- USC Libraries
- Research Guides
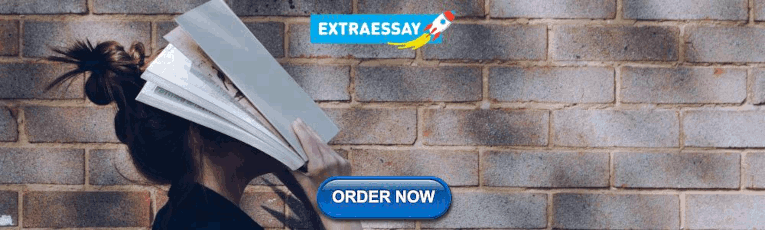
Organizing Your Social Sciences Research Paper
- Independent and Dependent Variables
- Purpose of Guide
- Design Flaws to Avoid
- Glossary of Research Terms
- Reading Research Effectively
- Narrowing a Topic Idea
- Broadening a Topic Idea
- Extending the Timeliness of a Topic Idea
- Academic Writing Style
- Applying Critical Thinking
- Choosing a Title
- Making an Outline
- Paragraph Development
- Research Process Video Series
- Executive Summary
- The C.A.R.S. Model
- Background Information
- The Research Problem/Question
- Theoretical Framework
- Citation Tracking
- Content Alert Services
- Evaluating Sources
- Primary Sources
- Secondary Sources
- Tiertiary Sources
- Scholarly vs. Popular Publications
- Qualitative Methods
- Quantitative Methods
- Insiderness
- Using Non-Textual Elements
- Limitations of the Study
- Common Grammar Mistakes
- Writing Concisely
- Avoiding Plagiarism
- Footnotes or Endnotes?
- Further Readings
- Generative AI and Writing
- USC Libraries Tutorials and Other Guides
- Bibliography
Definitions
Dependent Variable The variable that depends on other factors that are measured. These variables are expected to change as a result of an experimental manipulation of the independent variable or variables. It is the presumed effect.
Independent Variable The variable that is stable and unaffected by the other variables you are trying to measure. It refers to the condition of an experiment that is systematically manipulated by the investigator. It is the presumed cause.
Cramer, Duncan and Dennis Howitt. The SAGE Dictionary of Statistics . London: SAGE, 2004; Penslar, Robin Levin and Joan P. Porter. Institutional Review Board Guidebook: Introduction . Washington, DC: United States Department of Health and Human Services, 2010; "What are Dependent and Independent Variables?" Graphic Tutorial.
Identifying Dependent and Independent Variables
Don't feel bad if you are confused about what is the dependent variable and what is the independent variable in social and behavioral sciences research . However, it's important that you learn the difference because framing a study using these variables is a common approach to organizing the elements of a social sciences research study in order to discover relevant and meaningful results. Specifically, it is important for these two reasons:
- You need to understand and be able to evaluate their application in other people's research.
- You need to apply them correctly in your own research.
A variable in research simply refers to a person, place, thing, or phenomenon that you are trying to measure in some way. The best way to understand the difference between a dependent and independent variable is that the meaning of each is implied by what the words tell us about the variable you are using. You can do this with a simple exercise from the website, Graphic Tutorial. Take the sentence, "The [independent variable] causes a change in [dependent variable] and it is not possible that [dependent variable] could cause a change in [independent variable]." Insert the names of variables you are using in the sentence in the way that makes the most sense. This will help you identify each type of variable. If you're still not sure, consult with your professor before you begin to write.
Fan, Shihe. "Independent Variable." In Encyclopedia of Research Design. Neil J. Salkind, editor. (Thousand Oaks, CA: SAGE, 2010), pp. 592-594; "What are Dependent and Independent Variables?" Graphic Tutorial; Salkind, Neil J. "Dependent Variable." In Encyclopedia of Research Design , Neil J. Salkind, editor. (Thousand Oaks, CA: SAGE, 2010), pp. 348-349;
Structure and Writing Style
The process of examining a research problem in the social and behavioral sciences is often framed around methods of analysis that compare, contrast, correlate, average, or integrate relationships between or among variables . Techniques include associations, sampling, random selection, and blind selection. Designation of the dependent and independent variable involves unpacking the research problem in a way that identifies a general cause and effect and classifying these variables as either independent or dependent.
The variables should be outlined in the introduction of your paper and explained in more detail in the methods section . There are no rules about the structure and style for writing about independent or dependent variables but, as with any academic writing, clarity and being succinct is most important.
After you have described the research problem and its significance in relation to prior research, explain why you have chosen to examine the problem using a method of analysis that investigates the relationships between or among independent and dependent variables . State what it is about the research problem that lends itself to this type of analysis. For example, if you are investigating the relationship between corporate environmental sustainability efforts [the independent variable] and dependent variables associated with measuring employee satisfaction at work using a survey instrument, you would first identify each variable and then provide background information about the variables. What is meant by "environmental sustainability"? Are you looking at a particular company [e.g., General Motors] or are you investigating an industry [e.g., the meat packing industry]? Why is employee satisfaction in the workplace important? How does a company make their employees aware of sustainability efforts and why would a company even care that its employees know about these efforts?
Identify each variable for the reader and define each . In the introduction, this information can be presented in a paragraph or two when you describe how you are going to study the research problem. In the methods section, you build on the literature review of prior studies about the research problem to describe in detail background about each variable, breaking each down for measurement and analysis. For example, what activities do you examine that reflect a company's commitment to environmental sustainability? Levels of employee satisfaction can be measured by a survey that asks about things like volunteerism or a desire to stay at the company for a long time.
The structure and writing style of describing the variables and their application to analyzing the research problem should be stated and unpacked in such a way that the reader obtains a clear understanding of the relationships between the variables and why they are important. This is also important so that the study can be replicated in the future using the same variables but applied in a different way.
Fan, Shihe. "Independent Variable." In Encyclopedia of Research Design. Neil J. Salkind, editor. (Thousand Oaks, CA: SAGE, 2010), pp. 592-594; "What are Dependent and Independent Variables?" Graphic Tutorial; “Case Example for Independent and Dependent Variables.” ORI Curriculum Examples. U.S. Department of Health and Human Services, Office of Research Integrity; Salkind, Neil J. "Dependent Variable." In Encyclopedia of Research Design , Neil J. Salkind, editor. (Thousand Oaks, CA: SAGE, 2010), pp. 348-349; “Independent Variables and Dependent Variables.” Karl L. Wuensch, Department of Psychology, East Carolina University [posted email exchange]; “Variables.” Elements of Research. Dr. Camille Nebeker, San Diego State University.
- << Previous: Design Flaws to Avoid
- Next: Glossary of Research Terms >>
- Last Updated: Apr 9, 2024 1:19 PM
- URL: https://libguides.usc.edu/writingguide
Thank you for visiting nature.com. You are using a browser version with limited support for CSS. To obtain the best experience, we recommend you use a more up to date browser (or turn off compatibility mode in Internet Explorer). In the meantime, to ensure continued support, we are displaying the site without styles and JavaScript.
- View all journals
- Explore content
- About the journal
- Publish with us
- Sign up for alerts
- Published: 31 January 2022
The clinician’s guide to interpreting a regression analysis
- Sofia Bzovsky 1 ,
- Mark R. Phillips ORCID: orcid.org/0000-0003-0923-261X 2 ,
- Robyn H. Guymer ORCID: orcid.org/0000-0002-9441-4356 3 , 4 ,
- Charles C. Wykoff 5 , 6 ,
- Lehana Thabane ORCID: orcid.org/0000-0003-0355-9734 2 , 7 ,
- Mohit Bhandari ORCID: orcid.org/0000-0001-9608-4808 1 , 2 &
- Varun Chaudhary ORCID: orcid.org/0000-0002-9988-4146 1 , 2
on behalf of the R.E.T.I.N.A. study group
Eye volume 36 , pages 1715–1717 ( 2022 ) Cite this article
18k Accesses
9 Citations
1 Altmetric
Metrics details
- Outcomes research
Introduction
When researchers are conducting clinical studies to investigate factors associated with, or treatments for disease and conditions to improve patient care and clinical practice, statistical evaluation of the data is often necessary. Regression analysis is an important statistical method that is commonly used to determine the relationship between several factors and disease outcomes or to identify relevant prognostic factors for diseases [ 1 ].
This editorial will acquaint readers with the basic principles of and an approach to interpreting results from two types of regression analyses widely used in ophthalmology: linear, and logistic regression.
Linear regression analysis
Linear regression is used to quantify a linear relationship or association between a continuous response/outcome variable or dependent variable with at least one independent or explanatory variable by fitting a linear equation to observed data [ 1 ]. The variable that the equation solves for, which is the outcome or response of interest, is called the dependent variable [ 1 ]. The variable that is used to explain the value of the dependent variable is called the predictor, explanatory, or independent variable [ 1 ].
In a linear regression model, the dependent variable must be continuous (e.g. intraocular pressure or visual acuity), whereas, the independent variable may be either continuous (e.g. age), binary (e.g. sex), categorical (e.g. age-related macular degeneration stage or diabetic retinopathy severity scale score), or a combination of these [ 1 ].
When investigating the effect or association of a single independent variable on a continuous dependent variable, this type of analysis is called a simple linear regression [ 2 ]. In many circumstances though, a single independent variable may not be enough to adequately explain the dependent variable. Often it is necessary to control for confounders and in these situations, one can perform a multivariable linear regression to study the effect or association with multiple independent variables on the dependent variable [ 1 , 2 ]. When incorporating numerous independent variables, the regression model estimates the effect or contribution of each independent variable while holding the values of all other independent variables constant [ 3 ].
When interpreting the results of a linear regression, there are a few key outputs for each independent variable included in the model:
Estimated regression coefficient—The estimated regression coefficient indicates the direction and strength of the relationship or association between the independent and dependent variables [ 4 ]. Specifically, the regression coefficient describes the change in the dependent variable for each one-unit change in the independent variable, if continuous [ 4 ]. For instance, if examining the relationship between a continuous predictor variable and intra-ocular pressure (dependent variable), a regression coefficient of 2 means that for every one-unit increase in the predictor, there is a two-unit increase in intra-ocular pressure. If the independent variable is binary or categorical, then the one-unit change represents switching from one category to the reference category [ 4 ]. For instance, if examining the relationship between a binary predictor variable, such as sex, where ‘female’ is set as the reference category, and intra-ocular pressure (dependent variable), a regression coefficient of 2 means that, on average, males have an intra-ocular pressure that is 2 mm Hg higher than females.
Confidence Interval (CI)—The CI, typically set at 95%, is a measure of the precision of the coefficient estimate of the independent variable [ 4 ]. A large CI indicates a low level of precision, whereas a small CI indicates a higher precision [ 5 ].
P value—The p value for the regression coefficient indicates whether the relationship between the independent and dependent variables is statistically significant [ 6 ].
Logistic regression analysis
As with linear regression, logistic regression is used to estimate the association between one or more independent variables with a dependent variable [ 7 ]. However, the distinguishing feature in logistic regression is that the dependent variable (outcome) must be binary (or dichotomous), meaning that the variable can only take two different values or levels, such as ‘1 versus 0’ or ‘yes versus no’ [ 2 , 7 ]. The effect size of predictor variables on the dependent variable is best explained using an odds ratio (OR) [ 2 ]. ORs are used to compare the relative odds of the occurrence of the outcome of interest, given exposure to the variable of interest [ 5 ]. An OR equal to 1 means that the odds of the event in one group are the same as the odds of the event in another group; there is no difference [ 8 ]. An OR > 1 implies that one group has a higher odds of having the event compared with the reference group, whereas an OR < 1 means that one group has a lower odds of having an event compared with the reference group [ 8 ]. When interpreting the results of a logistic regression, the key outputs include the OR, CI, and p-value for each independent variable included in the model.
Clinical example
Sen et al. investigated the association between several factors (independent variables) and visual acuity outcomes (dependent variable) in patients receiving anti-vascular endothelial growth factor therapy for macular oedema (DMO) by means of both linear and logistic regression [ 9 ]. Multivariable linear regression demonstrated that age (Estimate −0.33, 95% CI − 0.48 to −0.19, p < 0.001) was significantly associated with best-corrected visual acuity (BCVA) at 100 weeks at alpha = 0.05 significance level [ 9 ]. The regression coefficient of −0.33 means that the BCVA at 100 weeks decreases by 0.33 with each additional year of older age.
Multivariable logistic regression also demonstrated that age and ellipsoid zone status were statistically significant associated with achieving a BCVA letter score >70 letters at 100 weeks at the alpha = 0.05 significance level. Patients ≥75 years of age were at a decreased odds of achieving a BCVA letter score >70 letters at 100 weeks compared to those <50 years of age, since the OR is less than 1 (OR 0.96, 95% CI 0.94 to 0.98, p = 0.001) [ 9 ]. Similarly, patients between the ages of 50–74 years were also at a decreased odds of achieving a BCVA letter score >70 letters at 100 weeks compared to those <50 years of age, since the OR is less than 1 (OR 0.15, 95% CI 0.04 to 0.48, p = 0.001) [ 9 ]. As well, those with a not intact ellipsoid zone were at a decreased odds of achieving a BCVA letter score >70 letters at 100 weeks compared to those with an intact ellipsoid zone (OR 0.20, 95% CI 0.07 to 0.56; p = 0.002). On the other hand, patients with an ungradable/questionable ellipsoid zone were at an increased odds of achieving a BCVA letter score >70 letters at 100 weeks compared to those with an intact ellipsoid zone, since the OR is greater than 1 (OR 2.26, 95% CI 1.14 to 4.48; p = 0.02) [ 9 ].
The narrower the CI, the more precise the estimate is; and the smaller the p value (relative to alpha = 0.05), the greater the evidence against the null hypothesis of no effect or association.
Simply put, linear and logistic regression are useful tools for appreciating the relationship between predictor/explanatory and outcome variables for continuous and dichotomous outcomes, respectively, that can be applied in clinical practice, such as to gain an understanding of risk factors associated with a disease of interest.
Schneider A, Hommel G, Blettner M. Linear Regression. Anal Dtsch Ärztebl Int. 2010;107:776–82.
Google Scholar
Bender R. Introduction to the use of regression models in epidemiology. In: Verma M, editor. Cancer epidemiology. Methods in molecular biology. Humana Press; 2009:179–95.
Schober P, Vetter TR. Confounding in observational research. Anesth Analg. 2020;130:635.
Article Google Scholar
Schober P, Vetter TR. Linear regression in medical research. Anesth Analg. 2021;132:108–9.
Szumilas M. Explaining odds ratios. J Can Acad Child Adolesc Psychiatry. 2010;19:227–9.
Thiese MS, Ronna B, Ott U. P value interpretations and considerations. J Thorac Dis. 2016;8:E928–31.
Schober P, Vetter TR. Logistic regression in medical research. Anesth Analg. 2021;132:365–6.
Zabor EC, Reddy CA, Tendulkar RD, Patil S. Logistic regression in clinical studies. Int J Radiat Oncol Biol Phys. 2022;112:271–7.
Sen P, Gurudas S, Ramu J, Patrao N, Chandra S, Rasheed R, et al. Predictors of visual acuity outcomes after anti-vascular endothelial growth factor treatment for macular edema secondary to central retinal vein occlusion. Ophthalmol Retin. 2021;5:1115–24.
Download references
R.E.T.I.N.A. study group
Varun Chaudhary 1,2 , Mohit Bhandari 1,2 , Charles C. Wykoff 5,6 , Sobha Sivaprasad 8 , Lehana Thabane 2,7 , Peter Kaiser 9 , David Sarraf 10 , Sophie J. Bakri 11 , Sunir J. Garg 12 , Rishi P. Singh 13,14 , Frank G. Holz 15 , Tien Y. Wong 16,17 , and Robyn H. Guymer 3,4
Author information
Authors and affiliations.
Department of Surgery, McMaster University, Hamilton, ON, Canada
Sofia Bzovsky, Mohit Bhandari & Varun Chaudhary
Department of Health Research Methods, Evidence & Impact, McMaster University, Hamilton, ON, Canada
Mark R. Phillips, Lehana Thabane, Mohit Bhandari & Varun Chaudhary
Centre for Eye Research Australia, Royal Victorian Eye and Ear Hospital, East Melbourne, VIC, Australia
Robyn H. Guymer
Department of Surgery, (Ophthalmology), The University of Melbourne, Melbourne, VIC, Australia
Retina Consultants of Texas (Retina Consultants of America), Houston, TX, USA
Charles C. Wykoff
Blanton Eye Institute, Houston Methodist Hospital, Houston, TX, USA
Biostatistics Unit, St. Joseph’s Healthcare Hamilton, Hamilton, ON, Canada
Lehana Thabane
NIHR Moorfields Biomedical Research Centre, Moorfields Eye Hospital, London, UK
Sobha Sivaprasad
Cole Eye Institute, Cleveland Clinic, Cleveland, OH, USA
Peter Kaiser
Retinal Disorders and Ophthalmic Genetics, Stein Eye Institute, University of California, Los Angeles, CA, USA
David Sarraf
Department of Ophthalmology, Mayo Clinic, Rochester, MN, USA
Sophie J. Bakri
The Retina Service at Wills Eye Hospital, Philadelphia, PA, USA
Sunir J. Garg
Center for Ophthalmic Bioinformatics, Cole Eye Institute, Cleveland Clinic, Cleveland, OH, USA
Rishi P. Singh
Cleveland Clinic Lerner College of Medicine, Cleveland, OH, USA
Department of Ophthalmology, University of Bonn, Bonn, Germany
Frank G. Holz
Singapore Eye Research Institute, Singapore, Singapore
Tien Y. Wong
Singapore National Eye Centre, Duke-NUD Medical School, Singapore, Singapore
You can also search for this author in PubMed Google Scholar
- Varun Chaudhary
- , Mohit Bhandari
- , Charles C. Wykoff
- , Sobha Sivaprasad
- , Lehana Thabane
- , Peter Kaiser
- , David Sarraf
- , Sophie J. Bakri
- , Sunir J. Garg
- , Rishi P. Singh
- , Frank G. Holz
- , Tien Y. Wong
- & Robyn H. Guymer
Contributions
SB was responsible for writing, critical review and feedback on manuscript. MRP was responsible for conception of idea, critical review and feedback on manuscript. RHG was responsible for critical review and feedback on manuscript. CCW was responsible for critical review and feedback on manuscript. LT was responsible for critical review and feedback on manuscript. MB was responsible for conception of idea, critical review and feedback on manuscript. VC was responsible for conception of idea, critical review and feedback on manuscript.
Corresponding author
Correspondence to Varun Chaudhary .
Ethics declarations
Competing interests.
SB: Nothing to disclose. MRP: Nothing to disclose. RHG: Advisory boards: Bayer, Novartis, Apellis, Roche, Genentech Inc.—unrelated to this study. CCW: Consultant: Acuela, Adverum Biotechnologies, Inc, Aerpio, Alimera Sciences, Allegro Ophthalmics, LLC, Allergan, Apellis Pharmaceuticals, Bayer AG, Chengdu Kanghong Pharmaceuticals Group Co, Ltd, Clearside Biomedical, DORC (Dutch Ophthalmic Research Center), EyePoint Pharmaceuticals, Gentech/Roche, GyroscopeTx, IVERIC bio, Kodiak Sciences Inc, Novartis AG, ONL Therapeutics, Oxurion NV, PolyPhotonix, Recens Medical, Regeron Pharmaceuticals, Inc, REGENXBIO Inc, Santen Pharmaceutical Co, Ltd, and Takeda Pharmaceutical Company Limited; Research funds: Adverum Biotechnologies, Inc, Aerie Pharmaceuticals, Inc, Aerpio, Alimera Sciences, Allergan, Apellis Pharmaceuticals, Chengdu Kanghong Pharmaceutical Group Co, Ltd, Clearside Biomedical, Gemini Therapeutics, Genentech/Roche, Graybug Vision, Inc, GyroscopeTx, Ionis Pharmaceuticals, IVERIC bio, Kodiak Sciences Inc, Neurotech LLC, Novartis AG, Opthea, Outlook Therapeutics, Inc, Recens Medical, Regeneron Pharmaceuticals, Inc, REGENXBIO Inc, Samsung Pharm Co, Ltd, Santen Pharmaceutical Co, Ltd, and Xbrane Biopharma AB—unrelated to this study. LT: Nothing to disclose. MB: Research funds: Pendopharm, Bioventus, Acumed—unrelated to this study. VC: Advisory Board Member: Alcon, Roche, Bayer, Novartis; Grants: Bayer, Novartis—unrelated to this study.
Additional information
Publisher’s note Springer Nature remains neutral with regard to jurisdictional claims in published maps and institutional affiliations.
Rights and permissions
Reprints and permissions
About this article
Cite this article.
Bzovsky, S., Phillips, M.R., Guymer, R.H. et al. The clinician’s guide to interpreting a regression analysis. Eye 36 , 1715–1717 (2022). https://doi.org/10.1038/s41433-022-01949-z
Download citation
Received : 08 January 2022
Revised : 17 January 2022
Accepted : 18 January 2022
Published : 31 January 2022
Issue Date : September 2022
DOI : https://doi.org/10.1038/s41433-022-01949-z
Share this article
Anyone you share the following link with will be able to read this content:
Sorry, a shareable link is not currently available for this article.
Provided by the Springer Nature SharedIt content-sharing initiative
Quick links
- Explore articles by subject
- Guide to authors
- Editorial policies

Have a language expert improve your writing
Run a free plagiarism check in 10 minutes, automatically generate references for free.
- Knowledge Base
- Methodology
- Types of Variables in Research | Definitions & Examples
Types of Variables in Research | Definitions & Examples
Published on 19 September 2022 by Rebecca Bevans . Revised on 28 November 2022.
In statistical research, a variable is defined as an attribute of an object of study. Choosing which variables to measure is central to good experimental design .
You need to know which types of variables you are working with in order to choose appropriate statistical tests and interpret the results of your study.
You can usually identify the type of variable by asking two questions:
- What type of data does the variable contain?
- What part of the experiment does the variable represent?
Table of contents
Types of data: quantitative vs categorical variables, parts of the experiment: independent vs dependent variables, other common types of variables, frequently asked questions about variables.
Data is a specific measurement of a variable – it is the value you record in your data sheet. Data is generally divided into two categories:
- Quantitative data represents amounts.
- Categorical data represents groupings.
A variable that contains quantitative data is a quantitative variable ; a variable that contains categorical data is a categorical variable . Each of these types of variable can be broken down into further types.
Quantitative variables
When you collect quantitative data, the numbers you record represent real amounts that can be added, subtracted, divided, etc. There are two types of quantitative variables: discrete and continuous .
Categorical variables
Categorical variables represent groupings of some kind. They are sometimes recorded as numbers, but the numbers represent categories rather than actual amounts of things.
There are three types of categorical variables: binary , nominal , and ordinal variables.
*Note that sometimes a variable can work as more than one type! An ordinal variable can also be used as a quantitative variable if the scale is numeric and doesn’t need to be kept as discrete integers. For example, star ratings on product reviews are ordinal (1 to 5 stars), but the average star rating is quantitative.
Example data sheet
To keep track of your salt-tolerance experiment, you make a data sheet where you record information about the variables in the experiment, like salt addition and plant health.
To gather information about plant responses over time, you can fill out the same data sheet every few days until the end of the experiment. This example sheet is colour-coded according to the type of variable: nominal , continuous , ordinal , and binary .
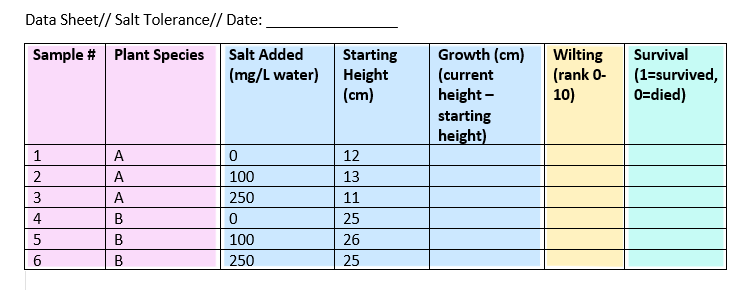
Prevent plagiarism, run a free check.
Experiments are usually designed to find out what effect one variable has on another – in our example, the effect of salt addition on plant growth.
You manipulate the independent variable (the one you think might be the cause ) and then measure the dependent variable (the one you think might be the effect ) to find out what this effect might be.
You will probably also have variables that you hold constant ( control variables ) in order to focus on your experimental treatment.
In this experiment, we have one independent and three dependent variables.
The other variables in the sheet can’t be classified as independent or dependent, but they do contain data that you will need in order to interpret your dependent and independent variables.
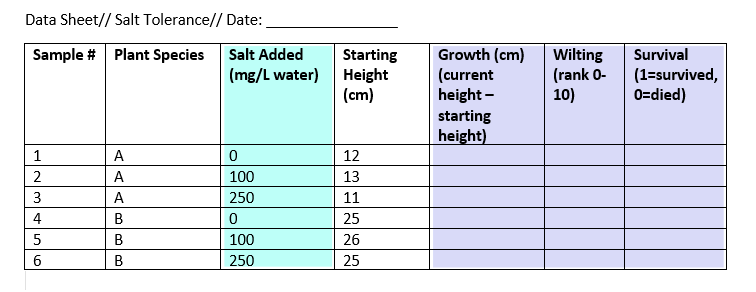
What about correlational research?
When you do correlational research , the terms ‘dependent’ and ‘independent’ don’t apply, because you are not trying to establish a cause-and-effect relationship.
However, there might be cases where one variable clearly precedes the other (for example, rainfall leads to mud, rather than the other way around). In these cases, you may call the preceding variable (i.e., the rainfall) the predictor variable and the following variable (i.e., the mud) the outcome variable .
Once you have defined your independent and dependent variables and determined whether they are categorical or quantitative, you will be able to choose the correct statistical test .
But there are many other ways of describing variables that help with interpreting your results. Some useful types of variable are listed below.
A confounding variable is closely related to both the independent and dependent variables in a study. An independent variable represents the supposed cause , while the dependent variable is the supposed effect . A confounding variable is a third variable that influences both the independent and dependent variables.
Failing to account for confounding variables can cause you to wrongly estimate the relationship between your independent and dependent variables.
Discrete and continuous variables are two types of quantitative variables :
- Discrete variables represent counts (e.g., the number of objects in a collection).
- Continuous variables represent measurable amounts (e.g., water volume or weight).
You can think of independent and dependent variables in terms of cause and effect: an independent variable is the variable you think is the cause , while a dependent variable is the effect .
In an experiment, you manipulate the independent variable and measure the outcome in the dependent variable. For example, in an experiment about the effect of nutrients on crop growth:
- The independent variable is the amount of nutrients added to the crop field.
- The dependent variable is the biomass of the crops at harvest time.
Defining your variables, and deciding how you will manipulate and measure them, is an important part of experimental design .
Cite this Scribbr article
If you want to cite this source, you can copy and paste the citation or click the ‘Cite this Scribbr article’ button to automatically add the citation to our free Reference Generator.
Bevans, R. (2022, November 28). Types of Variables in Research | Definitions & Examples. Scribbr. Retrieved 9 April 2024, from https://www.scribbr.co.uk/research-methods/variables-types/
Is this article helpful?
Rebecca Bevans
Other students also liked, a quick guide to experimental design | 5 steps & examples, quasi-experimental design | definition, types & examples, construct validity | definition, types, & examples.
- How it works
Types of Variables – A Comprehensive Guide
Published by Carmen Troy at August 14th, 2021 , Revised On October 26, 2023
A variable is any qualitative or quantitative characteristic that can change and have more than one value, such as age, height, weight, gender, etc.
Before conducting research, it’s essential to know what needs to be measured or analysed and choose a suitable statistical test to present your study’s findings.
In most cases, you can do it by identifying the key issues/variables related to your research’s main topic.
Example: If you want to test whether the hybridisation of plants harms the health of people. You can use the key variables like agricultural techniques, type of soil, environmental factors, types of pesticides used, the process of hybridisation, type of yield obtained after hybridisation, type of yield without hybridisation, etc.
Variables are broadly categorised into:
- Independent variables
- Dependent variable
- Control variable
Independent Vs. Dependent Vs. Control Variable
The research includes finding ways:
- To change the independent variables.
- To prevent the controlled variables from changing.
- To measure the dependent variables.
Note: The term dependent and independent is not applicable in correlational research as this is not a controlled experiment. A researcher doesn’t have control over the variables. The association and between two or more variables are measured. If one variable affects another one, then it’s called the predictor variable and outcome variable.
Example: Correlation between investment (predictor variable) and profit (outcome variable)
What data collection best suits your research?
- Find out by hiring an expert from ResearchProspect today!
- Despite how challenging the subject may be, we are here to help you.
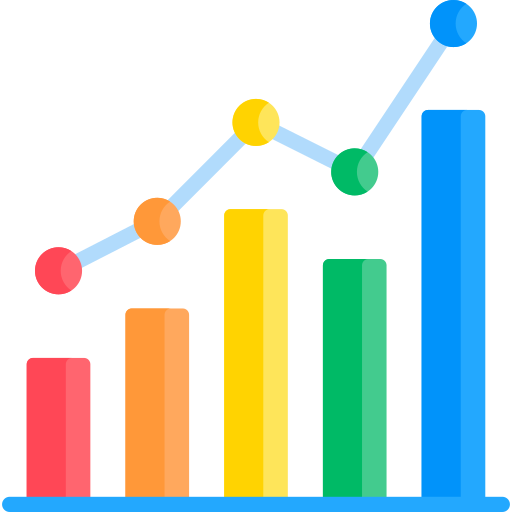
Types of Variables Based on the Types of Data
A data is referred to as the information and statistics gathered for analysis of a research topic. Data is broadly divided into two categories, such as:
Quantitative/Numerical data is associated with the aspects of measurement, quantity, and extent.
Categorial data is associated with groupings.
A qualitative variable consists of qualitative data, and a quantitative variable consists of a quantitative variable.
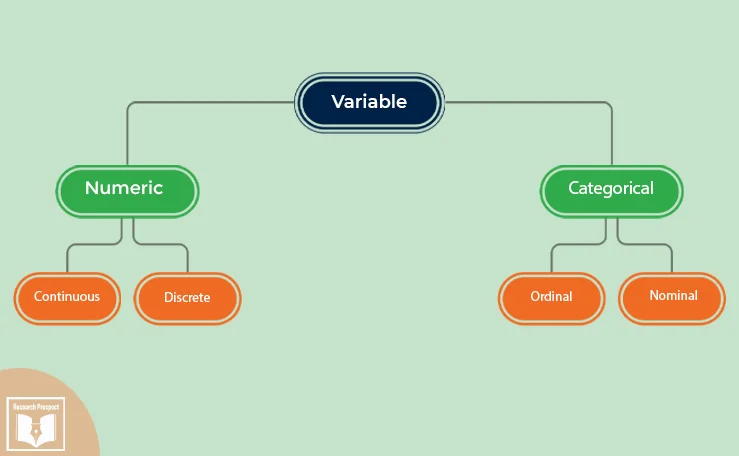
Quantitative Variable
The quantitative variable is associated with measurement, quantity, and extent, like how many . It follows the statistical, mathematical, and computational techniques in numerical data such as percentages and statistics. The research is conducted on a large group of population.
Example: Find out the weight of students of the fifth standard studying in government schools.
The quantitative variable can be further categorised into continuous and discrete.
Categorial Variable
The categorical variable includes measurements that vary in categories such as names but not in terms of rank or degree. It means one level of a categorical variable cannot be considered better or greater than another level.
Example: Gender, brands, colors, zip codes
The categorical variable is further categorised into three types:
Note: Sometimes, an ordinal variable also acts as a quantitative variable. Ordinal data has an order, but the intervals between scale points may be uneven.
Example: Numbers on a rating scale represent the reviews’ rank or range from below average to above average. However, it also represents a quantitative variable showing how many stars and how much rating is given.
Not sure which statistical tests to use for your data?
Let the experts at researchprospect do the daunting work for you..
Using our approach, we illustrate how to collect data, sample sizes, validity, reliability, credibility, and ethics, so you won’t have to do it all by yourself!
Other Types of Variables
It’s important to understand the difference between dependent and independent variables and know whether they are quantitative or categorical to choose the appropriate statistical test.
There are many other types of variables to help you differentiate and understand them.
Also, read a comprehensive guide written about inductive and deductive reasoning .
- Entertainment
- Online education
- Database management, storage, and retrieval
Frequently Asked Questions
What are the 10 types of variables in research.
The 10 types of variables in research are:
- Independent
- Confounding
- Categorical
- Extraneous.
What is an independent variable?
An independent variable, often termed the predictor or explanatory variable, is the variable manipulated or categorized in an experiment to observe its effect on another variable, called the dependent variable. It’s the presumed cause in a cause-and-effect relationship, determining if changes in it produce changes in the observed outcome.
What is a variable?
In research, a variable is any attribute, quantity, or characteristic that can be measured or counted. It can take on various values, making it “variable.” Variables can be classified as independent (manipulated), dependent (observed outcome), or control (kept constant). They form the foundation for hypotheses, observations, and data analysis in studies.
What is a dependent variable?
A dependent variable is the outcome or response being studied in an experiment or investigation. It’s what researchers measure to determine the effect of changes in the independent variable. In a cause-and-effect relationship, the dependent variable is presumed to be influenced or caused by the independent variable.
What is a variable in programming?
In programming, a variable is a symbolic name for a storage location that holds data or values. It allows data storage and retrieval for computational operations. Variables have types, like integer or string, determining the nature of data they can hold. They’re fundamental in manipulating and processing information in software.
What is a control variable?
A control variable in research is a factor that’s kept constant to ensure that it doesn’t influence the outcome. By controlling these variables, researchers can isolate the effects of the independent variable on the dependent variable, ensuring that other factors don’t skew the results or introduce bias into the experiment.
What is a controlled variable in science?
In science, a controlled variable is a factor that remains constant throughout an experiment. It ensures that any observed changes in the dependent variable are solely due to the independent variable, not other factors. By keeping controlled variables consistent, researchers can maintain experiment validity and accurately assess cause-and-effect relationships.
How many independent variables should an investigation have?
Ideally, an investigation should have one independent variable to clearly establish cause-and-effect relationships. Manipulating multiple independent variables simultaneously can complicate data interpretation.
However, in advanced research, experiments with multiple independent variables (factorial designs) are used, but they require careful planning to understand interactions between variables.
You May Also Like
A meta-analysis is a formal, epidemiological, quantitative study design that uses statistical methods to generalise the findings of the selected independent studies.
Inductive and deductive reasoning takes into account assumptions and incidents. Here is all you need to know about inductive vs deductive reasoning.
A hypothesis is a research question that has to be proved correct or incorrect through hypothesis testing – a scientific approach to test a hypothesis.
USEFUL LINKS
LEARNING RESOURCES
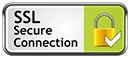
COMPANY DETAILS
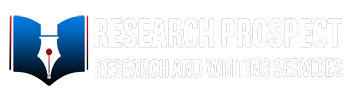
- How It Works

Research Variables 101
Independent variables, dependent variables, control variables and more
By: Derek Jansen (MBA) | Expert Reviewed By: Kerryn Warren (PhD) | January 2023
If you’re new to the world of research, especially scientific research, you’re bound to run into the concept of variables , sooner or later. If you’re feeling a little confused, don’t worry – you’re not the only one! Independent variables, dependent variables, confounding variables – it’s a lot of jargon. In this post, we’ll unpack the terminology surrounding research variables using straightforward language and loads of examples .
Overview: Variables In Research
What (exactly) is a variable.
The simplest way to understand a variable is as any characteristic or attribute that can experience change or vary over time or context – hence the name “variable”. For example, the dosage of a particular medicine could be classified as a variable, as the amount can vary (i.e., a higher dose or a lower dose). Similarly, gender, age or ethnicity could be considered demographic variables, because each person varies in these respects.
Within research, especially scientific research, variables form the foundation of studies, as researchers are often interested in how one variable impacts another, and the relationships between different variables. For example:
- How someone’s age impacts their sleep quality
- How different teaching methods impact learning outcomes
- How diet impacts weight (gain or loss)
As you can see, variables are often used to explain relationships between different elements and phenomena. In scientific studies, especially experimental studies, the objective is often to understand the causal relationships between variables. In other words, the role of cause and effect between variables. This is achieved by manipulating certain variables while controlling others – and then observing the outcome. But, we’ll get into that a little later…
The “Big 3” Variables
Variables can be a little intimidating for new researchers because there are a wide variety of variables, and oftentimes, there are multiple labels for the same thing. To lay a firm foundation, we’ll first look at the three main types of variables, namely:
- Independent variables (IV)
- Dependant variables (DV)
- Control variables
What is an independent variable?
Simply put, the independent variable is the “ cause ” in the relationship between two (or more) variables. In other words, when the independent variable changes, it has an impact on another variable.
For example:
- Increasing the dosage of a medication (Variable A) could result in better (or worse) health outcomes for a patient (Variable B)
- Changing a teaching method (Variable A) could impact the test scores that students earn in a standardised test (Variable B)
- Varying one’s diet (Variable A) could result in weight loss or gain (Variable B).
It’s useful to know that independent variables can go by a few different names, including, explanatory variables (because they explain an event or outcome) and predictor variables (because they predict the value of another variable). Terminology aside though, the most important takeaway is that independent variables are assumed to be the “cause” in any cause-effect relationship. As you can imagine, these types of variables are of major interest to researchers, as many studies seek to understand the causal factors behind a phenomenon.
Need a helping hand?
What is a dependent variable?
While the independent variable is the “ cause ”, the dependent variable is the “ effect ” – or rather, the affected variable . In other words, the dependent variable is the variable that is assumed to change as a result of a change in the independent variable.
Keeping with the previous example, let’s look at some dependent variables in action:
- Health outcomes (DV) could be impacted by dosage changes of a medication (IV)
- Students’ scores (DV) could be impacted by teaching methods (IV)
- Weight gain or loss (DV) could be impacted by diet (IV)
In scientific studies, researchers will typically pay very close attention to the dependent variable (or variables), carefully measuring any changes in response to hypothesised independent variables. This can be tricky in practice, as it’s not always easy to reliably measure specific phenomena or outcomes – or to be certain that the actual cause of the change is in fact the independent variable.
As the adage goes, correlation is not causation . In other words, just because two variables have a relationship doesn’t mean that it’s a causal relationship – they may just happen to vary together. For example, you could find a correlation between the number of people who own a certain brand of car and the number of people who have a certain type of job. Just because the number of people who own that brand of car and the number of people who have that type of job is correlated, it doesn’t mean that owning that brand of car causes someone to have that type of job or vice versa. The correlation could, for example, be caused by another factor such as income level or age group, which would affect both car ownership and job type.
To confidently establish a causal relationship between an independent variable and a dependent variable (i.e., X causes Y), you’ll typically need an experimental design , where you have complete control over the environmen t and the variables of interest. But even so, this doesn’t always translate into the “real world”. Simply put, what happens in the lab sometimes stays in the lab!
As an alternative to pure experimental research, correlational or “ quasi-experimental ” research (where the researcher cannot manipulate or change variables) can be done on a much larger scale more easily, allowing one to understand specific relationships in the real world. These types of studies also assume some causality between independent and dependent variables, but it’s not always clear. So, if you go this route, you need to be cautious in terms of how you describe the impact and causality between variables and be sure to acknowledge any limitations in your own research.
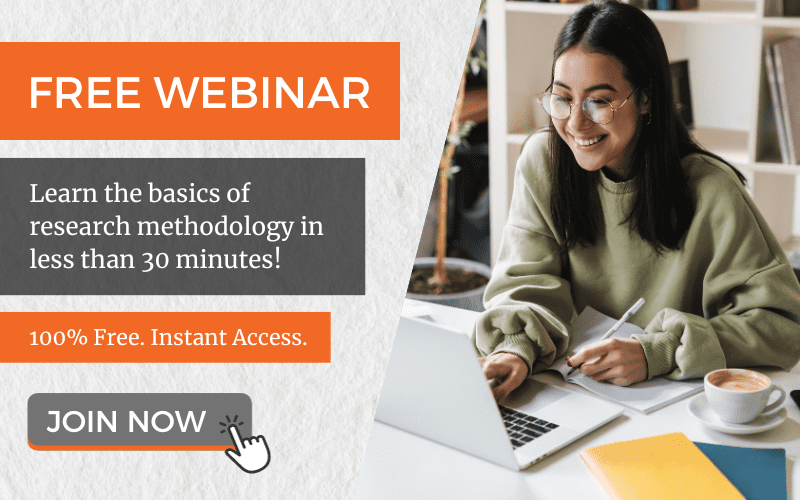
What is a control variable?
In an experimental design, a control variable (or controlled variable) is a variable that is intentionally held constant to ensure it doesn’t have an influence on any other variables. As a result, this variable remains unchanged throughout the course of the study. In other words, it’s a variable that’s not allowed to vary – tough life 🙂
As we mentioned earlier, one of the major challenges in identifying and measuring causal relationships is that it’s difficult to isolate the impact of variables other than the independent variable. Simply put, there’s always a risk that there are factors beyond the ones you’re specifically looking at that might be impacting the results of your study. So, to minimise the risk of this, researchers will attempt (as best possible) to hold other variables constant . These factors are then considered control variables.
Some examples of variables that you may need to control include:
- Temperature
- Time of day
- Noise or distractions
Which specific variables need to be controlled for will vary tremendously depending on the research project at hand, so there’s no generic list of control variables to consult. As a researcher, you’ll need to think carefully about all the factors that could vary within your research context and then consider how you’ll go about controlling them. A good starting point is to look at previous studies similar to yours and pay close attention to which variables they controlled for.
Of course, you won’t always be able to control every possible variable, and so, in many cases, you’ll just have to acknowledge their potential impact and account for them in the conclusions you draw. Every study has its limitations, so don’t get fixated or discouraged by troublesome variables. Nevertheless, always think carefully about the factors beyond what you’re focusing on – don’t make assumptions!
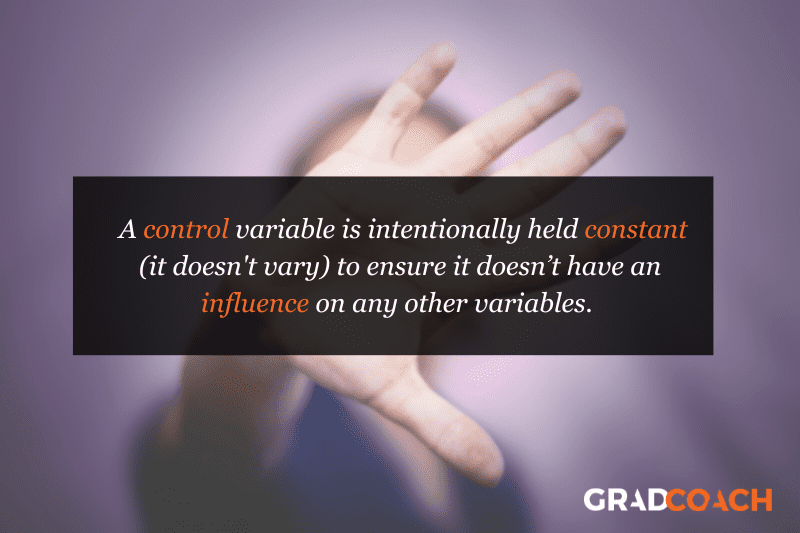
Other types of variables
As we mentioned, independent, dependent and control variables are the most common variables you’ll come across in your research, but they’re certainly not the only ones you need to be aware of. Next, we’ll look at a few “secondary” variables that you need to keep in mind as you design your research.
- Moderating variables
- Mediating variables
- Confounding variables
- Latent variables
Let’s jump into it…
What is a moderating variable?
A moderating variable is a variable that influences the strength or direction of the relationship between an independent variable and a dependent variable. In other words, moderating variables affect how much (or how little) the IV affects the DV, or whether the IV has a positive or negative relationship with the DV (i.e., moves in the same or opposite direction).
For example, in a study about the effects of sleep deprivation on academic performance, gender could be used as a moderating variable to see if there are any differences in how men and women respond to a lack of sleep. In such a case, one may find that gender has an influence on how much students’ scores suffer when they’re deprived of sleep.
It’s important to note that while moderators can have an influence on outcomes , they don’t necessarily cause them ; rather they modify or “moderate” existing relationships between other variables. This means that it’s possible for two different groups with similar characteristics, but different levels of moderation, to experience very different results from the same experiment or study design.
What is a mediating variable?
Mediating variables are often used to explain the relationship between the independent and dependent variable (s). For example, if you were researching the effects of age on job satisfaction, then education level could be considered a mediating variable, as it may explain why older people have higher job satisfaction than younger people – they may have more experience or better qualifications, which lead to greater job satisfaction.
Mediating variables also help researchers understand how different factors interact with each other to influence outcomes. For instance, if you wanted to study the effect of stress on academic performance, then coping strategies might act as a mediating factor by influencing both stress levels and academic performance simultaneously. For example, students who use effective coping strategies might be less stressed but also perform better academically due to their improved mental state.
In addition, mediating variables can provide insight into causal relationships between two variables by helping researchers determine whether changes in one factor directly cause changes in another – or whether there is an indirect relationship between them mediated by some third factor(s). For instance, if you wanted to investigate the impact of parental involvement on student achievement, you would need to consider family dynamics as a potential mediator, since it could influence both parental involvement and student achievement simultaneously.
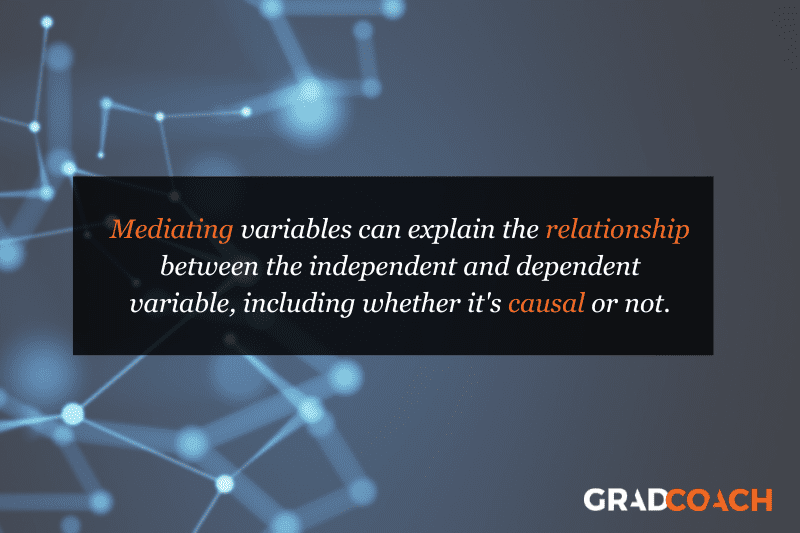
What is a confounding variable?
A confounding variable (also known as a third variable or lurking variable ) is an extraneous factor that can influence the relationship between two variables being studied. Specifically, for a variable to be considered a confounding variable, it needs to meet two criteria:
- It must be correlated with the independent variable (this can be causal or not)
- It must have a causal impact on the dependent variable (i.e., influence the DV)
Some common examples of confounding variables include demographic factors such as gender, ethnicity, socioeconomic status, age, education level, and health status. In addition to these, there are also environmental factors to consider. For example, air pollution could confound the impact of the variables of interest in a study investigating health outcomes.
Naturally, it’s important to identify as many confounding variables as possible when conducting your research, as they can heavily distort the results and lead you to draw incorrect conclusions . So, always think carefully about what factors may have a confounding effect on your variables of interest and try to manage these as best you can.
What is a latent variable?
Latent variables are unobservable factors that can influence the behaviour of individuals and explain certain outcomes within a study. They’re also known as hidden or underlying variables , and what makes them rather tricky is that they can’t be directly observed or measured . Instead, latent variables must be inferred from other observable data points such as responses to surveys or experiments.
For example, in a study of mental health, the variable “resilience” could be considered a latent variable. It can’t be directly measured , but it can be inferred from measures of mental health symptoms, stress, and coping mechanisms. The same applies to a lot of concepts we encounter every day – for example:
- Emotional intelligence
- Quality of life
- Business confidence
- Ease of use
One way in which we overcome the challenge of measuring the immeasurable is latent variable models (LVMs). An LVM is a type of statistical model that describes a relationship between observed variables and one or more unobserved (latent) variables. These models allow researchers to uncover patterns in their data which may not have been visible before, thanks to their complexity and interrelatedness with other variables. Those patterns can then inform hypotheses about cause-and-effect relationships among those same variables which were previously unknown prior to running the LVM. Powerful stuff, we say!
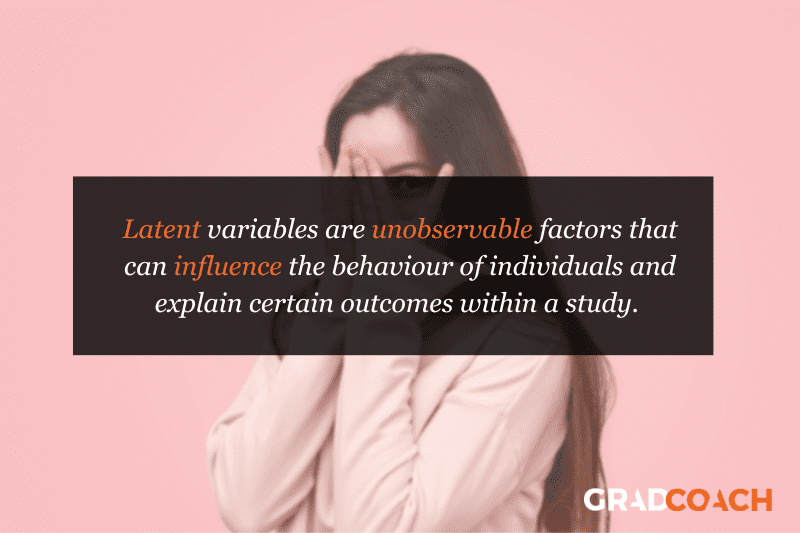
Let’s recap
In the world of scientific research, there’s no shortage of variable types, some of which have multiple names and some of which overlap with each other. In this post, we’ve covered some of the popular ones, but remember that this is not an exhaustive list .
To recap, we’ve explored:
- Independent variables (the “cause”)
- Dependent variables (the “effect”)
- Control variables (the variable that’s not allowed to vary)
If you’re still feeling a bit lost and need a helping hand with your research project, check out our 1-on-1 coaching service , where we guide you through each step of the research journey. Also, be sure to check out our free dissertation writing course and our collection of free, fully-editable chapter templates .
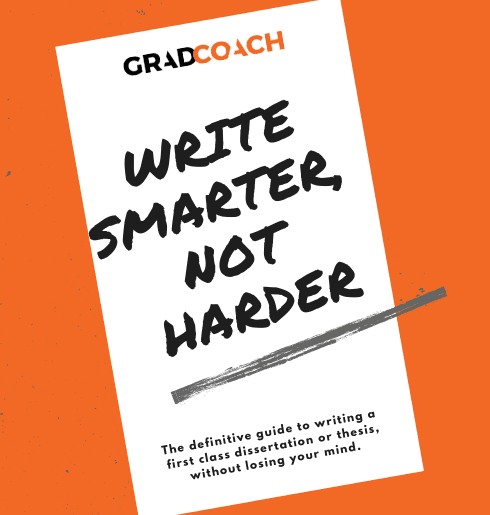
Psst… there’s more (for free)
This post is part of our dissertation mini-course, which covers everything you need to get started with your dissertation, thesis or research project.
You Might Also Like:
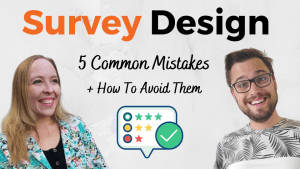
Very informative, concise and helpful. Thank you
Helping information.Thanks
practical and well-demonstrated
Very helpful and insightful
Submit a Comment Cancel reply
Your email address will not be published. Required fields are marked *
Save my name, email, and website in this browser for the next time I comment.
- Print Friendly
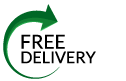
The Plagiarism Checker Online For Your Academic Work
Start Plagiarism Check
Editing & Proofreading for Your Research Paper
Get it proofread now
Online Printing & Binding with Free Express Delivery
Configure binding now
- Academic essay overview
- The writing process
- Structuring academic essays
- Types of academic essays
- Academic writing overview
- Sentence structure
- Academic writing process
- Improving your academic writing
- Titles and headings
- APA style overview
- APA citation & referencing
- APA structure & sections
- Citation & referencing
- Structure and sections
- APA examples overview
- Commonly used citations
- Other examples
- British English vs. American English
- Chicago style overview
- Chicago citation & referencing
- Chicago structure & sections
- Chicago style examples
- Citing sources overview
- Citation format
- Citation examples
- College essay overview
- Application
- How to write a college essay
- Types of college essays
- Commonly confused words
- Definitions
- Dissertation overview
- Dissertation structure & sections
- Dissertation writing process
- Graduate school overview
- Application & admission
- Study abroad
- Master degree
- Harvard referencing overview
- Language rules overview
- Grammatical rules & structures
- Parts of speech
- Punctuation
- Methodology overview
- Analyzing data
- Experiments
- Observations
- Inductive vs. Deductive
- Qualitative vs. Quantitative
- Types of validity
- Types of reliability
- Sampling methods
- Theories & Concepts
- Types of research studies
- Types of variables
- MLA style overview
- MLA examples
- MLA citation & referencing
- MLA structure & sections
- Plagiarism overview
- Plagiarism checker
- Types of plagiarism
- Printing production overview
- Research bias overview
- Types of research bias
- Example sections
- Types of research papers
- Research process overview
- Problem statement
- Research proposal
- Research topic
- Statistics overview
- Levels of measurment
- Frequency distribution
- Measures of central tendency
- Measures of variability
- Hypothesis testing
- Parameters & test statistics
- Types of distributions
- Correlation
- Effect size
- Hypothesis testing assumptions
- Types of ANOVAs
- Types of chi-square
- Statistical data
- Statistical models
- Spelling mistakes
- Tips overview
- Academic writing tips
- Dissertation tips
- Sources tips
- Working with sources overview
- Evaluating sources
- Finding sources
- Including sources
- Types of sources
Your Step to Success
Plagiarism Check within 10min
Printing & Binding with 3D Live Preview
Types of Variables in Research – Definition & Examples
How do you like this article cancel reply.
Save my name, email, and website in this browser for the next time I comment.
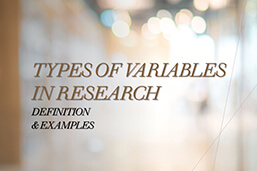
A fundamental component in statistical investigations is the methodology you employ in selecting your research variables. The careful selection of appropriate variable types can significantly enhance the robustness of your experimental design . This piece explores the diverse array of variable classifications within the field of statistical research. Additionally, understanding the different types of variables in research can greatly aid in shaping your experimental hypotheses and outcomes.
Inhaltsverzeichnis
- 1 Types of Variables in Research – In a Nutshell
- 2 Definition: Types of variables in research
- 3 Types of variables in research – Quantitative vs. Categorical
- 4 Types of variables in research – Independent vs. Dependent
- 5 Other useful types of variables in research
Types of Variables in Research – In a Nutshell
- A variable is an attribute of an item of analysis in research.
- The types of variables in research can be categorized into: independent vs. dependent , or categorical vs. quantitative .
- The types of variables in research (correlational) can be classified into predictor or outcome variables.
- Other types of variables in research are confounding variables , latent variables , and composite variables.
Definition: Types of variables in research
A variable is a trait of an item of analysis in research. Types of variables in research are imperative, as they describe and measure places, people, ideas , or other research objects . There are many types of variables in research. Therefore, you must choose the right types of variables in research for your study.
Note that the correct variable will help with your research design , test selection, and result interpretation.
In a study testing whether some genders are more stress-tolerant than others, variables you can include are the level of stressors in the study setting, male and female subjects, and productivity levels in the presence of stressors.
Also, before choosing which types of variables in research to use, you should know how the various types work and the ideal statistical tests and result interpretations you will use for your study. The key is to determine the type of data the variable contains and the part of the experiment the variable represents.
Types of variables in research – Quantitative vs. Categorical
Data is the precise extent of a variable in statistical research that you record in a data sheet. It is generally divided into quantitative and categorical classes.
Quantitative or numerical data represents amounts, while categorical data represents collections or groupings.
The type of data contained in your variable will determine the types of variables in research. For instance, variables consisting of quantitative data are called quantitative variables, while those containing categorical data are called categorical variables. The section below explains these two types of variables in research better.
Quantitative variables
The scores you record when collecting quantitative data usually represent real values you can add, divide , subtract , or multiply . There are two types of quantitative variables: discrete variables and continuous variables .
The table below explains the elements that set apart discrete and continuous types of variables in research:
Categorical variables
Categorical variables contain data representing groupings. Additionally, the data in categorical variables is sometimes recorded as numbers . However, the numbers represent categories instead of real amounts.
There are three categorical types of variables in research: nominal variables, ordinal variables , and binary variables . Here is a tabular summary.
It is worth mentioning that some categorical variables can function as multiple types. For example, in some studies, you can use ordinal variables as quantitative variables if the scales are numerical and not discrete.
Data sheet of quantitative and categorical variables
A data sheet is where you record the data on the variables in your experiment.
In a study of the salt-tolerance levels of various plant species, you can record the data on salt addition and how the plant responds in your datasheet.
The key is to gather the information and draw a conclusion over a specific period and filling out a data sheet along the process.
Below is an example of a data sheet containing binary, nominal, continuous , and ordinal types of variables in research.

Types of variables in research – Independent vs. Dependent
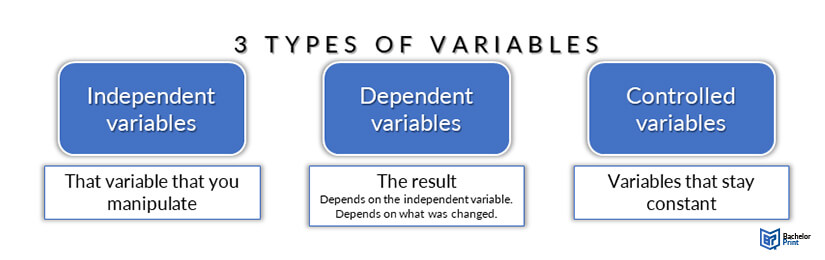
The purpose of experiments is to determine how the variables affect each other. As stated in our experiment above, the study aims to find out how the quantity of salt introduce in the water affects the plant’s growth and survival.
Therefore, the researcher manipulates the independent variables and measures the dependent variables . Additionally, you may have control variables that you hold constant.
The table below summarizes independent variables, dependent variables , and control variables .
Data sheet of independent and dependent variables
In salt-tolerance research, there is one independent variable (salt amount) and three independent variables. All other variables are neither dependent nor independent.
Below is a data sheet based on our experiment:
Types of variables in correlational research
The types of variables in research may differ depending on the study.
In correlational research , dependent and independent variables do not apply because the study objective is not to determine the cause-and-effect link between variables.
However, in correlational research, one variable may precede the other, as illness leads to death, and not vice versa. In such an instance, the preceding variable, like illness, is the predictor variable, while the other one is the outcome variable.
Other useful types of variables in research
The key to conducting effective research is to define your types of variables as independent and dependent. Next, you must determine if they are categorical or numerical types of variables in research so you can choose the proper statistical tests for your study.
Below are other types of variables in research worth understanding.
What is the definition for independent and dependent variables?
An autonomous or independent variable is the one you believe is the origin of the outcome, while the dependent variable is the one you believe affects the outcome of your study.
What are quantitative and categorical variables?
Knowing the types of variables in research that you can work with will help you choose the best statistical tests and result representation techniques. It will also help you with your study design.
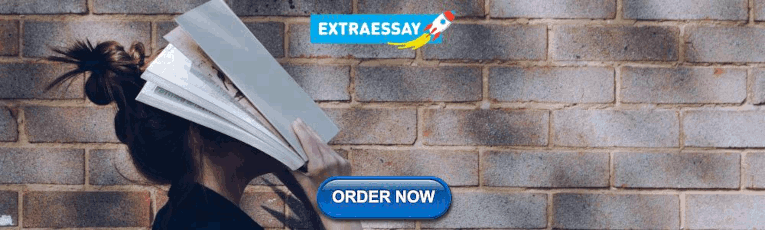
Discrete and continuous variables: What is their difference?
Discrete variables are types of variables in research that represent counts, like the quantities of objects. In contrast, continuous variables are types of variables in research that represent measurable quantities like age, volume, and weight.
We use cookies on our website. Some of them are essential, while others help us to improve this website and your experience.
- External Media
Individual Privacy Preferences
Cookie Details Privacy Policy Imprint
Here you will find an overview of all cookies used. You can give your consent to whole categories or display further information and select certain cookies.
Accept all Save
Essential cookies enable basic functions and are necessary for the proper function of the website.
Show Cookie Information Hide Cookie Information
Statistics cookies collect information anonymously. This information helps us to understand how our visitors use our website.
Content from video platforms and social media platforms is blocked by default. If External Media cookies are accepted, access to those contents no longer requires manual consent.
Privacy Policy Imprint
Have a language expert improve your writing
Run a free plagiarism check in 10 minutes, generate accurate citations for free.
- Knowledge Base
Methodology
Research Methods | Definitions, Types, Examples
Research methods are specific procedures for collecting and analyzing data. Developing your research methods is an integral part of your research design . When planning your methods, there are two key decisions you will make.
First, decide how you will collect data . Your methods depend on what type of data you need to answer your research question :
- Qualitative vs. quantitative : Will your data take the form of words or numbers?
- Primary vs. secondary : Will you collect original data yourself, or will you use data that has already been collected by someone else?
- Descriptive vs. experimental : Will you take measurements of something as it is, or will you perform an experiment?
Second, decide how you will analyze the data .
- For quantitative data, you can use statistical analysis methods to test relationships between variables.
- For qualitative data, you can use methods such as thematic analysis to interpret patterns and meanings in the data.
Table of contents
Methods for collecting data, examples of data collection methods, methods for analyzing data, examples of data analysis methods, other interesting articles, frequently asked questions about research methods.
Data is the information that you collect for the purposes of answering your research question . The type of data you need depends on the aims of your research.
Qualitative vs. quantitative data
Your choice of qualitative or quantitative data collection depends on the type of knowledge you want to develop.
For questions about ideas, experiences and meanings, or to study something that can’t be described numerically, collect qualitative data .
If you want to develop a more mechanistic understanding of a topic, or your research involves hypothesis testing , collect quantitative data .
You can also take a mixed methods approach , where you use both qualitative and quantitative research methods.
Primary vs. secondary research
Primary research is any original data that you collect yourself for the purposes of answering your research question (e.g. through surveys , observations and experiments ). Secondary research is data that has already been collected by other researchers (e.g. in a government census or previous scientific studies).
If you are exploring a novel research question, you’ll probably need to collect primary data . But if you want to synthesize existing knowledge, analyze historical trends, or identify patterns on a large scale, secondary data might be a better choice.
Descriptive vs. experimental data
In descriptive research , you collect data about your study subject without intervening. The validity of your research will depend on your sampling method .
In experimental research , you systematically intervene in a process and measure the outcome. The validity of your research will depend on your experimental design .
To conduct an experiment, you need to be able to vary your independent variable , precisely measure your dependent variable, and control for confounding variables . If it’s practically and ethically possible, this method is the best choice for answering questions about cause and effect.
Prevent plagiarism. Run a free check.
Your data analysis methods will depend on the type of data you collect and how you prepare it for analysis.
Data can often be analyzed both quantitatively and qualitatively. For example, survey responses could be analyzed qualitatively by studying the meanings of responses or quantitatively by studying the frequencies of responses.
Qualitative analysis methods
Qualitative analysis is used to understand words, ideas, and experiences. You can use it to interpret data that was collected:
- From open-ended surveys and interviews , literature reviews , case studies , ethnographies , and other sources that use text rather than numbers.
- Using non-probability sampling methods .
Qualitative analysis tends to be quite flexible and relies on the researcher’s judgement, so you have to reflect carefully on your choices and assumptions and be careful to avoid research bias .
Quantitative analysis methods
Quantitative analysis uses numbers and statistics to understand frequencies, averages and correlations (in descriptive studies) or cause-and-effect relationships (in experiments).
You can use quantitative analysis to interpret data that was collected either:
- During an experiment .
- Using probability sampling methods .
Because the data is collected and analyzed in a statistically valid way, the results of quantitative analysis can be easily standardized and shared among researchers.
Here's why students love Scribbr's proofreading services
Discover proofreading & editing
If you want to know more about statistics , methodology , or research bias , make sure to check out some of our other articles with explanations and examples.
- Chi square test of independence
- Statistical power
- Descriptive statistics
- Degrees of freedom
- Pearson correlation
- Null hypothesis
- Double-blind study
- Case-control study
- Research ethics
- Data collection
- Hypothesis testing
- Structured interviews
Research bias
- Hawthorne effect
- Unconscious bias
- Recall bias
- Halo effect
- Self-serving bias
- Information bias
Quantitative research deals with numbers and statistics, while qualitative research deals with words and meanings.
Quantitative methods allow you to systematically measure variables and test hypotheses . Qualitative methods allow you to explore concepts and experiences in more detail.
In mixed methods research , you use both qualitative and quantitative data collection and analysis methods to answer your research question .
A sample is a subset of individuals from a larger population . Sampling means selecting the group that you will actually collect data from in your research. For example, if you are researching the opinions of students in your university, you could survey a sample of 100 students.
In statistics, sampling allows you to test a hypothesis about the characteristics of a population.
The research methods you use depend on the type of data you need to answer your research question .
- If you want to measure something or test a hypothesis , use quantitative methods . If you want to explore ideas, thoughts and meanings, use qualitative methods .
- If you want to analyze a large amount of readily-available data, use secondary data. If you want data specific to your purposes with control over how it is generated, collect primary data.
- If you want to establish cause-and-effect relationships between variables , use experimental methods. If you want to understand the characteristics of a research subject, use descriptive methods.
Methodology refers to the overarching strategy and rationale of your research project . It involves studying the methods used in your field and the theories or principles behind them, in order to develop an approach that matches your objectives.
Methods are the specific tools and procedures you use to collect and analyze data (for example, experiments, surveys , and statistical tests ).
In shorter scientific papers, where the aim is to report the findings of a specific study, you might simply describe what you did in a methods section .
In a longer or more complex research project, such as a thesis or dissertation , you will probably include a methodology section , where you explain your approach to answering the research questions and cite relevant sources to support your choice of methods.
Is this article helpful?
Other students also liked, writing strong research questions | criteria & examples.
- What Is a Research Design | Types, Guide & Examples
- Data Collection | Definition, Methods & Examples
More interesting articles
- Between-Subjects Design | Examples, Pros, & Cons
- Cluster Sampling | A Simple Step-by-Step Guide with Examples
- Confounding Variables | Definition, Examples & Controls
- Construct Validity | Definition, Types, & Examples
- Content Analysis | Guide, Methods & Examples
- Control Groups and Treatment Groups | Uses & Examples
- Control Variables | What Are They & Why Do They Matter?
- Correlation vs. Causation | Difference, Designs & Examples
- Correlational Research | When & How to Use
- Critical Discourse Analysis | Definition, Guide & Examples
- Cross-Sectional Study | Definition, Uses & Examples
- Descriptive Research | Definition, Types, Methods & Examples
- Ethical Considerations in Research | Types & Examples
- Explanatory and Response Variables | Definitions & Examples
- Explanatory Research | Definition, Guide, & Examples
- Exploratory Research | Definition, Guide, & Examples
- External Validity | Definition, Types, Threats & Examples
- Extraneous Variables | Examples, Types & Controls
- Guide to Experimental Design | Overview, Steps, & Examples
- How Do You Incorporate an Interview into a Dissertation? | Tips
- How to Do Thematic Analysis | Step-by-Step Guide & Examples
- How to Write a Literature Review | Guide, Examples, & Templates
- How to Write a Strong Hypothesis | Steps & Examples
- Inclusion and Exclusion Criteria | Examples & Definition
- Independent vs. Dependent Variables | Definition & Examples
- Inductive Reasoning | Types, Examples, Explanation
- Inductive vs. Deductive Research Approach | Steps & Examples
- Internal Validity in Research | Definition, Threats, & Examples
- Internal vs. External Validity | Understanding Differences & Threats
- Longitudinal Study | Definition, Approaches & Examples
- Mediator vs. Moderator Variables | Differences & Examples
- Mixed Methods Research | Definition, Guide & Examples
- Multistage Sampling | Introductory Guide & Examples
- Naturalistic Observation | Definition, Guide & Examples
- Operationalization | A Guide with Examples, Pros & Cons
- Population vs. Sample | Definitions, Differences & Examples
- Primary Research | Definition, Types, & Examples
- Qualitative vs. Quantitative Research | Differences, Examples & Methods
- Quasi-Experimental Design | Definition, Types & Examples
- Questionnaire Design | Methods, Question Types & Examples
- Random Assignment in Experiments | Introduction & Examples
- Random vs. Systematic Error | Definition & Examples
- Reliability vs. Validity in Research | Difference, Types and Examples
- Reproducibility vs Replicability | Difference & Examples
- Reproducibility vs. Replicability | Difference & Examples
- Sampling Methods | Types, Techniques & Examples
- Semi-Structured Interview | Definition, Guide & Examples
- Simple Random Sampling | Definition, Steps & Examples
- Single, Double, & Triple Blind Study | Definition & Examples
- Stratified Sampling | Definition, Guide & Examples
- Structured Interview | Definition, Guide & Examples
- Survey Research | Definition, Examples & Methods
- Systematic Review | Definition, Example, & Guide
- Systematic Sampling | A Step-by-Step Guide with Examples
- Textual Analysis | Guide, 3 Approaches & Examples
- The 4 Types of Reliability in Research | Definitions & Examples
- The 4 Types of Validity in Research | Definitions & Examples
- Transcribing an Interview | 5 Steps & Transcription Software
- Triangulation in Research | Guide, Types, Examples
- Types of Interviews in Research | Guide & Examples
- Types of Research Designs Compared | Guide & Examples
- Types of Variables in Research & Statistics | Examples
- Unstructured Interview | Definition, Guide & Examples
- What Is a Case Study? | Definition, Examples & Methods
- What Is a Case-Control Study? | Definition & Examples
- What Is a Cohort Study? | Definition & Examples
- What Is a Conceptual Framework? | Tips & Examples
- What Is a Controlled Experiment? | Definitions & Examples
- What Is a Double-Barreled Question?
- What Is a Focus Group? | Step-by-Step Guide & Examples
- What Is a Likert Scale? | Guide & Examples
- What Is a Prospective Cohort Study? | Definition & Examples
- What Is a Retrospective Cohort Study? | Definition & Examples
- What Is Action Research? | Definition & Examples
- What Is an Observational Study? | Guide & Examples
- What Is Concurrent Validity? | Definition & Examples
- What Is Content Validity? | Definition & Examples
- What Is Convenience Sampling? | Definition & Examples
- What Is Convergent Validity? | Definition & Examples
- What Is Criterion Validity? | Definition & Examples
- What Is Data Cleansing? | Definition, Guide & Examples
- What Is Deductive Reasoning? | Explanation & Examples
- What Is Discriminant Validity? | Definition & Example
- What Is Ecological Validity? | Definition & Examples
- What Is Ethnography? | Definition, Guide & Examples
- What Is Face Validity? | Guide, Definition & Examples
- What Is Non-Probability Sampling? | Types & Examples
- What Is Participant Observation? | Definition & Examples
- What Is Peer Review? | Types & Examples
- What Is Predictive Validity? | Examples & Definition
- What Is Probability Sampling? | Types & Examples
- What Is Purposive Sampling? | Definition & Examples
- What Is Qualitative Observation? | Definition & Examples
- What Is Qualitative Research? | Methods & Examples
- What Is Quantitative Observation? | Definition & Examples
- What Is Quantitative Research? | Definition, Uses & Methods
"I thought AI Proofreading was useless but.."
I've been using Scribbr for years now and I know it's a service that won't disappoint. It does a good job spotting mistakes”
Help | Advanced Search
Economics > Econometrics
Title: caviar: categorical-variable embeddings for accurate and robust inference.
Abstract: Social science research often hinges on the relationship between categorical variables and outcomes. We introduce CAVIAR, a novel method for embedding categorical variables that assume values in a high-dimensional ambient space but are sampled from an underlying manifold. Our theoretical and numerical analyses outline challenges posed by such categorical variables in causal inference. Specifically, dynamically varying and sparse levels can lead to violations of the Donsker conditions and a failure of the estimation functionals to converge to a tight Gaussian process. Traditional approaches, including the exclusion of rare categorical levels and principled variable selection models like LASSO, fall short. CAVIAR embeds the data into a lower-dimensional global coordinate system. The mapping can be derived from both structured and unstructured data, and ensures stable and robust estimates through dimensionality reduction. In a dataset of direct-to-consumer apparel sales, we illustrate how high-dimensional categorical variables, such as zip codes, can be succinctly represented, facilitating inference and analysis.
Submission history
Access paper:.
- HTML (experimental)
- Other Formats

References & Citations
- Google Scholar
- Semantic Scholar
BibTeX formatted citation

Bibliographic and Citation Tools
Code, data and media associated with this article, recommenders and search tools.
- Institution
arXivLabs: experimental projects with community collaborators
arXivLabs is a framework that allows collaborators to develop and share new arXiv features directly on our website.
Both individuals and organizations that work with arXivLabs have embraced and accepted our values of openness, community, excellence, and user data privacy. arXiv is committed to these values and only works with partners that adhere to them.
Have an idea for a project that will add value for arXiv's community? Learn more about arXivLabs .

What Are Variables in a Research Paper?
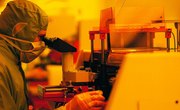
What Components Are Necessary for an Experiment to Be Valid?
Research papers will mention a variety of different variables, and, at first, these technical terms might seem difficult and confusing. But with a little practice, identifying these variables becomes second nature. Because they are sometimes not explicitly labeled in the research writeup, it is useful to have a real research paper on hand as you learn these terms, so you can get some hands-on practice at identifying them.
Independent Variable
The independent variable, also known as the IV, is the variable that the researchers are manipulating in an experiment or quasi-experiment. It is also the label given to the “criterion” variable in certain types of regression analysis. For example, if a researcher has two groups of people watch either a happy film or a sad film before giving an IQ test, the IV is the mood of the participants.
Dependent Variable
The dependent variable, or DV, is the one that is being measured by the researcher; it is the outcome variable. There is often confusion between the IV and the DV among new science students, but a good way to distinguish them is to remember that the outcome of measuring the DV is hypothesized to depend on the manipulation of the IV. In the above example, IQ was hypothesized to depend on the mood of the participants.
A covariate is a variable that the researchers include in an analysis to determine whether the IV is able to influence the DV over and above any effect the covariate might have. The classic example is when researchers take a baseline measurement, perform some manipulation, then take the measurement again. When they analyze this data, they will enter the baseline scores as a covariate, which will help cancel out any initial differences between the participants.
Extraneous Variables
An extraneous variable is a little different from the rest because it is not directly measured, or often even wanted, by the researchers. It is a variable that has an impact on the results of the experiment that the researchers didn't anticipate. For example, the heat of the room might be different between two groups of IQ tests, and the hot room might annoy people and affect their scores. Scientists try to control these variables, which means keeping them constant between groups.
Related Articles
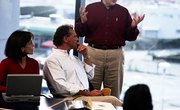
What Is Experimental Research Design?
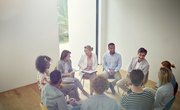
Why Do Experimental Procedures Include Control Samples?
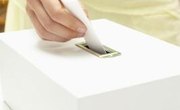
Independent Variables in Survey Methods
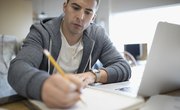
The Disadvantages of Logistic Regression
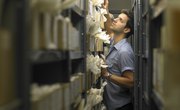
The Real Difference Between Reliability and Validity
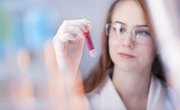
Qualitative and Quantitative Research Methods
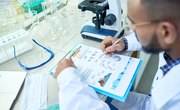
How to Evaluate Statistical Analysis
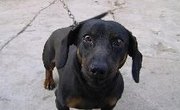
Ideas for Science Projects on Dog Behavior
- Laerd Statistics: Types of Variable
Warren Davies has been writing since 2007, focusing on bespoke projects for online clients such as PsyT and The Institute of Coaching. This has been alongside work in research, web design and blogging. A Linux user and gamer, warren trains in martial arts as a hobby. He has a Bachelor of Science and Master of Science in psychology, and further qualifications in statistics and business studies.

Advertisement
The Independent Variable vs. Dependent Variable in Research
- Share Content on Facebook
- Share Content on LinkedIn
- Share Content on Flipboard
- Share Content on Reddit
- Share Content via Email
In any scientific research, there are typically two variables of interest: independent variables and dependent variables. In forming the backbone of scientific experiments , they help scientists understand relationships, predict outcomes and, in general, make sense of the factors that they're investigating.
Understanding the independent variable vs. dependent variable is so fundamental to scientific research that you need to have a good handle on both if you want to design your own research study or interpret others' findings.
To grasp the distinction between the two, let's delve into their definitions and roles.
What Is an Independent Variable?
What is a dependent variable, research study example, predictor variables vs. outcome variables, other variables, the relationship between independent and dependent variables.
The independent variable, often denoted as X, is the variable that is manipulated or controlled by the researcher intentionally. It's the factor that researchers believe may have a causal effect on the dependent variable.
In simpler terms, the independent variable is the variable you change or vary in an experiment so you can observe its impact on the dependent variable.
The dependent variable, often represented as Y, is the variable that is observed and measured to determine the outcome of the experiment.
In other words, the dependent variable is the variable that is affected by the changes in the independent variable. The values of the dependent variable always depend on the independent variable.
Let's consider an example to illustrate these concepts. Imagine you're conducting a research study aiming to investigate the effect of studying techniques on test scores among students.
In this scenario, the independent variable manipulated would be the studying technique, which you could vary by employing different methods, such as spaced repetition, summarization or practice testing.
The dependent variable, in this case, would be the test scores of the students. As the researcher following the scientific method , you would manipulate the independent variable (the studying technique) and then measure its impact on the dependent variable (the test scores).
You can also categorize variables as predictor variables or outcome variables. Sometimes a researcher will refer to the independent variable as the predictor variable since they use it to predict or explain changes in the dependent variable, which is also known as the outcome variable.
When conducting an experiment or study, it's crucial to acknowledge the presence of other variables, or extraneous variables, which may influence the outcome of the experiment but are not the focus of study.
These variables can potentially confound the results if they aren't controlled. In the example from above, other variables might include the students' prior knowledge, level of motivation, time spent studying and preferred learning style.
As a researcher, it would be your goal to control these extraneous variables to ensure you can attribute any observed differences in the dependent variable to changes in the independent variable. In practice, however, it's not always possible to control every variable.
The distinction between independent and dependent variables is essential for designing and conducting research studies and experiments effectively.
By manipulating the independent variable and measuring its impact on the dependent variable while controlling for other factors, researchers can gain insights into the factors that influence outcomes in their respective fields.
Whether investigating the effects of a new drug on blood pressure or studying the relationship between socioeconomic factors and academic performance, understanding the role of independent and dependent variables is essential for advancing knowledge and making informed decisions.
Correlation vs. Causation
Understanding the relationship between independent and dependent variables is essential for making sense of research findings. Depending on the nature of this relationship, researchers may identify correlations or infer causation between the variables.
Correlation implies that changes in one variable are associated with changes in another variable, while causation suggests that changes in the independent variable directly cause changes in the dependent variable.
Control and Intervention
In experimental research, the researcher has control over the independent variable, allowing them to manipulate it to observe its effects on the dependent variable. This controlled manipulation distinguishes experiments from other types of research designs.
For example, in observational studies, researchers merely observe variables without intervention, meaning they don't control or manipulate any variables.
Context and Analysis
Whether it's intentional or unintentional, independent, dependent and other variables can vary in different contexts, and their effects may differ based on various factors, such as age, characteristics of the participants, environmental influences and so on.
Researchers employ statistical analysis techniques to measure and analyze the relationships between these variables, helping them to draw meaningful conclusions from their data.
We created this article in conjunction with AI technology, then made sure it was fact-checked and edited by a HowStuffWorks editor.
Please copy/paste the following text to properly cite this HowStuffWorks.com article:
Advertisement
A global analysis of habitat fragmentation research in reptiles and amphibians: what have we done so far?
- Review Paper
- Open access
- Published: 08 January 2023
- Volume 32 , pages 439–468, ( 2023 )
Cite this article
You have full access to this open access article
- W. C. Tan ORCID: orcid.org/0000-0002-6067-3528 1 ,
- A. Herrel ORCID: orcid.org/0000-0003-0991-4434 2 , 3 , 4 &
- D. Rödder ORCID: orcid.org/0000-0002-6108-1639 1
7489 Accesses
10 Citations
27 Altmetric
Explore all metrics
Habitat change and fragmentation are the primary causes of biodiversity loss worldwide. Recent decades have seen a surge of funding, published papers and citations in the field as these threats to biodiversity continue to rise. However, how research directions and agenda are evolving in this field remains poorly understood. In this study, we examined the current state of research on habitat fragmentation (due to agriculture, logging, fragmentation, urbanisation and roads) pertaining to two of the most threatened vertebrate groups, reptiles and amphibians. We did so by conducting a global scale review of geographical and taxonomical trends on the habitat fragmentation types, associated sampling methods and response variables. Our analyses revealed a number of biases with existing research efforts being focused on three continents (e.g., North America, Europe and Australia) and a surplus of studies measuring species richness and abundance. However, we saw a shift in research agenda towards studies utilising technological advancements including genetic and spatial data analyses. Our findings suggest important associations between sampling methods and prevalent response variables but not with the types of habitat fragmentation. These research agendas are found homogeneously distributed across all continents. Increased research investment with appropriate sampling techniques is crucial in biodiversity hotpots such as the tropics where unprecedented threats to herpetofauna exist.
Similar content being viewed by others
Habitat conservation research for amphibians: methodological improvements and thematic shifts.
Gentile Francesco Ficetola
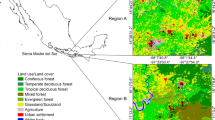
Effect of landscape composition and configuration on biodiversity at multiple scales: a case study with amphibians from Sierra Madre del Sur, Oaxaca, Mexico
Daniel G. Ramírez-Arce, Leticia M. Ochoa-Ochoa & Andrés Lira-Noriega
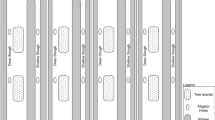
A dry future for the Everglades favors invasive herpetofauna
Hunter J. Howell, Giacomo L. Delgado, … Christopher A. Searcy
Avoid common mistakes on your manuscript.
Introduction
Habitat loss and fragmentation are the predominant causes underlying widespread biodiversity changes in terrestrial ecosystems (Fahrig 2003 ; Newbold et al. 2015 ). These processes may cause population declines by disrupting processes such as dispersal, gene flow, and survival. Over the past 30 years habitat loss and fragmentation have been suggested to have reduced biodiversity by up to 75% in different biomes around the world (Haddad et al. 2015 ). This is mainly due to the clearing of tropical forests, the expansion of agricultural landscapes, the intensification of farmland production, and the expansion of urban areas (FAO and UNEP 2020 ). The rate of deforestation and corresponding land conversions of natural habitats are happening rapidly and will continue to increase in the future at an accelerated rate, particularly in biodiversity hotspots (Deikumah et al. 2014 ; Habel et al. 2019 ; FAO and UNEP 2020 ).
For this reason, habitat fragmentation has been a central research focus for ecologists and conservationists over the past two decades (Fardila et al. 2017 ). However, habitat fragmentation consists of two different processes: loss of habitat and fragmentation of existing habitat (Fahrig 2003 ). The former simply means the removal of habitat, and latter is the transformation of continuous areas into discontinuous patches of a given habitat. In a radical review, Fahrig ( 2003 ) suggested that fragmentation per se, i.e., the breaking up of habitat after controlling for habitat loss, has a weaker or even no effect on biodiversity compared to habitat loss. She further recommended that the effects of these two components should be measured independently (Fahrig 2017 ). Despite being recognised as two different processes, researchers tend not to distinguish between their effects and commonly lump the combined consequences under a single umbrella term “habitat fragmentation” (Fahrig 2003 , 2017 ; Lindenmayer and Fischer 2007 ; Riva and Fahrig 2022 ). Nonetheless, fragmentation has been widely recognised in the literature and describes changes that occur in landscapes, including the loss of habitat (Hadley and Betts 2016 ). Hence, to avoid imprecise or inconsistent use of terminology and provide a holistic view of the effect of modified landscapes, we suggest the term “habitat fragmentation” to indicate any type of landscape change, both habitat loss and fragmentation throughout the current paper.
One main conundrum is that biodiversity decline does not occur homogeneously everywhere nor among all species (Blowes et al. 2019 ). Moreover, we should expect a global disparity in biodiversity responses to habitat fragmentation across different biomes (Newbold et al. 2020 ; Cordier et al. 2021 ). For example, tropical regions are predicted to have higher negative effects of habitat fragmentation than temperate regions. There are two possible reasons: a) higher intensification of land use change in the tropics (Barlow et al. 2018 ), and b) forest animals in the tropics are less likely to cross open areas (Lindell et al. 2007 ). Furthermore, individual species respond to landscape modification differently; some thrive whereas others decline (Fahrig 2003 ). Habitat specialists with broader habitat tolerance and wide-ranging distributions are most likely to benefit from increase landscape heterogeneity and more open and edge habitat (Hamer and McDonnell 2008 ; Newbold et al. 2014 ; Palmeirim et al. 2017 ). Therefore, appropriate response metrics should be used in measuring the effect of habitat fragmentation on biodiversity depending on the taxa group, biome and scale of study as patterns of richness can sometimes be masked by the abundance of generalist species (Riemann et al. 2015 ; Palmeirim et al. 2017 ).
Previous reviews have identified general patterns and responses of reptile and amphibian populations to habitat modification. They have been largely centred around specific types of habitat fragmentation: land use change (Newbold et al. 2020 ), logging (Sodhi et al. 2004 ), fragmentation per se (Fahrig 2017 ), urbanisation (Hamer and McDonnell 2008 ; McDonald et al. 2013 ), fire (Driscoll et al. 2021 ), and roads (Rytwinski and Fahrig 2012 ). Few reviews have, however, attempted a global synthesis of all types of land use changes and even fewer have addressed biases in geographical regions and taxonomical groups (but see Gardner et al. ( 2007 ) and Cordier et al. ( 2021 )). Gardner et al. ( 2007 ) synthesised the extant literature and focused on 112 papers on the consequences of habitat fragmentation on reptiles and amphibians published between 1945 and 2006. They found substantial biases across geographic regions, biomes, types of data collected as well as sampling design and effort. However, failure to report basic statistics by many studies prevented them from performing meta-analyses on research conclusions. More recently, a review by Cordier et al. ( 2021 ) conducted a meta-analysis based on 94 primary studies on the overall effects of land use changes through time and across the globe. Yet, there has been no comprehensive synthesis on the research patterns and agenda of published literature on habitat fragmentation associated with the recent advances of novel research tools and techniques. Therefore, our review may provide new insights of the evolution and biases in the field over the last decades and provide a basis for future research directions. Knowledge gaps caused by these biases could hamper the development of habitat fragmentation research and the implementation of effective strategies for conservation.
We aim to remedy this by examining research patterns for the two vertebrate classes Amphibia and Reptilia, at a global scale. We chose amphibians and reptiles for several reasons. First, habitat fragmentation research has been dominated by birds and mammals (Fardila et al. 2017 ). Reptiles and amphibians, on the other hand, are under-represented; together, they constitute only 10% of the studies (Fardila et al. 2017 ). Second, high proportions of amphibian and reptile species are threatened globally. To date, more than one third of amphibian (40%) and one in five reptile species (21%) are threatened with extinction (Stuart et al. 2004 ; Cox et al. 2022 ). Amphibians are known to be susceptible to land transformation as a result of their cryptic nature, habitat requirements, and reduced dispersal ability (Green 2003 ; Sodhi et al. 2008 ; Ofori‐Boateng et al. 2013 ; Nowakowski et al. 2017 ). Although poorly studied (with one in five species classified as data deficient) (Böhm et al. 2013 ), reptiles face the same threats as those impacting amphibians (Gibbons et al. 2000 ; Todd et al. 2010 ; Cox et al. 2022 ). Reptiles have small distributional ranges with high endemism compared to other vertebrates and as such are likely vulnerable to habitat fragmentation (Todd et al. 2010 ; Meiri et al. 2018 ). Third, both these groups are poikilotherms whose physiology makes them highly dependent on temperature and precipitation levels. Hence, they are very sensitive to changing thermal landscapes (Nowakowski et al. 2017 ).
Here, we first ask how is the published literature distributed across geographic regions and taxa? Is there a bias in the geographic distribution of species studied compared to known species? It is well known that conservation and research efforts are often concentrated in wealthy and English-speaking countries (Fazey et al. 2005 ), but has this bias improved over the years? Second, how are researchers conducting these studies? We assess whether certain sampling methods and response variables are associated to specific types of habitat fragmentation. Over the past decades new tools and techniques are constantly being discovered or developed. Combinations of methodologies are now shedding new light on biodiversity responses and consequences of habitat fragmentation. In particular, genetic techniques are useful in detecting changes in population structure, identifying isolated genetic clusters, and in estimating dispersal (Smith et al. 2016 ). Similarly, habitat occupancy and modelling can also provide powerful insights into dispersal (Driscoll et al. 2014 ). Remote sensing data are now used in analysing effects of area, edge, and isolation (Ray et al. 2002 ). Finally, how are these associations or research agendas distributed across space? We expect to find geographic structure of emerging agendas across the globe. For instance, we predict genetic studies to be located in North America and Europe but also in East Asian countries such as China and Japan as a result of their advancement in genetics (Forero et al. 2016 ). On the other hand, simple biodiversity response indicators which do not require extensive capacity building and application of advanced technologies are likely more used in developing regions of the world (Barber et al. 2014 ). These findings are valuable to evaluate and update the global status of our research on the effects of habitat fragmentation on herpetofauna and to suggest recommendations for conservation plans.
Materials and methods
Data collection.
We conducted the review according to the Preferred Reporting Items for Systematic Reviews and Meta-Analyses (PRISMA) guidelines (Fig. 1 ) (Moher et al. 2009 ). We conducted a comprehensive and exhaustive search using Web of Science to review published studies reporting the consequences of habitat fragmentation on amphibians and reptiles. We consulted the database in November 2019 by using two general search strings: (1) Habitat fragmentation AND (frog* OR amphib* OR salamander* OR tadpole*) (2) Habitat fragmentation AND (reptil* OR snake* OR lizard* OR turtle* OR crocodile*). This returned a total of 869 records from search (1) and 795 from search (2), with 1421 unique records remaining after duplicates were removed. We did not include “habitat loss” in our search term as it would only introduce unrelated articles focusing on biodiversity and conservation management instead of methodology and mechanistic approaches.
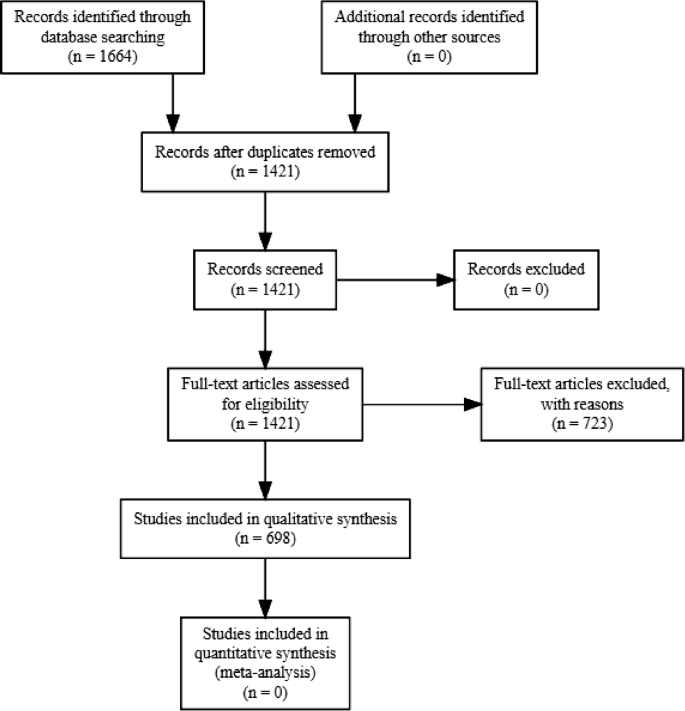
PRISMA flow-diagram of the study selection process
Throughout, we use the term papers to refer to individual journal article records. Out of the 1421 papers, we were unfortunately not able to locate seven papers from Acta Oecologica, Zoology: Analysis of Complex Systems, Israel Journal of Ecology and Evolution, Western North American Naturalist, Natural Areas Journal, Ecology, and the Herpetological Journal. We screened all articles from the title through the full text to determine whether they met our criteria for inclusion. To be included, studies needed to fulfil several criteria. First, papers needed to be peer-reviewed journal articles containing data collected on reptiles and/or amphibians at the species level (224 articles rejected because no species-specific data was available). Reviews and metastudies (n = 102) were excluded from the data analysis as they may represent duplicates as they are mainly based on data sets from other papers, but these form an integral part of our discussion. Furthermore, papers which do not provide data on contemporary time scales such as long-term (> 10, 000 years ago) changes on the paleo-spatial patterns (n = 59) were excluded. Because the effects of fragmentation per se have been measured inconsistently by many authors and have not been differentiated from habitat removal (Fahrig 2003 ), we consider any recent anthropogenic habitat degradation, and modification at patch or/and landscape scales during the Holocene as an effect of habitat fragmentation. Only papers which examined direct or indirect effects of habitat fragmentation were included in our analysis, regardless of the magnitude and direction. Papers which did not mention specific types of habitat fragmentation as the focus of their study (n = 338) were excluded.
Geographical and taxonomical distribution
Using the selected papers, we compiled a taxonomic and geographical database for each paper: (a) GPS or georeferenced location of the study site; (b) the focal group investigated (amphibian and/or reptile); (c) taxonomic groups (order, family, genus).
We listed the overall number of species studied covered by selected papers in each continent and compared them to the total number of currently described species. We obtained total described species of both reptiles and amphibians from the following sources: ReptileDatabase (Uetz et al. 2021 ) and AmphibiaWeb (AmphibiaWeb 2021 ). Then, we calculated the proportions of species covered by the selected papers compared to total number of described species for each continent. We did not update species nomenclature from selected papers as the mismatches from these potentially outdated taxonomies would be insignificant in our analyses.
Categorisation of papers
Each paper is classified into three main types of data collected: forms of habitat fragmentation, sampling methods, and response variables (Online Appendix 1). A paper can be classified into one or multiple categories in each type of data. The types of data and their following categories were:
Forms of habitat fragmentation
We recorded different types of habitat fragmentation from the selection of studies: (1) “Fragmentation” (includes patch isolation, edge and area effects); (2) “Agriculture” (includes any form of commercial and subsistence farming such as monocultures, plantations, and livestock farming); (3) “Logging” (e.g., agroforestry and silviculture); (4) “Mining” (presence of mining activities); (5) “Urbanisation” (includes presence of cities, towns or villages and parks created for recreational purposes); (6) “Road” (includes any vehicle roadway such as railways and highways) and (7) “Other types of habitat fragmentation” (e.g., fire, river dams, ditches, diseases, desertification etc.). Many studies deal with more than one type of habitat fragmentation. However, we made sure the selection for fragmentation forms is mainly based on the focus and wordings in the methodology section.
Sampling methods
We report trends in the design and sampling methods among the compiled studies over the last three decades. Due to the substantial variability in the level of sample design information reported by different studies, we narrowed them down into six general categories representing common sampling methods. Common methods used in estimating herpetofauna diversity (e.g., visual transect surveys, acoustic monitoring and trapping methods) were not included in the analyses due to their omnipresence in the data. The categories are:
(1) “Genetics” studies documented any use of codominant markers (i.e., allozymes and microsatellites), dominant markers [i.e., DNA sequences, random amplified polymorphic DNA (RAPDs) and amplified fragment length polymorphism (AFLPs)] to analyse genetic variability and gene diversity respectively. (2) “Direct tracking methods” studies measured potential dispersal distances or species movement patterns by means of radio telemetry, mark-recapture methods, or fluorescent powder tracking. (3) “Aerial photographs” studies reported the use of aerial photographs while (4) “GIS/Satellite image” studies described the use of satellite imagery and land cover data (i.e., Landsat) and GIS programs (e.g., QGIS and ArcGIS, etc.) in analysing spatial variables. (5) “Experimental” studies involved predictions tested through empirical studies, regardless if they occur naturally or artificially; in a natural or a captive environment. (6) “Prediction/simulation models” studies made use of techniques such as ecological niche models, habitat suitability (i.e., occurrence and occupancy models) and simulations for probability of survival and population connectivity.
Response variables
To further conceptualise how the effects of habitat fragmentation are measured, we assigned 12 biodiversity or ecological response variables. We recorded the type of data that was used in all selected studies: (1) “Species richness or diversity” which are measures of species richness, evenness or diversity (such as the Shannon–Wiener index) (Colwell 2009 ); (2) “Functional richness or species guilds” describes diversity indices based on functional traits (such as body size, reproductive modes, microhabitat association or taxonomic groups); (3) “Presence/absence” or species occupancy; (4) “Population” includes an estimation of population size or density (only when measured specifically in the paper). It includes genetic variation and divergence within and between populations; (5) “Abundance” or counts of individuals for comparison between habitat fragmentation type or species; (6) “Dispersal” takes into account any displacement or movement and can include indirect measurements of dispersal using genetic techniques; (7) “Breeding sites” which measures available breeding or reproduction sites; (8) “Fitness measure” are records of any physiological, ecological or behavioural changes; (9) “Interspecific interaction” depicts any interaction between species including competition and predation; (10) “Extinction or colonisation rate” counts the number of population extinctions or colonisations within a time period; (11) “Microhabitat preference” includes any direct observation made on an individual’s surrounding environmental features (substrate type, perch height, vegetation type, distance to cover etc.); (12) “Generalist or specialist comparison” involves any comparison made between generalist and specialist species. Generalists are able to thrive in various environments whereas specialists occupy a much narrower niche; (13) “Other response variables” can include road kill mortality counts, infection rate of diseases, injury, or any effect from introduced animals and a variety of other responses.
Data analysis
All statistical analyses were conducted in the open source statistical software package R 4.1.0 (R Core Team 2021 ). To gain a broad insight into our understanding of the complexity of habitat fragmentation we applied a Multiple Correspondence Analysis (MCA) (Roux and Rouanet 2004 ) and Hierarchical Clustering on Principle Components (HCPC) (Ward 1963 ) to investigate potential interactions between forms of habitat fragmentation, sampling methods and response variables. MCA is ideal for investigation of datasets with multiple categorical variables and exploration of unbiased relationships between these variables.
We first separate the dataset into papers concerning amphibians or reptiles. The MCA was performed using the MCA function from FactoMineR package of R version 3.1 (Lê et al. 2008 ). To identify subgroups (cluster) of similar papers within our dataset, we performed cluster analysis on our MCA results using HCPC. The cluster results are then visualised in factor map and dendrogram for easier interpretation using factoextra package. This allows us to identify categorical variables which have the highest effect within each cluster. Statistical analyses were considered significant at α = 0.05, while a p between 0.10 and 0.05 was considered as a tendency. The p-value is less than 5% when one category is significantly linked to other categories. The V tests show whether the category is over-expressed (positive values) or under-expressed (negative values) in the cluster (Lebart et al. 1995 ).
Results from the literature review were also analysed with VOSviewer, freeware for constructing and visualising bibliometric networks ( http://www.vosviewer.com/ ). The program uses clustering techniques to analyse co-authors, co-occurrence of keywords, citations, or co-citations (van Eck and Waltman 2014 ). First, we analyse co-authorships of countries to provide a geographical representation of groups of authors in various countries over the past 30 years. Each circle represents an author’s country and the size represents the collaboration frequency with other countries. The lines between the nodes represent the collaboration networks between the countries while the thickness of the lines indicates the collaboration intensities between them. Lastly, to complement the MCA and HCPC, we used VOSviewer to analyse a clustering solution of categories at an aggregate level. Aggregate clustering is a meta-clustering method to improve the robustness of clustering and does not require a priori information about the number of clusters. In this case, instead of author’s keywords, we used the co-occurrence of categories associated to each selected paper as input to run the software.
We identified a total of 698 papers published between January 1991 and November 2019 reporting consequences of habitat fragmentations corresponding to our selection criteria (Fig. 1 ). The complete list of studies included (hereafter termed “selected papers”) is available in Online Appendix 2. The distribution of these selected papers between focal groups and among continents was non-homogeneous (Fig. 2 ). Selected papers reviewed were predominantly studies which were conducted in North America 310 (44%) and Europe 131 (19%), but also from Oceania 104 (15%), South America 85 (12%), Asia 37 (5%) and Africa 31 (5%). For co-authorships between countries based on VOSviewer, the minimum document number of a country was set as 5 and a total of 21 and 14 countries met the threshold for amphibians and reptiles respectively (Fig. 3 ). For amphibians, countries in the American continent such as United States of America or USA (178 articles), Brazil (38 articles) and Canada (35 articles) have the largest research weight (Fig. 3 a). Authors from the USA have the largest international cooperation network, followed by Brazil. Australia and other European countries such as Germany, France and England also have high collaboration relationships with other countries. In contrast, reptile studies were mainly concentrated around two countries: the USA (139 articles) and Australia (86 articles) (Fig. 3 b). No other country from the rest of the world has more than 20 articles. While both the USA and Australia have the largest collaboration networks, Canada, Spain and Mexico are also highly cooperative with authors from other countries.
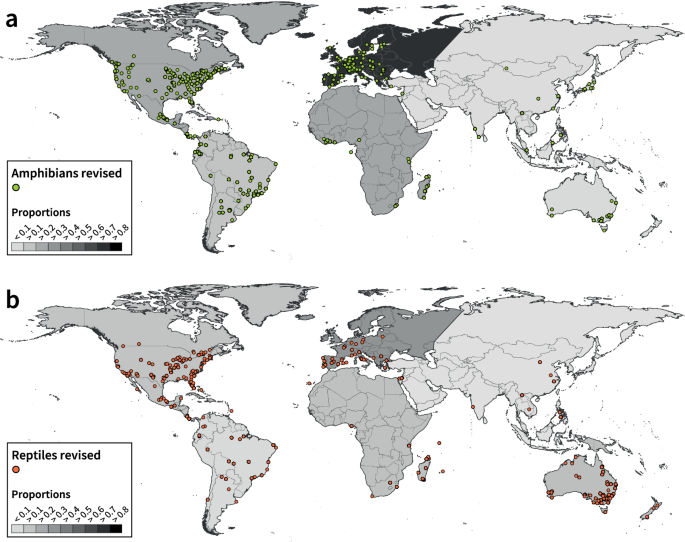
Map of study locations for a amphibians and b reptiles with each circle representing the study location of papers included in the review. The colour scale of the continents ranging from 0 – 0.9 indicates the proportions of amphibian and reptile species represented in the reviewed papers when compared to known species in the world (obtained from AmphibiaWeb and ReptileDatabase): a Europe (0.73), Africa (0.23), North America (0.23), South America (0.18), Oceania (0.07) and Asia (0.06) and b Europe (0.27), Oceania (0.18), Africa (0.12), North America (0.11), South America (0.09) and Asia (0.02)
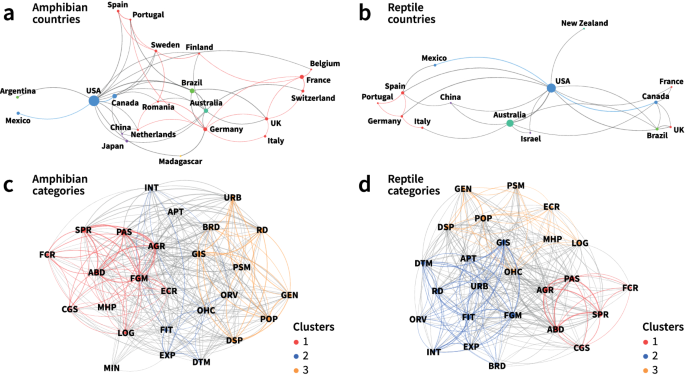
Co-authorship map of countries involved in habitat fragmentation research in a amphibians and b reptiles. The colours represent the continents countries belong to. Each circle represents an author’s country and the size represents the collaboration frequency with other countries. The lines between the nodes represent the collaboration networks between the countries while the thickness of the lines indicates the collaboration intensities between them. Category co-occurrence network maps for c amphibians and d reptiles. The colour represents the different cluster groups each category belongs to. Abbreviations for the categories in forms of habitat change: fragmentation (FGM), agriculture (AGR), Logging (LOG), Mining (MIN), Urbanisation (URB), road (RD), other habitat fragmentation (OHC); sampling methods: genetics (GEN), direct tracking method (DTM), aerial photographs (APT), GIS/ Satellite images (GIS), experimental (EXP), prediction/ simulation models (PSM) and response variables: species richness/ diversity (SPR), functional richness/ species guild (FCR), presence/ absence (PAS), population (POP), abundance (ABD), dispersal (DSP), breeding sites (BRD), fitness measure (FIT), interspecific interaction (INT),extinction/ colonisation rate (ECR), microhabitat preference (MHP), comparison between generalist and specialist (CGS), other response varialbes (ORV) (see also Online Appendix 1). Maps are created in VOSviewer
Overall, over half of all selected papers included only amphibians (376 papers; 54%), whilst 276 papers (39%) included only reptiles and 46 papers (7%) assessed both reptiles and amphibians. In relation to species richness, we identified 1490 amphibian species and 1199 reptile species across all papers; among which 141 taxa were not identified to species level but were still included in our analyses as taxonomic units analogous to species (Online Appendix 2). Among these species, more than half of the studied amphibians were found in South America (537; 38%) and North America (328; 23%), followed by Africa (297; 21%), Asia (137; 10%), Europe (77; 5%), and Oceania (51; 3%). Half of the reptile species studied were from North America (302; 25%) and Africa (278; 23%), with the other half consisting of species from Oceania (276; 23%), South America (200; 17%), Europe (76; 6%), and Asia (67; 6%).
When compared to the known species richness in the world, large portions of European species are studied while species from other continents were severely under-represented (Fig. 2 ). The proportions of amphibian species represented in papers were the highest in Europe (73%), while the proportions are much lower for Africa (23%), North America (23%), South America (18%), Oceania (7%) and Asia (6%) (Fig. 2 a). Among reptiles, Europe represents again the highest proportion of studied species (27%), followed by Oceania (18%), Africa (12%), North America (11%) and South America (8.9%) (Fig. 2 b). In contrast, of all Asian reptile species, only a mere 1.73% were included in the selected papers. The species coverage in our selected papers does not seem optimistic. Amphibians and reptiles each have only six families with more than half of the species covered (including three reptilian families containing one species in total). Meanwhile, 23 and 25 families remain fully neglected for amphibians and reptiles respectively (Figs. 4 – 5 ).
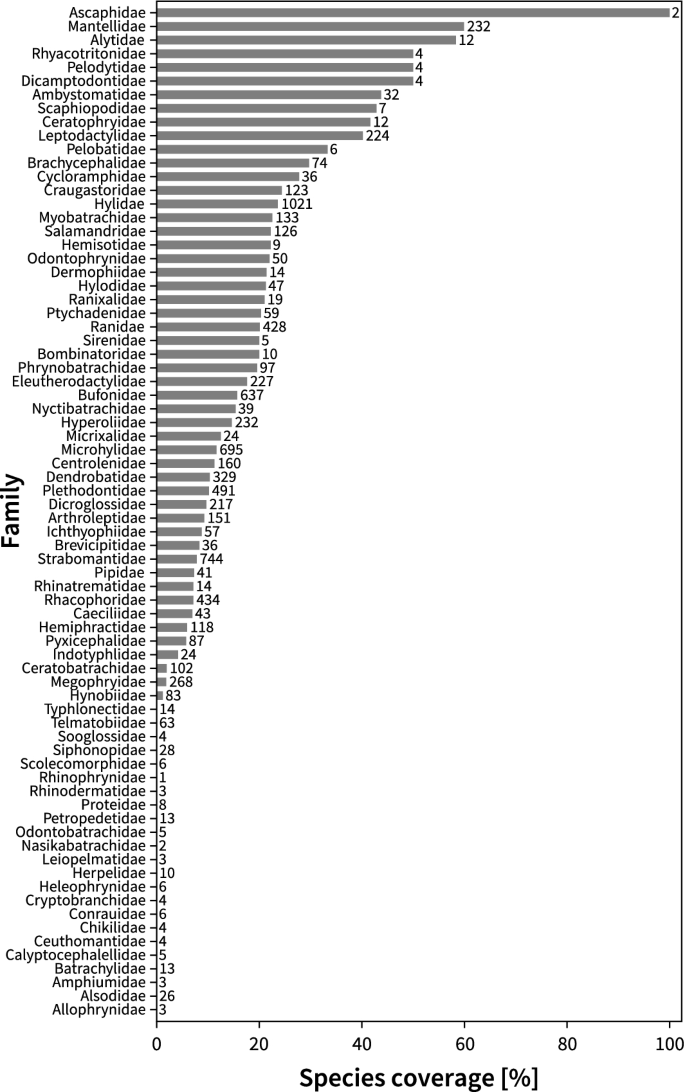
Species coverage for each taxonomic family in selected papers of amphibians. The numbers on each row indicate the total number of species known in its respective family (obtained from AmphibiaWeb 2021 )
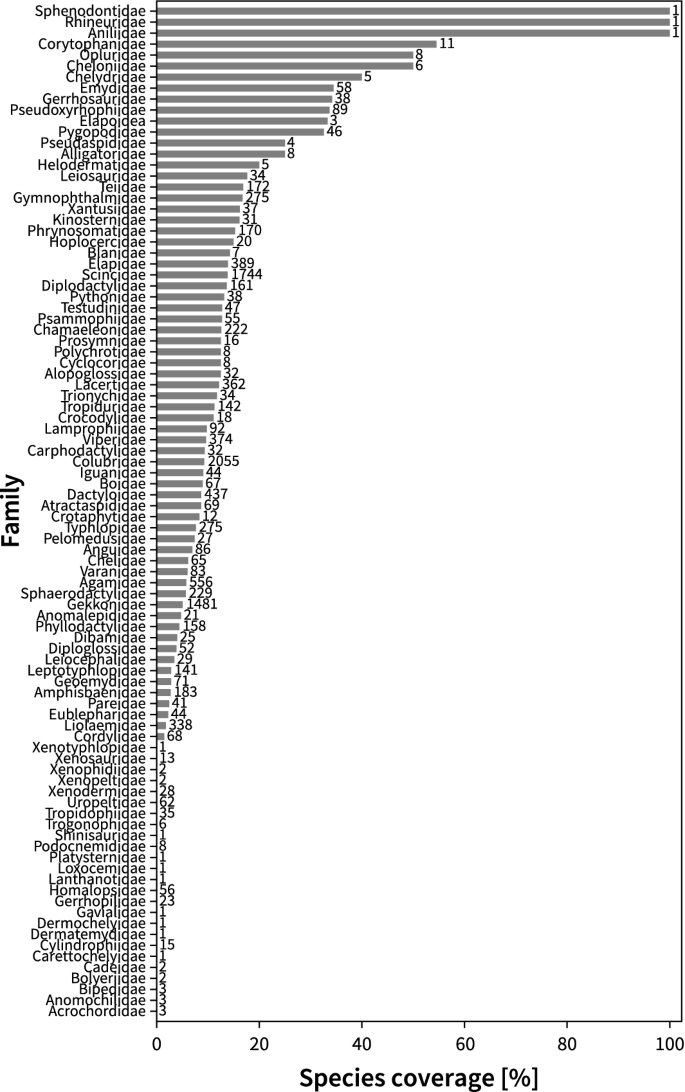
Species coverage for each taxonomic family in selected papers of reptiles. The numbers on each row indicate the total number of species known in its respective family (obtained from ReptileDatabase)
Multiple correspondence analysis provided important insights into underlying patterns in our data allowing us to visualise the relationship between forms of habitat fragmentation (Median = 1 [1–4]), sampling methods (Median = 1 [0–5]) and response variables (Median = 2 [1–6]). Percentage of variance (or eigenvalues) from MCA output represents the contribution of each dimension in explaining the observed patterns. The top ten new dimensions identified by MCA explained a total of 61.64% and 61.16% of the total variance for amphibians and reptiles respectively. The two dimensions with the highest variance percentages explained were found in the first (Dim1, 12.55%) and second (Dim2, 9.13%) dimensions in amphibians (Online Appendix 3–4). Genetics (sampling method; 13.73%) and population (response variable; 12.39%) contributed the most to Dim1, together with species richness (response variable;10.41%) and dispersal (response variable; 9.20%). For Dim2, experimental (sampling method; 14.38%) was the dominant variable, the rest was determined by GIS/Satellite images (sampling method; 9.71%), fitness measure (response variable; 9.12%) and urbanisation (form of fragmentation; 8.94%). For reptiles, the two dimensions explaining the most variation were the first (Dim1, 11.34%) and second (Dim2, 8.28%) dimensions (Online Appendix 3–4). The variables contributing the most to Dim1 were species richness (response variable; 15.51%), abundance (response variable; 10.11%), presence/absence (response variable; 6.97%) and genetics (sampling method; 6.39%). On the other hand, Dim2 was determined by interspecific interaction (response variable; 13.49%), genetics (12.79%), experimental methods (sampling method; 11.21%) and fitness measure (response variable; 10.94%). The contribution of each category to the definition of the dimensions is reported in Online Appendix 3. The categories identified in the MCA dimensions are subsequently used for building the distance matrix in the clustering analysis.
The HCPC analysis identified three clusters of variables for amphibians and reptiles (Online Appendix 5–6). The output of the HCPC analysis is reported in Online Appendix 7. V test represent the influence of variables in the cluster composition. In general, three clusters for both amphibians and reptiles appeared to be uniquely similar by definition of categories (Fig. 6 ). For amphibians, cluster 1 was defined by studies on species richness (p < 0.05, V test = 14.30) and presence/absence (p < 0.05, V test = 13.42), while cluster 2 was determined by experimental studies (p < 0.05, V test = 10.95) and fitness measures (p < 0.05, V test = 9.77). Cluster 3 was defined by genetics (p < 0.05, V test = 18.44) and population studies (p < 0.05, V test = 17.73) (Online Appendix 7). Abundance and functional richness were also unique to cluster 1; other response variables and direct tracking methods were important to cluster 2 and dispersal was present in cluster 3 even though these variables are less expressed (Fig. 6 a).
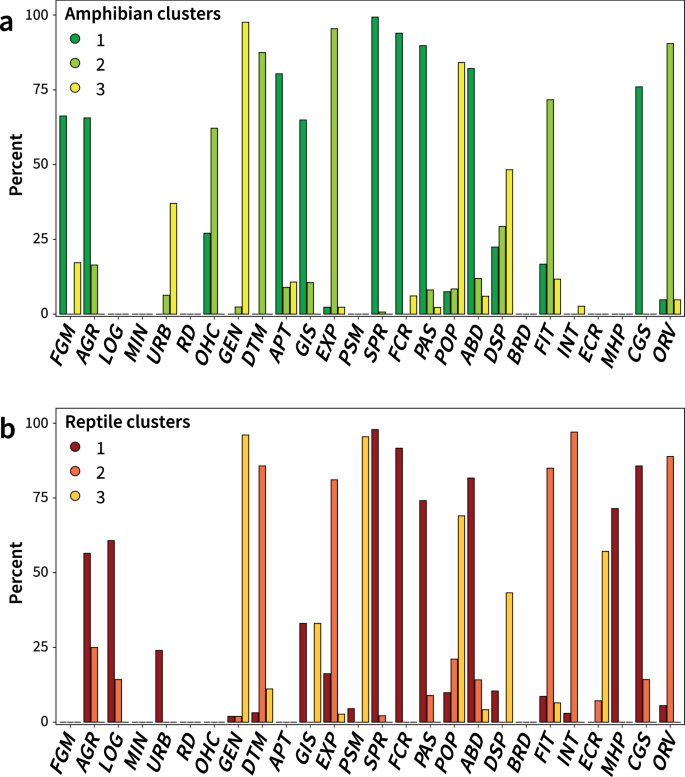
Percentage contribution of the categories contributing to the uniqueness of each cluster in amphibians (Dark green = 1, Bright green = 2, Bright yellow = 3) and reptiles (Dark red = 1, Orange = 2, Dark yellow = 3) based on the Cla/Mod results of HCPC (see Online Appendix 7). Abbreviations for the categories can be found in Fig. 3 and in Online Appendix 1
For reptiles, cluster 1 was represented by species richness (p < 0.05, V test = 14.26), abundance (p < 0.05, V test = 11.22) and presence absence (p < 0.05, V test = 8.55) papers, whereas cluster 2 was determined by papers on fitness measures (p < 0.05, V test = 10.99), direct tracking methods (p < 0.05, V test = 8.64) and interspecific interaction (p < 0.05, V test = 7.86), and cluster 3 was defined by genetics (p < 0.05, V test = 12.79), population (p < 0.05, V test = 9.95) and prediction/simulation models (p < 0.05, V test = 7.68) papers (Online Appendix 7). Although slightly less expressed in the clusters, papers using comparisons between generalist and specialist species and papers on functional richness were also unique to cluster 1; experimental methods and other response variables were heavily present in cluster 2, while dispersal studies were distinct to cluster 3 (Fig. 6 b).
Results from VOSviewer categories of both amphibians and reptiles appear to be similar to each other (Fig. 3 c, d). The clustering of the categories in the co-occurrence network maps confirms what we observed in the HCPC results (Fig. 6 ). In addition to geographical representation of study locations in (1), the corresponding clusters of selected papers are also mapped in Figs. 7 and 8 to investigate the spatial grouping patterns for the three clusters (see Online Appendix 8–9 for geographical representation for each category). We also plotted the temporal trend in Online Appendix 10 and 11. Overall, the three clusters are distributed homogeneously across the globe, but concentrated in the USA, Europe and south eastern Australia. Cluster 1 papers were found to be the most predominant cluster in amphibians (57% papers) across all continents (see Online Appendix 12; Fig. 7 ). When compared to other clusters, studies from this cluster are often conducted in Afrotropics, particularly Madagascar (100% papers), central (Costa Rica (60% papers) and Mexico (92% papers) and south America (80% papers) (Online Appendix 12, Figs. 7 , 8 ). On the other hand, cluster 2 papers appear to be more prevalent for reptile studies compared to amphibian studies, with a higher number of studies conducted across North America (65 to 51) and Australia (22 to 2) (Figs. 7 , 8 ). Lastly, a vast majority of cluster 3 papers were located in North America and Europe (both contributing to 79% of the papers) for amphibians and North America and Australia (both contributing to 84% of the papers) for reptiles (Online Appendix 12, Figs. 7 , 8 ). Publications from this cluster started to gain popularity from 2005 onwards, following similar increasing trends as cluster 2 (Online Appendix 10–11). Overall, except for cluster 1 in South America, most of the clusters in Asia and Africa appear to experience very little or no increase in publications over the years (Online Appendix 10–11).
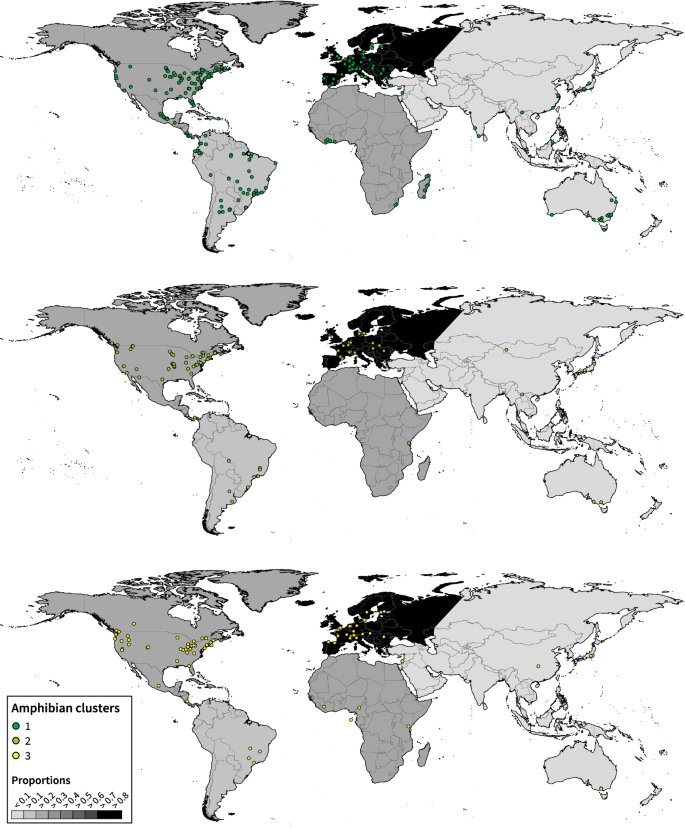
Map of the individual selected papers belonging to each cluster groups (Dark green = 1, Bright green = 2, Bright yellow = 3) for amphibians, with each circle representing the study location. The colour scale of the continents ranging from 0 to 0.9 indicates the proportions of amphibian species represented in the reviewed papers when compared to known species in the world (obtained from AmphibiaWeb)
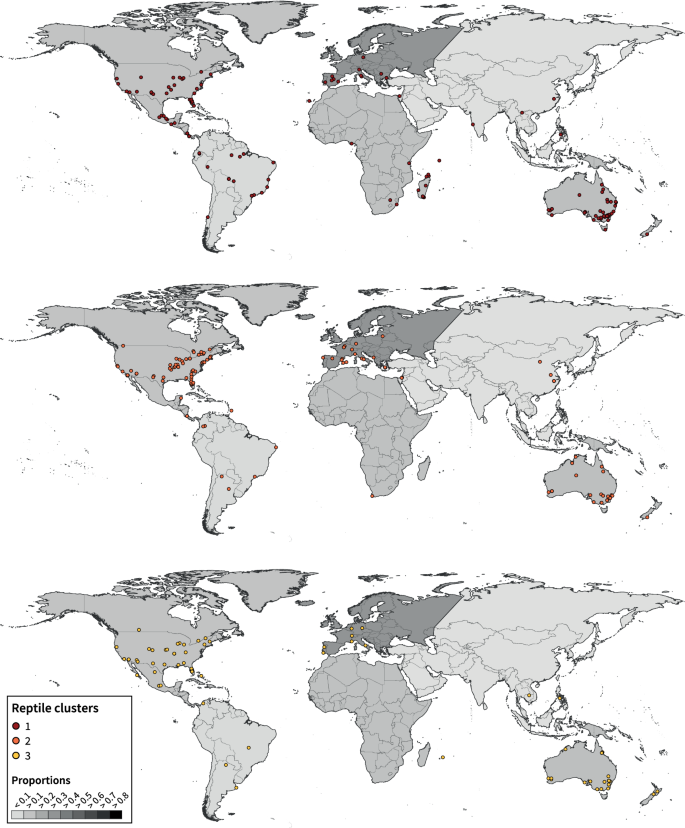
Map of the individual selected papers belonging to each cluster groups (Dark red=1, Orange=2, Dark yellow=3) for reptiles, with each circle representing the study location. The colour scale of the continents ranging from 0.0 – 0.9 indicates the proportions of reptile species represented in the reviewed papers when compared to known species in the world (obtained from ReptileDatabase).
Our review found no improvement in the geographical and taxonomic bias in habitat fragmentation studies for both reptiles and amphibians compared to earlier studies (Fardila et al. 2017 ). Yet, our study has made an effective contribution towards identifying major spatial gaps in habitat fragmentation studies over the past three decades (updating reviews such as Cushman 2006 ; Gardner et al. 2007 )). In particular, we found an overall increase in the number of studies measuring species richness and abundance throughout the years while population-level and genetics studies are still lacking in developing countries. Here, we discuss the issues of (1) biogeographical bias, (2) the extent and focus of habitat fragmentation research and (3) the limitations and knowledge gaps in habitat fragmentation research in herpetology and provide recommendations for future research.
Biogeographical bias
Geographic bias in research papers.
Given the research effort in relatively wealthy countries (Holmgren and Schnitzer 2004 ; Fazey et al. 2005 ) it is not surprising that more than half the papers concern North America and Europe, where there is strong prevalence of herpetological research. This pattern is also evident in other taxonomic groups and biological areas including invasion biology (Pyšek et al. 2008 ), biodiversity conservation (Trimble and Aarde 2012 ; Christie et al. 2020 ), and habitat fragmentation (Fardila et al. 2017 ). The USA alone contributed more than a third of the publications in terms of both authors and location of study (Fazey et al. 2005 ; Melles et al. 2019 ). English speaking countries including the USA, the United Kingdom, and Australia have dominated research output over the last 30 years (Melles et al. 2019 ). These patterns were reflected in the collaboration network maps generated by VOSviewer (Fig. 3 ). Similar hotspots found between who does the research (Fig. 3 ) and the study locations (Fig. 2 ) suggest that authors tend not to move much and only to study ecosystems near to where they are based (Meyer et al. 2015 ). One reason for this bias is the distance to field sites accentuated by the costs and time of travelling.
However, the near absence of studies from many parts of the world that are currently under extreme pressures of habitat loss and degradation are of great concern (Habel et al. 2019 ). We feel that the level of threat associated with habitat fragmentation in these continents is not proportional to the level of research attention required. Naturally biodiverse but less economically developed Southeast Asian and sub-Saharan countries will suffer greatest diversity losses in the next century (Newbold et al. 2015 ). If this persists at the current rate, biodiverse areas will likely disappear before new discoveries in those hotspots are made (Moura and Jetz 2021 ). Although conversely our study found that among other developing countries Brazil is currently conducting relatively more in-country amphibian studies and collaboration with other countries. However, how much of this information reaches decision makers and practitioners remains unknown. This is largely due to the lack of intermediary evidence bridges (Kadykalo et al. 2021 ). These intermediaries identify evidence summaries based on research and priorities and distribute them to practitioners, facilitating exchange of knowledge between and among researchers and practitioners (Holderegger et al. 2019 ; Kadykalo et al. 2021 ).
Geographic bias in focal groups
Congruent to results reported in Gardner et al. ( 2007 ), studies on amphibians were more abundant than studies on reptiles. Over the past years, there has been a strong focus on amphibian population declines. This was catalysed by the emergence of chytridiomycosis and global decline of amphibians (Fisher and Garner 2020 ). Amphibians, and predominantly frogs, are the principal focus of herpetological research, with the highest allocation of resources and the highest publication rates (Ferronato 2019 ). Another reason for this bias may be that amphibians serve as valuable indicators of environmental stress and degradation owing to their aquatic and terrestrial lifestyle and permeable skin (Green 2003 ). These attributes make them extremely sensitive to changes in temperature and precipitation as well as pollution (Sodhi et al. 2008 ). Lizards, also susceptible to temperature changes, however, are characterised by a high degree of endemism, restricted geographic ranges, late maturity, a long life-span and are thus very susceptible to population declines (Todd et al. 2010 ; Meiri et al. 2018 ). Certain groups of reptiles, such as worm lizards and blind snakes lead cryptic and solitary lives in contrast to the large breeding aggregations and choruses of, for example, frogs. Such characteristics make them difficult for researchers to study as they require large amount of search effort for little data (Thompson 2013 ).
- Taxonomic bias
We found a heightened geographical bias in the taxonomic coverage of studies. Given the sheer number of selected papers investigated, it is not surprising that the continents of North and South America cover more than half of the amphibian species studied whereas North America and Africa cover almost half of the reptile species studied. This trend broadly mirrors the geographic distribution pattern of the global described species in both these taxa (AmphibiaWeb 2021 ; Uetz et al. 2021 ). While a large proportion of the known European and North American families such as Alytidae and Ambystomatidae have been investigated (Fig. 4 ), species from other continents remain severely under-represented. Yet, the European continent represents only 2% of the described species globally. This high research intensity bias in low biodiverse regions of the world has been noted previously (Fazey et al. 2005 ). In general, reptiles and amphibians have been disproportionately poorly studied in the tropics and in developing areas despite that these areas show among the highest rates of deforestation and a corresponding rise in the number of threatened species (Böhm et al. 2013 ; Deikumah et al. 2014 ). These biodiverse areas largely consist of threatened species having restricted home ranges (Meiri et al. 2018 ). Even though we observed a great fraction of the species investigated in the Afrotropics (Vallan 2002 ; Hillers et al. 2008 ; Ofori‐Boateng et al. 2013 ; Riemann et al. 2015 ; Blumgart et al. 2017 ), especially Madagascar (see Mantellidae and Opluridae in Fig. 4 ), it seems insufficient when considering that an estimated 3.94 million hectares of forest area of the continent was cleared yearly over the last century (FAO and UNEP 2020 ). Further, biodiverse hotspots such as the neotropical regions and Indo-Malayan tropics have the highest chances of new species of amphibians and reptiles being discovered (Moura and Jetz 2021 ).
Being herpetofauna diversity hotspots, countries in South America and Asia are indeed understudied. Although Brazil has a high number of amphibian studies, less than one percent of known reptile species was studied in both continents (Fig. 2 ). A number of factors contribute to this lack of representation. First, there is an overwhelming number of new species being discovered every year in these hotspots (Moura et al. 2018 ; Moura and Jetz 2021 ). Furthermore, newly discovered species tend to belong to more secretive groups such as burrowing snakes, worm lizards and caecilians (Colli et al. 2016 ). Yet, these fossorial organisms are clearly neglected in fragmentation studies (see Fig. 4 – 5 ) with researchers focusing on well-known taxonomic groups (Böhm et al. 2013 ). On a positive note, despite having the country (Australia) with the highest reptile diversity (Uetz et al. 2021 ), Oceania represented a fair coverage of reptile diversity compared to other continents. Since 2001, there has been an increase of fragmentation studies in Australia (e.g., Brown 2001 ; Mac Nally and Brown 2001 ; Hazell et al. 2001 ) and there is a continuing increase in research output (Melles et al. 2019 ), contributing 85 out of 89 reviewed studies in Oceania over the last 30 years.
Extent and focus of research
Our findings showed important associations between methods and response metrics but not different forms of habitat fragmentation. This either suggests that researchers were not favouring any sampling method and response variable for evaluating the effects of certain habitat fragmentation or this pattern may occur due to a relatively even split of papers dealing with different forms or combinations of habitat fragmentation in the clusters. In general, species richness or diversity appears to explain most of the variation in our data ( see Online Appendix 4 ). While species richness remains a popular diversity metric employed in conservation biology (Online Appendix 12; also see Gardner et al. 2007 ), we also found an increasing trend in the use of genetic techniques for habitat fragmentation studies. More specifically in recent years, molecular genetics have become popular and are often studied together with population connectivity to capture species responses to habitat fragmentation ( see Online Appendix 4 ) (Keyghobadi 2007 ). The HCPC approach identified three main clusters of research fields which will be referred to as research agendas from here onwards. Contrary to our expectation, we did not find a global spatial pattern of research agendas, but instead a rather homogeneous distribution of papers, possibly due to the lack of selected studies which are found in developing countries outside USA, Europe and Australia (Figs. 7 , 8 ). This nevertheless indicates that different sampling methods are shared and used between leading herpetological experts from different countries and that there are continuing collaborations between countries, particularly in North America and Europe.
Below, we describe the research agendas and their corresponding categories (Fig. 6 ) that have contributed significantly to the study of habitat fragmentation for the past 30 years: (a) Agenda 1: Measures of direct individual species responses, (b) Agenda 2: Physiological and movement ecology, and (c) Agenda 3: Technology advancement in conservation research.
Agenda 1: Measures of direct individual species responses
We found that the majority of studies around the globe evaluated patterns of assemblage richness, species presence/absence, and abundance (Figs. 7 , 8 ). These simple patterns of richness, diversity and abundance are the most common responses measured because they provide a good indication of species response to habitat fragmentation and are easy to calculate (Colwell 2009 ). Although species richness does not consider abundance or biomass but treats each species as of equal importance to diversity, species evenness weighs each species by its relative abundance (Hill 1972). Further, composite measures like species diversity indices (e.g., Simpson’s 1/D or Shannon’s H) combine both richness and evenness in a single measure (Colwell 2009 ), preventing biases in results. However, directly measuring these species responses might not be ecologically relevant as they fail to account for patterns in species assemblage turnover. In fact, few selected papers (38 out of 697) in our study have attempted to categorise species into meaningful functional groups or guilds, despite that the categorisation of ecological functions such as habitat preference, taxonomic family, reproductive mode, and body size can be easily done (but see Knutson et al. 1999 ; Peltzer et al. 2006 ; Moreira and Maltchik 2014 ). Knutson et al.( 1999 ) was the first in our selected papers to group species with similar life-history characteristics into guilds and to examine their responses to landscape features. They observed negative associations between urban land use and anuran guilds. Analyses of guilds or functional groups can reveal contradictory results (but not always, see Moreira and Maltchik 2014 ). For example, the species richness of anurans in logged areas of West Africa is found to be as high as in primary habitat (Ernst et al. 2006 ). Yet, analyses of functional groups indicated significantly higher diversity in primary forest communities (Ernst et al. 2006 ). Similar differences were also observed for species with varying degrees of niche overlaps, habitat specialists, and for different continents (Ernst et al. 2006 ; Seshadri 2014 ). These results underline that species richness alone is a poor indicator of the functional value of species in the ecosystem as the relationships between functional diversity and species richness are inconsistent and can sometimes be redundant (functional diversity remains constant if assemblages are functionally similar; Riemann et al. 2017 ; Palmeirim et al. 2017 ; Silva et al. 2022 ). The results of some species richness studies may consequently provide misleading inferences regarding consequences of habitat fragmentation and conservation management (Gardner et al. 2007 ).
Although not substantially greater than the agendas 2 and 3, the measure of individual species responses has always been popular across the globe but also increasingly popular in the tropical and subtropical regions (e.g., South America and Africa; Online Appendix 10–11). For example, a research team led by Mark-Oliver Roedel from Germany has conducted numerous studies on Afrotropical amphibian communities (Hillers et al. 2008 ; Ofori‐Boateng et al. 2013 ; Riemann et al. 2017 ). Due to the higher biodiversity and species rarity in these regions compared to temperate areas, it is reasonable to expect a greater level of sampling effort in patterns of species richness, abundance, and guild assemblage to obtain comparisons of diversity with sufficient statistical power across different land use changes (Gardner et al. 2007 ). Access to highly specific expertise and most up to date methods and technology may not be available in these regions, and as such, study designs are limited to multispecies survey addressing simple patterns of diversity and species assemblages (Hetu et al. 2019 ). Unfortunately at the same time, these forest biomes holding the highest richness and abundance of amphibians and reptiles have showed consistent negative responses to land use changes (Cordier et al. 2021 ).
Agenda 2: physiological and movement ecology
We did not observe a strong association between occupancy and dispersal in our study. Perhaps this is because only a few papers investigated dispersal via habitat occupancy compared to the overwhelming proportions of papers examining the presence of species in response to habitat fragmentation in research agenda 1. Similarly, few studies measure dispersal with direct tracking methods, with the majority that discussed dispersal being based on indirect inferences, such as genetic divergence (see Fig. 3 c, d; Driscoll et al. 2014 ). Genetic approaches can be effective in situations where more direct approaches are not possible (Lowe and Allendorf 2010 ). For instance, using microsatellites and mitochondrial DNA, Buckland et al. ( 2014 ) found no migration occurring between isolated subpopulations of a forest day gecko ( Phelsuma guimbeaui ) in a fragmented forest and predicted a dramatic decrease in survival and allelic diversity in the next 50 years if no migration occurs (Buckland et al. 2014 ). In some cases, molecular markers also allow direct dispersal studies by assigning individuals to their parents or population of origin (Manel et al. 2005 ). However, there are limitations on when these techniques can be applied. Assignment tests require appropriate choices of molecular markers and sampling design to permit quantification of indices of dispersal (Broquet and Petit 2009 ; Lowe and Allendorf 2010 ). Parent–offspring analysis is constrained by the uncertainty in assessing whether offspring dispersal is completed at the time of sampling and sample size (Broquet and Petit 2009 ). Genetic tools may thus be best applied in combination with direct approaches because they contain complementary information (Lowe and Allendorf 2010 ; Safner et al. 2011 ; Smith et al. 2016 ).
Traditional approaches in habitat fragmentation research like radiotracking or capture-mark-recapture of animals can be effective in evaluating dispersal and ecological connectivity between populations. For example, based on mark-recapture data over a nine year period, facultative dispersal rates in an endangered amphibian ( Bombina variegata ) were found to be sex biased and relatively low from resulting patch loss (Cayuela et al. 2018 ). In our case, direct tracking methods are more commonly and effectively used in examining the impacts of habitat modification on changes in ecology directly relating to fitness (Fig. 6 ): home ranges (Price-Rees et al. 2013 ), foraging grounds (MacDonald et al. 2012 ) and survival rates (Breininger et al. 2012 ). Yet, such routine movements associated with resource exploitation do not reflect the biological reality and evolutionary consequences of how organisms change as landscape changes (Van Dyck and Baguette 2005 ). Instead, directed behavioural movements affecting dispersal processes (emigration, displacement or immigration) are crucial in determining the functional connectivity between populations in a fragmented landscape (Bonte et al. 2012 ). In one study, spotted salamanders Ambystoma maculatum tracked with fluorescent powder exhibited strong edge mediated behaviour when dispersing across borders between forest and field habitats and can perceive forest habitats from some distance (Pittman and Semlitsch 2013 ). Knowing such behaviour rules can improve predictions of the effects of habitat configuration on survival and dispersal. However, ongoing conversion of natural ecosystems to human modified land cover increases the need to consider various cover types that may be permeable to animal movements. As such, experimental approaches can be effective in examining the effect of matrix type on species movements as seen in our results (Fig. 6 ) (Rothermel and Semlitsch 2002 ; Mazerolle and Desrochers 2005 ). For example, researchers conducted experimental releases of post-metamorphic individuals of forest amphibians into different substrates and mapped the movements of paths and performance (Cline and Hunter Jr 2016 ). They showed that non-forest matrices with lower structural complexity influence the ability of frogs to travel across open cover and to orient themselves towards the forest from distances greater than 40–55 m. Therefore, it is inaccurate to assume matrix permeability to be uniform across all open-matrix types, particularly in amphibians (Cline and Hunter 2014 , 2016 ).
In addition, the ability to move and disperse is highly dependent on the range of external environments and internal physiological limits (Bonte et al. 2012 ), especially in reptiles and amphibians (Nowakowski et al. 2017 ). The study of physiological effects on movement was seen throughout our selected studies (Fig. 6 ). For example, higher temperatures and lower soil moisture in open habitats could increase evaporative water loss in salamanders (Rothermel and Semlitsch 2002 ). Other tests including interaction effects between landscape configuration and physiological constraints (e.g., dehydration rate Rothermel and Semlitsch 2002 ; Watling and Braga 2015 ); body size (Doherty et al. 2019 ) can be useful to better understand fitness and population persistence. We argue here that multidisciplinary projects examining movement physiology, behaviour and environmental constraints in addition to measuring distance moved are needed to progress this field.
Our results indicate a high bias of agenda 2 papers represented among developed countries, with a strong focus on reptiles compared to amphibians (Price-Rees et al. 2013 ; Doherty et al. 2019 ) (Online Appendix 12, Figs. 7 , 8 ). The adoption of direct tracking as well as genetic methods can be cost prohibitive in developing and poorer regions. However, cheaper and simpler methods to track individuals are increasing (Mennill et al. 2012 ; Cline and Hunter 2014 , 2016 ). Although existing application might not be ideal for reptiles and amphibians, new technologies for tagging and tracking small vertebrates are being developed including acoustic surveys and improved genetic methods (Broquet and Petit 2009 ; Mennill et al. 2012 ; Marques et al. 2013 ). While there are many improvements needed to obtain better quality dispersal data studies on movement ecology, reptiles and amphibians still only account for a mere 2.2% of the studies on dispersal when compared to plants and invertebrates which comprised over half of the studies based on a systematic review (Driscoll et al. 2014 ). Thus, we urge more studies to be conducted on these lesser-known taxa, especially in biodiverse regions. Given the limited dispersal in amphibians and reptiles, having a deeper understanding on their dispersal can be critical for the effective management and conservation of populations and metapopulations (Smith and Green 2005 ).
Agenda 3: technology advancement in conservation research
While community level approaches such as responses in species richness, occupancy, and abundance measure biodiversity response to habitat fragmentation, they are limited in inference because they do not reflect patterns of fitness across environmental gradients and landscape patterns. Instead, genetic structure at the population level can offer a higher resolution of species responses (Manel and Holderegger 2013 ). For instance, genetic erosion heavily affects the rate of species loss in many amphibian species (Allentoft and O’Brien 2010 ; Rivera‐Ortíz et al. 2015 ). Over the past decades we have seen a rapid increase in studies applying genetic analysis to assess the effects of habitat fragmentation (Keyghobadi 2007 ), reflecting the strength of these approaches. This growth is mostly evident in North America and Europe (but also Oceania for reptiles) ( Online Appendix 10–11). The availability of different genetic markers has been increasing, from microsatellites in the 1990s then shifting towards genotyping by sequencing (NGS) technologies that enable rapid genome-wide development (Allendorf et al. 2010 ; Monteiro et al. 2019 ). However, the study of population structure alone can lead to misleading results as environmental changes to species dynamics are not considered. The resistance imposed by landscape features on the dispersal of animals can ultimately shape gene flow and genetic structure (Bani et al. 2015 ; Pilliod et al. 2015 ; Monteiro et al. 2019 ).
To understand this, researchers combine genetic, land cover and climate variables to study the gene flow patterns across heterogeneous and fragmented landscapes (Manel and Holderegger 2013 ). Spatial analyses can be a powerful tool for monitoring biodiversity by quantifying environmental and landscape parameters. The growing interest in both landcover data and the rapid development of computer processing power prompted the development of new prediction methods, primarily in spatial models (Ray et al. 2002 ), ecological niche modelling (Urbina-Cardona and Loyola 2008 ; Tan et al. 2021 ), and landscape connectivity (Cushman et al. 2013 ; Ashrafzadeh et al. 2019 ). In some cases, niche models are useful in assessing the effectiveness of protected areas for endangered species (Urbina-Cardona and Loyola 2008 ; Tan et al. 2021 ).
The integration of genetic data in ecological niche models for recognising possible dispersal movements between populations were observed in our study (Fig. 3 c, d), especially in reptiles (Fig. 6b ). The hallmark of landscape genetics is the ability to estimate functional connectivity among populations and offer empirical approach of adaptive genetic variation in real landscapes to detect environmental factors driving evolutionary adaptation. The most common approach of landscape genetics is determining whether effective distances as determined by the presence of suitable habitat between populations, better predict genetic distances than do Euclidean distances (assuming spatially homogeneous landscape). However, straight-line geographic distance does not normally reflect true patterns of dispersal as landscape barriers or facilitators in a heterogeneous landscape could strongly affect gene flow (Emel and Storfer 2012 ; Fenderson et al. 2020 ). Therefore, in these cases, ecological distances or landscape resistance can often explain a greater deal of genetic variation between fragmented populations (Cushman 2006 ; Bani et al. 2015 ). Using a combination of habitat suitability modelling (e.g., Maxent, Phillips et al. 2017 ), multiple least-cost paths (LCPs) (Adriaensen et al. 2003 ) and the more recent circuit theory analysis (McRae et al. 2008 ) to investigate landscape resistance can be highly effective predicting potential pathways along which dispersal may occur, hence informing conservation management (Emel and Storfer 2012 ; Bani et al. 2015 ; Pilliod et al. 2015 ). To date, landscape genetics has been shown to be particularly useful in studying organisms with complex life histories (Emel and Storfer 2012 ; Shaffer et al. 2015 ). Yet, the applications of landscape genetics have been limited to contemporary patterns using modern genetic data. Few studies have benefitted from the inclusion of temporal genetic data (Fenderson et al. 2020 ). For example, historical DNA samples and heterochronous analyses could allow us to explore how anthropogenic impacts have affected past genetic diversity and population dynamics (Pacioni et al. 2015 ) and identify areas of future suitability of endangered animals in face of climate change (Nogués-Bravo et al. 2016 ). The possibility to investigate migration through spatiotemporal population connectivity can greatly improve the prediction of species responses under future landscape and climate change scenarios (Fenderson et al. 2020 ).
Population genetic and niche modelling studies for both taxa are rarely found in developing regions of the world, especially in Asia and Africa (Figs. 7 , 8 ). Even though conservation priorities are concentrated in these biodiverse regions, invaluable highly specific expertise such as conservation genetics and other contemporary methodologies might not be readily available due to lack of funding and infrastructure (Hetu et al. 2019 ). Thus, we encourage collaborations with the poorer countries initiated by foreign service providers from developed countries. Contrary to expectations, very few studies on conservation genetics were found in China and Japan despite their vast advances in genetic techniques. Fortunately, China has made substantial progress in the last 20 years in understanding human genetic history and interpreting genetic studies of human diseases (Forero et al. 2016 ) as well as biodiversity conservation (Wang et al. 2020 ), yet the same cannot be said for conservation genetics on reptiles and amphibians (Figs. 7 , 8 ), but see Fan et al. ( 2018 ) and Hu et al. ( 2021 ).
Limitations and knowledge gaps
The forms of habitat fragmentation which we categorised may not reflect the ecological impact in the real world as interactions between different habitat fragmentation forms were not accounted for. Although each of these forms of habitat fragmentation possesses serious environmental consequences, their combination could have severe synergistic impacts (Blaustein and Kiesecker 2002 ). For example, a fragmented landscape is not just reduced and isolated, but subject to other anthropogenic disturbances such as hunting, fire, invasive species, and pollution (Laurance and Useche 2009 ; Lazzari et al. 2022 ). Altered climatic conditions and emerging pathogens such as batrachochytrids can also interact with each other, and other threats (Fisher and Garner 2020 ). The use of habitat suitability models based on climatic scenarios, combined with hydrological and urbanisation models, are effective in detecting best to worst case scenarios and local extinctions, as shown for the spotted marsh frog ( Limnodynastes tasmaniensis ) (Wilson et al. 2013 ).
We acknowledge the bias of scientific research introduced from the limitation of search term to English-speaking literature on the geographic distribution of the papers we sampled (Konno et al. 2020 ; Angulo et al. 2021 ). In Latin American journals for example, we found a number of papers published in Spanish, but unfortunately, they did not fit the criteria of our selection (see Online Appendix 2). Conservation studies written in languages other than English are often published in local journals which do not normally go through international peer review.
The homogeneous distribution of the research agendas across geographical regions in our study may be explained by the lack of studies found in South America, Asia and Africa, preventing us to see a potentially dichotomous spatial pattern among the clusters. However, this reflects the current state of research and the challenges faced in less developed countries.
(4) Our study did not investigate whether habitat fragmentation has led to an improved or decreased biotic response. Predicting species response to habitat modification has been reviewed countless times (Rytwinski and Fahrig 2012 ; Driscoll et al. 2014 ; Doherty et al. 2020 ; Newbold et al. 2020 ; Cordier et al. 2021 ). Yet, these reviews often yield little or no general patterns (Doherty et al. 2020 ; Cordier et al. 2021 ). Response variables or traits measured are often found to be poor predictors of the impacts of habitat fragmentation. There are two possible explanations for this discrepancy. First, the strength and direction of the responses differs between species, ecophysiological groups (Rothermel and Semlitsch 2002 ), and phylogenetic or functional groups (Mazerolle and Desrochers 2005 ; Nowakowski et al. 2017 ). Second, responses in animals to different types of disturbance may be specific to the ecosystem where they live. Different biogeographic regions or biomes have different characteristics affecting local species (Lindell et al. 2007 ; Blowes et al. 2019 ; Newbold et al. 2020 ; Cordier et al. 2021 ).
Conclusions and recommendations
Our results underline promising research fields and geographic areas and may serve as a guideline or starting point for future habitat fragmentation studies. We suspect similar paradigms of geographic and thematic patterns to occur in other taxonomic groups.
Although studies dealing with habitat fragmentation impacts on mammals and birds are already widely recognised (Fardila et al. 2017 ), research on reptiles and amphibians has been lacking. We argue that amphibians and reptiles need more attention as they are equally or more threatened but highly neglected (Rytwinski and Fahrig 2012 ; Ferronato 2019 ; Cox et al. 2022 ).
Greater investment is required for studies in tropical and subtropical areas (Segovia et al. 2020 ), especially within the Asian continent. These areas are currently experiencing the highest rates of habitat loss (McDonald et al. 2013 ). Tropical specialists are further restricted to smaller geographic range sizes according to Rapoport’s rule which states that there is a positive latitudinal correlation with range size (Stevens 1989 ) (at least for amphibians in the Northern hemisphere where there is higher temperature and precipitation seasonality; Whitton et al. 2012 ). Having a small range size is often associated with negative responses to habitat modification (Doherty et al. 2020 ). Thus, more effort is needed in developing countries where the crisis is greatest and there is lack of funding and strong language barriers (Fazey et al. 2005 ). There is an urgent need to better integrate studies published in languages other than English with the broader international literature. Useful integration actions include training of local conservation biologists and promoting partnerships and research visits in these regions may have greater conservation consequences to understand global patterns of habitat modification (Meyer et al. 2015 ). Doing so will help remediate the sampling bias towards temperate generalists and will shed light on the fate of tropical specialists.
We encourage improved access to intermediary evidence-based conservation data (Kadykalo et al. 2021 ). Even when well-established genetic and genomic analyses have been proven to be promising area in herpetological conservation (Shaffer et al. 2015 ), there is a general lack of the transfer of knowledge between scientists and practitioners (Holderegger et al. 2019 ). As practitioners are generally interested in species monitoring and the evaluation of success of connectivity measures, an establishment of scientist-practitioner community to facilitate a platform for international exchange would help tremendously in future conservation planning and management (Holderegger et al. 2019 ).
Although different study designs and landscape measures have different strengths and limitations depending on the study objectives, we suggest reporting basic data to describe the effect of habitat fragmentation using standardised sampling methods, indices, and design (Holderegger et al. 2019 ). The results will allow future meta-analyses to be performed.
Incorporate remote sensing data, whenever possible, in studies involving habitat change and fragmentation. The use of niche modelling techniques combined with high resolution remote sensing has been instrumental in detecting potentially fragmented populations. With advances in landscape genomics, we are now able to examine the correlation between environmental factors and genomic data in natural populations (Manel and Holderegger 2013 ; Shaffer et al. 2015 ). Adopting such tools would be valuable in understanding how habitat amounts and configurations affect dispersal, survival, and population dynamics as well as the impacts of anthropogenic changes such as climate change (Shaffer et al. 2015 ).
Data availability
The datasets generated during the current study are available in Online Appendix 1. Codes used in the analyses are available from corresponding author on request.
Adriaensen F, Chardon JP, De Blust G et al (2003) The application of ‘least-cost’ modelling as a functional landscape model. Landsc Urban Plan 64:233–247. https://doi.org/10.1016/S0169-2046(02)00242-6
Article Google Scholar
Allendorf FW, Hohenlohe PA, Luikart G (2010) Genomics and the future of conservation genetics. Nat Rev Genet 11:697–709. https://doi.org/10.1038/nrg2844
Article CAS Google Scholar
Allentoft ME, O’Brien J (2010) Global amphibian declines, loss of genetic diversity and fitness: a review. Diversity 2:47–71. https://doi.org/10.3390/d2010047
AmphibiaWeb (2021) AmphibiaWeb. https://amphibiaweb.org/ . Accessed 22 Feb 2021
Angulo E, Diagne C, Ballesteros-Mejia L et al (2021) Non-English languages enrich scientific knowledge: the example of economic costs of biological invasions. Sci Total Environ 775:144441. https://doi.org/10.1016/j.scitotenv.2020.144441
Ashrafzadeh MR, Naghipour AA, Haidarian M et al (2019) Effects of climate change on habitat and connectivity for populations of a vulnerable, endemic salamander in Iran. Glob Ecol Conserv 19:e00637. https://doi.org/10.1016/j.gecco.2019.e00637
Bani L, Pisa G, Luppi M et al (2015) Ecological connectivity assessment in a strongly structured fire salamander ( Salamandra salamandra ) population. Ecol Evol 5:3472–3485. https://doi.org/10.1002/ece3.1617
Barber PH, Ablan-Lagman MCA, Ambariyanto, et al (2014) Advancing biodiversity research in developing countries: the need for changing paradigms. Bull Mar Sci 90:187–210. https://doi.org/10.5343/bms.2012.1108
Barlow J, França F, Gardner TA et al (2018) The future of hyperdiverse tropical ecosystems. Nature 559:517–526. https://doi.org/10.1038/s41586-018-0301-1
Blaustein AR, Kiesecker JM (2002) Complexity in conservation: lessons from the global decline of amphibian populations. Ecol Lett 5:597–608. https://doi.org/10.1046/j.1461-0248.2002.00352.x
Blowes SA, Supp SR, Antão LH et al (2019) The geography of biodiversity change in marine and terrestrial assemblages. Science 366:339–345. https://doi.org/10.1126/science.aaw1620
Blumgart D, Dolhem J, Raxworthy CJ (2017) Herpetological diversity across intact and modified habitats of Nosy Komba Island, Madagascar. J Nat Hist 51:625–642. https://doi.org/10.1080/00222933.2017.1287312
Böhm M, Collen B, Baillie JEM et al (2013) The conservation status of the world’s reptiles. Biol Conserv 157:372–385. https://doi.org/10.1016/j.biocon.2012.07.015
Bonte D, Van Dyck H, Bullock JM et al (2012) Costs of dispersal. Biol Rev 87:290–312. https://doi.org/10.1111/j.1469-185X.2011.00201.x
Breininger DR, Mazerolle MJ, Bolt MR et al (2012) Habitat fragmentation effects on annual survival of the federally protected eastern indigo snake: indigo snake survival. Anim Conserv 15:361–368. https://doi.org/10.1111/j.1469-1795.2012.00524.x
Broquet T, Petit EJ (2009) Molecular estimation of dispersal for ecology and population genetics. Annu Rev Ecol Evol Syst 40:193–216. https://doi.org/10.1146/annurev.ecolsys.110308.120324
Brown GW (2001) The influence of habitat disturbance on reptiles in a Box-Ironbark eucalypt forest of south-eastern Australia. Biodivers Conserv 10:161–176. https://doi.org/10.1023/A:1008919521638
Buckland S, Cole NC, Groombridge JJ et al (2014) High risks of losing genetic diversity in an endemic Mauritian gecko : implications for conservation. PLoS ONE 9:e93387. https://doi.org/10.1371/journal.pone.0093387
Cayuela H, Besnard A, Quay L et al (2018) Demographic response to patch destruction in a spatially structured amphibian population. J Appl Ecol 55:2204–2215. https://doi.org/10.1111/1365-2664.13198
Christie AP, Amano T, Martin PA et al (2020) The challenge of biased evidence in conservation. Conserv Biol 35:249–262. https://doi.org/10.1111/cobi.13577
Cline BB, Hunter ML Jr (2014) Different open-canopy vegetation types affect matrix permeability for a dispersing forest amphibian. J Appl Ecol 51:319–329. https://doi.org/10.1111/1365-2664.12197
Cline BB, Hunter ML Jr (2016) Movement in the matrix: substrates and distance-to-forest edge affect postmetamorphic movements of a forest amphibian. Ecosphere 7:e01202. https://doi.org/10.1002/ecs2.1202
Colli GR, Fenker J, Tedeschi LG et al (2016) In the depths of obscurity: Knowledge gaps and extinction risk of Brazilian worm lizards (Squamata, Amphisbaenidae). Biol Conserv 204:51–62. https://doi.org/10.1016/j.biocon.2016.07.033
Colwell R (2009) Biodiversity: concepts, patterns, and measurement. The Princeton guide to ecology. Princeton, Princeton University Press, pp 257–263
Chapter Google Scholar
Cordier JM, Aguilar R, Lescano JN et al (2021) A global assessment of amphibian and reptile responses to land-use changes. Biol Conserv 253:108863. https://doi.org/10.1016/j.biocon.2020.108863
Cox N, Young BE, Bowles P et al (2022) A global reptile assessment highlights shared conservation needs of tetrapods. Nature 605:285–290. https://doi.org/10.1038/s41586-022-04664-7
Cushman SA (2006) Effects of habitat loss and fragmentation on amphibians: a review and prospectus. Biol Conserv 128:231–240. https://doi.org/10.1016/j.biocon.2005.09.031
Cushman SA, Shirk AJ, Landguth EL (2013) Landscape genetics and limiting factors. Conserv Genet 14:263–274. https://doi.org/10.1007/s10592-012-0396-0
Deikumah JP, McAlpine CA, Maron M (2014) Biogeographical and taxonomic biases in tropical forest fragmentation research. Conserv Biol J Soc Conserv Biol 28:1522–1531. https://doi.org/10.1111/cobi.12348
Doherty TS, Fist CN, Driscoll DA (2019) Animal movement varies with resource availability, landscape configuration and body size: a conceptual model and empirical example. Landsc Ecol 34:603–614. https://doi.org/10.1007/s10980-019-00795-x
Doherty TS, Balouch S, Bell K et al (2020) Reptile responses to anthropogenic habitat modification: a global meta-analysis. Glob Ecol Biogeogr 29:1265–1279. https://doi.org/10.1111/geb.13091
Driscoll DA, Banks SC, Barton PS et al (2014) The trajectory of dispersal research in conservation biology. Syst Rev PLOS ONE 9:e95053. https://doi.org/10.1371/journal.pone.0095053
Driscoll DA, Armenteras D, Bennett AF et al (2021) How fire interacts with habitat loss and fragmentation. Biol Rev 96:976–998. https://doi.org/10.1111/brv.12687
Emel SL, Storfer A (2012) A decade of amphibian population genetic studies: synthesis and recommendations. Conserv Genet 13:1685–1689. https://doi.org/10.1007/s10592-012-0407-1
Ernst R, Linsenmair KE, Rödel M-O (2006) Diversity erosion beyond the species level: dramatic loss of functional diversity after selective logging in two tropical amphibian communities. Biol Conserv 133:143–155. https://doi.org/10.1016/j.biocon.2006.05.028
Fahrig L (2003) Effects of habitat fragmentation on biodiversity. Annu Rev Ecol Evol Syst 34:487–515. https://doi.org/10.1146/annurev.ecolsys.34.011802.132419
Fahrig L (2017) Ecological responses to habitat fragmentation per se. Annu Rev Ecol Evol Syst 48:1–23. https://doi.org/10.1146/annurev-ecolsys-110316-022612
Fan H, Hu Y, Wu Q et al (2018) Conservation genetics and genomics of threatened vertebrates in China. J Genet Genomics 45:593–601. https://doi.org/10.1016/j.jgg.2018.09.005
FAO and UNEP (2020) The State of the World’s Forests 2020: Forests, biodiversity and people. FAO and UNEP,
Fardila D, Kelly LT, Moore JL, McCarthy MA (2017) A systematic review reveals changes in where and how we have studied habitat loss and fragmentation over 20years. Biol Conserv 212:130–138. https://doi.org/10.1016/j.biocon.2017.04.031
Fazey I, Fischer J, Lindenmayer DB (2005) Who does all the research in conservation biology? Biodivers Conserv 14:917–934. https://doi.org/10.1007/s10531-004-7849-9
Fenderson LE, Kovach AI, Llamas B (2020) Spatiotemporal landscape genetics: investigating ecology and evolution through space and time. Mol Ecol 29:218–246. https://doi.org/10.1111/mec.15315
Ferronato B (2019) An assessment of funding and publication rates in herpetology. Herpetol J. https://doi.org/10.33256/hj29.4.264273
Fisher MC, Garner TWJ (2020) Chytrid fungi and global amphibian declines. Nat Rev Microbiol 18:332–343. https://doi.org/10.1038/s41579-020-0335-x
Forero DA, Wonkam A, Wang W et al (2016) Current needs for human and medical genomics research infrastructure in low and middle income countries. J Med Genet 53:438–440. https://doi.org/10.1136/jmedgenet-2015-103631
Gardner TA, Barlow J, Peres CA (2007) Paradox, presumption and pitfalls in conservation biology: the importance of habitat change for amphibians and reptiles. Biol Conserv 138:166–179. https://doi.org/10.1016/j.biocon.2007.04.017
Gibbons JW, Scott DE, Ryan TJ et al (2000) The Global Decline of Reptiles, Déjà Vu Amphibians: reptile species are declining on a global scale. Six significant threats to reptile populations are habitat loss and degradation, introduced invasive species, environmental pollution, disease, unsustainable use, and global climate change. Bioscience 50:653–666. https://doi.org/10.1641/0006-3568(2000)050[0653:TGDORD]2.0.CO;2
Green DM (2003) The ecology of extinction: population fluctuation and decline in amphibians. Biol Conserv 111:331–343. https://doi.org/10.1016/S0006-3207(02)00302-6
Habel JC, Rasche L, Schneider UA et al (2019) Final countdown for biodiversity hotspots. Conserv Lett 12:e12668. https://doi.org/10.1111/conl.12668
Haddad NM, Brudvig LA, Clobert J et al (2015) Habitat fragmentation and its lasting impact on Earth’s ecosystems. Sci Adv 1:e1500052. https://doi.org/10.1126/sciadv.1500052
Hadley AS, Betts MG (2016) Refocusing habitat fragmentation research using lessons from the last decade. Curr Landsc Ecol Rep 1:55–66. https://doi.org/10.1007/s40823-016-0007-8
Hamer AJ, McDonnell MJ (2008) Amphibian ecology and conservation in the urbanising world: a review. Biol Conserv 141:2432–2449. https://doi.org/10.1016/j.biocon.2008.07.020
Hazell D, Cunnningham R, Lindenmayer D et al (2001) Use of farm dams as frog habitat in an Australian agricultural landscape: factors affecting species richness and distribution. Biol Conserv 102:155–169. https://doi.org/10.1016/S0006-3207(01)00096-9
Hetu M, Koutouki K, Joly Y (2019) Genomics for All: International Open Science Genomics Projects and Capacity Building in the Developing World. Front Genet. https://doi.org/10.3389/fgene.2019.00095
Hillers A, Veith M, Rödel M-O (2008) Effects of forest fragmentation and habitat degradation on West African leaf-litter frogs. Conserv Biol 22:762–772. https://doi.org/10.1111/j.1523-1739.2008.00920.x
Holderegger R, Balkenhol N, Bolliger J et al (2019) Conservation genetics: linking science with practice. Mol Ecol 28:3848–3856. https://doi.org/10.1111/mec.15202
Holmgren M, Schnitzer SA (2004) Science on the rise in developing countries. PLOS Biol 2:e1. https://doi.org/10.1371/journal.pbio.0020001
Hu Y, Fan H, Chen Y et al (2021) Spatial patterns and conservation of genetic and phylogenetic diversity of wildlife in China. Sci Adv 7:eabd5725. https://doi.org/10.1126/sciadv.abd5725
Kadykalo AN, Buxton RT, Morrison P et al (2021) Bridging research and practice in conservation. Conserv Biol 35:1725–1737. https://doi.org/10.1111/cobi.13732
Keyghobadi NK (2007) The genetic implications of habitat fragmentation for animals. Can J Zool. https://doi.org/10.1139/Z07-095
Knutson MG, Sauer JR, Olsen DA et al (1999) Effects of Landscape Composition and Wetland Fragmentation on Frog and Toad Abundance and Species Richness in Iowa and Wisconsin, U.S.A. Conserv Biol 13:1437–1446. https://doi.org/10.1046/j.1523-1739.1999.98445.x
Konno K, Akasaka M, Koshida C et al (2020) Ignoring non-English-language studies may bias ecological meta-analyses. Ecol Evol 10:6373–6384. https://doi.org/10.1002/ece3.6368
Laurance WF, Useche DC (2009) Environmental synergisms and extinctions of tropical species. Conserv Biol 23:1427–1437. https://doi.org/10.1111/j.1523-1739.2009.01336.x
Lazzari J, Sato CF, Driscoll DA (2022) Traits influence reptile responses to fire in a fragmented agricultural landscape. Landsc Ecol 37:2363–2382. https://doi.org/10.1007/s10980-022-01417-9
Lê S, Josse J, Husson F (2008) FactoMineR: an R package for multivariate analysis. J Stat Softw 25:1–18. https://doi.org/10.18637/jss.v025.i01
Lebart L, Morineau A, Piron M (1995) Statistique exploratoire multidimensionnelle. Dunod Paris
Lindell CA, Riffell SK, Kaiser SA et al (2007) Edge responses of tropical and temperate birds. Wilson J Ornithol 119:205–220. https://doi.org/10.1676/05-133.1
Lindenmayer DB, Fischer J (2007) Tackling the habitat fragmentation panchreston. Trends Ecol Evol 22:127–132. https://doi.org/10.1016/j.tree.2006.11.006
Lowe WH, Allendorf FW (2010) What can genetics tell us about population connectivity? Mol Ecol 19:3038–3051. https://doi.org/10.1111/j.1365-294X.2010.04688.x
Mac Nally R, Brown GW (2001) Reptiles and habitat fragmentation in the box-ironbark forests of central Victoria, Australia: predictions, compositional change and faunal nestedness. Oecologia 128:116–125. https://doi.org/10.1007/s004420100632
MacDonald B, Lewison R, Madrak S et al (2012) Home ranges of East Pacific green turtles Chelonia mydas in a highly urbanized temperate foraging ground. Mar Ecol Prog Ser 461:211–221. https://doi.org/10.3354/meps09820
Manel S, Gaggiotti OE, Waples RS (2005) Assignment methods: matching biological questions with appropriate techniques. Trends Ecol Evol 20:136–142. https://doi.org/10.1016/j.tree.2004.12.004
Manel S, Holderegger R (2013) Ten years of landscape genetics. Trends Ecol Evol 28:614–621. https://doi.org/10.1016/j.tree.2013.05.012
Marques TA, Thomas L, Martin SW et al (2013) Estimating animal population density using passive acoustics. Biol Rev 88:287–309. https://doi.org/10.1111/brv.12001
Mazerolle MJ, Desrochers A (2005) Landscape resistance to frog movements. Can J Zool. https://doi.org/10.1139/z05-032
McDonald RI, Marcotullio PJ, Güneralp B (2013) Urbanization and Global Trends in Biodiversity and Ecosystem Services. In: Elmqvist T, Fragkias M, Goodness J et al (eds) Urbanization, Biodiversity and Ecosystem Services: Challenges and Opportunities: A Global Assessment. Springer, Dordrecht, pp 31–52
Google Scholar
McRae BH, Dickson BG, Keitt TH, Shah VB (2008) Using circuit theory to model connectivity in ecology, evolution, and conservation. Ecology 89:2712–2724. https://doi.org/10.1890/07-1861.1
Meiri S, Bauer AM, Allison A et al (2018) Extinct, obscure or imaginary: the lizard species with the smallest ranges. Divers Distrib 24:262–273. https://doi.org/10.1111/ddi.12678
Melles SJ, Scarpone C, Julien A et al (2019) Diversity of practitioners publishing in five leading international journals of applied ecology and conservation biology, 1987–2015 relative to global biodiversity hotspots. Écoscience 26:323–340. https://doi.org/10.1080/11956860.2019.1645565
Mennill DJ, Battiston M, Wilson DR et al (2012) Field test of an affordable, portable, wireless microphone array for spatial monitoring of animal ecology and behaviour. Methods Ecol Evol 3:704–712. https://doi.org/10.1111/j.2041-210X.2012.00209.x
Meyer C, Kreft H, Guralnick R, Jetz W (2015) Global priorities for an effective information basis of biodiversity distributions. Nat Commun 6:8221. https://doi.org/10.1038/ncomms9221
Moher D, Liberati A, Tetzlaff J et al (2009) Preferred reporting items for systematic reviews and meta-analyses: the PRISMA statement. PLoS Med 6:e1000097. https://doi.org/10.1371/journal.pmed.1000097
Monteiro WP, Veiga JC, Silva AR et al (2019) Everything you always wanted to know about gene flow in tropical landscapes (but were afraid to ask). PeerJ 7:e6446. https://doi.org/10.7717/peerj.6446
Moreira LFB, Maltchik L (2014) Does organic agriculture benefit anuran diversity in rice fields? Wetlands 34:725–733. https://doi.org/10.1007/s13157-014-0537-y
Moura MR, Jetz W (2021) Shortfalls and opportunities in terrestrial vertebrate species discovery. Nat Ecol Evol 5:631–639. https://doi.org/10.1038/s41559-021-01411-5
Moura MR, Costa HC, Peixoto MA et al (2018) Geographical and socioeconomic determinants of species discovery trends in a biodiversity hotspot. Biol Conserv 220:237–244. https://doi.org/10.1016/j.biocon.2018.01.024
Newbold T, Hudson LN, Phillips HRP et al (2014) A global model of the response of tropical and sub-tropical forest biodiversity to anthropogenic pressures. Proc R Soc B Biol Sci 281:20141371. https://doi.org/10.1098/rspb.2014.1371
Newbold T, Hudson LN, Hill SLL et al (2015) Global effects of land use on local terrestrial biodiversity. Nature 520:45–50. https://doi.org/10.1038/nature14324
Newbold T, Oppenheimer P, Etard A, Williams JJ (2020) Tropical and Mediterranean biodiversity is disproportionately sensitive to land-use and climate change. Nat Ecol Evol 4:1630–1638. https://doi.org/10.1038/s41559-020-01303-0
Nogués-Bravo D, Veloz S, Holt BG et al (2016) Amplified plant turnover in response to climate change forecast by Late Quaternary records. Nat Clim Change 6:1115–1119. https://doi.org/10.1038/nclimate3146
Nowakowski AJ, Watling JI, Whitfield SM et al (2017) Tropical amphibians in shifting thermal landscapes under land-use and climate change. Conserv Biol 31:96–105. https://doi.org/10.1111/cobi.12769
Ofori-Boateng C, Oduro W, Hillers A et al (2013) Differences in the Effects of Selective Logging on Amphibian Assemblages in Three West African Forest Types. Biotropica 45:94–101. https://doi.org/10.1111/j.1744-7429.2012.00887.x
Pacioni C, Hunt H, Allentoft ME et al (2015) Genetic diversity loss in a biodiversity hotspot: ancient DNA quantifies genetic decline and former connectivity in a critically endangered marsupial. Mol Ecol 24:5813–5828. https://doi.org/10.1111/mec.13430
Palmeirim AF, Vieira MV, Peres CA (2017) Herpetofaunal responses to anthropogenic forest habitat modification across the neotropics: insights from partitioning β-diversity. Biodivers Conserv 26:2877–2891. https://doi.org/10.1007/s10531-017-1394-9
Peltzer PM, Lajmanovich RC, Attademo AM, Beltzer AH (2006) Diversity of anurans across agricultural ponds in Argentina. In: Hawksworth DL, Bull AT (eds) Marine, Freshwater, and Wetlands Biodiversity Conservation. Springer, Dordrecht, pp 131–145
Phillips SJ, Anderson RP, Dudík M et al (2017) Opening the black box: an open-source release of Maxent. Ecography 40:887–893. https://doi.org/10.1111/ecog.03049
Pilliod DS, Arkle RS, Robertson JM et al (2015) Effects of changing climate on aquatic habitat and connectivity for remnant populations of a wide-ranging frog species in an arid landscape. Ecol Evol 5:3979–3994. https://doi.org/10.1002/ece3.1634
Pittman SE, Semlitsch RD (2013) Habitat type and distance to edge affect movement behavior of juvenile pond-breeding salamanders. J Zool 291:154–162. https://doi.org/10.1111/jzo.12055
Price-Rees SJ, Brown GP, Shine R (2013) Spatial ecology of bluetongue lizards ( Tiliqua spp.) in the Australian wet–dry tropics. Austral Ecol 38:493–503. https://doi.org/10.1111/j.1442-9993.2012.02439.x
Pyšek P, Richardson DM, Pergl J et al (2008) Geographical and taxonomic biases in invasion ecology. Trends Ecol Evol 23:237–244. https://doi.org/10.1016/j.tree.2008.02.002
R Core Team (2021) R: A language and environment for statistical computing
Ray N, Lehmann A, Joly P (2002) Modeling spatial distribution of amphibian populations: a GIS approach based on habitat matrix permeability. Biodivers Conserv 11:2143–2165. https://doi.org/10.1023/A:1021390527698
Riemann JC, Ndriantsoa SH, Raminosoa NR et al (2015) The value of forest fragments for maintaining amphibian diversity in Madagascar. Biol Conserv 191:707–715. https://doi.org/10.1016/j.biocon.2015.08.020
Riemann JC, Ndriantsoa SH, Rödel M-O, Glos J (2017) Functional diversity in a fragmented landscape — Habitat alterations affect functional trait composition of frog assemblages in Madagascar. Glob Ecol Conserv 10:173–183. https://doi.org/10.1016/j.gecco.2017.03.005
Riva F, Fahrig L (2022) Protecting many small patches will maximize biodiversity conservation for most taxa: the SS > SL principle. Preprints
Rivera-Ortíz FA, Aguilar R, Arizmendi MDC et al (2015) Habitat fragmentation and genetic variability of tetrapod populations. Anim Conserv 18:249–258. https://doi.org/10.1111/acv.12165
Rothermel BB, Semlitsch RD (2002) An Experimental investigation of landscape resistance of forest versus old-field habitats to emigrating juvenile amphibians. Conserv Biol 16:1324–1332. https://doi.org/10.1046/j.1523-1739.2002.01085.x
Roux BL, Rouanet H (2004) Geometric Data Analysis: From Correspondence Analysis to Structured Data Analysis. Springer Science, Dordrecht
Rytwinski T, Fahrig L (2012) Do species life history traits explain population responses to roads? A meta-analysis. Biol Conserv 147:87–98. https://doi.org/10.1016/j.biocon.2011.11.023
Safner T, Miaud C, Gaggiotti O et al (2011) Combining demography and genetic analysis to assess the population structure of an amphibian in a human-dominated landscape. Conserv Genet 12:161–173. https://doi.org/10.1007/s10592-010-0129-1
Segovia ALR, Romano D, Armsworth PR (2020) Who studies where? Boosting tropical conservation research where it is most needed. Front Ecol Environ 18:159–166. https://doi.org/10.1002/fee.2146
Seshadri KS (2014) Effects of Historical Selective Logging on Anuran Communities in a Wet Evergreen Forest, South India. Biotropica 46:615–623. https://doi.org/10.1111/btp.12141
Shaffer HB, Gidiş M, McCartney-Melstad E et al (2015) Conservation genetics and genomics of amphibians and reptiles. Annu Rev Anim Biosci 3:113–138. https://doi.org/10.1146/annurev-animal-022114-110920
Silva DJ, Palmeirim AF, Santos-Filho M et al (2022) Habitat Quality, Not Patch Size, Modulates Lizard Responses to Habitat Loss and Fragmentation in the Southwestern Amazon. J Herpetol 56:75–83. https://doi.org/10.1670/20-145
Smith MA, Green DM (2005) Dispersal and the metapopulation paradigm in amphibian ecology and conservation: are all amphibian populations metapopulations? Ecography 28:110–128. https://doi.org/10.1111/j.0906-7590.2005.04042.x
Smith AL, Landguth EL, Bull CM et al (2016) Dispersal responses override density effects on genetic diversity during post-disturbance succession. Proc R Soc B Biol Sci 283:20152934. https://doi.org/10.1098/rspb.2015.2934
Sodhi NS, Koh LP, Brook BW, Ng PKL (2004) Southeast Asian biodiversity: an impending disaster. Trends Ecol Evol 19:654–660. https://doi.org/10.1016/j.tree.2004.09.006
Sodhi NS, Bickford D, Diesmos AC et al (2008) Measuring the meltdown: drivers of global amphibian extinction and decline. PLoS ONE 3:e1636. https://doi.org/10.1371/journal.pone.0001636
Stevens GC (1989) The latitudinal gradient in geographical range: how so many species coexist in the tropics. Am Nat 133:240–256
Stuart SN, Chanson JS, Cox NA et al (2004) Status and trends of amphibian declines and extinctions worldwide. Science 306:1783–1786. https://doi.org/10.1126/science.1103538
Tan WC, Ginal P, Rhodin AGJ et al (2021) A present and future assessment of the effectiveness of existing reserves in preserving three critically endangered freshwater turtles in Southeast Asia and South Asia. Front Biogeogr. https://doi.org/10.21425/F5FBG50928
Thompson W (2013) Sampling Rare or Elusive Species: Concepts, Designs, and Techniques for Estimating Population Parameters. Island Press, Washington
Todd B, Willson J, Gibbons J (2010) The Global Status of Reptiles and Causes of Their Decline. Ecotoxicology of Amphibians and Reptiles. CRC Press, Boca Raton, pp 47–67
Trimble MJ, van Aarde RJ (2012) Geographical and taxonomic biases in research on biodiversity in human-modified landscapes. Ecosphere 3:art119. https://doi.org/10.1890/ES12-00299.1
Uetz P, Freed P, Aguilar R, Hošek J (2021) The Reptile Database. http://www.reptile-database.org/ . Accessed 6 Mar 2021
Urbina-Cardona JN, Loyola RD (2008) Applying niche-based models to predict endangered-hylid potential distributions: are neotropical protected areas effective enough? Trop Conserv Sci 1:417–445. https://doi.org/10.1177/194008290800100408
Vallan D (2002) Effects of anthropogenic environmental changes on amphibian diversity in the rain forests of Eastern Madagascar. J Trop Ecol 18:725–742
Van Dyck H, Baguette M (2005) Dispersal behaviour in fragmented landscapes: routine or special movements? Basic Appl Ecol 6:535–545. https://doi.org/10.1016/j.baae.2005.03.005
van Eck NJ, Waltman L (2014) Visualizing Bibliometric Networks. In: Ding Y, Rousseau R, Wolfram D (eds) Measuring Scholarly Impact: Methods and Practice. Springer International Publishing, Cham, pp 285–320
Wang W, Feng C, Liu F, Li J (2020) Biodiversity conservation in China: a review of recent studies and practices. Environ Sci Ecotechnology 2:100025. https://doi.org/10.1016/j.ese.2020.100025
Ward JH (1963) Hierarchical grouping to optimize an objective function. J Am Stat Assoc 58:236–244. https://doi.org/10.1080/01621459.1963.10500845
Watling JI, Braga L (2015) Desiccation resistance explains amphibian distributions in a fragmented tropical forest landscape. Landsc Ecol 30:1449–1459. https://doi.org/10.1007/s10980-015-0198-0
Whitton FJS, Purvis A, Orme CDL, Olalla-Tárraga MÁ (2012) Understanding global patterns in amphibian geographic range size: does Rapoport rule? Glob Ecol Biogeogr 21:179–190. https://doi.org/10.1111/j.1466-8238.2011.00660.x
Wilson JN, Bekessy S, Parris KM et al (2013) Impacts of climate change and urban development on the spotted marsh frog ( Limnodynastes tasmaniensis ). Austral Ecol 38:11–22. https://doi.org/10.1111/j.1442-9993.2012.02365.x
Download references
Acknowledgements
W.C. Tan was supported financially through a scholarship by the German Academic Exchange Service (DAAD). This work would not be possible without M. Flecks for his invaluable technical assistance with the figures.
Open Access funding enabled and organized by Projekt DEAL.
Author information
Authors and affiliations.
Herpetology Section, LIB, Museum Koenig, Bonn, Leibniz Institute for the Analysis of Biodiversity Change, Adenauerallee 127, 53113, Bonn, Germany
W. C. Tan & D. Rödder
UMR 7179 C.N.R.S/M.N.H.N., Département Adaptations du Vivant, Bâtiment d’Anatomie Comparée, 55 Rue Buffon, 75005, Paris, France
Department of Biology, Evolutionary Morphology of Vertebrates, Ghent University, K.L. Ledeganckstraat 35, 9000, Gent, Belgium
Department of Biology, University of Antwerp, Universiteitsplein 1, B-2610, Antwerpen, Belgium
You can also search for this author in PubMed Google Scholar
Contributions
WCT, AH, and DR contributed to the study idea and conception. Literature search and data collection were performed by WCT and data analysis by DR and WCT. The first draft of the manuscript was written by WCT and all authors critically revised on later versions. All authors read and approved the final manuscript.
Corresponding author
Correspondence to W. C. Tan .
Ethics declarations
Competing interests.
The authors declare no conflicts of interest.
Additional information
Communicated by Ricardo Correia.
Publisher's Note
Springer Nature remains neutral with regard to jurisdictional claims in published maps and institutional affiliations.
Online appendices
Below is the link to the electronic supplementary material.
Captions for appendices (PDF 288 kb)
Appendix 1(pdf 138 kb), appendix 2 (csv 3608 kb), appendix 3 (xlsx 47 kb), appendix 4 (pdf 113 kb), appendix 5 (pdf 293 kb), appendix 6 (pdf 80 kb), appendix 7 (xlsx 18 kb), appendix 8 (pdf 55343 kb), appendix 9 (pdf 55290 kb), appendix 10 (eps 5675 kb), appendix 11 (eps 5665 kb), appendix 12 (xlsx 13 kb), rights and permissions.
Open Access This article is licensed under a Creative Commons Attribution 4.0 International License, which permits use, sharing, adaptation, distribution and reproduction in any medium or format, as long as you give appropriate credit to the original author(s) and the source, provide a link to the Creative Commons licence, and indicate if changes were made. The images or other third party material in this article are included in the article's Creative Commons licence, unless indicated otherwise in a credit line to the material. If material is not included in the article's Creative Commons licence and your intended use is not permitted by statutory regulation or exceeds the permitted use, you will need to obtain permission directly from the copyright holder. To view a copy of this licence, visit http://creativecommons.org/licenses/by/4.0/ .
Reprints and permissions
About this article
Tan, W.C., Herrel, A. & Rödder, D. A global analysis of habitat fragmentation research in reptiles and amphibians: what have we done so far?. Biodivers Conserv 32 , 439–468 (2023). https://doi.org/10.1007/s10531-022-02530-6
Download citation
Received : 18 August 2022
Revised : 02 December 2022
Accepted : 09 December 2022
Published : 08 January 2023
Issue Date : February 2023
DOI : https://doi.org/10.1007/s10531-022-02530-6
Share this article
Anyone you share the following link with will be able to read this content:
Sorry, a shareable link is not currently available for this article.
Provided by the Springer Nature SharedIt content-sharing initiative
- Habitat change
- Herpetofauna
- Geographical bias
- Research agendas
- Systematic review
- Find a journal
- Publish with us
- Track your research

An official website of the United States government
The .gov means it’s official. Federal government websites often end in .gov or .mil. Before sharing sensitive information, make sure you’re on a federal government site.
The site is secure. The https:// ensures that you are connecting to the official website and that any information you provide is encrypted and transmitted securely.
- Publications
- Account settings
Preview improvements coming to the PMC website in October 2024. Learn More or Try it out now .
- Advanced Search
- Journal List
- Indian J Psychol Med
- v.43(2); 2021 Mar
A Student’s Guide to the Classification and Operationalization of Variables in the Conceptualization and Design of a Clinical Study: Part 1
Chittaranjan andrade.
1 Dept. of Clinical Psychopharmacology and Neurotoxicology, National Institute of Mental Health and Neurosciences, Bengaluru, Karnataka, India.
Students without prior research experience may not know how to conceptualize and design a study. This article explains how an understanding of the classification and operationalization of variables is the key to the process. Variables describe aspects of the sample that is under study; they are so called because they vary in value from subject to subject in the sample. Variables may be independent or dependent. Independent variables influence the value of other variables; dependent variables are influenced in value by other variables. A hypothesis states an expected relationship between variables. A significant relationship between an independent and dependent variable does not prove cause and effect; the relationship may partly or wholly be explained by one or more confounding variables. Variables need to be operationalized; that is, defined in a way that permits their accurate measurement. These and other concepts are explained with the help of clinically relevant examples.
Key Message:
This article explains the following concepts: Independent variables, dependent variables, confounding variables, operationalization of variables, and construction of hypotheses.
In any body of research, the subject of study requires to be described and understood. For example, if we wish to study predictors of response to antidepressant drugs (ADs) in patients with major depressive disorder (MDD), we might select patient age, sex, age at onset of MDD, number of previous episodes of depression, duration of current depressive episode, presence of psychotic symptoms, past history of response to ADs, and other patient and illness characteristics as potential predictors. These characteristics or descriptors are called variables. Whether or not the patient responds to AD treatment is also a variable. A solid understanding of variables is the cornerstone in the conceptualization and preparation of a research protocol, and in the framing of study hypotheses. This subject is presented in two parts. This article, Part 1, explains what independent and dependent variables are, how an understanding of these is important in framing hypotheses, and what operationalization of a variable entails.
Variables are defined as characteristics of the sample that are examined, measured, described, and interpreted. Variables are so called because they vary in value from subject to subject in the study. As an example, if we wish to examine the relationship between age and height in a sample of children, age and height are the variables of interest; their values vary from child to child. In the earlier example, patients vary in age, sex, duration of current depressive episode, and response to ADs. Variables are classified as dependent and independent variables and are usually analyzed as categorical or continuous variables.
Independent and Dependent Variables
Independent variables are defined as those the values of which influence other variables. For example, age, sex, current smoking, LDL cholesterol level, and blood pressure are independent variables because their values (e.g., greater age, positive for current smoking, and higher LDL cholesterol level) influence the risk of myocardial infarction. Dependent variables are defined as those the values of which are influenced by other variables. For example, the risk of myocardial infarction is a dependent variable the value of which is influenced by variables such as age, sex, current smoking, LDL cholesterol level, and blood pressure. The risk is higher in older persons, in men, in current smokers, and so on.
There may be a cause–effect relationship between independent and dependent variables. For example, consider a clinical trial with treatment (iron supplement vs placebo) as the independent variable and hemoglobin level as the dependent variable. In children with anemia, an iron supplement will raise the hemoglobin level to a greater extent than will placebo; this is a cause–effect relationship because iron is necessary for the synthesis of hemoglobin. However, consider the variables teeth and weight . An alien from outer space who has no knowledge of human physiology may study human children below the age of 5 years and find that, as the number of teeth increases, weight increases. Should the alien conclude that there is a cause–effect relationship here, and that growing teeth causes weight gain? No, because a third variable, age, is a confounding variable 1 – 3 that is responsible for both increase in the number of teeth and increase in weight. In general, therefore, it is more proper to state that independent variables are associated with variations in the values of the dependent variables rather than state that independent variables cause variations in the values of the dependent variables. For causality to be asserted, other criteria must be fulfilled; this is out of the scope of the present article, and interested readers may refer to Schunemann et al. 4
As a side note, here, whether a particular variable is independent or dependent will depend on the question that is being asked. For example, in a study of factors influencing patient satisfaction with outpatient department (OPD) services, patient satisfaction is the dependent variable. But, in a study of factors influencing OPD attendance at a hospital, OPD attendance is the dependent variable, and patient satisfaction is merely one of many possible independent variables that can influence OPD attendance.
Importance of Variables in Stating the Research Objectives
Students must have a clear idea about what they want to study in order to conceptualize and frame a research protocol. The first matters that they need to address are “What are my research questions?” and “What are my hypotheses?” Both questions can be answered only after choosing the dependent variables and then the independent variables for study.
In the case of a student who is interested in studying predictors of AD outcomes in patients with MDD, treatment response is the dependent variable and patient and clinical characteristics are possible independent variables. So, the selection of dependent and independent variables helps defines the objectives of the study:
- To determine whether sociodemographic variables, such as age and sex, predict the outcome of an episode of depression in MDD patients who are treated with an AD.
- To determine whether clinical variables, such as age at onset of depression, number of previous depressive episodes, duration of current depressive episode, and the presence of soft neurological signs, predict the outcome of an episode of depression in MDD patients who are treated with an AD.
Note that in a formal research protocol, the student will need to state all the independent variables and not merely list examples. The student may also choose to include additional independent variables, such as baseline biochemical, psychophysiological, and neuroradiological measures.
Importance of Variables in Framing Hypotheses
A hypothesis is a clear statement of what the researcher expects to find in the study. As an example, a researcher may hypothesize that longer duration of current depression is associated with poorer response to ADs. In this hypothesis, the duration of the current episode of depression is the independent variable and treatment response is the dependent variable. It should be obvious, now, that a hypothesis can also be defined as the statement of an expected relationship between an independent and a dependent variable . Or, expressed visually, (independent variable) (arrow) (dependent variable) = hypothesis.
It would be a waste of time and energy to do a study to examine only one question: whether duration of current depression predicts treatment response. So, it is usual for research protocols to include many independent variables and many dependent variables in the generation of many hypotheses, as shown in Table 1 . Pairing each variable in the “independent variable” column with each variable in the “dependent variable” column would result in the generation of these hypotheses. Table 2 shows how this is done for age. Sets of hypotheses can likewise be constructed for the remaining independent and dependent variables in Table 1 . Importantly, the student must select one of these hypotheses as the primary hypothesis; the remaining hypotheses, no matter how many they are, would be secondary hypotheses. It is necessary to have only one hypothesis as the primary hypothesis in order to calculate the sample size necessary for an adequately powered study and to reduce the risk of false positive findings in the analysis. 5 In rare situations, two hypotheses may be considered equally important and may be stated as coprimary hypotheses.
Independent Variables and Dependent Variables in a Study on Sociodemographic and Clinical Prediction of Response of Major Depressive Disorder to Antidepressant Drug Treatment
Combinations of Age with Dependent Variables in the Generation of Hypotheses
Operationalization of Variables
In Table 1 , suicidality is listed as an independent variable and severity of depression, as a dependent variable. These variables need to be operationalized; that is, stated in a way that explains how they will be measured. Table 3 presents three ways in which suicidality can be measured and four ways in which (reduction in) the severity of depression can be measured. Now, each way of measurement in the “independent variable” column can be paired with a way of measurement in the “dependent variable” column, making a total of 12 possible hypotheses. In like manner, the many variables listed in Table 1 can each be operationalized in several different ways, resulting in the generation of a very large number of hypotheses. As already stated, the student must select only one hypothesis as the primary hypothesis.
Possible Ways of Operationalization of Suicidality and Depression
HAM-D: Hamilton Depression Rating Scale, MADRS: Montgomery–Asberg Depression Rating Scale.
Much thought should be given to the operationalization of variables because variables that are carelessly operationalized will be poorly measured; the data collected will then be of poor quality, and the study will yield unreliable results. For example, socioeconomic status may be operationalized as lower, middle, or upper class, depending on the patient’s monthly income, on the total monthly income of the family, or using a validated socioeconomic status assessment scale that takes into consideration income, education, occupation, and place of residence. The student must choose the method that would best suit the needs of the study, and the method that has the greatest scientific acceptability. However, it is also permissible to operationalize the same variable in many different ways and to include all these different operationalizations in the study, as shown in Table 3 . This is because conceptualizing variables in different ways can help understand the subject of the study in different ways.
Operationalization of variables requires a consideration of the reliability and validity of the method of operationalization; discussions on reliability and validity are out of the scope of this article. Operationalization of variables also requires specification of the scale of measurement: nominal, ordinal, interval, or ratio; this is also out of the scope of the present article. Finally, operationalization of variables can also specify details of the measurement procedure. As an example, in a study on the use of metformin to reduce olanzapine-associated weight gain, we may state that we will obtain the weight of the patient but fail to explain how we will do it. Better would be to state that the same weighing scale will be used. Still better would be to state that we will use a weighing instrument that works on the principle of moving weights on a levered arm, and that the same instrument will be used for all patients. And best would be to add that we will weigh patients, dressed in standard hospital gowns, after they have voided their bladder but before they have eaten breakfast. When the way in which a variable will be measured is defined, measurement of that variable becomes more objective and uniform
Concluding Notes
The next article, Part 2, will address what categorical and continuous variables are, why continuous variables should not be converted into categorical variables and when this rule can be broken, and what confounding variables are.
Declaration of Conflicting Interests: The author declared no potential conflicts of interest with respect to the research, authorship, and/or publication of this article.
Funding: The author received no financial support for the research, authorship, and/or publication of this article.
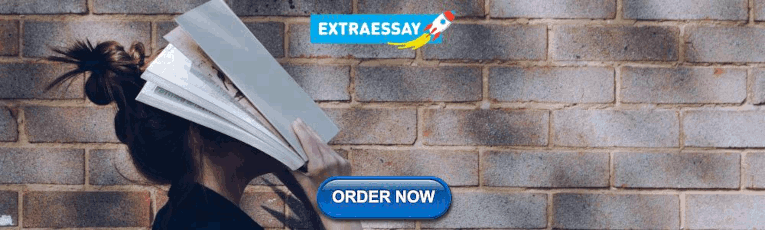
COMMENTS
So, it is usual for research protocols to include many independent variables and many dependent variables in the generation of many hypotheses, as shown in Table 1. Pairing each variable in the "independent variable" column with each variable in the "dependent variable" column would result in the generation of these hypotheses.
Designation of the dependent and independent variable involves unpacking the research problem in a way that identifies a general cause and effect and classifying these variables as either independent or dependent. The variables should be outlined in the introduction of your paper and explained in more detail in the methods section. There are no ...
Suitable statistical design represents a critical factor in permitting inferences from any research or scientific study.[1] Numerous statistical designs are implementable due to the advancement of software available for extensive data analysis.[1] Healthcare providers must possess some statistical knowledge to interpret new studies and provide up-to-date patient care. We present an overview of ...
Examples. Discrete variables (aka integer variables) Counts of individual items or values. Number of students in a class. Number of different tree species in a forest. Continuous variables (aka ratio variables) Measurements of continuous or non-finite values. Distance.
The purpose of research is to describe and explain variance in the world, that is, variance that. occurs naturally in the world or chang e that we create due to manipulation. Variables are ...
Researchers who instead decide to rely on observational data often attempt to deal with its weaknesses by cautiously avoiding causal language: They refer to "associations," "relationships," or tentative "links" between variables instead of clear cause-effect relationships, and they usually add a general disclaimer ("Of course, as the data were only observational, future ...
Abstract. This short "snippet" covers three important aspects related to statistics - the concept of variables, the importance, and practical aspects related to descriptive statistics and issues related to sampling - types of sampling and sample size estimation. Keywords: Biostatistics, descriptive statistics, sample size, variables.
The answer is written in length in the discussion section of the paper. Thus, the research question gives a preview of the different parts and variables of the study meant to address the problem posed in the research question.1 An excellent research question clarifies the research writing while facilitating understanding of the research topic ...
Distinguishing between independent and dependent variables can be tricky when designing a complex study or reading an academic research paper. A dependent variable from one study can be the independent variable in another study, so it's important to pay attention to research design. Here are some tips for identifying each variable type.
In a linear regression model, the dependent variable must be continuous (e.g. intraocular pressure or visual acuity), whereas, the independent variable may be either continuous (e.g. age), binary ...
Compare your paper to billions of pages and articles with Scribbr's Turnitin-powered plagiarism checker. Run a free check. ... Types of Variables in Research | Definitions & Examples. Published on 19 September 2022 by Rebecca Bevans. Revised on 28 November 2022. In statistical research, a variable is defined as an attribute of an object of ...
A variable is the characteristic or attribute of an individual, group, educational system, or the environment that is of interest in a research study. Variables can be straightforward and easy to ...
Moderating and mediating variables, or simply moderators and mediators, are related but distinct concepts in both general statistics and its application in psychology.A moderating variable is a variable that affects the relationship between two other variables. This effect is usually referred to as an interaction.The simplest case of an interaction can occur in analysis of variance (ANOVA).
Types of Variables in Research. Types of Variables in Research are as follows: Independent Variable. This is the variable that is manipulated by the researcher. It is also known as the predictor variable, as it is used to predict changes in the dependent variable. Examples of independent variables include age, gender, dosage, and treatment type ...
On the basis of Rocco (2010), Storberg-Walker's (2012) amended list on qualitative data analysis in research papers included the following: (a) the article should provide enough details so that reviewers could follow the same analytical steps; (b) the analysis process selected should be logically connected to the purpose of the study; and (c ...
It means one level of a categorical variable cannot be considered better or greater than another level. Example: Gender, brands, colors, zip codes. The categorical variable is further categorised into three types: Type of variable. Definition. Example. Dichotomous (Binary) Variable.
While the independent variable is the " cause ", the dependent variable is the " effect " - or rather, the affected variable. In other words, the dependent variable is the variable that is assumed to change as a result of a change in the independent variable. Keeping with the previous example, let's look at some dependent variables ...
A variable is an attribute of an item of analysis in research. The types of variables in research can be categorized into: independent vs. dependent, or categorical vs. quantitative. The types of variables in research (correlational) can be classified into predictor or outcome variables. Other types of variables in research are confounding ...
The paper is intended for the practicing statistician, whose routine work includes the development, estimation, and interpretation of statistical models for observational data to help answering research questions in life sciences. ... In applied research, variable selection methods have too often been misused, giving such data‐driven methods ...
Research methods are specific procedures for collecting and analyzing data. Developing your research methods is an integral part of your research design. When planning your methods, there are two key decisions you will make. First, decide how you will collect data. Your methods depend on what type of data you need to answer your research question:
CAVIAR: Categorical-Variable Embeddings for Accurate and Robust Inference. Social science research often hinges on the relationship between categorical variables and outcomes. We introduce CAVIAR, a novel method for embedding categorical variables that assume values in a high-dimensional ambient space but are sampled from an underlying manifold.
Independent Variable. The independent variable, also known as the IV, is the variable that the researchers are manipulating in an experiment or quasi-experiment. It is also the label given to the "criterion" variable in certain types of regression analysis. For example, if a researcher has two groups of people watch either a happy film or a ...
In any scientific research, there are typically two variables of interest: independent variables and dependent variables. In forming the backbone of scientific experiments, they help scientists understand relationships, predict outcomes and, in general, make sense of the factors that they're investigating.. Understanding the independent variable vs. dependent variable is so fundamental to ...
Categorical and Continuous Variables. Categorical variables are also known as discrete or qualitative variables. These are variables that are operationalized as categories. The value of the variable is expressed as a category count (also known as category frequency) for the sample, or as a cell count (or cell frequency) when the data are presented in a table.
Habitat change and fragmentation are the primary causes of biodiversity loss worldwide. Recent decades have seen a surge of funding, published papers and citations in the field as these threats to biodiversity continue to rise. However, how research directions and agenda are evolving in this field remains poorly understood. In this study, we examined the current state of research on habitat ...
To evaluate the impact of different momentum control variable (CV) schemes (CV5, the momentum control variable option with ψχ and CV7, the momentum control variable option with UV) on radar data assimilation (DA) in weather research and forecasting model data-assimilation (WRFDA) systems, a heavy snowfall in central and eastern regions of China, which started on 6 February 2022, was taken as ...
In any body of research, the subject of study requires to be described and understood. For example, if we wish to study predictors of response to antidepressant drugs (ADs) in patients with major depressive disorder (MDD), we might select patient age, sex, age at onset of MDD, number of previous episodes of depression, duration of current depressive episode, presence of psychotic symptoms ...
This paper reports on the bond behavior of glass fine aggregate reinforced concrete (GFARC) under chloride erosion, considering the chloride solution and glass fine aggregate (GFA) exchange rates as variable parameters. The 16 groups of specimens are designed to conduct central pull-out tests after chloride erosion. The experimental results are analyzed, such as the τ-s curve, ultimate bond ...