
An official website of the United States government
The .gov means it’s official. Federal government websites often end in .gov or .mil. Before sharing sensitive information, make sure you’re on a federal government site.
The site is secure. The https:// ensures that you are connecting to the official website and that any information you provide is encrypted and transmitted securely.
- Publications
- Account settings
Preview improvements coming to the PMC website in October 2024. Learn More or Try it out now .
- Advanced Search
- Journal List
- Front Med Technol

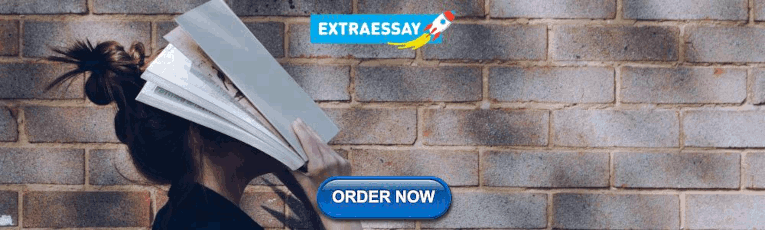
Present and future of gait assessment in clinical practice: Towards the application of novel trends and technologies
Abdul aziz hulleck.
1 Mechanical Engineering Department, Khalifa University, Abu Dhabi, United Arab Emirates
Dhanya Menoth Mohan
2 School of Mechanical and Aerospace Engineering, Monash University, Clayton Campus, Melbourne, Australia
Nada Abdallah
3 Weill Cornell Medicine, New York City, NY, United States
Marwan El Rich
Kinda khalaf.
4 Biomedical Engineering Department, Khalifa University, Abu Dhabi, United Arab Emirates
5 Health Engineering Innovation Center, Khalifa University, Abu Dhabi, United Arab Emirates
Despite being available for more than three decades, quantitative gait analysis remains largely associated with research institutions and not well leveraged in clinical settings. This is mostly due to the high cost/cumbersome equipment and complex protocols and data management/analysis associated with traditional gait labs, as well as the diverse training/experience and preference of clinical teams. Observational gait and qualitative scales continue to be predominantly used in clinics despite evidence of less efficacy of quantifying gait.
Research objective
This study provides a scoping review of the status of clinical gait assessment, including shedding light on common gait pathologies, clinical parameters, indices, and scales. We also highlight novel state-of-the-art gait characterization and analysis approaches and the integration of commercially available wearable tools and technology and AI-driven computational platforms.
A comprehensive literature search was conducted within PubMed, Web of Science, Medline, and ScienceDirect for all articles published until December 2021 using a set of keywords, including normal and pathological gait, gait parameters, gait assessment, gait analysis, wearable systems, inertial measurement units, accelerometer, gyroscope, magnetometer, insole sensors, electromyography sensors. Original articles that met the selection criteria were included.
Results and significance
Clinical gait analysis remains highly observational and is hence subjective and largely influenced by the observer's background and experience. Quantitative Instrumented gait analysis (IGA) has the capability of providing clinicians with accurate and reliable gait data for diagnosis and monitoring but is limited in clinical applicability mainly due to logistics. Rapidly emerging smart wearable technology, multi-modality, and sensor fusion approaches, as well as AI-driven computational platforms are increasingly commanding greater attention in gait assessment. These tools promise a paradigm shift in the quantification of gait in the clinic and beyond. On the other hand, standardization of clinical protocols and ensuring their feasibility to map the complex features of human gait and represent them meaningfully remain critical challenges.
1. Introduction
Changes in the signature of gait, or the unique sequential walking pattern in humans, reveal key information about the status and progression of numerous underlying health challenges, from neurological and musculoskeletal conditions to cardiovascular and metabolic disease, and to ageing-associated ambulatory dysfunction and trauma. Accurate reliable identification of gait patterns and characteristics in clinical settings, as well as monitoring and evaluating them over time, enable effective tailored treatment, inform predictive outcome assessment, and an allow for an overall better practice of precision medicine.
In clinical gait assessment, both a person's “ability” to walk and “how” the individual walks are highly relevant. The walking ability of a person is typically based on two main aspects: how far can an individual walk and what is his/her tolerance level ( 1 ). For example, for post stroke gait assessment, 3-, 6-, or 10 min walk tests are used, in addition to Functional Ambulation Category (FAC), Short Physical Performance Battery (SPPB), and/or Motor Assessment Scale (MAS). Other clinical subjective assessment scales include the Unified Parkinson Disease Rating Scale (UPDRS) the Scale for the Rating and Assessment of Ataxia (SARA)], the Alzheimer's Disease Assessment Scale (ADAS), the Expanded Disability Status Scale (EDSS) the High-level MobilitARTIy Assessment Tool (HiMAT), and the Dynamic Gait Index ( 2 ). The quality of gait or “how” the person walks, on the other hand, highly depends on the quantification of gait patterns and accurate identification of specific gait characteristics. Despite evidence of the advantages of quantitative instrumented gait analysis (IGA) in clinical practice and recommendations by the National Institute for Health and Clinical Excellence (NICE) ( 3 ) identifying IGA is the preferrable choice for “gait-improving surgery”, it remains not well leveraged in clinical settings due to the high cost/cumbersome equipment and complex protocols/data analysis associated with traditional gait labs, as well as diverse training, experience and preference of clinical teams ( 3 – 5 ). Moreover, the use of IGA by allied health professionals, such as physiotherapists, occupational therapists and orthotists, and training also remain non standardized and limited ( 5 – 7 ).
Observational gait analysis continues to be popular among clinicians due to its inherent simplicity, availability, and low cost ( 8 ). On the other hand, the validity, reliability, specificity, and responsiveness ( 9 , 10 ) of these qualitative methods are controversial and increasingly being questioned ( 6 ). Furthermore, there is evidence to suggest that subjective clinical assessment scales may not be sensitive to disease severity and specific characteristics and may limit understanding of underlying disease mechanisms, hence adversely impacting optimal treatment ( 11 ). Examples of such scales include Multiple Sclerosis (MS), where subjective measures, such as the Expanded Disability Status Scale (EDSS), the Multiple Sclerosis Severity Scale (MSSS), Multiple Sclerosis Walking Scale (MSWS), and Multiple Sclerosis Functional Composite (MSFC), continue to be widely used in clinical practice. These scales have been criticized for lack of sensitivity ( 12 ), high interrater variability ( 13 ), as well as being prone to practice effects and variability ( 14 , 15 ). Similarly, clinical assessment of Parkinson's disease (PD) using the Unified Parkinson's Disease Rating Scale (UPDRS) is subjective and largely dependent on the expertise and experience of the clinicians, as well as the severity of the disease ( 16 ). In Stroke patients, assessment tests such as Functional Ambulation Category (FAC), Short Physical Performance Battery (SPPB), and/or Motor Assessment Scale (MAS) are typically employed, along with qualitative observational/visual gait analysis (using naked eye or video images). Nevertheless, the validity, reliability, specificity, and responsiveness of these qualitative methods are questioned ( 9 ), and although they may be useful for the rudimentary evaluation of some gait parameters, they are not adequate for analyzing the multifaceted aspects of gait variability and complexity ( 17 ).
Instrumented gait analysis (IGA), which can provide accurate and precise quantitative measurement of gait patterns and characteristics, has long been the gold standard for gait assessment in research practice ( 18 ). IGA generally refers to the use of instrumentation to capture and analyze a variety of human gait parameters (spatiotemporal, kinematic, and kinetic measures). Traditional IGA systems include motion capture systems, and force plates, instrumented walkways, and treadmills, while more recent systems comprise of miniaturized wearable sensing system, computational platforms and modalities ( 18 ). Literature on the clinical applicability and efficacy of IGA indicates that IGA-based quantitative assessment can improve the diagnosis, outcome prediction, and rehabilitation of various gait impairments as compared to conventional observational scales and techniques for gait dysfunction in a wide spectrum of diseases including MS, PD, Stroke, and Cerebral Palsy ( 9 – 13 ). A recent review on the clinical efficacy of IGA confirms that there is strong evidence that 3-D gait analysis, or 3DGA; has the potential to alter and reinforce treatment decisions; increases confidence in treatment planning and agreement among clinicians; can better identify diagnostic groups and expected treatment outcomes; and overall can improve patient outcomes if recommendations are followed ( 19 ).
Emerging at an unprecedented rate, wearable sensing systems and associated computational modalities are rapidly transforming the quality and accessibility of healthcare, spanning multiple applications from neurology and orthopedics to cardiovascular, metabolic, and mental health. Magnetic (e.g., magnetometers), inertial measurement (e.g., accelerometers and gyroscopes), and force sensors (e.g., insole foot pressure) nowadays offer unprecedented data capture opportunities that can overcome limitations of non-wearable devices due to their low-cost, less setup-time and complexity, lightweight, and portability, making them ideal for out-of-lab and continuous monitoring in the clinic and beyond ( 20 ). Magneto-inertial measurement units (MIMUs), in conjunction with force pressure sensors, have the capability of capturing spatiotemporal, kinematic, and kinetic gait data ( 2 ) rendering the concept of a mobile gait lab a reality. Such labs can inherently overcome the limitations of IGA traditional labs, providing less costly and cumbersome tools with potential for gait assessment in natural environments (clinics, homes, sports arenas, etc.), user friendly interfaces, and the opportunity to provide continuous real-time feedback to clinicians and patients, as well as tele rehabilitation capabilities. In addition, wearable systems allow for easy synchronization with other physiological measurement systems, including EMG, ECG, and EEG, towards the acquisition of invaluable multimodal continuous physiological data in various settings.
This scoping review aims to provide a summary of the current state of clinical gait assessment, including shedding light on gait pathologies and clinical indices and scales, as well as a roadmap for the development of future gait mobile labs- highlighting the clinical validity and reliability of the latest devices and data interpretation algorithms. The word novel in the title of this review reflects recent emergence/implementation of the technologies reviewed and/or recent commercialization. This includes wearable technologies, as well as AI-driven computational platforms. The remainder of the review is structured as follows: Section 2 describes the adopted methodology, including the approach, search strategy and selection criteria. Section 3 details clinical gait pathologies, relevant parameters, as well as current clinical gait assessment tools, scales, and indices, while Section 4 presents gait assessment technologies applicable to clinical settings, including state-of-the-art imaging techniques and wearable technologies, algorithms, and novel AI-driven computational platforms. Section 5 deliberates on the concept of a mobile gait lab for clinical applications. Section 6 highlights the limitations, while Section 7 presents the conclusive remarks and future work.
This review is aimed at summarizing various clinical gait pathologies and associated parameters, applicable gait analysis techniques and gait indices, and the latest trends in wearables systems and algorithms. To address this broader research objective, the authors adopted a scoping review approach rather than a systematic review approach. As reported in ( 21 ), scoping reviews are ideal for addressing a broader scope with a more expansive inclusion criterion.
2.1. Search criteria
A keyword search was performed in PubMed, Web of Science, Medline, and ScienceDirect databases, using a combination of search terms from the following groups: 1. (normal gait OR pathological gait OR gait parameters OR gait indices), 2. (gait assessment OR gait analysis), 3. (wearable systems OR wearable algorithms), 4. (inertial measurement units OR accelerometer OR gyroscope OR magnetometer OR insole sensors OR electromyography sensors). No limit for the year of publication was set, however, the search was last updated in December 2021. Only articles written in the English language were considered in this review. In addition, the reference list of the included articles was checked to identify additional relevant publications meeting the inclusion criteria. The literature search and data extraction were carried out independently by two authors (AAH, DMM) and any inconsistencies and disagreements discrepancies were resolved through following discussions with the other authors (NA, MER, KK).
This scoping review included original published works and review articles which met the following inclusion/exclusion criteria: (i) studies addressing various gait disorders and associated gait parameters, (ii) studies focusing on instrumented gait analysis techniques and gait indices, (iii) studies evaluating the use, validity, and reliability of wearable-based gait measurement devices/systems for measuring gait events, and evaluating and assessing gait dysfunction, (iv) studies concerning the applicability of sensor fusion techniques and algorithms applicable for wearable-based systems with application to gait analysis. The title and/or abstract of the studies were initially screened for suitability. The full-text articles meeting the inclusion criteria were obtained for data extraction and synthesis. A flowchart explaining the same is shown in Figure 1 .

Publication selection process.
3. Clinical gait pathologies and parameters
3.1. normal gait cycle and parameters.
Normal gait can be defined as a series of rhythmic, systematic, and coordinated movements of the limbs and trunk that results in the forward advancement of the body's center of mass ( 22 ). A result of intricate dynamic interactions between the central nervous system and feedback mechanisms ( 23 ), walking is characterized by individual gait cycles and functional phases ( Figure 2 ). A gait cycle consists of two main phases, stance, and swing, which are further divided into five and three functional phases, respectively. The stance phase corresponds to the duration between heel strike and toe-off of the same foot, constituting approximately 60% of the gait cycle. The swing phase begins with toe-off and ends with heel contact of the same foot and occupies 40% of the cycle. As each functional phase contributes to successfully accomplishing the goal of walking, healthy gait involves cyclic and complementary movements of the limbs under control. It is characterized by stance stability; toe clearance during the swing; pre-positioning at swing; sufficient step length; as well as mechanical and metabolic efficiency ( 24 ). Table 1 provides gait parameter ranges based on studies on healthy adults. Determining an appropriate normal range for many of the features is highly challenging as individuals exhibit a wide range of gait patterns across different age groups and gender ( 17 ).

Normal gait cycle (adapted from 1 ).
Gait parameters for healthy individuals ( 1 ).
3.2. Gait parameters associated with pathology
Gait disorders are typically associated with deficits in the brain, spinal cord, peripheral nerves, muscles, joints, or bones. Some medical conditions leading to pathological gait include but not limited to muscular dystrophy, myelodysplasia, cerebral palsy, arthritis, osteoarthritis, head injury, lower limb amputation, multiple sclerosis, rheumatoid, spinal cord injury, parkinsonism, and stroke ( 25 ).
In neuromuscular conditions, the loss of central control affects the motion. In general, patients walk slower than healthy individuals and with compromised spatiotemporal, kinematic, and kinetic parameters. In older adults, a walking speed decline of 0.7% per year is observed, along with significant changes in cadence and step length. The aging population also exhibits lower knee extension at heel-strike and knee flexion during the swing phase ( 23 , 26 ). The following subsections describe some of the most common gait disorders and associated pathological parameters. The associated impacted parameters are summarized in Table 2 .
Effect of pathology on gait disorders.
3.2.1. Neurological gait disorders in elderly people
Gait ailments associated with aging lead to reduction in the quality of life and increased morbidity and mortality. Elderly patients exhibit complex gait disorders, and their dual task ability deteriorates due to a decline in their central resources ( 23 , 26 ).
Specific gait dysfunction noted in the elderly population are summarized as follows:
3.2.1.1. Hypokinetic-rigid gait disorders
Shuffling with a reduced step height and stride length characterizes hypokinetic gait disorder ( 27 ). Reduced arm swing with slow turning movements is also present in isolation. Festination, when patients use rapid small steps to maintain the feet beneath the forward moving trunk, is also observed. Ataxic elements include broad stance width and an increased variability in timing and amplitude of steps ( 27 ). Gait associated with underlying diseases, such as Parkinson's disease, cerebrovascular disease, and ventricular widening, is classified within hypokinetic-rigid gait disorders ( 27 , 28 ).
3.2.1.2. Cautious and careless gait
Defined as gait during which people move slowly with a wider base, and shorter stride, with minimal trunk movement, while the knees and elbows are bent. Whereas careless gait is when patients appear overly confident and walk insensitively fast. Careless gait is due to confusion and delirium associated with old age ( 27 ).
3.2.1.3. Dyskinetic gait or involuntary movements
Patients with post-anoxic encephalopathy exhibit bouncing gait and stance. This is also observed in patients with Parkinson's disease-causing excessive trunk movements contributing to falls. Several dystonic patients are reported to walk on their toes ( 27 , 28 ).
3.21.1.4. Psychogenic gait disorders
Gait dysfunction is common in elderly people due to adverse effects of drugs leading to extrapyramidal side-effects, sedation, orthostatic hypotension, behavioral abnormalities, or ataxia ( 27 , 28 ).
3.2.1.5. Fluctuating or episodic gait disorders
Elderly people often exhibit fluctuating or episodic gait disorder after exercise due to fatigue, and it might be an indication of underlying vascular or neurogenic limping. Freezing gait is part of hypokinetic-rigid syndrome ( 27 , 28 ).
3.2.2. Gait disorders in Parkinson's disease
PD is a neurological disorder which leads to cognition, where gait impairment deteriorates with disease progression, increasing reliance on cognition to control gait. Due to cognitive impairment with PD, the ability to compensate for gait disorders diminishes, leading to further gait impairment. PD is characterized by deficit in amplitude and gait speed, along with increased gait variability ( 29 ).
3.2.3. Gait in diabetic peripheral neuropathy
Neuropathy of motor, sensory, and autonomic components of the nervous system are one of the many complications of Type II Diabetes (T2D). An intact central and peripheral nervous system are essential to initiate and control healthy gait, along with sufficient muscle strength, bone, and joint movements in complete range for normal locomotion. Patients diagnosed with T2D take extra steps when walking in straight paths and during turns, along with an overall reduction in walking speed, step length, cadence, and fewer acceleration patterns as compared to age-matched healthy controls. Joint range of motion is also altered in T2D, where patients with diabetic peripheral neuropathy exhibit a reduced range of motion at the ankle joint in dorsi and plantar flexion and a reduced flexion and extension range of motion at the knee joint in both, as compared to non-diabetic people ( 24 ).
3.2.4. Post stroke gait
Hemiplegia after stroke contributes to significant reduction in gait performance. In stroke survivors, function of the cerebral cortex is usually impaired, whilst that of spinal cord is preserved. Dysfunction is typically demonstrated by a marked asymmetrical deficit. Decreased walking speed and cadence, in addition to longer gait cycle and double limb support as compared to healthy individuals. For hemiplegic stroke survivors, a reduced peak extension of the hip joint in late stance, varying peak lateral pelvis displacement, knee flexion and decreased plantarflexion of ankle at toe off are reported. The GRF (Ground Reaction Force) pattern is characterized as asymmetric, along with decreased amplitude of joint moments, at the lower limb joints on the paretic side ( 30 ).
3.2.5. Total hip arthroplasty (THA)
Large deficits in gait speed ( 31 ), stride length ( 32 , 33 ), sagittal hip range of motion ( 32 , 33 ), hip abduction moment-coronal plane ( 31 ), and negligible changes in transverse plane hip range of motion ( 31 ), deficiency in single limb support time ( 31 ), are reported in patients post THA as compared to healthy controls. Peak hip extension is typically reduced, whereas peak hip flexion remains similar as compared to controls. In addition, peak hip abduction moment is reduced along with peak hip external rotation moment ( 34 ).
3.3. Clinical gait assessment measures and indices
The use of observational gait analysis and subjective rating sales continues to be widespread in clinical settings, both as a diagnostic tool and as a prognostic measure, as previously mentioned. Although these techniques can be useful for the initial rudimentary evaluation of some gait parameters, the validity, reliability, specificity, and responsiveness of these qualitative methods are highly questionable. Researchers have therefore proposed various pathology-specific gait indices and summary measures ( 35 ) based on commercially available technologies with accepted levels of accuracy Table 3 . summarizes the current clinical gait summary measures, discrete and continuous gait indices, and non-linear approaches reported in literature, along with advantages and disadvantages.
Clinical gait measures and indices ( 123 , 124 ).
4. Gait assessment technologies applicable to clinical settings
In the past couple of decades, remarkable technological advancement has been witnessed in the field of gait assessment and analysis, particularly in gait assessment technology. Instrumented walkways, both portable and non-portable, became a good alternative to complicated, bulky and non-portable traditional gait labs. These systems (for example the Walkway and StrideWay from Tekscan Inc., Boston, United States) are now widely used in research and to a limited extent in clinical practice. They typically include low-profile floor walkway systems equipped with grids of embedded sensors below the surface, which record foot-strike patterns as a function of time and space as an individual walks across the platform, and dedicated software which computes the various spatiotemporal gait measures Although these instrumented mats involve less setup time and are generally simple to operate as compared to traditional IGA labs, they are expensive, restrictive to specific operational environment to over-ground trials ( 36 , 37 ).
Marker-based optical motion capture (Mocap) is another rapidly emerging technology effective for obtaining 3D kinematic movement data. Passive Mocap systems [e.g., Vicon (Vicon Motion Systems Ltd, Oxford, United Kingdom) and ELITE optoelectronic system (BTS S. p .A., Milano, Italy)], include retro-reflective markers (that reflect the light emitted by high-resolution infrared cameras) attached to specific anatomic landmarks. The location of the marker is identified by decoding the camera images. Here, the markers must be calibrated for identification before the recording session commences. Active Mocap systems (e.g., Optotrak motion capture system; Northern Digital Inc., Waterloo, Canada), on the other hand, use light-emitting diode (LED) markers (reflect their own light powered by a battery), which are automatically identified ( 38 , 39 ). In the context of clinical relevance, although such systems yield extremely accurate reliable data, operational factors including infrastructure, non-portability, high cost, additional time required for initial set-up and calibration, operational complexity, and restrictions to indoor setup impose hurdles to their functional deployment in clinics and rehabilitation centers ( 84 ). Recently, more portable cost-effective alternatives, such as Microsoft Kinect (based on a depth sensor-based markerless motion capture solution) became the application of choice ( 40 ).
Optoelectronic systems (e.g., Optogait®, Microgate, Italy) have also been used to capture spatiotemporal gait parameters. These mainly consist of a transmitting and a receiving bar containing an infrared light. Interruptions of the communication between the emitter and receiver are detected by the system to calculate the various gait parameters ( 41 ).
An evolution in the measurement of gait kinetic parameters can also be witnessed in the last two decades. These parameters include ground reaction forces, and intersegmental joint reaction forces, moments, and powers. Instrumented walkways offer dynamic plantar pressure mapping but are expensive and do not provide joint kinetic data. Force plates are also used in various gait analysis studies ( 38 , 39 , 42 ). These are able to provide intersegmental joint reaction forces by using the ground reaction forces measured along with inverse dynamics models (Winters book) Chen et al. ( 93 ) developed a novel remote sensing technology called “Electrostatic Field Sensing (EFS)” for measuring human gait including stepping, walking, and running, and further extended the work to post-stroke gait. This technology is credited with several advantages, such as being non-contact, affordable, and allows long-time monitoring ( 43 ). Shoe insole systems represent another category of gait quantification tools and techniques. These systems are designed to allow for the recording of both dynamic plantar pressure and spatiotemporal data. F-scan (Tekscan Inc., Boston, United States) is an ultra-thin in-shoe pressure measurement system utilizing Force-Sensitive Resistive films (FSR) technology ( 44 ).
The characteristics of different measurement systems applicable to clinical settings are summarized in Table 4 , and the pros and cons of these systems are listed in Table 5 .
Portable wearable gait assessment tools.
Pros and cons of different IGA systems.
Computational pipeline using computer vision techniques has been proposed as an ecological and precise method to quantify gait in children with neurodevelopmental disorders, along with the pose estimation software to obtain whole-body gait synchrony and balance ( 45 ). Speed, arm swing, postural control, and smoothness (or roughness) of movement features of gait for Parkinson's patients were extracted using videos processed by ordinal random forest classification model. Significant correlation between clinician labels and model estimates was reported, which provides gait impairment severity assessment in Parkinson's disease using single patient video, thereby reducing the need for sophisticated gait equipment ( 46 ). Computer vision-based gait assessment tools promise frequent gait monitoring using minimal resources ( 46 ). Deep learning to detect human subject in 2D images and then combining 3D sensing data to measure gait features has proven to be more robust than depth cameras in gait parameter acquisition ( 47 ).
4.1. Imaging techniques for gait assessment
As previously mentioned, marker-based optoelectronic systems are currently the most widely used systems in IGA among both research and clinical communities. On the other hand, one of the main sources of error inherent to these systems is the degree of movement of the skin, muscle, and other soft tissues, or the so- called soft tissue artifacts (STA), under the markers in relation to bony landmarks, hence violating the rigid body assumption underlying these methods ( 48 , 49 ). Moreover, STA varies by marker location in a unique and unpredictable manner, particularly during dynamic activities, which can make it unreliable for clinical applications ( 50 ).
Although not yet widespread in biomechanics, computer vision based markerless gait assessment methods offer a promising tool for gait assessment in research, as well clinical and sports biomechanics applications. By leveraging modern technologies, such as improved solvers, advanced image features and modern machine learning, markerless vision-based systems can reduce the required number of cameras, incorporating moving cameras, increasing the number of tracked individuals, and offering robust detection and fitting in diverse environments. On the other hand, issues such as accuracy and field-based feasibility remain to be addressed ( 51 ).
Three-dimensional imaging techniques have been successfully used to directly determine bone movements during walking as a gold reference standard to validate/improve current motion capture techniques ( 54 ). For example, researchers have resorted to quantifying STA by comparing with reference 3D kinematics of bone reconstructed from fluoroscopy-based tracking ( 53 ). Fluoroscopy has also emerged as a means for tracking position and orientation of underlying skeletal anatomy of the foot/ankle ( 54 ). Although single plane fluoroscopy yielded large errors when used to evaluate the accuracy of multi-segment foot models ( 49 ), dual fluoroscopy (DF) was found reliable and is considered as the current reference standard to compare joint angles ( 55 ). Combined with 2D/3D registration, video-fluoroscopy allows for accurate quantification of 3D joint motion free of STA ( 56 ). High-speed dual fluoroscopy (DF) has been reported to measure in-vivo bone motion of the foot and ankle with sub-millimeter and sub-degree errors ( 57 ). DF has also been used to evaluate multi-segment foot models and reported good agreement between DF and skin-marker data for the first metatarsal and sagittal plane measurements of the longitudinal arch ( 48 ).
Various researchers investigated the use of DF for clinical applications . In-vivo dual fluoroscopy was used to quantify the hip joint kinematics of patients with Femoroactabular impingement syndrome (FAIS) relative to asymptomatic, morphologically normal control participants during standing, level walking, incline walking and an unweighted functional activity. The kinematic position of the hip joint was obtained by registering projections of 3D computed Tomography models with DF images ( 58 ). Knee kinematic profiles were also obtained using 3D video-fluoroscopy and compared to actual and nominal flexion-extension, internal-external rotations, and antero-posterior translations profiles with optical mocap during stair climbing ( 59 ). Joint function for total talonavicular replacement after a complex articular fracture was evaluated using a full body gait analysis and 3D joint kinematics based on single-plane fluoroscopy ( 60 ). The 3D video fluoroscopic analysis was performed to assess joint motion of the replaced ankle ( 60 ). DF and CT imaging techniques were both employed to calculate in-vivo hip kinematics, along with model-based tracking, to compare the effect of different coordinate systems ( 61 ). Since marker-based systems are unable to accurately analyze talocrural or subtalar motion because the talus lacks palpable landmarks to place external markers ( 54 ), digitized video fluoroscopy was reportedly used to determine the sagittal plane motion of the medial longitudinal arch during dynamic gait ( 62 ). Characteristics of knee joint motion were also analyzed in 6DOF during treadmill walking using a dual fluoroscopy imaging system at different speeds ( 63 ).
DF uses anatomical landmarks visible on 3D CT reconstructions which substantially reduces errors due to STA ( 58 ). Computed tomography (CT) scans of participants are usually needed in DF to determine bone position from the DF images. Single plane fluoroscopy is restricted to 2D motion capture, while using a second FS allows for a full 3D analysis although a single gantry system has lower radiation than the biplane system with reported ionizing radiation levels of 10 µSv per trial ( 54 ). Stationary image intensifiers and static systems have a restricted field of view limiting their application to highly restricted movements ( 56 ). Moving fluoroscopes, consisting of a fluoroscopic unit mounted on a moving trolley which moves with the subject and is controlled by wire sensors to ensure that it remains in the field of view of the image intensifier ( 56 ), provide an enhanced field of view ideal for dynamic scenarios and moving joints.
Fluoroscopic systems designed for precise capture of bone movement and joint kinematics, unlike optical or inertial systems, are not yet commercially available, generally requiring in-house instrumentation and further performance evaluation. The evaluation would typically include determining the resolution of the hardware imaging chain, assessing how the hardware and software reduce or eliminate various distortions, and measuring static and dynamic accuracies and precisions based on precisely known motions and positions ( 64 ). Image quality is a major determinant of error in fluoroscopic applications ( 62 ). Pulse imaging of fluoroscopes, such as pulse width, limits image quality at a given frame rate. Increasing the pulse rate, which is function of pulse width, may add to radiation exposure, leading to an important tradeoff consideration between image quality and radiation exposure ( 63 ). Moving video-fluoroscopes reported lower gait velocity, step length, and cadence as compared to control conditions, indicating altered time distance parameters towards those of slow walking ( 56 ). So far, dynamic MRI used to define in- vivo talocrural and subtalar kinematics ( 65 ) does not allow data collection during normal gait.
Continued multidisciplinary collaborative efforts among biomechanists, imaging and computer vision experts, and clinicians are essential for fully leveraging these highly promising techniques in clinical applications.
4.2. Portable wearable systems for gait assessment
Wearable technology – the use of body-worn sensors to measure the characteristics of human locomotion, has recently emerged as an efficient, convenient, and most importantly, inexpensive option to quantitative gait analysis for both clinical and research-based applications ( Figure 3 ). In general, it uses individual sensor elements, such as accelerometers, gyroscopes, magneto resistive sensors, force/pressure sensors, goniometers, inclinometers, and electromyographic (EMG) sensors, or combined as an inertial measurement unit (IMU) ( 66 ). In comparison to conventional counterparts (e.g., walkway and camera based Mocap), wearable sensing enables continuous gait monitoring (> 2 h) outside the lab or clinic, allowing for replication of natural patterns of walking. Moreover, gait patterns over an ample distance could be measured as opposed to limited walking distance in a lab-based setting.

Wearable gait lab
Accelerometers are often used in gait analysis for assessing stability and risk of fall. In a study which used a single tri-axial accelerometer mounted on the sacrum to analyze the risk of fall among 80 participants, accelerometry-based techniques were found to be able to detect subjects with increased risk of fall by employing appropriate machine learning techniques ( 66 ). In ( 67 ), a 3D accelerometer attached to the lower back was used for stability assessment of older adults. The applicability of a single accelerometer, worn on the back was further examined in ( 68 ), which highlights promising results for implementation in routine clinical practices. Considerable work has also been carried out to assess the consistency of gait characteristics obtained from accelerometers, where discrepancies in sensors positioning yield to critical errors ( 69 ). Furthermore, in ( 70 ), the authors have provided a comprehensive review on the use of accelerometry-based gait analysis techniques and their application to clinical settings.
Gyroscopes are also increasingly employed for gait studies. These devices measure angular velocity and are often combined with accelerometers and other micro-electromechanical systems (MEMS) devices to enhance performance through sensor fusion techniques. They have found applications in step detection, gait event detection, segmental kinematics, and more. For instance, a single gyroscope placed in the instep of the foot was successfully used to detect gait events, including heel strike, foot flat, heel off, and toe-off ( 71 ). Another study involved two gyroscopes, mounted on the lower left and right side of the waist to calculate walking steps and step length ( 72 ).
Magnetometers measure the magnetic field direction and intensity at a specific point. In combination with other inertial sensors (accelerometers and gyroscopes), they form a so-called inertial measurement unit (IMU), which can produce a drift-free estimation of gait parameters ( 73 ). Sophisticated commercialized IMUs (Physiolog 5 IMU, Gait Up, Switzerland, MTw Awinda, Xsens Technologies B.V., Netherlands), as well as in-house developed systems, were equally used for gait studies ( 74 ). In the context of human motion analysis, IMUs are employed for several possible goals, for example, to estimate the joint angles ( 74 ), to detect the risk of fall in an elderly population, long term monitoring of activities and symptoms ( 75 ), measurement of gait events, spatiotemporal parameters ( 76 , 77 , 78 ), ground reaction forces and moments ( 79 ), and estimation of gait symmetry ( 80 ). Mariani et al. (2010) used IMUs to measure foot kinematics in a study involving both young and elderly and reported the suitability of the system to clinical practice ( 81 ). Parisi et al. developed a low-cost system with a single IMU attached to the lower trunk to examine the gait characteristics of both hemiparetic and normal control subjects through measurement of spatiotemporal parameters, which showed excellent correlation with the parameters obtained from a standard reference system ( 78 ).
Insole systems for gait measurement and analysis represent a major category, which is cost-effective, portable, and applicable for both indoor and outdoor settings. Over the years, various technologies were developed ( 82 ), tested, and commercialized. These include capacitive sensors (Pedar system, Novel GmbH, Germany) ( 83 ), force-sensing resistors (FSR) (F-Scan, Tekscan Inc., United States) ( 84 ), and piezoresistive sensors (FlexiForce system, Tekscan, United States and ParoTec system, Paromed, Germany) ( 82 ). Researchers have adopted different approaches about the design, fabrication, and applications of insole systems. Both prefabricated and in-house fabricated insole systems have been tested for healthy and pathological gait ( 85 , 86 ). Some studies have also integrated inertial measurement units (IMU) with shoe insoles to enhance their capabilities. Despite the fact that these shoe-based systems have successfully been used for various gait analysis applications, they suffer from some drawbacks, such as (i) distortion of the flexible contact surface due to repeated loading, which leads to changes in the sensor response, (ii) drift in the output due to prolonged load application that causes heat inside the shoe, and (iii) need for subject-specific calibration that may alter accuracy ( 87 ). Mancinelli et al. (2012) presented ActiveGait – a novel sensorized shoe system for real-time monitoring of gait deviations associated with Cerebral Palsy in children. They reported that the severity of gait deviations can be estimated with an accuracy greater than 80% using the features derived from the center of pressure trajectories gathered from the shoe system ( 88 ). In ( 87 ), the authors designed a novel flexible foot insole system using an optoelectronic sensing technology for monitoring plantar pressure deviations in real-time. The system consists of an array of 64 sensing elements and onboard electronics for signal processing and transmission. Experimental validation was conducted on healthy subjects while walking at self-selected slow and normal speed. A commercial force plate (AMTI, Watertown, United States) was used as a reference system for benchmarking. Jagos et al. (2017), on the other hand, developed the eSHOE, which consists of four FSR sensors, a three-axis accelerometer, and a three-axis gyroscope, and reported good agreement with the gait parameters obtained from the GAITRite mat ( 89 ). Various other studies have also examined the applicability of shoe-based systems for gait analysis ( 85 , 90 – 92 ).
Another class of sensors that found major applications in gait studies is electromyography (EMG) sensors. Surface EMG is a non-invasive technique used to measure muscle activity. In ( 93 ), Lee et al. proposed a method using EMG signals to obtain biometrics from gait for personal identification methods. Another study adopted EMG techniques to understand the co-contraction patterns of thigh muscle during free walking using surface EMG ( 94 ). These research efforts emphasize the importance of wearable sensors in the study of human gait. The wearable systems discussed in this section are summarized in Table 6 .
Instrumented gait analysis (IGA) systems and their features.
Although emerging new wearable technologies promise to enhance gait assessment and rehabilitation, there is limited research on the use of wearable technology to assess gait and mobility and its efficacy in clinical settings. According to a recently published review by Peters et. al. on the use of wearable technology to assess gait and mobility in stroke patients ( 95 ), most of the available studies are intervention studies conducted in laboratory settings that have used sensors to investigate change in cadence, step time variability, and gait speed. As wearable technologies continue to progress in affordability and accessibility, it is expected that such technologies would enable the gathering of movement-related data in “real-world” and various clinical settings. Importantly, these researchers indicated that so far only a limited number of studies examined reliability and validity of existing wearable devices, highlighting the need for more studies to examine psychometric and other properties when collecting gait and mobility information to determine which wearable technologies are most effective. Another recent review on the evaluation of the use of wearables in PD also indicates that novel technologies and wearables have the potential to enable early or differential diagnosis of PD, monitoring of motion state, prevention, or reduction of off-stage status, and assessing of movement complications. On the other hand, more research is required for the validation and the identification of more accurate markers of PD progression ( 96 ). Importantly, these authors warn that wearable devices may not be appropriate in cases of severe motor impairment, off-stage state, cognitive impairment, and for elderly patients and that further research is required for clinical validation.
4.3. Wearable-based gait computational algorithms
Besides sensor technology, sensor fusion algorithms play a critical role in predicting the accuracy/precision of these wearable-based systems. Most of the research has focused mainly on gait feature detection, daily physical activity monitoring, and gait data classification targeting disease diagnosis and user recognition. These algorithms are based on different data mining and AI technology, including machine learning, fuzzy computing, wavelet transforms, genetic algorithms, and data fusions. Alaqtash et al. ( 97 ) developed an intelligent fuzzy computational algorithm for characterizing gait in healthy, as well as impaired subjects. McCamley et al. established a method to calculate initial and final contact of gait using continuous wavelet transforms, employing waist-mounted inertial sensors ( 98 ). Another study cited the use of a single accelerometer mounted at the lower trunk and a corresponding algorithm to identify gait spatiotemporal parameters ( 68 ). A real-time gait event detection algorithm was proposed in ( 99 ) making use of adaptive decision rules. Further in ( 100 ), an original signal processing algorithm is developed to extract heel strike, toe strike, heel-off, and toe-off from an accelerometer positioned on the feet.
A novel gyroscope only (GO) algorithm was proposed in ( 101 ) to calculate knee angle through the integration of gyroscope-derived knee angular velocity. A zero-angle update algorithm was implemented to eliminate drift in the integral value. In addition, published work on noise-zero crossing (NZC) gait phase algorithm was also adapted. This method is applicable for continuous monitoring of gait data. Nukala et al. used support vector machines (SVM), KNN, binary decision trees (BDT), and backpropagation artificial neural network (BP-ANN) to classify the gait of patients from normal subjects, where features extracted from raw signals from gyroscopes and accelerometers were used as inputs. This study reported the highest overall classification accuracy of 100% with BP-ANN, 98% with SVM, 96% with KNN, and 94% with BDT ( 102 ).
Li et al. proposed DTW algorithm, sample entropy method, and empirical mode decomposition to calculate 3 main gait features of post-stroke subjects: symmetry, complexity character, and stepping stability. A k-nearest neighbor (KNN) classifier trained on the acquired features showed a promising result (area under the curve (AUC) of 0.94), which suggests the feasibility of such techniques to automatic gait analysis systems ( 43 ). Rastegari et al. employed a feature selection technique called maximum information gain minimum correlation (MIGMC) to extract gait data of subjects with Parkinson's Disease ( 103 ). The performance of several machine learning classifiers, including Support Vector Machines, Random Forest, AdaBoost, Bagging, and Naïve Bayes were also assessed to test the power of the feature set obtained.
The use of novel computational platforms, including Machine Learning, Support Vector Machine, and Neural Network approaches, are increasingly commanding greater attention in gait and rehabilitation research. Although their use in clinical settings are not yet well leveraged, these tools promise a paradigm shift in stroke gait quantification and rehabilitation, as they provide means for acquiring, storing and analyzing multifactorial complex gait data, while capturing its non-linear dynamic variability and offering the invaluable benefits of predictive analytics ( 1 ). A recent review article discussed the potential value of ML in gait analysis towards quantification and rehabilitation ( 104 ). The authors concluded that further evidence is required although preliminary data demonstrates that the control strategies for gait rehabilitation benefit from reinforcement learning and (deep) neural-networks due to their ability to capture participants' variability. This review paper demonstrated the success of ML techniques in detecting gait disorders, predicting rehabilitation length, and control of rehabilitation devices. Further work is needed for verification in clinical settings.
4.4. Data-driven gait rehabilitation in clinical settings
Quantitative gait assessment is invaluable towards disease-specific and patient specific rehabilitation/therapeutic interventions. Spatiotemporal, kinematic, and kinetic parameters obtained during instrumented gait assessment can help clinicians benchmark, devise strategies, and evaluate the effect of various rehabilitation interventions. Gait disorders not only affect these parameters, and patterns and time spent in the various gait phases, but can also highly impact gait symmetry, and regularity, depending on the disease and severity ( 105 ). Increasing evidence supports a data-driven physical rehabilitation approach to the treatment of functional gait disturbance ( 106 ). There are multiple examples in literature on the effective use of quantitative gait measures towards more effective data-driven rehabilitation. A recent review by Biase et al. ( 107 ) studied the most relevant technologies used to evaluate gait features and the associated algorithms that have shown promise to aid diagnosis and symptom monitoring towards rehabilitation in Parkinson's disease (PD) patients. They reported physical kinematic features of pitch, roll and yaw rotations of the foot during walking, based on which feature extraction and classification techniques, such as principal component analysis (PCA) and support vector machines (SVM) method were used to classify the PD patients. They also used gait features, including step duration, rise and fall gradients of the swing phase, as well as standard deviation of the minima as quantitative measures, for benchmarking and monitoring PD motor status during rehabilitation. Interestingly, this review sheds light on need to change the evaluated gait features as a function of disease progression. Another study was Pistacchi et al. ( 108 ) suggested spatiotemporal gait parameters, such as speed and step length, where reduced step length seems to be a specific feature of Parkinson's disease gait particularly in early disease stages. On the other hand, asymmetry, step shuffling, double-limb support and increased cadence are more common in mild to moderate stages, while advanced stages are more frequent freezing of gait (FOG) and motor blocks, reduced balance and postural control, motor fluctuations and dyskinesia ( 109 ). Researchers have also investigated the evaluation of ambulatory systems for gait analysis post hip replacement ( 110 ). They found gait characteristics such as stride length and velocity, as well as thigh and shank rotations different from healthy individuals and recommended their use to monitor post-surgical rehabilitation efficacy. Spatiotemporal gait parameters, such as step length, width and cadence have been used ( 111 ) to assess the effect of swing resistance and assistance rehabilitation on gait symmetry in hemiplegic patients. Investigators have also studied whether specific variables measured routinely at a rehabilitation center were predictors of gait performance of hemiparetic stroke patients ( 112 ). They found that motor control and balance were the best predictors of gait performance. A recent review article on assessment methods of post stroke gait suggests that multiple spatiotemporal, kinematic, and kinetic parameters can be useful in diagnosing post-stroke gait dysfunction and as quantitative measures to evaluate rehabilitation outcomes ( 1 ). Spatiotemporal characteristics of post-stroke gait include reduced step or stride length, increased step length on the hemiparetic side, wider base of support, greater toe-out angle, reduced walking speed and cadence. Stride time, stance period on both lower limb, and double support time are also increased, in addition to less time in stance and more time in swing phase for the paretic side, as well as asymmetries in spatial and temporal factors. Kinematic parameters associated with hemiplegic gait (reduced mean peak extension of the hip joint in late stance, alterations in the lateral displacement of the pelvis and flexion of the knee, and decreased plantarflexion of the ankle at toe-off, in addition to a significant decrease in peak hip and knee flexion during the swing phase, reduced knee extension prior to initial contact, as well as decreased ankle dorsiflexion during swing), and kinetic parameters (asymmetric patterns, as well as decreased amplitudes of the joint moments and joint powers at the hip, knee, and ankle joints on the paretic side) can be used as quantitative means to design and evaluate effective rehabilitation ( 113 – 115 ). IGA has also been successfully used to quantify and improve gait dysfunction associated with ageing and assess the risk of falling ( 116 ). Spatiotemporal gait parameters such as velocity, swing time, stride length, stride time- and double support time variability, as well as heel strike and toe off angles, and foot clearance, have been suggested as plausible indicative quantitative measures ( 116 ) to assess the risk of falling in elderly subjects. Inertial sensor-equipped shoes additionally provided heel strike and toe off angles, and foot clearance ( 116 ). The study ( 117 ) summarizes that multi-component exercise therapy which consisted of strength, ROM exercise, balance, flexibility and stretching exercises, circuit exercise training, and gait training was found to enhance gait function for individuals suffering with diabetic peripheral neuropathy compared to control groups using spatiotemporal gait parameters like velocity, cadence, step length, step time, double support time, stride length, stride time, ankle ROM. Gait assessment has potential to develop patient training paradigms for overcoming gait disorders ( 111 ).
5. Mobile gait lab for clinical applications and beyond
In recent decades, the healthcare field has witnessed a tremendous interest in the use of wearable sensing modalities and AI-driven data management/analysis techniques for patient diagnosis, monitoring, and rehabilitation. The portability, lightweight, ease of use, and high-power efficiency are some of the factors that promote applicability to a clinical platform.
There are few examples in literature demonstrating the potential success of using wearable-based systems for gait assessment in clinical settings. Prajapati et al. assessed the walking activity of inpatients with subacute stroke using commercial accelerometers attached above the ankle. They found that the walking bouts were shorter in duration and gait was more asymmetric ( 118 ). Studies have established test-retest reliability and accuracy of different sensor technologies; however, further validation trials are recommended prior to any clinical use. Hsu et al. assessed the test-retest reliability of an accelerometer-based system with infrared assist for measuring spatiotemporal parameters, including walking speed, step length, and cadence, as well as trunk control parameters, including gait symmetry, gait regularity, acceleration root mean square, and acceleration root mean square ratio of healthy subjects in hospital ( 119 ). This study showed excellent test-retest reliability of the parameters considered, and thus highlighting the reliability of an infrared assisted, trunk accelerometer-based device for clinical gait analysis. Another study investigated the concurrent validity and test-retest reliability of gait parameters (cadence, gait velocity, step time, step length, step time variability, and step time asymmetry) acquired from elderly subjects, using a tri-axial accelerometer attached to the center of body mass ( 120 ). In comparison to a reference GAITRite system, the acquired parameters showed good validity and reliability. Poitras et al. performed a systematic review of 42 studies assessing the reliability and validity of wearable sensors, specifically, IMUs, for quantifying the joint motion ( 121 ). Evidence suggests that IMU could be an alternative solution to an expensive motion capture system, as it shows good validity for lower-limb analysis involving fewer complex tasks. However, more work is needed to draw a better conclusion with regards to its reliability, as well as to standardize the protocol to get more accurate data in a clinical setting. Importantly, additional research efforts are needed to examine the responsiveness of wearables in free-living conditions in hospital settings.
6. Limitations
This review aimed to summarize available published work on the present and future of gait analysis in clinical settings. The focus was to highlight current systems, scales, and indices, as well as recent technology-driven gait characterization and analysis approaches and their applicability to clinical settings. Within this context, pathological gait associated with different disease, as well as ageing was briefly discussed. As such, this article may have not covered the complete spectrum of gait pathologies and associated parameters. A scoping (non-systematic) search methodology was selected to broaden the scope and integration of the three main aspects of focus (gait pathology, clinical assessment, recent tools, and technologies). In addition, we do not recommend any specific protocol over the other, as most of the papers incorporate different inclusion/exclusion criteria for subject selection, as well as different sampling sizes, which may render comparisons unrealistic.
7. Conclusive remarks and future work
This scoping review aimed to shed light on the status of gait assessment in clinical settings, as well as the state-of-the-art emerging tools and technologies and their potential clinical applicability. Clinical gait analysis continues to rely mainly on observational gait and quantitative scales and is hence subjective and suffers from variability and the lack of sensitivity influenced by the observer's background and experience. Based on the reviewed literature, quantitative IGA-based gait analysis, commonly used in research labs, has the capability of providing clinicians with accurate and reliable gait data for informed diagnosis and continuous monitoring. On the other hand, several factors, including high cost and infrastructure challenges; data variability, complexity, and multidimensionality; lack of sufficient knowledge and standardized training in clinical environments; and time constraints, continue to limit its wide-spread deployment. Rapidly emerging smart wearable technology and AI, including Machine Learning, Support Vector Machine, and Neural Network approaches, are increasingly playing a bigger role in gait assessment. Although their use in clinical settings is not yet well leveraged, these tools promise an unprecedented paradigm shift in the quantification of gait in the clinic and beyond, as they provide means for acquiring, storing, and analyzing multifactorial complex gait data, while capturing its non-linear dynamic variability and offering the invaluable benefits of predictive analytics.
Researchers are also paying increased attention to multisource and multi-modality sensor fusion approaches, which can further add value by integrating the output of multiple sensors to capture the complexity and variability of gait. Multimodality sensor fusion also allows for simultaneous monitoring of various physiological signals during locomotion, such as EMG, ECG, and EEG, where fusing these with various gait measures (spatiotemporal, kinematic, and kinetic) can shed light on underlying health conditions and disease etiology towards better informed outcome prediction and clinical decisions. As the volume of data from the variety of sensors, including electroencephalography, electro-oculography, electro-cardiography, and electromyography, motion capture and force sensors data, substantially increases, more AI-driven sophisticated data management and modeling are needed to quantify and interpret complex network AI/NN models. Models which include static and dynamic features, combined with sophisticated data reduction and individualized feature selection of the most relevant gait characteristics are needed to close the loop for this paradigm shift. Future work is warranted on a multidisciplinary level: to validate the clinical applicability and integration of the various sensing modalities, to ensure proper synchronization of the various systems for accurate continuous real-time monitoring, to develop and validate fast and reliable computational platforms, and to implement modular user-friendly interfaces easy to use in any environment.
In summary, instrumented gait analysis is a well-established tool for the quantitative assessment of gait dysfunction which could be effectively used for functional diagnosis, treatment/surgery/rehabilitation/planning, and progression monitoring for a wide spectrum of disease. The literature indicates that recent advancement in wearable technology and computationally advanced data analytics, including AI, can overcome the challenges of traditional gait labs, allowing for less costly, portable, and relatively simple gait testing protocols in clinical settings, as well as user-friendly data management, analysis, and interpretation computational platforms. On the other hand, the development of clinically driven standardized methodology and procedures is of paramount significance and remains largely unaddressed. These standardized practices should not only focus on quantitative gait diagnosis but should also incorporate sophisticated objective measures and 3-D dynamic gait profiles and markers for monitoring progress and outcome prediction and evaluation. Proper gait protocols should be devised and leveraged towards identifying gait characteristics that could be effectively used as early disease diagnostic markers. Importantly, training clinical teams at various levels, from doctors and surgeons to physiotherapists and other allied health professionals, on properly using these novel assessment and computational tools is equally important and warrants an equally rapid paradigm shift in training and practice in clinical settings towards patient-specific precise medicine.
This publication is based upon work supported by the HEIC at Khalifa University of Science and Technology.
Author contributions
AAH, MR and KK conceived the idea. AAH, DMM, KK and MR formulated the objective for this review. AAH designed the search strategy, conducted abstract screening and full text review, extracted the data, and drafted the manuscript. KK, NA, DMM, and AAH contributed to writing the manuscript. DMM and NA performed a part of the literature survey, including abstract screening, full text review, and data extraction. MR, and KK provided significant guidance on the content of the manuscript, overall supervision, and critical feedback. All authors contributed to the article and approved the submitted version.
Conflict of interest
The authors declare that the research was conducted in the absence of any commercial or financial relationships that could be construed as a potential conflict of interest.
Publisher's note
All claims expressed in this article are solely those of the authors and do not necessarily represent those of their affiliated organizations, or those of the publisher, the editors and the reviewers. Any product that may be evaluated in this article, or claim that may be made by its manufacturer, is not guaranteed or endorsed by the publisher.
Learn how UpToDate can help you.
Select the option that best describes you
- Medical Professional
- Resident, Fellow, or Student
- Hospital or Institution
- Group Practice
- Patient or Caregiver
- Find in topic
RELATED TOPICS
INTRODUCTION
The term "gait analysis" encompasses a broad spectrum of potential assessment strategies used to evaluate normal and abnormal gait, both walking and running. Such assessments range from simple observation to sophisticated computer analysis of biomechanics. This topic is intended to help general clinicians and primary care sports medicine physicians understand how to perform a basic analysis of a patient's normal walking and running gait and to recognize some common gait abnormalities. It is not intended to address all clinical variations. Such techniques as computer-assisted gait analysis, force platforms, pressure sensors, and other sophisticated modes of analysis are beyond the scope of this topic, as is the assessment of complex pathologic conditions, including cerebral palsy, other pediatric neuropathic gait patterns, and gait involving use of a prosthetic limb [ 2 ]. Specific injuries that may stem from abnormal gait patterns are reviewed in greater detail separately. (See "Running injuries of the lower extremities: Risk factors and prevention" and "Overview of foot anatomy and biomechanics and assessment of foot pain in adults" and "Overview of stress fractures" and "Stress fractures of the tibia and fibula" and "Hamstring muscle and tendon injuries" and "Patellofemoral pain" and "Iliotibial band syndrome" .)
GAIT CYCLE BASICS
● Stance phase: Period during gait when the foot is on the ground.
• Contact, including foot strike and early stance
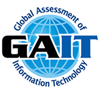
- Individuals
- Why GAIT is used in Corporate
Net One Systems Co., Ltd.
Hitachi solutions, ltd..
- KDDI Corporation
- ITOCHU Techno-Solutions Corporation
- Case Studies
GAIT is being used by a vast number of companies to monitor the skills of IT engineers and the results are being leveraged in training and professional development efforts.
Customer Profile
- Head Office: JP TOWER, 2-7-2 Marunouchi, Chiyoda-ku, Tokyo
- TEL: +81-3-6256-0600
- http://www.netone.co.jp/
- Date of Establishment: February 1, 1988
- Capital: 12,279 million yen
- Total Employee: 2,210 (Consolidated as of March 31, 2013)
- Main Business: ICT infrastructure related consulting, design, construction, operation, maintenance, etc.
- Head Office: 4-12-7 Higashishinagawa, Shinagawa-ku, Tokyo 140-0002, Japan
- TEL: +81-3-5780-2111
- http://www.hitachi-solutions.co.jp/
- Date of Establishment: September 21, 1970
- Capital: 38,758,139,571yen
- Total Employee: 15,452 (consolidated) * As of March 31, 2013
- Main Business: Software services, Information processing hardware sales
- Head Office:35th Floor, Tokyo Opera City Tower 3-20-2 Nishi-Shinjuku, Shinjuku-ku, Tokyo 163-1435, Japan
- TEL: +81-3-5333-6789
- http://www.mti.co.jp/
- Date of Establishment: August 12, 1996
- Capital: 22,562 million yen
- Total Employee: 802(Consolidated Dec. 31, 2013)
- Main Business: Content distribution for mobile phones
KDDI CORPORATION
- Head Office: Garden Air Tower, 3-10-10, Iidabashi, Chiyoda-ku Tokyo 102-8460, Japan
- TEL: +81-3-3347-0077
- http://www.kddi.com/english/
- Date of Establishment: June 1, 1984
- Capital: 141,851 million JPY
- Total Employee: 20,238 (consolidated base) As of March 31,2013
- Main Business: Telecom business
ITOCHU Techno-Solutions Corporation (CTC)
- Head Office:Kasumigaseki Bldg., 3-2-5, Kasumigaseki, Chiyoda-ku, Tokyo 100-6080
- TEL: +81-3-6203-5000
- URL: http://www.ctc-g.co.jp
- Date of Establishment: April 1, 1972
- Capital: 21,763 million yen
- Total Employee: CTC Group Total:7,238(As of April 1, 2012)
- Main Business:Sales, maintenance and support of computers and network systems; commissioned software development; information processing services; information services related to science and engineering; support; other
Inquiries about GAIT
Contact us for more information about adopting GAIT, its merits and detailed case studies.

GAIT is a global assessment tool designed to accurately and quantitatively evaluate the skills of IT engineers. GAIT’s assessment content covers seven fields spanning 22 categories, and the results offer clear insights into an engineer’s competency in IT. As of December 2014, GAIT had been implemented in over 160 countries and regions around the world.
Key Contents
Latest news.
- New Year’s Holiday Closing Notice December 1, 2021
- GAIT 2.0 Assessment summary is ready. November 9, 2021
- Notice of Scheduled Maintenance July 6, 2021
- Terms of Use
- Privacy Policy
- Information Security Policy
- Commitment to Impartiality
- Open access
- Published: 23 September 2022
Quantitative gait analysis in mild cognitive impairment, dementia, and cognitively intact individuals: a cross-sectional case–control study
- Sunee Bovonsunthonchai ORCID: orcid.org/0000-0003-1044-3174 1 ,
- Roongtiwa Vachalathiti ORCID: orcid.org/0000-0002-4626-1463 1 ,
- Vimonwan Hiengkaew ORCID: orcid.org/0000-0002-0842-6976 1 ,
- Mon S. Bryant ORCID: orcid.org/0000-0002-6016-8169 2 ,
- Jim Richards ORCID: orcid.org/0000-0002-4004-3115 3 &
- Vorapun Senanarong ORCID: orcid.org/0000-0002-2774-4187 4
BMC Geriatrics volume 22 , Article number: 767 ( 2022 ) Cite this article
2623 Accesses
8 Citations
Metrics details
Cognitive age-related decline is linked to dementia development and gait has been proposed to measure the change in brain function. This study aimed to investigate if spatiotemporal gait variables could be used to differentiate between the three cognitive status groups.
Ninety-three older adults were screened and classified into three groups; mild cognitive impairment (MCI) ( n = 32), dementia ( n = 31), and a cognitively intact ( n = 30). Spatiotemporal gait variables were assessed under single- and dual-tasks using an objective platform system. Effects of cognitive status and walking task were analyzed using a two-way ANCOVA. Sub-comparisons for between- and within-group were performed by one-way ANCOVA and Paired t-tests. Area Under the Curve (AUC) of Receiver Operating Characteristics (ROC) was used to discriminate between three groups on gait variables.
There were significant effects ( P < 0.05) of cognitive status during both single and dual-task walking in several variables between the MCI and dementia and between dementia and cognitively intact groups, while no difference was seen between the MCI and cognitively intact groups. A large differentiation effect between the groups was found for step length, stride length, and gait speed during both conditions of walking.
Conclusions
Spatiotemporal gait variables showed discriminative ability between dementia and cognitively intact groups in both single and dual-tasks. This suggests that gait could potentially be used as a clinical differentiation marker for individuals with cognitive problems.
Peer Review reports
Dementia affects approximately 47 million people worldwide, with 10 million new cases reported each year [ 1 ]. In East Asia, the prevalence of dementia is increasing which has been shown to be associated with cardiovascular risk factors [ 2 ] or exposure to air pollution [ 3 ]. In contrast, the declination of dementia incidence was reported in high-income countries such as the United States, the United Kingdom, Sweden, the Netherlands, France, and Iceland [ 2 , 4 ]. In Thailand, the majority of cases were caused by Alzheimer's disease (AD), followed by vascular dementia, dementia with Lewy bodies, frontotemporal dementia, and others [ 5 ]. Dementia is a group of different progressive diseases that currently has no cure and is arguably the most pressing public health challenge [ 2 , 6 ]. It is a syndrome that primarily affects older people and is characterized by a decline in cognitive performance that affects the individual's ability related to cognitive and physical functions [ 1 , 7 , 8 , 9 , 10 ]. Dementia and falls were reported as the top five causes of disability-adjusted life-years (DALYs) in people aged 75 and older [ 11 ]. This is an important cause of disability and dependency and has an impact on family and carers' physical, psychological and social well-being [ 1 , 6 , 12 ]. Among the various problems faced, individuals with dementia frequently experience gait and balance issues, which contribute to a significantly increased risk of falling [ 13 , 14 , 15 , 16 ], leading to fractures, hospitalization, functional dependence, or death [ 1 , 14 , 15 ].
Early detection of dementia is frequently challenging, as the functional deficits required for such diagnoses can be caused or exacerbated by comorbidities and social circumstances [ 17 , 18 , 19 ]. Progression of the disease escalates over time if individuals are misdiagnosed, which leads to inappropriate clinical management [ 10 , 20 , 21 , 22 ]. As a result, it is crucial to detect dementia quickly and accurately, as well as to monitor the disease progression. substantial evidence demonstrates that timely diagnosis and treatment can prevent the progression of dementia or its consequences [ 16 , 21 , 23 , 24 ]. A non-invasive, sensitive, and cost-effective marker is therefore required to help identify and classify individuals with cognitive decline [ 25 ].
Gait performance has been studied to determine its efficacy in screening individuals with cognitive dysfunction. Clinical and epidemiologic evidence supports the concept that gait is interrelated with cognitive ability in the elderly [ 26 , 27 , 28 , 29 ]. It has been reported that healthy younger individuals can preserve their primary walking when faced with a secondary cognitive task, whereas older adults have difficulty in preserving their gait performance during dual tasks, and this capability is greatly reduced in those with cognitive dysfunction [ 21 , 30 , 31 , 32 ]. An attempt to investigate the relationship between gait and cognition not only provides a better understanding of function but also provides an assessment of safety during movement. Decreased ability to walk during a single- or cognitive dual-task has been reported to be a contributing factor to falls in older adults and individuals with cognitive decline [ 33 , 34 ]. Dual-task gait assessment, where individuals have to perform a secondary attentional challenging task while walking, was shown to be more challenging as the tasks interfere with each other and divide the cortical control resources of the brain [ 35 ]. Gait modifications, such as reduced gait speed, rhythm, sway, instability, or stopping walking, can occur while performing the second task together, especially in individuals with cognitive decline [ 35 , 36 , 37 ]. In addition, a meta-analysis has demonstrated the importance of gait and cognition factors with the dual decline of gait speed and memory having the highest predictive risk of developing dementia among older individuals [ 31 ].
The role of using gait in classifying individuals with or without cognitive decline has been shown in the studies [ 16 , 38 , 39 , 40 , 41 , 42 , 43 , 44 , 45 , 46 , 47 ]. However, based on the different reports previously, the results varied from study to study. This may be a result of differences in participants' characteristics, measuring equipment, testing variables, and the walking data collection process. Yet most of the reported findings came from western countries [ 40 , 41 , 42 , 43 , 44 , 45 , 46 , 47 ] and less was done in Asian countries and reported on a small sample size [ 16 , 38 , 39 ]. Different biological structures, cultures, living behaviors, quality of life, pollution and environment, and education all could influence gait and cognitive profiles. Hence, data from the studies on different populations, especially people in Asian countries, are still needed. In addition, which gait variables are the most sensitive and practical to discriminate between groups of individuals with different cognitive levels is needed. This study therefore explored the use of gait profiles to differentiate people with different cognitive profiles within a South East Asian population.
Therefore, this study aimed to conduct a case–control study to differentiate individuals with and without cognitive impairment. We hypothesized that gait variables would be sensitive enough to differentiate between the groups with and without cognitive impairment, and these would be easier to detect under dual-task conditions.
Study design and recruitment
A cross-sectional case–control study with a convenience sampling method was used. Individuals living with MCI and dementia from the Memory Clinic, Siriraj hospital, Bangkok, Thailand were invited to participate in the study. The individuals who were cognitively intact were recruited from able-bodied individuals who live in Bangkok and metropolitan areas through word-of-mouth. All participants voluntarily participated in the study and were informed about the research objectives, benefits, and details before data collection. Once they understood and agreed to participate, project staff made an appointment for the date and time to measure gait and other related data.
Participants
Participants in the MCI, dementia, and cognitively intact groups were subjectively and physically examined by neurologists. Participants were community-dwelling middle and older adults with or without a diagnosis of MCI or dementia. Diagnosis of MCI and dementia was performed through the consensus of expert neurologists and psychologists who have routinely worked at Siriraj hospital based on the Diagnostic and Statistical Manual of Mental Disorders, 5 th edition (DSM-5) criteria [ 48 ] and the National Institute of Aging-Alzheimer’s Associaton criteria [ 49 ]. The DSM-5 criteria indicate the key symptoms used to distinguish MCI from dementia as the evidence of modest cognitive decline from a previous level of performance in one or more of the listed domains (cognitive deficits are not interfering with the independence of instrumental activities of daily living, cognitive deficits do not occur exclusively in the context of a delirium, and cognitive deficits are not primarily attributable to another mental disorder). The latter criteria indicate the preservation of independence in functional abilities and lack of significant impairment in social or occupational functioning for the MCI. Sub-types of MCI were defined based on the presence or absence of memory difficulties (amnestic and non-amnestic MCI). For the cognitively intact individuals, they had normal cognitive performance and were independent in their daily living activities. The exclusion criteria for all groups of participants were; severe cognitive function, unable to follow commands and instructions, serious infection, psychiatric disorder, epilepsy, and any other conditions that may affect the performance of the walking tasks such as severe pain, being blind, or deaf.
The study was conducted at the Neuro Computational Intelligence for Neuro-Cognitive disorders Laboratory (NN lab), Siriraj Medical Research Center (SiMR), Faculty of Medicine, Siriraj Hospital, Mahidol University, Bangkok, Thailand. Demographic data recorded included; sex, age, body weight and height, body mass index (BMI), year of education, cognitive function using the Montreal Cognitive Assessment (MoCA), disease duration of the cognitive decline, types, and underlying diseases. MoCA is one of the cognitive tools which was developed by Nasreddine et al. [ 50 ]. The total possible score of MoCA ranges from 0–30 scores assessing several cognitive domains (visuospatial/executive, naming, memory, attention, language, abstraction, delayed recall, and orientation). It has been tested for sensitivity and is widely used to investigate cognitive status [ 50 , 51 , 52 , 53 ]. In addition, the Functional Ambulatory Category (FAC) is a six-point scale used to evaluate functional gait status. The scale assesses the level of support that the patient requires during walking, regardless of whether or not an assistive device is used [ 54 ]. The ambulatory status could be classified into six levels, ranging from non-functional ambulator (FAC = 0) to independent ambulator (FAC = 5). This is a quick visual measurement of functional gait ability and is administered in individuals with dementia [ 55 ].
Spatiotemporal gait variables were then collected at 100 Hz using the Force Distribution Measurement (FDM) platform which had a length of 307 cm and width of 60.5 cm (Zebris Medical GmbH, Germany). The platform was installed in the middle part of a 5 m walkway and was synchronized with a video camera (SYNCCam) placed in front of the participants to check walking patterns for each trial. The FDM platform is widely used for gait assessment in various neurological conditions and was used as a gold standard to another proposed tool [ 56 , 57 , 58 , 59 ]. To remove the effect of the acceleration and deceleration phases of walking, gait data were recorded from the middle part of the walkway. Spatiotemporal gait data were extracted and analyzed using the WinFDM software (Zebris Medical GmbH, Germany, version 1.18.48).
For the single-task condition, the participants were asked to walk at their own usual walking pace on the walking platform. For the dual-task condition, to monitor the compliance of the testing, participants were asked to count a number backward by one out loud while walking at their usual walking pace [ 39 , 40 ]. A physical therapist walked slightly behind the participants to prevent slips, trips, and falls during the data collection. Three successful trials were recorded under each condition and the average of the three trials was used in the data analysis.
Spatiotemporal gait variables were used for the comparison and discrimination analyses. These included; foot rotation angle (deg), step length (cm), stride length (cm), step width (cm), stance phase as a percentage of the gait cycle (%GC)), loading response (%GC), single support phase (%GC), pre-swing (%GC), swing phase (%GC), double support phase (%GC), step time (s), stride time (s), cadence (steps/min), and gait speed (m/s).
Data analysis
SPSS version 26.0 (IBM Corp, USA) was used for all analyses. Participant characteristics and clinical variables were descriptively analyzed. A two-way ANCOVA was used to determine the main effects and interaction effects of the cognitive status groups (MCI, dementia, and cognitively intact) and walking task (single and dual). Comparisons of gait variables between the MCI, dementia, and cognitively intact groups during single- and dual-task walking were explored using one-way ANCOVA tests. Regarding age, sex, and BMI are the biological important factors that could affect gait variables, so these factors were used as the covariates in the analysis and the adjusted mean values were reported.
Comparisons of gait variables between single- and dual-task walking were performed within the groups using the Paired t-test. A Bonferroni correction for multiple comparisons was used for the two-way ANCOVA and one-way ANCOVA tests, and adjusted p -values were used for the Paired t-test analysis by dividing the p -value by the number of variables being tested or number of the test ( P < 0.05/14 or P < 0.004). The area under the curve (AUC) of the Receiver Operating Characteristics (ROC) was also used to determine the accuracy of discrete gait parameters between the MCI, dementia, and cognitively intact groups. Accuracy of AUC 0.5 was considered as no discrimination, 0.7–0.8 was considered acceptable, 0.8–0.9 was considered excellent, and more than 0.9 was considered outstanding [ 60 ].
Participant characteristics
Participant characteristics are presented in Table 1 . Ninety-three participants (70 females and 23 males) were included in this study and were classified into MCI ( n = 32), dementia ( n = 31), and cognitively intact ( n = 30) groups. No differences were found between the groups for sex, body weight, height, and body mass index, with an age range of 63.6 − 71.8 years and mean body weight and height of 56.7 − 63.0 kg and 155.9 − 158.4 cm, respectively. A greater number of females were seen in all three groups (68.75 − 86.67%). Age, year of education, and MoCA showed significant differences between the groups ( P < 0.05), with the cognitively intact group being the youngest (63.6 years) and the dementia group being the oldest (71.8 years). The cognitively intact group had the highest year of education (15.9 years) and the dementia group had the lowest (10.4 years). The greatest median MoCA score was seen in the cognitively intact group (28 scores), followed by the MCI group (25 scores), and the lowest was seen in the dementia group (14 scores). The mean onset duration of MCI was 3.38 years, with a similar number of individuals with amnestic and non-amnestic types ( n = 15 and n = 17), respectively. The mean onset duration of dementia was 4.68 years, with the most common type being Alzheimer's disease (AD) ( n = 20), followed by Frontotemporal Lobar Degeneration (FTLD) ( n = 6), Dementia with Lewy Bodies (DLB) ( n = 3), and Vascular dementia (VaD) ( n = 2). Hypertension was the only underlying disease that showed a difference between groups, with the dementia group having the highest number of individuals ( n = 19 or 65.52%), followed by the MCI group ( n = 17 or 60.71%), with only 15.3% in the cognitively intact group ( n = 4). No other differences were found for underlying diseases between the groups ( P > 0.05). All participants in this study could walk on the surface, as shown with the FAC score of 4 and 5 scores. It was found that most individuals with MCI and dementia were able to walk independently (FAC = 5).
The main effects and interactions of cognitive status and walking task on gait variables
The two-way ANCOVA demonstrated significant interaction effects for cognitive status (MCI, dementia, and cognitively intact) and walking task (single and dual) including step time ( P = 0.037, η 2 p = 0.038) and stride time ( P = 0.036, η 2 p = 0.038). Significant main effects of cognitive status were found in several variables; step length ( p < 0.001, η 2 p = 0.171), stride length ( p < 0.001, η 2 p = 0.171), step width ( P = 0.012, η 2 p = 0.050), stance phase ( P < 0.001, η 2 p = 0.114), loading response ( P < 0.001, η 2 p = 0.117), single support phase ( P < 0.001, η 2 p = 0.098), pre-swing ( P < 0.001, η 2 p = 0.116), swing phase ( P < 0.001, η 2 p = 0.114), double support phase ( P < 0.001, η 2 p = 0.112), step time ( P = 0.005, η 2 p = 0.060), stride time ( P = 0.005, η 2 p = 0.061), cadence ( P = 0.002, η 2 p = 0.069), and gait speed ( P < 0.001, η 2 p = 0.152).
Significant main effects of walking tasks were found in almost all gait variables including; step length ( P = 0.001, η 2 p = 0.061), stride length ( P = 0.001, η 2 p = 0.061), stance phase ( P = 0.001, η 2 p = 0.062), loading response ( P < 0.001, η 2 p = 0.072), single support phase ( P < 0.001, η 2 p = 0.079), pre-swing ( P < 0.001, η 2 p = 0.076), swing phase ( P = 0.001, η 2 p = 0.062), double support phase ( P < 0.001, η 2 p = 0.072), step time ( P < 0.001, η 2 p = 0.258), stride time ( P < 0.001, η 2 p = 0.259), cadence, ( P < 0.001, η 2 p = 0.284), and gait speed ( P < 0.001, η 2 p = 0.185).
Comparison of gait variables between the groups under the single and dual walking tasks
As shown in Table 2 , during single-task walking, there were significant differences ( P < 0.05) between the groups for step length, stride length, stance phase, loading response, single support phase, pre-swing, swing phase, double support phase, and gait speed. The post-hoc test showed differences between the MCI and dementia groups as well as between dementia and cognitively intact groups. During dual-task walking, significant differences were seen between the groups in all gait variables ( P < 0.05), except for foot rotation angle. The post-hoc test showed differences between the MCI and dementia groups as well as between dementia and cognitively intact groups in almost variables.
Comparisons of gait variables between single and dual-task walking within each group
Paired t-tests were used to compare gait variables between single and dual-task walking within each group separately as shown in Table 2 . A Bonferroni correction was used to adjust the significance level to allow for the number of comparisons. So, the P -value was set at < 0.05/14 or P < 0.004. Significant differences were found in almost gait variables ( P < 0.004) between single- and dual-task walking in the MCI and dementia groups, with exception of the foot rotation angle. For the cognitively intact group, differences were seen only in step length, stride length, step time, stride time, cadence, and gait speed.
Discriminating gait characteristics between the MCI, dementia, and cognitively intact groups
Discriminating gait characteristics between the groups are presented in Table 3 . Based on the accuracy value criteria of the AUC of ≥ 0.7 with a statistical significance of < 0.05, it was found that a single spatiotemporal gait variable differentiated between dementia and cognitively intact groups only. For the single-task walking, three variables including step length, stride length, and gait speed provided excellent discrimination levels between the groups (AUC = 0.823–0.849). During dual-task walking, excellent discrimination was found in the same set of gait variables similar to single-task walking and single support phase (AUC = 0.813–0.823), with the highest discrimination ability shown by gait speed.
No differences in demographic data were seen between the three groups, except for age, year of education, MoCA, and hypertension. The majority of participants in the MCI and dementia groups were female which was consistent with the findings of a previous systematic review [ 61 ]. Our findings revealed that the dementia group had the highest average age, which is not surprising given that the disease is more common in older people and its prevalence increases with age [ 1 , 61 ]. Individuals with AD who participated in our study were in the mild to severe stages with a large range of MoCA scores from 8 − 20. Considering the educational factor, it was not surprising that the dementia group had the lowest level of education. As reported previously, individuals with a higher level of education were more able to maintain cognitive ability than those with a lower level [ 62 ].
Among the comorbidities tested in this study, we found that MCI and dementia groups were more afflicted with hypertension and diabetes mellitus than the cognitively intact group. Hypertension is widely known as a major risk factor of damage to several organs, including the brain. Blood pressure variability and 24-h blood pressure profiles have been linked to cognitive impairment and/or silent cerebral diseases, such as silent cerebral infarction or white matter lesions, both of which are risk factors for cognitive impairment and dementia [ 63 ]. A certain number of MCI and dementia groups were diagnosed with diabetes mellitus ( n = 9 or 31% and n = 7 or 25%, respectively) and cerebrovascular disease ( n = 6 or 21% and n = 3 or 11%). Type 2 diabetes mellitus raises the risk of vascular dementia and Alzheimer's disease. The underlying mechanisms include the increase in neuronal insulin resistance, impaired insulin signaling, pro-inflammatory state, mitochondrial dysfunction, and vascular damage. All of which increase the deposition of β-amyloid, tau proteins, and GSK3β, leading to an earlier onset of dementia [ 64 ].
We found a decrease in gait performance in individuals with cognitive decline when compared with those who were cognitively intact. Specifically decreases in step length, stride length, single support phase, cadence, and gait speed; and increases in stance phase, loading response, pre-swing, double support, step time, and stride time. However, there was no change in the foot rotation angle and step width. Similar to previous findings, these measures of decline in gait performance were noticeable during single-task walking but showed greater deterioration when tested under a dual-task walking condition [ 36 , 40 , 65 , 66 , 67 ]. From our findings, there were significant differences in several gait variables between the MCI and dementia groups and between dementia and cognitively intact groups when tested under single- and dual-task walking. However, there were no differences in gait variables between the MCI and cognitively intact groups, whether testing under single- or dual-task walking. This may be the result of several factors, including 1) differences in the sample criteria and classification, 2) variability of the data observed by a larger standard error, even though the mean differences were similar between the groups, 3) dual-task walking test procedure, in which counting backward with one might challenge cognitive function to a lesser extent than other counting tasks such as subtracting by seven [ 68 ]. Subtraction with one was chosen in this study because the technique can be used to assess the majority of individuals with dementia. Although the method seems to be very simple, there were five individuals with dementia unable to perform this task. However, our counting method may not cognitively challenge a large number of individuals in the MCI group, thus the results cannot be differentiated from the cognitively intact group. A recent systematic review with meta-analysis. reported that the spatiotemporal gait variables (e.g., gait speed, cadence, stride length, stride time, stride time variability, and stance time) could be used to classify normal and dementia groups when tested using a single task. In addition, the dual-task could differentiate between these two groups using gait speed, stride length, and stride time variability only [ 69 ]. Another point of consideration is that the mean values of the gait variables in our study were substantially different from previous reports from Western countries, which may be due to racial, anthropometric, and sociocultural differences.
For the efficacy of using gait variables to compare individuals with different cognitive statuses, all gait variables except foot rotation angle showed significant differences between groups when testing gait under the dual-task condition. When testing with the single-task condition, most gait variables showed discriminating ability between groups, except foot rotation angle, step width, step time, stride time, and cadence. Based on the effect size criterion using the partial Eta square (η 2 p ), which are defined as small (> 0.01), medium (> 0.06), and large (> 0.14) effects. Step length, stride length, and gait speed had large effect sizes when assessed under both the single- and dual-task walking, while other variables (stance phase, loading response, single support phase, pre-swing, swing phase, and double support phase) had medium effect sizes when assessing under the single- and dual-task walking. In addition, medium effects were found in step width, step time, stride time, and cadence when tested under dual-task walking. This was consistent with several previous studies that reported a significant reduction in gait performance as evidenced by reduced step or stride length and reduced gait speed in individuals with cognitive decline compared to healthy controls [ 24 , 39 , 40 , 66 , 67 , 70 , 71 , 72 ]. In addition, gait performance was more impaired in the MCI group with episodic of memory decline [ 41 , 71 , 73 ]. However, the differences among studies were due to the characteristics of the participants, equipment, and procedure. For instance, the study done by Zhou et al. used a body-worn system to determine the most sensitive gait parameter in identifying older adults with and without cognitive frailty. The largest effect size was observed with dual-task gait speed with Cohen’s effect size d of 0.97, p < 0.001 only [ 67 ]. In contrast, another study reported that gait measures during a dual-task gait were not indicative of cognitive impairment in individuals with Parkinson’s disease [ 74 ].
Comparing gait data between single- and dual-task in each group, we found that walking abilities in all groups declined with the cognitive challenge of counting backward by one. Differences were found in most gait variables in both MCI and dementia groups. During the test, we observed that the dementia group demonstrated balance impairments (e.g., unsteady on feet, body swaying). Many of them had an obvious sway during gait with a markedly slower walking speed. This was confirmed by an increase in the double support phase when testing under the dual-task when compared to the single-task, which was 3.25 times higher in the dementia group compared to the cognitively intact group. Likewise, the MCI group was similarly altered and found to be 2.53 times higher than the cognitively intact group. Challenging the brain function by subtracting a number with one while walking seems easy for cognitively intact individuals. However, we found that cognitively intact individuals were still able to preserve function without modifying the phase-related gait variables, although cadence, temporal, and gait speed were found to decrease during the dual-task in this group.
In classifying the groups using the AUC test on different single gait variables as well as the combined variables. The dementia group was differentiated from the cognitively intact group by step length, stride length, single support phase, swing phase, and gait speed under the single- and dual-task walking tests. Cadence can also be used to differentiate these two groups if tested under the dual-task walking condition. Additionally, using the multiple computed gait variables can also be used to differentiate between dementia and cognitively intact groups as well as between the MCI and dementia groups. However, we did not find any variables which could classify the MCI group from the cognitively intact group.
Two previous studies used a wearable tool to differentiate gait in individuals with and without cognitive impairment [ 67 ] and among subtypes of dementia [ 75 ]. Zhou et al. reported that the single and dual-task gait speed could be used to differentiate cognitive frailty individuals with moderate accuracy (AUC of 0.73 for single-task and 0.76 for dual-task) [ 67 ]. MCI or dementia due to AD, dementia with Lewy bodies (DLB), and PD were able to be differentiated using 14 gait characteristics representing pace, variability, rhythm, asymmetry, and postural control [ 75 ]. Whereas this current study identified that all single gait variables, except foot rotation angle, showed the potential to differentiate cognitive statuses under both single and dual-task conditions.
This current study has some limitations as details of variability and asymmetry of gait characteristics were not determined. Gait variables should be analyzed in more diverse perspectives, such as intrapersonal gait variability and asymmetry or the difference between single- and dual-task. Although this study highlights interesting findings a longitudinal study should be performed to obtain a clearer link between the gait parameters and cognition. Comparisons of the data between different types of dementia as well as studying the relationship and underlying mechanism or process between gait and cognition through the mediator should be explored in the future.
Almost all single gait variables, except foot rotation angle and step width, showed the potential to differentiate between cognitive status under both single and dual-task conditions. The largest effects were found in step length, stride length, and in particular gait speed during both walking conditions. Gait speed is therefore recommended to be used to determine early clinical signs of people with cognitive problems, which should improve the accuracy of such diagnostic assessments and highlight the need for early management.
Availability of data and materials
The datasets generated and/or analyzed during the current study are not publicly available due to limitations of ethical approval involving the patient data and anonymity but are available from the corresponding author on reasonable request.
Abbreviations
- Mild cognitive impairment
Alzheimer's disease
Disability-adjusted life-years
Diagnostic and Statistical Manual of Mental Disorders, 5 th edition
Montreal Cognitive Assessment
Force Distribution Measurement
Area under the curve
Receiver operating characteristic
Taylor ME, Close JCT. Dementia. Handb Clin Neurol. 2018;159:303–21.
Article PubMed Google Scholar
Prince M, Ali GC, Guerchet M, Prina AM, Albanese E, Wu YT. Recent global trends in the prevalence and incidence of dementia, and survival with dementia. Alzheimers Res Ther. 2016;8(1):23.
Article PubMed PubMed Central Google Scholar
Ru M, Brauer M, Lamarque JF, Shindell D: Exploration of the global burden of dementia attributable to PM2.5: What do we know based on current evidence? Geohealth 2021, 5(5):e2020GH000356.
Mayer F, Remoli G, Bacigalupo I, Palazzesi I, Piscopo P, Bellomo G, Canevelli M, Corbo M, Vanacore N, Lacorte E. A systematic review of studies reporting a decreasing trend in the incidence and prevalence of dementia. Minerva Med. 2021;112(4):430–40.
Dharmasaroja PA, Assanasen J, Pongpakdee S, Jaisin K, Lolekha P, Phanasathit M, Cheewakriengkrai L, Chotipanich C, Witoonpanich P, Pitiyarn S, et al. Etiology of Dementia in Thai Patients. Dement Geriatr Cogn Dis Extra. 2021;11(1):64–70.
Burks HB, des Bordes JKA, Chadha R, Holmes HM, Rianon NJ: Quality of life assessment in older adults with dementia: A systematic review. Dement Geriatr Cogn Disord 2021:1–8.
Hausdorff JM, Buchman AS. What links gait speed and MCI with dementia? A fresh look at the association between motor and cognitive function. J Gerontol A Biol Sci Med Sci. 2013;68(4):409–11.
Lee NG, Kang TW, Park HJ. Relationship between balance, gait, and activities of daily living in older adults with dementia. Geriatr Orthop Surg Rehabil. 2020;11:2151459320929578.
Ohlin J, Gustafson Y, Littbrand H, Olofsson B, Toots A: Low or declining gait speed is associated with risk of developing dementia over 5 years among people aged 85 years and over. J Aging Phys Act 2021:1–8.
Montero-Odasso M, Speechley M, Muir-Hunter SW, Sarquis-Adamson Y, Sposato LA, Hachinski V, Borrie M, Wells J, Black A, Sejdic E, et al. Motor and cognitive trajectories before dementia: Results from Gait and Brain study. J Am Geriatr Soc. 2018;66(9):1676–83.
Diseases GBD, Injuries C. Global burden of 369 diseases and injuries in 204 countries and territories, 1990–2019: a systematic analysis for the Global Burden of Disease Study 2019. Lancet. 2020;396(10258):1204–22.
Article Google Scholar
Cantarero-Prieto D, Leon PL, Blazquez-Fernandez C, Juan PS, Cobo CS. The economic cost of dementia: A systematic review. Dementia (London). 2020;19(8):2637–57.
McGough EL, Logsdon RG, Kelly VE, Teri L. Functional mobility limitations and falls in assisted living residents with dementia: physical performance assessment and quantitative gait analysis. J Geriatr Phys Ther. 2013;36(2):78–86.
Taylor ME, Brodie MA, van Schooten KS, Delbaere K, Close JCT, Payne N, Webster L, Chow J, McInerney G, Kurrle SE, et al. Older people with dementia have reduced daily-life activity and impaired daily-Life gait when compared to age-sex matched controls. J Alzheimers Dis. 2019;71(s1):S125–35.
Taylor ME, Lasschuit DA, Lord SR, Delbaere K, Kurrle SE, Mikolaizak AS, Kvelde T, Close JCT. Slow gait speed is associated with executive function decline in older people with mild to moderate dementia: A one year longitudinal study. Arch Gerontol Geriatr. 2017;73:148–53.
Velayutham SG, Chandra SR, Bharath S, Shankar RG. Quantitative balance and gait measurement in patients with frontotemporal dementia and Alzheimer Diseases: A pilot study. Indian J Psychol Med. 2017;39(2):176–82.
Borders C, Sajjadi SA. Diagnosis and management of cognitive concerns in the oldest-old. Curr Treat Options Neurol. 2021;23(3):10.
Kondragunta J, Hirtz G. Gait parameter estimation of elderly people using 3D human pose estimation in early detection of dementia. Annu Int Conf IEEE Eng Med Biol Soc. 2020;2020:5798–801.
PubMed Google Scholar
Valkanova V, Ebmeier KP. What can gait tell us about dementia? Review of epidemiological and neuropsychological evidence. Gait Posture. 2017;53:215–23.
Doi T, Makizako H, Tsutsumimoto K, Hotta R, Nakakubo S, Makino K, Suzuki T, Shimada H. Combined effects of mild cognitive impairment and slow gait on risk of dementia. Exp Gerontol. 2018;110:146–50.
Montero-Odasso MM, Sarquis-Adamson Y, Speechley M, Borrie MJ, Hachinski VC, Wells J, Riccio PM, Schapira M, Sejdic E, Camicioli RM, et al. Association of dual-task gait with incident dementia in mild cognitive impairment: Results from the gait and brain study. JAMA Neurol. 2017;74(7):857–65.
van Iersel MB, Hoefsloot W, Munneke M, Bloem BR, Olde Rikkert MG. Systematic review of quantitative clinical gait analysis in patients with dementia. Z Gerontol Geriatr. 2004;37(1):27–32.
Schwenk M, Zieschang T, Englert S, Grewal G, Najafi B, Hauer K. Improvements in gait characteristics after intensive resistance and functional training in people with dementia: a randomised controlled trial. BMC Geriatr. 2014;14:73.
Verghese J, Wang C, Lipton RB, Holtzer R, Xue X. Quantitative gait dysfunction and risk of cognitive decline and dementia. J Neurol Neurosurg Psychiatry. 2007;78(9):929–35.
Varma VR, Ghosal R, Hillel I, Volfson D, Weiss J, Urbanek J, Hausdorff JM, Zipunnikov V, Watts A. Continuous gait monitoring discriminates community-dwelling mild Alzheimer’s disease from cognitively normal controls. Alzheimers Dement. 2021;7(1): e12131.
Google Scholar
Montero-Odasso M, Verghese J, Beauchet O, Hausdorff JM. Gait and cognition: a complementary approach to understanding brain function and the risk of falling. J Am Geriatr Soc. 2012;60(11):2127–36.
Parihar R, Mahoney JR, Verghese J: Relationship of gait and cognition in the elderly. Curr Transl Geriatr Exp Gerontol Rep 2013, 2(3): https://doi.org/10.1007/s13670-13013-10052-13677 .
Scherder E, Eggermont L, Swaab D, van Heuvelen M, Kamsma Y, de Greef M, van Wijck R, Mulder T. Gait in ageing and associated dementias; its relationship with cognition. Neurosci Biobehav Rev. 2007;31(4):485–97.
Valkanova V, Esser P, Demnitz N, Sexton CE, Zsoldos E, Mahmood A, Griffanti L, Kivimaki M, Singh-Manoux A, Dawes H, et al. Association between gait and cognition in an elderly population based sample. Gait Posture. 2018;65:240–5.
Ansai JH, Andrade LP, Rossi PG, Takahashi ACM, Vale FAC, Rebelatto JR. Gait, dual task and history of falls in elderly with preserved cognition, mild cognitive impairment, and mild Alzheimer’s disease. Braz J Phys Ther. 2017;21(2):144–51.
Tian Q, Resnick SM, Mielke MM, Yaffe K, Launer LJ, Jonsson PV, Grande G, Welmer AK, Laukka EJ, Bandinelli S, et al. Association of dual decline in memory and gait speed with risk for dementia among adults older than 60 years: A multicohort individual-level meta-analysis. JAMA Netw Open. 2020;3(2): e1921636.
Rojasavastera R, Bovonsunthonchai S, Hiengkaew V, Senanarong V. Action observation combined with gait training to improve gait and cognition in elderly with mild cognitive impairment A randomized controlled trial. Dement Neuropsychol. 2020;14(2):118–27.
Adam CE, Fitzpatrick AL, Leary CS, Hajat A, Phelan EA, Park C, Semmens EO: The association between gait speed and falls in community dwelling older adults with and without Mild Cognitive Impairment. Int J Environ Res Public Health 2021, 18(7).
Coelho FG, Stella F, de Andrade LP, Barbieri FA, Santos-Galduroz RF, Gobbi S, Costa JL, Gobbi LT. Gait and risk of falls associated with frontal cognitive functions at different stages of Alzheimer’s disease. Neuropsychol Dev Cogn B Aging Neuropsychol Cogn. 2012;19(5):644–56.
Yogev-Seligmann G, Hausdorff JM, Giladi N. The role of executive function and attention in gait. Mov Disord. 2008;23(3):329–42.
Maclean LM, Brown LJE, Khadra H, Astell AJ. Observing prioritization effects on cognition and gait: The effect of increased cognitive load on cognitively healthy older adults’ dual-task performance. Gait Posture. 2017;53:139–44.
Szturm T, Maharjan P, Marotta JJ, Shay B, Shrestha S, Sakhalkar V. The interacting effect of cognitive and motor task demands on performance of gait, balance and cognition in young adults. Gait Posture. 2013;38(4):596–602.
Meng X, Yu H, Tham MP. Gait phase detection in able-bodied subjects and dementia patients. Annu Int Conf IEEE Eng Med Biol Soc. 2013;2013:4907–10.
Lin YC, Hsu WC, Wu CK, Chang WH, Wu KP, Wong AM. Comparison of motor performance of upper and lower extremities in dual-task tests in patients with mild Alzheimer’s dementia. Aging Clin Exp Res. 2016;28(3):491–6.
Muir SW, Speechley M, Wells J, Borrie M, Gopaul K, Montero-Odasso M. Gait assessment in mild cognitive impairment and Alzheimer’s disease: the effect of dual-task challenges across the cognitive spectrum. Gait Posture. 2012;35(1):96–100.
Montero-Odasso M, Oteng-Amoako A, Speechley M, Gopaul K, Beauchet O, Annweiler C, Muir-Hunter SW. The motor signature of mild cognitive impairment: results from the gait and brain study. J Gerontol A Biol Sci Med Sci. 2014;69(11):1415–21.
Montero-Odasso M: Gait as a biomarker of cognitive impairment and dementia syndromes. Quo vadis? Eur J Neurol 2016, 23(3):437–438.
Beauchet O, Blumen HM, Callisaya ML, De Cock AM, Kressig RW, Srikanth V, Steinmetz JP, Verghese J, Allali G. Spatiotemporal gait characteristics associated with cognitive impairment: A multicenter cross-sectional study, the intercontinental “Gait, cognition & decline” initiative. Curr Alzheimer Res. 2018;15(3):273–82.
Article CAS PubMed Google Scholar
Boettcher LN, Hssayeni M, Rosenfeld A, Tolea MI, Galvin JE, Ghoraani B. Dual-task gait assessment and machine learning for early-detection of cognitive decline. Annu Int Conf IEEE Eng Med Biol Soc. 2020;2020:3204–7.
PubMed PubMed Central Google Scholar
de Oliveira SF, Ferreira JV, Placido J, Chagas D, Praxedes J, Guimaraes C, Batista LA, Laks J, Deslandes AC. Gait analysis with videogrammetry can differentiate healthy elderly, mild cognitive impairment, and Alzheimer’s disease: A cross-sectional study. Exp Gerontol. 2020;131: 110816.
Ghoraani B, Boettcher LN, Hssayeni MD, Rosenfeld A, Tolea MI, Galvin JE: Detection of Mild Cognitive Impairment and Alzheimer's Disease using dual-task gait assessments and machine learning. Biomed Signal Process Control 2021, 64.
Pieruccini-Faria F, Black SE, Masellis M, Smith EE, Almeida QJ, Li KZH, Bherer L, Camicioli R, Montero-Odasso M. Gait variability across neurodegenerative and cognitive disorders: Results from the Canadian Consortium of Neurodegeneration in Aging (CCNA) and the Gait and Brain Study. Alzheimers Dement. 2021;17(8):1317–28.
American Psychiatric Association. Diagnostic and statistical manual of mental disorders. 5th ed. Washington DC: American Psychiatric Publishing; 2013.
Book Google Scholar
Albert MS, DeKosky ST, Dickson D, Dubois B, Feldman HH, Fox NC, Gamst A, Holtzman DM, Jagust WJ, Petersen RC, et al. The diagnosis of mild cognitive impairment due to Alzheimer’s disease: recommendations from the National Institute on Aging-Alzheimer’s Association workgroups on diagnostic guidelines for Alzheimer’s disease. Alzheimers Dement. 2011;7(3):270–9.
Nasreddine ZS, Phillips NA, Bedirian V, Charbonneau S, Whitehead V, Collin I, Cummings JL, Chertkow H. The Montreal Cognitive Assessment, MoCA: a brief screening tool for mild cognitive impairment. J Am Geriatr Soc. 2005;53(4):695–9.
Davis DH, Creavin ST, Yip JL, Noel-Storr AH, Brayne C, Cullum S: Montreal Cognitive Assessment for the diagnosis of Alzheimer's disease and other dementias. Cochrane Database Syst Rev 2015(10):CD010775.
Pinto TCC, Santos MSP, Machado L, Bulgacov TM, Rodrigues-Junior AL, Silva GA, Costa MLG, Ximenes RCC, Sougey EB. Optimal cutoff scores for dementia and mild cognitive impairment in the Brazilian version of the Montreal Cognitive Assessment among the elderly. Dement Geriatr Cogn Dis Extra. 2019;9(1):44–52.
Tan JP, Li N, Gao J, Wang LN, Zhao YM, Yu BC, Du W, Zhang WJ, Cui LQ, Wang QS, et al. Optimal cutoff scores for dementia and mild cognitive impairment of the Montreal Cognitive Assessment among elderly and oldest-old Chinese population. J Alzheimers Dis. 2015;43(4):1403–12.
Holden MK, Gill KM, Magliozzi MR, Nathan J, Piehl-Baker L. Clinical gait assessment in the neurologically impaired. Reliability and meaningfulness Phys Ther. 1984;64(1):35–40.
CAS PubMed Google Scholar
Lee HH, Hong CT, Wu D, Chi WC, Yen CF, Liao HF, Chan L, Liou TH: Association between ambulatory status and functional disability in elderly people with dementia. Int J Environ Res Public Health 2019, 16(12).
Aung N, Bovonsunthonchai S, Hiengkaew V, Tretriluxana J, Rojasavastera R, Pheung-Phrarattanatrai A. Concurrent validity and intratester reliability of the video-based system for measuring gait poststroke. Physiother Res Int. 2020;25(1): e1803.
Bovonsunthonchai S, Witthiwej T, Ngamsombat C, Sathornsumetee S, Vachalathiti R, Muangpaisan W, Hengsomboon P, Thong-On S, Jankhum S, Yangyoo P. Effect of spinal tap test on the performance of sit-to-stand, walking, and turning in patients with idiopathic normal pressure hydrocephalus. Nagoya J Med Sci. 2018;80(1):53–60.
Pheung-phrarattanatrai A, Bovonsunthonchai S, Heingkaew V, Prayoonwiwat N, Chotik-anuchit S. Improvement of gait symmetry in patients with stroke by motor imagery. J Med Assoc Thai. 2015;98(Suppl 5):S113-118.
Bovonsunthonchai S, Vachalathiti R, Pisarnpong A, Khobhun F, Hiengkaew V. Spatiotemporal gait parameters for patients with Parkinson’s disease compared with normal individuals. Physiother Res Int. 2014;19(3):158–65.
Mandrekar JN. Receiver operating characteristic curve in diagnostic test assessment. J Thorac Oncol. 2010;5(9):1315–6.
Cao Q, Tan CC, Xu W, Hu H, Cao XP, Dong Q, Tan L, Yu JT. The prevalence of dementia: A systematic review and meta-analysis. J Alzheimers Dis. 2020;73(3):1157–66.
Lenehan ME, Summers MJ, Saunders NL, Summers JJ, Vickers JC. Relationship between education and age-related cognitive decline: a review of recent research. Psychogeriatrics. 2015;15(2):154–62.
Turana Y, Tengkawan J, Chia YC, Hoshide S, Shin J, Chen CH, Buranakitjaroen P, Nailes J, Park S, Siddique S, et al. Hypertension and dementia: A comprehensive review from the HOPE Asia Network. J Clin Hypertens (Greenwich). 2019;21(8):1091–8.
Bello-Chavolla OY, Antonio-Villa NE, Vargas-Vazquez A, Avila-Funes JA, Aguilar-Salinas CA. Pathophysiological mechanisms linking type 2 diabetes and dementia: Review of evidence from clinical, translational and epidemiological research. Curr Diabetes Rev. 2019;15(6):456–70.
Allali G, Kressig RW, Assal F, Herrmann FR, Dubost V, Beauchet O. Changes in gait while backward counting in demented older adults with frontal lobe dysfunction. Gait Posture. 2007;26(4):572–6.
Gillain S, Warzee E, Lekeu F, Wojtasik V, Maquet D, Croisier JL, Salmon E, Petermans J. The value of instrumental gait analysis in elderly healthy, MCI or Alzheimer’s disease subjects and a comparison with other clinical tests used in single and dual-task conditions. Ann Phys Rehabil Med. 2009;52(6):453–74.
Zhou H, Park C, Shahbazi M, York MK, Kunik ME, Naik AD, Najafi B: Digital biomarkers of cognitive frailty: The value of detailed gait assessment beyond gait speed. Gerontology 2021:1–10.
Bahureksa L, Najafi B, Saleh A, Sabbagh M, Coon D, Mohler MJ, Schwenk M. The impact of mild cognitive impairment on gait and balance: A systematic review and meta-analysis of studies using instrumented assessment. Gerontology. 2017;63(1):67–83.
Chiaramonte R, Cioni M. Critical spatiotemporal gait parameters for individuals with dementia: A systematic review and meta-analysis. Hong Kong Physiother J. 2021;41(1):1–14.
Allali G, Dubois B, Assal F, Lallart E, de Souza LC, Bertoux M, Annweiler C, Herrmann FR, Levy R, Beauchet O. Frontotemporal dementia: pathology of gait? Mov Disord. 2010;25(6):731–7.
Allali G, Annweiler C, Blumen HM, Callisaya ML, De Cock AM, Kressig RW, Srikanth V, Steinmetz JP, Verghese J, Beauchet O. Gait phenotype from mild cognitive impairment to moderate dementia: results from the GOOD initiative. Eur J Neurol. 2016;23(3):527–41.
Maquet D, Lekeu F, Warzee E, Gillain S, Wojtasik V, Salmon E, Petermans J, Croisier JL. Gait analysis in elderly adult patients with mild cognitive impairment and patients with mild Alzheimer’s disease: simple versus dual task: a preliminary report. Clin Physiol Funct Imaging. 2010;30(1):51–6.
Kuan YC, Huang LK, Wang YH, Hu CJ, Tseng IJ, Chen HC, Lin LF. Balance and gait performance in older adults with early-stage cognitive impairment. Eur J Phys Rehabil Med. 2021;57(4):560–7.
Gassner H, Marxreiter F, Steib S, Kohl Z, Schlachetzki JCM, Adler W, Eskofier BM, Pfeifer K, Winkler J, Klucken J. Gait and cognition in Parkinson’s Disease: Cognitive impairment is inadequately reflected by gait performance during dual task. Front Neurol. 2017;8:550.
Mc Ardle R, Del Din S, Galna B, Thomas A, Rochester L. Differentiating dementia disease subtypes with gait analysis: feasibility of wearable sensors? Gait Posture. 2020;76:372–6.
Download references
Acknowledgements
We thank the Memory Clinic at Siriraj Hospital and all participants who kindly gave their time and effort to the study, and Ms. Rattapha Ariyaudomkit , Ms. Htet Htet Hnin, and Ms. Hataitip Boonchum for data collection. In addition, we would like to thank Associate Professor Jaranit Kaewkungwal for his great advice on statistical analysis.
This work was funded by Thailand Science Research and Innovation (TSRI), Faculty of Medicine Siriraj Hospital and Faculty of Physical Therapy, Mahidol University, Bangkok, Thailand.
Author information
Authors and affiliations.
Faculty of Physical Therapy, Mahidol University, Nakhon Pathom, Thailand
Sunee Bovonsunthonchai, Roongtiwa Vachalathiti & Vimonwan Hiengkaew
Department of Physical Medicine and Rehabilitation, Baylor College of Medicine, Houston, TX, USA
Mon S. Bryant
Allied Health Research Unit, University of Central Lancashire, Preston, UK
Jim Richards
Division of Neurology, Faculty of Medicine Siriraj Hospital, Mahidol University, Bangkok, Thailand
Vorapun Senanarong
You can also search for this author in PubMed Google Scholar
Contributions
SB: conception and design of the study, data collection, data analysis and interpretation, manuscript drafting and revising, correspondence. RV: conception and design of the study, data analysis. VH: conception and design of the study, data analysis. MSB: manuscript drafting and revising. JR: manuscript drafting and revising. VS: conception and design of the study, data analysis, grant writing. All authors read and approved the final manuscript.
Corresponding author
Correspondence to Vorapun Senanarong .
Ethics declarations
Ethics approval and consent to participate.
The study was approved by the Siriraj Institutional Review Board (SIRB), Human Research Protection Unit, Faculty of Medicine Siriraj Hospital, Mahidol University (COA no: Si 779/2019). Participants were informed about the research objectives, benefits, and details and signed the informed consent approved by the Siriraj Institutional Review Board (SIRB), Human Research Protection Unit, Faculty of Medicine Siriraj Hospital, Mahidol University before participating in the study (COA no: Si 779/219). The study was conducted in accordance with the Declaration of Helsinki.
Consent for publication
Not applicable.
Competing interests
The authors declare they have no competing interests.
Additional information
Publisher’s note.
Springer Nature remains neutral with regard to jurisdictional claims in published maps and institutional affiliations.
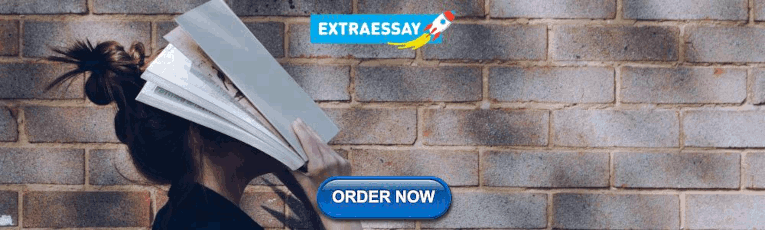
Rights and permissions
Open Access This article is licensed under a Creative Commons Attribution 4.0 International License, which permits use, sharing, adaptation, distribution and reproduction in any medium or format, as long as you give appropriate credit to the original author(s) and the source, provide a link to the Creative Commons licence, and indicate if changes were made. The images or other third party material in this article are included in the article's Creative Commons licence, unless indicated otherwise in a credit line to the material. If material is not included in the article's Creative Commons licence and your intended use is not permitted by statutory regulation or exceeds the permitted use, you will need to obtain permission directly from the copyright holder. To view a copy of this licence, visit http://creativecommons.org/licenses/by/4.0/ . The Creative Commons Public Domain Dedication waiver ( http://creativecommons.org/publicdomain/zero/1.0/ ) applies to the data made available in this article, unless otherwise stated in a credit line to the data.
Reprints and permissions
About this article
Cite this article.
Bovonsunthonchai, S., Vachalathiti, R., Hiengkaew, V. et al. Quantitative gait analysis in mild cognitive impairment, dementia, and cognitively intact individuals: a cross-sectional case–control study. BMC Geriatr 22 , 767 (2022). https://doi.org/10.1186/s12877-022-03405-9
Download citation
Received : 10 October 2021
Accepted : 23 August 2022
Published : 23 September 2022
DOI : https://doi.org/10.1186/s12877-022-03405-9
Share this article
Anyone you share the following link with will be able to read this content:
Sorry, a shareable link is not currently available for this article.
Provided by the Springer Nature SharedIt content-sharing initiative
- Classification
BMC Geriatrics
ISSN: 1471-2318
- Submission enquiries: [email protected]
- General enquiries: [email protected]
Click through the PLOS taxonomy to find articles in your field.
For more information about PLOS Subject Areas, click here .
Loading metrics
Open Access
Peer-reviewed
Research Article
Integrating a gait analysis test in hospital rehabilitation: A service design approach
Roles Conceptualization, Data curation, Investigation, Methodology, Visualization, Writing – original draft, Writing – review & editing
* E-mail: [email protected]
Affiliations IDERGO (Research and Development in Ergonomics) Research Group, I3A (Aragon Institute of Engineering Research), University of Zaragoza, Zaragoza, Spain, Department of Design and Manufacturing Engineering, University of Zaragoza, Zaragoza, Spain

Roles Conceptualization, Data curation, Investigation, Methodology, Supervision, Writing – original draft, Writing – review & editing
Affiliations HOWLab (Human Openware Research Lab) Research Group, I3A, University of Zaragoza, Zaragoza, Spain, GeoSpatiumLab, S.L. Zaragoza, Spain
Roles Conceptualization, Data curation, Investigation, Methodology, Project administration, Supervision, Writing – original draft, Writing – review & editing
Roles Conceptualization, Data curation, Investigation
Affiliations IDERGO (Research and Development in Ergonomics) Research Group, I3A (Aragon Institute of Engineering Research), University of Zaragoza, Zaragoza, Spain, Department of Health and Sports Sciences, University of Zaragoza, Zaragoza, Spain
Roles Conceptualization, Project administration, Supervision
Affiliation Rehabilitation and Physical Medicine Service, HUMS (Miguel Servet University Hospital), Zaragoza, Spain
Roles Conceptualization, Project administration, Supervision, Writing – review & editing
- Javier Marín,
- Teresa Blanco,
- José J. Marín,
- Alejandro Moreno,
- Elena Martitegui,
- Juan C. Aragüés
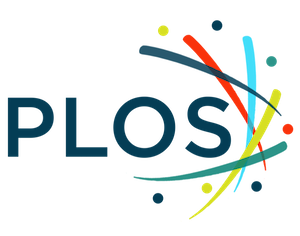
- Published: October 30, 2019
- https://doi.org/10.1371/journal.pone.0224409
- Reader Comments
Gait analysis with motion capture (MoCap) during rehabilitation can provide objective information to facilitate treatment decision making. However, designing a test to be integrated into healthcare services requires considering multiple design factors. The difficulty of integrating a ‘micro-service’ (gait test) within a ‘macro-service’ (healthcare service) has received little attention in the gait analysis literature. It is a challenge that goes beyond the gait analysis case study because service design methods commonly focus on the entire service design (macro-level).
This study aims to extract design considerations and generate guidelines to integrate MoCap technology for gait analysis in the hospital rehabilitation setting. Specifically, the aim is to design a gait test to assess the response of the applied treatments through pre- and post-measurement sessions.
We focused on patients with spasticity who received botulinum toxin treatment. A qualitative research design was used to investigate the integration of a gait analysis system based on inertial measurement units in a rehabilitation service at a reference hospital. The methodological approach was based on contrasted methodologies from the service design field, which materialise through observation techniques (during system use), semi-structured interviews, and workshops with healthcare professionals (13 patients, 10 ‘proxies’, and 6 doctors).
The analysis resulted in six themes: (1) patients’ understanding, (2) guiding the gait tests, (3) which professionals guide the gait tests, (4) gait test reports, (5) requesting gait tests (doctors and test guide communication), and the (6) conceptual design of the service with the gait test.
Conclusions
The extracted design considerations and guidelines increase the applicability and usefulness of the gait analysis technology, improving the link between technologists and healthcare professionals. The proposed methodological approach can also be useful for service design teams that deal with the integration of one service into another.
Citation: Marín J, Blanco T, Marín JJ, Moreno A, Martitegui E, Aragüés JC (2019) Integrating a gait analysis test in hospital rehabilitation: A service design approach. PLoS ONE 14(10): e0224409. https://doi.org/10.1371/journal.pone.0224409
Editor: Andrew Soundy, University of Birmingham, UNITED KINGDOM
Received: July 9, 2019; Accepted: October 11, 2019; Published: October 30, 2019
Copyright: © 2019 Marín et al. This is an open access article distributed under the terms of the Creative Commons Attribution License , which permits unrestricted use, distribution, and reproduction in any medium, provided the original author and source are credited.
Data Availability: All relevant data are within the manuscript and its Supporting Information files.
Funding: The authors received no specific funding for this work.
Competing interests: The authors have declared that no competing interests exist.
Although we are not conscious of its complexity, gait is a complex activity for human beings. It requires high motor control, and its pathologies have a harmful effect on personal autonomy and daily life activities [ 1 ]. Gait analysis with motion capture (MoCap) technology in the usual clinical practice is called ‘clinical gait analysis’ [ 2 ]; it is considered an important measurement in the rehabilitation field, where decision making on numerous treatments and interventions can benefit from objective information on the patient’s walking pattern [ 3 – 7 ]. Clinical gait analysis is especially important in the treatment of hemiparetic individuals after a stroke (or other causes), because they experience numerous impairments in walking skills that are reflected in the gait pattern [ 8 ].
In this regard, clinical gait analysis based on MoCap technology can be defined as the instrumented measurement of movement patterns that comprise walking and the associated interpretation of these patterns[ 2 ]. A MoCap instrumentation that is extensively used in biomedical research is optical technology (gold standard), which tracks the position of reflective surface markers with infrared cameras. Another instrumentation is technology based on inertial measurement units (IMU), which are electronic devices that measure rotations (rotation matrices, Euler angles, quaternions, etc.) by processing the signal of embedded sensors (accelerometers, gyroscopes, and magnetometers) [ 9 – 13 ]. Regarding their differences, optical technology is more accurate than IMU technology, which could present drift errors. However, it requires a camera infrastructure and sometimes presents shadowing problems [ 14 ]. In contrast, the IMU technology is more economical and portable and has been recently used in wearable technology [ 15 ], which could encourage cloud data processing and information exchange in the context of the Internet of things [ 16 ].
MoCap gait analysis is widely used in clinical research; however, certain factors have prevented the spread of this technology in hospital rehabilitation [ 17 , 18 ]. Nowadays, gait evaluation relies mostly on observational criteria. These systems involve high technical complexity, requiring the application of a strict protocol for accurate and repeatable measurements [ 2 , 19 , 20 ]. The massive amount of information they provide requires complex processing methods [ 8 , 21 , 22 ]. Currently, the professionals involved in this type of analysis must be highly qualified [ 2 ], which conflicts with the present needs for simplicity, usability, and intuitiveness [ 23 ].
Many of these factors have been identified by the cited researchers; however, despite being relevant, the difficulty of the ‘servitisation’ of gait analysis has received little attention in the literature [ 15 ]. Because of the challenges that servitisation poses, it should be added as an additional research objective.
Designing an integrated test in the biomedical field is particularly complex because multiple stakeholders have different needs. Solutions that involve all users (patients, doctors, therapists, etc.) in the context of the technology are required to make it useful, cost-effective, and truly usable [ 24 ]. This includes family members and people close to patients (proxies), who are especially affected by the situation that the patient experiences [ 25 , 26 ]. Therefore, we address a problem that goes beyond the development of the technology by investigating how to apply a certain technology (gait analysis) to a specific context (hospital rehabilitation services).
If we assume that the design object is a service and not only the technology that measures movement, the use of specific service design techniques [ 27 , 28 ] will allow us to predict failures, extract critical points, and consider the intangible and contextual part of the product [ 15 ]. These methods are aligned with the patient-centred care (PCC) philosophy, which is a priority in the healthcare field [ 29 ] and shares its philosophy with human-centred design (HCD) [ 30 ]. Despite the uncertainty in health services (timing, diagnoses, resources per patient, etc.), these methods are relevant for professionals to know exactly what their roles are and to work in a more optimal and orderly manner. To achieve this, the involvement of users in the design process is key [ 31 ].
However, these methods, although highly flexible, are commonly focused on the entire service design [ 27 , 30 ]. Service design methods and the theoretical basis of service-dominant logic [ 32 ] aim to guide service innovations and are focused on the perspective of the whole company. Wetter et al. [ 33 ] identified this situation as a ‘macro-level’ approach and asserted that, in some cases, it is not clear how to act at a more operational level with a ‘micro-level’ approach or how to guide specific actions and projects to favour service innovations. Thus, contributions are needed to connect design methods with real situations and problems, producing pragmatic, empirical, and micro-level approaches.
Based on this terminology, we face a scenario in which we incorporate what we call a ‘micro-service’ (a gait analysis test) within a more complex ‘macro-service’ (rehabilitation services). In terms of service design, this situation has particularities and implications that are essential to consider. How can the gait test be included in the hospital as an additional medical test? What additional materials must be designed, developed, or adapted for the gait test? How do the patient’s capabilities influence the design and guidance of the gait test? Which professionals will guide it? Is there a lack of certain professionals in the macro-service? In this regard, qualitative studies can provide a meaningful view of the perspectives, opinions, and priorities of the users (patients, healthcare professionals, and proxies) and reveal the underlying conceptual structure of their existing and/or desired interactions [ 34 – 36 ].
This article qualitatively evaluates a MoCap gait analysis system during its integration in a hospital rehabilitation environment, constructing the research through design methods. We focus on a specific case study of neurological patients with spasticity in the lower limbs who are treated with botulinum toxin. Thus, we present design considerations and guidelines for the improvement and adaptation of the system, which can be extrapolated to other scenarios at both the service design and hospital environment levels. In the results section, the guidelines are structured into six main themes. Finally, in the discussion section, we discuss the advantages and benefits that an integrated gait analysis test would introduce in rehabilitation services.
A qualitative research design was used to provide design considerations and guidelines to integrate the MoCap technology for gait analysis in a hospital rehabilitation service. The methodological approach was based on contrasted methodologies from the field of service design, which were materialised through observation techniques (during system use), semi-structured interviews, and workshops with healthcare professionals. A sample of 29 participants was analysed (13 patients, 10 ‘proxies’, and 6 doctors). The reporting of the results follows the Consolidated Criteria for Reporting Qualitative Research (COREQ) statement [ 37 ] ( S1 Table ).
Paradigmatic position
Within the paradigms ‘that guide disciplined research’ [ 38 ], the paradigmatic position of this research is in the field of interpretivism (aligned with qualitative research). In interpretivism, ‘reality’ is constructed in people’s minds and can be clearly understood through an interactive dialogue between the researcher and participant [ 39 ]. The interpretivism paradigm and the qualitative methods are naturally closer than quantitative methods to design practice [RW.ERROR—Unable to find reference:453]. According to Blanco et al. [ 40 ], not considering the qualitative perspective of end users could lead to suboptimal solutions in product and service designs. In our case, the research question of this study is concerned with providing an understanding of how to integrate MoCap gait analysis technology into the rehabilitation field according to the end user’s knowledge, experience, and expectations.
The experimental study was conducted after the formal approval of the local ethical committee (Bioethics Committee of Aragón Spain, CEICA; Act No. 12/2018). The study was conducted in accordance with relevant ethical guidelines, including a verbal explanation and written informed consent from the participants.
The gait analysis system
The MoCap system used in this study was the Move-Human Sensors system developed by the IDERGO research group, which includes a module for gait cycle analysis [ 15 , 41 ]. The first version of the system was implemented in 2007. Since then, it has been used in numerous public and private projects both in hospitals (healthcare field) and companies (ergonomics field). The system has been incorporating the concerns of the professionals involved in the projects (engineers, doctors, ergonomists, prevention technicians, etc.).
The measurement validity of the MoCap system is largely determined by the accuracy of the sensors it uses. In this case, it is based on wireless IMUs, specifically, the NGIMUs devices [ 42 ], which are placed on the patient’s body with elastic bands. The NGIMU sensors are calibrated by the manufacturer. They filter and process the signal internally to directly send the rotation information. The NGIMU sensors have been used and assessed in numerous publications, which have guaranteed their accuracy, as can be found on the manufacturer’s website [ 43 ].
The gait test of this study is aimed to be used as a medical test based on pre- and post-measurement sessions for the applied rehabilitation treatments. The generated reports show the changes that have occurred in each patient’s gait between the pre- and post-sessions. These reports can be used to make decisions about the treatments (continue treatment, change to another, increase the intensity, etc.). In this regard, it should be noted that this study does not focus on the gait report, how it is designed, or what information it contains. Instead, we present a more global perspective of the service design. However, because the gait report design is a relevant research issue, we have considered it in the fieldwork to extract participants’ expectations and research opportunities.
Hospital setting
The research setting was the Rehabilitation and Physical Medicine Service of the Miguel Servet University Hospital (HUMS) of Zaragoza, Spain, a reference hospital in the country. This service provides rehabilitative assistance to return the highest degree of functional capacity and independence to the patient as possible, favouring family, social, and work reinsertion. It is organised into areas of hospitalisation, outpatient consultation, and therapy (physiotherapy, occupational therapy, hydrotherapy, electrotherapy, and speech therapy).
Pathology and treatment
We were focused on evaluating the gait of patients with spasticity when they receive treatment with botulinum toxin. The choice of these patients makes it possible to extrapolate the results to other patients with more favourable physical or cognitive conditions. Spasticity is a symptom that affects a large group of patients after suffering from neurological damage. Negative effects include pain, decreased mobility, contractures, and muscle spasms, which can interfere with daily life activities and sleep to a greater or lesser degree. The causes are diverse; some of the best-known causes are stroke [ 44 ], multiple sclerosis [ 45 ], post-traumatic brain damage [ 44 ], spinal cord injury [ 46 ], cerebral palsy, amyotrophic lateral sclerosis, and polyradiculitis. In HUMS, more than 850 patients were admitted with stroke pathology in 2017, and 75% of these cases required attention and subsequent follow-up.
The botulinum toxin treatment aims to treat focal spasticity via muscle infiltration with reversible paralytic action after 4 to 6 months [ 44 , 47 , 48 ]. Although there are other possible treatments for spasticity, for this study, the observed efficacy, personalised patient needs (dose, muscles to be inoculated, etc.), relatively high cost, and widespread use of the treatment justify the treatment choice. The gait test can aid the doctor in decisions [ 49 ] regarding (1) continuing to apply the treatment, (2) detecting whether the infiltrated muscles are adequate, and (3) maintaining or modifying the dose.
Study design
Discover, define, design, and develop are the most common phases of service model development [ 28 ], constituting the global structure and philosophy that we follow. However, in this paper, as claimed in the literature [ 33 ], we delved into the specific methods related to our case study, providing the methodology design, reasoning, and how the specific methods were used and applied. Consistently, we contextualised the study design through a theoretical framework based on methods endorsed by the scientific community.
Our scenario started from an existing gait test based on IMU technology. In this context, we proposed a methodological approach to qualitatively assess three dimensions of the test, which gave rise to our research phases: (1) the user-product proximity effect, (2) the effect on and value in the service, and (3) user interactions. The theoretical framework of this approach was based on the following contrasted methodologies from the field of service design and HCD (which, as we have seen, is related to the PCC philosophy):
- The general vision was based on the Octopus methodology by Marin et al. [ 15 ], which aims to define design specifications to create MoCap devices from three points of view: product, software (information analysis), and service. As the design object is a service, this study focused on this approach.
- The phases within the methodological sequence were based on the Xassess evaluation methodology by Blanco et al. [ 40 ]. Because the starting point was an existing product (a gait analysis system), we inevitably faced the evaluation of the system. In this regard, Xassess proposed three evaluation strategies: (1) ‘complementation’ (each product dimension is evaluated with one qualitative or quantitative technique), (2) ‘triangulation’ (each dimension is evaluated with two or more parallel techniques), and (3) ‘combination’ (each dimension is evaluated with two or more successive techniques). As shown in Fig 1 , the methodological proposal followed a general strategy of triangulation (three parallel phases). Examining each phase separately, Phase 1 followed a triangulation strategy, and Phases 2 and 3 followed a combination strategy. Combination strategies offer advantages because each evaluation illuminates the following steps or techniques strategies, avoiding overlap and favouring the construction of knowledge on a solid basis.
- Finally, Phase 3 was based on Community, which Blanco [ 26 ] proposed as a methodology for the design of complex services with interrelationships between multiple users. Here, it was adapted to a workshop format for professionals from the health sector.
- PPT PowerPoint slide
- PNG larger image
- TIFF original image
https://doi.org/10.1371/journal.pone.0224409.g001
Table 1 shows the construction of the research methodology. Three assessment dimensions (phases) led to concrete research questions, which were answered with different user profiles (participants) through observation techniques, semi-structured interviews, and workshops.
https://doi.org/10.1371/journal.pone.0224409.t001
Regarding the participants mentioned in Table 1 , the relationship established with the doctors was possibly due to previous meetings in which they showed a shared interest in integrating a gait analysis system into their service. A relationship was established with the patients with the six doctors in Table 1 , who conducted the patient rehabilitation and recruited them face-to-face during the consultation. The interest in improving rehabilitation through gait analysis was communicated to the patients and proxies. No one refused to participate in the study.
The sample size of patients and proxies (phase 1) was determined by the concept of saturation , which was defined by Glaser and Strauss [ 50 ] and has been widely used in qualitative research. Saturation has been reached when adding more participants to the study does not generate additional insight or information. In this way, the measurement sessions were repeated until saturation was reached with 13 patients and 10 proxies. The sample size of doctors was six (Phases 1, 2, and 3), which corresponds to the number of physicians involved in the analysed rehabilitation service and the applied treatment.
In the following sections, each phase of the methodology is explained in depth, including the specific research objectives, participants, and study design.
Phase 1: User-product proximity effect
Phase 1 aims to learn from observing the use of the MoCap system in its context. The strategy in this step was to perform the gait test in the rehabilitation service, carrying out an observation focused on understanding the effect on the involved actors (patients, proxies, and professionals).
There were 26 observation sessions carried out with 29 users of different profiles: the patients ( n = 13) who performed the test, the proxies ( n = 10) who (in some cases) accompanied the patients and observed the test from nearby, and the doctors of the service ( n = 5 rehabilitation specialist doctors and 1 resident doctor) who were free to observe the gait test and talk with the patients or proxies. Each of the 13 patients with spasticity (7 men and 6 women; average age = 45.9 ± 19.8 years) underwent the gait test twice. The first test was performed a few minutes before receiving the botulinum toxin treatment, and a follow-up was performed a month later.
The patients had been diagnosed by the rehabilitation service of the hospital in the evaluation consultation and were selected for the study by meeting the following inclusion criteria:
- The patient can walk autonomously.
- The patient presents a dynamic or reducible contracture that alters motor function.
- A reduction in spasticity is expected to lead to a functional improvement with the treatment, according to the doctor’s previous experience.
- The patient has the possibility of receiving periodic controls to learn patterns of movement at home or complementary physiotherapy treatment.
- The patient is included in the indications of the technical sheet approved by the Spanish Agency of Medicines.
Table 2 includes the patient’s characteristics. The gait speed at the beginning of the patient’s evaluation has been included because it is an important indicator to represent the general state of health and is related to impairment, functionality, mobility, independence, autonomy, and comorbidity levels [ 51 , 52 ].
https://doi.org/10.1371/journal.pone.0224409.t002
According to the statement terminology of the COREQ [ 37 ], the sampling method was purposive in the case of the patients because they met the inclusion criteria and were of diverse ages and disease levels, according to the doctor’s criteria. Proxies were recruited through the snowball method because they were dependent on the patient section. Doctors were also purposively sampled because they represented different profiles (two heads, three specialists, and a resident doctor).
The gait test was carried out using two ‘test guides’, one engineer (JM), who managed the computer, and a physiotherapist (AM), who guided the patients and interacted with them. In each measurement session, the patients were instrumented with the MoCap sensors ( Fig 2 ) and walked naturally 6 m in a straight line at a self-selected speed. When the distance was completed, the patient turned around and walked back in the opposite direction. This operation was repeated to measure up to 25 strides. Only strides in a straight line were used, discarding any turns and start and stop zones. The duration of each test was 20 to 25 minutes.
The individual in this picture has given written informed consent (as outlined in the PloS consent form) to publish these case details.
https://doi.org/10.1371/journal.pone.0224409.g002
The observations during the test were carried out by the test guides. It was established that observers should focus on the following factors or dimensions: (1) physical and cognitive abilities (patients), (2) motivation (patients), (3) concentration (patients), (4) reactions to the test and technology including what they said, what they did, and how they responded (patients, relatives, and doctors), and (5) operational and technical problems (test guides). Field notes were made during the observation. Audio and visual recordings were not used because the pre-defined observation dimensions were considered enough to address the research objectives.
Phase 2: Effect and value in the service
Designing a system that aims to advise professionals in the rehabilitation process requires going beyond what happens only in the test session. Phase 2 evaluates the effect and value of the micro-service within the HUMS macro-service, seeking to obtain an overview of the general path followed by the patient according to the diagnostic and care decisions.
Regarding the participants involved in this phase, owing to their deep understanding of service performance, the participants were two doctors who were familiar with the gait test: (1) the head of the neurological rehabilitation section and (2) the head of the rehabilitation service.
First, a face-to-face semi-structured interview with the user (1) was conducted, addressing the issues collected in Table 3 . This allowed us to develop the first version of a graphic map that represents the flow of clinical decisions of the service with the new test introduced in it.
https://doi.org/10.1371/journal.pone.0224409.t003
Following a ‘combination’ strategy [ 40 ], in the second session, a workshop was held with both users (1 and 2) to evaluate the map of the service proposed after the first interview. The users had the opportunity to redefine and improve it to ensure that it reflected the entire process. The duration of each session was 40 minutes. They were pilot tested and conducted by JM and AM who recorded the audio.
Phase 3: User interactions
We assume that we are facing a complex and multi-user information network. For this reason, Phase 3 aims to define the information flows (connections) that must exist between the macro-service users for an adequate implementation of the gait test. These connections should allow the test implementation and should cover the relational needs of the Community methodology [ 17 ], improving the user experience, acceptance, and clinical effectiveness of the new gait test.
A workshop was held twice in succession with six doctors from the rehabilitation service, as shown in Table 1 , first with three doctors and later with another three others. They were pilot tested and conducted by JM and AM. The duration of each workshop was 100 minutes, and the audio was recorded.
The workshop challenge was ‘How could we develop a useful gait test for the rehabilitation service?’. The main task involved collaboratively drawing the users’ relational needs by connecting user groups using arrows (unidirectional or bidirectional) that represent information exchanges and/or interactions. To facilitate the process, pre-designed cards with icons of the involved professionals had been prepared. Initially, the organisers structured some user groups and connections as an example. The schemes created by the doctors during the sessions are shown in Fig 3 . In the second workshop, to reach a consensus between both workshops, following a ‘combination’ strategy [ 40 ], the researchers inquired about the differences from the map developed in the first session.
https://doi.org/10.1371/journal.pone.0224409.g003
Data analysis
Observational notes of Phase 1 and the audios of Phases 2 and 3 were transcribed and coded using the thematic analysis approach [ 53 ]. The full transcription was read several times separately by JM, JJM, and TB to identify differences and similarities of the content. Similar content was underlined in the same colour, and a descriptive concept (category) was assigned to each colour. Afterwards, the researchers discussed their reflections. Once a consensus was reached, the latent content and implicit messages of each category were described in the results section. According to the COREQ statement [ 37 ], the identified categories were derived from the data (i.e. they are inductive). To improve the understanding of the maps from Phases 2 and 3, they were laid out as simply and perceptibly as possible. It was necessary to hear the audios repeatedly to include all the comments in the maps, not just the handwritten information. Microsoft Word and Excel were used to manage the data, and Illustrator was used to create the figures.
Trustworthiness
To achieve scientific rigour in qualitative research, Guba and Lincoln [ 38 ] proposed including a section about the trustworthiness of the interpretations. An integral aspect of trustworthiness is maintaining a detailed audit trail. Thus, we recorded our reflective memos and analysis decisions throughout the study. Additionally, in this paper, we provide clear and thick descriptions of the context, data collection, and analysis process, which favour the reproducibility of the study. To consider different perspectives and avoid bias, members of the research team represented different professions, and three of them independently identified and agreed on the categories presented in the results section. Finally, the resulting maps from Phases 2 and 3 reduced the subjective nature of the paper because they are tangible and factual objects proposed by the participants, who constitute an additional perspective as experts on their own experience in the environment [ 31 ].
From the field research, design considerations and guidelines have been obtained and grouped in the following sections: (1) patients’ understanding, (2) guiding the gait test, (3) which professionals guide the gait tests, (4) gait test reports, (5) requesting gait tests (doctors and test guide communication), and the (6) conceptual design of the service with the gait test.
Patients’ understanding
The extreme diversity of the patients is evidenced. One of the most repeated phrases among doctors is ‘each patient is a whole world’ on a physical and cognitive level and in terms of the care that each one requires.
- The accessibility level of the gait test should be maximised .
Most patients receive other types of therapy than just those received in the hospital, such as therapies in elderly centres, associations, or private centres: ‘I've been going to rehabilitation since I can remember’. They are aware of their limitations and are realistic about their situation: ‘You can notice evolution for a while, but then you stabilise at a certain level’. ‘In winter, I can feel my muscles [are] more contracted’. However, we also observe how doctors try to lower the expectations that the proxies sometimes have with botulinum toxin therapy. They indicated that rehabilitation objectives that are too high or optimistic can lead to frustration and disinterest in the rehabilitation process.
- It should be assumed that not all patients will be able to recover their previous functional capacity. They usually know their own limitations. We should take this into account and be honest in relation to their chances of recovery .
Those who have decided to participate in this study go to rehabilitation voluntarily and have a clear motivation to recover; however, the degree and origin of this motivation are very diverse. Some of them transmit their willpower with their daily habits, ‘every morning I walk around the block’. ‘I am always trying to improve, stretching and moving, for example, while I cook or while I dress’. Those who are older show that they have recovered the motivation they had lost: ‘Recently, we went to the neurologist, and he explained the treatment to us. We have been encouraged to try. We had been disconnected from this world for many years’. In the youngest patients, motivation, strength, and interest usually come from family members, who are the protagonists in the interactions with the test guides and express their curiosity regarding new treatments and techniques.
- The motivation of the patients and proxies should be exploited and maximised as much as possible. The gait test should become a motivating and engaging element whether the results are positive or negative .
Guiding the gait test
How and who performs the test can be keys to its success. The test guides should ensure that patients walk with their usual pattern. In Fig 4 , we see the movement curve irregularities of (a) a patient who is not accustomed to walking in the test and (b) those of the same patient who became accustomed to walking in the test after crossing the corridor two or three times.
Femur angle with respect to the hip in the sagittal plane. (a) Before and (b) after the patient adapted to walking using the usual pattern.
https://doi.org/10.1371/journal.pone.0224409.g004
As a general rule, the concentration level of the patients during the test was high. They were previously silent, struggling, and looking at the ground as they walked. Even those with limited cognitive and communicative ability understood the explanations and satisfactorily executed the test guide instructions. However, there are factors that affect the concentration and are necessary to consider to accelerate the tests and avoid incidents that would require discarding the results.
The patient’s concentration is improved with a calm and constant environment without sudden noises, foot traffic, or conversations. Patients can sometimes lose concentration, become scared, and even stop walking when someone suddenly enters the room, when there is a slam, or when staff or family members converse, more so if the conversation deals with issues related to the patient. To create this environment, the test guides must empathise with the patient and their relatives, using appropriate language with clear, concrete, and predictable instructions, while avoiding technical terminology (e.g. using anatomical calibration functions: ‘Now I need you to be very still, like in a picture’).
- The role of the test guides is especially relevant. They should be able to extract the usual walking pattern of the patients. Access should be restricted to the room to achieve a calm and favourable environment during the performance of the test .
During the tests, the patients displayed different emotions and reactions from showing fear of pain to satisfaction or gratitude. Considering these reactions can provide value from the user-experience point of view. Below, the reactions are sorted in the order of appearance along the process.
- Mistrust, does it hurt? One of the first reactions and doubts that arose in both patients and family members was regarding whether the test was painful. They worried about whether the devices give electric shocks or punctures. Many of them had suffered from various painful treatments, and these doubts could be a reason for the initial rejection.
- Arriving late, anxiety . Some were nervous about being late for the tests, either due to not finding the room, transportation difficulties, or the schedules of the relatives who accompanied them. The doctors indicated that sometimes patients present anxiety for these reasons, and they must spend the first minutes of the consultation session reassuring them. Nonetheless, this was not the case in this study.
- Fatigue . Some patients, owing to their physical condition and especially their age, asked to rest as soon as they reached the room. In these cases, the sensors were placed and removed while they were sitting.
- Feeling observed . Once we placed the sensors, we saw how the patients joked with their relatives. Others, especially the younger ones, presented a certain shyness and appeared to feel uncomfortable, exposed, and observed.
- Gratitude . At the end of the test, gratitude reactions often arose: ‘It is a very difficult disease. It is comforting to see how people are working on this. We will help with everything that we can’. It is important that they feel they are part of the technological evolution in this field.
- Bond of trust in the second session . As a general rule, on the second day, the patients were more confident. They interacted more and had a greater link with the test guides. The most noticeable effect occurred in the young patients, who, as mentioned, were uncomfortable and shy on the first day.
- The test guides should explain the test properly and act effectively towards the different reactions that patients may present. Behaviour guidelines for test guides should be established .
Which professionals should guide the gait tests?
Due to the test characteristics, doctors concluded that two people are needed to run the test: one to guide the patient (place and remove sensors and give instructions to the patient) and another to operate the computer. Different health professionals have adequate training and capacity (doctors, nurses, physiotherapists, occupational therapists, etc.). According to healthcare professionals, in our environment, the test would be carried out by the nursing section: ‘Nursing is accustomed to doing this type of test; it falls within their competence’. ‘Certainly, there are tests performed by a doctor, for example, an endoscopy, but in that case, you are getting inside a human being. This test has nothing to do with that’.
Likewise, tests could also be performed by physiotherapists. These professionals are the most interested (along with doctors) in obtaining objective information on the gait pattern because they also apply therapies aimed at rehabilitating walking; however, the availability of these professionals is reduced, at least at HUMS. The final decision rests on the service head, on the professional availability of each hospital, and on the derived costs.
- The test should be conducted by two trained healthcare professionals. It would be feasible for nurses or physiotherapists to conduct the test. The decision depends on each service .
Gait test reports
Doctors have a negative perception of the results provided by the test. They consider the test to be far from their area of knowledge and difficult to interpret, requiring a high learning curve. They called it ‘the test of the engineers’, in some cases, showing concern and anxiety regarding who would interpret the data and how much time would be necessary to do so.
- The gait analysis test should provide a brief and easily interpreted report. Results must be communicated to patients in an understandable and personalised way to make more consensual decisions .
Defining the content and design of the test report is one of the challenges that must be addressed. According to the doctors’ opinion, the first test should serve to assess the functional state of the patient and, together with the rest of the information and inputs (clinical exploration, interview with the patient, clinical history, etc.), establish the rehabilitation objectives. Regarding the second test, they affirm that it should measure the treatment effects and allow the assessment of the achievement of the initial objectives: ‘We could try to assess if the patient has improved above what is expected, at what is expected, or below what is expected’.
- Future research should serve to detect the most representative and useful information for clinical decision making .
- With the first test, the rehabilitation objectives are established. With the second test, whether the treatment effects were as expected is assessed .
A general statement was made that the test report results should be available in digital format for consultation between the professionals involved in a patient’s rehabilitation. Consequently, the report should be uploaded and incorporated into the patient’s electronic medical record. In this regard, HUMS has its own intranet and another at the regional level. In our research, we refer to the electronic medical record without differentiating between these two. In this sense, the communication vehicle between doctors and therapists is the electronic rehabilitation plan, which allows prescribing treatments and tracking the patient by the involved physicians (renamed the electronic monitoring plan once the treatment begins). Uploading the gait test report to the electronic medical record would improve the doctor-therapist communication so they could share considerations about the results through the electronic rehabilitation plan and take more consensual decisions based on objective information.
- The doctor should be able to upload the gait test report in digital format to the electronic medical record .
Requesting gait tests: Doctors and test guide communication
Doctors agreed that it is not possible to run the test during consultation time. According to them, ‘it is the same as an X-ray or a blood test; I prescribe it, and I get the results back’. One of the questions that emerged in the workshops was how a doctor could request a test from the consultation. In this regard, doctors proposed the creation of a new agenda called the ‘gait test agenda’ with specific schedules and professionals assigned that would be shared between doctors and test guides and completed in the consultation.
- To cite prescribe patients to perform the test, it is proposed to create an electronic ‘gait test agenda’ shared between doctors and test guides .
Conceptual design of the service with the gait test
Based on the specifications obtained in the previous sections and according to the specific outputs of Phases 2 and 3 of the methodology ( Table 1 ), two schemes are presented that represent a conceptual proposal of the rehabilitation service that includes the new gait test in its operation. The first scheme, called the actuation flowchart ( Fig 5 ), shows the circuit of decisions that physicians must face during the spasticity rehabilitation process. The figure shows two different areas shaded in grey: consultation and intervention. A patient will go through both areas iteratively as many times as necessary. It also shows the gait test, which is accessed through two entryways:
- First gait entry . The test can be prescribed from a consultation in which the doctor considers that further information is needed. In this case, the test would have a diagnostic purpose. This option is parallel to the current possibility of requesting information from other services (e.g. an interconsultation request to obtain a psychological report).
- Second gait entry . It is possible to incorporate the gait tests during the intervention process, as long as it is planned from the consultation. In this case, the test would have a monitoring function. This option, based on pre- and post-treatment measurement sessions, is the one planned (methods section) to assist clinicians during the rehabilitation process.
Note that an item drawn with an empty circle indicates that the patient will undergo the process only if it is prescribed by the responsible physician (e.g. a patient may receive therapy sessions but not receive medical and nursing treatment sessions).
Figure elaborated by authors. Icons made by Freepick, Pause08, Pixel perfect and Srip from www.flaticon.com.
https://doi.org/10.1371/journal.pone.0224409.g005
The second scheme, developed following Community [ 26 ], represents the connections between the user profiles that interact with the gait test and its results ( Fig 6 ; arrows from 1 to 7). Dark arrows represent the information flows that need to be designed and implemented. Light coloured lines represent the macro-service connections, which our design will not influence, but it will have a certain influence on the gait test integration. The represented users are grouped according to the ‘network spaces’, areas of conceptual interaction with different objectives and themes where users communicate and interactions can take place (e.g. gait test session, management, etc.). We see how a user can be present in several ‘network spaces’.
Figure elaborated by authors. Icons made by Freepik, Smartline, Mynamepong, Pause08 and dDara from www.flaticon.com.
https://doi.org/10.1371/journal.pone.0224409.g006
Likewise, in the scheme, the materials to be developed are established (graphic documents, electronic agenda, cloud, etc.), which are also called ‘touch points’, that is, tangible or intangible elements that are in contact with the users. In line with the theory of service design, two conceptual interaction areas are also included, ‘backstage’ and ‘onstage’ [ 54 ]. Backstage is the invisible part for the user, where the processes that articulate the services take place. Onstage is the visible part that encompasses the target users.
Note that ‘developers’ have been included as temporary participants in the process because, although their permanent presence could be useful, their role during the first stages will be to process the information manually. Once the project evolves, and it is decided which information is the most adequate to facilitate the clinical evaluation, this process can be automated, and these users can be eliminated.
This study highlights an underlying need for MoCap gait analysis technology. Although it can generate individual objective outcome measures in usual clinical practice [ 55 ], further research is required for its effective and useful clinical application. In this framework, we apply a research methodology that starts from a specific concept: to design a gait test integrated into the hospital setting that provides objective information to support decision making regarding the rehabilitation process. We focus on the case study of neurological patients with disorders derived from spasticity who receive treatment with botulinum toxin. We have conducted a field investigation introducing a gait analysis system into the HUMS environment and evaluated it through observation, interviews, and workshops. As a result, design considerations have been obtained that can be useful for design teams that face these types of challenges in the future. Among these considerations, the micro-service (gait test) integrated into the macro-service (hospital rehabilitation service) has been conceptually defined through two infographics.
We assume that the proposed concept involves an extra effort for hospitals (economic and resource investment), for professionals (more workload and learning), and for patients and proxies (more visits to the hospital). However, the gait test would provide a substantial improvement in the quality of care, encouraging professional development and collaborative research among physicians. Likewise, the system requirements are relatively simple. With a short test duration and a short preparation time (20–25 min per patient), it does not require an exclusively dedicated room. For patients, it is not an invasive test, and it does not entail visiting centres other than the hospital where they usually receive treatments. The advantages and benefits that it would bring in rehabilitation services are described below:
- Sustain decisions at a clinical level . Having a test that provides objective data on the patient therapy response allows more precision in the decision-making process, including the option of not further intervening.
- Sustain decisions at the administrative and social levels . Inevitably, physicians are under pressure that comes with human responsibility, schedules, efficiency requirements, legal disputes, or limitations in resources.
- Improve treatment profitability . Some treatments entail significant costs to the public or private health entities involved, as in the case of treatment with botulinum toxin. The gait test can avoid applying those treatments that do not provide positive effects to certain patients.
- Facilitate communication between physicians . The test could unify gait evaluation and enhance the transmission of knowledge among health professionals.
- Positive feedback for the therapist . Objective information allows the clinical plan to be personalised for each patient, establishing more realistic objectives and generating a theoretical basis that could improve their evolution.
- Positive feedback for the patient . It may involve extra motivation for patients, encouraging confidence in the treatments and a greater perception of healthcare safety and trust.
- Improve medical and patient communication . Properly presented information can establish a doctor-patient communication pathway, improving the reasoning of patients (and relatives) and improving treatment decision making.
- Feed databases . Collecting gait information may have a practical effect to create predictions for new patients and facilitate diagnoses through machine-learning techniques.
- Enhance research among physicians . It can improve the collaborative research work among physicians, allowing the validation of new or modified treatments. Data from the database can be filtered for each investigation.
The mentioned benefits coincide with some concepts that McHorney and Tarlov [ 56 ] generically described for the healthcare field sector. They provide ways in which data from individual people in healthcare can be used, including to describe a patient’s health state, to monitor disease progression, or to standardize interactions between health care practitioners and patients [ 56 ]. In this regard, Cella et al. [ 57 ] summarised the concepts from McHorney and Tarlov [ 56 ] into two possible perspectives or uses that have similarities with the two gait test entries that we propose in Fig 5 . The first use (similar to the first gait entry) is clinical decision making under uncertainty: ‘estimating the likelihood that a patient will profit from a given intervention’. The second use (similar to the second gait entry) is clinical evaluation: ‘examining whether a given treatment makes a meaningful difference for an individual patient’.
Beyond the benefits that MoCap gait analysis would bring in rehabilitation services, this paper has two main strengths or contributions. First, it provides design considerations and guidelines to integrate a gait analysis test based on MoCap technology in the rehabilitation hospital setting, which is a novel contribution and can encourage the use of this technology in this field. In this regard, Martin et al. [ 58 ] encouraged researchers to realise studies that propose design recommendations based on HCD, especially in scenarios where users (in our case patients) are heterogeneous (as we see in patients with hemiplegia). This reasoning supports the presented results.
The second strength is proposing a reproducible methodological approach to integrate a ‘micro-service’ (gait test) within a ‘macro-service’ (rehabilitation service), which advances service design knowledge and may be applied to other case studies or other technologies. This methodological approach had great acceptance among physicians. They ensured that the sessions where they participated were useful, practical, and different from the usual way of working: ‘I see these types of sessions and workshops as essential. It is useful to clean up many useless connections and make them simpler. I like it [the map]; it’s clean and illustrative’.
The importance of properly designing the rehabilitation service is manifested in numerous investigations that seek to assess the patient’s experience (e.g. [ 59 , 60 ]). In this context, service design and the theoretical basis of service-dominant logic [ 32 ] have great importance. They allow one to address stakeholder’s needs and strengthen professional relationships. However, as Han et al. [ 28 ] indicated, it is necessary to consider the specific needs of the clinical environment when applying service design techniques. This is translated to our specific case study in the necessity of integrating a ‘micro-service’ (gait test) within a more complex ‘macro-service’ (rehabilitation service). The scenario of the micro-service integration has particularities that differentiate it from a mere application of service design methods, which concern the design of the service as a whole. In return, we focus our design on ‘one link in the chain’, a new gait test in a hospital rehabilitation service. According to Wetter et al. [ 33 ], this contributes to improving the theoretical framework of the service design because it is not actually clear how to act at a ‘micro-level’ approach to connect design methods with real situations and favour service innovations.
Additionally, certain weaknesses or limitations have been identified in this study. It should be noted that we focus on a relatively specific case (gait tests for patients with spasticity in a particular rehabilitation service) and on a limited sample of professionals who know the service and the treatment used. Thus, if one intends to integrate this or another similar system into another hospital, it should be considered that other centres may be different at the organisational level. Some concepts (mentioned throughout the results section), such as agendas, the involved personnel, the rehabilitation plan, or the patients under study, may be different; thus, certain sections may be adapted to the particular case. In this regard, it may be useful to rely on the research methodology presented, especially in Phases 2 and 3.
Another limitation concerns the (1) gait report (including processing backwards) and the (2) measurement validity of the MoCap system. Although they are not part of the objectives of this study, they are key questions for the system design to achieve successful integration in a hospital. Both topics have been extensively discussed in the literature, and we encourage further investigation. In this sense, this paper is focused on the service design, which complements and strengthens this area of research.
Additionally, it should be noted that this study is interpretivist and the research techniques used are qualitative. This intrinsically implies that the participants are those who experience, process, and label the ‘reality’ under investigation and transmit it to the researcher [ 39 ]. To do this, the participants rely on their individual experience, memories, and expectations [ 38 ]. This prevents the total objectivity of the study and complete neutrality.
In summary, although we focused on a relatively specific case (gait tests for patients with spasticity in a particular rehabilitation service), the proposed design recommendations are partially generalisable to other treatments or health centres. Additionally, the proposed methodology can be useful and reproducible to obtain design considerations to integrate a micro-service into a macro-service.
Nowadays, healthcare technology is developing more rapidly and efficiently and requires fewer resources. This research contributes to improving the application of this type of system and other Internet of things devices [ 16 ] that can be developed in the healthcare field of ‘smart health’ [ 61 , 62 ]. It is expected that future health devices (micro-services) could be more useful to hospital services (macro-services) by maintaining their use over time and improving their acceptance.
We conducted a field investigation introducing a gait analysis system into a hospital rehabilitation environment and evaluated it through observation, interviews, and workshops. We focused on patients with spasticity who received treatment with botulinum toxin. The main conclusion is that integrating a gait test into hospital rehabilitation services is beneficial for physicians, patients, and proxies, but to make it really applicable and useful, some design specifications should be considered. The design specifications and the methods applied to obtain them can be useful for both technology developers and healthcare professionals who seek to improve the quality of healthcare services.
Supporting information
S1 table. reporting according to the coreq criteria..
https://doi.org/10.1371/journal.pone.0224409.s001
Acknowledgments
The authors would like to acknowledge and thank Dr Nicolas Rivas, Dr Ricardo Jariod, Dr Inmaculada Salcedo, and Dr M. Teresa Zarraluqui for their suggestions, involvement in the project, and their interest. Likewise, the authors would like to thank all participants who voluntarily participated in this study.
- View Article
- PubMed/NCBI
- Google Scholar
- 9. Teunis Cloete and Cornie Scheffer. Benchmarking of a full-body inertial motion capture system for clinical gait analysis. 2008 30th Annual International Conference of the IEEE Engineering in Medicine and Biology Society: IEEE; 2008.
- 23. Tobias J. Uebbing. User experience in smart environments: design and prototyping. PhD. Thesis.University of Twente; 2016.
- 26. Blanco Teresa. Metodologías de diseño como plataforma para la x-disciplinaridad en proyectos tecnológicos: surfing disciplines. Universitat Politècnica de València; 2016.
- 27. R. Tassi. Dervice Design Tools Communication Methods Supporting Design Processes. Ph.D. ThesisPolitecnico di Milano, Milano, Italy; 2008.
- 41. Marín Zurdo J., Boné Pina M., Ros Mar R. and Martínez Gamarra M. Move‐Human Sensors: Sistema portátil de captura de movimiento humano basado en sensores inerciales, para el análisis de Lesiones Musculoesqueléticas y utilizable en entornos reales. Proceedings of the Sixth International Conference on Occupational Risk Prevention; 2008.
- 42. X-io technologies. Next Generation IMU (NGIMU). 2019 [Internet]; available from: http://x-io.co.uk/ngimu/ .
- 43. X-io technologies. Related scientific publications. 2019 [Internet]; available from: https://x-io.co.uk/publications/ .
- 50. Glaser BG, Strauss AL. Grounded theory: Strategies for qualitative research. Chicago, lL: Aldine Publishing Company 1967.
- 53. Patton MQ. Qualitative research and evaluation methods. Thousand Oaks. Cal.: Sage Publications 2002.
- 57. Cella David, Bullinger Monika, Scott Charles, Barofsky Ivan and Clinical Significance Consensus Meeting Group. Group vs individual approaches to understanding the clinical significance of differences or changes in quality of life. Mayo Clinic Proceedings: Elsevier; 2002.
Thank you for visiting nature.com. You are using a browser version with limited support for CSS. To obtain the best experience, we recommend you use a more up to date browser (or turn off compatibility mode in Internet Explorer). In the meantime, to ensure continued support, we are displaying the site without styles and JavaScript.
- View all journals
- My Account Login
- Explore content
- About the journal
- Publish with us
- Sign up for alerts
- Open access
- Published: 28 December 2023
Quantitative gait analysis and prediction using artificial intelligence for patients with gait disorders
- Nawel Ben Chaabane 1 , 2 ,
- Pierre-Henri Conze 1 , 3 ,
- Mathieu Lempereur 1 , 2 , 4 ,
- Gwenolé Quellec 1 ,
- Olivier Rémy-Néris 1 , 2 , 4 ,
- Sylvain Brochard 1 , 2 , 4 ,
- Béatrice Cochener 1 , 2 , 4 &
- Mathieu Lamard 1 , 2
Scientific Reports volume 13 , Article number: 23099 ( 2023 ) Cite this article
1487 Accesses
2 Altmetric
Metrics details
- Computer science
- Data mining
- Quality of life
Quantitative Gait Analysis (QGA) is considered as an objective measure of gait performance. In this study, we aim at designing an artificial intelligence that can efficiently predict the progression of gait quality using kinematic data obtained from QGA. For this purpose, a gait database collected from 734 patients with gait disorders is used. As the patient walks, kinematic data is collected during the gait session. This data is processed to generate the Gait Profile Score (GPS) for each gait cycle. Tracking potential GPS variations enables detecting changes in gait quality. In this regard, our work is driven by predicting such future variations. Two approaches were considered: signal-based and image-based. The signal-based one uses raw gait cycles, while the image-based one employs a two-dimensional Fast Fourier Transform (2D FFT) representation of gait cycles. Several architectures were developed, and the obtained Area Under the Curve (AUC) was above 0.72 for both approaches. To the best of our knowledge, our study is the first to apply neural networks for gait prediction tasks.
Similar content being viewed by others
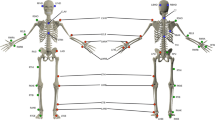
Gait analysis dataset of healthy volunteers and patients before and 6 months after total hip arthroplasty
Aurélie Bertaux, Mathieu Gueugnon, … Davy Laroche
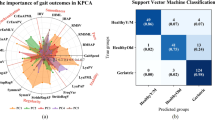
The detection of age groups by dynamic gait outcomes using machine learning approaches
Yuhan Zhou, Robbin Romijnders, … Claudine J. C. Lamoth
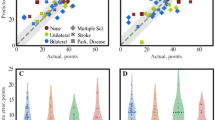
Machine learning based estimation of dynamic balance and gait adaptability in persons with neurological diseases using inertial sensors
Piergiuseppe Liuzzi, Ilaria Carpinella, … Andrea Mannini
Introduction
Gait disorders are described as any deviation from normal walking or gait 1 . Their prevalence among adults rises with age. In the elderly population over the age of 70 years, they are present in approximately 35% of patients 2 , 3 and in 72% of patients over 80 years 2 . These statistics take into account whether such disorders result from neurological etiologies or not, which can be determined through laboratory work, clinical presentation, and diagnostic testing 2 . In fact, gait disorders etiologies include neurological conditions (e.g., sensory or motor impairments), orthopedic abnormalities (e.g., osteoarthritis and skeletal deformities), and medical conditions (e.g., heart failure, respiratory insufficiency, peripheral arterial occlusive disease, obesity) 4 , 5 . Cerebral palsy, as a group of neurological disorders, affects about 2 in every 1000 newborns. Its prevalence reaches 5–8% among newborns with very low birth weights or very pre-term deliveries. Gait disturbances have a tremendous impact on patients, especially on their quality of life 1 : they complain most often of pain, joint stiffness, numbness, or weakness 6 . Neurological gait disorders, in particular, are associated with lower cognitive function, depressed mood, and diminished quality of life 7 . To have insight into patients’ conditions and therefore treat their gait disorders, clinicians historically used Observational Gait Analysis (OGA) 8 . OGA usually relies on a clinician’s observation freeze-framed techniques and video slow-motion replay to record and analyze a patient’s gait. It is subject to bias and has limited precision because it relies on the experience of the clinician. To overcome this limitation, Quantitative Gait Analysis (QGA) is considered. It uses instrumentation to quantify the gait cycle by recording temporal-spatial, kinematic, and kinetic data that is rarely gathered by observation. The challenge facing clinicians is to analyze a large amount of clinical data from QGA in order to determine the severity of the illness and select the most effective therapeutic strategy. It is a very tricky task because of the great disparity between patients (e.g., children and adults) and the diversity of their pathologies. In this context, our aim is to assist clinicians in analyzing this large amount of clinical data with an artificial intelligence applied to kinematics from QGA. The target objective is to go beyond objectively quantifying gait quality by predicting whether it will improve within the next visit. These predictions tend to help clinicians select the most effective treatment strategy. For this purpose, two approaches were considered: signal-based, which uses raw gait cycles, and image-based, which converts gait cycles into image-like representations, making them suitable for training image-based deep neural networks, especially pre-trained ones. In the signal-based approach, a Long Short Term Memory (LSTM) and a MultiLayer Perceptron (MLP) were designed from scratch. Their hyper-parameters were tuned with KerasTuner 9 . The obtained results were compared to five state-of-the-art architectures 10 , including Fully Convolutional neural Network (FCN), Residual Network (ResNet), Encoder, Time Le-Net (t-LeNet), and Transformer. For the two tailored and state-of-the-art architectures, the influence of data augmentation was studied. In the image-based approach, the first step was to map the time representation of 1D gait cycles to a 2D frequency representation using the two-dimensional Fast Fourier Transform (2D FFT). Then, the obtained 2D FFT images were processed with four pre-trained Convolutional Neural Networks (CNN): VGG16, ResNet34, EfficientNet_b0, and a Vision Transformer (ViT). The obtained results were compared to those of a tailored CNN with a much smaller number of parameters. The effectiveness of the proposed models was evaluated on a gait dataset collected from more than 700 patients.
Materials and methods
Data acquisition.
This study was carried out in accordance with the tenets of the Declaration of Helsinki and with the approval of the Brest, France hospital’s (CHRU’s) Ethics Committee. Patients had also signed an informed consent. Our work was conducted between 2021 and 2022. Data collected between June 2006 and June 2021 from 734 patients (115 adults and 619 children) who had undergone clinical 3D gait analysis were used. Their identities were preserved by respecting medical secret and protecting patient confidentiality. All data were recorded using the same motion analysis system (Vicon MX, Oxford Metrics, UK) and four force platforms (Advanced Mechanical Technology, Inc., Watertown, MA, USA) in the same motion laboratory (CHU Brest) between 2006 and 2022. The data collected by the 15 infrared cameras (sampling rate of 100 or 120 Hz) were synchronized with the ground reaction forces recorded by the force platforms (1000 Hz or 1200 Hz). The 16 markers were placed according to the protocol by Kadaba et al. 11 . Marker trajectories and ground reaction forces were dual-pass filtered with a low-pass Butterworth filter at a cut-off frequency of 6 Hz. After an initial calibration in the standing position, all patients were asked to walk at a self-selected speed along a 10m walkway.
Gait kinematics were processed using the Vicon Plug-in Gait model. Kinematics were time-normalized to stride duration, from 0 to 100% from initial contact (IC) to the next IC of the ipsilateral foot. Nine gait joint angles (kinematic gait variables) were used: anteversion/retroversion of the pelvis, rotation of the pelvis, pelvic tilt, flexion/extension of the hip, abduction/adduction of the hip, internal/external rotation of the hip, flexion/extension of the knee, plantar/dorsiflexion of the ankle, and the foot’s angle of progression. As a result, a gait cycle yielded 101 \(\times\) 9 measurements. Let \(E_{p,d}\) denote the gait session of patient p at datetime d . It can be written as follows:
where \({C_{ E_{p,d}}}^{k}\) is the k -th gait cycle of a gait session \(E_{p,d}\) and K the total number of gait cycles. Let \(c_{t,n}^{E_{p,d}^{k}}\) denote the gait cycle \({C_{E_{p,d}}}^{k}\) value at time step t and joint angle n . To keep notations simple, \(c_{t,n}^{E_{p,d}^{k}}\) is referred to as \(c_{t,n}\) in what follows. \({C_{E_{p,d}}}^{k}\) can simply be represented with a matrix of 101 lines and 9 columns, as follows:
The Gait Profile Score (GPS), a “walking behavior score”, was computed for each gait cycle from the previously described joint angles 12 , 13 , 14 . The GPS is a single index measure that summarizes the overall deviation of kinematic gait data relative to normative data. It can be decomposed to provide Gait Variable Scores (GVS) for nine key component kinematic gait variables, which are presented as a Movement Analysis Profile (MAP). The GVS corresponding to the n -th kinematic variable, GVS \(_{\textrm{n}}\) , is given by 15 , 16 , 17 :
where t is a specific point in the gait cycle, T its total number of points (typically equal to 101 18 , 19 ), \(c_{t,n}\) the value of the kinematic variable n at point t , and \(c_{t,n}^{\textrm{ref}}\) is its mean on the reference population (physiological normative). The GPS is obtained from the GVS scores 15 , 17 as follows:
where N is the total number of kinematic variables (equal to 9 by definition).
Gait database
We had a total of 1459 gait sessions from 734 patients (115 adults and 619 children). Each patient had an average of 1.988 gait sessions with a standard deviation of 1.515. 53,693 gait cycles were collected. Their average number per gait session is equal to 18 with a standard deviation of 6. Neurological conditions, notably cerebral palsy, are the most frequent etiologies, as we can see in Fig. 1 .
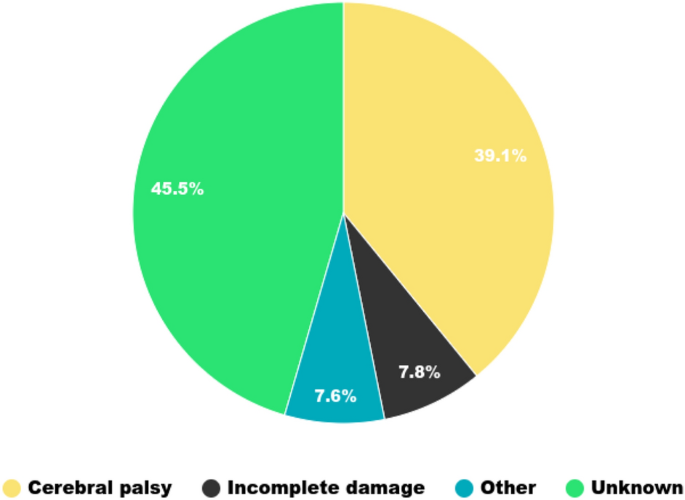
Etiologies pie chart.
The average patient age within the first gait session is equal to 14 years, with a standard deviation of 16 years. The time delay between the first and last gait session (for patients with more than one gait session, i.e., 319) is equal to 3.92 years on average with a standard deviation of 3.24 years. Directly consecutive gait sessions are, on average, separated by approximately 740 days, with a standard deviation of 577 days. The shortest (resp. longest) time delay was equal to 4 (resp. 4438) days. We had 1384 pairs of directly consecutive gait sessions belonging to 319 patients (the remaining patients were removed since they had only one gait session). Involved gait conditions are various: without any equipment, with a cane, with a rollator, with an orthosis, with a prosthesis.. Only pairs of gait sessions without equipment were selected in order to be in the same condition (79% of all available pairs, i.e. 1152). The first gait sessions in these pairs were used for training. Models were fed the gait cycles of these first gait sessions (i.e., 21,167 gait cycles in total).
GPS variation prediction
GPS variation prediction is similar enough to a Time Series Classification (TSC) issue that its proposed popular architectures should be adopted. Consecutive gait session pairs \((E_{p,d}, E_{p,d+\Delta d})\) were considered. For each gait cycle \({C_{ E_{p,d}}}^{k}\) of the current gait session \(E_{p,d}\) , a GPS variation \(\Delta {}GPS\) was computed using:
where \(GPS_{avg}(E_{p,d+\Delta d})\) is the average GPS per cycle of \(E_{p,d+\Delta d}\) and \(GPS({C_{ E_{p,d}}}^{k})\) the GPS of the current gait cycle \({C_{E_{p,d}}}^{k}\) . The average GPS per cycle \(GPS_{average}(E_{p,d})\) of a gait session \(E_{p,d}\) is simply equal to:
\(\Delta {}\)
GPS was ranked in a binary fashion. Either it is negative, in which case the patient’s gait improves (class 1), or it is positive, in which case the patient’s gait worsens (class 0). The metric used is the Area Under the Curve (AUC).
The distribution of patients between training, validation, and test groups is provided in Table 1 . Such a split put 73%, 12%, and 14% of total gait cycles within the training, validation, and test groups, respectively.
Signal-based approach
To be exhaustive, one MLP, one recurrent neural network (LSTM), one hybrid architecture (Encoder), several CNN architectures (FCN, ResNet, t-LeNet), and a one-dimensional Transformer 20 were included. The MLP and LSTM were designed and developed from scratch. Their hyper-parameters were optimized manually. FCN, ResNet, Encoder, and t-LeNet are among the most effective end-to-end discriminative architectures regarding the TSC state-of-the-art 10 . These methods were also compared to the Transformer, a more recent and popular architecture. The Transformer does not suffer from long-range context dependency issues compared to LSTM 21 . In addition, it is notable for requiring less training. The Adam optimizer 22 and binary cross-entropy loss were employed 23 .
For MLP, gait cycles were flattened so that the input length was equal to 909 time steps. The number of neurons was the same across all the fully connected layers. Many values of this number were tested to find the best structure for our task. In the same way, the number of layers was optimized. The corresponding architecture is shown in Fig. 2 .
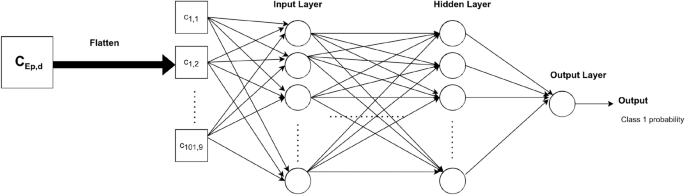
MLP architecture for prediction.
LSTM layers were stacked, and a dropout was added before the last layer to avoid overfitting. The corresponding architecture is shown in Fig. 3 .
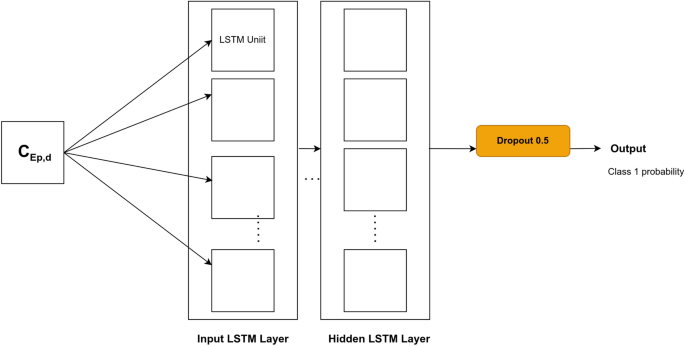
LSTM architecture for prediction.
For FCN, ResNet, Encoder and t-LeNet, the architectures proposed in Ref. 10 were considered. They are shown in Figs. 4 , 5 , 6 and 7 , respectively. We followed an existing implementation 24 to set up the Transformer.

FCN architecture for prediction.
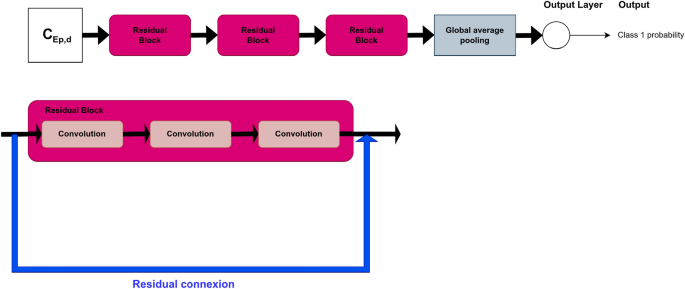
ResNet architecture for prediction.

Encoder architecture for prediction.
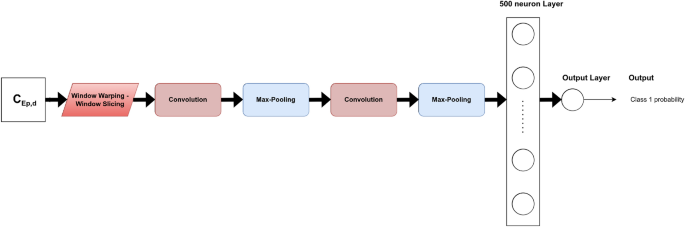
t-LeNet architecture for prediction.
Data augmentation
Different techniques of data augmentation were tested as a pre-processing step to avoid overfitting: jittering, scaling, window warping, permutation, and window slicing. Their hyperparameters were empirically optimized for each model. These are among the TSC literature’s most frequently utilized techniques, particularly when it comes from sensor data 10 .
Image-based approach
Image-based time series representation initiated a new branch of deep learning approaches that consider image transformation as an innovative pre-processing of feature engineering 25 . In an attempt to reveal features and patterns less visible in the one-dimensional sequence of the original time series, many transformation methods were developed to encode time series as input images.
In our study, sensor modalities are transformed to the visual domain using 2D FFT in order to utilize a set of pre-trained CNN models for transfer learning on the converted imagery data. The full workflow of our framework is represented in Fig. 8 .

Proposed \(\Delta GPS\) prediction workflow for the image-based approach.
2D FFT is used to work in the frequency domain or Fourier domain because it efficiently extracts features based on the frequency of each time step in the time series. It can be defined as:
where F ( u , v ) is the direct Fourier transform of the gait cycle. It is a complex function that shows the phase and magnitude of the signal in the frequency domain. u and v are the frequency space coordinates. The magnitude of the 2D FFT | F ( u , v )|, also known as the spectrum, is a two-dimensional signal that represents frequency information. Because the 2D FFT has translation and rotation attributes, the zero-frequency component can be moved to the center of | F ( u , v )| without losing any information, making the spectrum image more visible. The centralized FFT spectrums were computed and fed to the proposed deep learning models. A centralized FFT spectrum for a given gait cycle is represented in Fig. 9 .
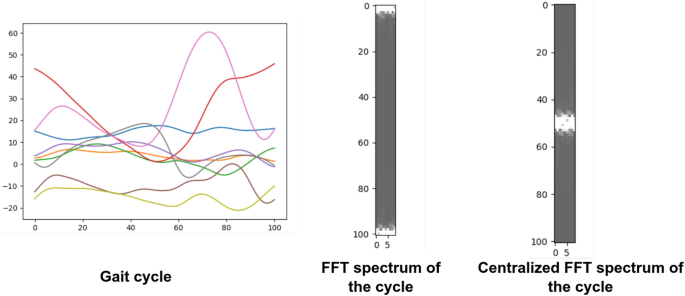
2D FFT for a given gait cycle. ( a ) The gait cycle; ( b ) FFT spectrum of the gait cycle; ( c ) Centralized FFT spectrum of the gait cycle.
Proposed deep learning models
Timm pre-trained models.
The Timm library’s 26 pre-trained VGG16, ResNet34, EfficientNet_b0, and the Vision Transformer ’vit_base_patch16_224’ were investigated. They were pre-trained on a large collection of images, in a supervised fashion. For the Transformer, the pre-training was at a resolution of \(224 \times 224\) pixels. Its input images were considered as a sequence of fixed-size patches (resolution \(16 \times 16\) ), which were linearly embedded.
Converting our grayscale images to RGB images was not necessary because Timm’s implementations support any number of input channels. The model’s minimum input size for VGG16 is \(32 \times 32\) . The image’s width dimension (N) equals 9, which is less than 32. In order to fit the minimum needed size, 2D FFT images were repeated 4 times in this width dimension. Transfer learning with fine-tuning methods was employed. One neuron’s final fully connected layer was used. In the same way that the top layers were trainable, all convolutional blocks were.
Two-dimensional 2D CNN
The pre-trained Timm models are deep and sophisticated, with many layers. As a result, a CNN model with fewer parameters, designed from scratch, was conceived. The number of used two-dimensional convolutional layers was a hyper-parameter to optimize in a finite range of values {1, 2, 3, 4, 5}. After the convolutional block, a dropout function was applied. Following that, two-dimensional max-pooling (MaxPooling2D) and batch normalization were used. The flattened output of the batch normalization was then fed to a dense layer of a certain number of neurons to tune. In order to predict the \(\Delta GPS\) , our model had a dense output layer with a single neuron. The corresponding architecture is shown in Fig. 10 .
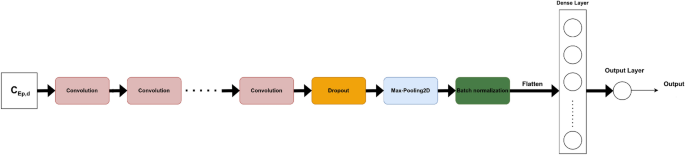
Tailored 2D CNN for prediction.
The following are all of the architecture hyper-parameters to tune: the number of convolutional layers (num_layers), the number of filters for each convolution layer (num_filters), the kernel size of each convolution layer (kernel_size), the dropout rate (dropout), the pooling size of the MaxPooling2D (pool_size), the number of neurons in the dense layer (units), and the learning rate (lr). Five models with a varying number of convolutional layers (from 1 to 5) were tested. For each of them, the rest of the hyper-parameters were tuned using KerasTuner 9 to maximize the validation AUC.
In this section, prediction results are presented in terms of AUC.
Without data augmentation
Results are given in Table 2 . They are homogeneous on the validation set. LSTM and MLP perform equally well on the validation set. ResNet has the highest val AUC (0.709) for the state-of-the-art architectures. FCN achieves a comparable result to ResNet with a val AUC of 0.705. Encoder, t-LeNet and Transformer perform nearly equally well, with a val AUC above 0.63.
The best model has 4 layers of 200 neurons each. It is referred to as MLP_4_200. It gives a val AUC equal to 0.717.
The best model has 4 LSTM layers of 500 units. It is referred to as LSTM_4_500. It gives a val AUC of 0.701.
For all the architectures used, an overfitting behavior with very quick convergence was exhibited. To mitigate this, 5 data augmentation techniques already presented were combined. The order of their application was chosen randomly for each training batch. The best data augmentation parameters were found for each architecture. Results are presented in Table 3 . Performances are slightly better after data augmentation except for the Transformer. In general, convergence is slower; it no longer appears in the first few epochs. FCN gives the best val AUC (0.723).
Table 4 presents the obtained results.
Despite the overfitting behavior of the pre-trained models, the test set’s results are nearly identical to those from the validation set. The tested Timm models all produced results that were comparable, with a val AUC of greater than 0.63. The model with the highest efficiency, the CNN trained entirely from scratch, gives a val AUC of 0.726. It has two convolutional layers, and its hyper-parameter values are as follows: num_filters = 4, kernel_size = 32, dropout = 0, pool_size = 8, units = 300 and lr = \(4.127 \times 10^{-4}\) . Transfer learning is unlikely to have made a significant contribution because our images are visually insufficiently meaningful. Besides, there are not enough large datasets (from the same domain) available within the community to carry out such a transfer learning task.
Discussion and conclusion
The goal of our study was to predict the \(\Delta GPS\) between two consecutive gait sessions in a binary fashion. If this variation is negative, gait quality gets better and vice versa. Globally, from scratch designed architectures gave slightly better results than state-of-the-art ones, which introduce too many parameters to optimize given the relatively small quantity of available data. As a result, a trade-off should be made between the amount of available training data, the complexity of the task, and performance. In the signal-based approach, in general, data augmentation techniques made some improvements in performance. Because of that, we suggest trying to find a way to improve their efficiency. In the image-based approach, developed from scratch CNN surpassed pre-trained Timm models. This can be explained by the fact that the source and target domains are so different. ROC (Receiver operating characteristic) curves for all models are presented in Fig. 11 .
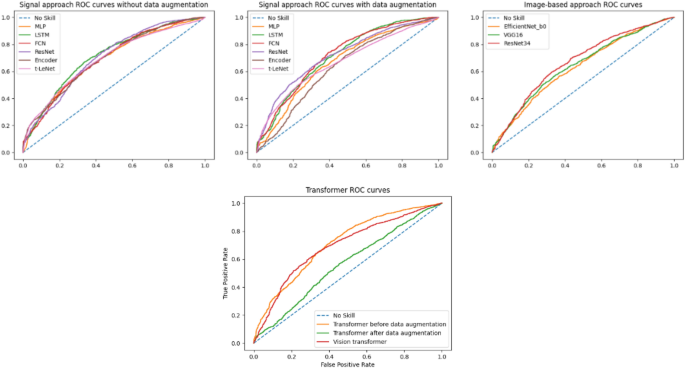
ROC curves for both approaches.
To have better insight into results, the ROC curves of the best models (i.e., FCN after data augmentation for the signal-based approach and CNN for the image-based one) were compared using the DeLong’s test. This revealed a p-value of \(2.316 \times 10^{-4}\) for the two ROC curves at hand, which means that the AUCs of both models are significantly different. In other words, the FCN model after data augmentation, with a val AUC of 0.723 and a test AUC of 0.717, is meaningfully better than the CNN model. This outcome proves that knowledge extraction is more efficient on raw signals than synthetic images. In summary, for both approaches, the prediction results are encouraging despite the complexity of such a prediction task on so heterogeneous data. The val AUC and test AUC are above 0.7 for both approaches.
One limitation of this study is the fact that we were not able to validate our findings on external datasets because we did not have any other external data at our disposal. Actually, we were unable to find any publicly-available medical databases.
Our future work will focus on taking the different pathologies into account. Ways of having more data should be thought about as well.
Data availability
The dataset used and analysed during the current study is available from the corresponding author on reasonable request.
Ataullah, A. H. M. & De Jesus, O. Gait Disturbances. in StatPearls (StatPearls Publishing, 2022).
Auvinet, B., Touzard, C., Montestruc, F., Delafond, A. & Goeb, V. Gait disorders in the elderly and dual task gait analysis: A new approach for identifying motor phenotypes. J. Neuroeng. Rehabilit. 14 , 1–14 (2017).
Google Scholar
Bishnoi, A. & Hernandez, M. E. Dual task walking costs in older adults with mild cognitive impairment: A systematic review and meta-analysis. Aging Mental Health 25 , 1618–1629 (2021).
Article PubMed Google Scholar
Pirker, W. & Katzenschlager, R. Gait disorders in adults and the elderly. Wiener Klinische Wochenschrift 129 , 81–95. https://doi.org/10.1007/s00508-016-1096-4 (2017).
Rodrigues, F., Domingos, C., Monteiro, D. & Morouço, P. A review on aging, sarcopenia, falls, and resistance training in community-dwelling older adults. Int. J. Environ. Res. Public Health 19 , 874 (2022).
Article PubMed PubMed Central Google Scholar
Osoba, M. Y., Rao, A. K., Agrawal, S. K. & Lalwani, A. K. Balance and gait in the elderly: A contemporary review. Laryngosc. Investig. Otolaryngol. 4 , 143–153 (2019).
Article Google Scholar
Rodríguez-Fernández, A., Lobo-Prat, J. & Font-Llagunes, J. M. Systematic review on wearable lower-limb exoskeletons for gait training in neuromuscular impairments. J. Neuroeng. Rehabilit. 18 , 1–21 (2021).
Ridao-Fernández, C., Pinero-Pinto, E., Chamorro-Moriana, G. et al. Observational gait assessment scales in patients with walking disorders: Systematic review. BioMed Res. Int . 2019 (2019).
O’Malley, T. et al. Kerastuner. https://github.com/keras-team/keras-tuner (2019).
Ismail Fawaz, H., Forestier, G., Weber, J., Idoumghar, L. & Muller, P. A. Deep learning for time series classification: A review. Data Mining Knowl. Discov. 33 , 917–963 (2019).
Article MathSciNet Google Scholar
Kadaba, M. P., Ramakrishnan, H. & Wootten, M. Measurement of lower extremity kinematics during level walking. J. Orthop. Res. 8 , 383–392 (1990).
Article CAS PubMed Google Scholar
Christian, J., Kröll, J. & Schwameder, H. Comparison of the classifier oriented gait score and the gait profile score based on imitated gait impairments. Gait Posture 55 , 49–54 (2017).
Holmes, S. J., Mudge, A. J., Wojciechowski, E. A., Axt, M. W. & Burns, J. Impact of multilevel joint contractures of the hips, knees and ankles on the gait profile score in children with cerebral palsy. Clin. Biomech. 59 , 8–14 (2018).
Robinson, L. et al. The relationship between the Edinburgh visual gait score, the gait profile score and gmfcs levels I–III. Gait Posture 41 , 741–743 (2015).
Baker, R. et al. The gait profile score and movement analysis profile. Gait Posture 30 , 265–269 (2009).
Barton, G. J., Hawken, M. B., Scott, M. A. & Schwartz, M. H. Movement deviation profile: A measure of distance from normality using a self-organizing neural network. Hum. Movement Sci. 31 , 284–294 (2012).
Jarvis, H. L. et al. The gait profile score characterises walking performance impairments in young stroke survivors. Gait Posture 91 , 229–234 (2022).
Winter, D. A. Biomechanics and motor control of human gait: normal, elderly and pathological (1991).
Zdero, R., Brzozowski, P., Schemitsch, E. H. et al. Experimental methods for studying the contact mechanics of joints. BioMed Res. Int. 2023 (2023).
Vaswani, A. et al. Attention is all you need. Adv. Neural Inform. Process. Syst . 30 (2017).
Dosovitskiy, A. et al. An image is worth 16x16 words: Transformers for image recognition at scale. arXiv preprint . arXiv:2010.11929 (2020).
Kingma, D. P. & Ba, J. Adam: A method for stochastic optimization. arXiv preprint . arXiv:1412.6980 (2014).
Ruby, U. & Yendapalli, V. Binary cross entropy with deep learning technique for image classification. Int. J. Adv. Trends Comput. Sci. Eng . 9 (2020).
GitHub-hsd1503/transformer1d: Pytorch implementation of transformer for 1D data—github.com. https://github.com/hsd1503/transformer1d/tree/master . [Accessed 29-11-2023].
Yang, C.-L., Chen, Z.-X. & Yang, C.-Y. Sensor classification using convolutional neural network by encoding multivariate time series as two-dimensional colored images. Sensors 20 , 168 (2019).
Article ADS CAS PubMed PubMed Central Google Scholar
Wightman, R. Pytorch image models. https://doi.org/10.5281/zenodo.4414861 (2019).
Download references
Acknowledgements
This study received funding from the French government via the national research agency (Agence Nationale de la Recherche) as part of the investment programme for the future (Programme d’Investissement d’avenir), under the reference ANR-17-RHUS-0005 (FollowKnee Project).
Author information
Authors and affiliations.
LaTIM UMR 1101 Laboratory, Inserm, Brest, France
Nawel Ben Chaabane, Pierre-Henri Conze, Mathieu Lempereur, Gwenolé Quellec, Olivier Rémy-Néris, Sylvain Brochard, Béatrice Cochener & Mathieu Lamard
Western Brittany University, Brest, France
Nawel Ben Chaabane, Mathieu Lempereur, Olivier Rémy-Néris, Sylvain Brochard, Béatrice Cochener & Mathieu Lamard
IMT Atlantique, Brest, France
Pierre-Henri Conze
University Hospital of Brest, Brest, France
Mathieu Lempereur, Olivier Rémy-Néris, Sylvain Brochard & Béatrice Cochener
You can also search for this author in PubMed Google Scholar
Contributions
N.B. conceived the experiments and wrote the manuscript, with the help of M.L.A., P.H.C. and G.Q. She conducted the experiments. M.L.E. gave deep insights into the database. O.R., S.B., B.C. provided clinical inputs. All authors helped supervise the project, discussed the results, commented on the manuscript, and provided critical feedback.
Corresponding author
Correspondence to Nawel Ben Chaabane .
Ethics declarations
Competing interests.
The authors declare no competing interests.
Additional information
Publisher's note.
Springer Nature remains neutral with regard to jurisdictional claims in published maps and institutional affiliations.
Rights and permissions
Open Access This article is licensed under a Creative Commons Attribution 4.0 International License, which permits use, sharing, adaptation, distribution and reproduction in any medium or format, as long as you give appropriate credit to the original author(s) and the source, provide a link to the Creative Commons licence, and indicate if changes were made. The images or other third party material in this article are included in the article’s Creative Commons licence, unless indicated otherwise in a credit line to the material. If material is not included in the article’s Creative Commons licence and your intended use is not permitted by statutory regulation or exceeds the permitted use, you will need to obtain permission directly from the copyright holder. To view a copy of this licence, visit http://creativecommons.org/licenses/by/4.0/ .
Reprints and permissions
About this article
Cite this article.
Ben Chaabane, N., Conze, PH., Lempereur, M. et al. Quantitative gait analysis and prediction using artificial intelligence for patients with gait disorders. Sci Rep 13 , 23099 (2023). https://doi.org/10.1038/s41598-023-49883-8
Download citation
Received : 06 July 2023
Accepted : 13 December 2023
Published : 28 December 2023
DOI : https://doi.org/10.1038/s41598-023-49883-8
Share this article
Anyone you share the following link with will be able to read this content:
Sorry, a shareable link is not currently available for this article.
Provided by the Springer Nature SharedIt content-sharing initiative
By submitting a comment you agree to abide by our Terms and Community Guidelines . If you find something abusive or that does not comply with our terms or guidelines please flag it as inappropriate.
Quick links
- Explore articles by subject
- Guide to authors
- Editorial policies
Sign up for the Nature Briefing newsletter — what matters in science, free to your inbox daily.

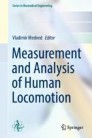
Measurement and Analysis of Human Locomotion pp 219–255 Cite as
Gait Analysis
- Vladimir Medved 3 ,
- Rodolfo Vastola 4 ,
- Daniele Albano 4 &
- Marko Pećina 5
- First Online: 23 January 2022
1040 Accesses
Part of the book series: Series in Biomedical Engineering ((BIOMENG))
Gait analysis can be considered a backbone of modern clinical locomotion biomechanics. Basic methodology, integrating kinematic, kinetic and myoelectric measurements in course of a subject's walking and subsequent interpretation of results is summarized and illustrated mainly with findings from our Zagreb and Salerno situated laboratories. In addition, pedobarography and portable oxygen consumption measurement system make desirable components of an equipment inventory for gait analysis. Physical examination and observational analysis precede measurement of several gait trials in a laboratory. Interpretation of measurement findings is the next step. Modern tendencies, pursued worldwide, incorporate individualized (subject-specific) neuro-musculo-skeletal modeling into a clinical procedure. Non-linear analysis of gait data emerges as yet another methodology of gait analysis. Alternative modern tendencies are finally pointed to whereby classical measurement instrumentation setup confined to laboratory environment is substituted by portable, so-called pervasive multisensor measurement solutions offering freedom of movement and aiming to replace classical approach, albeit with no success yet.
This is a preview of subscription content, log in via an institution .
Buying options
- Available as PDF
- Read on any device
- Instant download
- Own it forever
- Available as EPUB and PDF
- Compact, lightweight edition
- Dispatched in 3 to 5 business days
- Free shipping worldwide - see info
- Durable hardcover edition
Tax calculation will be finalised at checkout
Purchases are for personal use only
Nigg BM (1994) Selected historical highlights. In: Nigg B Herzog W (eds) Biomechanics of the musculo-skeletal system. New York, Wiley, pp 3–35
Google Scholar
Baker R (2006) Gait analysis methods in rehabilitation. J NeuroEng Rehab 3(1):4
Article Google Scholar
Gerber L, Rasch EK (2013) In memoriam: a tribute to Jacqueline Perry. Arch Phys Med Rehabil 94(2036):c1-e4
Baker R (2013) Measuring walking: a handbook of clinical gait analysis. Mac Keith Press, London
Baker R, Esquenazi A, Benedetti MG, Desloovere K (2016) Gait analysis: clinical facts. Eur J Phys Rehab Med 52(4):560–574
Gage JR, Schwartz MH, Koop SE, Novacheck TF (2009) The identification and treatment of gait problems in cerebral palsy, 2nd edn. McKeith Press, London
Perry J (1992) Gait analysis: normal and pathological function, Thoroafare, NJ, SLACK
Kaufman KR, Sutherland DH (2006) Kinematics of normal human walking (Chapter 3). In: Rose J, Gamble JG (eds) Human walking. 3rd edn. Lippincott Williams & Wilkins, Philadelphia, Pa, pp 33–51
Perry J, Antonelli DJ, Bontrager EL (1976) VA-Rancho gait analyzer, final project report, Rancho Los Amigos Hospital, Downey, California
Hannah R, Cousins S, Foort J (1978) The CARS-UBC electrogoniometer—a clinically viable tool, 7th Canadian Medical & Biological Engineering Conference
Medved V (1980) Initial evaluation of CARS-UBC electrogoniometer system. Biomechanics Laboratory, Division of Applied Sciences, Harvard University, technical note
Koopman B, Grootenboer HJ, de Jongh HJ (1995) An inverse dynamic model for the analysis, reconstruction and prediction of bipedal walking. J Biomech 28(11):1369–1376
Forner-Cordero A, Koopman HJFM, van der Helm FCT (2006) Inverse dynamics calculations during gait with restricted ground reaction force information from pressure insoles. Gait Posture 23(2):189–199
Chambers HG, Sutherland DH (2002) A practical guide to gait analysis. J Am Acad Orthop Surg 10(3):222–231
Kiseljak D, Bolčević F, Ćurko D, Pažin K, Ujaković F, Gruić I, Medved V (2017) The modified Thomas Test realized by optoelectronic kinematic measurement. Functional Neurology, Rehabilitation, and Ergonomics. 7(3):39–44 (and as abstract in: Book of Abstracts of the 2017 Conference on Movement: Brain, Body, Cognition (Leisman, Gerry (ur.)—Oxford University, Oxford, July 9–11, 2017. International association of functional neurology and rehabilitation, Oxford, UK, p 95)
Davis RB III, Ounpuu S, Tyburski D, Gage JR (1991) A gait analysis data collection and reduction technique. Hum Mov Sci 10(5):575–587
Kadaba MP, Ramakrishnan HK, Wootten ME, Gainey J, Gorton G, Cochran GVB (1989) Repeatability of kinematic, kinetic, and electromyographic data in normal adult gait. J Orthop Res 7(6):849–860
Heimer, Ž. (2005) Automatizirano kliničko mjerenje biomehanike i kineziologije hoda (Automated clinical measurement of biomechanics and kinesiology of gait). [master’s thesis]. [Zagreb (Croatia)]: University of Zagreb
Cappozzo A, Catani F, Della Croce U, Leardini A (1995) Position and orientation in space of bones during movement: anatomical frame definition and determination. Clin Biomech 10(4):171–178
Rabuffetti M, Crenna P (2004) A modular protocol for the analysis of movement in children. Gait Posture 20:S77–S78
Leardini A, Sawacha Z, Paolini G, Ingrosso S, Nativo R, Benedetti MG (2007) A new anatomically-based protocol for gait analysis in children. Gait Posture 26(4):560–571
Frigo C, Rabuffetti M, Kerrigan DC, Deming LC, Pedotti A (1998) Functionally oriented and clinically feasible quantitative gait analysis method. Med Biol Eng Comput 36(2):179–185
Di Prampero PE, Cappello A, Cappozzo A (Eds.) (2003) Bioingegneria della postura e del movimento. Patron
Manal K, Stanhope SJ (2004) A novel method for displaying gait and clinical movement analysis data. Gait Posture 20(2):222–226
Kasović M, Heimer Ž, Medved V (2011) Coloured signal coding in computerized human gait analysis. Proceedings 6th international scientific conference on kinesiology „Integrative power of kinesiology“, Opatija, Croatia, pp 155–157
Simon RS (2004) Quantification of human motion: gait analysis—benefits and limitations to its application to clinical problems. J Biomech 37:1869–1880
Wu G, Cavanaugh PR (2001) ISB recommendations for standardization in the reporting of kinematic data. J Biomech 28(10):1257–1261 (Appendix A)
Wu G, Siegler S, Allard P, Kirtley C, Leardini A, Rosenbaum D, Whittle M, D’Lima DD, Cristofolini L, Whittle H, Schmidt O, Stokes I (2002) Letter to the editor—ISB recommendation on definitions of joint coordinate system of various joints for the reporting of human joint motion—part I: ankle, hip, and spine. J Biomech 35(4):543–548 (Appendix B)
BTS GAITLAB—Analysis protocols davis protocol version 1.0.0 (2016) BTS S.p.A
Astone S, Mignone A, Albano D (2019) Comparison of gait kinematics and kinetics between qualified dancers and non-dancers. J Phys Educ Sport 19:2289–2291
Baker R, McGinley JL, Schwartz MH, Beynon S, Rozumalski A, Graham HK et al (2009) The gait profile score and movement analysis profile. Gait Posture 30:265–269
Schwartz MH, Rozumalski A (2008) The gait deviation index: a new comprehensive index of gait pathology. Gait Posture 28(3):351–357
Astone S, Mignone A, Coppola S (2020) Comparison of EMG profile during gait between qualified dancers and non dancers. RRISS 2nd edn, vol II, pp 80–84
Kovač I (2007) Biomehanička analiza hoda osoba s potkoljenom amputacijom opskrbljenih protezama s osloncem na ligament patele (Biomechanical analysis of walking in lower extremity amputees’ equipped with Patella Tendon Bearing (PTB) prostheses). [dissertation]. [Zagreb (Croatia)]: University of Zagreb
Jelić M, Kovač I (2002) Epidemiological analysis of war amputees. In: Book of abstracts—third ISPO Central and Eastern European Conference, Dubrovnik
Kovač I, Medved V, Ostojić Lj (2009) Ground reaction force analysis in traumatic transtibial amputees’ gait. Coll Antropol 33(Suppl. 2):107–114
Kovač I, Medved V, Ostojić Lj (2010) Spatial, temporal nad kinematic characteristics of traumatic transtibial amputees’ gait (2010) Coll Antropol 34(Suppl. 1):205–213
Kovač I, Medved V, Kasović M, Heimer Ž, Lužar-Stiffler V, Pećina M (2010) Instrumented joint mobility analysis in traumatic transtibial amputee patients. Period Biol 112(1):25–31
Gilleard WL, Crosbie J, Smith R (2002) Static trunk posture in sitting and standing during pregnancy and early postpartum. Arch Phys Med Rehabil 83(12):1739–1744
Cilio A (2019) Analisi biomeccanica del cammino in gravidanza (Biomechanical analysis of gait during pregnancy). [dissertation]. [Salerno (Italy)], University of Salerno
Perry J, Davids JR (1992) Gait analysis: normal and pathological function. J Pediatr Orthopaed 12(6):815
Whittle MW, Levine D, Richards J (2012) Whittle’s gait analysis. Churchill Livingstone, Elsevier
Skaggs DL, Rethlefsen SA, Kay RM, Dennis SW, Reynolds RAK, Tolo VT (2000) Variability in gait analysis interpretation. J Pediatr Orthopaed 20(6):759–764
Winter DA (1991) An elaborated strategy of clinical application of gait analysis (Gait Diagnostic Chart). In: Boenick U, Nader M, Mainka (eds) Gangbildanalyse-Stand der Messtechnik und Bedeutung für die Orthopadie-Technik. Duderstadt, Mecke Druck, pp 266–277
Gage JR, Stout JL (2009) Gait analysis: kinematics, kinetics, electromyography, oxygen consumption and pedobarography. In: Gage JR, Schwartz MH, Koop SE, Novacheck TF (2009) The identification and treatment of gait problems in cerebral palsy. 2nd edn. McKeith Press London, pp 260–284
Delp SL, Loan JP, Hoy MG, Zajac FE, Topp EL, Rosen JM (1990) An interactive graphics-based model of the lower extremity to study orthopaedic surgical procedures. IEEE Trans BME 37(8):757–766
Delp SL, Loan JP (1995) A graphics-based software system to develop and analyze models of musculoskeletal structures. Comput Biol Med 25(1):21–34
Seth A, Hicks JL, Uchida TK, Habib A, Dembia CL, Dunne JJ et al (2018) OpenSim: simulating musculoskeletal dynamics and neuromuscular control to study human and animal movement. PLoS Comput Biol 14(7):e1006223. https://doi.org/10.1371/journal.pcbi.1006223
Hicks JL, Schwartz MH, Delp SL (2009) Modeling and simulation of normal and pathological gait. In: Gage JR, Schwartz MH, Koop SE, Novacheck TF (2009) The identification and treatment of gait problems in cerebral palsy. 2nd edn. McKeith Press London, pp 285–305
Whittle MW (1993) Gait analysis. In: The soft tissues. Butterworth-Heinemann, pp 187–199
Perry J, Hoffer MM (1977) Preoperative and postoperative dynamic electromyography as an aid in planning tendon transfers in children with cerebral palsy. J Bone Joint Surg Am 59(4):531–537
Sutherland DH (1978) Gait analysis in cerebral palsy. Develop Med Child Neurol 20(6):807–813
Gage JR (1983) Gait analysis for decision-making in cerebral palsy. Bull Hosp Joint Dis Orthop Inst 43(2):147–163
Hicks R, Durinick N, Gage JR (1988) Differentiation of idiopathic toe-walking and cerebral palsy. J Pediatr Orthop 8(2):160–163
Gage JR, Perry J, Hicks RR, Koop S, Werntz JR (1987) Rectus femoris transfer to improve knee function of children with cerebral palsy. Develop Med Child Neurol 29(2):159–166
Sanders RD, Gillig PM (2010) Gait and its assessment in psychiatry. Psychiatry (Edgmont) 7(7):38–43
Bernstein, N. (1966). The co-ordination and regulation of movements. Pergamon-Press
Wurdeman SR, Myers SA, Jacobsen AL, Stergiou N (2014) Adaptation and prosthesis effects on stride-to-stride fluctuations in amputee gait. PloS one 9(6):e100125
Chen S, Lach J, Lo B, Yang G-Z (2016) Toward pervasive gait analysis with wearable sensors: a systematic review. IEEE J Biomed Health Inform 20(6):1521–1537
Rose J, Gamble JG (eds) (2006) Human walking. 3rd edn. Lippincott Williams & Wilkins, Philadelphia, Pa
McMahon TA (1984) Muscles, reflexes, and locomotion. Princeton University Press, Princeton, NJ
Book Google Scholar
Medved V (2001) Measurement of human locomotion. CRC Press Inc., Boca Raton, Fl
Tesio L, Rota V (2019) The motion of body center of mass during walking: a review oriented to clinical applications. Fron Neurol 10:999. https://doi.org/10.3389/fneur.2019.00999
Lu TW, Chang CF (2012) Biomechanics of human movement and its clinical applications. Kaohsiung J Med Sci 28:513–525
Download references
Author information
Authors and affiliations.
Faculty of Kinesiology, University of Zagreb, Horvaćanski zavoj 15, 10000, Zagreb, Croatia
Vladimir Medved
Department of Human, Philosophical and Educational Sciences, University of Salerno, Via Giovanni Paolo II, 132 - 84084, Fisciano (SA), Italy
Rodolfo Vastola & Daniele Albano
School of Medicine, University of Zagreb, Šalata 2, 10000, Zagreb, Croatia
Marko Pećina
You can also search for this author in PubMed Google Scholar
Corresponding author
Correspondence to Vladimir Medved .
Editor information
Editors and affiliations.
Faculty of Kinesiology, University of Zagreb, Zagreb, Croatia
Rights and permissions
Reprints and permissions
Copyright information
© 2021 Springer Nature Switzerland AG
About this chapter
Cite this chapter.
Medved, V., Vastola, R., Albano, D., Pećina, M. (2021). Gait Analysis. In: Medved, V. (eds) Measurement and Analysis of Human Locomotion. Series in Biomedical Engineering. Springer, Cham. https://doi.org/10.1007/978-3-030-79685-3_10
Download citation
DOI : https://doi.org/10.1007/978-3-030-79685-3_10
Published : 23 January 2022
Publisher Name : Springer, Cham
Print ISBN : 978-3-030-79684-6
Online ISBN : 978-3-030-79685-3
eBook Packages : Physics and Astronomy Physics and Astronomy (R0)
Share this chapter
Anyone you share the following link with will be able to read this content:
Sorry, a shareable link is not currently available for this article.
Provided by the Springer Nature SharedIt content-sharing initiative
- Publish with us
Policies and ethics
- Find a journal
- Track your research
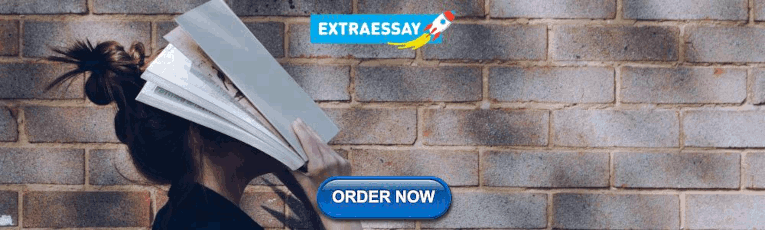
IMAGES
VIDEO
COMMENTS
This study showed excellent test-retest reliability of the parameters considered, and thus highlighting the reliability of an infrared assisted, trunk accelerometer-based device for clinical gait analysis. Another study investigated the concurrent validity and test-retest reliability of gait parameters (cadence, gait velocity, step time, step ...
Develop your skills in observational gait analysis in this virtual lecture series! Tracy and Will present this series based on our gait evaluation and interv...
If any changes occur, one can perform repeat gait analysis. Case Study Two: When Multiple Orthotic Accommodations Fail A 62-year-old male presented with a chronic ulcer on the plantar aspect of the right foot. This patient had previously undergone a pancreas/liver transplant because of the effects of chronic diabetes mellitus. Over the years of ...
Case Study. A boy aged 4 years 5 months pre- ... Gait analysis has been studied over the last few decades as the best way to objectively assess the technical outcome of a procedure designed to ...
Clinical gait analysis is the study of assessing gait disorders or abnormalities. [1]. Gait abnormalities can occur for a variety of reasons including. injuries, stroke, and neurological disorders ...
The gait cycle is the repetitive pattern of walking or running movement ( figure 1 ). Each complete gait cycle, or stride, begins when one foot makes initial contact with the ground, progressing through each phase of gait (see below), and ending when the same foot again makes contact. For walking, each stride is subdivided into a stance phase ...
A case study of a 24-year old male diagnosed with Alkaptonuria undergoing a routine gait analysis. A 3D motion capture with the Helen-Hayes marker set was used to quantify lower-limb joint kinematics during barefoot walking along a 10 m walkway at a self-selected pace. Additional 2D video data were recorded in the sagittal and frontal plane.
clinical gait analysis in Sect. 3. In Sect. 4, we describe our visual an-alytics approach and its underlying design decisions. Sect. 5 reports on the evaluation of the case study with our clinical collaboration partners on gait data from eight patients with CP. Sect. 6 discusses the clinicians' feedback, limitations, and future work and Sect. 7
Contact us for more information about adopting GAIT, its merits and detailed case studies. Contact Us. GAIT is a global assessment tool designed to accurately and quantitatively evaluate the skills of IT engineers. GAIT's assessment content covers seven fields spanning 22 categories, and the results offer clear insights into an engineer's ...
A case study of a 24-year old male diagnosed with Alkaptonuria undergoing a routine gait analysis. A 3D motion capture with the Helen-Hayes marker set was used to quantify lower-limb joint kinematics during barefoot walking along a 10 m walkway at a self-selected pace. Additional 2D video data were recorded in the sagittal and frontal plane.
The analysis of a person's gait may serve for examining that person's health status, functional mobility or progress in rehabilitation. In particular, the estimates of spatiotemporal parameters characterising the human gait—such as the self-selected walking speed, the variability of the length of steps and strides, and the relative durations of particular phases of the gait cycle ...
The biomechanics of the foot and ankle are intimately related to the knee, hip, and spine. Gait analysis is the systematic study of the human gait. Since gait is a mechanical process within a biological system, gait analysis allows us to put the knowledge of foot and ankle biomechanics to practical use. We cannot understand or interpret gait ...
Gait analysis is the systematic and quantitative instrumental assessment of the biomechanical measures of locomotion [], aimed at understanding the etiology of locomotor abnormalities and at assisting clinicians in their decisional process.Starting from the 1990s, gait analysis has turned from a purely academic discipline into a diagnostic tool, thus evolving into a process that embraces ...
Human gait is a periodic motion of body segments—the analysis of motion and related studies is termed gait analysis. Gait Analysis has gained much popularity because of its applications in clinical diagnosis, rehabilitation methods, gait biometrics, robotics, sports, and biomechanics. ... In the case of a normal gait, the step length of the ...
Background Cognitive age-related decline is linked to dementia development and gait has been proposed to measure the change in brain function. This study aimed to investigate if spatiotemporal gait variables could be used to differentiate between the three cognitive status groups. Methods Ninety-three older adults were screened and classified into three groups; mild cognitive impairment (MCI ...
It is a challenge that goes beyond the gait analysis case study because service design methods commonly focus on the entire service design (macro-level). Objective This study aims to extract design considerations and generate guidelines to integrate MoCap technology for gait analysis in the hospital rehabilitation setting. Specifically, the aim ...
Quantitative Gait Analysis (QGA) is considered as an objective measure of gait performance. In this study, we aim at designing an artificial intelligence that can efficiently predict the ...
In this case, the subject's skin at the electrode application points must be free of hair and oil, therefore it must be cleaned with alcohol and treated with a particular conductive solution ... The study of variability in gait analysis, in this perspective, seems promising for the identification and evaluation of adaptations of the ...
Gait analysis is the systematic study of animal locomotion, more specifically the study of human motion, using the eye and the brain of observers, augmented by instrumentation for measuring body movements, body mechanics, and the activity of the muscles. [1] Gait analysis is used to assess and treat individuals with conditions affecting their ...