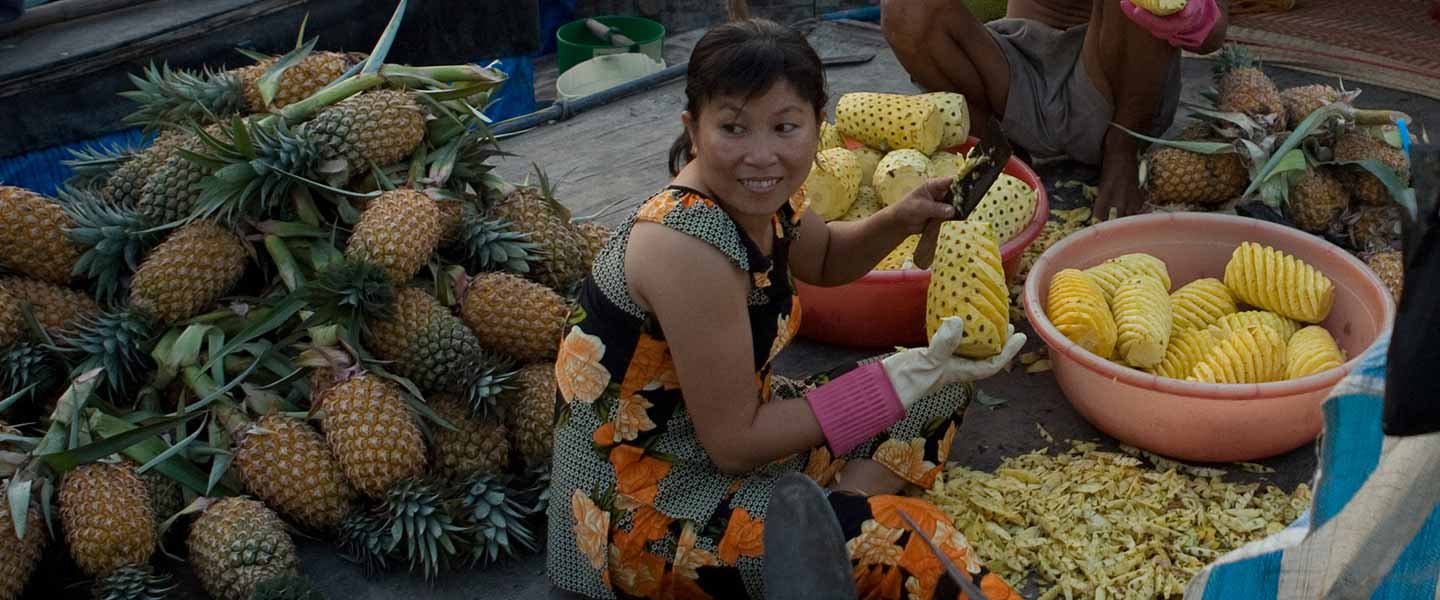
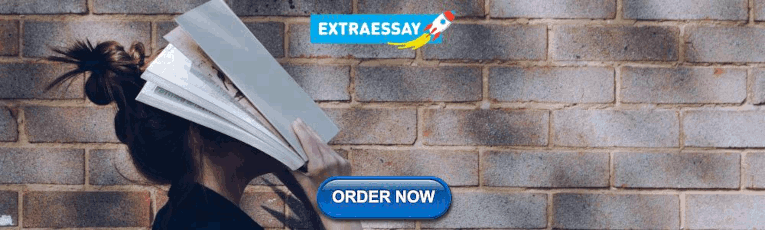
Agriculture and Food
Agriculture can help reduce poverty, raise incomes and improve food security for 80% of the world's poor, who live in rural areas and work mainly in farming. The World Bank Group is a leading financier of agriculture.
Healthy, sustainable and inclusive food systems are critical to achieve the world’s development goals. Agricultural development is one of the most powerful tools to end extreme poverty, boost shared prosperity, and feed a projected 10 billion people by 2050 . Growth in the agriculture sector is two to four times more effective in raising incomes among the poorest compared to other sectors.
Agriculture is also crucial to economic growth: accounting for 4% of global gross domestic product (GDP) and in some least developing countries, it can account for more than 25% of GDP .
But agriculture-driven growth, poverty reduction, and food security are at risk: Multiple shocks – from COVID-19 related disruptions to extreme weather, pests, and conflicts – are impacting food systems, resulting in higher food prices and growing hunger . Russia’s invasion of Ukraine has accelerated a global food crisis that is driving millions more into extreme poverty and hunger. Up to 783 million people faced hunger in 2022, which is 122 million more than before the COVID-19 pandemic. Of these, a quarter of a billion ( 258 million ) faced acute food insecurity.
The growing impact of climate change could further cut crop yields, especially in the world’s most food-insecure regions. At the same time, our food systems are responsible for about 30% of greenhouse gas emissions.
Current food systems also threaten the health of people and the planet and generate unsustainable levels of pollution and waste. One third of food produced globally is either lost or wasted. Addressing food loss and waste is critical to improving food and nutrition security, as well as helping to meet climate goals and reduce stress on the environment.
Risks associated with poor diets are also the leading cause of death worldwide . Millions of people are either not eating enough or eating the wrong types of food, resulting in a double burden of malnutrition that can lead to illnesses and health crises. Food insecurity can worsen diet quality and increase the risk of various forms of malnutrition, potentially leading to undernutrition as well as people being overweight and obese. An estimated 3 billion people in the world cannot afford a healthy diet.
Last Updated: Sep 19, 2023
The World Bank Group provides knowledge, advice, and financial resources in low- and middle-income countries to transform food systems to reduce poverty and achieve green, resilient, and inclusive development.
Our work in food and agriculture focuses on:
- Food and nutrition security , where we work with efforts to share information, and to rapidly provide resources where they are needed, while helping countries design the long-term reforms needed to build resilient food and nutrition systems.
- Climate-smart agriculture by working with client governments to provide solutions that address global climate priorities, while recognizing national contexts and development objectives.
- Data-driven digital agriculture by expand the frontier of financing and expertise for digital agriculture.
- Mobilizing capital for development in agriculture & food . We identify and leverage growth areas for productive investments, focusing on innovation and impact. And we design projects to ensure that financing boosts sustainable productivity gains, reaches smallholders and SMEs, and creates jobs to end poverty and hunger.
- Public policy and expenditure by working with governments to facilitate the adoption of more sustainable approaches, technologies, and practices, alongside policies that promote public and private sector investment.
- Sustainable and health diets to ensure that food can support a healthy population.
For fiscal year 2023, a total of $5,9 billion in new IBRD/IDA commitments to agriculture and related sectors has been approved. During that same period, investments, as part of new agriculture and food projects, directly supporting climate action have amounted to $3 billion.
As part of a comprehensive, global response to the food and nutrition crises, the World Bank is scaling up its short- and long-term responses. In May 2022, the World Bank made a commitment of making available $30 billion over a period of 15 months to tackle the crisis . We have surpassed that goal. The World Bank have scaled up our food and nutrition security response, to now making $45 billion available through a combination of $22 billion in new lending and $23 billion from existing portfolio. Our food and nutrition security portfolio now spans across 90 countries. It includes both short term interventions such as expanding social protection, also longer-term resilience such as boosting productivity and climate-smart agriculture.
Increasingly, the Bank supports country efforts to transform their food systems by taking a holistic look at public policies and spending for agriculture and food. A new Multi-Donor Trust Fund, Food Systems 2030 , provides a platform for change in this area.
In Angola, the Angola Commercial Agriculture Development Project , co-financed by the World Bank and the French Agency for Development, contributed to the government economic diversification agenda by supporting the transition from subsistence to a more market-oriented, competitive agriculture sector. The project helping producers or small and medium enterprises prepare and finance agriculture investments through technical assistance, grants, and de-risking via partial credit guarantees. As of August 2023, 101 projects have been approved, equivalent to about $26 million in agriculture investment. The project funded the first partial credit guarantees scheme dedicated to the agriculture sector in Angola – an innovation for country’s agribusiness sector – mobilizing so far $6.7 million in private bank financing.
In Argentina , the Bank supported 14,630 families who benefited from better socioeconomic inclusion. Under the project, 2,409 families accessed water for human and animal consumption, also irrigation; 7,499 rural families improved their productive capacity; and over 900 families accessed infrastructure, equipment and training that improved their marketing. Based on the model of productive alliances, 2,801 families from different regions became beneficiaries by linking their production with the markets. Among the funded activities, the production of honey, orchards, forage, livestock, nuts, spices, yerba mate and tea, among others, stand out.
In Armenia , the Bank supported 285,000 people to improve livestock farming and pasture management. Under the project, over 110,000 heads of livestock – about 17% of Armenia’s total livestock – received improved animal health services. The project was closed in 2022.
In Azerbaijan, the Agricultural Competitiveness Improvement Project , which ended in 2021, helped small and medium agribusinesses raise their productivity and sales by about 60% and 70% respectively. The project supported 70% of all livestock in the country through its animal disease-control program, also invested in seed research and processing to improve seed quality and production. The project also supported the privatization program on veterinary services, and provided financial support for over 200 agricultural enterprises. The project created 3,000 new jobs in the sector.
In Benin, between 2011-2021, the Agricultural Productivity and Diversification Project facilitated the adoption of productivity-enhancing technologies for 327,503 crop producers, leading to 135,549 hectares of land cultivated with improved technologies. The project interventions resulted in increased yields from 0.45 ton to 0.81 ton for cashew; from 1.2 tons to 2.97 tons for maize, from 4.0 tons to 6.2 tons for rice, and from 50 tons to 70 tons for pineapple. The project led to significant increases of milled rice and fish output. Combined with support for crop production and processing, support to exports has led to increases in the export of cashew and pineapple.
For the past 16 years, Bolivia has been developing a strategy to improve agricultural production and marketing through the productive alliances model. This model links small rural producers with markets, and facilitates their participation in value chains, and access to technical assistance and technology for better market access. Currently, over 2,600 productive alliances have been implemented, benefiting 107,308 producer families.
In Bhutan, a Bank-supported project supports the government's efforts to reduce rural poverty and high levels of malnutrition through climate-smart agriculture. Irrigation technology and greenhouses introduced through the project have helped farmers to increase their access to local and export markets. More than 6,500 people have increased the quality and quantity of produce like rice, maize, potato, vegetables, quinoa, citrus, apples, and potatoes, as well as high-value spices such as cardamom and ginger.
In Burkina Faso, the Bank supported the Burkina Faso Livestock Sector Development Project which ran from 2017 to 2022. By project completion, beneficiaries among selected value chains increased their yield by 8.4%. Yield increase for cattle, sheep, and egg production reached 6.76%, 11.93%, and 6.50%, respectively. Sales increased by 45% exceeding the target of a 30% increase. The volume of loans granted by partner financial institutions reached $5.02 million, exceeding the original target of $4.38 million. The project reached a total of 329,000 beneficiaries, out of which 138,314 were women and 112,573 were youth.
In the Central African Republic, through the Emergency Food Security Response project, 330,000 smallholder farmers received seeds, farming tools, and training in agricultural and post-harvest techniques. The project helped farmers boost their crop production and become more resilient to climate and conflict risks. Local food production increased by 250%, from 28,000 tons in September 2022 to 73,000 tons in June 2023. Moreover, 21,006 agricultural households received training on post-harvest loss management and provided equipment, such as mobile storage units, to enhance packaging of agricultural products, leading to higher selling prices.
In China, since 2014, a Bank-supported project has helped expand climate-smart agriculture. Better water-use efficiency on 44,000 hectares of farmland and new technologies have improved soil conditions and boosted production of rice by 12% and maize by 9%. More than 29,000 farmers’ cooperatives report higher incomes and increased climate resilience.
In Colombia, since 2010, the adoption of environmentally friendly silvopastoral production systems (SPS) for over 4,100 cattle ranches has converted 100,522 hectares of degraded pastures into more productive landscapes and captured 1,565,026 tons of CO2 equivalent. In addition, almost 40,000 hectares of pastureland were transformed to SPS and 4,640 hectares into intensive Silvopastoral Production Systems (iSPS). Moreover, 4,100 direct farmers beneficiaries, of which 17% were women, were trained in SPS and iSPS, and over 21,000 farmers, technicians and producers were also trained, visited demonstration farms, and participated in workshops and events and technology brigades. A network of 116 plant nurseries were also established, which produced around 3.1 million fodder trees that were delivered to beneficiary farmers.
In Cote d’Ivoire, between 2013 and 2017, the Agriculture Sector Project boosted the productivity of 200,000 farmers and rehabilitated 6,500 kilometers of rural roads which allowed farmers to more easily bring their products to market and reduce post-harvest losses. To aid the cashew industry, the Bank also supported a research program that helped disseminate 209 genotypes of high-performing trees and establish 18 nurseries. The Bank-financed project also helped leverage $27.5 million in private investment to boost productivity on at least 26,500 hectares.
In Croatia , the Bank supported the Ministry of Agriculture in building a National Agriculture and Rural Development Strategy that connects country needs and the EU Common Agricultural Policy.
In Djibouti , the Bank supported the construction of 112 water mobilization units, which improved water access for 9,762 households. The Bank also helped introduce hydroponic agriculture to 30 beneficiaries, rehabilitated 96 hectares of irrigated farmland, and produced 14,000 seedlings.
In Ethiopia, since 2015 a project has helped 2.3 million farmers with agricultural support services, agricultural research, small-scale irrigation, and market infrastructure development. In addition, almost 600,000 livestock and aquaculture farmers have been provided with various services (animal health, feeding, breeding and commercialization) and another 425,000 pastoralists and agro-pastoralists have been supported to build livelihoods resilience in the lowlands parts of the country.
In Guinea, the World Bank helps the government's efforts to adapt and mitigate climate shocks, build a resilient food system, and promote employment for youth and women. From 2018 to 2023, through the Guinea Integrated Agricultural Development Project , local farmers increased agriculture's productivity, sustainability, and profit. To help local communities, the project disseminated innovation packages with high-yielding seeds, helped improve irrigation, trained and mentored women and youth to access funds to create jobs, and increased income for beneficiaries. The project also promoted the use of climate-smart, gender-sensitive digital technologies with local producers. The project has reached 149,000 farmers (of whom 38% are women and 30% are youth). The project’s results include a 30% increase in yield of rice and maize; a 42% increase in commodity sales; a 47,470-hectare area covered by improved technologies; over 97,000 users of improved technologies, and more than 2,000 jobs created for women and youth. It also supported the national agriculture census and helped mitigate the impact of the COVID-19 pandemic by providing improved seeds, fertilizers, and farming kits to 40,000 vulnerable families.
In Honduras, since 2010 , 12,878 small farmers, of which 27% are women, have used productive alliances to improve productivity and access to markets, which has leveraged $33.5 million in finance from commercial banks and microfinance institutions. Under the project, gross sales of producer organizations rose by 24.3%. Also, support to Honduras’ Dry Corridor Alliance, has helped 12,202 households implement food security and agricultural business plans, and improved agricultural yields, nutrition, and food diversity of project beneficiaries.
In Bihar, India, the Bihar Transformative Development Project has supported 6.2 million women through 531,825 affinity based Self Help Groups (SHGs). The project, which closed in April 2023, helped Bihar become the state with the highest number of SHGs in the country, with 12.7 million women in over 1 million SHGs. In the project areas alone, the women SHGs saved over $473.5 million and leveraged $2.3 billion from the formal financial sector. In addition, over 500,000 women SHG members were supported through market-linked value chains.
In India, the Assam Agribusiness and Rural Transformation Project supported over 400,000 farm families and 1,270 businesses and over 100 of industry associations and producer organizations in improving their productivity and incomes and helping develop new marketing channels since 2017.
Since 2013, Bank support has strengthened Indonesia’s agricultural research system. Thirty-three Assessment Institute for Agricultural Technology centers now have the capacity to develop improved rice, vegetable, and fruit varieties. The project has supported 161 agricultural researchers through degree programs; upgraded 58 labs and 54 research stations; and funded 1,134 research activities, including 44 international research collaboration activities.
In Jamaica, an ongoing project since 2000 is strengthening value chains. The project emphasizes on the linkages between producers/service providers and buyers, to improve economies of scale for producer organizations, small agricultural enterprises and tourism clusters, and to mainstream climate resilience. The project introduces counter-seasonal production methods and technologies such as greenhouses, climate-smart agriculture, sustainable land and water management practices, small scale productive infrastructures such as cold storage facilities, local roads, alternative energy sources, among others. Around 9,000 people will benefit directly from these investments, of which 40% will be women and 30% youths.
In Kenya, since 2016, 1.5 million farmers , where over 60% are women, have increased their productivity , climate resilience and access to markets. The digital registry (including geo tagging) of these 1.5 million farmers enables them to access agro-weather and market advisories. In addition, the Bank is facilitating partnerships between the government and 26 ag-tech support agencies which enables almost 500,000 farmers to access a range of services (inputs, financial services and markets) by leveraging digital technologies.
In Kosovo , the Bank provided 775 grants to farmers and 103 grants to agri-processors to increase production capacities and enhance market competitiveness in the livestock and horticulture sector. This was done through upgrading facilities, adopting new technologies, and introducing food safety and environmental standards. Further, support was provided for the rehabilitation of irrigation schemes covering an area of 7,750 hectares which had an impact on the production, yield, quality, and variety of products cultivated in the area.
In Madagascar, since 2016 , the Bank has boosted the productivity of over 130,000 farmers. Sixty-thousand hectares of irrigated rice fields have been rehabilitated. The Bank also supported the cocoa sector through research, the development of certified seeds, and promotion of improved production and processing techniques. This allowed 4,000 cocoa producers to increase their incomes and increase production and export volumes by 50%. The Bank also financed the country’s largest land rights registration, facilitating the delivery of over 200,000 land certificates to farmers.
In Mali, the Food Security Resilience Project is distributing 15,000 metric tons of fertilizer and 4,500 tons of climate resilient seeds for over 400,000 farmers, of which 160,000 are women, in Segou, Koutiala and Sikasso. The Drylands Development Project funded activities including subprojects, agricultural infrastructure, cash transfers which benefitted over 150,000 agricultural households, equivalent to more than 1 million people, in the regions of the Sahel band, including Kayes, Koulikoro, Segou and Mopti.
In Mauritania, between 2016 and 2021, the intervention of the Sahel regional support project offered agricultural assets and services to more than 400,000 farmers/pastoralists, where nearly 30% are women. More than 1.9 million hectares of land under sustainable management practices, in addition to the construction of 133 vaccination parks and the realization of 118 water points (wells and boreholes) as well as other infrastructure of valorization and trade of animals were provided to agro-pastoralist communities. Additionally, from April 2023- June 2028, the Bank offered to support the Agriculture Development and Innovation Support Project (PADISAM) to improve land resources management and foster inclusive and sustainable commercial agriculture in selected areas of Mauritania. It is anticipated that by the end of the project, there will be 72,000 direct beneficiaries and about 5,000 Ha of land under sustainable landscape management practices.
In Moldova, since 2012 , the Bank has helped more than 7,500 farmers gain access to local and regional high-value markets for fresh fruit and vegetables and boosted land productivity through the promotion of sustainable land management practices on 120,000 hectares of farmland.
In Montenegro , since 2009, the Bank has supported almost 4,000 farmers working on orchards, vineyards, livestock and aromatic plants, 224 agro-processors, and 59 farmers working on processing on-farm complying with the European Union requirements for food safety and 278 agricultural households adopting agro-environmental measures, improving their competitiveness and sustainability.
In Nepal, the Bank-supported Nepal Poverty Alleviation Fund helped small farmers and rural poor people access microcredit, assets, services, and training. Since 2004, it has created over 30,000 community organizations and had an impact on more than 900,000 households.
In Nicaragua, between 2015 and 2019, food security in 563 communities along the Caribbean Coast was enhanced, benefiting 75,000 people. Nearly 8,400 families adopted improved agricultural technology and productivity increased by 65%.
In Niger , through the Climate Smart Agriculture Support Project , the World Bank supported over 370,000 farmers, where 145,000 of whom are women. The farmers benefited from the project’s investments in small and large-scale irrigation, improved climate-smart agriculture, and sustainable land management practices. Over 154,000 hectares of land were developed with sustainable land management practices, and 4,400 hectares of cropland were brought under irrigation. In collaboration with the International Crops Research Institute for the Semi-Arid Tropics and FAO, the project promoted good agriculture practices through farmer led e-extension services and technical assistance. The project investments led to significant increases in agriculture productivity: yields of cowpea, millet, and sorghum increased by 169, 164, and 142 percent, respectively. The project also strengthened the national climate information system by building the capacity of the National Meteorology Department (the project installed 30 meteorological stations and 600 rain gauges). Through its support to the Sahel Regional Center for Hydro and Agrometeorology, the project strengthened the early warning systems of national institutes such as National Meteorology and the National Hydrology Directorate.
In Nigeria, APPEALS Project was designed to enhance agricultural productivity of small and medium scale farmers and improve value addition along priority value chains. Since 2017, the project has demonstrated 204 improved technologies to 93,0009 farmers and continued to contribute to the national food basket across 11 value chains. Food crop production has surged, with 304,516 metric tons produced, representing 3.1% of the national output. Furthermore, the project has reached 61,171 farmers with processing assets to improve the quality of their produce. The project also trained 10,346 women and youth, including persons with disability, providing them with business, technical and life skills training, support to business planning and facilitation of business name registration, start-up grant to establish a commercially viable business, and mentorship to provide the beneficiaries with continued support from established agribusiness entrepreneurs. The project linked farmers to market through the facilitation of commercial partnerships resulting in a total of 327 business alliances with 147 off-takers already buying farmers’ produce across the 11 value chains, with a transaction worth of US$ 59.7 million. Similarly, the project has linked 200 agribusiness clusters to infrastructures which includes 55km rural farm access road, 75 aggregation and cottage processing centers, 102 solar-powered water intervention and energy supplies.
In Pakistan, in 2022, in response to the flood’s emergency, about 230,000 smallholder farmers received cash transfer support to winter cropping, more than 500 watercourses damaged by the floods were rehabilitated, 27,000 tents and 2.2 million mosquito nets were purchased. Women were provided with poultry and small ruminants restocking, tunnel farming as well as kitchen garden kits.
In Paraguay, since 2008, 20,863 farmers increased their agricultural income by at least 30% and 18,951 adopted improved agricultural practices, boosting the productivity of their land.
In Peru, since 2013, nearly 600 agricultural innovations have been identified and tested with the help of competitive matching grants. More than 110 of these innovations have been validated at the farm level, and as of September 2020, one or more of them have been adopted by nearly 32,000 producers.
In the Philippines, since 2015 , the Bank helped raise rural incomes, enhance farm and fishery productivity, improve market access and mainstream institutional and operational reforms, as well as science-based planning for agricultural commodities in 81 provinces. The project has benefitted a total of 323,501 people–46% of them women–with farm roads, irrigation, and agricultural enterprise projects, boosting incomes by up to 36%.
In Rwanda, since 2010, the Bank helped support more than 410,000 farmers – half are women – in improving their agricultural production by developing over 7,400 hectares for marshland irrigation, providing hillside irrigation on over 2,500 hectares, and several hundreds of farmers benefitted matching grants to support their investments in Farmer-Led Irrigation Development (FLID) technologies on over 1,200 hectares of their land. Interventions also included improving soil conservation and erosion on more than 39,000 hectares of hillside. Maize, rice, beans, and potato yields have all more than doubled and around 2.5 tons of vegetables are exported to Europe and the Middle-East every week from intervention areas, or locally, where more horticulture produce is sold to premium markets including 5-star hotels or the national airline, RwandAir. Less than two years after one of the Bank supported projects introduced greenhouse farming in its intervention areas to minimize the impacts of unfavorable weather conditions and better manage crop pests and diseases, by 2023, the demand for these technologies has seen a rapid increase in these areas and 132 units have been acquired and installed through the matching grants program under the project. Evidence shows relatively high revenues for farmers investing in greenhouse technology, with revenues increasing up to 15 times for vegetable growers.
In Tajikistan , the Bank supported the establishment of 545 farmer groups in horticulture value chains, specifically apricot, apple, pear, lemon, cucumber, and tomato, and dairy value chain benefiting a total of 13,516 farmers out of which 48% were women. The Bank also supported the establishment of 342 productive partnerships benefitting 4,340 smallholder farmers. A total of 21,882 beneficiaries achieved an increase in commercial activity. The project supported training for 13, 516 farmers, on value chain development.
In Tunisia, the Bank helped 113 remote rural villages improve land management practices on 37,000 hectares of land to increase productivity and improve 930 kilometers of rural roads serving some 160 villages.
In Uruguay, since 2014, climate-smart agriculture techniques have been adopted on 2.7 million hectares and adopted by 5,541 farmers, providing for a carbon sequestration potential of up to 9 million tons of CO2 annually.
In Uganda, since 2015 , the Agriculture Cluster Development Project’s e-voucher scheme has leveraged over $12 million of farmer investments enabling over 450,000 farm households access and use improved agro-inputs resulting in higher farm yields. Provision of matching grants has enhanced storage capacity by 55,000MT, acquiring value addition equipment and machinery thereby facilitating Producer Organizations to add value and undertake collective marketing. Additional infrastructure support addressing road chokes has also led to improved market access.
The Bank has also made investments into strengthening regulatory and administrative functions of the Ministry of Agriculture through the development of IT Platforms and tools facilitating timely planning and decision making.
In the Uganda Multi-Sectoral Food and Nutrition Security Project, the Bank has supported enhanced knowledge on nutrition resulting in improved household nutrition and incomes for 1.55 million direct project beneficiaries.
In Uzbekistan, the Horticulture Development Project has helped create, 34,520 jobs, including 13,124 for women; increase beneficiary productivity by 24% and profitability by 124%, including through entry into new export markets. The Livestock Sector Development Project supported a sub-loans benefitting a total of 560 large scale commercial livestock farmers, and a total of 135 value chain development projects benefiting 1,456 smallholder farmers (Dekhans). As a result of the project support, the share of improved and high yielding livestock breeds increased by 98.7%; thereby increasing milk and meat productivity by 33% and 38% respectively. A total of 3,659 livestock farmers acquired agricultural assets. In addition, the project created a total of 21,698 new jobs.
In Vietnam, since 2010, the Bank has promoted sustainable livelihoods by helping develop 9,000 “common interest groups” comprising over 15,500 households and partnering them with agricultural enterprises. The Bank also helped over 20,000 farmers improve their livestock production and benefited an additional 130,000 people through capacity building in food safety.
Under the West African Agricultural Productivity Program , the Bank supported a research and development effort that promoted technology generation, dissemination, and support to local farming systems in 13 ECOWAS countries. The project reached over 2.7 million beneficiaries, 41% of whom were women. It also generated 112 technologies that reached over 1,850,000 hectares.
Last Updated: Sep 25, 2023
The World Bank works with a range of partners to achieve ambitious development goals: transforming food systems, boosting food security and empowering smallholder farmers, to realize zero hunger and poverty by 2030.
The World Bank Group is a joint convener, with the G7 Presidency, of the Global Alliance for Food Security (GAFS) . A key outcome of the Global Alliance is the Global Food and Nutrition Security Dashboard , a key tool to fast-track a rapid response to the unfolding global food security crisis, designed to consolidate and present up-to-date data on food crisis severity, track global food security financing, and make available global and country-level research and analysis to improve coordination of the policy and financial response to the crisis.
The Bank hosts a Multi-Donor Trust Fund, Food Systems 2030 , that helps countries build better food systems, fostering healthy people, a healthy planet and healthy economies. The Trust Fund aims to deliver improved livelihoods and affordable, and nutritious diets for all, and progress towards the Sustainable Development Goals of zero poverty and hunger by 2030 and the climate goals of the Paris Agreement. Food Systems 2030 provides advice and analytical products to underpin policy options, funds to pilot innovative approaches, and information to build support for change in different country contexts. It engages with the private sector by supporting the design, piloting and de-risking of innovative public-private partnerships that advance development and climate goals.
The Global Agriculture and Food Security Program , a multilateral financing platform, is dedicated to improving food and nutrition security worldwide. Launched by the G20 in the wake of the global response to the 2007–08 food price crisis, GAFSP works to build sustainable and resilient agriculture and food systems in the world’s poorest and most vulnerable countries. Since its inception in 2010, the Program has mobilized more than US$2 billion in donor funds to reach more than 16.6 million people. GAFSP provides financial and technical resources – investment grants, technical assistance, concessional finance, and advisory services – to demand-driven projects along the food chain to accelerate the transformation of food systems at scale.
The World Bank leads the Food Systems, Land use and Restoration Global Platform (FOLUR) , financed by the Global Environment Facility, in partnership with UNDP, the UN Food and Agriculture Organization (FAO), the Global Landscapes Forum and the Food and Land-use Coalition. FOLUR is a $345 million, seven-year program that aims to improve the health and sustainability of landscapes that produce the world’s food. FOLUR targets sustainable production landscapes in 27 country projects for eight major commodities (livestock, cocoa, coffee, maize, palm oil, rice, soy, and wheat).
The World Bank chairs the System Council of CGIAR , a global partnership that advances cutting-edge science to reduce rural poverty, increase food security, improve human health and nutrition, and ensure sustainable management of natural resources.
For more information, contact Clare Murphy-McGreevey on [email protected].
AROUND THE BANK GROUP
Find out what the Bank Group's branches are doing on Agriculture.

STAY CONNECTED
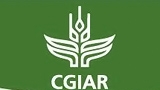
CGIAR Global Agricultural Research
CGIAR advances cutting-edge science to reduce rural poverty, increase food security, improve human health and nutrition, and ensure the sustainable management of natural resources.
Food Systems 2030
Food Systems 2030 is an Umbrella Multi-Donor Trust Fund that helps countries build better food systems by 2030. Food Systems 2030 helps countries rethink and transform their food systems from farm to fork, progressing ...
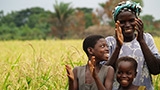
Global Agriculture and Food Security Program
The Global Agriculture and Food Security Program (GAFSP) finances investments that increase incomes and improve food and nutrition security in developing countries.
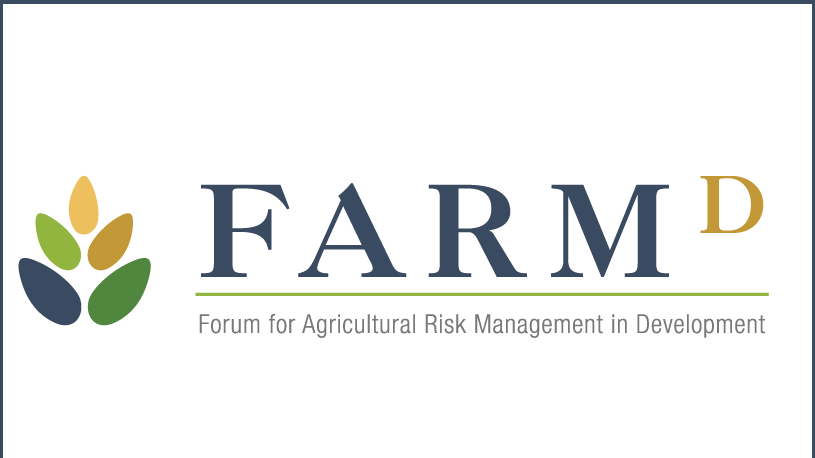
Forum for Agricultural Risk Management in Development
The Forum for Agricultural Risk Management in Development (FARMD) is a knowledge platform that provides information and best practices on agricultural risk management.
Additional Resources
Media inquiries.
This site uses cookies to optimize functionality and give you the best possible experience. If you continue to navigate this website beyond this page, cookies will be placed on your browser. To learn more about cookies, click here .
Thank you for visiting nature.com. You are using a browser version with limited support for CSS. To obtain the best experience, we recommend you use a more up to date browser (or turn off compatibility mode in Internet Explorer). In the meantime, to ensure continued support, we are displaying the site without styles and JavaScript.
- View all journals
- Explore content
- About the journal
- Publish with us
- Sign up for alerts
- Published: 02 November 2020
The global divide in data-driven farming
- Zia Mehrabi ORCID: orcid.org/0000-0001-9574-0420 1 , 2 , 3 ,
- Mollie J. McDowell ORCID: orcid.org/0000-0002-3642-4783 3 ,
- Vincent Ricciardi ORCID: orcid.org/0000-0002-6662-943X 1 , 2 ,
- Christian Levers ORCID: orcid.org/0000-0003-4810-9024 1 , 2 , 4 ,
- Juan Diego Martinez ORCID: orcid.org/0000-0003-4121-2045 1 , 2 ,
- Natascha Mehrabi 5 ,
- Hannah Wittman 2 , 3 ,
- Navin Ramankutty ORCID: orcid.org/0000-0002-3737-5717 1 , 2 &
- Andy Jarvis 6
Nature Sustainability volume 4 , pages 154–160 ( 2021 ) Cite this article
19k Accesses
64 Citations
263 Altmetric
Metrics details
- Agriculture
- Developing world
Big data and mobile technology are widely claimed to be global disruptive forces in agriculture that benefit small-scale farmers. Yet the access of small-scale farmers to this technology is poorly understood. We show that only 24–37% of farms of <1 ha in size are served by third generation (3G) or 4G services, compared to 74–80% of farms of >200 ha in size. Furthermore, croplands with severe yield gaps, climate-stressed locations and food-insecure populations have poor service coverage. Across many countries in Africa, less than ~40% of farming households have Internet access, and the cost of data remains prohibitive. We recommend a digital inclusion agenda whereby governments, the development community and the private sector focus their efforts to improve access so that data-driven agriculture is available to all farmers globally.
Similar content being viewed by others
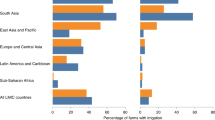
A scoping review of research funding for small-scale farmers in water scarce regions
Vincent Ricciardi, Abdrahmane Wane, … Zia Mehrabi
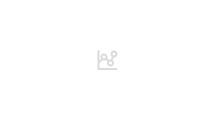
Responsible artificial intelligence in agriculture requires systemic understanding of risks and externalities
Asaf Tzachor, Medha Devare, … Seán Ó hÉigeartaigh
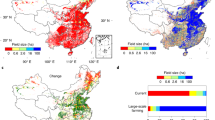
Consolidation of agricultural land can contribute to agricultural sustainability in China
Jiakun Duan, Chenchen Ren, … Baojing Gu
Data-driven interventions are widely suggested to be a disruptive force in global food systems 1 , 2 , 3 , 4 , 5 . The aim is to use big data and mobile technology to address issues as diverse as low crop yields 6 , poor human nutrition 7 , disease 8 and climate adaptation 1 . However, mobile network coverage, handset ownership and affordable mobile service subscriptions are necessary prerequisites to achieve these solutions. Although the footprints of mobile networks and mobile phone ownership have increased in recent years, and the costs of mobile data have declined 9 , substantial gaps in access remain. Nearly half of the world’s population is estimated to still be without Internet access 10 . There is concern that this digital divide could stall the realization of human rights 11 and the Sustainable Development Goals of the United Nations (UN), particularly those relating to education, equity, health and well-being 12 .
We define data-driven farming as the use of data to augment decision-making in farming systems and thus improve food system outcomes such as crop yields, profits, environmental sustainability and food security. The potential for data-driven farming to improve food systems has recently expanded due to advances in three key areas: data generation (for example, in mobile devices, field sensors, satellites and ‘farmers as sensors’), data processing and predictive analytics (for example, by using big data stacks, machine learning and deep learning), and human–computer interactions (that is, human-centric approaches to create experiences that improve the ease and use of insights through voice, text and images). Data-driven farming is promoted in areas such as climate services 13 , digital extension 14 , precision agriculture 3 , market information systems 15 and agricultural insurance 2 . There are many specific examples, including the IBM Watson Decision Platform for Agriculture, which uses performance geocomputation and a range of input data, such as high-resolution weather forecasts, for localized prescription farming and crop management 16 . Another example is the Nuru application of the UN Food and Agricultural Organization, which uses computer vision for in-field pest and disease diagnostics of major food security threats such as the damage caused by the fall armyworm 17 .
Recent synthesis has estimated that mobile-phone-based digital extension and price information systems in sub-Saharan Africa and India, some of which are data-driven, can modestly improve crop yields, increase adoption of recommended inputs, and raise farmer profits 14 . Basic benefits of the use of mobile technologies have also been reported in healthcare; for example, where improvements in patient outcomes and reduced healthcare costs have been documented 18 . However, it is poorly understood how the differential access to mobile technologies in farming communities might limit the vision for achieving data-driven farming, health and nutrition globally 19 . In particular, donors, governments and research organizations lack sufficient understanding of the spatial distribution of data services across farms, and how existing services intersect with farms that have the greatest need for support and areas which contain the most food-insecure populations 20 . This lack of understanding of the baseline for data-driven farming limits our ability to assess the feasibility, scalability and probable impact of investment in digital technology projects and interventions in food systems across the world.
Here, we report a global baseline assessment of the state of mobile coverage and access to data services in farming. We use the coverage of mobile services as a measure of availability, which is the basic infrastructural backbone needed for scaling data-driven solutions. We examine how this availability intersects with opportunities for raising productivity and delivering financial, climate and healthcare services. We then assess subnational patterns in mobile phone and Internet access across non-farming and farming populations, for a subset of countries for which subnational data are available. In these countries are found 75%, 88% and 46% of all farming households in Africa, Latin America and the Caribbean, and Asia, respectively. We consider our results within the context of the current needs for closing the digital divide in agriculture on a global scale.
Availability gaps
Our analysis shows that second generation (2G) services (suitable for voice and short message services (SMSs)) cover 84% of croplands globally, but this coverage is 62% for third generation (3G) and 42% for 4G services (Fig. 1a ). This pattern of unequal coverage is even more marked across farm size classes. Small farms have disproportionately slower services available to them than large farms (Fig. 1b ). Globally, only 37% and 24% of farms of <1 ha receive 3G and 4G services, respectively, whereas 80% and 74% of farms of >200 ha receive 3G and 4G services, respectively.
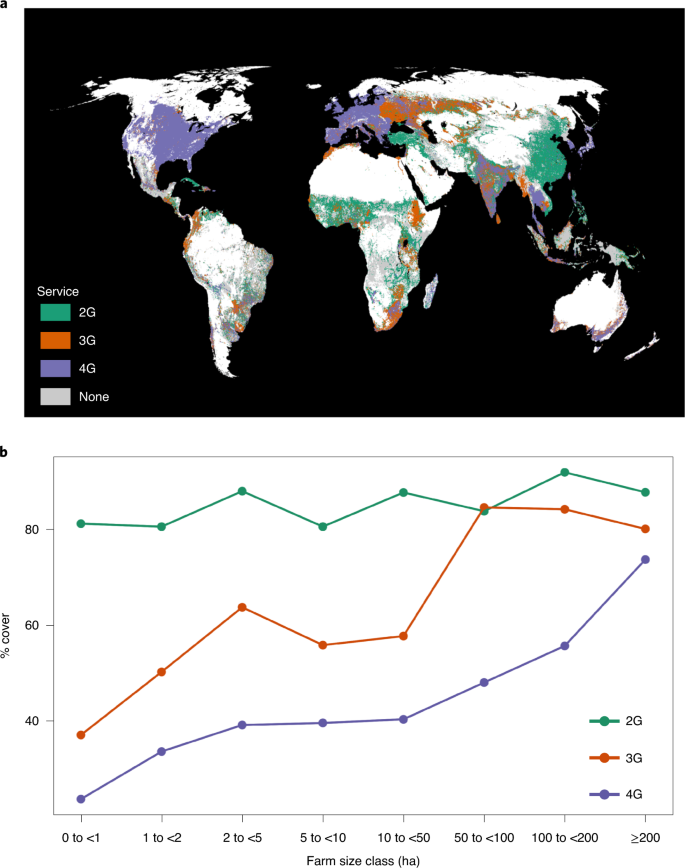
a , A global map showing 2G, 3G and 4G mobile coverage across croplands. Croplands not covered by any service are shown in grey. b , Percentage of global coverage across farm size classes. Cropland data 37 are centred on the year 2000. Coverage data are from April 2018 and were obtained from Mosaik Solutions (see Methods ).
Spatial heterogeneity in coverage is important. High-speed (that is, 3G and 4G) services are predominant in North America and Europe, whereas large coverage gaps exist in sub-Saharan Africa. Across the African continent, network coverage on cropland is only 33% for 3G and 9% for 4G services, and in Asia, it is 46% for 3G and 29% for 4G services. However, coverage in Asia varies by country. For example, the majority of India and Thailand are covered by 3G and 4G services, whereas large gaps exist in China, Vietnam and Mongolia (Fig. 1a ).
In terms of high-speed network coverage of areas with the greatest need for support, we find that, globally, coverage in areas affected by nitrogen-deficient soils and severe yield gaps is around 60% for 3G and 22% for 4G services. Coverage in areas that are most dependent on rainfall for crop production is 71% for 3G and 54% for 4G services. Coverage in arid croplands (environments that receive <250 mm annual rainfall) is 37% for 3G and 17% for 4G services (Table 1 ). Coverage gaps pose important obstacles for developing data-hungry nutrient advisories, climate services and financial services that need mobile Internet access.
We also assessed cross-cutting themes of food insecurity and the incidence of communicable diseases, which together represent compounding factors that increase population vulnerability alongside low crop productivity and climate stressors. These themes underpin efforts to develop mobile health services for rural populations. We find that, of individuals affected by food insecurity globally (as measured by the prevalence of childhood stunting 21 ), 61% are served by 3G and 45% are served by 4G. In Africa, the numbers are particularly low (52% and 22% for 3G and 4G, respectively). Globally, of individuals with malaria (those infected with Plasmodium falciparum ), only 37% have access to 3G services and 17% to 4G services. This lack of coverage for at-risk populations poses serious concerns not only for the development of mobile services for populations affected by existing nutritional burdens and diseases, but also for the response of wider populations to food security threats and health impacts of emerging diseases, including COVID-19.
Access gaps
Our analysis highlights differences in household ownership of mobile handsets. Although ownership of mobile phones by farming households in many locations throughout Asia and Latin America is at, or close to, 100%, we find that mobile phone ownership within African countries lags behind that of the rest of the world (Fig. 2a ). For example, in Angola, the Democratic Republic of the Congo (DRC), Madagascar and Burundi, the subnational averages for mobile phone ownership (for any kind of device) by farming households range between 34% and 51% (Fig. 2a ). The numbers are higher in other African countries, such as Malawi, Mozambique, Rwanda, Chad and Sierra Leone, nevertheless, more than 30% of farming households in these countries still do not own a mobile phone.
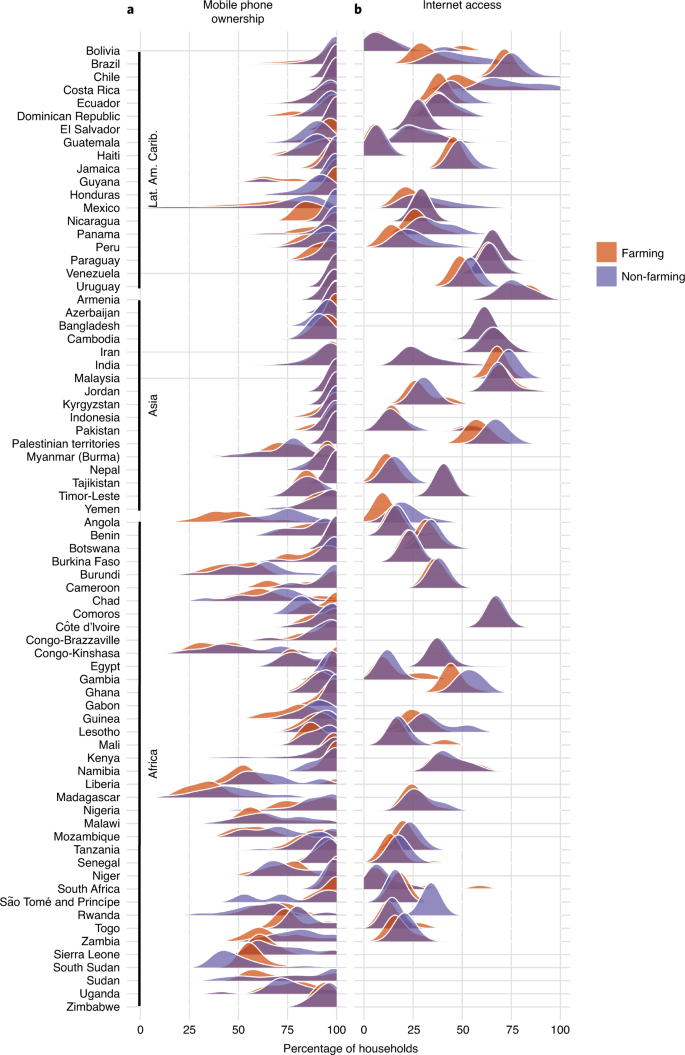
a , Subnational distributions of the percentage of mobile phone ownership in non-farming and farming households across Africa, Asia, and Latin America and the Caribbean ( n = 5,429 administrative units). b , Subnational distributions of the percentage of non-farming and farming households that have Internet access ( n = 4,905 administrative units). All data are centred in 2018. Lat. Am. Carib., Latin America and the Caribbean.
Furthermore, in some countries, farmer access to mobile phones is similar to, or exceeds, that of non-farmers, whereas in other countries farmers are less likely to own mobile phones than non-farmers. For example, in Angola, Madagascar and the DRC, ownership in non-farming populations is 24%, 12% and 11% (percentage points) higher, respectively, than in farming populations (Fig. 2a ). Other studies have pointed to a gender gap in mobile phone ownership, with women being 14% less likely to own one (and 38% less likely in South Asia). This means that there is not only a divide between farming and non-farming populations, but also between men and women within households 22 , and this poses a particular concern for inclusivity of interventions relating to primary care and child nutrition.
It is well known that Internet access across countries in Asia, Latin America and Africa still lags behind that in other regions of the world such as North America and Europe, but we show that Internet access also varies at the subnational level, and importantly, between farming and non-farming populations. Internet access is generally much lower than mobile handset ownership. For example, the average percentage of farming households with Internet access is low in India (31%), and even lower in Pakistan (21%) and Tajikistan (12%). Similarly, it is 25% in Mexico, 14% in Bolivia and just 5% in Haiti. Furthermore, with the exception of Côte d’Ivoire, Ghana and Namibia, we find that Internet access is less than 40% of households, on average, for the remaining African countries included in our analysis (Fig. 2b ). In Costa Rica, Brazil and Angola, the gap between Internet access for farmers and for the rest of the population at the subnational level is on average 19%, 15% and 11%, respectively (Fig. 2b ). Farmers in these locations are bearing the burden of the digital divide resulting from unequal Internet access, which is unaccounted for in aggregated statistics.
Farmers, particularly smallholders, not only depend on the installation of mobile networks and owning mobile handsets, but they must also pay for data access. However, the access problems of people living in poverty are often masked because the often-cited average costs of data are based on mean incomes 23 . We disaggregated the costs of accessing data for ten income groups across 83 countries in Africa, Asia, and Latin America and the Caribbean, and show that, for the poorest 10% of the population, the cost of fully engaging in the digital economy is still prohibitively high (Fig. 3 ). The situation is worst for some countries in sub-Saharan Africa (the Central African Republic, the DRC, Guinea-Bissau, Chad, Sierra Leone, Togo, Madagascar and Malawi) where the cost of a basic 1 GB monthly plan ranges from as much as 64% to 188% of the annual income of the poorest 10% of the population. Although incomes have risen and average Internet access costs have declined in Africa in recent years, the cost of a basic 1 GB monthly plan in the last quarter of 2018 was still estimated to be more than a quarter of the annual income of the poorest 10% in countries of the continent (Fig. 3 inset). To put this into perspective, the 2025 target adopted by the Broadband Commission for Sustainable Development is that entry-level broadband should be made available in all countries at 2% of gross national income per capita 24 .
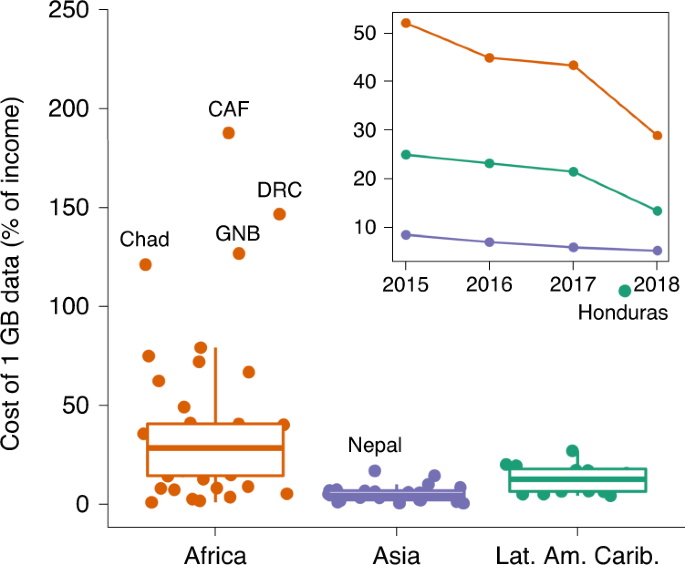
The cost of 1 GB of data per month as a percentage of annual income in Africa, Asia, and Latin America and the Caribbean in 2018. CAF, Central African Republic; GNB, Guinea-Bissau; Lat. Am. Carib., Latin America and the Caribbean. Inset, a time series of the median cost of 1 GB of data from 2015–2018 for Africa, Asia, and Latin America and the Caribbean. The vertical axis label of the inset graph is the same as for the main graph.
Key recommendations
Our analyses show that substantial gaps still exist in the availability of, and access to, data services for the world’s farming populations. These gaps preclude the participation of many smallholders in the latest technological innovations and need to be addressed if we are to move towards fairer, more inclusive and equitable food systems globally. Here, in light of these findings, we outline some key recommendations for governments, agricultural development organizations, funders, entrepreneurs and academics.
Invest in ‘last-mile infrastructure’ innovation
Closing the coverage gap will require continued innovation in three critical areas of last-mile infrastructure: energy (for example, moving from diesel generators to cost-efficient fuel cell generators or solar energy) 25 , cell towers (for example, installing ‘light towers’ with reduced complexity or deployment costs) and backhaul technologies (for example, complementing fibre optics with innovations such as scalable microwave ‘pay-as-you-grow’ solutions). Although efforts to bridge coverage gaps using these, as well as other more radical cross-cutting innovations that use satellites and wide-area deployments—such as Google’s project Loon, OneWeb, or SpaceX’s Starlink—exist, their success remains to be proven in many farming landscapes in the world, and particularly in the African continent. Such investments need to be supported by policies that lower regulatory friction, increase open competition and reduce capital risks for the private sector, especially in the early stages of deployment.
Increase handset affordability
Business model innovation will be required to provide handsets that are both affordable and capable of a seamless broadband experience. Existing business models include upfront payment for handsets (which is not possible for the poorest sectors of society), asset financing (which allows access to credit but has default risk) and third-party provision (by governments, non-governmental organizations and private companies that derive value from the user, but is often not sustainable). Average device costs in emerging markets remain high (approximately US$100) 26 . Affordability should be addressed from two perspectives. On the one hand, incomes need to be increased by financing business growth and job creation, particularly for women and underserved groups in rural communities 27 . On the other hand, device costs need to be reduced by blending business models that create value between state actors, financial institutions, mobile network operators, device manufacturers and content providers 24 . Here, national agricultural research services and research for development organizations can play important roles to assist in the design of localized solutions for farming populations.
Make data access universal
Innovation in infrastructure and business models can help to reduce the cost of deploying the last-mile infrastructure, which, in turn, should be reflected in the price of data for farmers. However, further actions will probably be required to ensure accessibility to all. Given the importance of mobile technology, to bring everyone online globally currently translates to universal access to mobile broadband. In addition, because the poorest farmers are unable to buy data, mobile broadband must be made a public good. Although a public and donor-funded Universal Service and Access Fund has been proposed to connect the poorest households in Africa to the Internet 24 , universal access should be made available to farmers globally.
Identify interim solutions
SMS-based advisories and alerts, and interactive voice response services that can run on low-end handsets, even if jointly owned or shared between community members, offer an important opportunity for addressing bundled issues of productivity, market connectivity, financial transfers, credit access, input use and within-season management, across large areas of farming landscapes globally 2 , 14 , 28 . These lean front-end solutions can be built on sophisticated back-end services that interface with the Internet and integrate remote sensing, ancillary data sets, and mechanistic and statistical prediction tools 2 . These interim solutions are essential for participation and inclusion, given current availability and access patterns.
Operationalize these findings
This baseline analysis assesses the digital divide in farming and regular operational updates will be required to track progress in access and availability of information and communications technology (ICT)-enabled solutions for agriculture. However, additional metrics, from availability and access to utilization, are also needed. Utilization depends on capacity building, skills transfer, digital literacy, cultural appropriateness of services and the agency to use services, particularly across ages and genders. We recommend core public research funding for this effort so that entrepreneurs, philanthropists and academics can better target their data-driven farming interventions, and so that the benefits of these interventions can be transparently assessed for positive farming and population health outcomes.
The idea of a technology revolution in agriculture has captured the minds of—and has secured funding from—donors, governments, national agricultural research services, development organizations, academic institutions and countless industry start-ups in recent years 1 , 2 , 3 , 4 , 5 . This vision is currently hindered by gaps in connectivity for farming populations. Emerging technologies such as 5G promise even more capabilities 29 , but the benefits for data-driven farming can never be fully realized as long as poor farmers are left behind 19 . A re-ignition of international efforts to deliver agricultural research for development is needed, this time not for the exchange and delivery of advanced seeds but for the exchange and delivery of data. This effort requires a fresh outlook, learning from the failures of previous revolutions in agriculture 30 , putting co-creation, knowledge sharing and farmer empowerment at the core, and seeking solutions that harmonize across the Sustainable Development Goals, particularly those related to education, gender inclusion, and access to water and energy 25 , 31 . The work presented here may provide a step in that direction and allows for the design of more targeted interventions for all those invested in solving farming problems by using data.
Details of the methods underlying specific components of this study are given below.
Coverage gaps
For the mobile service availability layers, we used a geographical information system data set, developed by Mosaik Solutions, on global mobile network coverage in 2018 for 910 entities running 2,391 networks in 229 countries and territories, and representing 2G (CDMA, 1XRTT, GSM, GPRS, EDGE), 3G (EVDO, UMTS, HDPA/+) and 4G (WiMax, LTE) services. This data set includes data provided directly by service operators, as well as data available on operator websites, in filings from regulatory bodies, and in the public domain, and is recognized as an industry standard reference 32 . Our map of smallholder farms was developed following the methods of ref. 33 and updated with the crowd-sourced field sizes of ref. 34 . Global layers representing nutrient deficits for 17 major crops 35 and aridity (annual precipitation <250 mm (ref. 36 )) were intersected with cropland data 37 (for aridity) and relevant crop types 38 (for nutrient deficiency) to create layers of cropland areas affected by each condition. Hotspots of yield gaps 39 were computed using the 90th percentile of the ratio of yield gaps to yield potentials. Hotspots of rainfall-dependent croplands were computed using the 90th percentile of the ratio of green water to total water used for crop production from ref. 40 . We also assessed the cross-cutting themes of food insecurity and the incidence of communicable diseases. We estimated the number of people affected by food insecurity using maps 21 that proxy food insecurity from the prevalence of childhood stunting, and we computed Africa-wide estimates using the updated data set of ref. 41 . We computed the number of people infected by P. falciparum using 2017 prevalence data from the Malaria Atlas Project ( https://malariaatlas.org/ ), and 2017 population estimates from LandScan 42 . For all layers, the coverage gap was computed as the proportion of each layer of cropland area and population classes covered by the 2G, 3G and 4G footprints.
We assessed access gaps in mobile phone ownership and Internet access across Asia, Africa, and Latin America and the Caribbean by compiling and harmonizing a sample of available subnationally representative micro-level data from the Demographic Health Surveys ( https://www.dhsprogram.com ), the Integrated Public Use Microdata Series ( https://www.ipums.org ), the Living Standards Measurement Study from the World Bank ( https://www.worldbank.org/en/programs/lsms ), and country-specific agricultural censuses, general household surveys and family panel studies (see Data availability for our accompanying data set). We define a farming household as one that owns usable land for agriculture or one in which a household member works in an agricultural occupation. Household mobile ownership is defined as a household that owns at least one mobile phone, and Internet access is defined as a household that has access to the Internet, through any device. Our sample represents farming and non-farming household-level mobile ownership in 70 countries (38 in Africa, 15 in Asia and 17 in Latin America and the Caribbean) and in 5,429 subnational units (941 in Africa, 848 in Asia and 3,640 in Latin America and the Caribbean). Our sample also represents farming and non-farming Internet access in 48 countries (20 in Africa, 11 in Asia and 17 in Latin America and the Caribbean) and 4,905 subnational units (522 in Africa, 931 in Asia and 3,452 in Latin America and the Caribbean).
To centre mobile phone ownership data, we used available panel data sets of ownership (from 2005 to 2017), for countries in each region (ten in Africa, five in Asia and two in Latin America and the Caribbean). We estimated time-dependent percentage point differences in ownership within each region and for farming versus non-farming populations. We used the following shape-constrained generalized additive mixed model 43 :
Here, y i is the observed number of households owning a mobile phone, n i is the number of households surveyed, and p i is the fraction of households that own a mobile phone; b il ( rf i t i ) are the l th monotonically increasing P-spline basis functions for year ( t ) and the region and farming versus non-farming populations ( rf ); q is the number of basis functions, β il are the spline coefficients; δ j are random intercepts (with variance \(\sigma^2_\delta\) ) to account for non-independence of observations in countries; and α terms are fixed intercepts representing differences in fractional ownership for each rf combination.
To centre Internet access data, we used national time-series data of individuals using the Internet (1990–2016) from the World Bank and the International Telecommunication Union ( https://www.data.worldbank.org/indicator ) to estimate time-dependent percentage point differences in access for each country between the observed years of sampling and a common focal year, using the following generalized additive model 44 :
Where y i is the observed proportion of individuals with Internet access, μ i is E( y ), ϕ is a precision parameter, b il ( ct i ) are l th cubic-spline basis functions for year ( t ) and country ( c ), and α terms are fixed intercepts representing differences in percentage access for each c .
We centred access data on the year 2018 and used the percentages of farming and non-farming households who own mobile phones or have Internet access at each subnational unit within a country. For visual comparison of access within countries and between farming and non-farming populations, we fit Gaussian probability density functions to the mobile phone and Internet access metrics using subnationally representative sampling units (see Data and Code availability for raw time-centred data underlying these plots).
Cost of data
To identify the costs of data for people living in poverty, the cost of 1 GB of prepaid mobile access as a percentage of income was obtained from the Alliance for Affordable Internet ( https://a4ai.org/ ) for 83 countries in Africa, Asia, and Latin America and the Caribbean. To disaggregate these data by income groups within each country, we used the income shares from the World Bank and re-computed the index of the Alliance for Affordable Internet using the income of the poorest decile of each country. To account for differences in sampling effort, we estimated the difference (a bias estimate) between the median regional cost of data in 2018 (the year with most complete coverage) and the median regional cost in 2018 when coverage equalled that of 2015–2018. We then bias corrected the time series by adjusting the median costs for the years 2015–2018 to known differences observed in 2018.
Data availability
The data sets used and created in this study are archived at the Zenodo Public Repository: https://doi.org/10.5281/zenodo.4082121 .
Code availability
All code for reproducing the results in this manuscript are archived at the Zenodo Public Repository: https://doi.org/10.5281/zenodo.4082121 .
Tricarico, D. & Darabian, N. mAgri—Weather Forecasting and Monitoring: Mobile Solutions for Climate Resilience (GSMA, 2016); https://go.nature.com/37lo5eU
Information and Communication Technology (ICT) in Agriculture: a Report to the G20 Agricultural Deputies (FAO, 2017).
King, A. Technology: the future of agriculture. Nature 544 , S21–S23 (2017).
Article CAS Google Scholar
World Bank Group Future of Food: Harnessing Digital Technologies to Improve Food System Outcomes (World Bank, 2019).
Kaka, N. et al. Digital India: Technology to Transform a Connected Nation (McKinsey Global Institute, 2019).
Eitzinger, A. et al. GeoFarmer: a monitoring and feedback system for agricultural development projects. Comput. Electron. Agric. 158 , 109–121 (2019).
Article Google Scholar
mNutrition: Addressing Hidden Hunger Through Mobile Messaging (CABI, 2017).
Asenso-Okyere, K., Asante, F. A., Tarekegn, J. & Andam, K. S. A review of the economic impact of malaria in agricultural development. Agric. Econ. 42 , 293–304 (2011).
Global Mobile Trends 2018. What’s Driving the Mobile Industry? (GSMA Intelligence, 2018); https://go.nature.com/2T41ZW9
Measuring the Information Society Report 2018 Vol. 1 (ITU Publications, 2018).
Szoszkiewicz, Ł. Internet Access as a New Human Right? State of the Art on the Threshold of 2020. Adam Mickiewicz Univ. Law Rev. 8 , 49–62 (2018).
Google Scholar
ITU Council contribution to the 2019 High-Level Political Forum on Sustainable Development (HLPF) Revision 3 to Document CWG-WSIS&SDG-33/15-E (ITU, 2019).
Dobardzic, S. et al. 2019 State of Climate Services: Agriculture and Food Security. Report No. WMO-No. 1242 (World Meteorological Organization, 2019).
Fabregas, R., Kremer, M. & Schilbach, F. Realizing the potential of digital development: the case of agricultural advice. Science 366 , eaay3038 (2019).
Building Agricultural Market Information Systems: a Literature Review (FAO, 2017).
IBM AI and cloud technology helps agriculture industry improve the world’s food and crop supply. IBM News Room (22 May 2019); https://go.nature.com/2T9nZiq
Nuru becomes African farmers’ newest ally against Fall Armyworm. FAO (25 June 2018); http://www.fao.org/news/story/en/item/1141889/icode/
Free, C. et al. The effectiveness of mobile-health technologies to improve health care service delivery processes: a systematic review and meta-analysis. PLoS Med. 10 , e1001363 (2013).
Mehrabi, Z., Jimenez, D. & Jarvis, A. Democratize access to digital agronomy. Nature 555 , 30 (2018).
Mattson, S. CIAT, World Bank and partners announce Digital Agriculture Country Profiles initiative. The Alliance of Biodiversity International and CIAT Blog (2019); https://go.nature.com/37xPHOj
FAO The State of the World’s Land and Water Resources for Food and Agriculture: Managing Systems at Risk (Taylor & Francis, 2011); https://doi.org/10.4324/9780203142837
Connected Women: Bridging the Gender Gap: Mobile Access and Usage in Low and Middle Income Countries (GSMA, 2015).
2019 Affordability Report (A4AI, 2019); https://a4ai.org/affordability-report/
Connecting Africa Through Broadband: a Strategy for Doubling Connectivity by 2021 and Reaching Universal Access by 2030 (United Nations Broadband Commission for Sustainable Development, 2019).
Handforth, C. Closing the Coverage Gap: How Innovation Can Drive Rural Connectivity (GSMA, 2019).
Karlsson, M. et al. Accelerating Affordable Smartphone Ownership in Emerging Markets (GSMA, 2017).
Investing in Rural Livelihoods to Eradicate Poverty and Create Shared Prosperity (IFAD, 2017).
ICT in Agriculture (Updated Edition): Connecting Smallholders to Knowledge, Networks, and Institutions (World Bank, 2017).
Understanding 5G: Perspectives on Future Technological Advancements in Mobile (GSMA Intelligence, 2014).
Pingali, P. Green revolution: impacts, limits, and the path ahead. Proc. Natl Acad. Sci. USA 109 , 12302–12308 (2012).
Maru, A. et al. Digital and Data-Driven Agriculture: Harnessing the Power of Data for Smallholders (Global Forum on Agricultural Research and Innovation, 2018).
20th Mobile Wireless Competition Report (FCC, 2017).
Herrero, M. et al. Farming and the geography of nutrient production for human use: a transdisciplinary analysis. Lancet Planet. Health 1 , e33–e42 (2017).
Lesiv, M. et al. Estimating the global distribution of field size using crowdsourcing. Glob. Change Biol. 25 , 174–186 (2019).
West, P. C. et al. Leverage points for improving global food security and the environment. Science 345 , 325–328 (2014).
Karger, D. N. et al. Climatologies at high resolution for the earth’s land surface areas. Sci. Data 4 , 170122 (2017).
Ramankutty, N., Evan, A. T., Monfreda, C. & Foley, J. A. Farming the planet: 1. Geographic distribution of global agricultural lands in the year 2000. Glob. Biogeochem. Cycles 22 , GB1003 (2008).
Monfreda, C., Ramankutty, N. & Foley, J. A. Farming the planet: 2. Geographic distribution of crop areas, yields, physiological types, and net primary production in the year 2000. Glob. Biogeochem. Cycles 22 , GB1022 (2008).
Mueller, N. D. et al. Closing yield gaps through nutrient and water management. Nature 490 , 254–257 (2012).
Portmann, F. T., Siebert, S. & Döll, P. MIRCA2000—Global monthly irrigated and rainfed crop areas around the year 2000: a new high-resolution data set for agricultural and hydrological modeling. Glob. Biogeochem. Cycles 24 , GB1011 (2010).
Osgood-Zimmerman, A. et al. Mapping child growth failure in Africa between 2000 and 2015. Nature 555 , 41–47 (2018).
Dobson, J. E., Bright, E. A., Coleman, P. R., Durfee, R. C. & Worley, B. A. LandScan: a global population database for estimating populations at risk. Photogramm. Eng. Remote Sens. 66 , 849–857 (2000).
Pya, N. & Wood, S. N. Shape constrained additive models. Stat. Comput. 25 , 543–559 (2015).
Hastie, T. J. & Tibshirani, R. Generalized additive models. Stat. Sci. 1 , 297–310 (1986).
Download references
Acknowledgements
Z.M. and N.R. were funded by NSERC Discovery Grant RGPIN-2017–04648. Z.M., H.W., V.R. and N.R. were funded by Social Sciences and Humanities Research Council Insight Grant 435-2015-1364, and H.W. by the Canadian Institutes of Health Research grant ROH-115207. Z.M. and H.W. received funds from the VPRI Research Excellence Cluster on Diversified Agroecosystems of the University of British Columbia. C.L. was funded by the Horizon 2020 research and innovation programme of the Euroipean Union under Marie Skłodowska-Curie grant agreement 796451. This work was implemented as part of the CGIAR Platform for Big Data in Agriculture, which is carried out with support from CGIAR Fund Donors and through bilateral funding agreements. For details, please visit https://www.cgiar.org/funders/ .
Author information
Authors and affiliations.
The UBC School of Public Policy and Global Affairs, University of British Columbia, Vancouver, British Columbia, Canada
Zia Mehrabi, Vincent Ricciardi, Christian Levers, Juan Diego Martinez & Navin Ramankutty
Institute for Resources, Environment and Sustainability, University of British Columbia, Vancouver, British Columbia, Canada
Zia Mehrabi, Vincent Ricciardi, Christian Levers, Juan Diego Martinez, Hannah Wittman & Navin Ramankutty
Center for Sustainable Food Systems, University of British Columbia, Vancouver, British Columbia, Canada
Zia Mehrabi, Mollie J. McDowell & Hannah Wittman
Department of Computational Landscape Ecology, Helmholtz Centre for Environmental Research - UFZ, Leipzig, Germany
Christian Levers
Vancouver, British Columbia, Canada
Natascha Mehrabi
CGIAR Research Program on Big Data in Agriculture, International Center for Tropical Agriculture, Cali, Colombia
Andy Jarvis
You can also search for this author in PubMed Google Scholar
Contributions
Z.M. conceived, designed and led the study. N.M. helped with concept development. Z.M., H.W., N.R. and A.J. helped to secure funding. M.J.M., V.R., C.L. and J.D.M. compiled and developed the underlying data sets. Z.M. conducted the analysis and wrote the paper. All authors provided comments on the paper.
Corresponding author
Correspondence to Zia Mehrabi .
Ethics declarations
Competing interests.
The authors declare no competing interests.
Additional information
Publisher’s note Springer Nature remains neutral with regard to jurisdictional claims in published maps and institutional affiliations.
Rights and permissions
Reprints and permissions
About this article
Cite this article.
Mehrabi, Z., McDowell, M.J., Ricciardi, V. et al. The global divide in data-driven farming. Nat Sustain 4 , 154–160 (2021). https://doi.org/10.1038/s41893-020-00631-0
Download citation
Received : 26 November 2019
Accepted : 22 September 2020
Published : 02 November 2020
Issue Date : February 2021
DOI : https://doi.org/10.1038/s41893-020-00631-0
Share this article
Anyone you share the following link with will be able to read this content:
Sorry, a shareable link is not currently available for this article.
Provided by the Springer Nature SharedIt content-sharing initiative
This article is cited by
The old, the new, or the old made new everyday counter-narratives of the so-called fourth agricultural revolution.
- David Christian Rose
- Anna Barkemeyer
- Dannielle Roche
Agriculture and Human Values (2023)
How can precision farming work on a small scale? A systematic literature review
- Tamás Mizik
Precision Agriculture (2023)
A typology of agricultural market information systems and its dimensions: Case studies of digital platforms
- Neeraj Singh
- Kushankur Dey
Electronic Markets (2023)
- Asaf Tzachor
- Medha Devare
- Seán Ó hÉigeartaigh
Nature Machine Intelligence (2022)
Urgently needed transition pathways toward sustainability in agriculture: the case of Persian lime (Citrus latifolia Tanaka) production in Veracruz, Mexico
- Susana Isabel Castillo-Martínez
- Julio Díaz-José
- Juan Guillermo Cruz-Castillo
Environment, Development and Sustainability (2022)
Quick links
- Explore articles by subject
- Guide to authors
- Editorial policies
Sign up for the Nature Briefing newsletter — what matters in science, free to your inbox daily.


Science Breakthroughs to Advance Food and Agricultural Research by 2030 (2019)
Chapter: 7 data science, 7 data science, 1. introduction.
The growing availability of data presents an opportunity to improve the resilience and efficiency of food and agricultural production on a scale unimaginable even one decade ago. The convergence of cloud computing, mobile, Internet of Things (IoT), and analytic technologies has resulted in an explosion of data that has been transformational in all sectors of the economy ( Cao, 2016 ). Approximately 90 percent of the data ever created were generated in the past 2 years ( Marr, 2018 ). By 2025, the amount of data created and copied annually is expected to grow to 1 trillion gigabytes ( Seagate, 2017 ). Understanding the connections between the biophysical and socioeconomic elements of agricultural systems through research depends on generating and analyzing massive amounts of data. Data will be at the center of the next revolution in food and agriculture ( UN, 2014 ).
The relationship between data, algorithms, and computing is symbiotic. In practice, (raw) data are processed by computers through mathematical models and algorithms into useable information. The value of data comes when they are analyzed to provide information that is used to make decisions based on insights and understandings derived from data. Advances in data storage, communications, and processing have led to new research methods and tools that were simply not possible just one decade ago ( NIH, 2018 ). Similarly, breakthroughs in agriculture will benefit from and compel state-of-the-art advances in the areas of data, algorithms, and computing. Machine learning and deep learning are two such examples, which will be further discussed in Section 3.1 .
Data science is the emerging field that sits at the nexus of data, algorithms, and computing. It is an interdisciplinary field of inquiry in which quantitative and analytical approaches, processes, and systems are developed and used to extract knowledge and insights from increasingly large and complex sets of data ( NIH, 2018 ). Data analysis has fostered knowledge creation for hundreds of years; achieving the science breakthroughs identified in this report will be spurred by data science.
In June 2018, the United States announced a new record in the global competition to build the world’s fastest supercomputer. The petascale machine called “Summit” can perform 200 petaflops (10 15 calculations) per second and is more than twice the speed of the incumbent at China’s National Supercomputing Center ( Top 500, 2018 ). The next horizon is exascale computing; a machine capable of executing 1 billion billion (10 9 × 10 9 = 10 18 ) calculations per second. Exascale computing will enable more complete and accurate representations in Earth systems modeling needed to improve our understanding of local to global scales for the food system and enable faster simulations that can, for example, more realistically mirror the speeds of biological processes ( McDermid et al., 2017 ).
Figure 7-1 shows a data analytics maturity curve that progresses from descriptive to predictive to prescriptive. In the early stages, information provided in the descriptive stage lends hindsight on what happened. The predictive stage is the next stage that provides insight into what will happen. The last stage of analytics maturity is prescriptive and enables foresight in what we can make happen. Much progress has been made in food and agricultural research in establishing descriptive analytics. Scientific breakthroughs in food and agricultural disciplines in the next 10-15 years will increasingly address the predictive and prescriptive levels of understanding.
Our understanding of the fundamental scientific underpinnings of the biological, chemical, physical, and socioeconomic elements of the food and agricultural system can benefit from better data access, data integration, and data analytics. The previous chapters have indicated specific instances where access to more data and data science can improve the resilience, efficiency, and sustainability of agriculture. This chapter describes the challenges of collecting, integrating, and analyzing a broad range of data types, and ensuring the quality of those data in near real time to afford the research breakthroughs needed. The chapter then proposes research needs and potential breakthroughs specific to data science for the food and agricultural enterprise.
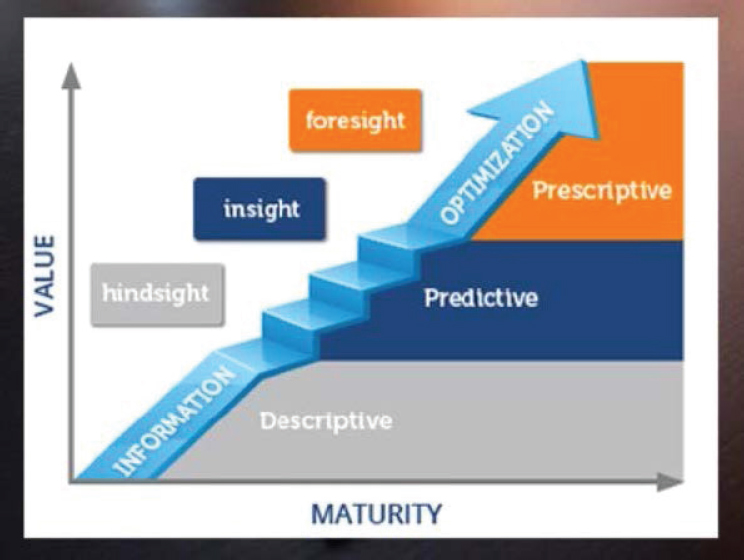
2. CHALLENGES
2.1 challenge 1: data heterogeneity and dimensionality.
The challenges and opportunities in agricultural and food data can be illustrated by the acronym GEMS, which represents the fact that agriculture yields are modeled as a function of genetic (G), environment (E), management (M), and socioeconomic (S) factors. To understand yield, there needs to be an understanding of the genetics of the plant or animal being cultivated, the environmental factors affecting growth, the management practices of the farm, and the socioeconomic factors, as well as an understanding of the complex interactions among these factors, represented as G × E × M × S (GEMS). Numerous environmental factors may be of interest (e.g., location, soil type, elevation, inclination, rainfall, precipitation, humidity, temperature, and dew point) and each may have positive or negative impacts on genotypes. GEMS data are in a wide variety of formats, in
different spatial and temporal scales, with different degrees of accuracy and precision that are challenging to harmonize for analysis and discovery ( Lu et al., 2016 ). Data curation and harmonization efforts currently constitute 80 percent of the effort related to data use ( Crowdflower, 2016 ).
The curation and analysis of GEMS data lead to the need for data standards and tools to manipulate and analyze the massive geospatial temporal datasets, and pushes the frontier of data, analytics, and computing systems (see Box 7-1 ). The complexities of data, such as the GEMS data, can be explored in terms of the four basic data attributes: volume, variety, velocity, and veracity (see Box 7-2 ).
Example: Improvements in Sensor Technology and Data Velocity Enabling Real-Time Continuous Monitoring in Agriculture. Novel sensors can provide accurate measures of soil, plant, animal, and food characteristics at a high frequency and data density and at any given time and location. The next generation of sensors will revolutionize the ability to deploy prescriptive solutions in near real time. For example, Figure 7-2 shows how georeferenced in situ soil sensors could be widely distributed across a farm, measure soil and plant nutrients, and display results in a colored geospatial map that allows farmers to determine site-specific actions (e.g., variable application of water and nutrients). Similarly, microelectromechanical technology is already being used to develop miniature sensors that
can be embedded into plant stems for measuring a plant’s water potential (the hydration relative to its growth and production yield) ( Pagay et al., 2014 ); this could replace traditional labor-intensive, destructive methods that provide only point-in-time measurements. Continuous measurements such as these will improve the calibration and performance of water- and nutrient-use models, and may lead to a new understanding of how plants use water and nutrients. Integrating these farm-scale sensor technologies with seasonal or hyper-local weather forecasts, or with measures of water availability in connected ecosystems via the IoT, creates opportunities to manage farm ecosystems integrated with natural ecosystems (e.g., ecohydrology). For vineyards, such an understanding could lead to more consistent production of high-quality wine grapes with certain flavor and
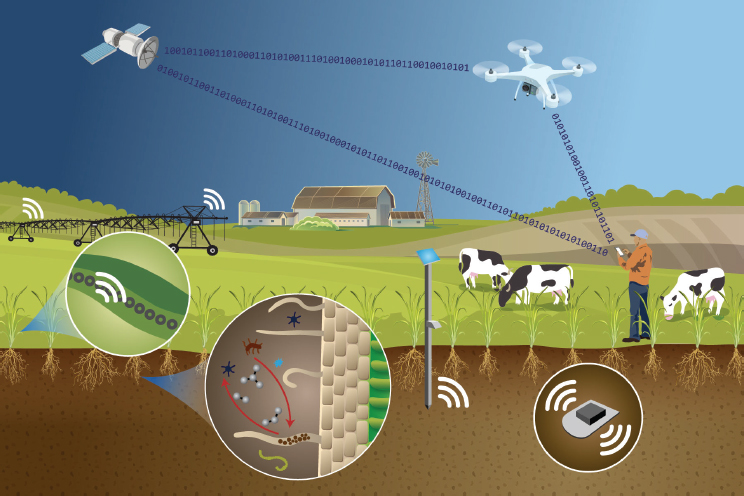
aroma profiles ( Campbell, 2016 ). Likewise, in planta crop health sensors may quantify biochemical changes in plants caused by an insect pest or a pathogen, alerting and enabling the producer to plan and deploy immediate site-specific control strategies before the infestation occurs and damage is visible and widespread across the field. Increased data velocity will enable dynamic control of agricultural equipment in motion in real time—such as precision planters, sprayers, and irrigation—and enable real-time continuous monitoring of individual livestock in a herd using wearable (and other) sensors for precision livestock applications, using advanced technologies such as microfluidics, sound analyzers, image-detection techniques, sweat and salivary sensing, serodiagnosis (diagnosis based on blood sera), and others. Creating efficient online monitoring systems in real time requires the ability to integrate all the available sensor data and run analysis in real time ( Neethirajan, 2017 ).
2.2 Challenge 2: Data Standards and Interoperability
Modern scientific research is becoming a more data-driven, interactive process between multiple stakeholders across the world ( EU SCAR, 2015 ). The term “e-science” has been coined to describe the potential of data-driven and computationally intensive processes to enhance manual laboratory work and fieldwork and reduce dependence on paper-based recording ( EU SCAR, 2015 ). E-science has the potential to enable worldwide collaboration in flexible teams using advanced tools, services, and data repositories. These can include distributed networks or grid computing, high-performance computing, visualizations, simulations, workflows, and provenance documentation ( EU SCAR, 2015 ).
Providing access to data can accelerate and democratize the scientific process. To do so would require infrastructure improvements for supporting the reuse of scientific data in the food and agricultural realm and enhancements for machines to automatically find and use data. While there are some notable cyberinfrastructure initiatives and data-sharing efforts under way (see Box 7-3 ), these efforts are only able to address some parts of the vast food system. As a set of universally agreed-upon guidelines referring to interoperability among systems or applications, standards support reuse. One of the major hurdles in data standardization and interoperability is the lack of sufficient knowledge representations (e.g., ontologies, semantic nets, and rules) ( Jonquet et al., 2018 ). Knowledge representations provide a representation that a computer system can utilize to solve complex tasks.
The FAIR data principles are a set of guiding principles that could facilitate data standardization and interoperability for scientific data management and stewardships. The principles are organized around four concepts: findable, accessible, interoperable, and reuseable ( Wilkinson et al., 2016 ). The FAIR principles are complementary to open-data philosophy—data can be open without being FAIR, and vice versa. Open data is the idea that some data should be freely available to everyone to use and republish as they wish, without restrictions from copyright, patents, or other mechanisms of control ( Auer et al., 2007 ). Government-funded awards often require researchers to open their data, but there is little guidance on best practices or dissemination for open data in the United States.
The existence of standards is an often-cited success factor for reuse of data and software ( Pasquetto et al., 2017 ). Standards can be administered in a variety of ways: created de facto by private organizations 1 or managed by nonprofit organizations. 2 The nonprofit consortium of businesses serv-
___________________
1 The computer file format PDF was created by Adobe in 1993 and was a de facto standard for many years before eventually becoming a formal ISO standard in 2005.
2 The nonprofit organization GS1 is best known for the barcode (universal product code) that is scanned by retailers more than 6 billion times every day.
ing the agriculture sector, AgGateway, has identified the need for standards as essential to promoting and enabling the industry’s transition to highly data-driven agricultural practices and is working to develop their own ( AgGateway, 2017 ; Smith, 2017 ).
2.3 Challenge 3: Data Privacy
Privacy is a confounding issue for food and agricultural data. In some domains, such as health care, data can be anonymized by removing or encrypting personally identifiable information. The best available privacy protection technology, differential privacy, is inadequate for agricultural
data because of its spatiotemporal nature ( Shekhar et al., 2017 ). Anonymizing geospatial data without distorting its granularity is a known problem which does not yet have an adequate solution. Aggregating data is a frequently used approach to anonymize farm- or field-level data. For instance, data from individual fields and farms are aggregated into county-level metrics that are made public. Aggregation limits the granularity of analysis, making intrafield or site-specific analysis impossible. For example, spatial data that are aggregated for anonymization purposes typically lack the high spatial resolution needed by biophysics-based models to accurately capture or predict the system responses.
3. SCIENTIFIC OPPORTUNITIES
There are emerging technologies related to data that can transform the food and agricultural system. In particular, there are four promising future areas: artificial intelligence, blockchain, IoT, and quantum computing.
3.1 Opportunity 1: Artificial Intelligence
Artificial intelligence (AI) refers to intelligence displayed by machines, as opposed to natural intelligence demonstrated by humans and other animals ( Bradford, 2017 ). The term AI is commonly used when a machine mimics cognitive functions that are typically associated with humans, such as planning, learning, reasoning, problem solving, knowledge representation, perception, motion, manipulation, social intelligence, and creativity. AI combines automation, robotics, and computer vision. Advances in statistics, faster computers, and access to large amounts of data have enabled advances in AI, particularly in the field of machine learning where significant progress has been made in the areas of image and pattern recognition, natural language understanding, and robotics.
The scale and complexity of the food and agricultural system make it well suited for AI, with large amounts of data available to train algorithms. Large amounts of data from ever-more-sophisticated experiments and models are being generated, and food and agricultural researchers are challenged with deciding what data to collect and how to best process the data generated. A 2016 analysis estimated that a new crop protection product requires the analysis of more than 160,000 compounds—the equivalent to more than 11 years of research and development and overall costs that exceed $280 million per commercial product ( Brayne et al., 2018 ). AI will enable new discoveries, not just in analyzing existing data but in augmenting human creativity around planning new experiments and accelerating discovery and improving efficiency.
There are promising applications and indicators for AI in agriculture. In selective breeding programs, the development of high-throughput automated phenotyping capabilities can speed the process of breeding to develop resilient yet high-yielding, high-quality crops. AI and machine-learning research on the massive amount of environmental, growth, and genetic data can help scientists, for example, elucidate the connections between system components, predict the yield of different varieties based on early-season plant attributes, and associate specific desirable traits with genetic markers ( Baxter et al., 2007 ). Robots can be designed to harvest crops at a higher volume and faster pace than human laborers, to monitor crop and soil health using computer vision and deep-learning algorithms that analyze data captured by drones, and to more accurately predict crop
yields using machine-learning models to better understand environmental impacts ( Sennar, 2017 ). Box 7-4 on robotic milking provides an example of integrating AI with sensor technologies in animal agriculture.
3.2 Opportunity 2: Blockchain
Blockchain is a recent technology advancement with potential for addressing the challenge of creating a more transparent, authentic, and trustworthy digital record of the journey that food and other physical products take across the supply chain ( Lougee, 2017 ). Blockchain technology arose out of the efforts to create the cybercurrency Bitcoin, but its potential goes well beyond its original purpose. As discussed in Chapter 4 , at its core, the blockchain is a shared, immutable ledger for recording the history of transactions ( IEEE, 2018a ). It uses a variety of technologies, including public–private key cryptography, distributed databases, decentralized processing, hash functions, and consensus algorithms ( Ge et al., 2017 ).
This could be valuable in creating transparency in a global food system with multiple disparate actors across the multitiered supply chain.
More information will be required to address research challenges on food and agricultural supply chains and a platform for studying how complex systems interact. Blockchain presents a potential new source of data and new platform to be leveraged to deliver advances toward transparency to the food system and transformations in food safety, fraud reduction, market access, waste reduction, and productivity gains ( Ahmed and ten Broek, 2017 ; Ge et al., 2017 ; Kim and Laskowski, 2017 ).
A systematic mapping study designed to understand the current research topics, challenges, and future directions of blockchain technology from a technical perspective examined 41 primary papers ( Yli-Huumo, 2016 ). It showed that most of the current research on blockchain technologies has been focused on security and privacy issues. Issues in scalability, such as performance and latency, need to be addressed in order to realize pervasive use of blockchain technology in the food system.
3.3 Opportunity 3: IoT
IoT is the network of physical devices embedded with electronics, software, sensors, actuators, and connectivity which enables these “things” to connect and exchange data creating opportunities for more direct integration of the physical world into computer-based systems, resulting in efficiency improvements, economic benefits, and reduced human exertions ( Wasik, 2013 ; Morgan, 2014 ). Among its advantages, IoT addresses the high cost of manual data collection, which impedes the adoption of beneficial data-driven technologies. IoT technologies enable seamless data collection from various sensors, cameras, and drones and are the foundation for intelligent systems, as illustrated in the example above and throughout this report. Farm applications of precision agriculture and precision livestock farming are prime examples of IoT; however, the influence of IoT spans the entire food system ( Wolfert et al., 2017 ).
IoT is still in its infancy in the food and agriculture sector ( Verdow et al., 2016 ). Realizing future subsystems within the food system where IoT devices are capturing and sending data, but in a time-sensitive and synchronized way, “could be stalled by our lack of effective methods to marry computers and networks with timing systems” ( NIST, 2015 ).
Challenging requirements for food and agriculture applications need to be addressed in both the technical and nontechnical arenas. For example, on-site applications in remote outdoor farming locations often lack Internet connectivity needed for communications. Easy access to power and durability and calibration are also essential. The FarmBeats system design explic-
itly accounts for weather-related power and Internet outages, which has enabled 6-month-long deployments in two U.S. farms ( Vasisht et al., 2017 ).
3.4 Opportunity 4: Quantum Computing
Quantum mechanics may play a vital role in biology, as quantum phenomena occur in biological systems. In 2007, it was discovered that photosynthesis operates though quantum coherence, giving rise to the new field of quantum biology ( Engel et al., 2007 ; Ball, 2011 ). Other examples of quantum phenomena relevant for food and agriculture include olfaction ( Turin, 2002 ), DNA mutation ( Lowdin, 1965 ), and Brownian motors in many cellular processes ( Krug et al., 2006 ). The emergence of quantum computing could be used to explore natural phenomena in the physical world—phenomena that traditional computing paradigms are ill suited to represent at scale.
Quantum mechanics is a subdiscipline of physics that explains the physical world and attempts to explore how it functions ( Feynman, 2002 ). Nature follows the laws of quantum mechanics, in which particles behave in “strange” ways. For example, caffeine molecules in a cup of coffee are difficult to model, and the detailed structure and properties are difficult to understand because the particles can take on more than one state and can even interact with other particles that are far away. The computers currently in use have not been able to take into account such complex properties because classical computers code information in bits that represent 0 or 1 values. On the other hand, quantum computers operate on the basis of qubits and incorporate two key principles of quantum physics: superposition and entanglement. Superposition allows a qubit to simultaneously take on the value of both 0 and 1, and entanglement allows qubits in a superposition to be correlated with one another (the state of one can be dependent on the other). Classical computers use a binary format as on-off switches, while quantum computers use qubits that can act as sophisticated on-off switches and can be used to solve more complex problems in quantum scales (including nature) ( IEEE, 2018b ).
Quantum computing technology is in its infancy. Many fundamental challenges exist, including developing long-living qubits for computation, scaling the number of qubit processors, and building effective quantum error correction. However, major efforts are under way across the globe in academia and industry and promising early results are unfolding ( Knight, 2017 ; Alibaba, 2018 ; Google, 2018 ; IBM Q, 2018 ; Intel, 2018 ; Microsoft, 2018 ). In 2016, the first publicly available quantum computing service on the cloud was made available. Quantum computers may lead to revolutionary breakthroughs in discovery of new materials for agriculture, agrochemical discovery, and artificial intelligence algorithms that could impact
food and agriculture. Molecular modeling has been identified as an area for exploration with quantum computing ( Emerging Technology from the arXiv, 2017 ). Another area in which quantum computing could help with is analyzing genomics data for plants, animals, or the soil microbiome. Quantum computing could make it possible to perform real-time analytics on various types of data (e.g., weather data at high spatial resolution) ( Accenture Labs, 2017 ; Popkin, 2017 ). This unique juncture in time provides an opportunity for food and agricultural research to help shape the next era of computing.
4. BARRIERS TO SUCCESS
Data science is a rapidly evolving field and data science skills for working with Big Data are in high demand in all sectors, including food and agricultural research and agricultural economics ( Woodward, 2016 ). Data science tools need to become more useable by non–computer science domain experts. Traditional agricultural and food science programs are evolving to better integrate the variety of disciplines needed; for instance, the digital agriculture initiative at Cornell University is aimed at generating innovating research at the intersection of agriculture, computing, and engineering ( Cornell University, 2018 ). New partnerships, prizes, and conferences are needed to spark convergence between those who are new to food and agriculture and current food and agricultural researchers. Initiatives such as the Syngenta Crop Challenge in Analytics awarded by the Analytics Society of INFORMS show promise in fostering much-needed cross-industry collaboration ( INFORMS, 2018 ). Reward systems for generating and sharing data in research environments may need to be considered, for example, creating incentive structures or changing the approach to tenure and promotion evaluations ( USDA-NIFA, 2017b ). It will be important to train a workforce that can manage, analyze, and manipulate large datasets and enable the workforce’s convergence with food and agricultural scientists. Furthermore, it may be necessary to develop a culture that supports and rewards sharing of data (including combinations of private and public data) by sets of communities of researchers, standardizes protocols, harmonizes experimental designs, and addresses ownership, privacy, and security concerns unique to the food and agricultural enterprise. Funding sources, public or private, need to be available for developing the standards and protocols that need to be harmonized for agricultural datasets to make them interoperable.
The collection of data from numerous stakeholders is required to create the pool of Big Data needed to well represent the multidimensional food system. To incentivize data sharing by individual stakeholders or researchers, reward systems may require new technologies that can iden-
tify and appropriately attribute value derived from the pool of Big Data to the individual contributions. These value attribution technologies may be conceptually similar in spirit to marketing attribution, the process of identifying a set of user actions (events, touchpoint) that contribute in some manner to a desired outcome, and then assigning a value to each of these ( Priest, 2018 ), but will need to account for the unique complexities in the food and agricultural domain.
Ownership of food and agricultural data can be a confounding issue, especially when it comes to IoT data. Ownership is a legal concept related to property, and U.S. law recognizes various categories of property (for example, real property, personal property, and intellectual property). However, data generated in the food and agricultural sectors may not belong in any of those categories of property. For example, farming data is a compilation of data generated from field operations (real property) using sensors on equipment (personal property) and represents intellectual property on maximizing yield. Questions emerge around legal ownership of data (which confers control) and how the data can be used. There are currently few legislative or judicial rulings for guidance. The American Farm Bureau Federation developed the Privacy and Security Principles for Farm Data ( AFBF, 2014 ), which lays out 13 data principles, but these are only guidelines. Users of data will need to consider the implications of data ownership, including protecting ownership of data, abiding by legal usage, and ensuring appropriate sharing with others. Ambiguity and uncertainty around ownership can cause inefficiencies and limit sharing and value creation.
5. RECOMMENDATIONS
Advances in data sciences can transform how data can be better collected, analyzed, and used for food and agricultural research. While there are many opportunities, the following actions merit high priority:
- Accelerate innovation by building a robust digital infrastructure that houses and provides FAIR (findable, accessible, interoperable, and reuseable) and open access to agri-food datasets.
- Develop a strategy for data science in food and agricultural research, and nurture the emerging area of agri-food informatics by adopting and influencing new developments in data science and information technology in food and agricultural research.
- Address privacy concerns and incentivize sharing of public, private, and syndicated data across the food and agricultural enterprise by investing anonymization, value attribution, and related technologies.
Accenture Labs. 2017. Innovating with Quantum Computing: Enterprise Experimentation Provides View into Future of Computing. Available at https://www.accenture.com/t00010101T0000000w/br-pt/acnmedia/PDF-45/Accenture-Innovating-QuantumComputing-Novo.pdf (accessed April 26, 2018).
AFBF (American Farm Bureau Federation). 2014. Privacy and Security Principles for Farm Data. Available at https://www.fb.org/issues/technology/data-privacy/privacy-and-security-principles-for-farm-data (accessed April 26, 2018).
AgGateway. 2017. AgGateway Releases Annual Report and 5-Year Plan: Enable Companies to Increase Efficiency, Agility and Profitability. Available at http://www.aggateway.org/Newsroom/2017PressReleases/AgGatewayReleasesAnnualReportand5-YearPlan.aspx (accessed April 26, 2018).
Ahmed, S., and N. ten Broek. 2017. Food supply: Blockchain could boost food security. Nature 550(7674):43.
Alibaba. 2018. Alibaba Cloud and CAS Launch One of the World’s Most Powerful Public Public Quantum Computing Services. Available at https://www.alibabacloud.com/pressroom/alibaba-cloud-and-cas-launch-one-of-the-worlds-most (accessed July 9, 2018).
Allan, G., J. Erdmann, A. Gustafson, A. Joglekar, M. Milligan, G. Onsongo, K. Pamulaparthy, P. Pardey, T. Prather, S. Senay, K. Silverstein, J. Wilgenbusch, Y. Zhang, and P. Zhou. 2017. G.E.M.S: An innovative agroinformatics data discovery and analysis platform. Available at https://rdmi.uchicago.edu/papers/08162017165531paperwilgenbusch081617.pdf (accessed July 9, 2018).
Auer, S. R., C. Bizer, G. Kobilarov, J. Lehmann, R. Cyganiak, and Z. Ives, Z. 2007. DBpedia: A Nucleus for a Web of Open Data. The Semantic Web . Lecture Notes in Computer Science. 4825. P. 722. doi: 10.1007/978-3-540-76298-0_52.
Ball, P. 2011. Physics of life: The dawn of quantum biology. Nature 474:272-274.
Baxter, I., M. Ouzzani, S. Orcun, B. Kennedy, S. S. Jandhyala, and D. E. Salt. 2007. Purdue Ionomics Information Management System. An integrated functional genomics platform. Plant Physiology 143(2):600-611.
Bradford, A. 2017. Empirical evidence: A Definition. LiveScience. Available at https://www.livescience.com/21456-empirical-evidence-a-definition.html (accessed July 9, 2018).
Brayne, S., S. McKellar, and K. Tzafestas. 2018. Artificial Intelligence in the Life Sciences & Patent Analytics: Market Developments and Intellectual Property Landscape. London: IP Pragmatics Ltd. Available at https://www.ip-pragmatics.com/media/1049/ip-pragmaticsartificial-intelligence-white-paper.pdf (accessed July 9, 2018).
Brouček, J., and P. Tongel’. 2015. Adaptability of dairy cows to robotic milking: A review. Slovak Journal of Animal Science 48(2):86-95.
Campbell, G. 2016. The Tensiometer: Micro-sized. Environmental Biophysics. Available at http://www.environmentalbiophysics.org/tensiometers-micro-sized (accessed July 9, 2018).
Cao, L. 2016. Data science and analytics: A new era. International Journal of Data Science and Analytics 1(1):1-2.
Centurion, C. 2015. Moving Along the Analytics Maturity Curve. River Logic Blog. Available at https://blog.riverlogic.com/moving-along-the-analytics-maturity-curve (accessed July 9, 2018).
Cornell University. 2018. Digital Agriculture: Cornell University Agricultural Experiment Station. Available at https://cuaes.cals.cornell.edu/digital-agriculture (accessed April 26, 2018).
Crowdflower. 2016. Data Science Report. Available at http://visit.crowdflower.com/rs/416ZBE-142/images/CrowdFlower_DataScienceReport_2016.pdf (accessed July 9, 2018).
de Koning, K. 2010. Automatic milking—Common practice on dairy farms. Pp. V59-V63 in Proceedings of the First North American Conference on Robotic Milking. Elora, ON, Canada: Precision Dairy Operators.
Emerging Technology from the arXiv. 2017. Google Reveals Blueprint for Quantum Supremacy. MIT Technology Review. Available at https://www.technologyreview.com/s/609035/ google-reveals-blueprint-for-quantum-supremacy (accessed July 9, 2018).
Engel, G. S., T. R. Calhoun, E. L. Read, T. K. Ahn, T. Mancal, Y. C. Cheng, R. E. Blakenship, and G. R. Fleming. 2007. Evidence for wavelike energy transfer through quantum coherence in photosynthetic systems. Nature 466:782-786.
EU SCAR (European Union Standing Committee on Agricultural Research). 2015. Agriculture Knowledge and Innovations Systems Towards the Future: A Foresight Paper. Strategic Working Group AKIS-3 Report. Available at https://ec.europa.eu/research/scar/pdf/akis-3_end_report.pdf (accessed March 15, 2018).
Feynman, R. 2002. Richard Feynman on quantum physics and computer simulation . Los Alamos Science No. 27. Los Alamos National Laboratory. Available at http://permalink.lanl.gov/object/tr?what=info:lanl-repo/lareport/LA-UR-02-4969-02 (accessed July 9, 2018).
Ge, L., C. Brewster, J. Spek, A. Smeenk, and J. Top. 2017. Blockchain for Agriculture and Food: Findings from the Pilot Study. Wageningen Economic Research. Available at https://www.wur.nl/upload_mm/b/3/d/df37c4ff-14f3-43b9-a34d-6ed8599d8aba_2017-112%20Ge_def.pdf (accessed July 9, 2018).
Google. 2018. A Preview of Bristlecone: Google’s New Quantum Processor. Available at https://ai.googleblog.com/2018/03/a-preview-of-bristlecone-googles-new.html (accessed July 9, 2018).
IBM Q. 2018. What Is Quantum Computing? Available at https://www.research.ibm.com/ibm-q/learn/what-is-quantum-computing (accessed March 15, 2018).
IEEE (Institute of Electrical and Electronics Engineers). 2018a. Blockchain Overview. Available at https://blockchain.ieee.org/about (accessed March 15, 2018).
IEEE. 2018b. Quantum Computers Strive to Break Out of the Lab. Available at https://spectrum.ieee.org/computing/hardware/quantum-computers-strive-to-break-out-of-the-lab (accessed March 15, 2018).
INFORMS (Institute for Operations Research and the Management Sciences). 2018. Syngenta Crop Challenge. Available at http://connect.informs.org/analytics/news-events/news-events-articles/news-events-news-events-articles-syngenta (accessed April 26, 2018).
Intel. 2018. Intel Starts Testing Smallest “Sping Qubit” Chip for Quantum Computing. Available at https://www.intc.com/investor-relations/investor-education-and-news/investor-news/press-release-details/2018/Intel-Starts-Testing-Smallest-Spin-Qubit-Chip-forQuantum-Computing/default.aspx (accessed March 15, 2018).
IOM and NRC (Institute of Medicine and National Research Council). 2015. A Framework for Assessing Effects of the Food System. Washington, DC: The National Academies Press.
Jonquet, C., A. Toulet, E. Amund, S. Aubin, E. D. Yeumo, V. Emonet, J. Graybeal, M. Laporte, M. A. Musen, V. Pesce, and P. Larmande. 2018. AgroPortal: A vocabulary and ontology repository for agronomy. Computers and Electronics in Agriculture 144:126-143.
Kim, H. M., and M. Laskowski. 2017. Agriculture on the blockchain: sustainable solutions for food, farmers, and financing. Available at http://dx.doi.org/10.2139/ssrn.3028164 .
Knight, W. 2017. IBM Raises the Bar with a 50-Qubit Quantum Computer. MIT Technology Review. Available at https://www.technologyreview.com/s/609451/ibm-raises-the-bar-with-a-50-qubit-quantum-computer (accessed March 15, 2018).
Krug, H., H. Brune, G. Schmid, U. Simon, V. Vogel, D. Wyrwa, H. Ernst, A. Grunwald, W. Grunwald, and H. Hofmann. 2006. Nanotechnology: Assessment and Perspectives. Springer-Verlag Berlin and Heidelberg GmbH & Co. K. Pp. 197-240.
Lougee, R. 2017. Are “Bytes” and “Blocks” the Secret Ingredients to Transforming Food Safety? SwissRe Institute. Available at http://institute.swissre.com/research/library/Food_Safety_Robin_Lougee.html (accessed March 15, 2018).
Lowdin, P. 1965. Quantum genetics and the aperiodic solid. Some aspects on the biological problems of heredity, mutations, aging and tumours in view of the quantum theory of the DNA molecule. Advances in Quantum Chemistry. Volume 2. Pp. 213-360. Academic Press.
Lu, S., L Shao, M. Freitag, L. Klein, J. Renwick, F. Marianno, C. Albrecht, and H. Hamann. 2016. IBM PAIRS Curated Big Data Service for Accelerated Geospatial Data Analytics and Discover, 2016 IEEE International Conference on Big Data. Available at https://ieeexplore.ieee.org/document/7840910 (accessed March 15, 2018).
Marr, B. 2018. How Much Data Do We Create Every Day? The Mind-Blowing Stats Everyone Should Read. Available at https://www.forbes.com/sites/bernardmarr/2018/05/21/how-much-data-do-we-create-every-day-the-mind-blowing-stats-everyone-should-read/#1d51008860ba (accessed July 9, 2018).
McDermid, S., L. Mearns, and A. Ruane. 2017. Representing agriculture in Earth systems models: Approaches and priorities for development. Journal of Advances 9(5):2230-2265.
Microsoft. 2018. Microsoft Quantum: Research. Available at https://www.microsoft.com/enus/research/lab/quantum (accessed March 15, 2018).
Morgan, J. 2014. A Simple Explanation of “The Internet of Things.” Available at https://www.forbes.com/sites/jacobmorgan/2014/05/13/simple-explanation-internet-things-thatanyone-can-understand/#958725c1d091 (accessed July 9, 2018).
Neethirajan, S. 2017. Recent advances in wearable sensors for animal health management. Sensing and Bio-Sensing Research 12:15-29.
NIH (National Institutes of Health). 2018. NIH Strategic Plan for Data Science. Available at https://commonfund.nih.gov/sites/default/files/NIH_Strategic_Plan_for_Data_Science_Final_508.pdf (accessed March 15, 2018).
NIST (National Institute of Standards and Technology). 2015. Lack of Effective Timing Signals Could Hamper “Internet of Things” Development. Available at https://www.nist.gov/news-events/news/2015/03/lack-effective-timing-signals-could-hamper-internet-thingsdevelopment (accessed March 15, 2018).
Pagay, V., M. Santiago, D. A. Sessoms, E. J. Huber, O. Vincent, A. Pharkya, T. N. Corso, A. N. Lakso, and A. D. Stroock. 2014. A microtensiometer capable of measuring water potentials below −10 MPa. Lab on a Chip 14(15):2806-2817.
Pasquetto, I., B. Randles, and C. Borgman. 2017. On the reuse of scientific data. Data Science Journal 16:8.
Popkin, G. 2017. Quantum computer simulates largest molecule yet, sparking hope of future drug discoveries. Science . Available at http://www.sciencemag.org/news/2017/09/quantum-computer-simulates-largest-molecule-yet-sparking-hope-future-drug-discoveries (accessed March 15, 2018).
Priest, C. 2018. How Machine Learning Can Help Marketers Measure Multi-Touch Attribution. Available at https://www.ama.org/partners/content/Pages/how-machine-learningcan-help-marketers-measure-multi-touch-attribution.aspx (accessed July 9, 2018).
Salfer, J., M. Endres, W. Lazarus, K. Minegishi, and B. Berning. 2017. Dairy Robotic Milking Systems—What Are the Economics? eXtension. Available at http://articles.extension.org/pages/73995/dairy-robotic-milking-systems-what-are-the-economics (accessed April 26, 2018).
Seagate. 2017. Data Age 2025. Available at https://www.seagate.com/www-content/our-story/trends/files/Seagate-WP-DataAge2025-March-2017.pdf (accessed March 15, 2018).
Sennar, K. 2017. AI in Agriculture—Present Applications and Impact. Techemergence. Available at https://www.techemergence.com/ai-agriculture-present-applications-impact (accessed March 15, 2018).
Shekhar, S., J. Colletti, F. Munoz-Arriola, L. Ramaswamy, C. Krintz, L. Varshney, and D. Richardson. 2017. Intelligent Infrastructure for Smart Agriculture: An Integrated Food, Energy and Water System. Computing Community Consortium white paper. Available at https://arxiv.org/ftp/arxiv/papers/1705/1705.01993.pdf (accessed March 15, 2018).
Smith, W. 2017. Uncertainty and Opportunity: Recent News Clips of Interest. Presentation, AgGateway 2017 Annual Conference: Efficiency-Opportunity-Profitability, November 6-9, 2017, San Diego, California.
Stephens, Z. D., S. Y. Lee, F. Faghri, R. H. Campbell, C. Zhai, M. J. Efron, R. Iyer, M. C. Schatz, S. Sinha, and G. E. Robinson. 2015. Big data: astronomical or genomical? PLoS Biology 13(7):e1002195.
Top 500. 2018. Available at https://www.top500.org/lists/2018/06 (accessed March 15, 2018).
Turin, L. 2002. A method for the calculation of odor character from molecular structure. Journal of Theoretical Biology 216(3):367-385.
UN (United Nations). 2014. A World That Counts. Available at http://www.undatarevolution.org/report (accessed March 15, 2018).
USDA-NIFA (U.S. Department of Agriculture’s National Institute of Food and Agriculture). 2017a. Big Data Yields Big Opportunities in Agriculture. Blog. Available at https://www.usda.gov/media/blog/2017/07/17/big-data-yields-big-opportunities-agriculture (accessed March 15, 2018).
USDA-NIFA. 2017b. Executive Summary: Changing the Face, Place, and Space of Agriculture. Available at https://nifa.usda.gov/sites/default/files/resource/Data%20Summit%20Summary.pdf (accessed March 15, 2018).
Vasisht, D., Z. Kapetanoic, J. Won, X. Jin, R. Chandra, A. Kapoor, S. Sinha, M. Sudarshan, and S. Stratman. 2017. FarmBeats: An IoT Platform for Data-Driven Agriculture. 14th USENIX Sympostium on Networked Systems Design and Implementation, March 27-29, 2017. Boston, MA. Available at https://www.usenix.org/system/files/conference/nsdi17/nsdi17-vasisht.pdf (accessed March 15, 2018).
Verdow, C., S. Wolfert, and B. Tekinerdogan. 2016. Internet of Things in Agriculture. Available at https://www.researchgate.net/publication/312164156_Internet_of_Things_in_ agriculture (accessed March 15, 2018).
Wasik, B. 2013. In the Programmable World, All Our Objects Will Act as One. Available at https://www.wired.com/2013/05/internet-of-things-2 (accessed July 9, 2018).
Wilkinson, M. D., M. Dumontier, I. J. Aalbersberg, G. Appleton, M. Axton, A. Baak, N. Blomberg, J.-W. Boiten, L. Bonino da Silva Santos, P. E. Bourne, J. Bouwman, A. J. Brookes, T. Clark, M. Crosas, I. Dillo, O. Dumon, S. Edmunds, C. T. Evelo, R. Finkers, A. Gonzalez-Beltran, A. J. G. Gray, P. Groth, C. Goble, J. S. Grethe, J. Heringa, P. A. C ’t Hoen, R. Hooft, T. Kuhn, R. Kok, J. Kok, S. J. Lusher, M. E. Martone, A. Mons, A. L. Packer, B. Persson, P. Rocca-Serra, M. Roos, R. van Schaik, S.-A. Sansone, E. Schultes, T. Sengstag, T. Slater, G. Strawn, M. A. Swertz, M. Thompson, J. van der Lei, E. van Mulligen, J. Velterop, A. Waagmeester, P. Wittenburg, K. Wolstencroft, J. Zhao, and B. Mons. 2016. The FAIR Guiding Principles for scientific data management and stewardship. Scientific Data 3:160018.
Wolfert, S., L. Ge, C. Verdouw, and M. Bogaardt. 2017. Big Data in smart framing—A review. Agricultural Systems 153:69-80.
Woodward, J. 2016. Big data and ag-analytics: An open source, open data platform for agricultural and environmental finance, insurance, and risk. Agricultural Finance Review 76(1):15-26.
Yang, Z., G. Yu, L. Di, B. Zhang, W. Han, and R. Mueller. 2013. Web service-based vegetation condition monitoring system—VegScape. Pp. 3638-3641 in 2013 IEEE International Geoscience and Remote Sensing Symposium (IGARSS) . Institute of Electrical and Electronics Engineers.
Yli-Huumo, J., D. Jo, and S. Choi. 2016. Where is current research on blockchain technology? A systematic review. PLoS ONE. Available at https://doi.org/10.1371/journal.pone.0163477 (accessed March 15, 2018).
For nearly a century, scientific advances have fueled progress in U.S. agriculture to enable American producers to deliver safe and abundant food domestically and provide a trade surplus in bulk and high-value agricultural commodities and foods. Today, the U.S. food and agricultural enterprise faces formidable challenges that will test its long-term sustainability, competitiveness, and resilience. On its current path, future productivity in the U.S. agricultural system is likely to come with trade-offs. The success of agriculture is tied to natural systems, and these systems are showing signs of stress, even more so with the change in climate.
More than a third of the food produced is unconsumed, an unacceptable loss of food and nutrients at a time of heightened global food demand. Increased food animal production to meet greater demand will generate more greenhouse gas emissions and excess animal waste. The U.S. food supply is generally secure, but is not immune to the costly and deadly shocks of continuing outbreaks of food-borne illness or to the constant threat of pests and pathogens to crops, livestock, and poultry. U.S. farmers and producers are at the front lines and will need more tools to manage the pressures they face.
Science Breakthroughs to Advance Food and Agricultural Research by 2030 identifies innovative, emerging scientific advances for making the U.S. food and agricultural system more efficient, resilient, and sustainable. This report explores the availability of relatively new scientific developments across all disciplines that could accelerate progress toward these goals. It identifies the most promising scientific breakthroughs that could have the greatest positive impact on food and agriculture, and that are possible to achieve in the next decade (by 2030).
Welcome to OpenBook!
You're looking at OpenBook, NAP.edu's online reading room since 1999. Based on feedback from you, our users, we've made some improvements that make it easier than ever to read thousands of publications on our website.
Do you want to take a quick tour of the OpenBook's features?
Show this book's table of contents , where you can jump to any chapter by name.
...or use these buttons to go back to the previous chapter or skip to the next one.
Jump up to the previous page or down to the next one. Also, you can type in a page number and press Enter to go directly to that page in the book.
Switch between the Original Pages , where you can read the report as it appeared in print, and Text Pages for the web version, where you can highlight and search the text.
To search the entire text of this book, type in your search term here and press Enter .
Share a link to this book page on your preferred social network or via email.
View our suggested citation for this chapter.
Ready to take your reading offline? Click here to buy this book in print or download it as a free PDF, if available.
Get Email Updates
Do you enjoy reading reports from the Academies online for free ? Sign up for email notifications and we'll let you know about new publications in your areas of interest when they're released.
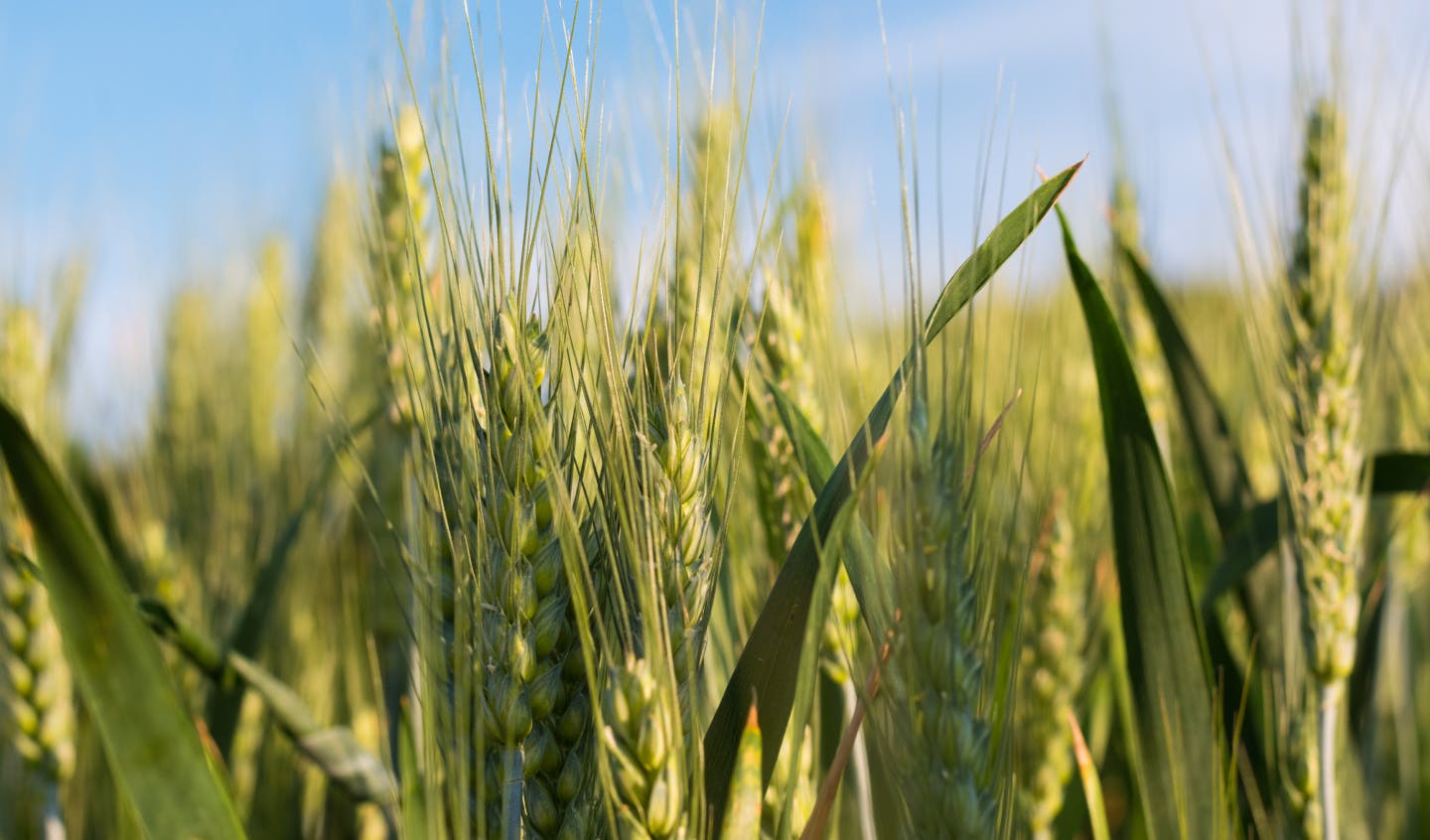
Driving Alberta’s agricultural landscape to new heights.
We are the largest producer-guided funder of agricultural research in Alberta. Together with industry partners, we push the boundaries of Alberta’s potential.
Funding Opportunities
Review current opportunities for funding innovative ideas
Funded Research
Curious about innovative research projects we've funded?
Together, we're driving Alberta agriculture to new heights
Big ideas are here; RDAR helps them grow.
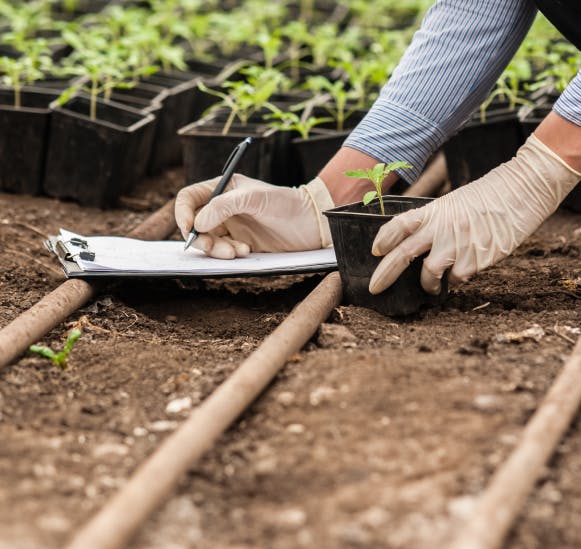
Our Strategy
Our evolving focus to cater to emerging issues in the industry, staying as a champion of the industry, connecting, powering, and driving innovation.
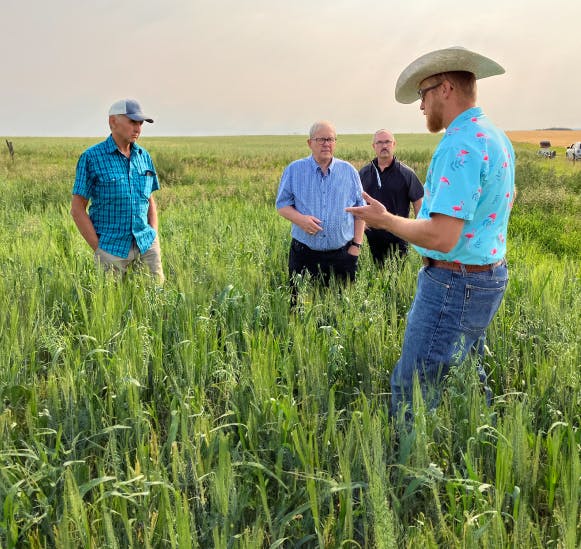
Research Priorities
Our approach involves a dynamic collaboration between producers, researchers, and innovators, tapping into the existing talent within the sector.
Research Outcomes
In fiscal 2022-23, we received 157 proposals, and had a 59% approval rate.
New approvals bring RDAR's portfolio to nearly 350 projects and growing. 240 projects were closed out in March 2023.
Number of dollars distributed between research and OFCAF programs during fiscal 2022-23.
Latest funded research
Imagine a world where innovation isn’t just a buzzword but a driving force, where convention is challenged, and new paths are forged. Big ideas are here; RDAR helps them grow.
Discover the latest happenings in agriculture.
Sign-up For Updates
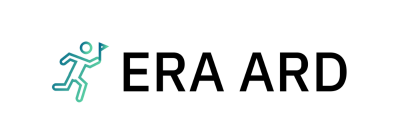
Crop Genetics for Agricultural Research: Enhancing Crop Improvement
Crop genetics plays a crucial role in agricultural research, as it offers valuable insights into enhancing crop improvement. By understanding the genetic makeup of crops, researchers can identify desirable traits and develop strategies to enhance their productivity, resilience, and nutritional value. For instance, consider a hypothetical scenario where scientists aim to improve drought tolerance in wheat plants by identifying key genes responsible for this trait. Through comprehensive genetic analysis, they could isolate specific genes associated with drought resistance, paving the way for targeted breeding programs and biotechnological interventions that lead to more resilient and productive wheat varieties.
In recent years, advancements in molecular biology techniques have revolutionized crop genetics research. With the advent of high-throughput sequencing technologies and bioinformatics tools, researchers can now analyze large-scale genomic data more efficiently than ever before. This enables them to unravel intricate relationships between genes and determine how different genetic variations contribute to various phenotypic traits. Moreover, these technological advancements facilitate gene editing approaches such as CRISPR-Cas9, allowing precise modifications within plant genomes. Such breakthroughs offer immense potential for accelerating crop improvement efforts by enabling scientists to directly manipulate target genes or introduce novel gene variants into existing cultivars.
Overall, crop genetics serves as an invaluable resource for agricultural research aimed at enhancing crop improvement. By By understanding the genetic basis of crops, researchers can develop new and improved varieties that are more resistant to diseases, pests, and environmental stresses. They can also enhance crop nutritional content, flavor, and shelf life. Additionally, crop genetics research can contribute to sustainable agriculture by reducing the reliance on chemical inputs and increasing resource use efficiency. Ultimately, this knowledge empowers farmers with better tools and options for achieving higher yields and ensuring food security in a changing climate.
Genetic Diversity in Crop Plants
Genetic diversity plays a crucial role in crop improvement by providing the raw material for breeding programs. It refers to the variety of genetic information present within a population or species, which can be harnessed to enhance traits such as yield, disease resistance, and environmental adaptability. To illustrate this concept, let us consider the example of maize (Zea mays), one of the world’s most important staple crops.
Maize exhibits immense genetic diversity due to its long history of cultivation and adaptation by indigenous farmers across different regions. This diversity is evident in various aspects, including morphology, physiology, and biochemical composition. For instance, certain maize landraces have been found to possess unique adaptations that enable them to thrive under extreme climatic conditions, such as drought or high temperatures.
Understanding and harnessing the genetic diversity in crop plants is essential for ensuring food security and sustaining agricultural productivity. The following bullet points highlight some key reasons why genetic diversity matters:
- Increased resilience : A diverse gene pool allows crops to better withstand biotic and abiotic stresses, reducing vulnerability to pests, diseases, and adverse environmental conditions.
- Improved nutritional quality : Genetic diversity enables the incorporation of desirable traits into crops, enhancing their nutritional value and promoting human health.
- Adaptation to changing environments : By preserving genetic variation through breeding programs, we can develop cultivars that are well-adapted to new challenges arising from climate change or evolving pest populations.
- Enhanced stability : Variability in genes ensures that there are always individuals with suitable characteristics available even when faced with unpredictable changes in growing conditions.
To further emphasize the significance of genetic diversity in crop plants, consider Table 1 below showcasing examples of inherent variations observed within different rice varieties:
Table 1: Examples of Inherent Variation in Rice Varieties
These illustrative examples demonstrate the diverse genetic characteristics that can be found within a single crop species. By harnessing this inherent variation, plant breeders can select for desirable traits to improve crop productivity and resilience.
In light of the importance of genetic diversity in crop improvement, understanding the methods for mapping genes responsible for specific traits becomes imperative. The subsequent section will delve into different techniques employed in genetic mapping in crops, enabling us to unravel the underlying mechanisms governing these valuable attributes without losing focus on their potential applications.
Methods for Genetic Mapping in Crops
Genetic Diversity in Crop Plants plays a crucial role in crop improvement. By understanding the genetic diversity within different varieties of crops, researchers can identify desirable traits and develop breeding strategies to enhance crop productivity and quality. One notable example is the study conducted on wheat cultivars, where researchers analyzed the genetic variations among different varieties of wheat to understand their resistance to various diseases and pests.
To effectively harness the potential of genetic diversity in crop plants for agricultural research, several methods are used for Genetic Mapping in Crops. These techniques allow scientists to pinpoint specific genes responsible for important agronomic traits such as yield, disease resistance, and drought tolerance. Through these methods, researchers can create detailed maps that highlight the location of genes associated with desired characteristics, enabling breeders to make informed decisions during plant breeding programs.
When conducting genetic mapping studies, there are four key points that need to be considered:
- Sample size: The larger the sample size, the more accurate the results will be.
- Marker selection: Careful selection of markers ensures that relevant regions of DNA are captured.
- Statistical analysis: Appropriate statistical tools help interpret data accurately and draw meaningful conclusions.
- Validation: Ensuring that identified genes or markers consistently produce desired traits across multiple environments is crucial before implementing them into breeding programs.
Moving forward from exploring genetic mapping techniques, Gene Editing Techniques in Crop Improvement offer promising avenues for advancing agriculture. With gene editing technologies like CRISPR-Cas9 becoming increasingly accessible and efficient, it is now possible to precisely modify target genes in crop plants. This opens up possibilities for enhancing traits such as disease resistance, nutrient utilization, and stress tolerance.
By utilizing these cutting-edge tools, scientists can accelerate the process of developing improved crop varieties with enhanced agronomic traits and resilience to environmental challenges without relying solely on traditional breeding methods.
Gene Editing Techniques in Crop Improvement
Enhancing Crop Improvement through Gene Editing Techniques
In recent years, gene editing techniques have emerged as powerful tools for crop improvement. By precisely modifying the genetic makeup of crops, scientists can create plants with desired traits that are better adapted to environmental conditions and possess enhanced agronomic characteristics. To illustrate the potential of these techniques, let us consider a hypothetical example: improving disease resistance in wheat.
Gene editing offers several advantages over traditional breeding methods when it comes to enhancing crop traits. First and foremost is its precision – specific genes can be targeted and modified, allowing for more accurate manipulation of desired traits. Furthermore, gene editing techniques enable researchers to introduce novel genes or modify existing ones without introducing foreign DNA into the plant’s genome. This reduces concerns related to regulatory approval and public acceptance.
To understand the various applications and implications of gene editing in crop improvement, we will explore the following aspects:
- Increased Disease Resistance : Gene editing allows for precise alterations in key genes responsible for disease susceptibility in crops. By modifying these genes, scientists can develop varieties that exhibit improved resistance against devastating pathogens.
- Improved Nutritional Content : Through gene editing, crops can be engineered to contain higher levels of essential nutrients such as vitamins, minerals, or proteins. This has the potential to address nutritional deficiencies prevalent in certain regions.
- Enhanced Stress Tolerance : Gene editing offers opportunities to enhance crop resilience under adverse environmental conditions such as drought, salinity, or extreme temperatures by manipulating genes involved in stress responses.
- Reduced Environmental Impact : Gene editing also enables the development of crops with reduced reliance on chemical inputs like pesticides or fertilizers by conferring natural defense mechanisms against pests and diseases.
These applications highlight how gene editing techniques hold immense promise for addressing pressing agricultural challenges while ensuring sustainable food production systems.
As we delve into the applications of marker-assisted selection in crop breeding, it becomes evident that gene editing techniques play a crucial role in revolutionizing agricultural research and contributing to global food security.
Applications of Marker-Assisted Selection in Crop Breeding
Advances in gene editing techniques for crop improvement.
In recent years, gene editing techniques have revolutionized the field of crop improvement. These powerful tools allow scientists to precisely modify an organism’s DNA, opening up new possibilities for enhancing crop traits and improving agricultural productivity. One striking example of the potential impact of gene editing is demonstrated by a hypothetical case study involving rice.
Imagine a scenario where researchers aim to develop rice varieties that are more resistant to drought. By using the CRISPR-Cas9 system, they can target specific genes involved in drought response pathways and introduce beneficial mutations or modifications. Through this approach, it becomes possible to enhance the plants’ ability to tolerate water scarcity without introducing foreign genetic material from unrelated species.
The applications of gene editing techniques in crop improvement are vast and varied. Here are some key aspects worth considering:
- Precision : Gene editing technologies offer unprecedented precision in modifying specific regions of a plant’s genome, minimizing off-target effects.
- Speed : Compared to traditional breeding methods, gene editing allows for faster development of improved crops by directly altering targeted genes.
- Flexibility : This technology enables precise modifications across various crops with different genetic backgrounds.
- Ethical considerations : The ethical implications of gene editing raise questions about regulation, intellectual property rights, and public acceptance.
To further illustrate the broad spectrum of advancements made through gene editing techniques, consider Table 1 below:
Table 1: Examples of Crop Traits Improved Using Gene Editing Techniques
As we delve deeper into the realm of crop genetics, it is essential to recognize the potential benefits and challenges associated with gene editing techniques. In the subsequent section, we will explore another powerful tool known as genomic selection for further enhancing crop improvement efforts.
[Transition Sentence]: Building upon the success of gene editing techniques, researchers have also embraced genomic selection as a promising approach in crop improvement.
Genomic Selection for Crop Improvement
Having explored the applications of marker-assisted selection in crop breeding, we now turn our attention to genomic selection as another powerful tool for enhancing crop improvement. The use of genomics and statistical modeling has revolutionized plant breeding by enabling breeders to predict the performance of plants based on their genetic makeup.
Genomic selection is a method that utilizes high-throughput genotyping technologies and phenotypic data to estimate the breeding values of individuals within a population. It involves training predictive models using large datasets consisting of both genotype and phenotype information. These models then allow breeders to select superior individuals without relying solely on direct phenotypic measurements, which can be time-consuming and costly.
To illustrate the potential impact of genomic selection, let us consider an example in maize breeding. Breeders are faced with the challenge of developing varieties that exhibit increased yield under drought conditions. By incorporating genomic selection into their breeding program, they can identify specific genetic markers associated with drought tolerance traits. This enables them to select individuals with desirable alleles even before exposing them to drought stress, significantly accelerating the breeding process.
The advantages offered by genomic selection in crop improvement are numerous:
- Enhanced efficiency: With genomic selection, breeders can make more accurate predictions about an individual’s performance early on in the breeding cycle.
- Increased genetic gain: By selecting individuals based on their estimated breeding values rather than directly observed phenotypes, breeders can exploit valuable but difficult-to-measure traits.
- Expanded diversity exploration: Genomic selection allows for better utilization of diverse germplasm resources by capturing allelic contributions from different populations or wild relatives.
- Accelerated development timeline: Genomic selection reduces the need for multiple generations of field evaluation, resulting in shorter breeding cycles and faster variety release.
Table: Key Advantages of Genomic Selection
In summary, genomic selection represents a significant advancement in crop improvement by harnessing the power of genomics and statistical modeling. By enabling breeders to select individuals based on their estimated breeding values, this approach enhances efficiency, increases genetic gain, explores expanded diversity, and accelerates the development timeline.
Building upon the potential offered by genomic selection, the role of biotechnology in crop genetics becomes increasingly crucial.
Role of Biotechnology in Crop Genetics
Building on the advancements in crop genetics, genomic selection has emerged as a powerful tool for enhancing crop improvement. By leveraging genomic data and statistical models, this approach enables breeders to predict an individual plant’s genetic potential more accurately.
Genomic selection is based on the principle that variations in DNA sequences contribute to phenotypic differences among plants. Through large-scale genotyping, researchers can identify thousands of markers across the genome associated with traits of interest such as yield, disease resistance, or nutrient content. This information is then used to train prediction models that estimate the breeding value of different individuals within a population.
One example illustrating the impact of genomic selection is its application in maize breeding programs. Researchers have successfully employed this technique to improve grain yield by selecting plants with favorable genetic variants associated with high productivity. By targeting specific regions of the genome through marker-assisted selection, breeders can accelerate the development of superior hybrids and cultivars.
To further understand the significance of genomic selection in crop improvement, consider the following emotional response evoking bullet points:
- Increased efficiency in developing improved varieties
- Enhanced precision in predicting desirable traits
- Accelerated breeding progress leading to faster release times
- Improved utilization of genetic resources for sustainable agriculture
In summary, genomic selection represents a major advancement in agricultural research as it provides breeders with valuable insights into an individual plant’s genetic makeup. By harnessing this knowledge effectively, breeders can expedite crop improvement efforts and develop superior varieties tailored to meet evolving challenges faced by modern agriculture.
Note: The section title ‘Role of Biotechnology in Crop Genetics’ will be addressed in the next section.
Related posts:
- Biotechnology in Agricultural Research for Development: Advancing Crop Improvement
- Crop Agronomy in Agricultural Research for Development: An Overview of Crop Improvement
Crop Improvement in Agricultural Research for Development: Enhancing Yield, Resilience, and Sustainability
Crop Physiology in Agricultural Research for Development: Enhancing Crop Improvement
Crop Diversification: Enhancing Adaptation to Climate Change in Agricultural Research for Development
Plant Breeding for Crop Improvement: Agricultural Research for Development
Genetic Engineering in Agricultural Research for Development: Crop Improvement
Crop Improvement in Agricultural Research for Development: Enhancing Yield,…
Comments are closed.
Welcome, Login to your account.
Recover your password.
A password will be e-mailed to you.

An official website of the United States government
The .gov means it’s official. Federal government websites often end in .gov or .mil. Before sharing sensitive information, make sure you’re on a federal government site.
The site is secure. The https:// ensures that you are connecting to the official website and that any information you provide is encrypted and transmitted securely.
- Publications
- Account settings
Preview improvements coming to the PMC website in October 2024. Learn More or Try it out now .
- Advanced Search
- Journal List

Artificial intelligence solutions enabling sustainable agriculture: A bibliometric analysis
Priya rani bhagat.
1 Institute of Agricultural and Food Economics, Hungarian University of Agriculture and Life Sciences, Gödöllő, Hungary
Farheen Naz
Robert magda.
2 North-West University, Vanderbijlpark, South Africa
Associated Data
The data for bibliometric analysis was extracted from SCOPUS database using relevant keywords provided within the study.
There is a dearth of literature that provides a bibliometric analysis concerning the role of Artificial Intelligence (AI) in sustainable agriculture therefore this study attempts to fill this research gap and provides evidence from the studies conducted between 2000–2021 in this field of research. The study is a systematic bibliographic analysis of the 465 previous articles and reviews done between 2000–2021 in relation to the utilization of AI in sustainable methods of agriculture. The results of the study have been visualized and presented using the VOSviewer and Biblioshiny visualizer software. The results obtained post analysis indicate that, the amount of academic works published in the field of AI’s role in enabling sustainable agriculture increased significantly from 2018. Therefore, there is conclusive evidence that the growth trajectory shows a significant climb upwards. Geographically analysed, the country collaboration network highlights that most number of studies in the realm of this study originate from China, USA, India, Iran, France. The co-author network analysis results represent that there are multi-disciplinary collaborations and interactions between prominent authors from United States of America, China, United Kingdom and Germany. The final framework provided from this bibliometric study will help future researchers identify the key areas of interest in research of AI and sustainable agriculture and narrow down on the countries where prominent academic work is published to explore co-authorship opportunities.
1. Introduction
As the world tries to battle through the novel challenges and obstacles caused by the Covid-19 pandemic and every sector faced detrimental impact of the pandemic at different stages of a business process. The agriculture sector is one of these sectors which faced several disruptions including labor supply for production, reduced transportation of agriculture produce, and risks associated with agriculture markets. Therefore, artificial intelligence (AI) systems and solutions will find contemporary applications in the field of agriculture and sustainable farming practices. Evidently, the pandemic is not a temporary disruption of normal but a milestone that will change the course of modern-history towards a sustainability driven society [ 1 , 2 ]. Sustainability is a multidimensional discipline that has been the popular research interest of scholars for the past few decades [ 3 , 4 ]. Because of its multidisciplinary attribute sustainability encompasses a wide variety of subjects ranging from climate, environment, green economy, food safety, sustainable agriculture, clean technologies etc. In a 2018 research article, Ávila et al. highlighted that in the field of education in sustainability, expansive research in every contributing domain is necessary to embark on the trail to global development [ 5 ].
The concept of “sustainable agriculture” has received increased attention in recent years with the rising technological improvements. In a 2020 keyword citation burst analysis on ecological modernization approach by Rocchi et al., “sustainable agriculture” consistently shows very high citation burst in the past and current literature [ 6 ]. Due to its high popularity among researchers the more recently published bibliometrics studies conduct in the realm of sustainable agriculture explore the interactions between sustainable agri-food systems with the economy, society, and policy making [ 7 ], agriculture systems modernization approach [ 6 ], big data in sustainable agriculture [ 8 ]. In terms of Artificial Intelligence in agriculture, recent articles provide bibliometric analysis of the crossover between remote sensing technologies and agriculture [ 9 ], advanced information and communication technology in agriculture [ 10 ], global trends in precision agriculture technology [ 11 ].
In the scope of this review, sustainable agriculture is defined as, the agricultural practices that ensure fulfillment of present day and future food and nutrition requirements of the society, while maximizing the net advantage towards the ecosystem, society, and earth when all its implication on costs and benefits are monitored [ 12 ]. Artificial intelligence (AI) was first coined by John McCarthy in 1956 and then many different definitions arise over the years but in the scope of this study, it is defined in the rational approach as a system that automates intelligent behaviour or acquires intelligence over time using computational programming and gives rational outputs to perform specific tasks without much human intervention [ 13 ].
The impact of the covid-19 pandemic on the agriculture sector is detrimental. There were various studies conducted in the past that observed the disruptions in the agriculture sector and provided sustainable solutions by employing AI technologies in creating sustainable agriculture. However, there is a dearth of studies that provided a state-of-the-art review of AI technologies application in sustainable agriculture. Therefore, this study was conducted to identify the current stage of knowledge concerning AI and sustainable agriculture and provided bibliometric and network analysis in this field. Also, this bibliometric research is motivated to bring together the highlights of the progress made in the application of AI in sustainable agriculture practices to inspire future applications of AI in this field. Hence, it is imperative for future researchers to reflect upon the past decade for academic literature in the field to be able to innovate according to the demands of the current pandemic situation [ 2 ]. This study attempts to compile, analyze, and identify the properties of the scholarly articles and reviews indexed in the Scopus database specifically for the keywords- artificial intelligence, Sustainable agriculture made available between the duration of 2000 to 2021. This study involves both quantifying and bringing out qualitative inferences from the size and features of previously published scholarly works from one or more source databases such as Scopus indexed database. The qualitative questions we intend to explore in the scope of this study are as follows:
- What is the number of scientific articles and reviews published between the year 2000–2021 in the scope of AI and sustainable agriculture?
- What is the growth trajectory in the number of works published in the area of AI and Sustainable agriculture?
- What is the geographic distribution of the research work produced around the world in the field of study?
- Which are the prominent journals and who are the eminent authors involved in the research of AI and sustainable agriculture?
Following this introduction, this article is structured in the following manner: in section 2 the materials and methods used are elaborated. Further, in section 2.1 the bibliometric analysis approach is explained, followed by section 3 which provides an overview and results, and finally, the article closes with sections 4, 5, and 6 providing a discussion about future research options, implications in the present and conclusion respectively.
2. Research methodology
The approach taken to conduct this study is a comprehensive bibliometric analysis of the previously published works of literature that incorporate the usage of AI in the field of sustainable agriculture between the duration of 2000 and 2021. A total of 637 articles were extracted from the Scopus database as a CVS file, out of which only 465 relevant journal articles and review papers were considered for maintaining the veracity of the resulting conclusions. Scopus as a source index, is highly regarded amongst academicians and researchers for searching legitimate scientific articles as it facilitates searching and extraction of specific keywords from the titles, citations, abstracts or keywords from the publications listed in the database [ 14 ].
Bibliometric analysis has been regarded as a reliable method to perform quantitative and empirical study and compilation of previously published works of literature in any field [ 15 ]. Pritchard in 1969 first vaguely defined bibliometrics as analyzing books, written documents, article or media communication by application of statistical and mathematical tools [ 16 ]. Broadus then in 1987 defined bibliometrics as “the quantitative study of physical published units, or of bibliographic units, or of the surrogates for either [ 17 ]". In the scope of this study it encompasses analysis methods such as citation network analysis, geographic network analysis, prominent countries and authors ranked and prominent word cloud. These tools provide a visual representation of the development of literature in the specified field over the course of time and highlight the impactful trending areas of research in the duration selected [ 18 ].
Visualization of the networks established from the large number of articles extracted is a key step in the bibliometric process which was done using multidimensional scaling there are various software tools available in the market like R package, iGraph package, VOSviewer and Biblioshiny [ 19 ]. Due to constant innovation and increased accessibility of advanced web-based and electronic bibliographic and referencing applications, the outputs from bibliometric analysis have greatly improved in quality [ 20 ]. Within the scope of this study, VOSviewer has been used to generate and visually represent the network of authors and countries as the VOSviewer platform provides the most suitable options for easily displaying the bibliometric maps and is easily understandable by any type of audience. Biblioshiny is used in the study to generate the prominent keyword cloud. It is an extension of the Bibliometrix R package web-interface used to visualize the clusters in the database.
2.1 Research method: Bibliometric study
The procedure followed in this study was standard comprehensive bibliometric analysis which starts from keyword search in the Scopus search syntax as shown in the first step of Fig 1 . The keywords indicate that articles and publications containing “Artificial Intelligence” or “Machine Learning” or “Robotics” etc. AND “Sustainable Agriculture” in their titles, keywords, or abstracts. The search was run, and the total number of articles obtained within the timespan of 2000 to 2021 and before any type of filtration was 637. The output was then refined for any redundancies. Thereafter, only journal articles, review papers, selecting only English papers, and within year 2000–2021 were considered for the study, bringing the total number of articles to 465.

This total consists of about 85.8% journal articles and 14.2% review papers. Further, about 46.6% of the publications were made during or after the onset of the Covid-19 pandemic. This set of indexes was then exported to Excel, VOSviewer, and Biblioshiny to obtain the network analysis, assessment of the growth trajectory, geographic distribution, identification of prominent authors, and keyword assessment. The ranking of country-wise publication of articles and citations were determined using Microsoft Excel features.
3. Results and overview
The Table 1 describes the important properties of the data set used for the study which helps in determining the overall scholarly or academic impact that has been made in the field of AI utilized in sustainable agriculture in the duration of 2000 to 2021. The total size of the literary works extracted for the study is 465. One of the most notable properties of this data set is that out of a total of 2005 authors who publish research in this field only 15 of them have produced single author documents. When compared to bibliometric analysis in other popular fields of academic study, these statistics have lower numbers which indicate that there is a need for more academic research and publication to provide knowledge and encourage the use of AI widely.
3.1 Year-wise article statistics
Further, Fig 2 is used to visually depict the growth trajectory and shift of research interest in the use of AI in sustainable agriculture. The academic literature in this discipline had steady but moderate growth from the year 2000 to 2015. After which evidently, there was a greater impact Industry 4.0 had on sustainable agriculture practices and hence we can see the trend gradually increasing since 2017 and we see an approximately 255.7% increase in Scopus indexed articles and review published from 2019 to 2020 and mid 2021. This growth confirms a surge in scholarly inclination towards addressing the uses of AI in sustainable agriculture practices.

3.2 Country wise article statistics
In terms of the geographic distribution of the literary works, as per Table 2 about 54.8% of the publications are produced from China ranking it at number 1 out of top 20. The next countries leading knowledge production in use of AI in sustainable agriculture are USA, India, Iran, and France. The rest of the list also comprises of highly developed Anglo-American-European nations such as, Italy, UK, Germany, Spain, Australia, Netherlands, Turkey, Canada, Switzerland and Portugal, and other developing Asian economies such as Malaysia, Indonesia and Pakistan.
3.3 Country wise citation statistics
The country wise citation ranking in Table 3 , shows USA and China received a maximum number of citations 1938 and 1141, respectively. Then, the following countries with a considerable number of received citations are UK, Netherlands, and Germany. Whereas, Algeria shows the highest average article citation at 68.
3.4 Ranking of key journals in the selected discipline
It is important for promising scholars to identify the role of prominent journals which are facilitating knowledge dissemination in the discipline of the use of AI in sustainable agriculture so that they can approach the industry leaders and foster further innovation and research potentials. In our analysis, we identified the top 20 journals that span into a wide variety of research disciplines ( Table 4 ). To highlight a few, Sustainable practices (Sustainability), agricultural economy (Agronomy, Agronomy For Sustainable Development), technology in agriculture (Computers and Electronics in Agriculture-81 papers), environmental research (Science of the Total Environment, Journal of Cleaner Production, Agricultural Water Management), emerging technologies (Remote Sensing, Applied Sciences), Multidisciplinary (IEEE Access), environmental and sustainability studies (Land Use Policy), Environmental Monitoring and Assessment (Agriculture Ecosystems and Environment, Ecological Indicators) and the list includes furthermore areas. This indicates that there is a growing inclination towards cross-disciplinary exploration in attaining AI systems that can help make agriculture more sustainable in the future.
3.5 Ranking of key authors in the selected discipline
Using biblioshiny package in R-software, we have generated Table 5 , which indicated the most prominent authors publishing in the domain of AI utilization in sustainable agriculture and farming. Based on the filtered database the papers, articles, and reviews are produced by 2005 authors in total. The top published authors come from China, USA, India, Iran and France based on their affiliate universities. The low number of articles even from the top authors suggest that this field of study is still in its emerging stages and increasing authorship and co-authorship has the potential of drawing further interest in this discipline.
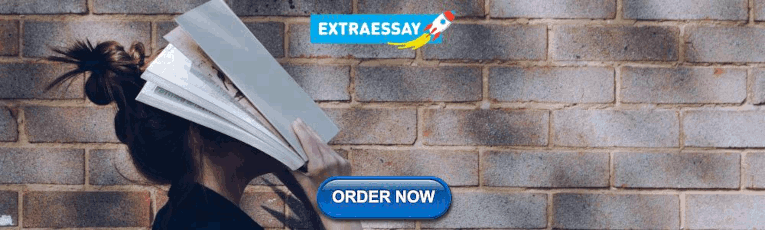
3.6 Ranking of key institutions in the selected discipline
The top ten affiliations by the number of articles produced are shown in Table 6 . It is essential to determine which distinguished organizations and universities are leading the research scenario in the field of AI utilization in sustainable agriculture and related disciplines. According to the output from the analysis, Wageningen University, Netherlands has produced 13 scientific articles in the scope of the topic under study. The following China Agricultural University, and Northwest A&F University are from China, and each have published 12 and 10 scientific articles, respectively.
3.7 Keyword analysis
The result of the keyword analysis using Biblioshiny shows precisely the various technologies within the scope of artificial intelligence which are being used in sustainable agriculture. These technologies are–machine learning, agricultural robots, neural networks, artificial neural networks, remote sensing, precision agriculture and support vectors machines. In addition to it, Fig 3 provided the major keywords and its occurrence in the selected articles. It is evident apart from the keywords of artificial intelligence and agriculture which are under study, the most projected key words are agricultural robots, decision making, machine learning, remote sensing, artificial neural systems, logic algorithms and other related technologies.

The projected set of keywords also helps in determining the various thematic areas in which the majority of the articles belong. The popular thematic areas determined are automation of agricultural production using AI, machine learning in the field of food production, modernization of farming methods using technology and weather forecasting solutions for sustainable agriculture.
3.8 Network analysis
The Fig 4 , is a VOSviewer generated network illustration of the most prominent published author collaborations for AI and Sustainable agriculture scientific articles that are indexed in Scopus from 2000–2021. The eight different color clusters represent the different domains and the interaction and collaboration of authors from different domains to produce multi-disciplinary scientific articles. The size of each circle denoted for each author represents the amount of academic literature, citations they have produced in the field of AI and sustainable agriculture respectively.

Based on the originating country of publication and using VOSviewer software the geographic network distribution is obtained in the form of Fig 5 . The network also reinforces the findings from the bibliometric analysis of the top 20 countries that produce scientific studies in the domain of AI and sustainable agriculture. The different colored clusters represent the various disciplines of study and their interaction with other disciplines in the scope of the study. Further, the circles representing China, the United States, India, the UK, and Germany confirm the earlier findings. On further exploration of Fig 5 , it is observed that the clusters created between the United States of America, European Union member states and United Kingdom are more dense and diverse as compared to the interaction with and amongst Asian countries.

4. Discussion
AI enhancements have made different sectors of production highly effective in doing so by providing sustainable solutions. Initially, the applications of AI technologies were intended to increase productivity but the trend had begun to gradually shift towards researching for sustainability and green technologies that would automate and reduce resource consumption in the production process. Jha et al. in their review of automation used in agriculture, highlighted that the younger farmers and producers are more inclined towards investing in automation technologies than the older generations [ 21 ]. Over utilization of pesticides and agrochemicals has led previously fertile lands to turn barren, this can be avoided by implementing Artificial Neural Network methods as suggested in the results obtained by Elahi et al. in their 2019 test on rice crops [ 22 ]. Studies have also emerged in utilization of data intelligence automation tools like extreme machine learning in precision farming decision support system to determine the accurate yield of crops of small holder farms of produce such as coffee [ 23 , 24 ].
The aim of this article is to compile and reach various inferences from the bibliometric analysis of the literature published in the duration of 2000–2021. The research is innovative and a novel study trying to explore the AI techniques utilised specifically in sustainable agriculture practices which have not been done before. The resulting research framework is intended to provide a basis for future research in an efficient and organised manner.
4.1 AI in sustainable agriculture
The use of AI in sustainable agriculture has the potential to transform aspects of farming such as image sensing for yield mapping, yield prediction, skilled and unskilled workforce, increasing yield and decision-support for farmers and producers [ 25 ]. Based on a 2019 article by Alreshidi, AI is widely being implemented in the following ways to make agriculture more sustainable, these are- climate monitoring, automatically climate-controlled greenhouses, crop quality monitoring, livestock management, predictive analysis and comprehensive farm management systems are a few [ 26 ]. The AI products that are highly in demand from customers of sustainable agriculture backgrounds are chatbots for help with information on farming practices, digital plant health diagnosis applications, remote sensing instruments and irrigation management solutions [ 27 ].
Klyushin & Tymoshenko, (2021) proposed an optimization approach for drip irrigation system optimization to attain sustainable agriculture by using AI methods [ 28 ]. On the other hand, Zhang et al. (2021b) emphasized on informatics and material science to find sustainable solutions in sustainable agriculture by using nanotechnology and AI [ 29 ]. Aggarwal & Singh (2021) asserted on technology assistance in precision farming and discussed the implications of AI and internet of things (IoT) in agriculture to assess water requirements, humidity, need for fertilizers etc [ 30 ]. Spanaki et al. (2021) addressed the issues concerning food security and proposed an AI technique as a solution by adopting a design science methodology [ 31 ]. Mohr & Kühl (2021) investigated the barriers in AI acceptance in agriculture and applied technology acceptance model [ 32 ]. Nevertheless, Mahto et al. (2021) used artificial neural network (ANN) to forecast prices of agriculture commodities and compared of performance of their model with ARIMA model for sustainable agriculture [ 33 ].
4.2 Machine learning in sustainable agriculture
Machine learning was defined in the 2018 book “Foundations of Machine Learning” as computational processes that utilize historic data and past experiences to modify, improve, repair and predict future performance accurately [ 34 ]. Machine learning in sustainable agriculture latest utilization is in optimizing supply chains [ 35 ], in-field monitoring [ 36 ], soil temperature prediction [ 37 ] and sustainable soil management [ 38 ]. The different types of machine learning technologies that can be implemented to foster sustainable production are decision trees, neural networks, polynomial predictive methods and K-nearest neighbors [ 39 ]. Traditional methods of soil suitability assessments for sustainable agriculture can prove to be expensive and time taking especially in remote areas where data about the properties of the soil is unavailable there machine learning technologies are gaining popularity for large scale land suitability assessments [ 40 ].
Qin et al. in 2018 successfully explored the predictive abilities of machine learning on estimating the economic optimum nitrogen rate for corn crops using data from 47 test conducted throughout the American corn belt in USA and found that more robust data was required to make accurate estimations using machine learning based models [ 41 ]. Liakos et al. in 2018 conducted a review of machine learning in agri-tech and found that the artificial and deep neural network method of machine learning was a popular choice across all categories of agriculture processes but specifically in the categories of livestock management, water management and soil management [ 42 ]. A 2019 comparative study between four different machine learning techniques by Ju et al., concluded that while conducting estimations on corn and soybean yields, Convolutional Neural Network or CNN was the most accurate [ 43 ].
4.3 Robotics in sustainable agriculture
Robotics in agriculture is mainly utilized to speed up repetitive and mundane tasks in the production process like spraying, mowing, seeding, harvesting, weed control, picking and finally in sorting products and packaging. Automation provided by robotics in combination with cloud computing, block-chain and big data has also found utility in supply chain of fresh produce. In the realm of making farming sustainable, field robots are used in precision farming by targeted weed control functions replacing treatment of crops and soil with harmful and excessive chemical sprays [ 44 ]. The concept of Agriculture 5.0 has been gaining momentum as a term used to define the incorporation of artificial intelligence and robotics in data-driven farming systems [ 45 ].
Sarri et al. in 2020 reported the results of SMASH project at its design stages of AgroBot with four modules combined to physically control weeds and protection of crops [ 46 ]. Robotic solutions are not only popular in the research community but also amongst the members of the industry who want to invest and implement these sustainable systems [ 47 ]. With the widespread utility, there is an emergence in new research interest in safe human-robot interactions in agricultural settings. Benos et al., express that due to automation and programmed robots, human safety is a concern and that efforts must be taken to make robots extra sensitive to perceiving human proximity and ensure risk-free work environment [ 48 ].
The article by Linaza et al., from 2021 summarises the recent research projects in European Union on the use of robotics and highlights that the use of robots can not only make it precise but also solve the labour shortages caused rise in the average age of farmers [ 49 ]. Furthermore, Mondejar et al., described that usage of robotics in agriculture can solve major food shortage issues and help achieve United Nations sustainable development goals without depleting non-renewable resources rapidly [ 50 , 51 ]. However, to bring the robotic technologies to the commercial level some barriers that must be overcome are improved speed and accuracy. There is a scarcity of research funding when compared to investment interests in industrial manufacturing and military equipment that’s why the body of study is small and the process from development to implementation is slow and on small scale [ 52 – 54 ].
4.4 Resulting observations made in the use of AI techniques in sustainable agriculture
There are a number of other AI solutions that can be implemented in ensuring sustainability in food production like predictive analytics, decision support systems, genomics tracing, artificial neural networks, fuzzy logic, neuro-fuzzy logic, Bayesian Network, and remote sensing. Studies have suggested that advanced bio-sensing technologies in sustainable agriculture will facilitate early diagnosis of diseases and plant pathogens even in asymptomatic plants hence reducing the loss of crops and production [ 55 ]. Unmanned Arial Vehicles or drones integrated with advanced machine learning help in continuous weed management enabling selection decisions and reducing herbicide diffusion in the environment [ 56 ]. Also, Rasmussen et al., in 2021 studied the effect of engaging unmanned arial vehicles which receive data from satellite imagery to perform weed mapping of a particular variety of weed and the result showed that it provides higher resolution images and makes an individual variety of weed to be detected in the crops [ 57 ].
AI and genomics research can enhance defect detection and improve production gains per unit of time with genetic historical data for making accurate predictions [ 58 – 60 ]. Artificial neural networks and fuzzy logic on the other hand are systems that can be continuously trained in making prediction models for sustainable agriculture [ 21 ]. A recent 2021 stimulation study by Pazouki, devised a system for surface irrigation using fuzzy expert systems and meta-heuristic optimization algorithms which enhanced the regular system and improved its practicality, performance and reduced the requirement for manual labour [ 61 ]. Hence proving the wide application of the system in irrigation and water management for sustainable agriculture.
On the other hand, the application of fuzzy logic was used in another study by Soylu and Çarman in 2021 where they developed an automatic fuzzy-based slip control system for tractors on the field by continuously recording how many times slippage occurred during the process of tilling, which proved to reduce slippage by 42% and reduced fuel consumption by 44% [ 62 ]. A few limitations of the artificial intelligence-based systems were highlighted in the recent 2021 study by Meroni et al., in which they stated that the difference between the accuracy of machine learning enabled systems and regular bench-marking systems were not significantly dispersed and the performance of machine learning systems reduced if the data was small [ 63 ].
Table 7 briefly discussed some recent studies conducted in the selected field of research. Based on previous literature and identified techniques used in providing solutions for sustainable agriculture, a research framework is developed in this study ( Fig 6 ).

Based on the results and further propositions from the above stated recent studies, we have attempted to propose a framework for optimizing future research in the utilization of AI technology in sustainable agriculture. We propose that, from Fig 6 depicted below, researchers can create novel topics and thematic areas utilizing the classification of operations performed in agriculture at various stages. Then choose relevant and correlatable AI technologies as in Fig 6 , that have been identified based on existing academic literature and research its application on the distinct processes identified in attaining sustainable agriculture practices.
The suggested framework can also provide a foundation to attract new researchers to the direction of researching this avenue as it is observed that the size of existing scholarly research is relatively very small between 2000–2021. There is an ethical need to motivate further scholastic interest in the development of modern technology usage in the food supply chain to ensure food safety for future generations owing to rapid depletion of resources and global environmental and climate changes.
5. Future research
The potential for future research is vast in the scope of sustainability studies involving advanced technologies and sustainable agriculture as these fields interact frequently in the market’s demand for newer innovations. In terms of bibliometric exploration of previous literary works, further scrutiny could be performed by using sophisticated R bibliometrix programming for functions such as co-occurrence analysis, co-citation analysis, cluster and stream analysis etc. The size of the dataset used for the studies can also be increased to include more technologies such as block chain, Internet of Things, cloud computing, and supply-chain solutions, which are extensively utilized in the agriculture industry. Further studies could also combine scholarly articles and reviews from more than one index source such as Scopus and Web of Science together for extracting a larger dataset. But this kind of dataset will require further steps to clean the dataset from duplications and redundant information.
6. Implications
The implications of the results from this study can be of significant impact as it provides the targeted scholars, readers and researchers with a comprehensive compilation of the body of research conducted previously in the area of sustainable agriculture and AI. The results imply that the interest in the exploration of diverse applications of AI in sustainable agriculture is steadily rising. Distinguished authors from multidisciplinary backgrounds and expertise are inclined towards combining it with other thematic domains around the world.
It also indicates that there is potential to publish more literary and research articles as the body of literature are not as large compared to other bibliometric studies. This article also brings the attention of the readers to this untapped opportunity, and they can get motivated to fill this research gap. The proposed research framework in Fig 6 , also has the potential to further provide a streamlined approach to building pertinent scholarly research topics in this particular thematic area.
7. Conclusion
In our analysis, we classified the keywords and used them to extract 674 relevant articles and reviews which gave us the following results: there is a rising academic interest in the field of AI usage in sustainable agriculture with a drastic improvement from 2019 to 2020. China, the USA and Australia are leaders in producing top works of literature and authors in the domain. These results were obtained by performing data cleansing and classifying the data set for network analysis using functions of Microsoft Excel, VOSviewer, and Biblioshiny. By analysing the results it can be determined that there is huge potential for the application of AI to attain sustainability, especially in predicting the yield, crop protection, climate control, crop genetic control, and produce supply-chain, wherein the prominent researchers and institutions need to collaborate further and form more networks to bring radical progress in the field.
As we proceed to the future, focusing on sustainability will dictate all aspects of life on earth. The first and foremost challenge with climate change will be ensuring food safety and availability for all. Although the green revolution and industrial revolution in the past had exponentially improved food production capacities, these approaches also exerted intolerable pressure on the agricultural lands, natural resources, and the ecosystem, the implications of which are unsustainable and irreversible. The 4th industrial revolution with its hi-tech capabilities promised sustainable alternatives to traditional agriculture practices which can potentially reduce or slow down the depletion of the earth’s resources. As the Covid-19 pandemic shocked and paused the usual shenanigans of every sector for a while, it is a good time to review the body of work in AI and sustainable agriculture before making our way forward.
Supporting information
Funding statement.
The author(s) received no specific funding for this work.
Data Availability

An official website of the United States government
Official websites use .gov A .gov website belongs to an official government organization in the United States.
Secure .gov websites use HTTPS A lock ( Lock A locked padlock ) or https:// means you’ve safely connected to the .gov website. Share sensitive information only on official, secure websites.

U.S. DEPARTMENT OF AGRICULTURE

Scientists Collaborate to Study the Cause and Development of Liver Abscesses in Cattle
Contact: Maribel Alonso Email: [email protected]
March 20, 2024
To effectively prevent liver abscesses in cattle, it is crucial to gain a complete understanding of the development of this condition and the bacteria responsible for causing the infection.
A collaborative effort between scientists from the USDA’s Agricultural Research Service (ARS), Texas Tech University, Kansas State University, and West Texas A&M University work on reliable and repeatable liver abscess models to learn the triggers for this costly condition in cattle that not only negatively impacts animal well-being but also causes liver condemnations and may lead to increased carcass trimming and an overall decrease in profitability.
The first successful model study , recently published in the Journal of Animal Science , is part of a series of studies conducted at the USDA-ARS Livestock Issues Research Unit in Lubbock, Texas, that aim to find solutions to a problem of concern for animal well-being that is costing the industry millions of dollars.
Cattle with liver abscesses can experience health problems and reduced growth and feed efficiency. However, cattle with liver abscesses don’t show clinical signs and are generally identified too late –at harvest. The economic losses associated with this condition in cattle can be as high as 400 million dollars annually. The knowledge gained from these models will help develop preventive interventions.
"After decades of studies, researchers haven't found an accurate way to predict nor diagnose liver abscesses, because of the complexity of the disease," said Rand Broadway , a research scientist with the Livestock Issues Research Unit. "Our study is a huge collaborative effort between USDA and academic partners to develop a liver abscess model in cattle that can help us better understand how liver abscess formation begins. We are constantly learning about the causes and development of these abscesses."
This model consistently showed a 50 percent prevalence, which is important for researchers to study liver abscess development and prevention strategies, particularly in calves entering the beef supply chain from dairy origin. In addition, the model continues to be improved in an effort to mimic “real-world” disease etiology while examining the physiological changes in the animal to better understand root causes of the disease.
Currently, the primary treatment to prevent liver abscesses in cattle has been in-feed antibiotics. However, antibiotics have come under more scrutiny by the general public, and alternatives to antibiotics are being sought.
"We are trying to ensure this model is effective and applicable to test non-antibiotic interventions in the future," added Broadway. "We seek to use the knowledge gained from these models to develop different alternative interventions, such as nutrition management strategies."
In the study, scientists work with dairy and ‘beef-dairy’ cross steers, the population of cattle that most commonly suffer from this infection. They tested two diets (a high grain-based and forage-based) and three bacteria commonly found in liver abscesses [ Fusobacterium necrophorum subsp. necrophorum, Trueperella pyogenes , and Salmonella enterica serovar Lubbock]. The results from the high-grain diet model were found to be more reliable, leading scientists to focus more on this model.
Most people associate liver abscesses in cattle with a high-energy diet. The theory is that when cattle are fed elevated grain levels, highly fermentable starch in the rumen is rapidly fermented by bacteria, causing a drop in rumen pH. This acidity causes damage to the rumen lining, allowing bacteria to travel into the blood, reaching the liver and other organs where they can cause infection. However, it is still unknown with accuracy the exact route that these bacteria take to cause infection or injury to the liver.
Scientists discovered that the bacteria associated with liver abscesses in cattle may not always originate from the rumen. An alternative route may be bacterial travel from the lower gastrointestinal tract. The research showed that in some cases, when these bacteria were not detected in the acidic rumen environment caused by a high-grain diet, no liver abscesses were detected. However, when scientists introduced bacteria directly to the rumen, they observed the formation of liver abscesses and were able to isolate the bacteria from the infected sites.
The study confirms that an acidotic diet, combined with bacterial inoculation in the rumen, can be used as a model to induce liver abscesses. However, further research is being conducted at USDA to determine the consistency of the model before it can be used to evaluate new interventions to prevent this complex infection.
The Agricultural Research Service is the U.S. Department of Agriculture's chief scientific in-house research agency. Daily, ARS focuses on solutions to agricultural problems affecting America. Each dollar invested in U.S. agricultural research results in $20 of economic impact.

share this!
March 25, 2024
This article has been reviewed according to Science X's editorial process and policies . Editors have highlighted the following attributes while ensuring the content's credibility:
fact-checked
trusted source
Food production using controlled environment agriculture and agrivoltaics systems could become the new normal
by Melissa Vidmar, Virginia Tech
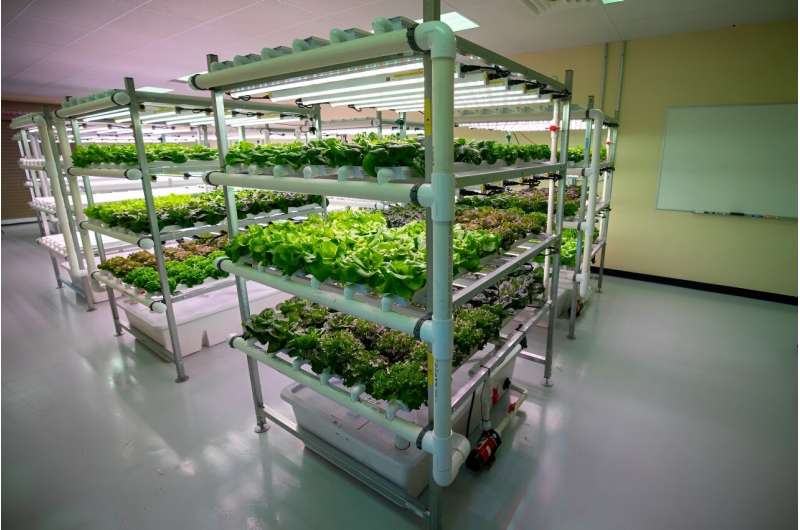
New research from the U.S. Department of Agriculture (USDA) and Virginia Tech suggests that technologies such as controlled environment agriculture and agrivoltaics may become part of the future of farming.
Controlled environment agriculture systems are typically enclosed or indoors. They include greenhouses, vertical farms, and hydroponics that use horticultural and engineering techniques beyond traditional, soil-based outdoor production. Agrivoltaics involve the use of land for both farming and solar photovoltaic energy generation.
A recent publication by USDA and Virginia Tech researchers highlights that these innovative techniques could increase yields, enhance nutrition, improve access to local foods, and provide better year-round access to fresh food than traditional large-scale outdoor production.
The research team found that even though these systems face technological and economic challenges , they are part of a long history of advancing technology for the U.S. agricultural sector.
Erik Dohlman led the team with Karen Maguire, Wilma Davis, Megan Husby, and Catharine Weber, all economists with the USDA's Economic Research Service. Virginia Tech researchers included John Bovay, assistant professor and Kohl Junior Faculty Fellow in the College of Agriculture and Life Sciences' Department of Agricultural and Applied Economics, and Yoonjung Lee, a graduate student in the department.
While these new technologies may not replace conventional production methods anytime soon, they have great potential to generate benefits, including renewable energy from agrivoltaic systems and reducing the risk of crop failure in extreme weather conditions.
"Part of the motivation for this project was to provide an overview of emerging technologies, particularly hydroponics and vertical farming," said Bovay, who is also affiliated faculty in the Center for Advanced Innovation in Agriculture.
"These industries have experienced tremendous growth over the last couple of decades, but official data collected and published by the USDA may not fully reflect the current technological and market situation. If controlled environment agriculture and indoor farming were to take off in the next decade, this could transform the consumer experience and possibly change the economic reality for traditional, soil-based farmers who grow crops that can also be grown in controlled environments and without soil."
Other research findings include the following:
- The number of individually controlled environment agriculture operations in the U.S. more than doubled to nearly 3,000 between 2009-19.
- The quantity of controlled environment crop production increased by 56 percent over that same period, from 502 million pounds to 786 million pounds.
- New technologies were used to produce a large share of total controlled environment agriculture production, with more than 60 percent of tomatoes, cucumbers, and lettuce grown using hydroponics in 2019.
- As of 2021, most of the approximately 300 agrivoltaics sites were solar farms planted with pollinator-friendly vegetative cover. Roughly 35 sites combined solar panels with vegetation that were grazed by sheep, and a few were co-located with specialty crop production, including blueberries.
Provided by Virginia Tech
Explore further
Feedback to editors

Invasive Pacific oyster proliferation during Blob marine heat wave portends similar events as seas warm
17 minutes ago

Scientists on the hunt for evidence of quantum gravity's existence at the South Pole

Albedo can reduce climate benefit of tree planting: New tool identifies locations with high climate-cooling potential

New roadmap to prevent pandemics centers on protecting biodiversity

Indonesia hunts clues as study suggests Javan tiger may still exist
2 hours ago

Tiniest 'starquakes' ever detected
3 hours ago

GPT-4 for identifying cell types in single cells matches and sometimes outperforms expert methods
14 hours ago

Stunning James Webb images show birth and death of massive stars
15 hours ago

Species diversity found to be directly correlated with productivity in eastern US forests
16 hours ago

Scientists deliver quantum algorithm to develop new materials and chemistry
Relevant physicsforums posts, are all biological catabolic reactions exergonic.
Mar 20, 2024
A First of Its Kind: A Calcium-based signal in the Human Brain
Mar 18, 2024
Biological culture and cultural biology
Mar 17, 2024
Potentially fatal dog parasite found in the Colorado River
Mar 15, 2024
Electrical potential difference and charge separation
Mar 14, 2024
Nick Lanes on Sean Carroll's podcast
Mar 11, 2024
More from Biology and Medical
Related Stories

Using artificial methods to grow crops could help solve global food insecurity
Aug 31, 2023

Vertical farming will play a role in future food production, expert says
Apr 12, 2022

Researchers seek to make solar energy and agricultural production more compatible with agrivoltaics
Oct 24, 2023

How shading crops with solar panels can improve farming, lower food costs and reduce emissions
Apr 27, 2023

Mixed-use solar and agricultural land is the silver bullet Alberta's Conservatives have wished for, says researcher
Sep 6, 2023

Made in the shade: Growing crops at solar farms yields efficiency
Mar 3, 2023
Recommended for you

Machine learning model demonstrates effect of public breeding on rice yields in climate change
17 hours ago

Stem cell model offers first glimpse of early human development
21 hours ago

Scientists discover how a motor protein helps cells move

Better phosphorus use can ensure its stocks last more than 500 years and boost global food production, study shows
23 hours ago

Researchers invent artificial intelligence model to design new superbug-fighting antibiotics
Mar 22, 2024

Researchers develop affordable, user-friendly method for single-cell reactions at the nanoliter level
Let us know if there is a problem with our content.
Use this form if you have come across a typo, inaccuracy or would like to send an edit request for the content on this page. For general inquiries, please use our contact form . For general feedback, use the public comments section below (please adhere to guidelines ).
Please select the most appropriate category to facilitate processing of your request
Thank you for taking time to provide your feedback to the editors.
Your feedback is important to us. However, we do not guarantee individual replies due to the high volume of messages.
E-mail the story
Your email address is used only to let the recipient know who sent the email. Neither your address nor the recipient's address will be used for any other purpose. The information you enter will appear in your e-mail message and is not retained by Phys.org in any form.
Newsletter sign up
Get weekly and/or daily updates delivered to your inbox. You can unsubscribe at any time and we'll never share your details to third parties.
More information Privacy policy
Donate and enjoy an ad-free experience
We keep our content available to everyone. Consider supporting Science X's mission by getting a premium account.
E-mail newsletter
Language selection
- Français fr
Supporting research and innovation in agriculture, with funding for 101 new projects
From: Agriculture and Agri-Food Canada
News release
The governments of Canada and Manitoba are providing $16.3 million in grant funding under the Sustainable Canadian Agricultural Partnership (Sustainable CAP) to support 101 projects under the Research and Innovation program over the next three years, federal Agriculture and Agri-Food Minister the Honourable Lawrence MacAulay and Manitoba Agriculture Minister Ron Kostyshyn announced today.
March 22, 2024 – Winnipeg, Manitoba – Agriculture and Agri-Food Canada
The funding will go towards research and capacity building to accelerate innovation in agriculture in Manitoba, supporting companies that are leading innovative research and putting Manitoba at the forefront of sustainable agriculture research.
Projects improve sustainability, resiliency and competitiveness of agriculture and agri-food sectors by accelerating the development and adoption of technologies and products that enhance efficiency and sustainability while addressing challenges such as climate change. Funding is available for stakeholders including primary producers, Agri-Processors, industry organizations, academic institutions and research bodies, industry service providers, and Indigenous governments, communities, and groups.
A call for letters of intent under the Research and Innovation program is now open for innovative industry-led research and development and/or capacity building project ideas for funding commencing in spring 2025. In April 2023 over $8.4 million was provided to support 4 7 p rojects and today an additional $ 7.9 m illion is being provided to 5 4 proj ects, for a total of 101 proje cts funded at $16 .3 milli on under the Research and Innovation program, the ministers added.
"Folks around the world are looking for more sustainable agri-food products and I know our producers can deliver. By investing in research and innovation across the value chain, we can address current and future challenges and help make sure the sector remains resilient, competitive, and efficient." - The Honourable Lawrence MacAulay, Minister of Agriculture and Agri-Food
"Research and innovation drives growth and economic development in rural Manitoba. Manitoba producers are leading the way with innovative research, putting Manitoba at the forefront of sustainable, environmentally friendly, and efficient agriculture. We're investing in them so they can better feed Manitoba and feed the world." - Ron Kostyshyn, Manitoba Minister of Agriculture
"Researchers at the University of Manitoba welcome this valuable support to develop sustainable and environmentally-friendly agricultural systems and tools that will benefit producers and consumers. The training opportunities enabled by these projects will ensure our students play a pivotal role in the future of the Canadian agricultural landscape." - Dr. Martin Scanlon, Dean, Faculty of Agricultural and Food Sciences, University of Manitoba
Quick facts
The Sustainable Canadian Agricultural Partnership is a 5-year, $3.5-billion investment by Canada's federal, provincial and territorial governments that supports Canada's agri-food and agri-products sectors. This includes $ 1 billio n in federal programs and activities and a $2. 5 bill ion commitment that is cost-shared 60% federally and 40% provincially-territorially for programs that are designed and delivered by provinces and territories.
Associated links
- Sustainable Canadian Agricultural Partnership - agriculture.canada.ca
- Province of Manitoba | Sustainable Canadian Agricultural Partnership (gov.mb.ca)
- Province of Manitoba | Research and Innovation
Francis Chechile Press Secretary Office of the Minister of Agriculture and Agri-Food [email protected]
Media Relations Agriculture and Agri-Food Canada Ottawa, Ontario 613-773-7972 1-866-345-7972 [email protected] Follow us on Twitter , Facebook , Instagram , and LinkedIn Web: Agriculture and Agri-Food Canada
Page details
Advertisement
Enhancing resilience in agricultural production systems with AI-based technologies
- Published: 11 August 2023
Cite this article
- Member Joy Usigbe 1 ,
- Senorpe Asem-Hiablie 2 ,
- Daniel Dooyum Uyeh 3 ,
- Olayinka Iyiola 4 ,
- Tusan Park 5 , 6 &
- Rammohan Mallipeddi 1
533 Accesses
Explore all metrics
Agricultural production systems play a crucial role in global societal sustenance as they provide the world's food, fuel, and fiber supplies. However, these systems face numerous challenges including climate change and resource depletion. Modern technologies powered by artificial intelligence (AI) can help address these challenges by contributing to revolutionizing agricultural production and building resilience. While there has been a growing body of research on AI-based technologies in agricultural production systems, comprehensive literature reviews on the potential of AI-based technologies in enhancing resilience, and sustainability in agricultural production systems is lacking to the extent of the authors’ knowledge. Additionally, some studies have focused on specific AI-based technologies such as internet of things, creating a gap in ascertaining the impact of the cumulative application of these techniques. This review aims to fill these gaps by exploring the trends in the emergence of AI technologies and applications in agricultural production systems. It also investigates the integration of these technologies into traditional farming operations and driving climate-smart agriculture (CSA). Data on automation systems, AI applications, and CSA were gathered from peer-reviewed publications, reports, and public databases. Two Natural Language Processing (NLP) tools were utilized: the Iris.ai application and an in-house NLP tool developed with Fast.ai-NLP (the Fast.ai deep learning library). The Iris.ai-NLP tool extracted a thousand papers between 1940 and 2021, while the Fast.ai-NLP extracted forty thousand papers from early 1900s to 2023. These extracted papers were finally revised to a concise reading list of a hundred and thirty four papers. Results showed that greater attention has been given to AI-based technologies and models that enhanced production systems. The collective application of AI-based techniques can improve food security and environmental sustainability by optimizing processes to increase yield and aiding in effective monitoring to decrease environmental emissions such as greenhouse gases. The analyzed studies using NLP tools showed how AI technologies could address limitations in the agricultural sector and contribute to improving productivity, resilience to climate change, and food security. Rapid implementation of these technologies in agricultural production systems worldwide has the potential to address challenges such as, resource degradation and depletion, skilled labor shortages, and high input costs.
This is a preview of subscription content, log in via an institution to check access.
Access this article
Price includes VAT (Russian Federation)
Instant access to the full article PDF.
Rent this article via DeepDyve
Institutional subscriptions
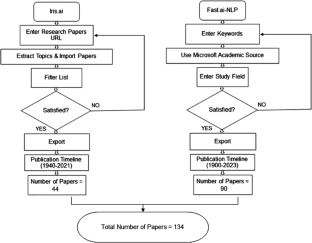
Similar content being viewed by others
IoT × AI: Introducing Agricultural Innovation for Global Food Production
Recent Advances and Challenges in AI for Sustainable Agricultural Systems
Network Analysis on Artificial Intelligence in Agriculture, a Bibliometric Review
Data availability.
Data sharing not applicable to this article as no datasets were generated or analyzed during the current study.
Abbasi, R., P. Martinez, and R. Ahmad, The digitization of agricultural industry–a systematic literature review on agriculture 4.0. Smart Agricultural Technology, 2022: pp 100042.
Abbasi, S., Choukolaei, H. A., & A,. (2023). systematic review of green supply chain network design literature focusing on carbon policy. Decision Analytics Journal . https://doi.org/10.1016/j.dajour.2023.100189
Article Google Scholar
Acosta, M., et al., (2020) Step-by-step process to mainstream gender in climate-smart agricultural initiatives in Guatemala.
Aggarwal, P. K., et al. (2018). The climate-smart village approach : Framework of an integrative strategy for scaling up adaptation options in agriculture. Ecology and Society, 23 , 14.
Ahmed, U., et al. (2021). A nutrient recommendation system for soil fertilization based on evolutionary computation. Computers and Electronics in Agriculture, 189 , 106407.
Ajani, O. S., et al. (2023). Greenhouse micro-climate prediction based on fixed sensor placements: A machine learning approach. Mathematics, 11 (14), 3052.
Amitrano, C., et al., Crop Management in Controlled Environment Agriculture (CEA) Systems Using Predictive Mathematical Models@. Sensors (Basel, Switzerland), 2020. 20 .
Anderson, N.W., Robotic watering unit. Google patents, 2012.
Arefi, A., et al. (2011). Recognition and localization of ripen tomato based on machine vision. Australian Journal of Crop Science, 5 , 1144–1149.
Google Scholar
Ashraf, M. A., Kondo, N., & Shiigi, T. (2011). Use of machine vision to sort tomato seedlings for grafting robot. Engineering in Agriculture, Environment and Food, 4 , 119–125.
Balducci, F., Impedovo, D., & Pirlo, G. (2018). Machine Learning Applications on Agricultural Datasets for Smart Farm Enhancement. Machines, 6 (3), 38.
Bannerjee, G., et al. (2018). Artificial intelligence in agriculture: A literature survey. International Journal of Scientific Research in Computer Science Applications and Management Studies, 7 (3), 1–6.
Barnea, E., Mairon, R., & Ben-Shahar, O. (2016). Colour-agnostic shape-based 3D fruit detection for crop harvesting robots. Biosystems Engineering, 146 , 57–70.
Barth, R., Hemming, J., & van Henten, E. J. (2016). Design of an eye-in-hand sensing and servo control framework for harvesting robotics in dense vegetation. Biosystems Engineering, 146 , 71–84.
Bawden, O., et al. (2017). Robot for weed species plan” specific management. Journal of Field Robotics, 34 , 1179–1199.
Bayati, M., & Fotouhi, R. (2018). A mobile robotic platform for crop monitoring. Advances in Robotics & Automation, 7 (1), 1000186.
Beal, C., et al., (2021) CCAFS Outcome Synthesis Report: Outcomes Achieved Within the Context of Climate-Smart Village Approach. CGIAR Research Program on Climate Change, Agriculture and Food Security Working Paper.
Binas, J., L. Luginbuehl, and Y. Bengio. Reinforcement learning for sustainable agriculture . in ICML 2019 Workshop Climate Change: How Can AI Help . 2019.
Bogue, R. (2020). Fruit picking robots: Has their time come? Industrial Robot: THe International Journal of Robotics Research and Application, 47 (2), 141–145.
Both, A.J., et al. (1997) Hydroponic lettuce production influenced by integrated supplemental light levels in a controlled environment agriculture facility: Experimental results .
Boursianis, A. D., et al. (2022). Internet of things (IoT) and agricultural unmanned aerial vehicles (UAVs) in smart farming: A comprehensive review. Internet of Things, 18 , 100187.
Brach, E.J., et al (1978). Use Of Laser Fluorescence To Study Lettuce Growth And Development Under Controlled Environment . in Optics & Photonics
Bronson, K. (2018). Smart farming: Including rights holders for responsible agricultural innovation. Technology Innovation Management Review, 8 , 7–14.
Cen, H., et al. (2016). Nondestructive detection of chilling injury in cucumber fruit using hyperspectral imaging with feature selection and supervised classification. Postharvest Biology and Technology, 111 , 352–361.
Chandavale, A., et al. (2019) Automated Systems for Smart Agriculture . in 2019 IEEE Pune Section International Conference (PuneCon) .
Chang, D.-H., & Islam, S. (2000). Estimation of Soil Physical Properties Using Remote Sensing and Artificial Neural Network. Remote Sensing of Environment, 74 , 534–544.
Chen, W.-H., Mattson, N. S., & You, F. (2022). Intelligent control and energy optimization in controlled environment agriculture via nonlinear model predictive control of semi-closed greenhouse. Applied Energy, 320 , 119334.
Article CAS Google Scholar
Cheng, B. and E.T. Matson. (2015) A Feature-Based Machine Learning Agent for Automatic Rice and Weed Discrimination . in ICAISC . 2015.
Chieochan, O., A. Saokaew, and E. Boonchieng, Internet of things (IOT) for smart solar energy: A case study of the smart farm at Maejo University. In: 2017 International Conference on Control, Automation and Information Sciences (ICCAIS), 2017: pp 262–267.
Cohen, A. R., et al. (2021). Dynamically controlled environment agriculture: Integrating machine learning and mechanistic and physiological models for sustainable food cultivation. ACS ES&T Engineering, 2 (1), 3–19.
Cornforth, I. S., & Sinclair, A. G. (1977). Two pot techniques for controlled-environment cabinets. New Zealand Journal of Experimental Agriculture, 5 , 189–192.
Culibrina, F.B. and E.P. Dadios. Smart farm using wireless sensor network for data acquisition and power control distribution . in 2015 International Conference on Humanoid, Nanotechnology, Information Technology,Communication and Control, Environment and Management (HNICEM) . 2015.
Deutsch, C. A., et al. (2018). Increase in crop losses to insect pests in a warming climate. Science, 361 (6405), 916–919.
Dorosh, P.A., A. Kennedy, and M. Torero, El Niño and cereal production shortfalls: Policies for resilience and food security in 2016 and beyond . 2016: Intl Food Policy Res Inst.
Dsouza, A., et al. (2023). Exploring the landscape of controlled environment agriculture research: A systematic scoping review of trends and topics. Agricultural Systems, 209 , 103673.
FAO, F., (2018) The impact of disasters and crises on agriculture and food security. Report.
FAO, F., (2020) How is COVID-19 affecting the fisheries and aquaculture food systems. Rome
Fernando, S., et al. (2020) Ai based greenhouse farming support system with robotic monitoring . in 2020 IEEE region 10 conference (tencon) . IEEE.
Fogg, L.W., et al. (1979) Apparatus for promoting growth of plants in an agricultural facility controlled environment .
Förch, W., et al., (2013) CCAFS Site Portfolio: Core Sites in the CCAFS Regions: East Africa, West Africa and South Asia.
Fynn, R. P., Roller, W. L., & Keener, H. M. (1989). A decision model for nutrition management in controlled environment agriculture. Agricultural Systems, 31 , 35–53.
Gagliardi, G., et al., (2021) An Internet of Things Solution for Smart Agriculture. Agronomy.
Gao, G., H.Y. Zhou, and X.M. Niu. (2012) An Intelligent Variable Spraying Decision-Making System Based on Fuzzy Neural Network for Greenhouse Mobile Robot
Gharpankar, S., Plant Leaf Disease Detection using Convolutional Neural Networks. Int. J. Res. Appl. Sci. Eng. Technol, 2020. 8 (11): p. 494–499.
Gianessi, L. P., & Reigner, N. P. (2007). The value of herbicides in US crop production. Weed Technology, 21 (2), 559–566.
Godfray, H. C. J., et al. (2010). Food security: the challenge of feeding 9 billion people. Science, 327 (5967), 812–818.
Hao, P., et al. (2015). Feature selection of time series MODIS data for early crop classification using random forest: A case study in Kansas, USA. Remote Sensing, 7 (5), 5347–5369.
Hastie, T.J., R. Tibshirani, and J.H. Friedman. (2001) The Elements of Statistical Learning: Data Mining, Inference, and Prediction, 2nd Edition . in Springer Series in Statistics .
Hauwiller, J.I. and Youxuan Jin (2001). Systems and method for creating agricultural decision and application maps for automated agricultural machines. Google patents.
Helm, J. M., et al. (2020). Machine learning and artificial intelligence: Definitions, applications, and future directions. Current Reviews in Musculoskeletal Medicine, 13 , 69–76.
Hemming, J., et al. (2014) A robot for harvesting sweet-pepper in greenhouses .
Hemming, S., et al. (2020). Cherry tomato production in intelligent greenhouses—Sensors and AI for control of climate, irrigation, crop yield, and quality. Sensors, 20 (22), 6430.
Hu, G., & You, F. (2022). Renewable energy-powered semi-closed greenhouse for sustainable crop production using model predictive control and machine learning for energy management. Renewable and Sustainable Energy Reviews, 168 , 112790.
Jin, X., et al. (2020). Artificial intelligence biosensors: Challenges and prospects. Biosensors & Bioelectronics, 165 , 112412.
Jirata, M., S. Grey, and E. Kilawe, Ethiopia climate-smart agriculture scoping study. FAO: Addis Ababa, Ethiopia, 2016.
Kakraliya, S. K., et al. (2021). Effect of climate-smart agriculture practices on climate change adaptation, greenhouse gas mitigation and economic efficiency of rice-wheat system in India. Agriculture, 11 (12), 1269.
Karimi, Y., et al. (2006). Application of support vector machine technology for weed and nitrogen stress detection in corn. Computers and Electronics in Agriculture, 51 (1–2), 99–109.
Kassim, M.R.M., I. Mat, and I.M. Yusoff, (2019) Applications of Internet of Things in Mushroom Farm Management. 2019 13th International Conference on Sensing Technology (ICST), pp 1–6.
Kestur, R., Meduri, A., & Narasipura, O. (2019). MangoNet: A deep semantic segmentation architecture for a method to detect and count mangoes in an open orchard. Engineering Applications of Artificial Intelligence, 77 , 59–69.
Khatri-Chhetri, A., et al. (2017). Farmers’ prioritization of climate-smart agriculture (CSA) technologies. Agricultural Systems, 151 , 184–191.
Khujamatov, K.E. and T. Toshtemirov. (2020) Wireless sensor networks based Agriculture 4.0: challenges and apportions . in 2020 international conference on information science and communications technologies (ICISCT) IEEE.
Kim, J. Y., et al. (2023). Open-source electronics for plant phenotyping and irrigation in controlled environment. Smart Agricultural Technology, 3 , 100093.
Landstrom, D.K., et al. (1983) Design of a solar controlled environment agriculture system (SCEAS) .
Li, X., et al., (2006) Embedded Wireless Network Control System: an Application of Remote Monitoring System for Greenhouse Environment. The Proceedings of the Multiconference on "Computational Engineering in Systems Applications",. 2 p. 1719–1722.
Lipper, L., et al. (2014). Climate-smart agriculture for food security. Nature Climate Change, 4 (12), 1068–1072.
Lottes, P., et al. (2017). UAV-based crop and weed classification for smart farming. IEEE International Conference on Robotics and Automation (ICRA), 2017 , 3024–3031.
Maroli, A., Narwane, V. S., & Gardas, B. B. (2021). Applications of IoT for achieving sustainability in agricultural sector: A comprehensive review. Journal of Environmental Management, 298 , 113488.
Marsland, S.R. (2009) Machine Learning - An Algorithmic Perspective . in Chapman and Hall / CRC machine learning and pattern recognition series .
Martín-Martín, A., et al. (2021). Google Scholar, Microsoft Academic, Scopus, Dimensions, Web of Science, and OpenCitations’ COCI: A multidisciplinary comparison of coverage via citations. Scientometrics, 126 (1), 871–906.
McNunn, G., et al. (2020). Climate smart agriculture opportunities for mitigating soil greenhouse gas emissions across the US Corn-Belt. Journal of Cleaner Production, 268 , 122240.
Mehta, P. (2016). Automation in agriculture: Agribot the next generation weed detection and herbicide sprayer-a review. Journal of Basic and Applied Engineering Research, 3 (3), 234–238.
Mendez, G.R., M.A. Md Yunus, and S.C. Mukhopadhyay, (2011) A WiFi based smart wireless sensor network for an agricultural environment. 2011 Fifth International Conference on Sensing Technology: p. 405–410.
Mikhaylov, A., et al. (2020). Global climate change and greenhouse effect. Entrepreneurship and Sustainability Issues, 7 (4), 2897.
Mohri, M., A. Rostamizadeh, and A.S. Talwalkar. (2012) Foundations of Machine Learning . in Adaptive computation and machine learning .
Muangprathub, J., et al. (2019). IoT and agriculture data analysis for smart farm. Computers and Electronics in Agriculture, 156 , 467–474.
Murphy, K.P., (2012) Machine learning: a probabilistic perspective , MIT press.
Naik, N.S., V.V. Shete, and S.R. Danve, (2016) Precision agriculture robot for seeding function. 2016 International Conference on Inventive Computation Technologies (ICICT), 2 : p. 1–3.
Naik, H. S., et al. (2017). A real-time phenotyping framework using machine learning for plant stress severity rating in soybean. Plant Methods, 13 (1), 1–12.
Nandeesh, T. and H. Kalpana. Smart multipurpose agricultural robot . in 2021 IEEE International Conference on Electronics, Computing and Communication Technologies (CONECCT) . 2021. IEEE.
Nandi, P.K. and M.A. Mahmood. An Automated Irrigation and Fertilization management System Using Fuzzy Logic . in 2021 5th International Conference on Electrical Information and Communication Technology (EICT) . 2021. IEEE.
Narasimham, S., & Subbarao, D. (2017). Relationship between climate change and agriculture–a review. International Journal of Science Environmental Technology, 6 (5), 3011–3025.
Nesteruk, S., et al. (2021). Image compression and plants classification using machine learning in controlled-environment agriculture: antarctic station use case. IEEE Sensors Journal, 21 , 17564–17572.
Niles, M. T., et al. (2020). The early food insecurity impacts of COVID-19. Nutrients, 12 (7), 2096.
Oberti, R., et al. (2016). Selective spraying of grapevines for disease control using a modular agricultural robot. Biosystems Engineering, 146 , 203–215.
Oerke, E., (2006) Crop Losses to Pests. Journal of Agricultural Science. Journal of Agricultural Science, Toronto, ON.
Ojango, J. M., et al. (2016). System characteristics and management practices for small ruminant production in “Climate Smart Villages” of Kenya. Animal Genetic Resources/resources Génétiques Animales/recursos Genéticos Animales, 58 , 101–110.
Organization, W.H., (2019) The state of food security and nutrition in the world 2019: Safeguarding against economic slowdowns and downturns . Vol. 2019: Food & Agriculture Org.
Ostovar, A., Ringdahl, O., & Hellström, T. (2018). Adaptive image thresholding of yellow peppers for a harvesting robot. Robotics, 7 (1), 11.
Pagliacci, F., et al. (2020). Drivers of farmers adoption and continuation of climate-smart agricultural practices A study from northeastern Italy. Science of the Total Environment, 710 , 136345.
Partel, V., Kakarla, S. C., & Ampatzidis, Y. (2019). Development and evaluation of a low-cost and smart technology for precision weed management utilizing artificial intelligence. Computers and Electronics in Agriculture, 157 , 339–350.
Partey, S.T., et al., Developing climate-smart agriculture to face climate variability in West Africa: Challenges and lessons learnt. Journal of Cleaner Production, 2018.
Paul, K., et al. (2022). Viable smart sensors and their application in data driven agriculture. Computers and Electronics in Agriculture, 198 , 107096.
Pilli, S.K., et al. eAGROBOT—A robot for early crop disease detection using image processing . in 2015 2nd International Conference on Electronics and Communication Systems (ICECS) . 2015. IEEE.
Pivoto, D., et al. (2018). Scientific development of smart farming technologies and their application in Brazil. Information Processing in Agriculture, 5 (1), 21–32.
Power, D.J., Decision support systems: concepts and resources for managers . 2002: Greenwood Publishing Group.
Qi, L., et al. (2016). Design of remote control system for automatic sprayer based on GPRS in greenhouse. Transactions of the Chinese Society of Agricultural Engineering, 32 (23), 51–57.
Ragaveena, S., Shirly Edward, A., & Surendran, U. (2021). Smart controlled environment agriculture methods: A holistic review. Reviews in Environmental Science and Bio/technology, 20 (4), 887–913.
Raihan, A., An econometric evaluation of the effects of economic growth, energy use, and agricultural value added on carbon dioxide emissions in Vietnam. Asia-Pacific Journal of Regional Science, 2023: pp 1–32.
Rath, T., & Kawollek, M. (2009). Robotic harvesting of Gerbera Jamesonii based on detection and three-dimensional modeling of cut flower pedicels. Computers and Electronics in Agriculture, 66 , 85–92.
Reddy Maddikunta, P. K., et al. (2021). Unmanned Aerial Vehicles in Smart Agriculture: Applications, Requirements, and Challenges. IEEE Sensors Journal, 21 , 17608–17619.
Rußwurm, M., & Körner, M. (2018). Multi-temporal land cover classification with sequential recurrent encoders. ISPRS International Journal of Geo-Information, 7 (4), 129.
Sakai, H., et al. (2013). Accurate Position Detecting during Asparagus Spear Harvesting using a Laser Sensor. Engineering in Agriculture, Environment and Food, 6 , 105–110.
Saleem, M. H., Potgieter, J., & Arif, K. M. (2021). Automation in agriculture by machine and deep learning techniques: A review of recent developments. Precision Agriculture, 22 , 2053–2091.
Sánchez-Hermosilla, J., et al. (2010) A Mechatronic Description of an Autonomous Mobile Robot for Agricultural Tasks in Greenhouses .
Sane, T.U. and T.U. Sane (2021). Artificial Intelligence and Deep Learning Applications in Crop Harvesting Robots -A Survey . in 2021 International Conference on Electrical, Communication, and Computer Engineering (ICECCE) .
Shaikh, T. A., Rasool, T., & Lone, F. R. (2022). Towards leveraging the role of machine learning and artificial intelligence in precision agriculture and smart farming. Computers and Electronics in Agriculture, 198 , 107119.
Shamshirband, S., et al. (2015). A multi-objective evolutionary algorithm for energy management of agricultural systems—a case study in Iran. Renewable and Sustainable Energy Reviews, 44 , 457–465.
Shi, X., et al. (2019). State-of-the-art internet of things in protected agriculture. Sensors, 19 (8), 1833.
Shirato, Y. and A. Hasebe, Climate Smart Agriculture for the Small-Scale Farmers in the Asian and Pacific Region . 2019: National Agriculture and Food Research Organization (NARO).
Singh, V., & Misra, A. K. (2017). Detection of plant leaf diseases using image segmentation and soft computing techniques. Information Processing in Agriculture, 4 (1), 41–49.
Sinha, S.K., et al. Plant identification using machine learning . in 2021 Asian conference on innovation in technology (ASIANCON) . 2021. IEEE.
Siopongco, J.D., R. Wassmann, and B.O. Sander, Alternate wetting and drying in Philippine rice production: feasibility study for a Clean Development Mechanism . 2013.
Song, H. and Y. He. Crop nutrition diagnosis expert system based on artificial neural networks . in Third International Conference on Information Technology and Applications (ICITA'05) . 2005. IEEE.
Srivastava, G., et al. (2014). Seed treatment with iron pyrite (FeS2) nanoparticles increases the production of spinach. RSC Advances, 4 , 58495–58504.
Suge, H. (1984). Re-examination on the role of vernalization and photoperiod in the flowering of brassica crops under controlled environment. Breeding Science, 34 , 171–180.
Suma, N., et al., IOT Based Smart Agriculture Monitoring System International Journal on Recent and Innovation Trends in Computing and Communication, 2017. 5 (2).
Sutton, R. S., & Barto, A. G. (2005). Reinforcement Learning: An Introduction. IEEE Transactions on Neural Networks, 16 , 285–286.
Tajik, S., Ayoubi, S., & Nourbakhsh, F. (2012). Prediction of soil enzymes activity by digital terrain analysis: Comparing artificial neural network and multiple linear regression models. Environmental Engineering Science, 29 (8), 798–806.
Takakura, T. SENSORS IN CONTROLLED ENVIRONMENT AGRICULTURE (CEA): MEASURING GROWTH AND DEVELOPMENT . 1992.
Talari, G., et al. (2022). State of the art review of Big Data and web-based Decision Support Systems (DSS) for food safety risk assessment with respect to climate change. Trends in Food Science & Technology, 126 , 192–204.
Talaviya, T., et al. (2020). Implementation of artificial intelligence in agriculture for optimisation of irrigation and application of pesticides and herbicides. Artificial Intelligence in Agriculture, 4 , 58–73.
Tao, W., et al. (2021). Review of the internet of things communication technologies in smart agriculture and challenges. Computers and Electronics in Agriculture, 189 , 106352.
Thomson, A., et al. (2022). Review of the potential for recycling CO2 from organic waste composting into plant production under controlled environment agriculture. Journal of Cleaner Production, 333 , 130051.
Uyeh, D. D., et al. (2023). Perspectives on the strategic importance of digitalization for Modernizing African Agriculture. Computers and Electronics in Agriculture, 211 , 107972.
Tobal, A., & Mokhtar, S. A. (2014). Weeds identification using Evolutionary Artificial Intelligence Algorithm. Journal of Computer Science, 10 (8), 1355–1361.
Tripathi, A. D., et al. (2019). Estimates for world population and global food availability for global health. The role of functional food security in global health (pp. 3–24). Elsevier.
Türk, A., E. Gunal, and U. Gurel, An Automation System Design for Greenhouses by Using DIY platforms . 2015.
Vincent, D. R., et al. (2019). Sensors driven AI-based agriculture recommendation model for assessing land suitability. Sensors, 19 (17), 3667.
Wang, J., et al. (2018). Effect of climate change on the yield of cereal crops: A review. Climate, 6 (2), 41.
Wheeler, T., & Von Braun, J. (2013). Climate change impacts on global food security. Science, 341 (6145), 508–513.
Yoon, C., et al. Implement smart farm with IoT technology . in 2018 20th International Conference on Advanced Communication Technology (ICACT) . 2018.
Zeng, H., et al. (2023). An IoT and Blockchain-based approach for the smart water management system in agriculture. Expert Systems, 40 (4), e12892.
Zhai, Z., et al., Decision support systems for agriculture 4.0: Survey and challenges. Computers and Electronics in Agriculture, 2020. 170 : p. 105256.
Zhang, B., et al. (2018). Challenges and solutions of optical-based nondestructive quality inspection for robotic fruit and vegetable grading systems: A technical review. Trends in Food Science & Technology, 81 , 213–231.
Download references
Acknowledgements
This work was supported by the Basic Science Research Program through the National Research Foundation of Korea (NRF) funded by the Ministry of Education (2021R1I1A3049810).
Author information
Authors and affiliations.
Department of Artificial Intelligence, School of Electronics Engineering, Kyungpook National University, Daegu 41566, Republic of Korea
Member Joy Usigbe & Rammohan Mallipeddi
Biotechnology, Shell International Exploration and Production Inc, Houston, TX, USA
Senorpe Asem-Hiablie
Department of Biosystems and Agricultural Engineering, Michigan State University, East Lansing, USA
Daniel Dooyum Uyeh
Department of Hydro Science and Engineering, Technische Universität Dresden, Dresden, Germany
Olayinka Iyiola
Department of Bio-Industrial Machinery Engineering, Kyungpook National University, Daegu, 41566, Republic of Korea
Smart Agriculture Innovation Center, Kyungpook National University, Daegu 41566, Republic of Korea
You can also search for this author in PubMed Google Scholar
Corresponding author
Correspondence to Rammohan Mallipeddi .
Ethics declarations
Conflict of interest.
The authors declare no conflict of interest.
Additional information
Publisher's note.
Springer Nature remains neutral with regard to jurisdictional claims in published maps and institutional affiliations.
Rights and permissions
Springer Nature or its licensor (e.g. a society or other partner) holds exclusive rights to this article under a publishing agreement with the author(s) or other rightsholder(s); author self-archiving of the accepted manuscript version of this article is solely governed by the terms of such publishing agreement and applicable law.
Reprints and permissions
About this article
Usigbe, M.J., Asem-Hiablie, S., Uyeh, D.D. et al. Enhancing resilience in agricultural production systems with AI-based technologies. Environ Dev Sustain (2023). https://doi.org/10.1007/s10668-023-03588-0
Download citation
Received : 28 February 2023
Accepted : 05 July 2023
Published : 11 August 2023
DOI : https://doi.org/10.1007/s10668-023-03588-0
Share this article
Anyone you share the following link with will be able to read this content:
Sorry, a shareable link is not currently available for this article.
Provided by the Springer Nature SharedIt content-sharing initiative
- Artificial intelligence
- Food security
- Climate-smart agriculture
- Controlled environments agriculture
- Natural language processing
- Autonomous growing
- Find a journal
- Publish with us
- Track your research
An official website of the United States government
Here’s how you know
Official websites use .gov A .gov website belongs to an official government organization in the United States.
Secure .gov websites use HTTPS A lock ( Lock Locked padlock icon ) or https:// means you’ve safely connected to the .gov website. Share sensitive information only on official, secure websites.
- News & Announcements
USDA Announces Up to $12 Million in Grant Funding Available to Promote U.S. Agricultural Products and Address Food Insecurity in Underserved Communities
Public affairs.
WASHINGTON, March 21, 2024 -- The U.S. Department of Agriculture (USDA) today announced up to $12 million in funding available to strengthen and explore new market opportunities for U.S. agricultural products and increase access to locally grown food in communities experiencing food insecurity. The funding is available through three grant programs administered by the Agricultural Marketing Service (AMS): the Acer Access and Development Program, the Federal State Marketing Improvement Program, and the Micro-Grants for Food Security Program.
“Each of these grant programs focus on a different area of the food system, but all work to support USDA’s goals to create new market opportunities that bring equity and financial stability to small farms and that rural and historically underserved communities have access to fresh, locally grown foods,” said USDA Under Secretary for Marketing and Regulatory Programs Jenny Lester Moffitt. “The projects funded through these programs will improve the nation’s food system by developing new products, supporting small-scale producers and improving healthy food access in food insecure communities.”
Acer Access and Development Program
This year, up to $6 million is available through the Acer Access and Development Program for projects that expand consumer awareness of the maple syrup industry and provide valuable resources to maple syrup producers. The program promotes the domestic maple syrup industry by funding research and education projects related to maple syrup production, natural resource sustainability in the maple syrup industry, and the marketing of maple syrup and maple-sap products. Acer funding is authorized by the 2018 Farm Bill and funded through annual appropriations.
Examples of projects previously awarded grants funds through the Acer Access and Development Program include:
- Stockton University used Acer Access and Development Program funding to help increase maple syrup production in New Jersey and the larger Mid-Atlantic region through the use of novel technology, landowner engagement, and sustainable forest management. The project fostered a new consumer base for maple products in a region where pure maple products are not embedded in the culture of the community.
- A West Virginia University Research Corp project used Acer Access and Development Program funding to assemble a team of experts in forest management, forest operations, forest pathology, landowner assistance, and maple syrup operations to create an integrated program designed to promote maple syrup production by educating forest landowners, foresters, and loggers on the nuances of southern sugarbush management. The program helped increase maple syrup production by increasing the number of maple trees tapped as landowners learn about these opportunities.
Federal State Marketing Improvement Program
Through the Federal State Marketing Improvement Program, up to $1 million in grant funding is available to support projects that explore new market opportunities for U.S. food and agricultural products and to encourage research and innovation aimed at improving marketing system efficiency and performance. The program supports state departments of agriculture, state agricultural experiment stations, and other appropriate state agencies. FSMIP is authorized by the Agricultural Marketing Act of 1946 and funded by annual appropriations.
One successful project that recently received funding through the Federal State Marketing Improvement program was managed by the University of Kentucky. The university received the grant to identify best practices in sustaining financially profitable relationships between local producers and restaurants. The project focused on developing strategies for verifying local purchasing, evaluating different systems for verifying businesses’ level of local sourcing, and gauging consumer willingness to pay for locally produced foods. By understanding the strategies of consumer engagement and local product incentivization and verification, the project developed best practices that state departments of agriculture are using to improve local-sourcing programs. These outcomes improve economic opportunities for farmers and extended rural economies.
Micro-Grants for Food Security Program
Additionally, up to $5 million is available through the Micro-Grants for Food Security Program to agricultural agencies in eligible states and territories to increase the quantity and quality of locally grown food in food insecure communities through small-scale gardening, herding, and livestock operations. The program focuses on food insecure communities in areas of the U.S. that have significant levels of food insecurity and import a significant quantity of foods. The agricultural agencies or departments competitively distribute the funds through subawards to eligible entities. The Micro-Grants for Food Security Program is authorized through the 2018 Farm Bill and funded by annual appropriations.
Through a subaward from the Alaska Department of Natural Resources, a family in Alaska recently received funding through the Micro-Grants for Food Security Program to increase food availability in their local community. The funding enabled the family to freeze dry Alaskan fruits and vegetables and purchase a high tunnel and freeze drier. As a result, they increased their gardening space and now provide biweekly food deliveries to families, supplying 18 people in their area.
Application Information
A Request for Applications (RFAs) for each program is available on the program webpages listed below. Applications must be submitted electronically through www.grants.gov by 11:59 p.m. ET on the date listed in the respective RFAs. Grant applications submitted after the due date will not be considered unless the applicant provides documentation of an extenuating circumstance that prevented their timely submission of the grant application. More information is available in the AMS Late and Non-Responsive Application Policy .
AMS encourages applications for initiatives that benefit smaller farms and ranches, new and beginning farmers and ranchers, underserved producers, veteran producers, low-income, and minority individuals, and underserved communities. For projects intending to serve these entities, applicants should engage and involve those beneficiaries when developing projects and applications.
USDA touches the lives of all Americans each day in so many positive ways. In the Biden-Harris Administration, USDA is transforming America’s food system with a greater focus on more resilient local and regional food production, fairer markets for all producers, ensuring access to safe, healthy and nutritious food in all communities, building new markets and streams of income for farmers and producers using climate smart food and forestry practices, making historic investments in infrastructure and clean energy capabilities in rural America, and committing to equity across the Department by removing systemic barriers and building a workforce more representative of America. To learn more, visit www.usda.gov .
Get the latest Agricultural Marketing Service news at www.ams.usda.gov/news or follow us on Twitter @USDA_AMS. You can also read about us on the USDA blog.
USDA is an equal opportunity provider, employer, and lender
Help | Advanced Search
Computer Science > Computer Vision and Pattern Recognition
Title: self-supervised backbone framework for diverse agricultural vision tasks.
Abstract: Computer vision in agriculture is game-changing with its ability to transform farming into a data-driven, precise, and sustainable industry. Deep learning has empowered agriculture vision to analyze vast, complex visual data, but heavily rely on the availability of large annotated datasets. This remains a bottleneck as manual labeling is error-prone, time-consuming, and expensive. The lack of efficient labeling approaches inspired us to consider self-supervised learning as a paradigm shift, learning meaningful feature representations from raw agricultural image data. In this work, we explore how self-supervised representation learning unlocks the potential applicability to diverse agriculture vision tasks by eliminating the need for large-scale annotated datasets. We propose a lightweight framework utilizing SimCLR, a contrastive learning approach, to pre-train a ResNet-50 backbone on a large, unannotated dataset of real-world agriculture field images. Our experimental analysis and results indicate that the model learns robust features applicable to a broad range of downstream agriculture tasks discussed in the paper. Additionally, the reduced reliance on annotated data makes our approach more cost-effective and accessible, paving the way for broader adoption of computer vision in agriculture.
Submission history
Access paper:.
- Download PDF
- HTML (experimental)
- Other Formats

References & Citations
- Google Scholar
- Semantic Scholar
BibTeX formatted citation

Bibliographic and Citation Tools
Code, data and media associated with this article, recommenders and search tools.
- Institution
arXivLabs: experimental projects with community collaborators
arXivLabs is a framework that allows collaborators to develop and share new arXiv features directly on our website.
Both individuals and organizations that work with arXivLabs have embraced and accepted our values of openness, community, excellence, and user data privacy. arXiv is committed to these values and only works with partners that adhere to them.
Have an idea for a project that will add value for arXiv's community? Learn more about arXivLabs .

Small changes can yield big savings in agricultural water use
While Hollywood and Silicon Valley love the limelight, California is an agricultural powerhouse, too. Agricultural products sold in the Golden State totaled $59 billion in 2022. But rising temperatures, declining precipitation and decades of over pumping may require drastic changes to farming. Legislation to address the problem could even see fields taken out of cultivation.
Fortunately, a study out of UC Santa Barbara suggests less extreme measures could help address California's water issues. Researchers combined remote sensing, big data and machine learning to estimate how much water crops use in the state's Central Valley. The results, published in Nature Communications , suggest that variation in efficiency due to farming practices could save as much water as switching crops or fallowing fields.
"There's an opportunity for less obtrusive methods of saving water to be more important than we originally thought," said lead author Anna Boser, a doctoral student at UCSB's Bren School of Environmental Science & Management. "So we might not have to make as many changes in land use as we originally thought."
California's fertile soils and Mediterranean climate enable farmers to cultivate high-value crops that just aren't viable in the rest of the country. Over a third of the country's vegetables, and nearly three-quarters of fruits and nuts, are grown in California, according to the state's Department of Food and Agriculture.
But many of these crops are quite thirsty. Agriculture accounts for around 80% of water used in California, explained co-author Kelly Caylor, a professor at the Bren School. "Declining groundwater levels and a changing climate put pressure on the availability of irrigation water, making it critical to determine how we can 'do more with less.'"
In 2014, Sacramento passed the Sustainable Groundwater Management Act (SGMA) to secure California's water resources. SGMA mandates that every groundwater basin in the state must be sustainable by 2040. Each basin created a local agency tasked with developing a plan to meet this goal. Mostly, that means ensuring that we don't pump more water out of the ground than what seeps in. We will need to reduce total groundwater use by 20% to 50% by 2040, depending on the basin, Boser said. But to accomplish this, we need an idea of how much water farms use, and what fraction of that actually makes it to crops.
Modeling water use
Scientists have a variety of methods to estimate the amount of water ascending from the Earth's surface to the atmosphere due to evaporation and transpiration through plant leaves. Notably, evaporation cools things down. "When we get hot, we sweat to cool off. The Earth does something similar," Boser said. Scientists look at how warm the ground is and see how much energy it's getting from sunlight and the atmosphere. If the ground is cooler than expected, it means some of that energy was used to turn water into vapor, which cools down that spot.
An evapotranspiration database called OpenET became publicly available in early 2023. It provides satellite-based evapotranspiration estimates for the western United States. But Boser was interested in the water being used specifically by crops. So, she compared transpiration in fallowed fields to active fields across the Central Valley. Subtracting evapotranspiration in fallow fields from total evapotranspiration yields the amount of water that crops are actually consuming.
Unfortunately for Boser, farmers don't fallow fields randomly. Often they'll take their lowest-yielding fields out of production. That creates systematic differences between fallowed and cultivated fields, which could skew Boser's analysis. So, she created a machine-learning model to conduct a weighted comparison between active and fallowed land, accounting for factors like location, topography and soil quality.
She trained the model on 60% of the areas and tested its results on 30%, fine tuning the algorithm until its predictions matched the actual conditions in these fields within 10 milliliters per square meter per day, on average. Now confident in her model, she applied it to the rest of California's Central Valley.
Encouraging results
Crop type only explained 34% of the variation in water consumption. "What that means is maybe we're overlooking some other ways that we could save water," Boser said. She continued to investigate the model, controlling for factors like location, topography, local climate, soil quality and orchard age (when applicable). Ultimately, a full 10% of crop transpiration could be saved if the top 50% of water users reduced their water consumption to match that of their median-consuming neighbors. Boser attributes these savings to differences in "farming practices."
Now, 10% might not sound like a lot, but it's comparable to a number of other interventions. The authors also estimated the effect of switching crops. If the same 50% of farmers switched to the median water-intensive crops for their area, agricultural evapotranspiration would drop by 10%.
Meanwhile, if the state took the top 5% most water-hungry fields out of production, the model says agricultural evapotranspiration would drop by, you guessed it: 10%. This suggests that addressing inefficiencies in farming practices could save as much water as switching crops or taking fields out of cultivation.
To be fair, the results from fallowing would affect only 5% of cultivated land, as opposed to 50% for crop changes and improved farming practices. "We're probably going to have to fall back on fallowing at least a little bit," Boser said, "but hopefully not as much as we were originally expecting."
The authors want to figure out what practices farmers are using that account for the 10% differences in crop water usage. Some examples include mulching, no-till planting, using drought-tolerant varietals, and deficit irrigation -- where you provide less water than the crop could theoretically consume. Deficit irrigation already yields good results in viticulture, where vintners find it can improve the quality of wine.
Changing irrigation practices could also help reduce water use. Irrigation efficiency accounts for the fraction of water a farm uses that actually gets consumed by crops. Inefficiencies include leakage, weed growth and evaporation in transport and in the field. These weren't within the scope of Boser's model, which only considers transpiration by the crops themselves. Inefficiencies happen before the water even gets to the plants.
According to Boser, up to 60% of the water a farm uses actually passes through the roots of its crops. Clearly there's plenty of potential gains in this area, though it isn't clear what efficiency is actually attainable, she said. "Irrigation efficiency is actually quite poorly understood."
Better characterizing this is on the team's to-do list. They hope to identify the causes of irrigation inefficiencies, quantify the efficiencies of different types of irrigation, and learn how climate and geography affects irrigation efficiency. All this will require collecting empirical data.
California is at a critical crossroads in water management. For the first time in its history, the state is putting in place regulations that require substantial reductions in groundwater extraction, including in regions where livelihoods depend on thirsty agricultural production.
"This paper uses novel, data-driven methods to show that, contrary to popular belief, there is large potential to cut water use in California agriculture without fallowing fields," said co-author Tamma Carleton, an assistant professor at UCSB's Bren School. "This raises the possibility that the state can continue its role as an agricultural powerhouse while also sustainably managing an essential natural resource."
- Agriculture and Food
- Food and Agriculture
- Drought Research
- Sustainability
- Resource Shortage
- Environmental Policies
- Land Management
- Agriculture
- Shifting cultivation
- Slash and burn
- Agroecology
- Winter storm
- Golden Retriever
- Organic farming
Story Source:
Materials provided by University of California - Santa Barbara . Original written by Harrison Tasoff. Note: Content may be edited for style and length.
Journal Reference :
- Anna Boser, Kelly Caylor, Ashley Larsen, Madeleine Pascolini-Campbell, John T. Reager, Tamma Carleton. Field-scale crop water consumption estimates reveal potential water savings in California agriculture . Nature Communications , 2024; 15 (1) DOI: 10.1038/s41467-024-46031-2
Cite This Page :
Explore More
- Amazing Archive of Ancient Human Brains
- Night-Time Light and Stroke Risk
- Toward Secure Quantum Communication Globally
- Artificial Nanofluidic Synapses: Memory
- 49 New Galaxies Discovered in Under Three Hours
- Rays Surprisingly Diverse 150 Million Years Ago
- Paint Coatings That Help You Feel Cool
- A Self-Cleaning Wall Paint
- Early Human Migration Out of Africa
- Unintended Consequences of Fire Suppression
Trending Topics
Strange & offbeat.
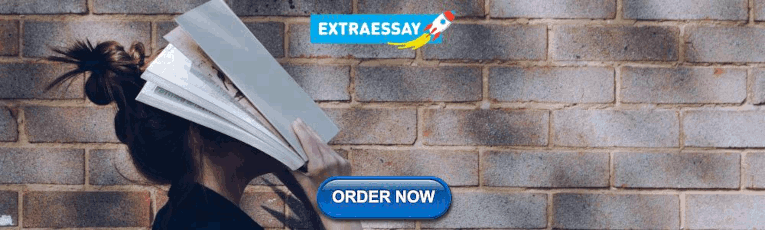
IMAGES
VIDEO
COMMENTS
Examples of high-payoff data-driven agriculture research include technical topics like measuring livestock feed intake and soil sensors, new methods for collecting and using the data, and management innovations in business models for data sharing and developing a trust-reinforcing regulatory framework. Public funding for research is lacking in ...
OFE research is demand driven, because the motivations of farmers to gain information relevant to their own farm drive the research process 14,16,17.OFE is a concrete, observable activity of clear ...
Improvements in agricultural production can help combat malnutrition, but aligning agriculture with nutrition objectives requires research and development specialists to contribute to integrated agriculture-health programs. Four comprehensive questions are chosen to promote health and nutrition-driven agriculture.
Data-driven digital agriculture by expand the frontier of financing and expertise for digital agriculture. ... In Ethiopia, since 2015 a project has helped 2.3 million farmers with agricultural support services, agricultural research, small-scale irrigation, and market infrastructure development. In addition, almost 600,000 livestock and ...
Agriculture is a major contributor to environmental degradation and climate change. At the same time, a growing human population with changing dietary preferences is driving ever increasing demand ...
The FSCI is filling that gap, having identified an indicator framework composed of 50 indicators that monitor agriculture and food systems at a global level, using existing data to enable immediate action. Repurposing existing data, rather than carrying out time-consuming new research, means policymakers have quick access to relevant information.
Environment, Development and Sustainability (2022) Big data and mobile technology are widely claimed to be global disruptive forces in agriculture that benefit small-scale farmers. Yet the access ...
Science Breakthroughs to Advance Food and Agricultural Research by 2030. Washington, DC: The National Academies Press. doi: 10.17226/25059. ... has identified the need for standards as essential to promoting and enabling the industry's transition to highly data-driven agricultural practices and is working to develop their own (AgGateway, 2017 ...
1 Data Analytics in Agriculture. By 2050, the world is expected to face a substantial increase in the global demand for food, necessitating a significant boost in food production by as much as 25% to 70% (Hunter et al. 2017 ); for this reason, it is crucial to double food production per hectare by the time the world population stabilizes around ...
Although this Special Issue, entitled "Data-Driven Agricultural Innovation Technology for Digital Agriculture", has closed, continued and in-depth research on data-based digital agriculture will significantly contribute to securing future food security for the world's population and solving pressing agricultural problems.
Data-driven agriculture has the potential to be part of the solution to achieving sustainable. learning and deep learning approaches in agriculture. These tools focus on developing. cation and ...
Edmonton, Alberta - RDAR (Results Driven Agriculture Research), a distribution partner for the Agriculture and Agri-Food Canada's (AAFC) On-Farm Climate Action Fund (OFCAF), is proud to have supported 1,280 projects on over a million acres of farm and ranch lands since its launch on August 4, 2022. Read More.
By Margaret B. Butler Last updated Nov 2, 2023. Crop genetics plays a crucial role in agricultural research, as it offers valuable insights into enhancing crop improvement. By understanding the genetic makeup of crops, researchers can identify desirable traits and develop strategies to enhance their productivity, resilience, and nutritional value.
Policy support and investments in research and development are essential to promote the widespread adoption of climate-smart farming technologies. In conclusion, the integration of advanced technology in agriculture is a game-changer for climate-smart farming. ... Kumar, P. (2024). Tech-Driven Agriculture: Adapting to Climate Challenges. In ...
Digital agriculture is often heralded as the next major wave of innovation in food and agriculture. Driven by big data, the intention is that digital agriculture will transform the entire research and development pipeline across agricultural value chains throughout the developed and developing worlds.
Advances in agricultural productivity have led to abundant and affordable food and fiber throughout most of the developed world. Public and private agricultural research has been the foundation and basis for much of this growth and development. ERS data, research, and analyses quantify agricultural productivity improvements and the sources of improvement, in the U.S. and globally.
Abstract. The future of agriculture stands at a crucial crossroads, necessitating a paradigm shift towards innovation, sustainability, and resilience. This comprehensive exploration navigates the ...
Stakeholders' most important reason to opt for a big data solution was its commercial value (n=23). Data, technology, and research drivers of change seem to be equally important to them (n=18, 19, 18). They are least driven by research (n=4) when considering to adopt a big data solution for making better decisions for their organizations.
- Data-driven Agriculture: According to research by NASSCOM and McKinsey, there is a $65 billion opportunity to be realized through enhancing 15 agriculture datasets, including soil health records, crop yields, weather, remote sensing, warehousing, land records, agriculture markets and pest images. The working group
There is a dearth of literature that provides a bibliometric analysis concerning the role of Artificial Intelligence (AI) in sustainable agriculture therefore this study attempts to fill this research gap and provides evidence from the studies conducted between 2000-2021 in this field of research. The study is a systematic bibliographic ...
Despite increased funding devoted to agricultural research and a growing body of agricultural scientists in the USA and globally, responses to both problems and opportunities in agriculture often cannot meet the challenges the industry faces quickly enough to avoid significant disruptions. ... Farmer-driven research: a key to accelerating ...
Agricultural expansion is recognized as a major driver of forest loss in the tropics. However, accurate data on the links between agriculture and tropical deforestation are lacking. Pendrill et al. synthesized existing research and datasets to quantify the extent to which tropical deforestation from 2011 to 2015 was associated with agriculture.
The Agricultural Research Service is the U.S. Department of Agriculture's chief scientific in-house research agency. Daily, ARS focuses on solutions to agricultural problems affecting America. Each dollar invested in U.S. agricultural research results in $20 of economic impact. Return to top. Last Modified: 3/20/2024
The research team found that even though these systems face technological and economic challenges, they are part of a long history of advancing technology for the U.S. agricultural sector.
The governments of Canada and Manitoba are providing $16.3 million in grant funding under the Sustainable Canadian Agricultural Partnership (Sustainable CAP) to support 101 projects under the Research and Innovation program over the next three years, federal Agriculture and Agri-Food Minister the Honourable Lawrence MacAulay and Manitoba Agriculture Minister Ron Kostyshyn announced today.
In agricultural research, ZigBee, Wi-Fi, and Bluetooth are the most commonly used WSN technologies (Khujamatov ... (2019). Sensors driven AI-based agriculture recommendation model for assessing land suitability. Sensors, 19(17), 3667. Article Google Scholar Wang, J., et al. (2018). Effect of climate change on the yield of cereal crops: A review
WASHINGTON, March 21, 2024-- The U.S. Department of Agriculture (USDA) today announced up to $12 million in funding available to strengthen and explore new market opportunities for U.S. agricultural products and increase access to locally grown food in communities experiencing food insecurity.The funding is available through three grant programs administered by the Agricultural Marketing ...
Computer vision in agriculture is game-changing with its ability to transform farming into a data-driven, precise, and sustainable industry. Deep learning has empowered agriculture vision to analyze vast, complex visual data, but heavily rely on the availability of large annotated datasets. This remains a bottleneck as manual labeling is error-prone, time-consuming, and expensive. The lack of ...
Agricultural products sold in the Golden State totaled $59 billion in 2022. But rising temperatures, declining precipitation and decades of over pumping may require drastic changes to farming.
Global climate change is not a future problem. Changes to Earth's climate driven by increased human emissions of heat-trapping greenhouse gases are already having widespread effects on the environment: glaciers and ice sheets are shrinking, river and lake ice is breaking up earlier, plant and animal geographic ranges are shifting, and plants and trees are blooming sooner.