Utilization of artificial intelligence in the banking sector: a systematic literature review
- Original Article
- Published: 11 August 2022
- Volume 28 , pages 835–852, ( 2023 )
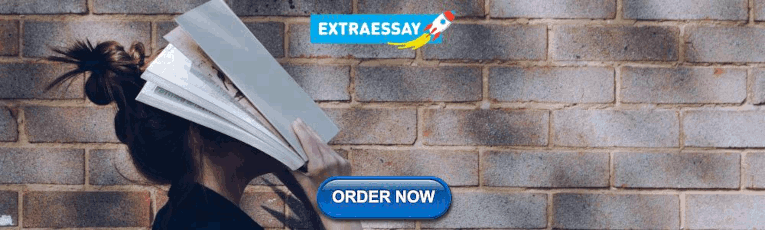
Cite this article
- Omar H. Fares ORCID: orcid.org/0000-0003-0950-0661 1 ,
- Irfan Butt 1 &
- Seung Hwan Mark Lee 1
30k Accesses
22 Citations
56 Altmetric
Explore all metrics
This study provides a holistic and systematic review of the literature on the utilization of artificial intelligence (AI) in the banking sector since 2005. In this study, the authors examined 44 articles through a systematic literature review approach and conducted a thematic and content analysis on them. This review identifies research themes demonstrating the utilization of AI in banking, develops and classifies sub-themes of past research, and uses thematic findings coupled with prior research to propose an AI banking service framework that bridges the gap between academic research and industry knowledge. The findings demonstrate how the literature on AI and banking extends to three key areas of research: Strategy, Process, and Customer. These findings may benefit marketers and decision-makers in the banking sector to formulate strategic decisions regarding the utilization and optimization of value from AI technologies in the banking sector. This study also provides opportunities for future research.
Similar content being viewed by others
Artificial Intelligence in Banking Systems: Trends and Possible Consequences of Implementation
The Impact of Artificial Intelligence on the Banking Industry Performance
The Role of Artificial Intelligence Techniques in the Digital Transformation of Jordanian Banking System
Avoid common mistakes on your manuscript.
Introduction
Digital innovations in the modern banking landscape are no longer discretionary for financial institutions; instead, they are becoming necessary for financial institutions to cope with an increasingly competitive market and changing customer expectations (De Oliveira Santini, 2018 ; Eren, 2021 ; Hua et al., 2019 ; Rajaobelina and Ricard, 2021 ; Valsamidis et al., 2020 ; Yang, 2009 ). In the era of modern banking, many new digital technologies have been driven by artificial intelligence (AI) as the key engine (Dobrescu and Dobrescu, 2018 ), leading to innovative disruptions of banking channels (e.g., automated teller machines, online banking, mobile banking), services (e.g., imaging of checks, voice recognition, chatbots), and solutions (e.g., AI investment advisors and AI credit selectors).
The application of AI in banking is across the board, with uses in the front office (voice assistants and biometrics), middle office (anti-fraud risk monitoring and complex legal and compliance workflows), and back office (credit underwriting with smart contracts infrastructure). Banks are expected to save $447 billion by 2023, by employing AI applications. Almost 80% of the banks in the USA are cognizant of the potential benefits offered by AI (Digalaki, 2022 ). Indeed, the emergence of AI has generated a wealth of opportunities and challenges (Malali and Gopalakrishnan, 2020 ). In the banking context, the use of AI has led to more seamless sales and has guided the development of effective customer relationship management systems (Tarafdar et al., 2019 ). While the focus in the past was on the automation of credit scoring, analyses, and the grants process (Mehrotra, 2019 ), capabilities evolved to support internal systems and processes as well (Caron, 2019 ).
The term AI was first used in 1956 by John McCarthy (McCarthy et al., 1956 ); it refers to systems that act and think like humans in a rational way (Kok et al., 2009 ). In the aftermath of the dot com bubble in 2000, the field of AI shifted toward Web 2.0. era in 2005, and the growth of data and availability of information encouraged more research in AI and its potential (Larson, 2021 ). More recently, technological advancements have opened the doors for AI to facilitate enterprise cognitive computing, which involves embedding algorithms into applications to support organizational processes (Tarafdar et al., 2019 ). This includes improving the speed of information analysis, obtaining more accurate and reliable data outputs, and allowing employees to perform high-level tasks. In recent years, AI-based technologies have been shown to be effective and practical. However, many corporate executives still lack knowledge regarding the strategic utilization of AI in their organizations. For instance, Ransbotham et al. ( 2017 ) found that 85% of business executives viewed AI as a key tool for providing businesses with a sustainable competitive advantage; however, only 39% had a strategic plan for the use of AI, due to the lack of knowledge regarding implementation of AI for their organizations.
Here, we systematically analyze the past and current state of AI and banking literature to understand how it has been utilized within the banking sector historically, propose a service framework, and provide clear future research opportunities. In the past, a limited number of systematic literature reviews have studied AI within the management discipline (e.g., Bavaresco et al., 2020 ; Borges et al., 2020 ; Loureiro et al., 2020 ; Verma et al., 2021 ). However, the current literature lacks either research scope and depth, and/or industry focus. In response, we seek to differentiate our study from prior reviews by providing a specific focus on the banking sector and a more comprehensive analysis involving multiple modes of analysis.
In light of this, we aim to address the following research questions:
What are the themes and sub-themes that emerge from prior literature regarding the utilization of AI in the banking industry?
How does AI impact the customer's journey process in the banking sector, from customer acquisition to service delivery?
What are the current research deficits and future directions of research in this field?
Methodology
Selection of articles.
Adhering to the best practices for conducting a Systematic Literature Review (SLR) (see Khan et al., 2003 ; Tranfield et al, 2003 ; Xiao and Watson, 2019 ), we began by selecting the appropriate database and identifying keywords, based on an in-depth review of the literature. Research papers were extracted from Web of Science (WoS) and Scopus. These databases were selected to complement one another and provide access to scholarly articles (Mongeon and Paul-Hus, 2016 ); this was also the first step in ensuring the inclusion of high-quality articles (Harzing and Alakangas, 2016 ). The following query was used to search the title, abstract, and keywords: “Artificial intelligence OR machine learning OR deep learning OR neural networks OR Intelligent systems AND Bank AND consumer OR customer OR user.” The keywords were selected, based on prior literature review, with the goal of covering various business functions, especially focusing on the banking sector (Loureiro et al., 2020 ; Verma et al., 2021 ; Borges et al., 2020 ; Bavaresco et al., 2020 ). The initial search criteria yielded 11,684 papers. These papers were then filtered by “English,” “article only” publications, and using the subject area filter of “Management, Business Finance, accounting and Business,” which resulted in 626 papers.
In this study, we used the preferred reporting method for systematic reviews and meta-analyses (PRISMA) to ensure that we follow the systematic approach and track the flow of data across different stages of the SLR (Moher et al., 2009 ). After extracting the articles, each of the 626 papers was given a distinctive ID number to help differentiate the papers; the ID number was maintained throughout the analysis process. The data were then organized using the following columns: “ID number,” “database source,” “Author,” “title,” “Abstract,” “keywords,” “Year,” Australian Business Deans Council (ABDC) Journals, “and keyword validation columns.”
The exclusion of papers was done systematically in the following manner: a) All duplicate papers in the database were eliminated (105 duplicates); b) as a second quality check, papers not published in ABDC journals (163 papers) were omitted to ensure a quality standard for inclusion in the review,Query a practice consistent with other recent SLRs (Goyal and Kumar, 2021 ; Nusair et al., 2019 ; Pahlevan-Sharif et al., 2019 ); c) in order to ensure the relevance of articles included, and following our research objectives, we excluded non-consumer-related papers, searching for consumers (consumer, customer, user) in the title, abstract, and keywords; this resulted in the removal of 314 papers; d) for the remaining 48 papers, a relevance check was manually conducted to determine whether the papers were indeed related to AI and banking. Papers that specifically focused on the technical computational process of AI were removed (4 papers). This process resulted in the selection of 44 articles for subsequent analyses.
Thematic analysis
A thematic analysis classifies the topics and subtopics being researched. It is a method for identifying, analyzing, and reporting patterns within data (Boyatzis, 1998 ). We followed Chatha and Butt ( 2015 ) to classify the articles into themes and sub-themes using manual coding. Second, we employed the Leximancer software to supplement the manual classification process. The use of these two approaches provides additional validity and quality to the research findings.
Leximancer is a text-mining software that provides conceptual and relational information by identifying concept occurrences and co-occurrences (Leximancer, 2019 ). After uploading all the 44 papers onto Leximancer, we added “English” to the stoplist, which removed words such as “or/and/like” that are not relevant to developing themes. We manually removed irrelevant filler words, such as “pp.,” “Figure,” and “re.” Finally, our results consisted of two maps: a) a conceptual map wherein central themes and concepts are identified, and b) a relational cloud map where a network of connections and relationships are drawn among concepts.
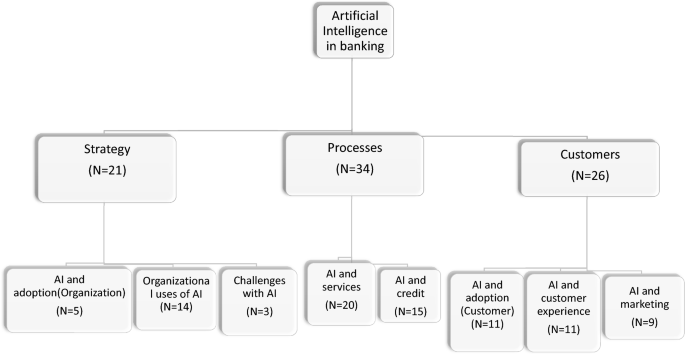
Thematic map
RQ 1: What are the themes and sub-themes that emerge from prior literature regarding the utilization of AI in the banking industry?
We began with a deductive approach to categorize articles into predetermined themes for the theme identification process. We then employed an inductive approach to identify the sub-themes and provide context for the primary themes (See Fig. 1 ). The procedure for determining the primary themes included, a) reviewing previous related systematic literature reviews (Bavaresco et al., 2020 ; Borges et al., 2020 ; Loureiro et al., 2020 ; Verma et al., 2021 ), b) identifying keywords and developing codes (themes) from selected papers; and c) reviewing titles, abstracts, and full papers, if needed, to identify appropriate allocation within these themes. Three primary themes were curated from the process: Strategy, Processes, and Customers (see Fig. 2 ).
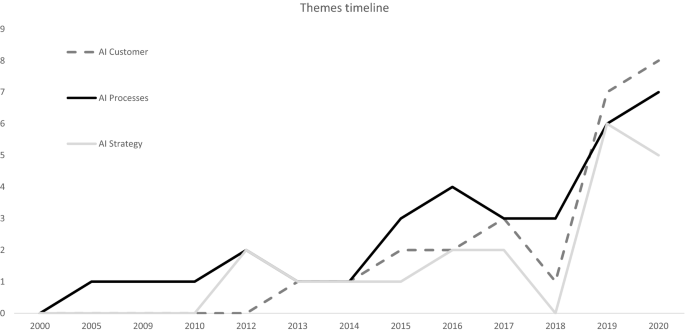
Themes by timeline
In the Strategy theme (21 papers), early research shows the potential uses and adoption of AI from an organizational perspective (e.g., Akkoç, 2012 ; Olson et al., 2012 ; Smeureanu et al., 2013 ). Data mining (an essential part of AI) has been used to predict bankruptcy (Olson et al., 2012 ) and to optimize risk models (Akkoç, 2012 ). The increasing use of AI-driven tools to drive organizational effectiveness creates greater business efficiency opportunities for financial institutions, as compared to traditional modes of strategizing and risk model development. The sub-theme Organizational use of AI (14 papers) covers a range of current activities wherein banks use AI to drive organizational value. These organizational uses include the use of AI to drive business strategies and internal business activities. Medhi and Mondal ( 2016 ) highlighted the use of an AI-driven model to predict outsourcing success. Our findings indicate the effectiveness of AI tools in driving efficient organizational strategies; however, there remain several challenges in implementing AI technologies, including the human resources aspect and the organizational culture to allow for such efficiencies (Fountain et al., 2019 ). More recently, there has been a noticeable focus on discussing some of the challenges associated with AI implementation in banking institutions (e.g., Jakšič and Marinč, 2019 ; Mohapatra, 2020 ). The sub-theme Challenges with AI (three papers) covers a range of challenges that organizations face, including the integration of AI in their organizations. Mohapatra ( 2020 ) characterizes some of the key challenges related to human–machine interactions to allow for the sustainable implementation of AI in banking. While much of the current research has focused on technology, our findings indicate that one of the main areas of opportunity in the future is related to adoption and integration. The sub-theme AI and adoption in financial institutions (six papers) covered a range of topics regarding motivation, and barriers to the adoption of AI technology from an organizational standpoint. Fountain et al. ( 2019 ) conceptually highlighted some barriers to organizational adoption, including workers’ fear, company culture, and budget constraints. Overall, in the Strategy theme, organizational uses of AI seemed to be the most prominent, which highlights the consistent focus on technology development compared with technology implementation. However, the literature remains limited in terms of discussions related to the organizational challenges associated with AI implementation.
In the Processes theme (34 papers), after the dot com bubble and with the emergence of Web 2.0, research on AI in the banking sector started to emerge. This could have been triggered by the suggested use of AI to predict stock market movements and stock selection (Kim and Lee, 2004 ; Tseng, 2003 ). At this stage, the literature on AI in the banking sector was related to its use in credit and loan analysis (Baesens et al., 2005 ; Ince and Aktan, 2009 ; Kao et al., 2012 ; Khandani et al., 2010 ). In the early stages of AI implementation, it is essential to develop fast and reliable AI infrastructure (Larson, 2021 ). Baesens et al. ( 2005 ) utilized a neural network approach to better predict loan defaults and early repayments. Ince and Aktan ( 2009 ) used a data mining technique to analyze credit scores and found that the AI-driven data mining approach was more effective than traditional methods. Similarly, Khandani et al. ( 2010 ) found machine-learning-driven models to be effective in analyzing consumer credit risk. The sub-theme, AI and credit (15 papers), covers the use of AI technology, such as machine learning and data mining, to improve credit scoring, analysis, and granting processes. For instance, Alborzi and Khanbabaei ( 2016 ) examined the use of data mining neural network techniques to develop a customer credit scoring model. Post-2013, there has been a noticeable increase in investigating how AI improves processes that go beyond credit analysis. The sub-theme AI and services (20 papers) covers the uses of AI for process improvement and enhancement. These process-related uses of technology include institutional uses of technology to improve internal service processes. For example, Soltani et al. ( 2019 ) examined the use of machine learning to optimize appointment scheduling time, and reduce service time. Overall, regarding the process theme, our findings highlight the usefulness of AI in improving banking processes; however, there remains a gap in practical research regarding the applied integration of technology in the banking system. In addition, while there is an abundance of research on credit risk, the exploration of other financial products remains limited.
In the Customer theme (26 papers), we uncovered the increasing use of AI as a methodological tool to better understand customer adoption of digital banking services. The sub-theme AI and Customer adoption (11 papers) covers the use of AI as a methodological tool to investigate customers’ adoption of digital banking technologies, including both barriers and motivational factors. For example, Arif et al. ( 2020 ) used a neural network approach to investigate barriers to internet-banking adoption by customers. Belanche et al. ( 2019 ) investigate factors related to AI-driven technology adoption in the banking sector. Payne et al. ( 2018 ) examine the drivers of the usage of AI-enabled mobile banking services. In addition, bank marketers have found an opportunity to use AI to better segment, target, and position their banking products and services. The sub-theme, AI and marketing (nine papers), covers the use of AI for different marketing activities, including customer segmentation, development of marketing models, and delivery of more effective marketing campaigns. For example, Smeureanu et al. ( 2013 ) proposed a machine learning technique to segment banking customers. Schwartz et al. ( 2017 ) utilized an AI-based method to examine the resource allocation in targeted advertisements. In recent years, there has been a noticeable trend in investigating how AI shapes customer experience (Soltani et al., 2019 ; Trivedi, 2019 ). The sub-theme of AI and customer experience (Papers 11) covers the use of AI to enhance banking experience and services for customers. For example, Trivedi ( 2019 ) investigated the use of chatbots in banking and their impact on customer experience.
Table 1 highlights the number of papers included in the themes and sub-themes. Overall, the papers related to Processes (77%) were the most frequently occurring, followed by Customer (59%) and Strategy-based (48%) papers. From 2013 onward, there was an increase in the inter-relation between all three areas of Strategy, Processes, and Customers. Since 2016, there has been a surge in research linking the themes of Processes and Customers. More recently, since 2017, papers combining Customers with Strategy have become more frequent.
Leximancer analysis
A Leximancer analysis was conducted on all the papers included in the study. This resulted in two major classifications and 56 distinct concepts. Here, a “concept” refers to a combination of closely related words. When referring to “concept co-occurrence,” we refer to the total number of times two concepts appear together. In comparison, the word association percentage refers to the conditional probability that two concepts will appear side-by-side.
Conceptual and relational analyses
Conceptual analysis refers to the analysis of data based on word frequency and word occurrence, whereas relational analysis refers to the analysis that draws connections between concepts and captures the co-occurrences between words (Leximancer, 2019 ). As Fig. 3 shows, the most prominent concept is “customer,” which provides additional credence to our customer theme. The concept “customer” appeared 2,231 times across all papers. For the concept “customer,” some of the key concept associations include satisfaction (324 co-occurrences and 64% word association), service (185 co-occurrences and 43% word association), and marketing (86 co-occurrences and 42% word association). This may imply the importance of utilizing AI in improving customer service and satisfaction, and in marketing to retain and grow the customer base. For instance, Trivedi ( 2019 ) examined the factors affecting chatbot satisfaction and found that information, system, and service quality, all have a significant positive association with it. Ekinci et al. ( 2014 ) proposed a customer lifetime value model, supported by a deep learning approach, to highlight key indicators in the banking sector. Xu et al. ( 2020 ) examined the effects of AI versus human customer service, and found that customers are more likely to use AI for low-complexity tasks, whereas a human agent is preferred for high-complexity tasks. It is worth noting that most of the research related to the customer theme has utilized a quantitative approach, with limited qualitative papers (i.e., four papers) in recent years.
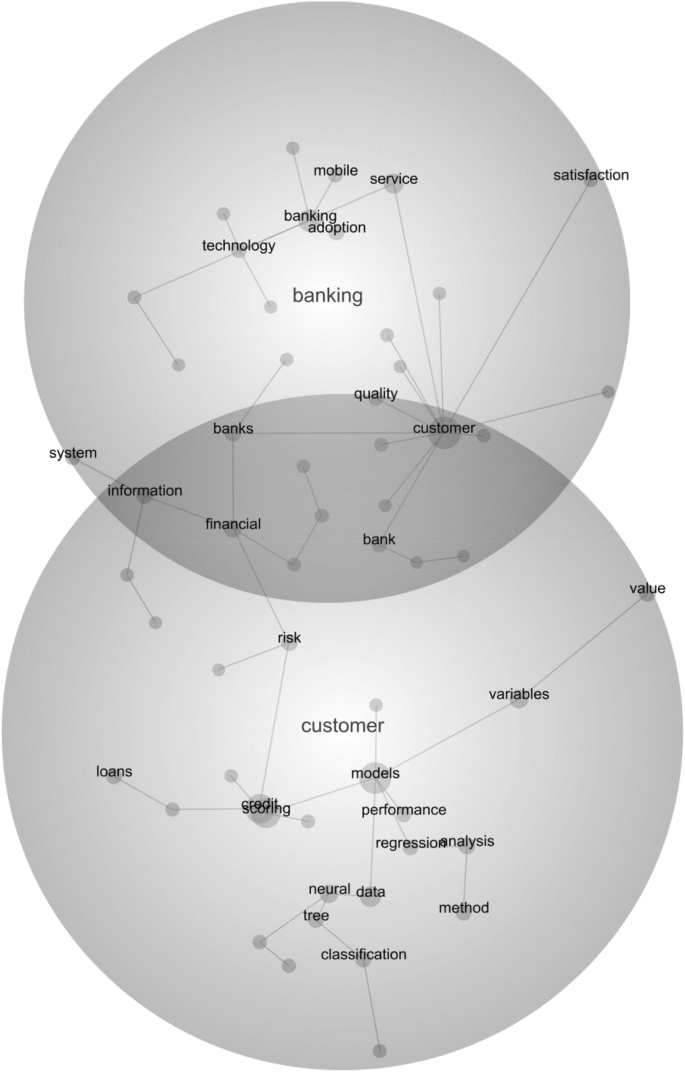
Concept map of content of all papers included in the study
Not surprisingly, the second most prominent concept is “banking,” which is expected as it is the sector that we are examining. The concept “banking” appeared 1,033 times across all the papers. In the “banking” concept, some of the key concept associations include mobile (248 co-occurrences and 88% word association), internet (152 co-occurrences and 82% word association), adoption (220 co-occurrences and 50% word association), and acceptance (71 co-occurrences and 42% word association). This implies the importance of utilizing AI in mobile- and internet-banking research, along with inquiries related to the adoption and acceptance of AI for such uses. Belanche et al. ( 2019 ) proposed a research framework to provide a deeper understanding of the factors driving AI-driven technology adoption in the banking sector. Payne et al. ( 2018 ) examined digital natives' comfort and attitudes toward AI-enabled mobile banking activities and found that the need for services, attitude toward AI, relative advantage, and trust had a significant positive association with the usage of AI-enabled mobile banking services.
Figure 4 highlights the concept associations and draws connections between concepts. The identification and classification of themes and sub-themes using the deductive method in thematic analysis, and the automated approach using Leximancer, provide a reliable and detailed overview of the prior literature.
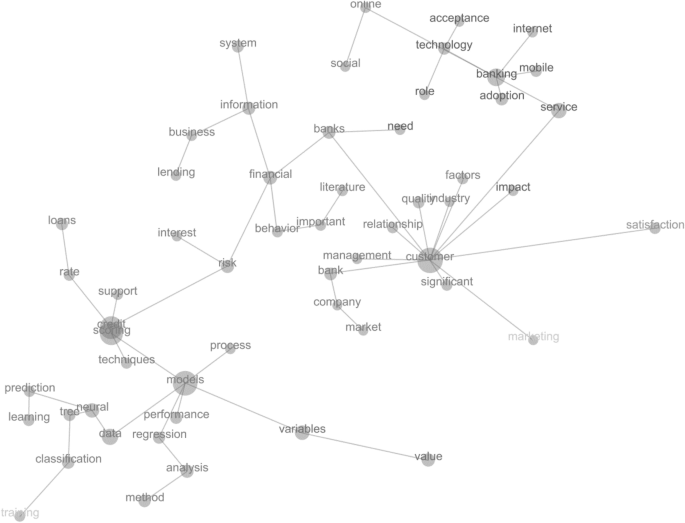
Cloud map of content of all papers included in the study
Customer credit solution application-service blueprint
RQ 2: How does AI impact the banking customer’s journey?
A service blueprint is a method that conceptualizes the customer journey while providing a framework for the front/back-end and support processes (Shostack, 1982 ). For a service blueprint to be effective, the core focus should be on the customer, and steps should be developed based on data and expertise (Bitner et al., 2008 ). As previously discussed, one of the key research areas, AI and banking, relates to credit applications and granting decisions; these are processes that directly impact customer accessibility and acquisition. Here, we develop and propose a Customer Credit Solution Application-Service Blueprint (CCSA) based on our earlier analyses.
Not only was the proposed design developed but the future research direction was also extracted from the articles included in this study. We also validated the framework through direct consultation with banking industry professionals. The CCSA model allows marketers, researchers, and banking professionals to gain a deeper understanding of the customer journey, understand the role of AI, provide an overview of future research directions, and highlight the potential for future growth in this field. As seen in Fig. 5 , we divided the service blueprint into four distinct segments: customer journey, front-stage, back-stage, and support processes. The customer journey is the first step in building a customer-centric blueprint, wherein we highlight the steps taken by customers to apply for a credit solution. The front-stage refers to how the customer interacts with a banking touchpoint (e.g., chatbots). Back-stage actions provide support to customer-facing front-stage actions. Support processes aid in internal organizational interactions and back-stage actions. This section lays out the steps for applying for credit solutions online and showcases the integration and use of AI in the process, with examples from the literature.
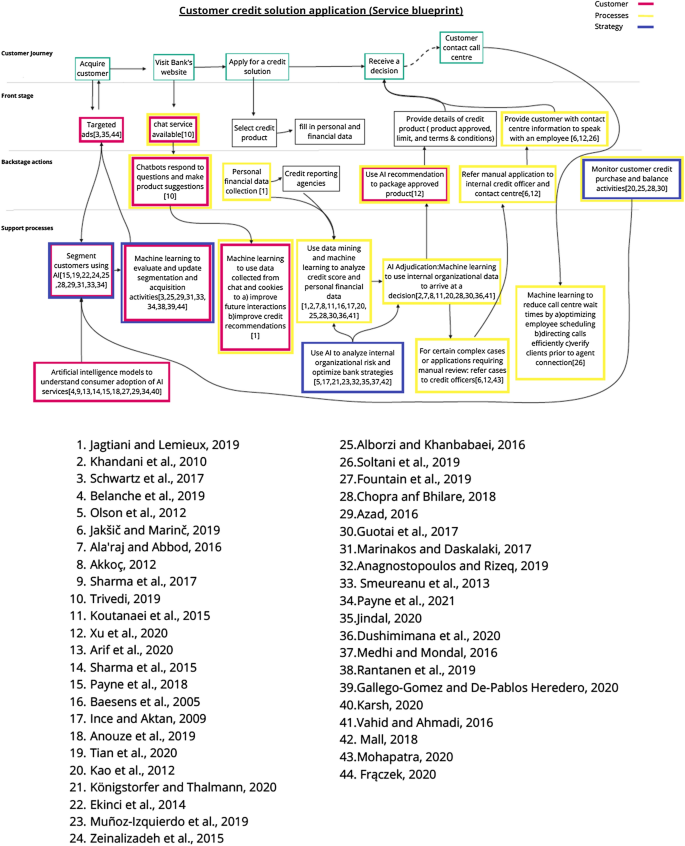
Customer credit solution application journey
Acquire customer
We begin from the initial step of customer acquisition, and proceed to credit decision, and post-decision (Broby, 2021 ). In the acquisition step, customers are targeted with the goal of landing them on the website and converting them to active customers. The front-stage includes targeted ads , where customers are exposed to ads that are tailored for them. For instance, Schwartz et al. ( 2017 ) utilized a multi-armed bandit approach for a large retail bank to improve customer acquisition, and proposed a method that allows bank marketers to maintain the balance between learning from advertisement data and optimizing advertisement investment. At this stage, the support processes focus on integrating AI as a methodological tool to better understand customers' banking adoption behaviors, in combination with utilizing machine learning to evaluate and update segmentation activities. The building block at this stage, is understanding the factors of online adoption. Sharma et al. ( 2017 ) used the neural network approach to investigate the factors influencing mobile banking adoption. Payne et al. ( 2018 ) examined digital natives' comfort and attitudes toward AI-enabled mobile banking activities. Markinos and Daskalaki ( 2017 ) used machine learning to classify bank customers based on their behavior toward advertisements.
Visit bank’s website & apply for a credit solution
At this stage, banking institutions aim to convert website traffic to credit solution applicants. The integration of robo-advisors will help customers select a credit solution that they can best qualify for, and which meets their banking needs. The availability of a robo-advisor can enhance the service offering, as it can help customers with the appropriate solution after gathering basic personal financial data and validating it instantly with credit reporting agencies. Trivedi ( 2019 ) found that information, system, and service quality are key to ensuring a seamless customer experience with the chatbot, with personalization moderating the constructs. Robo-advisors have task-oriented features (e.g., checking bank accounts) coupled with problem-solving features (e.g., processing credit applications). Following this, the data collected will be consistently examined through the use of machine learning to improve the offering and enhance customer experience. Jagtiani and Lemieux ( 2019 ) used machine learning to optimize data collected through different channels, which helps arrive at appropriate and inclusive credit recommendations. It is important to note that while the proposed process provides immense value to customers and banking institutions, many customers are hesitant to share their information; thus, trust in the banking institution is key to enhancing customer experience.
Receive a decision
After the data have been collected through the online channel, data mining and machine learning will aid in the analysis and provide optimal credit decisions. At this stage, the customer receives a credit decision through the robo-advisor. The traditional approaches for credit decisions usually take up to two weeks, as the application goes to the advisory network, then to the underwriting stage, and finally back to the customer. However, with the integration of AI, the customer can save time and be better informed by receiving an instant credit decision, allowing an increased sense of empowerment and control. The process of arriving at such decisions should provide a balance between managing organizational risk, maximizing profit, and increasing financial inclusion. For instance, Khandani et al. ( 2010 ) utilized machine learning techniques to build a model predicting customers' credit risk. Koutanaei et al. ( 2015 ) proposed a data mining model to provide more confidence in credit scoring systems. From an organizational risk standpoint, Mall ( 2018 ) used a neural network approach to examine the behavior of defaulting customers, so as to minimize credit risk, and increase profitability for credit-providing institutions.
Customer contact call center
At this stage, we outline the relationship between humans and AI. As Xu et al. ( 2020 ) found that customers prefer humans for high-complexity tasks, the integration of human employees for cases that require manual review is vital, as AI can make errors or misevaluate one of the C's of credit (Baiden, 2011 ). While AI provides a wealth of benefits for customers and organizations, we refer to Jakšič and Marinč's ( 2019 ) discussion that relationship banking still plays a key role in providing a competitive advantage for financial institutions. The integration of AI at this stage can be achieved by optimizing banking channels. For instance, banking institutions can optimize appointment scheduling time and reduce service time through the use of machine learning, as proposed by Soltani et al. ( 2019 ).
General discussion
Researchers have recognized the viable use of AI to provide enhanced customer service. As discussed in the CCSA service advice, facilities, such as robo-advisors, can aid in product selection, application for banking solutions, and time-saving in low-complexity tasks. As AI has been shown to be an effective tool for automating banking processes, improving customer satisfaction, and increasing profitability, the field has further evolved to examine issues pertaining to strategic insights. Recent research has been focused on investigating the use of AI to drive business strategies. For instance, researchers have examined the use of AI to simplify internal audit reports and evaluate strategic initiatives (Jindal, 2020 ; Muñoz-Izquierdo et al., 2019 ). The latest research also highlights the challenges associated with AI, whether from the perspective of implementation, culture, or organizational resistance (Fountain et al., 2019 ). Moreover, one of the key challenges uncovered in the CCSA is privacy and security concerns of customers in sharing their information. As AI technologies continue to grow in the banking sector, the privacy-personalization paradox has become a key research area that needs to be examined.
In addition, the COVID-19 pandemic has brought on a plethora of challenges in the implementation of AI in the banking sector. Although banks' interest in AI technologies remains high, the reduction in revenue has resulted in a decrease in short-term investment in AI technologies (Anderson et al., 2021 ). Wu and Olson ( 2020 ) highlight the need for banking institutions to continue investing in AI technologies to reduce future risks and enhance the integration between online and offline channels. From a customer perspective, COVID-19 has led to an uptick in the adoption of AI-driven services such as chatbots, E-KYC (Know your client), and robo-advisors (Agarwal et al., 2022 ).
Future research directions
RQ 3: What are the current research deficits and the future directions of research in this field?
Tables 2 , 3 , and 4 provide a complete list of recommendations for future research. These recommendations were developed by reviewing all the future research directions included in the 44 papers. We followed Watkins' ( 2017 ) rigorous and accelerated data reduction (RADaR) technique, which allows for an effective and systematic way to analyze and synthesize calls for future research (Watkins, 2017 ).
Regarding strategy, as AI continues to grow in the banking industry, financial institutions need to examine how internal stakeholders perceive the value of embracing AI, the role of leadership, and multiple other variables that impact the organizational adoption of AI. Therefore, we recommend that future research investigate the different factors (e.g., leadership role) that impact the organizational adoption of AI technologies. In addition, as more organizations use and accept AI, internal challenges emerge (Jöhnk et al., 2021 ). Thus, we recommend examining the different organizational challenges (e.g., organizational culture) associated with AI adoption.
Regarding processes, AI and credit is one of the areas that has been extensively explored since 2005 (Bhatore et al., 2020 ). We recommend expanding beyond the currently proposed models and challenging the underlying assumptions by exploring new aspects of risks presented with the introduction of AI technologies. In addition, we recommend the use of more practical case studies to validate new and existing models. Additionally, the growth of AI has evoked further exploration of how internal processes can be improved (Akerkar, 2019 ). For instance, we suggest investigating AI-driven models with other financial products/solutions (e.g., investments, deposit accounts, etc.).
Regarding customers, the key theories mentioned in the research papers included in the study are the Technology Acceptance Model (TAM) and diffusion of innovation theories (Anouze and Alamro, 2019 ; Azad, 2016 ; Belanche et al., 2019 ; Payne et al., 2018 ; Sharma et al., 2015 , 2017 ). However, as customers continue to become accustomed to AI, it may be imperative to develop theories that go beyond its acceptance and adoption. Thus, we recommend investigating different variables (e.g., social influence and user trends) and methods (e.g., cross-cultural studies) that impact customers' relationship with AI. The gradual shift toward its customer-centric utilization has prompted the exploration of new dimensions of AI that influence customer experience. Going forward, it is important to understand the impact of AI on customers and how it can be used to improve customer experience.
Limitations and implications
This study had several limitations. During our inclusion/exclusion criteria, it is plausible that some AI/banking papers may have been missed because of the specific keywords used to curate our dataset. In addition, articles may have been missed due to the time when the data were collected, such as Manrai and Gupta ( 2022 ), who examined investors' perceptions of robo-advisors. Second, regarding theme identification, there may be a potential bias toward selecting themes, which may lead to misclassification. In addition, we acknowledge that the papers were extracted only from the WoS and Scopus databases, which may limit our access to certain peer-reviewed outlets.
This research provides insights for practitioners and marketers in the North American banking sector. To assist in the implementation of AI-based decision-making, we encourage banking professionals to consider further refining their use of AI in the credit scoring, analysis, and granting processes to minimize risk, reduce costs, and improve customer experience. However, in doing so, we recommend using AI not only to improve internal processes but also as a tool (e.g., chatbots) to improve customer service for low-complexity tasks, thereby directing employees' efforts to other business-impacting activities. Moreover, we recommend using AI as a marketing segmentation tool to target customers for optimal solutions.
This study systematically reviewed the literature (44 papers) on AI and banking from 2005 to 2020. We believe that our findings may benefit industry professionals and decision-makers in formulating strategic decisions regarding the different uses of AI in the banking sector, and optimizing the value derived from AI technologies. We advance the field by providing a more comprehensive outlook specific to the area of AI and banking, reflecting the history and future opportunities for AI in shaping business strategies, improving logistics processes, and enhancing customer value.
Agarwal, P., Swami, S., & Malhotra, S. K. 2022. Artificial intelligence adoption in the post COVID-19 new-normal and role of smart technologies in transforming business: a review. Journal of Science and Technology Policy Management .
Akerkar, R. 2019. Employing AI in business. In artificial intelligence for business , 63–74. Cham: Springer.
Google Scholar
Akkoç, S. 2012. An empirical comparison of conventional techniques, neural networks and the three-stage hybrid adaptive neuro fuzzy inference system (ANFIS) model for credit scoring analysis: the case of Turkish credit card data. European Journal of Operational Research 222 (1): 168–178.
Article Google Scholar
Ala’raj, M., and M.F. Abbod. 2016. Classifiers consensus system approach for credit scoring. Knowledge-Based Systems 104: 89–105.
Alborzi, M., and M. Khanbabaei. 2016. Using data mining and neural networks techniques to propose a new hybrid customer behavior analysis and credit scoring model in banking services based on a developed RFM analysis method. International Journal of Business Information Systems 23 (1): 1–22.
Anagnostopoulos, I., and A. Rizeq. 2019. Confining value from neural networks: a sectoral study prediction of takeover targets in the US technology sector. Managerial Finance 45 (10–11): 1433–1457. https://doi.org/10.1108/MF-12-2017-0523 .
Anderson, J., Bholat, D., Gharbawi, M., & Thew, O. 2021. The impact of COVID-19 on artificial intelligence in banking. Bruegel-Blogs , NA-NA.
Anouze, A.L.M., and A.S. Alamro. 2019. Factors affecting intention to use e-banking in Jordan. International Journal of Bank Marketing 38: 86–112.
Arif, I., W. Aslam, and Y. Hwang. 2020. Barriers in adoption of internet banking: a structural equation modeling-neural network approach. Technology in Society 61: 101231.
Azad, M.A.K. 2016. Predicting mobile banking adoption in Bangladesh: a neural network approach. Transnational Corporations Review 8 (3): 207–214.
Baesens, B., T. Van Gestel, M. Stepanova, D. Van den Poel, and J. Vanthienen. 2005. Neural network survival analysis for personal loan data. Journal of the Operational Research Society 56 (9): 1089–1098.
Baiden, J.E. 2011. The 5 C's of Credit in the Lending Industry. Available at SSRN 1872804.
Bavaresco, R., D. Silveira, E. Reis, J. Barbosa, R. Righi, C. Costa, and C. Moreira. 2020. Conversational agents in business: a systematic literature review and future research directions. Computer Science Review 36: 100239.
Belanche, D., L.V. Casaló, and C. Flavián. 2019. Artificial intelligence in FinTech: understanding robo-advisors adoption among customers. Industrial Management Data Systems 119: 1411–1430.
Bhatore, S., L. Mohan, and Y.R. Reddy. 2020. Machine learning techniques for credit risk evaluation: A systematic literature review. Journal of Banking and Financial Technology 4 (1): 111–138.
Bitner, M.J., A.L. Ostrom, and F.N. Morgan. 2008. Service blueprinting: A practical technique for service innovation. California Management Review 50 (3): 66–94.
Borges, A.F., F.J. Laurindo, M.M. Spínola, R.F. Gonçalves, and C.A. Mattos. 2020. The strategic use of artificial intelligence in the digital era: Systematic literature review and future research directions. International Journal of Information Management 57: 102225.
Boyatzis, R.E. 1998. Transforming qualitative information: Thematic analysis and code development . Thousand Oaks, CA: Sage.
Broby, D. 2021. Financial technology and the future of banking. Financial Innovation 7 (1): 1–19.
Caron, M.S. 2019. The transformative effect of AI on the banking industry. Banking & Finance Law Review 34 (2): 169–214.
Chatha, K.A., and I. Butt. 2015. Themes of study in manufacturing strategy literature. International Journal of Operations & Production Management 35 (4): 604–698.
Chopra, A., and P. Bhilare. 2018. Application of ensemble models in credit scoring models. Business Perspectives and Research 6 (2): 129–141.
Daqar, M.A.A., and S. Arqawi. 2020. Fintech in the eyes of millennials and generation Z (the financial behavior and fintech perception). Banks and Bank Systems 15 (3): 20.
De Oliveira Santini, F., W.J. Ladeira, C.H. Sampaio, and M.G. Perin. 2018. Online banking services: A meta-analytic review and assessment of the impact of antecedents and consequents on satisfaction. Journal of Financial Services Marketing 23 (3): 168–178.
Digalaki, E. 2022. The impact of artificial intelligence in the banking sector & how AI is being used in 2022. https://www.businessinsider.com/ai-in-banking-report?r=US&IR=T
Dobrescu, E.M., and E.M. Dobrescu. 2018. Artificial intelligence (Ai)-the technology that shapes the world. Global Economic Observer 6 (2): 71–81.
Dushimimana, B., Y. Wambui, T. Lubega, and P.E. McSharry. 2020. Use of machine learning techniques to create a credit score model for airtime loans. Journal of Risk and Financial Management 13 (8): 180.
Ekinci, Y., N. Uray, and F. Ülengin. 2014. A customer lifetime value model for the banking industry: A guide to marketing actions. European Journal of Marketing 48 (3–4): 761–784.
Eren, B.A. 2021. Determinants of customer satisfaction in chatbot use: Evidence from a banking application in Turkey. International Journal of Bank Marketing 39 (2): 294–331.
Fountain, T., B. McCarthy, and T. Saleh. 2019. Building the AI-powered organization technology isn’t the biggest challenge, culture is. Harvard Business Review 97 (4): 62.
Frączek, B. 2020. A system to support the transparency of consumer credit offers. Journal of Risk and Financial Management 13 (12): 317.
Gallego-Gomez, C., and C. De-Pablos-Heredero. 2020. Artificial intelligence as an enabling tool for the development of dynamic capabilities in the banking industry. International Journal of Enterprise Information Systems (IJEIS) 16 (3): 20–33.
Goyal, K., and S. Kumar. 2021. Financial literacy: A systematic review and bibliometric analysis. International Journal of Consumer Studies 45 (1): 80–105.
Guotai, C., M.Z. Abedin, and F.E. Moula. 2017. Modeling credit approval data with neural networks: An experimental investigation and optimization. Journal of Business Economics and Management 18 (2): 224–240.
Harzing, A.W., and S. Alakangas. 2016. Google scholar, scopus and the web of science: A longitudinal and cross-disciplinary comparison. Scientometrics 106 (2): 787–804.
Hua, X., Y. Huang, and Y. Zheng. 2019. Current practices, new insights, and emerging trends of financial technologies. Industrial Management & Data Systems 119 (7): 1401–1410.
Ince, H., and B. Aktan. 2009. A comparison of data mining techniques for credit scoring in banking: A managerial perspective. Journal of Business Economics and Management 10 (3): 233–240.
Jagtiani, J., and C. Lemieux. 2019. The roles of alternative data and machine learning in fintech lending: Evidence from the LendingClub consumer platform. Financial Management 48 (4): 1009–1029.
Jakšič, M., and M. Marinč. 2019. Relationship banking and information technology: The role of artificial intelligence and FinTech. Risk Management 21 (1): 1–18.
Jindal, N. 2020. The impact of advertising and R&D on bankruptcy survival: A double-edged sword. Journal of Marketing 84 (5): 22–40.
Jöhnk, J., M. Weißert, and K. Wyrtki. 2021. Ready or not, AI comes—an interview study of organizational AI readiness factors. Business & Information Systems Engineering 63 (1): 5–20.
Kao, L.J., C.C. Chiu, and F.Y. Chiu. 2012. A Bayesian latent variable model with classification and regression tree approach for behavior and credit scoring. Knowledge-Based Systems 36: 245–252.
Khan, K.S., R. Kunz, J. Kleijnen, and G. Antes. 2003. Five steps to conducting a systematic review. Journal of the Royal Society of Medicine 96 (3): 118–121.
Khandani, A.E., A.J. Kim, and A.W. Lo. 2010. Consumer credit-risk models via machine-learning algorithms. Journal of Banking & Finance 34 (11): 2767–2787.
Kim, K.J., and W.B. Lee. 2004. Stock market prediction using artificial neural networks with optimal feature transformation. Neural Computing & Applications 13 (3): 255–260.
Kok, J.N., E.J. Boers, W.A. Kosters, P. Van der Putten, and M. Poel. 2009. Artificial intelligence: Definition, trends, techniques, and cases. Artificial Intelligence 1: 270–299.
Königstorfer, F., and S. Thalmann. 2020. Applications of artificial intelligence in commercial banks–a research agenda for behavioral finance. Journal of Behavioral and Experimental Finance 27: 100352.
Koutanaei, F.N., H. Sajedi, and M. Khanbabaei. 2015. A hybrid data mining model of feature selection algorithms and ensemble learning classifiers for credit scoring. Journal of Retailing and Consumer Services 27: 11–23.
Larson, E.J. 2021. The myth of artificial intelligence . In The Myth of Artificial Intelligence: Harvard University Press.
Book Google Scholar
Leximancer. (2019, November 25). Leximancer User Guide Release 5.0. Leximancer. https://static1.squarespace.com/static/539bebd7e4b045b6dc97e4f7/t/5e58d901137e3077d4409092/1582881372656/LeximancerUserGuide5.pdf .
Loureiro, S.M.C., J. Guerreiro, and I. Tussyadiah. 2020. Artificial intelligence in business: State of the art and future research agenda. Journal of Business Research . https://doi.org/10.1016/j.jbusres.2020.11.001 .
Malali, A.B., and S. Gopalakrishnan. 2020. Application of artificial intelligence and its powered technologies in the indian banking and financial industry: An overview. IOSR Journal of Humanities and Social Science 25 (4): 55–60.
Mall, S. 2018. An empirical study on credit risk management: The case of nonbanking financial companies. Journal of Credit Risk 14 (3): 49–66.
Manrai, R., and K.P. Gupta. 2022. Investor’s perceptions on artificial intelligence (AI) technology adoption in investment services in India. Journal of Financial Services Marketing . https://doi.org/10.1057/s41264-021-00134-9 .
Marinakos, G., and S. Daskalaki. 2017. Imbalanced customer classification for bank direct marketing. Journal of Marketing Analytics 5 (1): 14–30.
McCarthy, J., Minsky, M.L., & Rochester, N. 1956. The Dartmouth summer research project on artificial intelligence. Artificial intelligence: past, present, and future. Available at: http://www.dartmouth.edu/*vox/0607/ 0724/ai50.html
Medhi, P.K., and S. Mondal. 2016. A neural feature extraction model for classification of firms and prediction of outsourcing success: Advantage of using relational sources of information for new suppliers. International Journal of Production Research 54 (20): 6071–6081.
Mehrotra, A. (2019, April). Artificial Intelligence in Financial Services–Need to Blend Automation with Human Touch. In 2019 International Conference on Automation, Computational and Technology Management (ICACTM) (pp. 342–347). IEEE.
Mohapatra, S. 2020. Human and computer interaction in information system design for managing business. Information Systems and e-Business Management . https://doi.org/10.1007/s10257-020-00475-3 .
Moher, D., Liberati, A., Tetzlaff, J., Altman, D.G., & Prisma Group. 2009. Preferred reporting items for systematic reviews and meta-analyses: the PRISMA statement. PLoS medicine , 6(7), e1000097
Mongeon, P., and A. Paul-Hus. 2016. The journal coverage of Web of Science and Scopus: A comparative analysis. Scientometrics 106 (1): 213–228.
Muñoz-Izquierdo, N., M.D.M. Camacho-Miñano, M.J. Segovia-Vargas, and D. Pascual-Ezama. 2019. Is the external audit report useful for bankruptcy prediction? Evidence using artificial intelligence. International Journal of Financial Studies 7 (2): 20.
Nusair, K., I. Butt, and S.R. Nikhashemi. 2019. A bibliometric analysis of social media in hospitality and tourism research. International Journal of Contemporary Hospitality Management 31: 2691–2719.
Olson, D.L., D. Delen, and Y. Meng. 2012. Comparative analysis of data mining methods for bankruptcy prediction. Decision Support Systems 52 (2): 464–473.
Pahlevan-Sharif, S., P. Mura, and S.N. Wijesinghe. 2019. A systematic review of systematic reviews in tourism. Journal of Hospitality and Tourism Management 39: 158–165.
Payne, E.M., J.W. Peltier, and V.A. Barger. 2018. Mobile banking and AI-enabled mobile banking: The differential effects of technological and non-technological factors on digital natives’ perceptions and behavior. Journal of Research in Interactive Marketing 12 (3): 328–346.
Payne, E.H., J. Peltier, and V.A. Barger. 2021. Enhancing the value co-creation process: Artificial intelligence and mobile banking service platforms. Journal of Research in Interactive Marketing 15: 68–85.
Rajaobelina, L., and L. Ricard. 2021. Classifying potential users of live chat services and chatbots. Journal of Financial Services Marketing 26 (2): 81–94.
Ransbotham, S., Kiron, D., Gerbert, P., & Reeves, M. 2017. Reshaping business with artificial intelligence closing the gap between ambition and action. MIT Sloan Management Review , 59(1).
Rantanen, A., J. Salminen, F. Ginter, and B.J. Jansen. 2019. Classifying online corporate reputation with machine learning: A study in the banking domain. Internet Research 30: 45–66.
Schwartz, E.M., E.T. Bradlow, and P.S. Fader. 2017. Customer acquisition via display advertising using multi-armed bandit experiments. Marketing Science 36 (4): 500–522.
Sharma, S.K., S.M. Govindaluri, and S.M. Al Balushi. 2015. Predicting determinants of Internet banking adoption. Management Research Review 38 (7): 750–766.
Sharma, S.K., Govindaluri, S.M., Al-Muharrami, S., & Tarhini, A. 2017. A multi-analytical model for mobile banking adoption: a developing country perspective. Review of International Business and Strategy .
Shostack, G.L. 1982. How to design a service. European Journal of Marketing 16 (1): 49–63.
Smeureanu, I., G. Ruxanda, and L.M. Badea. 2013. Customer segmentation in private banking sector using machine learning techniques. Journal of Business Economics and Management 14 (5): 923–939.
Soltani, M., M. Samorani, and B. Kolfal. 2019. Appointment scheduling with multiple providers and stochastic service times. European Journal of Operational Research 277 (2): 667–683.
Tarafdar, M., C.M. Beath, and J.W. Ross. 2019. Using AI to enhance business operations. MIT Sloan Management Review 60 (4): 37–44.
Tian, Z., R.Y. Zhong, A. Vatankhah Barenji, Y.T. Wang, Z. Li, and Y. Rong. 2020. A blockchain-based evaluation approach for customer delivery satisfaction in sustainable urban logistics. International Journal of Production Research 59: 1–21.
Tranfield, D., D. Denyer, and P. Smart. 2003. Towards a methodology for developing evidence-informed management knowledge by means of systematic review. British Journal of Management 14 (3): 207–222.
Trivedi, J. 2019. Examining the customer experience of using banking Chatbots and its impact on brand love: The moderating role of perceived risk. Journal of Internet Commerce 18 (1): 91–111.
Tseng, C.C. (2003, July). Comparing artificial intelligence systems for stock portfolio selection. In The 9th international conference of computing in economics and finance (pp. 1–7).
Vahid, P.R., and A. Ahmadi. 2016. Modeling corporate customers’ credit risk considering the ensemble approaches in multiclass classification: Evidence from Iranian corporate credits. Journal of Credit Risk 12 (3): 71–95.
Valsamidis, S., Tsourgiannis, L., Pappas, D., & Mosxou, E. 2020. Digital banking in the new Era: Exploring customers' attitudes. In Business performance and financial institutions in Europe (pp. 91–104). Springer, Cham.
Verma, S., R. Sharma, S. Deb, and D. Maitra. 2021. Artificial intelligence in marketing: Systematic review and future research direction. International Journal of Information Management Data Insights 1: 100002.
Watkins, D.C. 2017. Rapid and rigorous qualitative data analysis: The “RADaR” technique for applied research. International Journal of Qualitative Methods 16 (1): 1609406917712131.
Wu, D.D., & Olson, D.L. 2020. The effect of COVID-19 on the banking sector. In Pandemic risk management in operations and finance (pp. 89–99). Springer, Cham.
Xiao, Y., and M. Watson. 2019. Guidance on conducting a systematic literature review. Journal of Planning Education and Research 39 (1): 93–112.
Xu, Y., C.H. Shieh, P. van Esch, and I.L. Ling. 2020. AI customer service: Task complexity, problem-solving ability, and usage intention. Australasian Marketing Journal 28 (4): 189–199.
Yang, A.S. 2009. Exploring adoption difficulties in mobile banking services. Canadian Journal of Administrative Sciences/revue Canadienne Des Sciences De L’administration 26 (2): 136–149.
Zeinalizadeh, N., A.A. Shojaie, and M. Shariatmadari. 2015. Modeling and analysis of bank customer satisfaction using neural networks approach. International Journal of Bank Marketing 33 (6): 717–732.
Download references
Author information
Authors and affiliations.
Ted Rogers School of Retail Management, Toronto Metropolitan University, 350 Victoria St, Toronto, ON, M5B 2K3, Canada
Omar H. Fares, Irfan Butt & Seung Hwan Mark Lee
You can also search for this author in PubMed Google Scholar
Corresponding author
Correspondence to Omar H. Fares .
Ethics declarations
Conflict of interest.
On behalf of all authors, the corresponding author states that there is no conflict of interest.
Additional information
Publisher's note.
Springer Nature remains neutral with regard to jurisdictional claims in published maps and institutional affiliations.
See Tables 2 , 3 and 4 .
Rights and permissions
Springer Nature or its licensor holds exclusive rights to this article under a publishing agreement with the author(s) or other rightsholder(s); author self-archiving of the accepted manuscript version of this article is solely governed by the terms of such publishing agreement and applicable law.
Reprints and permissions
About this article
Fares, O.H., Butt, I. & Lee, S.H.M. Utilization of artificial intelligence in the banking sector: a systematic literature review. J Financ Serv Mark 28 , 835–852 (2023). https://doi.org/10.1057/s41264-022-00176-7
Download citation
Received : 12 January 2022
Revised : 18 July 2022
Accepted : 27 July 2022
Published : 11 August 2022
Issue Date : December 2023
DOI : https://doi.org/10.1057/s41264-022-00176-7
Share this article
Anyone you share the following link with will be able to read this content:
Sorry, a shareable link is not currently available for this article.
Provided by the Springer Nature SharedIt content-sharing initiative
- Artificial intelligence
- Digital innovations
- Retail banking
- Customer journey map
- Systematic literature review
- Find a journal
- Publish with us
- Track your research
- Open access
- Published: 14 December 2021
Research evolution in banking performance: a bibliometric analysis
- S. M. Shamsul Alam 1 ,
- Mohammad Abdul Matin Chowdhury ORCID: orcid.org/0000-0001-6860-2305 1 &
- Dzuljastri Bin Abdul Razak 1
Future Business Journal volume 7 , Article number: 66 ( 2021 ) Cite this article
13k Accesses
8 Citations
Metrics details
Banking performance has been regarded as a crucial factor of economic growth. Banks collect deposits from surplus and provide loans to the investors that contribute to the total economic growth. Recent development in the banking industry is channelling the funds and participating in economic activities directly. Hence, academic researchers are gradually showing their concern on banking performance and its effect on economic growth. Therefore, this study aims to explore the academic researchers on this particular academic research article. By extracting data from the web of Science online database, this study employed the bibliometrix package (biblioshiny) in the ‘R’ and VOSviewer tool to conduct performance and science mapping analyses. A total of 1308 research documents were analysed, and 36 documents were critically reviewed. The findings exhibited a recent growth in academic publications. Three major themes are mainly identified, efficiency measurement, corporate governance effect and impact on economic growth. Besides, the content analysis represents the most common analysis techniques used in the past studies, namely DEA and GMM. The findings of this study will be beneficial to both bank managers and owners to gauge a better understanding of banking performance. Meanwhile, academic researchers and students may find the findings and suggestions to study in the banking area.
Introduction
The financial services formed a significant contributory trademark in the overall economic growth by stimulating employment, offering vast avenues for investment and services to the consumers and the society [ 1 ]. Thus, economic development is led by economic growth whereby required capital is provided by the financial services [ 2 ]. Suggestively, capital creation by the financial services industry through accumulation and mobilisation of resources is considered the most crucial economic growth strategy component [ 3 ]. The banking system associates with creating funds by accumulating funds from surplus and channelling them to the investors as credit; those exhibit excellent ideas to generate a surplus in the economy but lack the capital to implement such ideas [ 4 , 5 ]. Accordingly, the banking system plays a vital role to pledge the leading role of finance in economic development and promoting stable and healthy financial and economic development [ 6 ].
Banking performance has been regarded as a crucial factor of economic growth [ 7 ]. Efficiency and productivity change measures are rapidly used to evaluate banking performance. Academic researchers have been focusing on the efficiency and productivity of banking institutions for a long period, while economic growth is carried out in the discussions. Discovering research activities on banking efficiency and productivity in economic growth enables researchers to identify the local and international input to this particular discipline. More so, it will enable researchers to identify the ‘hot spots’ discussed by academic researchers and find the research gaps [ 8 ]. Indeed, banking performance in standings is a broad scientific topic, and estimating research activities might not be useful. For instance, research activities in this area extended to several constituents such as methodological approaches, banking approaches. In the current study, banking efficiency and productivity are considered as banking performance that contributes to the economic growth of an economy. Therefore, the main objective of this study is to explore the research activities of banking performance to economic growth. The investigation of banking performance research activities will enable the researchers to find the present directions of the research area and thus speculates the future research suggestions. Besides, it will also enable to expound the depth of past research activities and themes on banking performance relating to the economic growth measurements.
The use of the bibliometric method is appropriate to demonstrate the research shape and activity, volume and growth in a specific discipline [ 9 ]. A bibliometric method is a quantitative application of bibliometric data [ 10 ]. It analyses a wide-ranging quantity of published research articles employing the statistical tool to identify trends and citations or/and co-citations of a certain theme by year, author, country, journal, theory, method, and research constituent [ 11 ]. Significantly, this technique further distinguishes key research themes and active researchers, countries and institutions for future research planning and funding [ 12 ]. Scholars apply this method for several reasons: to reveal emerging trends in published research articles and journal performance, cooperation patterns, and research elements, and to reconnoitre the intellectual edifice of an exact domain in the existing literature [ 9 , 13 ].
Minimal studies have used bibliometric analysis related to banks. For instance, Violeta and Gordana have employed bibliometric analysis to spot the trends of DEA application in banking [ 14 ]. Another study conducted by Ikra et al. applied the bibliometric method to Islamic banking efficiency [ 15 ]. By an extensive search on the Scopus, Web of Science and Google Scholar, no such study was found related to bibliometric analysis on banking performance to the economic growth. Nevertheless, this study will be the first attempt to conduct bibliometric methods on the banking performance to the economic growth that could be the basis for future studies.
The findings of this study unfolded several contributions to both policymakers, bank managers and academic researchers. Firstly, the findings would benefit the policymakers regarding the contribution and trends of banking performance. It would allow them to take necessary initiatives to promote and improve banking performance, thus economic development. Meanwhile, bank managers may utilise the findings to strengthen their banking operations by acknowledging key factors that contributed to the performance. Finally, academic researchers are enabled to detect the current trend and topics related to the banking area that leads to further studies.
Bibliometric analysis has achieved enormous popularity in social sciences research in the current years [ 9 , 16 , 17 , 18 ]. The popularity of bibliometric analysis is observed from the development, accessibility and availability of software, for instance, Leximancer, Gephi, VOSviewer, Biblioshiny and publication databases (Web of Science and Scopus). Further, the rapid growth of bibliometric analysis in scientific production has emerged from business research to information science [ 9 ]. The popularity of bibliometric methodology in social science research is not a trend but moderately an image of its usefulness for constructing high research impact by handling excessive scientific data [ 9 ].
The bibliometric analysis is beneficial for briefing the trends in the research documents classifying ‘blind spots’ and ‘hot spots’, and finding a more inclusive understanding of the published research documents [ 19 ]. In detail, this analysis empowers the recognition of the most advanced (hot spots) and the less established topics (blind spots) within the documents that, shared with other bibliometric procedures, recommend future research avenues. The bibliometric analysis uncovers several ascriptions, such as unveiling emerging trends in documents and the performance of journals, research constituents and collaboration patterns and discovering the intellectual edifice of an exact domain in the existing literature [ 13 , 18 ]. The data that apply in this analysis incline to be immense (hundreds, thousands) and unbiased in nature (publications and citations number, keywords occurrences and topics). However, its explanations often depend on both subjective (thematic analysis) and objective (performance analysis) assessments formed through well-versed techniques and procedures [ 9 ]. Therefore, this study applied bibliometric analysis to examine the general perspective on banking performance and economic growth.
Two categories are mainly manifest in the bibliometric techniques, namely, performance and science mapping. Precisely, research elements’ contributions are accounted for in the performance analysis, while the connections between research elements are focused on science mapping [ 9 ]. This study follows performance analysis, science mapping and network analysis suggested by Donthu et al. [ 9 ].
Data extraction process
Two primary databases, the Web of Science and the Scopus, are commonly used in the bibliometric analysis [ 20 ]. Both databases are prominent for the peer-reviewed published research articles. The data for this analysis were a collection of bibliographic data from the Web of Science. The Web of Science (WoS) is a multidisciplinary online database providing access to several citation databases, namely Science Citation Index Expanded (SCIE), Social Sciences Citation Index (SSCI), Emerging Sources Citation Index (ESCI), Arts and Humanities Citation Index (AHCI), Conference Proceedings Citation Index, Index Chemicus and Current Chemical Reactions [ 18 , 21 ].
This study has applied a two-stage data extraction process, following Bretas and Alon, Alon et al. and Apriliyanti and Alon [ 16 , 22 , 23 ] as shown in Fig. 1 . The choice of the keywords is crucial to ensure that it covers the total body of published documents on banking performance and economic growth [ 21 ]. Accordingly, the selection of keywords was carried out by reviewing several abstracts and authors’ keywords in most related literature on the Web of Science. The selected keywords were executed in the WoS online database on 9 August 2021. A combination of keyword search terms was considered; (1) ‘banking performance*’ to nail all discrepancies of the term such as the role of the bank, bank efficiency, bank productivity, banking efficiency, banking productivity, banking performance, bank performance, upon refining the search by including only research articles from the categories; economics, business finance, business, management, operations research management, social sciences mathematical measures and documents written in English.
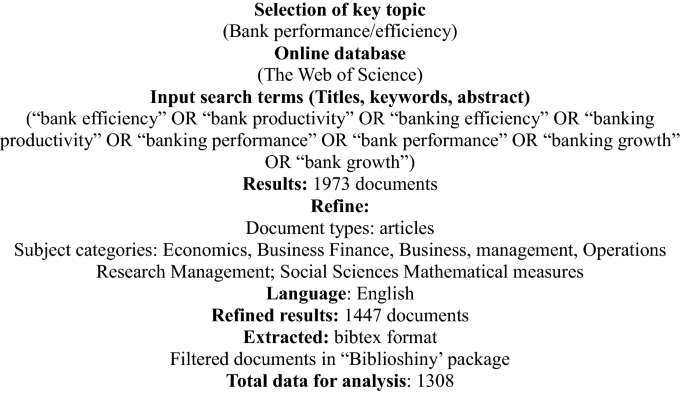
The second stage extracted raw data from the online database combined, checked for duplicate documents and merged using ‘R’. Further, the documents were filtered in the ‘biblioshiny’ tool to omit book chapters and conference proceedings. After the extraction process for the bibliometric analysis, several impactful documents were selected based on local and global citations to conduct content analysis. The content analysis allowed the researcher to identify the leading research scopes and trends. Further, it allows identifying the streams and recommendations for future studies [ 22 ]. A total of 36 documents were selected to conduct a comprehensive review and valuation of the documents.
Performance analysis
Performance analysis investigates the contributions of academic research elements to a particular discipline [ 24 ]. This analysis is naturally descriptive, which is the hallmark of bibliometric analysis [ 9 ]. It is a standard method in reviews to exhibit the performance of various research elements such as authors, countries, institutions and sources similar to the profile or background of respondents generally presented in empirical studies, albeit more statistically [ 9 , 18 ]. Many measures exist in the performance analysis; hence, the most protuberant measurements are publications number and citations per research constituent or year. The publication is considered productivity, whereby citation measures influence an impact [ 9 ]. Besides, citation per document and h -index associate both publications and citations with evaluating research performance [ 18 ].
Table 1 presents the publication’s performance of banking performance. The results show a total number of 1308 documents published from 1972 to the present. Among 2275 contributed authors, a total of 202 authors were solely, and 2106 authors collaborated to the publications. A total of 31,458 citations received by published documents lead to an average of 629.16 citations per year, while 775 in h -index and 1023 in g -index. Hence, the banking efficiency field acknowledged productivity of research published by an average of 26.16 documents per year whereby nearly two authors (CI = 1.9) published one article, and standardised collaboration is 0.43 (between 0 and 1).
The annual production of scientific publications on banking efficiency is presented in Fig. 2 . The first research article related to banking performance was published by Fraser and Rose [ 25 ], who studied the effect of new bank appearance in the market on bank performance. The annual growth of publications on banking performance or banking efficiency is recorded to 12.39%. The publications are significantly increasing in recent periods, especially from 2016 to the present. However, the mandated growth in publications is observed between 2004 and 2015, while earlier periods (1972–2003) were quite sluggish. In these consequences, academic researchers have started to focus on banking performance or banking efficiency in the recent period. As a result, it can be concluded that banking performance and its sphere are shaping upwards through the research contributions.
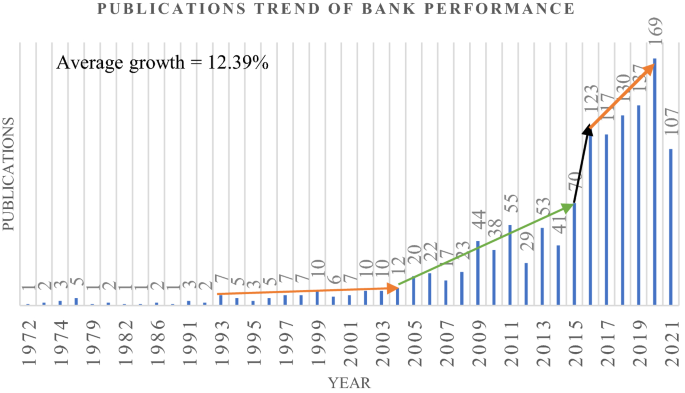
Annual Scientific production
Science mapping
Science mapping investigates the connections between research elements [ 26 ] that relates to the intellectual connections and structural networks within research constituents [ 9 ]. The science mapping includes citation analysis, bibliographic coupling, co-citation analysis, co-occurrence network, collaboration techniques. When combined with network analysis, these techniques are instrumental in exhibiting the research area’s bibliometric edifice and intellectual structure [ 27 ].
Citation analysis
The citation analysis is a fundamental approach for science mapping that runs on the assumption that citations reproduce intellectual contributions and impact the research horizons [ 28 ]. This analysis shows the impact of published documents by measuring the number of citations they received [ 9 ]. Accordingly, it enables the discovery of the most influential and informative documents in a research constituent. Thus, it allows gathering insights into that constituent’s intellectual dynamics [ 9 ]. Table 2 presents the top 20 impactful and influential documents in the field of banking performance or efficiency. The analysis has discovered that a total of 1112 documents (85%) out of 1308 documents received global citations. The global citations refer to the number of citations received in the overall Web of Science citations. However, 196 documents (about 15%) have not received any citation; meanwhile, 130 documents (about 10%) received only one citation. A document written by Berger An received the highest number (665) of citations which was published in 1997. The second most influential document was written by Seiford [51] received a total of 549 citations, followed by the document written by Back (2013) received 512 citations. In fact, a total of four documents written by Berger An rank in the top 20 impactful research articles in the field of banking performance or efficiency.
Factually, the majority of the documents without citations was published in a recent period. At the same time, the highly cited documents were published quite earlier. To detect the immediate influence of more recent documents is to apply the measurement of an average citation per year [ 29 ]. By evaluating the average citations per year, nine out of ten documents are also among the top 10 documents. Perpetually, Beck [45] holds the highest number of average citations per year (56.89), followed by Berger An (2013) ranked second position (51.44) and Beltratti A (2012) ranked the following position (48.40). Based on the citation analysis, it can be elucidated that Berger An is the most influential author in the banking efficiency research constituent.
Co-occurrence analysis
Co-occurrence analysis was projected by Callon et al. [ 30 ], considered as content analysis that is useful in plotting the strength of connotation within keywords in textual data. In other words, co-occurrence analysis is an approach that investigates the actual content of the document itself [ 9 ]. It maps the pertinent literature straight from the associations of keywords shared by research articles [ 24 , 27 , 31 , 32 ]. The co-occurrence analysis deduces words to appear recurrently in a cluster. It exhibits conceptual or semantic groups of various topics or sub-topics considered by research constituents [ 9 , 24 ]. Cobo and Herrera signified that spotted clusters could be applied with few objectives [ 24 ]. For instance, they can be applied to analyse their progression by gauging extension across successive subperiods and measuring the research area through performance analysis. Figure 3 displays the co-occurrence of keywords within the bank efficiency research constituent. As the focus of this research, bank performance represents the larger node associated with corporate governance, financial performance, financial crisis, nonperforming loans and others. In these scenarios, the red-coloured cluster depicts that these subtopics or variables are directly associated related to the bank performance theme due to repetitive co-occurrence of those words. Likewise, the green-coloured cluster represents a theme related to bank efficiency associated with performance and ownership. In the same cluster, the nonparametric data envelopment analysis is extensively used to measure commercial banks' technical and cost efficiency and productivity. Parametric stochastic frontier analysis is narrowly observed in efficiency measurements comparably. The green-coloured cluster depicts the determinants of bank profitability including other impactful variables such as risk, competition, corporate governance. This cluster applied panel data in order to examine performance, financial development as well as economic growth. Each of the cluster identifies the interacted themes used in the published documents using co-occurrence of keywords.
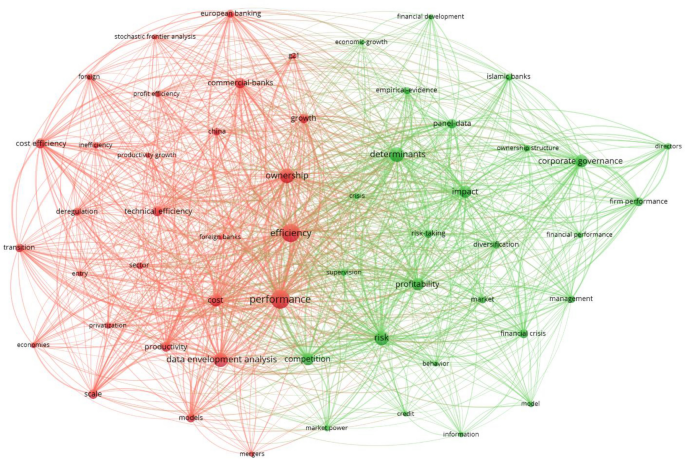
Co-occurrence of keywords, Tool: VOSviewer. Note the nodes represent the keywords, and the edges between words present their occurrence of interactions. Each colour of nodes represents a cluster/theme. The size of the node presents a greater frequency of occurrence
Collaboration networks
Collaboration analysis explores the associations within researchers in a particular constituent. It is a formal way of intellectual association among researchers [ 33 , 34 ]. Therefore, it is crucial to understand how researchers associate among themselves [ 9 ]. In the presence of growing theoretical and methodological complexity in research, intellectual networking (collaboration) has become commonplace [ 33 ]. Indeed, collaboration or interaction among researchers enables improvements in academic research; for instance, greater interactions among diverse researchers allow richer insights and greater clarity [ 35 ]. Researchers who collaborate form a network named ‘invisible collages’ whose research can help improve undertakings in the study field [ 36 ]. Figure 4 presents the collaboration network of authors those co-authored academic articles in banking efficiency. Based on the collaboration network, Wanke P (Universidade Federal do Rio de Janeiro) was the most collaborated author who co-authored with four authors from different institutions in different countries. At the same time, Matousek, R (University Kent), Hasan, I (Rensselaer Polytechnic Institute) and Mamatzakis, E (University of Sussex), have also exhibited as greater collaborative researchers. In these consequences, authors from different institutions and from different parts of the world are collaborating to the banking performance/efficiency field.
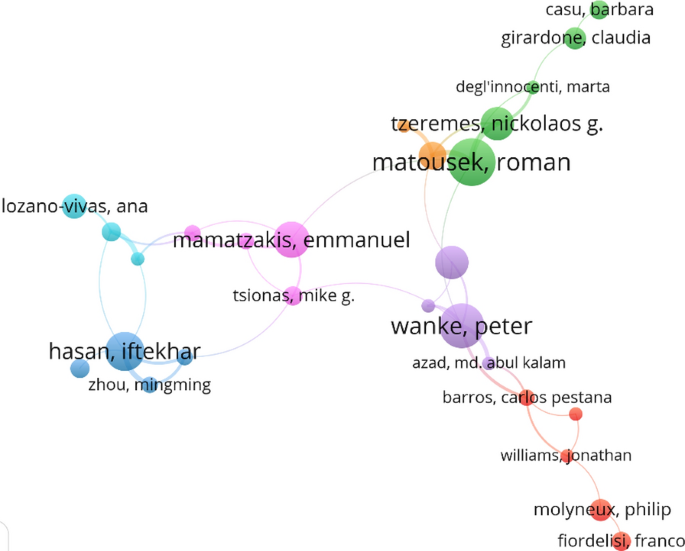
Source : VOSviewer. Note the nodes represent the authors, and the size represents the frequency of contribution, the colour presents a cluster or a particular group, and the link shows the link among authors that collaborated for research articles
Authors’ collaboration networks.
Bibliographic coupling
Co-authorship or collaborative networks within the authors and other crucial facets in the collaboration networks are the collaboration of author-affiliated countries and institutions [ 31 ]. Figure 5 exhibits the collaboration network within authors’ affiliated organisations. University Malaya and University Utara Malaysia, University Malaya and University Putra Malaysia, University Malaya and University Fed Rio de Janeiro all depict a strong collaboration network. In general, all the institutions display an embellishment among the institutions within the same region.
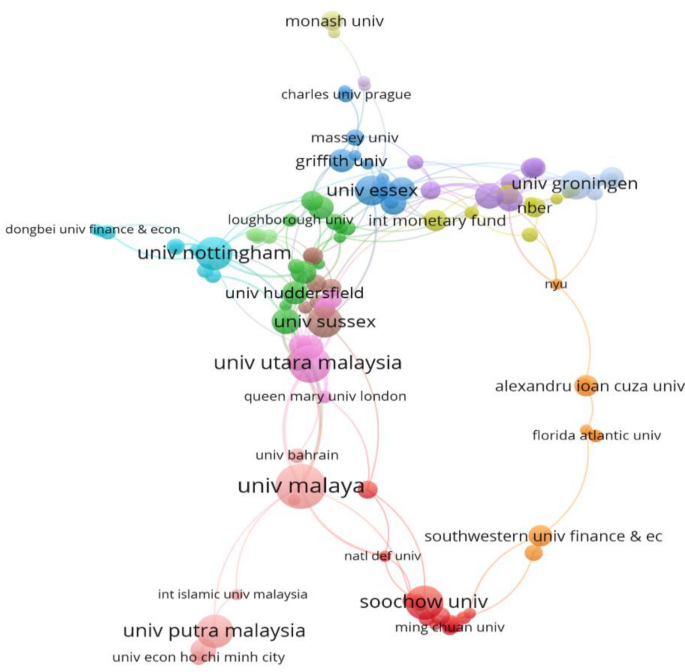
Source : VOSviewer
Bibliographic coupling of author-affiliated institutions.
Similar to co-authors’ affiliated institutions, the collaboration of authors’ country presents a steady association among authors’ connections that allow exploring comparative and concurrent research works. Figure 6 represents the network of collaborative authors’ affiliation countries. These countries include South Africa and the USA, England and the USA, Australia and the USA, Malaysia and the USA, Germany and the USA, representing a high proportion of authors’ affiliated institutions are in the USA with this country performing as a hub of co-authorship publications from 1972 to 2021.
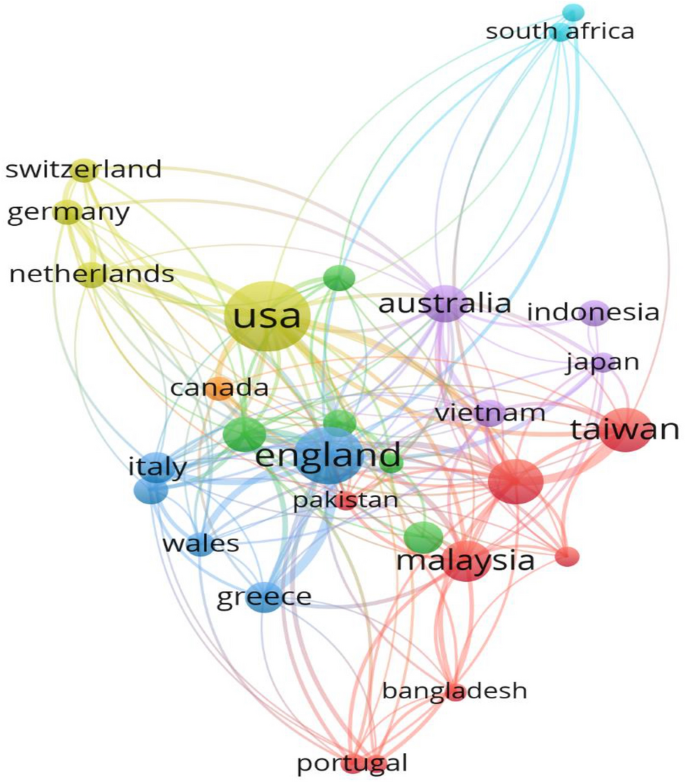
Collaborative authors’ affiliated countries
This study discusses trending themes based on the bibliometric findings and reviews of highly cited and most recent documents (see Appendix 1 ). It also indicated the type of study, theories, methods and main findings to suggest comprehensive future studies.
Research directions
Between 1991 and 2010, studies related to banking performance have posited several antecedents to banking performance. Figure 7 displays the trend topics based on author keywords that appeared between 1972 and 2010. Studies in this period mainly focused on mergers and acquisitions, information technology and transition economies that emerged from universal banking deregulation and bank privatisation. The financial crisis during 2008–2009 drew the attention of scholars to evaluate the banking performance. Idiosyncratically, this phenomenon has been acknowledged by researchers from 2010 to 2015, focusing on the role of corporate governance in the performance of the banking industry, including compensation, risk management, determinants of stock returns, capital buffer, productivity. Idiosyncratically, a vast of studies were conducted on Chinese commercial banks and the effect on their economic growth.
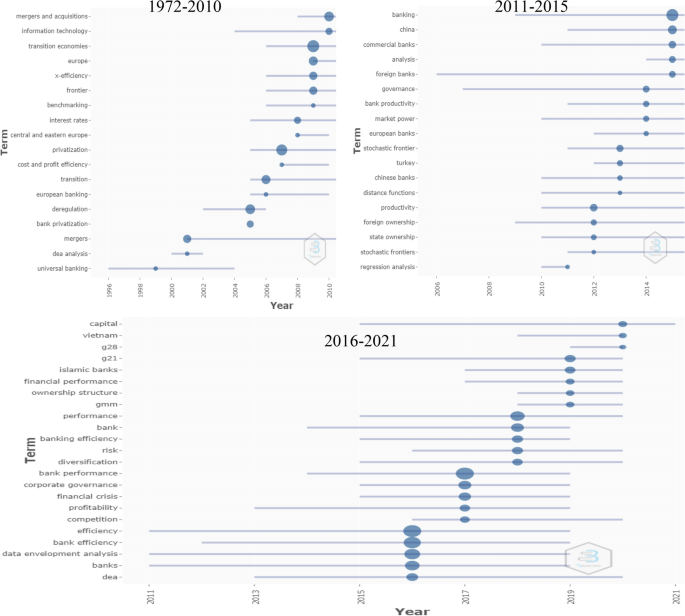
Source : Biblioshiny analysis. Note the frequency of terms selected 3 times for 1972–2010, 5 times for 2011–2015, 10 times for 2016–2021
Trend topics in different periods.
In the recent period (2016–2021), diverse factors posited in the studies that dominantly present a significant interest from banking scholars. While studies earlier mainly focusing on efficiency and its contributing factors, recent periods extended research directions to multiple constituents. For example, how banks diversified their services and the role of human capital efficiency to the banking performance [ 37 ]. Bose et al. employed the effect of green banking on the performance that underpins the inclusion of the environmental sustainability approach by the banking industry [ 38 ]. Meanwhile, Bhattacharyya et al. showed the effect of CSR expenditures and financial inclusion on the performance that define the social sustainability indicator of the banks [ 39 ]. Repeatedly, the role and structure of the board, categorisation of deposits and loans, risk exposures (business cycle), macroeconomic factors were also acknowledged in recent banking performance studies [ 40 , 41 , 42 , 43 ]. Idiosyncratically, scholars recently focus the components of sustainability of the banking industry from economic, environmental and social aspects [ 44 ]. Furthermore, the effect of banking and its stability on economic growth has been broadly carried out in the recent period. Moreover, the development of studies was taken into account, which implies the contribution to the economic growth of particular regions. Based on the earlier and recent studies, it is precisely observed the diversification of research constituents in relation to bank performance studies. Earlier studies (up to 2015) mainly measured banking performance or efficiency based on accounting measurements, while recent studies started to include market measurements principally based on stock returns performance. On the other hand, the rise of Islamic banking and finance influenced academic researchers to compare the business models [ 45 ], banking efficiencies [ 46 ] between conventional and Islamic banks, and efficiency for Islamic banks [ 5 ].
Based on the review of impactful documents published from 1990 to 2010, two particular objectives were identified: the effect of the board of directors or ownership on the bank performance [ 47 , 48 , 49 ] and measurement of efficiency, including cost and profit efficiency [ 50 , 51 , 52 ]. These constituents extended during 2011–2020 by the inclusion of risk-taking management [ 53 ], CEO incentives [ 54 ], contributing factors including capital, banking crises on banking performance [ 42 , 55 , 56 , 57 ]. Meanwhile, the Islamic banking system got crucial attention from academic researchers. Accordingly, several studies evaluated and compared efficiency between Islamic and conventional banks [ 45 , 58 , 59 ]. Nevertheless, the role of the banking industry in economic growth was included in the research constituents in the recent decade. For example, Xu, Santana and a few more scholars investigated the correlation between financial intermediation and economic growth [ 57 , 60 , 61 ]. In recent years, scholars extended the banking-related research constituents to diverse areas. The effect of human capital efficiency [ 37 ], green banking [ 38 ], CSR expenditures [ 39 ] and bank stability was included to measure banking performance. These extensions of research themes within banking performance studies posited a significant interest by academic researchers.
Apparently, almost all documents adopted the quantitative method in measuring banking performance research constituents. However, studies that measured banking efficiency mainly applied nonparametric analysis DEA [ 5 , 51 ], while SFA was adopted by limited studies [ 37 , 42 , 43 ]. On the other hand, regression analysis was predominantly applied to investigate banking performance from 1990 to 2010 [ 49 , 50 ]. In recent studies, academic researchers have vastly adopted GMM (generalised method of moments) to examine the contributing factors on banking performance [ 39 , 42 , 57 , 60 ]. These methods are dominating the banking-related studies throughout the publication periods. Over the periods, scholars have developed DEA applications in several categories, such as bootstrap, networking. Meanwhile, GMM with different approach (dynamic and system) techniques exploited panel data primarily extracted from Bankscope, Datastream, annual reports etc.
Main findings
Earlier, banking inefficiencies were substantially observed low, negatively affecting profitability and marketability [ 50 , 51 ]. This trend was continuously depicted in studies [ 52 ]. However, Berger et al. evidenced better efficiency for larger banks than smaller banks [ 50 ]. On the contrary, Seiford and Zhu posited an adverse effect of bank size on marketability [ 51 ]. More so, Rehman et al. found larger banks are less efficient than smaller banks [ 40 ]. Hence, Moudud-Ul-Huq posited diverse impacts of bank size and competition on performance [ 62 ]. So, banking size is deemed to have a substantial effect on the overall performance of banks. However, Adesina embellished that diversification of services and choices of management decisions on loans (nonperforming, debt issuances) [ 63 , 64 ] and deposits [ 41 ] affect the banking performance [ 37 ]. Moreover, board structure affects banking performance [ 40 , 65 ], while higher human capital efficiency enhances banking performance [ 37 ].
Generally, foreign-owned banks provide better service, greater profitability and are better efficient than local banks. This phenomenon was evidenced in several studies; for example, Bonin et al. and other scholars demonstrated that foreign-owned banks are more cost-efficient than other banks [ 48 ]. However, this trend did not exist for Islamic banks as local banks showed better efficiency than foreign peers [ 58 ] and more efficient than conventional [ 59 ]. Meanwhile, state-owned or government-owned commercial banks were less efficient and provided poorer services [ 48 , 49 , 52 ]. But these banks’ efficiency was higher than urban/rural banks during credit risk shock [ 41 ]. Nevertheless, banking efficiency and performance substantially depend on diversification of services, managerial adequacy, ownership, types and size.
Studies have evidenced financial development and thus the banking industry’s role in economic growth [ 60 ]. In the nineteenth century, the establishment of the savings bank demonstrated city growth in Prussia [ 66 ]. Potentially, banks provide investment capital to increase per capita GDP [ 43 ]. However, Haini documented a contrasting effect of banking development on economic growth through a push out of private investment due to high levels of the banking sector [ 67 ]. However, Stewart and Chowdhury proved that a stable banking sector lessens the negative impact of a crisis on GDP growth and provides economic resilience in both developed and developing countries. Overall, findings elaborated a crucial link between banking sector development and economic growth.
Future study suggestions
This study has recommended several scopes for future studies in the hybrid review, mainly through bibliometric findings and the structured review of impactful articles [ 11 ]. In other words, the recommendations for future studies are made by observing and analysing discussions on highly cited and recent cited documents. Overall findings and analyses raised several questions that need to be addressed for future studies.
Firstly, does the banking sector improve economic growth in the least developed countries? Prior studies mainly focused on developed and developing economies, but less attention was given to least developed countries. Secondly, vast studies investigated contributing factors of banking performance, while political instability has been ignored. Future studies might include political instability on the banking performance. Apart from it, nonperforming loans can be another addition to future studies, and even few studies documented it. Thirdly, how do banks perform during the pandemic crisis, for instance, COVID-19? The current pandemic crisis can be a significant factor in banking performance related to future studies, including efficiency, mortgages, loan recovery, deposits and business services. The studies can include consumer behaviour (due to restricted movements, safety measurements), green banking (online transaction and services), financial technologies (inclusion of nonbanking services) and the contribution or continuance of economic activities in the country during and after the pandemic crisis.
Significantly, prior studies have ignored the current trend of FinTech inclusion in banking performance. Fourthly, will FinTech takeover the banking services and diminish banks in the near future? Future studies may investigate the effect of FinTech applications on banking. More so, future studies may explore the banking industry’s barriers, challenges and threats due to FinTech growth. Fifthly, almost all studies employed quantitative analysis related to banking performance. Therefore, future studies may use qualitative methods to explore the opportunities and practices of banks and their performance. Sixthly, the majority of the studies either applied parametric or econometric techniques to investigate the bank performance. Recent developments in technologies and methods may provide easy and robust results in such related studies as using machine learning for data analysis and predicting banking efficiency and productivity determinants. Seventhly, past studies mostly followed the intermediation approach, which scarcely included production and operating approach measurement. Future studies may extend the efficiency analysis using productivity growth analysis. Further, the majority of the studies observed efficiency only. Future studies can include a productivity change index along with an efficiency analysis. Finally, GMM and regression were broadly applied to investigate the effect of antecedents of banking performance and link to economic growth. Future studies may adopt other advanced data analysis techniques such as partial least squares, structural equations and other econometric techniques.
Conclusions
The main purpose of this study is to explore the trends and research activities in banking performance and the economic growth research domain. To achieve this objective, a bibliometric analysis was applied and performed several analyses, namely citation, co-occurrence of keywords, the collaboration between authors and coupling between institutions and countries, and discussion by reviewing most cited and most recent influential research articles. This study presents the most common themes, sub-themes associated with highly cited documents and authors; furthermore, the content analysis identified the research directions, research objectives, methodologies, topics and findings.
Based on the reviewing literature, the efficiency theory, banking theory mainly intermediation approach and nonparametric technique, namely data envelopment analysis along with econometric method, regression was used in the published documents. The findings of this study, along with future study suggestions, could be beneficial to bankers as well as academic researchers and students studying banking performance and its role in the economy.
Limitations
The most crucial limitation in any bibliometric analysis is the database selection. It means selecting the data and the limits of its interpretation [ 68 ]. This study has three key limitations; firstly, it has chosen ‘Web of Science’, one of the largest online databases to gather data on banking performance research articles from 1972 to 2021 and refined based on subject categories and language (English). The database could be improved if other databases were included and also if book chapters and conference proceedings were added. Secondly, the selection of keywords; although selected keywords are deemed to be most relevant to encompass the majority of articles related to banking performance, there is always an opportunity to search further articles by using additional keywords. Lastly, this study could not conduct co-citation analysis due to the unavailability of cited documents in Web of Science data format.
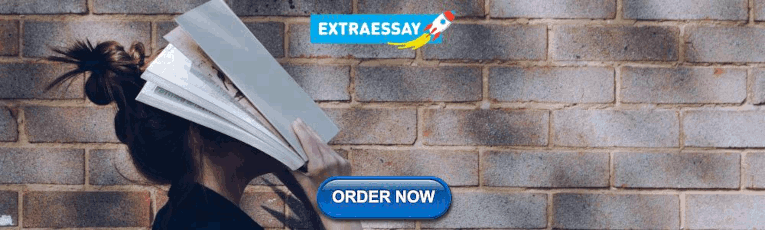
Availability of data and materials
The data collected from the Web of Science online database were saved on Microsoft excel and remained with authors. The data are available upon request.
Abbreviations
Data envelopment analysis
Generalized method of moments
- Web of Science
Collaboration index
Chief executive officer
Corporate social responsibility
Berger AN, Demsetz RS, Strahan PE (1999) The consolidation of the financial services industry: causes, consequences, and implications for the future. J Bank Finance 23(2):135–194. https://doi.org/10.1016/S0378-4266(98)00125-3
Article Google Scholar
Beckett A, Hewer P, Howcroft B (2000) An exposition of consumer behaviour in the financial services industry. Int J Bank Mark 18(1):15–26. https://doi.org/10.1108/02652320010315325
Berger AN (2000) The integration of the Financial Services Industry. North Am Actuar J 4(3):25–45. https://doi.org/10.1080/10920277.2000.10595922
Ndubuisi MN, Chikeziem FO (2014) Banks’ Credit as an instrument of economic growth in Nigeria. Int J Bus Law Res 2(2):25–33
Google Scholar
Chowdhury MAM, Haron R (2021) The efficiency of Islamic Banks in the Southeast Asia (SEA) region. Future Bus J 7(16):1–16. https://doi.org/10.1186/s43093-021-00062-z
Jiang Y, Li M, Xia P (2021) Bank supply chain efficiency analysis based on regional heterogeneity: a data-driven empirical study. Ind Manag Data Syst 121(4):940–963. https://doi.org/10.1108/IMDS-10-2019-0541
Moez D (2020) Does unsystematic risk management affect the relationship between banks’ performance and the objectives of Saudi Arabia Economic Vision 2030? Acad Account Financ Stud J 24(4):1–15
Sweileh WM, Al-Jabi SW, AbuTaha AS, Zyoud SH, Anayah FMA, Sawalha AF (2017) Bibliometric analysis of worldwide scientific literature in mobile - health: 2006–2016. BMC Med Inform Decis Mak 17(1):1–12. https://doi.org/10.1186/s12911-017-0476-7
Donthu N, Kumar S, Mukherjee D, Pandey N, Lim WM (2021) How to conduct a bibliometric analysis: an overview and guidelines. J Bus Res 133(May):285–296. https://doi.org/10.1016/j.jbusres.2021.04.070
Pritchard A (1969) Statistical bibliography or bibliometrics? J Doc 25(4):348–349
Paul J, Criado AR (2020) The art of writing literature review: what do we know and what do we need to know? Int Bus Rev 29(4):101717. https://doi.org/10.1016/j.ibusrev.2020.101717
Sweileh WM (2020) Bibliometric analysis of peer-reviewed literature on climate change and human health with an emphasis on infectious diseases. Glob Health 16(1):1–17. https://doi.org/10.1186/s12992-020-00576-1
Verma S, Gustafsson A (2020) Investigating the emerging COVID-19 research trends in the field of business and management: a bibliometric analysis approach. J Bus Res 118(January):253–261. https://doi.org/10.1016/j.jbusres.2020.06.057
Cvetkoska V, Savic G (2021) DEA in banking: analysis and visualization of bibliometric data. Data Envel Anal J 5(2):455–485. https://doi.org/10.1561/103.00000044
Ikra SS, Rahman MA, Wanke P, Azad MAK (2021) Islamic banking efficiency literature (2000–2020): a bibliometric analysis and research front mapping. Int J Islam Middle Eastern Finance Manag. https://doi.org/10.1108/imefm-05-2020-0226 ( ahead-of-p(ahead-of-print) )
Bretas VPG, Alon I (2021) Franchising research on emerging markets: bibliometric and content analyses. J Bus Res 133(May):51–65. https://doi.org/10.1016/j.jbusres.2021.04.067
Nobanee H (2021) A bibliometric review of big data in finance. Big Data 9(2):73–78. https://doi.org/10.1089/big.2021.29044.edi
Durieux V, Gevenois PA (2010) Bibliometric indicators: quality measurements of scientific publication. Radiology 255(2):342–351. https://doi.org/10.1148/radiol.09090626
Gaur A, Kumar M (2018) A systematic approach to conducting review studies: an assessment of content analysis in 25 years of IB research. J World Bus 53(2):280–289. https://doi.org/10.1016/j.jwb.2017.11.003
Aria M, Cuccurullo C (2017) bibliometrix: an R-tool for comprehensive science mapping analysis. J Informet 11(4):959–975. https://doi.org/10.1016/j.joi.2017.08.007
Bahoo S, Alon I, Paltrinieri A (2020) Sovereign wealth funds: past, present and future. Int Rev Financ Anal 67(October):101418. https://doi.org/10.1016/j.irfa.2019.101418
Alon I, Anderson J, Munim ZH, Ho A (2018) A review of the internationalization of Chinese enterprises. Asia Pac J Manag 35(3):573–605. https://doi.org/10.1007/s10490-018-9597-5
Apriliyanti ID, Alon I (2017) Bibliometric analysis of absorptive capacity. Int Bus Rev 26(5):896–907. https://doi.org/10.1016/j.ibusrev.2017.02.007
Cobo MJ, Herrera F (2011) An approach for detecting, quantifying, and visualizing the evolution of a research field: a practical application to the Fuzzy Sets Theory field. J Informet 5(1):146–166. https://doi.org/10.1016/j.joi.2010.10.002
Fraser DR, Rose PS (1972) Bank entry and bank performance. J Financ 27(1):65–78. https://doi.org/10.2307/2978504
Baker HK, Kumar S, Pandey N (2021) Forty years of the journal of futures markets: a bibliometric overview. J Futur Mark 41(7):1027–1054. https://doi.org/10.1002/fut.22211
Baker HK, Kumar S, Pandey N (2020) A bibliometric analysis of managerial finance: a retrospective. Manag Finance 46(11):1495–1517. https://doi.org/10.1108/MF-06-2019-0277
Appio FP, Cesaroni F, Di Minin A (2014) Visualizing the structure and bridges of the intellectual property management and strategy literature: a document co-citation analysis. Scientometrics 101(1):623–661. https://doi.org/10.1007/s11192-014-1329-0
Fahimnia B, Sarkis J, Davarzani H (2015) Green supply chain management: a review and bibliometric analysis. Int J Prod Econ 162:101–114. https://doi.org/10.1016/j.ijpe.2015.01.003
Callon M, Courtial J-P, Turner WA, Bauin S (1983) From translations to problematic networks: an introduction to co-word analysis. Soc Sci Inf 22(2):191–235. https://doi.org/10.1177/053901883022002003
Donthu N, Kumar S, Pattnaik D (2020) Forty-five years of Journal of Business Research: a bibliometric analysis. J Bus Res 109(October 2019):1–14. https://doi.org/10.1016/j.jbusres.2019.10.039
Emich KJ, Kumar S, Lu L, Norder K, Pandey N (2020) Mapping 50 years of small group research through small group research. Small Group Res 51(6):659–699. https://doi.org/10.1177/1046496420934541
Acedo FJ, Barroso C, Casanueva C, Galán JL (2006) Co-authorship in management and organizational studies: an empirical and network analysis. J Manag Stud 43(5):957–983. https://doi.org/10.1111/j.1467-6486.2006.00625.x
Cisneros L, Ibanescu M, Keen C, Lobato-Calleros O, Niebla-Zatarain J (2018) Bibliometric study of family business succession between 1939 and 2017: mapping and analyzing authors’ networks. Scientometrics. https://doi.org/10.1007/s11192-018-2889-1
Tahamtan I, SafipourAfshar A, Ahamdzadeh K (2016) Factors affecting number of citations: a comprehensive review of the literature. Scientometrics 107(3):1195–1225. https://doi.org/10.1007/s11192-016-1889-2
Crane D (1972) Invisible colleges: diffusion of knowledge in scientific communities. University of Chicago Press
Adesina KS (2021) How diversification affects bank performance: the role of human capital. Econ Model 94:303–319. https://doi.org/10.1016/j.econmod.2020.10.016
Bose S, Khan HZ, Monem RM (2021) Does green banking performance pay off? Evidence from a unique regulatory setting in Bangladesh. Corp Gov Int Rev 29(2):162–187. https://doi.org/10.1111/corg.12349
Bhattacharyya A, Wright S, Rahman ML (2021) Is better banking performance associated with financial inclusion and mandated CSR expenditure in a developing country? Account Finance 61(1):125–161. https://doi.org/10.1111/acfi.12560
Rehman RU, Zhang J, Naseem MA, Ahmed MI, Ali R (2021) Board independence and Chinese banking efficiency: a moderating role of ownership restructuring. Eurasian Bus Rev 11(3):517–536. https://doi.org/10.1007/s40821-020-00155-9
Yu MM, Lin CI, Chen KC, Chen LH (2021) Measuring Taiwanese bank performance: a two-system dynamic network data envelopment analysis approach. Omega (United Kingdom) 98:102145. https://doi.org/10.1016/j.omega.2019.102145
Moudud-Ul-Huq S (2019) The impact of business cycle on banks’ capital buffer, risk and efficiency: a dynamic GMM approach from a developing economy. Glob Bus Rev. https://doi.org/10.1177/0972150918817382
Chen X, Lu CC (2021) The impact of the macroeconomic factors in the bank efficiency: evidence from the Chinese city banks. North Am J Econ Finance 55:101294. https://doi.org/10.1016/j.najef.2020.101294
Buallay A, Fadel SM, Alajmi J, Saudagaran S (2020) Sustainability reporting and bank performance after financial crisis: evidence from developed and developing countries. Compet Rev 31(4):747–770. https://doi.org/10.1108/CR-04-2019-0040
Beck T, Demirgüç-kunt A, Merrouche O (2013) Islamic vs. conventional banking: business model, efficiency and stability. J Bank Finance 37(2):433–447. https://doi.org/10.1016/j.jbankfin.2012.09.016
Kamarudin F, Nordin BAA, Muhammad J, Hamid MAA (2014) Cost, revenue and profit efficiency of Islamic and conventional banking sector: empirical evidence from gulf cooperative council countries. Glob Bus Rev 15(1):1–24. https://doi.org/10.1177/0972150913515579
de Andres P, Vallelado E (2008) Corporate governance in banking: The role of the board of directors. J Bank Finance 32(12):2570–2580. https://doi.org/10.1016/j.jbankfin.2008.05.008
Micco A, Panizza U, Yañez M (2007) Bank ownership and performance. Does politics matter? J Bank Finance 31(1):219–241. https://doi.org/10.1016/j.jbankfin.2006.02.007
Bonin JP, Hasan I, Wachtel P (2005) Bank performance, efficiency and ownership in transition countries. J Bank Finance 29(1 SPEC. ISS.):31–53. https://doi.org/10.1016/j.jbankfin.2004.06.015
Berger AN, Hancock D, Humphrey DB (1993) Bank efficiency derived from the profit function. J Bank Finance 17(2–3):317–347. https://doi.org/10.1016/0378-4266(93)90035-C
Seiford LM, Zhu J (1999) Profitability and marketability of the Top 55 U.S. Commercial Banks. Manag Sci 45(9):1270–1288. https://doi.org/10.1287/mnsc.45.9.1270
Berger AN, Hasan I, Zhou M (2009) Bank ownership and efficiency in China: what will happen in the world’s largest nation? J Bank Finance 33(1):113–130. https://doi.org/10.1016/j.jbankfin.2007.05.016
Aebi V, Sabato G, Schmid M (2012) Risk management, corporate governance, and bank performance in the financial crisis. J Bank Finance 36(12):3213–3226. https://doi.org/10.1016/j.jbankfin.2011.10.020
Fahlenbrach R, Stulz RM (2011) Bank CEO incentives and the credit crisis. J Financ Econ 99(1):11–26. https://doi.org/10.1016/j.jfineco.2010.08.010
Beltratti A, Stulz RM (2012) The credit crisis around the globe: why did some banks perform better? J Financ Econ 105(1):1–17. https://doi.org/10.1016/j.jfineco.2011.12.005
Berger AN, Bouwman CHS (2013) How does capital affect bank performance during financial crisesα. J Financ Econ 109(1):146–176. https://doi.org/10.1016/j.jfineco.2013.02.008
Santana A (2020) The relationship between financial development and economic growth in Latin American countries: the role of banking crises and financial liberalization. Glob Econ J 20(04):1–26. https://doi.org/10.1142/S2194565920500232
Kamarudin F, Sufian F, Loong FW, Anwar NAM (2017) Assessing the domestic and foreign Islamic banks efficiency: insights from selected Southeast Asian countries. Future Bus J 3(1):33–46. https://doi.org/10.1016/j.fbj.2017.01.005
Chowdhury MAM, Haron R, Sulistyowati MIK, Masud Al MA (2020) The efficiency of commercial banks in Indonesia. Int J Econ Policy Emerg Econ 1(1):1–23. https://doi.org/10.1504/IJEPEE.2020.10034525
Xu H (2016) Financial intermediation and economic growth in China: new evidence from panel data. Emerg Mark Financ Trade 52(3):724–732. https://doi.org/10.1080/1540496X.2016.1116278
Zeqiraj V, Hammoudeh S, Iskenderoglu O, Tiwari AK (2020) Banking sector performance and economic growth: evidence from Southeast European countries. Post-Communist Econ 32(2):267–284. https://doi.org/10.1080/14631377.2019.1640988
Moudud-Ul-Huq S (2020) Does bank competition matter for performance and risk-taking? Empirical evidence from BRICS countries. Int J Emerg Mark 16(3):409–447. https://doi.org/10.1108/IJOEM-03-2019-0197
Berger AN, DeYoung R (1997) Problem loans and cost efficiency in commercial banks. J Bank Finance 21:849–870
Ryu D, Yu J (2021) Nonlinear effect of subordinated debt changes on bank performance. Finance Res Lett 38(January 2020):101496. https://doi.org/10.1016/j.frl.2020.101496
Tam OK, Liang HY, Chen SH, Liu B (2021) Do valued independent directors matter to commercial bank performance? Int Rev Econ Finance 71(April 2020):1–20. https://doi.org/10.1016/j.iref.2020.06.005
Lehmann-Hasemeyer S, Wahl F (2021) The German bank–growth nexus revisited: savings banks and economic growth in Prussia. Econ History Rev 74(1):204–222. https://doi.org/10.1111/ehr.13030
Haini H (2021) Examining the nonlinear impact of the banking sector on economic growth: evidence from China’s Provinces. J Chin Econ Bus Stud. https://doi.org/10.1080/14765284.2021.1943193
Xi J, Kraus S, Filser M, Kellermanns FW (2015) Mapping the field of family business research: past trends and future directions. Int Entrep Manag J 11(1):113–132. https://doi.org/10.1007/s11365-013-0286-z
Gaies B, Nabi M (2021) Banking crises and economic growth in developing countries: why privileging foreign direct investment over external debt? Bull Econ Res 73:736–761. https://doi.org/10.1111/boer.12271
Isnurhadi I, Adam M, Sulastri S, Andriana I, Muizzuddin M (2021) Bank capital, efficiency and risk: evidence from Islamic banks. J Asian Finance Econ Bus 8(1):841–850. https://doi.org/10.13106/jafeb.2021.vol8.no1.841
Kchikeche A, Khallouk O (2021) On the nexus between economic growth and bank-based financial development: evidence from Morocco. Middle East Dev J 13:245–264. https://doi.org/10.1080/17938120.2021.1930830
Li R, Li L, Zou P (2021) Credit risk shocks and banking efficiency: a study based on a bootstrap-DEA model with nonperforming loans as bad output. J Econ Stud 48(1):1–19. https://doi.org/10.1108/JES-08-2019-0395
Stewart R, Chowdhury M (2021) Banking sector distress and economic growth resilience: asymmetric effects. J Econ Asymmetries 24:e00218. https://doi.org/10.1016/j.jeca.2021.e00218
Download references
Acknowledgements
Author information, authors and affiliations.
Department of Finance, International Islamic University Malaysia, Jalan Gombak, 53100, Kuala Lumpur, Malaysia
S. M. Shamsul Alam, Mohammad Abdul Matin Chowdhury & Dzuljastri Bin Abdul Razak
You can also search for this author in PubMed Google Scholar
Contributions
MAMC conducted the data analysis. SMSA prepared the manuscript by contributing literature and discussion for this study. DBAR managed the data and edited the manuscript. All authors read and approved the final manuscript.
Corresponding author
Correspondence to Mohammad Abdul Matin Chowdhury .
Ethics declarations
Competing interest.
We have no conflicts of interest to disclose.
Additional information
Publisher's note.
Springer Nature remains neutral with regard to jurisdictional claims in published maps and institutional affiliations.
Appendix 1: Reviewed documents
Rights and permissions.
Open Access This article is licensed under a Creative Commons Attribution 4.0 International License, which permits use, sharing, adaptation, distribution and reproduction in any medium or format, as long as you give appropriate credit to the original author(s) and the source, provide a link to the Creative Commons licence, and indicate if changes were made. The images or other third party material in this article are included in the article's Creative Commons licence, unless indicated otherwise in a credit line to the material. If material is not included in the article's Creative Commons licence and your intended use is not permitted by statutory regulation or exceeds the permitted use, you will need to obtain permission directly from the copyright holder. To view a copy of this licence, visit http://creativecommons.org/licenses/by/4.0/ .
Reprints and permissions
About this article
Cite this article.
Alam, S.M.S., Chowdhury, M.A.M. & Razak, D.B.A. Research evolution in banking performance: a bibliometric analysis. Futur Bus J 7 , 66 (2021). https://doi.org/10.1186/s43093-021-00111-7
Download citation
Received : 24 August 2021
Accepted : 30 November 2021
Published : 14 December 2021
DOI : https://doi.org/10.1186/s43093-021-00111-7
Share this article
Anyone you share the following link with will be able to read this content:
Sorry, a shareable link is not currently available for this article.
Provided by the Springer Nature SharedIt content-sharing initiative
- Banking performance
- Banking efficiency
- Bibliometric analysis
- Biblioshiny
- Content analysis
- My Shodhganga
- Receive email updates
- Edit Profile
Shodhganga : a reservoir of Indian theses @ INFLIBNET
- Shodhganga@INFLIBNET
- Nims University Rajasthan
- Department of Management
Items in Shodhganga are licensed under Creative Commons Licence Attribution-NonCommercial-ShareAlike 4.0 International (CC BY-NC-SA 4.0).


- Commercial Lending
- Community Banking
- Compliance and Risk
- Cybersecurity
- Human Resources
- Mutual Funds
- Retail and Marketing
- Tax and Accounting
- Wealth Management
- Magazine Archive
- Newsletter Archive
- Sponsored Archive
- Podcast Archive

Financial Stability Oversight Council raises concerns about nonbank mortgage servicing

“[B]ecause NMCs focus almost exclusively on mortgage-related products and services, shocks to the mortgage market can lead to significant deterioration in NMC income, balance sheets and access to credit simultaneously,” the council concluded. “NMCs rely heavily on financing that can be repriced or canceled by the lender at times when the NMC is under financial stress. In addition to these liquidity and leverage vulnerabilities, NMCs face significant operational risk because mortgage servicing is complex and encompasses third-party and cybersecurity risks.”
The council said it supports efforts by state regulators and federal agencies to act within their authorities to promote safe and sound operations, address liquidity pressures in the event of stress, and ensure the continuity of servicing operations. It also encouraged Congress to promote greater stability in the mortgage market and the economy by addressing the risks outlined in the report.
Related Posts

Court blocks CFPB’s late fee rule from taking effect
ABA's Rob Nichols welcomed the ruling, which he said “will spare banks from having to immediately comply with a rule...

Consumer sentiment fell in May
The University of Michigan Consumer Sentiment Index decreased 12.7% in May compared to the month prior, landing at 67.4.

ABA Data Bank: Stronger corporate profits point to continued growth
With the majority of U.S. companies having reported their first-quarter earnings, earnings per share for S&P 500 companies grew a...

Fed report: Banking system sound, supervisory ratings drop for large banks
The U.S. banking system remains sound and resilient, with strong levels of capital and liquidity, according to the Federal Reserve’s...

FDIC issues regulatory relief guidance for Ohio, Nebraska
The FDIC released guidance with steps intended to provide regulatory relief to financial institutions and facilitate recovery in areas of...

OFAC revises blocked fund reporting rule
OFCA plans to update and amended its reporting and penalties regulations, including creating a specific “compliance release” procedure for banks...
Podcast: Maximizing business, client value from SBA loan programs
Crafting board committees to drive optimal risk governance, benefiting from advisory boards, is it time to kill the paper check, climate risk: threading the needle, unlocking the potential of marketing automation, sponsored content.

Sealing The Deal: Learn About Electronic Notarization in This Comprehensive Webinar Series

Digital Account Opening Best Practices That Will Boost Your Deposit Game

Why It May Be Time for Your Bank to Expand into Dealer Commercial Lending
Legal notice, podcast: is it time to kill the paper check, podcast: how a georgia community bank engaged employees at 3x the national rate, podcast: tackling big goals for financial literacy month, podcast: the ceo view from main street, podcast: the points guy on why credit card rewards matter.
American Bankers Association 1333 New Hampshire Ave NW Washington, DC 20036 1-800-BANKERS (800-226-5377) www.aba.com About ABA Privacy Policy Contact ABA

© 2024 American Bankers Association. All rights reserved.
We've detected unusual activity from your computer network
To continue, please click the box below to let us know you're not a robot.
Why did this happen?
Please make sure your browser supports JavaScript and cookies and that you are not blocking them from loading. For more information you can review our Terms of Service and Cookie Policy .
For inquiries related to this message please contact our support team and provide the reference ID below.
Mongolia’s Economy Recovering, Risks Remain: World Bank Report
ULAANBAATAR, May 10, 2024 — Mongolia’s economy is projected to grow by 4.8% in 2024 as an expanding mining sector, robust private consumption, and fiscal expansion offset a contraction of the agriculture sector caused by harsh climate conditions, according to the World Bank’s latest semi-annual Mongolia Economic Update.
Mongolia’s economy expanded by 7.1% in 2023, mainly driven by coal mining and related transportation services. The country’s medium-term growth outlook remains favorable, with economic growth expected to average 6.4% over 2025-2026, driven by an increase in production at Oyu Tolgoi, the country's largest copper mine, the report said.
The outlook is subject to significant downside risks. Globally, slower-than-expected growth, especially in China could dampen demand for Mongolian exports. An escalation of geopolitical tensions could result in higher prices for imported oil.
A weather phenomenon known as the dzud , characterized by extreme cold and heavy snowfall, has already caused Mongolia’s largest livestock losses since 2010 and is weighing on the agriculture sector. A more severe and prolonged dzud would lead to a greater contraction in agriculture production.
Domestically, fiscal expansion and rising household incomes are expected to elevate inflationary pressures in 2024.
While the recent mining-led recovery resulted in an improved macro-fiscal situation in 2023, structural reforms remain fundamental for achieving macroeconomic resilience and fostering sustainable and inclusive growth.
"The increasing frequency and intensity of natural disasters, as evidenced by the harsh winters over two consecutive years and last year’s floods, call for attention to institutions, policies, and investments to make the country more resilient to the adverse impacts of climate change," said World Bank Country Manager for Mongolia Taehyun Lee.
Download Report: Mongolia Economic Update, May 2024
This site uses cookies to optimize functionality and give you the best possible experience. If you continue to navigate this website beyond this page, cookies will be placed on your browser. To learn more about cookies, click here .
Watch CBS News
The Fed rate decision meeting is today. Here's their rate decision.
By Aimee Picchi
Edited By Alain Sherter
Updated on: May 1, 2024 / 3:45 PM EDT / CBS News
The Federal Reserve on Wednesday afternoon said it is holding its benchmark rate steady after an uptick in inflation, meaning that consumers aren't likely to see any near-term relief from high borrowing costs.
At year start, about 9 in 10 economists had forecast that the Fed would cut its benchmark rate at its May 1 meeting. Yet shifting economic winds and stubbornly high inflation have complicated policy makers' plans. On Wednesday, the Fed said it is keeping the federal funds rate in a range of 5.25% to 5.5%, the same level it has held since the central bank's July 2023 meeting .
The Fed is likely to hold off on cutting rates until later in 2024, with most experts now penciling the first rate reduction for the central bank's September or November meeting, FactSet's data shows. That means consumers are likely to continue grappling with higher costs for all types of loans, from credit cards to mortgages, even as the costs of goods and services remains elevated.
"The Fed has said time and time again that inflation would be really difficult to tame, and they are more than willing to keep rates high until inflation becomes more manageable," Jacob Channel, senior economist at LendingTree, told CBS MoneyWatch. "I understand why people are concerned, and perhaps a little upset, that the Fed isn't champing at the bit to cut rates."
But, he added, if the Fed cut rates prematurely and inflation ticked up even higher, that could make the economic situation worse for many consumers and businesses.
When is the Fed meeting this week?
The Federal Reserve's Open Market Committee announced its decision at 2 p.m. Eastern time. Fed Chair Jerome Powell will speak at a press conference at 2:30 p.m. to outline the central bank's economic outlook and answer questions about its decision.
When will the Fed cut interest rates?
Despite the Fed earlier this year penciling in three rate cuts in 2024 , Wall Street investors now forecast just a single cut.
At the heart of the issue is stubborn inflation , which has ticked upwards this year on higher costs for housing and gasoline, defying the Fed's efforts to tame prices. Consumer prices in March rose 3.5% on an annual basis, up from February's increase of 3.2% and January's bump of 3.1% on a year-over-year basis.
About half of economists are forecasting a cut at the Fed's September 18 meeting, while a majority are penciling in a cut at its November 7 meeting. It's likely those cuts could amount to one-quarter of a percentage point each, rather than a juicier cut of half a percentage point, Channel noted.
"It's not surprising that investor expectations for future rate cuts have drastically decreased," said Stephen J. Rich, CEO of Mutual of America Capital Management, in an email. "At this point, we see the potential of two cuts amounting to a half of a percentage point this year."
Solita Marcelli, Chief Investment Officer Americas at UBS Global Wealth Management, also thinks the Fed will have to keep rates higher for longer. She expects the Fed to pare its key short-term rate twice this year, likely starting in September, according to a research note.
How will the Fed's decision impact your money?
Brace for continued high borrowing costs, Channel said.
"In light of the meeting, we're probably going to have to get used to the average rate on a 30-year mortgage being above 7% again," he said. "Those 7% rates that people dread are probably going to stick around."
Credit card rates, which have reached record highs , aren't going to come down either, he noted.
"Borrowing money will remain relatively expensive for quite some time," Channel added. "We aren't going to wake up come August and rates will be back to zero."
If there's a silver lining to this, it's for savers, who now can find higher-interest savings accounts with yields above 5%, according to Ken Tumin, banking expert at DepositAccounts.com. Other savings vehicles like certificates of deposit can also offer juicy rates.
- Federal Reserve
Aimee Picchi is the associate managing editor for CBS MoneyWatch, where she covers business and personal finance. She previously worked at Bloomberg News and has written for national news outlets including USA Today and Consumer Reports.
More from CBS News
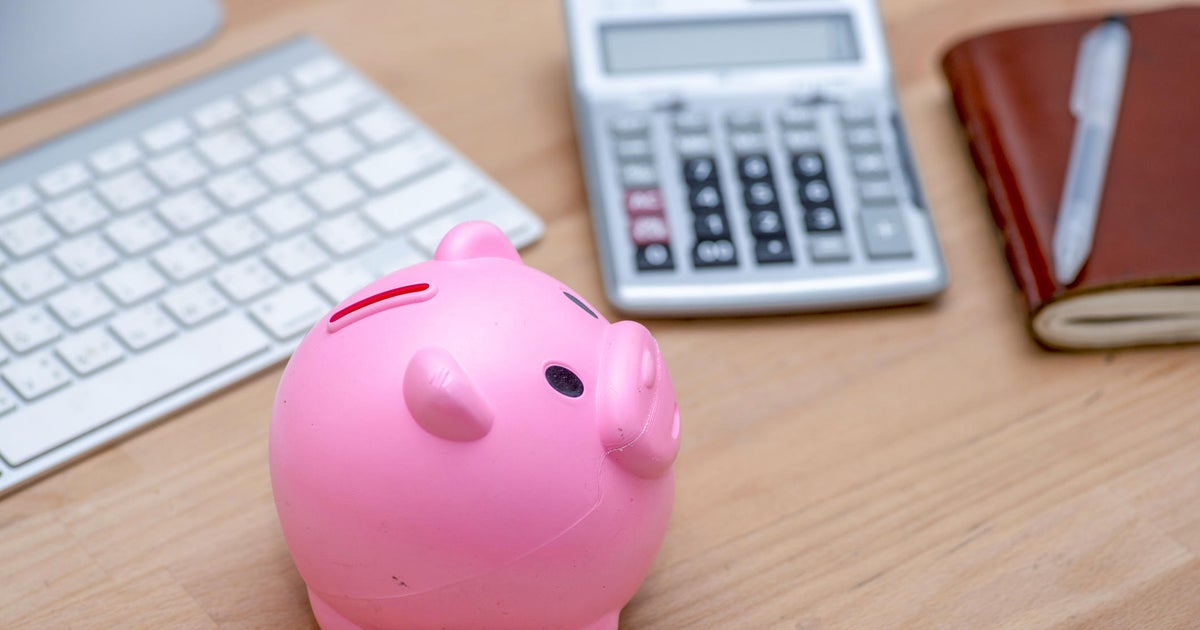
Why you should open a 6-month CD this May
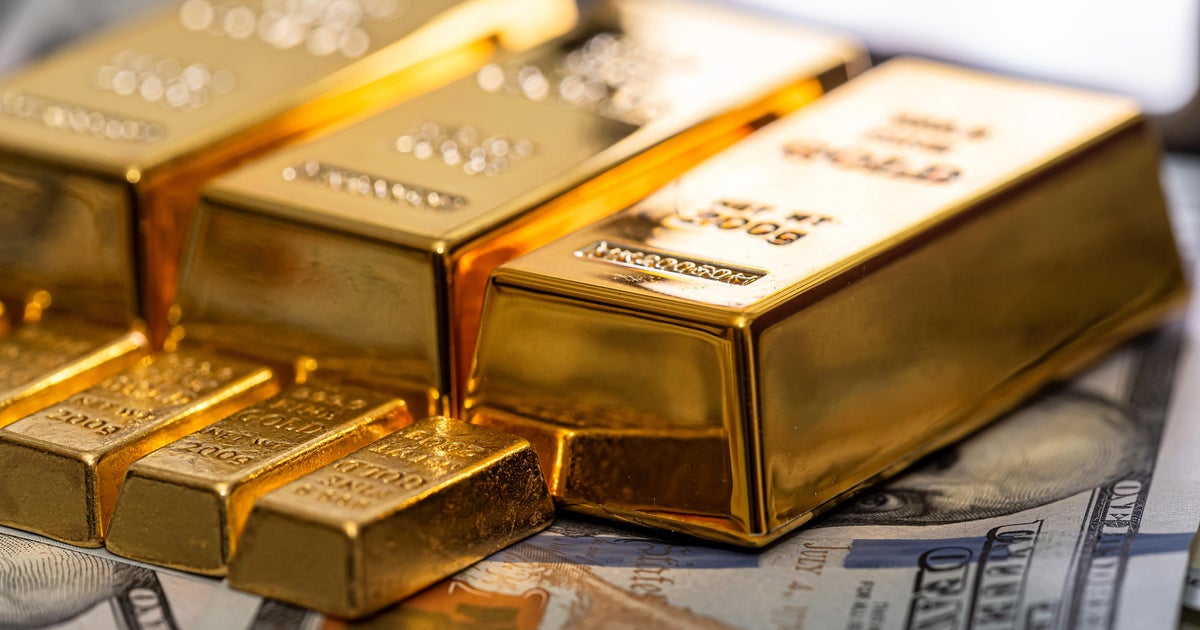
4 signs to invest in gold right now
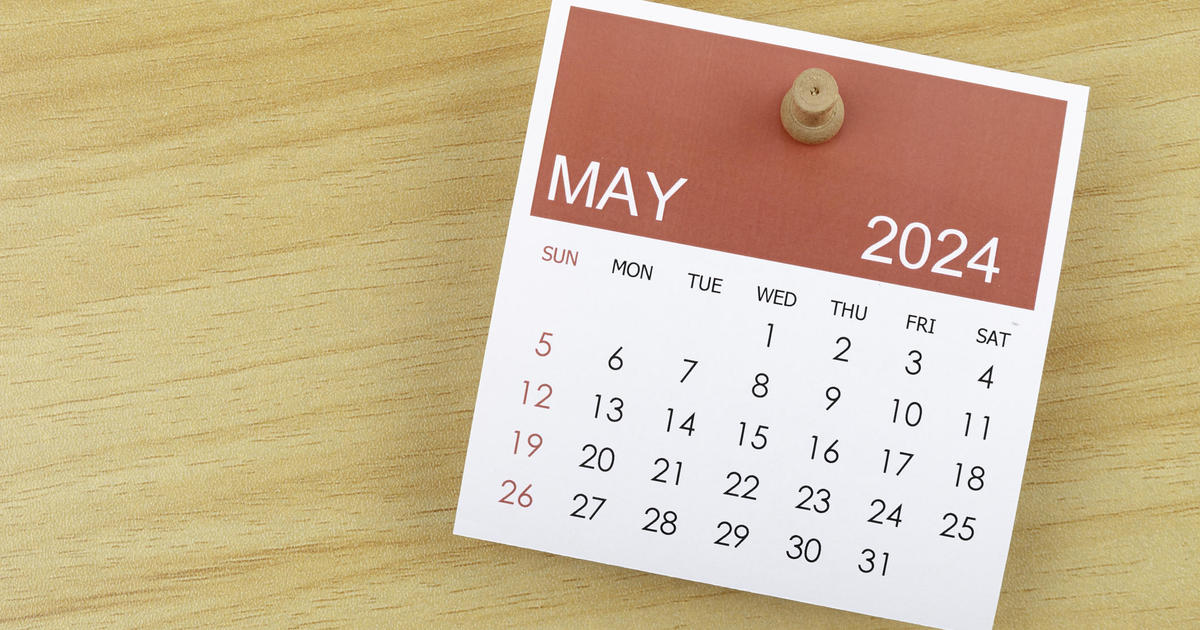
3 reasons to get a home equity loan before the May inflation report
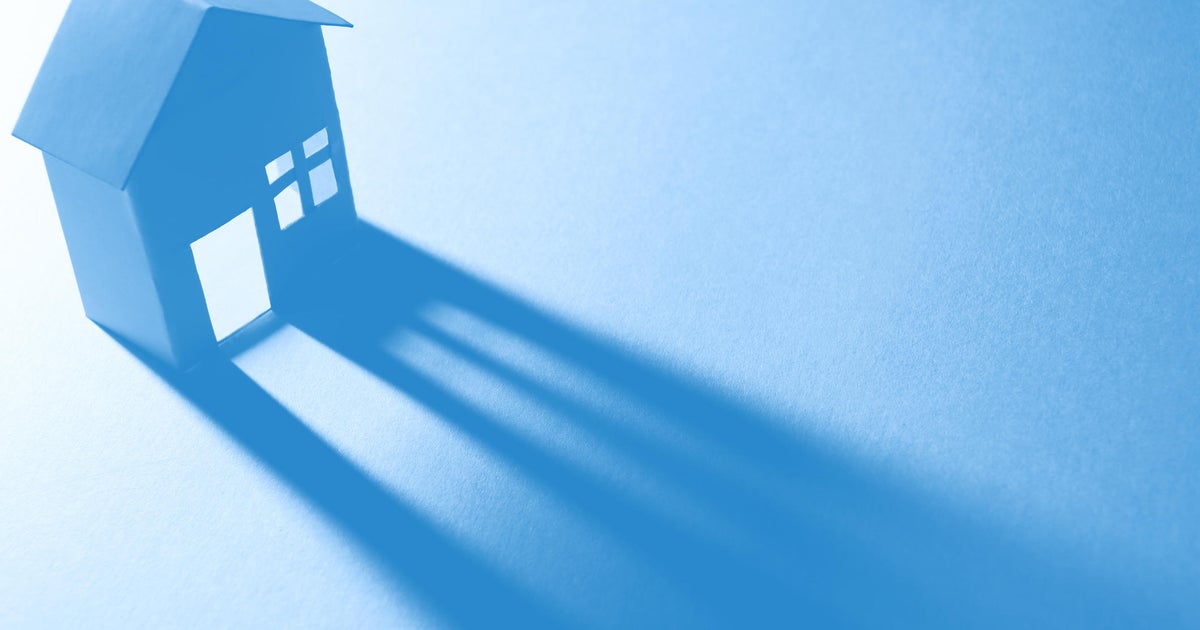
Can you use a HELOC to pay off your mortgage loan?

- Financeflux
- Latest Stories
- Submit Guest Post
- Press Releases
- Sponsored Posts
- Submit Your Content
- CRYPTO MARKETS
- Press Release
- Sponsored Post

JPMorgan Chase Warns Bank’s Assets May Be Seized in Russia After $1,460,000,000 Write-Off: Report

JPMorgan Chase is preparing for the prospect that its assets in Russia will officially be seized, according to a new report.
In a new filing, the bank says lawsuits in Russian and US courts may trigger an incoming seizure of the firm’s assets in the country, reports Reuters.
Two weeks ago, a Russian court sided with state-run lender VTB Bank in its push to recover $439.5 million that JPMorgan Chase allegedly froze in New York due to US sanctions.
The court ordered the seizure of JPMorgan’s accounts in Russia as well as all “movable and immovable property.”
Although it has not placed a dollar value on its assets in Russia, JPMorgan says the size of VTB’s claim exceeds the amount of capital that the US bank has in the country.
In April of 2022, following Russia’s invasion of Ukraine and wave of sanctions from the West, JPMorgan decided to write-off $1.46 billion in assets tied to Russia, along with assets that were “negatively exposed” to inflation.
Per the Associated Press,
“The bank said most of the Russian exposure was in its investment banking division as well as its asset management business…
JPMorgan Chief Financial Officer Jeremy Barnum said most of the Russian write downs were ‘name specific’ Russian companies and assets, as well as some Russian-related derivatives.”

Disclaimer: Opinions expressed at The Daily Hodl are not investment advice. Investors should do their due diligence before making any high-risk investments in Bitcoin, cryptocurrency or digital assets. Please be advised that your transfers and trades are at your own risk, and any losses you may incur are your responsibility. The Daily Hodl does not recommend the buying or selling of any cryptocurrencies or digital assets, nor is The Daily Hodl an investment advisor. Please note that The Daily Hodl participates in affiliate marketing.
Generated Image: Midjourney
Industry Announcements

Covering the future of finance, including macro, bitcoin, ethereum, crypto, and web 3. Categories Bitcoin • Ethereum • Trading • Altcoins • Futuremash • Financeflux • Blockchain • Regulators • Scams • HodlX • Press Releases
ABOUT US | EDITORIAL POLICY | PRIVACY POLICY TERMS AND CONDITIONS | CONTACT | ADVERTISE
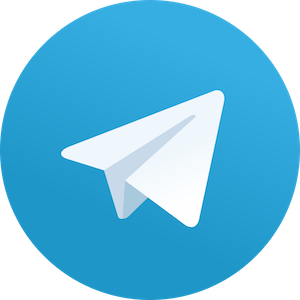
COPYRIGHT © 2017-2024 THE DAILY HODL
© 2023 The Daily Hodl
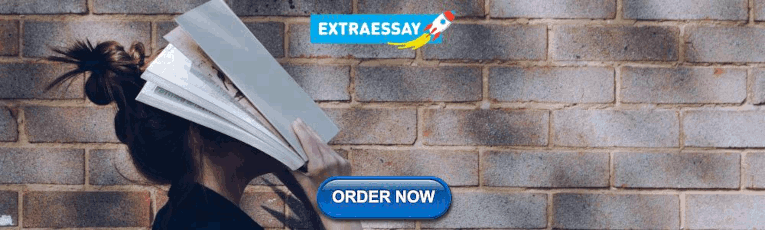
IMAGES
VIDEO
COMMENTS
A thesis submitted in partial fulfillment ... revolutionize all aspects of financial industries especially in the banking sector. New ... and Polsky's Banks and FinTech Report (2016), they found that 81% of bank respondents were currently collaborating with FinTech firms while 86% of those not currently collaborating were planning to within ...
Term and year when the thesis was submitted: Spring 2017 Number of pages: 42 The aim of the thesis is to measure the level of customer satisfaction for PAO "Sberbank". The purpose of the paper is to reveal the customers perception of the bank which would be important for the future growth of the company. Theoretical framework of the ...
focused on the banking development-to-economic growth dynamic. Through PCA, twenty-one banking variables measuring access, depth, efficiency, and stability are transformed into components to test the strength of the correlation between banking development and economic growth in Western, Central and Eastern Europe during the period (2004 - 2013).
Dissertation Advisors: Professor Jeremy Stein Professor Josh Lerner Author: Andrea Passalacqua Essays in Banking and Corporate Finance Abstract This dissertation studies the role of different types of frictions in preventing optimal
A Systematic Review on Banking Digit al Transformation. Riris Shanti 1, Wahyu Avianto 2, Wahyu Ari Wibowo 3. 1 ,2,3Sekolah Bisnis,Institute Pertanian Bogor. E-mail: [email protected] ...
A fintech is a form of financial technology. that can be wished for as an upcoming market for financial. services in the twenty-first century. The phrase was first. used to describe technology ...
15. Globally, banks entered the COVID -19 crisis better positioned to support the lending needs of the real economy. As documented by the BIS, the capital and liquidity buffers of banks at the onset of the cris is were substantially stronger than compared to the GFC (Borio, 2020; Lewrick et al., 2020).
ginning to intrude on traditional banking activities, it is still a relatively small industry overall. In 2021, the estimated global financial services amounted to $ 23,319.52 billion, while the size of the global Fintech activities that year was roughly $210 billion (figure 1), which indicated that even in the finance industry
Corporate Governance in the Banking and Finance Sector A Dissertation submitted to the School of Legal Studies University of Wolverhampton in fulfilment of the ...
This study provides a holistic and systematic review of the literature on the utilization of artificial intelligence (AI) in the banking sector since 2005. In this study, the authors examined 44 articles through a systematic literature review approach and conducted a thematic and content analysis on them. This review identifies research themes demonstrating the utilization of AI in banking ...
Banking performance has been regarded as a crucial factor of economic growth. Banks collect deposits from surplus and provide loans to the investors that contribute to the total economic growth. Recent development in the banking industry is channelling the funds and participating in economic activities directly. Hence, academic researchers are gradually showing their concern on banking ...
This paper examines the impact of financial sector policy announcements on bank stocks around the world during the onset of the COVID-19 crisis. Overall, we find that liquidity support, borrower assistance programs and monetary easing moderated the adverse impact from the crisis, but their impact varied considerably across banks and countries.
A common trend found in banking sector is that bigger banks are funding a lot of money in digital technologies for present as well as future works, however on the other hand various regional and small-scale banks are facing lots of problems in keeping up with the bigger banks due to their increased dependency on technology sellers. Hence, the ...
the University of Marmara with his thesis on 'fundamental approach to ethics in banking sector' 672 in 2006. He received his doctorate degree from the same university with his thesis ...
Cautious Resilience: The Impact of the Global Financial Crisis on Latin American and Caribbean Microfinance Institutions. Funded by MIF, CAF and Calmeadow, this MicroRate report provides a snapshot of how the impact of the global financial crisis evolved during the last quarter of 2008. The study combines data and….
Stamford University of Bangladesh. A THESIS REPORT ON A COMPARATIVE STUDY OF FINANCIAL PERFORMANCE OF BANKING SECTOR IN BANGLADESH- AN APPLICATION OF CAMELS RATING SYSTEM. A THESIS REPORT ON A COMPARATIVE STUDY OF FINANCIAL PERFORMANCE OF BANKING SECTOR IN BANGLADESH- AN APPLICATION OF CAMELS RATING SYSTEM. ii | P a g e. January 15, 2021. Ellina Mahbuba Shahid, Assistant Professor (Finance ...
actions simply, effectively, safely, and cheaply. The main aim of this thesis was to study the possible impacts of blockchain in banking industry along with its challenges and limitations. The outcome would include a good explanation of blockchain technology, how it works, fu-ture bank implementation and its challenges.
Effects of Covid-19 on the Banking Sector: The Market's Assessment. From the Document: Banks have been harder hit than most sectors since the unsettlingly rapid global spread of Covid-19 [coronavirus disease 2019] sent financial markets into a tailspin This Bulletin examines markets' assessment of banks' performance thus far The focus is on ...
Thesis Report on Banking Sector - Free download as PDF File (.pdf), Text File (.txt) or read online for free. Scribd is the world's largest social reading and publishing site.
The purpose of this thesis is to investigate the e ect of banking development on eco-nomic growth for Ethiopia. Using Autoregressive distributed lag, the relationship between banking development and economic growth is investigated. The banking sector indicator used in this study includes bank credit to private sector (PSC),
The aim of the thesis was to present the study on human resource management practice in banking sector. The key concept of human resource management is to manage each human resource working in the organization. This thesis consists of practice of human resource management from historical time period until now and in coming future.
Sonali Bank Limited is known as one of the Leading state-owned government running bank in the banking sector. They are doing tremendous works in the banking sector and also in the whole economy for progress. Through the internship program in SBL, I go to experience and have acknowledgements about their banking operations and corporate culture.
Federal Deposit Insurance Corporation Chairman Martin J. Gruenberg testifies at a Senate Banking, Housing and Urban Affairs Committee hearing on "Recent Bank Failures and the Federal Regulatory ...
In a new report on the nonbank mortgage servicing industry, the Financial Stability Oversight Council said that a patchwork of state laws and limited federal oversight have failed to address the risks that the sector poses to the U.S. financial system.
Number of banks weighing climate in provisions jumps in year Central bank facing push-back from executives on climate The number of European banks taking account of environmental risks in their reserves for loan losses has more than tripled in a year, signaling an initial win for the regulator in ...
Nonbank mortgage companies, which increasingly dominate the sector, pose unique risks and vulnerabilities that can weaken financial stability, according to a new Financial Stability Oversight ...
reportedly banking costed the banking sector a gross sum of more than R250 000 000. Furthermore, there had been an exponential increase in similar cases from January to August 2018, with an ...
ULAANBAATAR, May 10, 2024 — Mongolia's economy is projected to grow by 4.8% in 2024 as an expanding mining sector, robust private consumption, and fiscal expansion offset a contraction of the agriculture sector caused by harsh climate conditions, according to the World Bank's latest semi-annual Mongolia Economic Update.. Mongolia's economy expanded by 7.1% in 2023, mainly driven by ...
On Wednesday, the Fed said it is keeping the federal funds rate in a range of 5.25% to 5.5%, the same level it has held since the central bank's July 2023 meeting.
In a new filing, the bank says lawsuits in Russian and US courts may trigger an incoming seizure of the firm's assets in the country, reports Reuters. Two weeks ago, a Russian court sided with state-run lender VTB Bank in its push to recover $439.5 million that JPMorgan Chase allegedly froze in New York due to US sanctions.