6.1 Marketing Research and Big Data
Learning outcomes.
By the end of this section, you will be able to:
- 1 Define marketing research.
- 2 Explain how marketing information provides an understanding of the customer and the marketplace.
- 3 Explain the role of big data and the marketing information system.
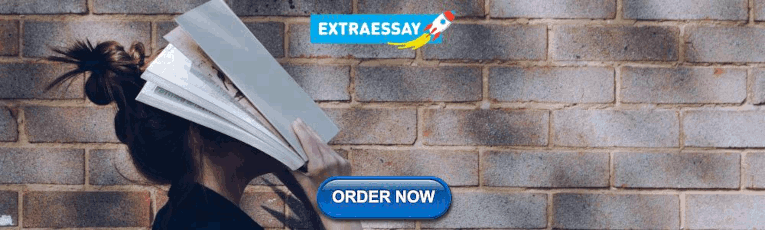
What Is Marketing Research?
Oftentimes people think of marketing as the process of communicating with a target market—sharing messages, products, and value with customers. However, marketers know that understanding customers, learning about their wants and needs, and developing a relationship with them is essential for success. Marketing research allows marketers to listen and assess the needs of the market, to understand what’s missing and how to reduce those gaps from their customers, target markets, and prospective clients. Managers need marketing information in order to make data-driven decisions rather than make assumptions about consumers. In this chapter we’re going to investigate the importance of marketing research, some strategies employed in the field, and how to complete the marketing research process.
Marketing research is the work to gather information and data about customers and markets. Let’s look at the definition, why it’s important, and the influx of big data.
Marketing Research Defined
According to the American Marketing Association (AMA) , marketing research “is the function that links the consumer, customer, and public to the marketer through information.” 2 Marketing research presents information gained through various sources as a resource for managers to make data-driven business decisions. Additionally, the AMA states that “marketing research specifies the information required to address these issues, designs the method for collecting information, manages and implements the data collection process, analyzes the results, and communicates the findings and their implications.” 3
The Importance of Marketing Information
Marketing information , also known as business intelligence, competitive intelligence, or marketing intelligence, is information about the market that helps to identify opportunities in the market. This information helps to determine a company’s strengths and weaknesses while also evaluating the external environment’s opportunities and threats. Ideas generated through analysis of marketing information support business decision-making from a long-term strategic approach to smaller issues at a tactical level.
Marketing information is essential for a company or organization to stay competitive and also meet the customer’s needs. As referenced above, LEGO used marketing research to gather information about how children use its product but also how parents felt about opportunities for their children. Universities and colleges use information gathered through marketing research to build next year’s recruitment materials by asking students their perceptions of the previous year’s items. Additionally, a fast-food restaurant might conduct an analysis of the time of day each of its items are more likely to be purchased. Obvious to most, coffee would probably be an item more likely ordered earlier in the day. Other items might not be as evident but could require different preparation times. By accounting for when each of these items is most likely to be ordered, the restaurant can plan its inventory and schedule of employees more efficiently.
Big Data and the Marketing Information System (MIS)
The amount of data currently available is not only vast but is growing at an exponential level every day, which describes the concept of big data. Big data is the countless number of records that continues in an increasing capacity and at a faster rate. Because of the constantly changing landscape of data, it’s difficult for companies to develop an approach for analyzing it. Big data is often described through the use of the three Vs: volume, velocity, and variety. The amount of data, or volume, is more than we have ever witnessed. Additionally, it is growing at a fast rate; the velocity is also ever increasing. We expect that the velocity of data will continue to surge exponentially as each day more users are contributing to the data available. Finally, there is the variety of data that is part of this enormous data set—because each user is contributing to the cache of data available, the diversity of data is just as unique as its creators.
Link to Learning
What is big data.
Big data is massive amounts of data. But what does that mean? Think of all the data a smartphone generates with texts, searches, emails, photos, etc. Now consider how many smartphone users there are in the world. Watch this video and learn how much data is generated on the internet each day and how it can be classified through the three Vs concept.
“Big data has become one of the most valuable assets held by enterprises, and virtually every large organization is making investments in big data initiatives.” 4 This statement was based on a 2021 NewVantage Partners survey of senior C-level executives at Fortune 1000 companies regarding their perceptions of big data and their utilization of its resources. NewVantage Partners learned that many companies are already pursuing this data, with 96 percent reporting that their companies have already had success using big data and artificial intelligence programs. Additionally, 99 percent of the executives surveyed are pursuing either new or existing big data programs. Why are companies so keen to invest in this source? It is estimated that the world creates approximately 2.5 quintillion bytes of data daily. This vast accumulation of data can be collected through various methods, such as point-of-sale databases, connected devices through the Internet of Things (IoT), third-party marketing research firms, social media, location data from mobile devices, or surveys. 5 Tapping into the resource generators is helpful for companies to understand their customers, employees, or others.
So, who are these data generators? They are everyone, like you and your friends, who post content on social media, engage with web content through streaming services, and share stories through web applications. In addition, businesses are contributing to the massive amount of content available. Extra data (volume), at a faster rate (velocity), and from a more widely diverse set of people (variety) explain why it is called “big” data. 6 So how do businesses and individuals capitalize on this vast data world?
In order to be able to utilize and make the most of the availability of business data, businesses use a marketing information system to collect, analyze, and report interesting findings from internal and external data of the company. This system is an ever-changing database of content that is used to support the company or organization’s marketing efforts. The marketing information system is the collection of data and requires actions taken by marketers to utilize the data saved.
Careers In Marketing
Market research analysts.
Market research analysts are needed in numerous industries, and according to the US Bureau of Labor and Statistics , the job outlook has a much higher rate of growth than average. Read about the skills needed, typical pay, education requirements, and career outlook in this article and watch this video about the various types of jobs available as a market research analyst.
Knowledge Check
It’s time to check your knowledge on the concepts presented in this section. Refer to the Answer Key at the end of the book for feedback.
- market intelligence
- management information system
- marketing research
- marketing information system
- survey data
- Market intelligence
- Management information system
- Marketing research
- It provides a guarantee of customer satisfaction.
- It identifies opportunities in the market to explore.
- It helps to quantify customer perceptions.
- It uncovers weaknesses within a company.
- Analyzing the product purchases of current customers
- Measuring the number of reactions to a social media post
- Examining a competitor’s database of information
- Surveying potential employees about why they are interested in working for a company
As an Amazon Associate we earn from qualifying purchases.
This book may not be used in the training of large language models or otherwise be ingested into large language models or generative AI offerings without OpenStax's permission.
Want to cite, share, or modify this book? This book uses the Creative Commons Attribution License and you must attribute OpenStax.
Access for free at https://openstax.org/books/principles-marketing/pages/1-unit-introduction
- Authors: Dr. Maria Gomez Albrecht, Dr. Mark Green, Linda Hoffman
- Publisher/website: OpenStax
- Book title: Principles of Marketing
- Publication date: Jan 25, 2023
- Location: Houston, Texas
- Book URL: https://openstax.org/books/principles-marketing/pages/1-unit-introduction
- Section URL: https://openstax.org/books/principles-marketing/pages/6-1-marketing-research-and-big-data
© Jan 9, 2024 OpenStax. Textbook content produced by OpenStax is licensed under a Creative Commons Attribution License . The OpenStax name, OpenStax logo, OpenStax book covers, OpenStax CNX name, and OpenStax CNX logo are not subject to the Creative Commons license and may not be reproduced without the prior and express written consent of Rice University.
What Is Big Data in Marketing? 22 Examples.

At first glance, marketing and big data might seem like an odd pair, but they’re actually very complementary. Marketing is all about effectively reaching different audiences, and data tells us what’s working and what’s not.
These days, there’s more available data than most businesses and marketing teams know what to do with, which can lead to new opportunities if that data is accurately interpreted and effectively deployed .
Why is Big Data Important in Marketing?
Big data is becoming a fundamental tool in marketing. Data constantly informs marketing teams of customer behaviors and industry trends, and is used to optimize future efforts, create innovative campaigns and build lasting relationships with customers.
How Is Big Data Used in Marketing?
Big data offers a snapshot of a business’ user base, as trends, preferences and behavior patterns can be revealed through data analysis. Companies use these data insights to drive marketing strategies and product decisions.
Customer Engagement and Retention
Big data regarding customers provides marketers details about user demographics, locations, and interests, which can be used to personalize the product experience and increase customer loyalty over time .
Marketing Optimization and Performance
Big data solutions can help organize data and pinpoint which marketing campaigns, strategies or social channels are getting the most traction. This lets marketers allocate marketing resources and reduce costs for projects that aren’t yielding as much revenue or meeting desired audience goals.
Competitor Tracking and Operation Adjustment
Big data can also compare prices and marketing trends among competitors to see what consumers prefer. Based on average industry standards, marketers can then adjust product prices, logistics and other operations to appeal to customers and remain competitive.
22 Big Data in Marketing Examples
The following companies offer unified views of data, entire customer databases, campaign optimization, strategy services and other crucial data services to make marketing as effective as possible.
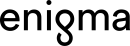
Focus: Customer engagement How it’s using big data: Enigma takes big data — meaning huge data sets accumulated from diverse sources —and uses AI to analyze it. Its focus is financial health and behavior in private small and medium sized businesses. The insights it gathers from this analysis are used in its sales and marketing platform, which uses dynamic data to increase spend efficiency and drive growth throughout the B2B customer lifecycle.
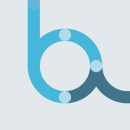
Focus: Customer engagement
How it’s using big data in marketing: BlueConic ’s customer data platform provides marketers with a single view of the customer. Unifying data from across channels and other sources, BlueConic gives marketer’s control over individual-level customer data. Consequently, companies can decrease bounce rates, enhance look-alike targeting and create more personal experiences for users.
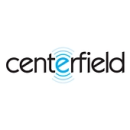
Centerfield
How it’s using big data in marketing: Centerfield develops intelligent big data-driven marketing and sales technology utilizing real time biddable media (RTB), automated call routing and customized scripting. Its proprietary platform, Dugout, combined with its sales and retention center delivers new customers at scale to many of the leading brands worldwide.
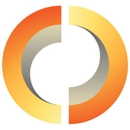
Core Digital Media
How it’s using big data in marketing: Core Digital Media utilizes predictive modeling to pinpoint hundreds of millions of daily online advertising impressions for its mortgage, home and auto brands. Of these impressions, Core determines optimal comparison-shopping paths and the expected value of these impressions for clients.
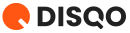
Focus: Marketing optimization
How it’s using big data in marketing: Serving market research agencies, brands and analytics companies, DISQO provides high quality consumer data based on data points from millions of opt-in consumers so clients can map and understand their customers’ digital journeys.
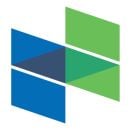
How it’s using big data in marketing: HAVI uses data to create supply chain solutions for the restaurant industry. With expertise in marketing ROI, customer and predictive analytics, HAVI helps its clients clean dissimilar and untidy data to create a single data source for use across the entire restaurant enterprise.
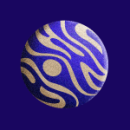
How it’s using big data in marketing: Howl is a creator platform for the customer-centric and product-obsessed. Creators of every size use Howl’s commerce and payments technology to get better rates and easy payments for their big ideas. Creators on Howl earn more when they open doors for others, and have the tools to turn their craft into a business.
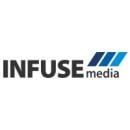
Infusemedia
How it’s using big data in marketing: INFUSEmedia creates demand generation solutions that include brand activation, intent-driven marketing, content marketing and lead-flow dashboards. The proprietary platform pulls from a worldwide database of over 15 million companies across more than 28 verticals (including technology, media, real estate, healthcare and consumer goods) to help B2B companies target audiences and drive interest.
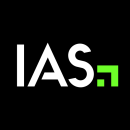
Integral Ad Science
How it’s using big data in marketing: Integral Ad Science (IAS) offers digital ad verification solutions, ensuring that ads are viewable by real people in safe and suitable environments. IAS’s mission is to be the global benchmark for trust and transparency in digital media quality for thousands of leading brands, publishers and platforms. IAS does this through data-driven technologies with actionable real-time signals and insight. IAS is part of the Vista Equity Partners portfolio of companies.
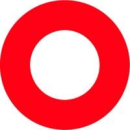
How it’s using big data in marketing: Ogury ’s advertising engine delivers comprehensive audience interests, brand performance, privacy protection and sustainability within one technology stack, built and optimized for mobile technology . Advertisers working with Ogury benefit from fully visible impactful ads, future-proof targeting and unwavering protection. Publishers enjoy the rewards of a respectful user experience, incremental revenues and premium demand with Ogury’s solutions.
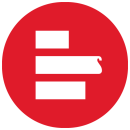
Supermetrics
How it’s using big data in marketing: Supermetrics is a platform focused on helping businesses streamline their marketing data. Whether the data destination is Snowflake, BigQuery or another platform, the company’s plug-in connectors can consolidate, automate and organize data.
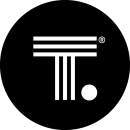
ThoughtSpot
How it’s using big data in marketing: ThoughtSpot lets any employee, regardless of tech background, glean important insights from company data. The search and AI analytics platform works across industries and teams so that everyone from marketing managers to customer care representatives can pull the data they need to understand customer usage, make better marketing decisions and improve customer loyalty.
Related 75 Big Data Companies to Know
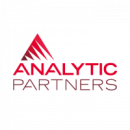
Analytic Partners
How it’s using big data in marketing: Analytic Partners is a marketing analytics company that assists clients in decision-making with its own data science technology, GPS Enterprise. The product has three functions: storing the data, uncovering the analytics and forecasting possible solutions. The platform also serves to answer questions like how to maximize a budget and what risks are associated with each solution.
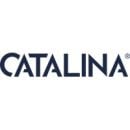
Catalina USA
How it’s using big data in marketing: Catalina combines an enormous database of shopper purchase history and a personalized advertising experience to ensure that retailers, CPG brands and agencies market to their audiences as effectively as possible. Catalina helps companies leverage data from millions of shoppers to drive personal messages based on current, past and future buying habits, resulting in more repeat trips from shoppers.
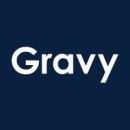
Gravy Analytics
How it’s using big data in marketing: Gravy Analytics uses location intelligence to give marketers more insight into where consumers go and what their interests are. Its Audiences product offers access to pseudonymous U.S. mobile user data, all to help brands in industries like hospitality, retail, finance and restaurants learn more about what current and potential customers want.
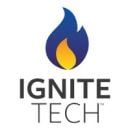
How it’s using big data in marketing: IgniteTech ’s Beckon provides marketing analytics software to help companies and brands optimize campaigns, analyze marketing and media performance, manage marketing data and more. Serving industries like consumer electronics, finance, retail, media and travel, Beckon’s solutions ensure that the data and efforts of marketing analysts and teams are visible across channels.
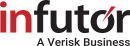
Infutor Data Solutions
How it’s using big data in marketing: Infutor Data Solutions provides consumer identity management solutions to enhance customer engagement and help companies better understand customers. Infutor’s ID Max solution uses an Identity Graph to create an up-to-date customer identity profile from inbound customer data. ID Max lets marketers create personalized campaigns, increase lead attribution and maintain more touch points.
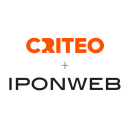
IPONWEB (acquired by Criteo)
How it’s using big data in marketing: IPONWEB designs ad exchange and digital advertising technologies. It offers businesses three solutions: BidSwitch, MediaGrid and Performance Platform, each of which is scalable and adaptable to business needs. They also assist in unlocking performance differences with other brands, optimizing bidstream performances and managing monetization and curation tools.
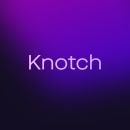
How it’s using big data in marketing: The Knotch platform is designed to help brands optimize digital content strategy by measuring analytics, user feedback and audience data while tracking competitor content for the purposes of comparison and website performance assessment.
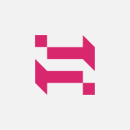
How it’s using big data in marketing: Simon Data ’s marketing solutions to help transform customer data into automated customer journeys. The platform integrates with first- and third-party data providers so marketing teams have a complete and unified view of customers in order to provide insights and meet needs.
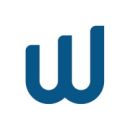
Wiland Inc.
How it’s using big data in marketing: Wiland blends data, business intelligence and marketing solutions to help companies grow audiences across channels. Working with clients in numerous industries, Wiland uses proprietary consumer transaction data and predictive analytics to produce highly responsive audiences.
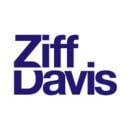
How it’s using big data in marketing: Ziff Davis ’ Performance Marketing data insights help marketing teams enhance campaigns and develop lead generation strategies to engage audiences. The company also aims to support businesses’ process standardization, data security and regulatory compliance efforts throughout campaign lifecycles.
Great Companies Need Great People. That's Where We Come In.
- Twin Cities
University of Minnesota
- Bachelor's Degrees
- Master's Degrees
- Doctorate Degrees
- Certificates
- Coursera Online Courses
- Licensing Programs
- Post-Secondary Enrollment Options (PSEO)
- Credit Online Courses
- Professional Development Online Courses
- Student Stories
- Health and Well-being
- Learn Online
A Guide to Data-Driven Marketing: How Big Data Is Transforming the Digital Marketing Landscape
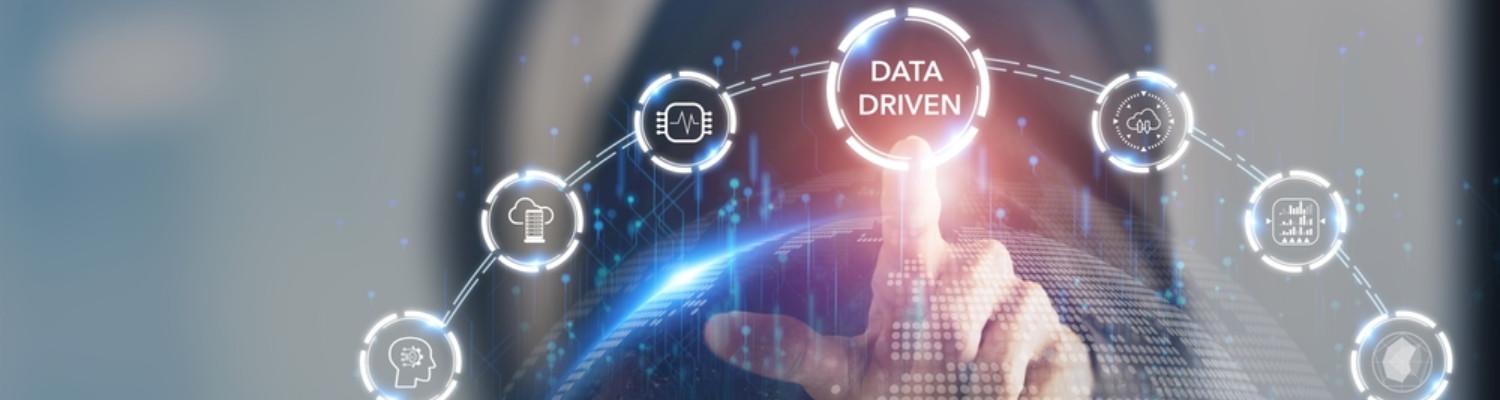
Big data drives modern marketing. Every purchase and online interaction plays into a complex and far-reaching system. This vast data landscape generates valuable consumer insights and can even predict how customers or clients will behave in the future. This has major implications for businesses, their marketing departments, and especially the digital marketing professionals responsible for data-driven campaigns.
While data-driven strategies can unlock a world of potential for modern marketing initiatives, there are still weaknesses to address. Some of these involve a simple desire to stick with the previous marketing status quo. Gartner's Senior Director Analyst Joseph Enever explains, "Better data won’t increase marketing analytics’ decision influence alone," adding that there's a need to "address the real challenges—cognitive biases and the need for a data-informed culture."
Comprehensive training can play a significant role in preparing the marketing leaders of tomorrow to leverage data-driven solutions. The Bachelor of Science in Marketing is a particularly appealing option, integrating technical know-how with still-important soft skills. To that end, we explain how far digital marketing analytics have come, what's next for predictive analytics in marketing, and how targeted degree programs can shape the data-dominant landscape of tomorrow.
The Emergence of Big Data in Marketing
Marketing has long been a data-driven field. As former Forbes council member Jon Simpson explains, this "process of analyzing data and using findings to influence decisions" has been present for centuries and has been especially prominent these past two decades. However, Savitha Namuduri tells Chief Data Officer Magazine that these "early days of digital marketing were marked by challenges in data capture and storage." Resolving these issues has been key to unlocking the power of big data.
The use of data in marketing first picked up speed during the 1980s, particularly with the early development of customer relationship management (CRM) systems. Soon after, the takeoff of the internet prompted immense increases in data collection and analysis, with even more opportunities arriving as social media entered the digital landscape. This evolved into a powerful blend of CRM and marketing automation, which streamline critical marketing and sales processes.
The wealth of data means little of it cannot be efficiently analyzed, but thankfully, robust tools, technologies, and methods have entered the picture. With cross-platform measurement opportunities and solutions such as media mix modeling (MMM) and multitouch attribution (MTA), big data is now a central component of digital marketing.
Personalization at Scale
Today's demanding consumers desire personalized messaging and services that reflect their unique needs and preferences. Data-driven solutions provide valuable opportunities to meet these lofty demands by revealing what users want, how they've behaved in the past, and what they are likely to respond to in the future.
Perhaps more importantly, big data makes it possible to bring this personalized approach to a broader population of consumers. Previously, personalized marketing strategies were notoriously time-consuming, to the point that their return on investment (ROI) was often limited. With improvements in AI-centric strategies, though, it is possible to efficiently collect and analyze data from a wider range of sources—and synthesize this data to produce actionable insights.
As the Adobe Communications Team explains, today's most successful marketing teams strive to use "the right data at the right time through an end-to-end personalization strategy at scale." This means avoiding data silos while promoting a company culture that embraces data and agile marketing.
Predictive Analytics in Decision-Making
Drawing on the power of past interactions or purchases to reveal how consumers might respond to future campaigns, predictive analytics plays an increasingly central role in today's digital marketing initiatives. These days, consumer behavior analysis largely revolves around two methods. We briefly mentioned these earlier and now describe them in greater detail:
- Media mix modeling (MMM) – Built on a strong foundation of aggregated data, MMM solutions track a broad spectrum of marketing activities, revealing how these influence ROI. Multilinear regression is a key component of this approach, as this shows how the dependent variable (like sales) is linked to independent variables (ad spend, for example).
- Multitouch attribution (MTA ) – Encompassing a range of consumer touchpoints, MTA grants fractional credit to various aspects of the customer journey to demonstrate the level of influence these channels or interactions have on eventual sales. This strategy can leverage data from numerous sources, including not only social media analytics and content marketing analytics but also paid search and even direct mail.
The Impact of Data Privacy Regulations
If the 2010s saw the rise of big data in digital marketing, the latter half of the decade and the first few years of the 2020s have produced considerable pushback in the form of strict data privacy regulations. We outline two of the most impactful below:
- General Data Protection Regulation (GDPR) – Implemented by the European Union but increasingly relevant in the United States, GDPR represents the world's toughest data protection rules, which, although vast in scope, center on one main goal: to give users more control over how their data is collected and used. Under GDPR, website visitors must explicitly consent to having their information gathered and must also be notified if sites are breached.
- California Consumer Privacy Act (CCPA) – Similar to GDPR, CCPA grants consumers extensive rights to dictate how their personal data is gathered and disseminated. Under this law, users are allowed to have their information deleted on request and cannot be penalized by businesses if they exercise these rights.
While these and other emerging regulations will have a huge impact on which types of data can be gathered and in what circumstances, they are not expected to slow the progress of big data in marketing. These days, many consumers are receptive to sharing their information but often want the chance to opt in or opt out. Furthermore, consent management systems and other privacy technologies promise to expedite the process of gaining consent.
Integrating Offline and Online Data
Data-driven marketing faces many challenges, and one of the most significant involves the integration of offline and online data. There are substantial volumes of both, and while online data tends to attract the most attention, disconnected offline data is also abundant. This can take various forms, such as purchase information, loyalty card history, and survey results.
When online and offline data are properly integrated, omnichannel optimization is well within reach. In addition, this approach is more likely to produce accurate consumer insights and audience profiles. Integration can produce exciting new revenue opportunities as well. Many organizations work with onboarding partners to streamline this process.
The Future of Data-Driven Marketing
The age of data-driven marketing has arrived. Some marketing firms and professionals have yet to catch up, however; every day that they fail to implement data-driven strategies, they yield even more advantages to their competitors.
As a greater share of organizations utilize data-driven solutions, the new focus will shift from whether these are used to how they are incorporated into campaigns and which technologies provide the best ROI. Artificial intelligence has a growing role to play in this, offering powerful opportunities to boost content optimization while helping forecast demand.
Moving forward, digital marketing professionals will be expected to master and embrace data-driven strategies while also getting comfortable working alongside AI solutions. Meanwhile, as GDPR, CCPA, and emerging legislation exert their influence, marketing teams will be more inclined to embrace first-party data, rather than relying on the third-party solutions of yesteryear. Other digital marketing trends to anticipate include:
- More touchpoints in the consumer journey — dozens or even hundreds for some consumers. This distribution of these touchpoints will change, too, moving away from linear sequences.
- Agile marketing solutions will leverage data-driven insights to allow teams to respond to challenges (and embrace new opportunities) in real time.
- Data science teams will be relied on to boost customer segmentation, predictive modeling, and channel optimization.
Learn More, Today
Are you ready to embrace new trends and technologies as they relate to digital marketing? First, you need to develop a robust skill set. The University of Minnesota’s Bachelor of Science in Marketing is an online marketing degree that provides a deep dive into a variety of fascinating topics, such as consumer behavior and international marketing. We also provide numerous hands-on learning opportunities to help you apply your newly developed skills. You can emerge feeling confident and excited about your prospects in the fast-paced field of digital marketing. Reach out today to get started.
- The Evolution of Digital Marketing Data — 1980s through the 2020s
- High-Tech Touchpoints Are Changing Customer Experience
- The Past, Present And Future Of Big Data In Marketing
- The History of Marketing Analytics
- General Data Protection Regulation (GDPR) Definition and Meaning
- The big reset: Data-driven marketing in the next normal
Home • Knowledge hub • Understanding the Role of Big Data in Market Research.
Understanding the Role of Big Data in Market Research.
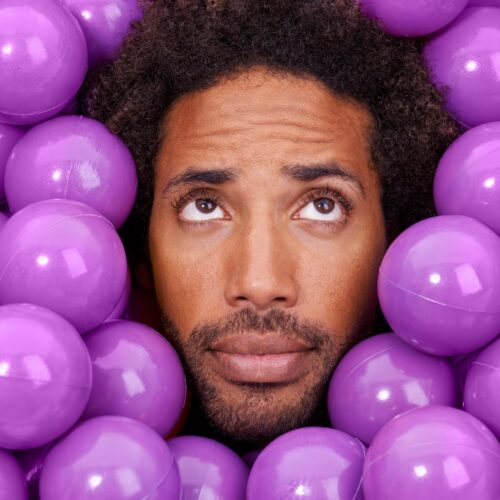
Big data refers to the massive amount of structured and unstructured data generated by various sources in our digital world, such as social media, e-commerce transactions, and mobile devices. This data is characterised by its sheer volume, velocity, and variety, making it difficult to process using traditional methods.
“Big data will become the basis for competitive advantage, replacing the traditional competitive advantage of having the best resources, the best people, or the best strategy.” – Ginni Rometty, CEO of IBM.
The role of big data in market research is crucial in providing businesses with valuable insights into consumer behaviour, preferences, and market trends. Market researchers use big data to analyse consumer data and understand their purchasing habits, preferences, and opinions, which helps businesses make informed decisions about product development, marketing, and sales strategies.
Big data also helps identify potential market opportunities and challenges and understand the effectiveness of marketing campaigns. By leveraging advanced analytical techniques, such as machine learning and predictive analytics, market researchers can uncover patterns and relationships in consumer data, which can help businesses tailor their products and services to meet the needs and preferences of their target market.
The term “big data” was first popularised in the late 1990s and early 2000s, but the concept of handling large amounts of data dates back to much earlier. Here is a rough timeline of the history of big data:
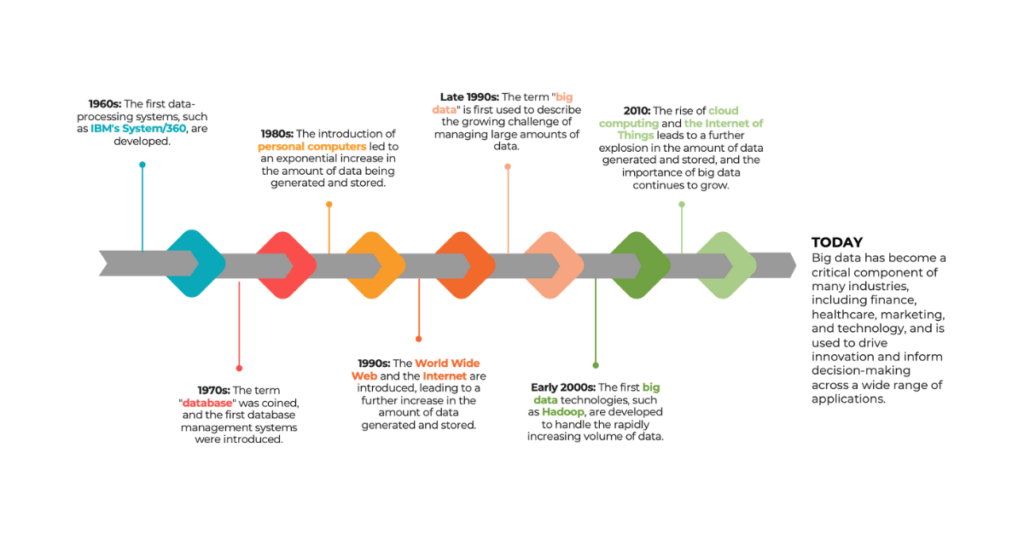
Get regular insights
Keep up to date with the latest insights from our research as well as all our company news in our free monthly newsletter.
- First Name *
- Last Name *
- Business Email *
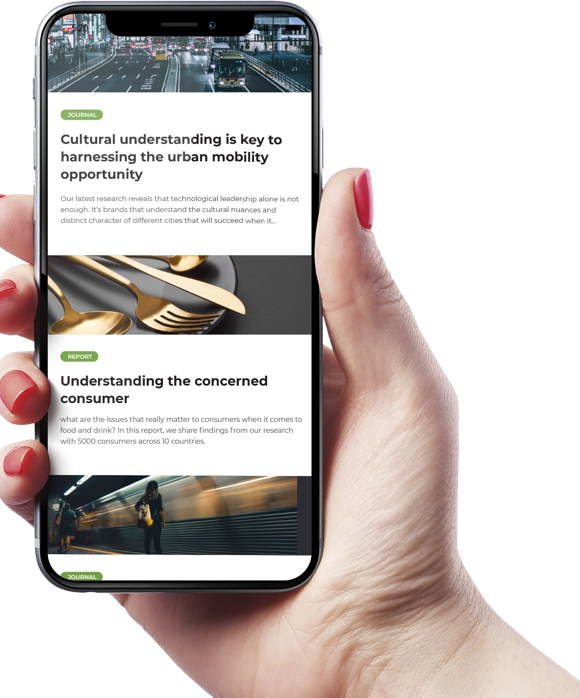
The outlook for big data is very positive, with demand for big data solutions expected to continue growing as brands seek to harness the value of their data and make more informed decisions.
Here are some of the key trends and factors that are shaping the future of big data:
- Continued Growth of Data: The amount of data being generated is continuing to grow at an exponential rate, driven by the proliferation of connected devices, the Internet of Things (IoT) , and the rise of new technologies such as artificial intelligence and machine learning.
- Wider Adoption of Cloud Computing: The trend towards cloud computing enables companies to store and process large amounts of data more efficiently and cost-effectively, driving the adoption of big data solutions.
- Increased Focus on Data Privacy: As consumers become more aware of the value of their personal data, there is a growing demand for solutions that allow them to control and protect their information.
- Advances in Artificial Intelligence and Machine Learning: The continued development of AI and machine learning makes it possible to extract more value from big data, enabling companies to gain new insights and make more informed decisions.
- Expansion into New Industries: Big data is no longer limited to tech-focused industries and is increasingly being adopted by a wider range of industries, including healthcare, retail, finance, and energy.
4 Ways Big Data is Changing Market Research
As previously mentioned, big data refers to large and complex datasets generated by various sources, including social media, e-commerce transactions, and mobile devices. The sheer volume, velocity, and variety of big data can make it difficult to process and analyse using traditional data processing techniques.
“Big data is more than just a matter of size; it is an opportunity to find insights in new and emerging types of data and content, to make your business more agile, and to answer questions that were previously considered beyond your reach.” – Tim O’Reilly, Founder, and CEO of O’Reilly Media.
Big data is changing the way market research is conducted in several ways. First, big data allows market researchers to gain insights into consumer behaviour and preferences at a scale that was previously not possible. With big data, researchers can track consumer interactions across multiple touchpoints, including online and offline behaviours, social media interactions, and purchase history.
Second, big data enables market researchers to gain more accurate and in-depth insights into consumer behaviour and preferences. With traditional market research methods, such as surveys and focus groups, it can be difficult to get a complete picture of consumer behaviour and preferences, as the sample size is often limited and the data is self-reported. With big data, researchers have access to a much larger and more diverse dataset, which can provide a more accurate and in-depth view of consumer behaviour and preferences.
Third, big data allows market researchers to conduct research in real-time, providing brands with insights into consumer behaviour and preferences as they happen. This will enable companies to respond quickly to changing consumer preferences and needs and make more informed decisions.
Finally, big data enables market researchers to use more advanced analytical techniques, such as machine learning and artificial intelligence, to gain deeper insights into consumer behaviour and preferences. With these techniques, researchers can analyse large and complex datasets, uncover patterns and correlations, and gain insights into consumer behaviour and preferences in a way that was previously not possible.
In conclusion, big data is changing the way market research is conducted by providing researchers with access to larger and more diverse datasets, enabling real-time research, and allowing for more advanced analytical techniques. As a result, companies can gain more accurate and in-depth insights into consumer behaviour and preferences and make more informed decisions.
The Benefits of Big Data
The use of big data in market research offers several benefits that can help brands gain a better understanding of their customers and make more informed decisions. Some of the key benefits of big data in market research include the following:
- Ability to gather and analyse vast amounts of data: One of the biggest benefits of big data in market research is the ability to gather and analyse vast amounts of data. With traditional market research methods, such as surveys and focus groups, it can be difficult to collect enough data to make accurate and informed decisions. However, with big data, researchers can gather and analyse vast amounts of data from a wide range of sources, including social media, e-commerce transactions, and mobile devices, providing a much more complete picture of consumer behaviour and preferences.
- Real-time insights: Another key benefit of big data in market research is the ability to gain real-time insights. Traditional market research methods can take weeks or even months to gather and analyse data, by which time consumer preferences and behaviours may have changed. With big data, researchers can gain real-time insights into consumer behaviour and preferences, allowing companies to respond quickly to changes in the market.
- Improved accuracy: Big data also provides a more accurate picture of consumer behaviour and preferences than traditional market research methods. With traditional methods, the sample size is often limited, and the data is self-reported, leading to biases and inaccuracies. With big data, researchers have access to a much larger and more diverse dataset, which can provide a more accurate view of consumer behaviour and preferences.
- Advanced analytical techniques: Finally, big data enables market researchers to use more advanced analytical methods, such as machine learning and artificial intelligence, to gain deeper insights into consumer behaviour and preferences. These techniques can help researchers uncover patterns and correlations in large and complex datasets, giving organizations a more in-depth understanding of their customers.
The Power of Predictive Analytics
Predictive analytics is a key component of big data and is increasingly used by companies to make informed business decisions. Predictive analytics involves statistical models, machine learning algorithms, and other techniques to analyse large and complex datasets and predict future events or trends.
In market research, predictive analytics can forecast consumer behaviour and preferences and predict the success of marketing campaigns, product launches, and other initiatives. By leveraging the power of predictive analytics, brandss can better understand their customers, make more informed decisions, and stay ahead of the competition.
One of the key advantages of predictive analytics is its ability to identify patterns and correlations in large and complex datasets. This allows brands to predict future consumer behaviour and preferences and identify key drivers of consumer behaviour. For example, predictive analytics can identify the factors influencing consumer purchasing decisions, such as brand loyalty, price sensitivity, and product quality.
Another advantage of predictive analytics is its ability to provide real-time insights. Traditional market research methods can take weeks or even months to gather and analyse data, by which time consumer preferences and behaviours may have changed. With predictive analytics, organisations can gain real-time insights into consumer behaviour and preferences, allowing them to respond quickly to changes in the market.
The Challenges of Big Data
Despite the many benefits of big data in market research, several challenges are associated with this approach. Some of the main challenges of big data include the following:
- The need for advanced data management systems: One of the biggest challenges of big data is the need for advanced data management systems. Traditional market research methods typically collect data in a centralised and structured format, making it easier to manage and analyse. However, with big data, data is often collected from a wide range of sources and in a variety of formats, making it more challenging to manage and analyse. As a result, companies must invest in advanced data management systems, such as data warehouses, data lakes, and cloud computing solutions, to effectively manage and analyse big data.
- The need for skilled data scientists: Another challenge of big data is the need for qualified data scientists. With big data, organisations must analyse vast amounts of data using advanced techniques, such as machine learning and artificial intelligence, which require a high level of expertise. As a result, companies must invest in training and development programs for their data scientists or partner with external firms with the necessary expertise to effectively leverage the power of big data.
- Data privacy and security concerns: With the increasing use of big data, there are also concerns about data privacy and security. With big data, organisations must collect and store vast amounts of personal data, which raises concerns about data privacy and security. As a result, companies must implement strong security measures and comply with data privacy regulations, such as the General Data Protection Regulation (GDPR), to protect personal data.
- Quality and accuracy of data: Another challenge of big data is the quality and accuracy of data. With big data, organisations must rely on data from a wide range of sources, including social media, e-commerce transactions, and mobile devices, which may only sometimes be accurate or up-to-date. As a result, companies must validate and clean the data they collect to ensure its accuracy and quality.
Big Data Gone Wrong
There are several examples of big data gone wrong that are worth mentioning. One such example is the Cambridge Analytica scandal, where the data analytics firm gained unauthorised access to the personal data of millions of Facebook users, which was then used to influence political elections. This scandal brought attention to the potential misuse of big data and the importance of ethical considerations in its use.
“Big data is not about the data. It’s about creating insights, making informed decisions, and driving outcomes.” – Tom Davenport, Professor of Information Technology and Management at Babson College .
Another example is the concept of “fake news,” which has become increasingly prevalent with the rise of big data. The vast amounts of information available through big data can make it difficult to distinguish between credible and non-credible sources, leading to the spread of false information and misleading insights.
Finally, big data can also perpetuate existing biases and discrimination if the data used to inform decision-making is not diverse and representative. For example, facial recognition technology has faced criticism for having higher error rates for people with darker skin tones due to a lack of diverse training data.
These examples highlight the importance of responsible and ethical use of big data in market research and the need for companies to consider the potential consequences of their actions when leveraging big data to inform business decisions.
Integrating Big Data with Traditional Research Methods
While big data in market research offers many benefits, it is also essential to integrate it with traditional research methods, such as surveys and focus groups, to achieve a comprehensive understanding of consumer behaviour. This integration can help organisations:
- Validate big data findings: By combining big data with traditional research methods, brands can validate the findings of big data and ensure the accuracy of their results. For example, by conducting surveys or focus groups, companies can gain insights into consumer attitudes and behaviours, which can be compared with the data collected from big data sources, such as social media or e-commerce transactions.
- Gain deeper insights into consumer behaviour: Integrating big data with traditional research methods can also help organisations gain deeper insights into consumer behaviour. For example, by combining big data with focus groups, brands can gain a complete understanding of consumer attitudes and motivations, which can help them make more informed decisions.
- Fill gaps in big data: Big data sources, such as social media and e-commerce transactions, only sometimes provide a complete picture of consumer behaviour. By integrating big data with traditional research methods, brands can fill gaps in their data and gain a full understanding of consumer behaviour.
- Enhance the reliability of results: Integrating big data with traditional research methods can also enhance the reliability of market research results. By combining multiple data sources, organisations can gain a more accurate and comprehensive understanding of consumer behaviour.
The Role of Artificial Intelligence and Machine Learning
Artificial intelligence (AI) and machine learning (ML) are rapidly becoming an important part of big data in market research. These technologies are often used to automate the analysis of large amounts of data, making it easier and faster to gain insights into consumer behaviour. Some of the ways in which AI and ML are used in market research include:
- Predictive modelling: AI and ML are used to create predictive models that can identify patterns and trends in big data. These models can be used to forecast consumer behaviour and make informed decisions.
- Sentiment analysis: AI and ML can also be used to perform sentiment analysis on social media data, making it possible to gain insights into consumer opinions and attitudes.
- Natural language processing: AI and ML are also used to perform natural language processing (NLP) on big data sources, such as customer reviews or surveys. NLP allows companies to analyse text data and gain insights into consumer behaviour.
In the future, AI technologies, such as ChatGPT, could play a significant role in market research. For example, ChatGPT could conduct virtual focus groups or customer interviews. This type of AI could provide a more natural and interactive experience for participants, making it easier to gain insights into consumer behaviour. Additionally, ChatGPT could automate customer feedback analysis, making it possible to gain insights into consumer behaviour in real-time.
Best Practices for Big Data Market Research
When conducting big data market research, it is essential to follow best practices to ensure the quality and accuracy of the data. Some of the best practices for big data market research include:
- Focus on data quality: The quality of the data is critical for making informed decisions. Organisations should focus on collecting high-quality data from reliable sources, such as customer surveys or transactional data. Additionally, it is essential to clean and validate the data to ensure accuracy.
- Ethical considerations: Big data market research raises significant ethical concerns like privacy and data security. Brands should be transparent about their data collection practices and obtain consent from participants. Additionally, it is crucial to secure and store data to protect sensitive information properly.
- Integration with traditional research methods: While big data provides valuable insights into consumer behaviour, it is important also to integrate it with traditional research methods, such as focus groups or customer interviews, to gain a comprehensive understanding of consumer behaviour.
- Data management and storage: The volume and complexity of big data requires advanced data management systems and storage solutions. Brands should invest in these technologies to ensure that they can efficiently store, manage, and analyse large amounts of data.
- Collaboration with data scientists: Organisations may need to collaborate with data scientists or other experts to analyse the data and extract insights. It is vital to work with experienced professionals to ensure that the data is analysed accurately and effectively.
Big Data in Action
Big data has been used in various industries to inform business decisions and improve market research. Here are a few examples:
- Retail: Big data has been used by retailers to analyse customer purchase patterns and improve inventory management. For example, retailers can use data on customer purchases to determine which products are in high demand and adjust their inventory accordingly.
- Healthcare: The healthcare industry uses big data to improve patient outcomes and reduce costs. For example, healthcare providers use patient health records and medical procedures data to identify trends and make treatment recommendations.
- Finance: Financial services companies use big data to improve risk management and fraud detection. For example, banks can use data on customer transactions to identify unusual patterns that may indicate fraudulent activity.
- Marketing: Marketers use big data to gain insights into consumer behaviour and target advertisements more effectively. For example, companies can analyse consumer searches and social media activity data to determine which products and services interest consumers.
These are just a few examples of how big data can inform business decisions and improve market research. As technology evolves and the amount of data generated continues to grow, we will likely see even more innovative uses of big data in the future.
Final thoughts and Key Takeaways
It is worth mentioning that the role of big data in market research is constantly evolving. As technology advances and the amount of data generated continues to grow, the opportunities to leverage big data in market research are only increasing.
“Big data, if used correctly, has the potential to change the face of market research forever. By harnessing the power of advanced analytics, market researchers can uncover new insights and trends that were previously hidden in the data.” – Raj De Datta, CEO and Co-Founder of Bloomreach.
One key trend in using big data for market research is the rise of omnichannel data. Omnichannel data refers to collecting data from various sources, including online and offline interactions, to understand consumer behaviour comprehensively. With the rise of the Internet of Things (IoT) and the increasing use of mobile devices, the amount of omnichannel data available for analysis is snowballing.
Another trend in using big data for market research is the increased focus on data privacy and ethics. With the growing amount of data being collected and analysed, companies must ensure that they respect consumers’ privacy and adhere to ethical standards.
Key Takeaways
- Big data refers to the vast amounts of structured and unstructured data generated by modern technologies, such as social media, online transactions, and IoT devices.
- The benefits of using big data in market research include gathering and analysing vast amounts of data in real-time, gaining deeper insights into consumer behaviour, and making more informed business decisions.
- Predictive analytics is a powerful tool in big data, as it can help brands identify trends and predict future behaviour.
- The use of big data in market research is not without its challenges, including the need for advanced data management systems, skilled data scientists, and ethical considerations.
- Integrating big data with traditional research methods, such as surveys and focus groups , can provide a comprehensive understanding of consumer behaviour and help companies make more informed decisions.
- AI and machine learning play a significant role in big data, as they can help process and analyse vast amounts of data and improve market research.
- Best practices for conducting big data market research include ensuring data quality, considering ethical considerations, and integrating big data with traditional research methods.
- Real-life examples of big data in action include its use in personalised marketing, identifying consumer trends, and predicting future behaviour.
- Despite the potential benefits of big data in market research, there are also possible consequences, including spreading false information, perpetuating existing biases and discrimination, and potential misuse of data. As such, market researchers must be aware of these potential consequences and ensure that they use big data in an ethical and responsible manner.
In conclusion, big data has already significantly impacted market research and is only becoming more important as technology advances, and the amount of data generated continues to grow. Market researchers who embrace big data and understand its potential benefits and challenges will be well-positioned to succeed in the future.
Helping brands uncover valuable insights
We’ve been working with Kadence on a couple of strategic projects, which influenced our product roadmap roll-out within the region. Their work has been exceptional in providing me the insights that I need. Senior Marketing Executive Arla Foods
Kadence’s reports give us the insight, conclusion and recommended execution needed to give us a different perspective, which provided us with an opportunity to relook at our go to market strategy in a different direction which we are now reaping the benefits from. Sales & Marketing Bridgestone
Kadence helped us not only conduct a thorough and insightful piece of research, its interpretation of the data provided many useful and unexpected good-news stories that we were able to use in our communications and interactions with government bodies. General Manager PR -Internal Communications & Government Affairs Mitsubishi
Kadence team is more like a partner to us. We have run a number of projects together and … the pro-activeness, out of the box thinking and delivering in spite of tight deadlines are some of the key reasons we always reach out to them. Vital Strategies
Kadence were an excellent partner on this project; they took time to really understand our business challenges, and developed a research approach that would tackle the exam question from all directions. The impact of the work is still being felt now, several years later. Customer Intelligence Director Wall Street Journal
Get In Touch
" (Required) " indicates required fields
Privacy Overview

Limited spots. Enroll now.
About the course.
Our eight-week, online Big Data in Marketing course will equip you with core conceptual frameworks and practical tools for exploring, managing, analyzing, and activating existing and emerging streams of data.
You Will Learn To:
- Identify and evaluate various types of marketing data, including emerging sources and attributes
- Align marketing data strategy to key business goals and develop KPIs to assess business impact
- Explain how marketing data can be utilized to address company-specific use cases and business objectives
- Evaluate data governance standards for consumer consent compliance and regulation
- Deploy strategic alignment and actions to accelerate company strategy
- Leverage your knowledge of the marketing data ecosystem to amplify strategy and support use cases
- Identify and develop strategic inputs into a marketing data strategy and plan
- Be awarded a credential from the University of Chicago and become part of the UChicago network
Career Outlook
Big data serves as the conduit through which marketers can establish closer proximity to consumers. By amassing and analyzing swathes of data, marketers are afforded a deeper look into customers’ patterns—knowledge that was previously out of reach. In the last few years, 90% of all the data that currently exists was created. The big data explosion has led to new approaches to marketing research and strategy. Diverse positions abound within this rapidly evolving field, especially in digital marketing, e-commerce, and multichannel marketing.
is the a verage base salary for a marketing data analyst in the United States
Source: Payscale
2.5 quintillion
bytes of data are created daily
Source: Forbes
is the anticipated growth in marketing research analysis positions from 2021 to 2031
U.S. Bureau of Labor Statistics
- Big Data Engineer
- Big Data Marketing Manager
- Business Intelligence Analyst
- Chief Marketing Officer
- CRO Specialist
- Customer Insights Analyst
- Digital Marketing Director
- Digital Marketing Engineer
- Growth Marketer
- Marketing Data Analyst
- Marketing Research Analyst
- Marketing Strategy Planner
- Pay-Per-Click (PPC) Manager
- SEO Specialist
Meet Your Instructors
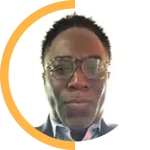
Edward Ramsey, BSc
Founder and Principal Consultant of 444 Ignite
Edward Ramsey has over twenty-five years of experience in advertising, media, and marketing services. As a senior executive, he leads enterprise initiatives to innovate services, grow revenue, build technologies, and drive business transformation.
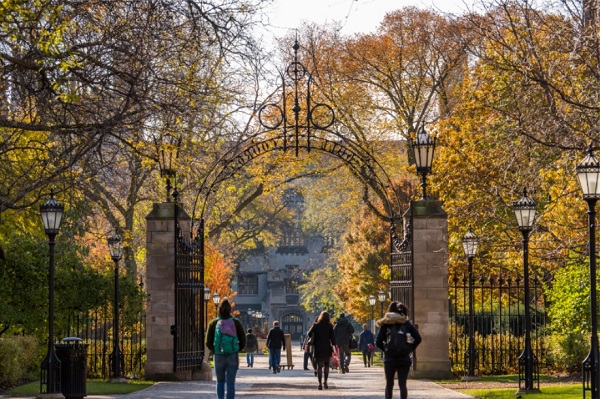
Do you have questions?
The University of Chicago Approach to Online Learning
Our online learning programs are crafted with your specific needs in mind. Courses combine e-learning with live, interactive sessions to strengthen your skill set while maximizing your time. We couple academic theory and business knowledge with practical, real-world application. Through online learning sessions, you will have an opportunity to grow your professional network and interact with University of Chicago instructors and your classmates.
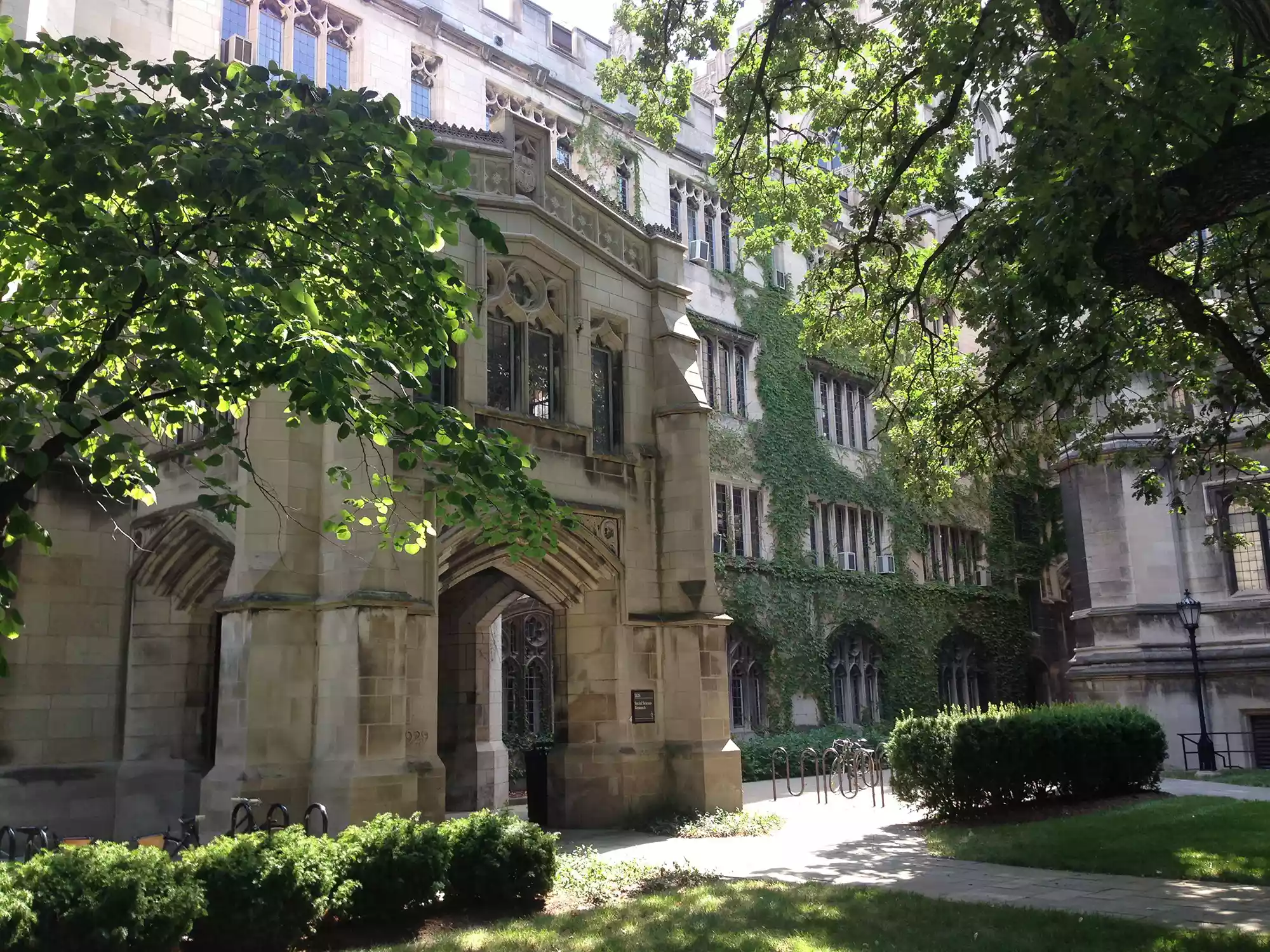
“By directly involving students in the challenge of securing the right data and exploring potential solutions, we’re equipping them with the foundational skills required to deliver tangible results to clients.” – Greg Green, PhD, Associate Senior Instructional Professor and Director of the MScA Program at the University of Chicago.
Register Now
Are you ready to take a leap forward and immerse yourself in a new learning experience? Register online or schedule a call to request more information. Enroll now and gain valuable knowledge and skills that will boost your career to the next level.
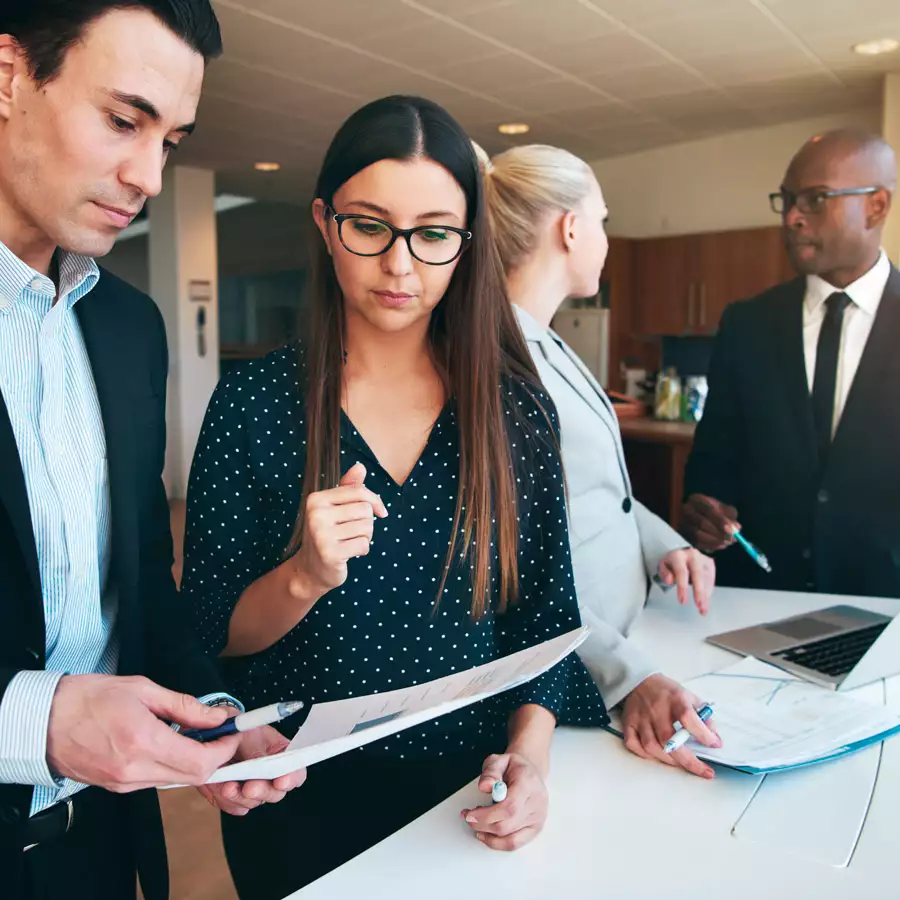
455 N. Cityfront Plaza Drive, Suite 950 Chicago, IL 60611, United States
Our professional certificates are recognized by industry leaders.
Unite your professional practice with our distinctive academic theory through immersive and impactful continuing education offerings.
Email: [email protected]
Phone: : 1.463.258.8174
- Privacy Policy
- Terms of Service
- Cookie Policy
- Data, AI, & Machine Learning
- Managing Technology
- Social Responsibility
- Workplace, Teams, & Culture
- AI & Machine Learning
- Diversity & Inclusion
- Big ideas Research Projects
- Artificial Intelligence and Business Strategy
- Responsible AI
- Future of the Workforce
- Future of Leadership
- All Research Projects
- AI in Action
- Most Popular
- The Truth Behind the Nursing Crisis
- Work/23: The Big Shift
- Coaching for the Future-Forward Leader
- Measuring Culture
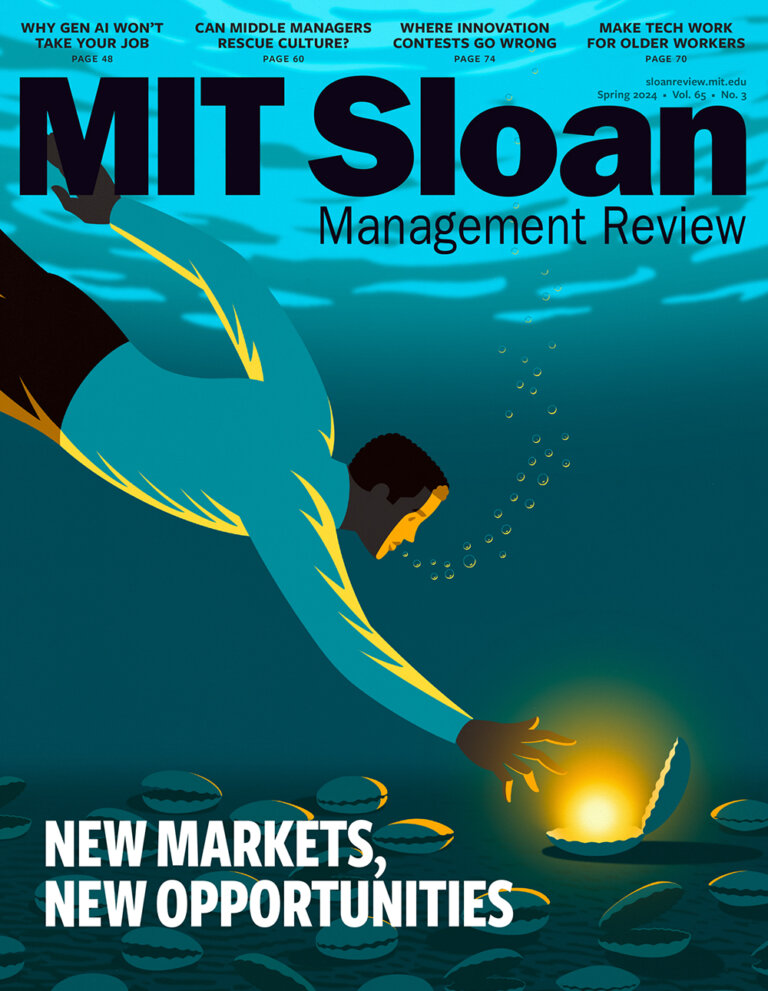
The spring 2024 issue’s special report looks at how to take advantage of market opportunities in the digital space, and provides advice on building culture and friendships at work; maximizing the benefits of LLMs, corporate venture capital initiatives, and innovation contests; and scaling automation and digital health platform.
- Past Issues
- Upcoming Events
- Video Archive
- Me, Myself, and AI
- Three Big Points
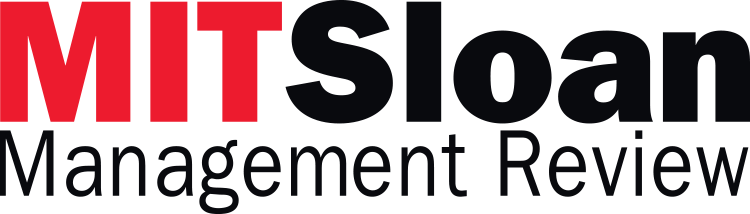
The Big Data Problem That Market Research Must Fix
Big data can support smart market research, but only if researchers embrace the basics of understanding what it is they want to measure — and how.
- Data, AI, & Machine Learning
- Marketing Strategy
- Analytics & Business Intelligence
- Data & Data Culture
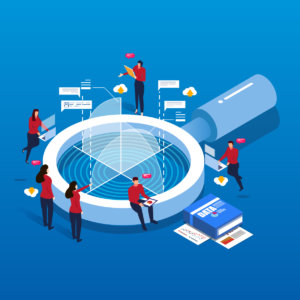
Today’s businesses see market data as a commodity. Readily accessible information about consumer activity and preferences allows market researchers to develop large data sets to mine for consumer insights. And indeed, a look through recent market research industry publications shows that discussions in the field have been dominated by a focus on data analysis.
But more often than not, insight into what customers really care about is hampered by the quality of the data being collected. Some market researchers conflate the idea of data quality with sample size, with the belief that reliability, validity, and other characteristics of “good measurement” derive solely from the amount of data collected. This is certainly not the case.
A heavy emphasis on data collection and analysis is irrelevant if it omits the first and most important step of market research — the design of the metrics. In the psychometric tradition, survey development and the construction of specific survey questions has been emphasized as the most important step in the research process. Unfortunately, this step is getting short shrift by most market researchers today.
Failing to assess the measures that are the foundation of business decisions poses a colossal risk. Making data-driven decisions based on poor measures can be infinitely worse than making decisions without data at all.
To help organizations think more critically about the measures they use to collect information about consumers, we’ve outlined four common misconceptions held by many market researchers and provide suggestions for how to break away from these mistaken beliefs.
Get Updates on Leading With AI and Data
Get monthly insights on how artificial intelligence impacts your organization and what it means for your company and customers.
Please enter a valid email address
Thank you for signing up
Privacy Policy
Belief in Measurement (Without Thinking About What We’re Measuring)
Historically, when market researchers wanted to measure a construct, such as how consumers feel about a particular brand (for example, “brand love”), they would ask respondents to rate questions that directly describe the construct, such as “How much do you love this brand?”
This kind of “measurement by describing” has its share of problems. For instance, many constructs are too abstract for regular consumers to report on in concrete terms. Think about how you’d reply if you were asked how much brand love you have for Tide laundry detergent. Most people couldn’t get more specific than reporting general approximations such as “a lot” or “a little.”
Researchers have begun to move toward methods that use self-reported data in better ways.
About the Authors
Ken Faro is a senior manager of research in the department of decision science at Hill Holliday, a Boston-based advertising company. He tweets @kennethrfarophd . Elie Ohana is a researcher in the department of decision science at Hill Holliday.
More Like This
Add a comment cancel reply.
You must sign in to post a comment. First time here? Sign up for a free account : Comment on articles and get access to many more articles.
The big reset: Data-driven marketing in the next normal
Between March and August 2020, one in five consumers switched brands, and seven in ten tried new digital shopping channels. The retail sector experienced ten years of growth in digital penetration in a matter of months. But the resulting surge in data has not provided marketers with substantially better understanding of their customers, because their companies’ outdated data modeling isn’t able to capture these shifts with the necessary granularity and speed.
Rather than using the data to try to better target customers and tailor messages, many marketers have reverted to mass communications and promotions. As one CMO told us, “I’ve largely retreated to mass marketing instead of data-driven marketing because customer behavior is changing so fast I can’t trust my historical data and models.”
But some marketers are accepting the data for the bounty it is and, rather than stepping back from precision marketing, are doubling down. A consumer-goods company, for example, anticipated that sales of beauty products would spike as communities eased out of lockdown. Marketing teams tracked reopenings on a county basis, using epidemiological statistics, municipal reporting, and traffic data to determine where to focus their media spend. These tactics drove a double-digit increase in sales.
Similar insights helped a business service provider get a jump on another emerging trend. Business registration and employment data showed that small healthcare providers in major metropolitan areas were growing at a much faster rate than other small and midsize businesses. Armed with that insight, the company created healthcare-specific product bundles and has launched paid media ads to target those businesses and locales. These moves, combined with other, similarly data-driven campaigns, are poised to increase sales in a core product by more than 10 percent.
Companies that hone their precision marketing in these ways can drive significant customer acquisition during periods of convulsive change. Capturing this opportunity, however, will require brands to update their modeling—from pulling in new sorts of data to retraining algorithms—in order to both keep pace with changing needs and expectations as well as anticipate shifts in customer behavior.
New challenges to account for
Precision-marketing models are trained to recognize and draw inferences from behavioral patterns. An algorithm might learn, for instance, that customers who make more than two visits to a store’s website within a two-week period are 30 percent more likely to make a purchase. Such indicators can trigger tailored offers to convert browsers into buyers, allowing marketers to direct their acquisition efforts and spend toward the most profitable segments.
But buyer behavior has changed significantly since the pandemic began, rendering the relationship rules baked into many existing data models invalid. Externalities that once seemed incidental, such as customer mobility, now have outsize importance. Is visitation down because customers can’t get to the store or because they no longer wish to shop there? Many marketing teams simply don’t know. A Fortune 100 CMO said, “The indicators for the new opportunities we face are not contained in our own data.”
Would you like to learn more about our Marketing & Sales Practice ?
In addition, while patterns exist, they are harder to discern—and even when discerned, they can feel ephemeral, such as communities opening up only to lock down again. To tease out salient behavioral indicators in time to act on them, marketers need continually refreshed data from a variety of sources and at a far more detailed level—looking as deeply as the city-block level in some cases. However, many companies tend to rely on internally derived customer data, using modeling tools that were not built to handle large volumes of data.
Two other issues compound the challenges facing marketers. McKinsey data show that marketing budgets have been slashed for most companies, with six of ten marketers reporting major cuts. “My budget has evaporated,” said one senior marketer. “We have barely enough to execute our ‘must do’ marketing, let alone experiment with new tactics.”
The other issue is the rapid, large-scale shift to remote working. Data-driven marketing works best in agile settings, where teams can test and iterate in sprints. But with nearly two-thirds of employees working from home, marketing leaders have found it difficult to create an effective cadence. “In the past we used to go all in on marketing opportunities by having a command-center-like war room,” said one Fortune 100 CMO, “but with everyone working remotely, we haven’t been able to react as fast as we have in the past.”
How to make modeling more precise when everything else is in flux
While other organizations may have retreated to mass marketing, those that upgrade their modeling can be far more effective in generating revenue. Here’s what they need to do.
Tap new (and better) data
Precision marketing is only as good as the data behind it. New models with old data are still likely to provide inaccurate results. To hone their insights, leaders in the new normal will take a wide-angle approach to data collection by gathering not only behavioral trends and location-based insights but also third-party analytics on their business, customers, and competitors to complement their in-house customer data. Companies starting this journey are finding the most value in incorporating epidemiological data from government sources and customer-mobility and sales data from third-party providers into their models. Companies that extend their data gathering in these ways can identify upticks in demand and where new customers are coming from, as well as assess which customers in their existing base have increased spending and where lapsed customers have gone.
Before it updated its modeling approach, for example, a retail chain could only tell how many customers it was gaining or losing. The company then decided to pull in cell-phone data to scan changes in their competitors’ net traffic. That analysis showed that many of the customers they were gaining during the pandemic were coming from more expensive, specialty players, while those they were losing were heading to cheaper, larger-format players. On the basis of this information, the retailer transformed its onboard and churn-prevention campaigns. They sent emails advertising higher-end offerings to customers transitioning from specialty stores while touting bargain-oriented products to value-oriented customers at risk of churn.
In another example, a business-services provider tapped into new third-party data sources that identify key moments in the small-business life cycle. In one such effort, the provider aggregated data sources that indicated, with a lag of only one day, when new companies were being launched during the turbulence of COVID-19. Their salespeople reached out immediately with products and messages tailored to the needs of newly formed companies, such as systems tools. These collective efforts increased sales productivity by more than 25 percent.
Robust data can also allow companies to generate better competitor insights. By comparing third-party assortment, sales, and promotional data to their own figures, for instance, marketers can evaluate the strength of different value propositions and see which elements resonate with different groups of customers. They can then provide these groups with tailored messaging, content, and offers.

Why every business needs a full-funnel marketing strategy
Invest in tech that learns at scale.
The increased uncertainty in the new normal requires marketers to get better at testing and faster at reacting. A more agile operating model is a key element in this, but it is also increasingly necessary to work with technology that learns at scale. This requires developing technology capabilities that can read and interpret signals of consumer intent and consumer responses to marketing messages and then feed them back into the marketing engine so it can learn what works and what doesn’t.
Marketers who really push the limits are using artificial intelligence (AI) to monitor campaigns and interrogate responses at a detailed level, to learn not only what works and what doesn’t but for which segments, at what times, and over which channels—and then to adjust their strategy based on those insights. Deriving those specific insights using standard analytics might take the average marketing organization several days. But AI-enabled monitoring can do this in minutes, sometimes seconds.
For example, a consumer services company launched consumer-retention campaigns as communities came out of lockdown. Their customary analytics, which could only assess campaigns in the aggregate, was only marginally effective. However, the organization piloted a new AI engine that could look deeply enough to evaluate responses at the core base statistical area (CBSA), which showed that the campaign was highly effective in specific niches with similar economic and epidemiological profiles. This AI engine will identify how the campaign’s performance patterns evolve, allowing marketers to configure the system so that nightly AI-driven analytics feed directly into the campaign’s targeting logic. This and similar campaigns are a crucial element in a broader data-driven marketing program that has helped the company increase its rate of testing more than fivefold.
Two keys to success: Investing savings and being agile
In order to derive value from these upgraded models, two actions are crucial.
Generate savings to invest in tech
While some companies are simply cutting budgets and retrenching across the board, others are finding it can be more beneficial to reduce spend in unproductive areas and reallocate the savings—as much as 10 to 20 percent of the overall budget, in some cases—into analytics. This requires a thorough but fast reevaluation of all marketing spend to see how the COVID-19 environment has affected ROI. Event sponsorships, traditional TV advertising, and programmatic display based on outdated terms are just a few areas where marketing performance is likely to have shifted significantly. One apparel retailer, for instance, found that the effectiveness of paid search has diminished sharply during the crisis, while social-media activity has been far more productive. Marketing leaders can free additional investment by also reusing and repurposing existing assets. The savings can then be redeployed to fund data-driven growth programs.
Deploy agile marketing in a remote setting
Agile practices are effective in allowing marketing teams to test consumer behaviors and react quickly to changes. While traditionally, agile teams were thought to perform best when working in the same place, the exigencies of the pandemic have required this approach to be rewired for remote work. Leading companies are converting physical war rooms into virtual ones, creating additional points of contact to support adherence to agile protocols (such as sprint check-ins by video, for example) and the use of collaboration tools. The best companies have gone a step further by integrating some of their vendor teams into their remote practices, including working with IT to create shared tools and compatibility guidelines to account for vendors’ different technologies.
Companies that get it right are showing impressive results. For example, a North American telco created a virtual war room that featured online work spaces and digital scrum boards for task and performance management. Sprint planning was conducted using rounds of progressive voting, with each vote spurring active debates on the merits of each test idea. They also held ceremonies using video stand-ups. The virtual war room not only improved test results but helped the team launch tests more than three times faster than their traditional, in-person setup.
Budgeting and operating practices need to be continually reviewed to support this remote agile model. Instead of quarterly or semi-annual planning sessions, marketing leaders should assess performance monthly to ensure that funding and resources are aligned with the biggest opportunities.
Organizations that prioritize their precision-marketing efforts can turn the COVID-19 crisis into a time of transformation. By capturing new data, searching for new behavioral relationships, and enabling rapid experimentation, marketers can seize granular growth opportunities and enter the recovery with significantly greater ROI and resilience.
Chase Bibby is an associate partner in McKinsey’s Boston office, Jonathan Gordon is a partner in the New York office, Gustavo Schüler is a partner in the Southern California office, and Eli Stein is a partner in the San Francisco office.
Explore a career with us
Related articles.
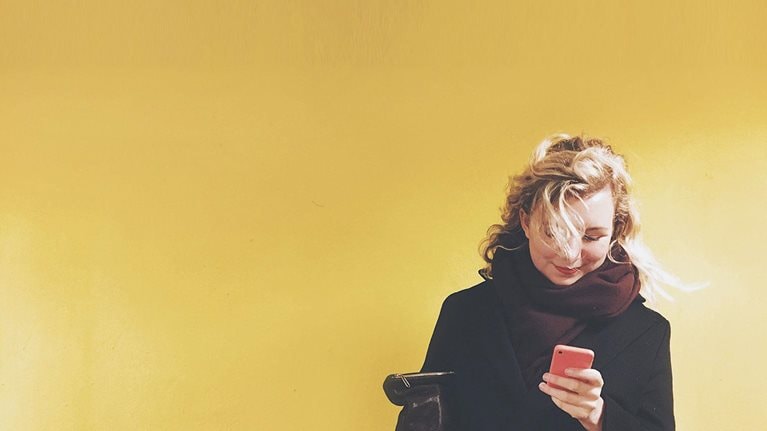
The future of personalization—and how to get ready for it
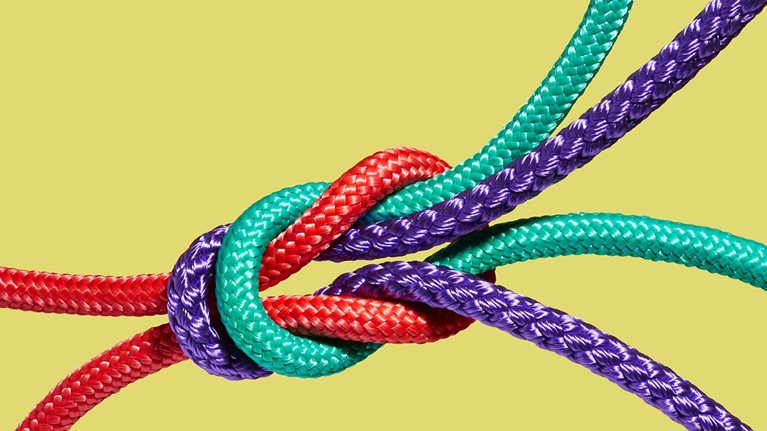
Unlocking growth with data-driven marketing and creativity
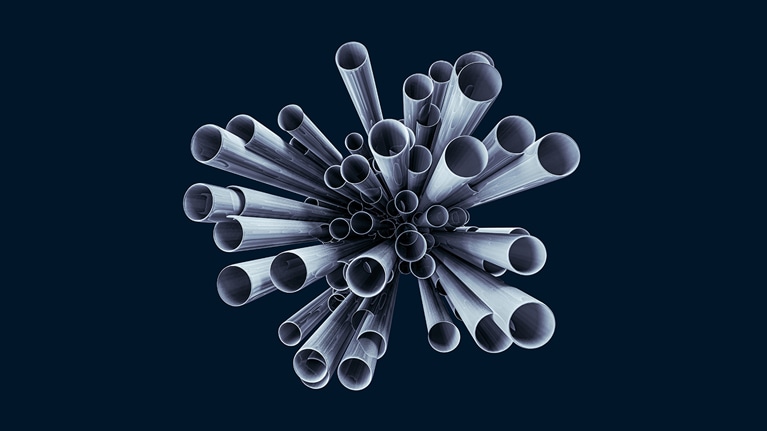
Think fast: How to accelerate e-commerce growth
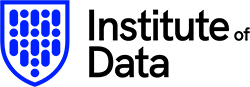
- New Zealand
- United Kingdom
How to Conduct Market Research in Digital Marketing

Market research is a vital component of digital marketing strategies.
Market research in digital marketing provides invaluable insights that help organizations understand their target audience, identify trends, and make informed decisions.
The importance of market research in digital marketing

It plays a crucial role in shaping digital marketing strategies. Market research in digital marketing helps businesses understand their customers, their preferences, and their behavior in the online space.
By analyzing market research data, organizations can identify opportunities, target the right audience, and create relevant content that resonates with potential customers.
Furthermore, market research in digital marketing helps organizations stay ahead of the competition by identifying industry trends, technological advancements, and consumer expectations.
It provides insights into consumer sentiment and allows organizations to tailor their marketing efforts accordingly.
The role of market research in shaping digital strategies
Market research acts as a blueprint for digital marketing strategies.
It guides businesses in setting objectives, defining target audiences, and identifying key performance indicators to measure success.
Market research in digital marketing helps marketers understand their customers’ needs and desires, allowing them to create products and services that genuinely serve their target market.
Moreover, market research helps businesses develop a competitive edge by highlighting gaps in the market and uncovering untapped opportunities.
By understanding customer pain points, preferences, and purchasing behavior, organizations can position themselves strategically and develop unique selling propositions that differentiate them from their competitors.
How market research influences digital marketing decisions
Market research provides the data and insights to make informed digital marketing decisions.
It helps businesses understand consumer behavior, preferences, and influences across various digital channels.
This information allows businesses to develop effective marketing strategies and allocate resources optimally.
For example, market research can help businesses identify the most effective digital marketing channels for reaching their target audience.
By analyzing demographic and psychographic data, businesses can determine which platforms their potential customers frequent and tailor their efforts accordingly.
This allows for targeted and cost-effective marketing campaigns, maximizing the return on investment.
The process of conducting digital marketing research
The process of conducting digital marketing research involves several crucial steps. These steps ensure that the research is thorough, accurate, and insightful.
Identifying your digital marketing research objectives
The first step in conducting digital marketing research is clearly defining your objectives.
What do you hope to achieve through your research?
Are you seeking to understand your target audience better?
Do you want to assess consumer sentiment towards your brand?
Defining your aims will guide the rest of your research process and ensure you gather the relevant data.
Choosing the right digital marketing research methods
Selecting the appropriate research methods is crucial once you have identified your research objectives.
You may choose to conduct surveys, interviews, or focus groups, depending on your objectives.
You may also utilize online tools to gather data on consumer behavior, such as website analytics or social media listening tools.
Choosing methods that align with your objectives and provide the most accurate and reliable data is important.
Analysing and interpreting digital marketing research data

Gathering data is only the first step. The true value of digital marketing research lies in analyzing and interpreting the data effectively.
By doing so, businesses can derive actionable insights to inform their marketing strategies and decision-making.
One key aspect of analyzing digital marketing research data is making sense of the findings.
Businesses can understand consumer behavior and preferences by identifying patterns, trends, and correlations.
This enables them to tailor their marketing efforts effectively to meet their customers’ needs.
Applying digital marketing research insights to strategy is another vital step.
Insights derived from research can refine marketing strategies, optimize campaigns, and improve overall digital marketing performance.
Making sense of digital marketing research findings
Analyzing data can be daunting, especially when dealing with large datasets. However, leveraging data analysis tools can simplify the process and give businesses meaningful insights.
Tools such as data visualization software can help businesses identify trends, patterns, and outliers, making it easier to draw valuable conclusions.
Applying digital marketing research insights to your strategy
The research findings should directly influence your digital marketing strategy.
By integrating the insights gained from the research, businesses can refine messaging, target specific customer segments, and personalize their marketing efforts.
This ensures that businesses deliver the right message to the right audience, leading to better engagement and, ultimately, more conversions.
Common challenges in digital marketing research and how to overcome them
Despite the numerous benefits of digital marketing research, there are several challenges that businesses may need help with.
By being aware of these challenges, organizations can take proactive steps to overcome them and improve the effectiveness of their research efforts.
Dealing with data overload in digital marketing research
In the digital age, businesses have access to vast amounts of data. While this can be advantageous, it can also be overwhelming.
Sorting through massive datasets and extracting relevant insights can be time-consuming and resource-intensive.
To overcome this challenge, businesses should employ data analysis tools to automate data processing and provide actionable insights.
Ensuring accuracy and reliability in digital marketing research
Ensuring the accuracy and reliability of market research in digital marketing is crucial for making informed decisions.
Organizations must use reliable data sources and validated research methods. It is also essential to check the quality of the data, eliminate biases, and ensure that the research sample is representative of the target audience.
By doing so, businesses can have confidence in the insights derived from the research.
The future of market research in digital marketing

The field of market research in digital marketing is continually evolving.
As technology advances and consumer behavior changes, businesses must adapt their research methodologies to stay ahead.
Here, we explore some emerging trends in digital marketing research and the impact of technology on the field.
Emerging trends in digital marketing research
One emerging trend in digital marketing research is artificial intelligence (AI).
AI-powered tools can analyze massive amounts of data, detect patterns, and generate insights at a speed and scale that humans cannot achieve.
This allows organizations to gain real-time insights and make data-driven decisions more efficiently.
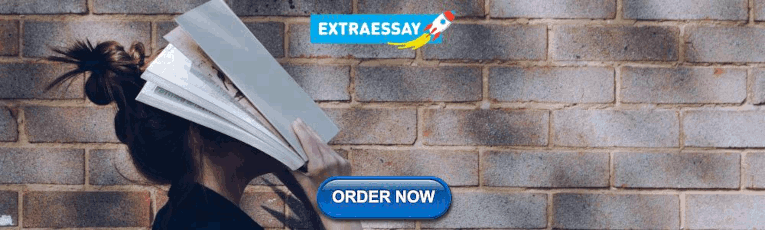
The impact of technology on digital marketing research
Technology has revolutionized the way businesses conduct market research in digital marketing.
From online surveys to social media listening tools , businesses have easier access to invaluable data.
Additionally, data analytics and machine learning advancements have made it possible to extract more meaningful insights from complex datasets.
The future of market research in digital marketing is undoubtedly intertwined with technology, and businesses must embrace these advancements to stay competitive.
Market research is core to digital marketing strategies. It helps businesses understand their target audience, make informed decisions, and gain a competitive edge.
By conducting research effectively and addressing common challenges, organizations can harness the power of market research to drive success in the digital marketing landscape.
As technology continues to shape the field, embracing emerging trends and leveraging technology will be crucial for organizations to remain ahead in the ever-evolving digital marketing arena .
Do you want to dive deeper into market research in digital marketing?
Are you ready to launch your career in digital marketing?
If so, we encourage you to explore the Institute of Data’s Digital Marketing & Artificial Intelligence program.
Alternatively, we invite you to book a complimentary call with a member of our team to discuss the Digital Marketing program in more detail.

Data Science for Developers

Why Is Network Security Important?

Why Network Security is Important?

The Importance of Network Security

How to Determine the Customer Lifetime Value

How to Determine Customer Acquisition Cost
© Institute of Data. All rights reserved.
Copy Link to Clipboard
- Customer Support
- Product Documentation
- Corporate Social Responsibility
- Diversity, Equality, Inclusion, and Belonging
- Academic Program
- Global Offices
- Support Portal
- Qlik Continuous Classroom
- Partner Portal
- Talend Cloud
- Talend Academy
Integrate, transform, analyze, and act on data

Qlik Staige
Bring your AI strategy to life with a trusted data foundation and actionable predictions

Integrations & Connectors
Connect and combine data from hundreds of sources
Featured Technology Partners

Data Integration and Quality
Build a trusted data foundation
Core Capabilities
- Data Streaming
- Application and API Integration
- Data Lake Creation
- Application Automation
- Data Warehouse Automation
- SAP Solutions
- Data Quality and Governance
- Stitch Data Loader
Guided Tour
Data Sources and Targets
Access and integrate the data you need to deliver greater business outcomes
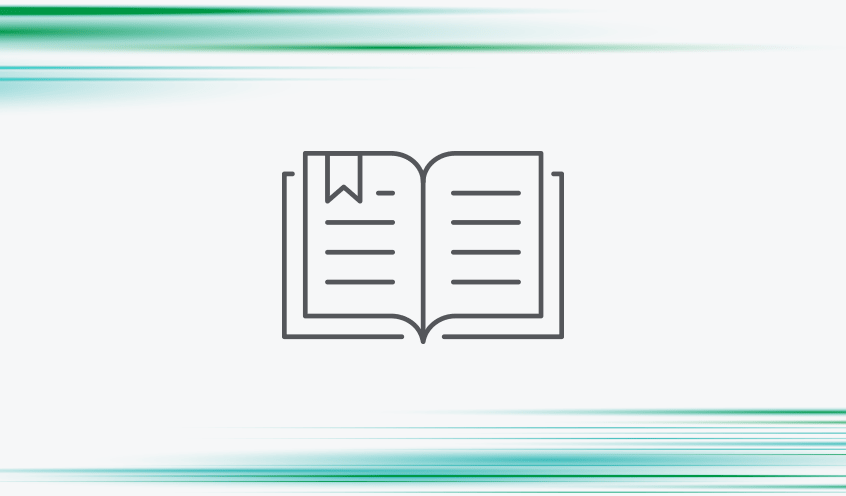
Data Integration Buyer's Guide: What to Look for in a Data Integration Solution
Take action with AI-powered insight
Embedded Analytics
- Augmented Analytics
- Visualizations and Dashboards
Try for Free
Data Sources
Connect and combine data from hundreds of sources to fuel your ever-evolving analytics needs
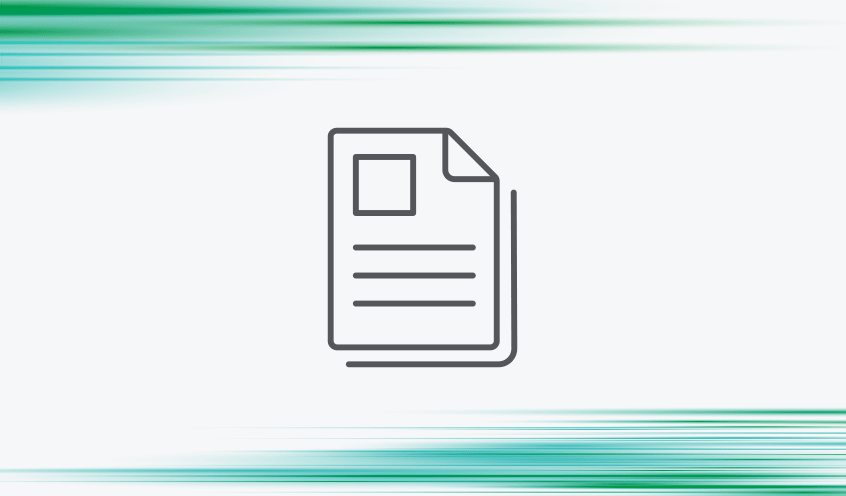
Qlik Platform Services for Analytics
Maximize the value of your data with AI
- Integration and Connectors
- Qlik Staige - Artificial Intelligence Built-in
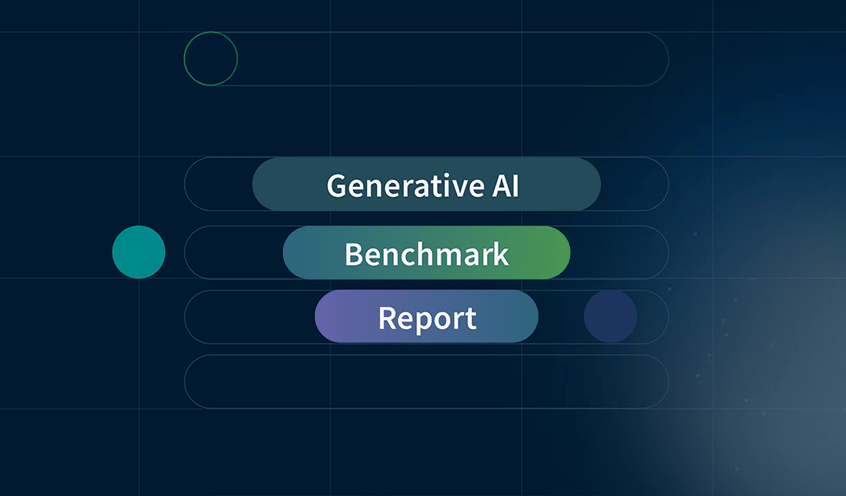
Generative AI Benchmark Report
All Data Integration and Quality Products
Qlik Cloud® Data Integration
Get a trusted data foundation to power your AI, ML, and analytics
Qlik Application Automation®
Automatically trigger informed action on most SaaS applications
Qlik Replicate®
Accelerate data replication, ingestion, and streaming.
Talend Data Fabric
Unify, integrate, and govern disparate data environments
Qlik Compose® for Data Lakes
Automate your data pipelines to create analytics-ready data sets
Talend Data Inventory
Find and improve data in a shared, collaborative workspace
Qlik Compose® for Data Warehouses
Automate the entire data warehouse lifecycle
Talend Data Preparation
Identify errors, and apply and share rules across massive datasets
Qlik Enterprise Manager®
Centrally configure, execute, and monitor replication and transformation
Talend Data Catalog
Understand the data flowing through your analytics pipelines
Qlik Gold Client®
Improve data management in your non-production SAP environments
Talend Data Stewardship
Define priorities and track progress on data projects
All Analytics Products
Qlik Cloud Analytics
All the power of Qlik analytics solutions in a cloud-based SaaS deployment.
Qlik Sense® - Client Managed
The on-premises solution for highly regulated industries.
All AI/ML Products
Bring machine learning to your analytics teams
Financial Services
Manufacturing
Consumer Products
Public Sector
Energy Utilities
US Government
Life Sciences
Communications
Product Intelligence
HR & People
Find a partner
Get the help you need to make your data work harder

Global System Integrators
Transform IT services, solution development, and delivery
- Data Integration and Quality Pricing Rapidly deliver trusted data to drive smarter decisions with the right data integration plan.
- Analytics Pricing Deliver better insights and outcomes with the right analytics plan.
- AI/ML Pricing Build and deploy predictive AI apps with a no-code experience.
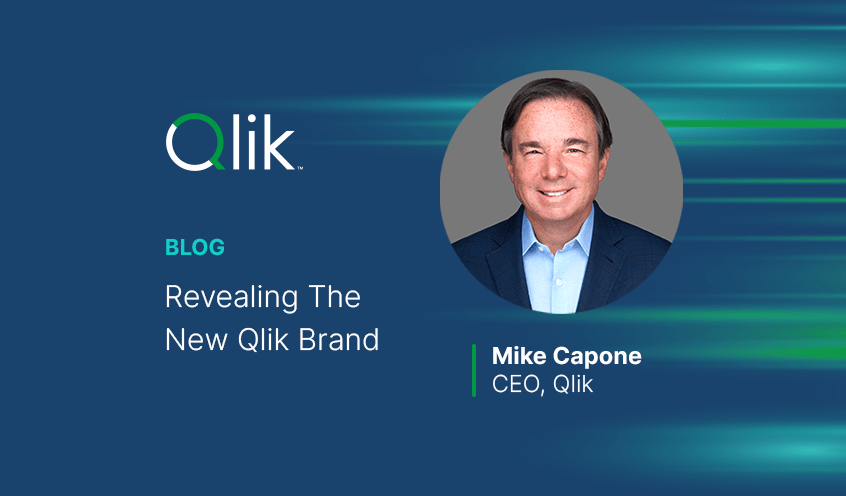
Hitting the Ground Running with Generative AI
Enter Qlik Staige – Helping customers unleash the full potential of Artificial Intelligence
The Path to Enterprises Maximizing AI Initiative Value
Artificial Intelligence
Act on insights with AI-powered analytics
Data Management
Collect, store, organize, and maintain data
Bring automated machine learning to analytics teams
Data Quality
Discover, manage, enhance, and regulate data
Data Fabric
Data Visualization
Make it easier to see trends and relationships in your data
Data Catalog
Find the data you need and evaluate its fitness for your use case
Integrate applications and data sources
Data Governance
Ensure data is trustworthy and consistent
Predictive Analytics
Predict future outcomes based on historical and current data
Data Literacy
Read, work with, analyze, and communicate with data.

Domino's Radically Improves Efficiency, Customer Service — and Sales with Real-time Data and Analytics
Urban Outfitters Reduces Store Level Reporting from Hours to Minutes
Data Research Went From Thousands of Hours to Near Real Time at Georgia-Pacific
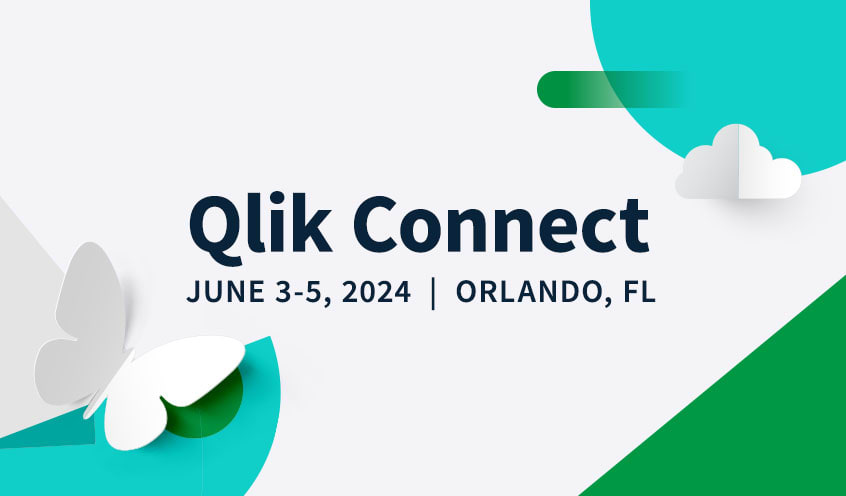
Visionary Voices Episode 2: Taking AI from Trends to Reality
Competitive Alert: Qlik Trends 2024: Data, Analytics, & AI Is Here!
Unleash Your Inner AI Hero
Customer Stories
More than 40,000 customers find answers with Qlik.
Analyst Reports
Read analyst reports for data integration and analytics.
Whitepapers and eBooks
Visit the Qlik Resource Library.
Visit the Qlik Webinar Library.
Visit the Qlik Video Library.
Datasheets & Brochures
Visit the Qlik Datasheet and Brochure Library.

AI analytics refers to the use of machine learning to automate processes, analyze data, derive insights, and make predictions or recommendations.
Business Intelligence
Data Analytics
Data Mining
Data Warehouse
Predictive Modeling
Community Overview
Welcome to the Qlik Community
Qlik Gallery
Get inspired by recent Qlik apps and discuss impacts with peers
Get support directly from a community of experts
Plot your path of engagement with Qlik
Vote for your favorite product ideas and suggest your own
Training Overview
World-class resources to adopt Qlik products and improve data literacy.
Instructor-Led Learning
Get interactive, hands-on learning with Qlik experts
Free Training
FREE courses and help, from basic to advanced
Literacy Program
Understand, analyze, and use data with confidence.
Self-Paced Learning
Get hundreds of self-paced training courses
Validate Your Skills
Validate knowledge and skills in Qlik products, analytics, and data literacy
- Why Qlik Turn your data into real business outcomes
- Technology Partners and Integrations Extend the value of Qlik data integration and analytics
- Data Integration
- All Products
- By Industry
- Solution Partners
Data Integration and Quality Pricing
Rapidly deliver trusted data to drive smarter decisions with the right data integration plan.
Analytics Pricing
Deliver better insights and outcomes with the right analytics plan.
AI/ML Pricing
Build and deploy predictive AI apps with a no-code experience.
- Topics and Trends
- Resource Library
Marketing Analytics
What it is, why you need it, and best practices. This guide provides definitions and practical advice to help you understand and establish world class marketing data analytics.
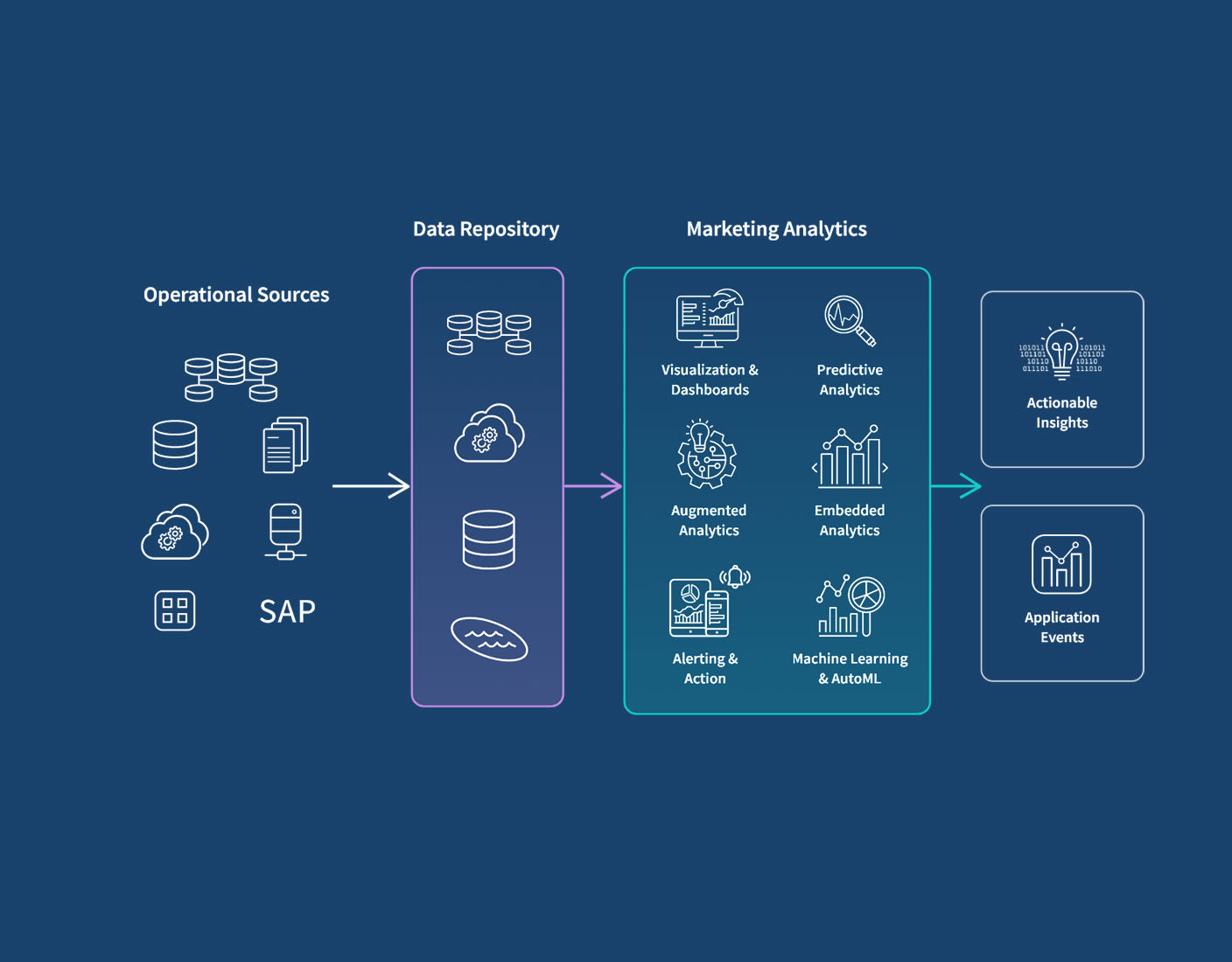
MARKETING ANALYTICS GUIDE
What is marketing analytics.
Marketing analytics is the practice of analyzing marketing data from multiple systems to gain insights and make informed decisions. It helps you evaluate the effectiveness of your marketing efforts, track campaign performance, and understand customer behavior. By utilizing data-driven insights, you can optimize your marketing strategies and drive business growth.
Marketing Analytics Benefits
Marketing analytics is crucial because it allows you to make data-driven decisions and measure the effectiveness of your marketing efforts. By analyzing data and metrics, you can gain valuable insights into customer behavior, preferences, and market trends. This information helps you optimize your marketing strategies, target the right audience, allocate resources efficiently, and ultimately drive better business outcomes.
Key benefits of marketing data analytics include:
Demonstrate ROI
Track how your marketing efforts impact pipeline creation and revenue using accurate campaign attribution. Marketing analytics helps you break down data silos and analyze an integrated data set to measure across the entire funnel.
Optimize Investment & Mix
Marketing data analysis using multi-touch attribution lets you close the loop between campaigns and revenue to optimize budget and resource investments in channels.
Improve Campaign Planning and Scheduling
Strategically plan and schedule marketing campaigns, ensuring optimal timing, channel selection, and resource allocation to maximize effectiveness and reach.
Improve Campaign Performance
Increase conversion rates and revenue by knowing how tests of ad creative, messaging and pricing are impacting sales, not just front-end metrics like impressions or click-through rates.
Enhance Workflow Efficiency
Optimizing marketing processes, systems, and workflows helps you identify bottlenecks, streamline operations, and enhance overall efficiency, resulting in improved productivity and resource utilization. This dashboard example identifies where prospects are getting stuck in your funnel.
Better Forecast and Track KPIs
Forecast and track your performance to goals for KPIs. Having your dashboards refresh automatically reduces your reporting time and predictive marketing analytics uses statistical modeling , data mining techniques and machine learning to make accurate forecasts based on historical and current data.
Other benefits of marketing data analysis include:
Customer segmentation involves dividing your company's customer base into distinct groups based on shared characteristics and behaviors to better target marketing efforts and tailor messages to specific segments.
Competitor analysis involves assessing and analyzing the strategies, tactics, strengths, and weaknesses of competitors in the market to gain insights and identify opportunities for differentiation and competitive advantage.
UX (User Experience) performance analytics focuses on evaluating and measuring the usability, effectiveness, and satisfaction of users when interacting with a company's digital assets or platforms, providing insights to improve user experiences and drive better engagement.
Customer lifetime value (CLV) analytics quantifies the total value a customer brings to your business over their entire relationship, helping you understand the long-term profitability of customers and enabling you to make informed decisions on customer acquisition, retention, and personalized marketing strategies.
How Marketing Analytics Works
Modern marketing data analytics is powered by a cloud-based, end-to-end data integration and analytics platform. This platform helps you manage data across its lifecycle as well as perform the necessary types of analytics.
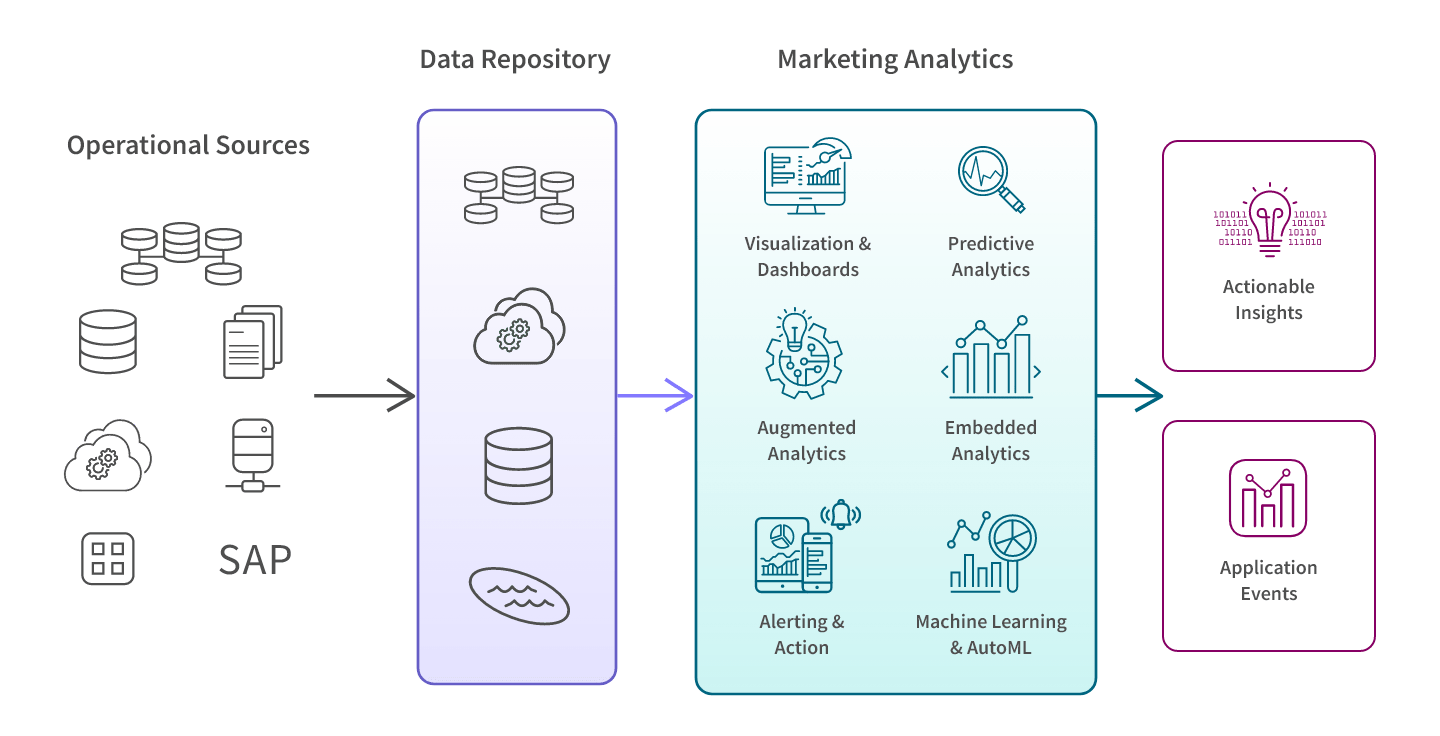
Let’s walk through the diagram above.
Data is sourced from your marketing operational systems such as ad platforms, CRM, marketing automation, web analytics, and IoT/product. Data from third-parties such as partners, data brokers, and merchants can also be included.
This data is extracted, transformed, and combined into a repository such as a data warehouse or data lake, typically in the cloud. This gives you a comprehensive view across the full customer lifecycle.
Your marketing analytics tool makes it easy for you to use this data to perform different types of analysis. For example, you could use predictive marketing analytics to project new customers for a campaign.
Marketing analytics software also allows you to create interactive visualizations and dashboards which help you identify patterns and develop insights into KPIs such as customer lifetime value, acquisition cost, and conversion rates. Some tools allow you to embed your analytics into other applications, allowing users to gain insights within their regular workflows.
Top tools go further and enable you to leverage AI analytics capabilities such as automated machine learning (AutoML), predictive analytics, and prescriptive analytics. Augmented analytics guides you by automatically surfacing insights and relationships in your data and providing recommendations of angles for you to explore.
This results in insights which you can action on and/or trigger alerts and actions in other systems.
Explore these top data analytics resources
Barc’s bi & analytics survey 23: qlik highlights.
BARC, a leading enterprise software analyst firm, talked to real BI users to analyze and compare leading BI and Analytics products. Users ranked Qlik Sense® #1 in Performance Satisfaction, Dashboards, and Project Length in its peer groups.
Get Qlik’s Highlights Report
Know the trends
Learn about the top 10 BI and data trends and how advanced analytics can help you navigate today’s fast-paced world.
Download Infographic
Marketing Analytics Types
Here are the four types of data analytics in marketing and the questions answered by each:
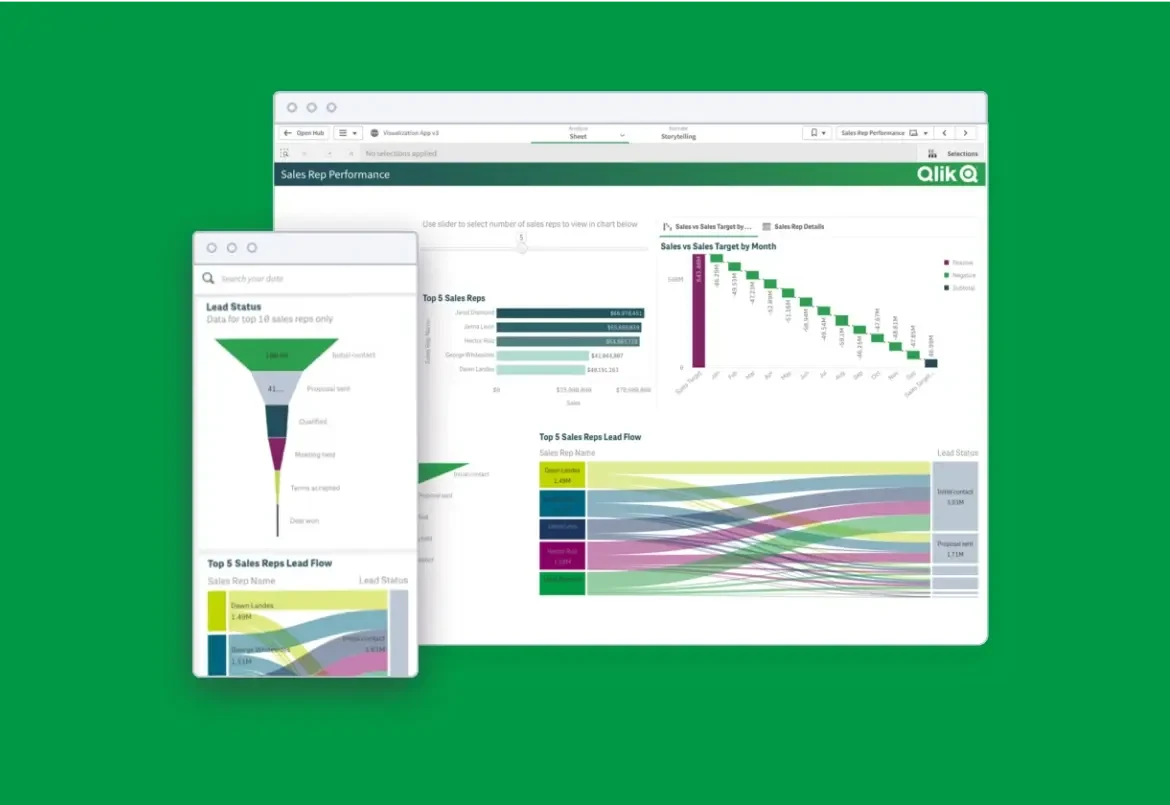
Modern Analytics Demo Videos
See how to explore information and quickly gain insights.
Combine data from all your sources
Dig into KPI visualizations and dashboards
Get AI-generated insights
Marketing Attribution Models
Marketing attribution models are used to understand and evaluate the impact of marketing channels and activities on customer conversions or sales. Different models offer varying perspectives and insights. You should choose the model that aligns best with your objectives and understanding of customer behavior.
Media Mix Models (MMM) are marketing attribution models that analyze historical data to determine the contribution and impact of various marketing channels on overall sales or conversions. They use statistical techniques to evaluate the effectiveness and ROI of different media channels and marketing activities, considering factors such as ad spend, reach, frequency, and market conditions.
Multi-Touch Attribution (MTA) models attribute credit for conversions or sales to multiple touchpoints along the customer journey. These models assign value to each interaction or touchpoint a customer has with marketing channels and activities, providing insights into the specific channels, campaigns, or tactics that influenced a conversion. MTA methods can vary, including rule-based models, time decay models, and algorithmic approaches.
First-Touch Attribution assigns all credit for a conversion or sale to the first interaction or touchpoint a customer had with a marketing channel. It emphasizes the initial touchpoint's role in capturing the customer's attention and initiating their journey.
Last-Touch Attribution attributes all credit for a conversion or sale to the last interaction or touchpoint a customer had before completing the desired action. It focuses on the final touchpoint's role in driving the customer to convert or make a purchase.
Linear Attribution distributes equal credit across all touchpoints a customer had during their journey. It assumes that each touchpoint contributes equally to the conversion or sale, regardless of its position in the customer journey.
Time Decay Attribution gives more credit to touchpoints that occur closer to the conversion or sale. It assumes that touchpoints closer in time to the conversion had a more significant influence on the customer's decision. Marketing attribution models are used to understand and evaluate the impact of marketing channels and activities on customer conversions or sales. Different models offer varying perspectives and insights. You should choose the model that aligns best with your objectives and understanding of customer behavior.
U-Shaped (Position-Based) Attribution assigns a higher percentage of credit to the first and last touchpoints, with the remaining credit distributed evenly among the touchpoints in between. It acknowledges the importance of both initial and final interactions in shaping the customer's journey.
What are examples of marketing analytics?
Examples of data analytics in marketing include analyzing website traffic and engagement metrics, conducting customer segmentation and profiling based on demographics and behaviors, measuring the return on investment (ROI) of marketing campaigns, tracking social media performance and sentiment analysis, and using predictive modeling to forecast future customer behavior and preferences.
What degree do you need for marketing analytics?
To pursue a career in marketing analytics, a bachelor's degree in fields such as marketing, business analytics, data science, statistics, or a related discipline is typically required. However, some positions may prefer or require a master's degree or higher level of education, particularly for advanced roles or in-depth data analysis. Additionally, gaining practical experience through internships, certifications, or specialized training in analytics tools and techniques can further enhance your job prospects in the field of marketing data analytics.
How is marketing analytics as a career?
Marketing analytics offers you a promising and rewarding career path. With the increasing availability of data and the growing importance of data-driven decision-making in marketing, there’s a high demand for professionals skilled in marketing data analytics. This career allows you to work at the intersection of marketing and data, leveraging analytical skills to extract insights, optimize marketing strategies, and drive business growth. You have the opportunity to work in diverse industries, collaborate with cross-functional teams, and contribute to key business decisions. It’s a dynamic field that offers continuous learning and growth opportunities as technology and analytical techniques evolve.
See Modern Analytics in Action
A business journal from the Wharton School of the University of Pennsylvania
Marketing the Future: How Data Analytics Is Changing
November 23, 2020 • 6 min read.
Data analytics is at an inflection point as growing concerns about security, privacy, bias, and regulation bump up against the benefits of artificial intelligence and machine learning.
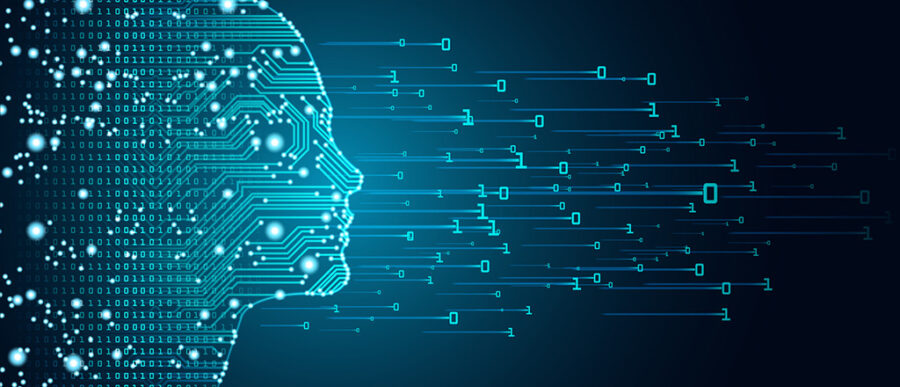
Data analytics helps marketers learn about their customers with target precision, from the movies they watch on Netflix to their favorite scoop of chocolate ice cream.
Data is ubiquitous, essential, and beneficial — except when it’s not.
Experts warn that data analytics is at an inflection point. Growing concerns about security risks, privacy, bias, and regulation are bumping up against all the benefits offered by machine learning and artificial intelligence. Layer those concerns on top of worries about the coronavirus pandemic and how it has rapidly changed consumer behavior, and the challenges become clear.
“What we’re seeing is a lot of chaos in terms of what is the right answer. And what we’re seeing is a change in strategy,” said Neil Hoyne , chief measurement strategist at Google and a senior fellow at Wharton Customer Analytics .
Hoyne said he’s in constant conversation with companies that are trying to figure out the future of data analysis. Google and other internet providers recently announced plans to phase out third-party cookies , which will strip marketers of a wealth of fine-grained information collected by tracking consumers across the web. Proactive companies are already pivoting, so they can be ready for a post-cookie, post-pandemic world.
“The companies that are going to win are the ones who are using data, not guessing,” said Hoyne, who spoke along with other industry and academic experts during a Nov. 17 virtual symposium, “ The Use of Analytics and AI in a Post-pandemic World .” The event was hosted by the nonprofit Marketing Science Institute , along with Wharton Customer Analytics and AI for Business at the Wharton School.
“The companies that are going to win are the ones who are using data, not guessing.” –Neil Hoyne
The symposium touched on a wide range of topics under the umbrella of data analytics while keeping sharp focus on what’s ahead in the evolving world of artificial intelligence.
“In trying to design the program today, we couldn’t ignore the obvious, which is 2020 has been the year of disruption and risks — and, hopefully, successful management of those risks,” said AI for Business Faculty Director Kartik Hosanagar , who is also a Wharton professor of operations, information and decisions.
The Risks and Rewards of Data
Much attention has been paid to all the impressive ways that AI and machine learning help companies by automating services, predicting patterns, and making recommendations that lead to greater sales and engagement. More than a third of Amazon’s sales come from its recommendation algorithm, for example, while YouTube’s algorithm drives 70% of the content watched on its platform.
But, Hosanagar said, the risks associated with AI need equal attention and priority from managers.
AI can create social, reputational, and regulatory risks, even for companies well-versed in technology. Amazon scrapped a recruiting software with a gender bias; Twitter shut down a Microsoft chatbot that “learned” how to post racists tweets; and Facebook was sued by the U.S. Department of Housing and Urban Development, which alleged the platform’s targeted advertising violates the Fair Housing Act by restricting who views housing ads.
“These are not small risks for the companies,” said Hosanagar, who strongly recommended business leaders create interdisciplinary teams to continuously monitor and evaluate data for bias.
Bias can be unwittingly baked into algorithms by the humans who create them. Symposium speaker Kalinda Ukanwa , a marketing professor at the University of Southern California’s Marshall School of Business, offered a powerful example to illustrate the problem. “Rebecca” applies for a loan with an online bank that uses AI to determine the loan. She is rejected, despite having good credit. But if she enters the same information with one difference — her gender — she is approved.
While the online bank may see an initial surge in business because of the ease of use, it may suffer long-term reputational effects. Months after Rebecca’s bad experience, she may tell her friend, “Jim,” not to bother applying for a loan at that bank because she didn’t get approved.
“Algorithm bias can be profitable in the short run, but unprofitable in the long run due to word of mouth reducing consumer demand,” Ukanwa said.
Still, she emphasized the value in data analytics. When it works well, it takes the guesswork out of decision-making and can lead to more equitable outcomes. But AI must be vigilantly monitored and tweaked. Sometimes, there’s an easy solution. In the bank loan example, simply dropping the gender input would have prevented the bias.
“Algorithm bias can be profitable in the short run, but unprofitable in the long run due to word of mouth reducing consumer demand.” –Kalinda Ukanwa
Raghuram Iyengar , Wharton marketing professor and faculty director for Wharton Customer Analytics, also cautioned marketers to consider how they deploy data analytics. Is it really needed to solve a problem? “I talk about this sometimes in my class: You don’t need a bazooka to get a fly,” he said.
Pushed by Pandemic Uncertainty
The current COVID-19 pandemic has disrupted business in unexpected ways, rendering obsolete some of the data analytics that were useful before consumers radically shifted their consumption patterns. Google’s Hoyne said smart companies are responding by moving from precision measurement to prediction. Instead of capturing more data, they are exploring what they can do with the data they already have. They also are shifting from third-party, cookie-based data to first-party data to establish more direct relationships with their customers.
He said companies are less interested in the historical tracking of consumer data because the past doesn’t matter now. And rising concern about privacy and regulation has companies examining how to make their data more transparent to customers, as well as more reliable and relevant.
These are incremental changes, not a major overhaul. “They just want to be a little bit better,” Hoyne said, calling that approach “refreshing” because it’s more sustainable for companies.
Barkha Saxena , chief data officer for social commerce site Poshmark, held up her firm as an example of flexibility in uncertain times. Data has always driven decisions at Poshmark, and the company has taken an integrated approach that allows it to be nimble during market changes. She shared a framework that could help other companies do the same: evaluate the data, execute the plan, learn what worked and what didn’t, then repeat.
“This is pretty much how you turn the data into an operating tool,” Saxena said.
She also encouraged a team mindset around data. It shouldn’t be sequestered in one department but shared across business functions.
“We have the foundation of very centralized, reliable, and easy-to-access data, but then it’s delivered to all the teams,” she said. “It allows for the data to be accessible to all the business users at the time of the decision.”
More From Knowledge at Wharton
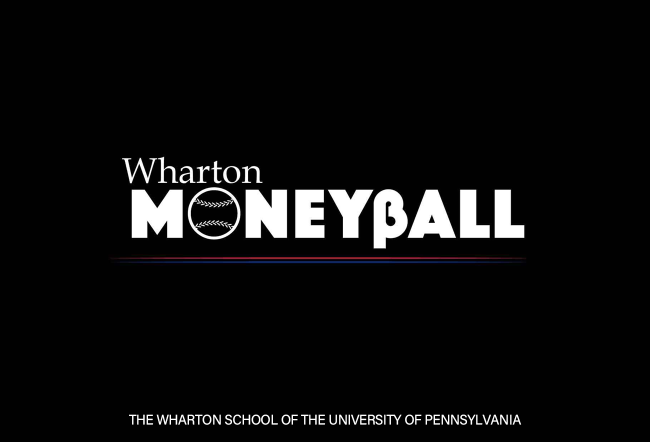
NHL Analytics with Micah McCurdy
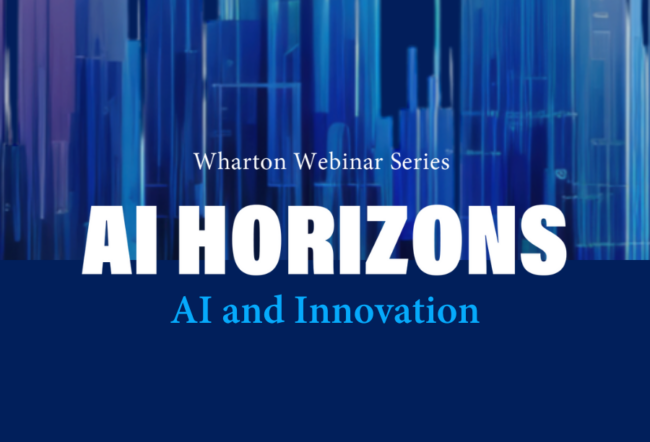
AI and Innovation: A Question of Quantity vs. Quality
Mlb spring training, looking for more insights.
Sign up to stay informed about our latest article releases.
Marketing Applications Using Big Data
- First Online: 27 May 2017
Cite this chapter
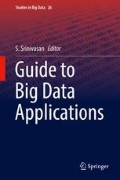
- S. Srinivasan 3
Part of the book series: Studies in Big Data ((SBD,volume 26))
4766 Accesses
Big Data applications abound in all disciplines. In this chapter we consider the practical applications of Big Data analytics in Marketing. Businesses spend significant human and financial resources in marketing their products and services to potential customers. In this chapter we look at how businesses use the data gathered from multiple sources and use that to promote their products and services to customers who are more likely to benefit from them. Typically, marketing involves communicating with the potential customer through multiple advertising and promotion mediums. Much of the information sent to the potential customer is either in print or electronic form. Businesses who use appropriate target marketing feel that a person within that market would eventually turn out to be a customer. With this in mind, the approaches businesses take are geared towards sending the right information to the right person. To gain this type of knowledge, businesses use extensive data from multiple sources. With the advancements in computing power, affordable resources and social media, businesses are in a better position to target their materials at the potential customer. Even though the cost of information dissemination is very small, if the information is sent to the wrong person then that person is not only going to discard the information but may resent being bombarded with unwanted information. In this chapter we show the various techniques real businesses use to target the right customer and send the information that will be used. In this effort Big Data techniques are helpful. We point out how the data was used in marketing and its success.
- Content marketing
- Multi-touch attribution
- Audience targeting
- Predictive analytics
- Forecasting
This is a preview of subscription content, log in via an institution to check access.
Access this chapter
- Available as PDF
- Read on any device
- Instant download
- Own it forever
- Available as EPUB and PDF
- Compact, lightweight edition
- Dispatched in 3 to 5 business days
- Free shipping worldwide - see info
- Durable hardcover edition
Tax calculation will be finalised at checkout
Purchases are for personal use only
Institutional subscriptions
An, M. (2016). Future of content marketing, hubspot . https://research.hubspot.com/reports/the-future-of-content-marketing . Accessed 20 October 2016.
Barnett, W. (1988). Four steps to forecast total market demand, Harvard Business Review, 66 (4), 28–38.
Google Scholar
Berman, R. (2015). Beyond the last touch: Attribution in online advertising, SSRN . http://ssrn.com/abstract=2384211 . Accessed 24 October 2016.
Berry, M., & Linoff, G. (1997). Data mining techniques: For marketing, sales, and customer support . New York: Wiley.
Brown, B., Chui, M., & Manyika, J. (2011). Are you ready for the era of Big Data? McKinsey Quarterly, 4 (1), 24–35.
BLS. (2000). Spending patterns by age . http://www.bls.gov/opub/btn/archive/spending-patterns-by-age.pdf Accessed 10 October 2016.
Chase, C. W. (2013). Using Big Data to enhance demand-driven forecasting and planning. Journal of Business Forecasting, 32 (2), 27–32.
MathSciNet Google Scholar
CMO Council. (2016). https://www.cmocouncil.org/facts-stats-categories.php?view=all&category =marketing-spend . Accessed 24 October 2016.
Cognizant. (2011). How predictive analytics elevate airlines customer-centricity and competitive advantage . https://www.cognizant.com/InsightsWhitepapers/How-Predictive-Analytics-Elevate-Airlines-Customer-Centricity-and-Competitive-Advantage.pdf . Accessed 10 October 2016.
du Plessis, C. (2015). Academic guidelines for content marketing: research-based recommendations for better practice. In LCBR European Marketing Conference, Lisbon (pp. 1–12).
Kaushik, A. (2010). Web analytics 2.0 . Hoboken, NJ: Sybex Publishing (a John Wiley Company).
Myerholtz, B., & Caffrey, H. (2014). Demand forecasting: Key to better supply chain performance, BCG perspectives . https://www.bcgperspectives.com/content/articles/supply_chain_consumer_retail_demand_forecasting_key_better_supply_chain_performance/ . Accessed 10 October 2016.
Pulizzi, J. (2010). B2B Content Marketing Benchmarks, Budgets and Trends, Content Marketing Institute . http://contentmarketinginstitute.com/2010/09/b2b-content-marketing . Accessed 26 October 2016.
Rancati, E., & Gordini, N. (2014). Content marketing metrics: Theoretical aspects and empirical evidence, European. Scientific Journal, 10 (34), 92–104.
Reichheld, F. (2003). The one number you need to grow. Harvard Business Review, 86 (12), 46–54.
Shao, X., & Li, L. (2011). Data-driven multi-touch attribution models. In Proceedings of the 17th ACMSIGKDD International Conference (pp. 258–264).
Turow, J., Hennessy, M., & Draper, N. (2015). The Tradeoff Fallacy: How marketers are misrepresenting American consumers and opening them up to exploitation, University of Pennsylvania Research Report.
Zhang, Y. (2014). Multi-touch attribution in online advertising with survival theory. In IEEE international conference on data mining (pp. 687–696).
Download references
Author information
Authors and affiliations.
Jesse H. Jones School of Business, Texas Southern University, Houston, TX, 77004, USA
S. Srinivasan
You can also search for this author in PubMed Google Scholar
Corresponding author
Correspondence to S. Srinivasan .
Editor information
Editors and affiliations.
Jesse H. Jones School of Business, Texas Southern University , Houston, Texas, USA
Rights and permissions
Reprints and permissions
Copyright information
© 2018 Springer International Publishing AG
About this chapter
Srinivasan, S. (2018). Marketing Applications Using Big Data. In: Srinivasan, S. (eds) Guide to Big Data Applications. Studies in Big Data, vol 26. Springer, Cham. https://doi.org/10.1007/978-3-319-53817-4_18
Download citation
DOI : https://doi.org/10.1007/978-3-319-53817-4_18
Published : 27 May 2017
Publisher Name : Springer, Cham
Print ISBN : 978-3-319-53816-7
Online ISBN : 978-3-319-53817-4
eBook Packages : Engineering Engineering (R0)
Share this chapter
Anyone you share the following link with will be able to read this content:
Sorry, a shareable link is not currently available for this article.
Provided by the Springer Nature SharedIt content-sharing initiative
- Publish with us
Policies and ethics
- Find a journal
- Track your research
- Publications
- NIM Marketing Intelligence Review
- Marketing and Data Science
Big Data in Market Research: Why More Data Does Not Automatically Mean Better Information
Bosch, V. (2016). Big Data in Market Research: Why More Data Does Not Automatically Mean Better Information. NIM Marketing Intelligence Review, 8(2), 56-63
Volker Bosch
Big data will change market research at its core in the long term because consumption of products and media can be logged electronically more and more, making it measurable on a large scale. Unfortunately, big data datasets are rarely representative, even if they are huge. Smart algorithms are needed to achieve high precision and prediction quality for digital and non-representative approaches. Also, big data can only be processed with complex and therefore error-prone software, which leads to measurement errors that need to be corrected.
Another challenge is posed by missing but critical variables. The amount of data can indeed be overwhelming, but it often lacks important information. The missing observations can only be filled in by using statistical data imputation. This requires an additional data source with the additional variables, for example a panel. Linear imputation is a statistical procedure that is anything but trivial. It is an instrument to “transport information,” and the higher the observed data correlates with the data to be imputed, the better it works. It makes structures visible even if the depth of the data is limited.
- Volker Bosch, Head of Marketing & Data Sciences, GfK SE, Nürnberg, Deutschland
Other articles of the MIR issue “Marketing and Data Science”
Here you can find more exciting articles of this issue.
To the entire issue
![[Translate to English:] [Translate to English:]](https://www.nim.org/fileadmin/_processed_/d/d/csm_16002_mir_02_2016_englisch_161027_Kap1_dc3b0c1bda.png)
Data, Data and Even More Data: Harvesting Insights from the Data Jungle
Increasing global digitalization brings huge amounts of data. Finding a successful way to handle all this data and to transform it into …
![[Translate to English:] [Translate to English:]](https://www.nim.org/fileadmin/_processed_/8/8/csm_16002_mir_02_2016_englisch_161027_Kap2_763e60ab89.png)
Marketing and Data Science: Together the Future Is Ours
The synergistic use of computer science and marketing science techniques offers the best avenue for knowledge development and improved …
![[Translate to English:] [Translate to English:]](https://www.nim.org/fileadmin/_processed_/1/c/csm_16002_mir_02_2016_englisch_161027_Kap3_6262d013a3.png)
On Storks and Babies: Correlation, Causality and Field Experiments
The explosion of available data has created much excitement among marketing practitioners about their ability to better understand the …
![[Translate to English:] [Translate to English:]](https://www.nim.org/fileadmin/_processed_/f/8/csm_16002_mir_02_2016_englisch_161027_Kap4_79cb20dfdd.png)
Tell Me Where You Are and I’ll Tell You What You Want: Using Location Data to Improve Marketing Decisions
Location data has become more and more accessible. Smartphone applications such as location-based services collect location data on a …
![[Translate to English:] [Translate to English:]](https://www.nim.org/fileadmin/_processed_/0/0/csm_16002_mir_02_2016_englisch_161027_Kap5_f209e865d2.png)
Using Big Data for Online Advertising Without Wastage: Wishful Dream, Nightmare or Reality?
Big data contains lots of information about consumers and allows companies real-time and data-assisted decision making to gain significant …
![[Translate to English:] [Translate to English:]](https://www.nim.org/fileadmin/_processed_/8/e/csm_16002_mir_02_2016_englisch_161027_Kap6_15f4771e1a.png)
The Art of Creating Attractive Consumer Experiences at the Right Time: Skills Marketers Will Need to Survive and Thrive
New technologies have made today’s marketing faster, more mobile, more location-based, more digital, more virtual, and more automatized …
![[Translate to English:] [Translate to English:]](https://www.nim.org/fileadmin/_processed_/8/b/csm_8_2_schmidberger_de_7a5cbec6aa.jpeg)
Interview: Data Analysis Trumps Specialist Advice - How Direct Banks Function
While many traditional retail banks are struggling with a business downturn, the direct bank market is enjoying steady and respectable …
- Go to NIM Publication Series
- Go to all MIR-Issues
No internet connection.
All search filters on the page have been cleared., your search has been saved..
- All content
- Dictionaries
- Encyclopedias
- Expert Insights
- Foundations
- How-to Guides
- Journal Articles
- Little Blue Books
- Little Green Books
- Project Planner
- Tools Directory
- Sign in to my profile My Profile
- Sign in Signed in
- My profile My Profile
- Popular Music
- Blog Analysis
- Skype Interviewing
- Social Media Scroll Back Method
- Asynchronous Online Communication for Qualitative Research
- Online Video Games Research
Big Data in Marketing Research and Analytics
- Social Media Data and Ethics in Social Research
- Text Mining
- Qualitative Analysis of Social Media Data
- Comics in Qualitative Research
- Ethnographies in Online Environments
- Email Diaries
- Digital Storytelling
- Smartphone Apps
- Web Surveying Design and Implementation
- Visual Elicitation in Interviews
- Multimodal Analysis
- Drawing as a Research Method
- Videography and Video Analysis
- Ethnographic Film
- Ethnomusicology
- Draw-and-Write Techniques
- Laboratory Ethnography
- Collage-Making
- Respondent-Generated Image Production
- Soundscapes
Discover method in the Methods Map
- By: G. Scott Erickson | Edited by: Paul Atkinson, Sara Delamont, Alexandru Cernat, Joseph W. Sakshaug & Richard A.Williams
- Publisher: SAGE Publications Ltd
- Publication year: 2019
- Online pub date: September 17, 2019
- Discipline: Marketing
- Methods: Big data , Marketing research , Data collection
- Length: 5k+ Words
- DOI: https:// doi. org/10.4135/9781526421036808804
- Online ISBN: 9781529746785 More information Less information
- What's Next
Big data and marketing analytics are concepts of increasing interest in a number of industries and a number of applications. The terms are used frequently in the business press but not always with a full understanding of the actual meaning or full implications. This entry covers some more precise definitions of terms, what those mean in marketing practice, and how they impact marketing research practice across exploratory, descriptive, and causal research designs.
Big data is commonly defined among scholars according to the “3 V’s” of volume, velocity, and variety (Laney, 2001). These are sometimes supplemented with veracity and value, encompassing “5 V’s.” The amount of data generated by information systems (volume), rapidly transferred to data users (velocity), and in different forms (variety) are all increasing at mind-boggling rates. Huge amounts of data can be available in real time not only as quantitative results but as text, pictures, audio, and video. The growth of interest in big data has mirrored the explosive changes in these descriptors (McAfee & Brynjolfsson, 2012).
The data are coming from several business processes. If one thinks of the value chain, data can be generated by supply chain and distribution systems (inbound and outbound logistics), manufacturing or service operations, marketing and sales activities (especially transactions and other customer interactions), and other communications such as digital media. The nature of the data, in a marketing context, is often nothing new but, again, the volume, velocity, and variety have changed considerably.
Transaction records, for example, have existed since people started selling things to one another, including everything from carbon paper copies to cash register receipts. In a big data world, however, identifiable consumers with all of their background data can now be tied to new purchases, contextual data (e.g., store location, time of day), and other joined data such as whatever is known to their credit card issuer. Similarly, media consumption has been tracked, often through sampling services such as Nielsen, for a very long time. But now media use and, again, a lot of the contextual and background data, can be tracked per individual across an entire population by services such as Netflix, Hulu, or your cable/satellite provider.
The rise of the Internet and mobile connections has enabled these applications while also creating new sources of data such as online behavior, app use, digital media use, and others. The bottom line is that firms are awash in data, extending the usefulness of some traditional marketing metrics and creating new ones. The opportunities for research are considerable.
The forerunner of much of this data revolution was loyalty programs. Even before the Internet, frequent flier and frequent stay programs, retail loyalty programs, and other initiatives creating close, one-on-one connections with consumers gathered considerable data. These data could be collected, monitored, and analyzed as a whole or by individual customer, opening the door to better descriptors of attitudes and behaviors, deeper understanding of each, and more accurate predictions of future behavior. Some knowledge of individual customers also became possible, including not only descriptions of activities but experimentation to determine response patterns to marketing initiatives.
In the pre-Internet era, pioneers in this area included Tesco and Caesars/Harrah’s. Tesco was an early adopter of a grocery loyalty card (Spethman, 2004). From information gathered through customer card usage, the firm could monitor transactions, uncovering broad-based buying patterns, and responses to marketing initiatives. On an individual level, the firm could make targeted offers, estimating at one time that it had over 4 million distinct offers that could be made to its 9 million club members. Similarly, Caesars developed a rewards card that tracked member behavior at its properties, including hotel stays, gaming behavior, restaurant patronage, and show attendance. Again, broad patterns could be tracked as well as individual behavior and both segment and individual responses to incentives. As technology improved, the firm could track gambling behavior, including betting levels, wins, and losses in real time (Welch & Westerman, 2013).
What newer technology has provided is an ability to treat more and more customers in this manner, in more and more interactions with the firm. Any circumstance in which individuals can be identified and tracked will allow similar data collection and similar analysis for marketing purposes. The most obvious area is on the web, tracking Internet usage, but ever greater use of loyalty programs, apps, digital media, and other circumstances also provide opportunities for this level of data collection. As noted at the start of this section, this can be an enormous volume of data, with identification down to the individual level. It is provided in real time, so that marketers can analyze the data and react immediately. And the data are of a wide variety not only quantitative data like number of visits or spending levels but text like social media chatter or customer comments, video (e.g., whether lines are forming at a service facility), or images (e.g., facial reactions to advertising). The implications for marketing research are dramatic.
Constant Monitoring
In understanding those implications, a couple of other aspects of big data and business analytics are important. Initially, as just discussed, organizations have the ability to monitor a tremendous amount of activity along the value chain, especially concerning interactions with customers. Marketing research has always noted the possibility of ongoing marketing information systems designed to continually collect specified data. The potential applications of such systems have been substantially enhanced by these new data collection abilities.
As such, a great deal of big data is simply collected and monitored. The development of dashboards, reporting important data in real time, has been a particularly notable aspect. As firms identify particularly critical data to monitor, these are often designated as key performance indicators and added to dashboards for particular marketing decision makers. So whether tracking sales, visits to websites, content downloads, or any number of other outcomes, big data systems are effective at providing specified data of interest on a regular basis, even down to real-time reports on a second-by-second basis.
Google Analytics, for example, provides a standard dashboard containing critical website data, including such ongoing figures as visits, bounce rate, average pages viewed, and time spent. Similarly, firms such as Spotify and Netflix can track usage, by individual and as segments, of content usage as well as individual user data attached to it. As a result, understanding what new music is being played at after-parties on Saturday nights in Stockholm or how an artist’s appearance on a chat show bumps download numbers is an easy talk for Spotify. Tracking how many users view a new series, when and if they drop out of it, and when they binge on multiple episodes is standard procedure for Netflix. Adding more specific user data to both reports is also straightforward for both. The key is the constant stream of data, then choosing what to monitor for marketing purposes.
Besides an ability to monitor data in real time, the continuous gathering and reporting also allows organizations to observe and act on longitudinal results. Trends, inflection points, and customer progressions can all be easily captured by systems designed to track ongoing data. Consultancy Sirius Decisions, for example, provides clients with a “waterfall” tool that uses Salesforce.com data to track individual accounts. Targets pass through multiple stages from prospecting to closing (and on to brand advocate in other contexts) with specific documented events moving them to each level. Again, target actions or reactions are easy to track with everyday data collection.
Constant monitoring also allows for easy experimentation. Premeasurement and postmeasurement can be gathered through routine data collection, and adding different variations on the experimental variable is simple. If a website wants to A/B test two versions of a landing page, for example, it can simply put them up, funnel users to each, and monitor with Google Analytics which has the biggest impact on time spent on site, click-through rates, or any other desired action.
The important point is that big data is constantly being collected. With some good planning, appropriate metrics can be identified and included. And, of course, new metrics can be added to the mix when desired. All of this allows a great deal of research to be pursued without necessarily launching a new project or adding new purposeful, primary data.
Predictive Analytics
The other unique aspect of big data falls more distinctly into the marketing analytics or marketing intelligence part of the equation. The huge databases constructed from continuous gathering can be further analyzed for useful insights. Approaches vary but are commonly referred to as predictive analytics, studying the data for similarities or correlations. Predictions can be made from regression, decision trees, neural networks, clustering analysis, or other means establishing relationships among variables.
The classic example of the power of predictive analytics came from a kerfuffle at Target when an enraged father objected to the store sending promotional offers for pregnancy products to his teenage daughter (Hill, 2012). It turned out she was pregnant, and Target knew before the father. The prediction came from a purposeful project Target initiated to identify expectant mothers based on purchase histories. Analytics staff identified 25 variables that provided predictions, with reportedly over 80% accuracy that a customer was pregnant. The identified variables provided strong correlations to the predictor variable, pregnancy.
Predictive analytics is what a number of high-profile big data users employ to add value to their customer offerings. When Amazon suggests other items you might wish to purchase, might wish to watch (Prime video), might want to listen to (Prime music), or so forth, it is employing predictive analytics based on your purchasing history, browsing history, and other personal variables it might have in its database. Spotify’s recommendations come from much the same place, clustering you with other subscribers with similar listening preferences. Netflix’s suggestions are made in a similar manner, identifying viewing tendencies in your history that match with other users and their likes and dislikes. If those like you have watched something you haven’t, that becomes a natural recommendation added to your account.
Predictive analytics typically requires highly trained computer scientists to design systems to gather, organize, and combine data from various sources as well as highly trained analysts who can use advanced statistical tools to search the data for nonobvious clusters of observations or correlations between variables. The role of the marketing researcher is to guide the team to a useful conclusion. In the Target case, for example, the marketers were particularly interested in pregnancy because it was identified as a major life event. Consumers often develop new attitudes and behaviors in response to such major life events, and a habit established early on can then persist. So gaining consumer loyalty early in the pregnancy can lead to a continuing relationship through birth and beyond. As such, the analytics team at Target was given specific instruction to identify variables correlated with pregnancy, and it was able to identify items such as large quantities of unscented lotions and specific nutritional supplements. Even though most of the data already existed in purchasing histories of customers, the specific research equation was answered with the deeper analysis of that data.
Implications for Marketing Research
A number of implications are immediately apparent from these trends, but some are more subtle. Again, according to the definitions of big data and how it is practiced, there is simply much more data already streaming into organizations (volume), in real time (velocity), and in all sorts of formats (variety). A long-standing guideline in marketing research has always been to assess existing secondary data before pursuing more costly and time-consuming primary data, and, increasingly, there is considerably more existing data already at hand. Advanced analytical methods are also available to study these much bigger, more varied databases to uncover new insights. But several other less obvious aspects of big data and business analytics also affect marketing research practice and how it might evolve in future years.
Initially, market researchers have always had a choice between communication and observation tools (or a combination, in some applications). While communication tools like surveys and focus groups could uncover unobservable phenomenon like motivations or intentions, observation tools were often seen as more reliable, not depending on accurate memory, truthfulness, or various potentially biasing factors. Based on the examples presented so far and the related universe of big data sources, we see that there is simply much more observation research now available to marketers. Observing transactions and all attached variables, observing customer communications or digital media activity, observing customer shopping behavior on the web or in a physical store, observing customer activities in a services environment (e.g., health care, entertainment), and other applications have become routine and incredibly detailed. Communications research still exists, as market researchers want to understand more of what goes on in customers’ minds. But that can be conducted separately and, in fact, with more prior understanding of what the actual behaviors might be and their context. Or communication and observation can be joined in the same study, as communication has also changed with newer technologies enabling big data.
Communication research itself has also seen some changes. The principal one is that many firms have extensive contact lists of customers. Whether through loyalty programs or just established accounts, one-time or frequent customers with attendant background and contextual data can be identified. When organizations do want to conduct surveys or focus groups, they have a natural and probably more willing population from which to draw respondents as all have an existing relationship with the firm. Caesars, for example, mentioned earlier concerning its loyalty program, claims to conduct 50,000 to 60,000 surveys every week among its loyalty card holders. So even when communication is a more appropriate approach than observation research, it can now be done relatively easily and quickly using these databases.
A related approach seeing considerable growth is the use of panels. Research firms such as Vision Critical are in the business of creating “influencer” panels for clients. Constructed from the considerable databases firms hold, the panels include users willing to communicate directly with the client firm; the panels provide instant feedback through surveys, focus groups, or other means. Discovery, Inc., the global television provider, for example, runs a panel of over 15,000 viewers. By utilizing the existing sample, the firm can conduct instant research on advertising or promotional initiatives, new show concepts, or similar topics. In the first year alone, Discovery conducted a survey every other day. The ability to conduct instant research, without drawing new samples every time, and immediately joining the results with existing data can be extremely valuable. There is some loss of representativeness if firms need data from potential customers rather than just current ones, but other research can fill those holes.
Both observation and communication research benefit from the ability to collect much larger samples or even involve full populations. The unobtrusiveness of the observation research makes it easy to collect considerable data from users, members, or random customers. The communications can also seem routine and/or a part of helping favored brand. Subjects often seem less concerned about anonymity. Furthermore, the ability to take in a wide variety and volume of observations or responses can also allow creative approaches such as gamification to the data-gathering process. All in all, research subjects can be more willing and more amenable to complex research designs.
Research Designs
Many of these implications relate most obviously to descriptive research designs. Unobtrusive data collection from large samples, potential combinations with existing data describing subjects, rapid collection, longitudinal capabilities, and other factors discussed in this entry have clear connections to descriptive research. And the huge databases themselves built up from regular collection and monitoring of business activities provide compelling descriptions of customers, activities, context, and just about anything of interest to marketers. Again, many of the most prominent examples of big data and marketing analytics derive from databases tailor-made for descriptive: Google and individuals’ web behavior, Facebook and users’ social media interactions, Spotify and listeners’ activities, Netflix or YouTube, and viewers’ activities, for example.
Causal/Experimental Research Designs
A little less obvious but also quite clear once one reflects on the examples is how big data can be used for experimental research designs. Indeed, the ease with which it can be used for experiments has caused an explosion in the number of applications. Traditionally, experiments were seen as the most conclusive research design, actually proving or disproving a hypothesis rather than just identifying insights (exploratory) or correlations (descriptive). Experiments, however, also tended to be the most time-consuming and costly, so they provided better information but also something for which one had to be willing to pay and wait.
That is not necessarily the case anymore. On-the-ground test markets can still take time as can beta tests, such as for new e-gaming titles or software. But even then, digital options are available, cutting both costs and time while providing easy collection of considerable data (e.g., details on gamers themselves combined with real-time feedback on game play, context). But even more options are available in day-to-day activities. This entry previously discussed big data’s volume, unobtrusiveness, and longitudinal capabilities. Related to experimental design, the timing of measurements such as preexperiment or postexperiment is just a matter of monitoring that specific data. If an experiment is particularly complex, multiple experimental or control groups can be populated and tracked without much additional bother. Since many of these experiments have to do with observed behavior or routine communications and are done quickly, many of the biases of experimentation are avoided (testing effect, instrument effect, selection effect, maturation, and history).
YouTube’s Unskippable Labs, for example, conduct experiments to try to improve the effectiveness of online advertising, particular looking for what variables can be manipulated to reduce the tendency of viewers to skip the ad as soon as possible. One effort included an artificial pizza brand, Doctor Fork, and looking at the variables of senses engaged (sight, hearing, perceived taste) and depicting actual eating (Ha, 2018). The latter is apparently a taboo in food advertising; potential customers are turned off by chewing. But because of the taboo, no one would test a real ad. Unskippable Labs could do it with its fake brand. Consequently, 33 versions of Doctor Fork ads were created and shown to different experimental groups totaling 20 million users. Given YouTube’s wide range of opportunities to screen the ads and viewers’ lack of awareness that the experiment was occurring, a complex, low-bias, high sample size experiment could be executed very quickly. In general, the results showed multiple senses engaged were better and “chomp and smile” was not the only positive reaction, chewing shots could be effective.
As the example suggests, experimentation is possible at any time when data collection is constant. Whether Facebook altering newsfeeds, OkCupid trying different match algorithms, or Amazon optimizing pricing by trying different levels at different times of day (and in response to competitor moves), experimentation is as straightforward as changing the key variable and measuring the results. Furthermore, although clearly skewed to online interactions in these examples, researchers are finding ways to use other data streams to measure other types of experiments. Loyalty cardholders, for example, were apt subjects even before the prevalence of the web, although modern technology has enhanced those abilities. But any firm with identifiable customers can feed promotional offers or other marketing initiatives to groups, then judge the effect based on behaviors captured through customer activity, whether that be facility visits, spending levels, or other metrics. This can even be done in-store through loyalty cards or shopping apps, either store-branded or general use such as Shopkick.
Looking at these diverse examples brings up a few other aspects of big data experimentation pertinent to this discussion. Initially, although sizable samples can be constructed and sizable experimental groups within even bigger total samples, the capability exists to test an entire population. An Amazon price change, for example, is just a matter of flipping a switch and could, conceivably, be offered to anyone who looks to buy that product, not just an identified sample. Or a firm with a database of customer accounts, whether loyalty card holders or just registered users, could offer a promotion to its entire database. Once again, the volumes can be substantial and, in fact, may include the entire population and render concerns about statistical extendibility somewhat moot.
At the other extreme, firms can also experiment on individual, identifiable customers. So Caesars can try different marketing initiatives to see what gets high-roller X into the casinos more frequently. Starbucks can see what specific offers get customer Y to spend more per store visit. Facebook can examine what kind of news feed or advertising gets user Z to spend more time on the platform. As before, this customized experimentation does not require any new data collection, just continued monitoring of what is already compiled.
Exploratory Research Designs
Perhaps the least likely research design to be impacted by big data is exploratory. As most approaches are small sample, in depth, and yield qualitative, unstructured data, large volumes of rapidly collected and processed results do not seem immediately applicable. But big data also has a role in exploratory.
Exploratory research design is where the aspect of big data variety is particularly relevant. While taking in quantitative descriptive and experimental data has a history, with big data only increasing the volume and velocity, unstructured data such as text, images, and video have been more limited. But with ever-cheaper storage and processing capacity in information systems, including the cloud and robust analysis tools that can handle such data variety, unstructured data can be a more important part of the equation.
Once again, observation particularly benefits from these capabilities as researchers can observe what is done (e.g., web browsing, app use, physical shopping patterns) and what is said (e.g., social media, reviews), easily converting the results into big data. Communication is possible as well, taking in full focus group records or customer interactions for storage and analysis.
In ethnographic studies, for example, researcher immersions can now extend to days, with data constantly collected from both video and conversations. The extended look at consumer lives can then be stored and studied or analyzed in depth. The nature of such ethnographic studies illustrates another capability found with the onset of big data that customer activities and behaviors can be observed, in depth, and then the subjects can be asked about them during or after the observation. If a consumer is browsing or shopping on the web, for example, all of that activity can be observed. But researchers can also ask about it, either in real time or after the fact: “Why did you click on that?” or “What were you thinking about when you made that choice?” Furthermore, the questions may be delivered and collected digitally (digital ethnographies), allowing a wider range of, perhaps, unstructured responses.
Such aspects allow studies that could be either exploratory or descriptive, somewhat blurring the lines between them—and that includes sample size. While exploratory studies would still be applied when researchers are looking for insights, unexpected results, or increased familiarity with the issue, big data capabilities provide the opportunity to collect qualitative and quantitative responses to those objectives from a wide range of sample sizes. Descriptive studies would still be used when seeking quantitative results to reliably describe population characteristics. As already seen in this entry, those sample sizes can be larger as well.
The ethnographies previously noted, for example, can be much easier to execute and then analyze, allowing more to be included, if researchers desire. Or advertising evaluations can be done as exploratory studies, seeking initial feedback about a new cut of the ad. Traditional exploratory approaches would include screening of the advertisement and either asking directly for comment or using an instrument to measure brand recall, brand preference, and creative likes/dislikes. Instead, other techniques can once again be employed to unobtrusively observe individuals, gathering considerable data on their physical reactions to the ads. Eye-tracking systems can gather data on visuals and what parts of an ad draw attention from the respondents’ eyes. Facial scanning systems can measure movements of respondents’ faces, including their mouths, eyes, brows, and other aspects to gauge happiness, surprise, concern, and multiple other reactions to ads. All of this can be gathered in real time, unobtrusively (respondents may know they are participating in research but not necessarily what is being measured), and across a considerable number of variables. It can be done to get initial reactions to ad concepts (exploratory), to describe ad response (descriptive), or even to test different versions (causal). In the end, the sample size can go quite large without necessarily adding a lot of cost.
The research plans can also include much more complex components. While projective techniques, even newer approaches such as deprivation studies, have been around for a very long time, the unstructured data gathering environments often limited their use to smaller samples. Here again, data gathering advances allow rapid gathering of subject responses, whether verbal or visual. Similarly, gamification elements in interviews, focus groups, or surveys can be more readily used as the data gathering easily handles less structured responses, even from larger samples.
Overall, advances in technology have allowed the collection and analysis of big data in many areas of marketing research. The impact is that much more data are available and cover many more variables than were previously available. The volume of data, the velocity at which it is collected and dispersed, and its wide varieties have opened up a number of possibilities for researchers. Continuous, unobtrusive data collection is not only possible but relatively straightforward. More complex research, collected more quickly, and retrieved from potentially larger samples (or even entire populations) is also executable and doesn’t necessarily cost more than traditional data gathering. Technology-enhanced marketing research utilizing big data is already impacting research and promises to continue to do so in dramatic ways in the future.
Sign in to access this content
Get a 30 day free trial, more like this, sage recommends.
We found other relevant content for you on other Sage platforms.
Have you created a personal profile? Login or create a profile so that you can save clips, playlists and searches
- Sign in/register
Navigating away from this page will delete your results
Please save your results to "My Self-Assessments" in your profile before navigating away from this page.
Sign in to my profile
Sign up for a free trial and experience all Sage Learning Resources have to offer.
You must have a valid academic email address to sign up.
Get off-campus access
- View or download all content my institution has access to.
Sign up for a free trial and experience all Sage Research Methods has to offer.
- view my profile
- view my lists
More From Forbes
How marketers are using qualitative data in the age of big data and ai.

- Share to Facebook
- Share to Twitter
- Share to Linkedin
Lacking a holistic understanding of their target audience limits marketers’ ability to create the most effective strategies. Yet they often prioritize the concrete metrics of quantitative data, such as sales numbers and website traffic that seem easier to measure and analyze, over subjective observations. Consequently, relying solely on quantitative data can lead marketers to overlook the human element of consumer behavior and miss out on valuable insights that only qualitative data can provide.
Qualitative data reveals vital information about customers’ emotions, motivations, preferences and perceptions. Through methods such as interviews and focus groups, marketers can gather rich, nuanced data that goes beyond mere numbers, illuminating a bigger picture that allows them to better tailor strategies, messaging and products to what consumers want. Below, 15 members of Forbes Agency Council explore the role that qualitative data can play in the era of big data and artificial intelligence, sharing how their agencies leverage it to better meet the needs and desires of target audiences.
1. To Get To The Quantitative Data
Without the qualitative data, you can’t get to the quantitative data. All the ad creatives, messaging, campaign structures and so forth that take place behind the scenes are going to feed the big data monster with the engagement, clicks and interactions that can be improved upon. AI helps us get 10 times more iterations and options—but nothing runs without the creative thought that fills the machine. - Bernard May , National Positions
2. To Create A Better User Experience
We use qualitative data to create a better UX for Web design projects. Through user testing, interviews and usability studies, we gain insights into user needs, preferences and pain points. This information guides the design process, ensuring that websites and digital platforms are not only functional, but also enjoyable to use, enhancing user satisfaction and engagement. - Goran Paun , ArtVersion
3. To Understand Sentiment And Brand Perception
Qualitative data adds depth to our understanding of consumer behaviors, emotions and motivations, complementing quantitative insights. Our agency uses it for sentiment analysis and brand perception studies to craft personalized messaging that resonates authentically with target audiences. - Pascal Wilpers , Streamerzone.gg
Best High-Yield Savings Accounts Of 2024
Best 5% interest savings accounts of 2024, 4. to discover unique, actionable insights.
Einstein said, “Not everything that counts can be counted, and not everything that can be counted counts.” In marketing, qualitative data is vital to understanding human emotions and decisions. Despite abundant data, actionable insights require human intuition and experience, especially in hyper-personalized marketing. Global Prairie excels at this by harnessing qualitative data to discover unique insights. - Tom Hileman , Global Prairie
Forbes Agency Council is an invitation-only community for executives in successful public relations, media strategy, creative and advertising agencies. Do I qualify?
5. To Provide Color And Context
Consider qualitative data as the color providing context and sentiment around the rigid boundaries of quantitative data. Often, both types of datasets are needed to derive business value and audience interest. Qualitative data is the “gut check” for the “instincts” you may follow while evaluating datasets. Data cannot always be simplified into a dashboard; sometimes it requires deep analysis. - Tyler Back , Mitosis
6. To Identify Pain Points, Gains And Jobs To Be Done
Customer interviews provide qualitative data that is increasingly vital for identifying pain points, gains and jobs to be done, as well as shaping your communication strategy. However, quantitative data from clients and prospects is also crucial. It informs us about what messages to convey, as well as where and how to communicate them more effectively. - Kate Vasylenko , 42DM Corporation
7. To Connect PR Efforts To Client Goals And Show ROI
Qualitative data is essential to connecting PR efforts to clients’ business goals and showing the ROI of our work. At Next PR, we go beyond reporting on quantitative KPIs, such as number of media placements, and instead focus on qualitative results that support clients’ objectives, such as leveraging Google Analytics to demonstrate how a media placement contributed to increased brand awareness. - Heather Kelly , Next PR
8. To Get Insights That Yield Marketing Recommendations
Qualitative data speaks to the emotion behind customers’ decision making. We use interviews, open-ended surveys, observational studies and focus groups to gather such data. Quantitative techniques capture numerical data (website visits, customer satisfaction scores), revealing trends and correlations in customer behavior. Combining both, we develop insights that yield marketing recommendations. - Robert Finlayson , Bold Marketing and Communications
9. To Understand Our Clients’ Audiences’ Motivations
AI allows us to analyze large sets of data to provide necessary context. The contextual frameworks need to be filled with emotions and insights beyond the numbers—in short, qualitative data. It’s all about storytelling, so leveraging user feedback and social listening helps us understand the motivations and preferences of our clients’ audiences and turn them into meaningful marketing strategies. - Christoph Kastenholz , Pulse Advertising
10. To Get As One-To-One As Possible In Our Messaging
Qualitative data is important, as it allows us to get as one-to-one as possible in our messaging and content. Right now, we’re seeing a lot of junk via email, LinkedIn and content in search engines and other places that, in many cases, is either wrong at worst or okay at best. Higher-quality data allows for brand messaging and content that meets the prospect or potential customer with value, not noise. - Corey Morris , Voltage
11. To Develop Briefs And Leverage Winning Insights
Qualitative data is increasingly precious in an age of data commoditization. Comments on social media are the new briefs, and invariably, the majority of winning insights are the consequence of qualitative inputs from customers. - Aasim Shaikh Zubaer , LPS Brands
12. To Understand Why What Works Is Working
Qualitative data goes deeper than quantitative data. It gives you the “why.” While big data and AI can tell you what works, understanding why it works will help your organization develop a larger story arc to guide customers across the full journey. - Alicia Arnold , AK Arnold
13. To Develop Richer Audience Personas
In the era of big data and AI, qualitative data helps marketers and advertisers gain a deeper understanding of customers’ motivations and preferences. Our agency plans to leverage it by developing richer audience personas, which can help clients get a clearer picture into their customers’ values, inspirations and challenges. - Jordan Edelson , Appetizer Mobile LLC
14. To Inform Campaigns And Evaluate Results
Qualitative data remains a crucial tool for marketers and advertisers. It plays a complementary and valuable role alongside quantitative data, offering deeper insights and richer understanding of consumers. Our platform uses it to conduct research and analysis, develop customer personas for our clients, inform campaign development and measure and evaluate campaign results. - Tanuj Joshi , Eulerity
15. To Accurately Model B2B Audiences
Qualitative data is incredibly important for B2B agencies such as Napier. For example, it’s often not possible to get statistically significant samples to accurately model audiences quantitatively. It’s also important to note that AI’s “pattern matching” capabilities are not quantitative analysis; in fact, I believe AI has introduced a golden age of qualitative data analysis. - Mike Maynard , Napier Partnership Limited
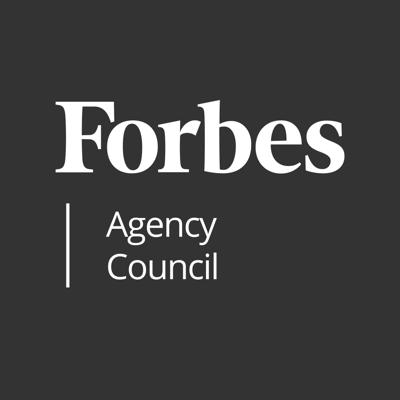
- Editorial Standards
- Reprints & Permissions
Industries Overview
Latest articles, generative ai’s actual use isn’t meeting its perceived marketing potential—yet, us adults are spending more time with digital video and less time with traditional tv, how marketers can do more with less after a rocky earnings season, home depot’s revamped retail media network simplifies campaigns, expands audience, vp says, navigating the digital frontier: strategic insights on tech investments | sponsored content, kids remember seeing ads on youtube over streaming, tiktok, exclusive: instagram's nano-influencers outperform—as do tiktok’s upper echelon of creators, where and how gen alpha consumes content: from youtube to netflix to roblox, a slight increase in minutes per day with media, thanks to another boost for digital, linkedin is testing a tiktok-like short-form video feed to boost user engagement, about emarketer, the digital healthcare consumer 2023, what providers and marketers need to know about the digital patient journey.
- Executive Summary
Digital healthcare usage was fast-tracked by the pandemic. But now, with so many tools in the market, providers and marketers must deliver differentiated experiences to stay competitive. They’ll need to know how and why patients use these tools—and how to resolve the pain points.
Key Question: Which digital health products and services are US consumers using, and how does usage differ across populations?
KEY STAT: About 46% of US consumers—an estimated 119 million patients—engage with their healthcare providers using a combination of patient portals, telehealth appointments, apps, and traditional in-person visits, per PYMNTS.
Here’s what’s in the full report
Exportable files for easy reading, analysis and sharing.
Reliable data in simple displays for presentations and quick decision making.
Table of Contents
- Consumer adoption of digital health tools is still growing.
- Who is the healthcare tech user?
- Patients demand an omnichannel experience.
- The patient healthcare journey often starts online.
- Patients are using social media to find health information.
- Patients believe digital health tools lead to better health.
- Consumers want doctors to embrace digital health tools.
- Healthcare is falling short on delivering a quality digital experience.
- Providers are at risk of losing patients if they don’t go digital.
- How can providers and marketers keep pace with the digital healthcare consumer?
- Media Gallery
Charts in This Report
Rajiv leventhal, contributors, access all charts and data.
Gain access to reliable data presented in clear and intelligible displays for quick understanding and decision making on the most important topics related to your industry
Industries →
Advertising & marketing.
- Social Media
- Content Marketing
- Email Marketing
- Browse All →
- Value-Based Care
- Digital Therapeutics
- Online Pharmacy
Ecommerce & Retail
- Ecommerce Sales
- Retail Sales
- Social Commerce
- Connected Devices
- Artificial Intelligence (AI)
Financial Services
- Wealth Management
More Industries
- Real Estate
- Customer Experience
- Small Business (SMB)
Geographies
- Asia-Pacific
- Central & Eastern Europe
- Latin America
- Middle East & Africa
- North America
- Western Europe
- Data Partnerships
Media Services
- Advertising & Sponsorship Opportunities
Free Content
- Newsletters
Contact Us →
Worldwide hq.
One Liberty Plaza 9th Floor New York, NY 10006 1-800-405-0844
Sales Inquiries
1-800-405-0844 [email protected]
We've detected unusual activity from your computer network
To continue, please click the box below to let us know you're not a robot.
Why did this happen?
Please make sure your browser supports JavaScript and cookies and that you are not blocking them from loading. For more information you can review our Terms of Service and Cookie Policy .
For inquiries related to this message please contact our support team and provide the reference ID below.
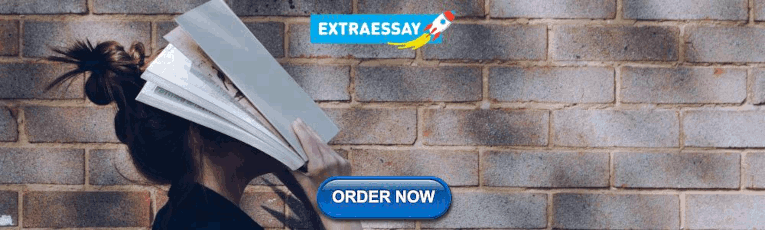
IMAGES
VIDEO
COMMENTS
Big Data, Bigger Marketing. Big data refers to the ever-increasing volume, velocity, variety, variability and complexity of information. For marketing organizations, big data is the fundamental consequence of the new marketing landscape, born from the digital world we now live in. The term "big data" doesn't just refer to the data itself; it ...
Marketing Research Defined. According to the American Marketing Association (AMA), marketing research "is the function that links the consumer, customer, and public to the marketer through information." 2 Marketing research presents information gained through various sources as a resource for managers to make data-driven business decisions.
22 Big Data in Marketing Examples. Da'Zhane Johnson and Margo Steines contributed reporting to this story. Great Companies Need Great People. That's Where We Come In. These big data marketing companies offer unified views of data, customer databases, campaign optimization and more to increase marketing effectiveness.
The benefits of using big data in market research include gathering and analyzing vast amounts of data in real-time, gaining deeper insights into consumer behavior, and making more informed business decisions. Predictive analytics is a powerful tool in big data, as it can help organizations identify trends and make predictions about future ...
Big data in marketing refers to the vast and complex sets of information generated by various sources, such as social media platforms, websites, and customer interactions. It encompasses both structured and unstructured data and includes text, images, video, and more. The sheer volume, velocity, and variety of data make it difficult to process ...
The Future Of Big Data-Based Marketing. By 2025, it's predicted more than 150 trillion gigabytes of data will need analysis. For marketing, this means that as time goes on, the need for advanced ...
Conclusion. In response to the blogosphere premise "that Big Data can replace survey research" our retort is that Big Data along with analytic techniques augments marketing research. Together, "Big Data" and marketing research represent a formidable toolkit in the hands of those who know how and when to exploit both to their full potential.
We discuss the impact of big data on modern marketing, emphasizing its role in consumer insights, predictive analytics, and personalization at scale. Read about the evolution of data usage, addresses data privacy regulations, and highlights the challenge of integrating offline and online data. The future of data-driven marketing involves increased use of AI, more touchpoints in consumer ...
The benefits of using big data in market research include gathering and analysing vast amounts of data in real-time, gaining deeper insights into consumer behaviour, and making more informed business decisions. Predictive analytics is a powerful tool in big data, as it can help brands identify trends and predict future behaviour. ...
Big Data in Marketing. Discover the core conceptual frameworks and practical tools you need to manage, analyze, and activate existing and emerging data streams to elevate your marketing campaigns. Start Date: February 26, 2024Students may register up to 7 days after the course start. Online learning with live, interactive sessions. USD $2,800.
The Internet's "footprint" has grown exponentially since the online world first became accessible to consumers in the late 1980s. By mid-2012, the Internet had reached 2.4 billion people, and while most developed markets are now mature with online penetration rates of 80 percent or more, it seems clear that emerging markets will ensure that Internet growth continues (e.g., Internet penetration ...
Big data can support smart market research, but only if researchers embrace the basics of understanding what it is they want to measure — and how. Today's businesses see market data as a commodity. Readily accessible information about consumer activity and preferences allows market researchers to develop large data sets to mine for consumer ...
Insights & Analytics. Translating consumer insights and big data into concrete initiatives that drive above-market growth. A company's ability to drive above-market growth hinges on the depth of its consumer insights and how well it translates those insights into effective action. Our area of work is made up of more than 130 dedicated ...
McKinsey data show that marketing budgets have been slashed for most companies, with six of ten marketers reporting major cuts. "My budget has evaporated," said one senior marketer. "We have barely enough to execute our 'must do' marketing, let alone experiment with new tactics.".
Market research provides the data and insights to make informed digital marketing decisions. It helps businesses understand consumer behavior, preferences, and influences across various digital channels. This information allows businesses to develop effective marketing strategies and allocate resources optimally.
Marketing analytics is the practice of analyzing marketing data from multiple systems to gain insights and make informed decisions. It helps you evaluate the effectiveness of your marketing efforts, track campaign performance, and understand customer behavior. By utilizing data-driven insights, you can optimize your marketing strategies and ...
Abstract. Big data analytics takes raw, real-time data and uses it to predict trends. Successful use of this data can have a powerful impact on a business's effectiveness and ultimately their bottom line. As the amount of data increases, the need for analytics is growing. This teaching study discusses the role of social media in data ...
How Data Analytics Can Help Deliver Social Good December 20, 2022 How Analytics Is Changing Finance November 29, 2022 Beyond Business: Humanizing ESG December 13, 2021
We broadly divide the nine studies into three themes: (1) Impact of social media (including studies that focus on Facebook, Snapchat, or Twitter) and text mining analyses, (2) Insights from survey-based data (in the context of healthcare and data marketing industry), and (3) New technologies and emerging research areas as summarized in Fig. 2.The next section offers a brief overview of the ...
Abstract. Big Data applications abound in all disciplines. In this chapter we consider the practical applications of Big Data analytics in Marketing. Businesses spend significant human and financial resources in marketing their products and services to potential customers. In this chapter we look at how businesses use the data gathered from ...
Reconsidering research methods on Big Data. Cutting edge technology and algorithms enable researchers to identify patterns mathematically without formal hypotheses (Anderson, 2008, Lycett, 2013). Taking advantage of these advancements, marketing researchers are encouraged to reevaluate the research methods associated with Big Data.
Discover strategies for marketing research teams to effectively manage and leverage big data without feeling overwhelmed. Prepare for the future with smart solutions.
Big data will change market research at its core in the long term because consumption of products and media can be logged electronically more and more, making it measurable on a large scale. Unfortunately, big data datasets are rarely representative, even if they are huge. Smart algorithms are needed to achieve high precision and prediction ...
Drawing as a Research Method; Videography and Video Analysis; Ethnographic Film; Ethnomusicology; Draw-and-Write Techniques; Qualitative Analysis of Social Media Data; Sensory Ethnography. Laboratory Ethnography; Collage-Making; Qualitative Research in Media Studies. Online Video Games Research; Big Data in Marketing Research and Analytics
2. To Create A Better User Experience. We use qualitative data to create a better UX for Web design projects. Through user testing, interviews and usability studies, we gain insights into user ...
Our research focuses on the five core coverage areas below. ... including full-length reports and data visualizations to equip you with actionable takeaways for better business decisions. PRO+. ... But now, with so many tools in the market, providers and marketers must deliver differentiated experiences to stay competitive. They'll need to ...
The Metaverse market may reach $783.3 billion in 2024 vs. $478.7 billion in 2020 representing a compound annual growth rate of 13.1%, based on our analysis and Newzoo, IDC, PWC, Statista and Two ...
Market Trend. Market Trend. The Big Picture; Stock Market Data; Stock Market Today; New? Start Here; ETF Market Strategy; IBD Digital: 2 Months for $20; Psychological Indicators; Stock Lists ...