Thank you for visiting nature.com. You are using a browser version with limited support for CSS. To obtain the best experience, we recommend you use a more up to date browser (or turn off compatibility mode in Internet Explorer). In the meantime, to ensure continued support, we are displaying the site without styles and JavaScript.
- View all journals
- Explore content
- About the journal
- Publish with us
- Sign up for alerts
- Review Article
- Published: 11 May 2000
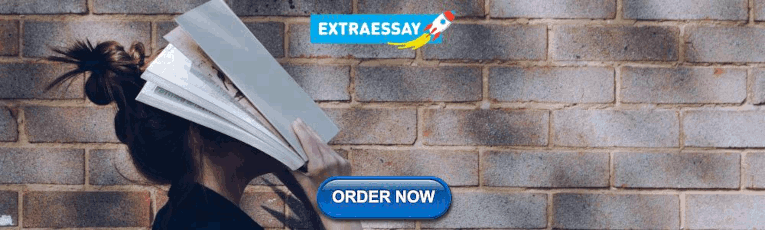
The diversity–stability debate
- Kevin Shear McCann 1
Nature volume 405 , pages 228–233 ( 2000 ) Cite this article
54k Accesses
1970 Citations
68 Altmetric
Metrics details
There exists little doubt that the Earth's biodiversity is declining. The Nature Conservancy, for example, has documented that one-third of the plant and animal species in the United States are now at risk of extinction. The problem is a monumental one, and forces us to consider in depth how we expect ecosystems, which ultimately are our life-support systems, to respond to reductions in diversity. This issue — commonly referred to as the diversity–stability debate — is the subject of this review, which synthesizes historical ideas with recent advances. Both theory and empirical evidence agree that we should expect declines in diversity to accelerate the simplification of ecological communities.
This is a preview of subscription content, access via your institution
Access options
Subscribe to this journal
Receive 51 print issues and online access
185,98 € per year
only 3,65 € per issue
Rent or buy this article
Prices vary by article type
Prices may be subject to local taxes which are calculated during checkout
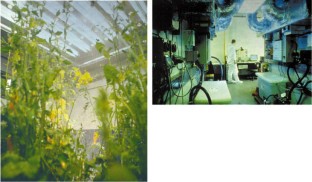
Similar content being viewed by others
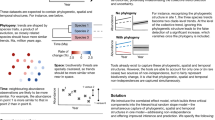
Revealing uncertainty in the status of biodiversity change
T. F. Johnson, A. P. Beckerman, … R. P. Freckleton
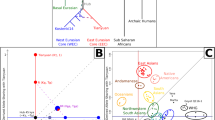
The Persian plateau served as hub for Homo sapiens after the main out of Africa dispersal
Leonardo Vallini, Carlo Zampieri, … Luca Pagani
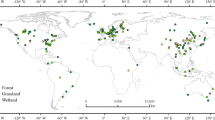
Meta-analysis shows the impacts of ecological restoration on greenhouse gas emissions
Tiehu He, Weixin Ding, … Quanfa Zhang
Riciardi, A. & Rasmussen, J. B. Extinction rates of North American freshwater fauna. Conserv. Biol. 13 , 1220 –1222 (2000).
Google Scholar
Reid, W. V. Strategies for conserving biodiversity. Environment 39 , 16–43 (1997).
Levin, S. Fragile Dominion: Complexity and the Commons (Helix books, Reading, MA, 1999).
Lodge, D. Biological invasions: lessons for ecology. Trends Ecol. Evol. 8 , 133–137 (1993).
CAS PubMed Google Scholar
Cohen, A. & Carlton, J. T. Accelerating invasion rate in a highly invaded estuary. Science 279 , 555 –558 (1998).
ADS CAS PubMed Google Scholar
Odum, E. P. Fundamentals of ecology (Saunders, Philadelphia, 1953 ).
Elton, C. S. Ecology of Invasions by Animals and Plants (Chapman & Hall, London, 1958).
MacArthur, R. H. Fluctuations of animal populations and a measure of community stability. Ecology 36 , 533–536 ( 1955).
May, R. M. Stability and complexity in model ecosystems (Princeton Univ. Press, 1973).
Gardner, M. R. & Ashby, W. R. Connectance of large dynamic (cybernetic) systems: critical values for stability. Nature 228 , 784 (1970).
Pimm, S. L. & Lawton, J. H. On feeding on more than one trophic level. Nature 275 , 542– 544 (1978).
ADS Google Scholar
Yodzis, P. The stability of real ecosystems. Nature 289 , 674–676 (1981).
Armstrong, R. A. & McGehee, R. Competitive exclusion . Am. Nat. 115 , 151–170 (1980).
MathSciNet Google Scholar
DeAngelis, D. & Waterhouse, J. C. Equilibrium and nonequilibrium concepts in ecological models. Ecol. Monogr. 57 , 1–21 (1987).
Michalski, J. & Arditi, R. in Advances in Environmental and Ecological Modelling (ed. Weill, A.), 1–20 (Elsevier, Paris,1999).
Huisman, J. & Weissing, F. J. Biodiversity of plankton by species oscillations and chaos. Nature 402 , 407–410 (1999).
McCann, K., Hastings, A. & Huxel, G. R. Weak trophic interactions and the balance of nature . Nature 395 , 794–798 (1998).
ADS CAS Google Scholar
Law, R., & Morton, D. Permanence and the assembly of ecological communities. Ecology 77 , 762– 775 (1996).
Hastings, A. & Higgins. K. Persistence of transients in spatially structured ecological models. Science 263 , 1133–1136 (1994).
Tilman, D. & Downing, J. A. Biodiversity and stability in grasslands. Nature 367 , 363– 365 (1994).
Tilman, D., Wedin, D. & Knops, J. Productivity and sustainability influenced by biodiversity in grassland ecosystems. Nature 379 , 718 –720 (1996).
Tilman, D. Biodiversity: population versus ecosystem stability. Ecology 77 , 350–363 (1996).
Schapfer, F. & Schmid, B. Ecosystem effects of biodiversity: a classification of hypotheses and exploration of empirical results. Ecol. Applic. 9 , 893–912 (1999).
Doak, D. F. et al . The statistical inevitability of stability-diversity relationships in community ecology. Am. Nat. 151 , 264– 276 (1998).
Tilman, D., Lehman, C. L. & Bristow, C. E. Diversity-stability relationships: statistical inevitability or ecological consequence. Am. Nat. 151 , 277–282 (1998).
Sankaran, M. & McNaughton, S. J. Determinants of biodiversity regulate compositional stability of communities. Nature 401 , 691–693 (1999).
Huston, M. A. Hidden treatments in ecological experiments: re-evaluating the ecosystem function of biodiversity. Oecologia 110 , 449– 460 (1997).
ADS PubMed Google Scholar
Tilman, D. et al . The influence of functional diversity and composition on ecosystem processes. Science 277 , 1300– 1302 (1997).
CAS Google Scholar
Hooper, D. U. & Vitousek, P. M. The effects of plant composition and diversity on ecosystem processes. Science 277 , 1302–1305 (1997).
Wardle, D. A. et al . Plant removals in perennial grassland: vegetation dynamics, decomposers, soil biodiversity, and ecosystem properties. Ecol. Monogr. 69 , 535–568 ( 1999).
van der Heijden, M. et al . Mycorrhizal fungal diversity determines plant biodiversity, ecosystem variability and productivity. Nature 396 , 69–72 (1998).
McNaughton, S. J. Ecology of a grazing ecosystem: the Serengeti. Ecol. Monogr. 55 , 259–294 (1985).
Lawton, J. H. Ecological experiments with model systems. Science 269 , 328–331 (1995).
McGrady-Steed, J., Harris, P. & Morin, P. J. Biodiversity regulates ecosystem predictability. Nature 390 , 162–165 ( 1997).
McGrady-Steed, J. & Morin, P. J. Biodiversity, density compensation, and the dynamics of populations and functional groups . Ecology 81 , 361–373 (2000).
Morin, P. J. & Lawler, S. P. Food web architecture and population dynamics: theory and empirical evidence. Annu. Rev. Ecol. System. 26 , 505–529 ( 1995).
Naeem, S. & Li, S. Biodiversity enhances ecosystem reliability . Nature 390 , 507–509 (1997).
Naeem, S. Species redundancy and ecosystem reliability. Conserv. Biol. 12 , 39–45 (1998).
Lawton, J. H. & Brown, V. K. in Biodiversity and Ecosystem Function (eds Schulze, E. D. & Mooney, H. A.), 255– 270 (Springer, New York,1993).
Yachi, S. & Loreau, M. Biodiversity and ecosystem functioning in a fluctuating environment: the insurance hypothesis. Proc. Natl Acad. Sci. USA 96 , 1463–1468 (1999).
Chesson, P. & Huntley, N. The roles of harsh and fluctuating conditions in the dynamics of ecological communities. Am. Nat. 150 , 519–553 ( 1997).
Winemiller, K. O. Spatial and temporal variation in tropical fish trophic networks. Ecol. Monogr. 60 , 331–367 (1990).
Polis, G. A. Complex trophic interactions in deserts: an empirical critique of food web theory. Am. Nat. 138 , 123– 155 (1991).
Polis, G. A. & Strong, D. Food web complexity and community dynamics. Am. Nat. 147 , 813– 846 (1996).
Strong, D. Are trophic cascades all wet? Differentiation and donor-control in speciose ecosystems. Ecology 73 , 747– 754 (1992).
Holt, R. D. in Multitrophic interactions (eds Begon, M., Gange, A. & Brown, V.) 333–350 (Chapman & Hall, London,1996).
McCann, K. & Hastings, A. Re-evaluating the omnivory-stability relationship in food webs. Proc. R. Soc. Lond. B 264 , 1249–1254 (1997).
Huxel, G. R. & McCann, K. Food web stability: the influence of trophic flows across habitats. Am. Nat. 152 , 460–469 (1998).
Post, D. M., Connors, E. & Goldberg, D. S. Prey preference by a top predator and the stability of linked food chains. Ecology 81 , 8– 14 (2000).
Yodzis, P. & Innes, S. Body-size and consumer-resource dynamics . Am. Nat. 139 , 1151–1175 (1992).
Chesson, J. The estimation and analysis of preference and its relationship to foraging models. Ecology 64 , 1297– 1304 (1983).
Kokkoris, G. D., Troumbis, A. Y. & Lawton, J. H. Patterns of species interaction strength in assembled theoretical competition communities. Ecol. Lett. 2 , 70–74 (1999).
Paine, R. T. Food-web analysis through field measurements of per capita interaction strengths . Nature 355 , 73–75 (1992).
Fagan, W. F. & Hurd, L. E. Hatch density variation of a generalist arthropod predator: population consequences and community impact. Ecology 75 , 2022–2032 ( 1994).
Goldwasser, L. & Roughgarden, J. Construction and analysis of a large Caribbean food web. Ecology 74 , 1216–1223 (1993).
Raffaelli, D. G. & Hall, S. J. in Food Webs: Integration of Patterns & Dynamics (eds Polis, G. A. & Winemiller, K. O.) 185–191 (Chapman & Hall, New York, 1996).
Wootton, J. T. Estimates and tests of per capita interaction strength: diet abundance and impact of intertidally foraging birds. Ecol. Monogr. 67 , 45–64 (1997).
Paine, R. T. Ecological determinism in the competition for space. Ecology 65 , 1339–1348 (1984).
Berlow, E. Strong effects of weak interactions in ecological communities. Nature 398 , 330–334 ( 1999).
Williamson, M. & Fitter, A. The varying success of invaders. Ecology 77 , 1661– 1666 (1996).
Vander Zanden, M. J., Casselman, J. M. & Rasmussen, J. B. Stable isotope evidence for the food web consequences of species invasions in lakes. Nature 401 , 464–467 (1999).
Fritts, T. H. & Rodda, G. H. The role of introduced species in the degradation of island ecosystems: a case history of Guam. Annu. Rev. Ecol. System. 29 , 113–140 . (1998).
Reinthal, P. N. & Kling, G. W. in Theory and Application in Fish Feeding Ecology (eds Stouder, D. J., Fresh, K. L. & Feller, R.) 296–313 (Univ. South Carolina Press, 1994).
Fagan, W. F. Omnivory as a stabilizing feature of natural communities. Am. Nat. 150 , 554–567 ( 1997).
de Ruiter, P. C., Neutel, A. & Moore, J. C. Energetics, patterns of interaction strengths, and stability in real ecosystems. Science 269 , 1257– 1260 (1995).
Holyoak, M. & Sachdev, S. Omnivory and the stability of simple food webs. Oecologia 117 , 413– 419 (1999).
Flaherty, D. Ecosystem trophic complexity and densities of the Williamette mite, Eotetranychus williamettei ewing (Acarina: Tetranychidae). Ecology 50 , 911–916 (1969).
Morin, P. Productivity, intraguild predation, and population dynamics in experimental food webs. Ecology 80 , 752– 760 (1999).
Luckinbill, L. S. Regulation, stability, and diversity in a model experimental microcosm. Ecology 60 , 1098–1102 ( 1979).
DeAngelis, D. Dynamics of Nutrient Recycling and Food Webs (Chapman & Hall, New York, 1992).
Andersen, T. Pelagic Nutrient Cycles: Herbivores as Sources and Sinks (Springer, New York, 1997).
Elser, J. J. & Urabe, J. The stoichiometry of consumer-driven nutrient recycling: theory, observations and consequences. Ecology 80 , 735–751 ( 1999).
Harding, S. P. Food web complexity enhances community stability and climate regulation in a geophysiological model. Tellus 51B , 815 –829 (1999).
Download references
Acknowledgements
This paper benefited from comments by D. Raffaelli. I also thank J. Rasmussen and P. Yodzis for conversations on this issue, and D. Kramer for providing a single comment that led me to a different viewpoint.
Author information
Authors and affiliations.
Department of Biology, McGill University, 1205 Docteur Penfield Avenue, Montreal, H3A 1B1, Quebec, Canada
Kevin Shear McCann
You can also search for this author in PubMed Google Scholar
Rights and permissions
Reprints and permissions
About this article
Cite this article.
McCann, K. The diversity–stability debate. Nature 405 , 228–233 (2000). https://doi.org/10.1038/35012234
Download citation
Issue Date : 11 May 2000
DOI : https://doi.org/10.1038/35012234
Share this article
Anyone you share the following link with will be able to read this content:
Sorry, a shareable link is not currently available for this article.
Provided by the Springer Nature SharedIt content-sharing initiative
This article is cited by
Predator mass mortality events restructure food webs through trophic decoupling.
- Simon P. Tye
- Samuel B. Fey
- Adam M. Siepielski
Nature (2024)
Education journal rankings: a diversity-based Author Affiliation Index assessment methodology
- Yan-Hong Yang
- Ying-Hui Shao
Scientometrics (2024)
Temporal variability of carabid beetles as a function of geography, environment, and species
- Tad A. Dallas
- Cleber Ten Caten
- Lauren A. Holian
Theoretical Ecology (2024)
Community ecological response to polycyclic aromatic hydrocarbons in Baiyangdian Lake based on an ecological model
Ecotoxicology (2024)
Resource-dependent biodiversity and potential multi-trophic interactions determine belowground functional trait stability
- Lingyue Zhu
Microbiome (2023)
By submitting a comment you agree to abide by our Terms and Community Guidelines . If you find something abusive or that does not comply with our terms or guidelines please flag it as inappropriate.
Quick links
- Explore articles by subject
- Guide to authors
- Editorial policies
Sign up for the Nature Briefing newsletter — what matters in science, free to your inbox daily.

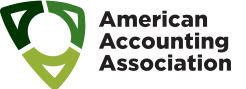
- Next Article
- INTRODUCTION
- LITERATURE REVIEW, BACKGROUND, AND HYPOTHESES DEVELOPMENT
- Background: KPMG's Aggressive Tax Shelters
- Hypotheses Development
- METHODOLOGY AND RESULTS
- Sample Size
- Empirical Model and Results
- Cross-Sectional Analyses
- Additional Analyses
- Competing Explanations
- CONCLUSIONS
The Insurance Hypothesis: An Examination of KPMG's Audit Clients around the Investigation and Settlement of the Tax Shelter Case
The authors thank Bill Baber and the participants of the Boston College, Illinois State University, The University of Arizona, and University of Central Florida accounting workshops for their contributions to this paper. They also acknowledge the financial support of the Boston College Carroll School of Management.
Editor's note: Accepted by Debra C. Jeter.
- Article contents
- Figures & tables
- Supplementary Data
- Peer Review
- Get Permissions
- Search Site
- View This Citation
- Add to Citation Manager
Darryl L. Brown , Susan Z. Shu , Billy S. Soo , Gregory M. Trompeter; The Insurance Hypothesis: An Examination of KPMG's Audit Clients around the Investigation and Settlement of the Tax Shelter Case. AUDITING: A Journal of Practice & Theory 1 November 2013; 32 (4): 1–24. https://doi.org/10.2308/ajpt-50515
Download citation file:
- Ris (Zotero)
- Reference Manager
Although prior literature has suggested that independent audits provide an implicit form of insurance against investor losses (the “insurance hypothesis”), it has been challenging to isolate the “insurance” effect. In this paper, we use a unique setting to examine this effect. In 2002, KPMG was investigated by the U.S. Department of Justice in relation to tax shelters sold by the firm. From then until early 2005, several news reports suggested that KPMG would be indicted and suffer potentially the same fate as Arthur Andersen. However, in August of 2005 KPMG entered into a deferred prosecution agreement with the U.S. Department of Justice, which ended widespread speculation of an impending federal indictment against the accounting firm. Because the investigation centered around tax services offered by the firm, we argue that the circumstances surrounding the investigation and settlement provide a natural setting to test the insurance value provided by auditors. We show that KPMG audit client firms experienced significant negative abnormal market returns when it appeared more likely that KPMG would face criminal charges, but earned significantly positive abnormal returns following news reports of an impending settlement. Further, these abnormal returns appear to be driven by KPMG client firms in greater financial distress or subject to greater litigation risk. These findings are consistent with the insurance hypothesis. Although we cannot completely eliminate other explanations such as an assurance effect or switching costs, we argue that such explanations are unlikely to drive our main findings.
Member Login
Citing articles via, email alerts, affiliations.
- Online ISSN 1558-7991
- Print ISSN 0278-0380
- Information for Authors
- Authorship Policy
- Plagiarism Policy
- Data Integrity Policy
- Citing Corrected Articles Policy
- Minimizing Overlapping Decision Rights Policy
- Prior Publication Policy
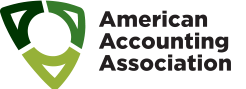
- Cookie Policy
- Accessibility
- Terms & Conditions
- Get Adobe Acrobat Reader
- © Copyright 1998 - 2023 American Accounting Association. All rights reserved.
This Feature Is Available To Subscribers Only
Sign In or Create an Account
Academia.edu no longer supports Internet Explorer.
To browse Academia.edu and the wider internet faster and more securely, please take a few seconds to upgrade your browser .
Enter the email address you signed up with and we'll email you a reset link.
- We're Hiring!
- Help Center
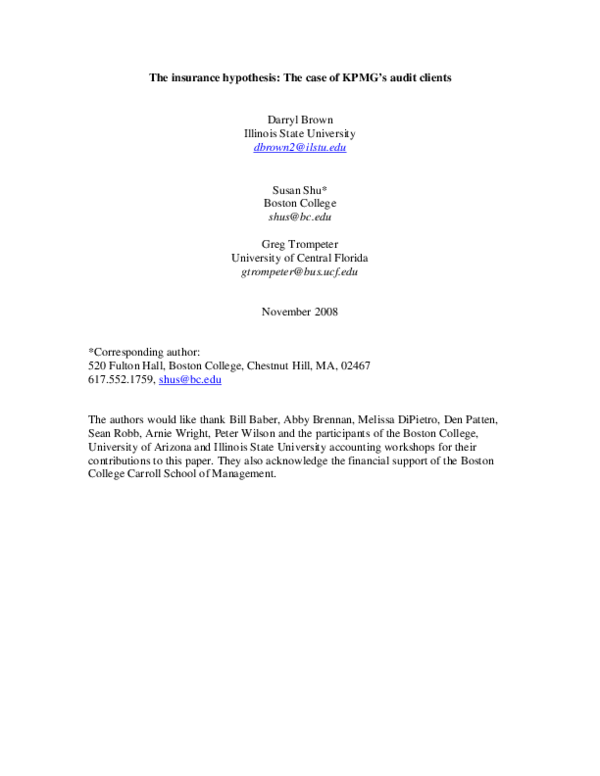
The Insurance Hypothesis: The Case of KPMG's Audit Clients

2000, SSRN Electronic Journal
Related Papers
AUDITING: A Journal of Practice & Theory
Susan Shu , Billy Soo
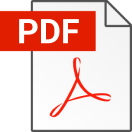
SSRN Electronic Journal
AUDITING: A Journal of Practice & Theory
Kevan Jensen
SUMMARY: This study examines the association between certain audit firm characteristics and audit firm litigation risk. Previous research shows a link between audit client characteristics and audit firm litigation risk. However, insurance companies do not make extensive use of financial information about individual audit clients to make risk assessments. Instead, they primarily use information about the audit firms themselves. Using data from a large insurance company, we examine the link between several audit firm characteristics and audit-related litigation. Based on a dichotomous measure of risk (existence of a lawsuit), we find that larger firms, firms experiencing rapid growth, firms that sue their clients, and firms with a history of problems all face greater litigation risk. Introducing a continuous measure of the cost of litigation we find, in addition to the previously mentioned risk factors, that firms with a prior history of regulatory problems and firms that choose small...
Mikhail Pevzner
Journal of Accounting and Public Policy
Marshall Geiger
The Indonesian Journal of Accounting Research
Sekar Mayangsari
John Morgan
Bharat Sarath
Jonathan Macey
RELATED PAPERS
Journal of Bangladesh Academy of Sciences
Saikh Shahjahan
dianita awaliyah
Giovanni Loureiro Raspantini
Revista Latinoamericana de Psicopatologia Fundamental
monica teixeira
Candelaria Gonzalez
Jurnal Administrasi Publik dan Bisnis
Dedy Afrizal
Marcia Perencin Tondato
East African Health Research Journal
Dinah Amongin
Journal of Travel Medicine
Boletín Científico CIOH
claudia rojas
Susi Setiawani
Interfaces Científicas - Humanas e Sociais
Patricia Nicolau Magris
Premjit Arpornmaeklong
School Mental Health
Jane Carroll
IOP Conference Series: Materials Science and Engineering
Filippos Azariadis
Nefrología Latinoamericana
Thais Forster
Retrovirology
Jolin Jolin
Journal on recent advances in pain
Depinder Kaur
Language Learning
Ron Thomson
Environmental Engineering and Management Journal
Revista Folios
PEDRO BERMUDEZ
Revista El Agora USB
Alfonso Insuasty Rodriguez
International Journal on Advanced Science, Engineering and Information Technology
Sigit Priyanto
Revista Caatinga
Pr.Francisco Viana Ribeiro Viana
Elisabete Maria da Graça Costa do NASCIMENTO
RELATED TOPICS
- We're Hiring!
- Help Center
- Find new research papers in:
- Health Sciences
- Earth Sciences
- Cognitive Science
- Mathematics
- Computer Science
- Academia ©2024
- Contributions
- Development Blog

The Insurance Hypothesis
Farming monoculture, such as in this corn field in Marqueseac (France), can lead to decreased net ecosystem CO2 intake, meaning land becomes less useful in the fight against climate change. According to the insurance hypothesis: “Biodiversity insures ecosystems against declines in their functioning because many species provide greater guarantees that some will maintain functioning even if others fail”. Put simply, given the same set of environmental conditions, more diverse plant ecosystems will tend to photosynthesise at a greater rate than ecosystems with fewer species present. Obviously, forests are very diverse, whereas crop fields are usually monocultures, meaning that only the one species of plant is present. In fact, any other plant species present are usually classified as ‘weeds’ and are, hence, destroyed by farmers post-haste.
The idea behind ‘the insurance hypothesis’ is simple: when different species are present, they are able to fulfil a variety of different ecological niches within a given ecosystem. By contrast, with monocultures, all of the individual plants are competing for the resources held within one specific ecological niche. Consequently, the overall rate of photosynthesis in biodiverse ecosystems tends to be much higher than that of monocultures, which means biodiverse ecosystems, usually natural ones, are much better at helping us tackle climate change.
In addition, monocultures are much more susceptible to disease than biodiverse ecosystems are. Plant viruses are usually specialised toward attacking a particular species, genus, or family of plants. Consequently, it is possible for one viral strain to destroy an entire monoculture plantation of biofuel crops, thus reducing the photosynthesis rate of this area of land to zero. In stark contrast to this, should a virus destroy any given type of plant within a biodiverse ecosystem, the gap created will quickly be filled by the other plant species present, meaning that overall rate of photosynthetic carbon dioxide uptake will remain high. Thus, the clearance of forests, in order to make way for crop plantations, is not only detrimental in terms of the animal species lost, for whom this forest was their habitat, but it can also cause a net increase in atmospheric carbon dioxide levels over time.
References:
Naeem, S. & Li, S. (1997). Biodiversity enhances ecosystem reliability. Nature 390 pp 507-509.
Yachi, S. & Loreau, M. (1999). Biodiversity and ecosystem productivity in a fluctuating environment: The insurance hypothesis. Proceedings of the National Academy of Sciences of the United States of America 96 (4) pp 1463-1468.
Back to top

An official website of the United States government
The .gov means it’s official. Federal government websites often end in .gov or .mil. Before sharing sensitive information, make sure you’re on a federal government site.
The site is secure. The https:// ensures that you are connecting to the official website and that any information you provide is encrypted and transmitted securely.
- Publications
- Account settings
Preview improvements coming to the PMC website in October 2024. Learn More or Try it out now .
- Advanced Search
- Journal List
- HHS Author Manuscripts

Biodiversity, productivity, and the spatial insurance hypothesis revisited
David w. shanafelt.
a Arizona State University, School of Life Sciences, PO Box 874601, Tempe, AZ 85287, USA
Ulf Dieckmann
b Schlossplatz 1, A-2361 Laxenburg, Austria; Ecology and Evolution. International Institute for Applied Systems Analysis
Matthias Jonas
c Schlossplatz 1, A-2361 Laxenburg, Austria; Advanced Systems Analysis. International Institute for Applied Systems Analysis
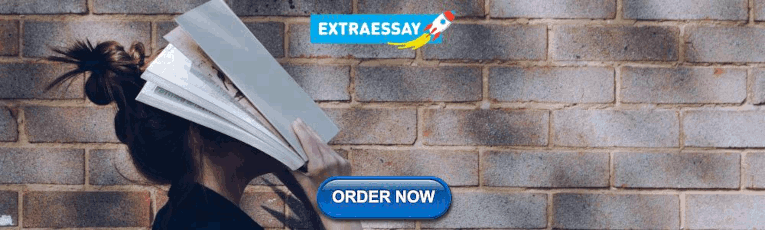
Oskar Franklin
d Schlossplatz 1, A-2361 Laxenburg, Austria; Ecosystem Services and Management. International Institute for Applied Systems Analysis
Michel Loreau
e Station d'Ecologie Expérimentale du CNRS à, 09200 Moulis, France; Centre for Biodiversity Theory and Modelling
Charles Perrings
Accelerating rates of biodiversity loss have led ecologists to explore the effects of species richness on ecosystem functioning and the flow of ecosystem services. One explanation of the relationship between biodiversity and ecosystem functioning lies in the spatial insurance hypothesis, which centers on the idea that productivity and stability increase with biodiversity in a temporally varying, spatially heterogeneous environment. However, there has been little work on the impact of dispersal where environmental risks are more or less spatially correlated, or where dispersal rates are variable. In this paper, we extend the original Loreau model to consider stochastic temporal variation in resource availability, which we refer to as “environmental risk,” and heterogeneity in species dispersal rates. We find that asynchronies across communities and species provide community-level stabilizing effects on productivity, despite varying levels of species richness. Although intermediate dispersal rates play a role in mitigating risk, they are less effective in insuring productivity against global (metacommunity-level) than local (individual community-level) risks. These results are particularly interesting given the emergence of global sources of risk such as climate change or the closer integration of world markets. Our results offer deeper insights into the Loreau model and new perspectives on the effectiveness of spatial insurance in the face of environmental risks.
1. Introduction
Accelerating rates of biodiversity loss have led ecologists to explore the effect of changes in species richness on ecosystem functioning, and the resulting flow of ecosystem services ( Cardinale et al., 2012 ). While some studies have evaluated the effect of species richness on mean levels of ecosystem functioning, most have focused on the impact of biodiversity on the variability of ecosystem functioning. 1 Several mechanisms have been proposed including overyielding ( Lehman and Tilman, 2001 ; Tilman, 1999 ), statistical averaging or the “portfolio effect” ( Cottingham et al., 2001 ; Doak et al., 1998 ; Isbell et al., 2009 ; Tilman et al., 1998 ), compensatory dynamics ( Gonzalez and Loreau, 2009 ; Lehman and Tilman, 2001 ) and the spatial insurance hypothesis ( Ives et al., 2000 ; McNaughton, 1977 ; Yachi and Loreau, 1999 ). 2 The last of these centers on the idea that the functional complementarity of species across space and time insures the system against environmental risk ( Loreau et al., 2003 ). Specifically, the greater the number and spatial distribution of species, and the greater the functional redundancy of species at particular locations, the more the system is protected against spatiotemporal environmental variability, including spatially distributed anthropogenic shocks. As the productivity of one species falls, others can fill its functional niche and maintain productivity. At the global scale, dispersal between communities provides source populations in which migrants may both replace extirpated local populations and maintain functional groups of species.
Empirical evidence on the role of spatial insurance in the relationship between biodiversity and the stability of productivity has been mixed. In microbial microcosms, community biomass and density have been shown to be more stable in systems with greater functional biodiversity ( Naeem and Li, 1997 ). Regional zooplankton biodiversity coupled with immigration has, for example, been shown to dampen the effects of temperature warming on net primary productivity ( Thompson and Shurin, 2012 ). In a broad scale statistical analysis, Valone and Barber (2008) tested for evidence of spatial insurance across multiple taxa. They found the greatest support for the hypothesis in plant taxa, but little or no support in rodent, avian, and ant systems. Other empirical studies have found that spatial insurance is less critical to system stability than other mechanisms such as statistical averaging or overyielding ( Aragon et al., 2011 ; Tilman et al., 1998 ). From a theoretical perspective, several papers have identified conditions in which spatial insurance might be expected to stabilize productivity ( Gonzalez et al., 2009 ; Ives et al., 2000 ; Loreau et al., 2003 ; Mouquet and Loreau, 2003 ). Mouquet and Loreau (2003) used a metacommunity approach to show that intermediate dispersal rates between communities experiencing asynchronous environmental fluctuations enhanced global and local biodiversity, productivity, and system stability. Species dispersal, as a mechanism for maintaining biodiversity, insures the metacommunity by stabilizing productivity.
Several theoretical papers have extended the spatial insurance hypothesis to consider the effects of competition structure ( Filotas et al., 2010 ; Loreau and de Mazancourt, 2013 ), species adaptation ( Urban, 2006 ), and trophic structure ( Ives et al., 2000 ). However, little has so far been done to investigate the effect of dispersal where environmental conditions and dispersal rates vary over space and time, as they do in most real ecosystems. In this paper, we re-evaluate the original model constructed by Loreau et al. (2003) and extend the model to consider stochastic temporal variation in resource availability, which we define as “environmental risk,” and consider the effect of heterogeneity in species dispersal rates.
Spatial variation in resource availability reflects differences in, for example, climatic conditions in distinct habitat patches or communities, while temporal variation reflects effects such as random fluctuations in temperature or precipitation. Climate change is projected to affect both temporal and spatial variation in conditions—increasing both the frequency of extreme climate events, and the spatial correlation between events (Intergovernmental Panel on Climate Change, 2013). One consequence is a change in the temporal consistency of resource availability. Availability of water (through droughts or flooding) and nutrients such as nitrogen or phosphorus (via erosion and/or deposition) may directly alter populations of primary producers or consumers, the effects of which cascade to organisms of other trophic levels. Another is that events occurring in one part of the world have an effect at spatial locations much further away. This is reflected in, for example, the growing intensity and global effects of El Niño and La Niña (Intergovernmental Panel on Climate Change, 2013). While research has been conducted to test the effect of stochastic variation in resource availability across space and time, less attention has been paid to their effects jointly.
Heterogeneity of species dispersal rates reflects two sets of processes that have been demonstrated to play roles in regulating biodiversity in real world systems. First, heterogeneity in dispersal rates between locations reflects the fact that some areas are naturally more strongly connected than others, and that the connections between areas are frequently directional. Air and water flows, for example, affect the direction of natural dispersal. This means that some locations will act as sink populations for dispersers, and others will act as sources. Source-sink dynamics have, for example, been shown to play a role in maintaining diversity in fisheries in economics ( Sanchirico and Wilen, 1999 ), and in conserving spatially distinct populations of wild species such as the checkerspot butterfly, ( Harrison et al., 1988 ) snowshoe hare, ( Griffin and Mills, 2009 ) and predatory reef fish, ( Russ and Alcala, 2011 ). Second, not all species disperse equally, naturally or by people. Anthropogenic dispersal through international trade and travel preferentially selects for species that are either the direct objects of trade, or incidentally incorporated in packaging, or as hitch hikers on the ships, planes, trains or trucks used to transport goods and people from place to place. Trade and travel is frequently cited as a major facilitator of the worldwide spread of invasive species ( Costello et al., 2007 ; Lenzen et al., 2012 ) and pathogens ( Kilpatrick, 2011 ; Smith et al., 2007 ; Tatem et al., 2006a ). The pattern of international trade and travel also determines where species are moved from and to, and in what quantities.
Introduced species have the potential to cause shifts in species composition, environmental processes, and the evolution of species populations ( Chisholm, 2012 ). We test the effect of species dispersal on productivity under local risk factors (affecting a single community) and global risk factors (affecting the whole metacommunity) that may alter both biodiversity and ecosystem functioning. We find that asynchronies across communities and species provide metacommunity-level stabilizing effects on productivity, despite variability in species richness. Our work provides new testable hypotheses about the effectiveness of spatial insurance when community level risks are more or less spatially correlated.
2. The Spatial Insurance Hypothesis: The Loreau Model
2.1 loreau model - construction.
We assume the same dynamics as Loreau et al. (2003) and Gonzalez et al. (2009) . Consider a meta-community with M communities and S species. Within each community, species compete for a single limiting resource of which the quantity consumed varies by species, environmental conditions (influencing how species consume the limiting resource), and time. Communities are coupled together by the natural dispersal of species. When dispersal is low, each community functions as a separate closed system; with high dispersal the entire metacommunity functions as a single patch.
Formally, the change in species biomass N and resource biomass R in the j th community is governed by the set of equations:
for species i = 1,2,…, S and communities j = 1,2,…, M at time t . Species are assumed to consume resources at the normalized rate c ij ( t ), convert resources to new biomass with efficiency e , and die at rate m . The limiting resource is assumed to increase in all communities by a fixed amount I and to be lost at a constant rate l . Initially, species are assumed to disperse between communities at a constant rate a . Species consumption of natural resources is a non-linear function of species-specific traits and environmental variation, fluctuating over time for each species in each community according to:
where H i is a dimensionless, species and community-dependent competition parameter such that H 1 = 1 and H i = H i − 1 − 1 M for i = 1,2,…, S . It is assumed that environmental conditions, E j , vary temporally, fluctuating over time as a sinusoidal function. The phase parameter, x j , is a random variable drawn from a uniform distribution [-2π, 2π], which shifts the environmental variation along its horizontal axis ( Figure 1 ). T determines the period of the environmental variation, and the subsequent periodicity of species consumption rates. We chose T = 40,000. In the absence of dispersal ( a = 0), a single set of species quickly drives all other species to extinction (see below). It is known that in such cases local species coexistence is impossible ( Armstrong and McGehee, 1980 ).

Community environmental variation (a) and species consumption (b) over time. (a) color denotes community number: black (community 1, x 1 = π /2), blue (community 2, x 2 = 0), red (community 3, x 3 = − π /2). The phase parameter, x j , shifts the environmental variation along the x-axis. (b) species consumption rates for community 1 indicated by color: black ( H 1 = 1), charcoal ( H 2 = 1/2), and light gray ( H 3 = 0). Consumption rate is determined by the interaction between the species competition parameter times environmental variation. Values of x j and H i were chosen to illustrate the full spectrum of potential environmental variation and consumption rate curves.
By construction, a single species will competitively exclude all others in a given community in the absence of dispersal. This will be the species that possesses the highest initial consumption rate, as measured by the interaction between the species competition parameter H and initial level of environmental variation. If there is dispersal such that the metacommunity begins to behave as a single community, the advantage lies with the most “generalist” species — defined as the species whose consumption rates are closest to the average over the course of the simulation period. This reflects the fact that “generalist” species are able to occupy a broad range of environments ( Futuyma and Moreno, 1988 ; Schluter, 2000 ). In a tightly coupled, high dispersal system the most “generalist” species will exclude all others across the metacommunity.
Productivity is measured as the average increment in species biomass per unit of time given by the first term on the right side of Eq. (1) :
System stability is then measured by the inverse of the coefficient of variation over time in productivity, a commonly used metric in ecology ( Tilman et al., 1998 ). 3
The original Loreau model made a number of assumptions. First, it assumed that communities initially contained the same set of species and differed only in their environmental variation over time as defined by the phase parameter x j . Species differed only in consumption rates that varied by community and time as a function of H i , x j , and t . Second, species were assumed to compete for a single limiting resource whose natural influx and loss rates were constant across time and communities. This could be thought of as water in a desert ecosystem or nitrogen in a forest ecosystem. Third, species competition arose solely from resource consumption; there was no direct interaction between different species within and across patches. Finally, species dispersed between communities at a constant rate. These assumptions simplified the analysis while providing a structure for species competition when environmental conditions varied. In the numerical experiments reported in this paper we relax certain of these assumptions in order to explore the effectiveness of dispersal in stabilizing productivity where environmental risk factors, e.g. stochastic variation in resource availability, are more or less spatially correlated. 4
Maintaining the approach of the original papers, the differential equation system in [ 1 ] and [ 2 ] was numerically simulated using an Euler approximation with a step size (Δ t ) equal to 0.08. The Euler approximation saves computation time compared to higher order estimators, particularly when the system of equations is unstable. (When M = 20 and S = 20, simulating [ 1 ] and [ 2 ] simultaneously solves a system of 400 equations.) Simulations were run for 800,000 iterations. Mean local and global biodiversity, as well as productivity, were measured every 4,000 iterations. Biodiversity was measured both by species richness and by Shannon-Wiener indices. 5 Average biodiversity, productivity, and stability values were generated from data in the last 200,000 iterations. To evaluate the insurance effect of dispersal where environmental risks are more or less spatially correlated we relaxed the assumption that resource influx is constant over time and across communities (see below). In order to test the effect of heterogeneity in species dispersal, dispersal rates were allowed to statically vary within the interval [0,1]. For each dispersal rate tested, a set of 50 simulations was run to generate new stochastic parameters. Species biomass was initially set at 10; resource biomass was set to the final (equilibrium) value of the previous simulation. 6 Species were assumed to be extinct if biomass fell below 0.10 units. This is meant to reflect a critical population threshold in which species are not able to recover due to demographic stochasticity, Allee effects and the like. For a list of model parameters, see Table 1 .
Parameter values for the Loreau et al. (2003) and Gonzalez et al. (2009) simulations.
Note that a value of “-” indicates a dimensionless parameter.
2.2 Loreau model - Results
At low dispersal rates (0 ≤ a < 0.001), each community functions as a separate closed system. Within each community, a single set of species with the highest initial consumption rate outcompetes all others for available resources leading to their extirpation. This leads to a high global (gamma) biodiversity but low local (alpha) biodiversity ( Figure 2 ). As the dispersal rate increases (within the range 0.001 ≤ a < 0.2), dispersal between communities allows local biodiversity to increase while global biodiversity is maintained. However, at high dispersal rates (0.2 ≤ a ≤ 1) the system becomes too closely coupled to maintain biodiversity locally or globally. The metacommunity functions as a single community and the set of species whose consumption rates are highest on average exclude all other species in the system. This produces the lowest global and local biodiversity.

Reproduction of biodiversity, mean net primary productivity, and stability results from the original Loreau model without spatial correlation and stochastic resource availability ( Gonzalez et al., 2009 ). (a) Mean regional (black) and local (gray) biodiversity; (b) average local Shannon biodiversity index; (c) mean productivity against local biodiversity; (d) mean productivity; and (e) mean temporal coefficient of variation of productivity. In (c), dotted lines and arrows indicate the trend in dispersal rate. Reported values are the average of 50 simulations. The dotted vertical line indicates the dispersal rate at which biodiversity reaches its maximum value. Model parameter values are found in Table 1 .
A relationship exists between biodiversity, mean productivity, and the stability of productivity across the metacommunity, where system stability is measured by the inverse of the coefficient of variation of productivity over time. At low dispersal rates, the metacommunity generates the lowest mean productivity and highest coefficient of variation in productivity (lowest system stability). The stability of productivity increases with dispersal up to a point, at which the system becomes too coupled and both mean productivity and the stability of productivity decline. At low and high dispersal rates, productivity and the stability of productivity depend solely on the competitive dominant species whose biomass fluctuates over time. At intermediate dispersal rates, the greater local biodiversity stabilizes productivity.
It is worth noting, however, that maximum productivity does not correspond to the maximum biodiversity ( Figures 2a, b, c, d ) - an observation not discussed in previous studies. Since productivity is maintained while biodiversity falls, the relative abundance of species must be changing. In fact, a trade-off exists between the degree of local biodiversity and aggregate species growth. With higher local biodiversity, more species exhibit growth but each grows at a lower rate than if fewer species were present. Maximum mean productivity is achieved when local biodiversity is relatively low, a significant proportion of biomass being accounted for by the most productive species. The latter condition is reflected in the Shannon diversity values on the left and right side of the productivity “hump” ( Figures 2b, d ). However, productivity differentials between species matter. A particular level of biodiversity does not guarantee a particular level of productivity. For example, the Loreau model experiences a species richness of 6 species at two dispersal rates, only one of which corresponds to maximum productivity ( Figure 2c ; see also species richness of 2 and 10 in Figures 2a, d ).
As the dispersal rate increases from 0 to 1 we observe a shift in the dominant species from the initial best competitor in each community to the “generalist” species that does the best in average conditions. When dispersal rates are either very low or very high, the meta-community is also characterized by low local biodiversity. But despite low biodiversity, productivity is higher at high than low dispersal rates. The average consumption rate of the “generalist” species is greater than that of the initially best competitor ( Figure 1 ). A dispersal rate increasing into the intermediate range allows the persistence of more species and a gradual extinction of the initial best competitor. When local and global biodiversity converge, the system exhibits the same species composition on the community and metacommunity scales ( Figure 2a ). In all cases, mean productivity peaks immediately after this convergence. At that point the system contains both the initially best competitors of several patches and the species that do best in average conditions.
3. Robustness and the Spatial Correlation of Risk: Extensions of the Loreau Model
The results reported by Loreau et al. (2003) , summarized above, provide a simple illustration of the spatial insurance hypothesis. They demonstrated how dispersal, as a mechanism to increase biodiversity, insures the system against asynchronous environmental fluctuations. In what follows we extend the model to consider factors that affect the spatial correlation of environmental risk, and the capacity of dispersal to stabilize productivity both at the level of individual communities and across the metacommunity.
3.1 Stochastic resource availability - Assumptions
Natural resources are rarely constant over time or space. To capture this variation we allow the natural resource influx, I , to vary stochastically over time, affecting the quantity of resources available for species consumption. This we define as “environmental risk.” 7 (Note that “environmental risk” affects the equation of motion for the resource and not variation in species consumption rates.) Several modeling options are available. Fluctuations of rainfall are often modeled as Poisson processes ( Rodriguez-Iturbe et al., 1987 ). Many biological processes, on the other hand, including the growth of organisms and populations, are characterized by either normal or lognormal distributions ( Mitzenmacher, 2004 ). Soil nutrients, for example, have been found to be both lognormally ( Vieira et al., 2011 ) and normally distributed ( Cusack et al., 2009 ). We chose I in [ 2 ] to be normally distributed with a mean equal to the value used by Gonzalez et al. (2009) . We tested the sensitivity of productivity to variation in the standard deviation of the distribution. Initially, we considered two polar cases: 1) all communities experience the same realization of I , which we call global environmental risk; and 2) each community possesses its own natural resource influx rate, which we call local environmental risk. This approach captures the degree of connectivity between communities (a risk event in a loosely/tightly connected system will affect few/many communities).
Formally, the “risk” of an outcome is the value of the outcome multiplied by the probability that it will occur. We take the value of outcomes to be the associated level of productivity, and tested the effect of different correlation coefficients of the probability distribution of the underlying environmental variables on productivity. Specifically, we consider two extreme cases of the spatial correlation of risks—local and global risk. Global risk implies that resource availability in each community is determined by the same set of environmental conditions, i.e. risks are perfectly correlated spatially. Local risk implies that communities are either far enough apart or sufficiently different in other respects that resource availability depends only on local environmental conditions, i.e. risks are uncorrelated spatially. We then tested intermediate levels of the spatial correlation of environmental risk by allowing rates of resource influx in individual patches to be more or less spatially correlated. Influx values for the patches were drawn from a multivariate normal distribution with the same mean and standard deviation as the global and local risk scenarios, but with varying values for the correlation coefficients. Parameters used to generate resource influx rates are presented in Table 2 .
Parameter values of model extensions.
Note that a value of “-” indicates a dimensionless parameter. In our first extension, resource influx rates, I , are drawn from a normal distribution with a mean μ I and covariance matrix composed of the standard deviation σ I (diagonals) and spatial correlation coefficient ρ I (off-diagonals). In our second extension, dispersal rates are drawn from a beta distribution where scale parameters are calculated using the average ( μ a ) and coefficient of variation ( COV a ) of the dispersal rate.
3.2 Stochastic resource availability - Results
Our primary result is summarized in Figure 3 . As in the original papers, we found that intermediate dispersal rates tend to stabilize productivity across the system. However, we also found that the stabilizing effect of dispersal depends strongly on the degree to which environmental risks are correlated across communities. Specifically, we found the stabilizing effect of dispersal to be weakest when resource availability is spatially perfectly correlated ( ρ I = 1) across communities ( Figure 3 ). In these circumstances all communities experience the same costs (benefits) of low (high) resource availability, and any compensation occurs temporally and at the level of the whole system. Periods of poor resource availability are compensated by periods of resource abundance. When environmental risks are not spatially correlated—implying that resource availability varies across communities—we found dispersal within the metacommunity to be more strongly stabilizing. A fall in productivity in one community where resource availability is low is compensated by an increase in productivity in other communities where resource availability is high. At intermediate levels of the spatial correlation of environmental risk, we found intermediate stabilizing effects of dispersal ( Figure 3 ).

Effect of spatial correlation ( ρ I ) of stochastic resource availability on the mean coefficient of variation of productivity. Reported values are the average of 50 simulations. Colors indicate the degree of spatial correlation: black, solid (global risk; perfect spatial correlation, ρ I = 1), brown ( ρ I = 0.7), purple ( ρ I = 0.4), blue ( ρ I = 0.2), red ( ρ I = 0.1), orange ( ρ I = 0.01), and black, dashed (local risk; no spatial correlation, ρ I = 0). The standard deviation of the resource availability ( σ I ) is given above each plot.
We found little or no change from the original Gonzalez et al. (2009) results on species richness or productivity. Despite stochasticity in resources, dispersal is able to maintain mean biodiversity and productivity but the latter experiences greater variation around its mean. By definition, stochasticity of resource flows increases the chance that resources will be above or below the mean - this should affect species abundances. In our model, species growth is linearly related to resource abundance (see equations [ 1 ] and [ 5 ]). Changes in R j will linearly scale the abundances of all species within the sub-community, other things being equal. 8 Higher resource influxes relieve competitive pressure on species (due to the greater resource availability), increasing both the abundance and diversity of species. Lower resource influxes reduce the abundance of the least competitive species, which increases the probability that those species will fall below the critical population threshold, leading to their extirpation. In addition, declines in resource influx intensify competitive pressure within communities. Under a global risk scenario, all communities share the same competitive pressure due to resource availability. As the spatial correlation between communities decreases (local risk scenario), asynchrony in resource abundance causes populations to grow in some communities and to crash in others. But in both global and local risk scenarios the “insuring” effect of dispersal maintains biodiversity and productivity.
3.3 Stochastic dispersal - Assumptions
The next effect we considered was the impact of variation in dispersal rates on global productivity and the stability of global productivity. To do this we assumed that some species disperse at higher rates than others (e.g. invasive species, trans-migratory species). Similarly we assumed that some locations are easier to reach than others (e.g. island communities, barrier zones). Both factors may have a significant effect on species coexistence. We therefore allowed species dispersal rates to vary 1) across species (each species possesses its own dispersal rate regardless of community) and 2) between communities (all species within a community have a single dispersal rate). The latter increases the capacity for communities to be a source (high dispersal) or sink (low dispersal) community for dispersing species, though the mean capacity remains the same as the original model. Dispersal rates in [ 1 ] were generated from a beta distribution with a mean value taken from Gonzalez et al. (2009) and a user-defined coefficient of variation. The beta distribution, bounded between [0, 1], is often used in modeling dispersal rates ( Wiley et al., 1989 ) and proportions ( Haskett et al., 1995 ). We tested several coefficients of variation ranging between [0, 1]. Values greater than one lead to negative scale parameter values. See Table 2 for a list of parameters used to generate dispersal rates.
3.4 Stochastic dispersal - results
Overall, heterogeneity in dispersal rates decreases system stability of the meta-community. Relaxing the homogeneity conditions on dispersal imposed in Loreau et al. (2003) and Gonzalez et al. (2009) removes the guaranteed balance between in- and out-migration, leading to potential extinctions of all species in some patches.
We found that mean productivity and the stability of productivity were both more robust to species heterogeneity than to community heterogeneity in dispersal rates, particularly at high dispersal rates ( Figure 4 ). At low to intermediate dispersal rates, variation in dispersal rates by species had a greater effect than when dispersal rates varied by community, although the qualitative pattern conforms to the original Gonzalez et al. (2009) result. At intermediate to high dispersal rates the metacommunity underwent significantly greater declines in productivity and stability when dispersal rates varied by community than by when they varied by species. When dispersal rates varied by community, communities with high species dispersal rates (“sources”) experienced greater rates of out-migration, which resulted in lower species abundances but also a release of competitive pressure within the community. However, if in-migration and new growth cannot compensate for out-migration, source communities will experience an exponential decline and eventual collapse of all species.

Effect of heterogeneous species dispersal varying by community (a-d) and species (e-h). (a, e) Mean regional (solid) and local (dashed) species richness; (b, f) mean productivity; (c, g) mean temporal coefficient of variation of productivity; and (d, h) mean local Shannon diversity index. Reported values are the average of 50 simulations. Color indicates the coefficient of variation in dispersal rates: black (original ( Gonzalez et al., 2009 ) result), blue ( CoV a = 0.1), red ( CoV a = 0.2), purple ( CoV a = 0.4), brown ( CoV a = 0.7), and orange ( CoV a = 1).
Low dispersal communities (“sinks”) experience more in-migration, but potentially lower productivity due to greater interspecific resource competition. Most growth of biomass therefore arises from the migration of species into the community. At the metacommunity level, biomass growth in low-productivity sink communities is balanced by biomass growth in high-productivity source communities. But as dispersal rates become more variable, the difference between the two types of communities becomes more pronounced and productivity and stability both decline ( Figure 4b, c ). 9 We found that local biodiversity remained lower than global biodiversity at higher dispersal rates.
Heterogeneity in dispersal rates decreases both the maximum attainable level of biodiversity, and the stability of productivity ( Figure 4c, g ). When dispersal is assumed to be constant across species and communities, communities experience synchrony in the exchange of species biomass. However, as dispersal rates vary between communities, some communities become sources while others become sinks. The direct effect on biodiversity is twofold. First, species that are able to persist in highly connected communities have a competitive advantage over other species on a system-level scale. Second, sink communities face internal competitive pressure on species biodiversity as in-migration places additional pressure on available resources.
Variation in dispersal rates between species generates lower biodiversity values than variation in dispersal by community. Species that disperse rapidly are at a competitive advantage over slow dispersers at the metacommunity level. We found a negative shift in the dispersal rate corresponding to the maximum biodiversity value. Even at low coefficients of variation, biodiversity peaked at lower dispersal rates than the homogeneous dispersal case. This implies that the competitive advantage of rapid dispersers is large enough to exclude slow or average dispersers across the metacommunity. Only a few rapid dispersers are required to alter community-level species dynamics significantly.
4. Discussion
Loreau et al. (2003) showed that dispersal can act to insure meta-communities against asynchronous temporal variation in environmental conditions. By increasing local and global species coexistence, dispersal increases mean productivity and the stability of productivity. Asynchronies in species per capita growth rates within and between communities maintain productivity despite temporal fluctuations in species consumption.
We extended these findings to show how the system performs in the face of environmental risk that may be more or less spatially correlated. That is, we showed how dispersal effectively insures the system against local and global risks in environmental resource availability. We also showed how the insurance effect of dispersal is affected by variation in rates of species dispersal. We found that dispersal promotes stability of productivity under local and global environmental resource stochasticity, but that its effectiveness differs substantially depending on the degree of the spatial correlation of risk. We found that the insurance effect on productivity is greatest when environmental risks across communities are not correlated. In other words, the insurance function of dispersal is greatest where risks are local. Low productivity communities are compensated by high productivity ones. Where the environmental risks experienced by each community are highly spatially correlated, the insurance effects of dispersal still exist but are significantly weaker. This result is consistent with the asynchrony literature ( Loreau and de Mazancourt, 2013 ). For instance, Loreau and de Mazancourt (2013) demonstrated analytically that asynchronies in species responses to environmental stochasticity stabilize community-level variation in species biomass. In source-sink and sink meta-populations, asynchronies in environmental fluctuations have been shown to have a stabilizing effect, increasing species persistence time in both theoretical and empirical applications ( Gonzalez and Holt, 2002 ; Matthews and Gonzalez, 2007 ; Roy et al., 2005 ). In periods of high resource abundance, sink populations experience an “inflationary effect” characterized by high per capita growth rates and outbreak dynamics.
We also found that variability in the rate at which species disperse between communities has quite different effects on the stability of productivity when rates differ by community than where they differ by species. When rates fluctuate across communities, intermediate dispersal is more strongly stabilizing than where rates fluctuate across species. Symmetrically high dispersal rates are more strongly destabilizing. Few studies have tested the consequences of biodiversity loss due to dispersal on productivity, although several have considered the effects of stochasticity in dispersal rates on biodiversity. Matias et al. (2013) observed similar decreases in local species richness when species dispersal rates are stochastic, as well as a shift in the peak diversity to high dispersal rates. Altering dispersal rates between communities has been shown to affect diversity. Altermatt et al. (2011) , in a Lotka-Volterra competition model testing the effects of dispersal and disturbance, found that adding directionality to dispersal significantly lowered biodiversity compared to a global dispersal case. Their results were supported by an empirical analysis of protist-rotifer microcosms. In a metacommunity model incorporating evolution and food web dynamics, Allhoff et al. (2015) found that both increasing and directing dispersal rates resulted in declines in regional diversity.
While the original Loreau model and this extension are highly abstract, they do have implications for the conditions in which dispersal would enhance ecosystem stability. This is particularly important because empirical experiments are difficult if not impossible to carry out at the scale of the Loreau model (though see Thompson and Shurin (2012 ) and Howeth and Leibold (2010) for examples in plankton metacommunities). From the Loreau model, an interconnected metacommunity in which sub-communities possess different “optimal” sets of species can maintain productivity and the stability of productivity through intermediate dispersal. We considered the effect of dispersal when environmental conditions are stochastic, fluctuating across communities or uniformly over the whole system. We also considered the effect of mean dispersal when dispersal rates are themselves stochastic, fluctuating either across communities or across species. While variability in environmental conditions leads to declines in system-wide biodiversity, we found little overall change in productivity. In other words, intermediate rates of species dispersal allow maintenance of mean productivity in highly variable environmental conditions, while simultaneously containing variation in that productivity. Moreover, they do so even at lower overall levels of biodiversity. Although there is a general consensus about the value of biodiversity in maintaining ecosystem functioning and the flow of ecosystem services, the level of biodiversity required to do this in particular cases is still unknown ( Cardinale et al., 2012 ; Hooper et al., 2005 ; Isbell et al., 2011 ; Loreau et al., 2001 ).
Our central finding is that the stabilizing effect of dispersal is more robust to local risk factors than to global risk factors. We found that variability in global resource availability significantly reduced the stability of productivity. Although intermediate dispersal has a role to play in mitigating this impact, it is less effective in protecting productivity against global risks than against local risks. This conclusion is interesting from a policy or management perspective because: (i) communities and ecosystems have become both more connected worldwide and more exposed to global risk events, (ii) global risk factors are on the rise, e.g. due to climate change, and (iii) dispersal rates are strongly affected by human activities.
The former is especially the case in production systems that are linked through international markets for inputs and outputs. In agriculture, for example, dispersal of cultivated crops is a result both of the development of global markets for foods, fuels and fibers, and of technological developments in plant breeding and more direct genetic engineering. For example, a major effect of the 20 th century Green Revolution, was the displacement of many of the 7000 plants previously cultivated worldwide by a handful of widely adapted high-yielding varieties distributed by a small number of seed companies ( Evenson and Gollin, 2003 ; Tisdell, 2003 ). The resulting homogenization of the global food supply ( Khoury et al., 2014 ) has significantly increased mean global yields, but has also increased temporal variability in yields as cultivated crops exhibit similar responses to changes in temperature, precipitation, disease, pests and other environmental disturbances.
It is also the case for natural systems. Anthropogenic dispersal of species through trade and travel has led to the homogenization of many ecosystems, which increases the spatial correlation of environmental risks and dispersal rates. Dispersal rates are significantly higher than they were, and local exposure to global risk factors has become more frequent. Human behavior has increased the connectivity of the world's ecosystems, causing declines in both the number and functional diversity of species ( Clavel et al., 2011 ; McKinney and Lockwood, 1999 ; Smart et al., 2006 ). Examples of invasive species being distributed globally and displacing native species are becoming more frequent in ecology ( Hulme, 2009 ). Global risk factors may include the use of nitrogen-rich agricultural fertilizers and the burning of fossil fuels which has led to rates of terrestrial nitrogen fixation almost double the natural rate, stressing many terrestrial and aquatic systems, and exacerbating climate change ( Canfield et al., 2010 ; Galloway et al., 2008 ; Moffat, 1998 ). Climate change in turn adds to the stress on local ecosystems by altering mean precipitation and temperature, water levels along coastal areas, and weather events ( Karl and Trenberth, 2003 ).
At the same time, the increasing connectivity of ecosystems as a result of globalization has affected the spatial distribution of environmental risk. Indeed, the dispersal of species is frequently the mechanism by which risks are transmitted from one location to another (Perrings et al. 2010). This is obvious in the case of infectious diseases of plants, animals or humans transmitted through trade or travel ( Tatem, 2009 ; Tatem et al., 2006a ; Tatem et al., 2006b ), but it may also occur through the effect of anthropogenic climate change on the range size of species ( Thomas and Ohlemüller, 2010 ). This mechanism, linking increased dispersal with risk globalization, may further aggravate the destabilizing effects of these two factors implied by our model. The potentially irreversible and severe consequences of these effects on both natural and human systems should warrant further evaluation of the spatial insurance hypothesis and the effect of global integration on the stability of ecological functioning.
- We revaluate the original Loreau spatial insurance model.
- We extend the Loreau model to consider stochastic temporal resource availability (“environmental risk”) and static, heterogeneous species dispersal.
- The stabilizing effect of dispersal is less effective when environmental risk is spatially correlated.
Despite declines in biodiversity and stability, dispersal is able to maintain productivity despite heterogeneity in species dispersal rates.
Acknowledgments
David Shanafelt and Charles Perrings acknowledge funding from the National Science Foundation grant 0639252, and the National Institute of General Medical Sciences (NIGMS) at the National Institutes of Health grant 1R01GM100471-01. Michel Loreau was supported by the TULIP Laboratory of Excellence (ANR-10-LABX-41). The paper's contents are solely the responsibility of the authors and do not necessarily represent the official views of NIGMS.
1 These mechanisms are typically broken down into selection and functional complementarity classes ( Loreau and Hector, 2001 ; Loreau, 2010 ; Loreau et al., 2012 ). Selection mechanisms involve the Darwinian selection of species that generate biodiversity such as niche specialization or differentiation. Mechanisms of functional complementarity focus on the interactions between species, which are in effect the consequences of selection mechanisms.
2 Many of these mechanisms are interlinked, implicitly derived from the same underlying concept ( Loreau, 2010 ). For instance, within a community of species, total community biomass will exhibit a variance that is a function of the variances of each individual species (statistical averaging) as well as the covariances between them (broadly termed the “covariance effect”) ( Lehman and Tilman, 2001 ). For a detailed review of the mechanisms contributing to biodiversity and stability, see ( Tilman, 1999 ; Lehman and Tilman, 2001 ; Loreau, 2010 ; Loreau and de Mazancourt, 2013 ).
3 In our study, stability is measured by the temporal variability in productivity. A high coefficient of variation implies an unstable system; a low coefficient of variation a stable one. A suite of stability measures could have been used including the persistence time of a species, resistance to disturbance (e.g. a change in species diversity or abundance after the introduction of an invader), the time for the system to return to a steady state after a disturbance event, or the size of the perturbation needed to dislodge the system from its current functional state (‘resilience’) ( Orians, 1975 ; Loeuille, 2012 ; Scheffer, 2012 ). Stability in one of these senses does not necessarily imply stability in another sense. We focus on a measure of stability that centers on productivity in a particular functional state.
4 We retain the assumptions that species dispersal is density-independent, and that all environmental variation involves the same period and amplitude. There is, however, good reason to believe that these may be too restrictive. Tradeoffs between a species' ability to disperse and colonize have been shown to be stabilizing mechanisms of diversity ( Kareiva and Wennergren, 1995 ). Similarly, an explicit spatial structure including more complex networks and degrees of connectivity would likely alter biodiversity and the system's ability to withstand external shocks (Gardner and Ashby, 1972; Boitani et al., 2007 ). Finally, empirical systems often possess dynamics that operate on different spatial and temporal scales, between both state variables and patches, that may create a “panarchy” of potential systems ( Gunderson and Holling, 2002 ). These are left for future work.
5 Biodiversity metrics were selected in order to capture changes in both the types of species (local and global species richness) and species abundance (Shannon index). A number of metrics exist to measure biodiversity, many of which are highly correlated ( Bandeira et al., 2013 ). See Humphries et al. (1995) for a review of diversity metrics and their application for conservation.
6 Altering the initial resource biomass causes a loss of the species coexistence result of Loreau et al. (2003) and Gonzalez et al. (2009) . However, the productivity and stability results are maintained. A discussion of the relationship between species richness, productivity, and dispersal rate is discussed in the proceeding section. See Haegeman and Loreau (2014) for a detailed analysis of the conditions under which the biodiversity-productivity result arises.
7 Other types of “environmental risk” could be stochastic disturbances that directly affect species biomass, such as extinction events or the removal of patches from the system ( Nee and May, 1992 ). However, these are beyond the scope of the current manuscript.
8 Certainly this result is not always the case in real-world systems. For example, increases in nitrogen and phosphorous in freshwater systems can lead to spikes in algae populations (consistent with our model) but, through resulting effects such as the reduction of oxygen, also cause system crashes at higher trophic levels. It is important to note that our model only considers a single trophic level and a single resource. Processes such as eutrophication operate on multiple spatial and temporal scales and across multiple trophic levels. In addition, while an increase in one resource, such as nutrients (eutrophication) reduces limitation and competition for that resource, it enhances limitation and competition for light, which may reduce diversity. Further investigation is warranted.
9 Due to high degrees of biomass influx caused by migration, sink populations force resource biomass to zero. In sources, species biomasses decay exponentially due to high rates of out-migration.
Publisher's Disclaimer: This is a PDF file of an unedited manuscript that has been accepted for publication. As a service to our customers we are providing this early version of the manuscript. The manuscript will undergo copyediting, typesetting, and review of the resulting proof before it is published in its final citable form. Please note that during the production process errors may be discovered which could affect the content, and all legal disclaimers that apply to the journal pertain.
Literature Cited
- Allhoff KT, Weiel EM, Rogge T, Drossel B. On the interplay of speciation and dispersal: An evolutionary food web model in space. Journal of Theoretical Biology. 2015; 366 :46–56. [ PubMed ] [ Google Scholar ]
- Altermatt F, Schreiber S, Holyoak M. Interactive effects of disturbance and dispersal directionality on species richness and composition in metacommunities. Ecology. 2011; 92 :859–870. [ PubMed ] [ Google Scholar ]
- Aragon R, Oesterheld M, Irisarri G, Texeira M. Stability of ecosystem functioning and diversity of grasslands at the landscape scale. Landscape Ecology. 2011; 26 :1011–1022. [ Google Scholar ]
- Armstrong RA, McGehee R. Competitive exclusion. The American Naturalist. 1980; 115 :151–170. [ Google Scholar ]
- Bandeira B, Jamet JL, Jamet D, Ginoux JM. Mathematical converges of biodiversity indices. Ecological Indicators. 2013; 29 :522–528. [ Google Scholar ]
- Boitani L, Falcucci A, Maiorano L, Rondinini C. Ecological networks as conceptual frameworks or operational tools in conservation. Conservation Biology. 2007; 21 :1414–1422. [ PubMed ] [ Google Scholar ]
- Canfield DE, Glazer AN, Falkowski PG. The evolution and future of Earth's nitrogen cycle. Science. 2010; 330 :192–196. [ PubMed ] [ Google Scholar ]
- Cardinale BJ, Duffy E, Gonzalez A, Hooper DU, Perrings C, Venail P, Narwani A, Mace GM, Tilman D, Wardle DA, Kinzig AP, Daily GC, Loreau M, Grace JB, Larigauderie A, Srivastava DS, Naeem S. Biodiversity loss and its impact on humanity. Nature. 2012; 486 :59–67. [ PubMed ] [ Google Scholar ]
- Chisholm R. The ecology, economics, and management of alien invasive species. In: Levin S, editor. Princeton Guide to Ecology. Princeton University Press; Princeton, New Jersey: 2012. [ Google Scholar ]
- Clavel J, Julliard R, Devictor V. Worldwide decline of specialist species: Toward a global functional homogenization? Frontiers in Ecology and the Environment. 2011; 9 :222–228. [ Google Scholar ]
- Costello C, Springborn M, McAusland C, Solow A. Unintended biological invasions: Does risk vary by trading partner? Journal of Environmental Economics and Management. 2007; 54 :262–276. [ Google Scholar ]
- Cottingham KL, Brown BL, Lennon JT. Biodiversity may regulate the temporal variability of ecological systems. Ecology Letters. 2001; 4 :72–85. [ Google Scholar ]
- Cusack D, Silver W, McDowell W. Biological Nitrogen Fixation in Two Tropical Forests: Ecosystem-Level Patterns and Effects of Nitrogen Fertilization. Ecosystems. 2009; 12 :1299–1315. [ Google Scholar ]
- Doak DF, Bigger D, Harding EK, Marvier MA, O'Malley RE, Thomson D. The statistical inevitability of stability-diversity relationships in community ecology. The American Naturalist. 1998; 151 :264–276. [ PubMed ] [ Google Scholar ]
- Evenson RE, Gollin D. Assessing the Impact of the Green Revolution, 1960 to 2000. Science. 2003; 300 :758–762. [ PubMed ] [ Google Scholar ]
- Filotas E, Grant M, Parrott L, Rikvold PA. The effect of positive interactions on community structure in a multi-species metacommunity model along an environmental gradient. Ecological Modelling. 2010; 221 :885–894. [ Google Scholar ]
- Futuyma DJ, Moreno G. The evolution of ecological specialization. Annual Review of Ecology, Evolution, and Systematics. 1988; 19 :207–233. [ Google Scholar ]
- Galloway JN, Townsend AR, Erisman JW, Bekunda M, Cai Z, Freney JR, Martinelli LA, Seitzinger SP, Sutton MA. Transformation of the nitrogen cycle: Recent trends, questions, and potential solutions. Science. 2008; 320 :889–892. [ PubMed ] [ Google Scholar ]
- Gardner MR, Ashby WR. Connectance of large dynamic (cybernetic) systems: Critical values for stability. Nature. 1970; 228 :784. [ PubMed ] [ Google Scholar ]
- Gonzalez A, Holt RD. The inflationary effects of environmental fluctuations in source-sink systems. Proceedings of the National Academy of the Sciences. 2002; 99 :14872–14877. [ PMC free article ] [ PubMed ] [ Google Scholar ]
- Gonzalez A, Loreau M. The causes and consequences of compensatory dynamics in ecological communities. Annual Review of Ecology, Evolution, and Systematics. 2009; 40 :393–414. [ Google Scholar ]
- Gonzalez A, Mouquet N, Loreau M. Biodiversity as spatial insurance: The effects of habitat fragmentation and dispersal on ecosystem functioning. In: Naeem S, et al., editors. Biodiversity, Ecosystem Functioning, and Human Wellbeing. Oxford University Press; 2009. [ Google Scholar ]
- Griffin PC, Mills LS. Sinks without borders: Snowshoe hare dynamics in a complex landscape. Oikos. 2009; 118 :1487–1498. [ Google Scholar ]
- Gunderson LH, Holling CS. Panarchy: Understanding transformations in human and natural systems. Island Press; Washington, D.C: 2002. [ Google Scholar ]
- Haegeman B, Loreau M. General relationships between consumer dispersal, resource dispersal and metacommunity diversity. Ecology Letters. 2014; 17 :175–184. [ PubMed ] [ Google Scholar ]
- Harrison S, Murphy D, Ehrlich PR. Distribution of the bay checkerspot butterfly, Euphydryas editha bayensis: Evidence for a metapopulation model. The American Naturalist. 1988; 132 :360–382. [ Google Scholar ]
- Haskett JD, Pachepsky YA, Acock B. Use of the beta distribution for parameterizing variability of soil properties at the regional level for crop yield simulation. Agricultural Systems. 1995; 48 :73–86. [ Google Scholar ]
- Hooper DU, Chapin FS, III, Ewel JJ, Hector A, Inchausti P, Lavorel S, Lawton JH, Lodge DM, Loreau M, Naeem S, Schmid B, Setala H, Symstad AJ, Vandermeer J, Wardle DA. Effects of biodiversity on ecosystem functioning: A consensus of current knowledge. Ecological Monographs. 2005; 75 :3–35. [ Google Scholar ]
- Howeth JG, Leibold MA. Species dispersal rates alter diversity and ecosystem stability in pond metacommunities. Ecology. 2010; 91 :2727–2741. [ PubMed ] [ Google Scholar ]
- Hulme PE. Trade, transport and trouble: Managing invasive species pathways in an era of globalization. Journal of Applied Ecology. 2009; 46 :10–18. [ Google Scholar ]
- Humphries CJ, Williams PH, Vane-Wright RI. Measuring biodiversity value for conservation. Annual Review of Ecology, Evolution, and Systematics. 1995; 26 :93–111. [ Google Scholar ]
- Intergovernmental Panel on Climate Change, Fifth assessment report: Climate change 2013. 2013 [ Google Scholar ]
- Isbell F, Calcagno V, Hector A, Connolly J, Harpole WS, Reich PB, Scherer-Lorenzen M, Schmid B, Tilman D, van Ruijven J, Weigelt A, Wilsey BJ, Zavaleta ES, Loreau M. High plant diversity is needed to maintain ecosystem services. Nature. 2011; 477 :199–203. [ PubMed ] [ Google Scholar ]
- Isbell FI, Polley HW, Wilsey BJ. Biodiversity, productivity and the temporal stability of productivity: Patterns and processes. Ecology Letters. 2009; 12 :443–451. [ PubMed ] [ Google Scholar ]
- Ives AR, Klug JL, Gross K. Stability and species richness in complex communities. Ecology Letters. 2000; 3 :399–411. [ Google Scholar ]
- Kareiva P, Wennergren U. Connecting landscape patterns to ecosystem and population processes. Nature. 1995; 373 :299–302. [ Google Scholar ]
- Karl TR, Trenberth KE. Modern global climate change. Science. 2003; 302 :1719–1723. [ PubMed ] [ Google Scholar ]
- Khoury CK, Bjorkman AD, Dempewolf H, Ramirez-Villegas J, Guarino L, Jarvis A, Rieseberg LH, Struik PC. Increasing homogeneity in global food supplies and the implications for food security. Proceedings of the National Academy of the Sciences. 2014; 111 :4001–4006. [ PMC free article ] [ PubMed ] [ Google Scholar ]
- Kilpatrick AM. Globalization, land use, and the invasion of West Nile virus. Science. 2011; 334 :323–327. [ PMC free article ] [ PubMed ] [ Google Scholar ]
- Lehman CL, Tilman D. Biodiversity, stability, and productivity in competitive communities. The American Naturalist. 2001; 156 :534–552. [ PubMed ] [ Google Scholar ]
- Lenzen M, Moran D, Kanemoto K, Foran B, Lobefaro L, Geschke A. International trade drives biodiversity threats in developing nations. Nature. 2012; 486 :109–112. [ PubMed ] [ Google Scholar ]
- Loeuille N. Evolution of communities and ecosystems. In: Levin S, editor. The Princeton Guide to Ecology. Princeton University Press; Princeton, New Jersey: 2012. [ Google Scholar ]
- Loreau M. From populations to ecosystems: Theoretical foundations for a new ecological synthesis. Princeton University Press; Princeton, New Jersey: 2010. [ Google Scholar ]
- Loreau M, Hector A. Partitioning selection and complementarity in biodiversity experiments. Nature. 2001; 412 :72–76. [ PubMed ] [ Google Scholar ]
- Loreau M, de Mazancourt C. Biodiversity and ecosystem stability: A synthesis of underlying mechanisms. Ecology Letters. 2013; 16 :106–115. [ PubMed ] [ Google Scholar ]
- Loreau M, Mouquet N, Gonzalez A. Biodiversity as spatial insurance in heterogeneous landscapes. Proceedings of the National Academy of the Sciences. 2003; 100 :12765–12770. [ PMC free article ] [ PubMed ] [ Google Scholar ]
- Loreau M, Sapijanskas J, Isbell F, Hector A. Niche and fitness differences relate the maintenance of diversity to ecosystem function: Comment. Ecology. 2012; 93 :1482–1487. [ PubMed ] [ Google Scholar ]
- Loreau M, Naeem S, Inchausti P, Bengtsson J, Grime JP, Hector A, Hooper DU, Huston MA, Raffaelli D, Schmid B, Tilman D, Wardle DA. Biodiversity and ecosystem functioning: Current knowledge and future challenges. Science. 2001; 294 :804–808. [ PubMed ] [ Google Scholar ]
- Matias MG, Mouquet N, Chase J. Dispersal stochasticity mediates species richness in source-sink metacommunities. Oikos. 2013; 122 :395–402. [ Google Scholar ]
- Matthews DP, Gonzalez A. The inflationary effects of environmental fluctuations ensure the persistence of sink metapopulations. Ecology. 2007; 88 :2848–2856. [ PubMed ] [ Google Scholar ]
- McCann KS. The diversity-stability debate. Nature. 2000; 405 :228–233. [ PubMed ] [ Google Scholar ]
- McKinney ML, Lockwood JL. Biotic homogenization: A few winners replacing many losers in the next mass extinction. Trends in Ecology and Evolution. 1999; 14 :450–453. [ PubMed ] [ Google Scholar ]
- McNaughton SJ. Diversity and stability of ecological communities: A comment on the role of empiricism in ecology. The American Naturalist. 1977; 111 :515–525. [ Google Scholar ]
- Mitzenmacher M. A Brief History of Generative Models for Power Law and Lognormal Distributions. Internet Mathematics. 2004; 1 :226–251. [ Google Scholar ]
- Moffat AS. Global nitrogen overload problem goes critical. Science. 1998; 279 :988–989. [ Google Scholar ]
- Mouquet N, Loreau M. Community patterns in source-sink metacommunities. The American Naturalist. 2003; 162 :544–557. [ PubMed ] [ Google Scholar ]
- Naeem S, Li S. Biodiversity enhances ecosystem reliability. Nature. 1997; 390 :507–509. [ Google Scholar ]
- Nee S, May RM. Dynamics of metapopulations: Habitat destruction and competitive coexistence. Journal of Animal Ecology. 1992; 61 :37–40. [ Google Scholar ]
- Orians GH. Diversity, stability and maturity in natural ecosystems. In: van Dobben WH, Lowe-McConnell RH, editors. Unifying Concepts in Ecology: Report of the plenary sessions of the First International Congress of Ecology. Dr W. Junk B.V. Publishers; The Hague, The Netherlands: 1975. [ Google Scholar ]
- Rodriguez-Iturbe I, Cox DR, Isham V. Some models for rainfall based on stochastic point processes. Proceedings of the Royal Society of London Series A, Mathematical and Physical. 1987; 410 :269–288. [ Google Scholar ]
- Roy M, Holt RD, Barfield M. Temporal autocorrelation can enhance the persistence and abundance of metapopulations comprised of coupled sinks. The American Naturalist. 2005; 166 :246–261. [ PubMed ] [ Google Scholar ]
- Russ GR, Alcala AC. Enhanced biodiversity beyond marine reserve boundaries: The cup spillith over. Ecological Applications. 2011; 21 :241–250. [ PubMed ] [ Google Scholar ]
- Sanchirico JN, Wilen J. Bioeconomics of spatial exploitation in a patchy environment. Journal of Environmental Economics and Management. 1999; 37 :129–150. [ Google Scholar ]
- Scheffer M. Alternative stable states and regime shifts in ecosystems. In: Levin S, editor. The Princeton Guide to Ecology. Princeton University Press; Princeton, New Jersey: 2012. [ Google Scholar ]
- Schluter D. The ecology of adaptive radiation. Oxford University Press; New York: 2000. [ Google Scholar ]
- Smart SM, Thompson K, Marrs RH, Le Duc MG, Maskell LC, Firbank LG. Biotic homogenization and changes in species diversity across human-modified ecosystems. Proceedings of the Royal Society of London Series B, Biological Sciences. 2006; 273 :2659–2665. [ PMC free article ] [ PubMed ] [ Google Scholar ]
- Smith KF, Sax DF, Gaines SD, Guernier V, Guegan J. Globalization of human infectious disease. Ecology. 2007; 88 :1903–1910. [ PubMed ] [ Google Scholar ]
- Tatem AJ. The worldwide airline network and the dispersal of exotic species: 2007-2010. Ecography. 2009; 34 :94–102. [ PMC free article ] [ PubMed ] [ Google Scholar ]
- Tatem AJ, Hay SS, Rogers DJ. Global traffic and disease vector dispersal. Proceedings of the National Academy of Sciences. 2006a; 103 :6242–6247. [ PMC free article ] [ PubMed ] [ Google Scholar ]
- Tatem AJ, Rogers DJ, Hay SI. Global transport networks and infectious disease spread. Advances in Parasitology. 2006b; 62 :293–343. [ PMC free article ] [ PubMed ] [ Google Scholar ]
- Thomas C, Ohlemüller R. Climate change and species distributions: an alien future? In: Perrings C, et al., editors. Bioinvasions and Globalization: Ecology, Economics, Management, and Policy. Oxford University Press; Oxford; 2010. pp. 19–29. [ Google Scholar ]
- Thompson PL, Shurin JB. Regional zooplankton biodiversity provides limited buffering of pond ecosystems against climate change. Journal of Animal Ecology. 2012; 81 :251–259. [ PubMed ] [ Google Scholar ]
- Tilman D. The ecological consequences of changes in biodiversity: A search for general principles. Ecology. 1999; 80 :1455–1474. [ Google Scholar ]
- Tilman D, Lehman CL, Bristow CE. Diversity-stability relationships: Statistical inevitability or ecological consequence? The American Naturalist. 1998; 151 :277–282. [ PubMed ] [ Google Scholar ]
- Tisdell C. Socioeconomic causes of loss of animal genetic diversity: analysis and assessment. Ecological Economics. 2003; 45 :365–376. [ Google Scholar ]
- Urban MC. Maladaptation and mass effects in a metacommunity: Consequences for species coexistence. The American Naturalist. 2006; 168 :28–40. [ PubMed ] [ Google Scholar ]
- Valone TJ, Barber NA. An empirical evaluation of the insurance hypothesis in diversity-stability models. Ecology. 2008; 89 :522–531. [ PubMed ] [ Google Scholar ]
- Vieira SA, Alves LF, Duarte-Neto PJ, Martins SC, Veiga LG, Scaranello MA, Picollo MC, Camargo PB, do Carmo JB, Neto ES, Santos FAM, Joly CA, Martinelli LA. Stocks of carbon and nitrogen and partitioning between above- and belowground pools in the Brazilian coastal Atlantic Forest elevation range. Ecology and Evolution. 2011; 1 :421–434. [ PMC free article ] [ PubMed ] [ Google Scholar ]
- Wiley JA, Herschkorn SJ, Padian NS. Heterogeneity in the probability of HIV transmission per sexual contact: The case of male-to-female transmission in penile-vaginal intercourse. Statistics in Medicine. 1989; 8 :93–102. [ PubMed ] [ Google Scholar ]
- Yachi S, Loreau M. Biodiversity and ecosystem productivity in a fluctuating environment: The insurance hypothesis. Proceedings of the National Academy of the Sciences. 1999; 96 :1463–1468. [ PMC free article ] [ PubMed ] [ Google Scholar ]

What Is A Research (Scientific) Hypothesis? A plain-language explainer + examples
By: Derek Jansen (MBA) | Reviewed By: Dr Eunice Rautenbach | June 2020
If you’re new to the world of research, or it’s your first time writing a dissertation or thesis, you’re probably noticing that the words “research hypothesis” and “scientific hypothesis” are used quite a bit, and you’re wondering what they mean in a research context .
“Hypothesis” is one of those words that people use loosely, thinking they understand what it means. However, it has a very specific meaning within academic research. So, it’s important to understand the exact meaning before you start hypothesizing.
Research Hypothesis 101
- What is a hypothesis ?
- What is a research hypothesis (scientific hypothesis)?
- Requirements for a research hypothesis
- Definition of a research hypothesis
- The null hypothesis
What is a hypothesis?
Let’s start with the general definition of a hypothesis (not a research hypothesis or scientific hypothesis), according to the Cambridge Dictionary:
Hypothesis: an idea or explanation for something that is based on known facts but has not yet been proved.
In other words, it’s a statement that provides an explanation for why or how something works, based on facts (or some reasonable assumptions), but that has not yet been specifically tested . For example, a hypothesis might look something like this:
Hypothesis: sleep impacts academic performance.
This statement predicts that academic performance will be influenced by the amount and/or quality of sleep a student engages in – sounds reasonable, right? It’s based on reasonable assumptions , underpinned by what we currently know about sleep and health (from the existing literature). So, loosely speaking, we could call it a hypothesis, at least by the dictionary definition.
But that’s not good enough…
Unfortunately, that’s not quite sophisticated enough to describe a research hypothesis (also sometimes called a scientific hypothesis), and it wouldn’t be acceptable in a dissertation, thesis or research paper . In the world of academic research, a statement needs a few more criteria to constitute a true research hypothesis .
What is a research hypothesis?
A research hypothesis (also called a scientific hypothesis) is a statement about the expected outcome of a study (for example, a dissertation or thesis). To constitute a quality hypothesis, the statement needs to have three attributes – specificity , clarity and testability .
Let’s take a look at these more closely.
Need a helping hand?
Hypothesis Essential #1: Specificity & Clarity
A good research hypothesis needs to be extremely clear and articulate about both what’ s being assessed (who or what variables are involved ) and the expected outcome (for example, a difference between groups, a relationship between variables, etc.).
Let’s stick with our sleepy students example and look at how this statement could be more specific and clear.
Hypothesis: Students who sleep at least 8 hours per night will, on average, achieve higher grades in standardised tests than students who sleep less than 8 hours a night.
As you can see, the statement is very specific as it identifies the variables involved (sleep hours and test grades), the parties involved (two groups of students), as well as the predicted relationship type (a positive relationship). There’s no ambiguity or uncertainty about who or what is involved in the statement, and the expected outcome is clear.
Contrast that to the original hypothesis we looked at – “Sleep impacts academic performance” – and you can see the difference. “Sleep” and “academic performance” are both comparatively vague , and there’s no indication of what the expected relationship direction is (more sleep or less sleep). As you can see, specificity and clarity are key.
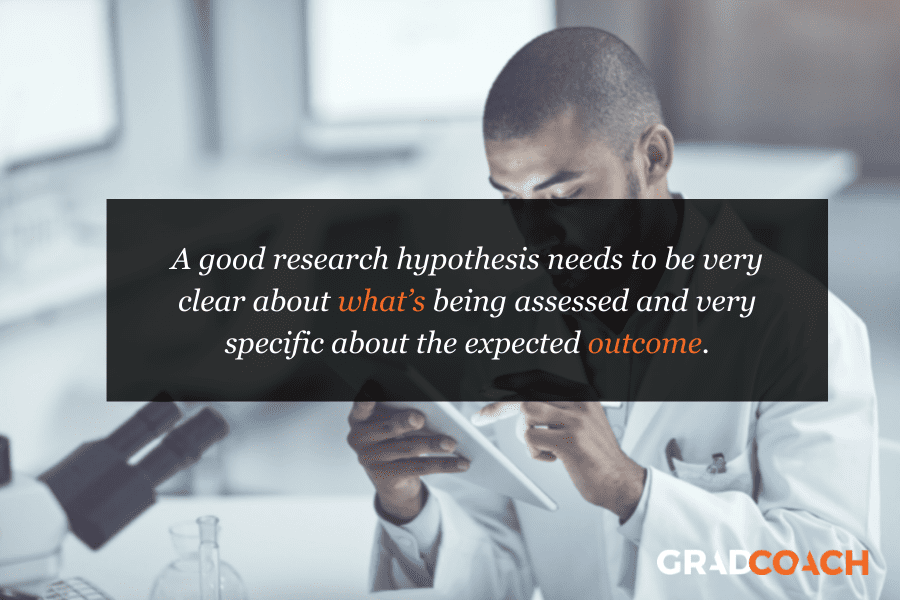
Hypothesis Essential #2: Testability (Provability)
A statement must be testable to qualify as a research hypothesis. In other words, there needs to be a way to prove (or disprove) the statement. If it’s not testable, it’s not a hypothesis – simple as that.
For example, consider the hypothesis we mentioned earlier:
Hypothesis: Students who sleep at least 8 hours per night will, on average, achieve higher grades in standardised tests than students who sleep less than 8 hours a night.
We could test this statement by undertaking a quantitative study involving two groups of students, one that gets 8 or more hours of sleep per night for a fixed period, and one that gets less. We could then compare the standardised test results for both groups to see if there’s a statistically significant difference.
Again, if you compare this to the original hypothesis we looked at – “Sleep impacts academic performance” – you can see that it would be quite difficult to test that statement, primarily because it isn’t specific enough. How much sleep? By who? What type of academic performance?
So, remember the mantra – if you can’t test it, it’s not a hypothesis 🙂
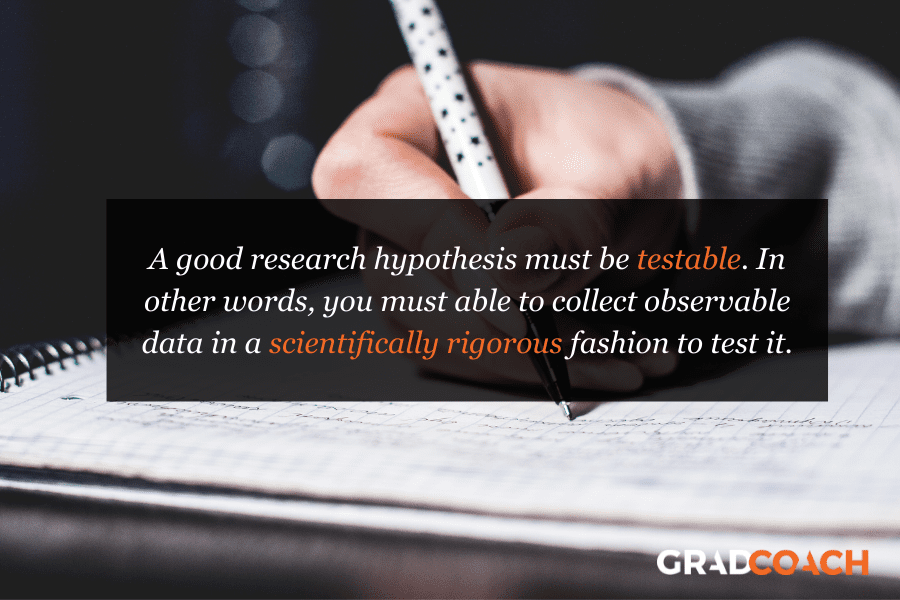
Defining A Research Hypothesis
You’re still with us? Great! Let’s recap and pin down a clear definition of a hypothesis.
A research hypothesis (or scientific hypothesis) is a statement about an expected relationship between variables, or explanation of an occurrence, that is clear, specific and testable.
So, when you write up hypotheses for your dissertation or thesis, make sure that they meet all these criteria. If you do, you’ll not only have rock-solid hypotheses but you’ll also ensure a clear focus for your entire research project.
What about the null hypothesis?
You may have also heard the terms null hypothesis , alternative hypothesis, or H-zero thrown around. At a simple level, the null hypothesis is the counter-proposal to the original hypothesis.
For example, if the hypothesis predicts that there is a relationship between two variables (for example, sleep and academic performance), the null hypothesis would predict that there is no relationship between those variables.
At a more technical level, the null hypothesis proposes that no statistical significance exists in a set of given observations and that any differences are due to chance alone.
And there you have it – hypotheses in a nutshell.
If you have any questions, be sure to leave a comment below and we’ll do our best to help you. If you need hands-on help developing and testing your hypotheses, consider our private coaching service , where we hold your hand through the research journey.
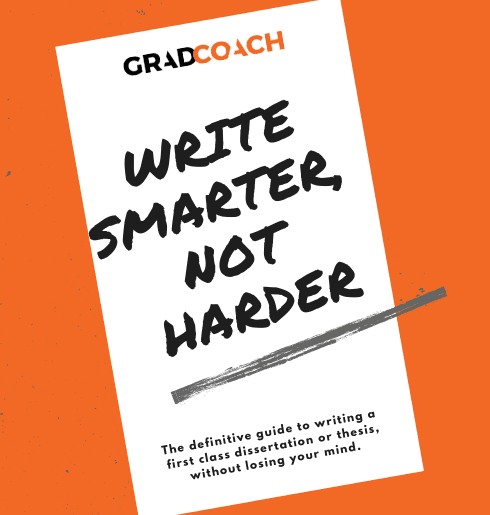
Psst… there’s more (for free)
This post is part of our dissertation mini-course, which covers everything you need to get started with your dissertation, thesis or research project.
You Might Also Like:
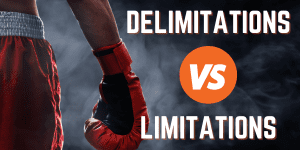
16 Comments
Very useful information. I benefit more from getting more information in this regard.
Very great insight,educative and informative. Please give meet deep critics on many research data of public international Law like human rights, environment, natural resources, law of the sea etc
In a book I read a distinction is made between null, research, and alternative hypothesis. As far as I understand, alternative and research hypotheses are the same. Can you please elaborate? Best Afshin
This is a self explanatory, easy going site. I will recommend this to my friends and colleagues.
Very good definition. How can I cite your definition in my thesis? Thank you. Is nul hypothesis compulsory in a research?
It’s a counter-proposal to be proven as a rejection
Please what is the difference between alternate hypothesis and research hypothesis?
It is a very good explanation. However, it limits hypotheses to statistically tasteable ideas. What about for qualitative researches or other researches that involve quantitative data that don’t need statistical tests?
In qualitative research, one typically uses propositions, not hypotheses.
could you please elaborate it more
I’ve benefited greatly from these notes, thank you.
This is very helpful
well articulated ideas are presented here, thank you for being reliable sources of information
Excellent. Thanks for being clear and sound about the research methodology and hypothesis (quantitative research)
I have only a simple question regarding the null hypothesis. – Is the null hypothesis (Ho) known as the reversible hypothesis of the alternative hypothesis (H1? – How to test it in academic research?
this is very important note help me much more
Trackbacks/Pingbacks
- What Is Research Methodology? Simple Definition (With Examples) - Grad Coach - […] Contrasted to this, a quantitative methodology is typically used when the research aims and objectives are confirmatory in nature. For example,…
Submit a Comment Cancel reply
Your email address will not be published. Required fields are marked *
Save my name, email, and website in this browser for the next time I comment.
- Print Friendly
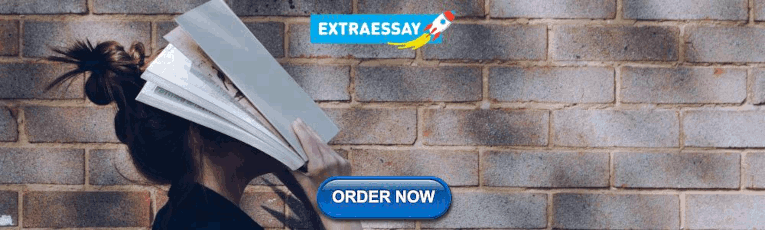
IMAGES
VIDEO
COMMENTS
Yachi & Loreau formalised this idea mathematically and introduced the insurance hypothesis, which posits that, in a variable environment, aggregate ecosystem properties (e.g. total biomass or production) will vary less in more diverse communities because declines in the performance or abundance of some species or phenotypes will be offset, at ...
This is a broad definition, ... the insurance hypothesis does not infer that diversity actively promotes stability. ... A simple food-web diagram depicting a strong consumer-resource ...
These findings are consistent with the insurance hypothesis. Although we cannot completely eliminate other explanations such as an assurance effect or switching costs, we argue that such explanations are unlikely to drive our main findings. Keywords: insurance hypothesis, KPMG, tax shelter.
The results reported by Loreau et al. (2003), summarized above, provide a simple illustration of the spatial insurance hypothesis. They demonstrated how dispersal, as a mechanism to increase biodiversity, insures the system against asynchronous environmental fluctuations. ... By definition, stochasticity of resource flows increases the chance ...
The insurance hypothesis so far has been an intuitive idea that increasing biodiversity insures ecosystems against declines in their functioning caused by environmental fluctuations (12, 14-16).Such an effect is expected because different species respond differently to environmental changes, hence the contribution of some species to ecosystem processes may decrease while that of others may ...
Although many have argued that independent audits implicitly provide clients with a form of insurance (the insurance hypothesis), there is limited empirical evidence to support the existence and magnitude of this function. In August of 2005, after months of intense negotiations and discussions, KPMG entered into a settlement agreement with the ...
The insurance hypothesis predicts a stabilizing effect of increasing species richness on community and ecosystem properties. Difference among species' responses to environmental fluctuations provides a general mechanism for the hypothesis. Previous experimental investigations of the insurance hypothesis have not examined this mechanism directly.
Assemblages contained two to six species censused on 5-12 routes over 10-39 years (Table 2; Appendix C). In all but one BBS assemblage, positive correlations outnumbered negative by more than two to one (Table 2). The percentage of positive correlations ranged from 56.3% to 94.2%, with the average median of 0.26.
The insurance hypothesis argues that a high degree of biodiversity, e.g., in the form of functional redundancy of different species, can safeguard ecosystems against anthropogenic environmental damage by, for example, compensating the loss of productivity of one species through filling its functional niche with other species (Loreau et al ...
Menon and Williams-The Insurance Hypothesis and Market Prices 329. In a typical auditor change situation, the investors continue to have rights against the. predecessor auditor. However, when L&H filed for bankruptcy protection, investors in client firms were restricted from recovering any present and potential claims.
Hypothesis 2 predicts that the insurance value will be the greatest for the riskiest firms (e.g., those in the top quartile). The results are consistent with expectations. For firms in the highest quartile of litigation risk, the abnormal return is 0.94%, highly significant at the 1% level.
Although many have argued that independent audits implicitly provide clients with a form of insurance (the insurance hypothesis), there is limited empirical evidence to support the existence and magnitude of this function. In August of 2005, after months of intense negotiations and discussions, KPMG entered into a settlement agreement with the Department of Justice, which ended widespread ...
The Insurance Hypothesis . Farming monoculture, such as in this corn field in Marqueseac (France), can lead to decreased net ecosystem CO2 intake, meaning land becomes less useful in the fight against climate change. ... The idea behind 'the insurance hypothesis' is simple: when different species are present, they are able to fulfil a ...
The results reported by Loreau et al. (2003), summarized above, provide a simple illustration of the spatial insurance hypothesis. They demonstrated how dispersal, as a mechanism to increase biodiversity, insures the system against asynchronous environmental fluctuations. ... By definition, stochasticity of resource flows increases the chance ...
planation is the insurance hypothesis. Investor losses that result from finan-cial statement misrepresentations may be wholly or partially recovered by suing auditors. In addressing the insurance hy-pothesis, the accounting literature posits that auditors provide a type of implicit insurance to users and inves-tors (Hill et aL, 1993). The ...
The hypothesis of the audit insurance needs can overall establish, but because of civil compensation mechanism is still not perfect and buyer's market and long-term pattern of the audit ...
monitoring hypothesis, the information hypothesis and the insurance hypothesis. Next these three roles will each be described to provide an overview of the different roles auditing can take in different environments. 6 Proceedings of the University of Vaasa. Teaching Aid Series 2.2.1 The monitoring hypothesis ...
The Insurance Hypothesis: An Examination of KPMG's Audit Clients around the Investigation and Settlement of the Tax Shelter Case Darryl L. Brown, Susan Z. Shu, Billy S. Soo, and Gregory M. Trompeter
SUMMARY: Although prior literature has suggested that independent audits provide an implicit form of insurance against investor losses (the "insurance hypothesis"), it has been challenging to isolate the "insurance" effect. In this paper, we use a unique setting to examine this effect. In 2002, KPMG was investigated by the U.S. Department of Justice in relation to tax shelters sold by ...
hypothesis (but they also note that it is not incons istent with the insurance hypothesis). 6 More recently, Weber et al. (2008) and Skinner and Srinivasan (2012) e xamine the assurance
9 Testing Full Insurance Early tests of the full insurance hypothesis were conducted using U.S. data by Mace [1991] and Cochrane [1991]. The rst test using developing country data was due to Townsend. The usual test of full insurance essentially proceeds from (1), and a simple parameter-ization of the utility function.
hypothesis: [noun] an assumption or concession made for the sake of argument. an interpretation of a practical situation or condition taken as the ground for action.
A research hypothesis (also called a scientific hypothesis) is a statement about the expected outcome of a study (for example, a dissertation or thesis). To constitute a quality hypothesis, the statement needs to have three attributes - specificity, clarity and testability. Let's take a look at these more closely.