
Statistics Made Easy
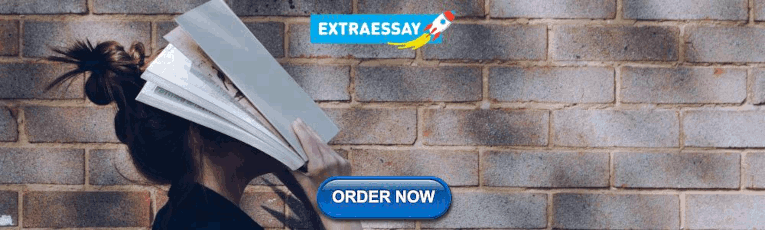
A Guide to Using Post Hoc Tests with ANOVA
An ANOVA is a statistical test that is used to determine whether or not there is a statistically significant difference between the means of three or more independent groups.
The hypotheses used in an ANOVA are as follows:
The null hypothesis (H 0 ): µ 1 = µ 2 = µ 3 = … = µ k (the means are equal for each group)
The alternative hypothesis: (Ha): at least one of the means is different from the others
If the p-value from the ANOVA is less than the significance level, we can reject the null hypothesis and conclude that we have sufficient evidence to say that at least one of the means of the groups is different from the others.
However, this doesn’t tell us which groups are different from each other. It simply tells us that not all of the group means are equal.
In order to find out exactly which groups are different from each other, we must conduct a post hoc test (also known as a multiple comparison test), which will allow us to explore the difference between multiple group means while also controlling for the family-wise error rate.
Technical Note: It’s important to note that we only need to conduct a post hoc test when the p-value for the ANOVA is statistically significant. If the p-value is not statistically significant, this indicates that the means for all of the groups are not different from each other, so there is no need to conduct a post hoc test to find out which groups are different from each other.
The Family-Wise Error Rate
As mentioned before, post hoc tests allow us to test for difference between multiple group means while also controlling for the family-wise error rate .
In a hypothesis test , there is always a type I error rate, which is defined by our significance level (alpha) and tells us the probability of rejecting a null hypothesis that is actually true. In other words, it’s the probability of getting a “false positive”, i.e. when we claim there is a statistically significant difference among groups, but there actually isn’t.
When we perform one hypothesis test, the type I error rate is equal to the significance level, which is commonly chosen to be 0.01, 0.05, or 0.10. However, when we conduct multiple hypothesis tests at once, the probability of getting a false positive increases.
For example, imagine that we roll a 20-sided dice. The probability that the dice lands on a “1” is just 5%. But if we roll two dice at once, the probability that one of the dice will land on a “1” increases to 9.75%. If we roll five dice at once, the probability increases to 22.6%.
The more dice we roll, the higher the probability that one of the dice will land on a “1.” Similarly, if we conduct several hypothesis tests at once using a significance level of .05, the probability that we get a false positive increases to beyond just 0.05.
Multiple Comparisons in ANOVA
When we conduct an ANOVA, there are often three or more groups that we are comparing to one another. Thus, when we conduct a post hoc test to explore the difference between the group means, there are several pairwise comparisons we want to explore.
For example, suppose we have four groups: A, B, C, and D. This means there are a total of six pairwise comparisons we want to look at with a post hoc test:
A – B (the difference between the group A mean and the group B mean) A – C A – D B – C B – D C – D
If we have more than four groups, the number of pairwise comparisons we will want to look at will only increase even more. The following table illustrates how many pairwise comparisons are associated with each number of groups along with the family-wise error rate:

Notice that the family-wise error rate increases rapidly as the number of groups (and consequently the number of pairwise comparisons) increases. In fact, once we reach six groups, the probability of us getting a false positive is actually above 50%!
This means we would have serious doubts about our results if we were to make this many pairwise comparisons, knowing that our family-wise error rate was so high.
Fortunately, post hoc tests provide us with a way to make multiple comparisons between groups while controlling the family-wise error rate.
Example: One-Way ANOVA with Post Hoc Tests
The following example illustrates how to perform a one-way ANOVA with post hoc tests.
Note: This example uses the programming language R, but you don’t need to know R to understand the results of the test or the big takeaways.
First, we’ll create a dataset that contains four groups (A, B, C, D) with 20 observations per group:
Next, we’ll fit a one-way ANOVA to the dataset:
From the ANOVA table output, we see that the F-statistic is 17.66 and the corresponding p-value is extremely small.
This means we have sufficient evidence to reject the null hypothesis that all of the group means are equal. Next, we can use a post hoc test to find which group means are different from each other.
We will walk through examples of the following post hoc tests:
Tukey’s Test – useful when you want to make every possible pairwise comparison
Holm’s Method – a slightly more conservative test compared to Tukey’s Test
Dunnett’s Correction – useful when you want to compare every group mean to a control mean, and you’re not interested in comparing the treatment means with one another.
Tukey’s Test
We can perform Tukey’s Test for multiple comparisons by using the built-in R function TukeyHSD() as follows:
Notice that we specified our confidence level to be 95%, which means we want our family-wise error rate to be .05. R gives us two metrics to compare each pairwise difference:
- Confidence interval for the mean difference (given by the values of lwr and upr )
- Adjusted p-value for the mean difference
Both the confidence interval and the p-value will lead to the same conclusion.
For example, the 95% confidence interval for the mean difference between group C and group A is (0.2813, 1.4309), and since this interval doesn’t contain zero we know that the difference between these two group means is statistically significant. In particular, we know that the difference is positive, since the lower bound of the confidence interval is greater than zero.
Likewise, the p-value for the mean difference between group C and group A is 0.0011, which is less than our significance level of 0.05, so this also indicates that the difference between these two group means is statistically significant.
We can also visualize the 95% confidence intervals that result from the Tukey Test by using the plot() function in R:

If the interval contains zero, then we know that the difference in group means is not statistically significant. In the example above, the differences for B-A and C-B are not statistically significant, but the differences for the other four pairwise comparisons are statistically significant.
Holm’s Method
Another post hoc test we can perform is holm’s method. This is generally viewed as a more conservative test compared to Tukey’s Test.
We can use the following code in R to perform holm’s method for multiple pairwise comparisons:
This test provides a grid of p-values for each pairwise comparison. For example, the p-value for the difference between the group A and group B mean is 0.20099.
If you compare the p-values of this test with the p-values from Tukey’s Test, you’ll notice that each of the pairwise comparisons lead to the same conclusion, except for the difference between group C and D. The p-value for this difference was .0505 in Tukey’s Test compared to .02108 in Holm’s Method.
Thus, using Tukey’s Test we concluded that the difference between group C and group D was not statistically significant at the .05 significance level, but using Holm’s Method we concluded that the difference between group C and group D was statistically significant.
In general, the p-values produced by Holm’s Method tend to be lower than those produced by Tukey’s Test.
Dunnett’s Correction
Yet another method we can use for multiple comparisons is Dunett’s Correction. We would use this approach when we want to compare every group mean to a control mean, and we’re not interested in comparing the treatment means with one another.
For example, using the code below we compare the group means of B, C, and D all to that of group A. So, we use group A as our control group and we aren’t interested in the differences between groups B, C, and D.
From the p-values in the output we can see the following:
- The difference between the group B and group A mean is not statistically significant at a significance level of .05. The p-value for this test is 0.4324 .
- The difference between the group C and group A mean is statistically significant at a significance level of .05. The p-value for this test is 0.0005 .
- The difference between the group D and group A mean is statistically significant at a significance level of .05. The p-value for this test is 0.00004 .
As we stated earlier, this approach treats group A as the “control” group and simply compares every other group mean to that of group A. Notice that there are no tests performed for the differences between groups B, C, and D because we aren’t interested in the differences between those groups.
A Note on Post Hoc Tests & Statistical Power
Post hoc tests do a great job of controlling the family-wise error rate, but the tradeoff is that they reduce the statistical power of the comparisons. This is because the only way to lower the family-wise error rate is to use a lower significance level for all of the individual comparisons.
For example, when we use Tukey’s Test for six pairwise comparisons and we want to maintain a family-wise error rate of .05, we must use a significance level of approximately 0.011 for each individual significance level. The more pairwise comparisons we have, the lower the significance level we must use for each individual significance level.
The problem with this is that lower significance levels correspond to lower statistical power. This means that if a difference between group means actually does exist in the population, a study with lower power is less likely to detect it.
One way to reduce the effects of this tradeoff is to simply reduce the number of pairwise comparisons we make. For example, in the previous examples we performed six pairwise comparisons for the four different groups. However, depending on the needs of your study, you may only be interested in making a few comparisons.
By making fewer comparisons, you don’t have to lower the statistical power as much.
It’s important to note that you should determine before you perform the ANOVA exactly which groups you want to make comparisons between and which post hoc test you will use to make these comparisons. Otherwise, if you simply see which post hoc test produces statistically significant results, that reduces the integrity of the study.
In this post, we learned the following things:
- An ANOVA is used to determine whether or not there is a statistically significant difference between the means of three or more independent groups.
- If an ANOVA produces a p-value that is less than our significance level, we can use post hoc tests to find out which group means differ from one another.
- Post hoc tests allow us to control the family-wise error rate while performing multiple pairwise comparisons.
- The tradeoff of controlling the family-wise error rate is lower statistical power. We can reduce the effects of lower statistical power by making fewer pairwise comparisons.
- You should determine beforehand which groups you’d like to make pairwise comparisons on and which post hoc test you will use to do so.

Published by Zach
Leave a reply cancel reply.
Your email address will not be published. Required fields are marked *
We've updated our Privacy Policy to make it clearer how we use your personal data. We use cookies to provide you with a better experience. You can read our Cookie Policy here.
Neuroscience News & Research
Stay up to date on the topics that matter to you
Post-Hoc Tests in Statistical Analysis

Complete the form below to unlock access to ALL audio articles.
What are post-hoc tests?
Post-hoc testing is carried out after a statistical analysis where you have performed multiple significance tests, ‘post-hoc’ coming from the Latin “after this”. Post-hoc analysis represents a way to adjust or ‘reinterpret’ results to account for the compounding uncertainty and risk of Type I error (more on that below) that is inherent in performing statistical tests. You may also see post-hoc tests referred to as multiple comparison tests (MCTs).
Significance testing
First, it may be helpful to recap what we mean by a significance test in statistics, and then explore how performing multiple tests may lead to spurious conclusions. Significance or hypothesis testing can be done with many types of data and in many different situations. The first step in performing one is to define a “null hypothesis”. We then calculate a p-value as a way of quantifying the strength of evidence against this null hypothesis. The p-value is the probability of observing a result as extreme, or more extreme, than that which you have observed if the null hypothesis were true. In other words, the probability that this result occurred due to chance. The smaller the p-value, the stronger the evidence against the null hypothesis.
For example, we may want to investigate whether mean systolic blood pressure differs between two groups of patients. We would test the null hypothesis (often written as H 0 ) that the two observed means are equal (no difference between the groups). We then calculate a test statistic, use a known theoretical distribution of that test statistic, and obtain and interpret the p-value that gives us an idea of the strength of evidence against the null hypothesis.
Correct interpretation of p-values can be a tricky business. While p-values exist on a continuum between 0 and 1, it is common to use an arbitrary cut-off value of 0.05 to represent a “statistically significant” result. The 0.05 significance level (or α level) can be useful for other purposes such as calculating the required sample size for a study.
What do post-hoc tests tell you?
Interpretation of multiple p-values becomes even trickier and this is the stage at which some researchers make use of post-hoc testing. If we test a null hypothesis that is in fact true, using the 0.05 significance level, there is a 0.95 probability of coming to the correct conclusion in accepting the null hypothesis. If we test two independent null hypotheses that are true, the probability of coming to the correct conclusion in accepting the null is now 0.95 x 0.95 = 0.90. Therefore, the more significance tests we perform together, the higher the compounding risk for us to mistakenly reject a null hypothesis that is in fact true (this is called a type I error or false positive – see Table 1 ). In other words, if we go on testing over and over, we will eventually find a “significant” result which is why care must be taken interpreting a p-value in the context of multiple tests. Moreover, at the 0.05 significance level, we might expect a significant result to be observed by chance alone once in every 20 significance tests. Post-hoc analysis, such as the Bonferroni test for multiple comparisons, aims to rebalance the compounding risk and adjust the p-values to reflect this risk of type I error. The Bonferroni test is in essence a series of t-tests performed on pairs of multiple groups being tested.
Other common post-hoc tests include the following:
- Tukey’s test – a common post-hoc test that makes adjustments to test statistics when comparing groups by calculating a Tukey’s Honest Significant Difference (HSD), an estimate of the difference between groups along with a confidence interval.
- Scheffe’s test – a test which also adjusts the test statistics for comparisons between groups and calculates a 95% confidence interval around the difference but in a more conservative way than Tukey’s test.
Less common post-hoc tests exist for various situations, summaries of which can be found here . These tests tend to give similar results and simply approach post-hoc analysis in different ways.
The Bonferroni test
Calculation of the Bonferroni test is done by simply taking the significance level at which you are conducting your hypothesis test (usually α=0.05) and dividing it by the number of separate tests performed. For example, if a researcher is investigating the difference between two treatments in 10 subgroups of patients (so 10 separate significance tests denoted by n ) the Bonferroni correction is calculated as α/ n = 0.05/10 = 0.005.
Hence, if any of the significance tests gave a p-value of <0.005 we would conclude that the test was significant at the 0.05 significance level and that there was evidence for a difference between the two treatments in that subgroup.
When not to use post-hoc tests?
As with many statistical procedures, there are disadvantages and even sometimes controversy attached to the use of post-hoc testing. Some statisticians prefer not to use post-hoc tests such as the Bonferroni test due to the inflation of the risk of type II error (not rejecting the null hypothesis when it is in fact false) as the type I error is adjusted, the implication that a comparison should be interpreted differently according to how many other tests are performed and the reliance on post-hoc testing in absence of a focused research question and approach to hypothesis testing.
Instead, it is suggested that a study should be designed to be specific about which subgroup differences or hypotheses are of interest before an analysis is performed so that conclusions are led by causal frameworks and prior knowledge rather than the data and chance alone. An example of this in practice might look like the preregistration of a clinical trial , in order for the researchers to pre-record and justify hypotheses and study design before analysis takes place. With careful study design, analysis planning and interpretation of findings, many statisticians and analysts avoid post-hoc testing without foregoing methodological rigor.
Moreover, since the aim of post-hoc testing is reinterpreting or setting a new criterion for reaching a ‘statistically significant’ finding, some argue that halting the use of post-hoc testing is compatible with the movement away from the concept of statistical significance more generally. P-values can and have been shown to mislead researchers, and an over-reliance on the somewhat arbitrary threshold of a statistically significant result (<0.05) often ignores the context – such as statistical assumptions, data quality, prior studies in the area and underlying mechanisms – in which these findings are reached.

Analysing Data using Linear Models
Chapter 11 post-hoc comparisons, 11.1 introduction.
Analysis of variance, as we have seen, can be used to test null-hypotheses about overall effects of certain factors (categorical variable) or combinations of factors (moderation). This is done with \(F\) -test statistics, with degrees of freedom that depend on the number of (combinations of) categories. The regression table, with \(t\) -tests in the output, can be used to compute specific contrasts, either the standard contrasts based on dummy coding, or contrasts based on alternative coding schemes.
In the previous chapter, all alternatives for specifying contrasts have been discussed in the context of specific research questions. It is important to make a distinction here between research questions that are posed before the data gathering and analysis, and research questions that pop up during the data analysis. Research questions of the first kind we call a priori (“at the outset”) questions, and questions of the second kind we call post hoc (“after the fact”) questions.
We’ve seen that the whole data analysis approach for inference is based on sampling distributions, for instance the sampling distribution of the \(t\) -statistic given that a population value equals 0. We then look at what \(t\) -value can be deemed large enough to reject the null-hypothesis (or to construct a confidence interval). Such a critical \(t\) -value is chosen in a way that if the null-hypothesis is true, it only happens in a low percentage of cases ( \(\alpha\) ) that we find a \(t\) -value more extreme than this critical value. This helps us to reject the null-hypothesis: we see something extreme that can’t be explained very well by the null-hypothesis.
However, if we look at a linear regression output table, we often see many \(t\) -values: one for the intercept, several for slope coefficients, and if the model includes moderation, we also may see several \(t\) -values for interaction effects. Every single \(t\) -value is based on a hypothesis that the null-hypothesis is true, that is, that the actual parameter value (or contrast) is 0 in the population. For every single \(t\) -test, we therefore know that if we would draw many many samples, in only \(\alpha\) % of the samples, we would find a \(t\) -value more extreme than the critical value (given that the null-hypothesis is true). But if we have for instance 6 different \(t\) -values in our output, how large is the probability that any of these 6 different \(t\) -values is more extreme than than the critical value?
Let’s use a very simple example. Let’s assume we have a relatively large data set, so that the \(t\) -distibution is very close to the normal distribution. When we assume we use two-sided testing with an \(\alpha\) of 5%, we know that the critical values for the null-hypothesis are \(-1.96\) and \(1.96\) . Imagine we have a dependent variable \(Y\) and a categorical independent variable \(X\) that consists of two levels, A and B. If we run a standard linear model on those variables \(Y\) and \(X\) , using dummy coding, we will see two parameters in the regression table: one for reference level A (labelled “(Intercept)”), and one coefficient for the difference between level B and A (labelled “XB”). Suppose that in reality, the population values for these parameters are both 0. That would mean that the two group means are equal to 0. When we do the research many times, drawing a large sample and repeat taking new samples 100 times, how many times will the intercept have a \(t\) -value more extreme than \(\pm 1.96\) ? Well, by definition, that frequency would be about 5, because we know that for the \(t\) -distribution, 5% of this distribution has values more extreme than \(\pm 1.96\) . Thus, if the intercept is truly 0 in the population, we will see a significant \(t\) -value in 5% of the samples.
The same is true for the second parameter “XB”: if this value is 0 in the population, then we will see a significant \(t\) -value for this parameter in the output in 5% of the samples.
Both of these events would be Type I errors: the kind of error that you make when you reject the null-hypothesis while it is actually true (see Chapter 2 ).
For any given sample, there can be either no Type I error, or there is one Type I error, of there are two Type I errors. Now, if the probability for a Type I error is 5% for a significant value for “(Intercept)”, and the probability is 5% for a type I error for “XB”, what then is the probability for at least one Type I error ?
This is a question for probability theory. If we assume that the Type I errors for the intercept and the slope are independent, we can use the binomial distribution (Ch. 3 ) and know that the probability of finding no Type I errors equals
\[P(errors = 0 | \alpha = 0.05) = {2 \choose 0} \times 0.05^0 \times (1-0.05)^2 = 0.95^2 = 0.9025\]
[Note: In case you skipped Chapter 3 , \(4 \choose 2\) is pronounced as “4 choose 2” and it stands for the number of combinations of two elements that you can have when you have four elements. For instance, if you have 4 letters A, B, C and D, then there are 6 possible pairs: AB, AC, AD, BC, BD, and CD. The general case of \(a \choose b\) can be computed by R using choose (a, b) . \(2 \choose 0\) is defined as 1 (there is only one way in which neither of the 2 tests results in a Type I error.)]
Therefore, since probabilities sum to 1, we know that the probability of at least one Type I error equals \(1- 0.9025= 0.0975\) .
We see that when we look at two \(t\) -tests in one analysis, the probability of a Type I error is no longer 5%, but almost twice that: 9.75%. This is under the assumption that the \(t\) -tests are independent of each other, which is often not the case. We will discuss what we mean with independent later. For now it suffices to know that the more null-hypotheses you test, the higher the risk of a Type I error.
For instance, if you have a categorical variable \(X\) with not two, but ten different groups, your regression output table will contain ten null-hypothesis tests: one for the intercept (reference category) and nine tests for the difference between the remaining groups and the reference group. In that case, the probability of at least one Type I error, if you perform each test with an \(\alpha\) of 5%, will be
\[1 - P(errors = 0| \alpha = 0.05) = 1 - { 10 \choose 0} \times 0.05^0 \times 0.95^{10} = 1 - 0.95^{10} = 0.4013\] And all this is only in the situation that you stick to the default output of a regression. Imagine that you not only test the difference between each group and the reference group, but that you also make many other contrasts: difference between group 2 and group 9, etcetera. If we would look at each possible pair among these ten groups, there would be \({10 \choose 2} = 144\) contrasts and consequently 144 \(t\) -tests. The probability of a Type I error will then be very close to 1, almost certainty!
The problem is further complicated by the fact that tests for all these possible pairs cannot be independent of each other. This is easy to see: If Jim is 5 centimetres taller than Sophie, and Sophie is 4 centimetres taller than Wendy, we already know the difference in height between Jim and Sophie: \(5 + 4 = 9\) centimetres. In other words, if you want to know the contrast between Jim and Wendy, you don’t need a new analysis: the answer is already there in the other two contrasts. Such a dependence in the contrasts that you estimate can lead to an even higher probability of a Type I error.
In order to get some grip on this dependence problem, we can use the so-called Bonferroni inequality . In the context of null-hypothesis testing, this states that the probability of at least one Type I error is less than or equal to \(\alpha\) times the number of contrasts J . This is called the upper bound.
\[P (errors > 0) = 1 - P (errors = 0) \leq J\alpha\]
This inequality is true whether two contrast are heavily dependent (as in the height example above) or only slightly, or not at all. For instance, if you have two contrasts in your output (the intercept and the slope), the probability of at least one Type I error equals 0.0975, but only if we assume these two contrasts are independent. In contrasts, if the two contrasts are dependent , we can use the Bonferroni inequality to know that the probability of at least one Type I error is less than or equal to \(0.05 \times 2 = 0.10\) . Thus, if there is dependency we know that the probability of at least one Type I error is at the most 0.10 (it could be less bad).
Note that if \(J\alpha > 1\) , then the upper bound is set equal to 1.
This Bonferroni upperbound can help us to take control over the overall probability of making Type I errors. Here we make a distinction between the test-wise Type I error rate, \(\alpha_{TW}\) , and the family-wise Type I error rate, \(\alpha_{FW}\) . Here, \(\alpha_{TW}\) is the probability of a Type I error used for one individual hypothesis test, and \(\alpha_{FW}\) is the probability of at least one Type I error among all tests performed. If we have a series of null-hypothesis tests, and if we want to have an overall probability of at most 5% (i.e., \(\alpha_{FW}=0.05\) ), then we should set the level for any individual test \(\alpha_{TW}\) at \(\alpha_{FW}/J\) . Then we know that the probability of at least one error is 5% or less.
Note that what is true here for null-hypothesis testing is also true for the calculation of confidence intervals. Also note that we should only look at output for which we have research questions. Below we see an example of how to apply these principles.
We use the ChickWeight data available in R. It is a data set on the weight of chicks over time, where the chicks are categorised into four different groups, each on a different feeding regime. Suppose we do a study on diet in chicks with one control group and three experimental groups. For each of these three experimental groups, we want to estimate the difference with the control condition (hence there are three research questions). We perform a regression analysis with dummy coding with the control condition (Diet 3) as the reference group to obtain these three contrasts. For the calculation of the confidence intervals, we want to have a family-wise Type I error rate of 0.05. That means that we need to have a test-wise Type I error rate of 0.05/3 = 0.0167. We therefore need to compute \(100-1.67 = 98.33\) % confidence intervals and we do null-hypothesis testing where we reject the null-hypothesis if \(p < 0.0167\) . The R code would look something like the following:
In the output we see the three contrasts that we need to answer our research questions. We can then report:
"We estimated the difference between each of the three experimental diet with the control diet. In order to control the family-wise Type I error rate and keep it below 5%, we used Bonferroni correction and chose a test-wise significance level of 0.0167 and computed 98.3% confidence intervals. The chicks on Diet 1 had a significantly lower weight than the chicks in the control conditions (Diet 3, \(b = -40.3\) , \(SE = 7.87\) , \(t(574) = -5.12\) , \(p < .001\) , 98.33% CI: -59.2, -21.4). The chicks on Diet 2 also had a lower weight than chicks on Diet 3, although the null-hypothesis could not be rejected ( \(b = -20.3\) , \(SE = 8.95\) , \(t(574) = -2.27\) , \(p = .024\) , 98.33% CI: -41.8, 1.15). The chicks on Diet 4 also had a weight not significantly different from chicks on Diet 3, ( \(b = -7.69\) , \(SE = 8.99\) , \(t(574) = -0.855\) , \(p = .039\) , 98.33% CI: -29.3, 13.9). "
Note that we do not report on the Intercept. Since we had no research question about the average weight of chicks on Diet 3, we ignore those results in the regression table, and divide the desired family-wise error rate by 3 (and not 4).
As we said, there are two kinds of research questions: a priori questions and post hoc questions. A priori questions are questions posed before the data collection. Often they are the whole reason why data were collected in the first place. Post hoc questions are questions posed during data analysis. When analysing data, some findings may strike you and they inspire you to do some more analyses. In order to explain the difference, let’s think of two different scenarios for analysing the ChickWeight data.
In Scenario 1, researchers are interested in the relationship between the diet and the weight of chicks. They see that in different farms, chicks show different mean sizes, and they are also on different diets. The researchers suspect that the weight differences are induced by the different diets, but they are not sure, because there are also many other differences between the farms (differences in climate, chicken breed and daily regime). In order to control for these other differences, the researchers pick one specific farm, they use one particular breed of chicken, and assign the chicks randomly to one of four diets. They reason that if they find differences between the four groups regarding weight, then diet is the factor responsible for those differences. Thus, their research question is: “Are there any differences in mean weight as a function of diet?” They run an ANOVA and find the following results:
They answer their (a priori) research question in the following way.
"We tested the null-hypothesis of equal mean weights in the four diet groups at an alpha of 5%, and found that it could be rejected, \(F(3, 574), p < .001\) . We conclude that diet does have an influence on the mean weight in chicks."
When analysing the data more closely, they also look at the mean weights per group.
They are struck by the relatively small difference in mean weight between Diet 4 and Diet 3. They are surprised because they know that one of them contains a lot more protein than the other. They are therefore curious to see whether the difference between Diet 4 and Diet 3 is actually significant. Moreover, they are very keen on finding out which Diets are different from each other, and which Diets are not different from each other. They decide to perform 6 additional \(t\) -tests: one for every possible pair of diets.
In this Scenario I, we see two kinds of research questions: the initial a priori question was whether Diet affects weight, and they answer this question with one F -test. The second question only arose during the analysis of the data. They look at the means, see some kind of pattern and want to know whether all means are different from each other. This follow-up question that arises during data analysis is a post hoc question.
Let’s look at Scenario II. A group of researchers wonder whether they can get chicks to grow more quickly using alternative diets. There is one diet, Diet 3, that is used in most farms across the world. They browse through the scientific literature and find three alternative diets. These alternative diets each have a special ingredient that makes the researchers suspect that they might lead to weight gain in chicks. The objective of their research is to estimate the differences between these three alternative diets and the standard diet, Diet 3. They use one farm and one breed of chicken and assign chicks randomly to one of the four diets. They perform the regression analysis with the dummy coding and reference group Diet 3 as shown above, and find that the differences between the three experimental diets and the standard diet are all negative: they show slower growth rather than faster growth. They report the estimates, the standard errors and the confidence intervals.
In general we can say that a priori questions can be answered with regular alphas and confidence intervals. For instance, if you state that your Type I error rate \(\alpha\) is set at 0.05, then you can use this \(\alpha = 0.05\) also for all the individual tests that you perform confidence intervals that you calculate. However, for post hoc questions, where your questions are guided by the results that you see, you should correct the test-wise error rate \(\alpha_{TW}\) in such a way that you control the family-wise error rate \(\alpha_{FW}\) .
Returning to the two scenarios, let’s look at the question whether Diet 4 differs from Diet 3. In Scenario I, this is a post hoc question, where in total you have 6 post hoc questions. You should therefore do the hypothesis test with an alpha of 0.05/6 = 0.0083, and/or compute a 99.17% confidence interval. In contrast, in Scenario II, the same question about Diets 4 and 3 is an a priori question, and can therefore be answered with an \(\alpha=5\) % and/or a 95% confidence interval.
Summarising, for post hoc questions you adjust your test-wise type I error rate, whereas you do not for a priori questions. The reason for this different treatment has to do with the dependency in contrasts that we talked about earlier. It also has to do with the fact that you only have a limited number of model degrees of freedom. In the example of the ChickWeight data, we have four groups, hence we can estimate only four contrasts. In the regression analysis with dummy coding, we see one contrast for the intercept and then three contrasts between the experimental groups and the reference group. Also if we use Helmert contrasts, we will only obtain four estimates in the output. This has to do with the dependency between contrasts: if you know that group A has a mean of 5, group B differs from group A by +2 and group C differs from group A by +3, you don’t need to estimate the difference between B and C any more, because you know that based on these numbers, the difference can only be +1. In other words, the contrast C to B totally depends on the contrast A versus B and A versus C. The next section discusses the dependency problem in more detail.
11.2 Independent (orthogonal) contrasts
Whether two contrasts are dependent is easily determined. Suppose we have \(J\) independent samples (groups), each containing values from a population of normally distributed values (assumption of normality). Each group is assumed to come from a population with the same variance \(\sigma^2_e\) (assumption of equal variance). For the moment also assume that the \(J\) groups have an equal sample size \(n\) . Any group \(j\) will have a mean \(\bar{Y}_j\) . Now imagine two contrasts among these means. The first contrast, \(L1\) , has the weights \(c_{1j}\) , and the second contrasts, \(L2\) , has the weights \(c_{2j}\) . Then we know that contrasts \(L1\) and \(L2\) are independent if
\[\sum_{j=1}^J c_{1j}c_{2j}=0\]
Thus, if you have \(J\) independent samples (groups), each of size \(n\) , one can decide if two contrasts are dependent by checking if the products of the weights sum to zero:
\[c_{11}c_{21} + c_{12}c_{22} + \dots + c_{1J}c_{2J} = 0\]
Another word for independent is orthogonal . Two contrasts are said to be orthogonal if the two contrasts are independent. Let’s look at some examples for a situation of four groups: one set of dependent contrasts and a set of orthogonal contrasts. For the first example, we look at default dummy coding. For contrast \(L1\) , we estimate the mean of group 1. Hence
\[L1 = \begin{bmatrix} 1 & 0 & 0 & 0 \end{bmatrix}\]
Let contrast \(L2\) be the contrast between group 2 and group 1:
\[L2 = \begin{bmatrix} -1 & 1 & 0 & 0 \end{bmatrix}\]
If we calculate the products of the weights, we get:
\[\sum_{j} c_{1j}c_{2j} = 1\times -1 + 0 \times 1 + 0 \times 0 + 0\times 0 = -1\] So we see that when we use dummy coding, the contrasts are not independent (not orthogonal).
For the second example, we look at Helmert contrasts. Helmert contrasts are independent (orthogonal). The Helmert contrast matrix for four groups looks like
\[L = \begin{bmatrix} \frac{1}{4} & \frac{1}{4} & \frac{1}{4} & \frac{1}{4} \\ -1 & 1 & 0 & 0 \\ -\frac{1}{2} & -\frac{1}{2} & 1 & 0 \\ -\frac{1}{3} & -\frac{1}{3} & -\frac{1}{3} & 1 \end{bmatrix}\]
For the first two contrasts, we see that the product of the weights equals zero:
\[\sum_{j} c_{1j}c_{2j} = \frac{1}{4} \times -1 + \frac{1}{4} \times 1 + \frac{1}{4} \times 0 + \frac{1}{4} \times 0 = 0\] Check for yourself and find that all four Helmert contrasts are independent of each other.
11.3 The number of independent contrasts is limited
Earlier we saw that there is only so much information you can gain from a data set. Once you have certain information, asking further questions leads to answers that depend on the answers already available.
This dependency has a bearing on the number of orthogonal comparisons that can be made with \(J\) group means. Given \(J\) independent sample means, there can be, apart from the grand mean, no more than \(J-1\) comparisons, without them being dependent on each other. This means that if you have \(J\) completely independent contrasts for \(J\) group means, it is impossible to find one more comparison which is also orthogonal to the first \(J\) ones.
This implies that if you ask more questions (i.e., ask for more contrasts) you should tread carefully. If you ask more questions, the answers to your questions will not be independent of each other (you are to some extent asking the same thing twice).
As an example, earlier we saw that if you know that group B differs from group A by +2 and group C differs from group A by -3, you don’t need to estimate the difference between B and C any more, because you know that based on these numbers, the difference can only be 5. In other words, the contrast C to B totally depends on the contrasts A versus B and A versus C. You can also see this in the contrast matrix for groups A, B and C below:
\[L = \begin{bmatrix} 1 & 0 & 0 \\ -1 & 1 & 0 \\ -1 & 0 & 1 \\ 0 & -1 & 1 \end{bmatrix} \]
The last contrast is dependent both on the third and the second contrast. Contrast \(L4\) can be calculated as \(L3 - L2\) by doing the calculation element-wise:
\[ \begin{bmatrix} -1 & 0 & 1 \end{bmatrix} - \begin{bmatrix} -1 & 1 & 0 \end{bmatrix} = \begin{bmatrix} 0 & -1 & 1 \end{bmatrix} \]
In other words, \(L4\) is a linear combination (weighted sum) of \(L2\) and \(L3\) : \(L4 = 1\times L3 - 1 \times L2\) . Statistically therefore, contrast \(L4\) is completely redundant given the contrasts \(L2\) and \(L3\) : it doesn’t provide any extra information.
It should however be clear that if you have a research question that can be answered with contrast \(L4\) , it is perfectly valid to make this contrast. However, you should realise that the number of independent research questions is limited. It is a wise idea to limit the number of research questions to the number of contrasts you can make: apart from the grand mean, you should make no more than \(J-1\) comparisons (your regression table should have no more than \(J\) parameters).
These contrasts that you specify belong to the a priori research question. Good research has a limited number of precisely worded research questions that should be answerable by a limited number of contrasts, usually 2 or 3, sometimes only 1. These can be answered by using the regular significance level. In social and behavioural sciences, oftentimes \(\alpha\) for each individual test or confidence interval equals 5%. However, the follow-up questions that arise only after the initial data analysis (computing means, plotting the data, etc.), should however be corrected to control the overall Type I error rate.
11.4 Fishing expeditions
Research and data analysis can sometimes be viewed as a fishing expedition. Imagine you fish the high seas for herring. Given your experience and what colleagues tell you (you browse the scientific literature, so to speak), you choose a specific location where you expect a lot of herring. By choosing this location, you maximise the probability of finding herring. This is analogous to the setting up of a data collection scheme where you maximise the probability of finding a statistically significant effect, or you maximise the precision of your estimates; in other words, you maximise statistical power (see Chapter 5 ). However, while fishing in that particular spot for herring, irrespective of whether you actually find herring, you find a lot of other fish and seafood. This is all coincidence, as you never planned to find these kinds of fish and seafood in your nets. The fact that you find a crab in your nets, might seem very interesting, but it should never be reported as if you were looking for that crab. You would have equally regarded it interesting if you had found a lobster, or a seahorse, or a baseball hat. You have to realise that it is pure random sampling error: you hang out your nets, and just pick up what’s there by chance. In research it works the same way: if you do a lot of statistical tests, or compute a large number of confidence intervals, you’re bound to find something that seems interesting, but is actually merely random noise due to sampling error. If the family-wise error rate is large, say 60%, then you cannot tell your family and friends ashore that the base-ball hat you found is very fascinating. Similarly, in research you have to control the number of Type I errors by adjusting the test-wise error rate in such a way that the family-wise error rate is low.
11.5 Several ways to define your post hoc questions
One question that often arises when we find that a categorical variable has an effect in an ANOVA, is to ask where this overall significant effect is coming from. For instance, we find that the four diets result in different mean weights in the chicks. This was demonstrated with an \(F\) -test at an \(\alpha\) of 5%. A follow-up question might then be, what diets are different from each other. You might then set up contrasts for all \({4 \choose 2} = 6\) possible pairs of the four diets.
Alternatively, you may specify your post hoc questions as simple or more complex contrasts in the same way as for your a priori questions, but now with no limit on how many. For instance, you may ask what alternative diets are significantly different from the standard diet (Diet 3). The number of comparisons is then limited to 3. Additionally, you might ask whether the alternative diets combined (grand mean of diets 1, 2 and 4) are significantly different from Diet 3.
Be aware, however, that the more comparisons you make, the more severe the correction must be to control the family-wise Type I error rate.
The analyses for the questions that you answer by setting up the entire data collection, and that are thus planned before the data collection (a priori), can be called confirmatory analyses. You would like to confirm the workings of an intervention, or you want to precisely estimate the size of a certain effect. Preferably, the questions that you have are statistically independent of each other, that is, the contrasts that you compute should preferably be orthogonal (independent).
In contrast, the analyses that you do for questions that arise while analysing the data (post hoc) are called exploratory analyses. You explore the data for any interesting patterns. Usually, while exploring data, a couple of questions are not statistically independent. Any interesting findings in these exploratory analyses could then be followed up by confirmatory analyses using a new data collection scheme, purposely set up to confirm the earlier findings. It is important to do that with a new or different sample, since the finding could have resulted from mere sampling error (i.e., a Type I error).
Also be aware of the immoral practice of \(p\) -hacking. \(P\) -hacking, sometimes referred to as selective reporting , is defining your research questions and setting up your analysis (contrasts) in such a way that you have as many significant results as possible. With p -hacking one presents their research in such a way that they find all these interesting results, ignoring the fact that they made a selection of the results based on what they saw in the data (post-hoc). For instance, their research was set up to find evidence for the workings of medicine A on the alleviation of migraine. Their study included a questionnaire on all sorts of other complaints and daily activities, for the sake of completeness. When analysing the results, they might find that the contrast between medicine A with placebo is not significant for migraine. But when exploring the data further, they find that medicine A was significantly better with regards to bloodpressure and the number of walks in the park. A \(p\) -hacker would write up the research as a study of the workings of medicine A on bloodpressure and walks in the park. This form of \(p\) -hacking is called cherry-picking : only reporting statistically significant findings and pretending you never set out to find the other things and not reporting them. Another \(p\) -hacking example would be to make a clever selection of the migraine data after which the effect becomes significant, for instance by filtering out the males in the study. Thus, \(p\) -hacking is the practice of trying to select the data or choose the method of analysis in such a way that the \(p\) -values in the report are as small as possible. The research questions are then changed from exploratory to confirmatory, without informing the reader.
11.6 Controlling the family-wise Type I error rate
There are several strategies that control the number of Type I errors. One is the Bonferroni method , where we adjust the test-wise error rate by dividing the family-wise error rate by the number of comparisons, \(\alpha_{TW} = \alpha_{FW} / J\) . This method is pretty conservative, in that the \(\alpha_{TW}\) becomes low with already a couple of comparisons, so that the statistical power to spot differences that also exist in the population becomes very low. The upside is that this method is easy to understand and perform. Alternative ways of addressing the problem are Scheffé’s procedure , and the Tukey HSD method. Of these two, Scheffé’s procedure is also relatively conservative (i.e., little statistical power). The logic of the Tukey HSD method is based on the probability that a difference between two group means is more than a critical value, by chance alone. This critical value is called Honestly Significant Difference (HSD) . We fix the probability of finding such a difference (or more) between the group means under the null-hypothesis at \(\alpha_{FW}\) . The details of the actual method will not be discussed here. Interested readers may refer to Wikipedia and references therein.
11.7 Post-hoc analysis in R
In general, post hoc contrasts can be done in the same way as in the previous chapter: specifying the contrasts in an \(\mathbf{L}\) matrix, taking the inverse and assigning the matrix to the variable in your model. Here, you are therefore limited to the number of levels of a factor: you can only have \(J-1\) new variables, apart from the intercept of 1s. You can then adjust \(\alpha\) yourself using Bonferroni. For instance if you want to have a family-wise type I error rate of 0.05, and you look at two post-hoc contrasts, you can declare a contrast significant if the corresponding \(p\) -value is less than 0.025.
There are also other options in R to get post hoc contrasts, where you can ask for as many comparisons as you want.
There are two ways in which you can control the overall Type I error rate: either by using an adjusted \(\alpha\) yourself (as above), or adjusting the \(p\) -value. For now we assume you generally want to test at an \(\alpha\) of 5%. But of course this can be any value.
In the first approach, you run the model and the contrast just as you would normally do. If the output contains answers to post hoc questions, you do not use \(\alpha = 0.05\) , but you use 0.05 divided by the number of tests that you inspect: \(\alpha_{TW}= \alpha_{FW}/k\) , with \(k\) being the number of tests you do.
For instance, if the output for a linear model with a factor with four levels contains the comparison on groups 1 and 2, and it applies to an a priori question, you simply report the statistics and concludes significance if the \(p < .05\) .
If the contrast pertains to a post hoc question and you compare all six possible pairs, you report the usual statistics and conclude significance if the \(p < \frac{0.05}{6}\) .
In the second approach, you can change the \(p\) -value itself: you multiply the plotted value by the number of comparisons and declare a difference to be significant if the corresponding adjusted \(p\) -value is less than 0.05. As an example, suppose you make six comparisons. Then you multiply the usual \(p\) -values by a factor 6: \(p_{adj}= 6p\) . Thus, if you see a \(p\) -value of 0.04, you compute \(p_{adj}\) to be 0.24 and conclude that the contrast is not significant. This is often done in R: the output yields \(adjusted\) \(p\) -values. Care should be taken with the confidence intervals: make sure that you know whether these are adjusted 95% confidence intervals or not. If not, then you should compute your own. Note that when you use the adjusted \(p\) -values, you should no longer adjust the \(\alpha\) . Thus, an adjusted \(p\) -value of 0.24 is not significant, because \(p_{adj}>.05\) .
In this section we will see how to perform post hoc comparisons in two situations: either with only one factor in your model, or when you have two factors in your model.
11.7.1 ANOVA with only one factor
We give an example of an ANOVA post hoc analysis with only one factor, using the data from the four diets. We first run an ANOVA to answer the primary research question whether diet has any effect on weight gain in chicks.
Seeing these results, noting that there is indeed a significant effect of diet, a secondary question pops up: “Which pairs of two diets show significant differences?” We answer that by doing a post hoc analysis, where we study each pair of diets, and control Type I error rate using the Bonferroni method. We can do that in the following way:
In the output we see six Bonferroni adjusted \(p\) -values for all six possible pairs. The column and row numbers refer to the levels of the Diet factor: Diet 3, Diet 1 and Diet 2 in the three columns, and Diet 1, Diet 2 and Diet 4 in the three rows. We see that all \(p\) -values are non significant ( \(p > .05\) ), except for two comparisons: the difference between Diet 3 and Diet 1 is significant, \(p < .001\) . as well as the difference between Diet 4 and Diet 1, \(p < .001\) .
"An analysis of variance showed that the mean weight was signficantly different for the four diets, \(F(3, 574) = 10.8, p < .001\) . We performed post hoc pair-wise comparisons, for all six possible pairs of diets. A family-wise Type I error rate of 0.05 was used, with Bonferroni correction. The difference between Diet 1 and Diet 3 was significant, and the difference between Diets 4 and 1 was significant. All other differences were not signficantly different from 0. "
11.7.2 ANOVA with two factors and moderation
In the previous subsection we did pair-wise comparisons in a one-way ANOVA (i.e., ANOVA with only one factor). In the previous chapter we also discussed how to set up contrasts in the situation of two factors that are modelled with interaction effects (Ch. 10 ). Let’s return to that example.
In the example, we were only interested in the gender effect for each of the education levels. That means only the last three lines are relevant.
In the simple_slopes() code we used the argument ci.width = 0.95 . That was because we hadn’t discussed post hoc analysis yet, nor adjustment of \(p\) or \(\alpha\) . In the case that we want to control the Type I error rate, we could use Bonferroni correction. We should then make the relevant \(p\) -values three times bigger than what they are uncorrected, because we are interested in three contrasts.
Confidence intervals should also be changed. For that we need to adjust the Type I error rate \(\alpha\) .
In the output we see adjusted confidence intervals (note that the \(p\) -values are the original ones). We conclude for our three contrasts that in the “school” group the females score 0.31 (adjusted 95% CI: -0.31, 0.94) higher than boys, in the “college” group females score 0.24 (adjusted 95% CI: -0.39, 0.86) higher than boys, and in the “university” group 0.89 (adjusted 95% CI: -1.49, -0.28) lower than the boys.
The same logic of adjusting \(p\) -values and adjusting confidence intervals can be applied in situations with numeric independent variables.
11.8 Take-away points
Your main research questions are generally very limited in number. If they can be translated into contrasts, we call these a priori contrasts.
Your a priori contrasts can be answered using a pre-set level of significance, in the social and behavioural sciences this is often 5% for \(p\) -values and using 95% for confidence intervals. No adjustment necessary.
This pre-set level of significance, \(\alpha\) , should be set before looking at the data (if possible before the collection of the data).
If you are looking at the data and want to answer specific research questions that arise because of what you see in the data (post hoc), you should use adjusted \(p\) -values and confidence intervals.
There are several ways of adjusting the test-wise \(\alpha\) s to obtain a reasonable family-wise \(\alpha\) : Bonferroni is the simplest method but rather conservative (low statistical power). Many alternative methods exist, among them are Scheffé’s procedure, and Tukey HSD method.
Key concepts
- Orthogonality/independence
- \(p\) -hacking
- Family-wise Type I error rate
- Test-wise Type I error rate
- Bonferroni correction
Introduction to Statistics and Data Analysis
18 apriori and post-hoc comparisons.
This chapter is about how to test hypotheses on data from ANOVA designs that are more specific than the omnibus test which just tests if the means are significantly different from each other. Examples include comparing just two of the means, or comparing one mean (e.g. a control condition) to all of the other means.
The main issue here is familywise error , discussed in the last chapter , which is the fact that the probability of making one or more Type I errors increases with the number of hypothesis tests you make. For example, if you run 10 independent hypothesis tests on your results, each with an alpha value of 0.05, the probability of getting at least one false positive would be:
\[1-(1-0.05)^{10} = 0.401\]
This number, 0.4013, is called the ‘familywise error rate’ or FW and is clearly unacceptably high. The methods described in this tutorial cover the various ways to control, or correct for, familywise error.
Specific hypothesis tests on ANOVA data fall into two categories, ‘A Priori’ and ‘post-hoc’.
A Priori tests are hypothesis tests that you planned on running before you started your experiment. Since there are many possible tests we could make, setting aside a list of just a few specific A Priori tests lets us correct for a much lower familywise error rate.
Post-hoc tests are hypothesis tests that you run after looking at your data. For example, you might want to go back and see if there is a significant difference between the highest and lowest means. Under the null hypothesis, the probability of rejecting a test on the most extreme difference between means will be much greater than \(\alpha\) . Or, perhaps we want to go crazy and compare all possible pairs of means.
18.1 One-Factor ANOVA Example:
We’ll go through A Priori and post-hoc tests with an example. Suppose you want to study the effect of background noise on test score. You randomly select 10 subjects for each of 5 conditions and have them take a standardized reading comprehension test with the following background noise: silence, white noise, rock music, classical music, and voices.
Throughout this chapter we’ll be referencing this same set of data. You can access it yourself at:
http://courses.washington.edu/psy524a/datasets/AprioriPostHocExample.csv
Your experiment generates the following statistics:
The results of the ANOVA are:
Here’s a plot of the means with error bars as the standard error of the mean:

18.2 A Priori Comparisons
An A Priori test is a hypothesis test that you had planned to make before you conducted the experiment.
18.2.1 t-test for two means
The simplest A Priori tests is a comparison between two means. In our example, suppose before we ran the experiment we had the prior hypothesis that there is a difference in mean test score between the silence and the voices conditions. This leads to comparing the means \(\overline{X}_{1} = 77.11\) and \(\overline{X}_{5} = 48.37\)
You’d think that we would simply conduct an independent measures two-tailed t-test using these two group means and variances, while ignoring all of the other conditions. But since we have \(MS_{within}\) , we should use this value since it’s a better estimate of the population variance than the pooled variance from just two groups (assuming homogeneity of variance).
The old t-statistic was (since we have equal sample size, n):
\[t=\frac{\overline{X}_{1}-\overline{X}_{5}}{\sqrt{\frac{s_{1}^2+s_{5}^2}{n}}} = \frac{77.11 - 48.37}{\sqrt{\frac{25.3^2 + 15^2}{10}}} = 3.091 \]
With \(df = (n-1)+(n-1) = 2n-2 = 18\) .
Instead, since we have mean-squared error within ( \(MS_w\) ), which is a better estimate of our population variance than \(s_{1}^2\) and \(s_{5}^2\) , we’ll use:
\[t= \frac{\overline{X}_{1}-\overline{X}_{5}}{\sqrt{\frac{2MS_{w}}{n}}}\]
The degrees of freedom is now N-k, since this is the df for \(SS_{within}\) .
For our example:
\[t= \frac{77.11 - 48.37}{\sqrt{\frac{(2)340.8}{10}}} = 3.4813\]
With df =N-k = 50 - 5 = 45, the p-value of t is 0.0011.
Since we planned on making this comparison ahead of time, and this is our only A Priori comparison, we can use this test to reject \(H_{0}\) and say that there is a difference in the mean test score between the silence and the voices conditions.
As an exercise, make a planned comparison t-test between the rock music and classical music conditions. You should get a t-value of 0.2919 and a p-value of 0.7717.
18.2.2 ‘Contrast’ for two means
Another way of thinking about the comparison we just made between the means from the silence and the voices conditions is to consider the numerator of our t-test as a ‘linear combination’ of means. A linear combination is simply a sum of weighted values. For this comparison, we assign a weight of 1 for the silence condition and a weight of -1 for the voices condition. All other means get zero weights. We use a lower case ‘psi’ ( \(\psi\) ) to indicate this weighted sum of means, with weights \(a_{i}\) for each mean, \(\bar{X}_{i}\) . For this example:
\[\psi = (1)(77.11) + (-1)(48.37) = 28.7400\]
The hypothesis test for contrasts can be done as either a t or an F test since when \(df_{bet}\) =1 F = \(t^{2}\) . We’ll use the F test in this chapter. The numerator of the F test is calculated with the following sums of squared error:
\[SS_{contrast} = \frac{{\psi}^2}{\sum{{(a_i^2/n_i)}}}\]
For equal sample sizes, like this example, this simplifies to:
\[SS_{contrast} = \frac{{\psi}^2}{(\sum{{a_i^2})/n}}\]
Which for our example is:
\[SS_{contrast} =\frac{28.74^2}{(1^2+(-1)^2)/10} = 4129.94\]
The mean squared error is always the sum of squared error divided by the degrees of freedom. The df for A Priori contrasts is always 1, so the numerator of the F test will be:
\[MS_{contrast} = \frac{SS_{contrast}}{1} = SS_{contrast}\]
The denominator of the F test for A Priori contrasts is the same denominator as for the omnibus F, or \(MS_{w}\) . So our F value is:
\[F(1,df_{within}) = \frac{MS_{contrast}}{MS_{within}}\]
\[F(1,45) = \frac{4129.94}{340.78} = 12.12\]
The p-value for this value of F is 0.0011, which is the same p-value as for the t-test above. That’s because our F statistic is equal to \(t^{2}\) ( \(12.12 = 3.48^{2}\) ).
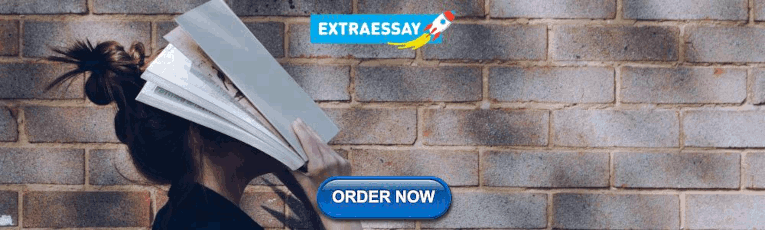
18.2.3 Contrast for groups of means
Contrasts also allow us to compare groups of means with other groups of means. In our example, suppose we have the prior hypothesis that music in general has a different effect on test scores than white noise. That is, we want to compare the average of the two music conditions (rock and classical) with the white noise condition.
Our weights will be 1 for the white noise condition, and -.5 for the rock and -.5 for the classical conditions, and zero for the remaining conditions. The corresponding linear combination of means is:
\[\psi = (1)(80.45) + (-0.5)(60.56) + (-0.5)(58.15) = 21.0950\]
You should convince yourself that this value, \(\psi\) , is the difference between the white noise condition and the average of the two music conditions. It should have an expected value of zero for the null hypothesis. If the sum of all the weights add up to zero then the expected value of the contrast will be zero under the null hypothesis.
The mean squared error for this contrast is:
\[SS_{contrast} = \frac{{\psi}^2}{(\sum{{a_i^2})/n}} = \frac{21.095^2}{\frac{(1)^2 +(-0.5)^2+(-0.5)^2}{10}} = 2966.66\]
As always for contrasts, \(df_{contrast} = 1\) , so \(MS_{contrast} = \frac{SS_{contrast}}{df_{contrast}} = SS_{contrast}\)
so our F statistic is:
\[F(1,45) = \frac{2966.66}{340.78} = 8.71\]
The p-value for this value of F is 0.005
18.2.3.1 Orthogonal contrasts and independence
We have now made two contrasts. We just compared the effects of white noise to the average of the effects of rock and classical music on test score. Before that we compared the silence and voices conditions. You should appreciate that these two contrasts are independent simply because they don’t share any groups in common.
Contrasts can be independent even if they share groups. Formally, two contrasts are independent if the sum of the products of their weights (the ‘dot product’) add up to zero. When this happens, the two contrasts are called orthogonal . In our example:
c1 = [1, 0, 0, 0, -1]
and our new contrast is
c2 = [0, 1, -1/2, -1/2, 0]
The sum of their products is 0:
(1)(0) + (0)(1) + (0)(-.5) + (0)(-.5) + (-1)(0) = 0
Another contrast that is orthogonal to the second one is:
c3 = [0, 0, 1, -1, 0]
This is because (0)(0) + (1)(0) + (-.5)(1) + (.5)(1) + (0)(0) = 0
What does this contrast test? It compares the test scores for the rock and classical music conditions. Notice that this contrast is also orthogonal to c1, the first contrast.
It turns out that there are exactly as many mutually orthogonal contrasts as there are degrees of freedom for the numerator of the omnibus (k-1). So there should be 4 orthogonal contrasts for our example (though this is not a unique set of 4 orthogonal contrasts). This leaves one more contrast. Can you think of it?
The answer is:
c4 = [1/2 -1/3 -1/3 -1/3 1/2]
Show that c4 is orthogonal to the other three. What is it comparing? It’s the mean of the silence and voices conditions compared to the mean of the other three conditions (white noise, rock and classical). We probably wouldn’t have had an A Priori hypothesis about this particular contrast.
Notice that since each contrast has one degree of freedom, the sum of degrees freedom across all possible contrasts is equal to the degrees of freedom of the omnibus. Likewise, it turns out that the sums of the \(SS_{contrast}\) across all orthogonal contrasts adds up to the \(SS_{bet}\) .
If two contrasts are orthogonal, then the two tests are ‘independent’. If two tests are independent, then the probability of rejecting one test does not depend of the probability of rejecting the other. An example of two contrasts that are not independent is comparing silence to white noise for the first contrast, and silence to rock music for the second contrast. You should see that if we happen to sample an extreme mean for the silence , then there will a high probability that both of the contrasts will be statistically significant. Even though both have a Type I error rate of \(\alpha\) , there is will be a positive correlation between the probability of rejecting the two tests.
Testing orthogonal contrasts on the same data set is just like running completely separate experiments. Since orthogonal contrasts are independent, we can easily calculate the familywise error rate:
\[FW = 1-(1-\alpha)^{n}\]
where \(n\) is the number of orthogonal contrasts.
If a set of tests are not independent, the familywise error rate still increases with the number of tests, but in more complicated ways that will be dealt with in the post-hoc comparison section below.
18.3 Contrasts with R
R doesn’t have any libraries to conduct contrasts, but it’s not too difficult to do them ‘by hand’. I’ve supplied some code to do it for you. First, though, lets’ load in the data set that we’ve been working with and compute the ANOVA. Although it’s a bit lengthy, we’ve covered this in the chapter on ANOVA as sums of squares:
Now that we have all of our ANOVA results stored in variables, we’re ready to compute contrasts. The coefficients for the four contrasts will be stored in a 4x5 matrix (4 rows by 5 columns since there are four contrasts and 5 ‘background noise’ levels):
We’re now ready to do the math to compute \(\psi\) , \(SS_{contrast}\) and the corresponding f-statistics and p-values. I’m not expecting you to be able to program this yourself - but it’s yours to have and will work on any set of contrasts that you define yourself. If your curious, the %*% means ‘element by element’ multiplication (like ’.*’ in Matlab if that helps) which is used to caluclate \(\psi\) .
The resulting table contrast.result looks like this:
18.3.1 Breaking down \(df_{between}\) with \(SS_{contrast}\)
For an ANOVA with \(k\) groups there will be \(k-1\) independent contrasts. These contrasts are not unique - there can be multiple sets of \(k-1\) orthogonal contrasts. But for any set, it turns out that \(SS_{between}\) is the sum the \(k-1\) \(SS_{contrast}\) values:
\[4129.94+2966.66+29.0405+159.578 = 7285.22 = SS_{between}\]
The intuition behind this is that the 4 contrasts are breaking down the total amount of variability between the means ( \(SS_{between}\) ) into separate independent components, each producing their own hypothesis test.
18.4 Controlling for familywise error rates
The most common way to control for familywise error is to simply lower the alpha value when making multiple independent comparisons. This comes at the expense of lowering the power for each individual test because, remember, decreasing alpha decreases power .
There is a variety and growing number of correction techniques, we’ll cover just a few here.
18.4.1 Bonferroni correction
Bonferroni correction is the easiest, oldest, and most common way to correct for familywise errors. All you do is lower alpha by dividing it by the number of comparisons. For example, if you want to make 4 comparisons and want a familywise error rate to be below 0.05, you simply test each comparison with an alpha value of 0.05/4 = 0.0125.
As you can see from our calculations, if you conduct an F-test on each of these contrasts, the corresponding p-values for these are: 0.0011, 0.005, 0.7717, and 0.4973. If we were to make all four A Priori comparisons, we’d need to adjust alpha to be 0.05/4 = 0.0125.
We’d therefore reject the null hypothesis for contrasts 1 and 2 but not for contrasts 3 and 4.
18.4.2 Šidák correction
Some software packages correct for familywise error using something called the Šidák correction. The result is almost exactly the same as the Bonferroni correction, but it’s worth mentioning here so you know what it means when you see a button for it in software packages like SPSS.
Remember, the familywise error rate is the probability of making one or more false positives. The Bonferroni correction is essentially assuming that the familywise error rate grows in proportion to the number of comparisons so we scale alpha down accordingly. But we know that the family wise error rate is \(1-(1-\alpha)^m\) , where m is the number of comparisons.
For example, for a Bonferroni correction with \(\alpha\) = .05 and 4 comparisons, we need to reduce the alpha value for each comparison to 0.05/4 = 0.0125. The familywise error rate is now
\[1-(1-0.0125)^4 = 0.049\]
It’s close to 0.05 but not exact. To bring the FW error rate up to 0.05 we need to use:
\[\alpha' = 1-(1-\alpha)^{1/m}\]
With 4 comparisons and \(\alpha\) = 0.05, the corrected alpha is:
\[\alpha' = 1-(1-0.05)^{1/4} = 0.01274\]
So instead of using an alpha of 0.0125 you’d use 0.01274. The difference between the Šidák correction and the Bonferroni correction is minimal but technically the Šidák correction sets the probability of getting one or more one false alarms to exactly alpha for independent tests.
18.4.2.1 Familywise error vs. ‘False Discovery Rate’
Familywise error is the probability of making one or more Type I errors. The Bonferroni and Sidac methods deal with familywise error by using a lower value of \(\alpha\) for each test so that the familywise error rate is \(\alpha\) . For example, a Bonferroni correction for a genetic test checks for 100 diseases with \(\alpha = 0.05\) , you need a p-value of less than \(\frac{0.05}{100} = 0.00050\) to reject any individual test. We’d be failing to detect a lot of diseases this way.
Statisticians have argued that this is way too conservative, especially when dealing with fields like genetics where there can be hundreds of statistical tests in a single experiment. While the Bonferroni and Šidák methods reduce the probability of one or more Type I errors to \(\alpha\) , the criterion is so stringent that if one or more type I errors are made, it’s almost always exactly one type I error that is made. In the genetic test example above, a simulation shows that using the Šidák correction, when one or more of the 100 tests are rejected, only 97.47 percent of the time exactly one test is rejected. That is, ‘one or more’ really means ‘just one’.
Instead, more recently statisticians are arguing that fixing the probability of correcting for familywise error is not the right goal. A better measure might be to correct for the overall proportion of Type I errors, which is called the ‘False Discovery Rate’, or FDR.
The trick to get the false discovery rate down to \(\alpha\) is to reject the ‘right’ set of hypothesis tests. Our best evidence of which are the right tests are to look at their p-values, and start with testing the most significant hypothesis tests. The next method, the Holm-Bonferroni procedure, does just that.
18.4.3 Holm-Bonferroni Multistage Procedure
The Holm-Bonferroni procedure is the simplest and most common of the FDR procedures. The procedure is best described by example. First, we rank-order our p-values from our multiple comparisons in from lowest to highest. From our four contrasts: 0.0011, 0.005, 0.4973, and 0.7717 for contrast numbers 1, 2, 4, and 3 respectively.
We start with the lowest p-value and compare it to the alpha that we’d use for the Bonferroni correction ( \(\frac{0.05}{4} = 0.0125\) ).
If our lowest p-value is less than this corrected alpha, then we reject the hypothesis for this contrast. If we fail to reject then we stop. For our example, p = 0.0011 is less than 0.0125, so we reject the corresponding contrast (number 1) and move on.
We then compare or next lowest p-value to a new corrected alpha. This time we divide alpha by 4-1=3 to get \(\frac{0.05}{3} = 0.0167\) , a less conservative value. If we reject this contrast, the we move on to the next p-value and the next corrected alpha \(\frac{0.05}{2}=0.025\) ).
This continues until we fail to reject a comparison, and then we stop. There’s not a clean package to do this in R, but here’s how you can do it on your own:
This isn’t a programming class, so I don’t expect you to fully understand the code. But it will work for your own set of contrasts. If you’re interested, however, see if you can follow how it works. It uses a while loop, which continues while the condition i<=m && !failed.yet is true. m is the number of contrasts, and i is an index that starts at 1 and increments after each rejected test. Each time through,the contrasts, ranked by their p-values, are compared to alpha/(m-i+1) which starts out at alpha/m for the first contrast, alpha/(m-1) for the second, etc.
The variable failed.yet is a logical (TRUE/FALSE) that starts out as FALSE and turns to true after the first contrast fails to reject. So !failed.yet starts out as true, allowing the while loop to continue until the first fail to reject, or until we run out of contrasts.
Multistage procedures like the Holm-Bonferroni are less conservative and therefore more powerful than the standard Bonferroni correction. They are less widely used probably because they’re more complicated. But as you’ve seen, computers can easily do these things for us.
There are other variants of this sort of multistage procedure, including sorting from highest to lowest p-values, and using a Sidac correction for each test instead of a Bonferroni correction. They all produce similar results and the field has not settled on one procedure in particular.
18.5 Post Hoc Comparisons
Now let’s get more exploratory and make some comparisons that we didn’t plan on making in the first place. These are called post hoc comparisons. For A Priori comparisons, we only needed to adjust for the FW rate associated with the number of planned comparisons. For post hoc comparisons, we need to adjust to not just the comparisons we feel like making, but for all possible comparisons of that type (e.g all possible pairwise comparisons or all possible contrasts).
18.5.1 The Tukey Test
The Tukey Test is a way of correcting for familywise error when testing all pairwise means. You can’t use a Bonferroni correction because not all comparisons are independent.
The test is based on the distribution that is expected when you specifically compare the largest and smallest mean. If the null hypothesis is true, the probability of a significant t-test for these most extreme means will be quite a bit greater than \(\alpha\) , which is the probability of rejecting any random pair of means.
The way for correcting for this inflated false positive rate when comparing the most extreme means is to use a statistic called the Studentized Range Statistic and goes by the letter q .
The statistic q is calculated as follows:
\[q = \frac{\bar{X}_l-\bar{X}_s}{\sqrt{\frac{MS_{within}}{n}}}\]
Where \(\overline{X}_{l}\) is the largest mean, \(\overline{X}_{s}\) is the smallest mean, and n is the sample size of each group (assuming that all sample sizes are equal). You’ll notice that the q statistic looks a lot like the t-statistic for a groups in an ANOVA:
\[t= \frac{\bar{X}_1-\bar{X}_2}{\sqrt{\frac{2MS_{w}}{n}}}\]
(For some annoying reason, the q statistic does not have the extra ‘2’ in the square root of the denominator so it’s not the same as t )
The q-statistic for our most extreme pairs of means is:
\[q = \frac{80.45 - 48.37} {\sqrt{\frac{340.7788}{10}}} = 5.4954\]
The q statistic has its own distribution. Like the t-statistic, it is broader than the normal distribution for small degrees of freedom. Also, q increases in width with increasing number of groups. That’s because as the number of possible comparisons increases, the difference between the extreme means increases.
R has the functions ptukey and qtukey to test the statistical significance of this difference of extreme means. ptukey needs our value of q , the number of groups, and \(df_{within}\) . For our pair of extreme means:
Importantly, even though we selected these two means after running the experiment, this p-value accounts for this bias.
In fact, almost magically, we can use this procedure to compare any or all pairs of means, and we won’t have to do any further correction for multiple comparisons.
18.5.2 Tukey’s ‘HSD’
Back in the chapter on effect sizes and confidence intervals we discussed how an alternative way to make a decision about \(H_{0}\) is to see if the null hypothesis is covered by the confidence interval. The same thing is often done with the Tukey Test.
Instead of finding a p-value from our q-statistic with ptukey , we’ll go the other way and find the value \(q_{crit}\) that covers the middle 95of the q distribution using qtukey . Since it’s a two-tailed test, we need to find q for the top 97.5%
We can go through the same logic that we did when we derived the equation for the confidence interval. If we assume that the observed difference between a pair of means, \(\overline{X}_i-\overline{X}_j\) is the true difference between the means, then we expect that in future experiments, we can expect to find a difference 95% of the time within:
\[\overline{X}_i-\overline{X}_j \pm q_{crit}\sqrt{\frac{MS_{w}}{n}}\] For our example:
\[ q_{crit}\sqrt{\frac{MS_{w}}{n}} = 4.0184\sqrt{\frac{340.7788}{10}} = 23.4579\]
This value, 23.4579 is called Tukeys’ honestly significant difference , or HSD . _Any pair of means that differs by more than this amount can be considered statistically significant at the level of \(\alpha\) .
The ‘H’ in Tukey’s HSD is for ‘honestly’ presumably because it takes into account all possible pairwise comparisons of means, so we’re being honest and accounting for familywise error.
We can use Tukey’s HSD to calculate a confidence interval by adding and subtracting it from the observed difference between means. Our most extreme means had a difference of
\[80.45 - 48.37 = 32.08\]
The 95% confidence interval for the difference between these means is:
\[(32.08 - 23.4579, 32.08 + 23.4579)\]
\[( 8.6221, 55.5379)\]
The lowest end of this interval is greater than zero, which is consistent with the fact that the p-value for the difference between means (0.0029) is less than \(\alpha\) = 0.05. If the difference between the means is significantly significant (with \(\alpha\) = 0.05), then the 95% confidence interval will never contain zero.
All this is easy to do in R using the tukeyHSD function. There’s just a little hack. It’s an old function which requires the output of an outdated ANOVA function called aov . But you can, instead, send in to tukeyHSD the output of lm after changing it’s class to aov . Here’s how you run the Tukey test on our example. We’ll run the lm function on our data, but instead of passing it into anova like we did before, we’ll change it’s class to ‘aov’ and pass it into TukeyHSD :
Here you’ll see p-values for all possible \(\frac{5 \times 4}{2} = 10\) comparisons. Can you find the row for the biggest difference between means?
You’ll also see the columns ‘lwr’ and ‘upr’. These are the ranges for the 95% confidence interval on the differences between the means. For all cases, if the p-value ‘p adj’ is less than .05, then the 95% confidence interval will not include zero. Is the confidence interval the same as our calculation? It’s off by a \(\pm\) sign flip because tukeyHSD decided to run the test with the opposite order of means (smallest - largest). Otherwise it’s the same, and it doesn’t matter anyway because it’s a two-tailed test. You should notice that the range between ‘lwr’ and ‘upr’ is always the same value of Tukey’s HSD = 23.4579.
18.5.3 Tukey-Kramer Test - for unequal sample sizes
The sample sizes must be equal when using the Tukey test. If the sample sizes varies across groups (called an ‘unbalanced design’) there is a modification of the Tukey test called the ‘Tukey-Kramer method’ which uses a different HSD depending upon which means are being compared.
Specifically, to compare means from group i to group j, with sample sizes \(n_{i}\) and \(n_{j}\) and variances \(s_{i}^{2}\) and \(s_{j}^{2}\) , the q-statistic is:
\[ q = \frac{\bar{X}_i-\bar{X}_j}{\sqrt{\frac{ \frac{s_{i}^{2}}{n_{i}} + \frac{s_{j}^{2}}{n_{j}}}{2}}}\] And replace \(df_{within}\) with:
\[df_{ij}= \frac{ \left(\frac{s_{i}^{2}}{n_{i}} + \frac{s_{j}^{2}}{n_{j}}\right)^{2}} { \frac{\left(\frac{s_{i}^{2}}{n_{i}}\right)^{2}}{n_{i}-1} + \frac{\left(\frac{s_{j}^{2}}{n_{j}}\right)^{2}}{n_{j}-1} }\] It’s kind of ugly, but here’s some R code that makes all possible paired comparisons based on our summary table mydata.summary that we computed above:
Which produces a nice table. I’ve rendered the table using the kable package, and colored the significant comparisons red.
Even though we ran the Tukey-Kramer test on the same data set, which has equal sample sizes, the p-values aren’t the same as for the regular Tukey Test. Usually, but not always, the Tukey-Kramer test will be less powerful (have larger p-values) than the Tukey Test because it is only using the variance for two means at a time which has a lower \(df\) than for the regular Tukey Test.
18.6 Dunnett’s Test for comparing one mean to all others
This is a post-hoc test designed specifically for comparing all means to a single control condition. For our example, it makes sense to compare all of our conditions to the silence condition. Dunnett’s test is a special case because (1) these comparisons are not independent and (2) there are fewer comparisons to correct for than for the Tukey Test since we’re testing only a subset of all possible pairs of means.
Dunnett’s test relies on finding critical values from a distribution that is related to the t-distribution called the Dunnett’s t Statistic . It’s easy to run Dunnett’s test in R with the function DunnetTest which requires the ‘DescTools’ library.
Let’s jump straight to the test, since it’s not likely that you’ll ever need to calculate p-values by hand with this test.
Let’s let the the first condition, the “silence” condition, be the control condition, so we’ll compare the other four means to this one ( \(\overline{X}_1 = 77.11)\)
Here’s how it works:
The table itself sits in the output under the field having the name of the control condition as a ‘matrix’. It’s useful to turn it into a data frame. For our example, use this:
Here’s the table formatted so that the significant values are in red:
These p-values are all corrected for familywise error, so no further correction is needed.
In general, this test is more powerful (gives lower p-values) than the Tukey Test, since fewer comparisons are being made.
18.7 The Sheffe’ Test: correcting for all possible contrasts
The Sheffe’ test allows for post-hoc comparisons across all possible contrasts (including non-orthogonal contrasts), not just means. Since there are many more possible contrasts than pairs of means, the Sheffe’ test has to control for more possible comparisons and is therefore more conservative and less powerful.
Remember, the F-test for a contrast is conducted by first computing the weighted sum of means:
\[\psi = \sum{a_i\bar{X}_i}\]
The \(SS_{contrast}\) (and \(MS_{contrast}\) ):
\[SS_{contrast} = MS_{contrast} =\frac{{\psi}^2}{\sum{{a_i^2/n_i}}}\]
and then the F statistic:
\[F(1,df_{w}) = \frac{MS_{contrast}}{MS_{w}}\]
The Sheffe’ test adjusts for multiple comparisons by multiplying the critical value of F for the original ‘omnibus’ test by k-1 (the number of groups minus one). In our example, the critical value of F for the omnibus test can be calculated using R’s qf function:
Our adjusted critical value for F is (4)(2.5787) = 10.315. Any contrast with an F-statistic greater than this can be considered statistically significant.
Instead of a critical value of F, we can convert F = 10.315 into a modified value of \(\alpha\) . Using R’s pf function we get:
Any contrast with a p-value less than 0.0024 is considered statistically significant. This is about 1 in 400 contrasts.
Remember, our four contrasts had weights:
c1 = [1 0 0 0 -1]
c2 = [0 1 -1/2 -1/2 0]
c3 = [0 0 1 -1 0]
The F values for these four contrasts are 12.12, 8.71, 0.09, and 0.47, and the four corresponding p-values are 0.0011, 0.005, 0.7717, and 0.4973.
Comparing these F values to our adjusted critical value of F, or comparing the p-values to 0.0024, lets us reject the null hypothesis for contrasts 1 but not for contrasts 2, 3 and 4. How does this compare to the A Priori Bonferroni test we used to compare these contrasts?
With the Sheffe’ test, the door is wide open to test any post-hoc comparison we want. Looking at the bar graph, it looks like there is a difference between the average of the silence, and white noise conditions, and the average of the rock music, classical music, and voices conditions. This would be a contrast with weights:
[1/2, 1/2, -1/3, -1/3, -1/3]
If you work this out you get an F value of 18.7686. This exceeds the Sheffe’-corrected F-value of 10.315 so we can say that there is a significant difference between these groups of means.
It feels like cheating - that we’re making stuff up. And yes, post-hoc tests are things you made up after you look at the data. I think if feels wrong because we’re so concerned about replicability and preregistration. But with the proper correction for multiple comparisons, this is totally fine. In fact, I’ll bet that most of the major discoveries on science have been made after noticing something interesting in the data that wasn’t anticipated. I would even argue that sticking strictly to your preregistered hypotheses could slow down the progress of science.
18.8 Summary
These notes cover just a few of the many A Priori and post hoc methods for controlling for multiple comparisons. Statistics is a relatively new and developing field of mathematics, so the standards for these methods are in flux. Indeed, SPSS alone provides a confusing array of post-hoc methods and allows you to run many or all of them at once. It is clearly wrong to run a bunch of post-hoc comparisons and then pick the comparison that suits you. This sort of post hoc post hoc analysis can lead to a sort of meta-familywise error rate.
It’s also not OK to treat a post hoc comparison with an A Priori method. You can’t go back and say “Oh yeah, I meant to make that comparison” if it hadn’t crossed your mind.
In the end, all of these methods differ by relatively small amounts. If the significance of your comparisons depend on which specific A Priori or post hoc test you choose, then you’re probably taking the .05 or .01 criterion too seriously anyway.
Post hoc analysis: use and dangers in perspective
Affiliation.
- 1 Department of Medicine and Therapeutics, Western Infirmary, Glasgow, UK.
- PMID: 8934374
- DOI: 10.1097/00004872-199609002-00006
DANGERS AND ADVANTAGES OF POST HOC ANALYSIS: Post hoc analysis is of major importance in the generation of hypotheses. However, the hypothesis is created by the analysis and has not been proved by any "experiment'. In some circumstances the conclusion derived from a post hoc analysis is entirely appropriate. For example, it was the only method used by Crick and Watson for determining the structure of DNA. In other circumstances, however, the results will be misleading. NEED FOR CAUTION WITH INTERPRETATION: The results of a post hoc analysis should be viewed with considerable scepticism and, in advance of confirmation by other appropriately designed prospective studies, should not be regarded as definitive proof.
Publication types
- Analysis of Variance*
- Case-Control Studies*
- Meta-Analysis as Topic*
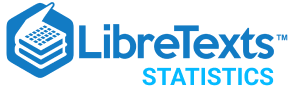
- school Campus Bookshelves
- menu_book Bookshelves
- perm_media Learning Objects
- login Login
- how_to_reg Request Instructor Account
- hub Instructor Commons
- Download Page (PDF)
- Download Full Book (PDF)
- Periodic Table
- Physics Constants
- Scientific Calculator
- Reference & Cite
- Tools expand_more
- Readability
selected template will load here
This action is not available.
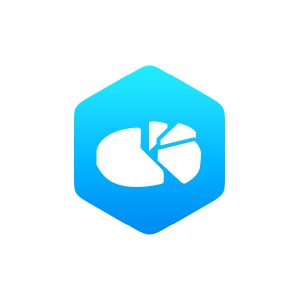
11.2: Pairwise Comparisons of Means (Post-Hoc Tests)
- Last updated
- Save as PDF
- Page ID 24072
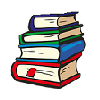
- Rachel Webb
- Portland State University
If you do in fact reject \(H_{0}\), then you know that at least two of the means are different. The ANOVA test does not tell which of those means are different, only that a difference exists. Most likely your sample means will be different from each other, but how different do they need to be for there to be a statistically significant difference?
To determine which means are significantly different, you need to conduct further tests. These post-hoc tests include the range test, multiple comparison tests, Duncan test, Student-Newman-Keuls test, Tukey test, Scheffé test, Dunnett test, Fisher’s least significant different test, and the Bonferroni test, to name a few. There are more options, and there is no consensus on which test to use. These tests are available in statistical software packages such as R, Minitab and SPSS.
One should never use two-sample \(t\)-tests from the previous chapter. This would inflate the type I error.
The probability of at least one type I error increases exponentially with the number of groups you are comparing. Let us assume that \(\alpha = 0.05\), then the probability that an observed difference between two groups that does not occur by chance is \(1 - \alpha = 0.95\). If two comparisons are made, the probability that the observed difference is true is no longer 0.95. The probability is \((1 - \alpha)^{2} = 0.9025\), and the P(Type I Error) = \(1 - 0.9025 = 0.0975\). Therefore, the P(Type I Error) occurs if \(m\) comparisons are made is \(1 - (1 - \alpha)m\).
For instance, if we are comparing the means of four groups: There would be \(m = {}_4 C_{2} = 6\) different ways to compare the 4 groups: groups (1,2), (1,3), (1,4), (2,3), (2,4), and (3,4). The P(Type I Error) = \(1 - (1 - \alpha)6 = 0.2649\). This is why a researcher should use ANOVA for comparing means, instead of independent \(t\)-tests.
There are many different methods to use. Many require special tables or software. We could actually just start with post-hoc tests, but they are a lot of work. If we run an ANOVA and we fail to reject the null hypothesis, then there is no need for further testing and it will save time if you were doing these steps by hand. Most statistical software packages give you the ANOVA table followed by the pairwise comparisons with just a change in the options menu. Keep in mind that Excel is not a statistical software and does not give pairwise comparisons.
We will use the Bonferroni Test, named after the mathematician Carlo Bonferroni. The Bonferroni Test uses the t-distribution table and is similar to previous t-tests that we have used, but adjusts \(\alpha\) to the number of comparisons being made.

The Bonferroni test is a statistical test for testing the difference between two population means (only done after an ANOVA test shows not all means are equal).
The formula for the Bonferroni test statistic is \(t = \dfrac{\bar{x}_{i} - \bar{x}_{j}}{\sqrt{\left( MSW \left(\frac{1}{n_{i}} + \frac{1}{n_{j}}\right) \right)}}\).
where \(\bar{x}_{i}\) and \(\bar{x}_{j}\) are the means of the samples being compared, \(n_{i}\) and \(n_{j}\) are the sample sizes, and \(MSW\) is the within-group variance from the ANOVA table.
The Bonferroni test critical value or p-value is found by using the t-distribution with within degrees of freedom \(df_{W} = N-k\), using an adjusted \(\frac{\alpha}{m}\) two-tail area under the t-distribution, where \(k\) = number of groups and \(m = {}_{k} C_{2}\), all the combinations of pairs out of \(k\) groups.
Critical Value Method
According to the ANOVA test that we previously performed, there does appear to be a difference in the average age of assistant professors \((\mu_{1})\), associate professors \((\mu_{2})\), and full professors \((\mu_{3})\) at this university.
.png?revision=1)
The hypotheses were:
\(H_{0}: \mu_{1} = \mu_{2} = \mu_{3}\)
\(H_{1}:\) At least one mean differs.
The decision was to reject \(H_{0}\), which means there is a significant difference in the mean age. The ANOVA test does not tell us, though, where the differences are. Determine which of the difference between each pair of means is significant. That is, test if \(\mu_{1} \neq \mu_{2}\), if \(\mu_{1} \neq \mu_{3}\), and if \(\mu_{2} \neq \mu_{3}\).
The alternative hypothesis for the ANOVA was “at least one mean is different.” There will be \({}_{3} C_{2} = 3\) subsequent hypothesis tests to compare all the combinations of pairs (Group 1 vs. Group 2, Group 1 vs. Group 3, and Group 2 vs. Group 3). Note that if you have 4 groups then you would have to do \({}_{4} C_{2} = 6\) comparisons, etc.
Use the t-distribution to find the critical value for the Bonferroni test. The total of all the individual sample sizes \(N = 21\) and \(k = 3\), and \(m = {}_{3} C_{2} = 3\), then the area for both tails would be \(\frac{\alpha}{m} = \frac{0.01}{m} = 0.003333\).
This is a two-tailed test so the area in one tail is \(\frac{0.003333}{2}\) with \(df_{W} = N-k = 21-3 = 18\) gives \(\text{C.V.} = \pm 3.3804\). The critical values are really far out in the tail so it is hard to see the shaded area. See Figure 11-3.
.png?revision=1)
Compare \(\mu_{1}\) and \(\mu_{2}\):
\(H_{0}: \mu_{1} = \mu_{2}\)
\(H_{1}: \mu_{1} \neq \mu_{2}\)
The test statistic is \(t = \frac{\bar{x}_{1} - \bar{x}_{2}}{\sqrt{\left( MSW \left(\frac{1}{n_{1}} + \frac{1}{n_{2}}\right) \right)}} = \frac{37 - 52}{\sqrt{\left(47.8889 \left(\frac{1}{7} + \frac{1}{7}\right) \right)}} = -4.0552\).
Compare the test statistic to the critical value. Since the test statistic \(-4.0552 < \text{critical value} = -3.3804\), we reject \(H_{0}\).
There is enough evidence to conclude that there is a difference in the average age of assistant and associate professors.
Compare \(\mu_{1}\) and \(\mu_{3}\):
\(H_{0}: \mu_{1} = \mu_{3}\)
\(H_{1}: \mu_{1} \neq \mu_{3}\)
The test statistic is \(t = \frac{\bar{x}_{1} - \bar{x}_{3}}{\sqrt{\left( MSW \left(\frac{1}{n_{1}} + \frac{1}{n_{3}}\right) \right)}} = \frac{37 - 54}{\sqrt{\left(47.8889 \left(\frac{1}{7} + \frac{1}{7}\right) \right)}} = -4.5958\).
Compare the test statistic to the critical value. Since the test statistic \(-4.5958 < \text{critical value} = -3.3804\), we reject \(H_{0}\).
Reject \(H_{0}\), since the test statistic is in the lower tail. There is enough evidence to conclude that there is a difference in the average age of assistant and full professors.
Compare \(\mu_{2}\) and \(\mu_{3}\):
\(H_{0}: \mu_{2} = \mu_{3}\)
\(H_{1}: \mu_{2} \neq \mu_{3}\)
The test statistic is \(t = \frac{\bar{x}_{2} - \bar{x}_{3}}{\sqrt{\left( MSW \left(\frac{1}{n_{2}} + \frac{1}{n_{3}}\right) \right)}} = \frac{52 - 54}{\sqrt{\left(47.8889 \left(\frac{1}{7} + \frac{1}{7}\right) \right)}} = -0.5407\)
Compare the test statistic to the critical value. Since the test statistic is between the critical values \(-3.3804 < -0.5407 < 3.3804\), we fail to reject \(H_{0}\).
Do not reject \(H_{0}\), since the test statistic is between the two critical values. There is enough evidence to conclude that there is not a difference in the average age of associate and full professors.
Note: you should get at least one group that has a reject \(H_{0}\), since you only do the Bonferroni test if you reject \(H_{0}\) for the ANOVA. Also, note that the transitive property does not apply. It could be that group 1 = group 2 and group 2 = group 3; this does not mean that group 1 = group 3.
P-Value Method
A research organization tested microwave ovens. At \(\alpha\) = 0.10, is there a significant difference in the average prices of the three types of oven?
.png?revision=1)
The ANOVA was run in Excel.
.png?revision=1)
To test if there is a significant difference in the average prices of the three types of oven, the hypotheses are:
Use the Excel output to find the p-value in the ANOVA table of 0.001019, which is less than \(\alpha\) so reject \(H_{0}\); there is at least one mean that is different in the average oven prices.
There is a statistically significant difference in the average prices of the three types of oven. Use the Bonferroni test p-value method to see where the differences are.
\(t = \frac{\bar{x}_{1} - \bar{x}_{2}}{\sqrt{\left( MSW \left(\frac{1}{n_{1}} + \frac{1}{n_{2}}\right) \right)}} = \frac{233.3333-203.125}{\sqrt{\left((1073.794 \left(\frac{1}{6} + \frac{1}{8}\right) \right)}} = 1.7070\)
To find the p-value, find the area in both tails and multiply this area by \(m\). The area to the right of \(t = 1.707\), using \(df_{W} = 19\), is 0.0520563. Remember these are always two-tail tests, so multiply this area by 2, to get both tail areas of 0.104113.
.png?revision=1)
Then multiply this area by \(m = {}_{3} C_{2} = 3\) to get a p-value = 0.3123.
.png?revision=1)
Since the p-value = \(0.3123 > \alpha = 0.10\), we do not reject \(H_{0}\). There is a statistically significant difference in the average price of the 1,000- and 900-watt ovens.
\(t = \frac{\bar{x}_{1} - \bar{x}_{3}}{\sqrt{\left( MSW \left(\frac{1}{n_{1}} + \frac{1}{n_{3}}\right) \right)}} = \frac{233.3333-155.625}{\sqrt{\left((1073.794 \left(\frac{1}{6} + \frac{1}{8}\right) \right)}} = 4.3910\)
Use \(df_{W}\) = 19 to find the p-value.
.png?revision=1)
Since the p-value = (tail areas)*3 = \(0.00094 < \alpha = 0.10\), we reject \(H_{0}\). There is a statistically significant difference in the average price of the 1,000- and 800-watt ovens.
\(t = \frac{\bar{x}_{2} - \bar{x}_{3}}{\sqrt{\left( MSW \left(\frac{1}{n_{2}} + \frac{1}{n_{3}}\right) \right)}} = \frac{203.125-155.625}{\sqrt{\left((1073.794 \left(\frac{1}{8} + \frac{1}{8}\right) \right)}} = 2.8991\)
Use \(df_{W} = 19\) to find the p-value (remember that these are always two-tail tests).
.png?revision=1)
Since the p-value = \(0.0276 < \alpha = 0.10\), we reject \(H_{0}\). There is a statistically significant difference in the average price of the 900- and 800-watt ovens.
There is a chance that after we multiply the area by the number of comparisons, the p-value would be greater than one. However, since the p-value is a probability we would cap the probability at one.
This is a lot of math! The calculators and Excel do not have post-hoc pairwise comparisons shortcuts, but we can use the statistical software called SPSS to get the following results. We will look specifically at interpreting the SPSS output for Example 11-4.
.png?revision=1)
The first table, labeled "Descriptives", gives descriptive statistics; the second table is the ANOVA table, and note that the p-value is in the column labeled Sig. The Multiple Comparisons table is where we want to look. There are repetitive pairs in the last table, just in a different order.
The first two rows in Figure 11-4 are comparing group 1 with groups 2 and 3. If we follow the first row across under the Sig. column, this gives the p-value = 0.312 for comparing the 1,000- and 900-watt ovens.
.png?revision=1)
The second row in Figure 11-4 compares the 1,000- and 800-watt ovens, p-value = 0.001.
.png?revision=1)
The third row in Figure 11-4 compares the 900- and 1000-watt ovens in the reverse order as the first row; note that the difference in the means is negative but the p-value is the same.
_(1).png?revision=1)
The fourth row in Figure 11-4 compares the 900- and 800-watt ovens, p-value = 0.028.
_(2).png?revision=1)
The last set of rows in Figure 11-4 are again repetitive and give the 800-watt oven compared to the 900- and 1000-watt ovens.
Keep in mind that post-hoc is defined as occurring after an event. A post-hoc test is done after an ANOVA test shows that there is a statistically significant difference. You should get at least one group that has a result of "reject \(H_{0}\)", since you only do the Bonferroni test if you reject \(H_{0}\) for the ANOVA.
Help | Advanced Search
Computer Science > Robotics
Title: temporal and semantic evaluation metrics for foundation models in post-hoc analysis of robotic sub-tasks.
Abstract: Recent works in Task and Motion Planning (TAMP) show that training control policies on language-supervised robot trajectories with quality labeled data markedly improves agent task success rates. However, the scarcity of such data presents a significant hurdle to extending these methods to general use cases. To address this concern, we present an automated framework to decompose trajectory data into temporally bounded and natural language-based descriptive sub-tasks by leveraging recent prompting strategies for Foundation Models (FMs) including both Large Language Models (LLMs) and Vision Language Models (VLMs). Our framework provides both time-based and language-based descriptions for lower-level sub-tasks that comprise full trajectories. To rigorously evaluate the quality of our automatic labeling framework, we contribute an algorithm SIMILARITY to produce two novel metrics, temporal similarity and semantic similarity. The metrics measure the temporal alignment and semantic fidelity of language descriptions between two sub-task decompositions, namely an FM sub-task decomposition prediction and a ground-truth sub-task decomposition. We present scores for temporal similarity and semantic similarity above 90%, compared to 30% of a randomized baseline, for multiple robotic environments, demonstrating the effectiveness of our proposed framework. Our results enable building diverse, large-scale, language-supervised datasets for improved robotic TAMP.
Submission history
Access paper:.
- HTML (experimental)
- Other Formats
References & Citations
- Google Scholar
- Semantic Scholar
BibTeX formatted citation

Bibliographic and Citation Tools
Code, data and media associated with this article, recommenders and search tools.
- Institution
arXivLabs: experimental projects with community collaborators
arXivLabs is a framework that allows collaborators to develop and share new arXiv features directly on our website.
Both individuals and organizations that work with arXivLabs have embraced and accepted our values of openness, community, excellence, and user data privacy. arXiv is committed to these values and only works with partners that adhere to them.
Have an idea for a project that will add value for arXiv's community? Learn more about arXivLabs .

An official website of the United States government
The .gov means it’s official. Federal government websites often end in .gov or .mil. Before sharing sensitive information, make sure you’re on a federal government site.
The site is secure. The https:// ensures that you are connecting to the official website and that any information you provide is encrypted and transmitted securely.
- Publications
- Account settings
Preview improvements coming to the PMC website in October 2024. Learn More or Try it out now .
- Advanced Search
- Journal List
- HHS Author Manuscripts

Amplifying the Noise: The Dangers of Post Hoc Power Analyses
Small sample sizes decrease statistical power, which is a study’s ability to detect a treatment effect when there is one to be detected. A power threshold of 80% is commonly used, indicating that statistical significance would be expected four of five times if the treatment effect is large enough to be clinically meaningful. This threshold may be difficult to achieve in surgical science, where practical limitations such as research budgets or rare conditions may make large sample sizes infeasible. Several researchers have used “post hoc” power calculations with observed effect sizes to demonstrate that studies are often underpowered and use this as evidence to advocate for lower power thresholds in surgical science. In this short commentary, we explain why post hoc power calculations are inappropriate and cannot differentiate between statistical noise and clinically meaningful effects. We use simulation analysis to demonstrate that lower power thresholds increase the risk of a false-positive result and suggest logical alternatives such as the use of larger p-values for hypothesis testing or qualitative research methods.
Bababekov et al . (2019) argue that commonly accepted guidelines for statistical power are inappropriate for surgical studies. 1 As evidence, the authors searched for randomized controlled trials and observational studies with human participants published in three top surgery journals from 2012 to 2016. They then conducted a post hoc power analysis, excluding studies which found significant effects or missing needed information. Not surprisingly, Bababekov et al . found these studies to be grossly underpowered. We believe the authors have mischaracterized the role of power analysis in study design. In this letter, we hope to correct the record and highlight some critical issues when relying on results from post hoc power analyses.
First, it is important to understand why a power analysis is conducted prospectively. Before a study begins, researchers should determine three pieces of information:
- The minimum effect size that could be considered clinically meaningful. For instance, a surgical intervention which reduces the likelihood of a hospital readmission within 30 dby 0.001% is not clinically meaningful regardless of statistical significance. 2
- The significance level that we will use (e.g., α = 0.10, 0.05, 0.01, and so on). This selection may be based on a variety of factors such as expected sample size, the number of statistical tests conducted, or what is common. The goal here is to minimize type I error: rejection of a true null hypothesis, also known as a “false positive” finding. 3
- The sample size required to reliably find the effect size significant at the selected significance level. Here we would like to minimize the probability of a type II error: failure to reject a false null hypothesis, also known as a ‘false negative’ finding. Naturally, we would like the power to equal 1.0 whenever the null hypothesis is false, but this is infeasible while keeping our significance level small. 4
The third step often involves a formal power analysis, where the researcher uses simulation analysis to estimate the required sample size. A power threshold of 80% is commonly used, indicating that if the minimum effect size is observed then our statistical test would find that effect significant (a “true positive”) four out of five times. We expect to fail to reject the null hypothesis (a false negative) the remaining one out of five times.
Bababekov et al . are correct when they note that the common power threshold of 80% is arbitrary. 1 This is not unlike the famous (or infamous) P -value threshold of 0.05, which was first proposed by Ronald Fisher in 1925 and has since become standard practice. 5 However, the authors made three fundamental errors when arguing to abandon the 80% power threshold.
First, encouraging more underpowered studies to proceed would simply increase the number of studies with nonsignificant findings. Alternatively, one could instead select a higher significance level (e.g., α = 0.10) when limited by small sample sizes. If sample sizes are sufficiently small, researchers could instead rely on descriptive statistics and qualitative comparisons without hypothesis testing (e.g., case reports). If the potential implications of the research on clinical practice are substantial and large sample sizes are infeasible, surgical journals could still consider these studies for publication.
Second, the authors’ arguments are tautological; if a study’s results are significant, then its findings are valuable, but if the study’s results are insignificant, then the study was simply underpowered and the findings are still valuable. Although it is true that clinically meaningful but statistically nonsignificant results may occur in underpowered studies, 6 the authors do not identify clinically meaningful thresholds to make this determination. Moreover, calculating post hoc power with observed effect sizes is simply a transformation of the P -value. The relationship between post hoc power and P -values is necessarily an inverse relationship. 4 This guarantees that calculating post hoc power with nonsignificant effect sizes will lead one to assert that the studies were “underpowered.”
Third, the authors misunderstand what statistical power refers to. It is a statement about the population being sampled. This is why statistical power is commonly calculated before conducting a study. Conducting a post hoc power calculation with observed effect sizes necessarily assumes that the effect size identified in the study is the true effect size in the population.
To illustrate the effect of these errors, we propose a stylized simulation. Let us assume the minimum clinically meaningful change in some surgical outcome X is 100 units. For simplicity, let us also assume there are three types of surgical interventions with varying effects on outcomes and these effects are measured with some error: those with clinically meaningful effects (μ = 100, σ = 20), those with less than clinically meaningful effects (μ = 40, σ = 20), and those having no effect (μ = 0, σ = 20). We simulated 100,000 interventions for each type and created density plots for their estimated effects (see Figure ). The area to the left of dashed line represents the rejection region at 80% power; 20% of studies with larger effects would fail to reject the null hypothesis, as would approximately 98.5% of studies with smaller effects and 99.994% of studies with no effect. If we calculate post hoc power for these insignificant studies, we will find approximately 38.6% power.

Density plots for the effects of simulated surgical interventions. Notes: The chart displays densities for the effects of simulated surgical interventions with big effects (μ = 100, σ = 20), small effects (μ = 100, σ = 20), or an ineffective intervention with noise-only (μ = 0, σ = 20). The dashed line represents the rejection region under 80% statistical power; observed effects to the right of this line are expected to be statistically significant.
When Bababekov et al . calculated post hoc power, their data included all three kinds of studies (excluding those with significant findings) and they found a median power of 16%. 1 This lack of statistical power is not unwanted, it is by design. We want to find clinically meaningful effects to be statistically significant, but not effects that are too small to be clinically meaningful or which are simply the result of noise in our effect estimates. Shifting the rejection region to the left (e.g., by accepting studies with higher P -values than 0.05) may result in more true positives and reduce type II error, but we would also expect to find more false positives and increase our type I error.
In conclusion, although we understand the challenges of small sample sizes in surgical science, we believe there are other more logical alternatives than abandonment of standard thresholds for prospective power analyses. These include selection of a higher significance level or omitting formal hypothesis testing. Analytical approaches under the latter option could rely instead on descriptive statistics or case studies. Researchers who calculate post hoc power analyses should be aware of the dangers in doing so; by “empowering the underpowered study”, they may simply be amplifying the noise.
Acknowledgments
Funding statement: This research did not receive any specific grant from funding agencies in the public, commercial, or not-for-profit sectors.
There are no conflicts of interest to disclose.
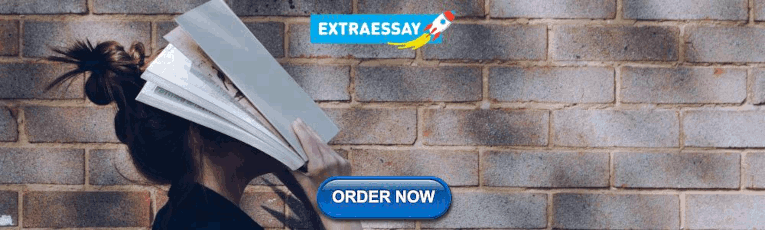
IMAGES
VIDEO
COMMENTS
Post hoc analysis. In a scientific study, post hoc analysis (from Latin post hoc, "after this") consists of statistical analyses that were specified after the data were seen. [1] [2] They are usually used to uncover specific differences between three or more group means when an analysis of variance (ANOVA) test is significant. [3]
This means we have sufficient evidence to reject the null hypothesis that all of the group means are equal. Next, we can use a post hoc test to find which group means are different from each other. We will walk through examples of the following post hoc tests: Tukey's Test - useful when you want to make every possible pairwise comparison
If the p-value from your ANOVA F-test or Welch's test is less than your significance level, you can reject the null hypothesis. Null: All group means are equal. Alternative: Not all group means are equal. ... As the number of comparisons increases, the post hoc analysis must lower the individual significance level even further. For our six ...
In research, there are different, overlapping ways in which the plan of analysis may be described. This article explains planned (prespecified) vs post hoc, primary vs secondary, hypothesis-driven vs exploratory, and subgroup and sensitivity analyses; intent-to-treat vs per-protocol vs completer analysis was explained in an earlier article in this column.
Tukey's Honest Significant Difference (HSD) is a very popular post hoc analysis. This analysis, like Bonferroni's, makes adjustments based on the number of comparisons, but it makes adjustments to the test statistic when running the comparisons of two groups. These comparisons give us an estimate of the difference between the groups and a ...
What do post-hoc tests tell you? Interpretation of multiple p-values becomes even trickier and this is the stage at which some researchers make use of post-hoc testing. If we test a null hypothesis that is in fact true, using the 0.05 significance level, there is a 0.95 probability of coming to the correct conclusion in accepting the null ...
Returning to the two scenarios, let's look at the question whether Diet 4 differs from Diet 3. In Scenario I, this is a post hoc question, where in total you have 6 post hoc questions. You should therefore do the hypothesis test with an alpha of 0.05/6 = 0.0083, and/or compute a 99.17% confidence interval.
This page titled 13.6: Post‐hoc Analysis - Tukey's Honestly Significant Difference (HSD) Test85 is shared under a CC BY-SA 4.0 license and was authored, remixed, and/or curated by Maurice A. Geraghty via source content that was edited to the style and standards of the LibreTexts platform; a detailed edit history is available upon request.
If we are able to reject the null hypothesis in the ANOVA F test, it tells us not all group means are equal. In other words, there are some differences among group means. ... Games-Howell test is the typical post hoc analysis. Like the analyses mentioned above, it makes appropriate adjustments based on the number of tests when comparing ...
This means we have sufficient evidence to reject the null hypothesis that all of the group means are equal. Next, we can use a post hoc test to find which group means are different from each other. We will walk through examples of the following post hoc tests: Tukey's Test - useful when you want to make every possible pairwise comparison
Tukey's Test. The purpose of Tukey's test is to figure out which groups in your sample differ. It uses the "Honest Significant Difference," a number that represents the distance between groups, to compare every mean with every other mean. Dunnett's correction. Like Tukey's this post hoc test is used to compare means.
Post-hoc tests are hypothesis tests that you run after looking at your data. For example, you might want to go back and see if there is a significant difference between the highest and lowest means. Under the null hypothesis, the probability of rejecting a test on the most extreme difference between means will be much greater than \(\alpha\) .
Post hoc power analysis identifies population-level parameters with sample-specific statistics and makes no conceptual sense. Analytically, such analysis can yield quite different power estimates that are difficult and can be misleading. To see this, consider again the problem to test the hypothesis in equation (1).
Answer: A post-hoc analysis involves looking at the data after a study has been concluded, and trying to find patterns that were not primary objectives of the study. In other words, all analyses that were not pre-planned and were conducted as 'additional' analyses after completing the experiment are considered to be post-hoc analyses. A post ...
DANGERS AND ADVANTAGES OF POST HOC ANALYSIS: Post hoc analysis is of major importance in the generation of hypotheses. However, the hypothesis is created by the analysis and has not been proved by any "experiment'. In some circumstances the conclusion derived from a post hoc analysis is entirely appropriate. For example, it was the only method ...
Writing up the post hoc test. Finally, having run the post hoc analysis to determine which groups are significantly different to one another, you might write up the result like this: Post hoc tests (using the Holm correction to adjust p) indicated that Joyzepam produced a significantly larger mood change than both Anxifree (p=.001) and the ...
Analysis of variance (ANOVA) tests the nonspecific null hypothesis that all three population means are equal. This nonspecific null hypothesis is sometimes called the omnibus null hypothesis. When the omnibus null hypothesis is rejected, the conclusion is that at least one population mean is different from at least one other mean. [ 2]
Thus, for post-hoc power analysis, we replace the alternative hypothesis in Equation 3 involving a known population effect size Δ with a set of candidate values for Δ with their candidacy described by the distribution N ... In contrast, the traditional hypothesis for post-hoc power analysis in Equation 7 treats ...
A priori theorizing is ignored, and virtually all theoretical analysis is developed post hoc to justify the hypotheses, which are presented as a priori in the introduction. The second type is known as suppressing loser hypotheses, in which disconfirmed hypotheses are dropped from the introduction.
The Bonferroni test is a statistical test for testing the difference between two population means (only done after an ANOVA test shows not all means are equal). The formula for the Bonferroni test statistic is t = ˉxi − ˉxj √(MSW(1 ni + 1 nj)). where ˉxi and ˉxj are the means of the samples being compared, ni and nj are the sample sizes ...
This is a crucial piece of information for the inferential conclusions of a post-hoc analysis because the result of a t-test is based on a comparison of the errors and, thus, how these are calculated plays a big role in the final result . ... If a hypothesis exists, comparing all sub-groups/sub-conditions with each other—as done with post-hoc ...
Recent works in Task and Motion Planning (TAMP) show that training control policies on language-supervised robot trajectories with quality labeled data markedly improves agent task success rates. However, the scarcity of such data presents a significant hurdle to extending these methods to general use cases. To address this concern, we present an automated framework to decompose trajectory ...
This is why statistical power is commonly calculated before conducting a study. Conducting a post hoc power calculation with observed effect sizes necessarily assumes that the effect size identified in the study is the true effect size in the population. To illustrate the effect of these errors, we propose a stylized simulation.