- Privacy Policy

Home » Quantitative Research – Methods, Types and Analysis
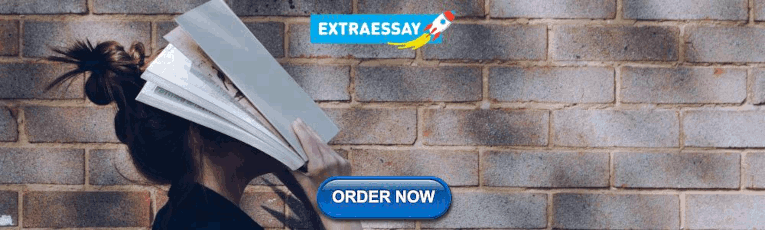
Quantitative Research – Methods, Types and Analysis
Table of Contents

Quantitative Research
Quantitative research is a type of research that collects and analyzes numerical data to test hypotheses and answer research questions . This research typically involves a large sample size and uses statistical analysis to make inferences about a population based on the data collected. It often involves the use of surveys, experiments, or other structured data collection methods to gather quantitative data.
Quantitative Research Methods

Quantitative Research Methods are as follows:
Descriptive Research Design
Descriptive research design is used to describe the characteristics of a population or phenomenon being studied. This research method is used to answer the questions of what, where, when, and how. Descriptive research designs use a variety of methods such as observation, case studies, and surveys to collect data. The data is then analyzed using statistical tools to identify patterns and relationships.
Correlational Research Design
Correlational research design is used to investigate the relationship between two or more variables. Researchers use correlational research to determine whether a relationship exists between variables and to what extent they are related. This research method involves collecting data from a sample and analyzing it using statistical tools such as correlation coefficients.
Quasi-experimental Research Design
Quasi-experimental research design is used to investigate cause-and-effect relationships between variables. This research method is similar to experimental research design, but it lacks full control over the independent variable. Researchers use quasi-experimental research designs when it is not feasible or ethical to manipulate the independent variable.
Experimental Research Design
Experimental research design is used to investigate cause-and-effect relationships between variables. This research method involves manipulating the independent variable and observing the effects on the dependent variable. Researchers use experimental research designs to test hypotheses and establish cause-and-effect relationships.
Survey Research
Survey research involves collecting data from a sample of individuals using a standardized questionnaire. This research method is used to gather information on attitudes, beliefs, and behaviors of individuals. Researchers use survey research to collect data quickly and efficiently from a large sample size. Survey research can be conducted through various methods such as online, phone, mail, or in-person interviews.
Quantitative Research Analysis Methods
Here are some commonly used quantitative research analysis methods:
Statistical Analysis
Statistical analysis is the most common quantitative research analysis method. It involves using statistical tools and techniques to analyze the numerical data collected during the research process. Statistical analysis can be used to identify patterns, trends, and relationships between variables, and to test hypotheses and theories.
Regression Analysis
Regression analysis is a statistical technique used to analyze the relationship between one dependent variable and one or more independent variables. Researchers use regression analysis to identify and quantify the impact of independent variables on the dependent variable.
Factor Analysis
Factor analysis is a statistical technique used to identify underlying factors that explain the correlations among a set of variables. Researchers use factor analysis to reduce a large number of variables to a smaller set of factors that capture the most important information.
Structural Equation Modeling
Structural equation modeling is a statistical technique used to test complex relationships between variables. It involves specifying a model that includes both observed and unobserved variables, and then using statistical methods to test the fit of the model to the data.
Time Series Analysis
Time series analysis is a statistical technique used to analyze data that is collected over time. It involves identifying patterns and trends in the data, as well as any seasonal or cyclical variations.
Multilevel Modeling
Multilevel modeling is a statistical technique used to analyze data that is nested within multiple levels. For example, researchers might use multilevel modeling to analyze data that is collected from individuals who are nested within groups, such as students nested within schools.
Applications of Quantitative Research
Quantitative research has many applications across a wide range of fields. Here are some common examples:
- Market Research : Quantitative research is used extensively in market research to understand consumer behavior, preferences, and trends. Researchers use surveys, experiments, and other quantitative methods to collect data that can inform marketing strategies, product development, and pricing decisions.
- Health Research: Quantitative research is used in health research to study the effectiveness of medical treatments, identify risk factors for diseases, and track health outcomes over time. Researchers use statistical methods to analyze data from clinical trials, surveys, and other sources to inform medical practice and policy.
- Social Science Research: Quantitative research is used in social science research to study human behavior, attitudes, and social structures. Researchers use surveys, experiments, and other quantitative methods to collect data that can inform social policies, educational programs, and community interventions.
- Education Research: Quantitative research is used in education research to study the effectiveness of teaching methods, assess student learning outcomes, and identify factors that influence student success. Researchers use experimental and quasi-experimental designs, as well as surveys and other quantitative methods, to collect and analyze data.
- Environmental Research: Quantitative research is used in environmental research to study the impact of human activities on the environment, assess the effectiveness of conservation strategies, and identify ways to reduce environmental risks. Researchers use statistical methods to analyze data from field studies, experiments, and other sources.
Characteristics of Quantitative Research
Here are some key characteristics of quantitative research:
- Numerical data : Quantitative research involves collecting numerical data through standardized methods such as surveys, experiments, and observational studies. This data is analyzed using statistical methods to identify patterns and relationships.
- Large sample size: Quantitative research often involves collecting data from a large sample of individuals or groups in order to increase the reliability and generalizability of the findings.
- Objective approach: Quantitative research aims to be objective and impartial in its approach, focusing on the collection and analysis of data rather than personal beliefs, opinions, or experiences.
- Control over variables: Quantitative research often involves manipulating variables to test hypotheses and establish cause-and-effect relationships. Researchers aim to control for extraneous variables that may impact the results.
- Replicable : Quantitative research aims to be replicable, meaning that other researchers should be able to conduct similar studies and obtain similar results using the same methods.
- Statistical analysis: Quantitative research involves using statistical tools and techniques to analyze the numerical data collected during the research process. Statistical analysis allows researchers to identify patterns, trends, and relationships between variables, and to test hypotheses and theories.
- Generalizability: Quantitative research aims to produce findings that can be generalized to larger populations beyond the specific sample studied. This is achieved through the use of random sampling methods and statistical inference.
Examples of Quantitative Research
Here are some examples of quantitative research in different fields:
- Market Research: A company conducts a survey of 1000 consumers to determine their brand awareness and preferences. The data is analyzed using statistical methods to identify trends and patterns that can inform marketing strategies.
- Health Research : A researcher conducts a randomized controlled trial to test the effectiveness of a new drug for treating a particular medical condition. The study involves collecting data from a large sample of patients and analyzing the results using statistical methods.
- Social Science Research : A sociologist conducts a survey of 500 people to study attitudes toward immigration in a particular country. The data is analyzed using statistical methods to identify factors that influence these attitudes.
- Education Research: A researcher conducts an experiment to compare the effectiveness of two different teaching methods for improving student learning outcomes. The study involves randomly assigning students to different groups and collecting data on their performance on standardized tests.
- Environmental Research : A team of researchers conduct a study to investigate the impact of climate change on the distribution and abundance of a particular species of plant or animal. The study involves collecting data on environmental factors and population sizes over time and analyzing the results using statistical methods.
- Psychology : A researcher conducts a survey of 500 college students to investigate the relationship between social media use and mental health. The data is analyzed using statistical methods to identify correlations and potential causal relationships.
- Political Science: A team of researchers conducts a study to investigate voter behavior during an election. They use survey methods to collect data on voting patterns, demographics, and political attitudes, and analyze the results using statistical methods.
How to Conduct Quantitative Research
Here is a general overview of how to conduct quantitative research:
- Develop a research question: The first step in conducting quantitative research is to develop a clear and specific research question. This question should be based on a gap in existing knowledge, and should be answerable using quantitative methods.
- Develop a research design: Once you have a research question, you will need to develop a research design. This involves deciding on the appropriate methods to collect data, such as surveys, experiments, or observational studies. You will also need to determine the appropriate sample size, data collection instruments, and data analysis techniques.
- Collect data: The next step is to collect data. This may involve administering surveys or questionnaires, conducting experiments, or gathering data from existing sources. It is important to use standardized methods to ensure that the data is reliable and valid.
- Analyze data : Once the data has been collected, it is time to analyze it. This involves using statistical methods to identify patterns, trends, and relationships between variables. Common statistical techniques include correlation analysis, regression analysis, and hypothesis testing.
- Interpret results: After analyzing the data, you will need to interpret the results. This involves identifying the key findings, determining their significance, and drawing conclusions based on the data.
- Communicate findings: Finally, you will need to communicate your findings. This may involve writing a research report, presenting at a conference, or publishing in a peer-reviewed journal. It is important to clearly communicate the research question, methods, results, and conclusions to ensure that others can understand and replicate your research.
When to use Quantitative Research
Here are some situations when quantitative research can be appropriate:
- To test a hypothesis: Quantitative research is often used to test a hypothesis or a theory. It involves collecting numerical data and using statistical analysis to determine if the data supports or refutes the hypothesis.
- To generalize findings: If you want to generalize the findings of your study to a larger population, quantitative research can be useful. This is because it allows you to collect numerical data from a representative sample of the population and use statistical analysis to make inferences about the population as a whole.
- To measure relationships between variables: If you want to measure the relationship between two or more variables, such as the relationship between age and income, or between education level and job satisfaction, quantitative research can be useful. It allows you to collect numerical data on both variables and use statistical analysis to determine the strength and direction of the relationship.
- To identify patterns or trends: Quantitative research can be useful for identifying patterns or trends in data. For example, you can use quantitative research to identify trends in consumer behavior or to identify patterns in stock market data.
- To quantify attitudes or opinions : If you want to measure attitudes or opinions on a particular topic, quantitative research can be useful. It allows you to collect numerical data using surveys or questionnaires and analyze the data using statistical methods to determine the prevalence of certain attitudes or opinions.
Purpose of Quantitative Research
The purpose of quantitative research is to systematically investigate and measure the relationships between variables or phenomena using numerical data and statistical analysis. The main objectives of quantitative research include:
- Description : To provide a detailed and accurate description of a particular phenomenon or population.
- Explanation : To explain the reasons for the occurrence of a particular phenomenon, such as identifying the factors that influence a behavior or attitude.
- Prediction : To predict future trends or behaviors based on past patterns and relationships between variables.
- Control : To identify the best strategies for controlling or influencing a particular outcome or behavior.
Quantitative research is used in many different fields, including social sciences, business, engineering, and health sciences. It can be used to investigate a wide range of phenomena, from human behavior and attitudes to physical and biological processes. The purpose of quantitative research is to provide reliable and valid data that can be used to inform decision-making and improve understanding of the world around us.
Advantages of Quantitative Research
There are several advantages of quantitative research, including:
- Objectivity : Quantitative research is based on objective data and statistical analysis, which reduces the potential for bias or subjectivity in the research process.
- Reproducibility : Because quantitative research involves standardized methods and measurements, it is more likely to be reproducible and reliable.
- Generalizability : Quantitative research allows for generalizations to be made about a population based on a representative sample, which can inform decision-making and policy development.
- Precision : Quantitative research allows for precise measurement and analysis of data, which can provide a more accurate understanding of phenomena and relationships between variables.
- Efficiency : Quantitative research can be conducted relatively quickly and efficiently, especially when compared to qualitative research, which may involve lengthy data collection and analysis.
- Large sample sizes : Quantitative research can accommodate large sample sizes, which can increase the representativeness and generalizability of the results.
Limitations of Quantitative Research
There are several limitations of quantitative research, including:
- Limited understanding of context: Quantitative research typically focuses on numerical data and statistical analysis, which may not provide a comprehensive understanding of the context or underlying factors that influence a phenomenon.
- Simplification of complex phenomena: Quantitative research often involves simplifying complex phenomena into measurable variables, which may not capture the full complexity of the phenomenon being studied.
- Potential for researcher bias: Although quantitative research aims to be objective, there is still the potential for researcher bias in areas such as sampling, data collection, and data analysis.
- Limited ability to explore new ideas: Quantitative research is often based on pre-determined research questions and hypotheses, which may limit the ability to explore new ideas or unexpected findings.
- Limited ability to capture subjective experiences : Quantitative research is typically focused on objective data and may not capture the subjective experiences of individuals or groups being studied.
- Ethical concerns : Quantitative research may raise ethical concerns, such as invasion of privacy or the potential for harm to participants.
About the author
Muhammad Hassan
Researcher, Academic Writer, Web developer
You may also like

Questionnaire – Definition, Types, and Examples

Case Study – Methods, Examples and Guide

Observational Research – Methods and Guide

Qualitative Research Methods

Explanatory Research – Types, Methods, Guide

Survey Research – Types, Methods, Examples
Have a language expert improve your writing
Run a free plagiarism check in 10 minutes, automatically generate references for free.
- Knowledge Base
- Methodology
- What Is Quantitative Research? | Definition & Methods
What Is Quantitative Research? | Definition & Methods
Published on 4 April 2022 by Pritha Bhandari . Revised on 10 October 2022.
Quantitative research is the process of collecting and analysing numerical data. It can be used to find patterns and averages, make predictions, test causal relationships, and generalise results to wider populations.
Quantitative research is the opposite of qualitative research , which involves collecting and analysing non-numerical data (e.g. text, video, or audio).
Quantitative research is widely used in the natural and social sciences: biology, chemistry, psychology, economics, sociology, marketing, etc.
- What is the demographic makeup of Singapore in 2020?
- How has the average temperature changed globally over the last century?
- Does environmental pollution affect the prevalence of honey bees?
- Does working from home increase productivity for people with long commutes?
Table of contents
Quantitative research methods, quantitative data analysis, advantages of quantitative research, disadvantages of quantitative research, frequently asked questions about quantitative research.
You can use quantitative research methods for descriptive, correlational or experimental research.
- In descriptive research , you simply seek an overall summary of your study variables.
- In correlational research , you investigate relationships between your study variables.
- In experimental research , you systematically examine whether there is a cause-and-effect relationship between variables.
Correlational and experimental research can both be used to formally test hypotheses , or predictions, using statistics. The results may be generalised to broader populations based on the sampling method used.
To collect quantitative data, you will often need to use operational definitions that translate abstract concepts (e.g., mood) into observable and quantifiable measures (e.g., self-ratings of feelings and energy levels).
Prevent plagiarism, run a free check.
Once data is collected, you may need to process it before it can be analysed. For example, survey and test data may need to be transformed from words to numbers. Then, you can use statistical analysis to answer your research questions .
Descriptive statistics will give you a summary of your data and include measures of averages and variability. You can also use graphs, scatter plots and frequency tables to visualise your data and check for any trends or outliers.
Using inferential statistics , you can make predictions or generalisations based on your data. You can test your hypothesis or use your sample data to estimate the population parameter .
You can also assess the reliability and validity of your data collection methods to indicate how consistently and accurately your methods actually measured what you wanted them to.
Quantitative research is often used to standardise data collection and generalise findings . Strengths of this approach include:
- Replication
Repeating the study is possible because of standardised data collection protocols and tangible definitions of abstract concepts.
- Direct comparisons of results
The study can be reproduced in other cultural settings, times or with different groups of participants. Results can be compared statistically.
- Large samples
Data from large samples can be processed and analysed using reliable and consistent procedures through quantitative data analysis.
- Hypothesis testing
Using formalised and established hypothesis testing procedures means that you have to carefully consider and report your research variables, predictions, data collection and testing methods before coming to a conclusion.
Despite the benefits of quantitative research, it is sometimes inadequate in explaining complex research topics. Its limitations include:
- Superficiality
Using precise and restrictive operational definitions may inadequately represent complex concepts. For example, the concept of mood may be represented with just a number in quantitative research, but explained with elaboration in qualitative research.
- Narrow focus
Predetermined variables and measurement procedures can mean that you ignore other relevant observations.
- Structural bias
Despite standardised procedures, structural biases can still affect quantitative research. Missing data , imprecise measurements or inappropriate sampling methods are biases that can lead to the wrong conclusions.
- Lack of context
Quantitative research often uses unnatural settings like laboratories or fails to consider historical and cultural contexts that may affect data collection and results.
Quantitative research deals with numbers and statistics, while qualitative research deals with words and meanings.
Quantitative methods allow you to test a hypothesis by systematically collecting and analysing data, while qualitative methods allow you to explore ideas and experiences in depth.
In mixed methods research , you use both qualitative and quantitative data collection and analysis methods to answer your research question .
Data collection is the systematic process by which observations or measurements are gathered in research. It is used in many different contexts by academics, governments, businesses, and other organisations.
Operationalisation means turning abstract conceptual ideas into measurable observations.
For example, the concept of social anxiety isn’t directly observable, but it can be operationally defined in terms of self-rating scores, behavioural avoidance of crowded places, or physical anxiety symptoms in social situations.
Before collecting data , it’s important to consider how you will operationalise the variables that you want to measure.
Reliability and validity are both about how well a method measures something:
- Reliability refers to the consistency of a measure (whether the results can be reproduced under the same conditions).
- Validity refers to the accuracy of a measure (whether the results really do represent what they are supposed to measure).
If you are doing experimental research , you also have to consider the internal and external validity of your experiment.
Hypothesis testing is a formal procedure for investigating our ideas about the world using statistics. It is used by scientists to test specific predictions, called hypotheses , by calculating how likely it is that a pattern or relationship between variables could have arisen by chance.
Cite this Scribbr article
If you want to cite this source, you can copy and paste the citation or click the ‘Cite this Scribbr article’ button to automatically add the citation to our free Reference Generator.
Bhandari, P. (2022, October 10). What Is Quantitative Research? | Definition & Methods. Scribbr. Retrieved 22 April 2024, from https://www.scribbr.co.uk/research-methods/introduction-to-quantitative-research/
Is this article helpful?
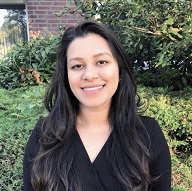
Pritha Bhandari
- USC Libraries
- Research Guides
Organizing Your Social Sciences Research Paper
- Quantitative Methods
- Purpose of Guide
- Design Flaws to Avoid
- Independent and Dependent Variables
- Glossary of Research Terms
- Reading Research Effectively
- Narrowing a Topic Idea
- Broadening a Topic Idea
- Extending the Timeliness of a Topic Idea
- Academic Writing Style
- Applying Critical Thinking
- Choosing a Title
- Making an Outline
- Paragraph Development
- Research Process Video Series
- Executive Summary
- The C.A.R.S. Model
- Background Information
- The Research Problem/Question
- Theoretical Framework
- Citation Tracking
- Content Alert Services
- Evaluating Sources
- Primary Sources
- Secondary Sources
- Tiertiary Sources
- Scholarly vs. Popular Publications
- Qualitative Methods
- Insiderness
- Using Non-Textual Elements
- Limitations of the Study
- Common Grammar Mistakes
- Writing Concisely
- Avoiding Plagiarism
- Footnotes or Endnotes?
- Further Readings
- Generative AI and Writing
- USC Libraries Tutorials and Other Guides
- Bibliography
Quantitative methods emphasize objective measurements and the statistical, mathematical, or numerical analysis of data collected through polls, questionnaires, and surveys, or by manipulating pre-existing statistical data using computational techniques . Quantitative research focuses on gathering numerical data and generalizing it across groups of people or to explain a particular phenomenon.
Babbie, Earl R. The Practice of Social Research . 12th ed. Belmont, CA: Wadsworth Cengage, 2010; Muijs, Daniel. Doing Quantitative Research in Education with SPSS . 2nd edition. London: SAGE Publications, 2010.
Need Help Locating Statistics?
Resources for locating data and statistics can be found here:
Statistics & Data Research Guide
Characteristics of Quantitative Research
Your goal in conducting quantitative research study is to determine the relationship between one thing [an independent variable] and another [a dependent or outcome variable] within a population. Quantitative research designs are either descriptive [subjects usually measured once] or experimental [subjects measured before and after a treatment]. A descriptive study establishes only associations between variables; an experimental study establishes causality.
Quantitative research deals in numbers, logic, and an objective stance. Quantitative research focuses on numeric and unchanging data and detailed, convergent reasoning rather than divergent reasoning [i.e., the generation of a variety of ideas about a research problem in a spontaneous, free-flowing manner].
Its main characteristics are :
- The data is usually gathered using structured research instruments.
- The results are based on larger sample sizes that are representative of the population.
- The research study can usually be replicated or repeated, given its high reliability.
- Researcher has a clearly defined research question to which objective answers are sought.
- All aspects of the study are carefully designed before data is collected.
- Data are in the form of numbers and statistics, often arranged in tables, charts, figures, or other non-textual forms.
- Project can be used to generalize concepts more widely, predict future results, or investigate causal relationships.
- Researcher uses tools, such as questionnaires or computer software, to collect numerical data.
The overarching aim of a quantitative research study is to classify features, count them, and construct statistical models in an attempt to explain what is observed.
Things to keep in mind when reporting the results of a study using quantitative methods :
- Explain the data collected and their statistical treatment as well as all relevant results in relation to the research problem you are investigating. Interpretation of results is not appropriate in this section.
- Report unanticipated events that occurred during your data collection. Explain how the actual analysis differs from the planned analysis. Explain your handling of missing data and why any missing data does not undermine the validity of your analysis.
- Explain the techniques you used to "clean" your data set.
- Choose a minimally sufficient statistical procedure ; provide a rationale for its use and a reference for it. Specify any computer programs used.
- Describe the assumptions for each procedure and the steps you took to ensure that they were not violated.
- When using inferential statistics , provide the descriptive statistics, confidence intervals, and sample sizes for each variable as well as the value of the test statistic, its direction, the degrees of freedom, and the significance level [report the actual p value].
- Avoid inferring causality , particularly in nonrandomized designs or without further experimentation.
- Use tables to provide exact values ; use figures to convey global effects. Keep figures small in size; include graphic representations of confidence intervals whenever possible.
- Always tell the reader what to look for in tables and figures .
NOTE: When using pre-existing statistical data gathered and made available by anyone other than yourself [e.g., government agency], you still must report on the methods that were used to gather the data and describe any missing data that exists and, if there is any, provide a clear explanation why the missing data does not undermine the validity of your final analysis.
Babbie, Earl R. The Practice of Social Research . 12th ed. Belmont, CA: Wadsworth Cengage, 2010; Brians, Craig Leonard et al. Empirical Political Analysis: Quantitative and Qualitative Research Methods . 8th ed. Boston, MA: Longman, 2011; McNabb, David E. Research Methods in Public Administration and Nonprofit Management: Quantitative and Qualitative Approaches . 2nd ed. Armonk, NY: M.E. Sharpe, 2008; Quantitative Research Methods. Writing@CSU. Colorado State University; Singh, Kultar. Quantitative Social Research Methods . Los Angeles, CA: Sage, 2007.
Basic Research Design for Quantitative Studies
Before designing a quantitative research study, you must decide whether it will be descriptive or experimental because this will dictate how you gather, analyze, and interpret the results. A descriptive study is governed by the following rules: subjects are generally measured once; the intention is to only establish associations between variables; and, the study may include a sample population of hundreds or thousands of subjects to ensure that a valid estimate of a generalized relationship between variables has been obtained. An experimental design includes subjects measured before and after a particular treatment, the sample population may be very small and purposefully chosen, and it is intended to establish causality between variables. Introduction The introduction to a quantitative study is usually written in the present tense and from the third person point of view. It covers the following information:
- Identifies the research problem -- as with any academic study, you must state clearly and concisely the research problem being investigated.
- Reviews the literature -- review scholarship on the topic, synthesizing key themes and, if necessary, noting studies that have used similar methods of inquiry and analysis. Note where key gaps exist and how your study helps to fill these gaps or clarifies existing knowledge.
- Describes the theoretical framework -- provide an outline of the theory or hypothesis underpinning your study. If necessary, define unfamiliar or complex terms, concepts, or ideas and provide the appropriate background information to place the research problem in proper context [e.g., historical, cultural, economic, etc.].
Methodology The methods section of a quantitative study should describe how each objective of your study will be achieved. Be sure to provide enough detail to enable the reader can make an informed assessment of the methods being used to obtain results associated with the research problem. The methods section should be presented in the past tense.
- Study population and sampling -- where did the data come from; how robust is it; note where gaps exist or what was excluded. Note the procedures used for their selection;
- Data collection – describe the tools and methods used to collect information and identify the variables being measured; describe the methods used to obtain the data; and, note if the data was pre-existing [i.e., government data] or you gathered it yourself. If you gathered it yourself, describe what type of instrument you used and why. Note that no data set is perfect--describe any limitations in methods of gathering data.
- Data analysis -- describe the procedures for processing and analyzing the data. If appropriate, describe the specific instruments of analysis used to study each research objective, including mathematical techniques and the type of computer software used to manipulate the data.
Results The finding of your study should be written objectively and in a succinct and precise format. In quantitative studies, it is common to use graphs, tables, charts, and other non-textual elements to help the reader understand the data. Make sure that non-textual elements do not stand in isolation from the text but are being used to supplement the overall description of the results and to help clarify key points being made. Further information about how to effectively present data using charts and graphs can be found here .
- Statistical analysis -- how did you analyze the data? What were the key findings from the data? The findings should be present in a logical, sequential order. Describe but do not interpret these trends or negative results; save that for the discussion section. The results should be presented in the past tense.
Discussion Discussions should be analytic, logical, and comprehensive. The discussion should meld together your findings in relation to those identified in the literature review, and placed within the context of the theoretical framework underpinning the study. The discussion should be presented in the present tense.
- Interpretation of results -- reiterate the research problem being investigated and compare and contrast the findings with the research questions underlying the study. Did they affirm predicted outcomes or did the data refute it?
- Description of trends, comparison of groups, or relationships among variables -- describe any trends that emerged from your analysis and explain all unanticipated and statistical insignificant findings.
- Discussion of implications – what is the meaning of your results? Highlight key findings based on the overall results and note findings that you believe are important. How have the results helped fill gaps in understanding the research problem?
- Limitations -- describe any limitations or unavoidable bias in your study and, if necessary, note why these limitations did not inhibit effective interpretation of the results.
Conclusion End your study by to summarizing the topic and provide a final comment and assessment of the study.
- Summary of findings – synthesize the answers to your research questions. Do not report any statistical data here; just provide a narrative summary of the key findings and describe what was learned that you did not know before conducting the study.
- Recommendations – if appropriate to the aim of the assignment, tie key findings with policy recommendations or actions to be taken in practice.
- Future research – note the need for future research linked to your study’s limitations or to any remaining gaps in the literature that were not addressed in your study.
Black, Thomas R. Doing Quantitative Research in the Social Sciences: An Integrated Approach to Research Design, Measurement and Statistics . London: Sage, 1999; Gay,L. R. and Peter Airasain. Educational Research: Competencies for Analysis and Applications . 7th edition. Upper Saddle River, NJ: Merril Prentice Hall, 2003; Hector, Anestine. An Overview of Quantitative Research in Composition and TESOL . Department of English, Indiana University of Pennsylvania; Hopkins, Will G. “Quantitative Research Design.” Sportscience 4, 1 (2000); "A Strategy for Writing Up Research Results. The Structure, Format, Content, and Style of a Journal-Style Scientific Paper." Department of Biology. Bates College; Nenty, H. Johnson. "Writing a Quantitative Research Thesis." International Journal of Educational Science 1 (2009): 19-32; Ouyang, Ronghua (John). Basic Inquiry of Quantitative Research . Kennesaw State University.
Strengths of Using Quantitative Methods
Quantitative researchers try to recognize and isolate specific variables contained within the study framework, seek correlation, relationships and causality, and attempt to control the environment in which the data is collected to avoid the risk of variables, other than the one being studied, accounting for the relationships identified.
Among the specific strengths of using quantitative methods to study social science research problems:
- Allows for a broader study, involving a greater number of subjects, and enhancing the generalization of the results;
- Allows for greater objectivity and accuracy of results. Generally, quantitative methods are designed to provide summaries of data that support generalizations about the phenomenon under study. In order to accomplish this, quantitative research usually involves few variables and many cases, and employs prescribed procedures to ensure validity and reliability;
- Applying well established standards means that the research can be replicated, and then analyzed and compared with similar studies;
- You can summarize vast sources of information and make comparisons across categories and over time; and,
- Personal bias can be avoided by keeping a 'distance' from participating subjects and using accepted computational techniques .
Babbie, Earl R. The Practice of Social Research . 12th ed. Belmont, CA: Wadsworth Cengage, 2010; Brians, Craig Leonard et al. Empirical Political Analysis: Quantitative and Qualitative Research Methods . 8th ed. Boston, MA: Longman, 2011; McNabb, David E. Research Methods in Public Administration and Nonprofit Management: Quantitative and Qualitative Approaches . 2nd ed. Armonk, NY: M.E. Sharpe, 2008; Singh, Kultar. Quantitative Social Research Methods . Los Angeles, CA: Sage, 2007.
Limitations of Using Quantitative Methods
Quantitative methods presume to have an objective approach to studying research problems, where data is controlled and measured, to address the accumulation of facts, and to determine the causes of behavior. As a consequence, the results of quantitative research may be statistically significant but are often humanly insignificant.
Some specific limitations associated with using quantitative methods to study research problems in the social sciences include:
- Quantitative data is more efficient and able to test hypotheses, but may miss contextual detail;
- Uses a static and rigid approach and so employs an inflexible process of discovery;
- The development of standard questions by researchers can lead to "structural bias" and false representation, where the data actually reflects the view of the researcher instead of the participating subject;
- Results provide less detail on behavior, attitudes, and motivation;
- Researcher may collect a much narrower and sometimes superficial dataset;
- Results are limited as they provide numerical descriptions rather than detailed narrative and generally provide less elaborate accounts of human perception;
- The research is often carried out in an unnatural, artificial environment so that a level of control can be applied to the exercise. This level of control might not normally be in place in the real world thus yielding "laboratory results" as opposed to "real world results"; and,
- Preset answers will not necessarily reflect how people really feel about a subject and, in some cases, might just be the closest match to the preconceived hypothesis.
Research Tip
Finding Examples of How to Apply Different Types of Research Methods
SAGE publications is a major publisher of studies about how to design and conduct research in the social and behavioral sciences. Their SAGE Research Methods Online and Cases database includes contents from books, articles, encyclopedias, handbooks, and videos covering social science research design and methods including the complete Little Green Book Series of Quantitative Applications in the Social Sciences and the Little Blue Book Series of Qualitative Research techniques. The database also includes case studies outlining the research methods used in real research projects. This is an excellent source for finding definitions of key terms and descriptions of research design and practice, techniques of data gathering, analysis, and reporting, and information about theories of research [e.g., grounded theory]. The database covers both qualitative and quantitative research methods as well as mixed methods approaches to conducting research.
SAGE Research Methods Online and Cases
- << Previous: Qualitative Methods
- Next: Insiderness >>
- Last Updated: Apr 24, 2024 10:51 AM
- URL: https://libguides.usc.edu/writingguide

Research Methods and Design
- Action Research
- Case Study Design
- Literature Review
- Quantitative Research Methods
- Qualitative Research Methods
- Mixed Methods Study
- Indigenous Research and Ethics This link opens in a new window
- Identifying Empirical Research Articles This link opens in a new window
- Research Ethics and Quality
- Data Literacy
- Get Help with Writing Assignments
Quantitative research methods
a method of research that relies on measuring variables using a numerical system, analyzing these measurements using any of a variety of statistical models, and reporting relationships and associations among the studied variables. For example, these variables may be test scores or measurements of reaction time. The goal of gathering this quantitative data is to understand, describe, and predict the nature of a phenomenon, particularly through the development of models and theories. Quantitative research techniques include experiments and surveys.
SAGE Research Methods Videos
What are the strengths of quantitative research.
Professor Norma T. Mertz briefly discusses qualitative research and how it has changed since she entered the field. She emphasizes the importance of defining a research question before choosing a theoretical approach to research.
This is just one segment in a series about quantitative methods. You can find additional videos in our SAGE database, Research Methods:

Videos covering research methods and statistics
Further Reading
- << Previous: Literature Review
- Next: Qualitative Research Methods >>
- Last Updated: Feb 6, 2024 9:20 AM
CityU Home - CityU Catalog

News alert: UC Berkeley has announced its next university librarian
Secondary menu
- Log in to your Library account
- Hours and Maps
- Connect from Off Campus
- UC Berkeley Home
Search form
Research methods--quantitative, qualitative, and more: overview.
- Quantitative Research
- Qualitative Research
- Data Science Methods (Machine Learning, AI, Big Data)
- Text Mining and Computational Text Analysis
- Evidence Synthesis/Systematic Reviews
- Get Data, Get Help!
About Research Methods
This guide provides an overview of research methods, how to choose and use them, and supports and resources at UC Berkeley.
As Patten and Newhart note in the book Understanding Research Methods , "Research methods are the building blocks of the scientific enterprise. They are the "how" for building systematic knowledge. The accumulation of knowledge through research is by its nature a collective endeavor. Each well-designed study provides evidence that may support, amend, refute, or deepen the understanding of existing knowledge...Decisions are important throughout the practice of research and are designed to help researchers collect evidence that includes the full spectrum of the phenomenon under study, to maintain logical rules, and to mitigate or account for possible sources of bias. In many ways, learning research methods is learning how to see and make these decisions."
The choice of methods varies by discipline, by the kind of phenomenon being studied and the data being used to study it, by the technology available, and more. This guide is an introduction, but if you don't see what you need here, always contact your subject librarian, and/or take a look to see if there's a library research guide that will answer your question.
Suggestions for changes and additions to this guide are welcome!
START HERE: SAGE Research Methods
Without question, the most comprehensive resource available from the library is SAGE Research Methods. HERE IS THE ONLINE GUIDE to this one-stop shopping collection, and some helpful links are below:
- SAGE Research Methods
- Little Green Books (Quantitative Methods)
- Little Blue Books (Qualitative Methods)
- Dictionaries and Encyclopedias
- Case studies of real research projects
- Sample datasets for hands-on practice
- Streaming video--see methods come to life
- Methodspace- -a community for researchers
- SAGE Research Methods Course Mapping
Library Data Services at UC Berkeley
Library Data Services Program and Digital Scholarship Services
The LDSP offers a variety of services and tools ! From this link, check out pages for each of the following topics: discovering data, managing data, collecting data, GIS data, text data mining, publishing data, digital scholarship, open science, and the Research Data Management Program.
Be sure also to check out the visual guide to where to seek assistance on campus with any research question you may have!
Library GIS Services
Other Data Services at Berkeley
D-Lab Supports Berkeley faculty, staff, and graduate students with research in data intensive social science, including a wide range of training and workshop offerings Dryad Dryad is a simple self-service tool for researchers to use in publishing their datasets. It provides tools for the effective publication of and access to research data. Geospatial Innovation Facility (GIF) Provides leadership and training across a broad array of integrated mapping technologies on campu Research Data Management A UC Berkeley guide and consulting service for research data management issues
General Research Methods Resources
Here are some general resources for assistance:
- Assistance from ICPSR (must create an account to access): Getting Help with Data , and Resources for Students
- Wiley Stats Ref for background information on statistics topics
- Survey Documentation and Analysis (SDA) . Program for easy web-based analysis of survey data.
Consultants
- D-Lab/Data Science Discovery Consultants Request help with your research project from peer consultants.
- Research data (RDM) consulting Meet with RDM consultants before designing the data security, storage, and sharing aspects of your qualitative project.
- Statistics Department Consulting Services A service in which advanced graduate students, under faculty supervision, are available to consult during specified hours in the Fall and Spring semesters.
Related Resourcex
- IRB / CPHS Qualitative research projects with human subjects often require that you go through an ethics review.
- OURS (Office of Undergraduate Research and Scholarships) OURS supports undergraduates who want to embark on research projects and assistantships. In particular, check out their "Getting Started in Research" workshops
- Sponsored Projects Sponsored projects works with researchers applying for major external grants.
- Next: Quantitative Research >>
- Last Updated: Apr 25, 2024 11:09 AM
- URL: https://guides.lib.berkeley.edu/researchmethods
Research Methodologies
Quantitative research methodologies.
- Qualitative Research Methodologies
- Systematic Reviews
- Finding Articles by Methodology
- Design Your Research Project
Library Help
What is quantitative research.
Quantitative methodologies use statistics to analyze numerical data gathered by researchers to answer their research questions. Quantitative methods can be used to answer questions such as:
- What are the relationships between two or more variables?
- What factors are at play in an environment that might affect the behavior or development of the organisms in that environment?
Quantitative methods can also be used to test hypotheses by conducting quasi-experimental studies or designing experiments.
Independent and Dependent Variables
In quantitative research, a variable is something (an intervention technique, a pharmaceutical, a temperature, etc.) that changes. There are two kinds of variables: independent variables and dependent variables . In the simplest terms, the independent variable is whatever the researchers are using to attempt to make a change in their dependent variable.
* This is a real, repeatable experiment you can try on your plants.
Correlational
Researchers will compare two sets of numbers to try and identify a relationship (if any) between two things.
- Köse S., & Murat, M. (2021). Examination of the relationship between smartphone addiction and cyberchondria in adolescents. Archives of Psychiatric Nursing, 35(6): 563-570.
- Pilger et al. (2021). Spiritual well-being, religious/spiritual coping and quality of life among the elderly undergoing hemodialysis: a correlational study. Journal of Religion, Spirituality & Aging, 33(1): 2-15.
Descriptive
Researchers will attempt to quantify a variety of factors at play as they study a particular type of phenomenon or action. For example, researchers might use a descriptive methodology to understand the effects of climate change on the life cycle of a plant or animal.
- Lakshmi, E. (2021). Food consumption pattern and body mass index of adolescents: A descriptive study. International Journal of Nutrition, Pharmacology, Neurological Diseases, 11(4), 293–297.
- Lin, J., Singh, S., Sha, L., Tan, W., Lang, D., Gašević, D., & Chen, G. (2022). Is it a good move? Mining effective tutoring strategies from human–human tutorial dialogues. Future Generation Computer Systems, 127, 194–207.
Experimental
To understand the effects of a variable, researchers will design an experiment where they can control as many factors as possible. This can involve creating control and experimental groups. The experimental group will be exposed to the variable to study its effects. The control group provides data about what happens when the variable is absent. For example, in a study about online teaching, the control group might receive traditional face-to-face instruction while the experimental group would receive their instruction virtually.
- Jinzhang Jia, Yinuo Chen, Guangbo Che, Jinchao Zhu, Fengxiao Wang, & Peng Jia. (2021). Experimental study on the explosion characteristics of hydrogen-methane premixed gas in complex pipe networks. Scientific Reports, 11(1), 1–11.
- Sasaki, R. et al. (2021). Effects of cryotherapy applied at different temperatures on inflammatory pain during the acute phase of arthritis in rats. Physical Therapy, 101(2), 1–9.
Quasi-Experimental/Quasi-Comparative
Researchers will attempt to determine what (if any) effect a variable can have. These studies may have multiple independent variables (causes) and multiple dependent variables (effects), but this can complicate researchers' efforts to find out if A can cause B or if X, Y, and Z are also playing a role.
- Jafari, A., Alami, A., Charoghchian, E., Delshad Noghabi, A., & Nejatian, M. (2021). The impact of effective communication skills training on the status of marital burnout among married women. BMC Women’s Health, 21(1), 1-10.
- Phillips, S. W., Kim, D.-Y., Sobol, J. J., & Gayadeen, S. M. (2021). Total recall?: A quasi-experimental study of officer’s recollection in shoot - don’t shoot simulators. Police Practice and Research, 22(3), 1229–1240.
Surveys can be considered a quantitative methodology if the researchers require their respondents to choose from pre-determined responses.
- Harries et al. (2021). Effects of the COVID-19 pandemic on medical students: A multicenter quantitative study. BMC Medical Education, 21(14), 1-8.
- Call : 801.863.8840
- Text : 801.290.8123
- In-Person Help
- Email a Librarian
- Make an Appointment
- << Previous: Home
- Next: Qualitative Research Methodologies >>
- Last Updated: Apr 25, 2024 2:32 PM
- URL: https://uvu.libguides.com/methods

Quantitative and Qualitative Research
- I NEED TO . . .
What is Quantitative Research?
- What is Qualitative Research?
- Quantitative vs Qualitative
- Step 1: Accessing CINAHL
- Step 2: Create a Keyword Search
- Step 3: Create a Subject Heading Search
- Step 4: Repeat Steps 1-3 for Second Concept
- Step 5: Repeat Steps 1-3 for Quantitative Terms
- Step 6: Combining All Searches
- Step 7: Adding Limiters
- Step 8: Save Your Search!
- What Kind of Article is This?
- More Research Help This link opens in a new window
Quantitative methodology is the dominant research framework in the social sciences. It refers to a set of strategies, techniques and assumptions used to study psychological, social and economic processes through the exploration of numeric patterns . Quantitative research gathers a range of numeric data. Some of the numeric data is intrinsically quantitative (e.g. personal income), while in other cases the numeric structure is imposed (e.g. ‘On a scale from 1 to 10, how depressed did you feel last week?’). The collection of quantitative information allows researchers to conduct simple to extremely sophisticated statistical analyses that aggregate the data (e.g. averages, percentages), show relationships among the data (e.g. ‘Students with lower grade point averages tend to score lower on a depression scale’) or compare across aggregated data (e.g. the USA has a higher gross domestic product than Spain). Quantitative research includes methodologies such as questionnaires, structured observations or experiments and stands in contrast to qualitative research. Qualitative research involves the collection and analysis of narratives and/or open-ended observations through methodologies such as interviews, focus groups or ethnographies.
Coghlan, D., Brydon-Miller, M. (2014). The SAGE encyclopedia of action research (Vols. 1-2). London, : SAGE Publications Ltd doi: 10.4135/9781446294406
What is the purpose of quantitative research?
The purpose of quantitative research is to generate knowledge and create understanding about the social world. Quantitative research is used by social scientists, including communication researchers, to observe phenomena or occurrences affecting individuals. Social scientists are concerned with the study of people. Quantitative research is a way to learn about a particular group of people, known as a sample population. Using scientific inquiry, quantitative research relies on data that are observed or measured to examine questions about the sample population.
Allen, M. (2017). The SAGE encyclopedia of communication research methods (Vols. 1-4). Thousand Oaks, CA: SAGE Publications, Inc doi: 10.4135/9781483381411
How do I know if the study is a quantitative design? What type of quantitative study is it?
Quantitative Research Designs: Descriptive non-experimental, Quasi-experimental or Experimental?
Studies do not always explicitly state what kind of research design is being used. You will need to know how to decipher which design type is used. The following video will help you determine the quantitative design type.
- << Previous: I NEED TO . . .
- Next: What is Qualitative Research? >>
- Last Updated: Dec 8, 2023 10:05 PM
- URL: https://libguides.uta.edu/quantitative_and_qualitative_research
University of Texas Arlington Libraries 702 Planetarium Place · Arlington, TX 76019 · 817-272-3000
- Internet Privacy
- Accessibility
- Problems with a guide? Contact Us.
Quantitative Research
- Reference work entry
- First Online: 13 January 2019
- Cite this reference work entry
- Leigh A. Wilson 2 , 3
4239 Accesses
4 Citations
Quantitative research methods are concerned with the planning, design, and implementation of strategies to collect and analyze data. Descartes, the seventeenth-century philosopher, suggested that how the results are achieved is often more important than the results themselves, as the journey taken along the research path is a journey of discovery. High-quality quantitative research is characterized by the attention given to the methods and the reliability of the tools used to collect the data. The ability to critique research in a systematic way is an essential component of a health professional’s role in order to deliver high quality, evidence-based healthcare. This chapter is intended to provide a simple overview of the way new researchers and health practitioners can understand and employ quantitative methods. The chapter offers practical, realistic guidance in a learner-friendly way and uses a logical sequence to understand the process of hypothesis development, study design, data collection and handling, and finally data analysis and interpretation.
This is a preview of subscription content, log in via an institution to check access.
Access this chapter
- Available as PDF
- Read on any device
- Instant download
- Own it forever
- Available as EPUB and PDF
- Durable hardcover edition
- Dispatched in 3 to 5 business days
- Free shipping worldwide - see info
Tax calculation will be finalised at checkout
Purchases are for personal use only
Institutional subscriptions
Babbie ER. The practice of social research. 14th ed. Belmont: Wadsworth Cengage; 2016.
Google Scholar
Descartes. Cited in Halverston, W. (1976). In: A concise introduction to philosophy, 3rd ed. New York: Random House; 1637.
Doll R, Hill AB. The mortality of doctors in relation to their smoking habits. BMJ. 1954;328(7455):1529–33. https://doi.org/10.1136/bmj.328.7455.1529 .
Article Google Scholar
Liamputtong P. Research methods in health: foundations for evidence-based practice. 3rd ed. Melbourne: Oxford University Press; 2017.
McNabb DE. Research methods in public administration and nonprofit management: quantitative and qualitative approaches. 2nd ed. New York: Armonk; 2007.
Merriam-Webster. Dictionary. http://www.merriam-webster.com . Accessed 20th December 2017.
Olesen Larsen P, von Ins M. The rate of growth in scientific publication and the decline in coverage provided by Science Citation Index. Scientometrics. 2010;84(3):575–603.
Pannucci CJ, Wilkins EG. Identifying and avoiding bias in research. Plast Reconstr Surg. 2010;126(2):619–25. https://doi.org/10.1097/PRS.0b013e3181de24bc .
Petrie A, Sabin C. Medical statistics at a glance. 2nd ed. London: Blackwell Publishing; 2005.
Portney LG, Watkins MP. Foundations of clinical research: applications to practice. 3rd ed. New Jersey: Pearson Publishing; 2009.
Sheehan J. Aspects of research methodology. Nurse Educ Today. 1986;6:193–203.
Wilson LA, Black DA. Health, science research and research methods. Sydney: McGraw Hill; 2013.
Download references
Author information
Authors and affiliations.
School of Science and Health, Western Sydney University, Penrith, NSW, Australia
Leigh A. Wilson
Faculty of Health Science, Discipline of Behavioural and Social Sciences in Health, University of Sydney, Lidcombe, NSW, Australia
You can also search for this author in PubMed Google Scholar
Corresponding author
Correspondence to Leigh A. Wilson .
Editor information
Editors and affiliations.
Pranee Liamputtong
Rights and permissions
Reprints and permissions
Copyright information
© 2019 Springer Nature Singapore Pte Ltd.
About this entry
Cite this entry.
Wilson, L.A. (2019). Quantitative Research. In: Liamputtong, P. (eds) Handbook of Research Methods in Health Social Sciences. Springer, Singapore. https://doi.org/10.1007/978-981-10-5251-4_54
Download citation
DOI : https://doi.org/10.1007/978-981-10-5251-4_54
Published : 13 January 2019
Publisher Name : Springer, Singapore
Print ISBN : 978-981-10-5250-7
Online ISBN : 978-981-10-5251-4
eBook Packages : Social Sciences Reference Module Humanities and Social Sciences Reference Module Business, Economics and Social Sciences
Share this entry
Anyone you share the following link with will be able to read this content:
Sorry, a shareable link is not currently available for this article.
Provided by the Springer Nature SharedIt content-sharing initiative
- Publish with us
Policies and ethics
- Find a journal
- Track your research
- Skip to main content
- Skip to primary sidebar
- Skip to footer
- QuestionPro

- Solutions Industries Gaming Automotive Sports and events Education Government Travel & Hospitality Financial Services Healthcare Cannabis Technology Use Case NPS+ Communities Audience Contactless surveys Mobile LivePolls Member Experience GDPR Positive People Science 360 Feedback Surveys
- Resources Blog eBooks Survey Templates Case Studies Training Help center

Home Market Research
Quantitative Research: What It Is, Practices & Methods
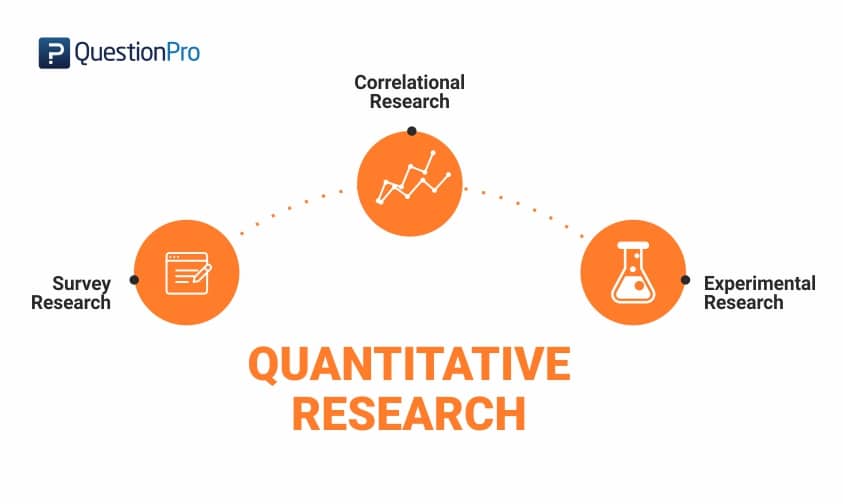
Quantitative research involves analyzing and gathering numerical data to uncover trends, calculate averages, evaluate relationships, and derive overarching insights. It’s used in various fields, including the natural and social sciences. Quantitative data analysis employs statistical techniques for processing and interpreting numeric data.
Research designs in the quantitative realm outline how data will be collected and analyzed with methods like experiments and surveys. Qualitative methods complement quantitative research by focusing on non-numerical data, adding depth to understanding. Data collection methods can be qualitative or quantitative, depending on research goals. Researchers often use a combination of both approaches to gain a comprehensive understanding of phenomena.
What is Quantitative Research?
Quantitative research is a systematic investigation of phenomena by gathering quantifiable data and performing statistical, mathematical, or computational techniques. Quantitative research collects statistically significant information from existing and potential customers using sampling methods and sending out online surveys , online polls , and questionnaires , for example.
One of the main characteristics of this type of research is that the results can be depicted in numerical form. After carefully collecting structured observations and understanding these numbers, it’s possible to predict the future of a product or service, establish causal relationships or Causal Research , and make changes accordingly. Quantitative research primarily centers on the analysis of numerical data and utilizes inferential statistics to derive conclusions that can be extrapolated to the broader population.
An example of a quantitative research study is the survey conducted to understand how long a doctor takes to tend to a patient when the patient walks into the hospital. A patient satisfaction survey can be administered to ask questions like how long a doctor takes to see a patient, how often a patient walks into a hospital, and other such questions, which are dependent variables in the research. This kind of research method is often employed in the social sciences, and it involves using mathematical frameworks and theories to effectively present data, ensuring that the results are logical, statistically sound, and unbiased.
Data collection in quantitative research uses a structured method and is typically conducted on larger samples representing the entire population. Researchers use quantitative methods to collect numerical data, which is then subjected to statistical analysis to determine statistically significant findings. This approach is valuable in both experimental research and social research, as it helps in making informed decisions and drawing reliable conclusions based on quantitative data.
Quantitative Research Characteristics
Quantitative research has several unique characteristics that make it well-suited for specific projects. Let’s explore the most crucial of these characteristics so that you can consider them when planning your next research project:
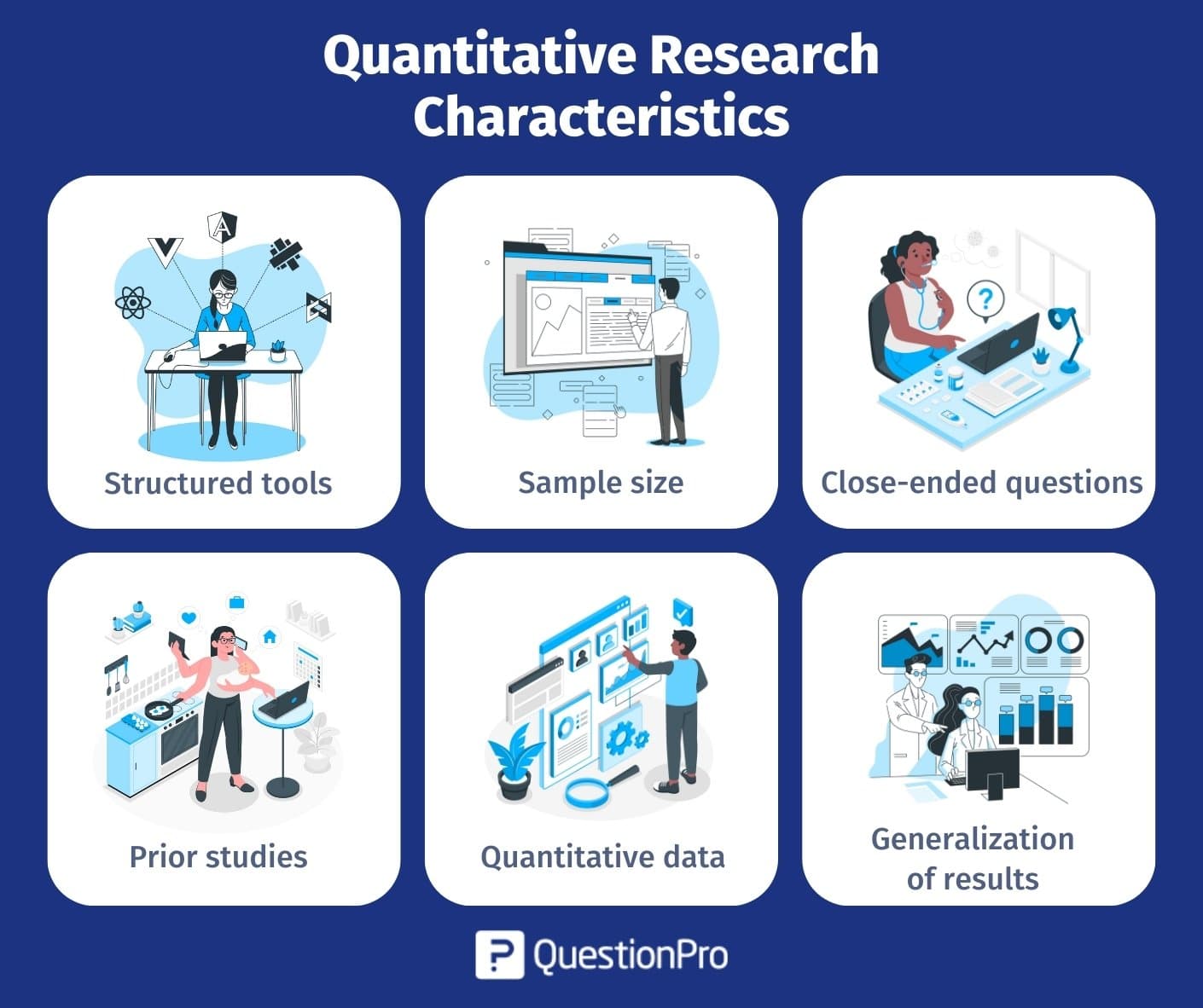
- Structured tools: Quantitative research relies on structured tools such as surveys, polls, or questionnaires to gather quantitative data . Using such structured methods helps collect in-depth and actionable numerical data from the survey respondents, making it easier to perform data analysis.
- Sample size: Quantitative research is conducted on a significant sample size representing the target market . Appropriate Survey Sampling methods, a fundamental aspect of quantitative research methods, must be employed when deriving the sample to fortify the research objective and ensure the reliability of the results.
- Close-ended questions: Closed-ended questions , specifically designed to align with the research objectives, are a cornerstone of quantitative research. These questions facilitate the collection of quantitative data and are extensively used in data collection processes.
- Prior studies: Before collecting feedback from respondents, researchers often delve into previous studies related to the research topic. This preliminary research helps frame the study effectively and ensures the data collection process is well-informed.
- Quantitative data: Typically, quantitative data is represented using tables, charts, graphs, or other numerical forms. This visual representation aids in understanding the collected data and is essential for rigorous data analysis, a key component of quantitative research methods.
- Generalization of results: One of the strengths of quantitative research is its ability to generalize results to the entire population. It means that the findings derived from a sample can be extrapolated to make informed decisions and take appropriate actions for improvement based on numerical data analysis.
Quantitative Research Methods
Quantitative research methods are systematic approaches used to gather and analyze numerical data to understand and draw conclusions about a phenomenon or population. Here are the quantitative research methods:
- Primary quantitative research methods
- Secondary quantitative research methods
Primary Quantitative Research Methods
Primary quantitative research is the most widely used method of conducting market research. The distinct feature of primary research is that the researcher focuses on collecting data directly rather than depending on data collected from previously done research. Primary quantitative research design can be broken down into three further distinctive tracks and the process flow. They are:
A. Techniques and Types of Studies
There are multiple types of primary quantitative research. They can be distinguished into the four following distinctive methods, which are:
01. Survey Research
Survey Research is fundamental for all quantitative outcome research methodologies and studies. Surveys are used to ask questions to a sample of respondents, using various types such as online polls, online surveys, paper questionnaires, web-intercept surveys , etc. Every small and big organization intends to understand what their customers think about their products and services, how well new features are faring in the market, and other such details.
By conducting survey research, an organization can ask multiple survey questions , collect data from a pool of customers, and analyze this collected data to produce numerical results. It is the first step towards collecting data for any research. You can use single ease questions . A single-ease question is a straightforward query that elicits a concise and uncomplicated response.
This type of research can be conducted with a specific target audience group and also can be conducted across multiple groups along with comparative analysis . A prerequisite for this type of research is that the sample of respondents must have randomly selected members. This way, a researcher can easily maintain the accuracy of the obtained results as a huge variety of respondents will be addressed using random selection.
Traditionally, survey research was conducted face-to-face or via phone calls. Still, with the progress made by online mediums such as email or social media, survey research has also spread to online mediums.There are two types of surveys , either of which can be chosen based on the time in hand and the kind of data required:
Cross-sectional surveys: Cross-sectional surveys are observational surveys conducted in situations where the researcher intends to collect data from a sample of the target population at a given point in time. Researchers can evaluate various variables at a particular time. Data gathered using this type of survey is from people who depict similarity in all variables except the variables which are considered for research . Throughout the survey, this one variable will stay constant.
- Cross-sectional surveys are popular with retail, SMEs, and healthcare industries. Information is garnered without modifying any parameters in the variable ecosystem.
- Multiple samples can be analyzed and compared using a cross-sectional survey research method.
- Multiple variables can be evaluated using this type of survey research.
- The only disadvantage of cross-sectional surveys is that the cause-effect relationship of variables cannot be established as it usually evaluates variables at a particular time and not across a continuous time frame.
Longitudinal surveys: Longitudinal surveys are also observational surveys , but unlike cross-sectional surveys, longitudinal surveys are conducted across various time durations to observe a change in respondent behavior and thought processes. This time can be days, months, years, or even decades. For instance, a researcher planning to analyze the change in buying habits of teenagers over 5 years will conduct longitudinal surveys.
- In cross-sectional surveys, the same variables were evaluated at a given time, and in longitudinal surveys, different variables can be analyzed at different intervals.
- Longitudinal surveys are extensively used in the field of medicine and applied sciences. Apart from these two fields, they are also used to observe a change in the market trend analysis , analyze customer satisfaction, or gain feedback on products/services.
- In situations where the sequence of events is highly essential, longitudinal surveys are used.
- Researchers say that when research subjects need to be thoroughly inspected before concluding, they rely on longitudinal surveys.
02. Correlational Research
A comparison between two entities is invariable. Correlation research is conducted to establish a relationship between two closely-knit entities and how one impacts the other, and what changes are eventually observed. This research method is carried out to give value to naturally occurring relationships, and a minimum of two different groups are required to conduct this quantitative research method successfully. Without assuming various aspects, a relationship between two groups or entities must be established.
Researchers use this quantitative research design to correlate two or more variables using mathematical analysis methods. Patterns, relationships, and trends between variables are concluded as they exist in their original setup. The impact of one of these variables on the other is observed, along with how it changes the relationship between the two variables. Researchers tend to manipulate one of the variables to attain the desired results.
Ideally, it is advised not to make conclusions merely based on correlational research. This is because it is not mandatory that if two variables are in sync that they are interrelated.
Example of Correlational Research Questions :
- The relationship between stress and depression.
- The equation between fame and money.
- The relation between activities in a third-grade class and its students.
03. Causal-comparative Research
This research method mainly depends on the factor of comparison. Also called quasi-experimental research , this quantitative research method is used by researchers to conclude the cause-effect equation between two or more variables, where one variable is dependent on the other independent variable. The independent variable is established but not manipulated, and its impact on the dependent variable is observed. These variables or groups must be formed as they exist in the natural setup. As the dependent and independent variables will always exist in a group, it is advised that the conclusions are carefully established by keeping all the factors in mind.
Causal-comparative research is not restricted to the statistical analysis of two variables but extends to analyzing how various variables or groups change under the influence of the same changes. This research is conducted irrespective of the type of relationship that exists between two or more variables. Statistical analysis plan is used to present the outcome using this quantitative research method.
Example of Causal-Comparative Research Questions:
- The impact of drugs on a teenager. The effect of good education on a freshman. The effect of substantial food provision in the villages of Africa.
04. Experimental Research
Also known as true experimentation, this research method relies on a theory. As the name suggests, experimental research is usually based on one or more theories. This theory has yet to be proven before and is merely a supposition. In experimental research, an analysis is done around proving or disproving the statement. This research method is used in natural sciences. Traditional research methods are more effective than modern techniques.
There can be multiple theories in experimental research. A theory is a statement that can be verified or refuted.
After establishing the statement, efforts are made to understand whether it is valid or invalid. This quantitative research method is mainly used in natural or social sciences as various statements must be proved right or wrong.
- Traditional research methods are more effective than modern techniques.
- Systematic teaching schedules help children who struggle to cope with the course.
- It is a boon to have responsible nursing staff for ailing parents.
B. Data Collection Methodologies
The second major step in primary quantitative research is data collection. Data collection can be divided into sampling methods and data collection using surveys and polls.
01. Data Collection Methodologies: Sampling Methods
There are two main sampling methods for quantitative research: Probability and Non-probability sampling .
Probability sampling: A theory of probability is used to filter individuals from a population and create samples in probability sampling . Participants of a sample are chosen by random selection processes. Each target audience member has an equal opportunity to be selected in the sample.
There are four main types of probability sampling:
- Simple random sampling: As the name indicates, simple random sampling is nothing but a random selection of elements for a sample. This sampling technique is implemented where the target population is considerably large.
- Stratified random sampling: In the stratified random sampling method , a large population is divided into groups (strata), and members of a sample are chosen randomly from these strata. The various segregated strata should ideally not overlap one another.
- Cluster sampling: Cluster sampling is a probability sampling method using which the main segment is divided into clusters, usually using geographic segmentation and demographic segmentation parameters.
- Systematic sampling: Systematic sampling is a technique where the starting point of the sample is chosen randomly, and all the other elements are chosen using a fixed interval. This interval is calculated by dividing the population size by the target sample size.
Non-probability sampling: Non-probability sampling is where the researcher’s knowledge and experience are used to create samples. Because of the researcher’s involvement, not all the target population members have an equal probability of being selected to be a part of a sample.
There are five non-probability sampling models:
- Convenience sampling: In convenience sampling , elements of a sample are chosen only due to one prime reason: their proximity to the researcher. These samples are quick and easy to implement as there is no other parameter of selection involved.
- Consecutive sampling: Consecutive sampling is quite similar to convenience sampling, except for the fact that researchers can choose a single element or a group of samples and conduct research consecutively over a significant period and then perform the same process with other samples.
- Quota sampling: Using quota sampling , researchers can select elements using their knowledge of target traits and personalities to form strata. Members of various strata can then be chosen to be a part of the sample as per the researcher’s understanding.
- Snowball sampling: Snowball sampling is conducted with target audiences who are difficult to contact and get information. It is popular in cases where the target audience for analysis research is rare to put together.
- Judgmental sampling: Judgmental sampling is a non-probability sampling method where samples are created only based on the researcher’s experience and research skill .
02. Data collection methodologies: Using surveys & polls
Once the sample is determined, then either surveys or polls can be distributed to collect the data for quantitative research.
Using surveys for primary quantitative research
A survey is defined as a research method used for collecting data from a pre-defined group of respondents to gain information and insights on various topics of interest. The ease of survey distribution and the wide number of people it can reach depending on the research time and objective makes it one of the most important aspects of conducting quantitative research.
Fundamental levels of measurement – nominal, ordinal, interval, and ratio scales
Four measurement scales are fundamental to creating a multiple-choice question in a survey. They are nominal, ordinal, interval, and ratio measurement scales without the fundamentals of which no multiple-choice questions can be created. Hence, it is crucial to understand these measurement levels to develop a robust survey.
Use of different question types
To conduct quantitative research, close-ended questions must be used in a survey. They can be a mix of multiple question types, including multiple-choice questions like semantic differential scale questions , rating scale questions , etc.
Survey Distribution and Survey Data Collection
In the above, we have seen the process of building a survey along with the research design to conduct primary quantitative research. Survey distribution to collect data is the other important aspect of the survey process. There are different ways of survey distribution. Some of the most commonly used methods are:
- Email: Sending a survey via email is the most widely used and effective survey distribution method. This method’s response rate is high because the respondents know your brand. You can use the QuestionPro email management feature to send out and collect survey responses.
- Buy respondents: Another effective way to distribute a survey and conduct primary quantitative research is to use a sample. Since the respondents are knowledgeable and are on the panel by their own will, responses are much higher.
- Embed survey on a website: Embedding a survey on a website increases a high number of responses as the respondent is already in close proximity to the brand when the survey pops up.
- Social distribution: Using social media to distribute the survey aids in collecting a higher number of responses from the people that are aware of the brand.
- QR code: QuestionPro QR codes store the URL for the survey. You can print/publish this code in magazines, signs, business cards, or on just about any object/medium.
- SMS survey: The SMS survey is a quick and time-effective way to collect a high number of responses.
- Offline Survey App: The QuestionPro App allows users to circulate surveys quickly, and the responses can be collected both online and offline.
Survey example
An example of a survey is a short customer satisfaction (CSAT) survey that can quickly be built and deployed to collect feedback about what the customer thinks about a brand and how satisfied and referenceable the brand is.
Using polls for primary quantitative research
Polls are a method to collect feedback using close-ended questions from a sample. The most commonly used types of polls are election polls and exit polls . Both of these are used to collect data from a large sample size but using basic question types like multiple-choice questions.
C. Data Analysis Techniques
The third aspect of primary quantitative research design is data analysis . After collecting raw data, there must be an analysis of this data to derive statistical inferences from this research. It is important to relate the results to the research objective and establish the statistical relevance of the results.
Remember to consider aspects of research that were not considered for the data collection process and report the difference between what was planned vs. what was actually executed.
It is then required to select precise Statistical Analysis Methods , such as SWOT, Conjoint, Cross-tabulation, etc., to analyze the quantitative data.
- SWOT analysis: SWOT Analysis stands for the acronym of Strengths, Weaknesses, Opportunities, and Threat analysis. Organizations use this statistical analysis technique to evaluate their performance internally and externally to develop effective strategies for improvement.
- Conjoint Analysis: Conjoint Analysis is a market analysis method to learn how individuals make complicated purchasing decisions. Trade-offs are involved in an individual’s daily activities, and these reflect their ability to decide from a complex list of product/service options.
- Cross-tabulation: Cross-tabulation is one of the preliminary statistical market analysis methods which establishes relationships, patterns, and trends within the various parameters of the research study.
- TURF Analysis: TURF Analysis , an acronym for Totally Unduplicated Reach and Frequency Analysis, is executed in situations where the reach of a favorable communication source is to be analyzed along with the frequency of this communication. It is used for understanding the potential of a target market.
Inferential statistics methods such as confidence interval, the margin of error, etc., can then be used to provide results.
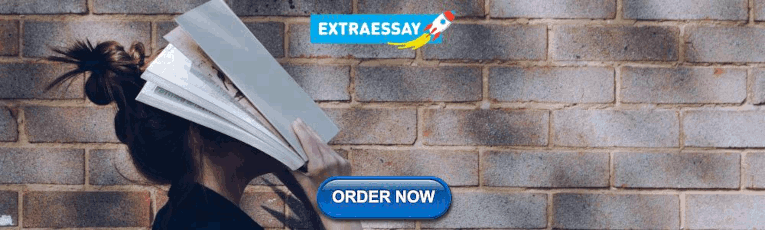
Secondary Quantitative Research Methods
Secondary quantitative research or desk research is a research method that involves using already existing data or secondary data. Existing data is summarized and collated to increase the overall effectiveness of the research.
This research method involves collecting quantitative data from existing data sources like the internet, government resources, libraries, research reports, etc. Secondary quantitative research helps to validate the data collected from primary quantitative research and aid in strengthening or proving, or disproving previously collected data.
The following are five popularly used secondary quantitative research methods:
- Data available on the internet: With the high penetration of the internet and mobile devices, it has become increasingly easy to conduct quantitative research using the internet. Information about most research topics is available online, and this aids in boosting the validity of primary quantitative data.
- Government and non-government sources: Secondary quantitative research can also be conducted with the help of government and non-government sources that deal with market research reports. This data is highly reliable and in-depth and hence, can be used to increase the validity of quantitative research design.
- Public libraries: Now a sparingly used method of conducting quantitative research, it is still a reliable source of information, though. Public libraries have copies of important research that was conducted earlier. They are a storehouse of valuable information and documents from which information can be extracted.
- Educational institutions: Educational institutions conduct in-depth research on multiple topics, and hence, the reports that they publish are an important source of validation in quantitative research.
- Commercial information sources: Local newspapers, journals, magazines, radio, and TV stations are great sources to obtain data for secondary quantitative research. These commercial information sources have in-depth, first-hand information on market research, demographic segmentation, and similar subjects.
Quantitative Research Examples
Some examples of quantitative research are:
- A customer satisfaction template can be used if any organization would like to conduct a customer satisfaction (CSAT) survey . Through this kind of survey, an organization can collect quantitative data and metrics on the goodwill of the brand or organization in the customer’s mind based on multiple parameters such as product quality, pricing, customer experience, etc. This data can be collected by asking a net promoter score (NPS) question , matrix table questions, etc. that provide data in the form of numbers that can be analyzed and worked upon.
- Another example of quantitative research is an organization that conducts an event, collecting feedback from attendees about the value they see from the event. By using an event survey , the organization can collect actionable feedback about the satisfaction levels of customers during various phases of the event such as the sales, pre and post-event, the likelihood of recommending the organization to their friends and colleagues, hotel preferences for the future events and other such questions.
What are the Advantages of Quantitative Research?
There are many advantages to quantitative research. Some of the major advantages of why researchers use this method in market research are:
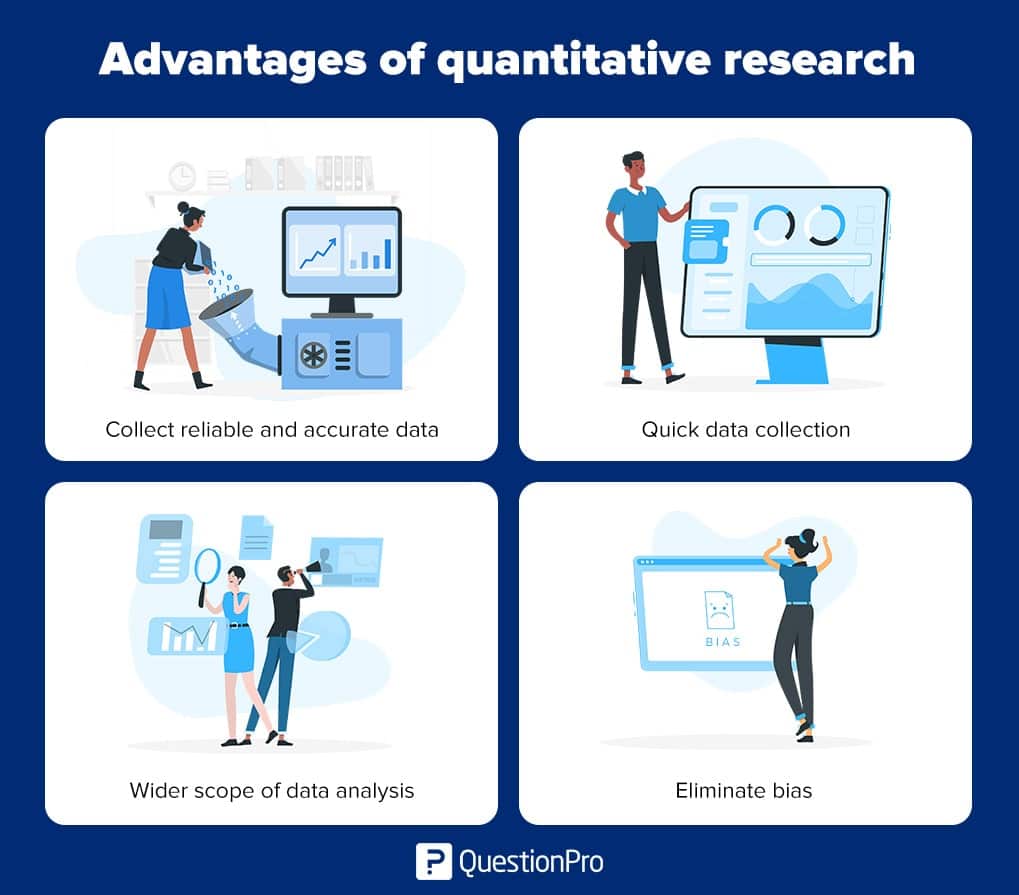
Collect Reliable and Accurate Data:
Quantitative research is a powerful method for collecting reliable and accurate quantitative data. Since data is collected, analyzed, and presented in numbers, the results obtained are incredibly reliable and objective. Numbers do not lie and offer an honest and precise picture of the conducted research without discrepancies. In situations where a researcher aims to eliminate bias and predict potential conflicts, quantitative research is the method of choice.
Quick Data Collection:
Quantitative research involves studying a group of people representing a larger population. Researchers use a survey or another quantitative research method to efficiently gather information from these participants, making the process of analyzing the data and identifying patterns faster and more manageable through the use of statistical analysis. This advantage makes quantitative research an attractive option for projects with time constraints.
Wider Scope of Data Analysis:
Quantitative research, thanks to its utilization of statistical methods, offers an extensive range of data collection and analysis. Researchers can delve into a broader spectrum of variables and relationships within the data, enabling a more thorough comprehension of the subject under investigation. This expanded scope is precious when dealing with complex research questions that require in-depth numerical analysis.
Eliminate Bias:
One of the significant advantages of quantitative research is its ability to eliminate bias. This research method leaves no room for personal comments or the biasing of results, as the findings are presented in numerical form. This objectivity makes the results fair and reliable in most cases, reducing the potential for researcher bias or subjectivity.
In summary, quantitative research involves collecting, analyzing, and presenting quantitative data using statistical analysis. It offers numerous advantages, including the collection of reliable and accurate data, quick data collection, a broader scope of data analysis, and the elimination of bias, making it a valuable approach in the field of research. When considering the benefits of quantitative research, it’s essential to recognize its strengths in contrast to qualitative methods and its role in collecting and analyzing numerical data for a more comprehensive understanding of research topics.
Best Practices to Conduct Quantitative Research
Here are some best practices for conducting quantitative research:
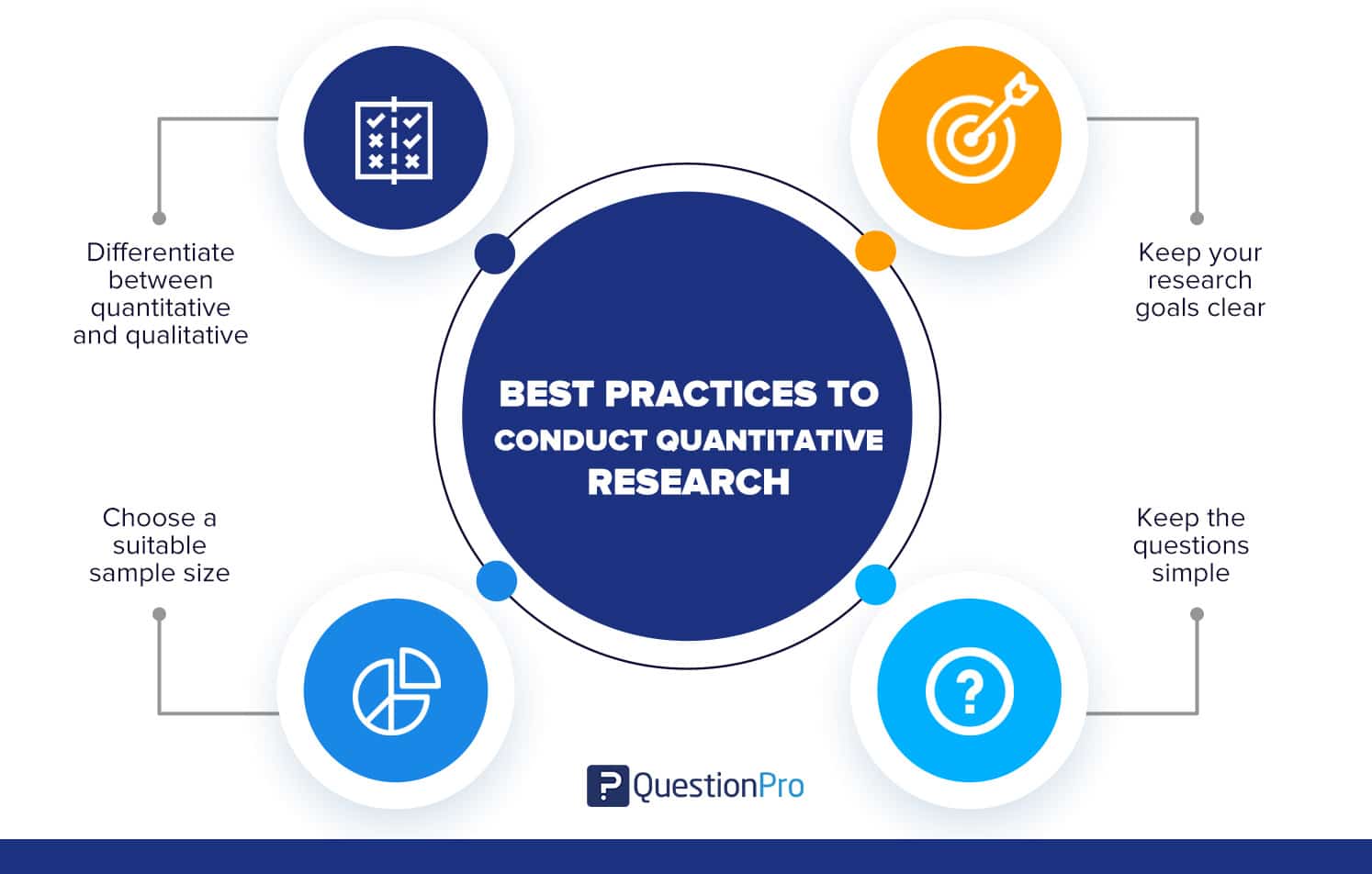
- Differentiate between quantitative and qualitative: Understand the difference between the two methodologies and apply the one that suits your needs best.
- Choose a suitable sample size: Ensure that you have a sample representative of your population and large enough to be statistically weighty.
- Keep your research goals clear and concise: Know your research goals before you begin data collection to ensure you collect the right amount and the right quantity of data.
- Keep the questions simple: Remember that you will be reaching out to a demographically wide audience. Pose simple questions for your respondents to understand easily.
Quantitative Research vs Qualitative Research
Quantitative research and qualitative research are two distinct approaches to conducting research, each with its own set of methods and objectives. Here’s a comparison of the two:
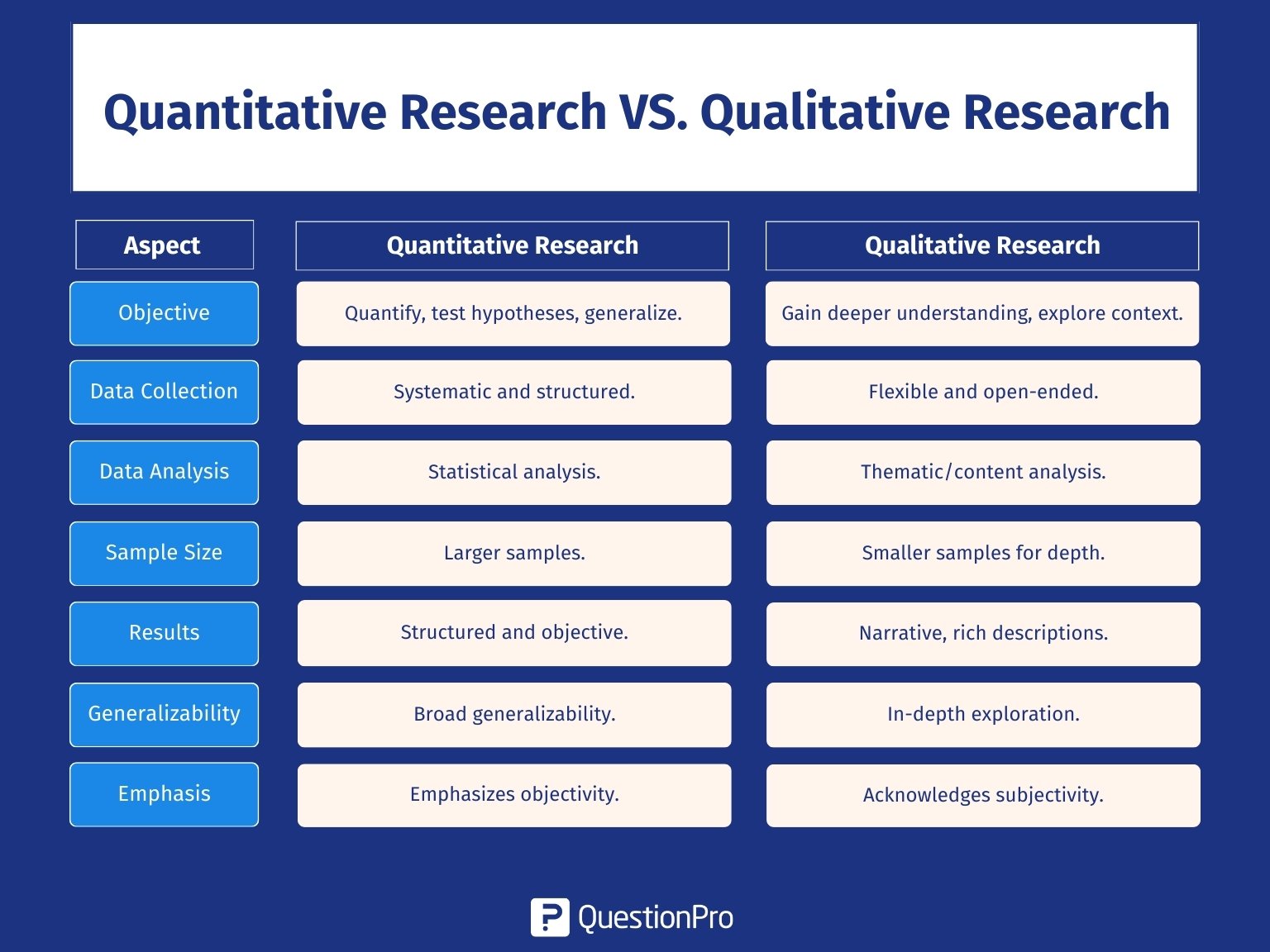
Quantitative Research
- Objective: The primary goal of quantitative research is to quantify and measure phenomena by collecting numerical data. It aims to test hypotheses, establish patterns, and generalize findings to a larger population.
- Data Collection: Quantitative research employs systematic and standardized approaches for data collection, including techniques like surveys, experiments, and observations that involve predefined variables. It is often collected from a large and representative sample.
- Data Analysis: Data is analyzed using statistical techniques, such as descriptive statistics, inferential statistics, and mathematical modeling. Researchers use statistical tests to draw conclusions and make generalizations based on numerical data.
- Sample Size: Quantitative research often involves larger sample sizes to ensure statistical significance and generalizability.
- Results: The results are typically presented in tables, charts, and statistical summaries, making them highly structured and objective.
- Generalizability: Researchers intentionally structure quantitative research to generate outcomes that can be helpful to a larger population, and they frequently seek to establish causative connections.
- Emphasis on Objectivity: Researchers aim to minimize bias and subjectivity, focusing on replicable and objective findings.
Qualitative Research
- Objective: Qualitative research seeks to gain a deeper understanding of the underlying motivations, behaviors, and experiences of individuals or groups. It explores the context and meaning of phenomena.
- Data Collection: Qualitative research employs adaptable and open-ended techniques for data collection, including methods like interviews, focus groups, observations, and content analysis. It allows participants to express their perspectives in their own words.
- Data Analysis: Data is analyzed through thematic analysis, content analysis, or grounded theory. Researchers focus on identifying patterns, themes, and insights in the data.
- Sample Size: Qualitative research typically involves smaller sample sizes due to the in-depth nature of data collection and analysis.
- Results: Findings are presented in narrative form, often in the participants’ own words. Results are subjective, context-dependent, and provide rich, detailed descriptions.
- Generalizability: Qualitative research does not aim for broad generalizability but focuses on in-depth exploration within a specific context. It provides a detailed understanding of a particular group or situation.
- Emphasis on Subjectivity: Researchers acknowledge the role of subjectivity and the researcher’s influence on the Research Process . Participant perspectives and experiences are central to the findings.
Researchers choose between quantitative and qualitative research methods based on their research objectives and the nature of the research question. Each approach has its advantages and drawbacks, and the decision between them hinges on the particular research objectives and the data needed to address research inquiries effectively.
Quantitative research is a structured way of collecting and analyzing data from various sources. Its purpose is to quantify the problem and understand its extent, seeking results that someone can project to a larger population.
Companies that use quantitative rather than qualitative research typically aim to measure magnitudes and seek objectively interpreted statistical results. So if you want to obtain quantitative data that helps you define the structured cause-and-effect relationship between the research problem and the factors, you should opt for this type of research.
At QuestionPro , we have various Best Data Collection Tools and features to conduct investigations of this type. You can create questionnaires and distribute them through our various methods. We also have sample services or various questions to guarantee the success of your study and the quality of the collected data.
Quantitative research is a systematic and structured approach to studying phenomena that involves the collection of measurable data and the application of statistical, mathematical, or computational techniques for analysis.
Quantitative research is characterized by structured tools like surveys, substantial sample sizes, closed-ended questions, reliance on prior studies, data presented numerically, and the ability to generalize findings to the broader population.
The two main methods of quantitative research are Primary quantitative research methods, involving data collection directly from sources, and Secondary quantitative research methods, which utilize existing data for analysis.
1.Surveying to measure employee engagement with numerical rating scales. 2.Analyzing sales data to identify trends in product demand and market share. 4.Examining test scores to assess the impact of a new teaching method on student performance. 4.Using website analytics to track user behavior and conversion rates for an online store.
1.Differentiate between quantitative and qualitative approaches. 2.Choose a representative sample size. 3.Define clear research goals before data collection. 4.Use simple and easily understandable survey questions.
MORE LIKE THIS
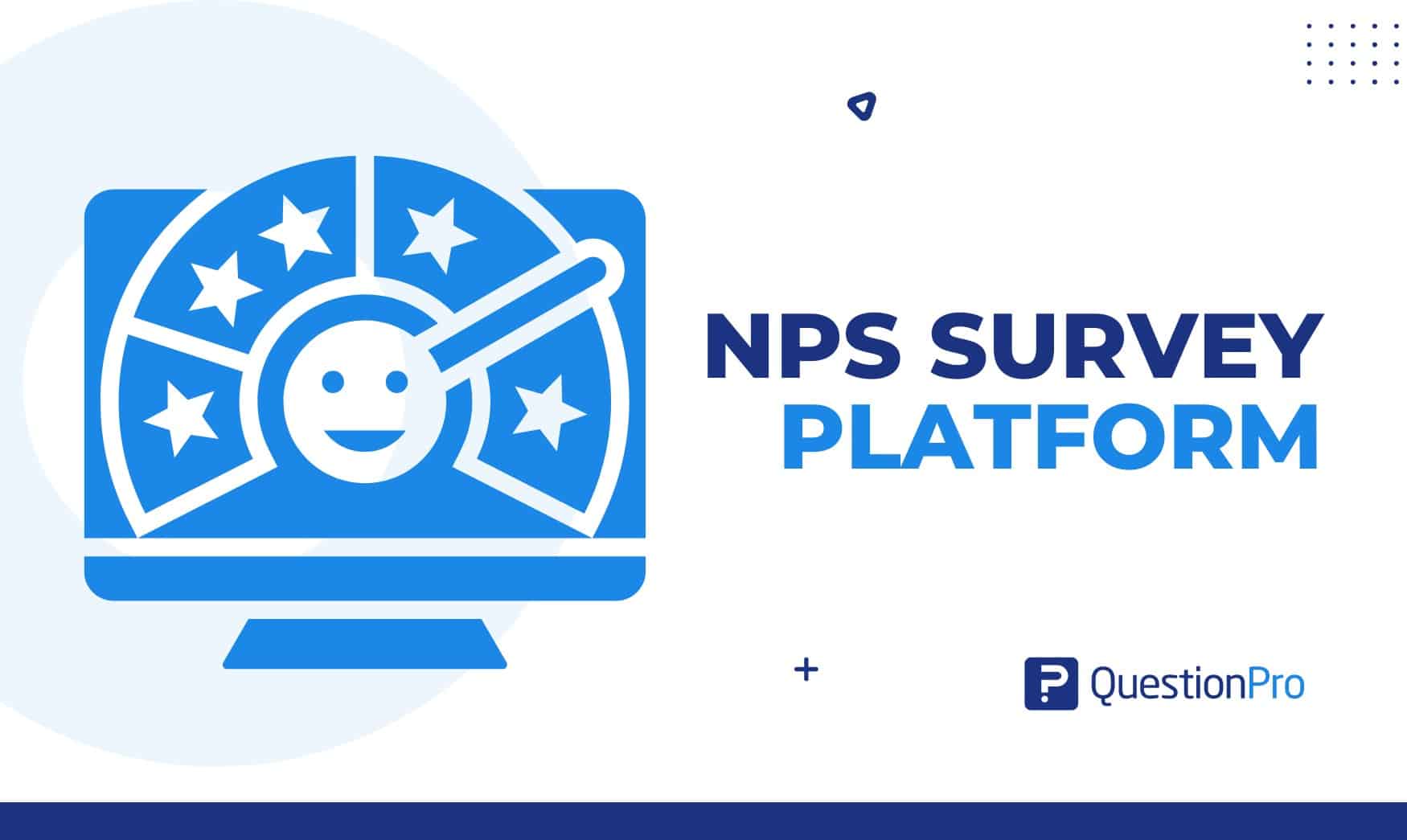
NPS Survey Platform: Types, Tips, 11 Best Platforms & Tools
Apr 26, 2024
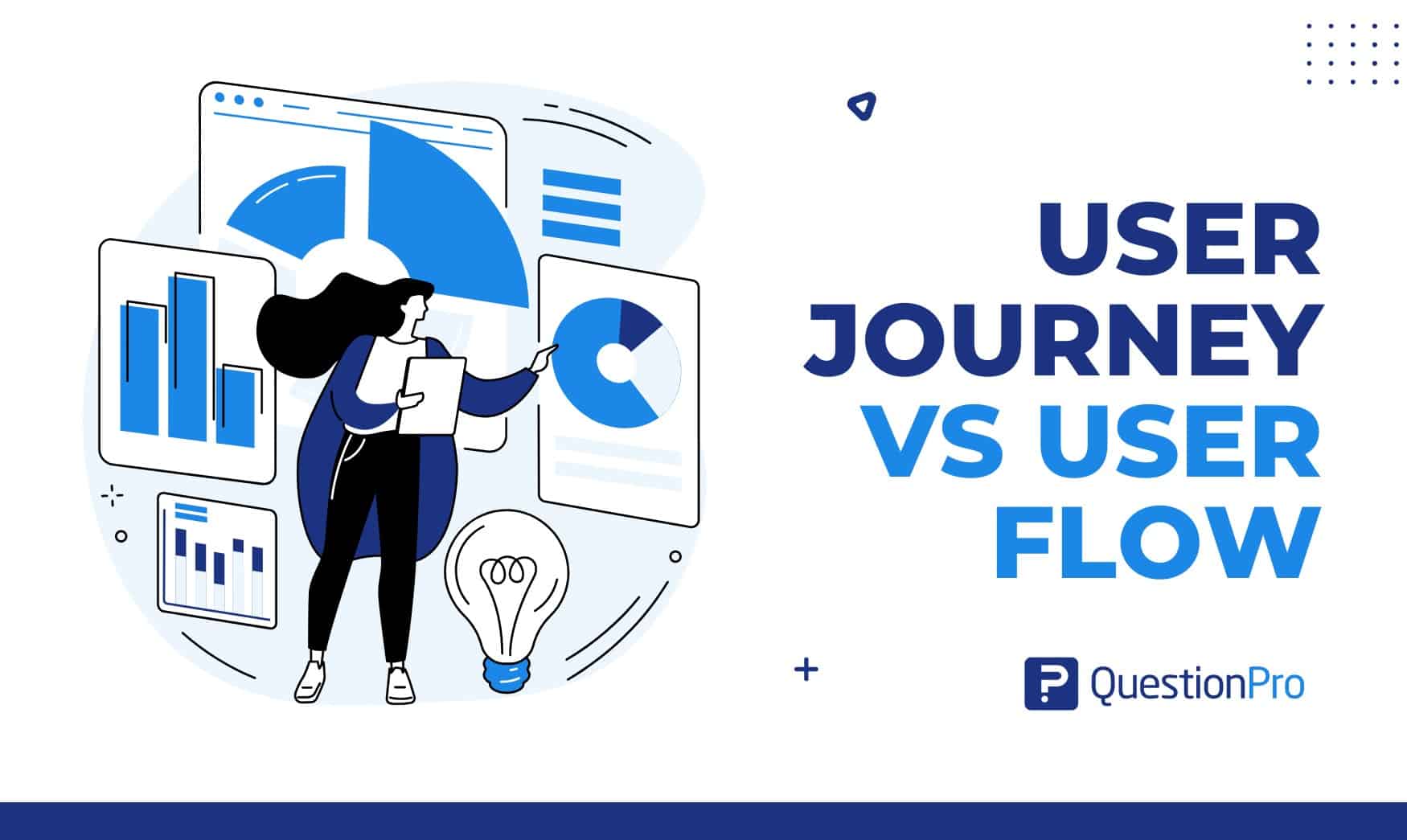
User Journey vs User Flow: Differences and Similarities
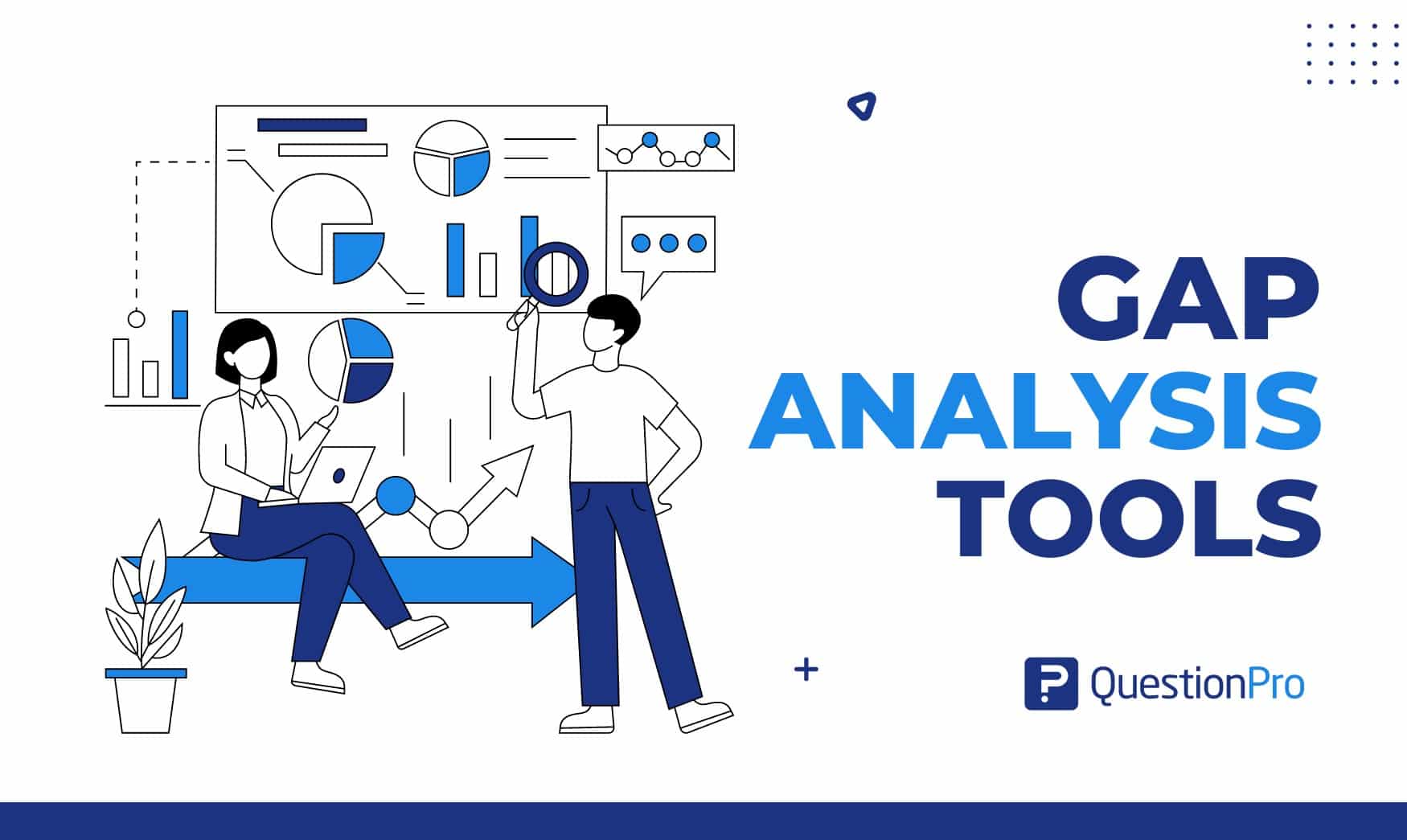
Best 7 Gap Analysis Tools to Empower Your Business
Apr 25, 2024
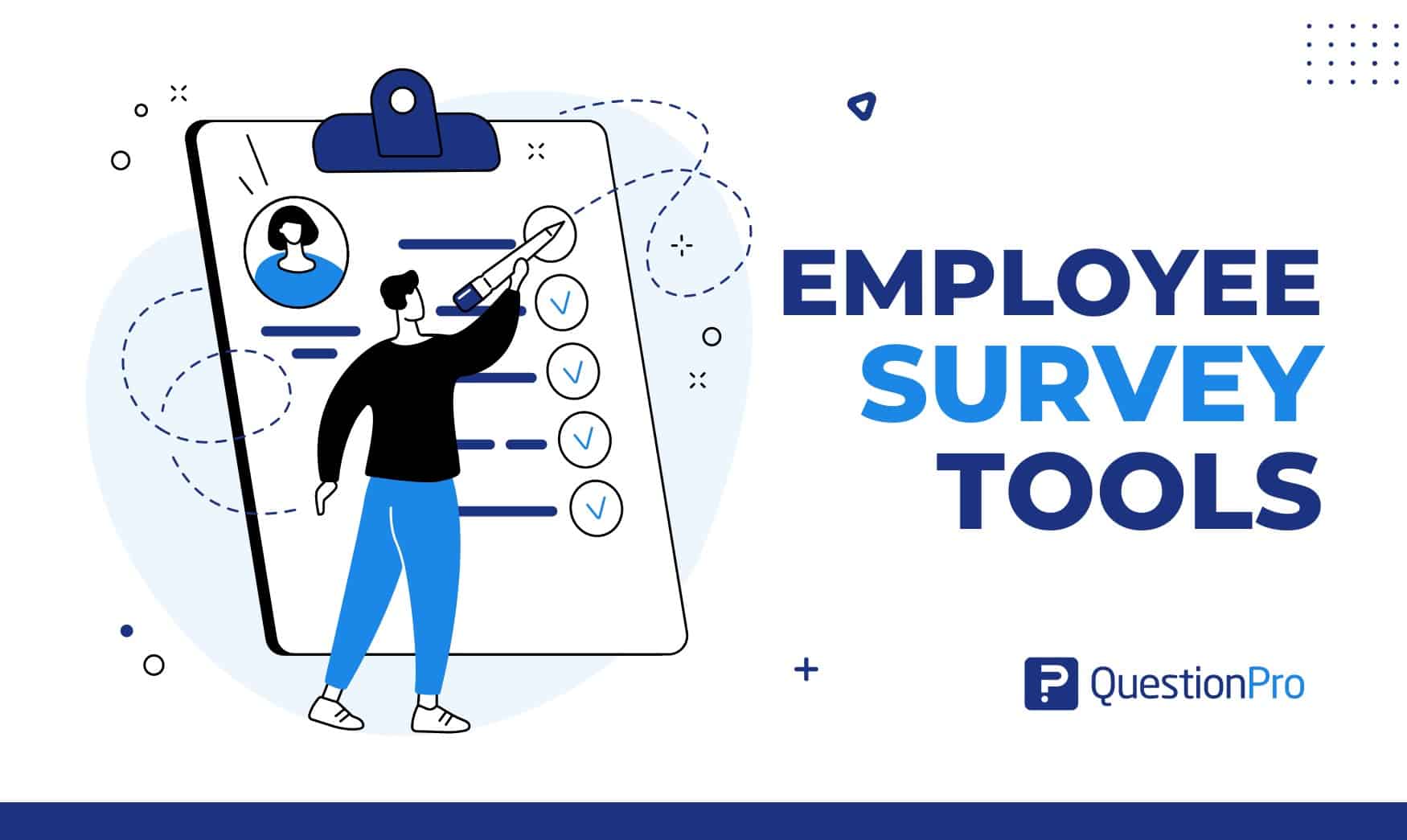
12 Best Employee Survey Tools for Organizational Excellence
Other categories.
- Academic Research
- Artificial Intelligence
- Assessments
- Brand Awareness
- Case Studies
- Communities
- Consumer Insights
- Customer effort score
- Customer Engagement
- Customer Experience
- Customer Loyalty
- Customer Research
- Customer Satisfaction
- Employee Benefits
- Employee Engagement
- Employee Retention
- Friday Five
- General Data Protection Regulation
- Insights Hub
- Life@QuestionPro
- Market Research
- Mobile diaries
- Mobile Surveys
- New Features
- Online Communities
- Question Types
- Questionnaire
- QuestionPro Products
- Release Notes
- Research Tools and Apps
- Revenue at Risk
- Survey Templates
- Training Tips
- Uncategorized
- Video Learning Series
- What’s Coming Up
- Workforce Intelligence

An official website of the United States government
The .gov means it’s official. Federal government websites often end in .gov or .mil. Before sharing sensitive information, make sure you’re on a federal government site.
The site is secure. The https:// ensures that you are connecting to the official website and that any information you provide is encrypted and transmitted securely.
- Publications
- Account settings
Preview improvements coming to the PMC website in October 2024. Learn More or Try it out now .
- Advanced Search
- Journal List
- J Korean Med Sci
- v.37(16); 2022 Apr 25

A Practical Guide to Writing Quantitative and Qualitative Research Questions and Hypotheses in Scholarly Articles
Edward barroga.
1 Department of General Education, Graduate School of Nursing Science, St. Luke’s International University, Tokyo, Japan.
Glafera Janet Matanguihan
2 Department of Biological Sciences, Messiah University, Mechanicsburg, PA, USA.
The development of research questions and the subsequent hypotheses are prerequisites to defining the main research purpose and specific objectives of a study. Consequently, these objectives determine the study design and research outcome. The development of research questions is a process based on knowledge of current trends, cutting-edge studies, and technological advances in the research field. Excellent research questions are focused and require a comprehensive literature search and in-depth understanding of the problem being investigated. Initially, research questions may be written as descriptive questions which could be developed into inferential questions. These questions must be specific and concise to provide a clear foundation for developing hypotheses. Hypotheses are more formal predictions about the research outcomes. These specify the possible results that may or may not be expected regarding the relationship between groups. Thus, research questions and hypotheses clarify the main purpose and specific objectives of the study, which in turn dictate the design of the study, its direction, and outcome. Studies developed from good research questions and hypotheses will have trustworthy outcomes with wide-ranging social and health implications.
INTRODUCTION
Scientific research is usually initiated by posing evidenced-based research questions which are then explicitly restated as hypotheses. 1 , 2 The hypotheses provide directions to guide the study, solutions, explanations, and expected results. 3 , 4 Both research questions and hypotheses are essentially formulated based on conventional theories and real-world processes, which allow the inception of novel studies and the ethical testing of ideas. 5 , 6
It is crucial to have knowledge of both quantitative and qualitative research 2 as both types of research involve writing research questions and hypotheses. 7 However, these crucial elements of research are sometimes overlooked; if not overlooked, then framed without the forethought and meticulous attention it needs. Planning and careful consideration are needed when developing quantitative or qualitative research, particularly when conceptualizing research questions and hypotheses. 4
There is a continuing need to support researchers in the creation of innovative research questions and hypotheses, as well as for journal articles that carefully review these elements. 1 When research questions and hypotheses are not carefully thought of, unethical studies and poor outcomes usually ensue. Carefully formulated research questions and hypotheses define well-founded objectives, which in turn determine the appropriate design, course, and outcome of the study. This article then aims to discuss in detail the various aspects of crafting research questions and hypotheses, with the goal of guiding researchers as they develop their own. Examples from the authors and peer-reviewed scientific articles in the healthcare field are provided to illustrate key points.
DEFINITIONS AND RELATIONSHIP OF RESEARCH QUESTIONS AND HYPOTHESES
A research question is what a study aims to answer after data analysis and interpretation. The answer is written in length in the discussion section of the paper. Thus, the research question gives a preview of the different parts and variables of the study meant to address the problem posed in the research question. 1 An excellent research question clarifies the research writing while facilitating understanding of the research topic, objective, scope, and limitations of the study. 5
On the other hand, a research hypothesis is an educated statement of an expected outcome. This statement is based on background research and current knowledge. 8 , 9 The research hypothesis makes a specific prediction about a new phenomenon 10 or a formal statement on the expected relationship between an independent variable and a dependent variable. 3 , 11 It provides a tentative answer to the research question to be tested or explored. 4
Hypotheses employ reasoning to predict a theory-based outcome. 10 These can also be developed from theories by focusing on components of theories that have not yet been observed. 10 The validity of hypotheses is often based on the testability of the prediction made in a reproducible experiment. 8
Conversely, hypotheses can also be rephrased as research questions. Several hypotheses based on existing theories and knowledge may be needed to answer a research question. Developing ethical research questions and hypotheses creates a research design that has logical relationships among variables. These relationships serve as a solid foundation for the conduct of the study. 4 , 11 Haphazardly constructed research questions can result in poorly formulated hypotheses and improper study designs, leading to unreliable results. Thus, the formulations of relevant research questions and verifiable hypotheses are crucial when beginning research. 12
CHARACTERISTICS OF GOOD RESEARCH QUESTIONS AND HYPOTHESES
Excellent research questions are specific and focused. These integrate collective data and observations to confirm or refute the subsequent hypotheses. Well-constructed hypotheses are based on previous reports and verify the research context. These are realistic, in-depth, sufficiently complex, and reproducible. More importantly, these hypotheses can be addressed and tested. 13
There are several characteristics of well-developed hypotheses. Good hypotheses are 1) empirically testable 7 , 10 , 11 , 13 ; 2) backed by preliminary evidence 9 ; 3) testable by ethical research 7 , 9 ; 4) based on original ideas 9 ; 5) have evidenced-based logical reasoning 10 ; and 6) can be predicted. 11 Good hypotheses can infer ethical and positive implications, indicating the presence of a relationship or effect relevant to the research theme. 7 , 11 These are initially developed from a general theory and branch into specific hypotheses by deductive reasoning. In the absence of a theory to base the hypotheses, inductive reasoning based on specific observations or findings form more general hypotheses. 10
TYPES OF RESEARCH QUESTIONS AND HYPOTHESES
Research questions and hypotheses are developed according to the type of research, which can be broadly classified into quantitative and qualitative research. We provide a summary of the types of research questions and hypotheses under quantitative and qualitative research categories in Table 1 .
Research questions in quantitative research
In quantitative research, research questions inquire about the relationships among variables being investigated and are usually framed at the start of the study. These are precise and typically linked to the subject population, dependent and independent variables, and research design. 1 Research questions may also attempt to describe the behavior of a population in relation to one or more variables, or describe the characteristics of variables to be measured ( descriptive research questions ). 1 , 5 , 14 These questions may also aim to discover differences between groups within the context of an outcome variable ( comparative research questions ), 1 , 5 , 14 or elucidate trends and interactions among variables ( relationship research questions ). 1 , 5 We provide examples of descriptive, comparative, and relationship research questions in quantitative research in Table 2 .
Hypotheses in quantitative research
In quantitative research, hypotheses predict the expected relationships among variables. 15 Relationships among variables that can be predicted include 1) between a single dependent variable and a single independent variable ( simple hypothesis ) or 2) between two or more independent and dependent variables ( complex hypothesis ). 4 , 11 Hypotheses may also specify the expected direction to be followed and imply an intellectual commitment to a particular outcome ( directional hypothesis ) 4 . On the other hand, hypotheses may not predict the exact direction and are used in the absence of a theory, or when findings contradict previous studies ( non-directional hypothesis ). 4 In addition, hypotheses can 1) define interdependency between variables ( associative hypothesis ), 4 2) propose an effect on the dependent variable from manipulation of the independent variable ( causal hypothesis ), 4 3) state a negative relationship between two variables ( null hypothesis ), 4 , 11 , 15 4) replace the working hypothesis if rejected ( alternative hypothesis ), 15 explain the relationship of phenomena to possibly generate a theory ( working hypothesis ), 11 5) involve quantifiable variables that can be tested statistically ( statistical hypothesis ), 11 6) or express a relationship whose interlinks can be verified logically ( logical hypothesis ). 11 We provide examples of simple, complex, directional, non-directional, associative, causal, null, alternative, working, statistical, and logical hypotheses in quantitative research, as well as the definition of quantitative hypothesis-testing research in Table 3 .
Research questions in qualitative research
Unlike research questions in quantitative research, research questions in qualitative research are usually continuously reviewed and reformulated. The central question and associated subquestions are stated more than the hypotheses. 15 The central question broadly explores a complex set of factors surrounding the central phenomenon, aiming to present the varied perspectives of participants. 15
There are varied goals for which qualitative research questions are developed. These questions can function in several ways, such as to 1) identify and describe existing conditions ( contextual research question s); 2) describe a phenomenon ( descriptive research questions ); 3) assess the effectiveness of existing methods, protocols, theories, or procedures ( evaluation research questions ); 4) examine a phenomenon or analyze the reasons or relationships between subjects or phenomena ( explanatory research questions ); or 5) focus on unknown aspects of a particular topic ( exploratory research questions ). 5 In addition, some qualitative research questions provide new ideas for the development of theories and actions ( generative research questions ) or advance specific ideologies of a position ( ideological research questions ). 1 Other qualitative research questions may build on a body of existing literature and become working guidelines ( ethnographic research questions ). Research questions may also be broadly stated without specific reference to the existing literature or a typology of questions ( phenomenological research questions ), may be directed towards generating a theory of some process ( grounded theory questions ), or may address a description of the case and the emerging themes ( qualitative case study questions ). 15 We provide examples of contextual, descriptive, evaluation, explanatory, exploratory, generative, ideological, ethnographic, phenomenological, grounded theory, and qualitative case study research questions in qualitative research in Table 4 , and the definition of qualitative hypothesis-generating research in Table 5 .
Qualitative studies usually pose at least one central research question and several subquestions starting with How or What . These research questions use exploratory verbs such as explore or describe . These also focus on one central phenomenon of interest, and may mention the participants and research site. 15
Hypotheses in qualitative research
Hypotheses in qualitative research are stated in the form of a clear statement concerning the problem to be investigated. Unlike in quantitative research where hypotheses are usually developed to be tested, qualitative research can lead to both hypothesis-testing and hypothesis-generating outcomes. 2 When studies require both quantitative and qualitative research questions, this suggests an integrative process between both research methods wherein a single mixed-methods research question can be developed. 1
FRAMEWORKS FOR DEVELOPING RESEARCH QUESTIONS AND HYPOTHESES
Research questions followed by hypotheses should be developed before the start of the study. 1 , 12 , 14 It is crucial to develop feasible research questions on a topic that is interesting to both the researcher and the scientific community. This can be achieved by a meticulous review of previous and current studies to establish a novel topic. Specific areas are subsequently focused on to generate ethical research questions. The relevance of the research questions is evaluated in terms of clarity of the resulting data, specificity of the methodology, objectivity of the outcome, depth of the research, and impact of the study. 1 , 5 These aspects constitute the FINER criteria (i.e., Feasible, Interesting, Novel, Ethical, and Relevant). 1 Clarity and effectiveness are achieved if research questions meet the FINER criteria. In addition to the FINER criteria, Ratan et al. described focus, complexity, novelty, feasibility, and measurability for evaluating the effectiveness of research questions. 14
The PICOT and PEO frameworks are also used when developing research questions. 1 The following elements are addressed in these frameworks, PICOT: P-population/patients/problem, I-intervention or indicator being studied, C-comparison group, O-outcome of interest, and T-timeframe of the study; PEO: P-population being studied, E-exposure to preexisting conditions, and O-outcome of interest. 1 Research questions are also considered good if these meet the “FINERMAPS” framework: Feasible, Interesting, Novel, Ethical, Relevant, Manageable, Appropriate, Potential value/publishable, and Systematic. 14
As we indicated earlier, research questions and hypotheses that are not carefully formulated result in unethical studies or poor outcomes. To illustrate this, we provide some examples of ambiguous research question and hypotheses that result in unclear and weak research objectives in quantitative research ( Table 6 ) 16 and qualitative research ( Table 7 ) 17 , and how to transform these ambiguous research question(s) and hypothesis(es) into clear and good statements.
a These statements were composed for comparison and illustrative purposes only.
b These statements are direct quotes from Higashihara and Horiuchi. 16
a This statement is a direct quote from Shimoda et al. 17
The other statements were composed for comparison and illustrative purposes only.
CONSTRUCTING RESEARCH QUESTIONS AND HYPOTHESES
To construct effective research questions and hypotheses, it is very important to 1) clarify the background and 2) identify the research problem at the outset of the research, within a specific timeframe. 9 Then, 3) review or conduct preliminary research to collect all available knowledge about the possible research questions by studying theories and previous studies. 18 Afterwards, 4) construct research questions to investigate the research problem. Identify variables to be accessed from the research questions 4 and make operational definitions of constructs from the research problem and questions. Thereafter, 5) construct specific deductive or inductive predictions in the form of hypotheses. 4 Finally, 6) state the study aims . This general flow for constructing effective research questions and hypotheses prior to conducting research is shown in Fig. 1 .

Research questions are used more frequently in qualitative research than objectives or hypotheses. 3 These questions seek to discover, understand, explore or describe experiences by asking “What” or “How.” The questions are open-ended to elicit a description rather than to relate variables or compare groups. The questions are continually reviewed, reformulated, and changed during the qualitative study. 3 Research questions are also used more frequently in survey projects than hypotheses in experiments in quantitative research to compare variables and their relationships.
Hypotheses are constructed based on the variables identified and as an if-then statement, following the template, ‘If a specific action is taken, then a certain outcome is expected.’ At this stage, some ideas regarding expectations from the research to be conducted must be drawn. 18 Then, the variables to be manipulated (independent) and influenced (dependent) are defined. 4 Thereafter, the hypothesis is stated and refined, and reproducible data tailored to the hypothesis are identified, collected, and analyzed. 4 The hypotheses must be testable and specific, 18 and should describe the variables and their relationships, the specific group being studied, and the predicted research outcome. 18 Hypotheses construction involves a testable proposition to be deduced from theory, and independent and dependent variables to be separated and measured separately. 3 Therefore, good hypotheses must be based on good research questions constructed at the start of a study or trial. 12
In summary, research questions are constructed after establishing the background of the study. Hypotheses are then developed based on the research questions. Thus, it is crucial to have excellent research questions to generate superior hypotheses. In turn, these would determine the research objectives and the design of the study, and ultimately, the outcome of the research. 12 Algorithms for building research questions and hypotheses are shown in Fig. 2 for quantitative research and in Fig. 3 for qualitative research.

EXAMPLES OF RESEARCH QUESTIONS FROM PUBLISHED ARTICLES
- EXAMPLE 1. Descriptive research question (quantitative research)
- - Presents research variables to be assessed (distinct phenotypes and subphenotypes)
- “BACKGROUND: Since COVID-19 was identified, its clinical and biological heterogeneity has been recognized. Identifying COVID-19 phenotypes might help guide basic, clinical, and translational research efforts.
- RESEARCH QUESTION: Does the clinical spectrum of patients with COVID-19 contain distinct phenotypes and subphenotypes? ” 19
- EXAMPLE 2. Relationship research question (quantitative research)
- - Shows interactions between dependent variable (static postural control) and independent variable (peripheral visual field loss)
- “Background: Integration of visual, vestibular, and proprioceptive sensations contributes to postural control. People with peripheral visual field loss have serious postural instability. However, the directional specificity of postural stability and sensory reweighting caused by gradual peripheral visual field loss remain unclear.
- Research question: What are the effects of peripheral visual field loss on static postural control ?” 20
- EXAMPLE 3. Comparative research question (quantitative research)
- - Clarifies the difference among groups with an outcome variable (patients enrolled in COMPERA with moderate PH or severe PH in COPD) and another group without the outcome variable (patients with idiopathic pulmonary arterial hypertension (IPAH))
- “BACKGROUND: Pulmonary hypertension (PH) in COPD is a poorly investigated clinical condition.
- RESEARCH QUESTION: Which factors determine the outcome of PH in COPD?
- STUDY DESIGN AND METHODS: We analyzed the characteristics and outcome of patients enrolled in the Comparative, Prospective Registry of Newly Initiated Therapies for Pulmonary Hypertension (COMPERA) with moderate or severe PH in COPD as defined during the 6th PH World Symposium who received medical therapy for PH and compared them with patients with idiopathic pulmonary arterial hypertension (IPAH) .” 21
- EXAMPLE 4. Exploratory research question (qualitative research)
- - Explores areas that have not been fully investigated (perspectives of families and children who receive care in clinic-based child obesity treatment) to have a deeper understanding of the research problem
- “Problem: Interventions for children with obesity lead to only modest improvements in BMI and long-term outcomes, and data are limited on the perspectives of families of children with obesity in clinic-based treatment. This scoping review seeks to answer the question: What is known about the perspectives of families and children who receive care in clinic-based child obesity treatment? This review aims to explore the scope of perspectives reported by families of children with obesity who have received individualized outpatient clinic-based obesity treatment.” 22
- EXAMPLE 5. Relationship research question (quantitative research)
- - Defines interactions between dependent variable (use of ankle strategies) and independent variable (changes in muscle tone)
- “Background: To maintain an upright standing posture against external disturbances, the human body mainly employs two types of postural control strategies: “ankle strategy” and “hip strategy.” While it has been reported that the magnitude of the disturbance alters the use of postural control strategies, it has not been elucidated how the level of muscle tone, one of the crucial parameters of bodily function, determines the use of each strategy. We have previously confirmed using forward dynamics simulations of human musculoskeletal models that an increased muscle tone promotes the use of ankle strategies. The objective of the present study was to experimentally evaluate a hypothesis: an increased muscle tone promotes the use of ankle strategies. Research question: Do changes in the muscle tone affect the use of ankle strategies ?” 23
EXAMPLES OF HYPOTHESES IN PUBLISHED ARTICLES
- EXAMPLE 1. Working hypothesis (quantitative research)
- - A hypothesis that is initially accepted for further research to produce a feasible theory
- “As fever may have benefit in shortening the duration of viral illness, it is plausible to hypothesize that the antipyretic efficacy of ibuprofen may be hindering the benefits of a fever response when taken during the early stages of COVID-19 illness .” 24
- “In conclusion, it is plausible to hypothesize that the antipyretic efficacy of ibuprofen may be hindering the benefits of a fever response . The difference in perceived safety of these agents in COVID-19 illness could be related to the more potent efficacy to reduce fever with ibuprofen compared to acetaminophen. Compelling data on the benefit of fever warrant further research and review to determine when to treat or withhold ibuprofen for early stage fever for COVID-19 and other related viral illnesses .” 24
- EXAMPLE 2. Exploratory hypothesis (qualitative research)
- - Explores particular areas deeper to clarify subjective experience and develop a formal hypothesis potentially testable in a future quantitative approach
- “We hypothesized that when thinking about a past experience of help-seeking, a self distancing prompt would cause increased help-seeking intentions and more favorable help-seeking outcome expectations .” 25
- “Conclusion
- Although a priori hypotheses were not supported, further research is warranted as results indicate the potential for using self-distancing approaches to increasing help-seeking among some people with depressive symptomatology.” 25
- EXAMPLE 3. Hypothesis-generating research to establish a framework for hypothesis testing (qualitative research)
- “We hypothesize that compassionate care is beneficial for patients (better outcomes), healthcare systems and payers (lower costs), and healthcare providers (lower burnout). ” 26
- Compassionomics is the branch of knowledge and scientific study of the effects of compassionate healthcare. Our main hypotheses are that compassionate healthcare is beneficial for (1) patients, by improving clinical outcomes, (2) healthcare systems and payers, by supporting financial sustainability, and (3) HCPs, by lowering burnout and promoting resilience and well-being. The purpose of this paper is to establish a scientific framework for testing the hypotheses above . If these hypotheses are confirmed through rigorous research, compassionomics will belong in the science of evidence-based medicine, with major implications for all healthcare domains.” 26
- EXAMPLE 4. Statistical hypothesis (quantitative research)
- - An assumption is made about the relationship among several population characteristics ( gender differences in sociodemographic and clinical characteristics of adults with ADHD ). Validity is tested by statistical experiment or analysis ( chi-square test, Students t-test, and logistic regression analysis)
- “Our research investigated gender differences in sociodemographic and clinical characteristics of adults with ADHD in a Japanese clinical sample. Due to unique Japanese cultural ideals and expectations of women's behavior that are in opposition to ADHD symptoms, we hypothesized that women with ADHD experience more difficulties and present more dysfunctions than men . We tested the following hypotheses: first, women with ADHD have more comorbidities than men with ADHD; second, women with ADHD experience more social hardships than men, such as having less full-time employment and being more likely to be divorced.” 27
- “Statistical Analysis
- ( text omitted ) Between-gender comparisons were made using the chi-squared test for categorical variables and Students t-test for continuous variables…( text omitted ). A logistic regression analysis was performed for employment status, marital status, and comorbidity to evaluate the independent effects of gender on these dependent variables.” 27
EXAMPLES OF HYPOTHESIS AS WRITTEN IN PUBLISHED ARTICLES IN RELATION TO OTHER PARTS
- EXAMPLE 1. Background, hypotheses, and aims are provided
- “Pregnant women need skilled care during pregnancy and childbirth, but that skilled care is often delayed in some countries …( text omitted ). The focused antenatal care (FANC) model of WHO recommends that nurses provide information or counseling to all pregnant women …( text omitted ). Job aids are visual support materials that provide the right kind of information using graphics and words in a simple and yet effective manner. When nurses are not highly trained or have many work details to attend to, these job aids can serve as a content reminder for the nurses and can be used for educating their patients (Jennings, Yebadokpo, Affo, & Agbogbe, 2010) ( text omitted ). Importantly, additional evidence is needed to confirm how job aids can further improve the quality of ANC counseling by health workers in maternal care …( text omitted )” 28
- “ This has led us to hypothesize that the quality of ANC counseling would be better if supported by job aids. Consequently, a better quality of ANC counseling is expected to produce higher levels of awareness concerning the danger signs of pregnancy and a more favorable impression of the caring behavior of nurses .” 28
- “This study aimed to examine the differences in the responses of pregnant women to a job aid-supported intervention during ANC visit in terms of 1) their understanding of the danger signs of pregnancy and 2) their impression of the caring behaviors of nurses to pregnant women in rural Tanzania.” 28
- EXAMPLE 2. Background, hypotheses, and aims are provided
- “We conducted a two-arm randomized controlled trial (RCT) to evaluate and compare changes in salivary cortisol and oxytocin levels of first-time pregnant women between experimental and control groups. The women in the experimental group touched and held an infant for 30 min (experimental intervention protocol), whereas those in the control group watched a DVD movie of an infant (control intervention protocol). The primary outcome was salivary cortisol level and the secondary outcome was salivary oxytocin level.” 29
- “ We hypothesize that at 30 min after touching and holding an infant, the salivary cortisol level will significantly decrease and the salivary oxytocin level will increase in the experimental group compared with the control group .” 29
- EXAMPLE 3. Background, aim, and hypothesis are provided
- “In countries where the maternal mortality ratio remains high, antenatal education to increase Birth Preparedness and Complication Readiness (BPCR) is considered one of the top priorities [1]. BPCR includes birth plans during the antenatal period, such as the birthplace, birth attendant, transportation, health facility for complications, expenses, and birth materials, as well as family coordination to achieve such birth plans. In Tanzania, although increasing, only about half of all pregnant women attend an antenatal clinic more than four times [4]. Moreover, the information provided during antenatal care (ANC) is insufficient. In the resource-poor settings, antenatal group education is a potential approach because of the limited time for individual counseling at antenatal clinics.” 30
- “This study aimed to evaluate an antenatal group education program among pregnant women and their families with respect to birth-preparedness and maternal and infant outcomes in rural villages of Tanzania.” 30
- “ The study hypothesis was if Tanzanian pregnant women and their families received a family-oriented antenatal group education, they would (1) have a higher level of BPCR, (2) attend antenatal clinic four or more times, (3) give birth in a health facility, (4) have less complications of women at birth, and (5) have less complications and deaths of infants than those who did not receive the education .” 30
Research questions and hypotheses are crucial components to any type of research, whether quantitative or qualitative. These questions should be developed at the very beginning of the study. Excellent research questions lead to superior hypotheses, which, like a compass, set the direction of research, and can often determine the successful conduct of the study. Many research studies have floundered because the development of research questions and subsequent hypotheses was not given the thought and meticulous attention needed. The development of research questions and hypotheses is an iterative process based on extensive knowledge of the literature and insightful grasp of the knowledge gap. Focused, concise, and specific research questions provide a strong foundation for constructing hypotheses which serve as formal predictions about the research outcomes. Research questions and hypotheses are crucial elements of research that should not be overlooked. They should be carefully thought of and constructed when planning research. This avoids unethical studies and poor outcomes by defining well-founded objectives that determine the design, course, and outcome of the study.
Disclosure: The authors have no potential conflicts of interest to disclose.
Author Contributions:
- Conceptualization: Barroga E, Matanguihan GJ.
- Methodology: Barroga E, Matanguihan GJ.
- Writing - original draft: Barroga E, Matanguihan GJ.
- Writing - review & editing: Barroga E, Matanguihan GJ.
ReviseSociology
A level sociology revision – education, families, research methods, crime and deviance and more!
Research Methods
Table of Contents
Last Updated on October 13, 2023 by Karl Thompson
Sociologists use a range of quantitative and qualitative, primary and secondary social research methods to collect data about society.
The main types of research method are:
- Social surveys (questionnaires and structured interviews)
- Experiments (Lab and Field)
- Unstructured interviews
- Partipant Observation
- Secondary qualitative data
- Official Statistics.
This page provides links to more in depth posts on all of the above research methods. It has primarily been written for students studying the A Level Sociology AQA 7192 specification, and incorporates Methods in the Context of Education.

Research Methods at a Glance – Key Concepts
Research Methods Top Ten Key Concepts – start here if you’re all at sea – includes simple explanations of terms such as validity, reliability, representativeness, Positivism and Interpretivism .
Research Methods A-Z Glossary – a more comprehensive index of the key terms you need to know for AS and A Level Sociology .

An Introduction to Research Methods
Without research methods there is no sociology!
This section covers the basics of the different types of research method and factors influencing choice of research methods, also the important distinction between Positivism and Interpretivism.
Research Methods in Sociology – An Introduction – d etailed class notes covering the basic types of research method available to sociologists such as social surveys, interviews, experiments, and observations
Factors Effecting Choice of Research Topic in Sociology – detailed class notes on the theoretical, ethical, and practical factors effecting the choice of research methods
Factors Effecting Choice of Research Method in Sociology – detailed class notes covering theoretical, practical and ethical factors and the nature of topic. NB choice of topic will affect choice of research method. Choice of topic and method are different issues!
Positivism and Interpretivism – Positivists generally prefer quantitative methods, Interpretivists prefer qualitative methods – this post consists of brief summary revision notes and revision diagrams outlining the difference between positivist and interpretivist approaches to social research.
Positivism, Sociology and Social Research – detailed class notes on the relationship between The Enlightenment, industrialisation and positivist sociology, which sees sociology as a science.
Stages of Social Research – detailed class notes covering research design, operationalising concepts, sampling, pilot studies, data collection and data analysis.
Outline and explain two practical problems which might affect social research (10) – A model answer to this exam question, which could appear on either paper 7191 (1) or 7191 (3).
Good Resources for Teaching and Learning Research Method s – simply links (with brief descriptions) which take you to a range of text books and web sites which focus on various aspects of quantitative and qualitative research methods. NB this post is very much a work in progress, being updated constantly.
Primary Quantitative Research Methods
social surveys.
An Introduction to Social Surveys – a brief introduction to the use of different types of survey in social research, including structured questionnaires and interviews and different ways of administering surveys such as online, by phone or face to face.
The advantages and disadvantages of social surveys in social research – detailed class notes covering the theoretical, practical and ethical strengths and limitations of social surveys. Generally, surveys are preferred by positivists and good for simple topics, but not so good for more complex topics which require a ‘human touch’ .
Structured Interviews in Social Research – Interviews are effectively one of the means of administering social surveys. This post covers the different contexts (types) of structured interview, and the stages of doing them. It also looks at the strengths, limitations and criticisms.
Experiments
An Introduction to Experiments in Sociology – a brief introduction covering definitions of key terms including hypotheses, dependent and independent variables and the Hawthorne Effect. NB sociologists don’t generally use experiments, especially not lab experiments, but you still need to know about them!
Laboratory Experiments in Sociology – detailed class notes on the strengths and limitations of laboratory experiments. Sociologists don’t generally use lab experiments, but examiners seem to ask questions about them more than other methods – one hypothesis for why is that sociology examiners have a burning hatred of teenagers.
Field Experiments in Sociology – detailed class notes on the strengths and limitations of field experiments. Field experiments take place in real life social settings so are more ‘sociological’ than lab experiments.
Seven Examples of Field Experiment for Sociology – class notes outlining a mixture of seven classic and contemporary field experiments relevant to various aspects of the AS and A level sociology syllabus .
Longitudinal Studies
Longitudinal Studies – These are interval studies designed to explore changes over a long period of time. Researchers start with a sample and keep going back to that same sample periodically – say every year, or every two years, to explore how and why changes occur.
The Seven Up Series – an in-depth look at Britain’s longest running and best loved Longitudinal study.
What Makes a Good Life ? – Lessons from a Longitudinal Study – This is one of the longest running Longitudinal studies in the world – the respondents were in their 20s when it started, now those who are still alive are in their 80s.
Primary Qualitative Research Methods
Primary qualitative research methods tend to be favoured by Interpretivists as they allow respondents to speak for themselves, and should thus yield valid data. However, because qualitative methods tend to involve the researcher getting more involved with the respondents, there is a risk that the subjective views of the researcher could interfere with the results, which could compromise both the validity and reliability of such methods.
Qualitative research methods also tend to be time consuming and so it can be difficult to to them with large samples of people.
Participant Observation
Overt and Covert Participant Observation – Participant Observation is where researchers take part in the life of respondents, sometimes for several months or even years, and try to ‘see the world through their eyes’. Overt research is where respondents know the researcher is doing research, covert is where the researcher is undercover.
The strengths and limitations of covert participant observation – sociologists don’t generally use covert participant observation because of the ethical problem of deception means they can’t get funding. This methods is more commonly used by journalists doing investigative reporting, or you could even say undercover police officers use it, and you can use these examples to illustrate the advantages and disadvantages of this method.
Some recent examples of sociological studies using participant observation – including Pearson’s covert research into football hooligans and Mears’s research into the modelling industry.
Non-Participant Observation – detailed class notes on non-participant observation. This is where the researcher observes from the sidelines and makes observations. Probably the most commonly used form of this is the OFSTED inspection.
Interviews in Social Research – This post consists of detailed class notes focusing strengths and limitations of mainly unstructured interviews, which are like a guided conversation that allow respondents the freedom to speak for themselves.
Secondary Research Methods
Official statistics.
Official Statistics in Sociology – class notes on the general strengths and limitations of official statistics, which are numerical data collected by the government. Examples include crime statistics, school league tables and education statistics.
Evaluating the Usefulness of Official Statistics – the UK government collects a wide variety of statistics, the validity of which can vary enormously. This post explores the validity of Religious belief statistics, crime and prison statistics, and immigration data, among other sources of data.
Cross National Comparisons – Comparing data across countries using official statistics can provide insight into the causes of social problems such as poverty, and war and conflict. This post looks at how you might go about doing this and the strengths and limitations of this kind of research.
Univariate Analysis in Quantitative Social Research – This involves looking at one variable at a time. This post covers the strengths and limitations of bar charts, pie charts and box plots.
Secondary Qualitative Data
Secondary Qualitative Data Analysis in Sociology – class notes covering private and public documents. Public documents include any written or visual document produced with an audience in mind, such things as government reports and newspapers, whereas private documents refer to personal documents such as diaries and letters which are not intended to be seen by their authors.
Content Analysis of the Media in Social Research – class notes covering formal content (quantitative) analysis and semiology .
Personal documents in social research – a more in-depth look at the strengths and limitations of using sources such as diaries and letters as sources of data.
Autobiographies in social research – Autobiographies are popular with the British public, but how useful are they as sources of data for the social researcher?
Sociology, Science and Value Freedom (Part of A2 Theory and Methods)
Sociology and Value Freedom – Detailed class notes .
Methods in Context – Research Methods Applied to Education
Field Experiments applied to Education – are Chinese Teaching Methods the Best? This is a summary of a documentary in which some students at one school undertook a Chinese style of teaching for 3 months, involving 12 hour days and ‘teach from the front techniques’. The students were then tested and their results compared to students from the same school who stuck to the traditional British way of teaching. The results may surprise you!
Participant Observation in Education – focusing on the work of Paul Willis and Mac An Ghail.
Non-Participant Observation in Education – focusing on OFSTED inspections, as these are probably the most commonly used of all methods in education .
The Strengths and Limitations of Education Statistics – This post discusses the strengths and limitations of results statistics. NB these may not be as valid as you think .
Evaluating the Usefulness of Secondary Qualitative Data to Research Education – there are lot of documents sociologists may use to research education, including school promotional literature and web sites, policy documents, written records on students, and, if they can access them, personal messages between students referring to what they think about school.
Focus on the AS and A Level Exams
Research Methods Practice Questions for A-level Sociology – you will get a 10 mark question on both papers SCLY1 and SCLY3 most likely asking you to ‘outline and explain’ the strengths and limitations of any of the main research methods. This post outlines some of the many variations.
Research Methods Essays – How to Write Them – general advice on writing research methods essays for the AS and A level sociology exams. This post covers the PET technique – Practical, Ethical and Theoretical.
Assess the Strengths of Using Participant Observation in Social Research (20) – example essay, top mark band.
Methods in Context Essay Template – a suggested gap fill essay plan on how to answer these challenging ‘applied research methods’ questions.
Methods in Context Mark Scheme – pared down mark scheme – easy to understand! It may surprise you to know that you can get up to 12/20 for just writing about the method, without even applying it to the question!
Outline and explain two advantages of overt compared to covert observation (10) – you might think that being undercover provides you with more valid data than when respondents know you are observing them, however, there are a few reasons why this might not be the case. This post explores why, and some of the other advantages overt has over covert observation. (Honestly, covert is a lot of hassle!). NB this post is written as a response to an exam style question .
Using Participant Observation to research pupils with behavourial difficulties (20) – a model answer for this methods in context style of essay.
For more links to methods and applied methods essays see my page – ‘ Exams, Essays and Short Answer Questions ‘.
Other Relevant Posts
Learning to Labour by Paul Willis – Summary and Evaluation of Research Methods .
How old are twitter users? – applied sociology – illustrates some of the problems us using social media to uncover social trends.
Twitter users by occupation and social class – applied sociology – illustrates some of the problems us using social media to uncover social trends.
Other posts and links will be forthcoming throughout 2020, check back soon .
Theory and Methods A Level Sociology Revision Bundle
If you like this sort of thing, then you might like my Theory and Methods Revision Bundle – specifically designed to get students through the theory and methods sections of A level sociology papers 1 and 3.

Contents include:
- 74 pages of revision notes
- 15 mind maps on various topics within theory and methods
- Five theory and methods essays
- ‘How to write methods in context essays’.
For better value I’ve bundled all of the above topics into six revision bundles , containing revision notes, mind maps, and exam question and answers, available for between £4.99 and £5.99 on Sellfy .

Best value is my A level sociology revision mega bundle – which contains the following:
- over 200 pages of revision notes
- 60 mind maps in pdf and png formats
- 50 short answer exam practice questions and exemplar answers
- Covers the entire A-level sociology syllabus, AQA focus.
Share this:
- Share on Tumblr
Thank you for visiting nature.com. You are using a browser version with limited support for CSS. To obtain the best experience, we recommend you use a more up to date browser (or turn off compatibility mode in Internet Explorer). In the meantime, to ensure continued support, we are displaying the site without styles and JavaScript.
- View all journals
- My Account Login
- Explore content
- About the journal
- Publish with us
- Sign up for alerts
- Open access
- Published: 24 April 2024
Quantitative analysis and stochastic modeling of osteophyte formation and growth process on human vertebrae based on radiographs: a follow-up study
- Tong Wu 1 ,
- Changxi Wang 1 , 3 &
- Kang Li 1 , 2 , 3
Scientific Reports volume 14 , Article number: 9393 ( 2024 ) Cite this article
120 Accesses
Metrics details
- Applied mathematics
- Disease prevention
- Musculoskeletal system
- Radiography
Osteophytes are frequently observed in elderly people and most commonly appear at the anterior edge of the cervical and lumbar vertebrae body. The anterior osteophytes keep developing and will lead to neck/back pain over time. In clinical practice, the accurate measurement of the anterior osteophyte length and the understanding of the temporal progression of anterior osteophyte growth are of vital importance to clinicians for effective treatment planning. This study proposes a new measuring method using the osteophyte ratio index to quantify anterior osteophyte length based on lateral radiographs. Moreover, we develop a continuous stochastic degradation model with time-related functions to characterize the anterior osteophyte formation and growth process on cervical and lumbar vertebrae over time. Follow-up data of anterior osteophytes up to 9 years are obtained for measurement and model validation. The agreement test indicates excellent reproducibility for our measuring method. The proposed model accurately fits the osteophyte growth paths. The model predicts the mean time to onset of pain and obtained survival function of the degenerative vertebrae. This research opens the door to future quantification and mathematical modeling of the anterior osteophyte growth on human cervical and lumbar vertebrae. The measured follow-up data is shared for future studies.
Similar content being viewed by others
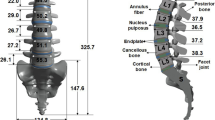
Analysis of the physiological load on lumbar vertebrae in patients with osteoporosis: a finite-element study
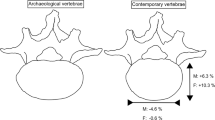
Temporal Trends in Vertebral Dimensions – a case study from Finland
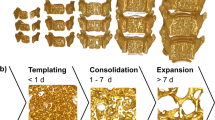
Micro-CT data of early physiological cancellous bone formation in the lumbar spine of female C57BL/6 mice
Introduction.
The spine is a crucial human body component that degenerates over time 1 . One of the most critical indicators of spinal degeneration is osteophyte formation, which is reported to be found in 20–30% of the elderly population and requires proper diagnosis and in-time interventions in clinical practice 2 , 3 , 4 .
Osteophytes most commonly appear at the anterior edges of the cervical and lumbar vertebral bodies 5 , 6 . They are not symptomatic early but would keep growing as the patient ages 7 . The anterior osteophytes that continuously protrude would result in neck/back pain at the point where they mechanically compress the spinal nerve roots or soft tissue structures 8 , 9 . In clinical practice, the oversized anterior osteophyte is a significant concern 2 , 5 . For patients with anterior osteophyte growing on the spine, lateral radiographs are mainly used for osteophyte length evaluation and diagnosis 4 . The accurate measurement of the anterior osteophyte length on lateral radiographs and the understanding of the temporal progression of anterior osteophyte growth are of vital importance to clinicians to develop effective treatment plans.
To date, two categories of measuring methods have been proposed to quantify the anterior osteophyte length on lateral radiographs. The first category 10 , 11 records the measured data directly without processing while the second category 12 uses an index to represent the osteophyte length. Regression models are mainly used to characterize the osteophyte growth process. Watanabe et al. 13 use a linear regression model to describe the relationship between the osteophyte index and age. Gender 14 , weight and BMI 15 , bone mass 16 , etc. are found to be the affecting factors of the osteophyte growth process.
The previous studies contribute to the understanding of the biology of osteophyte growth and may facilitate clinical prevention. However, they have the following three limitations. Firstly, existing methods of measuring anterior osteophyte length are not fully applicable in clinical practices since (i) the measurement steps are not well-defined and (ii) the proposed indicator cannot be applied to radiographs with different scale plates. Secondly, the previously used regression models fail to predict the time-related progression of the osteophyte growth process and the time to onset of pain (TTOOP) under uncertainties. Thirdly, to date, most of the studies on osteophytes are cross-sectional, where data is collected only once for each patient. Few longitudinal studies are available for understanding the temporal progression of osteophyte growth over an extended period.
The osteophyte formation and growth process is subject to uncertainties and may undergo significant variations. The specific degeneration initiation time is not a constant value but rather follows a distribution as it differs among the population due to factors such as incorrect posture and acute spinal injuries 17 . In addition, uncertainties exist during the osteophyte growth process since the biological effects may affect the growth rate of the osteophyte 18 and there are person-to-person variations due to their genetic and lifestyle differences 19 . Compared to the commonly used regression model, stochastic models that incorporate the temporal uncertainties and random factors are suitable for capturing the evolution of osteophyte formation and growth over time 20 .
Currently, a variety of stochastic models have been implemented in degradation analysis 21 . The survival function and the TTOOP can be obtained and the corresponding condition-based maintenance plans are scheduled for the systems subject to degradation to reduce the pain risk 22 . Despite their straightforward physical interpretations and tractable mathematical properties, the applications of stochastic models in characterizing the human spine degeneration process are still at their early stages.
In this study, a robust measuring method for anterior osteophyte length on lateral radiographs is proposed, which can be applied to spine degeneration quantification in clinical studies. Furthermore, we develop a stochastic model to characterize and predict the temporal progression of osteophyte formation and growth with high accuracy, validity and interpretability. Considering that the actual lifetime data are usually censored and aperiodic, the maximum likelihood estimation (MLE) method is used to estimate the parameters. The model is validated using long-term follow-up data. This work contributes not only to the understanding of the osteophyte growth process, but also to the survival assessment and prognostic care for degenerative spinal vertebrae in clinical practice.
Agreement of measurements
In this study, we use the osteophyte ratio index (ORI) to quantify the anterior osteophyte length on lateral radiographs. A robust measuring method is proposed as shown in Fig. 7 b legend. Four observers with different ages and experiences are included in the agreement tests to compare the robustness of the measuring methods proposed in this study and Walraevens et al. 12 ’s study. The results of agreement tests for the two measuring methods are shown in Table 1 .
Validation of the osteophyte formation and growth process model
The results of ORI measurements of the 23 cervical vertebrae samples and 74 lumbar vertebrae samples are shown in Figs. 1 a and 2 a respectively, where the x-axis represents the ages of patients, and the y-axis represents the corresponding ORIs. Based on the MLE functions described in Eq. ( 6 ), the parameters of the Weibull distribution are estimated as \(\left\{ {\hat{a} = {52}{\text{.5216}}, \, \hat{b} = {5}{\text{.7469}}} \right\}\) and \(\left\{ {\hat{a} = {5}4.9779, \, \hat{b} = {2}{\text{.3443}}} \right\}\) for cervical and lumbar vertebrae respectively. The actual osteophytes formation time of samples and the probability density function (PDF) of the fitted Weibull distribution for cervical and lumbar vertebrae are shown in Figs. 1 b and 2 b respectively.
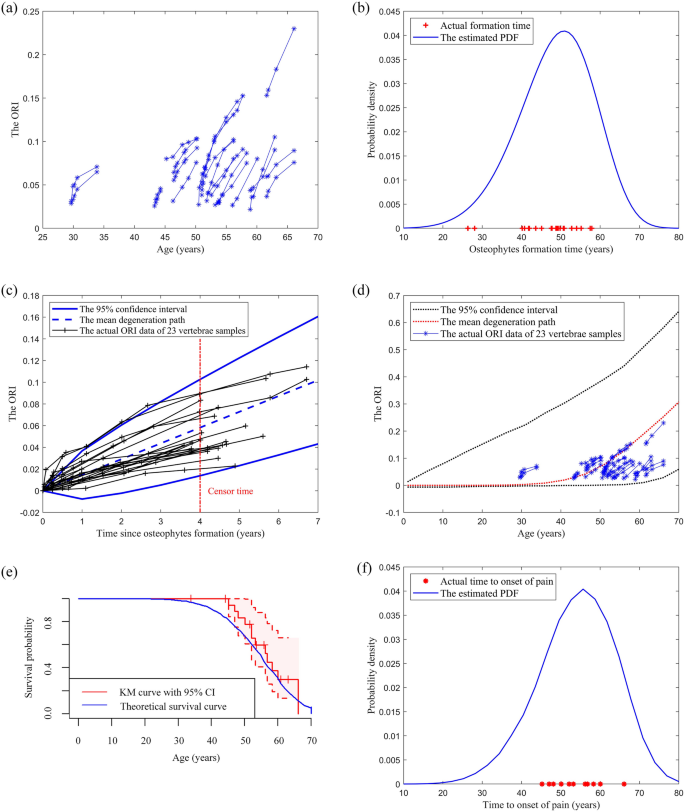
( a ) The osteophyte growth data of the 23 cervical vertebrae. ORI refers to the Osteophyte Ratio Index. ( b ) The validation of the Weibull distribution. The actual cervical osteophyte formation time is compared with the estimated probability density function (PDF). ( c ) The validation of the Wiener process. The actual cervical osteophyte growth paths are compared with the predicted confidence interval (CI). ( d ) The actual and predicted osteophyte formation and growth process of a cervical vertebra. ( e ) The comparison of the theoretical cervical vertebra’s survival function and the Kaplan–Meier (KM) curve of the actual data. ( f ) The comparison of the cervical vertebra’s time to onset of pain and the actual data with the estimated PDF.
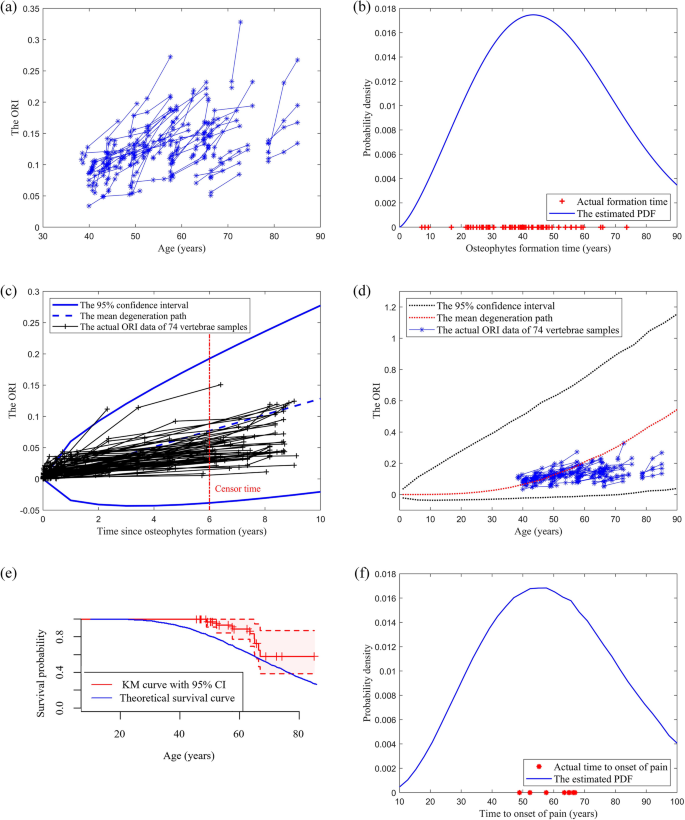
( a ) The osteophyte growth data of the 74 lumbar vertebrae. ORI refers to the Osteophyte Ratio Index. ( b ) The validation of the Weibull distribution. The actual lumbar osteophyte formation time is compared with the estimated probability density function (PDF). ( c ) The validation of the Wiener process. The actual lumbar osteophyte growth paths are compared with the predicted confidence interval (CI). ( d ) The actual and predicted osteophyte formation and growth process of a lumbar vertebra. ( e ) The comparison of the theoretical lumbar vertebra’s survival function and the Kaplan–Meier (KM) curve of the actual data. ( f ) The comparison of the lumbar vertebra’s time to onset of pain and the actual data with the estimated PDF.
Based on MLE functions described in Eq. ( 7 ), the parameters of the Wiener process are estimated as \(\left\{ {\hat{\mu } = {0}{\text{.0145553}}, \, \hat{\sigma } = {0}{\text{.0113259}}} \right\}\) and \(\left\{ {\hat{\mu } = {0}{\text{.012836}}, \, \hat{\sigma } = {0}{\text{.024040}}} \right\}\) for cervical and lumbar vertebrae respectively. Based on the parameters, the 95% confidence interval and the mean path of the predicted ORI of cervical and lumbar vertebrae are shown in Figs. 1 c and 2 c respectively, where the x-axis represents the duration since the osteophytes are formed, and the y-axis represents the corresponding ORIs.
Based on Eq. ( 2 ) and the estimated parameters, the mean and 95% confidence interval of the osteophyte length are obtained and shown in Figs. 1 d and 2 d for cervical and lumbar vertebrae respectively. The degeneration data are plotted in blue lines with asterisk markers.
Validation of the survival metrics
To validate the proposed survival metrics, we collected the X-ray image series datasets obtained at discrete time instants during the patients’ follow-up visits, where the ORI value of 0.08 is of the highest count over the 23 cervical vertebrae samples’ ORI series and the value of 0.15 is of the highest count over the 74 lumbar vertebrae samples’ ORI series. For illustration purposes, we assume that the pain threshold values are 0.08 and 0.15, which also conforms to the principles of statistics. The ages when the ORIs reach the pain thresholds are treated as the actual TTOOP values. Compared to other ORI values which only have one or two samples, the counts of 0.08 and 0.15 are higher and thus are more suitable to be used as the pain threshold values. Therefore, without loss of generality, we assume pain thresholds of 0.08 and 0.15 for cervical and lumbar vertebrae respectively to validate the survival metrics.
The theoretical survival curve of cervical/lumbar vertebrae under the pain threshold (i.e., the maximum clinically-acceptable osteophyte size) of 0.08/0.15 is obtained based on Eq. ( 4 ) and shown in Figs. 1 e and 2 e by a blue line. As this study involves survival data, Kaplan–Meier (KM) survival analysis is conducted and the corresponding KM curve of cervical/lumbar vertebrae is shown in Figs. 1 e and 2 e by a red line, which reflects the actual survival probability of cervical/lumbar vertebrae. The shaded pink region represents the 95% confidence interval of the KM curve.
The PDF of the TTOOP of cervical/lumbar vertebrae under the pain threshold of 0.08/0.15 is estimated based on Eq. ( 3 ) and shown in Figs. 1 f and 2 f by a blue line. The actual TTOOP is shown in Figs. 1 f and 2 f with red asterisk markers.
Based on Eq. ( 5 ), the mean time to onset of pain (MTTOOP) of the cervical/lumbar vertebra given the pain threshold of 0.08/0.15 are obtained. The theoretical and actual MTTOOP for cervical vertebra are 54.11 and 53.76 years respectively (percentage difference = 0.65%). The theoretical and actual MTTOOP for the lumbar vertebra are 60.40 and 60.66 years respectively (percentage difference = 0.43%).
Figures 1 b and 2 b indicate that the Weibull distribution can accurately characterize the osteophyte formation time of a cervical and lumbar vertebra. Note that all of the test ORI data of each cervical and lumbar vertebra after the censor time (i.e., the data series on the right of the red line) fall into the predicted confidence intervals in Figs. 1 c and 2 c. It shows that the Wiener process can accurately characterize the osteophyte growth of a cervical and lumbar vertebra. In Figs. 1 d and 2 d, all the actual data fall into the 95% CI. The large CI is due to the large variation and uncertainties of the osteophyte formation time and growth process among the population. It can increase the likelihood that the interval contains the mean response and also contributes to a more accurate and general prediction of the time to onset of pain for the population with large variations in degeneration performance. Figures 1 e and 2 e show that the theoretical survival curve derived from the proposed model accurately matches the actual survival curve under the pain-threshold assumption. It is observed from Figs. 1 f and 2 f that the distribution of the TTOOP obtained from the proposed model accurately characterizes the actual cases. In addition, the theoretical MTTOOP calculated from the proposed model fits the actual data precisely (i.e., The theoretical and actual MTTOOP for cervical vertebra are 54.11 and 53.76 years respectively (percentage difference = 0.65%); The theoretical and actual MTTOOP for the lumbar vertebra are 60.40 and 60.66 years respectively (percentage difference = 0.43%)). Results show that the model is of high accuracy, validity and interpretability.
According to Walraevens et al. 12 , there are no detailed guiding principles for finding the middle point on the irregularly shaped vertebra. As the measurements of osteophyte length are small in magnitude and highly sensitive to noises, measuring methods with ill-defined instructions may result in inaccurate conclusions and low repeatability in clinical practice. Thus, it is urgent to propose a novel robust osteophyte length indicator. In this paper, an indicator ORI (Eq. ( 1 )) with well-defined measuring steps (Fig. 7 b legend) is proposed for the quantification of anterior osteophyte length based on lateral radiographs. In the study of Walraevens et al. 12 , AB and CD (Fig. 7 c) are measured at the middle of the vertebral body to represent the anteroposterior diameter. However, as the anterior and posterior vertebra contour is curved, the determination of midpoints A/B/C/D is subjective. By comparison, parallel and tangent lines are more objective and suitable for measuring curved structures. In addition, since \(h_{0}\) is generally considered as unchanged during the spine degeneration process, the ratio function (Eq. ( 1 )) that divides the \(h_{1}\) by \(h_{0}\) in the same radiographic image can overcome the scale error caused by various generations of medical equipment and be applied to radiographs of different sizes to reflect the osteophyte size. Table 1 shows that the indicator ORI under the proposed well-defined measuring steps has excellent reliability (i.e., with ICC > 0.90 and low PD values). By comparison, the measurement in Walraevens , et al. 12 ’s study has lower ICC scores and higher PD values. This indicates that our method is more robust and can be reliably used in clinical practice and in related research to quantify the anterior osteophyte length.
Without an osteophyte growth prediction model for clinical reference, the current treatment plans for degenerative vertebrae are mainly made by clinicians and the treatment quality highly depends on their experience as shown in Fig. 3 a 9 . The treatment plans made by inexperienced clinicians may fail to provide interventions in time and result in unexpected pain. Studies reported that progressive symptoms caused by anterior osteophytes are easily missed during the early evaluation 25 . When severe and unexpected pain develops due to untimely treatment, surgery is required to remove the osteophyte 26 . The development of accurate and valid mathematical models of osteophyte growth is urgently needed to estimate the osteophyte formation time and growth rate, which can be used as a reference for clinical management in the early stage. In this paper, we develop a stochastic model to characterize and predict the temporal progression of osteophyte formation and growth under uncertainties. Compared to the existing stochastic models such as the Wiener processes 21 , Markov Chains 27 , Gamma processes 28 and Inverse Gaussian processes 29 , we characterize the initiation time of the osteophyte formation by Weibull distribution and incorporate it into the stochastic model by convolution. The proposed model with estimated parameters is especially suitable for characterizing osteophyte formation and growth. Previous studies mainly discuss the prevalence and symptoms of spinal osteophytes rather than quantitative prediction models that would assist prophylactic treatments. In this study, survival metrics can be derived from the stochastic model 20 . As the mean survival time and the pain risk of diseases are major concerns in clinical practice 30 , the derived survival metrics such as MTTOOP and survival function of the degenerated vertebrae can assist clinical decision-making. This study opens the door to the future application of stochastic models to predict degenerative changes in the human spine.
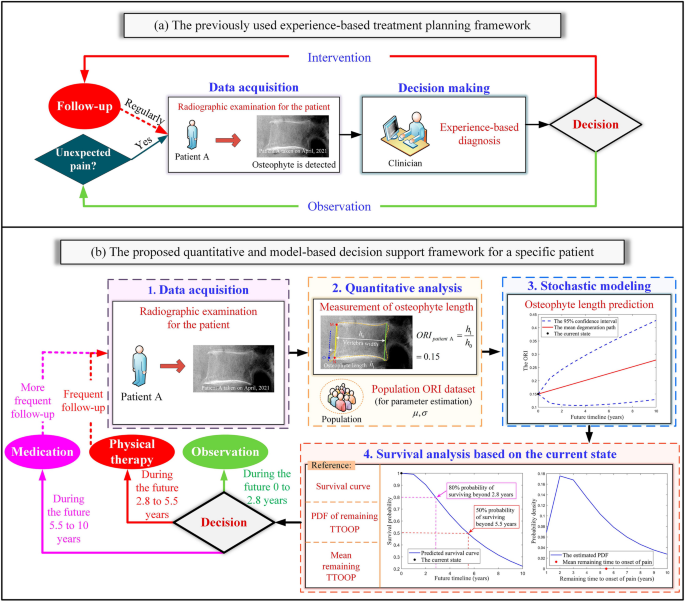
( a ) The existing experienced-based treatment planning framework. ( b ) The proposed quantitative and model-based decision support framework.
Our work has implications for clinical practices. As the proposed models incorporate temporal uncertainties, random factors and person-to-person variation that evolve in the osteophyte formation and growth process, they can provide population-level inferences for insurance companies to develop proper insurance policies accordingly for different populations. In addition, we propose a quantitative and model-based decision support framework with four steps as shown in Fig. 3 b. Step 1: patient A takes a radiographic examination and an osteophyte is detected. Step 2: the indicator ORI is measured to quantify the osteophyte length. Here we assume that the osteophyte is on patient A’s lumbar vertebra and its ORI value is measured to be 0.15 at that time. Step 3: based on the parameters estimated from the population data and the measured initial ORI value, the clinicians can predict how the mean and 95% CI of the patient’s ORI would progress in the future 10 years. Step 4: survival analysis is performed where the survival curve, the PDF plot of the remaining TTOOP and the mean value of the remaining TTOOP are available for the clinician’s reference. For instance, we assume that 80% and 50% are survival probability thresholds for treatment change. The threshold values can be modified by medical professionals. As the survival curve shows that there is an 80% probability of surviving beyond 2.8 years, clinicians can put patient A in observation during the future 0 to 2.8 years. Since the survival probability will decrease to 50% at 5.5 years, frequent follow-up and physical therapy can be scheduled for patient A during the future 2.8–5.5 years. More frequent Follow-up care and medication need to be scheduled during the future 5.5–10 years where the survival probability is under 50%. Note that the treatment plans can be modified in the next follow-up visit based on the patient’s remeasured ORI and the corresponding survival-analysis plots. Compared to the experience-based treatment shown in Fig. 3 a, the proposed quantitative and model-based framework in Fig. 3 b can provide quantitative prediction for clinicians to make more personalized treatment plans. The survival-analysis plots in Fig. 3 b are obtained under the assumed lumbar pain threshold of 0.22. In clinical practice, the pain threshold can be determined based on the criteria provided in the Pain Threshold Definition subsection or modified by the medical professionals. The corresponding survival-analysis plots can be obtained by running the codes in Supplementary B on the MATLAB Platform.
Previous studies are mainly cross-sectional in design and use point datasets to investigate the correlation of osteophyte length with age. For instance, in Chanapa et al. 8 ’s study, five age groups (15–35, 36–60, 61–75, 76–85, and > 85 years old) are used and the mean osteophyte length on the vertebral body of patients in each group is obtained as shown in Fig. 4 a. There is only one data point for each patient that reflects the osteophyte growth level in that group. However, the progressive changes in osteophyte length of the same patient’s vertebrae are unknown. In the study of 31 , 32 , although 2–3 years of follow-up data are collected, they are rather short periods considering the slow and progressive osteophyte growth process. As a result, little change in osteophyte growth is observed during the short periods of the studies 33 . With discrete-time datasets or short-term follow-up data, there is a lack of historical data for model fitting and validation. Long-term follow-up data are crucial for understanding osteophyte growth and developing predictive models. In this study, we obtained long-term time-series follow-up radiographs as illustrated in Fig. 4 b. To the best of the authors' knowledge, it is relatively new to apply time-series osteophyte data for the progression assessment of osteophyte growth. It helps to understand the temporal progression of osteophyte growth over an extended period and to develop models for osteophyte growth prediction.
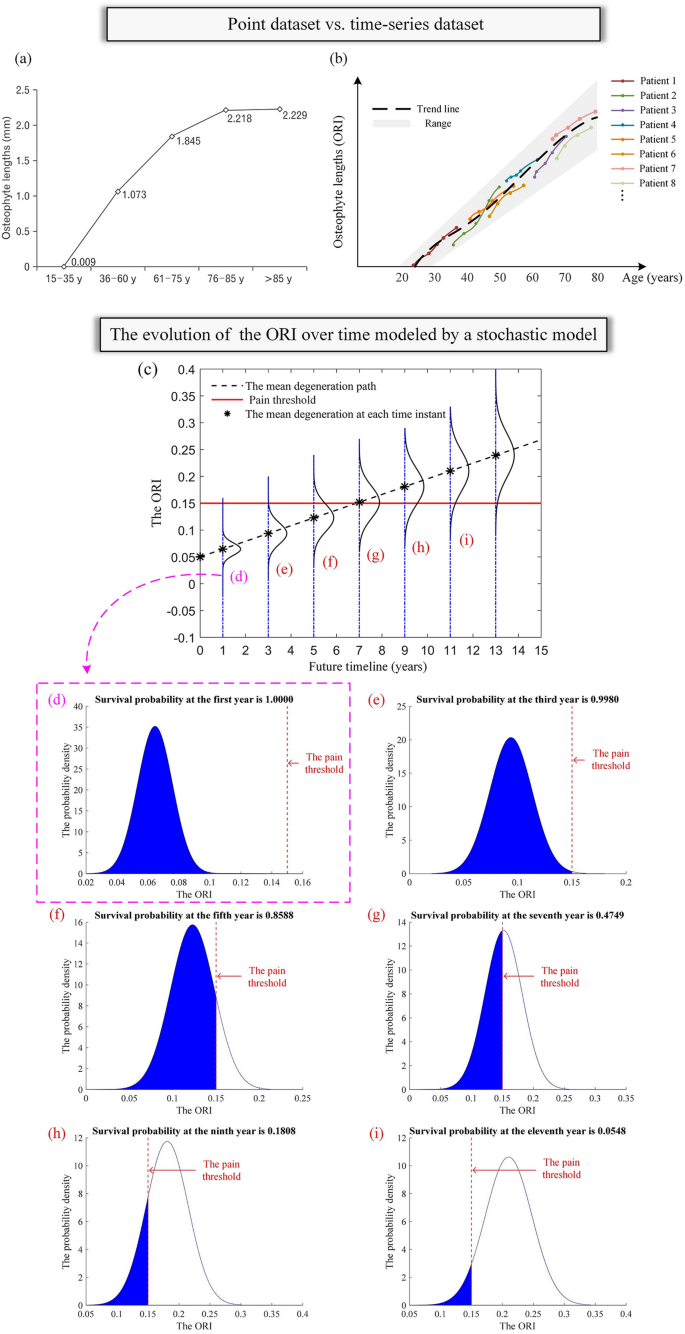
( a ) Point dataset in Chanapa et al. 8 ’s study. ( b ) Time-series dataset in our study. ( c ) The evolution of the PDFs of the ORI over time, assuming that the ORI at the current state is 0.05 and the pain threshold is 0.15 for illustration purposes. Survival probabilities of the vertebra at the first, third, fifth, seventh, ninth and eleventh year are shown in Figures ( d ), ( e ), ( f ), ( g ), ( h ) and ( i ), respectively.
In previous studies, deterministic models such as linear and logistic regression models are typically used in clinical analysis to predict the development of osteophyte-related diseases 34 , 35 , 36 , 37 . Compared to that, we used the stochastic process model which provides a range of estimates rather than point estimates. The range of estimates that, each of which is associated with a probability, can characterize the randomness and the temporal uncertainties associated with the evolution of the degeneration process. The uncertainties originate from both observable and unobservable factors including the variability of patients, the differences in medicine interventions, etc. The model uses variables to incorporate the random effects of those factors on the osteophyte growth process and therefore, is more appropriate to characterize and predict the osteophyte growth process than the deterministic models that only provide a certain value and neglect the person-to-person variation. Figure 4 c demonstrates the osteophyte growth progression modeled by the stochastic model—the Wiener process. The ORIs at a fixed time instant are normally distributed and the distribution of the ORI keeps evolving over time. The black-dashed line represents the most probable ORI growth path. When the pain threshold is 0.15 (indicated with the red-solid horizontal line in Fig. 4 c, the value is for illustrative purposes), the corresponding survival probability (i.e., survival function), which is the probability that the ORI is smaller than the pain threshold, keeps decreasing over time, as shown in Fig. 4 d–i. The distributions of the ORI in the future can be obtained based on the Wiener process. The medical professionals can provide more reasonable advice regarding the possible consequences and the corresponding probabilities given the current situation. To the best of the authors' knowledge, it is relatively new to apply stochastic models to predict osteophyte growth progression and obtain evaluation metrics that can provide valuable information for clinical decision-making.
Some limitations should be noted in our study. Firstly, the dataset includes the osteophyte formation and growth data of twenty-three cervical vertebrae and seventy-four lumbar vertebrae. Although the sample size is enough for parameter estimation and model validation, a larger quantity of data should be enrolled in future studies for more robust validation and more accurate estimation of the general population parameters. Secondly, this study proposes a basic model where the osteophyte formation and growth process are considered independent and the growth process is homogeneous. However, other behaviors, such as disc height reduction 38 , may occur simultaneously or successively with the osteophytes during the cervical and lumbar vertebrae degeneration and are reported to affect the osteophyte formation time and growth rate 39 . In addition, Gelse et al. 40 proposed that osteophyte growth shows a multi-stage pattern on the cell biological level. Therefore, both its association with other degenerative behaviors and its multi-stage behavior could be quantitatively investigated and enrolled into the future model for a more precise description (e.g., a narrower confidence interval) of osteophyte formation and growth process.
Figure 5 shows the workflow of our study. We develop a measuring method for the anterior osteophyte length and conduct an agreement test to show the robustness of our method. In addition, we develop a stochastic model for characterizing the osteophyte formation and growth process and derive the related survival metrics for clinical application purposes. Time-series radiographic datasets are obtained from the hospital for model validation. Based on the robust measuring method and accurate models, we propose a quantitative and model-based decision support framework for treatment planning of cervical and lumbar osteophytes in clinical practices. The detailed information is found in the subsequent subsections.
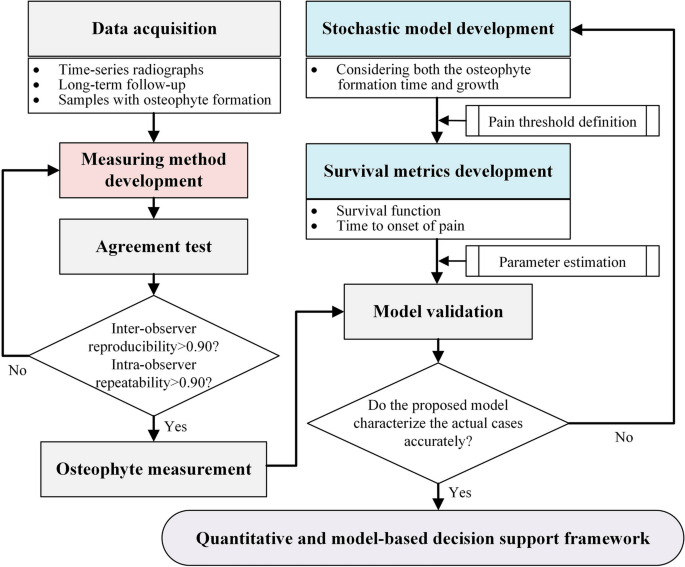
The workflow diagram.
Dataset description
This study focuses on the osteophyte formation time and time-dependent osteophyte growth on both cervical and lumbar vertebrae. Radiographic osteophyte formation and growth data collected from September 2009 to September 2021 are obtained from West China Hospital, Sichuan University. As the osteophytes most commonly form on the five cervical vertebrae C3 through C7 and rarely on C1 and C2 41 , we focus on the five levels C3-C7 to investigate the osteophyte formation and growth behavior. For lumbar vertebrae, as osteophytes are frequently reported to form on vertebrae L1 through L5 42 , the five levels are included in the dataset for lumbar osteophyte investigation. Males and females are reported to show similar patterns in osteophyte development 43 , so the gender is not considered in our study.
Figure 6 shows the database organization. Our dataset includes 29 radiographic series of cervical vertebrae from C3 to C7 (23 with osteophytes and 6 without osteophytes) and 103 radiographic series of lumbar vertebrae from L1 to L5 (74 with osteophytes and 29 without osteophytes), where each series corresponds to a vertebra and is collected during the patient’s follow-up visits as shown in Fig. 6 . The radiographic series of cervical and lumbar vertebra osteophytes are collected from 11 (at their first visit: mean age = 49.78 ± 8.72 years, age range = 29.65–61.62 years) and 33 (at their first visit: mean age = 53.07 ± 10.79 years, age range = 38.00–78.63 years) patients, respectively. One radiograph is taken for the vertebra and shows the osteophyte growth level of the vertebra during each visit. To protect the privacy of the patients, the specific dates of the radiographic images are not shown in Fig. 6 . The mean(max) follow-up time-span of patients with cervical and lumbar osteophytes are 4.39(6.71) and 6.77(9.13) years, respectively. Each series consists of over 3 follow-up visits. Radiographs are of high resolution and are viewed by RadiAnt DICOM Viewer.
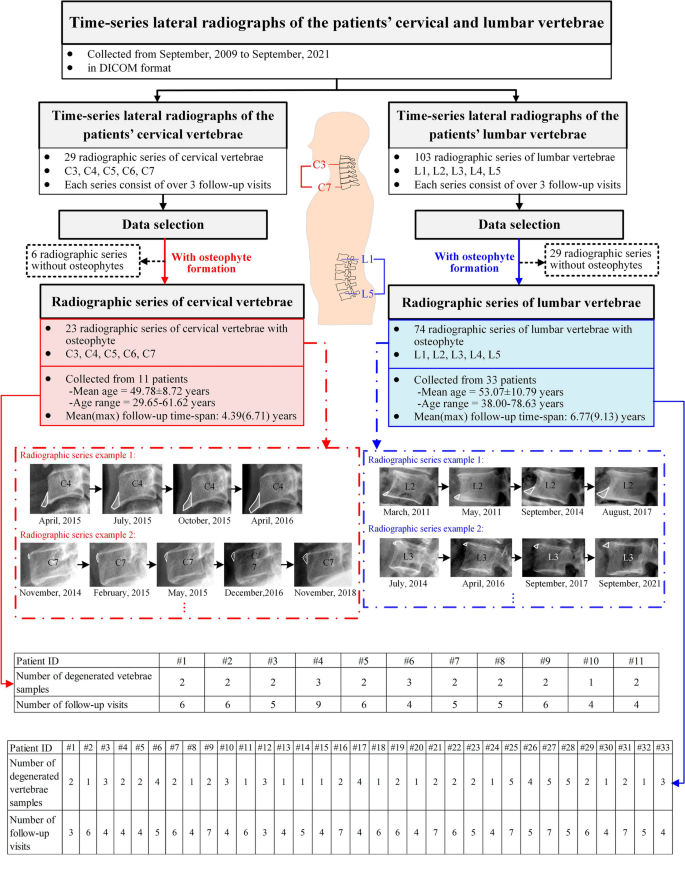
Database organization diagram.
Measurement of anterior osteophyte length based on lateral radiographs
Measuring methods.
In this study, a new indicator Osteophyte Ratio Index (ORI) is proposed as follows:
where \(h_{1}\) denotes the length of the osteophyte and \(h_{0}\) denotes the width of the cervical or lumbar vertebra body in the lateral view of the radiographic image.
The detailed measuring steps are in the Fig. 7 legend. The measurement is conducted with the RadiAnt DICOM Viewer 44 .
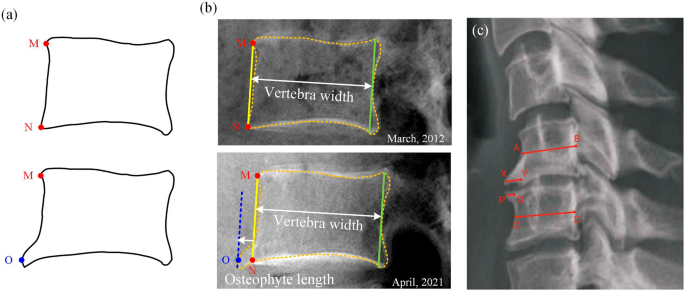
( a ) A normal vertebra (the top one) and a degenerated vertebra (the bottom one) with osteophyte in the anterior side (lower left-hand corner). Points M and N are the anterior edge points of the normal vertebra in the lateral view. Point O is the osteophyte tip in the lateral view. ( b ) Measurement of osteophyte length and vertebra width. An orange dashed line is drawn along the vertebra contour for illustrative purposes. The measurement method includes three steps. First, the points M and N are identified and a yellow line connecting the two points is drawn. A second line (green line) is drawn parallel to the yellow line and tangential to the innermost part of the right vertebra contour. The distance between the yellow and green lines is defined as the vertebra width (white double-sided arrow). A third line (blue dashed line) is drawn parallel to the yellow line and tangential to the osteophyte tip. The distance between the yellow and blue lines is defined as the osteophyte length (white single-sided arrow). ( c ) Measurement of osteophyte length in Walraevens et al. 12 ’s study. The osteophyte length (XY and PQ) is measured with respect to the anteroposterior diameter of the vertebral body (AB and CD, respectively). AB and CD are measured at the middle of the vertebral body.
Agreement test
Inter-observer reproducibility (i.e., the agreement between the measurements of two observers) and intra-observer repeatability (i.e., the agreement between the measurements of the same observer) of ORI are evaluated using the intra-class correlation coefficient (ICC) and percentage difference (PD) 45 . According to 46 , four observers (observers I, II, III and IV) are included to measure the lengths of anterior osteophytes using ORI independently from a subset of 50 randomly selected radiographs. Observers I, II, and III are a 35-year-old radiologic technologist, a 30-year-old orthopedist and a 23-year-old graduate student who majors in medical imaging technology, respectively. Observer IV is a 20-year-old undergraduate student who majors in engineering and has no clinical background. Each observer measures two rounds at a 2-week interval. The interpretation of ICC is based on the previous study 23 where ICC ≤ 0.50, 0.50 < ICC ≤ 0.75, 0.75 < ICC ≤ 0.90 and 0.90 < ICC refers to the poor, moderate, good and excellent agreement, respectively. It is reported that a smaller PD value indicates a lower difference and thus higher agreement 24 . For comparison, the same agreement test is performed by the same four observers on the index proposed in Walraevens et al. 12 ’s study.
Stochastic model development
The assumptions of the osteophyte formation and growth process of a single cervical/lumbar vertebra are made as follows: (1) After a random time, osteophyte forms on one vertebra due to factors such as aging and mechanical stresses; (2) The osteophyte continues to grow after the formation following a stochastic process; (3) Only the largest osteophyte at each vertebra is investigated. (4) The pain occurs when the length of its anterior osteophyte reaches the pain threshold. (5) As the osteophytes on cervical and lumbar spinal vertebrae show similar etiology and growth patterns 47 , their formation and growth are assumed to follow the same stochastic process but with different parameters. The cervical vertebra at different levels (i.e., C3 through C7), are similar in shape and function 48 , 49 and are treated as identical cervical subjects with the same parameters in our model. Likewise, lumbar vertebrae L1 to L5 are considered as identical lumbar subjects with the same parameters in our model as the degenerative behaviors are similar among the lumbar levels (i.e., L1 through L5) 50 .
The schematic diagram of the osteophyte formation and growth process is shown in Fig. 8 . Let \(\tau\) denote the osteophyte formation time of a vertebra. The degeneration status (i.e., osteophyte size) of the vertebra at time \(t\) is denoted as \(X\left( {t|\tau } \right)\) . Note that \(t - \tau\) is the length of time of its degeneration. The pain threshold \(c\) corresponds to the maximum acceptable osteophyte size of the vertebra and \(T\) corresponds to the time to onset of pain (TTOOP) when the degeneration status reaches \(c\) . Take the cervical vertebra as an example, pain occurs when a large anterior osteophyte compresses the pharyngeal wall (as illustrated in Fig. 8 ).
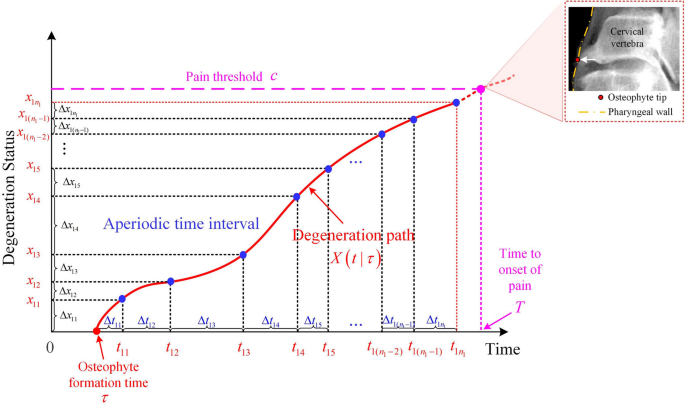
The schematic diagram of the osteophyte formation and growth on a single vertebra and the osteophyte growth data with aperiodic time interval.
Note that \(\tau\) is a random variable that reflects the biological properties of the cervical and lumbar vertebrae influenced by environmental and biological factors, physical activities, etc. In biomedical survival analysis, the two-parameter Weibull distribution is widely used to describe the time to develop a disease and is flexible in characterizing age-related diseases 51 . In this study, the osteophyte formation time since the patients' birthdays (i.e., age in years) is modeled with a Weibull distribution 52 .
In previous studies, a positive correlation has been found between the osteophyte index and age based on the linear regression models 13 . As it is observed that the osteophyte growth process has a linear trend and involves uncertainties, the widely implemented Wiener process 53 is suitable for characterizing the osteophyte growth path. Let \(X\left( {t|\tau } \right)\) denote the conditional osteophyte growth status of a cervical or lumbar vertebra at time \(t\) , given its osteophyte formation time \(\tau\) . For \(\forall t > \tau\) , \(X\left( {t|\tau } \right)\) follows a normal distribution \(N\left( {\mu \left( {t - \tau } \right),\sigma^{2} \left( {t - \tau } \right)} \right)\) .
In an osteophyte formation and growth process, the unconditional probability density function (PDF) of the degeneration status of a cervical or lumbar vertebra at time \(t\) is given by Eq. ( 2 ):
where \(a > 0\) and \(b > 0\) are the scale and shape parameters of the Weibull distribution. \(x\) is the degeneration status of the cervical or lumbar vertebra, the drift parameter \(\mu\) and the diffusion parameter \(\sigma\) correspond to the mean growth rate and the volatility of the osteophyte’s growth respectively.
Pain threshold definition
The anterior osteophyte that grows continuously on the cervical/lumbar vertebra may mechanically compress spinal nerve roots or soft tissue structures and lead to neck/back pain 8 , 9 . The pain threshold refers to the maximum clinically-acceptable osteophyte size. We assume that the patient with an osteophyte on cervical/lumbar vertebra that has an ORI value exceeding the pain threshold will experience neck/back pain. Given the pain threshold value \(c\) , the statistics including the time to onset of pain (TTOOP) distribution, survival function and mean time to onset of pain (MTTOOP) are derived based on the developed model. These statistics predict when the pain will occur and imply the probability that the patient will survive without pain. They can provide references for early intervention before the pain actually occurs.
In clinical practice, the pain thresholds are determined as 0.52 and 0.22 for cervical and lumbar vertebrae, respectively. The determination of the pain thresholds are as follows. According to the literature 54 , the anterior cervical osteophyte with an average length of over 10 mm will cause mechanical compression on the neck. Since the mean anteroposterior (AP) diameter of the cervical vertebral body is 19.13 mm 55 , we recommend the pain threshold \(c_{c} = 10/19.13 = 0.52\) for the cervical vertebra. Likewise, as Kojima et al. 56 propose that the anterior lumbar osteophyte length greater than 10 mm is associated with low back pain and the mean AP diameter of the lumbar vertebral body is 46.31 mm 57 , we recommend the pain threshold \(c_{l} = 10/46.31 = 0.22\) for lumbar vertebra. Note that the 0.52 and 0.22 are population-based recommended values, which are flexible and can be modified accordingly based on patient-specific characteristics. For instance, software or clinicians can measure the patient-specific AP diameter of the cervical/lumbar vertebral body on the patient’s lateral radiograph. The patient-specific pain threshold can be calculated by dividing the literature-recommended osteophyte length of 10 mm by the patient’s AP diameter.
Survival metrics development
Note that as the osteophyte growth follows a Wiener process with drift \(\mu\) and diffusion \(\sigma\) , TTOOP under pain threshold \(c\) after its formation follows an IG distribution \(\left( {T - \tau } \right) \sim IG\left( {\frac{c}{\mu },\frac{{c^{2} }}{{\sigma^{2} }}} \right)\) with a mean of \(\frac{c}{\mu }\) . Accordingly, the MTTOOP given its osteophyte formation time \(\tau\) is \(\frac{c}{\mu } + \tau\) .
The PDF of TTOOP given the pain threshold \(c\) is obtained as shown in Eq. ( 3 ):
where \(g\left( {t|\tau ,c} \right)\) denotes the conditional PDF of TTOOP of a cervical or lumbar vertebra given \(\tau\) is known.
The survival function and MTTOOP for a given threshold \(c\) are obtained in Eqs. ( 4 ) and ( 5 ):
where \(H\left( { \cdot |t,\tau } \right)\) denotes the conditional survival function of a cervical or lumbar vertebra at time \(t\) given \(\tau\) is known, \(\tau\) is the osteopyte formation time, \(E\left( {T|\tau ,c} \right)\) denotes the conditional TTOOP of a cervical or lumbar vertebra given \(\tau\) and \(c\) are known.
The codes for the calculation of Eqs. ( 2 ), ( 3 ), ( 4 ) and ( 5 ) are provided in Supplementary A for clinical application purposes. Clinicians can obtain the corresponding plots by running the program on the MATLAB Platform. In addition, the program can provide personalized prediction and survival analysis based on adjusted versions of the equations. The MATLAB codes are provided in Supplementary B.
Parameters estimation
The maximum likelihood estimation (MLE) algorithm is used to estimate the parameters of the model. The parameters of the proposed model include \(\left\{ {a,b,\mu ,\sigma } \right\}\) , where \(a,b\) are the parameters of the distribution of the osteophyte formation time, and \(\mu ,\sigma\) are the parameters that govern the osteophyte growth since the formation. It is assumed that the osteophyte formation and growth are independent and the likelihood functions for \(\left\{ {a,b} \right\}\) and \(\left\{ {\mu ,\sigma } \right\}\) are obtained independently. Parameters of the proposed model for cervical and lumbar vertebrae are estimated by the same method from separate data sources.
Parameters estimation of the distribution of osteophyte formation time
Assume the radiographic image series of a total of \(M\) cervical vertebrae are available. Among them, \(N\) vertebrae are found to have developed osteophyte over the data-collection period. Let \(\tau_{i}\) , \(1 \le i \le N\) denote the osteophyte formation time of the osteophyte on the \(i^{th}\) cervical vertebra, \(\tau_{k}\) , \(N + 1 \le k \le M\) denote the right-censoring time where the osteophyte has not developed on the cervical vertebra by the time of the last follow-up visit.
Taking the natural logarithm of the joint density:
The MLE estimators of \(a\) and \(b\) for the Weibull distribution are obtained by maximizing Eq. ( 6 ), which can be achieved by using the maxLik package in R.
As the actual radiographic images are not obtained continuously, the exact osteophyte formation time (i.e., the time when ORI begins to exceed zero) is not observed directly. In this study, 23 cervical vertebrae and 74 lumbar vertebrae developed osteophytes over the follow-up period. Since 6 cervical and 29 lumbar vertebrae have not developed osteophytes, the corresponding patient’s age at the last follow-up date is denoted as the right-censored osteophyte formation time 58 . As the overall osteophyte growth process has a linear trend 13 , the actual osteophyte formation time of each cervical/lumbar vertebra is estimated by linear interpolation based on its first and last observations. Both the actual and the right-censored osteophyte formation time are the INPUT for parameter estimation of the Weibull distribution.
Parameters estimation of the osteophyte growth process
The drift \(\mu\) and diffusion \(\sigma\) of the Wiener process are estimated as follows. Assume that a total of \(N\) cervical vertebrae with osteophyte growing on them are available. There are \(n_{i}\) measurements of osteophyte growth increment on the \(i^{th}\) cervical vertebra. Let \(\Delta x_{ij} \left( {1 \le i \le N,1 \le j \le n_{i} } \right)\) denote the \(j^{th}\) osteophyte growth increment on the \(i^{th}\) cervical vertebra and \(\Delta t_{ij} \left( {1 \le i \le N,1 \le j \le n_{i} } \right)\) denote the corresponding time interval for osteophyte growth.
Note that in reality, although periodic follow-up visits are suggested for patients with osteophyte growth on their cervical vertebrae, the follow-up visits are usually aperiodic. Accordingly, the obtained osteophyte growth observations are also aperiodic, as illustrated in Fig. 8 . The corresponding maximum likelihood estimators are obtained as follows.
The likelihood function of \(\mu\) and \(\sigma\) is:
where \(\Delta {\mathbf{x}}\) and \(\Delta {\mathbf{t}}\) are the vectors of the degeneration increments and time increments. The MLE estimators \(\left\{ {\hat{\mu },\hat{\sigma }} \right\}\) are obtained by maximizing the log-likelihood function using the maxLik package in R.
For the actual radiographic image datasets, all vertebrae samples with osteophytes developed are used for parameters estimation of the Wiener process. We censor the osteophyte growth data at time \(t = 4\) for cervical vertebrae and \(t = 6\) for lumbar vertebrae and use the data before the censor time for parameters estimation using MLE.
Ethical approval and consent to participate
This study was approved by the Institutional Review Board of West China Hospital. Written informed consent was obtained from all individual participants included in this study. We confirm that all methods were performed in accordance with the relevant guidelines and regulations.
Data availability
Summarized data have been presented and shared in this manuscript. The raw data that support the findings of this study are available from the West China Hospital but restrictions apply to the availability of these data, which were used under license for the current study, and so are not publicly available. Data are however available from the authors upon reasonable request and with permission of West China Hospital. Contact [email protected] to request the data from this study.
Kushchayev, S. V. et al. ABCs of the degenerative spine. Insights Imaging 9 (2), 253–274 (2018).
Article PubMed PubMed Central Google Scholar
Bone, R. C., Nahum, A. M. & Harris, A. S. Evaluation and correction of dysphagia-producing cervical osteophytosis. Laryngoscope 86 , 1–9 (2014).
Google Scholar
Klaassen, Z. et al. Vertebral spinal osteophytes. Anat. Sci. Int. 86 , 1–9 (2010).
Article PubMed Google Scholar
Song, A. R. et al. Surgical treatments on patients with anterior cervical hyperostosis-derived dysphagia. Ann. Rehabil. Med. 36 (5), 729–734 (2012).
Chanapa, P., Tohno, Y. & Mahakkanukrauh, P. Distribution and length of osteophytes in the lumbar vertebrae and risk of rupture of abdominal aortic aneurysms: A study of dry bones from Chiang Mai. Thailand. Anat. Cell Biol. 47 , 157–161 (2014).
Karasik, D. et al. Abdominal aortic calcification and exostoses at the hand and lumbar spine: the Framingham Study. Calcif. Tissue Int. 78 (1), 1–8 (2006).
Article CAS PubMed Google Scholar
Chan, A. Y., Mullin, J. P., Wathen, C. & Benzel, E. C. Degenerative spinal disease (cervical). In Principles of neurological surgery 4th edn 549–553 (Elsevier, Amsterdam, 2018).
Chapter Google Scholar
Chanapa, P., Yoshiyuki, T. & Mahakkanukrauh, P. Distribution and length of osteophytes in the lumbar vertebrae and risk of rupture of abdominal aortic aneurysms: A study of dry bones from Chiang Mai Thailand. Anat. Cell Biol. 47 (3), 157–161 (2014).
Al-Jafari, M. et al. Cervical spine osteophyte: A case report of an unusual presentation. Cureus 15 (9), e44762 (2023).
PubMed PubMed Central Google Scholar
Sekiya, I. et al. Medial tibial osteophyte width strongly reflects medial meniscus extrusion distance and medial joint space width moderately reflects cartilage thickness in knee radiographs. JMRI 56 (3), 824–834 (2022).
Moon, Y. et al. Factors correlated with the reducibility of varus deformity in knee osteoarthritis: An analysis using navigation guided TKA. Clin. Orthop. Surg. 5 , 36–43 (2013).
Walraevens, J. et al. Qualitative and quantitative assessment of degeneration of cervical intervertebral discs and facet. Eur. Spine J. Off. Publ. Eur. Spine Soc. Eur Spinal Deform. Soc. Eur. Sect. Cerv. Spine Res. Soc. 18 , 358–369 (2008).
Article Google Scholar
Watanabe, S. & Terazawa, K. Age estimation from the degree of osteophyte formation of vertebral columns in Japanese. Leg. Med. 8 (3), 156–160 (2006).
Resnick, D. & Niwayama, G. Degenerative disease of extraspinal locations . Diagnosis of Bone & Joint Disorders , 1263–1371 (1995).
Oishi, Y. et al. Lack of association between lumbar disc degeneration and osteophyte formation in elderly japanese women with back pain. Bone 32 (4), 405–411 (2003).
Hardcastle, S. A. et al. Osteophytes, enthesophytes, and high bone mass: a bone-forming triad with potential relevance in osteoarthritis. Arthritis Rheumatol. 66 (9), 2429–2439 (2014).
Chen, Y.R., Sung, K & Tharin, S. Symptomatic anterior cervical osteophyte causing dysphagia: Case report, imaging, and review of the literature . Cureus (2016).
Pelletier, J.-P. et al. Carprofen simultaneously reduces progression of morphological changes in cartilage and subchondral bone in experimental dog osteoarthritis. J RHEUMATOL. 27 , 2893–2902 (2000).
CAS PubMed Google Scholar
Wong, S., Chiu, K. Y. & Yan, C. H. Review article: Osteophytes. J. Orthop. Surg. 24 (3), 403–410 (2016).
Ye, Z. S. & Chen, N. The inverse Gaussian process as a degradation model. Technometrics 56 (3), 302–311 (2014).
Article MathSciNet Google Scholar
Si, X. S., Wang, W., Hu, C. H., Zhou, D. H. & Pecht, M. G. Remaining useful life estimation based on a nonlinear diffusion degradation process. IEEE Trans. Reliab. 61 (1), 50–67 (2012).
Lin, T. & Pham, H. A two-stage intervened decision system with state-dependent random inspection mechanisms. IEEE Trans. Comput. Soc. Syst. 6 (2), 365–376 (2019).
Koo, T. K. & Li, M. Y. A guideline of selecting and reporting intraclass correlation coefficients for reliability research. J. Chiropr. Med. 15 (2), 155–163 (2016).
Merino-Muñoz, P., Pérez-Contreras, J. & Aedo-Muñoz, E. The percentage change and differences in sport: a practical easy tool to calculate. Sport Perform. Sci. Rep. 118 , 446–450 (2020).
Li, C., Luo, W., Zhang, H., Zhao, J. & Gu, R. Case report: Diffuse idiopathic skeletal hyperostosis with ossification of the posterior longitudinal ligament in the cervical spine: A rare case with dysphagia and neurological deficit and literature review. Front. Surg. 9 , 963399 (2022).
Miyamoto, K. et al. Postsurgical recurrence of osteophytes causing dysphagia in patients with diffuse idiopathic skeletal hyperostosis. Eur. Spine J. 18 (11), 1652–1658 (2009).
Ossai, C. I., Boswell, B. & Davies, I. Markov chain modelling for time evolution of internal pitting corrosion distribution of oil and gas pipelines. Eng. Fail. Anal. 60 , 209–228 (2016).
Article CAS Google Scholar
Zhang, Y. Wiener and gamma processes overview for degradation modelling and prognostic (Norwegian university of science and technology, Trondheim, 2015).
Guo, J., Wang, C., Cabrera, J. & Elsayed, E. A. Improved inverse Gaussian process and bootstrap: Degradation and reliability metrics. Reliab. Eng. Syst. Saf. 178 , 269–277 (2018).
Quraishi, N. et al. Accuracy of the revised Tokuhashi score in predicting survival in patients with metastatic spinal cord compression (MSCC). Eur. Spine J. 22 (1), 21–26 (2013).
Article PubMed Central Google Scholar
Lane, N. E. et al. Running, osteoarthritis, and bone density: Initial 2-year longitudinal study. Am. J. Med. 88 (5), 452–459 (1990).
Felson, D. T. et al. Osteophytes and progression of knee osteoarthritis. Rheumatology (Oxford) 44 (1), 100–104 (2005).
Vilarrasa, N. et al. Evaluation of bone mineral density loss in morbidly obese women after gastric bypass: 3-Year follow-up. Obes. Surg. 21 (4), 465–472 (2011).
Figueiredo, C. P. et al. Quantification and impact of secondary osteoarthritis in patients with anti-citrullinated protein antibody-positive rheumatoid arthritis. A&R 68 (9), 2114–2121 (2016).
CAS Google Scholar
Kortekaas, M. C., Kwok, W.-Y., Reijnierse, M., Huizinga, T. W. J. & Kloppenburg, M. Osteophytes and joint space narrowing are independently associated with pain in finger joints in hand osteoarthritis. Ann. Rheum. Dis. 70 (10), 1835–1837 (2011).
Mathiessen, A., Slatkowsky-Christensen, B., Kvien, T. K., Haugen, I. K. & Hammer, H. B. Ultrasound-detected osteophytes predict the development of radiographic and clinical features of hand osteoarthritis in the same finger joints 5 years later. RMD Open 3 (2), e000505 (2017).
Neogi, T., Nevitt, M. C., Ensrud, K. E., Bauer, D. & Felson, D. T. The effect of alendronate on progression of spinal osteophytes and disc-space narrowing. Ann. Rheum. Dis. 67 (10), 1427–1430 (2008).
Harada, G. K. et al. Cervical spine MRI phenotypes and prediction of pain, disability and adjacent segment degeneration/disease after ACDF. J. Orthop. Res. 39 , 657 (2021).
Lipson, S. J. & Muir, H. Vertebral osteophyte formation in experimental disc degeneration. A&R 23 (3), 319–324 (1980).
Gelse, K., Söder, S., Eger, W., Diemtar, T. & Aigner, T. Osteophyte development–molecular characterization of differentiation stages. Osteoarthr. Cartil. 11 (2), 141–148 (2003).
Ezra, D., Hershkovitz, I., Salame, K., Alperovitch-Najenson, D. & Slon, V. Osteophytes in the cervical vertebral bodies (C3–C7)—Demographical perspectives. Anat. Rec. 302 (2), 226–231 (2019).
Kasai, Y., Kawakita, E., Sakakibara, T., Akeda, K. & Uchida, A. Direction of the formation of anterior lumbar vertebral osteophytes. BMC Musculoskelet. Disord. 10 (1), 4 (2009).
Snodgrass, J. J. Sex differences and aging of the vertebral column. J. Forensic Sci. 49 (3), 458–463 (2004).
Brühschwein, A. et al. Free DICOM-viewers for veterinary medicine: Survey and comparison of functionality and user-friendliness of medical imaging PACS-DICOM-viewer freeware for specific use in veterinary medicine practices. J. Dig. Imaging 33 (1), 54–63 (2020).
Ranganathan, P., Pramesh, C. S. & Aggarwal, R. Common pitfalls in statistical analysis: Measures of agreement. Perspect. Clin. Res. 8 (4), 187–191 (2017).
Landis, J. R. & Koch, G. G. The measurement of observer agreement for categorical data. Biometrics 33 (1), 159–174 (1977).
Klaassen, Z. et al. Vertebral spinal osteophytes. Anat. Sci. Int. 86 (1), 1–9 (2011).
Bland, J. H. & Boushey, D. R. Anatomy and physiology of the cervical spine. Semin Arthritis Rheum. 20 (1), 1–20 (1990).
Bazaldúa Cruz, J. J. et al. Morphometric study of cervical vertebrae C3–C7 in a population from Northeastern Mexico. INT. J. MORPHOL. 29 , 325–330 (2011).
Chaimongkhol, T., Thiamkaew, A. & Mahakkanukrauh, P. The characteristics of osteophyte around lumbar vertebral foramina associated with spinal stenosis. Anat. Cell Biol. 52 (2), 143–148 (2019).
Matsushita, S. et al. Lifetime data analysis of disease and aging by the weibull probability distribution. J. Clin. Epidemiol. 45 (10), 1165–1175 (1992).
Almalki, S. J. & Nadarajah, S. Modifications of the Weibull distribution: A review. Reliab. Eng. Syst. Saf. 124 , 32–55 (2014).
Huang, J. et al. Degradation modeling of mid-power white-light LEDs by using Wiener process. Opt. Express 23 (15), A966-978 (2015).
Article ADS CAS PubMed Google Scholar
Vodičar, M., Košak, R. & Vengust, R. Long-term results of surgical treatment for symptomatic anterior cervical osteophytes: A case series with review of the literature. Clin. Spine Surg. 29 (9), E482-e487 (2016).
Lu, J., Ebraheim, N. A., Yang, H., Rollins, J. & Yeasting, R. A. Anatomic bases for anterior spinal surgery: Surgical anatomy of the cervical vertebral body and disc space. Surg. Radiol. Anat. SRA 21 (4), 235–239 (1999).
Kojima, S. et al. Associations between degenerative lumbar scoliosis structures and pain distribution in adults with chronic low back pain. Healthcare (Basel, Switzerland) 11 (16), 2357 (2023).
PubMed Google Scholar
Shrestha, B. V. & Dhungana, S. P. Measurement of transverse and sagittal diameter of the lumbar vertebral canal in people from Western region of Nepal. Int. J. Infect. 2 , 55–58 (2013).
Klein, J. P. & Moeschberger, M. L. Survival analysis—techniques for censored and truncated data. In Statistics for biology and health 2nd edn 63–70 (Springer, New York, 2003).
Download references
This study was supported by National Natural Science Foundation of China (12201441), Sichuan Science and Technology Program (2023NSFSC1597), Med-X for informatics, Sichuan University (YGJC006), National Key Research and Development Program of China (2020YFB1711500, 2022YFC2407601), the 1·3·5 project for disciplines of excellence, West China Hospital, Sichuan University (ZYYC21004).
Author information
Authors and affiliations.
West China Biomedical Big Data Center, West China Hospital, Sichuan University, Chengdu, 610041, China
Tong Wu, Changxi Wang & Kang Li
Orthopedics Department of West China Hospital, Sichuan University, Chengdu, 610041, China
Sichuan University - Pittsburgh Institute, Sichuan University, Chengdu, 610207, China
Changxi Wang & Kang Li
You can also search for this author in PubMed Google Scholar
Contributions
Tong Wu contributed to the conception and design, data measurement, data analysis and interpretation and was a major contributor in writing the manuscript. Changxi Wang contributed to the acquisition of data, model construction and drafting/revisions of article. Kang Li contributed to the conception and design as well as final approval of the article. All authors read and approved the final manuscript.
Corresponding authors
Correspondence to Changxi Wang or Kang Li .
Ethics declarations
Competing interests.
The authors declare no competing interests.
Additional information
Publisher's note.
Springer Nature remains neutral with regard to jurisdictional claims in published maps and institutional affiliations.
Supplementary Information
Supplementary information., rights and permissions.
Open Access This article is licensed under a Creative Commons Attribution 4.0 International License, which permits use, sharing, adaptation, distribution and reproduction in any medium or format, as long as you give appropriate credit to the original author(s) and the source, provide a link to the Creative Commons licence, and indicate if changes were made. The images or other third party material in this article are included in the article's Creative Commons licence, unless indicated otherwise in a credit line to the material. If material is not included in the article's Creative Commons licence and your intended use is not permitted by statutory regulation or exceeds the permitted use, you will need to obtain permission directly from the copyright holder. To view a copy of this licence, visit http://creativecommons.org/licenses/by/4.0/ .
Reprints and permissions
About this article
Cite this article.
Wu, T., Wang, C. & Li, K. Quantitative analysis and stochastic modeling of osteophyte formation and growth process on human vertebrae based on radiographs: a follow-up study. Sci Rep 14 , 9393 (2024). https://doi.org/10.1038/s41598-024-60212-5
Download citation
Received : 28 June 2023
Accepted : 19 April 2024
Published : 24 April 2024
DOI : https://doi.org/10.1038/s41598-024-60212-5
Share this article
Anyone you share the following link with will be able to read this content:
Sorry, a shareable link is not currently available for this article.
Provided by the Springer Nature SharedIt content-sharing initiative
By submitting a comment you agree to abide by our Terms and Community Guidelines . If you find something abusive or that does not comply with our terms or guidelines please flag it as inappropriate.
Quick links
- Explore articles by subject
- Guide to authors
- Editorial policies
Sign up for the Nature Briefing: AI and Robotics newsletter — what matters in AI and robotics research, free to your inbox weekly.

- Study Protocol
- Open access
- Published: 22 April 2024
Implementation and early effects of medicaid policy interventions to promote racial equity in pregnancy and early childhood outcomes in Pennsylvania: protocol for a mixed methods study
- Marian Jarlenski 1 ,
- Evan Cole 1 ,
- Christine McClure 1 ,
- Sarah Sanders 2 ,
- Marquita Smalls 2 &
- Dara D Méndez 2
BMC Health Services Research volume 24 , Article number: 498 ( 2024 ) Cite this article
115 Accesses
Metrics details
There are large racial inequities in pregnancy and early childhood health within state Medicaid programs in the United States. To date, few Medicaid policy interventions have explicitly focused on improving health in Black populations. Pennsylvania Medicaid has adopted two policy interventions to incentivize racial health equity in managed care (equity payment program) and obstetric service delivery (equity focused obstetric bundle). Our research team will conduct a mixed-methods study to investigate the implementation and early effects of these two policy interventions on pregnancy and infant health equity.
Qualitative interviews will be conducted with Medicaid managed care administrators and obstetric and pediatric providers, and focus groups will be conducted among Medicaid beneficiaries. Quantitative data on healthcare utilization, healthcare quality, and health outcomes among pregnant and parenting people will be extracted from administrative Medicaid healthcare data. Primary outcomes are stakeholder perspectives on policy intervention implementation (qualitative) and timely prenatal care, pregnancy and birth outcomes, and well-child visits (quantitative). Template analysis methods will be applied to qualitative data. Quantitative analyses will use an interrupted time series design to examine changes over time in outcomes among Black people, relative to people of other races, before and after adoption of the Pennsylvania Medicaid equity-focused policy interventions.
Findings from this study are expected to advance knowledge about how Medicaid programs can best implement policy interventions to promote racial equity in pregnancy and early childhood health.
Peer Review reports
Rates of maternal and infant morbidity and mortality in the United States far exceed those of comparable nations [ 1 ]. The burdens of racist policies have produced vastly worse outcomes for Black and Native, relative to White, populations [ 2 ]. For example, Black and Native birthing people are more than three times as likely to experience pregnancy-related mortality compared to white birthing people [ 3 ]. For every pregnancy-related death, there are thousands of birthing people who experience severe morbidity; including stark racial disparities where Black populations are more likely to experience stroke or serious cardiovascular events sending them on a trajectory of adverse health outcomes beyond pregnancy [ 4 , 5 ]. We also see similar racial inequities for infant mortality and morbidity. These racial inequities are not adequately explained by individual behaviors or other socio-economic factors, but are a complex intersection of factors shaped by structural and social determinants [ 2 , 6 ], policies and institutions carrying out such policies [ 7 ]. There is a long history of structural racism that has resulted in practices and policies that have had a detrimental impact on Black and Indigenous populations in the United States [ 8 ].
State Medicaid programs are the largest single payer for pregnancy and birth in the US, covering 68% of births to Black people [ 9 ]. As such, Medicaid programs have great potential to implement structural interventions to advance racial equity in healthcare and health outcomes during pregnancy and postpartum [ 10 ]. Historically, Medicaid policies have promoted equality, that is, providing equal benefits to all regardless of the distribution of need [ 11 ]. An equity-focused policy approach, however, will direct resources toward improving health and well-being among those with the greatest need [ 12 ]. Unfortunately, a vast body of research conducted among Medicaid-enrolled populations shows that healthcare systems do not provide the same quality of obstetric care to Black people and other people of color, relative to white people [ 13 , 14 , 15 , 16 , 17 , 18 ].
Pennsylvania’s Medicaid program is the fourth-largest in the United States, with 3.5 million people enrolled and expenditures of $35.1 billion [ 19 , 20 ]. Past research in the Pennsylvania Medicaid program has demonstrated Black people were less able to access prenatal and postpartum care relative to those in other race groups [ 15 ]. Reporting from the Pennsylvania Maternal Mortality Commission shows that in more than half of the cases of pregnancy-associated deaths, the decadents were enrolled in Medicaid [ 21 ]. Similar to national figures, pregnancy-associated death was far more common among Black people vs. those of other races ( [ 21 ].
To ameliorate these racial disparities, Pennsylvania Medicaid is currently implementing two novel policies with the goal to advance racial equity in pregnancy and child health. The first, the equity incentive payment program, was initiated in 2020. The equity incentive payment program makes available approximately $26 million in Medicaid managed care organization (MCO) payments each year to plans that improve access to timely prenatal care and well-child visits among Black beneficiaries. The second is the maternity care bundled payment model, initiated in 2021, designed to provide incentives to obstetric providers across a wide range of pregnancy health outcomes and specifically incentivizes improvements among Black beneficiaries.
Although these policy approaches are unique, it is possible that other state Medicaid programs or other health insurers could learn from the policies and adapt or expand these approaches. Our research team will conduct a mixed-methods study to investigate the implementation and early effects of the two aforementioned policy changes on pregnancy and infant health equity. Our research aims are to: (1) evaluate implementation and early effects of the equity incentive payment program prenatal and early childhood healthcare outcomes and experiences among Black Medicaid beneficiaries; and (2) determine the extent to which an equity-focused maternity care bundled payment model affects racial equity in obstetric care and pregnancy health outcomes. To achieve these aims, we will draw on established partnerships between university researchers, community organizations, and policymakers to collect and analyze data. First, we will collect qualitative data with diverse stakeholders including Medicaid beneficiaries, MCO plan representatives, and pediatric and obstetric care clinicians to study implementation of these equity-focused policy changes. Second, we will use a community-partnered approach to develop a quantitative analysis plan of Medicaid administrative data for an estimated 167,000 birthing person-child dyads to estimate early effects of these policies. Our cross-disciplinary, community-engaged partnerships will enable us to triangulate how the healthcare policy structures of state Medicaid programs can be changed to promote racial equity in health.
Theoretical framework
The proposed research seeks to advance knowledge about the causes of, and structural interventions to improve, health and well-being among Black pregnant and parenting persons and their children in Medicaid. The theoretical model underlying this work is informed by foundational literature from a range of disciplines. First, it incorporates Critical Race Theory and Public Health Critical Race Praxis, which posit structural determinants, such as racism and other forms of oppression (e.g., sexism, classism, poverty), as fundamental causes of adverse social environments that interact to make certain populations more susceptible to illness and resulting in suboptimal health [ 22 , 23 , 24 , 25 , 26 ]. Second, it incorporates political science theory that dominant social definitions of populations shape group empowerment and resulting health policies and material benefits [ 27 ]. Third, it draws on new scholarship suggesting the necessity of studying social welfare policies with a critical race lens centering the beneficiaries’ lived experiences [ 11 , 28 , 29 ].
As depicted in Fig. 1 , our research project identifies both the Medicaid policy environment as well as the beneficiary experiences of the policy environment as upstream factors that influence healthcare organization and beneficiaries’ interaction with healthcare systems. In particular, we aim to facilitate and further enhance the connection between beneficiaries’ lived experiences and policy decision-makers through our collaboration with community partners. This connection can influence the policymaking process that shapes how care is delivered both at the managed care and healthcare provider levels. Healthcare utilization and quality are conceptualized as intermediate outcomes which may influence pregnancy and birth outcomes.
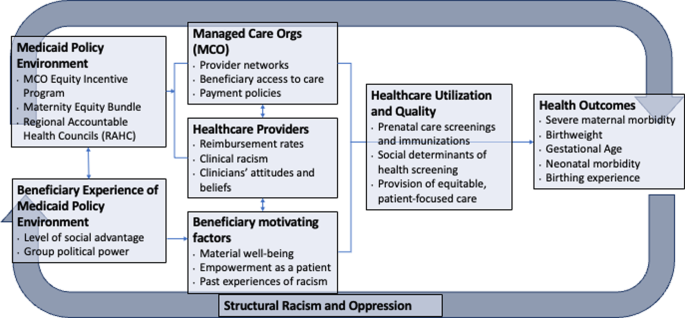
Conceptual model of the evaluation of structural interventions in Medicaid to promote racial equity in pregnancy and child health
Medicaid policy interventions
Nearly all Medicaid beneficiaries in Pennsylvania are enrolled in 1 of 8 Medicaid managed care plans, which manage the physical health care of enrollees and are subject to pay-for-performance requirements for healthcare quality measures. Currently, the Pennsylvania Medicaid program makes available 2% of total payments to MCO plans, contingent on MCO plan performance on 13 different healthcare quality metrics. An equity incentive payment program was added to this reimbursement scheme for two metrics in 2020: timely prenatal care and well-child visit utilization in the first 15 months of life (Fig. 2 ). Specifically, 2/13 (or 0.15%) of total payments are withheld for these two equity-focused metrics. MCO plans are assessed on overall performance and subsequently on the annual improvement on these measures among Black beneficiaries. MCO plans can be penalized (up to -0.12% of total payments) or rewarded (up to + 0.35% of total payments) for their performance on each of these two metrics.
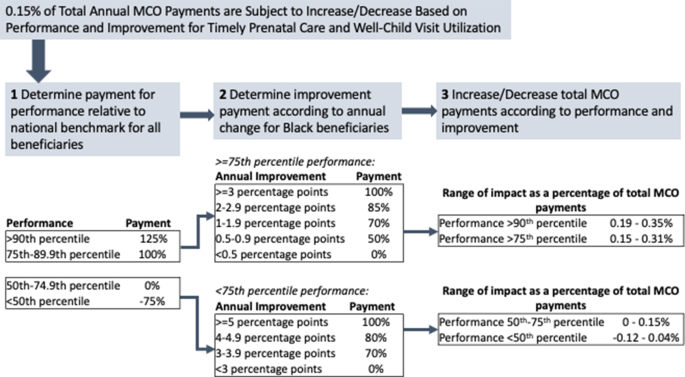
Pennsylvania Medicaid’s health equity incentive payment program will condition payments to managed care organizations based on overall performance as well as improvement among Black beneficiaries
Second, Pennsylvania Medicaid implemented a maternity care bundled payment model in 2021 that considers outcomes among Black beneficiaries (Fig. 3 ). Under maternity care bundled payment models, obstetric providers are incentivized to meet a total cost threshold and quality metrics for prenatal and delivery care [ 30 ]. Specifically, providers and payers agree on a target cost for low- or average-risk perinatal care, including pregnancy, delivery, and postpartum care. If total payments to providers are lower than the target cost while maintaining certain quality metrics, providers and payers share those savings. Under Pennsylvania’s new model, providers are able to achieve shared savings based on quality metric performance, as well as a health equity score reflecting performance on those metrics among Black beneficiaries.
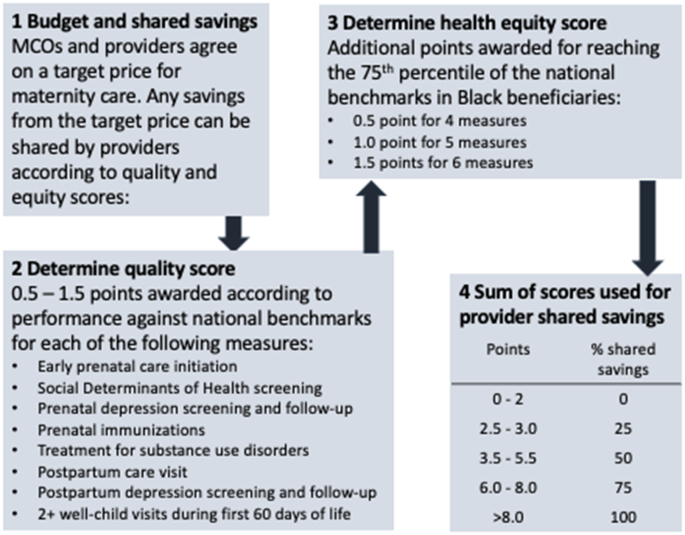
Pennsylvania Medicaid’s equity-focused maternity bundled payment model will allow for shared savings between obstetric care providers and managed care organizations, allowing for extra shared savings among providers whose Black patients experience better outcomes
Qualitative data Collection
To understand the interventions and responses to these policies, as well as related implementation barriers and facilitators, we will conduct interviews with each at least two representatives from each MCO ( n = 18). We will partner with colleagues from the Department of Human Services (DHS) to identify relevant MCO representatives. Interviews will elucidate MCOs’ perspectives, processes used by MCOs to design their interventions (e.g., review of existing evidence, input from community members or providers who serve them), anticipated effects, and sustainability of these payment policy changes. The goal is for some of the results of these interviews to inform our understanding of the implementation process which will be further explored in the interviews and focus groups with clinicians and Medicaid recipients.
In collaboration with the Community Health Advocates (CHA) program led by Healthy Start Pittsburgh, as well as other community and organizational partners across the state, we will recruit current and former Medicaid beneficiaries for focus group participation. We aim to recruit ∼ 50 community participants and will purposively oversample Black participants and will aim to recruit people of all ethnicities who identify as Black and multi-racial in order to achieve our aims of elucidating the experiences of Black parenting and pregnant people in Medicaid. Inclusion criteria are: current pregnancy or pregnant within the past 2 years; current or former enrollment in Pennsylvania Medicaid and/or Healthy Start; and ability to complete the interview in English.
Finally, we will partner with colleagues from DHS to identify pediatric and obstetric health professionals for interviews regarding the maternity bundled payment program and key outcomes related to the equity incentive payment. We will also use Medicaid administrative data to identify providers who serve Black beneficiaries and invite them to participate. We will aim to interview at least 20 obstetric and pediatric healthcare professionals to elucidate their perspectives on how structural racism in medicine affects patient outcomes, and the types of Medicaid policy changes that should be implemented.
We developed separate focus group/interview guides for community members, MCO leaders, and healthcare professionals. Each interview guide consists of open-ended questions to elucidate participants’ experiences with Medicaid; desired policy changes in Medicaid (among beneficiary participants); perceived steps that would be useful to combat anti-Black racism in healthcare and social services (especially among Black participants); and perspectives about the new Medicaid policies. Additionally, the interview guides ask demographic questions regarding gender identity, race, and ethnicity. We will first pilot-test the guide with our research partners and Healthy Start CHAs for clarity of question wording. All interviews will take place in-person in a private office space, or over the phone or videoconference, according to participants’ preferences and COVID-19 protocols. The interviewer will describe study objectives to each participant, obtain consent, and each interview will be audio-recorded and the interviewer will take notes throughout. Interview audio recordings will be transcribed verbatim, and transcripts spot-checked against the audio recordings for accuracy. The audio recording files will then be deleted to protect confidentiality of participants.
Qualitative data analysis
Study data will be analyzed and reported using the Consolidated Criteria for Reporting Qualitative Research (COREQ) Framework [ 31 ]. To analyze data, we will use template analysis, which combines features of deductive content analysis and inductive grounded theory, thereby allowing us to obtain specific information while also capturing any new or unanticipated themes [ 32 ]. Two coders will separately code the first 3 interview transcripts, meet to compare codes, discuss inconsistency in coding approaches, and then alter or add codes. This iterative process will be repeated until the coding scheme is fully developed. The coders will independently code all transcripts, and any coding discrepancies will be resolved via discussion. Once coding is complete, synthesis of content will begin by organizing codes under broader domains (meta-codes) as well as sub-codes. The primary analysis will be to convey qualitative data, including the use of illustrative quotes.
Quantitative healthcare data and analysis
Administrative healthcare data from the Pennsylvania Medicaid program, with linked birthing person-child dyads, will be used to create our quantitative analytic data. Medicaid data include a census of enrollment, hospital, outpatient/professional, pharmaceutical, and provider data for all beneficiaries in the Pennsylvania Medicaid program. Importantly, data contain self-reported race and ethnicity that is provided at the time of Medicaid enrollment (< 2% missing); as well as time-varying data on 9-digit ZIP code of residence. Data also include the amounts paid from Medicaid MCOs to healthcare providers for all medical services. Table 1 shows baseline data from Pennsylvania Medicaid-enrolled persons with a livebirth delivery in 2019, overall and stratified by race of the birthing person. We will also conduct sensitivity analyses to examine dyads that are multi-racial.
We will employ a comparative interrupted time series (ITS) analyses with a nonequivalent comparison group to estimate policy effects. Specifically, we will evaluate: (1) the extent to which the equity incentive payment program improved timely prenatal care and well-child visits among Black beneficiaries, relative to those of other races; and (2) the extent to which healthcare provider participation in the equity-focused maternity bundled payment model improved healthcare and health outcomes among Black beneficiaries, relative to those of other races.
For Aim 1, outcomes include binary measures of initiating prenatal care in the first trimester, and children receiving at least 6 well-child visits in the first six months of life. We will compare outcomes among Black beneficiaries relative to those of other racial groups, post- relative to pre- implementation of the equity incentive payment program. For Aim 2, outcomes include a composite of prenatal care quality measures (social determinants of health screening, prenatal and postpartum depression screening and follow-up, immunization, screening and treatment for substance use disorders, postpartum visit attendance), gestational age and birthweight, and severe maternal morbidity [ 33 ]. For both aims, multivariable regression models will control for maternal age, ethnicity, parity, ZIP code of residence, MCO plan enrollment, Medicaid eligibility category (expansion, pregnancy, disability, or others), and indices of obstetric and pediatric clinical comorbidities [ 34 , 35 ].
Sensitivity analyses
Analyses are designed to estimate early effects of the policies and should be interpreted alongside the qualitative results regarding policy implementation and beneficiary experiences. One assumption of ITS analyses is that our comparison groups approximate a counterfactual scenario for the intervention groups [ 36 , 37 , 38 ]. Although trends in Black-White inequities in pregnancy and child outcomes have, unfortunately, persisted over time [ 39 ], the COVID-19 pandemic has differentially burdened Black and Latina/x people relative to other race and ethnic groups [ 40 , 41 ]. Effects of the pandemic on pregnancy outcomes are only just beginning of what is to be explored [ 42 ]. It is therefore possible that we will not be able to disentangle policy effects from effects of COVID-19. To address this limitation, we will employ area-level rates of COVID-19 infection as control variables and for Aim 1 (equity incentive payment) we will conduct a sub-analysis investigating trends in 2021 vs. 2020. We chose to evaluate outcomes for Aim 2 (maternity care bundled payment) only in 2021, comparing the statistical intervention of race*provider. Finally, our qualitative analyses will provide context on differential impacts of COVID-19, which will inform interpretation of the quantitative results.
This study was approved by the University of Pittsburgh Institutional Review Board (Study # 23090108).
This mixed-methods research will investigate the extent to which changes in the Pennsylvania Medicaid program are associated with improvements in access to medical care and health outcomes among Black pregnant and birthing persons and their children. Our past research found that Black childbearing people in Pennsylvania Medicaid consistently experienced worse healthcare and health outcomes, compared to those of other racial and ethnic groups [ 43 , 44 ]. Racism in healthcare and other systems manifests in systematically worse access to and quality of care and other services for Black childbearing people [ 8 ]. In addition to suboptimal healthcare experiences, historical policies and practices such as residential redlining and segregation have resulted in lower wealth attainment in Black communities resulting in inequities in neighborhood factors and perinatal health [ 45 , 46 , 47 ].
The policies under study involve modifying common Medicaid reimbursement arrangements– namely, pay-for-performance programs and maternity care bundled payments. The policies are modified to embed financial incentives for Medicaid health plans and healthcare providers to improve the quality of care and health outcomes for Black pregnant and parenting persons and their children. These are the first such payment policies, to our knowledge, that explicitly aim to promote racial health equity with an explicit focus on addressing inequities that affect Black and Indigenous populations in Pennsylvania.
Interest from policymakers in payment reforms to improve health equity has increased recently; however, information on the implementation and effects of such models is sparse [ 48 , 49 ]. Although several state Medicaid programs have adopted maternity care bundled payment models, prior models have not considered racial inequities in pregnancy outcomes [ 30 , 50 ]. In 2012, Oregon adopted regional health equity coalitions as part of the state Medicaid program’s transition to Coordinated Care Organizations (CCOs). CCOs were required and given support to develop strategies that would address racial health disparities within the Medicaid population, and the regional health equity coalitions included underrepresented stakeholders to guide CCOs in the development of these interventions. While CCOs did reduce Black-white differences in primary care utilization and access to care within 3 years of policy implementation, it did not impact disparities in emergency department utilization [ 51 ]. The current research project will add to the extant evidence on how Medicaid programs can use policy to promote racial health equity.
Our study is limited in investigating the direct effects of the pandemic on racial inequities in perinatal and infant health and the intersections between the effects of the pandemic and the effects of the aforementioned Medicaid policies. However, we will have the ability to look at changes in outcomes over time. Additionally, these payment reform interventions focus largely on transforming the financing and delivery of healthcare, drawing attention to the structural and social determinants of health in the healthcare system. It is estimated that medical care contributes 10–20% to health outcomes; health and well-being are also shaped by factors such as environmental and socioeconomic conditions [ 52 ].
This study will contribute to the current body of knowledge about the recent interventions in Medicaid focused on racial equity. Specifically, findings will shed light on how the equity-focused obstetric care policies are being implemented and provide an evaluation of effects on health outcomes. These results can be used for future adaptions of the policy interventions or to inform the adoption of such equity-focused policies in different geographic regions of the United States.
Data availability
No datasets were generated or analysed during the current protocol study.
Abbreviations
Managed Care Organization
Community Health Advocate
Coordinated Care Organization
Alkema L, Chou D, Hogan D, Zhang S, Moller AB, Gemmill A, et al. Global, regional, and national levels and trends in maternal mortality between 1990 and 2015, with scenario-based projections to 2030: a systematic analysis by the UN Maternal Mortality Estimation Inter-agency Group. Lancet. 2016;387(10017):462–74.
Article PubMed Google Scholar
Wang E, Glazer KB, Howell EA, Janevic TM. Social determinants of pregnancy-related mortality and morbidity in the United States: a systematic review. Obstet Gynecol. 2020;135(4):896–915.
Article PubMed PubMed Central Google Scholar
Hoyert D. Maternal mortality rates in the United States, 2020. NCHS Health E-Stats; 2022.
Fingar K, Hambrick MM, Heslin KC, Moore JE. Trends and Disparities in Delivery Hospitalizations Involving Severe Maternal Morbidity, 2006–2015. Rockville, MD. 2018.
Thomas SB, Quinn SC, Butler J, Fryer CS, Garza MA. Toward a fourth generation of disparities research to achieve health equity. Annu Rev Public Health. 2011;32:399–416.
Crear-Perry J, Correa-de-Araujo R, Lewis Johnson T, McLemore MR, Neilson E, Wallace M. Social and Structural Determinants of Health Inequities in maternal health. J Womens Health (Larchmt). 2021;30(2):230–5.
Jones CP. Levels of racism: a theoretic framework and a gardener’s tale. Am J Public Health. 2000;90(8):1212–5.
Article CAS PubMed PubMed Central Google Scholar
Prather C, Fuller TR, Jeffries WLt, Marshall KJ, Howell AV, Belyue-Umole A, et al. Racism, African American Women, and their sexual and Reproductive Health: a review of historical and Contemporary Evidence and Implications for Health Equity. Health Equity. 2018;2(1):249–59.
Markus AR, Andres E, West KD, Garro N, Pellegrini C. Medicaid covered births, 2008 through 2010, in the context of the implementation of health reform. Womens Health Issues. 2013;23(5):e273–80.
Headen IE, Elovitz MA, Battarbee AN, Lo JO, Debbink MP. Racism and perinatal health inequities research: where we have been and where we should go. Am J Obstet Gynecol. 2022.
Michener JSM, Thurston C. From the Margins to the Center: A Bottom- Up Approach to Welfare State Scholarship. Perspectives on Politics. 2020:1–16.
Braveman PAE, Orleans T, Proctor D, Plough A. What is health equity? And what difference does a definition make? Princeton, NJ: Robert Wood Johnson Foundation; 2017.
Google Scholar
Thiel de Bocanegra H, Braughton M, Bradsberry M, Howell M, Logan J, Schwarz EB. Racial and ethnic disparities in postpartum care and contraception in California’s Medicaid program. Am J Obstet Gynecol. 2017;217(1):47. e1- e7.
Article Google Scholar
Gao YA, Drake C, Krans EE, Chen Q, Jarlenski MP. Explaining racial-ethnic disparities in the receipt of medication for opioid use disorder during pregnancy. J Addict Med. 2022.
Parekh N, Jarlenski M, Kelley D. Prenatal and Postpartum Care Disparities in a large Medicaid Program. Matern Child Health J. 2018;22(3):429–37.
Gavin NI, Adams EK, Hartmann KE, Benedict MB, Chireau M. Racial and ethnic disparities in the use of pregnancy-related health care among Medicaid pregnant women. Matern Child Health J. 2004;8(3):113–26.
Aseltine RH Jr., Yan J, Fleischman S, Katz M, DeFrancesco M. Racial and ethnic disparities in Hospital readmissions after Delivery. Obstet Gynecol. 2015;126(5):1040–7.
Wagner JL, White RS, Tangel V, Gupta S, Pick JS. Socioeconomic, racial, and ethnic disparities in Postpartum readmissions in patients with Preeclampsia: a multi-state analysis, 2007–2014. J Racial Ethn Health Disparities. 2019;6(4):806–20.
Kaiser Family Foundation. Total Monthly Medicaid/CHIP Enrollment and Pre-ACA Enrollment: Kaiser Family Foundation; 2022 [ https://www.kff.org/health-reform/state-indicator/total-monthly-medicaid-and-chip-enrollment/?currentTimeframe=0&sortModel=%7B%22colId%22:%22Location%22,%22sort%22:%22asc%22%7D .
Kaiser Family Foundation, Total Medicaid Spending. FY 2020 2022 [ https://www.kff.org/medicaid/state-indicator/total-medicaid-spending/?currentTimeframe=0&sortModel=%7B%22colId%22:%22Location%22,%22sort%22:%22asc%22%7D .
Pennsylvania Department of Health. Pregnancy-Associated Deaths in Pennsylvania, 2013–2018. Harrisburg, PA. 2020 December 2020.
Geronimus AT, Hicken MT, Pearson JA, Seashols SJ, Brown KL, Cruz TD. Do US Black women experience stress-related Accelerated Biological Aging? A Novel Theory and First Population-based test of black-white differences in telomere length. Hum Nat. 2010;21(1):19–38.
Marmot M. Health in an unequal world. Lancet. 2006;368(9552):2081–94.
Ekeke P, Mendez DD, Yanowitz TD, Catov JM. Racial differences in the biochemical effects of stress in pregnancy. Int J Environ Res Public Health. 2020;17:19.
Ford CL, Airhihenbuwa CO. Critical race theory, race equity, and public health: toward antiracism praxis. Am J Public Health. 2010;100(Suppl 1):S30–5.
Bailey ZD, Krieger N, Agenor M, Graves J, Linos N, Bassett MT. Structural racism and health inequities in the USA: evidence and interventions. Lancet. 2017;389(10077):1453–63.
Schneider AIH. Social Construction of Target populations: implications for politics and policy. Am Polit Sci Rev. 1993;87(2):334–47.
Michener J. Fragmented democracy: medicaid, federalism, and unequal politics. Cambridge, United Kingdom; New York, NY, USA: Cambridge University Press; 2018. xii, 226 pages p.
Ford CL, Airhihenbuwa CO. The public health critical race methodology: praxis for antiracism research. Soc Sci Med. 2010;71(8):1390–8.
Carroll C, Chernew M, Fendrick AM, Thompson J, Rose S. Effects of episode-based payment on health care spending and utilization: evidence from perinatal care in Arkansas. J Health Econ. 2018;61:47–62.
Tong A, Sainsbury P, Craig J. Consolidated criteria for reporting qualitative research (COREQ): a 32-item checklist for interviews and focus groups. Int J Qual Health Care. 2007;19(6):349–57.
Hsieh HF, Shannon SE. Three approaches to qualitative content analysis. Qual Health Res. 2005;15(9):1277–88.
Centers for Disease Control and Prevention. Severe Maternal Morbidity in the United States 2021 [ http://www.cdc.gov/reproductivehealth/maternalinfanthealth/severematernalmorbidity.html .
Bateman BT, Mhyre JM, Hernandez-Diaz S, Huybrechts KF, Fischer MA, Creanga AA, et al. Development of a comorbidity index for use in obstetric patients. Obstet Gynecol. 2013;122(5):957–65.
Feudtner C, Feinstein JA, Zhong W, Hall M, Dai D. Pediatric complex chronic conditions classification system version 2: updated for ICD-10 and complex medical technology dependence and transplantation. BMC Pediatr. 2014;14:199.
Ashenfelter OCD. Using the longitudinal structure of earnings to estimate the effect of training programs. Rev Econ Stat. 1985;67:648–60.
Donald SGLK. Inference with difference-in-differences and other panel data. Rev Econ Stat. 2007;89(2):221–33.
Imbens GWJ. Recent developments in the econometrics of program evaluation. J Econ Lit. 2009;47(1):5–86.
Goldfarb SS, Houser K, Wells BA, Brown Speights JS, Beitsch L, Rust G. Pockets of progress amidst persistent racial disparities in low birthweight rates. PLoS ONE. 2018;13(7):e0201658.
Prasannan L, Rochelson B, Shan W, Nicholson K, Solmonovich R, Kulkarni A et al. Social Determinants of Health and Coronavirus Disease 2019 in pregnancy: condensation: social determinants of health, including neighborhood characteristics such as household income and educational attainment, are associated with SARS-CoV-2 infection and severity of COVID-19 in pregnancy. Am J Obstet Gynecol MFM. 2021:100349.
Flores LE, Frontera WR, Andrasik MP, Del Rio C, Mondriguez-Gonzalez A, Price SA, et al. Assessment of the Inclusion of Racial/Ethnic Minority, female, and older individuals in Vaccine clinical trials. JAMA Netw Open. 2021;4(2):e2037640.
Janevic T, Glazer KB, Vieira L, Weber E, Stone J, Stern T, et al. Racial/Ethnic disparities in very Preterm Birth and Preterm Birth before and during the COVID-19 pandemic. JAMA Netw Open. 2021;4(3):e211816.
Krans EE, Kim JY, James AE 3rd, Kelley D, Jarlenski MP. Medication-assisted treatment use among pregnant women with opioid Use Disorder. Obstet Gynecol. 2019;133(5):943–51.
Parekh NJM, Kelley D. Disparities in access to care and emergency department utilization in a large Medicaid program. J Health Dispar Res Pract. 2018;11(4):1–13.
Assibey-Mensah V, Fabio A, Mendez DD, Lee PC, Roberts JM, Catov JM. Neighbourhood assets and early pregnancy cardiometabolic risk factors. Paediatr Perinat Epidemiol. 2019;33(1):79–87.
Mendez DD, Hogan VK, Culhane JF. Institutional racism, neighborhood factors, stress, and preterm birth. Ethn Health. 2014;19(5):479–99.
Mendez DD, Hogan VK, Culhane J. Institutional racism and pregnancy health: using Home Mortgage Disclosure act data to develop an index for mortgage discrimination at the community level. Public Health Rep. 2011;126(Suppl 3):102–14.
Huffstetler AN, Phillips RL. Jr. Payment structures that support Social Care Integration with Clinical Care: Social Deprivation indices and Novel Payment models. Am J Prev Med. 2019;57(6 Suppl 1):S82–8.
Anderson AC, O’Rourke E, Chin MH, Ponce NA, Bernheim SM, Burstin H. Promoting Health Equity and eliminating disparities through performance measurement and payment. Health Aff (Millwood). 2018;37(3):371–7.
Jarlenski M, Borrero S, La Charite T, Zite NB. Episode-based payment for Perinatal Care in Medicaid: implications for practice and policy. Obstet Gynecol. 2016;127(6):1080–4.
McConnell KJ, Charlesworth CJ, Meath THA, George RM, Kim H. Oregon’s emphasis on Equity Shows Signs of Early Success for Black and American Indian Medicaid Enrollees. Health Aff (Millwood). 2018;37(3):386–93.
Hood CM, Gennuso KP, Swain GR, Catlin BB. County Health rankings: relationships between determinant factors and Health outcomes. Am J Prev Med. 2016;50(2):129–35.
Download references
This study received funding from the National Institute of Nursing Research under award R01NR020670. The funder had no role in the study design, data collection or analysis, or decision to publish the study.
Author information
Authors and affiliations.
Department of Health Policy and Management, University of Pittsburgh School of Public Health, 130 DeSoto St, A619, 15261, Pittsburgh, PA, USA
Marian Jarlenski, Evan Cole & Christine McClure
Department of Epidemiology, University of Pittsburgh School of Public Health, Pittsburgh, PA, USA
Sarah Sanders, Marquita Smalls & Dara D Méndez
You can also search for this author in PubMed Google Scholar
Contributions
Jarlenski: Conceptualization; funding acquisition; investigation; methodology; supervision; writing-original draftCole: Conceptualization; data curation; investigation; resources; writing-reviewing and editingMcClure: Investigation; project administration; supervision; writing-reviewing and editingSanders: Investigation; methodology; visualization; writing-reviewing and editingSmalls: Investigation; project administration; visualization; writing-reviewing and editingMendez: Conceptualization; funding acquisition; investigation; validation; supervision; writing-original draft.
Corresponding author
Correspondence to Marian Jarlenski .
Ethics declarations
Ethical approval, competing interests.
The authors declare no competing interests.
Additional information
Publisher’s note.
Springer Nature remains neutral with regard to jurisdictional claims in published maps and institutional affiliations.
Rights and permissions
Open Access This article is licensed under a Creative Commons Attribution 4.0 International License, which permits use, sharing, adaptation, distribution and reproduction in any medium or format, as long as you give appropriate credit to the original author(s) and the source, provide a link to the Creative Commons licence, and indicate if changes were made. The images or other third party material in this article are included in the article’s Creative Commons licence, unless indicated otherwise in a credit line to the material. If material is not included in the article’s Creative Commons licence and your intended use is not permitted by statutory regulation or exceeds the permitted use, you will need to obtain permission directly from the copyright holder. To view a copy of this licence, visit http://creativecommons.org/licenses/by/4.0/ . The Creative Commons Public Domain Dedication waiver ( http://creativecommons.org/publicdomain/zero/1.0/ ) applies to the data made available in this article, unless otherwise stated in a credit line to the data.
Reprints and permissions
About this article
Cite this article.
Jarlenski, M., Cole, E., McClure, C. et al. Implementation and early effects of medicaid policy interventions to promote racial equity in pregnancy and early childhood outcomes in Pennsylvania: protocol for a mixed methods study. BMC Health Serv Res 24 , 498 (2024). https://doi.org/10.1186/s12913-024-10982-5
Download citation
Received : 12 December 2023
Accepted : 10 April 2024
Published : 22 April 2024
DOI : https://doi.org/10.1186/s12913-024-10982-5
Share this article
Anyone you share the following link with will be able to read this content:
Sorry, a shareable link is not currently available for this article.
Provided by the Springer Nature SharedIt content-sharing initiative
- Well-child visits
- Prenatal care
- Health policy
- Health equity
- Mixed methods
BMC Health Services Research
ISSN: 1472-6963
- General enquiries: [email protected]
Introduction:
“ Moscow – Post-Soviet Developments and Challenges” [1]
John O’Loughlin* and Vladimir Kolossov **
* University of Colorado , Boulder
** Russian Academy of Sciences, Moscow
Russia has always been a highly centralized state, with the capital playing an exceptional economic, social, cultural and political role. Paradoxically, the post-Soviet economic transition not only did not reduce this primacy, but on the contrary, considerably strengthen Moscow ’s hypertrophy. During the 1990s, political events in the capital (attempted coups d’etat in 1991 and 1993, the struggle between ” reformers” and left-wingers) decided the destiny of all Russia , with most regions only observing with anxiety. Though its ratio of Russia ’s total population is just less than 6%, the city contains more than half of all banking activity, more than one-fifth of retail trade, and one-third of wholesale trade. Moscow has, to a large extent, monopolized the functions of a mediator between the country and the world economy and has become by far the most important national node of financial flows. Even if the August 1998 financial crisis contributed to a certain improvement of the balance between the capital and the provinces, Moscow remains the major “exporter” of Russia ’s primary exports (oil, gas, timber, gold, etc.) It is being transformed into a true global city (Gritsai 1996, 1997; Taylor 2000).
The average per capita income in Moscow is much higher than in any other of the 88 regions in Russia, and more than twice as high as the second-highest, St. Petersburg. Moscow provides an example of post-Communist economic restructuring to the whole country and now contains the most sizeable new middle class. The streetscape of the capital has considerably changed during the last decade. In its downtown, contemporary offices are mushrooming, and historical buildings look fresh after recent renovation by private investors; at night, Moscow ’s main avenues are brightly illuminated by shining shop windows and advertising by global companies, provoking sharp envy from residents of many other Russian cities, which remain dark and suffer from municipal debts and power shortages. Is Moscow really the dominant player in the Russian economy, determining the orientation and the rates of national restructuring? How stable is the Moscow ’s “miracle”? What is the reverse side of the coin, the inequities and polarization that has become apparent in the past decade? These questions are increasingly at the center of discussions among politicians and academic specialists.
The economic and social costs of Moscow ’s rapid changes since 1991 are already clear. Moscow has definitely became a demographic “black hole”: mortality has exceeded fertility for a decade, the city’s population is getting older and the decrease is compensated only by labor migration from most of the former Soviet republics, from other Russian regions, and even from some third world countries. Most migrants live in Moscow illegally. These processes worsen the qualitative composition of population, create tensions in the labor and housing mark ets, and can potentially lead to ethnic and religious conflicts (see Vendina’s article in this issue). Though the social territorial differentiation in Moscow has not yet reach ed the scale of U.S. or even West European cities, the growing social polarization has already seen the creation of “gated communities” and can condemn the great majority of Muscovites to live in neglected and forgotten housing ghettos. Moreover, this polarization process risks perpetuating social contrasts in creating multi-standard systems of education and health care – separately for the richer and the poorer strata of society.
Moscow city authorities must solve other aggravations. One of the most serious, requiring huge investments, is the housing problem, specifically the reconstruction of the physically obsolete and dilapidated part of the housing stock, four-story apartment blocs ( Khrushchoby ) built in the 1960s. Moreover, the city lacks empty spaces for new developments and, thus, it is necessary to demolish old residential and industrial buildings or to shift industrial plants in order to intensify land uses. Another urgent problem is rapid automobilization, related to the insufficient capacity of the roads’ network and of parking; constant traffic jams already render downtown Moscow inaccessible by car on workdays. (See the paper in this issue by Bityukova and Argenbright on the pollution effects of the growth in car ownership). Against this background, slow development of public transportation caused by inadequate investment seems to be especially pressing. The city government does not possess the financial means to build highways such as the Third Ring and, at the same time, to invest in extending the Metro (subway) and other public transportation. The city authorities under the leadership of Yuri Luzhkov (mayor since 1992) has pledged to continue to invest in prestigious projects to maintain Moscow ’s image and competitiveness as the Russian capital and a new global city.
Like any major city, Moscow has a complex structure that needs to be considered at different levels. First, at the global and macro-regional (Central-East European) scale, Moscow ’s relations are dominantly economic as part of a world-city system (Taylor and Hoyler, 2000). Second, at the national scale, Moscow is both the federal capital and a subject of the Russian Federation – here the emphasis is on its primacy and balancing of the differentiation that is becoming more apparent in the country. Third, at the regional scale, Moscow ’s agglomeration over-reaches the capital’s city limits and issues about industrial re-location, regional transport, city-suburb relations, and the conversion of agricultural and forest zones to urban uses dominate. Fourth, at the city scale, the optimal combination that allows the city to assume its functions, to be competitive at the international scene while still meeting the needs of its population is still to be found. Finally, at the local (neighborhood) scale, Moscow is a relatively vast and heterogenous territorial unit, including 124 municipal districts ( rayoni ) (see Figure 1), which will soon become true local governments possessing not only their elected assemblies, but also their own budgets. Geographical distributions of housing, jobs, services, green spaces, etc. across these rayoni are likely to become more contentious and prominent.
To cope with the myriad of problems across a variety of scales, the city authorities need a long-term strategy. Obviously, the privileged 1990s situation of the capital can change rapidly, as the turmoil that occurred after the financial crisis of August 1998 showed. The Russian federal government refuses to cover even a small part of Moscow ’s expenses as the national capital. Some large companies (important taxpayers) have already moved their registered headquarters out from the city, and during the last two years city officials complain that the city budget is becoming very tight. Discussions about Moscow ’s economic future can be summarized as the choice between different models of development that depend in turn on the model followed by Russia as a whole. If Russia remains a dominant exporter of fuel and other raw materials and her manufacturing industry and high-tech industries decline further, there will obviously be much less opportunity for Moscow to realize its potential for innovation and to select a new, more balanced model of development. Simplifying, one can say that future of Moscow can be based on one of three options – a) traditional manufacturing; b) a service economy - mainly banking and trade, and c) science, information processing and high tech industry. In other words, Moscow can develop as the capital of an open economy, thus continuing current trends, or as the center of national modernization. Before the collapse of the Soviet Union , more than one million people in Moscow were engaged in pure and applied research (one-quarter of all Soviet scholars). Though this unique human capital is now considerably weakened, the Russian capital still ranks second among European cities by the number of citations in academic journals and monographs, thanks mainly to the sciences. To ensure a sustainable development, the city should promote these scientific innovation activities and extend them into high-tech manufacturing (Pchelintsev, 1999).
The authors of this special issue offer no solutions for the problems that Moscow is now facing. Rather, they analyze recent data showing the post-Soviet evolution of economic functions and social-territorial structures of the city from a perspective of its transformation into a global city. Radical shifts in the economic structure of the capital changed (but did not diminish) its impact on the urban environment. The theme of the changing sources of Moscow ’s environmental pollution (from stationary to automobiles) is developed in the paper by Viktoria Bityukova and Robert Argenbright . Olga Vendina devotes her article to social geographical developments among Moscow ’s ethnic minorities and the related issue of growing ghettoization . Elena Shomina, Vladimir Kolossov and Viktoria Shukhat analyze local community groups (non-governmental organizations) in Moscow as responses to changing neighborhood, especially housing conditions. In the first paper in this special issue, Vladimir Kolossov , Olga Vendina and John O’Loughlin examine the evidence for Moscow ’s claim to world-city status and the geography of business developments in the city since the end of the Soviet Union .
Soviet and Post-Soviet Research on Moscow .
Moscow attracted the attention of Soviet geographers who began to study the unique problems of the capital at the end of World War II. Human geographers were especially interested in studying the interaction between the capital and its region, and its impact on surrounding territories of Central Russia . They delimited Moscow ’s influence according to different criteria - the radius of daily and weekly cycles of activity, the number of second residences, etc.; the distribution of built-up areas, industry, services and recreational functions by sectors around the city by comparison to their environmental impact. The fundamental volume on these themes – Moscow’s Capital Region- the Territories, Structures, and Environment - written by a large group from the Institute of Geography of the Soviet (now Russian) Academy of Sciences, include interesting chapters that serve as a bench mark for the post-Soviet developments (Lappo, Goltz and Treivish 1988). Geographers gave much less attention to Moscow itself. The social-territorial structure of the city was hardly examined – partly because of the lack of reliable statistical data for micro-districts. The problems of Moscow ’s development were analyzed mainly by architects and urban planners, especially those serving at the Institute of Moscow ’s General Plan, whose reports are unavailable.
Some general works containing detailed information on urban life are available according to the respective periods. In particular, Yulian G. Saushkin , a patriarch of Soviet human geography and former chair of the Department of Human Geography of the USSR at Moscow State University, produced three books on Moscow (the last one appearing soon after his death with his former graduate student, Vera Glushkova) (Saushkin 1950, 1964, Saushkin and Glushkova 1983). In the late 1970s–early 1980s, new geographical approaches to the study of the local urban environment, the conditions of life and the functional organization of the municipal economy were developed, in particular, in a laboratory of the Institute of Geography founded by Yuri Medvedkov (now at Ohio State University). Contemporary quantitative methods (especially factorial ecology) were applied in the estimation of the quality of the urban environment (Barbash and Gutnov 1980; Barbash 1982) Examining patterns of distribution of residential areas and jobs, the number of requests to move into a specific area, its proximity to major transportation lines, the convenience of transport connections, the presence of city-wide services, the variety of employment opportunities, and the ecological situation was used as measures of attractiveness (Barbash 1984, Vasiliev and Privalova 1984). In the 1990s, a similar approach was used by Sidorov (1992).
In the post-Soviet period, the transition to the mark et economy challenged geographers and other social scientists with new theoretical and policy problems: economic restructuring, the development of the privatized housing mark et, social polarization, unemployment, and old and new social pathologies all came to the forefront of research. Despite publication of a number of innovative works, it is hardly possible to conclude that Russian human geographers met these challenges. However, the works of Vera Glushkova should be mentioned. She published a monograph devoted to the dynamics and the composition of Moscow’s population, the functions of the city and main branches of its economy, land-use and social problems (in particular, its religious geography) (Glushkova 1997, 1999). Glushkova also was one of the initiators and main authors of large, well- documented, illustrated volumes on history, geography and urbanism in Moscow (see, for instance, Kuzmin 2000, etc.). The second edition of the Encyclopaedia “ Moscow ” is especially worth of notice (Moskva, 1997). Relevant geographical information on the development of Moscow is also contained in key historical publications (Vinogradov 1997, Gorinov 1996, 1997).
A series of works on urbanism and architecture richly illustrated by detailed maps of the environment, roads, transportation, planning structure and functional zoning of the city appeared as a result of public discussions, the first steps in the discussion and adoption of the new General Plan of Moscow to 2020 (Arkhitectura... 1999, Moskva... 2000, Moskva..., 2001). Comprehenisve studies on the history of architecture and urbanism in the capital also appeared during the first post-Soviet decade (Kudriavtsev, 1994). Geographers participated during the 1990s in several important works on the environment in Moscow . In particular, Viktoria Bityukova wrote a thesis, where she studied both the “real” spatial distribution of polluters and their distribution by administrative-territorial units (Moskva... 1995; Bityukova 1996; Kuzmin 2000). Some environmental maps can be found on the official web-site of the government of Moscow ( www.mos.ru ).
Geographical studies of Moscow were given further impetus by the improvement of official statistics, as well as by the 1995 decision of the capital’s government to introduce a new instructional discipline in the city’s and region’s high schools – “knowledge of Moscow” ( “moskvovedenie” ). This educational policy spurred the appearance of a series of textbooks (for example, Alekseev et al ., 1996, 1997 ). Furthermore, the city government of Moscow initiated the publication of two monthly journals that contain the results of social studies of the city ( Simptom and Puls ). The Committee on Telecommunications and Mass Media of the Government of Moscow yearly publishes about a dozen small books on current social problems, but unfortunately, only a very limited number of copies are printed and are not available in most libraries.
Outside of Russia , in his key work, Timothy Colton (1995) analyzed the dynamics of the city’s boundaries, and its demographic, industrial and even political patterns in Moscow in the 20 th century. Many academic journals, including Post-Soviet Geography and Economy, regularly publish papers on the human geography of the Russian capital. GeoJournal prepared a special issue on Moscow and St. Petersburg ( Gdaniec , 1997; Kolossov, 1997; Vendina, 1997). In the field of social geography, Mozolin (1994) explained the intra-urban distribution of housing prices in terms of accessibility to the CBD and urban morphology. Bater (1994) and Daniell and Struyk (1994) analyzed the development of the housing mark et in Moscow and, in particular, the results of privatization of housing, the implementation of municipal housing programs under new conditions, and the construction of new one-family cottages by “new Russians”. Kirsanova (1996) widely used the results of the polls about evaluations and preferences of different parts of Moscow by Muscovites and analyzed their relations with the gender, age, education, and the place of socialization of respondents and with the types of housing. Vendina (1994, 1995, 1996, 1997), and Vendina and Kolossov (1996) related the transformations of functions and employment in different districts of Moscow in the post-socialist years with the pattern of housing prices on the secondary mark et, while Gritsai (1997) compared the first results of mark et transformations in Moscow, especially the development of business services, with the situation in some largest world metropolises.
Post-Soviet developments in Moscow of demographic and social indicators (natural population change, ethnic composition and the level of education, especially during the 1979-1989 intercensal period in the old administrative districts abolished in 1992), were examined by Rowland (1992). Differences between the inner and the outer zones of the city and the concentration of the educated people in the center and the south-west were also considered in the analysis of the outcomes of the first democratic elections in the capital (Berezkin et al. , 1990 and Colton, 1995). Bater, Degtyarev and Amelin (1995) and Kolossov (1996, 1997) showed that the voting behavior of Muscovites differs greatly from most other regions of the country and has stable territorial patterns, while the differences in electoral preferences between the west and south-west parts of the city with the remainder were defined and explained by O’Loughlin, Kolossov and Vendina, 1997. More recently, Pavlovskaya and Hanson (2001) reported on the effects on family life of changes in the retail structure and privatization of services in a rayon in central Moscow and Argenbright (2000) paints an evocative picture of the changing nature of Moscow ’s public spaces, from controlled Soviet to the contemporary free-for-all.
A visitor to Soviet Moscow would hardly recognize its successor. The center of Moscow increasingly looks like a Central European city, like Warsaw or Budapest , with expensive stores, gallerias, super mark ets, commercial offices, and a full array of retail services. Meanwhile, the streetscapes of Moscow ’s outer zones are hardly changed from Soviet times. As a small segment of the population of “new Russians” prosper in these times of frantic change, the majority of Moscovites struggle to make ends meet. In a sample of 3,500 Moscovites in April 2000, we found that only 22.8 % agreed with the statement that “things are not so bad, and it is possible to live”, while the majority (55.3%) said that “life is difficult but it’s possible to endure”. Another 13.3% said that “our condition makes it impossible to endure further.” And, it is worth re-emphasizing that Moscow is much wealthier than the rest of the country and should not be considered as a typical Russian city or even a harbinger of things to come for other large cities of the former Soviet Union .
The frenetic pace of commercial life in central Moscow and the associated rush to gentrification and re-development, quick money, criminal activity, banking and financial scandals, and great uncertainties has produced an “city on the make” ( Spector , 1997) . What is most startling is the contrast of these world-city functions and consequences to the daily lives of most of the population. The ‘dual city hypothesis” of Mollenkopf and Castells (1991), developed for New York City , seems increasingly apropos for Moscow . Of course, dramatic polarization is a persistent feature of many major Third World capitals but its appearance in Moscow is undoubtedly the quickest. Whether the growing gaps in Moscow - between rich and poor, between the segments of the population who are tied to the incorporation into the global economy and those whose livelihood is connected to state services or to small-scale local enterprise, and between the older generation whose world is still colored by their Soviet-era experiences and the post-Soviet generation – will magnify as they have for the past decade or will be eased is an important research question, not only for Moscovites and Russians, but for all societies undergoing rapid social and economic change in these globalized times. The papers in this special issue contribute to this research and offer a picture of the city after 10 years of post-Soviet change against which future developments can be compared.
Alekseev, Alexander I., ed. (1996) Moskvovedenie ( Moscow Studies). Moscow.: Ekopros .
Alekseev, Alexander I., Vera G. Glushkova and Galina Y. Lisenkova , eds. Geografia Moskvy i Moskovskoi oblasti (Geography of Moscow and of the Moscow Region). Moscow : Moskovskie Uchebniki , 1997.
Argenbright, Robert “Re making Moscow : New Places, New Selves." Geographical Review 89 (1): 1-22, January, 1999.
Arkhitektura , stroitelstvo , disain (Architecture, Construction Works and Design). Special Issue on the General Plan of Moscow to 2020, 4 (14), 1999.
Barbash , Natalia B. “Spatial Relations Among Places with Complementary Functions Within the City of Moscow .” Soviet Geography: Review and Translation , 23 , 2: 77-94, February, 1982.
Barbash , Natalia B. “ Privlekatelnost Razlichnykh Chastei Gorodskoi Sredy Dlia Gorozhan (Na Osnove Dannykh Obmena Zhiliem )” (The Attractivity of Different Parts of the Urban Environment for Residents (on the Basis of Data on Residential Exchange)” Izvestia Akademii Nauk SSSR: Seriya Ggeograficheskaya 5: 81-91, 1984.
Barbash , Natalia B. and Aleksei E. Gutnov “Urban Planning Aspects of the Spatial Organization of Moscow : An Application of Factorial Ecology.” Soviet Geography: Review and Translation, 21, 9: 557-574, November, 1980.
Bater , James H. “Housing Developments in Moscow in the 1990s”. Post-Soviet Geography, 35 , 6 : 309-328, June, 1994.
Bater , James H., Andrei A. Degtyarev , and Vladimir N. Amelin . “Politics in Moscow : Local Issues, Areas and Governance.” Political Geography, 14 , 8: 665-687, November, 1995.
Berezkin , Andrei V., Vladimir A. Kolossov, Marianna Pavlovskaya , Nicolai Petrov and Leonid Smirniagin . “The Geography of the 1989 Elections of People's Deputies of the USSR (Preliminary Results).” Soviet Geography , 30 , 8: 607-634, October 1989.
Bityukova , Viktoria R. Sotsialno-ekologicheskie problemy razvitia gorodskikh territorii ( na primere Moskvy ) (Social-Environmental Problems of Urban Territories’ Development: the Case of Moscow) Doctoral thesis. Moscow : Department of Geography, Moscow State University , 1996.
Colton, Timothy S. Moscow : Governing the Socialist Metropolis. Cambridge , MA : Harvard University Press, 1995.
Daniell , Jennifer and Ray Struyk , Housing Privatization in Moscow : Who Privatizes and Why . Cambridge , MA : Blackwell Publishers, 1994.
Federov , Dmitri “Telecommunication Computer Networks in Moscow .” Geojournal , 42 ,4: 433-448, August 1997.
Gdaniec , C. “Reconstruction in Moscow ’s Historic Centre: Conservation, Planning and Finance Strategies- the Example of the Ostozhenka District.” Geojournal , 42 , 4: 365-376, August 1997.
Glushkova , Vera G. Moskva . Istoria i Geografia ( Moscow : History and Geography). Moscow : Mir Publishers, 1997.
Glushkova , Vera G. Sotsialnyi Portret Moskvy na Poroge XXI Veka (A Social Portrait of Moscow at the Eve of the XXI Century). Moscow : Mysl Publishers, 1999.
Gorinov , Mikhail M., ed. Istoria Moskvy (History of Moscow ), vol. 1-4. Moscow : AOL, 1996, 1997.
Gritsai , Olga “ Postindustrialnye Sdvigi v Moskve : Kontseptsia Mirovykh Gorodov i Perestroika Ekonomicheskoi Struktury ” (The Post-Industrial Shifts in Moscow : the Concept of the World City and Economic Restructuring). Izvestia RAN, Seria Geograficheskaya 5: 90-97, 1996.
Gritsai , Olga “Business Services and Restructuring of Urban Space in Moscow .” Geojournal , 42 , 4:349-363, August 1997.
Gritsai , Olga “ Moscow under Globalization and Transition: Paths of Economic Restructuring.” Urban Geography , 18 , 2: 155-165, 1997.
Kirsanova 1996
Kolossov, Vladimir “Political Polarization at the National and the Intra-Urban Levels: The Role of Moscow in Russian Politics and Socio-Political Cleavages within the City,” GeoJournal , 42 , 4: 385-401, August, 1997.
Kolossov, Vladimir et Olga Vendina « Moscou, Retour a la Voie Mondiale. » Geographie et Culture, Metropolisation et Politique . Ed. Paul Claval and André-Louis Sanguin. Paris : L’Harmattan, 1997: 137-153
Kolossov, Vladimir, Olga Vendina, Nadezhda Borodulina , Elena Seredina , and Dmitri Fedorov “ Kontseptsia Informationnogo Goroda i Sozdanie Novoi Delovoi sredy v Moskve (The Concept of the Informational City and Formation of a New Business Environment in Moscow ).” Izvestia RAN, Seria Geograficheskaya , 5 : 141-156, 1998.
Kudriavtsev , Mikhail P., ed. Moskva – Tretii Rim: Istoriko-Gradostroitelnoye Issledovanie . ( Moscow – the Third Rome . A Historical-Urban Study). Moscow : SOL, 1994.
Kuzmin , Alexander V., ed. Gradostroitelstvo Moskvy . 90-e Gody XX Veka (Urbanism in Moscow . The 1990s). Moscow : Moskovskie Uchebniki i Kartolitografia , 2000.
Lappo , Georgi M., Golz , Grigori A., and Treivish , Andrei I. , eds. Mosckovskii Stolichnyi Region: Territorialnaya Struktura i Okuzhayushchaya Sreda ( Moscow Capital Region: the Territorial Structure and Environment). Moscow : Institute of Geography of the Academy of Sciences of the USSR , 1988.
Mollenkopf , John and Manuel Castells . Dual City : Urban Restructuring in New York . New York : Russell Sage Foundation, 1991.
Moskva i mockvichi : v kakoi okruzhayushchei srede my zhivem ? ( Moscow and Moscovites : In What Environment Do We Live?) Moscow : Institute of the Moscow General Plan, 1995.
Moskva ( Encyclopaedia of Moscow ). Moscow : Rossiiskaya Entsiklopedia , 1997.
Moskva : Vzgliad v Tretie Tysyacheletie ( Novyi Generalnyi Plan Razvitia i Ego Realizatsia ) ( Moscow : A View into the Third Millenium : the New General Plan of Development and Its Realization). Arkhitektura i stroitelstvo Moskvy (Architecture and Construction Works in Moscow ), special issue, 5-6, 2001.
Moskva 2000 ( Moskovskii spravochnik ). (Moscow 2000: A Moscow Reference Book). Moscow : Golden Be, 2000.
Mozolin , Mikhail. “The Geography of Housing Values in the Transformation to a Market Economy: A Case Study of Moscow .” Urban Geography, 15 , 2 : 107-127, March 1994.
O’Loughlin, John, Vladimir Kolossov and Olga Vendina “The Electoral Geographies of a Polarizing City: Moscow , 1993-1995” Post-Soviet Geography and Economics, 38 , 10: 567-600, December 1997.
Pavlovskaya , Marianna and Susan Hanson. “Privatization of the Urban Fabric: Gender and Local Geographies of Transition in Downtown Moscow .” Urban Geography, 22 , 1: 4-28, January 2001.
Pchelintsev , Oleg S . “ Moskva i Strategicheskoe Izmerenie Rossiiskikh Reform” ( Moscow and the Strategic Dimension of Russian Reforms)” Moskva na Fone Rossii i Mira: Problemy i Protivorechia Otnoshenii Stolitsy v Kontekste Rynochnoi Transformatsii (Moscow Against the Background of Russia and the World: Problems and Contradictions of the Capital’s Relations on the Context of Reforms). Leonid Vardomsky and Vladimir Mironov , eds . Moscow: Institute for International Economic and Political Studies and Institute of Geography of the Russian Academy of Science, 1999, pp. 13-31.
Rowland, Richard “Selected Urban Population Characteristics of Moscow .” Post-Soviet Geography, 33 , 2 : 569-590, March 1992.
Saushkin , Yulian G. Moskva ( Moscow ). Moscow : Geografgiz , 1950.
Saushkin , Yulian G. Moskva . Geograficheskaya Kharakteristika ( Moscow : A Geographical Characteristic). Moscow : Geografgiz , 1964.
Saushkin , Yulian G., and Vera G. Glushkova Moskva Sredi Gorodov Mira ( Moscow among the Cities of the World). Moscow : Mysl Publishers, 1983.
Sidorov , Dmitri “Variations in Perceived Level of Prestige of Residential Areas in the Former USSR .” Urban Geography , 13 , 4 : 355-373, July-August, 1992.
Stadelbauer , Jörg . “ Moskau . Post- sozialistische Megastadt im Transformationsprozess .” Geographische Rundschau , 48, 2: 113-120, February 1996.
Spector , Michael. “ Moscow on the make.” New York Times Magazine , June 1, 1997 .
Taylor, Peter J. “ World Cities and Territorial States under Conditions of Contemporary Globalization.” Political Geography, 19 , 1: 5-32, January 2000.
Taylor, Peter J. and Mark Hoyler . “The Spatial Order of European Cities under Conditions of Contemporary Globalization.” Tidjschrift voor Economische en Sociale Geografie , 91 , 2: 176-189, May 2000
Vasiliev , Georgi L., and Olga L. Privalova “A Social-Geographical Evaluation of Differences within a City.” Soviet Geography: Review and Translations, 25 , 7: 488-496, September 1984.
Vendina, Olga « Moscou: En Recherche d'Equilibre Entre la Planification et Laisser-Faire » Planification et Stratégies de Développement dans les Capitales Européennes. Christian Vandermotten, ed. Bruxelles: Universite Libre de Bruxelles, 1994, pp. 273-285.
Vendina, Olga « Moscou: les Rôles de la Ville et sa Genèse», Géographie et Cultures , 14 : 85-103, 1995.
Vendina, Olga “ Sotsialnaya Stratifikatsia v Moskve : Tsena Ekonomicheskikh Reform” (The Social Stratification in Moscow : the Cost of Economic Reform). Izvestia RAN , Seria Geograficheskaya , 5 : 98-113, 1996.
Vendina, Olga “Transformation Processes in Moscow and Intra-Urban Stratification of Population.” Geojournal 42 ,4:341 -347, August 1997.
Vendina, Olga and Vladimir Kolossov . “ Sotsialnaya Polarisatsia i Politicheskoe Povedenie Moskvichei ” (The Social Polarization and the Political Behavior of Moscovites ). Sociological Journal , 3 , 4: 164-176, 1996.
Vinogradov , Vladimir, ed. Moskva - 850 let. Istoria Moskvy ( Moscow - 850 years. History of Moscow ). Moscow : Moskovskie uchebniki (2 vols ), 1996-1997.
* Yablokov , Aleksei V., ed. O Sostoyanii Okruzhayushchei Sredy g. Moskvy v 1992 g. Gosudarstvennyi Doklad (About the State of Environment in Moscow in 1992 - The State Report). Moscow : Government of Moscow , 1993.
Figure SEQ Figure \* ARABIC 1 Location of Rayoni in Moscow
[1] Acknowledgements: This special issue benefited from the collaborative work of many individuals. In Boulder , Tom Dickinson prepared the final versions of all of the maps and Clionadh Raleigh helped with the bibliographic and grammatical elements of the project. Altinay Kuchukeeva assisted with the research on the papers and Jim Bell, now at the U.S. State Department, was involved in the project in the early stages. In Moscow , the project was assisted by xxxx , xxxxx , and xxx. The project was funded by a grant to Professor O’Loughlin by the National Science Foundation and by grants to Professor Kolossov by …….
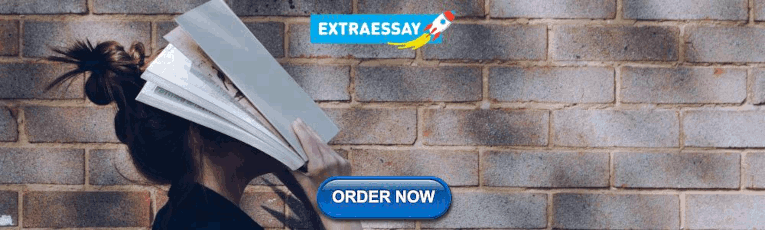
IMAGES
VIDEO
COMMENTS
Quantitative research methods. You can use quantitative research methods for descriptive, correlational or experimental research. In descriptive research, you simply seek an overall summary of your study variables.; In correlational research, you investigate relationships between your study variables.; In experimental research, you systematically examine whether there is a cause-and-effect ...
Quantitative Research Methods are as follows: Descriptive Research Design. Descriptive research design is used to describe the characteristics of a population or phenomenon being studied. This research method is used to answer the questions of what, where, when, and how. Descriptive research designs use a variety of methods such as observation ...
Quantitative research methods are used to observe events that affect a particular group of individuals, which is the sample population. In this type of research, diverse numerical data are collected through various methods and then statistically analyzed to aggregate the data, compare them, or show relationships among the data. ...
Quantitative research methods. You can use quantitative research methods for descriptive, correlational or experimental research. In descriptive research, you simply seek an overall summary of your study variables.; In correlational research, you investigate relationships between your study variables.; In experimental research, you systematically examine whether there is a cause-and-effect ...
Quantitative research is a research strategy that focuses on quantifying the collection and analysis of data. It is formed from a deductive approach where emphasis is placed on the testing of theory, ... Quantitative methods can be used to verify which of such hypotheses are true.
Quantitative methods emphasize objective measurements and the statistical, mathematical, or numerical analysis of data collected through polls, questionnaires, and surveys, or by manipulating pre-existing statistical data using computational techniques.Quantitative research focuses on gathering numerical data and generalizing it across groups of people or to explain a particular phenomenon.
Quantitative research methods. a method of research that relies on measuring variables using a numerical system, analyzing these measurements using any of a variety of statistical models, and reporting relationships and associations among the studied variables. For example, these variables may be test scores or measurements of reaction time.
About Research Methods. This guide provides an overview of research methods, how to choose and use them, and supports and resources at UC Berkeley. As Patten and Newhart note in the book Understanding Research Methods, "Research methods are the building blocks of the scientific enterprise. They are the "how" for building systematic knowledge.
Quantitative method is the collection and analysis of numerical data to answer scientific research questions. Quantitative method is used to summarize, average, find patterns, make predictions, and test causal associations as well as generalizing results to wider populations. It allows us to quantify effect sizes, determine the strength of ...
Quantitative methods can also be used to test hypotheses by conducting quasi-experimental studies or designing experiments. Independent and Dependent Variables In quantitative research, a variable is something (an intervention technique, a pharmaceutical, a temperature, etc.) that changes.
What is Quantitative Research? Quantitative methodology is the dominant research framework in the social sciences. It refers to a set of strategies, techniques and assumptions used to study psychological, social and economic processes through the exploration of numeric patterns.Quantitative research gathers a range of numeric data.
Quantitative research methods are concerned with the planning, design, and implementation of strategies to collect and analyze data. Descartes, the seventeenth-century philosopher, suggested that how the results are achieved is often more important than the results themselves, as the journey taken along the research path is a journey of discovery. . High-quality quantitative research is ...
Quantitative research, in contrast to qualitative research, deals with data that are numerical or that can be converted into numbers. The basic methods used to investigate numerical data are called 'statistics'. Statistical techniques are concerned with the organisation, analysis, interpretation and presentation of numerical data.
Quantitative research involves analyzing and gathering numerical data to uncover trends, calculate averages, evaluate relationships, and derive overarching insights. It's used in various fields, including the natural and social sciences. Quantitative data analysis employs statistical techniques for processing and interpreting numeric data.
The goal of quantitative research methods is to collect numerical data from a group of people, then generalize those results to a larger group of people to explain a phenomenon. Researchers generally use quantitative research when they want get objective, conclusive answers. For example, a chocolate brand may run a survey among a sample of ...
Unlike in quantitative research where hypotheses are usually developed to be tested, qualitative research can lead to both hypothesis-testing and hypothesis-generating outcomes.2 When studies require both quantitative and qualitative research questions, this suggests an integrative process between both research methods wherein a single mixed ...
Abstract. The aim of th is study i s to e xplicate the quanti tative methodology. The study established that. quantitative research de als with quantifying and analyzing variables in o rder to get ...
Research is a systematic way of collecting information to answer questions or solve problems. There are two primary research methods: qualitative and quantitative. We use these methods to collect and analyze data. In this tutorial, we'll explore the differences between qualitative and quantitative research methods.
A Level Sociology Research Methods | Revisesociology.com Sociologists use a range of quantitative and qualitative, primary and secondary social research methods to collect data about society. The main types of research method are: Social surveys (questionnaires and structured interviews) Experiments (Lab and Field) Unstructured interviews Partipant Observation Secondary qualitative data ...
Quantitative research is a type of research that focuses on collecting and analyzing numerical data to answer research questions. There are two main methods used to conduct quantitative research: 1. Primary Method. There are several methods of primary quantitative research, each with its own strengths and limitations.
The Altmetric Attention Score is a quantitative measure of the attention that a research article has received online. Clicking on the donut icon will load a page at altmetric.com with additional details about the score and the social media presence for the given article.
We surveyed quantitative and research methods instructors in graduate public affairs programs using the same survey instrument at two intervals 8 years apart and analyzed results from each period side-by-side. Findings indicate some stark differences in the content and delivery of these courses. Given the timing of the second survey—Spring ...
Based on the robust measuring method and accurate models, we propose a quantitative and model-based decision support framework for treatment planning of cervical and lumbar osteophytes in clinical ...
A mixed methods dissertation focuses on the collection of data and analyses it by combining the top two research approaches, i.e., quantitative and qualitative. Researchers have to follow a step-by-step detailed process to arrive at the results of a mixed research dissertation, as depicted in the ...
Our research team will conduct a mixed-methods study to investigate the implementation and early effects of these two policy interventions on pregnancy and infant health equity. Qualitative interviews will be conducted with Medicaid managed care administrators and obstetric and pediatric providers, and focus groups will be conducted among ...
Using the methods of analysis, synthesis, systematization, quantitative and qualitative characteristics of such objects and processes that determine the content of the selected aspects are
Furthermore, a case study is employed to illustrate the proposed method, results indicate that the failure of the whole underground system is caused by the insufficient safety reserve of surrounding rock element. In general, the proposed method provides theoretical guidance for quantitative stability analysis and design optimization of tunnels.
Contemporary quantitative methods (especially factorial ecology) were applied in the estimation of the quality of the urban environment (Barbash and Gutnov 1980; Barbash 1982) Examining patterns of distribution of residential areas and jobs, the number of requests to move into a specific area, its proximity to major transportation lines, the ...
Fundamentally, fluid flow through a rock fracture is governed by the Navier-Stokes equations, which. are a set of three coupled nonlinear partial di fferential equations. In the steady-state ...