
An official website of the United States government
The .gov means it’s official. Federal government websites often end in .gov or .mil. Before sharing sensitive information, make sure you’re on a federal government site.
The site is secure. The https:// ensures that you are connecting to the official website and that any information you provide is encrypted and transmitted securely.
- Publications
- Account settings
Preview improvements coming to the PMC website in October 2024. Learn More or Try it out now .
- Advanced Search
- Journal List
- HHS Author Manuscripts

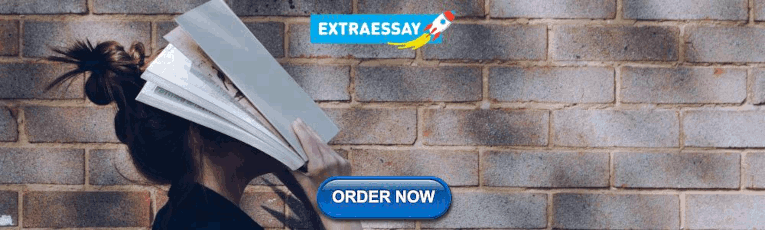
Single-Case Experimental Designs: A Systematic Review of Published Research and Current Standards
Justin d. smith.
Child and Family Center, University of Oregon
This article systematically reviews the research design and methodological characteristics of single-case experimental design (SCED) research published in peer-reviewed journals between 2000 and 2010. SCEDs provide researchers with a flexible and viable alternative to group designs with large sample sizes. However, methodological challenges have precluded widespread implementation and acceptance of the SCED as a viable complementary methodology to the predominant group design. This article includes a description of the research design, measurement, and analysis domains distinctive to the SCED; a discussion of the results within the framework of contemporary standards and guidelines in the field; and a presentation of updated benchmarks for key characteristics (e.g., baseline sampling, method of analysis), and overall, it provides researchers and reviewers with a resource for conducting and evaluating SCED research. The results of the systematic review of 409 studies suggest that recently published SCED research is largely in accordance with contemporary criteria for experimental quality. Analytic method emerged as an area of discord. Comparison of the findings of this review with historical estimates of the use of statistical analysis indicates an upward trend, but visual analysis remains the most common analytic method and also garners the most support amongst those entities providing SCED standards. Although consensus exists along key dimensions of single-case research design and researchers appear to be practicing within these parameters, there remains a need for further evaluation of assessment and sampling techniques and data analytic methods.
The single-case experiment has a storied history in psychology dating back to the field’s founders: Fechner (1889) , Watson (1925) , and Skinner (1938) . It has been used to inform and develop theory, examine interpersonal processes, study the behavior of organisms, establish the effectiveness of psychological interventions, and address a host of other research questions (for a review, see Morgan & Morgan, 2001 ). In recent years the single-case experimental design (SCED) has been represented in the literature more often than in past decades, as is evidenced by recent reviews ( Hammond & Gast, 2010 ; Shadish & Sullivan, 2011 ), but it still languishes behind the more prominent group design in nearly all subfields of psychology. Group designs are often professed to be superior because they minimize, although do not necessarily eliminate, the major internal validity threats to drawing scientifically valid inferences from the results ( Shadish, Cook, & Campbell, 2002 ). SCEDs provide a rigorous, methodologically sound alternative method of evaluation (e.g., Barlow, Nock, & Hersen, 2008 ; Horner et al., 2005 ; Kazdin, 2010 ; Kratochwill & Levin, 2010 ; Shadish et al., 2002 ) but are often overlooked as a true experimental methodology capable of eliciting legitimate inferences (e.g., Barlow et al., 2008 ; Kazdin, 2010 ). Despite a shift in the zeitgeist from single-case experiments to group designs more than a half century ago, recent and rapid methodological advancements suggest that SCEDs are poised for resurgence.
Single case refers to the participant or cluster of participants (e.g., a classroom, hospital, or neighborhood) under investigation. In contrast to an experimental group design in which one group is compared with another, participants in a single-subject experiment research provide their own control data for the purpose of comparison in a within-subject rather than a between-subjects design. SCEDs typically involve a comparison between two experimental time periods, known as phases. This approach typically includes collecting a representative baseline phase to serve as a comparison with subsequent phases. In studies examining single subjects that are actually groups (i.e., classroom, school), there are additional threats to internal validity of the results, as noted by Kratochwill and Levin (2010) , which include setting or site effects.
The central goal of the SCED is to determine whether a causal or functional relationship exists between a researcher-manipulated independent variable (IV) and a meaningful change in the dependent variable (DV). SCEDs generally involve repeated, systematic assessment of one or more IVs and DVs over time. The DV is measured repeatedly across and within all conditions or phases of the IV. Experimental control in SCEDs includes replication of the effect either within or between participants ( Horner et al., 2005 ). Randomization is another way in which threats to internal validity can be experimentally controlled. Kratochwill and Levin (2010) recently provided multiple suggestions for adding a randomization component to SCEDs to improve the methodological rigor and internal validity of the findings.
Examination of the effectiveness of interventions is perhaps the area in which SCEDs are most well represented ( Morgan & Morgan, 2001 ). Researchers in behavioral medicine and in clinical, health, educational, school, sport, rehabilitation, and counseling psychology often use SCEDs because they are particularly well suited to examining the processes and outcomes of psychological and behavioral interventions (e.g., Borckardt et al., 2008 ; Kazdin, 2010 ; Robey, Schultz, Crawford, & Sinner, 1999 ). Skepticism about the clinical utility of the randomized controlled trial (e.g., Jacobsen & Christensen, 1996 ; Wachtel, 2010 ; Westen & Bradley, 2005 ; Westen, Novotny, & Thompson-Brenner, 2004 ) has renewed researchers’ interest in SCEDs as a means to assess intervention outcomes (e.g., Borckardt et al., 2008 ; Dattilio, Edwards, & Fishman, 2010 ; Horner et al., 2005 ; Kratochwill, 2007 ; Kratochwill & Levin, 2010 ). Although SCEDs are relatively well represented in the intervention literature, it is by no means their sole home: Examples appear in nearly every subfield of psychology (e.g., Bolger, Davis, & Rafaeli, 2003 ; Piasecki, Hufford, Solham, & Trull, 2007 ; Reis & Gable, 2000 ; Shiffman, Stone, & Hufford, 2008 ; Soliday, Moore, & Lande, 2002 ). Aside from the current preference for group-based research designs, several methodological challenges have repressed the proliferation of the SCED.
Methodological Complexity
SCEDs undeniably present researchers with a complex array of methodological and research design challenges, such as establishing a representative baseline, managing the nonindependence of sequential observations (i.e., autocorrelation, serial dependence), interpreting single-subject effect sizes, analyzing the short data streams seen in many applications, and appropriately addressing the matter of missing observations. In the field of intervention research for example, Hser et al. (2001) noted that studies using SCEDs are “rare” because of the minimum number of observations that are necessary (e.g., 3–5 data points in each phase) and the complexity of available data analysis approaches. Advances in longitudinal person-based trajectory analysis (e.g., Nagin, 1999 ), structural equation modeling techniques (e.g., Lubke & Muthén, 2005 ), time-series forecasting (e.g., autoregressive integrated moving averages; Box & Jenkins, 1970 ), and statistical programs designed specifically for SCEDs (e.g., Simulation Modeling Analysis; Borckardt, 2006 ) have provided researchers with robust means of analysis, but they might not be feasible methods for the average psychological scientist.
Application of the SCED has also expanded. Today, researchers use variants of the SCED to examine complex psychological processes and the relationship between daily and momentary events in peoples’ lives and their psychological correlates. Research in nearly all subfields of psychology has begun to use daily diary and ecological momentary assessment (EMA) methods in the context of the SCED, opening the door to understanding increasingly complex psychological phenomena (see Bolger et al., 2003 ; Shiffman et al., 2008 ). In contrast to the carefully controlled laboratory experiment that dominated research in the first half of the twentieth century (e.g., Skinner, 1938 ; Watson, 1925 ), contemporary proponents advocate application of the SCED in naturalistic studies to increase the ecological validity of empirical findings (e.g., Bloom, Fisher, & Orme, 2003 ; Borckardt et al., 2008 ; Dattilio et al., 2010 ; Jacobsen & Christensen, 1996 ; Kazdin, 2008 ; Morgan & Morgan, 2001 ; Westen & Bradley, 2005 ; Westen et al., 2004 ). Recent advancements and expanded application of SCEDs indicate a need for updated design and reporting standards.
Many current benchmarks in the literature concerning key parameters of the SCED were established well before current advancements and innovations, such as the suggested minimum number of data points in the baseline phase(s), which remains a disputed area of SCED research (e.g., Center, Skiba, & Casey, 1986 ; Huitema, 1985 ; R. R. Jones, Vaught, & Weinrott, 1977 ; Sharpley, 1987 ). This article comprises (a) an examination of contemporary SCED methodological and reporting standards; (b) a systematic review of select design, measurement, and statistical characteristics of published SCED research during the past decade; and (c) a broad discussion of the critical aspects of this research to inform methodological improvements and study reporting standards. The reader will garner a fundamental understanding of what constitutes appropriate methodological soundness in single-case experimental research according to the established standards in the field, which can be used to guide the design of future studies, improve the presentation of publishable empirical findings, and inform the peer-review process. The discussion begins with the basic characteristics of the SCED, including an introduction to time-series, daily diary, and EMA strategies, and describes how current reporting and design standards apply to each of these areas of single-case research. Interweaved within this presentation are the results of a systematic review of SCED research published between 2000 and 2010 in peer-reviewed outlets and a discussion of the way in which these findings support, or differ from, existing design and reporting standards and published SCED benchmarks.
Review of Current SCED Guidelines and Reporting Standards
In contrast to experimental group comparison studies, which conform to generally well agreed upon methodological design and reporting guidelines, such as the CONSORT ( Moher, Schulz, Altman, & the CONSORT Group, 2001 ) and TREND ( Des Jarlais, Lyles, & Crepaz, 2004 ) statements for randomized and nonrandomized trials, respectively, there is comparatively much less consensus when it comes to the SCED. Until fairly recently, design and reporting guidelines for single-case experiments were almost entirely absent in the literature and were typically determined by the preferences of a research subspecialty or a particular journal’s editorial board. Factions still exist within the larger field of psychology, as can be seen in the collection of standards presented in this article, particularly in regard to data analytic methods of SCEDs, but fortunately there is budding agreement about certain design and measurement characteristics. A number of task forces, professional groups, and independent experts in the field have recently put forth guidelines; each has a relatively distinct purpose, which likely accounts for some of the discrepancies between them. In what is to be a central theme of this article, researchers are ultimately responsible for thoughtfully and synergistically combining research design, measurement, and analysis aspects of a study.
This review presents the more prominent, comprehensive, and recently established SCED standards. Six sources are discussed: (1) Single-Case Design Technical Documentation from the What Works Clearinghouse (WWC; Kratochwill et al., 2010 ); (2) the APA Division 12 Task Force on Psychological Interventions, with contributions from the Division 12 Task Force on Promotion and Dissemination of Psychological Procedures and the APA Task Force for Psychological Intervention Guidelines (DIV12; presented in Chambless & Hollon, 1998 ; Chambless & Ollendick, 2001 ), adopted and expanded by APA Division 53, the Society for Clinical Child and Adolescent Psychology ( Weisz & Hawley, 1998 , 1999 ); (3) the APA Division 16 Task Force on Evidence-Based Interventions in School Psychology (DIV16; Members of the Task Force on Evidence-Based Interventions in School Psychology. Chair: T. R. Kratochwill, 2003); (4) the National Reading Panel (NRP; National Institute of Child Health and Human Development, 2000 ); (5) the Single-Case Experimental Design Scale ( Tate et al., 2008 ); and (6) the reporting guidelines for EMA put forth by Stone & Shiffman (2002) . Although the specific purposes of each source differ somewhat, the overall aim is to provide researchers and reviewers with agreed-upon criteria to be used in the conduct and evaluation of SCED research. The standards provided by WWC, DIV12, DIV16, and the NRP represent the efforts of task forces. The Tate et al. scale was selected for inclusion in this review because it represents perhaps the only psychometrically validated tool for assessing the rigor of SCED methodology. Stone and Shiffman’s (2002) standards were intended specifically for EMA methods, but many of their criteria also apply to time-series, daily diary, and other repeated-measurement and sampling methods, making them pertinent to this article. The design, measurement, and analysis standards are presented in the later sections of this article and notable concurrences, discrepancies, strengths, and deficiencies are summarized.
Systematic Review Search Procedures and Selection Criteria
Search strategy.
A comprehensive search strategy of SCEDs was performed to identify studies published in peer-reviewed journals meeting a priori search and inclusion criteria. First, a computer-based PsycINFO search of articles published between 2000 and 2010 (search conducted in July 2011) was conducted that used the following primary key terms and phrases that appeared anywhere in the article (asterisks denote that any characters/letters can follow the last character of the search term): alternating treatment design, changing criterion design, experimental case*, multiple baseline design, replicated single-case design, simultaneous treatment design, time-series design. The search was limited to studies published in the English language and those appearing in peer-reviewed journals within the specified publication year range. Additional limiters of the type of article were also used in PsycINFO to increase specificity: The search was limited to include methodologies indexed as either quantitative study OR treatment outcome/randomized clinical trial and NOT field study OR interview OR focus group OR literature review OR systematic review OR mathematical model OR qualitative study.
Study selection
The author used a three-phase study selection, screening, and coding procedure to select the highest number of applicable studies. Phase 1 consisted of the initial systematic review conducted using PsycINFO, which resulted in 571 articles. In Phase 2, titles and abstracts were screened: Articles appearing to use a SCED were retained (451) for Phase 3, in which the author and a trained research assistant read each full-text article and entered the characteristics of interest into a database. At each phase of the screening process, studies that did not use a SCED or that either self-identified as, or were determined to be, quasi-experimental were dropped. Of the 571 original studies, 82 studies were determined to be quasi-experimental. The definition of a quasi-experimental design used in the screening procedure conforms to the descriptions provided by Kazdin (2010) and Shadish et al. (2002) regarding the necessary components of an experimental design. For example, reversal designs require a minimum of four phases (e.g., ABAB), and multiple baseline designs must demonstrate replication of the effect across at least three conditions (e.g., subjects, settings, behaviors). Sixteen studies were unavailable in full text in English, and five could not be obtained in full text and were thus dropped. The remaining articles that were not retained for review (59) were determined not to be SCED studies meeting our inclusion criteria, but had been identified in our PsycINFO search using the specified keyword and methodology terms. For this review, 409 studies were selected. The sources of the 409 reviewed studies are summarized in Table 1 . A complete bibliography of the 571 studies appearing in the initial search, with the included studies marked, is available online as an Appendix or from the author.
Journal Sources of Studies Included in the Systematic Review (N = 409)
Note: Each of the following journal titles contributed 1 study unless otherwise noted in parentheses: Augmentative and Alternative Communication; Acta Colombiana de Psicología; Acta Comportamentalia; Adapted Physical Activity Quarterly (2); Addiction Research and Theory; Advances in Speech Language Pathology; American Annals of the Deaf; American Journal of Education; American Journal of Occupational Therapy; American Journal of Speech-Language Pathology; The American Journal on Addictions; American Journal on Mental Retardation; Applied Ergonomics; Applied Psychophysiology and Biofeedback; Australian Journal of Guidance & Counseling; Australian Psychologist; Autism; The Behavior Analyst; The Behavior Analyst Today; Behavior Analysis in Practice (2); Behavior and Social Issues (2); Behaviour Change (2); Behavioural and Cognitive Psychotherapy; Behaviour Research and Therapy (3); Brain and Language (2); Brain Injury (2); Canadian Journal of Occupational Therapy (2); Canadian Journal of School Psychology; Career Development for Exceptional Individuals; Chinese Mental Health Journal; Clinical Linguistics and Phonetics; Clinical Psychology & Psychotherapy; Cognitive and Behavioral Practice; Cognitive Computation; Cognitive Therapy and Research; Communication Disorders Quarterly; Developmental Medicine & Child Neurology (2); Developmental Neurorehabilitation (2); Disability and Rehabilitation: An International, Multidisciplinary Journal (3); Disability and Rehabilitation: Assistive Technology; Down Syndrome: Research & Practice; Drug and Alcohol Dependence (2); Early Childhood Education Journal (2); Early Childhood Services: An Interdisciplinary Journal of Effectiveness; Educational Psychology (2); Education and Training in Autism and Developmental Disabilities; Electronic Journal of Research in Educational Psychology; Environment and Behavior (2); European Eating Disorders Review; European Journal of Sport Science; European Review of Applied Psychology; Exceptional Children; Exceptionality; Experimental and Clinical Psychopharmacology; Family & Community Health: The Journal of Health Promotion & Maintenance; Headache: The Journal of Head and Face Pain; International Journal of Behavioral Consultation and Therapy (2); International Journal of Disability; Development and Education (2); International Journal of Drug Policy; International Journal of Psychology; International Journal of Speech-Language Pathology; International Psychogeriatrics; Japanese Journal of Behavior Analysis (3); Japanese Journal of Special Education; Journal of Applied Research in Intellectual Disabilities (2); Journal of Applied Sport Psychology (3); Journal of Attention Disorders (2); Journal of Behavior Therapy and Experimental Psychiatry; Journal of Child Psychology and Psychiatry; Journal of Clinical Psychology in Medical Settings; Journal of Clinical Sport Psychology; Journal of Cognitive Psychotherapy; Journal of Consulting and Clinical Psychology (2); Journal of Deaf Studies and Deaf Education; Journal of Educational & Psychological Consultation (2); Journal of Evidence-Based Practices for Schools (2); Journal of the Experimental Analysis of Behavior (2); Journal of General Internal Medicine; Journal of Intellectual and Developmental Disabilities; Journal of Intellectual Disability Research (2); Journal of Medical Speech-Language Pathology; Journal of Neurology, Neurosurgery & Psychiatry; Journal of Paediatrics and Child Health; Journal of Prevention and Intervention in the Community; Journal of Safety Research; Journal of School Psychology (3); The Journal of Socio-Economics; The Journal of Special Education; Journal of Speech, Language, and Hearing Research (2); Journal of Sport Behavior; Journal of Substance Abuse Treatment; Journal of the International Neuropsychological Society; Journal of Traumatic Stress; The Journals of Gerontology: Series B: Psychological Sciences and Social Sciences; Language, Speech, and Hearing Services in Schools; Learning Disabilities Research & Practice (2); Learning Disability Quarterly (2); Music Therapy Perspectives; Neurorehabilitation and Neural Repair; Neuropsychological Rehabilitation (2); Pain; Physical Education and Sport Pedagogy (2); Preventive Medicine: An International Journal Devoted to Practice and Theory; Psychological Assessment; Psychological Medicine: A Journal of Research in Psychiatry and the Allied Sciences; The Psychological Record; Reading and Writing; Remedial and Special Education (3); Research and Practice for Persons with Severe Disabilities (2); Restorative Neurology and Neuroscience; School Psychology International; Seminars in Speech and Language; Sleep and Hypnosis; School Psychology Quarterly; Social Work in Health Care; The Sport Psychologist (3); Therapeutic Recreation Journal (2); The Volta Review; Work: Journal of Prevention, Assessment & Rehabilitation.
Coding criteria amplifications
A comprehensive description of the coding criteria for each category in this review is available from the author by request. The primary coding criteria are described here and in later sections of this article.
- Research design was classified into one of the types discussed later in the section titled Predominant Single-Case Experimental Designs on the basis of the authors’ stated design type. Secondary research designs were then coded when applicable (i.e., mixed designs). Distinctions between primary and secondary research designs were made based on the authors’ description of their study. For example, if an author described the study as a “multiple baseline design with time-series measurement,” the primary research design would be coded as being multiple baseline, and time-series would be coded as the secondary research design.
- Observer ratings were coded as present when observational coding procedures were described and/or the results of a test of interobserver agreement were reported.
- Interrater reliability for observer ratings was coded as present in any case in which percent agreement, alpha, kappa, or another appropriate statistic was reported, regardless of the amount of the total data that were examined for agreement.
- Daily diary, daily self-report, and EMA codes were given when authors explicitly described these procedures in the text by name. Coders did not infer the use of these measurement strategies.
- The number of baseline observations was either taken directly from the figures provided in text or was simply counted in graphical displays of the data when this was determined to be a reliable approach. In some cases, it was not possible to reliably determine the number of baseline data points from the graphical display of data, in which case, the “unavailable” code was assigned. Similarly, the “unavailable” code was assigned when the number of observations was either unreported or ambiguous, or only a range was provided and thus no mean could be determined. Similarly, the mean number of baseline observations was calculated for each study prior to further descriptive statistical analyses because a number of studies reported means only.
- The coding of the analytic method used in the reviewed studies is discussed later in the section titled Discussion of Review Results and Coding of Analytic Methods .
Results of the Systematic Review
Descriptive statistics of the design, measurement, and analysis characteristics of the reviewed studies are presented in Table 2 . The results and their implications are discussed in the relevant sections throughout the remainder of the article.
Descriptive Statistics of Reviewed SCED Characteristics
Note. % refers to the proportion of reviewed studies that satisfied criteria for this code: For example, the percent of studies reporting observer ratings.
Discussion of the Systematic Review Results in Context
The SCED is a very flexible methodology and has many variants. Those mentioned here are the building blocks from which other designs are then derived. For those readers interested in the nuances of each design, Barlow et al., (2008) ; Franklin, Allison, and Gorman (1997) ; Kazdin (2010) ; and Kratochwill and Levin (1992) , among others, provide cogent, in-depth discussions. Identifying the appropriate SCED depends upon many factors, including the specifics of the IV, the setting in which the study will be conducted, participant characteristics, the desired or hypothesized outcomes, and the research question(s). Similarly, the researcher’s selection of measurement and analysis techniques is determined by these factors.
Predominant Single-Case Experimental Designs
Alternating/simultaneous designs (6%; primary design of the studies reviewed).
Alternating and simultaneous designs involve an iterative manipulation of the IV(s) across different phases to show that changes in the DV vary systematically as a function of manipulating the IV(s). In these multielement designs, the researcher has the option to alternate the introduction of two or more IVs or present two or more IVs at the same time. In the alternating variation, the researcher is able to determine the relative impact of two different IVs on the DV, when all other conditions are held constant. Another variation of this design is to alternate IVs across various conditions that could be related to the DV (e.g., class period, interventionist). Similarly, the simultaneous design would occur when the IVs were presented at the same time within the same phase of the study.
Changing criterion design (4%)
Changing criterion designs are used to demonstrate a gradual change in the DV over the course of the phase involving the active manipulation of the IV. Criteria indicating that a change has occurred happen in a step-wise manner, in which the criterion shifts as the participant responds to the presence of the manipulated IV. The changing criterion design is particularly useful in applied intervention research for a number of reasons. The IV is continuous and never withdrawn, unlike the strategy used in a reversal design. This is particularly important in situations where removal of a psychological intervention would be either detrimental or dangerous to the participant, or would be otherwise unfeasible or unethical. The multiple baseline design also does not withdraw intervention, but it requires replicating the effects of the intervention across participants, settings, or situations. A changing criterion design can be accomplished with one participant in one setting without withholding or withdrawing treatment.
Multiple baseline/combined series design (69%)
The multiple baseline or combined series design can be used to test within-subject change across conditions and often involves multiple participants in a replication context. The multiple baseline design is quite simple in many ways, essentially consisting of a number of repeated, miniature AB experiments or variations thereof. Introduction of the IV is staggered temporally across multiple participants or across multiple within-subject conditions, which allows the researcher to demonstrate that changes in the DV reliably occur only when the IV is introduced, thus controlling for the effects of extraneous factors. Multiple baseline designs can be used both within and across units (i.e., persons or groups of persons). When the baseline phase of each subject begins simultaneously, it is called a concurrent multiple baseline design. In a nonconcurrent variation, baseline periods across subjects begin at different points in time. The multiple baseline design is useful in many settings in which withdrawal of the IV would not be appropriate or when introduction of the IV is hypothesized to result in permanent change that would not reverse when the IV is withdrawn. The major drawback of this design is that the IV must be initially withheld for a period of time to ensure different starting points across the different units in the baseline phase. Depending upon the nature of the research questions, withholding an IV, such as a treatment, could be potentially detrimental to participants.
Reversal designs (17%)
Reversal designs are also known as introduction and withdrawal and are denoted as ABAB designs in their simplest form. As the name suggests, the reversal design involves collecting a baseline measure of the DV (the first A phase), introducing the IV (the first B phase), removing the IV while continuing to assess the DV (the second A phase), and then reintroducing the IV (the second B phase). This pattern can be repeated as many times as is necessary to demonstrate an effect or otherwise address the research question. Reversal designs are useful when the manipulation is hypothesized to result in changes in the DV that are expected to reverse or discontinue when the manipulation is not present. Maintenance of an effect is often necessary to uphold the findings of reversal designs. The demonstration of an effect is evident in reversal designs when improvement occurs during the first manipulation phase, compared to the first baseline phase, then reverts to or approaches original baseline levels during the second baseline phase when the manipulation has been withdrawn, and then improves again when the manipulation in then reinstated. This pattern of reversal, when the manipulation is introduced and then withdrawn, is essential to attributing changes in the DV to the IV. However, maintenance of the effects in a reversal design, in which the DV is hypothesized to reverse when the IV is withdrawn, is not incompatible ( Kazdin, 2010 ). Maintenance is demonstrated by repeating introduction–withdrawal segments until improvement in the DV becomes permanent even when the IV is withdrawn. There is not always a need to demonstrate maintenance in all applications, nor is it always possible or desirable, but it is paramount in the learning and intervention research contexts.
Mixed designs (10%)
Mixed designs include a combination of more than one SCED (e.g., a reversal design embedded within a multiple baseline) or an SCED embedded within a group design (i.e., a randomized controlled trial comparing two groups of multiple baseline experiments). Mixed designs afford the researcher even greater flexibility in designing a study to address complex psychological hypotheses, but also capitalize on the strengths of the various designs. See Kazdin (2010) for a discussion of the variations and utility of mixed designs.
Related Nonexperimental Designs
Quasi-experimental designs.
In contrast to the designs previously described, all of which constitute “true experiments” ( Kazdin, 2010 ; Shadish et al., 2002 ), in quasi-experimental designs the conditions of a true experiment (e.g., active manipulation of the IV, replication of the effect) are approximated and are not readily under the control of the researcher. Because the focus of this article is on experimental designs, quasi-experiments are not discussed in detail; instead the reader is referred to Kazdin (2010) and Shadish et al. (2002) .
Ecological and naturalistic single-case designs
For a single-case design to be experimental, there must be active manipulation of the IV, but in some applications, such as those that might be used in social and personality psychology, the researcher might be interested in measuring naturally occurring phenomena and examining their temporal relationships. Thus, the researcher will not use a manipulation. An example of this type of research might be a study about the temporal relationship between alcohol consumption and depressed mood, which can be measured reliably using EMA methods. Psychotherapy process researchers also use this type of design to assess dyadic relationship dynamics between therapists and clients (e.g., Tschacher & Ramseyer, 2009 ).
Research Design Standards
Each of the reviewed standards provides some degree of direction regarding acceptable research designs. The WWC provides the most detailed and specific requirements regarding design characteristics. Those guidelines presented in Tables 3 , ,4, 4 , and and5 5 are consistent with the methodological rigor necessary to meet the WWC distinction “meets standards.” The WWC also provides less-stringent standards for a “meets standards with reservations” distinction. When minimum criteria in the design, measurement, or analysis sections of a study are not met, it is rated “does not meet standards” ( Kratochwill et al., 2010 ). Many SCEDs are acceptable within the standards of DIV12, DIV16, NRP, and in the Tate et al. SCED scale. DIV12 specifies that replication occurs across a minimum of three successive cases, which differs from the WWC specifications, which allow for three replications within a single-subject design but does not necessarily need to be across multiple subjects. DIV16 does not require, but seems to prefer, a multiple baseline design with a between-subject replication. Tate et al. state that the “design allows for the examination of cause and effect relationships to demonstrate efficacy” (p. 400, 2008). Determining whether or not a design meets this requirement is left up to the evaluator, who might then refer to one of the other standards or another source for direction.
Research Design Standards and Guidelines
Measurement and Assessment Standards and Guidelines
Analysis Standards and Guidelines
The Stone and Shiffman (2002) standards for EMA are concerned almost entirely with the reporting of measurement characteristics and less so with research design. One way in which these standards differ from those of other sources is in the active manipulation of the IV. Many research questions in EMA, daily diary, and time-series designs are concerned with naturally occurring phenomena, and a researcher manipulation would run counter to this aim. The EMA standards become important when selecting an appropriate measurement strategy within the SCED. In EMA applications, as is also true in some other time-series and daily diary designs, researcher manipulation occurs as a function of the sampling interval in which DVs of interest are measured according to fixed time schedules (e.g., reporting occurs at the end of each day), random time schedules (e.g., the data collection device prompts the participant to respond at random intervals throughout the day), or on an event-based schedule (e.g., reporting occurs after a specified event takes place).
Measurement
The basic measurement requirement of the SCED is a repeated assessment of the DV across each phase of the design in order to draw valid inferences regarding the effect of the IV on the DV. In other applications, such as those used by personality and social psychology researchers to study various human phenomena ( Bolger et al., 2003 ; Reis & Gable, 2000 ), sampling strategies vary widely depending on the topic area under investigation. Regardless of the research area, SCEDs are most typically concerned with within-person change and processes and involve a time-based strategy, most commonly to assess global daily averages or peak daily levels of the DV. Many sampling strategies, such as time-series, in which reporting occurs at uniform intervals or on event-based, fixed, or variable schedules, are also appropriate measurement methods and are common in psychological research (see Bolger et al., 2003 ).
Repeated-measurement methods permit the natural, even spontaneous, reporting of information ( Reis, 1994 ), which reduces the biases of retrospection by minimizing the amount of time elapsed between an experience and the account of this experience ( Bolger et al., 2003 ). Shiffman et al. (2008) aptly noted that the majority of research in the field of psychology relies heavily on retrospective assessment measures, even though retrospective reports have been found to be susceptible to state-congruent recall (e.g., Bower, 1981 ) and a tendency to report peak levels of the experience instead of giving credence to temporal fluctuations ( Redelmeier & Kahneman, 1996 ; Stone, Broderick, Kaell, Deles-Paul, & Porter, 2000 ). Furthermore, Shiffman et al. (1997) demonstrated that subjective aggregate accounts were a poor fit to daily reported experiences, which can be attributed to reductions in measurement error resulting in increased validity and reliability of the daily reports.
The necessity of measuring at least one DV repeatedly means that the selected assessment method, instrument, and/or construct must be sensitive to change over time and be capable of reliably and validly capturing change. Horner et al. (2005) discusses the important features of outcome measures selected for use in these types of designs. Kazdin (2010) suggests that measures be dimensional, which can more readily detect effects than categorical and binary measures. Although using an established measure or scale, such as the Outcome Questionnaire System ( M. J. Lambert, Hansen, & Harmon, 2010 ), provides empirically validated items for assessing various outcomes, most measure validation studies conducted on this type of instrument involve between-subject designs, which is no guarantee that these measures are reliable and valid for assessing within-person variability. Borsboom, Mellenbergh, and van Heerden (2003) suggest that researchers adapting validated measures should consider whether the items they propose using have a factor structure within subjects similar to that obtained between subjects. This is one of the reasons that SCEDs often use observational assessments from multiple sources and report the interrater reliability of the measure. Self-report measures are acceptable practice in some circles, but generally additional assessment methods or informants are necessary to uphold the highest methodological standards. The results of this review indicate that the majority of studies include observational measurement (76.0%). Within those studies, nearly all (97.1%) reported interrater reliability procedures and results. The results within each design were similar, with the exception of time-series designs, which used observer ratings in only half of the reviewed studies.
Time-series
Time-series designs are defined by repeated measurement of variables of interest over a period of time ( Box & Jenkins, 1970 ). Time-series measurement most often occurs in uniform intervals; however, this is no longer a constraint of time-series designs (see Harvey, 2001 ). Although uniform interval reporting is not necessary in SCED research, repeated measures often occur at uniform intervals, such as once each day or each week, which constitutes a time-series design. The time-series design has been used in various basic science applications ( Scollon, Kim-Pietro, & Diener, 2003 ) across nearly all subspecialties in psychology (e.g., Bolger et al., 2003 ; Piasecki et al., 2007 ; for a review, see Reis & Gable, 2000 ; Soliday et al., 2002 ). The basic time-series formula for a two-phase (AB) data stream is presented in Equation 1 . In this formula α represents the step function of the data stream; S represents the change between the first and second phases, which is also the intercept in a two-phase data stream and a step function being 0 at times i = 1, 2, 3…n1 and 1 at times i = n1+1, n1+2, n1+3…n; n 1 is the number of observations in the baseline phase; n is the total number of data points in the data stream; i represents time; and ε i = ρε i −1 + e i , which indicates the relationship between the autoregressive function (ρ) and the distribution of the data in the stream.
Time-series formulas become increasingly complex when seasonality and autoregressive processes are modeled in the analytic procedures, but these are rarely of concern for short time-series data streams in SCEDs. For a detailed description of other time-series design and analysis issues, see Borckardt et al. (2008) , Box and Jenkins (1970) , Crosbie (1993) , R. R. Jones et al. (1977) , and Velicer and Fava (2003) .
Time-series and other repeated-measures methodologies also enable examination of temporal effects. Borckardt et al. (2008) and others have noted that time-series designs have the potential to reveal how change occurs, not simply if it occurs. This distinction is what most interested Skinner (1938) , but it often falls below the purview of today’s researchers in favor of group designs, which Skinner felt obscured the process of change. In intervention and psychopathology research, time-series designs can assess mediators of change ( Doss & Atkins, 2006 ), treatment processes ( Stout, 2007 ; Tschacher & Ramseyer, 2009 ), and the relationship between psychological symptoms (e.g., Alloy, Just, & Panzarella, 1997 ; Hanson & Chen, 2010 ; Oslin, Cary, Slaymaker, Colleran, & Blow, 2009 ), and might be capable of revealing mechanisms of change ( Kazdin, 2007 , 2009 , 2010 ). Between- and within-subject SCED designs with repeated measurements enable researchers to examine similarities and differences in the course of change, both during and as a result of manipulating an IV. Temporal effects have been largely overlooked in many areas of psychological science ( Bolger et al., 2003 ): Examining temporal relationships is sorely needed to further our understanding of the etiology and amplification of numerous psychological phenomena.
Time-series studies were very infrequently found in this literature search (2%). Time-series studies traditionally occur in subfields of psychology in which single-case research is not often used (e.g., personality, physiological/biological). Recent advances in methods for collecting and analyzing time-series data (e.g., Borckardt et al., 2008 ) could expand the use of time-series methodology in the SCED community. One problem with drawing firm conclusions from this particular review finding is a semantic factor: Time-series is a specific term reserved for measurement occurring at a uniform interval. However, SCED research appears to not yet have adopted this language when referring to data collected in this fashion. When time-series data analytic methods are not used, the matter of measurement interval is of less importance and might not need to be specified or described as a time-series. An interesting extension of this work would be to examine SCED research that used time-series measurement strategies but did not label it as such. This is important because then it could be determined how many SCEDs could be analyzed with time-series statistical methods.
Daily diary and ecological momentary assessment methods
EMA and daily diary approaches represent methodological procedures for collecting repeated measurements in time-series and non-time-series experiments, which are also known as experience sampling. Presenting an in-depth discussion of the nuances of these sampling techniques is well beyond the scope of this paper. The reader is referred to the following review articles: daily diary ( Bolger et al., 2003 ; Reis & Gable, 2000 ; Thiele, Laireiter, & Baumann, 2002 ), and EMA ( Shiffman et al., 2008 ). Experience sampling in psychology has burgeoned in the past two decades as technological advances have permitted more precise and immediate reporting by participants (e.g., Internet-based, two-way pagers, cellular telephones, handheld computers) than do paper and pencil methods (for reviews see Barrett & Barrett, 2001 ; Shiffman & Stone, 1998 ). Both methods have practical limitations and advantages. For example, electronic methods are more costly and may exclude certain subjects from participating in the study, either because they do not have access to the necessary technology or they do not have the familiarity or savvy to successfully complete reporting. Electronic data collection methods enable the researcher to prompt responses at random or predetermined intervals and also accurately assess compliance. Paper and pencil methods have been criticized for their inability to reliably track respondents’ compliance: Palermo, Valenzuela, and Stork (2004) found better compliance with electronic diaries than with paper and pencil. On the other hand, Green, Rafaeli, Bolger, Shrout, & Reis (2006) demonstrated the psychometric data structure equivalence between these two methods, suggesting that the data collected in either method will yield similar statistical results given comparable compliance rates.
Daily diary/daily self-report and EMA measurement were somewhat rarely represented in this review, occurring in only 6.1% of the total studies. EMA methods had been used in only one of the reviewed studies. The recent proliferation of EMA and daily diary studies in psychology reported by others ( Bolger et al., 2003 ; Piasecki et al., 2007 ; Shiffman et al., 2008 ) suggests that these methods have not yet reached SCED researchers, which could in part have resulted from the long-held supremacy of observational measurement in fields that commonly practice single-case research.
Measurement Standards
As was previously mentioned, measurement in SCEDs requires the reliable assessment of change over time. As illustrated in Table 4 , DIV16 and the NRP explicitly require that reliability of all measures be reported. DIV12 provides little direction in the selection of the measurement instrument, except to require that three or more clinically important behaviors with relative independence be assessed. Similarly, the only item concerned with measurement on the Tate et al. scale specifies assessing behaviors consistent with the target of the intervention. The WWC and the Tate et al. scale require at least two independent assessors of the DV and that interrater reliability meeting minimum established thresholds be reported. Furthermore, WWC requires that interrater reliability be assessed on at least 20% of the data in each phase and in each condition. DIV16 expects that assessment of the outcome measures will be multisource and multimethod, when applicable. The interval of measurement is not specified by any of the reviewed sources. The WWC and the Tate et al. scale require that DVs be measured repeatedly across phases (e.g., baseline and treatment), which is a typical requirement of a SCED. The NRP asks that the time points at which DV measurement occurred be reported.
The baseline measurement represents one of the most crucial design elements of the SCED. Because subjects provide their own data for comparison, gathering a representative, stable sampling of behavior before manipulating the IV is essential to accurately inferring an effect. Some researchers have reported the typical length of the baseline period to range from 3 to 12 observations in intervention research applications (e.g., Center et al., 1986 ; Huitema, 1985 ; R. R. Jones et al., 1977 ; Sharpley, 1987 ); Huitema’s (1985) review of 881 experiments published in the Journal of Applied Behavior Analysis resulted in a modal number of three to four baseline points. Center et al. (1986) suggested five as the minimum number of baseline measurements needed to accurately estimate autocorrelation. Longer baseline periods suggest a greater likelihood of a representative measurement of the DVs, which has been found to increase the validity of the effects and reduce bias resulting from autocorrelation ( Huitema & McKean, 1994 ). The results of this review are largely consistent with those of previous researchers: The mean number of baseline observations was found to be 10.22 ( SD = 9.59), and 6 was the modal number of observations. Baseline data were available in 77.8% of the reviewed studies. Although the baseline assessment has tremendous bearing on the results of a SCED study, it was often difficult to locate the exact number of data points. Similarly, the number of data points assessed across all phases of the study were not easily identified.
The WWC, DIV12, and DIV16 agree that a minimum of three data points during the baseline is necessary. However, to receive the highest rating by the WWC, five data points are necessary in each phase, including the baseline and any subsequent withdrawal baselines as would occur in a reversal design. DIV16 explicitly states that more than three points are preferred and further stipulates that the baseline must demonstrate stability (i.e., limited variability), absence of overlap between the baseline and other phases, absence of a trend, and that the level of the baseline measurement is severe enough to warrant intervention; each of these aspects of the data is important in inferential accuracy. Detrending techniques can be used to address baseline data trend. The integration option in ARIMA-based modeling and the empirical mode decomposition method ( Wu, Huang, Long, & Peng, 2007 ) are two sophisticated detrending techniques. In regression-based analytic methods, detrending can be accomplished by simply regressing each variable in the model on time (i.e., the residuals become the detrended series), which is analogous to adding a linear, exponential, or quadratic term to the regression equation.
NRP does not provide a minimum for data points, nor does the Tate et al. scale, which requires only a sufficient sampling of baseline behavior. Although the mean and modal number of baseline observations is well within these parameters, seven (1.7%) studies reported mean baselines of less than three data points.
Establishing a uniform minimum number of required baseline observations would provide researchers and reviewers with only a starting guide. The baseline phase is important in SCED research because it establishes a trend that can then be compared with that of subsequent phases. Although a minimum number of observations might be required to meet standards, many more might be necessary to establish a trend when there is variability and trends in the direction of the expected effect. The selected data analytic approach also has some bearing on the number of necessary baseline observations. This is discussed further in the Analysis section.
Reporting of repeated measurements
Stone and Shiffman (2002) provide a comprehensive set of guidelines for the reporting of EMA data, which can also be applied to other repeated-measurement strategies. Because the application of EMA is widespread and not confined to specific research designs, Stone and Shiffman intentionally place few restraints on researchers regarding selection of the DV and the reporter, which is determined by the research question under investigation. The methods of measurement, however, are specified in detail: Descriptions of prompting, recording of responses, participant-initiated entries, and the data acquisition interface (e.g., paper and pencil diary, PDA, cellular telephone) ought to be provided with sufficient detail for replication. Because EMA specifically, and time-series/daily diary methods similarly, are primarily concerned with the interval of assessment, Stone and Shiffman suggest reporting the density and schedule of assessment. The approach is generally determined by the nature of the research question and pragmatic considerations, such as access to electronic data collection devices at certain times of the day and participant burden. Compliance and missing data concerns are present in any longitudinal research design, but they are of particular importance in repeated-measurement applications with frequent measurement. When the research question pertains to temporal effects, compliance becomes paramount, and timely, immediate responding is necessary. For this reason, compliance decisions, rates of missing data, and missing data management techniques must be reported. The effect of missing data in time-series data streams has been the topic of recent research in the social sciences (e.g., Smith, Borckardt, & Nash, in press ; Velicer & Colby, 2005a , 2005b ). The results and implications of these and other missing data studies are discussed in the next section.
Analysis of SCED Data
Visual analysis.
Experts in the field generally agree about the majority of critical single-case experiment design and measurement characteristics. Analysis, on the other hand, is an area of significant disagreement, yet it has also received extensive recent attention and advancement. Debate regarding the appropriateness and accuracy of various methods for analyzing SCED data, the interpretation of single-case effect sizes, and other concerns vital to the validity of SCED results has been ongoing for decades, and no clear consensus has been reached. Visual analysis, following systematic procedures such as those provided by Franklin, Gorman, Beasley, and Allison (1997) and Parsonson and Baer (1978) , remains the standard by which SCED data are most commonly analyzed ( Parker, Cryer, & Byrns, 2006 ). Visual analysis can arguably be applied to all SCEDs. However, a number of baseline data characteristics must be met for effects obtained through visual analysis to be valid and reliable. The baseline phase must be relatively stable; free of significant trend, particularly in the hypothesized direction of the effect; have minimal overlap of data with subsequent phases; and have a sufficient sampling of behavior to be considered representative ( Franklin, Gorman, et al., 1997 ; Parsonson & Baer, 1978 ). The effect of baseline trend on visual analysis, and a technique to control baseline trend, are offered by Parker et al. (2006) . Kazdin (2010) suggests using statistical analysis when a trend or significant variability appears in the baseline phase, two conditions that ought to preclude the use of visual analysis techniques. Visual analysis methods are especially adept at determining intervention effects and can be of particular relevance in real-world applications (e.g., Borckardt et al., 2008 ; Kratochwill, Levin, Horner, & Swoboda, 2011 ).
However, visual analysis has its detractors. It has been shown to be inconsistent, can be affected by autocorrelation, and results in overestimation of effect (e.g., Matyas & Greenwood, 1990 ). Visual analysis as a means of estimating an effect precludes the results of SCED research from being included in meta-analysis, and also makes it very difficult to compare results to the effect sizes generated by other statistical methods. Yet, visual analysis proliferates in large part because SCED researchers are familiar with these methods and are not only generally unfamiliar with statistical approaches, but lack agreement about their appropriateness. Still, top experts in single-case analysis champion the use of statistical methods alongside visual analysis whenever it is appropriate to do so ( Kratochwill et al., 2011 ).
Statistical analysis
Statistical analysis of SCED data consists generally of an attempt to address one or more of three broad research questions: (1) Does introduction/manipulation of the IV result in statistically significant change in the level of the DV (level-change or phase-effect analysis)? (2) Does introduction/manipulation of the IV result in statistically significant change in the slope of the DV over time (slope-change analysis)? and (3) Do meaningful relationships exist between the trajectory of the DV and other potential covariates? Level- and slope-change analyses are relevant to intervention effectiveness studies and other research questions in which the IV is expected to result in changes in the DV in a particular direction. Visual analysis methods are most adept at addressing research questions pertaining to changes in level and slope (Questions 1 and 2), most often using some form of graphical representation and standardized computation of a mean level or trend line within and between each phase of interest (e.g., Horner & Spaulding, 2010 ; Kratochwill et al., 2011 ; Matyas & Greenwood, 1990 ). Research questions in other areas of psychological science might address the relationship between DVs or the slopes of DVs (Question 3). A number of sophisticated modeling approaches (e.g., cross-lag, multilevel, panel, growth mixture, latent class analysis) may be used for this type of question, and some are discussed in greater detail later in this section. However, a discussion about the nuances of this type of analysis and all their possible methods is well beyond the scope of this article.
The statistical analysis of SCEDs is a contentious issue in the field. Not only is there no agreed-upon statistical method, but the practice of statistical analysis in the context of the SCED is viewed by some as unnecessary (see Shadish, Rindskopf, & Hedges, 2008 ). Traditional trends in the prevalence of statistical analysis usage by SCED researchers are revealing: Busk & Marascuilo (1992) found that only 10% of the published single-case studies they reviewed used statistical analysis; Brossart, Parker, Olson, & Mahadevan (2006) estimated that this figure had roughly doubled by 2006. A range of concerns regarding single-case effect size calculation and interpretation is discussed in significant detail elsewhere (e.g., Campbell, 2004 ; Cohen, 1994 ; Ferron & Sentovich, 2002 ; Ferron & Ware, 1995 ; Kirk, 1996 ; Manolov & Solanas, 2008 ; Olive & Smith, 2005 ; Parker & Brossart, 2003 ; Robey et al., 1999 ; Smith et al., in press ; Velicer & Fava, 2003 ). One concern is the lack of a clearly superior method across datasets. Although statistical methods for analyzing SCEDs abound, few studies have examined their comparative performance with the same dataset. The most recent studies of this kind, performed by Brossart et al. (2006) , Campbell (2004) , Parker and Brossart (2003) , and Parker and Vannest (2009) , found that the more promising available statistical analysis methods yielded moderately different results on the same data series, which led them to conclude that each available method is equipped to adequately address only a relatively narrow spectrum of data. Given these findings, analysts need to select an appropriate model for the research questions and data structure, being mindful of how modeling results can be influenced by extraneous factors.
The current standards unfortunately provide little guidance in the way of statistical analysis options. This article presents an admittedly cursory introduction to available statistical methods; many others are not covered in this review. The following articles provide more in-depth discussion and description of other methods: Barlow et al. (2008) ; Franklin et al., (1997) ; Kazdin (2010) ; and Kratochwill and Levin (1992 , 2010 ). Shadish et al. (2008) summarize more recently developed methods. Similarly, a Special Issue of Evidence-Based Communication Assessment and Intervention (2008, Volume 2) provides articles and discussion of the more promising statistical methods for SCED analysis. An introduction to autocorrelation and its implications for statistical analysis is necessary before specific analytic methods can be discussed. It is also pertinent at this time to discuss the implications of missing data.
Autocorrelation
Many repeated measurements within a single subject or unit create a situation that most psychological researchers are unaccustomed to dealing with: autocorrelated data, which is the nonindependence of sequential observations, also known as serial dependence. Basic and advanced discussions of autocorrelation in single-subject data can be found in Borckardt et al. (2008) , Huitema (1985) , and Marshall (1980) , and discussions of autocorrelation in multilevel models can be found in Snijders and Bosker (1999) and Diggle and Liang (2001) . Along with trend and seasonal variation, autocorrelation is one example of the internal structure of repeated measurements. In the social sciences, autocorrelated data occur most naturally in the fields of physiological psychology, econometrics, and finance, where each phase of interest has potentially hundreds or even thousands of observations that are tightly packed across time (e.g., electroencephalography actuarial data, financial market indices). Applied SCED research in most areas of psychology is more likely to have measurement intervals of day, week, or hour.
Autocorrelation is a direct result of the repeated-measurement requirements of the SCED, but its effect is most noticeable and problematic when one is attempting to analyze these data. Many commonly used data analytic approaches, such as analysis of variance, assume independence of observations and can produce spurious results when the data are nonindependent. Even statistically insignificant autocorrelation estimates are generally viewed as sufficient to cause inferential bias when conventional statistics are used (e.g., Busk & Marascuilo, 1988 ; R. R. Jones et al., 1977 ; Matyas & Greenwood, 1990 ). The effect of autocorrelation on statistical inference in single-case applications has also been known for quite some time (e.g., R. R. Jones et al., 1977 ; Kanfer, 1970 ; Kazdin, 1981 ; Marshall, 1980 ). The findings of recent simulation studies of single-subject data streams indicate that autocorrelation is a nontrivial matter. For example, Manolov and Solanas (2008) determined that calculated effect sizes were linearly related to the autocorrelation of the data stream, and Smith et al. (in press) demonstrated that autocorrelation estimates in the vicinity of 0.80 negatively affect the ability to correctly infer a significant level-change effect using a standardized mean differences method. Huitema and colleagues (e.g., Huitema, 1985 ; Huitema & McKean, 1994 ) argued that autocorrelation is rarely a concern in applied research. Huitema’s methods and conclusions have been questioned and opposing data have been published (e.g., Allison & Gorman, 1993 ; Matyas & Greenwood, 1990 ; Robey et al., 1999 ), resulting in abandonment of the position that autocorrelation can be conscionably ignored without compromising the validity of the statistical procedures. Procedures for removing autocorrelation in the data stream prior to calculating effect sizes are offered as one option: One of the more promising analysis methods, autoregressive integrated moving averages (discussed later in this article), was specifically designed to remove the internal structure of time-series data, such as autocorrelation, trend, and seasonality ( Box & Jenkins, 1970 ; Tiao & Box, 1981 ).
Missing observations
Another concern inherent in repeated-measures designs is missing data. Daily diary and EMA methods are intended to reduce the risk of retrospection error by eliciting accurate, real-time information ( Bolger et al., 2003 ). However, these methods are subject to missing data as a result of honest forgetfulness, not possessing the diary collection tool at the specified time of collection, and intentional or systematic noncompliance. With paper and pencil diaries and some electronic methods, subjects might be able to complete missed entries retrospectively, defeating the temporal benefits of these assessment strategies ( Bolger et al., 2003 ). Methods of managing noncompliance through the study design and measurement methods include training the subject to use the data collection device appropriately, using technology to prompt responding and track the time of response, and providing incentives to participants for timely compliance (for additional discussion of this topic, see Bolger et al., 2003 ; Shiffman & Stone, 1998 ).
Even when efforts are made to maximize compliance during the conduct of the research, the problem of missing data is often unavoidable. Numerous approaches exist for handling missing observations in group multivariate designs (e.g., Horton & Kleinman, 2007 ; Ibrahim, Chen, Lipsitz, & Herring, 2005 ). Ragunathan (2004) and others concluded that full information and raw data maximum likelihood methods are preferable. Velicer and Colby (2005a , 2005b ) established the superiority of maximum likelihood methods over listwise deletion, mean of adjacent observations, and series mean substitution in the estimation of various critical time-series data parameters. Smith et al. (in press) extended these findings regarding the effect of missing data on inferential precision. They found that managing missing data with the EM procedure ( Dempster, Laird, & Rubin, 1977 ), a maximum likelihood algorithm, did not affect one’s ability to correctly infer a significant effect. However, lag-1 autocorrelation estimates in the vicinity of 0.80 resulted in insufficient power sensitivity (< 0.80), regardless of the proportion of missing data (10%, 20%, 30%, or 40%). 1 Although maximum likelihood methods have garnered some empirical support, methodological strategies that minimize missing data, particularly systematically missing data, are paramount to post-hoc statistical remedies.
Nonnormal distribution of data
In addition to the autocorrelated nature of SCED data, typical measurement methods also present analytic challenges. Many statistical methods, particularly those involving model finding, assume that the data are normally distributed. This is often not satisfied in SCED research when measurements involve count data, observer-rated behaviors, and other, similar metrics that result in skewed distributions. Techniques are available to manage nonnormal distributions in regression-based analysis, such as zero-inflated Poisson regression ( D. Lambert, 1992 ) and negative binomial regression ( Gardner, Mulvey, & Shaw, 1995 ), but many other statistical analysis methods do not include these sophisticated techniques. A skewed data distribution is perhaps one of the reasons Kazdin (2010) suggests not using count, categorical, or ordinal measurement methods.
Available statistical analysis methods
Following is a basic introduction to the more promising and prevalent analytic methods for SCED research. Because there is little consensus regarding the superiority of any single method, the burden unfortunately falls on the researcher to select a method capable of addressing the research question and handling the data involved in the study. Some indications and contraindications are provided for each method presented here.
Multilevel and structural equation modeling
Multilevel modeling (MLM; e.g., Schmidt, Perels, & Schmitz, 2010 ) techniques represent the state of the art among parametric approaches to SCED analysis, particularly when synthesizing SCED results ( Shadish et al., 2008 ). MLM and related latent growth curve and factor mixture methods in structural equation modeling (SEM; e.g., Lubke & Muthén, 2005 ; B. O. Muthén & Curran, 1997 ) are particularly effective for evaluating trajectories and slopes in longitudinal data and relating changes to potential covariates. MLM and related hierarchical linear models (HLM) can also illuminate the relationship between the trajectories of different variables under investigation and clarify whether or not these relationships differ amongst the subjects in the study. Time-series and cross-lag analyses can also be used in MLM and SEM ( Chow, Ho, Hamaker, & Dolan, 2010 ; du Toit & Browne, 2007 ). However, they generally require sophisticated model-fitting techniques, making them difficult for many social scientists to implement. The structure (autocorrelation) and trend of the data can also complicate many MLM methods. The common, short data streams in SCED research and the small number of subjects also present problems to MLM and SEM approaches, which were developed for data with significantly greater numbers of observations when the number of subjects is fewer, and for a greater number of participants for model-fitting purposes, particularly when there are fewer data points. Still, MLM and related techniques arguably represent the most promising analytic methods.
A number of software options 2 exist for SEM. Popular statistical packages in the social sciences provide SEM options, such as PROC CALIS in SAS ( SAS Institute Inc., 2008 ), the AMOS module ( Arbuckle, 2006 ) of SPSS ( SPSS Statistics, 2011 ), and the sempackage for R ( R Development Core Team, 2005 ), the use of which is described by Fox ( Fox, 2006 ). A number of stand-alone software options are also available for SEM applications, including Mplus ( L. K. Muthén & Muthén, 2010 ) and Stata ( StataCorp., 2011 ). Each of these programs also provides options for estimating multilevel/hierarchical models (for a review of using these programs for MLM analysis see Albright & Marinova, 2010 ). Hierarchical linear and nonlinear modeling can also be accomplished using the HLM 7 program ( Raudenbush, Bryk, & Congdon, 2011 ).
Autoregressive moving averages (ARMA; e.g., Browne & Nesselroade, 2005 ; Liu & Hudack, 1995 ; Tiao & Box, 1981 )
Two primary points have been raised regarding ARMA modeling: length of the data stream and feasibility of the modeling technique. ARMA models generally require 30–50 observations in each phase when analyzing a single-subject experiment (e.g., Borckardt et al., 2008 ; Box & Jenkins, 1970 ), which is often difficult to satisfy in applied psychological research applications. However, ARMA models in an SEM framework, such as those described by du Toit & Browne (2001) , are well suited for longitudinal panel data with few observations and many subjects. Autoregressive SEM models are also applicable under similar conditions. Model-fitting options are available in SPSS, R, and SAS via PROC ARMA.
ARMA modeling also requires considerable training in the method and rather advanced knowledge about statistical methods (e.g., Kratochwill & Levin, 1992 ). However, Brossart et al. (2006) point out that ARMA-based approaches can produce excellent results when there is no “model finding” and a simple lag-1 model, with no differencing and no moving average, is used. This approach can be taken for many SCED applications when phase- or slope-change analyses are of interest with a single, or very few, subjects. As already mentioned, this method is particularly useful when one is seeking to account for autocorrelation or other over-time variations that are not directly related to the experimental or intervention effect of interest (i.e., detrending). ARMA and other time-series analysis methods require missing data to be managed prior to analysis by means of options such as full information maximum likelihood estimation, multiple imputation, or the Kalman filter (see Box & Jenkins, 1970 ; Hamilton, 1994 ; Shumway & Stoffer, 1982 ) because listwise deletion has been shown to result in inaccurate time-series parameter estimates ( Velicer & Colby, 2005a ).
Standardized mean differences
Standardized mean differences approaches include the common Cohen’s d , Glass’s Delta, and Hedge’s g that are used in the analysis of group designs. The computational properties of mean differences approaches to SCEDs are identical to those used for group comparisons, except that the results represent within-case variation instead of the variation between groups, which suggests that the obtained effect sizes are not interpretively equivalent. The advantage of the mean differences approach is its simplicity of calculation and also its familiarity to social scientists. The primary drawback of these approaches is that they were not developed to contend with autocorrelated data. However, Manolov and Solanas (2008) reported that autocorrelation least affected effect sizes calculated using standardized mean differences approaches. To the applied-research scientist this likely represents the most accessible analytic approach, because statistical software is not required to calculate these effect sizes. The resultant effect sizes of single subject standardized mean differences analysis must be interpreted cautiously because their relation to standard effect size benchmarks, such as those provided by Cohen (1988) , is unknown. Standardized mean differences approaches are appropriate only when examining significant differences between phases of the study and cannot illuminate trajectories or relationships between variables.
Other analytic approaches
Researchers have offered other analytic methods to deal with the characteristics of SCED data. A number of methods for analyzing N -of-1 experiments have been developed. Borckardt’s Simulation Modeling Analysis (2006) program provides a method for analyzing level- and slope-change in short (<30 observations per phase; see Borckardt et al., 2008 ), autocorrelated data streams that is statistically sophisticated, yet accessible and freely available to typical psychological scientists and clinicians. A replicated single-case time-series design conducted by Smith, Handler, & Nash (2010) provides an example of SMA application. The Singwin Package, described in Bloom et al., (2003) , is a another easy-to-use parametric approach for analyzing single-case experiments. A number of nonparametric approaches have also been developed that emerged from the visual analysis tradition: Some examples include percent nonoverlapping data ( Scruggs, Mastropieri, & Casto, 1987 ) and nonoverlap of all pairs ( Parker & Vannest, 2009 ); however, these methods have come under scrutiny, and Wolery, Busick, Reichow, and Barton (2010) have suggested abandoning them altogether. Each of these methods appears to be well suited for managing specific data characteristics, but they should not be used to analyze data streams beyond their intended purpose until additional empirical research is conducted.
Combining SCED Results
Beyond the issue of single-case analysis is the matter of integrating and meta-analyzing the results of single-case experiments. SCEDs have been given short shrift in the majority of meta-analytic literature ( Littell, Corcoran, & Pillai, 2008 ; Shadish et al., 2008 ), with only a few exceptions ( Carr et al., 1999 ; Horner & Spaulding, 2010 ). Currently, few proven methods exist for integrating the results of multiple single-case experiments. Allison and Gorman (1993) and Shadish et al. (2008) present the problems associated with meta-analyzing single-case effect sizes, and W. P. Jones (2003) , Manolov and Solanas (2008) , Scruggs and Mastropieri (1998) , and Shadish et al. (2008) offer four different potential statistical solutions for this problem, none of which appear to have received consensus amongst researchers. The ability to synthesize and compare single-case effect sizes, particularly effect sizes garnered through group design research, is undoubtedly necessary to increase SCED proliferation.
Discussion of Review Results and Coding of Analytic Methods
The coding criteria for this review were quite stringent in terms of what was considered to be either visual or statistical analysis. For visual analysis to be coded as present, it was necessary for the authors to self-identify as having used a visual analysis method. In many cases, it could likely be inferred that visual analysis had been used, but it was often not specified. Similarly, statistical analysis was reserved for analytic methods that produced an effect. 3 Analyses that involved comparing magnitude of change using raw count data or percentages were not considered rigorous enough. These two narrow definitions of visual and statistical analysis contributed to the high rate of unreported analytic method, shown in Table 1 (52.3%). A better representation of the use of visual and statistical analysis would likely be the percentage of studies within those that reported a method of analysis. Under these parameters, 41.5% used visual analysis and 31.3% used statistical analysis. Included in these figures are studies that included both visual and statistical methods (11%). These findings are slightly higher than those estimated by Brossart et al. (2006) , who estimated statistical analysis is used in about 20% of SCED studies. Visual analysis continues to undoubtedly be the most prevalent method, but there appears to be a trend for increased use of statistical approaches, which is likely to only gain momentum as innovations continue.
Analysis Standards
The standards selected for inclusion in this review offer minimal direction in the way of analyzing the results of SCED research. Table 5 summarizes analysis-related information provided by the six reviewed sources for SCED standards. Visual analysis is acceptable to DV12 and DIV16, along with unspecified statistical approaches. In the WWC standards, visual analysis is the acceptable method of determining an intervention effect, with statistical analyses and randomization tests permissible as a complementary or supporting method to the results of visual analysis methods. However, the authors of the WWC standards state, “As the field reaches greater consensus about appropriate statistical analyses and quantitative effect-size measures, new standards for effect demonstration will need to be developed” ( Kratochwill et al., 2010 , p.16). The NRP and DIV12 seem to prefer statistical methods when they are warranted. The Tate at al. scale accepts only statistical analysis with the reporting of an effect size. Only the WWC and DIV16 provide guidance in the use of statistical analysis procedures: The WWC “recommends” nonparametric and parametric approaches, multilevel modeling, and regression when statistical analysis is used. DIV16 refers the reader to Wilkinson and the Task Force on Statistical Inference of the APA Board of Scientific Affairs (1999) for direction in this matter. Statistical analysis of daily diary and EMA methods is similarly unsettled. Stone and Shiffman (2002) ask for a detailed description of the statistical procedures used, in order for the approach to be replicated and evaluated. They provide direction for analyzing aggregated and disaggregated data. They also aptly note that because many different modes of analysis exist, researchers must carefully match the analytic approach to the hypotheses being pursued.
Limitations and Future Directions
This review has a number of limitations that leave the door open for future study of SCED methodology. Publication bias is a concern in any systematic review. This is particularly true for this review because the search was limited to articles published in peer-reviewed journals. This strategy was chosen in order to inform changes in the practice of reporting and of reviewing, but it also is likely to have inflated the findings regarding the methodological rigor of the reviewed works. Inclusion of book chapters, unpublished studies, and dissertations would likely have yielded somewhat different results.
A second concern is the stringent coding criteria in regard to the analytic methods and the broad categorization into visual and statistical analytic approaches. The selection of an appropriate method for analyzing SCED data is perhaps the murkiest area of this type of research. Future reviews that evaluate the appropriateness of selected analytic strategies and provide specific decision-making guidelines for researchers would be a very useful contribution to the literature. Although six sources of standards apply to SCED research reviewed in this article, five of them were developed almost exclusively to inform psychological and behavioral intervention research. The principles of SCED research remain the same in different contexts, but there is a need for non–intervention scientists to weigh in on these standards.
Finally, this article provides a first step in the synthesis of the available SCED reporting guidelines. However, it does not resolve disagreements, nor does it purport to be a definitive source. In the future, an entity with the authority to construct such a document ought to convene and establish a foundational, adaptable, and agreed-upon set of guidelines that cuts across subspecialties but is applicable to many, if not all, areas of psychological research, which is perhaps an idealistic goal. Certain preferences will undoubtedly continue to dictate what constitutes acceptable practice in each subspecialty of psychology, but uniformity along critical dimensions will help advance SCED research.
Conclusions
The first decade of the twenty-first century has seen an upwelling of SCED research across nearly all areas of psychology. This article contributes updated benchmarks in terms of the frequency with which SCED design and methodology characteristics are used, including the number of baseline observations, assessment and measurement practices, and data analytic approaches, most of which are largely consistent with previously reported benchmarks. However, this review is much broader than those of previous research teams and also breaks down the characteristics of single-case research by the predominant design. With the recent SCED proliferation came a number of standards for the conduct and reporting of such research. This article also provides a much-needed synthesis of recent SCED standards that can inform the work of researchers, reviewers, and funding agencies conducting and evaluating single-case research, which reveals many areas of consensus as well as areas of significant disagreement. It appears that the question of where to go next is very relevant at this point in time. The majority of the research design and measurement characteristics of the SCED are reasonably well established, and the results of this review suggest general practice that is in accord with existing standards and guidelines, at least in regard to published peer-reviewed works. In general, the published literature appears to be meeting the basic design and measurement requirement to ensure adequate internal validity of SCED studies.
Consensus regarding the superiority of any one analytic method stands out as an area of divergence. Judging by the current literature and lack of consensus, researchers will need to carefully select a method that matches the research design, hypotheses, and intended conclusions of the study, while also considering the most up-to-date empirical support for the chosen analytic method, whether it be visual or statistical. In some cases the number of observations and subjects in the study will dictate which analytic methods can and cannot be used. In the case of the true N -of-1 experiment, there are relatively few sound analytic methods, and even fewer that are robust with shorter data streams (see Borckardt et al., 2008 ). As the number of observations and subjects increases, sophisticated modeling techniques, such as MLM, SEM, and ARMA, become applicable. Trends in the data and autocorrelation further obfuscate the development of a clear statistical analysis selection algorithm, which currently does not exist. Autocorrelation was rarely addressed or discussed in the articles reviewed, except when the selected statistical analysis dictated consideration. Given the empirical evidence regarding the effect of autocorrelation on visual and statistical analysis, researchers need to address this more explicitly. Missing-data considerations are similarly left out when they are unnecessary for analytic purposes. As newly devised statistical analysis approaches mature and are compared with one another for appropriateness in specific SCED applications, guidelines for statistical analysis will necessarily be revised. Similarly, empirically derived guidance, in the form of a decision tree, must be developed to ensure application of appropriate methods based on characteristics of the data and the research questions being addressed. Researchers could also benefit from tutorials and comparative reviews of different software packages: This is a needed area of future research. Powerful and reliable statistical analyses help move the SCED up the ladder of experimental designs and attenuate the view that the method applies primarily to pilot studies and idiosyncratic research questions and situations.
Another potential future advancement of SCED research comes in the area of measurement. Currently, SCED research gives significant weight to observer ratings and seems to discourage other forms of data collection methods. This is likely due to the origins of the SCED in behavioral assessment and applied behavior analysis, which remains a present-day stronghold. The dearth of EMA and diary-like sampling procedures within the SCED research reviewed, yet their ever-growing prevalence in the larger psychological research arena, highlights an area for potential expansion. Observational measurement, although reliable and valid in many contexts, is time and resource intensive and not feasible in all areas in which psychologists conduct research. It seems that numerous untapped research questions are stifled because of this measurement constraint. SCED researchers developing updated standards in the future should include guidelines for the appropriate measurement requirement of non-observer-reported data. For example, the results of this review indicate that reporting of repeated measurements, particularly the high-density type found in diary and EMA sampling strategies, ought to be more clearly spelled out, with specific attention paid to autocorrelation and trend in the data streams. In the event that SCED researchers adopt self-reported assessment strategies as viable alternatives to observation, a set of standards explicitly identifying the necessary psychometric properties of the measures and specific items used would be in order.
Along similar lines, SCED researchers could take a page from other areas of psychology that champion multimethod and multisource evaluation of primary outcomes. In this way, the long-standing tradition of observational assessment and the cutting-edge technological methods of EMA and daily diary could be married with the goal of strengthening conclusions drawn from SCED research and enhancing the validity of self-reported outcome assessment. The results of this review indicate that they rarely intersect today, and I urge SCED researchers to adopt other methods of assessment informed by time-series, daily diary, and EMA methods. The EMA standards could serve as a jumping-off point for refined measurement and assessment reporting standards in the context of multimethod SCED research.
One limitation of the current SCED standards is their relatively limited scope. To clarify, with the exception of the Stone & Shiffman EMA reporting guidelines, the other five sources of standards were developed in the context of designing and evaluating intervention research. Although this is likely to remain its patent emphasis, SCEDs are capable of addressing other pertinent research questions in the psychological sciences, and the current standards truly only roughly approximate salient crosscutting SCED characteristics. I propose developing broad SCED guidelines that address the specific design, measurement, and analysis issues in a manner that allows it to be useful across applications, as opposed to focusing solely on intervention effects. To accomplish this task, methodology experts across subspecialties in psychology would need to convene. Admittedly this is no small task.
Perhaps funding agencies will also recognize the fiscal and practical advantages of SCED research in certain areas of psychology. One example is in the field of intervention effectiveness, efficacy, and implementation research. A few exemplary studies using robust forms of SCED methodology are needed in the literature. Case-based methodologies will never supplant the group design as the gold standard in experimental applications, nor should that be the goal. Instead, SCEDs provide a viable and valid alternative experimental methodology that could stimulate new areas of research and answer questions that group designs cannot. With the astonishing number of studies emerging every year that use single-case designs and explore the methodological aspects of the design, we are poised to witness and be a part of an upsurge in the sophisticated application of the SCED. When federal grant-awarding agencies and journal editors begin to use formal standards while making funding and publication decisions, the field will benefit.
Last, for the practice of SCED research to continue and mature, graduate training programs must provide students with instruction in all areas of the SCED. This is particularly true of statistical analysis techniques that are not often taught in departments of psychology and education, where the vast majority of SCED studies seem to be conducted. It is quite the conundrum that the best available statistical analytic methods are often cited as being inaccessible to social science researchers who conduct this type of research. This need not be the case. To move the field forward, emerging scientists must be able to apply the most state-of-the-art research designs, measurement techniques, and analytic methods.
Acknowledgments
Research support for the author was provided by research training grant MH20012 from the National Institute of Mental Health, awarded to Elizabeth A. Stormshak. The author gratefully acknowledges Robert Horner and Laura Lee McIntyre, University of Oregon; Michael Nash, University of Tennessee; John Ferron, University of South Florida; the Action Editor, Lisa Harlow, and the anonymous reviewers for their thoughtful suggestions and guidance in shaping this article; Cheryl Mikkola for her editorial support; and Victoria Mollison for her assistance in the systematic review process.
Appendix. Results of Systematic Review Search and Studies Included in the Review
Psycinfo search conducted july 2011.
- Alternating treatment design
- Changing criterion design
- Experimental case*
- Multiple baseline design
- Replicated single-case design
- Simultaneous treatment design
- Time-series design
- Quantitative study OR treatment outcome/randomized clinical trial
- NOT field study OR interview OR focus group OR literature review OR systematic review OR mathematical model OR qualitative study
- Publication range: 2000–2010
- Published in peer-reviewed journals
- Available in the English Language
Bibliography
(* indicates inclusion in study: N = 409)
1 Autocorrelation estimates in this range can be caused by trends in the data streams, which creates complications in terms of detecting level-change effects. The Smith et al. (in press) study used a Monte Carlo simulation to control for trends in the data streams, but trends are likely to exist in real-world data with high lag-1 autocorrelation estimates.
2 The author makes no endorsement regarding the superiority of any statistical program or package over another by their mention or exclusion in this article. The author also has no conflicts of interest in this regard.
3 However, it should be noted that it was often very difficult to locate an actual effect size reported in studies that used statistical analysis. Although this issue would likely have added little to this review, it does inhibit the inclusion of the results in meta-analysis.
- Albright JJ, Marinova DM. Estimating multilevel modelsuUsing SPSS, Stata, and SAS. Indiana University; 2010. Retrieved from http://www.iub.edu/%7Estatmath/stat/all/hlm/hlm.pdf . [ Google Scholar ]
- Allison DB, Gorman BS. Calculating effect sizes for meta-analysis: The case of the single case. Behavior Research and Therapy. 1993; 31 (6):621–631. doi: 10.1016/0005-7967(93)90115-B. [ PubMed ] [ CrossRef ] [ Google Scholar ]
- Alloy LB, Just N, Panzarella C. Attributional style, daily life events, and hopelessness depression: Subtype validation by prospective variability and specificity of symptoms. Cognitive Therapy Research. 1997; 21 :321–344. doi: 10.1023/A:1021878516875. [ CrossRef ] [ Google Scholar ]
- Arbuckle JL. Amos (Version 7.0) Chicago, IL: SPSS, Inc; 2006. [ Google Scholar ]
- Barlow DH, Nock MK, Hersen M. Single case research designs: Strategies for studying behavior change. 3. New York, NY: Allyn and Bacon; 2008. [ Google Scholar ]
- Barrett LF, Barrett DJ. An introduction to computerized experience sampling in psychology. Social Science Computer Review. 2001; 19 (2):175–185. doi: 10.1177/089443930101900204. [ CrossRef ] [ Google Scholar ]
- Bloom M, Fisher J, Orme JG. Evaluating practice: Guidelines for the accountable professional. 4. Boston, MA: Allyn & Bacon; 2003. [ Google Scholar ]
- Bolger N, Davis A, Rafaeli E. Diary methods: Capturing life as it is lived. Annual Review of Psychology. 2003; 54 :579–616. doi: 10.1146/annurev.psych.54.101601.145030. [ PubMed ] [ CrossRef ] [ Google Scholar ]
- Borckardt JJ. Simulation Modeling Analysis: Time series analysis program for short time series data streams (Version 8.3.3) Charleston, SC: Medical University of South Carolina; 2006. [ Google Scholar ]
- Borckardt JJ, Nash MR, Murphy MD, Moore M, Shaw D, O’Neil P. Clinical practice as natural laboratory for psychotherapy research. American Psychologist. 2008; 63 :1–19. doi: 10.1037/0003-066X.63.2.77. [ PubMed ] [ CrossRef ] [ Google Scholar ]
- Borsboom D, Mellenbergh GJ, van Heerden J. The theoretical status of latent variables. Psychological Review. 2003; 110 (2):203–219. doi: 10.1037/0033-295X.110.2.203. [ PubMed ] [ CrossRef ] [ Google Scholar ]
- Bower GH. Mood and memory. American Psychologist. 1981; 36 (2):129–148. doi: 10.1037/0003-066x.36.2.129. [ PubMed ] [ CrossRef ] [ Google Scholar ]
- Box GEP, Jenkins GM. Time-series analysis: Forecasting and control. San Francisco, CA: Holden-Day; 1970. [ Google Scholar ]
- Brossart DF, Parker RI, Olson EA, Mahadevan L. The relationship between visual analysis and five statistical analyses in a simple AB single-case research design. Behavior Modification. 2006; 30 (5):531–563. doi: 10.1177/0145445503261167. [ PubMed ] [ CrossRef ] [ Google Scholar ]
- Browne MW, Nesselroade JR. Representing psychological processes with dynamic factor models: Some promising uses and extensions of autoregressive moving average time series models. In: Maydeu-Olivares A, McArdle JJ, editors. Contemporary psychometrics: A festschrift for Roderick P McDonald. Mahwah, NJ: Lawrence Erlbaum Associates Publishers; 2005. pp. 415–452. [ Google Scholar ]
- Busk PL, Marascuilo LA. Statistical analysis in single-case research: Issues, procedures, and recommendations, with applications to multiple behaviors. In: Kratochwill TR, Levin JR, editors. Single-case research design and analysis: New directions for psychology and education. Hillsdale, NJ, England: Lawrence Erlbaum Associates, Inc; 1992. pp. 159–185. [ Google Scholar ]
- Busk PL, Marascuilo RC. Autocorrelation in single-subject research: A counterargument to the myth of no autocorrelation. Behavioral Assessment. 1988; 10 :229–242. [ Google Scholar ]
- Campbell JM. Statistical comparison of four effect sizes for single-subject designs. Behavior Modification. 2004; 28 (2):234–246. doi: 10.1177/0145445503259264. [ PubMed ] [ CrossRef ] [ Google Scholar ]
- Carr EG, Horner RH, Turnbull AP, Marquis JG, Magito McLaughlin D, McAtee ML, Doolabh A. Positive behavior support for people with developmental disabilities: A research synthesis. Washington, DC: American Association on Mental Retardation; 1999. [ Google Scholar ]
- Center BA, Skiba RJ, Casey A. A methodology for the quantitative synthesis of intra-subject design research. Journal of Educational Science. 1986; 19 :387–400. doi: 10.1177/002246698501900404. [ CrossRef ] [ Google Scholar ]
- Chambless DL, Hollon SD. Defining empirically supported therapies. Journal of Consulting and Clinical Psychology. 1998; 66 (1):7–18. doi: 10.1037/0022-006X.66.1.7. [ PubMed ] [ CrossRef ] [ Google Scholar ]
- Chambless DL, Ollendick TH. Empirically supported psychological interventions: Controversies and evidence. Annual Review of Psychology. 2001; 52 :685–716. doi: 10.1146/annurev.psych.52.1.685. [ PubMed ] [ CrossRef ] [ Google Scholar ]
- Chow S-M, Ho M-hR, Hamaker EL, Dolan CV. Equivalence and differences between structural equation modeling and state-space modeling techniques. Structural Equation Modeling. 2010; 17 (2):303–332. doi: 10.1080/10705511003661553. [ CrossRef ] [ Google Scholar ]
- Cohen J. Statistical power analysis for the bahavioral sciences. 2. Hillsdale, NJ: Erlbaum; 1988. [ Google Scholar ]
- Cohen J. The earth is round (p < .05) American Psychologist. 1994; 49 :997–1003. doi: 10.1037/0003-066X.49.12.997. [ CrossRef ] [ Google Scholar ]
- Crosbie J. Interrupted time-series analysis with brief single-subject data. Journal of Consulting and Clinical Psychology. 1993; 61 (6):966–974. doi: 10.1037/0022-006X.61.6.966. [ PubMed ] [ CrossRef ] [ Google Scholar ]
- Dattilio FM, Edwards JA, Fishman DB. Case studies within a mixed methods paradigm: Toward a resolution of the alienation between researcher and practitioner in psychotherapy research. Psychotherapy: Theory, Research, Practice, Training. 2010; 47 (4):427–441. doi: 10.1037/a0021181. [ PubMed ] [ CrossRef ] [ Google Scholar ]
- Dempster A, Laird N, Rubin DB. Maximum likelihood from incomplete data via the EM algorithm. Journal of the Royal Statistical Society, Series B. 1977; 39 (1):1–38. [ Google Scholar ]
- Des Jarlais DC, Lyles C, Crepaz N. Improving the reporting quality of nonrandomized evaluations of behavioral and public health interventions: the TREND statement. American Journal of Public Health. 2004; 94 (3):361–366. doi: 10.2105/ajph.94.3.361. [ PMC free article ] [ PubMed ] [ CrossRef ] [ Google Scholar ]
- Diggle P, Liang KY. Analyses of longitudinal data. New York: Oxford University Press; 2001. [ Google Scholar ]
- Doss BD, Atkins DC. Investigating treatment mediators when simple random assignment to a control group is not possible. Clinical Psychology: Science and Practice. 2006; 13 (4):321–336. doi: 10.1111/j.1468-2850.2006.00045.x. [ CrossRef ] [ Google Scholar ]
- du Toit SHC, Browne MW. The covariance structure of a vector ARMA time series. In: Cudeck R, du Toit SHC, Sörbom D, editors. Structural equation modeling: Present and future. Lincolnwood, IL: Scientific Software International; 2001. pp. 279–314. [ Google Scholar ]
- du Toit SHC, Browne MW. Structural equation modeling of multivariate time series. Multivariate Behavioral Research. 2007; 42 :67–101. doi: 10.1080/00273170701340953. [ PubMed ] [ CrossRef ] [ Google Scholar ]
- Fechner GT. Elemente der psychophysik [Elements of psychophysics] Leipzig, Germany: Breitkopf & Hartel; 1889. [ Google Scholar ]
- Ferron J, Sentovich C. Statistical power of randomization tests used with multiple-baseline designs. The Journal of Experimental Education. 2002; 70 :165–178. doi: 10.1080/00220970209599504. [ CrossRef ] [ Google Scholar ]
- Ferron J, Ware W. Analyzing single-case data: The power of randomization tests. The Journal of Experimental Education. 1995; 63 :167–178. [ Google Scholar ]
- Fox J. TEACHER’S CORNER: Structural equation modeling with the sem package in R. Structural Equation Modeling: A Multidisciplinary Journal. 2006; 13 (3):465–486. doi: 10.1207/s15328007sem1303_7. [ CrossRef ] [ Google Scholar ]
- Franklin RD, Allison DB, Gorman BS, editors. Design and analysis of single-case research. Mahwah, NJ: Lawrence Erlbaum Associates; 1997. [ Google Scholar ]
- Franklin RD, Gorman BS, Beasley TM, Allison DB. Graphical display and visual analysis. In: Franklin RD, Allison DB, Gorman BS, editors. Design and analysis of single-case research. Mahway, NJ: Lawrence Erlbaum Associates, Publishers; 1997. pp. 119–158. [ Google Scholar ]
- Gardner W, Mulvey EP, Shaw EC. Regression analyses of counts and rates: Poisson, overdispersed Poisson, and negative binomial models. Psychological Bulletin. 1995; 118 (3):392–404. doi: 10.1037/0033-2909.118.3.392. [ PubMed ] [ CrossRef ] [ Google Scholar ]
- Green AS, Rafaeli E, Bolger N, Shrout PE, Reis HT. Paper or plastic? Data equivalence in paper and electronic diaries. Psychological Methods. 2006; 11 (1):87–105. doi: 10.1037/1082-989X.11.1.87. [ PubMed ] [ CrossRef ] [ Google Scholar ]
- Hamilton JD. Time series analysis. Princeton, NJ: Princeton University Press; 1994. [ Google Scholar ]
- Hammond D, Gast DL. Descriptive analysis of single-subject research designs: 1983–2007. Education and Training in Autism and Developmental Disabilities. 2010; 45 :187–202. [ Google Scholar ]
- Hanson MD, Chen E. Daily stress, cortisol, and sleep: The moderating role of childhood psychosocial environments. Health Psychology. 2010; 29 (4):394–402. doi: 10.1037/a0019879. [ PubMed ] [ CrossRef ] [ Google Scholar ]
- Harvey AC. Forecasting, structural time series models and the Kalman filter. Cambridge, MA: Cambridge University Press; 2001. [ Google Scholar ]
- Horner RH, Carr EG, Halle J, McGee G, Odom S, Wolery M. The use of single-subject research to identify evidence-based practice in special education. Exceptional Children. 2005; 71 :165–179. [ Google Scholar ]
- Horner RH, Spaulding S. Single-case research designs. In: Salkind NJ, editor. Encyclopedia of research design. Thousand Oaks, CA: Sage Publications; 2010. [ Google Scholar ]
- Horton NJ, Kleinman KP. Much ado about nothing: A comparison of missing data methods and software to fit incomplete data regression models. The American Statistician. 2007; 61 (1):79–90. doi: 10.1198/000313007X172556. [ PMC free article ] [ PubMed ] [ CrossRef ] [ Google Scholar ]
- Hser Y, Shen H, Chou C, Messer SC, Anglin MD. Analytic approaches for assessing long-term treatment effects. Evaluation Review. 2001; 25 (2):233–262. doi: 10.1177/0193841X0102500206. [ PubMed ] [ CrossRef ] [ Google Scholar ]
- Huitema BE. Autocorrelation in applied behavior analysis: A myth. Behavioral Assessment. 1985; 7 (2):107–118. [ Google Scholar ]
- Huitema BE, McKean JW. Reduced bias autocorrelation estimation: Three jackknife methods. Educational and Psychological Measurement. 1994; 54 (3):654–665. doi: 10.1177/0013164494054003008. [ CrossRef ] [ Google Scholar ]
- Ibrahim JG, Chen M-H, Lipsitz SR, Herring AH. Missing-data methods for generalized linear models: A comparative review. Journal of the American Statistical Association. 2005; 100 (469):332–346. doi: 10.1198/016214504000001844. [ CrossRef ] [ Google Scholar ]
- Institute of Medicine. Reducing risks for mental disorders: Frontiers for preventive intervention research. Washington, DC: National Academy Press; 1994. [ PubMed ] [ Google Scholar ]
- Jacobsen NS, Christensen A. Studying the effectiveness of psychotherapy: How well can clinical trials do the job? American Psychologist. 1996; 51 :1031–1039. doi: 10.1037/0003-066X.51.10.1031. [ PubMed ] [ CrossRef ] [ Google Scholar ]
- Jones RR, Vaught RS, Weinrott MR. Time-series analysis in operant research. Journal of Behavior Analysis. 1977; 10 (1):151–166. doi: 10.1901/jaba.1977.10-151. [ PMC free article ] [ PubMed ] [ CrossRef ] [ Google Scholar ]
- Jones WP. Single-case time series with Bayesian analysis: A practitioner’s guide. Measurement and Evaluation in Counseling and Development. 2003; 36 (28–39) [ Google Scholar ]
- Kanfer H. Self-monitoring: Methodological limitations and clinical applications. Journal of Consulting and Clinical Psychology. 1970; 35 (2):148–152. doi: 10.1037/h0029874. [ CrossRef ] [ Google Scholar ]
- Kazdin AE. Drawing valid inferences from case studies. Journal of Consulting and Clinical Psychology. 1981; 49 (2):183–192. doi: 10.1037/0022-006X.49.2.183. [ PubMed ] [ CrossRef ] [ Google Scholar ]
- Kazdin AE. Mediators and mechanisms of change in psychotherapy research. Annual Review of Clinical Psychology. 2007; 3 :1–27. doi: 10.1146/annurev.clinpsy.3.022806.091432. [ PubMed ] [ CrossRef ] [ Google Scholar ]
- Kazdin AE. Evidence-based treatment and practice: New opportunities to bridge clinical research and practice, enhance the knowledge base, and improve patient care. American Psychologist. 2008; 63 (3):146–159. doi: 10.1037/0003-066X.63.3.146. [ PubMed ] [ CrossRef ] [ Google Scholar ]
- Kazdin AE. Understanding how and why psychotherapy leads to change. Psychotherapy Research. 2009; 19 (4):418–428. doi: 10.1080/10503300802448899. [ PubMed ] [ CrossRef ] [ Google Scholar ]
- Kazdin AE. Single-case research designs: Methods for clinical and applied settings. 2. New York, NY: Oxford University Press; 2010. [ Google Scholar ]
- Kirk RE. Practical significance: A concept whose time has come. Educational and Psychological Measurement. 1996; 56 :746–759. doi: 10.1177/0013164496056005002. [ CrossRef ] [ Google Scholar ]
- Kratochwill TR. Preparing psychologists for evidence-based school practice: Lessons learned and challenges ahead. American Psychologist. 2007; 62 :829–843. doi: 10.1037/0003-066X.62.8.829. [ PubMed ] [ CrossRef ] [ Google Scholar ]
- Kratochwill TR, Hitchcock J, Horner RH, Levin JR, Odom SL, Rindskopf DM, Shadish WR. Single-case designs technical documentation. 2010 Retrieved from What Works Clearinghouse website: http://ies.ed.gov/ncee/wwc/pdf/wwc_scd.pdf . Retrieved from http://ies.ed.gov/ncee/wwc/pdf/wwc_scd.pdf .
- Kratochwill TR, Levin JR. Single-case research design and analysis: New directions for psychology and education. Hillsdale, NJ: Lawrence Erlbaum Associates, Inc; 1992. [ Google Scholar ]
- Kratochwill TR, Levin JR. Enhancing the scientific credibility of single-case intervention research: Randomization to the rescue. Psychological Methods. 2010; 15 (2):124–144. doi: 10.1037/a0017736. [ PubMed ] [ CrossRef ] [ Google Scholar ]
- Kratochwill TR, Levin JR, Horner RH, Swoboda C. Visual analysis of single-case intervention research: Conceptual and methodological considerations (WCER Working Paper No. 2011-6) 2011 Retrieved from University of Wisconsin–Madison, Wisconsin Center for Education Research website: http://www.wcer.wisc.edu/publications/workingPapers/papers.php .
- Lambert D. Zero-inflated poisson regression, with an application to defects in manufacturing. Technometrics. 1992; 34 (1):1–14. [ Google Scholar ]
- Lambert MJ, Hansen NB, Harmon SC. Developing and Delivering Practice-Based Evidence. John Wiley & Sons, Ltd; 2010. Outcome Questionnaire System (The OQ System): Development and practical applications in healthcare settings; pp. 139–154. [ Google Scholar ]
- Littell JH, Corcoran J, Pillai VK. Systematic reviews and meta-analysis. New York: Oxford University Press; 2008. [ Google Scholar ]
- Liu LM, Hudack GB. The SCA statistical system. Vector ARMA modeling of multiple time series. Oak Brook, IL: Scientific Computing Associates Corporation; 1995. [ Google Scholar ]
- Lubke GH, Muthén BO. Investigating population heterogeneity with factor mixture models. Psychological Methods. 2005; 10 (1):21–39. doi: 10.1037/1082-989x.10.1.21. [ PubMed ] [ CrossRef ] [ Google Scholar ]
- Manolov R, Solanas A. Comparing N = 1 effect sizes in presence of autocorrelation. Behavior Modification. 2008; 32 (6):860–875. doi: 10.1177/0145445508318866. [ PubMed ] [ CrossRef ] [ Google Scholar ]
- Marshall RJ. Autocorrelation estimation of time series with randomly missing observations. Biometrika. 1980; 67 (3):567–570. doi: 10.1093/biomet/67.3.567. [ CrossRef ] [ Google Scholar ]
- Matyas TA, Greenwood KM. Visual analysis of single-case time series: Effects of variability, serial dependence, and magnitude of intervention effects. Journal of Applied Behavior Analysis. 1990; 23 (3):341–351. doi: 10.1901/jaba.1990.23-341. [ PMC free article ] [ PubMed ] [ CrossRef ] [ Google Scholar ]
- Kratochwill TR, Chair Members of the Task Force on Evidence-Based Interventions in School Psychology. Procedural and coding manual for review of evidence-based interventions. 2003 Retrieved July 18, 2011 from http://www.sp-ebi.org/documents/_workingfiles/EBImanual1.pdf .
- Moher D, Schulz KF, Altman DF the CONSORT Group. The CONSORT statement: Revised recommendations for improving the quality of reports of parallel-group randomized trials. Journal of the American Medical Association. 2001; 285 :1987–1991. doi: 10.1001/jama.285.15.1987. [ PubMed ] [ CrossRef ] [ Google Scholar ]
- Morgan DL, Morgan RK. Single-participant research design: Bringing science to managed care. American Psychologist. 2001; 56 (2):119–127. doi: 10.1037/0003-066X.56.2.119. [ PubMed ] [ CrossRef ] [ Google Scholar ]
- Muthén BO, Curran PJ. General longitudinal modeling of individual differences in experimental designs: A latent variable framework for analysis and power estimation. Psychological Methods. 1997; 2 (4):371–402. doi: 10.1037/1082-989x.2.4.371. [ CrossRef ] [ Google Scholar ]
- Muthén LK, Muthén BO. Mplus (Version 6.11) Los Angeles, CA: Muthén & Muthén; 2010. [ Google Scholar ]
- Nagin DS. Analyzing developmental trajectories: A semiparametric, group-based approach. Psychological Methods. 1999; 4 (2):139–157. doi: 10.1037/1082-989x.4.2.139. [ PubMed ] [ CrossRef ] [ Google Scholar ]
- National Institute of Child Health and Human Development. Report of the National Reading Panel. Teaching children to read: An evidence-based assessment of the scientific research literature on reading and its implications for reading instruction (NIH Publication No. 00-4769) Washington, DC: U.S. Government Printing Office; 2000. [ Google Scholar ]
- Olive ML, Smith BW. Effect size calculations and single subject designs. Educational Psychology. 2005; 25 (2–3):313–324. doi: 10.1080/0144341042000301238. [ CrossRef ] [ Google Scholar ]
- Oslin DW, Cary M, Slaymaker V, Colleran C, Blow FC. Daily ratings measures of alcohol craving during an inpatient stay define subtypes of alcohol addiction that predict subsequent risk for resumption of drinking. Drug and Alcohol Dependence. 2009; 103 (3):131–136. doi: 10.1016/J.Drugalcdep.2009.03.009. [ PubMed ] [ CrossRef ] [ Google Scholar ]
- Palermo TP, Valenzuela D, Stork PP. A randomized trial of electronic versus paper pain diaries in children: Impact on compliance, accuracy, and acceptability. Pain. 2004; 107 (3):213–219. doi: 10.1016/j.pain.2003.10.005. [ PubMed ] [ CrossRef ] [ Google Scholar ]
- Parker RI, Brossart DF. Evaluating single-case research data: A comparison of seven statistical methods. Behavior Therapy. 2003; 34 (2):189–211. doi: 10.1016/S0005-7894(03)80013-8. [ CrossRef ] [ Google Scholar ]
- Parker RI, Cryer J, Byrns G. Controlling baseline trend in single case research. School Psychology Quarterly. 2006; 21 (4):418–440. doi: 10.1037/h0084131. [ CrossRef ] [ Google Scholar ]
- Parker RI, Vannest K. An improved effect size for single-case research: Nonoverlap of all pairs. Behavior Therapy. 2009; 40 (4):357–367. doi: 10.1016/j.beth.2008.10.006. [ PubMed ] [ CrossRef ] [ Google Scholar ]
- Parsonson BS, Baer DM. The analysis and presentation of graphic data. In: Kratochwill TR, editor. Single subject research. New York, NY: Academic Press; 1978. pp. 101–166. [ Google Scholar ]
- Parsonson BS, Baer DM. The visual analysis of data, and current research into the stimuli controlling it. In: Kratochwill TR, Levin JR, editors. Single-case research design and analysis: New directions for psychology and education. Hillsdale, NJ; England: Lawrence Erlbaum Associates, Inc; 1992. pp. 15–40. [ Google Scholar ]
- Piasecki TM, Hufford MR, Solham M, Trull TJ. Assessing clients in their natural environments with electronic diaries: Rationale, benefits, limitations, and barriers. Psychological Assessment. 2007; 19 (1):25–43. doi: 10.1037/1040-3590.19.1.25. [ PubMed ] [ CrossRef ] [ Google Scholar ]
- R Development Core Team. R: A language and environment for statistical computing. Vienna, Austria: R Foundation for Statistical Computing; 2005. [ Google Scholar ]
- Ragunathan TE. What do we do with missing data? Some options for analysis of incomplete data. Annual Review of Public Health. 2004; 25 :99–117. doi: 10.1146/annurev.publhealth.25.102802.124410. [ PubMed ] [ CrossRef ] [ Google Scholar ]
- Raudenbush SW, Bryk AS, Congdon R. HLM 7 Hierarchical Linear and Nonlinear Modeling. Scientific Software International, Inc; 2011. [ Google Scholar ]
- Redelmeier DA, Kahneman D. Patients’ memories of painful medical treatments: Real-time and retrospective evaluations of two minimally invasive procedures. Pain. 1996; 66 (1):3–8. doi: 10.1016/0304-3959(96)02994-6. [ PubMed ] [ CrossRef ] [ Google Scholar ]
- Reis HT. Domains of experience: Investigating relationship processes from three perspectives. In: Erber R, Gilmore R, editors. Theoretical frameworks in personal relationships. Mahwah, NJ: Erlbaum; 1994. pp. 87–110. [ Google Scholar ]
- Reis HT, Gable SL. Event sampling and other methods for studying everyday experience. In: Reis HT, Judd CM, editors. Handbook of research methods in social and personality psychology. New York, NY: Cambridge University Press; 2000. pp. 190–222. [ Google Scholar ]
- Robey RR, Schultz MC, Crawford AB, Sinner CA. Single-subject clinical-outcome research: Designs, data, effect sizes, and analyses. Aphasiology. 1999; 13 (6):445–473. doi: 10.1080/026870399402028. [ CrossRef ] [ Google Scholar ]
- Rossi PH, Freeman HE. Evaluation: A systematic approach. 5. Thousand Oaks, CA: Sage; 1993. [ Google Scholar ]
- SAS Institute Inc. The SAS system for Windows, Version 9. Cary, NC: SAS Institute Inc; 2008. [ Google Scholar ]
- Schmidt M, Perels F, Schmitz B. How to perform idiographic and a combination of idiographic and nomothetic approaches: A comparison of time series analyses and hierarchical linear modeling. Journal of Psychology. 2010; 218 (3):166–174. doi: 10.1027/0044-3409/a000026. [ CrossRef ] [ Google Scholar ]
- Scollon CN, Kim-Pietro C, Diener E. Experience sampling: Promises and pitfalls, strengths and weaknesses. Assessing Well-Being. 2003; 4 :5–35. doi: 10.1007/978-90-481-2354-4_8. [ CrossRef ] [ Google Scholar ]
- Scruggs TE, Mastropieri MA. Summarizing single-subject research: Issues and applications. Behavior Modification. 1998; 22 (3):221–242. doi: 10.1177/01454455980223001. [ PubMed ] [ CrossRef ] [ Google Scholar ]
- Scruggs TE, Mastropieri MA, Casto G. The quantitative synthesis of single-subject research. Remedial and Special Education. 1987; 8 (2):24–33. doi: 10.1177/074193258700800206. [ CrossRef ] [ Google Scholar ]
- Shadish WR, Cook TD, Campbell DT. Experimental and quasi-experimental designs for generalized causal inference. Boston, MA: Houghton Mifflin; 2002. [ Google Scholar ]
- Shadish WR, Rindskopf DM, Hedges LV. The state of the science in the meta-analysis of single-case experimental designs. Evidence-Based Communication Assessment and Intervention. 2008; 3 :188–196. doi: 10.1080/17489530802581603. [ CrossRef ] [ Google Scholar ]
- Shadish WR, Sullivan KJ. Characteristics of single-case designs used to assess treatment effects in 2008. Behavior Research Methods. 2011; 43 :971–980. doi: 10.3758/s13428-011-0111-y. [ PubMed ] [ CrossRef ] [ Google Scholar ]
- Sharpley CF. Time-series analysis of behavioural data: An update. Behaviour Change. 1987; 4 :40–45. [ Google Scholar ]
- Shiffman S, Hufford M, Hickcox M, Paty JA, Gnys M, Kassel JD. Remember that? A comparison of real-time versus retrospective recall of smoking lapses. Journal of Consulting and Clinical Psychology. 1997; 65 :292–300. doi: 10.1037/0022-006X.65.2.292.a. [ PubMed ] [ CrossRef ] [ Google Scholar ]
- Shiffman S, Stone AA. Ecological momentary assessment: A new tool for behavioral medicine research. In: Krantz DS, Baum A, editors. Technology and methods in behavioral medicine. Mahwah, NJ: Erlbaum; 1998. pp. 117–131. [ Google Scholar ]
- Shiffman S, Stone AA, Hufford MR. Ecological momentary assessment. Annual Review of Clinical Psychology. 2008; 4 :1–32. doi: 10.1146/annurev.clinpsy.3.022806.091415. [ PubMed ] [ CrossRef ] [ Google Scholar ]
- Shumway RH, Stoffer DS. An approach to time series smoothing and forecasting using the EM Algorithm. Journal of Time Series Analysis. 1982; 3 (4):253–264. doi: 10.1111/j.1467-9892.1982.tb00349.x. [ CrossRef ] [ Google Scholar ]
- Skinner BF. The behavior of organisms. New York, NY: Appleton-Century-Crofts; 1938. [ Google Scholar ]
- Smith JD, Borckardt JJ, Nash MR. Inferential precision in single-case time-series datastreams: How well does the EM Procedure perform when missing observations occur in autocorrelated data? Behavior Therapy. doi: 10.1016/j.beth.2011.10.001. (in press) [ PMC free article ] [ PubMed ] [ CrossRef ] [ Google Scholar ]
- Smith JD, Handler L, Nash MR. Therapeutic Assessment for preadolescent boys with oppositional-defiant disorder: A replicated single-case time-series design. Psychological Assessment. 2010; 22 (3):593–602. doi: 10.1037/a0019697. [ PubMed ] [ CrossRef ] [ Google Scholar ]
- Snijders TAB, Bosker RJ. Multilevel analysis: An introduction to basic and advanced multilevel modeling. Thousand Oaks, CA: Sage; 1999. [ Google Scholar ]
- Soliday E, Moore KJ, Lande MB. Daily reports and pooled time series analysis: Pediatric psychology applications. Journal of Pediatric Psychology. 2002; 27 (1):67–76. doi: 10.1093/jpepsy/27.1.67. [ PubMed ] [ CrossRef ] [ Google Scholar ]
- SPSS Statistics. Chicago, IL: SPSS Inc; 2011. (Version 20.0.0) [ Google Scholar ]
- StataCorp. Stata Statistical Software: Release 12. College Station, TX: StataCorp LP; 2011. [ Google Scholar ]
- Stone AA, Broderick JE, Kaell AT, Deles-Paul PAEG, Porter LE. Does the peak-end phenomenon observed in laboratory pain studies apply to real-world pain in rheumatoid arthritics? Journal of Pain. 2000; 1 :212–217. doi: 10.1054/jpai.2000.7568. [ PubMed ] [ CrossRef ] [ Google Scholar ]
- Stone AA, Shiffman S. Capturing momentary, self-report data: A proposal for reporting guidelines. Annals of Behavioral Medicine. 2002; 24 :236–243. doi: 10.1207/S15324796ABM2403_09. [ PubMed ] [ CrossRef ] [ Google Scholar ]
- Stout RL. Advancing the analysis of treatment process. Addiction. 2007; 102 :1539–1545. doi: 10.1111/j.1360-0443.2007.01880.x. [ PubMed ] [ CrossRef ] [ Google Scholar ]
- Tate RL, McDonald S, Perdices M, Togher L, Schultz R, Savage S. Rating the methodological quality of single-subject designs and N-of-1 trials: Introducing the Single-Case Experimental Design (SCED) Scale. Neuropsychological Rehabilitation. 2008; 18 (4):385–401. doi: 10.1080/09602010802009201. [ PubMed ] [ CrossRef ] [ Google Scholar ]
- Thiele C, Laireiter A-R, Baumann U. Diaries in clinical psychology and psychotherapy: A selective review. Clinical Psychology & Psychotherapy. 2002; 9 (1):1–37. doi: 10.1002/cpp.302. [ CrossRef ] [ Google Scholar ]
- Tiao GC, Box GEP. Modeling multiple time series with applications. Journal of the American Statistical Association. 1981; 76 :802–816. [ Google Scholar ]
- Tschacher W, Ramseyer F. Modeling psychotherapy process by time-series panel analysis (TSPA) Psychotherapy Research. 2009; 19 (4):469–481. doi: 10.1080/10503300802654496. [ PubMed ] [ CrossRef ] [ Google Scholar ]
- Velicer WF, Colby SM. A comparison of missing-data procedures for ARIMA time-series analysis. Educational and Psychological Measurement. 2005a; 65 (4):596–615. doi: 10.1177/0013164404272502. [ CrossRef ] [ Google Scholar ]
- Velicer WF, Colby SM. Missing data and the general transformation approach to time series analysis. In: Maydeu-Olivares A, McArdle JJ, editors. Contemporary psychometrics. A festschrift to Roderick P McDonald. Hillsdale, NJ: Lawrence Erlbaum; 2005b. pp. 509–535. [ Google Scholar ]
- Velicer WF, Fava JL. Time series analysis. In: Schinka J, Velicer WF, Weiner IB, editors. Research methods in psychology. Vol. 2. New York, NY: John Wiley & Sons; 2003. [ Google Scholar ]
- Wachtel PL. Beyond “ESTs”: Problematic assumptions in the pursuit of evidence-based practice. Psychoanalytic Psychology. 2010; 27 (3):251–272. doi: 10.1037/a0020532. [ CrossRef ] [ Google Scholar ]
- Watson JB. Behaviorism. New York, NY: Norton; 1925. [ Google Scholar ]
- Weisz JR, Hawley KM. Finding, evaluating, refining, and applying empirically supported treatments for children and adolescents. Journal of Clinical Child Psychology. 1998; 27 :206–216. doi: 10.1207/s15374424jccp2702_7. [ PubMed ] [ CrossRef ] [ Google Scholar ]
- Weisz JR, Hawley KM. Procedural and coding manual for identification of beneficial treatments. Washinton, DC: American Psychological Association, Society for Clinical Psychology, Division 12, Committee on Science and Practice; 1999. [ Google Scholar ]
- Westen D, Bradley R. Empirically supported complexity. Current Directions in Psychological Science. 2005; 14 :266–271. doi: 10.1111/j.0963-7214.2005.00378.x. [ CrossRef ] [ Google Scholar ]
- Westen D, Novotny CM, Thompson-Brenner HK. The empirical status of empirically supported psychotherapies: Assumptions, findings, and reporting controlled clinical trials. Psychological Bulletin. 2004; 130 :631–663. doi: 10.1037/0033-2909.130.4.631. [ PubMed ] [ CrossRef ] [ Google Scholar ]
- Wilkinson L The Task Force on Statistical Inference. Statistical methods in psychology journals: Guidelines and explanations. American Psychologist. 1999; 54 :694–704. doi: 10.1037/0003-066X.54.8.594. [ CrossRef ] [ Google Scholar ]
- Wolery M, Busick M, Reichow B, Barton EE. Comparison of overlap methods for quantitatively synthesizing single-subject data. The Journal of Special Education. 2010; 44 (1):18–28. doi: 10.1177/0022466908328009. [ CrossRef ] [ Google Scholar ]
- Wu Z, Huang NE, Long SR, Peng C-K. On the trend, detrending, and variability of nonlinear and nonstationary time series. Proceedings of the National Academy of Sciences. 2007; 104 (38):14889–14894. doi: 10.1073/pnas.0701020104. [ PMC free article ] [ PubMed ] [ CrossRef ] [ Google Scholar ]
Thank you for visiting nature.com. You are using a browser version with limited support for CSS. To obtain the best experience, we recommend you use a more up to date browser (or turn off compatibility mode in Internet Explorer). In the meantime, to ensure continued support, we are displaying the site without styles and JavaScript.
- View all journals
- Explore content
- About the journal
- Publish with us
- Sign up for alerts
- Published: 05 April 2024
Single-case experimental designs: the importance of randomization and replication
- René Tanious ORCID: orcid.org/0000-0002-5466-1002 1 ,
- Rumen Manolov ORCID: orcid.org/0000-0002-9387-1926 2 ,
- Patrick Onghena 3 &
- Johan W. S. Vlaeyen ORCID: orcid.org/0000-0003-0437-6665 1
Nature Reviews Methods Primers volume 4 , Article number: 27 ( 2024 ) Cite this article
46 Accesses
9 Altmetric
Metrics details
- Data acquisition
- Human behaviour
- Social sciences
Single-case experimental designs are rapidly growing in popularity. This popularity needs to be accompanied by transparent and well-justified methodological and statistical decisions. Appropriate experimental design including randomization, proper data handling and adequate reporting are needed to ensure reproducibility and internal validity. The degree of generalizability can be assessed through replication.
This is a preview of subscription content, access via your institution
Access options
Access Nature and 54 other Nature Portfolio journals
Get Nature+, our best-value online-access subscription
24,99 € / 30 days
cancel any time
Subscribe to this journal
Receive 1 digital issues and online access to articles
92,52 € per year
only 92,52 € per issue
Rent or buy this article
Prices vary by article type
Prices may be subject to local taxes which are calculated during checkout
Kazdin, A. E. Single-case experimental designs: characteristics, changes, and challenges. J. Exp. Anal. Behav. 115 , 56–85 (2021).
Article Google Scholar
Shadish, W. & Sullivan, K. J. Characteristics of single-case designs used to assess intervention effects in 2008. Behav. Res. 43 , 971–980 (2011).
Tanious, R. & Onghena, P. A systematic review of applied single-case research published between 2016 and 2018: study designs, randomization, data aspects, and data analysis. Behav. Res. 53 , 1371–1384 (2021).
Ferron, J., Foster-Johnson, L. & Kromrey, J. D. The functioning of single-case randomization tests with and without random assignment. J. Exp. Educ. 71 , 267–288 (2003).
Michiels, B., Heyvaert, M., Meulders, A. & Onghena, P. Confidence intervals for single-case effect size measures based on randomization test inversion. Behav. Res. 49 , 363–381 (2017).
Aydin, O. Characteristics of missing data in single-case experimental designs: an investigation of published data. Behav. Modif. https://doi.org/10.1177/01454455231212265 (2023).
De, T. K., Michiels, B., Tanious, R. & Onghena, P. Handling missing data in randomization tests for single-case experiments: a simulation study. Behav. Res. 52 , 1355–1370 (2020).
Baek, E., Luo, W. & Lam, K. H. Meta-analysis of single-case experimental design using multilevel modeling. Behav. Modif. 47 , 1546–1573 (2023).
Michiels, B., Tanious, R., De, T. K. & Onghena, P. A randomization test wrapper for synthesizing single-case experiments using multilevel models: a Monte Carlo simulation study. Behav. Res. 52 , 654–666 (2020).
Tate, R. L. et al. The single-case reporting guideline in behavioural interventions (SCRIBE) 2016: explanation and elaboration. Arch. Sci. Psychol. 4 , 10–31 (2016).
Google Scholar
Download references
Acknowledgements
R.T. and J.W.S.V. disclose support for the research of this work from the Dutch Research Council and the Dutch Ministry of Education, Culture and Science (NWO gravitation grant number 024.004.016) within the research project ‘New Science of Mental Disorders’ ( www.nsmd.eu ). R.M. discloses support from the Generalitat de Catalunya’s Agència de Gestió d’Ajusts Universitaris i de Recerca (grant number 2021SGR00366).
Author information
Authors and affiliations.
Experimental Health Psychology, Faculty of Psychology and Neuroscience, Maastricht University, Maastricht, the Netherlands
René Tanious & Johan W. S. Vlaeyen
Department of Social Psychology and Quantitative Psychology, Faculty of Psychology, University of Barcelona, Barcelona, Spain
Rumen Manolov
Methodology of Educational Sciences Research Group, Faculty of Psychology and Educational Science, KU Leuven, Leuven, Belgium
Patrick Onghena
You can also search for this author in PubMed Google Scholar
Corresponding author
Correspondence to René Tanious .
Ethics declarations
Competing interests.
The authors declare no competing interests.
Rights and permissions
Reprints and permissions
About this article
Cite this article.
Tanious, R., Manolov, R., Onghena, P. et al. Single-case experimental designs: the importance of randomization and replication. Nat Rev Methods Primers 4 , 27 (2024). https://doi.org/10.1038/s43586-024-00312-8
Download citation
Published : 05 April 2024
DOI : https://doi.org/10.1038/s43586-024-00312-8
Share this article
Anyone you share the following link with will be able to read this content:
Sorry, a shareable link is not currently available for this article.
Provided by the Springer Nature SharedIt content-sharing initiative
Quick links
- Explore articles by subject
- Guide to authors
- Editorial policies
Sign up for the Nature Briefing newsletter — what matters in science, free to your inbox daily.

- Subject List
- Take a Tour
- For Authors
- Subscriber Services
- Publications
- African American Studies
- African Studies
- American Literature
- Anthropology
- Architecture Planning and Preservation
- Art History
- Atlantic History
- Biblical Studies
- British and Irish Literature
- Childhood Studies
- Chinese Studies
- Cinema and Media Studies
- Communication
- Criminology
- Environmental Science
- Evolutionary Biology
- International Law
- International Relations
- Islamic Studies
- Jewish Studies
- Latin American Studies
- Latino Studies
- Linguistics
- Literary and Critical Theory
- Medieval Studies
- Military History
- Political Science
- Public Health
- Renaissance and Reformation
- Social Work
- Urban Studies
- Victorian Literature
- Browse All Subjects
How to Subscribe
- Free Trials
In This Article Expand or collapse the "in this article" section Single-Case Experimental Designs
Introduction, general overviews and primary textbooks.
- Textbooks in Applied Behavior Analysis
- Types of Single-Case Experimental Designs
- Model Building and Randomization in Single-Case Experimental Designs
- Visual Analysis of Single-Case Experimental Designs
- Effect Size Estimates in Single-Case Experimental Designs
- Reporting Single-Case Design Intervention Research
Related Articles Expand or collapse the "related articles" section about
About related articles close popup.
Lorem Ipsum Sit Dolor Amet
Vestibulum ante ipsum primis in faucibus orci luctus et ultrices posuere cubilia Curae; Aliquam ligula odio, euismod ut aliquam et, vestibulum nec risus. Nulla viverra, arcu et iaculis consequat, justo diam ornare tellus, semper ultrices tellus nunc eu tellus.
- Action Research
- Ambulatory Assessment in Behavioral Science
- Effect Size
- Mediation Analysis
- Path Models
- Research Methods for Studying Daily Life
Other Subject Areas
Forthcoming articles expand or collapse the "forthcoming articles" section.
- Data Visualization
- Remote Work
- Workforce Training Evaluation
- Find more forthcoming articles...
- Export Citations
- Share This Facebook LinkedIn Twitter
Single-Case Experimental Designs by S. Andrew Garbacz , Thomas R. Kratochwill LAST MODIFIED: 29 July 2020 DOI: 10.1093/obo/9780199828340-0265
Single-case experimental designs are a family of experimental designs that are characterized by researcher manipulation of an independent variable and repeated measurement of a dependent variable before (i.e., baseline) and after (i.e., intervention phase) introducing the independent variable. In single-case experimental designs a case is the unit of intervention and analysis (e.g., a child, a school). Because measurement within each case is conducted before and after manipulation of the independent variable, the case typically serves as its own control. Experimental variants of single-case designs provide a basis for determining a causal relation by replication of the intervention through (a) introducing and withdrawing the independent variable, (b) manipulating the independent variable across different phases, and (c) introducing the independent variable in a staggered fashion across different points in time. Due to their economy of resources, single-case designs may be useful during development activities and allow for rapid replication across studies.
Several sources provide overviews of single-case experimental designs. Barlow, et al. 2009 includes an overview for the development of single-case experimental designs, describes key considerations for designing and conducting single-case experimental design research, and reviews procedural elements, assessment strategies, and replication considerations. Kazdin 2011 provides detailed coverage of single-case experimental design variants as well as approaches for evaluating data in single-case experimental designs. Kratochwill and Levin 2014 describes key methodological features that underlie single-case experimental designs, including philosophical and statistical foundations and data evaluation. Ledford and Gast 2018 covers research conceptualization and writing, design variants within single-case experimental design, definitions of variables and associated measurement, and approaches to organize and evaluate data. Riley-Tillman and Burns 2009 provides a practical orientation to single-case experimental designs to facilitate uptake and use in applied settings.
Barlow, D. H., M. K. Nock, and M. Hersen, eds. 2009. Single case experimental designs: Strategies for studying behavior change . 3d ed. New York: Pearson.
A comprehensive reference about the process of designing and conducting single-case experimental design studies. Chapters are integrative but can stand alone.
Kazdin, A. E. 2011. Single-case research designs: Methods for clinical and applied settings . 2d ed. New York: Oxford Univ. Press.
A complete overview and description of single-case experimental design variants as well as information about data evaluation.
Kratochwill, T. R., and J. R. Levin, eds. 2014. Single-case intervention research: Methodological and statistical advances . New York: Routledge.
The authors describe in depth the methodological and analytic considerations necessary for designing and conducting research that uses a single-case experimental design. In addition, the text includes chapters from leaders in psychology and education who provide critical perspectives about the use of single-case experimental designs.
Ledford, J. R., and D. L. Gast, eds. 2018. Single case research methodology: Applications in special education and behavioral sciences . New York: Routledge.
Covers the research process from writing literature reviews, to designing, conducting, and evaluating single-case experimental design studies.
Riley-Tillman, T. C., and M. K. Burns. 2009. Evaluating education interventions: Single-case design for measuring response to intervention . New York: Guilford Press.
Focuses on accelerating uptake and use of single-case experimental designs in applied settings. This book provides a practical, “nuts and bolts” orientation to conducting single-case experimental design research.
back to top
Users without a subscription are not able to see the full content on this page. Please subscribe or login .
Oxford Bibliographies Online is available by subscription and perpetual access to institutions. For more information or to contact an Oxford Sales Representative click here .
- About Psychology »
- Meet the Editorial Board »
- Abnormal Psychology
- Academic Assessment
- Acculturation and Health
- Action Regulation Theory
- Addictive Behavior
- Adolescence
- Adoption, Social, Psychological, and Evolutionary Perspect...
- Advanced Theory of Mind
- Affective Forecasting
- Affirmative Action
- Ageism at Work
- Allport, Gordon
- Alzheimer’s Disease
- Analysis of Covariance (ANCOVA)
- Animal Behavior
- Animal Learning
- Anxiety Disorders
- Art and Aesthetics, Psychology of
- Artificial Intelligence, Machine Learning, and Psychology
- Assessment and Clinical Applications of Individual Differe...
- Attachment in Social and Emotional Development across the ...
- Attention-Deficit/Hyperactivity Disorder (ADHD) in Adults
- Attention-Deficit/Hyperactivity Disorder (ADHD) in Childre...
- Attitudinal Ambivalence
- Attraction in Close Relationships
- Attribution Theory
- Authoritarian Personality
- Bayesian Statistical Methods in Psychology
- Behavior Therapy, Rational Emotive
- Behavioral Economics
- Behavioral Genetics
- Belief Perseverance
- Bereavement and Grief
- Biological Psychology
- Birth Order
- Body Image in Men and Women
- Bystander Effect
- Categorical Data Analysis in Psychology
- Childhood and Adolescence, Peer Victimization and Bullying...
- Clark, Mamie Phipps
- Clinical Neuropsychology
- Clinical Psychology
- Cognitive Consistency Theories
- Cognitive Dissonance Theory
- Cognitive Neuroscience
- Communication, Nonverbal Cues and
- Comparative Psychology
- Competence to Stand Trial: Restoration Services
- Competency to Stand Trial
- Computational Psychology
- Conflict Management in the Workplace
- Conformity, Compliance, and Obedience
- Consciousness
- Coping Processes
- Correspondence Analysis in Psychology
- Counseling Psychology
- Creativity at Work
- Critical Thinking
- Cross-Cultural Psychology
- Cultural Psychology
- Daily Life, Research Methods for Studying
- Data Science Methods for Psychology
- Data Sharing in Psychology
- Death and Dying
- Deceiving and Detecting Deceit
- Defensive Processes
- Depressive Disorders
- Development, Prenatal
- Developmental Psychology (Cognitive)
- Developmental Psychology (Social)
- Diagnostic and Statistical Manual of Mental Disorders (DSM...
- Discrimination
- Dissociative Disorders
- Drugs and Behavior
- Eating Disorders
- Ecological Psychology
- Educational Settings, Assessment of Thinking in
- Embodiment and Embodied Cognition
- Emerging Adulthood
- Emotional Intelligence
- Empathy and Altruism
- Employee Stress and Well-Being
- Environmental Neuroscience and Environmental Psychology
- Ethics in Psychological Practice
- Event Perception
- Evolutionary Psychology
- Expansive Posture
- Experimental Existential Psychology
- Exploratory Data Analysis
- Eyewitness Testimony
- Eysenck, Hans
- Factor Analysis
- Festinger, Leon
- Five-Factor Model of Personality
- Flynn Effect, The
- Forensic Psychology
- Forgiveness
- Friendships, Children's
- Fundamental Attribution Error/Correspondence Bias
- Gambler's Fallacy
- Game Theory and Psychology
- Geropsychology, Clinical
- Global Mental Health
- Habit Formation and Behavior Change
- Health Psychology
- Health Psychology Research and Practice, Measurement in
- Heider, Fritz
- Heuristics and Biases
- History of Psychology
- Human Factors
- Humanistic Psychology
- Implicit Association Test (IAT)
- Industrial and Organizational Psychology
- Inferential Statistics in Psychology
- Insanity Defense, The
- Intelligence
- Intelligence, Crystallized and Fluid
- Intercultural Psychology
- Intergroup Conflict
- International Classification of Diseases and Related Healt...
- International Psychology
- Interviewing in Forensic Settings
- Intimate Partner Violence, Psychological Perspectives on
- Introversion–Extraversion
- Item Response Theory
- Law, Psychology and
- Lazarus, Richard
- Learned Helplessness
- Learning Theory
- Learning versus Performance
- LGBTQ+ Romantic Relationships
- Lie Detection in a Forensic Context
- Life-Span Development
- Locus of Control
- Loneliness and Health
- Mathematical Psychology
- Meaning in Life
- Mechanisms and Processes of Peer Contagion
- Media Violence, Psychological Perspectives on
- Memories, Autobiographical
- Memories, Flashbulb
- Memories, Repressed and Recovered
- Memory, False
- Memory, Human
- Memory, Implicit versus Explicit
- Memory in Educational Settings
- Memory, Semantic
- Meta-Analysis
- Metacognition
- Metaphor, Psychological Perspectives on
- Microaggressions
- Military Psychology
- Mindfulness
- Mindfulness and Education
- Minnesota Multiphasic Personality Inventory (MMPI)
- Money, Psychology of
- Moral Conviction
- Moral Development
- Moral Psychology
- Moral Reasoning
- Nature versus Nurture Debate in Psychology
- Neuroscience of Associative Learning
- Nonergodicity in Psychology and Neuroscience
- Nonparametric Statistical Analysis in Psychology
- Observational (Non-Randomized) Studies
- Obsessive-Complusive Disorder (OCD)
- Occupational Health Psychology
- Olfaction, Human
- Operant Conditioning
- Optimism and Pessimism
- Organizational Justice
- Parenting Stress
- Parenting Styles
- Parents' Beliefs about Children
- Peace Psychology
- Perception, Person
- Performance Appraisal
- Personality and Health
- Personality Disorders
- Personality Psychology
- Phenomenological Psychology
- Placebo Effects in Psychology
- Play Behavior
- Positive Psychological Capital (PsyCap)
- Positive Psychology
- Posttraumatic Stress Disorder (PTSD)
- Prejudice and Stereotyping
- Pretrial Publicity
- Prisoner's Dilemma
- Problem Solving and Decision Making
- Procrastination
- Prosocial Behavior
- Prosocial Spending and Well-Being
- Protocol Analysis
- Psycholinguistics
- Psychological Literacy
- Psychological Perspectives on Food and Eating
- Psychology, Political
- Psychoneuroimmunology
- Psychophysics, Visual
- Psychotherapy
- Psychotic Disorders
- Publication Bias in Psychology
- Reasoning, Counterfactual
- Rehabilitation Psychology
- Relationships
- Reliability–Contemporary Psychometric Conceptions
- Religion, Psychology and
- Replication Initiatives in Psychology
- Research Methods
- Risk Taking
- Role of the Expert Witness in Forensic Psychology, The
- Sample Size Planning for Statistical Power and Accurate Es...
- Schizophrenic Disorders
- School Psychology
- School Psychology, Counseling Services in
- Self, Gender and
- Self, Psychology of the
- Self-Construal
- Self-Control
- Self-Deception
- Self-Determination Theory
- Self-Efficacy
- Self-Esteem
- Self-Monitoring
- Self-Regulation in Educational Settings
- Self-Report Tests, Measures, and Inventories in Clinical P...
- Sensation Seeking
- Sex and Gender
- Sexual Minority Parenting
- Sexual Orientation
- Signal Detection Theory and its Applications
- Simpson's Paradox in Psychology
- Single People
- Single-Case Experimental Designs
- Skinner, B.F.
- Sleep and Dreaming
- Small Groups
- Social Class and Social Status
- Social Cognition
- Social Neuroscience
- Social Support
- Social Touch and Massage Therapy Research
- Somatoform Disorders
- Spatial Attention
- Sports Psychology
- Stanford Prison Experiment (SPE): Icon and Controversy
- Stereotype Threat
- Stereotypes
- Stress and Coping, Psychology of
- Student Success in College
- Subjective Wellbeing Homeostasis
- Taste, Psychological Perspectives on
- Teaching of Psychology
- Terror Management Theory
- Testing and Assessment
- The Concept of Validity in Psychological Assessment
- The Neuroscience of Emotion Regulation
- The Reasoned Action Approach and the Theories of Reasoned ...
- The Weapon Focus Effect in Eyewitness Memory
- Theory of Mind
- Therapies, Person-Centered
- Therapy, Cognitive-Behavioral
- Thinking Skills in Educational Settings
- Time Perception
- Trait Perspective
- Trauma Psychology
- Twin Studies
- Type A Behavior Pattern (Coronary Prone Personality)
- Unconscious Processes
- Video Games and Violent Content
- Virtues and Character Strengths
- Women and Science, Technology, Engineering, and Math (STEM...
- Women, Psychology of
- Work Well-Being
- Wundt, Wilhelm
- Privacy Policy
- Cookie Policy
- Legal Notice
- Accessibility
Powered by:
- [66.249.64.20|185.194.105.172]
- 185.194.105.172
Single-Case Experimental Designs
Single-case experimental designs are a family of experimental designs that are characterized by researcher manipulation of an independent variable and repeated measurement of a dependent variable before (i.e., baseline) and after (i.e., intervention phase) introducing the independent variable. In single-case experimental designs a case is the unit of intervention and analysis (e.g., a child, a school). Because measurement within each case is conducted before and after manipulation of the independent variable, the case typically serves as its own control. Experimental variants of single-case designs provide a basis for determining a causal relation by replication of the intervention through (a) introducing and withdrawing the independent variable, (b) manipulating the independent variable across different phases, and (c) introducing the independent variable in a staggered fashion across different points in time. Due to their economy of resources, single-case designs may be useful during development activities and allow for rapid replication across studies.
- Related Documents
A Synthesis of Elementary Mathematics Interventions: Comparisons of Students With Mathematics Difficulty With and Without Comorbid Reading Difficulty
In this synthesis, we reviewed 65 studies involving elementary students (i.e., grades 1–5) identified with mathematics difficulty (MD) in which authors implemented a mathematics intervention. Of these studies, we identified 33 group designs, 9 quasi-experimental designs, and 23 single-case designs. We aimed to synthesize performance differences between students with MD with and without reading difficulty (RD). We identified three categories of students for analysis: Students with MD+RD, MD-alone, or MD-nonspecified (i.e., no reading information provided). Overall, 80% of studies included students with MD-nonspecified, and the interventions for these students demonstrated strong effects. For the limited number of studies with students with MD+RD or MD-alone, intervention effects were strong for students with MD+RD and variable for students with MD-alone. In the three studies directly comparing the performance of students with MD+RD versus MD-alone, we noted differential patterns of performance. To tailor interventions to student need, more research must be conducted to understand whether students with MD with variable reading profiles respond differentially to mathematics intervention.
Markov Methodology to Improve Precision in the Assessment of Data-Intensive Single Case Designs
Single case designs that employ intensive longitudinal measures of health behavior have the potential to enable precise, nuanced evaluations of how individuals respond to interventions. Existing statistical tools typically focus on blunt, large effect outcomes such as changes in the level and/or slope of dependent variables over different intervention phases to arrive at a binary determination of whether an intervention was effective or not. This paper introduces a Markov-based, empirical transition method that offers a more comprehensive appraisal of interventions. The procedure projects a univariate time-series into discrete states and empirically determines the probability of transitioning from one state to another. Probabilities of state transition are computed separately for each intervention phase and are summarized in intervention phase-specific transition matrices. Comparing transition matrices illuminates intricate differences among intervention phases that can be quantified to estimate statistical significance via bootstrapping techniques. Analyses used to evaluate the face validity of findings are also discussed. This technique is demonstrated using three case studies from a quasi-SCD secondhand smoke reduction trial utilizing real-time air-particle sensors to discourage indoor cigarette smoking. The results enabled the identification of complex phenomena such avoidance and escape behavior in response to punitive contingencies. The Markov approach's ability to elucidate subtle behavioral details has not typically been feasible with standard methodologies, mainly due to historical limitations associated with infrequent repeated measures. The results suggest that Markov analyses can enhance the precision of outcomes provided by data-intensive single case designs, ultimately enabling the development of interventions uniquely tailored to specific individuals.
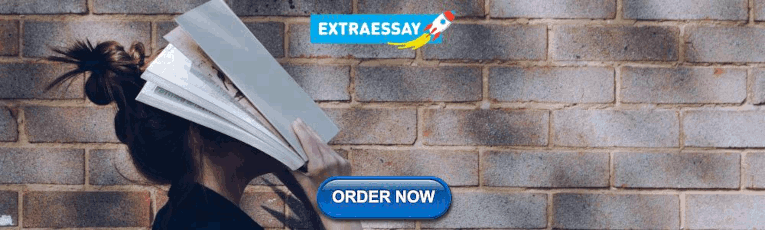
Consistent Methodological Errors Observed in Single-Case Studies: Suggested Guidelines
This article reviews current and consistent methodological problems that occur in research articles using single-case experimental designs. Problems discussed include (1) the use of pre- and quasi-experimental single-case designs, (2) the presentation and analysis of data, (3) the lack or inadequate demonstration of experimental reliability, and (4) the improper or ineffective use of generalization. The authors suggest ways of solving these problems and provide guidelines for avoiding their recurrence.
Applications of Single-Case Designs to Research with Visually Impaired Individuals
This article discusses single-case experimental designs as an alternative to group comparison designs in research with the visually impaired and presents the advantages and limitations of both approaches. In addition, the authors provide examples of some single-case strategies commonly used in behavioral research. Finally, they suggest that single-case research will have heuristic value in future investigations with the visually impaired.
Moving Forward Four Words at a Time: Effects of a Supplemental Preschool Vocabulary Intervention
Purpose This study evaluated the effects of an automated, small-group intervention designed to teach preschoolers challenging vocabulary words. Previous studies have provided evidence of efficacy. In this study, we evaluated the effects of the program after doubling the number of words taught from 2 to 4 words per book. Method Seventeen preschool children listened to 1 prerecorded book per week for 9 weeks. Each storybook had embedded, interactive lessons for 4 target vocabulary words. Each lesson provided repeated exposures to words and their definitions, child-friendly contexts, and multiple opportunities for children to respond verbally to instructional prompts. Participants were asked to define the weekly targeted vocabulary before and after intervention. A repeated acquisition single-case design was used to examine the effects of the books and embedded lessons on learning of target vocabulary words. Results Treatment effects were observed for all children across many of the books. Learning of at least 2 points (i.e., 1 word) was replicated for 74.5% of 149 books tested across the 17 participants. On average, children learned to define 47% of the target vocabulary words (17 out of 36). Conclusions Results support including 4 challenging words per book, as children learned substantially more words when 4 words were taught, in comparison to previous studies. Within an iterative development process, results of the current study take us 1 step closer to creating an optimal vocabulary intervention that supports the language development of at-risk children.
Supplemental Material for An Introduction to Modeling Longitudinal Data With Generalized Additive Models: Applications to Single-Case Designs
Comparing the transfer effects of three nonpharmacological interventions in children with ad/hd: a single-case experimental design.
The current study used behavioral and electroencephalograph measures to compare the transferability of cognitive training (CT), neurofeedback training (NFT), and CT combined with NFT in children with AD/HD. Following a multiple-baseline single-case experimental design, twelve children were randomized to a training condition. Each child completed a baseline phase, followed by an intervention phase. The intervention phase consisted of 20 sessions of at-home training. Tau-U analysis and standardized visual analysis were adopted to detect effects. CT improved inhibitory function, and NFT showed improved alpha activity and working memory. The combined condition, who was a reduced 'dose' of CT and NFT, did not show any improvements. The three conditions did not alleviate AD/HD symptoms. While CT and NFT may have near transfer effects, considering the lack of improvement in symptoms, this study does not support CT and NFT on their own as a treatment for children with AD/HD.
ANALISIS EFEKTIVITAS PROGRAM USAHA AGRIBISNIS PERDESAAN (PUAP) SERTA DAMPAKNYA TERHADAP TINGKAT PENDAPATAN PETANI (STUDI KASUS PADA GABUNGAN KELOMPOK TANI TELUK JAYA)
Study the effectivity analysis of Program Usaha Agribisnis Perdesaan (PUAP) and its impact on farmer income levels (case study of the Teluk Jaya Farmers Group Association) aims to 1. knowing how large the effectivity of PUAP funds is for income levels in poverty alleviation in Teluk Desa Sentosa Panai Hulu Subdistrict, 2. to analyze and determine the level of income of Gapoktan Teluk Jaya Farming Business in Teluk Sentosa, Panai Hulu Subdistrict before and after receiving PUAP assistance. The results showed that the effectivity of PUAP program funding is very effective and good, it can be seen from the results of the F test of 12.406 with a significant level of 0.000 while the F value of the table is 2.051. When compared to the value of F test (12.406) > Ftable (2.051) at α 5%, it was concluded that simultaneously the effectivity variable and the PUAP program had a positive effect. The increase in income of the Teluk Jaya Farmers Group in Teluk Sentosa after receiving PUAP funds can be known from the t table for the effectivity variable of 2.663 and the PUAP program for 1.270 with a significant value for each independent variable (2.051); (0.073). Whereas for the t table value in the distribution statistics t table with the level of test α = 5% and df1 = 27 of 2,051. Based on the criteria that if the value is t count> t table); namely (2.663> 2,051) (1,270> 2,051) so that it can be concluded that the effectivity variable partially has a positive and significant effect on the income level.Keywords : effectivity variable, income level, PUAP program
Selecting the proper Tau-U measure for single-case experimental designs: Development and application of a decision flowchart
Single-case experimental designs: reflections on conduct and analysis, export citation format, share document.
IResearchNet
Single-Case Experimental Design
Single-case experimental design, a versatile research methodology within psychology, holds particular significance in the field of school psychology. This article provides an overview of single-case experimental design, covering its definition, historical development, and key concepts. It delves into various types of single-case designs, including AB, ABA, and Multiple Baseline designs, illustrating their applications within school psychology. The article also explores data collection, analysis methods, and common challenges associated with this methodology. By highlighting its value in empirical research, this article underscores the enduring relevance of single-case experimental design in advancing the understanding and practice of school psychology.
Introduction
Single-case experimental design, a research methodology of profound importance in the realm of psychology, is characterized by its unique approach to investigating behavioral and psychological phenomena. Within this article, we will embark on a journey to explore the intricate facets of this research methodology and unravel its multifaceted applications, with a particular focus on its relevance in school psychology.
Academic Writing, Editing, Proofreading, And Problem Solving Services
Get 10% off with 24start discount code.
Single-case experimental design, often referred to as “N of 1” research, is a methodology that centers on the in-depth examination of individual subjects or cases. Unlike traditional group-based designs, this approach allows researchers to closely study and understand the nuances of a single participant’s behavior, responses, and reactions over time. The precision and depth of insight offered by single-case experimental design have made it an invaluable tool in the field of psychology, facilitating both clinical and experimental research endeavors.
One of the most compelling aspects of this research methodology lies in its applicability to school psychology. In educational settings, understanding the unique needs and challenges of individual students is paramount, and single-case experimental design offers a tailored and systematic way to address these issues. Whether it involves assessing the effectiveness of an intervention for a specific learning disability or studying the impact of a behavior modification program for a student with special needs, single-case experimental design equips school psychologists with a powerful tool to make data-driven decisions and individualized educational plans.
Throughout this article, we will delve into the foundations of single-case experimental design, exploring its historical evolution, key concepts, and core terminology. We will also discuss the various types of single-case designs, including AB, ABA, and Multiple Baseline designs, illustrating their practical applications within the context of school psychology. Furthermore, the article will shed light on the data collection methods and the statistical techniques used for analysis, as well as the ethical considerations and challenges that researchers encounter in single-case experiments.
In sum, this article aims to provide an in-depth understanding of single-case experimental design and its pivotal role in advancing knowledge in psychology, particularly within the field of school psychology. As we embark on this exploration, it is evident that single-case experimental design serves as a bridge between rigorous scientific inquiry and the real-world needs of individuals, making it an indispensable asset in enhancing the quality of psychological research and practice.
Understanding Single-Case Experimental Design
Single-Case Experimental Design (SCED), often referred to as “N of 1” research, is a research methodology employed in psychology to investigate behavioral and psychological phenomena with an emphasis on the individual subject as the primary unit of analysis. The primary purpose of SCED is to meticulously study the behavior, responses, and changes within a single participant over time. Unlike traditional group-based research, SCED is tailored to the unique characteristics and needs of individual cases, enabling a more in-depth understanding of the variables under investigation.
The historical background of SCED can be traced back to the early 20th century when researchers like B.F. Skinner pioneered the development of operant conditioning and experimental analysis of behavior. Skinner’s work laid the groundwork for single-case experiments by emphasizing the importance of understanding the functional relations between behavior and environmental variables. Over the decades, SCED has evolved and gained prominence in various fields within psychology, notably in clinical and school psychology. Its relevance in school psychology is particularly noteworthy, as it offers a systematic and data-driven approach to address the diverse learning and behavioral needs of students. School psychologists use SCED to design and assess individualized interventions, evaluate the effectiveness of specific teaching strategies, and make informed decisions about special education programs.
Understanding SCED involves familiarity with key concepts and terminology that underpin the methodology. These terms include:
- Baseline: The initial phase of data collection where the participant’s behavior is measured before any intervention is introduced. Baseline data serve as a point of reference for assessing the impact of subsequent interventions.
- Intervention: The phase in which a specific treatment, manipulation, or condition is introduced to the participant. The goal of the intervention is to bring about a change in the target behavior.
- Dependent Variables: These are the behaviors or responses under investigation. They are the outcomes that researchers aim to measure and analyze for changes across different phases of the experiment.
Reliability and validity are critical considerations in SCED. Reliability refers to the consistency and stability of measurement. In SCED, it is crucial to ensure that data collection procedures are reliable, as any variability can affect the interpretation of results. Validity pertains to the accuracy and truthfulness of the data. Researchers must establish that the dependent variable measurements are valid and accurately reflect the behavior of interest. When these principles are applied in SCED, it enhances the scientific rigor and credibility of the research findings, which is essential in both clinical and school psychology contexts.
This foundation of key concepts and terminology serves as the basis for designing, conducting, and interpreting single-case experiments, ensuring that the methodology maintains high standards of precision and integrity in the pursuit of understanding individual behavior and psychological processes.
Types of Single-Case Experimental Designs
The AB Design is one of the fundamental single-case experimental designs, characterized by its simplicity and effectiveness. In an AB Design, the researcher observes and measures a single subject’s behavior during two distinct phases: the baseline (A) phase and the intervention (B) phase. During the baseline phase, the researcher collects data on the subject’s behavior without any intervention or treatment. This baseline data serve as a reference point to understand the natural or typical behavior of the individual. Following the baseline phase, the intervention or treatment is introduced, and data on the subject’s behavior are collected again. The AB Design allows for the comparison of baseline data with intervention data, enabling researchers to determine whether the introduced intervention had a noticeable impact on the individual’s behavior.
AB Designs find extensive application in school psychology. For instance, consider a scenario where a school psychologist wishes to assess the effectiveness of a time-management training program for a student with attention deficit hyperactivity disorder (ADHD). During the baseline phase, the psychologist observes the student’s on-task behavior in the absence of any specific time-management training. Subsequently, during the intervention phase, the psychologist implements the time-management program and measures the student’s on-task behavior again. By comparing the baseline and intervention data, the psychologist can evaluate the program’s efficacy in improving the student’s behavior.
The ABA Design is another prominent single-case experimental design characterized by the inclusion of a reversal (A) phase. In this design, the researcher initially collects baseline data (Phase A), introduces the intervention (Phase B), and then returns to the baseline conditions (Phase A). The ABA Design is significant because it provides an opportunity to assess the reversibility of the effects of the intervention. If the behavior returns to baseline levels during the second A phase, it suggests a strong relationship between the intervention and the observed changes in behavior.
In school psychology, the ABA Design offers valuable insights into the effectiveness of interventions for students with diverse needs. For instance, a school psychologist may use the ABA Design to evaluate a behavior modification program for a student with autism spectrum disorder (ASD). During the first baseline phase (A), the psychologist observes the student’s behavior patterns. Subsequently, in the intervention phase (B), a behavior modification program is implemented. If the student’s behavior shows positive changes, this suggests that the program is effective. Finally, during the second baseline phase (A), the psychologist can determine if the changes are reversible, which informs decisions regarding the program’s ongoing use or modification.
The Multiple Baseline Design is a versatile single-case experimental design that addresses challenges such as ethical concerns or logistical constraints that might limit the use of reversal designs. In this design, researchers stagger the introduction of the intervention across multiple behaviors, settings, or individuals. Each baseline and intervention phase is implemented at different times for each behavior, allowing researchers to establish a cause-and-effect relationship by demonstrating that the intervention corresponds with changes in the specific behavior under investigation.
Within school psychology, Multiple Baseline Designs offer particular utility when assessing interventions for students in complex or sensitive situations. For example, a school psychologist working with a student who displays challenging behaviors may choose to implement a Multiple Baseline Design. The psychologist can introduce a behavior intervention plan (BIP) for different target behaviors, such as aggression, noncompliance, and self-injury, at different times. By measuring and analyzing changes in behavior across these multiple behaviors, the psychologist can assess the effectiveness of the BIP and make informed decisions about its implementation across various behavioral concerns. This design is particularly valuable when ethical considerations prevent the reversal of an effective intervention, as it allows researchers to demonstrate the intervention’s impact without removing a beneficial treatment.
Conducting and Analyzing Single-Case Experiments
In single-case experiments, data collection and measurement are pivotal components that underpin the scientific rigor of the research. Data are typically collected through direct observation, self-reports, or the use of various measuring instruments, depending on the specific behavior or variable under investigation. To ensure reliability and validity, researchers meticulously define and operationalize the target behavior, specifying how it will be measured. This may involve the use of checklists, rating scales, video recordings, or other data collection tools. In school psychology research, systematic data collection is imperative to make informed decisions about interventions and individualized education plans (IEPs). It provides school psychologists with empirical evidence to track the progress of students, assess the effectiveness of interventions, and adapt strategies based on the collected data.
Visual analysis is a core element of interpreting data in single-case experiments. Researchers plot the data in graphs, creating visual representations of the behavior across different phases. By visually inspecting the data, researchers can identify patterns, trends, and changes in behavior. Visual analysis is particularly well-suited for detecting whether an intervention has had a noticeable effect.
In addition to visual analysis, statistical methods are occasionally employed in single-case experiments to enhance the rigor of analysis. These methods include effect size calculations and phase change calculations. Effect size measures, such as Cohen’s d or Tau-U, quantify the magnitude of change between the baseline and intervention phases, providing a quantitative understanding of the treatment’s impact. Phase change calculations determine the statistical significance of behavior change across different phases, aiding in the determination of whether the intervention had a meaningful effect.
Visual analysis and statistical methods complement each other, enabling researchers in school psychology to draw more robust conclusions about the efficacy of interventions. These methods are valuable in making data-driven decisions regarding students’ educational and behavioral progress.
Single-case experimental designs are not without their challenges and limitations. Researchers must grapple with issues such as the potential for confounding variables, limited generalizability to other cases, and the need for careful control of extraneous factors. In school psychology, these challenges are compounded by the dynamic and diverse nature of educational settings, making it essential for researchers to adapt the methodology to specific contexts and populations.
Moreover, ethical considerations loom large in school psychology research. Researchers must adhere to strict ethical guidelines when conducting single-case experiments involving students. Informed consent, confidentiality, and the well-being of the participants are paramount. Ethical considerations are especially critical when conducting research with vulnerable populations, such as students with disabilities or those in special education programs. The ethical conduct of research in school psychology is pivotal to maintaining trust and ensuring the welfare of students and their families.
In conclusion, the application of single-case experimental design in school psychology research is a powerful approach for addressing individualized educational and behavioral needs. By emphasizing systematic data collection, employing visual analysis and statistical methods, and navigating the inherent challenges and ethical considerations, researchers can contribute to the advancement of knowledge in this field while ensuring the well-being and progress of the students they serve.
In conclusion, this article has provided a comprehensive exploration of Single-Case Experimental Design (SCED) and its vital role within the domain of school psychology. Key takeaways from this article underscore the significance of SCED as a versatile and invaluable research methodology:
First and foremost, SCED is a methodological cornerstone for investigating individual behavior and psychological phenomena. Through meticulous observation and data collection, it enables researchers to gain deep insights into the idiosyncratic needs and responses of students in educational settings.
The significance of SCED in school psychology is pronounced. It empowers school psychologists to design and assess tailored interventions, evaluate the effectiveness of educational programs, and make data-driven decisions that enhance the quality of education for students with diverse needs. Whether it’s tracking progress, assessing the efficacy of behavioral interventions, or individualizing education plans, SCED plays an instrumental role in achieving these goals.
Furthermore, the article has illuminated three primary types of single-case experimental designs: AB, ABA, and Multiple Baseline. These designs offer the flexibility to investigate the effects of interventions and assess their reversibility when required. Such methods have a direct and tangible impact on the daily practices of school psychologists, allowing them to optimize support and educational strategies.
The importance of systematic data collection and measurement, the role of visual analysis and statistical methods in data interpretation, and the acknowledgment of ethical considerations in school psychology research have been underscored. These aspects collectively serve as the foundation of SCED, ensuring the integrity and reliability of research outcomes.
As we look toward the future, the potential developments in SCED are promising. Advances in technology, such as wearable devices and digital data collection tools, offer new possibilities for precise and efficient data gathering. Additionally, the integration of SCED with other research methodologies, such as mixed-methods research, holds the potential to provide a more comprehensive understanding of students’ educational experiences.
In summary, Single-Case Experimental Design is a pivotal research methodology that bridges the gap between rigorous scientific inquiry and the real-world needs of students in school psychology. Its power lies in its capacity to assess, refine, and individualize interventions and educational plans. The continued application and refinement of SCED in school psychology research promise to contribute significantly to the advancement of knowledge and the enhancement of educational outcomes for students of all backgrounds and abilities. As we move forward, the integration of SCED with emerging technologies and research paradigms will continue to shape the landscape of school psychology research, leading to more effective and tailored interventions for the benefit of students and the field as a whole.
References:
- Barlow, D. H., & Nock, M. K. (2009). Why can’t we be more idiographic in our research? Perspectives on Psychological Science, 4(1), 19-21.
- Cook, B. G., & Schirmer, B. R. (2003). What is N of 1 research? Exceptionality, 11(1), 65-76.
- Cooper, J. O., Heron, T. E., & Heward, W. L. (2020). Applied behavior analysis (3rd ed.). Pearson.
- Kazdin, A. E. (1982). Single-case research designs: Methods for clinical and applied settings. Oxford University Press.
- Kratochwill, T. R., Hitchcock, J. H., Horner, R. H., Levin, J. R., Odom, S. L., Rindskopf, D. M., & Shadish, W. R. (2010). Single-case intervention research design standards. Remedial and Special Education, 31(3), 205-214.
- Levin, J. R., Ferron, J. M., Kratochwill, T. R., Forster, J. L., Rodgers, M. S., Maczuga, S. A., & Chinn, S. (2016). A randomized controlled trial evaluation of a research synthesis and research proposal process aimed at improving graduate students’ research competency. Journal of Educational Psychology, 108(5), 680-701.
- Morgan, D. L., & Morgan, R. K. (2009). Single-participant research design: Bringing science to managed care. Psychotherapy Research, 19(4-5), 577-587.
- Ottenbacher, K. J., & Maas, F. (1999). The effect of statistical methodology on the single subject design: An empirical investigation. Journal of Behavioral Education, 9(2), 111-130.
- Shadish, W. R., Cook, T. D., & Campbell, D. T. (2002). Experimental and quasi-experimental designs for generalized causal inference. Houghton Mifflin.
- Sidman, M. (1960). Tactics of scientific research: Evaluating experimental data in psychology. Basic Books.
- Vannest, K. J., Parker, R. I., Gonen, O., Adigüzel, T., & Bovaird, J. A. (2016). Single case research: web-based calculators for SCR analysis. Behavior Research Methods, 48(1), 97-103.
- Wilczynski, S. M., & Christian, L. (2008). Applying single-subject design for students with disabilities in inclusive settings. Pearson.
- Wong, C., Odom, S. L., Hume, K. A., Cox, A. W., Fettig, A., Kucharczyk, S., & Schultz, T. R. (2015). Evidence-based practices for children, youth, and young adults with autism spectrum disorder: A comprehensive review. Journal of Autism and Developmental Disorders, 45(7), 1951-1966.
- Kratochwill, T. R., & Levin, J. R. (2018). Single-case research design and analysis: New directions for psychology and education. Routledge.
- Hall, R. V., & Fox, L. (2015). The need for N of 1 research in special education. Exceptionality, 23(4), 225-233.
- Shadish, W. R., & Sullivan, K. J. (2011). Characteristics of single-case designs used to assess intervention effects in 2008. Behavior Research Methods, 43(4), 971-980.
- Campbell, D. T., & Stanley, J. C. (2015). Experimental and quasi-experimental designs for research. Ravenio Books.
- Kazdin, A. E. (2011). Single-case research designs: Methods for clinical and applied settings (2nd ed.). Oxford University Press.
- Therrien, W. J., & Bulawski, J. (2019). The use of single-case experimental designs in school psychology research: A systematic review. Journal of School Psychology, 73, 92-112.
- Gavidia-Payne, S., Little, E., & Schell, G. (2018). Single-case experimental design: Applications in developmental and behavioral science. Routledge.
- Open access
- Published: 11 April 2024
ESCO2’s oncogenic role in human tumors: a pan-cancer analysis and experimental validation
- Yue Huang 1 na1 ,
- Dapeng Chen 2 na1 ,
- Yamin Zhang 3 ,
- Zhiwen Zheng 1 ,
- Qingfeng Fu 1 ,
- Bocun Yi 1 ,
- Yuchen Jiang 1 ,
- Zhihong Zhang 1 &
- Jianqiang Zhu 1
BMC Cancer volume 24 , Article number: 452 ( 2024 ) Cite this article
Metrics details
Establishment of sister chromatid cohesion N-acetyltransferase 2 (ESCO2) is involved in the mitotic S-phase adhesins acetylation and is responsible for bridging two sister chromatids. However, present ESCO2 cancer research is limited to a few cancers. No systematic pan-cancer analysis has been conducted to investigate its role in diagnosis, prognosis, and effector function.
We thoroughly examined the ESCO2 carcinogenesis in pan-cancer by combining public databases such as The Cancer Genome Atlas (TCGA), Genotype-Tissue Expression Project (GTEx), UALCAN and Tumor Immune Single-cell Hub (TISCH). The analysis includes differential expression analysis, survival analysis, cellular effector function, gene mutation, single cell analysis, and tumor immune cell infiltration. Furthermore, we confirmed ESCO2’s impacts on clear cell renal cell carcinoma (ccRCC) cells’ proliferative and invasive capacities in vitro.
In our study, 30 of 33 cancer types exhibited considerably greater levels of ESCO2 expression in tumor tissue using TCGA and GTEx databases, whereas acute myeloid leukemia (LAML) exhibited significantly lower levels. Kaplan-Meier survival analyses in adrenocortical carcinoma (ACC), kidney chromophobe (KICH), kidney renal clear cell carcinoma (KIRC), kidney renal papillary cell carcinoma (KIRP), brain lower grade glioma (LGG), liver hepatocellular carcinoma (LIHC), lung adenocarcinoma (LUAD), mesothelioma (MESO), and pancreatic adenocarcinoma (PAAD) demonstrated that tumor patients with high ESCO2 expression have short survival periods. However, in thymoma (THYM), colon adenocarcinoma (COAD) and rectum adenocarcinoma (READ), ESCO2 was a favorable prognostic factor. Moreover, ESCO2 expression positively correlates with tumor stage and tumor size in several cancers, including LIHC, KIRC, KIRP and LUAD. Function analysis revealed that ESCO2 participates in mitosis, cell cycle, DNA damage repair, and other processes. CDK1 was identified as a downstream gene regulated by ESCO2. Furthermore, ESCO2 might also be implicated in immune cell infiltration. Finally, ESCO2’S knockdown significantly inhibited the A498 and T24 cells’ proliferation, invasion, and migration.
Conclusions
In conclusion, ESCO2 is a possible pan-cancer biomarker and oncogene that can reliably predict the prognosis of cancer patients. ESCO2 was also implicated in the cell cycle and proliferation regulation. In a nutshell, ESCO2 is a therapeutically viable and dependable target.
Peer Review reports
Introduction
Cancer in most nations gradually displaces cardiovascular disease as the leading cause of premature mortality [ 1 ]. According to statistics, there will be 19.3 million new cancer cases and almost 10 million cancer-related deaths in 2020. The global cancer burden is expected to reach 28.4 million patients by 2040 [ 2 ]. Cancer treatment has steadily transitioned into the era of precision therapy because of the advanced high-throughput sequencing technology. Advanced cancer patients frequently receive targeted therapy and immunotherapy in the clinic. For instance, palbociclib (a CDK4/6 inhibitor) in conjunction with letrozole (24.8 months) resulted in considerably longer progression-free survival than letrozole alone (14.5 months) in patients with previously untreated ER-positive, HER2-negative advanced breast cancer [ 3 ]. Due to the identification of molecular targets and the growing understanding of their cellular effects, small molecule inhibitors have been created as a primary therapeutic approach for cancer treatment. The discovery of new molecular targets may contribute to advancing combined therapies, eradicating drug resistance, and increasing therapeutic efficacy. Small molecule inhibitors have a bright future; thus, scientists should investigate novel biomarkers to find potential therapeutic targets.
The cell cycle is a tightly controlled process that supports genetic material replication and cell proliferation. One of the distinguishing characteristics of malignancies is abnormal cell proliferation brought on by cell cycle dysregulation [ 4 ]. Dysregulation of the cancer cell cycle facilitates cell proliferation, which is driven by excessive mitotic signaling, inhibitory checkpoint failure, or both. Scientists have demonstrated that it is effective to target proteins associated with the cell cycle in order to limit tumor growth. The most notable successes in targeting cell cycle mechanisms have been inhibitors of CDK4 and CDK6 [ 5 , 6 , 7 ]. The introduction of these CDK4/6 inhibitors for clinical use constitutes a milestone in the treatment of breast cancer and may have broad ramifications for the management of other tumor types [ 8 ]. Despite the current success of CDK4/6 inhibitors, cancer therapy that targets cell cycle proteins is still in its infancy. An in-depth exploration of cell cycle regulatory mechanisms and their role in cancer will guide current cancer treatments and identify new therapeutic targets.
Establishment of sister chromatid cohesion N-acetyltransferase 2 (ESCO2) was identified as an effective target for cancer therapy, which is a pivotal protein in the cell division process [ 9 ]. ESCO2, the human homolog of yeast ECO1, works on proliferating cell nuclear antigen (PCNA) to stimulate sister chromatid cohesion [ 10 , 11 ]. Acetylation of the SMC3 subunit of the adhesive protein via ESCO2 acetyltransferase facilitates sister chromatid cohesion, inhibiting cohesin release from chromatin [ 12 ]. The cohesin is a multiprotein complex whose typical role is to bind sister chromatids from S-phase to anaphase to prevent premature segregation of sister chromatids and to ensure equal segregation of chromosomes [ 13 , 14 ]. Hence, the presence of ESCO2 ensures correct chromosomal segregation and makes recombinant DNA repair possible. Recent research has demonstrated that ESCO2 is strongly linked to the formation of several cancer types [ 15 , 16 , 17 , 18 , 19 ]. ESCO2 knockdown in human gastric cancer cell lines in vitro significantly inhibited cell proliferation and induced apoptosis by regulating P53 [ 17 ]. Meanwhile, ESCO2 can promote LUAD cell proliferation and metastasis by promoting aerobic glycolysis [ 16 ]. Similarly, Fu et al. identified that 53BP1-MDS ring-like structure disruption caused by ESCO2 depletion in colorectal cancer cells, which reduced the effectiveness of non-homologous end joining (NHEJ) repair and made cancer cells more susceptible to chemotherapy [ 20 ]. However, Guo et al. discovered that ESCO2 overexpression in colon cancer reduced MMP2’s transcriptional activity to limit tumor metastasis [ 19 ]. ESCO2’s involvement in cancer is therefore controversial. Investigating ESCO2’s biological function in other malignancies is necessary. A pan-cancer analysis can investigate the oncogene in several malignancies, allowing oncogenes’ effector function and differential expression analyses.
We identified ESCO2’s abnormal expression value in tumor and normal samples by pan-cancer analysis and then validated its protein value in clinical tissues. Subsequently, we confirmed the value of ESCO2 in assessing prognosis and performed gene enrichment analysis, single cell analysis and immuno-infiltration analysis for ESCO2.Moreover, we conducted in vitro experiments to corroborate our bioinformatics findings. ESCO2 is a unique and useful biomarker and a prospective molecular target.
Materials and methods
Data preparation and differential expression analysis.
The Cancer Genome Atlas (TCGA) database ( https://portal.gdc.cancer.gov/ ), which has 11,315 total samples, was employed to download the bulk mRNA sequencing profiles of 33 distinct cancer samples and their corresponding normal tissues. All gene expression data were standardized using the Transcripts Per Kilobase of Exon Model per Million Mapped Reads (TPM) for all samples. In the meanwhile, we collected the relevant clinical information on cancer patients. The Progression Free Interval (PFI), Disease Free Interval (DFI), and Disease-Specific Survival (DSS) timings were retrieved from UCSC Xena( https://xena.ucsc.edu/ ), and the Overall Survival (OS) time was acquired from TCGA.
Then, using the TIMER [ 21 ] and Xena Shiny databases ( https://shiny.hiplot.cn/ucsc-xena-shiny/)-powerfu l online platforms that incorporated mRNA sequencing data from the TCGA and GTEx datasets [ 22 ]-we analyzed the ESCO2’s mRNA expression value between tumor and normal samples for 33 types of cancer. Furthermore, the ESCO2’s protein level was determined using the UALCAN online platform ( https://ualcan.path.uab.edu/index.html ). Finally, three pairs of paraffin-embedded kidney renal clear cell carcinoma (KIRC), bladder urothelial carcinoma (BLCA), and adjacent samples were collected from the Second Hospital of Tianjin Medical University. The pathologic type of these individuals was clear cell renal cell carcinoma (ccRCC, i.e., KIRC) or BLCA, and none of them received preoperative chemotherapy. More crucially, all patients have approved the use of the surgical material for academic research and publications. All methods were approved by The Institutional Review Committee and the Medical Ethics Committee of the Second Hospital of Tianjin Medical University. Written informed consents have been obtained from all subjects.
Survival analysis and relationship with clinical stage
We filtered the cancer patients to assess the ESCO2’s impacts on the cancer cases’ prognosis. (a) The average value of several samples from the same patient was thought to represent the ESCO2 expression level. (b) cancer patients with a follow-up time of zero were excluded. After that, the survival R package was applied to run the survival analysis. The survfit function was utilized to model the Kaplan-Meier survival curve. The best-cutoff point was discovered using the “surv cutpoint” function in a survminer R package, which can repeatedly check all viable cutting points to get the highest rank statistic. We next divided each cancer type’s patients into two groups using the appropriate cutoff point for gene expression. The Kaplan-Meier survival curves were compared based on the two-sided long-rank test. Moreover, we performed Receiver operating characteristic curve (ROC) testing to assess the ESCO2’s ability to predict cancer across all types. Then, we investigated the relationship between ESCO2’s expression level and clinical parameters, including tumor size, stage, and grade.
Functional enrichment analysis of ESCO2
We extracted the RNA sequencing profiles of cancer types where ESCO2 has prognostic value to investigate ESCO2’s carcinogenesis in malignancies. Hence, 12 cancer types, including thymoma (THYM), colon adenocarcinoma (COAD), rectum adenocarcinoma (READ), adrenocortical carcinoma (ACC), kidney chromophobe (KICH), KIRC, kidney renal papillary cell carcinoma (KIRP), brain lower grade glioma (LGG), liver hepatocellular carcinoma (LIHC), lung adenocarcinoma (LUAD), mesothelioma (MESO), and pancreatic adenocarcinoma (PAAD), were rolled into the next analysis. For each cancer type, individuals were grouped into ESCO2-high and ESCO2-low subgroups relied on the median value of ESCO2, respectively. After that, gene set enriched in two groups were detected by gene set enrichment analysis (GSEA). Gene sets, the 50 hall markers, were obtained from MSigDB [ 23 ] ( https://www.gsea-msigdb.org/gsea/msigdb ).
The hunt for ESCO2-related regulatory genes
The above data shows that ESCO2 is a negative oncogene in most cancers. Therefore, we extracted the RNA-seq matrix from KICH, KIRC, LGG, LIHC, LUAD, and PAAD to study ESCO2-related regulatory genes. ESCO2 had a negative impact on these cancer types, which also accounted for more than 150 cases. Similarly, we split patients into ESCO2-high and ESCO2-low groups based on the median value of ESCO2 in each cancer type. Following that, differentially expressed genes (DEGs) between ESCO2-high and the ESCO2-low group were performed using limma R packge [ 24 ]( p < 0.05, Log FC > 1). After identifying the intersecting genes of DEGs in each cancer, we calculated the association between ESCO2 and these DEGs by spearman’s correlation analysis for each cancer type, respectively ( p < 0.05, Cor > 0.4). Intersecting genes for these highly correlated genes were considered ESCO2-related regulatory genes. Then, using the clusterProfiler R [ 25 ] package, the Kyoto Encyclopedia of Genes and Genomes (KEGG) [ 26 , 27 , 28 ] and Gene Ontology (GO) pathway enrichment analyses were performed on ESCO2-related regulatory genes. Incorporating ESCO2-related regulatory genes into the String database ( https://cn.string-db.org/ ) to form a PPI network enables the identification of core genes.
Single-cell analysis of ESCO2 in cancers
Tumor Immune Single-cell Hub (TISCH, http://tisch.comp-genomics.org/home/ ) is a robust database created for multiple single-cell analyses containing nearly 190 single-cell databases. We estimated the ESCO2 expression level across multiple cancer types in each cell type. Our study focused on the distribution of ESCO2 in KIRC cell subpopulations. The expression levels of ESCO2 in each cell type were quantified and visualized by a heatmap, scatter diagrams, and violin plots. In addition to the single-cell dataset of KIRC contained in the TISCH database, we also downloaded additional single-cell sequencing data from GSE156632 [ 29 ] in the Gene Expression Omnibus (GEO) database to validate ESCO2 expression level in each cell types.
Cell culture and siRNA transfection
The Cell Resource Center Affiliated with the Chinese Academy of Medical Sciences provided the human ccRCC cell lines A498 and human BLCA cell lines T24. In a 5% CO 2 humidified cell incubator at 37 °C, A498 and T24 cells were grown in Dulbecco’s modified Eagle medium (DMEM) (Gibco BRL Life Technologies Inc., USA) with 10% FBS (Gibco BRL Life Technologies Inc., USA) and 1% penicillin-streptomycin (Hy-clone, CA, USA).
Small interfering RNAs (siRNAs) were ordered from GenePharma. The si-NC and si-ESCO2 siRNA were transfected into A498 and T24 cells with a Transfection reagent. When cells occupied 70–80% of a 50 mm Petri dish, transfection experiments were conducted; the whole medium was aspirated, and 2 mL of opti-MEM was applied to each well. The siRNA (GenePharma, Shanghai, China) and Transfection reagent (Cat#:L3000075, Thermo Fisher Scientific, United States) were added to 500 µL opti-MEM, mixed, and incubated for 20 min at room temperature. After that, the siRNA-Transfection reagent mixture was added to each well. Each well was switched to a complete medium 6 h after the transfection assay. The sequences for ESCO2 siRNA are as follows: si-ESCO2-1 (forward: GCAAAUCAAGGCUCACCAUTT; reverse: AUGGUGAGCCUUGAUUUGCTT) and si-ESCO2-2 (forward: CUCUUAGACCAGGAUUAUCTT; reverse: GAUAAUCCUGGUCUAAGAGUG).
qPCR and western blotting analysis
After the cells were transfected for 48 and 72 h, we harvested cells for RNA and protein extraction, respectively. Following the protocol, the total RNAs of cells were extracted using TRIzol reagents (Invitrogen). We then used cDNA reverse transcription kits to synthesize cDNA, and the mRNA expression level was calculated through qPCR assay in q225. We used the 2 − ΔΔ Ct method to calculate GAPDH as an internal reference for mRNA. The ESCO2’s sequences of primers are as follows: F, CACTGGGACGCACCCAAAA, R, CACTTGCCTTGTCGCAAAAG (Sangon biotech). The following are the primer sequences for the GAPDH gene.: F, CAGGAGGCATTGCTGATGAT, R, GAAGGCTGGGGCTCATTT (Sangon biotech).
The tissues or cells were collected and lysed in RIPA lysis buffer (Cat#:89,901, Thermo Fisher Scientific, USA) with supplementation of a protease inhibitor cocktail (Cat#:539,131, Roche, Switzerland) in the ice for 30 min. The total protein extracts were measured by the ABC method (Cat#:23,227, Thermo Fisher Scientific, USA), separated by 10% sodium dodecyl sulfate-polyacrylamide gel electrophoresis (SDS-PAGE), and transferred to the nitrocellulose membrane. The membrane was blocked using 5% non-fat milk or 5% BSA (Solarbio, Beijing, China), then incubated with primary antibodies anti- (ESCO2,Cat#:23525-1-AP, diluted 1:2000, Proteintech, USA; CDK1, Cat#:23525-1-AP, diluted 1:2000, Proteintech, USA). The proper secondary antibody is chosen based on the primary antibody’s type, including goat anti-mouse HRP-conjugated IgG (Cat#:SA00001-1, 1:2000 dilutions, Proteintech, USA) and goat anti-rabbit HRP-conjugated IgG (Cat#:SA00001-2, 1:2000 dilutions, Proteintech, USA). The target band signals were captured with the help of a BIO-RAD ChemiDoc XRS chemiluminescence system (Bio-Rad Inc., CA, USA). The densitometry of all Western blots was analysed using a Gel-Pro analyzer (Media Cybernetics, USA). Imagej software was used to perform quantitative analysis with β-actin as a control (1.52a, National Institutes of Health, Bethesda, MD, USA).
Cell proliferation and colony formation assay
Briefly, 2000 cells per well were planted onto 96-well plates for the cell proliferation assay, and transfection assay was conducted after cell fixation. Cells were cultured in the incubator for 12, 24, 48, and 72 h. After that, each well received 10 µL of Cell Counting Kit-8 reaction solution (CCK8, Cat#:CA1210, Solarbio Science & Technology Co., Ltd., Beijing, China) and was incubated for 1 h. The light absorption values (OD) for si-NC and si-ESCO2 groups were captured at 450 nm on a Varioskan Flash Multimode plate reader (Thermo Fisher Scientific Inc., USA).
Cancer cells per well were seeded onto a 12-well plate, and the medium was changed every two days. After being stained with 0.5% crystal violet (Cat#: C0121, Beyotime) for 20 min and fixed with 4% paraformaldehyde at room temperature for 10 min, colonies were counted 14 days later.
Ethynyl-2-Deoxyuridine (EdU) incorporation assay
Cancer cells were seeded in 3.5 cm confocal dishes (Thermo Fisher Scientific Inc., USA) with 50,000 cells per dish. An appropriate amount of 50 µM EdU (Cat#:K1077, ApexBio Technology LLC) medium was prepared by diluting the EdU solution with cell culture medium at a ratio of 1000:1.1 ml of 50 µM EdU medium was added after the entire medium had been aspirated, and it was then incubated for 2 h. After discarding the medium, it was washed with PBS, fixed with 4% paraformaldehyde, and then permeabilized with 0.5% TritonX-100 (Cat#:X100, Sigma-Aldrich, USA). 200 μm of 1x Apollo (Cat#:K1077, ApexBio Technology LLC) staining reaction solution was added to each dish, incubated in the dark for 30 min. Nuclei were stained with DAPI (Cat#:MBD0015, Sigma-Aldrich, USA) for 10 min. Using a Nikon ECLIPSE 80i fluorescence microscope, images were captured, and ImageJ was used to count the cell number (1.52a, National Institutes of Health, Bethesda, MD, USA).
Transwell migration and wound healing assay
Matrigel (BD Biosciences, Franklin Lakes, NJ, USA) was evenly spread in transwell chambers (Cat#: 3422, Corning Inc., Corning, NY, USA), and cells starved for 24 h were seeded in a serum-free medium in the upper chamber. Medium containing 10% FBS was added to the lower chamber as a catalyst. The upper chamber was removed and separated after roughly 24 h, then fixed with methanol for 10 min, rinsed with PBS, and stained with 0.1% crystal violet (Cat#:G1064, Solarbio Science & Technology Co., Ltd., Beijing, China). Under a microscope, cells that had been pierced were viewed and counted (CKX41, Olympus, Tokyo, Japan).
Cells were seeded uniformly in micro-insert 4-wells in a µ-dish 35 mm, high ibiTreat (ibidi GmbH, Germany). The culture inserts were removed when the cell density reached 90%. The 500 mm wide scratching gaps were washed with phosphate-buffered solution (PBS, Cat#:D1020, Solarbio Science & Technology Co., Ltd., Beijing, China) and were replaced with fresh medium. The dynamic changes of wells were recorded at 0, 12, 24 and 48 h on a microscope (Olympus, Japan).
Statistical analysis
Using the Wilcox test, comparisons between the two groups were made. For analyzing three or more groups, the one-way ANOVA test was performed. For all statistical calculations, R studio and GraphPad software were used. The threshold for significance was defined at P < 0.05.
ESCO2 expression level in various cancer
Typically, tumor tissue exhibits higher or lower oncogene expression levels than normal tissue. Using the TCGA database, our work found that ESCO2 is substantially expressed in the tumor tissue of most cancer types. However, the ESCO2 expression value did not significantly change in KICH, PAAD, pheochromocytoma and paraganglioma (PCPG), or READ (Fig. 1 A). Considering only a few normal samples available in the TCGA database, we combined the TCGA and GTEx databases. 30 of 33 cancer types exhibited considerably greater levels of ESCO2 expression in tumor tissue, whereas acute myeloid leukemia (LAML) exhibited significantly lower levels. In the ACC and PCPG, the ESCO2 expression levels of the tumor and normal tissues were similar (Fig. 1 B). ESCO2 expression was raised in most cancers, supporting its oncogene function.
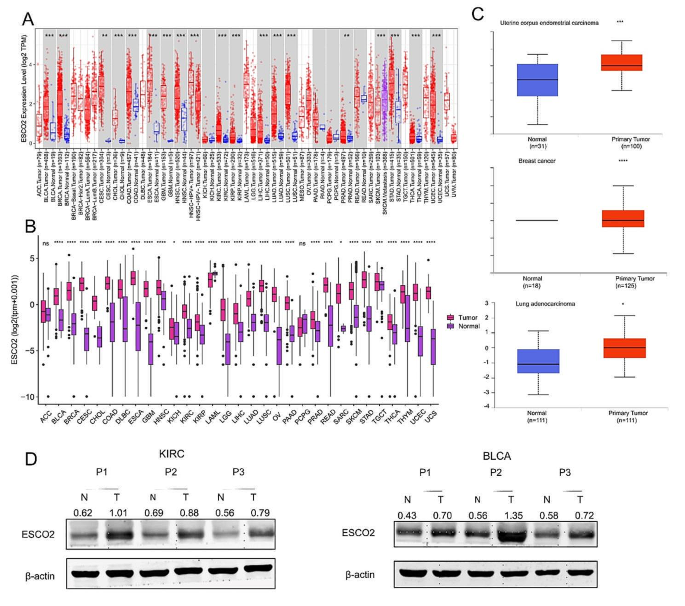
ESCO2 differential expression in pan-cancer. Comparison of ESCO2 expression between tumor and normal samples in TCGA database. Comparison of ESCO2 expression between tumor and normal samples in TCGA and GTEx database. (C) Comparison of ESCO2’s protein level between tumor and normal samples. (D) Western blot protein detection of the ESCO2 expression levels in adjacent normal tissues and paired cancer tissues, including KIRC and BLCA. The optical density ratio of bands represent objective proteins to band of β-actin was calculated
Furthermore, we evaluated the ESCO2 protein levels. We only found significantly higher protein levels of ESCO2 in tumor tissues of uterine corpus endometrial carcinoma (UCEC), breast invasive carcinoma (BRCA), and LUAD due to the UALCAN database’s limited inclusion of cancer types (Fig. 1 C). To further explore the alterations in ESCO2’s protein levels in carcinogenesis, we collected samples of KIRC and BLCA. In KIRC and BLCA, the protein level of tumor samples was noticeably greater than para-cancerous ones (Fig. 1 D).
ESCO2’s prognostic value across cancers
Using the TCGA database and focusing on OS, DFI, DSS, and PFI, we conducted a Kaplan-Meier survival analysis in each cancer to examine the association between ESCO2 and the cancer patient’s prognosis. Kaplan-Meier survival analyses in ACC, KICH, KIRC, KIRP, LGG, LIHC, LUAD, MESO, and PAAD demonstrated that tumor patients with high ESCO2 expression have short survival periods (Fig. 2 D, E, F, G, H, I, J, K and L). While ESCO2’s expression was linked to better outcomes in patients with THYM, COAD, and READ (Fig. 2 A, B and C). ROC analysis revealed that the ESCO2 performed well in predictive accuracy, with AUC values in ACC, KICH, KIRP, LGG, MESO, LIHC, and LUAD performing well (Figure S1 A). High ESCO2 expression was linked to a worse prognosis in cancer patients with KIRP, LIHC, and PAAD, according to Kaplan-Meier survival analysis for DFI (Figure S1 B). Similarly, as shown in Figures S2 and S3, cancer patients with increased ESCO2 expression had worse DSS and PFI across various malignancies. Our investigation revealed that ESCO2 might enhance tumor initiation and progression.
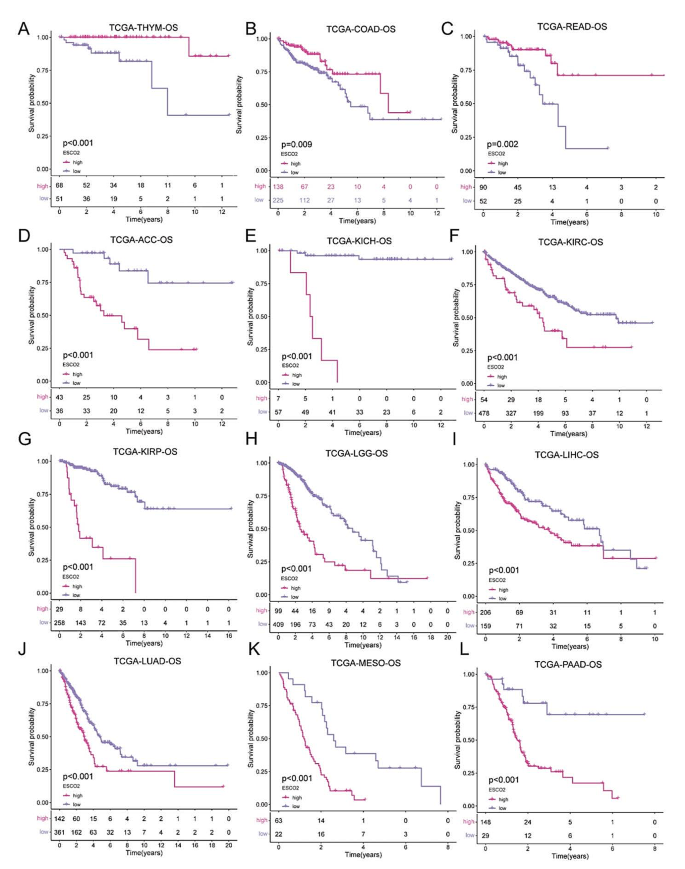
ESCO2’s Prognostic Value Across Cancers. (A–L) Kaplan–Meier analysis of the association between ESCO2 expression and OS
ESCO2 was positively related to tumor progression
To confirm the ESCO2 role in cancer progression, a correlation analysis was performed between the ESCO2’s mRNA value and clinical features. We discovered that ESCO2 expression was significantly increased in advanced tumor stage in several malignancies, including KICH, KIRC, KIRP, LUAD, and LIHC. On the contrary, ESCO2 expression was higher in lower tumor stages than in higher tumor stages in COAD (Fig. 3 A).
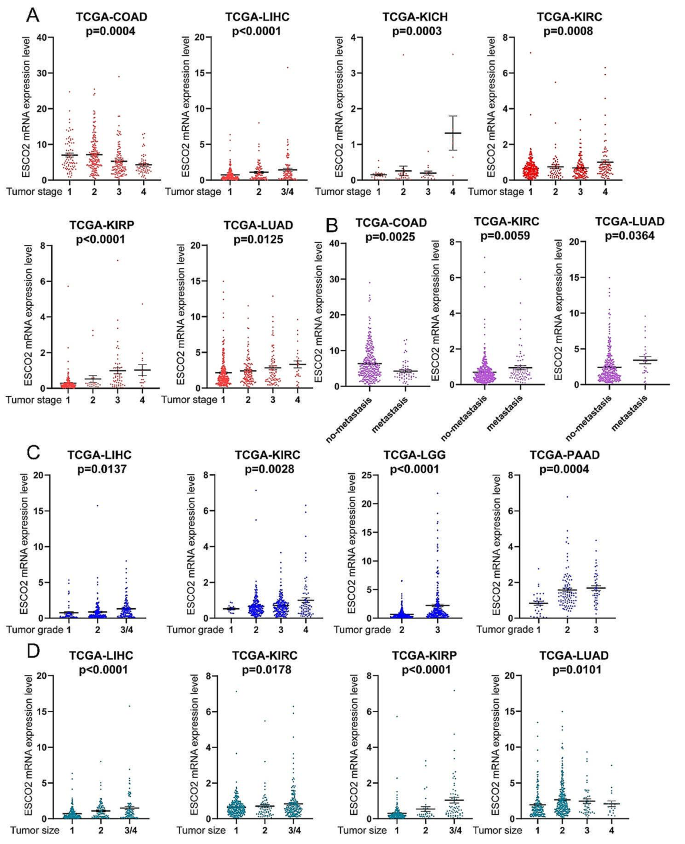
ESCO2 expression was positively correlated with tumor progression. Association between ESCO2 expression and tumor stage (A), tumor metastasis (B), tumor grade (C) and tumor size (D)
In LIHC, KIRC, LGG, and PAAD, ESCO2 expression was positively correlated with tumor grade (Fig. 3 C). Similarly, ESCO2 may also affect the tumor size: Tumor size and ESCO2 expression were positively linked in LIHC, KIRC, KIRP, and LUAD. (Figs. 3 D). Furthermore, ESCO2 was crucial for the metastasis of COAD, KIRC, and LUAD (Fig. 3 B). Tumor stage, size, and metastasis are the most widely used clinical indicators for evaluating the progression of cancer. Our investigation verified the connection between ESCO2 and the aforementioned indicators, indicating that ESCO2 is involved in tumor progression.
Analysis of ESCO2-related regulatory pathways
We gathered RNA sequencing profiles of cancer types where ESCO2 could significantly affect patients’ overall survival times to examine the ESCO2 oncogenic role in malignancies. For each cancer type described in the methods, cancer cases were grouped into ESCO2-high and -low subgroups according to the median value of ESCO2, respectively. Gene set enriched in two groups were then detected by gene set enrichment analysis. For visualization, we chose the top-10 NSE-ranked enriched pathways. Intriguingly, GSEA results were remarkably consistent across the 12 cancer types, indicating the validity of our findings (Fig. 4 A, B, C, D, E, F, G, H, I, J, K and L). Genes upregulated in the ESCO2-high group showed the E2F targets enrichment, G2M checkpoint, mitotic spindle and Mtorc1 signaling, which were associated with cell cycle and proliferation. Meanwhile, these ESCO2-high patients exhibited highly enriched DNA repair. In addition to cell proliferation-related pathways, glycolysis and fatty acid metabolism pathways were enriched in the ESCO2-high group in several cancer types. Furthermore, ESCO2 seemed to participate in the modulation of cancer inflammation, such as IL2 STAT5 signaling.
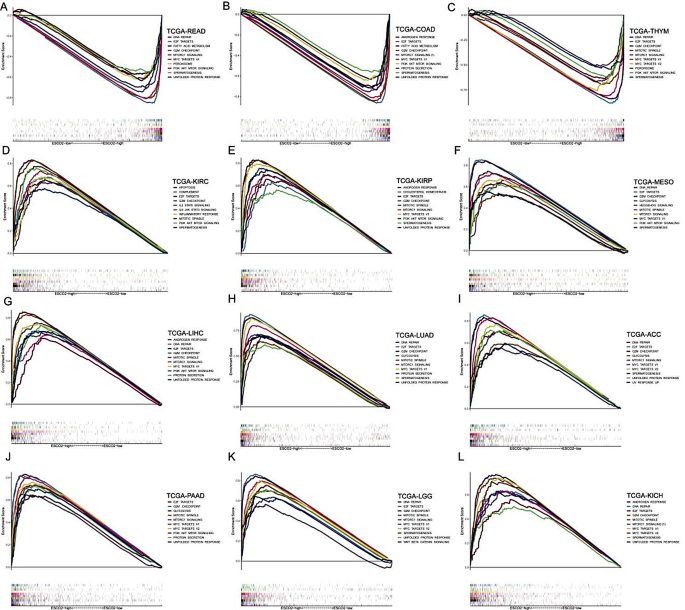
Results of gene set enrichment analysis (GSEA) based on the 50 hallmarks. (A–L) The GSEA analysis between ESCO2-high and ESCO2-low groups in READ, COAD, THYM, KIRC, KIRP, MESO, LIHC, LUAD, ACC, PAAD, LGG and KICH. Each panel’s left and right sides represent the enriched pathways of the ESCO2 high and low expression group, respectively
To investigate ESCO2-related regulatory genes, we divided cancer patients into ESCO2-high and ESCO2-low groups based on the median ESCO2 value in six cancer types specified in the materials and methods. We then obtained 2419 intersecting genes, which mainly enriched on histone binding, ATP hydrolysis activity and helicase activity (Fig. 5 A and B). The results of the GO analysis also confirmed that ESCO2 was primarily responsible for controlling cell growth. After spearman’s correlation analysis, we further identified the ESCO2-related regulatory genes. 249 ESCO2-related regulatory genes were collected in our study, which was highly correlated with ESCO2 across cancer types (Fig. 5 C). These ESCO2-related regulatory genes were primarily involved in cell-division-related processes, like the mitotic cell cycle and DNA metabolic processes (Fig. 5 F). We successfully identified the core genes by building the PPI network of ESCO2-related regulatory genes. The regulatory genes network’s nucleus is CDK1 (Fig. 5 D). Cell cycle kinase family member Cyclin-dependent kinase 1 (CDK1) significantly impacts cell cycle progression [ 30 , 31 ]. After the ESCO2 knockdown, we discovered that the CDK1 expression was significantly decreased (Fig. 5 E). Therefore, CDK1 may be an important signaling pathway regulated by ESCO2 in KIRC.
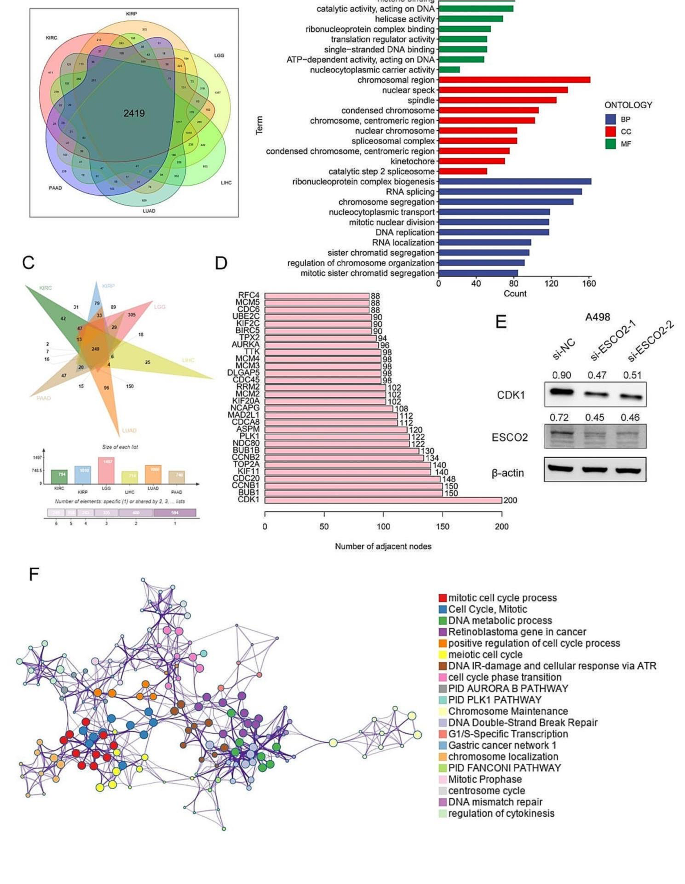
Analysis of ESCO2-related regulatory pathways. (A) The intersecting genes of DEGs between the ESCO2-high and ESCO2-low groups. (B) Gene Ontology analysis of the 2419 DEGs. FDR < 0.05 was considered significantly enriched. (C) The intersecting genes of DEGs with a high correlation to ESCO2 using spearman’s correlation analysis ( p < 0.05, Cor > 0.4). (D) Bar plot of the top 30 genes with the largest number of adjacent nodes in the PPI network of ESCO2-related regulatory genes. (E) Western blot results manifested the down-regulated expression of CDK1 compared with the si‐con group in A498 cells.The optical density ratio of bands represent objective proteins to band of β-actin was calculated. (F) The ESCO2-related regulatory gene functions were mainly enriched in mitotic cell cycle and DNA metabolic processes. The interactive network was constructed using the meta scape online platform
ESCO2 analysis at the single-cell level
Recent studies have shown that cell cycle proteins play an additional role in tumor development, affecting not only tumor cells but also their microenvironment and modulating anti-tumor immune responses [ 32 ]. For instance, inhibition of CDK4/6 enhances tumor cell immunogenicity through multiple mechanisms [ 7 ]. Meanwhile, CDK4/6 inhibitors substantially reduce the proliferation of regulatory T cells and encourage cytotoxic T cells to eliminate tumor cells [ 33 ]. Therefore, we next explored the ESCO2’s role in the anti-tumor immune response. By scRNA-seq, we can investigate the ESCO2 at the single-cell level. Using single-cell sequencing of COAD, KIRC, KICH, LIHC and PAAD, we observed that ESCO2 was expressed not only in malignant cells but also in endothelial and immune cells, including T cells, macrophages, NK cells and B cells (Fig. 6 A). Significantly, ESCO2 was strongly expressed in proliferating T cells. We found that ESCO2 was almost exclusively expressed in proliferating T cells in KIRC (Fig. 6 B). To further validate the results observed in the TICSH database, we subjected all quality single cells of KIRC(GSE156632) to single-cell processing procedures. Similarly, ESCO2 was significantly expressed in proliferating T cells, but we observed a higher expression of ESCO2 in tumor cells and macrophages as well (Fig. 6 C, D and E).
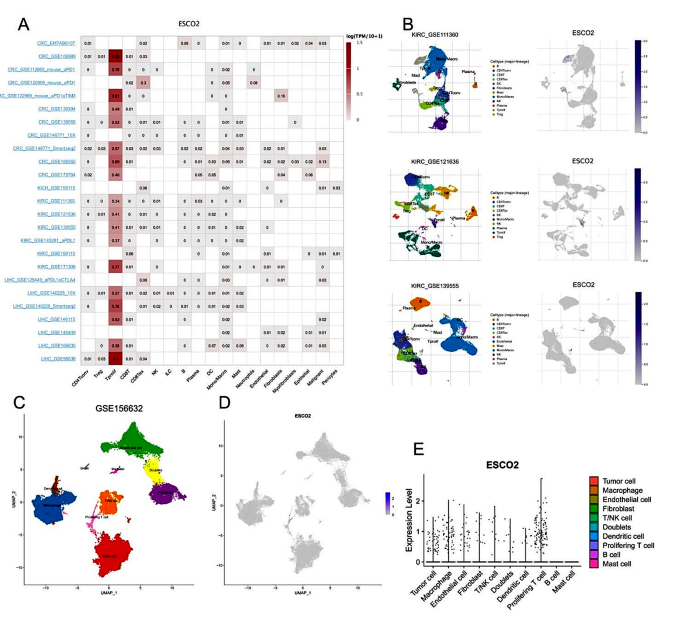
Single-Cell Analysis of ESCO2 in Cancers. (A) Summary of ESCO2 expression of 23 cell types in 31 single-cell datasets. (B) UMAP plots of all single cells of ccRCC patients, showing all cell types in the plot. (C) UMAP plot of all cell clusters in GSE156623 dataset. (D) UMAP plot and Violin plots(E) showing the expression of ESCO2 in each cell types
ESCO2 Promotes Human ccRCC and BLCA cell proliferation and invasion in vitro
To confirm ESCO2’s biological effector role in carcinogenesis, we first knocked it down in human ccRCC cells. qt-PCR and Western blotting results showed that ESCO2 was successfully knocked down (Fig. 7 A). Results from the CCK8 assay, colony formation assay, and EdU staining showed that the A498 cells’ ability to proliferate was significantly decreased by ESCO2 suppression than the control group (Fig. 7 B, C and F). This is consistent with our previous bioinformatic analysis that ESCO2 significantly regulates cell cycle progression. To further identify the potential role of ESCO2 in the invasive and migration of A498 cells. According to the findings of Transwell and Wound Healing, silencing ESCO2 inhibits invasion and migration (Fig. 7 D and E). To confirm ESCO2 as a potential pan-cancer biomarker, we further validated the carcinogenesis of ESCO2 in bladder cancer cell lines. According to the results of the experiments in vitro, the proliferative capacity of T24 cells was significantly inhibited after ESCO2’s knockdown (Fig. 8 A, B, C and F). Furthermore, the invasive ability of the T24 cells after knockdown of ESCO2 significantly decreased compared with the control group (Fig. 8 D and E). We confirmed, therefore, that ESCO2 is required for the proliferation and invasion of human ccRCC and BLCA cells.
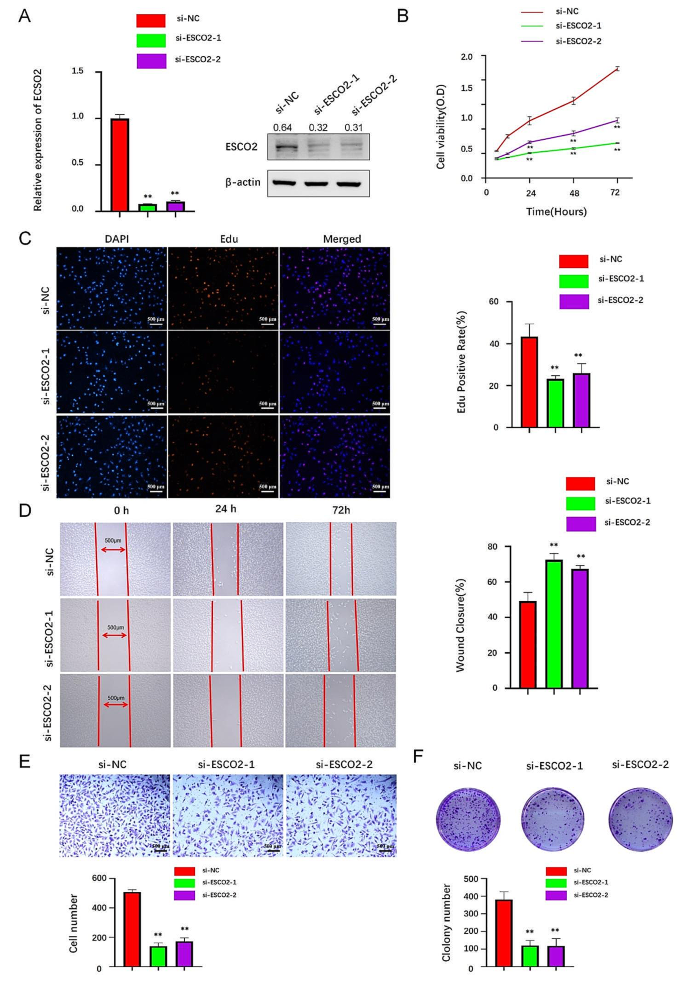
ESCO2 knockdown reduced cell proliferation, invasion, and migration in ccRCC. (A)A498 cells were transfected with si-ESCO2#1 and si-ESCO2#2, the level of ESCO2 was evaluated by qRT-PCR and Western blot. The optical density ratio of bands represent objective proteins to band of β-actin was calculated. (B) CCK8 analysis of cell viability in ESCO2-knockdown A498 cells at 0, 24, and 72 h, respectively, compared with the si‐con group. (C) EdU proliferation assay in A498 cells transduced with si-NC, si-ESCO2#1 or si-ESCO2#2. Scale bar = 500 μm. (D) Transwell and Wound Healing assay (E) revealed that ESCO2 silencing inhibited the invasion and migration ability. Scale bar = 500 μm. (F) ESCO2 silencing repressed colony formation in A498 cells. *: P < 0.05, **: P < 0.01, relative to untreated control
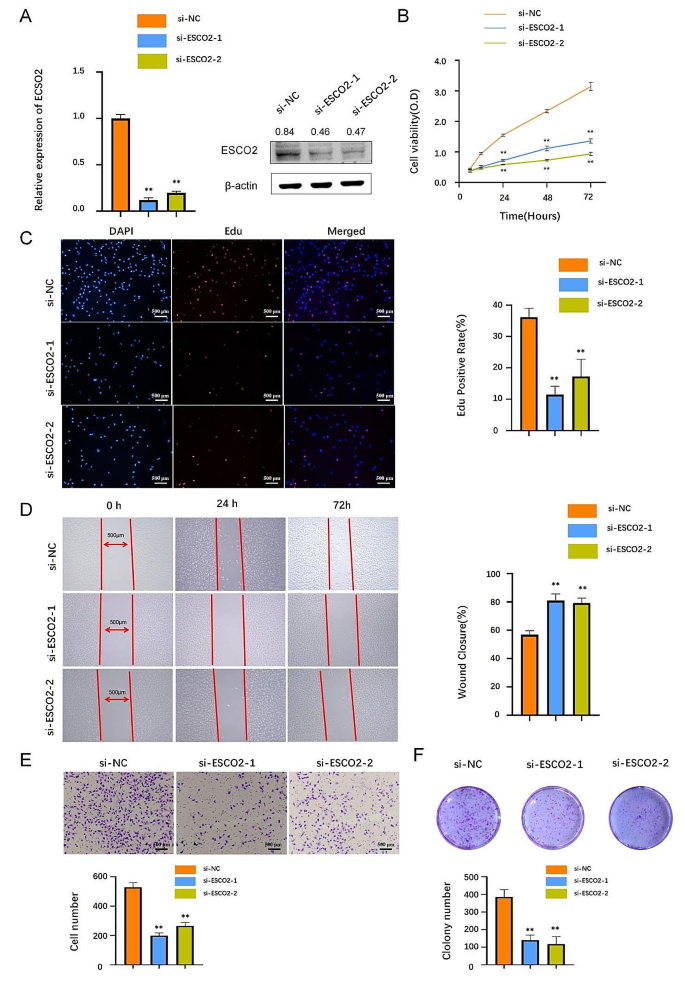
ESCO2 knockdown inhibited cell proliferation and invasion in BLCA cell lines. (A)T24 cells were transfected with si-ESCO2#1 and si-ESCO2#2, the level of ESCO2 was evaluated by qRT-PCR and Western blot. The optical density ratio of bands represent objective proteins to band of β-actin was calculated. (B) CCK8 analysis of cell viability in ESCO2-knockdown T24 cells at 0, 24, and 72 h, respectively, compared with the si‐con group. (C) EdU proliferation assay in T24 cells transduced with si-NC, si-ESCO2#1 or si-ESCO2#2. Scale bar = 500 μm. (D) Transwell and Wound Healing assay (E) revealed that ESCO2 silencing inhibited the invasion and migration ability. Scale bar = 500 μm. (F) ESCO2 silencing repressed colony formation in T24 cells
Cancer treatment has evolved significantly, with the introduction of immunotherapy and targeted medicines increasing patient survival rates for those with advanced or metastatic cancer, but overall clinical outcomes remain disappointing. The challenge of treating advanced cancers has inspired researchers to explore the underlying mechanisms that contribute to cancer growth, which will help identify potentially effective therapeutic targets. Unquestionably, one of the most promising therapeutic targets is cell cycle related protein. Palbociclib, the first CDK4/6-specific inhibitor, was created in 2004 and has proven effective against a variety of human cancer cell lines [ 34 ]. All three CDK4/6 inhibitors (palbociclib, ribociclib, and abemaciclib) are currently approved by the United States Food and Drug Administration (FDA) for the treatment of breast cancer. In addition, numerous inhibitors of cell-cycle proteins, including CDK9, CDK2, and CDK5, are in clinical trials [ 35 , 36 ]. Cell cycle regulating proteins such as CDCA4, CDCA8, and KIF2C have been linked to tumor growth and progression, affecting the proliferation, migration, invasion and metastasis of cancer cell lines [ 37 , 38 , 39 ]. ESCO2 was first reported in Roberts syndrome, whose inactivation mutation causes Roberts Syndrome [ 11 ]. Subsequent researchers have identified that ESCO2 is crucial in controlling cell mitosis and preserving genomic stability because it bridges two sister chromatids and is involved in the mitotic S-phase adhesins acetylation [ 40 , 41 ]. As described in the context, current researchers consider ESCO2 an excellent therapeutic target as an oncogene that promotes cancer development. However, current cancer research on ESCO2 is limited to a few cancers, such as lung, stomach, and colon cancers. No studies are focusing on ESCO2 in multiple cancers that can shed light on the similarities of ESCO2 in cancer. In this study, we identified that ESCO2 was a reliable biomarker for cancer patients and could accurately predict the cancer patient’s prognosis. Furthermore, ESCO2 participated in mitosis, the cell cycle, DNA damage repair, other processes, and tumor immune infiltration. Finally, we confirmed that ESCO2 is essential for the proliferation and invasion of human ccRCC and BLCA cells in vitro.
First, we evaluated the ESCO2’s mRNA expression value in 33 cancers by TCGA and GTEx databases. The analysis showed that ESCO2 expression was upregulated in most cancer tissues except LAML, ACC and PCPG. Due to post-transcriptional processing, protein and mRNA gene expression levels may differ. Thus, we validated ESCO2 protein levels in KIRC and BLCA clinical samples. ESCO2 protein values were considerably greater in malignant tissues than in para-cancerous ones, consistent with the mRNA analysis. These results confirmed that ESCO2 was upregulated in various cancers, suggesting a promising future for ESCO2 in cancer diagnosis. Additionally, OS, DSS, DFI, and PFI analyses all revealed that ESCO2 was significantly associated with cancer patient’s prognosis. Evaluated ESCO2 resulted in poorer prognosis in ACC, BLCA, BRCA, CESC, COAD, GBM, HNSC, KIRC, LGG, LIHC, LUAD, LUSC, and PAAD. However, in THYM, COAD and READ, ESCO2 was a favorable prognostic factor. ESCO2 has been shown to prevent cancer metastasis in a recent trial on colon cancer, which is consistent with the results of our bioinformatics research [ 19 ]. According to the findings above, ESCO2 is crucial in determining a cancer patient’s prognosis and can serve as a reliable prognostic biomarker. To some extent, tumor grading and clinical staging can show how tumors progress. Our results show that ESCO2 expression was positively associated with tumor stage and size in LIHC, KIRC, KIRP and LUAD. Furthermore, ESCO2 was crucial for the metastasis of COAD, KIRC, and LUAD. Our research identified that ESCO2 is a proto-oncogene that is linked to the development and progression of tumors.
Emerging research has confirmed that ESCO2 participated in apoptosis and cell proliferation-related pathways, such as the P53 and mTOR pathway [ 15 , 17 ]. Furthermore, ESCO2 could stimulate aerobic glycolysis in LUAD cells by upregulating PKM2 and downregulating PKM1 expression [ 16 ]. The GSEA results in our study were unexpectedly uniform among the 12 different cancers, indicating the validity of our findings. The cell cycle and proliferation-related E2F targets, G2M checkpoint, mitotic spindle, and Mtorc1 signaling were concentrated in the genes upregulated in the ESCO2-high group. These ESCO2-high patients also displayed greatly enhanced DNA repair. In sum up, our bioinformatics analysis confirmed ESCO2’s contribution to the control of cell proliferation and cycle. Therefore, we conducted experimental validation to verify the results of the bioinformatics analysis. We knocked down ESCO2 in A498 and T24 cells, and ESCO2’S knockdown significantly inhibited the proliferation, invasion, and migration of A498 and T24 cells. This result demonstrated that ESCO2’s role in cancer might be unified, further indicating the possibility that it could be an effective therapeutic target. Furthermore, we investigated its potential regulatory mechanisms. We found the core of the ESCO2-CDK1 regulatory network. When ESCO2 was inhibited, CDK1 expression decreased, demonstrating that CDK1 is a downstream gene controlled by ESCO2. Cell cycle progression is the central event in all proliferating cells and is primarily regulated by cell cycle-dependent kinases (CDK) [ 42 ].
CDK1 is the only CDK required for the G2-M transition and regulates G1 progression and G1-S transition [ 43 ]. In recent years, CDK1 has been suggested as a therapeutic target for cancer. CDK1 overexpression has been found in many cancers, including gastric cancer, ovarian cancer, oral squamous cell carcinoma, liver cancer, and breast cancer [ 44 ]. CDK1 inhibitors could be a potential small-molecule drug. CDK1 inhibitor RO3306 could improve the efficacy of sorafenib treatment by targeting cancer stem cells in a preclinical model of hepatocellular carcinoma [ 45 ]. In addition, Several CDK1 inhibitors, including Rigosertib (phase II/III) and Zotaraciclib, have begun phase I clinical trials for treating pancreatic cancer and glioma [ 46 , 47 ]. As a CDK1’s upstream regulatory gene, ESCO2 took part in the control of the cell cycle. Hence, ESCO2 is likely to be a promising therapeutic target in the future. Finally, single-cell analysis observed that ESCO2 was expressed not only in malignant cells but also in endothelial and immune cells, especially proliferating T cells. The GSEA results also indicate that ESCO2 is involved in cancer inflammation control, including IL2 STAT5 signaling, which raises the possibility that ESCO2 also controls the tumor immune microenvironment. Studies have shown that inhibition of cell cycle protein not only induce tumor cell cycle arrest, but also promote anti-tumor immunity. Inhibition of CDK4 /6, for example, increases levels of PD-L1 protein. More excitingly, combining CDK4/6 inhibitor with anti-PD-1 immunotherapy promotes tumor regression and significantly increases overall survival in mouse tumor models [ 48 ]. Therefore, research into ESCO2’s function in the tumor immune microenvironment is essential.
This study has the following limitations, though. First, this work does not apply any unique clinical cohorts to evaluate the diagnostic and prognostic significance of ESCO2 in cancer, despite its extensive use of sequencing data from public databases. Second, this study only performed ESCO2’s functional experiment in ccRCC cells; the upstream and downstream pathways of ESCO2 were not comprehensively examined, and the precise molecular mechanism of ESCO2 regulation is still unknown. Third, we did not conduct experiments in vivo, which is an important issue. We should attempt to resolve this issue in the future.
In conclusion, ESCO2 is a potential biomarker and oncogene for pan-cancer that can accurately predict the cancer patient’s prognosis in ACC, KICH, KIRC, KIRP, LGG, LIHC, LUAD, MESO, PAAD, THYM, COAD, and READ. Furthermore, our bioinformatics results discovered that ESCO2 is involved in cell division and cell cycle regulation and verified that ESCO2 is essential for the proliferation and invasion of human ccRCC and BLCA cells in vitro. In a nutshell, ESCO2 is a potential and reliable therapeutic target.
Data availability
The data analyzed in this study can be downloaded from the GEO (GSE156632, https://www.ncbi.nlm.nih.gov/geo/ ) and TCGA ( https://portal.gdc.cancer.gov/ ).
Abbreviations
differentially expressed genes
objective response rate
quality control
t-Distributed Stochastic Neighbor Embedding
Receiver operating characteristic curve
gene set enrichment analysis
Kyoto Encyclopedia of Genes and Genomes
tumor microenvironment
Bray F, Laversanne M, Weiderpass E, Soerjomataram I. The ever-increasing importance of cancer as a leading cause of premature death worldwide. Cancer. 2021;127(16):3029–30.
Article PubMed Google Scholar
Sung H, Ferlay J, Siegel RL, et al. Global Cancer statistics 2020: GLOBOCAN estimates of incidence and Mortality Worldwide for 36 cancers in 185 countries. CA Cancer J Clin. 2021;71(3):209–49.
Finn RS, Martin M, Rugo HS, et al. Palbociclib and Letrozole in Advanced breast Cancer. N Engl J Med. 2016;375(20):1925–36.
Article CAS PubMed Google Scholar
Matthews HK, Bertoli C, de Bruin R. Cell cycle control in cancer. Nat Rev Mol Cell Biol. 2022;23(1):74–88.
Weiss JM, Csoszi T, Maglakelidze M, et al. Myelopreservation with the CDK4/6 inhibitor trilaciclib in patients with small-cell lung cancer receiving first-line chemotherapy: a phase Ib/randomized phase II trial. Ann Oncol. 2019;30(10):1613–21.
Article CAS PubMed PubMed Central Google Scholar
Suski JM, Braun M, Strmiska V, Sicinski P. Targeting cell-cycle machinery in cancer. Cancer Cell. 2021;39(6):759–78.
Goel S, Bergholz JS, Zhao JJ. Targeting CDK4 and CDK6 in cancer. Nat Rev Cancer. 2022;22(6):356–72.
Wang R, Xu K, Gao F, Huang J, Guan X. Clinical considerations of CDK4/6 inhibitors in triple-negative breast cancer. Biochim Biophys Acta Rev Cancer. 2021;1876(2):188590.
Liu Z, Cheng X, Pang B, et al. Effects of ESCO2 or its methylation on the prognosis, clinical characteristics, immune microenvironment, and pathogenesis of low-grade glioma. Int Immunopharmacol. 2022;104:108399.
Bender D, Da Silva E, Chen J, et al. Multivalent interaction of ESCO2 with the replication machinery is required for sister chromatid cohesion in vertebrates. Proc Natl Acad Sci U S A. 2020;117(2):1081–9.
Vega H, Waisfisz Q, Gordillo M, et al. Roberts syndrome is caused by mutations in ESCO2, a human homolog of yeast ECO1 that is essential for the establishment of sister chromatid cohesion. Nat Genet. 2005;37(5):468–70.
Jevitt AM, Rankin BD, Chen J, Rankin S. The cohesin modifier ESCO2 is stable during DNA replication. Chromosome Res. 2023;31(1):6.
Michaelis C, Ciosk R, Nasmyth K. Cohesins: chromosomal proteins that prevent premature separation of sister chromatids. Cell. 1997;91(1):35–45.
Guacci V, Koshland D, Strunnikov A. A direct link between sister chromatid cohesion and chromosome condensation revealed through the analysis of MCD1 in S. Cerevisiae. Cell. 1997;91(1):47–57.
Wang QL, Liu L. Establishment of cohesion 1 homolog 2 facilitates cell aggressive behaviors and induces poor prognosis in renal cell carcinoma. J Clin Lab Anal. 2020;34(5):e23163.
Zhu HE, Li T, Shi S, Chen DX, Chen W, Chen H. ESCO2 promotes lung adenocarcinoma progression by regulating hnRNPA1 acetylation. J Exp Clin Cancer Res. 2021;40(1):64.
Chen H, Zhang L, He W, et al. ESCO2 knockdown inhibits cell proliferation and induces apoptosis in human gastric cancer cells. Biochem Biophys Res Commun. 2018;496(2):475–81.
Zhang T, Wang B, Gu B et al. Genetic and Molecular Characterization Revealed the Prognosis Efficiency of Histone Acetylation in Pan-Digestive Cancers. J Oncol. 2022. 2022: 3938652.
Guo XB, Huang B, Pan YH, Su SG, Li Y. ESCO2 inhibits tumor metastasis via transcriptionally repressing MMP2 in colorectal cancer. Cancer Manag Res. 2018;10:6157–66.
Fu J, Zhou S, Xu H, et al. ATM-ESCO2-SMC3 axis promotes 53BP1 recruitment in response to DNA damage and safeguards genome integrity by stabilizing cohesin complex. Nucleic Acids Res. 2023;51(14):7376–91.
Li T, Fu J, Zeng Z, et al. TIMER2.0 for analysis of tumor-infiltrating immune cells. Nucleic Acids Res. 2020;48(W1):W509–14.
The GTEx. Consortium atlas of genetic regulatory effects across human tissues. Science. 2020;369(6509):1318–30.
Article Google Scholar
Liberzon A, Birger C, Thorvaldsdóttir H, Ghandi M, Mesirov JP, Tamayo P. The Molecular signatures database (MSigDB) hallmark gene set collection. Cell Syst. 2015;1(6):417–25.
Ritchie ME, Phipson B, Wu D, et al. Limma powers differential expression analyses for RNA-sequencing and microarray studies. Nucleic Acids Res. 2015;43(7):e47.
Article PubMed PubMed Central Google Scholar
Yu G, Wang LG, Han Y, He QY. clusterProfiler: an R package for comparing biological themes among gene clusters. OMICS. 2012;16(5):284–7.
Kanehisa M, Goto S. KEGG: kyoto encyclopedia of genes and genomes. Nucleic Acids Res. 2000;28(1):27–30.
Kanehisa M. Toward understanding the origin and evolution of cellular organisms. Protein Sci. 2019;28(11):1947–51.
Kanehisa M, Furumichi M, Sato Y, Kawashima M, Ishiguro-Watanabe M. KEGG for taxonomy-based analysis of pathways and genomes. Nucleic Acids Res. 2023;51(D1):D587–92.
Zhang M, Zhai W, Miao J, et al. Single cell analysis reveals intra-tumour heterogeneity, microenvironment and potential diagnosis markers for clear cell renal cell carcinoma. Clin Transl Med. 2022;12(5):e713.
Izadi S, Nikkhoo A, Hojjat-Farsangi M, et al. CDK1 in breast Cancer: implications for theranostic potential. Anticancer Agents Med Chem. 2020;20(7):758–67.
Ren L, Yang Y, Li W, et al. CDK1 serves as a therapeutic target of adrenocortical carcinoma via regulating epithelial-mesenchymal transition, G2/M phase transition, and PANoptosis. J Transl Med. 2022;20(1):444.
Goel S, DeCristo MJ, Watt AC, et al. CDK4/6 inhibition triggers anti-tumour immunity. Nature. 2017;548(7668):471–5.
Deng J, Wang ES, Jenkins RW, et al. CDK4/6 inhibition augments Antitumor Immunity by enhancing T-cell activation. Cancer Discov. 2018;8(2):216–33.
Fry DW, Harvey PJ, Keller PR, et al. Specific inhibition of cyclin-dependent kinase 4/6 by PD 0332991 and associated antitumor activity in human tumor xenografts. Mol Cancer Ther. 2004;3(11):1427–38.
Diamond JR, Boni V, Lim E, et al. First-in-human dose-escalation study of cyclin-dependent kinase 9 inhibitor VIP152 in patients with Advanced malignancies shows early signs of clinical efficacy. Clin Cancer Res. 2022;28(7):1285–93.
Kumar SK, LaPlant B, Chng WJ, et al. Dinaciclib, a novel CDK inhibitor, demonstrates encouraging single-agent activity in patients with relapsed multiple myeloma. Blood. 2015;125(3):443–8.
Fang H, Sheng S, Chen B, et al. A Pan-cancer analysis of the oncogenic role of Cell Division Cycle-Associated protein 4 (CDCA4) in human tumors. Front Immunol. 2022;13:826337.
Xiang C, Sun WH, Ke Y, Yu X, Wang Y. CDCA8 contributes to the development and progression of thyroid Cancer through regulating CDK1. J Cancer. 2022;13(7):2322–35.
Wei S, Dai M, Zhang C, et al. KIF2C: a novel link between Wnt/β-catenin and mTORC1 signaling in the pathogenesis of hepatocellular carcinoma. Protein Cell. 2021;12(10):788–809.
Alomer RM, da Silva E, Chen J, et al. Esco1 and Esco2 regulate distinct cohesin functions during cell cycle progression. Proc Natl Acad Sci U S A. 2017;114(37):9906–11.
Sun H, Zhang J, Xin S, et al. Cul4-Ddb1 ubiquitin ligases facilitate DNA replication-coupled sister chromatid cohesion through regulation of cohesin acetyltransferase Esco2. PLoS Genet. 2019;15(2):e1007685.
Evan GI, Vousden KH. Proliferation, cell cycle and apoptosis in cancer. Nature. 2001;411(6835):342–8.
Santamaría D, Barrière C, Cerqueira A, et al. Cdk1 is sufficient to drive the mammalian cell cycle. Nature. 2007;448(7155):811–5.
Sofi S, Mehraj U, Qayoom H, et al. Targeting cyclin-dependent kinase 1 (CDK1) in cancer: molecular docking and dynamic simulations of potential CDK1 inhibitors. Med Oncol. 2022;39(9):133.
Wu CX, Wang XQ, Chok SH, et al. Blocking CDK1/PDK1/β-Catenin signaling by CDK1 inhibitor RO3306 increased the efficacy of sorafenib treatment by targeting cancer stem cells in a preclinical model of hepatocellular carcinoma. Theranostics. 2018;8(14):3737–50.
Wu J, Yuan Y, Long Priel DA, et al. Phase I study of Zotiraciclib in Combination with Temozolomide for patients with recurrent high-grade Astrocytomas. Clin Cancer Res. 2021;27(12):3298–306.
O’Neil BH, Scott AJ, Ma WW, et al. A phase II/III randomized study to compare the efficacy and safety of rigosertib plus gemcitabine versus gemcitabine alone in patients with previously untreated metastatic pancreatic cancer. Ann Oncol. 2015;26(9):1923–9.
Zhang J, Bu X, Wang H, et al. Cyclin D-CDK4 kinase destabilizes PD-L1 via cullin 3-SPOP to control cancer immune surveillance. Nature. 2018;553(7686):91–5.
Download references
Acknowledgements
We thank Yubo Feng for assistance with the statistical methods.
This work was supported by the National Natural Science Foundation of China (grant numbers: 22176142 and 22076138), Young Elite Scientists Sponsorship Program by Tianjin (No. TJSQNTJ-2020-07).
Author information
Yue Huang and Dapeng Chen contributed equally to this work.
Authors and Affiliations
Tianjin Institute of Urology, The Second Hospital of Tianjin Medical University, Tianjin, China
Yue Huang, Zhiwen Zheng, Qingfeng Fu, Bocun Yi, Yuchen Jiang, Zhihong Zhang & Jianqiang Zhu
Tianjin First Central Hospital Clinic Institute, Tianjin Medical University, Tianjin, 300192, China
Dapeng Chen
Department of Hepatobiliary Surgery, Tianjin First Central Hospital, School of Medicine, Nankai University, Tianjin, 300192, China
Yi Bai & Yamin Zhang
You can also search for this author in PubMed Google Scholar
Contributions
Conceptualization: YZ, ZZ, YB, and JZ. Methodology: YH, DC, ZZ, QF, and BY. Software: DC, ZZ, QF, and YJ. Validation: YH, DC, YB, and YJ. Writing-original draft: YH and DC. Writing-review and editing: YZ, ZZ, YB, and JZ. Funding acquisition: ZZ, JZ. Supervision: YZ and ZZ. All authors reviewed and revised the manuscript.
Corresponding authors
Correspondence to Zhihong Zhang or Jianqiang Zhu .
Ethics declarations
Ethics approval and consent to participate.
The authors confirm that all methods were conducted according to the principles of the Declaration of Helsinki and were approved by The Institutional Review Committee and the Medical Ethics Committee of the Second Hospital of Tianjin Medical University. Written informed consents have been obtained from all subjects.
Consent for publication
Not applicable.
Competing interests
The authors declare no competing interests.
Additional information
Publisher’s note.
Springer Nature remains neutral with regard to jurisdictional claims in published maps and institutional affiliations.
Electronic supplementary material
Below is the link to the electronic supplementary material.
Supplementary Material 1
Supplementary material 2, supplementary material 3, supplementary material 4, rights and permissions.
Open Access This article is licensed under a Creative Commons Attribution 4.0 International License, which permits use, sharing, adaptation, distribution and reproduction in any medium or format, as long as you give appropriate credit to the original author(s) and the source, provide a link to the Creative Commons licence, and indicate if changes were made. The images or other third party material in this article are included in the article’s Creative Commons licence, unless indicated otherwise in a credit line to the material. If material is not included in the article’s Creative Commons licence and your intended use is not permitted by statutory regulation or exceeds the permitted use, you will need to obtain permission directly from the copyright holder. To view a copy of this licence, visit http://creativecommons.org/licenses/by/4.0/ . The Creative Commons Public Domain Dedication waiver ( http://creativecommons.org/publicdomain/zero/1.0/ ) applies to the data made available in this article, unless otherwise stated in a credit line to the data.
Reprints and permissions
About this article
Cite this article.
Huang, Y., Chen, D., Bai, Y. et al. ESCO2’s oncogenic role in human tumors: a pan-cancer analysis and experimental validation. BMC Cancer 24 , 452 (2024). https://doi.org/10.1186/s12885-024-12213-w
Download citation
Received : 04 April 2023
Accepted : 01 April 2024
Published : 11 April 2024
DOI : https://doi.org/10.1186/s12885-024-12213-w
Share this article
Anyone you share the following link with will be able to read this content:
Sorry, a shareable link is not currently available for this article.
Provided by the Springer Nature SharedIt content-sharing initiative
- Single-cell
ISSN: 1471-2407
- Submission enquiries: [email protected]
- General enquiries: [email protected]
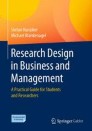
Research Design in Business and Management pp 141–170 Cite as
Single Case Research Design
- Stefan Hunziker 3 &
- Michael Blankenagel 3
- First Online: 10 November 2021
3809 Accesses
3 Citations
This chapter addresses the peculiarities, characteristics, and major fallacies of single case research designs. A single case study research design is a collective term for an in-depth analysis of a small non-random sample. The focus on this design is on in-depth. This characteristic distinguishes the case study research from other research designs that understand the individual case as a rather insignificant and interchangeable aspect of a population or sample. Also, researchers find relevant information on how to write a single case research design paper and learn about typical methodologies used for this research design. The chapter closes with referring to overlapping and adjacent research designs.
This is a preview of subscription content, log in via an institution .
Buying options
- Available as PDF
- Read on any device
- Instant download
- Own it forever
- Available as EPUB and PDF
Tax calculation will be finalised at checkout
Purchases are for personal use only
Baškarada, S. (2014). Qualitative case studies guidelines. The Qualitative Report, 19 (40), 1–25.
Google Scholar
Berg, B., & Lune, H. (2012). Qualitative research methods for the social sciences. Pearson.
Bryman, A. (2004). Social research methods (2nd ed.). Oxford University Press, 592.
Burns, R. B. (2000). Introduction to research methods. United States of America.
Creswell, J. W. (2013). Qualitative inquiry and research design. Choosing among five approaches (3rd ed.). SAGE.
Darke, P., Shanks, G., & Broadbent, M. (1998). Successfully completing case study research: Combining rigour, relevance and pragmatism. Inform Syst J, 8 (4), 273–289.
Article Google Scholar
Dey, I. (1999). Grounding grounded theory: Guidelines for qualitative inquiry . Academic Press.
Dick, B. (2005). Grounded theory: A thumbnail sketch. Retrieved 11 June 2021 from http://www.scu.edu.au/schools/gcm/ar/arp/grounded.html .
Dooley, L. M. (2002). Case study research and theory building. Advances in Developing Human Resources, 4 (3), 335–354.
Edmonds, W. A., & Kennedy, T. D. (2012). An applied reference guide to research designs: Quantitative, qualitative, and mixed methods . Thousand Oaks, CA: Sage.
Edmondson, A. & McManus, S. (2007). Methodological fit in management field research. The Academy of Management Review, 32 (4), 1155–1179.
Eisenhardt, K. M. (1989). Building theories from case study research. Academy of Management Review, 14 (4), 532–550.
Glaser, B., & Strauss, A. (1967). The discovery of grounded theory: Strategies for qualitative research . Sociology Press.
Flynn, B. B., Sakakibara, S., Schroeder, R. G., Bates, K. A., & Flynn, E. J. (1990). Empirical research methods in operations management. Journal of Operations Management, 9 (2), 250–284.
Flyvbjerg, B. (2006). Five misunderstandings about case-study research. Qualitative Inquiry, 12 (2), 219–245.
General Accounting Office (1990). Case study evaluations. Retrieved May 15, 2021, from https://www.gao.gov/assets/pemd-10.1.9.pdf .
Gomm, R. (2000). Case study method. Key issues, key texts . SAGE.
Halaweh, M. (2012). Integration of grounded theory and case study: An exemplary application from e-commerce security perception research. Journal of Information Technology Theory and Application (JITTA), 13 (1).
Hancock, D., & Algozzine, B. (2016). Doing case study research: A practical guide for beginning researchers (3rd ed.). Teachers College Press.
Hekkala, R. (2007). Grounded theory—the two faces of the methodology and their manifestation in IS research. In Proceedings of the 30th Information Systems Research Seminar in Scandinavia IRIS, 11–14 August, Tampere, Finland (pp. 1–12).
Hyett, N., Kenny, A., & Dickson-Swift, V. (2014). Methodology or method? A critical review of qualitative case study reports. International Journal of Qualitative Studies on Health and Well-Being, 9 , 23606.
Keating, P. J. (1995). A framework for classifying and evaluating the theoretical contributions of case research in management accounting. Journal of Management Accounting Research, 7 , 66.
Levy, J. S. (2008). Case studies: Types, designs, and logics of inference. Conflict Management and Peace Science, 25 (1), 1–18.
Meyer, J.-A., & Kittel-Wegner, E. (2002). Die Fallstudie in der betriebswirtschaftlichen Forschung und Lehre . Stiftungslehrstuhl für ABWL, insb. kleine und mittlere Unternehmen, Universität.
Mitchell, J. C. (1983). Case and situation analysis. The Sociological Review, 31 (2), 187–211.
Ng, Y. N. K. & Hase, S. (2008). Grounded suggestions for doing a grounded theory business research. Electronic Journal on Business Research Methods, 6 (2).
Ng. (2005). A principal-distributor collaboration moden in the crane industry. Ph.D. Thesis, Graduate College of Management, Southern Cross University, Australia.
Ridder, H.-G. (2016). Case study research. Approaches, methods, contribution to theory. Sozialwissenschaftliche Forschungsmethoden (vol. 12). Rainer Hampp Verlag.
Ridder, H.-G. (2017). The theory contribution of case study research designs. Business Research, 10 (2), 281–305.
Maoz, Z. (2002). Case study methodology in international studies: from storytelling to hypothesis testing. In F. P. Harvey & M. Brecher (Eds.). Evaluating methodology in international studies . University of Michigan Press.
May, T. (2011). Social research: Issues, methods and process . Open University Press/Mc.
Merriam, S. B. (2009). Qualitative research in practice: Examples for discussion and analysis .
Onwuegbuzie, A. J., Leech, N. L., & Collins, K. M. (2012). Qualitative analysis techniques for the review of the literature. Qualitative Report, 17 (56).
Piekkari, R., Welch, C., & Paavilainen, E. (2009). The case study as disciplinary convention. Organizational Research Methods, 12 (3), 567–589.
Stake, R. E. (1995). The art of case study research . Sage.
Stake, R. E. (2005). Qualitative case studies. The SAGE handbook of qualitative research (3rd ed.), ed. N. K. Denzin & Y. S. Lincoln (pp. 443–466).
Strauss, A. L., & Corbin, J. (1990). Basics of qualitative research: Grounded theory procedures and techniques . Sage publications.
Strauss, A. L., & Corbin, J. (1998). Basics of qualitative research techniques and procedures for developing grounded theory . Sage.
Tight, M. (2003). Researching higher education . Society for Research into Higher Education; Open University Press.
Tight, M. (2010). The curious case of case study: A viewpoint. International Journal of Social Research Methodology, 13 (4), 329–339.
Walsham, G. (2006). Doing interpretive research. European Journal of Information Systems, 15 (3), 320–330.
Welch, C., Piekkari, R., Plakoyiannaki, E., & Paavilainen-Mäntymäki, E. (2011). Theorising from case studies: Towards a pluralist future for international business research. Journal of International Business Studies, 42 (5), 740–762.
Woods, M. (2009). A contingency theory perspective on the risk management control system within Birmingham City Council. Management Accounting Research, 20 (1), 69–81.
Yin, R. K. (1994). Discovering the future of the case study. Method in evaluation research. American Journal of Evaluation, 15 (3), 283–290.
Yin, R. K. (2014). Case study research. Design and methods (5th ed.). SAGE.
Download references
Author information
Authors and affiliations.
Wirtschaft/IFZ – Campus Zug-Rotkreuz, Hochschule Luzern, Zug-Rotkreuz, Zug , Switzerland
Stefan Hunziker & Michael Blankenagel
You can also search for this author in PubMed Google Scholar
Corresponding author
Correspondence to Stefan Hunziker .
Rights and permissions
Reprints and permissions
Copyright information
© 2021 The Author(s), under exclusive license to Springer Fachmedien Wiesbaden GmbH, part of Springer Nature
About this chapter
Cite this chapter.
Hunziker, S., Blankenagel, M. (2021). Single Case Research Design. In: Research Design in Business and Management. Springer Gabler, Wiesbaden. https://doi.org/10.1007/978-3-658-34357-6_8
Download citation
DOI : https://doi.org/10.1007/978-3-658-34357-6_8
Published : 10 November 2021
Publisher Name : Springer Gabler, Wiesbaden
Print ISBN : 978-3-658-34356-9
Online ISBN : 978-3-658-34357-6
eBook Packages : Business and Economics (German Language)
Share this chapter
Anyone you share the following link with will be able to read this content:
Sorry, a shareable link is not currently available for this article.
Provided by the Springer Nature SharedIt content-sharing initiative
- Publish with us
Policies and ethics
- Find a journal
- Track your research
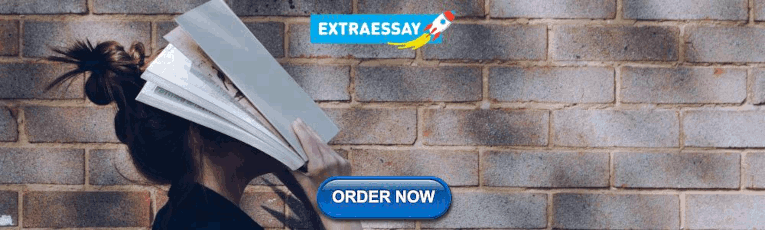
IMAGES
VIDEO
COMMENTS
The single-case experiment has a storied history in psychology dating back to the field's founders: Fechner (1889), Watson (1925), and Skinner (1938).It has been used to inform and develop theory, examine interpersonal processes, study the behavior of organisms, establish the effectiveness of psychological interventions, and address a host of other research questions (for a review, see ...
A systematic review of applied single-case research published between 2016 and 2018: study designs, randomization, data aspects, and data analysis. Behav. Res. 53 , 1371-1384 (2021).
General Overviews and Primary Textbooks. Several sources provide overviews of single-case experimental designs. Barlow, et al. 2009 includes an overview for the development of single-case experimental designs, describes key considerations for designing and conducting single-case experimental design research, and reviews procedural elements, assessment strategies, and replication considerations.
Abstract. Single-case experimental designs (SCEDs) represent a family of research designs that use experimental methods to study the effects of treatments on outcomes. The fundamental unit of analysis is the single case—which can be an individual, clinic, or community—ideally with replications of effects within and/or between cases.
Single-case designs (also called single-case experimental designs) are system of research design strategies that can provide strong evidence of intervention effectiveness by using repeated measurement to establish each participant (or case) as his or her own control. The flexibility of the designs, and the focus on the individual as the unit of ...
Tactics of Scientific Research (Sidman, 1960) provides a visionary treatise on single-case designs, their scientific underpinnings, and their critical role in understanding behavior. Since the foundational base was provided, single-case designs have proliferated especially in areas of application where they have been used to evaluate interventions with an extraordinary range of clients ...
This article systematically reviews the research design and methodological characteristics of single-case experimental design (SCED) research published in peer-reviewed journals between 2000 and 2010. SCEDs provide researchers with a flexible and viable alternative to group designs with large sample sizes. However, methodological challenges have precluded widespread implementation and ...
Single-case experimental designs have been used extensively with a variety of clinical populations and in a wide range of in-patient, outpatient, school, business, and community settings (Kazdin, 2011; Kratochwill & Levin, 2014).). "Single-case" can mislead because the designs are not necessarily restricted to the single case, that is, one individual.
This chapter addresses single-case research designs' peculiarities, characteristics, and significant fallacies. A single case research design is a collective term for an in-depth analysis of a small non-random sample. The focus of this design is in-depth. ... Here we can identify a significant difference to experimental research designs (see ...
Single-case experimental designs (SCEDs) have become a popular research methodology in educational science, psychology, and beyond. The growing popularity has been accompanied by the development of specific guidelines for the conduct and analysis of SCEDs. In this paper, we examine recent practices in the conduct and analysis of SCEDs by systematically reviewing applied SCEDs published over a ...
This article reviews current and consistent methodological problems that occur in research articles using single-case experimental designs. Problems discussed include (1) the use of pre- and quasi-experimental single-case designs, (2) the presentation and analysis of data, (3) the lack or inadequate demonstration of experimental reliability, and (4) the improper or ineffective use of ...
Single-case experimental designs (SCED) are experimental designs aiming at testing the effect of an intervention using a small number of patients (typically one to three), using repeated measurements, sequential (± randomized) introduction of an intervention and method-specific data analysis, including visual analysis and specific statistics.The aim of this paper is to familiarise ...
Identifying effective and efficient interventions for such a diverse group of clients and settings requires a flexible research approach. Single-case experimental designs (SCEDs) allow practitioners and researchers to answer experimental questions within the context of rigorous research designs. The purpose of this article is to highlight the ...
Abstract and Figures. Single-case experimental designs (SCEDs) represent a family of research designs that use experimental methods to study the effects of treatments on outcomes. The fundamental ...
Single-case experimental designs (SCEDs) provide a tool for rigorous evaluation of the effects of interventions at an individual level (Kratochwill et al. 2010) and can be used to identify and validate evidence-based practices (Horner et al. 2005).Consensus on what constitutes high-quality methodology exists for some aspects of SCED research (Manolov et al. 2014).
Single-case experimental design, often referred to as "N of 1" research, is a methodology that centers on the in-depth examination of individual subjects or cases. Unlike traditional group-based designs, this approach allows researchers to closely study and understand the nuances of a single participant's behavior, responses, and ...
Research and Methods. Johan W.S. Vlaeyen, ... Thomas R. Kratochwill, in Comprehensive Clinical Psychology (Second Edition), 2022 3.01.1.4 Utility of Single-Case Experimental Designs. Due to the increasing need of researchers, policy makers, public authorities, and private insurance companies to know which health care services are (cost-)effective, the question arises how best to obtain this ...
Case can refer to an individual person, classroom, school, business, entire city, or state. In this sense, case is the unit rather than the number of people involved. Single-case designs can evaluate the effects of interventions with large groups and address many of the questions posed in the group research that characterizes null-hypothesis ...
An ABA withdrawal/reversal single-case experimental design using concurrent multiple baselines and a four-week follow-up period was replicated across ten participants. The research design was developed in accordance with the Risk of Bias in N-of-1 Trials (RoBiNT) Scale (Tate et al., 2013; Supplementary Material
Purpose Establishment of sister chromatid cohesion N-acetyltransferase 2 (ESCO2) is involved in the mitotic S-phase adhesins acetylation and is responsible for bridging two sister chromatids. However, present ESCO2 cancer research is limited to a few cancers. No systematic pan-cancer analysis has been conducted to investigate its role in diagnosis, prognosis, and effector function. Methods We ...
A single case research design is not the same as researching a "case". Everything can be considered a "case" in most languages and certainly in English: a product, a patient, a business, an industry, a country, a currency, an ethnicity, a social group etc. Researching such a "case" does not make your research design a case study.