
- Previous Article
- Next Article
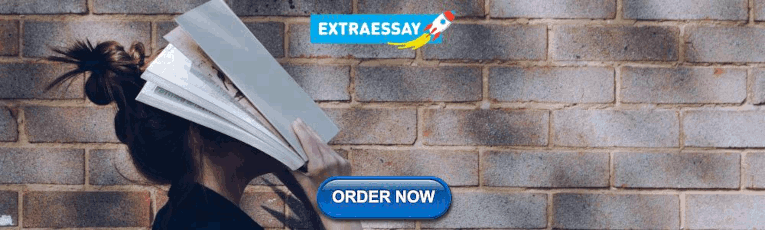
Promises and Pitfalls of Technology
Politics and privacy, private-sector influence and big tech, state competition and conflict, author biography, how is technology changing the world, and how should the world change technology.
- Split-Screen
- Article contents
- Figures & tables
- Supplementary Data
- Peer Review
- Open the PDF for in another window
- Guest Access
- Get Permissions
- Cite Icon Cite
- Search Site
Josephine Wolff; How Is Technology Changing the World, and How Should the World Change Technology?. Global Perspectives 1 February 2021; 2 (1): 27353. doi: https://doi.org/10.1525/gp.2021.27353
Download citation file:
- Ris (Zotero)
- Reference Manager
Technologies are becoming increasingly complicated and increasingly interconnected. Cars, airplanes, medical devices, financial transactions, and electricity systems all rely on more computer software than they ever have before, making them seem both harder to understand and, in some cases, harder to control. Government and corporate surveillance of individuals and information processing relies largely on digital technologies and artificial intelligence, and therefore involves less human-to-human contact than ever before and more opportunities for biases to be embedded and codified in our technological systems in ways we may not even be able to identify or recognize. Bioengineering advances are opening up new terrain for challenging philosophical, political, and economic questions regarding human-natural relations. Additionally, the management of these large and small devices and systems is increasingly done through the cloud, so that control over them is both very remote and removed from direct human or social control. The study of how to make technologies like artificial intelligence or the Internet of Things “explainable” has become its own area of research because it is so difficult to understand how they work or what is at fault when something goes wrong (Gunning and Aha 2019) .
This growing complexity makes it more difficult than ever—and more imperative than ever—for scholars to probe how technological advancements are altering life around the world in both positive and negative ways and what social, political, and legal tools are needed to help shape the development and design of technology in beneficial directions. This can seem like an impossible task in light of the rapid pace of technological change and the sense that its continued advancement is inevitable, but many countries around the world are only just beginning to take significant steps toward regulating computer technologies and are still in the process of radically rethinking the rules governing global data flows and exchange of technology across borders.
These are exciting times not just for technological development but also for technology policy—our technologies may be more advanced and complicated than ever but so, too, are our understandings of how they can best be leveraged, protected, and even constrained. The structures of technological systems as determined largely by government and institutional policies and those structures have tremendous implications for social organization and agency, ranging from open source, open systems that are highly distributed and decentralized, to those that are tightly controlled and closed, structured according to stricter and more hierarchical models. And just as our understanding of the governance of technology is developing in new and interesting ways, so, too, is our understanding of the social, cultural, environmental, and political dimensions of emerging technologies. We are realizing both the challenges and the importance of mapping out the full range of ways that technology is changing our society, what we want those changes to look like, and what tools we have to try to influence and guide those shifts.
Technology can be a source of tremendous optimism. It can help overcome some of the greatest challenges our society faces, including climate change, famine, and disease. For those who believe in the power of innovation and the promise of creative destruction to advance economic development and lead to better quality of life, technology is a vital economic driver (Schumpeter 1942) . But it can also be a tool of tremendous fear and oppression, embedding biases in automated decision-making processes and information-processing algorithms, exacerbating economic and social inequalities within and between countries to a staggering degree, or creating new weapons and avenues for attack unlike any we have had to face in the past. Scholars have even contended that the emergence of the term technology in the nineteenth and twentieth centuries marked a shift from viewing individual pieces of machinery as a means to achieving political and social progress to the more dangerous, or hazardous, view that larger-scale, more complex technological systems were a semiautonomous form of progress in and of themselves (Marx 2010) . More recently, technologists have sharply criticized what they view as a wave of new Luddites, people intent on slowing the development of technology and turning back the clock on innovation as a means of mitigating the societal impacts of technological change (Marlowe 1970) .
At the heart of fights over new technologies and their resulting global changes are often two conflicting visions of technology: a fundamentally optimistic one that believes humans use it as a tool to achieve greater goals, and a fundamentally pessimistic one that holds that technological systems have reached a point beyond our control. Technology philosophers have argued that neither of these views is wholly accurate and that a purely optimistic or pessimistic view of technology is insufficient to capture the nuances and complexity of our relationship to technology (Oberdiek and Tiles 1995) . Understanding technology and how we can make better decisions about designing, deploying, and refining it requires capturing that nuance and complexity through in-depth analysis of the impacts of different technological advancements and the ways they have played out in all their complicated and controversial messiness across the world.
These impacts are often unpredictable as technologies are adopted in new contexts and come to be used in ways that sometimes diverge significantly from the use cases envisioned by their designers. The internet, designed to help transmit information between computer networks, became a crucial vehicle for commerce, introducing unexpected avenues for crime and financial fraud. Social media platforms like Facebook and Twitter, designed to connect friends and families through sharing photographs and life updates, became focal points of election controversies and political influence. Cryptocurrencies, originally intended as a means of decentralized digital cash, have become a significant environmental hazard as more and more computing resources are devoted to mining these forms of virtual money. One of the crucial challenges in this area is therefore recognizing, documenting, and even anticipating some of these unexpected consequences and providing mechanisms to technologists for how to think through the impacts of their work, as well as possible other paths to different outcomes (Verbeek 2006) . And just as technological innovations can cause unexpected harm, they can also bring about extraordinary benefits—new vaccines and medicines to address global pandemics and save thousands of lives, new sources of energy that can drastically reduce emissions and help combat climate change, new modes of education that can reach people who would otherwise have no access to schooling. Regulating technology therefore requires a careful balance of mitigating risks without overly restricting potentially beneficial innovations.
Nations around the world have taken very different approaches to governing emerging technologies and have adopted a range of different technologies themselves in pursuit of more modern governance structures and processes (Braman 2009) . In Europe, the precautionary principle has guided much more anticipatory regulation aimed at addressing the risks presented by technologies even before they are fully realized. For instance, the European Union’s General Data Protection Regulation focuses on the responsibilities of data controllers and processors to provide individuals with access to their data and information about how that data is being used not just as a means of addressing existing security and privacy threats, such as data breaches, but also to protect against future developments and uses of that data for artificial intelligence and automated decision-making purposes. In Germany, Technische Überwachungsvereine, or TÜVs, perform regular tests and inspections of technological systems to assess and minimize risks over time, as the tech landscape evolves. In the United States, by contrast, there is much greater reliance on litigation and liability regimes to address safety and security failings after-the-fact. These different approaches reflect not just the different legal and regulatory mechanisms and philosophies of different nations but also the different ways those nations prioritize rapid development of the technology industry versus safety, security, and individual control. Typically, governance innovations move much more slowly than technological innovations, and regulations can lag years, or even decades, behind the technologies they aim to govern.
In addition to this varied set of national regulatory approaches, a variety of international and nongovernmental organizations also contribute to the process of developing standards, rules, and norms for new technologies, including the International Organization for Standardization and the International Telecommunication Union. These multilateral and NGO actors play an especially important role in trying to define appropriate boundaries for the use of new technologies by governments as instruments of control for the state.
At the same time that policymakers are under scrutiny both for their decisions about how to regulate technology as well as their decisions about how and when to adopt technologies like facial recognition themselves, technology firms and designers have also come under increasing criticism. Growing recognition that the design of technologies can have far-reaching social and political implications means that there is more pressure on technologists to take into consideration the consequences of their decisions early on in the design process (Vincenti 1993; Winner 1980) . The question of how technologists should incorporate these social dimensions into their design and development processes is an old one, and debate on these issues dates back to the 1970s, but it remains an urgent and often overlooked part of the puzzle because so many of the supposedly systematic mechanisms for assessing the impacts of new technologies in both the private and public sectors are primarily bureaucratic, symbolic processes rather than carrying any real weight or influence.
Technologists are often ill-equipped or unwilling to respond to the sorts of social problems that their creations have—often unwittingly—exacerbated, and instead point to governments and lawmakers to address those problems (Zuckerberg 2019) . But governments often have few incentives to engage in this area. This is because setting clear standards and rules for an ever-evolving technological landscape can be extremely challenging, because enforcement of those rules can be a significant undertaking requiring considerable expertise, and because the tech sector is a major source of jobs and revenue for many countries that may fear losing those benefits if they constrain companies too much. This indicates not just a need for clearer incentives and better policies for both private- and public-sector entities but also a need for new mechanisms whereby the technology development and design process can be influenced and assessed by people with a wider range of experiences and expertise. If we want technologies to be designed with an eye to their impacts, who is responsible for predicting, measuring, and mitigating those impacts throughout the design process? Involving policymakers in that process in a more meaningful way will also require training them to have the analytic and technical capacity to more fully engage with technologists and understand more fully the implications of their decisions.
At the same time that tech companies seem unwilling or unable to rein in their creations, many also fear they wield too much power, in some cases all but replacing governments and international organizations in their ability to make decisions that affect millions of people worldwide and control access to information, platforms, and audiences (Kilovaty 2020) . Regulators around the world have begun considering whether some of these companies have become so powerful that they violate the tenets of antitrust laws, but it can be difficult for governments to identify exactly what those violations are, especially in the context of an industry where the largest players often provide their customers with free services. And the platforms and services developed by tech companies are often wielded most powerfully and dangerously not directly by their private-sector creators and operators but instead by states themselves for widespread misinformation campaigns that serve political purposes (Nye 2018) .
Since the largest private entities in the tech sector operate in many countries, they are often better poised to implement global changes to the technological ecosystem than individual states or regulatory bodies, creating new challenges to existing governance structures and hierarchies. Just as it can be challenging to provide oversight for government use of technologies, so, too, oversight of the biggest tech companies, which have more resources, reach, and power than many nations, can prove to be a daunting task. The rise of network forms of organization and the growing gig economy have added to these challenges, making it even harder for regulators to fully address the breadth of these companies’ operations (Powell 1990) . The private-public partnerships that have emerged around energy, transportation, medical, and cyber technologies further complicate this picture, blurring the line between the public and private sectors and raising critical questions about the role of each in providing critical infrastructure, health care, and security. How can and should private tech companies operating in these different sectors be governed, and what types of influence do they exert over regulators? How feasible are different policy proposals aimed at technological innovation, and what potential unintended consequences might they have?
Conflict between countries has also spilled over significantly into the private sector in recent years, most notably in the case of tensions between the United States and China over which technologies developed in each country will be permitted by the other and which will be purchased by other customers, outside those two countries. Countries competing to develop the best technology is not a new phenomenon, but the current conflicts have major international ramifications and will influence the infrastructure that is installed and used around the world for years to come. Untangling the different factors that feed into these tussles as well as whom they benefit and whom they leave at a disadvantage is crucial for understanding how governments can most effectively foster technological innovation and invention domestically as well as the global consequences of those efforts. As much of the world is forced to choose between buying technology from the United States or from China, how should we understand the long-term impacts of those choices and the options available to people in countries without robust domestic tech industries? Does the global spread of technologies help fuel further innovation in countries with smaller tech markets, or does it reinforce the dominance of the states that are already most prominent in this sector? How can research universities maintain global collaborations and research communities in light of these national competitions, and what role does government research and development spending play in fostering innovation within its own borders and worldwide? How should intellectual property protections evolve to meet the demands of the technology industry, and how can those protections be enforced globally?
These conflicts between countries sometimes appear to challenge the feasibility of truly global technologies and networks that operate across all countries through standardized protocols and design features. Organizations like the International Organization for Standardization, the World Intellectual Property Organization, the United Nations Industrial Development Organization, and many others have tried to harmonize these policies and protocols across different countries for years, but have met with limited success when it comes to resolving the issues of greatest tension and disagreement among nations. For technology to operate in a global environment, there is a need for a much greater degree of coordination among countries and the development of common standards and norms, but governments continue to struggle to agree not just on those norms themselves but even the appropriate venue and processes for developing them. Without greater global cooperation, is it possible to maintain a global network like the internet or to promote the spread of new technologies around the world to address challenges of sustainability? What might help incentivize that cooperation moving forward, and what could new structures and process for governance of global technologies look like? Why has the tech industry’s self-regulation culture persisted? Do the same traditional drivers for public policy, such as politics of harmonization and path dependency in policy-making, still sufficiently explain policy outcomes in this space? As new technologies and their applications spread across the globe in uneven ways, how and when do they create forces of change from unexpected places?
These are some of the questions that we hope to address in the Technology and Global Change section through articles that tackle new dimensions of the global landscape of designing, developing, deploying, and assessing new technologies to address major challenges the world faces. Understanding these processes requires synthesizing knowledge from a range of different fields, including sociology, political science, economics, and history, as well as technical fields such as engineering, climate science, and computer science. A crucial part of understanding how technology has created global change and, in turn, how global changes have influenced the development of new technologies is understanding the technologies themselves in all their richness and complexity—how they work, the limits of what they can do, what they were designed to do, how they are actually used. Just as technologies themselves are becoming more complicated, so are their embeddings and relationships to the larger social, political, and legal contexts in which they exist. Scholars across all disciplines are encouraged to join us in untangling those complexities.
Josephine Wolff is an associate professor of cybersecurity policy at the Fletcher School of Law and Diplomacy at Tufts University. Her book You’ll See This Message When It Is Too Late: The Legal and Economic Aftermath of Cybersecurity Breaches was published by MIT Press in 2018.
Recipient(s) will receive an email with a link to 'How Is Technology Changing the World, and How Should the World Change Technology?' and will not need an account to access the content.
Subject: How Is Technology Changing the World, and How Should the World Change Technology?
(Optional message may have a maximum of 1000 characters.)
Citing articles via
Email alerts, affiliations.
- Special Collections
- Review Symposia
- Info for Authors
- Info for Librarians
- Editorial Team
- Emerging Scholars Forum
- Open Access
- Online ISSN 2575-7350
- Copyright © 2024 The Regents of the University of California. All Rights Reserved.
Stay Informed
Disciplines.
- Ancient World
- Anthropology
- Communication
- Criminology & Criminal Justice
- Film & Media Studies
- Food & Wine
- Browse All Disciplines
- Browse All Courses
- Book Authors
- Booksellers
- Instructions
- Journal Authors
- Journal Editors
- Media & Journalists
- Planned Giving
About UC Press
- Press Releases
- Seasonal Catalog
- Acquisitions Editors
- Customer Service
- Exam/Desk Requests
- Media Inquiries
- Print-Disability
- Rights & Permissions
- UC Press Foundation
- © Copyright 2024 by the Regents of the University of California. All rights reserved. Privacy policy Accessibility
This Feature Is Available To Subscribers Only
Sign In or Create an Account
Suggestions or feedback?
MIT News | Massachusetts Institute of Technology
- Machine learning
- Social justice
- Black holes
- Classes and programs
Departments
- Aeronautics and Astronautics
- Brain and Cognitive Sciences
- Architecture
- Political Science
- Mechanical Engineering
Centers, Labs, & Programs
- Abdul Latif Jameel Poverty Action Lab (J-PAL)
- Picower Institute for Learning and Memory
- Lincoln Laboratory
- School of Architecture + Planning
- School of Engineering
- School of Humanities, Arts, and Social Sciences
- Sloan School of Management
- School of Science
- MIT Schwarzman College of Computing
A comprehensive study of technological change
Press contact :.
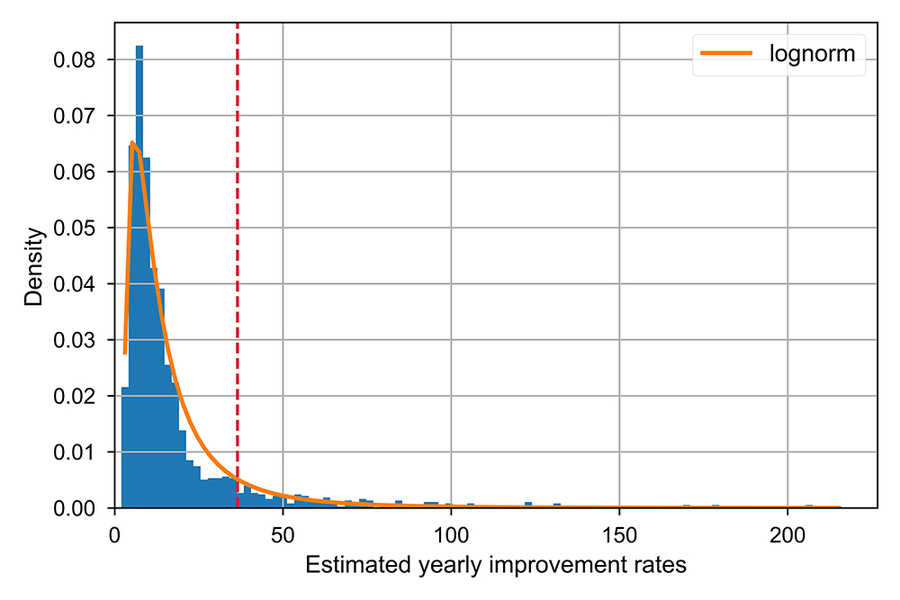
Previous image Next image
The societal impacts of technological change can be seen in many domains, from messenger RNA vaccines and automation to drones and climate change. The pace of that technological change can affect its impact, and how quickly a technology improves in performance can be an indicator of its future importance. For decision-makers like investors, entrepreneurs, and policymakers, predicting which technologies are fast improving (and which are overhyped) can mean the difference between success and failure.
New research from MIT aims to assist in the prediction of technology performance improvement using U.S. patents as a dataset. The study describes 97 percent of the U.S. patent system as a set of 1,757 discrete technology domains, and quantitatively assesses each domain for its improvement potential.
“The rate of improvement can only be empirically estimated when substantial performance measurements are made over long time periods,” says Anuraag Singh SM ’20, lead author of the paper. “In some large technological fields, including software and clinical medicine, such measures have rarely, if ever, been made.”
A previous MIT study provided empirical measures for 30 technological domains, but the patent sets identified for those technologies cover less than 15 percent of the patents in the U.S. patent system. The major purpose of this new study is to provide predictions of the performance improvement rates for the thousands of domains not accessed by empirical measurement. To accomplish this, the researchers developed a method using a new probability-based algorithm, machine learning, natural language processing, and patent network analytics.
Overlap and centrality
A technology domain, as the researchers define it, consists of sets of artifacts fulfilling a specific function using a specific branch of scientific knowledge. To find the patents that best represent a domain, the team built on previous research conducted by co-author Chris Magee, a professor of the practice of engineering systems within the Institute for Data, Systems, and Society (IDSS). Magee and his colleagues found that by looking for patent overlap between the U.S. and international patent-classification systems, they could quickly identify patents that best represent a technology. The researchers ultimately created a correspondence of all patents within the U.S. patent system to a set of 1,757 technology domains.
To estimate performance improvement, Singh employed a method refined by co-authors Magee and Giorgio Triulzi, a researcher with the Sociotechnical Systems Research Center (SSRC) within IDSS and an assistant professor at Universidad de los Andes in Colombia. Their method is based on the average “centrality” of patents in the patent citation network. Centrality refers to multiple criteria for determining the ranking or importance of nodes within a network.
“Our method provides predictions of performance improvement rates for nearly all definable technologies for the first time,” says Singh.
Those rates vary — from a low of 2 percent per year for the “Mechanical skin treatment — Hair removal and wrinkles” domain to a high of 216 percent per year for the “Dynamic information exchange and support systems integrating multiple channels” domain. The researchers found that most technologies improve slowly; more than 80 percent of technologies improve at less than 25 percent per year. Notably, the number of patents in a technological area was not a strong indicator of a higher improvement rate.
“Fast-improving domains are concentrated in a few technological areas,” says Magee. “The domains that show improvement rates greater than the predicted rate for integrated chips — 42 percent, from Moore’s law — are predominantly based upon software and algorithms.”
TechNext Inc.
The researchers built an online interactive system where domains corresponding to technology-related keywords can be found along with their improvement rates. Users can input a keyword describing a technology and the system returns a prediction of improvement for the technological domain, an automated measure of the quality of the match between the keyword and the domain, and patent sets so that the reader can judge the semantic quality of the match.
Moving forward, the researchers have founded a new MIT spinoff called TechNext Inc. to further refine this technology and use it to help leaders make better decisions, from budgets to investment priorities to technology policy. Like any inventors, Magee and his colleagues want to protect their intellectual property rights. To that end, they have applied for a patent for their novel system and its unique methodology.
“Technologies that improve faster win the market,” says Singh. “Our search system enables technology managers, investors, policymakers, and entrepreneurs to quickly look up predictions of improvement rates for specific technologies.”
Adds Magee: “Our goal is to bring greater accuracy, precision, and repeatability to the as-yet fuzzy art of technology forecasting.”
Share this news article on:
Related links.
- Sociotechnical Systems Research Center
- Institute for Data, Systems, and Society (IDSS)
Related Topics
- Technology and society
- Innovation and Entrepreneurship (I&E)
Related Articles
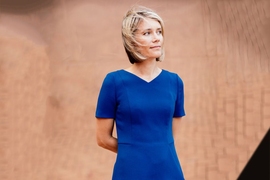
Shaping technology’s future
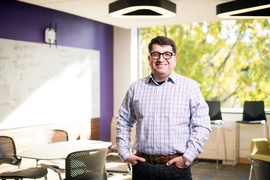
Tackling society’s big problems with systems theory
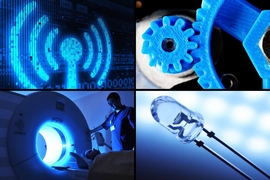
Patents forecast technological change
Previous item Next item
More MIT News
Study demonstrates efficacy of MIT-led Brave Behind Bars program
Read full story →
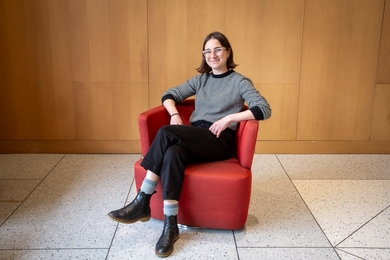
Bringing an investigator’s eye to complex social challenges
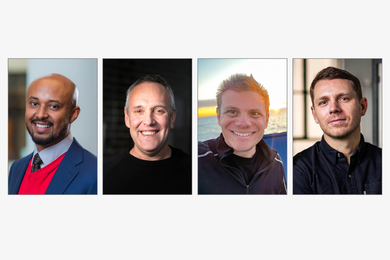
MIT announces 2024 Bose Grants
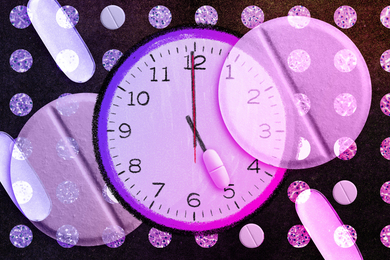
Circadian rhythms can influence drugs’ effectiveness
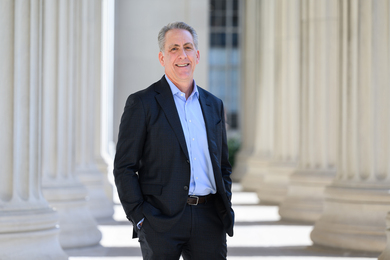
Ian Waitz named vice president for research
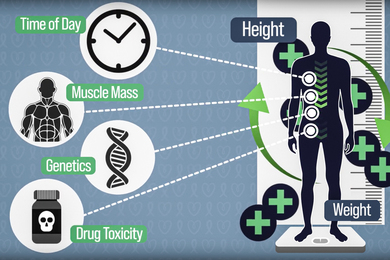
A closed-loop drug-delivery system could improve chemotherapy
- More news on MIT News homepage →
Massachusetts Institute of Technology 77 Massachusetts Avenue, Cambridge, MA, USA
- Map (opens in new window)
- Events (opens in new window)
- People (opens in new window)
- Careers (opens in new window)
- Accessibility
- Social Media Hub
- MIT on Facebook
- MIT on YouTube
- MIT on Instagram

An official website of the United States government
The .gov means it’s official. Federal government websites often end in .gov or .mil. Before sharing sensitive information, make sure you’re on a federal government site.
The site is secure. The https:// ensures that you are connecting to the official website and that any information you provide is encrypted and transmitted securely.
- Publications
- Account settings
Preview improvements coming to the PMC website in October 2024. Learn More or Try it out now .
- Advanced Search
- Journal List

Rise of the war machines: Charting the evolution of military technologies from the Neolithic to the Industrial Revolution
Peter turchin.
1 Complexity Science Hub, Vienna, Austria
2 University of Connecticut, Storrs, Connecticut, United States of America
3 University of Oxford, Oxford, England
Daniel Hoyer
4 Evolution Institute, Tampa, FL, United States of America
5 George Brown College, Toronto, Canada
Andrey Korotayev
6 HSE University, Moscow, Russia
7 Institute of Oriental Studies, Russian Academy of Sciences, Moscow, Russia
Nikolay Kradin
8 Institute of History, Archaeology and Ethnology, Far East Branch of the Russian Academy of Sciences, Vladivostok, Russia
Sergey Nefedov
9 Institute of History and Archeology of the Ural Branch of the Russian Academy of Sciences, Ural Federal University, Yekaterinburg, Russia
Gary Feinman
10 Field Museum of Natural History, Chicago, IL, United States of America
Jill Levine
Jenny reddish, enrico cioni, chelsea thorpe, james s. bennett.
11 University of Washington, Seattle, Washington, United States of America
Pieter Francois
Harvey whitehouse, associated data.
All relevant data are within the manuscript and its Supporting Information files. Additionally, all data are available alongside a preprint publication of this article, accessible at: https://osf.io/mkhde/
What have been the causes and consequences of technological evolution in world history? In particular, what propels innovation and diffusion of military technologies, details of which are comparatively well preserved and which are often seen as drivers of broad socio-cultural processes? Here we analyze the evolution of key military technologies in a sample of pre-industrial societies world-wide covering almost 10,000 years of history using Seshat : Global History Databank . We empirically test previously speculative theories that proposed world population size, connectivity between geographical areas of innovation and adoption, and critical enabling technological advances, such as iron metallurgy and horse riding, as central drivers of military technological evolution. We find that all of these factors are strong predictors of change in military technology, whereas state-level factors such as polity population, territorial size, or governance sophistication play no major role. We discuss how our approach can be extended to explore technological change more generally, and how our results carry important ramifications for understanding major drivers of evolution of social complexity.
Introduction
From simple sharpened stone projectiles in the Paleolithic to the weapons of mass destruction in the modern world, what have been the main factors driving the evolution of military technology? Many have argued that the evolution of military technologies is just one aspect of a much broader pattern of technological evolution driven by increasing size and interconnectedness among human societies [ 1 – 3 ]. Several cultural evolutionary theories, conversely, highlight military technologies as a special case, arguing that steep improvements in both offensive and defensive capabilities of technologies along with accompanying tactical and organizational innovations resulted in “Military Revolutions” (note the plural), which in turn had major ramifications on the rise and, of particular concern here, the spread of state formations globally [ 4 – 8 ] and the evolution of religion and other cultural phenomena [ 9 , 10 ]. But the evolutionary mechanisms underlying general technological innovation, adoption, and transmission (especially in pre-industrial societies) are not well understood. Moreover, available theories have drawn on evidence that is limited both in geographical scope and temporal depth and deployed in ways that are subject to selection bias. Here we explore a variety of factors that previous scholarship suggests may have played a role in the evolution of military technologies by systematically quantifying the effects of those factors for thousands of years of world history.
Earlier efforts to quantify levels of technological complexity in eastern and western ends of Eurasia [ 11 , 12 ] have been criticized for being unduly subjective [ 13 ], especially when it comes to measuring rates of innovation in military technology, and are obviously limited in spatial coverage. Here we propose an alternative methodology for quantifying technological evolution and expand the geographic scope from just these two broad regions to 35 “Natural Geographic Areas” across all ten major world regions, using Seshat : Global History Databank , a major resource for studying patterns of sociocultural evolution in world history (see Materials and Methods below).
This article has two related goals. The first is to establish broad spatio-temporal patterns in the evolution of military technologies in pre-industrial societies. By technological evolution we mean here the dynamics of uptake (and possible loss) of technologies used by societies at significant scale (rather than simply whether the technology was known at all), regardless of how that society came to acquire that technology (indigenous innovation or adoption from another culture). For those interested in the study of technological evolution in general, focusing specifically on military technologies in pre-industrial societies has many practical benefits. Warfare was one of the most intensive activities of human societies, leaving abundant traces in the archaeological and historical record.
The second goal is to explore why these important military technologies developed or were adopted in the places, at the times, and as part of the technological packages as we observe in the historical and archaeological record. There have been several theoretical conjectures (discussed below) about the main causal drivers of technological innovation that we test. As our approach will show, the pattern of military technological evolution shows great variation in time and space, with different regions assuming a leading role in innovation at different moments in time.
Delineating the possible causes and observed consequences of changes in levels of military technologies will have far-reaching implications for understanding the evolution of technology broadly. To encourage further progress towards that ultimate goal, we present here a detailed methodology for testing theories about technological change in human history. This paper serves as a crucial step along this path.
Theoretical background
Here, we review several competing theoretical perspectives on the evolution of technologies offered in the past. Technological change is one of the fundamental drivers in social and cultural evolution and of long-term economic growth [ 14 – 17 ]. Many have pointed to technology’s ramifying effects on warfare, state formation, and the development of information processing systems [ 1 , 18 – 22 ]. Military technologies and their widespread application in particular have been shown to foment rises in social complexity and to spur related ideological developments [ 23 – 27 ]. But what processes are responsible for the evolution–the development, spread, and cumulative adoption–of military technology globally and across time?
Following this link between military technologies and socio-cultural development, we might expect to find a positive feedback between technological innovation and population growth at the global scale [ 2 , 28 – 32 ] see also [ 33 – 36 ]. Indeed, a well-known and much discussed theory proposed by economist Michael Kremer and expanded by others suggests exactly this causal link [ 2 ]. According to Kremer, “high population spurs technological change because it increases the number of potential inventors.” Kremer notes that "this implication flows naturally from the nonrivalry of technology… The cost of inventing a new technology is independent of the number of people who use it. Thus, holding constant the share of resources devoted to research, an increase in population leads to an increase in technological change. Thus, in a larger population there will be proportionally more people lucky or smart enough to come up with new ideas" [ 2 : 685]. This innovation, in turn, can spur further population growth, creating a positive feedback loop between technological and population growth; for instance, the proliferation of iron axes facilitated the clearing of agricultural land from forests [ 37 ], while the iron ploughshare improved the quality of plowing allowing for increased productivity [ 38 ] and, hence, larger populations to develop further innovations. Note that what is described by Kremer is virtually identical with what David Christian calls “collective learning” [ 39 ].
This process was expressed mathematically by Taagepera, Kremer, Podlazov and Tsirel in the following way:
This equation states that the technological growth rate at a given moment in time ( dT / dt ) is proportional to the global population, N (the larger the population, the larger the number of potential inventors) and to the current technological level, T . The second factor is included in the model to reflect the assumption that the wider the existing technological base, the greater the number of inventions that can be made on its basis. This model explicitly refers to global population level, rather than regional or localized populations of specific societies. To account both for the effect of global population size and the existing stock of technology, the Taagepera-Kremer model assumes that the rate of technology growth is proportional to the product of these two quantities. Taagepera and Kremer did not test this hypothesis empirically in a direct way. Empirical tests of this hypothesis performed by other researchers, however, have found support [ 34 , 40 ]. Note that Kremer observed that these new technologies would, in turn, likely generate population growth, suggesting a positive feedback between technological innovation and population. Here, however, we are concerned only with the effect of population on the evolution of technology, rather than the reverse.
A limitation of such population-focused theories, however, is the assumption that world population can be treated as having been an integrated information-exchanging system for many centuries, if not millennia. To address this problem, world-systems analysts have advanced an additional cluster of hypotheses. Chase-Dunn and Hall, for example, distinguish four types of networks of world-system communications: bulk good networks, political-military networks, prestige good networks, and information networks (IN) [ 41 ]. Korotayev et al. [ 42 – 44 ] explicitly focus on INs as technological innovation diffusion networks, proposing that a systematic diffusion of technological innovations within a certain set of societies is a sufficient condition to consider them a “world-system”. Thus, an as-yet unexplored synthesis of these ideas is that, while population may be one factor in the pace and location of technological evolution, membership in such an information diffusion network may play an additional role in facilitating the exchange of ideas and propensity for wide-spread adoption of new technologies.
One important advantage of the population-driven model advocated by Kremer, Taagepera, and others is that it explicitly includes the effect of the existing stock of technologies on technological growth rate. The greater the existing stock, the greater number of new technologies the model expects to be developed in the next time period. Although this is only one, relatively straightforward, way to model the impact of the existing technology stock, there is substantial historical evidence to make it a strong contender to be tested empirically. For example, the improvement of metallurgy and metal processing led not only to the emergence of new tools such as iron ploughs, but also to the proliferation of various types of weapons—starting with knives, daggers, swords, battle axes, up to the appearance of rifles and artillery. Nevertheless, the model assumes that the means and knowledge to adapt and improve upon existing technologies are readily accessible as well as the organizational capacity to deploy these technologies at large scales, which are open questions requiring further scrutiny.
Further, once a military technology had proven advantageous in inter-state competition, there arose an existential pressure on nearby societies to adopt that technology as well, so as not to be left behind. This sort of mimetic diffusion has been observed with respect to key technologies such as horse-mounted warfare that spread initially among nomadic confederations and nearby agrarian societies located along the central Eurasian Steppe [ 45 – 48 ]. Indeed, the domestication of the horse and its use in the civil and military sphere–including both the material components of horse-mounted archery as well as the tactical and organizational means to wield these weapons–appear to be of particular importance in the evolution of technologies and social complexity during the pre-industrial era, improving transportation, agriculture, and military capacities alike [ 47 ]. Further, the creation of new and more lethal weapons in one society could force people in their “strike zone” [ 27 ] to invent more sophisticated defenses while also often adopting the offensive technology themselves, prompting further technological advances. Following the invention of increasingly powerful, armor-piercing projectiles from bows and crossbows, for instance, we tend to see the means of protection improved as well to include chain mail, scaled armor, and plate armor.
Similarly, some work suggests that location is a critical factor in this process, as societies on the periphery, or semi-periphery [ 41 ], of larger, more complex imperial states will tend to be hotbeds of innovation, as they have both the incentive to increase (typically military) capability to compete with regional powers as well as the requisite flexibility to explore more radical innovation by being removed from the institutionalized practices and path-dependencies experienced by the larger societies “locked in” to the tools and habits that won them their hegemony [ 41 , 49 , 50 ].
Overall, previous theoretical work suggests that the evolution of military technologies depends on the total number of potential innovators involved in this process, the connectedness of distinct centers of innovation as well as of spheres of inter-state competition, and on the already existing stock of technologies, especially such fundamental developments as metal processing and transportation. In Materials and Methods below we discuss how we operationalize an empirical test of these hypotheses.
Materials and methods
A general approach to quantifying the evolution of pre-industrial societies.
This article follows the general philosophy and procedures that have been developed by the Seshat : Global History Databank project [ 51 – 54 ]. The Seshat Databank stores large volumes of historical and archaeological data on a growing number of variables for past polities going back to the late Neolithic. Supplementary Information ( SI ) contains a detailed description of the core methods and workflows underpinning the Seshat project, including how we incorporate differing levels of uncertainty and disagreement and data quality procedures involving experts and research assistants. We make the data used for the analyses presented here available online through a DataBrowser site ( seshatdatabank.info/databrowser ) and we encourage scholars to make use of and to augment our dataset.
The principal unit for data collection and analysis is a polity, defined as any independent political unit ranging from autonomous villages (local communities) through simple and complex chiefdoms to states and empires, regardless of degree of centralization [ 51 , 52 ]. Our sample of historic polities was developed using a stratified sample of the globe using the concept of ‘Natural Geographic Area’ (NGA). An NGA is a fixed spatial location of roughly 100 x 100 km delimited by naturally occurring geographical features (for example, a river basin, a coastal plain, a mountain valley, or an island). All polities that occupied the NGA, or part thereof, at a century mark (e.g. 200 CE), are included in our sample. This strategy avoids oversampling (redundantly repeating information across time points) while still capturing meaningful changes in the variables of interest. Although this granularity is relatively coarse, it is suitable for uncovering macro-level patterns in societal dynamics and exploring pathways of cultural evolution [ 24 , 53 ]. The data used in the analyses presented here come from 373 historic polities covering 35 NGAs.
Aggregation of military technology data into “Warfare Characteristics”
We quantified the sophistication of war-making capacity by encoding 46 binary variables indicating the presence or absence of different military technologies by a polity. These variables were aggregated into six general categories, termed Warfare Characteristics (WCs): Metals used in producing weapons and armor, the variety of Projectiles and hand-held Weapons, the sophistication of Armor, the use of transport Animals, and different kinds of Defensive Fortifications. Finally these WCs were aggregated into a composite, temporal MilTech variable for each NGA. See SI for details of the aggregation.
Of the six WCs, two (Metal and Animal) have a much broader area of application than specifically warfare. In some analyses below we investigate another measure (CoreMil) that focuses more narrowly on the sophistication of core military technologies by aggregating only the Projectiles, Weapon, Armor, and Defense WCs. As described below, we explore the impact of the spread of Iron and Cavalry in particular. Because Iron and Cavalry are correlated with the Metals and Animals WCs, analyzing CoreMil allows us to disentangle any potentially spurious effects of these WCs on overall military technology.
Hypotheses to be tested: Defining predictor variables
Our review in Theoretical Background suggested that the evolution of military technologies may be a function of the total number of potential innovators, the connectedness of innovation/adoption centers, and/or the existing stocks of technology. We measure these various potential explanatory factors in the following ways:
Following Taagepera and Kremer, we proxy the number of potential innovators with the world population (WorldPop, defined as log(10) of the global population at time t ). We take data on the dynamics of world population during the Holocene from [ 55 ].
Connectedness is a harder variable to quantify. Here we build on the concept of IN used by Chase-Dunn and Hall and other world-systems theorists [ 34 , 43 ] who define the extent of any particular IN as the zone within which spatially and culturally distinct regions exchange information, so that technological innovations made in one society diffuse relatively rapidly (on the time scale of centuries) to all other societies within the system than to societies that may be close (spatially and culturally) but fall outside of the IN. As an example, the contacts between Western and Eastern Eurasia (mediated via Central Asia) in the third and especially the second millennia BCE led to the spread of multiple technological innovations between the western and eastern parts of Eurasia: wheat, cattle, horses, bronze metallurgy, wheeled chariots, among others [ 44 , 56 ]. Here, we constructed a predictor variable proxying the Centrality of each region within the evolving (eventually global) IN by calculating the distance between each of our NGAs and the system of Silk Routes that connected East and West Eurasia for the majority of the period under study [ 57 – 60 ]. Our measure of Centrality is the inverse of the distance between an NGA and the nearest node on the Silk Route (see Fig 1 ; and SI for details).

In addition to Centrality within the IN, we capture two additional kinds of connectivity, namely the possible influence of spatial proximity (Space) as well as cultural affinity (Phylogeny). These terms not only allow us to control for possible autocorrelations and phylogenetic effects in our response variable (see Dynamic Regression Analysis below), but can also carry important information about processes influencing the evolution of military technologies. In particular, Space captures the process by which technological innovations may travel between geographically proximate societies–separately from the possible mediating influence of an expanding IN described above–measuring the likelihood that neighboring regions will share similar levels of military technology. Phylogeny focuses on the cultural similarity between polities, however spatially close, proxied by the relatedness of their dominant languages.
Another possible factor in the evolution of technology identified in the theoretical review is the effect of the current technology stock. We measure this in two ways. First, we model MilTech as a temporal autoregressive process, in which past values of MilTech affect its future values (for the details of the statistical model, see the next section). Second, we focus on the potential effects of two specific fundamental technologies: horse-riding and iron smelting.
According to the Cavalry Revolution theory, the invention of effective horse-riding in the Pontic-Caspian steppes, combined with powerful recurved bows and iron-tipped arrows, triggered a process of military evolution that spread from the steppes south to the belt of farming societies over several centuries throughout the first millennia BCE and CE [ 8 , 47 , 61 ]. Specifically, the threat of nomadic warriors armed with this advanced (for the period) military technology spurred the development of counter-measures designed to mitigate the cavalry advantage, while also producing an incentive to adopt horse-riding and effective accompanying combat tactics in areas further and further away from the location of their initial invention within the Steppe. The history of the military use of the horse went through several stages: the use of the chariot, the development of riding, the formation of light auxiliary cavalry, the development of nomadic riding, the appearance of the hard saddle, armored cataphracts, stirrups and, finally, heavy cavalry—a major branch of troops across Afro-Eurasian societies between c. 550 and 1400 CE [ 62 ]. As a result, effective horse-riding had far-reaching consequences for the evolution of military technologies, and specifically armor, projectiles such as crossbows, and fortifications. We use the data from [ 63 ] to encode the Cavalry variable (see Fig 2 ).

Data from [ 63 ].
The effect of Iron is similarly widespread. Multiple authors [ 64 – 66 ] have suggested that the availability of iron had a major impact on the evolution of technologies, as this strong and malleable material served as an input for a host of important technologies, military and otherwise, throughout the period under investigation here. We use data from [ 67 ] to encode the Iron variable (see Fig 3 ).

Data from [ 67 ].
Note that these two variables, Cavalry and Iron, are highly correlated with each other (compare Figs Figs2 2 and and3) 3 ) and it may be difficult to estimate their effects separately (the problem of collinearity). To address this potential issue we created a synthetic variable, IronCav, that combines the two effects (by adding Cavalry and Iron together). IronCav, thus, takes the maximum value for societies with both mounted warfare and iron weapons, intermediate value for societies having one characteristic and not the other, and minimum for societies with neither characteristic. We explored with dynamic regressions whether IronCav turns out to be a better predictor than either of its constituent variables, reported below.
In addition to the theoretically-motivated predictors–WorldPop, Centrality, Iron, and Cavalry, along with our autocorrelation terms Space and Phylogeny–we explore other potential polity- and NGA-specific predictors to proxy interesting subsidiary hypotheses, as explained below. These measures are taken from previously published analyses using Seshat data [ 68 ] and enable us to reduce the potential “hidden variable” (or omitted variable bias) problem, which arises when analysis implicates X as a causal factor for Y , while in reality the true cause could be Z , with which X is closely correlated [ 69 , 70 ]. The additional predictor variables include the following:
- Social scale (Scale) represents the first principal component (PC) of the Seshat variables polity population , polity territory , population of the largest settlement , and the number of hierarchical levels . The hypothesis here is that larger and more complexly organized and productive societies (in both population, territory) will have more resources to both generate new inventions and to implement them, or adopt them from elsewhere, especially the costly ones like sophisticated siege engines or elaborate fortifications. This measure also reflects having larger shares of the population not mainly engaged in primary production, proxied by the population of the largest settlement [ 71 , 72 ]. Further, more stratified and administratively complex societies–measured by the number of levels in administrative, military, and settlement hierarchies (combined here as one measure of hierarchical levels–see SI )–are hypothesized to be better equipped to implement useful technologies along with developing or adopting effective tactical and organizational models at scale. Thus, by this logic, increases in military technology should occur preferentially in larger scale societies. Previous analysis [ 53 ] reveals that these four dimensions are highly correlated within the Seshat sample and so represent an effective cross-cultural measure of societal scale to explore this hypothesis.
- SocSoph (“social sophistication”) represents the first PC of the Seshat variables governance , infrastructure , information systems , and money . This measure likewise derives from previous analysis of the dimensions of social complexity [ 53 ], capturing the important non-scale institutional and informational aspects. The hypothesis here is that societies with more sophisticated, pre-existing mechanisms for the exchange and implementation of ideas will generate and/or adopt innovations into widespread use at a faster pace.
- Agricultural productivity (Agri) is the estimated yield of different regions, measured as tonnes per hectare of the major carbohydrate source consumed in each of our NGAs. These data are taken from the analyses in [ 73 ]. The term is included here to test the possibility that productivity affects the amount of resources that are available for technological advances.
Social scale and productivity, thus, give us two complementary views of the resource base that may drive the evolution of technology. Agri tracks the underlying material resource base in a given geographical region (our NGAs) needed to support development, including technological evolution. Social scale, on the other hand, is a measure of the territorial and population size of specific historical polities. Larger polities can gather resources from a large territory, including the human energy from large populations, even where agricultural productivity is low. Separately SocSoph represents the sophistication of infrastructure and exchange media that could conceivably facilitate the flow of ideas from invention (whether within or outside of the society) to widespread adoption.
Statistical analysis
In addition to standard correlational statistical analyses of our response and predictor variables, we used a general non-linear dynamic regression model to investigate factors affecting the evolution of military technology. This dynamic regression analysis distinguishes correlation from causation by estimating the influence potential causal factors at a previous time have on the response variable at a later time (known generally as Wiener-Granger causality [ 74 , 75 ]). While an improvement over ‘static’ correlations, where causal direction remains ambiguous, this method is, nevertheless, insufficient for making absolute claims of causality. Further scrutiny will be required to provide additional support for the provisional causal interpretations suggested below.
Our model takes the following form [ 70 ]:
Here Y i , t is the response variable (MilTech) for location (NGA) i at time t . We construct a spatio-temporal series for Seshat response and predictor variables by following polities (or quasi-polities, such as archaeologically attested cultures) that occupied a specific NGA at each century mark during the sampled period. Thus, the time step in the analysis is100 years.
On the right-hand side, a is the regression constant (intercept). The next term captures the influences of past history (“autoregressive terms”), with τ = 1, 2, … indexing time-lagged values of Y (as time is measured in centuries, Y i , t – 1 refers to the value of MilTech 100 years before t ).
The third term represents potential effects resulting from geographic diffusion using our Space term. We used a negative-exponential form to relate the distance between location i and location j , δ i , j , to the influence of j on i . Unlike a linear kernel, the negative-exponential does not become negative at very large δ i , j , instead approaching 0 smoothly. The third term, thus, is a weighted average of the response variable values in the vicinity of location i at the previous time step, with weights falling off to 0 as distance from i increases. Parameter d measures how steeply the influence falls with distance, and parameter c is a regression coefficient measuring the importance of geographic diffusion. For an overview of potential effects resulting from geographic diffusion, see [ 69 , 76 ]; for a description of how we avoided the problem of endogeneity, see [ 70 ].
The fourth term detects autocorrelations due to any shared cultural history at location i with other regions j using our Phylogeny variable. Here w represents the weight applied to the phylogenetic (linguistic) distance between locations (set to 1 if locations i and j share the same language, 0.5 if they are in the same linguistic genus, and 0.25 if they are in the same linguistic family). Linguistic genera and families were taken from The World Atlas of Language Structures and Glottolog [ 77 ].
The next term on the right-hand side represents the effects of the main predictor variables X k [ 70 ]; g k are regression coefficients. These variables (described in the previous section) are of primary interest because they enable us to test various theories about the evolution of MilTech against each other. Finally, ε i , t is the error term. We also include quadratic versions of these terms at a time lag (not shown) in order to explore non-linear responses to response and predictor factors.
Model selection (choosing which terms to include in the regression model) was accomplished by exhaustive search: regressing the response variable on all possible linear combinations of predictor variables. The degree of fit was quantified by the Akaike Information Criterion (AIC). Standard diagnostic tests were performed for the best-fitting models [ 70 ].
Missing values, estimate uncertainty, and expert disagreement in the predictors were dealt with by multiple imputation [ 78 , 79 ]. The response variable, MilTech, however, was not imputed as that can result in biased estimates [ 76 ]. For details of the multiple imputation procedure see SI . Because diagnostic tests indicated that the distribution of residuals are not gaussian, we used nonparametric bootstrap to estimate the P -values associated with various regression terms (see the SI for details). Additional robustness checks are similarly detailed in the SI .
Spatio-temporal patterns
We first examined the frequency distributions of the variables of interest and the cross-correlations between WCs, overall MilTech, which combines all WCs, as well as calendar Time and the various aspects of social complexity and productivity. As expected, we find that all WCs are closely correlated with each other and with the overall MilTech variables. Plotting MilTech as a function of time for each NGA ( Fig 4 ), we observe that there is a general upward trend, as expected. However, there are also periods when some technologies are lost, for a time. Most importantly, there is a great amount of variation between different geographic regions in the timing of MilTech increases. Interestingly, all WCs are more strongly correlated with the two dimensions of social complexity specified here–Scale and SocSoph–rather than with Time, suggesting that key drivers of MilTech evolution go beyond merely the additive nature of technology through the ‘march of time’. The nature of any causality between complexity and MilTech is discussed below.

MilTech trajectories in Seshat NGAs, divided by major world region: (A) Europe and Africa; (B) Western Asia; (C) East and SE Asia; (D) Americas and Oceania.
We next focus on “technology leaders”, NGAs that at some point in their history had the highest value of MilTech available at the time. Fig 5 shows them, roughly in the order that they achieved world leadership (note that this order is also affected by how far back in the past we have data). The hot spot of technological development, through either innovation or adoption, appears to roughly coincide with the “Imperial Belt” of the Old World, located just south of the Great Eurasian Steppe (and in places, impinging into it, as in Sogdiana), which can be seen by the location of the ‘leader’ NGAs (mapped in Fig 5 ).

This same territory also of course corresponds roughly to the path of the overland silk routes used in our analyses ( Fig 1 ). We return to this pattern below. Overall, the pattern is that most of the leading regions exhibit an increase in their overall MilTech levels roughly together and at a fairly regular, almost linear pace (after the 4 th millennium BCE), with late comers accelerating at various points to merge with leaders. This is seen clearly in this graph on the example of Sogdiana, but it is a general pattern discernible in the regional examples ( Fig 4 ).
We explored the “similarity” between NGAs by calculating the number of MilTech variables in each NGA shared with other NGAs at each time-step. As explained in SI (see Similarity Analysis and S1 Fig in S1 File ) we trace how NGAs join the expanding Eurasian (eventually global) IN by noting the time when they achieve a similarity index of 10, that is, when they share 10 or more specific MilTech variables with one or more other NGAs. As the histograms in S1 Fig in S1 File show, the first NGAs that achieve this threshold of similarity appear between 3000 and 2500 BCE. As time progresses, more and more NGAs cross this threshold. Fig 6 maps the expansion of this IN–initially restricted to central Eurasia but growing eventually into a global network–by color coding the date when the NGA cross this threshold. Thus, the similarity analysis reveals that different regions not only saw rapid increases in their overall level of MilTech, but these areas came increasingly to share specific technologies. A plausible interpretation for this pattern is that, as the IN expands, each new region accelerates its development of MilTech to join the level achieved by the network leaders, until, eventually, all regions in diverse areas around the globe adopt similar ‘MilTech packages’. Future work is needed to disentangle occasions where these late-comer regions adopt or adapt existing technologies from cases where ‘leader’ societies simply take over others, imposing their technologies (along with a host of other socio-political and cultural traits) onto this new regions.

NGAs are binned into 6 categories according to the earliest time they share 10 or more MilTech variables with another NGA, displayed by color: dark red = 2500 BCE or before; orange = between 2500 and 1500 BCE; yellow = between 1500 and 500 BCE; green = between 500 BCE and 500 CE; blue = after 500 CE; grey = did not display any similarities during our sample period. Unfilled red circles indicate Silk Route Nodes as in Fig 3 .
Dynamic regression results
The best fitting model from our general dynamic regression analysis is shown in Table 1 .
Estimate shows the standardized regression coefficients, which provide a direct measure of relative effects by the lagged predictors on the response variable. Thus, MilTech here represents the linear autoregressive term, AR(1). The column “t value” lists t-statistics, a measure of statistical significance of regression terms associated with various predictors. Pr(>|t|) is the statistical significance for regression assuming the Normal distribution of residuals, while Bootstrap estimated P is the result of nonparametric bootstrap that does not make this assumption.
Our analysis identifies the following variables as having the strongest causal influence on MilTech:
- Autocatalytic effects (the value of MilTech in the previous time step).
- Global population size (WorldPop).
- Connection to an expanding (eventually global) Information Network (Centrality).
- Spread of Iron+Cavalry (IronCav), revealing both the importance of prior technology stock on continued technological evolution as well as the incentive that these advances placed on societies within connected information and competition spheres to adopt or develop additional technologies in response.
- Cultural similarity (Phylogeny), revealing that polities linguistically similar to polities with high MilTech are more likely to have high MilTech themselves. This effect could be a result of either common inheritance or easier diffusion of technology between culturally similar polities, or, most likely, both.
- Productivity of the resource base (Agri).
Investigation of the effects of Cavalry and Iron as predictor variables indicate that either, separately, has a statistically significant effect of similar strength on the evolution of MilTech. The synthetic variable, IronCav, however, is a better predictor than either of its constituents. For this reason, the results here are reported for IronCav only.
We estimated how location with respect to the system of Silk Routes affects the evolution of MilTech in each region. Our measure of Centrality (inverse distance to the nearest Silk Route node) finds strong empirical support, although we ran analyses using alternate methods of proxying this type of spatial effect (see SI for details). Overall our best model predicts the level of military technology with regression coefficient of determination ( R 2 ) of 0.96. While some of this high predictability is a result of strong temporal autocorrelation, rerunning the regression omitting all autocorrelation terms nevertheless yields an R 2 of 0.72. Thus, more than 70% of the variation in MilTech is explained by WorldPop, Centrality, IronCav, and Agri.
We performed several supplemental analyses and robustness checks to detect any biases in our results. Several of these checks are discussed below and detailed in the SI .
Table 2 shows a comparison between the best fitting model and other models with Δ AIC ≤ 2. Strong effects are detected in these alternative models for all terms in the best model including Agri, which, though its standardized coefficient is the smallest, remains statistically significant at the conventional P < 0.05 level. However, additional robustness tests using multiple datasets built by random sampling from among the different WCs comprising the MilTech variable indicate that Agri is not always supported (see SI for details). Neither measures of social complexity, Scale and SocSoph, appear to have a consistent significant positive effect on MilTech evolution (they show up in several of the alternative models, but with small t -values and negative signs for Scale).
The table shows t-statistics associated with each of the predictors (column headings) for the best models with ΔAIC (the AIC difference with respect to the best model) less than 2. The best model by AIC is included as the top row. An empty entry indicates that the term associated with this predictor is not included in the model.
Further checks indicate that these results are robust to the inclusion of additional spatial and temporal autocorrelation effects: Neither geographic diffusion (Space) nor higher temporal lags ( τ = 2 centuries or greater) are significant. In addition, as we discussed in Materials and Methods , because our measure of MilTech includes the Metals and Animal WCs, which might confound the effect of IronCav due to a potential circularity, we re-ran the analysis using CoreMil, our measure of military technologies that does not include these WCs. This analysis yields essentially identical results (see SI ), thus suggesting that the effect of IronCav is not spurious.
What is remarkable is that neither Scale nor SocSoph variables, which characterize polities, have any detectable effect on the level of MilTech. Overall, these results suggest that MilTech evolves almost entirely as an exogenous variable: it is little, if at all, affected by such polity characteristics as the population, territory size, the sophistication of information systems or administrative institutions, or provision of infrastructure and public goods.
As noted, our dynamic regression approach cannot offer a definitive demonstration that the factors in the best model are the central causal forces driving the evolution of military technologies. These variables may simply be highly correlated with the ‘true’ causal factors, not included in our analyses, or the causal link may be indirect, as these factors, as well as MilTech, could be caused separately by additional factors whose effects were felt at different time-scales. We explored such a possible ‘hidden variable bias’ as much as possible through supplemental analyses of several variables for which we had reliable information. As our findings remain robust to various tests, we provisionally conclude that these results offer appealing and parsimonious causal explanation for the long-run and global evolution of military technologies. Future research will need to scrutinize whether these results hold up to the inclusion of additional factors and exploration using alternate statistical methods or mechanistic models perhaps using agent-based modelling [ 24 , 27 , 80 ].
Our goals were to investigate the global spatio-temporal evolution of key pre-industrial military technologies to illuminate the major forces driving the evolution of these critical tools, whether by innovation, adoption and adaption, or a combination of these processes. Further, our approach to testing theoretically-informed hypotheses against a broad and diverse set of empirical historical data taken from Seshat : Global History Databank serves as an example of how more general patterns of technological evolution can be explored in future research, as well as more fine-grained analyses seeking to distinguish these different processes or explore the pathways taken by individual regions or societies. Here we surveyed various causal hypotheses, which together suggested that the evolution of military technology would be a function of some combination of global population size, connectedness to information exchange networks, involvement in inter-state competition networks, and prior histories of technological innovation and adoption (especially major breakthroughs such as iron metallurgy and horse riding), along with, perhaps, various properties of polities and their resource base. We set out to test these theories empirically against the evidence from world history, using a stratified sample of polities in Seshat , dating from the Neolithic to the Industrial Revolutions.
While we found some empirical support for each of these hypotheses, no one theory alone accounted for the observed dynamics of military technology as well as a combination of the factors suggested by these various proposals. Our results not only explain why these theories have found support in previous studies, but also why a general understanding of the evolution of military technology has proven elusive. Our robust historical sample and extensive dynamic analyses allowed us to compare and combine elements of different theories proposed as critical drivers of military technology. Specifically, we found that global population size is a strong predictor of the subsequent levels of MilTech. While this result supports the Kremer-Taagepera model, it does not rule out other possible causal explanations based on additional variables, which, while correlated with global world population, could turn out to be a better predictor of MilTech. One such addition in future work could be to distinguish societies by their general affluence or social mobility [ 81 ], rather than treating populations as indistinguishable, which may play such a causal role driving both population increases and technological evolution.
Our analysis found that stock of prior technological innovations played an important role in the observed levels of military technology, not only from the autoregressive terms (again, supporting the Kremer-Taagepera model) but critically because the combination of iron metallurgy and horse riding had a particularly strong effect on innovation and adoption of militarily technologies in the periods under investigation here.
Importantly, we found that location within the central Eurasian IN was also a strong predictor of our response measure, in line with the insights of World Systems and cultural evolutionary theorists. This result supports the impact of being connected to other major centers of development and innovation, as well as being incorporated into spheres of inter-state competition.
However, it is noteworthy that geographic proximity between NGAs itself (proxied here by our Space measure) does not appear to be a strong predictor of the evolution of military technologies, contrary to what might be expected from certain cultural evolution theories and ideas of mimetic diffusion. This underscores the significance of iron and cavalry diffusion in particular, which have a strong effect on subsequent levels of MilTech, supporting previous work highlighting the unique role of the nomadic pastoralists of the Eurasian Steppe, early adopters of mounted archery tactics, in driving not only technological innovation among nearby agrarian populations, but in driving the expansion of social complexity and, relatedly, technological evolution throughout Afro-Eurasia [ 8 , 24 , 26 , 27 , 45 – 48 , 82 ]. The development of iron-smelting, as an input material for so many valuable weapons, appears to play a similarly crucial role [ 64 – 67 ]. These findings suggest that iron and cavalry were particularly critical technologies that conferred an important enough advantage that they fomented widespread adoption as well as sparked ‘arms races’ among competitors that included a host of other, related technologies as discussed above, which would explain the observed patterns.
This interpretation gains further support from our similarity analysis. Our main result indicate that the overall level of MilTech–measured with our aggregate MilTech score–generally rose over time (with some losses, noted above), with more and more regions coming to exhibit the same level of MilTech over time. Our similarity analysis unpacks this finding, demonstrating that not only did regions increasingly exhibit the same overall MilTech score, but they also came to share the same ‘packages’ of specific military technologies. Further, as expected, the regions with the highest combined similarity scores followed the same pattern as seen in the Centrality measure, as the NGAs closest to a Silk Route node both appeared as sharing MilTech variables with other NGAs earlier and continued to display similar MilTech packages with other NGAs that joined the IN over time, resulting in their larger combined scores (see Fig 4 ).
An interesting and somewhat surprising finding is that the properties of polities, including such seemingly important characteristics as their scale (population and territory) and sophistication (e.g., information systems), have no significant impact on the evolution of military technologies wielded by the polity (with the partial exception of Phylogeny, discussed below). We expected both scale (Scale) and non-scale (SocSoph) aspects of social complexity to play a significant role in these processes, due to an increased availability of populations and resources to put towards technological development as well as how developments in organizational and informational-exchange capacities could facilitate the adoption and adaption of existing technologies from elsewhere. However, these terms display no significant effect on subsequent levels of MilTech, suggesting that the level of technology characterizing a particular polity (whether invented or adopted) depends not on the polity’s characteristics, but rather on the characteristics of the inter-polity informational and competitive interaction spheres to which it belongs, along with the other factors identified above. The Arabian Peninsula, for example, despite being relatively low-scale in the early first millennium CE, adopts much of the ‘military package’ seen in other parts of Eurasia around 300 CE (see Fig 4 and the Similarity Analysis in the SI ), as it became increasingly incorporated into Silk Route trade connections via the Persian and Roman imperial systems, before becoming its own seat of imperial power with the rise of Islam a few centuries later.
The only polity-related term that is included in the best regression model is Phylogeny, which can reflect an operation of one of two (or both) processes: inheritance of technological sophistication from a “common ancestor” (for example, Italy and France inheriting technologies from the Roman Empire), or easier spread of innovations between culturally similar countries (such as between Romance-speaking Italy and France, or between Arabic-speaking Egypt and Mesopotamia). The latter process likely reflects the greater likelihood that an innovation developed in one polity will be more compatible with existing institutional, social, cultural, and economic systems of a culturally similar polity than those of a more distant one [ 83 ]. One major component of this effect might be that military technologies require specific tactical and organizational apparatus to wield effectively. Cultural similarity then could not only facilitate exchange of information about a new, useful technology across societies, but facilitate the spread of knowledge of and increase the ability to acquire these more ephemeral aspects accompanying the material components of this new technology. Alternatively, linguistically similar polities might have engaged in more frequent and intense competition, which could lead to a similar impact (likely correlated strongly with the IronCav and Centrality effects) on overall MilTech. This is less plausible than the other processes, however, as interstate competition has been shown to be most intense involving culturally dissimilar polities [ 45 , 47 , 61 ]. Additional study is needed to fully clarify the different possible causal forces driving this effect and to explore the possible causal role that each of these potential processes play in the overall development and spread of these key military technologies, along with technological evolution more generally. Nevertheless, the finding that Phylogeny is a significant predictor of MilTech further speaks to the importance of connection-mediated information exchange, over and above closeness in space.
Lastly, we find that agricultural productivity, measured here as per-hectare tons of major carbohydrates, displays a significant effect on subsequent levels of MilTech. While we had no strong theoretical motivations for this idea, we included the term in analyses to test the possibility that an increased resource base would impact technological development. Its inclusion in our best model suggests that a certain level of agriculture productivity may have been a necessary component in generating and adopting new technologies. Perhaps a more efficient productivity was required to support large enough populations not primarily employed in agriculture, or expanding a society’s general resource base and extractive capacity provided the raw materials and intermediate goods used in constructing key military technologies. As noted, however, this factor displays a much weaker effect compared to the others, and is least robust to supplemental analyses. Thus, this result must remain tentative. Exploring more deeply the impact of agricultural productivity on the evolution of technology stands out as an important avenue for future research.
While these findings constitute an important first step towards identifying some of the major long-term drivers of technological evolution in general, and in the domain of military capacity in particular, and finding broad support for previously somewhat speculative theories, there is still much to be done to build on this line of research. First, it would be desirable to extend the geographical coverage beyond the stratified global sample used in the present study, particularly relating to the phylogenic connections in the spread of existing technologies and the different possible processes that lead to this interesting effect. Second, it would be important to explore the downstream consequences of changes in military technology for other aspects of human life, including levels of peacefulness (or, alternatively, mortality rates due to violence), equality (e.g. distributions of wealth, rights of citizenry, levels of exploitation and oppression based on class or ethnicity) and public health (e.g. longevity, infant mortality, nutrition, infection rates, etc.). Third, our goal was to offer a preliminary exploration of some key causal forces proposed to support the evolution of military technology, ignoring differences between the initial innovation of new technologies and subsequent adoption by other societies. Future work is needed to pinpoint the source(s) of invention and distinguish advances made by innovation from advances by later spread to assess whether the same or different factors drive each of these separate processes. Fourth, additional potential drivers of technological innovation in general should be explored, over and above the effects of population size, connectivity, and existing stocks of critical innovations, as well as analyzing further the potential causal role played by rising agricultural productivity. These explorations would include factors impacting resource scarcity (e.g. due to drought, pestilence, and other natural disasters), more direct measures of intergroup competition (e.g. levels and intensities of external warfare, cultural distance between competitors, and other exogenous factors), identifying various different regional INs which might (partially) overlap in time and space.
Finally, it is important for future studies to ‘narrow in’ on the details of some of the more macro-level processes suggested by the present study. In particular, it will useful to explore the possible impact of regional-level factors along with a broader range of technological innovations within the polity (e.g. in energy, construction, transportation, and information sectors). Seshat data is relatively coarse, resolved here to 100-year intervals. While this granularity is well suited to exploring broad, global-level dynamics over thousands of years, it likely misses some of the nuances and outlying patterns. Future effort can hopefully generate more fine-grained temporal data allowing for meso- and even micro-level scrutiny of the pathways to technological evolution taken by different societies in various times and places. Alongside this, we require more qualitative investigation into the details of the specific items as well as the less material, tactical and managerial aspects of technological development employed in a host of specific historical cases.
Beyond the insights gained from these analyses on the development of military technologies over the very long-term, we hope that the approach presented here, which explores likely casual theories against a wide body of empirical data gathered by the Seshat project, will provide a roadmap to these important future studies, allowing scholars to delve deeper into not only the critical ‘Military Revolutions’ throughout history, but into the evolution of technology generally.
Supporting information
Acknowledgments.
The authors are grateful to Sergey Nefedov who reviewed data and provided helpful comments. We thank also Christopher Chase-Dunn, Peter Grimes, Gene Anderson, and the SetPol project for their constructive critique on earlier versions of the manuscript, as well as Jennifer Larson and Alan Covey for helpful comments on previous drafts. We gratefully acknowledge the contributions of our team of research assistants, post-doctoral researchers, consultants, and experts. Additionally, we have received invaluable assistance from our collaborators. Please see the Seshat website ( www.seshatdatabank.info ) for a comprehensive list of private donors, partners, experts, and consultants and their respective areas of expertise.
Funding Statement
This work was supported by: a John Templeton Foundation grant to the Evolution Institute, entitled "Axial-Age Religions and the Z-Curve of Human Egalitarianism" (HW, PF, PT); a Tricoastal Foundation grant to the Evolution Institute, entitled "The Deep Roots of the Modern World: The Cultural Evolution of Economic Growth and Political Stability" (PT); an Economic and Social Research Council Large Grant to the University of Oxford, entitled "Ritual, Community, and Conflict" (REF RES-060-25-0085) (HW); a grant from the European Union Horizon 2020 research and innovation programme (grant agreement No 644055 [ALIGNED, www.aligned-project.eu ]) (HW, PF); a European Research Council Advanced Grant to the University of Oxford, entitled “Ritual Modes: Divergent modes of ritual, social cohesion, prosociality, and conflict" (HW, PF); a grant from the Institute of Economics and Peace to develop a Historical Peace Index (HW, PF, PT, DH); and the program “Complexity Science,” which is supported by the Austrian Research Promotion Agency FFG under grant #873927 (PT).
Data Availability
- PLoS One. 2021; 16(10): e0258161.
Decision Letter 0
25 Jun 2021
PONE-D-21-17737
Rise of the War Machines: Charting the Evolution of Military Technologies from the Neolithic to the Industrial Revolution
Dear Daniel Hoyer,
I have received two reviewers' report on your manuscript and decided I would not wait for the third reviewer that I had invited. The two reviewers, I'm happy to say, concur that your manuscript is an important and valuable contribution to the cultural evolution literature. I share their point of view. Each reviewer offers specific recommendations that I would ask you to follow closely when revising the paper.
Reviewer 1 remarks that reducing the history of military technology to "hard" technologies (e.g. weapons or transportations) neglects important aspects of war tactics: "military doctrines, logistics, tactical skill, organizational structures, and ability to learn". In other words, your study captures the evolution of tactical "hardware", but not that of tactical "software", so to speak. They note that a more qualitative approach could capture this dimension. Please discuss this possible limitation of your study in the revision. Reviewer 1 also asks you to specify how your analysis can distinguish between technological change due to innovation vs. diffusion.
Reviewer 2 makes two specific and useful recommendations regarding your statistical analysis. The first is to make sure that the way you bundle military-technology related variables is justified. In other words, you should rule out the possibility that the effects you document are driven by one or a few outlier variables. Please implement the analysis they recommend, i.e., analysing random subsets of the 46 variables. Re-running your models with a subset of military technology variables is something you already do when you substitute "Core MilTech" for "MilTech". Reviewer 1 would like you to generalise this method to multiple, randomly selected subsamples. Reviewer 2 also suggests an alternative analysis for the impact of existing stock of technologies on the progress of military technologies.
In addition to these remarks, I have a number of editorial recommendations of my own, detailed below. Provided the revision appears to fulfil the reviewers' requirements and mine, I may not be sending it back for a new round of reviews.
My recommendations are either stylistic or related to the way statistical results are reported.
Concerning the reporting of results, my main concern is related to the repeated causality claims made in the manuscript. Given the data and methods, the results establish (at best) predictive causality in the sense of Granger. This type of causality cannot be equated with causality simpliciter , among other things because it does not rule our latent confounding effects. Please qualify all claims related to causality or causal inference.
I suspect there is a significant mistake in Table 1, which lists both MilTech and MilTech sq. as variables in the best-fitting model. This seems to contradict the description given on l. 301, but more importantly including two versions of the same variable in the same model raises obvious issues (multicollinearity, etc.). I suspect that you in fact tested 2 versions of the model, one with MilTech and the other with MilTech sq.
In general the manuscript in its current version assumes too much familiarity with the Seshat database and project, its organisation and its acronyms. Key constructs like "NGA" or "IN" are not explained or even glossed. Other constructs, like the "Scale" variable, will be familiar to readers who already read Seshat-based studies, but are only cursorily explained here (the characterisation given on l. 279 is insufficiently clear). For yet other variables like "SPC1" an explanation is promised but never provided (l. 349). There is also a tendency to use your own abbreviations for standard statistical constructs (e.g. using "delAIC" for ∆AIC or delta AIC). What is missing is not only a basic explanation of the Seshat lingo, but a sense of why the constructs were designed in the way that they are — for instance, why the Scale variable is a better measure of a polity's size than other possible measures.
Please submit your revised manuscript by Aug 09 2021 11:59PM. If you will need more time than this to complete your revisions, please reply to this message or contact the journal office at gro.solp@enosolp . When you're ready to submit your revision, log on to https://www.editorialmanager.com/pone/ and select the 'Submissions Needing Revision' folder to locate your manuscript file.
Please include the following items when submitting your revised manuscript:
- A rebuttal letter that responds to each point raised by the academic editor and reviewer(s). You should upload this letter as a separate file labeled 'Response to Reviewers'.
- A marked-up copy of your manuscript that highlights changes made to the original version. You should upload this as a separate file labeled 'Revised Manuscript with Track Changes'.
- An unmarked version of your revised paper without tracked changes. You should upload this as a separate file labeled 'Manuscript'.
If you would like to make changes to your financial disclosure, please include your updated statement in your cover letter. Guidelines for resubmitting your figure files are available below the reviewer comments at the end of this letter.
If applicable, we recommend that you deposit your laboratory protocols in protocols.io to enhance the reproducibility of your results. Protocols.io assigns your protocol its own identifier (DOI) so that it can be cited independently in the future. For instructions see: http://journals.plos.org/plosone/s/submission-guidelines#loc-laboratory-protocols . Additionally, PLOS ONE offers an option for publishing peer-reviewed Lab Protocol articles, which describe protocols hosted on protocols.io. Read more information on sharing protocols at https://plos.org/protocols?utm_medium=editorial-email&utm_source=authorletters&utm_campaign=protocols .
We look forward to receiving your revised manuscript.
Kind regards,
Olivier Morin
Academic Editor
Journal Requirements:
When submitting your revision, we need you to address these additional requirements.
1. Please ensure that your manuscript meets PLOS ONE's style requirements, including those for file naming. The PLOS ONE style templates can be found at
https://journals.plos.org/plosone/s/file?id=wjVg/PLOSOne_formatting_sample_main_body.pdf and
https://journals.plos.org/plosone/s/file?id=ba62/PLOSOne_formatting_sample_title_authors_affiliations.pdf
2. Please review your reference list to ensure that it is complete and correct. If you have cited papers that have been retracted, please include the rationale for doing so in the manuscript text, or remove these references and replace them with relevant current references. Any changes to the reference list should be mentioned in the rebuttal letter that accompanies your revised manuscript. If you need to cite a retracted article, indicate the article’s retracted status in the References list and also include a citation and full reference for the retraction notice.
3. Thank you for stating the following in the Competing Interests section:
"The authors have declared that no competing interests exist."
We note that one or more of the authors are employed by a commercial company: "Complexity Science Hub."
a) Please provide an amended Funding Statement declaring this commercial affiliation, as well as a statement regarding the Role of Funders in your study. If the funding organization did not play a role in the study design, data collection and analysis, decision to publish, or preparation of the manuscript and only provided financial support in the form of authors' salaries and/or research materials, please review your statements relating to the author contributions, and ensure you have specifically and accurately indicated the role(s) that these authors had in your study. You can update author roles in the Author Contributions section of the online submission form.
Please also include the following statement within your amended Funding Statement.
“The funder provided support in the form of salaries for authors [insert relevant initials], but did not have any additional role in the study design, data collection and analysis, decision to publish, or preparation of the manuscript. The specific roles of these authors are articulated in the ‘author contributions’ section.”
If your commercial affiliation did play a role in your study, please state and explain this role within your updated Funding Statement.
b) Please also provide an updated Competing Interests Statement declaring this commercial affiliation along with any other relevant declarations relating to employment, consultancy, patents, products in development, or marketed products, etc.
Within your Competing Interests Statement, please confirm that this commercial affiliation does not alter your adherence to all PLOS ONE policies on sharing data and materials by including the following statement: "This does not alter our adherence to PLOS ONE policies on sharing data and materials.” (as detailed online in our guide for authors http://journals.plos.org/plosone/s/competing-interests ) . If this adherence statement is not accurate and there are restrictions on sharing of data and/or materials, please state these. Please note that we cannot proceed with consideration of your article until this information has been declared.
Please include both an updated Funding Statement and Competing Interests Statement in your cover letter. We will change the online submission form on your behalf.
Please know it is PLOS ONE policy for corresponding authors to declare, on behalf of all authors, all potential competing interests for the purposes of transparency. PLOS defines a competing interest as anything that interferes with, or could reasonably be perceived as interfering with, the full and objective presentation, peer review, editorial decision-making, or publication of research or non-research articles submitted to one of the journals. Competing interests can be financial or non-financial, professional, or personal. Competing interests can arise in relationship to an organization or another person. Please follow this link to our website for more details on competing interests: http://journals.plos.org/plosone/s/competing-interests
[Note: HTML markup is below. Please do not edit.]
Reviewers' comments:
Reviewer's Responses to Questions
Comments to the Author
1. Is the manuscript technically sound, and do the data support the conclusions?
The manuscript must describe a technically sound piece of scientific research with data that supports the conclusions. Experiments must have been conducted rigorously, with appropriate controls, replication, and sample sizes. The conclusions must be drawn appropriately based on the data presented.
Reviewer #1: Partly
Reviewer #2: Yes
2. Has the statistical analysis been performed appropriately and rigorously?
Reviewer #1: Yes
3. Have the authors made all data underlying the findings in their manuscript fully available?
The PLOS Data policy requires authors to make all data underlying the findings described in their manuscript fully available without restriction, with rare exception (please refer to the Data Availability Statement in the manuscript PDF file). The data should be provided as part of the manuscript or its supporting information, or deposited to a public repository. For example, in addition to summary statistics, the data points behind means, medians and variance measures should be available. If there are restrictions on publicly sharing data—e.g. participant privacy or use of data from a third party—those must be specified.
4. Is the manuscript presented in an intelligible fashion and written in standard English?
PLOS ONE does not copyedit accepted manuscripts, so the language in submitted articles must be clear, correct, and unambiguous. Any typographical or grammatical errors should be corrected at revision, so please note any specific errors here.
5. Review Comments to the Author
Please use the space provided to explain your answers to the questions above. You may also include additional comments for the author, including concerns about dual publication, research ethics, or publication ethics. (Please upload your review as an attachment if it exceeds 20,000 characters)
Reviewer #1: This is a very valuable paper providing the most systematic large-n analysis to date of the evolution of military technology. It should acknowledge more clearly that large-n studies are just one of the tools needed for a proper explanation, but should definitely be published.
(Editor's note: I am here pasting Reviewer 1's attached comments since they may not be automatically added to this action letter for word limit reasons. OM)
This is a valuable paper, making a real contribution to a significant debate. My one reservation is that I think this kind of large-n survey usually has to be combined with more detailed work to explain something as complicated as military technologies. Military historians typically find that technology is only one part of effectiveness, and not always the most important part. The most interesting result in this paper is the lack of fit between SocSoph and technological gains (lines 455-56, 532-36), but I wonder how much of this is because technologies are little use without appropriate doctrine (the central point in Stephen Biddle’s book Military Power [2004]). Fig. 5 illustrates this—looking at the scores for Latium around 2kya, they’re not very different from other societies, because Roman military technology wasn’t actually that different from other Mediterranean, Middle Eastern, and European societies (as measured in the categories at lines 287-98 in the supporting material); however, the Roman army’s ability to apply these technologies transformed what they meant in practical terms, and that was probably determined primarily by Roman SocSoph. I also immediately think of the classical Greeks, whose military technologies were very ordinary but their application of them was extraordinary.
One difficulty for large-n surveys is that while technological categories are relatively easy to identify in the archaeological record, military doctrines, logistics, tactical skill, organizational structures, and ability to learn aren’t; and even when ancient and medieval writers describe such doctrines, there’s no obvious way to score them. This paper should absolutely be published, because there’ve been few (if any) large-n surveys of this level of sophistication, but the main point it illustrates is perhaps that large-n surveys are only the beginning of the study of warfare, and we have to follow up on their results with analytic narratives and case studies.
I also thought that the paper should distinguish more between technological innovation and diffusion. I’m currently reading two case studies of the Comanches, who probably had the best light cavalry in the world in the 18th century. They didn’t invent light cavalry, because there were no horses in North America to invent it with before the Spaniards brought them, but they did adopt and then adapt horse-herding and perfect styles of mounted fighting much better than the Apaches or even the Spaniards and Mexicans. They didn’t adopt or adapt firearms because their light-cavalry tactics made muzzle-loading muskets irrelevant. The Comanches were eventually defeated because Texans adopted and adapted Comanche tactics in the 1830s and combined them with revolvers and, later, breech-loading rifles that had been invented on the US East Coast. Neither the Comanche nor the Texans could have invented revolvers and rifles themselves, but the US Army failed to figure out how to use the new technologies until Texans put them together with tactics in new ways; but the final Comanche defeat in the 1870s depended on the US Army learning from the Texans and then exploiting the scale of US federal infrastructure.
These points aren’t just details that can be subsumed within a large-n model; we’re missing what really happened if we only see the coarse-grained technological/geographical narrative. The paper should be clearer that while a large-n survey is a necessary condition for a good explanation, it’s not sufficient, and should be treated as a starting point for other kinds of analysis.
One final detail: the throwaway comment on naval warfare on line 69 isn’t adequate.
Reviewer #2: This paper examines the long-term evolution of military technologies using the Seshat: Global History Databank. Creating a series of composite variables, which use simpler variables as a proxy for more complex ones such as Information Complexity or Military Technologies, the authors then test several theoretical claims in the literature. Several factors seem to significantly predict the advancement of Military Technology (MilTech), e.g., global population size, cultural similarity, and the spread of iron and cavalry. They also find variables that do not appear to dramatically influence of the level of military technology. Specifically, with the notable exception of phylogeny, characteristics such as the scale and sophistication of a polity appear to be non-significant predictors. This leads them to conclude that military technology evolves, for the most part, as an exogenous variable.
Overall, I applaud the authors for doing a very thorough and ambitious investigation in the evolution of military technology. I believe the paper will provide a catalyst for many follow up studies and serve a vital role of stimulating scientific debate. I do, however, have two general points that I’d like to make.
For my first point, I want to highlight a potential limitation of this approach, in that aggregation might mask a simpler explanation for the results of your model. That is, your predictors might be predicting only a subset of your 46 binary variables used to create your MilTech measure. One way to deal with this is to create alternative MilTech measures by randomly sampling from your set of 46 variables. What you do here is create multiple MilTech measures composed from different, randomly sampled subsets of your 46 variables. How much does this change your overall results in this study? It might be the case that what your model is predicting is a specific subset of technologies that disproportionately influence the overall result. If the result is not robust to these random subsets, then I believe it diminishes your claim that the variables are predicting military technology per se. Instead, it might be the case that your models are predicting a specific subset of the technologies, as opposed to an aggregate measure of overall military technology.
My second point relates to the variable for the existing stock of technologies. Here, you use the existing stock of technology as a proxy for the influence of current technological level on military technological evolution. This is done in two ways: as a temporal autoregressive process and focusing on horse riding and iron smelting. For the temporal autoregressive measure, you could also look at a related approach such as transfer entropy. The advantage of this is that it does not rely on using a single timeseries to predict its future state. Instead, you can use separate timeseries (X and Y), as predictors of one another. This tells you how much uncertainty is reduced in the future values of Y by knowing the past values of X given the past values of Y. It is a way of measuring the influence of one timeseries process (X) on another timeseries process (Y). I feel that this would help you disentangle the directionality of the relationship between some of your variables (as the measure is non-symmetric and X|Y does not equal Y|X). So, for instance, you could have the existing stock of technology as one timeseries and the miltech variable as another timeseries. You would predict that the information flow goes from the existing stock to miltech, but not necessarily the other way around.
Finally, a minor point is that for the results in Table 1, you should probably use scientific notation for extremely small p-values (especially for the MilTech and MilTech.sq p-values where you just have 0). In fact, after writing this comment, I noticed you already did this in your supplementary materials (so it should be easy to address).
6. PLOS authors have the option to publish the peer review history of their article ( what does this mean? ). If published, this will include your full peer review and any attached files.
If you choose “no”, your identity will remain anonymous but your review may still be made public.
Do you want your identity to be public for this peer review? For information about this choice, including consent withdrawal, please see our Privacy Policy .
Reviewer #1: No
Reviewer #2: No
[NOTE: If reviewer comments were submitted as an attachment file, they will be attached to this email and accessible via the submission site. Please log into your account, locate the manuscript record, and check for the action link "View Attachments". If this link does not appear, there are no attachment files.]
While revising your submission, please upload your figure files to the Preflight Analysis and Conversion Engine (PACE) digital diagnostic tool, https://pacev2.apexcovantage.com/ . PACE helps ensure that figures meet PLOS requirements. To use PACE, you must first register as a user. Registration is free. Then, login and navigate to the UPLOAD tab, where you will find detailed instructions on how to use the tool. If you encounter any issues or have any questions when using PACE, please email PLOS at gro.solp@serugif . Please note that Supporting Information files do not need this step.
Submitted filename: PONE-D-21-17737 comments.docx
Author response to Decision Letter 0
Our response to Reviewer and Editor comments are included in the uploaded file 'Response to Reviewers.docx'. We copy our responses below as well
Response to Editor
We thank both peer reviewers for their thoughtful and helpful comments as well as the Editor’s helpful summary and additional comments, and for allowing us the chance to revise our manuscript. Both reviewers had very favorable things to say about our analytical approach and description of results, each concluding that the paper will make a valuable contribution to the field.
The main concerns seemed to revolve around ensuring that we properly motivate our analytical approach and test the robustness of results to alternate methods, as well as properly qualifying our interpretations in light of the limitations of our methods. This later point is in line with the Editor’s main concern about our overly-ambitious causal inferences. We feel these are valid and quite useful critiques. We have made numerous edits and performed additional supplementary analyses to clarify our approach and interpretations and provide fuller support for our claims, while also highlighting the need for future work and alternative methods to further explore the evolution of military technology. Overall, our manuscript is much improved as a result of these comments, and we are grateful to the Reviewers for pointing out shortcomings in how we described and justified some of our central arguments.
Lastly, in the response to the original submission the PLOS editors requested that we add a Competing Interests Statement and amend the Funding Statement based on the idea that the Complexity Science Hub, Vienna (CSH), with which some of our coauthors are affiliated, is a commercial company. This is incorrect, as the CSH is a non-profit research institute based in Vienna. It is registered as a Verein (‘public association’) with the Austrian Vereinsbehoerden (you can find it listed as well with the Research Organization Registry, id: ror.org/023dz9m50 ). We have attached a file from the Austrian government regarding the CSH’s status. If you require further information about the CSH and its non-profit status, please contact Philipp Marxgut ( ta.ca.hsc@tugxram ), CSH Secretary General. I have reaffirmed the lack of competing interests with all coauthors.
Responses to Editor Comments
See below for responses to R1’s comments
Reviewer 2 makes two specific and useful recommendations regarding your statistical analysis. The first is to make sure that the way you bundle military-technology related variables is justified. In other words, you should rule out the possibility that the effects you document are driven by one or a few outlier variables. Please implement the analysis they recommend, i.e., analysing random subsets of the 46 variables. Re-running your models with a subset of military technology variables is something you already do when you substitute "Core MilTech" for "MilTech". Reviewer 1 would like you to generalise this method to multiple, randomly selected subsamples.
This is a very good suggestion. We implemented this by using a kind of bootstrap, generating multiple response variables by randomly resampling Warfare Components that make up our primary MilTech measure. We repeated the procedure 100 times to obtain and analyze the 100 best models by AIC. This analysis revealed that the most strongly supported terms found in the primary analysis remain supported, appearing in the best models in all or all-but-one instance. The only significant difference is the Agri term, which appeared in only 64 of the 100 cases using this bootstrap method.
This analysis suggests that randomly choosing WCs has a small effect on our results, with the exception of Agri. The implication is that different WCs can substitute for each other with little loss of information, something that was already hinted at with the comparison between MilTech and Core MilTech.
We have included this supplementary analysis in the SI (under the subheading ‘Testing bias in aggregation of MilTech variables’) with a description of results. We also added a discussion of this robustness check in the main text (in the Dynamic Regression Results section) and a paragraph in the Discussion detailing the more limited support that Agri receives as a key driver of MilTech compared to the other factors.
Reviewer 2 also suggests an alternative analysis for the impact of existing stock of technologies on the progress of military technologies.
See our response below.
Concerning the reporting of results, my main concern is related to the repeated causality claims made in the manuscript. Given the data and methods, the results establish (at best) predictive causality in the sense of Granger. This type of causality cannot be equated with causality simpliciter, among other things because it does not rule our latent confounding effects. Please qualify all claims related to causality or causal inference.
This is a very good point and we agree that our original language did not adequately convey the appropriate qualifications to our interpretation of results. We have edited text throughout the MS to make this more clear.
Specifically, we added an explanation in the Statistical Analysis section explaining how we use the dynamic analyses to support Granger-type causal inference, but stressing also that these inferences are partial and provisional and need further study to support. We have also added text at the end of the Results section clarifying that our interpretations are based on the predictive relationships between our (theoretically-motivated) predictor and response variables. We stress as well that our interpretations are provisional and subject to additional analyses and alternate approaches. Finally, we have modified text throughout the Discussion section to qualify the conclusiveness of our claims and highlight the suggestive nature of these results, requiring future work to explore further.
This is not a mistake. We routinely perform checks for nonlinearity in how predictors affect the response variable. This check showed that the relationship between MilTech(t+1) and MilTech(t) (that is, lagged value of the response variable) is curvilinear. We capture this nonlinearity with a quadratic term, MilTech (t) squared. There is a strong statistical support for this form, because both the linear and quadratic MilTech have high t-values, and P << 0.0001. For this reason, we retain both terms in the best-fitting model. The interpretation of this statistical result is that the evolution of MilTech is characterized by stabilizing selection, with the equilibrium trajectory set by the predictors. If a random perturbation moves MilTech level away from the equilibrium, the negative quadratic term ensures that MilTech returns to it.
We have added text in the Statistical Analysis section clarifying the inclusion of this square term. Detailing the statistical model, we now note: "We also include quadratic versions of these terms at a time lag (not shown) in order to explore non-linear responses to response and predictor factors. "
We are grateful for bringing to our attention this deficiency in our descriptions. We have modified text throughout to provide additional details about our methods and terms used as part of the Seshat project. Firstly, we explain our data coding procedure and define key terms early on in the main text (in A General Approach to Quantifying the Evolution of Pre-Industrial Societies subsection), and have added more detail to the descriptions of the factors used in analyses in Materials and Methods (‘Hypotheses to be Tested: Defining Predictor Variables’ subsection), particularly the Scale and SocSoph factors. We also clarified our NGA-based sampling procedure and edited text in the SI to clarify what the different factors were (e.g. the relationship between Scale and SocSoph to each other and as different dimensions of social complexity, as well as further detail on how and why we constructed the alternate response measure CoreMil).
Responses to Reviewer 1 Comments
This is a valuable paper, making a real contribution to a significant debate. My one reservation is that I think this kind of large-n survey usually has to be combined with more detailed work to explain something as complicated as military technologies. Military historians typically find that technology is only one part of effectiveness, and not always the most important part. The most interesting result in this paper is the lack of fit between SocSoph and technological gains (lines 455-56, 532-36), but I wonder how much of this is because technologies are little use without appropriate doctrine (the central point in Stephen Biddle’s book Military Power [2004]). Fig. 5 illustrates this—looking at the scores for Latium around 2kya, they’re not very different from other societies, because Roman military technology wasn’t actually that different from other Mediterranean, Middle Eastern, and European societies (as measured in the categories at lines 287-98 in the supporting material); however, the Roman army’s ability to apply these technologies transformed what they meant in practical terms, and that was probably determined primarily by Roman SocSoph. I also immediately think of the classical Greeks, whose military technologies were very ordinary but their application of them was extraordinary. One difficulty for large-n surveys is that while technological categories are relatively easy to identify in the archaeological record, military doctrines, logistics, tactical skill, organizational structures, and ability to learn aren’t; and even when ancient and medieval writers describe such doctrines, there’s no obvious way to score them. This paper should absolutely be published, because there’ve been few (if any) large-n surveys of this level of sophistication, but the main point it illustrates is perhaps that large-n surveys are only the beginning of the study of warfare, and we have to follow up on their results with analytic narratives and case studies.
This is an excellent point and we agree that the material components of technological development are only one part of the story. The tactical, organizational, and managerial aspects that go into how these technologies are wielded certainly play key roles in their effect. Here we are primarily interested in the evolution of the technologies themselves rather than the other aspects, both because, as R1 notes, coding these more ephemeral aspects is very difficult (though certainly possible using our approach to similar doctrinal ideological categories; e.g. Mullins et al. 2018, Whitehouse et al. forthcoming) and beyond the scope of what we could include here, but also because part of the motivation for the present study is to model an approach that could be extended to such follow-up questions.
We have added text in various places in the main text to make this explicit. In particular, we added to the Discussion section text noting the limitations of our approach and the relatively coarse detail of our data, remarking how more fine-grained study and qualitative investigation are important areas for future research.
It is worth noting too that we entirely agree that the lack of significant effect seen between SocSoph and our response measure is an important (and somewhat surprising) result. It may very well have been expected that the SocSoph measure would mediate the ability of societies to effectively wield technologies as R1 notes; namely, that societies with more sophisticated information storage and exchange systems would be better equipped to develop or adapt the tactical and organizational mechanisms that accompany material technologies. Yet, as noted we do not see a significant relationship between SocSoph and MilTech or any other polity-specific factors. As we discuss in the main text, we interpret this as suggesting that MilTech is driven largely by extra-polity processes. The accompanying tactics and other ephemeral aspects that accompany technological change may develop after the technologies themselves have been widely adopted, or evolve through separate processes; again, future work exploring tactical and organizational aspects of technological evolution would be needed to provide insight into this open question, which is beyond the scope of the present study but we feel is ripe for investigation using the Seshat approach evidenced here; we have added text at the end of the Discussion section remarking on this.
Finally, the tactical knowledge aspect of technological change may be reflected to some degree in our measure of cultural similarity (Phylogeny). Indeed, it may be that the tactical knowledge accompanying major technological developments (or standing on their own merits) is driven by external competition or other forces, and is then spread more effectively to culturally similar societies than dissimilar ones, since closeness in both language and socio-cultural systems could facilitate the adoption and adaption of these tactics. This may help to explain the result showing that Phylogeny is a significant predictor of subsequent MilTech, in addition to the benefits of exchanging information about the material and mechanical components of new technologies. We have added discussions to this effect in the Discussion section where we treat this finding. We feel that our overall findings are strengthened by this additional dimension and thank R1 for raising this very important and interesting aspect of our results that we had not fully considered before.
Mullins, Daniel Austin, Daniel Hoyer, Christina Collins, Thomas Currie, Kevin Feeney, Pieter François, Patrick E. Savage, Harvey Whitehouse, and Peter Turchin. “A Systematic Assessment of ‘Axial Age’ Proposals Using Global Comparative Historical Evidence.” American Sociological Review 83, no. 3 (May 8, 2018): 596–626. https://doi.org/10.1177/0003122418772567 .
Whitehouse, Harvey, Pieter François, Daniel Hoyer, Kevin Chekov Feeney, Enrico Cioni, Rosalind Purcell, Robert M. Ross, et al. “Big Gods Did Not Drive the Rise of Big Societies throughout World History.” OSF Preprints, April 1, 2021. https://doi.org/10.31219/osf.io/mbnvg .
I also thought that the paper should distinguish more between technological innovation and diffusion. I’m currently reading two case studies of the Comanches, who probably had the best light cavalry in the world in the 18th century. They didn’t invent light cavalry, because there were no horses in North America to invent it with before the Spaniards brought them, but they did adopt and then adapt horse-herding and perfect styles of mounted fighting much better than the Apaches or even the Spaniards and Mexicans. They didn’t adopt or adapt firearms because their light-cavalry tactics made muzzle-loading muskets irrelevant. The Comanches were eventually defeated because Texans adopted and adapted Comanche tactics in the 1830s and combined them with revolvers and, later, breech-loading rifles that had been invented on the US East Coast. Neither the Comanche nor the Texans could have invented revolvers and rifles themselves, but the US Army failed to figure out how to use the new technologies until Texans put them together with tactics in new ways; but the final Comanche defeat in the 1870s depended on the US Army learning from the Texans and then exploiting the scale of US federal infrastructure.
We entirely agree that the distinction between invention and later diffusion is a critical one to make to understand the histories of technological spread and use. However, as noted above we intend for this piece to be only the opening salvo in a line of work investigating the various aspects of technological evolution, military and more generally. We feel it is important to lay the foundation and model an approach to these such future work by focusing on technological evolution more broadly – which, as we define here, includes innovation as well as adoption and adaptation. This is why we conflate these different processes in this piece.
We have added text to make this more clear to readers. Specifically, in the Introduction we clarify how we define ‘technological evolution’ for the purposes of this study. We also clarify in the Results and Discussion sections that change in MilTech can occur through multiple mechanisms, e.g. when describing regional patterns in the pace of technological change (distinguishing early-adopters from late-joiners to the growing global information network) we explain that we do not distinguish innovation from later adoption. We also highlight that disentangling these process will be an important focus of future studies.
The example R1 cites is very interesting. From the perspective of the evolution of military technology broadly, this case actually illustrates well the processes identified by our analyses; various technologies (and tactics) not invented in North America are introduced as the area becomes entangled in the information exchange networks of western Europe (through conquest, settlement, and expansion) and then these become widespread in use within a context of fierce interstate competition. While our coarse-grained macro-level model can not predict which group would win out in this competition, the key point from this level of analysis is that information exchange and inter-group competition seem to have facilitated the spread of key military technologies (herding, mounted archery, hand-held firearms) into an area that did not have them previously
We, indeed, agree that more fine-grained and detailed study is a necessary component of reconstructing social dynamics, as noted above. We would argue though that neither the fine-grained nor the broader, macro-level approach reveals what ‘really happened’, rather that both are needed together to untangle these complicated patterns; these are complimentary, rather than competing approaches (we discuss this in for example in Turchin 2008 as well as Francois et al 2016). For the reasons detailed above, here our focus is on the macro-level patterns. We note this in the Materials and Methods section and have provided additional text in the Discussion section emphasizing that future work exploring more fine-grained data and qualitative case studies is needed to delve deeper into the broader patterns and processes suggested by the analyses here.
Turchin, Peter. “Arise ‘Cliodynamics.’” Nature 454, no. 7200 (2008): 34–35. https://doi.org/10.1038/454034a .
François, Pieter, J. G. Manning, Harvey Whitehouse, Rob Brennan, T. E. Currie, Kevin Feeney, and Peter Turchin. “A Macroscope for Global History. Seshat Global History Databank: A Methodological Overview.” Digital Humanities Quarterly 10, no. 4 (2016). http://www.digitalhumanities.org/dhq/vol/10/4/000272/000272.html .
One final detail: the throwaway comment on naval warfare on line 69 isn’t adequate.
This is a good point and we agree that this was a poorly articulated point. We have removed this ‘throwaway’ comment from the main text, which we believe reads more clearly now. We discuss our inclusion of primarily land-based, rather than specifically maritime, technologies in the SI (the Defining the Response Variable: coding military technologies in past societies section) which we feel is adequate to explain our approach to readers. We would however be willing to add a discussion on this in the main text, if that would be useful to readers.
Responses to Reviewer 2 Comments
This paper examines the long-term evolution of military technologies using the Seshat: Global History Databank. Creating a series of composite variables, which use simpler variables as a proxy for more complex ones such as Information Complexity or Military Technologies, the authors then test several theoretical claims in the literature. Several factors seem to significantly predict the advancement of Military Technology (MilTech), e.g., global population size, cultural similarity, and the spread of iron and cavalry. They also find variables that do not appear to dramatically influence of the level of military technology. Specifically, with the notable exception of phylogeny, characteristics such as the scale and sophistication of a polity appear to be non-significant predictors. This leads them to conclude that military technology evolves, for the most part, as an exogenous variable.
We thank R2 for this positive and encouraging assessment.
We addressed this point by the analysis and results described above (in our responses to the Editor). Note that randomly sampling from the 46 variables is not a feasible approach because of the way we construct our Warfare Characteristics (WCs). For example, if a society has iron technology, than we assume that it also has the knowhow to produce “simpler” technologies (copper, bronze). Similarly, if a society has crossbows, then it doesn’t matter whether they know atlatls. Thus, sampling randomly form the raw binary variables will not yield the desired test. Instead we implemented this suggestion by randomly sampling from the six WCs – please see the results above and the discussion of these results now included in the SI.
This is a good point and we have improved our explanation of the logic underlying our dynamic regression methodology. The approach we use is known among the econometricians as ‘Granger Causality’, as was pointed out by the Editor above. Transfer Entropy (TE) is a closely related approach; in fact, it was shown to be the equivalent to Granger Causality for Gaussian variables (Barnett et al. 2009). There is some discussion in the literature about which approach is better to take. TE is a nonparametric method, and so it doesn’t depend on the assumption of linearity. However, we test for nonlinearities and include nonlinear effects, where detected, using polynomials. On the downside, nonparametric methods require more data and have lower statistical power when variables are well-behaved. In our case, both inspection of scatter plots and formal regression results suggest that in most cases linear forms capture well the relationships between the response and predictors. And where this is not the case (as with the functional relationship between MilTech and its lag), the quadratic relationship appears to capture the curvature well (which is not huge, as visual inspection suggests).
To summarize, we believe that our approach is doing precisely what the reviewer would like us to do – exploring the full range of possible cross-relationships between our data – and we feel that using TE would not add any additional information, but would reduce the statistical power of results. For these reasons, we have not included a TE analysis or discussion of this decision in the main text or as a supplementary analysis
Barnett, L., A. B. Barrett and A. K. Seth (2009). "Granger Causality and Transfer Entropy Are Equivalent for Gaussian Variables." Physical Review Letters 103(23): 238701.
We thank R2 for pointing out this oversight. We have corrected this in Table 1 of the main text.
Submitted filename: Response to Reviewers.docx
Decision Letter 1
20 Sep 2021
PONE-D-21-17737R1
Dear Dr. Hoyer,
We’re pleased to inform you that your manuscript has been judged scientifically suitable for publication and will be formally accepted for publication once it meets all outstanding technical requirements.
Within one week, you’ll receive an e-mail detailing the required amendments. When these have been addressed, you’ll receive a formal acceptance letter and your manuscript will be scheduled for publication.
An invoice for payment will follow shortly after the formal acceptance. To ensure an efficient process, please log into Editorial Manager at http://www.editorialmanager.com/pone/ , click the 'Update My Information' link at the top of the page, and double check that your user information is up-to-date. If you have any billing related questions, please contact our Author Billing department directly at gro.solp@gnillibrohtua .
If your institution or institutions have a press office, please notify them about your upcoming paper to help maximize its impact. If they’ll be preparing press materials, please inform our press team as soon as possible -- no later than 48 hours after receiving the formal acceptance. Your manuscript will remain under strict press embargo until 2 pm Eastern Time on the date of publication. For more information, please contact gro.solp@sserpeno .
Additional Editor Comments (optional):
Acceptance letter
27 Sep 2021
Dear Dr. Hoyer:
I'm pleased to inform you that your manuscript has been deemed suitable for publication in PLOS ONE. Congratulations! Your manuscript is now with our production department.
If your institution or institutions have a press office, please let them know about your upcoming paper now to help maximize its impact. If they'll be preparing press materials, please inform our press team within the next 48 hours. Your manuscript will remain under strict press embargo until 2 pm Eastern Time on the date of publication. For more information please contact gro.solp@sserpeno .
If we can help with anything else, please email us at gro.solp@enosolp .
Thank you for submitting your work to PLOS ONE and supporting open access.
PLOS ONE Editorial Office Staff
on behalf of
Dr. Olivier Morin
A systematic review of the research framework and evolution of smart homes based on the internet of things
- Published: 15 April 2021
- Volume 77 , pages 597–623, ( 2021 )
Cite this article
- Yi Sun ORCID: orcid.org/0000-0003-4238-2943 1 &
- Shihui Li 2
1632 Accesses
11 Citations
Explore all metrics
The Internet of Things has brought unprecedented technological innovation to smart homes, but the impact of this change on technology and users is limited and dispersed. Based on a systematic literature review, the present research conducted a quantitative analysis of 2874 papers (published in 2008–2020) obtained from the Web of Science to bridge the research gap. The current research identifies the development status and trends, country distribution, and journal categories in this area. Then, based on the main topics covered by smart homes, we proposed a holistic research framework that integrates the infrastructure layer, the communications technology layer, the data analytics layer, and the user service layer. The framework analyzed wireless sensing networks, communication protocols, and security threats, as well as the activity identification process and user services, highlighting the lack of some degree of integration in this area. This study also discussed the evolution of hot spots in the field of smart homes and summarized potential future research directions. Finally, in the discussion section, this paper summarized the research contribution and compared the main proposed technical solutions. We hope this work will provide a solid basis for research and practical guidance for scholars and developers interested in smart homes based on the Internet of Things.
This is a preview of subscription content, log in via an institution to check access.
Access this article
Price excludes VAT (USA) Tax calculation will be finalised during checkout.
Instant access to the full article PDF.
Rent this article via DeepDyve
Institutional subscriptions
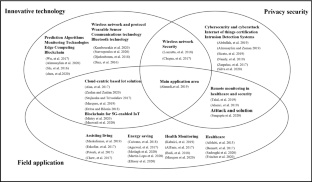
Similar content being viewed by others
The internet of things: a survey.
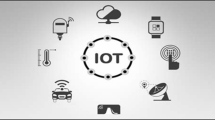
RETRACTED ARTICLE: A Review and State of Art of Internet of Things (IoT)
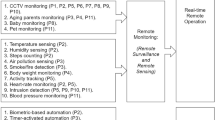
Usage and impact of the internet-of-things-based smart home technology: a quality-of-life perspective
Al-Fuqaha, A., Guizani, M., Mohammadi, M., Aledhari, M., & Ayyash, M. (2015). Internet of things: A survey on enabling technologies, protocols, and applications. IEEE Commun Surv TUTORIALS, 17 , 2347–2376.
Article Google Scholar
Machorro-Cano, I., Alor-Hernández, G., Paredes-Valverde, M. A., Rodríguez-Mazahua, L., Sánchez-Cervantes, J. L., & Olmedo-Aguirre, J. O. (2020). HEMS-IoT: a big data and machine learning-based smart home system for energy saving. Energies . https://doi.org/10.3390/en13051097 .
Alaa, M., Zaidan, A. A., Zaidan, B. B., Talal, M., & Kiah, M. L. M. (2017). A review of smart home applications based on Internet of Things. Journal of Network and Computer Applications, 97 , 48–65. https://doi.org/10.1016/j.jnca.2017.08.017 .
Almusaylim, Z. A., & Zaman, N. (2019). A review on smart home present state and challenges: linked to context-awareness internet of things (IoT). Wirel Netw, 25 , 3193–3204. https://doi.org/10.1007/s11276-018-1712-5 .
Alam, M. R., Reaz, M. B., & Ali, M. A. M. (2012). A review of smart homes-past, present, and Future. IEEE Transactions on Systems, Man, and Cybernetics Part C: Applications and Reviews, 42 , 1190–1203. https://doi.org/10.1109/tsmcc.2012.2189204 .
Gubbi, J., Buyya, R., Marusic, S., & Palaniswami, M. (2013). Internet of Things (IoT): A vision, architectural elements, and future directions. Futur Gener Comput Syst Int J ESCIENCE, 29 , 1645–1660. https://doi.org/10.1016/j.future.2013.01.010 .
Zhang, Q., Yin, M. L., & YJ, . (2007). A regular nine-prism array of patches for wireless LANs. IEICE Transactions on Communications, E-90-B (6), 1467–1473.
Dong, Y. Z., Gao, S., Luo, Q., Dong, S. W., & Wei, G. (2019). Filtering antennas for energy harvesting in wearable systems. Int J Numer Model Netw Devices Fields, 32 , 12. https://doi.org/10.1002/jnm.2661 .
Eltresy, N. A., Dardeer, O. M., Al-Habal, A., Elhariri, E., Abotaleb, A. M., Elsheakh, D. N., Khattab, A., Taie, S. A., Mostafa, H., Elsadek, H. A., & Abdallah, E. A. (2020). Smart home IoT system by using RF energy harvesting. J Sensors, 2020 , 1–14. https://doi.org/10.1155/2020/8828479 .
Roudjane, M., Bellemare-Rousseau, S., Khalil, M., Gorgutsa, S., Miled, A., & Messaddeq, Y. (2018). A portable wireless communication platform based on a multi-material fiber sensor for real-time breath detection. Sensors (Switzerland) . https://doi.org/10.3390/s18040973 .
Wang, L., An, H., Zhu, H., & Liu, W. (2020). MobiKey: mobility-based secret key generation in smart home. IEEE Internet of Things Journal, 7 , 7590–7600. https://doi.org/10.1109/JIOT.2020.2986399 .
Chen, M., Wan, J. F., & Li, F. (2012). Machine-to-machine communications: architectures, standards and applications. KSII Trans Int Inf Syst, 6 , 480–497. https://doi.org/10.3837/tiis.2012.02.002 .
Mistry, I., Tanwar, S., Tyagi, S., & Kumar, N. (2020). Blockchain for 5G-enabled IoT for industrial automation: a systematic review, solutions, and challenges. Mechanical Systems and Signal Processing, 135 , 21. https://doi.org/10.1016/j.ymssp.2019.106382 .
Lazaroiu C, Roscia M (2017) Smart district through IoT and Blockchain. In: 2017 IEEE 6th international conference on renewable energy research and applications (ICRERA), pp 454–461
Kong, S., Kim, Y., Ko, R., & Joo, S. K. (2015). Home appliance load disaggregation using cepstrum-smoothing-based method. IEEE Transactions on Consumer Electronics, 61 , 24–30. https://doi.org/10.1109/tce.2015.7064107 .
Barcelo, M., Correa, A., Llorca, J., Tulino, A. M., Vicario, J. L., & Morell, A. (2016). IoT-cloud service optimization in next generation smart environments. IEEE Journal on Selected Areas in Communications, 34 , 4077–4090. https://doi.org/10.1109/jsac.2016.2621398 .
Youngblood, G. M., & Cook, D. J. (2007). Data mining for hierarchical model creation. IEEE Transactions on Systems, Man, and Cybernetics Part C: Applications and Reviews, 37 , 561–572. https://doi.org/10.1109/tsmcc.2007.897341 .
Chen, L. M., Nugent, C. D., & Wang, H. (2012). A knowledge-driven approach to activity recognition in smart homes. IEEE Transactions on Knowledge and Data Engineering, 24 , 961–974. https://doi.org/10.1109/tkde.2011.51 .
Shi, W. S., Cao, J., Zhang, Q., Li, Y. H. Z., & Xu, L. Y. (2016). Edge computing: vision and challenges. IEEE Int Things J, 3 , 637–646. https://doi.org/10.1109/jiot.2016.2579198 .
Stellios, I., Kotzanikolaou, P., Psarakis, M., Alcaraz, C., & Lopez, J. (2018). A survey of IoT-enabled cyberattacks: assessing attack paths to critical infrastructures and services. IEEE Commun Surv Tutor, 20 , 3453–3495. https://doi.org/10.1109/comst.2018.2855563 .
Ferreira, M. P., Santos, J. C., Ribeiro de Almeida, M. I., & Reis, N. R. (2014). Mergers & acquisitions research: a bibliometric study of top strategy and international business journals, 1980–2010. Journal of Business Research, 67 , 2550–2558. https://doi.org/10.1016/j.jbusres.2014.03.015 .
Lutolf R (1992) Smart home concept and the integration of energy meters into a home based system. In: Seventh international conference on metering apparatus and tariffs for electricity supply 1992. IET, pp 277–278
Balta-Ozkan, N., Davidson, R., Bicket, M., & Whitmarsh, L. (2013). Social barriers to the adoption of smart homes. Energy Policy, 63 , 363–374. https://doi.org/10.1016/j.enpol.2013.08.043 .
De Silva, L. C., Morikawa, C., & Petra, I. M. (2012). State of the art of smart homes. Engineering Applications of Artificial Intelligence, 25 , 1313–1321.
Reinisch, C., Kofler, M., Iglesias, F., & Kastner, W. (2010). Think home energy efficiency in future smart homes. EURASIP J Embedded Syst, 2011 , 104617. https://doi.org/10.1155/2011/104617 .
Chan, M., Esteve, D., Escriba, C., & Campo, E. (2008). A review of smart homes—present state and future challenges. Comput Methods Progr Biomed, 91 , 55–81. https://doi.org/10.1016/j.cmpb.2008.02.001 .
Djedouboum, A. C., Ari, A. A. A., Gueroui, A. M., Mohamadou, A., & Aliouat, Z. (2018). Big data collection in large-scale wireless sensor networks. Sensors, 18 , 34. https://doi.org/10.3390/s18124474 .
Nkomo, M., Hancke, G. P., Abu-Mahfouz, A. M., Sinha, S., & Onumanyi, A. J. (2018). Overlay virtualized wireless sensor networks for application in industrial internet of things: a review. Sensors, 18 , 33. https://doi.org/10.3390/s18103215 .
Lonzetta, A. M., Cope, P., Campbell, J., Mohd, B. J., & Hayajneh, T. (2018). Security Vulnerabilities in bluetooth technology as used in IoT. Journal of Sensor and Actuator Networks, 7 , 26. https://doi.org/10.3390/jsan7030028 .
Stavropoulos, T. G., Papastergiou, A., Mpaltadoros, L., Nikolopoulos, S., & Kompatsiaris, I. (2020). IoT wearable sensors and devices in elderly care: a literature review. Sensors . https://doi.org/10.3390/s20102826 .
Chen, F., Xiao, Z., Cui, L., Lin, Q., Li, J., & Yu, S. (2020). Blockchain for Internet of things applications: a review and open issues. Journal of Network and Computer Applications, 172 , 102839. https://doi.org/10.1016/j.jnca.2020.102839 .
Alamri, M., Jhanjhi, N. Z., & Humayun, M. (2019). Blockchain for Internet of Things (IoT) research issues challenges & future directions: a review. Int J Comput Sci Netw Secur, 19 , 244–258.
Google Scholar
Chopra, G., Jha, R. K., & Jain, S. (2017). A survey on ultra-dense network and emerging technologies: Security challenges and possible solutions. Journal of Network and Computer Applications, 95 , 54–78. https://doi.org/10.1016/j.jnca.2017.07.007 .
Abdullah, T. A. A., Ali, W., Malebary, S., & Abdullah, A. A. (2019). A review of cyber security challenges, attacks and solutions for internet of things based smart home. Int J Comput Sci Netw Secur, 19 , 139–146.
Sicato, J. C. S., Sharma, P. K., Loia, V., & Park, J. H. (2019). VPNFilter malware analysis on cyber threat in smart home network. Applied Sciences, 9 , 20. https://doi.org/10.3390/app9132763 .
Zarpelao, B. B., Miani, R. S., Kawakani, C. T., & de Alvarenga, S. C. (2017). A survey of intrusion detection in Internet of Things. Journal of Network and Computer Applications, 84 , 25–37. https://doi.org/10.1016/j.jnca.2017.02.009 .
Nandy, T., Bin Idris, M. Y. I., Noor, R. M., Kiah, M. L. M., Lun, L. S., et al. (2019). Review on security of internet of things authentication mechanism. IEEE Access, 7 , 151054–151089. https://doi.org/10.1109/access.2019.2947723 .
Silva, F. S. D., Silva, E., Neto, E. P., Lemos, M., Neto, A. J. V., & Esposito, F. (2020). A taxonomy of DDoS attack mitigation approaches featured by SDN technologies in IoT scenarios. Sensors . https://doi.org/10.3390/s20113078 .
Zaidan, A. A., & Zaidan, B. B. (2020). A review on intelligent process for smart home applications based on IoT: coherent taxonomy, motivation, open challenges, and recommendations. Artificial Intelligence Review, 53 , 141–165. https://doi.org/10.1007/s10462-018-9648-9 .
Afolabi, A. O., Toivanen, P., Haataja, K., & Mykkanen, J. (2015). Systematic literature review on empirical results and practical implementations of healthcare recommender systems: lessons learned and a novel proposal. Int J Healthc Inf Syst Inform, 10 , 1–21. https://doi.org/10.4018/ijhisi.2015100101 .
Maskeliunas, R., Damasevicius, R., & Segal, S. (2019). A review of internet of things technologies for ambient assisted living environments. Futur Internet, 11 , 23. https://doi.org/10.3390/fi11120259 .
Eskofier, B. M., Lee, S. I., Baron, M., Simon, A., Martindale, C. F., Gassner, H., & Klucken, J. (2017). An overview of smart shoes in the internet of health things: gait and mobility assessment in health promotion and disease monitoring. Applied Sciences . https://doi.org/10.3390/app7100986 .
Potorti, F., Park, S., Jimenez Ruiz, A. R., Barsocchi, P., Girolami, M., Crivello, A., Lee, S. Y., Lim, J. H., Torres-Sospedra, J., Seco, F., Montoliu, R., Mendoza-Silva, G. M., Rubio, M. D. P., Losada-Gutierrez, C., Espinosa, F., & Macias-Guarasa, J. (2017). Comparing the performance of indoor localization systems through the EvAAL framework. Sensors . https://doi.org/10.3390/s17102327 .
Chew, I., Karunatilaka, D., Tan, C. P., & Kalavally, V. (2017). Smart lighting: the way forward? Reviewing the past to shape the future. Energy Build, 149 , 180–191. https://doi.org/10.1016/j.enbuild.2017.04.083 .
Talal, M., Zaidan, A. A., Zaidan, B. B., Albahri, A. S., Alamoodi, A. H., Albahri, O. S., Alsalem, M. A., Lim, C. K., Tan, K. L., Shir, W. L., & Mohammed, K. I. (2019). Smart home-based IoT for real-time and secure remote health monitoring of triage and priority system using body sensors: multi-driven systematic review. Journal of Medical Systems, 43 , 34. https://doi.org/10.1007/s10916-019-1158-z .
Bennett, J., Rokas, O., & Chen, L. M. (2017). Healthcare in the smart home: a study of past present and future. Sustainability, 9 , 23. https://doi.org/10.3390/su9050840 .
Kabalci, Y., Kabalci, E., Padmanaban, S., Holm-Nielsen, J. B., & Blaabjerg, F. (2019). Internet of things applications as energy internet in smart grids and smart environments. Electronics, 8 , 16. https://doi.org/10.3390/electronics8090972 .
Bedi, G., Venayagamoorthy, G. K., Singh, R., Brooks, R. R., & Wang, K. C. (2018). Review of Internet of Things (IoT) in electric power and energy systems. IEEE Int Things J, 5 , 847–870. https://doi.org/10.1109/jiot.2018.2802704 .
Motlagh, N. H., Mohammadrezaei, M., Hunt, J., & Zakeri, B. (2020). Internet of Things (IoT) and the energy sector. Energies . https://doi.org/10.3390/en13020494 .
Zaidan, A. A., Zaidan, B. B., Qahtan, M. Y., Albahri, O. S., Albahri, A. S., Alaa, M., Jumaah, F. M., Talal, M., Tan, K. L., Shir, W. L., & Lim, C. K. (2018). A survey on communication components for IoT-based technologies in smart homes. Telecommunication Systems, 69 , 1–25. https://doi.org/10.1007/s11235-018-0430-8 .
Kitchenham B (2007) Guidelines for performing systematic literature reviews in software engineering. Keele Univ Durham Univ Jt Rep
Souri, A., Navimipour, N. J., & Rahmani, A. M. (2018). Formal verification approaches and standards in the cloud computing: a comprehensive and systematic review. Comput Stand Interfaces, 58 , 1–22. https://doi.org/10.1016/j.csi.2017.11.007 .
Kitchenham, B., Brereton, O. P., Budgen, D., Turner, M., Bailey, J., & Linkman, S. (2009). Systematic literature reviews in software engineering—a systematic literature review. Inform Softw Technol, 51 , 7–15. https://doi.org/10.1016/j.infsof.2008.09.009 .
Zhao, X. B. (2017). A scientometric review of global BIM research: analysis and visualization. Automation in Construction, 80 , 37–47. https://doi.org/10.1016/j.autcon.2017.04.002 .
Muhuri, P., Shukla, A., & Abraham, A. (2019). Industry 4.0: a bibliometric analysis and detailed overview. Engineering Applications of Artificial Intelligence, 78 , 218–235. https://doi.org/10.1016/j.engappai.2018.11.007 .
Chen, C. M. (2006). CiteSpace II: detecting and visualizing emerging trends and transient patterns in scientific literature. Journal of the American Society for Information Science and Technology, 57 , 359–377. https://doi.org/10.1002/asi.20317 .
Qin, Y., Zhang, Q., & Liu, Y. (2020). Analysis of knowledge bases and research focuses of cerebral ischemia-reperfusion from the perspective of mapping knowledge domain. Brain Research Bulletin, 156 , 15–24.
Pan, W., Jian, L., & Liu, T. (2019). Grey system theory trends from 1991 to 2018: a bibliometric analysis and visualization. Scientometrics, 121 , 1407–1434. https://doi.org/10.1007/s11192-019-03256-z .
Small, H. (1973). Co-citation in the scientific literature: a new measure of the relationship between two documents. Journal of the Association for Information Science and Technology, 24 , 265–269.
Gubbi, J., Buyya, R., Marusic, S., & Palaniswami, M. (2013). Internet of Things (IoT): a vision, architectural elements, and future directions. Future Generation Computer Systems, 29 , 1645–1660. https://doi.org/10.1016/j.future.2013.01.010 .
Zanella, A., Bui, N., Castellani, A., Vangelista, L., & Zorzi, M. (2014). Internet of things for smart cities. IEEE Int Things J, 1 , 22–32. https://doi.org/10.1109/JIOT.2014.2306328 .
Islam, S. M. R., Kwak, D., Kabir, M. H., Hossain, M., & Kwak, K. (2015). The internet of things for health care: a comprehensive survey. IEEE Access, 3 , 678–708. https://doi.org/10.1109/ACCESS.2015.2437951 .
Riste Skastojkoska, B. L., & Trivodaliev, K. V. (2017). A review of Internet of Things for smart home: challenges and solutions. Journal of Cleaner Production, 140 , 1454–1464. https://doi.org/10.1016/j.jclepro.2016.10.006 .
Lyons, B. E., Austin, D., Seelye, A., Petersen, J., Yeargers, J., Riley, T., Sharma, N., Mattek, N., Wild, K., Dodge, H., & Kaye, J. A. (2015). Pervasive computing technologies to continuously assess Alzheimer’s disease progression and intervention efficacy. Front Aging Neurosci, 7 , 14. https://doi.org/10.3389/fnagi.2015.00102 .
Kambourakis, G., Kolias, C., Geneiatakis, D., Karopoulos, G., Makrakis, G. M., & Kounelis, I. (2020). A state-of-the-art review on the security of mainstream IoT wireless PAN protocol stacks. Symmetry (Basel), 12 , 579. https://doi.org/10.3390/SYM12040579 .
Nag, A., & Mukhopadhyay, S. C. (2015). Occupancy detection at smart home using real-time dynamic thresholding of flexiforce sensor. IEEE Sensors Journal, 15 , 4457–4463. https://doi.org/10.1109/jsen.2015.2421348 .
Suryadevara, N. K., & Mukhopadhyay, S. C. (2012). Wireless sensor network based home monitoring system for wellness determination of elderly. IEEE Sensors Journal, 12 , 1965–1972. https://doi.org/10.1109/jsen.2011.2182341 .
Nikoukar, A., Raza, S., Poole, A., Gunes, M., & Dezfouli, B. (2018). Low-power wireless for the internet of things: standards and applications. IEEE Access, 6 , 67893–67926. https://doi.org/10.1109/access.2018.2879189 .
Yang, J., Poellabauer, C., Mitra, P., & Neubecker, C. (2020). Beyond beaconing: emerging applications and challenges of BLE. Ad Hoc Networks, 97 , 12. https://doi.org/10.1016/j.adhoc.2019.102015 .
Solutions, N., Pathak, G., & Gutierrez, J. (2020). Security in low powered wide area networks: opportunities for software defined network-supported solutions. Electronics, 9 , 1195.
Awin, F. A., Alginahi, Y. M., Abdel-Raheem, E., & Tepe, K. (2019). Technical issues on cognitive radio-based internet of things systems: a survey. IEEE Access, 7 , 97887–97908. https://doi.org/10.1109/access.2019.2929915 .
Mbarek, B., Ge, M., & Pitner, T. (2020). Trust-based authentication for smart home systems. Wirel Personal Commun . https://doi.org/10.1007/s11277-020-07965-0 .
Kao, Y. S., Nawata, K., & Huang, C. Y. (2019). An exploration and confirmation of the factors influencing adoption of IoT-based wearable fitness trackers. International Journal of Environmental Research and Public Health, 16 , 31. https://doi.org/10.3390/ijerph16183227 .
Montori, F., Bedogni, L., Di Felice, M., & Bononi, L. (2018). Machine-to-machine wireless communication technologies for the Internet of Things: Taxonomy, comparison and open issues. Pervasive Mobile Comput, 50 , 56–81. https://doi.org/10.1016/j.pmcj.2018.08.002 .
Skocir, P., Kusek, M., & Jezic, G. (2017). Energy-efficient task allocation for service provisioning in machine-to-machine systems. Concurr Comput Exp, 29 , 21. https://doi.org/10.1002/cpe.4269 .
Jiang, Y. P., Chen, C. L. P., & Duan, J. W. (2016). A new practice-driven approach to develop software in a cyber-physical system environment. Enterp Inf Syst, 10 , 211–227. https://doi.org/10.1080/17517575.2014.939107 .
Capozucca, A., & Guelfi, N. (2010). Modelling dependable collaborative time-constrained business processes. Enterp Inf Syst, 4 , 153–214. https://doi.org/10.1080/17517571003753266 .
Hu, S. S., Tang, C. C., Liu, F., & Wang, X. J. (2016). A distributed and efficient system architecture for smart home. Int J Sens Networks, 20 , 119–130. https://doi.org/10.1504/ijsnet.2016.074701 .
Gowrishankar S, Madhu N, Basavaraju TG (2015) Role of BLE in proximity based automation of IoT: a practical approach. In: 2015 IEEE recent advances in intelligent computational systems (RAICS). IEEE, pp 400–405
Perumal, T., Ramli, A. R., & Leong, C. Y. (2011). Interoperability framework for smart home systems. IEEE Transactions on Consumer Electronics, 57 , 1607–1611.
Krishna MB, Verma A (2016) A framework of smart homes connected devices using internet of things. In: 2016 2nd international conference on contemporary computing and informatics (IC3I). IEEE, pp 810–815
Kim, J. E., Barth, T., Boulos, G., Yackovich, J., Beckel, C., & Mosse, D. (2017). Seamless integration of heterogeneous devices and access control in smart homes and its evaluation. Intell Build Int, 9 , 23–39. https://doi.org/10.1080/17508975.2015.1018116 .
Jara, A. J., Zamora-Izquierdo, M. A., & Skarmeta, A. F. (2013). Interconnection framework for mHealth and remote monitoring based on the internet of things. IEEE Journal on Selected Areas in Communications, 31 , 47–65. https://doi.org/10.1109/jsac.2013.Sup.0513005 .
Pham-Huu D-N, Nguyen V-H, Trinh V-A, Bui V-H, Pham H-A (2015) Towards an open framework for home automation development. In: 2015 international conference on advanced computing and applications (ACOMP). IEEE, pp 75–81
Iqbal, A., Ullah, F., Anwar, H., Kwak, K. S., Imran, M., Jamal, W., & Rahman, A. U. (2018). Interoperable Internet-of-Things platform for smart home system using Web-of-Objects and cloud. Sustain Chem Pharm, 38 , 636–646. https://doi.org/10.1016/j.scs.2018.01.044 .
Gambi, E., Montanini, L., Pigini, D., Ciattaglia, G., & Spinsante, S. (2018). A home automation architecture based on LoRa technology and Message Queue Telemetry Transfer protocol. Int J Distrib Sens Networks, 14 , 12. https://doi.org/10.1177/1550147718806837 .
Ande, R., Adebisi, B., Hammoudeh, M., & Saleem, J. (2020). Internet of Things: Evolution and technologies from a security perspective. Sustainable Chemistry and Pharmacy, 54 , 101728. https://doi.org/10.1016/j.scs.2019.101728 .
Perera, C., Ranjan, R., Wang, L., Khan, S. U., & Zomaya, A. Y. (2015). Big data privacy in the internet of things era. IT Prof, 17 , 32–39.
Singh, S., Sharma, P. K., & Park, J. H. (2017). SH-SecNet: an enhanced secure network architecture for the diagnosis of security threats in a smart home. Sustainability, 9 , 19. https://doi.org/10.3390/su9040513 .
Subahi, A., & Theodorakopoulos, G. (2019). Detecting IoT user behavior and sensitive information in encrypted IoT-app traffic. Sensors, 19 , 28. https://doi.org/10.3390/s19214777 .
Serror M, Henze M, Hack S, Schuba M, Wehrle K (2018) Towards in-network security for smart homes. In: Proceedings of the 13th international conference on availability, reliability and security. pp 1–8
OConnor TJ, Mohamed R, Miettinen M, Enck W, Reaves B, Sadeghi A-R (2019) HomeSnitch: behavior transparency and control for smart home IoT devices. In: Proceedings of the 12th conference on security and privacy in wireless and mobile networks, pp 128–138
Anthi, E., Williams, L., Slowinska, M., Theodorakopoulos, G., & Burnap, P. (2019). A supervised intrusion detection system for smart home IoT devices. IEEE Internet of Things Journal, 6 , 9042–9053. https://doi.org/10.1109/jiot.2019.2926365 .
Nobakht M, Sivaraman V, Boreli R (2016) A host-based intrusion detection and mitigation framework for smart home IoT using OpenFlow. In: 2016 11th international conference on availability, reliability and security (ARES). IEEE, pp 147–156
Sairam, R., Bhunia, S. S., Thangavelu, V., & Gurusamy, M. (2019). NETRA: enhancing IoT security using NFV-based edge traffic analysis. IEEE Sensors Journal, 19 , 4660–4671. https://doi.org/10.1109/jsen.2019.2900097 .
Banerjee, S., Odelu, V., Das, A. K., Srinivas, J., Kumar, N., Chattopadhyay, S., & Choo, K. K. R. (2019). A provably secure and lightweight anonymous user authenticated session key exchange scheme for internet of things deployment. IEEE Int Things J, 6 , 8739–8752. https://doi.org/10.1109/jiot.2019.2923373 .
Banerjee, S., Odelu, V., Das, A. K., Chattopadhyay, S., Rodrigues, J., & Park, Y. (2019). Physically secure lightweight anonymous user authentication protocol for internet of things using physically unclonable functions. IEEE Access, 7 , 85627–85644. https://doi.org/10.1109/access.2019.2926578 .
Kumar, P., Braeken, A., Gurtov, A., Iinatti, J., & Ha, P. H. (2017). Anonymous secure framework in connected smart home environments. IEEE Transactions on Information Forensics and Security, 12 , 968–979. https://doi.org/10.1109/tifs.2016.2647225 .
Yan, H. Y., Wang, Y., Jia, C. F., Li, J., Xiang, Y., & Pedrycz, W. (2019). IoT-FBAC: Function-based access control scheme using identity-based encryption in IoT. Futur Gener Comput Syst Int J Escience, 95 , 344–353. https://doi.org/10.1016/j.future.2018.12.061 .
Fernández-Caramés, T. M., & Fraga-Lamas, P. (2018). A review on the use of blockchain for the internet of things. IEEE Access, 6 , 32979–33001. https://doi.org/10.1109/ACCESS.2018.2842685 .
Han D, Kim H, Jang J (2017) Blockchain based smart door lock system. In: 2017 international conference on information and communication technology convergence (ICTC), pp 1165–1167
Dorri A, Kanhere SS, Jurdak R, Gauravaram P (2017) Blockchain for IoT security and privacy: the case study of a smart home. In: 2017 IEEE international conference on pervasive computing and communications workshops (PerCom Workshops), pp 618–623
Spathoulas, G., Giachoudis, N., Damiris, G. P., & Theodoridis, G. (2019). Collaborative blockchain-based detection of distributed denial of service attacks based on internet of things botnets. Futur Internet, 11 , 24. https://doi.org/10.3390/fi11110226 .
Dorri, A., Kanhere, S. S., Jurdak, R., & Gauravaram, P. (2019). LSB: a lightweight scalable blockchain for IoT security and anonymity. J Parallel Distrib Comput, 134 , 180–197. https://doi.org/10.1016/j.jpdc.2019.08.005 .
Sahni, Y., Cao, J. N., Zhang, S. G., & Yang, L. (2017). Edge mesh: a new paradigm to enable distributed intelligence in internet of things. IEEE Access, 5 , 16441–16458. https://doi.org/10.1109/access.2017.2739804 .
Lin, L., Liao, X. F., Jin, H., & Li, P. (2019). Computation offloading toward edge computing. Proceedings of the IEEE, 107 , 1584–1607. https://doi.org/10.1109/jproc.2019.2922285 .
Diaz, M., Martin, C., & Rubio, B. (2016). State-of-the-art, challenges, and open issues in the integration of Internet of things and cloud computing. J of Netw Comput Appl, 67 , 99–117. https://doi.org/10.1016/j.jnca.2016.01.010 .
Ni, J. B., Zhang, K., Lin, X. D., & Shen, X. M. (2018). Securing fog computing for internet of things applications: challenges and solutions. IEEE Commun Surv Tutorials, 20 , 601–628. https://doi.org/10.1109/comst.2017.2762345 .
Yassine, A., Singh, S., Hossain, M. S., & Muhammad, G. (2019). IoT big data analytics for smart homes with fog and cloud computing. Futur Gener Comput Syst Int J Escience, 91 , 563–573. https://doi.org/10.1016/j.future.2018.08.040 .
Dehury, C. K., & Sahoo, P. K. (2016). Design and implementation of a novel service management framework for IoT devices in cloud. Journal of Systems and Software, 119 , 149–161. https://doi.org/10.1016/j.jss.2016.06.059 .
Ganz, F., Puschmann, D., Barnaghi, P., & Carrez, F. (2015). A practical evaluation of information processing and abstraction techniques for the internet of things. IEEE Int Things J, 2 , 340–354. https://doi.org/10.1109/jiot.2015.2411227 .
Bulling, A., Blanke, U., & Schiele, B. (2014). A tutorial on human activity recognition using body-worn inertial sensors. ACM Computing Surveys, 46 , 1–33.
Nweke, H. F., Teh, Y. W., Al-Garadi, M. A., & Alo, U. R. (2018). Deep learning algorithms for human activity recognition using mobile and wearable sensor networks: State of the art and research challenges. Expert Systems with Applications, 105 , 233–261. https://doi.org/10.1016/j.eswa.2018.03.056 .
Das, S. K., Cook, D. J., Bhattacharya, A., Heierman, E. O., & Lin, T. Y. (2002). The role of prediction algorithms in the MavHome smart home architecture. IEEE Wireless Communications, 9 , 77–84. https://doi.org/10.1109/mwc.2002.1160085 .
Doctor, F., Hagras, H., & Callaghan, V. (2004). A fuzzy embedded agent-based approach for realizing ambient intelligence in intelligent inhabited environments. IEEE Trans Syst MAN Cybern Part A Syst Humans, 35 , 55–65.
Zhang, Y., Tian, G. H., Zhang, S. Y., & Li, C. C. (2020). A knowledge-based approach for multiagent collaboration in smart home: from activity recognition to guidance service. IEEE Transactions on Instrumentation and Measurement, 69 , 317–329. https://doi.org/10.1109/tim.2019.2895931 .
Ni, Q., Hernando, A. B. G., & de la Cruz, I. P. (2015). The elderly’s independent living in smart homes: a characterization of activities and sensing infrastructure survey to facilitate services development. Sensors, 15 , 11312–11362. https://doi.org/10.3390/s150511312 .
Schweizer D, Zehnder M, Wache H, Witschel H-F, Zanatta D, Rodriguez M (2015) Using consumer behavior data to reduce energy consumption in smart homes: applying machine learning to save energy without lowering comfort of inhabitants. In: 2015 IEEE 14th international conference on machine learning and applications (ICMLA). IEEE, pp 1123–1129
Kamal, S., Jalal, A., & Kim, D. (2016). Depth images-based human detection, tracking and activity recognition using spatiotemporal features and modified HMM. J Electr Eng Technol, 11 , 1857–1862. https://doi.org/10.5370/jeet.2016.11.6.1857 .
Sasakawa, D., Honma, N., Nakayama, T., & Iizuka, S. (2018). Human identification using MIMO array. IEEE Sensors Journal, 18 , 3183–3189. https://doi.org/10.1109/jsen.2018.2803157 .
Yu, L., Xie, W., Xie, D., Zou, Y., Zhang, D., Zhixin, S., Zhang, L., Zhang, Y., & Jiang, T. (2019). Deep reinforcement learning for smart home energy management. IEEE Internet of Things Journal . https://doi.org/10.1109/JIOT.2019.2957289 .
Tsirmpas, C., Anastasiou, A., Bountris, P., & Koutsouris, D. (2015). A new method for profile generation in an internet of things environment: an application in ambient-assisted living. IEEE Internet of Things Journal, 2 , 471–478. https://doi.org/10.1109/jiot.2015.2428307 .
Hassan, M. M., Uddin, M. Z., Mohamed, A., & Almogren, A. (2018). A robust human activity recognition system using smartphone sensors and deep learning. Futur Gener Comput Syst Int J Escience, 81 , 307–313. https://doi.org/10.1016/j.future.2017.11.029 .
Ye, J. A., & Dobson, S. (2010). Exploring semantics in activity recognition using context lattices. J Ambient Intell Smart Environ, 2 , 389–407. https://doi.org/10.3233/ais-2009-0082 .
Byrne, C. A., Collier, R., & O’Hare, G. M. P. (2018). A review and classification of assisted living systems. Inf Int Interdiscip J, 9 , 24. https://doi.org/10.3390/info9070182 .
Marques, G., Pitarma, R., Garcia, N. M., & Pombo, N. (2019). Internet of things architectures, technologies, applications, challenges, and future directions for enhanced living environments and healthcare systems: a review. Electronics, 8 , 27. https://doi.org/10.3390/electronics8101081 .
Konig, A., & Thongpull, K. (2015). Lab-on-spoon—a 3-D integrated hand-held multi-sensor system for low-cost food quality, safety, and processing monitoring in assisted-living systems. J Sensors Sens Syst, 4 , 63–75. https://doi.org/10.5194/jsss-4-63-2015 .
Shareef, H., Ahmed, M. S., Mohamed, A., & Al Hassan, E. (2018). Review on home energy management system considering demand responses, smart technologies, and intelligent controller. IEEE Access, 6 , 24498–24509. https://doi.org/10.1109/ACCESS.2018.2831917 .
Liu, X., Ivanescu, L., Kang, R., & Maier, M. (2012). Real-time household load priority scheduling algorithm based on prediction of renewable source availability. IEEE Transactions on Consumer Electronics, 58 , 318–326. https://doi.org/10.1109/TCE.2012.6227429 .
Paredes-Valverde, M. A., Alor-Hernandez, G., Garcia-Alcaraz, J. L., Salas-Zarate, M. D., Colombo-Mendoza, L. O., & Sanchez-Cervantes, J. L. (2020). IntelliHome: an internet of things-based system for electrical energy saving in smart home environment. Computational Intelligence . https://doi.org/10.1111/coin.12252 .
Marques, G., & Pitarma, R. (2016). An indoor monitoring system for ambient assisted living based on internet of things architecture. International Journal of Environmental Research and Public Health, 13 , 14. https://doi.org/10.3390/ijerph13111152 .
Marques, G., & Pitarma, R. (2019). A cost-effective air quality supervision solution for enhanced living environments through the internet of things. Electronics, 8 , 16. https://doi.org/10.3390/electronics8020170 .
Marques, G., & Pitarma, R. (2019). mHealth: indoor environmental quality measuring system for enhanced health and well-being based on internet of things. Journal of Sensor and Actuator Networks, 8 , 20. https://doi.org/10.3390/jsan8030043 .
Marques, G., Pires, I. M., Miranda, N., & Pitarma, R. (2019). Air quality monitoring using assistive robots for ambient assisted living and enhanced living environments through internet of things. Electronics, 8 , 18. https://doi.org/10.3390/electronics8121375 .
Fallahzadeh, R., Ghasemzadeh, H., & Shahrokni, A. (2018). Electronic assessment of physical decline in geriatric cancer patients. Current Oncology Reports, 20 , 11. https://doi.org/10.1007/s11912-018-0670-5 .
Ahanger, T. A., Tariq, U., Ibrahim, A., Ullah, I., & Bouterra, Y. (2020). Iot-inspired framework of intruder detection for smart home security systems. Electron, 9 , 1–17. https://doi.org/10.3390/electronics9091361 .
Khan, M., Silva, B. N., & Han, K. J. (2016). Internet of things based energy aware smart home control system. IEEE Access, 4 , 7556–7566. https://doi.org/10.1109/access.2016.2621752 .
Pars, A., Najafabadi, T. A., & Salmasi, F. R. (2019). A hierarchical smart home control system for improving load shedding and energy consumption: design and implementation. IEEE Sensors Journal, 19 , 3383–3390. https://doi.org/10.1109/jsen.2018.2880867 .
Tastan, M. (2019). Internet of things based smart energy management for smart home. KSII Trans Int Inf Syst, 13 , 2781–2798. https://doi.org/10.3837/tiis.2019.06.001 .
Piti, A., Verticale, G., Rottondi, C., Capone, A., & Lo Schiavo, L. (2017). The role of smart meters in enabling real-time energy services for households: the Italian case. Energies, 10 , 25. https://doi.org/10.3390/en10020199 .
Ghiani, G., Manca, M., Paterno, F., & Santoro, C. (2017). Personalization of context-dependent applications through trigger-action rules. ACM Trans Comput Interact, 24 , 33. https://doi.org/10.1145/3057861 .
Fogli, D., Peroni, M., & Stefini, C. (2017). ImAtHome: making trigger-action programming easy and fun. Journal of Visual Languages and Computing, 42 , 60–75. https://doi.org/10.1016/j.jvlc.2017.08.003 .
Hafidh, B., Al Osman, H., Arteaga-Falconi, J. S., Dong, H., & El Saddik, A. (2017). SITE: the simple internet of things enabler for smart homes. Ieee Access, 5 , 2034–2049. https://doi.org/10.1109/access.2017.2653079 .
Cabitza, F., Fogli, D., Lanzilotti, R., & Piccinno, A. (2017). Rule-based tools for the configuration of ambient intelligence systems: a comparative user study. Multimed Tools Appl, 76 , 5221–5241. https://doi.org/10.1007/s11042-016-3511-2 .
Shuhaiber, A., & Mashal, I. (2019). Understanding users’ acceptance of smart homes. Technology in Society, 58 , 9. https://doi.org/10.1016/j.techsoc.2019.01.003 .
Nikou, S. (2019). Factors driving the adoption of smart home technology: An empirical assessment. Telemat Inform, 45 , 12. https://doi.org/10.1016/j.tele.2019.101283 .
Pal, D., Funilkul, S., Charoenkitkarn, N., & Kanthamanon, P. (2018). Internet-of-things and smart homes for elderly healthcare: an end user perspective. IEEE Access, 6 , 10483–10496. https://doi.org/10.1109/access.2018.2808472 .
Dang, L. M., Piran, M. J., Han, D., Min, K., & Moon, H. (2019). A survey on internet of things and cloud computing for healthcare. Electronics, 8 , 49. https://doi.org/10.3390/electronics8070768 .
Yang, G., Xie, L., Mantysalo, M., Zhou, X. L., Pang, Z. B., Xu, L. D., Kao-Walter, S., Chen, Q., & Zheng, L. R. (2014). A health-IoT platform based on the integration of intelligent packaging, unobtrusive bio-sensor, and intelligent medicine box. IEEE Trans Ind Informatics, 10 , 2180–2191. https://doi.org/10.1109/tii.2014.2307795 .
Lee, Y. T., Hsiao, W. H., Lin, Y. S., & Chou, S. C. T. (2017). Privacy-preserving data analytics in cloud-based smart home with community hierarchy. IEEE Transactions on Consumer Electronics, 63 , 200–207. https://doi.org/10.1109/tce.2017.014777 .
Henze, M., Hermerschmidt, L., Kerpen, D., Haussling, R., Rumpe, B., & Wehrle, K. (2016). A comprehensive approach to privacy in the cloud-based Internet of Things. Futur Gener Comput Syst Int J Escience, 56 , 701–718. https://doi.org/10.1016/j.future.2015.09.016 .
Qolomany, B., Al-Fuqaha, A., Gupta, A., Benhaddou, D., Alwajidi, S., Qadir, J., & Fong, A. C. (2019). Leveraging machine learning and big data for smart buildings: a comprehensive survey. IEEE Access, 7 , 90316–90356. https://doi.org/10.1109/access.2019.2926642 .
Pekar, A., Mocnej, J., Seah, W. K. G., & Zolotova, I. (2020). Application domain-based overview of IoT network traffic characteristics. ACM Computing Surveys . https://doi.org/10.1145/3399669 .
Masek, P., Hosek, J., Zeman, K., Stusek, M., Kovac, D., Cika, P., Masek, J., Andreev, S., & Kropfl, F. (2016). Implementation of true IoT vision: survey on enabling protocols and hands-on experience. International Journal of Distributed Sensor Networks . https://doi.org/10.1155/2016/8160282 .
Liu, Y., Liu, X., Liu, A., Xiong, N. N., & Liu, F. (2019). A trust computing-based security routing scheme for cyber physical systems. ACM Trans Intell Syst Technol . https://doi.org/10.1145/3321694 .
Novo, O. (2018). Blockchain meets IoT: an architecture for scalable access management in IoT. IEEE Int Things J, 5 , 1184–1195. https://doi.org/10.1109/JIOT.2018.2812239 .
Pal, S., Rabehaja, T., Hitchens, M., Varadharajan, V., & Hill, A. (2020). On the design of a flexible delegation model for the internet of things using blockchain. IEEE Trans Ind Informatics, 16 , 3521–3530. https://doi.org/10.1109/TII.2019.2925898 .
Akpakwu, G. A., Silva, B. J., Hancke, G. P., & Abu-Mahfouz, A. M. (2018). A survey on 5G networks for the internet of things: communication technologies and challenges. IEEE Access, 6 , 3619–3647. https://doi.org/10.1109/ACCESS.2017.2779844 .
Dorri A, Kanhere SS, Jurdak R (2017) Towards an optimized blockchain for IoT. In: Proceedings of the second international conference on internet-of-things design and implementation. Association for Computing Machinery, New York, NY, USA, pp 173–178
Download references
This study was supported by grants from the National Natural Science Foundation of China (NSFC) (71802126), and a grant from the Shanghai Pujiang Program (18PJC060).
Author information
Authors and affiliations.
Department of Information Management and Information Systems, Shanghai University, Room 204, Shangda Road 99, Baoshan District, Shanghai, China
Department of Information Management and Information Systems, Shanghai University, Chengzhong Road 20, Jiading Town, Jiading District, Shanghai, China
You can also search for this author in PubMed Google Scholar
Corresponding author
Correspondence to Yi Sun .
Ethics declarations
Conflicts of interest.
Not applicable.
Additional information
Publisher's note.
Springer Nature remains neutral with regard to jurisdictional claims in published maps and institutional affiliations.
Rights and permissions
Reprints and permissions
About this article
Sun, Y., Li, S. A systematic review of the research framework and evolution of smart homes based on the internet of things. Telecommun Syst 77 , 597–623 (2021). https://doi.org/10.1007/s11235-021-00787-w
Download citation
Accepted : 01 April 2021
Published : 15 April 2021
Issue Date : July 2021
DOI : https://doi.org/10.1007/s11235-021-00787-w
Share this article
Anyone you share the following link with will be able to read this content:
Sorry, a shareable link is not currently available for this article.
Provided by the Springer Nature SharedIt content-sharing initiative
- Smart homes
- Systematic literature review
- Research framework
- Cluster and keywords analysis
- User perspective
- Find a journal
- Publish with us
- Track your research
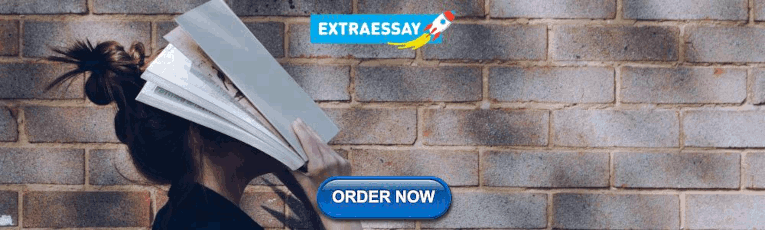
This paper is in the following e-collection/theme issue:
Published on 24.4.2024 in Vol 26 (2024)
Behavior Change Approaches in Digital Technology–Based Physical Rehabilitation Interventions Following Stroke: Scoping Review
Authors of this article:

- Helen J Gooch, BSc ;
- Kathryn A Jarvis, PhD ;
- Rachel C Stockley, PhD
Stroke Research Team, School of Nursing and Midwifery, University of Central Lancashire, Preston, United Kingdom
Corresponding Author:
Helen J Gooch, BSc
Stroke Research Team
School of Nursing and Midwifery
University of Central Lancashire
BB247 Brook Building
Victoria Street
Preston, PR1 2HE
United Kingdom
Phone: 44 1772894956
Email: [email protected]
Background: Digital health technologies (DHTs) are increasingly used in physical stroke rehabilitation to support individuals in successfully engaging with the frequent, intensive, and lengthy activities required to optimize recovery. Despite this, little is known about behavior change within these interventions.
Objective: This scoping review aimed to identify if and how behavior change approaches (ie, theories, models, frameworks, and techniques to influence behavior) are incorporated within physical stroke rehabilitation interventions that include a DHT.
Methods: Databases (Embase, MEDLINE, PsycINFO, CINAHL, Cochrane Library, and AMED) were searched using keywords relating to behavior change, DHT, physical rehabilitation, and stroke. The results were independently screened by 2 reviewers. Sources were included if they reported a completed primary research study in which a behavior change approach could be identified within a physical stroke rehabilitation intervention that included a DHT. Data, including the study design, DHT used, and behavior change approaches, were charted. Specific behavior change techniques were coded to the behavior change technique taxonomy version 1 (BCTTv1).
Results: From a total of 1973 identified sources, 103 (5%) studies were included for data charting. The most common reason for exclusion at full-text screening was the absence of an explicit approach to behavior change (165/245, 67%). Almost half (45/103, 44%) of the included studies were described as pilot or feasibility studies. Virtual reality was the most frequently identified DHT type (58/103, 56%), and almost two-thirds (65/103, 63%) of studies focused on upper limb rehabilitation. Only a limited number of studies (18/103, 17%) included a theory, model, or framework for behavior change. The most frequently used BCTTv1 clusters were feedback and monitoring (88/103, 85%), reward and threat (56/103, 54%), goals and planning (33/103, 32%), and shaping knowledge (33/103, 32%). Relationships between feedback and monitoring and reward and threat were identified using a relationship map, with prominent use of both of these clusters in interventions that included virtual reality.
Conclusions: Despite an assumption that DHTs can promote engagement in rehabilitation, this scoping review demonstrates that very few studies of physical stroke rehabilitation that include a DHT overtly used any form of behavior change approach. From those studies that did consider behavior change, most did not report a robust underpinning theory. Future development and research need to explicitly articulate how including DHTs within an intervention may support the behavior change required for optimal engagement in physical rehabilitation following stroke, as well as establish their effectiveness. This understanding is likely to support the realization of the transformative potential of DHTs in stroke rehabilitation.
Introduction
Digital health technologies (DHTs) comprise apps, programs, or software used in the health and social care systems [ 1 ]. They are considered to have almost unlimited potential to transform health care interventions and delivery and empower people to take a greater role in their own care and well-being [ 2 , 3 ].
Stroke is one of the leading causes of acquired disability worldwide, with around 12 million people experiencing a stroke each year [ 4 ]. Rehabilitation is a complex, multifaceted process [ 5 ] that facilitates those with health conditions and disabilities to participate in and gain independence in meaningful life roles [ 6 ]. It is considered an essential aspect of health care provision following a stroke [ 7 ] as a means to address poststroke impairments, which can involve motor, sensory, and cognitive functions. Changes in the ability to move due to impairment of both movement and sensory function are commonly experienced by people following a stroke [ 8 ] and are addressed by physical rehabilitation comprising regular, intensive practice and repetition of movements and tasks [ 9 , 10 ]. Conventional physical rehabilitation often struggles to deliver the intensity required to optimize recovery [ 11 ], and over recent years, there has been significant interest in the use of DHTs, such as virtual reality (VR), telerehabilitation, robotics, and activity monitors [ 12 - 16 ], to enhance and increase the intensity of rehabilitation. DHTs can provide a whole intervention or be used as a component of a wider intervention; the term DHT-based intervention has been used within this review to refer to both situations.
For many people who survive a stroke, rehabilitation requires individuals to engage in regular and frequent rehabilitative activities to achieve improvements in function and realize their optimal recovery. This necessitates adjustments to an individual’s behavior [ 17 ] over a sustained period of time. Changing behavior is a complex process and is underpinned by a variety of different theories, models, and frameworks [ 18 ], such as social cognitive theory [ 19 ] or the behavior change wheel framework [ 20 ]. Individual activities within a complex intervention that are designed to change behavior can be separated into replicable active components widely referred to as behavior change techniques (BCTs) [ 21 ]. Historically, labels applied to BCTs have lacked consensus, resulting in uncertainty and difficulty in comparing interventions. This has been addressed in the behavior change technique taxonomy version 1 (BCTTv1) [ 22 ], a classification system of 93 distinct BCTs clustered into 16 groups, which is a well-recognized tool to provide consistency with BCT reporting in interventions. DHTs provide an emerging opportunity to support the behavior change required within physical stroke rehabilitation interventions through facilitators that are embedded within the technology itself that aim to form, alter, or reinforce behaviors [ 23 ]. Understanding of this area is limited, with most literature exploring the use of DHTs to support behavior change focused on specific health-related behaviors such as physical activity or healthy eating [ 24 ] rather than as a core component of a type of rehabilitation intervention. Motivation is acknowledged to play an integral role in behavior change [ 25 ], and it is often assumed that DHTs provide motivation to engage with rehabilitation [ 26 ]. However, for this assumption to be realized, the DHTs must be able to support and deliver interventions that facilitate the vital changes in behavior needed to promote prolonged and sustained engagement in stroke rehabilitative activities. Imperative to this is understanding the theories, models, and frameworks that underpin interventions and the BCTs (active components) within the interventions [ 27 - 29 ]. The theories, models, and frameworks alongside the BCTs will be referred to hereinafter as approaches. Within the context of DHT-based physical stroke rehabilitation interventions, approaches to behavior change warrant further investigation.
Aim and Objectives
This scoping review aimed to identify if and how behavior change approaches are incorporated within DHT-based physical stroke rehabilitation interventions. Specifically, it sought to:
- Establish if behavior change theories, models, and frameworks, or BCTs, are described when reporting on DHT-based interventions that have been developed or evaluated for use in poststroke physical rehabilitation.
- Identify if behavior change theories, models, or frameworks underpin the interventions and which of these are being used.
- Identify if the BCTTv1 is being used to report BCTs within interventions.
- Determine which BCTs (based on the BCTTv1) can be identified within the interventions.
- Explore whether the type of technology influences the techniques used to change behaviors.
Review Methodology
A scoping review was completed and reported following established guidelines [ 30 , 31 ] and the Preferred Reporting of Systematic Reviews and Meta-Analyses Extension for Scoping Reviews (PRISMA-ScR; Multimedia Appendix 1 ) [ 32 ]. The protocol was registered with the Open Science Framework [ 33 ].
Eligibility Criteria
Any published sources that reported a completed primary research study in which a behavior change approach could be identified within a DHT-based physical stroke rehabilitation intervention were included ( Multimedia Appendix 2 ). Physical rehabilitation comprised interventions that addressed an impairment, or sequela of impairment, of sensory function and pain, neuromusculoskeletal and movement-related functions, or voice and speech, as defined by the International Classification of Functioning, Disability, and Health [ 34 ]. Completed primary research included all types of studies, both quantitative and qualitative, and no minimum sample size or intervention length was set. The BCTTv1 [ 22 ] was used to support the identification of BCTs within the interventions.
Information Sources and Search Strategy
A systematic database search was conducted in Embase, MEDLINE, PsycINFO, CINAHL, Cochrane Database of Systematic Reviews, CENTRAL Register of Controlled Trials, and AMED on March 21, 2023. The search was completed in collaboration with an information specialist who provided support with the development of the free text and thesaurus search terms, created the final search, adjusted the searches for the different databases, and ran the search. It consisted of 4 distinct search streams: behavior change, DHT, physical rehabilitation, and stroke, which were then combined ( Multimedia Appendix 3 ). Searches were restricted to the English language (due to review resources) and by date to search from 2001; the date restriction acknowledges the main time period of DHT growth [ 35 ], captures sources reported in systematic reviews of DHTs in stroke rehabilitation [ 12 - 16 ], and is reflected in other scoping literature exploring DHTs [ 24 ]. Additional sources were identified by hand searching, including scrutiny of the included source reference lists.
Selection of Sources of Evidence
The titles and abstracts of deduplicated sources from database searches and hand searches were independently screened by 2 reviewers, 1 of whom had completed the BCTTv1 web-based training package [ 36 ] to inform decisions made around the use of BCTs. Any conflicts were discussed, and if a consensus was not reached, the source was included for full-text screening. Attempts were made to locate a completed study publication from eligible conference abstracts, protocols, and trial registry entries. Full-text sources were screened independently by 2 reviewers, and disagreements were resolved by a third reviewer. Reasons for full-text exclusion were recorded. EndNote X9 software (Clarivate) and the Rayyan web tool software (Qatar Computing Research Institute) [ 37 ] were used to facilitate the source selection process.
Data Charting Process
A review-specific data charting tool was developed and initially piloted using 3 sources by 3 reviewers, and then further developed iteratively throughout the process [ 30 ]. Data charting was completed collectively by 2 reviewers. When several sources referred to a single study, these sources were grouped together for data charting, and if a source identified additional sources for further detail of the intervention (eg, a protocol or supplementary material), then this information was also used to support data charting.
The data charting tool was developed with reference to the Template for Intervention Description and Replication (TIDieR) checklist [ 27 ] and with a focus on the DHT-based intervention and behavior change approaches ( Multimedia Appendix 4 [ 14 , 38 - 40 ]). In the absence of a recognized predefined taxonomy for DHTs, the DHTs used in the sources were charted iteratively by the type of technology [ 41 ] from the information provided about the intervention. Over time, DHT categories emerged and were defined ( Multimedia Appendix 4 ). Discrete BCTs were identified from the intervention detail provided using the BCTTv1 [ 22 ] ( Multimedia Appendix 5 [ 42 ]). A pragmatic decision was made that the single reviewer who had completed the BCTTv1 web-based training package [ 36 ] would code the interventions to the BCTTv1. Any areas of uncertainty were discussed in detail among the review team.
Synthesis of Results
In accordance with the aims of a scoping review, formal assessments of methodological quality were not completed [ 30 , 31 ]. Findings were synthesized using descriptive statistics facilitated by SPSS Statistics 28.0.0.0 (IBM Corp) and Microsoft Excel (version 2208; Microsoft Corporation) and presented in text, table, and chart formats. The characteristics of the included sources, specifically participant numbers, age, and time since stroke, and intervention details, were summarized to provide contextual information for the review. Time since stroke was based on a published timeline framework [ 43 ], which describes the following phases: acute (1-7 days), early subacute (7 days to 3 months), late subacute (3-6 months), and chronic (greater than 6 months).
The behavior change theories, models, or frameworks underpinning the DHT-based interventions and sources where interventions had already been coded to the BCTTv1 were summarized. The use of individual BCTs, as coded by reviewers from intervention descriptions, was briefly summarized; however, the main focus of the BCT synthesis was completed by grouping the BCTs into the 16 BCTTv1 clusters, in order to provide an overview of their use across the sources and allow comparison with other reviews [ 44 , 45 ]. A cluster was only identified once per source, irrespective of the number of individual BCTs within that cluster. Relationships between BCTTv1 clusters and between DHT type and BCTTv1 clusters were descriptively explored. A relationship map was used to visually represent the strength of the connections between the BCTTv1 clusters, with a thicker line indicating that variables were more frequently reported together. No inferential statistical analysis was used.
From a total of 1973 sources screened, 357 full-text sources were assessed for eligibility, then after grouping sources that referred to a single study, 103 (5%) distinct sources were included in the review [ 46 - 148 ] ( Figure 1 ). Of the 245 sources excluded at full-text screening, 165 (67%) were excluded due to a lack of a behavior change approach.

Characteristics of Sources of Evidence
All sources of evidence were studies and will be referred to as such hereinafter. The number of studies in this field has rapidly increased over time ( Figure 2 ), from a single study in 2004 to 8 in 2022, with a peak of 15 in 2021. The majority (86/103, 83%) [ 47 - 51 , 53 - 56 , 58 , 59 , 61 , 63 - 68 , 71 - 86 , 89 - 95 , 97 - 105 , 107 , 109 , 111 , 112 , 114 , 115 , 117 - 126 , 128 - 136 , 138 - 148 ] were published in the past 10 years. Most studies took place in North America (41/103, 40%) [ 46 - 49 , 52 , 55 , 56 , 60 , 64 - 67 , 69 , 70 , 72 , 74 , 76 - 78 , 80 , 85 - 88 , 92 , 93 , 97 , 99 , 101 , 108 - 110 , 126 - 129 , 137 , 138 , 141 , 142 , 145 ] and Europe (35/103, 34%) [ 51 , 53 , 54 , 57 , 58 , 62 , 63 , 68 , 71 , 79 , 81 - 84 , 89 , 111 , 113 - 125 , 132 , 136 , 140 , 143 , 146 , 147 ], with the remainder in Asia (16/103, 16%) [ 50 , 59 , 61 , 91 , 94 , 95 , 98 , 100 , 102 - 104 , 107 , 135 , 139 , 144 , 148 ], Australasia (9/103, 9%) [ 75 , 96 , 105 , 106 , 112 , 130 , 131 , 133 , 134 ], Africa (1/103, 1%) [ 90 ], and a single multicontinental study (1/103, 1%) [ 73 ]. Almost half (45/103, 44%) the studies are reported as feasibility or pilot studies [ 49 , 56 , 58 , 64 , 66 , 68 , 69 , 72 - 74 , 76 , 77 , 79 , 82 - 84 , 89 , 90 , 92 , 93 , 95 , 97 , 100 - 104 , 106 , 108 , 114 , 116 , 117 , 119 , 122 , 124 - 126 , 131 , 134 , 136 , 138 , 139 , 141 , 143 , 147 ]. Other study designs included randomized controlled trials (20/103, 19%) [ 50 , 51 , 60 , 61 , 65 , 75 , 80 , 85 , 86 , 91 , 107 , 109 , 112 , 128 - 130 , 137 , 144 , 146 , 148 ], single session investigations (19/103, 18%) [ 47 , 52 , 57 , 59 , 71 , 78 , 87 , 88 , 98 , 110 , 115 , 118 , 120 , 123 , 127 , 132 , 133 , 135 , 142 ], nonrandomized experimental designs (13/103, 13%) [ 53 - 55 , 62 , 63 , 67 , 81 , 94 , 96 , 99 , 105 , 113 , 145 ], case studies (4/103, 4%) [ 46 , 48 , 70 , 140 ], and realist evaluations (2/103, 2%) [ 111 , 121 ].

Participants
There were a total of 2825 participants in the 103 included studies. Studies tended to be small, with a median of 16 participants and a range of 1-188. Only half (55/103, 53%) the studies [ 46 - 48 , 50 , 56 , 57 , 59 , 61 , 64 , 67 , 69 - 72 , 78 , 79 , 82 , 87 , 88 , 92 , 93 , 95 - 99 , 101 , 102 , 105 , 106 , 108 , 111 - 121 , 123 - 127 , 134 , 138 - 140 , 142 , 143 , 145 , 147 ] reported the minimum and maximum age of participants, which ranged from 17 to 99 years. Over three-quarters (83/103, 81%; 2508 participants) of studies reported the time since the onset of stroke. Of these 83 studies, 1 (1%; 48 participants) study [ 91 ] was conducted in the acute phase, 14 (17%; 504 participants) studies [ 60 , 61 , 68 , 74 , 79 , 92 , 100 , 102 , 109 , 114 , 133 , 144 , 146 , 148 ] were conducted in the early subacute phase, 11 (13%; 316 participants) studies [ 59 , 65 , 66 , 72 , 75 , 76 , 81 , 104 , 107 , 121 , 134 ] were conducted in the late subacute phase, and 57 (69%; 1640 participants) studies [ 46 , 48 , 49 , 51 , 53 , 54 , 57 , 63 , 64 , 67 , 69 , 70 , 73 , 78 , 80 , 82 , 84 , 85 , 88 , 89 , 93 - 99 , 101 , 103 , 105 , 106 , 108 , 111 - 113 , 117 - 120 , 122 - 125 , 127 - 131 , 136 - 142 , 145 , 147 ] were conducted in the chronic phase [ 43 ].
Study Intervention
An overview of study intervention characteristics is provided ( Table 1 ). Interventions were focused on upper limb rehabilitation in almost two-thirds (65/103, 63%) of the studies [ 46 - 49 , 51 , 54 - 59 , 62 - 65 , 68 , 71 , 72 , 74 , 75 , 77 - 81 , 85 - 88 , 92 , 95 , 96 , 99 , 101 - 103 , 105 - 108 , 110 , 112 , 113 , 116 - 118 , 121 , 123 - 125 , 127 , 128 , 132 , 133 , 135 - 137 , 139 - 142 , 144 - 147 ]. Nearly all interventions (96/103, 93%) [ 46 - 80 , 84 - 94 , 96 - 117 , 119 - 121 , 124 - 148 ] were delivered to individual participants, with over half (62/103, 60%) [ 46 - 50 , 53 - 58 , 60 , 61 , 64 - 70 , 72 , 74 - 77 , 79 , 80 , 82 - 86 , 89 , 90 , 93 , 94 , 96 , 97 , 99 , 101 , 105 , 111 , 112 , 116 , 117 , 119 - 122 , 126 , 129 - 131 , 134 , 136 , 138 , 139 , 141 , 143 - 145 , 147 ] delivered fully or partly in the participant’s homes. Two-thirds (70/103, 68%) of studies [ 46 - 50 , 52 - 54 , 57 , 60 , 62 , 63 , 65 - 74 , 76 - 84 , 86 - 93 , 98 , 100 , 102 , 104 , 108 , 109 , 112 - 115 , 117 , 118 , 120 , 122 - 125 , 129 - 131 , 135 - 138 , 140 - 142 , 144 - 146 , 148 ] included partial or full supervision of the intervention, with this predominately being provided face-to-face (48/70, 69%) [ 46 , 47 , 52 , 57 , 60 , 62 , 63 , 67 , 68 , 71 , 73 , 78 , 81 - 84 , 86 - 89 , 91 , 92 , 98 , 100 , 102 , 104 , 108 , 109 , 112 - 115 , 117 , 118 , 120 , 122 - 125 , 135 - 137 , 140 , 142 , 144 - 146 , 148 ]. Interventions lasted between a single session and 26 weeks.
Of the 103 studies, over half (n=57, 55%) of the studies [ 46 , 47 , 51 - 54 , 57 , 61 , 63 , 67 , 68 , 70 , 71 , 73 , 75 - 78 , 81 , 84 - 86 , 88 - 91 , 93 , 95 , 96 , 98 , 100 , 102 - 104 , 106 , 109 , 112 , 114 , 115 , 123 - 126 , 129 , 130 , 132 , 133 , 135 - 138 , 140 , 143 - 147 ] included 1 type of DHT, 30 (29%) studies [ 48 , 49 , 55 , 56 , 58 - 60 , 62 , 64 , 69 , 83 , 92 , 94 , 97 , 99 , 101 , 105 , 107 , 108 , 110 , 111 , 113 , 116 , 118 , 121 , 122 , 127 , 128 , 139 , 142 ] included 2 types, and 16 (16%) studies [ 50 , 65 , 66 , 72 , 74 , 79 , 80 , 82 , 87 , 117 , 119 , 120 , 131 , 134 , 141 , 148 ] included 3 types. VR was the most frequently used DHT (58/103, 56%) [ 46 - 49 , 51 - 53 , 57 , 59 , 62 , 63 , 65 , 66 , 69 , 71 , 72 , 74 , 77 , 78 , 80 , 81 , 84 - 89 , 92 , 95 , 96 , 98 , 102 - 104 , 106 , 112 , 113 , 115 , 117 - 120 , 123 - 128 , 132 , 135 - 137 , 140 , 142 , 143 , 146 - 148 ] followed by apps (31/103, 30%) [ 50 , 55 , 56 , 58 , 61 , 64 - 66 , 72 , 74 , 75 , 79 , 82 , 83 , 94 , 97 , 99 , 101 , 105 , 108 , 111 , 114 , 116 , 119 - 122 , 131 , 134 , 139 , 141 ]. Further information on intervention characteristics with detail on associated citations is available ( Multimedia Appendix 6 [ 46 - 148 ]).
a F2F: face-to-face.
b DHT: digital health technology.
c VR: virtual reality.
Behavior Change Theories, Models, and Frameworks
Most studies (93/103, 90%) [ 46 - 49 , 51 - 62 , 64 - 73 , 75 - 89 , 91 - 93 , 96 - 106 , 108 - 115 , 117 - 137 , 139 , 140 , 142 - 148 ] endeavored to link the intervention to behavior change; however, in the majority of these studies (75/93, 81%) [ 46 , 51 - 56 , 58 - 62 , 64 - 69 , 71 - 73 , 75 , 77 - 89 , 91 - 93 , 96 , 97 , 99 - 101 , 103 - 106 , 108 , 110 , 112 , 114 , 115 , 117 - 120 , 123 , 124 , 127 , 128 , 131 - 137 , 139 , 140 , 142 - 144 , 146 - 148 ], this explanation was centered on the reporting of the techniques perceived to change behaviors without direct reference to use of the BCTTv1 or on the reporting of a component of the intervention or the whole of the intervention as motivating. These explanations lack detail on how or why this influences behavior change. Examples of this included “the app also provided performance feedback, allowing the user to compare their current performance against their score from the previous session” (Bhattacharjya et al [ 56 ]) and “games motivate patients to engage in enjoyable play behavior” (Cramer et al [ 66 ]). A limited number of studies (18/103, 17%) [ 47 - 49 , 57 , 70 , 76 , 98 , 102 , 109 , 111 , 113 , 121 , 122 , 125 , 126 , 129 , 130 , 145 ] articulated 1 or more theories, models, or frameworks of behavior change. While it is acknowledged that the BCTTv1 is a taxonomy framework rather than a theoretical framework, for the purpose of this review, it has been included as a framework for behavior change. A total of 13 different theories, models, or frameworks were identified within these 18 studies, with social cognitive theory being the most frequently reported (6/18, 33%) [ 76 , 109 , 111 , 121 , 129 , 130 ], followed by the behavior change technique taxonomy (4/18, 22%) [ 48 , 49 , 122 , 129 ], game design theory (3/18, 17%) [ 47 , 57 , 125 ], operant conditioning (3/18, 17%) [ 47 , 98 , 121 ], and self-determination theory (3/18, 17%) [ 48 , 49 , 126 ]. Further information on behavior change theories, models, and frameworks, with details on associated citations, is available ( Multimedia Appendix 7 [ 47 - 49 , 57 , 70 , 76 , 98 , 102 , 109 , 111 , 113 , 121 , 122 , 125 , 126 , 129 , 130 , 145 ]).
Behavior Change Techniques
Despite 4 studies acknowledging the BCTTv1, explicit BCTTv1 codes were only reported in 2 studies (2/103, 2%) [ 48 , 122 ]. However, a third study (1/103, 1%) mapped the techniques used to change behavior directly to the transtheoretical model [ 145 ]. There was a median of 3 (range 1-14) individual BCTs coded per study, with a total of 383 BCTs across the 103 studies. The most frequently identified individual BCTs were feedback on behavior and nonspecific reward ( Multimedia Appendix 8 ).
There was also a median of 3 (range 1-8) BCTTv1 clusters per study, with a total of 288 clusters coded across the 103 studies. The most frequently used of the 16 possible clusters were feedback and monitoring (88/103, 85%) [ 46 - 60 , 62 - 69 , 71 - 74 , 76 , 78 - 80 , 82 - 92 , 94 - 106 , 108 - 113 , 116 , 117 , 119 - 129 , 134 - 146 , 148 ], reward and threat (56/103, 54%) [ 46 - 49 , 51 - 53 , 55 - 57 , 62 , 65 , 69 , 71 , 72 , 74 , 77 , 80 , 81 , 85 , 86 , 88 , 89 , 91 , 92 , 95 , 96 , 98 , 102 , 103 , 106 - 108 , 112 , 113 , 115 , 117 - 119 , 121 - 125 , 128 , 132 , 134 - 137 , 140 , 142 , 143 , 146 - 148 ], goals and planning (33/103, 32%) [ 49 , 58 , 60 , 65 - 68 , 70 , 72 , 74 , 76 , 79 , 80 , 82 , 83 , 90 , 91 , 93 , 94 , 97 , 100 , 109 , 111 , 112 , 121 , 122 , 126 , 129 , 130 , 134 , 138 , 141 , 145 ], and shaping knowledge (33/103, 32%) [ 46 , 48 , 50 , 53 - 56 , 58 , 60 , 61 , 64 - 72 , 74 , 75 , 86 , 94 , 97 , 101 - 103 , 108 , 111 , 113 , 114 , 120 , 129 - 131 , 139 - 141 ]. Other BCTTv1 clusters used were social support (24/103, 23%) [ 48 , 49 , 58 , 60 , 64 , 67 , 70 , 72 , 73 , 79 , 80 , 82 , 84 , 90 , 93 , 101 , 108 , 117 , 119 , 129 - 131 , 134 , 141 ], comparison of behavior (23/103, 22%) [ 46 , 50 , 53 , 54 , 60 , 61 , 64 - 66 , 74 , 75 , 81 , 86 , 101 , 104 , 111 , 114 , 118 , 122 , 123 , 125 , 131 , 139 ], associations (16/103, 15%) [ 58 , 60 , 65 , 66 , 68 , 75 , 80 , 83 , 87 , 90 , 110 , 120 , 131 , 133 , 139 , 144 ], repetition and substitution (6/103, 6%) [ 60 , 82 , 109 , 122 , 129 , 130 ], scheduled consequences (3/103, 3%) [ 47 , 80 , 88 ], natural consequences (2/103, 2%) [ 129 , 138 ], comparison of outcomes (2/103, 2%) [ 47 , 133 ], antecedents (1/103, 1%) [ 60 ], and self-belief (1/103, 1%) [ 70 ]. The clusters of regulation, identity, and covert learning were not identified. Within the context of the review, it was noted that the reward and threat cluster only included reward-based BCTs. A tabulated summary and graphical representation of the BCTTv1 clusters is available ( Multimedia Appendix 9 [ 46 - 148 ]).
The exploration of clusters that were reported together in an intervention ( Figure 3 ) identified the strongest relationship between the clusters of feedback and monitoring and reward and threat. Clear links were also identified between feedback and monitoring and 4 other clusters: goals and planning, shaping knowledge, social support, and comparison of behavior, and between the shaping knowledge and comparison of behavior clusters.

Behavior Change Techniques and Digital Health Technology
The feedback and monitoring cluster was reported most frequently for all types of DHT ( Figure 4 ), with the greatest proportion of this cluster in robotics (11/25, 44%) [ 59 , 62 , 87 , 92 , 110 , 113 , 117 , 127 , 128 , 142 , 148 ], VR (52/148, 35%) [ 46 - 49 , 51 - 53 , 57 , 59 , 62 , 63 , 65 , 66 , 69 , 71 , 72 , 74 , 78 , 80 , 84 - 89 , 92 , 95 , 96 , 98 , 102 - 104 , 106 , 112 , 113 , 117 , 119 , 120 , 123 - 135 - 137 , 140 , 142 , 143 , 146 , 148 ], and sensors (17/48, 35%) [ 50 , 55 , 56 , 87 , 94 , 99 , 101 , 105 , 108 , 110 , 111 , 116 , 119 - 121 , 134 , 141 ]. Robotics and VR also often used the reward and threat cluster (9/25, 36% [ 62 , 92 , 107 , 113 , 117 , 118 , 128 , 142 , 148 ] and 48/148, 32% [ 46 - 49 , 51 - 53 , 57 , 62 , 65 , 69 , 71 , 72 , 74 , 77 , 80 , 81 , 85 , 86 , 88 , 89 , 92 , 95 , 96 , 98 , 102 , 103 , 106 , 112 , 113 , 115 , 117 - 119 , 123 - 125 , 128 , 132 , 135 - 137 , 140 , 142 , 143 , 146 - 148 ], respectively), while the goals and planning cluster was a dominant second cluster in activity monitors (13/53, 25%) [ 67 , 68 , 76 , 79 , 80 , 82 , 91 , 100 , 109 , 122 , 129 , 138 , 145 ].

Summary of Evidence
This scoping review provides a comprehensive overview of approaches used to support changes in behavior in DHT-based physical stroke rehabilitation interventions. Research in this field is in its infancy, with the predominance of studies in this review being described as pilot or feasibility studies with limited participants.
Despite using comprehensive behavior change search terms, only a limited number (103/1973, 6%) of screened sources were included. Over two-thirds of full-text sources were excluded as they did not describe or refer to any behavior change theories, models, or frameworks or BCTs, suggesting that in general, explicit behavior change approaches are not reported as being integral to DHT-based physical stroke rehabilitation.
Only 18 (17%) of the 103 included studies articulated a theory, model, or framework to underpin the intervention, which aimed to change behavior, despite widely published recommendations about the importance of overt use of theory when developing, evaluating, and reporting interventions [ 27 , 29 ], including those related to behavior change [ 28 ]. The proportion of studies articulating a behavior change theory, model, or framework in this work is significantly lower than review findings in non-rehabilitation DHT-based interventions that have sought to influence specific behaviors such as physical activity or weight control [ 24 , 44 ]. These reviews have identified up to two-thirds of sources reporting a theory, model, or framework. However, our findings mirror the relative absence of behavior change theories, models, and frameworks in rehabilitation interventions more generally, irrespective of whether they use digital technology [ 149 ] or not [ 45 ], and it is widely recognized that the complex nature of rehabilitation often results in the essential characteristics of interventions being poorly defined [ 150 ]. Consistent with our findings in these other reviews, a variety of theories, models, and frameworks were found to underpin interventions, with social cognitive theory being the most frequently reported [ 24 , 44 , 45 , 149 ]. The explicit description of BCTs using the BCTTv1 within DHT-based physical stroke rehabilitation interventions is also poorly reported (2%), despite a significant proportion of the sources being dated after the publication of the BCTTv1 in 2013 [ 22 ]. This lack of acknowledgment of behavior change approaches impedes the accumulation of knowledge within this field.
It is important that both the underpinning theory and BCTs are reported so the mechanisms by which the BCTs elicit change can be better understood [ 21 ]. The general assumption that the motivational and captivating aspects of DHTs will promote prolonged and repeated engagement with rehabilitative activities, in particular in those DHTs that incorporate game design [ 151 ], risks suboptimal outcomes for patients and wasted investment of time and money if the mechanisms by which the DHT elicits change are not considered.
When exploring which BCT clusters featured within the reviewed DHT-based interventions, the findings relating to the commonly used clusters of feedback and monitoring, goals and planning, and shaping knowledge are consistent with findings from DHT-based interventions to change a specific behavior [ 44 ] and non-DHT–based rehabilitation [ 45 ]. However, a novel finding in our review was the frequent identification of the reward and threat cluster, although it was noted that all techniques related to reward and none to threat. A large number of studies in this review used VR technology, which frequently incorporates gamified tasks or gameplay. Reward is an integral part of game design theory alongside feedback [ 152 ], and so it is perhaps unsurprising that the feedback and monitoring, and reward and threat clusters dominated and an association between these 2 clusters was seen.
Limitations
Rehabilitation is a process that comprises multiple behaviors and so exploring approaches to change behavior within this context was complicated. There were challenges in searching and screening sources for inclusion as few studies explicitly reported approaches to change behavior, and there is a similarity in the vocabulary used within behavior change and other theoretical approaches (eg, “feedback,” which is used within motor learning). Similarly, only a very small proportion of studies explicitly reported BCTs within interventions. The lack of clear reporting of behavior change introduces the risk that sources may be omitted during both the searching and screening process highlighting the difficulty of comprehensively reviewing this field of work. An inclusive approach to screening reduced the risk of erroneously excluding sources, but it is perhaps inevitable that the sources included reflect those studies that have reported a behavior change approach rather than all studies that have used one.
This lack of clear BCT reporting also posed challenges for intervention coding. The use of the BCTTv1 aimed to ensure the review used a generalizable nomenclature to describe BCTs, and the 1 reviewer who had completed BCTTv1 training coded all the interventions. It is acknowledged that decisions made in the application of the BCTTv1 within the context of the review will have introduced some subjectivity in intervention coding, which will ultimately influence the review findings. Although the coding process could have been made more robust by having a second reviewer trained in the BCTTv1 also code the interventions, regular and extensive discussions between all members of the review team took place with the aim of ensuring consistency with the coding process. Clear documentation as to how the BCTTv1 was used within this review ( Multimedia Appendix 5 ) supports transparency as to the decisions made and the reproducibility of the review.
The absence of a recognized predefined taxonomy for DHTs posed a challenge when categorizing the DHT interventions, acknowledging that the distinction between the categories used to present the results is open to interpretation. A description of how the reviewers interpreted these categories is provided ( Multimedia Appendix 4 ).
Implications for Research
Future studies aimed at developing and evaluating DHT-based rehabilitation interventions, including those relating to physical stroke rehabilitation, need to ensure there is explicit recognition and reporting of the specific approaches used to change behavior, articulating both the theory on which the intervention is based and how the intervention plans to deliver the change in behavior using universally recognized terminology. This should be reported as part of a program theory and potential mechanisms of action, which are key parts of developing and evaluating complex interventions [ 29 ]. This detailed reporting would further support an understanding of how changes in behavior could be best enabled by DHT-based rehabilitation interventions and how this contributes to changes in patient outcomes. It would also enable further evaluation of the optimal behavioral components of interventions, enabling patients to use and clinicians to deliver the most effective DHT-based rehabilitative interventions. More generally, as the use of DHTs expands, there is an urgent need for some form of taxonomy to categorize and clearly define the different types of DHTs to facilitate consistent reporting, replication, and comparison of DHT-based interventions.
This novel and original review is the first to explore if and how approaches to change behavior are incorporated within DHT-based physical stroke rehabilitation. It demonstrates that a minority of studies report using approaches to change behavior within this context, despite these changes in behavior being vital to meet the demands of rehabilitative activities. Those who do report behavior change often lack the underpinning detail as to how the DHT-based intervention will facilitate these changes. In order for DHT-based interventions to realize their potential within rehabilitation and their impact on patient outcomes, approaches to change behavior must be embedded in the intervention and appropriately reported.
Acknowledgments
The authors would like to thank Catherine Harris (Information Specialist, University of Central Lancashire) for her assistance in developing the search strategy and running the searches, and Rebekah Murray (Undergraduate Research Intern, University of Central Lancashire) for her support with aspects of the screening and data charting process.
This work was funded by a UK Research and Innovation Future Leaders Fellowship (grant MR/T022434/1).
Data Availability
All data supporting this study are openly available from the University of Central Lancashire repository [ 153 ].
Authors' Contributions
RCS conceived the review focus and oversaw the work. HJG developed the review design and search strategy. HJG, KAJ, and RCS completed the screening of the identified sources. HJG and KAJ piloted the data charting tool. HJG completed the data charting, data analysis, and the initial manuscript draft. KAJ and RCS reviewed and made substantial contributions to the manuscript. All authors approved the final manuscript.
Conflicts of Interest
None declared.
Preferred Reporting of Systematic Reviews and Meta-Analyses Extension for Scoping Reviews (PRISMA-ScR) checklist.
Inclusion and exclusion criteria.
Full search strategy as used in Medline.
Data charting tool.
Review-specific behavior change technique taxonomy coding decisions.
Intervention characteristics (with associated references).
Behavior change theories, models, and frameworks reported (with associated references).
Individual behavior change techniques coded.
Behavior change technique taxonomy clusters identified (with associated references).
- Evidence standards framework for digital health technologies. National Institute of Clinical Excellence (NICE). 2018. URL: https://tinyurl.com/mpmrwrwx [accessed 2022-01-25]
- Castle-Clark S. The NHS at 70: What will new technology mean for the NHS and its patients? Kings Fund. 2018. URL: https://tinyurl.com/44k9fmat [accessed 2024-04-05]
- Topel E. The Topol review: preparing the healthcare workforce to deliver the digital future. Health Education England, NHS. 2019. URL: https://topol.hee.nhs.uk/ [accessed 2022-01-19]
- GBD 2019 Stroke Collaborators. Global, regional, and national burden of stroke and its risk factors, 1990-2019: a systematic analysis for the Global Burden of Disease Study 2019. Lancet Neurol. 2021;20(10):795-820. [ FREE Full text ] [ CrossRef ] [ Medline ]
- Wade DT. What is rehabilitation? An empirical investigation leading to an evidence-based description. Clin Rehabil. 2020;34(5):571-583. [ FREE Full text ] [ CrossRef ] [ Medline ]
- Rehabilitation. World Health Organisation. 2023. URL: https://www.who.int/news-room/fact-sheets/detail/rehabilitation [accessed 2023-05-04]
- Grefkes C, Fink GR. Recovery from stroke: current concepts and future perspectives. Neurol Res Pract. 2020;2:17. [ FREE Full text ] [ CrossRef ] [ Medline ]
- Lawrence ES, Coshall C, Dundas R, Stewart J, Rudd AG, Howard R, et al. Estimates of the prevalence of acute stroke impairments and disability in a multiethnic population. Stroke. 2001;32(6):1279-1284. [ FREE Full text ] [ CrossRef ] [ Medline ]
- National clinical guideline for stroke for the United Kingdom and Ireland. Intercollegiate Stroke Working Party. 2023. URL: https://tinyurl.com/yxy6b9tp [accessed 2023-05-02]
- Veerbeek JM, van Wegen E, van Peppen R, van der Wees PJ, Hendriks E, Rietberg M, et al. What is the evidence for physical therapy poststroke? a systematic review and meta-analysis. PLoS One. 2014;9(2):e87987. [ FREE Full text ] [ CrossRef ] [ Medline ]
- Lang CE, Macdonald JR, Reisman DS, Boyd L, Kimberley TJ, Schindler-Ivens SM, et al. Observation of amounts of movement practice provided during stroke rehabilitation. Arch Phys Med Rehabil. 2009;90(10):1692-1698. [ FREE Full text ] [ CrossRef ] [ Medline ]
- Laver KE, Lange B, George S, Deutsch JE, Saposnik G, Crotty M. Virtual reality for stroke rehabilitation. Cochrane Database Syst Rev. 2017;11(11):CD008349. [ FREE Full text ] [ CrossRef ] [ Medline ]
- Laver KE, Adey-Wakeling Z, Crotty M, Lannin NA, George S, Sherrington C. Telerehabilitation services for stroke. Cochrane Database Syst Rev. 2020;1(1):CD010255. [ FREE Full text ] [ CrossRef ] [ Medline ]
- Lynch EA, Jones TM, Simpson DB, Fini NA, Kuys SS, Borschmann K, et al. Activity monitors for increasing physical activity in adult stroke survivors. Cochrane Database Syst Rev. 2018;7(7):CD012543. [ FREE Full text ] [ CrossRef ] [ Medline ]
- Mehrholz J, Pohl M, Platz T, Kugler J, Elsner B. Electromechanical and robot-assisted arm training for improving activities of daily living, arm function, and arm muscle strength after stroke. Cochrane Database Syst Rev. 2018;9(9):CD006876. [ FREE Full text ] [ CrossRef ] [ Medline ]
- Mehrholz J, Thomas S, Kugler J, Pohl M, Elsner B. Electromechanical-assisted training for walking after stroke. Cochrane Database Syst Rev. 2020;10(10):CD006185. [ FREE Full text ] [ CrossRef ] [ Medline ]
- Jones F, Riazi A. Self-efficacy and self-management after stroke: a systematic review. Disabil Rehabil. 2011;33(10):797-810. [ CrossRef ] [ Medline ]
- Behaviour change: general approaches. National Institute of Clinical Excellence. UK. National Institute of Clinical Excellence (NICE); 2007. URL: https://tinyurl.com/yfthycch [accessed 2024-04-05]
- Bandura A. Social Foundations of Thought and Action: A Social Cognitive Theory. Englewood Cliffs, NJ. Prentice-Hall; 1986.
- Michie S, van Stralen MM, West R. The behaviour change wheel: a new method for characterising and designing behaviour change interventions. Implement Sci. 2011;6:42. [ FREE Full text ] [ CrossRef ] [ Medline ]
- Carey RN, Connell LE, Johnston M, Rothman AJ, de Bruin M, Kelly MP, et al. Behavior change techniques and their mechanisms of action: a synthesis of links described in published intervention literature. Ann Behav Med. 2019;53(8):693-707. [ FREE Full text ] [ CrossRef ] [ Medline ]
- Michie S, Richardson M, Johnston M, Abraham C, Francis J, Hardeman W, et al. The behavior change technique taxonomy (v1) of 93 hierarchically clustered techniques: building an international consensus for the reporting of behavior change interventions. Ann Behav Med. 2013;46(1):81-95. [ FREE Full text ] [ CrossRef ] [ Medline ]
- Oinas-Kukkonen H. A foundation for the study of behavior change support systems. Pers Ubiquit Comput. 2012;17(6):1223-1235. [ CrossRef ]
- Taj F, Klein MCA, van Halteren A. Digital health behavior change technology: bibliometric and scoping review of two decades of research. JMIR Mhealth Uhealth. 2019;7(12):e13311. [ FREE Full text ] [ CrossRef ] [ Medline ]
- West R, Michie S. A Guide to Development and Evaluation of Digital Behaviour Change Interventions in Healthcare. London. Silverback Publishing; 2016.
- Lewis GN, Rosie JA. Virtual reality games for movement rehabilitation in neurological conditions: how do we meet the needs and expectations of the users? Disabil Rehabil. 2012;34(22):1880-1886. [ CrossRef ] [ Medline ]
- Hoffmann TC, Glasziou PP, Boutron I, Milne R, Perera R, Moher D, et al. Better reporting of interventions: template for intervention description and replication (TIDieR) checklist and guide. BMJ. 2014;348:g1687. [ FREE Full text ] [ CrossRef ] [ Medline ]
- Michie S, Prestwich A. Are interventions theory-based? development of a theory coding scheme. Health Psychol. 2010;29(1):1-8. [ FREE Full text ] [ CrossRef ] [ Medline ]
- Skivington K, Matthews L, Simpson SA, Craig P, Baird J, Blazeby JM, et al. A new framework for developing and evaluating complex interventions: update of Medical Research Council guidance. BMJ. 2021;374:n2061. [ FREE Full text ] [ CrossRef ] [ Medline ]
- Peters MDJ, Godfrey CM, Khalil H, McInerney P, Parker D, Soares CB. Guidance for conducting systematic scoping reviews. Int J Evid Based Healthc. 2015;13(3):141-146. [ FREE Full text ] [ CrossRef ] [ Medline ]
- Peters MDJ, Godfrey CM, McInerney P, Munn Z, Tricco AC, Khalil H. Scoping Reviews (2020 version). In: Aromataris E, Munn Z, editors. JBI Manual for Evidence Synthesis. Adelaide, Australia. JBI; 2020. [ CrossRef ]
- Tricco AC, Lillie E, Zarin W, O'Brien KK, Colquhoun H, Levac D, et al. PRISMA Extension for Scoping Reviews (PRISMA-ScR): checklist and explanation. Ann Intern Med. 2018;169(7):467-473. [ FREE Full text ] [ CrossRef ] [ Medline ]
- Gooch H, Stockley R, Jarvis K. Behaviour change approaches within digital health technology-based interventions in physical rehabilitation following stroke: a scoping review protocol Version 2 081122. OSF Registries. 2022. URL: https://osf.io/yjn5g [accessed 2022-11-15]
- ICF checklist. World Health Organisation. 2003. URL: https://tinyurl.com/5fz6krew [accessed 2022-01-19]
- Hillyer M. How has technology changed—and changed us—in the past 20 years? World Economic Forum. 2020. URL: https://tinyurl.com/5n74wsym [accessed 2023-10-18]
- Online training. BCT Taxonomy v1. 2022. URL: http://www.bct-taxonomy.com/ [accessed 2022-03-08]
- Ouzzani M, Hammady H, Fedorowicz Z, Elmagarmid A. Rayyan-a web and mobile app for systematic reviews. Syst Rev. 2016;5(1):210. [ FREE Full text ] [ CrossRef ] [ Medline ]
- Wadley M, Bradbury M, Stockley R. Virtual reality. The Chartered Society of Physiotherapy. URL: https://www.csp.org.uk/professional-clinical/digital-physiotherapy/virtual-reality [accessed 2024-03-29]
- Cambridge dictionary. Cambridge University Press and Assessment. URL: https://tinyurl.com/2f2mx6kz [accessed 2024-03-29]
- Weber LM, Stein J. The use of robots in stroke rehabilitation: A narrative review. NeuroRehabilitation. 2018;43(1):99-110. [ CrossRef ] [ Medline ]
- English C, Ceravolo MG, Dorsch S, Drummond A, Gandhi DB, Green JH, et al. Telehealth for rehabilitation and recovery after stroke: state of the evidence and future directions. Int J Stroke. 2022;17(5):487-493. [ CrossRef ] [ Medline ]
- Taub E, Crago JE, Burgio LD, Groomes TE, Cook EW, DeLuca SC, et al. An operant approach to rehabilitation medicine: overcoming learned nonuse by shaping. J Exp Anal Behav. 1994;61(2):281-293. [ FREE Full text ] [ CrossRef ] [ Medline ]
- Bernhardt J, Hayward KS, Kwakkel G, Ward NS, Wolf SL, Borschmann K, et al. Agreed definitions and a shared vision for new standards in stroke recovery research: the Stroke Recovery and Rehabilitation Roundtable taskforce. Int J Stroke. 2017;12(5):444-450. [ FREE Full text ] [ CrossRef ] [ Medline ]
- Asbjørnsen RA, Smedsrød ML, Solberg Nes L, Wentzel J, Varsi C, Hjelmesæth J, et al. Persuasive system design principles and behavior change techniques to stimulate motivation and adherence in electronic health interventions to support weight loss maintenance: scoping review. J Med Internet Res. 2019;21(6):e14265. [ FREE Full text ] [ CrossRef ] [ Medline ]
- Bayly J, Wakefield D, Hepgul N, Wilcock A, Higginson IJ, Maddocks M. Changing health behaviour with rehabilitation in thoracic cancer: a systematic review and synthesis. Psychooncology. 2018;27(7):1675-1694. [ CrossRef ] [ Medline ]
- Alankus G, Proffitt R, Kelleher C, Engsberg J. Stroke therapy through motion-based games: a case study. ACM Trans Access Comput. 2011;4(1):1-35. [ CrossRef ]
- Alankus G, Kelleher C. Reducing compensatory motions in motion-based video games for stroke rehabilitation. Hum Comput Interact. 2015;30(3-4):232-262. [ CrossRef ]
- Allegue DR, Kairy D, Higgins J, Archambault PS, Michaud F, Miller W, et al. A personalized home-based rehabilitation program using exergames combined with a telerehabilitation app in a chronic stroke survivor: mixed methods case study. JMIR Serious Games. 2021;9(3):e26153. [ FREE Full text ] [ CrossRef ] [ Medline ]
- Allegue DR, Higgins J, Sweet SN, Archambault PS, Michaud F, Miller W, et al. Rehabilitation of upper extremity by telerehabilitation combined with exergames in survivors of chronic stroke: preliminary findings from a feasibility clinical trial. JMIR Rehabil Assist Technol. 2022;9(2):e33745. [ FREE Full text ] [ CrossRef ] [ Medline ]
- Asano M, Tai BC, Yeo FY, Yen SC, Tay A, Ng YS, et al. Home-based tele-rehabilitation presents comparable positive impact on self-reported functional outcomes as usual care: the Singapore Tele-technology Aided Rehabilitation in Stroke (STARS) randomised controlled trial. J Telemed Telecare. 2021;27(4):231-238. [ CrossRef ] [ Medline ]
- Ballester BR, Maier M, Mozo RMSS, Castañeda V, Duff A, Verschure PFMJ. Counteracting learned non-use in chronic stroke patients with reinforcement-induced movement therapy. J Neuroeng Rehabil. 2016;13(1):74. [ FREE Full text ] [ CrossRef ] [ Medline ]
- Bardack A, Bhandari P, Doggett J, Epstein M, Gagliolo N, Graff S, et al. EMG biofeedback videogame system for the gait rehabilitation of hemiparetic individuals. Digital Repository at the University of Maryland. 2010. URL: https://drum.lib.umd.edu/bitstream/handle/1903/10082/CHIP.pdf?sequence=1&isAllowed=y [accessed 2023-02-27]
- Bellomo RG, Paolucci T, Saggino A, Pezzi L, Bramanti A, Cimino V, et al. The WeReha Project for an innovative home-based exercise training in chronic stroke patients: a clinical study. J Cent Nerv Syst Dis. 2020;12:1179573520979866. [ FREE Full text ] [ CrossRef ] [ Medline ]
- Benvenuti F, Stuart M, Cappena V, Gabella S, Corsi S, Taviani A, et al. Community-based exercise for upper limb paresis: a controlled trial with telerehabilitation. Neurorehabil Neural Repair. 2014;28(7):611-620. [ FREE Full text ] [ CrossRef ] [ Medline ]
- Bhattacharjya S, Stafford MC, Cavuoto LA, Yang Z, Song C, Subryan H, et al. Harnessing smartphone technology and three dimensional printing to create a mobile rehabilitation system, mRehab: assessment of usability and consistency in measurement. J Neuroeng Rehabil. 2019;16(1):127. [ FREE Full text ] [ CrossRef ] [ Medline ]
- Bhattacharjya S, Cavuoto LA, Reilly B, Xu W, Subryan H, Langan J. Usability, usefulness, and acceptance of a novel, portable rehabilitation system (mRehab) using smartphone and 3D printing technology: mixed methods study. JMIR Hum Factors. 2021;8(1):e21312. [ FREE Full text ] [ CrossRef ] [ Medline ]
- Burke JW, McNeill MDJ, Charles DK, Morrow PJ, Crosbie JH, McDonough SM. Optimising engagement for stroke rehabilitation using serious games. Vis Comput. 2009;25(12):1085-1099. [ CrossRef ]
- Burridge JH, Lee ACW, Turk R, Stokes M, Whitall J, Vaidyanathan R, et al. Telehealth, wearable sensors, and the internet: will they improve stroke outcomes through increased intensity of therapy, motivation, and adherence to rehabilitation programs? J Neurol Phys Ther. 2017;41(Suppl 3):S32-S38. [ CrossRef ] [ Medline ]
- Cai S, Wei X, Su E, Wu W, Zheng H, Xie L. Online compensation detecting for real-time reduction of compensatory motions during reaching: a pilot study with stroke survivors. J Neuroeng Rehabil. 2020;17(1):58. [ FREE Full text ] [ CrossRef ] [ Medline ]
- Chumbler NR, Quigley P, Li X, Morey M, Rose D, Sanford J, et al. Effects of telerehabilitation on physical function and disability for stroke patients: a randomized, controlled trial. Stroke. 2012;43(8):2168-2174. [ FREE Full text ] [ CrossRef ] [ Medline ]
- Chung BPH, Chiang WKH, Lau H, Lau TFO, Lai CWK, Sit CSY, et al. Pilot study on comparisons between the effectiveness of mobile video-guided and paper-based home exercise programs on improving exercise adherence, self-efficacy for exercise and functional outcomes of patients with stroke with 3-month follow-up: a single-blind randomized controlled trial. Hong Kong Physiother J. 2020;40(1):63-73. [ FREE Full text ] [ CrossRef ] [ Medline ]
- Colombo R, Pisano F, Mazzone A, Delconte C, Micera S, Carrozza MC, et al. Design strategies to improve patient motivation during robot-aided rehabilitation. J Neuroeng Rehabil. 2007;4:3. [ FREE Full text ] [ CrossRef ] [ Medline ]
- Colomer C, Llorens R, Noé E, Alcañiz M. Effect of a mixed reality-based intervention on arm, hand, and finger function on chronic stroke. J Neuroeng Rehabil. 2016;13(1):45. [ FREE Full text ] [ CrossRef ] [ Medline ]
- Conroy SS, Harcum S, Keldsen L, Bever CT. Novel use of existing technology: a preliminary study of patient portal use for telerehabilitation. J Telemed Telecare. 2022;28(5):380-388. [ CrossRef ] [ Medline ]
- Cramer SC, Dodakian L, Le V, See J, Augsburger R, McKenzie A, et al. Efficacy of home-based telerehabilitation vs in-clinic therapy for adults after stroke: a randomized clinical trial. JAMA Neurol. 2019;76(9):1079-1087. [ FREE Full text ] [ CrossRef ] [ Medline ]
- Cramer SC, Dodakian L, Le V, McKenzie A, See J, Augsburger R, et al. A feasibility study of expanded home-based telerehabilitation after stroke. Front Neurol. 2020;11:611453. [ FREE Full text ] [ CrossRef ] [ Medline ]
- Danks KA, Roos MA, McCoy D, Reisman DS. A step activity monitoring program improves real world walking activity post stroke. Disabil Rehabil. 2014;36(26):2233-2236. [ FREE Full text ] [ CrossRef ] [ Medline ]
- Da-Silva RH, Moore SA, Rodgers H, Shaw L, Sutcliffe L, van Wijck F, et al. Wristband accelerometers to motivate arm exercises after Stroke (WAVES): a pilot randomized controlled trial. Clin Rehabil. 2019;33(8):1391-1403. [ CrossRef ] [ Medline ]
- Deng H, Durfee WK, Nuckley DJ, Rheude BS, Severson AE, Skluzacek KM, et al. Complex versus simple ankle movement training in stroke using telerehabilitation: a randomized controlled trial. Phys Ther. 2012;92(2):197-209. [ FREE Full text ] [ CrossRef ] [ Medline ]
- Deutsch JE, Maidan I, Dickstein R. Patient-centered integrated motor imagery delivered in the home with telerehabilitation to improve walking after stroke. Phys Ther. 2012;92(8):1065-1077. [ FREE Full text ] [ CrossRef ] [ Medline ]
- Dias P, Silva R, Amorim P, Lains J, Roque E, Pereira ISF, et al. Using virtual reality to increase motivation in poststroke rehabilitation. IEEE Comput Graph Appl. 2019;39(1):64-70. [ CrossRef ] [ Medline ]
- Dodakian L, McKenzie AL, Le V, See J, Pearson-Fuhrhop K, Quinlan EB, et al. A home-based telerehabilitation program for patients with stroke. Neurorehabil Neural Repair. 2017;31(10-11):923-933. [ FREE Full text ] [ CrossRef ] [ Medline ]
- Dorsch AK, Thomas S, Xu X, Kaiser W, Dobkin BH, SIRRACT investigators. SIRRACT: an international randomized clinical trial of activity feedback during inpatient stroke rehabilitation enabled by wireless sensing. Neurorehabil Neural Repair. 2015;29(5):407-415. [ FREE Full text ] [ CrossRef ] [ Medline ]
- Edwards D, Kumar S, Brinkman L, Ferreira IC, Esquenazi A, Nguyen T, et al. Telerehabilitation initiated early in post-stroke recovery: a feasibility study. Neurorehabil Neural Repair. 2023;37(2-3):131-141. [ FREE Full text ] [ CrossRef ] [ Medline ]
- Emmerson KB, Harding KE, Taylor NF. Home exercise programmes supported by video and automated reminders compared with standard paper-based home exercise programmes in patients with stroke: a randomized controlled trial. Clin Rehabil. 2017;31(8):1068-1077. [ CrossRef ] [ Medline ]
- Ezeugwu VE, Manns PJ. The feasibility and longitudinal effects of a home-based sedentary behavior change intervention after stroke. Arch Phys Med Rehabil. 2018;99(12):2540-2547. [ CrossRef ] [ Medline ]
- Fluet GG, Qiu Q, Patel J, Cronce A, Merians AS, Adamovich SV. Autonomous use of the home virtual rehabilitation system: a feasibility and pilot study. Games Health J. 2019;8(6):432-438. [ FREE Full text ] [ CrossRef ] [ Medline ]
- Foreman MH, Engsberg JR. A virtual reality tool for measuring and shaping trunk compensation for persons with stroke: design and initial feasibility testing. J Rehabil Assist Technol Eng. 2019;6:2055668318823673. [ FREE Full text ] [ CrossRef ] [ Medline ]
- Fusari G, Gibbs E, Hoskin L, Lawrence-Jones A, Dickens D, Crespo RF, et al. What is the feasibility and patient acceptability of a digital system for arm and hand rehabilitation after stroke? a mixed-methods, single-arm feasibility study of the 'OnTrack' intervention for hospital and home use. BMJ Open. 2022;12(9):e062042. [ FREE Full text ] [ CrossRef ] [ Medline ]
- Gauthier LV, Nichols-Larsen DS, Uswatte G, Strahl N, Simeo M, Proffitt R, et al. Video game rehabilitation for outpatient stroke (VIGoROUS): a multi-site randomized controlled trial of in-home, self-managed, upper-extremity therapy. EClinicalMedicine. 2022;43:101239. [ FREE Full text ] [ CrossRef ] [ Medline ]
- Goršič M, Cikajlo I, Goljar N, Novak D. A multisession evaluation of an adaptive competitive arm rehabilitation game. J Neuroeng Rehabil. 2017;14(1):128. [ FREE Full text ] [ CrossRef ] [ Medline ]
- Grau-Pellicer M, Lalanza JF, Jovell-Fernández E, Capdevila L. Impact of mHealth technology on adherence to healthy PA after stroke: a randomized study. Top Stroke Rehabil. 2020;27(5):354-368. [ CrossRef ] [ Medline ]
- Guidetti S, Gustavsson M, Tham K, Andersson M, Fors U, Ytterberg C. F@ce: a team-based, person-centred intervention for rehabilitation after stroke supported by information and communication technology—a feasibility study. BMC Neurol. 2020;20(1):387. [ FREE Full text ] [ CrossRef ] [ Medline ]
- Held JP, Ferrer B, Mainetti R, Steblin A, Hertler B, Moreno-Conde A, et al. Autonomous rehabilitation at stroke patients home for balance and gait: safety, usability and compliance of a virtual reality system. Eur J Phys Rehabil Med. 2018;54(4):545-553. [ FREE Full text ] [ CrossRef ] [ Medline ]
- Hernandez A, Bubyr L, Archambault PS, Higgins J, Levin MF, Kairy D. Virtual reality-based rehabilitation as a feasible and engaging tool for the management of chronic poststroke upper-extremity function recovery: randomized controlled trial. JMIR Serious Games. 2022;10(3):e37506. [ FREE Full text ] [ CrossRef ] [ Medline ]
- Hung NT, Paul V, Prakash P, Kovach T, Tacy G, Tomic G, et al. Wearable myoelectric interface enables high-dose, home-based training in severely impaired chronic stroke survivors. Ann Clin Transl Neurol. 2021;8(9):1895-1905. [ FREE Full text ] [ CrossRef ] [ Medline ]
- Johnson MJ, Shakya Y, Strachota E, Ahamed SI. Low-cost monitoring of patients during unsupervised robot/computer assisted motivating stroke rehabilitation. Biomed Tech (Berl). 2011;56(1):5-9. [ CrossRef ] [ Medline ]
- Johnson MJ, Van der Loos HFM, Burgar CG, Shor P, Leifer LJ. Experimental results using force-feedback cueing in robot-assisted stroke therapy. IEEE Trans Neural Syst Rehabil Eng. 2005;13(3):335-348. [ CrossRef ] [ Medline ]
- Jonsdottir J, Baglio F, Gindri P, Isernia S, Castiglioni C, Gramigna C, et al. Virtual reality for motor and cognitive rehabilitation from clinic to home: a pilot feasibility and efficacy study for persons with chronic stroke. Front Neurol. 2021;12:601131. [ FREE Full text ] [ CrossRef ] [ Medline ]
- Kamwesiga JT, Eriksson GM, Tham K, Fors U, Ndiwalana A, von Koch L, et al. A feasibility study of a mobile phone supported family-centred ADL intervention, F@ce, after stroke in Uganda. Global Health. 2018;14(1):82. [ FREE Full text ] [ CrossRef ] [ Medline ]
- Kanai M, Izawa KP, Kobayashi M, Onishi A, Kubo H, Nozoe M, et al. Effect of accelerometer-based feedback on physical activity in hospitalized patients with ischemic stroke: a randomized controlled trial. Clin Rehabil. 2018;32(8):1047-1056. [ CrossRef ] [ Medline ]
- Keeling AB, Piitz M, Semrau JA, Hill MD, Scott SH, Dukelow SP. Robot Enhanced Stroke Therapy Optimizes Rehabilitation (RESTORE): a pilot study. J Neuroeng Rehabil. 2021;18(1):10. [ FREE Full text ] [ CrossRef ] [ Medline ]
- Kessler D, Anderson ND, Dawson DR. Occupational performance coaching for stroke survivors delivered via telerehabilitation using a single-case experimental design. Br J Occup Ther. 2021;84(8):488-496. [ FREE Full text ] [ CrossRef ] [ Medline ]
- Kim DY, Kwon H, Nam KW, Lee Y, Kwon HM, Chung YS. Remote management of poststroke patients with a smartphone-based management system integrated in clinical care: prospective, nonrandomized, interventional study. J Med Internet Res. 2020;22(2):e15377. [ FREE Full text ] [ CrossRef ] [ Medline ]
- Kim J, Lee M, Kim Y, Eun SD, Yoon B. Feasibility of an individually tailored virtual reality program for improving upper motor functions and activities of daily living in chronic stroke survivors: a case series. Eur J Integr Med. 2016;8(5):731-737. [ CrossRef ]
- King M, Hijmans JM, Sampson M, Satherley J, Hale L. Home-based stroke rehabilitation using computer gaming. N Z J Physiother. 2012;40(3):128-134. [ FREE Full text ]
- Kringle EA, Setiawan IMA, Golias K, Parmanto B, Skidmore ER. Feasibility of an iterative rehabilitation intervention for stroke delivered remotely using mobile health technology. Disabil Rehabil Assist Technol. 2020;15(8):908-916. [ FREE Full text ] [ CrossRef ] [ Medline ]
- Kumar D, Sinha N, Dutta A, Lahiri U. Virtual reality-based balance training system augmented with operant conditioning paradigm. Biomed Eng Online. 2019;18(1):90. [ FREE Full text ] [ CrossRef ] [ Medline ]
- Langan J, Bhattacharjya S, Subryan H, Xu W, Chen B, Li Z, et al. In-home rehabilitation using a smartphone app coupled with 3D printed functional objects: single-subject design study. JMIR Mhealth Uhealth. 2020;8(7):e19582. [ FREE Full text ] [ CrossRef ] [ Medline ]
- Lawrie S, Dong Y, Steins D, Xia Z, Esser P, Sun S, et al. Evaluation of a smartwatch-based intervention providing feedback of daily activity within a research-naive stroke ward: a pilot randomised controlled trial. Pilot Feasibility Stud. 2018;4:157. [ FREE Full text ] [ CrossRef ] [ Medline ]
- Lawson S, Tang Z, Feng J. Supporting stroke motor recovery through a mobile application: a pilot study. Am J Occup Ther. 2017;71(3):7103350010p1-7103350010p5. [ CrossRef ] [ Medline ]
- Lee M, Pyun SB, Chung J, Kim J, Eun SD, Yoon BC. A further step to develop patient-friendly implementation strategies for virtual reality-based rehabilitation in patients with acute stroke. Phys Ther. 2016;96(10):1554-1564. [ FREE Full text ] [ CrossRef ] [ Medline ]
- Lee M, Son J, Kim J, Pyun SB, Eun SD, Yoon BC. Comparison of individualized virtual reality- and group-based rehabilitation in older adults with chronic stroke in community settings: a pilot randomized controlled trial. Eur J Integr Med. 2016;8(5):738-746. [ CrossRef ]
- Lee MM, Shin DC, Song CH. Canoe game-based virtual reality training to improve trunk postural stability, balance, and upper limb motor function in subacute stroke patients: a randomized controlled pilot study. J Phys Ther Sci. 2016;28(7):2019-2024. [ FREE Full text ] [ CrossRef ] [ Medline ]
- Levy T, Crotty M, Laver K, Lannin N, Killington M. Does the addition of concurrent visual feedback increase adherence to a home exercise program in people with stroke: a single-case series? BMC Res Notes. 2020;13(1):361. [ FREE Full text ] [ CrossRef ] [ Medline ]
- Lewis GN, Woods C, Rosie JA, McPherson KM. Virtual reality games for rehabilitation of people with stroke: perspectives from the users. Disabil Rehabil Assist Technol. 2011;6(5):453-463. [ CrossRef ] [ Medline ]
- Li X, Wang L, Miao S, Yue Z, Tang Z, Su L, et al. Sensorimotor rhythm-brain computer interface with audio-cue, motor observation and multisensory feedback for upper-limb stroke rehabilitation: a controlled study. Front Neurosci. 2022;16:808830. [ FREE Full text ] [ CrossRef ] [ Medline ]
- Lum PS, Taub E, Schwandt D, Postman M, Hardin P, Uswatte G. Automated Constraint-Induced Therapy Extension (AutoCITE) for movement deficits after stroke. J Rehabil Res Dev. 2004;41(3A):249-258. [ FREE Full text ] [ CrossRef ] [ Medline ]
- Mansfield A, Wong JS, Bryce J, Brunton K, Inness EL, Knorr S, et al. Use of accelerometer-based feedback of walking activity for appraising progress with walking-related goals in inpatient stroke rehabilitation: a randomized controlled trial. Neurorehabil Neural Repair. 2015;29(9):847-857. [ FREE Full text ] [ CrossRef ] [ Medline ]
- Matarić MJ, Eriksson J, Feil-Seifer DJ, Winstein CJ. Socially assistive robotics for post-stroke rehabilitation. J Neuroeng Rehabil. 2007;4:5. [ FREE Full text ] [ CrossRef ] [ Medline ]
- Mawson S, Nasr N, Parker J, Davies R, Zheng H, Mountain G. A personalized self-management rehabilitation system with an intelligent shoe for stroke survivors: a realist evaluation. JMIR Rehabil Assist Technol. 2016;3(1):e1. [ FREE Full text ] [ CrossRef ] [ Medline ]
- McNulty PA, Thompson-Butel AG, Faux SG, Lin G, Katrak PH, Harris LR, et al. The efficacy of Wii-based Movement Therapy for upper limb rehabilitation in the chronic poststroke period: a randomized controlled trial. Int J Stroke. 2015;10(8):1253-1260. [ CrossRef ] [ Medline ]
- Mihelj M, Novak D, Milavec M, Ziherl J, Olenšek A, Munih M. Virtual rehabilitation environment using principles of intrinsic motivation and game design. Presence: Teleop Virt Environ. 2012;21(1):1-15. [ CrossRef ]
- Mitchell C, Bowen A, Tyson S, Conroy P. A feasibility randomized controlled trial of ReaDySpeech for people with dysarthria after stroke. Clin Rehabil. 2018;32(8):1037-1046. [ FREE Full text ] [ CrossRef ] [ Medline ]
- Moan ME, Vonstad EK, Su X, Vereijken B, Solbjør M, Skjæret-Maroni N. Experiences of stroke survivors and clinicians with a fully immersive virtual reality treadmill exergame for stroke rehabilitation: a qualitative pilot study. Front Aging Neurosci. 2021;13:735251. [ FREE Full text ] [ CrossRef ] [ Medline ]
- Mountain G, Wilson S, Eccleston C, Mawson S, Hammerton J, Ware T, et al. Developing and testing a telerehabilitation system for people following stroke: issues of usability. J Eng Des. 2010;21(2-3):223-236. [ CrossRef ]
- Nijenhuis SM, Prange GB, Amirabdollahian F, Sale P, Infarinato F, Nasr N, et al. Feasibility study into self-administered training at home using an arm and hand device with motivational gaming environment in chronic stroke. J Neuroeng Rehabil. 2015;12:89. [ FREE Full text ] [ CrossRef ] [ Medline ]
- Novak D, Nagle A, Keller U, Riener R. Increasing motivation in robot-aided arm rehabilitation with competitive and cooperative gameplay. J Neuroeng Rehabil. 2014;11:64. [ FREE Full text ] [ CrossRef ] [ Medline ]
- Olafsdottir SA, Jonsdottir H, Bjartmarz I, Magnusson C, Caltenco H, Kytö M, et al. Feasibility of ActivABLES to promote home-based exercise and physical activity of community-dwelling stroke survivors with support from caregivers: a mixed methods study. BMC Health Serv Res. 2020;20(1):562. [ FREE Full text ] [ CrossRef ] [ Medline ]
- Olafsdottir SA, Jonsdottir H, Magnusson C, Caltenco H, Kytö M, Maye L, et al. Developing ActivABLES for community-dwelling stroke survivors using the Medical Research Council framework for complex interventions. BMC Health Serv Res. 2020;20(1):463. [ FREE Full text ] [ CrossRef ] [ Medline ]
- Parker J, Mawson S, Mountain G, Nasr N, Zheng H. Stroke patients' utilisation of extrinsic feedback from computer-based technology in the home: a multiple case study realistic evaluation. BMC Med Inform Decis Mak. 2014;14:46. [ FREE Full text ] [ CrossRef ] [ Medline ]
- Paul L, Wyke S, Brewster S, Sattar N, Gill JMR, Alexander G, et al. Increasing physical activity in stroke survivors using STARFISH, an interactive mobile phone application: a pilot study. Top Stroke Rehabil. 2016;23(3):170-177. [ CrossRef ] [ Medline ]
- Pereira F, Badia SBI, Jorge C, Cameirão MS. The use of game modes to promote engagement and social involvement in multi-user serious games: a within-person randomized trial with stroke survivors. J Neuroeng Rehabil. 2021;18(1):62. [ FREE Full text ] [ CrossRef ] [ Medline ]
- Perez-Marcos D, Chevalley O, Schmidlin T, Garipelli G, Serino A, Vuadens P, et al. Increasing upper limb training intensity in chronic stroke using embodied virtual reality: a pilot study. J Neuroeng Rehabil. 2017;14(1):119. [ FREE Full text ] [ CrossRef ] [ Medline ]
- Popović MD, Kostić MD, Rodić SZ, Konstantinović LM. Feedback-mediated upper extremities exercise: increasing patient motivation in poststroke rehabilitation. Biomed Res Int. 2014;2014:520374. [ FREE Full text ] [ CrossRef ] [ Medline ]
- Proffitt R, Lange B. Feasibility of a customized, in-home, game-based stroke exercise program using the Microsoft Kinect® sensor. Int J Telerehabil. 2015;7(2):23-34. [ FREE Full text ] [ CrossRef ] [ Medline ]
- Rosati G, Oscari F, Reinkensmeyer DJ, Secoli R, Avanzini F, Spagnol S, et al. Improving robotics for neurorehabilitation: enhancing engagement, performance, and learning with auditory feedback. IEEE Int Conf Rehabil Robot. 2011;2011:5975373. [ CrossRef ] [ Medline ]
- Rowe JB, Chan V, Ingemanson ML, Cramer SC, Wolbrecht ET, Reinkensmeyer DJ. Robotic assistance for training finger movement using a Hebbian model: a randomized controlled trial. Neurorehabil Neural Repair. 2017;31(8):769-780. [ FREE Full text ] [ CrossRef ] [ Medline ]
- Sakakibara BM, Lear SA, Barr SI, Goldsmith CH, Schneeberg A, Silverberg ND, et al. Telehealth coaching to improve self-management for secondary prevention after stroke: a randomized controlled trial of Stroke Coach. Int J Stroke. 2022;17(4):455-464. [ FREE Full text ] [ CrossRef ] [ Medline ]
- Saywell NL, Vandal AC, Mudge S, Hale L, Brown P, Feigin V, et al. Telerehabilitation after stroke using readily available technology: a randomized controlled trial. Neurorehabil Neural Repair. 2021;35(1):88-97. [ FREE Full text ] [ CrossRef ] [ Medline ]
- Scrivener K, Sewastenko J, Bouvier-Farrell A, MacDonald K, Van Rijn T, Tezak J, et al. Feasibility of a self-managed, video-guided exercise program for community-dwelling people with stroke. Stroke Res Treat. 2021;2021:5598100. [ FREE Full text ] [ CrossRef ] [ Medline ]
- Shah N, Amirabdollahian F, Basteris A. Designing motivational games for stroke rehabilitation. New Jersey. IEEE; 2014. Presented at: 7th International Conference on Human System Interactions (HSI); June 16-18, 2014;166-171; Costa da Caparica, Portugal. [ CrossRef ]
- Signal NEJ, McLaren R, Rashid U, Vandal A, King M, Almesfer F, et al. Haptic nudges increase affected upper limb movement during inpatient stroke rehabilitation: multiple-period randomized crossover study. JMIR Mhealth Uhealth. 2020;8(7):e17036. [ FREE Full text ] [ CrossRef ] [ Medline ]
- Simpson DB, Bird ML, English C, Gall SL, Breslin M, Smith S, et al. "Connecting patients and therapists remotely using technology is feasible and facilitates exercise adherence after stroke". Top Stroke Rehabil. 2020;27(2):93-102. [ CrossRef ] [ Medline ]
- Song X, van de Ven SS, Chen S, Kang P, Gao Q, Jia J, et al. Proposal of a wearable multimodal sensing-based serious games approach for hand movement training after stroke. Front Physiol. 2022;13:811950. [ FREE Full text ] [ CrossRef ] [ Medline ]
- Standen PJ, Threapleton K, Richardson A, Connell L, Brown DJ, Battersby S, et al. A low cost virtual reality system for home based rehabilitation of the arm following stroke: a randomised controlled feasibility trial. Clin Rehabil. 2017;31(3):340-350. [ FREE Full text ] [ CrossRef ] [ Medline ]
- Subramanian SK, Lourenço CB, Chilingaryan G, Sveistrup H, Levin MF. Arm motor recovery using a virtual reality intervention in chronic stroke: randomized control trial. Neurorehabil Neural Repair. 2013;27(1):13-23. [ FREE Full text ] [ CrossRef ] [ Medline ]
- Sullivan JE, Espe LE, Kelly AM, Veilbig LE, Kwasny MJ. Feasibility and outcomes of a community-based, pedometer-monitored walking program in chronic stroke: a pilot study. Top Stroke Rehabil. 2014;21(2):101-110. [ CrossRef ] [ Medline ]
- Toh SFM, Gonzalez PC, Fong KNK. Usability of a wearable device for home-based upper limb telerehabilitation in persons with stroke: a mixed-methods study. Digit Health. 2023;9:20552076231153737. [ FREE Full text ] [ CrossRef ] [ Medline ]
- Tsekleves E, Paraskevopoulos IT, Warland A, Kilbride C. Development and preliminary evaluation of a novel low cost VR-based upper limb stroke rehabilitation platform using Wii technology. Disabil Rehabil Assist Technol. 2016;11(5):413-422. [ CrossRef ] [ Medline ]
- Uswatte G, Taub E, Lum P, Brennan D, Barman J, Bowman MH, et al. Tele-rehabilitation of upper-extremity hemiparesis after stroke: proof-of-concept randomized controlled trial of in-home Constraint-Induced Movement therapy. Restor Neurol Neurosci. 2021;39(4):303-318. [ CrossRef ] [ Medline ]
- Valdés BA, Van der Loos HFM. Biofeedback vs. game scores for reducing trunk compensation after stroke: a randomized crossover trial. Top Stroke Rehabil. 2018;25(2):96-113. [ CrossRef ] [ Medline ]
- Wairagkar M, McCrindle R, Robson H, Meteyard L, Sperrin M, Smith A, et al. MaLT—combined motor and language therapy tool for brain injury patients using kinect. Methods Inf Med. 2017;56(2):127-137. [ FREE Full text ] [ CrossRef ] [ Medline ]
- Wei WXJ, Fong KNK, Chung RCK, Cheung HKY, Chow ESL. "Remind-to-Move" for promoting upper extremity recovery using wearable devices in subacute stroke: a multi-center randomized controlled study. IEEE Trans Neural Syst Rehabil Eng. 2019;27(1):51-59. [ CrossRef ] [ Medline ]
- Whitford M, Schearer E, Rowlett M. Effects of in home high dose accelerometer-based feedback on perceived and actual use in participants chronic post-stroke. Physiother Theory Pract. 2020;36(7):799-809. [ CrossRef ] [ Medline ]
- Widmer M, Held JPO, Wittmann F, Valladares B, Lambercy O, Sturzenegger C, et al. Reward during arm training improves impairment and activity after stroke: a randomized controlled trial. Neurorehabil Neural Repair. 2022;36(2):140-150. [ FREE Full text ] [ CrossRef ] [ Medline ]
- Wittmann F, Held JP, Lambercy O, Starkey ML, Curt A, Höver R, et al. Self-directed arm therapy at home after stroke with a sensor-based virtual reality training system. J Neuroeng Rehabil. 2016;13(1):75. [ FREE Full text ] [ CrossRef ] [ Medline ]
- Yuan Z, Peng Y, Wang L, Song S, Chen S, Yang L, et al. Effect of BCI-controlled pedaling training system with multiple modalities of feedback on motor and cognitive function rehabilitation of early subacute stroke patients. IEEE Trans Neural Syst Rehabil Eng. 2021;29:2569-2577. [ FREE Full text ] [ CrossRef ] [ Medline ]
- Chong MS, Sit JWH, Karthikesu K, Chair SY. Effectiveness of technology-assisted cardiac rehabilitation: a systematic review and meta-analysis. Int J Nurs Stud. 2021;124:104087. [ CrossRef ] [ Medline ]
- Hart T, Dijkers MP, Whyte J, Turkstra LS, Zanca JM, Packel A, et al. A theory-driven system for the specification of rehabilitation treatments. Arch Phys Med Rehabil. 2019;100(1):172-180. [ CrossRef ] [ Medline ]
- Deterding S, Dixon D, Khaled R, Nacke L. From game design elements to gamefulness: defining "gamification". New York, NY, US. Association for Computing Machinery; 2011. Presented at: Proceedings of the 15th International Academic MindTrek Conference: Envisioning Future Media Environments; September 28-30, 2011;9-15; Tampere, Finland. [ CrossRef ]
- Barrett N, Swain I, Gatzidis C, Mecheraoui C. The use and effect of video game design theory in the creation of game-based systems for upper limb stroke rehabilitation. J Rehabil Assist Technol Eng. 2016;3:2055668316643644. [ FREE Full text ] [ CrossRef ] [ Medline ]
- Welcome to UCLanData. University of Central Lancashire. URL: https://uclandata.uclan.ac.uk/383/ [accessed 2024-03-29]
Abbreviations
Edited by A Mavragani; submitted 15.05.23; peer-reviewed by M Broderick, G Sweeney, E Crayton, D Pogrebnoy; comments to author 11.10.23; revised version received 14.11.23; accepted 26.12.23; published 24.04.24.
©Helen J Gooch, Kathryn A Jarvis, Rachel C Stockley. Originally published in the Journal of Medical Internet Research (https://www.jmir.org), 24.04.2024.
This is an open-access article distributed under the terms of the Creative Commons Attribution License (https://creativecommons.org/licenses/by/4.0/), which permits unrestricted use, distribution, and reproduction in any medium, provided the original work, first published in the Journal of Medical Internet Research, is properly cited. The complete bibliographic information, a link to the original publication on https://www.jmir.org/, as well as this copyright and license information must be included.
Thank you for visiting nature.com. You are using a browser version with limited support for CSS. To obtain the best experience, we recommend you use a more up to date browser (or turn off compatibility mode in Internet Explorer). In the meantime, to ensure continued support, we are displaying the site without styles and JavaScript.
- View all journals
- Explore content
- About the journal
- Publish with us
- Sign up for alerts
Latest science news, discoveries and analysis
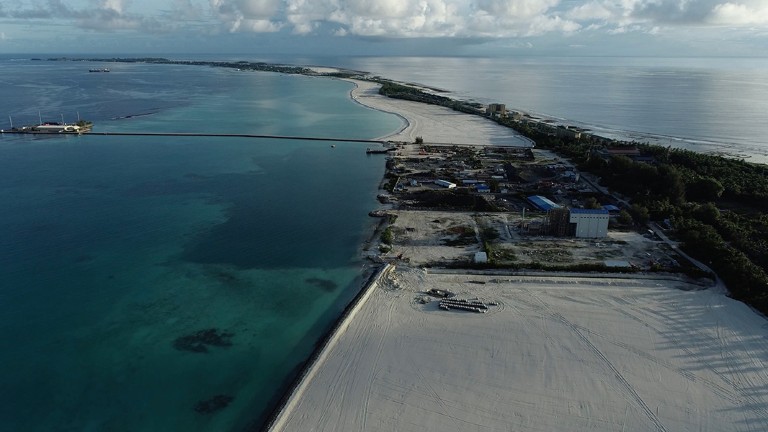
The Maldives is racing to create new land. Why are so many people concerned?
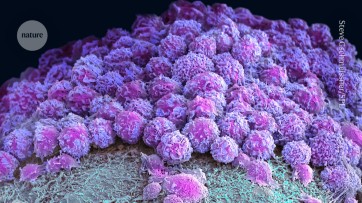
Mini-colon and brain 'organoids' shed light on cancer and other diseases
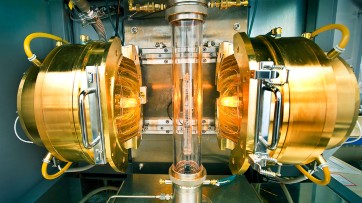
Retractions are part of science, but misconduct isn’t — lessons from a superconductivity lab
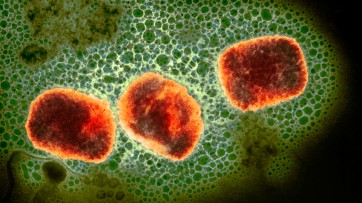
Monkeypox virus: dangerous strain gains ability to spread through sex, new data suggest
Dna from ancient graves reveals the culture of a mysterious nomadic people, atomic clock keeps ultra-precise time aboard a rocking naval ship, who redefines airborne transmission: what does that mean for future pandemics, ecologists: don’t lose touch with the joy of fieldwork chris mantegna, european ruling linking climate change to human rights could be a game changer — here’s how charlotte e. blattner.
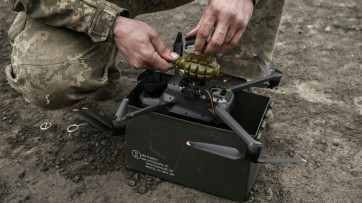
Lethal AI weapons are here: how can we control them?
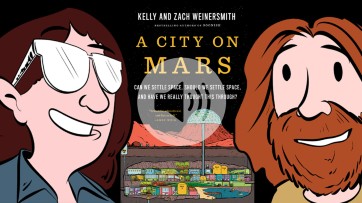
Living on Mars would probably suck — here's why
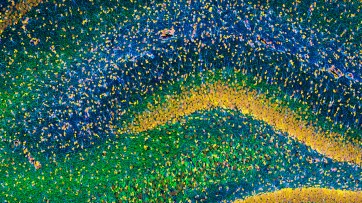
Dozens of genes are linked to post-traumatic stress disorder
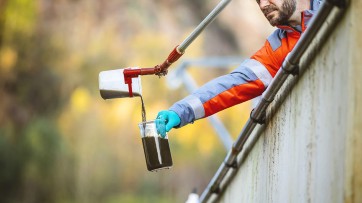
What toilets can reveal about COVID, cancer and other health threats
How gliding marsupials got their ‘wings’, plastic pollution: three numbers that support a crackdown, first glowing animals lit up the oceans half a billion years ago, how to freeze a memory: putting worms on ice stops them forgetting.
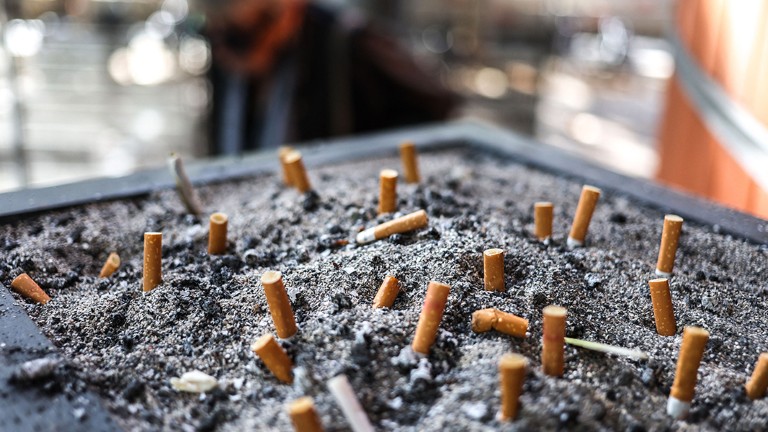
Any plan to make smoking obsolete is the right step
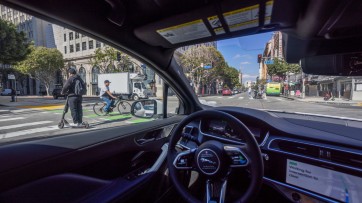
Will AI accelerate or delay the race to net-zero emissions?
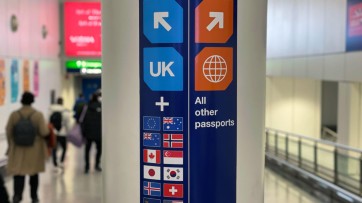
Citizenship privilege harms science
We must protect the global plastics treaty from corporate interference martin wagner, un plastics treaty: don’t let lobbyists drown out researchers, current issue.
Surprise hybrid origins of a butterfly species
Stripped-envelope supernova light curves argue for central engine activity, optical clocks at sea, research analysis.
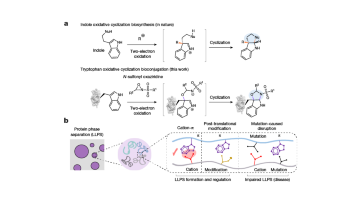
A chemical method for selective labelling of the key amino acid tryptophan
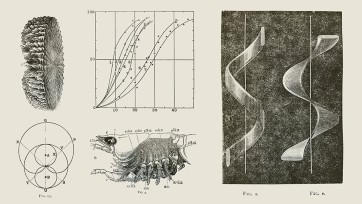
Charles Darwin investigates: the curious case of primrose punishment
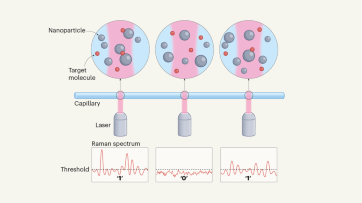
Nanoparticle fix opens up tricky technique to forensic applications
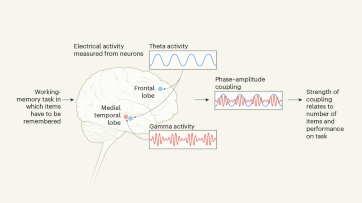
Coupled neural activity controls working memory in humans
Robust optical clocks promise stable timing in a portable package, targeting rna opens therapeutic avenues for timothy syndrome, bioengineered ‘mini-colons’ shed light on cancer progression, ancient dna traces family lines and political shifts in the avar empire.
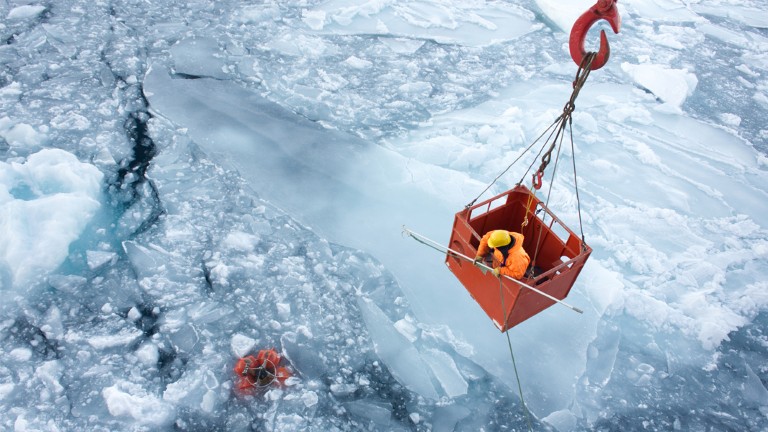
Breaking ice, and helicopter drops: winning photos of working scientists
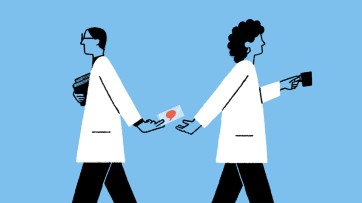
Shrouded in secrecy: how science is harmed by the bullying and harassment rumour mill
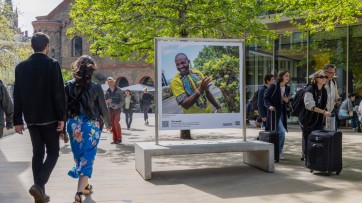
Londoners see what a scientist looks like up close in 50 photographs
How ground glass might save crops from drought on a caribbean island, deadly diseases and inflatable suits: how i found my niche in virology research, books & culture.
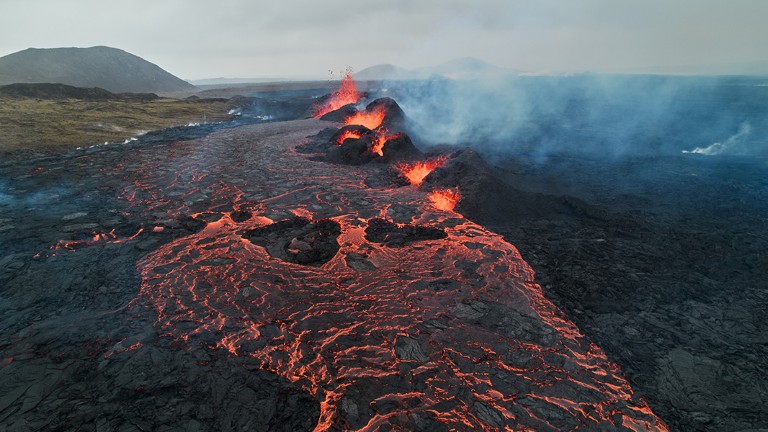
How volcanoes shaped our planet — and why we need to be ready for the next big eruption
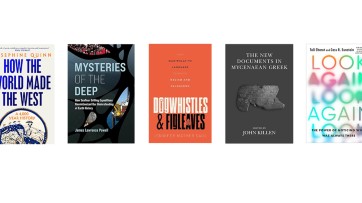
Dogwhistles, drilling and the roots of Western civilization: Books in brief
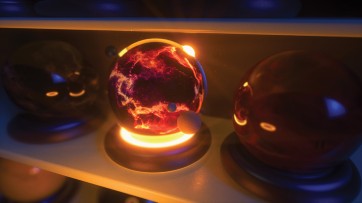
Cosmic rentals
Las boriqueñas remembers the forgotten puerto rican women who tested the first pill, dad always mows on summer saturday mornings, nature podcast.

Latest videos
Nature briefing.
An essential round-up of science news, opinion and analysis, delivered to your inbox every weekday.

Quick links
- Explore articles by subject
- Guide to authors
- Editorial policies
Regions & Countries
Religious landscape study.
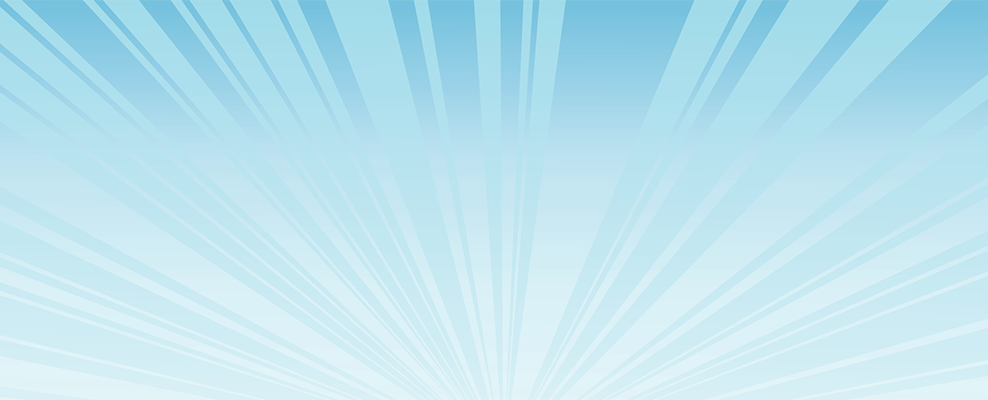
The RLS, conducted in 2007 and 2014, surveys more than 35,000 Americans from all 50 states about their religious affiliations, beliefs and practices, and social and political views. User guide | Report about demographics | Report about beliefs and attitudes
Explore religious groups in the U.S. by tradition, family and denomination
Explore religious affiliation data by state, region or select metro areas, northeastern states.
- Connecticut
- Massachusetts
- New Hampshire
- Pennsylvania
- Rhode Island
Southern States
- District of Columbia
- Mississippi
- North Carolina
- South Carolina
- West Virginia
Midwestern States
- North Dakota
- South Dakota
Western States
All metro areas.
- Atlanta Metro Area
- Baltimore Metro Area
- Boston Metro Area
- Chicago Metro Area
- Dallas/Fort Worth Metro Area
- Detroit Metro Area
- Houston Metro Area
- Los Angeles Metro Area
- Miami Metro Area
- Minneapolis/St. Paul Metro Area
- New York City Metro Area
- Philadelphia Metro Area
- Phoenix Metro Area
- Pittsburgh Metro Area
- Providence Metro Area
- Riverside, CA Metro Area
- San Diego Metro Area
- San Francisco Metro Area
- Seattle Metro Area
- St. Louis Metro Area
- Tampa Metro Area
- Washington, DC Metro Area
Topics & Questions
Demographic information.
- Race and Ethnicity
- Immigration Status
- Marital Status
- Parental Status
Beliefs and Practices
- Belief in God
- Importance of Religion
- Attendance at Religious Services
- Prayer Frequency
- Prayer Groups
- Feelings of Spiritual Wellbeing
- Feelings of Sense of Wonder
- Guidance on Right and Wrong
- Standards for Right and Wrong
- Reading Scripture
- Interpretation of Scripture
- Belief in Heaven
- Belief in Hell
Social and Political Views
- Political Party
- Political Ideology
- Size of Government
- Government Aid to the Poor
- Homosexuality
- Same-Sex Marriage
- Protecting the Environment
- Human Evolution
About Pew Research Center Pew Research Center is a nonpartisan fact tank that informs the public about the issues, attitudes and trends shaping the world. It conducts public opinion polling, demographic research, media content analysis and other empirical social science research. Pew Research Center does not take policy positions. It is a subsidiary of The Pew Charitable Trusts .
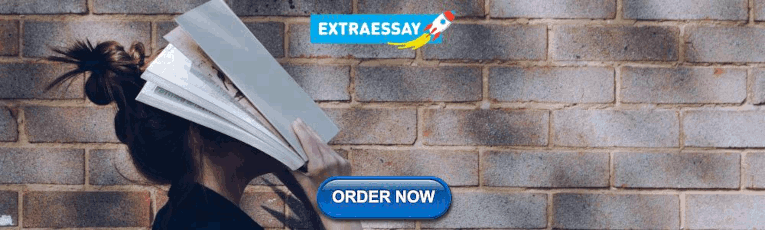
IMAGES
VIDEO
COMMENTS
This growing complexity makes it more difficult than ever—and more imperative than ever—for scholars to probe how technological advancements are altering life around the world in both positive and negative ways and what social, political, and legal tools are needed to help shape the development and design of technology in beneficial directions.
This paper presents a systematic outline of the development of 5G-related research until 2020 as revealed by over 10,000 science and technology publications. The exercise addresses the emergence, growth, and impact of this body of work and offers insights regarding disciplinary distribution, international performance, and historical dynamics.
Approached this way, the systematic literature review displays major research avenues of digital transformation that consider technology as the main driver of these changes. This paper qualitatively classifies the literature on digital business transformation into three different clusters based on technological, business, and societal impacts.
Within the field of digital technology education application research over the past two decades, Neil Selwyn stands as the most productive author, having published 15 papers garnering a total of ...
The evolution of technology is a central theme for management theory due to the transformative effect of technological change on societies, markets, industries, organizations, and individuals. Over the last decades, scholars from a broad range of theoretical and methodological traditions have generated a vast yet dispersed body of literature on technology evolution. We offer a comprehensive ...
Technology as a major determinant of organizational form and structure has been well acknowledged by academics for a long time (Thompson and Bates 1957; Woodward 1965; Scott 1992).Following a significant decline of interest in this relationship until the mid-1990s (Zammuto et al. 2007), innovations in information technologies (IT) and the rise of pre-internet technologies have revitalized its ...
Abstract. Evolution of technology is a stepwise advancement of a complex system of artifact, driven by interaction with sub-systems and other systems, considering technical choices, technical requirements and science advances, which generate new and/or improved products or processes for use or consumption to satisfy increasing needs and/or to solve complex problems of people in society.
To sum up, there is a close relationship between scientific research and technology development, and scientific research has a driving force for technology development. ... current researches related to technology evolution lack a more in-depth and complete exploration of the technology development trends. In this paper, an integrated method ...
Cellular wireless networks have come a long way since the first 1G system was introduced in 1981, with a new mobile generation appearing approximately every 10 years (Pathak 2013; Mishra 2018).In the past 30 years, the mobile industry has transformed society through 4 or 5 generations of technology revolution and evolution, namely 1G, 2G, 3G, and 4G networking technologies (Fig. 1). 1G gave us ...
Faculty of Business Studies and Economics, Chair of Innovation and Structural Change, University of Bremen, Germany. Abstract: This paper draws upon the evolutionary concepts of technological relatedness and knowledge complexity to enhance our understanding of the long-term evolution of Artificial Intelligence (AI).
New research from MIT aims to assist in the prediction of technology performance improvement using U.S. patents as a dataset. The study describes 97 percent of the U.S. patent system as a set of 1,757 discrete technology domains, and quantitatively assesses each domain for its improvement potential. "The rate of improvement can only be ...
The development of technology related to visual information processing (Marr, 1976) gradually makes computer vision a vital subfield of AI. Infinite attention is being paid to knowledge representation, and reasoning in this stage leads to new knowledge technology becoming the key to research. ... Methodology & research priorities. This paper ...
The paper draws mainly from the economics and human resources literature to describe trends in impact on jobs and skills development. It uses secondary sources and examples to explore policy options. This paper is structured as follows. The first section begins with a literature review of how technology impacts jobs and skills.
The primary research objectives of this paper are as under: RO1: - To study the need for digital technologies in education; ... Educational Technology Research and Development, 55 (3) (2007), pp. 301-314. CrossRef View in Scopus Google Scholar [71] A. Tlili, J. Zhang, Z. Papamitsiou, S. Manske, R. Huang, H.U. Hoppe.
Abstract. In wireless communication, Fifth Generation (5G) Technology is a recent generation of mobile networks. In this paper, evaluations in the field of mobile communication technology are presented. In each evolution, multiple challenges were faced that were captured with the help of next-generation mobile networks.
This paper proposes a reflection on the evolution of educational technologies and on its impact on school education. Over time, even though with different approaches, methods and tools, educational technology as a field of research has followed substantially two main directions. The first direction has resulted in studies on the new technical and conceptual skills required by digital ...
This paper will introduce education technology, evolution of technology and impact of education technology on the learning and teaching process. Discover the world's research 25+ million members
Theoretical background. Here, we review several competing theoretical perspectives on the evolution of technologies offered in the past. Technological change is one of the fundamental drivers in social and cultural evolution and of long-term economic growth [14-17].Many have pointed to technology's ramifying effects on warfare, state formation, and the development of information processing ...
Abstract and Figures. The technology evolution during industry 4.0 era is significantly impacting on consumer behaviour and expectation. This review started with the introduction of technology ...
Abstract. This literature review presents the History, Technology, and Applications of Natural Dialog Systems or simply chatbots. It aims to organize critical information that is a necessary background for further research activity in the field of chatbots. More specifically, while giving the historical evolution, from the generative idea to ...
Importance of technolog y in education. The role of technology in the field of education is four-. fold: it is included as a part of the curriculum, as an. instructional delivery system, as a ...
The Internet of Things has brought unprecedented technological innovation to smart homes, but the impact of this change on technology and users is limited and dispersed. Based on a systematic literature review, the present research conducted a quantitative analysis of 2874 papers (published in 2008-2020) obtained from the Web of Science to bridge the research gap. The current research ...
This paper is in the following e-collection/theme issue: Digital Health Reviews (1118) Web-based and Mobile Health Interventions (2968) Behavior Change (640) Innovations and Technology for Physical Activity Education (658) Postoperative Monitoring and Telemonitoring (101) Emerging Technologies for Rehabilitation (229) mHealth for Wellness, Behavior Change and Prevention (2645) mHealth for ...
Abstract. To reveal the origin of the IoT, evaluate its mainstream research topics, and discuss the challenges facing the IoT in the future, this paper conducts a bibliometric study of the IoT from 2000 to 2019. First, this paper presents a basic bibliometric overview of the IoT. Second, co-citation, coupling and cluster analysis methods are ...
Find breaking science news and analysis from the world's leading research journal.
The RLS, conducted in 2007 and 2014, surveys more than 35,000 Americans from all 50 states about their religious affiliations, beliefs and practices, and social and political views. User guide | Report about demographics | Report about beliefs and attitudes 1615 L St. NW, Suite 800 Washington, DC ...