Quantitative Research
- Reference work entry
- First Online: 13 January 2019
- Cite this reference work entry
- Leigh A. Wilson 2 , 3
4261 Accesses
4 Citations
Quantitative research methods are concerned with the planning, design, and implementation of strategies to collect and analyze data. Descartes, the seventeenth-century philosopher, suggested that how the results are achieved is often more important than the results themselves, as the journey taken along the research path is a journey of discovery. High-quality quantitative research is characterized by the attention given to the methods and the reliability of the tools used to collect the data. The ability to critique research in a systematic way is an essential component of a health professional’s role in order to deliver high quality, evidence-based healthcare. This chapter is intended to provide a simple overview of the way new researchers and health practitioners can understand and employ quantitative methods. The chapter offers practical, realistic guidance in a learner-friendly way and uses a logical sequence to understand the process of hypothesis development, study design, data collection and handling, and finally data analysis and interpretation.
This is a preview of subscription content, log in via an institution to check access.
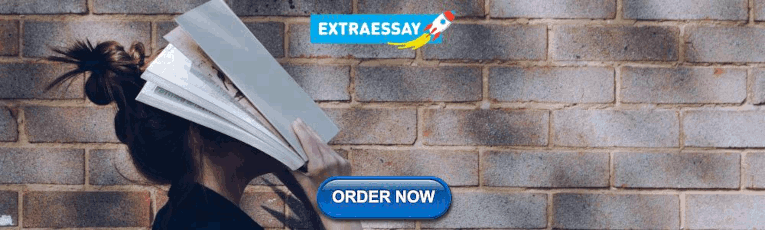
Access this chapter
- Available as PDF
- Read on any device
- Instant download
- Own it forever
- Available as EPUB and PDF
- Durable hardcover edition
- Dispatched in 3 to 5 business days
- Free shipping worldwide - see info
Tax calculation will be finalised at checkout
Purchases are for personal use only
Institutional subscriptions
Babbie ER. The practice of social research. 14th ed. Belmont: Wadsworth Cengage; 2016.
Google Scholar
Descartes. Cited in Halverston, W. (1976). In: A concise introduction to philosophy, 3rd ed. New York: Random House; 1637.
Doll R, Hill AB. The mortality of doctors in relation to their smoking habits. BMJ. 1954;328(7455):1529–33. https://doi.org/10.1136/bmj.328.7455.1529 .
Article Google Scholar
Liamputtong P. Research methods in health: foundations for evidence-based practice. 3rd ed. Melbourne: Oxford University Press; 2017.
McNabb DE. Research methods in public administration and nonprofit management: quantitative and qualitative approaches. 2nd ed. New York: Armonk; 2007.
Merriam-Webster. Dictionary. http://www.merriam-webster.com . Accessed 20th December 2017.
Olesen Larsen P, von Ins M. The rate of growth in scientific publication and the decline in coverage provided by Science Citation Index. Scientometrics. 2010;84(3):575–603.
Pannucci CJ, Wilkins EG. Identifying and avoiding bias in research. Plast Reconstr Surg. 2010;126(2):619–25. https://doi.org/10.1097/PRS.0b013e3181de24bc .
Petrie A, Sabin C. Medical statistics at a glance. 2nd ed. London: Blackwell Publishing; 2005.
Portney LG, Watkins MP. Foundations of clinical research: applications to practice. 3rd ed. New Jersey: Pearson Publishing; 2009.
Sheehan J. Aspects of research methodology. Nurse Educ Today. 1986;6:193–203.
Wilson LA, Black DA. Health, science research and research methods. Sydney: McGraw Hill; 2013.
Download references
Author information
Authors and affiliations.
School of Science and Health, Western Sydney University, Penrith, NSW, Australia
Leigh A. Wilson
Faculty of Health Science, Discipline of Behavioural and Social Sciences in Health, University of Sydney, Lidcombe, NSW, Australia
You can also search for this author in PubMed Google Scholar
Corresponding author
Correspondence to Leigh A. Wilson .
Editor information
Editors and affiliations.
Pranee Liamputtong
Rights and permissions
Reprints and permissions
Copyright information
© 2019 Springer Nature Singapore Pte Ltd.
About this entry
Cite this entry.
Wilson, L.A. (2019). Quantitative Research. In: Liamputtong, P. (eds) Handbook of Research Methods in Health Social Sciences. Springer, Singapore. https://doi.org/10.1007/978-981-10-5251-4_54
Download citation
DOI : https://doi.org/10.1007/978-981-10-5251-4_54
Published : 13 January 2019
Publisher Name : Springer, Singapore
Print ISBN : 978-981-10-5250-7
Online ISBN : 978-981-10-5251-4
eBook Packages : Social Sciences Reference Module Humanities and Social Sciences Reference Module Business, Economics and Social Sciences
Share this entry
Anyone you share the following link with will be able to read this content:
Sorry, a shareable link is not currently available for this article.
Provided by the Springer Nature SharedIt content-sharing initiative
- Publish with us
Policies and ethics
- Find a journal
- Track your research
- Privacy Policy

Home » Quantitative Research – Methods, Types and Analysis
Quantitative Research – Methods, Types and Analysis
Table of Contents

Quantitative Research
Quantitative research is a type of research that collects and analyzes numerical data to test hypotheses and answer research questions . This research typically involves a large sample size and uses statistical analysis to make inferences about a population based on the data collected. It often involves the use of surveys, experiments, or other structured data collection methods to gather quantitative data.
Quantitative Research Methods

Quantitative Research Methods are as follows:
Descriptive Research Design
Descriptive research design is used to describe the characteristics of a population or phenomenon being studied. This research method is used to answer the questions of what, where, when, and how. Descriptive research designs use a variety of methods such as observation, case studies, and surveys to collect data. The data is then analyzed using statistical tools to identify patterns and relationships.
Correlational Research Design
Correlational research design is used to investigate the relationship between two or more variables. Researchers use correlational research to determine whether a relationship exists between variables and to what extent they are related. This research method involves collecting data from a sample and analyzing it using statistical tools such as correlation coefficients.
Quasi-experimental Research Design
Quasi-experimental research design is used to investigate cause-and-effect relationships between variables. This research method is similar to experimental research design, but it lacks full control over the independent variable. Researchers use quasi-experimental research designs when it is not feasible or ethical to manipulate the independent variable.
Experimental Research Design
Experimental research design is used to investigate cause-and-effect relationships between variables. This research method involves manipulating the independent variable and observing the effects on the dependent variable. Researchers use experimental research designs to test hypotheses and establish cause-and-effect relationships.
Survey Research
Survey research involves collecting data from a sample of individuals using a standardized questionnaire. This research method is used to gather information on attitudes, beliefs, and behaviors of individuals. Researchers use survey research to collect data quickly and efficiently from a large sample size. Survey research can be conducted through various methods such as online, phone, mail, or in-person interviews.
Quantitative Research Analysis Methods
Here are some commonly used quantitative research analysis methods:
Statistical Analysis
Statistical analysis is the most common quantitative research analysis method. It involves using statistical tools and techniques to analyze the numerical data collected during the research process. Statistical analysis can be used to identify patterns, trends, and relationships between variables, and to test hypotheses and theories.
Regression Analysis
Regression analysis is a statistical technique used to analyze the relationship between one dependent variable and one or more independent variables. Researchers use regression analysis to identify and quantify the impact of independent variables on the dependent variable.
Factor Analysis
Factor analysis is a statistical technique used to identify underlying factors that explain the correlations among a set of variables. Researchers use factor analysis to reduce a large number of variables to a smaller set of factors that capture the most important information.
Structural Equation Modeling
Structural equation modeling is a statistical technique used to test complex relationships between variables. It involves specifying a model that includes both observed and unobserved variables, and then using statistical methods to test the fit of the model to the data.
Time Series Analysis
Time series analysis is a statistical technique used to analyze data that is collected over time. It involves identifying patterns and trends in the data, as well as any seasonal or cyclical variations.
Multilevel Modeling
Multilevel modeling is a statistical technique used to analyze data that is nested within multiple levels. For example, researchers might use multilevel modeling to analyze data that is collected from individuals who are nested within groups, such as students nested within schools.
Applications of Quantitative Research
Quantitative research has many applications across a wide range of fields. Here are some common examples:
- Market Research : Quantitative research is used extensively in market research to understand consumer behavior, preferences, and trends. Researchers use surveys, experiments, and other quantitative methods to collect data that can inform marketing strategies, product development, and pricing decisions.
- Health Research: Quantitative research is used in health research to study the effectiveness of medical treatments, identify risk factors for diseases, and track health outcomes over time. Researchers use statistical methods to analyze data from clinical trials, surveys, and other sources to inform medical practice and policy.
- Social Science Research: Quantitative research is used in social science research to study human behavior, attitudes, and social structures. Researchers use surveys, experiments, and other quantitative methods to collect data that can inform social policies, educational programs, and community interventions.
- Education Research: Quantitative research is used in education research to study the effectiveness of teaching methods, assess student learning outcomes, and identify factors that influence student success. Researchers use experimental and quasi-experimental designs, as well as surveys and other quantitative methods, to collect and analyze data.
- Environmental Research: Quantitative research is used in environmental research to study the impact of human activities on the environment, assess the effectiveness of conservation strategies, and identify ways to reduce environmental risks. Researchers use statistical methods to analyze data from field studies, experiments, and other sources.
Characteristics of Quantitative Research
Here are some key characteristics of quantitative research:
- Numerical data : Quantitative research involves collecting numerical data through standardized methods such as surveys, experiments, and observational studies. This data is analyzed using statistical methods to identify patterns and relationships.
- Large sample size: Quantitative research often involves collecting data from a large sample of individuals or groups in order to increase the reliability and generalizability of the findings.
- Objective approach: Quantitative research aims to be objective and impartial in its approach, focusing on the collection and analysis of data rather than personal beliefs, opinions, or experiences.
- Control over variables: Quantitative research often involves manipulating variables to test hypotheses and establish cause-and-effect relationships. Researchers aim to control for extraneous variables that may impact the results.
- Replicable : Quantitative research aims to be replicable, meaning that other researchers should be able to conduct similar studies and obtain similar results using the same methods.
- Statistical analysis: Quantitative research involves using statistical tools and techniques to analyze the numerical data collected during the research process. Statistical analysis allows researchers to identify patterns, trends, and relationships between variables, and to test hypotheses and theories.
- Generalizability: Quantitative research aims to produce findings that can be generalized to larger populations beyond the specific sample studied. This is achieved through the use of random sampling methods and statistical inference.
Examples of Quantitative Research
Here are some examples of quantitative research in different fields:
- Market Research: A company conducts a survey of 1000 consumers to determine their brand awareness and preferences. The data is analyzed using statistical methods to identify trends and patterns that can inform marketing strategies.
- Health Research : A researcher conducts a randomized controlled trial to test the effectiveness of a new drug for treating a particular medical condition. The study involves collecting data from a large sample of patients and analyzing the results using statistical methods.
- Social Science Research : A sociologist conducts a survey of 500 people to study attitudes toward immigration in a particular country. The data is analyzed using statistical methods to identify factors that influence these attitudes.
- Education Research: A researcher conducts an experiment to compare the effectiveness of two different teaching methods for improving student learning outcomes. The study involves randomly assigning students to different groups and collecting data on their performance on standardized tests.
- Environmental Research : A team of researchers conduct a study to investigate the impact of climate change on the distribution and abundance of a particular species of plant or animal. The study involves collecting data on environmental factors and population sizes over time and analyzing the results using statistical methods.
- Psychology : A researcher conducts a survey of 500 college students to investigate the relationship between social media use and mental health. The data is analyzed using statistical methods to identify correlations and potential causal relationships.
- Political Science: A team of researchers conducts a study to investigate voter behavior during an election. They use survey methods to collect data on voting patterns, demographics, and political attitudes, and analyze the results using statistical methods.
How to Conduct Quantitative Research
Here is a general overview of how to conduct quantitative research:
- Develop a research question: The first step in conducting quantitative research is to develop a clear and specific research question. This question should be based on a gap in existing knowledge, and should be answerable using quantitative methods.
- Develop a research design: Once you have a research question, you will need to develop a research design. This involves deciding on the appropriate methods to collect data, such as surveys, experiments, or observational studies. You will also need to determine the appropriate sample size, data collection instruments, and data analysis techniques.
- Collect data: The next step is to collect data. This may involve administering surveys or questionnaires, conducting experiments, or gathering data from existing sources. It is important to use standardized methods to ensure that the data is reliable and valid.
- Analyze data : Once the data has been collected, it is time to analyze it. This involves using statistical methods to identify patterns, trends, and relationships between variables. Common statistical techniques include correlation analysis, regression analysis, and hypothesis testing.
- Interpret results: After analyzing the data, you will need to interpret the results. This involves identifying the key findings, determining their significance, and drawing conclusions based on the data.
- Communicate findings: Finally, you will need to communicate your findings. This may involve writing a research report, presenting at a conference, or publishing in a peer-reviewed journal. It is important to clearly communicate the research question, methods, results, and conclusions to ensure that others can understand and replicate your research.
When to use Quantitative Research
Here are some situations when quantitative research can be appropriate:
- To test a hypothesis: Quantitative research is often used to test a hypothesis or a theory. It involves collecting numerical data and using statistical analysis to determine if the data supports or refutes the hypothesis.
- To generalize findings: If you want to generalize the findings of your study to a larger population, quantitative research can be useful. This is because it allows you to collect numerical data from a representative sample of the population and use statistical analysis to make inferences about the population as a whole.
- To measure relationships between variables: If you want to measure the relationship between two or more variables, such as the relationship between age and income, or between education level and job satisfaction, quantitative research can be useful. It allows you to collect numerical data on both variables and use statistical analysis to determine the strength and direction of the relationship.
- To identify patterns or trends: Quantitative research can be useful for identifying patterns or trends in data. For example, you can use quantitative research to identify trends in consumer behavior or to identify patterns in stock market data.
- To quantify attitudes or opinions : If you want to measure attitudes or opinions on a particular topic, quantitative research can be useful. It allows you to collect numerical data using surveys or questionnaires and analyze the data using statistical methods to determine the prevalence of certain attitudes or opinions.
Purpose of Quantitative Research
The purpose of quantitative research is to systematically investigate and measure the relationships between variables or phenomena using numerical data and statistical analysis. The main objectives of quantitative research include:
- Description : To provide a detailed and accurate description of a particular phenomenon or population.
- Explanation : To explain the reasons for the occurrence of a particular phenomenon, such as identifying the factors that influence a behavior or attitude.
- Prediction : To predict future trends or behaviors based on past patterns and relationships between variables.
- Control : To identify the best strategies for controlling or influencing a particular outcome or behavior.
Quantitative research is used in many different fields, including social sciences, business, engineering, and health sciences. It can be used to investigate a wide range of phenomena, from human behavior and attitudes to physical and biological processes. The purpose of quantitative research is to provide reliable and valid data that can be used to inform decision-making and improve understanding of the world around us.
Advantages of Quantitative Research
There are several advantages of quantitative research, including:
- Objectivity : Quantitative research is based on objective data and statistical analysis, which reduces the potential for bias or subjectivity in the research process.
- Reproducibility : Because quantitative research involves standardized methods and measurements, it is more likely to be reproducible and reliable.
- Generalizability : Quantitative research allows for generalizations to be made about a population based on a representative sample, which can inform decision-making and policy development.
- Precision : Quantitative research allows for precise measurement and analysis of data, which can provide a more accurate understanding of phenomena and relationships between variables.
- Efficiency : Quantitative research can be conducted relatively quickly and efficiently, especially when compared to qualitative research, which may involve lengthy data collection and analysis.
- Large sample sizes : Quantitative research can accommodate large sample sizes, which can increase the representativeness and generalizability of the results.
Limitations of Quantitative Research
There are several limitations of quantitative research, including:
- Limited understanding of context: Quantitative research typically focuses on numerical data and statistical analysis, which may not provide a comprehensive understanding of the context or underlying factors that influence a phenomenon.
- Simplification of complex phenomena: Quantitative research often involves simplifying complex phenomena into measurable variables, which may not capture the full complexity of the phenomenon being studied.
- Potential for researcher bias: Although quantitative research aims to be objective, there is still the potential for researcher bias in areas such as sampling, data collection, and data analysis.
- Limited ability to explore new ideas: Quantitative research is often based on pre-determined research questions and hypotheses, which may limit the ability to explore new ideas or unexpected findings.
- Limited ability to capture subjective experiences : Quantitative research is typically focused on objective data and may not capture the subjective experiences of individuals or groups being studied.
- Ethical concerns : Quantitative research may raise ethical concerns, such as invasion of privacy or the potential for harm to participants.
About the author
Muhammad Hassan
Researcher, Academic Writer, Web developer
You may also like

Questionnaire – Definition, Types, and Examples

Case Study – Methods, Examples and Guide

Observational Research – Methods and Guide

Qualitative Research Methods

Explanatory Research – Types, Methods, Guide

Survey Research – Types, Methods, Examples
How to Craft a Research Question
In the following we will work on crafting a successful research question. At this point, don't be committed to a methodology, and beware that you are not writing a question that unconsciously leans to a particular methodology.
The process you follow is critical, not the methodology, at least not yet.
In the following, you will:
- Learn the process of writing a successful research question.
- Apply this process to your own research topic and questions.
- What research questions are.
- Types of research questions and subquestions.
- Ways qualitative and quantitative questions differ.
- How hypotheses go with questions in quantitative methodology.
Let's get started.
Developing a Research Question
Learning to write good research questions is a skill that takes practice. Developing a research question is a developmental process. As you read the literature and gain a greater understanding about your research problem, you will rework your research question until you are able to focus more specifically on what you want to explore and learn about during the formal research process. Keep in mind that a question typically goes through several iterations. So don't worry if your first attempts may not be the final product. This is normal.
Getting Oriented to Research Questions
Let's get oriented first. The research question is what the researcher seeks to answer by collecting data. It is the foundation of the entire study, because the question embodies the method by which the research problem—called for by the existing literature—will be solved. It's simple: You are going to solve your research problem by collecting information, and you collect that information by asking about a specific question or set of questions.
Simply by reading a well-formed research question, you can usually tell:
- What concept(s), phenomena, or variables are going to be measured in quantitative research or described in qualitative research.
- What research design will be used.
- What the sample will consist of.
As we proceed you will need to have knowledge of a variety of research terms. If you don't recognize and understand these terms, there are handouts in the Dissertation Research Seminar Courseroom that you can refer to. We strongly encourage you to take notes as you work your way through this document, and make sure that you understand each section.
Let's work on quantitative questions first.
What Quantitative Research Questions Do
Quantitative research questions address the:
- description of variables being investigated,
- measurement of relationships between (at least two) variables,
- differences between two or more groups' scores on a variable or variables, and so on.
They also clearly identify the sample that will be questioned. Most importantly, they use the same language for these elements as the research problem used. Your research question needs to cover all three items.
Quantitative questions that are appropriate for a dissertation are worded to seek verification of a hypothesis, that is, a prediction. These predictions are based on the existing literature, and should be entirely consistent with the research problem (which comes from the literature).
Quantitative questions are written in two formats: conceptual and operational.
- Conceptual questions appear in chapter 1 of the proposal (and dissertation). They name the concepts being investigated as concepts.
- Operational questions name the concepts as variables, that is, as something that can vary or change; the research question is written operationally in chapter 3 of the proposal.
An example of Conceptual vs. Operational Questions
Let's take a subject like the relationship between depression and alcoholism as our example. First, here is a simple conceptual version:
What is the relationship between depression and alcoholism?
Okay. Now here is an example of an operational version of the same question:
Is there a statistically significant correlation between levels of depression and degree of alcoholism?
Notice three changes: between the conceptual and the operational versions of the question:
- The concept of "relationship" is operationalized as "a statistically significant correlation" (which is a specific, statistical form of a relationship).
- The concept "depression" is operationalized as "levels of depression." It might have been rewritten as "types of depression" too.
- The concept "alcoholism" is operationalized as "degree of alcoholism." It might have been written as "stage of alcoholism."
Because quantitative questions seek verification, a critical piece of the analysis will be to discover whether or not the results (e.g., the correlation between two variables) are due to chance or whether they can be considered real. Therefore, operational quantitative questions will always contain some way of asking about "statistical significance." They will not ask, "Is there a correlation between A and B?" They will ask, "Is there a statistically significant correlation …?"
Quantitative Main Questions and Subquestions
Quantitative studies usually pose more than one question. Indeed, all quantitative questions will have a set of subquestions. But some studies require additional main questions (and their subquestions).
Here's a conceptual question as an example:
Which psychological and organizational factors associated with employee burnout are most predictive of reduced productivity?
If it was found that a gap exists in the current literature as to what psychological and organizational factors are associated with employee burnout, then in that question there are really two questions:
- What are the psychological and organizational factors associated with employee burnout? And
- Which of those factors are most predictive of reduced productivity?
You'll note that the first question asks for a correlation, and the second one asks for a prediction. You can consider these to be two main questions, then.
Quantitative Main Questions Require Subquestions
Now, each quantitative main question requires subquestions. In qualitative methodology, you only have the main question and there is almost never any reason for more than one main question. But we're talking about quantitative here, so let's look at examples of subquestions. Take this conceptual main question:
Is being managed by an authoritarian manager a better predictor of employee burnout than being managed by a transformational manager?
Now we can transform that into an operational main question:
Is there a statistically significant difference in levels of burnout in employees managed by authoritarian managers compared with employees managed by transformational managers?
Before we can answer the main question, we need information on all the variables. First, we need to measure management style so we can create groups based on that concept:
- Who are the authoritarian managers?
- Who are the transformational managers?
Answering those two questions will allow us to group employees in either the authoritarian or the transformational group.
Next, we need to measure the levels of burnout in our two groups of employees:
- What are the levels of burnout in employees managed by authoritarian managers?
- What are the levels of burnout in employees managed by transformational managers?
Once we get the answers to those subquestions, we can proceed to the statistical analysis that will answer the main question about which management style better predicts employee burnout.
Main Questions are Complex, Subquestions Simpler
You will notice that in our examples, each subquestion describes a variable. Subquestions are almost always descriptive questions (and nearly all qualitative questions are descriptive). Very complex main questions asking for quite complicated statistical tests might require correlational subquestions to support the main analysis, but in general, subquestions typically are descriptive. Main questions, on the other hand, must, for a Capella dissertation, be at least correlational or predictive.
Let's see what these types of questions look like.
DESCRIPTIVE Quantitative Questions
Descriptive questions ask what a single measure is. For example:
What are the reading scores of 3rd graders receiving special education assistance in rural Minnesota schools?
Here, there is only one variable being described: reading scores. The most common subquestions are descriptive, obtaining the measures of each of the main question's variables.
Notice too, that a descriptive question can be framed conceptually or operationally:
- Conceptual version: What are the reading scores . . . ? is conceptual.
- Operational version: What are the mean reading scores . . .? is operational.
CORRELATIONAL Quantitative Questions
The word "quantitative" in the title of this section is actually redundant, because qualitative questions never ask for relationships between variables, or correlations. Correlational questions ask for a calculation of a relationship between two or more variables and its statistical significance. For example:
Is there a statistically significant correlation between time spent in the school bus each day and the reading scores of rural special education 3rd graders?
Usually, a dissertation will not be this simple. Successful dissertations ask somewhat more complicated questions, either asking about multiple variables or asking about a predictive or causal relationship. However, in a complex causal question, there may need to be some correlational subquestions in order to compare groups, for example. And of course, for each correlation, there will need to be two or more descriptive subquestions.
Here too, the correlational question can be framed either conceptually or operationally:
- Conceptual version: What is the relationship between . . .? is conceptual.
- Operational version: Is there a statistically significant correlation . . .? is operational.
DIFFERENCE (or PREDICTIVE) Questions
Difference questions form a set of questions that look for causal relationships between two or more conditions. Depending on the type of relationship being examined, different words are used. The general conceptual framework is:
Does A cause B?
The word "cause" has different meanings, and capturing those meanings will express a more precise question. For example,
What is the influence of A on B?
Other words indicate specific kinds of causal relationships, such as:
When the question asks for "effects," it is asking for a cause-effect relationship between or among variables.
The conceptual version of the causal or predictive questions is straightforward:
What is the effect of A on B?
Or you might ask, what is the influence of A on B? To what extent is B affected by A?
Does A predict B?
The operational version of the question needs to be carefully framed to detect precisely the kind of causal relationship you're interested in.
Is there a statistically significant difference between mean reading scores of rural special education 3rd graders who spend more than 60 minutes a day on the school bus as compared with those who spend fewer than 30 minutes a day on the school bus?
This operational question would be asked when a cause-effect relationship is suspected. If a statistically significant difference is found and if that difference is reasonably strong, the conclusion might be that time on the school bus affects, influences, impacts, predicts, perhaps even causes differences in the reading scores.
Quantitative Research Questions Measure Variables
Very simply, quantitative questions measure variables. We have found that a large number, perhaps a majority, of doctoral learners do not really understand variables, and this lack of understanding causes them significant time loss when writing their proposals. Remember, the research question is the "driver" of your methodology and design, and if you do not understand your variables, your question will be off-track and the design may be wrong.
If you have any confusion about any of these terms please take a minute and study the handout on variables available in the courseroom or refer to the discussions of variables in your statistics and research methods texts.
We have one more element to consider regarding quantitative research questions, namely, hypotheses.
Again, you may be familiar with hypotheses, but if you are not, please review your research methods and statistical textbooks to refresh your understanding. Discussing why hypotheses are necessary in quantitative research is beyond the scope of this particular document.
First, here are the characteristics of hypotheses.
- Hypotheses are declarative sentences, not questions.
There will be a statistically significant correlation between time spent in the school bus each day and the reading scores of rural special education 3rd graders.
- Hypotheses should mimic exactly the language and sentence structure of the research question. They should be exact transformations from the question into a declarative sentence, with no difference words, word order, or sentence structure.
- Hypotheses are only made for correlational questions and above.
- There are always two hypotheses for each research question, the
- Null hypothesis, which is conventionally stated first and declares that there will be no statistically significant finding, and the
- Alternate hypothesis, which declares that there will be a statistically significant finding.
Examples of the Hypotheses
The Null hypothes is states the research question in the negative. Using our 3rd grade reading example, the null hypothesis would be:
H 0 : There will be no statistically significant correlation between time spent in the school bus each day and the reading scores of rural special education 3rd graders.
The Alternate hypothesis states the research question in the positive. For example,
H 1 : There will be a statistically significant correlation between time spent in the school bus each day and the reading scores of rural special education 3rd graders.
Note how the two symbols for the null and the alternate are constructed. H 0 always indicates the null, and H 1 always indicates the alternate. H of course stands for hypothesis.
Having considered how to construct quantitative research questions, let's turn our attention to qualitative research questions.
Qualitative Research Seeks Discovery and Description
Qualitative research seeks discovery. Qualitative research questions often are chosen because the research problem indicates that little is known about the topic. Perhaps a great deal of statistical knowledge exists, but no one yet has inquired into how the people involved experience the topic. There are cases in which the topic is meaningful but no one yet has begun to investigate it. In both instances, the researcher decides to go into the field and discover how people describe their experience of the phenomenon.
In other words, qualitative research questions are always descriptive in some way or another and are written so that they obtain descriptive verbal information from participants.
Just like quantitative questions, the way the qualitative question is written suggests the specific qualitative design. For example:
- Qualitative case study questions: show a bounded system, ask for multiple sources of data, and seek "lessons learned."
- Grounded theory questions ask participants who have experienced some process to describe the process they experienced.
- Generic qualitative questions: ask people to share verbal information about experiences, events, opinions, attitudes, beliefs, and the like.
- Phenomenological questions ask participants to describe the lived experience of some psychological experience.
All qualitative questions inquire into descriptions, observations, and interpretations. They do not inquire into relationships between variables or seek causal explanations.
What Qualitative Questions Do
Qualitative research questions seek to discover the:
- Participant's verbal descriptions of a phenomenon being investigated, or
- Researcher's observations of the phenomenon being investigated, or
- An integrated interpretation of participant's descriptions and researcher's observations.
In only one qualitative design, namely Grounded theory, the researcher seeks to explain a process by relying on verbal descriptions of that process by participants who have undergone it. This is the one kind of qualitative design that goes beyond simple description.
Qualitative questions also identify the sample that will be questioned. Most importantly, they use the same language for these elements as were used in the research problem.
What Qualitative Questions Do Not Do
Qualitative questions do not:
- Measure variables or values. The data of qualitative inquiry are words or images only.
- Measure relationships between variables.
- Compare differences between scores or groups.
- Seek statistical significance.
As a result, qualitative questions also do not:
- Require operational versions.
- Require subquestions.
- Require hypotheses.
On a side note, many qualitative studies use interviews to collect their data, and of course, interviews will require that questions be asked. But these data collection questions are not our topic here. Here, we're concerned with how to craft the main research question only. So let's talk about that next.
Examples of Successful Qualitative Research Questions
Here are some examples of good qualitative questions, with the probable research design that would spring from them.
If one wished to learn how monks describe the everyday experience of being lonely, a good research question might be:
How do religious monks describe the lived experience of monastic loneliness?
This would lead to a phenomenological study.
Suppose the research problem is that it is not known how persons suffering from early-onset dementia come to terms with having the disorder. A research question for this might be:
How do persons diagnosed with early-onset Alzheimer's disease describe their processes of coming to terms with the disease?
Because this asks about a process, that is, a movement from one condition, being diagnosed, to another condition, coming to terms with the diagnosis, and because it asks for evidence from those who have experienced the process, it would trigger a Grounded theory study.
What if the research problem is that although much is known statistically about best treatments for a given psychological problem, too little is known about what the experience of receiving that treatment is like. For this, a good research question might be:
What can be learned from patients with dissociative identity disorder, their caregivers, and their families about the various aspects of the experience of inpatient treatment at a specialized large urban treatment facility?
Because this question asks for verbal information from a number of sources (patients, caregivers, and families), clearly identifies a "bounded system" (a specific facility for treating dissociative identity disorder), and asks for "lessons learned," it clearly specifies qualitative case study for its design.
One might be interested in what factors shape and inform the careers of successful business leaders. A good qualitative research question for this topic could be:
How do successful business leaders describe the forces, experiences, and influences that shaped and informed their careers?
Because the subject matter is about external factors—forces, experiences, and influences—and because the question does not flow naturally to the other designs, this would be an excellent generic qualitative inquiry question.
Words that Characterize Qualitative Research Questions
Here is a list of words that are commonly used in qualitative questions:
- Understand.
- Experience.
- Experiences.
- Perceive . . . or perceptions.
- Attitudes . . . beliefs . . . opinions.
All these share the common characteristics: that they inquire about subjective experience and require verbal answers.
Now you will have the opportunity to practice the working with the information from this document by actually craft your own research questions, one qualitative, one quantitative.
Doc. reference: phd_t2_u04s4_craftrq.html
To read this content please select one of the options below:
Please note you do not have access to teaching notes, coffee in capsules consumers’ behaviour: a quantitative study on attributes, consequences and values.
British Food Journal
ISSN : 0007-070X
Article publication date: 3 July 2020
Issue publication date: 24 December 2020
Coffee in capsules consumers’ behaviour depends not only on the products’ attributes, but also the consequences perceived by them and the alignment with their values. This paper aims to investigate the impacts of the Attributes of coffees in capsules on the consequences perceived by consumers concerning their consumption and the effects of these Consequences on consumers’ Values.
Design/methodology/approach
This study developed a scale for assessing the perception of consumers of coffee in capsules about Attributes, Consequences and Values (A-C-V) regarding its consumption. A link to this survey’s electronic questionnaire was posted on the social networks Facebook and Peabirus. This research sample is for convenience and accessibility and has 213 consumers of coffee in capsules. Structural Equation Modelling (SEM) was the statistical method used for data analysis.
Attributes have two sub-dimensions (Own attributes and Functional attributes), while Consequences have three sub-dimensions (Handling Benefits, Rational Benefits, Convenience Benefits) and Values have just one dimension. Also, SEM has shown a statistically significant positive relationship between A-C-V perceived by consumers of coffee in capsules. These results confirm the hypotheses developed based on the Means-End Chain Theory (MEC).
Originality/value
As academic contributions, this paper develops a structural model that quantitatively demonstrates the impacts of Attributes perceived by consumers of coffee in capsules on the Consequences of consumption and its effects on their Values. The present survey is the first in the literature that uses structural models contemplating A-C-V. As managerial contributions, this survey provides relevant information to the decision-making of several stakeholders of the chain of coffee in capsules.
- Attributes-consequences-values
- Coffee in capsules
- Agribusiness
- Means-end chain theory
- Consumer behaviour
- Food and drink
Acknowledgements
This paper is financed by Universidade Federal de Mato Grosso do Sul and by the National Funds provided by FCT-Foundation for Science and Technology through project UIDB/04020/2020.
Oliveira, A.S.d. , Souki, G.Q. , Gandia, R.M. and Vilas Boas, L.H.d.B. (2021), "Coffee in capsules consumers’ behaviour: a quantitative study on attributes, consequences and values", British Food Journal , Vol. 123 No. 1, pp. 191-208. https://doi.org/10.1108/BFJ-02-2020-0116
Emerald Publishing Limited
Copyright © 2020, Emerald Publishing Limited
Related articles
We’re listening — tell us what you think, something didn’t work….
Report bugs here
All feedback is valuable
Please share your general feedback
Join us on our journey
Platform update page.
Visit emeraldpublishing.com/platformupdate to discover the latest news and updates
Questions & More Information
Answers to the most commonly asked questions here

Quantitative Research
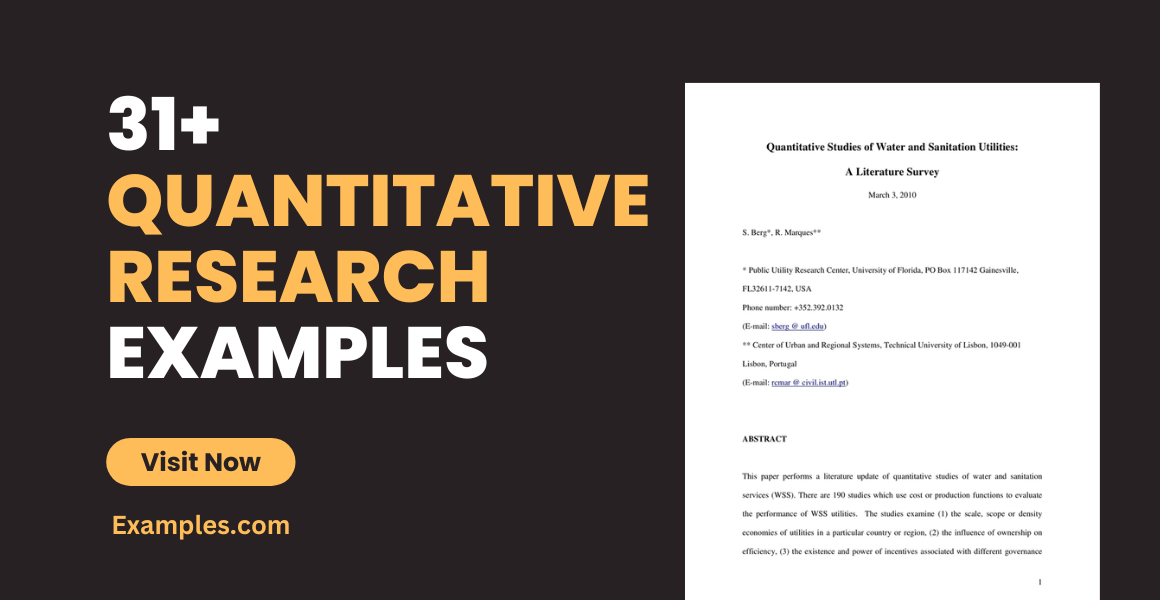
In conducting quantitative research, you need to make sure you have the right numbers and the correct values for specific variables. This is because quantitative research focuses more on numeric and logical results. Quantitative studies report and understand numerical data to make further analysis of a given phenomenon. This research organizes and computes statistics from current and prospect clients to make business forecasts for your company. Quantitative analysis examples also uses methods like polls, surveys, and sampling to gather information that can help complete your investigation.
31+ Quantitative Research Examples
Quantitative research demands focus and precision from the researcher. If you need a guide in doing your research, here are 10+ Quantitative research examples you can use.
1. Free Quantitative Research Flowchart Example
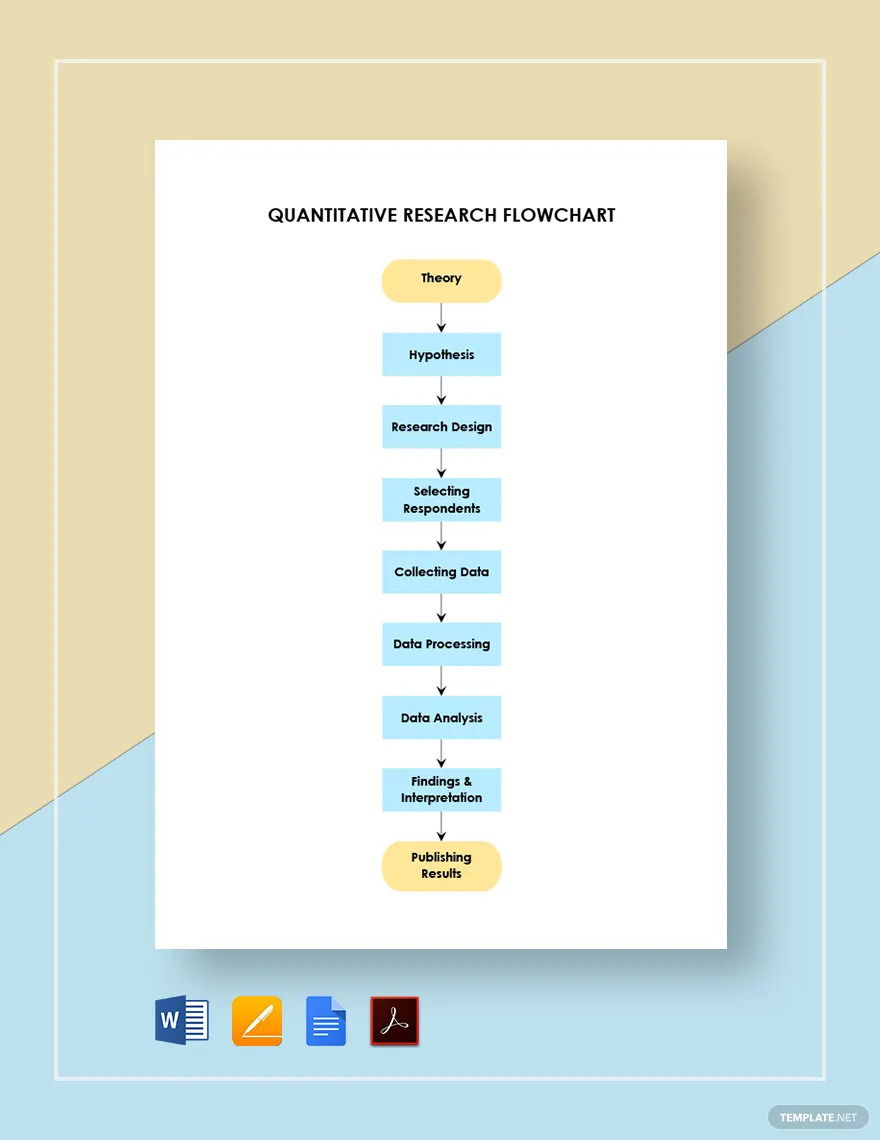
- Google Docs
- Apple Pages
Size: 80.2 KB Download
2. Free Quantitative Research Analyst Resume Example
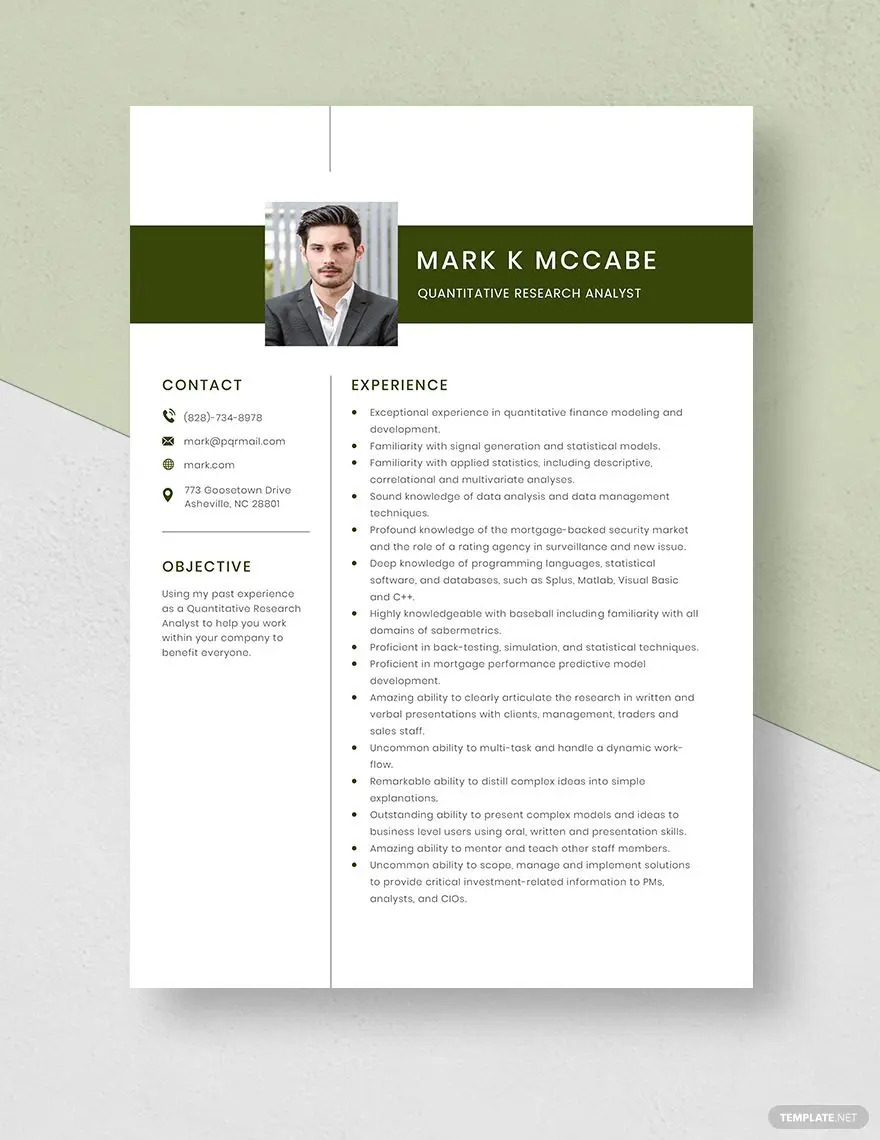
Size: 146 KB
3. Quantitative Research Review Template
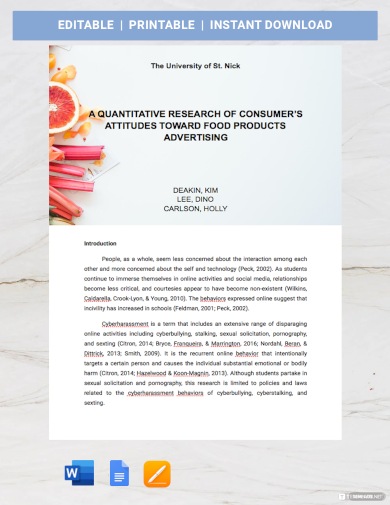
Size: 163 KB
4. Quantitative Research Plan Template
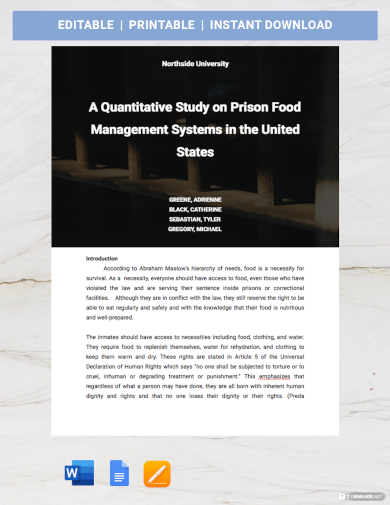
Size: 152 KB
5. Quantitative Research Descriptive Analysis Template
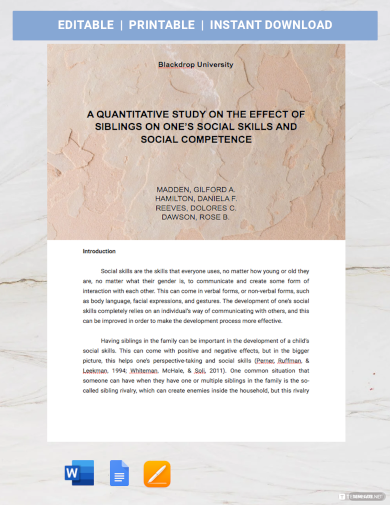
Size: 207 KB
6. Quantitative Research Checklist Template
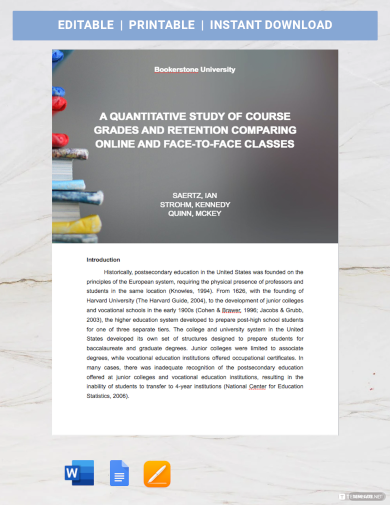
Size: 168 KB
7. Quantitative Research Survey Template
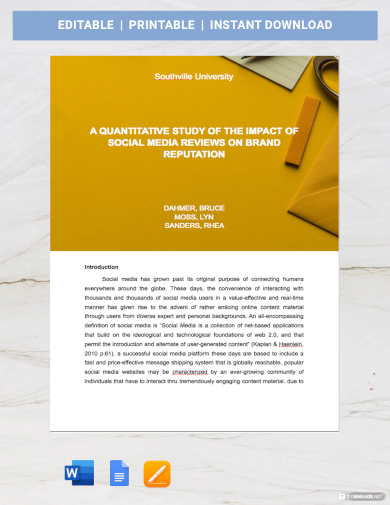
Size: 182 KB
8. Quantitative Research Data Analysis Template
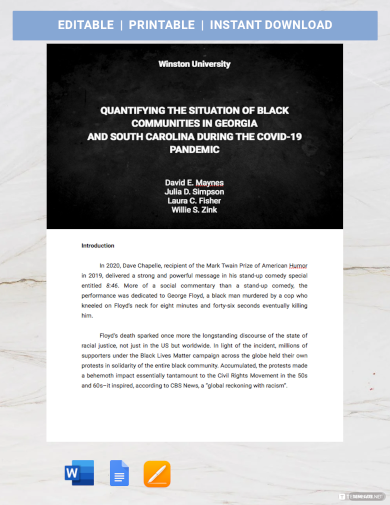
Size: 145 KB
9. Quantitative Research Guide Template
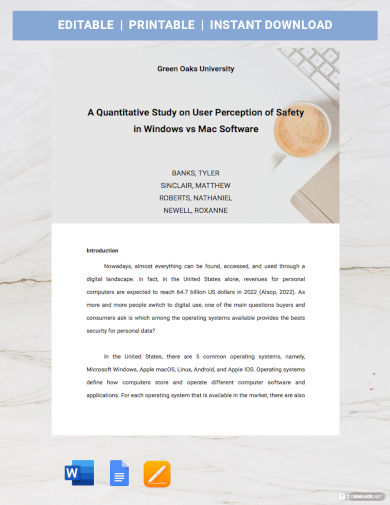
Size: 134 KB
10. Quantitative Research Proposal Template
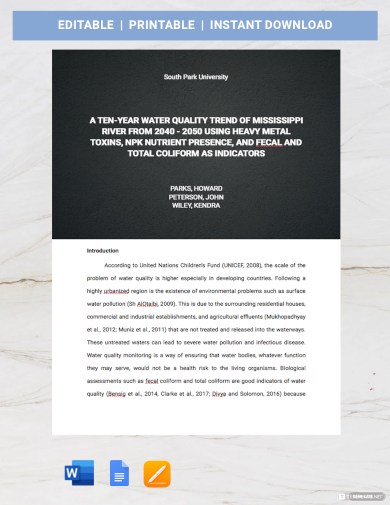
Size: 185 KB
11. Quantitative Research Question Template
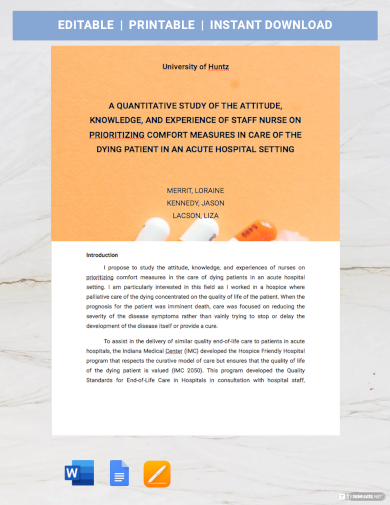
Size: 186 KB
12. Quantitative Research Literacy Template
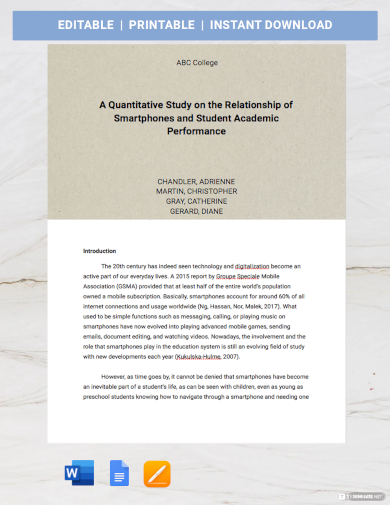
Size: 184 KB
13. Quantitative Research Correlation Template
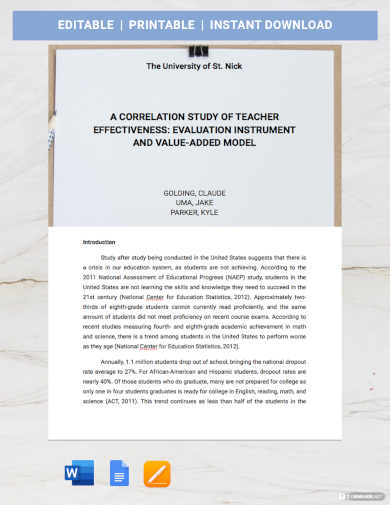
Size: 162 KB
14. Quantitative Research Template
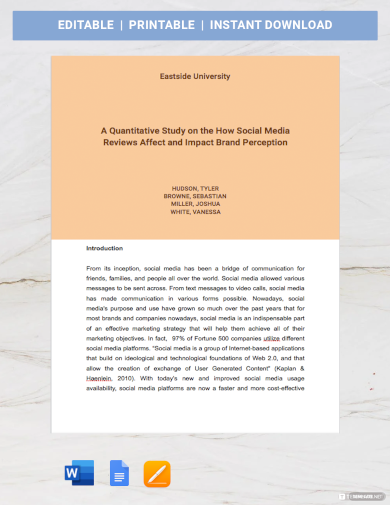
Size: 144 KB
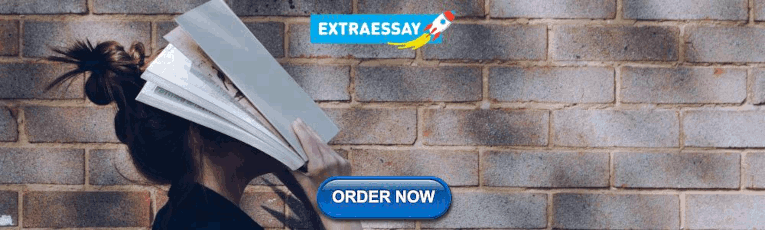
15. Quantitative Research Report Template
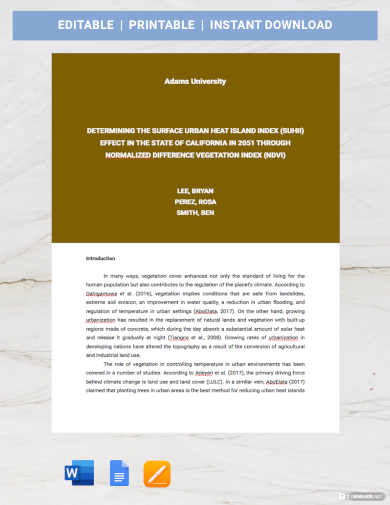
16. Simple Quantitative Research Template
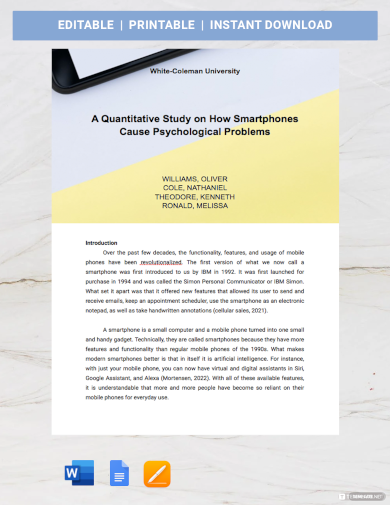
Size: 167 KB
17. Quantitative Research Paper Template
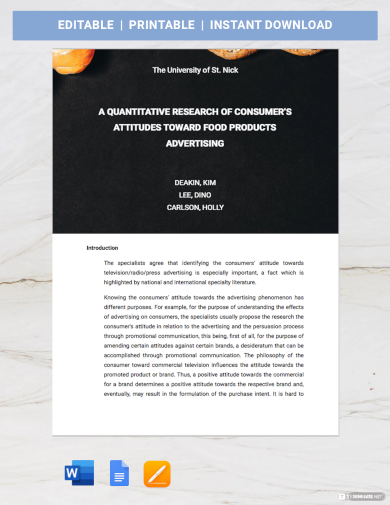
Size: 173 KB
18. Example of Quantitative Research
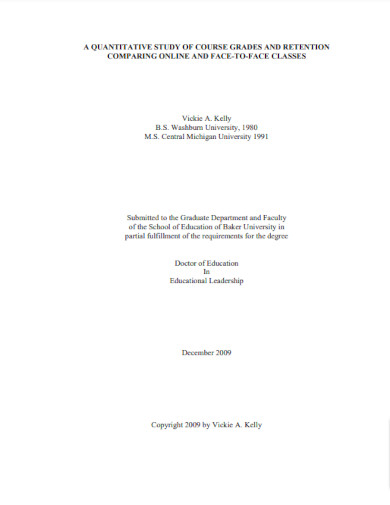
Size: 268 KB
19. Quantitative Research Design Examples
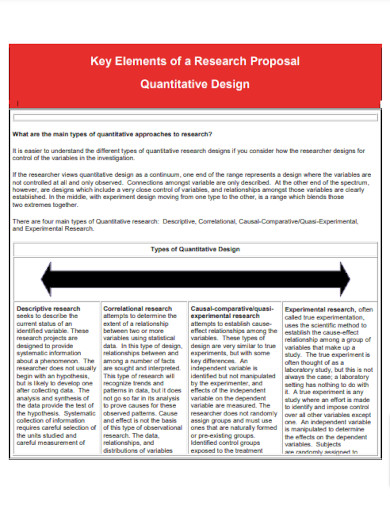
Size: 30 KB
20. Quantitative Research Examples for Students
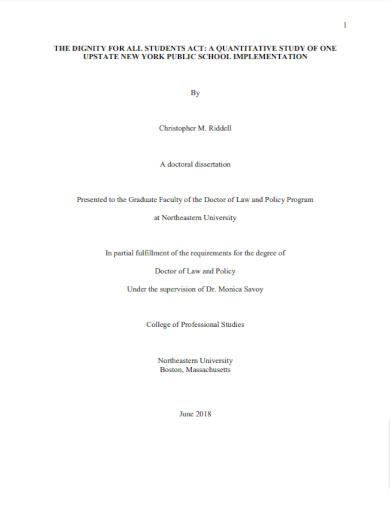
Size: 938 KB
21. Impact of Social Media Reviews on Brands Perception Example
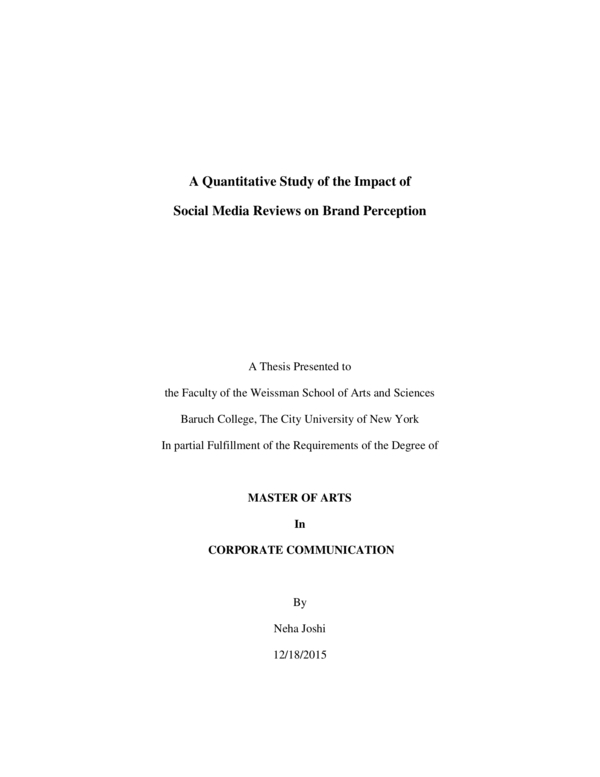
Size: 1.5 MB
In the age where likes, comments, and retweets measure the relevance of an entity online, brands make sure that their followers and customers have a positive perception of them on the web. The internet puts companies and individuals at a spot where the public eye sees reviews and comments about them. But how do these things affect the way people view a company’s branding? This quantitative study of the impact of social media reviews on brands perception answers that. Use this research as a guide in conducting your quantitative research.
22. Teacher Perceptions of Professional Learning Communities Example
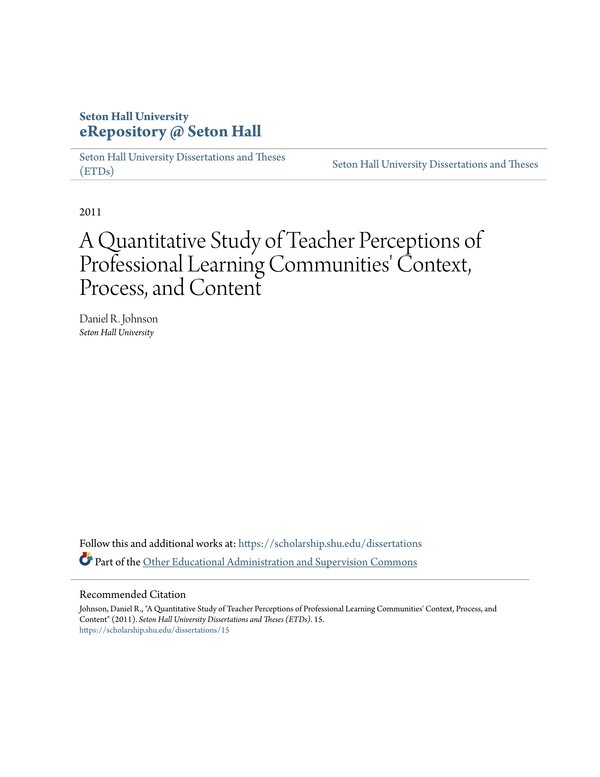
Size: 1.2 MB
Educators lead young minds to great success. That is why there are training programs examples and models where teachers can collaborate and share how they can improve students’ learning. Saying this, some do question the effectiveness of models such as Professional Learning Communities. Research called “A Quantitative Study of Teacher Perceptions of Professional Learning Communities’ Context, Process, and Content,” looks into these queries. If you are conducting your quantitative research, you can use this research as an example for your study. Format your content like this investigation for a foolproof thesis paper.
23. Quantitative Research On The Level of Social Media Addiction Example
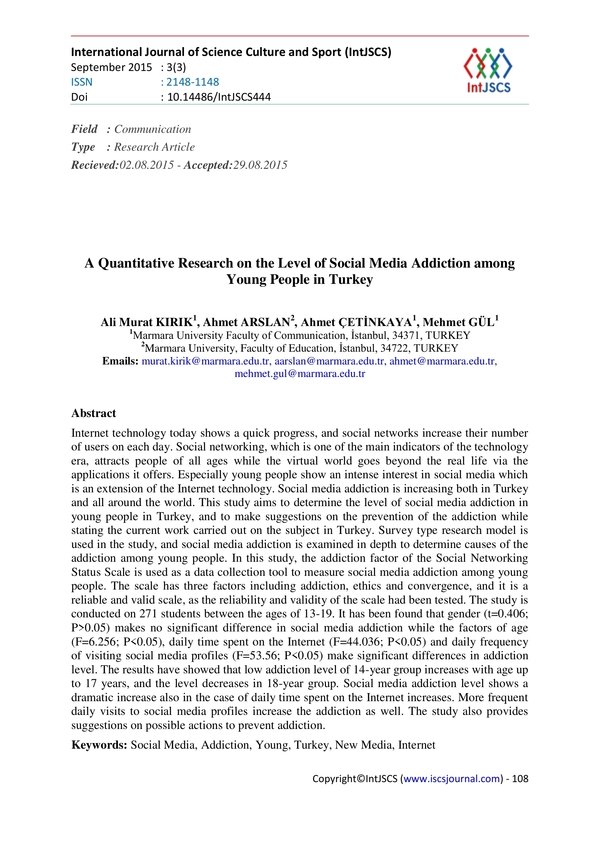
Size: 658.2 KB
The worldwide web is a being of wonder and mystery. That’s what makes it fascinating to young audiences. The internet helps them connect and interact with people through various social media platforms. With features and advancements that intrigue even the unexcited, addiction does become inevitable. An investigation in 2015 titled “A Quantitative Research on the Level of Social Media Addiction among Young People in Turkey” looks into the statistics of this problem. For your quantitative research, use this study as a guide in organizing and formatting your quantitative data.
24. Course Grades and Retention Comparing Online and Face-to-face Classes
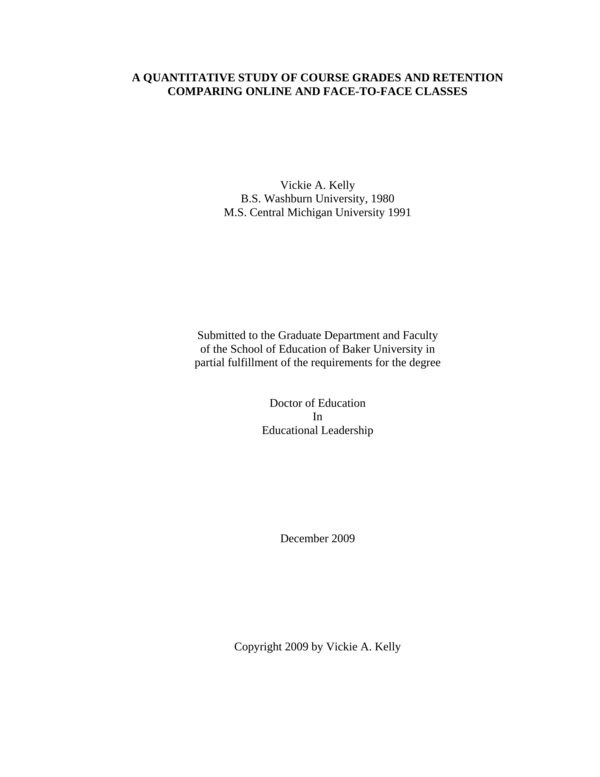
Size: 274.4 KB
Are you taking online classes, or are your classes held in a classroom? Do you believe there is a difference between online and face-to-face courses? There has always been a discussion between which education instructional method is more effective. Although both help students learn, some argue that the way they are taught makes an education gap. This quantitative study of course grades and retention comparing online and face-to-face classes can help answer your questions. It can also serve as a model in making your own quantitative research. Pattern your research design like this one now!
25. Free Nursing Quantitative Research Proposal Example
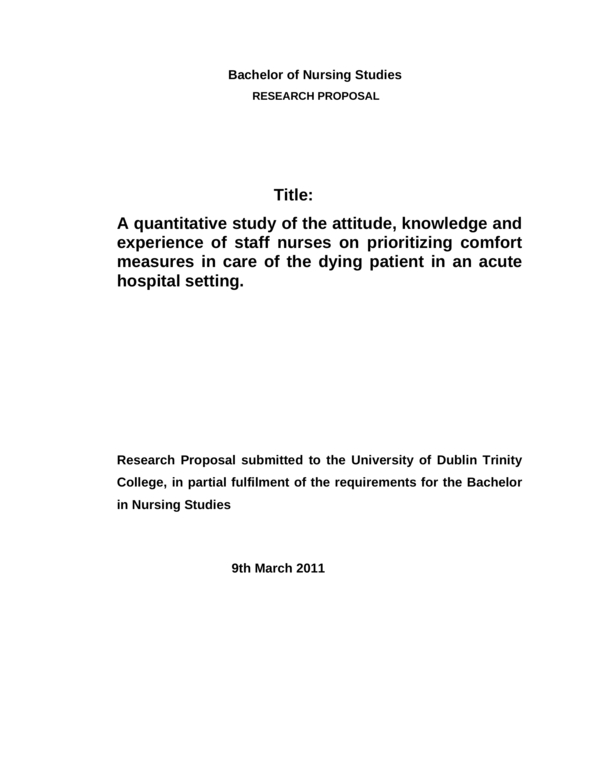
Size: 201.7 KB
One of a nurse’s primary duties is to assure patients are taken care of and attended to. Their line of work deals with peoples’ lives and health. This also means that they still need to address patients even if they’re close to death. In Ireland, a study called “A Quantitative Study of the Attitude, Knowledge, and Experience of Staff Nurses on Prioritizing Comfort measures in Care of the Dying Patient in an Acute Hospital Setting” was conducted. If you plan on undertaking any medical SWOT analysis , using this study as a guide would be beneficial for you.
26. Quantitative Research Of Consumer’s Attitude Towards Food Products Advertising
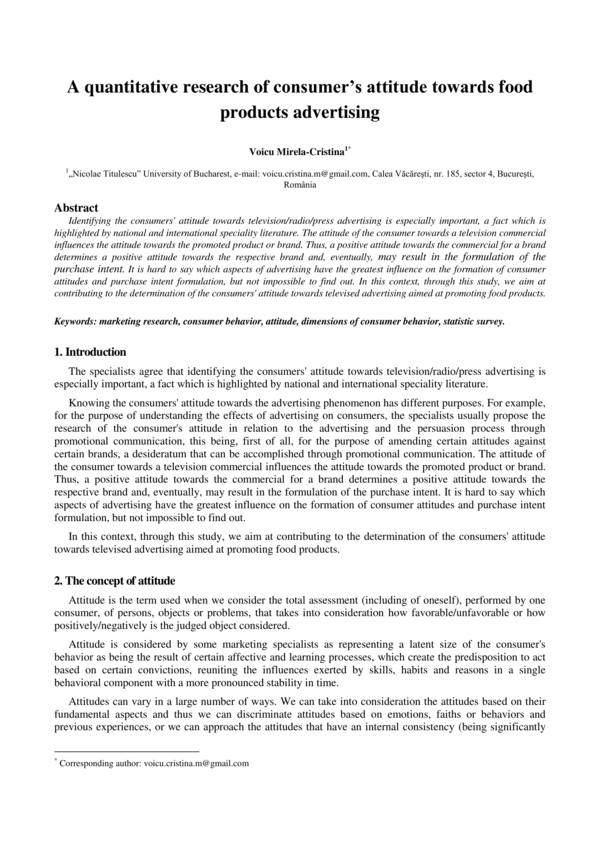
Size: 845.8 KB
In the corporate world, you can’t just start selling something without proper research. You first have to make sure that your products and services are relevant and marketable. The first step should be conducting marketing research. Marketing research can use either qualitative or quantitative data collection methods. But if you want to figure out how your clients react to your products and marketing strategy, this quantitative research of consumer’s attitude towards food products advertising could be your guide. You can even use this for your undergraduate research.
27. Free Effective Teacher Leadership Example
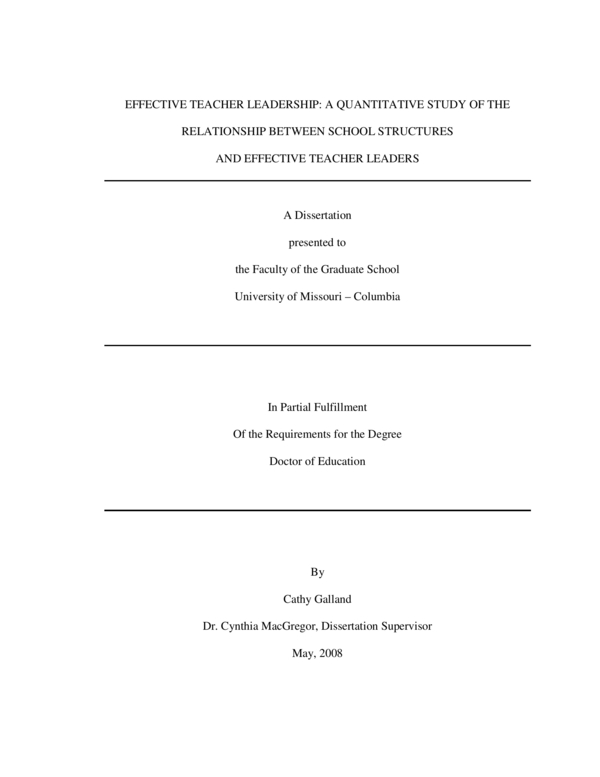
Size: 407.1 KB
Research projects have to be conducted with precision and accuracy, especially if it’s quantitative research. You need to make sure you get the right numbers to get valid results. In research called “Effective Teacher Leadership: A Quantitative Study of the Relationship Between School Structures and Effective Teacher Leaders,” quantitative data analysis is conducted to look into the school’s management plans. For your research, this would be a useful guide in doing comprehensive qualitative research. You can outline your investigations and even term papers using this as a sample.
28. Quantitative Studies of Water and Sanitation Utilities Example
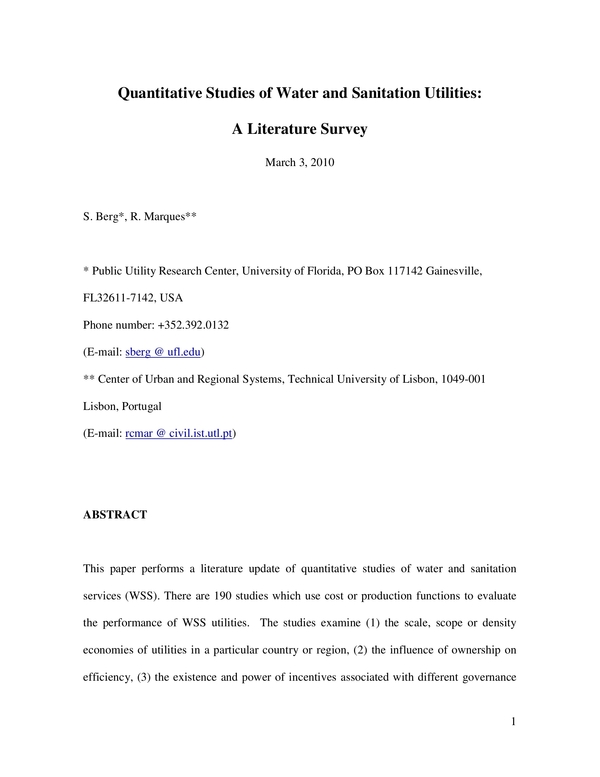
Size: 376 KB
Quantitative research is a method that studies numerical values. It follows a strict process of data collection. This type of research is used by different industries and even as undergraduate research. That is why the research design should reflect the nature of your research. It should look professional and comprehensive. But that doesn’t mean that your research project plan has to look dull. This study called “Quantitative Studies of Water and Sanitation Utilities: A Literature Survey” can be used as a sample. It’s research methodology utilizes surveys as a way to collect data needed for research.
29. Free Perceptions of First Year College Students Example
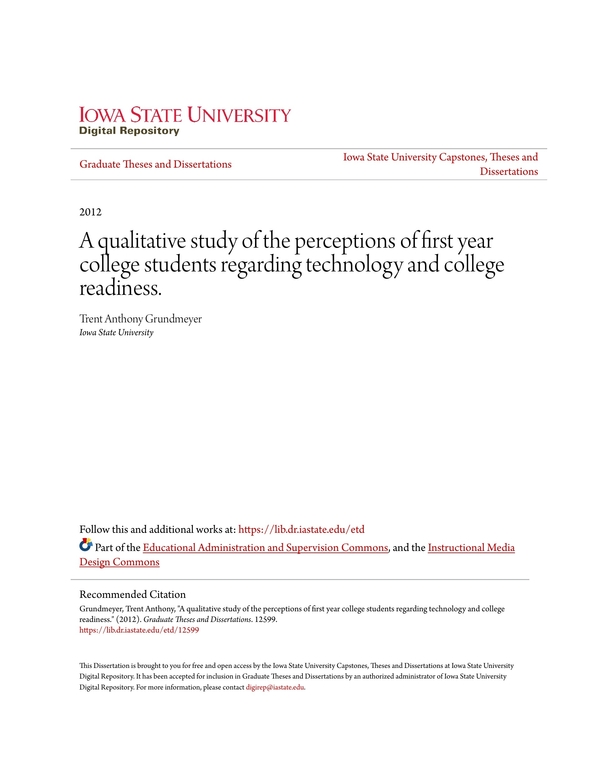
Do you want kids to be college-ready? Are you looking for a college planner to prepare high school kids for a higher level of education? The first year of college serves as an adjustment period for students. The way they cope and accustom themselves use different methods. That’s why you need a study to help you. If your research looks into college kids, this qualitative study of the perceptions of first-year college students regarding technology and college readiness could be your guide. Us it as an outline for the quantitative research you are conducting.
30. Free Qualitative Research Paper Example
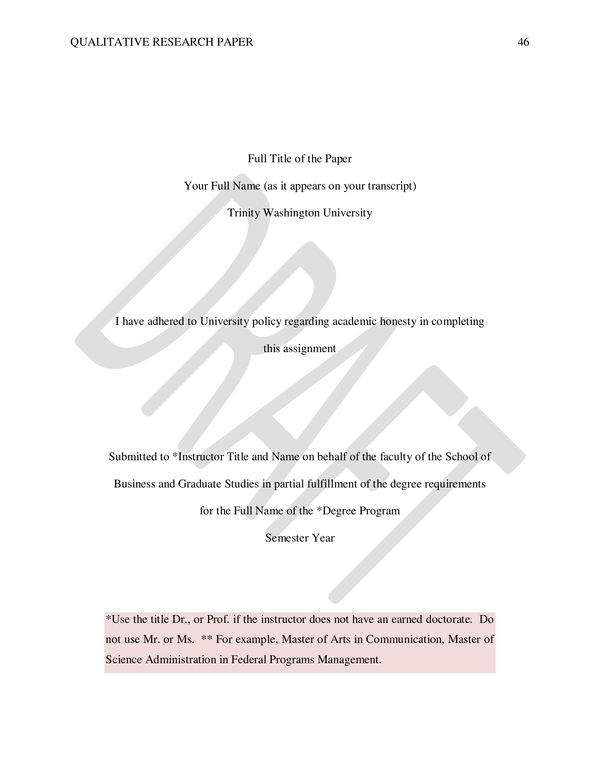
Size: 698.6 KB
Like any research, you must follow a particular format. A poorly organized study might give the impression of having unreliable data and results. You need to make sure your research is detailed and understandable. This applies significantly to quantitative project analysis example . This type of investigation urges researchers to be careful and efficient when gathering and analyzing information and statistics. Getting the wrong value can mess up your whole investigation. For your research, you can make use of this qualitative research paper as an outline. It details all the right parts needed in your research.
31. Quantitative Research For Health Programmes Example
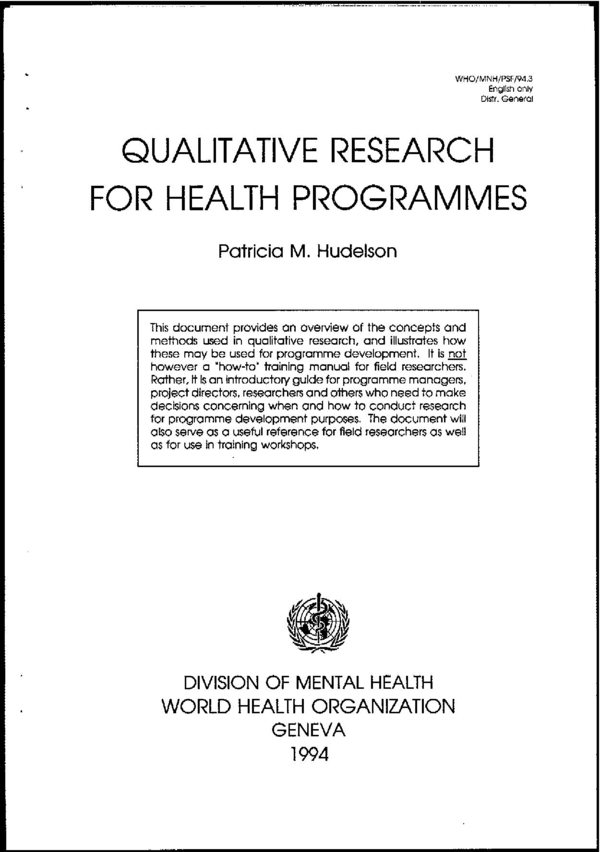
Size: 2.4 MB
If you are creating health newspapers and programs, you need to make sure you have the correct data. Your program will tackle a person’s health so you need to have the correct information as not to cause further complications. That’s also why you need to conduct quantitative research to get precise data. For your research, you can make this quantitative research for health programmes your guide. The World Health Organization uses it so you can be sure it is professionally made. Follow the formats on this document to make sure your research is high-quality.
What are the Quantitative research characteristics?
- Objective and Empirical: Quantitative research is based on objective and empirical observations, focusing on measurable, observable phenomena. It aims to collect data that can be analyzed statistically.
- Numerical Data: It primarily relies on numerical data, such as counts, measurements, percentages, and statistics, to draw conclusions and make comparisons.
- Structured and Controlled: Quantitative research is highly structured and controlled, with predefined methods and data collection procedures. Researchers follow standardized processes to ensure reliability and replicability.
- Large Sample Sizes: It often involves larger sample sizes to ensure statistical significance and generalizability. Sampling techniques are used to select representative samples from the population.
- Hypothesis-Driven: Quantitative research typically begins with a clear hypothesis or research question. Researchers aim to test hypotheses and draw conclusions based on data analysis.
- Quantitative Instruments: Researchers use various quantitative instruments, such as surveys, questionnaires, experiments, and structured observations, to collect data.
- Statistical Analysis: Data collected in quantitative research are subject to statistical analysis. Common statistical techniques include descriptive statistics, inferential statistics, regression analysis, and hypothesis testing.
- Objective Measurement: Measurements are typically objective and standardized to minimize bias and subjectivity. Instruments are designed to ensure consistency and reliability.
- Generalization: Quantitative research aims to generalize findings from a sample to a larger population. The results are often used to make broader conclusions and predictions.
- Numerical Results: Research findings are presented using numerical values, charts, graphs, and tables, making the results easily interpretable and comparable.
- Structured Questioning: Surveys and questionnaires used in quantitative research have structured questions with predefined response options to facilitate data collection and analysis.
- Replicability: Quantitative studies are designed to be replicable, allowing other researchers to conduct similar studies and verify or challenge the findings.
- Causality: While correlation can be established, quantitative research is suited for investigating causal relationships between variables by controlling for extraneous factors.
- Reductionist Approach: It often involves a reductionist approach, breaking down complex phenomena into measurable variables for analysis.
- Predefined Research Design: Quantitative research typically follows a predefined research design, including experimental designs, cross-sectional or longitudinal studies, and surveys.
- Validity and Reliability: Researchers pay careful attention to the validity (the accuracy of measurements) and reliability (the consistency of measurements) of data and instruments.
- Data-Based Conclusions: Conclusions in quantitative research are based on data analysis and statistical significance, emphasizing objectivity and evidence-based decision-making.
What are the 4 types of quantitative research?
1. Descriptive Research:
Descriptive research aims to describe and analyze a phenomenon, population, or variable. It provides a detailed account of the characteristics, behaviors, or attributes of a subject without manipulating it. Surveys, observational studies, and content analysis are often used in descriptive research.
2. Correlational Research:
Correlational research examines the relationship between two or more variables. It assesses how changes in one variable are associated with changes in another. The strength and direction of the relationship are measured using correlation coefficients. This type of research doesn’t establish causation but helps identify patterns and associations.
3. Experimental Research:
Experimental research is conducted to establish cause-and-effect relationships between variables. Researchers manipulate one or more independent variables to observe their impact on a dependent variable while controlling extraneous factors. Randomized controlled trials (RCTs) and laboratory experiments are common experimental research designs.
4. Quasi-Experimental Research:
Quasi-experimental research shares similarities with experimental research but lacks the full level of control over variables. In quasi-experiments, researchers often cannot use random assignment due to ethical or practical constraints. However, they still manipulate independent variables and measure their effects on dependent variables.
What is Quantitative Research vs Qualitative Research?
Which example demonstrates quantitative research.
Example 1: A study that surveys 1,000 consumers to determine the percentage who prefer Product A over Product B for a specific feature.
Example 1 demonstrates quantitative research because it involves collecting numerical data (the percentage of consumers) and relies on surveys, which are a common quantitative data collection method. This type of research is suitable for quantifying preferences and making statistical comparisons between products.
What are the advantages of quantitative research?
- Objectivity: Quantitative research is often highly structured and relies on empirical data, reducing the potential for bias and subjectivity. This enhances the objectivity of the research.
- Measurability: It allows for precise measurement of variables, making it easier to quantify and analyze data. This facilitates the comparison of findings across studies.
- Generalizability: Large sample sizes and statistical analysis enable researchers to generalize findings to a larger population, enhancing the external validity of the results.
- Replicability: Quantitative research is designed to be replicable, allowing other researchers to conduct similar studies and validate or challenge the findings.
- Data Analysis: Statistical analysis provides robust tools for testing hypotheses, identifying patterns, and drawing conclusions from data.
- Causality: It is well-suited for investigating causal relationships, as researchers can manipulate variables and control extraneous factors to establish cause-and-effect links.
- Efficiency: Surveys and questionnaires can collect data from a large number of participants efficiently. This is particularly useful for large-scale studies.
- Quantitative Comparison: It allows for direct comparison between groups or variables, facilitating the identification of differences and relationships.
- Data Precision: The use of standardized instruments and measurements results in precise and consistent data, reducing measurement errors.
- Data Visualization: Numerical data can be presented in charts, graphs, and tables, making it visually accessible and aiding in data interpretation.
- Decision Support: Quantitative research provides empirical evidence that can inform data-driven decision-making in various fields, including business, healthcare, and policy.
- Clear Findings: The structured nature of quantitative research often leads to clear and easily interpretable findings, which can be valuable for making informed conclusions.
- Resource Efficiency: While it may require substantial resources for data collection and analysis, quantitative research can be more cost-effective than qualitative research when dealing with large sample sizes.
General FAQ’s
What is quantitative research.
Quantitative research is a systematic approach to gathering and analyzing numerical data to understand and draw conclusions about a specific phenomenon or problem, often using statistical techniques.
What is the greatest strength of quantitative research?
The greatest strength of quantitative research is its ability to provide precise, objective, and statistically reliable data, enabling researchers to identify patterns, relationships, and make generalizable conclusions.
What is a common weakness of quantitative research?
A common weakness of quantitative research is its potential for oversimplification, as it may not capture the full complexity of human behavior or phenomena and may rely on limited predefined variables.
What are the risks of quantitative research?
Risks in quantitative research include the potential for data inaccuracies, oversimplification of complex phenomena, and overlooking unmeasurable factors, which can lead to biased or incomplete conclusions.
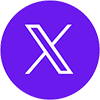
AI Generator
Text prompt
- Instructive
- Professional
10 Examples of Public speaking
20 Examples of Gas lighting
Academia.edu no longer supports Internet Explorer.
To browse Academia.edu and the wider internet faster and more securely, please take a few seconds to upgrade your browser .
Enter the email address you signed up with and we'll email you a reset link.
- We're Hiring!
- Help Center
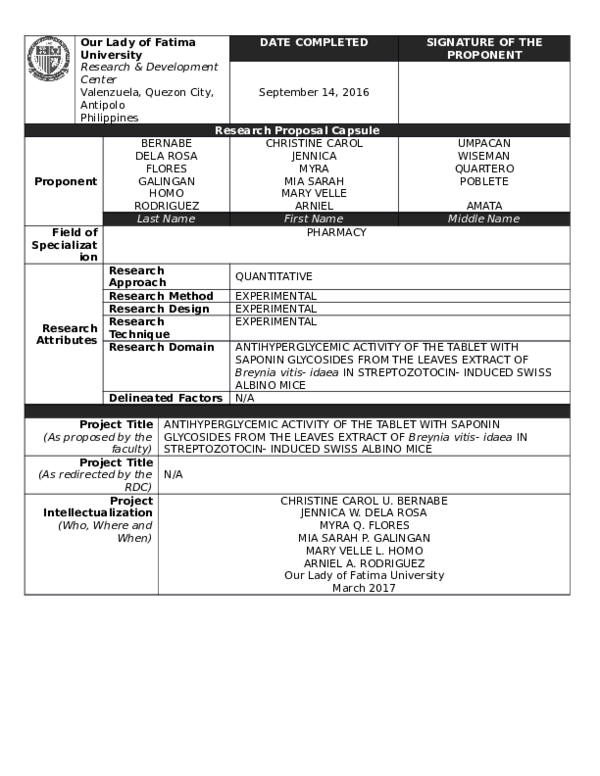
Research-Capsule

Related Papers
PharmaTutor Edu Labs
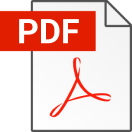
Liezel Alberto
Eirol Gomez
Thippeswamy SGowda
IJAR Indexing
The present work was carried out to study the effect of roots of Erythrina variegata and leaves of Breynia vitis-idaea on antioxidant enzymes levels in Alloxan induced diabetic rats. Alloxan (120 mg/kg, i.p) induced diabetic rats were treated with alcohol and aqueous extract at a dose levels of 300 and 600 mg/kg for 21 days. Antioxidant enzymes levels viz. Lipid peroxidation (LPO), Superoxide dismutase (SOD), Catalase (CAT) and Glutathione (GSH) were measured in liver homogenate. After 21 days of experimental period the level of reduced glutathione (GSH), superoxide dismutase (SOD) and catalase (CAT) significantly increase, while elevated level of lipid peroxidation (LPO) significantly decrease. It suggests that because of its antioxidant effects its administration may be useful in controlling the diabetic complications in experimental diabetic rats. So, it can be concluded that both the extracts of Erythrina variegata and Breynia vitis-idaea are very promising candidate for the design of new drugs based on its pharmacological effects of antioxidant adequacy.
Journal of Pharmacy and Bioallied Sciences
Janarra de Guzman
This study aims to investigate the saponin as anti-human head lice activity from Artocarpus altilis (Moracaea) extracts of dried twigs powder. The Artocarpus genus is known to produce a large number of secondary metabolites, and is specifically rich in phenylpropanoids such as flavonoids and flavones. Results of this study indicated that Artocarpus altilis (twigs) contain terpenoids, saponins, phenolic group, flavonoids, glycoside, steroids and tannins, while alkaloid test yielded negative result. Saponins are heterosides (substances containing in their structure one or more sugar molecule) of plant origin. This type of molecules has an interesting pesticide potential and this review constitutes and inventory of principal researches realized in this direction. Saponin acts as the active ingredient in the solution according to the present invention and with respect to hatched lice, it acts on the cell protoplasm, a liquid crystalline structure and changes it into a solid. As a result, holes are opened in the cell walls and the cell fluids flow out of the cells, thus, killing the lice. This study involves the formulation of a pediculicidal shampoo from the Artocarpus altilis twig extract using compatible excipients and the determination of its activity using Permethrin (Kwell) as positive control.
Ashok Silwal
The methanolic extract of herbal formulations were used to test their antidiabetic activity in Streptozotocin induced diabetic mice. Utrica dioica was used as single drug while one marketed polyherbal formulation “jameda churna” was also included for the comparative study. Diabetes was induced in albino mice by single i.p. injection of 50 mg/kg body weight of STZ and were given 200 mg/kg body weight of three different herbal formulation orally. All the formulations have shown potential in their role to reduce the blood glucose level. Polyherbal formulaion, which was rich in alkaloids, tanins and terpenoids, showed the higher activity on in-vitro and in-vivo than tri-herbal and single herbal formulations.
John Wendell
Liwayway Acero
—Calamansi or calamondin is very abundant and one of the sources of staple fruit juice in the Philippines. It is grown principally for its fruit juice, since it is widely known as good source of Vitamin C. However, the peels are thrown after the extraction of the juice. The medicinal use of the peel was still unknown to many Filipinos, thus this study focuses on the potential of calamansi peels in lowering blood sugar in streptozotocin induced Albino rats. Calamansi peels were dried, macerated, and the filtrate was subjected to rotary evaporator. The extract were diluted with distilled water and administered orally in Albino rats. Twenty Albino rats served as experimental animals. They are randomly assigned in two groups. The first group, or treatment 1, (10 animals) as the control wherein they only fed with rat pellets and drinking water. The second group-treatment 2 served as the experimental animals where calamansi peel extract solution was administered orally for the entire duration of the study. Baseline blood glucose, fasting blood sugar before Stretozotocin (STZ) induction and blood sugar after three days STZ induction of both treatments showed no significant result. Final blood sugar after five days of administration of the calamansi peel extract solution showed significant result. The result revealed that calamansi peel extract solution has the potential for lowering blood glucose in Albino Rats. This implies that calamansi peel extract solution could be used as herbal medicine to lower blood glucose.
RELATED PAPERS
jameskenneth bartolome
Dagmawi Tekeba
International Journal of Pharmacy and Pharmaceutical Sciences, 7(1):280-283
Y.C. Tripathi , Devesh Tewari , Pratibha Shukla
muhammad fakhruddin
Jaecel Joie Laygo
Vijayapandi Pandy
DONN URIEL BUENAVENTURA
International Research Journal of Pharmacy
NABARUN MUKHOPADHYAY
johana vallo
Md. Mustafizur Rahman
abdu khurgain
Mary Elizabeth Ochea
TILAMSIK: The Southern Luzon Journal of Arts and Sciences
Tilamsik: The Southern Luzon Journal of Arts and Sciences
Prof. Dr. Md. Moklesur Rahman Sarker
Christopher Larbie
Raven Santos
Philip Jay-ar Dimailig
Journal of Drug Discovery and Therapeutics
zubair labu
Research Paper
Rakib Hasan
JPR Solutions , Prof. Debidas Ghosh
Evidence-Based Complementary and Alternative Medicine
Antoine Brault , Hoda Eid
Molecular Nutrition & Food Research
Evidence-based Complementary and Alternative Medicine
Ganesh Halade , Bhushan Patwardhan , Ranjan Mogre
Wo Cuole Gutieliesi
Asian Plant Research Journal
Omoirri M O S E S Aziakpono
Naser al-Wabel
SP Balasubramani
Shenalynl Cerenas
Indra Tripathi
Revista Brasileira de Farmacognosia
Lais Araujo
International Journal of Pharmaceutical Archive
santosh Kumar Vaidya
Animal Research International
Journal of Ethnopharmacology
Mohammad Mosihuzzaman
Vivek Agrahari
Innoriginal International Journal of Sciences
GOPALASATHEESKUMAR K
Experimental and Toxicologic Pathology
Veeresh Veerapur
Dominic Aloc
- We're Hiring!
- Help Center
- Find new research papers in:
- Health Sciences
- Earth Sciences
- Cognitive Science
- Mathematics
- Computer Science
- Academia ©2024
Thank you for visiting nature.com. You are using a browser version with limited support for CSS. To obtain the best experience, we recommend you use a more up to date browser (or turn off compatibility mode in Internet Explorer). In the meantime, to ensure continued support, we are displaying the site without styles and JavaScript.
- View all journals
- My Account Login
- Explore content
- About the journal
- Publish with us
- Sign up for alerts
- Open access
- Published: 30 April 2024
Microbiome confounders and quantitative profiling challenge predicted microbial targets in colorectal cancer development
- Raúl Y. Tito ORCID: orcid.org/0000-0001-9660-7621 1 , 2 na1 ,
- Sara Verbandt 3 na1 ,
- Marta Aguirre Vazquez 3 ,
- Leo Lahti ORCID: orcid.org/0000-0001-5537-637X 1 , 4 ,
- Chloe Verspecht 1 , 2 ,
- Verónica Lloréns-Rico 1 , 2 , 5 ,
- Sara Vieira-Silva ORCID: orcid.org/0000-0002-4616-7602 1 , 6 , 7 ,
- Janine Arts 8 ,
- Gwen Falony 1 , 2 , 6 ,
- Evelien Dekker 9 ,
- Joke Reumers ORCID: orcid.org/0000-0001-5434-6515 10 ,
- Sabine Tejpar ORCID: orcid.org/0000-0003-3281-8643 3 na1 &
- Jeroen Raes ORCID: orcid.org/0000-0002-1337-041X 1 , 2 na1
Nature Medicine ( 2024 ) Cite this article
80 Altmetric
Metrics details
- Colon cancer
- Diagnostic markers
Despite substantial progress in cancer microbiome research, recognized confounders and advances in absolute microbiome quantification remain underused; this raises concerns regarding potential spurious associations. Here we study the fecal microbiota of 589 patients at different colorectal cancer (CRC) stages and compare observations with up to 15 published studies (4,439 patients and controls total). Using quantitative microbiome profiling based on 16S ribosomal RNA amplicon sequencing, combined with rigorous confounder control, we identified transit time, fecal calprotectin (intestinal inflammation) and body mass index as primary microbial covariates, superseding variance explained by CRC diagnostic groups. Well-established microbiome CRC targets, such as Fusobacterium nucleatum , did not significantly associate with CRC diagnostic groups (healthy, adenoma and carcinoma) when controlling for these covariates. In contrast, the associations of Anaerococcus vaginalis , Dialister pneumosintes , Parvimonas micra , Peptostreptococcus anaerobius , Porphyromonas asaccharolytica and Prevotella intermedia remained robust, highlighting their future target potential. Finally, control individuals (age 22–80 years, mean 57.7 years, standard deviation 11.3) meeting criteria for colonoscopy (for example, through a positive fecal immunochemical test) but without colonic lesions are enriched for the dysbiotic Bacteroides2 enterotype, emphasizing uncertainties in defining healthy controls in cancer microbiome research. Together, these results indicate the importance of quantitative microbiome profiling and covariate control for biomarker identification in CRC microbiome studies.
Colorectal cancer (CRC) incidence is steadily increasing 1 , especially in people under 50 years 2 . It is estimated that approximately 16 and approximately 14 individuals per 100,000 people in the United States and Belgium, respectively, die every year from CRC 3 . As medical interventions can effectively reduce CRC progression and associated mortality, it is imperative to identify individuals at increased risk.
Colonoscopies with polypectomy of adenomas reduce up to 90% of CRC risk 4 . Early identification of individuals with polyps would reduce the global burden of CRC. Yet, ascertainment of patients at an increased risk remains challenging, highlighting the need for population-wide screening.
Microbiota shifts have been associated with a wide array of disease phenotypes 5 . Some bacterial markers, such as Fusobacterium , have been reported enriched in lesions and stools of patients with CRC 6 , 7 , 8 , 9 , 10 , 11 , 12 , 13 , 14 across developing and developed countries 15 , suggesting a potential role for microbiome-based diagnostics and/or prognostics.
Although microbiome profiles are affected by multiple variables that may confound or compound biological phenomena, covariate control is far from standard. For example, moisture content, a proxy for transit time, remains uncontrolled despite showing the biggest explanatory power for overall gut microbiota variation in multiple cohorts 16 , 17 . Intestinal inflammation, measured as fecal calprotectin 18 , 19 that reflects increased neutrophil shedding into the intestinal lumen 20 , is more sensitive than fecal occult blood for identifying patients with CRC 21 , thus a potential untapped target for molecular stool CRC-screening 19 .
Relative microbiome profiling (RMP, taxon abundances are expressed in percentages) remains the dominant approach in microbiome research. However, given issues with compositionality 22 and interpretation of relative profiles 23 , the use of experimental and quantitative approaches is increasingly recommended 23 , 24 , 25 . This reduces both false-positive and false-negative rates in downstream analyses, thereby lowering the risk of erroneous interpretation of microbiome associations, and allows focusing clinical programs on biologically relevant targets 25 . Although quantitative microbiome profiling (QMP) facilitates normalized comparisons across different samples or conditions 24 , 25 , so far, no QMP CRC microbiota studies were performed.
In this Article, we address these two gaps in CRC microbiota studies: (1) to quantitively characterize the microbiota profile associated with malignant colonic transformation and (2) to identify microbiota covariates that may obscure biological phenomena behind microbiota-CRC associations. To this end, we examined the microbial profiles of 589 Belgian patients from Universitair Ziekenhuis Leuven (UZL) who warranted colonoscopies based on clinical presentations, including patients with CRC, and compared these to existing published datasets (total n = 4,439 patients and controls). To the best of our knowledge, this is the first large scale study of the gut microbiota across colonic cancer developmental stages that combines QMP analysis with extensive analysis of microbiota covariates to disentangle disease-associated from confounder-based signals to identify taxa specifically associated with CRC.
Intestinal inflammation is higher in patients with colorectal tumors
We recruited 650 volunteers referred for colonoscopy and colonic resections at UZL between 2017 and 2018 who provided a stool sample before the colonic procedure. Most participants were from the Flemish region of Belgium. For this study, cancer developmental stages were defined as diagnosis groups, and we classified participants into three groups according to a thorough colonoscopy and clinical assessment: (1) patients without evidence of colonic lesions (CTLs, n = 205), (2) patients with polyps (considering polyps as a precancerous lesion; n < 10 and size between 6 and 10 mm) (ADE, n = 337) and (3) patients with CRC ( n = 47; 2 (4%) stage 0, 14 (30%) stage I, 13 (28%) stage II, 11 (23%) stage III, 3 (6%) stage IV and 4 (9%) of undetermined stage). We excluded patients outside these criteria, as well as those with insufficient clinical and molecular data. The final Leuven CRC Progression Microbiome (LCPM) study cohort consisted of 589 patients. The most frequent indications for colonoscopy were either a positive fecal immunochemical test (FIT) or adenoma surveillance. Other indications included familial risk, abdominal symptoms and change in bowel habits (Fig. 1a and Supplementary Table 1 ). The study was registered at clinicaltrials.gov (NCT02947607).
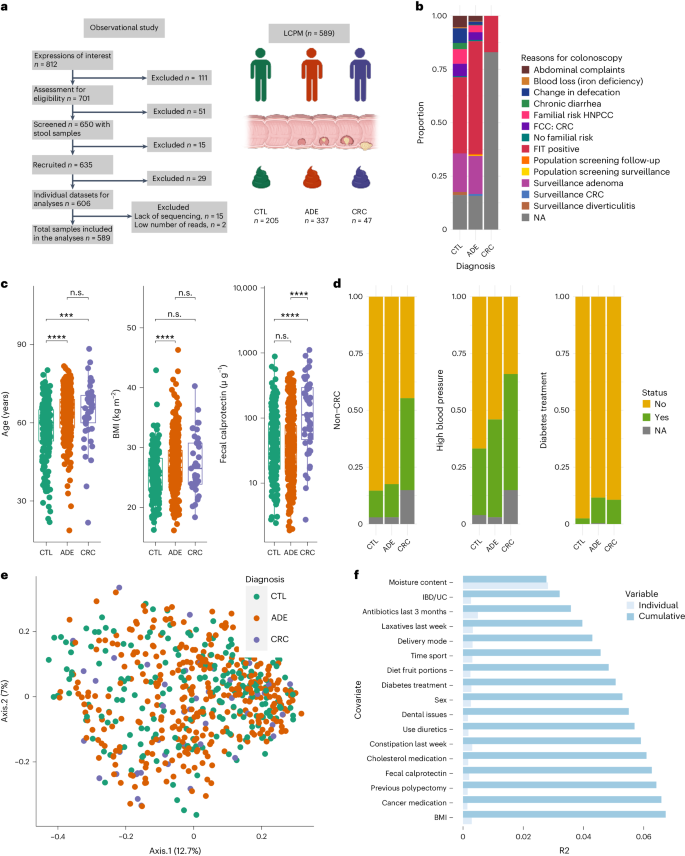
a , STROBE flowchart and cohort size. CTL represents patients without colonic lesions, ADE denotes patients with colonic polyps and CRC refers to patients with colorectal tumors (generated in BioRender.com ). b , Colonoscopy referral reasons for patients of the LCPM cohort: positive FIT, adenoma surveillance, familial risk cancer (FCC), hereditary nonpolyposis CRC (HNPCC) and changes in defecation. NA, denotes the proportion of patients without information. c , Age, BMI and calprotectin are associated with diagnosis groups. The patients without lesions were younger ( n = 589, two-sided KW test χ 2 = 35.77, adjusted P = 2.6 × 10 −7 ; phD tests) and had lower BMI ( n = 553, two-sided KW test χ 2 = 15.73, adjusted P = 1.9 × 10 −3 ; phD tests), while patients with tumors had higher fecal calprotectin levels ( n = 583, two-sided KW test χ 2 = 29.43, adjusted P = 3.0 × 10 −6 ; phD tests, adjusted *** P <0.001, ** P <0.01, * P <0.05 and n.s., non-significant P > 0.05; Supplementary Table 3 ). The box plot center represents the median value whiskers extend from the quartiles to the last data point within 1.5 times of the interquartile range, with outliers beyond. d , Previous non-CRC cancer, high blood pressure and diabetes treatment are associated with the distribution of diagnosis groups. The patients with CRC have a higher proportion of previous cancer (47.5% versus 15.0 % and 12.1%, two-sided CS test, CV effect size of 0.24, χ 2 = 31.65, d.f. of 2, adjusted P = 1.98 × 10 −2 ) and high blood pressure (60.0% versus 44.3% and 30.5%, CV of 0.17, two-sided CS test, χ 2 = 16.55, d.f. of 2, adjusted P = 1.98 × 10 −2 ) while the CTL group has the lowest proportion of patients with diabetes treatment (2.4% versus 10.3 and 10.6, two-sided CV effect size of 0.15, CS test, χ 2 = 13.79, d.f. of 2, adjusted P = 1.98 × 10 −2 ). e , PCoA on BCD representing QMP species-level microbiota variation in the LCPM cohort ( n = 589), PCoA1 (Axis.1) and PCoA2 (Axis.2) respectively explained 12.7% and 7% of the variance. Each dot represents one sample, colored by assigned diagnosis group. f , Cumulative effect sizes of significant covariates on microbiota community variation (cumulative bars; stepwise dbRDA on BCD) as compared to individual effect sizes (R 2 ) assuming covariate independence in the LCPM cohort ( n = 589; Supplementary Table 5 ). UC, ulcerative colitis.
Source data
We collected an extensive set of 165 universal metadata variables (nonspecific for any of the three groups) from each participant. After curation, we excluded variables that were colinear (if Pearson | r | > 0.8, we kept the variable with fewer missing data) or had incomplete data collection (variables missing more than 20% of the values). The final set consisted of 95 high-quality variables (Supplementary Table 2 ).
To identify metadata variables associated with diagnosis groups, we applied two statistical approaches: (1) nonparametric Kruskal–Wallis (KW) test and its η 2 effect size (Supplementary Table 3 ) for all numerical variables and (2) chi-square (CS) tests and Cramer’s V effect size (CV) (Supplementary Table 4 ) for categorical variables, followed by the Benjamini–Hochberg method for multiple testing correction (adjusted P ). We found eight variables associated with diagnosis groups (false discovery rate <5%), namely: age, body mass index (BMI), calprotectin, reported hours of sleep, previous cancer (including CRC), dental status (complete, partial and so on), diabetes treatment and high blood pressure (Supplementary Tables 3 and 4 ). The CTL patients were younger ( n = 589, KW test, η 2 = 0.058, χ 2 = 35.77, adjusted P = 2.6 × 10 −7 ; post hoc Dunn (phD) tests, adjusted P < 0.05 for CTL versus ADE or CRC groups), had a lower BMI ( n = 553, KW test, η 2 = 0.023, χ 2 = 15.73, adjusted P = 1.9 × 10 −3 ; phD tests, adjusted P < 0.05 for CTL versus ADE) and reported fewer hours of sleep than participants from the other two diagnosis groups ( n = 557, KW test, η 2 = 0.019, χ 2 = 13.41, adjusted P = 4.6 × 10 −3 ; phD tests, adjusted P < 0.05 for CTL versus ADE; Fig. 1 ; see Supplementary Table 3 for full results). Moisture content, an important microbiota covariate 16 , was not significant across diagnosis groups ( n = 589, KW test, η 2 = −0.001, χ 2 = 1.32, adjusted P = 7.0 × 10 −1 ).
The calprotectin levels were positively associated with malignant transformation. The patients with CRC showed higher intestinal inflammation, measured by fecal calprotectin 18 , 26 (Fig. 1a and Supplementary Table 3 ). Specifically, CRC exhibited higher levels (219.42 µg g −1 , range 2.74–1,114.42, n = 47) compared to ADE (70.24 µg g −1 , range 1.87–487.21, n = 337) or CTL (73.25 µg g −1 , range 2.42–884.82, n = 202) (Fig. 1a , N = 583, KW test, η 2 = 0.047, χ 2 = 29.43, adjusted P = 3.0 × 10 −6 ; phD tests, adjusted P < 0.05 for CRC versus CTL and CRC versus ADE). We also observed increased fecal calprotectin in patients reporting previous cancers (primarily breast and prostate cancer) (Wilcoxon ranksum (WR) test, W = 11,067, adjusted P = 4.1 × 10 −3 ), consumption of cancer medication (WR test, W = 3,671, adjusted P < 0.05), heartburn complaints (WR test, W = 11,067, adjusted P = 1.0 × 10 −10 ) and lower dietary fiber (WR test, W = 20,964, adjusted P = 3.3 × 10 −2 ).
The history of chronic diseases was distinct across diagnosis groups. The patients with CRC showed higher proportions of previous non-CRC cancer (47.5% versus 15.0 % and 12.1%, CS test, CV of 0.24, χ 2 = 31.65, d.f. of 2, adjusted P = 1.98 × 10 −2 ) and high blood pressure (60.0% versus 44.3% and 30.5%, CS test, CV of 0.17, χ 2 = 16.55, d.f. of 2, adjusted P = 1.98 × 10 −2 ) (Fig. 1b and Supplementary Table 4 ). The CTL group had the lowest diabetes treatment (2.4% versus 10.3% and 10.6%, CS test, CV of 0.15, χ 2 = 13.79, d.f. of 2, adjusted P = 1.98 × 10 −2 ) (Fig. 1b and Supplementary Table 4 ) and mostly complete dental sets (53.3% versus 35.2% and 32.5%, CS test, CV of 0.03, χ 2 = 30.78, d.f. of 10, adjusted P = 1.98 × 10 −2 ) (Supplementary Table 4 ).
Known confounders, not diagnosis groups, explain overall microbiota variation across CRC developmental stages
The influence of microbiota covariates and the quantitative amplitude of observed microbiota shifts are understudied in CRC. We combined sequencing data with flow cytometry measurements of fecal microbial load 23 to generate QMP data from our study cohort. 23 We studied the QMP variation in the context of the 94 potential covariates mentioned above (the 95th being microbial load) using established procedures 17 .
A principal coordinate analysis (PCoA; Fig. 1c ) on a species-level Bray–Curtis dissimilarity (BCD) matrix revealed no significant separation between diagnosis groups. Furthermore, no difference in total microbial load was found between groups ( n = 589, KW test, χ 2 = 0.68, adjusted P = 8.2 × 10 −1 ). Distance-based redundancy analysis (dbRDA) revealed 24 microbiota covariates associated with microbial variation in this cohort (Fig. 1d and Supplementary Table 5 ). We identified 17 nonredundant covariates that jointly explained 6.7% of microbiota compositional variation (Supplementary Table 5 ).
Consistent with previous reports 16 , 17 , moisture content exhibited the highest explanatory value (2.8%) of all covariates ( n = 589, stepwise dbRDA, R 2 = 2.8%, adjusted P = 2 × 10 −3 ). Intestinal bowel disease/ulcerative colitis (IBD/UC) status, a CRC-risk factor, possibly associated with its microbial dysbiotic community and intestinal inflammation 27 , was the second largest covariate. IBD/UC explained 0.4% of the microbiota variation ( n = 569, stepwise dbRDA, R 2 = 0.4%, adjusted P = 2 × 10 −3 ). Other top microbiota covariates included antibiotics and laxatives use (Fig. 1d ). Delivery mode (cesarean or natural birth) explained 0.3% variation ( n = 533, stepwise dbRDA, R 2 = 0.3%, adjusted P = 2 ×10 −3 ), although it is probably confounded by diet in this cohort (proportion of dietary vegetables; CS test, χ 2 = 33.09, d.f. of 14, P = 2.8 × 10 −3 , adjusted P < 0.05). Intestinal inflammation (fecal calprotectin) explained 0.2% ( n = 583, stepwise dbRDA, R 2 = 0.2%, adjusted P = 2.6 × 10 −2 ). In contrast with our previous study in the Flemish population (Flemish Gut Flora Project, FGFP) 17 , age did not explain microbiota variation ( n = 589, univariate dbRDA, R 2 = 0.2%, adjusted P = 5.9 × 10 −2 ). Surprisingly, the cancer diagnosis group (CTL, ADE and CRC), as a covariate, was not associated with microbial variation ( n = 589, univariate dbRDA, R 2 = 0.2%, adjusted P = 0.22; Supplementary Table 5 ).
Fusobacterium association with CRC stages disappears when controlling for confounders or when using QMP
Microbiota signals can be specific to taxonomic groups and, thus, not reflected in broad community shifts. While a multitude of microbial associations have been reported in CRC studies using RMP 6 , 7 , 8 , 13 , we used QMP to identify species whose absolute abundance associated with diagnosis groups. The comparisons were limited to the 138 species with a prevalence of greater than 5% in at least one of the diagnosis groups of the LCPM cohort (Supplementary Table 6 ). Only eight species showed significant differential abundance (absolute or relative) among diagnosis groups: Anaerococcus vaginalis ( Anaerococcus obesiensis ), Alistipes onderdonkii , Dialister pneumosintes , Fusobacterium nucleatum , Parvimonas micra , Peptostreptococcus anaerobius , Porphyromonas asaccharolytica and Prevotella intermedia (KW test, adjusted P < 0.05; Fig. 2a,b and Supplementary Table 7 ). While Fusobacterium nucleatum has been consistently associated with colorectal lesions across cohorts of diverse backgrounds 13 , 14 , in the LCPM cohort, Fusobacterium nucleatum absolute abundance was positively correlated with high fecal calprotectin levels (Spearman’s rank and Kendall’s tau correlations, adjusted P < 0.05; Fig. 2c , Extended Data Fig. 1 and Supplementary Table 8 ) and cancer progression (diagnosis groups) (KW test, η 2 = 0.010, adjusted P = 1.84 × 10 −5 ; phD test adjusted P = 8.80 × 10 −1 for CTL versus ADE, adjusted P = 3.84 × 10 −7 for CTL versus CRC and adjusted P = 3.84 × 10 −7 for ADE versus CRC; Fig. 2c and Supplementary Table 7 ). However, after deconfounding for calprotectin only or combined BMI, moisture content and calprotectin, and neither absolute nor relative Fusobacterium nucleatum abundance were associated with diagnosis (generalized linear model analysis of variance (ANOVA), n = 547, P > 0.05; Extended Data Fig. 2 ).
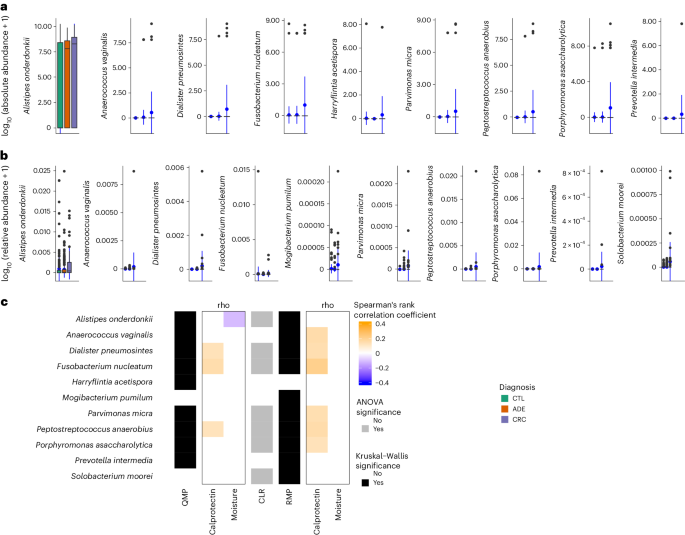
a , Nine species were identified with differential absolute abundance across diagnosis groups ( n = 589, KW test, adjusted P < 0.05; Supplementary Table 7 ). b , Ten species were identified with differential relative abundance across diagnosis groups ( n = 589, KW test, adjusted P < 0.05; Supplementary Table 7 ). The center of the box plot represents the median value of the data, and the whiskers extend from the quartiles to the last data point within 1.5 times of the interquartile range, with outliers beyond. The blue circles represent the mean. c , Biomarkers associations and their confounders. Species Spearman’s rank correlation with calprotectin levels and moisture proportions using QMP (first rho column panel) and RMP (second rho column panel) data. The effect size of the associations between species and calprotectin, moisture and diagnosis variables for QMP and RMP ( n = 589, Spearman’s rank correlation comparison, adjusted P < 0.05). Significant associations were tested using two-sided KW tests for QMP and RMP data and ANOVA for CLR data. The associations for Harryflintia acetispora , Parvimonas micra and Prevotella intermedia are sensitive to bias by the extreme values (absolute abundance) in the higher range. Removing these values leads to loss of significance. As rank-based approaches were used, it is not clear if this loss is due to the strength of the signal or the loss of power.
Multiple established CRC microbial markers are associated with transit time, intestinal inflammation and body mass index but not with CRC stages
The association of Fusobacterium abundance with fecal calprotectin urged us to investigate the influence of this confounder on previously reported CRC-associated genera, adding moisture content since it is the top microbiome covariate, and BMI, which showed differences among diagnosis groups.
To this end, we compiled a list of 89 CRC species-level markers from ten published cohorts 6 , 9 , 11 , 13 , 14 , 28 , 29 , 30 , 31 (including 1,633 samples) and 67 genera-level markers from 15 cohorts 6 , 7 , 8 , 9 , 11 , 12 , 13 , 14 , 15 , 28 , 29 , 30 , 31 , 32 (representing 4,439 samples). We used this compiled list of taxa as a criterion to test whether the CRC association of these taxa in our cohort is influenced by the target covariates. To reduce the impact of distinct statistical treatments, we downloaded the microbial profiles of nine out of ten studies at species level from the curated MetagenomicData 33 resource and analyzed them using the statistical component of our pipeline.
Spearman correlation between taxa abundances and the three focus covariates revealed strong associations between microbial targets and these confounders at the species (Extended Data Fig. 3a ) and genus level (Fig. 3b ). Most of these associations were replicated in an independent population cohort (FGFP), suggesting these associations are robust and not specifically linked to CRC (Extended Data Fig. 3 ). Moisture content, the known major covariate in microbiome studies 17 , is unsurprisingly associated with many taxa validated in both cohorts.
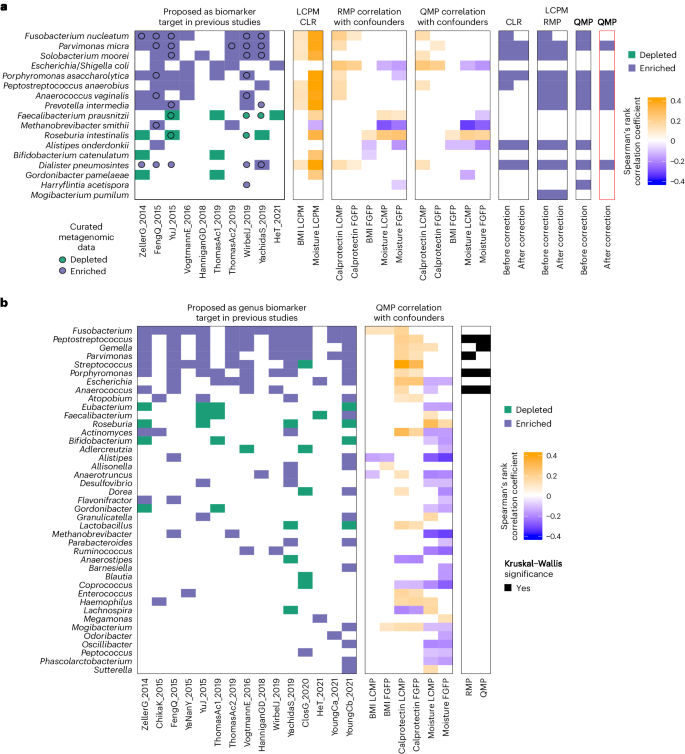
a , b , Species ( a ) and genera ( b ) previously reported in association with CRC (blue and green represent enrichment or depletion; the squares indicate reported in corresponding publications, while circles represent our reanalysis of the MetaPhlAn 3.0 profiles generated from the curatedMetagenomicData 33 of these cohorts using the statistical part of our pipeline). Graphic representation of Spearman’s rank correlation of pairwise analysis of fecal calprotectin, BMI, and moisture values against absolute species abundance (QMP) and RMP from the LCPM ( N = 589) and FGFP ( N = 1,045) cohorts (adjusted P < 0.05, Supplementary Table 8 ). The species enriched or depleted in relation to CRC diagnosis groups were tested using QMP, CLR and RMP data before ( n = 589, two-sided KW test and Spearman’s rank correlation comparison, adjusted P < 0.05) and after controlling for microbiota covariates (before adjustment for BMI, calprotectin and moisture; generalized linear model ANOVA, adjusted P < 0.05).
As we compiled the CRC-associated taxa from non-QMP studies, we conducted analyses using both RMP and QMP to assess whether confounder associations influence quantitative association of biomarkers or targets to diagnosis groups in LCPM. We found only 8% (6 out of 89) and 10% (9 out of 89) of species previously associated with CRC using QMP and RMP replicating after confounder control. Anaerococcus vaginalis , Dialister pneumosintes , Parvimonas micra , Peptostreptococcus anaerobius , Prevotella intermeia and Porphyromonas asaccharolytica , were identified by controlled QMP and RMP. Controlled QMP excluded Fusobacterium nucleatum and Alistipes onderdonkii , suggesting previous associations of these two species may be spurious (Fig. 3a ).
We identified eight species previously linked to CRC (that is, using QMP and/or RMP), including Fusobacterium nucleatum and Peptostreptococcus anaerobius , to be associated with inflammation (Fig. 3 and Supplementary Tables 8 and 9 ). This association was previously reported for only three out of the eight taxa above ( Escherichia , Fusobacterium and Streptococcus ) 24 . Further validation of this association was conducted using the FGFP (Extended Data Fig. 3 and Supplementary Tables 8 and 9 ).
Recognizing that inflammation is a risk factor, not a requirement, for CRC progression, we further investigated markers associated with diagnosis groups in relation to inflammatory status. To this end, we focused on a subset of 340 samples, which, regardless of their CRC status, exhibited normal levels of calprotectin (fecal calprotectin under 50 μg g −1 (ref. 34 )), indicating no evidence of local inflammation (112 CTL, 216 ADE and 12 CRC). Assessment of the 89 CRC species-level markers mentioned above confirmed that the association of three of the six replicating species ( Anaerococcus vaginalis , Prevotella intermedia and Porphyromonas asaccharolytica) is independent of intestinal inflammation (Supplementary Table 10 ).
Colonoscopy patients, with or without CRC, exhibit an excess of the Bacteroides2 enterotype
To study the LCPM cohort in a population context, we enterotyped participants using Dirichlet multinomial mixtures (DMM) on a genus matrix against the background of microbial variation as observed in the FGFP samples ( n = 1,045) 17 . Consistent with previous description of the Flemish population 23 , we identified four community types based on selecting the optimal number of clusters using the Bayesian Information Criterion (Fig. 4a,b and Extended Data Fig. 4 ), ‘Bacteroides1’ (Bact1), ‘Bacteroides2’ (Bact2), ‘Prevotella’ (Prev) and ‘Ruminococcaceae’ (Rum). The enterotype distribution was different between LCPM and FGFP (CS test, χ 2 = 34.3, d.f. of 3, adjusted P = 1.7 × 10 −7 ), but no differences were observed among diagnosis groups within the LCPM cohort (pairwise CS tests, adjusted P > 0.1). Pairwise comparisons of the prevalence of the dysbiotic Bact2 enterotype in the LCPM cohort diagnosis groups revealed that compared to the FGFP population, this enterotype was enriched in all CRC diagnosis groups (test of equal or given proportions, FGFP versus CTL: χ 2 = 15.09, d.f. of 1, adjusted P = 1.1 × 10 −4 ; FGFP versus ADE: χ 2 = 18.93, d.f. of 1, adjusted P = 2.4 × 10 −5 ; and FGFP versus CRC: χ 2 = 4.34, d.f. of 1, adjusted P = 3.4 × 10 −2 ). Although dysbiosis and CRC development were previously linked 13 , 35 , the high prevalence of this enterotype in the LCPM, even in samples from patients free of lesions, is unexpected. Consistent with previous reports 24 , 25 , the Bact2 enterotype in this group exhibited all hallmarks of dysbiosis: low cell count, low richness, higher calprotectin values, reduced butyrate producers and increased proinflammatory bacteria.
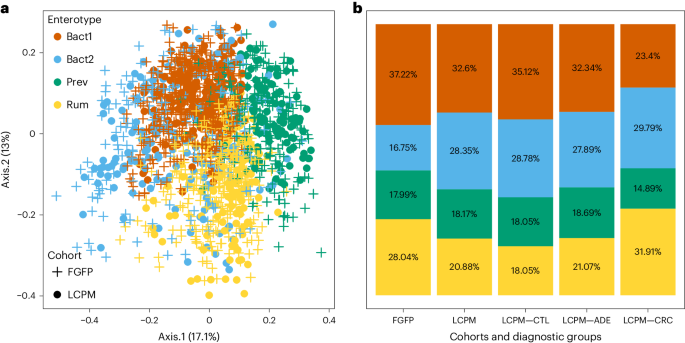
a , PCoA of interindividual differences (BCD) in relative microbiota profiles of the LCPM cohort ( n = 589 samples) using a cross-section of the Flemish population ( n = 1,045 samples) as a background dataset. PCoA1 (Axis.1) and PCoA2 (Axis.2) respectively explained 13% and 17.1% of the variance of microbiota at the genus level. b , Enterotype distribution across the FGFP, LCPM and LCPM diagnosis groups (CTL, ADE and CRC), increased prevalence of the Bact2 enterotype in the three groups from the LCPM cohort ( n = 589) as compared to FGFP samples ( n = 1,045); pairwise two-sided test of equal or given proportions ( P < 0.05).
Additional categorical variables appeared associated with the Bact2 enterotype. They included antibiotic consumption (CS test, χ 2 = 30.78, d.f. of 3, adjusted P = 2.1 × 10 −2 ), current treatment with anti-inflammatory medications (CS test, χ 2 = 30.78, d.f. of 3, adjusted P = 2.1 × 10 −2 ), diabetes treatment (CS test, χ 2 = 30.78, d.f. of 3, adjusted P = 3.3 × 10 −2 ), recent diarrhea (last week) (CS test, χ 2 = 30.78, d.f. of 3, adjusted P = 2.1 × 10 −2 ), history of gallstones (CS test, χ 2 = 30.78, d.f. of 3, adjusted P = 4.7 × 10 −2 ) and recent use of laxatives (last week) ( χ 2 = 30.78, d.f. of 3, adjusted P = 4.2 × 10 −2 ) (Supplementary Table 11 ).
While associations between the gut microbiota and CRC have been extensive, this is the first study using QMP and extensive metadata collection to systematically investigate microbiota covariates that potentially are masking or creating spurious associations between specific taxa and malignant transformation.
At first glance, this study yielded a gut microbial profile partially consistent with previous reports of CRC-associated taxa. Further analysis, however, suggested that many of the previously reported associations, including those of prominent biomarkers, such as Fusobacterium (nucleatum), are confounded by microbiota covariates. A total of 17 of 94 variables explained 6.7% of the observed variation. Of those, the moisture content had highest explanatory power (2.7%), greater than eight times that of the next covariate (IBD status). The explanatory power of fecal calprotectin was lower (0.2%) but significant; age and, most importantly, diagnosis groups were not.
Some associations were complex in nature. For example, BMI, consistent with previous reports, showed an association with both microbial composition 17 , 25 and cancer progression 36 , while others, such as age, suggested to modify the BMI-association with cancer progression 37 , were not significant in this cohort.
Inflammation is a known risk factor for CRC 38 , but its effect size in shaping the cancer-associated microbiota is yet to be described. Fecal calprotectin is a well-documented marker of intestinal local inflammation 39 , 40 and has been associated with cancer progression, probably having an effect on tumor development rather than on tumor initiation 41 . We observed participants with normal and elevated fecal calprotectin levels within each diagnosis group and covariate-controlled analysis of the LCPM cohort revealed that 8 and 19 CRC-associated markers, at the species and genus levels, respectively, associated with fecal calprotectin rather than with the diagnosis group. We replicated these observations in an independent cohort of apparently healthy individuals (FGFP).
High levels of fecal calprotectin have been associated with intestinal inflammatory pathologies 19 . However, when removing patients with IBD from our analysis, CRC diagnosis groups remained not significant, and the significance of Fusobacterium nucleatum , among other six species, was unaltered after differential abundance analysis. In patients with CRC, increased levels of fecal calprotectin (>50 µg g −1 stool 18 , 26 ) are directly associated with tumor presence, as the level decreases after tumor resection 42 . Here, fecal calprotectin was increased in CRC, consistent with previous associations between malignant transformation, local inflammation 43 and advanced tumor stages (T3 and T4) 42 . No difference in calprotectin levels was observed between CTL and ADE (mean 73.25 versus 70.24 µg g −1 ), suggesting that although no lesions are visible in the colon of the CTL group, they have a detectable level of local inflammation. The potential effect of local inflammation in shaping the colonic microbiota in the context of malignant transformation, or its potential confounding effect, remains largely obscure, as most studies surveying the association between gut microbiota and CRC, including meta-analysis 13 , 14 , do not control for local inflammation.
We argue that strict control of covariates is a must in any microbiota analysis assessing potential clinical associations, as for example, three of the species with repeated CRC association 11 , 13 , 14 , 28 , 29 , 30 , 32 , Escherichia coli , Fusobacterium nucleatun and Parvimonas micra , exhibit association with local inflammation, unfortunately uncontrolled for in previous studies, that may or may not be associated with cancer progression.
Fusobacterium nucleatum is one of the species that attracts more attention as there is a substantial body of work linking it to CRC 44 . In this study, Fusobacterium was enriched in patients with CRC. However, this apparent association disappears when the analysis is covariate controlled. Our study suggests that the association of Fusobacterium nucleatum to cancer may be driven by its association to intestinal inflammatory conditions; there are no differences in the abundance of Fusobacterium nucleatum across diagnostic groups once calprotectin is controlled for. These results suggest reassessment of the diagnostic utility of this marker. At the same time, our results do not mean that Fusobacterium nucleatum is not linked to CRC; they rather suggest that the reasons behind this association might be less straightforward than originally considered. They, thus, present a cautionary tale of the importance to control for covariates as the microbiome field moves forward. Given that inflammation is a risk factor for CRC but not a requirement 41 , potential use of Fusobacterium nucleatum as a marker of CRC development could fail to identify those cases of inflammation-independent cancer progression. While not yet commercialized, there are already publications proposing the use of microbial markers, including Fusobacterium nucleatum , for CRC screening 7 , 45 , which, in light of our results, raises concerns as uncontrolled variables may be obscuring actual biological mechanisms. We present evidence that purported CRC biomarkers, even those replicated in multiple studies, may suffer from the compounding or confounding effect of covariates, which in addition to the use of nonquantitative signals, may result in misleading conclusions on what diagnostic signals really mean—complicating the path towards potential clinical applications.
BMI, in combination or independent of inflammation, has been independently associated with changes in the gut microbiota 46 , which in turn are associated with increased risk of CRC 47 . Yet, microbial dysbiosis by itself does not explain the higher risk of colon cancer observed in the obese population 48 , indicating that the underlying process that associates obesity and CRC is more complex and demands further investigation.
Among four described gut enterotypes, the Bact2 enterotype is defined as a dysbiotic microbial profile 24 , 25 . Bact2 enrichment is observed in obesity 25 and in conditions such as PSC (Primary sclerosing cholangitis) and IBD 24 , further supporting the potential disease association of this enterotype. The analysis of the LCPM cohort revealed an excess of the Bact2 enterotype across all diagnosis subgroups, regardless of BMI.
Increased Bact2 prevalence in the no-lesions group compared to FGFP is particularly striking. While patients in the CTL group have no observable lesions, they may be considered at increased risk for colorectal perturbations based on clinical referrals (blood loss in the stool, familiar risk to colonic lesion and so on) that warranted colonoscopies—something that might also be reflected by their Bact2 enterotype. Of importance, ‘healthy’ biopsies included in CRC microbiome studies are often selected using colonoscopies with a negative result as the main criterium, posing a potential problem, as no other markers of colonic health are considered to qualify these healthy individuals. The reasons for the appearance of Bact2 in the no-lesion group are multifold, but these findings suggest that such individuals, while representing a useful category for biomarker discovery, may harbor an unhealthy gut ecosystem, from a microbial point of view.
There is a plethora of variables identified as modifiers of the gut microbiota. Yet, covariate control is far from standard and notably absent from most association studies. As intestinal microbial taxa are being nominated as potential biomarkers of malignant transformation, it is imperative to explore the influence of microbiota covariates as potential confounders or compounders of observed associations. Rather than denying previous associations, our analysis emphasizes the need for covariate-controlled analysis for any microbiota study aiming to establish clinical associations, as these covariates by themselves may explain most of the stool microbiota variation, independent of CRC status.
Out of the multiple taxa previously associated with CRC, six species remain significant after strict control of covariates in this quantitative cohort. Without denying other potential biomarkers, further studies are warranted on Anaerococcus vaginalis , Dialister pneumosintes , Parvimonas micra , Peptostreptococcus anaerobius , Prevotella intermedia and Porphyromonas asaccharolytica , as their reported association to CRC 6 , 7 is robust enough to remain independent of the method. Our data present a strong argument in favor of revisiting potential microbial associations with clinical phenotypes to ensure that the purported associations are not driven by uncontrolled covariates warranting further follow up of the mechanisms underlying these associations. Refining the approaches to discover microbial biomarkers will undoubtedly impact the microbiota field, facilitating the path towards the much-coveted clinical applications.
Limitations
We aim to identify taxa associated with malignant colonic transformation. While our cohort includes a set of participants without lesions, we make no claim that these are healthy controls, as there is an apparent increased incidence of gut dysbiosis in this group. Considering that all participants in this study had a medical need for a colonoscopy, there is an implicit increased risk to CRC. Thus, the present study cannot rule out that the group without polyps is undergoing potential molecular or cellular changes that are not detectable via colonoscopy. In addition, as this is a cross-sectional study, the term cancer progression is an extrapolation of what is seen at cancer development stages (operationalized here as diagnosis groups). We cannot rule out potential particularities of our cohort that may be contributing to our observations, as most studies do not report sufficient metadata for us to compare across cohorts. It is important to consider that certain taxonomic groups may not even be represented in current databases, and specific microbial species may require longer hypervariable regions or alternative sequencing approaches to achieve accurate species-level identification. Nonetheless, the V4 region for our cohort seems to be able to resolve species taxonomy of the biomarkers previously associated with CRC, as we show for the case of Fusobacterium .
Furthermore, it has been proposed that the potential diagnostic value of colonic microbial profiles goes beyond bacteria, as fungal and viral species have been proposed as CRC biomarkers 49 . We recognize that multidomain approaches to discover CRC biomarkers and longitudinal prospective studies to better study the dynamics of cancer progression are warranted to comprehensively inform cancer detection and treatment.
Participant recruitment
The LCPM project was an observational cross-sectional survey for which procedures were approved by the medical ethics committee of the UZL (ethical approval number S57084). Between 2017 and 2018, we recruited patients through the study nurse following a standardized procedure. Briefly, we invited patients scheduled for lower gastrointestinal endoscopy or abdominal surgery for CRC removal at the UZL were invited. After explaining the research project and if they expressed their agreement, participants signed an informed consent, and no compensation was offered. A set of stool sample collection material was provided.
Each patient completed an extensive questionnaire containing information about the date of sample collection, the consistency of the stool, diet, antibiotics usage, clinical symptoms or disease among other variables 17 , as well as an extensive medical and clinical questionnaire using the Websurvey service of KU Leuven.
As a validation cohort we included the FGFP 17 , a population-wide microbiota monitoring effort, representing one of the largest and best characterized fecal microbiota database currently available. Its extensive metadata including health and lifestyle allowed the identification of 69 factors associated with microbiota variation (microbiota covariates). The QMP transformation was conducted in parallel, with the same protocol, for both the FGFP and the LCPM cohorts.
CRC status classification
We invited patients referred for colonoscopy or colectomy to participate in the study. Those that consented were instructed to collect a stool sample at home, which was kept frozen using a sample kit provided by the research team. Upon completion of the medically necessary procedures (colonoscopy or colon resection), we stratified study participants into three diagnosis groups according to their clinical phenotype: (1) patients without evidence of lesions, (2) patients with polyps ( n < 10 and size between 6 and 10 mm) (ADE) and (3) patients with CRC. Patients whose clinical presentation did not fit any of these three groups were excluded from the study. Once the participants were included in the corresponding groups, extensive metadata was collected from their medical records as stated in the informed consent.
Sample collection
The stool samples of patients from UZL were collected as part of the LCPM project using aliquot ready mat without any buffer or preservative (Supplementary Fig. 1 ). The samples were kept at −20 °C freezers at the patients’ homes and brought to our laboratory on icepacks. Upon arrival, samples were stored in the Raes’ Lab at −80 °C until further analysis. Each stool sample had a temperature logger to make sure that, during the storage at home or transport to the laboratory, low stable temperature was maintained.
Stool sample analyses
Microbial load measurement by flow cytometry.
We determined microbial loads of stool samples of LCPM patients following published procedures 23 . We performed cell counting for all other samples in triplicate. Briefly, we dissolved 0.2 g frozen (−80 °C) aliquots in physiological solution to a total volume of 100 ml (8.5 g l −1 NaCl; VWR International). Subsequently, the slurry was diluted 1,000 times. The samples were filtered using a sterile syringe filter (pore size of 5 μm; Sartorius Stedim Biotech). Next, we stained 1 ml of the microbial cell suspension obtained with 1 μl SYBR Green I (1:100 dilution in dimethylsulfoxide; shaded for 15 min of incubation at 37 °C; 10,000 concentrate, Thermo Fisher Scientific) and monitored fluorescence events using the FL1 533/530 nm and FL3 >670 nm optical detectors of the C6 Accuri flow cytometer (BD Biosciences). In addition, forward and sideward scattered light was collected. The BD Accuri CFlow (v.1.0.264.21) software was used to gate and separate the microbial fluorescence events on the FL1/FL3 density plot from background events Supplementary Fig. 2 . A threshold value of 2,000 was applied on the FL1 channel. We evaluated the gated fluorescence events on the forward and sideward density plot, as to exclude remaining background events. We kept instrument and gating settings identical for all samples as described previously 24 . Based on the exact weight of the aliquots analyzed, we converted cell counts to microbial loads per gram of fecal material.
Fecal moisture content
We determined moisture content as the percentage of mass loss after lyophilization from 0.2 g frozen aliquots of nonhomogenized fecal material (−80 °C) as described previously 24 .
Fecal calprotectin measurement
We quantified fecal calprotectin concentrations using the fCAL ELISA Kit (Buhlmann). For patients and FGFP participants, we conducted analyses on frozen fecal material (−80 °C) as described previously 24 .
Microbiota phylogenetic profiling
Dna extraction and sequencing data preprocessing.
The fecal microbiota profile of the FGFP cohort was described previously 17 . For fecal DNA extraction and microbiota profiling of the new cohort, we followed the same protocols 17 .
The bacterial profiling was carried out as described previously 50 . Briefly, we extracted nucleic acids from frozen fecal aliquots using the MagAttract PowerMicrobiome DNA/RNA kit (Qiagen). We modified the manufacturer’s protocol by the addition of a heating step at 90 °C for 10 min after vortexing and excluding the steps where DNA is removed. For bacterial and archaeal characterization, we used 16S ribosomal RNA primers 515F (5′-GTGYCAGCMGCCGCGGTAA-3′) and 806R (5′-GGACTACNVGGGTWTCTAAT-3′) targeting the V4 region. These primers were modified to contain a barcode sequence between each primer and the Illumina adapter sequences to produce dual-barcoded libraries from the extracted DNA (dilution 1:10) in triplicate. Deep sequencing was performed on a MiSeq platform (2 × 250 paired end (PE) reads, Illumina). We randomized all samples and negative controls (polymerase chain reaction (PCR) and extraction controls) taken along for sequencing. After demultiplexing with sdm as part of the LotuS pipeline (v. 1.60) 51 without allowing for mismatches, we further analyzed fastq sequences per sample using DADA2 pipeline (v. 1.6) 52 . Briefly, we removed the primer sequences and the first ten nucleotides after the primer. After merging paired sequences and removing chimeras, we assigned taxonomy using formatted Silva set ‘SLV_nr99_v138.1’. We performed taxonomic assignments at the domain, class, order, family, genus and species levels were performed using the ‘assignTaxonomy’ function from the DADA2 R library, by a naive Bayesian classifier method with a minimum bootstrap confidence of 50, using the ‘silva_nr99_v138.1_wSpecies_train_set.fa.gz’ training database (Extended Data Fig. 5 ). Deep sequencing was performed on a MiSeq platform from the DADA2 R library with the formatted Silva SSU database ‘silva_species_assignment_v138.1.fa.gz’ to obtain species assignments for the amplicon sequence variants (ASVs). We labeled any unassigned ASVs at any taxonomic level, with the prefix ‘uc’ along with the assigned taxonomic level (not species level) to avoid the lack of labels.
Before the analyses, we removed sequences annotated to the class Chloroplast, family mitochondria or unknown archaea and bacteria from eukaryotic origin. phyloseq (v. 1.36.0) 53 and MicroViz (v. 0.11.0) 54 libraries were used for data curation and figure generation.
For the relative microbiome matrix, we transformed ASV counts to relative abundances. In other words, we divided ASV counts by the total counts of ASV per sample. We agglomerated ASV to species level using the phyloseq (v. 1.36.0) 53 function ‘tax_glom’.
We agglomerated ASV to the species level, and the abundance matrix was centered log-ratio (CLR)-transformed using ‘codaSeq.clr ’ in the CoDaSeq (v. 0.99.6) 55 using the minimum proportional abundance detected for each taxon for the imputation of zeros.
Workflow Assessment
We conducted a workflow assessment using (1) a commercial mock community, ZymoBIOMICS Gut, and (2) two Fusobacterium species: Fusobacterium hwasookii (THCT14E2) and Fusobacterium nucleatum (DSM 20482T). The assessment followed our standard methods, involving the amplification, sequencing and analysis of the extracted DNA. This evaluation aimed to assess the performance of our full methodology, as depicted in Extended Data Fig. 6 .
Quality control assessment for amplicon sequencing data (16S rRNA) using RMP
In short, we sequenced all samples in six MiSeq runs (Extended Data Fig. 7a ). Per each run, we used a set of internal controls to identify: 1) Technical variation within and between runs 1) Contamination events during the DNA extraction, 2) Contamination events during the amplification and sequencing procedures and, 3) Carry-over contamination at the sequencing facility and barcode crosstalk.
We amplified all samples, including biological material (stool samples), positive controls (DNA from a stool sample previously profiled and RS: nonhuman gut bacteria strain ‘ Runella slithyformis’ ), negative controls (negative control of extraction (NCE) and negative control during PCR (NCP)) in triplicate using a unique barcode combination, while omitting several barcode combinations to control for primer synthesis cross contamination. We used Runella slithyformis in duplicate within each sequencing library to detect barcode crosstalk during the sequencing procedure (Extended Data Fig. 7b ). This genus is not detected in human gut samples; therefore, we expected no Runella slithyformis reads in any of the stool samples analyzed. We determined technical variation based on the BCD of positive control samples (Extended Data Fig. 7c ). Finally, we included NCEs along the whole process from extraction to bioinformatic analysis. For amplification and sequencing contamination 56 , we used NCP and NCE (Extended Data Fig. 7d and Supplementary Table 12 ), and for carry-over contamination events, we used a different set of barcode combinations in consecutive MiSeq runs 56 .
We built the QMP matrix as described previously 23 . In brief, we downsized samples to even sampling depth, defined as the ratio between sampling size (16S rRNA gene copy number-corrected sequencing depth) and microbial load (the average total cell counts per gram of frozen fecal material; Supplementary Table 2 ). We imputed 16S rRNA genome copies (GC) numbers using RasperGade16S (v. 0.0.1) 57 , a new tool that utilizes a heterogeneous pulsed evolution model for predicting 16S rRNA GC. It not only predicts the GC but also provides confidence estimates for the predictions 57 . We used a minimum rarefied read count of less than 150 for QMP analyses. We converted rarefied ASV abundances into numbers of cells per gram. The QMP matrices had a final size of 589 samples for the study cohort and 1,045 samples for the FGFP validation cohort 17 . We agglomerate the QMP matrix at ASV level to species level using the phyloseq (v. 1.36.0) 53 function ‘tax_glom’. We used the resulting species QMP matrix for the main analysis.
Statistical analysis
We performed all statistical analyses with R (Version 4.2.1, RStudio v.2022.12.0 + 353, 86_64-apple-darwin17.0 (64-bit)) and packages phyloseq (v. 1.36.0) 53 , vegan (v. 2.6.2) 58 , coin(v. 1.4.2) 59 , effectsize (v. 0.8.3), vcd(1.4.11) 60 , DirichletMultinomial(v. 1.34.0) 61 , pairwiseAdonis (v. 0.4.1) and microbiome (v. 1.14.0) 62 . We used nonparametric statistical tests for robust comparisons among unbalanced groups. For multiple testing, we corrected all P values using the Benjamini–Hochberg method (reported as adjusted P ) as appropriate on lists ( n > 1) of features (for example, taxa–metadata or metadata–metadata associations) and also when performing multiple pairwise group ( n > 2) comparisons (for example, KW test with phD test).
Fecal microbiota derived features and visualization
We visualized microbiota interindividual variation by PCoA using BCD on the species QMP matrix 24 , 25 . All the rest of the microbiota derived features were calculated based on QMP. We determined the contribution of metadata variables to microbiota community variation (effect size) of each of 94 variables by dbRDA on a species-level BCD with the capscale function in the vegan package 58 . We visualized absolute abundance species as log10 (abundance +1). This was the same for relative abundance.
Microbiota and physiological features associations
We excluded from analyzes any taxa unclassified at the species level or present in less than 5% of samples per each diagnosis group (Supplementary Table 6 ). We used Spearman correlations for rank–order correlations, between continuous variables complemented by Kendall’s tau correlation, including species abundances, calprotectin values and moisture content. We used the Mann–Whitney U -test to test median differences of continuous variables between two different groups. For more than two groups, for example, for differential abundance analysis for QMP and RMP taxa versus diagnosis groups, we used the KW test with phD test. For differential abundance analysis among diagnosis groups and bacteria species abundances from CLR transformed data, we performed an ANOVA test.
We evaluated statistical differences in the proportions of categorical variables (enterotypes) between patient groups using pairwise CS tests. We tested for deconfounded microbiota contributions to the diagnosis groups variable by using a nested model comparison (ANOVA) of generalized linear models as follows:
[alternative model] glm1 = rank(abundance) + rank(calprotectin) + rank(moisture) + rank(BMI) + diagnosis, where the diagnosis groups were recoded as 1, 2 and 3 for patients without evidence of CTLs, patients with polyps and patients with CRC, respectively. We treated this variable as a continuous variable, translating the directional increase in disease progression, from healthy to lesions, in the colonic mucosa. For the nested model comparison, we used taxa abundances (quantitative or relative) as explanatory variables, the diagnosis groups variable as response variable and BMI, fecal calprotectin and moisture as covariates. Additionally, we employed rank-transformed modeling to perform nonparametric testing on data that is not normally distributed, such as species abundances.
Previous reported CRC microbial markers
To compile a list of published CRC markers that would define taxa that should be tested against covariates in our data set, we conducted a PubMed search query using the keywords ‘CRC AND microbiome AND stool AND human AND biomarkers’. We found ten studies that met our inclusion criteria, namely: (1) a sample size minimum of 60 and (2) the CRC biomarker described at the species level, with statistical significance, in the main text of the publication. We included this list of published biomarkers in our correlation analysis between taxa and the three main covariates (fecal calprotectin, BMI and moisture) within the LCPM cohort. A similar procedure was followed at the genus level, which included 15 studies found in our PubMed search.
CRC microbial markers identification
We performed differential abundance analyzes on nine different CRC shotgun datasets as part of ‘curatedMetagenomicData’ 33 using MetaPhlAn 3.0 profiles to compare the results while controlling for potential differences arising from the classification tools and statistical methods used in each independent study. The results of the meta-analysis are presented in Extended Data Fig. 8 and Supplementary Table 13 .
Enterotyping and visualization
Using the genus matrix (agglomerated and downsized to 10,000 reads), we enterotyped and calculated observed genus richness 53 , as already reported for previous studies 24 , 25 . For enterotyping (or community typing) based on the DMM approach we used R as described previously 61 . We performed enterotyping on a combined genus-level abundance RMP matrix including LCPM samples compiled with 1,045 samples originating from the FGFP 17 . The optimal number of Dirichlet components based on the Bayesian information criterion was four. The four clusters were named ‘Bact1’, ‘Bact2’, ‘Prev’ and ‘Rum’, as described previously 23 .
Reporting summary
Further information on research design is available in the Nature Portfolio Reporting Summary linked to this article.
Data availability
Raw amplicon sequencing data and metadata reported in this study have been deposited in European Nucleotide Archive with accession code EGAS00001007413 . FGFP 16S rRNA gene sequencing data and metadata are available at the European Genome-phenome Archive ( EGAS00001003296 ). The diagnosis metadata and processed microbiome data required for the reanalysis are provided as Supplementary Tables 1 and 14 , respectively. Formatted Silva set ‘SLV_nr99_v138.1’ files were downloaded from Zenodo via https://zenodo.org/records/4587955/files/silva_nr99_v138.1_wSpecies_train_set.fa.gz?download=1 (silva_nr99_v138.1_wSpecies_train_set.fa.gz) 63 and https://zenodo.org/records/4587955/files/silva_species_assignment_v138.1.fa.gz?download=1 (silva_species_assignment_v138.1.fa.gz) 63 . The nine CRC cohort MetaPhlAn 3.0 profiles were collected from curatedMetagenomicData, study names: FengQ_2015, HanniganGD_2017, ThomasAM_2018a, ThomasAM_2018b, VogtmannE_2016, WirbelJ_2018, YachidaS_2019 and YuJ_2015, ZellerG_2014 ( https://doi.org/10.18129/B9.bioc.curatedMetagenomicData ). Source data are provided with this paper.
Code availability
Analysis codes are available via Github at https://github.com/raeslab/QMP-Microbiome-CRC-confounders .
Yang, L. et al. Changes in colorectal cancer incidence by site and age from 1973 to 2015: a SEER database analysis. Aging Clin. Exp. Res. 33 , 1–10 (2020).
CAS Google Scholar
Keum, N. & Giovannucci, E. Global burden of colorectal cancer: emerging trends, risk factors and prevention strategies. Nat. Rev. Gastroenterol. Hepatol. 16 , 713–732 (2019).
Article PubMed Google Scholar
Araghi, M. et al. Global trends in colorectal cancer mortality: projections to the year 2035. Int. J. Cancer https://doi.org/10.1002/ijc.32055 (2018).
Rex, D. K. & Eid, E. Considerations regarding the present and future roles of colonoscopy in colorectal cancer prevention. Clin. Gastroenterol. Hepatol. 6 , 506–514 (2008).
Gupta, V. K. et al. A predictive index for health status using species-level gut microbiome profiling. Nat. Commun. 11 , 4635 (2020).
Article CAS PubMed PubMed Central Google Scholar
Yachida, S. et al. Metagenomic and metabolomic analyses reveal distinct stage-specific phenotypes of the gut microbiota in colorectal cancer. Nat. Med. 25 , 968–976 (2019).
Young, C. et al. Microbiome analysis of more than 2,000 NHSbowel cancer screening programme samples shows the potential to improve screening accuracy. Clin. Cancer Res. 27 , 2246–2254 (2021).
Clos-Garcia, M. et al. Integrative analysis of fecal metagenomics and metabolomics in colorectal cancer. Cancers https://doi.org/10.3390/cancers12051142 (2020).
Article PubMed PubMed Central Google Scholar
Yu, Y. N. et al. Berberine may rescue Fusobacterium nucleatum- induced colorectal tumorigenesis by modulating the tumor microenvironment. Oncotarget 6 , 32013–32026 (2015).
Yu, T. C. et al. Fusobacterium nucleatum promotes chemoresistance to colorectal cancer by modulating autophagy. Cell 170 , 548–563.e16 (2017).
He, T., Cheng, X. & Xing, C. The gut microbial diversity of colon cancer patients and the clinical significance. Bioengineered 12 , 7046–7060 (2021).
Kasai, C. et al. Comparison of human gut microbiota in control subjects and patients with colorectal carcinoma in adenoma: terminal restriction fragment length polymorphism and next-generation sequencing analyses. Oncol. Rep. 35 , 325–333 (2016).
Article CAS PubMed Google Scholar
Thomas, A. M. et al. Metagenomic analysis of colorectal cancer datasets identifies cross-cohort microbial diagnostic signatures and a link with choline degradation. Nat. Med. https://doi.org/10.1038/s41591-019-0405-7 (2019).
Wirbel, J. et al. Meta-analysis of fecal metagenomes reveals global microbial signatures that are specific for colorectal cancer. Nat. Med. https://doi.org/10.1038/s41591-019-0406-6 (2019).
Young, C. et al. The colorectal cancer-associated faecal microbiome of developing countries resembles that of developed countries. Genome Med. 13 , 1–13 (2021).
Article Google Scholar
Vandeputte, D. et al. Stool consistency is strongly associated with gut microbiota richness and composition, enterotypes and bacterial growth rates. Gut 65 , 57–62 (2016).
Falony, G. et al. Population-level analysis of gut microbiome variation. Science 352 , 560–564 (2016).
Poullis, A., Foster, R., Shetty, A., Fagerhol, M. K. & Mendall, M. A. Bowel inflammation as measured by fecal calprotectin: a link between lifestyle factors and colorectal cancer risk. Cancer Epidemiol. Biomarkers Prev. https://doi.org/10.1158/1055-9965.EPI-03-0160 (2004).
Högberg, C., Karling, P., Rutegård, J. & Lilja, M. Diagnosing colorectal cancer and inflammatory bowel disease in primary care: the usefulness of tests for faecal haemoglobin, faecal calprotectin, anaemia and iron deficiency. A prospective study. Scand. J. Gastroenterol. 52 , 69–75 (2017).
Schreuders, E. H., Grobbee, E. J., Spaander, M. C. W. & Kuipers, E. J. Advances in fecal tests for colorectal cancer screening. Curr. Treat. Options Gastroenterol. 14 , 152–162 (2016).
Røseth, A. G. et al. Faecal calprotectin: a novel test for the diagnosis of colorectal cancer? Scand. J. Gastroenterol. 28 , 1073–1076 (1993).
Gloor, G. B., Macklaim, J. M., Pawlowsky-Glahn, V. & Egozcue, J. J. Microbiomedatasets are compositional: and this is not optional. Front. Microbiol . 8 , 2224 (2017).
Vandeputte, D. et al. Quantitative microbiome profiling links gut community variation to microbial load. Nature 551 , 507–511 (2017).
Vieira-Silva, S. et al. Quantitative microbiome profiling disentangles inflammation-and bile duct obstruction-associated microbiota alterations across PSC/IBD diagnoses. Nat. Microbiol . 4 , 1826–1831(2019).
Vieira-Silva, S. et al. Statin therapy is associated with lower prevalence of gut microbiota dysbiosis. Nature https://doi.org/10.1038/s41586-020-2269-x (2020).
Tibble, J. A. & Bjarnason, I. Fecal calprotectin as an index of intestinal inflammation. Drugs Today https://doi.org/10.1358/dot.2001.37.2.614846 (2001).
Quaglio, A. E. V., Grillo, T. G., De Oliveira, E. C. S., Di Stasi, L. C. & Sassaki, L. Y. Gut microbiota, inflammatory bowel disease and colorectal cancer. World J. Gastroenterol. 28 , 4053–4060 (2022).
Zeller, G. et al. Potential of fecal microbiota for early-stage detection of colorectal cancer. Mol. Syst. Biol. 10 , 766 (2014).
Feng, Q. et al. Gut microbiome development along the colorectal adenoma–carcinoma sequence. Nat. Commun. 6 , 6528 (2015).
Vogtmann, E. et al. Colorectal cancer and the human gut microbiome: reproducibility with whole-genome shotgun sequencing. PLoS ONE 11 , e0155362 (2016).
Hannigan, G. D., Duhaime, M. B., Ruffin, M. T., Koumpouras, C. C. & Schloss, P. D. Diagnostic potential and interactive dynamics of the colorectal cancer virome. mBio 9 , e02248-18 (2018).
Yu, J. et al. Metagenomic analysis of faecal microbiome as a tool towards targeted non-invasive biomarkers for colorectal cancer. Gut 66 , 70–78 (2017).
Pasolli, E. et al. Accessible, curated metagenomic data through ExperimentHub. Nat. Methods 14 , 1023–1024 (2017).
Bjarnason, I. The use of fecal calprotectin in inflammatory bowel disease. Gastroenterol. Hepatol. 13 , 53–56 (2017).
Google Scholar
Dai, Z. et al. Multi-cohort analysis of colorectal cancer metagenome identified altered bacteria across populations and universal bacterial markers. Microbiome https://doi.org/10.1186/s40168-018-0451-2 (2018).
Zheng, R. et al. Body mass index (BMI) trajectories and risk of colorectal cancer in the PLCO cohort. Br. J. Cancer 119 , 130–132 (2018).
Carr, P. R. et al. Association of BMI and major molecular pathological markers of colorectal cancer in men and women. Am. J. Clin. Nutr. https://doi.org/10.1093/ajcn/nqz315 (2020).
Rutter, M. et al. Severity of inflammation is a risk factor for colorectal neoplasia in ulcerative colitis. Gastroenterology 126 , 451–459 (2004).
Costa, F. et al. Role of faecal calprotectin as non-invasive marker of intestinal inflammation. Digest. Liver Dis. 35 , 642–647 (2003).
Article CAS Google Scholar
Konikoff, M. R. & Denson, L. A. Role of fecal calprotectin as a biomarker of intestinal inflammation in inflammatory bowel disease. Inflamm. Bowel Dis. https://doi.org/10.1097/00054725-200606000-00013 (2006).
Terzić, J., Grivennikov, S., Karin, E. & Karin, M. Inflammation and colon cancer. Gastroenterology 138 , 2101–2114 (2010).
Lehmann, F. S. et al. Clinical and histopathological correlations of fecal calprotectin release in colorectal carcinoma. World J. Gastroenterol. https://doi.org/10.3748/wjg.v20.i17.4994 (2014).
Pathirana, W. G. W., Chubb, S. P., Gillett, M. J., & Vasikaran, S. D. Faecal calprotectin. Clin. Biochem. Rev. https://doi.org/10.1097/mpg.0000000000001847 (2018).
Bullman, S. et al. Analysis of Fusobacterium persistence and antibiotic response in colorectal cancer. Science 358 , 1443–1448 (2017).
Osman, M. A. et al. Parvimonas micra , Peptostreptococcus stomatis , Fusobacterium nucleatum and Akkermansia muciniphila as a four-bacteria biomarker panel of colorectal cancer. Sci. Rep. 11 , 1–12 (2021).
Turnbaugh, P. J. et al. A core gut microbiome in obese and lean twins. Nature 457 , 480–484 (2009).
Moghaddam, A. A., Woodward, M. & Huxley, R. Obesity and risk of colorectal cancer: a meta-analysis of 31 studies with 70,000 events. Cancer Epidemiol. Biomarkers Prev. 16 , 2533–2547 (2007).
Greathouse, K. L. et al. Gut microbiome meta-analysis reveals dysbiosis is independent of body mass index in predicting risk of obesity-associated CRC. BMJ Open Gastroenterol. https://doi.org/10.1136/bmjgast-2018-000247 (2019).
Liu, N. N. et al. Multi-kingdom microbiota analyses identify bacterial–fungal interactions and biomarkers of colorectal cancer across cohorts. Nat. Microbiol. 7 , 238–250 (2022).
Tito, R. Y. et al. Population-level analysis of Blastocystis subtype prevalence and variation in the human gut microbiota. Gut https://doi.org/10.1136/gutjnl-2018-316106 (2018).
Hildebrand, F., Tadeo, R., Voigt, A. Y., Bork, P. & Raes, J. LotuS: an efficient and user-friendly OTU processing pipeline. Microbiome 2 , 30 (2014).
Callahan, B. J. et al. DADA2: high-resolution sample inference from Illumina amplicon data. Nat. Methods 13 , 581–583 (2016).
McMurdie, P. J. & Holmes, S. phyloseq: an R package for reproducible interactive analysis and graphics of microbiome census data. PLoS ONE 8 , e61217 (2013).
Barnett, D., Arts, I. & Penders, J. microViz: an R package for microbiome data visualization and statistics. J. Open Source Softw. 6 , 3201 (2021).
Gloor, G. B., Wu, J. R., Pawlowsky-Glahn, V. & Egozcue, J. J. It’s all relative: analyzing microbiome data as compositions. Ann. Epidemiol. 26 , 322–329 (2016).
Seitz, V. et al. A new method to prevent carry-over contaminations in two-step PCR NGS library preparations. Nucleic Acids Res. https://doi.org/10.1093/nar/gkv694 (2015).
Gao, Y. & Wu, M. Accounting for 16S rRNA copy number prediction uncertainty and its implications in bacterial diversity analyses. ISME Commun. 3 , 59–67 (2023).
Oksanen, F. J. et al. Vegan: Community Ecology Package. R package Version 2.4-3 https://CRAN.R-project.org/package=vegan (2017).
Hothorn, T., Hornik, K., Van De Wiel, M. A. & Zeileis, A. A Lego system for conditional inference. Am. Stat. https://doi.org/10.1198/000313006×118430 (2006).
Friendly, M. & Institute, S. A. S. Visualizing Categorical Data (SAS Institute, 2000).
Holmes, I., Harris, K. & Quince, C. Dirichlet multinomial mixtures: generative models for microbial metagenomics. PLoS ONE 7 , e30126 (2012).
Shetty, S. A. & Lahti, L. Microbiome data science. J. Biosci. 44 , 1–6 (2019).
McLaren, M. R. & Callahan, B. J. Silva 138.1 prokaryotic SSU taxonomic training data formatted for DADA2. Zenodo https://doi.org/10.5281/zenodo.4587955 (2021).
Download references
Acknowledgements
We thank all study participants and the different staff members involved in the recruitment and execution of this project. We acknowledge L. Rymenans for her contribution to sample analysis. R.Y.T., S.V. and V.L.R. are funded by postdoctoral fellowships from the Research Fund–Flanders (1234321N, 12R6119N and 12V9421N, respectively). This work was funded by the Innovatie door Wetenschap en Technologie project ‘CRC_µBiome: characterization of human and microbial genetic components in premalignant adenoma and colorectal cancer’. The Raes lab is supported by Vlaams Instituut voor Biotechnologie (VIB), KU Leuven and the Rega Institute for Medical Research. The funders had no role in study design, data collection and analysis, decision to publish or preparation of the manuscript.
Author information
These authors contributed equally: Raúl Y. Tito, Sara Verbandt, Sabine Tejpar, Jeroen Raes.
Authors and Affiliations
Laboratory of Molecular Bacteriology, Department of Microbiology and Immunology, Rega Institute, Katholieke Universiteit Leuven, Leuven, Belgium
Raúl Y. Tito, Leo Lahti, Chloe Verspecht, Verónica Lloréns-Rico, Sara Vieira-Silva, Gwen Falony & Jeroen Raes
Center for Microbiology, Vlaams Instituut voor Biotechnologie, Leuven, Belgium
Raúl Y. Tito, Chloe Verspecht, Verónica Lloréns-Rico, Gwen Falony & Jeroen Raes
Digestive Oncology, Department of Oncology, Katholieke Universiteit Leuven, Leuven, Belgium
Sara Verbandt, Marta Aguirre Vazquez & Sabine Tejpar
Department of Computing, University of Turku, Turku, Finland
Systems Biology of Host–Microbiome Interactions Laboratory, Principe Felipe Research Center (CIPF), Valencia, Spain
Verónica Lloréns-Rico
Institute of Medical Microbiology and Hygiene and Research Center for Immunotherapy, University Medical Center of the Johannes Gutenberg-University Mainz, Mainz, Germany
Sara Vieira-Silva & Gwen Falony
Institute of Molecular Biology, Mainz, Germany
Sara Vieira-Silva
Oncology, Janssen Pharmaceutica NV, Beerse, Belgium
Janine Arts
Department of Gastroenterology and Hepatology, Amsterdam University Medical Centers, Amsterdam, the Netherlands
Evelien Dekker
Therapeutics Discovery, Janssen Pharmaceutica NV, Beerse, Belgium
Joke Reumers
You can also search for this author in PubMed Google Scholar
Contributions
This study was conceived by J.A., S.T., J. Reumers and J. Raes. The experiments were designed by R.Y.T. and J. Raes. The data were collected and curated by S.V., M.A.V., L.L., J. Reumers, V.L.R., S.V.S., G.F. and S.T. The molecular data were generated by C.V. and R.Y.T. The statistical analyses were planned and executed by R.Y.T. and J. Raes R.Y.T. and J. Raes drafted the manuscript. All authors revised the article and approved the final version for publication.
Corresponding author
Correspondence to Jeroen Raes .
Ethics declarations
Competing interests.
J.A. and J. Reumers are employees of Janssen Pharmaceutica NV. J. Raes and R.T. are inventors on the patent application WO2017109059A1 in the name of VIB VZW, Katholieke Universiteit Leuven, KU Leuven R&D and Universiteit Gent covering methods for detecting the presence or assessing the risk of development of inflammatory arthritis disease. J. Raes, S.V.S. and G.F. are inventors on the patent application PCT/EP2018/084920 in the name of VIB VZW, KAtholieke Universiteit Leuven, KU Leuven Research and Development and Vrije Universiteit Brussel covering microbiome features associated with inflammation described in Vieira-Silva et al. Nature Microbiology 2019. The other authors declare no competing interests.
Peer review
Peer review information.
Nature Medicine thanks Ruixin Zhu and the other, anonymous, reviewer(s) for their contribution to the peer review of this work. Primary Handling editor: Alison Farrell, in collaboration with the Nature Medicine team.
Additional information
Publisher’s note Springer Nature remains neutral with regard to jurisdictional claims in published maps and institutional affiliations.
Extended data
Extended data fig. 1 association of intestinal inflammation with fusobacterium nucleatum ..
Intestinal calprotectin levels associate Fusobacterium nucleatum absolute ( a) and relative ( b ) abundance in the LCMP. Two-sided Spearman rank correlation (adjP <0.05) and ‘x’ axes are log 10 transformed just for plotting. To rule out that the observed association is driven by a few samples with high abundance of Fusobacterium nucleatum, panel a has an insert of the plot removing samples with Fusobacterium nucleatum values above 1E8 cells per gram of stool. Best-fitting regression line in blue and 95% confidence interval shown in grey shading.
Extended Data Fig. 2 Fusobacterium nucleatum abundances before and after correction for intestinal calprotectin across diagnosis groups.
Absolute abundance of Fusobacterium nucleatum before ( a ) and after ( b ) correcting for intestinal calprotectin. Relative abundance of Fusobacterium nucleatum before ( c ) and after ( d ) correcting for intestinal calprotectin. The whiskers extend from the quartiles to the last data point within 1.5× of the interquartile range, with outliers beyond. The ‘y’ axes for (a) are log 10 transformed values (absolute abundance +1). The whiskers extend from the quartiles to the last data point within 1.5× of the interquartile range, with outliers beyond.
Extended Data Fig. 3 Spearman correlation between species abundance and microbiota covariates in the LCPM and FGFP cohorts.
Two-sided Spearman’s rank correlation comparison between absolute species abundance (QMP) and relative abundance (RMP) from the LCPM (N = 589 samples) and FGFP (N = 1045 samples) cohorts and a, BMI b, faecal calprotectin and c, moisture content values. Spearman correlation adjP < 0.05 (QMP and RMP, Supplementary Table 8 ).
Extended Data Fig. 4 Enterotype stratification by DMM community typing.
a , Identification of optimal number of clusters (Dirichlet components) in the LCPM cohort (n = 589) complemented with 1045 samples from the FGFP cohort, based on the Bayesian Information Criterion (BIC). b , Barplot representation of the average relative abundance of a few representative genera split into the four enterotypes identified by DMM community typing on the combined LCPM and FGFP cohorts (n = 1634).
Extended Data Fig. 5 Taxa assignation performance of the V4 amplicon marker in the LCPM.
a , Bootstrap values distribution across different ranks, b , Proportion of ASVs assigned from species to phylum, c , Proportion of ASVs assigned from species to phylum to each sample. The whiskers extend from the quartiles to the last data point within 1.5× of the interquartile range, with outliers beyond. The figure below (Panel a) illustrates our taxa assignation performance, showing that more than half of the ASVs were assigned to species level with bootstrap values above 80. Panel b shows the ASV assignation proportions from phylum (100%) to species level (50%). A comparison of proportions of ASVs assigned from each sample at different taxonomic levels revealed no significant differences in the distributions of assigned ASVs per sample across diagnosis groups, as indicated in panel c (KW test, p-values > 0.05). The center of the box plot represents the median value of the data, and the whiskers extend from the quartiles to the last data point within 1.5× of the interquartile range, with outliers beyond.
Extended Data Fig. 6 Performance of our methodology in small communities and isolated microorganisms.
a , Species composition of the ZymoBIOMICS gut controls, ten successfully identified species and b , two Fusobacterium species: Fusobacterium hwasookii (THCT14E2) and Fusobacterium nucleatum (DSM 20482T) were successfully identified using our methodology.
Extended Data Fig. 7 Quality control assessment for amplicon sequencing data (V4 16S rRNA gene).
a , The obtained reads for each sample are shown after processing with DADA2 (red and orange dashed lines represent 10, 000 and 1,000 reads, respectively; NCP: PCR negative control, NCE: DNA extraction Negative control, PC: positive control, and RS: Runella slithyformis control). b , Sequencing controls reveal the absence of barcode crosstalk. RS sequences serve as a marker for barcode crosstalk during sequencing. The absence of RS sequences in the samples without RS (no_RS) ruled out barcode crosstalk during the sequencing or PCR setup procedures. c , BCD among technical replicates demonstrating reproducibility. Pairwise comparisons between PC samples within and among MiSeq runs showed values under 0.2 (depicted by the pointed blue line). The center of the box plot represents the median value of the data, and the whiskers extend from the quartiles to the last data point within 1.5× of the interquartile range, with outliers beyond. d , Species composition of negative controls is presented, indicating the relative abundance and prevalence of the top 20 species. None of the species detected with differential abundance using QMP, RMP or CLR were found as background contaminants. Non-significant differences in bacteria composition were observed among DNA sequencing runs (Padj > 0.05, pairwiseAdonis test). A full list of detected species is available in Supplementary Table 12 . Of note, DI18R24 is not shown as the negative controls (NCE and NCP) did not produce reads.
Extended Data Fig. 8 Species and genera associated with CRC on a subset of the curatedMetagenomicData.
After performing our differential abundance procedure on the MataPhalAn 3.0 profiles downloaded from the curatedMetagenomicData, 108 species ( a ) and 63 genera ( b ) were identified across the 9 metagenomics datasets.
Supplementary information
Supplementary information.
Supplementary Figs. 1 and 2 and Tables 1–14.
Reporting Summary
Supplementary tables 1–14.
Supplementary Table 1. Reasons for the colonoscopy referral of the LCPM cohort. Supplementary Table 2. LCMP cohort variable names, 95 variables plus enterotypes. Supplementary Table 3. Associations between continuous variables and cancer progression (KW test with phD tests. N is specified for each test, and statistical significance was derived from two-sided testing and adjusted for multiple testing (adjusted P , Benjamini–Hochberg method)). Supplementary Table 4. Associations between categorical variables and cancer progression (two-sided CS test; statistical significance was derived from two-sided testing and adjusted for multiple testing (adjusted P , Benjamini–Hochberg method)). Supplementary Table 5. Microbiome variation in the LCMP cohort. Independent and cumulative contribution of metadata variables to species-level microbiome variation (dbRDA and stepwise dbRDA; false discovery rate by Benjamini–Hochberg). Cumulative explanatory power and significance level of the included variables are reported. Supplementary Table 6. List of species excluded and included from the analysis. Supplementary Table 7. Differences in absolute (QMP) and relative (RMP) species abundances over diagnostic groups LCMP cohort ( n = 589, KW, phD test; statistical significance was derived from two-sided testing and adjusted for multiple testing (adjusted P , Benjamini–Hochberg method)). Supplementary Table 8. Associations between species abundances (QMP and RMP) and BMI, intestinal calprotectin and moisture in the LCPM cohort ( n = 589, Spearman and Kendall’s tau; statistical significance was derived from two-sided testing and adjusted for multiple testing (adjusted P , Benjamini–Hochberg method)). Supplementary Table 9. Associations between species abundances (QMP and RMP) and BMI, intestinal calprotectin and moisture in the FGFP cohort ( n = 1,045, Spearman; statistical significance was derived from two-sided testing and adjusted for multiple testing (adjusted P , Benjamini–Hochberg method)). Supplementary Table 10. Differences in absolute (QMP) and relative (RMP) species abundances over diagnostic groups in the LCMP cohort subset with normal levels of fecal calprotectin ( n = 340 (112 PWoL, 216 PWP and 12 PWT, KW and adjusted for multiple testing (adjusted P , Benjamini–Hochberg method)). Supplementary Table 11. Associations between categorical variables and enterotype distribution (two-sided CS test; statistical significance was derived from two-sided testing and adjusted for multiple testing (adjusted P , Benjamini–Hochberg method)). Supplementary Table 12. Full list of the species detected in the negative controls (NCE and NCP). Supplementary Table 13. Differences in relative abundances of species profiles from MetaPhlAn 3.0 between CRC and controls from nine published CRC cohorts from the curatedMetagenomicData ( n = 1,254, two-sided Wilcoxon signed-rank test and adjusted for multiple testing (adjusted P , Benjamini–Hochberg method)). Supplementary Table 14. Absolute taxonomic abundances at species level in the LCMP cohort ( n = 589).
Source Data Fig. 1
Statistical source data.
Source Data Fig. 2
Source data fig. 3, source data fig. 4, rights and permissions.
Open Access This article is licensed under a Creative Commons Attribution 4.0 International License, which permits use, sharing, adaptation, distribution and reproduction in any medium or format, as long as you give appropriate credit to the original author(s) and the source, provide a link to the Creative Commons licence, and indicate if changes were made. The images or other third party material in this article are included in the article’s Creative Commons licence, unless indicated otherwise in a credit line to the material. If material is not included in the article’s Creative Commons licence and your intended use is not permitted by statutory regulation or exceeds the permitted use, you will need to obtain permission directly from the copyright holder. To view a copy of this licence, visit http://creativecommons.org/licenses/by/4.0/ .
Reprints and permissions
About this article
Cite this article.
Tito, R.Y., Verbandt, S., Aguirre Vazquez, M. et al. Microbiome confounders and quantitative profiling challenge predicted microbial targets in colorectal cancer development. Nat Med (2024). https://doi.org/10.1038/s41591-024-02963-2
Download citation
Received : 18 November 2022
Accepted : 29 March 2024
Published : 30 April 2024
DOI : https://doi.org/10.1038/s41591-024-02963-2
Share this article
Anyone you share the following link with will be able to read this content:
Sorry, a shareable link is not currently available for this article.
Provided by the Springer Nature SharedIt content-sharing initiative
Quick links
- Explore articles by subject
- Guide to authors
- Editorial policies
Sign up for the Nature Briefing newsletter — what matters in science, free to your inbox daily.

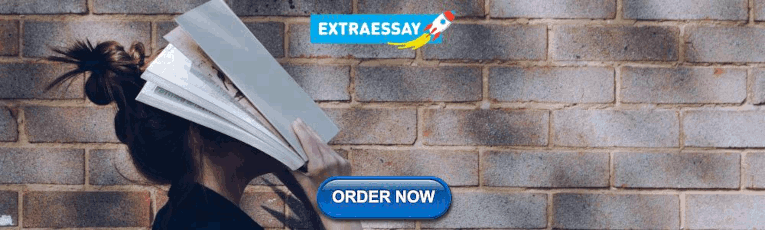
IMAGES
VIDEO
COMMENTS
A Sample Quantitative Research Proposal Written in the APA 6th Style. [Note: This sample proposal is based on a composite of past proposals, simulated information and references, and material I've included for illustration purposes - it is based roughly on a fairly standard research proposal; I say roughly because there is no one set way of ...
The capsule summary is written precisely by summarizing the Abstract to hit those four elements listed above in under 50 words. Here is a fictitious example of a capsule summary: A double-blind randomized crossover trial was conducted to determine if NewDrug is better than OldDrug as treatment for chronic brainfog in overworked scientific ...
INTRODUCTION. Scientific research is usually initiated by posing evidenced-based research questions which are then explicitly restated as hypotheses.1,2 The hypotheses provide directions to guide the study, solutions, explanations, and expected results.3,4 Both research questions and hypotheses are essentially formulated based on conventional theories and real-world processes, which allow the ...
the topic of the research proposal This section should discuss design, participant, instrument, Procedure, analysis and Ethical Consideration. This refers to the end results Expected upon. completion of the research. The output needs. to be identified to highlight impact and importance of the research. EXPECTED OUTPUT. This section indicate the ...
Example: 1.Value chain analysis 2.Profiling 3.Prototyping (6) Expected Output/s (The term "OUTPUT" means an activity, effort, and/or associated work product related to project goals and objectives that will be produced or provided over a period of time or by a specified date. Outputs may be quantitative or
Revised on June 22, 2023. Quantitative research is the process of collecting and analyzing numerical data. It can be used to find patterns and averages, make predictions, test causal relationships, and generalize results to wider populations. Quantitative research is the opposite of qualitative research, which involves collecting and analyzing ...
Quantitative research is a type of research that focuses on collecting and analyzing numerical data to answer research questions. There are two main methods used to conduct quantitative research: 1. Primary Method. There are several methods of primary quantitative research, each with its own strengths and limitations.
Mixed-methods research is a flexible approach, where the research design is determined by what we want to find out rather than by any predetermined epistemological position. In mixed-methods research, qualitative or quantitative components can predominate, or both can have equal status. 1.4. Units and variables.
Quantitative research Quantitative methods allow us to learn about the world by quantifying some variation(s) in it. Example: how do suicide rates vary across demographic categories (Durkheim)? In order to learn about the world, we use inference: General definition: "Using facts you know to learn about facts you don't know" (Gary King)
Quantitative research methods are concerned with the planning, design, and implementation of strategies to collect and analyze data. Descartes, the seventeenth-century philosopher, suggested that how the results are achieved is often more important than the results themselves, as the journey taken along the research path is a journey of discovery. . High-quality quantitative research is ...
Template: Capsule Research - Free download as Word Doc (.doc / .docx), PDF File (.pdf), Text File (.txt) or read online for free. Sample template of Research Capsule
Quantitative research is the process of collecting and analyzing numerical data to describe, predict, or control variables of interest. This type of research helps in testing the causal relationships between variables, making predictions, and generalizing results to wider populations. The purpose of quantitative research is to test a predefined ...
When collecting and analyzing data, quantitative research deals with numbers and statistics, while qualitative research deals with words and meanings. Both are important for gaining different kinds of knowledge. Quantitative research. Quantitative research is expressed in numbers and graphs. It is used to test or confirm theories and assumptions.
during the Research Methods course. If the capsule format is approved, full proposal development will follow. B. RESEARCH PROPOSAL (FULL-BLOWN) A research proposal (full-blown) is a document that shows a comprehensive justification for doing the research study and a detailed description of the whole research process.
Research Proposal Capsule_sample - Free download as Word Doc (.doc / .docx), PDF File (.pdf), Text File (.txt) or read online for free. This study show how to make capsule
Examples of Quantitative Research. Here are some examples of quantitative research in different fields: Market Research: A company conducts a survey of 1000 consumers to determine their brand awareness and preferences. The data is analyzed using statistical methods to identify trends and patterns that can inform marketing strategies.
Simply by reading a well-formed research question, you can usually tell: What concept (s), phenomena, or variables are going to be measured in quantitative research or described in qualitative research. What research design will be used. What the sample will consist of. As we proceed you will need to have knowledge of a variety of research ...
This research sample is for convenience and accessibility and has 213 consumers of coffee in capsules. Structural Equation Modelling (SEM) was the statistical method used for data analysis.
This research sample is for convenience and accessibility and has 213 consumers of coffee in capsules. Structural Equation Modelling (SEM) was the statistical method used for data analysis.,Attributes have two sub-dimensions (Own attributes and Functional attributes), while Consequences have three sub-dimensions (Handling Benefits, Rational ...
View SAMPLE-RESEARCH-CAPSULE-JPO.pdf from DOUS DAF 203 at Don Mariano Marcos Memorial State University. Reference Code Revision Number Date Effective FM-GRAD-041.3 0 July 1, 2019 Saint Louis ... They noted that the elementary method of conducting research was quantitative, but recently, qualitative method of research has also gained momentum ...
31+ Quantitative Research Examples. Quantitative research demands focus and precision from the researcher. If you need a guide in doing your research, here are 10+ Quantitative research examples you can use. 1. Free Quantitative Research Flowchart Example. Details. File Format. MS Word. Google Docs.
Alloxan (120 mg/kg, i.p) induced diabetic rats were treated with alcohol and aqueous extract at a dose levels of 300 and 600 mg/kg for 21 days. Antioxidant enzymes levels viz. Lipid peroxidation (LPO), Superoxide dismutase (SOD), Catalase (CAT) and Glutathione (GSH) were measured in liver homogenate. After 21 days of experimental period the ...
9781841849768_C013.indd 348 6/15/2017 5:51:59 PM. Analytical Testing and Evaluation of Capsules. 10°C for 1 6-18 h in a standard bott le, adjusted for a depressi on of 4 m m using a 12. 7-mm ...
Equity indicators as defined in flood-risk research. Despite our relatively large sample of quantitative studies (99 studies), we find few measurements that meet our standard for indicators ...
Despite substantial progress in cancer microbiome research, recognized confounders and advances in absolute microbiome quantification remain underused; this raises concerns regarding potential ...