Click through the PLOS taxonomy to find articles in your field.
For more information about PLOS Subject Areas, click here .
Loading metrics
Open Access
Peer-reviewed
Research Article
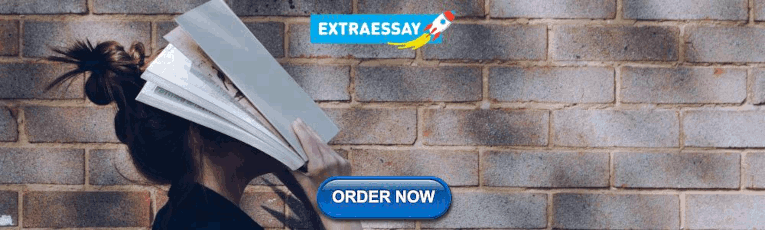
Measures of empathy and compassion: A scoping review
Contributed equally to this work with: Cassandra Vieten, Caryn Kseniya Rubanovich, Lora Khatib, Meredith Sprengel, Chloé Tanega
Roles Conceptualization, Data curation, Formal analysis, Funding acquisition, Investigation, Methodology, Project administration, Supervision, Validation, Visualization, Writing – original draft, Writing – review & editing
* E-mail: [email protected]
Affiliations Centers for Integrative Health, Department of Family Medicine, University of California, San Diego, San Diego, California, United States of America, Clarke Center for Human Imagination, School of Physical Sciences, University of California, San Diego, San Diego, California, United States of America

Roles Data curation, Formal analysis, Investigation, Methodology, Writing – original draft, Writing – review & editing
Affiliations Department of Psychiatry, University of California, San Diego, San Diego, California, United States of America, San Diego State University/University of California San Diego Joint Doctoral Program in Clinical Psychology, San Diego, San Diego, California, United States of America, T. Denny Sanford Institute for Empathy and Compassion, University of California, San Diego, San Diego, California, United States of America, T. Denny Sanford Center for Empathy and Technology, University of California, San Diego, San Diego, California, United States of America
Roles Conceptualization, Data curation, Formal analysis, Investigation, Visualization, Writing – review & editing
Affiliation Department of Psychiatry, University of California, San Diego, San Diego, California, United States of America
Roles Data curation, Investigation, Methodology, Project administration, Software, Writing – review & editing
Affiliation Human Factors, Netherlands Organisation for Applied Scientific Research (TNO), Soesterberg, The Netherlands
Roles Data curation, Formal analysis, Investigation, Project administration, Validation, Visualization, Writing – review & editing
Affiliation Clarke Center for Human Imagination, School of Physical Sciences, University of California, San Diego, San Diego, California, United States of America
Roles Data curation, Investigation, Validation, Writing – review & editing
¶ ‡ CP, PV, AM, GC, AJL, MTS, LE and CB also contributed equally to this work.
Affiliations U.S. Department of Veteran Affairs, VA Boston Healthcare System, Boston, Massachusetts, United States of America, Department of Psychiatry, Boston University Chobanian & Avedisian School of Medicine, Boston, Massachusetts, United States of America
Affiliation Compassion Clinic, San Diego, California, United States of America
Roles Writing – review & editing
Affiliations Department of Psychiatry, University of California, San Diego, San Diego, California, United States of America, VA San Diego Center of Excellence for Stress and Mental Health, San Diego, California, United States of America
Affiliation VA San Diego Center of Excellence for Stress and Mental Health, San Diego, California, United States of America
Roles Conceptualization, Writing – review & editing
Affiliations Department of Psychiatry, University of California, San Diego, San Diego, California, United States of America, VA San Diego Center of Excellence for Stress and Mental Health, San Diego, California, United States of America, Herbert Wertheim School of Public Health and Human Longevity Science, University of California, San Diego, San Diego, California, United States of America
Affiliation Departments of Family Medicine and Medicine (Bioinformatics), School of Medicine, University of California, San Diego, San Diego, California, United States of America
Affiliations Department of Psychiatry, University of California, San Diego, San Diego, California, United States of America, T. Denny Sanford Institute for Empathy and Compassion, University of California, San Diego, San Diego, California, United States of America, T. Denny Sanford Center for Empathy and Compassion Training in Medical Education, University of California, San Diego, San Diego, California, United States of America
Affiliations Department of Psychiatry, University of California, San Diego, San Diego, California, United States of America, T. Denny Sanford Institute for Empathy and Compassion, University of California, San Diego, San Diego, California, United States of America, T. Denny Sanford Center for Empathy and Technology, University of California, San Diego, San Diego, California, United States of America, T. Denny Sanford Center for Empathy and Compassion Training in Medical Education, University of California, San Diego, San Diego, California, United States of America
- Cassandra Vieten,
- Caryn Kseniya Rubanovich,
- Lora Khatib,
- Meredith Sprengel,
- Chloé Tanega,
- Craig Polizzi,
- Pantea Vahidi,
- Anne Malaktaris,
- Gage Chu,
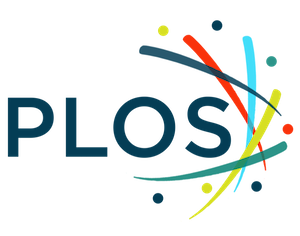
- Published: January 19, 2024
- https://doi.org/10.1371/journal.pone.0297099
- Reader Comments
Evidence to date indicates that compassion and empathy are health-enhancing qualities. Research points to interventions and practices involving compassion and empathy being beneficial, as well as being salient outcomes of contemplative practices such as mindfulness. Advancing the science of compassion and empathy requires that we select measures best suited to evaluating effectiveness of training and answering research questions. The objective of this scoping review was to 1) determine what instruments are currently available for measuring empathy and compassion, 2) assess how and to what extent they have been validated, and 3) provide an online tool to assist researchers and program evaluators in selecting appropriate measures for their settings and populations. A scoping review and broad evidence map were employed to systematically search and present an overview of the large and diverse body of literature pertaining to measuring compassion and empathy. A search string yielded 19,446 articles, and screening resulted in 559 measure development or validation articles reporting on 503 measures focusing on or containing subscales designed to measure empathy and/or compassion. For each measure, we identified the type of measure, construct being measured, in what context or population it was validated, response set, sample items, and how many different types of psychometrics had been assessed for that measure. We provide tables summarizing these data, as well as an open-source online interactive data visualization allowing viewers to search for measures of empathy and compassion, review their basic qualities, and access original citations containing more detail. Finally, we provide a rubric to help readers determine which measure(s) might best fit their context.
Citation: Vieten C, Rubanovich CK, Khatib L, Sprengel M, Tanega C, Polizzi C, et al. (2024) Measures of empathy and compassion: A scoping review. PLoS ONE 19(1): e0297099. https://doi.org/10.1371/journal.pone.0297099
Editor: Ipek Gonullu, Ankara University Faculty of Medicine: Ankara Universitesi Tip Fakultesi, TURKEY
Received: July 5, 2023; Accepted: December 21, 2023; Published: January 19, 2024
Copyright: © 2024 Vieten et al. This is an open access article distributed under the terms of the Creative Commons Attribution License , which permits unrestricted use, distribution, and reproduction in any medium, provided the original author and source are credited.
Data Availability: All relevant data are within the manuscript and its Supporting Information files.
Funding: CV received a grant from the T. Denny Sanford Institute for Empathy and Compassion at https://empathyandcompassion.ucsd.edu/ . Co-authors included faculty members affiliated with the T. Denny Sanford Institute who were involved in study design and reviewing/editing the manuscript. Other than that, the funders had no role in study design, data collection and analysis, decision to publish, or preparation of the manuscript.
Competing interests: The authors have declared that no competing interests exist.
Introduction
Historically, psychological assessment has overwhelmingly focused on measuring human struggles, difficulties, and pathologies. However, converging evidence indicates that positive emotions and prosocial qualities are just as important for improving overall well-being as stress, depression, and anxiety are to detracting from health and well-being [ 1 ]. Across fields—from medicine, mental health care, and education to economics, business and organizational development—there is a growing emphasis on investigating prosocial constructs such as compassion and empathy [ 2 ].
Compassion, or the heartfelt wish to reduce the suffering of self and others, promotes social connection and is an important predictor of overall quality of life [ 2 ] and well-being [ 3 ]. Empathy, or understanding and vicariously sharing other people’s positive emotions, is related to prosocial behaviors (e.g., helping, giving, emotional support), positive affect, quality of life, closeness, trust, and relationship satisfaction [ 4 ]. Compassion and empathy improve parenting [ 5 ], classroom environments [ 6 ], and teacher well-being [ 7 ]. Compassionate love toward self and others is associated with disease outcomes as well, such as increased long-term survival rates in patients with HIV [ 8 ]. Self-compassion refers to being gentle, supportive, and understanding toward ourselves in instances of perceived failure, inadequacy, or personal suffering [ 9 ]. Research indicates that self-compassion appears to reduce anxiety, depression, and rumination [ 10 ], and increase psychological well-being and connections with others [ 11 , 12 ]. Both compassion and self-compassion appear to protect against stress [ 13 ] and anxiety [ 10 ].
In healthcare professionals, empathy is associated with patient satisfaction, diagnostic accuracy, adherence to treatment recommendations, clinical outcomes, clinical competence, and physician retention [ 14 – 16 ]. Importantly, it is also linked to reduced burnout, medical errors, and malpractice claims [ 17 ]. However, evidence indicates that empathy declines during medical training and residency [ 18 – 20 ]. This may present an opportunity to improve many aspects of healthcare by identifying ways to maintain or enhance empathy during medical training. It is also important to note that while empathy is beneficial for patients, the effects on healthcare professionals are more complicated. A distinction can be drawn between positive empathy and/or compassion versus over-empathizing , which can lead to what has been termed “compassion fatigue” and/or burnout.
Disentangling these relationships through scientific investigation requires selecting measures and instruments capable of capturing these nuances. In addition, growing evidence that empathy and compassion can be improved through training [ 21 , 22 ] relies on selection or development of measures that can assess the effectiveness of such training. While empathy and compassion training for healthcare professionals has shown positive outcomes, it still requires improvement. For example, in a recent systematic review, only 9 of 23 empathy education studies in undergraduate nursing samples demonstrated practical improvements in empathy [ 23 ]. Another systematic review of 103 compassion interventions in the healthcare context [ 24 ] identified a number of limitations such as focusing on only a single domain of compassion; inadequately defining compassion; assessing the constructs exclusively by self-report; and not evaluating retention, sustainability, and translation to clinical practice over time: all related to how compassion and empathy are conceptualized and measured. The researchers recommend that such interventions should “be grounded in an empirically-based definition of compassion; use a competency-based approach; employ multimodal teaching methods that address the requisite attitudes, skills, behaviors, and knowledge within the multiple domains of compassion; evaluate learning over time; and incorporate patient, preceptor, and peer evaluations” (p. 1057). Improving conceptualization and measurement of compassion and empathy are crucial to advancing effective training.
Conceptualizing compassion and empathy
Compassion and empathy are complex constructs, and therefore challenging to operationalize and measure. Definitions of compassion and empathy vary, and while they are often used interchangeably, they are distinct constructs [ 25 ]. Like many other constructs, both compassion and empathy can be conceptualized at state and/or trait levels: people can have context-dependent experiences of empathy or compassion (i.e., state), or can have a general tendency to be empathic or compassionate (i.e., trait). The constructs of empathy and compassion each have multiple dimensions: affective, cognitive, behavioral, intentional, motivational, spiritual, moral and others. In addition to their multidimensionality, compassion and empathy are crowded by multiple adjacent constructs with which they overlap to varying degrees, such as kindness, caring, concern, sensitivity, respect, and a host of behaviors such as listening, accurately responding, patience, and so on.
Strauss et al. [ 26 ] conducted a systematic review of measures of compassion, and by combining the definitions of compassion among the few existing instruments at the time, proposed five elements of compassion: recognizing suffering, understanding the universality of human suffering, feeling for the person suffering, tolerating uncomfortable feelings, and motivation to act/acting to alleviate suffering. Gilbert [ 27 ] proposed that compassion consists of six attributes: sensitivity, sympathy, empathy, motivation/caring, distress tolerance, and non-judgement.
Likewise, empathy has been conceptualized as having at least four elements (as measured by the Interpersonal Reactivity Index [ 28 ] for example): perspective-taking (i.e., taking the point of view of others), fantasy (i.e., imagining or transposing oneself into the feelings and actions of others), empathic concern (i.e., accessing other-oriented feelings of sympathy or concern) and personal distress (i.e., or unease in intense interpersonal interactions). Early work by Wiseman [ 29 ] used a concept analysis approach identifying four key domains of empathy: seeing the world the way others see it, understanding their feelings, being non-judgmental, and communicating or expressing that understanding. Other conceptualizations of empathy [ 30 ] include subdomains of affective reactivity (i.e., being emotionally affected by others), affective ability (i.e., others tell me I’m good at understanding them), affective drive (i.e., I try to consider the other person’s feelings), cognitive drive (i.e., trying to understand or imagine how someone else feels), cognitive ability (i.e., I’m good at putting myself in another person’s shoes), and social perspective taking. De Waal and Preston [ 31 ] propose a “Russian doll” model of empathy, in which evolutionary advances in empathy layer one on top of the next, resulting in their definition of empathy as “emotional and mental sensitivity to another’s state, from being affected by and sharing in this state to assessing the reasons for it and adopting the other’s point of view” (p. 499).
Compassion is conceptualized as generally positive, and “more is better” in terms of health and well-being. Empathy on the other hand can lead to positive outcomes such as empathic concern, compassion, and prosocial motivations and behaviors, whereas unregulated empathic distress can be aversive, decrease helping behaviors, and lead to burnout [ 32 ]. Compassion and empathy also appear to differ in underlying brain structure [ 33 ] as well as brain function [ 34 ]. Terms such as “compassion fatigue” are more accurately characterized as empathy fatigue, and some evidence indicates that compassion can actually counteract negative aspects of empathy [ 35 ].
When assessing compassion and empathy, it is often important to measure their opposites, or constructs that present barriers to experiencing and expressing compassion or empathy. Personal distress, for example, can be confused for empathy but in fact is a “self-focused, aversive affective reaction” to encountering another person’s suffering, accompanied by the desire to “alleviate one’s own, but not the other’s distress” [ 36 , p.72]. Personal distress is viewed as a barrier to true compassion, and experienced chronically, is associated with burnout (i.e. exhaustion, cynicism, and inefficacy due to feeling frenetic/overloaded, underchallenged/indifferent, or worn-out/neglected [ 37 ]).
Other constructs that have been measured as barriers to compassion include lack of empathy or empathy impairment, apathy, coldness, judgmental attitudes toward specific populations or conditions, and fear of compassion. In sum, compassion and empathy are not so much singular constructs as multi-faceted collections of cognitions, affects, motivations and behaviors. When researchers or program evaluators consider the best ways to assess empathy and compassion, they must often attend to measuring these constructs as well.
Past systematic reviews focused on measurement of empathy and compassion sought to (1) review definitions [ 26 , 38 ]; (2) evaluate measurement methods [ 39 ]; (3) assess psychometric properties [ 40 ]; (4) provide quality ratings [ 26 , 41 , 42 ]; and/or (5) recommend gold standard measures [ 26 , 43 ]. To our knowledge, this review is the first scoping review focused on capturing the wide array of instruments measuring empathy, compassion, and adjacent constructs.
We conducted a scoping review and broad evidence map (as opposed to a systematic review or meta-analysis) for several reasons. Whereas systematic reviews attempt to collate empirical evidence from a relatively smaller number of studies pertaining to a focused research question, scoping reviews are designed to employ a systematic search and article identification method to answer broader questions about a field of study. As such, this scoping review provides a large and diverse map of the available measures across this family of constructs and measurement methodology, with the primary goal of aiding researchers and program evaluators in selecting measures appropriate for their setting.
Another unique feature of this scoping review is a data visualization that we have developed to help readers navigate the findings. This interactive tool is called the Compassion and Empathy Measures Interactive Data Visualization (CEM-IDV) ( https://imagination.ucsd.edu/compassionmeasures/ ).
The aims of this scoping review were achieved, including 1) identifying existing measures of empathy and compassion, 2) providing an overview of the evidence for validity of these measures, and 3) providing an online tool to assist researchers and program evaluators in searching for and selecting the most appropriate instruments to evaluate empathy, compassion, and/or adjacent constructs, based on their specific context, setting, or population.
The objective of this project was to capture all peer-reviewed published research articles that were focused on developing, or assessing the psychometric properties of, instruments measuring compassion and empathy and overlapping constructs, such as self-compassion, theory of mind, perspective taking, vicarious pain, caring, the doctor-patient relationship, emotional cues, sympathy, tenderness and emotional intelligence. We included only articles that were specifically focused on measure development or validation, and therefore did not include articles that may have developed idiosyncratic ways of assessing compassion or empathy in service to conducting experiments. We included self-report assessments, observational ratings or behavioral coding schemes, and tasks. This review was conducted according to the PRISMA statement for scoping reviews [ 44 ]. The population, concept, and context (PCC) for this scoping review were 1) population: adults and children, 2) concepts: compassion and empathy, and 3) context: measures/questionnaires for English-speaking populations (behavioral measures and tasks in all languages).
Eligibility criteria
Articles were included if they focused on development or psychometric validation/evaluation of whole or partial scales, tasks, or activities designed to measure empathy, compassion, or synonymous or adjacent constructs. Conference proceedings and abstracts as well as grey-literature were excluded from this review, as were articles in languages other than English or reporting on self-report scales that were in languages other than English. Behavioral tasks or observational measures that were conducted in languages other than English, but were reported in English and could be utilized in an English-speaking context, were included. Papers were excluded if they were in a language other than English, did not include human participants, or did not focus on reporting on development or psychometric validation of measures of compassion, empathy, or adjacent constructs.
Information sources
To identify the peer-reviewed literature reporting on the psychometric properties of measures of empathy and compassion, the following databases were searched: PubMed, Embase, PsychInfo, CINAHL, and Sociological Abstracts. See Table 1 to review the search terms and strategy applied for each database. All databases were searched in October 2020 and again in May 2023 by a reference librarian trained in systematic and scoping reviews at the University of California, San Diego library.
- PPT PowerPoint slide
- PNG larger image
- TIFF original image
https://doi.org/10.1371/journal.pone.0297099.t001
Abstracts of the articles identified through the search were uploaded to Covidence [ 45 , 46 ]. Covidence is a web-based collaboration software platform that streamlines the production of systematic and other literature reviews. Each article was screened by two reviewers and any conflicts reviewed in team meetings until the team reached 90% agreement. Thereafter, one screener included or excluded each abstract.
Full text screening
After articles were screened in, full text for all articles tagged as “Measure Development/Validation” were uploaded to the system. The project coordinator (MS) reviewed all articles that were included to ensure that they were tagged appropriately and that all articles reporting on development or validation of measures or assessments of psychometric properties were included in this review.
Each article was reviewed for its general characteristics and psychometric evaluation/validation data reported. General data extracted from each article included: the article title, full citation, abstract, type of study, the name of the scale/assessment/measure, the author’s definition of the construct(s) being measured (if stated), the specific purpose of the scale (context and population, such as “a scale for measuring nurses’ compassion in patient interactions”), whether the measure was conceptualized as assessing state or trait (or neither or both); whether the scale was self-report, peer-report, or expert observer/coder; the validation population, number, gender proportion, and location; and any reviewer notes.
See Table 2 for the psychometric data extracted from each article. In this scoping review we did not evaluate or record/analyze the results of the psychometric evaluations or validations. We only recorded whether or not they had been completed. Because some members of the team did not have enough experience/training to properly identify psychometric evaluations or assessments, data extraction was completed using two data extraction forms (i.e., one for general data and one for psychometric data) constructed in Survey Planet [ 47 ]. A group of four experienced coders completed both the general and psychometric data extraction forms, and a group of six less experienced coders completed only the general data extraction form with an experienced coder completing the psychometric data extraction form.
https://doi.org/10.1371/journal.pone.0297099.t002
Once the data were extracted, they were reviewed by the research coordinator or principal investigator and combined into a spreadsheet. After combining, the answers were reviewed by a team of four additional reviewers to ensure that the information extracted was correct. These four reviewers received additional training on how to confirm that the appropriate information was extracted from the article as well as how to clean the information in a systematic way.
Systematic literature search
A total of 29,119 articles were identified and 9,673 duplicates were removed, resulting in 19,446 titles/abstracts screened for eligibility ( Fig 1 ). A total of 10,553 full-text articles were assessed for inclusion based on the criteria previously described. A total of 6,023 articles were included in the final sample. Of these articles, 559 reported on the development or validation of a measure of empathy and/or compassion, 1,059 identified biomarkers of empathy and/or compassion, and 3,936 used a measure or qualitative interview of empathy or compassion in the respective study. This scoping review reports on the 559 measure development/validation articles.
https://doi.org/10.1371/journal.pone.0297099.g001
Measure development and validation studies
An overview of the 503 measures of empathy or compassion that were developed, validated, or psychometrically evaluated in the 559 articles can be found in the S1 Table . The majority of the studies ( n = 181) used a student population for development and/or validation. Student populations included undergraduate students, nursing students, and medical students. A total of 136 studies used samples of general, healthy adults (18 and older). Eighty-three (83) studies developed and/or validated a measure using health care workers, mostly comprising physicians and nursing staff. A total of 66 studies reported on a combined sample of populations such as clinicians and patients. There were 63 studies that used a patient population (e.g., cancer patients, surgical patients). A total of 34 studies used samples of individuals in other specific professions (e.g., military personnel), 32 used youth and adolescent samples (5–18 years old), 18 included older adults/aging populations, while 28 used samples in mental health care related professions (e.g., therapists). Nine studies used samples in other specific populations (e.g., spouses of depressed patients).
The number of possible psychometric assessments was 13 (see list below), and the total types of psychometric assessments reported for each measure ranged from 0 to 12. On average, each measure reported four types of psychometric assessments being completed. The measures with the highest number of psychometric assessments reported included the Interpersonal Reactivity Index (IRI) and the Self-Compassion Scale (SCS) with 12 psychometric assessments each. All scales with eight or more psychometric assessments reported in the articles we located can be found in S2 Table .
In regards to the type of psychometric assessments reported, a total of 409 studies assessed internal consistency, 342 used construct validity, 316 used factor analysis or principal component analysis, 299 assessed convergent validity, 218 used confirmatory factor analysis, 187 evaluated content validity, 165 tested for discriminant/divergent validity, 108 assessed test re-test reliability, 71 measured interrater reliability, 69 tested for predictive validity, 68 used structural equation modeling, 38 controlled for or examined correlations with social desirability, and 6 used a biased responding assessment or “lie” scale. Eighty studies performed other advanced statistics.
Measures of empathy and compassion
A total of 503 measures of compassion and empathy were identified in the literature. S3 Table is sorted alphabetically by the name of the measure, and includes a description of each measure, year developed, type of measure, subscales (if applicable), administration time (if provided), number of items, sample items, and response set. The majority of the scales were developed in the past decade (since 2013). Most of the measures identified were self-report scales (412 scales). Fifty-three (53) were peer/corollary report measures (descriptions of target individuals’ thoughts, feelings, motives, or behaviors), and 38 were behavioral/expert coder measures (someone who has been trained to assess target’s thoughts, feelings motives or behaviors). There were 370 measures with subscales and 133 measures without subscales. The number of items of each scale varied widely from 1 item to 567 items. The average number of items was 32 (SD = 45.2) and the median was 21 items. Most authors did not report on the estimated time it would take to complete the measure.
Interactive data visualization
Data visualizations are graphical representations of data designed to communicate key aspects of complex datasets [ 48 ]. Interactive data visualizations allow users to search, filter, and otherwise manipulate views of the data, and are increasingly being used for healthcare decision making [ 49 ]. We used Google Data Studio to create an online open-access interactive data visualization ( Fig 2 ) displaying the results of this scoping review. Access it at: https://imagination.ucsd.edu/compassionmeasures/
https://doi.org/10.1371/journal.pone.0297099.g002
The purpose of this Compassion and Empathy Measures Interactive Data Visualization (CEM-IDV) is to assist health researchers and program evaluators in selecting appropriate measures of empathy and compassion based on a number of parameters, as well as learning more about how these constructs are currently being conceptualized. Visualization parameters include: number of types of psychometric assessments completed (1–12) on the y-axis, number of items on the x-axis (with measures with over 70 items appearing on a separate display, not shown in Fig 2 ), and the bubble size indicating the number of participants in the validation studies. Search filters include Population in which the measure has been validated (e.g. students, healthcare workers, general adults), Construct (e.g. empathy, compassion, caring, self-compassion), and Type of Measure (e.g. self-report, behavioral/expert coder). Users can also search measures by name of the parent measure. For example, there are multiple versions of the Jefferson Scale of Empathy (JSE) (e.g., for physicians, for nurses, for medical students). To retrieve all articles reporting on any version of the JSE, one would search for the parent measure (i.e., “Jefferson Scale of Empathy”). If a measure does not have multiple versions (for example, the Griffith Empathy Measure), this search would yield all articles on that single version.
A robust science of compassion and empathy relies on effective measures. This scoping review examined the broad literature of peer-reviewed published research articles that either developed, or assessed the psychometric properties of, instruments measuring compassion and empathy. The review also includes overlapping and related constructs such as self-compassion, theory of mind, perspective-taking, vicarious pain, caring, the doctor-patient relationship, emotional cues, sympathy, tenderness, and emotional intelligence.
Our review indicates that the field of measuring compassion and empathy is maturing. Strides have been made in recent years in conceptualization, definition, and assessment of compassion and empathy. Since the time of earlier critical reviews of measurement of compassion and empathy, several measures have gained more psychometric support: S2 Table shows that 34 measures have been subjected to 9 or more types of psychometric validation. Multiple measures in this review demonstrate consistent reliability and validity along with many other strengths.
Newer measures align more closely with experimental, theoretical and methodological advances in understanding the various components of compassion and empathy. For example, the newer Empathic Expressions Scale [ 50 ] recognizes that actual empathy behaviors are different from cognitive and affective aspects of empathy. In another example, increasing understanding of the role of warmth and affection as an important component of empathy has led to the development of the Warmth/Affection Coding System (WACS) [ 51 ]. That measure also includes both micro- and macro-social observations, recognizing that implicit and explicit behaviors are important for assessment.
As measurement becomes more precise, assessments have also reflected increasing understanding of the differences between compassion and empathy, and the interaction between the two. For example, the Compassion Scale [ 52 ] subscales include kindness, common humanity, mindfulness and indifference (reverse-scored), whereas the family of the Jefferson Scale(s) of Empathy include compassion as well as “standing in the patient’s shoes” and “understanding the client’s perspective.” Recognizing recent research on how compassion could temper consequences of empathic distress such as burnout, it becomes important for researchers and program evaluators to not only avoid conflating the two, but also measure both separately.
Empathy and compassion in specific circumstances for specific populations have also been developed, such as the Body Compassion Questionnaire [ 53 ] with clear relevance for adolescents and young adults, as well as those with eating and body-dysmorphic disorders, or the modified 5-Item Compassion Measure [ 54 ] created specifically for patients to assess provider compassion during emergency room visits.
In our review, we included self-report assessments, peer/corollary observational measures, and behavioral tasks/expert coder measures, for adults and children in English-speaking populations. A discussion of the utility of each of these types of measures follows, along with a rubric for measure selection that researchers and program evaluators can use with the assistance of the tables and/or CEM-IDV online tool.
Self-report measures
The vast majority of measures of empathy and compassion are self-report measures (surveys, questionnaires, or items asking people to report on their own compassion and empathy). While perhaps the most efficient way to assess large numbers of participants, historically self-assessments of compassion and empathy have been riddled with challenges. Over a decade ago, Gerdes et al. [ 38 ] in their review of the literature noted that:
In addition to a multitude of definitions, different researchers have employed a host of disparate ways to measure empathy (Pederson, 2009). A review of the literature pertaining to empathy reveals that as a result of these inconsistencies, conceptualisations and measurement techniques for empathy vary so widely that it is difficult to engage in meaningful comparisons or make significant conclusions about how we define and measure this key component of human behaviour. (pp. 2327).
While a 2007 systematic review of 36 measures of empathy identified eight instruments demonstrating evidence of reliability, internal consistency, and validity [ 40 ], a systematic review of 12 measures of empathy used in nursing contexts [ 41 ] revealed low-quality scores (scoring 2–8 on a scale of 14), concluding that none of the measures were both psychometrically and conceptually satisfactory.
Our scoping review did not assess psychometric robustness other than the number of psychometric assessments completed, but a 2022 systematic review of measures of compassion [ 26 ] continued to reveal low-quality ratings (ranging from 2 to 7 out of 14) due to poor internal consistency for subscales, insufficient evidence for factor structure and/or failure to examine floor/ceiling effects, test-retest reliability, or discriminant validity. They concluded that “currently no psychometrically robust self- or observer-rated measure of compassion exists, despite widespread interest in measuring and enhancing compassion towards self and others” (pp. 26).
Several issues have been identified as potentially explaining shortcomings of compassion and empathy measures. For example, definitions of compassion and empathy vary widely in scholarly and popular vernacular, which can lead to variability in respondents’ perceptions. In addition to issues of semantics, the vast majority of compassion and empathy measures are face valid, relying on questions such as “I feel for others when they are suffering,” or “When I see someone who is struggling, I want to help.” These questions can increase the risk for social desirability bias (i.e., the tendency to give overly positive self-descriptions either to others or within themselves) and other response biases. Indeed, feeling uncompassionate can be quite difficult to admit, requiring not only a large degree of self-reflection and insight, but also an ability to manage the cognitive dissonance, shame, or embarrassment that could accompany such an admission. This difficulty may be particularly true among healthcare professionals.
Using self-report measures to assess the impact of compassion-focused interventions can also be confounded by mere exposure and demand characteristics, particularly when compared to standard-of-care or wait-list controls. In other words, after spending eight-weeks learning about and practicing compassion, it is not surprising that one might more frequently endorse items with respect to compassion due to increased familiarity with the concept, or implicit desire to satisfy experimenters, as opposed to increased compassionate states or behaviors. On the other hand, interventions could paradoxically result in people more accurately rating themselves lower on these outcomes once they investigate more thoroughly their own levels of, and barriers to, compassion and empathy, potentially masking improvements.
Peer/corollary and behavioral/expert coder measures
With increasing technological, statistical, and conceptual sophistication, we can innovate new measures that can increase validity by triangulating more objective measures with self-perceptions. In fact, multiple measures using observation and ratings by peers, patients, or trained/expert behavioral coders have been developed to do just that. We identified 61 measures utilizing observational measures or peer/corollary reports, some involving a spouse, friend, supervisor, client or patient completing a questionnaire, rating form or checklist regarding their observations of that person. These measures may also include ratings of a live or recorded interaction by someone who has been trained to assess, or is an expert in assessing, compassion or empathy behaviors. Compassion or empathy behaviors include verbalizations and signals such as eye contact, tone of voice, or body language. Similarly, qualitative coding of transcribed narratives, interactions, or responses to interview questions or vignettes can be conducted with human qualitative coders, which is increasingly supported by artificial intelligence.
These methods have the clear benefit of avoiding self-report biases and providing richer data for each individual (for use in admissions or competency exams for instance). However, they can be labor intensive, can introduce potential changes in behavior due to knowing one is being observed, and can introduce another layer of subjectivity on the part of the observer/rater (which can be overcome in part by measures of agreement between two or more raters). They also tend to have fewer psychometric assessments testing their validity or reliability than other measures.
Behavioral tasks
Laboratory-based behavioral tasks have been useful for assessing empathy and compassion under controlled conditions while reducing self-report biases and taking less time than qualitative/observational measures. These lab protocols involve exposure to stimuli designed to induce empathy and compassion or related constructs. For example, respondents might view a video-recorded vignette that reliably results in responses to seeing another person who is suffering [ 55 ] or write a letter to a prison inmate who has committed a violent crime [ 56 ]. Game theory has been used to create tasks focused on giving people options to share with, withhold from, or penalize others with cash, points, or goods. These are used to assess prosocial behaviors and constructs adjacent to empathy and compassion such as altruism and generosity [ 57 ].
The association of these implicit measures of compassion and empathy with real-world settings or with subjective perceptions of empathy and compassion is unknown. A meta-analysis of 85 studies ( N = 14,327) indicates that self-report cognitive empathy scores account for only approximately 1% of the variance in behavioral cognitive empathy assessments [ 58 ]. This finding could demonstrate the superiority of implicit measures and a rather damning verdict for the accuracy of self-perceptions, or could imply that these different types of measures are capturing very different constructs (a problem that exists across many psychosocial versus behavioral measures, see [ 59 ]).
Selecting measures
Our review revealed that there is not one or even a few measures of empathy and compassion that are best across all situations. Rather than providing overarching recommendations, therefore, we emphasize that measurement is context-dependent. As such, we recommend a series of questions researchers and program evaluators might ask themselves when selecting a measure.
We encourage readers to use the online CEM-IDV as a decision-aid tool to identify the best measure for their specific needs. To select the most appropriate instrument(s), we offer the following questions (in a suggested order) to provide guidance:
- Which precise domains of empathy, compassion, or adjacent constructs do you want to measure? For example, is it the participant’s experience of empathy, or a skill or behavior? See the “General Construct” dropdown menu. Because definitions of empathy, compassion and related constructs are often imprecise, investigate whether the sample items, factors, and authors’ definition of the construct matches the outcome or variable you actually want to measure.
- What measurement type is best suited to answering your research/evaluation question, or what is feasible for your setting and sample size? For example, if you have limited time or a large sample size, you may prefer a self-report survey, whereas if you are concerned about self-report bias, you might consider a direct observation or behavioral task/expert coder measure. Use S1 Table to examine measures by type of measure, or use the “Type of Measure” filter in the CEM-IDV.
- What measure length, number of items, or time it takes to complete the assessment is feasible for the study? Refer to the X-axis of the CEM-IDV tool.
- What population (s) are you working with? Use the population filter to explore whether the measures you are considering have been validated in those populations.
- Do you want to differentiate the domain you are measuring from other adjacent constructs , such as sympathy or altruism, or distinguish between empathy and compassion? Select and include measures of each construct in order to make this distinction. Finally, now that you have selected several candidate measures, ask:
- How valid and reliable is the measure? Use S1 Table or the Y-axis of CEM-IDV tool to determine which psychometric assessments have been completed, and click on the measure in the table below to review the full text of the papers to discover the strength of those assessments, as well as familiarizing oneself with the recent literature on the measure. Evidence for the validity, factor structure, or length of measures is often hotly debated, and it can be that a measure has been improved or its interpretation cautioned by recent literature.
For example, imagine you are conducting a study of emergency room outcomes, including number of admissions, time from registration to discharge, and patient satisfaction. You would like to include emergency-room healthcare-provider empathy and/or compassion as a potential predictor or mediator of outcomes. After reviewing the literature on the topic and the definitions, you decide that compassion is the specific domain you are most interested in (Question 1). Because you are aware of the limitations of self-report measures, you decide not to use a self-report measure. You recognize that peer-reports, behavioral tasks, or expert coders are not appropriate for the fast-paced environment and number of interactions, but decide that patient reports of provider compassion would be ideal (Question 2). You recognize that the questionnaire must be brief, given the existing measurement burden and limited time participants have (Question 3). The population is emergency room clinicians and patients (Question 4). In this case, you are not interested in differentiating compassion from other similar constructs because that is not relevant to the question you are trying to answer: whether emergency room physician compassion predicts or mediates patient outcomes (Question 5).
In this case, you might use the CEM-IDV tool to select the population “Patients” and the construct “Compassion.” Your search yields eight potential measures, and upon reviewing each, you find that the 5-item Compassion Scale [ 54 ] has sample items that reflect what you are hoping to measure and was validated with emergency room patients and their clinicians. It demonstrates good reliability and validity and is an excellent choice for your project.
Strengths and limitations
This scoping review has several strengths. First, it covers a wide breadth of literature on ways to assess empathy, compassion, and adjacent constructs using different types of measures (i.e., self-report, peer/corollary report, and behavioral/expert coder). Second, the findings were integrated into an accessible interactive data visualization tool designed to help researchers/program evaluators identify the most suitable measure(s) for their context. Third, the review team included individuals with expertise in conducting reviews, with the project manager having received formal training in best practices for systematic reviews, and an experienced data librarian helping to develop the search string and conduct the literature search. Fourth, the literature search was conducted without a start date limitation, thus capturing all measures published prior to October 2020. Fifth, the review team employed a comprehensive consensus process to establish study inclusion/exclusion criteria and utilized state-of-the-art review software, Covidence, to support the process of screening and data extraction.
There are also several limitations to consider. First, our literature search was limited to five databases (i.e., PubMed, Embase, PsychInfo, CINAHL, and Sociological Abstracts), and excluded grey literature, conference proceedings/abstracts, and measures not written in English. We also included only articles specifically focused on development and/or psychometric validation of measures. Thus, it is possible we missed relevant measures. Second, although we captured how frequently a measure was validated and the types of available psychometric evidence for each measure, we did not review the quality of the evidence. Measures with greater numbers of psychometric assessments may not necessarily be the most appropriate in all contexts or for particular settings, and psychometric studies can lead to conflicting results/interpretations. Importantly, the number of psychometric assessments might be skewed in favor of older measures that have existed in the scientific literature longer, and allegiance biases are possible. Thus, we reiterate that readers would benefit most from using the questions recommended above when selecting measures. Third, this scoping review provides a static snapshot of available measures through October 2020 and does not include measures that may have been published after that time.
Finally, the scoping review does not identify gold-standard measures to use. While systematic reviews typically include quality assessments, scoping reviews do not. Rather, scoping reviews seek to present an overview of a potentially large and diverse body of literature pertaining to a topic. As such, this review did not evaluate the quality of design, appraise the strength of the evidence, or synthesize reliability or validity results for each study. It may therefore include multiple studies that may have weak designs, low power, or evidence inadequate to the conclusions drawn.
Given the multitude of problems facing society (e.g., violence and war, social injustices and inequities, mental health crises), learning how to cultivate compassion and empathy towards self and others is one of the most pressing topics for science to address. Furthermore, studies of compassion, empathy, and adjacent constructs rely on the use of appropriate measures, which are often difficult to select due to inconsistent definitions and susceptibility to biases. Our scoping review identified and reviewed numerous measures of compassion, empathy, and adjacent constructs, extracting the qualities of each measure to create an interactive data visualization tool. This tool is intended to assist researchers and program evaluators in searching for and selecting the most appropriate instruments to evaluate empathy, compassion, and adjacent constructs based on their specific context, setting, or population. It does not replace reviewers’ own critical evaluation of the instruments.
How a construct is measured reflects how it is being defined and conceptualized. Reviewing the subscales/factors and individual items that make up each measure sheds light on how each of these measures conceptualizes empathy and compassion. Ongoing research by our team is using these subscales, factors and items across measures to construct a conceptual map of compassion and empathy, which will be reported in a future paper. In the meantime, a useful feature of the CEM-IDV is that the list of articles yielded by searches includes subscales and sample items from each measure/article. These allow for a snapshot of how each measure or its authors have defined the constructs being assessed.
Future directions for measurement of empathy and compassion should consider incorporating advances in measurement and technology, and strive to bring together two or more assessment methods such as self-report, peer or patient reports, expert observation, implicit tasks, and biomarkers/physiological data to provide a more well-rounded picture of compassion and empathy. Innovations such as voice analysis and automated facial expression recognition may hold promise. Brief measures dispersed across multiple time points such as ecological momentary assessment and daily experience sampling may be useful. In conjunction with mobile technology and wearables, artificial intelligence and machine-learning data processing, could facilitate these formerly labor and time-intensive assessment methods.
Supporting information
S1 table. measure populations and psychometric assessments..
https://doi.org/10.1371/journal.pone.0297099.s001
S2 Table. Measures with 8+ psychometric assessments.
https://doi.org/10.1371/journal.pone.0297099.s002
S3 Table. Measures of compassion and empathy.
https://doi.org/10.1371/journal.pone.0297099.s003
Acknowledgments
Thank you to Omar Shaker for his work to create the online interactive data visualization.
- View Article
- Google Scholar
- PubMed/NCBI
- 6. Hart S, Hodson VK. The compassionate classroom: Relationship based teaching and learning. 1st ed. PuddleDancer Press; 2004.
- 27. Gilbert P. Compassion focused therapy: Distinctive features. Routledge/Taylor & Francis Group; 2010.
- 36. Eisenberg N, Eggum ND. Empathic responding: Sympathy and personal distress. In: Decety J, Ickes W, editors. The social neuroscience of empathy [Internet]. The MIT Press; 2009. Chapter 6. https://doi.org/10.7551/mitpress/9780262012973.003.0007
- 45. Covidence Systematic Review Software [software]. Veritas Health Innovation. Available from: www.covidence.org .
- 49. Zhu Z, Hoon HB, Teow KL. Interactive data visualization techniques applied to healthcare decision making. In: Wang B, Li R, Perrizo W, editors. Big Data Analytics in Bioinformatics and Healthcare. IGI Global; 2015. Chapter 3. https://doi.org/10.4018/978-1-4666-6611-5.ch003
Cognitive and Affective Empathy Relate Differentially to Emotion Regulation
- RESEARCH ARTICLE
- Open access
- Published: 15 November 2021
- Volume 3 , pages 118–134, ( 2022 )
Cite this article
You have full access to this open access article
- Nicholas M. Thompson 1 ,
- Carien M. van Reekum 1 &
- Bhismadev Chakrabarti 1
14k Accesses
15 Citations
39 Altmetric
Explore all metrics
The constructs of empathy (i.e., understanding and/or sharing another’s emotion) and emotion regulation (i.e., the processes by which one manages emotions) have largely been studied in relative isolation of one another. To better understand the interrelationships between their various component processes, this manuscript reports two studies that examined the relationship between empathy and emotion regulation using a combination of self-report and task measures. In study 1 ( N = 137), trait cognitive empathy and affective empathy were found to share divergent relationships with self-reported emotion dysregulation. Trait emotion dysregulation was negatively related to cognitive empathy but did not show a significant relationship with affective empathy. In the second study ( N = 92), the magnitude of emotion interference effects (i.e., the extent to which inhibitory control was impacted by emotional relative to neutral stimuli) in variants of a Go/NoGo and Stroop task were used as proxy measures of implicit emotion regulation abilities. Trait cognitive and affective empathy were differentially related to both task metrics. Higher affective empathy was associated with increased emotional interference in the Emotional Go/NoGo task; no such relationship was observed for trait cognitive empathy. In the Emotional Stroop task, higher cognitive empathy was associated with reduced emotional interference; no such relationship was observed for affective empathy. Together, these studies demonstrate that greater cognitive empathy was broadly associated with improved emotion regulation abilities, while greater affective empathy was typically associated with increased difficulties with emotion regulation. These findings point to the need for assessing the different components of empathy in psychopathological conditions marked by difficulties in emotion regulation.
Similar content being viewed by others
Individual differences in emotion regulation moderate the associations between empathy and affective distress.
Philip A. Powell
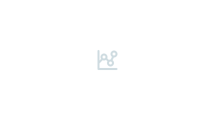
Trait-level emotion regulation and emotional awareness predictors of empathic accuracy
Nathaniel S. Eckland & Tammy English
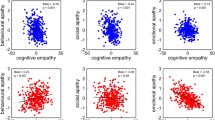
Individual differences in empathy are associated with apathy-motivation
Patricia L. Lockwood, Yuen-Siang Ang, … Molly J. Crockett
Avoid common mistakes on your manuscript.
Our social lives often rely upon the linked processes of how we manage our own emotions and respond to the emotions of others. Yet, until recently the constructs of empathy (i.e., understanding and/or sharing another’s emotion) and emotion regulation (i.e., the processes by which one manages emotions) have largely been studied in relative isolation of one another. Despite the growing research interest in this area, fundamental questions remain regarding the nature of the interrelationships between the various component processes that comprise empathy and emotion regulation. To address these gaps in current knowledge, this manuscript reports two studies which examined how trait empathy is associated with self-report (study 1) and task-based (study 2) measures of emotion regulation.
Empathy is commonly conceptualised as comprising two dimensions: affective empathy (the ability to share others’ emotions), and cognitive empathy (the ability to infer/ understand others’ emotional experiences; Chakrabarti & Baron-Cohen, 2006 ; Decety & Jackson, 2004 ; Singer & Lamm, 2009 ). Emotion regulation refers to the various processes that can be employed in order to influence one’s own emotions (Gross, 2015 ). A close relationship between empathy and emotion regulation has been proposed by previous theoretical accounts, including our own (e.g., Decety, 2010 ; Schipper & Peterman, 2013 ; Thompson et al., 2019 ; Zaki, 2014 ). Of the handful of empirical studies that have explored this relationship, most have focused on the moderating role of emotion regulation on the association between affective empathy and different ‘empathic outcomes’, such as empathic concern (i.e., sympathy), personal distress, and prosocial behaviours (e.g., Brethel-Haurwitz et al., 2020 ; Lockwood et al., 2014 ; Lopez-Perez & Ambrona, 2015 ; Okun et al., 2000 ). While not their primary focus, such studies provide evidence suggestive of a direct relationship between empathy and emotion regulation. For example, one’s self-reported capacity to understand others’ emotions is positively related to the habitual use of more adaptive emotion regulation strategies such as reappraisal (Lockwood et al., 2014 ; Powell, 2018 ; Tully et al., 2016 ). Similarly, self-reported perspective-taking ability (a process associated with cognitive empathy) is negatively related to trait measures of emotion dysregulation (Contardi et al., 2016 ; Eisenberg & Okun, 1996 ; Okun et al., 2000 ).
Some of this prior work suggests a potential overlap in the cognitive control processes that underlie key abilities associated with these constructs. The ability to attribute an emotional/mental state to another individual (i.e., cognitive empathy) requires various cognitive control processes, which facilitate the necessary coordination of self and other representations (e.g., Carlson et al., 2004 ; Decety & Sommerville, 2003 ; Hansen, 2011 ; Mutter et al., 2006 ). Similar cognitive control processes have been implicated in emotion regulation, where they underlie the ability to exert control over one’s affective responses and behaviours (e.g., Buhle et al., 2014 ; Hendricks & Buchanan, 2016 ; McRae et al., 2012 ; Schmeichel & Demaree, 2010 ).
While the aforementioned evidence would suggest that greater cognitive empathy may facilitate emotion regulation, there is reason to expect that increased affective empathy could in fact hinder these processes. Individuals with higher affective empathy exhibit an increased facial mimicry response to others’ emotions (Sonnby-Borgstrom, 2002 ; Dimberg et al., 2011 ), which has been shown to relate to heightened resonance with the mimicked emotion (Hatfield et al., 1993 ; Laird et al., 1994 ; Wild et al., 2001 ). Additionally, individuals with greater affective empathy may be predisposed to experience emotions with a greater intensity and/or frequency (Davis, 1983 ; Eisenberg et al., 2000 ; Rueckert et al., 2011 ; Sato et al., 2013 ). Given that emotions can have a detrimental effect on the efficiency of cognitive control processes (Hare et al., 2008 ; Tottenham et al., 2011 ), it is possible that the increased emotional reactivity associated with higher levels of affective empathy could interfere with one’s ability to engage the often demanding processes necessary for emotion regulation .
Building upon prior work, the current research adopted a multi-method approach in which we examined the relationship between empathy and emotion regulation using a combination of self-report and more objective performance-based measures. Study 1 examined how trait cognitive empathy and affective empathy are associated with self-reported difficulties with emotion regulation (i.e., emotion dysregulation). Study 2 examined how the same trait empathy measure relates to task metrics that index emotion regulation abilities. Based on the findings discussed above, it was predicted that higher cognitive empathy would be associated with improved emotion regulation, whereas the opposite relationship would be observed for affective empathy.
Various abilities should be taken into account when considering an individual’s capacity for emotion regulation, including (1) awareness/understanding of one’s emotions, (2) the capacity to select and implement appropriate regulation strategies to manage emotions across diverse contexts, and (3) the ability to monitor the extent to which regulatory efforts successfully generate the desired modulation of emotion (Bonanno & Burton, 2013 ; Gohm & Clore, 2002 ; Gratz & Roemer, 2004 ; Gross, 2015 ; Koole et al., 2015 ). In this first study, we examined how trait cognitive empathy and affective empathy are related to a self-report measure of emotion regulation that assesses difficulties with emotion regulation across each of the abilities highlighted above (Kaufman et al., 2016 ). We predicted that self-reported emotion dysregulation would be negatively related to trait cognitive empathy but show a positive relationship with trait affective empathy.
Participants
An a priori sample size estimation was conducted using G*power 3.1 (Faul et al., 2007 ). Based on an expected correlation coefficient of ~ 0.3 (Contardi et al., 2016 ; Lockwood et al., 2014 ), a minimum sample of 67 was required to detect such effects at an alpha level of p = 0.05, with a power of 0.80. To account for the potential of data loss due to incomplete responses in the online survey, we aimed to collect a sample of approximately N = 100. A sample of 137 participants (101 female), with a mean age (± SD) of 20.6 years (± 3.01), was recruited from the University of Reading campus via the school of psychology research panel. Questionnaires were completed online and participants were awarded course credit for participation. Ethical approval was obtained from the University of Reading research ethics committee. This study was conducted as part of a larger project and included an additional questionnaire measure of emotion regulation (the ERQ; Gross & John, 2003 ), the data from which are not reported here.
Trait empathy was measured using the Questionnaire of Cognitive and Affective Empathy (QCAE; Reniers et al., 2011 ). The QCAE is a 31-item self-report questionnaire assessing respondents’ capacity to understand and resonate with the emotions of others. It comprises five subscales which track onto the two dimensions of empathy (cognitive and affective). The cognitive empathy dimension assesses one’s propensity to take another’s perspective and accurately infer their state (e.g., “When I am upset at someone, I usually try to ‘put myself in his shoes’ for a while”; “I can sense if I am intruding, even if the other person does not tell me”). The affective empathy dimension comprises items assessing respondents’ tendency to resonate with others’ emotions (e.g., “I am happy when I am with a cheerful group and sad when the others are glum”; “It affects me very much when one of my friends seems upset”). Participants rate their response to each item using a 4-point scale, ranging from “strongly disagree” to “strongly agree”; higher values reflect greater trait empathy. Cronbach’s alpha was high for both empathy dimensions (α Cognitive empathy = 0.88; α Affective empathy = 0.82). The cognitive and affective subscales of the QCAE were positively correlated, rho (135) = 0.42 (CI 95% [0.27, 0.55]), p < 0.001.
Emotion Dysregulation
Trait emotion dysregulation was measured using the Difficulties in Emotion Regulation-short form (DERS-SF; Kaufman et al., 2016 ). The DERS-SF (henceforth, DERS) is an 18-item questionnaire assessing difficulties in six aspects of emotion regulation: (1) awareness of emotions (awareness), (2) clarity/understanding of emotions (clarity), (3) acceptance of emotions (non-acceptance), (4) capacity to maintain goal-directed behaviours in emotional situations (goals), (5) ability to exert control over one’s emotional impulses (impulse), and (6) ability to effectively manage one’s emotional responses (strategies). Respondents report the frequency with which they experience difficulties in these aspects of emotion regulation using a 5-point Likert scale, where 1 = almost never (0–10% of the time) and 5 = almost always (91–100% of the time); higher ratings reflect increased emotion dysregulation. The sum of the six subscales provides a total score reflecting overall levels of emotion dysregulation (DERS-Total). Within this sample, the DERS-Total metric and each subscale thereof demonstrated acceptable to high Cronbach’s alpha: α DERS-Total = 0.91; α Awareness = 0.79; α Clarity = 0.86; α Acceptance = 0.82; α Goals = 0.89; α Impulse = 0.89; α Strategies = 0.83.
Data Reduction and Analysis
The relationship between empathy and emotion dysregulation was examined using bivariate correlations. Normality of each variable was assessed using Kolmogorov–Smirnov tests. As some variable distributions showed significant deviation from normality, Spearman’s rho is reported for all correlations in order to enable their direct comparability. All correlations are reported as two-tailed, with an alpha level of p = 0.05. Data were analysed using SPSS version 27 (IBM Corp., Armonk, N.Y., USA) and Jamovi version 1.6 ( https://www.jamovi.org ). To ensure that the observed results were not unduly influenced by a small number of outlier cases, the results following the removal of univariate and bivariate outliers are reported in supplementary material ( S1 ).
Descriptive statistics for all variables used in the correlation analysis are reported in Table 1 (descriptive statistics for the DERS subscales are reported in supplementary material, S2 ). Affective empathy was not significantly correlated with DERS-Total, rho (135) = 0.13 (CI 95% [− 0.04, 0.29]), p = 0.14. Cognitive empathy showed a small significant negative correlation with DERS-Total, rho (135) = − 0.18 (CI 95% [− 0.34, − 0.01]), p = 0.04. These two correlations were significantly different from each other, Steiger’s Z = − 3.36, p < 0.001 (Fig. 1 ).
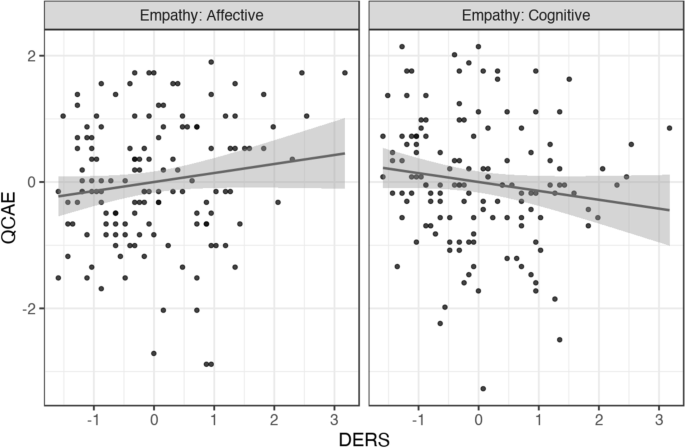
Scatterplot showing the relationship between Z -transformed trait cognitive/affective empathy (QCAE) and Z -transformed emotion dysregulation (DERS-Total). Affective empathy (left panel) was not significantly correlated with DERS-Total; cognitive empathy (right panel) was negatively correlated with DERS-Total
To better understand the relationship between cognitive/affective empathy and trait emotion dysregulation, we conducted exploratory correlations testing the relationship between both empathy dimensions and each subscale of the DERS. Cognitive empathy was significantly negatively related to the awareness subscale and showed a trend-level negative relationship with the impulse control subscale. Affective empathy was significantly positively related to the goals and strategies subscales and showed a trend-level positive relationship with non-acceptance. There was also a significant negative relationship between affective empathy and the awareness subscale. Steiger’s tests demonstrated that, with the exception of the clarity subscale, the cognitive and affective dimensions of empathy showed significantly different relationships with each subscale of the DERS. False Discovery Rate (FDR)–corrected correlation results and associated Z statistics are reported in Table 2 .
The results of study 1 highlight several points of divergence between trait cognitive empathy and affective empathy in terms of their relationship with self-reported emotion dysregulation. Cognitive empathy was more negatively related to difficulties with emotional awareness than affective empathy. Significant differences between these empathy components were also observed in relation to other subscales of DERS measuring difficulties with impulse control (impulse), maintaining focus on goal-directed behaviours (goals), and managing/accepting one’s emotions (strategies and non-acceptance, respectively). Many of these abilities are thought to be dependent upon more ‘implicit’ forms of emotion regulation, which refer to regulatory processes that can be enacted with little or no reliance upon conscious effortful control or monitoring (Gyurak et al., 2011 ; Mauss et al., 2007 ). Consequently, in this second study, we examined how these same measures of trait cognitive and affective empathy are associated with performance on two tasks that assess implicit emotion regulation abilities.
Prior work has highlighted the deleterious impact of emotional stimuli on cognitive control processes (e.g., Jasinska et al., 2012 ; Padmala et al., 2011 ; Reeck & Egner, 2011 ; Tottenham et al., 2011 ). While definitions of emotion regulation often emphasise more deliberate and effortful processes, regulating the early influence of emotions on cognitive control is largely dependent upon more implicit processes, which also play a key role in emotion regulation in daily life (Gyurak et al., 2011 ; Mauss et al., 2007 ).
Emotional variants of well-known cognitive control tasks are commonly used to assess attentional biases and the interaction between emotion and cognition. Such tasks have also proven useful for assessing more implicit emotion regulation abilities (see review by Buhle et al., 2010 ). In these paradigms, performance is compared across conditions in which distracting stimuli are either emotional or non-emotional, thereby indexing the extent to which performance is disrupted by emotional information (a phenomenon henceforth referred to as ‘emotion interference effects’). The assumption underlying this approach is that individuals with better implicit emotion regulation abilities will exhibit a reduced magnitude of emotion interference effects relative to their less well-regulated counterparts (Etkin et al., 2010 ; Koole & Rothermund, 2011 ; Zhang & Lu, 2012 ). In the present study, we used two paradigms that have been used in previous research to assess implicit emotion regulation: the emotional go/nogo and the emotional Stroop (henceforth Emo-GNG and Emo-Stroop, respectively). While the term implicit is sometimes used in reference to regulation paradigms wherein the processing of emotional information is entirely irrelevant to task performance (Zhang & Lu, 2012 ), we use the term slightly more broadly to refer to tasks in which regulatory processes may be initiated without any explicit instruction/intention (Yiend et al., 2008 ).
Prior studies using the Emo-GNG have demonstrated that emotional nogo stimuli tend to induce more commission errors (i.e., false alarms) relative to neutral nogo stimuli (Hare et al., 2005 , 2008 ; Tottenham et al., 2011 ; Zhang et al., 2016 ). Similarly, numerous emotional variants of the Stroop task (Stroop, 1935 ) have demonstrated the heightened potential for emotional stimuli to disrupt cognitive control processes relative to neutral stimuli, indexed by increased response times (RT) and/or decreased accuracy (Etkin et al., 2006 ; Haas et al., 2006 ; Stenberg et al., 1998 ). These emotion interference effects are often exhibited to a greater extent, or in some cases, only, in individuals with high trait anxiety (e.g., Kalanthroff et al., 2016 ; Richards et al., 1992 ; see also Buhle et al., 2010 ).
One prior study found that improved performance on a task measuring cognitive empathy–related processes was associated with a greater ability to ignore irrelevant distractors in an Emo-Stroop (Bradford, 2015 ). However, this study did not test the relationship between affective empathy and the Emo-Stroop. Additionally, while there is some evidence to suggest that affective empathy is associated with a heightened sensitivity to emotional stimuli (such as in attentional blink tasks, e.g., Kanske et al., 2013 ), prior work has failed to find any direct evidence for a relationship between affective empathy and interference effects in Emo-Stroop tasks (Hofelich & Preston, 2012 ).
It is important to note that the trait empathy measures used in these prior studies have been criticised for conflating the cognitive and affective dimensions of empathy with dissociable constructs, such as sympathy (Reniers et al., 2011 ). Furthermore, many studies that have used Emo-Stroop variants lacked a neutral control condition, which makes it difficult to determine the extent to which any RT difference between the congruent and incongruent conditions is driven by emotion interference effects (on incongruent trials) or emotion facilitation effects (on congruent trials). Accordingly, the current study includes a neutral control condition to enable the examination of emotional interference independently of facilitation. As prior work has demonstrated the potential for both positively and negatively valenced stimuli to attract attention and disrupt cognitive processing (e.g., Hare et al., 2005 ; Pratto & John, 1991 ), both tasks included positive and negative emotional facial expressions in order to increase the generalizability of the results.
It was expected that emotional stimuli would disrupt inhibitory control processes, as indexed by decreased performance relative to neutral stimuli (i.e., increased emotion interference effects). Based on the literature discussed in the general introduction, it was predicted that trait cognitive and affective empathy would be differentially related to the magnitude of these emotion interference effects. Given evidence suggestive of overlap in the cognitive control processes underlying abilities related to cognitive empathy and emotion regulation (e.g., Buhle et al., 2014 ; Decety & Sommerville, 2003 ; Hansen, 2011 ; Hendricks & Buchanan, 2016 ), it was predicted that cognitive empathy would be negatively related to the magnitude of emotion interference effects. Conversely, based on evidence that affective empathy is positively associated with increased spontaneous mimicry and emotional reactivity (Davis, 1983 ; Eisenberg et al., 2000 ; Hatfield et al., 1993 ; Laird et al., 1994 ; Wild et al., 2001 ), it was predicted that trait affective empathy would show a positive relationship with the magnitude of emotion interference effects.
As in study 1, an a priori sample size estimation was conducted using G*power 3.1 (Faul et al., 2007 ). Based on an expected correlation coefficient of ~ 0.3 (Contardi et al., 2016 ; Lockwood et al., 2014 ), a minimum sample of 67 was required to detect such effects at an alpha level of p = 0.05, with a power of 0.80. To account for potential data loss due to non-responders and data outliers, we sought to obtain a sample of approximately N = 100. Ninety-two right-handed participants (78 females) were recruited from the undergraduate psychology population at the University of Reading. All participants were recruited through the online research panel and received course credit for participation. The mean age (± SD) was 19.86 (± 2.39). The two tasks were administered as part of a lab session, with the order of task completion counterbalanced. This study was conducted as part of a larger project and included an additional questionnaire measure of emotion regulation (the ERQ; Gross & John, 2003 ), the data from which are not reported here.
Following data quality checks (see “Data Reduction and Analysis” for details), thirteen participants’ data were removed from the Emo-GNG, and nine participants’ data were removed from the Emo-Stroop, leaving a final sample of N = 79 (71 females; mean age ± SD = 19.95 ± 2.55) and N = 83 (69 female; mean age ± SD = 19.93 ± 2.50) for these two tasks, respectively. The QCAE was completed online; Cronbach’s alpha was high for both QCAE subscales (α Affective Empathy = 0.79; α Cognitive Empathy = 0.88). Following case removals based on the Emo-GNG and Emo-Stroop task data, the cognitive and affective subscales of the QCAE were positively correlated in both instances, rho (77) = 0.38 (CI 95% [0.18, 0.56]), p < 0.001; rho (81) = 0.36 (CI 95% [0.15, 0.53]), p = 0.001.
Materials and Procedure
Face stimuli were taken from the Nimstim Face Stimulus Set (Tottenham et al., 2009 ; www.macbrain.org ) and comprised photographs of six female (identity numbers: 1, 3, 6, 8, 9, 10) and six male (identity numbers: 21, 22, 23, 24, 28, 34) actors. Different male and female actors displaying fearful expressions were used for the practice block. Each image was converted to greyscale with the dimensions 256 × 329 pixels. The facial expressions included in the task were the closed-mouth versions of happy, sad, disgusted, and calm (i.e., neutral). These emotions were selected in order to include stimuli depicting expressions of positive, neutral, and negative valence, which were likely to differ in terms of the approach/avoid tendencies they elicit (Seidel et al., 2010 ; Tottenham et al., 2011 ). The same actors were used for each facial expression, and the frequency with which each stimulus was presented was balanced across conditions.
The Emo-GNG task lasted approximately 25 min. This included a practice block comprising 12 trials, followed by 6 experimental blocks each comprising 48 trials. Upon completion of a block, a holding screen was presented until participants pressed a key to continue; the order in which the blocks were completed was randomised. In each block, an emotional target (go) or distractor (nogo) was always paired with a calm target/distractor, such that if an emotional face was the go stimulus, a calm face was the nogo stimulus, and vice versa. Each block contained one emotion type only, alongside the neutral (calm) expressions. At the start of each block, participants were told which emotion represented the go stimulus and were instructed to respond to these targets by pressing ‘0’ on the keypad with their right index finger. Participants were not told what the nogo expression would be but were instructed to respond as quickly as possible (while maintaining accuracy) to the target expression and to withhold responding for any other expression (full verbatim instructions for the Emo-GNG task are presented in supplementary material, S3 ).
To induce a prepotent tendency to respond, 73% (35 trials) of the trials in each block were go trials, and 27% (13 trials) were nogo trials. As in previous Emo-GNG tasks (Durston et al., 2002 ; Hare et al., 2005 ), trial order was pseudorandom and parametrically balanced to control for the number of go stimuli preceding each nogo stimulus and to ensure that nogo trials occurred equally across the early, middle, and late stages of a block. Stimuli were presented in the centre of the screen for 500 ms at a size of 7.2 cm wide 9.2 cm high. A white fixation cross positioned centrally atop a black background was presented during each interstimulus interval (ISI), which was jittered, ranging from 2,000 to 6,000 ms (M ± SD = 3,708 ms ± 1211 ms). Following the onset of a stimulus there was a 2,000 ms window in which responses were recorded (go trials in which a response was not made within this time window were classed as misses). A schematic of the trial structure is depicted in Fig. 2 .
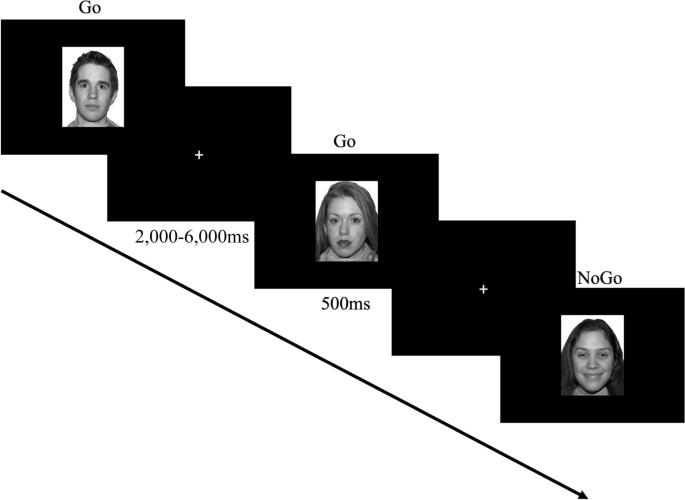
Schematic of the Emo-GNG task events. This example shows three trials in the calm-happy block; participants were instructed to respond as quickly as possible to frequent “go” faces while withholding responses to infrequent “nogo” faces
Face stimuli comprised the same male and female actors used in the Emo-GNG. The facial expressions used in the Emo-Stroop were angry, happy, and calm (i.e., neutral). Each image was converted to greyscale, with the dimensions of 256 × 329 pixels. Different actors (identities: 7, 14, 20, 38) and emotional expressions (fearful) were used for the practice trials. For each face, the word “angry” or “happy” was superimposed over the bridge of the nose (so as not to obscure any features) in capitalized Arial font at a size of 30 with 10% transparency. The stimuli were presented centrally on the monitor at a size of 13 cm high by 10 cm wide.
The Emo-Stroop took approximately 15 min to complete. This comprised 16 practice trials, followed by three experimental blocks each comprising 48 trials. The blocks were emotion-specific (i.e., angry face, happy face, calm face), and contained an equal number of trials in which the word was “happy” or “angry”. Trial and block orders were randomised. The task was 2-AFC, with participants instructed to respond by pressing the 1 or 2 key on the keyboard with the index and middle finger of their right hand depending upon whether the word was positive (i.e., “happy”) or negative (i.e., “angry”; button mappings were counterbalanced across the sample). Full verbatim instructions for the Emo-Stroop task are presented in supplementary material, S3 .
Each stimulus was presented for 500 ms, followed by a fixation screen comprising a white cross presented centrally atop a black background. The duration of the fixation screen was jittered, ranging from 4,500 to 6,000 ms (M ± SD = 5,250 ms ± 565 ms). Responses were recorded within a 2,500 ms window, which incorporated the 500 ms stimulus presentation and 2,000 ms of the post-stimulus fixation screen. Any trials in which the participant failed to respond within this time window were classed as incorrect. A schematic of the Emo-Stroop task structure is depicted in Fig. 3 .
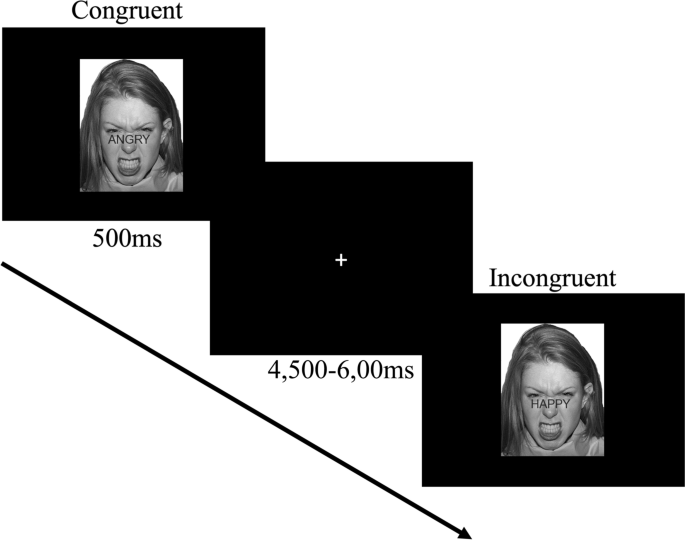
Schematic of events in the Emo-Stroop. This figure depicts an example of a congruent and an incongruent stimulus in the angry face block
Bivariate correlations were used to examine the relationship between trait cognitive/affective empathy and the emotion interference effect metrics from the Emo-GNG and Emo-Stroop (see task-specific sections below for details of how these metrics were calculated). Normality of each variable was assessed using Kolmogorov–Smirnov tests. As some variable distributions showed significant deviation from normality, Spearman’s rho is reported for all correlations in order to enable their direct comparability. All correlations are reported as two-tailed, with an alpha level of p = 0.05. Data were analysed using SPSS version 27 (IBM Corp., Armonk, N.Y., USA) and Jamovi version 1.6 ( https://www.jamovi.org ). To ensure that the observed results were not unduly influenced by a small number of outlier cases, the same analyses reported in the “ Results ” were conducted following the removal of univariate and bivariate outlier cases (see supplementary material, S4 ). Descriptive statistics for all variables included in the correlation analyses are reported in supplementary material ( S5 ).
Participants with a mean hit rate (HR) or false alarm rate (FAR) that deviated from the group mean by more than 3*SD were removed as outliers (12 participants). Visual inspection of these data confirmed that these participants had failed to correctly follow task instructions in at least one block (e.g., they always/never responded across all trials and/or evidently confused the go/nogo stimuli). Further, one participant was removed because the necessary questionnaire data was incomplete, leaving a final sample of N = 79, which was subject to analysis.
The key index of task performance on the Emo-GNG was D-prime, which was calculated by subtracting the z -transformed FAR from the z -transformed HR. As D-prime calculations cannot be performed for values of 1 (i.e., 100% HR/FAR) or 0 (i.e., 0% HR/FAR), any such values were transformed using the formula: 1/(2 N) for values of 0, and 1–1/(2 N) for values of 1. D-prime data were analysed using a repeated-measures ANOVA with trial type (Emo-NoGo/Calm-NoGo) and emotion (happy, sad, disgust) as within-subjects factors. Greenhouse Geisser corrected values are reported where the assumption of sphericity was not satisfied.
The key metric extracted from this task for the correlation analysis was the difference in mean D-prime between the calm-nogo and emo-nogo conditions (calm-nogo D-prime minus emo-nogo D-prime). This metric was termed the “emotion interference effect”. As the different facial expression pairings were balanced across these two conditions, this metric controls for variability in participants’ ability to discriminate between the calm and emotional faces, providing a direct measure of the extent to which their performance was affected by emotional nogo stimuli.
An unexpected error in the task program resulted in data for a substantial number of trials being lost for two participants; these cases were removed prior to analysis. Two participants who failed to complete the necessary questionnaire data were also removed. To ensure data quality, participants with an overall mean accuracy more than 3*SD below the group mean were removed (5 cases), leaving a final sample of N = 83, which was subject to analysis. Incorrect trials (mean number of trials removed per participant = 12.28, SD = 8.07) and correct trials in which the RT deviated from the group mean by more than 3*SD were removed (mean number of trials removed per participant = 8.08, SD = 10.65). The mean number of trials per block following all removals was 41.21 (SD = 4.76).
The dependent variable in the Emo-Stroop was RT for correct trials. These data were analysed using a repeated-measures ANOVA, with face (angry, happy, calm) and word (angry, happy) as within-subjects factors. Greenhouse Geisser corrected values are reported where the assumption of sphericity was not satisfied. Given the inclusion of a neutral (calm face) control condition, this paradigm enabled the assessment of interference (resulting from incongruent task-irrelevant emotional faces) independently of facilitation (resulting from congruent task-irrelevant emotional faces) effects. The emotion interference effect metric was calculated by subtracting the mean RT for the neutral control condition from the mean RT for the incongruent condition.
A repeated-measures ANOVA demonstrated a significant main effect of trial type, F (1, 78) = 96.60, p < 0.001, η p 2 = 0.55, and emotion, F (1.83, 142.88) = 70.91, p < 0.001, η p 2 = 0.48. A significant trial type by emotion interaction was also observed, F (2, 156) = 32.52, p < 0.001, η p 2 = 0.29. D-prime was significantly higher for calm ( M ± SD = 2.76 ± 0.51) relative to emotional ( M ± SD = 2.19 ± 0.61) nogo trials ( p < 0.001; Fig. 4 , left panel). The post hoc pairwise comparisons for the main effect of emotion and the trial type by emotion interaction are presented in supplementary material ( S6 ).
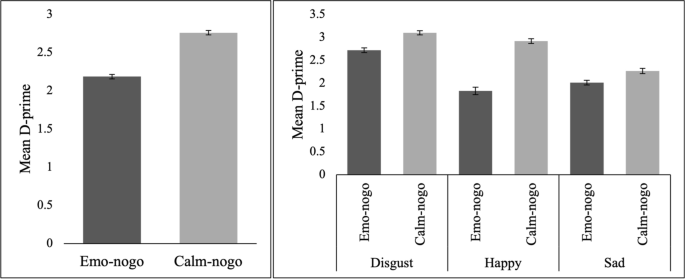
Mean D-prime for Emo-NoGo and Calm-NoGo conditions on the left. Mean D-prime for Emo-NoGo and Calm-NoGo trials for each emotion on the right (see supplementary material S6 for accompanying results). Error bars depict ± 1 within-subjects SEM
Trait affective empathy was positively correlated with the GNG emotion interference effect, rho (77) = 0.34 (CI 95% [0.12, 0.52]), p = 0.003. Cognitive empathy was not significantly related to the GNG emotion interference effect, rho (77) = 0.14 (CI 95% [-0.08, 0.35]), p = 0.22. The difference between these correlations was not significant, Steiger’s Z = − 1.63, p = 0.10 (two-tailed; Fig. 5 ).
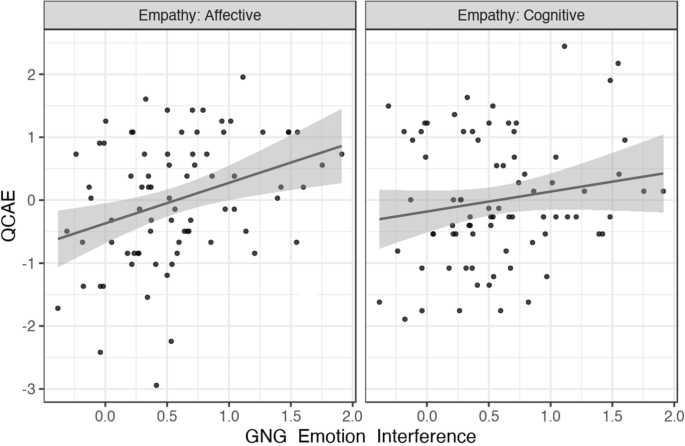
Scatterplot showing the relationship between z -transformed cognitive/affective empathy and the Emo-GNG emotion interference effect (calm-nogo D-prime minus emo-nogo D-prime). Affective empathy (left panel) showed a significant positive correlation with the emotion interference effect; cognitive empathy (right panel) was not significantly related to the emotion interference effect
Given that affective empathy has been found to be associated with improved emotion discrimination under conditions of brief stimulus exposure (e.g., Kang et al., 2017), it is possible that the correlation between affective empathy and the emotion interference effect could have been driven by a higher D-prime in the calm-nogo condition (i.e., improved HR when responding to emotional go targets). A significant negative correlation between affective empathy and D-prime in the emo-nogo condition was observed, rho (77) = − 0.24 (CI 95% [− 0.44, − 0.02]), p = 0.04; affective empathy showed no relationship with D-prime in the calm-nogo condition, rho (77) = 0.05 (CI 95% [− 0.17, 0.27]), p = 0.64. These results suggest that the correlation between affective empathy and the emotion interference effect was driven by increased emotional interference in the emo-nogo condition, rather than improved performance in the calm-nogo condition. Cognitive empathy was not significantly related to D-prime in either the emo-nogo, rho (77) = − 0.13 (CI 95% [− 0.30, 0.10]), p = 0.26, or the calm-nogo condition, rho (77) = − 0.02 (CI 95% [− 0.23, 0.21]), p = 0.90.
Mean accuracy was 91.47% ( SD = 5.6), which confirms that participants were able to complete the task as instructed. A repeated-measures ANOVA examining the effect of face (angry, happy, calm) and word (angry, happy) on the dependent variable RT demonstrated a significant main effect of word, F (1, 82) = 19.05, p < 0.001, η p 2 = 0.19, with shorter RTs for “happy” compared to “angry”, but no main effect of face, F (2, 164) = 0.13, p = 0.88, η p 2 = 0.002. The hypothesised face by word interaction was at trend-level, F (2, 164) = 2.31, p = 0.10, η p 2 = 0.03. In the calm face condition, RT for the word happy ( M ± SD = 593.44 ms ± 101.22 ms) was significantly shorter than for the word angry ( M ± SD = 603.64 ms ± 100.28 ms; p = 0.04). Similarly, in the happy face condition, RT for the word happy ( M ± SD = 586.80 ms ± 102.06 ms) was significantly shorter than for the word angry ( M ± SD = 608.65 ± 101.07 ms; p < 0.001). However, in the angry face condition, the difference between RT for the word happy ( M ± SD = 591.75 ms ± 98.13 ms) and the word angry ( M ± SD = 600.19 ms ± 98.64 ms) did not reach significance ( p = 0.11; Fig. 6 , left panel).
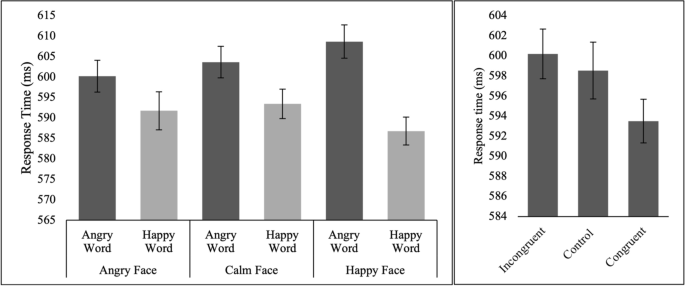
Mean RT across each condition in the Emo-Stroop task (left panel). Mean RT for the incongruent, congruent, and control conditions (right panel). Error bars depict ± 1 within-subjects SEM
In sum, some evidence of emotion interference effects was observed, which reduced the positive word RT advantage in the angry face condition. To test more directly for interference effects, paired-samples t -tests were conducted to compare RT across the congruent, incongruent, and control conditions. No significant differences were observed between the control and incongruent conditions ( t (82) = 0.34, p = 0.73). A trend-level difference was observed between the congruent and incongruent conditions, t (82) = 1.82, p = 0.07 (two-tailed; Fig. 6 , right panel).
As per our hypothesis, we examined how trait empathy was associated with individual differences in the magnitude of the emotion interference effect. It is worth noting that the lack of a significant effect at the group level does not necessarily refute the potential presence of significant individual differences (see Hedge et al., 2018 for a relevant discussion). Indeed, prior studies have demonstrated that Emo-Stroop interference and incongruency effects may often be observable only in certain individuals/groups, such as those with high trait/clinical anxiety (e.g., Kalanthroff et al., 2016 ; Richards et al., 1992 ).
Trait cognitive empathy was negatively correlated with the Emo-Stroop emotion interference effect, rho (81) = − 0.24 (CI 95% [− 0.43, − 0.03]), p = 0.03. In contrast, affective empathy showed no relationship with this emotion interference effect, rho (81) = 0.003 (CI 95% [− 0.21, 0.22]), p = 0.98. The difference between these correlations was approaching the threshold for significance, Steiger’s Z = − 1.95, p = 0.05 (two-tailed; Fig. 7 ).
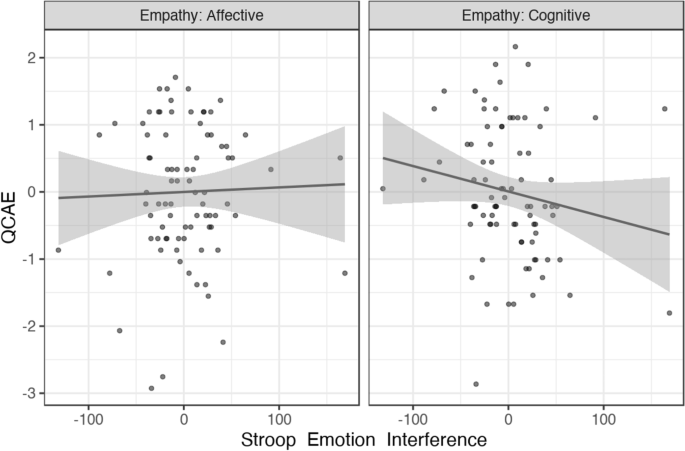
Scatterplot showing the relationship between z -transformed cognitive/affective empathy and the Emo-Stroop emotion interference effect (incongruent RT minus control RT). The emotion interference effect was not related to affective empathy (left panel) but showed a significant negative relationship with cognitive empathy (right panel)
Across two studies, the relationship between trait empathy and emotion regulation was examined. It was predicted that greater cognitive empathy would be associated with improved emotion regulation (i.e., reduced emotion dysregulation), whereas greater affective empathy would be associated with increased difficulties in emotion regulation (i.e., increased emotion dysregulation). These predictions were largely borne out by the data, with a few caveats as discussed below.
In study 1, trait cognitive and affective empathy showed divergent patterns in their relationships with self-reported emotion dysregulation. Cognitive empathy was negatively related to overall levels of emotion dysregulation, suggesting that those with greater cognitive empathy experience fewer difficulties with emotion regulation. In contrast, affective empathy did not show a significant relationship with overall levels of emotion dysregulation. With the exception of the Clarity subscale, trait cognitive empathy and affective empathy shared significantly different relationships with each subscale of the DERS. These findings are broadly consistent with those reported by Contardi et al. ( 2016 ), who observed similar relationships between trait empathy and emotion dysregulation in a sample of Italian students. Our findings provide support for this work by replicating the findings in a UK sample; furthermore, the exploratory subscale analysis provides greater specificity as to the relationship that cognitive and affective empathy share with different aspects of emotion dysregulation.
Building upon the findings of study 1, the second study examined how the same trait empathy measures were associated with performance-based metrics of emotion regulation. We used the magnitude of emotion interference effects (i.e., the extent to which inhibitory control was affected by emotional relative to neutral stimuli) in an Emo-GNG and an Emo-Stroop task as a proxy measure of participants' implicit emotion regulation abilities. Affective empathy was positively correlated with the emotion interference effect in the Emo-GNG, which suggests that higher affective empathy was associated with greater difficulty regulating impulsive behaviours in the presence of emotional distractors. In contrast, no relationship was observed between trait cognitive empathy and the Emo-GNG emotion interference effect. In the Emo-Stroop, higher cognitive empathy was associated with reduced emotional interference, which suggests that individuals with higher cognitive empathy were more efficient in regulating the potential interference caused by task-irrelevant emotional faces. No relationship was observed between affective empathy and the Emo-Stroop interference effect.
On the basis of prior literature, we had predicted that the emotion interference effects from both tasks would show a negative relationship with cognitive empathy, and a positive relationship with affective empathy. However, this hypothesis was only partially supported, since cognitive and affective empathy were associated with different emotion interference effects. We speculate that the Emo-GNG and Emo-Stroop tasks used in study 2 may be assessing different processes relevant to emotion regulation, which could have differential relevance to cognitive and affective empathy, respectively. It has been asserted that the GNG task assesses response inhibition whereas the Stroop assesses conflict resolution, which reflect related but dissociable aspects of cognitive control (Nee et al., 2007 ; Swick et al., 2011 ; see also supplementary material S7 , where we report no inter-relationship between these task metrics). The differential results for these tasks could reflect the extent to which they each provide a ‘pure’ measure of a unitary component of cognitive control. While the GNG provides a relatively pure measure of inhibition, the Stroop may also assess other aspects of cognitive control not captured by the GNG, such as shifting and updating (Miyake et al., 2000 ).
Additionally, these divergent findings may be driven by differences in the task-relevance of the emotional face stimuli in these two tasks. Given that the faces in the Emo-Stroop were always task-irrelevant, it could be that participants were able to focus their attention more fixedly on the target words, reducing the likelihood for trait affective empathy to modulate task effects. As participants were required to actively attend to the faces in order to perform successfully on the Emo-GNG task, such an approach would not have been possible. A recent study found that affective empathy was associated with greater self-reported ‘attentional focusing’ abilities but poorer ‘attentional shifting’ abilities (Goodhew & Edwards, 2020 ), which is consistent with the above interpretation.
Despite the caveats mentioned above, study 2 task results provide evidence to suggest that higher trait cognitive empathy is associated with improved implicit emotion regulation abilities, whereas higher trait affective empathy may be associated with a diminished capacity to regulate emotional influences on cognitive control processes. Given the differences in the way in which emotion regulation was assessed across studies 1 and 2, we are cautious in making strong generalizability inferences from their results. That being said, the findings of study 2 are broadly consistent with those of study 1, where higher cognitive empathy was associated with reduced emotion dysregulation. While affective empathy was not significantly related to overall emotion dysregulation in study 1, it did show positive relationships with subscales assessing difficulties in certain aspects of emotion regulation, such as maintaining focus on goal-directed behaviours.
While the relationships between the two dimensions of empathy and facets of self-reported emotion dysregulation were broadly in opposing directions, cognitive and affective empathy were both negatively associated with difficulties with emotional awareness. This observation is consistent with the interpretation that individuals with greater empathy show a heightened awareness and understanding of their own emotional experiences (Frith & Frith, 2003 ; Happé & Frith, 1996 ; Hobson, 2010 ; Rieffe & Camodeca, 2016 ), which is a key competency supporting adaptive emotion regulation (Gratz & Roemer, 2004 ; Gross, 2015 ).
Prior theoretical and empirical work suggests that the cognitive component of empathy is reliant upon various cognitive control processes, which support the ability to take another’s perspective and make accurate inferences about their mental/emotional state (Carlson et al., 2004 ; Decety & Sommerville, 2003 ; Hansen, 2011 ; Mutter et al., 2006 ). Given that many forms of adaptive emotion regulation are reliant upon similar processes and neural architecture to those that support cognitive empathy (Buhle et al., 2014 ; Hendricks & Buchanan, 2016 ; McRae et al., 2012 ; Schmeichel & Demaree, 2010 ), the results of the current studies could reflect the fact that higher cognitive empathy is associated with improved efficiency of the cognitive control processes that also underlie the ability to regulate one’s emotions.
Rather than making any causal attributions to the observed association between cognitive empathy and emotion regulation, we believe that the relationship between these constructs is likely bi-directional in nature. Greater cognitive empathy abilities may support emotion regulation and vice versa. For instance, in an emotional situation, an individual with heightened emotion regulation abilities may have greater cognitive resources available with which to make accurate inferences about others’ states. Similarly, an individual who is more adept in the cognitively demanding task of taking others’ perspectives, may be better able to adopt a more distanced self-perspective in emotional situations, which could facilitate the use of more adaptive regulation strategies such as reappraisal (Kross & Ayduk, 2011 ; Ochsner & Gross, 2008 ; Wallace-Hadrill & Kamboj, 2016 ).
In contrast to cognitive empathy, affective empathy was positively associated with emotion interference effects in the Emo-GNG task and showed positive relationships with DERS subscales that assessed difficulties in managing emotions and maintaining focus on goal-directed behaviours. These findings could reflect a heightened propensity for spontaneous facial mimicry (SFM) and increased emotional reactivity in individuals with higher affective empathy (Eisenberg et al., 1989 ; Davis, 1983 ; Rueckert et al., 2011 ; Sonnby-Borgstrom, 2002 ; Sato et al., 2013 ; Wild et al., 2001 ), which results in increased interference on cognitive control processes necessary for certain aspects of emotion regulation (Tottenham et al., 2011 ).
The present findings represent an important step towards further elucidating the relationship between the various component processes associated with empathy and emotion regulation. However, it is worth noting the limitations of the current work that future studies could address. First, while emotion regulation was assessed using a combination of self-report trait measures and more objective performance-based metrics, in both studies, empathy was assessed using a self-report questionnaire. While helpful in understanding respondents’ self-perceptions of their own abilities (Dziobek et al., 2008 ), given that many of the processes associated with empathy are thought to occur on an implicit level (e.g., Decety & Jackson, 2004 ; Pfeifer et al., 2008 ; Singer & Lamm, 2009 ), one could argue that certain features of this construct may be difficult to assess accurately via introspection (Kagan, 1988 ). Further, self-report empathy measures rely upon retrospective self-reporting, which may leave them susceptible to inaccuracies and/or response biases (Moskowitz, 1986 ) such as socially desirable responding (Gerdes et al., 2010 ; Paulhus, 1991 ). A further limitation of using only trait measures is that they may not accurately capture ability-based components of empathy, which may be more amenable to measurement using performance-based task approaches. Indeed, prior work highlights a lack of convergence between trait and task measures of empathy (e.g., Melchers et al., 2015 ), which could suggest that these approaches are assessing slightly distinct latent constructs.
While the difference metrics we derived from the tasks in study 2 were designed to minimise the impact of individual differences in general cognitive control, it would be useful for future work to examine the relationship that cognitive and affective empathy share with emotional as well as non-emotional versions of similar cognitive control tasks. Additionally, given the evidence of positive relationships between intelligence and cognitive control abilities (e.g., Checa & Fernandez-Berrocal, 2015 ; Shamosh & Gray, 2008 ), controlling for IQ in future work may also help to shed more light on the potential mechanisms that underlie the relationships observed in these studies.
An additional caveat comes from the lack of an overall emotion interference effect in the Emo-Stroop task. While it does not preclude us from drawing inferences about individual differences, it points to the possibility that the condition-related variability in this version of the task may be significantly smaller than the between-subject variability (Hedge et al., 2018 ). The decision to use the affective faces, rather than the affective words, as the distractor stimuli was based on prior work which demonstrated greater interference effects for face relative to word distractors (Beall & Herbert, 2008 ). However, other studies have observed contrary results (Ovaysikia et al., 2011 ), and future work might seek to examine the relative differences in condition-related effects and between-subject variability in these effects across different Emo-Stroop task variants.
Finally, consideration should be given to our characterization of these tasks as implicit regulation. The explicit goal for these tasks was to respond as quickly and accurately as possible. To achieve this explicit goal, participants would have had to regulate the potential interference caused by the emotional distractors. According to Braunstein et al.’s ( 2017 ) framework, such ‘incidental’ regulation would be considered implicit. Accordingly, we characterize both of these tasks as assessing implicit emotion regulation. The distinction between implicit and explicit processes is by no means a binary one (Gyurak et al., 2011 ; Koole et al., 2015 ), and it would be useful for future work to examine how cognitive and affective empathy relate to regulatory processes that lie at different points along the continuum between implicit and explicit. Similarly, future work should take into account whether different stimuli used as emotional distractors (e.g., IAPS images, threat of shock) yield similar associations with empathy.
In conclusion, the current studies provide new evidence on the relationship that the cognitive and affective dimensions of empathy share with emotion regulation. While greater cognitive empathy was broadly associated with improved emotion regulation abilities based on both self-report and task-based measures, greater affective empathy was associated with increased difficulties with specific aspects of emotion regulation. Given the myriad subprocesses with which both of these constructs are related, coupled with the diverse range of tools used in their assessment, future work should seek to test the generalizability of these findings by examining these relationships using different trait and/or task measures of empathy and emotion regulation to those used in the current studies. An additional avenue for future research would be to explore these relationships in populations known to exhibit atypicalities in empathy and/or emotion regulation, such as individuals with autism spectrum conditions or psychopathy.
Baron-Cohen, S., Wheelwright, S., & Jolliffe, T. (1997). Is there a “language of the eyes”? Evidence from normal adults, and adults with autism or asperger syndrome. Visual Cognition, 4 (3), 311–331. https://doi.org/10.1080/713756761
Article Google Scholar
Beall, P. M., & Herbert, A. M. (2008). The face wins: Stronger automatic processing of affect in facial expressions than words in a modified Stroop task. Cognition and Emotion, 22 (8), 1613–1642. https://doi.org/10.1080/02699930801940370
Blair, K. S., Smith, B. W., Mitchell, D. G. V., Morton, J., Vythilingam, M., Pessoa, L., & Blair, R. J. R. (2007). Modulation of emotion by cognition and cognition by emotion. NeuroImage, 35 (1), 430–440. https://doi.org/10.1016/j.neuroimage.2006.11.048
Article PubMed Google Scholar
Bonanno, G. A., & Burton, C. L. (2013). Regulatory flexibility: An individual differences perspective on coping and emotion regulation. Perspectives on Psychological Science, 8 (6), 591–612. https://doi.org/10.1177/1745691613504116
Bradford, E. E. F., Jentzsch, I., & Gomez, J. C. (2015). From self to social cognition: Theory of mind mechanisms and their relation to executive functioning. Cognition, 138 , 21–34. https://doi.org/10.1016/j.cognition.2015.02.001
Braunstein, L. M., Gross, J. J., & Ochsner, K. N. (2017). Explicit and implicit emotion regulation A multi level framework. Social Cognitive and Affective Neuroscience, 12 (10), 1545–1557. https://doi.org/10.1093/scan/nsx096
Article PubMed PubMed Central Google Scholar
Breiter, H. C., Etcoff, N. L., Whalen, P. J., Kennedy, W. A., Rauch, S. L., Buckner, R. L., & Rosen, B. R. (1996). Response and habituation of the human amygdala during visual processing of facial expression. Neuron, 17 (5), 875–887. https://doi.org/10.1016/S0896-6273(00)80219-6
Brethel-Haurwitz, K. M., Stoianova, M., & Marsh, A. A. (2020). Empathic emotion regulation in prosocial behaviour and altruism. Cognition and Emotion, 34 (8), 1532–1548. https://doi.org/10.1080/02699931.2020.1783517
Buhle, J. T., Silvers, J. A., Wager, T. D., Lopez, R., Onyemekwu, C., Kober, H., Weber, J., & Ochsner, K. N. (2014). Cognitive reappraisal of emotion: A meta-analysis of human neuroimaging studies. Cerebral Cortex, 24 , 2981–2990. https://doi.org/10.1093/cercor/bht154
Buhle, J. T., Wager, T. D, & Smith, E. (2010). Using the Stroop task to study emotion regulation. In R. R. Hassin, K. N. Ochsner, & Y Trope (Eds), Oxford series in social cognition and social neuroscience. Self control in society, mind, and brain (p. 93–113). Oxford University Press. https://doi.org/10.1093/acprof:oso/9780195391381.003.0006
Campos, J. J., Frankel, C. B., & Camras, L. (2004). On the nature of emotion regulation. Child Development, 75 (2), 377–394. https://doi.org/10.1111/j.1467-8624.2004.00681.x
Carlson, S. M., Moses, L. J., & Claxton, L. J. (2004). Individual differences in executive functioning and theory of mind: An investigation of inhibitory control and planning ability. Journal of Experimental Child Psychology, 87 (4), 299–319. https://doi.org/10.1016/j.jecp.2004.01.002
Carlson, S. M., & Moses, L. J. (2001). Individual differences in inhibitory control and children’s theory of mind. Child Development, 72 (4), 1032–1053. https://doi.org/10.1111/1467-8624.00333
Chakrabarti, B., & Baron-Cohen, S. (2006). Empathizing: Neurocognitive developmental mechanisms and individual differences. Progress in Brain Research, 156 , 403–417. https://doi.org/10.1016/S0079-6123(06)56022-4
Checa, P., & Fernández-Berrocal, P. (2015). The role of intelligence quotient and emotional intelligence in cognitive control processes. Frontiers in Psychology, 6 , 1853. https://doi.org/10.3389/fpsyg.2015.01853
Contardi, A., Imperatori, C., Penzo, I., Del Gatto, C., & Farina, B. (2016). The association among difficulties in emotion regulation, hostility, and empathy in a sample of young Italian adults. Frontiers in Psychology , 7 . https://doi.org/10.3389/fpsyg.2016.01068
Davis, M. H. (1983). Measuring individual differences in empathy: Evidence for a multidimensional approach. Journal of Personality and Social Psychology, 44 (1), 113–126. https://doi.org/10.1037/0022-3514.44.1.113
Decety, J., & Jackson, P. L. (2004). The functional architecture of human empathy. Behavioral and Cognitive Neuroscience Reviews, 3 (2), 71–100. https://doi.org/10.1177/1534582304267187
Decety, J., & Sommerville, J. A. (2003). Shared representations between self and other: A social cognitive neuroscience view. Trends in Cognitive Sciences, 7 (12), 527–533. https://doi.org/10.1016/j.tics.2003.10.004
Decety, J. (2010). The neurodevelopment of empathy in humans. Developmental Neuroscience, 32 (4), 257–267. https://doi.org/10.1159/000317771
Dimberg, U., Andréasson, P., & Thunberg, M. (2011). Emotional empathy and facial reactions to facial expressions. Journal of Psychophysiology, 25 (1), 26–31. https://doi.org/10.1027/0269-8803/a000029
Durston, S., Thomas, K. M., Yang, Y., Ulug, A. M., Zimmerma, R. D., & Casey, B. J. (2002). A neural basis for the development of inhibitory control. Developmental Science, 5 (4), 9–1. https://doi.org/10.1111/1467-7687.00235
Dziobek, I., Rogers, K., Fleck, S., Bahnemann, M., Heekeren, H. R., Wolf, O. T., & Convit, A. (2008). Dissociation of cognitive and emotional empathy in adults with Asperger syndrome using the Multifaceted Empathy Test (MET). Journal of Autism and Developmental Disorders, 38 (3), 464–473. https://doi.org/10.1007/s10803-007-0486-x
Eisenberg, N., Fabes, R. A., Miller, P. A., Fultz, J., Shell, R., Mathy, R. M., & Reno, R. R. (1989). Relation of sympathy and personal distress to prosocial behavior: A multimethod study. Journal of Personality and Social Psychology, 57 (1), 55–66. https://doi.org/10.1037//0022-3514.57.1.55
Eisenberg, N., & Okun, M. A. (1996). The relations of dispositional regulation and emotionality to elders’ empathy-related responding and affect while volunteering. Journal of Personality, 64 (1), 157–183. https://doi.org/10.1111/j.1467-6494.1996.tb00818.x
Eisenberg, N., Fabes, R. A., Guthrie, I. K., & Reiser, M. (2000). Dispositional emotionality and regulation: Their role in predicting quality of social functioning. Journal of Personality and Social Psychology, 78 (1), 136–157. https://doi.org/10.1037/0022-3514.78.1.136
Etkin, A., Egner, T., Peraza, D. M., Kandel, E. R., & Hirsch, J. (2006). Resolving emotional conflict: A role for the rostral anterior cingulate cortex in modulating activity in the amygdala. Neuron, 51 (6), 871–882. https://doi.org/10.1016/j.neuron.2006.07.029
Etkin, A., Prater, K. E., Hoeft, F., Menon, V., & Schatzberg, A. F. (2010). Failure of anterior cingulate activation and connectivity with the amygdala during implicit regulation of emotional processing in generalized anxiety disorder. The American Journal of Psychiatry, 167 (5), 545–554. https://doi.org/10.1176/appi.ajp.2009.09070931
Faul, F., Erdfelder, E., Lang, A.-G., & Buchner, A. (2007). G*Power 3: A flexible statistical power analysis program for the social, behavioral, and biomedical sciences. Behavior Research Methods, 39 (2), 175–191. https://doi.org/10.3758/BF03193146
Flynn, E. (2007). The role of inhibitory control in false belief understanding. Infant and Child Development, 16 (1), 53–69. https://doi.org/10.1002/icd.500
Frith, U., & Happé, F. (2005). Autism spectrum disorder. Current Biology, 15 (19), 786–790. https://doi.org/10.1016/j.cub.2005.09.033
Frith, U., & Frith, C. D. (2003). Development and neurophysiology of mentalizing. Philosophical Transactions of the Royal Society of London. Series B, Biological Sciences , 358 (1431), 459–473. https://doi.org/10.1098/rstb.2002.1218
Gerdes, K. E., Segal, E. A., & Lietz, C. A. (2010). Conceptualising and measuring empathy. The British Journal of Social Work, 40 (7), 2326–2343. https://doi.org/10.1093/bjsw/bcq048
Gohm, C. L., & Clore, G. L. (2002). Four latent traits of emotional experience and their involvement in well-being, coping, and attributional style. Cognition and Emotion, 16 (4), 495–518. https://doi.org/10.1080/02699930143000374
Goodhew, S. C., & Edwards, M. (2020). Attentional control both helps and harms empathy. Cognition, 206 , 104505. https://doi.org/10.1016/j.cognition.2020.104505
Gratz, K. L., & Roemer, L. (2004). Multidimensional assessment of emotion regulation and dysregulation: Development, factor structure, and initial validation of the difficulties in emotion regulation scale. Journal of Psychopathology and Behavioral Assessment, 26 (1), 41–54. https://doi.org/10.1023/B:JOBA.0000007455.08539.94
Greenberg, D. M., Kolasi, J., Hegsted, C. P., Berkowitz, Y., & Jurist, E. L. (2017). Mentalized affectivity: A new model and assessment of emotion regulation. PLoS ONE, 12 (10), e0185264. https://doi.org/10.1371/journal.pone.0185264
Gross, J. J. (2002). Emotion regulation: Affective, cognitive, and social consequences. Psychophysiology , 39 (3), 281–291. https://doi.org/10.1017/s0048577201393198
Gross, J. J. (2015). Emotion regulation: Current status and future prospects. Psychological Inquiry, 26 (1), 1–26. https://doi.org/10.1080/1047840X.2014.940781
Gross, J. J., & Feldman Barrett, L. F. (2011). Emotion generation and emotion regulation: One or two depends on your point of view. Emotion Review, 3 (1), 8–16. https://doi.org/10.1177/1754073910380974
Gross, J. J., & John, O. P. (2003). Individual differences in two emotion regulation processes: Implications for affect, relationships, and well-being. Journal of Personality and Social Psychology, 85 , 348–362. https://doi.org/10.1037/0022-3514.85.2.348
Gyurak, A., Gross, J. J., & Etkin, A. (2011). Explicit and implicit emotion regulation: A dual-process framework. Cognition & Emotion, 25 (3), 400–412. https://doi.org/10.1080/02699931.2010.544160
Haas, B. W., Omura, K., Constable, R. T., & Canli, T. (2006). Interference produced by emotional conflict associated with anterior cingulate activation. Cognitive, Affective, & Behavioral Neuroscience, 6 (2), 152–156. https://doi.org/10.3758/CABN.6.2.152
Hansen, S. (2011). Inhibitory control and empathy-related personality traits: Sex-linked associations. Brain and Cognition, 76 (3), 364–368. https://doi.org/10.1016/j.bandc.2011.04.004
Happe, F., & Frith, U. (1996). Theory of mind and social impairment in children with conduct disorder. British Journal of Developmental Psychology, 14 (4), 385–398. https://doi.org/10.1111/j.2044-835x.1996.tb00713.x
Hare, T. A., Tottenham, N., Davidson, M. C., Glover, G. H., & Casey, B. J. (2005). Contributions of amygdala and striatal activity in emotion regulation. Biological Psychiatry, 57 (6), 624–632. https://doi.org/10.1016/j.biopsych.2004.12.038
Hare, T. A., Tottenham, N., Galvan, A., Voss, H. U., Glover, G. H., & Casey, B. J. (2008). Biological substrates of emotional reactivity and regulation in adolescence during an emotional go-nogo task. Biological Psychiatry, 63 (10), 927–934. https://doi.org/10.1016/j.biopsych.2008.03.015015
Hatfield, E., Cacioppo, J. T., & Rapson, R. L. (1993). Emotional contagion. Current Directions in Psychological Science, 2 (3), 96–99. https://doi.org/10.1111/1467-8721.ep10770953
Hedge, C., Powell, G., & Sumner, P. (2018). The reliability paradox: Why robust cognitive tasks do not produce reliable individual differences. Behaviour Research Methods, 50 , 1166–1186. https://doi.org/10.3758/s13428-017-0935-1
Hendricks, M. A., & Buchanan, T. W. (2016). Individual differences in cognitive control processes and their relationship to emotion regulation. Cognition & Emotion, 30 (5), 912–924. https://doi.org/10.1080/02699931.2015.1032893
Hobson, R. P. (2010). Explaining autism Ten reasons to focus on the developing self. Autism. The International Journal of Research and Practic, 14 (5), 391–407. https://doi.org/10.1177/1362361310364142
Hofelich, A. J., & Preston, S. D. (2012). The meaning in empathy: Distinguishing conceptual encoding from facial mimicry, trait empathy, and attention to emotion. Cognition & Emotion, 26 (1), 119–128. https://doi.org/10.1080/02699931.2011.559192
Huizinga, M., Dolan, C. V., & van der Molen, M. W. (2006). Age-related change in executive function: Developmental trends and a latent variable analysis. Neuropsychologia, 44 (11), 2017–2036. https://doi.org/10.1016/j.neuropsychologia.2006.01.010
Jasinska, A. J., Yasuda, M., Rhodes, R. E., Wang, C., & Polk, T. A. (2012). Task difficulty modulates the impact of emotional stimuli on neural response in cognitive-control regions. Frontiers in Psychology , 3 . https://doi.org/10.3389/fpsyg.2012.00345
Kagan, J. (1988). The meanings of personality predicates. American Psychologist, 43 (8), 614–620. https://doi.org/10.1037/0003-066X.43.8.614
Kalanthroff, E., Henik, A., Derakshan, N., Usher., M. (2016). Anxiety, emotional distraction, and attentional control in the Stroop task. Emotion, 16 (3), 293–300. https://doi.org/10.1037/emo0000129
Kanske, P., Schönfelder, S., & Wessa, M. (2013). Emotional modulation of the attentional blink and the relation to interpersonal reactivity. Frontiers in Human Neuroscience , 7 . https://doi.org/10.3389/fnhum.2013.00641
Kaufman, E. A., Xia, M., Fosco, G. M., Yaptangco, M., Skidmore, C. R., & Crowell, S. E. (2016). The Difficulties in Emotion Regulation Scale Short Form (DERS-SF): Validation and Replication in Adolescent and Adult Samples. Journal of Psychopathology and Behavioral Assessment, 38 (3), 443–455. https://doi.org/10.1007/s10862-015-9529-3
Killgore, W. D., & Yurgelun-Todd, D. A. (2001). Sex differences in amygdala activation during the perception of facial affect. NeuroReport, 12 (11), 2543–2547. https://doi.org/10.1097/00001756-200108080-00050
Koole, S. L., & Rothermund, K. (2011). “I feel better but I don’t know why”: The psychology of implicit emotion regulation. Cognition & Emotion, 25 (3), 389–399. https://doi.org/10.1080/02699931.2010.550505
Koole, S. L., Webb, T., & Sheeran, P. (2015). Implicit emotion regulation: Feeling better without knowing why. Current Opinion in Psychology, 3 , 6–10. https://doi.org/10.1016/j.copsyc.2014.12.027
Kross, E., & Ayduk, O. (2011). Making meaning out of negative experiences by selfdistancing. Current Directions in Psychological Science, 20 (3), 187–191. https://doi.org/10.1177/0963721411408883
Laird, J. D., Alibozak, T., Davainis, D., Deignan, K., Fontanella, K., Hong, J., & Pacheco, C. (1994). Individual differences in the effects of spontaneous mimicry on emotional contagion. Motivation and Emotion, 18 (3), 231–247. https://doi.org/10.1007/BF02254830
Lei, Y., Dou, H., Liu, Q., Zhang, W., Zhang, Z., & Li, H. (2017). Automatic processing of emotional words in the absence of awareness: The critical role of P2. Frontiers in Psychology , 8 . https://doi.org/10.3389/fpsyg.2017.00592
Lockwood, P. L., Bird, G., Bridge, M., & Viding, E. (2013). Dissecting empathy: High levels of psychopathic and autistic traits are characterized by difficulties in different social information processing domains. Frontiers in Human Neuroscience , 7 . https://doi.org/10.3389/fnhum.2013.00760
Lockwood, P. L., Seara-Cardoso, A., & Viding, E. (2014). Emotion regulation moderates the association between empathy and prosocial behavior. PLoS ONE, 9 (5), e96555. https://doi.org/10.1371/journal.pone.0096555
López-Pérez, B., & Ambrona, T. (2015). The role of cognitive emotion regulation on the vicarious emotional response. Motivation and Emotion, 39 (2), 299–308. https://doi.org/10.1007/s11031-014-9452-z
MacDonald, H. Z., & Price, J. L. (2019). The role of emotion regulation in the relationship between empathy and internalizing symptoms in college students. Mental Health & Prevention, 13 , 43–49. https://doi.org/10.1016/j.mhp.2018.11.004
Mauss, I. B., Bunge, S. A., & Gross, J. J. (2007). Automatic emotion regulation. Social and Personality Psychology Compass, 1 (1), 146–167. https://doi.org/10.1111/j.1751-9004.2007.00005.x
McRae, K., Gross, J. J., Weber, J., Robertson, E. R., Sokol-Hessner, P., Ray, R. D., & Ochsner, K. N. (2012). The development of emotion regulation: An fMRI study of cognitive reappraisal in children, adolescents and young adults. Social Cognitive and Affective Neuroscience, 7 (1), 11–22. https://doi.org/10.1093/scan/nsr093
Melchers, M., Montag, C., Markett, S., & Reuter, M. (2015). Assessment of empathy via self-report and behavioural paradigms: Data on convergent and discriminant validity. Cognitive Neuropsychiatry, 20 (2), 157–171. https://doi.org/10.1080/13546805.2014.991781
Miyake, A., Friedman, N. P., Emerson, M. J., Witzki, A. H., Howerter, A., & Wager, T. D. (2000). The unity and diversity of executive functions and their contributions to complex “Frontal Lobe” tasks: A latent variable analysis. Cognitive Psychology., 41 (1), 49–100. https://doi.org/10.1006/cogp.1999.073
Morooka, T., Ogino, T., Takeuchi, A., Hanafusa, K., Oka, M., & Ohtsuka, Y. (2012). Relationships between the color-word matching Stroop task and the Go/NoGo task: Toward multifaceted assessment of attention and inhibition abilities of children. Acta Medica Okayama, 66 (5), 377–386. https://doi.org/10.18926/AMO/49385
Morris, J. S., Frith, C. D., Perrett, D. I., Rowland, D., Young, A. W., Calder, A. J., & Dolan, R. J. (1996). A differential neural response in the human amygdala to fearful and happy facial expressions. Nature, 383 (6603), 812–815. https://doi.org/10.1038/383812a0
Moskowitz, D. S. (1986). Comparison of self-reports, reports by knowledgeable informants, and behavioral observation data. Journal of Personality, 54 (1), 294–317. https://doi.org/10.1111/j.1467-6494.1986.tb00396.x
Mutter, B., Alcorn, M. B., & Welsh, M. (2006). Theory of mind and executive function: Working-memory capacity and inhibitory control as predictors of false-belief task performance. Perceptual and Motor Skills, 102 (3), 819–835. https://doi.org/10.2466/pms.102.3.819-835
Nee, D. E., Wager, T. D., & Jonides, J. (2007). Interference resolution: Insights from a meta-analysis of neuroimaging tasks. Cognitive, Affective, & Behavioral Neuroscience, 7 (1), 1–17. https://doi.org/10.3758/CABN.7.1.1
Ochsner, K. N., & Gross, J. J. (2008). Cognitive emotion regulation: Insights from social cognitive and affective neuroscience. Current Directions in Psychological Science, 17 (2), 153–158. https://doi.org/10.1111/j.1467-8721.2008.00566.x
Okun, M. A., Shepard, S. A., & Eisenberg, N. (2000). The relations of emotionality and regulation to dispositional empathy-related responding among volunteers-in-training. Personality and Individual Differences, 28 (2), 367–382. https://doi.org/10.1016/S0191-8869(99)00107-5
Ólafsson, R. P., Smári, J., Guðmundsdóttir, F., Ólafsdóttir, G., Harðardóttir, H. L., & Einarsson, S. M. (2011). Self-reported attentional control with the Attentional Control Scale: Factor structure and relationship with symptoms of anxiety and depression. Journal of Anxiety Disorders, 25 (6), 777–782. https://doi.org/10.1016/j.janxdis.2011.03.013
Olatunji, B. O., Ciesielski, B. G., Armstrong, T., Zhao, M., & Zald, D. H. (2011). Making something out of nothing: Neutral content modulates attention in generalized anxiety disorder. Depression and Anxiety, 28 (5), 427–434. https://doi.org/10.1002/da.20806
Ovaysikia, S., Tahir, K. A., Chan, J. L., & DeSouza, J. F. X. (2011). Word wins over face: Emotional stroop effect activates the frontal cortical network. Frontiers in Human Neuroscience , 4 . https://doi.org/10.3389/fnhum.2010.00234
Padmala, S., Bauer, A., & Pessoa, L. (2011). Negative emotion impairs conflict-driven executive control. Frontiers in Psychology , 2 . https://doi.org/10.3389/fpsyg.2011.00192
Paulhus, D. L. (1991). Measurement and control of response bias. In J. P. Robinson, J.P., Shaver, P. R., & Wrightsman, L. S. (Eds), Measures of Social Psychological Attitudes, Vol. 1. Measures of personality and social psychological attitudes (pp. 17–59). https://doi.org/10.1016/B978-0-12-590241-0.50006-X
Pfeifer, J. H., Iacoboni, M., Mazziotta, J. C., & Dapretto, M. (2008). Mirroring others’ emotions relates to empathy and interpersonal competence in children. NeuroImage, 39 (4), 2076–2085. https://doi.org/10.1016/j.neuroimage.2007.10.032
Powell, P. A. (2018). Individual differences in emotion regulation moderate the associations between empathy and affective distress. Motivation and Emotion, 42 (4), 602–613. https://doi.org/10.1007/s11031-018-9684-4
Pratto, F., & John, O. P. (1991). Automatic vigilance: The attention-grabbing power of negative social information. Journal of Personality and Social Psychology, 61 (3), 380–391. https://doi.org/10.1037/0022-3514.61.3.380
Reeck, C., & Egner, T. (2011). Affective privilege: Asymmetric interference by emotional distracters. Frontiers in Psychology , 2 . https://doi.org/10.3389/fpsyg.2011.00232
Reniers, R. L. E. P., Corcoran, R., Drake, R., Shryane, N. M., & Völlm, B. A. (2011). The QCAE: A questionnaire of cognitive and affective empathy. Journal of Personality Assessment, 93 (1), 84–95. https://doi.org/10.1080/00223891.2010.528484
Richards, A., French, C. C., Johnson, W., Naparstek, J., & Williams, J. (1992). Effects of mood manipulation and anxiety on performance of an emotional Stroop task. British Journal of Psychology , 83 (Pt 4) , 479–491. https://doi.org/10.1111/j.2044-8295.1992.tb02454.x
Rieffe, C., & Camodeca, M. (2016). Empathy in adolescence: Relations with emotion awareness and social roles. British Journal of Developmental Psychology, 34 (3), 340–353. https://doi.org/10.1111/bjdp.12133
Rive, M. M., van Rooijen, G., Veltman, D. J., Phillip, M. L., Schene, A. H., & Ruhé, H. G. (2013). Neural correlates of dysfunctional emotion regulation in major depressive disorder. A systematic review of neuroimaging studies. Neuroscience and Biobehavioral Reviews, 37 (10), 2529–2553. https://doi.org/10.1016/j.neubiorev.2013.07.018
Rueckert, L., Branch, B., & Doan, T. (2011). Are gender differences in empathy due to differences in emotional reactivity? Psychology, 02 (06), 574–578. https://doi.org/10.4236/psych.2011.26088
Sato, W., Fujimura, T., Kochiyama, T., & Suzuki, N. (2013). Relationships among facial mimicry, emotional experience, and emotion recognition. PLoS ONE, 8 (3), e57889. https://doi.org/10.1371/journal.pone.0057889
Schipper, M., & Petermann, F. (2013). Relating empathy and emotion regulation: Do deficits in empathy trigger emotion dysregulation? Social Neuroscience, 8 (1), 101–107. https://doi.org/10.1080/17470919.2012.761650
Schmeichel, B. J., & Demaree, H. A. (2010). Working memory capacity and spontaneous emotion regulation: High capacity predicts self-enhancement in response to negative feedback. Emotion, 10 (5), 739–744. https://doi.org/10.1037/a0019355
Seidel, E. M., Habel, U., Finkelmeyer, A., Schneider, F., Gur, R. C., & Derntl, B. (2010). Implicit and explicit behavioural tendencies in male and female depression. Psychiatry Research, 177 , 124–130. https://doi.org/10.1016/j.psyhres.2010.02.001
Shamosh, N. A., & Gray, J. R. (2008). Delay discounting and intelligence: A meta-analysis. Intelligence, 36 (4), 289–305. https://doi.org/10.1016/j.intell.2007.09.004
Singer, T., & Lamm, C. (2009). The social neuroscience of empathy. Annals of the New York Academy of Sciences, 1156 , 81–96. https://doi.org/10.1111/j.1749-6632.2009.04418.x
Sonnby-Borgström, M. (2002). Automatic mimicry reactions as related to differences in emotional empathy. Scandinavian Journal of Psychology, 43 (5), 433–443. https://doi.org/10.1111/1467-9450.00312
Stenberg, G., Wiking, S., & Dahl, M. (1998). Judging words at face value: Interference in a word processing task reveals automatic processing of affective facial expressions. Cognition and Emotion, 12 (6), 755–782. https://doi.org/10.1080/026999398379420
Stroop, J. R. (1935). Studies of interference in serial verbal reactions. Journal of Experimental Psychology, 18 (6), 643–662. https://doi.org/10.1037/h0054651
Swick, D., Ashley, V., & Turken, U. (2011). Are the neural correlates of stopping and not going identical? Quantitative meta-analysis of two response inhibition tasks. NeuroImage, 56 (3), 1655–1665. https://doi.org/10.1016/j.neuroimage.2011.02.070
Taylor, J. G., & Fragopanagos, N. F. (2005). The interaction of attention and emotion. Neural Networks, 18 (4), 353–369. https://doi.org/10.1016/j.neunet.2005.03.005
Thompson, N. M., Uusberg, A., Gross, J. J. and Chakrabarti, B. (2019) Empathy and emotion regulation: An integrative account. In: Srinivasan, N. (Ed.), Emotion and Cognition. Progress in Brain Research, 247. Elsevier, pp. 273–304. https://doi.org/10.1016/bs.pbr.2019.03.024
Tottenham, N., Hare, T. A., & Casey, B. J. (2011). Behavioral assessment of emotion discrimination, emotion regulation, and cognitive control in childhood, adolescence, and adulthood. Frontiers in Psychology , 2 . https://doi.org/10.3389/fpsyg.2011.00039
Tottenham, N., Tanaka, J. W., Leon, A. C., McCarry, T., Nurse, M., Hare, T. A., & Nelson, C. (2009). The NimStim set of facial expressions: Judgments from untrained research participants. Psychiatry Research, 168 (3), 242–249. https://doi.org/10.1016/j.psychres.2008.05.006
Tully, E. C., Ames, A. M., Garcia, S. E., & Donohue, M. R. (2016). Quadratic associations between empathy and depression as moderated by emotion dysregulation. The Journal of Psychology, 150 (1), 15–35. https://doi.org/10.1080/00223980.2014.992382
Wallace-Hadrill, S. M. A., & Kamboj, S. K. (2016). The impact of perspective change as a cognitive reappraisal strategy on affect: A systematic review. Frontiers in Psychology, 7 , 1715. https://doi.org/10.3389/fpsyg.2016.01715
Whalen, P. J., Rauch, S. L., Etcoff, N. L., McInerney, S. C., Lee, M. B., & Jenike, M. A. (1998). Masked presentations of emotional facial expressions modulate amygdala activity without explicit knowledge. The Journal of Neuroscience, 18 (1), 411–418. https://doi.org/10.1523/JNEUROSCI.18-01-00411.1998
Wild, B., Erb, M., & Bartels, M. (2001). Are emotions contagious? Evoked emotions while viewing emotionally expressive faces: Quality, quantity, time course and gender differences. Psychiatry Research, 102 (2), 109–124. https://doi.org/10.1016/S0165-1781(01)00225-6
Yiend, J., Mathews, A., Weston, B., Dunn, B. D., Cusack, R., & Mackintosh, B. (2008). An investigation of the implicit control of the processing of negative pictures. Emotion, 8 (6), 828–837. https://doi.org/10.1037/a0014146
Yu, C.L., & Chou, T.-L. (2018). A dual route model of empathy: A neurobiological prospective. Frontiers in Psychology , 9 . https://doi.org/10.3389/fpsyg.2018.02212
Zaki, J. (2014). Empathy: A motivated account. Psychological Bulletin, 140 (6), 1608–1647. https://doi.org/10.1037/a0037679
Zhang, W., & Lu, J. (2012). Time course of automatic emotion regulation during a facial Go/Nogo task. Biological Psychology, 89 (2), 444–449. https://doi.org/10.1016/j.biopsycho.2011.12.011
Zhang, W., Ding, Q., Cheng, N., Wei, Q., Zhao, C., Zhang, P., Li, X., & Li, H. (2016). The development of automatic emotion regulation in an implicit emotional Go/NoGo paradigm and the association with depressive symptoms and anhedonia during adolescence. Neuroimage Clinical, 11 , 116–123. https://doi.org/10.1016/j.nicl.2016.01.018
Download references
Acknowledgements
The authors would like to thank Esther Olaniyi, George Holland, Jessica Neal-Reidy, Jordan Fearon-Brown, Lauren Weaver, and Michael Jeanne Caguiat for their help with data collection.
Author information
Authors and affiliations.
School of Psychology and Clinical Language Sciences, University of Reading, Reading, RG6 6AL, UK
Nicholas M. Thompson, Carien M. van Reekum & Bhismadev Chakrabarti
You can also search for this author in PubMed Google Scholar
Corresponding author
Correspondence to Bhismadev Chakrabarti .
Ethics declarations
This research was supported by a Medical Research Council (MRC) doctoral studentship (MR/J003980/1) awarded to NMT. BC was supported by the Leverhulme Trust (Grant No: PLP-2015–329), and Medical Research Council UK (Grant No: MR/P023894/1).
Data Availability
The datasets analysed during the current studies are available in the University of Reading Data Archive, https://doi.org/10.17864/1947.305 .
Ethical Approval
This study was performed in line with the principles of the Declaration of Helsinki. Approval was granted by the School of Psychology Research Ethics Committee at the University of Reading (2015–136-BC). All participants provided informed consent.
Conflicts of Interest
The authors declare no competing interests.
Author Contributions
NMT contributed to study design, data collection/analysis, and manuscript preparation. CVR and BC contributed to study design and manuscript preparation.
Additional information
Handling Editor: Shigehiro Oishi
Supplementary Information
Below is the link to the electronic supplementary material.
Supplementary file1 (DOCX 23 KB)
Rights and permissions.
Open Access This article is licensed under a Creative Commons Attribution 4.0 International License, which permits use, sharing, adaptation, distribution and reproduction in any medium or format, as long as you give appropriate credit to the original author(s) and the source, provide a link to the Creative Commons licence, and indicate if changes were made. The images or other third party material in this article are included in the article's Creative Commons licence, unless indicated otherwise in a credit line to the material. If material is not included in the article's Creative Commons licence and your intended use is not permitted by statutory regulation or exceeds the permitted use, you will need to obtain permission directly from the copyright holder. To view a copy of this licence, visit http://creativecommons.org/licenses/by/4.0/ .
Reprints and permissions
About this article
Thompson, N.M., van Reekum, C.M. & Chakrabarti, B. Cognitive and Affective Empathy Relate Differentially to Emotion Regulation. Affec Sci 3 , 118–134 (2022). https://doi.org/10.1007/s42761-021-00062-w
Download citation
Received : 22 January 2021
Accepted : 15 June 2021
Published : 15 November 2021
Issue Date : March 2022
DOI : https://doi.org/10.1007/s42761-021-00062-w
Share this article
Anyone you share the following link with will be able to read this content:
Sorry, a shareable link is not currently available for this article.
Provided by the Springer Nature SharedIt content-sharing initiative
- Emotion regulation
- Social cognition
- Find a journal
- Publish with us
- Track your research
ORIGINAL RESEARCH article
Adaptive empathy: empathic response selection as a dynamic, feedback-based learning process.
- 1 Department of Psychology, University of Haifa, Haifa, Israel
- 2 Integrated Brain and Behavior Research Center, Haifa, Israel
- 3 Department of Cognitive Sciences, University of Haifa, Haifa, Israel
Empathy allows us to respond to the emotional state of another person. Considering that an empathic interaction may last beyond the initial response, learning mechanisms may be involved in dynamic adaptation of the reaction to the changing emotional state of the other person. However, traditionally, empathy is assessed through sets of isolated reactions to another's distress. Here we address this gap by focusing on adaptive empathy, defined as the ability to learn and adjust one's empathic responses based on feedback. For this purpose, we designed a novel paradigm of associative learning in which participants chose one of two empathic strategies (reappraisal or distraction) to attenuate the distress of a target person, where one strategy had a higher probability of relieving distress. After each choice, participants received feedback about the success of their chosen strategy in relieving the target person's distress, which they could use to inform their future decisions. The results show that the participants made more accurate choices in the adaptive empathy condition than in a non-social control condition, pointing to an advantage for learning from social feedback. We found a correlation between adaptive empathy and a trait measure of cognitive empathy. These findings indicate that the ability to learn about the effectiveness of empathic responses may benefit from incorporating mentalizing abilities. Our findings provide a lab-based model for studying adaptive empathy and point to the potential contribution of learning theory to enhancing our understanding of the dynamic nature of empathy.
Introduction
Empathy allows us to share emotions and understand the mental and affective states of others. While definitions of empathy may vary, one of the main objectives of empathic capabilities is to be able to respond to the emotional state of another person in order to alleviate that person's distress ( 1 ). Empathy has been shown to play a major role in promoting well-being ( 2 ), enhancing parenting skills ( 3 ), and supporting emotional development ( 4 ). There is strong evidence that empathy is a fundamental contributor to other-oriented prosocial behavior ( 5 ). Indeed, Zaki and Williams ( 1 ) suggested that empathy is apparent in the interpersonal emotion regulation cycle, as the distressed target evokes an empathic reaction in the observer, who may thus help the suffering person. Although empathic reactions can be covert and not communicated to others, e.g., change in mood, emotions, and thoughts, they are often overt, e.g., detectable facial or body expression, verbal response, and are conveyed back to the target. While empathic reactions do not necessarily lead to action, in many contexts of empathic interactions between an empathizer and a distressed target, they are the driver of prosocial responses. Also, the empathic interaction does not necessarily end with the initial empathic response. After feedback from the target, an individual's empathic responses may change, generating a process we refer to as adaptive empathy ( 6 ). Since we focus on empathic responses which are manifested in social interactions over time, the covert empathic reactions are beyond our scope, and from now and on we will focus on overt responses only, i.e., responses that are communicated to the distressed target. We currently do not know how the adaptive empathy process unfolds and how it is related to other learning processes and to trait empathy. Here we set out to examine adaptive empathy as a unique facet of empathy.
Despite a long tradition of studying empathy in social interactions in the field of social psychology ( 7 , 8 ), most known paradigms measuring empathy involve one-shot, passive observation of a suffering target. Current studies rely either on directly asking individuals to evaluate their trait empathy or to assess their state empathy ( 9 ). These studies facilitated the essential behavioral and neural differentiation of empathy components and provided several classifications of empathic abilities, the most prevalent of which is the distinction between emotional and cognitive empathy ( 10 , 11 ). Emotional empathy includes sharing of another's emotions, as well as emotional contagion, a condition in which one feels emotions detected in others ( 12 – 15 ). Cognitive empathy involves mentalizing and identifying another's thoughts and feelings ( 16 ), understanding another's perspective ( 11 ), as well as inferring and attributing mental states or traits to specific persons ( 17 , 18 ). Mentalizing, also known as Theory of Mind (ToM), is important because of the assumption that other people's mental states determine their actions and influence their interactions ( 18 , 19 ). Mentalizing is affected by culture and developmental stage ( 17 , 20 ) and requires high-order cognitive abilities, such as cognitive flexibility ( 21 , 22 ) and episodic memory ( 23 ). Both empathy components (emotional and cognitive) appear to operate independently on behavioral and neural levels, while an empathic response may encompass both processes or either one, depending on the context ( 11 , 24 ). Notably, both types of empathy may affect the dynamic process of adaptive empathy. The sharing of another's emotional state serves as a trigger for the empathic interaction, hence, emotional empathy may be essential in contexts that include affective empathic responses such as empathic touch and facial expressions ( 25 ). By means of mentalizing the state of the distressed person, cognitive empathy may help the empathizer evaluate the effectiveness of responses before reacting and thus choose the appropriate response for the specific person in distress, or learn the most effective one over time. Cognitive empathy may therefore be most relevant in contexts where one suggests emotional regulation strategies to alleviate distress, using verbal communication for example, which is the context of the current experiments.
In line with the view that empathic responses are dynamic and adapted to the needs of the target, Shamay-Tsoory and Hertz ( 6 ) proposed examining empathy in the context of interactions between empathizer and target over time. Adaptive empathy is the process through which an empathizer detects the effects of his or her initial empathic response and adapts this response accordingly, i.e., learns what is the most effective response strategy. The core of this approach sees empathy as taking place along a feedback cycle, in which the probability of providing a specific empathic response changes within an interaction according to the feedback ( 9 , 16 , 26 ). This cycle can endure over multiple incidents of distress relief during an interpersonal ( 27 ) or therapeutic relationship ( 28 ). This feedback cycle is akin to many other well-studied learning paradigms ( 29 , 30 ). Considering that the empathic response aims to diminish distress ( 2 , 3 ), learning mechanisms may be involved in dynamic adaptation and tailoring of the response to the specific person we interact with. Learning in the social domain bears some similarities to learning in a non-social domain in terms of the general computations that drive learning, though social learning has also been shown to operate differently ( 16 , 31 ). For example, when playing against humans as opposed to computers, participants preferred generosity over maximizing their reward ( 32 ). Moreover, recent evidence suggests that decisions in a social context are made by integrating multiple types of inferences about one's own rewards, others' rewards, and others' mental states ( 33 , 34 ). Social learning processes have also been shown to be related to trait empathy. For example, high cognitive empathy correlated with the dynamics of learning about options that maximize rewards for others ( 35 ) and with increased prosocial tendencies ( 36 ). Moreover, higher levels of cognitive trait empathy predicted better emotion regulation by a long-term romantic partner, suggesting that the ability to understand the partner's point of view, i.e., mentalization, is an important factor in distress relief ( 37 ).
Here we aim to characterize adaptive empathy as a learning process. Our first goal was to compare adaptive empathy to other types of learning in terms of accuracy. Our main hypothesis was that during adaptive empathy participants will demonstrate an overall learning pattern resembling other statistical learning paradigms. Nevertheless, we also had a non-directional hypothesis, according to which learning the empathic responses would be distinct from non-social learning. We further sought to evaluate the relationship between adaptive empathy and traditional cognitive and emotional empathy measures. Since adjusting the empathy reaction in response to feedback must involve cognitive empathy elements, such as mentalizing and inference of the other's mental state, we hypothesized that in the adaptive empathy condition, but not in other conditions, learning accuracy would be associated with cognitive empathy. We further assumed that performance in the adaptive empathy condition would not be correlated with emotional empathy.
To test these hypotheses, we developed a novel experimental paradigm of two-choice associative learning, as an adaptation of the classical behavioral paradigm “two-armed bandit task.” In this task the participants must make repeated choices among options (bandit arms), learning about the statistical relations between choices and expected outcomes. Such tasks are often used in learning and decision-making studies, demonstrating the abilities of participants to learn about the most rewarding action and adjust their behavior accordingly ( 29 , 38 – 41 ). In our paradigm, over multiple encounters, on each trial participants chose one of two empathic strategies (reappraisal or distraction) to attenuate the distress of a target. Following each choice, they observed the effect of their empathic response on the target's emotional state, such that the feedback could inform their future decisions. To pinpoint differences between empathic learning and other types of learning, participants also completed two control conditions involving learning about targets' food preference (social control) and the likely location of a monetary reward (non-social control). This paradigm allowed us to evaluate the relationship between adaptive empathy and learning in other contexts, and control for non-social associative learning skills, as well as assess the link between adaptive empathy and the individual's trait empathy.
Materials and Methods
Participants.
For the study, which was conducted online, we recruited 199 participants [77 male, aged 39.3 ± 14 (mean ± std); 121 female, aged 35.2 ± 13.4] using the Prolific platform (December 16, 2020). The study was approved by the University of Haifa, Faculty of Social Sciences Research Ethics Committee (Project ID Number: 100/21), and the experiment was conducted in accordance with relevant guidelines and regulations. All participants were screened for neurological disorders. Due to technical issues, choice data were corrupted for 15 participants and therefore discarded in further data analysis. Furthermore, 21 participants were excluded from the study due to insufficient effort invested in the task: failure to complete the task within a reasonable time limit (inactive over half an hour during the task); always selected the same side or the same option; performance below 30% accuracy in one of the three blocks. This level of performance was chosen to avoid excluding participants that had difficulties in learning in one of the blocks, which are meaningful and relevant to our expected differences. Therefore, our final sample size for the analysis was n = 163. This sample size was sufficient to allow detection of a moderate effect size of individual difference (ρ = 0.2, β = 0.8).
Adaptive Empathy Task
In the adaptive empathy task, the paradigm included three conditions: adaptive empathy, social control, and non-social control. Each condition included 20 trials in which participants had to choose between two options and learn which is more likely to lead to a desirable outcome ( Figure 1 ). In each condition, the participant interacted with one person/room over 20 trials. For example, a participant could make 20 decisions to alleviate person 1 distress in the adaptive empathy condition, 20 decisions regarding food courses for person 3 in the social-control condition, and 20 closet choices in room 2 in the non-social condition. The targets in each condition were counterbalanced across participants. The gender of the target person matched the participant's gender. The order of the conditions was randomized between participants. Progress within and between the trials was self-paced. The task was developed using JS and HTML (see Figure 1A for sample screens, the code is freely available in the Open Science Framework https://osf.io/dgt5e/ ).
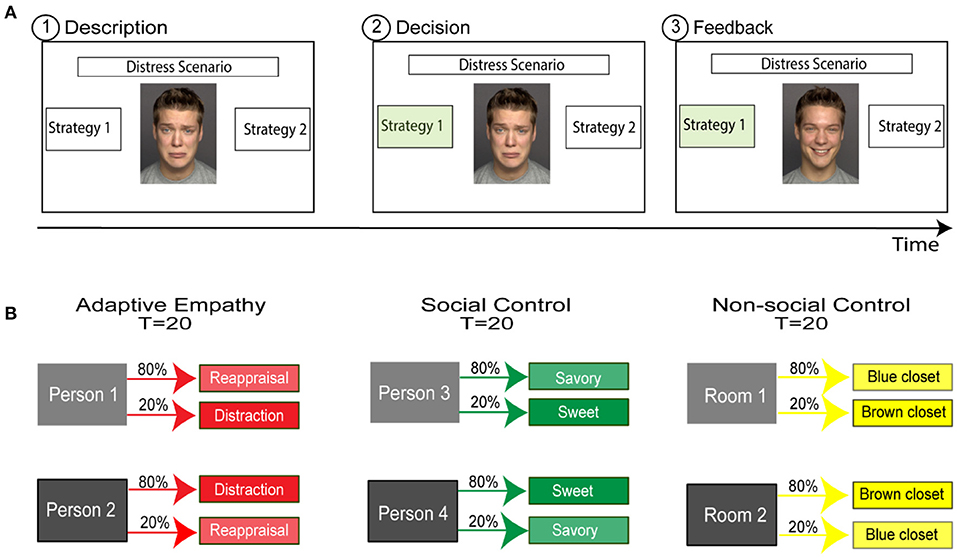
Figure 1 . Experimental Design. (A) A sample trial in the adaptive empathy condition. Participants had to choose between two options and learn which one was more likely to lead to a desirable outcome. Each trial consisted of three stages: (1) Participants were shown a picture of a person with a sad facial expression, together with a textual description of the current cause of the person's distress. Textual descriptions of two empathic responses corresponding to two different emotion regulation strategies were also provided. (2) Participants chose one of two responses. (3) Feedback was provided regarding the effect of the chosen strategy, as indicated by the person's face changing to a happy expression or remaining sad. (B) Overall experimental design of the adaptive empathy task. The task included three experimental learning conditions carried out by all participants. In each condition, participants learned about one person/room. The order of the blocks and the preferred strategy learned in each block were randomized across participants.
Adaptive Empathy Condition
In this condition, participants were shown 20 distress-related scenarios entailing a target person. Each trial began with a picture of the person with a sad facial expression, alongside a textual description of the current cause of the person's distress (description stage) (e.g., “Ben and his girlfriend broke up”). While viewing the scenario, participants were instructed to select one of two responses aimed at diminishing the distress of the targets. The responses were two types of emotion regulation strategies (distraction vs. reappraisal): “Let's go camping on the beach, maybe set up a campfire and take a swim” (distraction strategy) or “The relationship depends on both of you; maybe she just needed some me time” (reappraisal strategy). Finally, the participant observed the effect of the chosen strategy, indicated by the person's face changing to a happy expression or remaining sad (feedback stage). Unbeknownst to the participants, one strategy was more likely to relieve the target, with a probability of 0.8, while the other strategy had a relief probability of 0.2. About half the participants (86) interacted with a target that preferred reappraisal, while 77 participants interacted with a target that preferred distraction (see Figure 1B ).
Social Control Condition
In this control condition, the participant was required to learn about a target person's food preferences over 20 trials. Each trial began with a picture of the person with a neutral facial expression, alongside a textual description of a restaurant where the participants were supposedly present. The participant was offered two types of dishes (savory main course and sweet dessert), e.g., “Chop steak freshly ground and smothered with grilled mushrooms, onions and savory garlic sauce” or “Crepes with Nutella, strawberry, cherry, apple or apricot rich jam and ice cream,” and had to choose one that would please the target. Finally, the participant observed the effect of the chosen dish on the target, as indicated by the target's face changing to a happy expression or remaining neutral (feedback stage). One type of dish had a higher probability ( p = 0.8) of pleasing the target, while the other had a low probability of pleasing the target ( p = 0.2; see Figure 1B ).
Non-social Control Condition
In this control condition, the participant was required to learn which of two closets is more likely to contain a monetary reward over 20 trials. On each trial, after selecting a closet, the participant observed the effect of the choice (closet), indicated by whether the opened closet contained the money or was empty (feedback stage). One closet was more likely to contain the monetary reward than the other ( p = 0.8 vs. p = 0.2) (see Figure 1B ).
Paradigm and Stimuli
The facial stimuli shown to each participant were taken from the FACES Life Span Database of Facial Expressions, with their obtained permission ( 42 ). Only neutral, sad, and happy facial expressions for younger men and women were selected from the database.
The distress scenarios were taken from everyday life situations related to relationships, work, daily routines, and the like. The choice of emotion regulation strategies was based on a wide range of studies suggesting that cognitive reappraisal and expressive suppression (distraction) are widely used as emotion regulation strategies. Reappraisal is defined as changing the way one thinks about a situation, thus changing its emotional impact, while distraction is a strategy that involves inhibiting the emotion ( 43 – 46 ). The restaurant types were chosen according to popular categories found online. 1 The dish descriptions were taken and adjusted from various online restaurant menus, according to the type of restaurant.
To create a similar reading load, all the strategies (emotion regulation and dish descriptions) consisted of 15 words on average. The stimuli were tested and confirmed in a pilot study with independent reviewers.
Questionnaire of Cognitive and Affective Empathy
Based on a contemporary theoretical model of empathy, we chose the Questionnaire of Cognitive and Affective Empathy [QCAE; ( 47 )] as the tool to assess participants' levels of trait cognitive and affective (emotional) empathy. The QCAE consists of 31 items grouped into two scales of cognitive and affective (emotional) empathy. The cognitive empathy (CE) scale includes two subscales: perspective taking (PT) - the ability to see a situation from another person's perspective (e.g., “I can easily tell if someone else wants to enter a conversation”); online simulation (OS) - the ability to understand and mentally represent or imagine how another person is feeling (e.g., “Before criticizing somebody, I try to imagine how I would feel if I was in their place”). The affective empathy (AE) scale includes three subscales: emotion contagion (EC) - the automatic mirroring of emotions of others (e.g., “I am happy when I am with a cheerful group and sad when the others are glum”); peripheral responsivity (PER)—the emotional reaction to the mental states of others in a detached social context (e.g., “I often get deeply involved with the feelings of a character in a film, play, or novel”); and proximal responsivity (4 items)—the emotional reaction to the moods of others in a physically or emotionally close social context (e.g., “I often get emotionally involved with my friends' problems”). Items are rated on a 4-point Likert scale ranging from 1 = “strongly disagree” to 4 = “strongly agree.” Higher scores indicate greater empathy.
Participants were recruited using the Prolific platform and performed the experimental task online on their own computers, using a mouse to input their choices (smartphones or similar devices were blocked). They began by reading information about the experiment, signing an informed consent form, and answering several demographic questions (age, gender, and level of education). The participants were paid a fixed monetary compensation of £4 for their participation and were promised a performance-based bonus of £1 maximum for making correct choices across all experimental conditions. The central part of the experiment, i.e., the Adaptive Empathy Task, followed. The task average duration across participants was 8.2 min (SD = 3.2 min; MIN = 4.2 min; MAX = 24.8 min). The durations per block are detailed in Supplementary Table 2.3 . Upon completing the task, participants were asked to complete the empathy scales questionnaire (QCAE).
Statistical analyses were conducted using R version 4.0.1 ( 48 ), with the following packages: rstatix ( 49 ), afex ( 50 ), and jtools ( 51 ). Differences in accuracy between conditions were examined by a one-way repeated-measures ANOVA, followed-up by a post-hoc paired-samples t -test to determine the origin of the differences. A Welch t -test for unequal variances was conducted to compare means between two preferred strategies by different targets within each condition, considering two independent samples of participants receiving one of the two targets. To directly examine the relationship between adaptive empathy and trait empathy scales, we applied separate linear regression models. Participants' accuracy in each block, as well as the difference in accuracy between adaptive empathy and a non-social control block, served as dependent variables, while empathy scores served as independent variables.
Learning Accuracy Between Conditions and According to Preferred Strategy
The participants performed on average above chance-level (50%), suggesting learning of emotion regulation preferences, food preferences, and money location (see Figure 2A ). We also compared the learning accuracy between the conditions, applying a one-way repeated-measures ANOVA at three levels of a within-subjects variable block type (adaptive empathy, social control, and non-social control). This analysis revealed a significant difference in average learning accuracy between conditions [ F (2, 324) = 6.43 ** , p = 0.002, η P 2 = 0.038]. Follow-up post-hoc paired t -tests showed that the highest accuracy emerged in the social control condition (M = 76.72, SD = 16.22), which was significantly higher than the accuracy levels in the non-social control condition [ t (162) = 3.73, p < 0.001, d = 0.29], which exhibited the lowest learning accuracy (M = 70.46, SD = 18.19). In line with our prediction, accuracy in the adaptive empathy condition (M = 74.20, SD = 15.64) was significantly higher than in the non-social condition [ t (162) = 2.03, p = 0.04, d = 0.16] (see Figure 2B ). We further compared performance within the adaptive empathy condition, showing that the mean accuracy for the reappraisal strategy was 78.31 (SD = 13.25), whereas the mean accuracy for the distraction strategy was 69.61 (SD = 16.87). The Welch two-sample t -test showed that the difference was statistically significant, t (143.9) = 3.633, p < 0.001, d = 0.57. No such differences were found between strategies in the other conditions (see Figure 2C ).
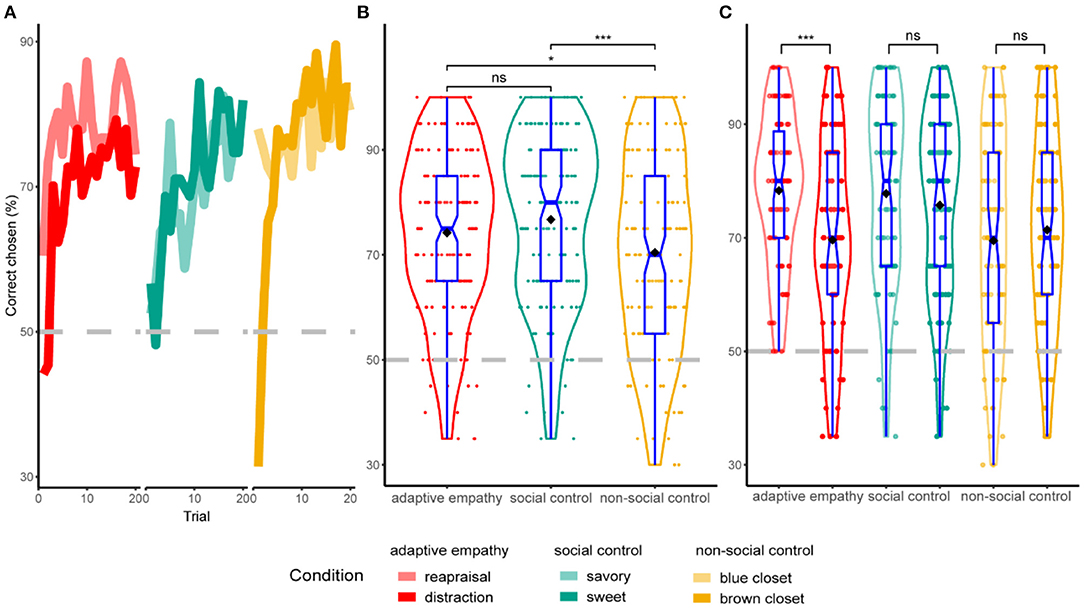
Figure 2 . Learning accuracy between conditions. (A) Group-level learning curves showing choice behavior in the three learning conditions. Trials are averaged over the three conditions for adaptive empathy, social control, and non-social control. Dashed lines indicate chance level. (B) Comparison of accuracy between the three conditions. Participants exhibited significantly higher learning accuracy in social vs. non-social and in adaptive empathy vs. non-social control conditions. (C) Comparison of learning accuracy within each condition, between strategies preferred by the target. Participants exhibited significantly higher learning accuracy when learning that targets preferred reappraisal over distraction. * p ≤ 0.05, *** p ≤ 0.001, ns, not significant.
Relationship Between Trait Empathy and Adaptive Empathy
We tested whether individuals' cognitive empathy rates were uniquely associated with adaptive empathy. In separate linear regression analyses, the two cognitive empathy subscales were entered as potential predictor variables, gender, and age as control variables, and learning accuracy at each condition was entered as the single dependent variable (see Figure 3 ). Consistent with our predictions, the analyses revealed that the online simulation subscale ( 47 ), a measure of trait empathy that probes the tendency to understand and imagine how another person is feeling, was positively associated with learning accuracy in the adaptive empathy condition [β = 0.67 ± 0.28, t (158) = 2.39, p = 0.02]. Such an association was not found for the social control and non-social control conditions, indicating that online simulation makes a unique contribution to adaptive empathy (see Figure 3A ). We directly compared the difference in slopes between the adaptive empathy and non-social conditions, by subtracting each participant's accuracy in the adaptive empathy condition from the accuracy in the non-social condition, and regressing this difference against the cognitive subscales. The linear regression results showed that the difference in accuracy was significantly correlated with the online simulation subscale, such that those high in this subscale exhibited a larger gap in performance between adaptive empathy and non-social control conditions [β = 0.83 ± 0.43, t (158) = 1.96, p = 0.05]. The perspective taking subscale was also positively correlated with the difference in accuracies between adaptive empathy and the non-social conditions [β = 0.75 ± 0.35, t (158) = 2.12, p = 0.04] (see Figures 3B,D ; Supplementary Results-Simple Linear Regression Tables in Supplementary Material ).
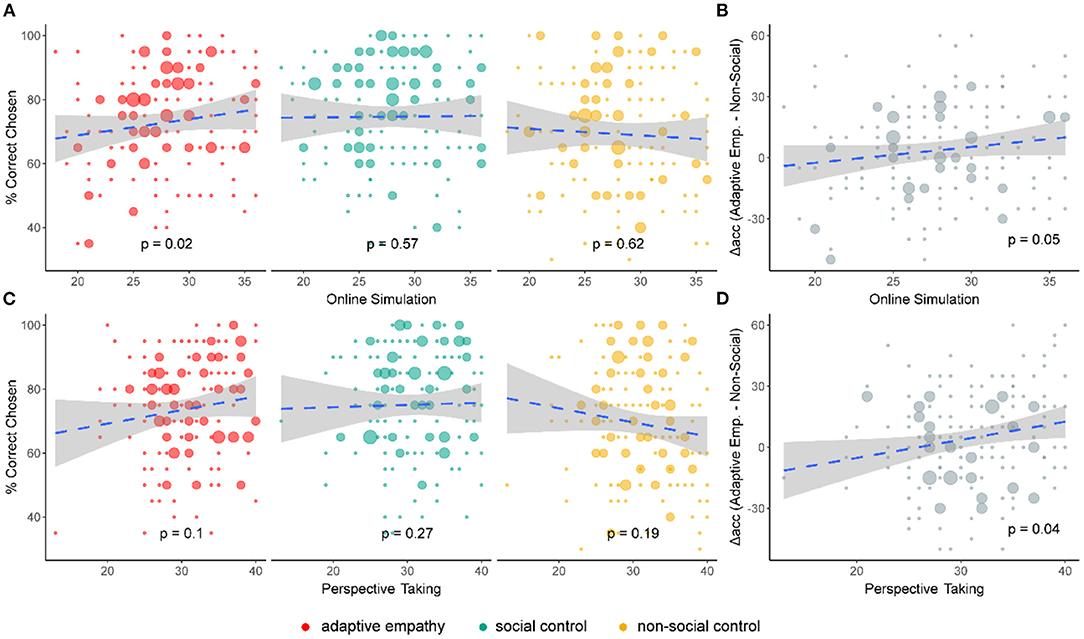
Figure 3 . Cognitive empathy subscales. (A) Correlations between task conditions and cognitive empathy trait: participants high on the online simulation subscale exhibited higher performance in adaptive empathy. There was no such correlation in other conditions. (B) Correlation of difference in accuracy between adaptive empathy and non-social control with cognitive empathy trait: participants high in online simulation had a larger gap in accuracy between the two conditions. (C) Same as (A) , but for the perspective taking subscale. No correlation was found with performance in any of the conditions. (D) Same as (B) , but for the perspective taking subscale: participants high in perspective taking had a larger gap in accuracy between the two conditions. Dashed lines indicate the fitted linear regression, gray areas indicate a 95% confidence interval.
We conducted another set of linear regression analyses by entering the three emotional empathy subscales as potential predictor variables, gender and age as control variables, and learning accuracy in each condition as the single dependent variable (see Figure 4 ). No correlation was found between emotional empathy and performance in the adaptive empathy and social control conditions. However, emotional empathy scores - emotion contagion (EC), proximal responsivity (PRR), and peripheral responsivity (PER) - exhibited a negative association with learning accuracy in the non-social condition [β = −1.5 ± 0.57, t (158) = −2.62, p = 0.01; β = −1.83 ± 0.59, t (158) = −3.09, p = 0.002; β = −1.33 ± 0.6, t (158) = −2.2, p = 0.03, respectively] (see Figures 4A,C,E ; Supplementary Results-Simple Linear Regression Tables in Supplementary Material ). In other words, higher levels of emotional empathy had a detrimental effect on learning in the non-social condition. Here, the PRR and PER subscale scores also predicted the difference between adaptive empathy accuracy and the non-social condition, such that higher trait empathy predicted a larger gap in accuracy between the conditions (see Figures 4D,F ; Supplementary Results-Simple Linear Regression Tables in Supplementary Material ).
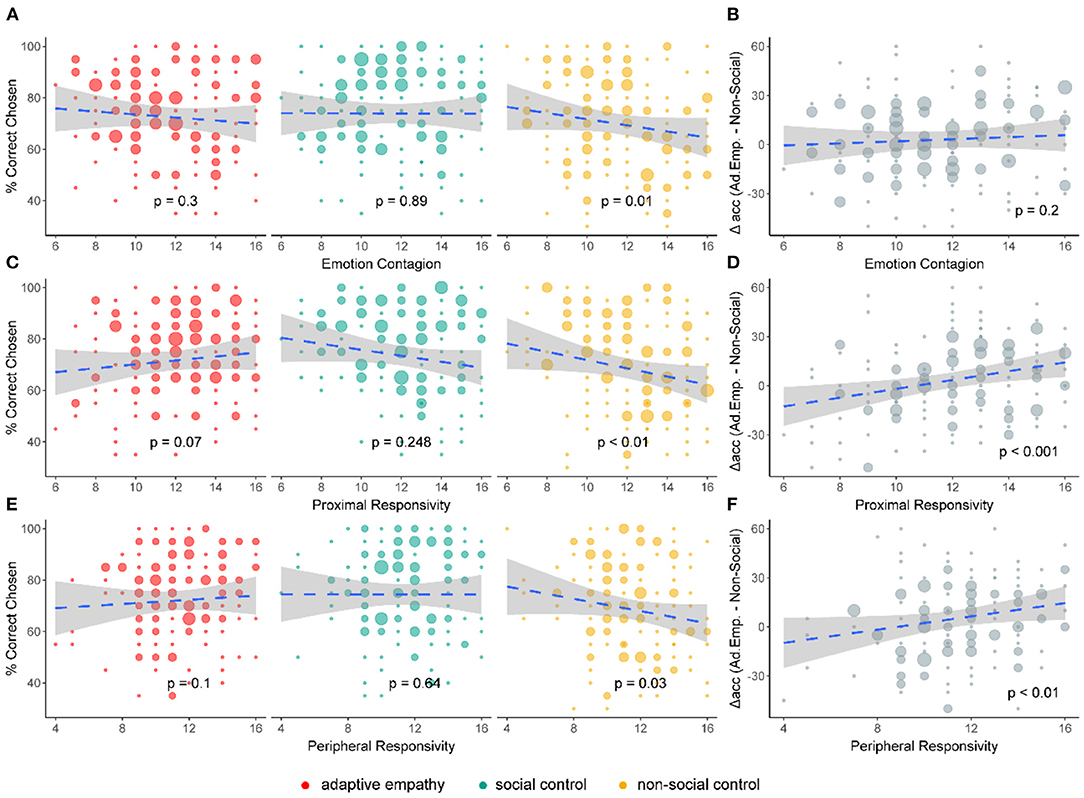
Figure 4 . Emotional empathy subscales. (A) Correlations between task conditions and emotional empathy trait: participants high in emotional contagion exhibited a deficit in learning in the non-social control condition. No such deficit was found for other conditions. (B) Correlation of difference in accuracy between adaptive empathy and non-social control with emotional empathy trait: there was no gap between conditions across emotion contagion scores. (C) Same as (A) , but for the proximal responsivity subscale, showing a learning deficit in non-social control conditions for higher scores. (D) Same as (B) , but for the proximal responsivity subscale: participants high in proximal responsivity had a larger gap in accuracy between the two conditions. (E) Same as (A) , but for the peripheral responsivity subscale, showing a learning deficit in non-social control conditions for higher scores. (F) Same as (B) , but for the peripheral responsivity subscale: participants high in peripheral responsivity exhibited a larger gap in accuracy between the two conditions.
The present study investigated adaptive empathy, i.e., the way participants learned and adapted their empathic responses according to the impact of these responses on a target person and the way this learning process corresponded with trait empathy measurements. We found a significant difference in choice accuracy between social and non-social conditions, as participants were more accurate in their choices of empathic responses and food preferences than in their choices of reward locations. This suggests that learning in the social domain is comparable to or even superior to non-social learning, even though the social domain involved more complex scenarios and option descriptions. Furthermore, within the adaptive empathy condition, performance was significantly higher when the target person preferred reappraisal rather than distraction. No such differences emerged in the other conditions. We observed an association between adaptive empathy and traditional empathy measures. In line with our hypothesis, the analysis revealed that cognitive empathy, and specifically its online simulation subscale, correlated with performance in the adaptive empathy condition only. The emotional empathy trait's subscales were not correlated with performance in the adaptive empathy condition, but were found to be negatively associated with performance in the non-social control condition. These results indicate that adaptive empathy is comparable to other learning processes and is linked to cognitive empathy abilities, at least when learning about the effectiveness of emotion regulation strategies. These findings suggest that adaptive empathy may be an important facet of empathy, which may influence the dynamics and outcomes of social interactions.
Our findings of higher accuracy levels in the social conditions support the idea that learning in the social domain is somewhat different than in other, non-social domains ( 34 ). The notion of privileged learning in the social domain has been examined from different perspectives, among them cognitive (i.e., which cognitive processes are involved in this process) and motivational (i.e., what are the goals or intentions of the learner) ( 52 ). From the cognitive perspective, while social and non-social learning may depend on the same basic learning mechanisms ( 53 ), learning about people may incorporate prior, human-specific, expectations, such as consistency of people's traits over time and expectations about how people may respond to different actions based on previous encounters and our own experiences ( 16 , 54 ), which we do not use when learning about the location of money rewards (as an example). For instance, in the case of learning about people's moral behaviors, the attribution of selfish behavior to a person's character was found to be more volatile than the attribution of moral or prosocial behaviors ( 55 ). Such a bias was not observed when learning about the resource-sharing decisions of non-human agents. Hence, the distinction found in social vs. non-social learning may not be due to differences in basic learning mechanisms per se , but rather result from our mentalizing capacity or theory of mind, in the form of a socially specific cognitive module that is present when learning from a social partner ( 34 , 56 ). Mentalizing and employing an internal model of human mind may make learning about other people, i.e., reasoning and forming predictions about them, easier than learning about abstract associations ( 16 , 54 ).
The correlation observed here between cognitive empathy and performance in the adaptive empathy condition supports the role of mentalizing in social learning. Higher levels of cognitive empathy ability, and specifically its online simulation subscale, were linked with enhanced ability to adapt one's empathic response based on feedback from the target person. The online simulation subscale developed by Reniers et al. ( 47 ) is defined as the capacity to simulate other people's feelings and is relatively similar to perspective taking from the Interpersonal Reactivity Index [IRI; ( 57 )]. However, as suggested by Heym et al. ( 58 ), this scale seems to encompass not only imagining how other people feel, but also how they think and may act, i.e., simulating other people's mental states (both thoughts and feelings) and spontaneously adopting their psychological point of view, which resembles the traditional conceptualization of mentalizing ( 17 ). Mentalizing may greatly aid the iterative process of interpersonal emotion regulation, i.e., adaptive empathy, as it involves learning and adjusting one's expectations of another person's behavior and determining which course of action will have a more relieving effect on a specific person ( 16 – 19 ). Moreover, a previous study showed that individuals with high scores on the online simulation subscale learned equally fast for the benefit of others as for their own benefit, as opposed to those who scored low on this subscale and learned slower for others ( 59 ). This is in line with our finding that people who scored high on the online simulation subscale are better in learning about emotion regulation preferences of others than are individuals with low scores on this subscale.
In addition to mentalizing, learning about humans integrates prior biases and assumptions ( 33 ). Such prior expectations about other people may explain the difference found in adaptive empathy accuracy between the two emotion regulation strategies, as reappraisal strategy was more readily learned than distraction strategy. The use of reappraisal rather than distraction to regulate emotions is widely considered to be associated with well-being. Researchers have also suggested that reappraisal is more effective and has healthier emotional, cognitive and social consequences than distraction ( 60 , 61 ). Hence, learning that reappraisal rather than distraction is the most effective strategy may be easier due to common knowledge about the success of this strategy in coping with negative emotions ( 62 ). In addition, the scenarios presented to the participants were low-intensity distress situations. Previous findings showed that individuals prefer to regulate emotions using reappraisal in such situations, compared with high-intensity distress situations, in which they prefer to use distraction ( 43 ).
Another factor shaping social learning is motivation, which may also explain the differences in performance observed here. Although empathy is an effortful process that people sometimes tend to avoid ( 63 , 64 ), it may still be affected by stronger motivational factors, e.g., approach motives ( 65 ), than the demand to find a monetary reward. Perhaps the evaluative feedback, e.g., the emotional response in the form of a smiling or sad face, is considered more valuable than a reward in the form of money in a closet ( 34 ). Our results indicate that those high in emotional empathy displayed lower performance in the abstract value-based condition, but when their learning was associated with people, their performance level remained intact. If we consider a target person's emotional responses as motivating learning, high emotional empathy may be more affected by the target's emotional responses. That is, the participant may be more affected by sad/happy facial expressions and more driven to learn the most effective strategy. Another motivation to learn in a social context may be the desire to maintain a social connection ( 66 ). According to the “Need-to-belong” theory ( 67 ), the motivation to form social relationships shapes cognition and behavior and may be an essential factor when operating in a social interaction context rather than in an abstract one. Studies show that people are willing to pay more to reduce the pain of others than to reduce their own pain ( 68 ).
Another possible explanation for differences in accuracy between social and non-social conditions, and for the negative correlation between non-social performance and emotional empathy levels, may be rooted in empathizing-systemizing theory ( 69 ). According to this theory, strong empathizers are good at understanding the social world. These individuals show an advantage in emotion recognition and social sensitivity, while strong systemizers are detail-oriented, good at understanding how things work and excel at solving technical problems. Previous research on social information processing by empathizers and systemizers revealed that empathizers, in contrast to systemizers, had stronger activation in brain areas related to emotional empathy during emotional empathy tasks ( 70 ). Our findings offer additional support for the fact that highly empathic individuals exhibit poor performance when it comes to problems in the physical world.
Potential Limitations
The current study was designed to examine adaptive empathy empirically by means of a novel experimental task that allows comparison of the empathic learning process to other, well-established learning paradigms. As such, it uses a computerized task that is somewhat distanced from real-life social interactions. In such context, emotional empathy traits effect on adaptive empathy may be limited. It may therefore be that when using a face-to-face paradigm, where social cues and empathic responses such as touch, tone of voice, and facial expressions are available, emotional empathy may have a greater influence on adaptive empathy. Another limitation has to do with the non-social condition used here. This condition was designed to be similar to learning paradigms in the non-social literature. It differed both in its abstract action-outcome association of money in closets compared with the more concrete social conditions (food leads to satisfaction, emotion regulation leads to distress relief) and in the cognitive demands of recognizing the different strategies. The adaptive empathy condition involves a demanding request to detect different empathic response strategies presented in text, and the food choices were menu items presented in text, and the underlying strategy (distraction/reappraisal or savory/sweet) had to be inferred. However, in line with previous studies, in the non-social condition, the participant had to choose between two closets, which were identical in each trial. The finding that accuracy was lower in the non-social condition may therefore stem from participants finding the social conditions more engaging. While we address the effect of motivation in the social conditions, highlighting the negative correlation of emotional empathy and accuracy in the non-social condition, and we use additional social-control condition, future studies should aspire to use more engaging non-social control conditions. Future studies may adapt our current task to track specific aspects of adaptive empathy, such as differentiating the roles of expectations and motivation in adaptive empathy and studying it in different contexts.
This research provides a new approach to viewing empathy as a dynamic, feedback-based process. Taking the dynamic dimension of empathy into account can enhance our understanding of the empathy construct, for example by examining the relationship between adaptive empathy and other prosocial and empathic skills, such as prosocial learning and prosocial tendencies. Our work indicates that adaptive empathy is indeed comparable to other learning processes, and therefore future studies may draw on the vast body of findings, paradigms, and models used in learning research to better characterize this process. In addition, adaptive empathy was linked with trait empathy measures. Such a link may be useful in examining how the social deficits present in different psychopathologies are related to aspects of the adaptive process, for example, due to malfunctioning in emotional identification or mentalization.
Data Availability Statement
The datasets presented in this study can be found in online repositories. The names of the repository/repositories and accession number(s) can be found below: the Open Science Framework ( https://osf.io/dgt5e/ ).
Ethics Statement
The studies involving human participants were reviewed and approved by The University of Haifa, Faculty of Social Sciences Research Ethics Committee. The participants provided their written informed consent to participate in this study.
Author Contributions
EK, SS-T, and UH: conceptualization, writing–review, and editing. EK: analysis, methodology, software, and writing–original draft. SS-T and UH: supervision. EK and UH: visualization. All authors contributed to the article and approved the submitted version.
UH was supported by the National Institute of Psychobiology in Israel (211-19-20) and by the Israel Science Foundation (1532/20).
Conflict of Interest
The authors declare that the research was conducted in the absence of any commercial or financial relationships that could be construed as a potential conflict of interest.
Supplementary Material
The Supplementary Material for this article can be found online at: https://www.frontiersin.org/articles/10.3389/fpsyt.2021.706474/full#supplementary-material
1. ^ https://en.wikipedia.org/wiki/Category:Restaurants_by_type
1. Zaki J, Williams WC. Interpersonal emotion regulation. Emotion . (2013) 13:803–10. doi: 10.1037/a0033839
CrossRef Full Text | Google Scholar
2. Morelli SA, Lieberman MD, Zaki J. The emerging study of positive empathy. Soc Personal Psychol Compass . (2015) 9:57–68. doi: 10.1111/spc3.12157
3. Atzil S, Gao W, Fradkin I, Barrett LF. Growing a social brain. Nat Hum Behav . (2018) 2:624–36. doi: 10.1038/s41562-018-0384-6
4. Gonzalez-Liencres C, Shamay-Tsoory SG, Brüne M. Towards a neuroscience of empathy: ontogeny, phylogeny, brain mechanisms, context and psychopathology. Neurosci Biobehav Rev . (2013) 37:1537–48. doi: 10.1016/j.neubiorev.2013.05.001
PubMed Abstract | CrossRef Full Text | Google Scholar
5. Eisenberg N, Eggum ND, Di Giunta L. Empathy-related responding: associations with prosocial behavior, aggression, and intergroup relations. Soc Issues Policy Rev . (2010) 4:143–80. doi: 10.1111/j.1751-2409.2010.01020.x
6. Hertz U, Shamay-Tsoory S. Adaptive empathy: a model for learning empathic responses based on feedback. PsyArXiv . (2021) [Preprint]. doi: 10.31234/osf.io/juc87
7. Thomas G, Fletcher GJO, Lange C. On-line empathic accuracy in marital interaction. J Pers Soc Psychol . (1997) 72:839–50. doi: 10.1037/0022-3514.72.4.839
8. Stinson L, Ickes W. Empathic accuracy in the interactions of male friends versus male strangers. J Pers Soc Psychol . (1992) 62:787–97. doi: 10.1037/0022-3514.62.5.787
9. Main A, Walle EA, Kho C, Halpern J. The interpersonal functions of empathy: a relational perspective. Emot Rev . (2017) 9:358–66. doi: 10.1177/1754073916669440
10. Eres R, Decety J, Louis WR, Molenberghs P. Individual differences in local gray matter density are associated with differences in affective and cognitive empathy. Neuroimage . (2015) 117:305–310. doi: 10.1016/j.neuroimage.2015.05.038
11. Shamay-Tsoory SG. The neural bases for empathy. Neurosci . (2011) 17:18–24. doi: 10.1177/1073858410379268
12. Zaki J, Ochsner K. The neuroscience of empathy: progress, pitfalls and promise. Nat Neurosci . (2012) 15:675–80. doi: 10.1038/nn.3085
13. Hatfield E, Cacioppo JT, Rapson RL. Emotional Contagion Cambridge University Press (1993). doi: 10.1017/CBO9781139174138
14. Bernhardt BC, Singer T. The neural basis of empathy. Annu Rev Neurosci . (2012) 35:1–23. doi: 10.1146/annurev-neuro-062111-150536
15. Jospe K, Flöe A, Lavidor M. The interaction between embodiment and empathy in facial expression recognition. Soc Cogn Affect Neurosci . (2018) 13:203–15. doi: 10.1093/scan/nsy005
16. Tamir DI, Thornton MA. Modeling the predictive social mind. Trends Cogn Sci . (2018) 22:201–12. doi: 10.1016/j.tics.2017.12.005
17. Frith U, Frith CD. Development and neurophysiology of mentalizing. Philos Trans R Soc London Ser B Biol Sci . (2003) 358:459–73. doi: 10.1098/rstb.2002.1218
18. Premack D, Woodruff G. Does the chimpanzee have a theory of mind? Behav Brain Sci . (1978) 1:515–26. doi: 10.1017/S0140525X00076512
19. Frith CD, Frith U. The neural basis of mentalizing. Neuron . (2006) 50:531–4. doi: 10.1016/j.neuron.2006.05.001
20. Heyes CM, Frith CD. The cultural evolution of mind reading. Science . (2014) 344:1243091. doi: 10.1126/science.1243091
21. Decety J, Jackson PL. The functional architecture of human empathy. Behav Cogn Neurosci Rev . (2004) 3:71–100. doi: 10.1177/1534582304267187
22. Shamay-Tsoory SG, Tomer R, Goldsher D, Berger BD, Aharon-Peretz J. Impairment in cognitive and affective empathy in patients with brain lesions: anatomical and cognitive correlates. J Clin Exp Neuropsychol . (2004) 26:1113–27. doi: 10.1080/13803390490515531
23. Perry D, Hendler T, Shamay-Tsoory SG. Projecting memories: the role of the hippocampus in emotional mentalizing. Neuroimage . (2011) 54:1669–76. doi: 10.1016/j.neuroimage.2010.08.057
24. Shamay-Tsoory SG, Aharon-Peretz J, Perry D. Two systems for empathy: a double dissociation between emotional and cognitive empathy in inferior frontal gyrus versus ventromedial prefrontal lesions. Brain . (2009) 132:617–27. doi: 10.1093/brain/awn279
25. Englis BG, Vaughan KB, Lanzetta JT. Conditioning of counter-empathetic emotional responses. J Exp Soc Psychol . (1982) 18:375–91. doi: 10.1016/0022-1031(82)90060-9
26. Zaki J. Empathy: a motivated account. Psychol Bull . (2014) 140:1608–47. doi: 10.1037/a0037679
27. Goldstein P, Weissman-Fogel I, Dumas G, Shamay-Tsoory SG. Brain-to-brain coupling during handholding is associated with pain reduction. Proc Natl Acad Sci . (2018) 115:E2528–37. doi: 10.1073/pnas.1703643115
28. Barrett-Lennard GT. The empathy cycle: refinement of a nuclear concept. J Couns Psychol . (1981) 28:91–100. doi: 10.1037/0022-0167.28.2.91
29. Lockwood PL, Klein-Flügge MC. Computational modelling of social cognition and behaviour-a reinforcement learning primer. Soc Cogn Affect Neurosci . (2020) nsaa040. doi: 10.1093/scan/nsaa040
30. Zhang L, Lengersdorff L, Mikus N, Gläscher J, Lamm C. Using reinforcement learning models in social neuroscience: frameworks, pitfalls and suggestions of best practices. Soc Cogn Affect Neurosci . (2020) 15:695–707. doi: 10.1093/scan/nsaa089
31. Farmer H, Hertz U, Hamilton AFC. The neural basis of shared preference learning. Soc Cogn Affect Neurosci . (2019) 14:1061–1072. doi: 10.1093/scan/nsz076
32. Hackel LM, Mende-Siedlecki P, Amodio DM. Reinforcement learning in social interaction: the distinguishing role of trait inference. J Exp Soc Psychol . (2020) 88:103948. doi: 10.1016/j.jesp.2019.103948
33. Suzuki S, Adachi R, Dunne S, Bossaerts P, O'Doherty JP. Neural mechanisms underlying human consensus decision-making. Neuron. (2015) 86:591–602. doi: 10.1016/j.neuron.2015.03.019
34. Ho MK, MacGlashan J, Littman ML, Cushman F. Social is special: a normative framework for teaching with and learning from evaluative feedback. Cognition . (2017) 167:91–106. doi: 10.1016/j.cognition.2017.03.006
35. Lockwood PL. The anatomy of empathy: vicarious experience and disorders of social cognition. Behav Brain Res . (2016) 311:255–66. doi: 10.1016/j.bbr.2016.05.048
36. Lockwood PL, Seara-Cardoso A, Viding E. Emotion regulation moderates the association between empathy and prosocial behavior. PLoS ONE . (2014) 9:e96555. doi: 10.1371/journal.pone.0096555
37. Levy-Gigi E, Shamay-Tsoory SG. Help me if you can: evaluating the effectiveness of interpersonal compared to intrapersonal emotion regulation in reducing distress. J Behav Ther Exp Psychiatry . (2017) 55:33–40. doi: 10.1016/j.jbtep.2016.11.008
38. Hertz U, Bahrami B, Keramati M. Stochastic satisficing account of confidence in uncertain value-based decisions. PLoS ONE . (2018) 13:1–23. doi: 10.1371/journal.pone.0195399
39. Niv Y. Learning task-state representations. Nat Neurosci . (2019) 22:1544–53. doi: 10.1038/s41593-019-0470-8
40. Behrens TEJ, Woolrich MW, Walton ME, Rushworth MFS. Learning the value of information in an uncertain world. Nat Neurosci . (2007) 10:1214–21. doi: 10.1038/nn1954
41. Daw ND, O'Doherty JP, Dayan P, Seymour B, Dolan RJ. Cortical substrates for exploratory decisions in humans. Nature . (2006) 441:876–9. doi: 10.1038/nature04766
42. Ebner NC, Riediger M, Lindenberger U. FACES-a database of facial expressions in young, middle-aged, and older women and men: development and validation. Behav Res Methods . (2010) 42:351–62. doi: 10.3758/BRM.42.1.351
43. Sheppes G, Scheibe S, Suri G, Gross JJ. Emotion-regulation choice. Psychol Sci. (2011) 22:1391–6. doi: 10.1177/0956797611418350
44. Marroquín B. Interpersonal emotion regulation as a mechanism of social support in depression. Clin Psychol Rev . (2011) 31:1276–90. doi: 10.1016/j.cpr.2011.09.005
45. Gross JJ, John OP. Individual differences in two emotion regulation processes: implications for affect, relationships, and well-being. J Pers Soc Psychol . (2003) 85:348–62. doi: 10.1037/0022-3514.85.2.348
46. Webb TL, Miles E, Sheeran P. Dealing with feeling: a meta-analysis of the effectiveness of strategies derived from the process model of emotion regulation. Psychol Bull . (2012) 138:775–808. doi: 10.1037/a0027600
47. Reniers RLEP, Corcoran R, Drake R, Shryane NM, Völlm BA. The QCAE: a questionnaire of cognitive and affective empathy. J Pers Assess . (2011) 93:84–95. doi: 10.1080/00223891.2010.528484
48. R Core Team. R: A Language and Environment for Statistical Computing . (2020). Available online at: https://www.r-project.org/ (accessed July 6, 2021).
Google Scholar
49. Kassambara A. rstatix: Pipe-Friendly Framework for Basic Statistical Tests (2020). Available online at: https://cran.r-project.org/package=jtools (accessed July 6, 2021).
50. Singmann H, Bolker B, Westfall J, Aust F, Ben-Shachar MS. afex: Analysis of Factorial Experiments (2021). Available online at: https://cran.r-project.org/package=afex (accessed July 6, 2021).
51. Long JA. jtools: Analysis and Presentation of Social Scientific Data . (2020). Available online at: https://cran.r-project.org/package=jtools (accessed July 6, 2021).
52. Lockwood PL, Apps MAJ, Chang SWC. Is there a ‘Social' brain? implementations and algorithms. Trends Cogn Sci . (2020) 24:802–13. doi: 10.1016/j.tics.2020.06.011
53. Heyes C. What's social about social learning? J Comp Psychol . (2012) 126:193–202. doi: 10.1037/a0025180
54. Saxe R, Kanwisher N. People thinking about thinking people: the role of the temporo-parietal junction in “theory of mind.” Neuroimage . (2003) 19:1835–42. doi: 10.1016/S1053-8119(03)00230-1
55. Siegel JZ, Mathys C, Rutledge RB, Crockett MJ. Beliefs about bad people are volatile. Nat Hum Behav . (2018) 2:750–6. doi: 10.1038/s41562-018-0425-1
56. Koster-Hale J, Saxe R. Theory of mind: a neural prediction problem. Neuron . (2013) 79:836–48. doi: 10.1016/j.neuron.2013.08.020
57. Davis MH. Measuring individual differences in empathy: evidence for a multidimensional approach. J Pers Soc Psychol . (1983) 44:113–26. doi: 10.1037/0022-3514.44.1.113
58. Heym N, Firth J, Kibowski F, Sumich A, Egan V, Bloxsom CAJ. Empathy at the heart of darkness: empathy deficits that bind the dark triad and those that mediate indirect relational aggression. Front Psychiatry . (2019) 10:95. doi: 10.3389/fpsyt.2019.00095
59. Lockwood PL, Apps MAJ, Valton V, Viding E, Roiser JP. Neurocomputational mechanisms of prosocial learning and links to empathy. Proc Natl Acad Sci . (2016) 113:9763–8. doi: 10.1073/pnas.1603198113
60. Gross JJ. Emotion regulation in adulthood: timing is everything. Curr Dir Psychol Sci . (2001) 10:214–9. doi: 10.1111/1467-8721.00152
61. John OP, Gross JJ. Healthy and unhealthy emotion regulation: personality processes, individual differences, and life span development. J Pers . (2004) 72:1301–34. doi: 10.1111/j.1467-6494.2004.00298.x
62. Nezlek JB, Kuppens P. Regulating positive and negative emotions in daily life. J Pers . (2008) 76:561–80. doi: 10.1111/j.1467-6494.2008.00496.x
63. Lockwood PL, Hamonet M, Zhang SH, Ratnavel A, Salmony FU, Husain M, et al. Prosocial apathy for helping others when effort is required. Nat Hum Behav . (2017) 1:1–10. doi: 10.1038/s41562-017-0131
64. Contreras-Huerta LS, Pisauro MA, Apps MAJ. Effort shapes social cognition and behaviour: a neuro-cognitive framework. Neurosci Biobehav Rev . (2020) 118:426–39. doi: 10.1016/j.neubiorev.2020.08.003
65. Weisz E, Zaki J. Motivated empathy: a social neuroscience perspective. Curr Opin Psychol . (2018) 24:67–71. doi: 10.1016/j.copsyc.2018.05.005
66. Pickett CL, Gardner WL, Knowles M. Getting a cue: the need to belong and enhanced sensitivity to social cues. Personal Soc Psychol Bull . (2004) 30:1095–107. doi: 10.1177/0146167203262085
67. Baumeister RF. Need-to-belong theory. In: Handbook of Theories of Social Psychology . London: SAGE Publications Ltd (2012). p. 121–40.
68. Crockett MJ, Kurth-Nelson Z, Siegel JZ, Dayan P, Dolan RJ. Harm to others outweighs harm to self in moral decision making. Proc Natl Acad Sci. (2014) 111:17320–5. doi: 10.1073/pnas.1408988111
69. Baron-Cohen S. Autism: the empathizing-systemizing (E-S) theory. Ann N Y Acad Sci . (2009) 1156:68–80. doi: 10.1111/j.1749-6632.2009.04467.x
70. Riekki T, Svedholm-Häkkinen AM, Lindeman M. Empathizers and systemizers process social information differently. Soc Neurosci . (2018) 13:616–27. doi: 10.1080/17470919.2017.1368700
Keywords: empathy, cognitive empathy, online simulation, social cognition, learning, reward, decision-making
Citation: Kozakevich Arbel E, Shamay-Tsoory SG and Hertz U (2021) Adaptive Empathy: Empathic Response Selection as a Dynamic, Feedback-Based Learning Process. Front. Psychiatry 12:706474. doi: 10.3389/fpsyt.2021.706474
Received: 07 May 2021; Accepted: 29 June 2021; Published: 22 July 2021.
Reviewed by:
Copyright © 2021 Kozakevich Arbel, Shamay-Tsoory and Hertz. This is an open-access article distributed under the terms of the Creative Commons Attribution License (CC BY) . The use, distribution or reproduction in other forums is permitted, provided the original author(s) and the copyright owner(s) are credited and that the original publication in this journal is cited, in accordance with accepted academic practice. No use, distribution or reproduction is permitted which does not comply with these terms.
*Correspondence: Elena Kozakevich Arbel, elen.kozakevich@gmail.com
Disclaimer: All claims expressed in this article are solely those of the authors and do not necessarily represent those of their affiliated organizations, or those of the publisher, the editors and the reviewers. Any product that may be evaluated in this article or claim that may be made by its manufacturer is not guaranteed or endorsed by the publisher.
Thank you for visiting nature.com. You are using a browser version with limited support for CSS. To obtain the best experience, we recommend you use a more up to date browser (or turn off compatibility mode in Internet Explorer). In the meantime, to ensure continued support, we are displaying the site without styles and JavaScript.
- View all journals
Empathy articles from across Nature Portfolio
Empathy is a social process by which a person has an understanding and awareness of another's emotions and/or behaviour, and can often lead to a person experiencing the same emotions. It differs from sympathy, which involves concern for others without sharing the same emotions as them.
Latest Research and Reviews
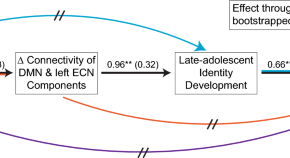
Diverse adolescents’ transcendent thinking predicts young adult psychosocial outcomes via brain network development
- Rebecca J. M. Gotlieb
- Xiao-Fei Yang
- Mary Helen Immordino-Yang
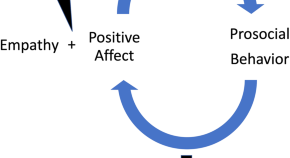
The effect of psilocybin on empathy and prosocial behavior: a proposed mechanism for enduring antidepressant effects
- Kush V. Bhatt
- Cory R. Weissman
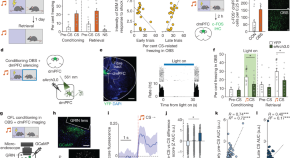
A distinct cortical code for socially learned threat
Studies in mice show that observational fear learning is encoded by neurons in the dorsomedial prefrontal cortex in a manner that is distinct from the encoding of fear learned by direct experience.
- Shana E. Silverstein
- Ruairi O’Sullivan
- Andrew Holmes
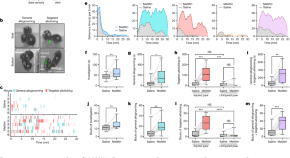
Cortical regulation of helping behaviour towards others in pain
A study describes the role of the anterior cingulate cortex in coding and regulating helping behaviour exhibited by mice towards others experiencing pain.
- Mingmin Zhang
- Ye Emily Wu
- Weizhe Hong
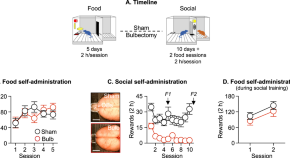
Social odor choice buffers drug craving
- Kimberly M. Papastrat
- Cody A. Lis
- Marco Venniro
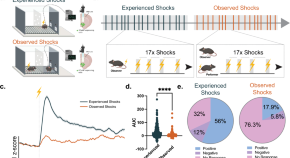
Nucleus accumbens core single cell ensembles bidirectionally respond to experienced versus observed aversive events
- Oyku Dinckol
- Noah Harris Wenger
- Munir Gunes Kutlu
News and Comment
Natural primate neurobiology.
A new study captures nearly the full repertoire of primate natural behaviour and reveals that highly distributed cortical activity maintains multifaceted dynamic social relationships.
- Jake Rogers
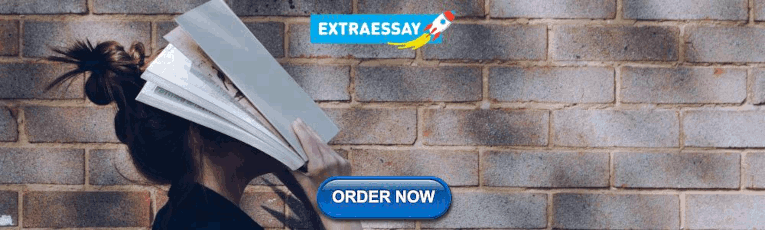
Your pain in my brain
- Helena Hartmann
Comforting in mice
- Sachin Ranade
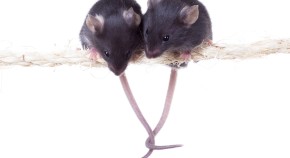
Feeling another’s pain
Projections from the anterior cingulate cortex to the nucleus accumbens are required for the social transfer of pain or analgesia in mice.
- Darran Yates
Taking action: empathy and social interaction in rats
- Sam A. Golden
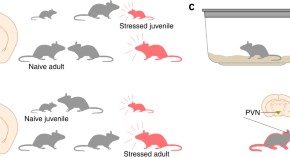
Stress and sociability
Humans and animals can react to the affective state of others in distress. However, exposure to a stressed partner can trigger stress-related adaptations. Two studies shed light on the mechanisms underlying the behavioral responses toward stressed individuals and on the synaptic changes associated with social transmission of stress.
- Dana Rubi Levy
- Ofer Yizhar
Quick links
- Explore articles by subject
- Guide to authors
- Editorial policies


‘I Feel Your Pain’: The Neuroscience of Empathy
- Developmental Psychology
- Forecasting
- Neuroscience
- Sensory Systems
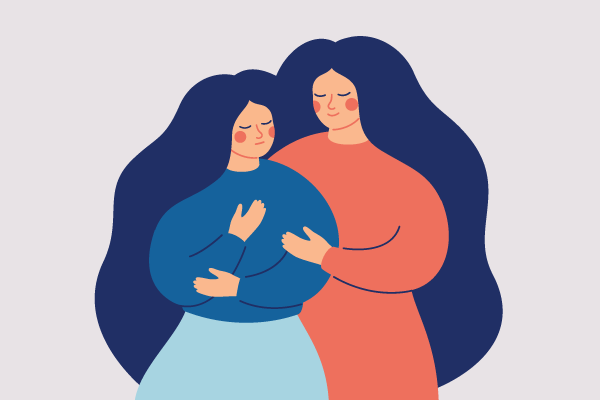
Whether it’s watching a friend get a paper cut or staring at a photo of a child refugee, observing someone else’s suffering can evoke a deep sense of distress and sadness — almost as if it’s happening to us. In the past, this might have been explained simply as empathy, the ability to experience the feelings of others, but over the last 20 years, neuroscientists have been able to pinpoint some of the specific regions of the brain responsible for this sense of interconnectedness. Five scientists discussed the neuroscience behind how we process the feelings of others during an Integrative Science Symposium chaired by APS Fellow Piotr Winkielman (University of California, San Diego) at the 2017 International Convention of Psychological Science in Vienna.
Mirroring the Mind
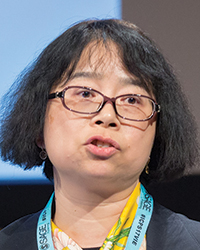
Cultural emphasis on ingroups and outgroups may create an “empathy gap” between people of different races and nationalities, says Ying-yi Hong .
“When we witness what happens to others, we don’t just activate the visual cortex like we thought some decades ago,” said Christian Keysers of the Netherlands Institute for Neuroscience in Amsterdam. “We also activate our own actions as if we’d be acting in similar ways. We activate our own emotions and sensations as if we felt the same.”
Through his work at the Social Brain Lab, Keysers, together with Valeria Gazzola, has found that observing another person’s action, pain, or affect can trigger parts of the same neural networks responsible for executing those actions and experiencing those feelings firsthand. Keysers’ presentation, however, focused on exploring how this system contributes to our psychology. Does this mirror system help us understand what goes on in others? Does it help us read their minds? Can we “catch” the emotions of others?
To explore whether the motor mirror system helps us understand the inner states behind the actions of others, Keysers in one study asked participants to watch a video of a person grasping toy balls hidden within a large bin. In one condition, participants determined whether or not the person in the video hesitated before selecting a ball (a theory-of-mind task). Using transcranial magnetic stimulation (TMS) in combination with fMRI, Keysers showed that interfering with the mirror system impaired people’s ability to detect the level of confidence of others, providing evidence that this system indeed contributes to perceiving the inner states of others. Performing fMRI and TMS on other brain regions such as the temporoparietal junction (TPJ) further suggests that this motor simulation in the mirror system is then sent onward to more cognitive regions in the TPJ.
“Very rapidly, we got this unifying notion that when you witness the states of others you replicate these states in yourself as if you were in their shoes, which is why we call these activities ‘vicarious states,’” Keysers said.
Studies have suggested that this ability to mentalize the experiences of others so vividly can lead us to take prosocial steps to reduce their pain, but Keysers also wanted to investigate the depth of this emotional contagion — how and to what extent we experience other people’s suffering. To do this, Keysers’ lab studied two very different populations: human psychopaths and rats.
While witnessing the pain of others is correlated with activity in the insula, which is thought to contribute to self-awareness by integrating sensory information, and the anterior cingulate cortex (ACC), which is associated with decision making and impulse control, the researchers found that psychopaths who passively observed an aggressor twisting someone’s hand exhibited significantly less brain activity than their neurotypical peers. When the psychopathic individuals were asked to attempt to empathize with the person in the video, however, their brain activity increased to baseline levels.
This suggests that the current model of empathy as a one-dimensional scale with empathic individuals at one end and psychopaths at the other may be overly simplistic, Keysers said.
“Psychopaths are probably equally high on ability, it’s just that they don’t recruit this spontaneously, so their propensity is modified,” he explained.
These findings could lead to more effective interventions for psychopathic individuals, as well as to future research into where people with autism spectrum disorders may fall on these axes.
Shared Pain
Studies of emotional contagion in animal models have allowed researchers to further examine the role of deep brain activity, which can be difficult to neurostimulate in humans. Keysers’ work with rats has found that these animals are more likely to freeze after watching another rat receive an electric shock if they themselves had been shocked in the past.
Inhibiting a region analogous to the ACC in the rats’ brains reduced their response to another rat’s distress, but not their fear of being shocked themselves, suggesting that the area deals specifically with socially triggered fear, Keysers said.
Claus Lamm, University of Vienna, investigates the processes that regulate firsthand pain and those that cause empathy for pain through numerous studies on the influence of painkillers.
In these experiments, participants who took a placebo “painkiller” reported lower pain ratings after receiving a shock than did those in the control group. When those same participants watched a confederate get shocked, they reported a similar drop in their perception of the actor’s pain.
“If you reduce people’s self-experienced pain, if you induce analgesia, that not only helps people to deal with their own pain, but it also reduces empathy for the pain of another person,” Lamm said.
On the neural level, Lamm said, fMRI scans showed that people in the placebo group displayed lower levels of brain activity in the anterior insula and mid cingulate cortex in both cases. These results were further confirmed in another study that compared participants who received only the painkiller placebo with those who received both the placebo and naltrexone, an opioid antagonist that prevents the brain from regulating pain.
This resulted in a “complete reversal” of the placebo effect, causing participants to report both their own pain and the pain of others at near baseline rates, supporting Lamm’s previous claims about the pain system’s role in empathy.
“This suggests that empathy for pain is grounded in representing others’ pain within one’s own pain systems,” Lamm said.
The Self/Other Divide
Empathy may not give us a full sense of someone else’s experiences, however. When observers in one of Keysers’ studies were given the opportunity to pay to reduce the severity of the electric shocks a confederate was about to receive, on average participants paid only enough to reduce her pain by 50%.
Lamm studied this self/other distinction through a series of experiments that measured people’s emotional egocentricity bias. To do so, participants were presented with visuo-tactile stimulation that was either congruent or incongruent with that of a partner under fMRI. In an incongruent pair, for example, one participant might be presented with an image of a rose and be touched with something that felt like a rose, while the other was shown a slug and touched with a slimy substance.
Participants’ own emotions were found to color their perception of other people’s affect at a relatively low rate — however, when researchers inhibited the right supramarginal gyrus (rSMG), a region of the brain previous associated mainly with language processing, this egocentricity bias increased, suggesting that the rSMG may be responsible for maintaining a self/other divide, Lamm said.
“Empathy not only requires a mechanism for sharing emotions, but also for keeping them separate. Otherwise we are getting ‘contaged,’ emotionally distressed and so on,” he said.
The rate of rSMG activation also changes significantly across a lifetime, Lamm added, with the area’s developmental trajectory causing emotional egocentricity to be more common in adolescents and the elderly.
Developing Division
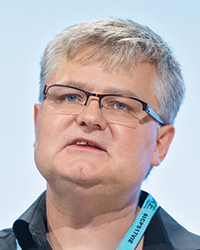
Researchers are working to unite neuroscientific and psychological perspectives on feelings, empathy, and identity, says Piotr Winkielman .
Rebecca Saxe (Massachusetts Institute of Technology) said her work with developmental psychology confirms this trend. In one series of experiments, Saxe monitored the brain networks that 3- to 5-year-old children used to consider a character’s mind (the temporoparietal junction, posterior cingulate, and prefrontal cortex) and body (the secondary somatosensory cortex, insula, middle frontal gyrus, and ACC) throughout a short film.
Saxe found that while these brain regions may interact with each other, there were no points of overlap between the mind and body networks’ activities.
“When we’re getting information from the same source and about the same people, we still nevertheless impose a kind of dualism where we alternate between considering what their bodies feel like and the causes of their minds,” Saxe said.
Furthermore, Saxe and her colleagues found that while these networks were more distinct in children who were able to pass an explicit-false-belief task (e.g., if Sally puts her sandwich on a shelf and her friend moves it to the desk, where will she look for it?), the division was present in participants of all ages.
“Most people have treated explicit false belief as if it were the milestone,” Saxe said. “Actually, the false-belief task is just one measure of a much more continuous developmental change as children become increasingly sophisticated in their thinking about other people’s minds.”
Next, Saxe scaled this experiment down to test the theory of mind of infants as young as 6 months, this time measuring their response to children’s facial expressions, outdoor scenes, and visual static. This time period may be key to understanding the neuropsychology of empathy because most of the brain’s cognitive development happens within the first year of life, she explained.
“A baby’s brain is more different from a 3-year-old’s brain than a 3-year-old’s brain is from a 33-year-old’s brain,” Saxe said.
Under fMRI, the infants’ brains were found to have many of the same regional responses that allow adults to distinguish between faces and scenes. Their brains didn’t show any regional preferences for objects and bodies, however.
This level of regional specificity suggests that the Kennard Principle, the theory that infants’ brains possess such resilience and plasticity because the cortex hasn’t specialized yet, may be only partially true. There does appear to be some functional organization of social process, Saxe said, with gradually increasing specialization as the child ages.
Empathy in Action
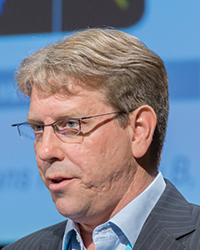
Brian D. Knutson says analysis of individuals’ brain activity when considering a purchase may be predictive of aggregate market choices.
On the surface, neuroforecasting sounds like a concept that would be right at home in the world of Philip K. Dick’s Minority Report — a science fiction thriller about a society that stops crime before it happens based on the brainwaves of three mutant “precogs” — said APS Fellow Brian D. Knutson (Stanford University), but someday it could play a very real role in the future of economics.
Knutson’s research on the brain mechanisms that influence choice homes in on three functional targets: the nucleus accumbens (NAcc) for gain anticipation, the anterior insula for loss anticipation, and the medial prefrontal cortex (mPFC) for value integration.
Using fMRI, Knutson was able to predict participants’ purchases in a simulated online shopping environment on the basis of brain activations in these areas. Before participants chose to buy a product, increased activity in the NAcc and mPFC was paired with a decrease in the insula, while the reverse was true of trials in which participants chose not to make a purchase.
“This was very exciting to me as a psychologist to be able to say, ‘Wow, we can take activity out of the brain and, not knowing anything else about who it is and what product they’re seeing, we can predict choice,’” Knutson said.
His economist colleagues weren’t as impressed: They were interested in market activity, not individual choice. Knutson said he accepted this challenge by applying his neuroanaylsis to large-scale online markets such as Kiva and Kickstarter.
Knutson asked 30 participants to rate the appeal and neediness of loan requests on Kiva and found that posts with photos of people displaying a positive affect were most likely to trigger the increased NAcc activity that caused them to make a purchase — or in this case, a loan. More importantly, the averaged choices of those participants forecasted the loan appeal’s success on the internet. Two similar studies involving Kickstarter campaigns also suggested a link between NAcc activity and aggregate market activity.
While brain activity doesn’t scale perfectly to aggregate choice, Knutson said, some components of decision making, such as affective responses, may be more generalizable than others.
“The paradox may be that the things that make you most consistent as an individual, that best predict your choices, may not be the things that make your choices conform to those of others. We may be able to deconstruct and decouple those components in the brain,” Knutson said.
Global Empathy
The neuroanatomy of our brains may allow us to feel empathy for another’s experiences, but it can also stop us from making cross-cultural connections, said APS Fellow Ying-yi Hong (Chinese University of Hong Kong).
“Despite all these neurobiological capabilities enabling us to empathize with others, we still see cases in which individuals chose to harm others, for example during intergroup conflicts or wars,” Hong said.
This may be due in part to the brain’s distinction between in-group and out-group members, she explained. People have been found to show greater activation in the amygdala when viewing fearful faces of their own race, for example, and less activation in the ACC when watching a needle prick the face of someone of a different race.
The cultural mixing that accompanies globalization can heighten these responses, Hong added. In one study, she and her colleagues found that melding cultural symbols (e.g., combining the American and Chinese flags, putting Chairman Mao’s head on the Lincoln Memorial, or even presenting images of “fusion” foods) can elicit a pattern of disgust in the anterior insula of White Americans similar to that elicited by physical contaminant objects such as insects.
These responses can also be modulated by cultural practices, Hong said. One study comparing the in-group/out-group bias in Korea, a more collectivist society, and the United States, a more individualistic society, found that more interdependent societies may foster a greater sense of in-group favoritism in the brain.
Further research into this empathy gap should consider not just the causal relationship between neural activation and behavior, she said, but the societal context in which they take place.
“What I want to propose,” Hong said, “is that maybe there is another area that we can also think about, which is the culture, the shared lay theories, values, and norms.”
There is some fantastic research going on in empathy. From an evolutionary point of view however it’s important to distinguish an evolved motivation system from a competency. Empathy is a competency not a motivation. Empathy can be used for both benevolent but also malevolent motives. And psychopaths have a competency for empathy but what they lack is mammalian caring motivation. Insofar as part of the reproductive strategy of the psychopath is to exploit others and even threaten them then having a brain that turns off distress to the suffering they cause would be an advantage to them. Psychopaths are much more likely to be prepared to harm others to get what they want. Mammalian caring motivation, when guided by higher cognitive processes and human empathy gives rise to compassion. Without empathy compassion would be tricky but without compassion you can still have empathic competencies
Gilbert, P. (2017). Compassion as a social mentality: An evolutionary approach. In: P. Gilbert (ed). Compassion: Concepts, Research and Applications. (p. 31-68). London: Routledge
Gilbert. P. (2015). The evolution and social dynamics of compassion Journal of Social & Personality Psychology Compass, 9, 239–254. DOI: 10.1111/spc3.12176
Catarino, F., Gilbert, P., McEwan., K & Baião, R. (2014). Compassion motivations: Distinguishing submissive compassion from genuine compassion and its association with shame, submissive behaviour, depression, anxiety and stress Journal of Social and Clinical Psychology, 33, 399-412.
Gilbert, P., Catarino, F., Sousa, J., Ceresatto, L., Moore, R., & Basran, J. (2017). Measuring competitive self-focus perspective taking, submissive compassion and compassion goals. Journal of Compassionate Health Care, 4(1), 5
Very interesting article. The research behind what links our empathy to our actions determining the agenda is fascinating. As social creatures, we seem to inhibit empathetic tendencies naturally in our genetic makeup when studied. Since we have the highest empathetic behavior compared to other animals, who also show empathetic behavior, I wonder if it falls more on our social norms. What we consider relatable is worthy of our empathy. If we don’t relate, we may be less inclined to put ourselves in the other position.
I have what I call empathy pain. It radiates an aching pain in my legs and I can barely stand it. I’ve googled it in attempts to validate it is real. It seems people either do not believe me or can’t understand stand when I tell them it makes my legs ache. Seeing someone’s cuts, surgical incisions, bloody wounds. I can’t describe all the triggers, but I can 100% say the pain I feel in response is intense, even when they say “oh, it didn’t hurt” or “it’s not hurting”. Well, it hurt ME seeing it.
I am currently writing a literature review for my psychology course in University, based on what I am writing about I believe you may have Mirror Touch Synesthesia. This condition is characterized by viewing others being touched and feeling tactile sensations, and this seems quite similar to what you shared. I would recommend doing a bit of research on MTS, and see if it relates to you.
Since I was 7 years old I felt others pain Then I thought everyone could . I came to realize I feel so much more than most . I feel what I see, I feel what I hear. My sensitive to touch is more like pain but my pain level is very high, I can take a lot of pain.
What about feeling pain or illness without observing it or even having knowledge of someone else’s pain? Such as the phenomenon of twins. I’m looking for research of this outside of the twin sibling relationship.
When carrying out functional mapping of the amygdala cortex by means of electrical stimulation in one of my patients with focal epileptic seizures who was being evaluated for resective epilepsy surgery of the orbitofrontal, opercular, and anterior insular cortex the stimulation caused the patient to reminisce over video films he had seen of cartoons (animaniacs) as a child, at the same time empathizing with the suffering of those characters. I had probably activated a limbic pathway connected to the limen insulae where I was administering electrical stimulation at that time. The visual imagery stopped as soon as the stimulus train was over but the patient still empathized with the cartoon characters for about 20 seconds after the stimulation was over and reported his feelings to me.
Wow… I thought I was alone in the way I feel everyone’s pain and joy. I find that I can not watch scenes of torture or violence on tv, thus I hate most movies, unless it’s a children flick. I get pulled into every story I read. On 911 I thought my heart really was breaking, it consumed my entire body. I can’t watch history shows of Pearl Harbor, or nazis. If I do, sometimes those images stay with me for years and come back as nightmares. It’s not easy living with this in today’s world.
APS regularly opens certain online articles for discussion on our website. Effective February 2021, you must be a logged-in APS member to post comments. By posting a comment, you agree to our Community Guidelines and the display of your profile information, including your name and affiliation. Any opinions, findings, conclusions, or recommendations present in article comments are those of the writers and do not necessarily reflect the views of APS or the article’s author. For more information, please see our Community Guidelines .
Please login with your APS account to comment.

Scientists Discuss How to Study the Psychology of Collectives, Not Just Individuals
In a set of articles appearing in Perspectives on Psychological Science, an international array of scientists discusses how the study of neighborhoods, work units, activist groups, and other collectives can help us better understand and respond to societal changes.

Artificial Intelligence: Your Thoughts and Concerns
APS members weigh in on the biggest opportunities and/or ethical challenges involving AI within the field of psychological science. Will we witness vast and constructive cross-fertilization—or “a dystopian cyberpunk corporation-led hellscape”?

Hearing is Believing: Sounds Can Alter Our Visual Perception
Audio cues can not only help us to recognize objects more quickly but can even alter our visual perception. That is, pair birdsong with a bird and we see a bird—but replace that birdsong with a squirrel’s chatter, and we’re not quite so sure what we’re looking at.
Privacy Overview
Search form (GSE) 1
The psychology of emotional and cognitive empathy.
The study of empathy is an ongoing area of major interest for psychologists and neuroscientists in many fields, with new research appearing regularly.
Empathy is a broad concept that refers to the cognitive and emotional reactions of an individual to the observed experiences of another. Having empathy increases the likelihood of helping others and showing compassion. “Empathy is a building block of morality—for people to follow the Golden Rule, it helps if they can put themselves in someone else’s shoes,” according to the Greater Good Science Center , a research institute that studies the psychology, sociology, and neuroscience of well-being. “It is also a key ingredient of successful relationships because it helps us understand the perspectives, needs, and intentions of others.”
Though they may seem similar, there is a clear distinction between empathy and sympathy. According to Hodges and Myers in the Encyclopedia of Social Psychology , “Empathy is often defined as understanding another person’s experience by imagining oneself in that other person’s situation: One understands the other person’s experience as if it were being experienced by the self, but without the self actually experiencing it. A distinction is maintained between self and other. Sympathy, in contrast, involves the experience of being moved by, or responding in tune with, another person.”
Emotional and Cognitive Empathy
Researchers distinguish between two types of empathy. Especially in social psychology, empathy can be categorized as an emotional or cognitive response. Emotional empathy consists of three separate components, Hodges and Myers say. “The first is feeling the same emotion as another person … The second component, personal distress, refers to one’s own feelings of distress in response to perceiving another’s plight … The third emotional component, feeling compassion for another person, is the one most frequently associated with the study of empathy in psychology,” they explain.
It is important to note that feelings of distress associated with emotional empathy don’t necessarily mirror the emotions of the other person. Hodges and Myers note that, while empathetic people feel distress when someone falls, they aren’t in the same physical pain. This type of empathy is especially relevant when it comes to discussions of compassionate human behavior. There is a positive correlation between feeling empathic concern and being willing to help others. “Many of the most noble examples of human behavior, including aiding strangers and stigmatized people, are thought to have empathic roots,” according to Hodges and Myers. Debate remains concerning whether the impulse to help is based in altruism or self-interest.
The second type of empathy is cognitive empathy. This refers to how well an individual can perceive and understand the emotions of another. Cognitive empathy, also known as empathic accuracy, involves “having more complete and accurate knowledge about the contents of another person’s mind, including how the person feels,” Hodges and Myers say. Cognitive empathy is more like a skill: Humans learn to recognize and understand others’ emotional state as a way to process emotions and behavior. While it’s not clear exactly how humans experience empathy, there is a growing body of research on the topic.
How Do We Empathize?
Experts in the field of social neuroscience have developed two theories in an attempt to gain a better understanding of empathy. The first, Simulation Theory, “proposes that empathy is possible because when we see another person experiencing an emotion, we ‘simulate’ or represent that same emotion in ourselves so we can know firsthand what it feels like,” according to Psychology Today .
There is a biological component to this theory as well. Scientists have discovered preliminary evidence of “mirror neurons” that fire when humans observe and experience emotion. There are also “parts of the brain in the medial prefrontal cortex (responsible for higher-level kinds of thought) that show overlap of activation for both self-focused and other-focused thoughts and judgments,” the same article explains.
Some experts believe the other scientific explanation of empathy is in complete opposition to Simulation Theory. It’s Theory of Mind, the ability to “understand what another person is thinking and feeling based on rules for how one should think or feel,” Psychology Today says. This theory suggests that humans can use cognitive thought processes to explain the mental state of others. By developing theories about human behavior, individuals can predict or explain others’ actions, according to this theory.
While there is no clear consensus, it’s likely that empathy involves multiple processes that incorporate both automatic, emotional responses and learned conceptual reasoning. Depending on context and situation, one or both empathetic responses may be triggered.
Cultivating Empathy
Empathy seems to arise over time as part of human development, and it also has roots in evolution. In fact, “Elementary forms of empathy have been observed in our primate relatives, in dogs, and even in rats,” the Greater Good Science Center says. From a developmental perspective, humans begin exhibiting signs of empathy in social interactions during the second and third years of life. According to Jean Decety’s article “The Neurodevelopment of Empathy in Humans ,” “There is compelling evidence that prosocial behaviors such as altruistic helping emerge early in childhood. Infants as young as 12 months of age begin to comfort victims of distress, and 14- to 18-month-old children display spontaneous, unrewarded helping behaviors.”
While both environmental and genetic influences shape a person’s ability to empathize, we tend to have the same level of empathy throughout our lives, with no age-related decline. According to “Empathy Across the Adult Lifespan: Longitudinal and Experience-Sampling Findings,” “Independent of age, empathy was associated with a positive well-being and interaction profile .”
And it’s true that we likely feel empathy due to evolutionary advantage : “Empathy probably evolved in the context of the parental care that characterizes all mammals. Signaling their state through smiling and crying, human infants urge their caregiver to take action … females who responded to their offspring’s needs out-reproduced those who were cold and distant,” according to the Greater Good Science Center. This may explain gender differences in human empathy.
This suggests we have a natural predisposition to developing empathy. However, social and cultural factors strongly influence where, how, and to whom it is expressed. Empathy is something we develop over time and in relationship to our social environment, finally becoming “such a complex response that it is hard to recognize its origin in simpler responses, such as body mimicry and emotional contagion,” the same source says.
Psychology and Empathy
In the field of psychology, empathy is a central concept. From a mental health perspective, those who have high levels of empathy are more likely to function well in society, reporting “larger social circles and more satisfying relationships,” according to Good Therapy , an online association of mental health professionals. Empathy is vital in building successful interpersonal relationships of all types, in the family unit, workplace, and beyond. Lack of empathy, therefore, is one indication of conditions like antisocial personality disorder and narcissistic personality disorder. In addition, for mental health professionals such as therapists, having empathy for clients is an important part of successful treatment. “Therapists who are highly empathetic can help people in treatment face past experiences and obtain a greater understanding of both the experience and feelings surrounding it,” Good Therapy explains.
Exploring Empathy
Empathy plays a crucial role in human, social, and psychological interaction during all stages of life. Consequently, the study of empathy is an ongoing area of major interest for psychologists and neuroscientists in many fields, with new research appearing regularly. Lesley University’s online bachelor’s degree in Psychology gives students the opportunity to study the field of human interaction within the broader spectrum of psychology.
Related Articles & Stories
Read more about our students, faculty, and alumni.
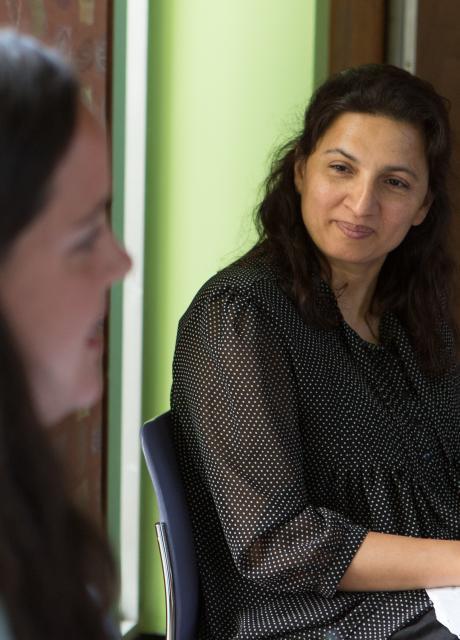
6 Critical Skills for Counselors
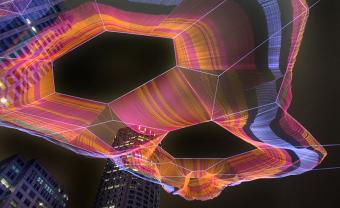
Janet Echelman ’97
Becoming a champion for the deaf community.
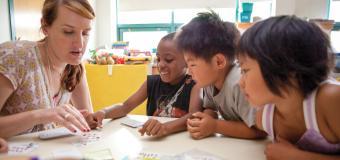
6 Ways Educators Can Prevent Bullying
- Research article
- Open access
- Published: 12 January 2021
Empathy in nurse-patient interaction: a conversation analysis
- Yijin Wu ORCID: orcid.org/0000-0002-2505-6575 1
BMC Nursing volume 20 , Article number: 18 ( 2021 ) Cite this article
40k Accesses
25 Citations
3 Altmetric
Metrics details
Considerable attention has been drawn to empathy in nursing and the concept of empathy has firmly been embedded in nursing discourse. However, little has been known about the details of how nurses express empathy to their patients. In this study, we aim to conduct a qualitative study of actual nurse-patient conversations through which empathy was achieved.
The data in this study was based on audio-recording of sessions of conversations between participating nurses and patients in two Chinese hospitals. The participants in this study involved 6 female nurses and 14 patients. Based on Bachelor’s (1988) categorization of empathy, this study described and analyzed the actual empathic sequences in nursing conversations in an attempt to demonstrate how nursing empathy was interactionally achieved using the method of conversation analysis. Conversation analysis (CA), focusing on the study of talk in interaction, is a useful method for the qualitative analysis of empathic talk in nursing.
By drawing on prior theoretical work as well as on empathic sequence in nursing, this study described and analyzed some of the conversational resources nurses and patients used in achieving empathy. It has been shown that empathy can be interactionally and sequentially achieved in actual sequences of talk. Specifically, nursing empathy is a collaboratively constructed action instead of the nurse’s own committed action, which is produced in specific interactional contexts.
Conversation analysis is a very useful method for describing and analyzing the nurse-patient interaction, especially for studying empathy in nursing care. The sequences in this study present example of exemplary empathic interaction between nurses and patients, which might shed some light on how nurses express empathy to their patients. Also, this study could help to increase the understanding of the mirco-process of empathy in nursing and contribute to improving nursing communicative skills.
Peer Review reports
As a fundamental element for nursing care [ 1 ], empathy is closely connected with the caring process in nursing [ 2 ]. Nursing empathy could be characterized by nurses’ ability to understand the feeling, experiences or psychosocial ability of their patients [ 3 ]. Empathy has an important role to play in establishing a positive nurse-patient relationship and offering favourable nursing care [ 4 ]. Nurses who show understanding of their patients’ illness experiences will find their relationship enhanced [ 5 ]. Research on nurse-patient communication demonstrates that nurse empathy could provide high-quality care to their patients [ 6 ]. Empathy is the essence of all nurse-patient interaction [ 7 , 8 , 9 ], and should be seen as an important clinical indicator for offering high-quality nursing care [ 10 ].
Moreover, an empathic relationship between nurses and patients could contribute to improved clinical outcomes. Norman (1996) reported that empathy is beneficial to the delivery of care for the elderly mentally ill [ 11 ]. Reynolds and Scott (2000) reported that nursing empathy could contribute to patient’s positive responses such as relief from pain, improved pulse, and emotional self-disclosure [ 12 ]. Williams (1979) found that the elderly patients received nursing empathy would experience a statistically significant improvement of self-concept [ 13 ]. It has also been found that nurse empathy could reduce the cancer patient’s anxiety, depression, and hostility significantly [ 14 ].
Considerable attention has been drawn to empathy in nursing and the concept of empathy has firmly been embedded in nursing discourse [ 15 ]. However, there have little research available that examines how nursing empathy occur in Chinese nursing care. To the best of our knowledge, this study is the first qualitative research on empathy in nurse-patient communication in Chinese nursing care. So far, Bachelor’s (1988) work on empathy has been considered as one of the most systematic and influential contributions to the study of the types of empathy in psychology [ 16 , 17 ]. In an analysis of how clients perceive the therapist empathy, Bachelor (1988) identified that therapist empathy can be classified into four types, namely cognitive, affective, sharing, and nurturant empathy [ 18 ]. In present study, on the basis of Bachelor’s categorisation of empathy, we will examine if this categorisation is available for identifying and analyzing empathy in nurse-patient communication. It was relatively unproblematic to apply Bachelor’s categorization of empathy to study empathy in other fields, because her system is based on a large scale empirical research [ 16 ]. In the present study, using naturally occurring nurse-patient conversation, the author will describe and analyze the interactional sequences whereby empathy is achieved and explore the details of how nurses show empathy to their patients following the framework for categorization of empathy proposed by Bachelor (1988). The research questions were the following: 1). How do nurses and their patients collaborate in producing conversations which could show nursing empathy? 2). How nursing empathy was interactionally achieved in actual sequences of talk?
Patient and public involvement
This study was conducted as part of a larger prospective study that deals with the process of nurse-patient communication in China. The data in this study was based on audio-recording of sessions of conversation between participating nurses and patients in two Chinese hospitals. All data in this study consisted of 230 min of nurse-patient conversation, which involved 6 nurses and 14 patients. All nurses involved in this study were female. They aged between 22 to 35 years old. Among the 14 patients, 10 are male, and the remaining 4 patients are female. They aged between 38 to 65 years old. Nurses involved have more than two years of experience in nursing care. The inclusion criteria for patients were 1) ≥18 years of age; 2) clear consciousness; 3) with good communication ability; 3) hospitalized. Informed consent was obtained from all participating nurses and patients. The length of nursing care ranged from 12 to 30 min. The audio-recordings were transcribed verbatim. The spoken data are Chinese, which is translated into English. Here, the data were presented in English.
Data analysis
Conversation analysis is an approach to the study of the social organization of ‘naturally occurring conversation’, or ‘talk-in-interaction’ through a detailed characterization and analysis of target data collected by audio or video recording equipment [ 19 , 20 ]. Special focus is given to the identification and description of various types of interactional sequences in the course of social interaction. Sequentiality is characterized by relations of nextness between utterances (turns-at-talk), that is, the speaker’s ongoing utterance displays his or her understanding of the preceding speaker’s utterance [ 19 ]. This kind of relation between turns is “endemic to the organization of conversation” [ 20 ] and as such, it is the backbone of the possibility of intersubjective understanding between human beings [ 21 , 22 ]. Conversation Analysis, as the study of talk-in-interaction, should therefore have much to say about empathic interactional sequences in nursing care. Jones (2003) argues that the most notable strength of CA in nursing research lies in its ability to uncover the dynamic interactional order that occurs in much nurse-patient interaction whilst providing some guarantee that ‘analytic considerations will not arise as artifacts of intuitive idiosyncrasy, selective attention or recollection, or experimental design’ [ 23 ]. Also, using the CA approach to study nurse-patient interaction offers us a good opportunity to recognize the importance of managing communication exchanges in nursing care such as admission interviews with the aim of successfully integrating the patient’s experience into the nursing assessment [ 24 ]. Methodologically speaking, qualitative approach to empathy will be able to build a sound basis for theory development in this area of nursing care [ 25 ].
In this study, the detailed transcripts involve the sequential nature of nurses’ empathic response to clients’ utterances. The data were transcribed using Jefferson’s system [ 26 ]. Selecting target instances is also an important step in conducting conversation analytical study [ 27 ]. Specifically, each extract should contain utterance showing empathy and its preceding and following turns related to empathy.
Using the method of conversation analysis, this study would conduct a turn-by-turn analysis of how these interactional practices display empathy in sequences. Data analysis involves not only what is occurring in the extract but also how it is relevant to the overall interaction. Particular actions which occurred prior to the selected excerpt are also discussed. Describing particular actions which occurred prior to the selected excerpt could contribute to a better understanding of why and how nurses express empathy.
Cognitive empathy
Utterances used by the therapist to demonstrate understanding of the thoughts, feeling, or behaviour of the patient are identified as “cognitive empathy” [ 18 ]. An instance of cognitive empathy was shown in extract 1 where the nurse offers a candidate understanding of what the patient is feeling. Extract 1 is taken from an interaction between a female nurse and a male patient who suffered from pneumoconiosis. This session occurs when the nurse makes her rounds. In this sequence, it is the nurse that initiates an exchange by making an assumption that the patient seems not to be happy. The nurse has arrived at this assumption through the observation that the patient has a negative facial expression. In line 2, the patient makes an explanation why he does not look happy. In the ensuing talk, the nurse responds to the patient’s troubles talk with an acknowledgment token“En” (uhm ) repeated twice, displaying his supportive orientation toward the troubles talk in line 2. Immediately following this, the nurse’s demonstration of cognitive empathy occurs in line 4, where the nurse offers an alternative way to pin down the patient’s ongoing feeling, that is, replacing ‘cannot feel happy’ with ‘feel down’. In this sense, the nurse attempt to provide a more specific description of the patient’s unhappiness following the onset of disease and thus seems to have more accurate knowledge about the contents of the patient’s ongoing feeling. In line 5, the nurse encourages the patient to keep a good mood which could contribute to his recovery. Using an agreement token “right”, the patient shows his acceptance of empathy and encouragement the nurse offers in lines 4 and 5. In this extract, it seems that the nurse accurately perceives and understands the patient’s feeling and communicates that understanding back to the patient successfully, thus displaying cognitive empathy.
01 Nurse: You seems not to be happy.
02 Patient:My health got (.) really bad, I cannot feel happy.
03 Nurse: Uhm uhm.
04 people feel down when they are (.) not in a good health,
05 but you should keep in a good mood that can help aid your recovery.
06 nurse: right
Extract 2 shows a sequence where the nurse displays a candidate understanding of what the patient is thinking. In line 1, the nurse suggests that the patient should not do that work again. In line 2, the patient responds with a full acknowledgment of the nurse’s recommendation, which can be shown by the fact that once he goes back home, he will change his job to another one. In what follows, the nurse gives the patient a supportive feedback on what he has said. In lines 4 and 5, the patient says that health is much more important than money and thus he will change his job after his hospital discharge, implying that the way for him to earn money was at the expense of his health. The nurse’s expression of cognitive empathy occurs in line 6, where she provides an upshot formulation of the patient’s preceding utterance. Several CA studies have suggested that formulation is one conversational action that regularly functions as a vehicle for empathetic responses [ 27 , 28 , 29 ]. In a formulation, one speaker (in this case, the nurse) shows his or her understanding of the other’s (in this case, the patient’s) preceding utterances by proposing a rephrased version of it [ 30 ]. In this study, formulation refers to the act that nurses try to show a candidate understanding of what has just been said by clients. By means of formulation, the nurse rephrases the patient’s current thought that health is much more important than money. In line 7, the patient receives and acknowledges the patient’s expression of empathy by means of laughing. What the patient says in line 8 indicates that he shows high degree of agreement with the empathic response the nurse made in line 6.
01 Nurse: Don’t do that work again.
02 Patient: Uh, once I go back home, I will (.) change my job.
03 Nurse: Change it to another one, change it to another one.
04 Patient: Uh, health is much more important than money.
05 and I will change-change my job after my hospital discharge.
06 Nurse: Health is a priceless wealth.
07 Patient: Ha ha ha.
08 It does not make any sense to earn much more money without health
Sharing empathy
Sharing empathy is understood as the sequences where the therapist displays that he/she has something in common with the patient, specifically, his/her personal opinions or experiences are similar to the patient’s ongoing situation and thereby the patient does not feel alone [ 18 ]. In Extract 3, a patient who has trouble in sleeping is discussing his symptom with the nurse. The nurse initiates the topic by an indirect speech act, that is, she has heard that the patient had trouble in sleeping. The information about the patient’s sleep problem may come from the attending doctor or the medical record. In line 2, the patient offers a candidate understanding of the cause for his symptom. In line 3, the nurse first repeats the utterance produced by the patient in line 2. Here, it is an extreme case formulation “quite right”, justifying the patient’s self-assessment of the cause for his sleeping problem. Immediately following the justification, the nurse shows that she also has the same problem as the patient has, that is, she also has trouble in sleeping in that she works too much at night, thereby expressing ‘sharing empathy’. In this sense, by expressing a sense of shared understanding, the nurse may prevent the patient from feeling alone and isolated. Towards the end of line 4, the nurse chooses to use “We”, which is a “we-inclusive” pronoun, including both the nurse and the patient, which aims to shorten the psychological distance between them and bind them into a temporary alliance, contributing further to indicating her shared understanding of the patient’s problem. At this point, it can be seen that the nurse and patient are in perfect alignment with each other in terms of sleeping problem. It could be suggested that sharing empathy can strengthen the nurse-patient relationship and put them on equal footing.
01Nurse: I heard that you have not been (.)sleeping well, right?
02Patient: Uhm, (0.5) it may be connected with (.) working at night.
03 Nurse:Quite right, because of working at night too much, I also have sleeping problem.
04 We are prone to sleep problems due to working too much at night.
5 Patient: Right, right.
Affective empathy
Affective empathy occurs in the sequences where the therapist shows that he/she partakes of the same feelings the client is personally experiencing at that moment [ 18 ]. In the following extract, an instance of affective empathy is presented. In this instance, the patient has been hospitalized for almost one month. In line 1, the patient asks the nurse when he could be discharged from the hospital. In the same turn, he continues to say that he cannot eat well and sleep well, which indicates that he is annoyed over hospitalization. Here, the patient’s negative emotion hides behind the literal meaning of his utterance. Coulehan et al’s (2001) report that in some cases the patient present an indirect expression of suffering or emotion,which are embedded in quasi-factual statements [ 31 ]. In this sequence, the nurse does not ignore the patient’s underlying concerns, but empathetically responds to his negative emotion. In lines 3–5, the nurse says that she experienced a similar troubling situation as the patient last year, namely, being hospitalized for three weeks. Thus, she could deeply understand that it is inconvenient for the patient to be in the hospital for a long time. It is clear that the nurse share the same feeling as the patient, namely, being uncomfortable in the hospital for a long time. In this way, the patient seems to describe it as ‘your pain in my heart’. This could contribute to help the nurse build emotional connections with the patient. The nurse thus expresses her empathy and partakes in the patient’s feelings. The patient’s “ye:ah” in line 6 serves to indicate that the patient has received the nurse’s expression of empathy and also functions as a confirmation of the nurse’s statement that she also feels inconvenient. After this, the patient continues to say an utterance “all things are not convenient”, showing that what the patient feels is consistent with the empathic statement the nurse made in line 3. It thus can be seen that communicating empathy is just as important as care delivery.
01 Patient: When could I (.) be discharged from hospital?
02 I cannot eat well and sleep well in the hospital.
03 Nurse:I were hospitalized for three weeks last year.
04 I could (.) deeply understand that it is downright inconvenient for you to be in.
05 the hospital for a long time.
06 Patient:ye::ah, all things are not convenient
Nurturant empathy
The final type of empathy that will be presented and described here is that of ‘nurturant empathy’, which is characterized by the therapist being supportive, security-providing or totally attentive [ 15 ]. In the following extract, an instance of nurturant empathy is demonstrated. In line 1, the patient tells the nurse that she worries about the surgery that will be performed tomorrow. The nurse follows up on this by stating that she feels assured because the doctor, Prof. Zhang, who will do surgery for her tomorrow, is a skillful practitioner. It appears that the nurse’s contribution conceives the patient’s concern as unreasonable and thus the nurse is confident about providing security to tomorrow’s surgery. In line 4, the patient uses two minimal responses “uh” and an acknowledgment token “that is right” to show her receipt of the nurse’s expression of nurturant empathy. In line 5, the nurse says that 300 patients similar to her condition have received successful surgeries in this department last year. The nurse attempts to convince the patient that she would just like the other 300 cured patients in performing her surgery. In this sense, drawing on the previous successful experiences of other patients, the nurse is very confident about the patient’s surgery. In other words, what the nurse states here contributes to displaying her optimistic stance toward tomorrow’s surgery, which in itself may achieve a therapeutic effect [ 32 ]. The patient’s utterance “If so, I will not be worried about tomorrow’s surgery.” in line 7 can be seen as a favorable response to the nurse’s empathic utterance in lines 5 and 6, and as an indication of nurturant empathy as a good nursing strategy achieving good effect on the patient’s concern.
01Patient: I am going to (.) receive a surgery tomorrow. I am very scared.
02Nurse: Please be at ease, Prof. Zhang will perform the surgery (.) for you.
03 He is a skillful doctor
04Patient: Uhm uhm, that’s right.
05Nurse: 300 patients similar to your condition are at our department last year.
06 They all have received successful surgery
07Patient: Uh, (0.5) if so, I will not be worried about tomorrow’s surgery.
Empathy is regarded as an important component in nursing care. However, little work has been done to examine how nurse and patient interact with each other in sequences of talk through which empathy is achieved. As Jones (2003) puts it, CA is a research approach that could accurately capture the contribution of both participants within nurse-patient interaction. CA could reveal the interactional process of empathy in interaction [ 30 ]. Thus, the method of conversation analysis is a useful analytical approach to the study of empathy in nursing. In this study, drawing on the method of conversation analysis, four types of empathic interactional sequences are characterized and analyzed, namely cognitive empathy, affective empathy, sharing empathy and nurturant empathy. Our study suggests that “empathy” establishes a caring environment in which nurses not only express understanding of what the patient is experiencing, but also aligns with patients. The present study suggests that to a certain degree empathy could contribute to a smooth sequential development and improved nursing outcome. The sequences in this study present example of exemplary empathic interaction between nurse and patient and the naturally occurring data provides very useful guidelines for professional development of clinical nurses. It is no doubt that CA provides a new way for observing and understanding nurse-patient interaction. It unfolds the sequential organization in the nurse-patient in detail and explicates the practices and interactional sequences through which the nursing care is carried out. It has also been shown in our study that empathy in nursing care can be interactively achieved in actual sequences of talk which was produced by the nurse’s and patient’s collaborative teamwork. A conversation analytic approach presents a turn-by-turn analysis of how empathy unfolds in the course of nurse-patient interaction.
Limitations
The method of conversation analysis used in this study has several limitations. For instance, we are not able to relate the use of conversational resources to the interactional outcomes, i.e. in terms of patient’s satisfaction. Furthermore, the research findings in this study do not concern all aspects of empathy in nursing. This study just addresses aspects of empathy that characterized by Bachelor (1988) [ 18 ]. In other words, this study does not examine other types of empathy which are not covered by Bachelor’ (1988) categorization. Moreover, there were only female nurses involved in this study, which may not have demonstrated the male nurses’ way of expressing empathy. Another limitation concerns data collection which was only conducted in two hospitals, thus raising concerns of displaying nurses’ way of expressing empathy working in different levels of hospitals.
CA is an inductive, micro-analytic, and predominantly qualitative method for studying language as it is used in social interaction. The microanalytic approach used in this study is available for understanding the interactional features of nursing empathy. Specifically, we could have a clear understanding of how nurse empathy was initiated, how nurse empathy was expressed and how nurse empathy was responded to by patients’ talk. In this sense, Conversation analysis is a very useful method for describing and analyzing the nurse-patient interaction, especially for studying empathy in nursing care. Thus, we call for more attention to be paid to the role of conversation analysis in nursing care.
Availability of data and materials
The transcribed data are available on reasonable request from the corresponding author.
Abbreviations
- Conversation analysis
McEwen M, Wills E. Theoretical basis for nursing. 2nd ed. Philadelphia: Lippincott Williams & Wikins; 2007.
Google Scholar
Kunyk D, Olson JK. Clarification of conceptualizations of empathy. J Adv Nurs. 2001;35:317–25.
Article CAS Google Scholar
Wu Y. Empathy in psychotherapy: Using conversation analysis to explore the therapists’ empathic interaction with clients. Southern Afr Linguistics Appl Language Stud. 2019;37(3):232–46.
Article Google Scholar
Wade GH, Kasper N. Nursing students’ perceptions of instructor caring: an instrument based on Watson’s Theory of Transpersonal Caring. J Nurs Educ. 2006;45:162–8.
Tyner R. Elements of empathic care for dying patients and their families. Nurs Clin North Am. 1985;20:393–401.
CAS PubMed Google Scholar
Derksen F, et al. Empathy: what does it mean for GPs? A qualitative study. Fam Pract. 2014;30:1–7.
Peplau H. Interpersonal Relations in Nursing: A Conceptual Frame of Reference for Psychodynamic Nursing. New York: G.P. Putnam; 1952.
Kalish BJ. What is empathy? Am J Nurs. 1973;73:1548–52.
Benner P, Wrubel J. The primacy of Caring: Stress and Coping in Health and Illness. Menlo Park, CA: Addison-Wesley; 1989.
Neumann M, Bensing J, Mercer S, Ernstmann N, Ommen O, Pfaff HP. Analyzing the “nature” and “specific effectiveness” of clinical empathy: A theoretical overview and contribution towards a theory-based research agendas. Patient Educ Counseling. 2009;74:339–46.
Norman KD. The role of empathy in the care of dementia. J Psychiatric Ment Health Nurs. 1996;3:313–7.
Reynolds W, Scott B. Do nurses and other professional helpers normally display much empathy. J Adv Nurs. 2000;31:226–34.
Williams L. Empathic communication and its effect on client outcome. Issues Ment Health Nurs. 1979;2:15–26.
La Monica EL, Wolf RM, Madea AR, et al. Empathy and nursing care outcomes. Scholarly Inquiry Nurs Pract. 1987;1:197–213.
Williams J, Stickley T. Empathy and nurse education. Nurse Educ Today. 2010;30:752–5.
Wynn R. Empathy in general practice consultations: A qualitative analysis. Epidemiologia e Psichiatria Sociale. 2005;14:163–9.
Wynn R, Wynn M. Empathy as an interactionally achieved phenomenon in psychotherapy: Characteristics of some conversational resources. J Pragmatics. 2006;38:1385–97.
Bachelor A. How clients perceive empathy: a content analysis of ‘received’ emnpathy. Psychotherapy. 1988;25:227–40.
Schegloff EA. Conveying who you are: The presentation of self, strictly speaking. In: Enfield NJ, Stivers T, editors. Person reference in interaction: Linguistic, cultural, and social perspectives. Cambridge: Cambridge University Press; 2007. p. 123–48.
Schegloff EA. Sequence organization on in interaction: A primer in conversation analysis: Cambridge,UK: Cambridge University Press; 2007.
Heritage J. Garfinkel and ethnomethodology. Cambridge: Polity Press; 1984.
Schegloff EA. Repair after next turn: The last structurally provided defense of intersubjectivity in conversation. Am J Sociol. 1992;97:1295–345.
Heritage J. A change-of-state token and aspects of its sequential placement. In: Atkinson JM, Heritage J, editors. Structures of Social Action: Studies in Conversation Analysis. Cambridge: Cambridge University Press; 1984. p. 299–345.
Jones A. Nurses talking to patients: exploring conversation analysis as a means of researching nurse-patient communication. Int J Nurs Stud. 2003;40:609–19.
Kristjánsdóttir G. Empathy: therapeutic phenomenon in nursing care. J Clin Nurs. 1992;1:131–40.
Atkinson JM, Heritage J. Structures of social action: Studies in conversation analysis. Cambridge, UK: Cambridge University Press; 1984.
Beach WA, Dixson CN. Revealing moments: Formulating understanding of adverse experiences in a health appraisal interview. Soc Sci Med. 2001;52:25–44.
Hepburn A, Potter J. Crying receipts: Time, empathy, and institutional practice. Res Language Soc Interact. 2007;40:89–116.
Pudlinkski C. Doing empathy and sympathy: Caring responses to troubles telling on a peer support line. Discourse Stud. 2005;7:267–88.
Heritage J, Watson R. Formulation as conversational objects. In: Psathas G, editor. Everyday language. Studies in ethnomethodology. New York: Irvington; 1979. p. 123–62.
Coulehan JL, Platt FW, Egener B, Frankel R, Lin C, Lown B, Salazar WH. “Let me see if i have this right ...”: words that help build empathy. Ann Intern Med. 2001;135:221–7.
Priebe S, Gruyters T. The importance of the first three days: predictors of treatment outcome in depressed in-patients. Br J Clin Psychol. 1995;34:229–36.
Download references
Acknowledgements
The author expresses gratitude to nurses and patients who consent to participate in this study.
No funding was obtained for this study.
Author information
Authors and affiliations.
School of Translation Studies/Editorial Office for Medical Humanities in the Developing World, Qufu Normal University, No.80, Yantaibei Road, Donggang District, Rizhao, China
You can also search for this author in PubMed Google Scholar
Contributions
YW conceived the study and wrote the manuscript. The author read and approved the final manuscript.
Corresponding author
Correspondence to Yijin Wu .
Ethics declarations
Ethics approval and consent to participate.
This study was approved by the ethics committee of Center for neurolinguistics, Qufu Normal University. Written consent was obtained from all participants.
Consent for publication
Not applicable.
Competing interests
The authors declare that they have no competing interests.
Additional information
Publisher’s note.
Springer Nature remains neutral with regard to jurisdictional claims in published maps and institutional affiliations.
Rights and permissions
Open Access This article is licensed under a Creative Commons Attribution 4.0 International License, which permits use, sharing, adaptation, distribution and reproduction in any medium or format, as long as you give appropriate credit to the original author(s) and the source, provide a link to the Creative Commons licence, and indicate if changes were made. The images or other third party material in this article are included in the article's Creative Commons licence, unless indicated otherwise in a credit line to the material. If material is not included in the article's Creative Commons licence and your intended use is not permitted by statutory regulation or exceeds the permitted use, you will need to obtain permission directly from the copyright holder. To view a copy of this licence, visit http://creativecommons.org/licenses/by/4.0/ . The Creative Commons Public Domain Dedication waiver ( http://creativecommons.org/publicdomain/zero/1.0/ ) applies to the data made available in this article, unless otherwise stated in a credit line to the data.
Reprints and permissions
About this article
Cite this article.
Wu, Y. Empathy in nurse-patient interaction: a conversation analysis. BMC Nurs 20 , 18 (2021). https://doi.org/10.1186/s12912-021-00535-0
Download citation
Received : 13 July 2020
Accepted : 02 January 2021
Published : 12 January 2021
DOI : https://doi.org/10.1186/s12912-021-00535-0
Share this article
Anyone you share the following link with will be able to read this content:
Sorry, a shareable link is not currently available for this article.
Provided by the Springer Nature SharedIt content-sharing initiative
- Nurse-patient interaction
BMC Nursing
ISSN: 1472-6955
- General enquiries: [email protected]
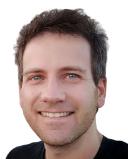
Forgiveness
Strong evidence for the link between empathy and forgiveness, summarizing our replication registered report of mccullough et al..
Posted April 11, 2024 | Reviewed by Tyler Woods
- The Importance of Forgiveness
- Find a therapist near me
- Our ability to forgive others is closely tied to the level of empathy we feel towards them.
- We conducted a replication of McCullough et al. (1997) and the empathy model of forgiveness.
- Replication was done as a Registered Report with Peer Community in Registered Report, a revolution in science.
This post was written by Timothy Chan, a graduate of the University of Hong Kong who led the replication of the classic McCullough et al. (1997 ; 1998) discussed in this post. Gilad Feldman was Timothy's thesis advisor, is the corresponding author on the joint article, and edited this post for Psychology Today.
We are all familiar with the concept of forgiveness , but what leads us to forgive someone who has hurt us deeply? This question has fascinated countless scholars, theologians, and even psychologists. Some propose that we require a motive to forgive, while others suggest that receiving an apology from the offender is necessary for forgiveness.
The empathy model of forgiveness
In 1997, Prof. McCullough and his colleagues proposed the empathy model of forgiveness, which has been one of the most widely cited models in the field of psychology when it comes to explaining why we forgive. The essence of this model is straightforward.
Forgiveness is not an instant process. Instead, our ability to forgive others is closely tied to the level of empathy we feel towards them. The conscious decision to forgive is strongly influenced by the emotion of empathy we experience towards the person who has wronged us. The figure below illustrates the original empathy model of forgiveness.
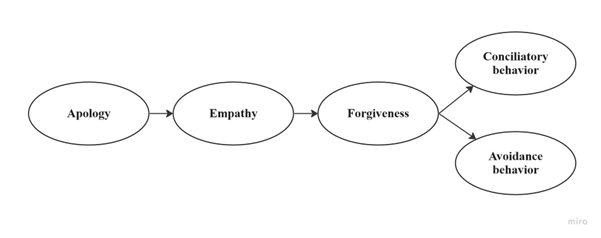
Empathy acts as a mediator between dispositional and environmental variables, influencing their causal effects on forgiving. Also, forgiveness represents a motivational shift that encourages constructive actions, such as conciliation towards the offender, while discouraging destructive actions, such as avoidance, following an interpersonal offense.
The theory sounds straightforward, and it has been scientifically tested by McCullough and his colleagues. I believe you might wonder why we replicated a research study that was conducted two decades ago instead of pursuing a novel research topic.
The replication crisis in science
I want to bring your attention to one of the most pressing and concerning issues for the scientific community: the replication crisis . Replicability is a cornerstone of modern science, ensuring its reliability and credibility. However, in the past decade, we realized that we cannot replicate many of the findings in published articles.
In the field of psychological science, many psychologists have been working to rebuild the credibility of the discipline as part of a "science reform" movement. New initiatives like the Center of Open Science and several "Many Labs" mass-scholar collaborations began a systematic attempt to replicate some of the classic studies in the field that were considered solid and heavily cited in influential research. To the community's surprise, many of the classics in reputable journals did not replicate well, with many showing much weaker effects compared to the published articles ( Feldman, 2023 ). These prompted other fields to embark on similar initiatives, with the most recent disappointing findings from cancer biology, which has very low reproducibility and replication rates (Errington et al., 2021).
How I embarked on my journey of replication studies
One of the professors at the University of Hong Kong, Gilad Feldman, set up the CORE team (Collaborative Open Science and Meta-Research), and with students in his classes and under his supervision, the team conducted over 120 replications of classics in social psychology and decision-making .
When I first learned about this crisis in Gilad’s lecture as an undergraduate student, I was genuinely shocked. It made me realize that many theories I learned in my introductory psychology courses could be groundless. Yet, these theories are still presented as "truths" in psychology that students are expected to memorize. Subsequently, I explored if there was anything I could do to change the situation. That's when I decided to conduct a replication study as my thesis, under Gilad’s supervision.
I reviewed numerous classic studies in the field of social psychology that had not been directly replicated before but were frequently cited in other journals, or even served as the foundation for many other theories. Finally, I decided to replicate and extend two significant papers published by McCullough and his team in 1997 and 1998, which focused on explaining interpersonal forgiveness.

In our replication, we followed the original study’s plan in data collection and examined the correlations among variables. We also added an extension, aiming to go beyond correlational evidence to achieve a randomized experiment. We, therefore, manipulated affective empathy in recall tasks, examining the causal relationship between empathy and forgiveness.
Result: A highly successful replication and extension study
This replication was highly successful, and the results aligned closely with the original research: Empathy was positively associated with forgiveness. Apology was positively associated with empathy. The association between forgiveness and conciliation motivation was weaker than in the original article. However, the correlation between forgiveness and avoidance motivation remained about the same in the replication. Furthermore, forgiveness was negatively associated with revenge motivation.
In our extension, we found support for the causal influence of empathy on forgiveness. Additionally, through the manipulation of emotional empathy, we also found support for the causal influence of empathy on the perception of apology, providing scientific evidence for the role of empathy in shaping how individuals perceive apologies.
Publishing registered reports with Peer Community in Registered Reports (PCI-RR)
Lastly, I would like to share my experience of conducting a replication study as a Registered Report with "Peer Community in Registered Report" (PCI-RR).
Registered Reports are a publication process now adopted by over 350 journals in psychological science and beyond, in which Stage 1 journal peer review occurs on a pre-registration plan prior to any data collection. Once the preregistration passes peer review and is approved, the journal grants authors an in-principle acceptance, guaranteeing the publication of the article provided that data collection and analysis follow the intended plan. This emphasizes design, methods, and rigor, addressing issues of reviewers' and publication bias with the publication of findings regardless of data collection outcomes, with the Stage 2 peer review focused solely on ensuring adherence to the Stage 1 plan (or documenting deviations).
PCI-RR is a new initiative launched in 2021 that builds on Registered Reports and is aimed at further improvement. It provides community open-identified peer-review on posted Registered Report Stage 1 preprints, led by open-science trained recommenders (editors), with in-principle acceptance from the community endorsed for publication by a variety of open-science supportive journals. This initiative also includes an option for a "scheduled review" path which allows timely community expert open-science supportive peer review within two weeks, short enough to fit into an academic year thesis schedule. PCI-RR is nothing short of a revolution in science.
My fellow undergraduate guided thesis students and I, working with Gilad Feldman, submitted our theses to PCI-RR and have been accepted for publication following the community's approval of our pre-registration plan.
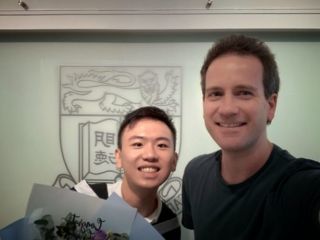
The Best Learning Experience for Researchers
PCI-RR has profound implications for our research community, and I believe it is one of the best learning experiences for all early career researchers. Through PCI-RR, we can receive direct feedback from field experts regarding the research plans. This feedback not only provides constructive criticism (which, although sometimes critical, is essential for improvement) but also occurs at an early stage when we can still address issues and improve.
Throughout this amazing journey, I am grateful to have a chance to engage in direct conversations and learn from all the outstanding researchers, authors, and reviewers. Their expertise positively influenced my understanding of psychological science. Completing my thesis as a Registered Report on replication provided me with invaluable knowledge, reinforcing my commitment to endorse true open science. Let's continue to prioritize transparency, exercise caution, and approach our work with confidence and humility.
For more information, please see my talk about the project and a thesis as a submission to PCIRR:
Chan, C., & Feldman, G. (2024). The impact of Empathy on Forgiveness: Replication and extensions Registered Report of McCullough et al. (1997)'s Study 1. Endorsed by Peer Community in Registered Reports. DOI: 10.24072/pci.rr.100444 [ PCIRR Stage 2 recommendation/Open peer review ] [ PCIRR Stage 1 recommendation/Open peer review ] [ Preprint ] [ Open materials/data/code ]
McCullough, M. E., Worthington, E. L., & Rachal, K. C. (1997). Interpersonal Forgiving in Close Relationships. Journal of Personality and Social Psychology , 73(2), 321–336. https://doi.org/10.1037/0022-3514.73.2.321
McCullough, M. E., Rachal, K. C., Sandage, S. J., Worthington, E. L., Brown, S. W., & Hight, T. L. (1998). Interpersonal Forgiving in Close Relationships: II. Theoretical Elaboration and Measurement. Journal of Personality and Social Psychology , 75(6), 1586–1603. https://doi.org/10.1037/0022-3514.75.6.1586
Errington, T. M., Mathur, M., Soderberg, C. K., Denis, A., Perfito, N., Iorns, E., & Nosek, B. A. (2021). Investigating the replicability of preclinical cancer biology. Elife , 10 , e71601. https://doi.org/10.7554/eLife.71601
Feldman, G. (2023). "Time for a science reform". Talk given at University of Hong Kong. https://doi.org/10.17605/OSF.IO/2CTFJ
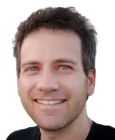
Gilad Feldman, Ph.D. , is an assistant professor with the psychology department at the University of Hong Kong.
- Find a Therapist
- Find a Treatment Center
- Find a Psychiatrist
- Find a Support Group
- Find Teletherapy
- United States
- Brooklyn, NY
- Chicago, IL
- Houston, TX
- Los Angeles, CA
- New York, NY
- Portland, OR
- San Diego, CA
- San Francisco, CA
- Seattle, WA
- Washington, DC
- Asperger's
- Bipolar Disorder
- Chronic Pain
- Eating Disorders
- Passive Aggression
- Personality
- Goal Setting
- Positive Psychology
- Stopping Smoking
- Low Sexual Desire
- Relationships
- Child Development
- Therapy Center NEW
- Diagnosis Dictionary
- Types of Therapy
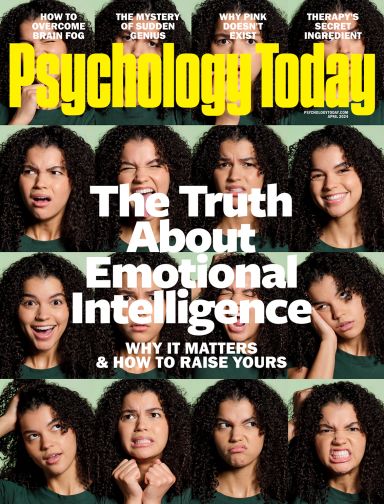
Understanding what emotional intelligence looks like and the steps needed to improve it could light a path to a more emotionally adept world.
- Coronavirus Disease 2019
- Affective Forecasting
- Neuroscience
- Introduction
- Conclusions
- Article Information
A, Measured with a numerical rating scale for the typical pain level in the 7 days prior to each encounter, with scores ranging from 0 to 10. B, Measured with the Roland-Morris Disability Questionnaire, with scores ranging from 0 to 24. Higher scores on both assessments indicate worse outcomes for pain and function. Physicians were classified as very empathic if their Consultation and Relational Empathy (CARE) measure scores were 30 or higher and as slightly empathic if their scores were 29 or lower. Scores range from 10 to 50, with higher scores indicating greater physician empathy. Slightly empathic physicians made up approximately the lowest quartile on the CARE measure. Summary measures and P values are for the entire 12-month period adjusted for time and empathy × time interaction. Error bars represent 95% CIs. SEP indicates slightly empathic physician; VEP, very empathic physician.
Health-related quality of life was measured using the Patient-Reported Outcomes Measurement Information System with 29 items. Each deficit measure included 4 ordinal-scale items that were scored and normed according to the US general population to yield mean (SD) scores of 50 (10). The sole exception was sleep disturbance, which was normed using a calibration sample enriched with patients having chronic illness. Higher scores indicate worse outcomes. Physicians were classified as very empathic if their Consultation and Relational Empathy (CARE) measure scores were 30 or higher and as slightly empathic if their scores were 29 or lower. Scores ranged from 10 to 50, with higher scores indicating greater physician empathy. Slightly empathic physicians made up approximately the lowest quartile on the CARE measure. Summary measures and P values are for the entire 12-month period adjusted for time and empathy × time interaction. Error bars represent 95% CIs. SEP indicates slightly empathic physician; VEP, very empathic physician.
eTable 1. Outcomes Based on Physician Empathy (CARE Measure Score), Time, and Empathy × Time Interaction
eTable 2. Clinical Relevance of Outcomes Based on Physician Empathy Group (VEP vs SEP), Time, and Empathy × Time Interaction
eTable 3. Outcomes Based on Physician Empathy (CARE Measure Score), Time, Empathy × Time Interaction, and the Full Array of Covariates
eTable 4. Clinical Relevance of Outcomes Based on Physician Empathy Group (VEP vs SEP), Time, Empathy × Time Interaction, and the Full Array of Covariates
eTable 5. Clinical Relevance of Outcomes Based on Physician Empathy Group (Greater vs Lesser Physician Empathy), Time, and Empathy × Time Interaction According to Alternative Cut Points for Physician Empathy
eTable 6. Clinical Relevance of Outcomes Based on Physician Empathy Group (Greater vs Lesser Physician Empathy), Time, Empathy × Time Interaction, and the Full Array of Covariates According to Alternative Cut Points for Physician Empathy
Data Sharing Statement
See More About
Sign up for emails based on your interests, select your interests.
Customize your JAMA Network experience by selecting one or more topics from the list below.
- Academic Medicine
- Acid Base, Electrolytes, Fluids
- Allergy and Clinical Immunology
- American Indian or Alaska Natives
- Anesthesiology
- Anticoagulation
- Art and Images in Psychiatry
- Artificial Intelligence
- Assisted Reproduction
- Bleeding and Transfusion
- Caring for the Critically Ill Patient
- Challenges in Clinical Electrocardiography
- Climate and Health
- Climate Change
- Clinical Challenge
- Clinical Decision Support
- Clinical Implications of Basic Neuroscience
- Clinical Pharmacy and Pharmacology
- Complementary and Alternative Medicine
- Consensus Statements
- Coronavirus (COVID-19)
- Critical Care Medicine
- Cultural Competency
- Dental Medicine
- Dermatology
- Diabetes and Endocrinology
- Diagnostic Test Interpretation
- Drug Development
- Electronic Health Records
- Emergency Medicine
- End of Life, Hospice, Palliative Care
- Environmental Health
- Equity, Diversity, and Inclusion
- Facial Plastic Surgery
- Gastroenterology and Hepatology
- Genetics and Genomics
- Genomics and Precision Health
- Global Health
- Guide to Statistics and Methods
- Hair Disorders
- Health Care Delivery Models
- Health Care Economics, Insurance, Payment
- Health Care Quality
- Health Care Reform
- Health Care Safety
- Health Care Workforce
- Health Disparities
- Health Inequities
- Health Policy
- Health Systems Science
- History of Medicine
- Hypertension
- Images in Neurology
- Implementation Science
- Infectious Diseases
- Innovations in Health Care Delivery
- JAMA Infographic
- Law and Medicine
- Leading Change
- Less is More
- LGBTQIA Medicine
- Lifestyle Behaviors
- Medical Coding
- Medical Devices and Equipment
- Medical Education
- Medical Education and Training
- Medical Journals and Publishing
- Mobile Health and Telemedicine
- Narrative Medicine
- Neuroscience and Psychiatry
- Notable Notes
- Nutrition, Obesity, Exercise
- Obstetrics and Gynecology
- Occupational Health
- Ophthalmology
- Orthopedics
- Otolaryngology
- Pain Medicine
- Palliative Care
- Pathology and Laboratory Medicine
- Patient Care
- Patient Information
- Performance Improvement
- Performance Measures
- Perioperative Care and Consultation
- Pharmacoeconomics
- Pharmacoepidemiology
- Pharmacogenetics
- Pharmacy and Clinical Pharmacology
- Physical Medicine and Rehabilitation
- Physical Therapy
- Physician Leadership
- Population Health
- Primary Care
- Professional Well-being
- Professionalism
- Psychiatry and Behavioral Health
- Public Health
- Pulmonary Medicine
- Regulatory Agencies
- Reproductive Health
- Research, Methods, Statistics
- Resuscitation
- Rheumatology
- Risk Management
- Scientific Discovery and the Future of Medicine
- Shared Decision Making and Communication
- Sleep Medicine
- Sports Medicine
- Stem Cell Transplantation
- Substance Use and Addiction Medicine
- Surgical Innovation
- Surgical Pearls
- Teachable Moment
- Technology and Finance
- The Art of JAMA
- The Arts and Medicine
- The Rational Clinical Examination
- Tobacco and e-Cigarettes
- Translational Medicine
- Trauma and Injury
- Treatment Adherence
- Ultrasonography
- Users' Guide to the Medical Literature
- Vaccination
- Venous Thromboembolism
- Veterans Health
- Women's Health
- Workflow and Process
- Wound Care, Infection, Healing
Get the latest research based on your areas of interest.
Others also liked.
- Download PDF
- X Facebook More LinkedIn
Licciardone JC , Tran Y , Ngo K , Toledo D , Peddireddy N , Aryal S. Physician Empathy and Chronic Pain Outcomes. JAMA Netw Open. 2024;7(4):e246026. doi:10.1001/jamanetworkopen.2024.6026
Manage citations:
© 2024
- Permissions
Physician Empathy and Chronic Pain Outcomes
- 1 Department of Family Medicine, University of North Texas Health Science Center at Fort Worth, Fort Worth
- 2 University of North Texas Health Science Center at Fort Worth, Fort Worth
- 3 School of Nursing, Johns Hopkins University, Baltimore, Maryland
Question Is physician empathy associated with the outcomes of patients with chronic pain?
Findings In this cohort study that included 1470 adults with chronic low back pain, patients treated by very empathic physicians reported having significantly better and clinically relevant outcomes pertaining to pain, function, and health-related quality of life over 12 months compared with patients treated by slightly empathic physicians. Physician empathy was more strongly associated with favorable outcomes than were nonpharmacological treatments, opioid therapy, and lumbar spine surgery.
Meaning These findings suggest that physician empathy is an important aspect of the patient-physician relationship and was associated with better outcomes among patients with chronic pain.
Importance Empathy is an aspect of the patient-physician relationship that may be particularly important in patients with chronic pain.
Objective To measure the association of physician empathy with pain, function, and health-related quality of life (HRQOL) among patients with chronic low back pain.
Design, Setting, and Participants This cohort study included adult enrollees from the Pain Registry for Epidemiological, Clinical, and Interventional Studies and Innovation national pain research registry. Study dates were from April 1, 2016, to July 25, 2023, with up to 12 months of follow-up.
Exposure Physician empathy was assessed with the Consultation and Relational Empathy measure and dichotomized to yield very empathic physician and slightly empathic physician groups.
Main Outcomes and Measures Main outcomes were patient-reported pain, function, and HRQOL measured with a numerical rating scale for low back pain intensity, the Roland-Morris Disability Questionnaire for back-related disability, and the Patient-Reported Outcomes Measurement Information System for HRQOL deficits pertaining to anxiety, depression, fatigue, sleep disturbance, and pain interference. Data were collected at 5 quarterly encounters from registry enrollment through 12 months and analyzed with generalized estimating equations, including multivariable models to measure temporal trends and to adjust for baseline and longitudinal covariates.
Results Among the 1470 patients, the mean (SD) age was 53.1 (13.2) years, and 1093 (74.4%) were female. Patients completed 5943 encounters in which multivariable analyses demonstrated that greater physician empathy was inversely associated with pain intensity (β = −0.014; 95% CI, −0.022 to −0.006; P < .001), back-related disability (β = −0.062; 95% CI, −0.085 to −0.040; P < .001), and HRQOL deficits on each measure (eg, pain interference: β = −0.080; 95% CI, −0.111 to −0.049; P < .001). Correspondingly, compared with the slightly empathic physician group, the very empathic physician group reported lower mean pain intensity (6.3; 95% CI, 6.1-6.5 vs 6.7; 95% CI, 6.5-6.9; P < .001), less mean back-related disability (14.9; 95% CI, 14.2-15.6 vs 16.8; 95% CI, 16.0-17.6; P < .001), and fewer HRQOL deficits on each measure (eg, fatigue: 57.3; 95% CI, 56.1-58.5 vs 60.4; 95% CI, 59.0-61.7; P < .001). All physician empathy group differences were clinically relevant, with Cohen d statistics ranging from 0.21 for pain intensity to 0.30 for back-related disability, fatigue, and pain interference. Physician empathy was associated with more favorable outcomes than non-pharmacological treatments, opioid therapy, and lumbar spine surgery.
Conclusions and Relevance In this cohort study of adult patients with chronic pain, physician empathy was associated with better outcomes over 12 months. Greater efforts to cultivate and improve physician empathy appear warranted.
The patient-physician relationship is fundamental to the practice of medicine. Although there is no agreement on how to define or study empathy, 1 empathic opportunities arising during medical encounters may be missed, thereby posing a threat to the patient-physician relationship. 2 Physician empathy may enhance patient adherence to treatment and improve clinical outcomes. A systematic review of randomized trials involving artificially manipulated practitioner empathy compared with usual care found modest patient benefits for several clinical conditions. 3 Nevertheless, the review was limited by variability of interventions, practitioners, patients, and conditions, thereby yielding high statistical heterogeneity and low-quality evidence. Moreover, empathy outcomes were often assessed immediately after an encounter and never beyond 3 weeks.
Studies of physician empathy generally rely on observational research in a more natural setting. These involve physician self-assessed empathy or patient-perceived empathy. Unlike the latter, the former often measures physician attitudes about empathy rather than empathy itself. 4 Because no correlation between physician and patient ratings of empathy has been observed, patients must be involved in assessing physician empathy. 5 - 7 The Consultation and Relational Empathy (CARE) measure is the primary patient rating instrument for physician empathy, 7 owing to its reliability 8 and internal validity and consistency. 9 The CARE measure may capture subtle nuances of patient interactions with physicians, thereby confirming its value in assessing relational components of empathy. 6
The patient-physician relationship is vital among patients with chronic low back pain (CLBP) because patients often feel isolated, misunderstood, or stigmatized when an underlying cause of pain cannot be identified. 10 A large correlation between physician empathy and satisfaction was reported immediately following a pain clinic consultation, 11 and physician empathy was strongly associated with satisfaction among patients with CLBP even after controlling for confounders. 12 A therapeutic alliance between such patients and physical therapists led to better pain and function outcomes over 8 weeks. 13 Improved physician empathy over 3 months also was associated with better pain and health-related quality-of-life (HRQOL) outcomes among patients attending pain clinics. 14 Because it is unclear whether these results would be sustained in general medical settings over time, we aimed to assess the association of patient-reported physician empathy with CLBP outcomes in such settings over 12 months.
We conducted a cohort study using participants enrolled in the Pain Registry for Epidemiological, Clinical, and Interventional Studies and Innovation (PRECISION) from April 1, 2016, to July 25, 2023. The registry offers screening throughout the contiguous US using social media advertising. 15 Those screened were eligible for the study if they were aged 21 to 79 years, had CLBP (≥3 months), had a physician who provided CLBP usual care, and had sufficient English-language proficiency to complete case report forms. Exclusion criteria were being pregnant or residing at an institutional facility. Hereinafter, the terms participants and patients may be used interchangeably to refer to registry participants who were patients of treating physicians in this study. Study participants provided self-reported data at registry enrollment and for up to 4 subsequent quarterly encounters over 12 months using a digital research platform for electronic data capture. This precluded missing item responses during completed encounters. Participants who missed consecutive encounters were considered lost to follow-up and disenrolled from the registry; however, available data were retained for analysis. To minimize potential objections to participation, the registry does not collect sensitive data such as income or health insurance coverage. It also does not collect names, demographic descriptors, or practice characteristics of physicians. Participants and, by extension, their physicians were blinded to research questions studied herein. This research was approved by the North Texas Institutional Review Board, and all participants provided written informed consent. This study followed the Strengthening the Reporting of Observational Studies in Epidemiology ( STROBE ) reporting guideline. 16
Patients reported their physician’s empathy at enrollment using the CARE measure. 8 , 9 It included 10 items about characteristics pertaining to physician empathy during medical encounters using an ordinal rating scale (score), including 1 for poor; 2, fair; 3, good; 4, very good; and 5, excellent. It was scored as the sum of responses to each item, ranging from 10 to 50, with higher scores indicating greater physician empathy. During development, the CARE measure demonstrated face and content validity and high internal validity (Cronbach α, 0.93), although scores were positively skewed (about one-fourth of patients reported a maximum physician empathy score). 8 It was perceived by patients and physicians to be relevant to general medical encounters across different populations. 9 Because CARE measure scores are positively skewed, we transformed them using a dichotomous variable theoretically based on its rating descriptors. Physicians whose scores were 30 or higher (ie, rated as good, very good, or excellent on most items) were classified as very empathic physicians (VEPs), whereas those whose scores were 29 or lower (ie, rated as poor or fair on most items) were classified as slightly empathic physicians (SEPs). The latter physicians made up approximately the lowest quartile of CARE measure scores. Data were analyzed only for encounters in which patients reported continuously having the same physician they had at enrollment. We assessed long-term stability of CARE measure scores among patients who retained the same physician 24 months later, when it was next administered.
The outcomes were patient-reported pain, function, and HRQOL. Pain intensity was measured with a numerical rating scale for the typical pain level in the 7 days prior to each encounter, ranging from 0 to 10. Back-related disability, or function, on the encounter date was measured with the Roland-Morris Disability Questionnaire, and scores ranged from 0 to 24 to reflect difficulties that patients with CLBP may experience on each of its items. 17 Because pain intensity may not adequately capture the overall experience of patients living with and negatively affected by CLBP, 18 - 20 we assessed HRQOL deficits using the Patient-Reported Outcomes Measurement Information System with 29 items. 21 This involved measures of anxiety, depression, fatigue, sleep disturbance, and pain interference. Each measure included 4 ordinal-scale items that were scored and normed according to the US general population to yield mean (SD) scores of 50 (10). The sole exception was sleep disturbance, which was normed using a calibration sample enriched with patients having chronic illness. Higher scores on all assessments represented worse outcomes for pain, function, and HRQOL.
Comprehensive data pertaining to CLBP were collected at enrollment, 15 and a series of baseline variables were selected to characterize patients and control for potential confounding. Sociodemographic characteristics included age, sex, race, ethnicity, and educational level. Health history included cigarette smoking status, musculoskeletal comorbidities (herniated disc, sciatica, osteoarthritis, and osteoporosis), and general medical comorbidities (hypertension, heart disease, diabetes, asthma, and depression). The CLBP history was characterized by ongoing duration (<1, 1-5, or >5 years). Data on CLBP treatment included nonpharmacological treatments ever used (exercise therapy, yoga, massage therapy, spinal manipulation, acupuncture, and cognitive behavioral therapy), opioid therapy, and lumbar spine surgery. Current opioid use and lumbar spine surgery were measured at each encounter. Race categories included American Indian or Alaska Native, Asian, Black or African American, Native Hawaiian or Other Pacific Islander, and White. (Racial categories other than White were combined in statistical analyses because small numbers in some racial categories yielded imprecise estimates that precluded meaningful interpretation.) Ethnicity categories included Hispanic and non-Hispanic. Categories were ascertained by self-report and were included in the study to serve as covariates used to adjust for potential confounding in the statistical analyses.
Patient characteristics according to physician empathy group were described and compared using number (percentage) or mean (SD). Generalized estimating equation (GEE) models were used initially to assess associations among CARE measure scores and each outcome over 12 months, including a time variable (number of quarterly intervals) to assess temporal trends. All GEE models used an autoregressive AR(1) correlation structure and fixed effects and included an empathy × time interaction term. For more tangible assessments of clinical relevance, GEE models also compared outcomes of the VEP vs SEP groups. The GEE analyses were repeated using a comprehensive multivariable model that included baseline and longitudinal data to adjust for potential confounding in addition to measuring time effects. Diagnostic plots, including normal Q-Q plots of Pearson residuals, supported appropriateness of GEE modeling and did not identify influential outliers. Clinically relevant differences in CARE measure scores and outcomes between VEP and SEP groups were assessed using thresholds for the magnitude of Cohen d (small effect: Cohen d = 0.2; medium effect: Cohen d = 0.5; and large effect: Cohen d = 0.8), 22 with positive Cohen d statistics favoring the VEP group. Sensitivity analyses were performed by repeating the aforementioned analyses with alternative CARE measure score cut points to classify physician empathy.
Statistical power was estimated with the General Linear Mixed Model Power and Sample Size program for repeated measures designs 23 and involved hypothesized mean differences in outcomes between VEP and SEP groups during 5 encounters over 12 months. Our sample size was sufficiently large to exceed 95% statistical power in detecting clinically important differences (Cohen d ≥ 0.2 in magnitude) between VEP and SEP groups on all outcomes in a wide variety of scenarios involving the correlation among outcome variables, base correlation of outcome variables at successive encounters within patients, and decay rate of the base correlation with increased time between encounters. The study was not designed to detect significant empathy × time interactions, owing to uncertainties about the nature of potential interactions (reversed, fully attenuated, or partially attenuated) and thresholds for clinical relevance. 24 Data were managed and analyzed using SPSS Statistics, version 29 (IBM Inc). Hypotheses were assessed at the α level of .05 using 2-sided testing.
A total of 1470 patients were studied, including 1133 (77.1%) in the VEP group and 337 (22.9%) in the SEP group. The mean (SD) age of patients was 53.1 (13.2) years, 1093 (74.4%) were female, and 377 (25.6%) were male. The mean (SD) CARE measure score at enrollment was 38.4 (11.6). The mean (SD) CARE measure scores for the VEP and SEP groups were 43.7 (6.6) vs 20.6 (5.9) ( P < .001; Cohen d = 3.57). The CARE measure scores were remarkably stable among 319 patients with 24-month follow-up (mean difference, −0.02; 95% CI, −1.32 to 1.29; P = .98; Cohen d = 0). The mean CARE measure score among 120 patients (8.2%) lost to follow-up was 38.5 (95% CI, 36.3-40.6) vs 38.4 (95% CI, 37.8-39.0) for the remaining patients ( P = .95; Cohen d = 0.01). Baseline characteristics of the VEP and SEP groups were generally comparable, although there were marginally significant differences indicating that SEP group patients were more likely to be current smokers and to report a history of herniated disc, sciatica, and depression ( Table 1 ).
Patients completed 5943 encounters, including 1470, 1296, 1192, 989, and 996 at successive encounters over 12 months. In GEE models that included only empathy, time, and empathy × time as explanatory variables for outcomes over 12 months, the CARE measure score was inversely associated with pain intensity (β = −0.013; 95% CI, −0.022 to −0.005; P = .003), back-related disability (β = −0.066; 95% CI, −0.091 to −0.040; P < .001), and each HRQOL deficit (eg, depression: β = −0.106; 95% CI, −0.150 to −0.062; P < .001) (eTable 1 in Supplement 1 ). Pain intensity decreased over time (β = −0.094; 95% CI, −0.185 to −0.003; P = .04), whereas back-related disability and HRQOL deficits did not change over time. There were generally no empathy × time interaction effects observed in these or subsequent analyses throughout the study.
Mean pain intensity in the VEP group was 5.7 (95% CI, 5.6-5.8) vs 6.2 (95% CI, 6.0-6.3) in the SEP group ( P = .002) ( Figure 1 A). Similarly, mean back-related disability was 13.5 (95% CI, 13.1-13.8) in the VEP group vs 15.6 (95% CI, 15.0-16.1) in the SEP group ( P < .001) ( Figure 1 B). Mean scores on each HRQOL deficit measure were also lower in the VEP group compared with the SEP group ( Figure 2 ); the score for mean anxiety in the VEP group was 54.6 (95% CI, 54.1-55.1) vs 57.5 (95% CI, 56.5-58.4) in the SEP group ( P < .001) and for sleep disturbance, 56.6 (95% CI, 56.2-57.0) in the VEP group vs 59.1 (95% CI, 58.3-59.8) in the SEP group ( P < .001). All between-group differences pertaining to pain, function, and HRQOL yielded Cohen d statistics that met criteria for clinical relevance, ranging from 0.22 for pain intensity to 0.36 for fatigue (eTable 2 in Supplement 1 ).
In GEE models that included empathy, time, empathy × time, and the full array of explanatory variables for outcomes over 12 months, the CARE measure score was inversely associated with pain intensity (β = −0.014; 95% CI, −0.022 to −0.006; P < .001), back-related disability (β = −0.062; 95% CI, −0.085 to −0.040; P < .001), and each HRQOL deficit (eg, pain interference: β = −0.080; 95% CI, −0.111 to −0.049; P < .001) (eTable 3 in Supplement 1 ). Mean pain intensity in the VEP group was 6.3 (95% CI, 6.1-6.5) vs 6.7 (95% CI, 6.5-6.9) in the SEP group ( P < .001) (eTable 4 in Supplement 1 ). Similarly, mean back-related disability was 14.9 (95% CI, 14.2-15.6) in the VEP group vs 16.8 (95% CI, 16.0-17.6) in the SEP group ( P < .001). Mean scores on each HRQOL deficit measure were also lower in the VEP group compared with the SEP group (eg, fatigue: 57.3; 95% CI, 56.1-58.5 in the VEP group vs 60.4; 95% CI, 59.0-61.7 in the SEP group; P < .001). All between-group differences pertaining to pain, function, and HRQOL remained clinically relevant, with Cohen d statistics ranging from 0.21 for pain intensity to 0.30 for back-related disability, fatigue, and pain interference.
Greater levels of physician empathy were generally associated with better outcomes in pain, function, and HRQOL in analyses that were unadjusted (eTable 5 in Supplement 1 ) and adjusted for multiple covariates (eTable 6 in Supplement 1 ) in addition to time. The favorability of having a more empathic physician generally increased as the CARE measure score cut point for greater vs lesser physician empathy decreased. The greatest group differences, which were observed for patients having physicians with CARE measure scores 20 or higher vs 19 or lower, involved Cohen d statistics ranging from 0.29 for pain intensity to 0.56 for fatigue (eTable 5 in Supplement 1 ) and from 0.24 for anxiety to 0.46 for fatigue (eTable 6 in Supplement 1 ).
Although the outcomes of having a VEP exceeded the threshold for clinical relevance in models that were covariate unadjusted (eTable 2 in Supplement 1 ) and covariate adjusted (eTable 4 in Supplement 1 ), they were classified as small effects in the main analyses, and no empathy × time interaction effects were observed. However, the magnitude of physician empathy effects exceeded those reported for nonpharmacological treatments, current opioid use, and lumbar spine surgery, as demonstrated by their respective β coefficients ( Table 2 and Table 3 ). Moreover, unlike physician empathy, patients receiving these 3 treatments generally reported worse outcomes. For example, current opioid use was associated with greater pain intensity (β = 0.351; 95% CI, 0.221-0.481; P < .001) and back-related disability (β = 1.205; 95% CI, 0.870-1.541; P < .001), and lumbar spine surgery was associated with greater back-related disability (β = 0.992; 95% CI, 0.344-1.639; P = .003) ( Table 2 ). Furthermore, although not significant, empathy × time interaction effects trended to favor the VEP group for pain, function, and all HRQOL outcomes except sleep disturbance.
In this cohort study of adults with CLBP, physician empathy was inversely associated with pain intensity, back-related disability, and HRQOL deficits in all main analyses, including those that controlled for time effects and a wide array of sociodemographic and clinical covariates at baseline and also for current opioid use and lumbar spine surgery over 12 months. Moreover, all VEP compared with SEP group differences were clinically relevant. Similar trends were observed in sensitivity analyses that measured outcomes after altering cut points for classifying physician empathy. Empathy is an essential aspect of the patient-physician relationship in delivering patient-centered care. It is particularly important in pain medicine in which traditional, hard outcome measures are not often available, and softer outcomes driven by patient perceptions of pain, function, and HRQOL are generally the rule. Patients who somatize their chronic pain may be more likely to discuss psychosocial issues with VEPs, thereby directing diagnostic and therapeutic efforts down more rewarding paths that enhance compliance and outcomes. 25
Although our findings suggest that greater physician empathy should be encouraged during encounters for chronic pain, there is a longstanding debate about whether it can or should be taught. 26 One view is that physician empathy cannot be achieved in the patient-physician relationship and questions attempts to measure it. 27 An alternative view is that it is a skill that, although partly genetic, can be purposefully grown, broadened, and fine-tuned through life experiences to improve medical care. 28 An aspirational view is that empathy should not be limited to individual practitioners but should be incorporated within the broader domain of health care systems. 29 Such systems would be structured and organized to facilitate empathic health care delivery using macro-level decisions involving political and jurisdictional considerations.
Medical students and residents often become less empathic during education and training, owing to a greater perceived need for patient detachment and reliance on technology. The challenges of contemporary medicine, including electronic medical records and time constraints, may also contribute to an erosion of empathy among seasoned physicians. 30 Although more research is needed on interventions to cultivate physician empathy, 31 a randomized clinical trial of training grounded in the neurobiology of empathy demonstrated significant improvements in patient-reported empathy among resident physicians. 32 Our sensitivity analysis findings suggest that the greatest improvements in pain-related outcomes may be achieved by targeting for intervention those physicians comprising the lowest decile of empathy. More research involving rigorous designs is needed to determine if greater physician empathy improves clinical outcomes.
Strengths of our study include recruiting participants through a national registry, longitudinal follow-up for 12 months, and multivariable adjustment of outcomes for potential confounders. We also measured empathy as perceived by patients rather than self-reported by physicians. By contrast, a systematic review of interventions to cultivate physician empathy found that 58 of 64 studies, including most of the least rigorous studies, used physician self-reported measures of empathy. 31 Finally, we only included patients who retained the same physician during all encounters, and CARE measure scores among registry participants with 24 months of follow-up indicated that physician empathy remained remarkably stable over time.
There are also limitations of this study. First, although patients resembled adults with CLBP in the National Health and Nutrition Examination Survey on characteristics such as age, sex, educational level, cigarette smoking status, and medical comorbidities, 33 PRECISION is not a population-based registry, and results may be subject to participant volunteer bias. Moreover, we excluded participants who were unable to complete case report forms in English. Second, although patients included only those with a physician who provided CLBP usual care, factors such as physician demographic characteristics, racial concordance, specialty, and clinical setting were not measured. Third, the registry did not collect sensitive participant data such as income or health insurance coverage. Thus, we relied on educational level as a surrogate measure for socioeconomic factors in our models with covariate adjustment. Fourth, we cannot rule out that patient ratings of physician empathy at enrollment may have been affected by preexisting pain, function, or HRQOL. However, adjusting for baseline values of these outcome variables would have negated prior benefits in these domains that may have been attributable to physician empathy. Finally, all data pertaining to explanatory and outcome variables were self-reported and not otherwise corroborated.
In this cohort study of patients with chronic pain, physician empathy was associated with better longitudinal outcomes in pain, function, and HRQOL over 12 months, including in multivariable analyses that controlled for time effects and a comprehensive array of covariates that included current opioid use and lumbar spine surgery throughout the study. The outcomes of having a VEP were clinically relevant and better than those associated with nonpharmacological treatments, opioid therapy, and lumbar spine surgery. Physician empathy is an important aspect of the patient-physician relationship among those with chronic pain. Greater efforts to cultivate and improve physician empathy appear warranted in this population.
Accepted for Publication: February 13, 2024.
Published: April 11, 2024. doi:10.1001/jamanetworkopen.2024.6026
Open Access: This is an open access article distributed under the terms of the CC-BY License . © 2024 Licciardone JC et al. JAMA Network Open .
Corresponding Author: John C. Licciardone, DO, MS, MBA, University of North Texas Health Science Center at Fort Worth, 3500 Camp Bowie Blvd, Fort Worth, TX 76107 ( [email protected] ).
Author Contributions: Dr Licciardone had full access to all of the data in the study and takes responsibility for the integrity of the data and the accuracy of the data analysis.
Concept and design: All authors.
Acquisition, analysis, or interpretation of data: All authors.
Drafting of the manuscript: All authors.
Critical review of the manuscript for important intellectual content: All authors.
Statistical analysis: All authors.
Administrative, technical, or material support: Licciardone, Tran.
Supervision: Licciardone, Aryal.
Conflict of Interest Disclosures: None reported.
Data Sharing Statement: See Supplement 2 .
- Register for email alerts with links to free full-text articles
- Access PDFs of free articles
- Manage your interests
- Save searches and receive search alerts

- SUGGESTED TOPICS
- The Magazine
- Newsletters
- Managing Yourself
- Managing Teams
- Work-life Balance
- The Big Idea
- Data & Visuals
- Reading Lists
- Case Selections
- HBR Learning
- Topic Feeds
- Account Settings
- Email Preferences
Advice for the Unmotivated
- Robin Abrahams
- Boris Groysberg

How to reignite your enthusiasm for work
Employee disengagement is rampant in the workplace. We’ve all experienced it as customers encountering unhelpful retail clerks and as colleagues dealing with apathetic teammates. But what happens when you yourself feel dead at work?
This article describes what you as an individual can do to sustain your motivation or recover it, even in the most stultifying of jobs. After synthesizing research on this challenge and experimenting with various strategies, the authors have developed a process for recharging yourself called DEAR.
The first step is to detach and objectively analyze your situation so that you can make wise choices about it, instead of reacting in a fight-or-flight way. At day’s end, review what went well at your job and then mentally disconnect from it to give yourself a break. Meditation and exercise can help you do that and will improve your mood and cognitive function. Next, show empathy. Practice self-care, make friends, recognize the accomplishments of others, seek their views, and help them. Research shows that this combats burnout. Third, take action: achieve small wins, invest in rewarding outside activities, redefine your responsibilities, and turn uninteresting tasks into games. Ask yourself how someone you admire would behave in your situation, and dress in a way that projects confidence. Last, reframe your thinking: Focus on the informal roles you enjoy at work, your job’s higher-order purpose, and how others benefit from your work. All these techniques will improve your mental health and increase the energy you bring to your job—even if it is not what you’d like it to be.
In virtually everyone’s career, there comes a time when motivation and interest vanish. The usual tasks feel tedious. It’s hard to muster the energy for new projects. Though we go through the motions of being good employees or managers, we’re not really “there.” We become ghosts or zombies: the working dead.
- Robin Abrahams is a research associate at Harvard Business School.
- BG Boris Groysberg is a professor of business administration in the Organizational Behavior unit at Harvard Business School and a faculty affiliate at the school’s Race, Gender & Equity Initiative. He is the coauthor, with Colleen Ammerman, of Glass Half-Broken: Shattering the Barriers That Still Hold Women Back at Work (Harvard Business Review Press, 2021). bgroysberg
Partner Center

An official website of the United States government
The .gov means it’s official. Federal government websites often end in .gov or .mil. Before sharing sensitive information, make sure you’re on a federal government site.
The site is secure. The https:// ensures that you are connecting to the official website and that any information you provide is encrypted and transmitted securely.
- Publications
- Account settings
Preview improvements coming to the PMC website in October 2024. Learn More or Try it out now .
- Advanced Search
- Journal List
- v.7(4); Fall 2003

An Overview of Empathy
Empathy is a powerful communication skill that is often misunderstood and underused. Initially, empathy was referred to as “bedside manner”; now, however, authors and educators consider empathetic communication a teachable, learnable skill that has tangible benefits for both clinician and patient: Effective empathetic communication enhances the therapeutic effectiveness of the clinician-patient relationship. Appropriate use of empathy as a communication tool facilitates the clinical interview, increases the efficiency of gathering information, and honors the patient.
Introduction
That the medical care experience is enhanced by effective communication between clinicians and their patients is a well established fact. Byproducts of this enhanced communication include improved health outcomes, 1 better patient compliance, 2 reduction in medical-legal risk, 3 – 5 and improved satisfaction of clinicians and patients. 6 , 7 Of all the elements involved in effective communication, empathy seems to be the component that is most powerful yet is easily overlooked—and some commentators have asserted that in medical practice the importance of empathy cannot be overemphasized. 8
What is Empathy?
The origin of the word empathy dates back to the 1880s, when German psychologist Theodore Lipps coined the term “einfuhlung” (literally, “in-feeling”) to describe the emotional appreciation of another’s feelings. Empathy has further been described as the process of understanding a person’s subjective experience by vicariously sharing that experience while maintaining an observant stance. 9 Empathy is a balanced curiosity leading to a deeper understanding of another human being; stated another way, empathy is the capacity to understand another person’s experience from within that person’s frame of reference. 10
Even more simply stated, empathy is the ability to “put oneself in another’s shoes.” In an essay entitled “Some Thoughts on Empathy,” Columbia University psychiatrist Alberta Szalita stated, “I view empathy as one of the important mechanisms through which we bridge the gap between experience and thought.” A few sentences earlier in her essay, she had emphasized that … “[empathy is] consideration of another person’s feelings and readiness to respond to his [or her] needs … without making his [or her] burden one’s own.” 11:p151
Can Empathy Be Taught?
Unfortunately, many physicians were trained in the world of “Find it and Fix it” medicine, a world where empathetic communication was only an afterthought—if this behavior was considered at all. Empathy was known as “bedside manner,” a quality considered innate and impossible to acquire—either you were born with it or you weren’t. More recently, greater emphasis has been placed on empathy as a communication tool of substantial importance in the medical interview, and many experts now agree that empathy and empathetic communication are teachable, learnable skills. 12 , 13 As we might therefore expect, empathy is the cornerstone of several communication models, including “The Four Habits” model (Invest in the Beginning, Elicit the Patient’s Perspective, Demonstrate Empathy, Invest in the End) developed by The Permanente Medical Group’s Terry Stein with Richard Frankel; 14 “The 4 E’s” (Engage, Empathize, Educate, and Enlist) model used by the Bayer Institute for Health Care Communication; 15 the “PEARLS” (Partnership, Empathy, Apology, Respect, Legitimization, Support) framework adopted by the American Academy on Physician and Patient; 16 and other models. 17 , 18
Many medical schools have developed curricula with a strong focus on physician-patient communication and empathy. Delivery of these curricula begins early in the students’ training. At the University of Colorado Health Sciences Center, this curriculum is known as the “Foundations of Doctoring” program, a curriculum whose teaching staff includes several physicians and trainers from the Colorado Permanente Medical Group (CPMG). CPMG has also developed an eight-hour clinician-patient communication course based on The Four Habits model which is offered to all newly hired physicians in the Kaiser Permanente (KP) Colorado Region. In this course, plenty of time is set aside to explore empathy and to practice empathetic communication with patients selected according to standard criteria.
Practical Empathetic Communication
Making practical use of an otherwise esoteric concept such as empathy requires division of the concept into its simplest elements. As outlined by Frederic Platt, 19 key steps to effective empathy include:
- recognizing presence of strong feeling in the clinical setting (ie, fear, anger, grief, disappointment);
- pausing to imagine how the patient might be feeling;
- stating our perception of the patient’s feeling (ie, “I can imagine that must be …” or “It sounds like you’re upset about …”);
- legitimizing that feeling;
- respecting the patient’s effort to cope with the predicament; and
- offering support and partnership (ie, “I’m committed to work with you to …” or “Let’s see what we can do together to …”).
Being a psychiatrist or mental health expert is not necessary for using empathetic communication; the only requirement is an awareness of opportunities for empathy as they arise during the interview with a patient. This type of opportunity arises from a patient’s emotion (either directly expressed or implied): This emotion creates the opportunity for an empathetic response by the physician. In a study by Wendy Levinson et al, 20 116 office visits to primary care and surgical physicians were audiotaped and transcribed to look at the frequency of empathy opportunities or “clues.” More than half of visits in each setting included one or more clues. In more than half of cases, patients presented these clues not overtly but in more subtle ways. Unfortunately, physicians responded to those clues in only 38% of surgical cases and in only 21% of primary care cases and frequently missed opportunities to adequately acknowledge a patient’s feelings. 20 Clues are often hidden in the fabric of discussion about medical problems and thus may be easily missed by physicians who are busy attending to biomedical details of diagnosis and management. In fact, when opportunities for empathy are missed by physicians, patients tend to offer them again, sometimes repeatedly. This phenomenon can lead to longer, more frustrating interviews, return visits, and “doctor shopping” by patients who feel dismissed or alienated.
After an opportunity for empathy has been presented, the clinician should consider offering a gesture or statement of empathy. Statements that facilitate empathy have been categorized as queries, clarifications, and responses. 21 Examples of each are as follows:
- “Can you tell me more about that?”
- “What has this been like for you?”
- “How has all of this made you feel?”
- “Let me see if I’ve gotten this right … ”
- “Tell me more about … ”
- “I want to make sure I understand what you’ve said … ”
- “Sounds like you are … ”
- “I imagine that must be … ”
- “I can understand that must make you feel … ”
Ideally, after perceiving the clinician’s statement of empathy, the patient expresses agreement or confirmation (“You got it, Doc!” or “Yeah, that’s exactly how I feel”). When we have not understood the patient’s experience exactly, we must allow the patient to correct our perception. Use of the Hypothesis-Test-Feedback Loop allows the patient to clarify his or her experience and thus allow the physician to restate an empathetic statement that originally missed its mark. The following exchange is an example of this Hypothesis-Test-Feedback Loop used in the doctor-patient encounter:
Patient: I am sick and tired of living with these headaches. No one has been able to help me, and none of the medications are working. Doctor (stating the hypothesis): I can see that you are frustrated by the lack of improvement in your symptoms. Patient (giving feedback): Yeah, but I’m really more worried that we’re missing something serious. I’ve got a wife and kids who are depending on me. Doctor (correcting the hypothesis): So, it sounds like you’re really more concerned that something serious could be going on that is causing these headaches. Patient (closing the empathy loop): Yes, exactly.
In this example, the physician makes an empathetic statement (hypothesis) about what he or she surmises is the chief aspect of the patient’s experience: frustration about an unrelenting headache. When the hypothesis is tested, the patient clarifies that although frustrated, he is mainly experiencing worry about the situation. Armed with this feedback, the physician restates the hypothesis back to the patient, who lets the physician know that he or she “got it exactly right.”
Barriers to Giving Empathy
Because empathy is such a powerful communication skill, we might suppose that clinicians would scramble to learn about and use it at every available opportunity. However, this is not necessarily the case. Clinicians have many reasons for not offering empathy to patients. An informal survey of practicing clinicians participating in a recent clinician-patient communication course revealed misgivings (and misconceptions) about empathetic communication. Concerns mentioned included:
- “There is not enough time during the visit to give empathy.”
- “It is not relevant, and I’m too busy focusing on the acute medical problem.”
- “Giving empathy is emotionally exhausting for me.”
- “I don’t want to open that Pandora’s box.”
- “I haven’t had enough training in empathetic communication.”
- “I’m concerned that if I use up all my empathy at work I won’t have anything left for my family.”
In our experience, empathy facilitates the clinical interview, increases efficiency of gathering information, and honors the patient. Empathy need not be awkward nor emotionally exhausting; unlike sympathy, empathy does not require emotional effort on the part of the clinician. An appropriate statement or gesture of empathy takes only a moment and can go a long way to enhance rapport, build positive relationships, and even improve difficult ones. Studies have shown that when opportunities for empathy were repeatedly missed, visits tended to be longer and more frustrating for both physician and patient. 18 , 20 Conversely, empathy may save time and expense and often is a cost-effective method of facilitating early diagnosis and proper treatment. 10
Empathy Versus Sympathy (and Versus Pity)
Despite some divergent opinion on the matter, we may propose a subtle but important distinction between empathy and sympathy.
Whereas empathy is used by skilled clinicians to enhance communication and delivery of care, sympathy can be burdensome and emotionally exhausting and can lead to burnout. Sympathy implies feeling shared with the sufferer as if the pain belonged to both persons: We sympathize with other human beings when we share and suffer with them. It would stand to reason, therefore, that completely shared suffering can never exist between physician and patient; otherwise, the physician would share the patient’s plight and would therefore be unable to help.
Empathy is concerned with a much higher order of human relationship and understanding: engaged detachment. In empathy, we “borrow” another’s feelings to observe, feel, and understand them— but not to take them onto ourselves. By being a participant-observer, we come to understand how the other person feels. An empathetic observer enters into the equation and then is removed.
Harry Wilmer 22 summarizes these three emotions—Empathy, Sympathy, and Pity—as follows:
- Pity describes a relationship which separates physician and patient. Pity is often condescending and may entail feelings of contempt and rejection.
- Sympathy is when the physician experiences feelings as if he or she were the sufferer. Sympathy is thus shared suffering.
- Empathy is the feeling relationship in which the physician understands the patient’s plight as if the physician were the patient. The physician identifies with the patient and at the same time maintains a distance. Empathetic communication enhances the therapeutic effectiveness of the clinician-patient relationship.
Empathy is a powerful, efficient communication tool when used appropriately during a medical interview. Empathy extends understanding of the patient beyond the history and symptoms to include values, ideas, and feelings. Benefits of improved empathetic communication are tangible for both physician and patient.
Acknowledgments
Ilene Kasper, MS, and Andrew M Lum, MD of Kaiser Permanente Colorado; and Brian Dwinnell, MD, and Frederic W Platt, MD, FACP, of the University of Colorado Health Sciences Center, reviewed the article.
James T Hardee, MD , Obtained his medical degree from the University of New Mexico School of Medicine, where he also completed his Internal Medicine residency. He joined the Colorado Permanente Medical Group in 1998 and is currently on the CPMG Board of Directors. He is the physician lead for Clinician-Patient Communication in the Colorado Region. E-mail: [email protected] .

Two Things Stand
Life is mostly froth and bubble, Two things stand like stone, Kindness in another’s trouble, Courage in your own. Adam Lindsay Gordon, 1833–1870, poet
April 2, 2024
Eclipse Psychology: When the Sun and Moon Align, So Do We
How a total solar eclipse creates connection, unity and caring among the people watching
By Katie Weeman
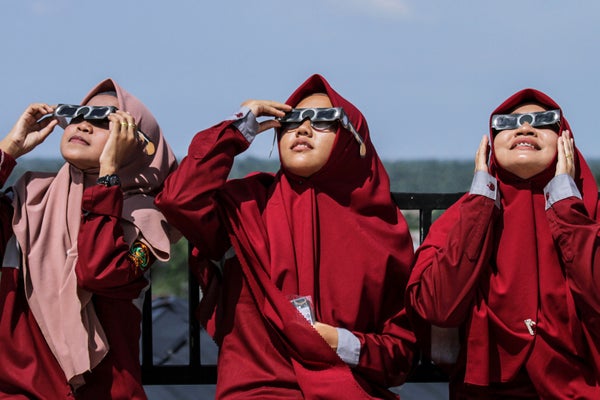
Students observing a partial solar eclipse on June 21, 2020, in Lhokseumawe, Aceh Province, Indonesia.
NurPhoto/Getty Images
This article is part of a special report on the total solar eclipse that will be visible from parts of the U.S., Mexico and Canada on April 8, 2024.
It was 11:45 A.M. on August 21, 2017. I was in a grassy field in Glendo, Wyo., where I was surrounded by strangers turned friends, more than I could count—and far more people than had ever flocked to this town, population 210 or so. Golden sunlight blanketed thousands of cars parked in haphazard rows all over the rolling hills. The shadows were quickly growing longer, the air was still, and all of our faces pointed to the sky. As the moon progressively covered the sun, the light melted away, the sky blackened, and the temperature dropped. At the moment of totality, when the moon completely covered the sun , some people around me suddenly gasped. Some cheered; some cried; others laughed in disbelief.
Exactly 53 minutes later, in a downtown park in Greenville, S.C., the person who edited this story and the many individuals around him reacted in exactly the same ways.
On supporting science journalism
If you're enjoying this article, consider supporting our award-winning journalism by subscribing . By purchasing a subscription you are helping to ensure the future of impactful stories about the discoveries and ideas shaping our world today.
When a total solar eclipse descends—as one will across Mexico, the U.S. and Canada on April 8—everyone and everything in the path of totality are engulfed by deep shadow. Unlike the New Year’s Eve countdown that lurches across the globe one blocky time zone after another, the shadow of totality is a dark spot on Earth that measures about 100 miles wide and cruises steadily along a path, covering several thousand miles in four to five hours. The human experiences along that path are not isolated events any more than individual dominoes are isolated pillars in a formation. Once that first domino is tipped, we are all linked into something bigger—and unstoppable. We all experience the momentum and the awe together.
When this phenomenon progresses from Mexico through Texas, the Great Lakes and Canada on April 8, many observers will describe the event as life-changing, well beyond expectations. “You feel a sense of wrongness in those moments before totality , when your surroundings change so rapidly,” says Kate Russo, an author, psychologist and eclipse chaser. “Our initial response is to ask ourselves, ‘Is this an opportunity or a threat?’ When the light changes and the temperature drops, that triggers primal fear. When we have that threat response, our whole body is tuned in to taking in as much information as possible.”
Russo, who has witnessed 13 total eclipses and counting, has interviewed eclipse viewers from around the world. She continues to notice the same emotions felt by all. They begin with that sense of wrongness and primal fear as totality approaches. When totality starts, we feel powerful awe and connection to the world around us. A sense of euphoria develops as we continue watching, and when it’s over, we have a strong desire to seek out the next eclipse.
“The awe we feel during a total eclipse makes us think outside our sense of self. It makes you more attuned to things outside of you,” says Sean Goldy, a postdoctoral fellow at the department of psychiatry and behavioral sciences at Johns Hopkins University.
Goldy and his team analyzed Twitter data from nearly 2.9 million people during the 2017 total solar eclipse. They found that people within the path of totality were more likely to use not only language that expressed awe but also language that conveyed being unified and affiliated with others. That meant using more “we” words (“us” instead of “me”) and more humble words (“maybe” instead of “always”).
“During an eclipse, people have a broader, more collective focus,” Goldy says. “We also found that the more people expressed awe, the more likely they were to use those ‘we’ words, indicating that people who experience this emotion feel more connected with others.”
This connectivity ties into a sociological concept known as “collective effervescence,” Russo and Goldy say. When groups of humans come together over a shared experience, the energy is greater than the sum of its parts. If you’ve ever been to a large concert or sporting event, you’ve felt the electricity generated by a hive of humans. It magnifies our emotions.
I felt exactly that unified feeling in the open field in Glendo, as if thousands of us were breathing as one. But that’s not the only way people can experience a total eclipse.
During the 2008 total eclipse in Mongolia “I was up on a peak,” Russo recounts. “I was with only my husband and a close friend. We had left the rest of our 25-person tour group at the bottom of the hill. From that vantage point, when the shadow came sweeping in, there was not one man-made thing I could see: no power lines, no buildings or structures. Nothing tethered me to time: It could have been thousands of years ago or long into the future. In that moment, it was as if time didn’t exist.”
Giving us the ability to unhitch ourselves from time—to stop dwelling on time is a unique superpower of a total eclipse. In Russo’s work as a clinical psychologist, she notices patterns in our modern-day mentality. “People with anxiety tend to spend a lot of time in the future. And people with depression spend a lot of time in the past,” she says. An eclipse, time and time again, has the ability to snap us back into the present, at least for a few minutes. “And when you’re less anxious and worried, it opens you up to be more attuned to other people, feel more connected, care for others and be more compassionate,” Goldy says.
Russo, who founded Being in the Shadow , an organization that provides information about total solar eclipses and organizes eclipse events around the world, has experienced this firsthand. Venue managers regularly tell her that eclipse crowds are among the most polite and humble: they follow the rules; they pick up their garbage—they care.
Eclipses remind us that we are part of something bigger, that we are connected with something vast. In the hours before and after totality you have to wear protective glasses to look at the sun, to prevent damage to your eyes. But during the brief time when the moon blocks the last of the sun’s rays, you can finally lower your glasses and look directly at the eclipse. It’s like making eye contact with the universe.
“In my practice, usually if someone says, ‘I feel insignificant,’ that’s a negative thing. But the meaning shifts during an eclipse,” Russo says. To feel insignificant in the moon’s shadow instead means that your sense of self shrinks, that your ego shrinks, she says.
The scale of our “big picture” often changes after witnessing the awe of totality, too. “When you zoom out—really zoom out—it blows away our differences,” Goldy says. When you sit in the shadow of a celestial rock blocking the light of a star 400 times its size that burns at 10,000 degrees Fahrenheit on its surface, suddenly that argument with your partner, that bill sitting on your counter or even the differences among people’s beliefs, origins or politics feel insignificant. When we shift our perspective, connection becomes boundless.
You don’t need to wait for the next eclipse to feel this way. As we travel through life, we lose our relationship with everyday awe. Remember what that feels like? It’s the way a dog looks at a treat or the way my toddler points to the “blue sky!” outside his car window in the middle of rush hour traffic. To find awe, we have to surrender our full attention to the beauty around us. During an eclipse, that comes easily. In everyday life, we may need to be more intentional.
“Totality kick-starts our ability to experience wonder,” Russo says. And with that kick start, maybe we can all use our wonderment faculties more—whether that means pausing for a moment during a morning walk, a hug or a random sunset on a Tuesday. In the continental U.S., we won’t experience another total eclipse until 2044. Let’s not wait until then to seek awe and connection.
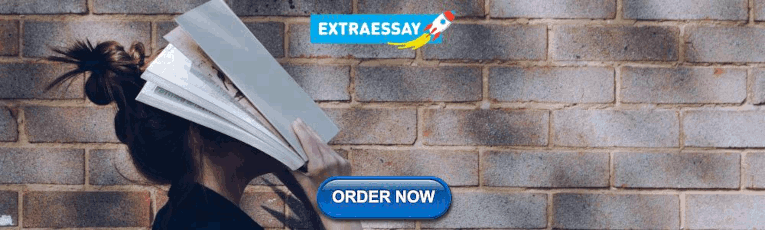
IMAGES
VIDEO
COMMENTS
In the past, empathy was considered an inborn trait that could not be taught, but research has shown that this vital human competency is mutable and can be taught to health-care providers. The evidence for patient-rated empathy improvement in physicians has been demonstrated in pilot and retention studies (3,4) and a randomized controlled trial .
For example, research by C. Daniel Batson, PhD, a professor emeritus of social psychology at the University of Kansas, suggests empathy can motivate people to help someone else in need (Altruism in Humans, Oxford University Press, 2011), and a 2019 study suggests empathy levels predict charitable donation behavior (Smith, K. E., et al.,
The current article is an integrative and analytical literature review on the concept and meaning of empathy in health and social care professionals. Empathy, i.e., the ability to understand the personal experience of the patient without bonding with them, constitutes an important communication skill for a health professional, one that includes ...
Empathy is a Hardwired Capacity. Research in the neurobiolgy of empathy has changed the perception of empathy from a soft skill to a neurobiologically based competency ().The theory of inner imitation of the actions of others in the observer has been supported by brain research. Functional magnetic resonance imaging now demonstrates the existence of a neural relay mechanism that allows ...
In general, authors consider empathy as the competence of a physician to understand the patient's situation, perspective, and feelings; to communicate that understanding and check its accuracy; and to act on that understanding in a helpful therapeutic way. It has an affective, a cognitive, and a behavioural dimension. 1, 21 - 24.
Evidence to date indicates that compassion and empathy are health-enhancing qualities. Research points to interventions and practices involving compassion and empathy being beneficial, as well as being salient outcomes of contemplative practices such as mindfulness. Advancing the science of compassion and empathy requires that we select measures best suited to evaluating effectiveness of ...
Abstract. Empathy is one of the most consistent outcome predictors in contemporary psychotherapy research. The function of empathy is particularly important for the development of a positive therapeutic relationship: patients report positive therapeutic experiences when they feel understood, safe, and able to disclose personal information to their therapists.
A General Theoretical Perspective on Empathy. Historically, two distinct lines of research have evolved around empathy (for an overview see, e.g., Baron-Cohen & Wheelwright, 2004; Davis, 1983).First, from the affective perspective, empathy describes the emotional reactions to another person's affective experiences.According to Eisenberg and Miller (), this means that one experiences the same ...
The constructs of empathy (i.e., understanding and/or sharing another's emotion) and emotion regulation (i.e., the processes by which one manages emotions) have largely been studied in relative isolation of one another. To better understand the interrelationships between their various component processes, this manuscript reports two studies that examined the relationship between empathy and ...
E MPATHY: A REVIEW OF THE CONCEPT. 2. Abstract. The inconsistent definition of empathy has had a negative impact on both research and. practice. The aim of this paper is to review and critically ...
1 Department of Psychology, University of Haifa, Haifa, Israel; 2 Integrated Brain and Behavior Research Center, Haifa, Israel; 3 Department of Cognitive Sciences, University of Haifa, Haifa, Israel; Empathy allows us to respond to the emotional state of another person. Considering that an empathic interaction may last beyond the initial response, learning mechanisms may be involved in dynamic ...
Empathy articles from across Nature Portfolio. Empathy is a social process by which a person has an understanding and awareness of another's emotions and/or behaviour, and can often lead to a ...
Claus Lamm, University of Vienna, investigates the processes that regulate firsthand pain and those that cause empathy for pain through numerous studies on the influence of painkillers. In these experiments, participants who took a placebo "painkiller" reported lower pain ratings after receiving a shock than did those in the control group.
Given the complexity of what encompasses the phenomenological experience of empathy, investigation of its neurobiological underpinnings would be worthless without breaking down this construct into component processes (fig. (fig.1). 1).In spite of reports in the popular press that give the appealing, yet wrong, notion that the organization of psychological phenomena maps in a 1:1 fashion into ...
1. As the reader sees, we put quotation marks around the word "empathy." By so doing, we emphasize that empathy is an abstract concept. Repeated repetition of the term empathy without qualification inevitably brings risk of reification and, obviously, confusion. However, for reading ease we drop the quotation marks in the remainder of this article and mainly use the preferred terms empathy ...
Empathy is a broad concept that refers to the cognitive and emotional reactions of an individual to the observed experiences of another. Having empathy increases the likelihood of helping others and showing compassion. "Empathy is a building block of morality—for people to follow the Golden Rule, it helps if they can put themselves in ...
Background Considerable attention has been drawn to empathy in nursing and the concept of empathy has firmly been embedded in nursing discourse. However, little has been known about the details of how nurses express empathy to their patients. In this study, we aim to conduct a qualitative study of actual nurse-patient conversations through which empathy was achieved. Methods The data in this ...
This replication was highly successful, and the results aligned closely with the original research: Empathy was positively associated with forgiveness. Apology was positively associated with empathy.
The research team discussed and reviewed the results of an initial scoping search. We developed a strategy using four groups of search terms. These were: empathy OR empathic (group 1) AND questionnaire OR measure OR measurement OR scale (group 2) AND child OR children OR adolescent OR youth (group 3) AND validity OR validation (group 4).
All physician empathy group differences were clinically relevant, with Cohen d statistics ranging from 0.21 for pain intensity to 0.30 for back-related disability, fatigue, and pain interference. Physician empathy was associated with more favorable outcomes than non-pharmacological treatments, opioid therapy, and lumbar spine surgery.
Next, show empathy. Practice self-care, make friends, recognize the accomplishments of others, seek their views, and help them. Research shows that this combats burnout.
Empathy is a powerful, efficient communication tool when used appropriately during a medical interview. Empathy extends understanding of the patient beyond the history and symptoms to include values, ideas, and feelings. Benefits of improved empathetic communication are tangible for both physician and patient. 1.
This article is part of a special report on the total solar eclipse that will be visible from parts of the U.S., Mexico and Canada on April 8, 2024.. It was 11:45 A.M. on August 21, 2017. I was in ...