Rigorous primary research
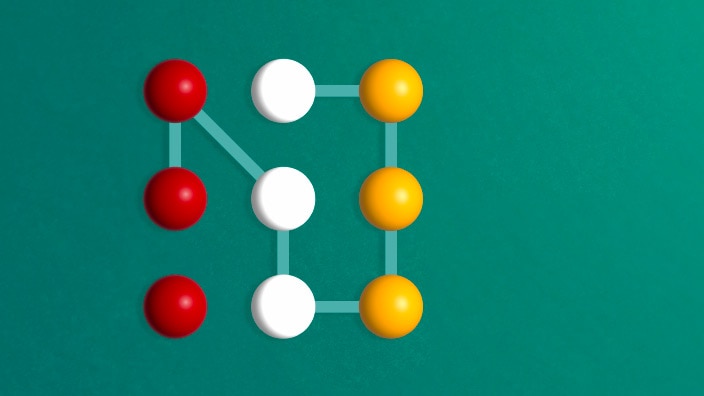
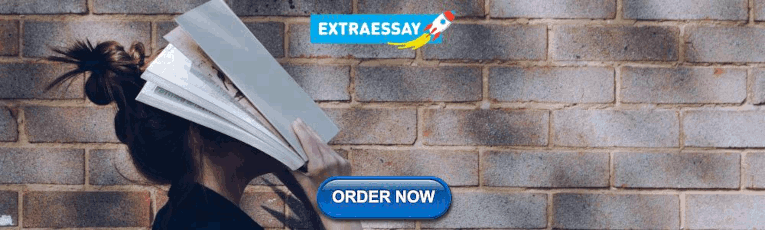
A framework for allocating to cash: risk, horizon, and funding level
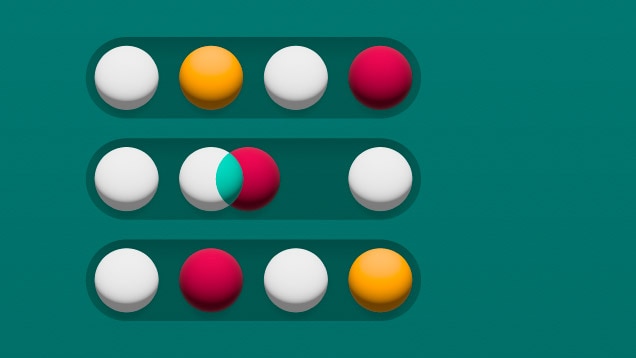
A Rational Multi-Asset Portfolio Rebalancing Decision-Making Framework
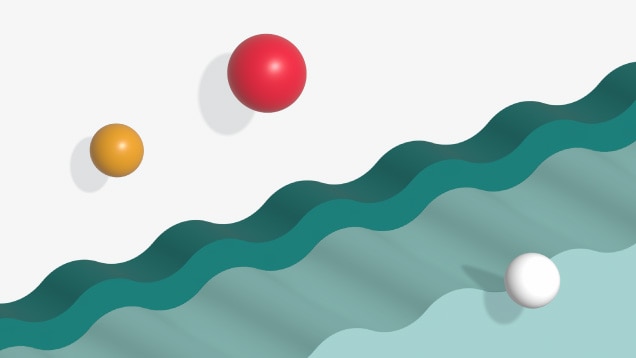
Resilient Liquidity: The Robust Reaction of Equity Volume and Spreads to Market Volatility
Filter by topic
Sort by date
Financial wellness & planning
May 26, 2022
For many families, planning for college costs is a top financial priority. A Byzantine landscape of consumer choices, loans, tuition discounting, and public and private aid, however, makes it difficult to answer key questions like "How much will this cost us?” This is especially so for young families, for whom future spending needs are necessarily vague. Vanguard researchers explore how understanding the dynamics of financial aid and net pricing can help parents set a realistic savings target early on and shape a savings plan that evolves with them.
Portfolio considerations
May 20, 2022
A number of factors have fueled the growth of index investing around the world. “The case for low-cost index-fund investing,” a newly revised research paper from Vanguard’s Investment Strategy Group, explores these factors. The paper discusses why the authors expect that index investing will continue to be effective over the long term.
April 14, 2022
The past few decades were a good time for spending from a retirement portfolio. What about the next 30 years? The apparent shifts in capital market conditions seen since the start of 2022 led us to examine sustainable withdrawal rates. Looking at historical return environments our authors developed three scenarios, with rates from 2.8% to 3.3%.
April 13, 2022
Vanguard believes private corporate pension plans should consider a liability-driven investing (LDI) program in the fixed income portion of their portfolio. Based on our analysis, a customized mix of U.S. investment-grade credit bonds and Treasuries aligns well with that liability. Such a mix has historically helped plan sponsors mitigate risk.
March 29, 2022
Personalized indexing (PI) is a flexible portfolio management solution that can track a personalized index and harvest capital losses for tax-saving purposes. This paper presents a portfolio construction plan using PI, touching on how to optimally implement tax-loss harvesting and allocate to PI in the presence of other assets.
August 10, 2022
Investors typically decide whether to convert to a Roth IRA from a traditional IRA by comparing their current and expected future marginal tax rates. The traditional wisdom has been that higher future tax rates make conversion more desirable and lower ones make it less so. Vanguard researchers describe a break-even tax rate (BETR) that yields a more accurate view of what future tax rate would make an investor indifferent to a conversion.
March 15, 2022
New Vanguard research indicates that investors are frequently using non-total market index funds to build portfolios with active exposures, according to “How investors use passive for active,” a paper authored by Vanguard’s Jan-Carl Plagge, Haifeng Wang, and Jim Rowley. The paper was published in The Journal of Beta Investment Strategies .
Behavioral research
February 23, 2022
Investors prefer some aspects of human advice over digital but perceive value in all advice services. Technology can help human advisors scale their businesses while strengthening their uniquely human value proposition.
February 02, 2022
Vanguard shares our current views on best practices for U.S. private-sector pension plan asset allocation. We highlight the value of identifying three targets—the return-seeking asset allocation, interest rate hedge ratio, and credit spread hedge ratio—that can be the foundation for a robust, customized portfolio construction process.
December 15, 2021
Retirees often eschew investing for total returns, relying instead on dividends and interest from high-income-producing assets to support spending from ad hoc portfolios. We introduce a method for building high-income portfolios that maximize the investor’s utility of wealth based on total returns while accounting for yield preferences.
Economics & markets
December 13, 2021
Our annual forecast explains why we expect the global economy to slow, leaving full-year 2022 growth, in our base case, of 4.8%. It also explains why our 10-year market outlook is guarded, as reflected in the low-single-digit range of expected returns for a globally diversified, 60% stock/40% bond portfolio.
November 19, 2021
Tax-loss harvesting (TLH) has been promoted as a “free lunch” for increasing investment returns. Vanguard researchers explore the potential for TLH and demonstrate how to customize a TLH strategy for optimal benefits. A case study is also featured that illustrates how the benefits of TLH can vary considerably from investor to investor.
November 15, 2021
Historically low yields on most global fixed income investments are likely to result in low returns over the next decade, leading some investors to question the allocation to bonds in multi-asset portfolios. In this paper, Vanguard researchers demonstrate the value of bonds as “shock absorbers” for portfolios when equity markets are under stress.
October 29, 2021
This paper examines the effect of automatic escalation on defined contribution plan contribution rates and provides insights into plan designs that maximize participants’ chance of savings at rates necessary for a secure retirement. The motivations of participants to override automatic deferral rates and annual increases are also explored.
October 11, 2021
This study explores how factoring in health care cost uncertainty can benefit retirement planning by enabling the development of more customized plans. Vanguard researchers employ a stochastic health care model to offer more realistic insights into how longevity and health care-related risks affect the viability of spending plans in retirement.
September 08, 2021
Growth in pension liabilities, along with new disclosure requirements and market-based accounting standards, have led defined benefit pension plan sponsors to consider corporate finances as they manage their plans. This paper discusses how pension plan risk can affect corporate finances and the strategies sponsors can use to mitigate that risk.
October 08, 2021
Parents and students face distinct challenges when financing higher education expenses. This research paper discusses financial aid options, tax credits, and 529 savings plans and other savings vehicles, as well as the need to plan strategically and understand how college spending decisions affect one's broader financial situation.
September 28, 2021
With interest rates and inflation expected to rise, the negative stock/bond correlation that supports the diversification properties of a balanced portfolio could also be at risk. In this paper, researchers find that negative correlation will continue and the diversification benefits of a 60% stock/40% bond portfolio will persist.
Vanguard’s Megatrends series examines fundamental shifts in the global economic landscape that are likely to affect the financial services industry and broader society. In this edition, Vanguard researchers explore China’s future as an economic powerhouse and its broad implications for global growth, geopolitics, and financial markets.
September 23, 2021
Vanguard’s Megatrends series examines fundamental shifts in the global economic landscape that are likely to affect the financial services industry and broader society. In this paper, Vanguard economists examine global trade growth and conclude that growth will likely slow, as it has since the global financial crisis, but not decline.
September 13, 2021
Vanguard researchers classify the economic and market environment into five states: recession, recovery, expansion, slowdown, and high inflation. This paper discusses the characteristics, valuations, and return expectations of each and concludes that investors willing to take on active risk should adopt a dynamic, state-dependent approach.
Amid a backdrop of highly accommodative monetary and fiscal policies, how likely are persistently higher rates of inflation in the United States for 2022 and beyond? In this paper, Vanguard researchers present the findings of Vanguard’s state-of-the-art forecasting model to project core inflation over the next two years.
August 30, 2021
Employing machine-learning, Vanguard researchers found that government bonds have historically acted as a counterbalance in an equity/bond portfolio during low-rate periods. Although in some months both equities and bonds fall, the analysis suggests that this can be thought of as market noise and distinctly different from typical outcomes.
Most target-date fund series offer a single post-retirement asset allocation designed to help retirees replace a reasonable portion of pre-retirement income. In this paper, Vanguard researchers offer a framework demonstrating that adding a second asset allocation could support additional goals for those with the resources and desire to pursue them.
A Vanguard survey shows that most investors underestimate the risk of mild cognitive impairment. In addition, while most respondents had some plans to mitigate the effects of cognitive decline, they were less likely to focus on the transfer of control of their finances, the timing of which can have significant implications for financial well-being.
August 05, 2021
This paper examines developments in the fixed income markets before and during the liquidity event precipitated by the coronavirus pandemic and the accompanying economic shock to the global financial markets. Vanguard calls for fixed income market participants and policymakers to make recommendations to improve market resiliency in the future.
July 23, 2021
This research looks at the withdrawal patterns, rates, and frequencies of more than two million Vanguard retail households at or near retirement age. It finds that only 52% took at least one withdrawal during the three-year study period.
Asset classes
Vanguard researchers examine the range of frequencies, durations, and magnitudes of underperformance experienced by active fixed income funds. The findings: Nearly all outperforming active fixed income funds experience periods of underperformance, although significant drawdowns over long periods are less likely than they are for active equity funds.
July 14, 2021
This paper explores the relationship of the number of fixed income holdings in a fund to systemic risk and alpha.
July 06, 2021
Vanguard researchers analyze the results of a survey of Vanguard investors' expectations for U.S. stock returns throughout the COVID market crisis. The results indicate that expectations follow market activity in close lockstep, shifting in tandem with recent performance rather than rising and falling in a more rational or contrary manner.
June 25, 2021
The Financial Independence, Retire Early (F.I.R.E.) movement relies on the 4% rule to determine withdrawals over 40 to 50 years in retirement. But that rule was designed for a 30-year horizon. This paper illustrates how F.I.R.E. investors can improve their chances of financing an early retirement by employing Vanguard’s investing principles.
June 11, 2021
This paper presents a framework, developed by Vanguard and Mercer, to forecast health care costs for U.S. retirees. Vanguard researchers outline key health care cost factors and personal considerations and frame expenses as an annual cost rather than a lifetime lump sum, enabling retirees to plan accordingly during their retirement years.
June 07, 2021
This supplement to How America Saves delves deeper into defined contribution plan landscape, combining comprehensive data on plan design and participant behavior with practical advice and insights from Vanguard's leading retirement plan experts to offer recommendations to improve plan efficacy and employee participation.
"How America Saves" is the industry's definitive report on American's retirement savings habits. The 2021 edition details how automatic plan features and target-date funds helped millions of defined contribution plans participants adhere to their financial goals, even amid severe market volatility resulting from the coronavirus pandemic.
June 02, 2021
While the global economy recovers from the pandemic, central bank policy rates are expected to lift off starting in 2023 as quantitative easing unwinds. But how will that affect bond yields? Vanguard's model suggests that long-term government bond yields will increase only modestly as ultra-accommodative monetary policy normalizes.
June 01, 2021
Critical to constructing a portfolio for a specific goal is selecting a combination of assets that offers the best chance of meeting that objective. This paper reviews the decisions investors face when constructing a globally diversified portfolio and discusses the importance of broad asset allocation and diversification within sub-asset classes.
May 28, 2021
Although one-third of participants in Vanguard defined contribution plans had access to a self-directed brokerage option in 2020, only 1 percent of those participants chose to use it. On average, brokerage participants invest half of their account balance in the brokerage option.
April 19, 2021
The past ten years have been tremendous for growth stocks, but we do not expect that trend to continue. This research presents the results of our fair value model for the ratio of value to growth stocks. These results suggest that value will outperform growth over the next ten years by as much as the equity risk premium.
April 12, 2021
Researchers and policymakers have suggested that annuities can help retirees manage the risk of outliving their assets. Vanguard research finds, however, that most retirees are reluctant to annuitize and decide against exchanging liquid wealth for guaranteed income in order to retain future financial flexibility.
May 24, 2019
In this paper, Vanguard researchers examine investing behavior in 1.8 million 529 plan accounts holding $53 billion in assets. The findings: Accounts using automatic investment methods exhibit the most consistent savings behavior, and those using age-based allocations have substantially less portfolio risk than self-directed ones.
October 11, 2018
Technology advances have spawned fear that they eliminate jobs. New Vanguard research finds more room for optimism.
February 19, 2019
ETFs can be useful tools for investors building their portfolios. A Vanguard commentary explores their role in portfolio construction and in providing market liquidity and market information.
June 23, 2022
A new Megatrends paper explores equilibrium interest rates, the forces that shape them, and where they may be headed.
June 16, 2022
Our experts provide insights into who has retired during the pandemic and whether these retirees might need to return to the workforce.
June 28, 2022
Recent Vanguard research indicates that gender diversity on a fund’s investment team may offer performance benefits. The findings underscore the benefit of evaluating the diversity of an investment management team with the same rigor we do portfolio diversification, recognizing the meaningful impact that both can have on fund performance.
July 06, 2022
Even the most groundbreaking technological innovations can experience a lag between discovery and the implementation of a commercial application. That lag—along with current social, economic, and technological conditions—can have vast implications on the broader economy.
July 13, 2022
We summarize in two pages the conditions of five major economic blocs and provide 10-year return forecasts for major asset classes.
July 27, 2022
A Vanguard white paper looks at ways to tailor portfolios to address specific investor goals and preferences.
March 29, 2019
This paper presents a methodology for estimating the trading volume on U.S. exchanges attributable to index funds, index ETFs, and other indexing strategies. Vanguard researchers estimate that index funds and index ETFs currently account for approximately 1% of overall trading volume on U.S. exchanges, well below the more widely quoted 5% to 7%.
August 04, 2022
Using four hypothetical case studies, a new Vanguard research paper, The Value of Personalized Advice , provides insights into how to think about and measure the value of financial advice for individual clients.
September 14, 2022
Regulation, fee, and technology trends in the advice industry may get all the attention, but it’s still soft skills and relationships that will distinguish advisors from others. Learn more from the latest in the Vanguard Advisor’s Alpha series.
September 15, 2022
A new Vanguard research paper sheds light on how investors’ health state can affect their asset allocation and consumption in retirement.
November 09, 2022
Vanguard Financial Advice Model (VFAM) is a new approach to financial planning, assessing combinations of strategies and their relative value for an investor.
November 08, 2022
A new Vanguard research paper outlines an approach to determining life insurance needs that could save thousands of dollars while minimizing the chances that coverage falls short of those needs.
October 31, 2022
A recently updated research paper makes the investment case for Vanguard target-date funds and offers insights into the role these strategies can play in the portfolios of different types of investors.
October 27, 2022
As direct indexing accelerates in popularity among investors, one of its measurable benefits is the ability to implement a systematic tax-loss harvesting (TLH) strategy. Vanguard researchers examine the value of different modes of TLH for direct indexing investors to determine which is the most effective. The paper was published in The Journal of Beta Investment Strategies .
October 24, 2022
Our research shows that an annual rebalancing is optimal for investors who don’t participate in tax-loss harvesting or where maintaining tight tracking to the multiasset benchmark portfolio is not a concern.
October 06, 2022
Understanding your health care priorities and available coverage types can help you choose a Medicare coverage option, according to Vanguard researchers.
September 22, 2022
Americans donated $471 billion to charitable organizations in 2020. Vanguard research explores the three key elements of a successful giving plan: When to give, what to give, and how to give.
June 06, 2019
Our Megatrends series examines fundamental shifts in the global economy likely to affect the financial services industry and society. In this edition, Vanguard explores the economic implications of an unprecedented shift in the age of global populations toward a smaller young-age cohort and a larger old-age cohort.
January 31, 2018
In this article published in The Journal of Portfolio Management in 2018, Vanguard researchers describe an enhancement to a standard approach of forecasting U.S. stock market returns. Starting with the cyclically adjusted price-to-earnings (CAPE) ratio, they incorporate bond yields, inflation, and volatility into the calculations. The authors find that results from this “fair-value” CAPE forecasting model, while not perfect, are about 40%–50% more accurate than conventional methods for 10-year-ahead stock market forecasts since 1960.
August 14, 2023
Key components for efficient tax savings: threshold planning, capital gains, income exclusions, and deductions.
July 27, 2023
Our updated research examines saving and investing behavior in 1.9 million 529 plan education savings accounts.
July 05, 2023
Updates are provided on our 2023 outlook for growth, inflation, and central bank policies, plus 10-year asset class return forecasts.
June 15, 2023
In its 22nd edition, How America Saves provides an in-depth examination of the retirement plan data of nearly 5 million defined contribution plan participants.
May 16, 2023
Learn how our target enrollment glidepath can help maximize risk-adjusted value for investors saving for college.
May 12, 2023
Our research explores practical ways to build savings for an emergency while balancing long-term financial goals.
April 28, 2023
Selectively adding a credit tilt to the bond portion of a portfolio can enhance long-term expected returns.
April 04, 2023
This paper highlights some of the generational differences we have observed in our recordkeeping data over a 15-year period as a result of automatic solutions. We answer the question: How have generational patterns of saving and investing changed in DC plans?
March 28, 2023
Our research offers a framework to help maximize wealth by taking advantage of your investment options alongside repaying debt.
March 01, 2023
This paper compares the performance of cost averaging with lump-sum investing as strategies for investing cash.
February 27, 2023
Relocating to a cheaper housing market can help retirees bolster retirement readiness, Vanguard research finds.
February 15, 2023
Our research considers ways to measure inflation based on the specific circumstances of an individual and how to build portfolios that seek to minimize the risks of rising costs.
February 01, 2023
A small commodities position may improve an investor’s outcome while adding resilience to a portfolio.
January 31, 2023
Best way to hedge a portfolio against inflation? Research suggests a method but not without its own risks.
January 23, 2023
Pension plan sponsors considering a risk transfer should look beyond immediate benefits and consider the impact on the management of the remainder of the plan, according to Vanguard experts.
January 09, 2023
The prospect of saving for long-term financial planning goals can be overwhelming at times given its enormity and uncertainty. Vanguard’s guide to financial wellness offers a blueprint for achieving financial flexibility to meet both short- and long-term goals.
December 13, 2022
Studies of ESG investment strategies have tried to identify an ESG-related factor to explain performance, but Vanguard research reveals that the performance of many ESG investment strategies is largely driven by well-known traditional sources of risk. We found quite a bit of variability in the exposure to and influence of the factors explored.
December 12, 2022
Our base case for 2023 includes a global recession brought about by policymakers’ efforts to control inflation. Unemployment is likely to rise and consumer demand weaken, but central banks may still miss their targets of 2% inflation in 2023.
November 30, 2022
Sustainable withdrawal rates can vary considerably, depending on the levels of desired bequests, portfolio depletion risk, and asset allocation, highlighting the need for customization rather than relying on a single rule of thumb. Learn more about the feasible ranges.
November 21, 2022
There’s no place like home, even when it comes to stock investing. Recent Vanguard research discusses investor local bias, what that disposition means for a portfolio, and how a few small tweaks can alleviate a portfolio’s concentration risk.
November 16, 2022
Time-varying asset allocation can help build diversified, tax-efficient portfolios, Vanguard researchers say.
October 23, 2020
What is the value of tax-loss harvesting? Examining more than 80,000 investors, Vanguard analysts demonstrate that outcomes of equity-based tax-loss harvesting vary significantly across investor characteristics and market environments. The expected results range from no benefit or even negative returns to gains of more than 1% annually.
August 08, 2022
A new Vanguard research paper, Revisiting the conventional wisdom regarding asset location , takes a second look at best practices for asset location and whether they are appropriate for investors.
March 11, 2021
The Vanguard Life-Cycle Investing Model is a proprietary model for glide-path construction that can assist in the creation of custom investment portfolios for retirement and nonretirement goals. This paper describes the model, the benefits of a customizable, goals-based approach, and practical applications in product design and advice methodology.
April 10, 2024
While holding cash can provide a sense of security, it can come at a cost.
January 05, 2024
Our research into years of daily stock-level data suggests that U.S. equities are resilient to periods of volatility and that liquidity remains available under all market conditions.
December 12, 2023
A return to sound money, with interest rates above inflation, is the best financial market development in 20 years.
November 17, 2023
In this paper, Vanguard espouses four simple and enduring principles to help investors achieve long-term success. Goals: Create clear, appropriate investment goals. Balance: Keep a balanced and diversified mix of investments. Costs: Minimize costs. Discipline: Maintain perspective and long-term discipline.
November 15, 2023
Our research looks at the role factors can play in shaping a portfolio’s performance and risk profile.
November 01, 2023
Vanguard research considers investors who may benefit from target portfolios using valuation-aware, time-varying return forecasts.
October 20, 2023
We consider the “loss harvest profile” of direct indexing over time, focusing on volatility and cash contributions.
October 13, 2023
Vanguard research shows how to optimize after-tax returns through placement of equity subclasses across accounts.
October 03, 2023
A Vanguard analysis assesses retirement readiness for a nationally representative sample of American workers.
September 15, 2023
The benefits of HSAs aren’t limited to just covering medical expenses, according to updated Vanguard research.
September 06, 2023
Negative convexity has rejoined duration and credit as central considerations in active muni investing.
Vanguard is the trusted name in investing. Since our founding in 1975, we’ve put investors first.
- Open access
- Published: 28 August 2020
Short-term stock market price trend prediction using a comprehensive deep learning system
- Jingyi Shen 1 &
- M. Omair Shafiq ORCID: orcid.org/0000-0002-1859-8296 1
Journal of Big Data volume 7 , Article number: 66 ( 2020 ) Cite this article
266k Accesses
161 Citations
91 Altmetric
Metrics details
In the era of big data, deep learning for predicting stock market prices and trends has become even more popular than before. We collected 2 years of data from Chinese stock market and proposed a comprehensive customization of feature engineering and deep learning-based model for predicting price trend of stock markets. The proposed solution is comprehensive as it includes pre-processing of the stock market dataset, utilization of multiple feature engineering techniques, combined with a customized deep learning based system for stock market price trend prediction. We conducted comprehensive evaluations on frequently used machine learning models and conclude that our proposed solution outperforms due to the comprehensive feature engineering that we built. The system achieves overall high accuracy for stock market trend prediction. With the detailed design and evaluation of prediction term lengths, feature engineering, and data pre-processing methods, this work contributes to the stock analysis research community both in the financial and technical domains.
Introduction
Stock market is one of the major fields that investors are dedicated to, thus stock market price trend prediction is always a hot topic for researchers from both financial and technical domains. In this research, our objective is to build a state-of-art prediction model for price trend prediction, which focuses on short-term price trend prediction.
As concluded by Fama in [ 26 ], financial time series prediction is known to be a notoriously difficult task due to the generally accepted, semi-strong form of market efficiency and the high level of noise. Back in 2003, Wang et al. in [ 44 ] already applied artificial neural networks on stock market price prediction and focused on volume, as a specific feature of stock market. One of the key findings by them was that the volume was not found to be effective in improving the forecasting performance on the datasets they used, which was S&P 500 and DJI. Ince and Trafalis in [ 15 ] targeted short-term forecasting and applied support vector machine (SVM) model on the stock price prediction. Their main contribution is performing a comparison between multi-layer perceptron (MLP) and SVM then found that most of the scenarios SVM outperformed MLP, while the result was also affected by different trading strategies. In the meantime, researchers from financial domains were applying conventional statistical methods and signal processing techniques on analyzing stock market data.
The optimization techniques, such as principal component analysis (PCA) were also applied in short-term stock price prediction [ 22 ]. During the years, researchers are not only focused on stock price-related analysis but also tried to analyze stock market transactions such as volume burst risks, which expands the stock market analysis research domain broader and indicates this research domain still has high potential [ 39 ]. As the artificial intelligence techniques evolved in recent years, many proposed solutions attempted to combine machine learning and deep learning techniques based on previous approaches, and then proposed new metrics that serve as training features such as Liu and Wang [ 23 ]. This type of previous works belongs to the feature engineering domain and can be considered as the inspiration of feature extension ideas in our research. Liu et al. in [ 24 ] proposed a convolutional neural network (CNN) as well as a long short-term memory (LSTM) neural network based model to analyze different quantitative strategies in stock markets. The CNN serves for the stock selection strategy, automatically extracts features based on quantitative data, then follows an LSTM to preserve the time-series features for improving profits.
The latest work also proposes a similar hybrid neural network architecture, integrating a convolutional neural network with a bidirectional long short-term memory to predict the stock market index [ 4 ]. While the researchers frequently proposed different neural network solution architectures, it brought further discussions about the topic if the high cost of training such models is worth the result or not.
There are three key contributions of our work (1) a new dataset extracted and cleansed (2) a comprehensive feature engineering, and (3) a customized long short-term memory (LSTM) based deep learning model.
We have built the dataset by ourselves from the data source as an open-sourced data API called Tushare [ 43 ]. The novelty of our proposed solution is that we proposed a feature engineering along with a fine-tuned system instead of just an LSTM model only. We observe from the previous works and find the gaps and proposed a solution architecture with a comprehensive feature engineering procedure before training the prediction model. With the success of feature extension method collaborating with recursive feature elimination algorithms, it opens doors for many other machine learning algorithms to achieve high accuracy scores for short-term price trend prediction. It proved the effectiveness of our proposed feature extension as feature engineering. We further introduced our customized LSTM model and further improved the prediction scores in all the evaluation metrics. The proposed solution outperformed the machine learning and deep learning-based models in similar previous works.
The remainder of this paper is organized as follows. “ Survey of related works ” section describes the survey of related works. “ The dataset ” section provides details on the data that we extracted from the public data sources and the dataset prepared. “ Methods ” section presents the research problems, methods, and design of the proposed solution. Detailed technical design with algorithms and how the model implemented are also included in this section. “ Results ” section presents comprehensive results and evaluation of our proposed model, and by comparing it with the models used in most of the related works. “ Discussion ” section provides a discussion and comparison of the results. “ Conclusion ” section presents the conclusion. This research paper has been built based on Shen [ 36 ].
Survey of related works
In this section, we discuss related works. We reviewed the related work in two different domains: technical and financial, respectively.
Kim and Han in [ 19 ] built a model as a combination of artificial neural networks (ANN) and genetic algorithms (GAs) with discretization of features for predicting stock price index. The data used in their study include the technical indicators as well as the direction of change in the daily Korea stock price index (KOSPI). They used the data containing samples of 2928 trading days, ranging from January 1989 to December 1998, and give their selected features and formulas. They also applied optimization of feature discretization, as a technique that is similar to dimensionality reduction. The strengths of their work are that they introduced GA to optimize the ANN. First, the amount of input features and processing elements in the hidden layer are 12 and not adjustable. Another limitation is in the learning process of ANN, and the authors only focused on two factors in optimization. While they still believed that GA has great potential for feature discretization optimization. Our initialized feature pool refers to the selected features. Qiu and Song in [ 34 ] also presented a solution to predict the direction of the Japanese stock market based on an optimized artificial neural network model. In this work, authors utilize genetic algorithms together with artificial neural network based models, and name it as a hybrid GA-ANN model.
Piramuthu in [ 33 ] conducted a thorough evaluation of different feature selection methods for data mining applications. He used for datasets, which were credit approval data, loan defaults data, web traffic data, tam, and kiang data, and compared how different feature selection methods optimized decision tree performance. The feature selection methods he compared included probabilistic distance measure: the Bhattacharyya measure, the Matusita measure, the divergence measure, the Mahalanobis distance measure, and the Patrick-Fisher measure. For inter-class distance measures: the Minkowski distance measure, city block distance measure, Euclidean distance measure, the Chebychev distance measure, and the nonlinear (Parzen and hyper-spherical kernel) distance measure. The strength of this paper is that the author evaluated both probabilistic distance-based and several inter-class feature selection methods. Besides, the author performed the evaluation based on different datasets, which reinforced the strength of this paper. However, the evaluation algorithm was a decision tree only. We cannot conclude if the feature selection methods will still perform the same on a larger dataset or a more complex model.
Hassan and Nath in [ 9 ] applied the Hidden Markov Model (HMM) on the stock market forecasting on stock prices of four different Airlines. They reduce states of the model into four states: the opening price, closing price, the highest price, and the lowest price. The strong point of this paper is that the approach does not need expert knowledge to build a prediction model. While this work is limited within the industry of Airlines and evaluated on a very small dataset, it may not lead to a prediction model with generality. One of the approaches in stock market prediction related works could be exploited to do the comparison work. The authors selected a maximum 2 years as the date range of training and testing dataset, which provided us a date range reference for our evaluation part.
Lei in [ 21 ] exploited Wavelet Neural Network (WNN) to predict stock price trends. The author also applied Rough Set (RS) for attribute reduction as an optimization. Rough Set was utilized to reduce the stock price trend feature dimensions. It was also used to determine the structure of the Wavelet Neural Network. The dataset of this work consists of five well-known stock market indices, i.e., (1) SSE Composite Index (China), (2) CSI 300 Index (China), (3) All Ordinaries Index (Australian), (4) Nikkei 225 Index (Japan), and (5) Dow Jones Index (USA). Evaluation of the model was based on different stock market indices, and the result was convincing with generality. By using Rough Set for optimizing the feature dimension before processing reduces the computational complexity. However, the author only stressed the parameter adjustment in the discussion part but did not specify the weakness of the model itself. Meanwhile, we also found that the evaluations were performed on indices, the same model may not have the same performance if applied on a specific stock.
Lee in [ 20 ] used the support vector machine (SVM) along with a hybrid feature selection method to carry out prediction of stock trends. The dataset in this research is a sub dataset of NASDAQ Index in Taiwan Economic Journal Database (TEJD) in 2008. The feature selection part was using a hybrid method, supported sequential forward search (SSFS) played the role of the wrapper. Another advantage of this work is that they designed a detailed procedure of parameter adjustment with performance under different parameter values. The clear structure of the feature selection model is also heuristic to the primary stage of model structuring. One of the limitations was that the performance of SVM was compared to back-propagation neural network (BPNN) only and did not compare to the other machine learning algorithms.
Sirignano and Cont leveraged a deep learning solution trained on a universal feature set of financial markets in [ 40 ]. The dataset used included buy and sell records of all transactions, and cancellations of orders for approximately 1000 NASDAQ stocks through the order book of the stock exchange. The NN consists of three layers with LSTM units and a feed-forward layer with rectified linear units (ReLUs) at last, with stochastic gradient descent (SGD) algorithm as an optimization. Their universal model was able to generalize and cover the stocks other than the ones in the training data. Though they mentioned the advantages of a universal model, the training cost was still expensive. Meanwhile, due to the inexplicit programming of the deep learning algorithm, it is unclear that if there are useless features contaminated when feeding the data into the model. Authors found out that it would have been better if they performed feature selection part before training the model and found it as an effective way to reduce the computational complexity.
Ni et al. in [ 30 ] predicted stock price trends by exploiting SVM and performed fractal feature selection for optimization. The dataset they used is the Shanghai Stock Exchange Composite Index (SSECI), with 19 technical indicators as features. Before processing the data, they optimized the input data by performing feature selection. When finding the best parameter combination, they also used a grid search method, which is k cross-validation. Besides, the evaluation of different feature selection methods is also comprehensive. As the authors mentioned in their conclusion part, they only considered the technical indicators but not macro and micro factors in the financial domain. The source of datasets that the authors used was similar to our dataset, which makes their evaluation results useful to our research. They also mentioned a method called k cross-validation when testing hyper-parameter combinations.
McNally et al. in [ 27 ] leveraged RNN and LSTM on predicting the price of Bitcoin, optimized by using the Boruta algorithm for feature engineering part, and it works similarly to the random forest classifier. Besides feature selection, they also used Bayesian optimization to select LSTM parameters. The Bitcoin dataset ranged from the 19th of August 2013 to 19th of July 2016. Used multiple optimization methods to improve the performance of deep learning methods. The primary problem of their work is overfitting. The research problem of predicting Bitcoin price trend has some similarities with stock market price prediction. Hidden features and noises embedded in the price data are threats of this work. The authors treated the research question as a time sequence problem. The best part of this paper is the feature engineering and optimization part; we could replicate the methods they exploited in our data pre-processing.
Weng et al. in [ 45 ] focused on short-term stock price prediction by using ensemble methods of four well-known machine learning models. The dataset for this research is five sets of data. They obtained these datasets from three open-sourced APIs and an R package named TTR. The machine learning models they used are (1) neural network regression ensemble (NNRE), (2) a Random Forest with unpruned regression trees as base learners (RFR), (3) AdaBoost with unpruned regression trees as base learners (BRT) and (4) a support vector regression ensemble (SVRE). A thorough study of ensemble methods specified for short-term stock price prediction. With background knowledge, the authors selected eight technical indicators in this study then performed a thoughtful evaluation of five datasets. The primary contribution of this paper is that they developed a platform for investors using R, which does not need users to input their own data but call API to fetch the data from online source straightforward. From the research perspective, they only evaluated the prediction of the price for 1 up to 10 days ahead but did not evaluate longer terms than two trading weeks or a shorter term than 1 day. The primary limitation of their research was that they only analyzed 20 U.S.-based stocks, the model might not be generalized to other stock market or need further revalidation to see if it suffered from overfitting problems.
Kara et al. in [ 17 ] also exploited ANN and SVM in predicting the movement of stock price index. The data set they used covers a time period from January 2, 1997, to December 31, 2007, of the Istanbul Stock Exchange. The primary strength of this work is its detailed record of parameter adjustment procedures. While the weaknesses of this work are that neither the technical indicator nor the model structure has novelty, and the authors did not explain how their model performed better than other models in previous works. Thus, more validation works on other datasets would help. They explained how ANN and SVM work with stock market features, also recorded the parameter adjustment. The implementation part of our research could benefit from this previous work.
Jeon et al. in [ 16 ] performed research on millisecond interval-based big dataset by using pattern graph tracking to complete stock price prediction tasks. The dataset they used is a millisecond interval-based big dataset of historical stock data from KOSCOM, from August 2014 to October 2014, 10G–15G capacity. The author applied Euclidean distance, Dynamic Time Warping (DTW) for pattern recognition. For feature selection, they used stepwise regression. The authors completed the prediction task by ANN and Hadoop and RHive for big data processing. The “ Results ” section is based on the result processed by a combination of SAX and Jaro–Winkler distance. Before processing the data, they generated aggregated data at 5-min intervals from discrete data. The primary strength of this work is the explicit structure of the whole implementation procedure. While they exploited a relatively old model, another weakness is the overall time span of the training dataset is extremely short. It is difficult to access the millisecond interval-based data in real life, so the model is not as practical as a daily based data model.
Huang et al. in [ 12 ] applied a fuzzy-GA model to complete the stock selection task. They used the key stocks of the 200 largest market capitalization listed as the investment universe in the Taiwan Stock Exchange. Besides, the yearly financial statement data and the stock returns were taken from the Taiwan Economic Journal (TEJ) database at www.tej.com.tw/ for the time period from year 1995 to year 2009. They conducted the fuzzy membership function with model parameters optimized with GA and extracted features for optimizing stock scoring. The authors proposed an optimized model for selection and scoring of stocks. Different from the prediction model, the authors more focused on stock rankings, selection, and performance evaluation. Their structure is more practical among investors. But in the model validation part, they did not compare the model with existed algorithms but the statistics of the benchmark, which made it challenging to identify if GA would outperform other algorithms.
Fischer and Krauss in [ 5 ] applied long short-term memory (LSTM) on financial market prediction. The dataset they used is S&P 500 index constituents from Thomson Reuters. They obtained all month-end constituent lists for the S&P 500 from Dec 1989 to Sep 2015, then consolidated the lists into a binary matrix to eliminate survivor bias. The authors also used RMSprop as an optimizer, which is a mini-batch version of rprop. The primary strength of this work is that the authors used the latest deep learning technique to perform predictions. They relied on the LSTM technique, lack of background knowledge in the financial domain. Although the LSTM outperformed the standard DNN and logistic regression algorithms, while the author did not mention the effort to train an LSTM with long-time dependencies.
Tsai and Hsiao in [ 42 ] proposed a solution as a combination of different feature selection methods for prediction of stocks. They used Taiwan Economic Journal (TEJ) database as data source. The data used in their analysis was from year 2000 to 2007. In their work, they used a sliding window method and combined it with multi layer perceptron (MLP) based artificial neural networks with back propagation, as their prediction model. In their work, they also applied principal component analysis (PCA) for dimensionality reduction, genetic algorithms (GA) and the classification and regression trees (CART) to select important features. They did not just rely on technical indices only. Instead, they also included both fundamental and macroeconomic indices in their analysis. The authors also reported a comparison on feature selection methods. The validation part was done by combining the model performance stats with statistical analysis.
Pimenta et al. in [ 32 ] leveraged an automated investing method by using multi-objective genetic programming and applied it in the stock market. The dataset was obtained from Brazilian stock exchange market (BOVESPA), and the primary techniques they exploited were a combination of multi-objective optimization, genetic programming, and technical trading rules. For optimization, they leveraged genetic programming (GP) to optimize decision rules. The novelty of this paper was in the evaluation part. They included a historical period, which was a critical moment of Brazilian politics and economics when performing validation. This approach reinforced the generalization strength of their proposed model. When selecting the sub-dataset for evaluation, they also set criteria to ensure more asset liquidity. While the baseline of the comparison was too basic and fundamental, and the authors did not perform any comparison with other existing models.
Huang and Tsai in [ 13 ] conducted a filter-based feature selection assembled with a hybrid self-organizing feature map (SOFM) support vector regression (SVR) model to forecast Taiwan index futures (FITX) trend. They divided the training samples into clusters to marginally improve the training efficiency. The authors proposed a comprehensive model, which was a combination of two novel machine learning techniques in stock market analysis. Besides, the optimizer of feature selection was also applied before the data processing to improve the prediction accuracy and reduce the computational complexity of processing daily stock index data. Though they optimized the feature selection part and split the sample data into small clusters, it was already strenuous to train daily stock index data of this model. It would be difficult for this model to predict trading activities in shorter time intervals since the data volume would be increased drastically. Moreover, the evaluation is not strong enough since they set a single SVR model as a baseline, but did not compare the performance with other previous works, which caused difficulty for future researchers to identify the advantages of SOFM-SVR model why it outperforms other algorithms.
Thakur and Kumar in [ 41 ] also developed a hybrid financial trading support system by exploiting multi-category classifiers and random forest (RAF). They conducted their research on stock indices from NASDAQ, DOW JONES, S&P 500, NIFTY 50, and NIFTY BANK. The authors proposed a hybrid model combined random forest (RF) algorithms with a weighted multicategory generalized eigenvalue support vector machine (WMGEPSVM) to generate “Buy/Hold/Sell” signals. Before processing the data, they used Random Forest (RF) for feature pruning. The authors proposed a practical model designed for real-life investment activities, which could generate three basic signals for investors to refer to. They also performed a thorough comparison of related algorithms. While they did not mention the time and computational complexity of their works. Meanwhile, the unignorable issue of their work was the lack of financial domain knowledge background. The investors regard the indices data as one of the attributes but could not take the signal from indices to operate a specific stock straightforward.
Hsu in [ 11 ] assembled feature selection with a back propagation neural network (BNN) combined with genetic programming to predict the stock/futures price. The dataset in this research was obtained from Taiwan Stock Exchange Corporation (TWSE). The authors have introduced the description of the background knowledge in detail. While the weakness of their work is that it is a lack of data set description. This is a combination of the model proposed by other previous works. Though we did not see the novelty of this work, we can still conclude that the genetic programming (GP) algorithm is admitted in stock market research domain. To reinforce the validation strengths, it would be good to consider adding GP models into evaluation if the model is predicting a specific price.
Hafezi et al. in [ 7 ] built a bat-neural network multi-agent system (BN-NMAS) to predict stock price. The dataset was obtained from the Deutsche bundes-bank. They also applied the Bat algorithm (BA) for optimizing neural network weights. The authors illustrated their overall structure and logic of system design in clear flowcharts. While there were very few previous works that had performed on DAX data, it would be difficult to recognize if the model they proposed still has the generality if migrated on other datasets. The system design and feature selection logic are fascinating, which worth referring to. Their findings in optimization algorithms are also valuable for the research in the stock market price prediction research domain. It is worth trying the Bat algorithm (BA) when constructing neural network models.
Long et al. in [ 25 ] conducted a deep learning approach to predict the stock price movement. The dataset they used is the Chinese stock market index CSI 300. For predicting the stock price movement, they constructed a multi-filter neural network (MFNN) with stochastic gradient descent (SGD) and back propagation optimizer for learning NN parameters. The strength of this paper is that the authors exploited a novel model with a hybrid model constructed by different kinds of neural networks, it provides an inspiration for constructing hybrid neural network structures.
Atsalakis and Valavanis in [ 1 ] proposed a solution of a neuro-fuzzy system, which is composed of controller named as Adaptive Neuro Fuzzy Inference System (ANFIS), to achieve short-term stock price trend prediction. The noticeable strength of this work is the evaluation part. Not only did they compare their proposed system with the popular data models, but also compared with investment strategies. While the weakness that we found from their proposed solution is that their solution architecture is lack of optimization part, which might limit their model performance. Since our proposed solution is also focusing on short-term stock price trend prediction, this work is heuristic for our system design. Meanwhile, by comparing with the popular trading strategies from investors, their work inspired us to compare the strategies used by investors with techniques used by researchers.
Nekoeiqachkanloo et al. in [ 29 ] proposed a system with two different approaches for stock investment. The strengths of their proposed solution are obvious. First, it is a comprehensive system that consists of data pre-processing and two different algorithms to suggest the best investment portions. Second, the system also embedded with a forecasting component, which also retains the features of the time series. Last but not least, their input features are a mix of fundamental features and technical indices that aim to fill in the gap between the financial domain and technical domain. However, their work has a weakness in the evaluation part. Instead of evaluating the proposed system on a large dataset, they chose 25 well-known stocks. There is a high possibility that the well-known stocks might potentially share some common hidden features.
As another related latest work, Idrees et al. [ 14 ] published a time series-based prediction approach for the volatility of the stock market. ARIMA is not a new approach in the time series prediction research domain. Their work is more focusing on the feature engineering side. Before feeding the features into ARIMA models, they designed three steps for feature engineering: Analyze the time series, identify if the time series is stationary or not, perform estimation by plot ACF and PACF charts and look for parameters. The only weakness of their proposed solution is that the authors did not perform any customization on the existing ARIMA model, which might limit the system performance to be improved.
One of the main weaknesses found in the related works is limited data-preprocessing mechanisms built and used. Technical works mostly tend to focus on building prediction models. When they select the features, they list all the features mentioned in previous works and go through the feature selection algorithm then select the best-voted features. Related works in the investment domain have shown more interest in behavior analysis, such as how herding behaviors affect the stock performance, or how the percentage of inside directors hold the firm’s common stock affects the performance of a certain stock. These behaviors often need a pre-processing procedure of standard technical indices and investment experience to recognize.
In the related works, often a thorough statistical analysis is performed based on a special dataset and conclude new features rather than performing feature selections. Some data, such as the percentage of a certain index fluctuation has been proven to be effective on stock performance. We believe that by extracting new features from data, then combining such features with existed common technical indices will significantly benefit the existing and well-tested prediction models.
The dataset
This section details the data that was extracted from the public data sources, and the final dataset that was prepared. Stock market-related data are diverse, so we first compared the related works from the survey of financial research works in stock market data analysis to specify the data collection directions. After collecting the data, we defined a data structure of the dataset. Given below, we describe the dataset in detail, including the data structure, and data tables in each category of data with the segment definitions.
Description of our dataset
In this section, we will describe the dataset in detail. This dataset consists of 3558 stocks from the Chinese stock market. Besides the daily price data, daily fundamental data of each stock ID, we also collected the suspending and resuming history, top 10 shareholders, etc. We list two reasons that we choose 2 years as the time span of this dataset: (1) most of the investors perform stock market price trend analysis using the data within the latest 2 years, (2) using more recent data would benefit the analysis result. We collected data through the open-sourced API, namely Tushare [ 43 ], mean-while we also leveraged a web-scraping technique to collect data from Sina Finance web pages, SWS Research website.
Data structure
Figure 1 illustrates all the data tables in the dataset. We collected four categories of data in this dataset: (1) basic data, (2) trading data, (3) finance data, and (4) other reference data. All the data tables can be linked to each other by a common field called “Stock ID” It is a unique stock identifier registered in the Chinese Stock market. Table 1 shows an overview of the dataset.
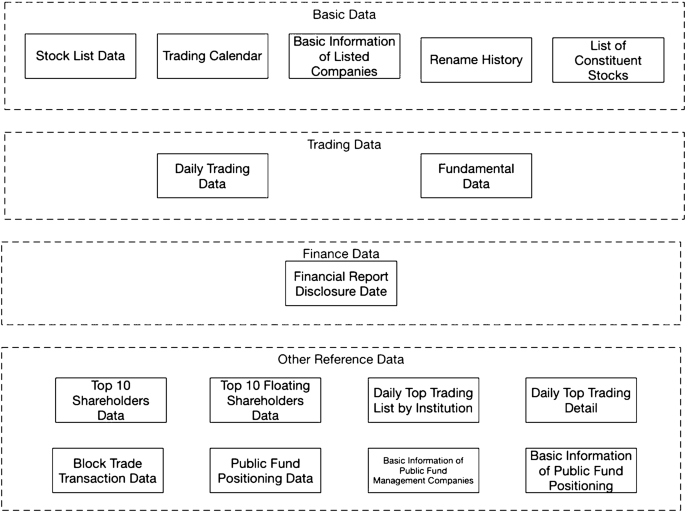
Data structure for the extracted dataset
The Table 1 lists the field information of each data table as well as which category the data table belongs to.
In this section, we present the proposed methods and the design of the proposed solution. Moreover, we also introduce the architecture design as well as algorithmic and implementation details.
Problem statement
We analyzed the best possible approach for predicting short-term price trends from different aspects: feature engineering, financial domain knowledge, and prediction algorithm. Then we addressed three research questions in each aspect, respectively: How can feature engineering benefit model prediction accuracy? How do findings from the financial domain benefit prediction model design? And what is the best algorithm for predicting short-term price trends?
The first research question is about feature engineering. We would like to know how the feature selection method benefits the performance of prediction models. From the abundance of the previous works, we can conclude that stock price data embedded with a high level of noise, and there are also correlations between features, which makes the price prediction notoriously difficult. That is also the primary reason for most of the previous works introduced the feature engineering part as an optimization module.
The second research question is evaluating the effectiveness of findings we extracted from the financial domain. Different from the previous works, besides the common evaluation of data models such as the training costs and scores, our evaluation will emphasize the effectiveness of newly added features that we extracted from the financial domain. We introduce some features from the financial domain. While we only obtained some specific findings from previous works, and the related raw data needs to be processed into usable features. After extracting related features from the financial domain, we combine the features with other common technical indices for voting out the features with a higher impact. There are numerous features said to be effective from the financial domain, and it would be impossible for us to cover all of them. Thus, how to appropriately convert the findings from the financial domain to a data processing module of our system design is a hidden research question that we attempt to answer.
The third research question is that which algorithms are we going to model our data? From the previous works, researchers have been putting efforts into the exact price prediction. We decompose the problem into predicting the trend and then the exact number. This paper focuses on the first step. Hence, the objective has been converted to resolve a binary classification problem, meanwhile, finding an effective way to eliminate the negative effect brought by the high level of noise. Our approach is to decompose the complex problem into sub-problems which have fewer dependencies and resolve them one by one, and then compile the resolutions into an ensemble model as an aiding system for investing behavior reference.
In the previous works, researchers have been using a variety of models for predicting stock price trends. While most of the best-performed models are based on machine learning techniques, in this work, we will compare our approach with the outperformed machine learning models in the evaluation part and find the solution for this research question.
Proposed solution
The high-level architecture of our proposed solution could be separated into three parts. First is the feature selection part, to guarantee the selected features are highly effective. Second, we look into the data and perform the dimensionality reduction. And the last part, which is the main contribution of our work is to build a prediction model of target stocks. Figure 2 depicts a high-level architecture of the proposed solution.
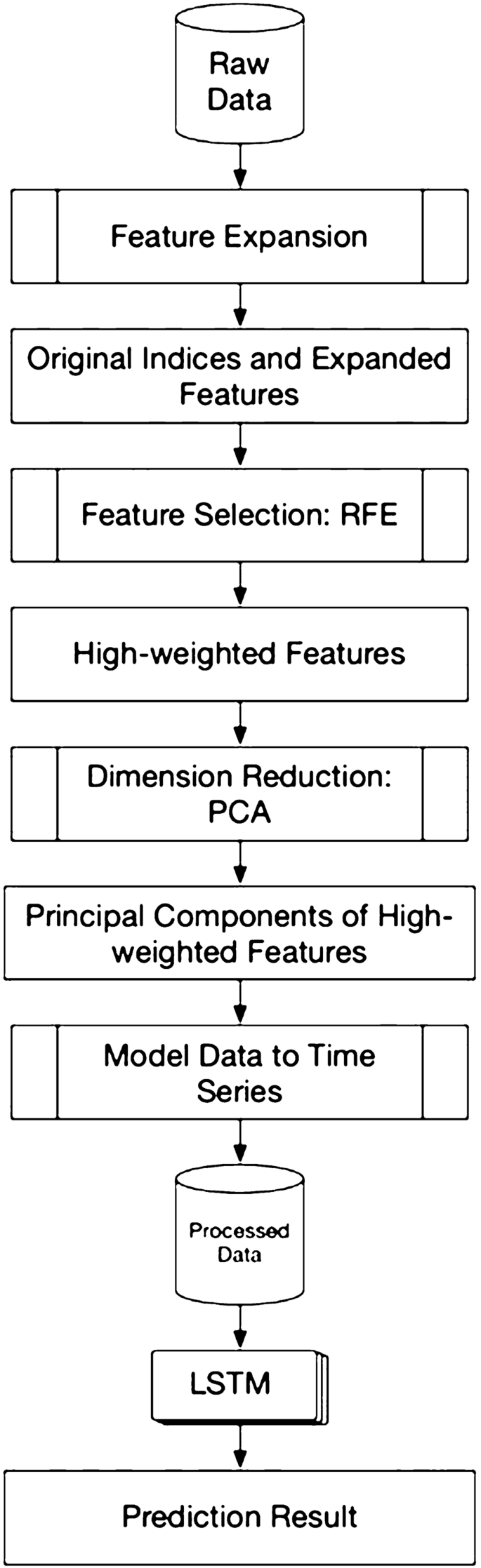
High-level architecture of the proposed solution
There are ways to classify different categories of stocks. Some investors prefer long-term investments, while others show more interest in short-term investments. It is common to see the stock-related reports showing an average performance, while the stock price is increasing drastically; this is one of the phenomena that indicate the stock price prediction has no fixed rules, thus finding effective features before training a model on data is necessary.
In this research, we focus on the short-term price trend prediction. Currently, we only have the raw data with no labels. So, the very first step is to label the data. We mark the price trend by comparing the current closing price with the closing price of n trading days ago, the range of n is from 1 to 10 since our research is focusing on the short-term. If the price trend goes up, we mark it as 1 or mark as 0 in the opposite case. To be more specified, we use the indices from the indices of n − 1 th day to predict the price trend of the n th day.
According to the previous works, some researchers who applied both financial domain knowledge and technical methods on stock data were using rules to filter the high-quality stocks. We referred to their works and exploited their rules to contribute to our feature extension design.
However, to ensure the best performance of the prediction model, we will look into the data first. There are a large number of features in the raw data; if we involve all the features into our consideration, it will not only drastically increase the computational complexity but will also cause side effects if we would like to perform unsupervised learning in further research. So, we leverage the recursive feature elimination (RFE) to ensure all the selected features are effective.
We found most of the previous works in the technical domain were analyzing all the stocks, while in the financial domain, researchers prefer to analyze the specific scenario of investment, to fill the gap between the two domains, we decide to apply a feature extension based on the findings we gathered from the financial domain before we start the RFE procedure.
Since we plan to model the data into time series, the number of the features, the more complex the training procedure will be. So, we will leverage the dimensionality reduction by using randomized PCA at the beginning of our proposed solution architecture.
Detailed technical design elaboration
This section provides an elaboration of the detailed technical design as being a comprehensive solution based on utilizing, combining, and customizing several existing data preprocessing, feature engineering, and deep learning techniques. Figure 3 provides the detailed technical design from data processing to prediction, including the data exploration. We split the content by main procedures, and each procedure contains algorithmic steps. Algorithmic details are elaborated in the next section. The contents of this section will focus on illustrating the data workflow.
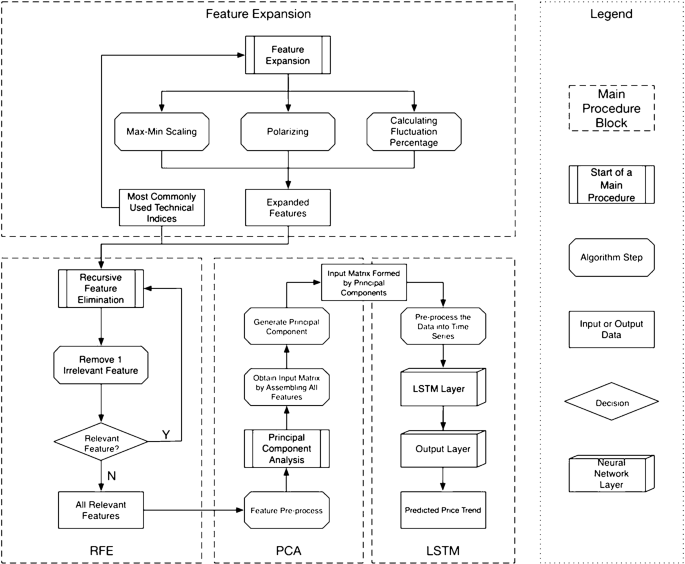
Detailed technical design of the proposed solution
Based on the literature review, we select the most commonly used technical indices and then feed them into the feature extension procedure to get the expanded feature set. We will select the most effective i features from the expanded feature set. Then we will feed the data with i selected features into the PCA algorithm to reduce the dimension into j features. After we get the best combination of i and j , we process the data into finalized the feature set and feed them into the LSTM [ 10 ] model to get the price trend prediction result.
The novelty of our proposed solution is that we will not only apply the technical method on raw data but also carry out the feature extensions that are used among stock market investors. Details on feature extension are given in the next subsection. Experiences gained from applying and optimizing deep learning based solutions in [ 37 , 38 ] were taken into account while designing and customizing feature engineering and deep learning solution in this work.
Applying feature extension
The first main procedure in Fig. 3 is the feature extension. In this block, the input data is the most commonly used technical indices concluded from related works. The three feature extension methods are max–min scaling, polarizing, and calculating fluctuation percentage. Not all the technical indices are applicable for all three of the feature extension methods; this procedure only applies the meaningful extension methods on technical indices. We choose meaningful extension methods while looking at how the indices are calculated. The technical indices and the corresponding feature extension methods are illustrated in Table 2 .
After the feature extension procedure, the expanded features will be combined with the most commonly used technical indices, i.e., input data with output data, and feed into RFE block as input data in the next step.
Applying recursive feature elimination
After the feature extension above, we explore the most effective i features by using the Recursive Feature Elimination (RFE) algorithm [ 6 ]. We estimate all the features by two attributes, coefficient, and feature importance. We also limit the features that remove from the pool by one, which means we will remove one feature at each step and retain all the relevant features. Then the output of the RFE block will be the input of the next step, which refers to PCA.
Applying principal component analysis (PCA)
The very first step before leveraging PCA is feature pre-processing. Because some of the features after RFE are percentage data, while others are very large numbers, i.e., the output from RFE are in different units. It will affect the principal component extraction result. Thus, before feeding the data into the PCA algorithm [ 8 ], a feature pre-processing is necessary. We also illustrate the effectiveness and methods comparison in “ Results ” section.
After performing feature pre-processing, the next step is to feed the processed data with selected i features into the PCA algorithm to reduce the feature matrix scale into j features. This step is to retain as many effective features as possible and meanwhile eliminate the computational complexity of training the model. This research work also evaluates the best combination of i and j, which has relatively better prediction accuracy, meanwhile, cuts the computational consumption. The result can be found in the “ Results ” section, as well. After the PCA step, the system will get a reshaped matrix with j columns.
Fitting long short-term memory (LSTM) model
PCA reduced the dimensions of the input data, while the data pre-processing is mandatory before feeding the data into the LSTM layer. The reason for adding the data pre-processing step before the LSTM model is that the input matrix formed by principal components has no time steps. While one of the most important parameters of training an LSTM is the number of time steps. Hence, we have to model the matrix into corresponding time steps for both training and testing dataset.
After performing the data pre-processing part, the last step is to feed the training data into LSTM and evaluate the performance using testing data. As a variant neural network of RNN, even with one LSTM layer, the NN structure is still a deep neural network since it can process sequential data and memorizes its hidden states through time. An LSTM layer is composed of one or more LSTM units, and an LSTM unit consists of cells and gates to perform classification and prediction based on time series data.
The LSTM structure is formed by two layers. The input dimension is determined by j after the PCA algorithm. The first layer is the input LSTM layer, and the second layer is the output layer. The final output will be 0 or 1 indicates if the stock price trend prediction result is going down or going up, as a supporting suggestion for the investors to perform the next investment decision.
Design discussion
Feature extension is one of the novelties of our proposed price trend predicting system. In the feature extension procedure, we use technical indices to collaborate with the heuristic processing methods learned from investors, which fills the gap between the financial research area and technical research area.
Since we proposed a system of price trend prediction, feature engineering is extremely important to the final prediction result. Not only the feature extension method is helpful to guarantee we do not miss the potentially correlated feature, but also feature selection method is necessary for pooling the effective features. The more irrelevant features are fed into the model, the more noise would be introduced. Each main procedure is carefully considered contributing to the whole system design.
Besides the feature engineering part, we also leverage LSTM, the state-of-the-art deep learning method for time-series prediction, which guarantees the prediction model can capture both complex hidden pattern and the time-series related pattern.
It is known that the training cost of deep learning models is expansive in both time and hardware aspects; another advantage of our system design is the optimization procedure—PCA. It can retain the principal components of the features while reducing the scale of the feature matrix, thus help the system to save the training cost of processing the large time-series feature matrix.
Algorithm elaboration
This section provides comprehensive details on the algorithms we built while utilizing and customizing different existing techniques. Details about the terminologies, parameters, as well as optimizers. From the legend on the right side of Fig. 3 , we note the algorithm steps as octagons, all of them can be found in this “ Algorithm elaboration ” section.
Before dive deep into the algorithm steps, here is the brief introduction of data pre-processing: since we will go through the supervised learning algorithms, we also need to program the ground truth. The ground truth of this research is programmed by comparing the closing price of the current trading date with the closing price of the previous trading date the users want to compare with. Label the price increase as 1, else the ground truth will be labeled as 0. Because this research work is not only focused on predicting the price trend of a specific period of time but short-term in general, the ground truth processing is according to a range of trading days. While the algorithms will not change with the prediction term length, we can regard the term length as a parameter.
The algorithmic detail is elaborated, respectively, the first algorithm is the hybrid feature engineering part for preparing high-quality training and testing data. It corresponds to the Feature extension, RFE, and PCA blocks in Fig. 3 . The second algorithm is the LSTM procedure block, including time-series data pre-processing, NN constructing, training, and testing.
Algorithm 1: Short-term stock market price trend prediction—applying feature engineering using FE + RFE + PCA
The function FE is corresponding to the feature extension block. For the feature extension procedure, we apply three different processing methods to translate the findings from the financial domain to a technical module in our system design. While not all the indices are applicable for expanding, we only choose the proper method(s) for certain features to perform the feature extension (FE), according to Table 2 .
Normalize method preserves the relative frequencies of the terms, and transform the technical indices into the range of [0, 1]. Polarize is a well-known method often used by real-world investors, sometimes they prefer to consider if the technical index value is above or below zero, we program some of the features using polarize method and prepare for RFE. Max-min (or min-max) [ 35 ] scaling is a transformation method often used as an alternative to zero mean and unit variance scaling. Another well-known method used is fluctuation percentage, and we transform the technical indices fluctuation percentage into the range of [− 1, 1].
The function RFE () in the first algorithm refers to recursive feature elimination. Before we perform the training data scale reduction, we will have to make sure that the features we selected are effective. Ineffective features will not only drag down the classification precision but also add more computational complexity. For the feature selection part, we choose recursive feature elimination (RFE). As [ 45 ] explained, the process of recursive feature elimination can be split into the ranking algorithm, resampling, and external validation.
For the ranking algorithm, it fits the model to the features and ranks by the importance to the model. We set the parameter to retain i numbers of features, and at each iteration of feature selection retains Si top-ranked features, then refit the model and assess the performance again to begin another iteration. The ranking algorithm will eventually determine the top Si features.
The RFE algorithm is known to have suffered from the over-fitting problem. To eliminate the over-fitting issue, we will run the RFE algorithm multiple times on randomly selected stocks as the training set and ensure all the features we select are high-weighted. This procedure is called data resampling. Resampling can be built as an optimization step as an outer layer of the RFE algorithm.
The last part of our hybrid feature engineering algorithm is for optimization purposes. For the training data matrix scale reduction, we apply Randomized principal component analysis (PCA) [ 31 ], before we decide the features of the classification model.
Financial ratios of a listed company are used to present the growth ability, earning ability, solvency ability, etc. Each financial ratio consists of a set of technical indices, each time we add a technical index (or feature) will add another column of data into the data matrix and will result in low training efficiency and redundancy. If non-relevant or less relevant features are included in training data, it will also decrease the precision of classification.
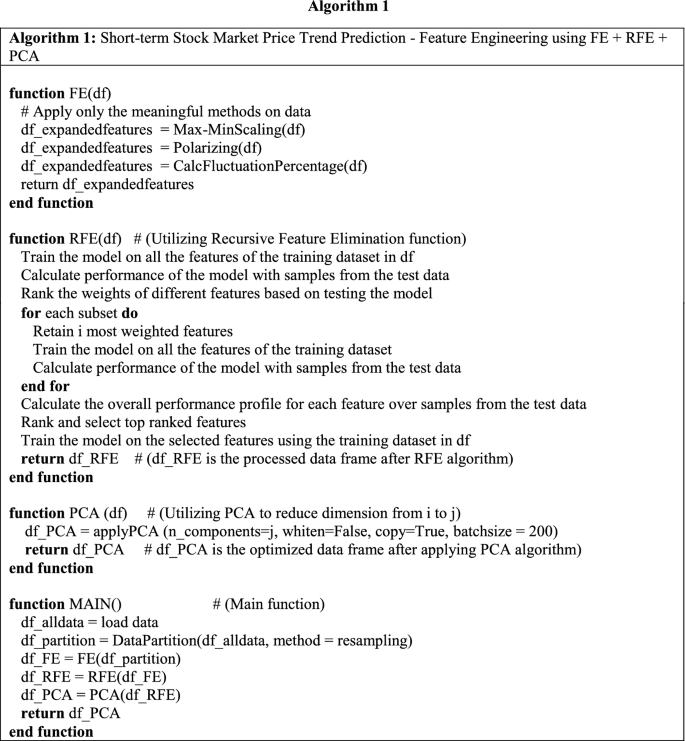
The above equation represents the explanation power of principal components extracted by PCA method for original data. If an ACR is below 85%, the PCA method would be unsuitable due to a loss of original information. Because the covariance matrix is sensitive to the order of magnitudes of data, there should be a data standardize procedure before performing the PCA. The commonly used standardized methods are mean-standardization and normal-standardization and are noted as given below:
Mean-standardization: \(X_{ij}^{*} = X_{ij} /\overline{{X_{j} }}\) , which \(\overline{{X_{j} }}\) represents the mean value.
Normal-standardization: \(X_{ij}^{*} = (X_{ij} - \overline{{X_{j} }} )/s_{j}\) , which \(\overline{{X_{j} }}\) represents the mean value, and \(s_{j}\) is the standard deviation.
The array fe_array is defined according to Table 2 , row number maps to the features, columns 0, 1, 2, 3 note for the extension methods of normalize, polarize, max–min scale, and fluctuation percentage, respectively. Then we fill in the values for the array by the rule where 0 stands for no necessity to expand and 1 for features need to apply the corresponding extension methods. The final algorithm of data preprocessing using RFE and PCA can be illustrated as Algorithm 1.
Algorithm 2: Price trend prediction model using LSTM
After the principal component extraction, we will get the scale-reduced matrix, which means i most effective features are converted into j principal components for training the prediction model. We utilized an LSTM model and added a conversion procedure for our stock price dataset. The detailed algorithm design is illustrated in Alg 2. The function TimeSeriesConversion () converts the principal components matrix into time series by shifting the input data frame according to the number of time steps [ 3 ], i.e., term length in this research. The processed dataset consists of the input sequence and forecast sequence. In this research, the parameter of LAG is 1, because the model is detecting the pattern of features fluctuation on a daily basis. Meanwhile, the N_TIME_STEPS is varied from 1 trading day to 10 trading days. The functions DataPartition (), FitModel (), EvaluateModel () are regular steps without customization. The NN structure design, optimizer decision, and other parameters are illustrated in function ModelCompile () .
Some procedures impact the efficiency but do not affect the accuracy or precision and vice versa, while other procedures may affect both efficiency and prediction result. To fully evaluate our algorithm design, we structure the evaluation part by main procedures and evaluate how each procedure affects the algorithm performance. First, we evaluated our solution on a machine with 2.2 GHz i7 processor, with 16 GB of RAM. Furthermore, we also evaluated our solution on Amazon EC2 instance, 3.1 GHz Processor with 16 vCPUs, and 64 GB RAM.
In the implementation part, we expanded 20 features into 54 features, while we retain 30 features that are the most effective. In this section, we discuss the evaluation of feature selection. The dataset was divided into two different subsets, i.e., training and testing datasets. Test procedure included two parts, one testing dataset is for feature selection, and another one is for model testing. We note the feature selection dataset and model testing dataset as DS_test_f and DS_test_m, respectively.
We randomly selected two-thirds of the stock data by stock ID for RFE training and note the dataset as DS_train_f; all the data consist of full technical indices and expanded features throughout 2018. The estimator of the RFE algorithm is SVR with linear kernels. We rank the 54 features by voting and get 30 effective features then process them using the PCA algorithm to perform dimension reduction and reduce the features into 20 principal components. The rest of the stock data forms the testing dataset DS_test_f to validate the effectiveness of principal components we extracted from selected features. We reformed all the data from 2018 as the training dataset of the data model and noted as DS_train_m. The model testing dataset DS_test_m consists of the first 3 months of data in 2019, which has no overlap with the dataset we utilized in the previous steps. This approach is to prevent the hidden problem caused by overfitting.
Term length
To build an efficient prediction model, instead of the approach of modeling the data to time series, we determined to use 1 day ahead indices data to predict the price trend of the next day. We tested the RFE algorithm on a range of short-term from 1 day to 2 weeks (ten trading days), to evaluate how the commonly used technical indices correlated to price trends. For evaluating the prediction term length, we fully expanded the features as Table 2 , and feed them to RFE. During the test, we found that different length of the term has a different level of sensitive-ness to the same indices set.
We get the close price of the first trading date and compare it with the close price of the n _ th trading date. Since we are predicting the price trend, we do not consider the term lengths if the cross-validation score is below 0.5. And after the test, as we can see from Fig. 4 , there are three-term lengths that are most sensitive to the indices we selected from the related works. They are n = {2, 5, 10}, which indicates that price trend prediction of every other day, 1 week, and 2 weeks using the indices set are likely to be more reliable.
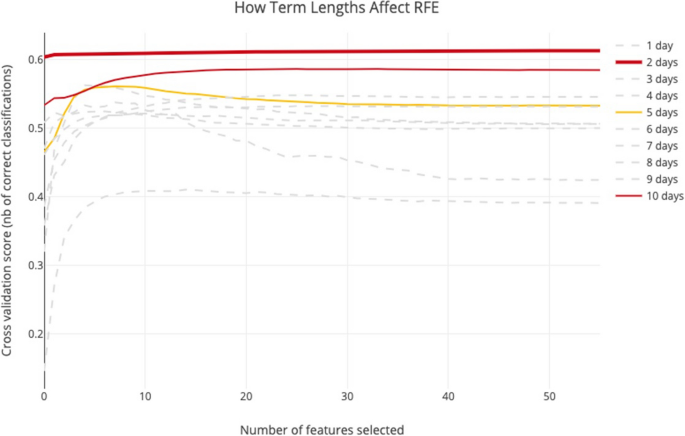
How do term lengths affect the cross-validation score of RFE
While these curves have different patterns, for the length of 2 weeks, the cross-validation score increases with the number of features selected. If the prediction term length is 1 week, the cross-validation score will decrease if selected over 8 features. For every other day price trend prediction, the best cross-validation score is achieved by selecting 48 features. Biweekly prediction requires 29 features to achieve the best score. In Table 3 , we listed the top 15 effective features for these three-period lengths. If we predict the price trend of every other day, the cross-validation score merely fluctuates with the number of features selected. So, in the next step, we will evaluate the RFE result for these three-term lengths, as shown in Fig. 4 .
We compare the output feature set of RFE with the all-original feature set as a baseline, the all-original feature set consists of n features and we choose n most effective features from RFE output features to evaluate the result using linear SVR. We used two different approaches to evaluate feature effectiveness. The first method is to combine all the data into one large matrix and evaluate them by running the RFE algorithm once. Another method is to run RFE for each individual stock and calculate the most effective features by voting.
Feature extension and RFE
From the result of the previous subsection, we can see that when predicting the price trend for every other day or biweekly, the best result is achieved by selecting a large number of features. Within the selected features, some features processed from extension methods have better ranks than original features, which proves that the feature extension method is useful for optimizing the model. The feature extension affects both precision and efficiency, while in this part, we only discuss the precision aspect and leave efficiency part in the next step since PCA is the most effective method for training efficiency optimization in our design. We involved an evaluation of how feature extension affects RFE and use the test result to measure the improvement of involving feature extension.
We further test the effectiveness of feature extension, i.e., if polarize, max–min scale, and calculate fluctuation percentage works better than original technical indices. The best case to leverage this test is the weekly prediction since it has the least effective feature selected. From the result we got from the last section, we know the best cross-validation score appears when selecting 8 features. The test consists of two steps, and the first step is to test the feature set formed by original features only, in this case, only SLOWK, SLOWD, and RSI_5 are included. The next step is to test the feature set of all 8 features we selected in the previous subsection. We leveraged the test by defining the simplest DNN model with three layers.
The normalized confusion matrix of testing the two feature sets are illustrated in Fig. 5 . The left one is the confusion matrix of the feature set with expanded features, and the right one besides is the test result of using original features only. Both precisions of true positive and true negative have been improved by 7% and 10%, respectively, which proves that our feature extension method design is reasonably effective.
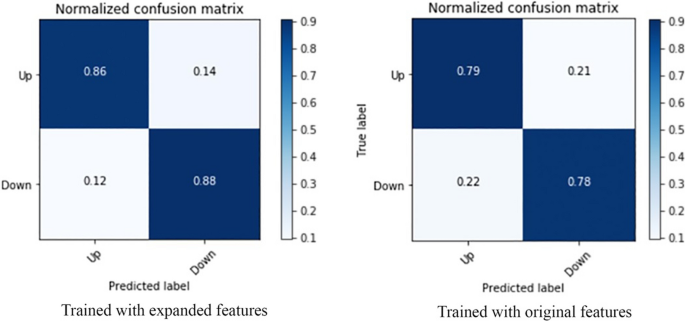
Confusion matrix of validating feature extension effectiveness
Feature reduction using principal component analysis
PCA will affect the algorithm performance on both prediction accuracy and training efficiency, while this part should be evaluated with the NN model, so we also defined the simplest DNN model with three layers as we used in the previous step to perform the evaluation. This part introduces the evaluation method and result of the optimization part of the model from computational efficiency and accuracy impact perspectives.
In this section, we will choose bi-weekly prediction to perform a use case analysis, since it has a smoothly increasing cross-validation score curve, moreover, unlike every other day prediction, it has excluded more than 20 ineffective features already. In the first step, we select all 29 effective features and train the NN model without performing PCA. It creates a baseline of the accuracy and training time for comparison. To evaluate the accuracy and efficiency, we keep the number of the principal component as 5, 10, 15, 20, 25. Table 4 recorded how the number of features affects the model training efficiency, then uses the stack bar chart in Fig. 6 to illustrate how PCA affects training efficiency. Table 6 shows accuracy and efficiency analysis on different procedures for the pre-processing of features. The times taken shown in Tables 4 , 6 are based on experiments conducted in a standard user machine to show the viability of our solution with limited or average resource availability.
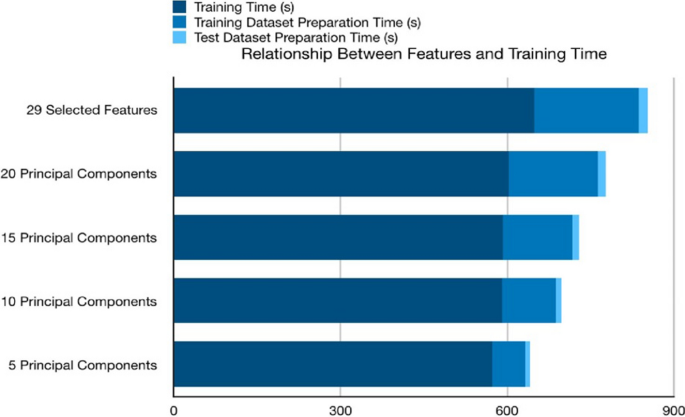
Relationship between feature number and training time
We also listed the confusion matrix of each test in Fig. 7 . The stack bar chart shows that the overall time spends on training the model is decreasing by the number of selected features, while the PCA method is significantly effective in optimizing training dataset preparation. For the time spent on the training stage, PCA is not as effective as the data preparation stage. While there is the possibility that the optimization effect of PCA is not drastic enough because of the simple structure of the NN model.
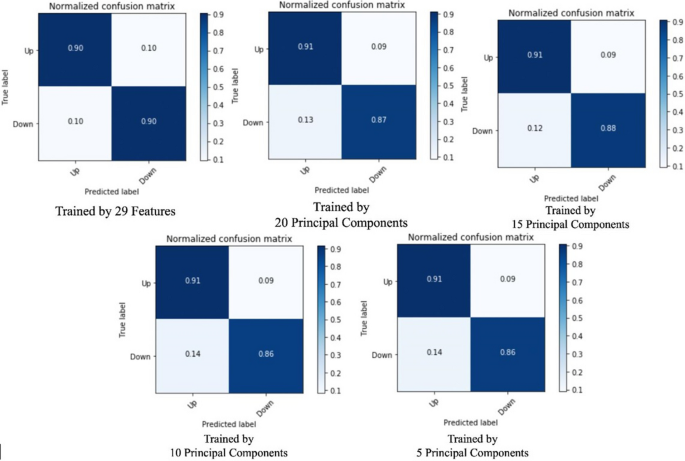
How does the number of principal components affect evaluation results
Table 5 indicates that the overall prediction accuracy is not drastically affected by reducing the dimension. However, the accuracy could not fully support if the PCA has no side effect to model prediction, so we looked into the confusion matrices of test results.
From Fig. 7 we can conclude that PCA does not have a severe negative impact on prediction precision. The true positive rate and false positive rate are barely be affected, while the false negative and true negative rates are influenced by 2% to 4%. Besides evaluating how the number of selected features affects the training efficiency and model performance, we also leveraged a test upon how data pre-processing procedures affect the training procedure and predicting result. Normalizing and max–min scaling is the most commonly seen data pre-procedure performed before PCA, since the measure units of features are varied, and it is said that it could increase the training efficiency afterward.
We leveraged another test on adding pre-procedures before extracting 20 principal components from the original dataset and make the comparison in the aspects of time elapse of training stage and prediction precision. However, the test results lead to different conclusions. In Table 6 we can conclude that feature pre-processing does not have a significant impact on training efficiency, but it does influence the model prediction accuracy. Moreover, the first confusion matrix in Fig. 8 indicates that without any feature pre-processing procedure, the false-negative rate and true negative rate are severely affected, while the true positive rate and false positive rate are not affected. If it performs the normalization before PCA, both true positive rate and true negative rate are decreasing by approximately 10%. This test also proved that the best feature pre-processing method for our feature set is exploiting the max–min scale.
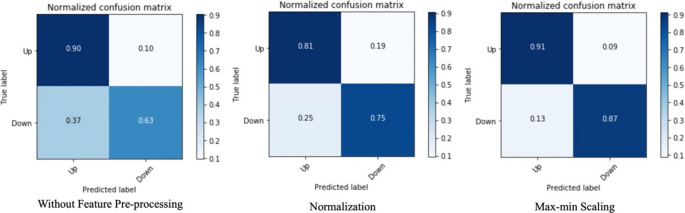
Confusion matrices of different feature pre-processing methods
In this section, we discuss and compare the results of our proposed model, other approaches, and the most related works.
Comparison with related works
From the previous works, we found the most commonly exploited models for short-term stock market price trend prediction are support vector machine (SVM), multilayer perceptron artificial neural network (MLP), Naive Bayes classifier (NB), random forest classifier (RAF) and logistic regression classifier (LR). The test case of comparison is also bi-weekly price trend prediction, to evaluate the best result of all models, we keep all 29 features selected by the RFE algorithm. For MLP evaluation, to test if the number of hidden layers would affect the metric scores, we noted layer number as n and tested n = {1, 3, 5}, 150 training epochs for all the tests, found slight differences in the model performance, which indicates that the variable of MLP layer number hardly affects the metric scores.
From the confusion matrices in Fig. 9 , we can see all the machine learning models perform well when training with the full feature set we selected by RFE. From the perspective of training time, training the NB model got the best efficiency. LR algorithm cost less training time than other algorithms while it can achieve a similar prediction result with other costly models such as SVM and MLP. RAF algorithm achieved a relatively high true-positive rate while the poor performance in predicting negative labels. For our proposed LSTM model, it achieves a binary accuracy of 93.25%, which is a significantly high precision of predicting the bi-weekly price trend. We also pre-processed data through PCA and got five principal components, then trained for 150 epochs. The learning curve of our proposed solution, based on feature engineering and the LSTM model, is illustrated in Fig. 10 . The confusion matrix is the figure on the right in Fig. 11 , and detailed metrics scores can be found in Table 9 .
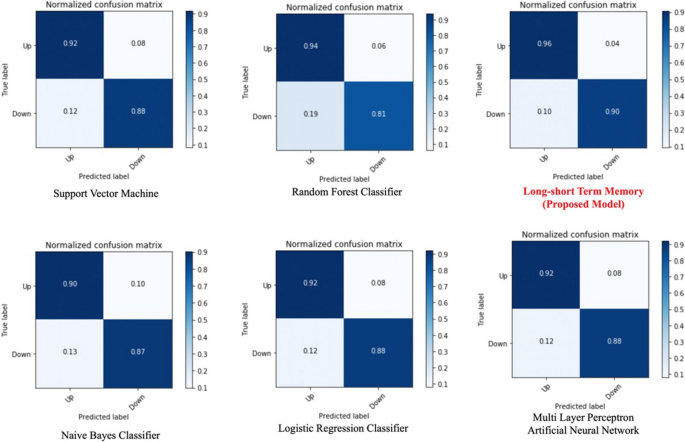
Model prediction comparison—confusion matrices
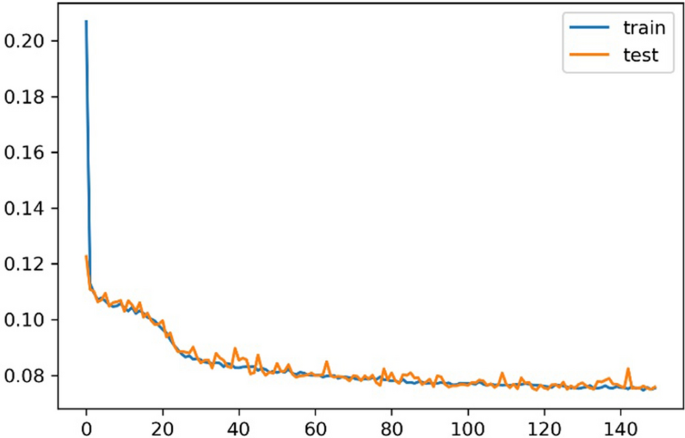
Learning curve of proposed solution
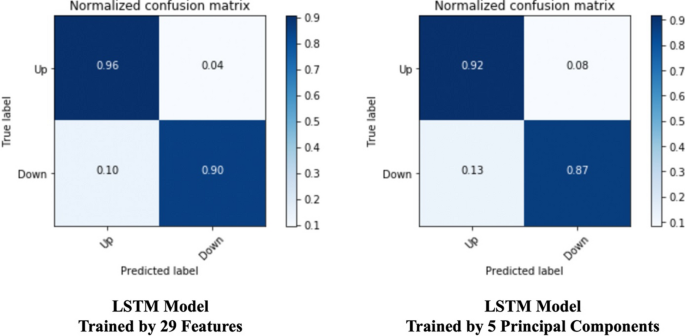
Proposed model prediction precision comparison—confusion matrices
The detailed evaluate results are recorded in Table 7 . We will also initiate a discussion upon the evaluation result in the next section.
Because the resulting structure of our proposed solution is different from most of the related works, it would be difficult to make naïve comparison with previous works. For example, it is hard to find the exact accuracy number of price trend prediction in most of the related works since the authors prefer to show the gain rate of simulated investment. Gain rate is a processed number based on simulated investment tests, sometimes one correct investment decision with a large trading volume can achieve a high gain rate regardless of the price trend prediction accuracy. Besides, it is also a unique and heuristic innovation in our proposed solution, we transform the problem of predicting an exact price straight forward to two sequential problems, i.e., predicting the price trend first, focus on building an accurate binary classification model, construct a solid foundation for predicting the exact price change in future works. Besides the different result structure, the datasets that previous works researched on are also different from our work. Some of the previous works involve news data to perform sentiment analysis and exploit the SE part as another system component to support their prediction model.
The latest related work that can compare is Zubair et al. [ 47 ], the authors take multiple r-square for model accuracy measurement. Multiple r-square is also called the coefficient of determination, and it shows the strength of predictor variables explaining the variation in stock return [ 28 ]. They used three datasets (KSE 100 Index, Lucky Cement Stock, Engro Fertilizer Limited) to evaluate the proposed multiple regression model and achieved 95%, 89%, and 97%, respectively. Except for the KSE 100 Index, the dataset choice in this related work is individual stocks; thus, we choose the evaluation result of the first dataset of their proposed model.
We listed the leading stock price trend prediction model performance in Table 8 , from the comparable metrics, the metric scores of our proposed solution are generally better than other related works. Instead of concluding arbitrarily that our proposed model outperformed other models in related works, we first look into the dataset column of Table 8 . By looking into the dataset used by each work [ 18 ], only trained and tested their proposed solution on three individual stocks, which is difficult to prove the generalization of their proposed model. Ayo [ 2 ] leveraged analysis on the stock data from the New York Stock Exchange (NYSE), while the weakness is they only performed analysis on closing price, which is a feature embedded with high noise. Zubair et al. [ 47 ] trained their proposed model on both individual stocks and index price, but as we have mentioned in the previous section, index price only consists of the limited number of features and stock IDs, which will further affect the model training quality. For our proposed solution, we collected sufficient data from the Chinese stock market, and applied FE + RFE algorithm on the original indices to get more effective features, the comprehensive evaluation result of 3558 stock IDs can reasonably explain the generalization and effectiveness of our proposed solution in Chinese stock market. However, the authors of Khaidem and Dey [ 18 ] and Ayo [ 2 ] chose to analyze the stock market in the United States, Zubair et al. [ 47 ] performed analysis on Pakistani stock market price, and we obtained the dataset from Chinese stock market, the policies of different countries might impact the model performance, which needs further research to validate.
Proposed model evaluation—PCA effectiveness
Besides comparing the performance across popular machine learning models, we also evaluated how the PCA algorithm optimizes the training procedure of the proposed LSTM model. We recorded the confusion matrices comparison between training the model by 29 features and by five principal components in Fig. 11 . The model training using the full 29 features takes 28.5 s per epoch on average. While it only takes 18 s on average per epoch training on the feature set of five principal components. PCA has significantly improved the training efficiency of the LSTM model by 36.8%. The detailed metrics data are listed in Table 9 . We will leverage a discussion in the next section about complexity analysis.
Complexity analysis of proposed solution
This section analyzes the complexity of our proposed solution. The Long Short-term Memory is different from other NNs, and it is a variant of standard RNN, which also has time steps with memory and gate architecture. In the previous work [ 46 ], the author performed an analysis of the RNN architecture complexity. They introduced a method to regard RNN as a directed acyclic graph and proposed a concept of recurrent depth, which helps perform the analysis on the intricacy of RNN.
The recurrent depth is a positive rational number, and we denote it as \(d_{rc}\) . As the growth of \(n\) \(d_{rc}\) measures, the nonlinear transformation average maximum number of each time step. We then unfold the directed acyclic graph of RNN and denote the processed graph as \(g_{c}\) , meanwhile, denote \(C(g_{c} )\) as the set of directed cycles in this graph. For the vertex \(v\) , we note \(\sigma_{s} (v)\) as the sum of edge weights and \(l(v)\) as the length. The equation below is proved under a mild assumption, which could be found in [ 46 ].
They also found that another crucial factor that impacts the performance of LSTM, which is the recurrent skip coefficients. We note \(s_{rc}\) as the reciprocal of the recurrent skip coefficient. Please be aware that \(s_{rc}\) is also a positive rational number.
According to the above definition, our proposed model is a 2-layers stacked LSTM, which \(d_{rc} = 2\) and \(s_{rc} = 1\) . From the experiments performed in previous work, the authors also found that when facing the problems of long-term dependency, LSTMs may benefit from decreasing the reciprocal of recurrent skip coefficients and from increasing recurrent depth. The empirical findings above mentioned are useful to enhance the performance of our proposed model further.
This work consists of three parts: data extraction and pre-processing of the Chinese stock market dataset, carrying out feature engineering, and stock price trend prediction model based on the long short-term memory (LSTM). We collected, cleaned-up, and structured 2 years of Chinese stock market data. We reviewed different techniques often used by real-world investors, developed a new algorithm component, and named it as feature extension, which is proved to be effective. We applied the feature expansion (FE) approaches with recursive feature elimination (RFE), followed by principal component analysis (PCA), to build a feature engineering procedure that is both effective and efficient. The system is customized by assembling the feature engineering procedure with an LSTM prediction model, achieved high prediction accuracy that outperforms the leading models in most related works. We also carried out a comprehensive evaluation of this work. By comparing the most frequently used machine learning models with our proposed LSTM model under the feature engineering part of our proposed system, we conclude many heuristic findings that could be future research questions in both technical and financial research domains.
Our proposed solution is a unique customization as compared to the previous works because rather than just proposing yet another state-of-the-art LSTM model, we proposed a fine-tuned and customized deep learning prediction system along with utilization of comprehensive feature engineering and combined it with LSTM to perform prediction. By researching into the observations from previous works, we fill in the gaps between investors and researchers by proposing a feature extension algorithm before recursive feature elimination and get a noticeable improvement in the model performance.
Though we have achieved a decent outcome from our proposed solution, this research has more potential towards research in future. During the evaluation procedure, we also found that the RFE algorithm is not sensitive to the term lengths other than 2-day, weekly, biweekly. Getting more in-depth research into what technical indices would influence the irregular term lengths would be a possible future research direction. Moreover, by combining latest sentiment analysis techniques with feature engineering and deep learning model, there is also a high potential to develop a more comprehensive prediction system which is trained by diverse types of information such as tweets, news, and other text-based data.
Abbreviations
Long short term memory
Principal component analysis
Recurrent neural networks
Artificial neural network
Deep neural network
Dynamic Time Warping
Recursive feature elimination
Support vector machine
Convolutional neural network
Stochastic gradient descent
Rectified linear unit
Multi layer perceptron
Atsalakis GS, Valavanis KP. Forecasting stock market short-term trends using a neuro-fuzzy based methodology. Expert Syst Appl. 2009;36(7):10696–707.
Article Google Scholar
Ayo CK. Stock price prediction using the ARIMA model. In: 2014 UKSim-AMSS 16th international conference on computer modelling and simulation. 2014. https://doi.org/10.1109/UKSim.2014.67 .
Brownlee J. Deep learning for time series forecasting: predict the future with MLPs, CNNs and LSTMs in Python. Machine Learning Mastery. 2018. https://machinelearningmastery.com/time-series-prediction-lstm-recurrent-neural-networks-python-keras/
Eapen J, Bein D, Verma A. Novel deep learning model with CNN and bi-directional LSTM for improved stock market index prediction. In: 2019 IEEE 9th annual computing and communication workshop and conference (CCWC). 2019. pp. 264–70. https://doi.org/10.1109/CCWC.2019.8666592 .
Fischer T, Krauss C. Deep learning with long short-term memory networks for financial market predictions. Eur J Oper Res. 2018;270(2):654–69. https://doi.org/10.1016/j.ejor.2017.11.054 .
Article MathSciNet MATH Google Scholar
Guyon I, Weston J, Barnhill S, Vapnik V. Gene selection for cancer classification using support vector machines. Mach Learn 2002;46:389–422.
Hafezi R, Shahrabi J, Hadavandi E. A bat-neural network multi-agent system (BNNMAS) for stock price prediction: case study of DAX stock price. Appl Soft Comput J. 2015;29:196–210. https://doi.org/10.1016/j.asoc.2014.12.028 .
Halko N, Martinsson PG, Tropp JA. Finding structure with randomness: probabilistic algorithms for constructing approximate matrix decompositions. SIAM Rev. 2001;53(2):217–88.
Article MathSciNet Google Scholar
Hassan MR, Nath B. Stock market forecasting using Hidden Markov Model: a new approach. In: Proceedings—5th international conference on intelligent systems design and applications 2005, ISDA’05. 2005. pp. 192–6. https://doi.org/10.1109/ISDA.2005.85 .
Hochreiter S, Schmidhuber J. Long short-term memory. J Neural Comput. 1997;9(8):1735–80. https://doi.org/10.1162/neco.1997.9.8.1735 .
Hsu CM. A hybrid procedure with feature selection for resolving stock/futures price forecasting problems. Neural Comput Appl. 2013;22(3–4):651–71. https://doi.org/10.1007/s00521-011-0721-4 .
Huang CF, Chang BR, Cheng DW, Chang CH. Feature selection and parameter optimization of a fuzzy-based stock selection model using genetic algorithms. Int J Fuzzy Syst. 2012;14(1):65–75. https://doi.org/10.1016/J.POLYMER.2016.08.021 .
Huang CL, Tsai CY. A hybrid SOFM-SVR with a filter-based feature selection for stock market forecasting. Expert Syst Appl. 2009;36(2 PART 1):1529–39. https://doi.org/10.1016/j.eswa.2007.11.062 .
Idrees SM, Alam MA, Agarwal P. A prediction approach for stock market volatility based on time series data. IEEE Access. 2019;7:17287–98. https://doi.org/10.1109/ACCESS.2019.2895252 .
Ince H, Trafalis TB. Short term forecasting with support vector machines and application to stock price prediction. Int J Gen Syst. 2008;37:677–87. https://doi.org/10.1080/03081070601068595 .
Jeon S, Hong B, Chang V. Pattern graph tracking-based stock price prediction using big data. Future Gener Comput Syst. 2018;80:171–87. https://doi.org/10.1016/j.future.2017.02.010 .
Kara Y, Acar Boyacioglu M, Baykan ÖK. Predicting direction of stock price index movement using artificial neural networks and support vector machines: the sample of the Istanbul Stock Exchange. Expert Syst Appl. 2011;38(5):5311–9. https://doi.org/10.1016/j.eswa.2010.10.027 .
Khaidem L, Dey SR. Predicting the direction of stock market prices using random forest. 2016. pp. 1–20.
Kim K, Han I. Genetic algorithms approach to feature discretization in artificial neural networks for the prediction of stock price index. Expert Syst Appl. 2000;19:125–32. https://doi.org/10.1016/S0957-4174(00)00027-0 .
Lee MC. Using support vector machine with a hybrid feature selection method to the stock trend prediction. Expert Syst Appl. 2009;36(8):10896–904. https://doi.org/10.1016/j.eswa.2009.02.038 .
Lei L. Wavelet neural network prediction method of stock price trend based on rough set attribute reduction. Appl Soft Comput J. 2018;62:923–32. https://doi.org/10.1016/j.asoc.2017.09.029 .
Lin X, Yang Z, Song Y. Expert systems with applications short-term stock price prediction based on echo state networks. Expert Syst Appl. 2009;36(3):7313–7. https://doi.org/10.1016/j.eswa.2008.09.049 .
Liu G, Wang X. A new metric for individual stock trend prediction. Eng Appl Artif Intell. 2019;82(March):1–12. https://doi.org/10.1016/j.engappai.2019.03.019 .
Liu S, Zhang C, Ma J. CNN-LSTM neural network model for quantitative strategy analysis in stock markets. 2017;1:198–206. https://doi.org/10.1007/978-3-319-70096-0 .
Long W, Lu Z, Cui L. Deep learning-based feature engineering for stock price movement prediction. Knowl Based Syst. 2018;164:163–73. https://doi.org/10.1016/j.knosys.2018.10.034 .
Malkiel BG, Fama EF. Efficient capital markets: a review of theory and empirical work. J Finance. 1970;25(2):383–417.
McNally S, Roche J, Caton S. Predicting the price of bitcoin using machine learning. In: Proceedings—26th Euromicro international conference on parallel, distributed, and network-based processing, PDP 2018. pp. 339–43. https://doi.org/10.1109/PDP2018.2018.00060 .
Nagar A, Hahsler M. News sentiment analysis using R to predict stock market trends. 2012. http://past.rinfinance.com/agenda/2012/talk/Nagar+Hahsler.pdf . Accessed 20 July 2019.
Nekoeiqachkanloo H, Ghojogh B, Pasand AS, Crowley M. Artificial counselor system for stock investment. 2019. ArXiv Preprint arXiv:1903.00955 .
Ni LP, Ni ZW, Gao YZ. Stock trend prediction based on fractal feature selection and support vector machine. Expert Syst Appl. 2011;38(5):5569–76. https://doi.org/10.1016/j.eswa.2010.10.079 .
Pang X, Zhou Y, Wang P, Lin W, Chang V. An innovative neural network approach for stock market prediction. J Supercomput. 2018. https://doi.org/10.1007/s11227-017-2228-y .
Pimenta A, Nametala CAL, Guimarães FG, Carrano EG. An automated investing method for stock market based on multiobjective genetic programming. Comput Econ. 2018;52(1):125–44. https://doi.org/10.1007/s10614-017-9665-9 .
Piramuthu S. Evaluating feature selection methods for learning in data mining applications. Eur J Oper Res. 2004;156(2):483–94. https://doi.org/10.1016/S0377-2217(02)00911-6 .
Qiu M, Song Y. Predicting the direction of stock market index movement using an optimized artificial neural network model. PLoS ONE. 2016;11(5):e0155133.
Scikit-learn. Scikit-learn Min-Max Scaler. 2019. https://scikit-learn.org/stable/modules/generated/sklearn.preprocessing.MinMaxScaler.html . Retrieved 26 July 2020.
Shen J. Thesis, “Short-term stock market price trend prediction using a customized deep learning system”, supervised by M. Omair Shafiq, Carleton University. 2019.
Shen J, Shafiq MO. Deep learning convolutional neural networks with dropout—a parallel approach. ICMLA. 2018;2018:572–7.
Google Scholar
Shen J, Shafiq MO. Learning mobile application usage—a deep learning approach. ICMLA. 2019;2019:287–92.
Shih D. A study of early warning system in volume burst risk assessment of stock with Big Data platform. In: 2019 IEEE 4th international conference on cloud computing and big data analysis (ICCCBDA). 2019. pp. 244–8.
Sirignano J, Cont R. Universal features of price formation in financial markets: perspectives from deep learning. Ssrn. 2018. https://doi.org/10.2139/ssrn.3141294 .
Article MATH Google Scholar
Thakur M, Kumar D. A hybrid financial trading support system using multi-category classifiers and random forest. Appl Soft Comput J. 2018;67:337–49. https://doi.org/10.1016/j.asoc.2018.03.006 .
Tsai CF, Hsiao YC. Combining multiple feature selection methods for stock prediction: union, intersection, and multi-intersection approaches. Decis Support Syst. 2010;50(1):258–69. https://doi.org/10.1016/j.dss.2010.08.028 .
Tushare API. 2018. https://github.com/waditu/tushare . Accessed 1 July 2019.
Wang X, Lin W. Stock market prediction using neural networks: does trading volume help in short-term prediction?. n.d.
Weng B, Lu L, Wang X, Megahed FM, Martinez W. Predicting short-term stock prices using ensemble methods and online data sources. Expert Syst Appl. 2018;112:258–73. https://doi.org/10.1016/j.eswa.2018.06.016 .
Zhang S. Architectural complexity measures of recurrent neural networks, (NIPS). 2016. pp. 1–9.
Zubair M, Fazal A, Fazal R, Kundi M. Development of stock market trend prediction system using multiple regression. Computational and mathematical organization theory. Berlin: Springer US; 2019. https://doi.org/10.1007/s10588-019-09292-7 .
Book Google Scholar
Download references
Acknowledgements
This research is supported by Carleton University, in Ottawa, ON, Canada. This research paper has been built based on the thesis [ 36 ] of Jingyi Shen, supervised by M. Omair Shafiq at Carleton University, Canada, available at https://curve.carleton.ca/52e9187a-7f71-48ce-bdfe-e3f6a420e31a .
NSERC and Carleton University.
Author information
Authors and affiliations.
School of Information Technology, Carleton University, Ottawa, ON, Canada
Jingyi Shen & M. Omair Shafiq
You can also search for this author in PubMed Google Scholar
Contributions
Yes. All authors read and approved the final manuscript.
Corresponding author
Correspondence to M. Omair Shafiq .
Ethics declarations
Competing interests.
The authors declare that they have no competing interests.
Additional information
Publisher's note.
Springer Nature remains neutral with regard to jurisdictional claims in published maps and institutional affiliations.
Rights and permissions
Open Access This article is licensed under a Creative Commons Attribution 4.0 International License, which permits use, sharing, adaptation, distribution and reproduction in any medium or format, as long as you give appropriate credit to the original author(s) and the source, provide a link to the Creative Commons licence, and indicate if changes were made. The images or other third party material in this article are included in the article's Creative Commons licence, unless indicated otherwise in a credit line to the material. If material is not included in the article's Creative Commons licence and your intended use is not permitted by statutory regulation or exceeds the permitted use, you will need to obtain permission directly from the copyright holder. To view a copy of this licence, visit http://creativecommons.org/licenses/by/4.0/ .
Reprints and permissions
About this article
Cite this article.
Shen, J., Shafiq, M.O. Short-term stock market price trend prediction using a comprehensive deep learning system. J Big Data 7 , 66 (2020). https://doi.org/10.1186/s40537-020-00333-6
Download citation
Received : 24 January 2020
Accepted : 30 July 2020
Published : 28 August 2020
DOI : https://doi.org/10.1186/s40537-020-00333-6
Share this article
Anyone you share the following link with will be able to read this content:
Sorry, a shareable link is not currently available for this article.
Provided by the Springer Nature SharedIt content-sharing initiative
- Deep learning
- Stock market trend
- Feature engineering
SYSTEMATIC REVIEW article
Covid and world stock markets: a comprehensive discussion.
- 1 Department of Management Sciences, Lahore College for Women University, Lahore, Pakistan
- 2 Department of Management Sciences, National University of Modern Languages, Islamabad, Pakistan
The COVID-19 outbreak has disturbed the victims' economic conditions and posed a significant threat to economies worldwide and their respective financial markets. The majority of the world stock markets have suffered losses in the trillions of dollars, and international financial institutions were forced to reduce their forecasted growth for 2020 and the years to come. The current research deals with the impact of the COVID-19 pandemic on the global stock markets. It has focused on the contingent effects of previous and current pandemics on the financial markets. It has also elaborated on the pandemic impact on diverse pillars of the economy. Irrespective of all these destructive effects of the pandemic, still hopes are there for a sharp rise and speedy improvement in global stock markets' performance.
Introduction
The world is experiencing the worst health and economic disaster in the shape of COVID-19 pandemic. Dealing with this pandemic is the most challenging task being faced by human beings since the Second World War ( Maqsood et al., 2021 ). Coronavirus has pushed the markets toward the danger zone. The market panic has been started. This disease is contagious even before it shows obvious symptoms. It is quite difficult to hold people in quarantine in this outbreak. That's the narrative, and we haven't gotten very far into it yet. So, the potential for market disruption because of a scary narrative is quite high.
—Robert James Shriller, Nobel Memorial Prize Winner in Economic Sciences, 2013.
The epidemiological perspectives are not required to be understood here. Currently, well-informed individuals ought to have some know-how about the basics of contagious diseases. Times of fear are also times of rumor and misinformation; knowledge is the antidote ( Baldwin et al., 2020 ). The COVID-19 outbreak was officially reported in the Wuhan City of China in December 2019 and covered all continents of this globe other than Antarctica ( Hui et al., 2020 ). COVID-19 is a distinctive black swan event, and we are unaware of its existence, expansion, breadth, depth, magnitude, and even its disappearance ( He P. et al., 2020 ; He Q. et al., 2020 ). World Health Organization (WHO) officially declared COVID-19 a pandemic on 11th March 2020 ( Cucinotta and Vanelli, 2020 ). The pandemic has severely hit global economies ( Shafi et al., 2020 ). It has disrupted the life and lifestyle of almost everyone ( Aqeel et al., 2021 ). Almost no one has been left untouched. Another pandemic of information and misinformation is keeping pace with it during this pandemic, spreading fear and anxiety ( Koley and Dhole, 2020 ). The outbreak has changed the outlook of this globe within no time at all. Human beings are struggling with the long-lasting effects of this disease and the unforgettable reality of their existence which has never happened before. The pandemic affected more than 107 million people, with around 2.3 million causalities, and the numbers of cases are escalated day by day. The alarming point is the growth factor of this disease, where 100 contaminated cases create another 10,000 within a very limited time ( Bagchi et al., 2020 ).
The people from this generation have seen wars. They have seen the collapse of the Soviet Union. They have seen extremely dangerous terrorist attacks. They have seen the burst of financial bubbles, and they have seen the effects of climate change. However, they had not seen anything like the coronavirus before. A similar case has not existed for more than one hundred years. They were not ready for it, and they did not know how to respond to it. Since it was something that no one had any prior experience with, the pandemic has also led to reconsidering some things which were always previously thought either right or wrong ( Sharma, 2021 ).
The COVID-19 pandemic has spread globally, has made millions of people sick, and triggered an international response spearheaded by the World Health Organization to stop its spread. From Wuhan, China, it spread like wildfire. The virus has now visited almost every nation in the world, bringing helplessness and death with it. None are spared, and in some way or another, almost everyone has become a victim. In a recent message, the WHO warned that the worst is yet to come. The coronavirus has not only triggered disease and death, and it has affected almost every aspect human life. There is a long list of disruptions to daily life in the cities and states with lockdown, global sporting events, weddings, social events, post-poned ceremonies; all this has elicited the global crisis. Moreover, industries worldwide have been affected; stock markets have been reported in record downfall; airlines, travel, tourism, and hospitality sectors are the major victims of this pandemic. A significant disaster is job loss in various sectors ( Koley and Dhole, 2020 ).
Crucial and groundbreaking strategies are required to protect not only human lives but also to safeguard economies and uplift economic growth and financial health. Nations are exposed to a global health crisis, the like of which has not occurred for a century. This crisis is killing human beings, enhancing human distress, and upsetting the lives of individuals. This can be considered a sort of human, social, and economic crisis ( Mishra, 2020 ). The best efforts by governments from every country have failed to halt its spread: cities were put under lockdown; people were advised to stay at home; international borders were closed; travel bans at local, national and international level were imposed; markets, schools, universities and shopping complexes were closed. Quarantine and self-isolation have been advised to stop the spread of COVID-19. The virus has triggered an unprecedented global crisis which led the WHO to provide technical guidance for government authorities, healthcare workers, and other key stakeholders to respond to community spread ( Koley and Dhole, 2020 ).
In the intermingled economies, the Covid-19 pandemic came as a global distress that affects both the demand and supply side concurrently. Rapidly growing infectivity limits labor supply and badly affects productivity, whereas supply disruptions are also caused by social distancing, lockdowns and industry closures. On the other hand, disruption on the demand side is caused by reduced consumption, unemployment, and income loss and these economic prospects result in reduced company investment. The unpredictability about the path, instance, enormity and impact of Covid-19 could create a vicious cycle of redundancy, less consumption, and business closures, leading to financial distress. To identify and determine this extraordinary shock is the key challenge for the experiential analysis of this pandemic. The unprecedented nature of COVID-19 makes it difficult to recognize its non-linear effects, cross-country spillovers, and quantify unobserved factors to compose forecasts ( Chudik et al., 2020 ).
International institutions including the FAO, ILO, IFAD, and WHO jointly declared this pandemic a global challenge to food systems, public health, trade, and industry. Overwhelming social and economic disruptions put tens of millions of individuals at risk of falling below the poverty line. According to another approximation, by the end of this year, the number of undernourished people could increase by up to 32 million, which are ~690 million at present. It also poses an existential threat to a considerable number of business ventures. The world has a 3.3 billion workforce and ~50% of which are near to being unemployed. Significant individuals are informal workers with limited access to productive assets, quality health, and the majority lack social protection. Due to lockdowns, they lost their means to earn money and became incapable of feeding themselves and their families because for most their daily food depends upon their daily wages earned. Such a devastating effect on the entire food chain has exposed the vulnerability of this pandemic. Farmers have no access to markets, nor can buy inputs or sell their output and result in a reduced harvest. In addition to market shutdown, trade limitations, border closures, and detention measures dislocate food supply chains nationally and internationally, which badly influenced a healthy diet. Small scale farmers are the soft target of COVID-19 and placed nutrition and food security of the most marginalized population under threat, as income producers fall ill, die, or otherwise lose their work ( Chriscaden, 2020 ).
It is still difficult to understand the recovery due to the development of vaccines. To understand corona's economic impact, the following charts and maps exhibit real statistics so far.
Impact on Jobs
A report published by the OECD (2020) shows the impact of COVID-19 and containment measures on OECD economies where people were prohibited from going to work, resulting in a significant drop in business activity and extraordinary job losses. In some countries, millions have been moved to reduced hours and most people worked up to ten times fewer hours. Moreover, the rate of entire job loss is also very high. Some people are more exposed to this pandemic than others. As young people and women workers are at greater risk due to less secured and unskilled jobs. They are also associated with the industries most affected by this unprecedented shock, including restaurants, cafés and tourism.
Causing Recession
Worldwide economic downturn caused by the COVID-19 pandemic forced the Organization for Economic Cooperation and Development (OECD), International Monetary Fund (IMF), and World Bank (WB) to revise their forecasts and reported a significant decline in the projected rate of growth in late 2019 and mid-2020. Such deterioration can be seen in the IMF figures in which global economic growth forecasts declined from +3.4% to −4.4% during October 2019 and October 2020. In the same way, OECD also revised its forecast and lowered the growth rate from positive 2.9% in November to −4.5% in September 2020. In June 2020, OECD anticipated the blow of another wave of infections.
Impact on Travel
The travel industry is one of those acutely damaged industries due to lockdowns, border closures, and abandoned flight operations. Airlines are not only canceling flights, but customers also restrict themselves from holidays and business trips. A recently discovered subsequent wave of COVID-19 has forced national and international airlines to promulgate new travel restrictions and tighten their policies. While providing data of 2020, Flight tracking service Flight Radar 24 reveals a huge hit in number of flights worldwide and requires a long way to recover ( Jones et al., 2021 ).
Impact on Tourism
Tourism is another badly affected industry due to this unprecedented pandemic. The World Tourism Organization, also known as UNWTO (2020) marked this pandemic as a serious threat to the travel and tourism sector. Many jurisdictions put restrictions on international travel to restrict the spread of the virus; some fully closed their borders, resulting in a massive decline in demand. In 2020, tourism reported a loss of ~1 billion tourists, equivalent to US$ 1.1 trillion in international tourism receipts. This decline in international tourism could cause an ~$2 trillion loss in global GDP, over 2% of the global GDP in 2019. While predicting a rebound in the global tourism industry, UNWTO presented an extended scenario for the year 2021 to the year 2024. According to them, global tourism will start recovery from the second half of the year 2021 but it will take 2.5 to 4 years to return to 2019.
Impact on Stock Markets
The capital markets are at the front line of any country's economy, and the stock markets are considered the indicator of any economy ( He P. et al., 2020 ; He Q. et al., 2020 ). The COVID-19 outbreak has disturbed the victims' economic conditions and posed a significant threat to the worldwide economies and their respective financial markets ( Barro et al., 2020 ; Ramelli and Wagner, 2020 ). The majority of the world stock markets have suffered in terms of trillion-dollar losses ( Lyócsa et al., 2020 ) and international financial institutions were forced to reduce their forecasted growth for 2020 and the years to come ( Boone et al., 2020 ). The root cause of this severe decline is the exposure of stock markets to several risks, for instance, the global financial crisis of 2008, which had pushed these markets in a melting position ( Dang and Nguyen, 2020 ). The current pandemic has affected the global stock markets significantly compared to the SARS virus, which was spread in 2003 as China has got tremendous development in comparison to the last 17 years and recognized as a leading economy of the world and also a global production hub, manufacturing the highly demanded technology products ( Alameer et al., 2019 ).
Effects of Previous Pandemics on Stock Markets
Scholars have argued that previous pandemics triggered fragile stock markets ( Chen et al., 2018 ) and impeded stock market participants' decision-making capacity by reducing their active involvement in stock market trading ( Dong and Heo, 2014 ). The literature has provided empirical evidence of the stock market reactions to significant systematic events. The research has shown the cyclical nature of the stock market reactions and the factors that affected the stock markets ( Keating, 2001 ). The historical performance of stock markets has been documented in the previous literature regarding influenza and other major epidemics. Similarly, the scholars have examined the influences of significant events on the stock markets, i.e., Severe Acute Respiratory Syndrome (SARS), ( Chen et al., 2018 ), natural disasters ( Caporale et al., 2019 ), corporate events ( Ranju and Mallikarjunappa, 2019 ), public news, and political events ( Bash and Alsaifi, 2019 ). Some other studies have also demonstrated that SARS in 2003 weakened the Taiwanese economy ( Chen et al., 2007 ) and regional stock markets ( Chen et al., 2018 ).
The previous studies have comprehensively examined the association between outbreaks and stock market performance. Kalra et al. (1993) investigated the disaster of the Soviet Chernobyl nuclear power plant. Delisle (2003) recognized that effects were of greater intensity after SARS (2003) than the Asian financial crisis. Nippani and Washer (2004) investigated the effects of SARS on global financial markets and found that it influenced the markets of China and Vietnam. Lee and and McKibbin (2004) reported the strong effect of SARS on human beings and financial integration. Loh (2006) explained a robust linkage between SARS and airline stocks performance in Canada, China, Hong Kong, Singapore, and Thailand and illustrated that the stocks of the aviation sector are more sensitive than non-aviation stocks. MckKibbin and Sidorenko (2006) investigated the influenza epidemic's impact on the global economy's growth by considering its diverse magnitudes like slight, moderate and intense. Moreover, Chen et al. (2007) noticed the negative effects of SARS on the hotel industry's stock prices in Taiwan. They also investigated the significant influence of SARS on the four major stock markets of Asia and China. Nikkinen et al. (2008) discovered the impact of the 9/11 incident on the global stock prices; however, the markets recovered rapidly. Al Rjoub (2009) also studied the influence of financial crisis on stock market.
Besides, Kaplanski and Levy (2010) studied the effect of aviation accidents on stock returns and established that price fluctuations are sensitive to such incidents. Al Rjoub (2011) and Al Rjoub and Azzam (2012) investigated the impact of the Mexican tequila crisis (1994), Asian-Russian financial crisis (1997–98), 9/11 incident, Iraq war (2004), financial crisis (2005), and global financial crisis (2008–09) on the stock compensation behavior in Jordan's Stock Exchange. Righi and Ceretta (2011) established the positive effect of the European debt crisis (2010) on European markets' risk aptitude, especially the German, French, and British markets. Schwert (2011) explored the variabilities in the prices of US stocks during the financial crisis. Mctier et al. (2011) found the negative impact of Flu on the intensity of trading activities and stock returns in the USA. Besides, Rengasamy (2012) examined the effect of Eurozone sovereign debt-related policy announcements, development rewards, and stock market volatility on Brazil, Russia, India, China, and South Africa. Karlsson and Nilsson (2014) found the negative impact of the 1918 Spanish flu epidemic on capital returns. Lanfear et al. (2018) conducted a study to explore the effect of cyclones on stock returns, and they observed the effect of emergencies on stock returns. Chen et al. (2018) examined the influence of SARS on Asian financial markets.
Brief Overview of Literature
Studies have elaborated on the performance of global stock markets affecting the COVID-19 outbreak ( Ahmar and del Val, 2020 ; Al-Awadhi et al., 2020 ; Liu et al., 2020 ; Zhang et al., 2020 ). The pandemic has decreased investors' confidence level in the stock market as the market uncertainty was very high ( Liu et al., 2020 ). Iyke (2020) explained that COVID-19 has robust and continual negative effects on the global economy. Ahmar and del Val (2020) used ARIMA and SutteARIMA and forecasted the short-term impact of COVID-19 on Spain's IBEX index. They further explained that SutteARIMA is the better statistical measure in forecasting such impact.
Moreover, Alam et al. (2020) explained that pandemic has greatly hit Australia's capital market right from the start of 2020. The stock market has shown a bearish trend, though some sectors were at high risk and others have performed well. The researchers have focused on initial volatility and sectoral returns in eight different sectors. They have analyzed the data using the event study method and 10-days window for the official announcements of COVID-19 events in Australia. The findings revealed that some sectors performed well on the day of the announcement. Simultaneously, some others also showed good performance after the announcement except the transportation sector, which performed poorly.
The pandemic has posed severe challenges to the global economies ( Wang et al., 2021 ) and it has also created mental health issues ( Abbas et al., 2021 ). Chowdhury et al. (2020) examined the impact of COVID-19 on economic activities and stock markets worldwide. The study has targeted 12 countries from four continents from January-April 2020 by using the panel data. The stock market impact was measured using the event study method, and economic impact was measured using the panel vector autoregressive model. The results showed the extremely negative effects of pandemic variables on stock returns. Singh et al. (2020) investigated the influence of COVID-19 on the stock markets of G-20 states. The study used an event study for measuring abnormal returns and panel data to describe the causes of abnormal returns. The data consisted of 58 days of post-COVID period news provided by international media and 120 days before the event. The findings exhibited the significant negative abnormal returns during the event days. Liu et al. (2020) also examined the pandemic's effect on the most affected countries' stock markets by using the event study. The researchers revealed the negative effects of COVID-19 on the stock markets' performance.
He P. et al. (2020) and He Q. et al. (2020) also used the event study method to explore the impact of COVID-19 on Chinese industries and stock market performance. It has been observed that some industries were severely affected by the pandemic (mining, environment etc.). However, some other industries have faced limited effects of an outbreak (manufacturing, education etc.). Machmuddah et al. (2020) used the event study method to observe consumer goods' share prices before and after COVID-19. The data about daily stock prices and stock trade volume has been collected before and after the pandemic. Significant differences have been observed between daily closing prices and stock trade volume before and after the pandemic. Liu et al. (2020) used an event study method to study the short term impact of the outbreak on the stock market indices of 21 countries strongly affected by pandemic (Italy, UK, Germany etc.). Asian countries have taken the severe negative effect of the pandemic as compared to other states. Khatatbeh et al. (2020) also applied the event study method to discover the impact of COVID-19 on some targeted countries' stock indices by employing the daily stock prices and found a significant negative impact on returns.
Al-Awadhi et al. (2020) investigated the association of pandemic and stock market outcomes in the Chinese stock market. The findings showed the effect of pandemic cases and deaths on the stock returns of different organizations. Baker et al. (2020) claimed that COVID-19 strongly affects the US stock market compared to previous epidemics, including the Spanish Flu. Eighteen market jumps were observed from February-March 2020. The market jumps were considered to be the largest ones since 1990. The causes behind such jumps were the lockdowns and production cut. Ozili and Arun (2020) described that COVID-19 uncertainty and the fear of losing profit have resulted in 6 trillion USD in the global stock market on 24th February 2020. Similarly, the S&P 500 index has faced a loss of 5 trillion US dollar. The research also demonstrated the significant influence the pandemic on the opening, highest, and lowest stock indices in the US. Ngwakwe (2020) illustrated the influence of COVID-19 outbreak on some targeted stock indices (SSE, Euronext, and DJIA) by collecting the data for 50 days before and 50 days within the pandemic. The differential effects of the pandemic were observed in different stock markets. DJIA stock returns were decreased, SSE increased, however, S&P 500 index and Euronext 100 revealed insignificant effects.
He P. et al. (2020) and He Q. et al. (2020) examined the direct effects of COVID-19 spillovers on the stock market. The daily return data has collected from China, Italy, South Korea, France, Spain, Germany, Japan and the USA. The findings showed the negative short term effects of COVID-19 on the stock indices. Zhang et al. (2020) elaborated the impact of pandemic fear on the pattern of systematic risk and country-specific risk in the global financial markets. They explained the volatile nature of financial markets and the huge impact of uncertain market conditions on financial market risk. Sobieralski (2020) evaluated the effect of COVID-19 on employment and the aviation industry. The stock returns of China and US stocks have declined at a record level. Qin et al. (2020) investigated the influence of outbreak on oil markets.
Sansa (2020) explained the association between COVID-19 recorded cases and financial markets systems of SSE and DJIA during March 2020. Aslam et al. (2020) studied the impact of COVID-19 on 56 global stock market (developed, developing, emerging, and frontier) indices by using the network method. Topcu and Gulal (2020) have discovered a huge impact on Asian markets as compared to European markets. Ashraf (2020) explained that confirmed cases more strongly affect the stock market than deaths. Czech et al. (2020) used the TGARCH model and found the negative impact of COVID-19 on Visegrad stock market indices. They discovered that stock markets were seriously affected when the disease's nature was changed from epidemic to pandemic.
Zhang et al. (2020) also investigated the influence of COVID-19 on the stock markets of 10 countries. It was concluded that European stock markets showed connectivity during the outbreak; however, US markets could not show a leading role before and during the pandemic. Okorie and Lin (2021) discovered the occurrence of financial contagion during the pandemic. Corbet et al. (2020) presented some interesting insights. They illustrated that pandemic greatly affected the companies having names related to the virus, although these companies were not related to the virus.
The current research work basically pertains to the comprehensive discussion about the past present and future of world stock markets. For the sake of achieving the research aims, it has also presented a somehow brief yet inclusive debate about the happenings in the renowned stock markets. It has focused on the major market indices belong to different regions and also it has attempted to explain the actual position of some famous indices with the help of underlying real time data based graphs. Its major contribution is presenting the diverse opinions of traditional and behavioral finance regarding the behavior of stock market participants.
A General Debate About Stock Markets Performance
The global stock markets have been reported for their record decline. On 23 March 2020 the S&P 500 Index witnessed an usual drop of 35% compared to the record high on 18 February 2020. In no time at all, the intensity of this record fall became comparable with the financial crisis of 2008, black Monday of 1987, and the great depression of October-November, 1929 ( Helppie McFall, 2011 ). Fernandes (2020) also explained that the US S&P 500 index went down to 30% during March 2020. He further described that the UK and Germany's stock markets were noticed for their worst performance than the US market. The returns of these two markets were fallen by 37 and 33%, respectively. However, the worst performers in the global stock markets were Brazil (−48%) and Columbia (−47%).
Japan's market index dropped more than 20% compared to the record high values of December 2019. S&P 500 Index and Dow Jones share points were declined by 20% in March 2020. The Nikkei Index also reported the same downfall. The Colombo Stock Exchange witnessed a 9% drop in share value and experienced three market halts during mid-March 2020. The Indonesian stock market followed a similar decline. In April 2020, the index was opened with a 64.06 points decline. The UK-FTSE index plunged by 29.72%. The DAX (Germany) index was dropped by 33.37%, CAC (France) by 33.63%, NIKKEI (Japan) by 26.85%, and SUNSEX (India) want down by 17.74% ( Machmuddah et al., 2020 ). Shanghai Composite went down to 2,660.17 points on 23rd March 2020, showing a decline of 12.49% compared to December 2019. KOSPI touched the peak level of 2,204.21 points on 27th December 2019 and dropped to the lowest point of 1,457.64 on 19th March 2020, showing a drop of 33.87%. The BSE SENSEX reported the highest points of 41,681.54 on 20th December 2019. BSE SENSEX plunged to 25,981 points on 23rd March 2020 due to the COVID-19 outbreak, demonstrating a decline of 37.66%. FTSE 100 showed an upward trend on 27th December 2019 with a record index of 7,644.90 points, but it reflected the downward trend followed by a pandemic with an index value of 4,993.89 34.67% decline. The NASDAQ 100 Index reached 8,778.31 points on 26th December 2019 and observed the negative effects of the COVID outbreak by touching 7006.92 points with a declining trend of 20.17%. Moreover, MOEX revealed a bullish trend on 27th December 2019 with an index value of 3,050.47 points and reflected the effects of COVID-19 by reaching 2,112.64 points with the corresponding decline of 30.74%.
Besides, FTSE MIB reached the record level of 24,003.64 points on 20th December 2019 and then touched 14,894.44 points due to pandemic on 12th March 2020 with a declining rate of 37.94%. Nikkei 225 demonstrated an upward trend with the peak value of 24,066.12 on 17th December 2019 and represented the lowest range of 16,552.83 points following the pandemic on 19th March 2020 with the corresponding decline of 31.21%. CAC 40 represented 6,037.39 points on 27th December 2019, consequently faced the sharp jerk of 37.80% on 18th March 2020. DAX exhibited an ascending trend on 16th December 2020 with a peak value of 13,407.66, with the corresponding decline of 8,441.71 on 18th March 2020, signifying an increase of 37.04%. Moving forward, S&P/TSX jumped to 17,180.15 on 24th December 2019 and showed the devastating effects of COVID-19 with the sharp decline of 34.64% on 23rd March 2020. Besides, FTS/JSE reflected 3,513.21 points on 20th November 2020 and affected by the outbreak with a decline of 36.37% on 23rd March 2020 (Investopedia).
However, the global stock markets regained and demonstrated a bullish trend during the days of April 2020. The S&P 500 index increased by 29% and regained the strong position it had held in August 2019 ( Cox et al., 2020 ). Shanghai Composite index further increased by 8.22% in May 2020. KOSPI index showed a bullish trend and the index increased by 27.05%. Similarly, BSE SENSEX recaptured its position and touched 33,717.62 points on 30th April 2020, representing the rise of 22.94%. FTSE 100 secured an 18.33% increase, and the index targeted 6,115.25 points on 29th April 2020. NASDAQ 100 touched 9,485.02 points on 20th May 2020 with the respective rise of 26.12%. MOEX showed an upward trend with a 74.64% increase on 13th April 2020. Also, BOVESPA regained by 23.56% on 29th April 2020 and touched 83.170.80 points. FTSE MIB upbeat and reached 18,067.29 on 29th April 2020. On 20th May 2020, NIKKEI Index climbed at 20,595.15 points, reflecting an increase of 19.62%. Moreover, CAC 40 revived by 19.61% on 29th April 2020. DAX invigorated with the 24.79% increase on 20th May 2020. S&P/TSX touched 15,228 points on 29th April 2020, FTSE/JSE recovered by 27.09% on 20th May 2020, beating the outbreak's negative effect (Investopedia).
The stock market indices worldwide have been categorized in terms of Major Stock Indices, Global Stock Indices, and World Stock Indices etc. The Major World Stock market indices as well as their respective countries have been presented in the Table 1 .
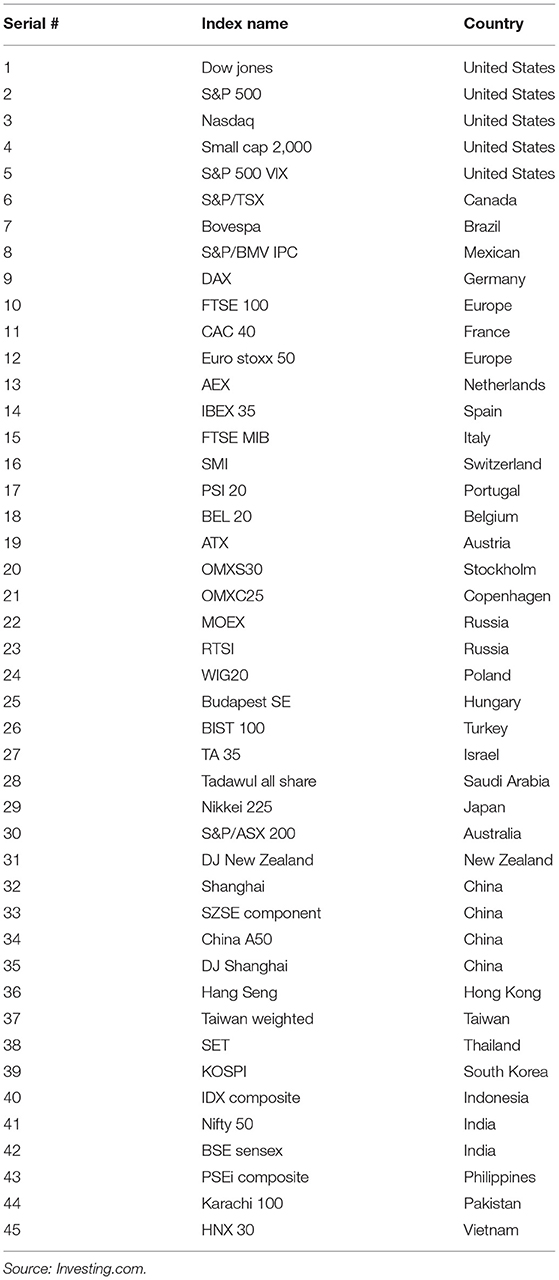
Table 1 . Major world market indices.
Graphical Representation of Some Leading Indices
Source of all figures: tradingeconomics.com .
Figure 1 represents the stock market performance of the S&P ASX 50 index of Australia. It can be seen that the index was performing well-during January 2020, when COVID-19 was at its initial phase. However, March seemed to be a nightmare, when the index plunged and reached the lowest level as COVID-19 spread rapidly and hit a majority of the nations. But the index revived during April 2020, and a gradually limited bullish trend was observed. In-spite of such revival, the index could not reach its peak as the world is still facing the 3rd wave of the pandemic.
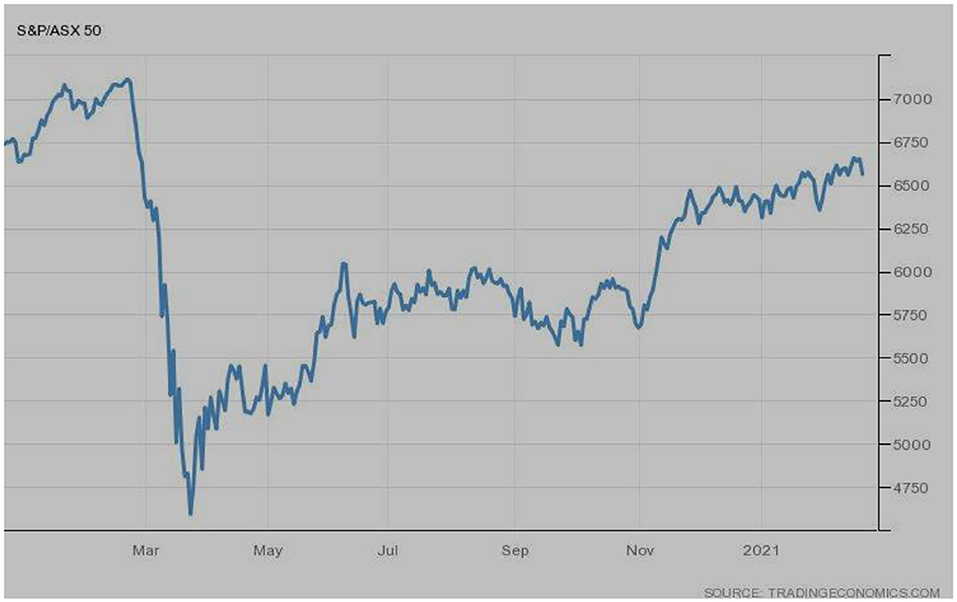
Figure 1 . S&P ASX 50 (Australia). Source: tradingeconomics.com . Reproduced with permission.
Figure 2 exhibits the stock market conditions of DAX Germany. The stock market did perform well-until February 2020, it showed a bearish trend in March 2020, followed by a gradual increase, and finally, it realized the position as it was before the pandemic.
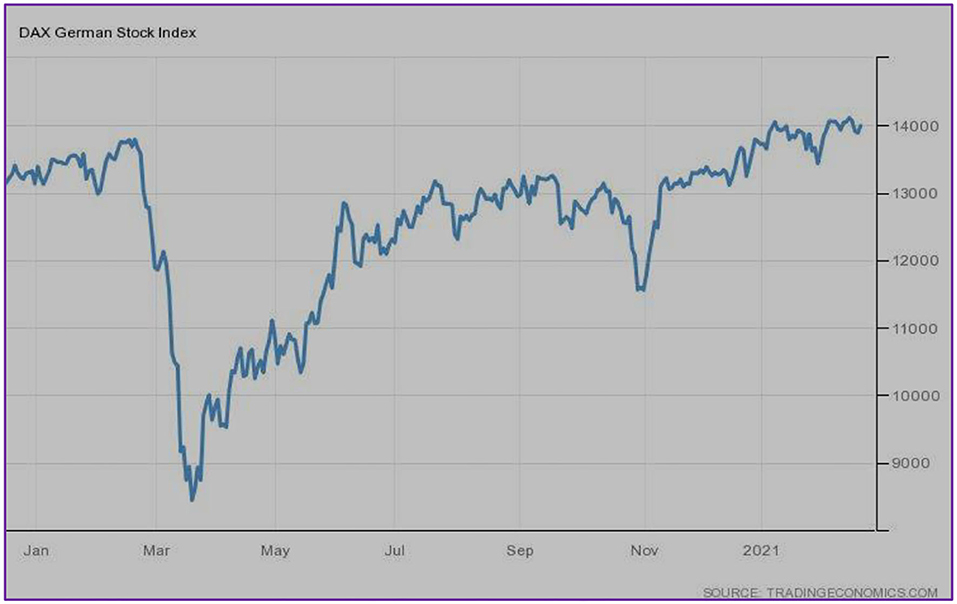
Figure 2 . DAX (Germany). Source: tradingeconomics.com . Reproduced with permission.
Figure 3 demonstrates the stock market situation of Dow Jones Industrial Averages, one of the USA's leading indices. The same situation was observed just like previous indices. The bullish trend was observed before March 2020, followed by the bearish trend during March-April, 2020. Index regained slowly, and revival leads to the extreme upward movements.
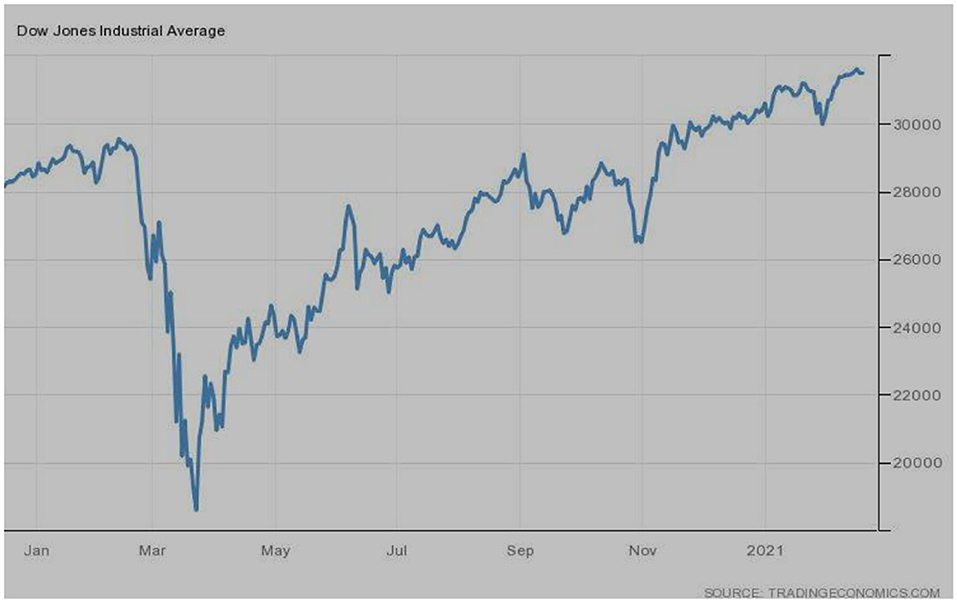
Figure 3 . Dow Jones industrial averages (USA). Source: tradingeconomics.com . Reproduced with permission.
Figure 4 illustrates the stock market trend of CAC 40, the index of France. The index was at its peak during February 2020. However, a sudden jerk was observed during March 2020, and the index touched the lowest points. The index recovered quite slowly, and to date, it could not recover its previous position. The fluctuations in the index can still be noticed.
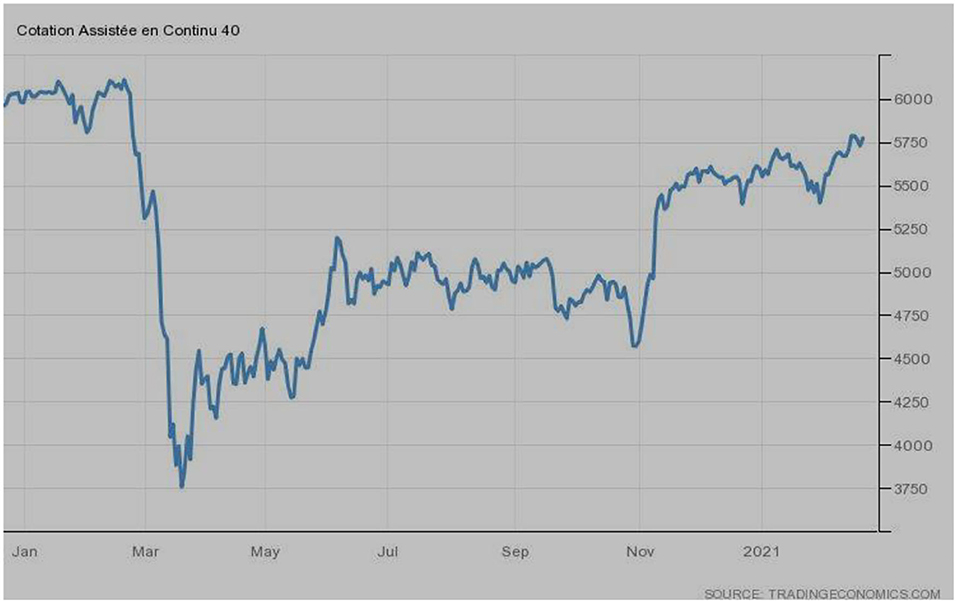
Figure 4 . CAC40 (France). Source: tradingeconomics.com . Reproduced with permission.
The variations in the FTSE 100 index of Europe's market conditions can be seen in Figure 5 . The bullish trend can be observed before March 2020, followed by the extreme bearish trend. The index went to the historical lowest points during March 2020. The upward movements were started during April 2020; however, slow movements were there, and the index is still in a slow recovery phase.
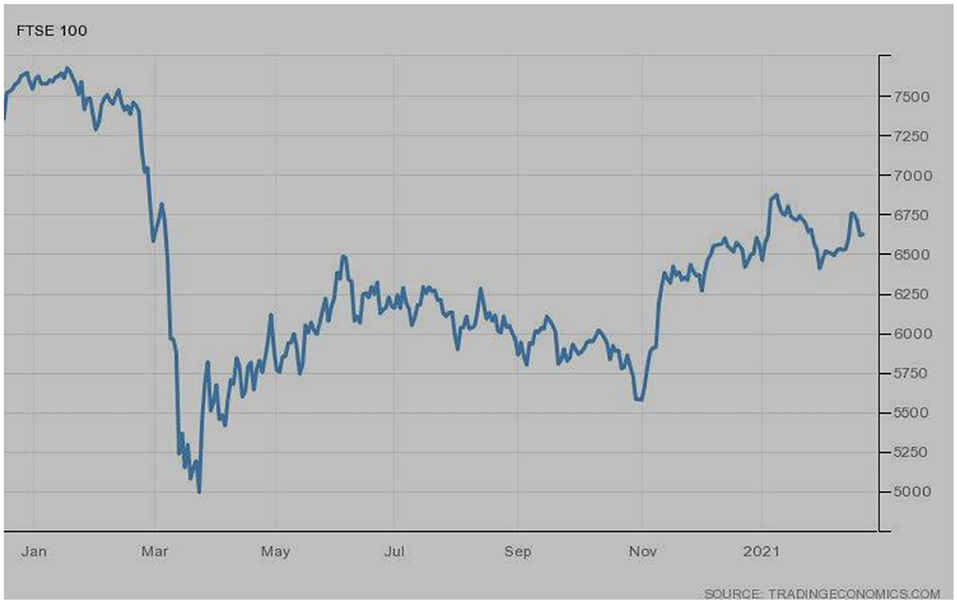
Figure 5 . FTSE-100 (Europe). Source: tradingeconomics.com . Reproduced with permission.
SENSEX index is a famous stock market index of India. Figure 6 is representing its performance. In January 2020, though the index was not performing very well, it faced the effects of COVID-19 during March 2020. The extreme slow revival was observed after March, and the index remains at the same pace. However, the gradual upward trends lead the index to its highest peak in 2021, as shown in the figure.
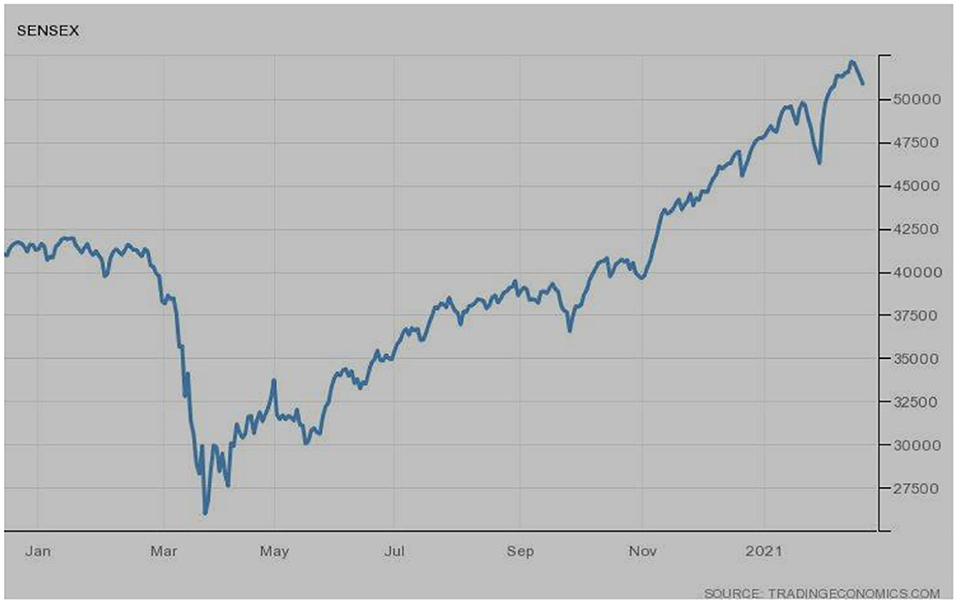
Figure 6 . SENSEX (India). Source: tradingeconomics.com . Reproduced with permission.
Figure 7 depicts Japan's famous index, i.e., the Nikkei 225. The index was in the recovery phase during January 2020; however, a bearish trend was observed from the outbreak. After March 2020, the recovery phase was there, but static movements were observed. Nevertheless, these slow recoveries finally touched the highest peak in 2021.
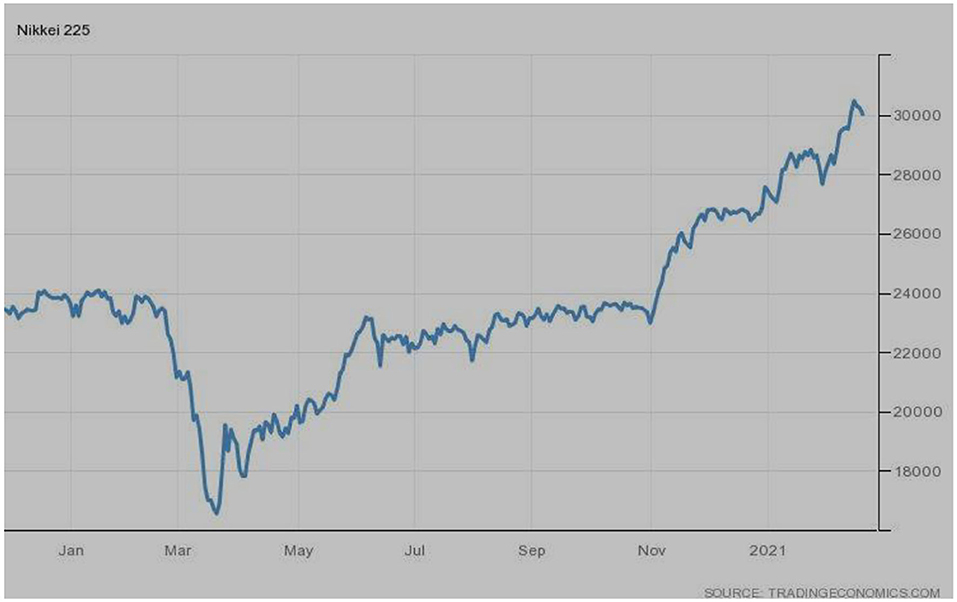
Figure 7 . Nikkei-225 (Japan). Source: tradingeconomics.com . Reproduced with permission.
Figure 8 deals with the NASDAQ stock market performance, one of the USA's leading indices. During the start of 2020, its performance was below average, ultimately reaching the lowest points in March 2020 as per the COVID-19 effects. The index escalates gradually, and to date, it jumped and touched the peak level.
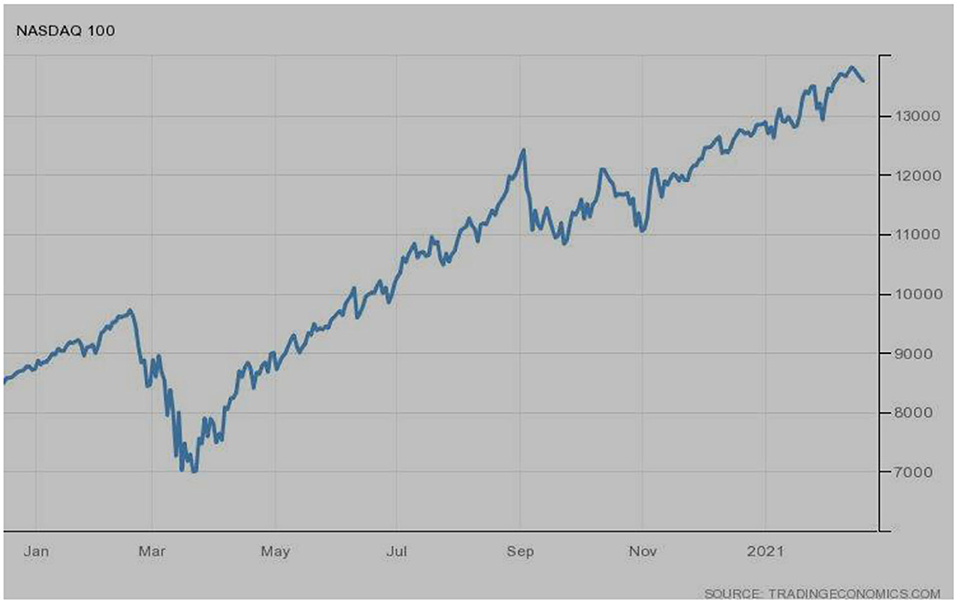
Figure 8 . NASDAQ (USA). Source: tradingeconomics.com . Reproduced with permission.
Referring to Figure 9 , the PSX-100 of Pakistan was performing well-before the sharp rise of COVID-19 in Pakistan. However, the month of March 2020 proved to be a terrible one; the index plunged and touched the lowest level. A slow revival was observed, which ultimately hit the highest points during 2021, as showing in the Figure 9 .
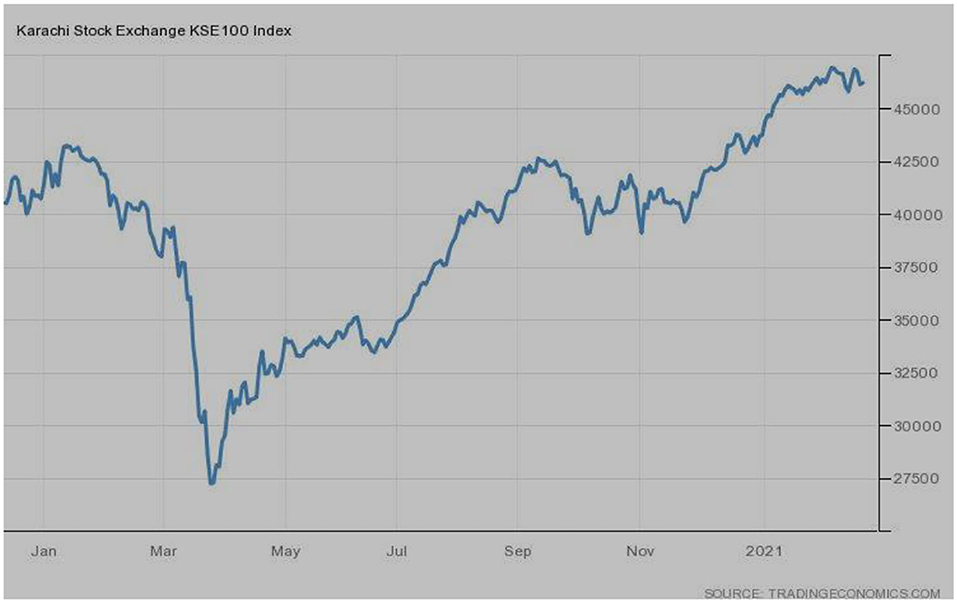
Figure 9 . PSX-100 (Pakistan). Source: tradingeconomics.com . Reproduced with permission.
The performance of the S&P 500 index, a prominent index of the USA, seems to be similar to the NASDAQ stock market index. However, before COVID and the recovery phase after March 2020 is better than the NASDAQ index. Currently, the index has reached the highest level as shown in Figure 10 .
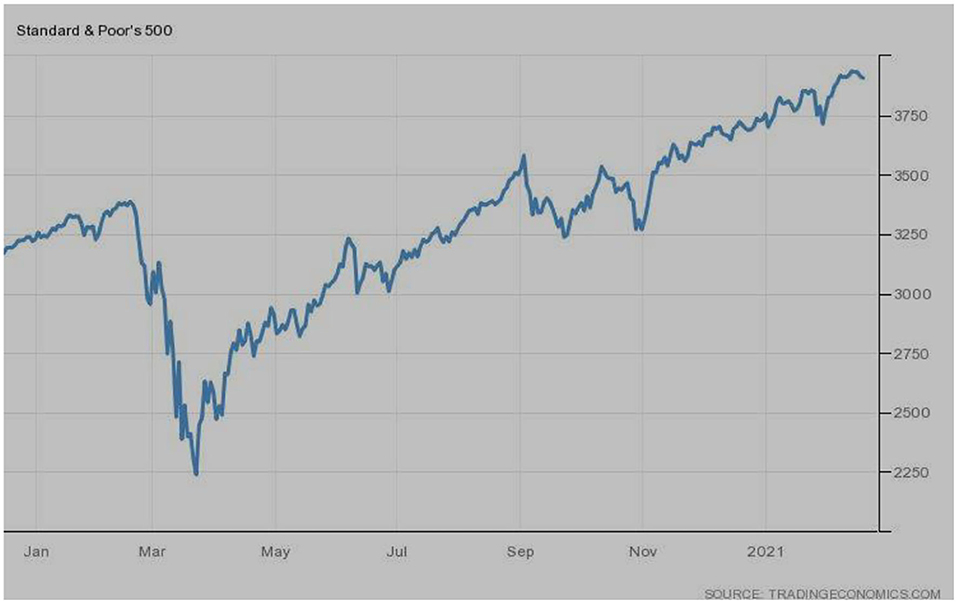
Figure 10 . S&P 500 (USA). Source: tradingeconomics.com . Reproduced with permission.
Figure 11 exhibits the Shanghai Stock Exchange Composite index of China, the origin of the COVID-19 pandemic. The SSE index is the outperformer index of China; however, it was severely affected by the pandemic. The index plunged from the start of the outbreak up to June 2020, followed by a sharp rise and now, with the gradual increase, the index has reached the maximum points.
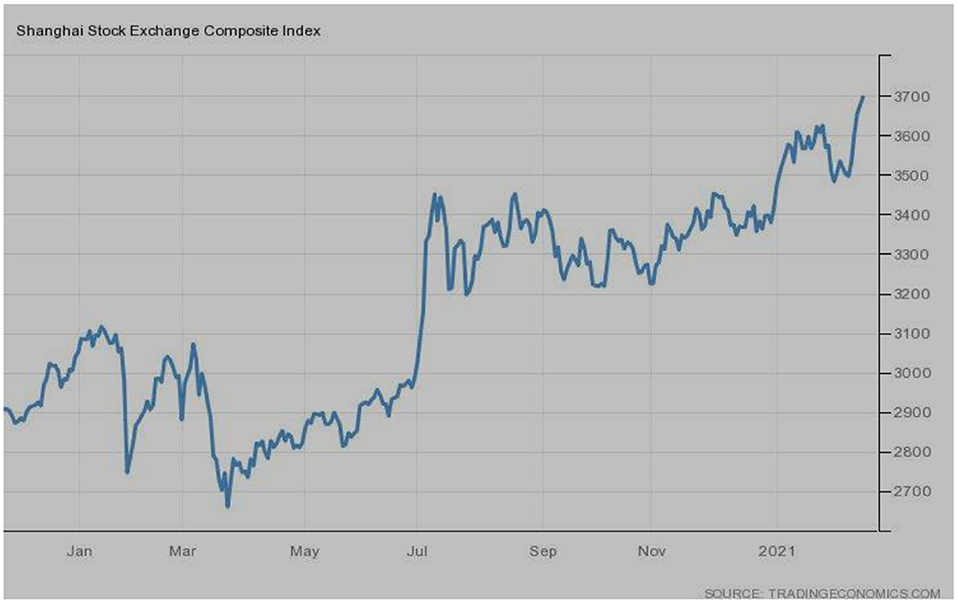
Figure 11 . SSE composite (China). Source: tradingeconomics.com . Reproduced with permission.
Implications for Stock Market Participants
The current study has some implications for market participants and policymakers, i.e., investors, managers, corporations, and governments. The investors must have market know how to invest their resources in favorable avenues even during the contingent market conditions, which have just happened during COVID-19. The investors can take guidance from the mangers and policymakers in this regard. In this way, investors can take rational decision making for their investments. Moreover, investors can generate their portfolios and risk management strategies. Besides, investors must focus on diversification to avoid losses during the pandemic situation.
The managers are the key stakeholders of financial markets; they have experience with stocks' risky nature during the pandemic and can take preventive measures accordingly. The mangers can increase the confidence level of investors, which lead them to make long term investments.
Governments can also play a vital role in assisting with the outbreak in tax rebates and interest-free loans. Governments can even facilitate the national markets by relaxing the lending policies and providing short-term loans on relaxing terms. Governments can conduct surveys and can assist investors in reducing their uncertainty.
Policymakers can develop successful methodologies for balancing financial investments during the outbreak. For this, they can focus on understanding the dynamics of stock markets in devising effective strategies. Besides, policymakers can integrate policies to cope-up with the financial and economic impacts of the COVID-19 outbreak. The emphasis must be on the improvement of stock market stability.
Unlocking the Future in Post-COVID-19 World
The aim of realizing sustainable growth, a big challenge for all economies, has been underscored by the novel virus. The pandemic has directed that economies are not goal-oriented in terms of their aspiration; they are required to achieve the milestones of a robust global economy. The greatest accomplishments are always achieved through the heights of determination. History is always there to provide lessons for the future. Nearly 75 years ago, amid World War II and one of Britain's most difficult hours, Winston Churchill inspired the whole nation not with the slogans to “reconsider what is achievable” but with a firm determination to “never surrender.” Now at the most difficult time of the century, we are required to continue our fight for what the world needs instead of reconsidering the sustainable development goals. This crisis requires a determined global effort to “build back better” by making a big reset to reach where we were before ( Kharas and and McArthur, 2020 ). Moreover, the leaders of the world must draw a new course of action for improving the functioning of international financial and monetary system to make it strong enough to cope with any such crisis in future ( Coulibaly and Prasad, 2020 ). The stock markets had to face the worst situation in the last 30 years, business operations have abruptly failed, and various economic sectors have been critically affected. However, the best point that came out of the COVID-19 pandemic is the businesses' pressure to be innovative and redefine their operations. One example is the tech community, which has been progressing to facilitate the community in adopting the technology to deal with the pandemic's challenges. Such technological innovations assist specific divisions of organizations or even the whole organizations to carry on their operations irrespective of the current contingent situation. Certainly, the world and the multinational business models will face diverse post-virus issues. Following the COVID-19 pandemic, the nations will observe new policies relating to restructuring and operational strategies, i.e., strategic workforce planning including remote staff planning, flexible conventions, workers proficiency, best practices and HR strategies; Crisis response and business continuity planning, risk control strategies and measures; Financial resources to weather future unforeseen events; Cloud-enabled IT infrastructure (and the attendant improved cybersecurity procedures); and the Redundant sourcing of necessities (inventory, materials and individuals), ( David, 2020 ).
The prospects of the stock markets and the economies are based on the availability and accessibility of the vaccine. The optimism about the vaccine has revitalized the investors' appetite regarding hotels, energy firms, and airlines. However, some others have been brutally affected by the pandemic and are forced to sell their respective market shares. Stock markets are largely dealing with the sentiment that tomorrow may be better than today, leading to a fundamental and perhaps enduring sea change. The development of more vaccines would pave the way for more optimism. What this result demonstrates is that while the virus is not yet beaten, it is beatable. That ray of light has lit up stock markets around the world. As usual, some stock market participants are there to look for something else to worry about ( Jack, 2020 ).
The current study deals with the impact of the COVID-19 pandemic on the global stock markets. It has focused on the contingent effects of previous and current pandemics on the financial markets. It has also elaborated on the impact of the pandemic on diverse pillars of the economy. The pandemic has severely hit the worldwide markets and posited challenges for economists, policymakers, head of states, international financial institutions, regulatory authorities, and health institutions to deal with the long-lasting effects of the outbreak. It has opened our eyes to concentrate our efforts to protect the future health of citizens and the also financial issues. In the current pandemic situation, the stock markets faced the effects of Covid-19, and this back-and-forth is ongoing. The majority of the world stock markets have suffered trillion-dollar losses ( Lyócsa et al., 2020 ). International financial institutions like IMP and World Bank have been forced to reduce their forecasted growth for 2020 and the years to come ( Boone et al., 2020 ). The global stock markets have been reported for their record decline. The month of March 2020 saw an unusual drop in most worldwide indices like the S&P 500 Index, NASDAQ, NIKKEI, SSE composite, CAC-40; DAX etc. However, the global stock markets regained and demonstrated the bullish trend during the days of April 2020. Irrespective of all these cyclical effects of the pandemic, still hopes are there for the sharp rise and speedy improvements in global stock markets' performance. Moreover, these past events have become a key for mankind to get insights for better future planning ( Su et al., 2021 ).
Behavioral vs. Conventional Finance
The two polar aspects of finance i.e., traditional finance and behavioral finance have also shed light on the psychology of investors during the COVID-19 pandemic. As far as traditional finance is concerned, investors behave rationally. The rational attitude of investors restricts them from imitating the decisions of others. Investors get the basic facts and figures about the stock markets through their own efforts, resultantly the fear of avoiding future losses compel the investors to sell their stocks and the market shows bearish trend ( Jabeen and Rizavi, 2021 ). The same has happened in the world's stock markets during the peak of pandemic. There have been sudden jerks observed around the global stock markets ( Jabeen and Farhan, 2020 ).
On the other hand, behavioral finance is supposed to consist of a set of theories which focus on the irrationality of investors. The viewpoint of irrationality of investors is the foundation of behavioral finance. The irrational aspect of investors forces them to follow the decision of other investors by setting aside their own information. In such context, investors have confidence on the decision of other investors as they feel that others may possess better information skills ( Jabeen and Rizavi, 2021 ). As a result the panic market conditions lead investors to blindly follow the others to protect their investment and market also depicts the bearish trend, the one which has been seen during the COVID-19 outbreak.
This debate has proven that both the traditional finance and behavioral finance have provided the same mechanism during the COVID-19 pandemic, irrespective of the fact that these pillars of finance deal with the opposing behaviors of investors i.e., rational and irrational. In both of the scenarios, the investors have sale their shares and resultantly the bearish trend has been observed.
Data Availability Statement
The raw data supporting the conclusions of this article will be made available by the authors, without undue reservation.
Author Contributions
All authors listed have made a substantial, direct and intellectual contribution to the work, and approved it for publication.
Conflict of Interest
The authors declare that the research was conducted in the absence of any commercial or financial relationships that could be construed as a potential conflict of interest.
Publisher's Note
All claims expressed in this article are solely those of the authors and do not necessarily represent those of their affiliated organizations, or those of the publisher, the editors and the reviewers. Any product that may be evaluated in this article, or claim that may be made by its manufacturer, is not guaranteed or endorsed by the publisher.
Abbas, J., Wang, D., Su, Z., and Ziapour, A. (2021). The role of social media in the advent of COVID-19 pandemic: crisis management, mental health challenges and implications. Risk Manag. Healthc. Policy 14, 1917–1932. doi: 10.2147/RMHP.S284313
PubMed Abstract | CrossRef Full Text | Google Scholar
Ahmar, A. S., and del Val, E. B. (2020). SutteARIMA: short-term forecasting method, a case: Covid-19 and stock market in Spain. Sci. Total Environ. 729:138883. doi: 10.1016/j.scitotenv.2020.138883
Al Rjoub, S. A. (2011). Business cycles, financial crises, and stock volatility in Jordan stock exchange. Int. J. Econ. Perspect. 5:21. doi: 10.2139/ssrn.1461819
CrossRef Full Text | Google Scholar
Al Rjoub, S. A., and Azzam, H. (2012). Financial crises, stock returns and volatility in an emerging stock market: the case of Jordan. Journal of Economic Studies 39, 178–211. doi: 10.1108/01443581211222653
Al Rjoub, S. A. M. (2009). Business cycles, financial crises, and stock volatility in Jordan stock exchange. Soc. Sci. Electron. Publish. 31, 127–132.
Google Scholar
Alam, M. M., Wei, H., and Wahid, A. N. (2020). COVID-19 outbreak and sectoral performance of the Australian stock market: an event study analysis. Curr. Protocols 60, 482-495. doi: 10.1111/1467-8454.12215
Alameer, Z., Elaziz, M. A., Ewees, A. A., Ye, H., and Jianhua, Z. (2019). Forecasting copper prices using hybrid adaptive neuro-fuzzy inference system and genetic algorithms. Nat. Resour. Res. 28, 1385–1401. doi: 10.1007/s11053-019-09473-w
Al-Awadhi, A. M., Alsaifi, K., Al-Awadhi, A., and Alhammadi, S. (2020). Death and contagious infectious diseases: impact of the COVID-19 virus on stock market returns. J. Behav. Exp. Finance 27:100326. doi: 10.1016/j.jbef.2020.100326
Aqeel, M., Shuja, k,. H., Abbas, J., and Ziapour, A. (2021). The influence of illness perception, anxiety, and depression disorders on student mental health during COVID-19 outbreak in Pakistan: a web-based cross sectional survey. Int. J. Hum. Rights Healthc. 14, 1–20. doi: 10.1108/IJHRH-10-2020-0095
Ashraf, B. N. (2020). Stock markets' reaction to COVID-19: cases or fatalities? Res. Int. Bus. Finance 54:101249. doi: 10.1016/j.ribaf.2020.101249
Aslam, F., Mohmand, Y. T., Ferreira, P., Memon, B. A., Khan, M., and Khan, M. (2020). Network analysis of global stock markets at the beginning of the coronavirus disease (Covid-19) outbreak. Borsa Istanb. Rev. 20, 49-61. doi: 10.1016/j.bir.2020.09.003
Bagchi, B., Chatterjee, S., Ghosh, R., and Dandapat, D. (2020). Coronavirus outbreak and the great lockdown: impact on oil prices and major stock markets across the globe (Heidelberg; Singapore: Springer), 112. doi: 10.1007/978-981-15-7782-6
Baker, S. R., Bloom, N., Davis, S. J., Kost, K. J., Sammon, M. C., and Viratyosin, T. (2020). The unprecedented stock market impact of COVID-19 (No. w26945). Natl. Bur. Econ. Res . doi: 10.3386/w26945
Baldwin, R. E., and Weder, B. (2020). Economics in the Time of COVID-19 . Washington, DC: CEPR Press.
Barro, R. J., Ursua, J. F., and Weng, J. (2020). The coronavirus and the great influenza epidemic: lessons from the “Spanish Flu” for the coronavirus' potential effects on mortality and economic activity. Natl. Bur. Econ. Res . doi: 10.3386/w26866
Bash, A., and Alsaifi, K. (2019). Fear from uncertainty: an event study of Khashoggi and stock market returns. J. Behav. Exp. Finance 23, 54–58. doi: 10.1016/j.jbef.2019.05.004
Boone, L., Haugh, D., Pain, N., Salins, V., and Boone, L. (2020). Tackling the Fallout from COVID-19. Economics in the Time of COVID-19 (London: CEPR Press), 37.
Caporale, G. M., Plastun, A., and Makarenko, I. (2019). Force majeure events and stock market reactions in Ukraine. Invest. Manag. Financ. Innov. 16, 334–345. doi: 10.21511/imfi.16(1)0.2019.26
Chen, M. H., Jang, S. S., and Kim, W. G. (2007). The impact of the SARS outbreak on Taiwanese hotel stock performance: an event-study approach. Int. J. Hosp. Manag. 26, 200–212. doi: 10.1016/j.ijhm.2005.11.004
Chen, M. P., Lee, C. C., Lin, Y. H., and Chen, W. Y. (2018). Did the SARS epidemic weaken the integration of Asian stock markets? Evidence from smooth time-varying cointegration analysis. Econ. Res. Ekon. Istraz. 3, 908–926. doi: 10.1080/1331677X.2018.1456354
Chowdhury, E. K., Khan, I. I., and Dhar, B. K. (2020). Catastrophic impact of Covid-19 on the global stock markets and economic activities. Bus. Soc. Rev. 1–24. doi: 10.1111/basr.12219
Chriscaden, K. (2020). Impact of COVID-19 on People's Livelihoods, Their Health and our Food Systems [Joint statement by ILO, FAO, IFAD and WHO] . Available online at: https://www.who.int/news/item/13-10-2020-impact-of-covid-19-on-people's-livelihoods-their-health-and-our-food-systems (accessed February 13, 2021).
Chudik, A., Mohaddes, K., Pesaran, M. H., Raissi, M., and Rebucci, A. (2020). Economic Consequences of Covid-19: A Counterfactual Multi-Country analysis. VoxEU.Org . Available online at: https://voxeu.org/article/economic-consequences-covid-19-multi-country-analysis (accessed October 19, 2020).
Corbet, S., Hou, Y., Hu, Y., Lucey, B., and Oxley, L. (2020). Aye Corona! The contagion effects of being named Corona during the COVID-19 pandemic. Finance Res. Lett. 38:2020. doi: 10.2139/ssrn.3561866
Coulibaly, B., and Prasad, E. (2020). “The international monetary and financial system: how to fit it for purpose?,” in Reimagining the Global Economy: Building Back Better in a Post-COVID-19 World . Washington, DC: The Brookings Institution.
Cox, J., Greenwald, D. L., and Ludvigson, S. C. (2020). What explains the COVID-19 stock market. Natl. Bur. Econ. Res . doi: 10.3386/w27784
Cucinotta, D., and Vanelli, M. (2020). WHO declares COVID-19 a pandemic. Acta Biomed. Ateneo Parm. 9, 157–160. doi: 10.23750/abm.v91i1.9397
Czech, K., Wielechowsk, M., Kotyza, P., Benešová, I., and Laputková, A. (2020). Shaking stability: COVID-19 impact on the Visegrad group countries' financial markets. Sustain. Times 12:6282. doi: 10.3390/su12156282
Dang, T. L., and Nguyen, T. M. H. (2020). Liquidity risk and stock performance during the financial crisis. Res. Int. Bus. Finance 52:101165. doi: 10.1016/j.ribaf.2019.101165
David, S. (2020). International Business Models for a Post-COVID-19 World HLB. HLB The Global Advisory and Accounting Network . Available online at: https://www.hlb.global/international-business-models-for-a-post-covid-19-world/ (accessed February 23, 2021).
Delisle, J. (2003). SARS, greater China, and the pathologies of globalization and transition. Orbis 47, 587–604. doi: 10.1016/S0030-4387(03)00076-0
Dong, G. N., and Heo, Y. (2014). Flu epidemic, limited attention and analyst forecast behavior. Limited Attent. Analyst Forecast Behav. doi: 10.2139/ssrn.3353255
Fernandes, N. (2020). Economic effects of coronavirus outbreak (COVID-19) on the world economy . doi: 10.2139/ssrn.3557504
He, P., Sun, Y., Zhang, Y., and Li, T. (2020). COVID−19's impact on stock prices across different sectors—an event study based on the chinese stock market. Emerg. Mark. Finance Trade 56, 2198–2212. doi: 10.1080/1540496X.2020.1785865
He, Q., Liu, J., Wang, S., and Yu, J. (2020). The impact of COVID-19 on stock markets. Econ. Political Stud. 8, 275–288. doi: 10.1080/20954816.2020.1757570
Helppie McFall, B. (2011). Crash and wait? The impact of the great recession on the retirement plans of older Americans. Am. Econ. Rev. 101, 40–44. doi: 10.1257/aer.101.3.40
Hui, D. S., Azhar, D. I., Madani, T. A., Ntoumi, F., Kock, R., Dar, O., et al. (2020). The continuing 2019 nCoV epidemic threat of novel coronaviruses to global health—the latest 2019 novel coronavirus outbreak in Wuhan, China. Int. J. Infect. Dis. 91, 264–266. doi: 10.1016/j.ijid.2020.01.009
Iyke, B. N. (2020). The disease outbreak channel of exchange rate return predictability: evidence from COVID-19. Emerg. Mark. Finance Trade 56, 2277–2297. doi: 10.1080/1540496X.2020.1784718
Jabeen, S., and Rizavi, S. S. (2021). Long term and short term herding prospects: evidence from Pakistan stock exchange. Abasyn J. Manag. Sci. 14, 119–144. doi: 10.34091/AJSS.14.1.08
Jabeen, S., and Farhan, M. (2020). COVID-19: the pandemic's impact on economy and stock markets. J. Manag. Sci. 14, 29–43.
Jack, S. (2020). Covid-19: Global Stock Markets Rocket on Vaccine Hopes. BBC News . Available online at: https://www.bbc.com/news/business-54874108 (accessed November 9, 2020).
Jones, L., Palumbo, D., and Brown, D. (2021). Coronavirus: How the Pandemic has Changed the World Economy. BBC News . Available online at: https://www.bbc.com/news/business-51706225 (accessed January 24, 2021).
Kalra, R., Henderson, G. V., and Raines, G. A. (1993). Effects of the chernobyl nuclear accident on utility share prices. Q. J. Bus. Econ. 32, 52–77.
Kaplanski, G., and Levy, H. (2010). Sentiment and stock prices: the case of aviation disasters. J. Financ. Econ. 95, 174–201. doi: 10.1016/j.jfineco.2009.10.002
Karlsson, M., and Nilsson, S. (2014). The impact of the 1918 Spanish flu epidemic on economic performance in Sweden: an investigation into the consequences of an extraordinary mortality shock. J. Health Econ. 36, 1–9. doi: 10.1016/j.jhealeco.2014.03.005
Keating, J. (2001). An investigation into the cyclical incidence of dengue feve. Soc. Sci. Med. 53, 1587–1597. doi: 10.1016/S0277-9536(00)00443-3
Kharas, H., and McArthur, J. W. (2020). “Sustainable development goals: how can they be a handrail for recovery?,” in Reimagining the Global Economy: Building Back Better in a Post-COVID-19 World (Washington, DC. The Brookings Institution). Available online at: https://www.brookings.edu/multi-chapter-report/reimagining-the-global-economy-building-back-better-in-a-post-covid-19-world
Khatatbeh, I. N., Hani, M. B., and Abu-Alfoul, M. N. (2020). The impact of COVID-19 pandemic on global stock markets: an event study. Int. J. Econ. Bus. 8, 505–514. doi: 10.35808/ijeba/602
Koley, T. K., and Dhole, M. (2020). COVID-19 pandemic: The Deadly Coronavirus Outbreak in the 21st Century, 1st Edn . Oxfordshire: Routledge. doi: 10.4324/9781003095590
Lanfear, M. G., Lioui, A., and Siebert, M. G. (2018). Market anomalies and disaster risk: evidence from extreme weather events. J. Financial Mark. 46:100477. doi: 10.1016/j.finmar.2018.10.003
Lee, J. W., and McKibbin, W. J. (2004). “Estimating the global economic costs of SARS,” in Learning from SARS: Preparing for the Next Disease Outbreak , eds S. Knobler, A. Mahmoud, and S. Lemon, et al. (Washington, DC: National Academies Press).
Liu, H., Manzoor, A., Wang, C., Zhang, L., and Manzoor, Z. (2020). The COVID-19 outbreak and affected countries stock markets response. Int. J. Environ. Res. Public Health 17:2800. doi: 10.3390/ijerph17082800
Loh, E. (2006). The impact of SARS on the performance and risk profile of airline stocks. Int. J. Transp. Econ. 33, 401–422.
Lyócsa, Š., Baumöhl, E., Výrost, T., and Molná, P. (2020). Fear of the coronavirus and the stock markets. Finance Res. Lett. 36:101735. doi: 10.1016/j.frl.2020.101735
Machmuddah, Z., Utomo, S.t., Suhartono, E., Ali, S., and Ghulam, W. A. (2020). Stock market reaction to COVID-19: evidence in customer goods sector with the implication for open innovation. J. Open Innov.: Technol. Mark. Complex. 6:99. doi: 10.3390/joitmc6040099
Maqsood, A., Abbas, J., Rehman, G., and Mubeen, R. (2021). The paradigm shift for educational system continuance in the advent of COVID-19 pandemic: mental health challenges and reflections. Curr. Res. Behav. Sci. 2, 1–5. doi: 10.1016/j.crbeha.2020.100011
MckKibbin, W. J., and Sidorenko, A. A. (2006). Global macroeconomic consequences of pandemic influenza. 79.
Mctier, B. C., Tse, Y., and Wald, J. K. (2011). Do stock markets catch the flu? J. Financ. Quant. Anal. 48, 979–1000. doi: 10.1017/S0022109013000239
Mishra, M. K. (2020). The World After COVID-19 and Its Impact on Global Economy . Kiel: ZBW–Leibniz Information Centre for Economics. Available online at: https://www.econstor.eu/handle/10419/215931
Ngwakwe, C. V. (2020). Effect of COVID-19 pandemic on global stock market values: a differential analysis. Economica 16, 261–275.
Nikkinen, J., Omran, M. M., and Sahlstr, M. P. (2008). Stock returns and volatility following the september 11 attacks: evidence from 53 equity markets. Int. Rev. Financial Anal. 17, 27–46. doi: 10.1016/j.irfa.2006.12.002
Nippani, S., and Washer, K. M. (2004). SARS: a non-event for affected countries' stock markets? Appl. Financial Econ. 14, 1105–1110 doi: 10.1080/0960310042000310579
OECD (2020). OECDEmployment Outlook 2020 Facing the Jobs Crisis. Paris: OECD. Available online at: http://www.oecd.org/employment-outlook/2020 (accessed February 13, 2021).
Okorie, D. I., and Lin, B. (2021). Stock markets and the COVID-19 fractal contagion effects. Finance Res. Lett. 38:101640.
PubMed Abstract | Google Scholar
Ozili, P. K., and Arun, T. (2020). Spillover of COVID-19: impact on the global economy. doi: 10.2139/ssrn.3562570
Qin, M., Zhang, Y. C., and Su, C. W. (2020). The essential role of pandemics: a fresh insight into the oil market. Energy Res. Lett. 1, 1–6. doi: 10.46557/001c.13166
Ramelli, S., and Wagner, A. F. (2020). Feverish stock price reactions to COVID-19. Rev. Corp. Finance Stud. 9, 622–655. doi: 10.1093/rcfs/cfaa012
Ranju, P. K., and Mallikarjunappa, T. (2019). Spillover effect of MandA announcements on acquiring firms' rivals: evidence from India. Glob. Bus. Rev. 20, 692–707. doi: 10.1177/0972150919837080
Rengasamy, E. (2012). Sovereign debt crisis in the euro zone and its impact on the BRICS's stock index returns and volatility. Econ. Finance Rev. 2, 37–46. Retrieved from: https://tradingeconomics.com/pakistan/stock-market (accessed February 21, 2021).
Righi, M. B., and Ceretta, P. S. (2011). Analyzing the structural behavior of volatility in the major European markets during the Greek crisis. Econ. Bull . 31, 3016–3029.
Sansa, N. A. (2020). The impact of the COVID-19 on the financial markets: evidence from China and USA. Electron. Res. J. Soc. Sci. Human. 2:11. doi: 10.2139/ssrn.3567901
Schwert, G. W. (2011). Stock volatility during the recent financial crisis. Eur. Financ. Manag. 17, 789–805. doi: 10.1111/j.1468-036X.2011.00620.x
Shafi, M., Liu, J., and Ren, W. (2020). Impact of COVID-19 pandemic on micro, small, and medium-sized enterprises operating in Pakistan. Res. Glob. 2:100018. doi: 10.1016/j.resglo.2020.100018
Sharma, P. (2021). Coronavirus News, Markets and AI: The COVID-19 Diaries . Oxfordshire: Routledge. doi: 10.4324/9781003138976-1
Singh, B., Dhall, R., Narang, S., and Rawat, S. (2020). The outbreak of COVID-19 and stock market responses: an event study and panel data analysis for G-20 countries. Glob. Bus. Rev. 1–26. doi: 10.1177/0972150920957274
Sobieralski, J. B. (2020). Covid-19 and airline employment: insights from historical uncertainty shocks to the industry. Transp. Res. Interdiscip. Perspect. 5:100123. doi: 10.1016/j.trip.2020.100123
Su, Z., McDonnell, D., Cheshmehzangi, A., Abbas, J., Li, X., and Cai, Y. (2021). The promise and perils of Unit 731 data. BMG Glob. Health 6, 1–4. doi: 10.1136/bmjgh-2020-004772
Topcu, M., and Gulal, O. S. (2020). The impact of COVID-19 on emerging stock markets. Finance Res. Lett. 36, 101691.
UNWTO (2020). Impact Assessment of the COVID-19 Outbreak on International Tourism UNWTO . Available online at: https://www.unwto.org/impact-assessment-of-the-covid-19-outbreak-on-international-tourism (accessed February 13, 2021).
Wang, C., Wang, D., Duan, K., and Mubeen, R. (2021). Global financial crisis, smart lockdown strategies, and the COVID-19 spillover impacts: a global perspective implications from Southeast Asia. Front. Psychiatry 12:643783. doi: 10.3389/fpsyt.2021.643783
Zhang, D., Hu, M., and Ji, Q. (2020). Financial markets under the global pandemic of COVID-19. Finance Res. Lett. 36:101528. doi: 10.1016/j.frl.2020.101528
Keywords: COVID-19, stock markets, market indices, behavioral finance, SARS
Citation: Jabeen S, Farhan M, Zaka MA, Fiaz M and Farasat M (2022) COVID and World Stock Markets: A Comprehensive Discussion. Front. Psychol. 12:763346. doi: 10.3389/fpsyg.2021.763346
Received: 23 August 2021; Accepted: 30 September 2021; Published: 28 February 2022.
Reviewed by:
Copyright © 2022 Jabeen, Farhan, Zaka, Fiaz and Farasat. This is an open-access article distributed under the terms of the Creative Commons Attribution License (CC BY) . The use, distribution or reproduction in other forums is permitted, provided the original author(s) and the copyright owner(s) are credited and that the original publication in this journal is cited, in accordance with accepted academic practice. No use, distribution or reproduction is permitted which does not comply with these terms.
*Correspondence: Muhammad Farhan, muhammad.farhan@numl.edu.pk
Disclaimer: All claims expressed in this article are solely those of the authors and do not necessarily represent those of their affiliated organizations, or those of the publisher, the editors and the reviewers. Any product that may be evaluated in this article or claim that may be made by its manufacturer is not guaranteed or endorsed by the publisher.

An official website of the United States government
The .gov means it’s official. Federal government websites often end in .gov or .mil. Before sharing sensitive information, make sure you’re on a federal government site.
The site is secure. The https:// ensures that you are connecting to the official website and that any information you provide is encrypted and transmitted securely.
- Publications
- Account settings
Preview improvements coming to the PMC website in October 2024. Learn More or Try it out now .
- Advanced Search
- Journal List
- Entropy (Basel)

Stock Market Volatility and Return Analysis: A Systematic Literature Review
Roni bhowmik.
1 School of Economics and Management, Jiujiang University, Jiujiang 322227, China
2 Department of Business Administration, Daffodil International University, Dhaka 1207, Bangladesh
Shouyang Wang
3 Academy of Mathematics and Systems Science, Chinese Academy of Sciences, Beijing 100080, China; nc.ca.ssma@gnawys
In the field of business research method, a literature review is more relevant than ever. Even though there has been lack of integrity and inflexibility in traditional literature reviews with questions being raised about the quality and trustworthiness of these types of reviews. This research provides a literature review using a systematic database to examine and cross-reference snowballing. In this paper, previous studies featuring a generalized autoregressive conditional heteroskedastic (GARCH) family-based model stock market return and volatility have also been reviewed. The stock market plays a pivotal role in today’s world economic activities, named a “barometer” and “alarm” for economic and financial activities in a country or region. In order to prevent uncertainty and risk in the stock market, it is particularly important to measure effectively the volatility of stock index returns. However, the main purpose of this review is to examine effective GARCH models recommended for performing market returns and volatilities analysis. The secondary purpose of this review study is to conduct a content analysis of return and volatility literature reviews over a period of 12 years (2008–2019) and in 50 different papers. The study found that there has been a significant change in research work within the past 10 years and most of researchers have worked for developing stock markets.
1. Introduction
In the context of economic globalization, especially after the impact of the contemporary international financial crisis, the stock market has experienced unprecedented fluctuations. This volatility increases the uncertainty and risk of the stock market and is detrimental to the normal operation of the stock market. To reduce this uncertainty, it is particularly important to measure accurately the volatility of stock index returns. At the same time, due to the important position of the stock market in the global economy, the beneficial development of the stock market has become the focus. Therefore, the knowledge of theoretical and literature significance of volatility are needed to measure the volatility of stock index returns.
Volatility is a hot issue in economic and financial research. Volatility is one of the most important characteristics of financial markets. It is directly related to market uncertainty and affects the investment behavior of enterprises and individuals. A study of the volatility of financial asset returns is also one of the core issues in modern financial research and this volatility is often described and measured by the variance of the rate of return. However, forecasting perfect market volatility is difficult work and despite the availability of various models and techniques, not all of them work equally for all stock markets. It is for this reason that researchers and financial analysts face such a complexity in market returns and volatilities forecasting.
The traditional econometric model often assumes that the variance is constant, that is, the variance is kept constant at different times. An accurate measurement of the rate of return’s fluctuation is directly related to the correctness of portfolio selection, the effectiveness of risk management, and the rationality of asset pricing. However, with the development of financial theory and the deepening of empirical research, it was found that this assumption is not reasonable. Additionally, the volatility of asset prices is one of the most puzzling phenomena in financial economics. It is a great challenge for investors to get a pure understanding of volatility.
A literature reviews act as a significant part of all kinds of research work. Literature reviews serve as a foundation for knowledge progress, make guidelines for plan and practice, provide grounds of an effect, and, if well guided, have the capacity to create new ideas and directions for a particular area [ 1 ]. Similarly, they carry out as the basis for future research and theory work. This paper conducts a literature review of stock returns and volatility analysis based on generalized autoregressive conditional heteroskedastic (GARCH) family models. Volatility refers to the degree of dispersion of random variables.
Financial market volatility is mainly reflected in the deviation of the expected future value of assets. The possibility, that is, volatility, represents the uncertainty of the future price of an asset. This uncertainty is usually characterized by variance or standard deviation. There are currently two main explanations in the academic world for the relationship between these two: The leverage effect and the volatility feedback hypothesis. Leverage often means that unfavorable news appears, stock price falls, leading to an increase in the leverage factor, and thus the degree of stock volatility increases. Conversely, the degree of volatility weakens; volatility feedback can be simply described as unpredictable stock volatility that will inevitably lead to higher risk in the future.
There are many factors that affect price movements in the stock market. Firstly, there is the impact of monetary policy on the stock market, which is extremely substantial. If a loose monetary policy is implemented in a year, the probability of a stock market index rise will increase. On the other hand, if a relatively tight monetary policy is implemented in a year, the probability of a stock market index decline will increase. Secondly, there is the impact of interest rate liberalization on risk-free interest rates. Looking at the major global capital markets, the change in risk-free interest rates has a greater correlation with the current stock market. In general, when interest rates continue to rise, the risk-free interest rate will rise, and the cost of capital invested in the stock market will rise simultaneously. As a result, the economy is expected to gradually pick up during the release of the reform dividend, and the stock market is expected to achieve a higher return on investment.
Volatility is the tendency for prices to change unexpectedly [ 2 ], however, all kinds of volatility is not bad. At the same time, financial market volatility has also a direct impact on macroeconomic and financial stability. Important economic risk factors are generally highly valued by governments around the world. Therefore, research on the volatility of financial markets has always been the focus of financial economists and financial practitioners. Nowadays, a large part of the literature has studied some characteristics of the stock market, such as the leverage effect of volatility, the short-term memory of volatility, and the GARCH effect, etc., but some researchers show that when adopting short-term memory by the GARCH model, there is usually a confusing phenomenon, as the sampling interval tends to zero. The characterization of the tail of the yield generally assumes an ideal situation, that is, obeys the normal distribution, but this perfect situation is usually not established.
Researchers have proposed different distributed models in order to better describe the thick tail of the daily rate of return. Engle [ 3 ] first proposed an autoregressive conditional heteroscedasticity model (ARCH model) to characterize some possible correlations of the conditional variance of the prediction error. Bollerslev [ 4 ] has been extended it to form a generalized autoregressive conditional heteroskedastic model (GARCH model). Later, the GARCH model rapidly expanded and a GARCH family model was created.
When employing GARCH family models to analyze and forecast return volatility, selection of input variables for forecasting is crucial as the appropriate and essential condition will be given for the method to have a stationary solution and perfect matching [ 5 ]. It has been shown in several findings that the unchanged model can produce suggestively different results when it is consumed with different inputs. Thus, another key purpose of this literature review is to observe studies which use directional prediction accuracy model as a yardstick from a realistic point of understanding and has the core objective of the forecast of financial time series in stock market return. Researchers estimate little forecast error, namely measured as mean absolute deviation (MAD), root mean squared error (RMSE), mean absolute error (MAE), and mean squared error (MSE) which do not essentially interpret into capital gain [ 6 , 7 ]. Some others mention that the predictions are not required to be precise in terms of NMSE (normalized mean squared error) [ 8 ]. It means that finding the low rate of root mean squared error does not feed high returns, in another words, the relationship is not linear between two.
In this manuscript, it is proposed to categorize the studies not only by their model selection standards but also for the inputs used for the return volatility as well as how precise it is spending them in terms of return directions. In this investigation, the authors repute studies which use percentage of success trades benchmark procedures for analyzing the researchers’ proposed models. From this theme, this study’s authentic approach is compared with earlier models in the literature review for input variables used for forecasting volatility and how precise they are in analyzing the direction of the related time series. There are other review studies on return and volatility analysis and GARCH-family based financial forecasting methods done by a number of researchers [ 9 , 10 , 11 , 12 , 13 ]. Consequently, the aim of this manuscript is to put forward the importance of sufficient and necessary conditions for model selection and contribute for the better understanding of academic researchers and financial practitioners.
Systematic reviews have most notable been expanded by medical science as a way to synthesize research recognition in a systematic, transparent, and reproducible process. Despite the opportunity of this technique, its exercise has not been overly widespread in business research, but it is expanding day by day. In this paper, the authors have used the systematic review process because the target of a systematic review is to determine all empirical indication that fits the pre-decided inclusion criteria or standard of response to a certain research question. Researchers proved that GARCH is the most suitable model to use when one has to analysis the volatility of the returns of stocks with big volumes of observations [ 3 , 4 , 6 , 9 , 13 ]. Researchers observe keenly all the selected literature to answer the following research question: What are the effective GARCH models to recommend for performing market volatility and return analysis?
The main contribution of this paper is found in the following four aspects: (1) The best GARCH models can be recommended for stock market returns and volatilities evaluation. (2) The manuscript considers recent papers, 2008 to 2019, which have not been covered in previous studies. (3) In this study, both qualitative and quantitative processes have been used to examine the literature involving stock returns and volatilities. (4) The manuscript provides a study based on journals that will help academics and researchers recognize important journals that they can denote for a literature review, recognize factors motivating analysis stock returns and volatilities, and can publish their worth study manuscripts.
2. Methodology
A systematic literature examination of databases should recognize as complete a list as possible of relevant literature while keeping the number of irrelevant knocks small. The study is conducted by a systematic based literature review, following suggestions from scholars [ 14 , 15 ]. This manuscript was led by a systematic database search, surveyed by cross-reference snowballing, as demonstrated in Figure 1 , which was adapted from Geissdoerfer et al. [ 16 ]. Two databases were selected for the literature search: Scopus and Web-of-Science. These databases were preferred as they have some major depositories of research and are usually used in literature reviews for business research [ 17 ].

Literature review method.
At first stage, a systematic literature search is managed. The keywords that were too broad or likely to be recognized in literature-related keywords with other research areas are specified below. As shown in Table 1 , the search string “market return” in ‘Title‘ respectively “stock market return”, “stock market volatility”, “stock market return volatility”, “GARCH family model* for stock return”, “forecasting stock return”, and GARCH model*, “financial market return and volatility” in ‘Topic’ separately ‘Article title, Abstract, Keywords’ were used to search for reviews of articles in English on the Elsevier Scopus and Thomson Reuters Web-of-Science databases. The asterisk (*) is a commonly used wildcard symbol that broadens a search by finding words that start with the same letters.
Literature search strings for database.
At second stage, suitable cross-references were recognized in this primary sample by first examining the publications’ title in the reference portion and their context and cited content in the text. The abstracts of the recognized further publications were examined to determine whether the paper was appropriate or not. Appropriate references were consequently added to the sample and analogously scanned for appropriate cross-references. This method was continual until no additional appropriate cross-references could be recognized.
At the third stage, the ultimate sample was assimilated, synthesized, and compiled into the literature review presented in the subsequent section. The method was revised a few days before the submission.
Additionally, the list of affiliation criteria in Table 2 , which is formed on discussions of the authors, with the summaries of all research papers were independently checked in a blind system method. Evaluations were established on the content of the abstract, with any extra information unseen, and were comprehensive rather than exclusive. In order to check for inter-coder dependability, an initial sample of 30 abstracts were studied for affiliation by the authors. If the abstract was not satisfactorily enough, the whole paper was studied. Simply, 4.61 percent of the abstract resulted in variance between the researchers. The above-mentioned stages reduced the subsequent number of full papers for examination and synthesis to 50. In order to recognize magnitudes, backgrounds, and moderators, these residual research papers were reviewed in two rounds of reading.
Affiliation criteria.
3. Review of Different Studies
In this paper, a large amount of articles were studied but only a few were well thought out to gather the quality developed earlier. For every published article, three groups were specified. Those groups were considered as index and forecast time period, input elements, econometric models, and study results. The first group namely “index and forecast time period with input elements” was considered since market situation like emerging, frontier, and developed markets which are important parameters of forecast and also the length of evaluation is a necessary characteristic for examining the robustness of the model. Furthermore, input elements are comparatively essential parameters for a forecast model because the analytical and diagnostic ability of the model is mainly supported on the inputs that a variable uses. In the second group, “model” was considered forecast models proposed by authors and other models for assessment. The last group is important to our examination for comparing studies in relationships of proper guiding return and volatility, acquired by using recommended estimate models, named the “study results” group.
Measuring the stock market volatility is an incredibly complex job for researchers. Since volatility tends to cluster, if today’s volatility is high, it is likely to be high tomorrow but they have also had an attractive high hit rate with major disasters [ 4 , 7 , 11 , 12 ]. GARCH models have a strong background, recently having crossed 30 years of the fast progress of GARCH-type models for investigating the volatility of market data. Literature of eligible papers were clustered in two sub groups, the first group containing GARCH and its variations model, and the second group containing bivariate and other multivariate GARCH models, summarized in a table format for future studies. Table 3 explains the review of GARCH and its variations models. The univariate GARCH model is for a single time series. It is a statistical model that is used to analyze a number of different kinds of financial data. Financial institutions and researchers usually use this model to estimate the volatility of returns for stocks, bonds, and market indices. In the GARCH model, current volatility is influenced by past innovation to volatility. GARCH models are used to model for forecast volatility of one time series. The most widely used GARCH form is GARCH (1, 1) and this has some extensions.
Different literature studies based on generalized autoregressive conditional heteroskedastic (GARCH) and its variations models.
Notes: APARCH (Asymmetric Power ARCH), AIC (Akaike Information Criterion), OHLC (Open-High-Low-Close Chart), NSE (National Stock Exchange of India), EWMA (Exponentially Weighted Moving Average), CGARCH (Component GARCH), BDS (Brock, Dechert & Scheinkman) Test, ARCH-LM (ARCH-Lagrange Multiplier) test, VAR (Vector Autoregression) model, VEC (Vector Error Correction) model, ARFIMA (Autoregressive Fractional Integral Moving Average), FIGARCH (Fractionally Integrated GARCH), SHCI (Shanghai Stock Exchange Composite Index), SZCI (Shenzhen Stock Exchange Component Index), ADF (Augmented Dickey–Fuller) test, BSE (Bombay Stock Exchange), and PGARCH (Periodic GARCH) are discussed.
In a simple GARCH model, the squared volatility σ t 2 is allowed to change on previous squared volatilities, as well as previous squared values of the process. The conditional variance satisfies the following form: σ t 2 = α 0 + α 1 ϵ t − 1 2 + … + α q ϵ t − q 2 + β 1 σ t − 1 2 + … + β p σ t − p 2 where, α i > 0 and β i > 0 . For the GARCH model, residuals’ lags can substitute by a limited number of lags of conditional variances, which abridges the lag structure and in addition the estimation method of coefficients. The most often used GARCH model is the GARCH (1, 1) model. The GARCH (1, 1) process is a covariance-stationary white noise process if and only if α 1 + β < 1 . The variance of the covariance-stationary process is given by α 1 / ( 1 − α 1 − β ) . It specifies that σ n 2 is based on the most recent observation of φ t 2 and the most recent variance rate σ n − 1 2 . The GARCH (1, 1) model can be written as σ n 2 = ω + α φ n − 1 2 + β σ n − 1 2 and this is usually used for the estimation of parameters in the univariate case.
Though, GARCH model is not a complete model, and thus could be developed, these developments are detected in the form of the alphabet soup that uses GARCH as its key component. There are various additions of the standard GARCH family models. Nonlinear GARCH (NGARCH) was proposed by Engle and Ng [ 18 ]. The conditional covariance equation is in the form: σ t 2 = γ + α ( ε t − 1 − ϑ σ t − 1 ) 2 + β σ t − 1 2 , where α , β , γ > 0 . The integrated GARCH (IGARCH) is a restricted version of the GARCH model, where the sum of all the parameters sum up to one and this model was introduced by Engle and Bollerslev [ 19 ]. Its phenomenon might be caused by random level shifts in volatility. The simple GARCH model fails in describing the “leverage effects” which are detected in the financial time series data. The exponential GARCH (EGARCH) introduced by Nelson [ 5 ] is to model the logarithm of the variance rather than the level and this model accounts for an asymmetric response to a shock. The GARCH-in-mean (GARCH-M) model adds a heteroskedasticity term into the mean equation and was introduced by Engle et al. [ 20 ]. The quadratic GARCH (QGARCH) model can handle asymmetric effects of positive and negative shocks and this model was introduced by Sentana [ 21 ]. The Glosten-Jagannathan-Runkle GARCH (GJR-GARCH) model was introduced by Glosten et al. [ 22 ], its opposite effects of negative and positive shocks taking into account the leverage fact. The threshold GARCH (TGARCH) model was introduced by Zakoian [ 23 ], this model is also commonly used to handle leverage effects of good news and bad news on volatility. The family GARCH (FGARCH) model was introduced by Hentschel [ 24 ] and is an omnibus model that is a mix of other symmetric or asymmetric GARCH models. The COGARCH model was introduced by Klüppelberg et al. [ 25 ] and is actually the stochastic volatility model, being an extension of the GARCH time series concept to continuous time. The power-transformed and threshold GARCH (PTTGARCH) model was introduced by Pan et al. [ 26 ], this model is a very flexible model and, under certain conditions, includes several ARCH/GARCH models.
Based on the researchers’ articles, the symmetric GARCH (1, 1) model has been used widely to forecast the unconditional volatility in the stock market and time series data, and has been able to simulate the asset yield structure and implied volatility structure. Most researchers show that GARCH (1, 1) with a generalized distribution of residual has more advantages in volatility assessment than other models. Conversely, the asymmetry influence in stock market volatility and return analysis was beyond the descriptive power of the asymmetric GARCH models, as the models could capture more specifics. Besides, the asymmetric GARCH models can incompletely measure the effect of positive or negative shocks in stock market return and volatility, and the GARCH (1, 1) comparatively failed to accomplish this fact. In asymmetric effect, the GJR-GARCH model performed better and produced a higher predictable conditional variance during the period of high volatility. In addition, among the asymmetric GARCH models, the reflection of EGARCH model appeared to be superior.
Table 4 has explained the review of bivariate and other multivariate GARCH models. Bivariate model analysis was used to find out if there is a relationship between two different variables. Bivariate model uses one dependent variable and one independent variable. Additionally, the Multivariate GARCH model is a model for two or more time series. Multivariate GARCH models are used to model for forecast volatility of several time series when there are some linkages between them. Multivariate model uses one dependent variable and more than one independent variable. In this case, the current volatility of one time series is influenced not only by its own past innovation, but also by past innovations to volatilities of other time series.
Different literature studies based on bivariate and other multivariate GARCH models.
The most recognizable use of multivariate GARCH models is the analysis of the relations between the volatilities and co-volatilities of several markets. A multivariate model would create a more dependable model than separate univariate models. The vector error correction (VEC) models is the first MGARCH model which was introduced by Bollerslev et al. [ 66 ]. This model is typically related to subsequent formulations. The model can be expressed in the following form: v e c h ( H t ) = ℂ + ∑ j = 1 q X j v e c h ( ϵ t − j ϵ t − j ' ) + ∑ j = 1 p Y j v e c h ( H t − j ) where v e c h is an operator that stacks the columns of the lower triangular part of its argument square matrix and H t is the covariance matrix of the residuals. The regulated version of the VEC model is the DVEC model and was also recommended by Bollerslev et al. [ 66 ]. Compared to the VEC model, the estimation method proceeded far more smoothly in the DVEC model. The Baba-Engle-Kraft-Kroner (BEKK) model was introduced by Baba et al. [ 67 ] and is an innovative parameterization of the conditional variance matrix H t . The BEKK model accomplishes the positive assurance of the conditional covariance by conveying the model in a way that this property is implied by the model structure. The Constant Conditional Correlation (CCC) model was recommended by Bollerslev [ 68 ], to primarily model the conditional covariance matrix circuitously by estimating the conditional correlation matrix. The Dynamic Conditional Correlation (DCC) model was introduced by Engle [ 69 ] and is a nonlinear mixture of univariate GARCH models and also a generalized variety of the CCC model. To overcome the inconveniency of huge number of parameters, the O-GARCH model was recommended by Alexander and Chibumba [ 70 ] and consequently developed by Alexander [ 71 , 72 ]. Furthermore, a multivariate GARCH model GO-GARCH model was introduced by Bauwens et al. [ 73 ].
The bivariate models showed achieve better in most cases, compared with the univariate models [ 85 ]. MGARCH models could be used for forecasting. Multivariate GARCH modeling delivered a realistic but parsimonious measurement of the variance matrix, confirming its positivity. However, by analyzing the relative forecasting accuracy of the two formulations, BEKK and DCC, it could be deduced that the forecasting performance of the MGARCH models was not always satisfactory. By comparing it with the other multivariate GARCH models, BEKK-GARCH model was comparatively better and flexible but it needed too many parameters for multiple time series. Conversely, for the area of forecasting, the DCC-GARCH model was more parsimonious. In this regard, it was significantly essential to balance parsimony and flexibility when modeling multivariate GARCH models.
The current systematic review has identified 50 research articles for studies on significant aspects of stock market return and volatility, review types, and GARCH model analysis. This paper noticed that all the studies in this review used an investigational research method. A literature review is necessary for scholars, academics, and practitioners. However, assessing various kinds of literature reviews can be challenging. There is no use for outstanding and demanding literature review articles, since if they do not provide a sufficient contribution and something that is recent, it will not be published. Too often, literature reviews are fairly descriptive overviews of research carried out among particular years that draw data on the number of articles published, subject matter covered, authors represented, and maybe methods used, without conducting a deeper investigation. However, conducting a literature review and examining its standard can be challenging, for this reason, this article provides some rigorous literature reviews and, in the long run, to provide better research.
4. Conclusions
Working on a literature review is a challenge. This paper presents a comprehensive literature which has mainly focused on studies on return and volatility of stock market using systematic review methods on various financial markets around the world. This review was driven by researchers’ available recommendations for accompanying systematic literature reviews to search, examine, and categorize all existing and accessible literature on market volatility and returns [ 16 ]. Out of the 435 initial research articles located in renowned electronic databases, 50 appropriate research articles were extracted through cross-reference snowballing. These research articles were evaluated for the quality of proof they produced and were further examined. The raw data were offered by the authors from the literature together with explanations of the data and key fundamental concepts. The outcomes, in this research, delivered future magnitudes to research experts for further work on the return and volatility of stock market.
Stock market return and volatility analysis is a relatively important and emerging field of research. There has been plenty of research on financial market volatility and return because of easily increasing accessibility and availability of researchable data and computing capability. The GARCH type models have a good model on stock market volatilities and returns investigation. The popularity of various GARCH family models has increased in recent times. Every model has its specific strengths and weaknesses and has at influence such a large number of GARCH models. To sum up the reviewed papers, many scholars suggest that the GARCH family model provides better results combined with another statistical technique. Based on the study, much of the research showed that with symmetric information, GARCH (1, 1) could precisely explain the volatilities and returns of the data and when under conditions of asymmetric information, the asymmetric GARCH models would be more appropriate [ 7 , 32 , 40 , 47 , 48 ]. Additionally, few researchers have used multivariate GARCH model statistical techniques for analyzing market volatility and returns to show that a more accurate and better results can be found by multivariate GARCH family models. Asymmetric GARCH models, for instance and like, EGARCH, GJR GARCH, and TGARCH, etc. have been introduced to capture the effect of bad news on the change in volatility of stock returns [ 42 , 58 , 62 ]. This study, although short and particular, attempted to give the scholar a concept of different methods found in this systematic literature review.
With respect to assessing scholars’ articles, the finding was that rankings and specifically only one GARCH model was sensitive to the different stock market volatilities and returns analysis, because the stock market does not have similar characteristics. For this reason, the stock market and model choice are little bit difficult and display little sensitivity to the ranking criterion and estimation methodology, additionally applying software is also another matter. The key challenge for researchers is finding the characteristics in stock market summarization using different kinds of local stock market returns, volatility detection, world stock market volatility, returns, and other data. Additional challenges are modeled by differences of expression between different languages. From an investigation perception, it has been detected that different authors and researchers use special datasets for the valuation of their methods, which may put boundary assessments between research papers.
Whenever there is assurance that scholars build on high accuracy, it will be easier to recognize genuine research gaps instead of merely conducting the same research again and again, so as to progress better and create more appropriate hypotheses and research questions, and, consequently, to raise the standard of research for future generation. This study will be beneficial for researchers, scholars, stock exchanges, regulators, governments, investors, and other concerned parties. The current study also contributes to the scope of further research in the area of stock volatility and returns. The content analysis can be executed taking the literature of the last few decades. It determined that a lot of methodologies like GARCH models, Johansen models, VECM, Impulse response functions, and Granger causality tests are practiced broadly in examining stock market volatility and return analysis across countries as well as among sectors with in a country.
Author Contributions
R.B. and S.W. proposed the research framework together. R.B. collected the data, and wrote the document. S.W. provided important guidance and advice during the process of this research. All authors have read and agreed to the published version of the manuscript.
This research received no external funding.
Conflicts of Interest
The authors declare no conflict of interest.
- Browse All Articles
- Newsletter Sign-Up
Investment →
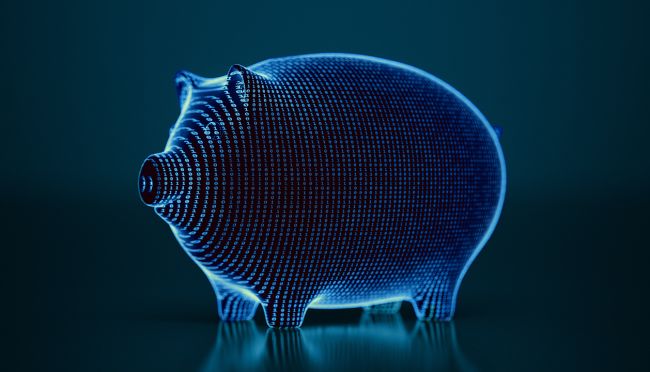
- 17 Aug 2023
- Research & Ideas
‘Not a Bunch of Weirdos’: Why Mainstream Investors Buy Crypto
Bitcoin might seem like the preferred tender of conspiracy theorists and criminals, but everyday investors are increasingly embracing crypto. A study of 59 million consumers by Marco Di Maggio and colleagues paints a shockingly ordinary picture of today's cryptocurrency buyer. What do they stand to gain?
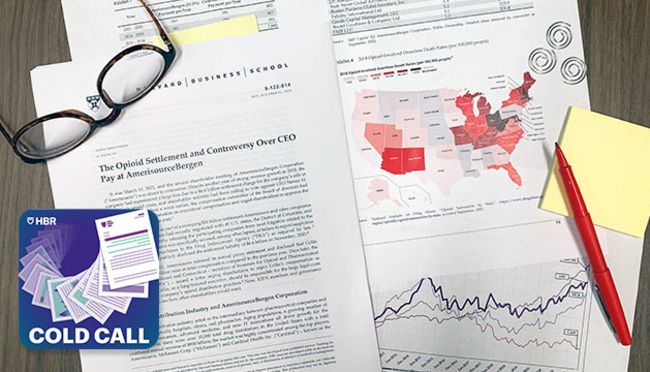
- 06 Jun 2023
- Cold Call Podcast
The Opioid Crisis, CEO Pay, and Shareholder Activism
In 2020, AmerisourceBergen Corporation, a Fortune 50 company in the drug distribution industry, agreed to settle thousands of lawsuits filed nationwide against the company for its opioid distribution practices, which critics alleged had contributed to the opioid crisis in the US. The $6.6 billion global settlement caused a net loss larger than the cumulative net income earned during the tenure of the company’s CEO, which began in 2011. In addition, AmerisourceBergen’s legal and financial troubles were accompanied by shareholder demands aimed at driving corporate governance changes in companies in the opioid supply chain. Determined to hold the company’s leadership accountable, the shareholders launched a campaign in early 2021 to reject the pay packages of executives. Should the board reduce the executives’ pay, as of means of improving accountability? Or does punishing the AmerisourceBergen executives for paying the settlement ignore the larger issue of a business’s responsibility to society? Harvard Business School professor Suraj Srinivasan discusses executive compensation and shareholder activism in the context of the US opioid crisis in his case, “The Opioid Settlement and Controversy Over CEO Pay at AmerisourceBergen.”
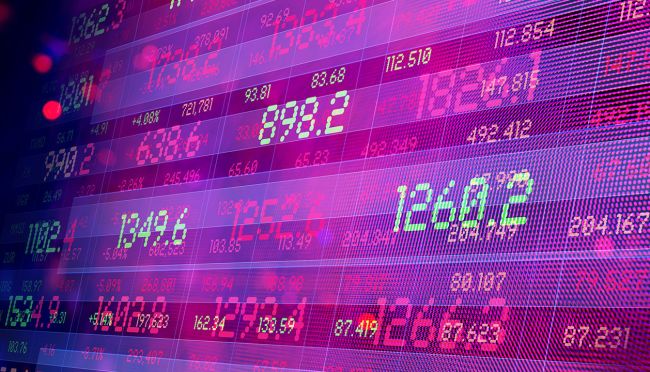
- 11 Apr 2023
Is Amazon a Retailer, a Tech Firm, or a Media Company? How AI Can Help Investors Decide
More companies are bringing seemingly unrelated businesses together in new ways, challenging traditional stock categories. MarcAntonio Awada and Suraj Srinivasan discuss how applying machine learning to regulatory data could reveal new opportunities for investors.
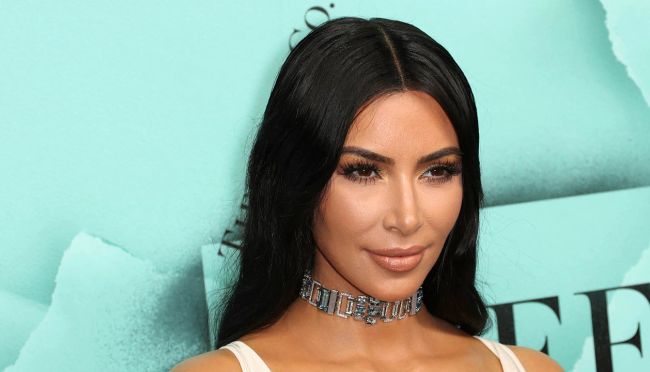
- 07 Apr 2023
When Celebrity ‘Crypto-Influencers’ Rake in Cash, Investors Lose Big
Kim Kardashian, Lindsay Lohan, and other entertainers have been accused of promoting crypto products on social media without disclosing conflicts. Research by Joseph Pacelli shows what can happen to eager investors who follow them.
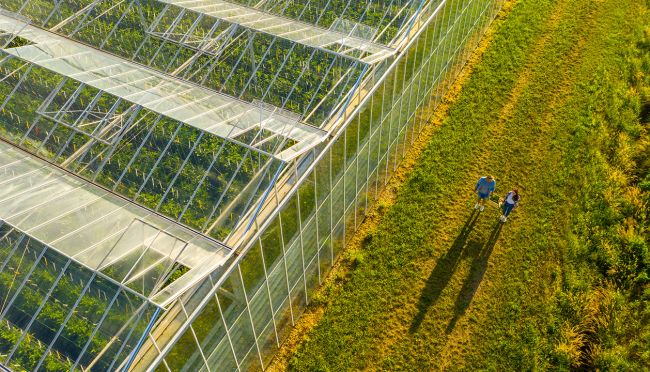
- 23 Mar 2023
As Climate Fears Mount, More Investors Turn to 'ESG' Funds Despite Few Rules
Regulations and ratings remain murky, but that's not deterring climate-conscious investors from paying more for funds with an ESG label. Research by Mark Egan and Malcolm Baker sizes up the premium these funds command. Is it time for more standards in impact investing?
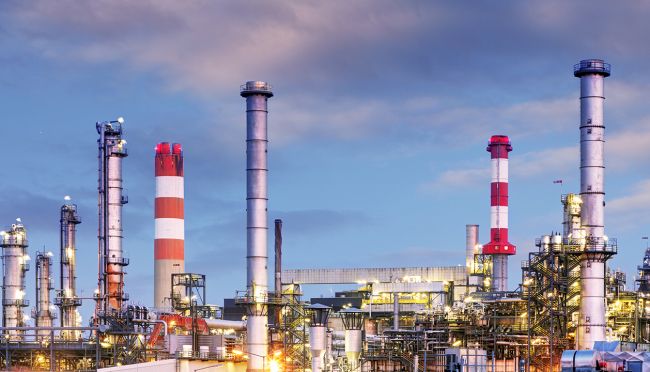
- 16 Feb 2023
ESG Activists Met the Moment at ExxonMobil, But Did They Succeed?
Engine No. 1, a small hedge fund on a mission to confront climate change, managed to do the impossible: Get dissident members on ExxonMobil's board. But lasting social impact has proved more elusive. Case studies by Mark Kramer, Shawn Cole, and Vikram Gandhi look at the complexities of shareholder activism.
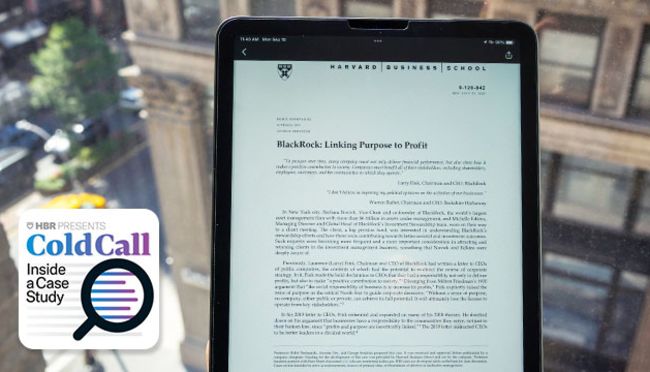
- 20 Sep 2022
Larry Fink at BlackRock: Linking Purpose to Profit
In 2014, Larry Fink started writing letters to the leaders of some of the largest publicly listed companies, urging them to consider the importance of environmental, social, and governance (ESG) issues. Fink is the chairman and CEO of BlackRock, one of the largest asset management houses in the world. The firm’s success was rooted in its cost-effective, passive investment products that rely more on tracking indices and funds. But Fink wanted his firm to engage with the companies in which they invest and hold them accountable for their social and environmental impacts. What role should investors play in urging business leaders to take environmental, social, and governance issues more seriously and enforcing compliance? Harvard Business School professor George Serafeim discusses the merits of Fink’s approach, the importance of corporate investments in ESG themes, and how to lead a company driven by purpose and profit in his case, “BlackRock: Linking Purpose to Profit,” and his new book Purpose and Profit: How Business Can Lift Up The World.
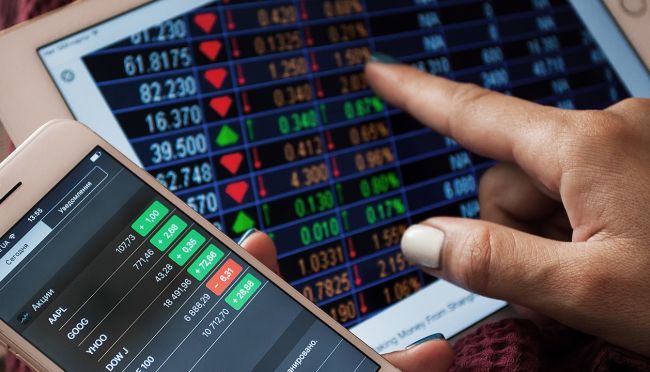
- 21 Jul 2022
Did Pandemic Stimulus Funds Spur the Rise of 'Meme Stocks'?
Remember the GameStop stock frenzy? Research by Robin Greenwood and colleagues shows how market speculation can flare up when you combine stimulus funds, trading platforms, and plain old boredom.
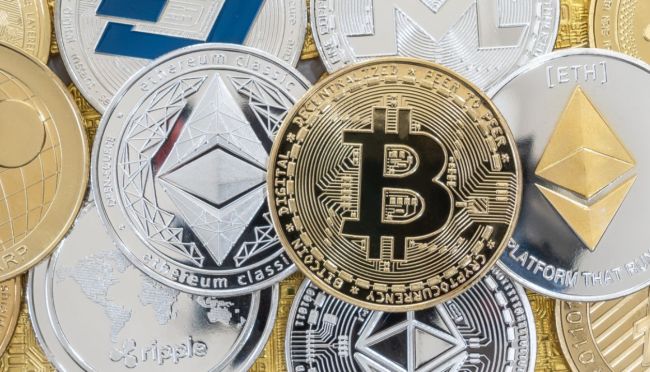
- 18 Jul 2022
After the 'Crypto Crash,' What's Next for Digital Currencies?
After soaring to dizzying levels, Bitcoin and other cryptocurrencies have lost more than half of their value in recent months. Scott Duke Kominers discusses crypto's volatility, potential for regulation, and why these digital assets are likely here to stay.
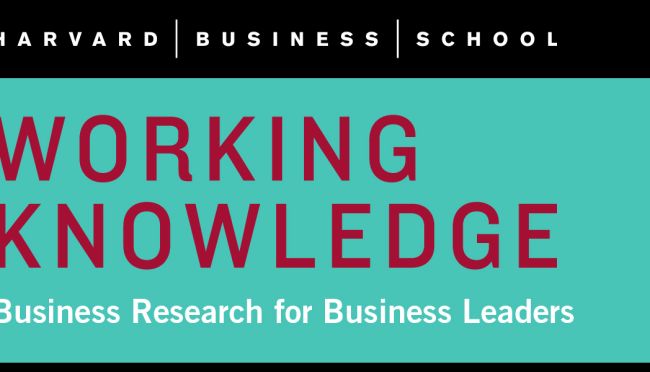
- 13 Oct 2020
- Working Paper Summaries
Fencing Off Silicon Valley: Cross-Border Venture Capital and Technology Spillovers
This study of foreign corporate investment transactions from 32 countries between 1976 and 2015 finds these investments pose a trade-off: While they support young firms in pursuing innovations they could not otherwise afford, they also generate knowledge for the foreign investors.
- 20 Aug 2020
The “best ideas” in investment managers’ portfolios generate statistically and economically significant risk-adjusted returns over time, and they systematically outperform other positions in the portfolios. Investors can gain substantially if managers choose less-diversified portfolios that tilt more towards their best ideas.
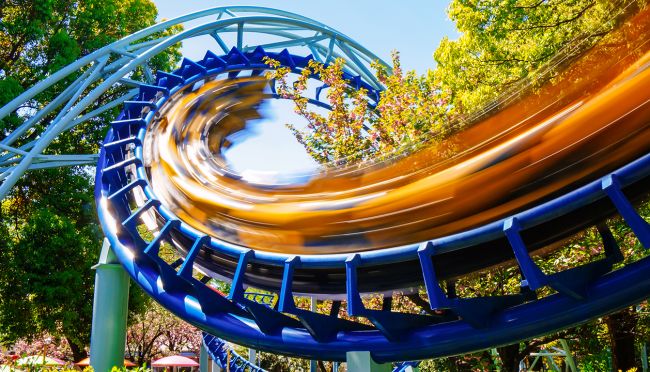
- 12 Aug 2020
Why Investors Often Lose When They Sue Their Financial Adviser
Forty percent of American investors rely on financial advisers, but the COVID-19 market rollercoaster may have highlighted a weakness when disputes arise. The system favors the financial industry, says Mark Egan. Open for comment; 0 Comments.
- 29 Jul 2020
Two Case Studies on the Financing of Forest Conservation
Case studies about The Conservation Fund and Sonen Capital highlight three broad lessons about fresh approaches to the ownership and management of forestland.
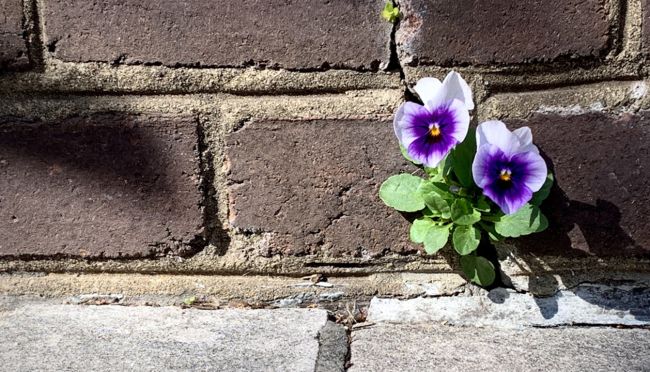
- 07 Jul 2020
Market Investors Pay More for Resilient Companies
During a market collapse, investors will pay up for companies considered resilient in their response, according to George Serafeim. Open for comment; 0 Comments.
- 12 Jun 2020
Corporate Resilience and Response During COVID-19
Investors look for evidence during a market crisis that a company is resilient. This study includes findings that challenge the notion that companies need to adopt practices that hurt their employees because investors want them to do so.
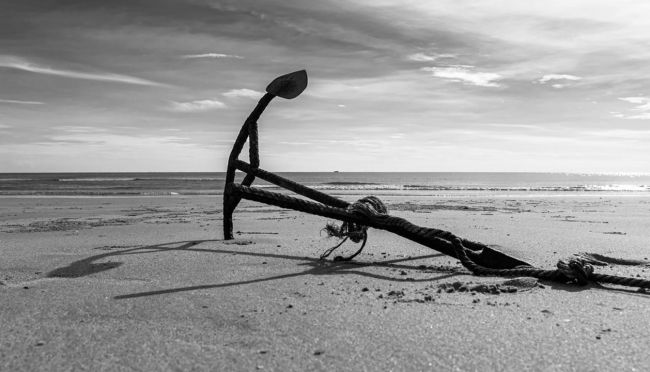
- 05 Jun 2020
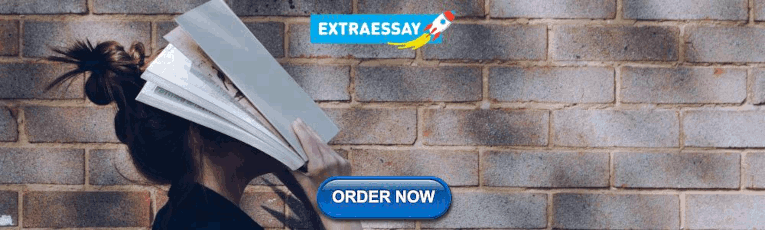
How Anchor Investors Help Impact Funds Succeed
3Questions A startup fund's ability to attract a major first investor is a signal to others that the investment pool is just fine for entering. Shawn Cole and Rob Zochowski answer questions about anchor investors. Open for comment; 0 Comments.
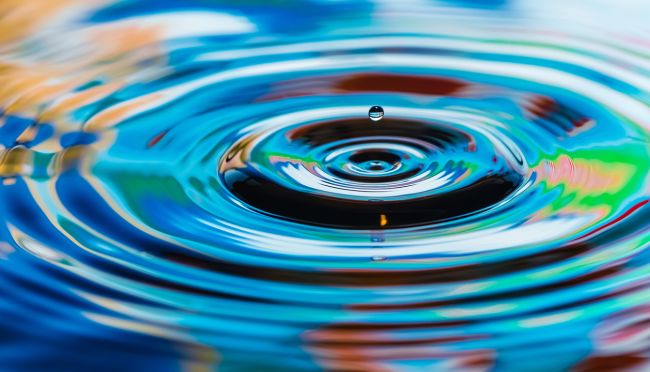
- 09 Apr 2020
How Social Entrepreneurs Can Increase Their Investment Impact
Grants or investments? Philanthropic organizations have multiple funding tools available, but choosing the wrong one can dilute the benefits, according to research by Benjamin N. Roth. Open for comment; 0 Comments.
- 09 Mar 2020
Impact Investing: A Theory of Financing Social Entrepreneurship
The author provides a formal definition of organizational sustainability and characterizes the situations in which a social enterprise should be sustainable. The analysis then delineates when an investment in a social enterprise delivers superior impact to a grant.
- 18 Feb 2020
A Preliminary Framework for Product Impact-Weighted Accounts
Although there is growing interest in environmental, social, and governance measurement, the impact of company operations is emphasized over product use. A framework like this one that captures a product’s reach, accessibility, quality, optionality, environmental use emissions, and end of life recyclability allows for a systematic methodology that can be applied to companies across many industries.
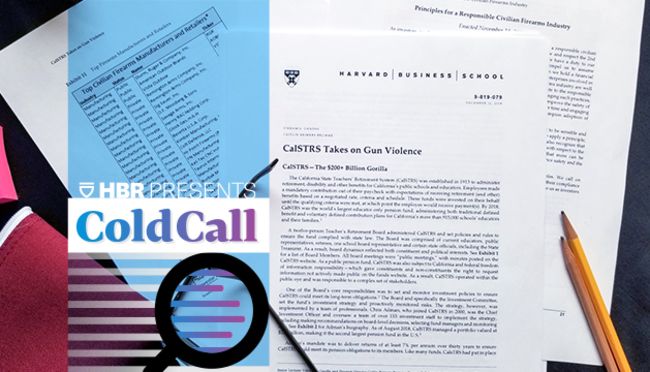
- 03 Dec 2019
Why CalSTRS Chooses to Engage with the Gun Industry
Should large institutional investors divest or engage if they have an issue with a company? In a recent case study, Vikram Gandhi discusses how CalSTRS, the $200 billion pension plan for California public school teachers, chose to engage with gun makers and retailers. Open for comment; 0 Comments.
Visualization and forecasting of stock’s closing price using machine learning
- Published: 10 February 2024
Cite this article
- Aditi Gupta 1 ,
- Akansha . 1 ,
- Khushboo Joshi 1 ,
- Madhu Patel 1 &
- Vibha Pratap ORCID: orcid.org/0000-0002-0479-8473 1
90 Accesses
1 Altmetric
Explore all metrics
Stock market investments have become an essential part of our lives as they offer a means of growing wealth and securing financial stability for individuals and businesses alike. However, predicting and investing in a stock is a complex method and demands significant levels of knowledge, proficiency, and skill. Stock market prediction is the act of analyzing historical information and trends in the market in order to make informed forecasts about the potential future worth of a given stock or instrument that is traded on a financial instrument exchange. When making stock predictions, most stockbrokers use both fundamental and technical analysis and time series analysis. The study focuses on the implementation of Multi-Linear Regression, LSTM, CNN, and LSTM + GRU based Machine learning techniques using technical analysis to predict stock’s closing values from the NYSE, and NASDAQ markets for multiple days. The dataset has been taken from Yahoo, of 10-year span. The factors taken into consideration for predicting stock prices are open, close, low, and high. The model’s effectiveness is measured using common strategic metrics like RMSE, MSE, MAE, and R2. A lower value for these variables suggests that the models are good at forecasting stock closing prices. After conducting a comprehensive evaluation, we found that LSTM + GRU model performs the best among the tested models for predicting multiple days, followed by CNN and LSTM. The tested models demonstrate a remarkable level of accuracy in predicting stock market prices. This research work provides a valuable contribution to the fields of financial and technical analysis in the stock market research community.
This is a preview of subscription content, log in via an institution to check access.
Access this article
Price includes VAT (Russian Federation)
Instant access to the full article PDF.
Rent this article via DeepDyve
Institutional subscriptions
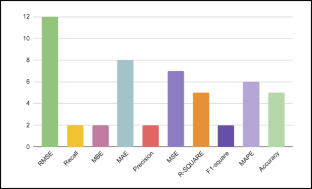
Similar content being viewed by others
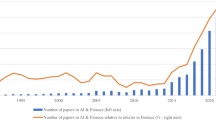
Artificial intelligence in Finance: a comprehensive review through bibliometric and content analysis
Salman Bahoo, Marco Cucculelli, … Jasmine Mondolo
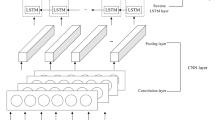
A CNN-BiLSTM-AM method for stock price prediction
Wenjie Lu, Jiazheng Li, … Lele Qin
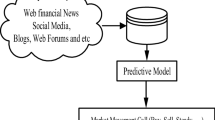
A systematic review of fundamental and technical analysis of stock market predictions
Isaac Kofi Nti, Adebayo Felix Adekoya & Benjamin Asubam Weyori
Data availability
We used publicly available datasets from Yahoo Finance ( https://finance.yahoo.com ) (for the time period April 2013 to 31 March 2023).
Fathali Z, Kodia Z, Ben Said L (2022) Stock market prediction of Nifty 50 index applying machine learning techniques. Appl Artif Intell 36(1):2111134
Article Google Scholar
Gupta A, Joshi K, Patel M, Pratap MV (2023) Stock prices prediction using machine learning. In: 2023 2nd International Conference for Innovation in Technology (INOCON), . IEEE, pp 1–7. https://doi.org/10.1109/INOCON57975.2023.10101226
Aldhyani TH, Alzahrani A (2022) Framework for predicting and modeling stock market prices based on deep learning algorithms. Electronics 11(19):3149
What Is Stock Price Prediction? Wikipedia contributors, “Stock market prediction,” Wikipedia, The Free Encyclopedia, 03-Apr-2023. [Online]. Available: https://en.wikipedia.org/w/index.php?title=Stock_market_prediction&oldid=1147956250 . Accessed 15 Apr 2023
De Prado ML (2018) Advances in financial machine learning. John Wiley & Sons
Google Scholar
Hilpisch Y (2014) Python for finance: analyze big financial data. " O'Reilly Media, Inc."
Omar AB, Huang S, Salameh AA, Khurram H, Fareed M (2022) Stock market forecasting using the random forest and deep neural network models before and during the COVID-19 period. Front Environ Sci 10:917047. https://doi.org/10.3389/fenvs.2022.917047
Xiao D, Su J (2022) Research on stock price time series prediction based on deep learning and autoregressive integrated moving average. Sci Program. https://doi.org/10.1155/2022/4758698
Shen J, Shafiq MO (2020) Short-term stock market price trend prediction using a comprehensive deep learning system. J Big Data 7(1):1–33
Article CAS Google Scholar
Chandola D, Mehta A, Singh S, Tikkiwal VA, Agrawal H (2023) Forecasting directional movement of stock prices using deep learning. Ann Data Sci 10(5):1361–1378
Ji X, Wang J, Yan Z (2021) A stock price prediction method based on deep learning technology. Int J Crowd Sci 5(1):55–72
Wei J, Xu Q, He C (2022) Deep learning of predicting closing price through historical adjustment closing price. Proc Comput Sci 202:379–384
Sen J, Mehtab S (2021) Design and analysis of robust deep learning models for stock price prediction. Mach Learn–Algoritms Model Appl 15–46. https://doi.org/10.5772/intechopen.99982
Bhandari HN, Rimal B, Pokhrel NR, Rimal R, Dahal KR, Khatri RK (2022) Predicting stock market index using LSTM. Mach Learn Appl 9:100320
de la Fuente AR, Sanchez-Ramirez E, Calderón-Alvarado MP, Segovia-Hernandez JG, Hernández-Vargas EA (2022) Development of Deep Learning Architectures for Forecasting Distillation Columns Dynamic Behavior of Biobutanol Purification. In Computer Aided Chemical Engineering (vol 51, pp 49–54). Elsevier
Yujun Y, Yimei Y, Jianhua X (2020) A hybrid prediction method for stock price using LSTM and ensemble EMD. Complexity 2020:1–16
Article ADS Google Scholar
Saxena S (2021) Introduction to gated recurrent unit (GRU). Analytics Vidhya. [Online]. Available: https://www.analyticsvidhya.com/blog/2021/03/introduction-to-gated-recurrent-unit-gru/ . [Accessed: 30-Apr-2023]
Zhou GB, Wu J, Zhang CL, Zhou ZH (2016) Minimal gated unit for recurrent neural networks. Int J Autom Comput 13(3):226–234. https://doi.org/10.1007/s11633-016-1006-2
Download references
Author information
Authors and affiliations.
Department of CSE, Indira Gandhi Delhi Technical University for Women, Delhi, India
Aditi Gupta, Akansha ., Khushboo Joshi, Madhu Patel & Vibha Pratap
You can also search for this author in PubMed Google Scholar
Corresponding author
Correspondence to Vibha Pratap .
Ethics declarations
Conflict of interest.
The authors declare that they have no conflict of interest.
Additional information
Publisher's note.
Springer Nature remains neutral with regard to jurisdictional claims in published maps and institutional affiliations.
Rights and permissions
Springer Nature or its licensor (e.g. a society or other partner) holds exclusive rights to this article under a publishing agreement with the author(s) or other rightsholder(s); author self-archiving of the accepted manuscript version of this article is solely governed by the terms of such publishing agreement and applicable law.
Reprints and permissions
About this article
Gupta, A., ., A., Joshi, K. et al. Visualization and forecasting of stock’s closing price using machine learning. Multimed Tools Appl (2024). https://doi.org/10.1007/s11042-024-18376-9
Download citation
Received : 11 May 2023
Revised : 11 November 2023
Accepted : 19 January 2024
Published : 10 February 2024
DOI : https://doi.org/10.1007/s11042-024-18376-9
Share this article
Anyone you share the following link with will be able to read this content:
Sorry, a shareable link is not currently available for this article.
Provided by the Springer Nature SharedIt content-sharing initiative
- Machine learning
- Multiple-day prediction
- Find a journal
- Publish with us
- Track your research
What Hundreds of Economic News Events Say About Belief Overreaction in the Stock Market
We measure the nature and severity of a variety of belief distortions in market reactions to hundreds of economic news events using a new methodology that synthesizes estimation of a structural asset pricing model with algorithmic machine learning to quantify bias. We estimate that investors systematically overreact to perceptions about multiple fundamental shocks in a macro-dynamic system, generating asymmetric compositional effects when several counteracting shocks occur simultaneously in real-world events. We show that belief overreaction to all shocks can lead the market to over- or underreact to events, amplifying or dampening volatility.
Bianchi and Ludvigson received financial support from the National Science Foundation under Grant 2116641. The views expressed herein are those of the authors and do not necessarily reflect the views of the National Bureau of Economic Research.
MARC RIS BibTeΧ
Download Citation Data
Working Groups
Conferences, more from nber.
In addition to working papers , the NBER disseminates affiliates’ latest findings through a range of free periodicals — the NBER Reporter , the NBER Digest , the Bulletin on Retirement and Disability , the Bulletin on Health , and the Bulletin on Entrepreneurship — as well as online conference reports , video lectures , and interviews .
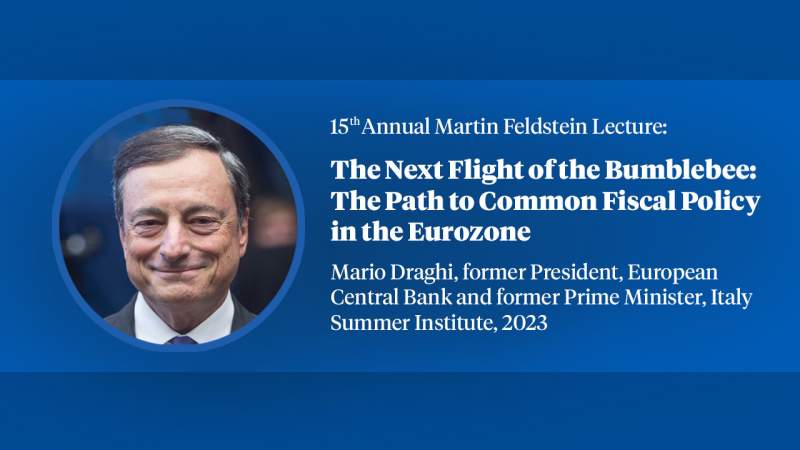
share this!
April 10, 2024
This article has been reviewed according to Science X's editorial process and policies . Editors have highlighted the following attributes while ensuring the content's credibility:
fact-checked
trusted source
Psychological health of investors and stock market linked, study finds
by Ball State University
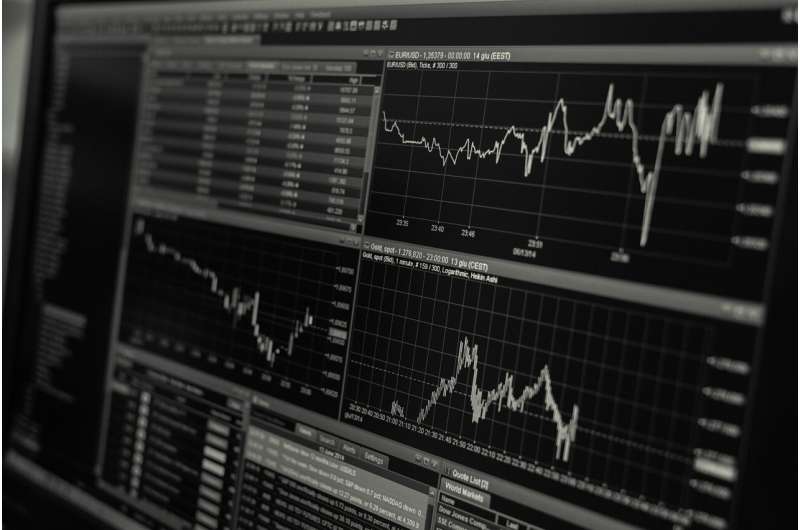
A recent study by two Ball State University faculty members has found a clear and robust link between local stock market downturns and an increase in antidepressant use among investors.
"This relationship, unaffected by local economic conditions, suggests that investment losses directly impact investors' psychological health," said Dr. Chang Liu, assistant professor of finance, who co-authored the research paper with Dr. Maoyong Fan, professor of economics. The paper, "Stock Market and the Psychological Health of Investors," was recently published in The Financial Review .
Utilizing in their study a national individual-level medical dataset and the home bias in investment, the researchers also concluded that "the effect intensifies in areas with higher per capita dividend income, suggesting a direct relationship between higher stock ownership and stronger responses," and confirmed that "portfolio losses, not local economic conditions, are responsible for increased antidepressant usage during market downturns."
"The financial stress from stock market declines not only affects mental health but also leads to physical health problems such as insomnia and depression, indicating its broad societal consequences," Dr. Liu added.
"Amid stock market volatilities, the surge in mental health issues calls for a proactive approach to investor support and education," Dr. Fan said.
"Our findings advocate for the inclusion of mental health resources in financial advising, especially during times of market turbulence. And our study highlights the need for policy and education actions to mitigate mental health risks associated with stock market participation, ensuring investors are prepared emotionally and financially for market changes."
Findings in the research paper also support the loss aversion hypothesis, noting that positive stock returns do not influence antidepressant usage.
Provided by Ball State University
Explore further
Feedback to editors

New study finds potential targets at chromosome ends for degenerative disease prevention
11 hours ago

Scientists discover first nitrogen-fixing organelle

Traces of DNA in the stomachs of predatory snails provide new insights into the ecology of placozoans

New study reveals novel approach for combating 'resting' bacteria

Researchers develop method to extract useful proteins from beer-brewing leftovers
12 hours ago

Scientists find blue light makes buildings more deadly to migrating birds

Ant pheromones may help protect hikers and campers from ticks

Trapped in the middle: Billiards with memory framework leads to mathematical questions

What do bird dreams sound like?

Scientists develop biofortified rice to combat nutrient deficiencies
13 hours ago
Relevant PhysicsForums posts
Cover songs versus the original track, which ones are better.
5 hours ago
For WW2 buffs!
6 hours ago
Who is your favorite Jazz musician and what is your favorite song?
7 hours ago
Biographies, history, personal accounts
10 hours ago
Which ancient civilizations are you most interested in?
17 hours ago
Etymology of a Curse Word
18 hours ago
More from Art, Music, History, and Linguistics
Related Stories

Stock market returns track the strength of the dollar
Mar 29, 2022

Entrepreneurs' stock losses bruise their businesses
Feb 22, 2024

Quantum mechanics model unveils hidden patterns in stock markets
Jan 9, 2024

Daily movie theater ticket sales can predict stock market returns
Oct 12, 2022

Expert says multistate foodborne illness outbreaks impact restaurant stock price, public perception
Feb 2, 2024

Investors less likely to sell losing stocks when entire portfolio is at a loss, research finds
Sep 13, 2023
Recommended for you

Building footprints could help identify neighborhood sociodemographic traits
Apr 10, 2024

Are the world's cultures growing apart?

First languages of North America traced back to two very different language groups from Siberia
Apr 9, 2024

Can the bias in algorithms help us see our own?

The 'Iron Pipeline': Is Interstate 95 the connection for moving guns up and down the East Coast?

Public transit agencies may need to adapt to the rise of remote work, says new study
Let us know if there is a problem with our content.
Use this form if you have come across a typo, inaccuracy or would like to send an edit request for the content on this page. For general inquiries, please use our contact form . For general feedback, use the public comments section below (please adhere to guidelines ).
Please select the most appropriate category to facilitate processing of your request
Thank you for taking time to provide your feedback to the editors.
Your feedback is important to us. However, we do not guarantee individual replies due to the high volume of messages.
E-mail the story
Your email address is used only to let the recipient know who sent the email. Neither your address nor the recipient's address will be used for any other purpose. The information you enter will appear in your e-mail message and is not retained by Phys.org in any form.
Newsletter sign up
Get weekly and/or daily updates delivered to your inbox. You can unsubscribe at any time and we'll never share your details to third parties.
More information Privacy policy
Donate and enjoy an ad-free experience
We keep our content available to everyone. Consider supporting Science X's mission by getting a premium account.
E-mail newsletter
How Quickly Do Prices Respond to Monetary Policy?
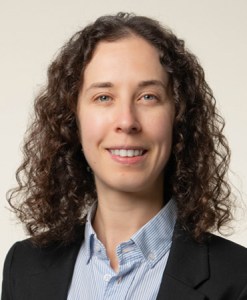
Download PDF (238 KB)
FRBSF Economic Letter 2024-10 | April 8, 2024
With inflation still above the Federal Reserve’s 2% objective, there is renewed interest in understanding how quickly federal funds rate hikes typically affect inflation. Beyond monetary policy’s well-known lagged effect on the economy overall, new analysis highlights that not all prices respond with the same strength or speed. Results suggest that inflation for the most responsive categories of goods and services has come down substantially from recent highs, likely due in part to more restrictive monetary policy. As a result, the contributions of these categories to overall inflation have fallen.
Monetary policy affects inflation with a lag. This means that, although interest rates react quickly when the Federal Reserve raises the federal funds rate, the effects on inflation are slower and indirect. Higher interest rates increase borrowing costs, slowing investment and overall demand, which ultimately eases the pressure on prices. Understanding the timing and strength of this mechanism is key for policymakers.
Many researchers have estimated the speed and strength of the economy’s response to monetary policy, notably Romer and Romer (2004). The focus is typically a broader measure of inflation, such as headline or core, which reflects an average across many goods and services. However, not all prices of the component goods and services react to monetary policy in the same way. For example, food and energy prices, which are excluded from core but included in headline inflation, often move more in response to global market fluctuations, such as changes in international oil prices, rather than to changes in domestic monetary policy.
In this Economic Letter , we estimate how prices of different goods and services respond to changes in the federal funds rate and use those estimates to build a monetary policy-responsive inflation index. We find substantial variation in how prices react to monetary policy, which suggests that understanding the makeup of overall inflation can provide insights into the transmission of monetary policy to inflation. The extent to which categories that are more responsive to the federal funds rate contribute to inflation affects how much slowing in economic activity is needed to reduce overall inflation. Our analysis indicates that recent ups and downs of inflation have been focused in categories that are most sensitive to monetary policy. Inflation rates for the most sensitive categories—and their contributions to headline inflation—rose from the first half of 2020 through mid-2022, reaching a higher peak than headline inflation, and then began to decline. The inflation rate for this most responsive group of goods and services categories is now close to its pre-2020 rate. Our findings suggest that the Fed’s rate hikes that began in March 2022 are exerting downward pressure on prices and will continue to do so in the near term. Our estimated lags are consistent with the view that the full effects of past policy tightening are still working their way through the economy.
Measuring how prices react to monetary policy
To understand which goods and services are most responsive to monetary policy, we need to determine how their prices react to changes in the federal funds rate, the Federal Reserve’s main policy rate. Because the Federal Reserve adjusts the federal funds rate target in response to macroeconomic developments, including inflation, we use a transformation of the federal funds rate in our estimation. This transformed series, developed by Romer and Romer (2004) and updated by Wieland and Yang (2020), captures the differences between Federal Reserve staff forecasts and the chosen target rate, leaving only policy shocks, or movements in the federal funds rate that are not driven by actual or anticipated changes in economic conditions. We use this series as a so-called instrument for the federal funds rate, such that our results can account for how the federal funds rate itself, rather than its transformation, affects inflation.
We use an approach developed by Jordà (2005) that compares two forecasts—with and without rate shocks—to estimate how the federal funds rate affects price movements over time. Specifically, we estimate the relationship between the federal funds rate and the cumulative percent change in prices, controlling for recent trends in the federal funds rate, inflation, and economic activity. Repeating this estimation over multiple horizons produces a forecast comparison, or impulse response function, that gives us an estimate of the expected percent change in prices following a rate increase. For example, applying this method to the headline personal consumption expenditures (PCE) price index indicates that four years after a 1 percentage point increase in the federal funds rate, overall prices are typically about 2.5% below what they would have been without the rate increase.
Creating a policy-responsive inflation index
We estimate impulse response functions separately for the 136 goods and services categories that collectively make up headline PCE inflation. Figure 1 shows examples of the largest cumulative percent price declines over a four-year period in response to a 1 percentage point increase in the federal funds rate. The goods and services categories selected as examples account for large shares of total expenditures in headline PCE inflation. We also include one example of the few categories where prices do not decline, higher education, shown as a small positive value.
Figure 1 Reaction to a policy rate increase: Selected PCE categories
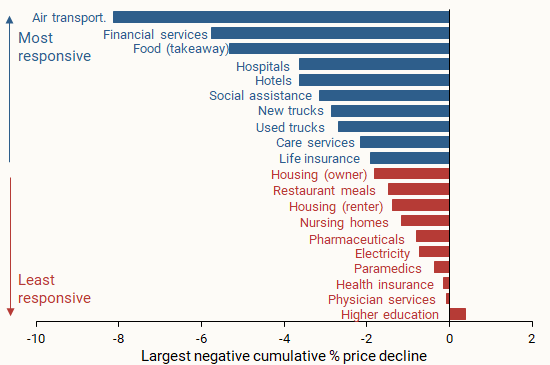
The takeaway from Figure 1 is that headline PCE inflation is made up of categories that differ in their responsiveness to increases in the federal funds rate. Some respond more strongly, such as those with larger typical cumulative price declines, while others respond less strongly, such as those with smaller typical price declines. Focusing on the most responsive categories can shed light on how monetary policy has influenced the path of inflation over the post-pandemic period. We use our results to divide the categories into two groups of goods and services. The most responsive group (blue bars) contains goods and services whose largest cumulative percent price decline over a four-year window is in the top 50% of all such declines. The least responsive group (red bars) contains goods and services in the bottom 50%.
Following the methods in Shapiro (2022), we use these two groups, along with the share of total expenditures for each good or service, to create two new aggregate PCE inflation measures. Figure 2 shows their 12-month percent changes over time. The blue shading marks the period from mid-2019 until early 2020 when the Federal Reserve lowered the federal funds rate. The vertical yellow line marks the start of the most recent tightening cycle in March 2022. Inflation in the most responsive categories (blue line) is more volatile than overall headline PCE inflation (green line) from the Bureau of Economic Analysis (BEA), and inflation in the least responsive categories is less volatile (red line).
Figure 2 Most and least responsive inflation rates
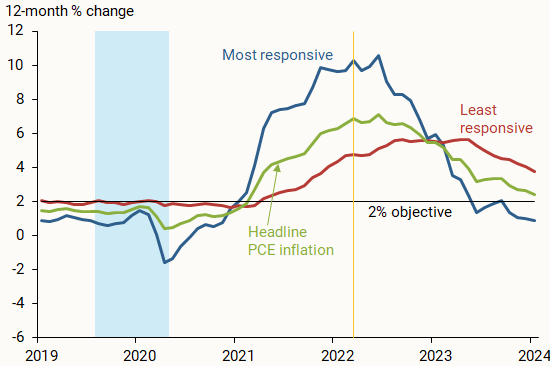
After the start of the 2020 recession, inflation rates for both categories rose but have since come down from their recent peaks. This pattern is particularly pronounced for the most responsive inflation group, for which inflation peaked at 10.5% in mid-2022 and has fallen to 0.9% as of January 2024; this is just under its average of 1% from 2012, when the Federal Reserve officially adopted a numerical inflation objective, to 2019. Inflation in the least responsive group peaked later, in early 2023, and has fallen only slightly to 3.8% as of January 2024; it remains well above its 2012–2019 average of 1.8%.
How does policy-responsive inflation react to rate increases?
The inflation rates of categories in the most and least responsive groups can move for reasons beyond changes in the federal funds rate, such as global or national macroeconomic developments. To assess the specific role of policy rate increases, we use the methodology described earlier to estimate how the most and least responsive inflation groups tend to react to rate hikes.
The results in Figure 3 suggest that an increase in the federal funds rate typically starts exerting downward pressure on the most responsive prices after about 18 months, when the line showing the impulse response function falls below zero. Month-to-month price changes start falling after a little over a year, depicted when the slope drops below zero and stays negative. This is quicker than the response of overall headline prices from the BEA (not shown), which becomes negative after a little over 24 months and shows month-to-month declines after about 18 months.
Figure 3 Reaction of most and least responsive prices to rate hikes
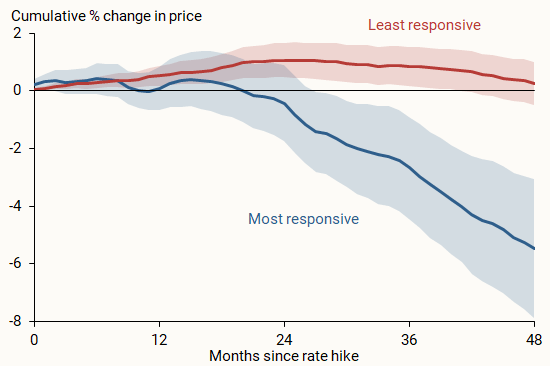
Because we grouped inflation categories based on the size of their response, there is not necessarily a tie-in to the speed of each categories’ change. However, our results suggest that looking at the most responsive goods and services may also be a useful way of assessing how quickly monetary policy affects inflation.
Applying the typical impact timing of the most responsive group of goods and services to the most recent tightening cycle, shown by the federal funds rate line in Figure 4, leads to several conclusions. First, rate cuts from 2019 to early 2020 could have contributed upward price pressures starting in mid- to late 2020 and thus could explain some of the rise in inflation over this period. Second, the tightening cycle that began in March 2022 likely started putting downward pressure on prices in mid-2023 and will continue to do so in the near term. This is consistent with the view that the full effects of monetary policy tightening have yet to be felt. Finally, though inflation for the most responsive categories has been falling since mid-2022, the early part of this decline was likely to have been driven more by changes in prevailing economic conditions than by policy tightening, given estimated policy lags. Some research has considered whether policy lags have shortened (see, for example, Doh and Foerster 2021); however, because inflation began falling mere months after the first rate hike, the drop in inflation may have been too soon to be caused by policy action.
Figure 4 Headline inflation contributions and the federal funds rate
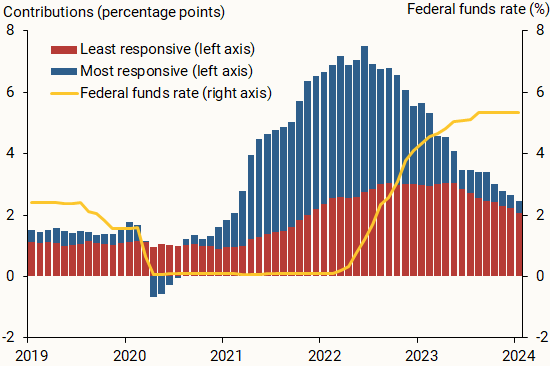
Our findings in this Letter are useful for broadening our understanding of how monetary policy affects inflation. For example, if inflation and the contributions to overall headline inflation are high in a set of categories that are more responsive to monetary policy, as was the case in early 2022, then rate hikes during the most recent tightening cycle are likely to continue to reduce inflation due to policy lags. On the other hand, though inflation in the least responsive categories may come down because of other economic forces, less inflation is currently coming from categories that are most responsive to monetary policy, perhaps limiting policy impacts going forward.
Doh, Taeyoung, and Andrew T. Foerster. 2022. “ Have Lags in Monetary Policy Transmission Shortened? ” FRB Kansas City Economic Bulletin (December 21).
Jordà, Òscar. 2005. “Estimation and Inference of Impulse Responses by Local Projections.” American Economic Review 95(1), pp. 161–182.
Romer, Christina, and David Romer. 2004. “A New Measure of Monetary Shocks: Derivation and Implications.” American Economic Review 94(4), pp. 1,055–1,084.
Shapiro, Adam. 2022. “ A Simple Framework to Monitor Inflation .” FRB San Francisco Working Paper 2020-29.
Wieland, Johannes, and Mu‐Jeung Yang. 2020. “Financial Dampening.” Journal of Money, Credit and Banking 52(1), pp. 79–113.
Opinions expressed in FRBSF Economic Letter do not necessarily reflect the views of the management of the Federal Reserve Bank of San Francisco or of the Board of Governors of the Federal Reserve System. This publication is edited by Anita Todd and Karen Barnes. Permission to reprint portions of articles or whole articles must be obtained in writing. Please send editorial comments and requests for reprint permission to [email protected]
Advertisement
Supported by
Stock Market Surges to Start the Year: 22 Record Highs in 3 Months
The S&P 500, rising 10 percent, set the pace as investors looked forward to interest rate cuts.
- Share full article

By Joe Rennison
It’s been a blistering start to the year for the stock market.
The S&P 500 , one of the most widely watched stock indexes in the world, has risen more than 10 percent over the first three months of 2024, buoyed by 22 record highs.
Roughly 40 percent of the stocks in the index are trading above where they were 12 months ago. And even when the index has lost ground, it hasn’t been by much, with only three days so far in 2024 in which the S&P 500 has fallen more than 1 percent by the close.
The move has been driven by renewed appetite for stocks. Investors in March poured roughly $50 billion into funds that buy stocks in the United States, according to data from EPFR Global.
A modest rally in January, based on expectations the Federal Reserve would start cutting interest rates this year has given way to more widespread optimism that the central bank could bring inflation down to its target of 2 percent without inflicting too much damage on the economy — the long-hoped-for “soft landing.”
A fresh reading on inflation and spending released on Friday was in line with economists’ expectations, reinforcing the prevailing forecasts for Fed rate moves. “We don’t need to be in a hurry to cut,” Jerome H. Powell, the Fed chair, said at an event on Friday .
In the markets, exuberance has spread to the riskier corners of the financial system. Bitcoin continues to trade above $70,000, a threshold it reached for the first time this month after regulators made it easier for ordinary investors to buy funds that track the price of the cryptocurrency. At the same time, mergers and takeovers have surged and the public debuts of Reddit and Trump Media were greeted with big pops in share price on their first day of trading. And in credit markets, where investors finance companies via bonds and loans, the demand to borrow and the desire to lend have swelled — a sign of optimism over the outlook for corporate America.
Even with the Fed contemplating cutting interest rates as many as three times this year, by as much as three-quarters of a percentage point in total, the returns on offer to investors remain well above those found elsewhere around the globe, helping keep money flowing into the United States.
“I am seeing it from all over the world,” said Andrew Brenner, head of international fixed income at National Alliance Securities.
But Mr. Brenner also sees reason for caution. Cracks are emerging in the economy, with consumer finances beginning to wane. Credit card debt has been rising, and the number of people behind on their car loans has surged at the quickest pace in more than a decade. Some companies are also beginning to struggle, with the number defaulting on their debts more than doubling last year, according to S&P Global.
The Russell 2000 index of smaller companies, a measure of firms more susceptible to the ebb and flow of the domestic economy, also rose over the first three months of the year, but by just 4.3 percent. It’s a reminder that the biggest companies are driving the stock market higher — especially those surfing the wave of optimism over artificial intelligence.
“Stocks are working for people right now,” Mr. Brenner said. “I just wonder how long until we run into some trouble.”
The so-called Magnificent Seven group of stocks that drove the market higher last year continued to have an outsize impact, responsible for almost 40 percent of the S&P 500’s rise over the first three months, according to data from Howard Silverblatt at S&P.
However, steep drops for Apple and Tesla meant that an even smaller cohort of companies — Nvidia , Meta, Amazon and Microsoft — pushed the market to new heights. They were responsible for half of the index’s gain on their own.
“Earnings are good, interest rates are off their peak and employment remains high, with consumers willing to spend their paychecks,” Mr. Silverblatt said. “So the market continues up.”
Joe Rennison writes about financial markets, a beat that ranges from chronicling the vagaries of the stock market to explaining the often-inscrutable trading decisions of Wall Street insiders. More about Joe Rennison
- a. Send us an email
- b. Anonymous form
- Buyer's Guide
- Upcoming Products
- Tips / Contact Us
- Podcast Instagram Facebook Twitter Mastodon YouTube Notifications RSS Newsletter
Apple Researchers Reveal New AI System That Can Beat GPT-4
Apple researchers have developed an artificial intelligence system named ReALM (Reference Resolution as Language Modeling) that aims to radically enhance how voice assistants understand and respond to commands.
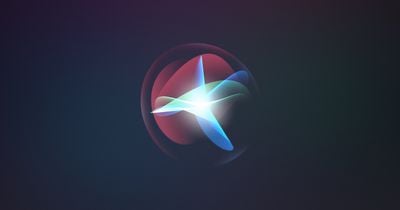
Reference resolution is an important part of natural language understanding, enabling users to use pronouns and other indirect references in conversation without confusion. For digital assistants, this capability has historically been a significant challenge, limited by the need to interpret a wide range of verbal cues and visual information. Apple's ReALM system seeks to address this by converting the complex process of reference resolution into a pure language modeling problem. In doing so, it can comprehend references to visual elements displayed on a screen and integrate this understanding into the conversational flow.
ReALM reconstructs the visual layout of a screen using textual representations. This involves parsing on-screen entities and their locations to generate a textual format that captures the screen's content and structure. Apple researchers found that this strategy, combined with specific fine-tuning of language models for reference resolution tasks, significantly outperforms traditional methods, including the capabilities of OpenAI's GPT-4.
ReALM could enable users to interact with digital assistants much more efficiently with reference to what is currently displayed on their screen without the need for precise, detailed instructions. This has the potential to make voice assistants much more useful in a variety of settings, such as helping drivers navigate infotainment systems while driving or assisting users with disabilities by providing an easier and more accurate means of indirect interaction.
Apple has now published several AI research papers. Last month, the company revealed a new method for training large language models that seamlessly integrates both text and visual information. Apple is widely expected to unveil an array of AI features at WWDC in June.
Get weekly top MacRumors stories in your inbox.
Top Rated Comments

enabling users to use pronouns and other indirect references in conversation without confusion.

It's good if AI understands "Can you repeat that?" properly. /thread

Popular Stories
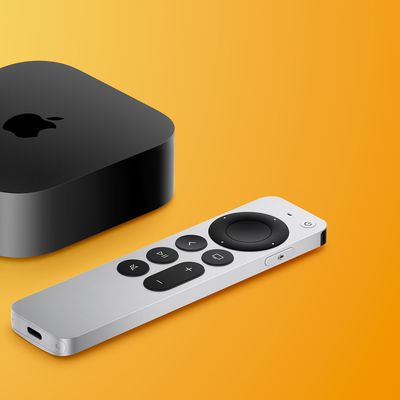
When to Expect a New Apple TV to Launch
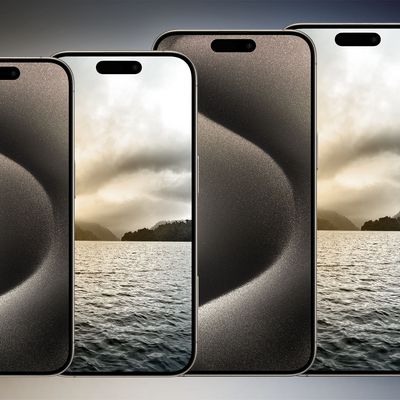
Alleged iPhone 16 Battery Details Show Smaller Capacity for One Model
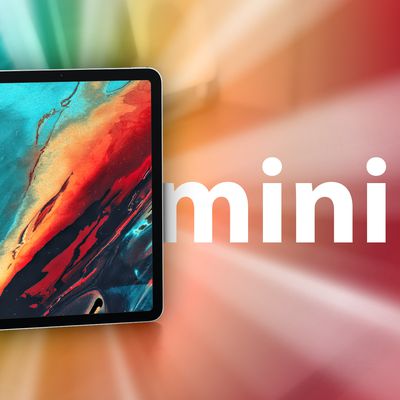
When to Expect the Next iPad Mini and Low-End iPad Models to Launch
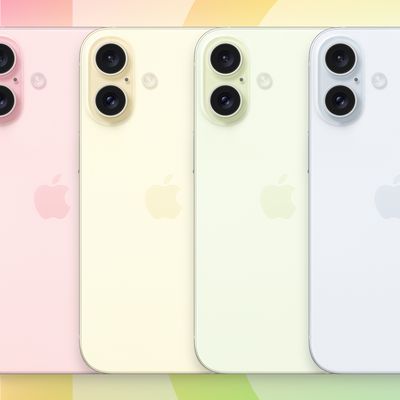
iPhone 16 Plus Rumored to Come in These 7 Colors
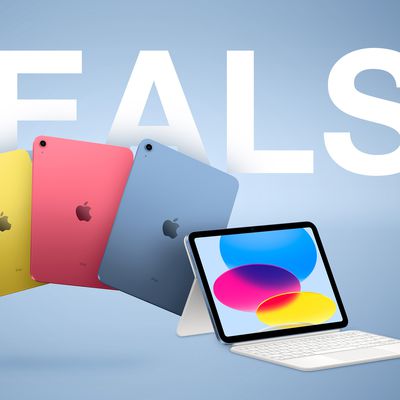
Best Buy Introduces Record Low Prices Across Every 10th Gen iPad
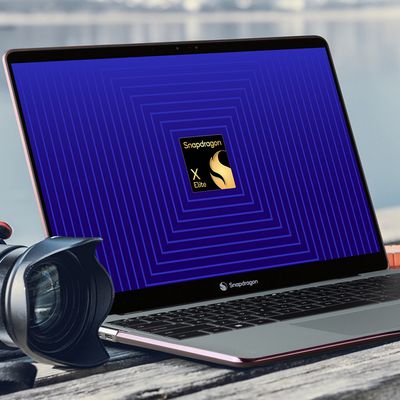
Microsoft Says Windows Laptops With Snapdragon X Elite Will Be Faster Than M3 MacBook Air
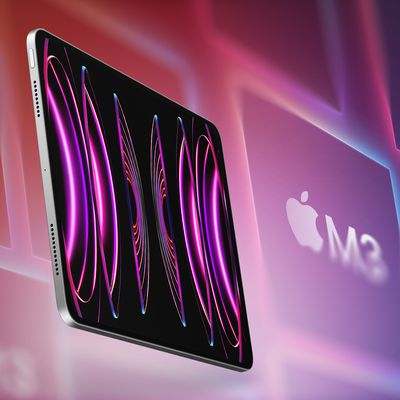
Apple Event for New iPads Still Considered 'Unlikely' Following Delays
Next article.
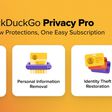
Our comprehensive guide highlighting every major new addition in iOS 17, plus how-tos that walk you through using the new features.
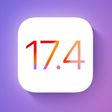
App Store changes for the EU, new emoji, Podcasts transcripts, and more.
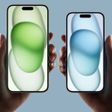
Get the most out your iPhone 15 with our complete guide to all the new features.
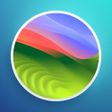
A deep dive into new features in macOS Sonoma, big and small.
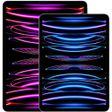
Revamped models with OLED displays, M3 chip, and redesigned Magic Keyboard accessory.
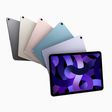
Updated 10.9-inch model and new 12.9-inch model, M2 chip expected.
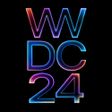
Apple's annual Worldwide Developers Conference will kick off with a keynote on June 10.
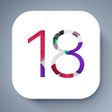
Expected to see new AI-focused features and more. Preview coming at WWDC in June with public release in September.
Other Stories
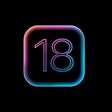
6 hours ago by Tim Hardwick
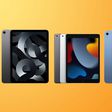
8 hours ago by Tim Hardwick
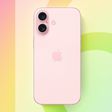
1 day ago by Tim Hardwick
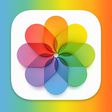
1 day ago by MacRumors Staff
- Asia Pacific
- Latin America
- Middle East & Africa
- North America
- Australia & New Zealand
Mainland China
- Hong Kong SAR, China
- Philippines
- Taiwan, China
- Channel Islands
- Netherlands
- Switzerland
- United Kingdom
- Saudi Arabia
- South Africa
- United Arab Emirates
- United States
From startups to legacy brands, you're making your mark. We're here to help.
- Innovation Economy Fueling the success of early-stage startups, venture-backed and high-growth companies.
- Midsize Businesses Keep your company growing with custom banking solutions for middle market businesses and specialized industries.
- Large Corporations Innovative banking solutions tailored to corporations and specialized industries.
- Commercial Real Estate Capitalize on opportunities and prepare for challenges throughout the real estate cycle.
- Community Impact Banking When our communities succeed, we all succeed. Local businesses, organizations and community institutions need capital, expertise and connections to thrive.
- International Banking Power your business' global growth and operations at every stage.
- Client Stories
Prepare for future growth with customized loan services, succession planning and capital for business equipment.
- Asset Based Lending Enhance your liquidity and gain the flexibility to capitalize on growth opportunities.
- Equipment Financing Maximize working capital with flexible equipment and technology financing.
- Trade & Working Capital Experience our market-leading supply chain finance solutions that help buyers and suppliers meet their working capital, risk mitigation and cash flow objectives.
- Syndicated Financing Leverage customized loan syndication services from a dedicated resource.
- Employee Stock Ownership Plans Plan for your business’s future—and your employees’ futures too—with objective advice and financing.
Institutional Investing
Serving the world's largest corporate clients and institutional investors, we support the entire investment cycle with market-leading research, analytics, execution and investor services.
- Institutional Investors We put our long-tenured investment teams on the line to earn the trust of institutional investors.
- Markets Direct access to market leading liquidity harnessed through world-class research, tools, data and analytics.
- Prime Services Helping hedge funds, asset managers and institutional investors meet the demands of a rapidly evolving market.
- Global Research Leveraging cutting-edge technology and innovative tools to bring clients industry-leading analysis and investment advice.
- Securities Services Helping institutional investors, traditional and alternative asset and fund managers, broker dealers and equity issuers meet the demands of changing markets.
- Financial Professionals
- Liquidity Investors
Providing investment banking solutions, including mergers and acquisitions, capital raising and risk management, for a broad range of corporations, institutions and governments.
- Center for Carbon Transition J.P. Morgan’s center of excellence that provides clients the data and firmwide expertise needed to navigate the challenges of transitioning to a low-carbon future.
- Corporate Finance Advisory Corporate Finance Advisory (“CFA”) is a global, multi-disciplinary solutions team specializing in structured M&A and capital markets. Learn more.
- Development Finance Institution Financing opportunities with anticipated development impact in emerging economies.
- Sustainable Solutions Offering ESG-related advisory and coordinating the firm's EMEA coverage of clients in emerging green economy sectors.
- Mergers and Acquisitions Bespoke M&A solutions on a global scale.
- Capital Markets Holistic coverage across capital markets.
- Capital Connect
- In Context Newsletter from J.P. Morgan
- Director Advisory Services
Accept Payments
Explore Blockchain
Client Service
Process Payments
Manage Funds
Safeguard Information
Banking-as-a-service
Send Payments
- Partner Network
A uniquely elevated private banking experience shaped around you.
- Banking We have extensive personal and business banking resources that are fine-tuned to your specific needs.
- Investing We deliver tailored investing guidance and access to unique investment opportunities from world-class specialists.
- Lending We take a strategic approach to lending, working with you to craft the fight financing solutions matched to your goals.
- Planning No matter where you are in your life, or how complex your needs might be, we’re ready to provide a tailored approach to helping your reach your goals.
Whether you want to invest on your own or work with an advisor to design a personalized investment strategy, we have opportunities for every investor.
- Invest on your own Unlimited $0 commission-free online stock, ETF and options trades with access to powerful tools to research, trade and manage your investments.
- Work with our advisors When you work with our advisors, you'll get a personalized financial strategy and investment portfolio built around your unique goals-backed by our industry-leading expertise.
- Expertise for Substantial Wealth Our Wealth Advisors & Wealth Partners leverage their experience and robust firm resources to deliver highly-personalized, comprehensive solutions across Banking, Lending, Investing, and Wealth Planning.
- Why Wealth Management?
- Retirement Calculators
- Market Commentary
Who We Serve
Explore a variety of insights.
Global Research
- Newsletters
Insights by Topic
Explore a variety of insights organized by different topics.
Insights by Type
Explore a variety of insights organized by different types of content and media.
- All Insights
We aim to be the most respected financial services firm in the world, serving corporations and individuals in more than 100 countries.
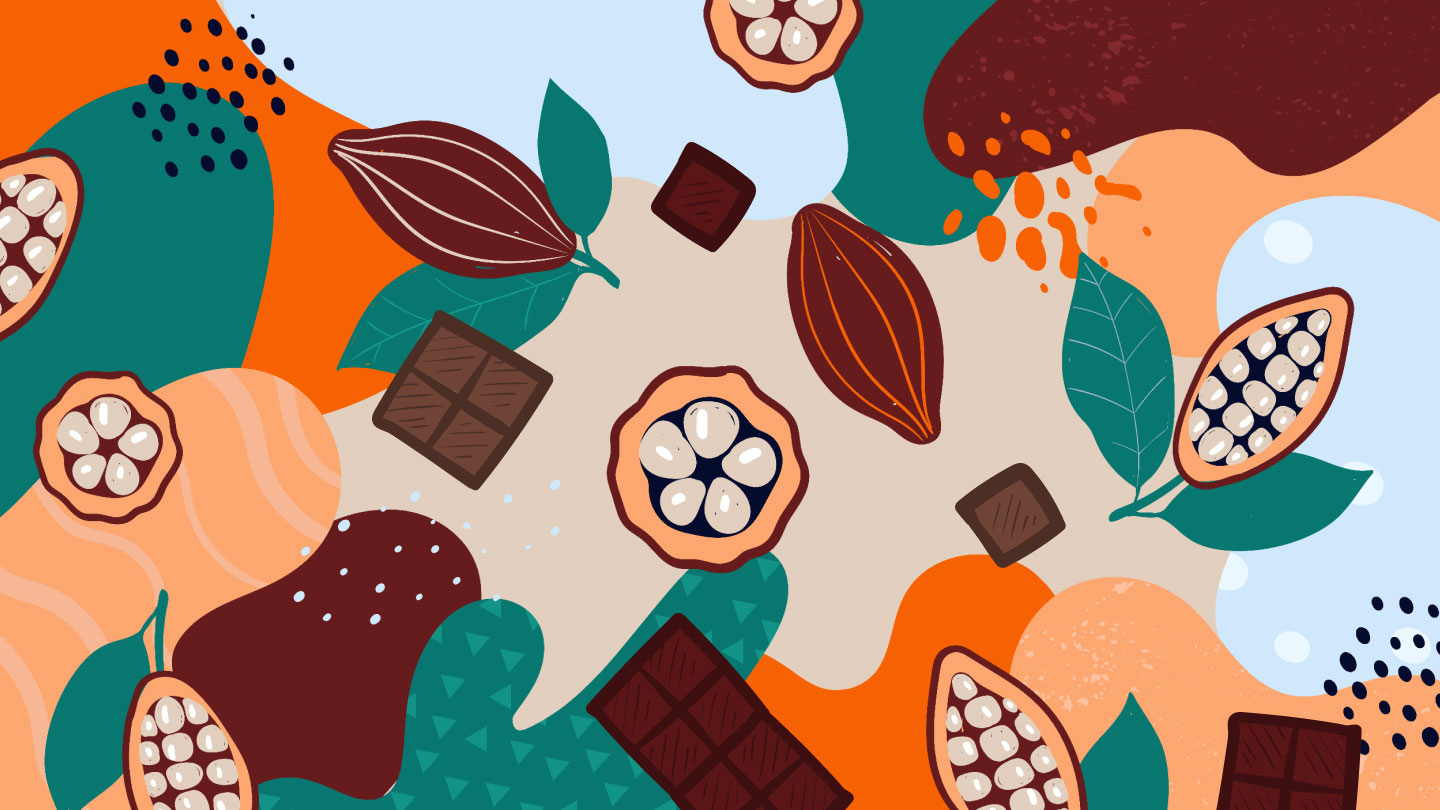
Key takeaways
- Cocoa prices are rising due to a global supply shortage, chronic underinvestment in cocoa farms and investor speculation.
- Chocolate brands are grappling with the impact of higher cocoa costs, and this is resulting in price hikes and shrinkflation.
- J.P. Morgan Research projects cocoa prices will come down slightly over the medium term, tracking around the $6,000 mark.
Cocoa prices have skyrocketed in recent months, reaching historical highs of nearly $10,000 per metric ton in March 2024. What’s driving this unprecedented increase? How will this impact chocolate brands? And where is the market headed?
Why are cocoa prices rising?
The rise in cocoa prices is largely due to a global cocoa shortage. Climate change -induced drought has ravaged crops in West Africa, which contributes around 80% of the world’s cocoa output. According to the International Cocoa Organization, global cocoa supply will decline by almost 11% over the 2023/2024 season.
There are also deep-rooted structural issues at play, including chronic underinvestment in cocoa farms. Today, the crop is still largely cultivated by smallholder farmers, many of whom struggle to make a living income and lack the means to reinvest in their land — which translates to lower yields over time. “Cocoa is a market where the grower produces a very high-value good but receives a very low share of the actual value chain. As a result, replanting rates are very low and cocoa trees are ageing,” said Tracey Allen, an Agricultural Commodities Strategist at J.P. Morgan.
This is all further compounded by investor speculation, which is driving prices up. “What was a structurally led, supply-side issue has been exacerbated by dry weather and a strong Harmattan [a season in West Africa]. It has now manifested in an investor-driven parabolic move in prices, especially over the last six weeks,” Allen added. “For instance, non-commercial investors now hold over 60% of total open interest across cocoa futures and options in the New York market, which is an historical high. Consumers are now scrambling to hedge forward exposure in thin liquidity.”
Chocolate sales have declined in recent months
Non-seasonal unit sales of chocolate have declined year-over-year since 2022. On the other hand, prices have increased year-over-year.
“We have seen some shifting away from chocolate to other products, whether that’s cookies or salty snacks. We think chocolate’s losing a bit of share, so consumers are certainly reacting to higher prices.”
Ken Goldman
Lead Equity Research Analyst for U.S. Food Producers and Food Retailers, J.P. Morgan
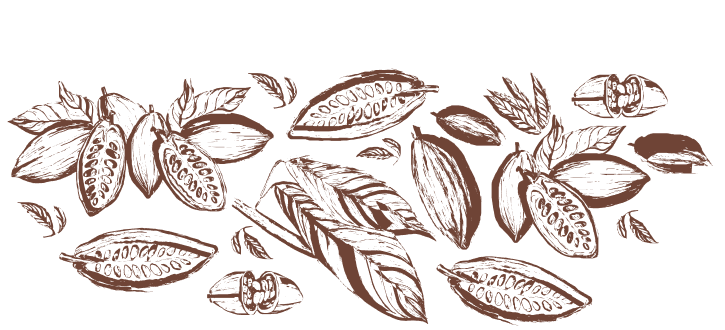
What’s the impact on chocolate makers?
Chocolate brands are grappling with the impact of higher cocoa costs, and many are passing on the burden to consumers in the form of price hikes.
“In the U.S., Hershey has been very clear that list pricing is still one of the most important arrows in their quiver to offset inflation . Over the next year or two, they will probably pass on more cocoa inflation, and consumers will see higher prices for their chocolate as a result,” said Ken Goldman, Lead Equity Research Analyst for U.S. Food Producers and Food Retailers at J.P. Morgan. “I’m sure Hershey’s competitors like Mars and Lindt are going to do the same — it’s just a matter of time.”
This could, however, dampen consumer demand. “Companies will have to be careful about how they manage the elasticity of demand for chocolate, especially as consumers might not be able to take the price hikes needed to offset the huge increase in cocoa costs. We see an impact on volume whenever prices are raised, even in other categories that are deemed inelastic,” said Celine Pannuti, Head of European Staples & Beverages at J.P. Morgan.
Indeed, chocolate sales have already taken a hit. “In the U.S., snacks in general have been slumping a little bit over the last two months, likely because they are a little bit more discretionary in nature. Within the category itself, we have seen some shifting away from chocolate to other products, whether that’s cookies or salty snacks,” Goldman added. “We think chocolate’s losing a bit of share, so consumers are certainly reacting to higher prices.”
In a bid to regain market share, some manufacturers are innovating with recipes that call for less cocoa — for instance, chocolate bars containing a higher proportion of fruits and nuts — while others are reducing the size of their products. “We’ve seen cases of shrinkflation, so for the same price, the chocolate bar is much smaller,” Pannuti noted. “But while these are some avenues that companies are taking, consumers will ultimately have to face up to higher prices.”
“Companies will have to be careful about how they manage the elasticity of demand for chocolate, especially as consumers might not be able to take the price hikes needed to offset the huge increase in cocoa costs.”
Celine Pannuti
Head of European Staples & Beverages, J.P. Morgan
Will cocoa prices continue to surge?
While J.P. Morgan Research projects cocoa prices will remain elevated, they are expected to come down slightly over the medium term, tracking around the $6,000 mark.
“The market has been well overshot, so I wouldn’t be surprised if prices move off the peak before stabilizing at an elevated level, until there is a genuine supply-side response,” Allen noted.
The latter could materialize thanks to global climate patterns , which could increase yields in some cocoa-producing regions. The ongoing El Niño phenomenon (the warming of sea surface temperatures) is gradually weakening, which could herald a transition to La Niña (the cooling of sea surface temperatures) in the summer. “Under this scenario, weather threats would diminish and we should receive improved rainfall across West Africa and Asia,” Allen said. “This would be supportive of main crop output from over 80% of the world’s cocoa-producing countries including Côte d’Ivoire and Ghana, paving the way for cocoa prices to ease off the historic highs. Increasing cacao plantings will be critical to boost longer-term supply.”
How are rising cocoa prices impacting chocolate brands?
Cocoa prices have soared to an all-time high due to a global supply shortage. What’s the impact on chocolate makers, and what are they doing to adapt? Here, Ken Goldman, Lead Equity Research Analyst for U.S. Food Producers and Food Retailers, and Celine Pannuti, Head of European Staples and Beverages, share their thoughts with Shirley Wang, a credit analyst covering the investment-grade and high-yield consumer and retail sector.
How are Rising Cocoa Prices Impacting Chocolate Brands?
Shirley Wang: Cocoa prices have reached multi-decade highs due to a global supply shortage. How is this effecting chocolate makers? And is consumer demand suffering as a result? Welcome to Research Recap on J.P. Morgan's Making Sense Podcast channel. I'm Shirley Wang, a credit analyst covering the investment-grade and high-yield consumer and retail sector at J.P. Morgan. Today, I'm joined by my colleagues, Ken Goldman, lead equity research analyst for US food producers and food retailers, and Céline Pannuti, head of European staples and beverages, and we're here to discuss all things chocolate. Ken and Céline, thanks so much for joining us today.
Ken Goldman: Thank you for having us.
Céline Pannuti: Thank you so much.
Shirley Wang: To kick things off, Ken, can you start us off by providing some context? How is the ongoing cocoa shortage affecting the prices of chocolate on supermarket shelves?
Ken Goldman: Up and about to go up more. Chocolate prices are high, obviously, like every other food item, but whereas most food inflation, we're probably done with and prices will remain high, because cocoa is rising so much, chocolate prices will have to rise more. Now, this may take some time. Hershey, for example, has said that right now they are not taking a lot of incremental list pricing, meaning it won't raise the actual price that they charge pre-discount to their customers. But they've also been very clear recently that list pricing is still one of the most important arrows in their quiver to offset inflation. And so, I think over the next year, two years, they will pass on as much as they possibly can of cocoa inflation. And as a result we as consumers we'll see higher prices for our chocolate. And I'm sure Hershey's competitors like Mars and Lindt and everyone else who's big in the United States is going to do the same. It's just a matter of time.
Shirley Wang: And Céline, what are you noticing from some of the companies you cover?
Céline Pannuti: From my standpoint, I concur with what Ken said. Nestlé, which I cover, is active in chocolate. They own Kit Kat, for example. We've seen pricing for them really accelerating by the last two years, but what you're referring to is really a huge spike in cocoa prices that we are seeing now in the last four, five months. And that will take time to work its way through. But effectively, there will be more pricing to be taken. At the same time, I think companies will have to be careful on how they manage the elasticity because I presume consumers won't be able to take as much pricing as what they probably need to take to offset this huge increase in cocoa prices.
Shirley Wang: Great, thanks for that. So, both of you mentioned pricing. But, apart from that how else are companies within your coverage being affected by the higher cocoa prices. Are they using less chocolate in their recipes for example?
Ken Goldman: So, some of the companies that I cover, I think you will see them lean a little bit in their marketing away from some chocolate items, right? And it's tough for Mondelēz because most of what they sell outside the US is chocolate. But Hershey has items like Twizzlers and Jolly Ranchers, and they can certainly try and shift some of their consumer consumption toward those confectionery items that are not based on chocolate. And as we think about the ingredients themselves and the products themselves, yeah, it's possible, right? It's possible to use a little bit less cocoa. I don't think these companies really wanna do that, but at times in the past, maybe the distant past, some of these companies that we cover have shifted that mix a little bit. There's also a way to lean people more toward the chocolate bars that have nuts in them and fruit in them, or add more fruit and nuts. So if you're a fan of chocolate bars that have all those sorts of croutons in them, that's the kind of side benefit you get from this. But in general, I think it's safe to say that there's only so much the companies can do in terms of the product itself to offset cocoa inflation.
Céline Pannuti: Yeah, I think the innovation will be a way to try to re-engineer some of those recipes. What we've seen as well is shrinkflation. So for the same price of a chocolate bar, the size of that bar will be much smaller. So that sometimes has infuriated consumers. Those clearly could be some of the avenues that our companies are going to take, but agreed that ultimately you will have to face up to a much higher price nonetheless.
Shirley Wang: So looking at the consumer, are people buying less chocolate products as a result, or is demand still resilient?
Ken Goldman: So snacks in general in the United States have been slumping a little bit for the last two months. Both sweet snacks and salty have been off a little bit more than usual as a percentage of total food at home sales in the US. It's a little surprising. And there's some controversy or some questions about why that is. Some people might think that it's because these are a little bit more discretionary in nature, right? It's not a meal to have a chocolate bar or a bag of potato chips. And if you as a consumer go into a grocery store and you have $50 to spend, and the cost of everything else that you must have in your basket has gone up, you may not have an extra dollar for a candy bar. So that's a broader answer. In terms of chocolate specifically, we have seen a little bit of shifting away within snacks in general, from chocolate to other products, whether it's cookies or salty snacks a little bit. So within snacking in general, we think that chocolate's losing a little bit of share as well. So consumers are certainly reacting to higher prices. It's one of the reasons why I think some of the companies that we cover are a little hesitant to take as much pricing as they normally would at this time. In a typical environment, either Hershey or Mars may have already taken more price. Now, Hershey has other reasons not to take price right now. They're implementing a new ERP system. They don't wanna complicate things as they do that, but it's a little surprising that some of their competitors haven't taken pricing either. And I think that's a large part because of some of the elasticity we've seen in the category.
Shirley: Celine, do you have anything you’d like to add on that?
Céline Pannuti: What I would say concerning, the volume performance for Nestlé specifically, we've seen that post-COVID where the chocolate or their confectionery business had been impacted by less impulse obviously during COVID, we've really seen a re-acceleration with the reopening. So we have had two good years of better volume growth and at the same time companies have raised prices. So probably the category on the global basis has been quite resilient. But like Ken said, the question mark going forward is how much more consumers can take in terms of price increases. It is true that it's a treat. But what we've seen in other categories, like coffee for instance, is that even in categories that are deemed inelastic, when prices are raised so much, we started to see this impact in volume. So I think we have to be a bit careful about what seems to have been quite resilient volume over the past couple of years if our corporates need to raise prices further. And I would expect that yes, maybe consumers could be a bit more choosy because price points ultimately would lead to them looking at alternatives, some other categories could benefit in that.
Shirley Wang: Great. So, Ken, and Céline, you've mentioned somewhat uncertain volume and strong pricing for chocolate by manufacturers passed through cost inflation, as well as mixed consumer sentiment. As we wrap up today’s podcast, how do you see the overall impact for food manufacturers?
Ken Goldman: Well, I think, you know, higher inflation in general for food producers presents a challenge, right? It's not always easy to pass on all of your higher costs to your customers. They don't wanna always take it because then they have to pass it on to their consumers. And especially when you have recession-like symptoms for lack of a better word, among lower-end consumers, it creates even more of a challenge. So, certainly we've seen Hershey guide to flat earnings for 2024. A large part of that is because of cocoa inflation and potentially because of their limited ability to pass it on immediately. As we look to 2025, a lot of my clients, institutional investors, have become concerned that higher cocoa will affect Hershey's EPS in 2025 as well. And the, the, the reason it's so far out is Hershey and other food companies, including Mondelēz, typically don't buy most of their cocoa needs on spot markets. They will buy them on future markets. Hershey has said they will go anywhere from 3 to 24 months out in terms of their purchases. So it's difficult for us to be precise in forecasting exactly when a higher inflation will hit their income statement, but at some point down the road, and a lot of investors think it's 2025, they will have another headwind incrementally above what they have this year, and they'll have to figure out how to deal with that, but it's not going to be something they can fully offset.
Céline Pannuti: Yeah. And, and as far as I'm concerned, Nestlé only has 10% of their sales tied to confectionery, so it's not exactly relevant on the overall EPS growth, but effectively they have been constrained in terms of their EPS delivery in the past for higher cost inflation. So I would imagine that the confectionery profit pool is probably going to be constrained even if it's only a certain percentage of the total for Nestlé.
Shirley Wang: Ken and Céline, thanks so much for sharing your thoughts today.
Ken Goldman: Thank you.
Céline Pannuti: Thank you.
Shirley Wang: And thank you to all our listeners for tuning in. We hope you join us again next time.
[END OF EPISODE]
Related insights
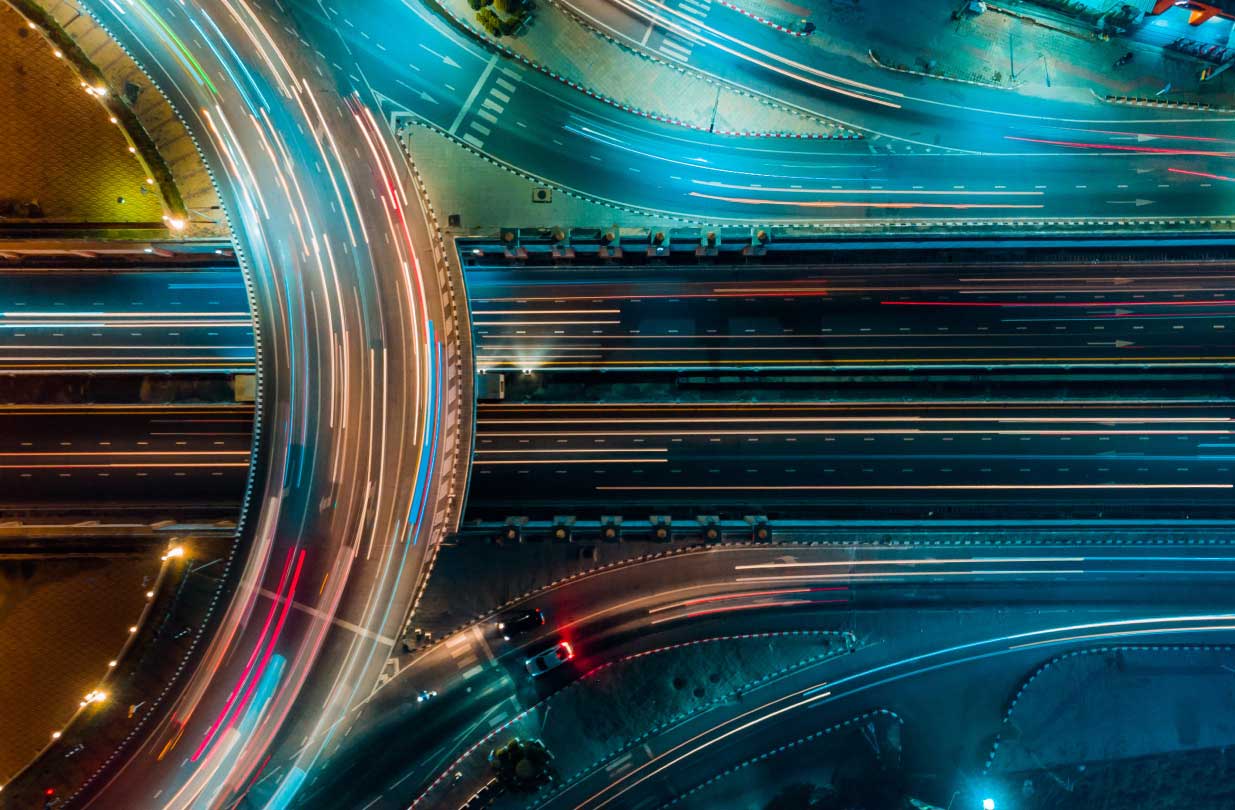
Leveraging cutting-edge technology and innovative tools to bring clients industry-leading analysis and investment advice.
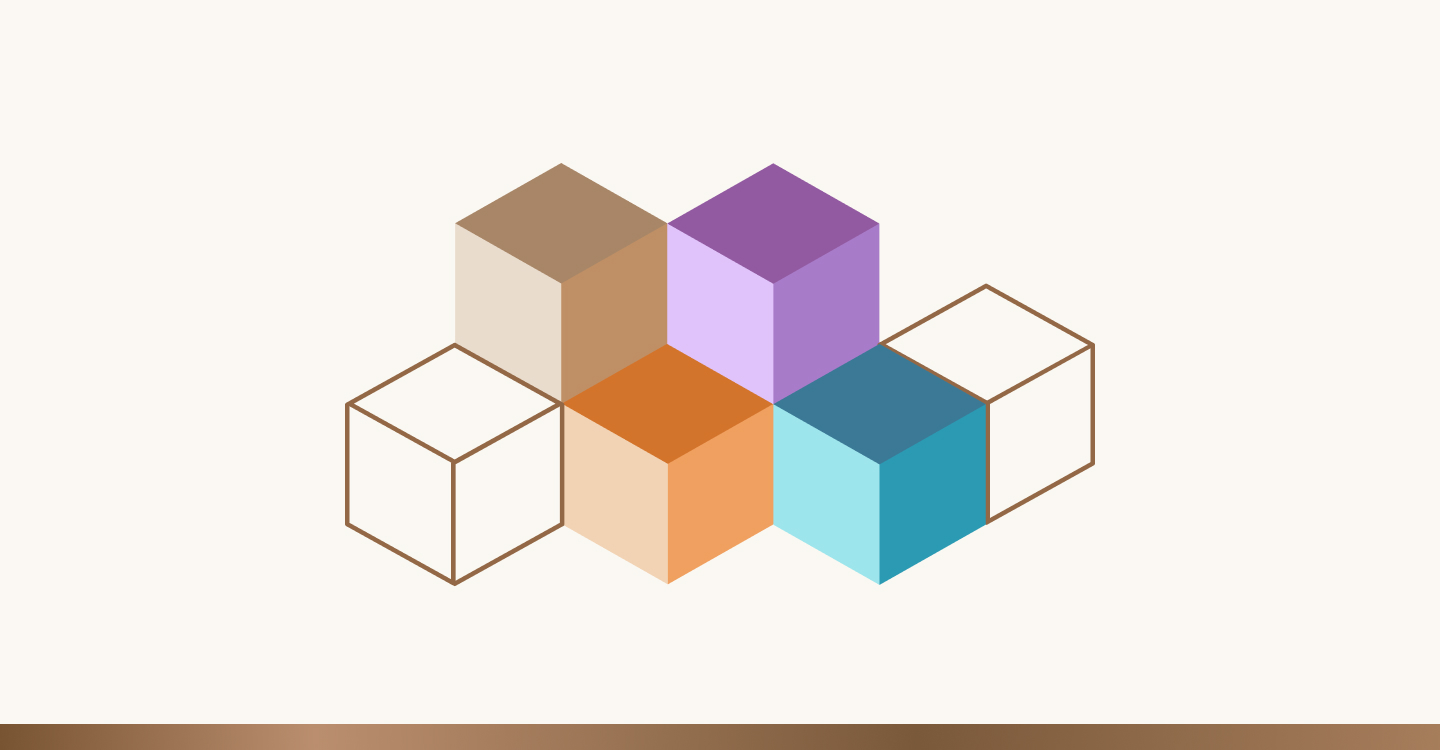
16:12 - Economy
Research Recap | El Niño and the impact on agriculture
July 26, 2023
In this episode of Research Recap, discover how El Niño will affect crop yields and other commodities.
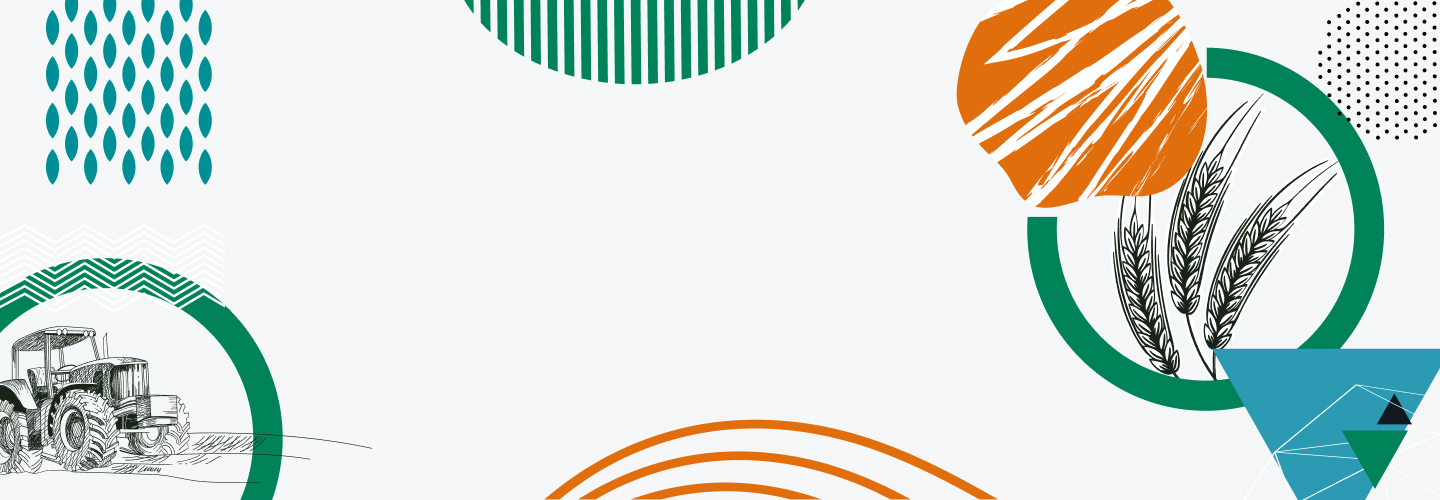
Climate change and the impact on agriculture
August 02, 2022
How is climate change and extreme weather affecting crop production and prices? J.P. Morgan Research examines the effects on agricultural commodities.
This communication is provided for information purposes only. Please read J.P. Morgan research reports related to its contents for more information, including important disclosures. JPMorgan Chase & Co. or its affiliates and/or subsidiaries (collectively, J.P. Morgan) normally make a market and trade as principal in securities, other financial products and other asset classes that may be discussed in this communication. This communication has been prepared based upon information, including market prices, data and other information, from sources believed to be reliable, but J.P. Morgan does not warrant its completeness or accuracy except with respect to any disclosures relative to J.P. Morgan and/or its affiliates and an analyst's involvement with any company (or security, other financial product or other asset class) that may be the subject of this communication. Any opinions and estimates constitute our judgment as of the date of this material and are subject to change without notice. Past performance is not indicative of future results. This communication is not intended as an offer or solicitation for the purchase or sale of any financial instrument. J.P. Morgan Research does not provide individually tailored investment advice. Any opinions and recommendations herein do not take into account individual client circumstances, objectives, or needs and are not intended as recommendations of particular securities, financial instruments or strategies to particular clients. You must make your own independent decisions regarding any securities, financial instruments or strategies mentioned or related to the information herein. Periodic updates may be provided on companies, issuers or industries based on specific developments or announcements, market conditions or any other publicly available information. However, J.P. Morgan may be restricted from updating information contained in this communication for regulatory or other reasons. Clients should contact analysts and execute transactions through a J.P. Morgan subsidiary or affiliate in their home jurisdiction unless governing law permits otherwise. This communication may not be redistributed or retransmitted, in whole or in part, or in any form or manner, without the express written consent of J.P. Morgan. Any unauthorized use or disclosure is prohibited. Receipt and review of this information constitutes your agreement not to redistribute or retransmit the contents and information contained in this communication without first obtaining express permission from an authorized officer of J.P. Morgan.
You're now leaving J.P. Morgan
J.P. Morgan’s website and/or mobile terms, privacy and security policies don’t apply to the site or app you're about to visit. Please review its terms, privacy and security policies to see how they apply to you. J.P. Morgan isn’t responsible for (and doesn’t provide) any products, services or content at this third-party site or app, except for products and services that explicitly carry the J.P. Morgan name.
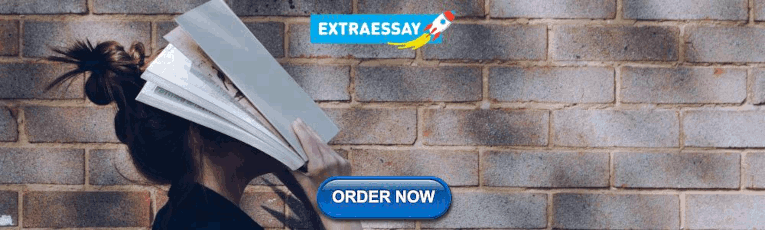
IMAGES
VIDEO
COMMENTS
This paper presents an updated systematic review of the state of the art in the stock market forecast considering fundamental and technical analysis from 2014 to 2018. ... after 2009, there has been produced a significant amount of new research work on stock market prediction using techniques from the family of neural networks. At the same time ...
The stock market is a key pivot in every growing and thriving economy, and every investment in the market is aimed at maximising profit and minimising associated risk. As a result, numerous studies have been conducted on the stock-market prediction using technical or fundamental analysis through various soft-computing techniques and algorithms. This study attempted to undertake a systematic ...
Stock market predictions use mathematical strategies and learning tools. This paper provides a complete overview of 30 research papers recommending methods that include calculation methods, ML algorithms, performance parameters, and outstanding journals. The studies are selected based on research questions.
To our knowledge, there has been very limited research on the variables included during the model building process for stock market prediction. In this paper, we focus on a systematic review of the literature in financial market forecasting with a focus on the data included in the studies and the statistical and machine learning methods used ...
This research paper discusses financial aid options, tax credits, and 529 savings plans and other savings vehicles, as well as the need to plan strategically and understand how college spending decisions affect one's broader financial situation. ... Our research into years of daily stock-level data suggests that U.S. equities are resilient to ...
The purpose of this study is to identify the key aspects that have been studied in the area of stock market liquidity, accumulate their important findings, and also provide a quantitative categorization of reviewed literature that will facilitate in conducting further research. The study analyzes relevant research papers published after the ...
Finally, this paper shows the findings on unaddressed open problems and gives suggestions for future work. The aim of this study is to survey the main stock market analysis models, text representation techniques for financial market prediction, shortcomings of existing techniques, and propose promising directions for future research.
Research papers as well as online sources tackling this problem were reviewed, a brief list of the same is included as part of ref-erences. 1.1 Literature Review Early research on Stock Market Prediction was based on Random walk and Efficient Market Hypothesis (EMH). Numerous studies like Gallagher, Kavussanos, Butler, show that stock market ...
In this research paper, we have tried to cover three of the latest and the most effective techniques based on Machine learning to forecast stock market prices precisely. ... The main aim of the research was to predict stock prices for the 7 stocks in the duration of 15 days period from 21 September 2016 to 11 October 2016 without referring to ...
The widespread usage of machine learning in different mainstream contexts has made deep learning the technique of choice in various domains, including finance. This systematic survey explores various scenarios employing deep learning in financial markets, especially the stock market. A key requirement for our methodology is its focus on research papers involving backtesting. That is, we ...
In the era of big data, deep learning for predicting stock market prices and trends has become even more popular than before. We collected 2 years of data from Chinese stock market and proposed a comprehensive customization of feature engineering and deep learning-based model for predicting price trend of stock markets. The proposed solution is comprehensive as it includes pre-processing of ...
The rest of this paper proceeds in the following structure. Part 2 reviews the literature that explores the mechanism of interest rate on the stock price. Part 3 proceeds to offer a theoretical analysis and further elaborates the relationship among interest rate, stock price, and the macroeconomy. ... (SEE) stock markets. Economic Research ...
The research also demonstrated the significant influence the pandemic on the opening, highest, and lowest stock indices in the US. Ngwakwe (2020) illustrated the influence of COVID-19 outbreak on some targeted stock indices (SSE, Euronext, and DJIA) by collecting the data for 50 days before and 50 days within the pandemic.
public. This resulted in a far higher volume of research papers studying the prediction of the stock market based on the technical analysis approaches. As one of the early studies in this field, at the beginning of the 90s, Kimoto et al: [9] worked on a feed-forward neural network (NN) algorithm [10] to predict the stock market
In this paper, we try to address empirically the broader question of how the stock market affects investment. We identify four theories that ... Since Robert Shiller's demonstration of the excess volatility of stock market prices, research on the efficiency of financial markets has exploded.4 In subsequent work, Shiller suggested that fads and ...
The paper concludes by highlighting the potential of Python for advanced stock market analysis and the need for further research to enhance the tool's functionalities in this regard. Overall, this research paper demonstrates how Python can extract insights from stock market data efficiently and effectively.
The current systematic review has identified 50 research articles for studies on significant aspects of stock market return and volatility, review types, and GARCH model analysis. This paper noticed that all the studies in this review used an investigational research method.
This study of foreign corporate investment transactions from 32 countries between 1976 and 2015 finds these investments pose a trade-off: While they support young firms in pursuing innovations they could not otherwise afford, they also generate knowledge for the foreign investors. 20 Aug 2020. Working Paper Summaries.
with numerous research papers published each year addressing this topic. A main reason for this growing interest is the success of deep learning in applications ranging from speech recognition to image classification and natural language processing. Considering the complexity of financial
A stock exchange, also called a securities exchange or. bourse is the name given to the facility for engaging in buying and selling of shares of. stock or bonds or other financial instruments. For ...
This article aims to build a model using Recurrent Neural Networks (RNN) and especially Long-Short Term Memory model (LSTM) to predict future stock market values. The main objective of this paper is to see in which precision a Machine learning algorithm can predict and how much the epochs can improve our model. © 2020 The Authors.
In paper [], the proposed model created three different deep learning algorithms using CNN, LSTM, and RNN to predict the NIFTY 50 index's stock returns.For situations requiring sequential data, these popular methods perform better than traditional deep learning techniques. The NIFTY 50 index uses time-varying prediction algorithms that rely only on past data to anticipate stock prices.
Stock prediction is an extremely difficult and complex endeavor since stock values can fluctuate abruptly owing to a variety of reasons, making the stock market incredibly unpredictable.This paper ...
We measure the nature and severity of a variety of belief distortions in market reactions to hundreds of economic news events using a new methodology that synthesizes estimation of a structural asset pricing model with algorithmic machine learning to quantify bias. We estimate that investors ...
From the cross-market perspective, this paper investigates crude oil volatility index (OVX) forecasts by proposing a hybrid method, which combines the data-driven SVR technique and parametric models. In terms of parametric models, we utilize GARCH-type models with jumps, and the forecasting effects of five non-parametric jumps (including ...
The paper, "Stock Market and the Psychological Health of Investors," was recently published in The Financial Review. ... research finds. Sep 13, 2023. Recommended for you.
With inflation still above the Federal Reserve's 2% objective, there is renewed interest in understanding how quickly federal funds rate hikes typically affect inflation. Beyond monetary policy's well-known lagged effect on the economy overall, new analysis highlights that not all prices respond with the same strength or speed. Results suggest that inflation for the most responsive ...
It's been a blistering start to the year for the stock market. The S&P 500, one of the most widely watched stock indexes in the world, has risen more than 10 percent over the first three months ...
Apple has now published several AI research papers. Last month, ... Apple's CEO Tim Cook this week sold 196,410 shares of the company's stock, which had a total value of approximately $33.2 ...
Unlimited $0 commission-free online stock, ETF and options trades with access to powerful tools to research, trade and manage your investments. Work with our advisors When you work with our advisors, you'll get a personalized financial strategy and investment portfolio built around your unique goals-backed by our industry-leading expertise.