align with your study objectives, such as experiments, surveys, or observational studies. The selected methods enable you to test your research hypothesis effectively.
Creating a research hypothesis usually takes more than one try. Expect to make changes as you collect data. It’s normal to test and say no to a few hypotheses before you find the right answer to your research question.
Testing and Evaluating Hypotheses
Testing hypotheses is a really important part of research. It’s like the practical side of things. Here, real-world evidence will help you determine how different things are connected. Let’s explore the main steps in hypothesis testing:
- State your research hypothesis.
Before testing, clearly articulate your research hypothesis. This involves framing both a null hypothesis, suggesting no significant effect or relationship, and an alternative hypothesis, proposing the expected outcome.
- Collect data strategically.
Plan how you will gather information in a way that fits your study. Make sure your data collection method matches the things you’re studying.
Whether through surveys, observations, or experiments, this step demands precision and adherence to the established methodology. The quality of data collected directly influences the credibility of study outcomes.
- Perform an appropriate statistical test.
Choose a statistical test that aligns with the nature of your data and the hypotheses being tested. Whether it’s a t-test, chi-square test, ANOVA, or regression analysis, selecting the right statistical tool is paramount for accurate and reliable results.
- Decide if your idea was right or wrong.
Following the statistical analysis, evaluate the results in the context of your null hypothesis. You need to decide if you should reject your null hypothesis or not.
- Share what you found.
When discussing what you found in your research, be clear and organized. Say whether your idea was supported or not, and talk about what your results mean. Also, mention any limits to your study and suggest ideas for future research.
The Role of QuestionPro to Develop a Good Research Hypothesis
QuestionPro is a survey and research platform that provides tools for creating, distributing, and analyzing surveys. It plays a crucial role in the research process, especially when you’re in the initial stages of hypothesis development. Here’s how QuestionPro can help you to develop a good research hypothesis:
- Survey design and data collection: You can use the platform to create targeted questions that help you gather relevant data.
- Exploratory research: Through surveys and feedback mechanisms on QuestionPro, you can conduct exploratory research to understand the landscape of a particular subject.
- Literature review and background research: QuestionPro surveys can collect sample population opinions, experiences, and preferences. This data and a thorough literature evaluation can help you generate a well-grounded hypothesis by improving your research knowledge.
- Identifying variables: Using targeted survey questions, you can identify relevant variables related to their research topic.
- Testing assumptions: You can use surveys to informally test certain assumptions or hypotheses before formalizing a research hypothesis.
- Data analysis tools: QuestionPro provides tools for analyzing survey data. You can use these tools to identify the collected data’s patterns, correlations, or trends.
- Refining your hypotheses: As you collect data through QuestionPro, you can adjust your hypotheses based on the real-world responses you receive.
A research hypothesis is like a guide for researchers in science. It’s a well-thought-out idea that has been thoroughly tested. This idea is crucial as researchers can explore different fields, such as medicine, social sciences, and natural sciences. The research hypothesis links theories to real-world evidence and gives researchers a clear path to explore and make discoveries.
QuestionPro Research Suite is a helpful tool for researchers. It makes creating surveys, collecting data, and analyzing information easily. It supports all kinds of research, from exploring new ideas to forming hypotheses. With a focus on using data, it helps researchers do their best work.
Are you interested in learning more about QuestionPro Research Suite? Take advantage of QuestionPro’s free trial to get an initial look at its capabilities and realize the full potential of your research efforts.
LEARN MORE FREE TRIAL
MORE LIKE THIS
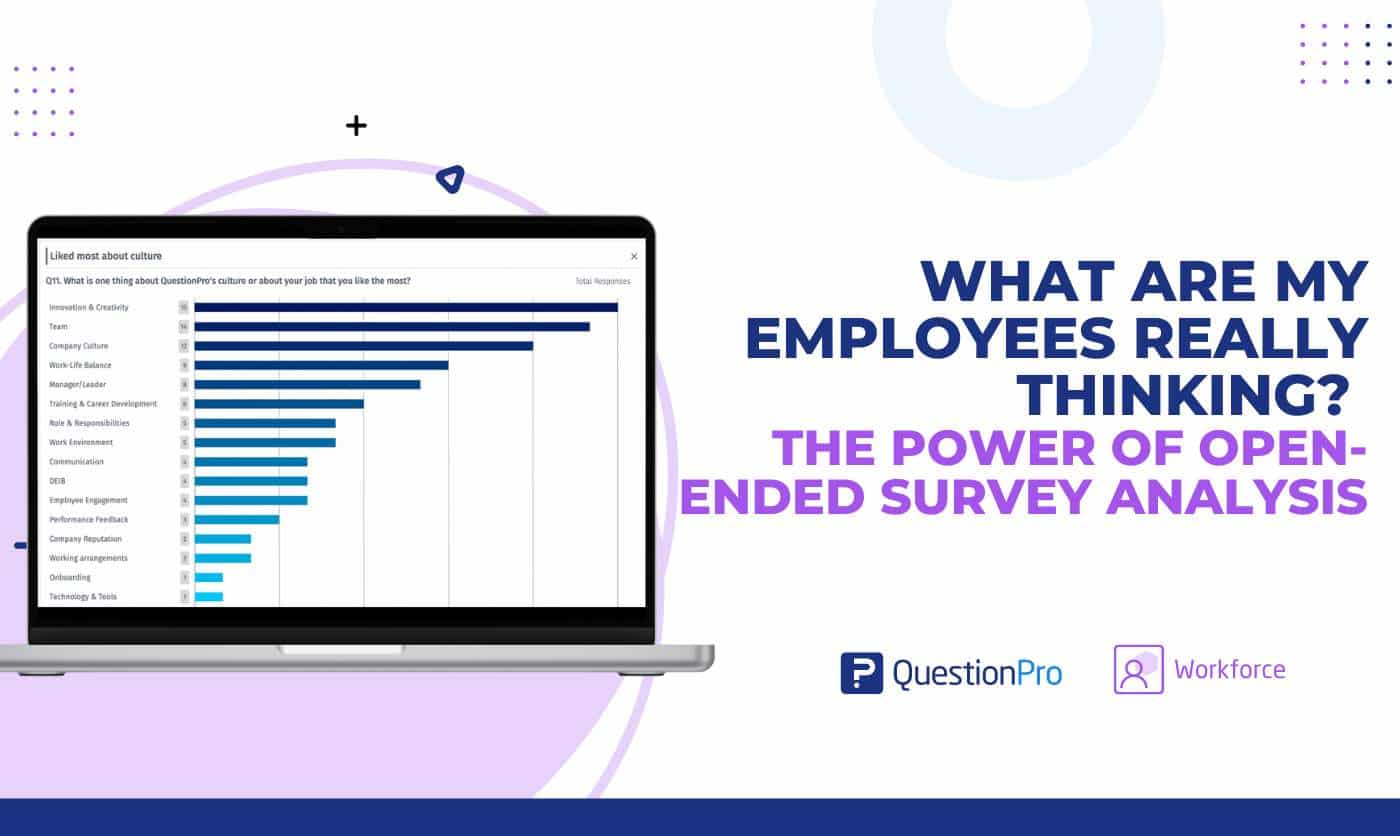
What Are My Employees Really Thinking? The Power of Open-ended Survey Analysis
May 24, 2024
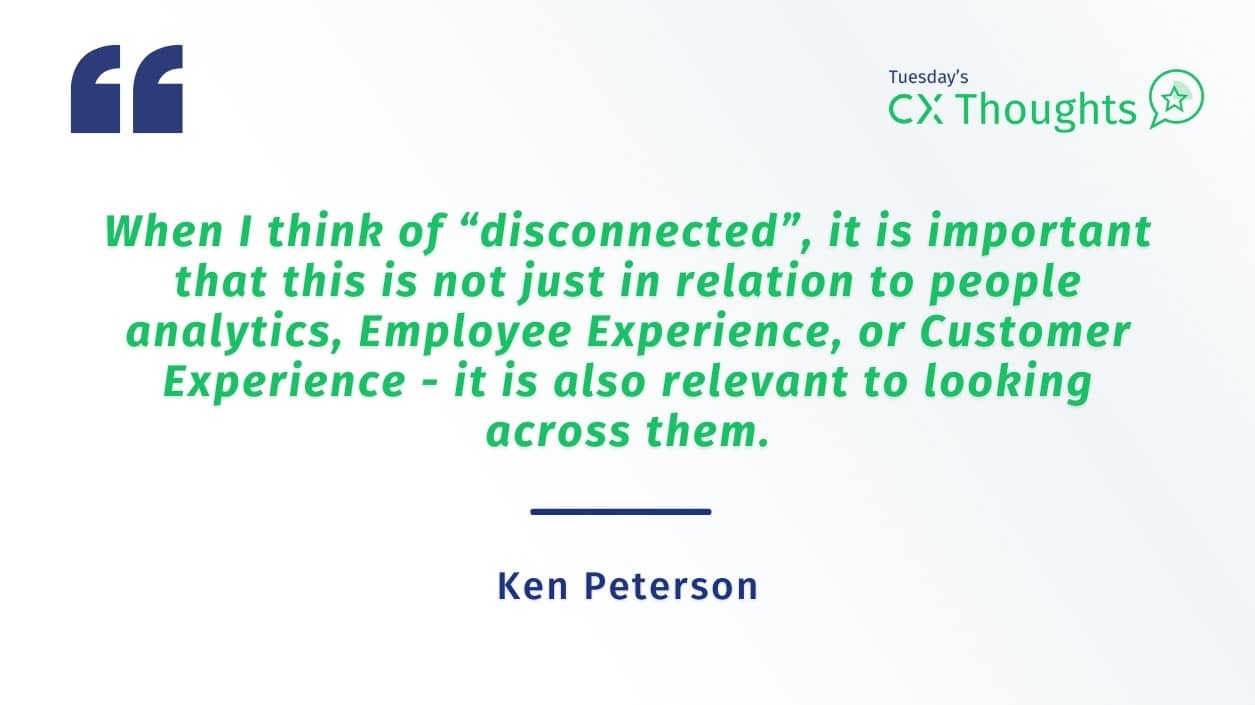
I Am Disconnected – Tuesday CX Thoughts
May 21, 2024
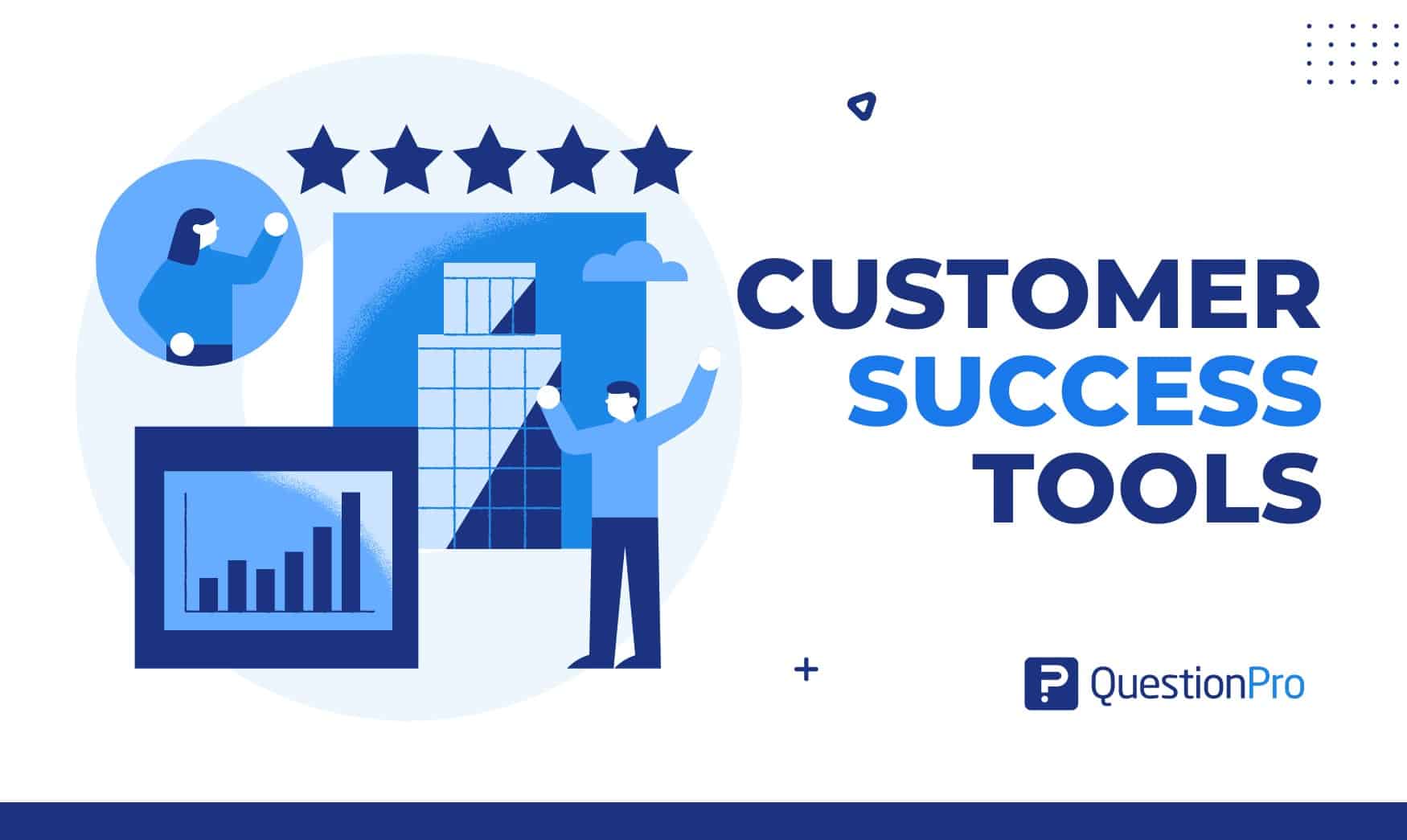
20 Best Customer Success Tools of 2024
May 20, 2024
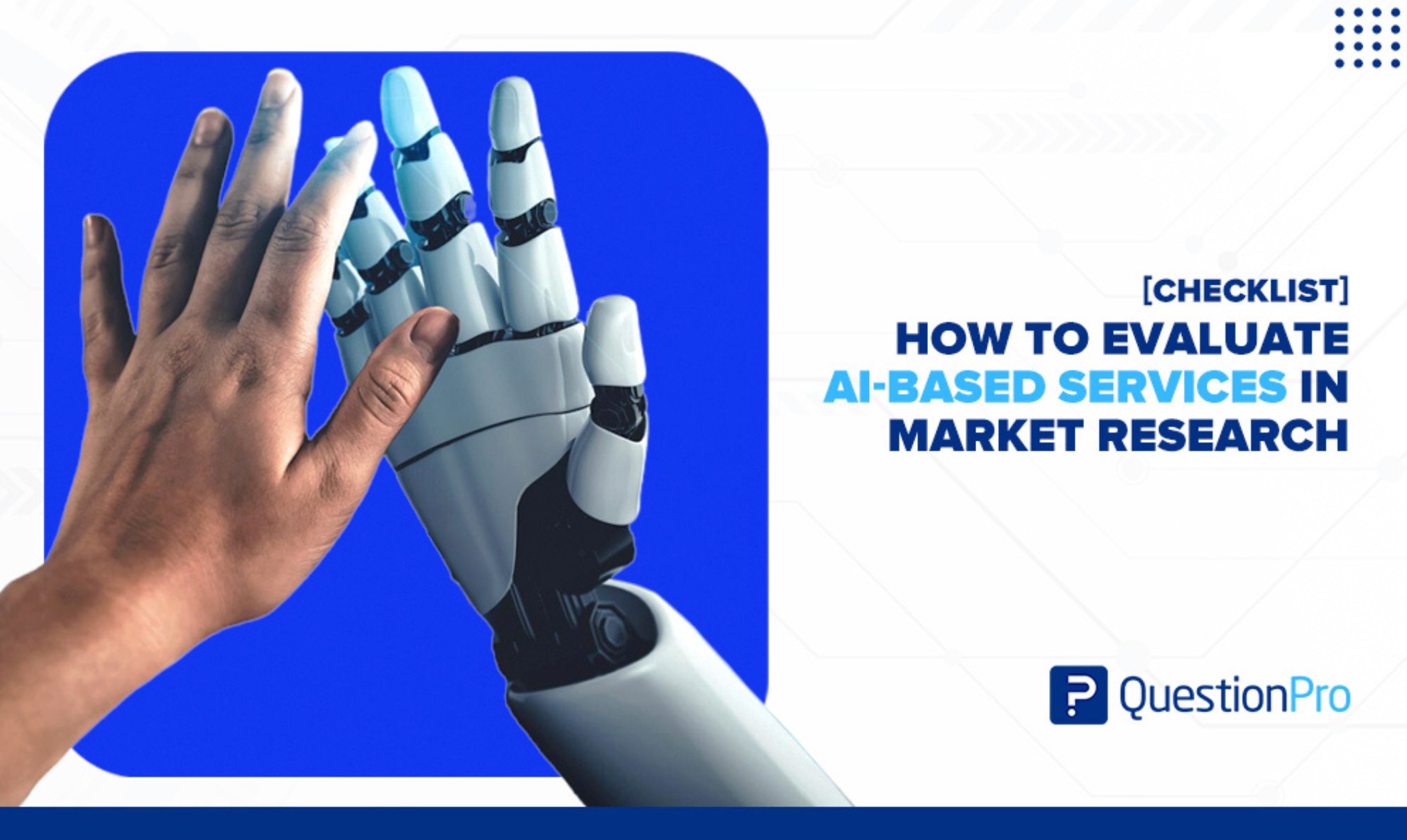
AI-Based Services Buying Guide for Market Research (based on ESOMAR’s 20 Questions)
Other categories.
- Academic Research
- Artificial Intelligence
- Assessments
- Brand Awareness
- Case Studies
- Communities
- Consumer Insights
- Customer effort score
- Customer Engagement
- Customer Experience
- Customer Loyalty
- Customer Research
- Customer Satisfaction
- Employee Benefits
- Employee Engagement
- Employee Retention
- Friday Five
- General Data Protection Regulation
- Insights Hub
- Life@QuestionPro
- Market Research
- Mobile diaries
- Mobile Surveys
- New Features
- Online Communities
- Question Types
- Questionnaire
- QuestionPro Products
- Release Notes
- Research Tools and Apps
- Revenue at Risk
- Survey Templates
- Training Tips
- Uncategorized
- Video Learning Series
- What’s Coming Up
- Workforce Intelligence

What Is A Research (Scientific) Hypothesis? A plain-language explainer + examples
By: Derek Jansen (MBA) | Reviewed By: Dr Eunice Rautenbach | June 2020
If you’re new to the world of research, or it’s your first time writing a dissertation or thesis, you’re probably noticing that the words “research hypothesis” and “scientific hypothesis” are used quite a bit, and you’re wondering what they mean in a research context .
“Hypothesis” is one of those words that people use loosely, thinking they understand what it means. However, it has a very specific meaning within academic research. So, it’s important to understand the exact meaning before you start hypothesizing.
Research Hypothesis 101
- What is a hypothesis ?
- What is a research hypothesis (scientific hypothesis)?
- Requirements for a research hypothesis
- Definition of a research hypothesis
- The null hypothesis
What is a hypothesis?
Let’s start with the general definition of a hypothesis (not a research hypothesis or scientific hypothesis), according to the Cambridge Dictionary:
Hypothesis: an idea or explanation for something that is based on known facts but has not yet been proved.
In other words, it’s a statement that provides an explanation for why or how something works, based on facts (or some reasonable assumptions), but that has not yet been specifically tested . For example, a hypothesis might look something like this:
Hypothesis: sleep impacts academic performance.
This statement predicts that academic performance will be influenced by the amount and/or quality of sleep a student engages in – sounds reasonable, right? It’s based on reasonable assumptions , underpinned by what we currently know about sleep and health (from the existing literature). So, loosely speaking, we could call it a hypothesis, at least by the dictionary definition.
But that’s not good enough…
Unfortunately, that’s not quite sophisticated enough to describe a research hypothesis (also sometimes called a scientific hypothesis), and it wouldn’t be acceptable in a dissertation, thesis or research paper . In the world of academic research, a statement needs a few more criteria to constitute a true research hypothesis .
What is a research hypothesis?
A research hypothesis (also called a scientific hypothesis) is a statement about the expected outcome of a study (for example, a dissertation or thesis). To constitute a quality hypothesis, the statement needs to have three attributes – specificity , clarity and testability .
Let’s take a look at these more closely.
Need a helping hand?
Hypothesis Essential #1: Specificity & Clarity
A good research hypothesis needs to be extremely clear and articulate about both what’ s being assessed (who or what variables are involved ) and the expected outcome (for example, a difference between groups, a relationship between variables, etc.).
Let’s stick with our sleepy students example and look at how this statement could be more specific and clear.
Hypothesis: Students who sleep at least 8 hours per night will, on average, achieve higher grades in standardised tests than students who sleep less than 8 hours a night.
As you can see, the statement is very specific as it identifies the variables involved (sleep hours and test grades), the parties involved (two groups of students), as well as the predicted relationship type (a positive relationship). There’s no ambiguity or uncertainty about who or what is involved in the statement, and the expected outcome is clear.
Contrast that to the original hypothesis we looked at – “Sleep impacts academic performance” – and you can see the difference. “Sleep” and “academic performance” are both comparatively vague , and there’s no indication of what the expected relationship direction is (more sleep or less sleep). As you can see, specificity and clarity are key.
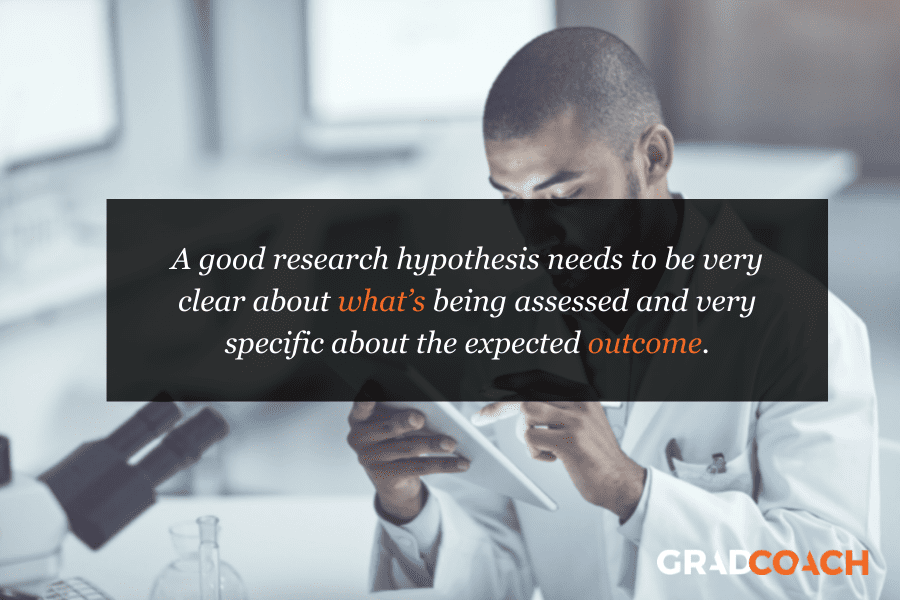
Hypothesis Essential #2: Testability (Provability)
A statement must be testable to qualify as a research hypothesis. In other words, there needs to be a way to prove (or disprove) the statement. If it’s not testable, it’s not a hypothesis – simple as that.
For example, consider the hypothesis we mentioned earlier:
Hypothesis: Students who sleep at least 8 hours per night will, on average, achieve higher grades in standardised tests than students who sleep less than 8 hours a night.
We could test this statement by undertaking a quantitative study involving two groups of students, one that gets 8 or more hours of sleep per night for a fixed period, and one that gets less. We could then compare the standardised test results for both groups to see if there’s a statistically significant difference.
Again, if you compare this to the original hypothesis we looked at – “Sleep impacts academic performance” – you can see that it would be quite difficult to test that statement, primarily because it isn’t specific enough. How much sleep? By who? What type of academic performance?
So, remember the mantra – if you can’t test it, it’s not a hypothesis 🙂
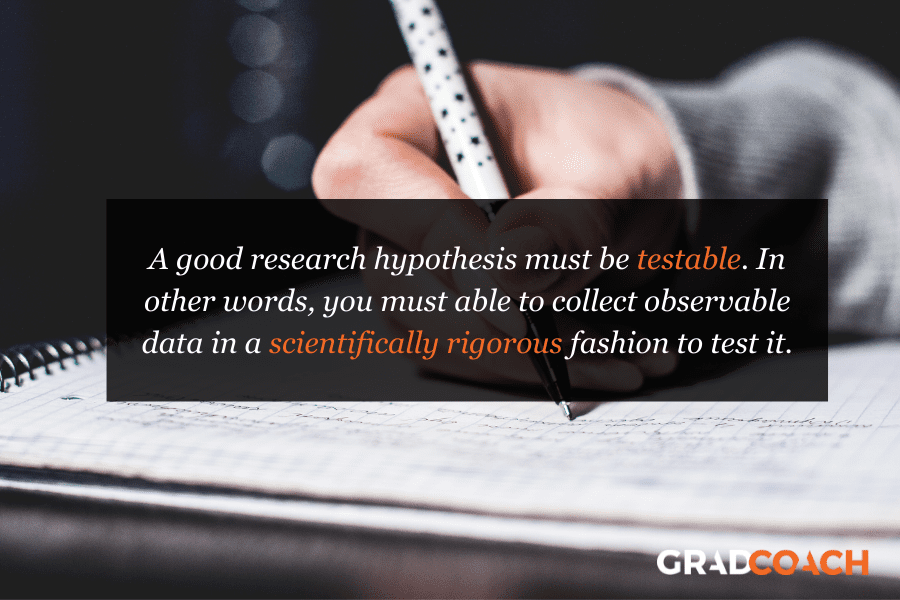
Defining A Research Hypothesis
You’re still with us? Great! Let’s recap and pin down a clear definition of a hypothesis.
A research hypothesis (or scientific hypothesis) is a statement about an expected relationship between variables, or explanation of an occurrence, that is clear, specific and testable.
So, when you write up hypotheses for your dissertation or thesis, make sure that they meet all these criteria. If you do, you’ll not only have rock-solid hypotheses but you’ll also ensure a clear focus for your entire research project.
What about the null hypothesis?
You may have also heard the terms null hypothesis , alternative hypothesis, or H-zero thrown around. At a simple level, the null hypothesis is the counter-proposal to the original hypothesis.
For example, if the hypothesis predicts that there is a relationship between two variables (for example, sleep and academic performance), the null hypothesis would predict that there is no relationship between those variables.
At a more technical level, the null hypothesis proposes that no statistical significance exists in a set of given observations and that any differences are due to chance alone.
And there you have it – hypotheses in a nutshell.
If you have any questions, be sure to leave a comment below and we’ll do our best to help you. If you need hands-on help developing and testing your hypotheses, consider our private coaching service , where we hold your hand through the research journey.
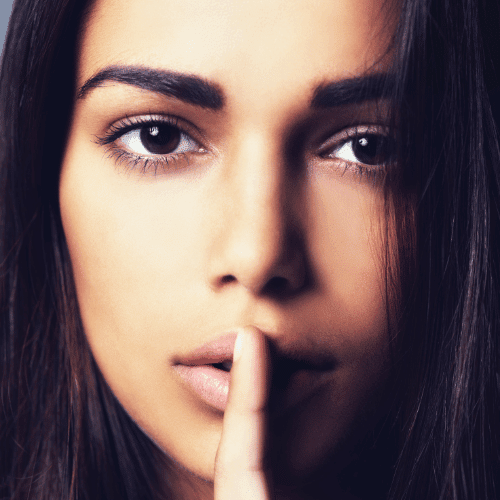
Psst... there’s more!
This post was based on one of our popular Research Bootcamps . If you're working on a research project, you'll definitely want to check this out ...
You Might Also Like:
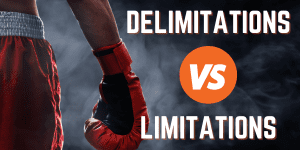
16 Comments
Very useful information. I benefit more from getting more information in this regard.
Very great insight,educative and informative. Please give meet deep critics on many research data of public international Law like human rights, environment, natural resources, law of the sea etc
In a book I read a distinction is made between null, research, and alternative hypothesis. As far as I understand, alternative and research hypotheses are the same. Can you please elaborate? Best Afshin
This is a self explanatory, easy going site. I will recommend this to my friends and colleagues.
Very good definition. How can I cite your definition in my thesis? Thank you. Is nul hypothesis compulsory in a research?
It’s a counter-proposal to be proven as a rejection
Please what is the difference between alternate hypothesis and research hypothesis?
It is a very good explanation. However, it limits hypotheses to statistically tasteable ideas. What about for qualitative researches or other researches that involve quantitative data that don’t need statistical tests?
In qualitative research, one typically uses propositions, not hypotheses.
could you please elaborate it more
I’ve benefited greatly from these notes, thank you.
This is very helpful
well articulated ideas are presented here, thank you for being reliable sources of information
Excellent. Thanks for being clear and sound about the research methodology and hypothesis (quantitative research)
I have only a simple question regarding the null hypothesis. – Is the null hypothesis (Ho) known as the reversible hypothesis of the alternative hypothesis (H1? – How to test it in academic research?
this is very important note help me much more
Trackbacks/Pingbacks
- What Is Research Methodology? Simple Definition (With Examples) - Grad Coach - […] Contrasted to this, a quantitative methodology is typically used when the research aims and objectives are confirmatory in nature. For example,…
Submit a Comment Cancel reply
Your email address will not be published. Required fields are marked *
Save my name, email, and website in this browser for the next time I comment.
- Print Friendly
REVIEW article
What exactly is universal grammar, and has anyone seen it.

- Department of Humanities, Northumbria University, Newcastle upon Tyne, UK
Universal Grammar (UG) is a suspect concept. There is little agreement on what exactly is in it; and the empirical evidence for it is very weak. This paper critically examines a variety of arguments that have been put forward as evidence for UG, focussing on the three most powerful ones: universality (all human languages share a number of properties), convergence (all language learners converge on the same grammar in spite of the fact that they are exposed to different input), and poverty of the stimulus (children know things about language which they could not have learned from the input available to them). I argue that these arguments are based on premises which are either false or unsubstantiated. Languages differ from each other in profound ways, and there are very few true universals, so the fundamental crosslinguistic fact that needs explaining is diversity, not universality. A number of recent studies have demonstrated the existence of considerable differences in adult native speakers’ knowledge of the grammar of their language, including aspects of inflectional morphology, passives, quantifiers, and a variety of more complex constructions, so learners do not in fact converge on the same grammar. Finally, the poverty of the stimulus argument presupposes that children acquire linguistic representations of the kind postulated by generative grammarians; constructionist grammars such as those proposed by Tomasello, Goldberg and others can be learned from the input. We are the only species that has language, so there must be something unique about humans that makes language learning possible. The extent of crosslinguistic diversity and the considerable individual differences in the rate, style and outcome of acquisition suggest that it is more promising to think in terms of a language-making capacity, i.e., a set of domain-general abilities, rather than an innate body of knowledge about the structural properties of the target system.
Introduction
The Universal Grammar (UG) hypothesis—the idea that human languages, as superficially diverse as they are, share some fundamental similarities, and that these are attributable to innate principles unique to language: that deep down, there is only one human language ( Chomsky, 2000a , p. 7)—has generated an enormous amount of interest in linguistics, psychology, philosophy, and other social and cognitive sciences. The predominant approach in linguistics for almost 50 years ( Smith, 1999 , p. 105: described it as “unassailable”), it is now coming under increasing criticism from a variety of sources. In this paper, I provide a critical assessment of the UG approach. I argue that there is little agreement on what UG actually is; that the arguments for its existence are either irrelevant, circular, or based on false premises; and that there are fundamental problems with the way its proponents address the key questions of linguistic theory.
What Exactly is UG?
Universal Grammar is usually defined as the “system of categories, mechanisms and constraints shared by all human languages and considered to be innate” ( O’Grady et al., 1996 , p. 734; cf. also Chomsky, 1986 , p. 3, 2007 , p. 1; Pesetsky, 1999 , p. 476). These are generally thought to include formal universals (e.g., principles, i.e., general statements which specify the constraints on the grammars of human languages, and parameters, which specify the options for grammatical variation between languages) as well as substantive universals (e.g., lexical categories and features). There is very little agreement, however, on what these actually are.
Chomsky (1986) sees UG as “an intricate and highly constrained structure” (p. 148) consisting of “various subsystems of principles” (p. 146). These include “X-bar theory, binding theory, Case theory, theta theory, bounding theory … and so forth – each containing certain principles with a limited degree of parametric variation. In addition there are certain overriding principles such as the projection principle, FI (full interpretation), and the principles of licensing… [UG also contains] certain concepts, such as the concept of domain … and the related notions of c-command and government” (p. 102). However, every major development in the theory since then was accompanied by very substantial revisions to the list of proposed universals. Thus the list of UG principles is quite different when we move to the Barriers period, and radically different in Minimalism (see below).
With respect to parameters, very few scholars have even attempted to give a reasonably comprehensive inventory of what these are. Two rare exceptions are Baker (2001) , who discusses 10 parameters, and Fodor and Sakas (2004) , who list 13. In both cases, the authors stress that the list is far from complete; but it is interesting to note that only three parameters occur on both lists ( Tomasello, 2005 ; see also Haspelmath, 2007 ). There is no agreement even on approximately how many parameters there are: thus Pinker (1994 , p. 112) claims that there are “only a few”; Fodor (2003 , p. 734) suggests that there are “perhaps 20”; according to Roberts and Holmberg (2005 , p. 541), the correct figure is probably “in the region of 50–100.” However, if, following Kayne (2005) , we assume that there is a parameter associated with every functional element, the number of parameters must be considerably larger than this. Cinque and Rizzi (2008) , citing Heine and Kuteva’s (2002) work on grammaticalization targets, estimate that there are about 400 functional categories. According to Shlonsky (2010 , p. 424), even this may be a low estimate. Shlonsky (2010) also suggests that “[e]very feature is endowed with its own switchboard, consisting of half a dozen or so binary options” (p. 425), which implies that there are thousands of parameters.
Things are no better when we consider substantive universals. While most generative linguists agree that the inventory of lexical categories includes N, V, and A, there is little agreement on what the functional categories are (see Newmeyer, 2008 ; Corbett, 2010 ; Pullum and Tiede, 2010 ; Boeckx, 2011 ). Newmeyer (2008) surveys some of the relevant literature and concludes:
“There is no way to answer this question that would satisfy more than a small number of generativists. It seems fair to say that categories are proposed for a particular language when they appear to be needed for that language, with little thought as to their applicability to the grammar of other languages. My guess is that well over two hundred have been put forward in current work in the principles-and-parameters tradition.” (p. 51)
The situation, Newmeyer (2008) observes, is even less clear when it comes to features:
“Even more than for categories, features tend to be proposed ad hoc in the analysis of a particular language when some formal device is needed to distinguish one structure (or operation on a particular structure) from another. As a result, supplying even a provisional list of what the set of universal distinctive syntactic features might be seems quite hopeless.” (p. 53)
Thus, some linguists see UG as a very elaborate structure, consisting of a large number of principles, parameters, and categories. At the other extreme, we have the strong minimalist thesis, according to which UG may comprise just the structure-building operation Merge (cf. Chomsky, 2004 , 2012 ; Berwick et al., 2011 ). It seems that the only point of agreement amongst proponents of UG is that it exists; they do not agree on what it actually contains. What evidence, then, is there for the existence of specifically linguistic innate knowledge? I turn to this question in the next section.
Arguments for UG
Over the years, a number of arguments have been put forward in support of the UG hypothesis. These include the following:
(1) Language Universals: (All) human languages share certain properties.
(2) Convergence: Children are exposed to different input yet converge on the same grammar.
(3) Poverty of the Stimulus: Children acquire knowledge for which there is no evidence in the input.
(4) No Negative Evidence: Children know which structures are ungrammatical and do not acquire overgeneral grammars in spite of the fact that they are not exposed to negative evidence.
(5) Species Specificity: We are the only species that has language.
(6) Ease and Speed of Child Language Acquisition: Children learn language quickly and effortlessly, on minimal exposure.
(7) Uniformity: All children acquiring language go through the same stages in the same order.
(8) Maturational Effects: Language acquisition is very sensitive to maturational factors and relatively insensitive to environmental factors.
(9) Dissociations between Language and Cognition: Some clinical populations have (relatively) normal language and impaired cognition; some have impaired cognition and (relatively) normal language.
(10) Neurological Separation: Different brain circuits are responsible for representing/processing linguistic and non-linguistic information.
Arguments 1–4 are generally regarded as the most powerful ones; 5–10 are subsidiary in the sense they only provide support for the idea of innateness of language general, rather than the innateness of a specific aspect of linguistic organization, and they are also open to other interpretations. I begin by evaluating the subsidiary arguments, and then move on to the more powerful ones.
Species Specificity
“To say that language is not innate is to say that there is no difference between my granddaughter, a rock and a rabbit. In other words, if you take a rock, a rabbit and my granddaughter and put them in a community where people are talking English, they’ll all learn English. If people believe that, then they believe that language is not innate. If they believe that there is a difference between my granddaughter, a rabbit, and a rock, then they believe that language is innate.” ( Chomsky, 2000b , p. 50)
Clearly, there is something unique about human biological make-up that makes it possible for humans, and only humans, to acquire language. However, nobody disputes this, so in the passage quoted above Chomsky is fighting a straw man. The crucial question is whether the relevant knowledge or abilities are language-specific or whether they can be attributed to more general cognitive processes—and this is far from clear.
There are a number of other characteristics which appear to be specific to our species. These include collaboration, cultural learning, the use of complex tools, and—surprisingly—the use of pointing and others means of drawing attention to particular features of the immediate environment, such as holding objects up for others to see. 1 This suggests there may be a more fundamental difference between humans and the rest of the animal kingdom. As Tomasello et al. (2005) put it, “saying that only humans have language is like saying that only humans build skyscrapers, when the fact is that only humans (among primates) build freestanding shelters at all” (p. 690). Tomasello et al. (2005) argue that language is a consequence of the basic human ability to recognize others’ communicative intentions and to engage in joint attention, which also underlies other cultural achievements.
The ability to read and share intentions, including communicative intentions—i.e., theory of mind in the broad sense—is important for language for two reasons. First, it enables the language learner to understand what language is for : an animal that did not understand that other individuals have beliefs and intentions different from its own would have little use for language. Secondly, it provides the learner with a vital tool for learning language. In order to learn a language, one must acquire a set of form-meaning conventions; and to acquire these, learners must be able to guess at least some of the meanings conveyed by the utterances they hear.
The human ability to read and share intentions may not explain subjacency effects—the existence of other differences between humans and other species does not entail lack of UG, just as species specificity does not entail its existence. The point is that arguments for the innateness of language in a general sense (what Scholz and Pullum, 2002 call “general nativism”) do not constitute arguments for the innateness of UG (“linguistic nativism”) if UG is taken to be a specific body of linguistic knowledge. In other words, the fact that we are the only species that has language does not entail that we have innate knowledge of subjacency.
Ease and Speed of Child Language Acquisition
It has been often suggested that children acquire grammatical systems of enormous complexity rapidly and effortlessly on the basis of very little evidence, and by “mere exposure,” that is to say, without explicit teaching (see, for example, Chomsky, 1962 , p. 529, 1976 , p. 286, 1999 ; Guasti, 2002 , p. 3). In fact, they get vast amounts of language experience. If we assume that language acquisition begins at age 1 and ends at age 5 and that children are exposed to language for 8 h a day, they get 11680 h of exposure (4 × 365 × 8 = 11680). At 3600 input words per hour (the average number of words heard by the children in the Manchester corpus), 2 this amounts to over 42 million words over 4 years.
Note that this is a rather conservative estimate: we know that language development begins before age 1 ( Jusczyk, 1997 ; Karmiloff and Karmiloff-Smith, 2001 ) and continues throughout childhood and adolescence ( Nippold, 1998 ; Berman, 2004 , 2007 ; Nippold et al., 2005 ; Kaplan and Berman, 2015 ); moreover, children are exposed to language—through utterances directed to them, utterances directed to other people present, radio and television, and later school, reading and the internet almost every waking hour of their lives.
Furthermore, we know that “mere exposure” is not enough—as demonstrated by studies of hearing children of deaf parents ( Todd and Aitchison, 1980 ; Sachs et al., 1981 ; see also Dąbrowska, 2012 , for some observations on the effects of the quality of the input). Consider, for example, Jim—one of children studied by Sachs et al. (1981) . In early childhood, Jim had very little contact with hearing adults but watched television quite frequently, and occasionally played with hearing children. His parents used sign language when addressing each other, but did not sign to the children. At age 3;9 (3 years and 9 months)—the beginning of the study—Jim had very poor comprehension of spoken language, and severe articulation problems. His utterances were very short, with an MLU (mean length of utterance) of 2.9—typical for a child aged about 2;9. He had low use of grammatical morphemes, producing them in only 37% of obligatory contexts, while MLU-matched controls supplied them 64–81% of the time; and many of his utterances had clearly deviant syntax (My mommy my house ǝ play ball; House ǝ chimney my house ǝ my chimney). And, interestingly, although he was exposed to ASL at home, he did not sign. Jim’s spoken language improved rapidly once he began interacting with adults on a one-on-one basis, and by age 6;11, he performed above age level on most measures—showing that he was not language impaired. Thus, although he was exposed to both spoken English (through television and occasional interaction with other children) and to ASL (though observing his parents), Jim did not acquire either language until he was given an opportunity to interact with competent users.
Some researchers (e.g., Stromswold, 2000 ; Guasti, 2002 ) have suggested that children acquire language in a very similar manner, going through the same stages at approximately the same ages, in spite of the fact that they are exposed to different input. Stromswold (2000) , for instance, observes that
“Within a given language, the course of language acquisition is remarkably uniform…. Most children say their first referential words at 9 to 15 months… and for the next 6-8 months, children typically acquire single words fairly slowly until they have acquired approximately 50 words…. Once children have acquired 50 words, their vocabularies often increase rapidly…. At around 18 to 24 months, children learning morphologically impoverished languages such as English begin combining words to form two-word utterances…. Children acquiring such morphologically impoverished languages gradually begin to use sentences longer than two words; but for several months their speech often lacks phonetically unstressed functional category morphemes such as determiners, auxiliary verbs, and verbal and nominal inflectional endings …. Gradually, omissions become rarer until children are between three and four years old, at which point the vast majority of English-speaking children’s utterances are completely grammatical.” (p. 910)
This uniformity, Stromswold argues, indicates that the course of language acquisition is strongly predetermined by an innate program.
There are several points to be made in connection with this argument. First, many of the similarities that Stromswold mentions are not very remarkable: we do not need UG to explain why children typically (though by no means always) produce single word utterances before they produce word combinations, or why frequent content words are acquired earlier than function words. Secondly, the age ranges she gives (e.g., 9–15 months for first referential words) are quite wide: 6 months is a very long time for an infant. Thirdly, the passage describes typical development, as evidenced by qualifiers like “most children,” “typically,” “often”—so the observations are not true of all children. Finally, by using qualifiers like “within a given language” and limiting her observations to “children acquiring morphologically impoverished languages” Stromswold implicitly concedes the existence of crosslinguistic differences. These are quite substantial: children acquiring different languages have to rely on different cues, and this results in different courses of development ( Bavin, 1995 ; Jusczyk, 1997 ; Lieven, 1997 ); and they often acquire “the same” constructions at very different ages. For example, the passive is acquired quite late by English speaking children—typically (though by no means always—see below) by age 4 or 5, and even later—by about 8—by Hebrew-speaking children ( Berman, 1985 ). However, children learning languages in which the passive is more frequent and/or simpler master this construction much earlier—by about 2;8 in Sesotho ( Demuth, 1989 ) and as early as 2;0 in Inuktitut ( Allen and Crago, 1996 ).
Even within the same language, contrary to Stromswold’s claims, there are vast individual differences both in the rate and course of language development ( Bates et al., 1988 ; Richards, 1990 ; Shore, 1995 ; Goldfield and Snow, 1997 ; Peters, 1997 ; Huttenlocher, 1998 ). Such differences are most obvious, and easiest to quantify, in lexical development. The comprehension vocabularies of normally developing children of the same age can differ tenfold or more ( Benedict, 1979 ; Goldfield and Reznick, 1990 ; Bates et al., 1995 ). There are also very large differences in the relationship between a child’s expressive and receptive vocabulary early in development: some children are able to understand over 200 words before they start producing words themselves, while others are able to produce almost all the words they know ( Bates et al., 1995 ). Children also differ with regard to the kinds of words they learn in the initial stages of lexical development. “Referential” children initially focus primarily on object labels (i.e., concrete nouns), while “expressive” children have more varied vocabularies with more adjectives and verbs and some formulaic phrases such as thank you , not now , you’re kidding , don’t know ( Nelson, 1973 , 1981 ). Last but not least, there are differences in the pattern of growth. Many children do go through the “vocabulary spurt” that Stromswold alludes to some time between 14 and 22 months, but about a quarter do not, showing a more gradual growth pattern with no spurt ( Goldfield and Reznick, 1990 ).
Grammatical development is also far from uniform. While some children begin to combine words as early as 14 months, others do not do so until after their second birthday ( Bates et al., 1995 ), with correspondingly large differences in MLU later in development—from 1.2 to 5.0 at 30 months ( Wells, 1985 ). Some children learn to inflect words before they combine them into larger structures, while others begin to combine words before they are able to use morphological rules productively ( Smoczyńska, 1985 , p. 618; Thal et al., 1996 ). Some children are very cautious learners who avoid producing forms they are not sure about, while others are happy to generalize on the basis of very little evidence. This results in large differences in error rates ( Maratsos, 2000 ). Considerable individual differences have also been found in almost every area of grammatical development where researchers have looked for them, including word order ( Clark, 1985 ), case marking ( Dąbrowska and Szczerbiński, 2006 ), the order of emergence of grammatical morphemes ( Brown, 1973 ), auxiliary verbs ( Wells, 1979 ; Richards, 1990 ; Jones, 1996 ), questions ( Gullo, 1981 ; Kuczaj and Maratsos, 1983 ; de Villiers and de Villiers, 1985 ), passives ( Horgan, 1978 ; Fox and Grodzinsky, 1998 ), and multiclause sentences ( Huttenlocher et al., 2002 ).
Children also differ in their learning “styles” ( Peters, 1977 ; Nelson, 1981 ; Peters and Menn, 1993 ). “Analytic” (or “referential”) children begin with single words, which they articulate reasonably clearly and consistently. “Holistic” (or “expressive”) children, on the other hand, begin with larger units which have characteristic stress and intonation patterns, but which are often pronounced indistinctly, and sometimes consist partly or even entirely of filler syllables such as [dadada]. Peters (1977) argues that holistic children attempt to approximate the overall shape of the target utterance while analytic children concentrate on extracting and producing single words. These different starting points determine how the child “breaks into” grammar, and therefore have a substantial effect on the course of language development. Analytic children must learn how to combine words to form more complex units. They start by putting together content words, producing telegraphic utterances such as there doggie or doggie eating . Later in development they discover that different classes of content words require specific function words and inflections (nouns take determiners, verbs take auxiliaries, and tense inflections, etc.), and gradually learn to supply these. Holistic children, in contrast, must segment their rote-learned phrases and determine how each part contributes to the meaning of the whole. Unlike analytic children, they sometimes produce grammatical morphemes very early in acquisition, embedded in larger unanalysed or only partially analyzed units; or they may use filler syllables as place-holders for grammatical morphemes. As their systems develop, the fillers gradually acquire more phonetic substance and an adult-like distribution, and eventually evolve into function words of the target language ( Peters and Menn, 1993 ; Peters, 2001 ). Thus, while both groups of children eventually acquire similar grammars, they get there by following different routes. 3
Maturational Effects
Language acquisition is sometimes claimed to be “highly sensitive to maturational factors” and “surprisingly insensitive to environmental factors” ( Fodor, 1983 , p. 100; see also Gleitman, 1981 ; Crain and Lillo-Martin, 1999 ; Stromswold, 2000 ), which, these researchers suggest, indicates that the language faculty develops, or matures, according to a biologically determined timetable.
The claim that language acquisition is insensitive to environmental factors is simply incorrect, as demonstrated by the vast amount of research showing that both the amount and quality of input have a considerable effect on acquisition—particularly for vocabulary, but also for grammar (e.g., Huttenlocher, 1998 ; Huttenlocher et al., 2002 ; Ginsborg, 2006 ; Hoff, 2006 ). There is no doubt that maturation also plays a very important role—but this could be due to the development of the cognitive prerequisites for language ( Slobin, 1973 , 1985 ; Tomasello, 2003 ) rather than the maturation of the language faculty. Likewise, while it is possible that critical/sensitive period effects are due to UG becoming inaccessible at some point in development, they could also arise as a result of older learners’ greater reliance on declarative memory ( Ullman, 2006 ), developmental changes in working memory capacity ( Newport, 1990 ), or entrenchment of earlier learning ( Elman et al., 1996 ; MacWhinney, 2008 ). Thus, again, the existence of maturational effects does not entail the existence of an innate UG: they are, at best, an argument for general innateness, not linguistic innateness.
Dissociations between Language and Cognition
A number of researchers have pointed out that some individuals (e.g., aphasics and children with Specific Language Impairment) show severe language impairment and relatively normal cognition, while others (e.g., individuals with Williams syndrome (WS), or Christopher, the “linguistic savant” studied by Smith and Tsimpli, 1995 ) show the opposite pattern: impaired cognition but good language skills. The existence of such a double dissociation suggests that language is not part of “general cognition”—in other words, that it depends at least in part on a specialized linguistic “module.”
The existence of double dissociations in adults is not particularly informative with regard to the innateness issue, however, since modularization can be a result of development ( Paterson et al., 1999 ; Thomas and Karmiloff-Smith, 2002 ); hence, the fact that language is relatively separable in adults does not entail innate linguistic knowledge. On the other hand, the developmental double dissociation between specific language impairment (SLI) and WS, is, on the face of it, much more convincing. There are, however, several reasons to be cautious in drawing conclusions from the observed dissociations.
First, there is growing evidence suggesting that WS language is impaired, particularly early in development ( Karmiloff-Smith et al., 1997 ; Brock, 2007 ; Karmiloff-Smith, 2008 ). Children with WS begin talking much later than typically developing children, and their language develops along a different trajectory. Adolescents and adults with WS show deficits in all areas of language: syntax ( Grant et al., 2002 ), morphology ( Thomas et al., 2001 ), phonology ( Grant et al., 1997 ), lexical knowledge ( Temple et al., 2002 ), and pragmatics ( Laws and Bishop, 2004 ). Secondly, many, perhaps all, SLI children have various non-linguistic impairments ( Leonard, 1998 ; Tallal, 2003 ; Lum et al., 2010 )—making the term Specific Language Impairment something of a misnomer. Thus the dissociation is, at best, partial: older WS children and adolescents have relatively good language in spite of a severe cognitive deficit; SLI is a primarily linguistic impairment.
More importantly, it is debatable whether we are really dealing with a double dissociation in this case. Early reports of the double dissociation between language and cognition in Williams and SLI were based on indirect comparisons between the two populations. For instance, Pinker (1999) discusses a study conducted by Bellugi et al. (1994) , which compared WS and Down’s syndrome adolescents and found that the former have much better language skills, and van der Lely’s work on somewhat younger children with SLI ( van der Lely, 1997 ; van der Lely and Ullman, 2001 ), which found that SLI children perform less well than typically developing children. However, a study which compared the two populations directly ( Stojanovik et al., 2004 ) suggests rather different conclusions. Stojanovik et al. (2004) gave SLI and WS children a battery of verbal and non-verbal tests. As expected, the SLI children performed much better than the WS children on all non-verbal measures. However, there were no differences between the two groups on the language tests—in fact, the SLI children performed slightly better on some measures, although the differences were not statistically significant. Clearly, one cannot argue that language is selectively impaired in SLI and intact in WS if we find that the two populations’ performance on the same linguistic tests is indistinguishable.
To summarize: There is evidence of a partial dissociation in SLI children, who have normal IQ and below-normal language—and, as pointed out earlier, a variety of non-linguistic impairments which may the underlying cause of their linguistic deficit. There is, however, no evidence for a dissociation in Williams syndrome: WS children’s performance on language tests is typically appropriate for their mental age, and well below their chronological age.
Neurological Separation
The fact that certain parts of the brain—specifically, the perisylvian region including Broca’s area, Wernicke’s area and the angular gyrus—appear to be specialized for language processing has led some researchers (e.g., Pinker, 1995 ; Stromswold et al., 1996 ; Stromswold, 2000 , p. 925; Musso et al., 2003 ) to speculate that they may constitute the neural substrate for UG. Intriguing though such proposals are, they face a number of problems. First, the language functions are not strongly localized: many other areas outside the classical “language areas” are active during language processing; and, conversely, the language areas may also be activated during non-linguistic processing ( Stowe et al., 2005 ; Anderson, 2010 ; see, however, Fedorenko et al., 2011 ). More importantly, studies of neural development clearly show that the details of local connectivity in the language areas (as well as other areas of the brain) are not genetically specified but emerge as a result of activity and their position in the larger functional networks in the brain ( Elman et al., 1996 ; Müller, 2009 ; Anderson et al., 2011 ; Kolb and Gibb, 2011 ). Because of this, human brains show a high amount of plasticity, and other areas of the brain can take over if the regions normally responsible for language are damaged. In fact, if the damage occurs before the onset of language, most children develop normal conversational skills ( Bates et al., 1997 ; Aram, 1998 ; Bates, 1999 ; Trauner et al., 2013 ), although language development is often delayed ( Vicari et al., 2000 ), and careful investigations do sometimes reveal residual deficits in more complex aspects of language use ( Stiles et al., 2005 ; Reilly et al., 2013 ). Lesions sustained in middle and late childhood typically leave more lasting deficits, although these are relatively minor ( van Hout, 1991 ; Bishop, 1993 ; Martins and Ferro, 1993 ). In adults, the prospects are less good, but even adults typically show some recovery ( Holland et al., 1996 ), due partly to regeneration of the damaged areas and partly to shift to other areas of the brain, including the right hemisphere ( Karbe et al., 1998 ; Anglade et al., 2014 ). Thus, while the neurological evidence does suggest that certain areas of the brain are particularly well-suited for language processing, there is no evidence that these regions actually contain a genetically specified preprint blueprint for grammar.
Language Universals
Generative linguists have tended to downplay the differences between languages and emphasize their similarities. In Chomsky’s (2000a) words,
“… in their essential properties and even down to fine detail, languages are cast to the same mold. The Martian scientist might reasonably conclude that there is a single human language, with differences only at the margins.” (p. 7)
Elsewhere ( Chomsky, 2004 , p. 149) he describes human languages as “essentially identical.” Stromswold (1999) expresses virtually the same view:
“In fact, linguists have discovered that, although some languages seem, superficially, to be radically different from other languages …, in essential ways all human languages are remarkably similar to one another.” (p. 357)
This view, however, is not shared by most typologists (cf. Croft, 2001 ; Haspelmath, 2007 ; Evans and Levinson, 2009 ). Evans and Levinson (2009) , for example, give counterexamples to virtually all proposed universals, including major lexical categories, major phrasal categories, phrase structure rules, grammaticalized means of distinguishing between subjects and objects, use of verb affixes to signal tense and aspect, auxiliaries, anaphora, and WH movement, and conclude that
“….languages differ so fundamentally from one another at every level of description (sound, grammar, lexicon, meaning) that it is very hard to find any single structural property they share. The claims of Universal Grammar … are either empirically false, unfalsifiable or misleading in that they refer to tendencies rather than strict universals.” (p. 429)
Clearly, there is a fundamental disagreement between generative linguists like Chomsky and functionalists like Evans and Levinson (2009) . Thus, it is misleading to state that “linguists have discovered that … in essential ways all human languages are remarkably similar to one another”; it would have been more accurate to prefix such claims with a qualifier such as “some linguists think that….”
One reason for the disagreement is that generative and functional linguists have a very different view of language universals. For the functionalists, universals are inductive generalizations about observable features of language, discovered by studying a large number of unrelated languages—what some people call descriptive, or “surface” universals. The generativists’ universals, on the other hand, are cognitive or “deep” universals, which are highly abstract and cannot be derived inductively from observation of surface features. As Smolensky and Dupoux (2009) argue in their commentary on Evans and Levinson’s paper,
“Counterexamples to des-universals are not counterexamples to cog-universals … a hypothesised cog-universal can only be falsified by engaging the full apparatus of the formal theory.” (p. 468)
This is all very well—but how exactly do we “engage the full apparatus of the formal theory”? The problem with deep universals is that in order to evaluate them, one has to make a number of subsidiary (and often controversial) assumptions which in turn depend on further assumptions—so the chain of reasoning is very long indeed (cf. Hulst, 2008 ; Newmeyer, 2008 ). This raises obvious problems of falsifiability. Given that most deep universals are parameterized, that they may be parameterized “invisibly,” and that some languages have been argued to be exempt from some universals (cf. Newmeyer, 2008 ), it is not clear what would count as counterevidence for a proposed universal.
The issue is particularly problematic for substantive universals. The predominant view of substantive universals (lexical categories, features, etc.,) is that they are part of UG, but need not be used by all languages: in other words, UG makes available a list of categories, and languages “select” from this list. But as Evans and Levinson (2009) point out,
“… the claim that property X is a substantive universal cannot be falsified by finding a language without it, because the property is not required in all of them. Conversely, suppose we find a new language with property Y, hitherto unexpected: we can simply add it to the inventory of substantive universals…. without limits on the toolkit, UG is unfalsifiable.” (p. 436)
Apart from issues of falsifiability, the fact that deep universals are theory internal has another consequence, nicely spelled out by Tomasello (1995) :
“Many of the Generative Grammar structures that are found in English can be found in other languages—if it is generative grammarians who are doing the looking. But these structures may not be found by linguists of other theoretical persuasions because these structures are defined differently, or not recognised at all, in other linguistic theories.” (p. 138)
In other words, deep universals may exist—but they cannot be treated as evidence for the theory, because they are assumed by the theory.
Returning to the more mundane, observable surface universals: although absolute universals are very hard to find, there is no question that there are some very strong universal tendencies, and these call for an explanation. Many surface universals have plausible functional explanations ( Comrie, 1983 ; Hawkins, 2004 ; Haspelmath, 2008 ). It is also possible that they derive from a shared protolanguage or that they are in some sense “innate,” i.e., that they are part of the initial state of the language faculty—although existing theories of UG do not fare very well in explaining surface universals ( Newmeyer, 2008 ).
Generative linguists’ focus on universals has shifted attention from what may be the most remarkable property of human languages—their diversity. Whatever one’s beliefs about UG and the innateness hypothesis, it is undeniable that some aspects of our knowledge—the lexicon, morphological classes, various idiosyncratic constructions, i.e., what generative linguists sometimes refer to as the “periphery”—must be learned, precisely because they are idiosyncratic and specific to particular languages. These aspects of our linguistic knowledge are no less complex (in fact, in some cases considerably more complex) than the phenomena covered by “core” grammar, and mastering them requires powerful learning mechanisms. It is possible, then, that the cognitive mechanisms necessary to learn about the periphery may suffice to learn core grammar as well ( Menn, 1996 ; Culicover, 1999 ; Dąbrowska, 2000a ).
Convergence
“… it is clear that the language each person acquires is a rich complex construction hopelessly underdetermined by the fragmentary evidence available [to the learner]. Nevertheless individuals in a speech community have developed essentially the same language. This fact can be explained only on the assumption that these individuals employ highly restrictive principles that guide the construction of the grammar.” ( Chomsky, 1975 , p. 11)
“The set of utterances to which any child acquiring a language is exposed is equally compatible with many distinct descriptions. And yet children converge to a remarkable degree on a common grammar, with agreement on indefinitely many sentences that are novel. Mainly for this reason, Chomsky proposed that the child brings prior biases to the task.” ( Lidz and Williams, 2009 , p. 177)
“The explanation that is offered must also be responsive to other facts about the acquisition process; in particular, the fact that every child rapidly converges on a grammatical system that is equivalent to everyone else’s, despite a considerable latitude in linguistic experience – indeed, without any relevant experience in some cases. Innate formal principles of language acquisition are clearly needed to explain these basic facts.” ( Crain et al., 2009 , p. 124)
As illustrated by these passages, the (presumed) fact that language learners converge on the same grammar despite having been exposed to different input is often regarded as a powerful argument for an innate UG. It is interesting to note that all three authors quoted above simply assume that learners acquire essentially the same grammar: the convergence claim is taken as self-evident, and is not supported with any evidence. However, a number of recent studies which have investigated the question empirically found considerable individual differences in how much adult native speakers know about the grammar of their language, including inflectional morphology ( Indefrey and Goebel, 1993 ; Dąbrowska, 2008 ), a variety of complex syntactic structures involving subordination ( Dąbrowska, 1997 , 2013 ; Chipere, 2001 , 2003 ), and even simpler structures such as passives and quantified noun phrases ( Dąbrowska and Street, 2006 ; Street, 2010 ; Street and Dąbrowska, 2010 , 2014 ; for recent reviews, see Dąbrowska, 2012 , 2015 ).
For example, Street and Dąbrowska (2010) tested adult native English speakers’ comprehension of simple sentences with universal quantifiers such as (1–2) and unbiased passives (3); the corresponding actives (4) were a control condition.
(1) Every toothbrush is in a mug.
(2) Every mug has a toothbrush in it.
(3) The girl was hugged by the boy.
(4) The girl hugged the boy.
Participants listened to each test sentence and were asked to select the matching picture from an array of two. For the quantifier sentences the pictures depicted objects and containers in partial one-to-one correspondence (e.g., three mugs, each with a toothbrush in it plus an extra toothbrush; three mugs, each with a toothbrush in it plus an extra mug). For actives and passives, the pictures depicted a transitive event (e.g., a girl hugging a boy and a boy hugging a girl).
Experiment 1 tested two groups, a high academic attainment (HAA) group, i.e., postgraduate students, and a low academic attainment (LAA) group, who worked as shelf-stackers, packers, assemblers, or clerical workers and who had no more than 11 years of formal education. The HAA participants consistently chose the target picture in all four conditions. The LAA participants were at ceiling on actives, 88% correct on passives, 78% on simple locatives with quantifiers, and 43% correct (i.e., at chance) on possessive locatives with quantifiers. The means for the LAA group mask vast differences between participants: individual scores in this group ranged from 0 to 100% for the quantifier sentences and from 33 to 100% for passives.
Street and Dąbrowska argue that the experiment reveals differences in linguistic knowledge (competence), not performance, pointing out that the picture selection task has minimal cognitive demands (and can be used with children as young as 2 to test simpler structures); moreover, all participants, including the LAA group, were at ceiling on active sentences, showing that they had understood the task, were cooperative, etc. (For further discussion of this issue, see Dąbrowska, 2012 .)
Experiment 2 was a training study. LAA participants who had difficulty with all three of the experimental constructions (i.e., those who scored no more than 4 out of 6 correct on each construction in the pre-test) were randomly assigned to either a passive training group or a quantifier training group. The training involved an explicit explanation of the target construction followed by practice with feedback. Subsequently, participants were given a series of post-tests: immediately after training, a week later, and 12 weeks after training. The results revealed that performance improved dramatically after training, but only on the construction trained, and that the effects of training were long-lasting—that is to say, the participants performed virtually at ceiling even on the last post-test. This indicates that the participants were not language impaired, and that their poor performance on the pre-test is attributable to lack of knowledge rather than failure to understand the instructions or to cooperate with the experimenter.
The existence of individual differences in linguistic attainment is not, of course, incompatible with the existence of innate predispositions and biases. In fact, we know that differences in verbal ability are heritable ( Stromswold, 2001 ; Misyak and Christiansen, 2011 ), although it is clear that environmental factors also play an important role (see Dąbrowska, 2012 ). However, the Street and Dąbrowska experiments as well as other studies mentioned earlier in this section suggest that the convergence argument is based on a false premise. Native speakers do not converge on the same grammar: there are, in fact, considerable differences in how much speakers know about some of the basic constructions of their native language.
Poverty of the Stimulus and Negative Evidence
The most famous, and most powerful, argument for UG is the poverty of the stimulus argument: the claim that children have linguistic knowledge which could not have been acquired from the input which is available to them:
“…every child comes to know facts about the language for which there is no decisive evidence from the environment. In some cases, there appears to be no evidence at all.” ( Crain, 1991 )
“People attain knowledge of the structure of their language for which no evidence is available in the data to which they are exposed as children.” ( Hornstein and Lightfoot, 1981 , p. 9)
“Universal Grammar provides representations that support deductions about sentences that fall outside of experience…. These abstract representations drive the language learner’s capacity to project beyond experience in highly specific ways.” ( Lidz and Gagliardi, 2015 )
The textbook example of the poverty of the stimulus is the acquisition of the auxiliary placement rule in English Y/N questions (see, for example, Chomsky, 1972 , 2012 ; Crain, 1991 ; Lasnik and Uriagereka, 2002 ; Berwick et al., 2011 ). On hearing pairs of sentences such as (5a) and (5b) a child could infer the following rule for deriving questions:
Hypothesis A: Move the auxiliary to the beginning of the sentence.
However, such a rule would incorrectly derive (6b), although the only grammatical counterpart of (6a) is (6c).
5a The boy will win.
5b Will the boy win?
6a The boy who can swim will win.
6b *Can the boy who swim will win?
6c Will the boy who can swim win?
In order to acquire English, the child must postulate a more complex, structure dependent rule:
Hypothesis B: Move the first auxiliary after the subject to the beginning of the sentence.
Crucially, the argument goes, children never produce questions such as (6b), and they know that such sentences are ungrammatical; furthermore, it has been claimed that they know this without ever being exposed to sentences like (6c) (see, for example, Piattelli-Palmarini, 1980 , p. 40, pp. 114–115; Crain, 1991 ).
A related issue, sometimes conflated with poverty of the stimulus, is lack of negative evidence. Language learners must generalize beyond the data that they are exposed to, but they must not generalize too much. A learner who assumed an overly general grammar would need negative evidence—evidence that some of the sentences that his or her grammar generates are ungrammatical—to bring the grammar in line with that of the speech community. Since such evidence is not generally available, learners’ generalizations must be constrained by UG ( Baker, 1979 ; Marcus, 1993 ).
Let us begin with the negative evidence problem. Several observations are in order. First, while parents do not reliably correct their children’s errors, children do get a considerable amount of indirect negative evidence in the form of requests for clarification and adult reformulations of their erroneous utterances. Moreover, a number of studies have demonstrated that children understand that requests for clarification and recasts are negative evidence, and respond appropriately, and that corrective feedback results in improvement in the grammaticality of child speech ( Demetras et al., 1986 ; Saxton et al., 1998 ; Saxton, 2000 ; Chouinard and Clark, 2003 ). Negative evidence can also be inferred from absence of positive evidence: a probabilistic learner can distinguish between accidental non-occurrence and a non-occurrence that is statistically significant, and infer that the latter is ungrammatical ( Robenalt and Goldberg, in press ; Scholz and Pullum, 2002 , 2006 ; Stefanowitsch, 2008 ).
Secondly, as Cowie (2008) points out, the acquisition of grammar is not the only area where we have to acquire knowledge about what is not permissible without the benefit of negative evidence. We face exactly the same problem in lexical learning and learning from experience generally: few people have been explicitly told that custard is not ice-cream, and yet somehow they manage to learn this. Related to this, children do make overgeneralization errors—including morphological overgeneralizations like bringed and gooder and overgeneralizations of various sentence level constructions (e.g., I said her no , She giggled me ), and they do recover from them (cf. Bowerman, 1988 ). Thus, the question isn’t “What sort of innate constraints must we assume to prevent children from overgeneralizing?” but rather “How do children recover from overgeneralization errors?”—and there is a considerable amount of research addressing this very issue (see, for example, Brooks and Tomasello, 1999 ; Brooks et al., 1999 ; Tomasello, 2003 ; Ambridge et al., 2008 , 2009 , 2011 ; Boyd and Goldberg, 2011 ).
Let us return to the poverty of the stimulus argument. The structure of the argument may be summarized as follows:
(1) Children know certain things about language.
(2) To learn them from the input, they would need access to data of a particular kind.
(3) The relevant data is not available in the input, or not frequent enough in the input to guarantee learning.
(4) Therefore, the knowledge must be innate.
As with any deductive argument, the truth of the conclusion (4) depends on the validity of the argument itself and the truth of the premises. Strikingly, most expositions of the poverty of the stimulus argument in the literature do not take the trouble to establish the truth of the premises: it is simply assumed. In a well-known critique of the POS argument, Pullum and Scholz (2002) analyze four linguistic phenomena (plurals inside compounds, anaphoric one , auxiliary sequences, auxiliary placement in Y/N questions) which are most often used to exemplify it, and show that the argument does not hold up: in all four cases, either the generalization that linguists assumed children acquired is incorrect or the relevant data is present in the input, or both. With respect to the auxiliary placement rule, for example, Pullum and Scholz (2002) estimate that by age 3, most children will have heard between 7500 and 22000 utterances that falsify the structure independent rule.
Lasnik and Uriagereka (2002) and others argue that Pullum and Scholz (2002) have missed the point: knowing that sentences like (6c) are grammatical does not entail that sentences like (6b) are not; and it does not tell the child how to actually form a question. They point out that “not even the fact that [6c] is grammatical proves that something with the effect of hypothesis B is correct ( and the only possibility [my italics]), hence does not lead to adult knowledge of English” ( Lasnik and Uriagereka, 2002 ; p. 148), and conclude that “children come equipped with a priori knowledge of language… because it is unimaginable [my italics] how they could otherwise acquire the complexities of adult language” (pp. 149–150).
Note that Lasnik and Uriagereka (2002) have moved beyond the original poverty of the stimulus argument. They are not arguing merely that a particular aspect of our linguistic knowledge must be innate because the relevant data is not available to learners (poverty of the stimulus); they are making a different argument, which Slobin (cited in Van Valin, 1994 ) refers to as the “argument from the poverty of the imagination”: “I can’t imagine how X could possibly be learned from the input; therefore, it must be innate.” Appeals to lack of imagination are not very convincing, however. One can easily construct analogous arguments to argue for the opposite claim: “I can’t imagine how X could have evolved (or how it could be encoded in the genes); therefore, it must be learned.” Moreover, other researchers may be more imaginative.
The Construction Grammar Approach
Lasnik and Uriagereka (2002) conclude their paper with a challenge to non-nativist researchers to develop an account of how grammar could be learned from positive evidence. The challenge has been taken up by a number of constructionist researchers ( Tomasello, 2003 , 2006 ; Dąbrowska, 2004 ; Goldberg, 2006 ; for reviews, see Diessel, 2013 ; Matthews and Krajewski, 2015 ). Let us begin by examining how a constructionist might account for the acquisition of the auxiliary placement rule.
Case Study: The Acquisition of Y/N Questions by Naomi
Consider the development of Y/N questions with the auxiliary can in one particular child, Naomi (see Dąbrowska, 2000b , 2004 , 2010a , also discussed data for two other children from the CHILDES database). 4 The first recorded questions with can appeared in Naomi’s speech at age 1;11.9 (1 year, 11 months and 9 days) and were correctly inverted:
1; 11.9 can I get down? [repeated 4x]
1; 11.9 can I get up?
Seven days later there are some further examples, but this time the subject is left out, although it is clear from the context that the subject is Naomi herself:
1; 11.16 can eat it ice cream?
1; 11.16 can lie down? [repeated 2x]
In total, there are 56 tokens of this “permission formula” in the corpus, 25 with explicit subjects.
The early questions with can are extremely stereotypical: the auxiliary is always placed at the beginning of the sentence (there are no “uninverted” questions), and although the first person pronoun is often left out, the agent of the action is invariably Naomi herself. There are other interesting restrictions on her usage during this period. For example, in Y/N interrogatives with can , if she explicitly refers to herself, she always uses the pronoun I (25 tokens)—never her name. In contrast, in other questions (e.g., the formulas What’s Nomi do? , What’s Nomi doing? , and Where’s Nomi? —45 tokens in total) she always refers to herself as Nomi . Furthermore, while she consistently inverts in first person questions with can and could , all the other Y/N questions with first person subjects are uninverted.
As the formula is analyzed, usage becomes more flexible. Two weeks after the original can I…? question, a variant appears with could instead of can :
1; 11.21 could do this?
2; 0.3 could I throw that?
Five weeks later, we get the first question with a subject other than I :
2; 0.28 can you draw eyes?
The transcripts up to this point contain 39 questions with can , including 10 with explicit subjects.
So we see a clear progression from an invariant formula ( Can I get down? ) through increasingly abstract formulaic frames ( Can I + ACTION? ABILITY VERB + I + ACTION?) to a fairly general constructional schema in which none of the slots is tied to particular lexical items (ABILITY VERB + PERSON + ACTION?).
Questions with other auxiliaries follow different developmental paths. Not surprisingly, the first interrogatives with will were requests ( will you ACTION? ); this was later generalized to questions about future actions, and to other agents ( will PERSON ACTION?). The earliest interrogatives with do were offers of a specific object ( do you want THING? ). This was later generalized to do you ACTION? ; but for a long time, Naomi used “ do support” almost exclusively with second person subjects.
Thus, Naomi started with some useful formulas such as request for permission ( Can I ACTION? ), request that the addressee do something for her ( Will you ACTION? ), and offers of an object ( Do you want THING? ). These were gradually integrated into a network of increasingly general constructional schemas. The process is depicted schematically in Figure 1 . The left hand side of the figure shows the starting point of development: formulaic phrases. The boxes in the second columns represent low-level schemas which result from generalizations over specific formulaic phrases. The schemas contain a slot for specifying the type of activity; this must be filled by a verb phrase containing a plain verb. The schemas in the third column are even more abstract, in that they contain two slots, one for the activity and one for the agent; they can be derived by generalizing over the low-level schemas. Finally, on the far right, we have a fully abstract Y/N question schema. The left-to-right organization of the figure represents the passage of time, in the sense that concrete schemas developmentally precede more abstract ones. However, the columns are not meant to represent distinct stages, since the generalizations are local: for example, Noami acquired the Can NP VP? schema about 6 months before she started to produce Will you VP? questions. Thus, different auxiliaries followed different developmental patterns, and, crucially, there is no evidence that she derived questions from structures with declarative-like word order at any stage, as auxiliaries in declaratives were used in very different ways. It is also important to note that the later, more abstract schemas probably do not replace the early lexically specific ones: there is evidence that the two continue to exist side by side in adult speakers ( Langacker, 2000 ; Dąbrowska, 2010b ).
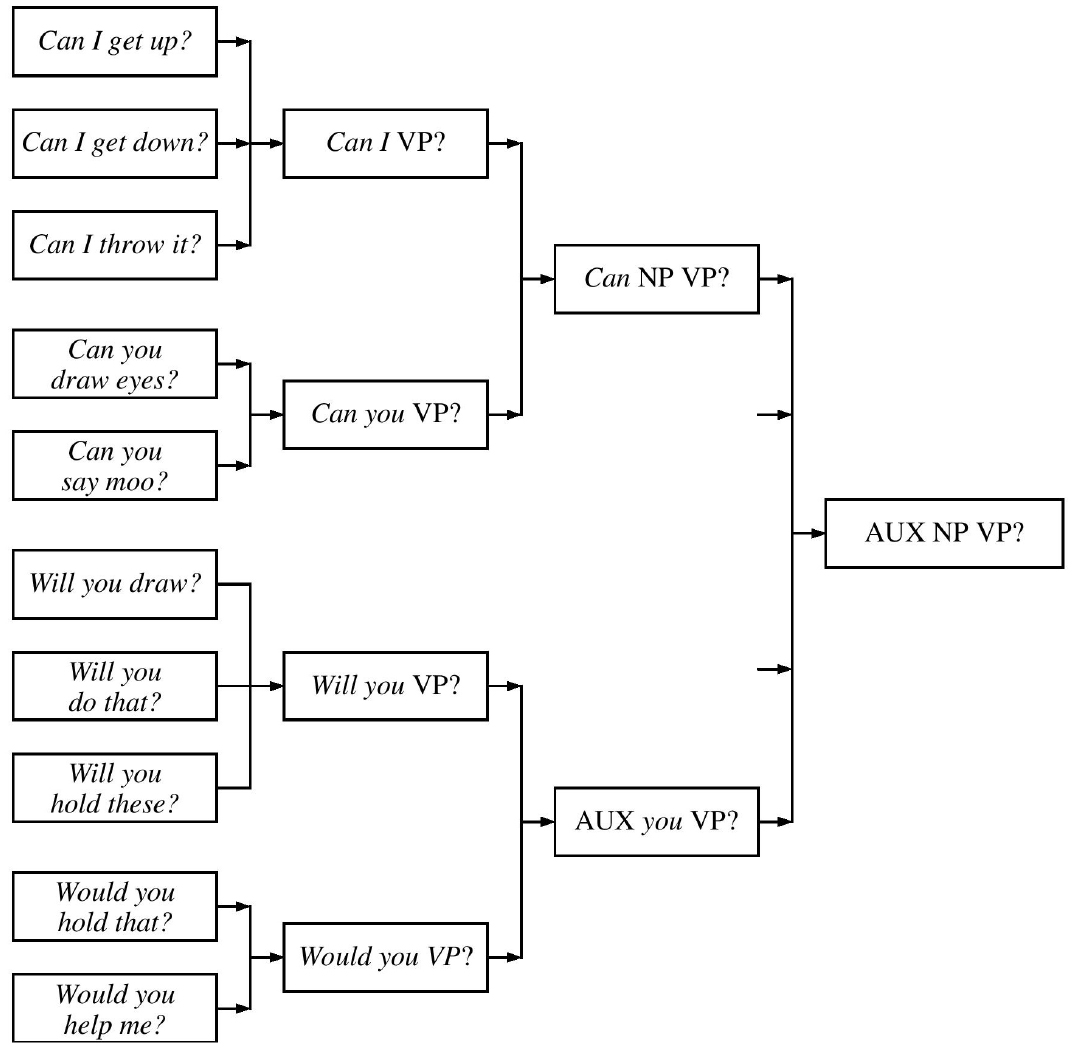
Figure 1. Progressive schematization. Labels like NP are VP in the figure are used merely for convenience: we need not assume that the child has abstract syntactic categories, particularly in the early stages of acquisition. The slots in early formulas are defined in semantic terms and may be frame specific, e.g., the VP slot in the Can I VP? formula can be filled with any expression referring to “something I would like to do.” For ease of exposition, I am also ignoring the difference between grounded (tensed) and untensed verbs.
Dąbrowska and Lieven (2005) , using data from eight high-density developmental corpora, show that young children’s novel questions can be explained by appealing to lexically specific units which can be derived from the child’s linguistic experience. Dąbrowska (2014) argues that such units can also account for the vast majority of adult utterances, at least in informal conversation.
One might object that, since the slots in the formulas can be filled by words or phrases, this approach assumes that the child knows something about constituency. This is true; note, however, that constituency is understood differently in this framework: not as a characteristic of binary branching syntactic trees with labeled nodes, but merely an understanding that some combinations of words function as a unit when they fill a particular slot in a formula. In the constructionist approach, constituency is an emergent property of grammar rather than something that is present from the start, and it is sometimes fluid and variable (cf. Langacker, 1997 ). Constituency in this sense—i.e., hierarchical organization—is something that is a general property of many cognitive structures and is not unique to language.
Understanding Language, Warts, and All
Languages are shot through with patterns. The patterns exist at all levels: some are very general, others quite low-level. Languages are also shot through with idiosyncrasies: constructional idioms, lexical items which do not fit easily into any grammatical class, irregular morphology. The generative program focuses on uncovering the deepest, most fundamental generalizations, and relegates the low-level patterns and idiosyncrasies—which are regarded as less interesting—to the periphery. But low-level patterns are a part of language, and a satisfactory theory of language must account for them as well as more general constructions.
Construction grammar began as an attempt to account for constructional idioms such as the X-er the Y-er (e.g., The more the merrier ; The bigger they come, the harder they fall —see Fillmore et al., 1988 ) and what’s X doing Y? (e.g., What’s this fly doing in my soup? , What are you doing reading my diary? —see Kay and Fillmore, 1999 ). Such constructional idioms have idiosyncratic properties which are not predictable from general rules or principles, but they are productive: we can create novel utterances based on the schema. As construction grammar developed, it quickly became apparent that whatever mechanisms were required to explain low-level patterns could also account for high-level patterns as a special case: consequently, as Croft (2001) put it, “the constructional tail has come to wag the syntactic dog” (p. 17). As suggested earlier, the same is true of acquisition: the learning mechanisms that are necessary to learn relational words can also account for the acquisition of more abstract constructions.
Back to Poverty of the Stimulus
It is important to note that the way the poverty-of-the-stimulus problem is posed (e.g., “how does the child know that the auxiliary inside the subject cannot be moved?”) presupposes a generative account of the phenomena (i.e., interrogatives are derived from declarative-like structures by moving the auxiliary). The problem does not arise in constructionist accounts, which do not assume movement.
More generally, generativist and constructionist researchers agree about the basic thrust of the POS argument: the child cannot learn about the properties of empty categories, constraints on extraction, etc., from the input. What they disagree about is the conclusion that is to be drawn from this fact. For generative researchers, the fact that some grammatical principles or notions are unlearnable entails that they must be part of an innate UG. Constructionist researchers, on the other hand, draw a completely different conclusion: if X cannot be learned from the input, then we need a better linguistic theory—one that does not assume such an implausible construct.
Thus, one of the basic principles of the constructionist approach is that linguists should focus on developing “child-friendly” grammars ( Langacker, 1987 , 1991 , 2008 ; Goldberg, 2003 ; Tomasello, 2003 , 2006 ; Dąbrowska, 2004 ) rather than postulate an innate UG. Construction grammar attempts to capture all that speakers know about their language in terms of constructions—form-meaning pairings which can be simple or complex and concrete or partially or entirely schematic (i.e., they can contain one or more “slots” which can be elaborated by more specific units, allowing for the creation of novel expressions). Most construction grammar researchers also assume that children prefer relatively concrete, lexically-specific patterns which can be easily inferred from the input; more schematic patterns emerge later in development, as a result of generalization over the concrete units acquired earlier ( Johnson, 1983 ; Dąbrowska, 2000b ; Tomasello, 2003 , 2006 ; Diessel, 2004 ). Crucially, the mechanisms required to learn constructional schemas are also necessary to acquire relational terms such as verbs and prepositions ( Dąbrowska, 2004 , 2009 ). Since we know that children are able to learn the meanings and selectional restrictions of verbs and prepositions, it follows that they are able to learn constructional schemas as well.
As we have seen, contemporary views on what is or is not in UG are wildly divergent. I have also argued that, although many arguments have been put forward in favor of some kind of an innate UG, there is actually very little evidence for its existence: the arguments for the innateness of specific linguistic categories or principles are either irrelevant (in that they are arguments for general innateness rather than linguistic innateness), based on false premises, or circular.
Some generative linguists respond to criticisms of this kind by claiming that UG is an approach to doing linguistics rather than a specific hypothesis. For example, Nevins et al. (2009) in their critique of Everett’s work on Pirahã, assert that
“The term Universal Grammar (UG), in its modern usage, was introduced as a name for the collection of factors that underlie the uniquely human capacity for language—whatever they may turn out to be …. There are many different proposals about the overall nature of UG, and continuing debate about its role in the explanation of virtually every linguistic phenomenon. Consequently, there is no general universal-grammar model for which [Everett’s claims] could have consequences – only a wealth of diverse hypotheses about UG and its content.” (p. 357)
This view contrasts sharply with other assessments of the UG enterprise. Chomsky (2000a) , for instance, claims that the Principles and Parameters framework was “highly successful” (p. 8), that it “led to an explosion of inquiry into a very broad range of typologically diverse languages, at a level of depth not previously envisioned” ( Chomsky, 2004 , p. 11), and that it was “the only real revolutionary departure in linguistics maybe in the last several thousand years, much more so than the original work in generative grammar” ( Chomsky, 2004 , p. 148). If Nevins et al. (2009) are right in their assertion that the UG literature is no more than a collection of proposals which, as a set, do not make any specific empirical predictions about languages, then such triumphalist claims are completely unjustified.
Is it a fruitful approach? (Or perhaps a better question might be: Was it a fruitful approach?) It was certainly fruitful in the sense that it generated a great deal of debate. Unfortunately, it does not seem to have got us any closer to answers to the fundamental questions that it raised. One could regard the existing disagreements about UG as a sign of health. After all, debate is the stuff of scientific inquiry: initial hypotheses are often erroneous; it is by reformulating and refining them that we gradually get closer to the truth. However, the kind of development we see in UG theory is very different from what we see in the natural sciences. In the latter, the successive theories are gradual approximations to the truth. Consider an example discussed by Asimov (1989) . People once believed that the earth is flat. Then, ancient Greek astronomers established that it was spherical. In the seventeenth century, Newton argued that it was an oblate spheroid (i.e., slightly squashed at the poles). In the twentieth century, scientists discovered that it is not a perfect oblate spheroid: the equatorial bulge is slightly bigger in the southern hemisphere. Note that although the earlier theories were false, they clearly approximated the truth: the correction in going from “sphere” to “oblate spheroid,” or from “oblate spheroid” to “slightly irregular oblate spheroid” is much smaller than when going from “flat” to “spherical.” And while “slightly irregular oblate spheroid” may not be entirely accurate, we are extremely unlikely to discover tomorrow that the earth is conical or cube-shaped. We do not see this sort of approximation in work in the UG approach: what we see instead is wildly different ideas being constantly proposed and abandoned. After more than half a century of intensive research we are no nearer to understanding what UG is than we were when Chomsky first used the term.
This lack of progress, I suggest, is a consequence of the way that the basic questions are conceptualized in the UG approach, and the strategy that it adopts in attempting to answer them. Let us consider a recent example. Berwick et al. (2011) list four factors determining the outcome of language acquisition:
(1) innate, domain-specific factors;
(2) innate, domain-general factors;
(3) external stimuli;
(4) natural law.
They go on to assert that the goal of linguistic theory is to explain how these factors “conspire to yield human language” (p. 1223), and that “on any view, (1) is crucial, at least in the initial mapping of external data to linguistic experience” (p. 1209).
There are three problems with this approach. First, it assumes that innate language-specific factors are “crucial.” It may well be that this is true; however, such a statement should be the outcome of a research program, not the initial assumption.
Secondly, Berwick et al. (2011) appear to assume that the four types of factors are separate and isolable: a particular principle can be attributed to factor 1, 2, 3, or 4. The problem is that one cannot attribute specific properties of complex systems to individual factors, since they emerge from the interaction of various factors ( Elman et al., 1996 ; Bates, 2003 ; MacWhinney, 2005 ). Asking whether a particular principle is “innate” or due to “external stimuli” is meaningless—it is both: genes and the environment interact in myriad ways at different levels (molecular, cellular, at the level of the organism, and in the external environment, both physical and social). Asking whether something is “domain general” or “domain specific” may be equally unhelpful. Presumably everybody, including the staunchest nativists, agrees that (the different components of) what we call the language faculty arose out of some non-linguistic precursors. Bates (2003) argues that language is “a new machine built out of old parts”; she also suggests that the “old parts” (memory consolidation, motor planning, attention) “have kept their day jobs” ( Bates, 1999 ). However, it is perfectly possible that they have undergone further selection as a result of the role they play in language, so that language is now their “day job,” although they continue to “moonlight” doing other jobs.
Finally, Berwick et al. (2011) like most researchers working in the UG tradition, assume that one can determine which aspects of language can be attributed to which factor by ratiocination rather than empirical enquiry: “the best overall strategy for identifying the relative contributions of (1–4) to human linguistic knowledge is to formulate POS arguments that reveal a priori assumptions that theorists can reduce to more basic linguistic principles” (p. 1210). This “logical” approach to language learnability is a philosophical rather than a scientific stance, somewhat reminiscent of Zeno’s argument that motion could not exist. Zeno of Elea was an ancient Greek philosopher who “proved,” through a series of paradoxes (Achilles and the tortoise, the dichotomy argument, the arrow in flight), that motion is an illusion. However, Zeno’s paradoxes, intriguing as they are, are not a contribution to the study of physics: in fact, we would not have modern physics if we simply accepted his argument.
Virtually everyone agrees that there is something unique about humans that makes language acquisition possible. There is a growing consensus, even in the generativist camp, that the “big mean UG” of the Principles and Parameters model is not tenable: UG, if it exists, is fairly minimal, 5 and most of the interesting properties of human languages arise through the interaction of innate capacities and predispositions and environmental factors. This view has long been part of the constructivist outlook ( Piaget, 1954 ; Bates and MacWhinney, 1979 ; Karmiloff-Smith, 1992 ; MacWhinney, 1999 , 2005 ; O’Grady, 2008 , 2010 ), and it is encouraging to see the two traditions in cognitive science are converging, to some extent at least.
The great challenge is to understand exactly how genes and environment interact during individual development, and how languages evolve and change as a result of interactions between individuals. To do this, it is crucial to examine interactions at different levels. Genes do not interact with the primary linguistic data: they build proteins which build brains which learn to “represent” language and the external environment by interacting with it via the body. It is unlikely that we will be able to tease apart the contribution of the different factors by ratiocination: the interactions are just too complex, and they often lead to unexpected results ( Thelen and Smith, 1994 ; Elman et al., 1996 ; Bates, 2003 ; MacWhinney, 2005 ). We have already made some headway in this area. Further progress will require empirical research and the coordinated efforts of many disciplines, from molecular biology to psychology and linguistics.
Conflict of Interest Statement
The author declares that the research was conducted in the absence of any commercial or financial relationships that could be construed as a potential conflict of interest.
- ^ Our nearest relatives, the great apes, do not point and do not understand pointing gestures ( Tomasello, 1999 ; Tomasello et al., 2005 ). Dogs do understand human pointing, which is believed to be a consequence of domestication ( Hare et al., 2002 ); they do not, however, use pointing gestures themselves. “Pointing” dogs do not intentionally point things out to others: they merely look at the game, enabling the human hunter to follow their line of sight.
- ^ The Manchester corpus is described in Theakston et al. (2001) and is available from CHILDES ( MacWhinney, 1995 ).
- ^ It should be emphasized that these styles are idealizations. Most children use a mixture of both strategies, although many have a clear preference for one or the other.
- ^ Naomi’s linguistic development was recorded by Sachs (1983) . The transcripts are available from the CHILDES database ( MacWhinney, 1995 ).
- ^ In fact, Roberts and Holmberg (2011) suggest that “UG does not have to be seen as either language-specific or human-specific,” thus capitulating on the central claims of the UG approach. Note that this dilutes the innateness hypothesis to the point where it becomes trivial: if UG is neither language specific nor human specific, then saying that it exists amounts to saying that we are different from rocks.
Allen, S. E. M., and Crago, M. B. (1996). Early passive acquisition in Inuktitut. J. Child Lang. 23, 129–155. doi: 10.1017/S0305000900010126
PubMed Abstract | CrossRef Full Text | Google Scholar
Ambridge, B., Pine, J. M., and Rowland, C. F. (2011). Children use verb semantics to retreat from overgeneralization errors: a novel verb grammaticality judgment study. Cogn. Linguist. 22, 303–324. doi: 10.1515/cogl.2011.012
CrossRef Full Text | Google Scholar
Ambridge, B., Pine, J. M., Rowland, C. F., Jones, R. L., and Clark, V. (2009). A semantics-based approach to the ‘no negative-evidence’ problem. Cogn. Sci. 33, 1301–1316. doi: 10.1111/j.1551-6709.2009.01055.x
Ambridge, B., Pine, J. M., Rowland, C. F., and Young, C. R. (2008). The effect of verb semantic class and verb frequency (entrenchment) on children’s and adults’ graded judgements of argument-structure overgeneralization errors. Cognition 106, 87–129. doi: 10.1016/j.cognition.2006.12.015
Anderson, M. L. (2010). Neural reuse: a fundamental organizational principle of the brain. Behav. Brain Sci. 33, 245–313. doi: 10.1017/S0140525X10000853
Anderson, V., Spencer-Smith, M., and Wood, A. (2011). Do children really recover better? Neurobehavioural plasticity after early brain insult. Brain 134, 2197–2221. doi: 10.1093/brain/awr103
Anglade, C., Thiel, A., and Ansaldo, A. I. (2014). The complementary role of the cerebral hemispheres in recovery from aphasia after stroke: a critical review of literature. Brain Inj. 28, 138–145. doi: 10.3109/02699052.2013.859734
Aram, D. M. (1998). “Acquired aphasia in children,” in Acquired Aphasia , 3rd Edn, ed. M. T. Sarno (San Diego, CA: Academic Press), 451–480.
Google Scholar
Asimov, I. (1989). The relativity of wrong. Skept. Inq. 14, 35–44.
Baker, C. L. (1979). Syntactic theory and the projection problem. Linguist. Inq. 10, 533–581.
Baker, M. C. (2001). The Atoms of Language . New York, NY: Basic Books.
Bates, E. (1999). Language and the infant brain. J. Commun. Disord. 32, 195–205. doi: 10.1016/S0021-9924(99)00015-5
Bates, E. (2003). “On the nature and nurture of language,” in Frontiere Della Biologia. Il Cervello Di Homo Sapiens , eds E. Bizzi, P. Calissano, and V. Volterra (Rome: Istituto della Enciclopedia Italiana fondata da Giovanni Trecanni), 241–265.
Bates, E., Bretherton, I., and Snyder, L. (1988). From First Words to Grammar: Individual Differences and Dissociable Mechanisms . Cambridge: Cambridge University Press.
Bates, E., Dale, P. S., and Thal, D. (1995). “Individual differences and their implications for theories of language development,” in The Handbook of Child Language , eds P. Fletcher and B. MacWhinney (Oxford: Blackwell), 96–151.
Bates, E., and MacWhinney, B. (1979). “A functionalist approach to the acquisition of grammar,” in Developmental Pragmatics , eds E. Ochs and B. B. Schieffelin (New York, NY: Academic Press), 167–209.
Bates, E., Thal, D., Trauner, D., Fenson, J., Aram, D., Eisele, J., et al. (1997). From first words to grammar in children with focal brain injury. Dev. Neuropsychol. 13, 447–476. doi: 10.1080/87565649709540682
Bavin, E. L. (1995). Language acquisition in crosslinguistic perspective. Annu. Rev. Anthropol. 24, 373–396. doi: 10.1146/annurev.an.24.100195.002105
Bellugi, U., Wang, P. P., and Jernigan, T. L. (1994). “Williams syndrome: an unusual neuropsychological profile,” in Atypical Cognitive Deficits in Developmental Disorders , eds S. H. Broman and J. Grafman (Hillsdale, NJ: Lawrence Erlbaum), 23–56.
Benedict, H. (1979). Early lexical development: comprehension and production. J. Child Lang. 6, 183–200. doi: 10.1017/S0305000900002245
Berman, R. A. (1985). “The acquisition of Hebrew,” in The Crosslinguistic Study of Language Acquisition , ed. D. I. Slobin (Hillsdale, NJ: Erlbaum), 255–371.
Berman, R. A. (ed.). (2004). Language Development Across Childhood and Adolescence . Amsterdam: Benjamins.
Berman, R. A. (2007). “Developing linguistic knowledge and language use across adolescence,” in Blackwell Handbook of Language Development , eds E. Hoff and M. Shatz (Oxford: Blackwell Publishing).
Berwick, R. C., Pietroski, P., Yankama, B., and Chomsky, N. (2011). Poverty of the stimulus revisited. Cogn. Sci. 35, 1207–1242. doi: 10.1111/j.1551-6709.2011.01189.x
Bishop, D. (1993). “Language development after focal brain damage,” in Language Development in Exceptional Circumstances , eds D. Bishop and K. Mogford (Hove: Lawrence Erlbaum), 203–219.
Boeckx, C. (2011). “Features: perspectives on a key notion in linguistics,” in Journal of Linguistics , Vol. 47, eds A. Kibort and G. G. Corbett (Oxford: Oxford University Press), 522–524.
Bowerman, M. (1988). “The ‘no negative evidence’ problem,” in Explaining Language Universals , ed. J. A. Hawkins (Oxford: Basil Blackwell), 73–101.
Boyd, J. K., and Goldberg, A. E. (2011). Learning what NOT to say: the role of statistical preemption and categorization in a-adjective production. Language 87, 55–83. doi: 10.1353/lan.2011.0012
Brock, J. (2007). Language abilities in Williams syndrome: a critical review. Dev. Psychopathol. 19, 97–127. doi: 10.1017/S095457940707006X
Brooks, P. J., and Tomasello, M. (1999). How children constrain their argument structure constructions. Language 75, 720–738. doi: 10.2307/417731
Brooks, P., Tomasello, M., Lewis, L., and Dodson, K. (1999). Children’s overgeneralization of fixed-transitivity verbs: the entrenchment hypothesis. Child Dev. 70, 1325–1337. doi: 10.1111/1467-8624.00097
Brown, R. (1973). A First Language. The Early Stages . Cambridge, MA: Harvard University Press.
Chipere, N. (2001). Native speaker variations in syntactic competence: implications for first language teaching. Lang. Aware. 10, 107–124. doi: 10.1080/09658410108667029
Chipere, N. (2003). Understanding Complex Sentences: Native Speaker Variations in Syntactic Competence . Basingstoke: Palgrave.
Chomsky, N. (1962). “Explanatory models in linguistics,” in Logic, Methodology, and Philosophy of Science , eds E. Nagel, P. Suppes, and A. Tarski (Stanford, CA: Stanford University Press), 528–550.
Chomsky, N. (1972). Language and Mind . New York, NY: Harcourt Brace Jovanovich.
Chomsky, N. (1975). Reflections on Language . New York, NY: Pantheon.
Chomsky, N. (1976). “Problems and mysteries in the study of human language,” in Language in Focus: Foundations, Methods and Systems. Essays in Memory of Yehoshua Bar-Hillel , ed. A. Kasher (Dordrecht: D. Reidel), 281–357.
Chomsky, N. (1986). Knowledge of Language: Its Nature, Origin and Use . New York, NY: Praeger.
Chomsky, N. (1999). “On the nature, use, and acquisition of language,” in Handbook of Child Language Acquisition , eds W. C. Ritchie and T. K. Bhatia (San Diego, CA: Academic Press), 33–54.
Chomsky, N. (2000a). New Horizons in the Study of Language and Mind . Cambridge: Cambridge University Press.
Chomsky, N. (2000b). The Architecture of Language . New Delhi: Oxford University Press.
Chomsky, N. (2004). The Generative Enterprise Revisited: Discussions with Riny Huybregts, Henk van Riemsdijk, Naoki Fukui and Mihoko Zushi . New York, NY: Mouton de Gruyter.
Chomsky, N. (2007). “Approaching UG from below,” in Interfaces + Recursion = Language?: Chomsky’s Minimalism and the View from Syntax-Semantics , eds U. Sauerland and H. Gartner (New York, NY: Mouton de Gruyter), 1–29.
Chomsky, N. (2012). Poverty of stimulus: unfinished business. Stud. Chin. Linguist. 33, 3–16.
Chouinard, M. M., and Clark, E. V. (2003). Adult reformulations of child errors as negative evidence. J. Child Lang. 30, 637–669. doi: 10.1017/S0305000903005701
Cinque, G., and Rizzi, L. (2008). The cartography of syntactic structures. Stud. Linguist. 2, 42–58.
Clark, E. V. (1985). “The acquisition of romance, with special reference to French,” in The Crosslinguistic Study of Language Acquisition , ed. D. I. Slobin (Hillsdale, NJ: Erlbaum), 687–782.
Comrie, B. (1983). Form and function in explaining language universals. Linguistics 21, 87–103. doi: 10.1515/ling.1983.21.1.87
Corbett, G. G. (2010). “Features: essential notions,” in Features: Perspectives on a Key Notion in Linguistics , eds A. Kibort and G. G. Corbett (Oxford: Oxford University Press), 17–36.
Cowie, F. (2008). “Innateness and language,” in Stanford Encyclopedia of Philosophy-Stanford Encyclopedia of Philosophy , ed. E. N. Zalta. http://plato.stanford.edu/entries/innateness-language/
Crain, S. (1991). Language acquisition in the absence of experience. Behav. Brain Sci. 14, 597–650. doi: 10.1017/S0140525X00071491
Crain, S., and Lillo-Martin, D. (1999). An Introduction to Linguistic Theory and Language Acquisition . Malden, MA: Blackwell.
Crain, S., Thornton, R., and Murasugi, K. (2009). Capturing the evasive passive. Lang. Acquis. 16, 123–133. doi: 10.1080/10489220902769234
Croft, W. (2001). Radical Construction Grammar: Syntactic Theory in Typological Perspective . Oxford: Oxford University Press.
Culicover, P. W. (1999). Syntactic Nuts: Hard Cases, Syntactic Theory and Language Acquisition . Oxford: Oxford University Press.
Dąbrowska, E. (1997). The LAD goes to school: a cautionary tale for nativists. Linguistics 35, 735–766. doi: 10.1515/ling.1997.35.4.735
Dąbrowska, E. (2000a). “Could a Chomskyan child learn Polish? The logical argument for learnability,” in New Directions in Language Development and Disorders , eds M. R. Perkins and S. Howard (New York, NY: Plenum), 85–96.
Dąbrowska, E. (2000b). From formula to schema: the acquisition of English questions. Cogn. Linguist. 11, 83–102.
Dąbrowska, E. (2004). Language, Mind and Brain. Some Psychological and Neurological Constraints on Theories of Grammar . Edinburgh: Edinburgh University Press.
Dąbrowska, E. (2008). The effects of frequency and neighbourhood density on adult speakers’ productivity with Polish case inflections: an empirical test of usage-based approaches to morphology. J. Mem. Lang. 58, 931–951. doi: 10.1016/j.jml.2007.11.005
Dąbrowska, E. (2009). “Words as constructions,” in New Directions in Cognitive Linguistics , eds V. Evans and S. Pourcel (Amsterdam: John Benjamins), 201–223.
Dąbrowska, E. (2010a). “Formulas in the acquisition of English interrogatives: a case study,” in Lingua Terra Cognita II: A Festschrift for Roman Kalisz , eds D. Stanulewicz, T. Z. Wolanski, and J. Redzimska. (Gdańsk: Wydawnictwo Uniwersytetu Gdańskiego), 675–702.
Dąbrowska, E. (2010b). “The mean lean grammar machine meets the human mind: empirical investigations of the mental status of rules,” in Cognitive Foundations of Linguistic Usage Patterns. Empirical Approaches , eds H.-J. Schmid and S. Handl (Berlin: Mouton de Gruyter), 151–170.
Dąbrowska, E. (2012). Different speakers, different grammars: individual differences in native language attainment. Linguist. Approaches Biling. 2, 219–253. doi: 10.1075/lab.2.3.01dab
Dąbrowska, E. (2013). Functional constraints, usage, and mental grammars: a study of speakers’ intuitions about questions with long-distance dependencies. Cogn. Linguist. 24, 633–665. doi: 10.1515/cog-2013-0022
Dąbrowska, E. (2014). Recycling utterances: a speaker’s guide to sentence processing. Cogn. Linguist. 25, 617–653. doi: 10.1515/cog-2014-0057
Dąbrowska, E. (2015). “Individual differences in grammatical knowledge,” in Handbook of Cognitive Linguistics , eds E. Dąbrowska and D. Divjak (Berlin: De Gruyter Mouton), 649–667.
Dąbrowska, E., and Lieven, E. (2005). Towards a lexically specific grammar of children’s question constructions. Cogn. Linguist. 16, 437–474. doi: 10.1515/cogl.2005.16.3.437
Dąbrowska, E., and Street, J. (2006). Individual differences in language attainment: comprehension of passive sentences by native and non-native English speakers. Lang. Sci. 28, 604–615. doi: 10.1016/j.langsci.2005.11.014
Dąbrowska, E., and Szczerbiński, M. (2006). Polish children’s productivity with case marking: the role of regularity, type frequency, and phonological coherence. J. Child Lang. 33, 559–597. doi: 10.1017/S0305000906007471
Demetras, M. J., Post, K. N., and Snow, C. E. (1986). Feedback to first language learners: the role of repetitions and clarification questions. J. Child Lang. 13, 275–292. doi: 10.1017/S0305000900008059
Demuth, K. (1989). Maturation and the acquisition of the Sesotho passive. Language 65, 56–80. doi: 10.2307/414842
de Villiers, J. G., and de Villiers, P. A. (1985). “The acquisition of English,” in The Crosslinguistic Study of Language Acquisition , Vol. 1, The Data , ed. D. I. Slobin (Hillsdale, NJ: Lawrence Erlbaum), 27–140.
Diessel, H. (2004). The Acquisition of Complex Sentences . Cambridge: Cambridge University Press.
Diessel, H. (2013). “Construction grammar and first language acquisition,” in The Oxford Handbook of Construction Grammar , eds T. Hoffmann and G. Trousdale (Oxford: Oxford University Press), 347–364.
Elman, J. L., Bates, E., Johnson, M. H., Karmiloff-Smith, A., Parisi, D., and Plunkett, K. (1996). Rethinking Innateness: A Connectionist Perspective on Development . Cambridge, MA: MIT Press.
Evans, N., and Levinson, S. (2009). The myth of language universals. Behav. Brain Sci. 32, 429–492. doi: 10.1017/S0140525X0999094X
Fedorenko, E., Behr, M. K., and Kanwisher, N. (2011). Functional specificity for high-level linguistic processing in the human brain. Proc. Natl. Acad. Sci. U.S.A. 108, 16428–16433. doi: 10.1073/pnas.1112937108
Fillmore, C. J., Kay, P., and O’connor, M. C. (1988). Regularity and idiomaticity in grammatical constructions: the case of let alone. Language 64, 501–538. doi: 10.2307/414531
Fodor, J. A. (1983). The Modularity of Mind . Cambridge, MA: MIT Press.
Fodor, J. D. (2003). “Setting syntactic parameters,” in The Handbook of Contemporary Syntactic Theory , eds M. Baltin and C. Collins (Oxford: Blackwell), 730–767.
Fodor, J. D., and Sakas, W. G. (2004). “Evaluating models of parameter setting,” in BUCLD 28: Proceedings of the 28th Annual Boston University Conference on Language Development , eds A. Brugos, L. Micciulla, and C. E. Smith (Somerville, MA: Cascadilla Press), 1–27.
Fox, D., and Grodzinsky, Y. (1998). Children’s passive: a view from the by-phrase. Linguist. Inq. 29, 311–332. doi: 10.1162/002438998553761
Ginsborg, J. (2006). “The effects of socio-economic status on children’s language acquisition and use,” in Language and Social Disadvantage , eds J. Clegg and J. Ginsborg (Chichester: John Wiley & Sons), 9–27.
Gleitman, L. R. (1981). Maturational determinants of language growth. Cognition 10, 103–114. doi: 10.1016/0010-0277(81)90032-9
Goldberg, A. E. (2003). Constructions: a new theoretical approach to language. Trends Cogn. Sci. 7, 219–224. doi: 10.1016/S1364-6613(03)00080-9
Goldberg, A. E. (2006). Constructions at Work. The Nature of Generalization in Language . Oxford: Oxford University Press.
Goldfield, B. A., and Reznick, J. S. (1990). Early lexical acquisition: rate, content and vocabulary spurt. J. Child Lang. 17, 171–184. doi: 10.1017/S0305000900013167
Goldfield, B. A., and Snow, C. E. (1997). “Individual differences: implications for the study of language acquisition,” in The Development of Language , 4th Edn, ed. J. B. Gleason (Boston, MA: Allyn and Bacon), 317–347.
Grant, J., Karmiloff-Smith, A., Gathercole, S. E., Paterson, S., Howlin, P., Davies, M., et al. (1997). Phonological short-term memory and its relationship to language in Williams syndrome. J. Cogn. Neuropsychol. 2, 81–99. doi: 10.1080/135468097396342
Grant, J., Valian, V., and Karmiloff-Smith, A. (2002). A study of relative clauses in Williams syndrome. J. Child Lang. 29, 403–416. doi: 10.1017/S030500090200510X
Guasti, M. T. (2002). Language Acquisition: The Growth of Grammar . Cambridge, MA: MIT Press.
Gullo, D. F. (1981). Social class differences in preschool children’s comprehension of WH-questions. Child Dev. 52, 736–740.
Hare, B., Brown, M., Williamson, C., and Tomasello, M. (2002). The domestication of social cognition in dogs. Science 298, 1634–1636. doi: 10.1126/science.1072702
Haspelmath, M. (2007). Pre-established categories don’t exist: consequences for language description and typology. Linguist. Typol. 11, 119–132. doi: 10.1515/lingty.2007.011
Haspelmath, M. (2008). “Parametric versus functional explanations of syntactic universals,” in The Limits of Syntactic Variation , ed. T. Biberauer (Amsterdam: Benjamins), 75–10.
Hawkins, J. A. (2004). Efficiency and Complexity in Grammars . Oxford: Oxford University Press.
Heine, B., and Kuteva, T. (2002). World Lexicon of Grammaticalization . Cambridge: Cambridge University Press.
Hoff, E. (2006). How social contexts support and shape language development. Dev. Rev. 26, 55–88. doi: 10.1016/j.dr.2005.11.002
Holland, A. L., Fromm, D. S., Deruyter, F., and Stein, M. (1996). Treatment efficacy: aphasia. J. Speech Hear. Res. 39, S27–S36. doi: 10.1044/jshr.3905.s27
Horgan, D. (1978). The development of the full passive. J. Child Lang. 5, 65–80. doi: 10.1017/S030500090000194X
Hornstein, N., and Lightfoot, D. (1981). “Introduction,” in Explanation in Linguistics: The Logical Problem of Language Acquisition , eds N. Hornstein and D. Lightfoot (London: Longman), 9–31.
Hulst, H. V. D. (2008). On the question of linguistic universals. Linguist. Rev. 25, 1–34. doi: 10.1515/TLIR.2008.001
Huttenlocher, J. (1998). Language input and language growth. Prev. Med. 27, 195–199. doi: 10.1006/pmed.1998.0301
Huttenlocher, J., Vasilyeva, M., Cymerman, E., and Levine, S. (2002). Language input and child syntax. Cognit. Psychol. 45, 337–374. doi: 10.1016/S0010-0285(02)00500-5
Indefrey, P., and Goebel, R. (1993). “The learning of weak noun declension in German: children vs. artificial network models,” Proceedings of the Fifteenth Annual Conference of the Cognitive Science Society , (Hillsdale, NJ: Erlbaum), 575–580.
Johnson, C. E. (1983). The development of children’s interrogatives: from formulas to rules. Pap. Rep. Child Lang. Dev. 22, 108–115.
Jones, M. J. (1996). A Longitudinal and Methodological Investigation of Auxiliary Verb Development . Ph.D. thesis, University of Manchester, Manchester.
Jusczyk, P. (1997). The Discovery of Spoken Language . Cambridge, MA: MIT Press.
Kaplan, D., and Berman, R. (2015). Developing linguistic flexibility across the school years. First Lang. 35, 27–53. doi: 10.1177/0142723714566335
Karbe, H., Thiel, A., Weber-Luxemberger, G., Herholz, K., Kessler, J., and Heiss, W. (1998). Brain plasticity in poststroke aphasia: what is the contribution of the right hemisphere? Brain Lang. 64, 215–230. doi: 10.1006/brln.1998.1961
Karmiloff, K., and Karmiloff-Smith, A. (2001). Pathways to Language. From Fetus to Adolescent . Cambridge, MA: Harvard University Press.
Karmiloff-Smith, A. (1992). Beyond Modularity. A Developmental Perspective on Cognitive Science . Cambridge, MA: MIT Press.
Karmiloff-Smith, A. (2008). “Research into Williams syndrome: the state of the art,” in Handbook of Developmental Cognitive Neuroscience , eds C. A. Nelson and M. Luciana. (Cambridge, MA: MIT Press), 691–699.
Karmiloff-Smith, A., Grant, J., Bethoud, I., Davies, M., Howlin, P., and Udwin, O. (1997). Language and Williams syndrome: how intact is ‘intact’? Child Dev. 68, 246–262. doi: 10.2307/1131848
Kay, P., and Fillmore, C. J. (1999). Grammatical constructions and linguistic generalizations: the What’s X doing Y construction. Language 75, 1–33. doi: 10.2307/417472
Kayne, R. S. (2005). “Some notes on comparative syntax, with particular reference to English and French,” in The Oxford Handbook of Comparative Syntax , eds G. Cinque and R. S. Kayne. (Oxford: Oxford University Press), 3–69.
Kolb, B., and Gibb, R. (2011). Brain plasticity and behaviour in the developing brain. J. Can. Acad. Child. Adolesc. Psychiatry 20, 265–276.
PubMed Abstract | Google Scholar
Kuczaj, S. A., and Maratsos, M. P. (1983). Initial verbs in yes-no questions: a different kind of general grammatical category? Dev. Psychol. 19, 440–444. doi: 10.1037/0012-1649.19.3.440
Langacker, R. W. (1987). Foundations of Cognitive Grammar , Vol. 1, Theoretical Prerequisites . Stanford, CA: Stanford University Press.
Langacker, R. W. (1991). Foundations of Cognitive Grammar , Vol. 2, Descriptive Application . Stanford, CA: Stanford University Press.
Langacker, R. W. (1997). Constituency, dependency, and conceptual grouping. Cogn. Linguist. 8, 1–32. doi: 10.1515/cogl.1997.8.1.1
Langacker, R. W. (2000). “A dynamic usage-based model,” in Usage-Based Models of Language , eds M. Barlow and S. Kemmer (Stanford, CA: CSLI Publications), 1–63.
Langacker, R. W. (2008). Cognitive Grammar: A Basic Introduction . Oxford: Oxford University Press.
Lasnik, H., and Uriagereka, J. (2002). On the poverty of the challenge. Linguist. Rev. 19, 147–150. doi: 10.1515/tlir.19.1-2.147
Laws, G., and Bishop, D. V. M. (2004). Pragmatic language impairment and social deficits in Williams syndrome: a comparison with Down’s syndrome and specific language impairment. Int. J. Lang. Commun. Disord. 39, 45–64. doi: 10.1080/13682820310001615797
Leonard, L. B. (1998). Children with Specific Language Impairment . Cambridge, MA: MIT Press.
Lidz, J., and Gagliardi, A. (2015). How nature meets nurture: universal grammar and statistical learning. Ann. Rev. Linguist . 1, 333–353. doi: 10.1146/annurev-linguist-030514-125236
Lidz, J., and Williams, A. (2009). Constructions on holiday. Cogn. Linguist. 20, 177–189. doi: 10.1515/COGL.2009.011
Lieven, E. V. (1997). “Variation in a crosslinguistic context,” in The Crosslinguistic Study of Language Acquisition , Vol. 5, Expanding the Contexts , ed. D. I. Slobin (Mahwah, NJ: Lawrence Erlbaum), 199–263.
Lum, J., Kidd, E., Davis, S., and Conti-Ramsden, G. (2010). Longitudinal study of declarative and procedural memory in primary school-aged children. Aust. J. Psychol. 62, 139–148. doi: 10.1080/00049530903150547
MacWhinney, B. (1995). The CHILDES Project: Tools for Analyzing Talk . Hillsdale, NJ: Lawrence Erlbaum.
MacWhinney, B. (ed.). (1999). The Emergence of Language . Mahwah, NJ: Lawrence Erlbaum.
MacWhinney, B. (2005). The emergence of linguistic form in time. Conn. Sci. 17, 191–211. doi: 10.1080/09540090500177687
MacWhinney, B. (2008). “A unified model,” in Handbook of Cognitive Linguistics and Second Language Acquisition , eds P. Robinson and N. C. Ellis (New York, NY: Routledge), 341–371.
Maratsos, M. (2000). More overregularizations after all: new data and discussion on Marcus, Pinker, Ullmann, Hollander, Rosen & Xu. J. Child Lang. 27, 183–212. doi: 10.1017/S0305000999004067
Marcus, G. F. (1993). Negative evidence in language acquisition. Cognition 46, 53–85. doi: 10.1016/0010-0277(93)90022-N
Martins, I. P., and Ferro, J. M. (1993). Acquired childhood aphasia: a clinicoradiological study of 11 stroke patients. Aphasiology 7, 489–495. doi: 10.1080/02687039308248624
Matthews, D., and Krajewski, G. (2015). “First language acquisition,” in Handbook of Cognitive Linguistics , eds E. Dąbrowska and D. Divjak (Berlin: De Gruyter Mouton), 649–667.
Menn, L. (1996). Evidence children use: learnability and the acquisition of grammatical morphemes. Berkeley Linguist. Soc. 22, 481–497.
Misyak, J. B., and Christiansen, M. H. (2011). “Genetic variation and individual differences in language,” in Experience, Variation and Generalization: Learning a First Language , eds E. V. Clark and I. Arnon (Amsterdam: John Benjamins), 223–238.
Müller, R.-A. (2009). “Language universals in the brain: how linguistic are they?” in Language Universals , eds M. H. Christiansen, C. Collins and S. Edelman (Oxford: Oxford University Press), 224–253.
Musso, M., Moro, A., Glauche, V., Rijntjes, M., Reichenbach, J., Büchel, C., et al. (2003). Broca’s area and the language instinct. Nat. Neurosci. 6, 774–781. doi: 10.1038/nn1077
Nelson, K. (1973). Structure and strategy in learning to talk. Monogr. Soc. Res. Child Dev 38, 1–135. doi: 10.2307/1165788
Nelson, K. (1981). Individual differences in language development: implications for development and language. Dev. Psychol. 17, 170–187. doi: 10.1037/0012-1649.17.2.170
Nevins, A., Pesetsky, D., and Rodrigues, C. (2009). Pirahã exceptionality: a reassessment. Language 85, 355–404. doi: 10.1353/lan.0.0107
Newmeyer, F. J. (2008). Universals in syntax. Linguist. Rev. 25, 35–82. doi: 10.1515/TLIR.2008.002
Newport, E. L. (1990). Maturational constraints on language learning. Cogn. Sci. 14, 11–28. doi: 10.1207/s15516709cog1401_2
Nippold, M. A. (1998). Later Language Development: The School-Age and Adolescent Years . Austin, TX: Pro-ed.
Nippold, M. A., Hesketh, L. J., Duthie, J. K., and Mansfield, T. C. (2005). Conversational versus expository discourse: a study of syntactic development in children, adolescents and adults. J. Speech Lang. Hear. Res. 48, 1048–1064. doi: 10.1044/1092-4388(2005/073)
O’Grady, W. (2008). The emergentist program. Lingua 118, 447–464. doi: 10.1016/j.lingua.2006.12.001
O’Grady, W. (2010). “An emergentist approach to syntax,” in The Oxford Handbook of Linguistic Analysis , eds H. Narrog and B. Heine (Oxford: Oxford University Press), 257–283.
O’Grady, W., Dobrovolsky, M., and Katamba, F. (1996). Contemporary Linguistics. An Introduction . London: Longman.
Paterson, S. J., Brown, J. H., Gsödl, M. H., Johnson, M. H., and Karmiloff-Smith, A. (1999). Cognitive modularity and genetic disorders. Science 286, 2355–2358. doi: 10.1126/science.286.5448.2355
Pesetsky, D. (1999). “Linguistic universals and Universal Grammar,” in The MIT Encyclopedia of the Cognitive Sciences , eds R. A. Wilson and F. C. Keil (Cambridge, MA: MIT Press).
Peters, A. M. (1977). Language learning strategies: does the whole equal the sum of the parts? Language 53, 560–573. doi: 10.2307/413177
Peters, A. M. (1997). “Language typology, individual differences and the acquisition of grammatical morphemes,” in The Crosslinguistic Study of Language Acquisition , ed. D. I. Slobin (Hillsdale, NJ: Erlbaum), 136–197.
Peters, A. M. (2001). Filler syllables: what is their status in emerging grammar? J. Child Lang. 28, 229–242. doi: 10.1017/S0305000900004438
Peters, A. M., and Menn, L. (1993). False starts and filler syllables: ways to learn grammatical morphemes. Language 69, 742–777. doi: 10.2307/416885
Piaget, J. (1954). The Construction of Reality in the Child . New York, NY: Basic Books.
Piattelli-Palmarini, M. (1980). Language Learning: The Debate between Jean Piaget and Noam Chomsky . Cambridge, MA: Harvard University Press.
Pinker, S. (1994). The Language Instinct. The New Science of Language and Mind . London: Penguin Books.
Pinker, S. (1995). “Facts about human language relevant to its evolution,” in Origins of the Human Brain , eds J.-P. Changeux and J. Chavaillon (Oxford: Clarendon Press), 262–283.
Pinker, S. (1999). Words and Rules. The Ingredients of Language . London: Weidenfeld and Nicolson.
Pullum, G. K., and Scholz, B. C. (2002). Empirical assessment of stimulus poverty arguments. Linguist. Rev. 19, 9–50. doi: 10.1515/tlir.19.1-2.9
Pullum, G. K., and Tiede, H.-J. (2010). “Inessential features and expressive power of descriptive metalanguages,” in Features: Perspectives on a Key Notion in Linguistics , eds A. Kibort and G. G. Corbett (Oxford: Oxford University Press).
Reilly, J. S., Wasserman, S., and Appelbaum, M. (2013). Later language development in narratives in children with perinatal stroke. Dev. Sci. 16, 67–83. doi: 10.1111/j.1467-7687.2012.01192.x
Richards, B. J. (1990). Language Development and Individual Differences: A Study of Auxiliary Verb Learning . Cambridge: Cambridge University Press. doi: 10.1017/CBO9780511519833
Robenalt, C., and Goldberg, A. E. (in press). Judgment and frequency evidence for statistical preemption: it is relatively better to vanish than to disappear a rabbit, but a lifeguard can equally well backstroke or swim children to shore. Cogn. Linguist .
Roberts, I., and Holmberg, A. (2005). “On the role of parameters in universal grammar: a reply to Newmeyer,” in Organizing Grammar: Linguistic Studies in Honor of Henk Van Riemsdijk , eds H. Broekhuis, N. Corver, R. Huybregts, U. Kleinhenz, and J. Koster (Berlin: Mouton de Gruyter), 538–553.
Roberts, I., and Holmberg, A. (2011). Past and future approaches to linguistic variation: why doubt the existence of UG? Paper Presented at The Past and Future of Universal Grammar, University of Durham , Durham.
Sachs, J. (1983). “Talking about the there and then: The emergence of displaced reference in parent-child discourse,” in Children’s Language , ed. K. E. Nelson. (Hillsdale, NJ: Lawrence Erlbaum), 1–28.
Sachs, J., Bard, B., and Johnson, M. L. (1981). Language learning with restricted input: case studies of two hearing children of deaf parents. Appl. Psycholinguist. 2, 33–54. doi: 10.1017/S0142716400000643
Saxton, M. (2000). Negative evidence and negative feedback: immediate effects on the grammaticality of child speech. First Lang . 20, 221–252. doi: 10.1177/014272370002006001
Saxton, M., Kulcsar, B., Marshall, G., and Rupra, M. (1998). Longer-term effects of corrective input: an experimental approach. J. Child Lang. 25, 701–721. doi: 10.1017/S0305000998003559
Scholz, B. C., and Pullum, G. K. (2002). Searching for arguments to support linguistic nativism. Linguis. Rev. 19, 185–223. doi: 10.1515/tlir.19.1-2.185
Scholz, B. C., and Pullum, G. K. (2006). “Irrational nativist exuberance,” in Contemporary Debates in Cognitive Science , ed. R. J. Stainton (Malden, MA: Blackwell Publishing), 59–80.
Shlonsky, U. (2010). The cartographic enterprise in syntax. Lang. Linguist. Compass 4, 417–429. doi: 10.1111/j.1749-818X.2010.00202.x
Shore, C. M. (1995). Individual Differences in Language Development . Thousand Oaks, CA: Sage Publications. doi: 10.4135/9781483327150
Slobin, D. I. (1973). “Cognitive prerequisites for the development of grammar,” in Studies in Child Language Development , eds C. A. Ferguson and D. I. Slobin (New York: Holt, Rinehart and Winston), 175–208.
Slobin, D. I. (1985). “Crosslinguistic evidence for the language-making capacity,” in The Crosslinguistic Study of Language Acquisition , Vol. 2, Theoretical Issues , ed. D. I. Slobin (Hillsdale, NJ: Lawrence Erlbaum Associates), 1157–1255.
Smith, N. (1999). Chomsky: Ideas and Ideals . Cambridge: Cambridge University Press. doi: 10.1017/CBO9781139163897
Smith, N., and Tsimpli, I. M. (1995). The Mind of a Savant. Language Learning and Modularity . Oxford: Blackwell.
Smoczyńska, M. (1985). “The acquisition of Polish,” in The Crosslinguistic Study of Language Acquisition , Vol. 1, The Data , ed. D. I. Slobin (Hillsdale, NJ: Lawrence Erlbaum), 595–683.
Smolensky, P., and Dupoux, E. (2009). Universals in cognitive theories of language. Behav. Brain Sci. 32, 468–469. doi: 10.1017/S0140525X09990586
Stefanowitsch, A. (2008). Negative entrenchment: a usage-based approach to negative evidence. Cogn. Linguist. 19, 513–531. doi: 10.1515/COGL.2008.020
Stiles, J., Reilly, J. S., Paul, B., and Moses, P. (2005). Cognitive development following early brain injury: evidence for neural adaptation. Trends. Cogn. Sci. 9, 136–143.
Stojanovik, V., Perkins, M., and Howard, S. (2004). Williams Syndrome and Specific Language Impairment do not support claims for developmental double dissociations. J. Neurolinguist. 17, 403–424. doi: 10.1016/j.jneuroling.2004.01.002
Stowe, L. A., Haverkort, M., and Zwarts, H. (2005). Rethinking the neurological basis of language. Lingua 115, 997–1042. doi: 10.1016/j.lingua.2004.01.013
Street, J. (2010). Individual Differences in Comprehension of Passives and Universal Quantifiers by Adult Native Speakers of English . Ph.D. thesis, University of Sheffield, Sheffield.
Street, J., and Dąbrowska, E. (2010). More individual differences in language attainment: how much do adult native speakers of English know about passives and quantifiers? Lingua 120, 2080–2094. doi: 10.1016/j.lingua.2010.01.004
Street, J., and Dąbrowska, E. (2014). Lexically specific knowledge and individual differences in adult native speakers’ processing of the English passive. Appl. Psycholinguist. 35, 97–118. doi: 10.1017/S0142716412000367
Stromswold, K. (1999). “Cognitive and neural aspects of language acquisition,” in What Is Cognitive Science ? eds E. Lepore and Z. Pylyshyn (Oxford: Blackwell), 356–400.
Stromswold, K. (2000). “The cognitive neuroscience of language acquisition,” in The New Cognitive Neurosciences , ed. M. S. Gazzaniga (Cambridge, MA: MIT Press), 909–932.
Stromswold, K. (2001). The heritability of language: a review and meta-analysis of twin, adoption and linkage studies. Language 77, 647–723. doi: 10.1353/lan.2001.0247
Stromswold, K., Caplan, D., Alpert, N., and Rausch, S. (1996). Localization of syntactic processing by positron emission tomography. Brain Lang. 51, 452–473. doi: 10.1006/brln.1996.0024
Tallal, P. (2003). Language learning disabilities: integrating research approaches. Curr. Dir. Psychol. Sci. 12, 206–211. doi: 10.1046/j.0963-7214.2003.01263.x
Temple, C. M., Almazan, M., and Sherwood, S. (2002). Lexical skills in Williams syndrome: a cognitive neuropsychological analysis. J. Neurolinguist. 15, 463–495. doi: 10.1016/S0911-6044(01)00006-9
Thal, D. J., Bates, E., Zappia, M. J., and Oroz, M. (1996). Ties between lexical and grammatical development: evidence from early talkers. J. Child Lang. 23, 349–368. doi: 10.1017/S0305000900008837
Theakston, A. L., Lieven, E. V., Pine, J. M., and Rowland, C. F. (2001). The role of performance limitations in the acquisition of verb argument structure: an alternative account. J. Child Lang. 28, 127–152. doi: 10.1017/S0305000900004608
Thelen, E., and Smith, L. B. (1994). A Dynamic Systems Approach to the Development of Cognition and Action . Cambridge, MA: MIT Press.
Thomas, M., and Karmiloff-Smith, A. (2002). Are developmental disorders like cases of adult brain damage? Implications from connectionist modeling. Behav. Brain Sci. 25, 727–788. doi: 10.1017/S0140525X02000134
Thomas, M. S. C., Grant, J., Barham, Z., Gsödl, M., Laing, E., Lakusta, L., et al. (2001). Past tense formation in Williams syndrome. Lang. Cogn. Processes 16, 143–176. doi: 10.1080/01690960042000021
Todd, P., and Aitchison, J. (1980). Learning language the hard way. First Lang. 1, 122–140. doi: 10.1177/014272378000100203
Tomasello, M. (1995). Language is not an instinct. Cogn. Dev. 10, 131–156. doi: 10.1016/0885-2014(95)90021-7
Tomasello, M. (1999). The Cultural Origins of Human Cognition . Cambridge, MA: Harvard University Press.
Tomasello, M. (2003). Constructing a Language: A Usage-Based Theory of Child Language Acquisition . Cambridge, MA: Harvard University Press.
Tomasello, M. (2005). Beyond formalities: the case of language acquisition. Linguist. Rev. 22, 183–197. doi: 10.1515/tlir.2005.22.2-4.183
Tomasello, M. (2006). Construction grammar for kids. Constructions SV1–SV11, 1–23.
Tomasello, M., Carpenter, M., Call, J., Behne, T., and Moll, H. (2005). Understanding and sharing intentions: the origins of cultural cognition. Behav. Brain Sci. 28, 675–691. doi: 10.1017/S0140525X05000129
Trauner, D. A., Eshagh, K., Ballantyne, A. O., and Bates, E. (2013). Early language development after peri-natal stroke. Brain Lang. 127, 399–403. doi: 10.1016/j.bandl.2013.04.006
Ullman, M. T. (2006). The declarative/procedural model and the shallow structure hypothesis. Appl. Psycholinguist. 27, 97–105.
van der Lely, H. (1997). Language and cognitive development in a grammatical SLI boy: modularity and innateness. J. Neurolinguist. 10, 75–107. doi: 10.1016/S0911-6044(97)00011-0
van der Lely, H. K. J., and Ullman, M. (2001). Past tense morphology in specifically language impaired children and normally developing children. Lang. Cogn. Processes 16, 177–217. doi: 10.1080/01690960042000076
van Hout, A. (1991). “Outcome of acquired aphasia in childhood: prognosis factors,” in Acquired Aphasia in Children. Acquisition and Breakdown of Language in the Developing Brain , eds I. Pavão Martins, A. Castro-Caldas, H. R. Van Dongen, and A. Van Hout (Dordrecht: Kluwer), 163–169. doi: 10.1007/978-94-011-3582-5_13
Van Valin, R. D. Jr. (1994). “Extraction restrictions, competing theories and the argument from the poverty of the stimulus,” in The Reality of Linguistic Rules , eds S. D. Lima, R. Corrigan, and G. K. Iverson (Amsterdam: Benjamins), 243–259.
Vicari, S., Albertoni, A., Chilosi, A. M., Cipriani, P., Cioni, G., and Bates, E. (2000). Plasticity and reorganization during language development in children with early brain injury. Cortex 36, 31–46. doi: 10.1016/S0010-9452(08)70834-7
Wells, G. (1979). “Learning and using the auxiliary verb in English,” in Language Development , ed. V. Lee (London: Croom Helm), 250–270.
Wells, G. (1985). Language Development in the Preschool Years . Cambridge: Cambridge University Press.
Keywords: Universal Grammar, language universals, poverty of the stimulus, convergence, individual differences, language acquisition, construction grammar, linguistic nativism
Citation: Dąbrowska E (2015) What exactly is Universal Grammar, and has anyone seen it? Front. Psychol. 6:852. doi: 10.3389/fpsyg.2015.00852
Received: 19 February 2015; Accepted: 08 June 2015; Published: 23 June 2015.
Reviewed by:
Copyright © 2015 Dąbrowska. This is an open-access article distributed under the terms of the Creative Commons Attribution License (CC BY) . The use, distribution or reproduction in other forums is permitted, provided the original author(s) or licensor are credited and that the original publication in this journal is cited, in accordance with accepted academic practice. No use, distribution or reproduction is permitted which does not comply with these terms.
*Correspondence: Ewa Dąbrowska, Department of Humanities, Faculty of Arts, Design and Social Sciences, Northumbria University, Newcastle upon Tyne NE1 8ST, UK, [email protected]
Disclaimer: All claims expressed in this article are solely those of the authors and do not necessarily represent those of their affiliated organizations, or those of the publisher, the editors and the reviewers. Any product that may be evaluated in this article or claim that may be made by its manufacturer is not guaranteed or endorsed by the publisher.

An official website of the United States government
The .gov means it’s official. Federal government websites often end in .gov or .mil. Before sharing sensitive information, make sure you’re on a federal government site.
The site is secure. The https:// ensures that you are connecting to the official website and that any information you provide is encrypted and transmitted securely.
- Publications
- Account settings
Preview improvements coming to the PMC website in October 2024. Learn More or Try it out now .
- Advanced Search
- Journal List

The Sapir-Whorf Hypothesis and Probabilistic Inference: Evidence from the Domain of Color
Emily cibelli.
1 Department of Linguistics, Northwestern University, Evanston, IL 60208, United States of America
2 Department of Linguistics, University of California, Berkeley, CA 94720, United States of America
3 Cognitive Science Program, University of California, Berkeley, CA 94720, United States of America
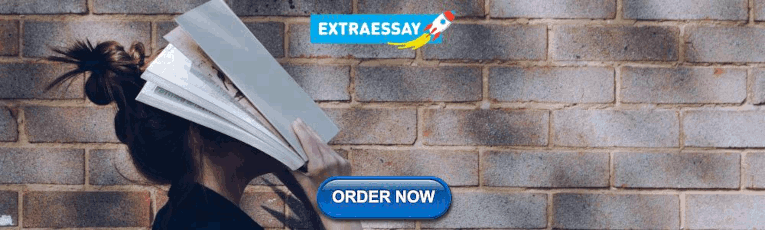
Joseph L. Austerweil
4 Department of Psychology, University of Wisconsin, Madison, WI 53706, United States of America
Thomas L. Griffiths
5 Department of Psychology, University of California, Berkeley, CA 94720, United States of America
Terry Regier
Conceived and designed the experiments: EC YX JLA TLG TR. Performed the experiments: EC YX JLA. Analyzed the data: EC YX JLA. Wrote the paper: TR EC YX.
Associated Data
All relevant data are available within the paper and/or at: https://github.com/yangxuch/probwhorfcolor This GitHub repository is mentioned in the paper.
The Sapir-Whorf hypothesis holds that our thoughts are shaped by our native language, and that speakers of different languages therefore think differently. This hypothesis is controversial in part because it appears to deny the possibility of a universal groundwork for human cognition, and in part because some findings taken to support it have not reliably replicated. We argue that considering this hypothesis through the lens of probabilistic inference has the potential to resolve both issues, at least with respect to certain prominent findings in the domain of color cognition. We explore a probabilistic model that is grounded in a presumed universal perceptual color space and in language-specific categories over that space. The model predicts that categories will most clearly affect color memory when perceptual information is uncertain. In line with earlier studies, we show that this model accounts for language-consistent biases in color reconstruction from memory in English speakers, modulated by uncertainty. We also show, to our knowledge for the first time, that such a model accounts for influential existing data on cross-language differences in color discrimination from memory, both within and across categories. We suggest that these ideas may help to clarify the debate over the Sapir-Whorf hypothesis.
Introduction
The Sapir-Whorf hypothesis [ 1 , 2 ] holds that our thoughts are shaped by our native language, and that speakers of different languages therefore think about the world in different ways. This proposal has been controversial for at least two reasons, both of which are well-exemplified in the semantic domain of color. The first source of controversy is that the hypothesis appears to undercut any possibility of a universal foundation for human cognition. This idea sits uneasily with the finding that variation in color naming across languages is constrained, such that certain patterns of color naming recur frequently across languages [ 3 – 5 ], suggesting some sort of underlying universal basis. The second source of controversy is that while some findings support the hypothesis, they do not always replicate reliably. Many studies have found that speakers of a given language remember and process color in a manner that reflects the color categories of their language [ 6 – 13 ]. Reinforcing the idea that language is implicated in these findings, it has been shown that the apparent effect of language on color cognition disappears when participants are given a verbal [ 7 ] (but not a visual) interference task [ 8 , 11 , 12 ]; this suggests that language may operate through on-line use of verbal representations that can be temporarily disabled. However, some of these findings have a mixed record of replication [ 14 – 17 ]. Thus, despite the substantial empirical evidence already available, the role of language in color cognition remains disputed.
An existing theoretical stance holds the potential to resolve both sources of controversy. On the one hand, it explains effects of language on cognition in a framework that retains a universal component, building on a proposal by Kay and Kempton [ 7 ]. On the other hand, it has the potential to explain when effects of language on color cognition will appear, and when they will not—and why. This existing stance is that of the “category adjustment” model of Huttenlocher and colleagues [ 18 , 19 ]. We adopt this stance, and cast color memory as inference under uncertainty, instantiated in a category adjustment model, following Bae et al. [ 20 ] and Persaud and Hemmer [ 21 ]. The model holds that color memory involves the probabilistic combination of evidence from two sources: a fine-grained representation of the particular color seen, and the language-specific category in which it fell (e.g. English green ). Both sources of evidence are represented in a universal perceptual color space, yet their combination yields language-specific bias patterns in memory, as illustrated in Fig 1 . The model predicts that such category effects will be strongest when fine-grained perceptual information is uncertain. It thus has the potential to explain the mixed pattern of replications of Whorfian effects in the literature: non-replications could be the result of high perceptual certainty.

A stimulus is encoded in two ways: (1) a fine-grained representation of the stimulus itself, shown as a (gray) distribution over stimulus space centered at the stimulus’ location in that space, and (2) the language-specific category (e.g. English “green”) in which the stimulus falls, shown as a separate (green) distribution over the same space, centered at the category prototype. The stimulus is reconstructed by combining these two sources of information through probabilistic inference, resulting in a reconstruction of the stimulus (black distribution) that is biased toward the category prototype. Adapted from Fig 11 of Bae et al. (2015) [ 20 ].
In the category adjustment model, both the fine-grained representation of the stimulus and the category in which it falls are modeled as probability distributions over a universal perceptual color space. The fine-grained representation is veridical (unbiased) but inexact: its distribution is centered at the location in color space where the stimulus itself fell, and the variance of that distribution captures the observer’s uncertainty about the precise location of the stimulus in color space, with greater variance corresponding to greater uncertainty. Psychologically, such uncertainty might be caused by noise in perception itself, by memory decay over time, or by some other cause—and any increase in such uncertainty is modeled by a wider, flatter distribution for the fine-grained representation. The category distribution, in contrast, captures the information about stimulus location that is given by the named category in which the stimulus fell (e.g. green for an English-speaking observer). Because named color categories vary across languages, this category distribution is assumed to be language-specific—although the space over which it exists is universal. The model infers the original stimulus location by combining evidence from both of these distributions. As a result, the model tends to produce reconstructions of the stimulus that are biased away from the actual location of the stimulus and toward the prototype of the category in which it falls.
As illustrated in Fig 2 , this pattern of bias pulls stimuli on opposite sides of a category boundary in opposite directions, producing enhanced distinctiveness for such stimuli. Such enhanced distinctiveness across a category boundary is the signature of categorical perception, or analogous category effects in memory. On this view, language-specific effects on memory can emerge from a largely universal substrate when one critical component of that substrate is language-specific: the category distribution.

Model reconstructions tend to be biased toward category prototypes, yielding enhanced distinctiveness for two stimuli that fall on different sides of a category boundary. Categories are shown as distributions in green and blue; stimuli are shown as vertical black lines; reconstruction bias patterns are shown as arrows.
If supported, the category adjustment model holds the potential to clarify the debate over the Sapir-Whorf hypothesis in three ways. First, it would link that debate to independent principles of probabilistic inference. In so doing, it would underscore the potentially important role of uncertainty , whether originating in memory or perception, in framing the debate theoretically. Second, and relatedly, it would suggest a possible reason why effects of language on color memory and perception are sometimes found, and sometimes not [ 17 ]. Concretely, the model predicts that greater uncertainty in the fine-grained representation—induced for example through a memory delay, or noise in perception—will lead to greater influence of the category, and thus a stronger bias in reproduction. The mirror-image of this prediction is that in situations of relatively high certainty in memory or perception, there will be little influence of the category, to the point that such an influence may not be empirically detectable. Third, the model suggests a way to think about the Sapir-Whorf hypothesis without jettisoning the important idea of a universal foundation for cognition.
Closely related ideas appear in the literature on probabilistic cue integration [ 22 – 25 ]. For example, Ernst and Banks [ 24 ] investigated perceptual integration of cues from vision and touch in judging the height of an object. They found that humans integrate visual and haptic cues in a statistically optimal fashion, modulated by cue certainty. The category adjustment model we explore here can be seen as a form of probabilistic cue integration in which one of the cues is a language-specific category.
The category adjustment model has been used to account for category effects in various domains, including spatial location [ 18 , 26 ], object size [ 19 , 27 ], and vowel perception [ 28 ]. The category adjustment model also bears similarities to other theoretical accounts of the Sapir-Whorf hypothesis that emphasize the importance of verbal codes [ 7 , 8 ], and the interplay of such codes with perceptual representations [ 29 – 31 ]. Prior research has linked such category effects to probabilistic inference, following the work of Huttenlocher and colleagues [ 18 , 19 ]. Roberson and colleagues [ 32 ] invoked the category adjustment model as a possible explanation for categorical perception of facial expressions, but did not explore a formal computational model; Goldstone [ 33 ] similarly referenced the category adjustment model with respect to category effects in the color domain. Persaud and Hemmer [ 21 , 34 ] explored bias in memory for color, and compared empirically obtained memory bias patterns from English speakers with results predicted by a formally specified category adjustment model, but did not link those results to the debate over the Sapir-Whorf hypothesis, and did not manipulate uncertainty. More recently, a subsequent paper by the same authors and colleagues [ 35 ] explored category-induced bias in speakers of another language, Tsimané, and did situate those results with respect to the Sapir-Whorf hypothesis, but again did not manipulate uncertainty. Most recently, Bae et al. [ 20 ] extensively documented bias in color memory in English speakers, modeled those results with a category-adjustment computational model, and did manipulate uncertainty—but did not explore these ideas relative to the Sapir-Whorf hypothesis, or to data from different languages.
In what follows, we first present data and computational simulations that support the recent finding that color memory in English speakers is well-predicted by a category adjustment model, with the strength of category effects modulated by uncertainty. We then show, to our knowledge for the first time, that a category adjustment model accounts for influential existing cross-language data on color that support the Sapir-Whorf hypothesis.
In this section we provide general descriptions of our analyses and results. Full details are supplied in the section on Materials and Methods.
Study 1: Color reconstruction in English speakers
Our first study tests the core assumptions of the category adjustment model in English speakers. In doing so, it probes questions that were pursued by two studies that appeared recently, after this work had begun. Persaud and Hemmer [ 21 ] and Bae et al. [ 20 ] both showed that English speakers’ memory for a color tends to be biased toward the category prototype of the corresponding English color term, in line with a category adjustment model. Bae et al. [ 20 ] also showed that the amount of such bias increases when subjects must retain the stimulus in memory during a delay period, compared to when there is no such delay, as predicted by the principles of the category adjustment model. In our first study, we consider new evidence from English speakers that tests these questions, prior to considering speakers of different languages in our following studies.
English-speaking participants viewed a set of hues that varied in small steps from dark yellow to purple, with most hues corresponding to some variety of either green or blue. We collected two kinds of data from these participants: bias data and naming data. Bias data were based on participants’ non-linguistic reconstruction of particular colors seen. Specifically, for each hue seen, participants recreated that hue by selecting a color from a color wheel, either while the target was still visible ( Fig 3A : simultaneous condition), or from memory after a short delay ( Fig 3B : delayed condition). We refer to the resulting data as bias data, because we are interested in the extent to which participants’ reconstructions of the stimulus color are biased away from the original target stimulus. Afterwards, the same participants indicated how good an example of English green (as in Fig 3C ) and how good an example of English blue each hue was. We refer to these linguistic data as naming data.

Screenshots of example trials illustrating (A) simultaneous reconstruction, (B) delayed reconstruction, and (C) green goodness rating.
Fig 4 shows both naming and bias data as a function of target hue. The top panel of the figure shows the naming data and also shows Gaussian functions corresponding to the English color terms green and blue that we fitted to the naming data. Bias data were collected for only a subset of the hues for which naming data were collected, and the shaded region in the top panel of Fig 4 shows that subset, relative to the full range of hues for naming data. We collected bias data only in this smaller range because we were interested specifically in bias induced by the two color terms blue and green , and colors outside the shaded region seemed to us to clearly show some influence of neighboring categories such as yellow and purple . The bottom panel of the figure shows the bias data, plotted relative to the prototypes (means) of the fitted Gaussian functions for green and blue . It can be seen that reconstruction bias appears to be stronger in the delayed than in the simultaneous condition, as predicted, and that—especially in the delayed condition—there is an inflection in the bias pattern between the two category prototypes, suggesting that bias may reflect the influence of each of the two categories. The smaller shaded region in this bottom panel denotes the subset of these hues that we subsequently analyzed statistically, and to which we fit models. We reduced the range of considered hues slightly further at this stage, to ensure that the range was well-centered with respect to the two relevant category prototypes, for green and blue , as determined by the naming data.

In both top and bottom panels, the horizontal axis denotes target hue, ranging from yellow on the left to purple on the right. Top panel (naming data): The solid green and blue curves show, for each target hue, the average goodness rating for English green and blue respectively, as a proportion of the maximum rating possible. The dashed green and blue curves show Gaussian functions fitted to the naming goodness data. The dotted vertical lines marked at the bottom with green and blue squares denote the prototypes for green and blue , determined as the means of the green and blue fitted Gaussian functions, respectively. The shaded region in the top panel shows the portion of the spectrum for which bias data were collected. Bottom panel (bias data): Solid curves denote, for each target hue, the average reconstruction bias for that hue, such that positive values denote reconstruction bias toward the purple (here, right) end of the spectrum, and negative values denote reconstruction bias toward the yellow (here, left) end of the spectrum. Units for the vertical axis are the same as for the horizontal axis, which is normalized to length 1.0. The black and red curves show bias under simultaneous and delayed response, respectively. Blue stars at the top of the bottom panel mark hues for which there was a significant difference in the magnitude of bias between simultaneous and delayed conditions. The shaded region in the bottom panel shows the portion of the data that was analyzed statistically, and to which models were fit. In both panels, error bars represent standard error of the mean.
The absolute values (magnitudes) of the bias were analyzed using a 2 (condition: simultaneous vs. delayed) × 15 (hues) repeated measures analysis of variance. This analysis revealed significantly greater bias magnitude in the delayed than in the simultaneous condition. It also revealed that bias magnitude differed significantly as a function of hue, as well as a significant interaction between the factors of hue and condition. The blue stars in Fig 4 denote hues for which the difference in bias magnitude between the simultaneous and delayed conditions reached significance. The finding of greater bias magnitude in the delayed than in the simultaneous condition is consistent with the proposal that uncertainty is an important mediating factor in such category effects, as argued by Bae et al. [ 20 ]. It also suggests that some documented failures to find such category effects could in principle be attributable to high certainty, a possibility that can be explored by manipulating uncertainty.
We wished to test in a more targeted fashion to what extent these data are consistent with a category adjustment model in which a color is reconstructed based in part on English named color categories. To that end, we compared the performance of four models against these data; only one of these models considered both of the relevant English color categories, green and blue . As in Fig 1 , each model contains a fine-grained but inexact representation of the perceived stimulus, and (for most models) a representation of one or more English color categories. Each model predicts the reconstruction of the target stimulus from its fine-grained representation of the target together with any category information. Category information in the model is specified by the naming data. Each model has a single free parameter, corresponding to the uncertainty of the fine-grained representation; this parameter is fit to bias data.
- The null model is a baseline model that predicts hue reconstruction based only on the fine-grained representation of the stimulus, with no category component.
- The 1-category (green) model predicts hue reconstruction based on the fine-grained representation of the stimulus, combined with a representation of only the green category, derived from the green naming data.
- The 1-category (blue) model predicts hue reconstruction based on the fine-grained representation of the stimulus, combined with a representation of only the blue category, derived from the blue naming data.
- The 2-category model predicts hue reconstruction based on the fine-grained representation of the stimulus, combined with representations of both the green and blue categories.
If reproduction bias reflects probabilistic inference from a fine-grained representation of the stimulus itself, together with any relevant category, we would expect the 2-category model to outperform the others. The other models have access either to no category information at all (null model), or to category information for only one of the two relevant color categories (only one of green and blue ). The 2-category model in contrast combines fine-grained stimulus information with both of the relevant categories ( green and blue ); this model thus corresponds most closely to a full category adjustment model.
Fig 5 redisplays the data from simultaneous and delayed reconstruction, this time with model fits overlaid. The panels in the left column show data from simultaneous reconstruction, fit by each of the four models, and the panels in the right column analogously show data and model fits from delayed reconstruction. Visually, it appears that in the case of delayed reconstruction, the 2-category model fits the data at least qualitatively better than competing models: it shows an inflection in bias as the empirical data do, although not as strongly. For simultaneous reconstruction, the 2-category model fit is also reasonable but visually not as clearly superior to the others (especially the null model) as in the delayed condition.

Left column: Bias from simultaneous reconstruction, fit by each of the four models. The empirical data (black lines with error bars) in these four panels are the same, and only the model fits (red lines) differ. Within each panel, the horizontal axis denotes target hue, and the vertical axis denotes reconstruction bias. The green and blue prototypes are indicated as vertical lines with green and blue squares at the bottom. Right column: delayed reconstruction, displayed analogously.
Table 1 reports quantitative results of these model fits. The best fit is provided by the 2-category model, in both the simultaneous and delayed conditions, whether assessed by log likelihood (LL) or by mean squared errror (MSE). In line with earlier studies [ 20 , 21 ], these findings demonstrate that a category adjustment model that assumes stimulus reconstruction is governed by relevant English color terms provides a reasonable fit to data on color reconstruction by English speakers. The category adjustment model fits well both when the category bias is relatively slight (simultaneous condition), and when the bias is stronger (delayed condition).
LL = log likelihood (higher is better). MSE = mean squared error (lower is better). The best value in each row is shown in bold .
Study 2: Color discrimination across languages
The study above examined the categories of just one language, English, whereas the Sapir-Whorf hypothesis concerns cross-language differences in categorization, and their effect on cognition and perception. Empirical work concerning this hypothesis has not specifically emphasized bias in reconstruction, but there is a substantial amount of cross-language data of other sorts against which the category adjustment model can be assessed. One method that has been extensively used to explore the Sapir-Whorf hypothesis in the domain of color is a two-alternative forced choice (2AFC) task. In such a task, participants first are briefly shown a target color, and then shortly afterward are shown that same target color together with a different distractor color, and are asked to indicate which was the color originally seen. A general finding from such studies [ 8 – 10 ] is that participants exhibit enhanced discrimination for pairs of colors that would be named differently in their native language. For example, in such a 2AFC task, speakers of English show enhanced discrimination for colors from the different English categories green and blue , compared with colors from the same category (either both green or both blue ) [ 8 ]. In contrast, speakers of the Berinmo language, which has named color categories that differ from those of English, show enhanced discrimination across Berinmo category boundaries, and not across those of English [ 9 ]. Thus color discrimination in this task is enhanced at the boundaries of native language categories, suggesting an effect of those native language categories on the ability to discriminate colors from memory.
Considered informally, this qualitative pattern of results appears to be consistent with the category adjustment model, as suggested above in Fig 2 . We wished to determine whether such a model would also provide a good quantitative account of such results, when assessed using the specific color stimuli and native-language naming patterns considered in the empirical studies just referenced.
We considered cross-language results from two previous studies by Debi Roberson and colleagues, one that compared color memory in speakers of English and Berinmo, a language of Papua New Guinea [ 9 ], and another that explored color memory in speakers of Himba, a language of Namibia [ 10 ]. Berinmo and Himba each have five basic color terms, in contrast with eleven in English. The Berinmo and Himba color category systems are similar to each other in broad outline, but nonetheless differ noticeably. Following these two previous studies, we considered the following pairs of categories in these three languages:
- the English categories green and blue ,
- the Berinmo categories wor (covering roughly yellow, orange, and brown), and nol (covering roughly green, blue, and purple), and
- the Himba categories dumbu (covering roughly yellow and beige) and burou (covering roughly green, blue, and purple).
These three pairs of categories are illustrated in Fig 6 , using naming data from Roberson et al. (2000) [ 9 ] and Roberson et al. (2005) [ 10 ]. It can be seen that the English green - blue distinction is quite different from the Berinmo wor - nol and the Himba dumbu - burou distinctions, which are similar but not identical to each other. The shaded regions in this figure indicate specific colors that were probed in discrimination tasks. The shaded (probed) region that straddles a category boundary in Berinmo and Himba falls entirely within the English category green , and the shaded (probed) region that straddles a category boundary in English falls entirely within the Berinmo category nol and the Himba category burou , according to naming data in Fig 1 of Roberson et al. (2005) [ 10 ]. The empirical discrimination data in Fig 7 are based on those probed colors [ 9 , 10 ], and show that in general, speakers of a language tend to exhibit greater discrimination for pairs of colors that cross a category boundary in their native language, consistent with the Sapir-Whorf hypothesis.

The English categories green and blue (top panel), the Berinmo categories wor and nol (middle panel), and the Himba categories dumbu and burou (bottom panel), plotted against a spectrum of hues that ranges from dark yellow at the left, through green, to blue at the right. Colored squares mark prototypes: the shared prototype for Berinmo wor and Himba dumbu , and the prototypes for English green and blue ; the color of each square approximates the color of the corresponding prototype. For each language, the dotted-and-dashed vertical lines denote the prototypes for the two categories from that language, and the dashed vertical line denotes the empirical boundary between these two categories. Black curves show the probability of assigning a given hue to each of the two native-language categories, according to the category component of a 2-category model fit to each language’s naming data. The shaded regions mark the ranges of colors probed in discrimination tasks; these two regions are centered at the English green - blue boundary and the Berinmo wor - nol boundary. Data are from Roberson et al. (2000) [ 9 ] and Roberson et al. (2005) [ 10 ].

Top panels: Discrimination from memory by Berinmo and English speakers for pairs of colors across and within English and Berinmo color category boundaries. Empirical data are from Table 11 of Roberson et al. (2000:392). Empirical values show mean proportion correct 2AFC memory judgments, and error bars show standard error. Model values show mean model proportion correct 2AFC memory judgments after simulated reconstruction with native-language categories. Model results are range-matched to the corresponding empirical values, such that the minimum and maximum model values match the minimum and maximum mean values in the corresponding empirical dataset, and other model values are linearly interpolated. Bottom panels: Discrimination from memory by Himba and English speakers for pairs of colors across and within English and Himba color category boundaries, compared with model results based on native-language categories. Empirical data are from Table 6 of Roberson et al. (2005:400); no error bars are shown because standard error was not reported in that table.
We sought to determine whether the 2-category model explored above could account for these data. To that end, for each language, we created a version of the 2-category model based on the naming data for that language. Thus, we created an English model in which the two categories were based on empirical naming data for green and blue , a Berinmo model in which the two categories were based on empirical naming data for wor and nol , and a Himba model in which the two categories were based on empirical naming data for dumbu and burou . The black curves in Fig 6 show the probability of assigning a given hue to each of the two native-language categories, according to the category component of a 2-category model fit to each language’s naming data. Given this category information, we simulated color reconstruction from memory for the specific colors considered in the empirical studies [ 9 , 10 ] (the colors in the shaded regions in Fig 6 ). We did so separately for the cases of English, Berinmo, and Himba, in each case fitting a model based on naming data for a given language to discrimination data from speakers of that language. As in Study 1, we fit the model parameter corresponding to the uncertainty of fine-grained perceptual representation to the empirical non-linguistic (here discrimination) data, and we used a single value for this parameter across all three language models. The model results are shown in Fig 7 , beside the empirical data to which they were fit. The models provide a reasonable match to the observed cross-language differences in discrimination. Specifically, the stimulus pairs for which empirical performance is best are those that cross a native-language boundary—and these are stimulus pairs for which the corresponding model response is strongest.
Although not shown in the figure, we also conducted a followup analysis to test whether the quality of these fits was attributable merely to model flexibility, or to a genuine fit between a language’s category system and patterns of discrimination from speakers of that language. We did this by switching which language’s model was fit to which language’s discrimination data. Specifically, we fit the model based on Berinmo naming to the discrimination data from English speakers (and vice versa), and fit the model based on Himba naming to the discrimination data from English speakers (and vice versa), again adjusting the model parameter corresponding to the uncertainty of the fine-grained perceptual representation to the empirical discrimination data. The results are summarized in Table 2 . It can be seen that the discrimination data are fit better by native-language models (that is, models with a category component originally fit to that language’s naming data) than by other-language models (that is, models with a category component originally fit to another language’s naming data). These results suggest that cross-language differences in discrimination may result from category-induced reconstruction bias under uncertainty, guided by native-language categories.
The best value in each row is shown in bold . Data are fit better by native-language models than by other-language models.
Study 3: Within-category effects
Although many studies of categorical perception focus on pairs of stimuli that cross category boundaries, there is also evidence for category effects within categories. In a 2AFC study of categorical perception of facial expressions, Roberson and colleagues [ 32 ] found the behavioral signature of categorical perception (or more precisely in this case, categorical memory): superior discrimination for cross-category than for within-category pairs of stimuli. But in addition, they found an interesting category effect on within-category pairs, dependent on order of presentation. For each within-category pair they considered, one stimulus of the pair was always closer to the category prototype (the “good exemplar”) than the other (the “poor exemplar”). They found that 2AFC performance on within-category pairs was better when the target was the good exemplar (and the distractor was therefore the poor exemplar) than when the target was the poor exemplar (and the distractor was therefore the good exemplar)—even though the same stimuli were involved in the two cases. Moreover, performance in the former (good exemplar) case did not differ significantly from cross-category performance. Hanley and Roberson [ 36 ] subsequently reanalyzed data from a number of earlier studies that had used 2AFC tasks to explore cross-language differences in color naming and cognition, including those reviewed and modeled in the previous section. Across studies and across domains, including color, they found the same asymmetrical within-category effect originally documented for facial expressions.
This within-category pattern may be naturally explained in category-adjustment terms, as shown in Fig 8 , and as argued by Roberson and colleagues [ 32 ]. The central idea is that because the target is held in memory, it is subject to bias toward the prototype in memory, making discrimination of target from distractor either easier or harder depending on which of the two stimuli is the target. Although this connection with the category adjustment model has been made in the literature in general conceptual terms [ 32 ], followup studies have been theoretically focused elsewhere [ 31 , 36 ], and the idea has not to our knowledge been tested computationally using the specific stimuli and naming patterns involved in the empirical studies. We sought to do so.

The category adjustment model predicts: (top panel, good exemplar) easy within-category discrimination in a 2AFC task when the initially-presented target t is closer to the prototype than the distractor d is; (bottom panel, poor exemplar) difficult within-category discrimination with the same two stimuli when the initially-presented target t is farther from the prototype than the distractor d is. Category is shown as a distribution in blue; stimuli are shown as vertical black lines marked t and d; reconstruction bias patterns are shown as arrows.
The empirical data in Fig 9 illustrate the within-category effect with published results on color discrimination by speakers of English, Berinmo, and Himba. In attempting to account for these data, we considered again the English, Berinmo, and Himba variants of the 2-category model first used in Study 2, and also retained from that study the parameter value corresponding to the uncertainty of the fine-grained perceptual representation, in the case of native-language models. We simulated reconstruction from memory of the specific colors examined in Study 2. Following the empirical analyses, this time we disaggregated the within-category stimulus pairs into those in which the target was a good exemplar of the category (i.e. the target was closer to the prototype than the distractor was), vs. those in which the target was a poor exemplar of the category (i.e. the target was farther from the prototype than the distractor was). The model results are shown in Fig 9 , and match the empirical data reasonably well, supporting the informal in-principle argument of Fig 8 with a more detailed quantitative analysis.

Across: stimulus pair crosses the native-language boundary; GE: within-category pair, target is the good exemplar; PE: within-category pair, target is the poor exemplar. Empirical data are from Figs 2 (English: 10-second retention interval), 3 (Berinmo), and 4 (Himba) of Hanley and Roberson [ 36 ]. Empirical values show mean proportion correct 2AFC memory judgments, and error bars show standard error. Model values show mean model proportion correct 2AFC memory judgments after simulated reconstruction using native-language categories, range-matched as in Fig 7 . English model compared with English data: 0.00002 MSE; Berinmo model compared with Berinmo data: 0.00055 MSE; Himba model compared with Himba data: 0.00087 MSE.
Conclusions
We have argued that the debate over the Sapir-Whorf hypothesis may be clarified by viewing that hypothesis in terms of probabilistic inference. To that end, we have presented a probabilistic model of color memory, building on proposals in the literature. The model assumes both a universal color space and language-specific categorical partitionings of that space, and infers the originally perceived color from these two sources of evidence. The structure of this model maps naturally onto a prominent proposal in the literature that has to our knowledge not previously been formalized in these terms. In a classic early study of the effect of language on color cognition, Kay and Kempton [ 7 ] interpret Whorf [ 2 ] as follows:
Whorf […] suggests that he conceives of experience as having two tiers: one, a kind of rock bottom, inescapable seeing-things-as-they-are (or at least as human beings cannot help but see them), and a second, in which [the specific structures of a given language] cause us to classify things in ways that could be otherwise (and are otherwise for speakers of a different language).
Kay and Kempton argue that color cognition involves an interaction between these two tiers. The existence of a universal groundwork for color cognition helps to explain why there are constraints on color naming systems across languages [ 3 – 5 , 37 ]. At the same time, Kay and Kempton acknowledge a role for the language-specific tier in cognition, such that “there do appear to be incursions of linguistic categorization into apparently nonlinguistic processes of thinking” (p. 77). These two tiers map naturally onto the universal and language-specific components of the model we have explored here. This structure offers a straightforward way to think about effects of language on cognition while retaining the idea of a universal foundation underpinning human perception and cognition. Thus, this general approach, and our model as an instance of it, offer a possible resolution of one source of controversy surrounding the Sapir-Whorf hypothesis: taking that hypothesis seriously need not entail a wholesale rejection of important universal components of human cognition.
The approach proposed here also has the potential to resolve another source of controversy surrounding the Sapir-Whorf hypothesis: that some findings taken to support it do not replicate reliably (e.g. in the case of color: [ 15 – 17 ]). Framing the issue in terms of probabilistic inference touches this question by highlighting the theoretically central role of uncertainty , as in models of probabilistic cue integration [ 24 ]. We have seen stronger category-induced bias in color memory under conditions of greater delay and presumably therefore greater uncertainty (Study 1, and [ 20 ]). This suggests that in the inverse case of high certainty about the stimulus, any category effect could in principle be so small as to be empirically undetectable, a possibility that can be pursued by systematically manipulating uncertainty. Thus, the account advanced here casts the Sapir-Whorf hypothesis in formal terms that suggest targeted and quantitative followup tests. A related theoretical advantage of uncertainty is that it highlights an important level of generality: uncertainty could result from memory, as explored here, but it could also result from noise or ambiguity in perception itself, and on the view advanced here, the result should be the same.
The model we have proposed does not cover all aspects of language effects on color cognition. For example, there are documented priming effects [ 31 ] which do not appear to flow as naturally from this account as do the other effects we have explored above. However, the model does bring together disparate bodies of data in a simple framework, and links them to independent principles of probabilistic inference. Future research can usefully probe the generality and the limitations of the ideas we have explored here.
Materials and Methods
Code and data supporting the analyses reported here are available at https://github.com/yangxuch/probwhorfcolor.git .
The basic model we consider is shown in Fig 10 , which presents in graphical form the generative process behind Fig 1 above. Our model follows in general outline that of Bae et al. [ 20 ], but the formalization of inference within this structure more closely follows Feldman et al.’s [ 28 ] model of category effects in vowel perception. In our model, the perception of a stimulus S = s produces a fine-grained memory M , and a categorical code c . We wish to obtain a reconstruction s ^ of the original stimulus S = s , by combining evidence from the two internal representations M and c that s has produced. That reconstruction is derived as follows:
Because hue is a circular dimension, the components p ( M | S ) and p ( S | c ) could be modeled using circular normal or von Mises distributions, as was done by Bae et al. [ 20 ]. However each of our studies treats only a restricted subsection of the full hue circle, and for that reason we instead model these representations using normal distributions.

The perception of stimulus S = s produces a fine-grained memory M , and a categorical code c specifying the category in which s fell. We wish to reconstruct the original stimulus S = s , given M and c .
p ( M | S ) represents the fine-grained memory trace M of the original stimulus S = s . We model this as a normal distribution with mean μ m at the location of the original stimulus s , and with uncertainty captured by variance σ m 2 :
This is an unbiased representation of the original stimulus s because μ m = s .
p ( S | c ) captures the information about the location of stimulus S that is given by the categorical code c . We again model this as a normal distribution, this time centered at the prototype μ c of category c , with variance σ c 2 :
This assumes that there is a single categorical code c , and we use this assumption in some of our model variants below. However in other cases we will capture the fact that more than one category may be applicable to a stimulus. In such cases we assume that the perceiver knows, for each category c , the applicability π ( c ) of that category for the observed stimulus s . We model this as:
where p ( S = s | c ) is given by Eq (4) above, and p ( c ) is assumed to be uniform.
We consider three variants of this basic model, described below in order of increasing complexity: the null model, the 1-category model, and the 2-category model. For each model, we take the predicted reconstruction s ^ of a given stimulus S = s to be the expected value of the posterior distribution:
The null model assumes that reconstruction is based only on the fine-grained memory, with no category influence. This model is derived from Eq (2) by assuming that the memory component p ( M | S ) is as defined above, and the category component p ( S | c ) is uniform, yielding:
The predicted reconstruction for this model is given by the expected value of this distribution, namely:
where we have assumed μ m = s , the originally observed stimulus. This model predicts no category-induced bias: the reconstruction of the stimulus S = s is simply the value of the stimulus s itself.
1-category model
The 1-category model assumes that reconstruction is based both on fine-grained memory and on information from a single category, e.g. English green . This model is derived from Eq (2) by assuming that both the memory component p ( M | S ) and the category component p ( S | c ) are as defined above, yielding:
where we have assumed μ m = s , the originally observed stimulus. This equation parallels Eq (7) of Feldman et al. [ 28 ]. This model produces a reconstruction that is a weighted average of the original stimulus value s and the category prototype μ c , with weights determined by the relative certainty of each of the two sources of information. The same weighted average is also central to Ernst and Banks’ [ 24 ] study of cue integration from visual and haptic modalities. That study was based on the same principles we invoke here, and our model—like that of Feldman et al.—can be viewed as a probabilistic cue integration model in which one of the two cues being integrated is a category, rather than a cue from a different modality.
2-category model
The 2-category model is similar to the 1-category model, but instead of basing its reconstruction on a single category c , it bases its reconstruction on two categories c 1 and c 2 (e.g. English green and blue ). It does so by averaging together the reconstruction provided by the 1-category model for c 1 and the reconstruction provided by the 1-category model for c 2 , weighted by the applicability π ( c ) of each category c to the stimulus:
Here, p ( S | M , c ) inside the sum is given by the 1-category model specified in Eq (9) , and π ( c ) is the applicability of category c to the observed stimulus s as specified above in Eq (5) . Our equation here parallels Eq (9) of Feldman et al. [ 28 ] who similarly take a weighted average over 1-category models in their model of category effects in speech perception. The predicted reconstruction for this model is given by the expected value of this distribution, namely:
assuming as before that μ m = s , the original stimulus value. This equation follows Feldman et al. [ 28 ] Eq (10).
Fitting models to data
For each model, any category parameters μ c and σ c 2 are first fit to naming data. The single remaining free parameter σ m 2 , corresponding to the uncertainty of fine-grained memory, is then fit to non-linguistic bias or discrimination data, with no further adjustment of the category parameters. Although this two-step process is used in all of our studies, it is conducted in slightly different ways across studies; we supply study-specific details below in our presentation of each study. All model fits were done using fminsearch in Matlab.
Participants
Twenty subjects participated in the experiment, having been recruited at UC Berkeley. All subjects were at least 18 years of age, native English speakers, and reported normal or corrected-to-normal vision, and no colorblindness. All subjects received payment or course credit for participation.
Informed consent was obtained verbally; all subjects read an approved consent form and verbally acknowledged their willingness to participate in the study. Verbal consent was chosen because the primary risk to subjects in this study was for their names to be associated with their response; this approach allowed us to obtain consent and collect data without the need to store subjects’ names in any form. Once subjects acknowledged that they understood the procedures and agreed to participate by stating so to the experimenter, the experimenter recorded their consent by assigning them a subject number, which was anonymously linked to their data. All study procedures, including those involving consent, were overseen and approved by the UC Berkeley Committee for the Protection of Human Subjects.
Stimuli were selected by varying a set of hues centered around the blue - green boundary, holding saturation and lightness constant. Stimuli were defined in Munsell coordinate space, which is widely used in the literature we engage here (e.g. [ 9 , 10 ]). All stimuli were at lightness 6 and saturation 8. Hue varied from 5Y to 10P, in equal hue steps of 2.5. Colors were converted to xyY coordinate space following Table I(6.6.1) of Wyszecki and Stiles (1982) [ 38 ]. The colors were implemented in Matlab in xyY; the correspondence of these coordinate systems in the stimulus set, as well as approximate visualizations of the stimuli, are reported in Table 3 .
All stimuli were presented at lightness 6, saturation 8 in Munsell space.
We considered three progressively narrower ranges of these stimuli for different aspects of our analyses, in an attempt to focus the analyses on a region that is well-centered relative to the English color categories green and blue . We refer to these three progressively narrower ranges as the full range , the medium range , and the focused range . We specify these ranges below, together with the aspects of the analysis for which each was used.
- Full range: We collected naming data for green and blue relative to the full range, stimuli 1-27, for a total of 27 stimuli. We fit the category components of our models to naming data over this full range.
- Medium range: We collected bias data for a subset of the full range, namely the medium range, stimuli 5-23, for a total of 19 stimuli. We considered this subset because we were interested in bias induced by the English color terms green and blue , and we had the impression, prior to collecting naming or bias data, that colors outside this medium range had some substantial element of the neighboring categories yellow and purple .
- Focused range: Once we had naming data, we narrowed the range further based on those data, to the focused range, stimuli 5-19, for a total of 15 stimuli. The focused range extends between the (now empirically assessed) prototypes for green and blue , and also includes three of our stimulus hues on either side of these prototypes, yielding a range well-centered relative to those prototypes, as can be seen in the bottom panel of Fig 4 above. We considered this range in our statistical analyses, and in our modeling of bias patterns.
Experimental procedure
The experiment consisted of four blocks. The first two blocks were reconstruction (bias) tasks: one simultaneous block and one delay block. In the simultaneous block ( Fig 3A ), the subject was shown a stimulus color as a colored square (labeled as “Original” in the figure), and was asked to recreate that color in a second colored square (labeled as “Target” in the figure) as accurately as possible by selecting a hue from a color wheel. The (“Original”) stimulus color remained on screen while the subject selected a response from the color wheel; navigation of the color wheel would change the color of the response (“Target”) square. The stimulus square and response square each covered 4.5 degrees of visual angle, and the color wheel covered 11.1 degrees of visual angle. Target colors were drawn from the medium range of stimuli (stimuli 5—23 of Table 3 ). The color wheel was constructed based on the full range of stimuli (stimuli 1—27 of Table 3 ), supplemented by interpolating 25 points evenly in xyY coordinates between each neighboring pair of the 27 stimuli of the full range, to create a finely discretized continuum from yellow to purple, with 677 possible responses. Each of the 19 target colors of the medium range was presented five times per block in random order, for a total of 95 trials per block. The delay block ( Fig 3B ) was similar to the simultaneous block but with the difference that the stimulus color was shown for 500 milliseconds then disappeared, then a fixation cross was shown for 1000 milliseconds, after which the subject was asked to reconstruct the target color from memory, again using the color wheel to change the color of the response square. The one colored square shown in the final frame of Fig 3B is the response square that changed color under participant control. The order of the simultaneous block and delay block were counterbalanced by subject. Trials were presented with a 500 millisecond inter-trial interval.
Several steps were taken to ensure that responses made on the color wheel during the reconstruction blocks were not influenced by bias towards a particular spatial position. The position of the color wheel was randomly rotated up to 180 degrees from trial to trial. The starting position of the cursor was likewise randomly generated for each new trial. Finally, the extent of the spectrum was jittered one or two stimuli (2.5 or 5 hue steps) from trial to trial, which had the effect of shifting the spectrum slightly in the yellow or the purple direction from trial to trial. This was done to ensure that the blue - green boundary would not fall at a consistent distance from the spectrum endpoints on each trial.
The second two blocks were naming tasks. In each, subjects were shown each of the 27 stimuli of the full range five times in random order, for a total of 135 trials per block. On each trial, subjects were asked to rate how good an example of a given color name each stimulus was. In one block, the color name was green , in the other, the color name was blue ; order of blocks was counterbalanced by subject. To respond, subjects positioned a slider bar with endpoints “Not at all [green/blue]” and “Perfectly [green/blue]” to the desired position matching their judgment of each stimulus, as shown above in Fig 3C . Responses in the naming blocks were self-paced. Naming blocks always followed reconstruction blocks, to ensure that repeated exposure to the color terms green and blue did not bias responses during reconstruction.
The experiment was presented in Matlab version 7.11.0 (R2010b) using Psychtoolbox (version 3) [ 39 – 41 ]. The experiment was conducted in a dark, sound-attenuated booth on an LCD monitor that supported 24-bit color. The monitor had been characterized using a Minolta CS100 colorimeter. A chin rest was used to ensure that each subject viewed the screen from a constant position; when in position, the base of the subject’s chin was situated 30 cm from the screen.
As part of debriefing after testing was complete, each subject was asked to report any strategies they used during the delay block to help them remember the target color. Summaries of each response, as reported by the experimenter, are listed in Table 4 .
When subjects gave specific examples of color terms used as memory aids, they are reported here.
Color spectrum
We wished to consider our stimuli along a 1-dimensional spectrum such that distance between two colors on that spectrum approximates the perceptual difference between those colors. To this end, we first converted our stimuli to CIELAB color space. CIELAB is a 3-dimensional color space designed “in an attempt to provide coordinates for colored stimuli so that the distance between the coordinates of any two stimuli is predictive of the perceived color difference between them” (p. 202 of [ 42 ]). The conversion to CIELAB was done according to the equations on pp. 167-168 of Wyszecki and Stiles (1982) [ 38 ], assuming 2 degree observer and D65 illuminant. For each pair of neighboring colors in the set of 677 colors of our color wheel, we measured the distance (Δ E ) betwen these two colors in CIELAB space. We then arranged all colors along a 1-dimensional spectrum that was scaled to length 1, such that the distance between each pair of neighboring colors along that spectrum was proportional to the CIELAB Δ E distance between them. This CIELAB-based 1-dimensional spectrum was used for our analyses in Study 1, and an analogous spectrum for a different set of colors was used for our analyses in Studies 2 and 3.
Statistical analysis
As a result of the experiment detailed above, we obtained bias data from 20 participants, for each of 19 hues (the medium range), for 5 trials per hue per participant, in each of the simultaneous and delayed conditions. For analysis purposes, we restricted attention to the focused range of stimuli (15 hues), in order to consider a region of the spectrum that is well-centered with respect to green and blue , as we are primarily interested in bias that may be induced by these two categories. We wished to determine whether the magnitude of the bias differed as a function of the simultaneous vs. delayed condition, whether the magnitude of the bias varied as a function of hue, and whether there was an interaction between these two factors. To answer those questions, we conducted a 2 (condition: simultaneous vs. delayed) × 15 (hues) repeated measures analysis of variance (ANOVA), in which the dependent measure was the absolute value of the reproduction bias (reproduced hue minus target hue), averaged across trials for a given participant at a given target hue in a given condition. The ANOVA included an error term to account for across-subject variability. We found a main effect of condition, with greater bias magnitude in the delayed than in the simultaneous condition [ F (1, 19) = 61.61, p < 0.0001], a main effect of hue [ F (14, 266) = 4.565, p < 0.0001], and an interaction of hue and condition [ F (14, 266) = 3.763, p < 0.0001]. All hue calculations were relative to the CIELAB-based spectrum detailed in the preceding section.
We then conducted paired t-tests at each of the target hues, comparing each participant’s bias magnitude for that hue (averaged over trials) in the simultaneous condition vs. the delayed condition. Blue asterisks at the top of Fig 4 mark hues for which the paired t-test returned p < 0.05 when applying Bonferroni corrections for multiple comparisons.
Modeling procedure
We considered four models in accounting for color reconstruction in English speakers: the null model, a 1-category model for which the category was green , a 1-category model for which the category was blue , and a 2-category model based on both green and blue .
We fit these models to the data in two steps. We first fit any category parameters (the means μ c and variances σ c 2 for any categories c ) to the naming data. We then fit the one remaining free parameter ( σ m 2 ), which captures the uncertainty of fine-grained memory, to the bias data, without further adjusting the category parameters. We specify each of these two steps below.
We fit a Gaussian function to the goodness naming data for green , and another Gaussian function to the data for blue , using maximum likelihood estimation. The fitted Gaussian functions can be seen, together with the data to which they were fit, in the top panel of Fig 4 . This process determined values for the category means μ c and category variances σ c 2 for the two categories green and blue .
For each of the four variants of the category adjustment model outlined above (null, 1-category green, 1-category blue, and 2-category), we retained the category parameter settings resulting from the above fit to the naming data. We then obtained a value for the one remaining free parameter σ m 2 , corresponding to the uncertainty of fine-grained memory, by fitting the model to the bias data via maximum likelihood estimation, without further adjusting the category parameters.
Empirical data
The empirical data considered for this study were drawn from two sources: the study of 2AFC color discrimination by speakers of Berinmo and English in Experiment 6a of Roberson et al. (2000) [ 9 ], and the study of 2AFC color discrimination by speakers of Himba and English in Experiment 3b of Roberson et al. (2005) [ 10 ]. In both studies, two sets of color stimuli were considered, all at value (lightness) level 5, and chroma (saturation) level 8. Both sets varied in hue by increments of 2.5 Munsell hue steps. The first set of stimuli was centered at the English green - blue boundary (hue 7.5BG), and contained the following seven hues: 10G, 2.5BG, 5BG, 7.5BG, 10BG, 2.5B, 5B. The second set of stimuli was centered at the Berinmo wor - nol boundary (hue 5GY), and contained the following seven hues: 7.5Y, 10Y, 2.5GY, 5GY, 7.5GY, 10GY, 2.5G. Stimuli in the set that crossed an English category boundary all fell within a single category in Berinmo ( nol ) and in Himba ( burou ), and stimuli in the set that crossed a Berinmo category boundary also crossed a Himba category boundary ( dumbu - burou ) but all fell within a single category in English ( green ), according to naming data in Fig 1 of Roberson et al. (2005) [ 10 ]. Based on specifications in the original empirical studies [ 9 , 10 ], we took the pairs of stimuli probed to be those presented in Table 5 .
Any stimulus pair that includes a boundary color is considered to be a cross-category pair. All hues are at value (lightness) level 5, and chroma (saturation) level 8. 1s denotes a 1-step pair; 2s denotes a 2-step pair.
Based on naming data in Fig 1 of Roberson et al. 2005 [ 10 ], we took the prototypes of the relevant color terms to be:
English green prototype = 10GY
English blue prototype = 10B
Berinmo wor prototype = 5Y
Berinmo nol prototype = 5G
Himba dumbu prototype = 5Y
Himba burou prototype = 10G
Fig 6 above shows a spectrum of hues ranging from the Berinmo wor prototype (5Y) to the English blue prototype (10B) in increments of 2.5 Munsell hue steps, categorized according to each of the three languages we consider here. These Munsell hues were converted to xyY and then to CIELAB as above, and the positions of the hues on the spectrum were adjusted so that the distance between each two neighboring hues in the spectrum is proportional to the CIELAB Δ E distance between them. We use this CIELAB-based spectrum for our analyses below. The two shaded regions on each spectrum in Fig 6 denote the two target sets of stimuli identified above.
The discrimination data we modeled were drawn from Table 11 of Roberson et al. (2000:392) [ 9 ] and Table 6 of Roberson et al. (2005:400) [ 10 ].
We considered three variants of the 2-category model: an English blue - green model, a Berinmo wor - nol model, and a Himba dumbu - burou model. As in Study 1, we fit each model to the data in two steps. For each language’s model, we first fit the category component of that model to naming data from that language. Because color naming differs across these languages, this resulted in three models with different category components. For each model, we then retained and fixed the resulting category parameter settings, and fit the single remaining parameter, corresponding to memory uncertainty, to discrimination data. We detail these two steps below.
For the naming data, we modeled the probability of applying category name c to stimulus i as:
where p ( c ) is assumed to be uniform, and f ( i | c ) is a non-normalized Gaussian function corresponding to category c , with mean μ c and variance σ c 2 . There were two categories c for each model, e.g. wor and nol in the case of the Berinmo model. Category means μ c were set to the corresponding category prototypes shown above (e.g. μ c for Berinmo nol corresponded to 5G), and category variances σ c 2 were left as free parameters. We then adjusted these free category variances to reproduce the empirical boundary between the two categories c 1 and c 2 for that language, as follows. Sweeping from left ( c 1 ) to right ( c 2 ), we took the model’s boundary between c 1 and c 2 to be the first position i on the spectrum for which p ( c 1 | i ) ≤ p ( c 2 | i ); we refer to this as the model crossover point . We measured the distance in the CIELAB-based spectrum between the model crossover point and the empirical category boundary, and adjusted the category variances σ c 1 2 and σ c 2 2 so as to minimize that distance. This was done separately for each language’s model. Fig 6 shows the resulting fits of category components to naming data for each of the three languages.
We then simulated performance in the 2AFC discrimination task for each stimulus pair in Table 5 , by each model, as follows. Given a pair of stimuli, one stimulus was taken to be the target t and therefore held in memory, and the other taken to be the distractor d . We took the reconstruction r for the target stimulus t to be the expected value of the posterior for the 2-category model:
We then measured, along the hue spectrum in question, the distance dist ( r , t ) between the reconstruction r and the target t , and the distance dist ( r , d ) between the reconstruction r and the distractor d . We converted each of these two distances to a similarity score:
and modeled the proportion correct choice as:
These equations are based on Luce’s [ 43 ] (pp. 113-114) model of choice behavior. For each pair of stimuli, each stimulus was once taken to be the target, and once taken to be the distractor, and the results averaged to yield a mean discrimination score for that pair. Scores were then averaged across all pairs listed as within-category pairs, and separately for all pairs listed as cross-category pairs. These scores were range-matched to the empirical data, in an attempt to correct for other factors that could affect performance, such as familiarity with such tasks, etc.; such external factors could in principle differ substantially across participant pools for the three languages modeled. We measured MSE between the model output and the data so treated, and adjusted the remaining parameter σ m 2 , corresponding to memory uncertainty, so as to minimize this MSE. This entire process was conducted two times. The first time, each language’s model was fit to that same language’s discrimination data. Then, to test whether native-language categories allow a better fit than the categories of another language, we fit the Berinmo model to the English discrimination data (and vice versa), and the Himba model to the English discrimination data (and vice versa).
The empirical data considered for this study are those of Figs 2 (English green/blue , 10 second delay), 3 (Berinmo wor/nol ), and 4 (Himba dumbu/borou ) of Hanley and Roberson (2011) [ 36 ]. These data were originally published by Roberson and Davidoff (2000) [ 8 ], Roberson et al. (2000) [ 9 ], and Roberson et al. (2005) [ 10 ], respectively. The Berinmo and Himba stimuli and data were the same as in our Study 2, but the English stimuli and data reanalyzed by Hanley and Roberson (2011) [ 36 ] Fig 2 were instead drawn from Table 1 of Roberson and Davidoff (2000) [ 8 ], reproduced here in Table 6 , and used for the English condition of this study. These stimuli for English were at lightness (value) level 4, rather than 5 as for the other two languages. We chose to ignore this difference for modeling purposes.
Any stimulus pair that includes a boundary color is considered to be a cross-category pair. All hues are at value (lightness) level 4, and chroma (saturation) level 8. 1s denotes a 1-step pair; 2s denotes a 2-step pair.
All modeling procedures were identical to those of Study 2, with the exception that GE (target = good exemplar) and PE (target = poor exemplar) cases were disaggregated, and analyzed separately.
Acknowledgments
We thank Roland Baddeley, Paul Kay, Charles Kemp, Steven Piantadosi, and an anonymous reviewer for their comments.
Funding Statement
This research was supported by the National Science Foundation ( www.nsf.gov ) under grants DGE-1106400 (EC) and SBE-1041707 (YX, TR). Publication was made possible in part by support from the Berkeley Research Impact Initiative (BRII) sponsored by the UC Berkeley Library. The funders had no role in study design, data collection and analysis, decision to publish, or preparation of the manuscript.
Data Availability
- Scientific Methods
What is Hypothesis?
We have heard of many hypotheses which have led to great inventions in science. Assumptions that are made on the basis of some evidence are known as hypotheses. In this article, let us learn in detail about the hypothesis and the type of hypothesis with examples.
A hypothesis is an assumption that is made based on some evidence. This is the initial point of any investigation that translates the research questions into predictions. It includes components like variables, population and the relation between the variables. A research hypothesis is a hypothesis that is used to test the relationship between two or more variables.
Characteristics of Hypothesis
Following are the characteristics of the hypothesis:
- The hypothesis should be clear and precise to consider it to be reliable.
- If the hypothesis is a relational hypothesis, then it should be stating the relationship between variables.
- The hypothesis must be specific and should have scope for conducting more tests.
- The way of explanation of the hypothesis must be very simple and it should also be understood that the simplicity of the hypothesis is not related to its significance.
Sources of Hypothesis
Following are the sources of hypothesis:
- The resemblance between the phenomenon.
- Observations from past studies, present-day experiences and from the competitors.
- Scientific theories.
- General patterns that influence the thinking process of people.
Types of Hypothesis
There are six forms of hypothesis and they are:
- Simple hypothesis
- Complex hypothesis
- Directional hypothesis
- Non-directional hypothesis
- Null hypothesis
- Associative and casual hypothesis
Simple Hypothesis
It shows a relationship between one dependent variable and a single independent variable. For example – If you eat more vegetables, you will lose weight faster. Here, eating more vegetables is an independent variable, while losing weight is the dependent variable.
Complex Hypothesis
It shows the relationship between two or more dependent variables and two or more independent variables. Eating more vegetables and fruits leads to weight loss, glowing skin, and reduces the risk of many diseases such as heart disease.
Directional Hypothesis
It shows how a researcher is intellectual and committed to a particular outcome. The relationship between the variables can also predict its nature. For example- children aged four years eating proper food over a five-year period are having higher IQ levels than children not having a proper meal. This shows the effect and direction of the effect.
Non-directional Hypothesis
It is used when there is no theory involved. It is a statement that a relationship exists between two variables, without predicting the exact nature (direction) of the relationship.
Null Hypothesis
It provides a statement which is contrary to the hypothesis. It’s a negative statement, and there is no relationship between independent and dependent variables. The symbol is denoted by “H O ”.
Associative and Causal Hypothesis
Associative hypothesis occurs when there is a change in one variable resulting in a change in the other variable. Whereas, the causal hypothesis proposes a cause and effect interaction between two or more variables.
Examples of Hypothesis
Following are the examples of hypotheses based on their types:
- Consumption of sugary drinks every day leads to obesity is an example of a simple hypothesis.
- All lilies have the same number of petals is an example of a null hypothesis.
- If a person gets 7 hours of sleep, then he will feel less fatigue than if he sleeps less. It is an example of a directional hypothesis.
Functions of Hypothesis
Following are the functions performed by the hypothesis:
- Hypothesis helps in making an observation and experiments possible.
- It becomes the start point for the investigation.
- Hypothesis helps in verifying the observations.
- It helps in directing the inquiries in the right direction.
How will Hypothesis help in the Scientific Method?
Researchers use hypotheses to put down their thoughts directing how the experiment would take place. Following are the steps that are involved in the scientific method:
- Formation of question
- Doing background research
- Creation of hypothesis
- Designing an experiment
- Collection of data
- Result analysis
- Summarizing the experiment
- Communicating the results
Frequently Asked Questions – FAQs
What is hypothesis.
A hypothesis is an assumption made based on some evidence.
Give an example of simple hypothesis?
What are the types of hypothesis.
Types of hypothesis are:
- Associative and Casual hypothesis
State true or false: Hypothesis is the initial point of any investigation that translates the research questions into a prediction.
Define complex hypothesis..
A complex hypothesis shows the relationship between two or more dependent variables and two or more independent variables.
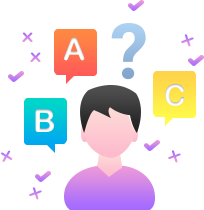
Put your understanding of this concept to test by answering a few MCQs. Click ‘Start Quiz’ to begin!
Select the correct answer and click on the “Finish” button Check your score and answers at the end of the quiz
Visit BYJU’S for all Physics related queries and study materials
Your result is as below
Request OTP on Voice Call
Leave a Comment Cancel reply
Your Mobile number and Email id will not be published. Required fields are marked *
Post My Comment

Register with BYJU'S & Download Free PDFs
Register with byju's & watch live videos.
Problem, Hypothesis, and Research Questions
- First Online: 16 September 2022
Cite this chapter
- Matthias Ripp 14
Part of the book series: Heritage Studies ((HEST))
136 Accesses
The main problem that this work aims to address is that there is no universal method to use urban heritage as a resource for development. This chapter sets out the foundations of this research: the identified problem, hypothesis, and research questions. Setting the context of the established problem, Sect. 2.1 explores the potential benefits of urban heritage and introduces the scope of the research while also justifying its necessity. Then, in Sect. 2.2, based on the key research problem, the main hypothesis and a solution to address the problem are elaborated, along with the research questions that guide this work. The role of the preparational phase in projects for heritage-based urban developments is explained, and how the proposed metamodel can be used in this project phase and for other purposes is further elaborated.
This is a preview of subscription content, log in via an institution to check access.
Access this chapter
- Available as EPUB and PDF
- Read on any device
- Instant download
- Own it forever
- Compact, lightweight edition
- Dispatched in 3 to 5 business days
- Free shipping worldwide - see info
- Durable hardcover edition
Tax calculation will be finalised at checkout
Purchases are for personal use only
Institutional subscriptions
Barile, S., & Saviano, M. (2015). From the management of cultural heritage to the governance of the cultural heritage system. In G. M. Golinelli (Ed.), Cultural heritage and value creation (pp. 71–103). Springer.
Google Scholar
Bos, L. (2015). Towards an EU urban agenda . Ministry of the Interior and Kingdom Relations of the Netherlands. Retrieved 02 Jan 2020 from https://agendastad.nl/wp-content/uploads/2015/02/ROM-special-EAS-ENG.pdf
City of Regensburg, Ripp, M., & Scheffler, N. (2011). HerO – Heritage as opportunity. The road to success: Integrated management of historic towns – Guidebook . https://www.regensburg.de/fm/464/HerO%20Guidebook%20EN.pdf
Cultural Heritage Counts for Europe. (2015). Cultural Heritage Counts for Europe. Full report . http://www.encatc.org/culturalheritagecountsforeurope/wp-content/uploads/2015/06/CHCfE_FULL-REPORT_v2.pdf
Dostal, P., & Hampl, M. (2007). Systemic geographical approach, methodological plurality, uncertainties and risks. In P. Dostal & J. Langhammer (Eds.), Modeling natural environment and society (pp. 29–44).
European Commission. (2014). Towards an integrated approach to cultural heritage for Europe. Communication from the Commission to the European Parliament, the Council, the European Economic and Socla Commitee and the Commitee of the Regions. Council Document 12150/14 . https://www.eumonitor.eu/9353000/1/j9vvik7m1c3gyxp/vjlot9swvzyt
European Commission. (2015). Getting cultural heritage to work for Europe. Report of the Horizon 2020 Expert Group on Cultural Heritage . https://op.europa.eu/en/publication-detail/-/publication/b01a0d0a-2a4f-4de0-88f7-85bf2dc6e004
European Commission. (2016). Urban agenda for the EU “Pact of Amsterdam”. Agreed at the informal meeting of EU Ministers responsible for urban matters on 30 May 2016 in Amsterdam, The Netherlands . http://urbanagendaforthe.eu/wp-content/uploads/2016/05/Pact-of-Amsterdam_v7_WEB.pdf
Griggs, D., Stafford-Smith, M., Gaffney, O., Rockström, J., Öhman, M. C., Shyamsundar, P., Steffen, W., Glaser, G., Kanie, N., & Noble, I. (2013). Policy: Sustainable development goals for people and planet. Nature, 495 (7441), 305–307.
Article Google Scholar
Grosskurth, J., & Rotmans, J. (2005). The scene model: Getting a grip on sustainable development in policy making. Environment, Development and Sustainability, 7 , 135–151.
Gustafsson, C. (2009). The Halland Model. A Trading zone for building conservation in concert with labour market policy and the construction industry, aiming at regional sustainable development .
Kostakis, I., Lolos, S., & Doulgeraki, C. (2020). Cultural heritage led growth: Regional evidence from Greece (1998–2016) .
Lewis, R., Arthurs, K., & British Council. (2018). Cultural heritage for inclusive growth . https://www.britishcouncil.org/sites/default/files/bc_chig_report_final.pdf
Miles, S., & Paddison, R. (2005). Introduction: The rise and rise of culture-led urban regeneration. Urban Studies, 42 (5–6), 833–839.
Ripp, M. (2018a). Heritage as a system and process that belongs to local communities. Reframing the role of local communities and stakeholders . Council of Europe/Faro Convention Workshop, Fontecchio. https://rm.coe.int/heritage-as-a-system-and-process-that-belongs-to-local-communities-mr-/16807bc255
Ripp, M. (2018b). Baukulturelles Erbe – Ressource für nachhaltige städtische Entwicklung. In: PLANERIN – Sharing Heritage , (2), 19–23.
Ripp, M., & Rodwell, D. (2015). The geography of urban heritage. The Historic Environment: Policy & Practice, 6 (3), 240–276.
Ripp, M., & Stein, P. (2018). Applying the Faro convention principles to deliver heritage-based urban development: The COMUS-project. Community-led urban strategies in historic towns . Council of Europe. http://rm.coe.int/applying-the-faro-convention-principles-to-deliver-heritage-based-urba/168078827f
Ruoss, E. (2016). Opportunities to leverage World Heritage Sites for local development in the Alps. Journal on Protected Mountain Areas Research and Management, 8 (1), 53–61.
Rypkema, D. D. (2005). The economics of historic preservation: A community leader’s guide (S. National Trust for Historic Preservation in the United, Ed., 2nd ed.).
Sandholz, S. (2017). Urban centres in Asia and Latin America. Heritage and identities in changing urban landscapes . Springer.
Book Google Scholar
Sulzer, J. (2007). Revitalisierender Städtebau. Werte (Schriftenreihe Stadtentwicklung und Denkmalpflege Bd. 5). Verlag der Wissenschaften.
Throsby, D. (2001). Economics and culture . Cambridge University Press.
Throsby, D. (2013). Assessment of value in heritage regulation. In I. Rizzo & A. Mignosa (Eds.), Handbook on the economics of cultural heritage (pp. 456–469). Edward Elgar Publishing.
Chapter Google Scholar
UN Habitat. (2014). The state of African cities 2014. Re-imagining sustainable urban transitions . https://unhabitat.org/state-of-african-cities-2014-re-imagining-sustainable-urban-transitions
Unesco. (2016). Culture: Urban future; global report on culture for sustainable urban development . https://unesdoc.unesco.org/ark:/48223/pf0000245999
Van Gigch, J. P. (1991). System design modeling and metamodeling . Plenum Press.
Winter, T. (2013). Clarifying the critical in critical heritage studies. International Journal of Heritage Studies, 19 (6), 532–545.
World Bank. (2001). Cultural heritage and development: A framework for action in the Middle East and North Africa . World Bank. http://www.loc.gov/catdir/toc/fy02/2001026833.html
Download references
Author information
Authors and affiliations.
Brandenburg University of Technology Cottbus–Senftenberg, Cottbus, Germany
Matthias Ripp
You can also search for this author in PubMed Google Scholar
Corresponding author
Correspondence to Matthias Ripp .
Rights and permissions
Reprints and permissions
Copyright information
© 2022 The Author(s), under exclusive license to Springer Nature Switzerland AG
About this chapter
Ripp, M. (2022). Problem, Hypothesis, and Research Questions. In: A Metamodel for Heritage-based Urban Development. Heritage Studies. Springer, Cham. https://doi.org/10.1007/978-3-031-08238-2_2
Download citation
DOI : https://doi.org/10.1007/978-3-031-08238-2_2
Published : 16 September 2022
Publisher Name : Springer, Cham
Print ISBN : 978-3-031-08237-5
Online ISBN : 978-3-031-08238-2
eBook Packages : Social Sciences Social Sciences (R0)
Share this chapter
Anyone you share the following link with will be able to read this content:
Sorry, a shareable link is not currently available for this article.
Provided by the Springer Nature SharedIt content-sharing initiative
- Publish with us
Policies and ethics
- Find a journal
- Track your research
Thank you for visiting nature.com. You are using a browser version with limited support for CSS. To obtain the best experience, we recommend you use a more up to date browser (or turn off compatibility mode in Internet Explorer). In the meantime, to ensure continued support, we are displaying the site without styles and JavaScript.
- View all journals
- Explore content
- About the journal
- Publish with us
- Sign up for alerts
- NEWS AND VIEWS
- 16 December 2020
- Correction 21 January 2021
AI weighs in on debate about universal facial expressions
- Lisa Feldman Barrett 0
Lisa Feldman Barrett is in the Department of Psychology, Northeastern University College of Science, Boston, Massachusetts 02115, USA.
You can also search for this author in PubMed Google Scholar
When you are angry, do you scowl, cry or even laugh? To what extent do your facial movements depend on the situation you are in — whether you are in a formal meeting, say, or at home with your family? And do other people around the world express anger in such situations in the same way? These questions are at the centre of a contentious scientific debate about the nature of emotion that has raged for more than a century. Writing in Nature , Cowen et al. 1 enter the fray. The authors used a type of machine learning to investigate whether people adopt the same facial expressions in similar contexts across cultures. Their results are sure to be the subject of lively discussion.
Access options
Access Nature and 54 other Nature Portfolio journals
Get Nature+, our best-value online-access subscription
24,99 € / 30 days
cancel any time
Subscribe to this journal
Receive 51 print issues and online access
185,98 € per year
only 3,65 € per issue
Rent or buy this article
Prices vary by article type
Prices may be subject to local taxes which are calculated during checkout
Nature 589 , 202-203 (2021)
doi: https://doi.org/10.1038/d41586-020-03509-5
Updates & Corrections
Correction 21 January 2021 : The original version of this News & Views described a control experiment as assessing the raters; instead it assessed the DNN.
Cowen, A. S. et al. Nature 589 , 251–257 (2021).
Article Google Scholar
Shariff, A. F. & Tracy, J. L. Curr. Direct. Psychol. Sci. 20 , 395–399 (2011).
Cosmides, L. & Tooby, J. in Handbook of Emotions 2nd edn (eds Lewis, M. & Haviland-Jones, J. M.) 91–115 (Guilford, 2000).
Google Scholar
Russell, J. A. Psychol. Bull. 115 , 102–141 (1994).
Article PubMed Google Scholar
Barrett, L. F., Adolphs, R., Marsella, S., Martinez, A. M. & Pollak, S. D. Psychol. Sci. Public Interest 20 , 1–68 (2019).
Gendron, M., Crivelli, C. & Barrett, L. F. Curr. Direct. Psychol. Sci. 27 , 211–219 (2018).
Gendron, M. et al. Sci. Rep. 10 , 3867 (2020).
Rychlowska, M. et al. Proc. Natl Acad. Sci. USA 112 , E2429–E2436 (2015).
Aviezer, H. et al. Psychol. Sci. 19 , 724–732 (2008).
Hoemann, K. et al. Emotion 19 , 1292–1313 (2019).
Gendron, M. & Barrett, L. F. in The Science of Facial Expression (eds Fernández-Dols, J.-M. & Russell, J. A.) 15–36 (Oxford Univ. Press, 2017).
Srinivasan, R. & Martinez, A. M. IEEE Trans. Affective Comput . https://doi.org/10.1109/TAFFC.2018.2887267 (IEEE, 2018).
Download references
Reprints and permissions
Related Articles
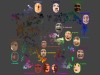
See all News & Views
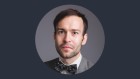
Why role-playing games can spur climate action
World View 22 MAY 24
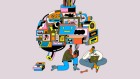
How does ChatGPT ‘think’? Psychology and neuroscience crack open AI large language models
News Feature 14 MAY 24
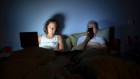
Is the Internet bad for you? Huge study reveals surprise effect on well-being
News 12 MAY 24
Professor, Division Director, Translational and Clinical Pharmacology
Cincinnati Children’s seeks a director of the Division of Translational and Clinical Pharmacology.
Cincinnati, Ohio
Cincinnati Children's Hospital & Medical Center
Data Analyst for Gene Regulation as an Academic Functional Specialist
The Rheinische Friedrich-Wilhelms-Universität Bonn is an international research university with a broad spectrum of subjects. With 200 years of his...
53113, Bonn (DE)
Rheinische Friedrich-Wilhelms-Universität
Recruitment of Global Talent at the Institute of Zoology, Chinese Academy of Sciences (IOZ, CAS)
The Institute of Zoology (IOZ), Chinese Academy of Sciences (CAS), is seeking global talents around the world.
Beijing, China
Institute of Zoology, Chinese Academy of Sciences (IOZ, CAS)
Full Professorship (W3) in “Organic Environmental Geochemistry (f/m/d)
The Institute of Earth Sciences within the Faculty of Chemistry and Earth Sciences at Heidelberg University invites applications for a FULL PROFE...
Heidelberg, Brandenburg (DE)
Universität Heidelberg
Postdoc: deep learning for super-resolution microscopy
The Ries lab is looking for a PostDoc with background in machine learning.
Vienna, Austria
University of Vienna
Sign up for the Nature Briefing newsletter — what matters in science, free to your inbox daily.
Quick links
- Explore articles by subject
- Guide to authors
- Editorial policies
September 7, 2016
15 min read
Evidence Rebuts Chomsky’s Theory of Language Learning
Much of Noam Chomsky’s revolution in linguistics—including its account of the way we learn languages—is being overturned
By Paul Ibbotson & Michael Tomasello
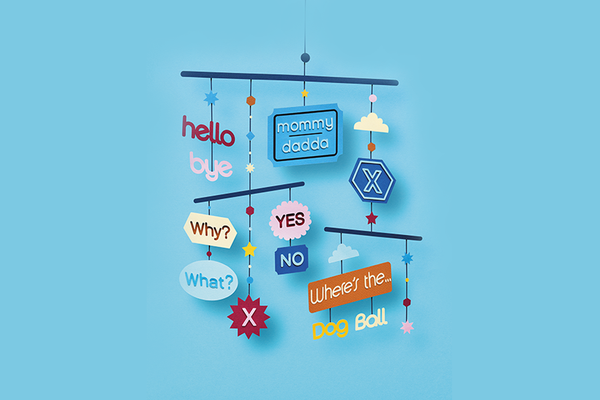
Owen Gildersleeve
The idea that we have brains hardwired with a mental template for learning grammar—famously espoused by Noam Chomsky of the Massachusetts Institute of Technology—has dominated linguistics for almost half a century. Recently, though, cognitive scientists and linguists have abandoned Chomsky’s “universal grammar” theory in droves because of new research examining many different languages—and the way young children learn to understand and speak the tongues of their communities. That work fails to support Chomsky’s assertions. The research suggests a radically different view, in which learning of a child’s first language does not rely on an innate grammar module. Instead the new research shows that young children use various types of thinking that may not be specific to language at all—such as the ability to classify the world into categories (people or objects, for instance) and to understand the relations among things. These capabilities, coupled with a unique human ability to grasp what others intend to communicate, allow language to happen. The new findings indicate that if researchers truly want to understand how children, and others, learn languages, they need to look outside of Chomsky’s theory for guidance.
This conclusion is important because the study of language plays a central role in diverse disciplines—from poetry to artificial intelligence to linguistics itself; misguided methods lead to questionable results. Further, language is used by humans in ways no animal can match; if you understand what language is , you comprehend a little bit more about human nature.
Chomsky’s first version of his theory, put forward in the mid-20th century, meshed with two emerging trends in Western intellectual life. First, he posited that the languages people use to communicate in everyday life behaved like mathematically based languages of the newly emerging field of computer science. His research looked for the underlying computational structure of language and proposed a set of procedures that would create “well-formed” sentences. The revolutionary idea was that a computerlike program could produce sentences real people thought were grammatical. That program could also purportedly explain the way people generated their sentences. This way of talking about language resonated with many scholars eager to embrace a computational approach to ... well ... everything.
On supporting science journalism
If you're enjoying this article, consider supporting our award-winning journalism by subscribing . By purchasing a subscription you are helping to ensure the future of impactful stories about the discoveries and ideas shaping our world today.
As Chomsky was developing his computational theories, he was simultaneously proposing that they were rooted in human biology. In the second half of the 20th century, it was becoming ever clearer that our unique evolutionary history was responsible for many aspects of our unique human psychology, and so the theory resonated on that level as well. His universal grammar was put forward as an innate component of the human mind—and it promised to reveal the deep biological underpinnings of the world’s 6,000-plus human languages. The most powerful, not to mention the most beautiful, ideas in science reveal hidden unity underneath surface diversity, and so this paradigm held immediate appeal.
But evidence has overtaken Chomsky’s theory, which has been inching toward a slow death for years. It is dying so slowly because, as physicist Max Planck once noted, older scholars tend to hang on to the old ways: “Science progresses one funeral at a time.”
In the Beginning
The earliest incarnations of universal grammar in the 1960s took the underlying structure of “standard average European” languages as their starting point—the ones spoken by most of the linguists working on them. Thus, the universal grammar program operated on chunks of language, such as noun phrases (“The nice dogs”) and verb phrases (“like cats”).
Fairly soon, however, linguistic comparisons among multiple languages began rolling in that did not fit with this neat schema. Some native Australian languages, such as Warlpiri, had grammatical elements scattered all over the sentence—noun and verb phrases that were not “neatly packaged” so that they could be plugged into Chomsky’s universal grammar—and some sentences had no verb phrase at all.
These so-called outliers were difficult to reconcile with the universal grammar that was built on examples from European languages. Other exceptions to Chomsky’s theory came from the study of “ergative” languages, such as Basque or Urdu, in which the way a sentence subject is used is very different from that in many European languages, again challenging the idea of a universal grammar.
These findings, along with theoretical linguistic work, led Chomsky and his followers to a wholesale revision of the notion of universal grammar during the 1980s. The new version of the theory, called principles and parameters, replaced a single universal grammar for all the world’s languages with a set of “universal” principles governing the structure of language. These principles manifested themselves differently in each language. An analogy might be that we are all born with a basic set of tastes (sweet, sour, bitter, salty and umami) that interact with culture, history and geography to produce the present-day variations in world cuisine. The principles and parameters were a linguistic analogy to tastes. They interacted with culture (whether a child was learning Japanese or English) to produce today’s variation in languages as well as defined the set of human languages that were possible.
Languages such as Spanish form fully grammatical sentences without the need for separate subjects—for example, Tengo zapatos (“I have shoes”), in which the person who has the shoes, “I,” is indicated not by a separate word but by the “o” at the end of the verb. Chomsky contended that as soon as children encountered a few sentences of this type, their brains would set a switch to “on,” indicating that the sentence subject should be dropped. Then they would know that they could drop the subject in all their sentences.
The “subject-drop” parameter supposedly also determined other structural features of the language. This notion of universal principles fits many European languages reasonably well. But data from non-European languages turned out not to fit the revised version of Chomsky’s theory. Indeed, the research that had attempted to identify parameters, such as the subject-drop, ultimately led to the abandonment of the second incarnation of universal grammar because of its failure to stand up to scrutiny.
More recently, in a famous paper published in Science in 2002, Chomsky and his co-authors described a universal grammar that included only one feature, called computational recursion (although many advocates of universal grammar still prefer to assume there are many universal principles and parameters). This new shift permitted a limited number of words and rules to be combined to make an unlimited number of sentences.
The endless possibilities exist because of the way recursion embeds a phrase within another phrase of the same type. For example, English can embed phrases to the right (“John hopes Mary knows Peter is lying”) or embed centrally (“The dog that the cat that the boy saw chased barked”). In theory, it is possible to go on embedding these phases infinitely. In practice, understanding starts to break down when the phrases are stacked on top of one another as in these examples. Chomsky thought this breakdown was not directly related to language per se. Rather it was a limitation of human memory. More important, Chomsky proposed that this recursive ability is what sets language apart from other types of thinking such as categorization and perceiving the relations among things. He also proposed recently this ability arose from a single genetic mutation that occurred between 100,000 and 50,000 years ago.
As before, when linguists actually went looking at the variation in languages across the world, they found counterexamples to the claim that this type of recursion was an essential property of language. Some languages—the Amazonian Pirahã, for instance—seem to get by without Chomskyan recursion.
As with all linguistic theories, Chomsky’s universal grammar tries to perform a balancing act. The theory has to be simple enough to be worth having. That is, it must predict some things that are not in the theory itself (otherwise it is just a list of facts). But neither can the theory be so simple that it cannot explain things it should. Take Chomsky’s idea that sentences in all the world’s languages have a “subject.” The problem is that the concept of a subject is more like a “family resemblance” of features than a neat category. About 30 different grammatical features define the characteristics of a subject. Any one language will have only a subset of these features—and the subsets often do not overlap with those of other languages.
Chomsky tried to define the components of the essential tool kit of language—the kinds of mental machinery that allow human language to happen. Where counterexamples have been found, some Chomsky defenders have responded that just because a language lacks a certain tool—recursion, for example—does not mean that it is not in the tool kit. In the same way, just because a culture lacks salt to season food does not mean salty is not in its basic taste repertoire. Unfortunately, this line of reasoning makes Chomsky’s proposals difficult to test in practice, and in places they verge on the unfalsifiable.
Death Knells
A key flaw in Chomsky’s theories is that when applied to language learning, they stipulate that young children come equipped with the capacity to form sentences using abstract grammatical rules. (The precise ones depend on which version of the theory is invoked.) Yet much research now shows that language acquisition does not take place this way. Instead young children begin by learning simple grammatical patterns; then, gradually, they intuit the rules behind them bit by bit.
Thus, young children initially speak with only concrete and simple grammatical constructions based on specific patterns of words: “Where’s the X?”; “I wanna X”; “More X”; “It’s an X”; “I’m X-ing it”; “Put X here”; “Mommy’s X-ing it”; “Let’s X it”; “Throw X”; “X gone”; “Mommy X”; “I Xed it”; “Sit on the X”; “Open X”; “X here”; “There’s an X”; “X broken.” Later, children combine these early patterns into more complex ones, such as “Where’s the X that Mommy Xed?”
Many proponents of universal grammar accept this characterization of children’s early grammatical development. But then they assume that when more complex constructions emerge, this new stage reflects the maturing of a cognitive capacity that uses universal grammar and its abstract grammatical categories and principles.
Noam-enclature
Noam Chomsky took the linguistics community by storm more than 50 years ago. The idea was simple. Underlying language is a set of rules innate to every child that generates grammatical sentences from the earliest age. Chomsky set out to define those rules and how they work. Without this universal grammar, he thought, it would be impossible for a child to learn any language. In the ensuing years, Chomsky’s theory has gradually been challenged by new theories asserting that language is acquired as children discern patterns in the language they hear around them.

Illustration by Lucy Reading-Ikkanda
For example, most universal grammar approaches postulate that a child forms a question by following a set of rules based on grammatical categories such as “What (object) did (auxiliary) you (subject) lose (verb)?” Answer: “I (subject) lost (verb) something (object).” If this postulate is correct, then at a given developmental period children should make similar errors across all wh-question sentences alike. But children’s errors do not fit this prediction. Many of them early in development make errors such as “Why he can’t come?” but at the same time as they make this error—failing to put the “can’t” before the “he”—they correctly form other questions with other wh-words and auxiliary verbs, such as the sentence “What does he want?”
Experimental studies confirm that children produce correct question sentences most often with particular wh-words and auxiliary verbs (often those with which they have most experience, such as “What does ...”), while continuing to make errors with question sentences containing other (often less frequent) combinations of wh-words and auxiliary verbs: “Why he can’t come?”
The main response of universal grammarians to such findings is that children have the competence with grammar but that other factors can impede their performance and thus both hide the true nature of their grammar and get in the way of studying the “pure” grammar posited by Chomsky’s linguistics. Among the factors that mask the underlying grammar, they say, include immature memory, attention and social capacities.
Yet the Chomskyan interpretation of the children’s behavior is not the only possibility. Memory, attention and social abilities may not mask the true status of grammar; rather they may well be integral to building a language in the first place. For example, a study co-authored by one of us (Ibbotson) showed that children’s ability to produce a correct irregular past tense verb—such as “Every day I fly, yesterday I flew ” (not “flyed”)—was associated with their ability to inhibit a tempting response that was unrelated to grammar. (For example, to say the word “moon” while looking at a picture of the sun.) Rather than memory, mental analogies, attention and reasoning about social situations getting in the way of children expressing the pure grammar of Chomskyan linguistics, those mental faculties may explain why language develops as it does.
As with the retreat from the cross-linguistic data and the tool-kit argument, the idea of performance masking competence is also pretty much unfalsifiable. Retreats to this type of claim are common in declining scientific paradigms that lack a strong empirical base—consider, for instance, Freudian psychology and Marxist interpretations of history.
Even beyond these empirical challenges to universal grammar, psycholinguists who work with children have difficulty conceiving theoretically of a process in which children start with the same algebraic grammatical rules for all languages and then proceed to figure out how a particular language—whether English or Swahili—connects with that rule scheme. Linguists call this conundrum the linking problem, and a rare systematic attempt to solve it in the context of universal grammar was made by Harvard University psychologist Steven Pinker for sentence subjects. Pinker’s account, however, turned out not to agree with data from child development studies or to be applicable to grammatical categories other than subjects. And so the linking problem—which should be the central problem in applying universal grammar to language learning—has never been solved or even seriously confronted.
An Alternative View
All of this leads ineluctably to the view that the notion of universal grammar is plain wrong. Of course, scientists never give up on their favorite theory, even in the face of contradictory evidence, until a reasonable alternative appears. Such an alternative, called usage-based linguistics, has now arrived. The theory, which takes a number of forms, proposes that grammatical structure is not innate. Instead grammar is the product of history (the processes that shape how languages are passed from one generation to the next) and human psychology (the set of social and cognitive capacities that allow generations to learn a language in the first place). More important, this theory proposes that language recruits brain systems that may not have evolved specifically for that purpose and so is a different idea to Chomsky’s single-gene mutation for recursion.
In the new usage-based approach (which includes ideas from functional linguistics, cognitive linguistics and construction grammar), children are not born with a universal, dedicated tool for learning grammar. Instead they inherit the mental equivalent of a Swiss Army knife: a set of general-purpose tools—such as categorization, the reading of communicative intentions, and analogy making, with which children build grammatical categories and rules from the language they hear around them.
For instance, English-speaking children understand “The cat ate the rabbit,” and by analogy they also understand “The goat tickled the fairy.” They generalize from hearing one example to another. After enough examples of this kind, they might even be able to guess who did what to whom in the sentence “The gazzer mibbed the toma,” even though some of the words are literally nonsensical. The grammar must be something they discern beyond the words themselves, given that the sentences share little in common at the word level.
The meaning in language emerges through an interaction between the potential meaning of the words themselves (such as the things that the word “ate” can mean) and the meaning of the grammatical construction into which they are plugged. For example, even though “sneeze” is in the dictionary as an intransitive verb that only goes with a single actor (the one who sneezes), if one forces it into a ditransitive construction—one able to take both a direct and indirect object—the result might be “She sneezed him the napkin,” in which “sneeze” is construed as an action of transfer (that is to say, she made the napkin go to him). The sentence shows that grammatical structure can make as strong a contribution to the meaning of the utterance as do the words. Contrast this idea with that of Chomsky, who argued there are levels of grammar that are free of meaning entirely.
The concept of the Swiss Army knife also explains language learning without any need to invoke two phenomena required by the universal grammar theory. One is a series of algebraic rules for combining symbols—a so-called core grammar hardwired in the brain. The second is a lexicon—a list of exceptions that cover all of the other idioms and idiosyncrasies of natural languages that must be learned. The problem with this dual-route approach is that some grammatical constructions are partially rule-based and also partially not—for example, “Him a presidential candidate?!” in which the subject “him” retains the form of a direct object but with the elements of the sentence not in the proper order. A native English speaker can generate an infinite variety of sentences using the same approach: “Her go to ballet?!” or “That guy a doctor?!” So the question becomes, are these utterances part of the core grammar or the list of exceptions? If they are not part of a core grammar, then they must be learned individually as separate items. But if children can learn these part-rule, part-exception utterances, then why can they not learn the rest of language the same way? In other words, why do they need universal grammar at all?
In fact, the idea of universal grammar contradicts evidence showing that children learn language through social interaction and gain practice using sentence constructions that have been created by linguistic communities over time. In some cases, we have good data on exactly how such learning happens. For example, relative clauses are quite common in the world’s languages and often derive from a meshing of separate sentences. Thus, we might say, “My brother.... He lives over in Arkansas.... He likes to play piano.” Because of various cognitive-processing mechanisms—with names such as schematization, habituation, decontextualization and automatization—these phrases evolve over long periods into a more complex construction: “My brother, who lives over in Arkansas, likes to play the piano.” Or they might turn sentences such as “I pulled the door, and it shut” gradually into “I pulled the door shut.”
What is more, we seem to have a species-specific ability to decode others’ communicative intentions—what a speaker intends to say. For example, I could say, “She gave/bequeathed/sent/loaned/sold the library some books” but not “She donated the library some books.” Research has now shown that there are several mechanisms that lead children to constrain these types of inappropriate analogies. For example, children do not make analogies that make no sense. So they would never be tempted to say “She ate the library some books.” In addition, if children hear quite often “She donated some books to the library,” then this usage preempts the temptation to say “She donated the library some books.”
Such constraining mechanisms vastly cut down the possible analogies a child could make to those that align the communicative intentions of the person he or she is trying to understand. We all use this kind of intention reading when we understand “Can you open the door for me?” as a request for help rather than an inquiry into door-opening abilities.
Chomsky allowed for this kind of “pragmatics”—how we use language in context—in his general theory of how language worked. Given how ambiguous language is, he had to. But he appeared to treat the role of pragmatics as peripheral to the main job of grammar. In a way, the contributions from usage-based approaches have shifted the debate in the other direction to how much pragmatics can do for language before speakers need to turn to the rules of syntax.
Usage-based theories are far from offering a complete account of how language works. Meaningful generalizations that children make from hearing spoken sentences and phrases are not the whole story of how children construct sentences either—there are generalizations that make sense but are not grammatical (for example, “He disappeared the rabbit”). Out of all the possible meaningful yet ungrammatical generalizations children could make, they appear to make very few. The reason seems to be they are sensitive to the fact that the language community to which they belong conforms to a norm and communicates an idea in just “this way.” They strike a delicate balance, though, as the language of children is both creative (“I goed to the shops”) and conformative to grammatical norms (“I went to the shops”). There is much work to be done by usage-based theorists to explain how these forces interact in childhood in a way that exactly explains the path of language development.
A Look Ahead
At the time the Chomskyan paradigm was proposed, it was a radical break from the more informal approaches prevalent at the time, and it drew attention to all the cognitive complexities involved in becoming competent at speaking and understanding language. But at the same time that theories such as Chomsky’s allowed us to see new things, they also blinded us to other aspects of language. In linguistics and allied fields, many researchers are becoming ever more dissatisfied with a totally formal language approach such as universal grammar—not to mention the empirical inadequacies of the theory. Moreover, many modern researchers are also unhappy with armchair theoretical analyses, when there are large corpora of linguistic data—many now available online—that can be analyzed to test a theory.
The paradigm shift is certainly not complete, but to many it seems that a breath of fresh air has entered the field of linguistics. There are exciting new discoveries to be made by investigating the details of the world’s different languages, how they are similar to and different from one another, how they change historically, and how young children acquire competence in one or more of them.
Universal grammar appears to have reached a final impasse. In its place, research on usage-based linguistics can provide a path forward for empirical studies of learning, use and historical development of the world’s 6,000 languages.”
Paul Ibbotson is a lecturer in language development at the Open University, based in England.
Michael Tomasello is a professor of psychology and neuroscience at Duke University and emeritus director of the Max Planck Institute for Evolutionary Anthropology in Leipzig, Germany.
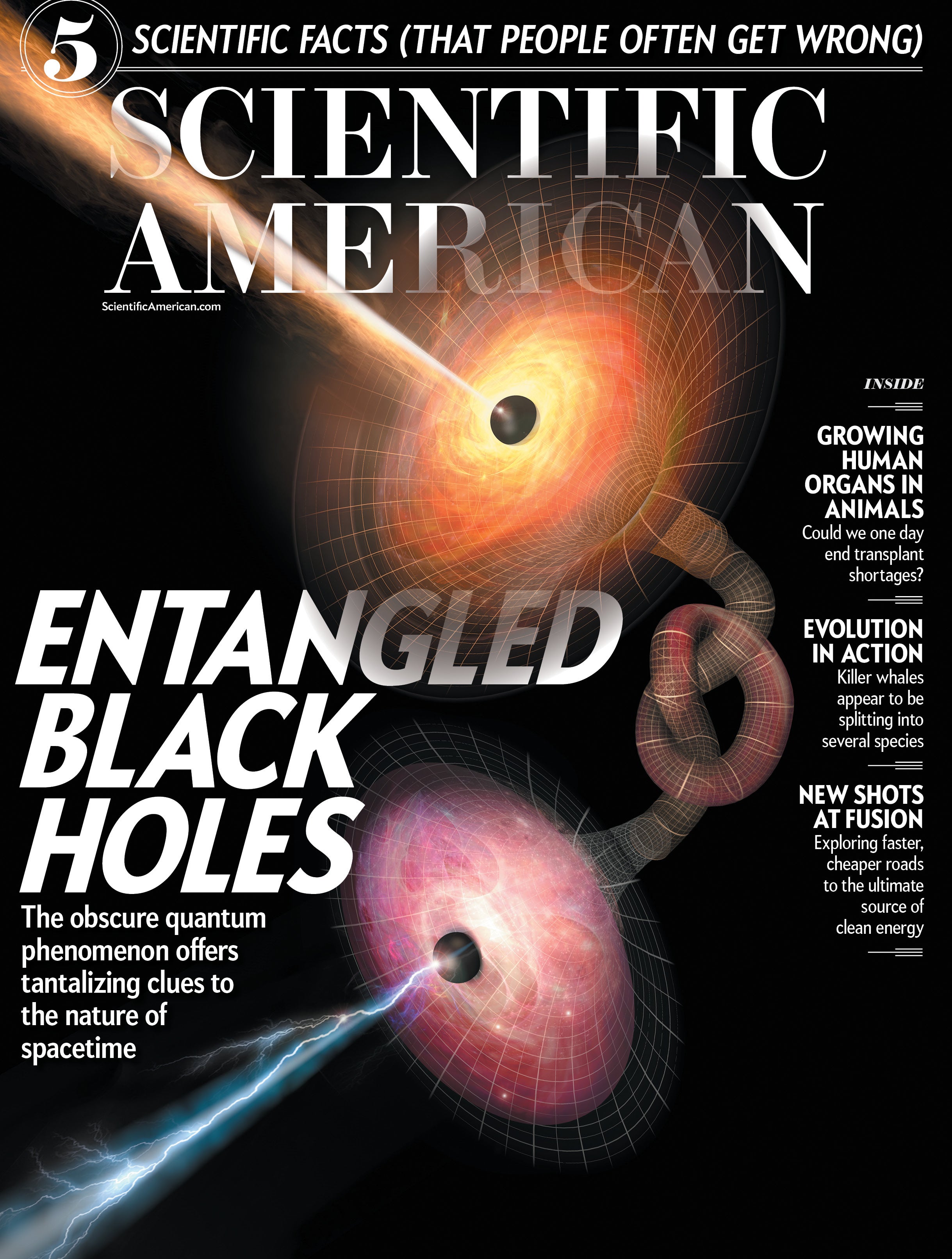
The Experience
- Career Impact
- Inclusion and Belonging
- Global Opportunities
More about Kellogg
- History & Legacy
- Convocation Ceremony
Degree Programs
- Full-Time MBA
- Executive MBA
- Master in Management
- Evening & Weekend MBA
- Certificate Program for Undergraduates
- Which Program is Right for Me?
- Academic Calendars
Executive Education
- Online Programs
- Programs for Individuals
- Nonprofit Programs
- Programs for Groups
- The Kellogg Advantage
- Contact Executive Education
- Request a Brochure
- Find a Program
- Alumni Network
- Career Journeys
- Global Impact
- Student Stories
- Applying to Kellogg
Publications and blogs
- Kellogg Magazine
- Kellogg Insight
- See All News + Stories
Academics + Research
- Faculty Directory
- Research Centers
- Case Studies
- Faculty Teaching Awards
- Academic Departments
- Research + Books
- Faculty Recruiting
Academic expertise
- Data Analytics
- Family Business
- Leadership & Organizations
- Social Impact
- Entrepreneurship
- Full-Time MBA Admissions
- Evening & Weekend MBA Admissions
- Executive MBA Admissions
Additional resources
- Master in Management Admissions
- PhD / Doctoral Admissions
- Undergraduate Certificate Admissions
- Admissions Events
- Financial Aid Office
- Log into my account portal
- Companies + Recruiters
Kellogg creates first research institute applying complexity sciences to unlock new understandings of societal, market and business issues
- Kellogg News
Northwestern University’s Kellogg School of Management announced today the creation of the Ryan Institute on Complexity to be led by distinguished faculty in the areas of physics, economics and sociology. The Ryan Institute will comprise a revolutionary research lab designed to change the way business, markets and societal issues are studied, combining multidisciplinary talents and quantitative sciences in new ways to tackle bigger problems faster. Funded by a $25 million gift from the Ryan Family Foundation, the Ryan Institute will place Kellogg and Northwestern at the forefront of solving increasingly complex societal, business and market challenges by harnessing the power of big data and artificial intelligence. The institute will provide Kellogg students with an understanding of powerful new quantitative approaches to problem solving. The field of complexity science had a watershed moment in 2021 when the Nobel Prize in Physics was awarded in this area for the first time. But complexity science’s potential in the realm of business is just starting to be tapped, and the possibilities are vast — in better understanding everything from the intertwined forces driving a nation’s innovativeness to the inner workings of a global team dynamic. The goal is to improve understanding of how the success of organizations, regions and nations build from their deep and rich interconnections. With this focus, the Ryan Institute will be the first of its kind to be housed in a business school. It will gather leaders from academia and industry in diverse fields, along with PhD students and postdoctoral fellows to collectively study the future of quantitative sciences, complex systems and the complex issues affecting businesses and society. Initial areas of focus include the power of social networks, the secrets of invention and human-machine partnerships. Unique to the institute will be a “dry lab” setting typically found in the hard sciences, which will enable scholars to work with big data and the quantitative tools needed to study social and business phenomena on a large scale. This approach holds the promise of generating deeper and more generalizable insights into society’s biggest questions, such as understanding human-machine partnerships at the forefront of generative AI. A key pillar of study will focus on leveraging large language models (LLMs) with the belief that generative AI will not replace humans, but those who understand AI will replace those who don’t. Given the explosion of big data and the rapid acceleration of AI, there are now opportunities to better understand the world in ways that previously were subject only to hypothesis. “Kellogg has always taken an interdisciplinary approach to open new areas,” said Kellogg Dean Francesca Cornelli. “Our vision is bold — that complexity science shall permeate every aspect of business, with the most effective leaders succeeding through understanding and managing a system’s connectivity. Thanks to the generosity of the Ryan Family, Kellogg will drive a new field and best prepare the leaders of the future.” “We are thrilled to support the establishment of this revolutionary research institute that will place Kellogg and Northwestern University at the forefront of the study of complexity science,” said Pat Ryan, Jr (’97 JD, MBA) of the Ryan Family Foundation. “Cutting-edge analytical approaches can now unlock previously unimaginable understandings of our complex world that will be transformational for business and society.”
The Ryan Institute will be led by three Kellogg professors who are all highly recognized leaders in their fields, as well as frequent collaborators: Dashun Wang , a physicist and Professor of Management and Organizations; Ben Jones , an economist and the Gordon and Llura Gund Family Professor of Entrepreneurship and Professor of Strategy; and Brian Uzzi , a sociologist and the Richard L. Thomas Professor of Leadership and Organizational Change. “If we can integrate complexity science and this fundamental thinking from physics and the natural sciences and apply it to business and markets, developing thought leadership and training future leaders in the school — that could be a very meaningful contribution to society,” said Wang. Research and tools emerging from the Ryan Institute will also drive the creation of unique curriculum at Kellogg, including the first PhD training program in a business school helping to train future thought leaders in complexity. The Institute will also collaborate closely with other Northwestern schools and institutions. The Ryan Institute will hold an annual conference as a premier, global convening of thought leaders in the study of complexity. The Ryan Institute Conference will bring together academia and industry to provide opportunities for collaboration across leaders in the field. About the Ryan Family Foundation and Pat & Shirley Ryan family The Ryan family [Pat '59, '09 H ('97, '00 P) and Shirley '61, '19 H ('97, '00 P); Pat '97 JD, MBA and Lydia; Rob '00 JD, MBA and Jennifer; and Corbett] has made deep and broad philanthropic investments across the institution including nanotechnology, the musical arts, the Ryan Family Scholars Program for high-achieving, low-income students with exceptional leadership potential, and the ongoing efforts to Rebuild Ryan Field. The Patrick G. and Shirley W. Ryan Family Foundation is the philanthropic arm of the Ryan family. Patrick G. Ryan is a 1959 Northwestern graduate. He received his undergraduate degree in business from what was then called the School of Business and now is named the Kellogg School of Management. He also received an honorary degree from the University in 2009 in appreciation for his 14 years of service as chairman of Northwestern’s Board of Trustees. In 2013, he was inducted into Northwestern’s Athletics Hall of Fame. Shirley Welsh Ryan is a 1961 Northwestern graduate. She received her undergraduate degree in English Literature from what was then called the College of Arts and Sciences and is now named the Weinberg College of Arts and Sciences. In 2019, Northwestern awarded Mrs. Ryan the honorary title of Doctor of Humane Letters. Mr. Ryan is distinguished as one of the nation’s most successful entrepreneurs and prominent civic leaders. His first business venture while a student involved selling scrapbooks to fellow students, which paid for his Northwestern education. Mr. Ryan founded and served for 41 years as CEO of Aon Corporation, the leading global provider of risk management, insurance and reinsurance brokerage. At the time of his retirement, Aon had nearly $8 billion in annual revenue with more than 500 offices in 120 countries. In 2010, Mr. Ryan founded Ryan Specialty, a service provider of specialty products and solutions for insurance brokers, agents and carriers. The firm provides distribution, underwriting, product development, administration and risk management services by acting as a wholesale broker and a managing underwriter. Mr. Ryan currently serves as chairman and CEO of Ryan Specialty Holdings, Inc., which completed its initial public offering in July 2021. The firm’s shares trade on the New York Stock Exchange under the symbol “RYAN.” Mr. Ryan is distinct in having founded and built two major New York Stock Exchange traded companies. Mr. Ryan is a member of the Chicago Business Hall of Fame, and a member and past president of the Economic Club of Chicago. He also is a member of the International Insurance Hall of Fame and the Automotive Hall of Fame, a member and past chairman of Northwestern’s Board of Trustees, a recipient of the esteemed Horatio Alger Award and a member of the American Academy of Arts and Sciences. He is a former director of numerous public multi-national corporations as well as many major cultural and not-for-profit organizations. Shirley Welsh Ryan is founder of Pathways.org, which is used by 40 million parents and healthcare professionals annually through its video-based website and social media in every country except North Korea. Three hundred U.S. institutions of higher learning use Pathway.org’s free materials. Mrs. Ryan’s pioneering work to empower every infant’s fullest physical development has won numerous awards. Two U.S. presidents have appointed her to the National Council on Disability in Washington D.C., which advises the U.S. Congress on disability policy. In 2017, Pathways.org merged with the Shirley Ryan Ability Lab, acclaimed for 32 years as the number one U.S. rehabilitation hospital by U.S. News and World Report. The Pathways.org Medical Round Table (P.M.R.T.), created in 1990, is the first Infant Milestone Chart of typical and atypical development to be endorsed by the American Academy of Pediatrics (A.A.P.). All Pathways.org material is in accord with the leadership of P.M.R.T. and A.A.P. Mrs. Ryan is a strong believer in the power of early infant detection, therapeutic intervention, universal accessibility, and the concept that all children can learn. She serves on the boards of University of Notre Dame, the Lyric Opera of Chicago, the Art Institute of Chicago, the Chicago Council on Global Affairs, Alain Locke Charter School and WTTW-PBS. She also has served on the boards of the Kennedy Center for Performing Arts in Washington D.C. and Ronald McDonald House Charities; has chaired the Chicago Community Trust; and founded the Lincoln Park Zoo Women’s Board. For 46 years, Mrs. Ryan has led a Northwestern graduate-level course entitled Learning for Life. Mrs. Ryan has been awarded honorary doctorates from Northwestern, the University of Notre Dame and the University of Illinois at Chicago. She also has received the Chicago History Museum Award for Distinction in Civic Leadership. In addition to earning her B.A. from Northwestern, Mrs. Ryan studied at the Sorbonne of the University of Paris and the École du Louvre in Paris. In addition to Mr. and Mrs. Ryan, the Ryan Family includes Northwestern Trustee Pat ’97 JD, MBA and Lydia; Rob ’00 JD, MBA and Jennifer; and Corbett.
More From Forbes
Women are taking more mental health days. here’s how to combat stress and burnout.
- Share to Facebook
- Share to Twitter
- Share to Linkedin
Lonely sad woman deep in thoughts sitting daydreaming or waiting for someone in the living room ... [+] with a serious expression, she is pensive and suffering from insomnia sitting on couch
Mental health-related leaves of absence have skyrocketed. New research from ComPsych , a mental health service provider, finds that mental health-related absences were up 300% between 2017 and 2023, and up 33% in 2023 alone. What's more, the research finds that this increase is largely driven by women. In 2023, 69% of mental health leaves of absence were taken by women.
In fact, time off for mental health is so essential that a Chinese supermarket recently made waves by implementing a policy that allows its employees to take up to 10 "unhappy days" each year. A better solution would be to create a culture and attitude toward well-being that the time off for mental health isn't needed. Until that happens, it's not surprising that companies continue to see an increase in mental health-related leaves.
Dr. Richard Chaifetz, a leading expert in mental health and the CEO of ComPsych, discusses the current state of mental health in the workplace, the unique challenges faced by women, and solutions companies can implement to better support their people.
Anxiety overtakes depression as the top mental health concern
One of the most intriguing insights from the research is the shift in the primary reasons people seek mental health support. For the first time, anxiety has overtaken depression as the top concern, with depression falling to the fifth position. Stress, relationship issues, and general psychological issues round out the top five. This shift, Dr. Chaifetz explained, might be influenced by changing social perceptions. "People are more comfortable saying they're anxious, given the current societal context. Anxiety is a much more palatable thing to report."
The data backs up Dr. Chaifetz's hunch. About half of all people are comfortable talking about mental health at work — though that percentage isn't nearly as high as it should be, but still a good sign of how past workplace taboo topics are receding. One theory regarding the increase isn't that things have suddenly gotten worse; rather, people are now in more psychologically safe work environments so they can speak up and take the time they would have previously been hesitant to request.
Why Is Chief Boden Leaving Chicago Fire Eamonn Walker s Exit Explained
Biden vs. trump 2024 election polls: biden leads trump by only single digits in new york, latest survey shows, here are the major allegations against sean diddy combs as cassie ventura breaks silence on attack video.
"Now, people are much more comfortable talking about going to see a therapist," says Dr. Chaifetz. "In fact, it's in vogue. How many people do you see at a party talking about their therapist and discussing their problems? Even when we even talk with our customers, they'll sit in meetings with and just start talking about their mental health problems as openly as they would talk about breaking an ankle."
Another key factor driving the rise of anxiety might come down to paperwork — Dr. Chaifetz suspects inaccurate diagnostic labeling could be at play. "Relationship issues have always been high and often get coded in different ways, but when you get into the underlying issues, there may be a depression or anxiety that's driving the larger relationship issues."
What's driving the surge in women taking mental health leave?
The dramatic increase in women taking mental health leave perhaps reflects broader workplace trends in gender dynamics that have yet to be resolved. For example, the 2023 McKinsey Women in the Workplace report found that women experience microaggressions at a significantly higher rate than men, which contributes to feelings of stress and anxiety at work. The report goes on to say that women who experience microaggressions at work are three times more likely to think about quitting their jobs, and four times more likely to almost always be burned out.
Another hypothesis is that perhaps women are taking an extended break in the hopes that they can still salvage the work situation if they just have a moment to breathe, versus outright quitting. Decisions on whether to quit or stay in role are always challenging, but often by the time it's even a question to leave, some part of the subconscious has already made the decision.
4 ways to improve mental health at work
Given that recent research from Headspace found that 83% of respondents said that their stress primarily comes from work, employers have a duty of care to improve mental health. Dr. Chaifetz pointed to several organizations that have been recognized for their outstanding mental health and wellness programs. "We have an awards program recognizing companies with the best wellness programs," Dr. Chaifetz said. "These organizations are leading the way in mental health support, demonstrating that it's possible to create a supportive and healthy workplace environment." The 2023 winners include NVIDIA NVIDIA SPDR Dow Jones Industrial Average ETF Trust , Gallagher, Bayer and more.
1: Resource your teams properly
Most workplace-caused stress comes from unsustainable workloads. Until companies fix that, the rest of the mental health efforts are likely to have a minimal impact (if any).
2: Show a more vulnerable side of your leaders
Have leaders share their own mental health journeys and encourage others in the business to share their stories, as well. Host a panel on mental health, where leaders and others in the business talk openly about their struggles, fears around speaking up, anxieties they have faced, and what their "aha" moment was for knowing it was time to make changes.
3: Normalize talking about mental health
Get as many people as possible included in the conversation, and most importantly, create a place for sharing these stories in a way that feels authentic to your company. For example, several years ago, Facebook ran a campaign called #OpenUp that encouraged people to share stories. It was underpinned by imagery of butterflies — a universal symbol of hope. Collect and house the stories that are surfaced so people can browse and feel less alone — and be encouraged to share their own, too.
4: Encourage care for others as part of your core values and culture
Check in with your peers. EY had a famous campaign – 'r u okay? — that encouraged colleagues to check in with each other just by asking that one simple question. Go a step further and create a list of conversation starters for people to use to check in with each other. This gives gentle prompts on questions people can ask colleagues if they want to check in — but aren't sure how to do so appropriately or sensitively.
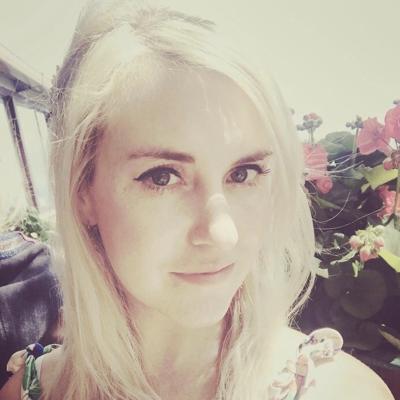
- Editorial Standards
- Reprints & Permissions
Join The Conversation
One Community. Many Voices. Create a free account to share your thoughts.
Forbes Community Guidelines
Our community is about connecting people through open and thoughtful conversations. We want our readers to share their views and exchange ideas and facts in a safe space.
In order to do so, please follow the posting rules in our site's Terms of Service. We've summarized some of those key rules below. Simply put, keep it civil.
Your post will be rejected if we notice that it seems to contain:
- False or intentionally out-of-context or misleading information
- Insults, profanity, incoherent, obscene or inflammatory language or threats of any kind
- Attacks on the identity of other commenters or the article's author
- Content that otherwise violates our site's terms.
User accounts will be blocked if we notice or believe that users are engaged in:
- Continuous attempts to re-post comments that have been previously moderated/rejected
- Racist, sexist, homophobic or other discriminatory comments
- Attempts or tactics that put the site security at risk
- Actions that otherwise violate our site's terms.
So, how can you be a power user?
- Stay on topic and share your insights
- Feel free to be clear and thoughtful to get your point across
- ‘Like’ or ‘Dislike’ to show your point of view.
- Protect your community.
- Use the report tool to alert us when someone breaks the rules.
Thanks for reading our community guidelines. Please read the full list of posting rules found in our site's Terms of Service.
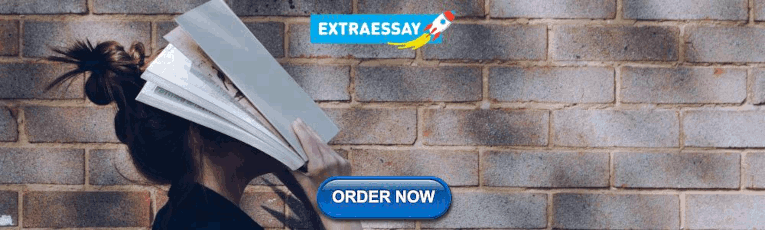
COMMENTS
A research hypothesis, in its plural form "hypotheses," is a specific, testable prediction about the anticipated results of a study, established at its outset. It is a key component of the scientific method. Hypotheses connect theory to data and guide the research process towards expanding scientific understanding.
A hypothesis is a tentative statement about the relationship between two or more variables. It is a specific, testable prediction about what you expect to happen in a study. It is a preliminary answer to your question that helps guide the research process. Consider a study designed to examine the relationship between sleep deprivation and test ...
A research hypothesis helps test theories. A hypothesis plays a pivotal role in the scientific method by providing a basis for testing existing theories. For example, a hypothesis might test the predictive power of a psychological theory on human behavior. It serves as a great platform for investigation activities.
Developing a hypothesis (with example) Step 1. Ask a question. Writing a hypothesis begins with a research question that you want to answer. The question should be focused, specific, and researchable within the constraints of your project. Example: Research question.
The Royal Society - On the scope of scientific hypotheses (Apr. 24, 2024) scientific hypothesis, an idea that proposes a tentative explanation about a phenomenon or a narrow set of phenomena observed in the natural world. The two primary features of a scientific hypothesis are falsifiability and testability, which are reflected in an "If ...
3. Simple hypothesis. A simple hypothesis is a statement made to reflect the relation between exactly two variables. One independent and one dependent. Consider the example, "Smoking is a prominent cause of lung cancer." The dependent variable, lung cancer, is dependent on the independent variable, smoking. 4.
A research hypothesis (also called a scientific hypothesis) is a statement about the expected outcome of a study (for example, a dissertation or thesis). To constitute a quality hypothesis, the statement needs to have three attributes - specificity, clarity and testability. Let's take a look at these more closely.
A hypothesis is a statement of the researcher's expectation or prediction about relationship among study variables. The research process begins and ends with the hypothesis. It is core to the ...
A Universal Hypothesis Test. ... This line of research began over 50 y ago by Darling and Robbins , Robbins , Robbins and Siegmund , and Lai (46, 47). In fact, for testing point nulls, the running MLE (or nonanticipating) martingale was suggested in passing by Wald (ref. 48, equation 10:10) and analyzed in depth by refs. 45 and 49 ...
Formulating Hypotheses for Different Study Designs. Generating a testable working hypothesis is the first step towards conducting original research. Such research may prove or disprove the proposed hypothesis. Case reports, case series, online surveys and other observational studies, clinical trials, and narrative reviews help to generate ...
However, a disconfirming instance is not possible when a universal hypothesis is true. This, of course, is in keeping with Popper's ... For decades, the standard approach taken by most studies in psychology has been null hypothesis testing. Research has sought to provide evidence that the null hypothesis is not true, and there is a relation ...
The scientific method is an empirical method for acquiring knowledge that has characterized the development of science since at least the 17th century. The scientific method involves careful observation coupled with rigorous scepticism, because cognitive assumptions can distort the interpretation of the observation.Scientific inquiry includes creating a hypothesis through inductive reasoning ...
Introduction. The Universal Grammar (UG) hypothesis—the idea that human languages, as superficially diverse as they are, share some fundamental similarities, and that these are attributable to innate principles unique to language: that deep down, there is only one human language (Chomsky, 2000a, p. 7)—has generated an enormous amount of interest in linguistics, psychology, philosophy, and ...
The first source of controversy is that the hypothesis appears to undercut any possibility of a universal foundation for human cognition. This idea sits uneasily with the finding that variation in color naming across languages is constrained, such that certain patterns of color naming recur frequently across languages [ 3 - 5 ], suggesting ...
What is Hypothesis? A hypothesis is an assumption that is made based on some evidence. This is the initial point of any investigation that translates the research questions into predictions. It includes components like variables, population and the relation between the variables.
The main problem that this work aims to address is that there is no universal method to use urban heritage as a resource for development. This chapter sets out the foundations of this research: the identified problem, hypothesis, and research questions. Setting the context of the established problem, Sect. 2.1 explores the potential benefits of ...
Universal grammar (UG), in modern linguistics, is the theory of the innate biological component of the language faculty, usually credited to Noam Chomsky.The basic postulate of UG is that there are innate constraints on what the grammar of a possible human language could be. When linguistic stimuli are received in the course of language acquisition, children then adopt specific syntactic rules ...
Sometime in our evolutionary past, the hypothesis goes, our ancestors capitalized on specific, universal emotional expressions as a means of communicating those challenges to one another, helping ...
Universal grammar appears to have reached a final impasse. In its place, research on usage-based linguistics can provide a path forward for empirical studies of learning, use and historical ...
The partial availability of Universal Grammar in second language acquisition: the 'failed functional features hypothesis'. Second Language Research 13 : 187-226 Haznedar, B. (1997).
The review identified eight studies that met the inclusion criteria. The results reported a lack of empirical research, leading to a recommendation to conduct research that used UDL principles; however, the review indicated a lack of empirical research applying the Universal Design Instruction (UDI) in higher education settings.
This article examines how changes in linguistic theory affect the debate between the fundamental difference hypothesis and the access-to-Universal Grammar (UG) approach to SLA.
Academics + Research Where world-renowned faculty and cutting-edge research converge to fuel innovation and drive impact. ... there are now opportunities to better understand the world in ways that previously were subject only to hypothesis. ... universal accessibility, and the concept that all children can learn. She serves on the boards of ...
New research from ComPsych, a mental health service provider, finds that mental health-related absences were up 300% between 2017 and 2023, and up 33% in 2023 alone. What's more, the research ...