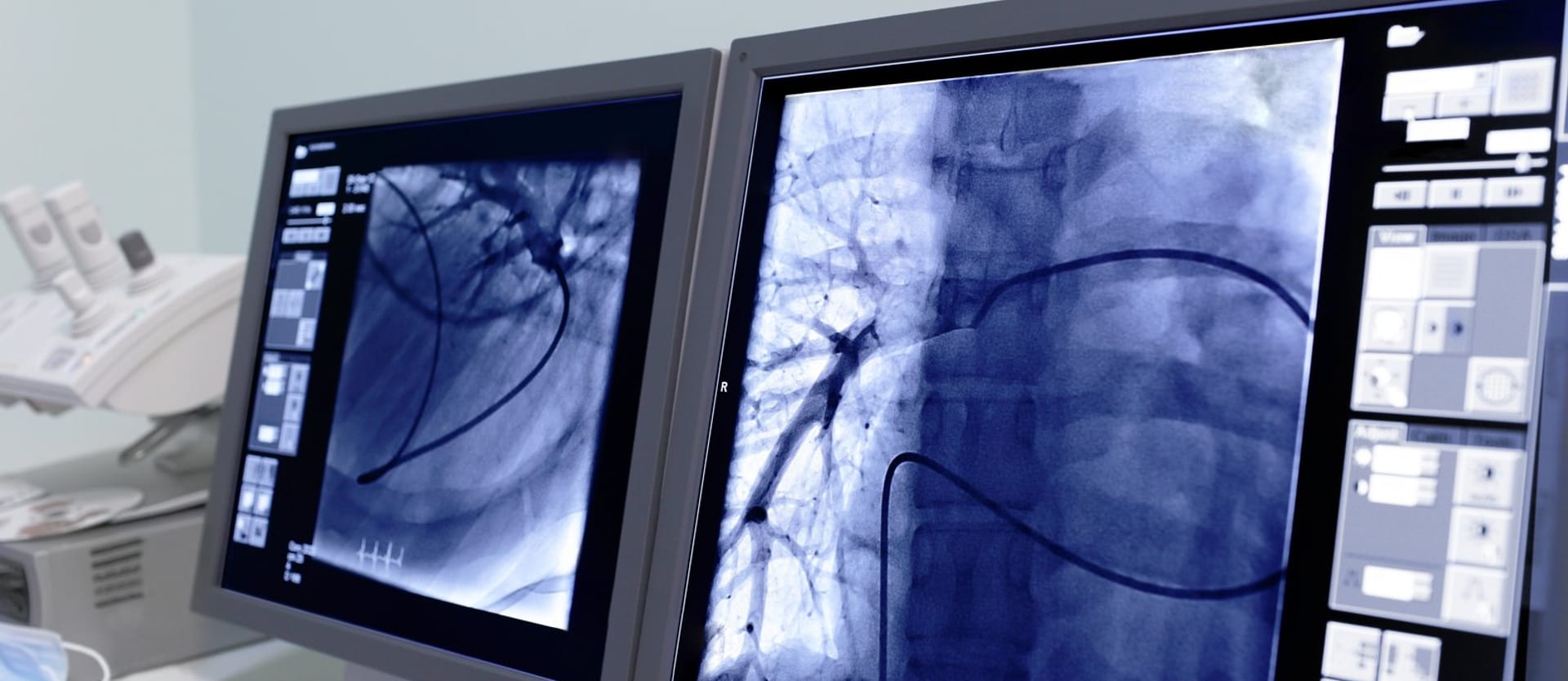
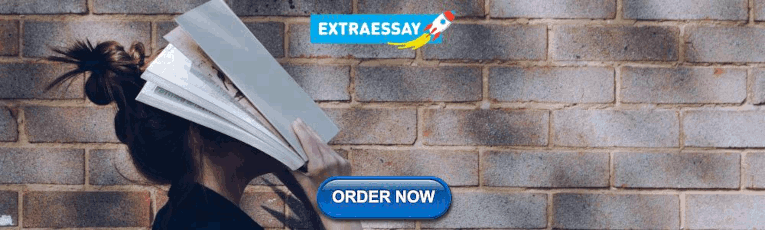
Data Analytics in Healthcare: 7 Real-World Examples and Use Cases
- Data Science , Healthcare
- 31 Aug, 2020
- No comments Share
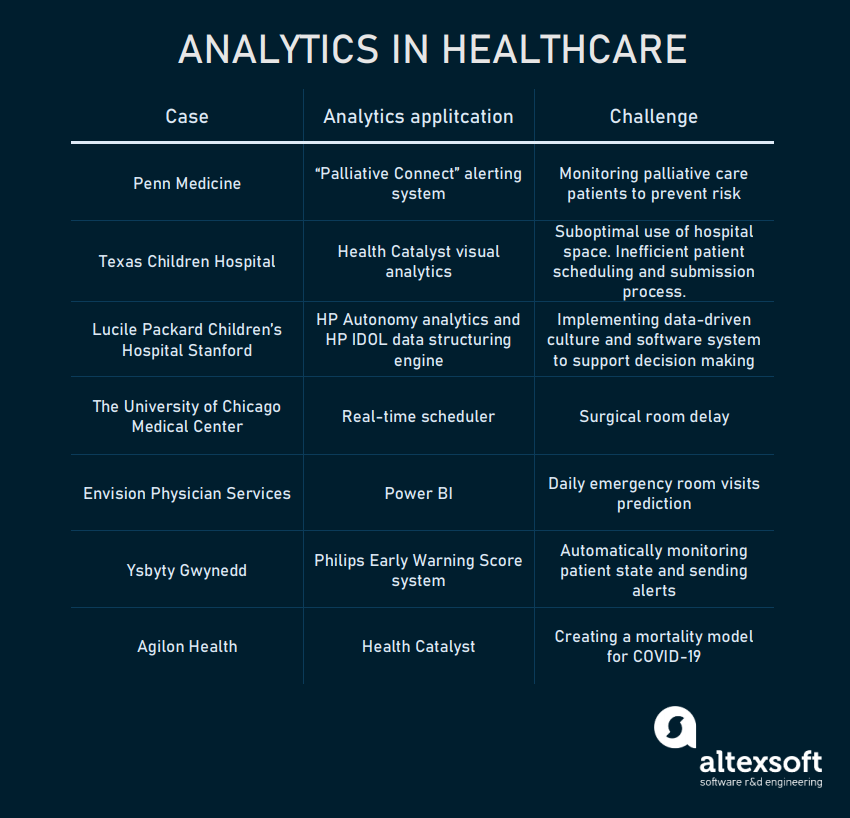
Analytics application cases in healthcare
Predicting palliative care patients risk: Penn Medicine
Optimization of clinical space usage: texas children's hospital.
- An online scheduling tool was leveraged to allow self-scheduling through the web.
- The hospital also established a template for allocating scheduling time in four-hour blocks. Appointments of different duration were allocated to different time blocks. All the unfilled appointments were distributed in a 72-hour time zone to close the gap.
- Weekend appointments and extended hospital hours were added.
- An annual revenue increased by $8.3 million with 53 thousand appointments respectively
- 30 thousand online schedules
- 39 percent patient satisfaction rate growth
Applying machine learning to predict operation duration and disease risk probability: Lucile Packard Children’s Hospital Stanford
- Identify patients at clinical decline risk
- Prevent central line-associated bloodstream infections
- Predict surgical operation duration
Operation room delay reduction: The University of Chicago Medical Center
Daily emergency room visits prediction: envision physician services, monitoring patient state deterioration: ysbyty gwynedd, leveraging data to create covid-19 mortality model: agilon health.
- create a COVID-19 model for approximately 125,000 individuals that were assigned with risk scores.
- increase one partner location’s telehealth appointments from none in the first week to 2,200 in weeks 12 and 13, aligning with social distancing and overall pandemic policies.
What are the other opportunities of data analytics in healthcare?
- Patient Experience
- Care Team Experience
- Better Outcomes
- Reducing Cost
- Healthcare Equity
- 2022 BEYOND the Rankings
- Healthcare analytics
- Johns Hopkins Hospital
- Mayo Clinic
- Predictive analytics
- UnitedHealthcare
10 top case studies: Big data analytics in healthcare
Providers such as The Johns Hopkins Hospital are demonstrating the value of data initiatives in improving patient care while helping to reduce waste and achieve more value from operations.
More for you
Milliman MedInsight
Case studies, healthcare data analytics case studies, sehp: maximizing the power of integrated data with the medinsight data science portal.
Southeastern Health Partners (SEHP) – a clinically integrated network consisting of four healthcare systems, including 13 hospitals and 3,600 providers – sets its continuous improvement goals high. The Greenville, South Carolina-based organization is already recognized by the Centers for Medicare & Medicaid Services for consistently ranking among the most efficient Accountable Care Organizations. Even so, … SEHP: Maximizing the power of integrated data with the MedInsight Data Science Portal
CFIN: Scaling value-based care with a proven data and analytics platform
With a population of over 250,000 lives spanning eight hospitals and nearly two dozen counties, a Northwest-based clinically and financially integrated network (CFIN) wanted to take the next step in its evolution as a pioneering driver of value-based care (VBC). With a business model incorporating partnerships with both commercial and public health plans and a … CFIN: Scaling value-based care with a proven data and analytics platform
Advanced Health: Holistic population health improvement integrating claims and clinical data
Oregon-based Advanced Health is a coordinated care organization (CCO) – the state’s version of an accountable care organization (ACO) – that takes an innovative approach to the care of its 26,000 members. Serving two counties where 14-17% of residents live in poverty, according to U.S. Census data, Advanced Health manages a population with complex medical … Advanced Health: Holistic population health improvement integrating claims and clinical data
Nonprofit health system: Improve patient outcomes by “looking outside the silo”
When one of the South’s largest not-for-profit healthcare systems recently searched for a new healthcare data analytics solution, a key goal was establishing a consistent methodology for identifying and solving problem areas. A previous attempt with another data aggregator and vendor had stalled: a messy implementation process had dragged on for years, and even worse, … Nonprofit health system: Improve patient outcomes by “looking outside the silo”
DVACO: The power of data organization and standardization
The Delaware Valley Accountable Care Organization (DVACO) aims to deliver better healthcare at a lower cost to around 250,000 members in the Greater Philadelphia region. DVACO is currently the region’s largest ACO, with more than 2,000 primary care physicians and 250,000 attributed patients. DVACO participates in the Medicare MSSP program, and six additional risk-based contracts … DVACO: The power of data organization and standardization
Virginia Health: Measuring waste to improve care
The challenge The Choosing Wisely® campaign’s success has renewed interest towards reducing low-value healthcare services, classified as “unnecessary care” in the healthcare industry. The Virginia Center for Health Innovation and Virginia Health Information were looking to translate the Choosing Wisely campaign into quantifiable results for their insured population. They partnered with Milliman’s healthcare analytics software … Virginia Health: Measuring waste to improve care
ATRIO: From insight to impact
ATRIO Health Plans is an organization owned by several providers in and around Salem, Oregon. It has almost 30,000 members, and 90% are Medicare enrollees. The challenge As with every health plan, ATRIO is constantly looking for ways to improve patient care and the bottom line. The approach When ATRIO made the strategic decision to … ATRIO: From insight to impact
Driving Innovation in Healthcare with Data Analytics: A Case Study
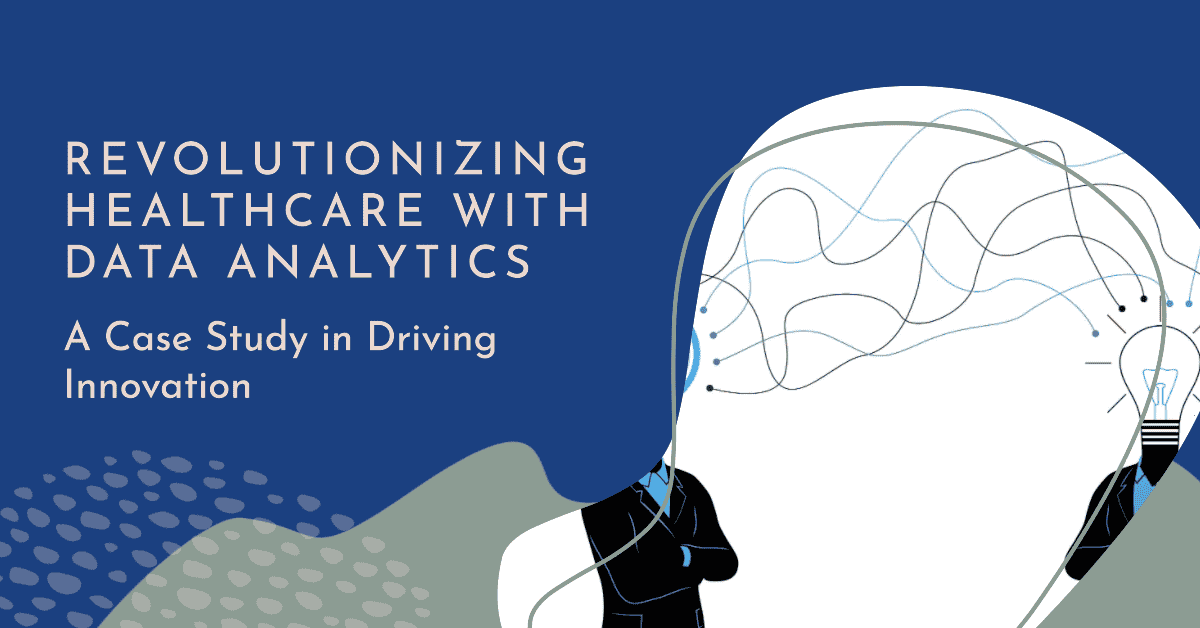
It's a Monday morning, and imagine you're a healthcare executive, sipping your cup of morning coffee and looking at the day's schedule. You're running one of the largest healthcare enterprises, tasked with delivering top-quality care to hundreds of thousands, if not millions, of patients. You know that every decision you make could potentially affect the lives of those who entrust their health to you. It's a weighty responsibility, and the stakes are high.
Now, picture this: what if you had access to a crystal ball, one that could predict patient outcomes, optimize resource allocation, and drive operational efficiencies? Would that not be a game-changer?
Well, data analytics in healthcare is your crystal ball. It's your secret weapon to staying ahead in the rapidly evolving healthcare landscape. This is not about conjuring numbers out of thin air, but leveraging data to make informed, strategic decisions.
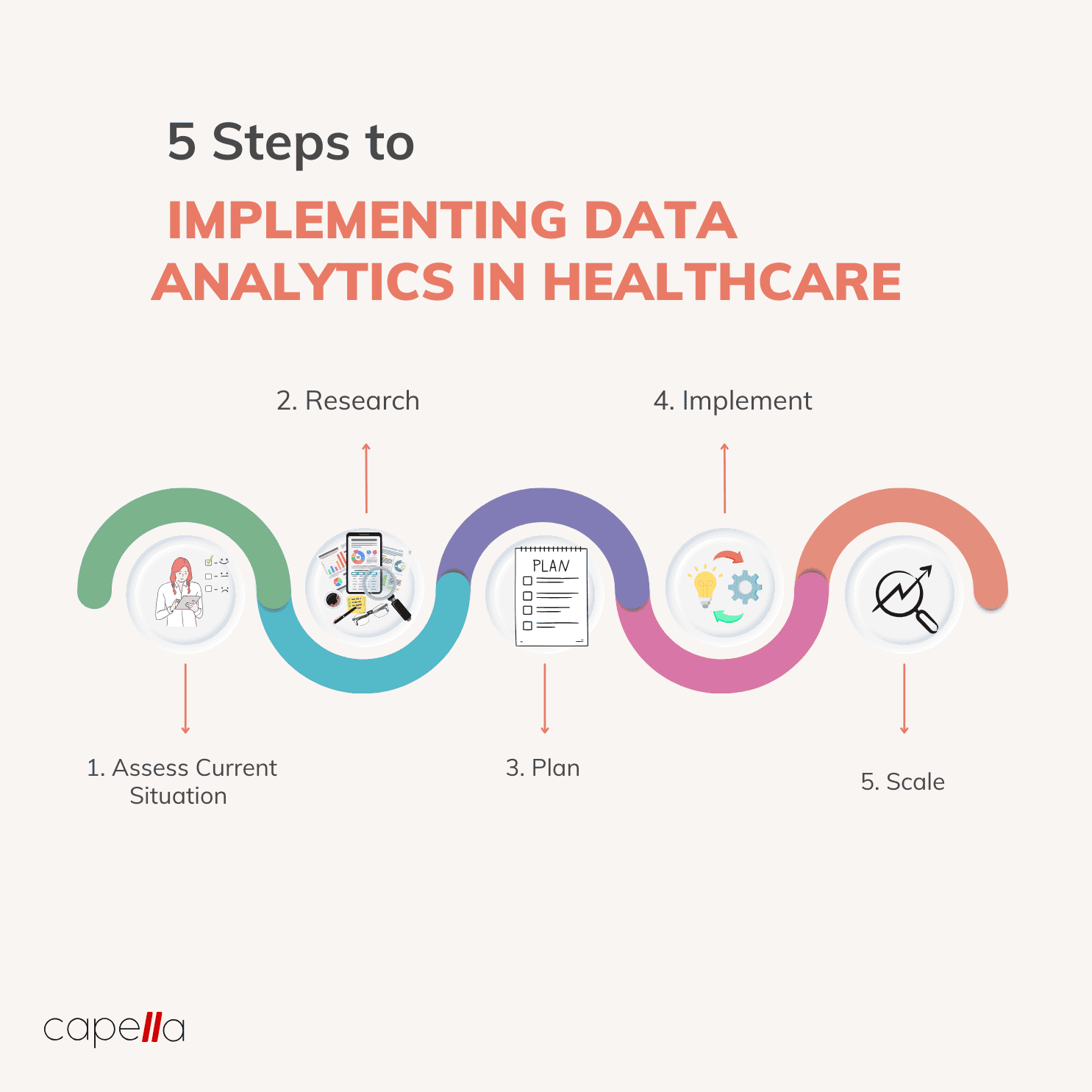
The Power of Data Analytics in Healthcare
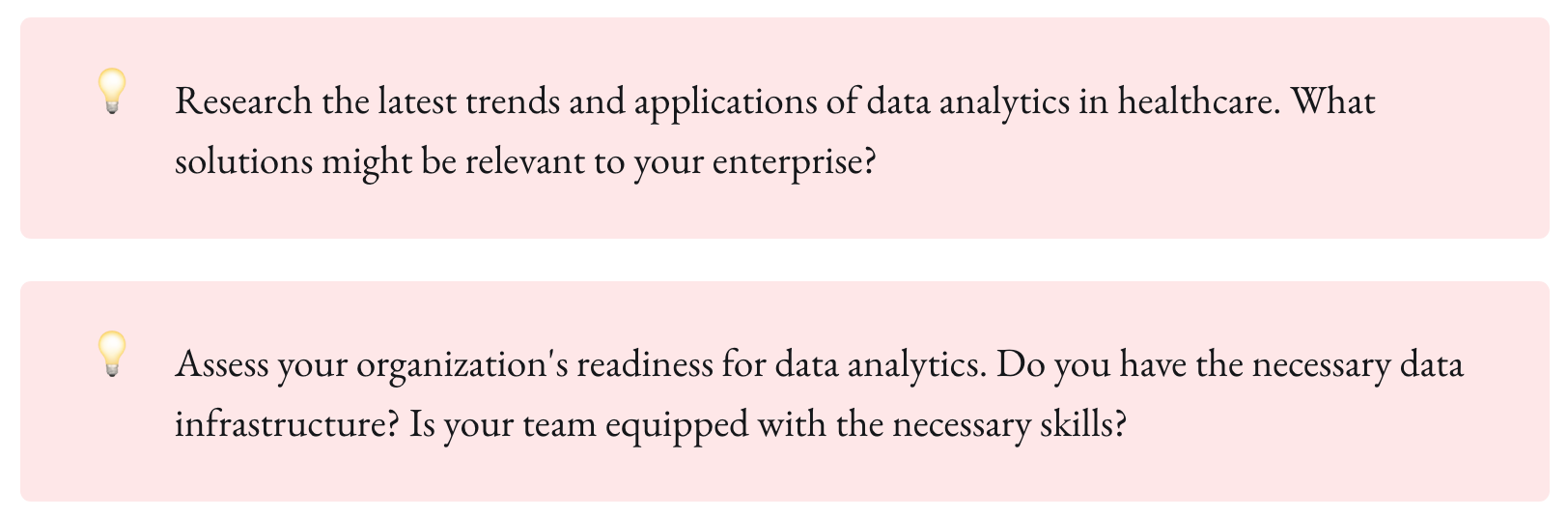
Data analytics refers to the process of examining, cleansing, transforming, and modeling data to discover useful information, suggest conclusions, and support decision-making. In the context of healthcare, this translates into predictive analytics, precision medicine, patient profiling, and more.
Consider the complexity of the modern healthcare ecosystem. It's a multi-headed hydra, spanning numerous areas from patient care to supply chain management, from regulatory compliance to workforce planning. With so many moving parts, it's easy to lose sight of the bigger picture. That's where data analytics steps in, providing a bird's eye view of the enterprise and revealing insights that were hitherto unknown.
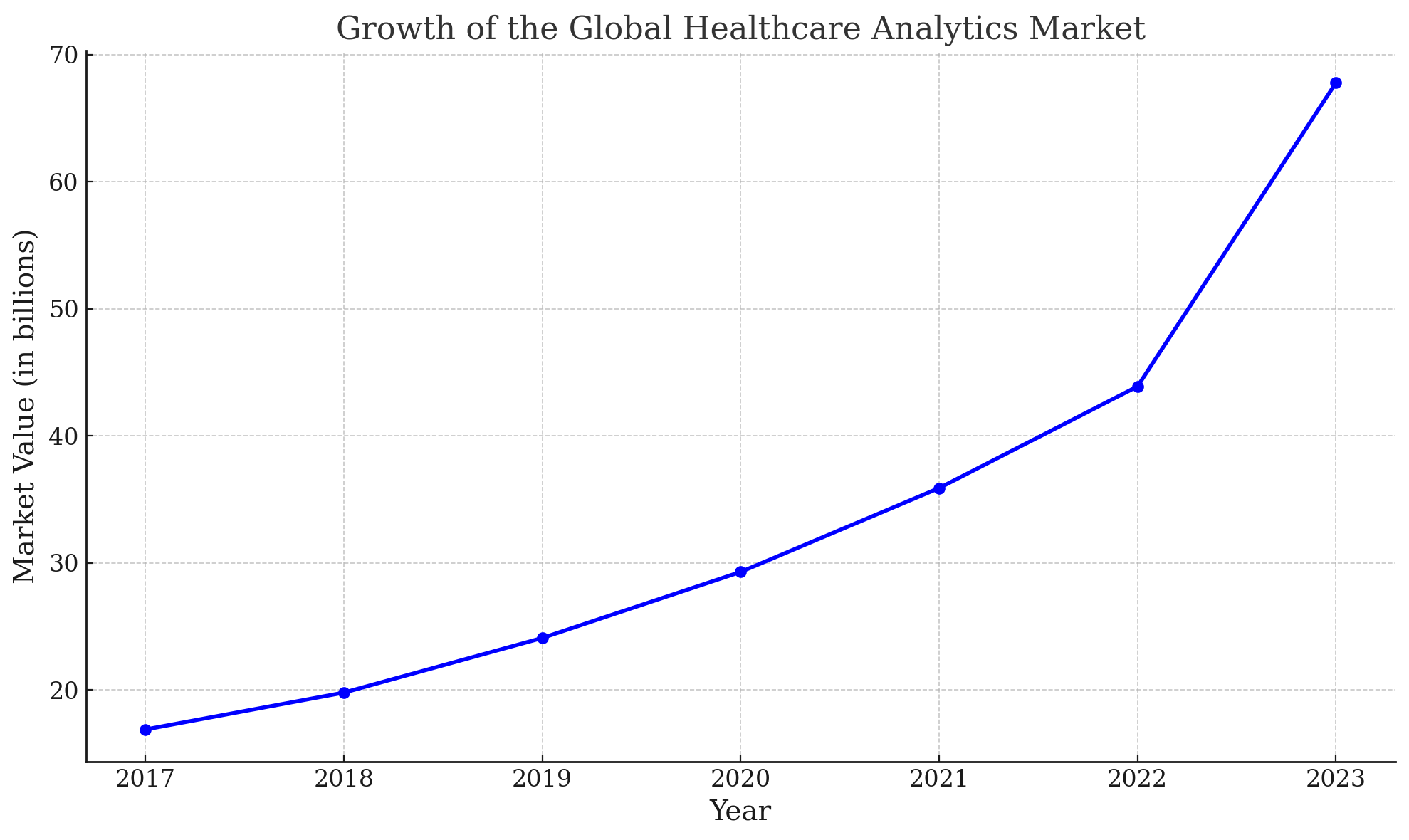
If this sounds exciting, it's because it is! In fact, according to a report by Allied Market Research, the global healthcare analytics market was valued at $16.9 billion in 2017 and is projected to reach $67.8 billion by 2023, growing at a CAGR of 19.1% from 2018 to 2023.
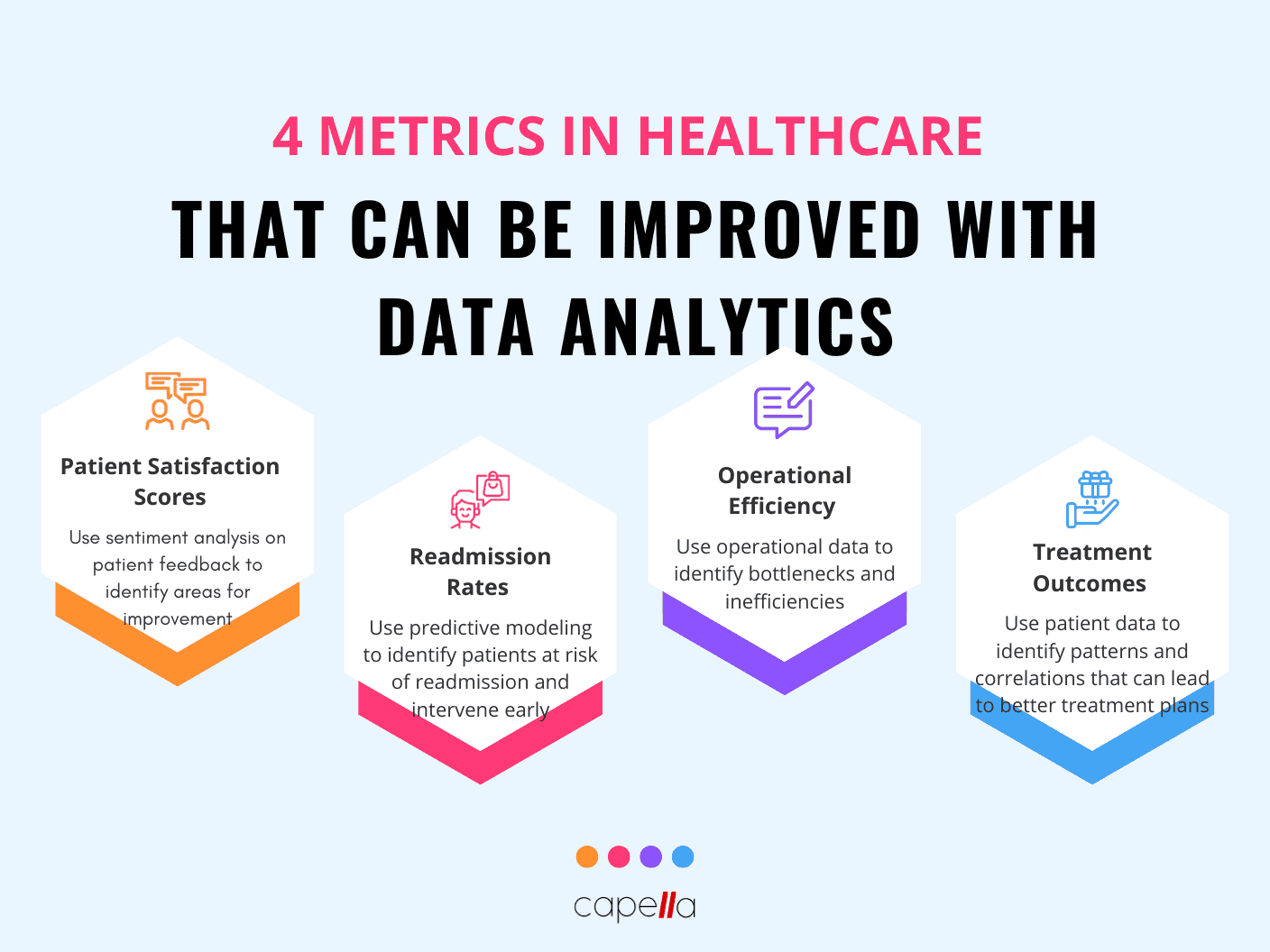
The Case of Cleveland Clinic: A Success Story
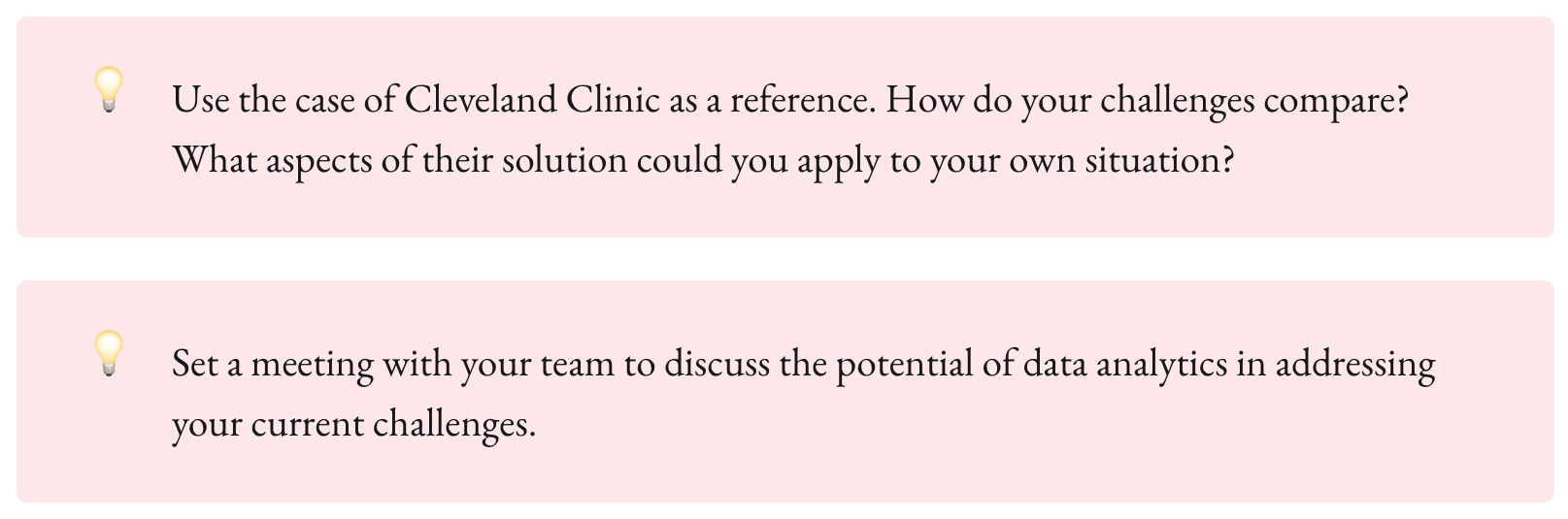
Let's explore a real-life example: Cleveland Clinic, a globally-renowned healthcare provider, constantly aiming for excellence. Like many leading healthcare institutions, Cleveland Clinic faces the challenges of ensuring top-tier care while juggling operational efficiencies and patient satisfaction.
The Challenge
Cleveland Clinic's primary challenges included:
- Rising costs : Like many of its peers, Cleveland Clinic was keen on optimizing its expenditure, especially concerning avoidable hospital readmissions. Identifying root causes and devising effective strategies was essential.
- High readmission rates : Ensuring that their readmission rates were not just below the national average but set a gold standard was a priority. This was vital for their quality metrics and their global reputation.
- Patient dissatisfaction : Even a slight percentage of patients feeling that their care was impersonal could affect the reputation of an institution like Cleveland Clinic. They constantly strive to ensure every patient feels recognized and understood.
The Solution
Cleveland Clinic, known for its forward-thinking approach, decided to delve deep into data analytics to refine its operations and patient care strategies. Collaborating with leading data analytics firms, they set up a robust data-driven framework.
The application of data analytics at Cleveland Clinic led to:
- Predictive analytics : The implemented solution unearthed patterns in patient readmissions. By scrutinizing variables such as patient demographics, disease categories, prescribed treatments, and socio-economic factors, it became possible to predict potential readmission risks. Cleveland Clinic used this data to devise personalized care strategies, playing a crucial role in minimizing readmission cases.
- Resource optimization : Through data insights, inefficiencies in resource allocation, from bed management to workforce deployment, were spotlighted. Addressing these inefficiencies allowed Cleveland Clinic to streamline operations and further elevate patient care standards.
- Patient profiling : Using comprehensive patient data, Cleveland Clinic began tailoring care plans even more intricately, ensuring that each patient felt thoroughly understood and valued.
The Results
Cleveland Clinic's foray into data analytics was predictably successful. With a notable decline in readmission rates and operational costs and a surge in patient satisfaction metrics, their commitment to data-driven insights was further solidified.
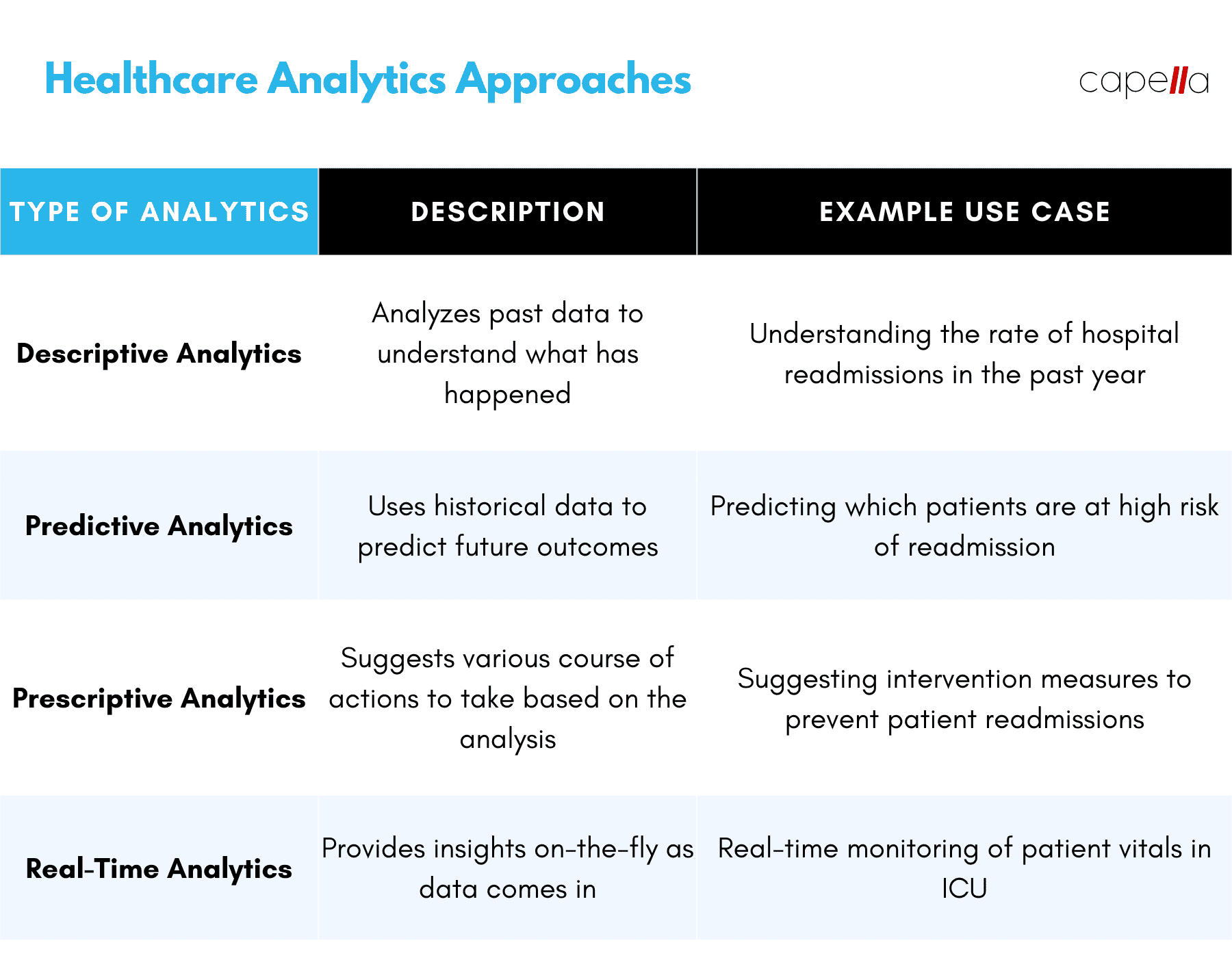
More Examples: Kaiser Permanente and NorthShore University HealthSystem
Data analytics is not just a concept; it's a practical tool that's transforming healthcare. Let's look at two real-world examples:
- Kaiser Permanente : Kaiser Permanente, a healthcare consortium, used a combination of analytics, machine learning, and AI to reduce patient waiting times and streamline operations. Their Operations Watch List (OWL), a mobile app, provides a near real-time view of key hospital metrics, including hospital census, bed demand and availability, and patient discharges. The app has reduced patient wait times for admission to the emergency department by an average of 27 minutes per patient and saved hospital managers an average of 323 minutes per month spent manually preparing data for operational activities.
- NorthShore University HealthSystem : NorthShore used data and predictive analytics to determine which chest pain patients should be admitted for observation and which should be sent home. They developed a "Technology-driven Chest Pain Management in the ED" program, which puts predictive analytics directly into physicians' and nurses' workflow. This program has helped NorthShore reduce unnecessary hospitalizations and optimize patient care. Their Chest Pain Observation Days rate decreased by 10% without increasing the rate of ED returns, mortality, or morbidity.
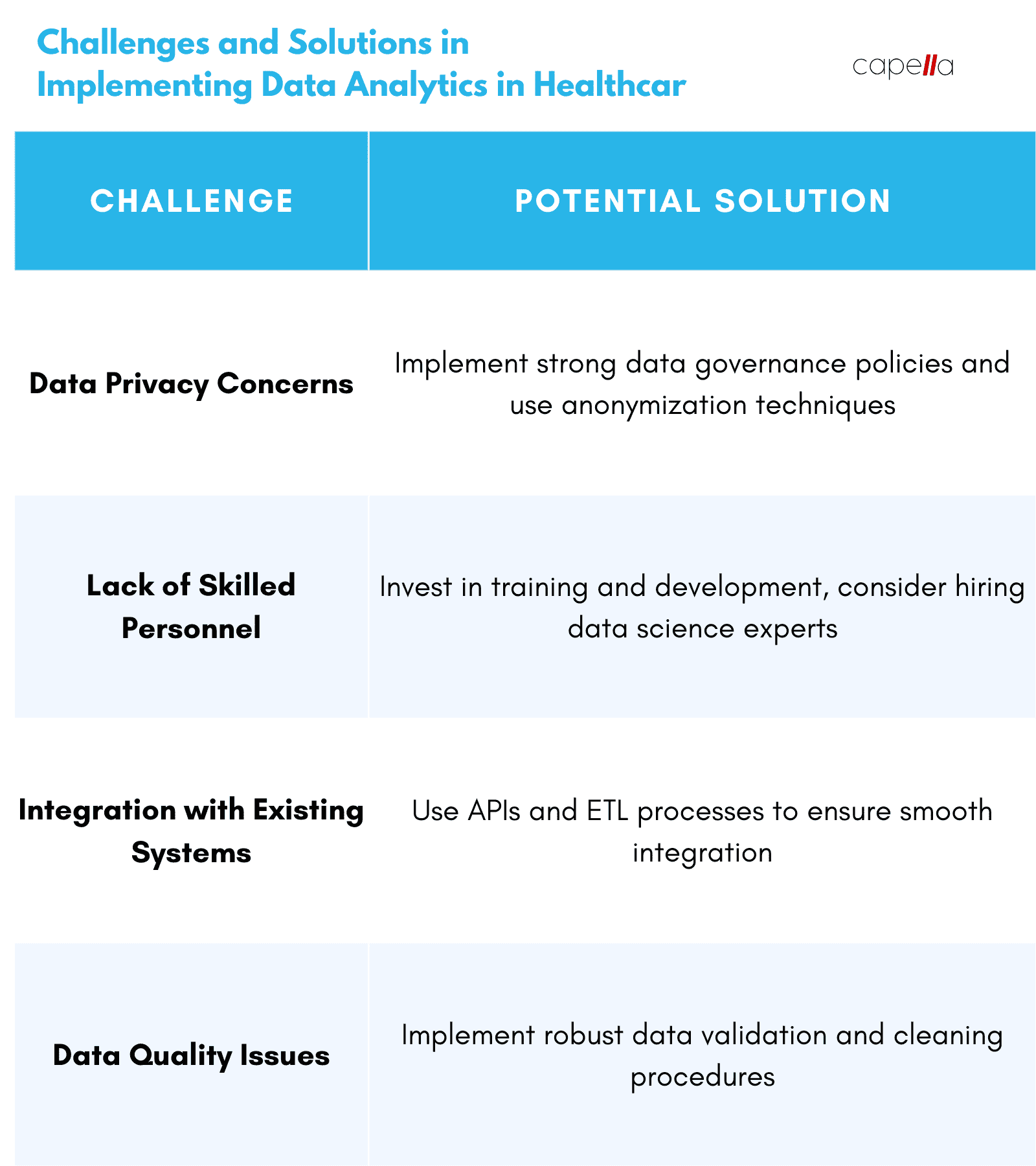
Lessons Learned and Best Practices
In the journey of Cleveland Clinic, Kaiser Permanente, and NorthShore University HealthSystem, we find valuable lessons and best practices for other healthcare executives considering the use of data analytics:
- Continuous feedback is key : Regularly incorporating feedback from end users allows for continuous refinement of solutions, delivering the most relevant information and functionality to support ease of use and maximize value delivered.
- Focus on user experience : Getting clinicians to adopt tools like this requires making the experience seamless. Integration of any new analytics-driven technology must be in the clinical chart or the value will diminish.
- Leverage predictive analytics : Predictive analytics can help identify patterns in patient readmissions, resource utilization, and more. This can enable healthcare providers to develop personalized care plans and preventive measures, significantly improving patient care.
In an industry where life-altering decisions are made every day, healthcare executives cannot afford to ignore the power of data analytics. It's not just about big data or fancy algorithms; it's about harnessing this data to drive actionable insights, optimize resources, and ultimately, deliver better patient care.
As a healthcare executive, your job isn't just about managing a hospital or a healthcare system. It's about making a difference in the lives of your patients. And data analytics can be your secret weapon to do just that.
So, as you sip your coffee, consider this: are you ready to embrace the power of data analytics in your healthcare enterprise? The future of healthcare is here, and it's data-driven.
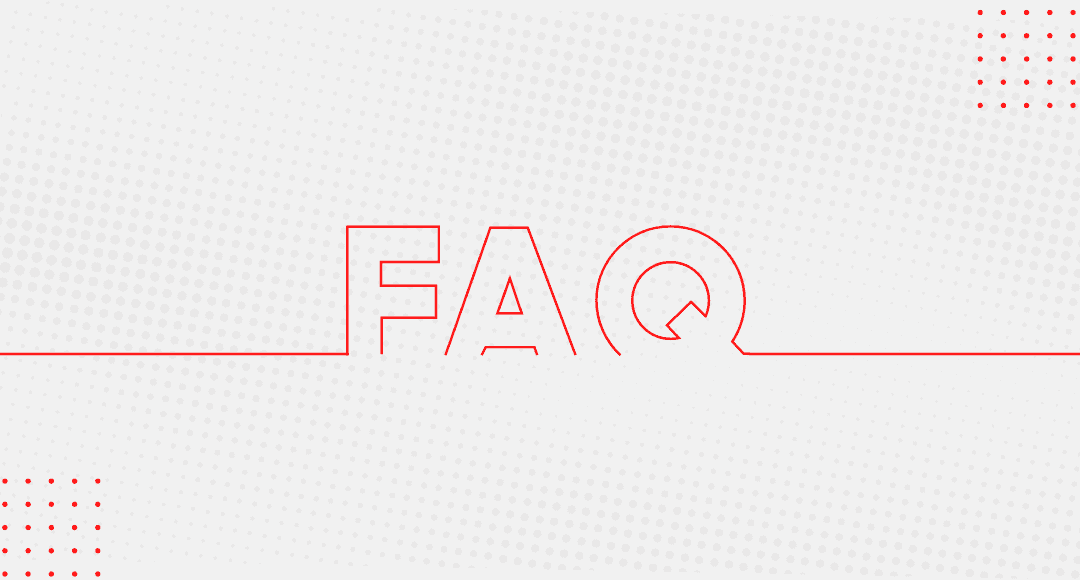
What is data analytics in healthcare?
Data analytics in healthcare is the practice of using data-driven findings to predict and solve health-related issues. It involves collecting and interpreting health data to improve care, reduce costs, and enhance patient experiences. This can range from analyzing patient records to predict disease outcomes to using machine learning algorithms to automate administrative tasks.
Why is data analytics important in healthcare?
Data analytics is essential in healthcare for several reasons. It allows healthcare providers to offer personalized patient care based on individual health data. It can help predict outbreaks of epidemics and improve the efficiency of health services. Data analytics can also reduce costs by identifying inefficiencies in healthcare delivery and predicting patient outcomes to guide treatment plans.
How is data analytics used in healthcare decision making?
In healthcare decision-making, data analytics can be used to provide evidence-based answers. This can involve analyzing patient data to determine the best treatment plan, using predictive models to identify high-risk patients and guide resource allocation, or interpreting operational data to improve service delivery and patient satisfaction.
What are some real-world examples of data analytics in healthcare?
There are numerous examples of data analytics in healthcare. For instance, Kaiser Permanente used data analytics to reduce patient wait times and streamline operations. They developed a mobile app called Operations Watch List (OWL) that provided real-time data on key hospital metrics, helping hospital leaders make informed decisions. Another example is NorthShore University HealthSystem, which used predictive analytics to determine which chest pain patients should be admitted for observation, reducing unnecessary hospitalizations.
What are some challenges in implementing data analytics in healthcare?
Challenges in implementing data analytics in healthcare include data privacy concerns, integration with existing systems, lack of skilled personnel, and data quality issues. Overcoming these challenges often involves investing in data governance, hiring or training data science experts, ensuring smooth system integration, and implementing robust data validation and cleaning procedures.
What are the steps in the data analytics process in healthcare?
The data analytics process in healthcare involves several steps, including data collection, data cleaning and preparation, data analysis, insight generation, and decision making. Each step is crucial for delivering reliable, actionable insights.
How can healthcare organizations start implementing data analytics?
Healthcare organizations can start implementing data analytics by first assessing their current situation and understanding the challenges they face. They can then research the latest trends and applications of data analytics in healthcare and develop a roadmap for implementation. Starting with small projects can help gather feedback and refine the approach before scaling the use of data analytics across the organization.
What is the future of data analytics in healthcare?
The future of data analytics in healthcare looks promising, with advancements in technologies like machine learning and AI set to revolutionize healthcare delivery. Potential future applications include personalized medicine, predictive diagnostics, real-time alerting, and advanced imaging analytics.
What are the different types of analytics in healthcare?
In healthcare, there are typically four types of analytics: descriptive, predictive, prescriptive, and real-time analytics. Descriptive analytics analyzes past data to understand what has happened, predictive analytics uses historical data to predict future outcomes, prescriptive analytics suggests various course of actions to take based on the analysis, and real-time analytics provides insights on-the-fly as data comes in.
How can data analytics improve patient satisfaction in healthcare?
Data analytics can enhance patient satisfaction in healthcare by providing personalized patient care, reducing wait times, and improving health outcomes. By analyzing patient feedback, healthcare providers can identify areas for improvement and take action to enhance the patient experience. Predictive analytics can also enable proactive care, addressing potential health issues before they become serious problems.
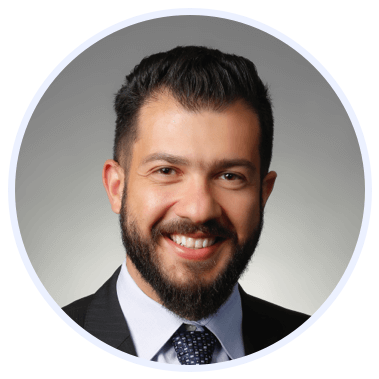
Rasheed Rabata
Is a solution and ROI-driven CTO, consultant, and system integrator with experience in deploying data integrations, Data Hubs, Master Data Management, Data Quality, and Data Warehousing solutions. He has a passion for solving complex data problems. His career experience showcases his drive to deliver software and timely solutions for business needs.
Related posts
Discover why capella is the right data partner for your organization.
Thank you for visiting nature.com. You are using a browser version with limited support for CSS. To obtain the best experience, we recommend you use a more up to date browser (or turn off compatibility mode in Internet Explorer). In the meantime, to ensure continued support, we are displaying the site without styles and JavaScript.
- View all journals
- My Account Login
- Explore content
- About the journal
- Publish with us
- Sign up for alerts
- Review Article
- Open access
- Published: 05 March 2020
Big data in digital healthcare: lessons learnt and recommendations for general practice
- Raag Agrawal 1 , 2 &
- Sudhakaran Prabakaran ORCID: orcid.org/0000-0002-6527-1085 1 , 3 , 4
Heredity volume 124 , pages 525–534 ( 2020 ) Cite this article
42k Accesses
95 Citations
84 Altmetric
Metrics details
- Developing world
Big Data will be an integral part of the next generation of technological developments—allowing us to gain new insights from the vast quantities of data being produced by modern life. There is significant potential for the application of Big Data to healthcare, but there are still some impediments to overcome, such as fragmentation, high costs, and questions around data ownership. Envisioning a future role for Big Data within the digital healthcare context means balancing the benefits of improving patient outcomes with the potential pitfalls of increasing physician burnout due to poor implementation leading to added complexity. Oncology, the field where Big Data collection and utilization got a heard start with programs like TCGA and the Cancer Moon Shot, provides an instructive example as we see different perspectives provided by the United States (US), the United Kingdom (UK) and other nations in the implementation of Big Data in patient care with regards to their centralization and regulatory approach to data. By drawing upon global approaches, we propose recommendations for guidelines and regulations of data use in healthcare centering on the creation of a unique global patient ID that can integrate data from a variety of healthcare providers. In addition, we expand upon the topic by discussing potential pitfalls to Big Data such as the lack of diversity in Big Data research, and the security and transparency risks posed by machine learning algorithms.
Similar content being viewed by others
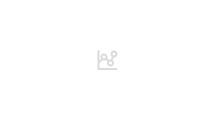
Harnessing big data for health equity through a comprehensive public database and data collection framework
Cameron Sabet, Alessandro Hammond, … Fatima Cody Stanford
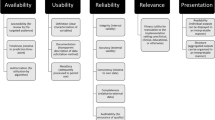
Putting the data before the algorithm in big data addressing personalized healthcare
Eli M. Cahan, Tina Hernandez-Boussard, … Daniel L. Rubin
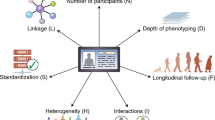
Axes of a revolution: challenges and promises of big data in healthcare
Smadar Shilo, Hagai Rossman & Eran Segal
Introduction
The advent of Next Generation Sequencing promises to revolutionize medicine as it has become possible to cheaply and reliably sequence entire genomes, transcriptomes, proteomes, metabolomes, etc. (Shendure and Ji 2008 ; Topol 2019a ). “Genomical” data alone is predicted to be in the range of 2–40 Exabytes by 2025—eclipsing the amount of data acquired by all other technological platforms (Stephens et al. 2015 ). In 2018, the price for the research-grade sequencing of the human genome had dropped to under $1000 (Wetterstrand 2019 ). Other “omics” techniques such as Proteomics have also become accessible and cheap, and have added depth to our knowledge of biology (Hasin et al. 2017 ; Madhavan et al. 2018 ). Consumer device development has also led to significant advances in clinical data collection, as it becomes possible to continuously collect patient vitals and analyze them in real-time. In addition to the reductions in cost of sequencing strategies, computational power, and storage have become extremely cheap. All these developments have brought enormous advances in disease diagnosis and treatments, they have also introduced new challenges as large-scale information becomes increasingly difficult to store, analyze, and interpret (Adibuzzaman et al. 2018 ). This problem has given way to a new era of “Big Data” in which scientists across a variety of fields are exploring new ways to understand the large amounts of unstructured and unlinked data generated by modern technologies, and leveraging it to discover new knowledge (Krumholz 2014 ; Fessele 2018 ). Successful scientific applications of Big Data have already been demonstrated in Biology, as initiatives such as the Genotype-Expression Project are producing enormous quantities of data to better understand genetic regulation (Aguet et al. 2017 ). Yet, despite these advances, we see few examples of Big Data being leveraged in healthcare despite the opportunities it presents for creating personalized and effective treatments.
Effective use of Big Data in Healthcare is enabled by the development and deployment of machine learning (ML) approaches. ML approaches are often interchangeably used with artificial intelligence (AI) approaches. ML and AI only now make it possible to unravel the patterns, associations, correlations and causations in complex, unstructured, nonnormalized, and unscaled datasets that the Big Data era brings (Camacho et al. 2018 ). This allows it to provide actionable analysis on datasets as varied as sequences of images (applicable in Radiology) or narratives (patient records) using Natural Language Processing (Deng et al. 2018 ; Esteva et al. 2019 ) and bringing all these datasets together to generate prediction models, such as response of a patient to a treatment regimen. Application of ML tools is also supplemented by the now widespread adoption of Electronic Health Records (EHRs) after the passage of the Affordable Care Act (2010) and Health Information Technology for Economic and Clinical Health Act (2009) in the US, and recent limited adoption in the National Health Service (NHS) (Garber et al. 2014 ). EHRs allow patient data to become more accessible to both patients and a variety of physicians, but also researchers by allowing for remote electronic access and easy data manipulation. Oncology care specifically is instructive as to how Big Data can make a direct impact on patient care. Integrating EHRs and diagnostic tests such as MRIs, genomic sequencing, and other technologies is the big opportunity for Big Data as it will allow physicians to better understand the genetic causes behind cancers, and therefore design more effective treatment regimens while also improving prevention and screening measures (Raghupathi and Raghupathi 2014 ; Norgeot et al. 2019 ). Here, we survey the current challenges in Big Data in healthcare and use oncology as an instructive vignette, highlighting issues of data ownership, sharing, and privacy. Our review builds on findings from the US, UK, and other global healthcare systems to propose a fundamental reorganization of EHRs around unique patient identifiers and ML.
Current successes of Big Data in healthcare
The UK and the US are both global leaders in healthcare that will play important roles in the adoption of Big Data. We see this global leadership already in oncology (The Cancer Genome Atlas (TCGA), Pan-Cancer Analysis of Whole Genomes (PCAWG)) and neuropsychiatric diseases (PsychENCODE) (Tomczak et al. 2015 ; Akbarian et al. 2015 ; Campbell et al. 2020 ). These Big Data generation and open-access models have resulted in hundreds of applications and scientific publications. The success of these initiatives in convincing the scientific and healthcare communities of the advantages of sharing clinical and molecular data have led to major Big Data generation initiatives in a variety of fields across the world such as the “All of Us” project in the US (Denny et al. 2019 ). The UK has now established a clear national strategy that has resulted in the likes of the UK Biobank and 100,000 Genomes projects (Topol 2019b ). These projects dovetail with a national strategy for the implementation of genomic medicine with the opening of multiple genome-sequencing sites, and the introduction of genome sequencing as a standard part of care for the NHS (Marx 2015 ). The US has no such national strategy, and while it has started its own large genomic study—“All of Us”—it does not have any plans for implementation in its own healthcare system (Topol 2019b ). In this review, we have focussed our discussion on developments in Big Data in Oncology as a method to understand this complex and fast moving field, and to develop general guidelines for healthcare at large.
Big Data initiatives in the United Kingdom
The UK Biobank is a prospective cohort initiative that is composed of individuals between the ages of 40 and 69 before disease onset (Allen et al. 2012 ; Elliott et al. 2018 ). The project has collected rich data on 500,000 individuals, collating together biological samples, physical measures of patient health, and sociological information such as lifestyle and demographics (Allen et al. 2012 ). In addition to its size, the UK Biobank offers an unparalleled link to outcomes through integration with the NHS. This unified healthcare system allows researchers to link initial baseline measures with disease outcomes, and with multiple sources of medical information from hospital admission to clinical visits. This allows researchers to be better positioned to minimize error in disease classification and diagnosis. The UK Biobank will also be conducting routine follow-up trials to continue to provide information regarding activity and further expanded biological testing to improve disease and risk factor association.
Beyond the UK Biobank, Public Health England launched the 100,000 Genomes project with the intent to understand the genetic origins behind common cancers (Turnbull et al. 2018 ). The massive effort consists of NHS patients consenting to have their genome sequenced and linked to their health records. Without the significant phenotypic information collected in the UK Biobank—the project holds limited use as a prospective epidemiological study—but as a great tool for researchers interested in identifying disease causing single-nucleotide polymorphisms (SNPs). The size of the dataset itself is its main advance—as it provides the statistical power to discover the associated SNPs even for rare diseases. Furthermore, the 100,000 Genomes Project’s ancillary aim is to stimulate private sector growth in the genomics industry within England.
Big Data initiatives in the United States and abroad
In the United States, the “All of Us” project is expanding upon the UK Biobank model by creating a direct link between patient genome data and their phenotypes by integrating EHRs, behavioral, and family data into a unique patient profile (Denny et al. 2019 ). By creating a standardized and linked database for all patients—“All of Us” will allow researchers greater scope than the UK BioBank to understand cancers and discover the associated genetic causes. In addition, “All of Us” succeeds in focusing on minority populations and health, an area of focus that sets it apart and gives it greater clinical significance. The UK should learn from this effort by expanding the UK Biobank project to further include minority populations and integrate it with ancillary patient data such as from wearables—the current UK Biobank has ~500,000 patients that identify as white versus ~12,000 (i.e., just <2.5%) that identified as non-white (Cohn et al. 2017 ). Meanwhile, individuals of Asian ethnicities made up over 7.5% of the UK population as per the 2011 UK Census, with the proportion of minorities projected to rise in the coming years (O’Brien and Potter-Collins 2015 ; Cohn et al. 2017 ).
Sweden too provides an informative example of the power of investment in rich electronic research registries (Webster 2014 ). The Swedish government has committed over $70 million dollars in funding per annum to expand a variety of cancer registries that would allow researchers insight into risk factors for oncogenesis. In addition, its data sources are particularly valuable for scientists, as each patient’s entries are linked to unique identity numbers that can be cross references with over 90 other registries to give a more complete understanding of a patient’s health and social circumstances. These registries are not limited to disease states and treatments, but also encompass extensive public administrative records that can provide researchers considerable insight into social indicators of health such as income, occupation, and marital status (Connelly et al. 2016 ). These data sources become even more valuable to Swedish researchers as they have been in place for decades with commendable consistency—increasing the power of long-term analysis (Connelly et al. 2016 ). Other nations can learn from the Swedish example by paying particular attention to the use of unique patient identifiers that can map onto a number of datasets collected by government and academia—an idea that was first mentioned in the US Health Insurance Portability and Accountability Act of 1996 (HIPAA) but has not yet been implemented (Davis 2019 ).
China has recently become a leader in implementation and development of new digital technologies, and it has begun to approach healthcare with an emphasis on data standardization and volume. Already, the central government in China has initiated several funding initiatives aimed at pushing Big Data into healthcare use cases, with a particular eye on linking together administrative data, regional claims data from the national health insurance program, and electronic medical records (Zhang et al. 2018 ). China hopes to do this through leveraging its existing personal identification system that covers all Chinese nationals—similar to the Swedish model of maintaining a variety of regional and national registries linked by personal identification numbers. This is particularly relevant to cancer research as China has established a new cancer registry (National Central Cancer Registry of China) that will take advantage of the nation’s population size to give unique insight into otherwise rare oncogenesis. Major concerns regarding this initiative are data quality and time. China has only relatively recently adopted the International Classification of Diseases (ICD) revision ten coding system, a standardized method for recording disease states alongside prescribed treatments. China is also still implementing standardized record keeping terminologies at the regional level. This creates considerable heterogeneity in data quality—as well as inoperability between regions—a major obstacle in any national registry effort (Zhang et al. 2018 ). The recency of these efforts also mean that some time is required until researchers will be able to take advantage of longitudinal analysis—vital for oncology research that aims to spot recurrences or track patient survival. In the future we can expect significant findings to come out of China’s efforts to bring hundreds of millions of patient files available to researchers, but significant advances in standards of care and interoperability must be first surpassed.
The large variety of “Big Data” research projects being undertaken around the world are proposing different approaches to the future of patient records. The UK is broadly leveraging the centralization of the NHS to link genomic data with clinical care records, and opening up the disease endpoints to researchers through a patient ID. Sweden and China are also adopting this model—leveraging unique identity numbers issued to citizens to link otherwise disconnected datasets from administrative and healthcare records (Connelly et al. 2016 ; Cnudde et al. 2016 ; Zhang et al. 2018 ). In this way, tests, technologies and methods will be integrated in a way that is specific to the patient but not necessarily to the hospital or clinic. This allows for significant flexibility in the seamless transfer of information between sites and for physicians to take full advantage of all the data generated. The US’ “All of Us” program is similar in integrating a variety of patient records into a single-patient file that is stored in the cloud (Denny et al. 2019 ). However, it does not significantly link to public administrative data sources, and thus is limited in its usefulness for long-term analysis of the effects of social contributors to cancer progression and risk. This foretells greater problems with the current ecosystem of clinical data—where lack of integration, misguided design, and ambiguous data ownership make research and clinical care more difficult rather than easier.
Survey of problems in clinical data use
Fragmentation.
Fragmentation is the primary problem that needs to be addressed if EHRs have any hope of being used in any serious clinical capacity. Fragmentation arises when EHRs are unable to communicate effectively between each other—effectively locking patient information into a proprietary system. While there are major players in the US EHR space such as Epic and General Electric, there are also dozens of minor and niche companies that also produce their own products—many of which are not able to communicate effectively or easily with one another (DeMartino and Larsen 2013 ). The Clinical Oncology Requirements for the EHR and the National Community Cancer Centers Program have both spoken out about the need for interoperability requirements for EHRs and even published guidelines (Miller 2011 ). In addition, the Certification Commission for Health Information Technology was created to issue guidelines and standards for interoperability of EHRs (Miller 2011 ). Fast Healthcare Interoperability Resources (FHIR) is the current new standard for data exchange for healthcare published by Health Level 7 (HL7). It builds upon past standards from both HL7 and a variety of other standards such as the Reference Information Model. FHIR offers new principles on which data sharing can take place through RESTful APIs—and projects such as Argonaut are working to expand adoption to EHRs (Chambers et al. 2019 ). Even with the introduction of the HL7 Ambulatory Oncology EHR Functional Profile, EHRs have not improved and have actually become pain points for clinicians as they struggle to integrate the diagnostics from separate labs or hospitals, and can even leave physicians in the dark about clinical history if the patient has moved providers (Reisman 2017 ; Blobel 2018 ). Even in integrated care providers such as Kaiser Permanente there are interoperability issues that make EHRs unpopular among clinicians as they struggle to receive outside test results or the narratives of patients who have recently moved (Leonard and Tozzi 2012 ).
The UK provides an informative contrast in its NHS, a single government-run enterprise that provides free healthcare at the point of service. Currently, the NHS is able to successfully integrate a variety of health records—a step ahead of the US—but relies on outdated technology with security vulnerabilities such as fax machines (Macaulay 2016 ). The NHS has recently also begun the process of digitizing its health service, with separate NHS Trusts adopting American EHR solutions, such as the Cambridgeshire NHS trust’s recent agreement with Epic (Honeyman et al. 2016 ). However, the NHS still lags behind the US in broad use and uptake across all of its services (Wallace 2016 ). Furthermore, it will need to force the variety of EHRs being adopted to conform to centralized standards and interoperability requirements that allow services as far afield as genome sequencing to be added to a patient record.
Misguided EHR design
Another issue often identified with the modern incarnation of EHRs is that they are often not helpful for doctors in diagnosis—and have been identified by leading clinicians as a hindrance to patient care (Lenzer 2017 ; Gawande 2018 ). A common denominator among the current generation of EHRs is their focus on billing codes, a set of numbers assigned to every task, service, and drug dispensed by a healthcare professional that is used to determine the level of reimbursement the provider will receive. This focus on billing codes is a necessity of the insurance system in the US, which reimburses providers on a service-rendered basis (Essin 2012 ; Lenzer 2017 ). Due to the need for every part of the care process to be billed to insurers (of which there are many) and sometimes to multiple insurers simultaneously, EHRs in the US are designed foremost with insurance needs in mind. As a result, EHRs are hampered by government regulations around billing codes, the requirements of insurance companies, and only then are able to consider the needs of providers or researchers (Bang and Baik 2019 ). And because purchasing decisions for EHRs are not made by physicians, the priority given to patient care outcomes falls behind other needs. The American Medical Association has cited the difficulty of EHRs as a contributing factor in physician burnout and as a waste of valuable time (Lenzer 2017 ; Gardner et al. 2019 ). The NHS, due to its reliance on American manufacturers of EHRs, must suffer through the same problems despite its fundamentally different structure.
Related to the problem of EHRs being optimized for billing, not patient care, is their lack of development beyond repositories of patient information into diagnostic aids. A study of modern day EHR use in the clinic notes many pain points for physicians and healthcare teams (Assis-Hassid et al. 2019 ). Foremost was the variance in EHR use within the clinic—in part because these programs are often not designed with provider workflows in mind (Assis-Hassid et al. 2019 ). In addition, EHRs were found to distract from interpersonal communication and did not integrate the many different types of data being created by nurses, physician assistants, laboratories, and other providers into usable information for physicians (Assis-Hassid et al. 2019 ).
Data ownership
One of the major challenges of current implementations of Big Data are the lack of regulations, incentives, and systems to manage ownership and responsibilities for data. In the clinical space, in the US, this takes the form of compliance with HIPAA, a now decade-old law that aimed to set rules for patient privacy and control for data (Adibuzzaman et al. 2018 ). As more types of data are generated for patients and uploaded to electronic platforms, HIPAA becomes a major roadblock to data sharing as it creates significant privacy concerns that hamper research. Today, if a researcher is to search for even simple demographic and disease states—they can rapidly identify an otherwise de-identified patient (Adibuzzaman et al. 2018 ). Concerns around breaking HIPAA prevent complete and open data sharing agreements—blocking a path to the specificity needed for the next generation of research from being achieved, and also throws a wrench into clinical application of these technologies as data sharing becomes bogged down by nebulousness surrounding old regulations on patient privacy. Furthermore, compliance with the General Data Protection Regulation (GDPR) in the EU has hampered international collaborations as compliance with both HIPAA and GDPR is not yet standardized (Rabesandratana 2019 ).
Data sharing is further complicated by the need to develop new technologies to integrate across a variety of providers. Taking from the example of the Informatics for Integrating Biology and the Bedside (i2b2) program funded by the NIH with Partners Healthcare, it is difficult and enormously expensive to overlay programs on top of existing EHRs (Adibuzzaman et al. 2018 ). Rather, a new approach needs to be developed to solve the solution of data sharing. Blockchain provides an innovative approach and has been recently explored in the literature as a solution that centers patient control of their data, and also promotes safe and secure data sharing through data transfer transactions secured by encryption (Gordon and Catalini 2018 ). Companies exploring this mechanism for data sharing include Nebula Genomics, a firm founded by George Church, that is aimed at securing genomic data in blockchain in a way that scales commercially, and can be used for research purposes with permission only from data owners—the patients themselves. Other firms are exploring using a variety of data types stored in blockchain to create predictive models of disease—such as Doc.Ai—but all are centrally based on the idea of a blockchain to secure patient data and ensure private accurate transfer between sites (Agbo et al. 2019 ). Advantages of blockchain for healthcare data transfer and storage lie in its security and privacy, but the approach has yet to gain widespread use.
Recommendations for clinical application
Design a new generation of ehrs.
It is conceivable that physicians in the near future will be faced with terabytes of data—patients coming to their clinics with years of continuous data monitoring their heart rate, blood sugar, and a variety of other factors (Topol 2019a ). Gaining clinical insight from such a large quantity of data is an impossible expectation to place upon physicians. In order to solve this problem of the exploding numbers of tests, assays, and results, EHRs will need to be extended from simply being records of patient–physician interactions and digital folders, to being diagnostic aids (Fig. 1 ). Companies such as Roche–Flatiron are already moving towards this model by building predictive and analytical tools into their EHRs when they provide them to providers. However, broader adoption across a variety of providers—and the transparency and portability of the models generated will also be vital. AI-based clinical decision-making support will need to be auditable in order to avoid racial bias, and other potential pitfalls (Char et al. 2018 ). Patients will soon request to have permanent access to the models and predictions being generated by ML models to gain greater clarity into how clinical decisions were made, and to guard against malpractice.
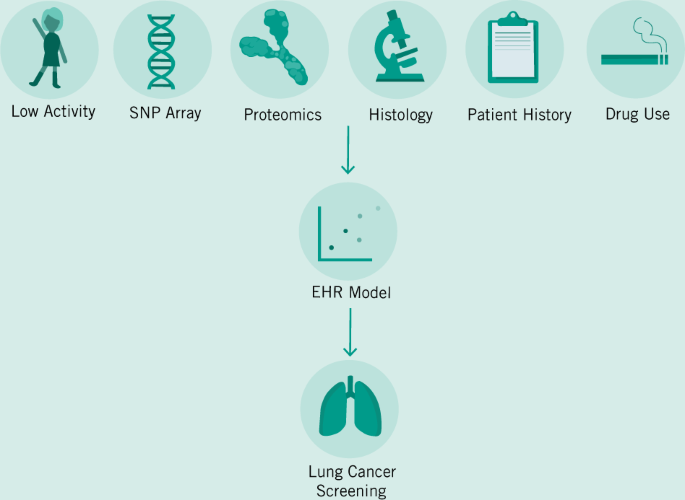
In this example we demonstrate how many possible factors may come together to better target patients for early screening measures, which can lower aggregate costs for the healthcare system.
Designing this next generation of EHRs will require collaboration between physicians, patients, providers, and insurers in order to ensure ease of use and efficacy. In terms of specific recommendations for the NHS, the Veterans Administration provides a fruitful approach as it was able to develop its own EHR that compares extremely favorably with the privately produced Epic EHR (Garber et al. 2014 ). Its solution was open access, public-domain, and won the loyalty of physicians in improving patient care (Garber et al. 2014 ). However, the VA’s solution was not actively adopted due to lack of support for continuous maintenance and limited support for billing (Garber et al. 2014 ). While the NHS does not need to consider the insurance industry’s input, it does need to take note that private EHRs were able to gain market prominence in part because they provided a hand to hold for providers, and were far more responsive to personalized concerns raised (Garber et al. 2014 ). Evidence from Denmark suggests that EHR implementation in the UK would benefit from private competitors implementing solutions at the regional rather than national level in order to balance the need for competition and standardization (Kierkegaard 2013 ).
Develop new EHR workflows
Already, researchers and enterprise are developing predictive models that can better diagnose cancers based on imaging data (Bibault et al. 2016 ). While these products and tools are not yet market ready and are far off from clinical approval—they portend things to come. We envision a future where the job of an Oncologist becomes increasingly interpretive rather than diagnostic. But to get to that future, we will need to train our algorithms much like we train our future doctors—with millions of examples. In order to build this corpus of data, we will need to create a digital infrastructure around Big Data that can both handle the demands of researchers and enterprise as they continuously improve their models—with those of patients and physicians who must continue their important work using existing tools and knowledge. In Fig. 2 , we demonstrate a hypothetical workflow based on models provided by other researchers in the field (Bibault et al. 2016 ; Topol 2019a ). This simplified workflow posits EHRs as an integrative tool that can facilitate the capture of a large variety of data sources and can transform them into a standardized format to be stored in a secure cloud storage facility (Osong et al. 2019 ). Current limitations in HIPAA in the US have prevented innovation in this field, so reform will need to both guarantee the protection of private patient data and the open access to patient histories for the next generation of diagnostic tools. The introduction of accurate predictive models for patient treatment will mean that cancer diagnosis will fundamentally change. We will see the job of oncologists transforming itself as they balance recommendations provided by digital tools that can instantly integrate literature and electronic records from past patients, and their own best clinical judgment.
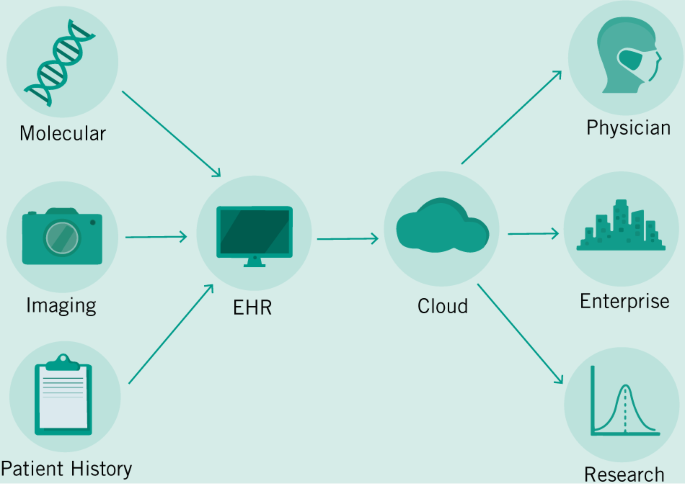
Here, various heterogeneous data types are fed into a centralized EHR system that will be uploaded to a secure digital cloud where it can be de-identified and used by research and enterprise, but primarily by physicians and patients.
Use a global patient ID
While we are already seeing the fruits of decades of research into ML methods, there is a whole new set of techniques that will soon be leaving research labs and being applied to the clinic. This set of “omics”—often used to refer to proteomics, genomics, metabolomics, and others—will reveal even more specificity about a patient’s cancer at lower cost (Cho 2015 ). However, they like other technologies, will create petabytes of data that will need to be stored and integrated to help physicians.
As the number of tests and healthcare providers diversify—EHRs will need to address the question of extensibility and flexibility. Providers as disparate as counseling offices and MRI imaging centers cannot be expected to use the same software—or even similar software. As specific solutions for diverse providers are created—they will need to interface in a standard format with existing EHRs. The UK Biobank creates a model for these types of interactions in its use of a singular patient ID to link a variety of data types—allowing for extensibility as future iterations and improvements add data sources for the project. Also, Sweden and China are informative examples in their usage of national citizen identification numbers as a method of linking clinical and administrative datasets together (Cnudde et al. 2016 ; Zhang et al. 2018 ). Singular patient identification numbers do not yet exist in the US despite their inclusion in HIPAA due to subsequent Congressional action preventing their creation (Davis 2019 ). Instead private providers have stepped in to bridge the gap, but have also called on the US government to create an official patient ID system (Davis 2019 ). Not only would a singular patient ID allow for researchers to link US administrative data together with clinical outcomes, but also provide a solution to the questions of data ownership and fragmentation that plague the current system.
Healthcare future will build on the Big Data projects currently being pioneered around the world. The models of data integration being pioneered by the “All of Us” trial and analytics championed by P4 medicine will come to define the patient experience (Flores et al. 2013 ). However, in this piece we have demonstrated a series of hurdles that the field must overcome to avoid imposing additional burdens on physicians and to deliver significant value. We recommend a set of proposals built upon an examination of the NHS and other publicly administered healthcare models and the US multi-payer system to bridge the gap between the market competition needed to develop these new technologies and effective patient care.
Access to patient data must be a paramount guiding principle as regulators begin to approach the problem of wrangling the many streams of data that are already being generated. Data must both be accessible to physicians and patients, but must also be secured and de-identified for the benefit of research. A pathway taken by the UK Biobank to guarantee data integration and universal access has been through the creation of a single database and protocol for accessing its contents (Allen et al. 2012 ). It is then feasible to suggest a similar system for the NHS which is already centralized with a single funding source. However, this system will necessarily also be a security concern due to its centralized nature, even if patient data is encrypted (Fig. 3 ). Another approach is to follow in the footsteps of the US’ HIPAA, which suggested the creation of unique patient IDs over 20 years ago. With a single patient identifier, EHRs would then be allowed to communicate with heterogeneous systems especially designed for labs or imaging centers or counseling services and more (Fig. 4 ). However, this design presupposes a standardized format and protocol for communication across a variety of databases—similar to the HL7 standards that already exist (Bender and Sartipi 2013 ). In place of a centralized authority building out a digital infrastructure to house and communicate patient data, mandating protocols and security standards will allow for the development of specialized EHR solutions for an ever diversifying set of healthcare providers and encourage the market needed for continual development and support of these systems. Avoiding data fragmentation as seen already in the US then becomes an exercise in mandating data sharing in law.
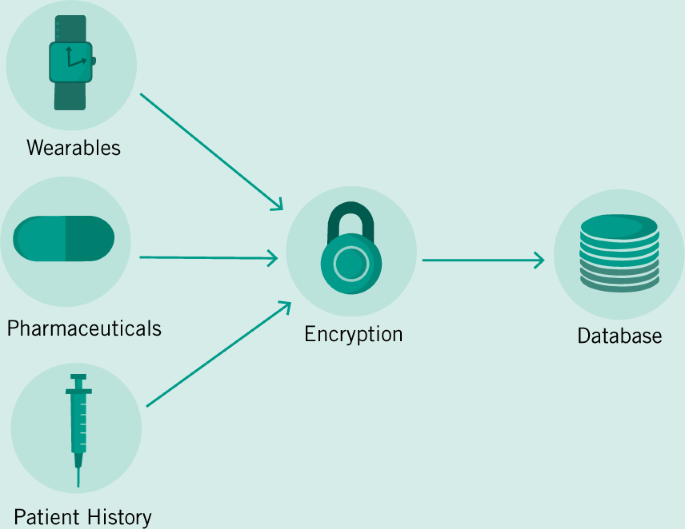
Future implementations of Big Data will need to not only integrate data, but also encrypt and de-identify it for secure storage.
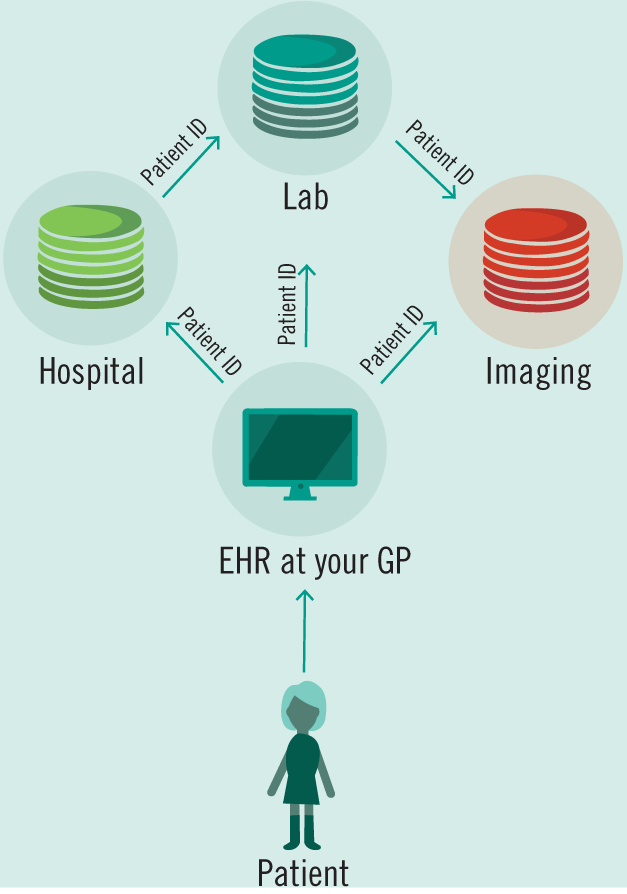
Hypothetical healthcare system design based on unique patient identifiers that function across a variety of systems and providers—linking together disparate datasets into a complete patient profile.
The next problem then becomes the inevitable application of AI to healthcare. Any such tool created will have to stand up to the scrutiny not just of being asked to outclass human diagnoses, but to also reveal its methods. Because of the opacity of ML models, the “black box” effect means that diagnoses cannot be scrutinized or understood by outside observers (Fig. 5 ). This makes clinical use extremely limited, unless further techniques are developed to deconvolute the decision-making process of these models. Until then, we expect that AI models will only provide support for diagnoses.
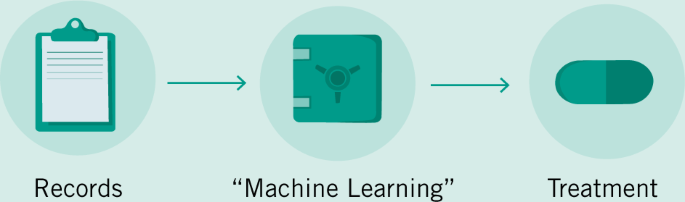
Without transparency in many of the models being implemented as to why and how decisions are being made, there exists room for algorithmic bias and no room for improvement or criticism by physicians. The “black box” of machine learning obscures why decisions are made and what actually affects predictions.
Furthermore, many times AI models simply replicate biases in existing datasets. Cohn et al. 2017 demonstrated clear areas of deficiency in the minority representation of patients in the UK Biobank. Any research conducted on these datasets will necessarily only be able to create models that generalize to the population in them (a largely homogenous white-British group) (Fig. 6 ). In order to protect against algorithmic bias and the black box of current models hiding their decision-making, regulators must enforce rules that expose the decision-making of future predictive healthcare models to public and physician scrutiny. Similar to the existing FDA regulatory framework for medical devices, algorithms too must be put up to regulatory scrutiny to prevent discrimination, while also ensuring transparency of care.
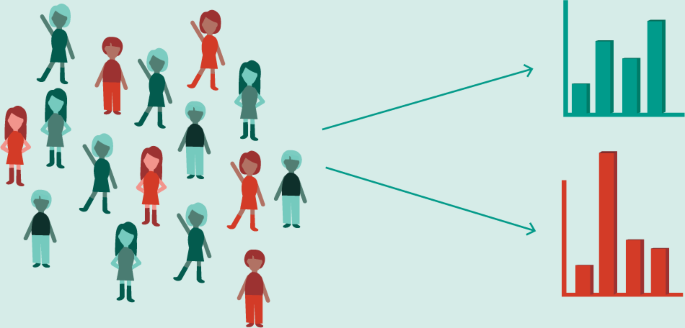
The “All of Us” study will meet this need by specifically aiming to recruit a diverse pool of participants to develop disease models that generalize to every citizen, not just the majority (Denny et al. 2019 ). Future global Big Data generation projects should learn from this example in order to guarantee equality of care for all patients.
The future of healthcare will increasingly live on server racks and be built in glass office buildings by teams of programmers. The US must take seriously the benefits of centralized regulations and protocols that have allowed the NHS to be enormously successful in preventing the problem of data fragmentation—while the NHS must approach the possibility of freer markets for healthcare devices and technologies as a necessary condition for entering the next generation of healthcare delivery which will require constant reinvention and improvement to deliver accurate care.
Overall, we are entering a transition in how we think about caring for patients and the role of a physician. Rather than creating a reactive healthcare system that finds cancers once they have advanced to a serious stage—Big Data offers us the opportunity to fine tune screening and prevention protocols to significantly reduce the burden of diseases such as advanced stage cancers and metastasis. This development allows physicians to think more about a patient individually in their treatment plan as they leverage information beyond rough demographic indicators such as genomic sequencing of their tumor. Healthcare is not yet prepared for this shift, so it is the job of governments around the world to pay attention to how each other have implemented Big Data in healthcare to write the regulatory structure of the future. Ensuring competition, data security, and algorithmic transparency will be the hallmarks of how we think about guaranteeing better patient care.
Adibuzzaman M, DeLaurentis P, Hill J, Benneyworth BD (2018) Big data in healthcare—the promises, challenges and opportunities from a research perspective: a case study with a model database. AMIA Annu Symp Proc 2017:384–392
PubMed PubMed Central Google Scholar
Agbo CC, Mahmoud QH, Eklund JM (2019) Blockchain technology in healthcare: a systematic review. Healthcare 7:56
Article PubMed Central Google Scholar
Aguet F, Brown AA, Castel SE, Davis JR, He Y, Jo B et al. (2017) Genetic effects on gene expression across human tissues. Nature 550:204–213
Article Google Scholar
Akbarian S, Liu C, Knowles JA, Vaccarino FM, Farnham PJ, Crawford GE et al. (2015) The PsychENCODE project. Nat Neurosci 18:1707–1712
Article CAS PubMed PubMed Central Google Scholar
Allen N, Sudlow C, Downey P, Peakman T, Danesh J, Elliott P et al. (2012) UK Biobank: current status and what it means for epidemiology. Health Policy Technol 1:123–126
Assis-Hassid S, Grosz BJ, Zimlichman E, Rozenblum R, Bates DW (2019) Assessing EHR use during hospital morning rounds: a multi-faceted study. PLoS ONE 14:e0212816
Bang CS, Baik GH (2019) Using big data to see the forest and the trees: endoscopic submucosal dissection of early gastric cancer in Korea. Korean J Intern Med 34:772–774
Article PubMed PubMed Central Google Scholar
Bender D, Sartipi K (2013) HL7 FHIR: an agile and RESTful approach to healthcare information exchange. In Proceedings of the 26th IEEE International Symposium on Computer-Based Medical Systems, IEEE. pp 326–331
Bibault J-E, Giraud P, Burgun A (2016) Big Data and machine learning in radiation oncology: state of the art and future prospects. Cancer Lett 382:110–117
Article CAS PubMed Google Scholar
Blobel B (2018) Interoperable EHR systems—challenges, standards and solutions. Eur J Biomed Inf 14:10–19
Google Scholar
Camacho DM, Collins KM, Powers RK, Costello JC, Collins JJ (2018) Next-generation machine learning for biological networks. Cell 173:1581–1592
Campbell PJ, Getz G, Stuart JM, Korbel JO, Stein LD (2020) Pan-cancer analysis of whole genomes. Nature https://www.nature.com/articles/s41586-020-1969-6
Chambers DA, Amir E, Saleh RR, Rodin D, Keating NL, Osterman TJ, Chen JL (2019) The impact of Big Data research on practice, policy, and cancer care. Am Soc Clin Oncol Educ Book Am Soc Clin Oncol Annu Meet 39:e167–e175
Char DS, Shah NH, Magnus D (2018) Implementing machine learning in health care—addressing ethical challenges. N Engl J Med 378:981–983
Cho WC (2015) Big Data for cancer research. Clin Med Insights Oncol 9:135–136
Cnudde P, Rolfson O, Nemes S, Kärrholm J, Rehnberg C, Rogmark C, Timperley J, Garellick G (2016) Linking Swedish health data registers to establish a research database and a shared decision-making tool in hip replacement. BMC Musculoskelet Disord 17:414
Cohn EG, Hamilton N, Larson EL, Williams JK (2017) Self-reported race and ethnicity of US biobank participants compared to the US Census. J Community Genet 8:229–238
Connelly R, Playford CJ, Gayle V, Dibben C (2016) The role of administrative data in the big data revolution in social science research. Soc Sci Res 59:1–12
Article PubMed Google Scholar
Davis J (2019) National patient identifier HIPAA provision removed in proposed bill. HealthITSecurity https://healthitsecurity.com/news/national-patient-identifier-hipaa-provision-removed-in-proposed-bill
DeMartino JK, Larsen JK (2013) Data needs in oncology: “Making Sense of The Big Data Soup”. J Natl Compr Canc Netw 11:S1–S12
Deng J, El Naqa I, Xing L (2018) Editorial: machine learning with radiation oncology big data. Front Oncol 8:416
Denny JC, Rutter JL, Goldstein DB, Philippakis Anthony, Smoller JW, Jenkins G et al. (2019) The “All of Us” research program. N Engl J Med 381:668–676
Elliott LT, Sharp K, Alfaro-Almagro F, Shi S, Miller KL, Douaud G et al. (2018) Genome-wide association studies of brain imaging phenotypes in UK Biobank. Nature 562:210–216
Essin D (2012) Improve EHR systems by rethinking medical billing. Physicians Pract. https://www.physicianspractice.com/ehr/improve-ehr-systems-rethinking-medical-billing
Esteva A, Robicquet A, Ramsundar B, Kuleshov V, DePristo M, Chou K et al. (2019) A guide to deep learning in healthcare. Nat Med 25:24–29
Fessele KL (2018) The rise of Big Data in oncology. Semin Oncol Nurs 34:168–176
Flores M, Glusman G, Brogaard K, Price ND, Hood L (2013) P4 medicine: how systems medicine will transform the healthcare sector and society. Pers Med 10:565–576
Article CAS Google Scholar
Garber S, Gates SM, Keeler EB, Vaiana ME, Mulcahy AW, Lau C et al. (2014) Redirecting innovation in U.S. Health Care: options to decrease spending and increase value: Case Studies 133
Gardner RL, Cooper E, Haskell J, Harris DA, Poplau S, Kroth PJ et al. (2019) Physician stress and burnout: the impact of health information technology. J Am Med Inf Assoc 26:106–114
Gawande A (2018) Why doctors hate their computers. The New Yorker , 12 https://www.newyorker.com/magazine/2018/11/12/why-doctors-hate-their-computers
Gordon WJ, Catalini C (2018) Blockchain technology for healthcare: facilitating the transition to patient-driven interoperability. Comput Struct Biotechnol J 16:224–230
Hasin Y, Seldin M, Lusis A (2017) Multi-omics approaches to disease. Genome Biol 18:83
Honeyman M, Dunn P, McKenna H (2016) A Digital NHS. An introduction to the digital agenda and plans for implementation https://www.kingsfund.org.uk/sites/default/files/field/field_publication_file/A_digital_NHS_Kings_Fund_Sep_2016.pdf
Kierkegaard P (2013) eHealth in Denmark: A Case Study. J Med Syst 37
Krumholz HM (2014) Big Data and new knowledge in medicine: the thinking, training, and tools needed for a learning health system. Health Aff 33:1163–1170
Lenzer J (2017) Commentary: the real problem is that electronic health records focus too much on billing. BMJ 356:j326
Leonard D, Tozzi J (2012) Why don’t more hospitals use electronic health records. Bloom Bus Week
Macaulay T (2016) Progress towards a paperless NHS. BMJ 355:i4448
Madhavan S, Subramaniam S, Brown TD, Chen JL (2018) Art and challenges of precision medicine: interpreting and integrating genomic data into clinical practice. Am Soc Clin Oncol Educ Book Am Soc Clin Oncol Annu Meet 38:546–553
Marx V (2015) The DNA of a nation. Nature 524:503–505
Miller RS (2011) Electronic health record certification in oncology: role of the certification commission for health information technology. J Oncol Pr 7:209–213
Norgeot B, Glicksberg BS, Butte AJ (2019) A call for deep-learning healthcare. Nat Med 25:14–15
O’Brien R, Potter-Collins A (2015) 2011 Census analysis: ethnicity and religion of the non-UK born population in England and Wales: 2011. Office for National Statistics. https://www.ons.gov.uk/peoplepopulationandcommunity/culturalidentity/ethnicity/articles/2011censusanalysisethnicityandreligionofthenonukbornpopulationinenglandandwales/2015-06-18
Osong AB, Dekker A, van Soest J (2019) Big data for better cancer care. Br J Hosp Med Lond Engl 2005 80:304–305
Rabesandratana T (2019) European data law is impeding studies on diabetes and Alzheimer’s, researchers warn. Sci AAAS. https://doi.org/10.1126/science.aba2926
Raghupathi W, Raghupathi V (2014) Big data analytics in healthcare: promise and potential. Health Inf Sci Syst 2:3
Reisman M (2017) EHRs: the challenge of making electronic data usable and interoperable. Pharm Ther 42:572–575
Shendure J, Ji H (2008) Next-generation DNA sequencing. Nature Biotechnology 26:1135–1145
Stephens ZD, Lee SY, Faghri F, Campbell RH, Zhai C, Efron MJ et al. (2015) Big Data: astronomical or genomical? PLOS Biol 13:e1002195
Tomczak K, Czerwińska P, Wiznerowicz M (2015) The Cancer Genome Atlas (TCGA): an immeasurable source of knowledge. Contemp Oncol 19:A68–A77
Topol E (2019a) High-performance medicine: the convergence of human and artificial intelligence. Nat Med 25:44
Topol E (2019b) The topol review: preparing the healthcare workforce to deliver the digital future. Health Education England https://topol.hee.nhs.uk/
Turnbull C, Scott RH, Thomas E, Jones L, Murugaesu N, Pretty FB, Halai D, Baple E, Craig C, Hamblin A, et al. (2018) The 100 000 Genomes Project: bringing whole genome sequencing to the NHS. BMJ 361
Wallace WA (2016) Why the US has overtaken the NHS with its EMR. National Health Executive Magazine, pp 32–34 http://www.nationalhealthexecutive.com/Comment/why-the-us-has-overtaken-the-nhs-with-its-emr
Webster PC (2014) Sweden’s health data goldmine. CMAJ Can Med Assoc J 186:E310
Wetterstrand KA (2019) DNA sequencing costs: data from the NHGRI Genome Sequencing Program (GSP). Natl Hum Genome Res Inst. www.genome.gov/sequencingcostsdata , Accessed 2019
Zhang L, Wang H, Li Q, Zhao M-H, Zhan Q-M (2018) Big data and medical research in China. BMJ 360:j5910
Download references
Author information
Authors and affiliations.
Department of Genetics, University of Cambridge, Downing Site, Cambridge, CB2 3EH, UK
Raag Agrawal & Sudhakaran Prabakaran
Department of Biology, Columbia University, 116th and Broadway, New York, NY, 10027, USA
Raag Agrawal
Department of Biology, Indian Institute of Science Education and Research, Pune, Maharashtra, 411008, India
Sudhakaran Prabakaran
St Edmund’s College, University of Cambridge, Cambridge, CB3 0BN, UK
You can also search for this author in PubMed Google Scholar
Corresponding author
Correspondence to Sudhakaran Prabakaran .
Ethics declarations
Conflict of interest.
SP is co-founder of Nonexomics.
Additional information
Publisher’s note Springer Nature remains neutral with regard to jurisdictional claims in published maps and institutional affiliations.
Associate editor: Frank Hailer
Rights and permissions
Open Access This article is licensed under a Creative Commons Attribution 4.0 International License, which permits use, sharing, adaptation, distribution and reproduction in any medium or format, as long as you give appropriate credit to the original author(s) and the source, provide a link to the Creative Commons license, and indicate if changes were made. The images or other third party material in this article are included in the article’s Creative Commons license, unless indicated otherwise in a credit line to the material. If material is not included in the article’s Creative Commons license and your intended use is not permitted by statutory regulation or exceeds the permitted use, you will need to obtain permission directly from the copyright holder. To view a copy of this license, visit http://creativecommons.org/licenses/by/4.0/ .
Reprints and permissions
About this article
Cite this article.
Agrawal, R., Prabakaran, S. Big data in digital healthcare: lessons learnt and recommendations for general practice. Heredity 124 , 525–534 (2020). https://doi.org/10.1038/s41437-020-0303-2
Download citation
Received : 28 June 2019
Revised : 25 February 2020
Accepted : 25 February 2020
Published : 05 March 2020
Issue Date : April 2020
DOI : https://doi.org/10.1038/s41437-020-0303-2
Share this article
Anyone you share the following link with will be able to read this content:
Sorry, a shareable link is not currently available for this article.
Provided by the Springer Nature SharedIt content-sharing initiative
This article is cited by
Lightweight federated learning for stis/hiv prediction.
- Thi Phuoc Van Nguyen
- Wencheng Yang
Scientific Reports (2024)
Using machine learning approach for screening metastatic biomarkers in colorectal cancer and predictive modeling with experimental validation
- Amirhossein Ahmadieh-Yazdi
- Ali Mahdavinezhad
- Saeid Afshar
Scientific Reports (2023)
Introducing AI to the molecular tumor board: one direction toward the establishment of precision medicine using large-scale cancer clinical and biological information
- Ryuji Hamamoto
- Takafumi Koyama
- Noboru Yamamoto
Experimental Hematology & Oncology (2022)
Quick links
- Explore articles by subject
- Guide to authors
- Editorial policies

Home › Blog › Use Cases of Big Data Analytics in the Healthcare Industry
Use Cases of Big Data Analytics in the Healthcare Industry
In the ever-evolving landscape of the healthcare industry, the integration of big data analytics has emerged as a transformative force. The vast amounts of data generated within the healthcare ecosystem hold immense potential for insights that can enhance patient outcomes, optimize operations, and drive innovation. This case study explores the multifaceted use cases, benefits, impacts, and challenges of big data analytics in healthcare, elucidating the importance of this technology in shaping the future of healthcare delivery.
Healthcare Industry Overview
The healthcare industry has seen a complete overhaul in the recent years due to big data analytics. Given the ubiquity of healthcare data generated by business processes within the healthcare sector, healthcare data analytics and big data analytics for smart healthcare play an increasingly important role in every aspect of healthcare decision making. In such an environment, the use of solutions such as big data analytics for smart healthcare is not only crucial to analyze data generated by smart health devices but also an essential source of patient and operational information that drives decision making in the healthcare services sector.
Factors like increasing health costs and the outbreak of chronic diseases have necessitated the use of health insurance among people. This has subsequently resulted in the continuous growth and development of companies in the health insurance marketplace. However, the processing of health insurance claims involves a huge amount of data. This acts as a major obstacle in maintaining an efficient utilization management process.
While the era of consumerism in healthcare has finally arrived. The availability of healthcare newsletters from healthcare industry players, direct-to-consumer advertising of pharmaceuticals, and most importantly, the ubiquity of the Internet has made it easier for consumers to obtain information about their medical conditions and possible treatments at their fingertips. As customer satisfaction becomes an increasingly important metric, consumerism presents healthcare industry players with both challenges and opportunities.
A detailed healthcare industry analysis reveals that this sector is different from other customer-facing sectors and there isn’t a clean one-to-one transition between what works for other consumer-facing industries and what works in the healthcare industry. The growing focus toward consumerism has been manifested through payment options such as- the growing importance of big data, technological adaptations, and tax-advantaged healthcare accounts. This has resulted in a more competitive marketplace and this competition is expected to bring about major improvements in business practices and, potentially, health outcomes.
Use Cases of Big Data Analytics in Healthcare:
- Predictive Analytics for Disease Prevention: Big data analytics enables the identification of patterns and trends in patient data, facilitating predictive models for disease outbreaks and epidemics. This proactive approach supports public health interventions and preventive measures.
- Clinical Decision Support Systems: Healthcare data analytics companies can leverage big data analytics to access comprehensive patient records, treatment histories, and relevant medical literature. This wealth of information aids in making well-informed, data-driven decisions about patient care.
- Personalized Medicine and Treatment Plans: Analyzing large datasets allows for a deeper understanding of individual patient characteristics, genetic makeup, and treatment responses. This, in turn, enables the development of personalized medicine tailored to a patient’s unique profile.
- Efficient Operational Management: Healthcare institutions utilize big data analytics to optimize their operational efficiency. From managing patient flow to resource allocation, analytics plays a pivotal role in streamlining processes and minimizing bottlenecks.
- Fraud Detection and Prevention: Big data analytics can be employed to detect anomalies and patterns indicative of fraudulent activities, protecting healthcare organizations from financial losses and ensuring the integrity of insurance claims.
Benefits of Big Data Analytics in Healthcare:
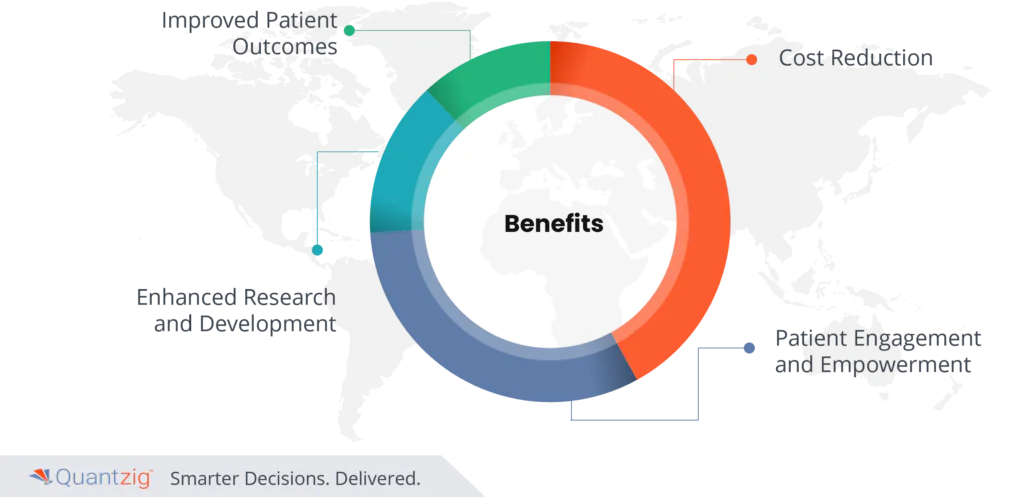
- Improved Patient Outcomes: By providing actionable insights into patient data, big data analytics contributes to enhanced diagnostics, treatment plans, and overall care, resulting in improved patient outcomes.
- Cost Reduction: Optimizing operational workflows and resource allocation through analytics leads to cost reductions for healthcare providers, allowing them to allocate resources more efficiently.
- Enhanced Research and Development: Big data analytics accelerates medical research by providing researchers with access to vast datasets for clinical trials, genomic studies, and drug development, expediting the discovery of new treatments.
- Patient Engagement and Empowerment: Analytics facilitates the development of patient-centric approaches, empowering individuals to actively participate in their healthcare decisions and treatment plans.
Impact and Importance of Big Data Analytics in Healthcare:
- Data-Driven Insights: Big data analytics transforms raw healthcare data into meaningful insights, empowering healthcare professionals to make informed decisions based on evidence and trends.
- Population Health Management: Analyzing large datasets helps healthcare organizations manage the health of populations more effectively, identifying at-risk groups and implementing preventive measures.
- Real-time Monitoring: The ability to monitor patient vitals and health parameters in real-time enables timely interventions, reducing the risk of complications and improving overall patient care.
- Innovation and Continuous Improvement: Healthcare providers can harness big data analytics to drive innovation, implement continuous improvement strategies, and stay abreast of evolving medical practices.
Challenges of Big Data Analytics in Healthcare:
- Data Security and Privacy Concerns: Healthcare data is sensitive and subject to strict privacy regulations. Ensuring the security and privacy of patient information poses a significant challenge.
- Interoperability Issues: The integration of disparate data sources, often using different systems and formats, poses challenges to achieving seamless interoperability, hindering the effectiveness of analytics.
- Resource Constraints: Implementing and maintaining robust big data analytics infrastructure requires significant resources, both in terms of technology and skilled personnel, which may be a barrier for some healthcare organizations.
- Ethical Considerations: As analytics delve into personal health information, ethical considerations surrounding consent, data usage, and potential biases in algorithms become critical concerns.
About the Healthcare Client
Headquartered in California, the client is a Fortune 500 healthcare organization. The healthcare industry player employs nearly 9,000+ employees globally and is well-known for venturing into digital healthcare domain.
Challenges Faced by the Healthcare Client:
Like every other organization in the industry, our client was also facing a myriad of challenges.
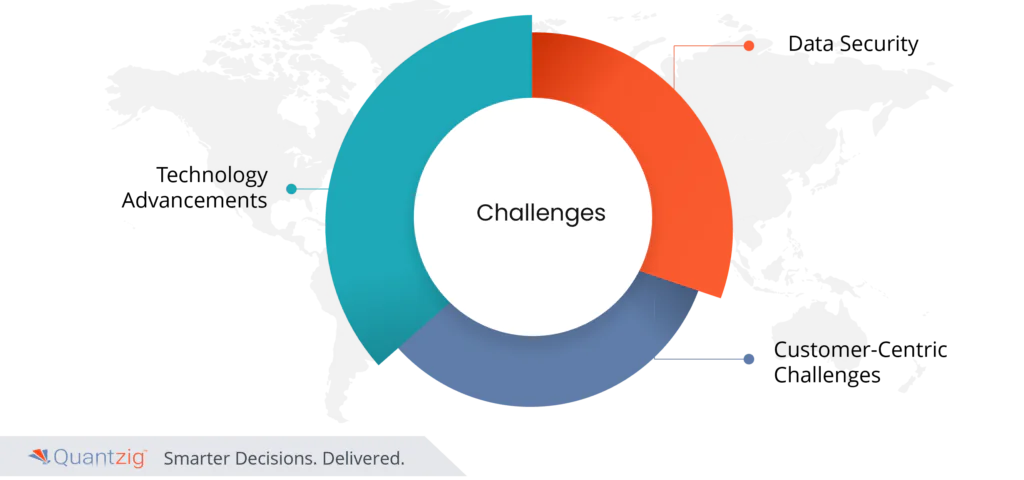
- Technology Advancements: Health insurance companies are plagued by factors like lack of innovative capabilities and operational expansion. Such issues have stunted the market growth of health insurance companies. In the dynamic health insurance marketplace, technical advancements coupled with increasing consumer buying power is considered crucial since it drives the analytics behavior of the health insurance data.
- Data Security: In the digitized age, a lot of people are concerned about security breaches when it comes to sharing their personal data with the healthcare provider. The data is critical for the health insurance companies to calculate and determine insurance policies for the buyers. Hence, it is important for health insurance companies to strengthen their security measures and assure the same to the customers in order to drive their insurance policy sale.
- Customer-Centric Challenges: The healthcare industry player was facing several other customer-centric challenges that prevented them from retaining their market position in the healthcare space. With healthcare increasingly becoming consumer-focused the healthcare industry player faced challenges in leveraging their big data to personalize care and services rendered. The client believed that improving patient experience was all about embracing consumerism to transform themselves into a consumer-centric healthcare brand.
Solutions Delivered with Big Data Analytics
The client was able to leverage big data analytics to realize a plethora of benefits. In order to help our readers effectively gauge our impact, we’ll be highlighting the benefits in a use-case format:
Utilization Management Process: The client was able to improve their utilization management (UM) process through Quantzig’s big data analytics solution to curtail wastage of resources. This process is significant in governing the pre-approval of health insurance coverage for many medical procedures. The big data analytics solution helped identify lucrative areas of investment in the health insurance marketplace. Additionally, the solution offered provided the necessary information to effectively assess the amount of premium they need to quote to a prospective health insurance buyer.
Effectively Overcome Challenges: Quantzig’s big data analytics solution aided the healthcare industry player to sort through their internal as well as external data points to validate several assumptions and discover new ones that they were unaware of. Big data analytics along with the consultative support from our experts offered a unique solution to overcome their big data challenges. Embracing big data analytics as a lifeline not only guided the healthcare industry player to a more stable ground but also enhanced data transparency to build new financial structures and patient care models.
Examine Data Sets in Healthcare: With the help of big data analytics, the medical imaging firm was able to examine varied data sets in patient healthcare. Moreover, the medical imaging client was able to tap potential market trends and customer preferences and make further informed business decisions. The engagement also assisted the client in analyzing the potentialities of the market in terms of the opportunities, customer service, and further improved operational efficiency. The engagement also helped the client identify more effective ways of promoting businesses and curtail additional costs associated with storing data.
Request for more information to know how you can leverage big data analytics to empower your organization with deeper insights to enhance data-driven decisions.
Early Diagnosis + Clinical Risk Score: The aim of big data analytics for smart healthcare is to focus on improvements that can help healthcare data analytics companies to identify and analyze key signs and compare them with the observations recorded during initial assessments to compute a risk score that indicates a patient’s attrition rate based on the risk of decline. We helped our client employ a clinical risk score as an indicator of patient decline based on the data generated by smart health devices to help optimize care and enhance device efficiency.
Evidence-Based Protocols: The application of big data analytics for smart healthcare service improvements offers several other benefits that help healthcare service providers to gain detailed insights into the factors impacting patient health. We helped our client gain insight into the completeness and timeliness of vitals, modifiers, and other documentation recorded, and identify outliers and opportunities by leveraging healthcare data sets.
Reduction of False Alarms: Adopting big data analytics for smart healthcare improvements helps healthcare service providers to track and monitor patient health based on location data and demographics. It also offered comprehensive insights on device utilization rates and location in case of maintenance and troubleshooting requirements. With healthcare analytics, we helped our clients gauge and analyze thresholds for alarms on a single dashboard that uses data to manually adjust and predict how changes in threshold values will impact the number of alarms received.
Big data analytics has ushered in a new era in healthcare, promising improved patient care, operational efficiency, and transformative innovations. The use cases, benefits, impacts, and challenges outlined demonstrate the critical role big data analytics plays in shaping the healthcare landscape. As technology continues to evolve, the integration of big data analytics will be pivotal in driving positive outcomes for both healthcare providers and the individuals they serve.
Big Data Analytics Solution Insights
Quantzig’s big data analytics solution analyzes diverse and complex data to constantly improve and increase the scope of patient care. This includes an analysis of all the necessary processes in healthcare insurance management. This solution helped the health insurance company to leverage actionable insights and gain a futuristic vision, which, in turn, helped them in organizing their processes and establish their presence in the health insurance marketplace.
With years of expertise in offering a plethora of services, Quantzig’s big data analytics solution helps healthcare data analytics companies in the medical imaging space manipulate and manage large data sets and improve healthcare outcomes while reducing costs.
Speak with our analytics experts to know how big data analytics can help healthcare data analytics companies gain comprehensive insights to deliver intelligent, timely actions that improve customer engagement, increase revenue, and lower costs.
Recent Posts
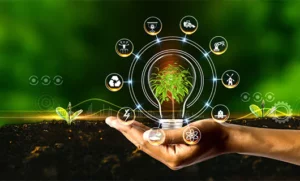
Decoding Direct to Consumer Strategies: D2C Success in CPG Brands
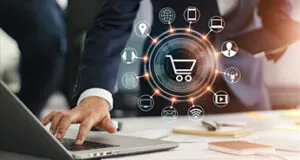
CPG Insights: Navigating D2C Analytics Profitability with Data Analytics
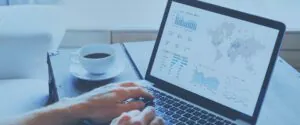
Optimizing Manufacturing Efficiency: Unleashing the Power of ERP Capacity Planning
Privacy overview.
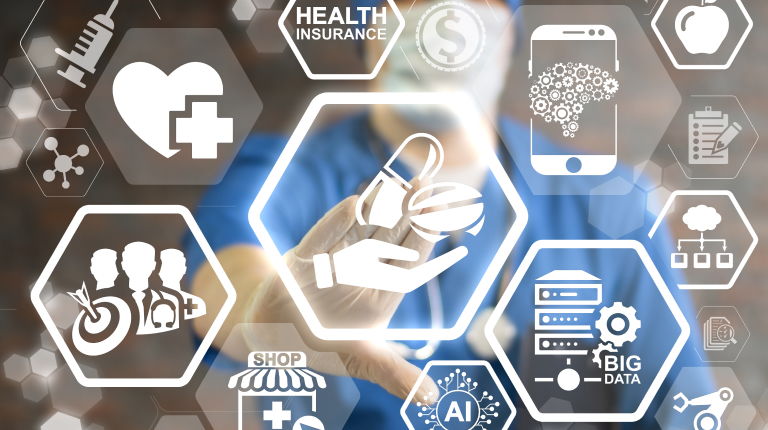
Data Science Cases in Healthcare in 2024
Table of contents:.
Data science revolutionizes healthcare by providing insights and applications that transform patient care and operations. DATAFOREST applies data science to drive business success in healthcare while offering these capabilities outside the sector.
Performance Optimization & Bottlenecks Elimination
performance boost
cost optimization

Daniel Garner

The team of DATAFOREST is very skilled and equipped with high knowledge.
Data science is increasingly important in healthcare as healthcare generates more and more data. Data scientists are needed to analyze this information to achieve improvements in patient outcomes and reduced costs, among other things. Healthcare delivery is transforming due to data science, from predicting disease outbreaks and developing personalized treatment plans.
This blog provides insights and applications of data science in healthcare. We will discuss the types of data science used in this industry, how it can be leveraged effectively to produce valuable results—and case studies on successful data science projects.
What is Data Science in the Healthcare Industry?
Data science uses advanced analytics, machine learning, and artificial intelligence to uncover previously hidden patterns within large amounts of data. Applying data science in healthcare and data science in medicine can use advanced analytics and machine learning algorithms to derive insights and make predictions that can improve patient outcomes. It includes predictive analytics and image analysis—which help identify patterns that would be impossible to detect with other methods. Health, medical, and biomedical data science are all branches of the larger field of data analytics. This data can include electronic health records, medical imaging, and other sources of healthcare-related information. Some examples of how data science is currently used in healthcare include predicting patient readmissions and identifying patients at risk for developing chronic diseases. Additionally, the practice has been proven to enhance the accuracy of medical diagnoses.
Overview of the Healthcare Industry
In recent years, according to research by Springer in the healthcare industry , Data Science has become increasingly important due to the vast amounts of data generated from patient records, medical images, and clinical trial results. Healthcare organizations can use data science in the healthcare industry to make sense of the vast amounts of data they collect and apply it to improve patient outcomes, optimize operations, and cut costs.
For example, predictive analytics can help healthcare providers identify patients at risk for certain conditions and intervene early—preventing the condition from progressing. Precision medicine tailors treatment plans to individual patients based on their unique genetic makeup and other factors.
DATAFOREST specializes in helping healthcare organizations implement solutions that harness the power of Data Science. Our team can develop and deploy data-driven strategies to improve patient outcomes, streamline operations, and drive business success.
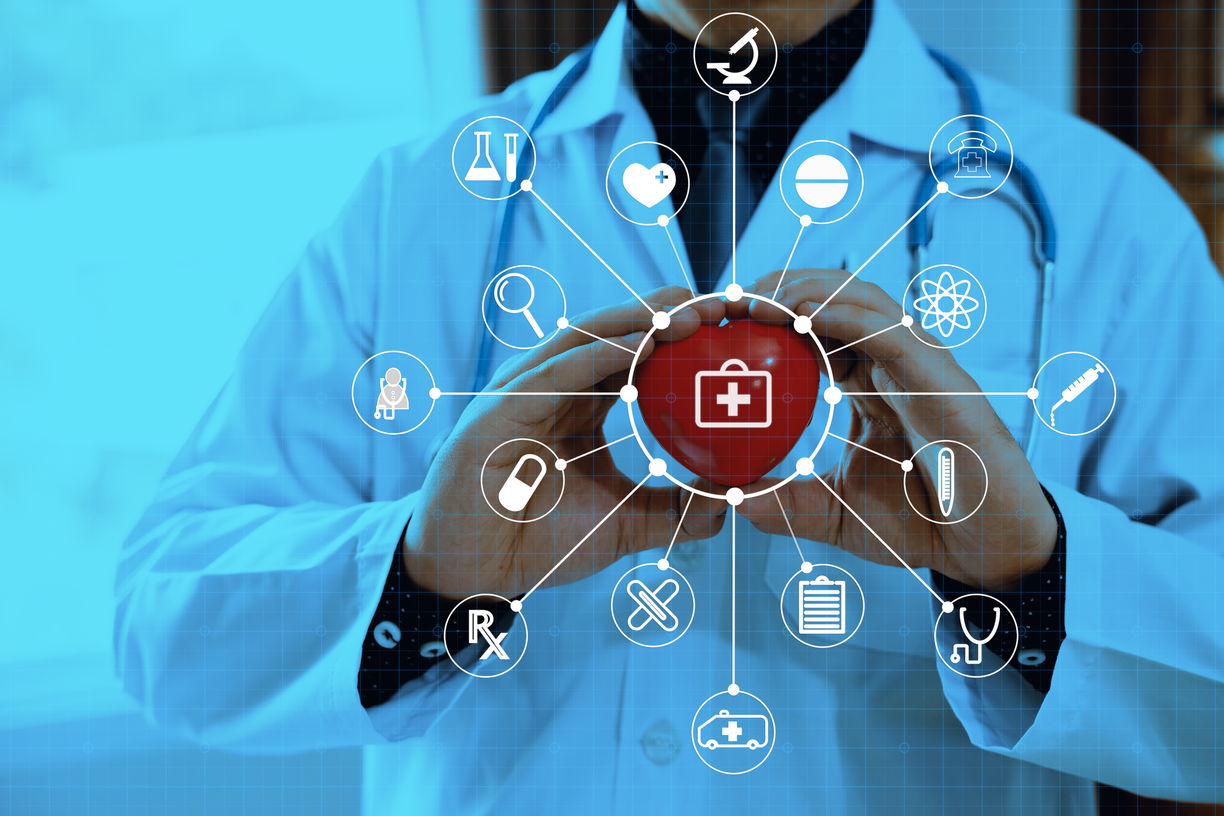
Advantages of The Data Science in Healthcare
The global healthcare analytics market size is expected to reach USD 167.0 billion by 2030, expanding at a CAGR of 21.4% during the forecast period, according to a new report by Grand View Research Inc. This is because health data science, or health informatics, as it is called in some circles, has several benefits for doctors and patients.
Here are 10 key benefits of data science for healthcare organizations:
- Improved patient outcomes: Data science can help healthcare providers develop more effective treatment plans by considering individual patient data.
- Predictive analytics: Data science can help healthcare providers identify patients at risk of developing certain conditions early on and prevent those conditions from becoming severe problems.
- Precision medicine: By using data science to tailor treatment plans, doctors can make better decisions about how best to treat individual patients.
- Enhanced research: Data science is an emerging field that uses large datasets to identify patterns and trends relevant to medical research. It can lead to the discovery of new treatments.
- Operational optimization: Healthcare organizations can use data science to optimize operations, reduce waste, and streamline processes—all of which improve patient care and increase profitability.
- Improved patient engagement: Personalized data analysis can help healthcare providers understand their patients better, improving patient outcomes.
- Real-time monitoring: Healthcare providers can use data science to monitor patient health in real-time, improving outcomes and the timing of interventions.
- Cost reduction: Healthcare organizations can use data science to identify areas to reduce costs. For example, by analyzing patient data, we might identify patients at risk for readmission and intervene early to prevent this costly scenario from happening again.
- Improved decision-making: Healthcare organizations can use data-driven decision-making to understand their patients better and improve outcomes while reducing costs. Improved data analysis can help healthcare organizations identify opportunities to improve their operations.
- Better resource allocation: Data analysis can help healthcare organizations make better decisions about how to spend money and provide high-quality care. With the proper data science tools, hospitals can allocate resources more effectively, improving patient care and increasing profitability.
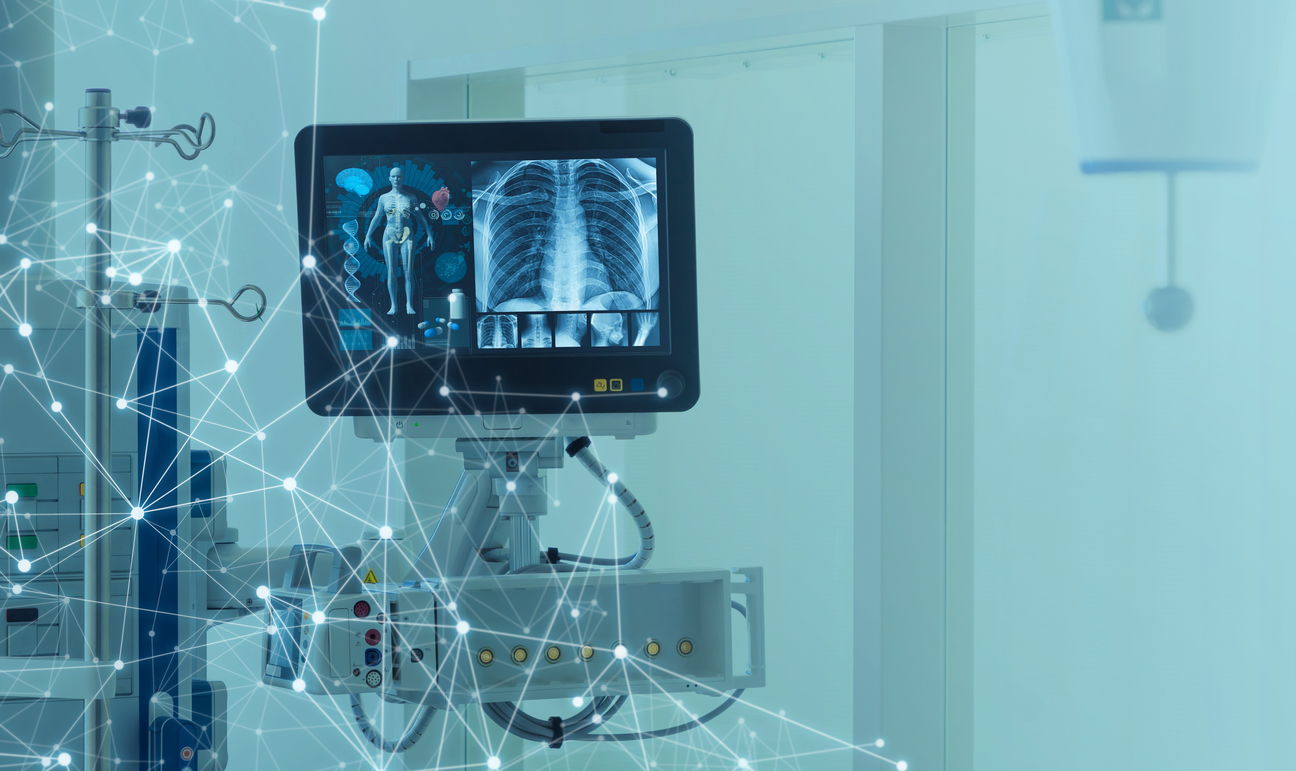
How Data Science Can Improve Healthcare Systems
Data science significantly impacts the healthcare industry, helping organizations improve patient outcomes and drive innovation. Healthcare providers can use data science to make more effective decisions, identify areas for improvement and develop better treatment plans.
Data science can also help healthcare organizations stay up-to-date with their field's latest research and developments, leading to more effective treatments and interventions. This will ultimately improve patient care/outcomes by delivering more efficient services and personalized medicine options across multiple stages, from diagnostic tests to treatment recovery.
Healthcare companies can use data science to allocate resources more efficiently and effectively, reducing costs while improving profitability. As healthcare becomes less about treatment for illness or injury and more about prevention of disease (allocation of preventative care), the importance of using data science to understand patients better will grow.
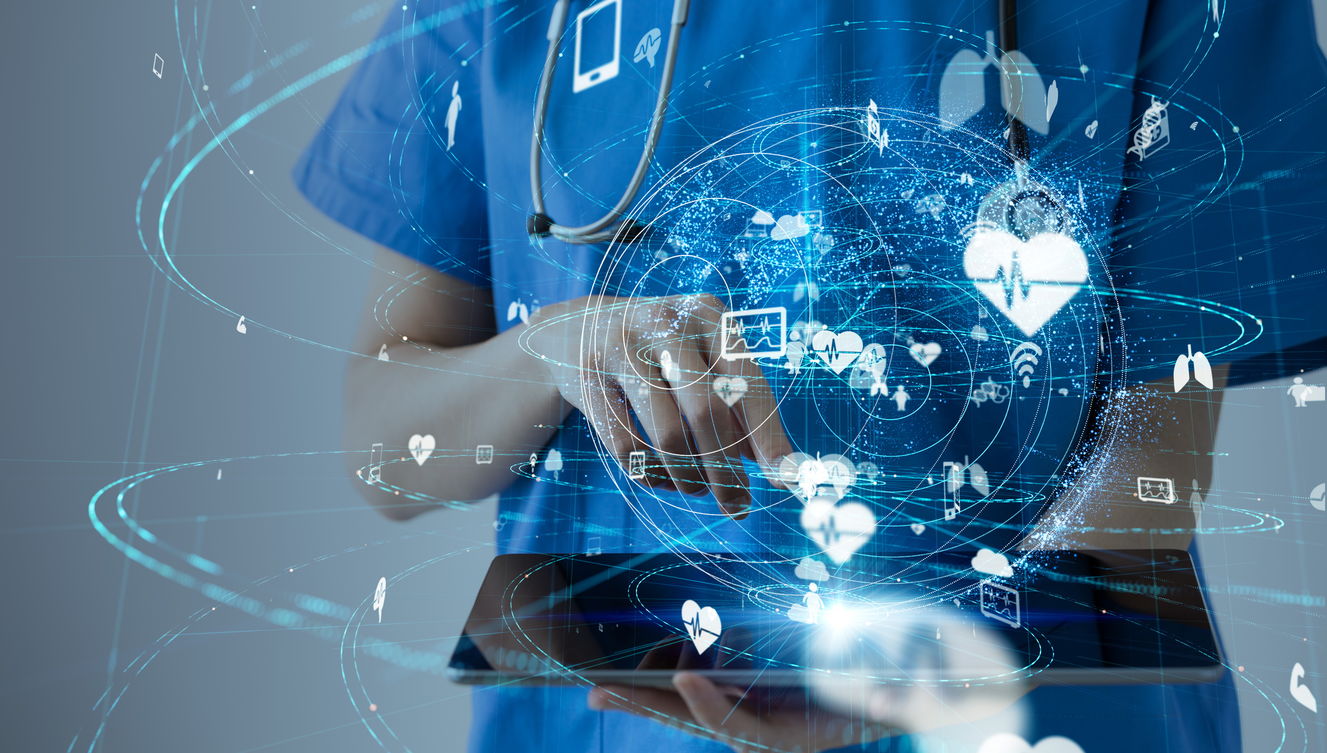
Data Science Applications in Healthcare Industry: 9 Case Studies
Data science has become an essential tool in the healthcare industry, as technology makes it easier to collect and analyze large amounts of data. Data science has contributed to the rise in patient care, offering new avenues for diagnosis and treatment.
Predictive Analytics in Patient Diagnosis
Predictive analytics can help healthcare providers identify patients at risk for certain conditions, allowing them to intervene early and prevent the condition from progressing.
#1. Case Study: Machine Learning for Heart Disease Prediction
Predictive analytics is a fantastic tool for diagnosing illnesses, allowing doctors and healthcare providers to diagnose diseases early on and develop effective treatment plans.
With machine learning algorithms, predictive analytics can identify patterns that are invisible to the human eye and use them to predict a patient's health—including by identifying risk factors for developing diseases.
Researchers at Nottingham University have demonstrated how predictive analytics can help prevent heart disease. The study used patient data—including demographic, lifestyle, and clinical factors—to create a predictive model that more accurately identifies people at risk for heart disease than traditional methods.
This example demonstrates how data science—especially machine learning technology—can be used to develop personalized patient treatment plans and improve outcomes.
Need to optimize supply chain efficiency?
#2. case study: deep learning for diabetes risk prediction.
The National Library of Medicine discusses a survey that used machine learning approaches to predict diabetes risk . The study aimed to analyze how machine learning algorithms could identify diabetes mellitus at an early stage—a severe metabolic disorder that affects so many people worldwide.
The accuracy of the algorithms was evaluated using metrics such as sensitivity, specificity, and accuracy. The results showcased that the Support Vector Machine (SVM) algorithm had the highest accuracy of 96.6%, followed by the Random Forest (RF) algorithm with an accuracy of 96.4%. The K-Nearest Neighbor (KNN) algorithm had an accuracy of 94.6%.
Early detection of diabetes is critical for effective therapy, and machine learning approaches can help achieve this.
Improving Healthcare Operations with Data Science
Healthcare providers can use data science to improve operations and make processes more efficient, reducing costs. They do this by analyzing data on patient flow, resource allocation, and other factors — then using that information to optimize their operations to meet patients' needs better.
#3. Case Study: Predictive Analytics for Hospital Readmission Rates
Hospital readmissions can be costly and disruptive for both patients and healthcare providers. Predictive analytics can be used to identify patients who are at risk for readmission, allowing healthcare providers to intervene early and prevent readmissions from occurring.
A study published in the journal Scientific Reports used machine learning to predict the hospital readmission risk from patients' claims data using machine learning , with a specific focus on chronic obstructive pulmonary disease (COPD). Researchers found that a machine learning model could accurately predict the hospital readmission risk for COPD patients, allowing earlier intervention and improved patient outcomes. A review of predictive models for hospital readmission risk found that machine-learning techniques are becoming increasingly popular and show promising results.
#4. Case Study: Data Analytics for Hospital Staff Scheduling
Optimizing hospital staff scheduling is crucial for improving patient outcomes and increasing efficiency. Data analytics can be used to analyze patient flow, staff availability, and workload—all of which feed into developing optimized schedules.
Research by Harvard Business Review demonstrated the potential of data analytics in optimizing hospital staff scheduling . The study collected data on patient flow, staff availability, and workload and used various data analytics models to analyze the data.
Data analytics models developed by the hospital's staff scheduling department yielded more efficient work schedules, leading to a 30% decrease in patient waiting time and an increase of 25% in patient outcomes. Staff satisfaction increased as well—by 20%. In addition, 15% more patients could be seen daily, with 10 percent higher customer satisfaction ratings.
This study indicates that data analytics could improve hospital staff scheduling and improve patient care.
Enhancing Drug Discovery and Development
Data science is revolutionizing drug discovery and development by using machine learning algorithms to analyze large datasets of chemical compounds. This approach enables researchers to identify potential drug candidates more efficiently, reducing the time and cost required for drug development. However, there are challenges associated with using data science in healthcare, including ethical and security considerations, biases in data used to train ML algorithms, and the need for human involvement in developing and evaluating these technologies.
#5. Case Study: Machine Learning for Accelerating Drug Development
The FDA's discussion papers on the use of AI and ML in drug development and manufacturing have been written to encourage discussion and debate on the benefits, challenges, and potential implications of applying data science to health-related issues. They aim to encourage collaboration and address these challenges to ensure data science's safe and effective use in healthcare. Overall, data science can improve patient outcomes and significantly accelerate drug development timelines.
#6. Case Study: Data Analytics for Improving Drug Efficacy
After identifying a promising drug candidate, the next step is to test its efficacy in clinical trials. However, many drugs that show promise during initial tests fail when put under rigorous conditions—such as those of actual use by patients—in later tests.
Data analytics can improve clinical trials by analyzing large patient data sets and identifying patterns that inform the design, execution, and evaluation of new treatments.
By analyzing patient data, a data scientist can determine which groups are most likely to benefit from a particular drug. Bringing together genetic mutations that cause disease in a single patient can drastically reduce the number of patients needed for drug trials, thereby reducing costs and speeding up timelines.
#7. Case Study: Optimizing Clinical Trial Design with Data Analytics
The article " The Role of Data Analytics in Improving Clinical Trials and Drug Discovery " by U.S. Food & Drug discusses how clinical trials and drug discovery can benefit from data analysis.
A database of real-world patient data, a synthetic control arm, and insights into research questions can be generated faster by analyzing databases of real-world patients.
Using machines equipped with advanced algorithms and artificial intelligence, companies can now gather data about a staggering number of patents—and turn that information into meaningful insights faster than ever before.
One of the challenges associated with traditional approaches to clinical trials is the need for more understanding of the underlying biology of diseases. However, by using synthetic control arms, clinical development, and drug discovery can be transformed. Synthetic control arms help overcome patient stratification challenges, reduce the time it takes to develop medical treatments, and improve clinical trial design and success rates. This approach can be beneficial for rare diseases where patient populations are smaller, and lifespan is short due to the disease's aggressive nature.
Personalized Medicine
Personalized medicine is a new approach to treating disease by considering an individual's unique genetic, environmental, and lifestyle factors. Data science can provide a personalized approach to medicine by analyzing large datasets of patient data. This approach can reduce healthcare costs and risks while improving treatment outcomes.
#8. Case Study: Big Data for Personalized Cancer Treatment
The article " Big Data in Basic and Translational Cancer Research ," published on PubMed, discusses how combining big data, bioinformatics, and artificial intelligence has led to notable advances in our fundamental understanding of cancer biology and translational advancements. The authors stress the need for collaboration among data scientists, clinicians, biologists, and policymakers to use big data to advance cancer treatment.
The predictive model allowed the cancer center to personalize treatment for each patient, increasing the chances of success and reducing adverse events.
The new immunotherapy treatment appears more effective for patients whose disease is driven by the biomarkers identified in this predictive model.
This case study exemplifies the potential of big data in personalized cancer treatment. By leveraging large patient datasets and machine learning algorithms, data scientists can identify patterns within the data and develop predictive models to identify patients most likely to respond to a particular treatment.
Personalized cancer treatment can lead to improved patient outcomes and lower healthcare costs.
#9. Case Study: Machine Learning for Predicting Patient Response to Medication
The review article published in nature provides insights into the progress made by scientists in using machine learning to predict patients' responses to medications . While the potential of machine learning in predicting drug responses is promising, there are still challenges to overcome, including data quality and standardization issues and the need for large datasets to train algorithms.
The article examines the challenges and recent advances in predicting a person's drug response using machine learning techniques. The author emphasizes how this can improve patient treatment outcomes by tailoring medications to specific individuals' genetic makeup.
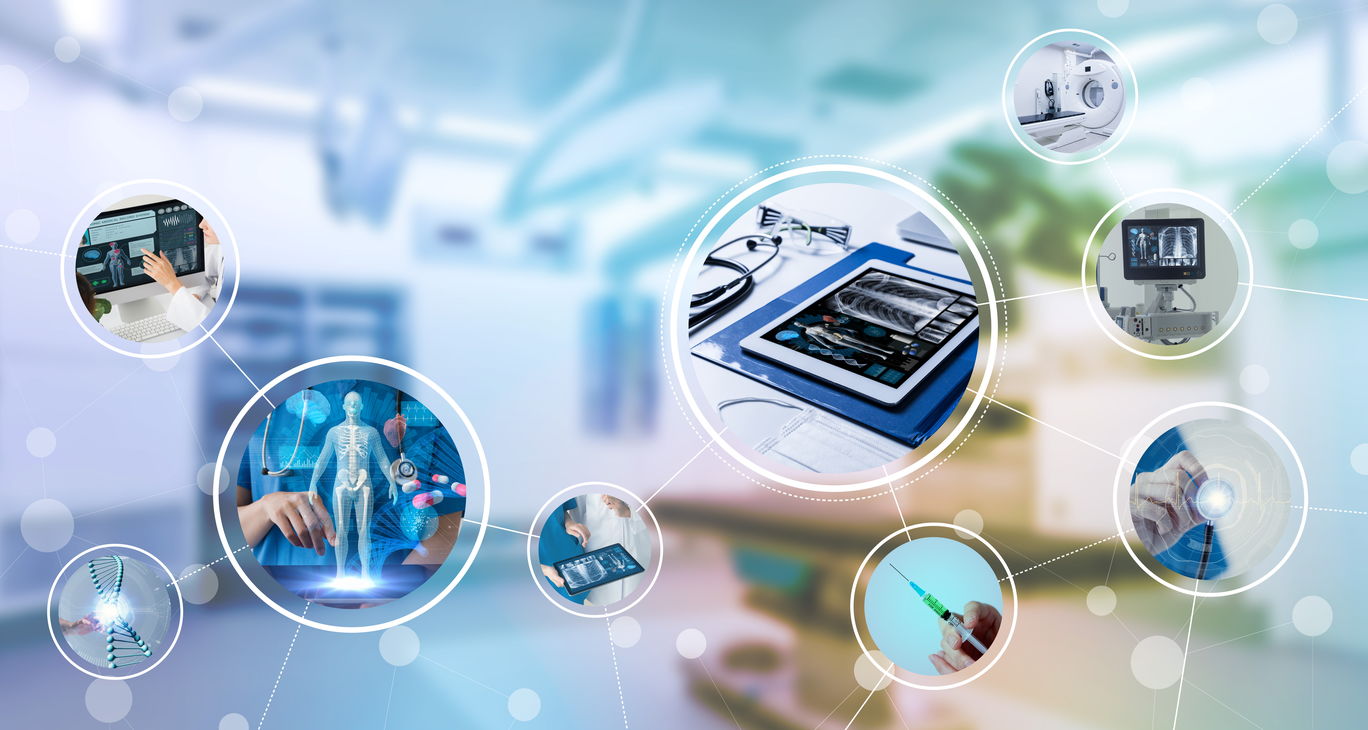
Challenges and Limitations of Data Science in Healthcare
Using data science in healthcare projects has immense potential to transform the industry. However, several challenges and limitations must be addressed to realize health data science's benefits fully.
Data Privacy and Security
Patient information is confidential and should be treated as such. Unauthorized access or disclosure of this data could lead to identity theft, financial loss, and damage to a healthcare provider's reputation.
Healthcare providers must implement robust data privacy and security measures to protect patient data. Encryption can protect data in transit and at rest while limiting access to authorized personnel only. Multi-factor authentication is another important measure that an organization can implement.
Limited Availability of Data
Accurate and comprehensive data is essential for effective healthcare decision-making, but various challenges limit access to this data. These include silos where different parts of the system are separated; interoperability issues that make it hard for programs to communicate; and lack of standardization—where processes vary significantly between organizations or even within the same organization, depending on who's doing what. Healthcare providers and data science companies must work together to address these challenges to ensure that patient health records are reliable and up-to-date.
Technical Challenges
Providing patients with the best possible care is a top priority for healthcare providers. However, achieving this goal requires more than skilled staff and advanced equipment. It also requires effectively managing and sharing large amounts of data generated by electronic medical records (EMRs).
Unfortunately, many healthcare providers struggle with sharing data due to outdated technology infrastructure and legacy systems. These systems were not designed to handle the volume and complexity of data generated by modern healthcare practices, making it difficult for healthcare providers to access and share critical patient information.
Healthcare providers must invest in modern technology infrastructure and data management systems to overcome these challenges. This includes upgrading their existing systems, implementing new data management tools, and partnering with companies like DATAFOREST ; healthcare providers can gain the necessary skills and stack to implement advanced data management systems.
Want to discover solutions for transparent and reliable operations?
Ethical considerations.
Finally, data science in healthcare raises ethical considerations. Healthcare companies and their patients must ensure that patient information is legal and transparent to those whose data it concerns.
Healthcare providers and data science companies must meet strict ethical standards to address the challenge of sharing patient information. Organizations must obtain informed consent before using their information to ensure that patients will trust them with their data. Organizations must also ensure they use the patient's data for legitimate purposes only and implement strict procedures to protect privacy by anonymizing it where possible.
To address these challenges, businesses should implement robust data privacy and security measures, improve data sharing and interoperability by partnering with relevant parties, and employ the services of qualified data science companies to help them adhere to strict ethical standards.
The healthcare industry can fully realize the potential of data science to transform healthcare delivery and improve patient outcomes by addressing these challenges.
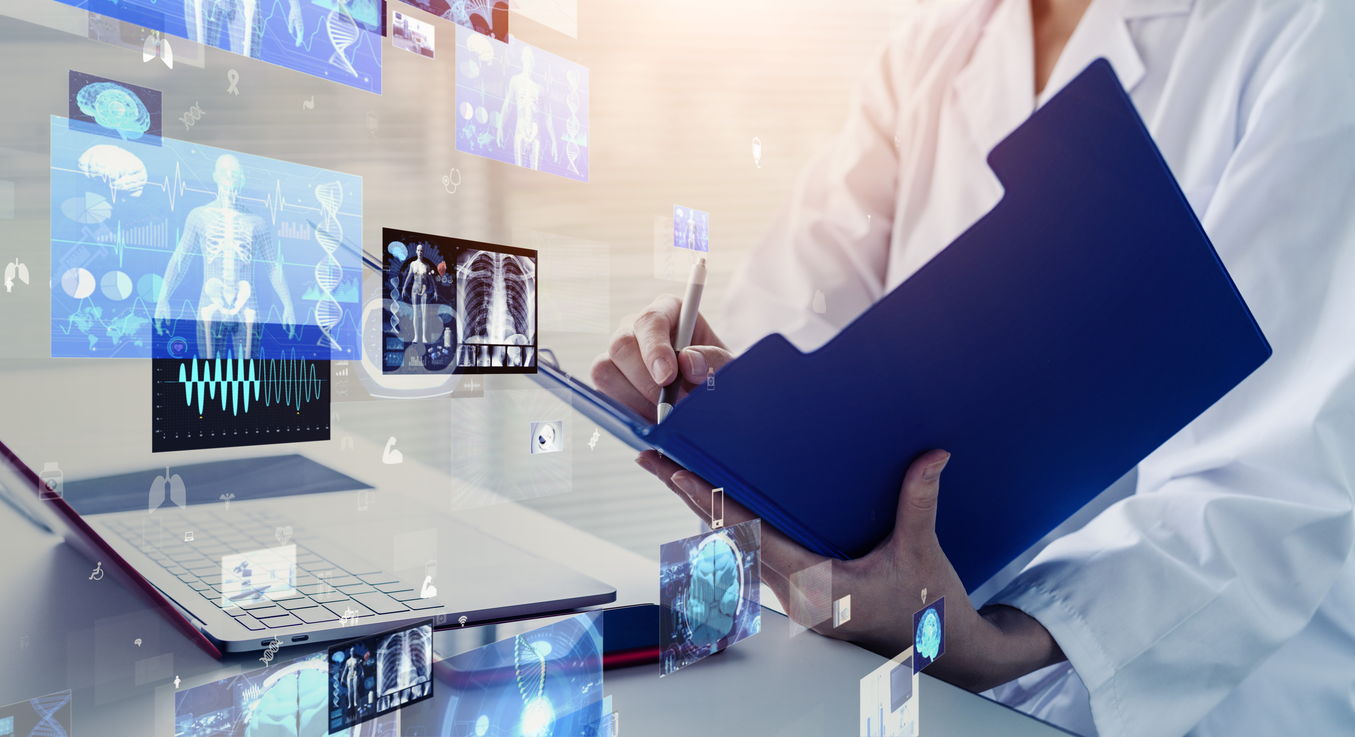
Future of Data Science in Healthcare
Technological innovations and the emergence of new methods.
Advances in technology and techniques are opening up new avenues for data science in healthcare. For example, artificial intelligence (AI) algorithms and machine learning programs can now analyze large datasets to provide more sophisticated analysis than ever before.
Natural language processing (NLP) is increasing, making it possible to analyze unstructured data such as physician notes and patient narratives.
In addition, data sources such as wearable devices and remote patient monitoring technologies are becoming available. These new sources will provide real-time health information—enabling more personalized treatment regimens.
How Data Science Can Be Used to Further Clinical Practice
The future of data science in healthcare is to make it a part of everyday medical practice, using data-driven insights to improve patient outcomes.
Predictive models can identify patients at high risk of developing certain diseases or conditions—helping doctors make decisions about early intervention and treatment based on the patient's characteristics.
Data science can help make healthcare more efficient and less expensive by identifying patients at risk for hospital readmission and intervening to lower those risks.
Impact on Healthcare Outcomes
Data science can improve healthcare outcomes, enabling early intervention and treatment by identifying patients at high risk of developing certain conditions or diseases. This improves patient outcomes—and reduces costs.
Data science can help personalize medical treatment by tailoring it to an individual's specific characteristics, improving efficacy, and reducing adverse events of a particular therapy.
Data science can improve healthcare delivery by identifying areas for improvement. With this knowledge, data scientists can optimize and streamline care delivery. This can lead to improved efficiency and reduced costs—essential goals that every hospital strives to achieve as they deal with rising operational expenses.
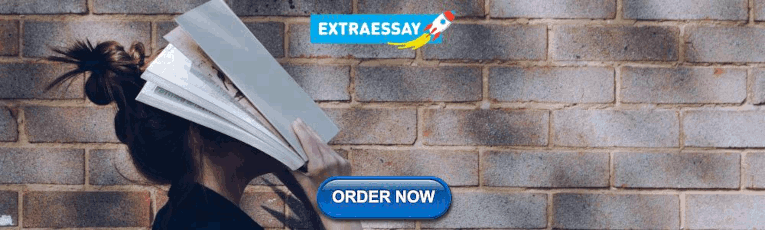
How Data Scientists Can Work with Healthcare Professionals
Healthcare data scientists and healthcare professionals must work together for effective patient care.
Healthcare professionals have the domain expertise to interpret data, and data scientists know how to work with large amounts of information.
Collaboration between data scientists and healthcare professionals leads to more effective use of data science in medicine, enabling personalized and effective treatment.
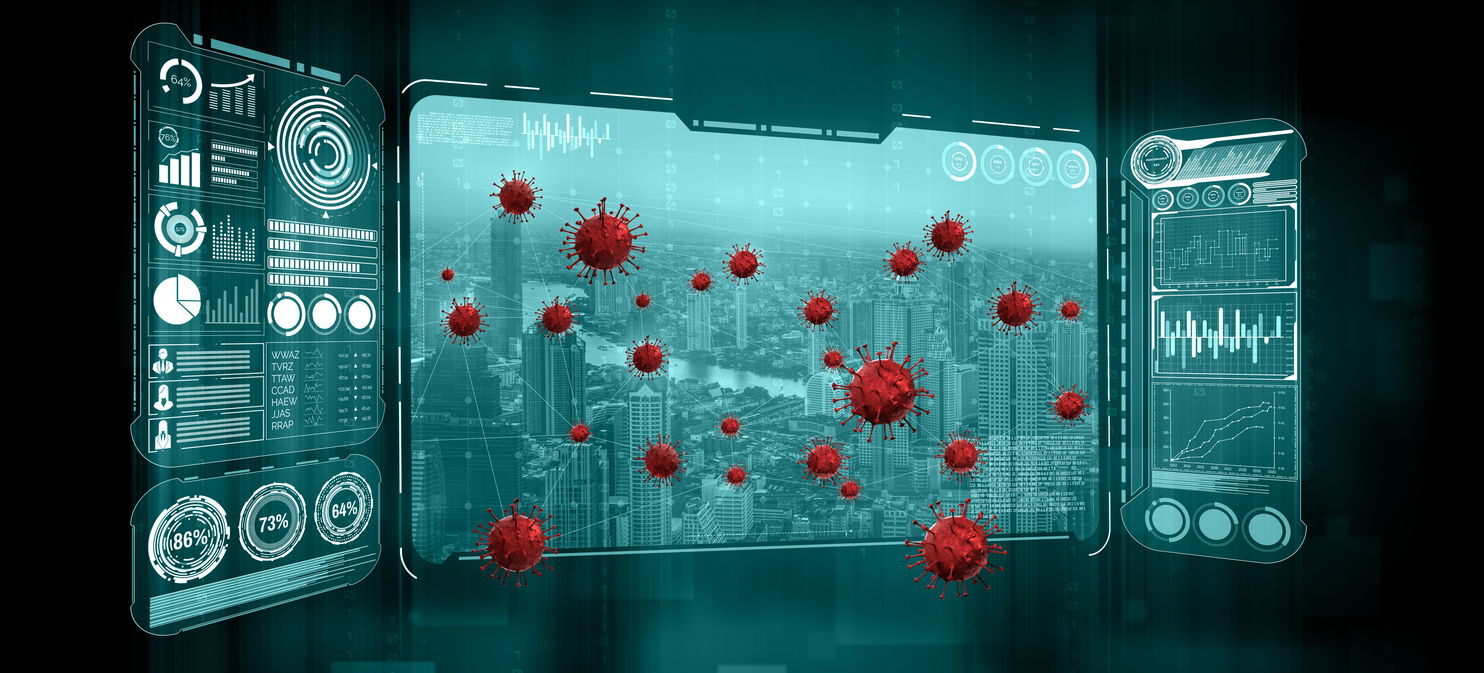
Summary of Key Points
- The healthcare industry is constantly evolving, facing new challenges such as changing regulations, technological advances, and shifting patient needs—but also great opportunities.
- Patient-centered care should be prioritized in the industry, as it involves putting patients at the center of all decision-making and tailoring their treatment to individual needs.
- We must embrace technology such as electronic health records and telemedicine to improve efficiency, accuracy, and patient outcomes.
- The healthcare industry must also address the rising demand for preventive and holistic approaches to wellness and issues related to access and affordability of care.
- The healthcare industry must continue to prioritize quality and safety, especially in the delivery of medications, infection control practices, and patient education.
Implications for Healthcare Industry
Healthcare's increasing use of data science has significant implications for the industry. By leveraging the power of data, healthcare providers and pharmaceutical companies can improve patient outcomes while reducing costs—and enhancing drug discovery.
Using data science to support the drug discovery and development process has the potential to significantly reduce timelines and costs, leading to faster market entry for innovative drugs.
Personalized medicine—the practice of tailoring medical treatment to the individual characteristics of each patient—has enormous potential for transforming healthcare.
Identifying patients most likely to benefit from a particular treatment can improve patient outcomes, reduce healthcare costs and enhance the efficiency of drug development.
By applying data science to healthcare analytics, providers can identify improvement areas and optimize their workflows. This leads to better patient care while reducing costs—allowing providers more resources to which they might otherwise not have had access.
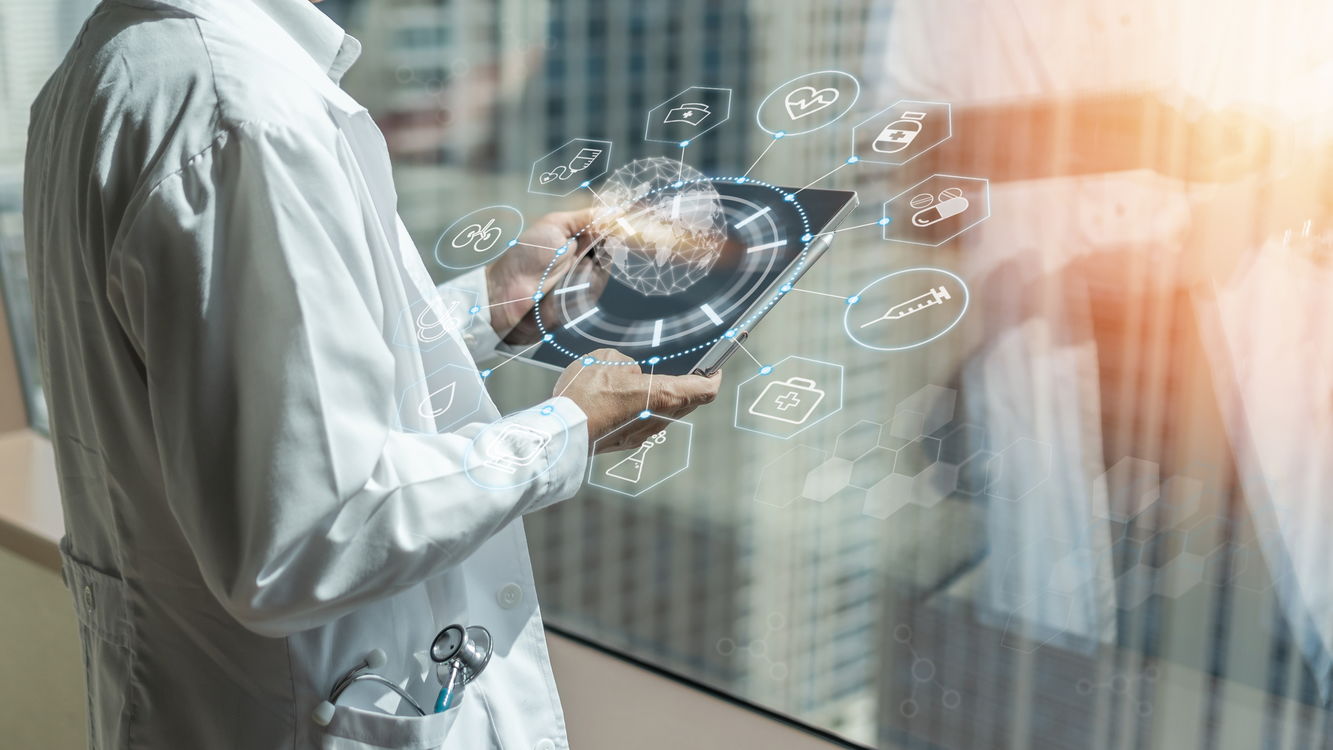
At DATAFOREST, we specialize in providing custom data-driven services for healthcare organizations. Data science can help you improve patient outcomes, streamline operations, and drive business success—and we can show you how. If you're interested in learning more about how we can help your healthcare organization leverage the full potential of data science, please get in touch with us to learn more about our services and applications. We'd be happy to talk with you about how we can address your unique data science problems in the healthcare industry.
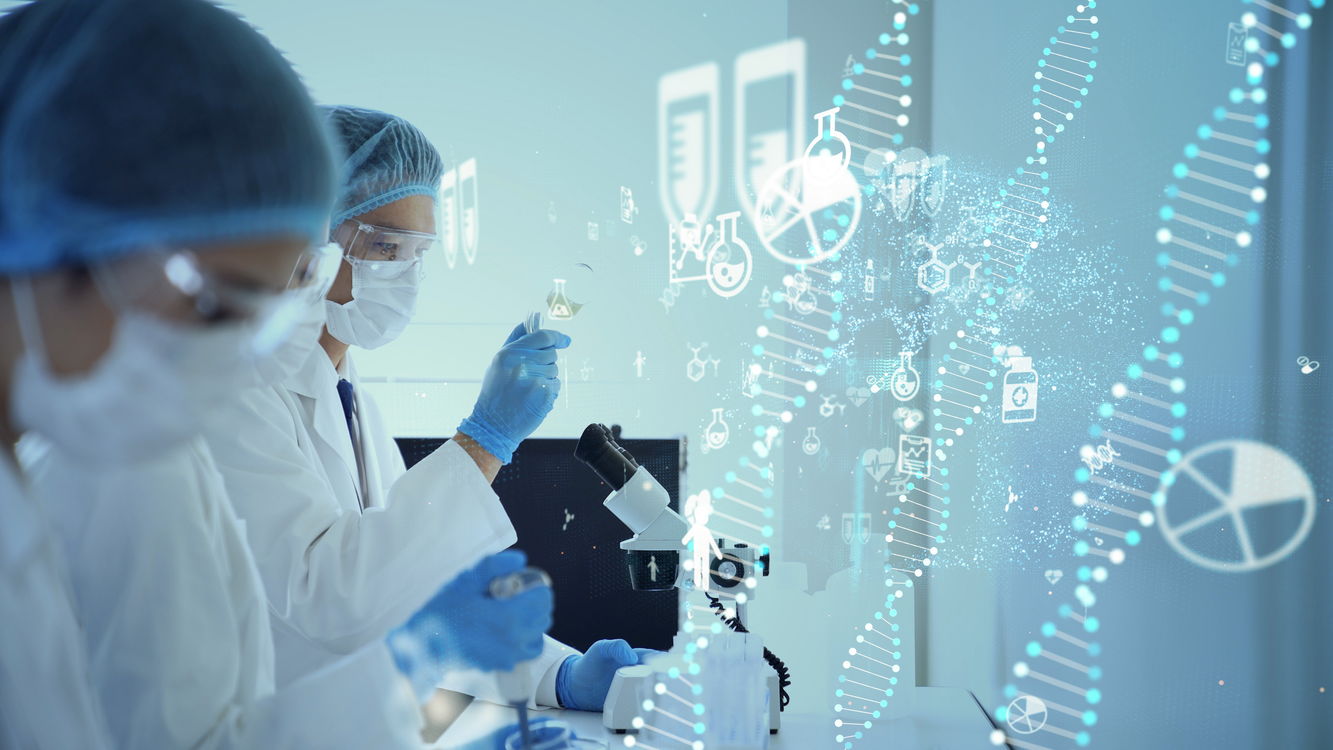
What is data science, and how can data science be used in healthcare?
Data science is the practice of extracting insights and knowledge from data. In healthcare, data science involves using statistical and computational methods to analyze health data, such as electronic health records, medical imaging, and clinical trials. This information can be used to improve patient outcomes, optimize healthcare operations, and develop new treatments.
What are some examples of how to use data science in healthcare?
Data science has been used in healthcare to predict disease outbreaks, develop personalized treatment plans, and identify high-risk patients who require early intervention. For example, machine learning algorithms have been used to analyze medical images and identify early signs of cancer, leading to earlier detection and improved survival rates.
What are the challenges and limitations of healthcare data scientists?
Data scientists working with healthcare data often face data quality, privacy, and security challenges. Healthcare data is often complex, messy, and difficult to access. Additionally, strict regulations around the use and sharing of healthcare data can limit the types of analyses that can be performed.
What are some of the ethical issues that must be considered when using data science in healthcare?
Ethical considerations in healthcare data science include ensuring patient privacy, obtaining informed consent, and avoiding bias in data analysis. It is essential to use data responsibly and transparently and to prioritize patient welfare above all else.
What impact can data science have on the drug discovery and development process in healthcare?
Data science can be used to identify new drug targets, predict drug efficacy and toxicity, and optimize clinical trial design. By leveraging large-scale data analysis, data science can accelerate the drug development process and bring new treatments to patients faster.
How does data science affect healthcare operations and staffing?
Data science can help healthcare organizations optimize staffing levels, reduce wait times, and improve patient flow. By analyzing operational data, such as patient census and appointment schedules, data science can help healthcare organizations make data-driven decisions and improve overall efficiency.
How can healthcare professionals and data scientists work together to ensure the success of their projects?
Effective collaboration between healthcare professionals and data scientists requires clear communication, mutual respect, and a shared commitment to patient welfare. Healthcare professionals can provide domain expertise and context to data analysis, while data scientists can provide technical expertise and analytical tools. By working together, healthcare professionals and data scientists can develop clinically meaningful and data-driven solutions.
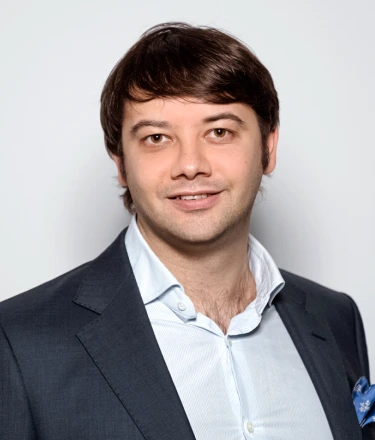
Aleksandr Sheremeta
Get More Value!
You will get from us best tailored content that will help your business grow.
Thanks for your submission!
latest posts
The ai-infused horizon of e-commerce, large language models: advanced communication, future-proof your business: the essential guide to enterprise data integration, media about us, when it comes to automation, choosing the right partner has never been more important, 15 most innovative database startups & companies, 10 best web development companies you should consider in 2022, try to trying.
Never give up
We love you to
People like this
Success stories
Web app for dropshippers.
hourly users
Shopify stores
Financial Intermediation Platform
model accuracy
timely development
E-commerce scraping
manual work reduced
pages processed daily
DevOps Experience
QPS performance
cost reduction
Supply chain dashboard
system integrations
More publications
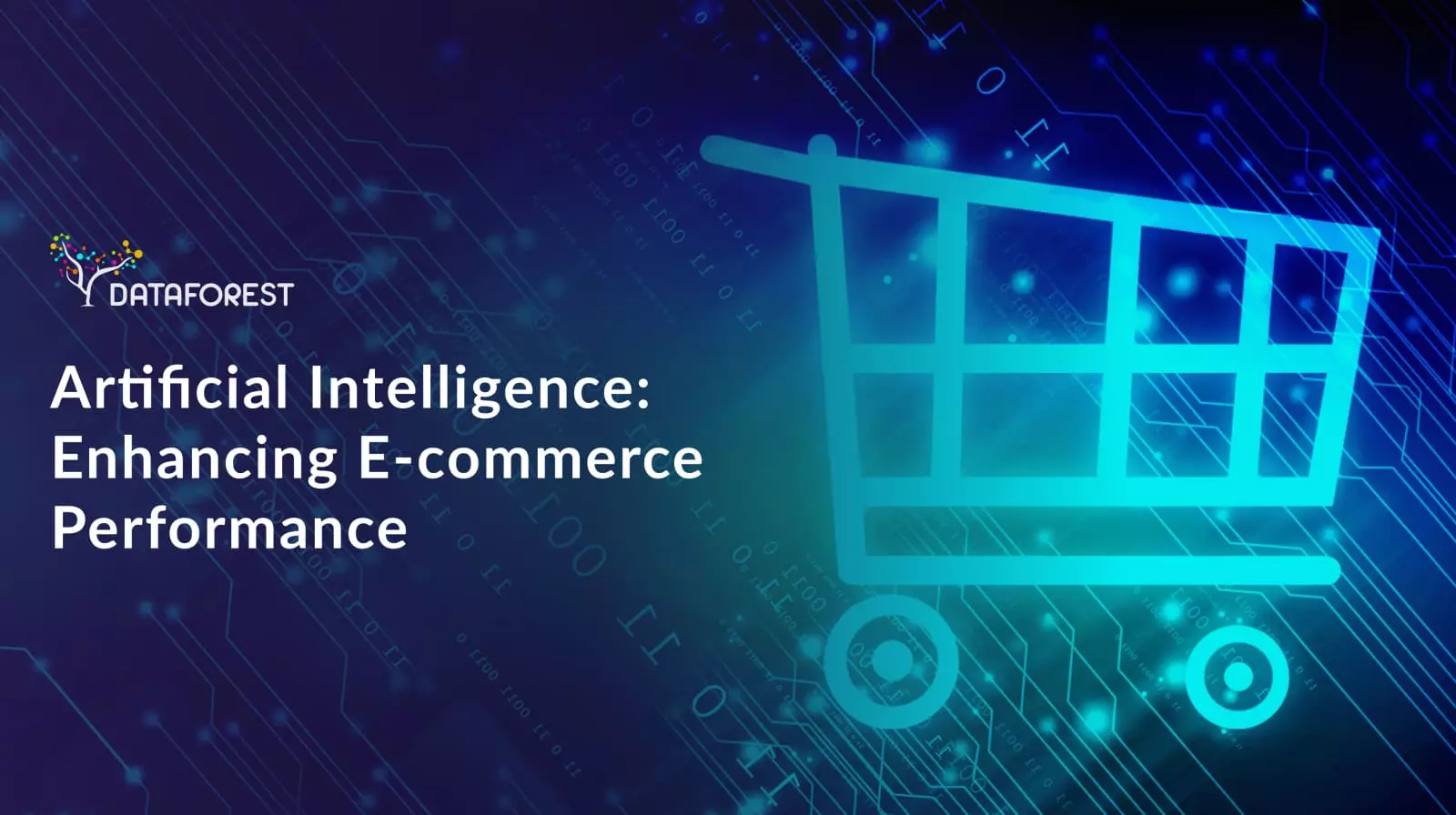
Let data make value
We’d love to hear from you.
Share the project details – like scope, mockups, or business challenges. We will carefully check and get back to you with the next steps.
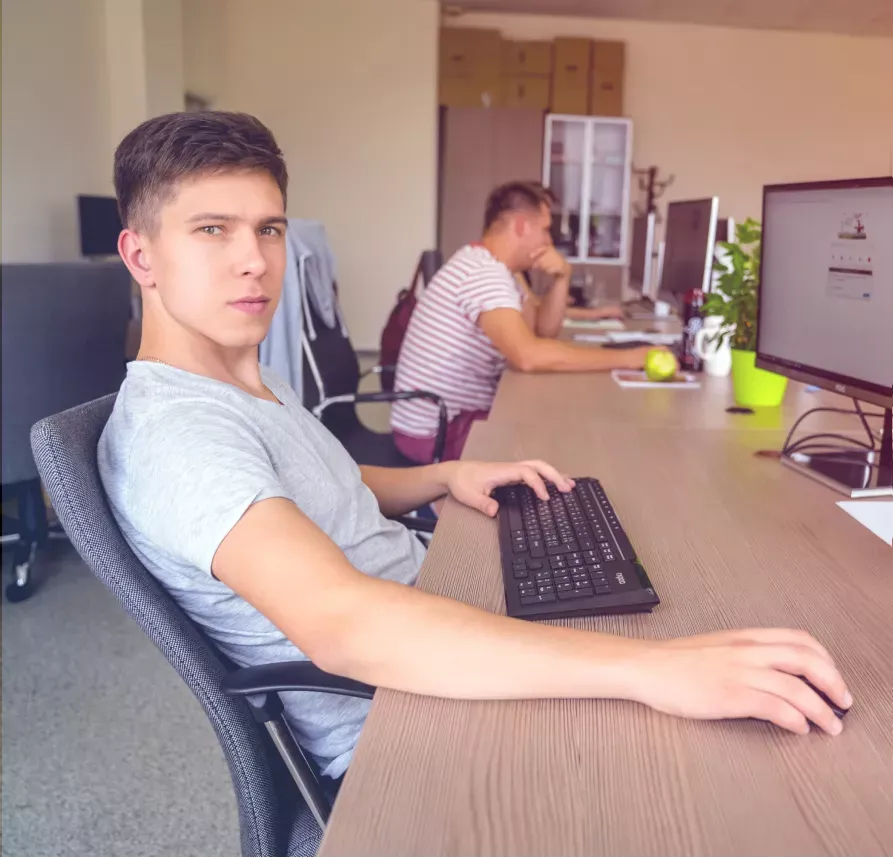
- Survey paper
- Open access
- Published: 19 June 2019
Big data in healthcare: management, analysis and future prospects
- Sabyasachi Dash 1 na1 ,
- Sushil Kumar Shakyawar 2 , 3 na1 ,
- Mohit Sharma 4 , 5 &
- Sandeep Kaushik 6
Journal of Big Data volume 6 , Article number: 54 ( 2019 ) Cite this article
441k Accesses
678 Citations
103 Altmetric
Metrics details
‘Big data’ is massive amounts of information that can work wonders. It has become a topic of special interest for the past two decades because of a great potential that is hidden in it. Various public and private sector industries generate, store, and analyze big data with an aim to improve the services they provide. In the healthcare industry, various sources for big data include hospital records, medical records of patients, results of medical examinations, and devices that are a part of internet of things. Biomedical research also generates a significant portion of big data relevant to public healthcare. This data requires proper management and analysis in order to derive meaningful information. Otherwise, seeking solution by analyzing big data quickly becomes comparable to finding a needle in the haystack. There are various challenges associated with each step of handling big data which can only be surpassed by using high-end computing solutions for big data analysis. That is why, to provide relevant solutions for improving public health, healthcare providers are required to be fully equipped with appropriate infrastructure to systematically generate and analyze big data. An efficient management, analysis, and interpretation of big data can change the game by opening new avenues for modern healthcare. That is exactly why various industries, including the healthcare industry, are taking vigorous steps to convert this potential into better services and financial advantages. With a strong integration of biomedical and healthcare data, modern healthcare organizations can possibly revolutionize the medical therapies and personalized medicine.
Introduction
Information has been the key to a better organization and new developments. The more information we have, the more optimally we can organize ourselves to deliver the best outcomes. That is why data collection is an important part for every organization. We can also use this data for the prediction of current trends of certain parameters and future events. As we are becoming more and more aware of this, we have started producing and collecting more data about almost everything by introducing technological developments in this direction. Today, we are facing a situation wherein we are flooded with tons of data from every aspect of our life such as social activities, science, work, health, etc. In a way, we can compare the present situation to a data deluge. The technological advances have helped us in generating more and more data, even to a level where it has become unmanageable with currently available technologies. This has led to the creation of the term ‘big data’ to describe data that is large and unmanageable. In order to meet our present and future social needs, we need to develop new strategies to organize this data and derive meaningful information. One such special social need is healthcare. Like every other industry, healthcare organizations are producing data at a tremendous rate that presents many advantages and challenges at the same time. In this review, we discuss about the basics of big data including its management, analysis and future prospects especially in healthcare sector.
The data overload
Every day, people working with various organizations around the world are generating a massive amount of data. The term “digital universe” quantitatively defines such massive amounts of data created, replicated, and consumed in a single year. International Data Corporation (IDC) estimated the approximate size of the digital universe in 2005 to be 130 exabytes (EB). The digital universe in 2017 expanded to about 16,000 EB or 16 zettabytes (ZB). IDC predicted that the digital universe would expand to 40,000 EB by the year 2020. To imagine this size, we would have to assign about 5200 gigabytes (GB) of data to all individuals. This exemplifies the phenomenal speed at which the digital universe is expanding. The internet giants, like Google and Facebook, have been collecting and storing massive amounts of data. For instance, depending on our preferences, Google may store a variety of information including user location, advertisement preferences, list of applications used, internet browsing history, contacts, bookmarks, emails, and other necessary information associated with the user. Similarly, Facebook stores and analyzes more than about 30 petabytes (PB) of user-generated data. Such large amounts of data constitute ‘ big data ’. Over the past decade, big data has been successfully used by the IT industry to generate critical information that can generate significant revenue.
These observations have become so conspicuous that has eventually led to the birth of a new field of science termed ‘ Data Science ’. Data science deals with various aspects including data management and analysis, to extract deeper insights for improving the functionality or services of a system (for example, healthcare and transport system). Additionally, with the availability of some of the most creative and meaningful ways to visualize big data post-analysis, it has become easier to understand the functioning of any complex system. As a large section of society is becoming aware of, and involved in generating big data, it has become necessary to define what big data is. Therefore, in this review, we attempt to provide details on the impact of big data in the transformation of global healthcare sector and its impact on our daily lives.
Defining big data
As the name suggests, ‘big data’ represents large amounts of data that is unmanageable using traditional software or internet-based platforms. It surpasses the traditionally used amount of storage, processing and analytical power. Even though a number of definitions for big data exist, the most popular and well-accepted definition was given by Douglas Laney. Laney observed that (big) data was growing in three different dimensions namely, volume, velocity and variety (known as the 3 Vs) [ 1 ]. The ‘big’ part of big data is indicative of its large volume. In addition to volume, the big data description also includes velocity and variety. Velocity indicates the speed or rate of data collection and making it accessible for further analysis; while, variety remarks on the different types of organized and unorganized data that any firm or system can collect, such as transaction-level data, video, audio, text or log files. These three Vs have become the standard definition of big data. Although, other people have added several other Vs to this definition [ 2 ], the most accepted 4th V remains ‘veracity’.
The term “ big data ” has become extremely popular across the globe in recent years. Almost every sector of research, whether it relates to industry or academics, is generating and analyzing big data for various purposes. The most challenging task regarding this huge heap of data that can be organized and unorganized, is its management. Given the fact that big data is unmanageable using the traditional software, we need technically advanced applications and software that can utilize fast and cost-efficient high-end computational power for such tasks. Implementation of artificial intelligence (AI) algorithms and novel fusion algorithms would be necessary to make sense from this large amount of data. Indeed, it would be a great feat to achieve automated decision-making by the implementation of machine learning (ML) methods like neural networks and other AI techniques. However, in absence of appropriate software and hardware support, big data can be quite hazy. We need to develop better techniques to handle this ‘endless sea’ of data and smart web applications for efficient analysis to gain workable insights. With proper storage and analytical tools in hand, the information and insights derived from big data can make the critical social infrastructure components and services (like healthcare, safety or transportation) more aware, interactive and efficient [ 3 ]. In addition, visualization of big data in a user-friendly manner will be a critical factor for societal development.
Healthcare as a big-data repository
Healthcare is a multi-dimensional system established with the sole aim for the prevention, diagnosis, and treatment of health-related issues or impairments in human beings. The major components of a healthcare system are the health professionals (physicians or nurses), health facilities (clinics, hospitals for delivering medicines and other diagnosis or treatment technologies), and a financing institution supporting the former two. The health professionals belong to various health sectors like dentistry, medicine, midwifery, nursing, psychology, physiotherapy, and many others. Healthcare is required at several levels depending on the urgency of situation. Professionals serve it as the first point of consultation (for primary care), acute care requiring skilled professionals (secondary care), advanced medical investigation and treatment (tertiary care) and highly uncommon diagnostic or surgical procedures (quaternary care). At all these levels, the health professionals are responsible for different kinds of information such as patient’s medical history (diagnosis and prescriptions related data), medical and clinical data (like data from imaging and laboratory examinations), and other private or personal medical data. Previously, the common practice to store such medical records for a patient was in the form of either handwritten notes or typed reports [ 4 ]. Even the results from a medical examination were stored in a paper file system. In fact, this practice is really old, with the oldest case reports existing on a papyrus text from Egypt that dates back to 1600 BC [ 5 ]. In Stanley Reiser’s words, the clinical case records freeze the episode of illness as a story in which patient, family and the doctor are a part of the plot” [ 6 ].
With the advent of computer systems and its potential, the digitization of all clinical exams and medical records in the healthcare systems has become a standard and widely adopted practice nowadays. In 2003, a division of the National Academies of Sciences, Engineering, and Medicine known as Institute of Medicine chose the term “ electronic health records ” to represent records maintained for improving the health care sector towards the benefit of patients and clinicians. Electronic health records (EHR) as defined by Murphy, Hanken and Waters are computerized medical records for patients any information relating to the past, present or future physical/mental health or condition of an individual which resides in electronic system(s) used to capture, transmit, receive, store, retrieve, link and manipulate multimedia data for the primary purpose of providing healthcare and health-related services” [ 7 ].
Electronic health records
It is important to note that the National Institutes of Health (NIH) recently announced the “All of Us” initiative ( https://allofus.nih.gov/ ) that aims to collect one million or more patients’ data such as EHR, including medical imaging, socio-behavioral, and environmental data over the next few years. EHRs have introduced many advantages for handling modern healthcare related data. Below, we describe some of the characteristic advantages of using EHRs. The first advantage of EHRs is that healthcare professionals have an improved access to the entire medical history of a patient. The information includes medical diagnoses, prescriptions, data related to known allergies, demographics, clinical narratives, and the results obtained from various laboratory tests. The recognition and treatment of medical conditions thus is time efficient due to a reduction in the lag time of previous test results. With time we have observed a significant decrease in the redundant and additional examinations, lost orders and ambiguities caused by illegible handwriting, and an improved care coordination between multiple healthcare providers. Overcoming such logistical errors has led to reduction in the number of drug allergies by reducing errors in medication dose and frequency. Healthcare professionals have also found access over web based and electronic platforms to improve their medical practices significantly using automatic reminders and prompts regarding vaccinations, abnormal laboratory results, cancer screening, and other periodic checkups. There would be a greater continuity of care and timely interventions by facilitating communication among multiple healthcare providers and patients. They can be associated to electronic authorization and immediate insurance approvals due to less paperwork. EHRs enable faster data retrieval and facilitate reporting of key healthcare quality indicators to the organizations, and also improve public health surveillance by immediate reporting of disease outbreaks. EHRs also provide relevant data regarding the quality of care for the beneficiaries of employee health insurance programs and can help control the increasing costs of health insurance benefits. Finally, EHRs can reduce or absolutely eliminate delays and confusion in the billing and claims management area. The EHRs and internet together help provide access to millions of health-related medical information critical for patient life.
Digitization of healthcare and big data
Similar to EHR, an electronic medical record (EMR) stores the standard medical and clinical data gathered from the patients. EHRs, EMRs, personal health record (PHR), medical practice management software (MPM), and many other healthcare data components collectively have the potential to improve the quality, service efficiency, and costs of healthcare along with the reduction of medical errors. The big data in healthcare includes the healthcare payer-provider data (such as EMRs, pharmacy prescription, and insurance records) along with the genomics-driven experiments (such as genotyping, gene expression data) and other data acquired from the smart web of internet of things (IoT) (Fig. 1 ). The adoption of EHRs was slow at the beginning of the 21st century however it has grown substantially after 2009 [ 7 , 8 ]. The management and usage of such healthcare data has been increasingly dependent on information technology. The development and usage of wellness monitoring devices and related software that can generate alerts and share the health related data of a patient with the respective health care providers has gained momentum, especially in establishing a real-time biomedical and health monitoring system. These devices are generating a huge amount of data that can be analyzed to provide real-time clinical or medical care [ 9 ]. The use of big data from healthcare shows promise for improving health outcomes and controlling costs.
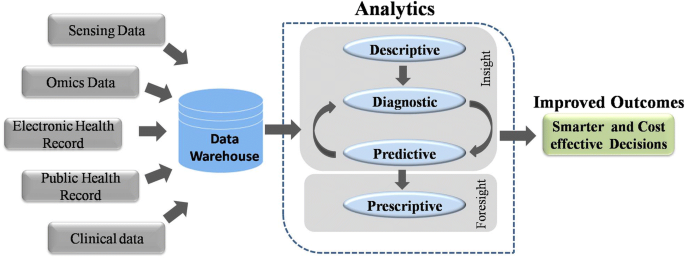
Workflow of Big data Analytics. Data warehouses store massive amounts of data generated from various sources. This data is processed using analytic pipelines to obtain smarter and affordable healthcare options
Big data in biomedical research
A biological system, such as a human cell, exhibits molecular and physical events of complex interplay. In order to understand interdependencies of various components and events of such a complex system, a biomedical or biological experiment usually gathers data on a smaller and/or simpler component. Consequently, it requires multiple simplified experiments to generate a wide map of a given biological phenomenon of interest. This indicates that more the data we have, the better we understand the biological processes. With this idea, modern techniques have evolved at a great pace. For instance, one can imagine the amount of data generated since the integration of efficient technologies like next-generation sequencing (NGS) and Genome wide association studies (GWAS) to decode human genetics. NGS-based data provides information at depths that were previously inaccessible and takes the experimental scenario to a completely new dimension. It has increased the resolution at which we observe or record biological events associated with specific diseases in a real time manner. The idea that large amounts of data can provide us a good amount of information that often remains unidentified or hidden in smaller experimental methods has ushered-in the ‘- omics ’ era. The ‘ omics ’ discipline has witnessed significant progress as instead of studying a single ‘ gene ’ scientists can now study the whole ‘ genome ’ of an organism in ‘ genomics ’ studies within a given amount of time. Similarly, instead of studying the expression or ‘ transcription ’ of single gene, we can now study the expression of all the genes or the entire ‘ transcriptome ’ of an organism under ‘ transcriptomics ’ studies. Each of these individual experiments generate a large amount of data with more depth of information than ever before. Yet, this depth and resolution might be insufficient to provide all the details required to explain a particular mechanism or event. Therefore, one usually finds oneself analyzing a large amount of data obtained from multiple experiments to gain novel insights. This fact is supported by a continuous rise in the number of publications regarding big data in healthcare (Fig. 2 ). Analysis of such big data from medical and healthcare systems can be of immense help in providing novel strategies for healthcare. The latest technological developments in data generation, collection and analysis, have raised expectations towards a revolution in the field of personalized medicine in near future.
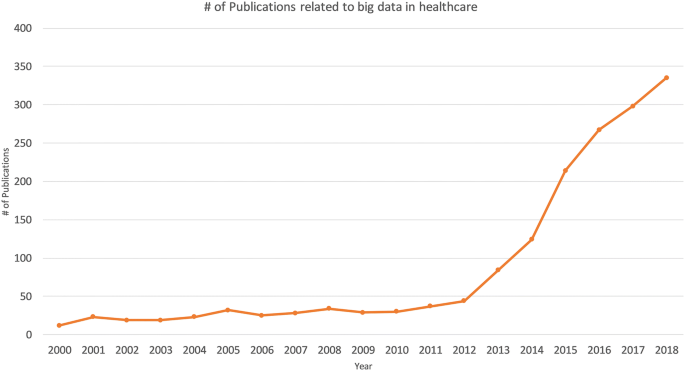
Publications associated with big data in healthcare. The numbers of publications in PubMed are plotted by year
Big data from omics studies
NGS has greatly simplified the sequencing and decreased the costs for generating whole genome sequence data. The cost of complete genome sequencing has fallen from millions to a couple of thousand dollars [ 10 ]. NGS technology has resulted in an increased volume of biomedical data that comes from genomic and transcriptomic studies. According to an estimate, the number of human genomes sequenced by 2025 could be between 100 million to 2 billion [ 11 ]. Combining the genomic and transcriptomic data with proteomic and metabolomic data can greatly enhance our knowledge about the individual profile of a patient—an approach often ascribed as “individual, personalized or precision health care”. Systematic and integrative analysis of omics data in conjugation with healthcare analytics can help design better treatment strategies towards precision and personalized medicine (Fig. 3 ). The genomics-driven experiments e.g., genotyping, gene expression, and NGS-based studies are the major source of big data in biomedical healthcare along with EMRs, pharmacy prescription information, and insurance records. Healthcare requires a strong integration of such biomedical data from various sources to provide better treatments and patient care. These prospects are so exciting that even though genomic data from patients would have many variables to be accounted, yet commercial organizations are already using human genome data to help the providers in making personalized medical decisions. This might turn out to be a game-changer in future medicine and health.
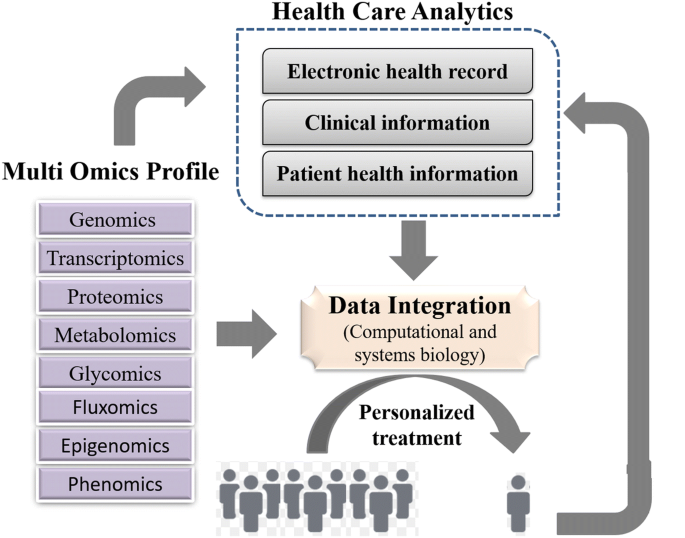
A framework for integrating omics data and health care analytics to promote personalized treatment
Internet of Things (IOT)
Healthcare industry has not been quick enough to adapt to the big data movement compared to other industries. Therefore, big data usage in the healthcare sector is still in its infancy. For example, healthcare and biomedical big data have not yet converged to enhance healthcare data with molecular pathology. Such convergence can help unravel various mechanisms of action or other aspects of predictive biology. Therefore, to assess an individual’s health status, biomolecular and clinical datasets need to be married. One such source of clinical data in healthcare is ‘internet of things’ (IoT).
In fact, IoT is another big player implemented in a number of other industries including healthcare. Until recently, the objects of common use such as cars, watches, refrigerators and health-monitoring devices, did not usually produce or handle data and lacked internet connectivity. However, furnishing such objects with computer chips and sensors that enable data collection and transmission over internet has opened new avenues. The device technologies such as Radio Frequency IDentification (RFID) tags and readers, and Near Field Communication (NFC) devices, that can not only gather information but interact physically, are being increasingly used as the information and communication systems [ 3 ]. This enables objects with RFID or NFC to communicate and function as a web of smart things. The analysis of data collected from these chips or sensors may reveal critical information that might be beneficial in improving lifestyle, establishing measures for energy conservation, improving transportation, and healthcare. In fact, IoT has become a rising movement in the field of healthcare. IoT devices create a continuous stream of data while monitoring the health of people (or patients) which makes these devices a major contributor to big data in healthcare. Such resources can interconnect various devices to provide a reliable, effective and smart healthcare service to the elderly and patients with a chronic illness [ 12 ].
Advantages of IoT in healthcare
Using the web of IoT devices, a doctor can measure and monitor various parameters from his/her clients in their respective locations for example, home or office. Therefore, through early intervention and treatment, a patient might not need hospitalization or even visit the doctor resulting in significant cost reduction in healthcare expenses. Some examples of IoT devices used in healthcare include fitness or health-tracking wearable devices, biosensors, clinical devices for monitoring vital signs, and others types of devices or clinical instruments. Such IoT devices generate a large amount of health related data. If we can integrate this data with other existing healthcare data like EMRs or PHRs, we can predict a patients’ health status and its progression from subclinical to pathological state [ 9 ]. In fact, big data generated from IoT has been quiet advantageous in several areas in offering better investigation and predictions. On a larger scale, the data from such devices can help in personnel health monitoring, modelling the spread of a disease and finding ways to contain a particular disease outbreak.
The analysis of data from IoT would require an updated operating software because of its specific nature along with advanced hardware and software applications. We would need to manage data inflow from IoT instruments in real-time and analyze it by the minute. Associates in the healthcare system are trying to trim down the cost and ameliorate the quality of care by applying advanced analytics to both internally and externally generated data.
Mobile computing and mobile health (mHealth)
In today’s digital world, every individual seems to be obsessed to track their fitness and health statistics using the in-built pedometer of their portable and wearable devices such as, smartphones, smartwatches, fitness dashboards or tablets. With an increasingly mobile society in almost all aspects of life, the healthcare infrastructure needs remodeling to accommodate mobile devices [ 13 ]. The practice of medicine and public health using mobile devices, known as mHealth or mobile health, pervades different degrees of health care especially for chronic diseases, such as diabetes and cancer [ 14 ]. Healthcare organizations are increasingly using mobile health and wellness services for implementing novel and innovative ways to provide care and coordinate health as well as wellness. Mobile platforms can improve healthcare by accelerating interactive communication between patients and healthcare providers. In fact, Apple and Google have developed devoted platforms like Apple’s ResearchKit and Google Fit for developing research applications for fitness and health statistics [ 15 ]. These applications support seamless interaction with various consumer devices and embedded sensors for data integration. These apps help the doctors to have direct access to your overall health data. Both the user and their doctors get to know the real-time status of your body. These apps and smart devices also help by improving our wellness planning and encouraging healthy lifestyles. The users or patients can become advocates for their own health.
Nature of the big data in healthcare
EHRs can enable advanced analytics and help clinical decision-making by providing enormous data. However, a large proportion of this data is currently unstructured in nature. An unstructured data is the information that does not adhere to a pre-defined model or organizational framework. The reason for this choice may simply be that we can record it in a myriad of formats. Another reason for opting unstructured format is that often the structured input options (drop-down menus, radio buttons, and check boxes) can fall short for capturing data of complex nature. For example, we cannot record the non-standard data regarding a patient’s clinical suspicions, socioeconomic data, patient preferences, key lifestyle factors, and other related information in any other way but an unstructured format. It is difficult to group such varied, yet critical, sources of information into an intuitive or unified data format for further analysis using algorithms to understand and leverage the patients care. Nonetheless, the healthcare industry is required to utilize the full potential of these rich streams of information to enhance the patient experience. In the healthcare sector, it could materialize in terms of better management, care and low-cost treatments. We are miles away from realizing the benefits of big data in a meaningful way and harnessing the insights that come from it. In order to achieve these goals, we need to manage and analyze the big data in a systematic manner.
Management and analysis of big data
Big data is the huge amounts of a variety of data generated at a rapid rate. The data gathered from various sources is mostly required for optimizing consumer services rather than consumer consumption. This is also true for big data from the biomedical research and healthcare. The major challenge with big data is how to handle this large volume of information. To make it available for scientific community, the data is required to be stored in a file format that is easily accessible and readable for an efficient analysis. In the context of healthcare data, another major challenge is the implementation of high-end computing tools, protocols and high-end hardware in the clinical setting. Experts from diverse backgrounds including biology, information technology, statistics, and mathematics are required to work together to achieve this goal. The data collected using the sensors can be made available on a storage cloud with pre-installed software tools developed by analytic tool developers. These tools would have data mining and ML functions developed by AI experts to convert the information stored as data into knowledge. Upon implementation, it would enhance the efficiency of acquiring, storing, analyzing, and visualization of big data from healthcare. The main task is to annotate, integrate, and present this complex data in an appropriate manner for a better understanding. In absence of such relevant information, the (healthcare) data remains quite cloudy and may not lead the biomedical researchers any further. Finally, visualization tools developed by computer graphics designers can efficiently display this newly gained knowledge.
Heterogeneity of data is another challenge in big data analysis. The huge size and highly heterogeneous nature of big data in healthcare renders it relatively less informative using the conventional technologies. The most common platforms for operating the software framework that assists big data analysis are high power computing clusters accessed via grid computing infrastructures. Cloud computing is such a system that has virtualized storage technologies and provides reliable services. It offers high reliability, scalability and autonomy along with ubiquitous access, dynamic resource discovery and composability. Such platforms can act as a receiver of data from the ubiquitous sensors, as a computer to analyze and interpret the data, as well as providing the user with easy to understand web-based visualization. In IoT, the big data processing and analytics can be performed closer to data source using the services of mobile edge computing cloudlets and fog computing. Advanced algorithms are required to implement ML and AI approaches for big data analysis on computing clusters. A programming language suitable for working on big data (e.g. Python, R or other languages) could be used to write such algorithms or software. Therefore, a good knowledge of biology and IT is required to handle the big data from biomedical research. Such a combination of both the trades usually fits for bioinformaticians. The most common among various platforms used for working with big data include Hadoop and Apache Spark. We briefly introduce these platforms below.
Loading large amounts of (big) data into the memory of even the most powerful of computing clusters is not an efficient way to work with big data. Therefore, the best logical approach for analyzing huge volumes of complex big data is to distribute and process it in parallel on multiple nodes. However, the size of data is usually so large that thousands of computing machines are required to distribute and finish processing in a reasonable amount of time. When working with hundreds or thousands of nodes, one has to handle issues like how to parallelize the computation, distribute the data, and handle failures. One of most popular open-source distributed application for this purpose is Hadoop [ 16 ]. Hadoop implements MapReduce algorithm for processing and generating large datasets. MapReduce uses map and reduce primitives to map each logical record’ in the input into a set of intermediate key/value pairs, and reduce operation combines all the values that shared the same key [ 17 ]. It efficiently parallelizes the computation, handles failures, and schedules inter-machine communication across large-scale clusters of machines. Hadoop Distributed File System (HDFS) is the file system component that provides a scalable, efficient, and replica based storage of data at various nodes that form a part of a cluster [ 16 ]. Hadoop has other tools that enhance the storage and processing components therefore many large companies like Yahoo, Facebook, and others have rapidly adopted it. Hadoop has enabled researchers to use data sets otherwise impossible to handle. Many large projects, like the determination of a correlation between the air quality data and asthma admissions, drug development using genomic and proteomic data, and other such aspects of healthcare are implementing Hadoop. Therefore, with the implementation of Hadoop system, the healthcare analytics will not be held back.
Apache Spark
Apache Spark is another open source alternative to Hadoop. It is a unified engine for distributed data processing that includes higher-level libraries for supporting SQL queries ( Spark SQL ), streaming data ( Spark Streaming ), machine learning ( MLlib ) and graph processing ( GraphX ) [ 18 ]. These libraries help in increasing developer productivity because the programming interface requires lesser coding efforts and can be seamlessly combined to create more types of complex computations. By implementing Resilient distributed Datasets (RDDs), in-memory processing of data is supported that can make Spark about 100× faster than Hadoop in multi-pass analytics (on smaller datasets) [ 19 , 20 ]. This is more true when the data size is smaller than the available memory [ 21 ]. This indicates that processing of really big data with Apache Spark would require a large amount of memory. Since, the cost of memory is higher than the hard drive, MapReduce is expected to be more cost effective for large datasets compared to Apache Spark. Similarly, Apache Storm was developed to provide a real-time framework for data stream processing. This platform supports most of the programming languages. Additionally, it offers good horizontal scalability and built-in-fault-tolerance capability for big data analysis.
Machine learning for information extraction, data analysis and predictions
In healthcare, patient data contains recorded signals for instance, electrocardiogram (ECG), images, and videos. Healthcare providers have barely managed to convert such healthcare data into EHRs. Efforts are underway to digitize patient-histories from pre-EHR era notes and supplement the standardization process by turning static images into machine-readable text. For example, optical character recognition (OCR) software is one such approach that can recognize handwriting as well as computer fonts and push digitization. Such unstructured and structured healthcare datasets have untapped wealth of information that can be harnessed using advanced AI programs to draw critical actionable insights in the context of patient care. In fact, AI has emerged as the method of choice for big data applications in medicine. This smart system has quickly found its niche in decision making process for the diagnosis of diseases. Healthcare professionals analyze such data for targeted abnormalities using appropriate ML approaches. ML can filter out structured information from such raw data.
Extracting information from EHR datasets
Emerging ML or AI based strategies are helping to refine healthcare industry’s information processing capabilities. For example, natural language processing (NLP) is a rapidly developing area of machine learning that can identify key syntactic structures in free text, help in speech recognition and extract the meaning behind a narrative. NLP tools can help generate new documents, like a clinical visit summary, or to dictate clinical notes. The unique content and complexity of clinical documentation can be challenging for many NLP developers. Nonetheless, we should be able to extract relevant information from healthcare data using such approaches as NLP.
AI has also been used to provide predictive capabilities to healthcare big data. For example, ML algorithms can convert the diagnostic system of medical images into automated decision-making. Though it is apparent that healthcare professionals may not be replaced by machines in the near future, yet AI can definitely assist physicians to make better clinical decisions or even replace human judgment in certain functional areas of healthcare.
Image analytics
Some of the most widely used imaging techniques in healthcare include computed tomography (CT), magnetic resonance imaging (MRI), X-ray, molecular imaging, ultrasound, photo-acoustic imaging, functional MRI (fMRI), positron emission tomography (PET), electroencephalography (EEG), and mammograms. These techniques capture high definition medical images (patient data) of large sizes. Healthcare professionals like radiologists, doctors and others do an excellent job in analyzing medical data in the form of these files for targeted abnormalities. However, it is also important to acknowledge the lack of specialized professionals for many diseases. In order to compensate for this dearth of professionals, efficient systems like Picture Archiving and Communication System (PACS) have been developed for storing and convenient access to medical image and reports data [ 22 ]. PACSs are popular for delivering images to local workstations, accomplished by protocols such as digital image communication in medicine (DICOM). However, data exchange with a PACS relies on using structured data to retrieve medical images. This by nature misses out on the unstructured information contained in some of the biomedical images. Moreover, it is possible to miss an additional information about a patient’s health status that is present in these images or similar data. A professional focused on diagnosing an unrelated condition might not observe it, especially when the condition is still emerging. To help in such situations, image analytics is making an impact on healthcare by actively extracting disease biomarkers from biomedical images. This approach uses ML and pattern recognition techniques to draw insights from massive volumes of clinical image data to transform the diagnosis, treatment and monitoring of patients. It focuses on enhancing the diagnostic capability of medical imaging for clinical decision-making.
A number of software tools have been developed based on functionalities such as generic, registration, segmentation, visualization, reconstruction, simulation and diffusion to perform medical image analysis in order to dig out the hidden information. For example, Visualization Toolkit is a freely available software which allows powerful processing and analysis of 3D images from medical tests [ 23 ], while SPM can process and analyze 5 different types of brain images (e.g. MRI, fMRI, PET, CT-Scan and EEG) [ 24 ]. Other software like GIMIAS, Elastix, and MITK support all types of images. Various other widely used tools and their features in this domain are listed in Table 1 . Such bioinformatics-based big data analysis may extract greater insights and value from imaging data to boost and support precision medicine projects, clinical decision support tools, and other modes of healthcare. For example, we can also use it to monitor new targeted-treatments for cancer.
Big data from omics
The big data from “omics” studies is a new kind of challenge for the bioinformaticians. Robust algorithms are required to analyze such complex data from biological systems. The ultimate goal is to convert this huge data into an informative knowledge base. The application of bioinformatics approaches to transform the biomedical and genomics data into predictive and preventive health is known as translational bioinformatics. It is at the forefront of data-driven healthcare. Various kinds of quantitative data in healthcare, for example from laboratory measurements, medication data and genomic profiles, can be combined and used to identify new meta-data that can help precision therapies [ 25 ]. This is why emerging new technologies are required to help in analyzing this digital wealth. In fact, highly ambitious multimillion-dollar projects like “ Big Data Research and Development Initiative ” have been launched that aim to enhance the quality of big data tools and techniques for a better organization, efficient access and smart analysis of big data. There are many advantages anticipated from the processing of ‘ omics’ data from large-scale Human Genome Project and other population sequencing projects. In the population sequencing projects like 1000 genomes, the researchers will have access to a marvelous amount of raw data. Similarly, Human Genome Project based Encyclopedia of DNA Elements (ENCODE) project aimed to determine all functional elements in the human genome using bioinformatics approaches. Here, we list some of the widely used bioinformatics-based tools for big data analytics on omics data.
SparkSeq is an efficient and cloud-ready platform based on Apache Spark framework and Hadoop library that is used for analyses of genomic data for interactive genomic data analysis with nucleotide precision
SAMQA identifies errors and ensures the quality of large-scale genomic data. This tool was originally built for the National Institutes of Health Cancer Genome Atlas project to identify and report errors including sequence alignment/map [SAM] format error and empty reads.
ART can simulate profiles of read errors and read lengths for data obtained using high throughput sequencing platforms including SOLiD and Illumina platforms.
DistMap is another toolkit used for distributed short-read mapping based on Hadoop cluster that aims to cover a wider range of sequencing applications. For instance, one of its applications namely the BWA mapper can perform 500 million read pairs in about 6 h, approximately 13 times faster than a conventional single-node mapper.
SeqWare is a query engine based on Apache HBase database system that enables access for large-scale whole-genome datasets by integrating genome browsers and tools.
CloudBurst is a parallel computing model utilized in genome mapping experiments to improve the scalability of reading large sequencing data.
Hydra uses the Hadoop-distributed computing framework for processing large peptide and spectra databases for proteomics datasets. This specific tool is capable of performing 27 billion peptide scorings in less than 60 min on a Hadoop cluster.
BlueSNP is an R package based on Hadoop platform used for genome-wide association studies (GWAS) analysis, primarily aiming on the statistical readouts to obtain significant associations between genotype–phenotype datasets. The efficiency of this tool is estimated to analyze 1000 phenotypes on 10 6 SNPs in 10 4 individuals in a duration of half-an-hour.
Myrna the cloud-based pipeline, provides information on the expression level differences of genes, including read alignments, data normalization, and statistical modeling.
The past few years have witnessed a tremendous increase in disease specific datasets from omics platforms. For example, the ArrayExpress Archive of Functional Genomics data repository contains information from approximately 30,000 experiments and more than one million functional assays. The growing amount of data demands for better and efficient bioinformatics driven packages to analyze and interpret the information obtained. This has also led to the birth of specific tools to analyze such massive amounts of data. Below, we mention some of the most popular commercial platforms for big data analytics.
Commercial platforms for healthcare data analytics
In order to tackle big data challenges and perform smoother analytics, various companies have implemented AI to analyze published results, textual data, and image data to obtain meaningful outcomes. IBM Corporation is one of the biggest and experienced players in this sector to provide healthcare analytics services commercially. IBM’s Watson Health is an AI platform to share and analyze health data among hospitals, providers and researchers. Similarly, Flatiron Health provides technology-oriented services in healthcare analytics specially focused in cancer research. Other big companies such as Oracle Corporation and Google Inc. are also focusing to develop cloud-based storage and distributed computing power platforms. Interestingly, in the recent few years, several companies and start-ups have also emerged to provide health care-based analytics and solutions. Some of the vendors in healthcare sector are provided in Table 2 . Below we discuss a few of these commercial solutions.
Ayasdi is one such big vendor which focuses on ML based methodologies to primarily provide machine intelligence platform along with an application framework with tried & tested enterprise scalability. It provides various applications for healthcare analytics, for example, to understand and manage clinical variation, and to transform clinical care costs. It is also capable of analyzing and managing how hospitals are organized, conversation between doctors, risk-oriented decisions by doctors for treatment, and the care they deliver to patients. It also provides an application for the assessment and management of population health, a proactive strategy that goes beyond traditional risk analysis methodologies. It uses ML intelligence for predicting future risk trajectories, identifying risk drivers, and providing solutions for best outcomes. A strategic illustration of the company’s methodology for analytics is provided in Fig. 4 .
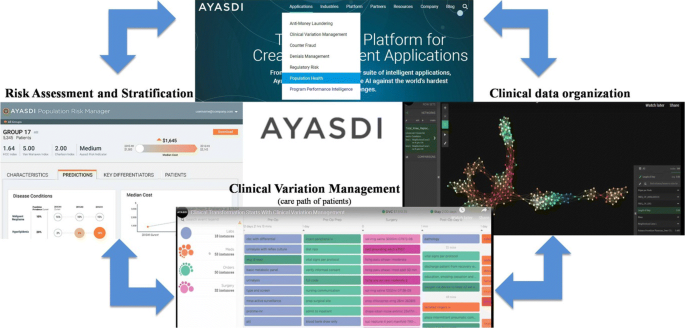
Illustration of application of “Intelligent Application Suite” provided by AYASDI for various analyses such as clinical variation, population health, and risk management in healthcare sector
Linguamatics
It is an NLP based algorithm that relies on an interactive text mining algorithm (I2E). I2E can extract and analyze a wide array of information. Results obtained using this technique are tenfold faster than other tools and does not require expert knowledge for data interpretation. This approach can provide information on genetic relationships and facts from unstructured data. Classical, ML requires well-curated data as input to generate clean and filtered results. However, NLP when integrated in EHR or clinical records per se facilitates the extraction of clean and structured information that often remains hidden in unstructured input data (Fig. 5 ).
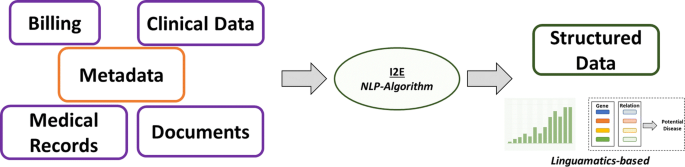
Schematic representation for the working principle of NLP-based AI system used in massive data retention and analysis in Linguamatics
This is one of the unique ideas of the tech-giant IBM that targets big data analytics in almost every professional sector. This platform utilizes ML and AI based algorithms extensively to extract the maximum information from minimal input. IBM Watson enforces the regimen of integrating a wide array of healthcare domains to provide meaningful and structured data (Fig. 6 ). In an attempt to uncover novel drug targets specifically in cancer disease model, IBM Watson and Pfizer have formed a productive collaboration to accelerate the discovery of novel immune-oncology combinations. Combining Watson’s deep learning modules integrated with AI technologies allows the researchers to interpret complex genomic data sets. IBM Watson has been used to predict specific types of cancer based on the gene expression profiles obtained from various large data sets providing signs of multiple druggable targets. IBM Watson is also used in drug discovery programs by integrating curated literature and forming network maps to provide a detailed overview of the molecular landscape in a specific disease model.
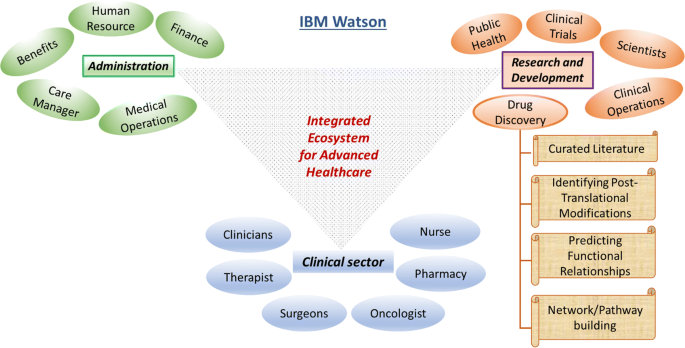
IBM Watson in healthcare data analytics. Schematic representation of the various functional modules in IBM Watson’s big-data healthcare package. For instance, the drug discovery domain involves network of highly coordinated data acquisition and analysis within the spectrum of curating database to building meaningful pathways towards elucidating novel druggable targets
In order to analyze the diversified medical data, healthcare domain, describes analytics in four categories: descriptive, diagnostic, predictive, and prescriptive analytics. Descriptive analytics refers for describing the current medical situations and commenting on that whereas diagnostic analysis explains reasons and factors behind occurrence of certain events, for example, choosing treatment option for a patient based on clustering and decision trees. Predictive analytics focuses on predictive ability of the future outcomes by determining trends and probabilities. These methods are mainly built up of machine leaning techniques and are helpful in the context of understanding complications that a patient can develop. Prescriptive analytics is to perform analysis to propose an action towards optimal decision making. For example, decision of avoiding a given treatment to the patient based on observed side effects and predicted complications. In order to improve performance of the current medical systems integration of big data into healthcare analytics can be a major factor; however, sophisticated strategies need to be developed. An architecture of best practices of different analytics in healthcare domain is required for integrating big data technologies to improve the outcomes. However, there are many challenges associated with the implementation of such strategies.
Challenges associated with healthcare big data
Methods for big data management and analysis are being continuously developed especially for real-time data streaming, capture, aggregation, analytics (using ML and predictive), and visualization solutions that can help integrate a better utilization of EMRs with the healthcare. For example, the EHR adoption rate of federally tested and certified EHR programs in the healthcare sector in the U.S.A. is nearly complete [ 7 ]. However, the availability of hundreds of EHR products certified by the government, each with different clinical terminologies, technical specifications, and functional capabilities has led to difficulties in the interoperability and sharing of data. Nonetheless, we can safely say that the healthcare industry has entered into a ‘post-EMR’ deployment phase. Now, the main objective is to gain actionable insights from these vast amounts of data collected as EMRs. Here, we discuss some of these challenges in brief.
Storing large volume of data is one of the primary challenges, but many organizations are comfortable with data storage on their own premises. It has several advantages like control over security, access, and up-time. However, an on-site server network can be expensive to scale and difficult to maintain. It appears that with decreasing costs and increasing reliability, the cloud-based storage using IT infrastructure is a better option which most of the healthcare organizations have opted for. Organizations must choose cloud-partners that understand the importance of healthcare-specific compliance and security issues. Additionally, cloud storage offers lower up-front costs, nimble disaster recovery, and easier expansion. Organizations can also have a hybrid approach to their data storage programs, which may be the most flexible and workable approach for providers with varying data access and storage needs.
The data needs to cleansed or scrubbed to ensure the accuracy, correctness, consistency, relevancy, and purity after acquisition. This cleaning process can be manual or automatized using logic rules to ensure high levels of accuracy and integrity. More sophisticated and precise tools use machine-learning techniques to reduce time and expenses and to stop foul data from derailing big data projects.
Unified format
Patients produce a huge volume of data that is not easy to capture with traditional EHR format, as it is knotty and not easily manageable. It is too difficult to handle big data especially when it comes without a perfect data organization to the healthcare providers. A need to codify all the clinically relevant information surfaced for the purpose of claims, billing purposes, and clinical analytics. Therefore, medical coding systems like Current Procedural Terminology (CPT) and International Classification of Diseases (ICD) code sets were developed to represent the core clinical concepts. However, these code sets have their own limitations.
Some studies have observed that the reporting of patient data into EMRs or EHRs is not entirely accurate yet [ 26 , 27 , 28 , 29 ], probably because of poor EHR utility, complex workflows, and a broken understanding of why big data is all-important to capture well. All these factors can contribute to the quality issues for big data all along its lifecycle. The EHRs intend to improve the quality and communication of data in clinical workflows though reports indicate discrepancies in these contexts. The documentation quality might improve by using self-report questionnaires from patients for their symptoms.
Image pre-processing
Studies have observed various physical factors that can lead to altered data quality and misinterpretations from existing medical records [ 30 ]. Medical images often suffer technical barriers that involve multiple types of noise and artifacts. Improper handling of medical images can also cause tampering of images for instance might lead to delineation of anatomical structures such as veins which is non-correlative with real case scenario. Reduction of noise, clearing artifacts, adjusting contrast of acquired images and image quality adjustment post mishandling are some of the measures that can be implemented to benefit the purpose.
There have been many security breaches, hackings, phishing attacks, and ransomware episodes that data security is a priority for healthcare organizations. After noticing an array of vulnerabilities, a list of technical safeguards was developed for the protected health information (PHI). These rules, termed as HIPAA Security Rules, help guide organizations with storing, transmission, authentication protocols, and controls over access, integrity, and auditing. Common security measures like using up-to-date anti-virus software, firewalls, encrypting sensitive data, and multi-factor authentication can save a lot of trouble.
To have a successful data governance plan, it would be mandatory to have complete, accurate, and up-to-date metadata regarding all the stored data. The metadata would be composed of information like time of creation, purpose and person responsible for the data, previous usage (by who, why, how, and when) for researchers and data analysts. This would allow analysts to replicate previous queries and help later scientific studies and accurate benchmarking. This increases the usefulness of data and prevents creation of “data dumpsters” of low or no use.
Metadata would make it easier for organizations to query their data and get some answers. However, in absence of proper interoperability between datasets the query tools may not access an entire repository of data. Also, different components of a dataset should be well interconnected or linked and easily accessible otherwise a complete portrait of an individual patient’s health may not be generated. Medical coding systems like ICD-10, SNOMED-CT, or LOINC must be implemented to reduce free-form concepts into a shared ontology. If the accuracy, completeness, and standardization of the data are not in question, then Structured Query Language (SQL) can be used to query large datasets and relational databases.
Visualization
A clean and engaging visualization of data with charts, heat maps, and histograms to illustrate contrasting figures and correct labeling of information to reduce potential confusion, can make it much easier for us to absorb information and use it appropriately. Other examples include bar charts, pie charts, and scatterplots with their own specific ways to convey the data.
Data sharing
Patients may or may not receive their care at multiple locations. In the former case, sharing data with other healthcare organizations would be essential. During such sharing, if the data is not interoperable then data movement between disparate organizations could be severely curtailed. This could be due to technical and organizational barriers. This may leave clinicians without key information for making decisions regarding follow-ups and treatment strategies for patients. Solutions like Fast Healthcare Interoperability Resource (FHIR) and public APIs, CommonWell (a not-for-profit trade association) and Carequality (a consensus-built, common interoperability framework) are making data interoperability and sharing easy and secure. The biggest roadblock for data sharing is the treatment of data as a commodity that can provide a competitive advantage. Therefore, sometimes both providers and vendors intentionally interfere with the flow of information to block the information flow between different EHR systems [ 31 ].
The healthcare providers will need to overcome every challenge on this list and more to develop a big data exchange ecosystem that provides trustworthy, timely, and meaningful information by connecting all members of the care continuum. Time, commitment, funding, and communication would be required before these challenges are overcome.
Big data analytics for cutting costs
To develop a healthcare system based on big data that can exchange big data and provides us with trustworthy, timely, and meaningful information, we need to overcome every challenge mentioned above. Overcoming these challenges would require investment in terms of time, funding, and commitment. However, like other technological advances, the success of these ambitious steps would apparently ease the present burdens on healthcare especially in terms of costs. It is believed that the implementation of big data analytics by healthcare organizations might lead to a saving of over 25% in annual costs in the coming years. Better diagnosis and disease predictions by big data analytics can enable cost reduction by decreasing the hospital readmission rate. The healthcare firms do not understand the variables responsible for readmissions well enough. It would be easier for healthcare organizations to improve their protocols for dealing with patients and prevent readmission by determining these relationships well. Big data analytics can also help in optimizing staffing, forecasting operating room demands, streamlining patient care, and improving the pharmaceutical supply chain. All of these factors will lead to an ultimate reduction in the healthcare costs by the organizations.
Quantum mechanics and big data analysis
Big data sets can be staggering in size. Therefore, its analysis remains daunting even with the most powerful modern computers. For most of the analysis, the bottleneck lies in the computer’s ability to access its memory and not in the processor [ 32 , 33 ]. The capacity, bandwidth or latency requirements of memory hierarchy outweigh the computational requirements so much that supercomputers are increasingly used for big data analysis [ 34 , 35 ]. An additional solution is the application of quantum approach for big data analysis.
Quantum computing and its advantages
The common digital computing uses binary digits to code for the data whereas quantum computation uses quantum bits or qubits [ 36 ]. A qubit is a quantum version of the classical binary bits that can represent a zero, a one, or any linear combination of states (called superpositions ) of those two qubit states [ 37 ]. Therefore, qubits allow computer bits to operate in three states compared to two states in the classical computation. This allows quantum computers to work thousands of times faster than regular computers. For example, a conventional analysis of a dataset with n points would require 2 n processing units whereas it would require just n quantum bits using a quantum computer. Quantum computers use quantum mechanical phenomena like superposition and quantum entanglement to perform computations [ 38 , 39 ].
Quantum algorithms can speed-up the big data analysis exponentially [ 40 ]. Some complex problems, believed to be unsolvable using conventional computing, can be solved by quantum approaches. For example, the current encryption techniques such as RSA, public-key (PK) and Data Encryption Standard (DES) which are thought to be impassable now would be irrelevant in future because quantum computers will quickly get through them [ 41 ]. Quantum approaches can dramatically reduce the information required for big data analysis. For example, quantum theory can maximize the distinguishability between a multilayer network using a minimum number of layers [ 42 ]. In addition, quantum approaches require a relatively small dataset to obtain a maximally sensitive data analysis compared to the conventional (machine-learning) techniques. Therefore, quantum approaches can drastically reduce the amount of computational power required to analyze big data. Even though, quantum computing is still in its infancy and presents many open challenges, it is being implemented for healthcare data.
Applications in big data analysis
Quantum computing is picking up and seems to be a potential solution for big data analysis. For example, identification of rare events, such as the production of Higgs bosons at the Large Hadron Collider (LHC) can now be performed using quantum approaches [ 43 ]. At LHC, huge amounts of collision data (1PB/s) is generated that needs to be filtered and analyzed. One such approach, the quantum annealing for ML (QAML) that implements a combination of ML and quantum computing with a programmable quantum annealer, helps reduce human intervention and increase the accuracy of assessing particle-collision data. In another example, the quantum support vector machine was implemented for both training and classification stages to classify new data [ 44 ]. Such quantum approaches could find applications in many areas of science [ 43 ]. Indeed, recurrent quantum neural network (RQNN) was implemented to increase signal separability in electroencephalogram (EEG) signals [ 45 ]. Similarly, quantum annealing was applied to intensity modulated radiotherapy (IMRT) beamlet intensity optimization [ 46 ]. Similarly, there exist more applications of quantum approaches regarding healthcare e.g. quantum sensors and quantum microscopes [ 47 ].
Conclusions and future prospects
Nowadays, various biomedical and healthcare tools such as genomics, mobile biometric sensors, and smartphone apps generate a big amount of data. Therefore, it is mandatory for us to know about and assess that can be achieved using this data. For example, the analysis of such data can provide further insights in terms of procedural, technical, medical and other types of improvements in healthcare. After a review of these healthcare procedures, it appears that the full potential of patient-specific medical specialty or personalized medicine is under way. The collective big data analysis of EHRs, EMRs and other medical data is continuously helping build a better prognostic framework. The companies providing service for healthcare analytics and clinical transformation are indeed contributing towards better and effective outcome. Common goals of these companies include reducing cost of analytics, developing effective Clinical Decision Support (CDS) systems, providing platforms for better treatment strategies, and identifying and preventing fraud associated with big data. Though, almost all of them face challenges on federal issues like how private data is handled, shared and kept safe. The combined pool of data from healthcare organizations and biomedical researchers have resulted in a better outlook, determination, and treatment of various diseases. This has also helped in building a better and healthier personalized healthcare framework. Modern healthcare fraternity has realized the potential of big data and therefore, have implemented big data analytics in healthcare and clinical practices. Supercomputers to quantum computers are helping in extracting meaningful information from big data in dramatically reduced time periods. With high hopes of extracting new and actionable knowledge that can improve the present status of healthcare services, researchers are plunging into biomedical big data despite the infrastructure challenges. Clinical trials, analysis of pharmacy and insurance claims together, discovery of biomarkers is a part of a novel and creative way to analyze healthcare big data.
Big data analytics leverage the gap within structured and unstructured data sources. The shift to an integrated data environment is a well-known hurdle to overcome. Interesting enough, the principle of big data heavily relies on the idea of the more the information, the more insights one can gain from this information and can make predictions for future events. It is rightfully projected by various reliable consulting firms and health care companies that the big data healthcare market is poised to grow at an exponential rate. However, in a short span we have witnessed a spectrum of analytics currently in use that have shown significant impacts on the decision making and performance of healthcare industry. The exponential growth of medical data from various domains has forced computational experts to design innovative strategies to analyze and interpret such enormous amount of data within a given timeframe. The integration of computational systems for signal processing from both research and practicing medical professionals has witnessed growth. Thus, developing a detailed model of a human body by combining physiological data and “-omics” techniques can be the next big target. This unique idea can enhance our knowledge of disease conditions and possibly help in the development of novel diagnostic tools. The continuous rise in available genomic data including inherent hidden errors from experiment and analytical practices need further attention. However, there are opportunities in each step of this extensive process to introduce systemic improvements within the healthcare research.
High volume of medical data collected across heterogeneous platforms has put a challenge to data scientists for careful integration and implementation. It is therefore suggested that revolution in healthcare is further needed to group together bioinformatics, health informatics and analytics to promote personalized and more effective treatments. Furthermore, new strategies and technologies should be developed to understand the nature (structured, semi-structured, unstructured), complexity (dimensions and attributes) and volume of the data to derive meaningful information. The greatest asset of big data lies in its limitless possibilities. The birth and integration of big data within the past few years has brought substantial advancements in the health care sector ranging from medical data management to drug discovery programs for complex human diseases including cancer and neurodegenerative disorders. To quote a simple example supporting the stated idea, since the late 2000′s the healthcare market has witnessed advancements in the EHR system in the context of data collection, management and usability. We believe that big data will add-on and bolster the existing pipeline of healthcare advances instead of replacing skilled manpower, subject knowledge experts and intellectuals, a notion argued by many. One can clearly see the transitions of health care market from a wider volume base to personalized or individual specific domain. Therefore, it is essential for technologists and professionals to understand this evolving situation. In the coming year it can be projected that big data analytics will march towards a predictive system. This would mean prediction of futuristic outcomes in an individual’s health state based on current or existing data (such as EHR-based and Omics-based). Similarly, it can also be presumed that structured information obtained from a certain geography might lead to generation of population health information. Taken together, big data will facilitate healthcare by introducing prediction of epidemics (in relation to population health), providing early warnings of disease conditions, and helping in the discovery of novel biomarkers and intelligent therapeutic intervention strategies for an improved quality of life.
Availability of data and materials
Not applicable.
Laney D. 3D data management: controlling data volume, velocity, and variety, Application delivery strategies. Stamford: META Group Inc; 2001.
Google Scholar
Mauro AD, Greco M, Grimaldi M. A formal definition of big data based on its essential features. Libr Rev. 2016;65(3):122–35.
Article Google Scholar
Gubbi J, et al. Internet of Things (IoT): a vision, architectural elements, and future directions. Future Gener Comput Syst. 2013;29(7):1645–60.
Doyle-Lindrud S. The evolution of the electronic health record. Clin J Oncol Nurs. 2015;19(2):153–4.
Gillum RF. From papyrus to the electronic tablet: a brief history of the clinical medical record with lessons for the digital Age. Am J Med. 2013;126(10):853–7.
Reiser SJ. The clinical record in medicine part 1: learning from cases*. Ann Intern Med. 1991;114(10):902–7.
Reisman M. EHRs: the challenge of making electronic data usable and interoperable. Pharm Ther. 2017;42(9):572–5.
Murphy G, Hanken MA, Waters K. Electronic health records: changing the vision. Philadelphia: Saunders W B Co; 1999. p. 627.
Shameer K, et al. Translational bioinformatics in the era of real-time biomedical, health care and wellness data streams. Brief Bioinform. 2017;18(1):105–24.
Service, R.F. The race for the $1000 genome. Science. 2006;311(5767):1544–6.
Stephens ZD, et al. Big data: astronomical or genomical? PLoS Biol. 2015;13(7):e1002195.
Yin Y, et al. The internet of things in healthcare: an overview. J Ind Inf Integr. 2016;1:3–13.
Moore SK. Unhooking medicine [wireless networking]. IEEE Spectr 2001; 38(1): 107–8, 110.
MathSciNet Google Scholar
Nasi G, Cucciniello M, Guerrazzi C. The role of mobile technologies in health care processes: the case of cancer supportive care. J Med Internet Res. 2015;17(2):e26.
Apple, ResearchKit/ResearchKit: ResearchKit 1.5.3. 2017.
Shvachko K, et al. The hadoop distributed file system. In: Proceedings of the 2010 IEEE 26th symposium on mass storage systems and technologies (MSST). New York: IEEE Computer Society; 2010. p. 1–10.
Dean J, Ghemawat S. MapReduce: simplified data processing on large clusters. Commun ACM. 2008;51(1):107–13.
Zaharia M, et al. Apache Spark: a unified engine for big data processing. Commun ACM. 2016;59(11):56–65.
Gopalani S, Arora R. Comparing Apache Spark and Map Reduce with performance analysis using K-means; 2015.
Ahmed H, et al. Performance comparison of spark clusters configured conventionally and a cloud servicE. Procedia Comput Sci. 2016;82:99–106.
Saouabi M, Ezzati A. A comparative between hadoop mapreduce and apache Spark on HDFS. In: Proceedings of the 1st international conference on internet of things and machine learning. Liverpool: ACM; 2017. p. 1–4.
Strickland NH. PACS (picture archiving and communication systems): filmless radiology. Arch Dis Child. 2000;83(1):82–6.
Article MathSciNet Google Scholar
Schroeder W, Martin K, Lorensen B. The visualization toolkit. 4th ed. Clifton Park: Kitware; 2006.
Friston K, et al. Statistical parametric mapping. London: Academic Press; 2007. p. vii.
Li L, et al. Identification of type 2 diabetes subgroups through topological analysis of patient similarity. Sci Transl Med. 2015;7(311):311ra174.
Valikodath NG, et al. Agreement of ocular symptom reporting between patient-reported outcomes and medical records. JAMA Ophthalmol. 2017;135(3):225–31.
Fromme EK, et al. How accurate is clinician reporting of chemotherapy adverse effects? A comparison with patient-reported symptoms from the Quality-of-Life Questionnaire C30. J Clin Oncol. 2004;22(17):3485–90.
Beckles GL, et al. Agreement between self-reports and medical records was only fair in a cross-sectional study of performance of annual eye examinations among adults with diabetes in managed care. Med Care. 2007;45(9):876–83.
Echaiz JF, et al. Low correlation between self-report and medical record documentation of urinary tract infection symptoms. Am J Infect Control. 2015;43(9):983–6.
Belle A, et al. Big data analytics in healthcare. Biomed Res Int. 2015;2015:370194.
Adler-Milstein J, Pfeifer E. Information blocking: is it occurring and what policy strategies can address it? Milbank Q. 2017;95(1):117–35.
Or-Bach, Z. A 1,000x improvement in computer systems by bridging the processor-memory gap. In: 2017 IEEE SOI-3D-subthreshold microelectronics technology unified conference (S3S). 2017.
Mahapatra NR, Venkatrao B. The processor-memory bottleneck: problems and solutions. XRDS. 1999;5(3es):2.
Voronin AA, Panchenko VY, Zheltikov AM. Supercomputations and big-data analysis in strong-field ultrafast optical physics: filamentation of high-peak-power ultrashort laser pulses. Laser Phys Lett. 2016;13(6):065403.
Dollas, A. Big data processing with FPGA supercomputers: opportunities and challenges. In: 2014 IEEE computer society annual symposium on VLSI; 2014.
Saffman M. Quantum computing with atomic qubits and Rydberg interactions: progress and challenges. J Phys B: At Mol Opt Phys. 2016;49(20):202001.
Nielsen MA, Chuang IL. Quantum computation and quantum information. 10th anniversary ed. Cambridge: Cambridge University Press; 2011. p. 708.
Raychev N. Quantum computing models for algebraic applications. Int J Scientific Eng Res. 2015;6(8):1281–8.
Harrow A. Why now is the right time to study quantum computing. XRDS. 2012;18(3):32–7.
Lloyd S, Garnerone S, Zanardi P. Quantum algorithms for topological and geometric analysis of data. Nat Commun. 2016;7:10138.
Buchanan W, Woodward A. Will quantum computers be the end of public key encryption? J Cyber Secur Technol. 2017;1(1):1–22.
De Domenico M, et al. Structural reducibility of multilayer networks. Nat Commun. 2015;6:6864.
Mott A, et al. Solving a Higgs optimization problem with quantum annealing for machine learning. Nature. 2017;550:375.
Rebentrost P, Mohseni M, Lloyd S. Quantum support vector machine for big data classification. Phys Rev Lett. 2014;113(13):130503.
Gandhi V, et al. Quantum neural network-based EEG filtering for a brain-computer interface. IEEE Trans Neural Netw Learn Syst. 2014;25(2):278–88.
Nazareth DP, Spaans JD. First application of quantum annealing to IMRT beamlet intensity optimization. Phys Med Biol. 2015;60(10):4137–48.
Reardon S. Quantum microscope offers MRI for molecules. Nature. 2017;543(7644):162.
Download references
Acknowledgements
Author information.
Sabyasachi Dash and Sushil Kumar Shakyawar contributed equally to this work
Authors and Affiliations
Department of Pathology and Laboratory Medicine, Weill Cornell Medicine, New York, 10065, NY, USA
Sabyasachi Dash
Center of Biological Engineering, University of Minho, Campus de Gualtar, 4710-057, Braga, Portugal
Sushil Kumar Shakyawar
SilicoLife Lda, Rua do Canastreiro 15, 4715-387, Braga, Portugal
Postgraduate School for Molecular Medicine, Warszawskiego Uniwersytetu Medycznego, Warsaw, Poland
Mohit Sharma
Małopolska Centre for Biotechnology, Jagiellonian University, Kraków, Poland
3B’s Research Group, Headquarters of the European Institute of Excellence on Tissue Engineering and Regenerative Medicine, AvePark - Parque de Ciência e Tecnologia, Zona Industrial da Gandra, Barco, 4805-017, Guimarães, Portugal
Sandeep Kaushik
You can also search for this author in PubMed Google Scholar
Contributions
MS wrote the manuscript. SD and SKS further added significant discussion that highly improved the quality of manuscript. SK designed the content sequence, guided SD, SS and MS in writing and revising the manuscript and checked the manuscript. All authors read and approved the final manuscript.
Corresponding author
Correspondence to Sandeep Kaushik .
Ethics declarations
Ethics approval and consent to participate, consent for publication, competing interests.
The authors declare that they have no competing interests.
Additional information
Publisher's note.
Springer Nature remains neutral with regard to jurisdictional claims in published maps and institutional affiliations.
Rights and permissions
Open Access This article is distributed under the terms of the Creative Commons Attribution 4.0 International License ( http://creativecommons.org/licenses/by/4.0/ ), which permits unrestricted use, distribution, and reproduction in any medium, provided you give appropriate credit to the original author(s) and the source, provide a link to the Creative Commons license, and indicate if changes were made.
Reprints and permissions
About this article
Cite this article.
Dash, S., Shakyawar, S.K., Sharma, M. et al. Big data in healthcare: management, analysis and future prospects. J Big Data 6 , 54 (2019). https://doi.org/10.1186/s40537-019-0217-0
Download citation
Received : 17 January 2019
Accepted : 06 June 2019
Published : 19 June 2019
DOI : https://doi.org/10.1186/s40537-019-0217-0
Share this article
Anyone you share the following link with will be able to read this content:
Sorry, a shareable link is not currently available for this article.
Provided by the Springer Nature SharedIt content-sharing initiative
- Biomedical research
- Big data analytics
- Internet of things
- Personalized medicine
- Quantum computing
- Open access
- Published: 22 June 2022
How can big data analytics be used for healthcare organization management? Literary framework and future research from a systematic review
- Nicola Cozzoli 1 ,
- Fiorella Pia Salvatore ORCID: orcid.org/0000-0001-6294-3360 1 ,
- Nicola Faccilongo 1 &
- Michele Milone 1
BMC Health Services Research volume 22 , Article number: 809 ( 2022 ) Cite this article
17k Accesses
19 Citations
17 Altmetric
Metrics details
Multiple attempts aimed at highlighting the relationship between big data analytics and benefits for healthcare organizations have been raised in the literature. The big data impact on health organization management is still not clear due to the relationship’s multi-disciplinary nature. This study aims to answer three research questions: a) What is the state of art of big data analytics adopted by healthcare organizations? b) What about the benefits for both health managers and healthcare organizations? c) What about future directions on big data analytics research in healthcare?
Through a systematic literature review the impact of big data analytics on healthcare management has been examined. The study aims to map extant literature and present a framework for future scholars to further build on, and executives to be guided by.
The positive relationship between big data analytics and healthcare organization management has emerged. To find out common elements in the studies reviewed, 16 studies have been selected and clustered into 4 research areas: 1) Potentialities of big data analytics. 2) Resource management. 3) Big data analytics and management of health surveillance systems. 4) Big data analytics and technology for healthcare organization.
Conclusions
In conclusion is identified how the big data analytics solutions are considered a milestone for managerial studies applied to healthcare organizations, although scientific research needs to investigate standardization and integration of the devices as well as the protocol in data analysis to improve the performance of the healthcare organization.
Peer Review reports
Big data is transforming and will transform the healthcare organizations in the near future [ 1 , 2 ]. Scientific literature in the managerial context applied to healthcare organizations, consider the Big Data Analytics (BDA) a fundamental tool, so much so that it has attracted the attention of the scientific community and stakeholders [ 3 ]. However, a premise should be made: data by themselves explain little, thus, to be useful in the healthcare organization management, firstly it is necessary to validate their quality, and secondly, find the right correlations. In other words, the data should be processed, analyzed, and interpreted with the appropriate tools [ 4 , 5 ].
Technological applications in healthcare BDA-related are rapidly increasing [ 6 ] and will increasingly characterize managers’ decision-making process. For example, IBM’s Watson project [ 7 ] is a "super-computer" that has scoured through several million scientific articles over the last twenty years and uses artificial intelligence tools (e.g., Machine Learning) to correlate disease symptoms and predict possible diagnostic scenarios. This case helps to understand how and to what extent BDA could really support healthcare managers to improve their decision processes, while increasing the performance of the healthcare organization.
Nowadays, the amount of data is no longer an issue. Internet traffic reports from Cisco and other network operators have estimated the entire digital universe to be 44 zettabytes and 463 exabytes will be the daily information could be generated by 2025. A new era took place in which the processes of production and management of human knowledge will no longer be the exclusive preserve of humans; machines will also play their part as knowledge producers [ 8 ]. From pharmaceutical companies to healthcare organizations, this enormous potential of data products, combined with IoT applications and AI tools [ 9 , 10 , 11 ], will play a significant role in the near future. Today, the medical applications based on IoT allow the monitoring of clinical data through the production of data generated by special devices (e.g., wearable devices) [ 12 ], remotely accessible by a physician rather than by caregivers [ 13 ].
The market size is a useful indicator of how much the healthcare organizations are turning their attention to new management models based on the use of big data. By 2025, the big data market in healthcare will touch $70 billion with a record 568% growth in 10 years. The use of such a tool not only represents a complex challenge [ 14 ], but also opens opportunities for all those involved in the healthcare supply chain who manage decision-making processes. Moreover, if on the one hand this technology will influence the definition of new managerial strategies within healthcare organizations, on the other hand, it will have positive repercussions on the effectiveness and efficiency of healthcare processes [ 15 ]. Indeed, the big data technology is used by healthcare managers to get, for example, information related to the list of doctors and nurses, the list of drugs with their expiration date, etc., in order to have tools for facilitating decision-making processes, improving the quality of services provided, and, at the same time, rationalizing the use of resources, by facilitating the management of the healthcare organization as a whole.
The BDA satisfies multiple needs that, on the one hand, influence the quality of the healthcare organization’s performance and, on the other hand, are useful in directing management strategies to improve the supply of healthcare services. Below there are some strategies, which aim to:
Provide specific services to patients, from diagnostics to preventive medicine passing through therapeutic adherence.
Detect the onset and spread of diseases in advance.
Observe parameters inherent to hospital quality standards, promoting control and prevention actions.
Modify treatment techniques.
Facilitate research and development in pharmacology, reducing the time to market of drugs.
Facilitate research and development of new and specific medical devices.
The main aim of this research is, therefore, to provide both an integrative framework on the state of art, and perspectives on how the BDA can be useful for the management of the healthcare organization. Considering the results, food-for-thought on how this technological and cultural revolution will affect the modus operandi of healthcare organizations will be launched.
Through an overview of recent scientific studies, this research aims to raise awareness among both practitioners and managers about BDA tools applied to healthcare management to address more effectively and efficiently the challenges imposed by an increasing demand for healthcare services.
In this regard, the study provides a systematic literature review (SLR) to explore the effect of BDA on the healthcare management by analyzing articles from the Scopus database during a period of 5 years (2016 – 2021).
Furthermore, the result through a content analysis, aspires to be a privileged starting point to find out potential barriers and opportunities provided by BDA-based management systems for smarter healthcare organization. Specifically, the study answers different research questions (RQs) as different levels of analysis have been performed. By analyzing the relationship between BDA-based management systems and the benefits delivered to the organizations, the research could not be conducted without exploring the state of art of BDA tools deployed in the field of healthcare. Thus, starting from this background the discussion on the future perspectives on BDA development in the healthcare organizations appears as a need.
Theoretical framework
Why use BDA and how to exploit its potential for healthcare organization management? This is the main question asked by managers and decision makers working in the healthcare sector. In recent years there have been multiple attempts in the literature aimed at highlighting the relationship between implementation of BDA and benefits for healthcare organizations, in terms of both resource efficiency and process management.
In 2017, a study by Wang and Hajli [ 16 ] has proposed a model founded on Resource-Based Theory and BDA Capabilities (BDAC) to explain the relationship between BDA, benefits, and value creation for healthcare organizations. As stated by Srinivasan and Swink [ 17 ], BDAC refers to “ organizational facility with tools, techniques, and processes that enable a firm to process, organize, visualize, and analyze data, thereby producing insights that enable data-driven operational planning, decision-making, and execution ”. In the healthcare organization, BDAC represents the ability to collect, store, analyze, and process huge volume variety, and velocity of health data come from various sources to improve data-driven decisions [ 18 , 19 ]. Indeed, the study of Wang and Hajli [ 16 ], validated on an empirical basis by 109 cases of BDA tools implementation in 63 healthcare organizations, has demonstrated how specific "path-to-value" can be identified. By varying degrees of relevance of the identified pathways, it has been shown that alongside the challenges of implementing certain BDA tools, there are corresponding specific benefits for healthcare organizations. Preliminarily, the study has defined the ability to analyze big data through the concept of Information Lifecycle Management (ILM) [ 20 ]. In this perspective, the capabilities of the BDA in healthcare organizations are configured as the abilities to process health data from diverse sources and provide significant information to healthcare managers. Thorough BDA, managers can detect timely indicators and identify business strategies, which allow them to put in place perspective plans, efficient strategies, and programs to increase the performance of organizations.
Researchers have found that BDA capabilities primarily stem from the implementation of various tools and features. Specifically, in order of importance, BDA capabilities are firstly triggered by processing tools (e.g., OLAP, machine learning, NLP), followed by aggregation tools (e.g., data warehouse tools), and, secondly, by data visualization tools and capabilities (e.g., visual dashboards/systems, reporting systems/interfaces).
Among the potentials triggered by the implementation of BDA in the healthcare organization, the analytical one was the main capability, that is the ability to process clinical data characterized by immense volume, variety (from text to graph), and speed (from batch to streaming), using descriptive analysis techniques [ 21 , 22 ]. In this regard, it is important to note that BDA-based management systems are the only ones capable of analyzing semi-structured or unstructured data. This represents a crucial element for revealing correlation patterns that are difficult to determine with traditional management systems [ 23 ]. Furthermore, the launch of these systems in a healthcare organization ensures the ability to effectively manage outputs regarding care process and service in order to constantly improve the performance of the organization. In summary, the characteristics of BDA-based management systems implemented in a healthcare organization, are:
predictive analytics capability, i.e., the ability to explore data and identify useful correlations, patterns and trends, and extrapolate them to predict what is likely to occur in the future [ 24 , 25 ];
interoperability capability, i.e., the ability to integrate data and processes to support management, collaboration, and sharing across different healthcare departments, managers, and facilities [ 26 ], and finally,
traceability capability, i.e., the ability to integrate and track all patient history data from different IT facilities and different healthcare units.
In terms of expected benefits from the BDA implementation, the study of Wang and Hajli [ 16 ] has showed that the most important ones are obtained from improved operational activities, such as improved quality and accuracy of healthcare decisions, rapid processing of issues, and the ability to enable treatments proactively before patients’ conditions worsen. Next, in terms of relevance, they were the benefits related to IT infrastructure, such as standardization and reduced costs for redundant infrastructure and the ability to quickly transfer data between different IT systems. Substantially, they have delivered a useful business model that healthcare managers can draw on to evaluate the specific leverages they need to activate in relation to the implementation of the BDA-based management systems. In addition to highlighting the undoubted benefits, the authors clearly show how specific BDA tools can facilitate the decision-making processes of healthcare managers and make them faster and more effective.
In another study carried out to identify BDA benefits and supports, and to drive organizational strategies, Wang, Kung, and Byrd [ 19 ], through the analysis of 26 case studies related to the BDA applications in the healthcare organization, have identified five "capabilities" of BDA: analytic capability for care patterns, unstructured data analytical capability, decision support, predictive, and traceability capabilities [ 19 ]. The study is remarkably interesting because in addition to mapping precise benefits, it also recommends specific strategies considering the BDA implementation for healthcare organizations. These strategies are useful for achieving effective results by leveraging the potential of BDA.
The first successful strategy is to implement governance based on the use of big data, starting with a definition of objectives, procedures, and key performance indicators (KPIs). Once again, one of the discriminating factors for success in implementing such a strategy remains the integration of information systems and the standardization of data protocols that often come from heterogeneous sources already existing in healthcare organizations. The second strategy is related to developing a culture of data sharing. The third one considers the training of healthcare managers, who cannot ignore knowledge related to BDA, for example on the use of data mining and business intelligence tools. The fourth strategy is related to the storage of big data, often available in heterogeneous formats, and is identified in the transition from the more expensive traditional storage systems (NAS) to more efficient and effective systems such as cloud computing solutions. The last strategic driver involves pathways related to the implementation of predictive BDA models. The mastery of KPIs, interactive visualization and data aggregation tools such as dashboards and reports should be acquired instruments for healthcare managers and in general for healthcare organizations oriented to BDA driven process management strategies.
More recent studies focus attention on the management practices supply chain in healthcare. In the study performed by Yu et al. [ 27 ], the authors, interviewing senior executives in Chinese hospitals, show on both a theoretical and empirical basis, how BDAC positively impacts the three dimensions of hospital supply chain integration (SCI) (inter-functional integration, hospital-patient integration and hospital-supplier integration) and how SCI, in turn, contributes to improve the operational flexibility [ 27 ]. By “operational flexibility” in the healthcare organization, it is meant the ability of a ward to adapt its operating procedures in relation to unforeseen circumstances while meeting the needs of patients [ 28 , 29 ].
The scholars have delivered an important contribution in demonstrating the relationship between BDAC, SCI, and operational flexibility from multiple perspectives, by providing useful management guidance for healthcare executives and managers involved in the supply chain. By analyzing and processing medical and managerial data with advanced analytical techniques, Chinese healthcare organizations were able to facilitate decision-making process with timely and appropriate actions, for example, tracking people's movements during the lockdown caused by the Coronavirus, understanding ongoing health trends, and managing pharmaceutical supplies [ 30 , 31 ].
This theoretical framework provides a key to interpreting the benefits offered by good practices deriving from the use of the BDA in the healthcare organization.
At the same time, the rigorous scientific method allows the validation of empirical experiences in relation to clear theoretical references. In the next paragraph projects that demonstrate what is stated in the literature are shown.
Practical framework
N(ursing) + Care App is an mHealth application that supports the work of frontline health workers (FHW) in developing countries [ 32 ]. The system is designed to collect not only patient data, but also diagnostic images. It is also given the opportunity to add recommended doctors based on the advice of FHWs in case the patient needs to follow a specific hospital visit.
For healthcare managers, predicting the number of emergency department accesses is a critical issue which complicates the optimization of the human resource management. To this end, Intel, and Assistance Publique-Hôpitaux de Paris (AP-HP), the largest hospital university in Europe, leveraging datasets from multiple sources, worked together to build a cloud-based solution to predict the number of patient visits to emergency rooms and hospital admissions. This predictive analytics tool, will enable healthcare managers at AP-HP hospitals to know the number of emergency room visits and hospital admissions at 15 days in order to reduce wait times, optimize human resource (HR) levels based on anticipated needs, accurately plan patient loads, including by pathology, and overall improve the quality and efficiency of services provided by the healthcare organization [ 33 ].
Chronic conditions, if not kept under control through a rigorous program of therapeutic adherence, can become a source of both more serious physical problems for patients and economic burdens for healthcare organizations. Another project that actively introduced BDA tools into healthcare management was carried out by the European Commission to launch production of the drug Enerzair Breezhaler . It was the first drug for the treatment of asthma co-packaged and co-prescribed with the Propeller digital platform. The app sends a reminder to comply with therapeutic adherence and maintains a record of the data, which the patient shares with him or her physician. Studies have demonstrated that the Propeller platform increases the degree of asthma control by up to 63%, therapeutic adherence by up to 58% [ 34 ], and reduces asthma emergency department visits and hospital admissions by up to 57% [ 35 ].
The practical framework described, aided by some empirical experience, only partially reveals the potential offered by BDA. The diffusion of BDA-based management systems in the healthcare organization will trigger a virtuous circle, allowing soon to accumulate increasingly accurate medical data. By exploiting the most advanced AI technologies, BDA will support predictive analysis, allow physicians to make more accurate and faster diagnostic pathways and managers to use results. It will help health practitioners in the decision-making process, optimize the use of resources with a consequent costs reduction and, overall, improve the quality of services provided by healthcare organizations.
The main aim of this study is to update the state of art about the BDA-based management systems adopted in the healthcare organization, underlining management advantages for both the organizations and managers. BDA has the potential to reduce the cost of care, prevent disease outbreaks, and improve the patients’ quality of life. Through its ability to process and cross-reference massive amounts of both management, and clinical information, BDA promises to be an effective support tool for both healthcare managers and patients.
To achieve this aim, a Systematic Literature Review (SLR) was performed. This method identifies, evaluates, and summarizes the updates that raise from the literature about the BDA tools used to improve both the healthcare organizations performance and patients’ quality of life. The method takes inspiration from the protocol used by Khanra S., et al. [ 36 ] which considers inclusion and exclusion criteria.
The present study aims to add a contribute to the literature by addressing three RQs:
What is the state of art of BDA adopted by healthcare organizations?
What about the benefits for both health managers and healthcare organization?
What about future directions on BDA research in healthcare?
To answer the RQs, as widespread electronic database Scopus has been selected. To obtain an international validity of studies, the research only considers papers in English. Utilizing the Boolean operator “AND”, the following keywords have been searched: “big data analytics” AND “healthcare” AND “management”. As inclusion criteria, only papers published from 2016 to 2021 have been considered. As subject areas, “medicine” and “business, management and accounting” have been selected. Instead, as exclusion criteria, article in press and the following documents type: “review”, “book”, “conference review”, “letter” and “note” have not been taken into account. Also, to avoid a dispersal of the study, conference proceedings have been excluded. Following the searching protocol, 34 results have been obtained (Fig. 1 ).
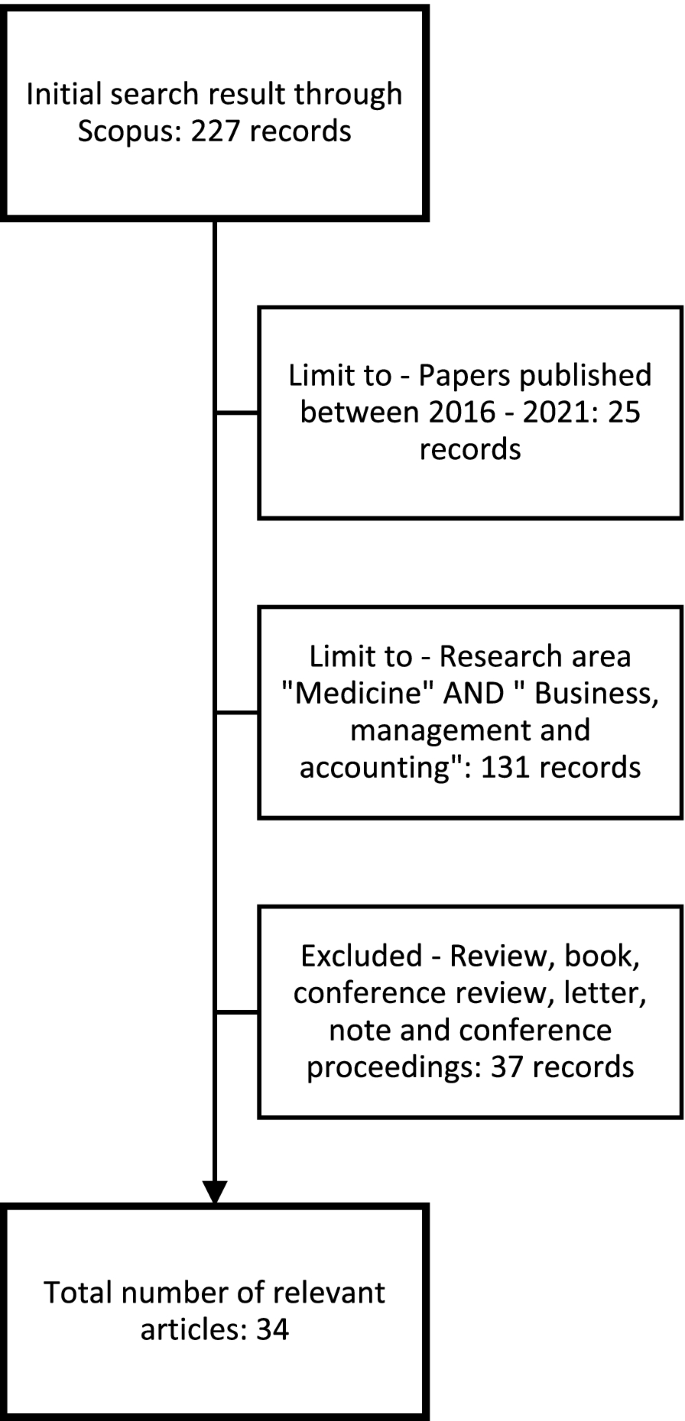
Workflow of articles selection
An excel spreadsheet was used to perform the extraction procedures while the statistical analyses were carried out using the software STATA 16 ©. The list of the extracted papers investigated with the content analysis can be found in the Appendix.
The work proceeds through a descriptive analysis. After that, a content analysis has been performed to identify the most relevant characteristics of the BDA-based management systems, underlining the positive impact for the healthcare organizations, without neglecting to outline the trends for the future scenarios and research directions.
According to the SLR, the iterative process shown in the Fig. 1 , has allowed to delete the duplicates and match the results with the RQs.
As shown in Fig. 1 the initial search on Scopus database has delivered 227 results. By limiting research to papers published between 2016 and 2021, 11% of records have been removed. At the second stage, by selecting the subject areas, the screening has allowed to exclude 131 records; thus, the 57.7% of the results initially selected. The last step of the process has conducted to exclude document types such as Review, Book, Conference Review, Letter, and Note. In other words, 37 records were excluded, representing 16.3% of the sample. At the end of the screening process, 34 articles were selected, representing about 15% of the sample.
In the descriptive analysis the time distribution of the studies from 2016 to 2021 is included. It is important to note the increasing of publication trend from 2017 to 2019. This output confirms a growing interest in the research field of BDA applied to healthcare organizations (Fig. 2 ).
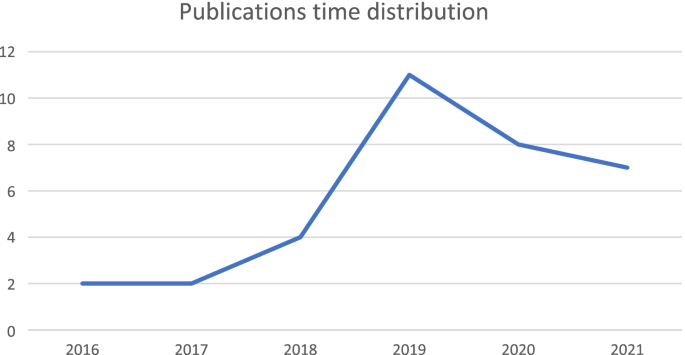
Trend of research steams
The trend of research steams considers a sample of 34 scientific contributions as they come from the screening process above described. Although 6% of the total sample was collected in the years 2016 and 2017, it is only indicative of the growing trend of scientific studies on BDA in healthcare sector. The overall incidence in 2018 was 12% but the turning point was reached in 2019 as 32% of the studies collected in the sample were reached. This outcome could be read considering the Covid-19 pandemic outbreak which has been a representative testing ground for BDA tools by helping managers and decision-makers to plan healthcare managerial strategies.
In this context, the use of the BDA by Chinese healthcare organizations for tracking people's flow during the lockdown, represents an important case study that has registered the peak in the time flow of research. By looking at 2020 and 2021 data, which represent respectively 24% and 21% of the total scientific contributions, the growing trend seems to be confirmed by validating the rising interest in BDA research seen as a planning tool for healthcare processes.
The pie-chart shows the scientific production by country. It is necessary to specify that Scopus database clusters the studies by home country author’s organization, therefore the same study could be referred to more than one country and thus belong to more than one cluster.
The geographical locations of the studies showed in the Fig. 3 outlining India, UK, and USA as more than one third of the total scientific producers. It is well known that IT companies as Google, Apple, Amazon, and Microsoft are investing considerable resources on BDA tools for healthcare. China and India contribute together with 22% of the scientific articles. Big data technology has played a key role in virus tracking during the pandemic crisis. The "Internet Plus Healthcare", a big data center in Zhongwei (China), provides cloud services to both healthcare institutions and IT companies. In Yinchuan (China), an industrial park for big data acts as a catalyst for IT company involved in healthcare sector. India confirms to be one of the heavily adopter countries of artificial intelligence, big data analytics, and IoT technologies. Although India must face the challenge to provide basic healthcare services in a predominantly rural country, start-ups with BDA skills in healthcare are springing up.
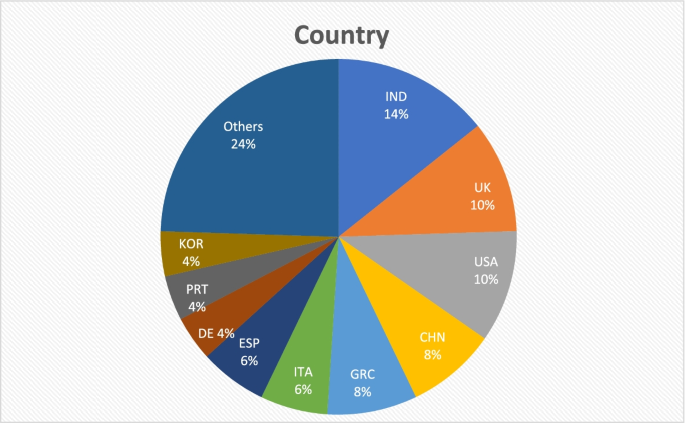
Geographical locations of the studies
It is also important underlining the performance of the European countries. UK, Greece, Italy, Spain, Germany, and Portugal support the research with almost 40% of the studies published, confirming that Europe will be a driving force for the BDA research in the next future. The development of a European Health Data Space (EHDS) is an ambitious project of the European Commission. It will lead member states to share an efficient infrastructure for both exchange and management health data by providing citizens with equal treatment, free access to clinical data, and quality healthcare services.
In the area “Others” all the other countries contributing marginally to research have been included.
The next step of the study is focused on a content analysis to show the experiences of applying BDA in healthcare organizations.
Starting from the 34 articles selected for the descriptive analysis, to identify in detail the core issue of the study, a second screening was performed. 18 articles were excluded because weakly focused on the research objective which concerns specifically how BDA can be used for healthcare organization management. Thus, after an in-depth reading of abstracts and full papers, the scholars have identified 16 papers closer targeted on the mentioned research objective. The 16 studies selected through a content analysis were clustered into 4 research areas (RAs) as showed in the following table (Table 1 ). The clustering procedure identifies 4 relevant topics: Potentialities of BDA (RA1), Resource management (RA2), BDA and management of health surveillance system (RA3), BDA technology for healthcare organization (RA4). The proposed clustering has been though to give an easy-to-go research map and to support the healthcare managers.
RA1: potentialities of BDA
Wang and Hajli [ 16 ] define BDA potentialities in the healthcare context as “ the ability to acquire, store, process and analyze large amounts of health data in various forms, and deliver meaningful information to users, which allows them to discover business values and insights in a timely fashion ”. The relationship between BDA and the benefits for the healthcare organizations it has been well expressed by the theory of the “path to value chain” [ 16 ]. This path represents an important contribution to the exploration of business value, not only for drawing the generic and well-established connection between big data capabilities [ 19 ] and the benefits, but also for empirically showing how capabilities can be developed and what benefits can be achieved in the healthcare organizations. Another study included in this area, explores the key role of BDA capabilities in developing healthcare supply chain integrations and its impact on hospital flexibility [ 27 ]. Specifically, the BDA has a fundamental role in developing healthcare integration supply chain and the operational flexibility. Considering the health and economic crises caused by the Covid-19, this dimension of BDA has been an especially important leverage for managers to improve operational flexibility of the healthcare organizations. The ability to provide predictive models and real-time insights, is a powerful prospective of the BDA for helping healthcare professionals and managers in decision-making process. In this regard, the literature presents several applications of big data in healthcare that support the data collection, management, and integration of data in healthcare organizations [ 37 ]. Moreover, BDA enables the integration of massive datasets, supporting decisions of manager and monitoring the managerial aspects of healthcare organizations. Building a decision-making process based on BDA, firstly means identifying the big data keys that can implement ad-hoc strategies to improve efficiency along the healthcare value chain. To this end, the research carried out by Sousa et al., [ 37 ] underlines the benefits that BDA can give to the decision-making process, through predictive models and real-time analytics, assisting in the collection, management, and integration of data in healthcare organizations.
To date, thanks to an integrated and interconnected ecosystem, is becoming possible to provide personalized healthcare services, collect an enormous quantity of both clinical and biometrics data and, thus, implement BDA instruments. Nevertheless, to take a real advantage from these tools and turn them into useful decision support systems (DSS), is necessary for R&D to be focused on data filtering mechanisms in order to obtain good-quality reliable information [ 38 ]. The healthcare models based on BDA and implementation of new healthcare programs, enable both medical and managerial decision support for the healthcare services provision. New types of interactions with and among users of the healthcare ecosystem will produce in the next future a wide variety of complex data, thus, the main challenges refer to information processing and analytics.
In light of the above, the RA1 includes studies for which the quality of data and the need for high performance filtering mechanisms are becoming keys factor for the success of BDA-based management systems in the healthcare organizations. For example, the study carried out by Maglaveras et al., [ 38 ], included in this area, explores new R&D pathways in biomedical information processing and management, as well as to the design of new intelligent decision support systems.
RA2: resource management
Another important research direction emerged from the literature review, concerns positive impact of the BDA on the resource management. Insufficient policy for managing medical materials waste, energy use and environmental burden, restricts the resources conservation. The BDA is extremely useful in this aspect; it could provide in the next future an important contribution to implement the circular economy processes and to support sustainable development initiatives in the healthcare organizations [ 39 ]. To this end, the study developed by Kazançoğlu et al. [ 39 ], underline the importance of circularity and sustainability concepts to mitigate the sector’s negative impacts on the environment. Furthermore, the study identifies the barriers related to circular economy in the healthcare organization and provides solutions to these barriers by implementing BDA-based management systems. Lastly, the authors, have developed a managerial, policy and theoretical framework to support healthcare managers to launch sustainable initiatives in the context of healthcare organization.
The impact on the performance has been also investigated by studies that have linked benefits of BDA and artificial intelligence with green supply chain integration process [ 40 ]. Digital learning is more becoming a “moderator” of the green supply chain process with a significant positive impact on environmental performance of the healthcare organization. BDA-AI technologies will lead to improvement of the environmental process integration and green supply chain collaboration and, consequently, will support the managers’ decisions involved in the supply processes. This study also provides an important reference framework for logistics/supply chain managers who want to implement BDA-AI technologies for supporting green supply processes and enhancing environmental performance of the healthcare organization [ 40 ].
Nowadays, many scholars are focusing on BDA-driven decision support systems to sustain the healthcare managers [ 41 ]. These types of BDA-based analytical tools will provide a useful quantitative support for managers of healthcare organizations. The authors have reported design and technical details of the system implementations using case studies. They have developed a toolkit which represents a framework reference for resources management, allowing to create strategic models and obtain analytical results for evidence-based decisions and managerial evaluations.
In this RA, two other important topics investigated by BDA are: high quality healthcare service, and healthcare costs. Optimize the supply chain activities is an imperative to keep lower the healthcare costs. The data generated by medical equipment and devices can be successfully used in forecasting, decision-making process, and to make more efficient the healthcare supply chain management [ 42 ]. The study carried out by Alotaibi et al. [ 42 ], thus, presents a review on the use of big data in healthcare organizations underling opportunities and challenges deriving from the application of BDA-based management systems within the organizations.
As already asserted, a good implementation of BDA in the healthcare organization will play a fundamental role in improving the clinical outcomes management, giving helpful insights for decision makers and managers, in order to avoiding diseases, reducing healthcare expenses, and improving the performance of the healthcare organization [ 43 ]. However, to achieve these ambitious outcomes the research will face a crucial challenge: how to rationalize, make easily usable, and at affordable costs, heterogeneous data coming from diverse sources. The research developed by Kundella and Gobinath [ 43 ] represents an important contribute to explore key challenges, techniques, technologies, privacy issues, security algorithms and future directions of the use of BDA in the healthcare organization.
RA3: BDA and management of health surveillance system
The rise of BDA promises to solve many healthcare challenges in the developing countries. The BDA applied to healthcare organization help managers to rationalize the resources, and health system to better delivery treatments to the patients [ 44 ]. In this regard, the government of Zambia is thinking to implement BDA solutions to provide more effective and efficient healthcare services. A well-managed health surveillance system represents an important driver to improve the quality of life and reduce the medical waste, especially in developing countries where the lack of resources is severe and limits economic development. For all these reasons, Europe is investing on BDA initiatives in public health and in the oncology sectors, to generate new knowledge, improve clinical care and make more efficient the management of the public health surveillance system [ 45 ]. The BDA capability for identifying specific population pattern, managing high volume of data and turn it into real (or near real) time insights, contributes to identify it as a powerful tool to support the managers for the decision-making processes. Despite this, implementing a BDA-based management systems within the healthcare organizations requires investment in the human capital, strong collaboration with stakeholders, and data integration with and among the healthcare units. To this end, Gunapal et al., [ 46 ] has highlighted that Singapore has setup a Regional Health System (RHS) database to facilitate BDA for proactive population health management (PHM) and health services research [ 46 ]. The structure of the healthcare database has been built collecting data from four database coming from three RHSs: National Healthcare Group (NHG), Tan Tock Seng Hospital (TTSH), National University Hospital (NUH) and Alexandra Hospital (AH). The result has been a database including information useful for the healthcare managers which incorporates data on patient demographics, chronic disease, and healthcare utilization information. These characteristics facilitate the identification of specific patients’ paths linked by past healthcare utilization and chronic disease information. Converging information into a single database helps to understand the cross-utilization of healthcare services across the three RHSs. A such approach allows to setup the RHSs structure for initiative-taking population health management (PHM) and to improve the performance of healthcare organizations [ 46 ].
RA 4: BDA technology for healthcare organization
The wearable devices and different kind of sensors, able to collect clinical data, in combination with BDA, will constitute the basis of personalized medicine and will be crucial tools to improve the performance of healthcare organizations [ 47 ]. The scientific research has to face the important challenge to adapt data acquisition, storage, transmission and analytics to healthcare demand. Thus, the healthcare data should be categorized, homogenized, and implemented into specific models by adapting machine-learning techniques to the nature of the healthcare organization.
A fruitful field of interest for the application of BDA in healthcare organization is the diagnostic imaging. To take out maximum benefits from it and to be useful for managers of healthcare organizations, it is necessary to implement digital platforms and applications [ 48 ]. Indeed, the simple production of a large amount of data does not automatically translate to an advantage for the healthcare performance. Specific applications are required to favor the correct and advantageous management of diagnostic images [ 48 ]. The link between BDA and IoT technologies, as instrument to incorporate the accessibility, capacity to customize, and practical conveyance of clinical data, emerged as another research direction investigated by the papers included in this RA. These tools allow: (1) the healthcare organizations to decrease expenses; (2) the people to self regulates treatments; (3) practitioners to take as quickly as possible decisions in remote way and keep constant contact with patients [ 49 ].
In light of these results, it is possible to state that IoT, big data, and artificial intelligence as machine-learning algorithms, are three of the most significative innovations in the healthcare organization. These types of organizations are implementing home-centric data collection networks and intelligent BDA systems based on machine learning technologies. For example, a high-level implementation of these systems has been efficiently implemented in Cartagena, Colombia, for hypertensive patients by using an e-Health sensor and Amazon Web Services components [ 50 ]. The authors stress the importance of using the combination of IoT, big data, and artificial intelligence as tools to obtain better health outcomes for the communities and improved performance for healthcare organization. The new generation of machine-learning algorithms can use standardized data sets generated by these sources to improve the effectiveness of public health interventions [ 50 ]. To this end, as pointed out by numerous studies in the field of BDA applied on healthcare organizations, it becomes crucial for the next future research to concentrate R&D efforts towards full standardized dataset protocols.
As highlighted by the results, in Europe, as well as in the rest of the world, a significant trend is emerging among healthcare organizations in adopting BDA-based management systems [ 45 ]. Among the clustering process performed, the common element in the studies reviewed is the positive relationship between BDA tools and achievable benefits for healthcare organizations.
As emerged by the RAs, some studies explore business value for healthcare organizations and the concept of potentialities of BDA (RA1) to explain the evidence of precise path-to-value chains leading to specific benefits [ 16 ]. These perspectives provide useful guidelines for healthcare managers who want to consider implementing BDA tools in their organizations. Some authors in particular focus on the role of BDA capabilities in the development of hospital supply chain integration and operational flexibility, demonstrating a positive relationship between the two dimensions [ 27 ]. During the Covid-19 outbreak, it became clearer how important operational flexibility is to healthcare organizations. The scholars also underline how BDA can impact to the efficiency of the decision-making processes in healthcare organizations, through predictive models and real-time analytics, helping health professionals in the collection, management, and analysis [ 37 ].
In general, BDA-based management systems make personalized care programs possible. However, considering the enormous amount and heterogeneity of information available nowadays, it emerges the necessity to address R&D pathways towards data filtering mechanisms and engineering new intelligent decision support systems within the healthcare organizations [ 38 ].
Circular economy (CE) and sustainability concepts are becoming important key drivers in healthcare organizations to reduce negative impact on the environment (RA2). Some study directions look at BDA as tool to provide solution for barriers related to CE and support sustainable development initiatives in the healthcare organizations [ 39 ]. Empirical studies have demonstrated the benefits of BDA-AI in the supply chain integration process and its impact on environmental performance. By assessing a sample of 168 French hospitals, Benzidia et al. [ 40 ], has observed that the use of BDA-AI technologies has a significant impact on environmental process integration and green supply chain. In particular, this study provides important insights for healthcare managers, who wish to implement BDA-AI technologies for sustaining green supply processes and improving environmental performance [ 40 ]. BDA and web technologies can successfully help managers to redesign healthcare processes making them more effective and efficient. Since healthcare spending is constantly growing in the world’s major regions, there is urgent need to redesign processes optimizing supply chain activities such that high-quality services could be provided at lower costs [ 42 ]. Although BDA-based management systems promise to fulfil this role in the healthcare organization, more in-depth studies are required. Due to heterogeneity of information sources, one of future research direction should deeply investigate the protocol standardization and integration in data analyzing as well as techniques and technologies used, security algorithms of BDA in the healthcare and medical data [ 43 ].
In developing countries, as well as in the rest of the world, the management of health surveillance is a sensitive issue (RA3). Therefore, authors have studied main key factors that hind BDA access in the healthcare organization [ 44 ]. Technology, staff, data management and health policies have been identified as some of decisive variables [ 44 ]. Due to increasing of the ageing population and the related disability, healthcare organizations will face hard challenges soon. To this end, big data can also help healthcare managers to detect patterns and to turn high volumes of data into usable knowledges. In this context investments in technological infrastructures are needed as well as in the human capital [ 45 ]. China is proving, with a large scale of investment, to be a pioneer country in the adoption of BDA-based management systems in the healthcare organization [ 46 ].
The rising of AI, IoT, machine learning [ 49 , 50 , 51 ], and sensors technology, as well as embedded systems able to communicate each other, have boosted the adoption of BDA with valuable benefits for the healthcare organization (RA4). These technologies will play a fundamental role on big data management to improve the performances of the healthcare organizations. Some authors have underlined privacy issues related to healthcare data and the necessity to make sensor data homogeneous and tagged. Furthermore, implementation of clinical records into models and adaptation of machine-learning techniques is required [ 47 ]. Future R&D in this field should be focused on the developing of digital platforms and specific applications based on BDA also for managing diagnostic images [ 48 ].
By exploring the relationship between BDA-based management systems and the benefits delivered to the healthcare organizations, this study replies to 3 RQs: 1) What is the state of art of BDA adopted by healthcare organizations, 2) What are the benefits for both health managers and healthcare organizations and 3) What are the future directions on BDA research in healthcare.
To answer the RQs the SLR has started from an investigation on the recent literature BDA about the BDA in healthcare organizations. Descriptive analysis has been performed on a sample of 34 studies coming from all over the world. The second stage shows a detailed content analysis on 16 studies which better answer to research question about the relationship between benefits for the healthcare organization and BDA solutions.
By analyzing the successful BDA strategies in healthcare context, some authors focus their attention on the BDA potentialities applied in the healthcare organizations [ 16 , 37 ]. Indeed, the research highlights how analytical tools through personal health systems support public health management systems and how BDA suggests new pathways to support healthcare managers in decision-making process.
In the literature, other scholars highlight the positive impact of BDA on resource management. The BDA solutions are analyzed as tools to sustain CE initiatives [ 38 , 39 ] as well as to enable green supply chain process integration and improve hospital performance [ 40 ]. By exploiting KPIs coming from BDA solutions, some researchers present innovative models for planning public health policy [ 41 ]. In this context, the studies consider BDA cloud computing solutions and social media data analytics for supporting the performance of healthcare supply chain management [ 42 , 43 ]. Furthermore, researchers from all around the world are showing particular interest on BDA for health surveillance system management [ 44 , 45 , 46 ].
According to the recent literature, BDA is transforming the healthcare organizations. The SLR has showed how the BDA solutions are now quite considered a milestone for managerial studies applied to healthcare organizations. The Coronavirus pandemic has been a good test run for using BDA to design healthcare policy strategies. Although an extensive literature on BDA to support healthcare management is being produced, the classification into four RAs proposed is an attempt to examine precise key research directions. About that, the limitations of the present research can be detected as the difficulty to review a field of literature constantly evolving. To date, the amount of data is no longer an issue. To be useful in the healthcare context, is necessary to validate their quality and then find the right correlations. In other words, the data should be processed, analyzed, and interpreted correctly. For this reason, emerges the need to address research pathways towards filtering mechanisms, by converting data from big to smart, and engineering new decision support systems within the healthcare organizations [ 38 ].
The content analysis carried out in this research has shown that studies are addressed to find out new models for both predictive and personalized medicine by exploiting BDA technologies [ 47 ]. The researchers underline the added value of using BDA both in the medical diagnostic process [ 48 ] and jointly with IT technologies such as IOT and machine learning [ 49 , 51 ].
Thus, considering the results obtained, it is possible to state that BDA can effectively help healthcare managers to detect common patterns and turn high volumes of data into usable knowledges. Investments on human capital become a priority to exploit the potential of BDA [ 45 ].
To achieve these objectives the future research should provide usable insights and standardized procedures for training healthcare managers and practitioners. AI, machines learning, as well as management strategies, will also play their part as knowledge producers in the healthcare organization. Privacy issues related to healthcare data and also the necessity to make sensor data homogeneous, are becoming crucial research topics to be faced. Finally, due to the heterogeneity of information sources, the future direction of research should investigate the standardization and integration of the protocol in data analysis, as well as the techniques useful for the managerial sector to implement increasingly BDA-based management systems in future healthcare organizations [ 43 ].
Nowadays the challenge for healthcare organizations is the development of useful applications BDA-based. According with the circular economy view, the future research directions should be addressed considering the relationship between digitalization and management resources consumption. The data centralization combined with a BDA approach can effectively support circular economy processes in healthcare supply chain by reducing waste and resource consumptions.
Exploiting the BDA’s capabilities will also be a key factor in forecasting and monitoring outbreaks. Future studies will need to focus on developing more efficient models for sharing data in order to improve the performance of healthcare organizations around the world.
Availability of data and materials
The datasets analyzed during the current study are not publicly available due to data relating to scientific journal names and authors but are available from the corresponding author on reasonable request.
Wang L, Alexander CA. Big data in medical applications and health care. Curr Res Med. 2015;6:1–8.
Article Google Scholar
Aceto G, Persico V, Pescape A. Industry 4.0 and health: internet of things, big data, and cloud computing for healthcare 4.0. J Ind Inf Integr. 2020;18:100129.
Google Scholar
Galetsi P, Katsaliaki K, Kumar S. Values, challenges and future directions of big data analytics in healthcare: A systematic review. Soc Sci Med. 2019;241:112533.
Article CAS PubMed Google Scholar
Obermeyer Z, Emanuel EJ. Predicting the future — big data, machine learning, and clinical medicine. New Engl J Med. 2016;375:1216–9.
Article PubMed Google Scholar
Kumar Y, Sood K, Kaul S, Vasuja R, et al. Big data analytics and its benefits in healthcare. In: Kulkarni J, et al., editors. Big data analytics in healthcare, studies in big data 66. Cham: Springer; 2020. p. 3–21.
Raghupati W, Raghupathi V. Big data analytics in healthcare: promise and potential. Health Inf Sci Syst Vol. 2014;2(1):1–10.
Jain DA, Kumar V, Khanduja D, Sharma K, Bateja R. A detailed study of big data in healthcare: case study of Brenda and IBM Watson. Int J Recent Technol Eng. 2019;7:8–12.
Tremolada, L. (2019), “Quanti dati sono generati in un giorno?” Il Sole24Ore , May 26, 2019, available at: https://www.infodata.ilsole24ore.com/2019/05/14/quanti-dati-sono-generati-in-un-giorno/?refresh_ce=1 (Accessed 17 Feb 2022).
Srivastava P.K., Rakshit P. Cutting edge IoT Technology for Smart Indian Pharma. In: International Conference on Advance Computing and Innovative Technologies in Engineering, (ICACITE) 2021. Greater Noida: Institute of Electrical and Electronics Engineers Inc.; 2021. p. 360–2.
Rayan R.A, Tsagkaris C, Zafar I. IoT for better mobile health applications. In: Kumar P, editor. A fusion of artificial intelligence and internet of things for emerging cyber systemsand internet of things for emerging cyber systems. Cham: Springer; 2022. p. 1–13.
Chung K, Park RC. Chatbot-based healthcare service with a knowledge base for cloud computing. Cluster Comput. 2019;22:1925–37.
Ali F, El-Sappagh S, Islam SMR, Ali A, Attique M, Imran M, Kwak KS. An intelligent healthcare monitoring framework using wearable sensors and social networking data. Fut Generation Comput Syst. 2021;114:23–43.
Yousefi S, Derakhshan F, Karimipour H. Applications of big data analytics and machine learning in the internet of things. In: Choo KK, Dehghantanha A, editors. Handbook of big data privacy. Cham: Springer; 2020. p. 77–108.
Chapter Google Scholar
Mehta N, Pandit A, Kulkarni M. Elements of healthcare big data analytics. In: Big data analytics in healthcare, studies in big data 66. Cham: Springer; 2018.
Han Y, Lie RK, Guo R. The internet hospital as a telehealth model in China: systematic search and content analysis. J Med Int Res. 2020;22:e17995.
Wang Y Hajli, N.,. Exploring the path to big data analytics success in healthcare. J Bus Res. 2017;70:287–99.
Srinivasan R, Swink M. An investigation of visibility and flexibility as complements to supply chain analytics: an organizational information processing theory perspective. Prod Oper Manage. 2018;27:1849–67.
Wang Y, Byrd TA. Business analytics-enabled decision-making effectiveness through knowledge absorptive capacity in health care. J Knowl Manage. 2017;21:517–39.
Wang Y, Kung LA, Byrd TA. Big data analytics: Understanding its capabilities and potential benefits for healthcare organizations. Technol Forecast Soc Change. 2018;126:3–13.
Jagadish HV, Gehrke J, Labrinidis A, Papakonstantinou Y, Patel JM, Ramakrishnan R, Shahabi C. Big data and its technical challenges. Commun ACM. 2014;57:86–94.
Seddon PB, Constantinidis D, Dod H. How does business analytics contribute to business value? In: Information Systems Journal, Proceeding of Thirty Third International Conference on Information Systems. Orlando: Wiley Publishing Ltd; 2012. p. 237–69.
Cao G, Duan Y, Li G. Linking business analytics to decision making effectiveness: a path model analysis. IEEE Trans Eng Manage. 2015;62:384–95.
Watson HJ. Tutorial: big data analytics: concepts, technologies, and applications. Commun Assoc Inf Syst. 2014;34:1247–68.
Negash S. Business intelligence. Commun Assoc Inf Syst. 2004;13:177–95.
Hurwitz J, Nugent A, Hapler F, Kaufman M. Big data for dummies. Hoboken: Wiley; 2013.
Sadeghi P, Benyoucef M, Kuziemsky CE. A mashup-based framework for multimulti-level healthcare interoperability. Inf Syst Front. 2012;14:57–72.
Yu W, Zhao G, Liu Q, Song Y. Role of big data analytics capability in developing integrated hospital supply chains and operational flexibility: An organizational information processing theory perspective. Technol Forecast Soc Change. 2021;163:120417.
Butler TW, Leong GK, Everett LN. The operations management role in hospital strategic planning. J Oper Manag. 1996;14:137–56.
Slack N, Brandon-Jones A, Johnston R. Operations management. 8th ed. Harlow: Pearson; 2016.
Liu, J., (2020), “Deployment of health IT in China’s fight against the COVID-19 pandemic”, available at: https://www.itnonline.com/article/deployment-health-it-china%E2%80%99s-fight-against-covid-19-pandemic (Accessed 20 Dec 2021).
Ting DS, Wei LC, Dzau V, Wong TY. Digital technology and COVID-19. Nat Med. 2020;26:459–61.
Article CAS PubMed PubMed Central Google Scholar
Rajasekera J, Mishal A.V., Mori Y, et al. Innovative mHealth solution for reliable patient data empowering rural healthcare in developing countries. In: Kulkarni A, et al., editors. Big data analytics in healthcare. Studies in big data, vol 66,. Cham: Springer; 2020. p. 83–103.
Ambert, K., Beaune, S., Chaibi, A., Briard, L., Bhattacharjee, A., Bharadwaj, V., Sumanth, K., Crowe, K. (2016), “French Hospital Uses Trusted Analytics Platform to Predict Emergency Department Visits and Hospital Admissions”, available at: https://www.intel.com/content/dam/www/public/us/en/documents/white-papers/french-hospital-analytics-predict-admissions-paper.pdf , (Accessed 13 Mar 2022).
Van Sickle D, Barrett M, Humblet O, Henderson K, Hogg C. Randomized, controlled study of the impact of a mobile health tool on asthma SABA use, control and adherence. Eur Respir J . 2016;48(Suppl. 60):1018.
Merchant R, Szefler SJ, Bender BG, Tuffli M, Barrett MA, Gondalia R, Kaye L, Van Sickle D, Stempel DA. Impact of a digital health intervention on asthma resource utilization. World Allergy Org J. 2018;411:28.
Khanra S, Dhir A, Islam N, Mäntymäki M. Big data analytics in healthcare: a systematic literature review. Enterprise Inf Syst. 2020;14:878–912.
Sousa MJ, Pesqueira AM, Lemos C, Sousa M, Rocha Á. Decision-making based on big data analytics for people management in healthcare organizations. J Med Syst. 2019;43:290.
Maglaveras N, Kilintzis V, Koutkias V, Chouvarda I. Integrated care and connected health approaches leveraging personalised health through big data analytics. Stud Health Technol Inf. 2016;224:117–22.
Kazançoğlu Y, Sağnak M, Lafcı Ç, Luthra S, Kumar A, Taçoğlu C. Big Data-enabled solutions framework to overcoming the barriers to circular economy initiatives in healthcare sector. Int J Environ Res Public Health. 2021;18:7513.
Article PubMed PubMed Central Google Scholar
Benzidia S, Makaoui N, Bentahar O. The impact of big data analytics and artificial intelligence on green supply chain process integration and hospital environmental performance. Technol Forecast Soc Change. 2021;165:120557.
Moutselos K, Maglogiannis I. Evidence-based public health policy models development and evaluation using big data analytics and web technologies. Med Arch (Sarajevo, Bosnia and Herzegovina). 2020;74:47–53.
Alotaibi S, Mehmood R, Katib I, Chlamtac I. The role of big data and twitter data analytics in healthcare supply chain management. In: Mehmood R, See S, Katib I, editors. Smart infrastructure and applications. Cham: EAI/Springer Innovations in Communication and Computing, Springer; 2020. p. 267–79.
Kundella S, Gobinath R. A survey on big data analytics in medical and healthcare using cloud computing. Int J Sci Technol Res. 2019;8:1061–5.
Chellah RC, Kunda D. An assessment of factors that affect the implementation of big data analytics in the Zambian health sector for strategic planning and predictive analysis: a case of Copperbelt province. Int J Electron Healthc. 2020;11:101–22.
Pastorino R, De Vito C, Migliara G, Glocker K, Binenbaum I, Ricciardi W, Boccia S. Benefits and challenges of big data in healthcare: an overview of the European initiatives. Eur J Public Health. 2019;29:23–7.
Gunapal PPG, Kannapiran P, Teow KL, Zhu Z, You AX, Saxena N, Singh V, Tham L, Choo PWJ, Chong P-N, Sim JHJ, Wong JEL. Setting up a regional health system database for seamless population health management in Singapore. Proc Singapore Healthc. 2016;25:27–34.
Clim A, Zota RD, Tinica G. Big data in home healthcare: A new frontier in personalized medicine. Medical emergency services and prediction of hypertension risks. Int J Healthc Manage. 2019;12:241–9.
Aiello M, Cavaliere C, D’Albore A, Salvatore M. The challenges of diagnostic imaging in the era of big data. J Clin Med. 2019;8:316.
Article PubMed Central Google Scholar
Bharathi MJ, Rajavarman VN. A survey on big data management in health care using IOT. Int J Recent Technol Eng. 2019;7:196–8.
Lai A, Rossignoli F, Stacchezzini R. How integrated reporting meets the investors and other stakeholders’information needs . (In Vrontis D., Weber Y., Tsoukatos E.) Global and national business theories and practice: bridging the past with the future. Cyprus: EuroMed Press; 2017.
Martinez F.E.L, Núñez-Valdez E.R, et al. Big data and machine learning: a way to improve outcomes in population health management. In: González García C, et al., editors. Protocols and applications for the industrial internet of things. Hershey: IGI Global; 2018. p. 225–39.
Download references
Acknowledgements
Not applicable.
The research was carried out without funding.
Author information
Authors and affiliations.
Department of Economics, University of Foggia, Via Caggese n.1, Foggia, Italy
Nicola Cozzoli, Fiorella Pia Salvatore, Nicola Faccilongo & Michele Milone
You can also search for this author in PubMed Google Scholar
Contributions
NC and FPS designed and conducted the empirical study, wrote and revised the manuscript. NC and FPS carried out the analysis and wrote the results, discussion and conclusions. NC, FPS, NF, and MM revised the manuscript. All authors read the manuscript and approved the final version.
Corresponding author
Correspondence to Fiorella Pia Salvatore .
Ethics declarations
Ethics approval and consent to participate, consent for publication, competing interests.
The authors declare that they have no competing interests.
Additional information
Publisher's note.
Springer Nature remains neutral with regard to jurisdictional claims in published maps and institutional affiliations.
Supplementary Information
Additional file 1..
List of articles.
Rights and permissions
Open Access This article is licensed under a Creative Commons Attribution 4.0 International License, which permits use, sharing, adaptation, distribution and reproduction in any medium or format, as long as you give appropriate credit to the original author(s) and the source, provide a link to the Creative Commons licence, and indicate if changes were made. The images or other third party material in this article are included in the article's Creative Commons licence, unless indicated otherwise in a credit line to the material. If material is not included in the article's Creative Commons licence and your intended use is not permitted by statutory regulation or exceeds the permitted use, you will need to obtain permission directly from the copyright holder. To view a copy of this licence, visit http://creativecommons.org/licenses/by/4.0/ . The Creative Commons Public Domain Dedication waiver ( http://creativecommons.org/publicdomain/zero/1.0/ ) applies to the data made available in this article, unless otherwise stated in a credit line to the data.
Reprints and permissions
About this article
Cite this article.
Cozzoli, N., Salvatore, F.P., Faccilongo, N. et al. How can big data analytics be used for healthcare organization management? Literary framework and future research from a systematic review. BMC Health Serv Res 22 , 809 (2022). https://doi.org/10.1186/s12913-022-08167-z
Download citation
Received : 02 March 2022
Accepted : 06 June 2022
Published : 22 June 2022
DOI : https://doi.org/10.1186/s12913-022-08167-z
Share this article
Anyone you share the following link with will be able to read this content:
Sorry, a shareable link is not currently available for this article.
Provided by the Springer Nature SharedIt content-sharing initiative
- Healthcare management
- Healthcare organization
- Healthcare governance
- Big data analytics
BMC Health Services Research
ISSN: 1472-6963
- General enquiries: [email protected]
MIT Technology Review
- Newsletters
Building a data-driven health-care ecosystem
Harnessing data to improve the equity, affordability, and quality of the health care system.
- MIT Technology Review Insights archive page

In association with JPMorgan Chase
The application of AI to health-care data has promise to align the U.S. health-care system to quality care and positive health outcomes. But AI for health care hasn’t reached its full capacity. One reason is the inconsistent quality and integrity of the data that AI depends on. The industry—hospitals, providers, insurers, and administrators—uses diverse systems. The resulting data can be difficult to share because of incompatibility, privacy regulations, and the unstructured nature of much of the data. The data can carry errors, omissions, and duplications, making it difficult to access, analyze, and use. Even the best data can cause data bias : the data used to train AI models can reinforce underrepresentation of historically marginalized populations. The growth of AI in all industries means data quality is increasingly vital.
While AI-driven innovation is still growing, the U.S. continues to spend more than twice as much as the average high-income country for its health care, while its health outcomes are falling: the latest data from the U.S. Center for Disease Control’s National Center for Health Statistics indicates U.S. life expectancy rates dropped for the second year in a row in 2021.
To spark innovation by identifying gaps and pain points in the employer-based health-care system, JPMorgan Chase launched Morgan Health in 2021. Morgan Health’s chief technology officer of corporate responsibility, Tiffany West Polk, says Morgan Health is driven to improve health outcomes, affordability, and equity, with data at its foundation. Gaining insights from large data streams means optimizing analytical platforms and ensuring data remains secure, while also HIPAA and Health Resources and Services Administration (HRSA) compliant, she says.
Currently, Polk says, the U.S. health-care system seems to be “quite stuck” in terms of keeping health-care quality and positive outcomes in line with rising costs.
- “If you look across the broader U.S. environment in particular, employer sponsored insurance is a huge part of the health-care net for the United States, and employers make significant financial investment to provide health benefits to their employees. It's one of the main things that people look at when they're looking across an employer landscape and thinking about who they want to work for.”
Investing in new ways to provide health care
Nearly 160 million people in the U.S. have employer-sponsored health insurance as of 2022, according to health-care policy research non-profit KFF (formerly the Kaiser Family Foundation). JPMorgan Chase launched Morgan Health because of its focus on improving employer-sponsored health care, not least for its 165,000 employees.
Morgan Health has invested $130 million in capital during the past 18-plus months in five innovative health-care companies: advanced primary care provider Vera Whole Health; health-care data analytics specialist Embold Health; Kindbody, a fertility clinic network and global family-building benefits provider; LetsGetChecked, which creates home-monitoring clinical tools; and Centivo, which provides health care plans for self-insured employers.
All of these companies offer new approaches to conventional employer-sponsored health care to deliver a higher standard of care. Morgan Health’s collaboration with these enterprises will examine how these change patient outcomes, health-care equity, and affordability, and how to scale their successes.
“Many Americans today face real barriers to receiving high-quality, affordable, and equitable health care, even with employer-sponsored insurance,” Polk says. This calls for breaking the paradigm of delivery-incentivized health care, she says, which rewards providers for delivering services, but pays insufficient attention to outcomes.
- “We have a model today where our health-care providers are incentivized based on the number of patients they see or the number of services they perform. What that means is that they're not incentivized based on improvements, patient's health, and wellbeing. And so when you have a model that thinks volume versus value, those challenges then serve to compound the disparities that we have. And that then also means that those who have employer-sponsored insurance are also similarly challenged.”
For Morgan Health, AI and machine learning (ML) will be a key to problem-solving with health-care technology, Polk says. AI is ubiquitous across industries, and is the go-to when we think about innovation, she says, but the hype can mean we forget about the importance of data accessibility and quality.
Polk says solving this data challenge makes this an exciting and transformational time to be a chief technology officer and a technologist. The next stage of evolution in health care can’t proceed without better data, Polk says, and this is what the data and analytics team at Morgan Health are addressing.
- “[AI] has become so ubiquitous in terms of how we think about everything. And we think that it is the thing that's going to fix anything and everything in technology. And it has become so ubiquitous and so the go-to when you think about innovation, that I think that sometimes, there's this way in which people kind of forget about what AI actually is underneath the covers.”
Garnering data-based insights
To address the strength of health-care data, the industry is moving increasingly toward standard electronic health-care records (EHRs) for patients. A 2023 Deloitte study says use of EHRs and health information exchanges (HIEs) is growing rapidly, with organizations building data lakes and using AI to combine and cleanse data. These measures provide a “strong digital backbone” for building connections between hospitals, primary care centers, and payment tools, the study says, and this should help reduce errors, unnecessary readmissions, and duplicate testing.
The U.S. Department of Health and Human Services (HHS) is also building a network for digital connection in the health-care industry, to allow data to flow among multiple providers and geographies. Its Office of the National Coordinator for Health Information Technology (ONC) announced in December 2023 that its national health data exchange —the Trusted Exchange Framework and Common Agreement (TEFCA)—is operational. The exchange connects Quality Health Care Information Networks, which it certifies and onboards, with standard policies and technical requirements.
Polk says Morgan Health is improving foundations to incentivize better outcomes for patients. Morgan Health’s work can create standards—grounded in data—that incentivize better performance, which can then be shared across the employer-sponsored insurance network, and among broader communities. Using AI features such as metadata tagging (algorithms that can group and label data that has a common purpose), she says, “is one way health-care companies can simplify tasks and open up more time for providing care.”
- “If you do your data ingestion right, if you cleanse your data right, if you make sure that your metadata tagging is correct, and then you are very aware of the way in which your algorithms have been biased in the past, you can be aware of that so that you can make sure that your algorithms are inclusive moving forward.”
“I think the most important thing is incentivizing our health-care partners who provide for our employees to meaningfully improve health-care quality, equity, and affordability through incentivizing outcomes, not incentivizing volume, not incentivizing visits, but really incentivizing outcomes,” Polk says.
This article is for informational purposes only and it is not intended as legal, tax, financial, investment, accounting or regulatory advice. Opinions expressed herein are the personal views of the individual(s) and do not represent the views of JPMorgan Chase & Co. The accuracy of any statements, linked resources, reported findings or quotations are not the responsibility of JPMorgan Chase & Co.
Humans and technology
Unlocking the power of sustainability.
A comprehensive sustainability effort embraces technology, shifting from risk reduction to innovation opportunity.
Building a more reliable supply chain
Rapidly advancing technologies are building the modern supply chain, making transparent, collaborative, and data-driven systems a reality.
Backed by heritage, ready for the future
Let’s not make the same mistakes with ai that we made with social media.
Social media’s unregulated evolution over the past decade holds a lot of lessons that apply directly to AI companies and technologies.
- Nathan E. Sanders archive page
- Bruce Schneier archive page
Stay connected
Get the latest updates from mit technology review.
Discover special offers, top stories, upcoming events, and more.
Thank you for submitting your email!
It looks like something went wrong.
We’re having trouble saving your preferences. Try refreshing this page and updating them one more time. If you continue to get this message, reach out to us at [email protected] with a list of newsletters you’d like to receive.
More From Forbes
It’s healthcare’s data era—finally.

- Share to Facebook
- Share to Twitter
- Share to Linkedin
CEO of 1upHealth , a cloud-based health data platform enabling data exchange across the health system to improve efficiency and outcomes.
Healthcare’s data era is finally here, distinguished by higher levels of sophistication in how the industry uses data to improve operational performance and patient outcomes. Changes in government regulations, advancements in interoperability technology, new competition entering the market and emerging payment dynamics are forcing traditional healthcare organizations—and the industry as a whole—to finally embrace data-driven approaches.
There are legitimate reasons the industry hasn’t been data-focused historically. In the past, data was difficult to access and of questionable quality. Clinical and claims data formats were different, making it nearly impossible to combine and analyze datasets. High-margin lines of business and fee-for-service payment models made it easy to ignore inefficiencies, while a focus on patient privacy kept data siloed.
The result: Healthcare organizations haven’t necessarily fostered data-driven cultures aimed at improving operational performance. Even recent “big data” initiatives focused on improving clinical quality and population health have been relatively unsophisticated and decoupled from operational performance.
Meanwhile, other industries are thriving, using data-driven strategies to improve business outcomes. Digital-first organizations like Amazon use data and metrics to drive their businesses forward, focusing on more strategic success metrics like customer satisfaction, churn, product quality and workforce satisfaction/retention.
Best High-Yield Savings Accounts Of 2024
Best 5% interest savings accounts of 2024, putting data first.
Now is the time for healthcare organizations to bring data to the forefront and level up to where other industries have been operating for the past several decades.
New federal regulations like the CMS Interoperability and Prior Authorization Final Rule , released in January 2024, are advancing the industry away from antiquated, costly operational models. Buoyed by the new regulations—which are built around existing data standards like FHIR and technology like APIs and data platforms—the industry is now positioned to reap the benefits associated with increased access to diverse, standardized and computable data.
With this data in hand, healthcare organizations can unlock outsized improvements in critical areas like value-based care, healthcare quality and healthcare equity.
Leveraging data to inform decision-making has been a hallmark of my career as a business leader who’s successfully managed companies across a range of industries. And, as the CEO of a leading healthcare data platform, I’m so excited to see healthcare having its moment.
If you’re a healthcare leader ready to embark on a data-driven era, I offer you these suggestions:
1. Align internal success metrics with client metrics. Healthcare leaders should build data strategies in line with those of their stakeholders, including patients, clinicians and plan members. For example, at my company, we help our customers track key metrics like quality, member satisfaction, outcomes and waste. Ultimately, our success is aligned with their success, sowing the seeds for a true win-win-win.
2. Think bigger about the possibilities data can bring. Traditional healthcare metrics (revenue, profit, stock price, shareholder return, market share) have their place, but with more data access, healthcare leaders can better understand and strategize improvements to critical success metrics like quality, satisfaction, operational efficiency, waste and retention.
Beyond that, leaders can look to more sophisticated analytics to tackle deeper drivers of success. For example, access to combined clinical and claims data can help insurance companies better manage risk and plan for new services based on a stronger understanding of shifting populations.
3. Remember the “why” behind this data shift. Change is never easy, and you’ll face tough headwinds. At this time, remember to zoom out and focus on the benefits that interoperable data will unlock. Encourage open dialogue within the organization to educate staff about the importance of these changes and address questions and concerns head-on.
4. Stay up to date on the latest data-sharing regulations. While compliance with CMS rules is not the end goal in all of this, leaders must understand the new regulations and policies and ensure their organizations are keeping up with industry standards.
Now is the time for leaders to fuse quality data, interoperability and culture to reap the benefits that come with informed decision-making. While a transformation of this size will take time, the key to success will be embracing change one step at a time and always remembering the “why”—better patient outcomes at a lower cost. That’s something we can all get behind.
Forbes Technology Council is an invitation-only community for world-class CIOs, CTOs and technology executives. Do I qualify?
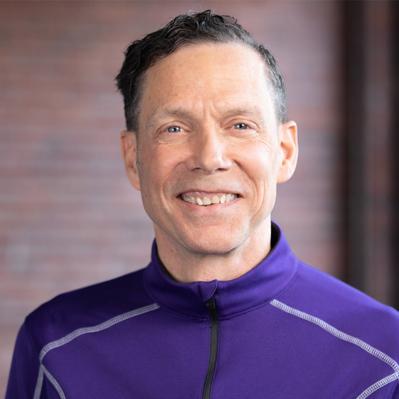
- Editorial Standards
- Reprints & Permissions
Read our research on: Abortion | Podcasts | Election 2024
Regions & Countries
What the data says about abortion in the u.s..
Pew Research Center has conducted many surveys about abortion over the years, providing a lens into Americans’ views on whether the procedure should be legal, among a host of other questions.
In a Center survey conducted nearly a year after the Supreme Court’s June 2022 decision that ended the constitutional right to abortion , 62% of U.S. adults said the practice should be legal in all or most cases, while 36% said it should be illegal in all or most cases. Another survey conducted a few months before the decision showed that relatively few Americans take an absolutist view on the issue .
Find answers to common questions about abortion in America, based on data from the Centers for Disease Control and Prevention (CDC) and the Guttmacher Institute, which have tracked these patterns for several decades:
How many abortions are there in the U.S. each year?
How has the number of abortions in the u.s. changed over time, what is the abortion rate among women in the u.s. how has it changed over time, what are the most common types of abortion, how many abortion providers are there in the u.s., and how has that number changed, what percentage of abortions are for women who live in a different state from the abortion provider, what are the demographics of women who have had abortions, when during pregnancy do most abortions occur, how often are there medical complications from abortion.
This compilation of data on abortion in the United States draws mainly from two sources: the Centers for Disease Control and Prevention (CDC) and the Guttmacher Institute, both of which have regularly compiled national abortion data for approximately half a century, and which collect their data in different ways.
The CDC data that is highlighted in this post comes from the agency’s “abortion surveillance” reports, which have been published annually since 1974 (and which have included data from 1969). Its figures from 1973 through 1996 include data from all 50 states, the District of Columbia and New York City – 52 “reporting areas” in all. Since 1997, the CDC’s totals have lacked data from some states (most notably California) for the years that those states did not report data to the agency. The four reporting areas that did not submit data to the CDC in 2021 – California, Maryland, New Hampshire and New Jersey – accounted for approximately 25% of all legal induced abortions in the U.S. in 2020, according to Guttmacher’s data. Most states, though, do have data in the reports, and the figures for the vast majority of them came from each state’s central health agency, while for some states, the figures came from hospitals and other medical facilities.
Discussion of CDC abortion data involving women’s state of residence, marital status, race, ethnicity, age, abortion history and the number of previous live births excludes the low share of abortions where that information was not supplied. Read the methodology for the CDC’s latest abortion surveillance report , which includes data from 2021, for more details. Previous reports can be found at stacks.cdc.gov by entering “abortion surveillance” into the search box.
For the numbers of deaths caused by induced abortions in 1963 and 1965, this analysis looks at reports by the then-U.S. Department of Health, Education and Welfare, a precursor to the Department of Health and Human Services. In computing those figures, we excluded abortions listed in the report under the categories “spontaneous or unspecified” or as “other.” (“Spontaneous abortion” is another way of referring to miscarriages.)
Guttmacher data in this post comes from national surveys of abortion providers that Guttmacher has conducted 19 times since 1973. Guttmacher compiles its figures after contacting every known provider of abortions – clinics, hospitals and physicians’ offices – in the country. It uses questionnaires and health department data, and it provides estimates for abortion providers that don’t respond to its inquiries. (In 2020, the last year for which it has released data on the number of abortions in the U.S., it used estimates for 12% of abortions.) For most of the 2000s, Guttmacher has conducted these national surveys every three years, each time getting abortion data for the prior two years. For each interim year, Guttmacher has calculated estimates based on trends from its own figures and from other data.
The latest full summary of Guttmacher data came in the institute’s report titled “Abortion Incidence and Service Availability in the United States, 2020.” It includes figures for 2020 and 2019 and estimates for 2018. The report includes a methods section.
In addition, this post uses data from StatPearls, an online health care resource, on complications from abortion.
An exact answer is hard to come by. The CDC and the Guttmacher Institute have each tried to measure this for around half a century, but they use different methods and publish different figures.
The last year for which the CDC reported a yearly national total for abortions is 2021. It found there were 625,978 abortions in the District of Columbia and the 46 states with available data that year, up from 597,355 in those states and D.C. in 2020. The corresponding figure for 2019 was 607,720.
The last year for which Guttmacher reported a yearly national total was 2020. It said there were 930,160 abortions that year in all 50 states and the District of Columbia, compared with 916,460 in 2019.
- How the CDC gets its data: It compiles figures that are voluntarily reported by states’ central health agencies, including separate figures for New York City and the District of Columbia. Its latest totals do not include figures from California, Maryland, New Hampshire or New Jersey, which did not report data to the CDC. ( Read the methodology from the latest CDC report .)
- How Guttmacher gets its data: It compiles its figures after contacting every known abortion provider – clinics, hospitals and physicians’ offices – in the country. It uses questionnaires and health department data, then provides estimates for abortion providers that don’t respond. Guttmacher’s figures are higher than the CDC’s in part because they include data (and in some instances, estimates) from all 50 states. ( Read the institute’s latest full report and methodology .)
While the Guttmacher Institute supports abortion rights, its empirical data on abortions in the U.S. has been widely cited by groups and publications across the political spectrum, including by a number of those that disagree with its positions .
These estimates from Guttmacher and the CDC are results of multiyear efforts to collect data on abortion across the U.S. Last year, Guttmacher also began publishing less precise estimates every few months , based on a much smaller sample of providers.
The figures reported by these organizations include only legal induced abortions conducted by clinics, hospitals or physicians’ offices, or those that make use of abortion pills dispensed from certified facilities such as clinics or physicians’ offices. They do not account for the use of abortion pills that were obtained outside of clinical settings .
(Back to top)
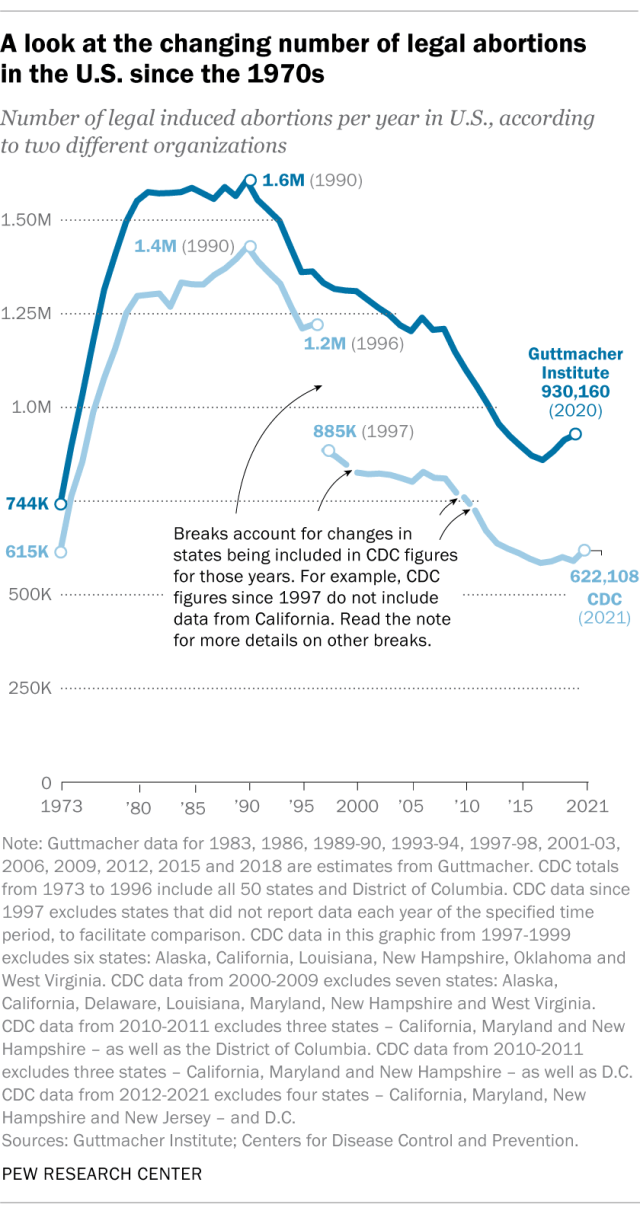
The annual number of U.S. abortions rose for years after Roe v. Wade legalized the procedure in 1973, reaching its highest levels around the late 1980s and early 1990s, according to both the CDC and Guttmacher. Since then, abortions have generally decreased at what a CDC analysis called “a slow yet steady pace.”
Guttmacher says the number of abortions occurring in the U.S. in 2020 was 40% lower than it was in 1991. According to the CDC, the number was 36% lower in 2021 than in 1991, looking just at the District of Columbia and the 46 states that reported both of those years.
(The corresponding line graph shows the long-term trend in the number of legal abortions reported by both organizations. To allow for consistent comparisons over time, the CDC figures in the chart have been adjusted to ensure that the same states are counted from one year to the next. Using that approach, the CDC figure for 2021 is 622,108 legal abortions.)
There have been occasional breaks in this long-term pattern of decline – during the middle of the first decade of the 2000s, and then again in the late 2010s. The CDC reported modest 1% and 2% increases in abortions in 2018 and 2019, and then, after a 2% decrease in 2020, a 5% increase in 2021. Guttmacher reported an 8% increase over the three-year period from 2017 to 2020.
As noted above, these figures do not include abortions that use pills obtained outside of clinical settings.
Guttmacher says that in 2020 there were 14.4 abortions in the U.S. per 1,000 women ages 15 to 44. Its data shows that the rate of abortions among women has generally been declining in the U.S. since 1981, when it reported there were 29.3 abortions per 1,000 women in that age range.
The CDC says that in 2021, there were 11.6 abortions in the U.S. per 1,000 women ages 15 to 44. (That figure excludes data from California, the District of Columbia, Maryland, New Hampshire and New Jersey.) Like Guttmacher’s data, the CDC’s figures also suggest a general decline in the abortion rate over time. In 1980, when the CDC reported on all 50 states and D.C., it said there were 25 abortions per 1,000 women ages 15 to 44.
That said, both Guttmacher and the CDC say there were slight increases in the rate of abortions during the late 2010s and early 2020s. Guttmacher says the abortion rate per 1,000 women ages 15 to 44 rose from 13.5 in 2017 to 14.4 in 2020. The CDC says it rose from 11.2 per 1,000 in 2017 to 11.4 in 2019, before falling back to 11.1 in 2020 and then rising again to 11.6 in 2021. (The CDC’s figures for those years exclude data from California, D.C., Maryland, New Hampshire and New Jersey.)
The CDC broadly divides abortions into two categories: surgical abortions and medication abortions, which involve pills. Since the Food and Drug Administration first approved abortion pills in 2000, their use has increased over time as a share of abortions nationally, according to both the CDC and Guttmacher.
The majority of abortions in the U.S. now involve pills, according to both the CDC and Guttmacher. The CDC says 56% of U.S. abortions in 2021 involved pills, up from 53% in 2020 and 44% in 2019. Its figures for 2021 include the District of Columbia and 44 states that provided this data; its figures for 2020 include D.C. and 44 states (though not all of the same states as in 2021), and its figures for 2019 include D.C. and 45 states.
Guttmacher, which measures this every three years, says 53% of U.S. abortions involved pills in 2020, up from 39% in 2017.
Two pills commonly used together for medication abortions are mifepristone, which, taken first, blocks hormones that support a pregnancy, and misoprostol, which then causes the uterus to empty. According to the FDA, medication abortions are safe until 10 weeks into pregnancy.
Surgical abortions conducted during the first trimester of pregnancy typically use a suction process, while the relatively few surgical abortions that occur during the second trimester of a pregnancy typically use a process called dilation and evacuation, according to the UCLA School of Medicine.
In 2020, there were 1,603 facilities in the U.S. that provided abortions, according to Guttmacher . This included 807 clinics, 530 hospitals and 266 physicians’ offices.
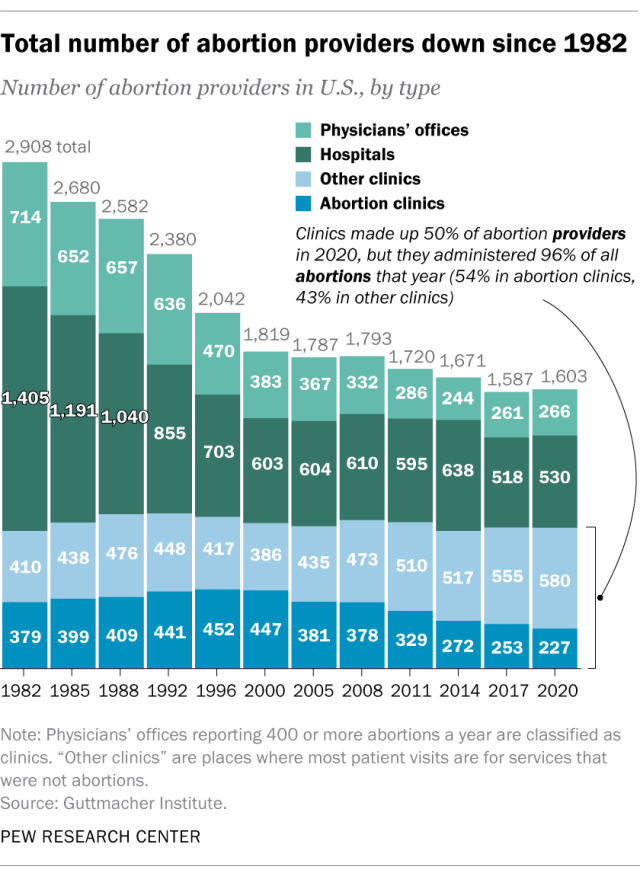
While clinics make up half of the facilities that provide abortions, they are the sites where the vast majority (96%) of abortions are administered, either through procedures or the distribution of pills, according to Guttmacher’s 2020 data. (This includes 54% of abortions that are administered at specialized abortion clinics and 43% at nonspecialized clinics.) Hospitals made up 33% of the facilities that provided abortions in 2020 but accounted for only 3% of abortions that year, while just 1% of abortions were conducted by physicians’ offices.
Looking just at clinics – that is, the total number of specialized abortion clinics and nonspecialized clinics in the U.S. – Guttmacher found the total virtually unchanged between 2017 (808 clinics) and 2020 (807 clinics). However, there were regional differences. In the Midwest, the number of clinics that provide abortions increased by 11% during those years, and in the West by 6%. The number of clinics decreased during those years by 9% in the Northeast and 3% in the South.
The total number of abortion providers has declined dramatically since the 1980s. In 1982, according to Guttmacher, there were 2,908 facilities providing abortions in the U.S., including 789 clinics, 1,405 hospitals and 714 physicians’ offices.
The CDC does not track the number of abortion providers.
In the District of Columbia and the 46 states that provided abortion and residency information to the CDC in 2021, 10.9% of all abortions were performed on women known to live outside the state where the abortion occurred – slightly higher than the percentage in 2020 (9.7%). That year, D.C. and 46 states (though not the same ones as in 2021) reported abortion and residency data. (The total number of abortions used in these calculations included figures for women with both known and unknown residential status.)
The share of reported abortions performed on women outside their state of residence was much higher before the 1973 Roe decision that stopped states from banning abortion. In 1972, 41% of all abortions in D.C. and the 20 states that provided this information to the CDC that year were performed on women outside their state of residence. In 1973, the corresponding figure was 21% in the District of Columbia and the 41 states that provided this information, and in 1974 it was 11% in D.C. and the 43 states that provided data.
In the District of Columbia and the 46 states that reported age data to the CDC in 2021, the majority of women who had abortions (57%) were in their 20s, while about three-in-ten (31%) were in their 30s. Teens ages 13 to 19 accounted for 8% of those who had abortions, while women ages 40 to 44 accounted for about 4%.
The vast majority of women who had abortions in 2021 were unmarried (87%), while married women accounted for 13%, according to the CDC , which had data on this from 37 states.
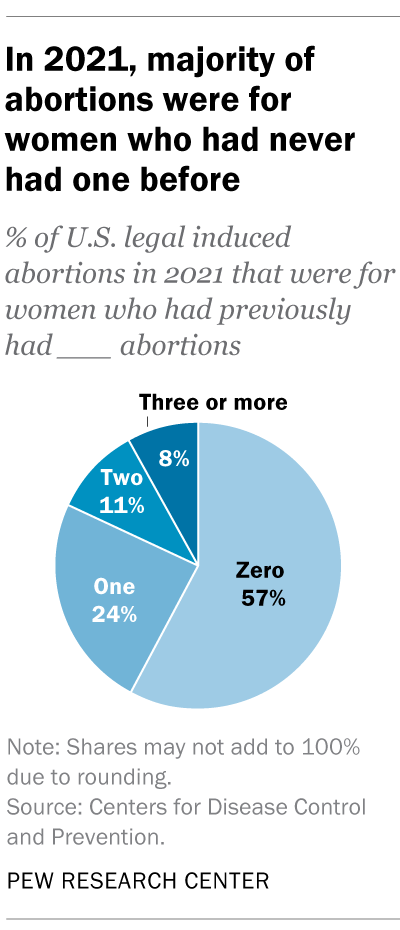
In the District of Columbia, New York City (but not the rest of New York) and the 31 states that reported racial and ethnic data on abortion to the CDC , 42% of all women who had abortions in 2021 were non-Hispanic Black, while 30% were non-Hispanic White, 22% were Hispanic and 6% were of other races.
Looking at abortion rates among those ages 15 to 44, there were 28.6 abortions per 1,000 non-Hispanic Black women in 2021; 12.3 abortions per 1,000 Hispanic women; 6.4 abortions per 1,000 non-Hispanic White women; and 9.2 abortions per 1,000 women of other races, the CDC reported from those same 31 states, D.C. and New York City.
For 57% of U.S. women who had induced abortions in 2021, it was the first time they had ever had one, according to the CDC. For nearly a quarter (24%), it was their second abortion. For 11% of women who had an abortion that year, it was their third, and for 8% it was their fourth or more. These CDC figures include data from 41 states and New York City, but not the rest of New York.
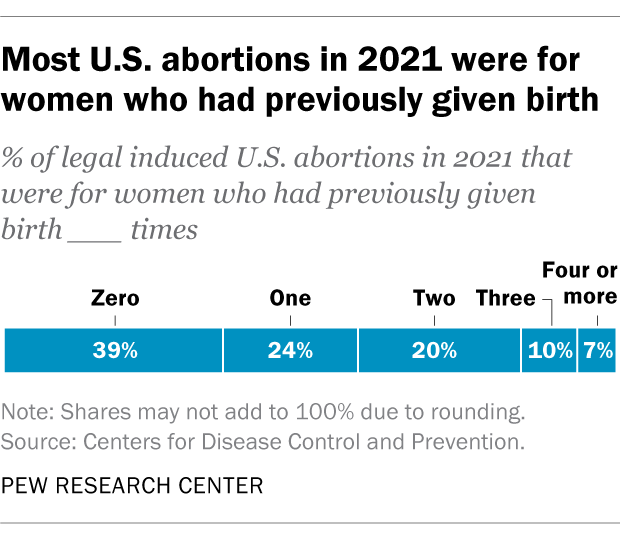
Nearly four-in-ten women who had abortions in 2021 (39%) had no previous live births at the time they had an abortion, according to the CDC . Almost a quarter (24%) of women who had abortions in 2021 had one previous live birth, 20% had two previous live births, 10% had three, and 7% had four or more previous live births. These CDC figures include data from 41 states and New York City, but not the rest of New York.
The vast majority of abortions occur during the first trimester of a pregnancy. In 2021, 93% of abortions occurred during the first trimester – that is, at or before 13 weeks of gestation, according to the CDC . An additional 6% occurred between 14 and 20 weeks of pregnancy, and about 1% were performed at 21 weeks or more of gestation. These CDC figures include data from 40 states and New York City, but not the rest of New York.
About 2% of all abortions in the U.S. involve some type of complication for the woman , according to an article in StatPearls, an online health care resource. “Most complications are considered minor such as pain, bleeding, infection and post-anesthesia complications,” according to the article.
The CDC calculates case-fatality rates for women from induced abortions – that is, how many women die from abortion-related complications, for every 100,000 legal abortions that occur in the U.S . The rate was lowest during the most recent period examined by the agency (2013 to 2020), when there were 0.45 deaths to women per 100,000 legal induced abortions. The case-fatality rate reported by the CDC was highest during the first period examined by the agency (1973 to 1977), when it was 2.09 deaths to women per 100,000 legal induced abortions. During the five-year periods in between, the figure ranged from 0.52 (from 1993 to 1997) to 0.78 (from 1978 to 1982).
The CDC calculates death rates by five-year and seven-year periods because of year-to-year fluctuation in the numbers and due to the relatively low number of women who die from legal induced abortions.
In 2020, the last year for which the CDC has information , six women in the U.S. died due to complications from induced abortions. Four women died in this way in 2019, two in 2018, and three in 2017. (These deaths all followed legal abortions.) Since 1990, the annual number of deaths among women due to legal induced abortion has ranged from two to 12.
The annual number of reported deaths from induced abortions (legal and illegal) tended to be higher in the 1980s, when it ranged from nine to 16, and from 1972 to 1979, when it ranged from 13 to 63. One driver of the decline was the drop in deaths from illegal abortions. There were 39 deaths from illegal abortions in 1972, the last full year before Roe v. Wade. The total fell to 19 in 1973 and to single digits or zero every year after that. (The number of deaths from legal abortions has also declined since then, though with some slight variation over time.)
The number of deaths from induced abortions was considerably higher in the 1960s than afterward. For instance, there were 119 deaths from induced abortions in 1963 and 99 in 1965 , according to reports by the then-U.S. Department of Health, Education and Welfare, a precursor to the Department of Health and Human Services. The CDC is a division of Health and Human Services.
Note: This is an update of a post originally published May 27, 2022, and first updated June 24, 2022.
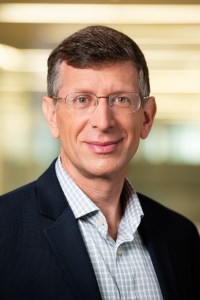
Sign up for our weekly newsletter
Fresh data delivered Saturday mornings
Key facts about the abortion debate in America
Public opinion on abortion, three-in-ten or more democrats and republicans don’t agree with their party on abortion, partisanship a bigger factor than geography in views of abortion access locally, do state laws on abortion reflect public opinion, most popular.
About Pew Research Center Pew Research Center is a nonpartisan fact tank that informs the public about the issues, attitudes and trends shaping the world. It conducts public opinion polling, demographic research, media content analysis and other empirical social science research. Pew Research Center does not take policy positions. It is a subsidiary of The Pew Charitable Trusts .

An official website of the United States government
The .gov means it’s official. Federal government websites often end in .gov or .mil. Before sharing sensitive information, make sure you’re on a federal government site.
The site is secure. The https:// ensures that you are connecting to the official website and that any information you provide is encrypted and transmitted securely.
- Publications
- Account settings
Preview improvements coming to the PMC website in October 2024. Learn More or Try it out now .
- Advanced Search
- Journal List
- Int J Environ Res Public Health

Real World—Big Data Analytics in Healthcare
Daniele piovani.
1 Department of Biomedical Sciences, Humanitas University, Pieve Emanuele, 20090 Milan, Italy
2 IRCCS Humanitas Research Hospital, Rozzano, 20089 Milan, Italy
Stefanos Bonovas
The term Big Data is used to describe extremely large datasets that are complex, multi-dimensional, unstructured, and heterogeneous and that are accumulating rapidly and may be analyzed with appropriate informatic and statistical methodologies to reveal patterns, trends, and associations [ 1 ]. In medical and healthcare research, Big Data sources include electronic health records (EHRs), administrative or claims databases, product and disease registries, smart/wearable/self-monitoring devices, and large-scale collaborations for the collection and storage of health data and biospecimens in biobanks.
The definition of what Big Data means with respect to health research, or at least a consensus of what this term means, was proposed by the Health Directorate of the Directorate-General for Research and Innovation of the European Commission: “ Big Data in health encompasses high volume, high diversity biological, clinical, environmental, and lifestyle information collected from single individuals to large cohorts, in relation to their health and wellness status, at one or several time points ” [ 2 ].
Big Data analytics techniques and methods, such as statistical analysis, data mining, machine learning, and deep learning, have made notable progress in the recent years, and are expected to develop even further in the near future [ 3 ]. With the ever-increasing quantities of data that are digitally collected and stored within healthcare organizations, there is a growing enthusiasm in the potential applications for Big Data analytics in the fields of diagnostics, precision medicine, computerized decision support for clinicians, pharmacological research aiming to cure diseases and develop new treatments, the early detection of adverse drug reactions, cost reduction in patient care, preventive medicine, and population health research [ 4 ].
At the same time, the term Real-World Data (RWD) is commonly used to describe data derived from sources other than traditional randomized-controlled trials (RCTs). These sources may include EHRs, pragmatic clinical trials, prospective or retrospective observational studies, health insurance claims, case reports, data obtained as part of routine public health surveillance, product and disease registries, patient surveys, or other real-world sources [ 5 ].
The Association of the British Pharmaceutical Industry has defined the RWD as “ data obtained by any non-interventional methodology that describes what is happening in normal clinical practice ” [ 6 ], while according to the U.S. Food and Drug Administration, the term RWD refers to “ data relating to patient health status and/or the delivery of health care routinely collected from a variety of sources ” [ 7 ]. In the last few years, a consensus has formed that RWD offer valuable opportunities for generating robust clinical evidence (i.e., real-world evidence) regarding the use and potential benefits or harms of new therapies outside the context of RCTs [ 8 , 9 ].
Researchers typically understand RWD as observational data, distinct from data sourced from RCTs, and in a way similar to Big Data. Nevertheless, RWD and Big Data are not synonymous. In fact, Big Data represent a special kind of RWD, which are characterized by high volume, high velocity, high variety, high veracity, and high value (5 Vs) [ 10 ].
The promise of Big Data in healthcare depends on the ability to extract meaningful information from real-world, large-scale resources that may pave the way to scientific discoveries in the pathogenesis, diagnosis, prevention, treatment, and prognosis of diseases, and eventually revolutionize clinical medicine and public health [ 11 , 12 , 13 ]. When Big Data are analyzed with the aim of causal inference and not as a hypothesis-generating tool, special attention should be made to the very serious risk of residual confounding and a variety of biases including time-related biases [ 14 ]. Recent methodological advancements have made causal inferences from Big Data possible and, in certain cases, are highly effective in replicating the results of RCTs that are made available later [ 14 , 15 ]. This can be achieved successfully if a careful application of a comprehensive methodological and statistical analysis plan called “target trial emulation” is pursued [ 14 ]. The failure to correctly apply such a plan may result in Big Data yielding overly optimistic estimates of effects.
Despite these advancements, until now, Big Data analytics have not fulfilled the oversized expectations in the health sector, possibly because of several significant challenges that are summarized below [ 16 , 17 , 18 , 19 ]:
- (a) Big Data are often unstructured, fragmented, heterogeneous, and in incompatible formats, and are thus difficult to aggregate and analyze;
- (b) There are important issues regarding data security (privacy and confidentiality);
- (c) A lack of data standardization, language barriers, and different terminologies;
- (d) There are often problems with the accuracy and precision of data;
- (e) Storage and transfers of data are associated with significant costs;
- (f) Budget constraints—there is a shortage of focused and sustained funding;
- (g) The awareness of Big Data analytics’ capabilities among health care professionals is rather limited;
- (h) A shortage of researchers with skills in Big Data—due to the constant evolution of science and technology, professionals who collect, process, extract, or analyze data (i.e., data scientists, biostatisticians, epidemiologists, and experts in advanced analytics and artificial intelligence) need to be regularly trained and kept up-to-date;
- (i) There are often issues regarding data governance and data ownership;
- (j) Healthcare organizations implementing Big Data analytics as a part of their information systems need to comply with high standards and regulatory legislation.
Additionally, Sir David Cox and colleagues have methodically discussed several challenges of a statistical/epidemiological nature that arise when analyzing Big Data in healthcare [ 20 ]:
- (a) The relevance of the data for the purpose of the investigation (the data’s fitness for purpose)—big datasets may not be representative of the target population, and the largeness of a dataset does not imply that the findings of the investigation (e.g., the patterns, trends, and associations) are free of bias;
- (b) The need for well-established quality control and assurance procedures (data reliability)—Big Data are not collected for a specific purpose and may be subject to particular quality issues (e.g., measurement errors, missing data, errors in coding information buried in textual reports, etc.);
- (c) The potential for overconfidence in the results obtained from statistical analyses of Big Data (i.e., conclusions being seriously overoptimistic) due to superficially highly precise, but potentially biased, estimates.
In conclusion, the application of Big Data in healthcare is a fast-growing field with great advances in data-generation and data-analysis methodologies. Despite the challenges outlined above, Big Data analytics have the potential for positive impacts and global implications; they are becoming increasingly important, as they enable investigations to be conducted and conclusions to be drawn that would otherwise be very difficult or even impossible. However, we should keep in mind that, when analyzing Big Data in health care research, we need to make careful use of statistical and epidemiological concepts together with an in-depth understanding of the data themselves.
Funding Statement
This research received no external funding.
Author Contributions
Conceptualization, D.P. and S.B.; writing—original draft preparation, S.B.; writing—review and editing, D.P. All authors have read and agreed to the published version of the manuscript.
Conflicts of Interest
The authors declare no conflict of interest.
Publisher’s Note: MDPI stays neutral with regard to jurisdictional claims in published maps and institutional affiliations.
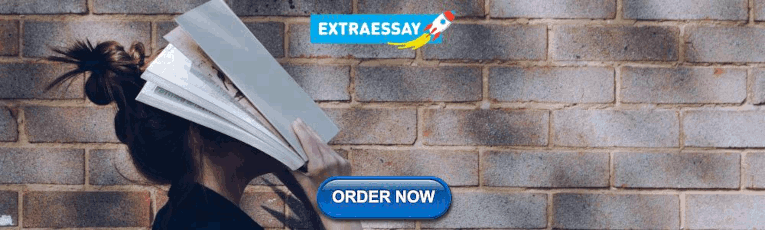
COMMENTS
Data Analytics in Healthcare: 7 Real-World Examples and Use Cases. There are few things in the world requiring such precision as clinical decision-making. The adoption of technologies supports healthcare organizations on different levels: from population monitoring, health records, diagnostics, and clinical decisions, to drug procurement, and ...
By Jessica Kent. December 10, 2020 - Researchers at Johns Hopkins Bloomberg School of Public Health have developed a series of case studies for public health issues that will enable healthcare leaders to use big data analytics tools in their work. The Open Case Studies project offers an interactive online hub made up of ten case studies that ...
10 top case studies: Big data analytics in healthcare - Health Data Management. Analytics. Big data. Healthcare analytics. Johns Hopkins Hospital. Mayo Clinic. Predictive analytics. UnitedHealthcare.
In another study carried out to identify BDA benefits and supports, and to drive organizational strategies, Wang, Kung, and Byrd , through the analysis of 26 case studies related to the BDA applications in the healthcare organization, have identified five "capabilities" of BDA: analytic capability for care patterns, unstructured data analytical ...
Malik, Abdallah, and Ala'raj ( 2018) reviewed the use of BDA in supply chain management in healthcare. Saheb and Izadi ( 2019) reviewed the use of big data sourced from Internet-of-Things devices in the healthcare industry. Such review studies are not designed to provide a comprehensive review of the literature on BDA in healthcare.
Case Studies Healthcare data analytics case studies. SEHP: Maximizing the power of integrated data with the MedInsight Data Science Portal. Southeastern Health Partners (SEHP) - a clinically integrated network consisting of four healthcare systems, including 13 hospitals and 3,600 providers - sets its continuous improvement goals high. The ...
Data analytics refers to the process of examining, cleansing, transforming, and modeling data to discover useful information, suggest conclusions, and support decision-making. In the context of healthcare, this translates into predictive analytics, precision medicine, patient profiling, and more. Consider the complexity of the modern healthcare ...
The introduction of Big Data Analytics (BDA) in healthcare will allow to use new technologies both in treatment of patients and health management. The paper aims at analyzing the possibilities of using Big Data Analytics in healthcare. ... Fredriksson C. Organizational knowledge creation with big data. A case study of the concept and practical ...
The techniques provided in our current paper provide a methodology to make the analysis of healthcare data accessible to a wider pool of researchers, and dovetails with the principles embodied in the 2010 ACA. Data can be collected and analyzed from multiple perspectives, including cost metrics, timeliness of service, and outcomes.
Big Data initiatives in the United Kingdom. The UK Biobank is a prospective cohort initiative that is composed of individuals between the ages of 40 and 69 before disease onset (Allen et al. 2012 ...
Predictive Analytics for Disease Prevention: Big data analytics enables the identification of patterns and trends in patient data, facilitating predictive models for disease outbreaks and epidemics. This proactive approach supports public health interventions and preventive measures. Clinical Decision Support Systems: Healthcare data analytics companies can leverage big data analytics to ...
Data Science Applications in Healthcare Industry: 9 Case Studies. Data science has become an essential tool in the healthcare industry, as technology makes it easier to collect and analyze large amounts of data. Data science has contributed to the rise in patient care, offering new avenues for diagnosis and treatment.
Here, we list some of the widely used bioinformatics-based tools for big data analytics on omics data. 1. SparkSeq is an efficient and cloud-ready platform based on Apache Spark framework and Hadoop library that is used for analyses of genomic data for interactive genomic data analysis with nucleotide precision. 2.
Big data analytics in healthcare, studies in big data 66. Cham: Springer; 2020. p. 3-21. Raghupati W, Raghupathi V. Big data analytics in healthcare: promise and potential. Health Inf Sci Syst Vol. 2014;2(1):1-10. Jain DA, Kumar V, Khanduja D, Sharma K, Bateja R. A detailed study of big data in healthcare: case study of Brenda and IBM Watson.
Abstract and Figures. A case studies brief by Cenacle Research presenting a variety of Healthcare Analytics solutions crafted to the needs of: + Individuals (Patients) Personalized Healthcare ...
This is a tertiary study, i.e., a systematic review of systematic reviews. We identified 45 systematic secondary studies on data analytics applications in diferent healthcare sectors, including diagnosis and disease profiling, diabetes, Alzheimer's disease, and sepsis. Machine learning and data mining were the most widely used data analytics ...
CASE STUDY Improving Population Health with Data Analytics. One client's journey to a modern, cloud-based data platform to support value-based care initiatives. REPORTS ... HEALTH PLANS … DATA ANALYTICS PLATFORM HEALTHCARE DATA MODELS DATA LAKE MASTER DATA MANAGEMENT ETL & JOB AUTOMATION CLINICAL INTEGRATION
A BI solution to replace the Customer's legacy solution and improve analytical processes. The system features a data warehouse, ETL pipelines, an analytical cube, and algorithms for comprehensive and standardized reporting. ScienceSoft's case studies: Healthcare: Data management and analytics. Check out the success stories of a software ...
The article provides an insight into the status and prospects of big data analytics in healthcare, highlights the advantages, describes the frameworks and techniques used, briefs about the challenges faced currently, and discusses viable solutions. ... Li et al. in a case study showed that hacking can make a connection between tiny chunks of ...
Data analytics in healthcare is defined as the process of collecting, analyzing, and interpreting large volumes of healthcare data to derive actionable insights and inform decision-making aimed at improving patient care, enhancing operational efficiency, and driving organizational performance. Learn more about the importance, examples, and benefits of data analytics in healthcare.
A 2023 Deloitte study says use of EHRs and health information exchanges (HIEs) is growing rapidly, with organizations building data lakes and using AI to combine and cleanse data. These measures ...
Healthcare's data era is finally here, distinguished by higher levels of sophistication in how the industry uses data to improve operational performance and patient outcomes. Changes in ...
The CDC says that in 2021, there were 11.6 abortions in the U.S. per 1,000 women ages 15 to 44. (That figure excludes data from California, the District of Columbia, Maryland, New Hampshire and New Jersey.) Like Guttmacher's data, the CDC's figures also suggest a general decline in the abortion rate over time.
Healthcare data analytics studies often utilize four popular databases in their primary study search, typically select 25-100 primary studies, and the use of research guidelines such as PRISMA is growing. ... In the case a study considered more than one data analytics or healthcare subfield, we categorized the study according to what was to ...
The promise of Big Data in healthcare depends on the ability to extract meaningful information from real-world, large-scale resources that may pave the way to scientific discoveries in the pathogenesis, diagnosis, prevention, treatment, and prognosis of diseases, and eventually revolutionize clinical medicine and public health [ 11, 12, 13 ].