The Historiography of Scientific Revolutions: A Philosophical Reflection
- Living reference work entry
- Later version available View entry history
- First Online: 16 May 2023
- Cite this living reference work entry
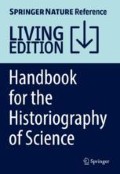
- Yafeng Shan ORCID: orcid.org/0000-0001-7736-1822 3
Part of the book series: Historiographies of Science ((HISTSC))
183 Accesses
Scientific revolution has been one of the most controversial topics in the history and philosophy of science. Yet there has been no consensus on what is the best unit of analysis in the historiography of scientific revolutions. Nor is there a consensus on what best explains the nature of scientific revolutions. This chapter provides a critical examination of the historiography of scientific revolutions. It begins with a brief introduction to the historical development of the concept of scientific revolution, followed by an overview of the five main philosophical accounts of scientific revolutions. It then challenges two historiographical assumptions of the philosophical analyses of scientific revolutions.
This is a preview of subscription content, log in via an institution to check access.
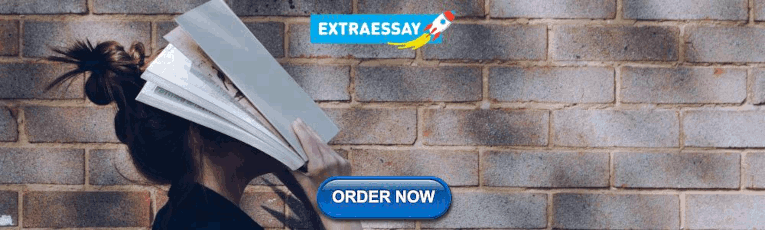
Access this chapter
Institutional subscriptions
Bateson W (1902) Mendel’s principles of heredity: a Defence. Cambridge University Press, Cambridge
Book Google Scholar
Bowler PJ (1989) The Mendelian revolution: the emergence of Hereditarian concepts in modern science and society. The Athlone Press, London
Google Scholar
Burtt EA (1925) The metaphysical foundations of modern physical science. Kegan Paul, Trench, Trubner & Co., Ltd., London
Butterfield H (1949) The Origins of Modern Science 1300–1800. G. Bell & Sons, London
Cawdrey R (1604) A table alphabeticall, London
Chang H (2012) Is water H2O? Evidence, realism and pluralism. Springer, Dordrecht
Chang H (2014) Epistemic activities and Systems of Practice: units of analysis in philosophy of science after the practical turn. In: Soler L, Zwart S, Lynch M, Israel-Jost V (eds) Science after the practice turn in the philosophy, history and social studies of science. Routledge, New York/London, pp 67–79
Clairaut A-C (1754) Du Systeme Du Monde, Dans Les Principes de La Gravitation Universelle. Suite Des Memoires de Mathematique, et Dephysique, Tires Des Registres de l’Academie Royale Des Sciences de l’annee 2:465
Cohen IB (1985) Revolution in science. Harvard University Press, Cambridge, MA
Correns C (1900) G. Mendels Regel Über Das Verhalten Der Nachkommenschaft Der Rassenbastarde. Berichte Der Deutschen Botanischen Gesellschaft 18(4):158–168
Article Google Scholar
Crombie AC (1994) Styles of scientific thinking in the European tradition. Gerald Duckworth & Company, London
Dampier WC (1929) A history of science. Cambridge University Press, Cambridge
Darden L (1991) Theory change in science: strategies from Mendelian genetics. Oxford University Press, Oxford
Darden L (2005) Relations among Fields: Mendelian, Cytological and Molecular Mechanisms. Stud Hist Philos Biol Biomed Sci Stud Hist Phi Part C 36(2 Spec. Iss.):349–371
Darden L, Craver CF (2002) Strategies in the Interfield discovery of the mechanism of protein synthesis. Stud Hist Phil Biol Biomed Sci 33(1):1–28
Darden L, Maull N (1977) Interfield theories. Philos Sci 44(1):43–64
de Vries H (1889) Intracellulare Pangenesis. Gustav Fischer, Jean
de Vries H (1900a) Das Spaltungsgesetz Der Bastarde (Vorlaufige Mittheilung). Berichte Der Deutschen Botanischen Gesellschaft 18(3):83–90
de Vries H (1900b) Sur La Loi de Disjonction Des Hybrides. Comptes Rendus de I’Academie Des Sciences (Paris) 130:845–847
Delage Y (1903) L’hérédité et Les Grands Problèmes de La Biologie Générale, 2nd edn. Schleicher Frères, Paris
Diderot D (1754) Pensées Sur l’interpretation de La Nature
Encyclopaedia Britannica (1771) Vol 3. Edinburgh: A. Bell and C. MacFarquhar
Enfantin P, Saint-Simon H (1858) Science de l’homme, Physiologie Religieuse. Librairie Victor Masson, Paris
Fleck L (1935) Entstehung Und Entwicklung Einer Wissenschaftlichen Tatsache: Einführung in Die Lehre Vom Denkstil Und Denkkollektiv. Benno Schwabe & Co., Basel
Florio J (1611) Queen Anna’s New World of words, or, Dictionarie of the Italian and English tongues. Melch. Bradwood, London
Galton F (1889) Natural inheritance. Macmillan & Company, London/New York
Giere RN (2006) Scientific Perspectivism. The University of Chicago Press, Chicago/London
Hacking I (1994) Styles of scientific thinking or reasoning: a new analytic tool for historians and philosophers of the sciences. In: Gavroglu K, Christianidis J, Nicolaidis E (eds) Trends in the historiography of science. Kluwer Academic Publishers, Dordrecht, pp 31–48
Chapter Google Scholar
Hall AR (1954) The Scientific Revolution 1500–1800. Longmans Green and CO, London
Johnson S (1755) Dictionary of the English language. Longman, Hurts, Rees, Orme, and Brown, London
Kitcher P (1984) 1953 and all that: a tale of two sciences. Philos Rev 93(3):335–373
Kitcher P (1989) Explanatory unification and the causal structure of the world. In: Kitcher P, Salmon WC (eds) Scientific explanation. University of Minnesota Press, Minneapolis, MN, pp 410–505
Koyré A (1939) Études Galiléennes. Hermann, Paris
Kuhn TS (1962) The structure of scientific revolutions, 1st edn. The University of Chicago Press, Chicago, IL
Kuhn TS (1970a) Logic of discovery or psychology of research? In: Lakatos I, Musgrave A (eds) Criticism and the growth of knowledge. Cambridge University Press, Cambridge, pp 1–23
Kuhn TS (1970b) The structure of scientific revolutions, 2nd edn. University of Chicago Press, Chicago, IL
Lakatos I (1968) Criticism and the methodology of scientific research Programmes. Proc Aristot Soc 69:149–186
Lakatos I (1970) History of science and its rational reconstructions. PSA: Proceedings of the Biennial Meeting of the Philosophy of Science Association 1970:91–136
Lakatos I (1978) Falsification and the methodology of scientific research Programmes. In: Worrall J, Currie G (eds) The methodology of scientific research Programme. Cambridge University Press, Cambridge, pp 8–101
Laudan L (1977) Progress and its problems: toward a theory of scientific growth. University of California Press, Berkeley/Los Angeles
Laudan L (1981) A confutation of convergent realism. Philos Sci 48(1):19–49
Lavoisier AL (1790) Elements of chemistry (trans: Robert K). William Creech, London
MacLaurin C (1748) An account of sir Isaac Newton’s philosophical discoveries, London
Massimi M (2018) Four kinds of perspectival truth. Philos Phenomenol Res 96(2):342–359
Mendel G (1866) Versuche Über Pflanzenhybriden. Verhandlungen Des Naturforschenden Vereins Brünn IV (1865) (Abhandlungen): 3–47
Morgan TH (1926) The theory of the gene. Yale University Press, New Haven, CT
Müller-Wille S (2021) Gregor Mendel and the history of heredity. In: Dietrich MR, Borrello ME, Harman O (eds) Handbook of the historiography of biology. Springer, Cham, pp 105–126
Musgrave A (1976) Why did oxygen supplant phlogiston? Research Programmes in the chemical revolution. In: Howson C (ed) Sciences, method and appraisal in the physical sciences. Cambridge University Press, Cambridge, pp 181–209
Nagel E (1961) The structure of science: problems in the logic of scientific explanation, vol 29. Harcourt, Brace & World, New York, p 716
Olby RC (1985) Origins of Mendelism, 2nd edn. University of Chicago Press, Chicago, IL
Ornstein M (1913) The role of the scientific societies in the seventeenth century. Columbia University
Pearson K (1903) The law of ancestral heredity. Biometrika 2(2):211–229
Popper K (1959) The logic of scientific discovery, 1st edn. Hutchinson & Co., London
Popper K (1963) Truth, rationality, and the growth of scientific knowledge. In: Conjectures and refutations: the growth of scientific knowledge. Routledge and Kegan Paul, London, pp 215–250
Priestley J (1796) Experiments and observations relating to the analysis of Atmospherical air: also, farther experiments relating to the generation of air from water. To which are added, considerations on the doctrine of phlogiston, and the decomposition of water. Philadelphia
Psillos S (1999) Scientific realism: how science tracks truth. Routledge, London
Randall JH (1926) The making of the modern mind. Houghton, Mifflin and Company, Boston, MA
Renouvier C (1864) Essais de Critique Générale. Troisième Essai. Les Principes de La Nature. Ladrange, Paris
Robinson JH (1921) The scientific revolution. In: The mind in the making. Harper & Brothers Publishers, New York/London, pp 151–157
Sankey H (1994) The incommensurability thesis. Avebury, Aldershot
Schmaus W (2023) Cournot and Renouvier on scientific revolutions. J Gen Philos Sci 54:7–17
Shan Y (2020a) Doing integrated history and philosophy of science: a case study of the origin of genetics, Boston Studies in the Philosophy and History of Science, 1st edn. Springer, Cham
Shan Y (2020b) Kuhn’s ‘wrong turning’ and legacy today. Synthese 197(1):381–406
Shan Y (2021) Beyond Mendelism and biometry. Stud Hist Phil Sci 89:155–163
Shan Y (2022) The functional approach: scientific Progress as increased usefulness. In: Shan Y (ed) New Philosophical Perspectives on scientific Progress. Routledge, New York, pp 46–61
Smith P (1930) The scientific revolution. In: A history of modern culture. George Routledge & Sons, Ltd., London, pp 144–178
Stanford PK (2006) Exceeding our grasp. Oxford University Press, Oxford
Temple W (1731) Of Health and Long Life. In: The Works of Sir William Temple, Bart, London, pp 272–289
Waters CK (2004) What was classical genetics? Stud Hist Philos Sci Part A 35(4):783–809
Weismann A (1892) Das Keimplasma: Eine Theorie Der Vererbung. Gustav Fischer, Jena
Weldon WFR (1905) Theory of inheritance (unpublished). UCL Library, London
Whewell W (1847) The philosophy of the inductive sciences. A new edit, vol 2. John W. Parker, London
Woodbridge HE (1940) Sir William Temple: the man and his work. Modern Language Association, New York
Worrall J (1989) Structural realism: the best of both worlds? Dialectica 43(1–2):99–124
Wray KB (2011) Kuhn’s evolutionary social ppistemology. Cambridge University Press, Cambridge
Download references
Acknowledgements
An early draft of this chapter was presented at Evidence Seminar, University of Kent on 24 May 2022. I thank the audience there for the helpful comments and discussion.
Author information
Authors and affiliations.
Hong Kong University of Science and Technology, Hong Kong, China
Yafeng Shan
You can also search for this author in PubMed Google Scholar
Corresponding author
Correspondence to Yafeng Shan .
Editor information
Editors and affiliations.
Universidade Federal de Minas Gerais, Belo Horizonte, Brazil
Mauro L. Condé
Universidade Federal de Goiás, Goiânia, Brazil
Marlon Salomon
Rights and permissions
Reprints and permissions
Copyright information
© 2023 Springer Nature Switzerland AG
About this entry
Cite this entry.
Shan, Y. (2023). The Historiography of Scientific Revolutions: A Philosophical Reflection. In: Condé, M.L., Salomon, M. (eds) Handbook for the Historiography of Science. Historiographies of Science. Springer, Cham. https://doi.org/10.1007/978-3-030-99498-3_12-1
Download citation
DOI : https://doi.org/10.1007/978-3-030-99498-3_12-1
Received : 11 August 2022
Accepted : 14 December 2022
Published : 16 May 2023
Publisher Name : Springer, Cham
Print ISBN : 978-3-030-99498-3
Online ISBN : 978-3-030-99498-3
eBook Packages : Springer Reference Humanities, Soc. Sciences and Law
- Publish with us
Policies and ethics
Chapter history
DOI: https://doi.org/10.1007/978-3-030-99498-3_12-2
DOI: https://doi.org/10.1007/978-3-030-99498-3_12-1
- Find a journal
- Track your research
If you're seeing this message, it means we're having trouble loading external resources on our website.
If you're behind a web filter, please make sure that the domains *.kastatic.org and *.kasandbox.org are unblocked.
To log in and use all the features of Khan Academy, please enable JavaScript in your browser.
World History Project - 1750 to the Present
Course: world history project - 1750 to the present > unit 3.
- BEFORE YOU WATCH: Origins of the Industrial Revolution
- WATCH: Origins of the Industrial Revolution
- READ: Scale of the Industrial Revolution
READ: The Scientific Revolution
- READ: The Industrial Revolution
- BEFORE YOU WATCH: Coal, Steam, and the Industrial Revolution
- WATCH: Coal, Steam, and the Industrial Revolution
- Origins of the Industrial Revolution
First read: preview and skimming for gist
Second read: key ideas and understanding content.
- What is the usual story of the Scientific Revolution?
- How does the author challenge the usual story of the Scientific Revolution?
- Who participated in the Scientific Revolution?
- What were some negative social effects of the Scientific Revolution?
- Does the author think the Scientific Revolution caused the Industrial Revolution?
Third read: evaluating and corroborating
- You just read an article about scale and the Industrial Revolution. In that article, the author questioned whether the Industrial Revolution happened in Britain because of local or global factors. What do you think explains the emergence of the Scientific Revolution in Europe during the sixteenth and seventeenth centuries? Was this the result of local or global processes?
- Using the networks frame, explain why the Scientific Revolution happened in Europe and how it might have led to the Industrial Revolution.
The Scientific Revolution
Was it revolutionary, was it european, whose revolution, did it cause the industrial revolution.
- The word other can refer to the otherness of marginalize people. Anyone not belonging to the most powerful or privileged class can be a type of “other” due to race, gender, religion, socio-economic status, etc.
- It’s hard to say exactly when people started thinking about race, but it’s definitely not a natural and ancient idea. Of course, people had a sense of others outside their community, who they often looked down upon, but that wasn’t the same as seeing people as different races. For Europeans in the medieval period, humans were sorted into Christians, Jews, and heathens.
Want to join the conversation?
- Upvote Button navigates to signup page
- Downvote Button navigates to signup page
- Flag Button navigates to signup page
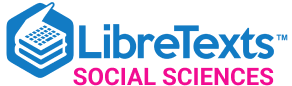
- school Campus Bookshelves
- menu_book Bookshelves
- perm_media Learning Objects
- login Login
- how_to_reg Request Instructor Account
- hub Instructor Commons
- Download Page (PDF)
- Download Full Book (PDF)
- Periodic Table
- Physics Constants
- Scientific Calculator
- Reference & Cite
- Tools expand_more
- Readability
selected template will load here
This action is not available.
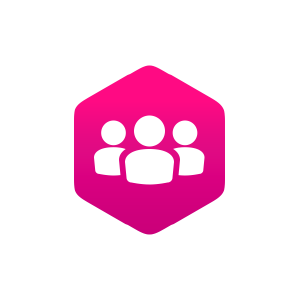
11.5: Scientific Revolution
- Last updated
- Save as PDF
- Page ID 132401
Figure of the heavenly bodies — An illustration of the Ptolemaic geocentric system by Portuguese cosmographer and cartographer Bartolomeu Velho, 1568 (Bibliothèque Nationale, Paris) Source: https://commons.wikimedia.org/wiki/F...Velho_1568.jpg
Roots of the Scientific Revolution
The word "revolution" connotes a period of turmoil and social upheaval where ideas about the world change severely and a completely new era of academic thought is ushered in. Therefore, the Scientific Revolution (1543 – 1687) describes quite accurately what took place in the scientific community. Medieval scientific philosophy was abandoned in favor of the new methods proposed by Bacon, Galileo, Descartes, and Newton. The importance of experimentation to the scientific method was reaffirmed. The importance of God to science was for the most part invalidated. Last, the pursuit of science itself (rather than philosophy) gained validity on its own terms. The change to the medieval idea of science occurred for four reasons:
- Seventeenth-century scientists and philosophers were able to collaborate with members of the mathematical and astronomical communities to effect advances in all fields.
- Scientists realized the inadequacy of medieval experimental methods for their work and so felt the need to devise new methods (some of which we use today).
- Academics had access to a legacy of European, Greek, and Middle Eastern scientific philosophy they could use as a starting point (either by disproving or building on the theorems).
- Groups like the British Royal Society helped validate science as a field by providing an outlet for the publication of scientists' work.
These changes were not immediate, nor did they directly create the experimental method used today. But they did represent a step toward Enlightenment thinking (with an emphasis on reason) that was revolutionary for the time.
Science and Philosophy Before the Revolution
At the end of the sixteenth century, "natural philosopher" carried much more academic clout. So, the majority of the research on scientific theory was conducted not in the scientific realm, but in philosophy, where "scientific methods" like empiricism and teleology were promoted.
In the 17th century, empiricism and teleology existed as remnants of medieval thought that were utilized by philosophers. Empiricism is a theory that reality consists solely of what one physically experiences. Teleology is the idea that phenomena exist only because they have a purpose, i.e. because God wills them to do so. Both these theories did not have a need for fact-gathering, hypothesis writing, and controlled experimentation.
Robert Boyle (1627–1691) , an Irish-born English scientist, was an early supporter of the scientific method and the founder of modern chemistry. Source: https://commons.wikimedia.org/wiki/F...bert_Boyle.jpg
The defining feature of the scientific revolution lies in how much scientific thought changed during a period of only a century. Modern experimental methods incorporate Francis Bacon's focus on the use of controlled experiments and inductive reasoning, Galileo's emphasis on incorporation of established laws from all disciplines (math, astronomy, chemistry, biology, physics) in coming to a conclusion through mechanism, and Newton's method of composition. Each successive method strengthened the validity of the next.
Francis Bacon (1561-1626)
Bacon represents the first step away from sixteenth-century thinking, in that he denied the validity of empiricism. He preferred inductive reasoning (drawing a general conclusion from a set of specific observations) to deductive reasoning (making an inference based on widely-accepted facts or premises).
He emphasized the necessity of fact-gathering as a first step in the scientific method , which could then be followed by carefully recorded and controlled (unbiased) experimentation. Further, experimentation should not be conducted to simply "see what happens" but "as a way of answering specific questions." Moreover, the main purpose of science was the betterment of human society and that experimentation should be applied to hard, real situations rather than to Aristotelian abstract ideas. His work influenced advances in chemistry and biology through the 18th century.
Justus Sustermans - Portrait of Galileo Galilei, 1636
Galileo Galilei (1564-1642)
Galileo argued that "an explanation of a scientific problem is truly begun when it is reduced to its basic terms of matter and motion," because only the most basic events occur because of one axiom.
For example, one can demonstrate the concept of "acceleration" in the laboratory with a ball and a slanted board. However, according to Galileo's reasoning, one would have to utilize the concepts of many different disciplines: the physics-based concepts of time and distance, the idea of gravity, force, and mass, or even the chemical composition of the element that is accelerating. All of these components could be individually broken down to their smallest elements in order for a scientist to fully understand the item as a whole.
This "mechanic" or "systemic" approach partially removed the burden of fact-gathering emphasized by Bacon. Galileo's experimental method aided advances in chemistry and biology by allowing biologists to explain the work of a muscle or any body function using existing ideas of motion, matter, energy, and other basic principles.
Robert Boyle (1627-1691)
Even though he made progress in the field of chemistry through Baconian experimentation, Boyle remained drawn to teleological explanations for scientific phenomena. For example, Boyle believed that because God establish some rules of motion and nature, phenomena must exist to serve a certain purpose within that established order.
Sir Isaac Newton (1643-1747)
Newton composed a set of four rules for scientific reasoning stated in his famed Principia . His analytical method and laws lent well to experimentation with mathematical physics, where conclusions "could then be confirmed by direct observation. " Newton also refined Galileo's experimental method by making experiments and observations, followed by inducted conclusions that could only be overturned by the realization of other, more substantiated truths. Essentially, through his physical and mathematical approach to experimental design, Newton established a clear distinction between "natural philosophy" and "physical science. "
All of these natural philosophers built upon the work of their contemporaries. This collaboration became even simpler with the establishment of professional societies for scientists that published journals and provided forums for scientific discussion.
In Aristotelian metaphysics, abstractions dominated. All entities were understood as following rules based on their essences. Each substance had a proper or natural place. In astronomy, space was divided into two main regions. The stars resided in the higher region and were fixed in space. The planets and the moon resided in the lower region and revolved around the Earth. The sun also revolved around the Earth. Celestial and earthly motion was understood in terms of bodies seeking their natural resting places.
By the completion of the Scientific Revolution, most Aristotelian notions were overthrown. Space was reconceived as isotropic and non-hierarchical. Matter was seen as compositionally consistent throughout the universe. Motion was understood in terms of forces acting upon otherwise inert bodies. Finally, with Isaac Newton, a single constant called "gravity" was introduced as the fundamental operative force of the entire universe.
Nicolaus Copernicus (1473–1543) was a mathematician, lawyer, physician, and classicist. He was also a polyglot, a fluent speaker and writer in several languages, including Latin, Polish, German, Greek, and Italian. Most importantly for posterity, Copernicus was the astronomer credited with founding the field of modern astronomy.
Copernicus's major work, On the Revolutions of the Heavenly Spheres , was published in 1543, the year of his death. In this great work, he made a radical transformation of the system developed by Ptolemy (ca. 87–150 CE). Contrary to the Ptolemaic system, Copernicus posited a heliocentric model wherein the Earth and other planets, including Mercury, Venus, Mars, Jupiter, and Saturn, were carried by spheres around a stationary sun.
De revolutionibus, p. 9. Heliocentric model of the solar system in Copernicus' manuscript Source: https://en.Wikipedia.org/wiki/File:D...script_p9b.jpg
In the Ptolemaic system, the Earth had been figured as the stationary center of the universe around which the planets and sun revolved. In the Copernican system, on the other hand, the Earth was merely "another planet," that is, a "wandering star. "
Because his philosophy and theology held that God created only perfect order and harmony, Copernicus envisioned the planetary revolutions as perfectly circular. In order to account for some locations of planets and moons, he retained the auxiliary theory of epicycles that had been a part of the Ptolemaic system. In Copernicus's use of epicycles, the planets also circled the Earth while circling the sun.
Like a majority of Europeans of his time, Copernicus was an adherent of the Catholic Church. Yet a heliocentric universe ran contrary to the Christian, Earth-centered cosmology. That's why his On the Revolutions of the Heavenly Spheres was not published until the end of his life.
While Copernicus's ideas were revolutionary at the time, they did not cause a revolution in the study of astronomy until taken up by Galileo Galilei and Johannes Kepler several years later.
Jan Matejko-Astronomer Copernicus-Conversation with God Oil painting by the Polish artist Jan Matejko, finished in 1873, depicting Nicolaus Copernicus observing the heavens from a balcony by a tower near the cathedral in Frombork. Source: https://en.Wikipedia.org/wiki/Jan_Ma...n_with_God.jpg
While accepting the central feature of Copernicus's model—a stationary sun and revolving planets—Johannes Kepler (1571- 1640) threw out the idea of epicycles. In their place, he introduced a wholly new conception of the universe that is still essentially accepted today. In his book The New Astronomy (1609), his "elliptical thesis" boldly declared that the planets, including the Earth, revolve around a stationary sun in ellipses, rather than in perfect circles.
Under Aristotle's metaphysics, an object's movement toward its "natural" resting place accounted for its motion. Kepler introduced the modern notion of physical forces as the causes of motion. For Kepler, a planet or other lifeless object is without an internal or active force of its own. Motion is derived only from external forces acting on the object. This particular insight was essential for Isaac Newton's breakthrough formulation of the law of gravity.
Attribution: Material modified from CK-12 6.3 The Scientific Revolution
What is a scientific hypothesis?
It's the initial building block in the scientific method.
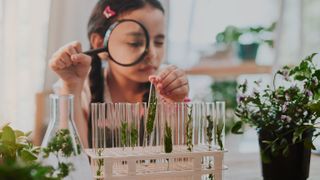
Hypothesis basics
What makes a hypothesis testable.
- Types of hypotheses
- Hypothesis versus theory
Additional resources
Bibliography.
A scientific hypothesis is a tentative, testable explanation for a phenomenon in the natural world. It's the initial building block in the scientific method . Many describe it as an "educated guess" based on prior knowledge and observation. While this is true, a hypothesis is more informed than a guess. While an "educated guess" suggests a random prediction based on a person's expertise, developing a hypothesis requires active observation and background research.
The basic idea of a hypothesis is that there is no predetermined outcome. For a solution to be termed a scientific hypothesis, it has to be an idea that can be supported or refuted through carefully crafted experimentation or observation. This concept, called falsifiability and testability, was advanced in the mid-20th century by Austrian-British philosopher Karl Popper in his famous book "The Logic of Scientific Discovery" (Routledge, 1959).
A key function of a hypothesis is to derive predictions about the results of future experiments and then perform those experiments to see whether they support the predictions.
A hypothesis is usually written in the form of an if-then statement, which gives a possibility (if) and explains what may happen because of the possibility (then). The statement could also include "may," according to California State University, Bakersfield .
Here are some examples of hypothesis statements:
- If garlic repels fleas, then a dog that is given garlic every day will not get fleas.
- If sugar causes cavities, then people who eat a lot of candy may be more prone to cavities.
- If ultraviolet light can damage the eyes, then maybe this light can cause blindness.
A useful hypothesis should be testable and falsifiable. That means that it should be possible to prove it wrong. A theory that can't be proved wrong is nonscientific, according to Karl Popper's 1963 book " Conjectures and Refutations ."
An example of an untestable statement is, "Dogs are better than cats." That's because the definition of "better" is vague and subjective. However, an untestable statement can be reworded to make it testable. For example, the previous statement could be changed to this: "Owning a dog is associated with higher levels of physical fitness than owning a cat." With this statement, the researcher can take measures of physical fitness from dog and cat owners and compare the two.
Types of scientific hypotheses
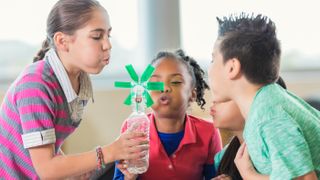
In an experiment, researchers generally state their hypotheses in two ways. The null hypothesis predicts that there will be no relationship between the variables tested, or no difference between the experimental groups. The alternative hypothesis predicts the opposite: that there will be a difference between the experimental groups. This is usually the hypothesis scientists are most interested in, according to the University of Miami .
For example, a null hypothesis might state, "There will be no difference in the rate of muscle growth between people who take a protein supplement and people who don't." The alternative hypothesis would state, "There will be a difference in the rate of muscle growth between people who take a protein supplement and people who don't."
If the results of the experiment show a relationship between the variables, then the null hypothesis has been rejected in favor of the alternative hypothesis, according to the book " Research Methods in Psychology " (BCcampus, 2015).
There are other ways to describe an alternative hypothesis. The alternative hypothesis above does not specify a direction of the effect, only that there will be a difference between the two groups. That type of prediction is called a two-tailed hypothesis. If a hypothesis specifies a certain direction — for example, that people who take a protein supplement will gain more muscle than people who don't — it is called a one-tailed hypothesis, according to William M. K. Trochim , a professor of Policy Analysis and Management at Cornell University.
Sometimes, errors take place during an experiment. These errors can happen in one of two ways. A type I error is when the null hypothesis is rejected when it is true. This is also known as a false positive. A type II error occurs when the null hypothesis is not rejected when it is false. This is also known as a false negative, according to the University of California, Berkeley .
A hypothesis can be rejected or modified, but it can never be proved correct 100% of the time. For example, a scientist can form a hypothesis stating that if a certain type of tomato has a gene for red pigment, that type of tomato will be red. During research, the scientist then finds that each tomato of this type is red. Though the findings confirm the hypothesis, there may be a tomato of that type somewhere in the world that isn't red. Thus, the hypothesis is true, but it may not be true 100% of the time.
Scientific theory vs. scientific hypothesis
The best hypotheses are simple. They deal with a relatively narrow set of phenomena. But theories are broader; they generally combine multiple hypotheses into a general explanation for a wide range of phenomena, according to the University of California, Berkeley . For example, a hypothesis might state, "If animals adapt to suit their environments, then birds that live on islands with lots of seeds to eat will have differently shaped beaks than birds that live on islands with lots of insects to eat." After testing many hypotheses like these, Charles Darwin formulated an overarching theory: the theory of evolution by natural selection.
"Theories are the ways that we make sense of what we observe in the natural world," Tanner said. "Theories are structures of ideas that explain and interpret facts."
- Read more about writing a hypothesis, from the American Medical Writers Association.
- Find out why a hypothesis isn't always necessary in science, from The American Biology Teacher.
- Learn about null and alternative hypotheses, from Prof. Essa on YouTube .
Encyclopedia Britannica. Scientific Hypothesis. Jan. 13, 2022. https://www.britannica.com/science/scientific-hypothesis
Karl Popper, "The Logic of Scientific Discovery," Routledge, 1959.
California State University, Bakersfield, "Formatting a testable hypothesis." https://www.csub.edu/~ddodenhoff/Bio100/Bio100sp04/formattingahypothesis.htm
Karl Popper, "Conjectures and Refutations," Routledge, 1963.
Price, P., Jhangiani, R., & Chiang, I., "Research Methods of Psychology — 2nd Canadian Edition," BCcampus, 2015.
University of Miami, "The Scientific Method" http://www.bio.miami.edu/dana/161/evolution/161app1_scimethod.pdf
William M.K. Trochim, "Research Methods Knowledge Base," https://conjointly.com/kb/hypotheses-explained/
University of California, Berkeley, "Multiple Hypothesis Testing and False Discovery Rate" https://www.stat.berkeley.edu/~hhuang/STAT141/Lecture-FDR.pdf
University of California, Berkeley, "Science at multiple levels" https://undsci.berkeley.edu/article/0_0_0/howscienceworks_19
Sign up for the Live Science daily newsletter now
Get the world’s most fascinating discoveries delivered straight to your inbox.
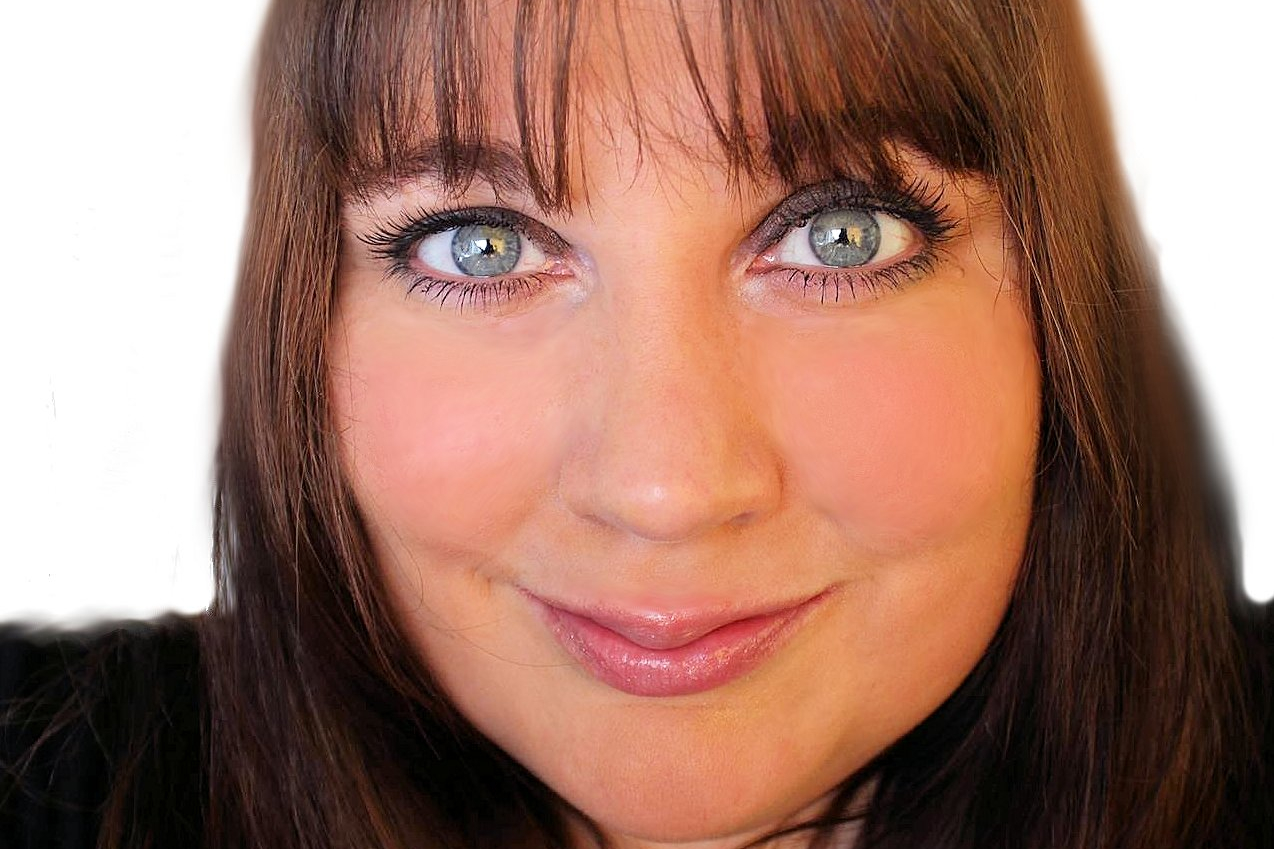
Part of the San Andreas fault may be gearing up for an earthquake
Antarctica is covered in volcanoes, could they erupt?
Extremely rare marsupial mole that 'expertly navigates' sand dunes spotted in Western Australia
Most Popular
By Anna Gora December 27, 2023
By Anna Gora December 26, 2023
By Anna Gora December 25, 2023
By Emily Cooke December 23, 2023
By Victoria Atkinson December 22, 2023
By Anna Gora December 16, 2023
By Anna Gora December 15, 2023
By Anna Gora November 09, 2023
By Donavyn Coffey November 06, 2023
By Anna Gora October 31, 2023
By Anna Gora October 26, 2023
- 2 How and where to watch the April 8 solar eclipse online for free
- 3 NASA engineers discover why Voyager 1 is sending a stream of gibberish from outside our solar system
- 4 Giant coyote killed in southern Michigan turns out to be a gray wolf — despite the species vanishing from region 100 years ago
- 5 When is the next total solar eclipse after 2024 in North America?
- 2 Giant 'toe biter' water bugs discovered in Cyprus for the 1st time
- 3 When is the next total solar eclipse after 2024 in North America?
- 4 Watch live! The total solar eclipse has begun over North America.
- 5 8,200-year-old campsite of 'Paleo-Archaic' peoples discovered on US Air Force base in New Mexico
Revolutions in Science & the Enlightenment
The scientific revolution, roots of the scientific revolution.
The scientific revolution, which emphasized systematic experimentation as the most valid research method, resulted in developments in mathematics, physics, astronomy, biology, and chemistry. These developments transformed the views of society about nature.
Learning Objectives
Outline the changes that occurred during the Scientific Revolution that resulted in developments towards a new means for experimentation
Key Takeaways
- The scientific revolution was the emergence of modern science during the early modern period, when developments in mathematics, physics, astronomy, biology (including human anatomy), and chemistry transformed societal views about nature.
- The change to the medieval idea of science occurred for four reasons: collaboration, the derivation of new experimental methods, the ability to build on the legacy of existing scientific philosophy, and institutions that enabled academic publishing.
- Under the scientific method, which was defined and applied in the 17th century, natural and artificial circumstances were abandoned and a research tradition of systematic experimentation was slowly accepted throughout the scientific community.
- During the scientific revolution, changing perceptions about the role of the scientist in respect to nature, and the value of experimental or observed evidence, led to a scientific methodology in which empiricism played a large, but not absolute, role.
- As the scientific revolution was not marked by any single change, many new ideas contributed. Some of them were revolutions in their own fields.
- Science came to play a leading role in Enlightenment discourse and thought. Many Enlightenment writers and thinkers had backgrounds in the sciences, and associated scientific advancement with the overthrow of religion and traditional authority in favor of the development of free speech and thought.
- empiricism : A theory stating that knowledge comes only, or primarily, from sensory experience. It emphasizes evidence, especially the kind of evidence gathered through experimentation and by use of the scientific method.
- Galileo : An Italian thinker (1564-1642) and key figure in the scientific revolution who improved the telescope, made astronomical observations, and put forward the basic principle of relativity in physics.
- Baconian method : The investigative method developed by Sir Francis Bacon. It was put forward in Bacon’s book Novum Organum (1620), (or New Method), and was supposed to replace the methods put forward in Aristotle’s Organon. This method was influential upon the development of the scientific method in modern science, but also more generally in the early modern rejection of medieval Aristotelianism.
- scientific method : A body of techniques for investigating phenomena, acquiring new knowledge, or correcting and integrating previous knowledge, through the application of empirical or measurable evidence subject to specific principles of reasoning. It has characterized natural science since the 17th century, consisting in systematic observation, measurement, and experiment, and the formulation, testing, and modification of hypotheses.
- British Royal Society : A British learned society for science; possibly the oldest such society still in existence, having been founded in November 1660.
The scientific revolution was the emergence of modern science during the early modern period, when developments in mathematics, physics, astronomy, biology (including human anatomy), and chemistry transformed societal views about nature. The scientific revolution began in Europe toward the end of the Renaissance period, and continued through the late 18th century, influencing the intellectual social movement known as the Enlightenment. While its dates are disputed, the publication in 1543 of Nicolaus Copernicus ‘s De revolutionibus orbium coelestium ( On the Revolutions of the Heavenly Spheres ) is often cited as marking the beginning of the scientific revolution.
The scientific revolution was built upon the foundation of ancient Greek learning and science in the Middle Ages, as it had been elaborated and further developed by Roman/Byzantine science and medieval Islamic science. The Aristotelian tradition was still an important intellectual framework in the 17th century, although by that time natural philosophers had moved away from much of it. Key scientific ideas dating back to classical antiquity had changed drastically over the years, and in many cases been discredited. The ideas that remained (for example, Aristotle ‘s cosmology, which placed the Earth at the center of a spherical hierarchic cosmos, or the Ptolemaic model of planetary motion) were transformed fundamentally during the scientific revolution.
The change to the medieval idea of science occurred for four reasons:
- Seventeenth century scientists and philosophers were able to collaborate with members of the mathematical and astronomical communities to effect advances in all fields.
- Scientists realized the inadequacy of medieval experimental methods for their work and so felt the need to devise new methods (some of which we use today).
- Academics had access to a legacy of European, Greek, and Middle Eastern scientific philosophy that they could use as a starting point (either by disproving or building on the theorems).
- Institutions (for example, the British Royal Society) helped validate science as a field by providing an outlet for the publication of scientists’ work.
New Methods
Under the scientific method that was defined and applied in the 17th century, natural and artificial circumstances were abandoned, and a research tradition of systematic experimentation was slowly accepted throughout the scientific community. The philosophy of using an inductive approach to nature (to abandon assumption and to attempt to simply observe with an open mind) was in strict contrast with the earlier, Aristotelian approach of deduction, by which analysis of known facts produced further understanding. In practice, many scientists and philosophers believed that a healthy mix of both was needed—the willingness to both question assumptions, and to interpret observations assumed to have some degree of validity.
During the scientific revolution, changing perceptions about the role of the scientist in respect to nature, the value of evidence, experimental or observed, led towards a scientific methodology in which empiricism played a large, but not absolute, role. The term British empiricism came into use to describe philosophical differences perceived between two of its founders—Francis Bacon, described as empiricist, and René Descartes, who was described as a rationalist. Bacon’s works established and popularized inductive methodologies for scientific inquiry, often called the Baconian method , or sometimes simply the scientific method. His demand for a planned procedure of investigating all things natural marked a new turn in the rhetorical and theoretical framework for science, much of which still surrounds conceptions of proper methodology today. Correspondingly, Descartes distinguished between the knowledge that could be attained by reason alone (rationalist approach), as, for example, in mathematics, and the knowledge that required experience of the world, as in physics.
Thomas Hobbes, George Berkeley, and David Hume were the primary exponents of empiricism, and developed a sophisticated empirical tradition as the basis of human knowledge. The recognized founder of the approach was John Locke, who proposed in An Essay Concerning Human Understanding (1689) that the only true knowledge that could be accessible to the human mind was that which was based on experience.
Many new ideas contributed to what is called the scientific revolution. Some of them were revolutions in their own fields. These include:
- The heliocentric model that involved the radical displacement of the earth to an orbit around the sun (as opposed to being seen as the center of the universe). Copernicus’ 1543 work on the heliocentric model of the solar system tried to demonstrate that the sun was the center of the universe. The discoveries of Johannes Kepler and Galileo gave the theory credibility and the work culminated in Isaac Newton’s Principia, which formulated the laws of motion and universal gravitation that dominated scientists’ view of the physical universe for the next three centuries.
- Studying human anatomy based upon the dissection of human corpses, rather than the animal dissections, as practiced for centuries.
- Discovering and studying magnetism and electricity, and thus, electric properties of various materials.
- Modernization of disciplines (making them more as what they are today), including dentistry, physiology, chemistry, or optics.
- Invention of tools that deepened the understating of sciences, including mechanical calculator, steam digester (the forerunner of the steam engine), refracting and reflecting telescopes, vacuum pump, or mercury barometer.
The Shannon Portrait of the Hon. Robert Boyle F. R. S. (1627-1691): Robert Boyle (1627-1691), an Irish-born English scientist, was an early supporter of the scientific method and founder of modern chemistry. Boyle is known for his pioneering experiments on the physical properties of gases, his authorship of the Sceptical Chymist, his role in creating the Royal Society of London, and his philanthropy in the American colonies.
The Scientific Revolution and the Enlightenment
The scientific revolution laid the foundations for the Age of Enlightenment, which centered on reason as the primary source of authority and legitimacy, and emphasized the importance of the scientific method. By the 18th century, when the Enlightenment flourished, scientific authority began to displace religious authority, and disciplines until then seen as legitimately scientific (e.g., alchemy and astrology) lost scientific credibility.
Science came to play a leading role in Enlightenment discourse and thought. Many Enlightenment writers and thinkers had backgrounds in the sciences, and associated scientific advancement with the overthrow of religion and traditional authority in favor of the development of free speech and thought. Broadly speaking, Enlightenment science greatly valued empiricism and rational thought, and was embedded with the Enlightenment ideal of advancement and progress. At the time, science was dominated by scientific societies and academies, which had largely replaced universities as centers of scientific research and development. Societies and academies were also the backbone of the maturation of the scientific profession. Another important development was the popularization of science among an increasingly literate population. The century saw significant advancements in the practice of medicine, mathematics, and physics; the development of biological taxonomy; a new understanding of magnetism and electricity; and the maturation of chemistry as a discipline, which established the foundations of modern chemistry.
Isaac Newton’s Principia, developed the first set of unified scientific laws
Newton’s Principia formulated the laws of motion and universal gravitation, which dominated scientists’ view of the physical universe for the next three centuries. By deriving Kepler’s laws of planetary motion from his mathematical description of gravity, and then using the same principles to account for the trajectories of comets, the tides, the precession of the equinoxes, and other phenomena, Newton removed the last doubts about the validity of the heliocentric model of the cosmos. This work also demonstrated that the motion of objects on Earth and of celestial bodies could be described by the same principles. His laws of motion were to be the solid foundation of mechanics.
Physics and Mathematics
In the 16th and 17th centuries, European scientists began increasingly applying quantitative measurements to the measurement of physical phenomena on the earth, which translated into the rapid development of mathematics and physics.
Distinguish between the different key figures of the scientific revolution and their achievements in mathematics and physics
- The philosophy of using an inductive approach to nature was in strict contrast with the earlier, Aristotelian approach of deduction, by which analysis of known facts produced further understanding. In practice, scientists believed that a healthy mix of both was needed—the willingness to question assumptions, yet also to interpret observations assumed to have some degree of validity. That principle was particularly true for mathematics and physics.
- In the 16th and 17th centuries, European scientists began increasingly applying quantitative measurements to the measurement of physical phenomena on the earth.
- The Copernican Revolution, or the paradigm shift from the Ptolemaic model of the heavens to the heliocentric model with the sun at the center of the solar system, began with the publication of Copernicus’s De revolutionibus orbium coelestium, and ended with Newton’s work over a century later.
- Galileo showed a remarkably modern appreciation for the proper relationship between mathematics, theoretical physics, and experimental physics. His contributions to observational astronomy include the telescopic confirmation of the phases of Venus, the discovery of the four largest satellites of Jupiter, and the observation and analysis of sunspots.
- Newton’s Principia formulated the laws of motion and universal gravitation, which dominated scientists’ view of the physical universe for the next three centuries. He removed the last doubts about the validity of the heliocentric model of the solar system.
- The electrical science developed rapidly following the first discoveries of William Gilbert.
- scientific method : A body of techniques for investigating phenomena, acquiring new knowledge, or correcting and integrating previous knowledge that apply empirical or measurable evidence subject to specific principles of reasoning. It has characterized natural science since the 17th century, consisting in systematic observation, measurement, and experiment, and the formulation, testing, and modification of hypotheses.
- Copernican Revolution : The paradigm shift from the Ptolemaic model of the heavens, which described the cosmos as having Earth stationary at the center of the universe, to the heliocentric model with the sun at the center of the solar system. Beginning with the publication of Nicolaus Copernicus’s De revolutionibus orbium coelestium, contributions to the “revolution” continued, until finally ending with Isaac Newton’s work over a century later.
- scientific revolution : The emergence of modern science during the early modern period, when developments in mathematics, physics, astronomy, biology (including human anatomy), and chemistry transformed societal views about nature. It began in Europe towards the end of the Renaissance period, and continued through the late 18th century, influencing the intellectual social movement known as the Enlightenment.
Introduction
Under the scientific method that was defined and applied in the 17th century, natural and artificial circumstances were abandoned, and a research tradition of systematic experimentation was slowly accepted throughout the scientific community. The philosophy of using an inductive approach to nature—to abandon assumption and to attempt to simply observe with an open mind—was in strict contrast with the earlier, Aristotelian approach of deduction, by which analysis of known facts produced further understanding. In practice, many scientists (and philosophers) believed that a healthy mix of both was needed—the willingness to question assumptions, yet also to interpret observations assumed to have some degree of validity. That principle was particularly true for mathematics and physics. René Descartes, whose thought emphasized the power of reasoning but also helped establish the scientific method, distinguished between the knowledge that could be attained by reason alone (rationalist approach), which he thought was mathematics, and the knowledge that required experience of the world, which he thought was physics.
Mathematization
To the extent that medieval natural philosophers used mathematical problems, they limited social studies to theoretical analyses of local speed and other aspects of life. The actual measurement of a physical quantity, and the comparison of that measurement to a value computed on the basis of theory, was largely limited to the mathematical disciplines of astronomy and optics in Europe. In the 16th and 17th centuries, European scientists began increasingly applying quantitative measurements to the measurement of physical phenomena on Earth.
The Copernican Revolution
While the dates of the scientific revolution are disputed, the publication in 1543 of Nicolaus Copernicus’s De revolutionibus orbium coelestium ( On the Revolutions of the Heavenly Spheres ) is often cited as marking the beginning of the scientific revolution. The book proposed a heliocentric system contrary to the widely accepted geocentric system of that time. Tycho Brahe accepted Copernicus’s model but reasserted geocentricity. However, Tycho challenged the Aristotelian model when he observed a comet that went through the region of the planets. This region was said to only have uniform circular motion on solid spheres, which meant that it would be impossible for a comet to enter into the area. Johannes Kepler followed Tycho and developed the three laws of planetary motion. Kepler would not have been able to produce his laws without the observations of Tycho, because they allowed Kepler to prove that planets traveled in ellipses, and that the sun does not sit directly in the center of an orbit, but at a focus. Galileo Galilei came after Kepler and developed his own telescope with enough magnification to allow him to study Venus and discover that it has phases like a moon. The discovery of the phases of Venus was one of the more influential reasons for the transition from geocentrism to heliocentrism. Isaac Newton’s Philosophiæ Naturalis Principia Mathematica concluded the Copernican Revolution. The development of his laws of planetary motion and universal gravitation explained the presumed motion related to the heavens by asserting a gravitational force of attraction between two objects.
Other Advancements in Physics and Mathematics
Galileo was one of the first modern thinkers to clearly state that the laws of nature are mathematical. In broader terms, his work marked another step towards the eventual separation of science from both philosophy and religion, a major development in human thought. Galileo showed a remarkably modern appreciation for the proper relationship between mathematics, theoretical physics, and experimental physics. He understood the parabola, both in terms of conic sections and in terms of the ordinate (y) varying as the square of the abscissa (x). He further asserted that the parabola was the theoretically ideal trajectory of a uniformly accelerated projectile in the absence of friction and other disturbances.
Newton’s Principia formulated the laws of motion and universal gravitation, which dominated scientists’ view of the physical universe for the next three centuries. By deriving Kepler’s laws of planetary motion from his mathematical description of gravity, and then using the same principles to account for the trajectories of comets, the tides, the precession of the equinoxes, and other phenomena, Newton removed the last doubts about the validity of the heliocentric model of the cosmos. This work also demonstrated that the motion of objects on Earth, and of celestial bodies, could be described by the same principles. His prediction that Earth should be shaped as an oblate spheroid was later vindicated by other scientists. His laws of motion were to be the solid foundation of mechanics; his law of universal gravitation combined terrestrial and celestial mechanics into one great system that seemed to be able to describe the whole world in mathematical formulae. Newton also developed the theory of gravitation. After the exchanges with Robert Hooke, English natural philosopher, architect, and polymath, he worked out proof that the elliptical form of planetary orbits would result from a centripetal force inversely proportional to the square of the radius vector.
The scientific revolution also witnessed the development of modern optics. Kepler published Astronomiae Pars Optica ( The Optical Part of Astronomy ) in 1604. In it, he described the inverse-square law governing the intensity of light, reflection by flat and curved mirrors, and principles of pinhole cameras, as well as the astronomical implications of optics, such asparallax and the apparent sizes of heavenly bodies. Willebrord Snellius found the mathematical law of refraction, now known as Snell’s law, in 1621. Subsequently, Descartes showed, by using geometric construction and the law of refraction (also known as Descartes’ law), that the angular radius of a rainbow is 42°. He also independently discovered the law of reflection. Finally, Newton investigated the refraction of light, demonstrating that a prism could decompose white light into a spectrum of colors, and that a lens and a second prism could recompose the multicolored spectrum into white light. He also showed that the colored light does not change its properties by separating out a colored beam and shining it on various objects.
Portrait of Galileo Galilei by Giusto Sustermans, 1636
Galileo Galilei (1564-1642) improved the telescope, with which he made several important astronomical discoveries, including the four largest moons of Jupiter, the phases of Venus, and the rings of Saturn, and made detailed observations of sunspots. He developed the laws for falling bodies based on pioneering quantitative experiments, which he analyzed mathematically.
Dr. William Gilbert, in De Magnete , invented the New Latin word electricus from ἤλεκτρον ( elektron ), the Greek word for “amber.” Gilbert undertook a number of careful electrical experiments, in the course of which he discovered that many substances were capable of manifesting electrical properties. He also discovered that a heated body lost its electricity, and that moisture prevented the electrification of all bodies, due to the now well-known fact that moisture impaired the insulation of such bodies. He also noticed that electrified substances attracted all other substances indiscriminately, whereas a magnet only attracted iron. The many discoveries of this nature earned for Gilbert the title of “founder of the electrical science.”
Robert Boyle also worked frequently at the new science of electricity, and added several substances to Gilbert’s list of electrics. In 1675, he stated that electric attraction and repulsion can act across a vacuum. One of his important discoveries was that electrified bodies in a vacuum would attract light substances, this indicating that the electrical effect did not depend upon the air as a medium. He also added resin to the then known list of electrics. By the end of the 17th Century, researchers had developed practical means of generating electricity by friction with an anelectrostatic generator, but the development of electrostatic machines did not begin in earnest until the 18th century, when they became fundamental instruments in the studies about the new science of electricity. The first usage of the word electricity is ascribed to Thomas Browne in 1646 work. In 1729, Stephen Gray demonstrated that electricity could be “transmitted” through metal filaments.
Treasures of the RAS: Starry Messenger by Galileo Galilei : In 1610, Galileo published this book describing his observations of the sky with a new invention – the telescope. In it he describes his discovery of the moons of Jupiter, of stars too faint to be seen by the naked eye, and of mountains on the moon. The book was the first scientific publication to be based on data from a telescope. It was an important step towards our modern understanding of the solar system. The Latin title is Sidereus Nuncius , which translates as Starry Messenger , or Sidereal Message .
Though astronomy is the oldest of the natural sciences, its development during the scientific revolution entirely transformed societal views about nature by moving from geocentrism to heliocentrism.
Assess the work of both Copernicus and Kepler and their revolutionary ideas
- The development of astronomy during the period of the scientific revolution entirely transformed societal views about nature. The publication of Nicolaus Copernicus ‘ De revolutionibus in 1543 is often seen as marking the beginning of the time when scientific disciplines gradually transformed into the modern sciences as we know them today.
- Copernican heliocentrism is the name given to the astronomical model developed by Copernicus that positioned the sun near the center of the universe, motionless, with Earth and the other planets rotating around it in circular paths, modified by epicycles and at uniform speeds.
- For over a century, few astronomers were convinced by the Copernican system. Tycho Brahe went so far as to construct a cosmology precisely equivalent to that of Copernicus, but with the earth held fixed in the center of the celestial sphere, instead of the sun. However, Tycho’s idea also contributed to the defense of the heliocentric model.
- In 1596, Johannes Kepler published his first book, which was the first to openly endorse Copernican cosmology by an astronomer since the 1540s. Kepler’s work on Mars and planetary motion further confirmed the heliocentric theory.
- Galileo Galilei designed his own telescope, with which he made a number of critical astronomical observations. His observations and discoveries were among the most influential in the transition from geocentrism to heliocentrism.
- Isaac Newton developed further ties between physics and astronomy through his law of universal gravitation, and irreversibly confirmed and further developed heliocentrism.
- Copernicus : A Renaissance mathematician and astronomer (1473-1543), who formulated a heliocentric model of the universe which placed the sun, rather than the earth, at the center.
- epicycles : The geometric model used to explain the variations in speed and direction of the apparent motion of the moon, sun, and planets in the Ptolemaic system of astronomy.
- Copernican heliocentrism : The name given to the astronomical model developed by Nicolaus Copernicus and published in 1543. It positioned the sun near the center of the universe, motionless, with Earth and the other planets rotating around it in circular paths, modified by epicycles and at uniform speeds. It departed from the Ptolemaic system that prevailed in western culture for centuries, placing Earth at the center of the universe.
The Emergence of Modern Astronomy
While astronomy is the oldest of the natural sciences, dating back to antiquity, its development during the period of the scientific revolution entirely transformed the views of society about nature. The publication of the seminal work in the field of astronomy, Nicolaus Copernicus ‘ De revolutionibus orbium coelestium ( On the Revolutions of the Heavenly Spheres ) published in 1543, is, in fact, often seen as marking the beginning of the time when scientific disciplines, including astronomy, began to apply modern empirical research methods, and gradually transformed into the modern sciences as we know them today.
The Copernican Heliocentrism
Copernican heliocentrism is the name given to the astronomical model developed by Nicolaus Copernicus and published in 1543. It positioned the sun near the center of the universe, motionless, with Earth and the other planets rotating around it in circular paths, modified by epicycles and at uniform speeds. The Copernican model departed from the Ptolemaic system that prevailed in western culture for centuries, placing Earth at the center of the universe. Copernicus’ De revolutionibus marks the beginning of the shift away from a geocentric (and anthropocentric) universe with Earth at its center. Copernicus held that Earth is another planet revolving around the fixed sun once a year, and turning on its axis once a day. But while he put the sun at the center of the celestial spheres, he did not put it at the exact center of the universe, but near it. His system used only uniform circular motions, correcting what was seen by many as the chief inelegance in Ptolemy’s system.
From 1543 until about 1700, few astronomers were convinced by the Copernican system. Forty-five years after the publication of De Revolutionibus , the astronomer Tycho Brahe went so far as to construct a cosmology precisely equivalent to that of Copernicus, but with Earth held fixed in the center of the celestial sphere instead of the sun. However, Tycho challenged the Aristotelian model when he observed a comet that went through the region of the planets. This region was said to only have uniform circular motion on solid spheres, which meant that it would be impossible for a comet to enter into the area. Following Copernicus and Tycho, Johannes Kepler and Galileo Galilei, both working in the first decades of the 17th century, influentially defended, expanded and modified the heliocentric theory.
Johannes Kepler
Johannes Kepler was a German scientist who initially worked as Tycho’s assistant. In 1596, he published his first book, the Mysterium cosmographicum , which was the first to openly endorse Copernican cosmology by an astronomer since the 1540s. The book described his model that used Pythagorean mathematics and the five Platonic solids to explain the number of planets, their proportions, and their order. In 1600, Kepler set to work on the orbit of Mars, the second most eccentric of the six planets known at that time. This work was the basis of his next book, the Astronomia nova (1609). The book argued heliocentrism and ellipses for planetary orbits, instead of circles modified by epicycles. It contains the first two of his eponymous three laws of planetary motion (in 1619, the third law was published). The laws state the following:
- All planets move in elliptical orbits, with the sun at one focus.
- A line that connects a planet to the sun sweeps out equal areas in equal times.
- The time required for a planet to orbit the sun, called its period, is proportional to long axis of the ellipse raised to the 3/2 power. The constant of proportionality is the same for all the planets.
Galileo Galilei
Galileo Galilei was an Italian scientist who is sometimes referred to as the “father of modern observational astronomy.” Based on the designs of Hans Lippershey, he designed his own telescope, which he had improved to 30x magnification. Using this new instrument, Galileo made a number of astronomical observations, which he published in the Sidereus Nuncius in 1610. In this book, he described the surface of the moon as rough, uneven, and imperfect. His observations challenged Aristotle ’s claim that the moon was a perfect sphere, and the larger idea that the heavens were perfect and unchanging. While observing Jupiter over the course of several days, Galileo noticed four stars close to Jupiter whose positions were changing in a way that would be impossible if they were fixed stars. After much observation, he concluded these four stars were orbiting the planet Jupiter and were in fact moons, not stars. This was a radical discovery because, according to Aristotelian cosmology, all heavenly bodies revolve around Earth, and a planet with moons obviously contradicted that popular belief. While contradicting Aristotelian belief, it supported Copernican cosmology, which stated that Earth is a planet like all others.
In 1610, Galileo also observed that Venus had a full set of phases, similar to the phases of the moon, that we can observe from Earth. This was explainable by the Copernican system, which said that all phases of Venus would be visible due to the nature of its orbit around the sun, unlike the Ptolemaic system, which stated only some of Venus’s phases would be visible. Due to Galileo’s observations of Venus, Ptolemy’s system became highly suspect and the majority of leading astronomers subsequently converted to various heliocentric models, making his discovery one of the most influential in the transition from geocentrism to heliocentrism.
Heliocentric model of the solar system, Nicolas Copernicus, De revolutionibus, p. 9, from an original edition, currently at the Jagiellonian University in Cracow, Poland
Copernicus was a polyglot and polymath who obtained a doctorate in canon law and also practiced as a physician, classics scholar, translator, governor, diplomat, and economist. In 1517 he derived a quantity theory of money–a key concept in economics–and in 1519, he formulated a version of what later became known as Gresham’s law (also in economics).
Uniting Astronomy and Physics: Isaac Newton
Although the motions of celestial bodies had been qualitatively explained in physical terms since Aristotle introduced celestial movers in his Metaphysics and a fifth element in his On the Heavens , Johannes Kepler was the first to attempt to derive mathematical predictions of celestial motions from assumed physical causes. This led to the discovery of the three laws of planetary motion that carry his name.
Isaac Newton developed further ties between physics and astronomy through his law of universal gravitation. Realizing that the same force that attracted objects to the surface of Earth held the moon in orbit around the Earth, Newton was able to explain, in one theoretical framework, all known gravitational phenomena. Newton’s Principia (1687) formulated the laws of motion and universal gravitation, which dominated scientists’ view of the physical universe for the next three centuries. By deriving Kepler’s laws of planetary motion from his mathematical description of gravity, and then using the same principles to account for the trajectories of comets, the tides, the precession of the equinoxes, and other phenomena, Newton removed the last doubts about the validity of the heliocentric model of the cosmos. This work also demonstrated that the motion of objects on Earth and of celestial bodies could be described by the same principles. His laws of motion were to be the solid foundation of mechanics; his law of universal gravitation combined terrestrial and celestial mechanics into one great system that seemed to be able to describe the whole world in mathematical formulae.
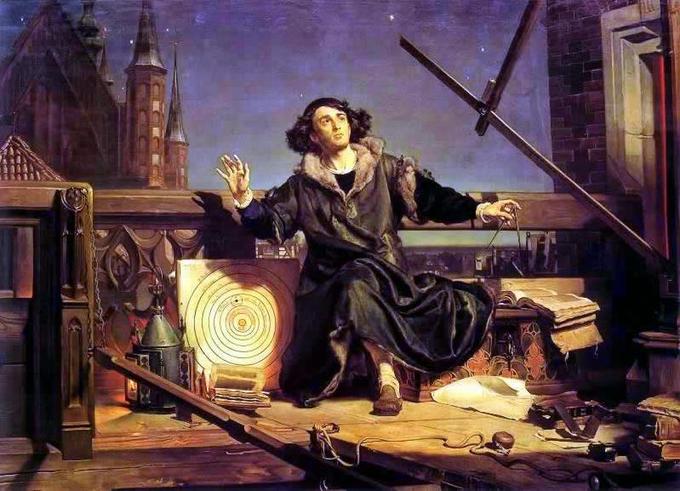
Jan Matejko, Astronomer Copernicus, or Conversations with God, 1873: Oil painting by the Polish artist Jan Matejko depicting Nicolaus Copernicus observing the heavens from a balcony by a tower near the cathedral in Frombork. Currently, the painting is in the collection of the Jagiellonian University of Cracow, which purchased it from a private owner with money donated by the Polish public.
Johannes Kepler Biography (1571-1630) : Johannes Kepler was a German astronomer and mathematician, who played an important role in the 17th century scientific revolution.
- Jo Kent, The Impact of the Scientific Revolution: A Brief History of the Experimental Method in the 17th Century. June 12, 2014. Provided by : OpenStax CNX. Located at : http://cnx.org/content/m13245/1.1/ . License : CC BY: Attribution
- Royal Society. Provided by : Wikipedia. Located at : http://en.wikipedia.org/wiki/Royal_Society . License : CC BY-SA: Attribution-ShareAlike
- Age of Enlightenment. Provided by : Wikipedia. Located at : https://en.wikipedia.org/wiki/Age_of_Enlightenment . License : CC BY-SA: Attribution-ShareAlike
- Scientific method. Provided by : Wikipedia. Located at : https://en.wikipedia.org/wiki/Scientific_method . License : CC BY-SA: Attribution-ShareAlike
- Baconian method. Provided by : Wikipedia. Located at : https://en.wikipedia.org/wiki/Baconian_method . License : CC BY-SA: Attribution-ShareAlike
- Scientific revolution. Provided by : Wikipedia. Located at : https://en.wikipedia.org/wiki/Scientific_revolution . License : CC BY-SA: Attribution-ShareAlike
- Renu00e9 Descartes. Provided by : Wikipedia. Located at : https://en.wikipedia.org/wiki/Ren%C3%A9_Descartes . License : CC BY-SA: Attribution-ShareAlike
- Science in the Age of Enlightenment. Provided by : Wikipedia. Located at : https://en.wikipedia.org/wiki/Science_in_the_Age_of_Enlightenment . License : CC BY-SA: Attribution-ShareAlike
- Galileo Galilei. Provided by : Wikipedia. Located at : https://en.wikipedia.org/wiki/Galileo_Galilei . License : CC BY-SA: Attribution-ShareAlike
- NewtonsPrincipia.jpg. Provided by : Wikipedia. Located at : https://en.wikipedia.org/wiki/Scientific_revolution#/media/File:NewtonsPrincipia.jpg . License : CC BY-SA: Attribution-ShareAlike
- The Shannon Portrait of the Hon Robert Boyle. Provided by : Wikipedia. Located at : http://en.wikipedia.org/wiki/File:The_Shannon_Portrait_of_the_Hon_Robert_Boyle.jpg . License : Public Domain: No Known Copyright
- Robert Boyle. Provided by : Wikipedia. Located at : http://en.wikipedia.org/wiki/Robert_Boyle . License : CC BY-SA: Attribution-ShareAlike
- Scientific Revolution. Provided by : Wikipedia. Located at : http://en.wikipedia.org/wiki/Scientific_revolution . License : CC BY-SA: Attribution-ShareAlike
- Isaac Newton. Provided by : Wikipedia. Located at : http://en.wikipedia.org/wiki/Sir_Isaac_Newton . License : CC BY-SA: Attribution-ShareAlike
- Copernican Revolution. Provided by : Wiktionary. Located at : https://en.wikipedia.org/wiki/Copernican_Revolution . License : CC BY-SA: Attribution-ShareAlike
- Robert Hooke. Provided by : Wikipedia. Located at : https://en.wikipedia.org/wiki/Robert_Hooke . License : CC BY-SA: Attribution-ShareAlike
- Treasures of the RAS: Starry Messenger by Galileo Galilei. Located at : http://www.youtube.com/watch?v=WlA_UYtt21c . License : Public Domain: No Known Copyright . License Terms : Standard YouTube license
- Justus Sustermans - Portrait of Galileo Galilei, 1636. Provided by : Wikipedia. Located at : http://en.wikipedia.org/wiki/File:Justus_Sustermans_-_Portrait_of_Galileo_Galilei,_1636.jpg . License : Public Domain: No Known Copyright
- Copernican Revolution. Provided by : Wikipedia. Located at : https://en.wikipedia.org/wiki/Copernican_Revolution . License : CC BY-SA: Attribution-ShareAlike
- History of astronomy. Provided by : Wikipedia. Located at : https://en.wikipedia.org/wiki/History_of_astronomy . License : CC BY-SA: Attribution-ShareAlike
- Nicolaus Copernicus. Provided by : Wikipedia. Located at : http://en.wikipedia.org/wiki/Nicolaus_Copernicus . License : CC BY-SA: Attribution-ShareAlike
- The New Astronomy and Cosmology of the Scientific Revolution: Nicolaus Copernicus, Tycho Brahe, and Johannes Kepler. Provided by : Saylor. Located at : https://en.wikipedia.org/wiki/Copernican_heliocentrism . License : CC BY: Attribution
- Deferent and epicycle. Provided by : Wikipedia. Located at : http://en.wikipedia.org/wiki/Deferent_and_epicycle . License : CC BY-SA: Attribution-ShareAlike
- Astronomer Copernicus, or Conversations with God. Provided by : Wikipedia. Located at : http://en.wikipedia.org/wiki/Astronomer_Copernicus,_or_Conversations_with_God . License : CC BY-SA: Attribution-ShareAlike
- Jan Matejko Astronomer Copernicus Conversation with God.. Provided by : Wikipedia. Located at : http://en.wikipedia.org/wiki/Jan_Matejko%23mediaviewer/File:Jan_Matejko-Astronomer_Copernicus-Conversation_with_God.jpg . License : Public Domain: No Known Copyright
- Johannes Kepler Biography (1571-1630). Located at : http://www.youtube.com/watch?v=VB3V36LJpGc . License : Public Domain: No Known Copyright . License Terms : Standard YouTube license
- De Revolutionibus manuscript p9b. Provided by : Wikipedia. Located at : http://en.wikipedia.org/wiki/File:De_Revolutionibus_manuscript_p9b.jpg . License : Public Domain: No Known Copyright
- Herman Boerhaave. Provided by : Wikipedia. Located at : https://en.wikipedia.org/wiki/Herman_Boerhaave . License : CC BY-SA: Attribution-ShareAlike
- Humorism. Provided by : Wikipedia. Located at : https://en.wikipedia.org/wiki/Humorism . License : CC BY-SA: Attribution-ShareAlike
- History of medicine. Provided by : Wikipedia. Located at : https://en.wikipedia.org/wiki/History_of_medicine . License : CC BY-SA: Attribution-ShareAlike
- William Harvey. Provided by : Wikipedia. Located at : http://en.wikipedia.org/wiki/William_Harvey . License : CC BY-SA: Attribution-ShareAlike
- Medical Renaissance. Provided by : Wikipedia. Located at : http://en.wikipedia.org/wiki/Medical_Renaissance . License : CC BY-SA: Attribution-ShareAlike
- Andreas Vesalius. Provided by : Wikipedia. Located at : http://en.wikipedia.org/wiki/Andreas_Vesalius . License : CC BY-SA: Attribution-ShareAlike
- Galen. Provided by : Wikipedia. Located at : http://en.wikipedia.org/wiki/Galen . License : CC BY-SA: Attribution-ShareAlike
- Ambroise Paru00e9. Provided by : Wikipedia. Located at : http://en.wikipedia.org/wiki/Ambroise_Par%C3%A9 . License : CC BY-SA: Attribution-ShareAlike
- Vesalius01. Provided by : Wikipedia. Located at : http://en.wikipedia.org/wiki/File:Vesalius01.jpg . License : Public Domain: No Known Copyright
- Vesalius Fabrica p174. Provided by : Wikipedia. Located at : http://en.wikipedia.org/wiki/Andreas_Vesalius%23mediaviewer/File:Vesalius_Fabrica_p174.jpg . License : Public Domain: No Known Copyright
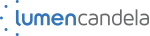
Privacy Policy

- Table of Contents
- Random Entry
- Chronological
- Editorial Information
- About the SEP
- Editorial Board
- How to Cite the SEP
- Special Characters
- Advanced Tools
- Support the SEP
- PDFs for SEP Friends
- Make a Donation
- SEPIA for Libraries
- Entry Contents
Bibliography
Academic tools.
- Friends PDF Preview
- Author and Citation Info
- Back to Top
Scientific Revolutions
The topic of scientific revolutions has been philosophically important since Thomas Kuhn’s account in The Structure of Scientific Revolutions (1962, 1970). Kuhn’s death in 1996 and the fiftieth anniversary of Structure in 2012 have renewed attention to the issues raised by his work. It is controversial whether or not there have been any revolutions in the strictly Kuhnian sense. It is also controversial what exactly a Kuhnian revolution is, or would be. Although talk of revolution is often exaggerated, most analysts agree that there have been transformative scientific developments of various kinds, whether Kuhnian or not. However, there is considerable disagreement about their import. The existence and nature of scientific revolutions is a topic that raises a host of fundamental questions about the sciences and how to interpret them, a topic that intersects most of the major issues that have concerned philosophers of science and their colleagues in neighboring science and technology studies disciplines. Even if the so-called Scientific Revolution from Copernicus to Newton fits the attractive, Enlightenment picture of the transition from feudalism to modernity (a claim that is also contested), the putative revolutions in mature sciences (e.g., relativity and quantum mechanics) challenge this Enlightenment vision of permanent rational and methodological standards underlying objective sciences and technologies that lead society along the path of progress toward the truth about the world. Today’s scientific realists are the most visible heirs of this picture. Although many philosophers and philosophically or historically reflective scientists had commented on the dramatic developments in twentieth-century physics, it was not until Kuhn that such developments seemed so epistemologically and ontologically damaging as to seriously challenge traditional conceptions of science—and hence our understanding of knowledge acquisition generally. Why it was Kuhn’s work and its timing that made the major difference are themselves interesting questions for investigation, given that others (e.g., Wittgenstein, Fleck, Bachelard, Polanyi, Toulmin, and Hanson) had already broached important “Kuhnian” themes.
Was there a Scientific Revolution that replaced pre-scientific thinking about nature and society and thus marked the transition to modernity? Which later developments, if any, are truly revolutionary? Are attributions of revolution usually a sign of insufficient historiographical understanding? In any case, how are such episodes to be explained historically and epistemologically? Are they contingent, that is, historical accidents and thus perhaps avoidable; or are they somehow necessary to a “progressive” science? And, if so, why? Is there an overall pattern of scientific development? If so, is it basically one of creative displacement, as Kuhn claimed? Do all revolutions have the same structure and function, or are there diverse forms of rupture, discontinuity, or rapid change in science? Do they represent great leaps forward or, on the contrary, does their existence undercut the claim that science progresses? Does the existence of revolutions in mature sciences support a postmodern or “post-critical” (Polanyi) rather than a modern, neo-Enlightenment conception of science in relation to other human enterprises? Does their existence support a strongly constructionist versus a realist conception of scientific knowledge claims? Are revolutions an exercise in rationality or are they so excessive as to be labeled irrational? Do they invite epistemological relativism? What are the implications of revolution for science policy? This entry will survey some but not all of these issues.
1. The Problems of Revolution and Innovative Change
2.1 scientific revolution as a topic for historiography of science, 2.2 scientific revolution as a topic for philosophy, 2.3 criteria for identifying scientific revolutions.
- 3.1 Kuhn’s Early Model of Mature Scientific Development
3.2 Revolution as Incommensurable Paradigm Change
3.3 progress through revolutions, 3.4 revolution or evolution, 4. kuhn’s later account of scientific revolutions, 5.1 thomas kuhn: kantian or hegelian, 5.2 the german neo-kantian tradition, 5.3 the french discontinuity theorists, 5.4 kuhn’s relation to the germanic and french traditions, 6.1 some alternative conceptions of scientific revolution, 6.2 some biological cases, 6.3 nonlinear dynamics, 6.4 the essential tension between tradition and innovation, other internet resources, related entries.
The difficulties in identifying and conceptualizing scientific revolutions involve many of the most challenging issues in epistemology, methodology, ontology, philosophy of language, and even value theory. With revolution we immediately confront the problem of deep, possibly noncumulative, conceptual and practical change, now in modern science itself, a locus that Enlightenment thinkers would have found surprising. And since revolution is typically driven by new results, or by a conceptual-cum-social reorganization of old ones, often highly unexpected, we also confront the hard problem of understanding creative innovation. Third, major revolutions supposedly change the normative landscape of research by altering the goals and methodological standards of the enterprise, so we face also the difficult problem of relating descriptive claims to normative claims and practices, and changes in the former to changes in the latter.
Comparing the world of business innovation and economic theory provides a perspective on the difficulty of these problems, for both the sciences and the industrial technologies change rapidly and sometimes deeply (in the aforementioned ways), thanks to what might be termed “innovation push”—both the pressure to innovate (to find and solve new problems, thereby creating new designs) and the pressure to accommodate innovation (see, e.g., Christensen 1997; Christensen and Raynor, 2003; Arthur 2009). In a market economy, as in science, there is a premium on change driven by innovation. Yet most economists have treated innovations as exogenous factors—as accidental, economically contingent events that come in from outside the economic system to work their effects. It is surprising that only recently has innovation become a central topic of economic theorists. Decades ago, the Austrian-American economist Joseph Schumpeter characterized economic innovation as
the process of industrial mutation—if I may use that biological term—that incessantly revolutionizes the economic structure from within , incessantly destroying the old one, incessantly creating a new one. This process of Creative Destruction is the essential fact about capitalism. [1942, chap. VII; Schumpeter’s emphasis]
Unfortunately, economists largely ignored this sort of claim (made also by a few others) until the recent development of economic growth theory (e.g., Robert Solow, Paul Romer, and W. Brian Arthur: see Beinhocker 2006 and Warsh 2006). The result was an inability of economic models to account for economic innovation endogenously and, thereby, to gain an adequate understanding of the generation of economic wealth.
The parallel observation holds for philosophy of science. Here, too, the leading philosophers of science until the 1960s—the logical empiricists and the Popperians—rejected innovation as a legitimate topic, even though it is the primary intellectual driver of scientific change and producer of the wealth of skilled knowledge that results. The general idea is that the so-called context of discovery, the context of creatively constructing new theories, experimental designs, etc., is only of historical and psychological interest, not epistemological interest, and that the latter resides in the epistemic status of the “final products” of investigation. On this view, convincing confirmation or refutation of a claim enables scientists to render an epistemic judgment that detaches it from its historical context. This judgment is based on the logical relations of theories and evidence rather than on history or psychology. According to this traditional view, there exists a logic of justification but not a logic of discovery. The distinction has nineteenth-century antecedents (Laudan 1980). Cohen and Nagel (1934) contended that to take historical path into account as part of the epistemic assessment was to confuse historical questions with logical questions and thereby to commit what they called a “genetic fallacy.” However, the context of discovery / context of justification distinction (or family of distinctions) is often attributed to Reichenbach (1938). (See the entry on Reichenbach . For recent discussion see Schickore and Steinle, 2006.)
Today there are entire academic industries devoted to various aspects of the topic of scientific revolutions, whether political or scientific, yet we have no adequate general theory or model of revolutions in either sphere. This article will focus on Thomas Kuhn’s conception of scientific revolutions, which relies partly on analogies to political revolution and to religious conversion. Kuhn’s is by far the most discussed account of scientific revolutions and did much to reshape the field of philosophy of science, given his controversial claims about incommensurability, rationality, objectivity, progress, and realism. For a general account of Kuhn’s work, see the entry on Kuhn . See also Hoyningen-Huene (1993), and Bird (2001).
2. History of the Concept of Scientific Revolution
What history lies behind the terms ‘revolution’ and ‘scientific revolution’? The answer is an intriguing mix of accounts of physical phenomena, political fortunes, and conceptions of chance, fate, and history. Originally a term applying to rotating wheels and including the revolution of the celestial bodies (as in Copernicus’ title: De Revolutionibus Orbium Coelestium ) and, more metaphorically, the wheel of fortune, ‘revolution’ was eventually transferred to the political realm. The term later returned to science at the metalevel, to describe developments within science itself (e.g., “the Copernican Revolution”). Christopher Hill, historian of seventeenth-century Britain and of the so-called English Revolution in particular, writes:
Conventional wisdom has it that the word ‘revolution’ acquired its modern political meaning only after 1688. Previously it had been an astronomical and astrological term limited to the revolution of the heavens, or to any complete circular motion. [Hill 1990, 82]
Hill himself dates the shift to the governmental realm somewhat earlier, pointing out that the notion of over turning was also present in groups of reformers who aspired to return human society to an earlier, ideal state: over turning as re turning. This conception of revolution as overturning was compatible with a cyclical view of history as a continuous process.
It was in the socio-political sphere that talk of revolution as a successful uprising and overturning became common. In this sense, a revolution is a successful revolt, ‘revolution’ being an achievement or product term, whereas ‘to revolt’ is a process verb. The fully modern conception of revolution as involving a break from the past—an abrupt, humanly-made overturning rather than a natural overturning—depended on the linear, progressive conception of history that perhaps originated in the Italian Renaissance, gained strength during the Protestant Reformation and the two later English revolutions, and became practically dogma among the champions of the scientific Enlightenment. The violent English Revolution of the 1640s gave political revolution a bad name, whereas the Glorious Revolution of 1688, a bloodless, negotiated compromise, reversed this reputation.
When did the term ‘revolution’ become a descriptor of specifically scientific developments? In the most thorough treatment of the history of the concept of scientific revolution, I. B. Cohen (1985) notes that the French word revolution was being used in early eighteenth-century France to mark significant developments. By mid-century it was pretty clear that Clairaut, D’Alembert, Diderot and others sometimes applied the term to scientific developments, including Newton’s achievement but also to Descartes’ rejection of Aristotelian philosophy. Cohen fails to note that Émilie Du Châtelet preceded them, in her Institutions de Physique of 1740, where she distinguished scientific from political revolutions (Châtelet and Zinnser 2009, p. 118). However, the definition of revolution in the Encyclopédie of the French philosophes was still political. Toward the end of the century, Condorcet could speak of Lavoisier as having brought about a revolution in chemistry; and, indeed, Lavoisier and his associates also applied the term to their work, as did Cuvier to his. Meanwhile, of course, Kant, in The Critique of Pure Reason (first edition 1781), spoke of his “Copernican Revolution” in philosophy. In fact, Cohen (1985) and Ian Hacking (2012) credit Kant with originating the idea of a scientific revolution, although Kant had read Du Châtelet. Interestingly, for Kant (1798) political revolutions are, by nature, unlawful, whereas Locke, in his social contract theory, had permitted them under special circumstances.
It was during the twentieth century that talk of scientific revolutions slowly gained currency. One can find scientists using the term occasionally. For example, young Einstein, in a letter to his friend Habicht, describes his new paper on light quanta as “very revolutionary” (Klein 1963, 59). The idea of radical breaks was foreign to such historians of science as Pierre Duhem and George Sarton, but Alexandre Koyré, in Études Galiléennes (1939), rejected inductivist history, interpreting the work of Galileo as a sort of Platonic intellectual transformation. (See Zambelli (2016) for a revealing account of Koyré’s own background.) In The Origins of Modern Science: 1300–1800 (1949 and later editions), widely used as a course text, Herbert Butterfield, a political historian working mainly from secondary sources, wrote a compact summary of the Scientific Revolution, one that emphasized the importance of conceptual transformation rather than the infusion of new empirical information. The anti-whiggism that he had advocated in his The Whig Interpretation of History (1931) became a major constraint on the new historiography of science, especially in the Anglophone world. In Origins , Butterfield applied the revolution label not only to the Scientific Revolution and to several of its components but also to “The Postponed Revolution in Chemistry” (a chapter title), as if it were a delayed component of the Scientific Revolution. His history ended there. A revolution for Butterfield is a major event that founds a scientific field. Taken together, these revolutions founded modern science. As the title of his book suggests, he was concerned with origins, not with what comes after the founding. In the Introduction he famously (or notoriously) stated that the Scientific Revolution
outshines everything since the rise of Christianity and reduces the Renaissance and Reformation to the rank of mere episodes, mere internal displacements, within the system of medieval Christendom.
For Butterfield, the Scientific Revolution was a watershed event on the scale of total human history, an event that, somewhat ironically and somewhat like Christianity according to its believers, enabled the sciences, to some degree, to escape from history and thereby to become exceptional among human endeavors. Subsequently, A. Rupert Hall, a full-fledged historian of science who worked from primary sources, published The Scientific Revolution: 1500–1800 (Hall 1954). Soon many other scholars spoke of the Scientific Revolution, the achievements of the period from Copernicus to Newton, including such luminaries as Kepler, Galileo, Bacon, Descartes, Huygens, Boyle, and Leibniz.
Then Thomas Kuhn and Paul Feyerabend challenged received views of science and made talk of revolutionary breaks and incommensurability central to the emerging new field of history and philosophy of science. They asserted that major conceptual changes lay in the future of mature, modern sciences as well as in their past. Kuhn (1962, ch. IX) contended that there will be no end to scientific revolutions as long as systematic scientific investigation continues, for they are a necessary vehicle of ongoing scientific progress–necessary to break out of dated conceptual frameworks. In other words, there are both founding revolutions, in something like Butterfield’s sense of threshold events to maturity, and a never-ending series of later revolutions within an ongoing field, no matter how mature it is. However, soon after Structure , Kuhn had second thoughts and eventually abandoned the Butterfield conception of revolution, on the ground that even his so-called preparadigm schools had their paradigms (Kuhn 1974, 460, note 4; details below). So multiple Kuhnian paradigms in long-term competition now became possible.
The Scientific Revolution was the topic around which the field of history of science itself came to maturity. Kuhn’s popularization of the idea that even the mature natural sciences undergo deep conceptual change stimulated much general intellectual interest in the history of science during the 1960s and 1970s. The revolution frame of reference was also a boon to historiographical narrative itself (see Cohen 1985 and Nickles 2006). And by challenging the received, quasi-foundational, Enlightenment conception of science, history of science and related philosophies of science gained great cultural significance for a time.
In recent decades, however, many historians have contested even the claim that there was a single, coherent development appropriately called “the Scientific Revolution.” Steven Shapin (1996, 1) captured the unease in his opening sentence: “There was no such thing as the Scientific Revolution, and this is a book about it.” Everyone agrees that a series of rapid developments of various kinds took place during the period in question, but the operative word here is ‘various’. One difficulty is that no one has succeeded in capturing a 150-year (or more) period of work in an insightful, widely accepted characterization that embraces the important changes in theory, method, practices, instrumentation, social organization, and social status ranging over such a wide variety of projects. The very attempt has come to seem reductionist. Older styles of historical writing were characterized by grand narratives such as “the mechanization of the world picture” (Dijksterhuis 1961; original, Dutch edition, 1950) and humanity’s passage from subjective superstition to objectivity and mathematical precision (Gillispie 1960). Philosophically oriented writers attempted to find unity and progress in terms of the discovery of a new, special scientific method. Today even most philosophers of science dismiss the claim that there exists a powerful, general, scientific method, the discovery of which explains the Scientific Revolution and the success of modern science. Quite the contrary: effective scientific methods are themselves the product of painstaking work at the frontier—scientific results methodized—and are hence typically laden with the technical content of the specialty in question. There is no content-neutral, thereby general and timeless method that magically explains how those results were achieved (Schuster and Yeo 1986, Nickles 2009).
Continuity theorists such as Pierre Duhem (1914), John Herman Randall (1940), A. C. Crombie (1959, 1994), and more recent historians such as Peter Dear (2001) have pointed out a second major difficulty in speaking of “the Scientific Revolution.” It is hard to locate the sharp break from medieval and Renaissance practices that discontinuity historians from Koyré to Kuhn have celebrated. When examined closely in their own cultural context, all the supposed revolutionaries are found to have had one foot in the old traditions and to have relied heavily on the work of predecessors. In this vein, J. M. Keynes famously remarked that Newton was “the last of the magicians,” not the first of the age of reason (Keynes 1947). Still, most historians and philosophers would agree that the rate of change of scientific development increased notably during this period. Hence, Shapin, despite his professional reservations, could still write an instructive, synthetic book about the Scientific Revolution. The most thorough appraisal of historiographical treatments of the Scientific Revolution is H. Floris Cohen’s (1994).
The Scientific Revolution supposedly encompassed all of science or natural philosophy, as it then existed, with major social implications, as opposed to more recent talk of revolutions within particular technical fields. Have there been other multidisciplinary revolutions? Some have claimed the existence of a “second scientific revolution” in the institutional structure of the sciences in the decades around 1800, especially in France, others (including Kuhn 1977a, ch. 3) of a multidisciplinary revolution in the “Baconian sciences” (chemistry, electricity, magnetism, heat, etc.) during roughly the same time period. Enrico Bellone 1980), Kuhn, and others Kuhn have focused on the tremendous increase in mathematical abstraction and sophistication during the early-to-mid nineteenth century that essentially created what we know as mathematical physics. Still others have claimed that there was a general revolution in the sciences in the decades around 1900. (See also Cohen 1985, chap. 6, for discussion of these claims.)
For many historians, ‘the Scientific Revolution’ now describes a topic area rather than a clearly demarcated event. They find it safer to divide the Scientific Revolution into several more topic- and project-specific developments. However, in their unusually comprehensive history of science textbook, Peter Bowler and Iwan Morus (2005) query of practically every major development they discuss whether or not it was a genuine revolution at all, at least by Kuhnian standards. More recently, David Wootton’s (2015) is a revisionist account that returns to a more heroic understanding of the Scientific Revolution.
Commitment to the existence of deep scientific change does not, for all experts, equate to a commitment to the existence of revolutions in Kuhn’s sense. Consider the historically–oriented philosopher Stephen Toulmin (1953, 1961, 1972), who wrote of “ideals of natural order,” principles so basic that they are normally taken for granted during an epoch but that are subject to eventual historical change. Such was the change from the Aristotelian to the Newtonian conception of inertia. Yet Toulmin remained critical of revolution talk. Although the three influential college course texts that he co-authored with June Goodfield recounted the major changes that resulted in the development of several modern sciences (Toulmin and Goodfield 1961, 1962, 1965), these authors could write, already about the so-called Copernican Revolution:
We must now look past the half-truths of this caricature, to what Copernicus attempted and what he in fact achieved. For in science, as in politics, the term ‘revolution’—with its implication that a whole elaborate structure is torn down and reconstructed overnight—can be extremely misleading. In the development of science, as we shall see, thorough-going revolutions are just about out of the question. [1961, 164]
The Toulmin and Goodfield quotation invites us to ask, When did talk of scientific revolutions enter philosophy of science in a significant way? And the answer seems to be: there is a sprinkling of uses of the term ‘scientific revolution’ and its cognates prior to Kuhn, but these were ordinary expressions that did not yet have the status of a technical term.
Given the prominence of the topic today, it is surprising that we do not find the term in Philipp Frank’s account of the positivist discussion group in Vienna in the early twentieth century. However, Frank (1957) does speak of their perception of a “crisis” in modern physics caused by the undermining of classical mechanics by special relativity and quantum mechanics, and it was common to speak of this or that worldview or world picture ( Weltanschauung , Weltbild ), e.g., the electromagnetic vs. the Einsteinian vs. the mechanical picture. Nor do we find talk of scientific revolutions in the later Vienna Circle, even after the diaspora following the rise of Hitler. The technical term does not appear in Karl Popper’s Logik der Forschung (1934) nor in his 1959 English expansion of that work as The Logic of Scientific Discovery , at least not important enough to be indexed. Hans Reichenbach (1951) speaks rather casually of the revolutions in physics. The technical term is not in Ernest Nagel’s The Structure of Science (1961). Nor is it in Stephen Pepper’s World Hypotheses (1942). It plays no significant role in N. R. Hanson’s Patterns in Discovery (1958), despite its talk of the theory-ladenness of observation and perceptual Gestalt switches. Meanwhile, there were, of course, a few widely-read works in the background that spoke of major ontological changes associated with the rise of modern science, especially E. A. Burtt’s Metaphysical Foundations of Modern Physical Science (1924). Burtt’s book influenced Koyré, who, in turn, influenced Kuhn.
In his retrospective autobiographical lecture at Cambridge in 1953, Popper did refer to the dramatic political and intellectual events of his youth as revolutionary:
[T]he air was full of revolutionary slogans and ideas, and new and often wild theories. Among the theories which interested me Einstein’s theory of relativity was no doubt by far the most important. The others were Marx’s theory of history, Freud’s psycho-analysis, and Alfred Adler’s so-called ‘individual psychology’. [Popper 1957]
And during the 1960s and 1970s, Popper indicated that, according to his “critical approach” to science and philosophy, all science should be revolutionary—revolution in permanence. But this was a tame conception of revolution compared to Kuhn’s, given Popper’s two logical criteria for a progressive new theory: (a) it must logically conflict with its predecessor and overthrow it; yet (b) “a new theory, however revolutionary, must always be able to explain fully the success of its predecessor” (Popper 1975). As we shall see, Kuhn’s model of revolution rejects both these constraints (depending on how one interprets his incommensurability claim) as well as the idea of progress toward final, big, theoretical truths about the universe. Kuhn dismissed Popper’s notion of revolution in perpetuity as a contradiction in terms, on the ground that a revolution is something that overthrows a long and well–established order, in violation of the rules of that order. Kuhn (1970) also vehemently rejected Popper’s doctrine of falsification, which implied that a theory could be rejected in isolation, without anything to replace it. According to Popper, at any time there may be several competing theories being proposed and subsequently refuted by failed empirical tests—rather like several balloons being launched, over time, and then being shot down, one by one. Popper’s view thus faces the difficulty, among others, of explaining the long-term coherence that historians find in scientific research.
Beginning in the 1960s, several philosophers and historians addressed this difficulty by proposing the existence of larger units (than theories) of and for analysis. Kuhn’s paradigms, Imre Lakatos’s research programmes, Larry Laudan’s research traditions (Lakatos 1970, Laudan 1977), and the widespread use of terms such as ‘conceptual scheme’, ‘conceptual framework’, ‘worldview’, and Weltanschauung (Suppe 1974) instanced this felt need for larger-sized units among Anglo-American writers, as had Toulmin’s old concept of ideals of natural order. These stable formations correspondingly raised the eventual prospect of larger-scale instabilities, for an abrupt change in such a formation would surely be more dramatic, more revolutionary, than a Popperian theory change. However, none of the other writers endorsed Kuhn’s radical conception of scientific revolution. Meanwhile, Michel Foucault 1963, 1966, 1969, 1975), working in a French tradition, was positing the existence of “discursive formations” or epistemes , sets of deep-structural cultural rules that define the limits of discourse during a period. Section 5 returns to this theme.
I. B. Cohen (1985, chap. 2) lays down four historical tests, four necessary conditions, for the correct attribution of a revolution. First, the scientists involved in the development must perceive themselves as revolutionaries, and relevant contemporaries must agree that a revolution is underway. Second, documentary histories must count it as a revolution. Third, later historians and philosophers must agree with this attribution and, fourth, so must later scientists working in that field or its successors. By including both reports from the time of the alleged revolution and later historiographical judgments, Cohen excludes people who claimed in their day to be revolutionaries but who had insufficient impact on the field to sustain the judgment of history. He also guards against whiggish, post hoc attributions of revolution to people who had no idea that they were revolutionaries. His own four examples of big scientific revolutions all have an institutional dimension: The Scientific Revolution featured the rise of scientific societies and journals, the second was the aforementioned revolution in measurement from roughly 1800 to 1850 (which Kuhn, too, called “the second scientific revolution”; 1977, 220). Third is the rise of university graduate research toward the end of that century. Fourth is the post-World War II explosion in government funding of science and its institutions.
Cohen sets the bar high. Given Copernicus’ own conservatism and the fact that few people paid attention to his work for half a century, the Copernican achievement was not a revolution by Cohen’s lights. Or if there was a revolution, should it not be attributed to Kepler, Galileo, and Descartes? This thought further problematizes the notion of revolution, for science studies experts as well as scientists themselves know that scientific and technological innovation can be extremely nonlinear in the sense that a seemingly small, rather ordinary development may eventually open up an entire new domain of research problems or a powerful new approach. Consider Planck’s semi-classical derivation of the empirical blackbody radiation law in 1900, which, under successively deeper theoretical derivations by himself and (mainly) others over the next two and a half decades, became a pillar of the revolutionary quantum theory. As Kuhn (1978) shows, despite the flood of later attributions to Planck, it is surprisingly difficult, on historical and philosophical grounds, to justify the claim that he either was, or saw himself as, a revolutionary in 1900 and for many years thereafter. (Kuhn 2000b offers a short summary.) Augustine Brannigan (1981) and Robert Olby (1985) defend similar claims about Mendel’s alleged discovery of Mendelian inheritance.
These examples suggest that Cohen’s account of scientific revolution (and Kuhn’s) is tied too closely to the idea of political revolution in placing so much weight on the intentions of the generators. In the last analysis, many would agree, revolution, like speciation in biology, is a retrospective judgment, a judgment of eventual consequences, not something that is always directly observable as such in its initial phases, e.g., in the stated the intentions of its authors. On the other hand, a counterintuitive implication of this consequentialist view of revolutions is that there can be revolution without revolt (assuming that revolt is a deliberate course of action), revolutionary work without authors, so to speak, or at least revolutionary in eventual meaning despite the authors’ intentions. Then why not just speak of evolution rather than revolution in such cases? For, as we know by analogy from evolutionary biology, in the long run evolution can be equally transformative, even moreso (see below).
A related point is that, insofar as revolutions are highly nonlinear, it is difficult to ascribe to them any particular reason or cause; for, as indicated, the triggering events can be quite ordinary work, work that unexpectedly opens up new vistas for exploration. A small cause may have an enormous effect. To be sure, the state of the relevant scientific system must be such that the events do function as triggers, but we need not expect that such a system always be readily identifiable as one in crisis in Kuhn’s sense. Rather, the highly nonlinear revolutionary developments can be regarded as statistical fluctuations out of a “noisy” background of ordinary work. At any rate, on this view it is a mistake to think that explaining revolutions requires locating a momentous breakthrough (Nickles 2012a and b).
What of the common requirement that revolutions be rapid, event-like, unlike the century-and-a half-long Scientific Revolution? Brad Wray (2011, 42f) answers that there is no reason that a revolution need be an abrupt event. What is important is how thoroughgoing the change is and that it be change at the community level rather than a Gestalt switch experienced by certain individuals. (After the original publication of Structure , Kuhn acknowledged his confusion in attributing Gestalt switches to the community as a whole as well as to individuals.) On Wray’s view, evolution and revolution are not necessarily opposed categories. And with this understanding, the Toulmin and Goodfield comment quoted above becomes compatible with revolutionary transformation, which, not surprisingly, takes time to become thoroughgoing. Meanwhile, the Butterfield quotation suggests that what counts as a striking change is a matter of historical scale. By our lights today, 150 years is a long time; but, against the long sweep of total human history, a change of the magnitude of the Scientific Revolution was quite rapid. Perhaps today’s rapid pace of scientific and technological innovation makes us impatient with slower-scaled developments in the past. And it is surely the case the some of the slow, large-scale transformations now underway are scarcely visible to us.
Finally, what of Butterfield’s criterion of broader social impacts? Kuhn retained this criterion in The Copernican Revolution , but revolutions increasingly become changes in specialist communities in his later work, since those communities insulate themselves from the larger society. In the chapter on the invisibility of revolutions in Structure , Kuhn tells us that a tiny subspecialty can undergo a revolution that looks like a cumulative change even to neighboring fields of the same scientific discipline. In this respect Kuhn remained an internalist.
3. Kuhn’s Early Account of Scientific Revolutions
Although virtually no one in the science studies fields accepts Kuhn’s model in Structure as correct in detail, there has been a revival of interest in his views since his death and, more recently, in connection with the fiftieth anniversary in 2012 of the book’s original publication. Some examples are: the fiftieth anniversary edition of Structure itself, including a valuable introduction by Ian Hacking; Kuhn (2000a), a collection that records the later evolution of Kuhn’s thought; Sankey (1997); Bitbol (1997); Fuller (2000); Bird (2001); Friedman (2001); Andersen (2001); Sharrock and Read (2002); Nickles (2003a); González (2004); Soler et al. (2008); Agazzi (2008); Gattei (2008); Torres (2010); Wray (2011), Kindi and Arabatzis (2012), De Langhe (2013), Marcum (2015), and Richards and Daston (2016). Kuhn on revolutions has helped to shape many symposia on scientific realism and related matters, for example, Soler (2008) on contingency in the historical development of science and Rowbottom and Bueno (2011) on Bas van Fraassen’s (2002) treatment of stance, voluntarism, and the viability of empiricism. Since Kuhn’s work is discussed in some detail in other contributions to this Encyclopedia (see, especially, “Kuhn, Thomas”, and “The Incommensurability of Scientific Theories”), a brief account will suffice here. For a detailed reading guide to Structure , consult Preston (2008).
3.1 Kuhn’s Early Model of Scientific Development
According to Kuhn in Structure , a loosely characterized group of activities, often consisting of competing schools, becomes a mature science when a few concrete problem solutions provide models for what good research is (or can be) in that domain. These exemplary problems-cum-solutions become the basis of a “paradigm” that defines what it is to do “normal science.” As its name suggests, normal science is the default state of a mature science and of the community of researchers who constitute it. The paradigm informs investigators what their domain of the world is like and practically guarantees that all legitimate problems can be solved in its terms. Normal science is convergent rather than divergent: it actively discourages revolutionary initiatives and essentially novel (unexpected) discoveries, for these threaten the paradigm. However, normal research is so detailed and focused that it is bound to turn up anomalous experimental and theoretical results, some of which will long resist the best attempts to resolve them. Given the historical contingencies involved in the formation of guiding paradigms as well as the fallibility of all investigators, it would be incredibly improbable for everything to end up working perfectly. According to Kuhn, anomalies are therefore to be expected. Historically, all paradigms and theory complexes face anomalies at all times. If and when persistent efforts by the best researchers fail to resolve the anomalies, the community begins to lose confidence in the paradigm and a crisis period ensues in which serious alternatives can now be entertained. If one of these alternatives shows sufficient promise to attract a dominant group of leading researchers away from the old paradigm, a paradigm shift or paradigm change occurs—and that is a Kuhnian revolution. The radicals accomplish this by replacing the former set of routine problems and problem-solving techniques (exemplars) by a new set of exemplars, making the old practices seem defective, or at least old fashioned.
The new paradigm overturns the old by displacing it as no longer a competent guide to future research. In the famous (or notorious)chapter X of Structure , Kuhn claims that the change is typically so radical that the two paradigms cannot be compared against the same goals and methodological standards and values. Moreover, the accompanying meaning shift of key terms, such as ‘simultaneous’, ‘mass’, and ‘force’ in physics, leads to communication breakdown. In effect, scientists on different sides of a paradigm debate “live in different worlds.” Kuhn speaks of scientists experiencing a kind of gestalt switch or religious conversion experience. The heated rhetoric of debate and the resulting social reorganization, he says, resemble those of a political revolution. “Like the choice between political institutions, that between competing paradigms proves to be a choice between incompatible modes of community life” (1970, 94). The comparison of scientific with political revolutions should not surprise, given the entangled history of the term ‘revolution’, but claiming such close similarity enraged philosophical and cultural critics of Kuhn.
The typical paradigm change does not involve a large infusion of new empirical results, Kuhn tells us (chs. IX and X). Rather, it is a conceptual reorganization of otherwise familiar materials, as in the relativity revolution. A paradigm change typically changes goals, standards, linguistic meaning, key scientific practices, the way both the technical content and the relevant specialist community are organized, and the way scientists perceive the world. (For the often neglected practices dimension in Kuhn’s account, see Rouse, 2003.) Nor can we retain the old, linear, cumulative conception of scientific progress characteristic of Enlightenment thinking; for, Kuhn insists, attempts to to show that the new paradigm contains the old, either logically or in some limit or under some approximation, will be guilty of a fallacy of equivocation. The meaning change reflects the radical change in the assumed ontology of the world. A second Kuhnian objection to cumulative progress is what has come to be called “Kuhn loss” (see Post 1971, 229, n. 38). Rarely does the new paradigm solve all of the problems that its predecessor apparently solved. So even in this sense the new paradigm fails completely to enclose the old. The consequence, according to Kuhn, is that attempts to defend continuous, cumulative scientific progress by means of theory reduction or even a correspondence relationship (e.g., a limiting relationship) between a theory and its predecessor must fail. Revolutions produce discontinuities.
Given all these changes, Kuhn claimed that the two competing paradigms are “incommensurable”, a technical term that he repeatedly attempted to clarify. Traditional appeals to empirical results and logical argument are insufficient to resolve the debate. For details of the incommensurability debate, see the entry “The Incommensurability of Scientific Theories.” as well as Hoyningen-Huene and Sankey (2001) as a sample of the large literature on incommensurability.
Naturally, many thinkers of a logical empiricist or Popperian bent, or simply of an Enlightenment persuasion, were shocked by these claims and responded with a barrage of criticism—as if Kuhn had committed a kind of sacrilege by defiling the only human institution that could be trusted to provide the objective truth about the world. Today there is fairly wide agreement that some of Kuhn’s claims no longer look so radical. Meanwhile, Kuhn himself was equally shocked by the vehemence of the attacks and (to his mind) the willful distortion of his views (see, e.g., Lakatos and Musgrave 1970). In later papers and talks, he both clarified his views and softened some of his more radical claims. Critics reacted to the radical views of Paul Feyerabend (1962, 1975) in a somewhat similar manner. (For details, see the entry “Feyerabend, Paul.”)
Given that cyclic theories of history have, for the most part, long given way to linear, progressive accounts, readers may be surprised at Kuhn critic, physicist Stephen Weinberg’s comment that Kuhn’s overall model is still, in a sense, cyclic (Weinberg 2001). In fact, Kuhn himself had already recognized this. After the founding paradigm in Kuhn’s account in Structure , we have normal science under a paradigm, then crisis, then revolution, then a new paradigm—a development that brings back a new period of normal science. At this abstract level of description, the model is indeed cyclic, but of course the new paradigm heads the science in question in a new direction rather than returning it to a previous state. Other commentators, including Marxists, have regarded Kuhn’s mechanism as dialectical, as illustrated by the succession of self-undermining developments in the theory of light, from a Newtonian particle theory to a wave theory to a new kind of wave-particle duality. (For the dialectical interpretation see especially Krajewski 1977 and Nowak 1980 on the idealizational approach to science, as originated by Karl Marx.)
Somewhat ironically, Kuhn’s attempt to revolutionize the epistemology of science has had a wider socio-cultural impact than many scientific revolutions themselves. While some of Kuhn’s doctrines step into the postmodern era, he still had a foot in the Enlightenment, which helps to explain his dismay at the critical reaction to his work and to radical developments in the new-wave sociology of science of the 1970s and ‘80s. For, unlike many postmodernists (some of whom make use of his work), Kuhn retained a scientific exceptionalism. He did not doubt that the sciences have been uniquely successful since the Scientific Revolution. For him, unlike for many of his critics, revolutions in his radical sense were great epistemological leaps forward rather than deep scientific failures. On the science policy front, he intended his work to help preserve the integrity of this socially valuable enterprise. It is on science policy issues that Steve Fuller is most critical of Kuhn (Fuller 2000).
The general problem presented by Kuhn’s critique of traditional philosophy of science is that, although the various sciences have been successful, we do not understand how they have accomplished this or even how to characterize this success. Enlightenment-style explanations have failed. For example, Kuhn and Feyerabend (1975), preceded by Popper, were among the first philosophers to expose the bankruptcy of the claim that it was the discovery of a special scientific method that explains that success, a view that is still widely taught in secondary schools today. And that conclusion (one that cheered those postmodernists who regard scientific progress as an illusion) left Kuhn and the science studies profession with the problem of how science really does work. To explain how and why it had been so successful became an urgent problem for him—again, a problem largely rejected as bogus by many science studies scholars other than philosophers.
Another of Kuhn’s declared tasks in Structure was to solve the problem of social order for mature science, that is, how cohesive modern science (especially normal science) is possible (Barnes 1982, 2003). Yet another was to bring scientific discovery back into philosophical discussion by endogenizing it in his model, while denying the existence of a logic of discovery. Whereas the logical empiricists and Popper had excluded discovery issues from philosophy of science in favor of theory of confirmation or corroboration, Kuhn was critical of confirmation theory and supportive of historical and philosophical work on discovery. He argued that discoveries are temporally and cognitively structured and that they are an essential component of an epistemology of science. In Kuhnian normal science the problems are so well structured and the solutions so nearly guaranteed in terms of the resources of the paradigm that the problems reduce to puzzles (Nickles 2003b). Kuhn kept things under control there by denying that normal scientists seek essential innovation, for, as indicated above, major, unexpected discoveries threaten the extant paradigm and hence threaten crisis and revolution. So, even in normal science, Kuhn had to admit that major discoveries are unexpected challenges to the reigning paradigm. They are anomalous, even exogenous in the sense that they come as shocks from outside the normal system.
But this is the working scientists’ point of view. As noted, normal science is bound to turn up difficulties that resist resolution, at least some of which are sooner or later recognized by the community. In Kuhn’s own view, as a historian and philosopher standing high above the fray, it is deliberate, systematic normal research that will most readily sow the seeds of revolution and hence of rapid scientific progress. According to the old musicians’ joke, the fastest way to Carnegie Hall is slow practice. For Kuhn the fastest way to revolutionary innovation is intensely detailed normal science.
When it comes to revolution on Kuhn’s account, the social order breaks down dramatically. And here his strategy of taming creative normal research so as to make room for articulated discovery (the reduction of research problems to puzzles) also breaks down. Kuhn had to acknowledge that he had no idea how the scientists in extraordinary research contexts manage to come up with brilliant new ideas and techniques. This failure exacerbated his problem of explaining what sort of continuity underlies the revolutionary break that enables us to identify the event as a revolution within an ongoing field of inquiry. As he later wrote:
Even those who have followed me this far will want to know how a value-based enterprise of the sort I have described can develop as a science does, repeatedly producing powerful new techniques for prediction and control. To that question, unfortunately, I have no answer at all…. [1977b, 332]
Kuhn’s work on scientific revolutions raises difficult questions about whether science progresses and, if so, in what that progress consists. Kuhn asks (p. 60), “Why is progress a perquisite reserved almost exclusively for the activities we call science” and not for art, political theory, or philosophy? Early critics took him to deny scientific progress, because he rejected the traditional correspondence theory of truth and the related idea of cumulative progress toward a representational truth waiting out there for science to find it. For Kuhn the internalist, the technical goals of science are endogenously generated and change over time, rapidly during revolutions. Yet, somewhat paradoxically, Kuhn regarded revolutions as the most progressive components of his model of science. Unfortunately, he was not able to articulate fully in what that progress consists, given the issues of truth, incommensurability and Kuhn loss, a problem that those who reject convergent scientific realism still face. However, problem-solving know-how and success, including predictive precision, are major components of his answer. “[T]he unit of scientific achievement is the solved problem....” (p. 169). In a retreat from his most radical statements, Kuhn responded to critics by saying that we do possess a general set of scientific values that enables us, usually pretty easily, to order scientific works in historical time according to the degree in which they realize these values. A new paradigm, he says, must always treat successfully a serious anomaly left by the old one as well as opening up new questions for fruitful investigation.
Kuhn’s emphasis on scientific practices, relative to the philosophical state of play in the 1960s, takes up some of the slack left by the rejection of strong realism. His emphasis on skilled practice may have been influenced by Michael Polanyi’s Personal Knowledge (1958), with its “tacit knowing” component, although Kuhn denied that he found Polanyi’s account appealing (see, e.g., Baltas et al., 2000, pp. 296f).
If there have been so many revolutions, then why did the world have to wait for Kuhn to see them? Because, he said, they are largely invisible. For, after a revolution, the winners rewrite the history of science to make it look as if the present paradigm is the brilliant but rational sequel to previous work. The implication is that only someone of Kuhn’s historical sensitivity could be expected to notice this. (Skeptical critics reply that Kuhn invented the problem for which he had a solution.) Indeed, in his large book on the history of the early quantum theory (Kuhn 1978), he moved the origin of the quantum theory revolution forward five years, from Planck in 1900 to Einstein and Ehrenfest in 1905. Revisionist historiography by whiggish scientists, he claimed, had smoothed out the actual history by crediting Planck with a solution that he actually rejected at the time to a problem that he did not then have—and by diminishing the truly radical contribution of Einstein. Kuhn’s move again raises the question whether the authors of a revolution must knowingly break from the received research tradition.
At the end of Structure , Kuhn drew an analogy between the development of science and evolutionary biology. This was surprising, since ‘evolution’ is commonly employed as a contrast term to ‘revolution’. Kuhn’s main point was that evolution ramifies rather than progressing toward a final goal, yet its degree of specialization through speciation can be regarded as a sort of progress, a progress from a historically existing benchmark rather than a progress toward a preordained, speculative goal. So specialization is an indicator of progress. As for revolutions, they correspond to macromutations.
The process described in Section XII as the resolution of revolutions is the selection by conflict within the scientific community of the fittest way to practice future science. The net result of a sequence of such revolutionary selections, separated by periods of normal research, is the wonderfully adapted set of instruments we call modern scientific knowledge. Successive stages in that developmental process are marked by an increase in articulation and specialization. And the entire process may have occurred, as we now suppose biological evolution did, without benefit of a set goal… . [1970, 172f]
At the time, it was striking that Kuhn compared revolutionary transitions, rather than normal scientific developments, with evolutionary change. It seems clear that he did not consider revolution and evolution to be mutually incompatible. But keep in mind that, for him, normal science represents periods of stasis, whereas revolutions are short, highly creative periods that more closely resemble the exploration by random trial and error (p. 87) that we associate with biological evolution. Examined on a minute time scale, however, normal science arguably also involves a (more constrained) variation and selection process, as scientific practitioners search for ways to articulate the paradigm. So, given the inevitability of evolution under such conditions, Kuhn’s treatment of normal science would appear to be too static. The important point here is that, in Kuhn’s view revolution and evolution are compatible when considered on the correct time scales (see Wray 2011 and Kuukkanen 2012). Examined from afar, revolutions are simply the more noteworthy episodes in the evolution of the sciences. Examined up close, they (like discoveries in general for Kuhn) have a detailed structure that is evolutionary, even something as revolutionary as the quantum theory (Kuhn 1978).
But how, then, the reader is entitled to ask, can Kuhn accommodate the sharp discontinuities that he advertised in chapter X of the book? We cannot equate revolutions simply with speciation in Darwin’s own sense, given that Darwin’s favorite mechanism of speciation was anagenesis, not cladogenesis—just the long-term gradual evolution within a single line rather than the splitting of lines. Interestingly, the later Kuhn will opt for cladogenesis.
As many commentators have pointed out, the theory of punctuated equilibrium of Niles Eldredge and Stephen Jay Gould (1992) raises the question of evolution versus revolution, now precisely in the biological (paleontological) context. In fact, Gould and Eldredge were themselves influenced by Kuhn, whom Gould once described as “a dear friend”; but they denied that they had deliberately fashioned themselves as Kuhnian revolutionaries (Gould 1997). Gould and Eldredge end their later review article on punctuated equilibrium by remarking:
[C]ontemporary science has massively substituted notions of indeterminacy, historical contingency, chaos and punctuation for previous convictions about gradual, progressive, predictable determinism. These transitions have occurred in field after field; Kuhn’s celebrated notion of scientific revolutions is, for example, a punctuation theory for the history of scientific ideas. [1993, 227]
Here, as with Kuhn, those who resist Gould’s attempt to sound so revolutionary as to be contrary to Darwin’s phyletic gradualism note that it is only on a geological timescale that such developments as the Cambrian explosion appear to be episodic. When examined on the timescale of the biological generations of the life forms in question, the development is evolutionary—more rapid evolution than during other periods, to be sure, but still evolutionary.
Stuart Kauffman (1993) and Brian Goodwin (1994) defended reorganization in the form of self-organization as the primary macro-biological mechanism, with evolutionary adaptation adding only the finishing touches. Gould and Richard Lewontin had raised this possibility in their famous paper of 1979, “The Spandrels of San Marco and the Panglossian Paradigm.” Applied to the development of science, this view implies that revolutions determine the overall shape, while ordinary scientific work applies the adaptive microevolution. Meanwhile, Michael Ruse (1989) defended the view that the Darwinian paradigm (with its emphasis on function and adaptation) and the punctuated equilibrium paradigm (with its emphasis on Germanic ideas of form and internal constraints) are complementary.
David Hull ended his book, Science as a Process (1988), with the remark that the book can be regarded as an attempt to fulfill both Kuhn’s and Toulmin’s ambitions to provide a evolutionary account of scientific development. Hull’s is the most thoroughgoing attempt to date to provide an evolutionary account of scientific practice, at least in a specific field. However, nothing like Kuhnian paradigms, normal science, and revolutions are to be found in Hull’s book, and this was deliberate on his part. Whereas Kuhn originally said that paradigms correspond one-to-one with scientific communities, Hull rejected Kuhn’s idea of a scientific community as too loose. A scientific community, he said, does not consist of people who merely happen to agree on certain things (anymore than the members of a species are individuals who happen to share a set of traits). Mere consensus is not enough. Rather, communities are tightly causally linked in the right sorts of ways, just as species are. There is no community of biologists or even of evolutionary biologists but only a patchwork of cliques. It is here, locally, that the seeds of innovation are sown, most of which are weeded out in a selective process by the larger group of specialists. Hull’s is a story of the socio-cultural evolution of science without revolution.
In “The Road Since Structure,” his 1990 Presidential Address to the Philosophy of Science Association, Kuhn reported on a book in progress, a project that would remain unfinished at his death. (See also Kuhn 1993.) In this and other fragments of that work, he develops the biological metaphor broached at the end of Structure . He retains his old parallel to biological evolution, that science progresses or evolves away from its previous forms rather than toward a final truth about the world; but he now extends the biological analogy by regarding scientific specialties themselves as akin to biological species that carve out research and teaching niches for themselves. In the process, he significantly modifies his conception of scientific revolutions and attendant claims concerning crises and incommensurable breaks. No longer do we hear of revolutions as paradigm change, certainly not in the sense of large paradigms. In fact, Kuhn prefers to speak of “developmental episodes” instead of revolutions. However, he does retain something of his original idea of small paradigms, the concrete problem solutions that he had termed “exemplars” in the “Postscript-1969” to Structure . Most revolutions, he tells us, are not major discontinuities in which a successor theory overturns and replaces its predecessor. Rather, they are like (allopatric) biological speciation, in which a group of organisms becomes reproductively isolated from the main population.
[R]evolutions, which produce new divisions between fields in scientific development, are much like episodes of speciation in biological evolution. The biological parallel to revolutionary change is not mutation, as I thought for many years, but speciation. And the problems presented by speciation (e.g., the difficulty in identifying an episode of speciation until some time after it has occurred, and the impossibility even then, of dating the time of its occurrence) are very similar to those presented by revolutionary change and by the emergence and individuation of new scientific specialties. … Though I greet the thought with mixed feelings, I am increasingly persuaded that the limited range of possible partners for fruitful intercourse is the essential precondition for what is known as progress in both biological development and the development of knowledge. When I suggested earlier that incommensurability, properly understood, could reveal the source of the cognitive bite and authority of the sciences, its role as an isolating mechanism was prerequisite to the topic I had principally in mind. … [Kuhn 2000, 98–99]
In short, specialization is speciation, a scientific progress heightens communication breakdown. Experts doing similar kinds of research come to realize that their use of key taxonomic terms no longer jibes with mainline usage, in the sense that what Kuhn calls “the no overlap principle” is violated: the group is using a taxonomic hierarchy for crucial kind terms and the associated categories that is incompatible with that of the established tradition. The group splits off and forms a distinct specialty with its own professional journals, conferences, etc., while leaving the rest of the field largely intact. The incommensurability is now a local, community-licensed, taxonomic one that creates something of a barrier to communication with neighboring specialties. One thinks, for example, of the way different biological specialties employ the species concept itself, and the concept of gene. This linguistic sensitivity as a group identifier permits the kind of fullness of communication, both linguistic and practical, within the group that Kuhn had stressed already in Structure and thus permits the group to progress more rapidly. This multiplication of specialties is the key to Kuhn’s new conception of scientific progress. Two recent books that directly engage these issues are Andersen et al. (2006) and Wray (2011), the first from a cognitive science point of view, the second with emphasis on Kuhn’s social epistemology. See also Nersessian (2003, 2008) and Kuukkanen (2012).
Another striking fact about Kuhn’s last project is the demotion to “a historical perspective” of the history of science as a detailed source of data or phenomena upon which philosophers of science should draw. While Structure was already a curious mix of an inductive, history-based, bottom-up approach to modeling scientific development and a more formal, top-down approach based on Kuhn’s Kantianism (see below), he now concludes that his core position follows from first principles alone.
With much reluctance I have increasingly come to feel that this process of specialization, with its consequent limitation on communication and community, is inescapable, a consequence of first principles. Specialization and the narrowing of the range of expertise now look to me like the necessary price of increasingly powerful cognitive tools. [2000, p. 98]
Thus Kuhn’s new emphasis is on synchronic revolutions, in which a field splits into subfields, rather than on the diachronic replacement of one paradigm complex by another that his early account featured. His conception of a science is therefore less monolithic. A vibrant field such as evolutionary biology can tolerate several distinct species concepts at the same time, a fact that contributes rather than detracts from its vibrancy. The overall result is a less tightly integrated, less dogmatic conception of normal science under an overarching paradigm, a view that has implications also for the necessity and size of future revolutions. For no longer need an esoteric discrepancy get the leverage to trigger a crisis that eventuates in the replacement of an entire, tightly integrated system. In this respect, Kuhn’s conception of physics becomes somewhat closer to Godfrey-Smith’s characterization of progress in biology as “a deluge” rather than a full-scale Kuhnian revolution (see below and Godfrey-Smith 2007). Given that progress in biological evolution is better regarded as the remarkable proliferation of intricate, useful design rather than movement toward a goal, the explicit parallels that Kuhn draws to biological evolution suggest that he is moving toward the same conception of scientific progress as some see in biological evolution—as the proliferation of adaptive design. We may know more about his final position once more of the book manuscript, left incomplete at his death, is published.
5. Larger Formations and Historical A Prioris: The Germanic and French Traditions
We pass now from the smaller-scale revolutions of Kuhn’s later work to large-scale movements that, in several cases, exceed the bounds of the early Kuhnian paradigms and the revolutionary transformations linking them. Other thinkers have gone even further than Kuhn, by positing the existence of cognitive formations that are both broader and deeper than his. One prominent line of thought here is the neo-Kantian one up through Reichenbach and Carnap, discussed and further developed by Michael Friedman (2001, 2003). Another, not entirely distinct, idea is that of a thought style or discursive formation found variously in such writers as Ludwik Fleck (1935), Alistair Crombie (1994), Michel Foucault (1969), and Ian Hacking (1990, 2002, 2012). Especially in the accounts of Foucault and Hacking, new conceptual spaces are constructed and may “crystallize” (Hacking) rapidly. Once they become canonical, they seem to be such obvious frameworks for making true or false claims that the corresponding categories of thought and action appear to be given as part of the nature of things, as written in the language of nature, so to speak, when they are in fact a product of the cultural conditioning of our socio-cognitive systems. In the limit we project our deeply ingrained cultural categories not only onto our world as we encounter it but also onto all (historically) conceivable worlds. The historical change in question, once called to our attention, seems revolutionary—in a manner that is both broader and deeper than the transition to a new paradigm within a particular scientific specialty. Once again, the magnitude of the change is practically invisible to all but the most sensitive archeologist of knowledge. Feyerabend was alive to this perspective in his work on Galileo. But, unlike his treatment of the Copernican Revolution (Kuhn 1957), Kuhn’s revolutions in Structure and beyond are more limited in scope, typically occurring more or less wholly within a single discipline. Nor is it obvious that the emergence of a new thought style must overturn a distinct predecessor. Of course, we should not regard social constructionist / deconstructionist projects (whether or not deliberately designed) as, automatically, ongoing, enlightened processes that “unfreeze” the stones inherited from the past; for it was these very processes that created essentialist constructions in the first place. The claim is that our constructions today are no different.
The four main characteristics of Hacking’s “big revolutions” are that they lead to whole new interdisciplinary complexes, replete with new social institutions and corresponding social changes, and they alter that period’s overall take on, or “feel” for, the universe. For critical discussion of Hacking on styles of reasoning, see Kusch (2010) and Scortino (2016). For more on Hacking, see section 5.3 below.
Kuhn several times described himself as “a Kantian with moveable categories.” Hoyningen-Huene (1993) provides a broadly Kantian interpretation of Kuhn (endorsed by Kuhn himself), as does Friedman (2001, 2003, 2010). Given the historical approach of Structure , other commentators have likened Kuhn to Hegel instead of Kant. And given the early Kuhn’s view that scientific reason is manifested more clearly in historical change as well as in normal scientific practices than in symbolic logical structures, Kuhn’s early theory of scientific change can be termed very broadly Hegelian. Also fitting the broadly Hegelian frame is Kuhn’s internalist account of normal scientific research as sowing the seeds of its own destruction through unintended innovation, resulting eventually in a kind of dialectical conflict that drives the enterprise forward. However, there is no Hegelian Spirit lurking within Kuhn’s model, nor a permanent logic of science as its replacement as there was for Lakatos (Lakatos 1970; Hoyningen-Hühne 1993; Bird 2008; Worrall 2003, 2008; Nickles 2009).
Kuhn disliked being compared to Hegel, whose work he found obscure and characterized by a non-naturalistic philosophy of history, but it is worth commenting further on the partial resemblance. Both Kant and Hegel rejected naïve empiricism, according to which all human knowledge arises, somehow, from the accumulative aggregation of individual sensations or perceptions. Kant argued that we need transcendental structures such as a system of processing rules in order to organize sensory input into something coherent and intelligible, e.g., as physical objects interacting causally in space and time. These were Kant’s forms of intuition (in space and time) and categories (substance, causality, etc.). They represent the human mind’s contribution to knowledge. (In this regard Kant can be regarded as a forerunner of cognitive psychology.) Thus, our experience of the world is shaped to fit a priori forms, and this is where the Kantian version of idealism enters the picture: the world of human experience is not the world of ultimate reality (Kant’s unknowable, noumenal world of things-in-themselves); rather, it is a world shaped by our own cognitive structures.
Hegel, one of the founders of the deep conception of historical change broadly characteristic of nineteenth-century German scholarship, proceeded to historicize Kant’s innovation, in effect by historicizing Kant’s categories. They are not inborn, permanent, and universal; on the contrary, they are socio-historically acquired or lost and hence differ from one historical epoch to another. People living in different epochs cognize the world differently. It is tempting to read the Kuhn of Structure as further relativizing and localizing Hegel to specific scientific domains and their paradigms. Kuhn’s model provides endogenous, dynamic mechanisms of (inadvertent) scientific innovations that sow the seeds of the paradigm’s eventual destruction in a vaguely Hegelian sort of dialectical process. It thus becomes possible to experience a change in categorical scheme within one’s own lifetime—the victory of the new paradigm being the small-scale scientific counterpart of Hegel watching Napoleon march in victory through the streets of Jena! Thus it is tempting to regard Kuhnian revolutions as Hegelian revolutions writ small.
Nonetheless, in terms of historical genealogy, Kuhn is better aligned with the Kantian tradition, especially the neo-Kantian relativization of Kant. Interestingly, some logical empiricists (especially Reichenbach) were influenced by the neo-Kantianism of the German Marburg School of philosophy to develop a historically relativized but constitutive a priori (see below and Friedman 2001.)
There is a long historical gap between Kant/Hegel and Kuhn, and this space is not empty. Many other thinkers, especially those in the various nineteenth-century idealist traditions, were Kantian or Hegelian or neo-Hegelian or neo-Kantian opponents of empiricist positions that they considered naïve, such as that of John Stuart Mill. The neo-Kantian label applies even to prominent logical positivists of the Vienna Circle and logical empiricists of the Berlin Circle, who have too often been caricatured as simple, cumulative empiricists. As Friedman (2001) and others have shown, several founders of twentieth-century academic philosophy of science extended the neo-Kantian attack on simple empiricism. The basic idea here is that, just as Kant regarded any account of perception and knowledge as naïvely empiricist insofar as it left no room for underlying cognitive organizing principles, so any account of the sciences that provided no analogous underlying social-cognitive framework was a continuation of simple empiricism, i.e., a version of “positivism.” In this particular respect, W. V. Quine’s “Two Dogmas of Empiricism” and Word and Object (Quine 1951, 1960) were throwbacks to simple empiricism in their attempt to eliminate the Kantian formal component.
The German Marburg School of Hermann Cohen, Paul Natorp, and Ernst Cassirer was especially important in the emergence of modern philosophy of science in the form of the logical positivism and logical empiricism. Friedman (2001 and elsewhere) has explored its influence on young Reichenbach’s attempt to interpret the significance of the new relativity theory. Rudolf Carnap had been influenced by Ernst Cassirer, among others. (See the entries on logical empiricism, Reichenbach, Carnap, Cohen, Natorp, and Cassirer.) Cassirer’s central theme was the fundamental epistemological implications of the replacement of Aristotle’s subject-predicate logic and substance ontology by the new relational logic of the modern, mathematical-functional approach to nature. This could not be a priori in Kant’s original sense, since the emergence of non-Euclidean geometry had shown that there are alternative organizing principles. But the very fact that we still needed organizing structures that are constitutive or definitive of the cognitive enterprise in question meant that Kant was still basically correct. Like Moritz Schlick, the first leader of the Vienna Circle, Reichenbach of the Berlin school parlayed his engagement with relativity theory and non-Euclidean geometries into a conception of the “relativized a priori.” All of this by around 1920, with the younger Carnap’s views developing during the 1920s and ‘30s. In the USA, meanwhile, C. I. Lewis (1929) was defending his “pragmatic a priori.”
In his famous paper of 1950, “Empiricism, Semantics, and Ontology,” Carnap made his two-tiered view of inquiry quite explicit. Starting from the problem of the existence of abstract entities, Carnap distinguished internal questions, that is, questions that can arise and be answered within a particular logico-linguistic framework, from external questions, that is, meta-level questions about which framework to prefer. External questions cannot be answered in the same, disciplined manner as internal, for choice of framework is ultimately a pragmatic decision based on the expected fertility of using one framework rather than another. Although it is difficult to equate Carnap’s fruitfulness decisions with Kuhn’s revolutionary breaks (Kuhn 1993, 313f), Carnap regarded his vision of science as similar to Kuhn’s, and he liked the manuscript of Structure that Kuhn submitted to the International Encyclopedia of Unified Science , as George Reisch (1991) has shown. Thus the clash of Kuhn’s work with that of Carnap and the positivist movement has been exaggerated.
Although both defended two-tiered conceptions of inquiry, there are important differences between Kuhn and Carnap (as Friedman, 2001, 2003, 2010, among others, observes). For Carnap, as for Reichenbach, the choice of framework or coordinating definitions was conventional, a matter of convenience or heuristic fertility, whereas for committed Kuhnian normal scientists the foundational tenets of their paradigm are deep truths about the world, principles not subject to empirical test. (However, in a crisis situation, fertility becomes a key element in theory and paradigm choice.) In Carnap’s frameworks these were explicit systems of logical rules, whereas Kuhn’s account of normal science largely jettisoned rule-based knowledge in favor of a kind of case-based tacit knowledge, the cases being the concrete exemplars. Third, Kuhn himself emphasized that his approach was historical, whereas Carnap’s was not. Although a Carnapian change from one logical framework to another could, in principle, be quite revolutionary, Carnap himself never emphasized this point, suggested nothing of Kuhn’s radical discontinuity, and was simply not interested in the history of science.
Meanwhile, Friedman himself has extensively developed the idea of historically contingent but constitutive a prioris (e.g., 2001, 2003, 2008). He is sympathetic to Kuhn’s view that revolutions occur when the constitutive principles change. From the old point of view, there is disruptive and incommensurability, but defenders of the new viewpoint manages to establish a kind of continuity. Friedman goes well beyond Kuhn in stressing the role of philosophical ideas in establishing this continuity.
Since deep conceptual revolutions or paradigm-shifts are a fact of scientific life (and, I would argue, a necessity), we are never in a position to make our present constitutive principles as truly universal principles of human reason–as fixed once and for all throughout the evolution of science.
Another development that appeals to Germanic themes in its criticism of naive empiricism is the idealization movement of the Poznań School in Poland, associated especially with Leszek Nowak. This group regards science as developing in an idealizational and dialectical manner, ideas that they trace back to Karl Marx’s analysis of economics, inspired by his own study of Galileo’s fruitful use of abstract, idealized models against the Aristotelians. The Poznań group regards idealization as the secret to modern science and finds it remarkable that virtually all previous analytic philosophy of science remains Aristotelian in treating proposed laws and theories not as ideal models but as true or false statements directly about the real world (Nowak 1980 and later writings; see also Krajewsky 1977). As models, these constructions must be concretized to some degree before they can be applied to the real world. While the idealizationists tend to reject Kuhnian revolutions as too discontinuous and irrational, they do see a resemblance to their internalistic, dialectical conception of scientific development. For them a revolution consists in a new theory or model that reveals a previously unnoticed idealizing assumption built into its predecessor, a change that alters scientists’ conception of what is essential versus peripheral to the domain of phenomena in question. Hence there can be a significant change of world-conception.
There is some affiliation of Poznań with the European structuralist account of theories, based on a set-theoretical analysis of theory structure and theory relations. Kuhn himself was much attracted to Joseph Sneed’s approach (Sneed 1971), soon extended by Wolfgang Stegmüller (1974/1976) and others. Given the informality of Kuhn’s own approach and his explicit shunning of rules and rational reconstructions, his attraction to the structuralist line was initially puzzling. However, the structuralists were and are interested in intertheory relations, and models are central to their non-sentential conception of theories. These are models in the formal sense, but Kuhn found insightful connections to his own use of models in the form of exemplars. For both Kuhn and the structuralists it is the collection of exemplars or models, not an abstract statement of a theory, that carries the weight in scientific inquiry.
Already the early Kuhn, especially in the postscript to the second edition of Structure , largely abandoned the traditional conception of theories as deductive systems, even in physics, and substituted informal collections of models of various, exemplary kinds, along with a toolbox of expert practices for constructing and applying them (Cartwright 1983, Giere 1988, Teller 2008). He always liked Margaret Masterson’s remark that “a paradigm [in the sense of preferred models or exemplars] is what you use when the theory is not there” (Baltas et al. 2000, 300). Such a view, like Nowak’s, anticipates the move from theory-centered to model-centered accounts of scientific work. However, Kuhn’s normal scientific practitioners presumably hold the models to be true in their original application, as is the grand theory incorporated in the paradigm, whereas today the emphasis is on modeling practices across the sciences, in which the models are almost always known in advance to be false because of their employment of idealizations, approximations, abstractions, etc.
Meanwhile, important French thinkers had already taken a historical approach, one that explicitly characterizes science as a series of breaks or coupures . The principal genealogy includes Léon Brunschvicg, Gaston Bachelard and his student, Georges Canguilhem, and the latter’s student, Michel Foucault. Neither Kuhn’s historicism nor his talk of revolutionary breaks was news to the French (Gutting, 2001, 2003). The French tradition of science studies, going back to Auguste Comte and including later figures such as Pierre Duhem and Henri Poincaré, possessed a historical dimension that positivism lost after Ernst Mach, as it became logical positivism. However, the French and Germanic traditions have some roots in common. As Gutting points out, Brunschvicg, like Émile Meyerson, was a science-oriented idealist. For him the mind is not a passive wax tablet; rather, it actively forges internal links among ideas, yet it is also often surprised by the resistant exteriority of the natural world. Against traditional metaphysics, philosophy of science should limit itself to what the science of the time allows—but not dogmatically so. For dogmatic attempts to extract timeless principles and limitations (such as Kant’s denial of the possibility of non-Euclidean geometry) may soon be embarrassed by further scientific advances. Einstein’s general theory of relativity exemplifies the revolutionary nature of the most impressive developments.
Bachelard, French physicist and philosopher-historian of science, also believed that only by studying history of science can we gain an adequate understanding of human reason. He stressed the importance of epistemological breaks or discontinuities ( coupures épistémologiques ). In Le Nouvel Esprit Scientifique (1934), Bachelard argued that the worldview of classical physics, valuable in its own time, eventually became an obstacle to future progress in physics. Hence a break was needed. Here, then, we already find the idea that a successful theory can lose its luster by being considered exhausted of its resources and thus lacking in fertility. Like Brunschvicg, Bachelard held that a defensible, realist philosophy had to be based on the science of its day. Hence, scientific revolutions have (and ought to have) brought about epistemological revolutions. The reality we posit, he said, ought to be that of the best science but with the realization that our concepts are active constructs of our own minds, not imported from nature’s own language, as it were. Future mental activity as well as future empirical findings are likely to require another rupture. As Gutting points out, Bachelard’s account of discontinuity was not as radical as Kuhn’s. Bachelard was willing to speak of progress toward the truth. He made much of the fact that successor frameworks, such as non-Euclidean geometry or quantum physics, retain key predecessor results as special cases and, in effect, contextualize them.
Canguilhem was more interested in the biological and health sciences than Bachelard and gave great attention to the distinction between the normal and the pathological, a distinction that does not arise in physical science. For this and other reasons, in his view, we can expect no reduction of biology to physics. Canguilhem provided a more nuanced conception of obstacles and ruptures, noting, for example, that an approach such as vitalism that constitutes an obstacle in one domain of research can simultaneously play a positive role elsewhere, as in helping biological scientists to resist reductive thinking. Here we find context sensitivities and heuristic resources difficult to capture in terms of a context- and content-neutral logic of science such as the logical empiricists espoused.
Bachelard and Canguilhem also had less disruptive conceptions of scientific objectivity and scientific closure than Kuhn. Canguilhem criticized Kuhn’s (alleged) view that rational closure could not amount to more than group consensus. Both Frenchmen emphasized the importance of norms and denied that disciplinary agreement was as weak as Kuhnian consensus. Kuhn replied to this sort of objection (in “Postscript” and elsewhere) that his scientific communities do possess shared values, that their agreement is not something arbitrary, say, as whipped up by political ideologues.
Foucault’s archaeology of knowledge (Foucault 1966, 1969) posits a distinction between a superstructure of deliberately made observations, claims, and arguments and a deep structure, most elements of which we are probably unconscious. Once again we meet a two-level account. Writes Hacking:
Foucault used the French word connaissance to stand for such items of surface knowledge while savoir meant more than science; it was a frame, postulated by Foucault, within which surface hypotheses got their sense. Savoir is not knowledge in the sense of a bunch of solid propositions. This “depth” knowledge is more like a postulated set of rules that determine what kinds of sentences are going to count as true or false in some domain. The kinds of things to be said about the brain in 1780 are not the kinds of things to be said a quarter-century later. That is not because we have different beliefs about brains, but because “brain” denotes a new kind of object in the later discourse, and occurs in different sorts of sentences. [2002, 77]
Given the influence of Foucault, we may also locate our discussion of Hacking’s own work on historical ontology here (Hacking 2002). Hacking (1975, 1990, 1995, 2012) has studied in depth the emergence of probability theory and (later) of statistical thinking and the construction of the modern self as key examples of what he terms “historical ontology.” He acknowledges inspiration from both Foucault’s discursive formations and Crombie’s styles of thinking (Crombie 1994), with a dose of Feyerabend thrown into the mix. Like Kuhn (and Friedman), Hacking returns to Kant’s “how possible?” question, the answer to which sets out the necessary conditions for a logical space of reasons in which practitioners can make true or false claims about objects and pose research questions about them. Hacking, too, historicizes the Kantian conception. He likes the term ‘historical a priori’, which Canguilhem once applied to the work of his erstwhile student, Foucault.
The historical a priori points at conditions whose dominion is as inexorable, there and then, as Kant’s synthetic a priori . Yet they are at the same time conditioned and formed in history, and can be uprooted by later, radical, historical transformations. T. S. Kuhn’s paradigms have some of the character of a historical a priori . [Hacking 2002, 5] … [S]cientific styles of thinking & doing are not good because they find out the truth. They have become part of our standards for what it is, to find out the truth. They establish criteria of truthfulness. … Scientific reason, as manifested in Crombie’s six genres of inquiry, has no foundation. The styles are how we reason in the sciences. To say that these styles of thinking & doing are self-authenticating is to say that they are autonomous: they do not answer to some other, higher, or deeper, standard of truth and reason than their own. To repeat: No foundation. The style does not answer to some external canon of truth independent of itself. [2012, 605; Hacking’s emphasis]
Hacking describes changes in historical a prioris as “significant singularities during which the coordinates of ‘scientific objectivity’ are rearranged” (2002, 6).
Although reminiscent of Kuhn’s positions in some ways, there are striking differences. As noted above, Hacking’s constructed formations are much broader than Kuhn’s. Thus he feels free to employ telling bits of popular culture in laying out his claims, and he admits to being whiggish in starting from the present and working backward to find out how we got here. Moreover, in mature, modern science, unlike Kuhnian paradigms, several of Hacking’s styles of thinking and doing can exist side by side, e.g., the laboratory and hypothetical modeling traditions. Yet people living before and after the historical crystallization of a style would find each other mutually unintelligible. Hacking recognizes that Kuhnian problems of relativism (rather than subjectivism) lurk in such positions. “Just as statistical reasons had no force for the Greeks, so one imagines a people for whom none of our reasons for belief have force” (2002, 163). This sort of incommensurability is closer to Feyerabend’s extreme cases (as in the ancient Greek astronomers versus their Homeric predecessors) than to Kuhn’s “no common measure” (2002, chap. 11). This sort of unintelligibility runs deeper than a Kuhnian translation failure. It is not a question of determining which old style statements match presumed new style truths; rather, it is a question of the conditions for an utterance to make a claim that is either true or false at all. Writes Hacking,
Many of the recent but already classical philosophical discussions of such topics as incommensurability, indeterminacy of translation, and conceptual schemes seem to discuss truth where they ought to be considering truth-or-falsehood. [2002, 160]
By contrast, Kuhnian paradigms include a set of positive assertions about the world. Yet Kuhn himself was attracted by Hacking’s way of putting the point about truth-and-falsity (Kuhn 2000, p. 99).
To what extent was Kuhn indebted to these thinkers? As noted above, he took Kant but not Hegel very seriously. He was largely self-taught in philosophy of science. Among his contemporaries, he was familiar with Popper but not in any detail with the various strains of logical positivism and logical empiricism, in particular the positions of Carnap and Reichenbach. Apparently, he was only slightly acquainted with the work of Bachelard while writing Structure , and they never engaged in a fruitful interchange (Baltas et al. 2000, 284f). Kuhn did acknowledge, in print and in his classes, the crucial influence on his historical and philosophical thinking of the two Russian émigrés, Émile Meyerson, author of Identité et Realité (1908) and Alexandre Koyré, especially his Études Galiléenes (1939), and that of Annaliese Maier, the German historian of medieval and early modern science. He had read Ludwik Fleck’s Genesis and Development of a Scientific Fact (originally published in German in 1935) and Michael Polanyi’s Personal Knowledge (1958) and had had some discussion with Polanyi (Baltas et al. 2000, 296). Kuhn was also indebted to Wittgenstein, early (“The limits of my language are the limits of my world,” 1922, 148) and late (on language games and forms of life). (See Sharrock and Read 2002, the introduction to Harris 2005, and Kindi 2010 for Kuhn’s relation to Wittgenstein and others.) He knew something of Toulmin’s work.
Kuhn more than anyone in the Anglo-American world pointed out the need for larger-sized units than individual theories in making sense of modern science. Nonetheless, as we have seen, others in the Teutonic and Francophone worlds had previously postulated even larger socio-intellectual units and correspondingly deeper changes than Kuhn’s, on somewhat different scales of intellectual space and time. If we think of authors such as the Annales historian Fernand Braudel, with his distinct time-scales, we recognize that the attribution of transformative change clearly depends heavily on the choice of time-scale and on how fine- or course-grained is our approach. Hacking (2002, 76) makes this point with reference to the French context:
There are two extremes in French historiography. The Annales school went in for long-term continuities or slow transitions—“the great silent motionless bases that traditional history has covered with a thick layer of events” (to quote from the first page of Foucault’s 1969 Archeology of Knowledge ). Foucault takes the opposite tack, inherited from Gaston Bachelard, Georges Canguilhem, and Louis Althusser. He posits sharp discontinuities in the history of knowledge.
Although Kuhn emphasized the importance of skilled scientific practice, his paradigms remained closer to the articulate surface of scientific culture than Foucault’s discursive formations, which are better located in the unconscious than in the Kuhnian subconscious. Foucault does not speak of revolution.
6. Other Revolution Claims and Examples
Oliver Wendell Holmes, Jr. (1861) remarked that “Revolutions never follow precedents nor furnish them.” Given the unpredictability, the nonlinearity, the seeming uniqueness of revolutions, whether political or scientific, it is therefore surprising to find Thomas Kuhn attempting to provide a General Theory of Scientific Revolutions (Kindi 2005). Early Kuhn did seem to believe that there is a single, underlying pattern to the development of mature sciences that is key to their success, and late Kuhn a different pattern. Has either early or late Kuhn found such a pattern, or has he imposed his own philosophical structure on the vagaries and vicissitudes of history? Kuhn’s Kantianism always did live in tension with his historicism, and in his late work (e.g., 2000c) he surprisingly gave up the pretense of deriving his pattern of taxonomic change and speciation from history of science, on the ground that it largely followed “from first principles.”
Numerous philosophers, scientists, and other commentators have made claims about scientific change that differ from Kuhn’s. (For a recent selection see Soler et al. 2008.) Some, as we have seen, are skeptical of revolution talk altogether, others of Kuhn’s in particular. Still others accept that some revolutions are Kuhnian but deny that all of them are. One common criticism is that not all revolutionary advances are preceded by an acute crisis, that is, by major failures of preceding research. Kuhn himself allowed for exceptions already in Structure . Another is that revolutionary changes need not involve discontinuities in all of Kuhn’s levels at once (especially Laudan 1984). Yet another is that there need be little logical or linguistic discontinuity. A rapid, seemingly transformative change in research practices may involve simply a marked gain in data accessibility or accuracy or computational processing ability via new instrumentation or experimental design. And on the later Kuhn’s own view, revolution need not be a game of creative destruction. Only a few examples can be considered here.
Do revolutions consist, according to Kuhn, of major new materials (experimental facts, theories, models, instruments, techniques) entering a scientific domain or, instead, of a major restructuring or rearrangement of materials, practices, and community affiliations already present? Kuhn states that the relativity revolution might serve as
a prototype for revolutionary reorientation in the sciences. Just because it did not involve the introduction of additional objects or concepts, the transition from Newtonian to Einsteinian mechanics illustrates with particular clarity the scientific revolution as a displacement of the conceptual network through which scientists view the world. [1970, 102]
The reader may find this claim confusing, however, because in the just-preceding paragraphs Kuhn had emphasized the ontological and conceptual changes of precisely this revolution, e.g., the radical change in the concept of mass. Einstein’s masses are not Newtonian masses, he insisted. They are newly introduced entities; hence, we may infer, new content. Yet Kuhn surely does have a point worth saving, in that relativity theory still deals with most of the same kinds of phenomena and problems as classical mechanics and employs immediate successors to the classical concepts. But, if so, then reorganization of familiar materials implies a disciplinary continuity through revolution that Kuhn minimized.
That reorganization dominates Kuhn’s conception of revolutions is apparent throughout his work. As a young scholar he had an epiphany when Aristotle’s seemingly radically false or unintelligible claims suddenly came together for him as a coherent, comprehensive worldview. This experience became Kuhn’s psychological model for revolutionary transformation from one paradigm to its successor and informed his later talk of Gestalt switches. But he also emphasized that revolution involves social reorganization of the field (not merely the cognitive reorganization of an individual), from one form of scientific life to another, incompatible with it. By implication, his structural or formal conception of revolution excluded the alternative idea of revolution as extraordinary bursts in substantive content.
In Conceptual Revolutions , Paul Thagard (1992) retains something of Kuhn’s idea of conceptual transformation and the more specific idea of taxonomic transformation. He distinguishes two kinds of reclassification, in terms of the language of tree structures used in computer science: branch jumping and tree switching. Branch jumping reclassifies or relocates something to another branch of the same tree, e.g., reclassifying the whale as a mammal rather than a fish, the earth as a planet, or Brownian motion as a physical rather than a biological phenomenon. New branches can appear and old branches can be eliminated. Meanwhile, tree switching replaces an entire classification tree by a different tree structure based on different principles of classification, as when Darwin replaced the static classification tree of Linnaeus by one based on evolutionary genealogy and when Mendeleev replaced alternative classification systems of the chemical elements by his own table. Taking a computational approach to philosophy of science, Thagard employs his computer program ECHO to reconstruct and evaluate several historical cases of alleged conceptual revolution and arrives at a tamer conception of revolutionary breaks than Kuhn’s.
The Cognitive Structure of Scientific Revolutions by Hanne Andersen, Peter Barker, and Xiang Chen (2006) also devotes a good deal of attention to cognition and categorization issues, in a defense of the later Kuhn’s approach. The work of cognitive psychologist Lawrence Barsalou and of philosopher-historian Nancy Nersessian (the founder of the “cognitive historical” approach to science) plays a significant role in their account. Nersessian herself (2003, 2008) emphasizes model-based reasoning. These are no longer static cases or exemplars, for they possess an internal dynamic.
Howard Margolis (1993) distinguishes two kinds of revolutions, depending on which kinds of problems they solve. Those revolutions that bridge gaps, he contends, differ from those that surmount or penetrate or somehow evade barriers. His focus is on barriers, a neglected topic even though it fits Kuhn’s account of cognition well. Margolis develops Kuhnian themes in terms of deeply ingrained “habits of mind.” While such habits are necessary for efficient scientific work within any specialty discipline, they constitute barriers to alternative conceptions. More broadly, deeply ingrained cultural habits of mind can close off opportunities that, according to the perspective of later generations, were staring them in the face. Margolis is struck by the apparent fact that all the materials for Copernicus’ new model of the solar system had been available, in scattered form, for centuries. No new gap-crossing developments were needed. He concludes that, rather than a gap to be bridged, the problem was a cognitive barrier that needed to be removed, a barrier that blocked expert mathematical astronomers from bringing together, as mutually relevant, what turned out to be the crucial premises, and then linking them in the tight way that Copernicus did. If Margolis’ account of the Copernican Revolution is correct, it provides an example of revolution as holistic reorganization of available materials, hence the non-piecemeal, noncumulative nature of revolutions. The developments that lead to a barrier’s removal can be minor and, as in the case of Copernicus, even quite peripheral to the primary subject matter that they ultimately help to transform. Here one thinks of a model popular with mystery writers, where an everyday observation leads to a sudden change in perspective.
Davis Baird (2004) contends that there can be revolutions in practice that are not conceptual revolutions. He emphasizes the knowledge embodied in skills and in instruments themselves. His central example is analytic chemistry.
There is little doubt that analytical chemistry has undergone a radical change. The practice of the analyst, who now deals with large, expensive equipment, is different than it was in 1930. Modern instrumental methods are by and large more sensitive and accurate, have lower limits of detection, and require smaller samples; different kinds of analyses can be performed. Analytical chemistry is much less a science of chemical separations and much more a science of determining and deploying the physical properties of substances. … The revolutionary phase of Thomas S. Kuhn’s Structure of Scientific Revolutions starts with a crisis, a problem that the established methods of normal science cannot solve (Kuhn [1962] 1970; 1996, ch. 5). There was no such crisis in analytical chemistry. While one might imagine that analytical chemistry underwent a change of paradigm, there was no crisis that provoked this change. Pre-1930 analytical chemists did not bemoan the inability of their chemistry to solve certain problems. Instead, new methods were developed that could solve established “solved” problems, but solve them better: more efficiently, with smaller samples, greater sensitivity, and lower limits of detection. These changes in analytical chemistry do not suffer from any kind of incommensurability: today, one can easily enough understand what analytical chemists were doing in 1900–although the idea that the analytical chemist is one who can quantitatively manufacture pure chemicals is startling on first encounter. … The transformation in analytical chemistry passes all of Cohen’s tests.
Recently, Rogier De Langhe (2012, 2014a and b, 2017) has been developing a broadly Kuhnian, two-process account of science from an economics standpoint. Instead of doing a series of historical cases, De Langhe and colleagues are developing algorithms to detect subtle patterns in the large citation databases now available. De Langhe employs economic arguments to illuminate such themes as the division of cognitive labor, models of scientific progress, and scientists’ decisions about whether to specialize or to innovate.
The account of the dynamics of science in Structure ill fit the rapid splitting and recombining of fields in the post-World War II era of Big Science, as Kuhn recognized. So he excluded from his account the division and recombination of already mature fields such as happened with the emergence of biochemistry. (This exclusion is troubling, given the universal thrust of his account. It is as if Kuhn admitted that his account applies only to a particular historical period that is now largely past; yet he also wrote as if the normal-revolutionary model would apply to mature disciplines into the long future.) In his later work he did devote careful attention to the division of fields into specialties and subspecialties (see §5). However, he still gave little attention to the more-or-less reverse process of new fields coming into existence by combinations of previously distinct fields as well as to cross- and trans-disciplinary research, in which a variety of different specialists somehow succeed in working together (Galison 1997, Kellert 2008, Andersen 2013).
And what can we make, on Kuhn’s account, of the explosion of work in molecular biology following the Watson-Crick discovery, in 1953, of the chemical structure of DNA and the development of better laboratory equipment and techniques? Molecular genetics quickly grew into the very general field of molecular biology. Less than two decades after Watson and Crick, Gunther Stent could already write in his 1971 textbook:
How times have changed! Molecular genetics has … grown from the esoteric specialty of a small, tightly knit vanguard into an elephantine academic discipline whose basic doctrines today form part of the primary school science curriculum. [Stent 1971, ix]
There is something paradigmatic about molecular biology and also something revolutionary about its rapid progress and expansion. It is not clear how to characterize this and similar developments. Was this a Kuhnian revolution? It did involve major social and intellectual reorganization, one that conflicted with the previous ones in some respects but without undermining the Darwinian paradigm. Quite the contrary. Or is molecular biology more like a style of scientific practice than a paradigm? Such an explosive development as molecular biology hardly fits Kuhn’s description of steady, normal scientific articulation of the new paradigm by puzzle solving. Instead, it seems better to regard it as a large toolkit of methods or techniques applicable to several specialty fields rather than as an integrative theory-framework within one field.
Should we then focus on practices rather than on integrative theories in our interpretation of Kuhnian paradigms? The trouble with this move is that practices can also change so rapidly that it is tempting to speak of revolutionary transformations of scientific work even though there is little change in the overarching theoretical framework (see Part II of Soler et al. 2008). Moreover, as Baird (2004) points out, the rapid replacement of old practices by new is often a product of efficiency rather than intellectual incompatibility. Why continue to do gene sequencing by hand when automated processing in now available? Replacement can also be a product of change in research style, given that, as Kuhn already recognized, scientific communities are cultural communities.
Similar points can be made about the rise of statistical physics, mentioned above in relation to Hacking’s work. (See also Brush 1983 and Porter 1986.) This was an explosion of work within the classical mechanical paradigm rather than a slow, puzzle-by-puzzle articulation of precisely that paradigm in its own previous terms. Or was it? For Kuhn himself recognized that modern mathematical physics only came into existence starting around 1850 and that Maxwellian electrodynamics was a major departure from the strictly Newtonian paradigm. In any case, there was much resistance among physicists to the new style of reasoning. The kinetic theory of gases quickly grew into statistical mechanics, which leapt the boundaries of its initial specialty field. New genres as well as new styles of mathematical-physical thinking quickly replaced old—and displaced the old generation of practitioners. Yet on Kuhn’s official theory of science it was all just “classical mechanics.”
Furthermore, the biological and chemical sciences do not readily invite a Kuhnian analysis, given the usual, theory-centered interpretation of Kuhn. For biological fields rarely produce lawful theories of the kind supposedly found in physics. Indeed, it is controversial whether there exist distinctly biological laws at all. And yet the biological sciences have advanced so rapidly that their development cries out for the label ‘revolutionary’.
What of the emerging field of evolutionary-developmental biology (evo-devo)? It is too soon to know whether future work in this accelerating field will merely complete evolutionary biology rather than displacing it. It does seem unlikely that it will amount to a complete, revolutionary overturning of the Darwinian paradigm. (Kuhn might reply that the discovery of homeobox genes overturned a smaller paradigm based on the expectation that the genetic makeup of different orders of organisms would have little in common at the relevant level of description.) And if it complements the Darwinian paradigm, then evo-devo is, again, surely too big and too rapidly advancing to be considered a mere, piecemeal, puzzle-solving articulation of that paradigm. Based on work to date, evo-devo biologist Sean B. Carroll, for example, holds precisely the complement view—complementary yet revolutionary:
Evo-Devo constitutes the third major act in a continuing evolutionary synthesis. Evo-Devo has not just provided a critical missing piece of the Modern Synthesis—embryology—and integrated it with molecular genetics and traditional elements such as paleontology. The wholly unexpected nature of some of its key discoveries and the unprecedented quality and depth of evidence it has provided toward settling previously unresolved questions bestow it with a revolutionary character. [2005, 283]
Kuhn treated a scientific field (and perhaps science as a whole) as a system with a far more interesting internal dynamics than either Popper or the logical empiricists had proposed. The famous opening paragraphs of Structure read as though Kuhn had analyzed a historical time series and extracted a pattern from it inductively as the basis for his model of scientific development. The broadly cyclic nature of this pattern immediately jumps out at dynamical systems theorists. Yet despite this perhaps promising start as an early dynamical modeler of science, Kuhn apparently paid little attention to the explosion of work in nonlinear dynamics that began with “chaos” theory and widened into such areas as complex adaptive systems and network theory. This is unfortunate, since the new developments might have provided valuable tools for articulating his own ideas.
For example, it would appear that, as Kuhnian normal science becomes more robust in the sense of closing gaps, tightening connections, and thereby achieving multiple lines of derivation and hence mutual reinforcement of many results. However, that very fact makes normal science increasingly fragile, less resilient to shocks, and more vulnerable to cascading failure (Nickles 2008). Kuhn claimed, contrary to the expectations of scientific realists, that there would be no end to scientific revolutions in ongoing, mature sciences, with no reason to believe that such revolutions would gradually diminish in size as these sciences continued to mature. But it would seem to follow from his model that he could have made a still stronger point. For Kuhn’s position in Structure arguably implies that, when considering a single field over time, future revolutions can occasionally be even larger than before. The reason is that just mentioned: as research continues filling gaps and further articulating the paradigm, normal science becomes more tightly integrated but also forges tighter links to relevant neighboring fields. Taking these developments into account predicts that Kuhnian normal science should evolve toward an ever more critical state in which something that was once an innocuous anomaly can now trigger a cascade of failures (Nickles 2012a and b), sometimes rather quickly. For there will be little slack left to absorb such discrepancies. If so, then we have an important sort of dynamical nonlinearity even in normal science, which means that Kuhnian normal science itself is more dynamic, less static, than he made it out to be.
It seems clear that Kuhnian revolutions are bifurcations in the nonlinear dynamical sense, and it seems plausible to think that Kuhnian revolutions may have a fat-tailed or power-law distribution (or worse) when their size is plotted over time on an appropriate scale. Each of these features is a “hallmark of nonlinear dynamics” (Hooker 2011A, 5; 2011B, 850, 858). To elaborate a bit: one intriguing suggestion coming from work in nonlinear dynamics is that scientific changes may be like earthquakes and many other phenomena (perhaps including punctuated equilibrium events of the Gould-Eldredge sort as well as mass extinction events in biology) in following a power-law distribution in which there are exponentially fewer changes of a given magnitude than the number of changes in the next lower category. For example, there might be only one magnitude 5 change (or above) for every ten magnitude 4 changes (on average over time), as in the Gutenberg-Richter scale for earthquakes. If so, then scientific revolutions would be scale free, meaning that large revolutions in the future are more probable than a Gaussian normal distribution would predict. Such a conclusion would have important implications for the issue of scientific realism.
To be sure, working out such a timescale of revolutions and their sizes in the history of science would be difficult and controversial, but Nicholas Rescher (1978, 2006) has begun the task in terms of ranking scientific discoveries and studying their distribution over time. Derek Price (1963) had previously introduced quantitative historical considerations into history of science, pointing out, among many other things, the exponential increase in the number of scientists and quantity of their publications since the Scientific Revolution. Such an exponential increase, faster than world population increase, obviously cannot continue forever and, in fact, was already beginning to plateau in industrialized nations in the 1960s. Among philosophers, Rescher was probably the first to analyze aggregate data concerning scientific innovation, arguing that, as research progresses, discoveries of a given magnitude become more difficult. Rescher concludes that we must eventually expect a decrease in the rate of discovery of a given magnitude and hence, presumably, a similar decrease in the rate of scientific revolutions. Although he does not mention Schumpeter in this work, he expresses a similar view:
Scientific progress in large measure annihilates rather than enlarges what has gone before—it builds the new on the foundations of the ruins of the old. Scientific theorizing generally moves ahead not by addition and enlargement but by demolition and replacement . [1978, p. 82, Rescher’s emphasis]
This broadly Kuhnian position position on the number and magnitude of revolutions contrasts sharply with Butterfield’s, who saw revolutions only as founding revolutions, and also with that of those epistemological realists who grant that revolutionary conceptual and practical changes have occurred but who believe that they will become successively smaller in the future as science approaches the true theory. Kuhn’s own later position, in which specialties are insulated from one another by taxonomic incommensurability, presents us with a somewhat less integrated conception of science and thus one less subject to large-scale revolutionary disruption. Since we can regard scientific practices and organization as highly designed technological systems, the work of Charles Perrow and others on technological risk is relevant here. (See Perrow 1984 for entry into this approach.)
Margolis (1993) notes the importance of the phenomenon of “contagion,” in which new ideas or practices suddenly reach a kind of social tipping point and spread rapidly. Contagion is, of course, necessary for a revolt to succeed as a revolution. Today, contagion is a topic being studied carefully by network theorists and popularized by Malcolm Gladwell’s The Tipping Point (2000). Steven Strogatz, Duncan Watts, and Albert-László Barabási are among the new breed of network theorists who are developing technical accounts of “phase changes” resulting from the growth and reorganization of networks, including social networks of science—a topic dear to the early Kuhn’s heart as he struggled with the themes of Structure (see Strogatz, 2003, chap. 10; Watts 1999; Newman 2001; Barabási 2002; Buchanan 2002).
Does the emergence to prominence of “chaos theory” (nonlinear dynamics) itself constitute a scientific revolution and, if so, is it a distinctly Kuhnian revolution? In recent years several writers, including both scientists and science writers, have attempted to link Kuhn’s idea of revolutionary paradigm shifts to the emergence of chaos theory, complexity theory, and network theory (e.g., Gleick 1987, chap. 2, on the chaos theory revolution; Ruelle 1991, chap. 11; Jen in Cowan et al. 1999, 622f, on complexity theory; and Buchanan 2002, 47, on network theory). Interestingly, some authors reapply these ideas to Kuhn’s account itself, theoretically construing revolutionary paradigm shifts as phase changes or as nonlinear jumps from one strange attractor or one sort of network structure to another.
Steven Kellert (1993) considers and rejects the claim that chaos theory represents a Kuhnian revolution. Although it does provide a new set of research problems and standards and, to some degree, transforms our worldview, it does not overturn and replace an entrenched theory. Kellert argues that chaos theory does not even constitute the emergence of a new, mature science rather than an extension of standard mechanics, although it may constitute a new style of reasoning.
Kellert’s position hangs partly on how we construe theories. If a theory is just a toolbox of models, something like an integrated collection of Kuhnian exemplars (Giere 1988, Teller 2008), then the claim for a revolutionary theory development of some kind becomes more plausible. For nonlinear dynamics highlights a new set of models and the strange attractors that characterize their behaviors. In addition, complex systems theorists often stress the holistic, anti-reductive, emergent nature of the systems they study, by contrast with the linear, Newtonian paradigm. Kuhn wrote that one way in which normal science articulates its paradigm is by “permitting the solution of problems to which it had previously only drawn attention.” But had not classical dynamics suppressed rather than drawn attention to the problems of chaos theory and the various sorts of complexity theory and network theory that are much studied today? Still, it is easy to agree with Kellert that this case does not fit Kuhn’s account neatly. To some readers it suggests that a more pluralistic conception of scientific revolutions than Kuhn’s is needed.
Kellert also questions whether traditional dynamics was really in a special state of crisis prior to the recent emphasis on nonlinear dynamics, for difficulties in dealing with nonlinear phenomena have been apparent almost from the beginning. Since Kuhn himself emphasized, against Popper, that all theories face anomalies at all times, it is unfortunately all too easy, after an apparently revolutionary development, to point back and claim crisis.
Kuhn’s work called attention to what he called “the essential tension” between tradition and innovation (Kuhn 1959, 1977a). While he initially claimed that his model applied only to mature natural sciences such as physics, chemistry, and parts of biology, he believed that the essential tension point applies, in varying degrees, to all enterprises that place a premium on creative innovation. His work thereby raises interesting questions, such as which kinds of social structures make revolution necessary (by contrast with more continuous varieties of transformative change) and whether those that do experience revolutions tend to be more progressive by some standard.
Some analysts agree that casting the net more widely might shed comparative light on scientific change, and that Kuhn’s model is too restrictive even when applied only to the mature sciences. We have already met several alternative conceptions of transformative change in the sciences. Kuhn believed that innovation in the arts was often too divergent fully to express the essential tension. By contrast, the sciences, he claimed, do not seek innovation for its own sake, at least normal scientists do not.
But what about technological innovation (which is often closely related to mature science) and what about business enterprise more generally? There are, of course, important differences between the products of basic scientific research and commercial products and services, but there are enough similarities to make comparison worthwhile—the more so with today’s emphasis on translational science. And in the sciences as well as economic life there would seem to be other forms of displacement than the logical and epistemological forms commonly recognized by philosophers of science. Consider the familiar economic phenomenon of obsolescence, including cases that lead to major social reorganization as technological systems are improved. Think of algorithmic data mining and statistical computation, robotics, and the automation to be found in any modern biological laboratory. In The Innovator’s Dilemma (1997), economist Clayton Christensen denies that major technological breakthroughs are either necessary or sufficient for disruptive innovation. In that and later work he distinguishes sustaining technologies that make incremental improvements of a company’s sales leaders from two kinds of disruptive technologies. “New-market disruptions” appeal to a previously non-existent market, whereas “low-market” or “low-end disruptions” provide simpler and cheaper ways to do things than do the leading products and services. Such companies can sometimes scale up their more efficient processes to displace the major players, as did Japanese steel makers to the big U.S. corporations. There would seem to be parallels in the history of science.
Speaking of technological developments, philosophers, including Kuhn, have undervalued a major source of transformative developments, namely, material culture, specifically the development of new instruments. There is, however, a growing literature in history and sociology of science and technology. A good example is Andy Pickering’s discussion of the conception and construction of the large cloud chamber at Lawrence Berkeley Laboratory (Pickering 1995). Pickering’s Constructing Quarks (1984), Peter Galison’s How Experiments End (1987) and Image and Logic (1997), and Sharon Traweek’s Beamtimes and Lifetimes (1988) describe the cultures that grew up around the big machines and big theories of high-energy physics in the U.S., Europe, and Japan. As he himself recognized, Kuhn’s model of rapid change runs into increasing difficulty with the Big Science of the World War II era and beyond. But a similar point extends to smaller-scale material practices as documented by much recent research, as in Baird (2004), discussed above. One line of fruitful investigation has been that of the Social Construction of Technology (SCOT) program of Trevor Pinch and Wiebe Bijker (see Bijker et al. 1987 and a good deal of later work). Such work takes place on all scales.
In Structure and later writings, Kuhn locates revolutionary change both at the logico-semantical and methodological level (incompatibility between successor and predecessor paradigm) and at the level of form of community life and practice. But does the latter always require the former? Perhaps expressions such as “the problem of conceptual change” and “breaking out of the old conceptual framework” have led philosophers to over-intellectualize historical change. As we know from the history of economics and business, one form of life can replace another in various ways without being based directly upon a logical or semantic incompatibility. The old ways may be not wrong but simply obsolete, inefficient, out of fashion—destroyed by a process that requires more resources than simple logical relations to understand it. There can be massive displacement by non-logical means. Many have argued that Kuhn’s semantic holism, with its logical-relational underpinnings, led him to underappreciate how flexible scientists and technologists can be at the frontiers of research (Galison 1997). Having distinguished the working scientists’ point of view from those of the historian and the philosopher, looking down from above, he proceeded to confuse them. Retrospectively, as many commentators have noted, we can view Kuhn on scientific revolutions as a transitional figure, more indebted to logical empiricist conceptions of logic, language, and meaning than he could have recognized at the time, while departing sharply from the logical empiricists and Popper in other respects.
- Agazzi, E., 2008, Le rivoluzioni scientifiche e il mondo moderno , Milan: Fondazione Achille e Giulia Boroli.
- Andersen, H., 2001, On Kuhn , Belmont, CA: Wadsworth.
- –––, 2013, “The Second Essential Tension: On Tradition and Innovation in Interdisciplinary Research,” Topoi , 32: 3–8.
- –––, P. Barker, and X. Chen, 2006, The Cognitive Structure of Scientific Revolutions , Cambridge: Cambridge University Press.
- Arthur, W. B., 2009, The Nature of Technology: What It Is and How It Evolves , New York: Free Press.
- Bachelard, G., 1934, Le nouvel esprit scientifique , Paris: PUF; translated as The New Scientific Spirit , A. Goldhammer (trans.), Boston: Beacon Press, 1984.
- Baird, D., 2004, Thing Knowledge: A Philosophy of Scientific Instruments , Berkeley: University of California Press.
- Baltas, A., K. Gavroglu, and V. Kindi, 2000, “A Discussion with Thomas S. Kuhn,” in Kuhn (2000a), pp. 255–323.
- Barabási, A-L., 2002, Linked: The New Science of Networks , Cambridge, MA: Perseus.
- Barnes, B., 1982, T. S. Kuhn and Social Science , New York: Columbia University Press.
- –––, 2003, “Thomas Kuhn and the Problem of Social Order in Science,” in Nickles (2003a), pp. 122–141.
- Bijker, W., T. Hughes, and T. Pinch (eds.), 1987, The Social Construction of Technological Systems: New Directions in the Sociology and History of Technology , Cambridge, MA: MIT Press.
- Beinhocker, E., 2006, The Origin of Wealth , Cambridge, MA: Harvard Business School.
- Bellone, E., 1980, A World on Paper: Studies on the Second Scientific Revolution , Cambridge, MA: MIT Press.
- Bird, A., 2001, Thomas Kuhn , Princeton: Princeton University Press.
- –––, 2008, “The Historical Turn in the Philosophy of Science,” in Psillos and Curd (2008), pp. 66–77.
- Bitbol, M., 1997, “En quoi consiste la ‘Révolution Quantique’?,” Revue Internationale de Systématique 11: 215–239.
- Bowler, P., and I. Morus, 2005, Making Modern Science: A Historical Survey , Chicago: University of Chicago Press.
- Brannigan, A., 1981, The Social Basis of Scientific Discoveries , Cambridge: Cambridge University Press.
- Brush, S., 1983, Statistical Physics and the Atomic Theory of Matter , Princeton: Princeton University Press.
- Buchanan, M., 2002, Nexus: Small Worlds and the Groundbreaking Science of Networks . New York: Norton.
- Burtt, E. A., 1924, The Metaphysical Foundations of Modern Physical Science: A Historical and Critical Essay , London: Kegan Paul, Trench, Trübner.
- Butterfield, H., 1931, The Whig Interpretation of History , London: Bell.
- –––, 1949, The Origins of Modern Science: 1300–1800 , London: Bell.
- Carnap, R., 1950, “Empiricism, Semantics, and Ontology,” Revue Internationale de Philosophie , 4: 20–40; reprinted, in Meaning and Necessity: A Study in Semantics and Modal Logic (Supplement), Chicago: University of Chicago Press, 1956.
- Carroll, S. B., 2005, Endless Forms Most Beautiful: The New Science of Evo Devo , New York: Norton.
- –––, 2005, Endless Forms Most Beautiful: The New Science of Evo Devo , New York: Norton.
- Christensen, C., 1997, The Innovator’s Dilemma: When New Technologies Cause Great Firms to Fail , Cambridge, MA: Harvard Business School Press.
- –––, and M. Raynor, 2003, The Innovator’s Solution , Cambridge, MA: Harvard Business School Press.
- Cohen, H. F., 1994, The Scientific Revolution: A Historiographical Inquiry , Chicago: University of Chicago Press.
- Cohen, I. B., 1985, Revolution in Science , Cambridge: Harvard University Press.
- Cohen, M. R., and E. Nagel, 1934, An Introduction to Logic and Scientific Method , New York: Harcourt, Brace & World.
- Cowan, G., D. Pines, and D. Meltzer (eds.), 1999, Complexity: Metaphors, Models, and Reality , Cambridge, MA: Perseus Books.
- Crombie, A. C., 1959, Medieval and Early Modern Science , Garden City, NY: Doubleday.
- –––, 1994, Styles of Scientific Thinking in the European Tradition , 3 vols., London: Duckworth.
- De Langhe, R., 2012, “A Comparison of Two Models of Scientific Progress,” Studies in History and Philosophy of Science , 46: 94–99.
- –––, 2014a, “A Unified Model of the Division of Cognitive Labor,” Philosophy of Science , 81(3): 444–459.
- –––, 2014b, “To Specialize or to Innovate? An Internalist Account of Pluralistic Ignorance in Economics,” Synthese , 191: 2499–2511.
- –––, 2017, “Towards the Discovery of Scientific Revolutions in Scientometric Data,” Scientometrics , 110: 505–519.
- ––– (ed.), 2013, Topoi , 32 (1), special issue on Kuhn after fifty years.
- Dear, P., 2001, Revolutionizing the Sciences: European Knowledge and Its Ambitions, 1500–1700 , Princeton: Princeton University Press.
- Dijksterhuis, E. J., 1961, The Mechanization of the World Picture , Oxford: Oxford University Press. Original Dutch edition, 1950.
- DiSalle, R., 2002, “Reconsidering Kant, Friedman, Logical Positivism, and the Exact Sciences”, Philosophy of Science , 69: 191–211.
- Domski, M., and M. Dickson (eds.), 2010, Discourse on a New Method: Reinvigorating the Marriage of History and Philosophy of Science , Chicago: Open Court.
- Doppelt, G., 1978, “Kuhn’s Epistemological Relativism: An Interpretation and Defense,” Inquiry 21: 33–86.
- Du Châtelet, É, 1740, Institutions de Physique , translated as Foundations as Physics , Paris.
- –––, and J. Zinnser (eds.), 2009, Selected Philosophical and Scientific Writings , Chicago: University of Chicago Press.
- Duhem, P., 1914, Théorie Physique: Son Objet, Sa Structure , 2nd ed., Paris. Published in English translation as The Aim and Structure of Physical Theory by Princeton University Press, 1954.
- Feyerabend, P., 1962, “Explanation, Reduction, and Empiricism,” in Scientific Explanation, Space, and Time , H. Feigl and G. Maxwell (eds.), Minneapolis: University of Minnesota Press, pp. 28–97.
- –––, 1975, Against Method: Outline of an Anarchistic Theory of Knowledge , London: New Left Books.
- Fleck, L., 1935, Entstehung und Entwicklung einer wissenschaftliche Tatsache: Einführung in die Lehre von Denkstil und Denkkollecktiv , Basel: Benno Schwabe, translated as Genesis and Development of a Scientific Fact , Chicago: University of Chicago Press, 1979.
- Foucault, M., 1963, Naissance de la Clinique , Paris: Presses Universitaires de France, translated as The Birth of the Clinic , New York: Pantheon, 1973.
- –––, 1966, Les Mots et les Choses , Paris: Gallimard, translated as The Order of Things , New York: Vintage, 1973.
- –––, 1969, L’Archéologie du Savoir , Paris: Gallimard, translated as The Archaeology of Knowledge , New York: Harper and Row, 1972.
- –––, 1975, Surveiller et Punir , Paris: Gallimard, translated as Discipline and Punish , New York: Pantheon, 1977.
- Frank, P., 1957, Philosophy of Science: The Link between Science and Philosophy , Englewood Cliffs, NJ: Prentice-Hall.
- Friedman, M., 2001, Dynamics of Reason , Stanford: CSLI Publications.
- –––, 2003, “Kuhn and Logical Empiricism,” in Nickles (2003a), pp. 19–44.
- –––, 2010, “Synthetic History Reconsidered,” in Domski and Dickson (2010), pp. 571–813.
- Fuller, S., 2000, Thomas Kuhn: A Philosophical History for Our Times . Chicago: University of Chicago Press.
- Galison, P., 1987, How Experiments End , Chicago: University of Chicago Press.
- –––, 1997, Image and Logic: A Material Culture of Microphysics . Chicago: University of Chicago Press.
- Gattei, S., 2008, Thomas Kuhn’s “Linguistic Turn” and the Legacy of Logical Empiricism: Incommensurability, Rationality and the Search for Truth , Aldershot, UK: Ashgate.
- Giere, R., 1988, Explaining Science: A Cognitive Approach , Chicago: University of Chicago Press.
- Gillispie, C., 1960, The Edge of Objectivity , Princeton: Princeton University Press.
- Gladwell, M., 2000, The Tipping Point: How Little Things Can Make a Big Difference , New York: Little Brown.
- Gleick, J., 1987, Chaos: Making a New Science , New York: Viking Penguin.
- Godfrey-Smith, P. 2007, “Is It a Revolution?” (essay review of E. Jablonka and M. Lamb, Evolution in Four Dimensions ), Biology and Philosophy 22 (3): 429–437.
- González, W. J. (ed.), 2004, Análisis de Thomas Kuhn: Las Revoluciones Cientificas , Madrid, Trotta.
- Goodwin, B., 1994, How the Leopard Changed Its Spots: The Evolution of Complexity , New York: Charles Scribner’s Sons.
- Gould, S. J., 1997, “Evolution: The Pleasures of Pluralism,” New York Review of Books 44 (11, 26 June): 47–52.
- –––, and R. Lewontin, 1979, “The Spandrels of San Marco and the Panglossian Paradigm,” Proceedings of the Royal Society of London 205: 281–288.
- –––, and N. Eldredge, 1992, “Punctuated Equilibria: An Alternative to Phyletic Gradualism,” in Models in Paleobiology , T. Schopf (ed.), San Francisco: Freeman-Cooper, pp. 82–115.
- Gutting, G., 2001, French Philosophy in the Twentieth Century , Cambridge: Cambridge University Press.
- –––, 2003, “Thomas Kuhn and French Philosophy of Science,” in Nickles (2003a), pp. 45–64.
- Hacking, I., 1975, The Emergence of Probability , Cambridge: Cambridge University Press.
- –––, 1990, The Taming of Chance , Cambridge: Cambridge University Press.
- –––, 1995, Rewriting the Soul , Princeton: Princeton University Press.
- –––, 2002, Historical Ontology , Cambridge, MA: Harvard University Press.
- –––, 2012, “‘Language, Truth and Reason’ 30 Years Later,” Studies in History and Philosophy of Science A 43: 599–609. [This issue of the journal is devoted to critical articles on Hacking’s styles of reasoning project.]
- Hanson, N. R., 1958, Patterns of Discovery , Cambridge: Cambridge University Press.
- Harris, R. A. (ed.), 2005, Rhetoric and Incommensurability , West Lafayette, IN: Parlor Press.
- Holmes, O. W., Jr., 1861, Letter to Felton, quoted by L. Menand, The Metaphysical Club , New York: Farrar, Straus and Giroux, 2001, p. 33.
- Hooker, C., 2011, “Introduction to Philosophy of Complex Systems: Parts A and B,” in Hooker (ed.), Philosophy of Complex Systems (Volume 10, Handbook of the Philosophy of Science ), Amsterdam: North-Holland, pp. 3–90 and 841–909.
- Hoyningen-Huene, P., 1993, Reconstructing Scientific Revolutions: Thomas S. Kuhn’s Philosophy of Science , Chicago: University of Chicago Press.
- –––, and H. Sankey (eds.), 2001, Incommensurability and Related Matters , Dordrecht: Kluwer.
- Hull, D., 1988, Science as a Process: An Evolutionary Account of the Social and Conceptual Development of Science , Chicago: University of Chicago Press.
- Jablonka, E., and M. Lamb, 2005, Evolution in Four Dimensions: Genetic, Epigenetic, Behavioral, and Symbolic Variation in the History of Life , Cambridge, MA: MIT Press.
- Kant, I., 1781, Critique of Pure Reason , German original, Kritik der reinen Vernunft , Riga.
- –––, 1798, Anthropology from a Pragmatic Point of View , trans. R. Louden, Cambridge: Cambridge University Press, 2006.
- Kauffman, S., 1993, The Origins of Order: Self-Organization and Selection in Evolution , Oxford: Oxford University Press.
- Kellert, S., 1993, “A Philosophical Evaluation of the Chaos Theory ‘Revolution,’” in PSA 1992, vol. 2, D. Hull, M. Forbes, and K. Okruhlik (eds.), East Lansing, MI: Philosophy of Science Association, pp. 33–49.
- –––, 2008, Borrowed Knowledge: Chaos Theory and the Challenge of Learning across Disciplines , Chicago: University of Chicago Press.
- Keynes, J. M., 1947, “Newton, the Man,” Proceedings of the Royal Society Newton Tercentenary Celebrations , 15–19 July 1946, Cambridge, Cambridge University Press.
- Kindi, V., 2005, “The Relation of History of Science to Philosophy of Science in The Structure of Scientific Revolutions and Kuhn’s Later Philosophical Work,” Perspectives on Science , 13: 495–530.
- –––, 2010, “Novelty and Revolution in Art and Science: The Influence of Kuhn on Cavell,” Perspectives on Science , 18: 284–310.
- –––, and T. Arabatzis (eds.), 2012, Kuhn’s The Structure of Scientific Revolutions Revisited , Chicago: University of Chicago Press.
- Klein, M. 1983, “Einstein’s First Paper on Quanta,” The Natural Philosopher 2: 59–86.
- Koyré, A., 1939, Études Galiléenes, Paris: Hermann, translated as Galileo Studies , Atlantic Highlands, NJ: Humanities Press, 1978.
- Krajewski, W., 1977, Correspondence Principle and Growth of Science , Dordrecht: Reidel.
- Kuhn, T. S., 1957, The Copernican Revolution , Cambridge, MA: Harvard University Press.
- –––, 1959, “The Essential Tension: Tradition and Innovation in Scientific Research,” reprinted in Kuhn (1977a), pp. 225–239.
- –––, 1962, The Structure of Scientific Revolutions , Chicago: University of Chicago Press, 2nd ed., enlarged with “Postscript–1969,” 1970; fiftieth anniversary edition with introduction by Ian Hacking, 2012. (Page references are to the 2nd edition.)
- –––, 1970, “Logic of Discovery or Psychology of Research?”, in I. Lakatos and A. Musgrave (eds.), Criticism and the Growth of Knowledge , Cambridge: Cambridge University Press, pp. 1–22. Reprinted in Kuhn (1977a), pp. 266–292.
- –––, 1974, “Second Thoughts on Paradigms,” in Suppe (1974). Reprinted in Kuhn (1977a), pp. 293–319.
- –––, 1977a, The Essential Tension , Chicago: University of Chicago Press.
- –––, 1977b, “Objectivity, Value Judgment, and Theory Choice,” in Kuhn (1977a), pp. 320–339.
- –––, 1978, Black-Body Theory and the Quantum Discontinuity, 1894–1912 , Oxford: Clarendon Press, 1978.
- –––, 1993, “Afterwords,” in P. Horwich (ed.), World Changes: Thomas Kuhn and the Nature of Science , Cambridge, MA: MIT Press, pp. 311–341. Reprinted in Kuhn (2000a), pp. 224–252.
- –––, 2000a, The Road Since Structure , J. Conant and J. Haugeland (eds.), Chicago: University of Chicago Press (a collection of Kuhn’s last philosophical essays).
- –––, 2000b, “What Are Scientific Revolutions?,” in Kuhn (2000a), pp. 13–32, originally published in 1987.
- –––, 2000c, “The Road since Structure,” in Kuhn (2000a), pp. 90–120).
- Kusch, M., 2010, “Hacking’s Historical Epistemology: A Critique of Styles of Reasoning”, Studies in History and Philosophy of Science , 41: 158–173.
- Kuukkanen, J-M., 2012, “Revolution as Evolution: The Concept of Evolution in Kuhn’s Philosophy,” in Kindi and Arabatzis (2012), pp. 134–152.
- Lakatos, I., 1970, “Falsification and the Methodology of Scientific Research Programmes,” in Lakatos and Musgrave (1970), pp. 91–195.
- –––, and Musgrave, A. (eds.), 1970, Criticism and the Growth of Knowledge . Cambridge: Cambridge University Press.
- Laudan, L., 1977, Progress and Its Problems , Berkeley: University of California Press.
- –––, 1980, “Why Was the Logic of Discovery Abandoned?,” in T. Nickles (ed.), Scientific Discovery, Logic, and Rationality , Dordrecht: Reidel, pp. 173–183.
- –––, 1984, Science and Values , Berkeley: University of California Press.
- Lewis, C. I., 1929, Mind and the World Order , New York: Scribners.
- Marcum, J., 2015, Thomas Kuhn’s Revolutions: A Historical and Evolutionary Philosophy of Science? , London: Bloomsbury Academic.
- Margolis, H., 1993, Paradigms and Barriers: How Habits of Mind Govern Scientific Beliefs , Chicago: University of Chicago Press.
- Meyerson, É., 1908, Identité et Realité , Paris: Alcan. Translated as Identity and Reality , London: Allen and Unwin, 1930.
- Nagel, E., 1961, The Structure of Science: Problems in the Logic of Explanation , New York: Harcourt, Brace & World.
- Nersessian, N., 2003, “Kuhn, Conceptual Change, and Cognitive Science,” in Nickles (2003a), pp. 178–211.
- –––, 2008, Creating Scientific Concepts , Cambridge, MA: MIT Press.
- Newman, M., 2001, “The Structure of Scientific Collaboration Networks,” Proceedings of the National Academy of Science , 98: 404–409.
- Nickles, T. (ed.), 2003a, Thomas Kuhn , Cambridge: Cambridge University Press.
- –––, 2003b, “Normal Science: From Logic to Case-Based and Model-Based Reasoning,” in Nickles (2003a), pp. 142–177.
- –––, 2006, “Scientific Revolutions,” in The Philosophy of Science: An Encyclopedia , vol. 2, S. Sarkar and J. Pfeifer (eds.), New York: Routledge, pp. 754–765.
- –––, 2008, “Disruptive Scientific Change,” in Soler et al. (2008), pp. 349–377.
- –––, 2009, “The Strange Story of Scientific Method,” in J. Meheus and T. Nickles (eds.), Models of Discovery and Creativity , Dordrecht: Springer, pp. 167–207.
- –––, 2012a, “Dynamic Robustness and Design in Nature and Artifact,” in L. Soler, E. Trizio, T. Nickles, and W. C. Wimsatt (eds.), Characterizing the Robustness of Science: After the Practice Turn in Philosophy of Science , Dordrecht: Springer, pp. 329–360.
- Nowak, L., 1980, The Structure of Idealization , Dordrecht: Reidel.
- Olby, R., 1985, Mendelism , 2nd ed., Chicago: University of Chicago Press.
- Pepper, S., 1942, World Hypotheses: A Study in Evidence , Berkeley: University of California Press.
- Perrow, C., 1984, Normal Accidents: Living with High-Risk Technologies , New York: Basic Books.
- Pickering, A., 1984, Constructing Quarks: A Sociological History of Particle Physics , Chicago: University of Chicago Press.
- –––, 1995, The Mangle of Practice: Time, Agency and Science , Chicago: The University of Chicago Press.
- Polanyi, M., 1958, Personal Knowledge: Towards a Post-Critical Philosophy . Chicago: University of Chicago Press.
- Popper, K. R., 1957, “Philosophy of Science: A Personal Report,” in C. A. Mace (ed.), British Philosophy at Mid-Century , London: Allen & Unwin, pp. 155–191. Reprinted with the title “Science: Conjectures and Refutations” in Popper’s Conjectures and Refutations: The Growth of Scientific Knowledge , New York: Basic Books, 1963, pp. 33–65.
- –––, 1959, The Logic of Scientific Discovery , London: Hutchinson. Expanded translation of Logik der Forschung , 1934.
- –––, 1975, “The Rationality of Scientific Revolutions” (Herbert Spencer Lecture), in R. Harré (ed.), Problems of Scientific Revolution , Oxford: Oxford University Press, pp. 72–101.
- Porter, T., 1986, The Rise of Statistical Thinking: 1820–1900 , Princeton: Princeton University Press.
- Post, H. R., 1971, “Correspondence, Invariance, and Heuristics: In Praise of Conservative Induction,” Studies in History and Philosophy of Science 2: 213–255.
- Preston, J., 2008, Kuhn’s The Structure of Scientific Revolutions: A Reader’s Guide , London: Continuum.
- Price, D., 1963, Big Science, Little Science , New York: Columbia University Press.
- Psillos, S., and M. Curd (eds.), 2008, The Routledge Companion to Philosophy of Science , London: Routledge.
- Quine, W. V., 1951, “Two Dogmas of Empiricism,” The Philosophical Review 60: 20–43, reprinted in his From a Logical Point of View , Cambridge, MA: Harvard University Press, 1953, pp. 20–46.
- –––, 1960, Word and Object , Cambridge, MA: MIT Press.
- Reichenbach, H., 1938, Experience and Prediction , Chicago: University of Chicago Press.
- –––, 1951, The Rise of Scientific Philosophy , Berkeley: University of California Press.
- Reisch, G., 1991, “Did Kuhn Kill Logical Empiricism?,” Philosophy of Science 58: 264–277.
- Rescher, N., 1978, Scientific Progress , Oxford: Blackwell.
- –––, 2006, Epistemetrics , Cambridge: Cambridge University Press.
- Richards, R., and L. Daston (eds.), 2016, Kuhn’s ’Structure of Scientific Revolutions‘ at Fifty: Reflections on a Science Classic , Chicago: University of Chicago Press.
- Rouse, J., 2003, “Kuhn’s Philosophy of Scientific Practice,” in Nickles (2003a), pp. 101–121.
- Rowbottom, D., and O. Bueno (eds.), 2011, Synthese , 178.1: 1–169, special issue on stance and rationality.
- Ruelle, D., 1991, Chance and Chaos , Princeton: Princeton University Press.
- Ruse, M., 1989, The Darwinian Paradigm , London: Routledge.
- Sankey, H., 1997, Rationality, Relativism and Incommensurability , Aldershot, UK: Ashgate.
- Schickore, J., and F. Steinle (eds.), 2006, Revisiting Discovery and Justification , Dordrecht: Springer.
- Schumpeter, J., 1942, Capitalism, Socialism, and Democracy , New York: Harper.
- Schuster, J., and R. Yeo (eds.), 1986, The Politics and Rhetoric of Scientific Method , Dordrecht: Reidel.
- Sciortino, L., 2017, “On Ian Hacking’s Notion of a Style of Reasoning”, Erkenntnis , 82: 243–264.
- Shapin, S., 1996, The Scientific Revolution , Chicago: University of Chicago Press.
- Sharrock, W., and R. Read, 2002, Kuhn: Philosopher of Scientific Revolution , Cambridge: Polity Press.
- Sneed, J., 1971, The Logical Structure of Mathematical Physics , Dordrecht: Reidel; 2nd edition, 1979.
- Soler, L. (ed.), 2008, Studies in History and Philosophy of Science A 39:221–264, special issue on contingency vs. inevitability in science.
- –––, H. Sankey, and P. Hoyningen-Huene (eds.), 2008, Rethinking Scientific Change and Theory Comparison: Stabilities, Ruptures, Incommensurabilities? Dordrecht: Springer.
- Stegmüller, W., 1974, Theorie und Erfahrung , vol. 2 of Probleme und Resultate der Wissenschafstheorie und Analytische Philosophie , Berlin: Springer-Verlag. Part 2 of vol. 2 appeared in English translation as The Structure and Dynamics of Physical Theories , New York: Springer-Verlag, 1976.
- Stent, G., 1971, Molecular Genetics: An Introductory Narrative , San Francisco: W. H. Freeman.
- Strogatz, S., 2003, Sync: The Emerging Science of Spontaneous Order , New York: Hyperion.
- Suppe, F. (ed.), 1974, The Structure of Scientific Theories , Urbana-Champaign: University of Illinois Press, 2nd ed. 1977.
- Thagard, P., 1992, Conceptual Revolutions , Princeton: Princeton University Press.
- Toulmin, S., 1953, The Philosophy of Science: An Introduction , London: Hutchinson.
- –––, 1961, Foresight and Understanding , Bloomington: Indiana University Press.
- –––, 1972, Human Understanding, vol. 1: The Collective Use and Evolution of Concepts . Oxford: Clarendon Press.
- ––– and June Goodfield, 1961, The Fabric of the Heavens: The Development of Astronomy and Dynamics , New York: Harper.
- –––, 1962, The Architecture of Matter , New York: Harper.
- –––, 1965, The Discovery of Time , New York: Harper.
- Traweek, S., 1988, Beamtimes and Lifetimes: The World of High Energy Physicists , Cambridge, MA: Harvard University Press.
- van Fraassen, B., 2002, The Empirical Stance , New Haven, CT: Yale University Press.
- Warsh, D., 2006, Knowledge and the Wealth of Nations: A Story of Economic Discovery , New York: Norton.
- Watts, D., 1999, Small Worlds: The Dynamics of Networks Between Order and Randomness , Princeton: Princeton University Press.
- Weinberg, S., 2001, Facing Up: Science and its Cultural Adversaries , Cambridge, MA: Harvard University Press.
- Wittgenstein, L., 1922, Tractatus Logico-Philosophicus , London: Routledge & Kegan Paul.
- Wootton, D., 2015, The Invention of Science: A New History of the Scientific Revolution , London: Penguin.
- Worrall, J., 2003, “Normal Science and Dogmatism, Paradigms and Progress: Kuhn ‘versus’ Popper and Lakatos,” in Nickles (2003a), pp. 65–100.
- –––, 2008, “Theory-Change in Science,” in Psillos and Curd (2008), pp. 281–291.
- Wray, K. B., 2011, Kuhn’s Evolutionary Social Epistemology , New York: Cambridge University Press.
- Zambelli, P., 2016, Alexandre Koyré in Incognito , Firenze: Leo Olschki Editore.
How to cite this entry . Preview the PDF version of this entry at the Friends of the SEP Society . Look up topics and thinkers related to this entry at the Internet Philosophy Ontology Project (InPhO). Enhanced bibliography for this entry at PhilPapers , with links to its database.
[Please contact the author with suggestions.]
Carnap, Rudolf | Châtelet, Émilie du | choice, dynamic | empiricism: logical | Feyerabend, Paul | Fleck, Ludwik | Foucault, Michel | incommensurability: of scientific theories | Kuhn, Thomas | models in science | physics: intertheory relations in | physics: structuralism in | Popper, Karl | rationality: historicist theories of | Reichenbach, Hans | relativism | science: unity of | scientific progress | scientific realism | value: incommensurable
Acknowledgement
Thanks to Katherine Brading for the reference to Émilie Du Châtelet.
Copyright © 2017 by Thomas Nickles
- Accessibility
Support SEP
Mirror sites.
View this site from another server:
- Info about mirror sites
The Stanford Encyclopedia of Philosophy is copyright © 2024 by The Metaphysics Research Lab , Department of Philosophy, Stanford University
Library of Congress Catalog Data: ISSN 1095-5054
A Short History of the Scientific Revolution
- Famous Inventions
- Famous Inventors
- Patents & Trademarks
- Invention Timelines
- Computers & The Internet
- American History
- African American History
- African History
- Ancient History and Culture
- Asian History
- European History
- Latin American History
- Medieval & Renaissance History
- Military History
- The 20th Century
- Women's History
Human history is often framed as a series of episodes, representing sudden bursts of knowledge. The Agricultural Revolution , the Renaissance , and the Industrial Revolution are just a few examples of historical periods where it is generally thought that innovation moved more rapidly than at other points in history, leading to huge and sudden shake-ups in science, literature, technology, and philosophy. Among the most notable of these is the Scientific Revolution, which emerged just as Europe was awakening from an intellectual lull referred to by historians as the dark ages.
The Pseudo-Science of the Dark Ages
Much of what was considered known about the natural world during the early middle ages in Europe dated back to the teachings of the ancient Greeks and Romans. And for centuries after the downfall of the Roman empire, people still generally didn’t question many of these long-held concepts or ideas, despite the many inherent flaws.
The reason for this was because such “truths” about the universe were widely accepted by the Catholic church, which so happened to be the main entity responsible for the widespread indoctrination of western society at the time. Also, challenging church doctrine was tantamount to heresy back then and thus doing so ran the risk of being trialed and punished for pushing counter ideas.
An example of a popular but unproven doctrine was the Aristotelian laws of physics. Aristotle taught that the rate at which an object fell was determined by its weight since heavier objects fell faster than lighter ones. He also believed that everything beneath the moon was comprised of four elements: earth, air, water, and fire.
As for astronomy, Greek astronomer Claudius Ptolemy’s earth-centric celestial system, in which heavenly bodies such as the sun, moon, planets and various stars all revolved around the earth in perfect circles, served as the adopted model of planetary systems. And for a time, Ptolemy’s model was able to effectively preserve the principle of an earth-centered universe as it was fairly accurate in predicting the motion of the planets.
When it came to the inner workings of the human body, the science was just as error-ridden. The ancient Greeks and Romans used a system of medicine called humorism, which held that illnesses were the result of an imbalance of four basic substances or “humors.” The theory was related to the theory of the four elements. So blood, for instance, would correspond with air and phlegm corresponded with water.
Rebirth and Reformation
Fortunately, the church would, over time, begin to lose its hegemonic grip on the masses. First, there was the Renaissance, which, along with spearheading a renewed interest in the arts and literature, led to a shift toward more independent thinking. The invention of the printing press also played an important role as it greatly expanded literacy as well as enabled readers to reexamine old ideas and belief systems.
And it was around this time, in 1517 to be exact, that Martin Luther, a monk who was outspoken in his criticisms against the Catholic Church's reforms, authored his famous "95 theses" that listed all of his grievances. Luther promoted his 95 theses by printing them out on a pamphlet and distributing them among the crowds. He also encouraged churchgoers to read the bible for themselves and opened the way for other reform-minded theologians such as John Calvin.
The Renaissance, along with Luther’s efforts, which led to a movement known as the Protestant Reformation , would both serve to undermine the church’s authority on all matters that were essentially mostly pseudoscience. And in the process, this burgeoning spirit of criticism and reform made it so that the burden of proof became more vital to understanding the natural world, thus setting the stage for the scientific revolution.
Nicolaus Copernicus
In a way, you can say that the scientific revolution started out as the Copernican Revolution. The man who started it all, Nicolaus Copernicus , was a Renaissance mathematician and astronomer who was born and raised in the Polish city of Toruń. He attended the University of Cracow, later continuing his studies in Bologna, Italy. This is where he met astronomer Domenico Maria Novara and the two soon began exchanging scientific ideas that often challenged the long-accepted theories of Claudius Ptolemy.
Upon returning to Poland, Copernicus took up a position as a canon. Around 1508, he quietly started developing a heliocentric alternative to Ptolemy’s planetary system. To correct some of the inconsistencies that made it insufficient to predict planetary positions, the system he eventually came up with placed the Sun at the center instead of the Earth. And in Copernicus’ heliocentric solar system, the speed in which Earth and other planets circled the Sun was determined by their distance from it.
Interestingly enough, Copernicus wasn’t the first to suggest a heliocentric approach to understanding the heavens. The ancient Greek astronomer Aristarchus of Samos, who lived in the third century B.C., had proposed a somewhat similar concept much earlier that never quite caught on. The big difference was that Copernicus’ model proved to be more accurate at predicting the movements of the planets.
Copernicus detailed his controversial theories in a 40-page manuscript titled Commentariolus in 1514 and in De revolutionibus orbium coelestium ("On the Revolutions of the Heavenly Spheres"), which was published right before his death in 1543. Not surprisingly, Copernicus’ hypothesis enraged the Catholic church, which eventually banned De revolutionibus in 1616.
Johannes Kepler
Despite the Church’s indignation, Copernicus’ heliocentric model generated a lot of intrigue among scientists. One of these people who developed a fervent interest was a young German mathematician named Johannes Kepler . In 1596, Kepler published Mysterium cosmographicum (The Cosmographic Mystery), which served as the first public defense of Copernicus’ theories.
The problem, however, was that Copernicus’ model still had its flaws and was not completely accurate in predicting planetary motion. In 1609, Kepler, whose main work was coming up with a way to account for the way Mars’ would periodically move backward, published Astronomia nova (New Astronomy). In the book, he theorized that planetary bodies didn’t orbit the Sun in perfect circles as Ptolemy and Copernicus had both assumed, but rather along an elliptical path.
Besides his contributions to astronomy, Kepler made other notable discoveries. He figured out that it was refraction that allows for the eyes’ visual perception and used that knowledge to develop eyeglasses for both nearsightedness and farsightedness. He was also able to describe how a telescope worked. And what’s less known was that Kepler was able to calculate the birth year of Jesus Christ.
Galileo Galilei
Another contemporary of Kepler’s who also bought into the notion of a heliocentric solar system and was the Italian scientist Galileo Galilei . But unlike Kepler, Galileo didn’t believe that planets moved in an elliptical orbit and stuck with the perspective that planetary motions were circular in some way. Still, Galileo’s work produced evidence that helped bolster the Copernican view and in the process further undermine the church’s position.
In 1610, using a telescope he built himself, Galileo began fixing its lens on the planets and made a series of important discoveries. He found that the moon was not flat and smooth, but had mountains, craters and valleys. He spotted spots on the sun and saw that Jupiter had moons that orbited it, rather than the Earth. Tracking Venus, he found that it had phases like the Moon, which proved that the planet rotated around the sun.
Much of his observations contradicted the established Ptolemic notion that all planetary bodies revolved around the Earth and instead supported the heliocentric model. He published some of these earlier observations in the same year under the title Sidereus Nuncius (Starry Messenger). The book, along with subsequent findings led many astronomers to convert to Copernicus’ school of thought and put Galileo in very hot water with the church.
Yet despite this, in the years that followed, Galileo continued his “heretical” ways, which would further deepen his conflict with both the Catholic and Lutheran church. In 1612, he refuted the Aristotelian explanation of why objects floated on water by explaining that it was due to the object’s weight relative to the water and not because an object’s flat shape.
In 1624, Galileo got permission to write and publish a description of both the Ptolemic and Copernican systems under the condition that he does not do so in a manner that favors the heliocentric model. The resulting book, “Dialogue Concerning the Two Chief World Systems” was published in 1632 and was interpreted to have violated the agreement.
The church quickly launched the inquisition and put Galileo on trial for heresy. Though he was spared harsh punishment after admitting to have supported Copernican theory, he was put under house arrest for the remainder of his life. Still, Galileo never stopped his research, publishing several theories until his death in 1642.
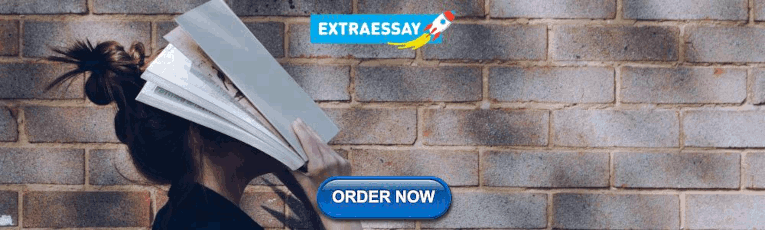
Isaac Newton
While both Kepler and Galileo’s work helped to make a case for the Copernican heliocentric system, there was still a hole in the theory. Neither can adequately explain what force kept the planets in motion around the sun and why they moved this particular way. It wasn’t until several decades later that the heliocentric model was proven by the English mathematician Isaac Newton .
Isaac Newton, whose discoveries in many ways marked the end of the Scientific Revolution, can very well be considered among one of the most important figures of that era. What he achieved during his time has since become the foundation for modern physics and many of his theories detailed in Philosophiae Naturalis Principia Mathematica (Mathematical Principles of Natural Philosophy) has been called the most influential work on physics.
In Principa , published in 1687, Newton described three laws of motion that can be used to help explain the mechanics behind elliptical planetary orbits. The first law postulates that an object that is stationary will remain so unless an external force is applied to it. The second law states that force is equal to mass times acceleration and a change in motion is proportional to the force applied. The third law simply stipulates that for every action there is an equal and opposite reaction.
Although it was Newton’s three laws of motion, along with law of universal gravitation, that ultimately made him a star among the scientific community, he also made several other important contributions to the field of optics, such as building he first practical reflecting telescope and developing a theory of color.
- Explore Johannes Kepler's Laws of Motion
- Trace the Earliest History of Astronomy
- Understanding Cosmology and Its Impact
- Biography of Johannes Kepler, Pioneering German Astronomer
- Claudius Ptolemy: Astronomer and Geographer from Ancient Egypt
- What Is Astronomy and Who Does It?
- Aristarchus of Samos: An Ancient Philosopher With Modern Ideas
- The History of Gravity
- Profile of Tycho Brahe, Danish Astronomer
- 14 Notable European Scientists Throughout History
- Explore Earth - Our Home Planet
- A Historical Timeline of Rockets
- Newton's Law of Gravity
- Biography of Isaac Newton, Mathematician and Scientist
- What Is a Paradigm Shift?
- What are Rotation and Revolution?
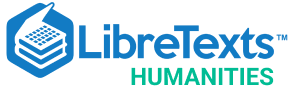
- school Campus Bookshelves
- menu_book Bookshelves
- perm_media Learning Objects
- login Login
- how_to_reg Request Instructor Account
- hub Instructor Commons
- Download Page (PDF)
- Download Full Book (PDF)
- Periodic Table
- Physics Constants
- Scientific Calculator
- Reference & Cite
- Tools expand_more
- Readability
selected template will load here
This action is not available.
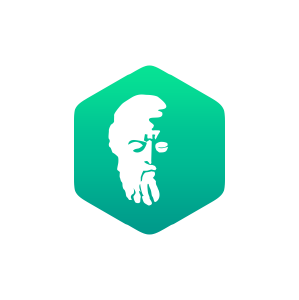
9.6: Roots of the Scientific Revolution
- Last updated
- Save as PDF
- Page ID 85879
During the 1500s and 1600s, a handful of brilliant individuals laid the foundations for science as we know it today. Some historians consider the development of modern science the most important event in the intellectual history of humankind.

The series of events that led to the birth of modern science is called the Scientific Revolution. It occurred between about 1540 and 1700. Why would the birth of science be called a “revolution”? The answer is that science was a radical new idea. It was a completely different way of looking at the world.
Before the Scientific Revolution, most educated people who studied the world took guidance from the explanations given by authorities like ancient Greek writers and Catholic Church officials. After the Scientific Revolution, educated people placed more importance on what they observed and less on what they were told. They gained knowledge by observing the world around them and coming up with logical explanations for what they saw.
Understanding Science
Science is a particular way of gaining knowledge about the world. In fact, the word science comes from a Latin word meaning “knowledge” or “understanding.” Science starts with observation. Scientists observe, or look at, the world. By observing the world they can identify facts about it. A famous scientist once said, “Science is built up with facts, as a house is with stones. But a collection of facts is no more a science than a pile of stones is a house.”
So scientists do more than identify facts. They use logic to explain the facts they have observed. The explanations scientists develop based on these facts are called theories. Theories are not accepted on faith. They must be tested to see if they are true. Scientists design experiments to test their theories. If the experiments keep showing that the theory makes sense, the theory is kept. If the experiments do not support the theory, scientists try a new theory. In this way, scientists learn more about the world.
As you can see, scientific knowledge is based on observations, facts, and logical ideas, or theories, about them. Before the Scientific Revolution, this method of gaining knowledge was uncommon.
Roots of the Revolution
Some of the main ideas of science had been expressed long before the Scientific Revolution. In fact, some of the basic ideas of science are ancient.
Greek Thinkers
Many Greek thinkers expressed ideas that, today, we would call scientific. The great philosopher Aristotle, for example, wrote about astronomy, geography, and many other fields. But his greatest contribution to science was the idea that people should observe the world carefully and draw logical conclusions about what they see. The use of observation and logic, as you have just read, is important in gaining scientific knowledge.
Another Greek thinker was Ptolemy (TAHL-uh-mee), an ancient astronomer. He studied the skies, recorded his observations, and offered theories to explain what he saw. Ptolemy was also a geographer who made the best maps of his time. His maps were based on observations of the real world. Aristotle, Ptolemy, and other Greek thinkers were rationalists, people who looked at the world in a rational, or reasonable and logical, way. During the Renaissance, Europeans studied the works of Greek rationalists. As a result, they began to view the world in a rational way. They began to think like scientists.

Preserving Ancient Knowledge
European scholars could study ancient Greek writings because of the work of others. Muslim scholars translated Greek writings into Arabic. They studied them for centuries and added their own new ideas. Later, the Arabic versions were translated into Latin, which was read in Europe. This work preserved ancient knowledge and spread interest in science to Europe.
Other religious scholars also played a role in preserving Greek ideas. The Jewish scholar Maimonides (my-MAHN-uh-deez) studied and wrote about Aristotle, trying to unite his work with Jewish ideas. The Christian scholar Thomas Aquinas tried to unite the work of Aristotle with Christian ideas. Other Christian scholars studied Greek ideas in Europe’s universities.
Developments in Europe
An interactive or media element has been excluded from this version of the text. You can view it online here: http://pb.libretexts.org/hwcii/?p=168
The Scientific Revolution was not just the result of European scholars studying ancient Greek writings. Developments in Europe also helped bring about the Scientific Revolution. One development that helped lead to the Scientific Revolution was the growth of humanism during the Renaissance. Humanist artists and writers spent much of their time studying the natural world. This interest in the natural world carried forward into the Scientific Revolution.
Another development was a growing interest in alchemy (AL-kuh-mee). Alchemy was a forerunner of chemistry. Alchemists experimented with various natural substances. They were best known for trying to change other metals into gold. Although they failed at that, alchemists succeeded in using experiments to learn more about how nature worked.
All of these developments—the interest in ancient Greek writings, the growth of humanism, the experiments of alchemists—came together in the early 1500s to bring about the Scientific Revolution.
Home 02 – NEW DISCOVERIES

Then an event took place that caused Europeans to doubt some of what the Greeks had said. In 1492, Christopher Columbus sailed west across the Atlantic Ocean in hopes of reaching Asia. As a guide, he took the map of the world that Ptolemy had created. Columbus never reached Asia because he ran into North America instead. Within a few years voyages of exploration made it clear that there was an entire continent that Europeans hadn’t even known existed.
This discovery stunned Europeans. This continent was not on Ptolemy’s map. Ptolemy was wrong. Observation of the real world had disproved the teachings of an ancient authority. Soon, European scholars began to question the accuracy of other Greek authorities. More and more, observations the Europeans made did not fit with what the authorities had described. Such observations helped lead to the Scientific Revolution.
Home 03 – ASTRONOMY
In 1543 an astronomer published a book that contradicted what a Greek authority had written. Many historians think the publication of this book marks the beginning of the Scientific Revolution.
Nicolaus Copernicus
The book thought to have marked the beginning of the Scientific Revolution was written by a Polish astronomer, Nicolaus Copernicus (kuh-PUHR-ni-kuhs). His 1543 book was called On the Revolution of the Celestial Spheres. Copernicus was familiar with Ptolemy’s theories and writings. Ptolemy had written that the earth was the center of the universe and that the sun and other planets orbited, or circled around, the earth. For 1,400 years, people accepted this belief as fact.
As Copernicus studied the movements of the planets, however, what Ptolemy stated made less and less sense to him. If the planets were indeed orbiting the earth, they would have to be moving in very complex patterns. So Copernicus tried a different explanation for what he observed in the sky. Copernicus asked, What if the planets actually orbited the sun? Suddenly, complex patterns weren’t necessary to make sense of what Copernicus observed. Instead, simple circular orbits would account for the planets’ movements.
What Copernicus had done was practice science. Instead of trying to make his observations fit an old idea, he came up with a different idea—a different theory—to explain what he observed. Copernicus never proved his theory, but the Scientific Revolution had begun.
Brahe and Kepler
Another important astronomer of the Scientific Revolution was Tycho Brahe (TYOO-koh BRAH-huh). Brahe, who was Danish, spent most of his life observing the stars. In the late 1500s, he charted the positions of more than 750 of them.
What Brahe did, however, was less important than how he did it. Brahe emphasized the importance of careful observation and detailed, accurate records. Careful recording of information is necessary so that other scientists can use what has previously been learned. In this way, Brahe made an important contribution to modern science.
Brahe was assisted by the German astronomer Johannes Kepler. Later, Kepler tried to map the orbits of the planets. But Kepler ran into a problem. According to his observations, the planet Mars did not move in a circle as he expected it to. Kepler knew that Copernicus had stated that the orbits of the planets were circular. But Kepler’s observations showed that Copernicus was mistaken. In 1609 Kepler wrote that Mars—and all other planets—moved in elliptical, or oval, orbits instead of circular ones. Here was a new theory that fit the observed facts.
Kepler’s work helped prove Copernicus’s theory that the planets orbit the sun. In fact, Kepler became one of the first scientists to speak out in support of Copernicus. Kepler continued to study the planets for the rest of his life. His basic ideas about the planets’ movements are still accepted by scientists today.
Home 04 – GALILEO GALILEI
Galileo Galilei (gal-uh-LEE-oh gal-uh-LAY) was one of the most important scientists of the Scientific Revolution. He was the first person to study the sky with a telescope. With his telescope, Galileo discovered craters and mountains on the moon. He also discovered that moons orbit Jupiter.
Galileo was interested in more than astronomy, however. He also was interested in such things as how falling objects behave. Today, we use the term mechanics for the study of objects and motion.
Galileo’s biggest contribution to the development of science was the way he learned about mechanics. Instead of just observing things in nature, he set up experiments to test what he observed. Galileo was the first scientist to routinely use experiments to test his theories. For this, he is remembered as the father of experimental science.
Home 05 – SIR ISAAC NEWTON
The high point of the Scientific Revolution was marked by the publication of a remarkable book. This book, published in 1687, was Principia Mathematica. Its author was the English scientist Sir Isaac Newton. Newton was one of the greatest and most influential scientists who ever lived. Newton studied and simplified the work of earlier scientists. In doing so, he:
- reviewed everything scientists had been learning,
- coupled it with his own observations and ideas, and
- identified four theories that described how the physical world worked.
Some of his theories have been proven so many times that they are no longer called theories, but laws.
One of Newton’s laws is called the law of gravity. You may know that gravity is the force that attracts objects to each other. It’s the force that makes a dropped apple fall to the ground and that keeps the planets in orbit around the sun.
Newton’s other three laws are called the laws of motion. They describe how objects move in space. You may have heard of one of them: “For every action there is an equal and opposite reaction.” Newton proposed that the universe was like a huge machine. Within this machine, all objects follow the laws he identified. In short, Newton explained how the physical world worked—and he was correct. Newton’s laws became the foundation of nearly all scientific study until the 1900s.
Newton also invented calculus, an advanced form of mathematics that scientists use to solve complex problems. For this, and for his laws of motion, Newton is remembered as a great scientist.
Home 06 – NEW INVENTIONS
Around 1590, a Dutch lens maker named Zacharias Janssen invented a simple microscope. The first person to use a microscope as a scientific instrument, though, was the Dutch scientist Antoni van Leeuwenhoek (LAY-ven-hook) in the mid-1600s. Examining a drop of pond water with his microscope, he saw tiny plants and animals not visible to the naked eye.
In 1593, Galileo invented the thermometer. Thermometers are used to measure temperature. About 50 years later an Italian doctor developed a more accurate model than Galileo’s.
The telescope was probably invented by a Dutch lens maker in 1608. The next year, Galileo built a much-improved telescope that he used to make his important astronomical discoveries.
In 1643, the Italian scientist Evange-lista Torricelli invented the barometer. A barometer is a scientific instrument that measures air pressure. Barometers are used to help forecast the weather.
These instruments—the microscope, the thermometer, the telescope, and the barometer—are very common today. In fact, you have probably used at least one of them yourself. But when they were invented, they were dramatic advances in technology. They gave scientists the tools they needed to make more accurate observations of the world and to conduct experiments. They were the tools of the Scientific Revolution.
Home 07 – THE SCIENTIFIC METHOD
Today scientists use a procedure called the scientific method when doing their research. The scientific method is a step-by-step method for performing experiments and other scientific research.
The scientific method combines Bacon’s idea of a systematic scientific process, Descartes’s insistence on proof and clear reasoning, and the work of other scientists. Using the scientific method, scientists have learned more about the universe in the few hundred years since the Scientific Revolution than in all of the thousands of years that came before. Because of this, the basics of the scientific method—observation and experimentation—are considered the main principles of modern science.
1. Stating the problem. The problem is often a question that begins with why. For example, Copernicus’s problem today would be stated, “Why do the planets move as they do?”
2. Gathering information. This can involve reading what other scientists have written and making observations.
3. Forming a hypothesis. A hypothesis is a solution that the scientist proposes to solve the problem. A hypothesis differs from a theory in that a hypothesis has not yet been tested.
4. Testing the hypothesis by performing experiments.
5. Recording and analyzing data gathered from the experiments.
6. Drawing conclusions from the data collected.
After scientists have concluded their experiments, they typically publish their results. This sharing of ideas is very important for two reasons. First, publishing results lets other scientiststry to reproduce the experiments. By reproducing experiments, scientists can determine whether the results are the same. If they are, they can be reasonably sure that the results are accurate. Second, publishing results spreads scientific knowledge. New scientific knowledge builds on previous knowledge. Sir Isaac Newton once wrote, “If I have seen further it is by standing on the shoulders of Giants.”
Home 08 – SCIENCE, GOVERNMENT, AND SOCIETY
Some of the most important effects of the Scientific Revolution had nothing to do with science at all. When philosophers began applying scientific thought to other areas of human life, they came up with some startling new ideas.
The Power of Reason
By the end of the Scientific Revolution, one thing had become clear to many European thinkers: human reason, or logical thought, was a powerful tool. After all, scientists using reason had made many discoveries about the universe in a relatively short time. Since reason had proven itself as a way to learn some of nature’s great secrets, might reason also be used to solve the problems facing people? Philosophers decided to use reason when they considered society’s problems like poverty and war, or what type of government is best. This use of reason to consider the problems of society led philosophers to look at the world in a new way. They thought they could use reason to determine how to improve society.
Democratic Ideas
One way in which scientists thought they could improve society was by changing its government. Scientists’ use of reason and logic during the Scientific Revolution helped pave the way for the beginnings of democratic thought in Europe. As scientists like Sir Isaac Newton studied the world, they discovered laws that governed nature. In time, some scientists began to think that there must be laws that governed human behavior as well. Once people learned what these laws were, the scientists argued, they could improve their lives and their societies. But the idea that people’s lives were governed by laws had a deeper meaning as well. If all people were governed by the same laws, then it stood to reason that all people must be equal. This idea of the equality of all people was a fundamental step in the development of democratic ideas in Europe.

Home 09 – SCIENCE AND RELIGION
The Roman Catholic Church was a powerful force in Europe during the time of the Scientific Revolution. The birth and growth of science led to conflicts between scientists and the Church.
Reason for Conflict
There were two related parts to the conflict between science and the Church. The first was that the new science was putting forth ideas that contradicted Church teachings. For example, Copernicus’s idea that the earth orbited the sun contradicted the Church teaching that the earth was at the center of the universe.
A second part of the conflict was related to the first. When people contradicted the Church’s teachings, they weakened the Church. Church officials were afraid that questioning even one Church teaching might lead to more and more questions about the Church. People might even start to doubt key elements of the faith. Church officials feared this would undermine the Church’s influence.
The Trial of Galileo
The conflict between science and the Church was illustrated by a trial. Galileo published a book that supported the view that the planets orbit the sun. For this, he was put on trial by the Inquisition, a Church court that investigated people who questioned Church authority. Catholic officials insisted that Galileo publicly reject his findings and accept Catholic teachings that the earth was the center of the universe and did not move. Under threat of torture, Galileo agreed. Still, legend has it that as Galileo left his trial, he muttered, “And yet it does move.” Although he is remembered for opposing this Church teaching, Galileo was a devout Catholic. He believed that experimentation was a search for an understanding of God’s creation.

Knowledge and Belief
Many of the scientists you have been reading about held views similar to Galileo’s. For the scientists of the Scientific Revolution, science and traditional religious beliefs could exist at the same time. Nicolaus Copernicus served as a Church official. Sir Isaac Newton saw a close connection between science and religion. For example, Newton believed that all forces in nature were actions directed by God.
Despite the conflicts, science developed rapidly after the Scientific Revolution. Scientists made—and continue to make—countless discoveries. Scientific knowledge has changed human life dramatically and touches your life every day. Therefore, the Scientific Revolution ranks as one of the most influential events in history.
Home 10 – AUDIO RESOURCES
Home 11 – full youtube videos, home galileo – battle for the heavens – pbs, home sir isaac newton – gravity.
- Roots of the Scientific Revolution. Located at : https://mrgrayhistory.wikispaces.com/UNIT+12+-+THE+SCIENTIFIC+REVOLUTION . License : CC BY-SA: Attribution-ShareAlike
Want to create or adapt books like this? Learn more about how Pressbooks supports open publishing practices.
22 MAKING CONNECTIONS BETWEEN IDEAS: The Scientific Revolution
The scientific revolution, which emphasized systematic experimentation as the most valid research method, resulted in developments in mathematics, physics, astronomy, biology, and chemistry.
The Scientific Revolution
The scientific revolution was the emergence of modern science during the early modern period, when developments in mathematics, physics, astronomy, biology (including human anatomy), and chemistry transformed societal views about nature. The scientific revolution began in Europe toward the end of the Renaissance period, and continued through the late 18th century, influencing the intellectual social movement known as the Enlightenment. While its dates are disputed, the publication in 1543 of Nicolaus Copernicus ‘s De revolutionibus orbium coelestium ( On the Revolutions of the Heavenly Spheres ) is often cited as marking the beginning of the scientific revolution.
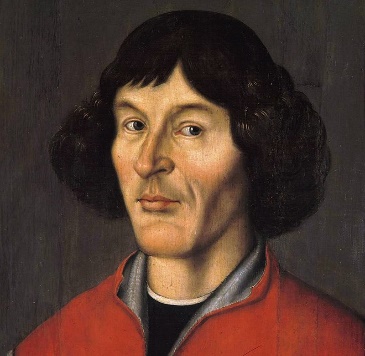
A portrait of Nicolaus Copernicus, 1580. (Source: Wikimedia)
The scientific revolution was built upon the foundation of ancient Greek learning and science in the Middle Ages, as it had been elaborated and further developed by Roman/Byzantine science and medieval Islamic science. The Aristotelian tradition was still an important intellectual framework in the 17th century, although by that time natural philosophers had moved away from much of it. Key scientific ideas dating back to classical antiquity had changed drastically over the years, and in many cases been discredited. The ideas that remained (for example, Aristotle ‘s cosmology, which placed the Earth at the center of a spherical hierarchic cosmos, or the Ptolemaic model of planetary motion) were transformed fundamentally during the scientific revolution.
The change to the medieval idea of science occurred for four reasons:
- Seventeenth century scientists and philosophers were able to collaborate with members of the mathematical and astronomical communities to effect advances in all fields.
- Scientists realized the inadequacy of medieval experimental methods for their work and (out of necessity) devised new methods of observation and evaluation.
- Academics had access to a legacy of European, Greek, and Middle Eastern scientific philosophy that they could use as a starting point (either by disproving or building on the theorems).
- Institutions (for example, the British Royal Society) helped validate science as a field by providing an outlet for the publication of scientists’ work.
A drawing of Gresham College where members of the British Royal Society met. (Source: Wikimedia)
New Methods
Under the scientific method that was defined and applied in the 17th century, natural and artificial circumstances were abandoned, and a research tradition of systematic experimentation was slowly accepted throughout the scientific community. The philosophy of using an inductive approach to nature (to abandon assumption and to attempt to simply observe with an open mind) was in strict contrast with the earlier, Aristotelian approach of deduction, by which analysis of known facts produced further understanding. In practice, many scientists and philosophers believed that a healthy mix of both was needed—the willingness to both question assumptions, and to interpret observations assumed to have some degree of validity.
During the scientific revolution, changing perceptions about the role of the scientist in respect to nature, the value of evidence, experimental or observed, led towards a scientific methodology in which empiricism played a large, but not absolute, role. The term British empiricism came into use to describe philosophical differences perceived between two of its founders—Francis Bacon, described as empiricist, and René Descartes, who was described as a rationalist. Bacon’s works established and popularized inductive methodologies for scientific inquiry, often called the Baconian method , or sometimes simply the scientific method. His demand for a planned procedure of investigating all things natural marked a new turn in the rhetorical and theoretical framework for science, much of which still surrounds conceptions of proper methodology today. Correspondingly, Descartes distinguished between the knowledge that could be attained by reason alone (rationalist approach), as, for example, in mathematics, and the knowledge that required experience of the world, as in physics.
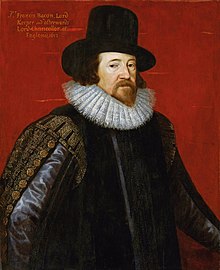
Francis Bacon was a pivotal figure in establishing the scientific method. This portrait was painted by Frans Pourbus the Younger in 1617 (Source: Wikimedia)
Thomas Hobbes, George Berkeley, and David Hume were the primary exponents of empiricism, and developed a sophisticated empirical tradition as the basis of human knowledge. The recognized founder of the approach was John Locke, who proposed in An Essay Concerning Human Understanding (1689) that the only true knowledge that could be accessible to the human mind was that which was based on experience.
Many new ideas contributed to what is called the scientific revolution. Some of them were revolutions in their own fields. These include:
- The heliocentric model that involved the radical displacement of the earth to an orbit around the sun (as opposed to being seen as the center of the universe). Copernicus’ 1543 work on the heliocentric model of the solar system tried to demonstrate that the sun was the center of the universe. The discoveries of Johannes Kepler and Galileo gave the theory credibility and the work culminated in Isaac Newton’s Principia, which formulated the laws of motion and universal gravitation that dominated scientists’ view of the physical universe for the next three centuries.
- Studying human anatomy based upon the dissection of human corpses, rather than the animal dissections, as practiced for centuries.
- Discovering and studying magnetism and electricity, and thus, electric properties of various materials.
- Modernization of disciplines (making them more as what they are today), including dentistry, physiology, chemistry, or optics.
- Invention of tools that deepened the understating of sciences, including mechanical calculator, steam digester (the forerunner of the steam engine), refracting and reflecting telescopes, vacuum pump, or mercury barometer.
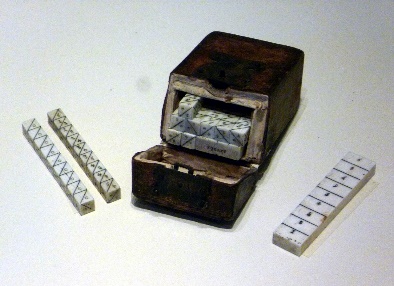
An ivory set of “Napier’s Bones,” an early calculating device invented by John Napier (Source: Wikimedia)
Physics and Mathematics
In the 16th and 17th centuries, European scientists began increasingly applying quantitative measurements to the measurement of physical phenomena on the earth, which translated into the rapid development of mathematics and physics.
Under the scientific method that was defined and applied in the 17th century, natural and artificial circumstances were abandoned, and a research tradition of systematic experimentation was slowly accepted throughout the scientific community. The philosophy of using an inductive approach to nature—to abandon assumption and to attempt to simply observe with an open mind—was in strict contrast with the earlier, Aristotelian approach of deduction, by which analysis of known facts produced further understanding. That principle was particularly true for mathematics and physics. René Descartes, whose thought emphasized the power of reasoning but also helped establish the scientific method, distinguished between the knowledge that could be attained by reason alone (rationalist approach), which he thought was mathematics, and the knowledge that required experience of the world, which he thought was physics.
Mathematization
To the extent that medieval natural philosophers used mathematical problems, they limited social studies to theoretical analyses of local speed and other aspects of life. The actual measurement of a physical quantity, and the comparison of that measurement to a value computed on the basis of theory, was largely limited to the mathematical disciplines of astronomy and optics in Europe. In the 16th and 17th centuries, European scientists began increasingly applying quantitative measurements to the measurement of physical phenomena on Earth.
Galileo Galilei (1564-1642) improved the telescope, with which he made several important astronomical discoveries, including the four largest moons of Jupiter, the phases of Venus, and the rings of Saturn, and made detailed observations of sunspots. He developed the laws for falling bodies based on pioneering quantitative experiments, which he analyzed mathematically.
Galileo was one of the first modern thinkers to clearly state that the laws of nature are mathematical. In broader terms, his work marked another step towards the eventual separation of science from both philosophy and religion, a major development in human thought. Galileo showed a remarkably modern appreciation for the proper relationship between mathematics, theoretical physics, and experimental physics.
Newton’s Principia formulated the laws of motion and universal gravitation, which dominated scientists’ view of the physical universe for the next three centuries. By deriving Kepler’s laws of planetary motion from his mathematical description of gravity, and then using the same principles to account for the trajectories of comets, the tides, the precession of the equinoxes, and other phenomena, Newton removed the last doubts about the validity of the model of the universe that placed the sun (and not the earth) at the center. This work also demonstrated that the motion of objects on Earth, and of celestial bodies, could be described by the same principles. His laws of motion were to be the solid foundation of mechanics; his law of universal gravitation combined terrestrial and celestial mechanics into one great system that seemed to be able to describe the whole world in mathematical formulae. Newton also developed the theory of gravitation.
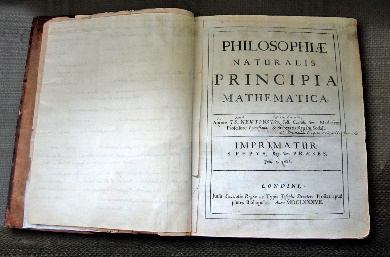
The title page of an early copy of Newton’s Principia. (Source: Wikimedia)
The scientific revolution also witnessed the development of modern optics. Johannes Kepler published Astronomiae Pars Optica ( The Optical Part of Astronomy ) in 1604. In it, he described the inverse-square law governing the intensity of light, reflection by flat and curved mirrors, and principles of pinhole cameras, as well as the astronomical implications of optics, such a parallax and the apparent sizes of heavenly bodies. Willebrord Snellius found the mathematical law of refraction, now known as Snell’s law, in 1621. Subsequently, Descartes showed, by using geometric construction and the law of refraction (also known as Descartes’ law), that the angular radius of a rainbow is 42°. He also independently discovered the law of reflection. Finally, Newton investigated the refraction of light, demonstrating that a prism could decompose white light into a spectrum of colors, and that a lens and a second prism could recompose the multicolored spectrum into white light. He also showed that the colored light does not change its properties by separating out a colored beam and shining it on various objects.
Dr. William Gilbert, in De Magnete , invented the New Latin word electricus from elektron , the Greek word for “amber.” Gilbert undertook a number of careful electrical experiments, in the course of which he discovered that many substances were capable of manifesting electrical properties. He also discovered that a heated body lost its electricity, and that moisture prevented the electrification of all bodies, due to the fact that moisture impaired the insulation of such bodies. He also noticed that electrified substances attracted all other substances indiscriminately, whereas a magnet only attracted iron. The many discoveries of this nature earned for Gilbert the title of “founder of the electrical science.”
Robert Boyle also worked frequently at the new science of electricity and added several substances to Gilbert’s list of electrics. In 1675, he stated that electric attraction and repulsion can act across a vacuum. One of his important discoveries was that electrified bodies in a vacuum would attract light substances, this indicating that the electrical effect did not depend upon the air as a medium. He also added resin to the then known list of electrics. By the end of the 17th Century, researchers had developed practical means of generating electricity by friction with an an electrostatic generator, but the development of electrostatic machines did not begin in earnest until the 18th century, when they became fundamental instruments in the studies about the new science of electricity. The first usage of the word electricity is ascribed to Thomas Browne in 1646 work. In 1729, Stephen Gray demonstrated that electricity could be “transmitted” through metal filaments.
Though astronomy is the oldest of the natural sciences, its development during the scientific revolution entirely transformed societal views about nature by moving from geocentrism (the idea that the earth is the center of the universe) to heliocentrism (the idea that the sun is the center of the universe).
The Emergence of Modern Astronomy
While astronomy is the oldest of the natural sciences, dating back to antiquity, its development during the period of the scientific revolution entirely transformed the views of society about nature. As we have already seen, the publication Nicolaus Copernicus ‘ De revolutionibus orbium coelestium ( On the Revolutions of the Heavenly Spheres ) published in 1543, is, in fact, often seen as marking the beginning of the time when scientific disciplines, including astronomy, began to apply modern empirical research methods, and gradually transformed into the modern sciences as we know them today.
Copernican heliocentrism is the name given to the astronomical model developed by Nicolaus Copernicus and published in 1543. It positioned the sun near the center of the universe, motionless, with Earth and the other planets rotating around it in circular paths, modified by epicycles and at uniform speeds. The Copernican model departed from the Ptolemaic system that prevailed in western culture for centuries, placing Earth at the center of the universe. Copernicus’ De revolutionibus marks the beginning of the shift away from a geocentric (and anthropocentric) universe with Earth at its center. Copernicus held that Earth is another planet revolving around the fixed sun once a year and turning on its axis once a day. But while he put the sun at the center of the celestial spheres, he did not put it at the exact center of the universe, but near it. His system used only uniform circular motions, correcting what was seen by many as the chief inelegance in Ptolemy’s system.
Johannes Kepler
Johannes Kepler was a German scientist. In 1596, he published his first book, the Mysterium cosmographicum , which was the first to openly endorse Copernican cosmology by an astronomer since the 1540s. The book described his model that used Pythagorean mathematics and the five Platonic solids to explain the number of planets, their proportions, and their order.
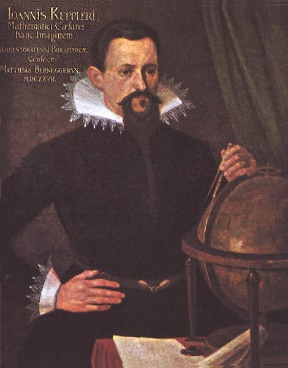
A portrait of Johannes Kepler (Source: Wikimedia)
In 1600, Kepler set to work on the orbit of Mars, the second most eccentric of the six planets known at that time. This work was the basis of his next book, the Astronomia nova (1609). The book argued heliocentrism and ellipses for planetary orbits, instead of circles modified by epicycles. It contains the first two of his eponymous three laws of planetary motion (in 1619, the third law was published). The laws state the following:
- All planets move in elliptical orbits, with the sun at one focus.
- A line that connects a planet to the sun sweeps out equal areas in equal times.
- The time required for a planet to orbit the sun, called its period, is proportional to long axis of the ellipse raised to the 3/2 power. The constant of proportionality is the same for all the planets.
Galileo Galilei
Galileo Galilei was an Italian scientist who is sometimes referred to as the “father of modern observational astronomy.” Based on the designs of Hans Lippershey, he designed his own telescope, which he had improved to 30x magnification. Using this new instrument, Galileo made a number of astronomical observations, which he published in the Sidereus Nuncius in 1610. In this book, he described the surface of the moon as rough, uneven, and imperfect. His observations challenged Aristotle ’s claim that the moon was a perfect sphere, and the larger idea that the heavens were perfect and unchanging. While observing Jupiter over the course of several days, Galileo noticed four stars close to Jupiter whose positions were changing in a way that would be impossible if they were fixed stars. After much observation, he concluded these four stars were orbiting the planet Jupiter and were in fact moons, not stars. This was a radical discovery because, according to Aristotelian cosmology, all heavenly bodies revolve around Earth, and a planet with moons obviously contradicted that popular belief. While contradicting Aristotelian belief, it supported Copernican cosmology, which stated that Earth is a planet like all others.
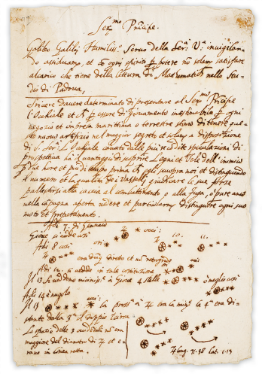
On this page, Galileo first noted the moons of Jupiter. (Source: Wikimedia)
In 1610, Galileo also observed that Venus had a full set of phases, similar to the phases of the moon, that we can observe from Earth. This was explainable by the Copernican system, which said that all phases of Venus would be visible due to the nature of its orbit around the sun, unlike the Ptolemaic system, which stated only some of Venus’s phases would be visible. Due to Galileo’s observations of Venus, Ptolemy’s system became highly suspect, and the majority of leading astronomers subsequently converted to various heliocentric models, making his discovery one of the most influential in the transition from geocentrism to heliocentrism.
Uniting Astronomy and Physics: Isaac Newton
Isaac Newton developed further ties between physics and astronomy through his law of universal gravitation. Realizing that the same force that attracted objects to the surface of Earth held the moon in orbit around the Earth, Newton was able to explain, in one theoretical framework, all known gravitational phenomena. Newton’s Principia (1687) formulated the laws of motion and universal gravitation, which dominated scientists’ view of the physical universe for the next three centuries. By deriving Kepler’s laws of planetary motion from his mathematical description of gravity, and then using the same principles to account for the trajectories of comets, the tides, the precession of the equinoxes, and other phenomena, Newton removed the last doubts about the validity of the heliocentric model of the cosmos. This work also demonstrated that the motion of objects on Earth and of celestial bodies could be described by the same principles. His laws of motion were to be the solid foundation of mechanics; his law of universal gravitation combined terrestrial and celestial mechanics into one great system that seemed to be able to describe the whole world in mathematical formulae.
The Renaissance had brought an intense focus on varied scholarship to Europe in the 15 th century. A major effort to translate the Arabic and Greek scientific works into Latin emerged, and Europeans gradually became experts not only in the ancient writings of the Romans and Greeks, but also in the contemporary writings of Islamic scientists. During the 16 th and 17 th centuries, experimental investigation, particularly in the field of dissection and body examination, advanced the knowledge of human anatomy. Other developments of the period also contributed to the modernization of medical research, including printed books that allowed for a wider distribution of medical ideas and anatomical diagrams, more open attitudes of Renaissance humanism, and the Church’s diminishing impact on the teachings of the medical profession and universities. In addition, the invention and popularization of microscope in the 17th century greatly advanced medical research.
Human Anatomy
The writings of ancient Roman physician Galen had dominated European thinking in medicine. Galen’s understanding of anatomy and medicine was principally influenced by the then-current theory of humorism (also known as the four humors: black bile, yellow bile, blood, and phlegm), as advanced by ancient Greek physicians, such as Hippocrates. His theories dominated and influenced western medical science for more than 1,300 years. His anatomical reports, based mainly on dissection of monkeys and pigs, remained uncontested until 1543, when printed descriptions and illustrations of human dissections were published in the seminal work De humani corporis fabrica by Andreas Vesalius, who first demonstrated the mistakes in the Galenic model. His anatomical teachings were based upon the dissection of human corpses, rather than the animal dissections that Galen had used as a guide. Vesalius’ work emphasized the priority of dissection and what has come to be called the “anatomical” view of the body, seeing human internal functioning as an essentially corporeal structure filled with organs arranged in three-dimensional space. This was in stark contrast to many of the anatomical models used previously.
Further groundbreaking work was carried out by William Harvey, who published De Motu Cordis in 1628. Harvey made a detailed analysis of the overall structure of the heart, going on to an analysis of the arteries, showing how their pulsation depends upon the contraction of the left ventricle, while the contraction of the right ventricle propels its charge of blood into the pulmonary artery. He noticed that the two ventricles move together almost simultaneously and not independently like had been thought previously by his predecessors. Harvey also estimated the capacity of the heart, how much blood is expelled through each pump of the heart, and the number of times the heart beats in a half an hour. From these estimations, he went on to prove how the blood circulated in a circle through the body.
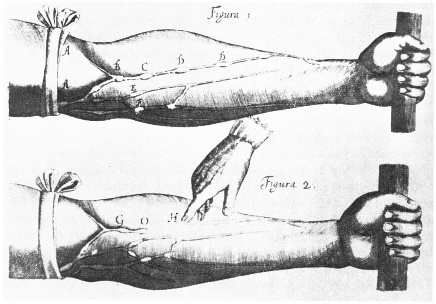
Image of veins from William Harvey’s De Motu Cordis. (Source: Wikimedia)
Other Medical Advances
Various other advances in medical understanding and practice were made. French surgeon Ambroise Paré (c. 1510-1590) is considered one of the fathers of surgery and modern forensic pathology, and a pioneer in surgical techniques and battlefield medicine, especially in the treatment of wounds. He was also an anatomist who invented several surgical instruments and was part of the Parisian Barber Surgeon guild. Paré was also an important figure in the progress of obstetrics in the middle of the 16th century.
Herman Boerhaave (1668-1738), a Dutch botanist, chemist, Christian humanist and physician of European fame, is regarded as the founder of clinical teaching and of the modern academic hospital. He is sometimes referred to as “the father of physiology,” along with the Venetian physician Santorio Santorio (1561-1636), who introduced the quantitative approach into medicine, and with his pupil Albrecht von Haller (1708-1777). He is best known for demonstrating the relation of symptoms to lesions and, in addition, he was the first to isolate the chemical urea from urine. He was the first physician that put thermometer measurements to clinical practice.
Bacteria and protists were first observed with a microscope by Antonie van Leeuwenhoek in 1676, initiating the scientific field of microbiology.
French physician Pierre Fauchard started dentistry science as we know it today, and he has been named “the father of modern dentistry.” He is widely known for writing the first complete scientific description of dentistry, Le Chirurgien Dentiste ( “The Surgeon Dentist” ), published in 1728. The book described basic oral anatomy and function, signs and symptoms of oral pathology, operative methods for removing decay and restoring teeth, periodontal disease (pyorrhea), orthodontics, replacement of missing teeth, and tooth transplantation.
A Brief History of the World Since 1500 Copyright © by John Mack is licensed under a Creative Commons Attribution-NonCommercial-ShareAlike 4.0 International License , except where otherwise noted.
Share This Book
Francis Bacon and the Scientific Revolution
The Four Humors, from Deutche Kalendar, 1498 (Pierpont Morgan Library)
How do we know that something is true?
The word science comes from the latin root scientia , meaning knowledge. But where does the knowledge that makes up science come from? How do you ever really know that something is true?
For instance, modern science tell us that some types of disease spread through tiny organisms. Medieval people believed instead that sickness arose from an imbalance of the body’s four humors. How do we know with certainty that modern science is correct? Microscopes enable us to see the germs that cause sickness, but when we look through microscopic lenses to examine microbes, how do we know our understanding of what they are and what they are doing is true? Of course, medieval philosophers did not have microscopic lenses—but if they did, they very likely would have disagreed with our modern understanding of disease. Believing in the inaccuracy of the human senses, and moreover of the human mind’s inability to correctly judge anything, medieval knowledge instead privileged ancient texts as the best way of making sense of the world.
Sir Francis Bacon
Francis Bacon, c. 1622, oil on canvas, 470 x 610 cm (Dulwich Picture Gallery)
In 1620, around the time that people first began to look through microscopes, an English politician named Sir Francis Bacon developed a method for philosophers to use in weighing the truthfulness of knowledge. While Bacon agreed with medieval thinkers that humans too often erred in interpreting what their five senses perceived, he also realized that people’s sensory experiences provided the best possible means of making sense of the world. Because humans could incorrectly interpret anything they saw, heard, smelled, tasted, or felt, Bacon insisted that they must doubt everything before assuming its truth.
Testing hypotheses
In order to test potential truths, or hypotheses, Bacon devised a method whereby scientists set up experiments to manipulate nature, and attempt to prove their hypotheses wrong. For example, in order to test the idea that sickness came from external causes, Bacon argued that scientists should expose healthy people to outside influences such as coldness, wetness, or other sick people to discover if any of these external variables resulted in more people getting sick. Knowing that many different causes for sickness might be missed by humans who are unable or unwilling to perceive them, Bacon insisted that experiments must be consistently repeated before truth can be known: a scientist must show that patients exposed to a specific variable more frequently got sick again, and again, and again.
Frontispiece for the Opere di Galileo Galilei , 1656, etching, 17.8 x 24.9 (The Museum of Fine Arts, Houston). Galileo is shown kneeling before personifications of mathematics (holding a compass), astronomy (with the crown of stars) and optics.
Although modern scientists have revised many of the truths subsequently adopted by Bacon and his contemporaries, we still utilize the method of proving knowledge to be true via doubt and experimentation that Bacon laid out in 1620. Bacon’s philosophical work marks a very significant breakthrough for the study of the world around us, but it is important to stress that this method of investigation was not completed in a vacuum. Rather, Bacon’s work should be seen as a part of a widespread cultural revolution accelerated by the rise of the printing press in the fifteenth century.
Importance of the printing press
Advances in the ability to disseminate new ideas by making standardized letters, numbers, and diagrams repeatable allowed for an unprecedented level of cooperation among philosophers who could now build on each other’s ideas over long periods of time. It would be difficult to overstate the effect of the print revolution. Astronomers such as Copernicus and Galileo began to share and build upon their experiments, and religious reformers began to publicize new (and increasingly radical) Protestant ideas. In a mutually beneficial relationship the Protestant Reformation and the Scientific Revolution encouraged philosophers to discover all they could about nature as a way to learn more about God, an undertaking that promoted a break with past authorities.
A direct engagement with nature
Artisans and craftspeople soon began engaging in the new “natural philosophy,” exemplifying the fact that a monumental shift in what constituted evidence for truth was under way. Not only did renaissance artisans create lenses to see, tools to measure, and artworks to replicate the natural world, but by the sixteenth century, they began to publish philosophical treatises asserting that through the imitation and reproduction of nature in their arts, they were able to achieve a state of direct engagement with nature. Rather than taking knowledge from ancient sources, they argued that true knowledge came from direct experience. Alchemists likewise prioritized direct engagement with nature. In using fire to divide elements into their “smallest” components (and discovering that there were more than four of them), alchemists promoted the revolutionary idea that observation of nature itself, rather than reliance on ancient authorities, provided the best foundation for knowledge.
Attributed to Bernard Palissy, Oval Basin , c. 1550, lead-glazed earthenware, 18 7/8 x 14 1/2″ (The J. Paul Getty Museum)
The Royal Society
These new ideas crystallized with the work of Francis Bacon. In his work as a politician he called for the development of an institution that would promote and regulate the acquisition of knowledge derived from observation. After considerable delay, caused by a civil war and the execution of King Charles I, the Royal Society for Improving Natural Knowledge was founded in 1660. A gentleman’s club composed of tinkering aristocrats, the Royal Society promoted Bacon’s principles of exact observation and measurement of experiments in its periodical Philosophical Transactions of the Royal Society, generally credited as being the first scientific journal.
Frontispiece to Thomas Sprat, The History of the Royal-Society of London , etching by Winceslaus Hollar, after John Evelyn, 1667. “The book was a manifesto of the Society’s aims and methods….primarily aimed at the king in the (unrealised) hope that he would fund their future activities. The frontispiece flatters Charles II by presenting him as a classical bust being wreathed by an allegorical figure of Fame. The Society President, Viscount Brouncker, points to the Latin inscription ‘Charles II founder and Patron of the Royal Society.’ Francis Bacon, gesturing towards an array of scientific instruments, is indentified as the ‘Renewer of Arts’.” (National Portrait Gallery, London)
Once Bacon’s philosophies regarding experimentation and observation came to be accepted, people began using them to harness nature for profit. The study of nature came to be less about changing traditional attitudes and beliefs, and more about stimulating the economy. By the end of the following century, the Scientific Revolution had given birth to an Industrial Revolution which dramatically transformed the daily lives of people around the world. Western society has been moving forward on Bacon’s model for the past three hundred years. Perhaps though, we are in danger of forgetting the vital role doubt played in Bacon’s philosophy. Even with powerful microscopes, there is still a lot that human senses miss.
Additional resources:
In Our Time podcast on Baconian Science
Cite this page
Your donations help make art history free and accessible to everyone!
- Search Menu
- Browse content in A - General Economics and Teaching
- Browse content in A1 - General Economics
- A11 - Role of Economics; Role of Economists; Market for Economists
- Browse content in B - History of Economic Thought, Methodology, and Heterodox Approaches
- Browse content in B4 - Economic Methodology
- B49 - Other
- Browse content in C - Mathematical and Quantitative Methods
- Browse content in C0 - General
- C00 - General
- C01 - Econometrics
- Browse content in C1 - Econometric and Statistical Methods and Methodology: General
- C10 - General
- C11 - Bayesian Analysis: General
- C12 - Hypothesis Testing: General
- C13 - Estimation: General
- C14 - Semiparametric and Nonparametric Methods: General
- C18 - Methodological Issues: General
- Browse content in C2 - Single Equation Models; Single Variables
- C21 - Cross-Sectional Models; Spatial Models; Treatment Effect Models; Quantile Regressions
- C23 - Panel Data Models; Spatio-temporal Models
- C26 - Instrumental Variables (IV) Estimation
- Browse content in C3 - Multiple or Simultaneous Equation Models; Multiple Variables
- C30 - General
- C31 - Cross-Sectional Models; Spatial Models; Treatment Effect Models; Quantile Regressions; Social Interaction Models
- C32 - Time-Series Models; Dynamic Quantile Regressions; Dynamic Treatment Effect Models; Diffusion Processes; State Space Models
- C35 - Discrete Regression and Qualitative Choice Models; Discrete Regressors; Proportions
- Browse content in C4 - Econometric and Statistical Methods: Special Topics
- C40 - General
- Browse content in C5 - Econometric Modeling
- C52 - Model Evaluation, Validation, and Selection
- C53 - Forecasting and Prediction Methods; Simulation Methods
- C55 - Large Data Sets: Modeling and Analysis
- Browse content in C6 - Mathematical Methods; Programming Models; Mathematical and Simulation Modeling
- C63 - Computational Techniques; Simulation Modeling
- C67 - Input-Output Models
- Browse content in C7 - Game Theory and Bargaining Theory
- C71 - Cooperative Games
- C72 - Noncooperative Games
- C73 - Stochastic and Dynamic Games; Evolutionary Games; Repeated Games
- C78 - Bargaining Theory; Matching Theory
- C79 - Other
- Browse content in C8 - Data Collection and Data Estimation Methodology; Computer Programs
- C83 - Survey Methods; Sampling Methods
- Browse content in C9 - Design of Experiments
- C90 - General
- C91 - Laboratory, Individual Behavior
- C92 - Laboratory, Group Behavior
- C93 - Field Experiments
- C99 - Other
- Browse content in D - Microeconomics
- Browse content in D0 - General
- D00 - General
- D01 - Microeconomic Behavior: Underlying Principles
- D02 - Institutions: Design, Formation, Operations, and Impact
- D03 - Behavioral Microeconomics: Underlying Principles
- D04 - Microeconomic Policy: Formulation; Implementation, and Evaluation
- Browse content in D1 - Household Behavior and Family Economics
- D10 - General
- D11 - Consumer Economics: Theory
- D12 - Consumer Economics: Empirical Analysis
- D13 - Household Production and Intrahousehold Allocation
- D14 - Household Saving; Personal Finance
- D15 - Intertemporal Household Choice: Life Cycle Models and Saving
- D18 - Consumer Protection
- Browse content in D2 - Production and Organizations
- D20 - General
- D21 - Firm Behavior: Theory
- D22 - Firm Behavior: Empirical Analysis
- D23 - Organizational Behavior; Transaction Costs; Property Rights
- D24 - Production; Cost; Capital; Capital, Total Factor, and Multifactor Productivity; Capacity
- Browse content in D3 - Distribution
- D30 - General
- D31 - Personal Income, Wealth, and Their Distributions
- D33 - Factor Income Distribution
- Browse content in D4 - Market Structure, Pricing, and Design
- D40 - General
- D41 - Perfect Competition
- D42 - Monopoly
- D43 - Oligopoly and Other Forms of Market Imperfection
- D44 - Auctions
- D47 - Market Design
- D49 - Other
- Browse content in D5 - General Equilibrium and Disequilibrium
- D50 - General
- D51 - Exchange and Production Economies
- D52 - Incomplete Markets
- D53 - Financial Markets
- D57 - Input-Output Tables and Analysis
- Browse content in D6 - Welfare Economics
- D60 - General
- D61 - Allocative Efficiency; Cost-Benefit Analysis
- D62 - Externalities
- D63 - Equity, Justice, Inequality, and Other Normative Criteria and Measurement
- D64 - Altruism; Philanthropy
- D69 - Other
- Browse content in D7 - Analysis of Collective Decision-Making
- D70 - General
- D71 - Social Choice; Clubs; Committees; Associations
- D72 - Political Processes: Rent-seeking, Lobbying, Elections, Legislatures, and Voting Behavior
- D73 - Bureaucracy; Administrative Processes in Public Organizations; Corruption
- D74 - Conflict; Conflict Resolution; Alliances; Revolutions
- D78 - Positive Analysis of Policy Formulation and Implementation
- Browse content in D8 - Information, Knowledge, and Uncertainty
- D80 - General
- D81 - Criteria for Decision-Making under Risk and Uncertainty
- D82 - Asymmetric and Private Information; Mechanism Design
- D83 - Search; Learning; Information and Knowledge; Communication; Belief; Unawareness
- D84 - Expectations; Speculations
- D85 - Network Formation and Analysis: Theory
- D86 - Economics of Contract: Theory
- D89 - Other
- Browse content in D9 - Micro-Based Behavioral Economics
- D90 - General
- D91 - Role and Effects of Psychological, Emotional, Social, and Cognitive Factors on Decision Making
- D92 - Intertemporal Firm Choice, Investment, Capacity, and Financing
- Browse content in E - Macroeconomics and Monetary Economics
- Browse content in E0 - General
- E00 - General
- E01 - Measurement and Data on National Income and Product Accounts and Wealth; Environmental Accounts
- E02 - Institutions and the Macroeconomy
- E03 - Behavioral Macroeconomics
- Browse content in E1 - General Aggregative Models
- E10 - General
- E12 - Keynes; Keynesian; Post-Keynesian
- E13 - Neoclassical
- Browse content in E2 - Consumption, Saving, Production, Investment, Labor Markets, and Informal Economy
- E20 - General
- E21 - Consumption; Saving; Wealth
- E22 - Investment; Capital; Intangible Capital; Capacity
- E23 - Production
- E24 - Employment; Unemployment; Wages; Intergenerational Income Distribution; Aggregate Human Capital; Aggregate Labor Productivity
- E25 - Aggregate Factor Income Distribution
- Browse content in E3 - Prices, Business Fluctuations, and Cycles
- E30 - General
- E31 - Price Level; Inflation; Deflation
- E32 - Business Fluctuations; Cycles
- E37 - Forecasting and Simulation: Models and Applications
- Browse content in E4 - Money and Interest Rates
- E40 - General
- E41 - Demand for Money
- E42 - Monetary Systems; Standards; Regimes; Government and the Monetary System; Payment Systems
- E43 - Interest Rates: Determination, Term Structure, and Effects
- E44 - Financial Markets and the Macroeconomy
- Browse content in E5 - Monetary Policy, Central Banking, and the Supply of Money and Credit
- E50 - General
- E51 - Money Supply; Credit; Money Multipliers
- E52 - Monetary Policy
- E58 - Central Banks and Their Policies
- Browse content in E6 - Macroeconomic Policy, Macroeconomic Aspects of Public Finance, and General Outlook
- E60 - General
- E62 - Fiscal Policy
- E66 - General Outlook and Conditions
- Browse content in E7 - Macro-Based Behavioral Economics
- E71 - Role and Effects of Psychological, Emotional, Social, and Cognitive Factors on the Macro Economy
- Browse content in F - International Economics
- Browse content in F0 - General
- F00 - General
- Browse content in F1 - Trade
- F10 - General
- F11 - Neoclassical Models of Trade
- F12 - Models of Trade with Imperfect Competition and Scale Economies; Fragmentation
- F13 - Trade Policy; International Trade Organizations
- F14 - Empirical Studies of Trade
- F15 - Economic Integration
- F16 - Trade and Labor Market Interactions
- F18 - Trade and Environment
- Browse content in F2 - International Factor Movements and International Business
- F20 - General
- F21 - International Investment; Long-Term Capital Movements
- F22 - International Migration
- F23 - Multinational Firms; International Business
- Browse content in F3 - International Finance
- F30 - General
- F31 - Foreign Exchange
- F32 - Current Account Adjustment; Short-Term Capital Movements
- F34 - International Lending and Debt Problems
- F35 - Foreign Aid
- F36 - Financial Aspects of Economic Integration
- Browse content in F4 - Macroeconomic Aspects of International Trade and Finance
- F40 - General
- F41 - Open Economy Macroeconomics
- F42 - International Policy Coordination and Transmission
- F43 - Economic Growth of Open Economies
- F44 - International Business Cycles
- Browse content in F5 - International Relations, National Security, and International Political Economy
- F50 - General
- F51 - International Conflicts; Negotiations; Sanctions
- F52 - National Security; Economic Nationalism
- F55 - International Institutional Arrangements
- Browse content in F6 - Economic Impacts of Globalization
- F60 - General
- F61 - Microeconomic Impacts
- F63 - Economic Development
- Browse content in G - Financial Economics
- Browse content in G0 - General
- G00 - General
- G01 - Financial Crises
- G02 - Behavioral Finance: Underlying Principles
- Browse content in G1 - General Financial Markets
- G10 - General
- G11 - Portfolio Choice; Investment Decisions
- G12 - Asset Pricing; Trading volume; Bond Interest Rates
- G14 - Information and Market Efficiency; Event Studies; Insider Trading
- G15 - International Financial Markets
- G18 - Government Policy and Regulation
- Browse content in G2 - Financial Institutions and Services
- G20 - General
- G21 - Banks; Depository Institutions; Micro Finance Institutions; Mortgages
- G22 - Insurance; Insurance Companies; Actuarial Studies
- G23 - Non-bank Financial Institutions; Financial Instruments; Institutional Investors
- G24 - Investment Banking; Venture Capital; Brokerage; Ratings and Ratings Agencies
- G28 - Government Policy and Regulation
- Browse content in G3 - Corporate Finance and Governance
- G30 - General
- G31 - Capital Budgeting; Fixed Investment and Inventory Studies; Capacity
- G32 - Financing Policy; Financial Risk and Risk Management; Capital and Ownership Structure; Value of Firms; Goodwill
- G33 - Bankruptcy; Liquidation
- G34 - Mergers; Acquisitions; Restructuring; Corporate Governance
- G38 - Government Policy and Regulation
- Browse content in G4 - Behavioral Finance
- G40 - General
- G41 - Role and Effects of Psychological, Emotional, Social, and Cognitive Factors on Decision Making in Financial Markets
- Browse content in G5 - Household Finance
- G50 - General
- G51 - Household Saving, Borrowing, Debt, and Wealth
- Browse content in H - Public Economics
- Browse content in H0 - General
- H00 - General
- Browse content in H1 - Structure and Scope of Government
- H10 - General
- H11 - Structure, Scope, and Performance of Government
- Browse content in H2 - Taxation, Subsidies, and Revenue
- H20 - General
- H21 - Efficiency; Optimal Taxation
- H22 - Incidence
- H23 - Externalities; Redistributive Effects; Environmental Taxes and Subsidies
- H24 - Personal Income and Other Nonbusiness Taxes and Subsidies; includes inheritance and gift taxes
- H25 - Business Taxes and Subsidies
- H26 - Tax Evasion and Avoidance
- Browse content in H3 - Fiscal Policies and Behavior of Economic Agents
- H31 - Household
- Browse content in H4 - Publicly Provided Goods
- H40 - General
- H41 - Public Goods
- H42 - Publicly Provided Private Goods
- H44 - Publicly Provided Goods: Mixed Markets
- Browse content in H5 - National Government Expenditures and Related Policies
- H50 - General
- H51 - Government Expenditures and Health
- H52 - Government Expenditures and Education
- H53 - Government Expenditures and Welfare Programs
- H54 - Infrastructures; Other Public Investment and Capital Stock
- H55 - Social Security and Public Pensions
- H56 - National Security and War
- H57 - Procurement
- Browse content in H6 - National Budget, Deficit, and Debt
- H63 - Debt; Debt Management; Sovereign Debt
- Browse content in H7 - State and Local Government; Intergovernmental Relations
- H70 - General
- H71 - State and Local Taxation, Subsidies, and Revenue
- H73 - Interjurisdictional Differentials and Their Effects
- H75 - State and Local Government: Health; Education; Welfare; Public Pensions
- H76 - State and Local Government: Other Expenditure Categories
- H77 - Intergovernmental Relations; Federalism; Secession
- Browse content in H8 - Miscellaneous Issues
- H81 - Governmental Loans; Loan Guarantees; Credits; Grants; Bailouts
- H83 - Public Administration; Public Sector Accounting and Audits
- H87 - International Fiscal Issues; International Public Goods
- Browse content in I - Health, Education, and Welfare
- Browse content in I0 - General
- I00 - General
- Browse content in I1 - Health
- I10 - General
- I11 - Analysis of Health Care Markets
- I12 - Health Behavior
- I13 - Health Insurance, Public and Private
- I14 - Health and Inequality
- I15 - Health and Economic Development
- I18 - Government Policy; Regulation; Public Health
- Browse content in I2 - Education and Research Institutions
- I20 - General
- I21 - Analysis of Education
- I22 - Educational Finance; Financial Aid
- I23 - Higher Education; Research Institutions
- I24 - Education and Inequality
- I25 - Education and Economic Development
- I26 - Returns to Education
- I28 - Government Policy
- Browse content in I3 - Welfare, Well-Being, and Poverty
- I30 - General
- I31 - General Welfare
- I32 - Measurement and Analysis of Poverty
- I38 - Government Policy; Provision and Effects of Welfare Programs
- Browse content in J - Labor and Demographic Economics
- Browse content in J0 - General
- J00 - General
- J01 - Labor Economics: General
- J08 - Labor Economics Policies
- Browse content in J1 - Demographic Economics
- J10 - General
- J12 - Marriage; Marital Dissolution; Family Structure; Domestic Abuse
- J13 - Fertility; Family Planning; Child Care; Children; Youth
- J14 - Economics of the Elderly; Economics of the Handicapped; Non-Labor Market Discrimination
- J15 - Economics of Minorities, Races, Indigenous Peoples, and Immigrants; Non-labor Discrimination
- J16 - Economics of Gender; Non-labor Discrimination
- J18 - Public Policy
- Browse content in J2 - Demand and Supply of Labor
- J20 - General
- J21 - Labor Force and Employment, Size, and Structure
- J22 - Time Allocation and Labor Supply
- J23 - Labor Demand
- J24 - Human Capital; Skills; Occupational Choice; Labor Productivity
- Browse content in J3 - Wages, Compensation, and Labor Costs
- J30 - General
- J31 - Wage Level and Structure; Wage Differentials
- J33 - Compensation Packages; Payment Methods
- J38 - Public Policy
- Browse content in J4 - Particular Labor Markets
- J40 - General
- J42 - Monopsony; Segmented Labor Markets
- J44 - Professional Labor Markets; Occupational Licensing
- J45 - Public Sector Labor Markets
- J48 - Public Policy
- J49 - Other
- Browse content in J5 - Labor-Management Relations, Trade Unions, and Collective Bargaining
- J50 - General
- J51 - Trade Unions: Objectives, Structure, and Effects
- J53 - Labor-Management Relations; Industrial Jurisprudence
- Browse content in J6 - Mobility, Unemployment, Vacancies, and Immigrant Workers
- J60 - General
- J61 - Geographic Labor Mobility; Immigrant Workers
- J62 - Job, Occupational, and Intergenerational Mobility
- J63 - Turnover; Vacancies; Layoffs
- J64 - Unemployment: Models, Duration, Incidence, and Job Search
- J65 - Unemployment Insurance; Severance Pay; Plant Closings
- J68 - Public Policy
- Browse content in J7 - Labor Discrimination
- J71 - Discrimination
- J78 - Public Policy
- Browse content in J8 - Labor Standards: National and International
- J81 - Working Conditions
- J88 - Public Policy
- Browse content in K - Law and Economics
- Browse content in K0 - General
- K00 - General
- Browse content in K1 - Basic Areas of Law
- K14 - Criminal Law
- K2 - Regulation and Business Law
- Browse content in K3 - Other Substantive Areas of Law
- K31 - Labor Law
- Browse content in K4 - Legal Procedure, the Legal System, and Illegal Behavior
- K40 - General
- K41 - Litigation Process
- K42 - Illegal Behavior and the Enforcement of Law
- Browse content in L - Industrial Organization
- Browse content in L0 - General
- L00 - General
- Browse content in L1 - Market Structure, Firm Strategy, and Market Performance
- L10 - General
- L11 - Production, Pricing, and Market Structure; Size Distribution of Firms
- L13 - Oligopoly and Other Imperfect Markets
- L14 - Transactional Relationships; Contracts and Reputation; Networks
- L15 - Information and Product Quality; Standardization and Compatibility
- L16 - Industrial Organization and Macroeconomics: Industrial Structure and Structural Change; Industrial Price Indices
- L19 - Other
- Browse content in L2 - Firm Objectives, Organization, and Behavior
- L21 - Business Objectives of the Firm
- L22 - Firm Organization and Market Structure
- L23 - Organization of Production
- L24 - Contracting Out; Joint Ventures; Technology Licensing
- L25 - Firm Performance: Size, Diversification, and Scope
- L26 - Entrepreneurship
- Browse content in L3 - Nonprofit Organizations and Public Enterprise
- L33 - Comparison of Public and Private Enterprises and Nonprofit Institutions; Privatization; Contracting Out
- Browse content in L4 - Antitrust Issues and Policies
- L40 - General
- L41 - Monopolization; Horizontal Anticompetitive Practices
- L42 - Vertical Restraints; Resale Price Maintenance; Quantity Discounts
- Browse content in L5 - Regulation and Industrial Policy
- L50 - General
- L51 - Economics of Regulation
- Browse content in L6 - Industry Studies: Manufacturing
- L60 - General
- L62 - Automobiles; Other Transportation Equipment; Related Parts and Equipment
- L63 - Microelectronics; Computers; Communications Equipment
- L66 - Food; Beverages; Cosmetics; Tobacco; Wine and Spirits
- Browse content in L7 - Industry Studies: Primary Products and Construction
- L71 - Mining, Extraction, and Refining: Hydrocarbon Fuels
- L73 - Forest Products
- Browse content in L8 - Industry Studies: Services
- L81 - Retail and Wholesale Trade; e-Commerce
- L83 - Sports; Gambling; Recreation; Tourism
- L84 - Personal, Professional, and Business Services
- L86 - Information and Internet Services; Computer Software
- Browse content in L9 - Industry Studies: Transportation and Utilities
- L91 - Transportation: General
- L93 - Air Transportation
- L94 - Electric Utilities
- Browse content in M - Business Administration and Business Economics; Marketing; Accounting; Personnel Economics
- Browse content in M1 - Business Administration
- M11 - Production Management
- M12 - Personnel Management; Executives; Executive Compensation
- M14 - Corporate Culture; Social Responsibility
- Browse content in M2 - Business Economics
- M21 - Business Economics
- Browse content in M3 - Marketing and Advertising
- M31 - Marketing
- M37 - Advertising
- Browse content in M4 - Accounting and Auditing
- M42 - Auditing
- M48 - Government Policy and Regulation
- Browse content in M5 - Personnel Economics
- M50 - General
- M51 - Firm Employment Decisions; Promotions
- M52 - Compensation and Compensation Methods and Their Effects
- M53 - Training
- M54 - Labor Management
- Browse content in N - Economic History
- Browse content in N0 - General
- N00 - General
- N01 - Development of the Discipline: Historiographical; Sources and Methods
- Browse content in N1 - Macroeconomics and Monetary Economics; Industrial Structure; Growth; Fluctuations
- N10 - General, International, or Comparative
- N11 - U.S.; Canada: Pre-1913
- N12 - U.S.; Canada: 1913-
- N13 - Europe: Pre-1913
- N17 - Africa; Oceania
- Browse content in N2 - Financial Markets and Institutions
- N20 - General, International, or Comparative
- N22 - U.S.; Canada: 1913-
- N23 - Europe: Pre-1913
- Browse content in N3 - Labor and Consumers, Demography, Education, Health, Welfare, Income, Wealth, Religion, and Philanthropy
- N30 - General, International, or Comparative
- N31 - U.S.; Canada: Pre-1913
- N32 - U.S.; Canada: 1913-
- N33 - Europe: Pre-1913
- N34 - Europe: 1913-
- N36 - Latin America; Caribbean
- N37 - Africa; Oceania
- Browse content in N4 - Government, War, Law, International Relations, and Regulation
- N40 - General, International, or Comparative
- N41 - U.S.; Canada: Pre-1913
- N42 - U.S.; Canada: 1913-
- N43 - Europe: Pre-1913
- N44 - Europe: 1913-
- N45 - Asia including Middle East
- N47 - Africa; Oceania
- Browse content in N5 - Agriculture, Natural Resources, Environment, and Extractive Industries
- N50 - General, International, or Comparative
- N51 - U.S.; Canada: Pre-1913
- Browse content in N6 - Manufacturing and Construction
- N63 - Europe: Pre-1913
- Browse content in N7 - Transport, Trade, Energy, Technology, and Other Services
- N71 - U.S.; Canada: Pre-1913
- Browse content in N8 - Micro-Business History
- N82 - U.S.; Canada: 1913-
- Browse content in N9 - Regional and Urban History
- N91 - U.S.; Canada: Pre-1913
- N92 - U.S.; Canada: 1913-
- N93 - Europe: Pre-1913
- N94 - Europe: 1913-
- Browse content in O - Economic Development, Innovation, Technological Change, and Growth
- Browse content in O1 - Economic Development
- O10 - General
- O11 - Macroeconomic Analyses of Economic Development
- O12 - Microeconomic Analyses of Economic Development
- O13 - Agriculture; Natural Resources; Energy; Environment; Other Primary Products
- O14 - Industrialization; Manufacturing and Service Industries; Choice of Technology
- O15 - Human Resources; Human Development; Income Distribution; Migration
- O16 - Financial Markets; Saving and Capital Investment; Corporate Finance and Governance
- O17 - Formal and Informal Sectors; Shadow Economy; Institutional Arrangements
- O18 - Urban, Rural, Regional, and Transportation Analysis; Housing; Infrastructure
- O19 - International Linkages to Development; Role of International Organizations
- Browse content in O2 - Development Planning and Policy
- O23 - Fiscal and Monetary Policy in Development
- O25 - Industrial Policy
- Browse content in O3 - Innovation; Research and Development; Technological Change; Intellectual Property Rights
- O30 - General
- O31 - Innovation and Invention: Processes and Incentives
- O32 - Management of Technological Innovation and R&D
- O33 - Technological Change: Choices and Consequences; Diffusion Processes
- O34 - Intellectual Property and Intellectual Capital
- O38 - Government Policy
- Browse content in O4 - Economic Growth and Aggregate Productivity
- O40 - General
- O41 - One, Two, and Multisector Growth Models
- O43 - Institutions and Growth
- O44 - Environment and Growth
- O47 - Empirical Studies of Economic Growth; Aggregate Productivity; Cross-Country Output Convergence
- Browse content in O5 - Economywide Country Studies
- O52 - Europe
- O53 - Asia including Middle East
- O55 - Africa
- Browse content in P - Economic Systems
- Browse content in P0 - General
- P00 - General
- Browse content in P1 - Capitalist Systems
- P10 - General
- P16 - Political Economy
- P17 - Performance and Prospects
- P18 - Energy: Environment
- Browse content in P2 - Socialist Systems and Transitional Economies
- P26 - Political Economy; Property Rights
- Browse content in P3 - Socialist Institutions and Their Transitions
- P37 - Legal Institutions; Illegal Behavior
- Browse content in P4 - Other Economic Systems
- P48 - Political Economy; Legal Institutions; Property Rights; Natural Resources; Energy; Environment; Regional Studies
- Browse content in P5 - Comparative Economic Systems
- P51 - Comparative Analysis of Economic Systems
- Browse content in Q - Agricultural and Natural Resource Economics; Environmental and Ecological Economics
- Browse content in Q1 - Agriculture
- Q10 - General
- Q12 - Micro Analysis of Farm Firms, Farm Households, and Farm Input Markets
- Q13 - Agricultural Markets and Marketing; Cooperatives; Agribusiness
- Q14 - Agricultural Finance
- Q15 - Land Ownership and Tenure; Land Reform; Land Use; Irrigation; Agriculture and Environment
- Q16 - R&D; Agricultural Technology; Biofuels; Agricultural Extension Services
- Browse content in Q2 - Renewable Resources and Conservation
- Q25 - Water
- Browse content in Q3 - Nonrenewable Resources and Conservation
- Q32 - Exhaustible Resources and Economic Development
- Q34 - Natural Resources and Domestic and International Conflicts
- Browse content in Q4 - Energy
- Q41 - Demand and Supply; Prices
- Q48 - Government Policy
- Browse content in Q5 - Environmental Economics
- Q50 - General
- Q51 - Valuation of Environmental Effects
- Q53 - Air Pollution; Water Pollution; Noise; Hazardous Waste; Solid Waste; Recycling
- Q54 - Climate; Natural Disasters; Global Warming
- Q56 - Environment and Development; Environment and Trade; Sustainability; Environmental Accounts and Accounting; Environmental Equity; Population Growth
- Q58 - Government Policy
- Browse content in R - Urban, Rural, Regional, Real Estate, and Transportation Economics
- Browse content in R0 - General
- R00 - General
- Browse content in R1 - General Regional Economics
- R11 - Regional Economic Activity: Growth, Development, Environmental Issues, and Changes
- R12 - Size and Spatial Distributions of Regional Economic Activity
- R13 - General Equilibrium and Welfare Economic Analysis of Regional Economies
- Browse content in R2 - Household Analysis
- R20 - General
- R23 - Regional Migration; Regional Labor Markets; Population; Neighborhood Characteristics
- R28 - Government Policy
- Browse content in R3 - Real Estate Markets, Spatial Production Analysis, and Firm Location
- R30 - General
- R31 - Housing Supply and Markets
- R38 - Government Policy
- Browse content in R4 - Transportation Economics
- R40 - General
- R41 - Transportation: Demand, Supply, and Congestion; Travel Time; Safety and Accidents; Transportation Noise
- R48 - Government Pricing and Policy
- Browse content in Z - Other Special Topics
- Browse content in Z1 - Cultural Economics; Economic Sociology; Economic Anthropology
- Z10 - General
- Z12 - Religion
- Z13 - Economic Sociology; Economic Anthropology; Social and Economic Stratification
- Advance Articles
- Editor's Choice
- Author Guidelines
- Submission Site
- Open Access Options
- Self-Archiving Policy
- Why Submit?
- About The Quarterly Journal of Economics
- Editorial Board
- Advertising and Corporate Services
- Journals Career Network
- Dispatch Dates
- Journals on Oxford Academic
- Books on Oxford Academic

Article Contents
I. introduction, ii. a simple framework for discovery, iii. application and data, iv. the surprising importance of the face, v. algorithm-human communication, vi. evaluating these new hypotheses, vii. conclusion, data availability.
- < Previous
Machine Learning as a Tool for Hypothesis Generation *
- Article contents
- Figures & tables
- Supplementary Data
Jens Ludwig, Sendhil Mullainathan, Machine Learning as a Tool for Hypothesis Generation, The Quarterly Journal of Economics , Volume 139, Issue 2, May 2024, Pages 751–827, https://doi.org/10.1093/qje/qjad055
- Permissions Icon Permissions
While hypothesis testing is a highly formalized activity, hypothesis generation remains largely informal. We propose a systematic procedure to generate novel hypotheses about human behavior, which uses the capacity of machine learning algorithms to notice patterns people might not. We illustrate the procedure with a concrete application: judge decisions about whom to jail. We begin with a striking fact: the defendant’s face alone matters greatly for the judge’s jailing decision. In fact, an algorithm given only the pixels in the defendant’s mug shot accounts for up to half of the predictable variation. We develop a procedure that allows human subjects to interact with this black-box algorithm to produce hypotheses about what in the face influences judge decisions. The procedure generates hypotheses that are both interpretable and novel: they are not explained by demographics (e.g., race) or existing psychology research, nor are they already known (even if tacitly) to people or experts. Though these results are specific, our procedure is general. It provides a way to produce novel, interpretable hypotheses from any high-dimensional data set (e.g., cell phones, satellites, online behavior, news headlines, corporate filings, and high-frequency time series). A central tenet of our article is that hypothesis generation is a valuable activity, and we hope this encourages future work in this largely “prescientific” stage of science.
Science is curiously asymmetric. New ideas are meticulously tested using data, statistics, and formal models. Yet those ideas originate in a notably less meticulous process involving intuition, inspiration, and creativity. The asymmetry between how ideas are generated versus tested is noteworthy because idea generation is also, at its core, an empirical activity. Creativity begins with “data” (albeit data stored in the mind), which are then “analyzed” (through a purely psychological process of pattern recognition). What feels like inspiration is actually the output of a data analysis run by the human brain. Despite this, idea generation largely happens off stage, something that typically happens before “actual science” begins. 1 Things are likely this way because there is no obvious alternative. The creative process is so human and idiosyncratic that it would seem to resist formalism.
That may be about to change because of two developments. First, human cognition is no longer the only way to notice patterns in the world. Machine learning algorithms can also find patterns, including patterns people might not notice themselves. These algorithms can work not just with structured, tabular data but also with the kinds of inputs that traditionally could only be processed by the mind, like images or text. Second, data on human behavior is exploding: second-by-second price and volume data in asset markets, high-frequency cellphone data on location and usage, CCTV camera and police bodycam footage, news stories, children’s books, the entire text of corporate filings, and so on. The kind of information researchers once relied on for inspiration is now machine readable: what was once solely mental data is increasingly becoming actual data. 2
We suggest that these changes can be leveraged to expand how hypotheses are generated. Currently, researchers do of course look at data to generate hypotheses, as in exploratory data analysis, but this depends on the idiosyncratic creativity of investigators who must decide what statistics to calculate. In contrast, we suggest capitalizing on the capacity of machine learning algorithms to automatically detect patterns, especially ones people might never have considered. A key challenge is that we require hypotheses that are interpretable to people. One important goal of science is to generalize knowledge to new contexts. Predictive patterns in a single data set alone are rarely useful; they become insightful when they can be generalized. Currently, that generalization is done by people, and people can only generalize things they understand. The predictors produced by machine learning algorithms are, however, notoriously opaque—hard-to-decipher “black boxes.” We propose a procedure that integrates these algorithms into a pipeline that results in human-interpretable hypotheses that are both novel and testable.
While our procedure is broadly applicable, we illustrate it in a concrete application: judicial decision making. Specifically we study pretrial decisions about which defendants are jailed versus set free awaiting trial, a decision that by law is supposed to hinge on a prediction of the defendant’s risk ( Dobbie and Yang 2021 ). 3 This is also a substantively interesting application in its own right because of the high stakes involved and mounting evidence that judges make these decisions less than perfectly ( Kleinberg et al. 2018 ; Rambachan et al. 2021 ; Angelova, Dobbie, and Yang 2023 ).
We begin with a striking fact. When we build a deep learning model of the judge—one that predicts whether the judge will detain a given defendant—a single factor emerges as having large explanatory power: the defendant’s face. A predictor that uses only the pixels in the defendant’s mug shot explains from one-quarter to nearly one-half of the predictable variation in detention. 4 Defendants whose mug shots fall in the bottom quartile of predicted detention are 20.4 percentage points more likely to be jailed than those in the top quartile. By comparison, the difference in detention rates between those arrested for violent versus nonviolent crimes is 4.8 percentage points. Notice what this finding is and is not. We are not claiming the mug shot predicts defendant behavior; that would be the long-discredited field of phrenology ( Schlag 1997 ). We instead claim the mug shot predicts judge behavior: how the defendant looks correlates strongly with whether the judge chooses to jail them. 5
Has the algorithm found something new in the pixels of the mug shot or simply rediscovered something long known or intuitively understood? After all, psychologists have been studying people’s reactions to faces for at least 100 years ( Todorov et al. 2015 ; Todorov and Oh 2021 ), while economists have shown that judges are influenced by factors (like race) that can be seen from someone’s face ( Arnold, Dobbie, and Yang 2018 ; Arnold, Dobbie, and Hull 2020 ). When we control for age, gender, race, skin color, and even the facial features suggested by previous psychology research (dominance, trustworthiness, attractiveness, and competence), none of these factors (individually or jointly) meaningfully diminishes the algorithm’s predictive power (see Figure I , Panel A). It is perhaps worth noting that the algorithm on its own does rediscover some of the signal from these features: in fact, collectively these known features explain |$22.3\%$| of the variation in predicted detention (see Figure I , Panel B). The key point is that the algorithm has discovered a great deal more as well.

Correlates of Judge Detention Decision and Algorithmic Prediction of Judge Decision
Panel A summarizes the explanatory power of a regression model in explaining judge detention decisions, controlling for the different explanatory variables indicated at left (shaded tiles), either on their own (dark circles) or together with the algorithmic prediction of the judge decisions (triangles). Each row represents a different regression specification. By “other facial features,” we mean variables that previous psychology research suggests matter for how faces influence people’s reactions to others (dominance, trustworthiness, competence, and attractiveness). Ninety-five percent confidence intervals around our R 2 estimates come from drawing 10,000 bootstrap samples from the validation data set. Panel B shows the relationship between the different explanatory variables as indicated at left by the shaded tiles with the algorithmic prediction itself as the outcome variable in the regressions. Panel C examines the correlation with judge decisions of the two novel hypotheses generated by our procedure about what facial features affect judge detention decisions: well-groomed and heavy-faced.
Perhaps we should control for something else? Figuring out that “something else” is itself a form of hypothesis generation. To avoid a possibly endless—and misleading—process of generating other controls, we take a different approach. We show mug shots to subjects and ask them to guess whom the judge will detain and incentivize them for accuracy. These guesses summarize the facial features people readily (if implicitly) believe influence jailing. Although subjects are modestly good at this task, the algorithm is much better. It remains highly predictive even after controlling for these guesses. The algorithm seems to have found something novel beyond what scientists have previously hypothesized and beyond whatever patterns people can even recognize in data (whether or not they can articulate them).
What, then, are the novel facial features the algorithm has discovered? If we are unable to answer that question, we will have simply replaced one black box (the judge’s mind) with another (an algorithmic model of the judge’s mind). We propose a solution whereby the algorithm can communicate what it “sees.” Specifically, our procedure begins with a mug shot and “morphs” it to create a mug shot that maximally increases (or decreases) the algorithm’s predicted detention probability. The result is pairs of synthetic mug shots that can be examined to understand and articulate what differs within the pairs. The algorithm discovers, and people name that discovery. In principle we could have just shown subjects actual mug shots with higher versus lower predicted detention odds. But faces are so rich that between any pair of actual mug shots, many things will happen to be different and most will be unrelated to detention (akin to the curse of dimensionality). Simply looking at pairs of actual faces can, as a result, lead to many spurious observations. Morphing creates counterfactual synthetic images that are as similar as possible except with respect to detention odds, to minimize extraneous differences and help focus on what truly matters for judge detention decisions.
Importantly, we do not generate hypotheses by looking at the morphs ourselves; instead, they are shown to independent study subjects (MTurk or Prolific workers) in an experimental design. Specifically, we showed pairs of morphed images and asked participants to guess which image the algorithm predicts to have higher detention risk. Subjects were given both incentives and feedback, so they had motivation and opportunity to learn the underlying patterns. While subjects initially guess the judge’s decision correctly from these morphed mug shots at about the same rate as they do when looking at “raw data,” that is, actual mug shots (modestly above the |$50\%$| random guessing mark), they quickly learn from these morphed images what the algorithm is seeing and reach an accuracy of nearly |$70\%$| . At the end, participants are asked to put words to the differences they see across images in each pair, that is, to name what they think are the key facial features the algorithm is relying on to predict judge decisions. Comfortingly, there is substantial agreement on what subjects see: a sizable share of subjects all name the same feature. To verify whether the feature they identify is used by the algorithm, a separate sample of subjects independently coded mug shots for this new feature. We show that the new feature is indeed correlated with the algorithm’s predictions. What subjects think they’re seeing is indeed what the algorithm is also “seeing.”
Having discovered a single feature, we can iterate the procedure—the first feature explains only a fraction of what the algorithm has captured, suggesting there are many other factors to be discovered. We again produce morphs, but this time hold the first feature constant: that is, we orthogonalize so that the pairs of morphs do not differ on the first feature. When these new morphs are shown to subjects, they consistently name a second feature, which again correlates with the algorithm’s prediction. Both features are quite important. They explain a far larger share of what the algorithm sees than all the other variables (including race and skin color) besides gender. These results establish our main goals: show that the procedure produces meaningful communication, and that it can be iterated.
What are the two discovered features? The first can be called “well-groomed” (e.g., tidy, clean, groomed, versus unkept, disheveled, sloppy look), and the second can be called “heavy-faced” (e.g., wide facial shape, puffier face, wider face, rounder face, heavier). These features are not just predictive of what the algorithm sees, but also of what judges actually do ( Figure I , Panel C). We find that both well-groomed and heavy-faced defendants are more likely to be released, even controlling for demographic features and known facial features from psychology. Detention rates of defendants in the top and bottom quartile of well-groomedness differ by 5.5 percentage points ( |$24\%$| of the base rate), while the top versus bottom quartile difference in heavy-facedness is 7 percentage points (about |$30\%$| of the base rate). Both differences are larger than the 4.8 percentage points detention rate difference between those arrested for violent versus nonviolent crimes. Not only are these magnitudes substantial, these hypotheses are novel even to practitioners who work in the criminal justice system (in a public defender’s office and a legal aid society).
Establishing whether these hypotheses are truly causally related to judge decisions is obviously beyond the scope of the present article. But we nonetheless present a few additional findings that are at least suggestive. These novel features do not appear to be simply proxies for factors like substance abuse, mental health, or socioeconomic status. Moreover, we carried out a lab experiment in which subjects are asked to make hypothetical pretrial release decisions as if they were a judge. They are shown information about criminal records (current charge, prior arrests) along with mug shots that are randomly morphed in the direction of higher or lower values of well-groomed (or heavy-faced). Subjects tend to detain those with higher-risk structured variables (criminal records), all else equal, suggesting they are taking the task seriously. These same subjects, though, are also more likely to detain defendants who are less heavy-faced or well-groomed, even though these were randomly assigned.
Ultimately, though, this is not a study about well-groomed or heavy-faced defendants, nor are its implications limited to faces or judges. It develops a general procedure that can be applied wherever behavior can be predicted using rich (especially high-dimensional) data. Development of such a procedure has required overcoming two key challenges.
First, to generate interpretable hypotheses, we must overcome the notorious black box nature of most machine learning algorithms. Unlike with a regression, one cannot simply inspect the coefficients. A modern deep-learning algorithm, for example, can have tens of millions of parameters. Noninspectability is especially problematic when the data are rich and high dimensional since the parameters are associated with primitives such as pixels. This problem of interpretation is fundamental and remains an active area of research. 6 Part of our procedure here draws on the recent literature in computer science that uses generative models to create counterfactual explanations. Most of those methods are designed for AI applications that seek to automate tasks humans do nearly perfectly, like image classification, where predictability of the outcome (is this image of a dog or a cat?) is typically quite high. 7 Interpretability techniques are used to ensure the algorithm is not picking up on spurious signal. 8 We developed our method, which has similar conceptual underpinnings to this existing literature, for social science applications where the outcome (human behavior) is typically more challenging to predict. 9 To what degree existing methods (as they currently stand or with some modification) could perform as well or better in social science applications like ours is a question we leave to future work.
Second, we must overcome what we might call the Rorschach test problem. Suppose we, the authors, were to look at these morphs and generate a hypothesis. We would not know if the procedure played any meaningful role. Perhaps the morphs, like ink blots, are merely canvases onto which we project our creativity. 10 Put differently, a single research team’s idiosyncratic judgments lack the kind of replicability we desire of a scientific procedure. To overcome this problem, it is key that we use independent (nonresearcher) subjects to inspect the morphs. The fact that a sizable share of subjects all name the same discovery suggests that human-algorithm communication has occurred and the procedure is replicable, rather than reflecting some unique spark of creativity.
At the same time, the fact that our procedure is not fully automatic implies that it will be shaped and constrained by people. Human participants are needed to name the discoveries. So whole new concepts that humans do not yet understand cannot be produced. Such breakthroughs clearly happen (e.g., gravity or probability) but are beyond the scope of procedures like ours. People also play a crucial role in curating the data the algorithm sees. Here, for example, we chose to include mug shots. The creative acquisition of rich data is an important human input into this hypothesis generation procedure. 11
Our procedure can be applied to a broad range of settings and will be particularly useful for data that are not already intrinsically interpretable. Many data sets contain a few variables that already have clear, fixed meanings and are unlikely to lead to novel discoveries. In contrast, images, text, and time series are rich high-dimensional data with many possible interpretations. Just as there is an ocean of plausible facial features, these sorts of data contain a large set of potential hypotheses that an algorithm can search through. Such data are increasingly available and used by economists, including news headlines, legislative deliberations, annual corporate reports, Federal Open Market Committee statements, Google searches, student essays, résumés, court transcripts, doctors’ notes, satellite images, housing photos, and medical images. Our procedure could, for example, raise hypotheses about what kinds of news lead to over- or underreaction of stock prices, which features of a job interview increase racial disparities, or what features of an X-ray drive misdiagnosis.
Central to this work is the belief that hypothesis generation is a valuable activity in and of itself. Beyond whatever the value might be of our specific procedure and empirical application, we hope these results also inspire greater attention to this traditionally “prescientific” stage of science.
We develop a simple framework to clarify the goals of hypothesis generation and how it differs from testing, how algorithms might help, and how our specific approach to algorithmic hypothesis generation differs from existing methods. 12
II.A. The Goals of Hypothesis Generation
What criteria should we use for assessing hypothesis generation procedures? Two common goals for hypothesis generation are ones that we ensure ex post. First is novelty. In our application, we aim to orthogonalize against known factors, recognizing that it may be hard to orthogonalize against all known hypotheses. Second, we require that hypotheses be testable ( Popper 2002 ). But what can be tested is hard to define ex ante, in part because it depends on the specific hypothesis and the potential experimental setups. Creative empiricists over time often find ways to test hypotheses that previously seemed untestable. 13 To these, we add two more: interpretability and empirical plausibility.
What do we mean by empirically plausible? Let y be some outcome of interest, which for simplicity we assume is binary, and let h ( x ) be some hypothesis that maps the features of each instance, x , to [0,1]. By empirical plausibility we mean some correlation between y and h ( x ). Our ultimate aim is to uncover causal relationships. But causality can only be known after causal testing. That raises the question of how to come up with ideas worth causally testing, and how we would recognize them when we see them. Many true hypotheses need not be visible in raw correlations. Those can only be identified with background knowledge (e.g., theory). Other procedures would be required to surface those. Our focus here is on searching for true hypotheses that are visible in raw correlations. Of course not every correlation will turn out to be a true hypothesis, but even in those cases, generating such hypotheses and then invalidating them can be a valuable activity. Debunking spurious correlations has long been one of the most useful roles of empirical work. Understanding what confounders produce those correlations can also be useful.
We care about our final goal for hypothesis generation, interpretability, because science is largely about helping people make forecasts into new contexts, and people can only do that with hypotheses they meaningfully understand. Consider an uninterpretable hypothesis like “this set of defendants is more likely to be jailed than that set,” but we cannot articulate a reason why. From that hypothesis, nothing could be said about a new set of courtroom defendants. In contrast an interpretable hypothesis like “skin color affects detention” has implications for other samples of defendants and for entirely different settings. We could ask whether skin color also affects, say, police enforcement choices or whether these effects differ by time of day. By virtue of being interpretable, these hypotheses let us use a wider set of knowledge (police may share racial biases; skin color is not as easily detected at night). 14 Interpretable descriptions let us generalize to novel situations, in addition to being easier to communicate to key stakeholders and lending themselves to interpretable solutions.
II.B. Human versus Algorithmic Hypothesis Generation
Human hypothesis generation has the advantage of generating hypotheses that are interpretable. By construction, the ideas that humans come up with are understandable by humans. But as a procedure for generating new ideas, human creativity has the drawback of often being idiosyncratic and not necessarily replicable. A novel hypothesis is novel exactly because one person noticed it when many others did not. A large body of evidence shows that human judgments have a great deal of “noise.” It is not just that different people draw different conclusions from the same observations, but the same person may notice different things at different times ( Kahneman, Sibony, and Sunstein 2022 ). A large body of psychology research shows that people typically are not able to introspect and understand why we notice specific things those times we do notice them. 15
There is also no guarantee that human-generated hypotheses need be empirically plausible. The intuition is related to “overfitting.” Suppose that people look at a subset of all data and look for something that differentiates positive ( y = 1) from negative ( y = 0) cases. Even with no noise in y , there is randomness in which observations are in the data. That can lead to idiosyncratic differences between y = 0 and y = 1 cases. As the number of comprehensible hypotheses gets large, there is a “curse of dimensionality”: many plausible hypotheses for these idiosyncratic differences. That is, many different hypotheses can look good in sample but need not work out of sample. 16
In contrast, supervised learning tools in machine learning are designed to generate predictions in new (out-of-sample) data. 17 That is, algorithms generate hypotheses that are empirically plausible by construction. 18 Moreover, machine learning can detect patterns in data that humans cannot. Algorithms can notice, for example, that livestock all tend to be oriented north ( Begall et al. 2008 ), whether someone is about to have a heart attack based on subtle indications in an electrocardiogram ( Mullainathan and Obermeyer 2022 ), or that a piece of machinery is about to break ( Mobley 2002 ). We call these machine learning prediction functions m ( x ), which for a binary outcome y map to [0, 1].
The challenge is that most m ( x ) are not interpretable. For this type of statistical model to yield an interpretable hypothesis, its parameters must be interpretable. That can happen in some simple cases. For example, if we had a data set where each dimension of x was interpretable (such as individual structured variables in a tabular data set) and we used a predictor such as OLS (or LASSO), we could just read the hypotheses from the nonzero coefficients: which variables are significant? Even in that case, interpretation is challenging because machine learning tools, built to generate accurate predictions rather than apportion explanatory power across explanatory variables, yield coefficients that can be unstable across realizations of the data ( Mullainathan and Spiess 2017 ). 19 Often interpretation is much less straightforward than that. If x is an image, text, or time series, the estimated models (such as convolutional neural networks) can have literally millions of parameters. The models are defined on granular inputs with no particular meaning: if we knew m ( x ) weighted a particular pixel, what have we learned? In these cases, the estimated model m ( x ) is not interpretable. Our focus is on these contexts where algorithms, as black-box models, are not readily interpreted.
Ideally one might marry people’s unique knowledge of what is comprehensible with an algorithm’s superior capacity to find meaningful correlations in data: to have the algorithm discover new signal and then have humans name that discovery. How to do so is not straightforward. We might imagine formalizing the set of interpretable prediction functions, and then focus on creating machine learning techniques that search over functions in that set. But mathematically characterizing those functions is typically not possible. Or we might consider seeking insight from a low-dimensional representation of face space, or “eigenfaces,” which are a common teaching tool for principal components analysis ( Sirovich and Kirby 1987 ). But those turn out not to provide much useful insight for our purposes. 20 In some sense it is obvious why: the subset of actual faces is unlikely to be a linear subspace of the space of pixels. If we took two faces and linearly interpolated them the resulting image would not look like a face. Some other approach is needed. We build on methods in computer science that use generative models to generate counterfactual explanations.
II.C. Related Methods
Our hypothesis generation procedure is part of a growing literature that aims to integrate machine learning into the way science is conducted. A common use (outside of economics) is in what could be called “closed world problems”: situations where the fundamental laws are known, but drawing out predictions is computationally hard. For example, the biochemical rules of how proteins fold are known, but it is hard to predict the final shape of a protein. Machine learning has provided fundamental breakthroughs, in effect by making very hard-to-compute outcomes computable in a feasible timeframe. 21
Progress has been far more limited with applications where the relationship between x and y is unknown (“open world” problems), like human behavior. First, machine learning here has been useful at generating unexpected findings, although these are not hypotheses themselves. Pierson et al. (2021) show that a deep-learning algorithm is better able to predict patient pain from an X-ray than clinicians can: there are physical knee defects that medicine currently does not understand. But that study is not able to isolate what those defects are. 22 Second, machine learning has also been used to explore investigator-generated hypotheses, such as Mullainathan and Obermeyer (2022) , who examine whether physicians suffer from limited attention when diagnosing patients. 23
Finally, a few papers take on the same problem that we do. Fudenberg and Liang (2019) and Peterson et al. (2021) have used algorithms to predict play in games and choices between lotteries. They inspected those algorithms to produce their insights. Similarly, Kleinberg et al. (2018) and Sunstein (2021) use algorithmic models of judges and inspect those models to generate hypotheses. 24 Our proposal builds on these papers. Rather than focusing on generating an insight for a specific application, we suggest a procedure that can be broadly used for many applications. Importantly, our procedure does not rely on researcher inspection of algorithmic output. When an expert researcher with a track record of generating scientific ideas uses some procedure to generate an idea, how do we know whether the result is due to the procedure or the researcher? By relying on a fixed algorithmic procedure that human subjects can interface with, hypothesis generation goes from being an idiosyncratic act of individuals to a replicable process.
III.A. Judicial Decision Making
Although our procedure is broadly applicable, we illustrate it through a specific application to the U.S. criminal justice system. We choose this application partly because of its social relevance. It is also an exemplar of the type of application where our hypothesis generation procedure can be helpful. Its key ingredients—a clear decision maker, a large number of choices (over 10 million people are arrested each year in the United States) that are recorded in data, and, increasingly, high-dimensional data that can also be used to model those choices, such as mug shot images, police body cameras, and text from arrest reports or court transcripts—are shared with a variety of other applications.
Our specific focus is on pretrial hearings. Within 24–48 hours after arrest, a judge must decide where the defendant will await trial, in jail or at home. This is a consequential decision. Cases typically take 2–4 months to resolve, sometimes up to 9–12 months. Jail affects people’s families, their livelihoods, and the chances of a guilty plea ( Dobbie, Goldin, and Yang 2018 ). On the other hand, someone who is released could potentially reoffend. 25
While pretrial decisions are by law supposed to hinge on the defendant’s risk of flight or rearrest if released ( Dobbie and Yang 2021 ), studies show that judges’ decisions deviate from those guidelines in a number of ways. For starters, judges seem to systematically mispredict defendant risk ( Jung et al. 2017 ; Kleinberg et al. 2018 ; Rambachan 2021 ; Angelova, Dobbie, and Yang 2023 ), partly because judges overweight the charge for which people are arrested ( Sunstein 2021 ). Judge decisions can also depend on extralegal factors like race ( Arnold, Dobbie, and Yang 2018 ; Arnold, Dobbie, and Hull 2020 ), whether the judge’s favorite football team lost ( Eren and Mocan 2018 ), weather ( Heyes and Saberian 2019 ), the cases the judge just heard ( Chen, Moskowitz, and Shue 2016 ), and if the hearing is on the defendant’s birthday ( Chen and Philippe 2023 ). These studies test hypotheses that some human being was clever enough to think up. But there remains a great deal of unexplained variation in judges’ decisions. The challenge of expanding the set of hypotheses for understanding this variation without losing the benefit of interpretability is the motivation for our own analysis here.
III.B. Administrative Data
We obtained data from Mecklenburg County, North Carolina, the second most populated county in the state (over 1 million residents) that includes North Carolina’s largest city (Charlotte). The county is similar to the rest of the United States in terms of economic conditions (2021 poverty rates were |$11.0\%$| versus |$11.4\%$| , respectively), although the share of Mecklenburg County’s population that is non-Hispanic white is lower than the United States as a whole ( |$56.6\%$| versus |$75.8\%$| ). 26 We rely on three sources of administrative data: 27
The Mecklenburg County Sheriff’s Office (MCSO) publicly posts arrest data for the past three years, which provides information on defendant demographics like age, gender, and race, as well as the charge for which someone was arrested.
The North Carolina Administrative Office of the Courts (NCAOC) maintains records on the judge’s pretrial decisions (detain, release, etc.).
Data from the North Carolina Department of Public Safety includes information about the defendant’s prior convictions and incarceration spells, if any.
We also downloaded photos of the defendants from the MCSO public website (so-called mug shots), 28 which capture a frontal view of each person from the shoulders up in front of a gray background. These images are 400 pixels wide by 480 pixels high, but we pad them with a black boundary to be square 512 × 512 images to conform with the requirements of some of the machine learning tools. In Figure II , we give readers a sense of what these mug shots look like, with two important caveats. First, given concerns about how the overrepresentation of disadvantaged groups in discussions of crime can contribute to stereotyping ( Bjornstrom et al. 2010 ), we illustrate the key ideas of the paper using images for non-Hispanic white males. Second, out of sensitivity to actual arrestees, we do not wish to display actual mug shots (which are available at the MCSO website). 29 Instead, the article only shows mug shots that are synthetic, generated using generative adversarial networks as described in Section V.B .

Illustrative Facial Images
This figure shows facial images that illustrate the format of the mug shots posted publicly on the Mecklenberg County, North Carolina, sheriff’s office website. These are not real mug shots of actual people who have been arrested, but are synthetic. Moreover, given concerns about how the overrepresentation of disadvantaged groups in discussions of crime can exacerbate stereotyping, we illustrate the our key ideas using images for non-Hispanic white men. However, in our human intelligence tasks that ask participants to provide labels (ratings for different image features), we show images that are representative of the Mecklenberg County defendant population as a whole.
These data capture much of the information the judge has available at the time of the pretrial hearing, but not all of it. Both the judge and the algorithm see structured variables about each defendant like defendant demographics, current charge, and prior record. Because the mug shot (which the algorithm uses) is taken not long before the pretrial hearing, it should be a reasonable proxy for what the judge sees in court. The additional information the judge has but the algorithm does not includes the narrative arrest report from the police and what happens in court. While pretrial hearings can be quite brief in many jurisdictions (often not more than just a few minutes), the judge may nonetheless hear statements from police, prosecutors, defense lawyers, and sometimes family members. Defendants usually have their lawyers speak for them and do not say much at these hearings.
We downloaded 81,166 arrests made between January 18, 2017, and January 17, 2020, involving 42,353 unique defendants. We apply several data filters, like dropping cases without mugshots ( Online Appendix Table A.I ), leaving 51,751 observations. Because our goal is inference about new out-of-sample (OOS) observations, we partition our data as follows:
A train set of N = 22,696 cases, constructed by taking arrests through July 17, 2019, grouping arrests by arrestee, 30 randomly selecting |$70\%$| to the training-plus-validation data set, then randomly selecting |$70\%$| of those arrestees for the training data specifically.
A validation set of N = 9,604 cases used to report OOS performance in the article’s main exhibits, consisting of the remaining |$30\%$| in the combined training-plus-validation data frame.
A lock-box hold-out set of N = 19,009 cases that we did not touch until the article was accepted for final publication, to avoid what one might call researcher overfitting: we run lots of models over the course of writing the article, and the results on the validation data set may overstate our findings. This data set consists of the N = 4,759 valid cases for the last six months of our data period (July 17, 2019, to January 17, 2020) plus a random sample of |$30\%$| of those arrested before July 17, 2019, so that we can present results that are OOS with respect to individuals and time. Once this article was officially accepted, we replicated the findings presented in our main exhibits (see Online Appendix D and Online Appendix Tables A.XVIII–A.XXXII ). We see that our core findings are qualitatively similar. 31
Descriptive statistics are shown in Table I . Relative to the county as a whole, the arrested population substantially overrepresents men ( |$78.7\%$| ) and Black residents ( |$69.4\%$| ). The average age of arrestees is 31.8 years. Judges detain |$23.3\%$| of cases, and in |$25.1\%$| of arrests the person is rearrested before their case is resolved (about one-third of those released). Randomization of arrestees to the training versus validation data sets seems to have been successful, as shown in Table I . None of the pairwise comparisons has a p -value below .05 (see Online Appendix Table A.II ). A permutation multivariate analysis of variance test of the joint null hypothesis that the training-validation differences for all variables are all zero yields p = .963. 32 A test for the same joint null hypothesis for the differences between the training sample and the lock-box hold-out data set (out of sample by individual) yields a test statistic of p = .537.
Summary Statistics for Mecklenburg County NC Data, 2017–2020
Notes. This table reports descriptive statistics for our full data set and analysis subsets, which cover the period January 18, 2017, through January 17, 2020, from Mecklenburg County, NC. The lock-box hold-out data set consists of data from the last six months of our study period (July 17, 2019–January 17, 2020) plus a subset of cases through July 16, 2019, selected by randomly selecting arrestees. The remainder of the data set is then randomly assigned by arrestee to our training data set (used to build our algorithms) or to our validation set (which we use to report results in the article’s main exhibits). For additional details of our data filters and partitioning procedures, see Online Appendix Table A.I . We define pretrial release as being released on the defendant’s own recognizance or having been assigned and then posting cash bail requirements within three days of arrest. We define rearrest as experiencing a new arrest before adjudication of the focal arrest, with detained defendants being assigned zero values for the purposes of this table. Arrest charge categories reflect the most serious criminal charge for which a person was arrested, using the FBI Uniform Crime Reporting hierarchy rule in cases where someone is arrested and charged with multiple offenses. For analyses of variance for the test of the joint null hypothesis that the difference in means across each variable is zero, see Online Appendix Table A.II .
III.C. Human Labels
The administrative data capture many key features of each case but omit some other important ones. We solve these data insufficiency problems through a series of human intelligence tasks (HITs), which involve having study subjects on one of two possible platforms (Amazon’s Mechanical Turk or Prolific) assign labels to each case from looking at the mug shots. More details are in Online Appendix Table A.III . We use data from these HITs mostly to understand how the algorithm’s predictions relate to already-known determinants of human decision making, and hence the degree to which the algorithm is discovering something novel.
One set of HITs filled in demographic-related data: ethnicity; skin tone (since people are often stereotyped on skin color, or “colorism”; Hunter 2007 ), reported on an 18-point scale; the degree to which defendants appear more stereotypically Black on a 9-point scale ( Eberhardt et al. 2006 show this affects criminal justice decisions); and age, to compare to administrative data for label quality checks. 33 Because demographics tend to be easy for people to see in images, we collect just one label per image for each of these variables. To confirm one label is enough, we repeated the labeling task for 100 images but collected 10 labels for each image; we see that additional labels add little information. 34 Another data quality check comes from the fact that the distributions of skin color ratings do systematically differ by defendant race ( Online Appendix Figure A.III ).
A second type of HIT measured facial features that previous psychology research has shown affect human judgments. The specific set of facial features we focus on come from the influential study by Oosterhof and Todorov (2008) of people’s perceptions of the facial features of others. When subjects are asked to provide descriptions of different faces, principal components analysis suggests just two dimensions account for about |$80\%$| of the variation: (i) trustworthiness and (ii) dominance. We also collected data on two other facial features shown to be associated with real-world decisions like hiring or whom to vote for: (iii) attractiveness and (iv) competence ( Frieze, Olson, and Russell 1991 ; Little, Jones, and DeBruine 2011 ; Todorov and Oh 2021 ). 35
We asked subjects to rate images for each of these psychological features on a nine-point scale. Because psychological features may be less obvious than demographic features, we collected three labels per training–data set image and five per validation–data set image. 36 There is substantial variation in the ratings that subjects assign to different images for each feature (see Online Appendix Figure A.VI ). The ratings from different subjects for the same feature and image are highly correlated: interrater reliability measures (Cronbach’s α) range from 0.87 to 0.98 ( Online Appendix Figure A.VII ), similar to those reported in studies like Oosterhof and Todorov (2008) . 37 The information gain from collecting more than a few labels per image is modest. 38 For summary statistics, see Online Appendix Table A.IV .
Finally, we also tried to capture people’s implicit or tacit understanding of the determinants of judges’ decisions by asking subjects to predict which mug shot out of a pair would be detained, with images in each pair matched on gender, race, and five-year age brackets. 39 We incentivized study subjects for correct predictions and gave them feedback over the course of the 50 image pairs to facilitate learning. We treat the first 10 responses per subject as a “learning set” that we exclude from our analysis.
The first step of our hypothesis generation procedure is to build an algorithmic model of some behavior, which in our case is the judge’s detention decision. A sizable share of the predictable variation in judge decisions comes from a surprising source: the defendant’s face. Facial features implicated by past research explain just a modest share of this predictable variation. The algorithm seems to have found a novel discovery.
IV.A. What Drives Judge Decisions?
We begin by predicting judge pretrial detention decisions ( y = 1 if detain, y = 0 if release) using all the inputs available ( x ). We use the training data set to construct two separate models for the two types of data available. We apply gradient-boosted decision trees to predict judge decisions using the structured administrative data (current charge, prior record, age, gender), m s ( x ); for the unstructured data (raw pixel values from the mug shots), we train a convolutional neural network, m u ( x ). Each model returns an estimate of y (a predicted detention probability) for a given x . Because these initial steps of our procedure use standard machine learning methods, we relegate their discussion to the Online Appendix .
We pool the signal from both models to form a single weighted-average model |$m_p(x)=[\hat{\beta _s} m_s(x) + \hat{\beta _u} m_u(x)]$| using a so-called stacking procedure where the data are used to estimate the relevant weights. 40 Combining structured and unstructured data is an active area of deep-learning research, often called fusion modeling ( Yuhas, Goldstein, and Sejnowski 1989 ; Lahat, Adali, and Jutten 2015 ; Ramachandram and Taylor 2017 ; Baltrušaitis, Ahuja, and Morency 2019 ). We have tried several of the latest fusion architectures; none improve on our ensemble approach.
Judge decisions do have some predictable structure. We report predictive performance as the area under the receiver operating characteristic curve, or AUC, which is a measure of how well the algorithm rank-orders cases with values from 0.5 (random guessing) to 1.0 (perfect prediction). Intuitively, AUC can be thought of as the chance that a uniformly randomly selected detained defendant has a higher predicted detention likelihood than a uniformly randomly selected released defendant. The algorithm built using all candidate features, m p ( x ), has an AUC of 0.780 (see Online Appendix Figure A.X ).
What is the algorithm using to make its predictions? A single type of input captures a sizable share of the total signal: the defendant’s face. The algorithm built using only the mug shot image, m u ( x ), has an AUC of 0.625 (see Online Appendix Figure A.X ). Since an AUC of 0.5 represents random prediction, in AUC terms the mug shot accounts for |$\frac{0.625-0.5}{0.780-0.5}=44.6\%$| of the predictive signal about judicial decisions.
Another common way to think about predictive accuracy is in R 2 terms. While our data are high dimensional (because the facial image is a high-dimensional object), the algorithm’s prediction of the judge’s decision based on the facial image, m u ( x ), is a scalar and can be easily included in a familiar regression framework. Like AUC, measures like R 2 and mean squared error capture how well a model rank-orders observations by predicted probabilities, but R 2 , unlike AUC, also captures how close predictions are to observed outcomes (calibration). 41 The R 2 from regressing y against m s ( x ) and m u ( x ) in the validation data is 0.11. Regressing y against m u ( x ) alone yields an R 2 of 0.03. So depending on how we measure predictive accuracy, around a quarter ( |$\frac{0.03}{0.11}=27.3\%)$| to a half ( |$44.6\%$| ) of the predicted signal about judges’ decisions is captured by the face.
Average differences are another way to see what drives judges’ decisions. For any given feature x k , we can calculate the average detention rate for different values of the feature. For example, for the variable measuring whether the defendant is male ( x k = 1) versus female ( x k = 0), we can calculate and plot E [ y | x k = 1] versus E [ y | x k = 0]. As shown in Online Appendix Figure A.XI , the difference in detention rates equals 4.8 percentage points for those arrested for violent versus nonviolent crimes, 10.2 percentage points for men versus women, and 4.3 percentage points for bottom versus top quartile of skin tone, which are all sizable relative to the baseline detention rate of |$23.3\%$| in our validation data set. By way of comparison, average detention rates for the bottom versus top quartile of the mug shot algorithm’s predictions, m u ( x ), differ by 20.4 percentage points.
In what follows, we seek to understand more about the mug shot–based prediction of the judge’s decision, which we refer to simply as m ( x ) in the remainder of the article.
IV.B. Judicial Error?
So far we have shown that the face predicts judges’ behavior. Are judges right to use face information? To be precise, by “right” we do not mean a broader ethical judgment; for many reasons, one could argue it is never ethical to use the face. But suppose we take a rather narrow (exceedingly narrow) formulation of “right.” Recall the judge is meant to make jailing decisions based on the defendant’s risk. Is the use of these facial characteristics consistent with that objective? Put differently, if we account for defendant risk differences, do these facial characteristics still predict judge decisions? The fact that judges rely on the face in making detention decisions is in itself a striking insight regardless of whether the judges use appearance as a proxy for risk or are committing a cognitive error.
At first glance, the most straightforward way to answer this question would be to regress rearrest against the algorithm’s mug shot–based detention prediction. That yields a statistically significant relationship: The coefficient (and standard error) for the mug shot equals 0.6127 (0.0460) with no other explanatory variables in the regression versus 0.5735 (0.0521) with all the explanatory variables (as in the final column, Table III ). But the interpretation here is not so straightforward.
The challenge of interpretation comes from the fact that we have only measured crime rates for the released defendants. The problem with having measured crime, not actual crime, is that whether someone is charged with a crime is itself a human choice, made by police. If the choices police make about when to make an arrest are affected by the same biases that might afflict judges, then measured rearrest rates may correlate with facial characteristics simply due to measurement bias. The problem created by having measures of rearrest only for released defendants is that if judges have access to private information (defendant characteristics not captured by our data set), and judges use that information to inform detention decisions, then the released and detained defendants may be different in unobservable ways that are relevant for rearrest risk ( Kleinberg et al. 2018 ).
With these caveats in mind, at least we can perform a bounding exercise. We created a predictor of rearrest risk (see Online Appendix B ) and then regress judges’ decisions on predicted rearrest risk. We find that a one-unit change in predicted rearrest risk changes judge detention rates by 0.6103 (standard error 0.0213). By comparison, we found that a one-unit change in the mug shot (by which we mean the algorithm’s mug shot–based prediction of the judge detention decision) changes judge detention rates by 0.6963 (standard error 0.0383; see Table III , column (1)). That means if the judges were reacting to the defendant’s face only because the face is a proxy for rearrest risk, the difference in rearrest risk for those with a one-unit difference in the mug shot would need to be |$\frac{0.6963}{0.6103} = 1.141$| . But when we directly regress rearrest against the algorithm’s mug shot–based detention prediction, we get a coefficient of 0.6127 (standard error 0.0460). Clearly 0.6127 < 1.141; that is, the mug shot does not seem to be strongly related enough to rearrest risk to explain the judge’s use of it in making detention decisions. 42
Of course this leaves us with the second problem with our data: we only have crime data on the released. It is possible the relationship between the mug shot and risk could be very different among the |$23.3\%$| of defendants who are detained (which we cannot observe). Put differently, the mug shot–risk relationship among the |$76.7\%$| of the defendants who are released is 0.6127; and let A be the (unknown) mug shot–risk relationship among the jailed. What we really want to know is the mug shot–risk relationship among all defendants, which equals (0.767 · 0.6127) + (0.233 · A ). For this mug shot–risk relationship among all defendants to equal 1.141, A would need to be 2.880, nearly five times as great among the detained defendants as among the released. This would imply an implausibly large effect of the mug shot on rearrest risk relative to the size of the effects on rearrest risk of other defendant characteristics. 43
In addition, the results from Section VI.B call into question that these characteristics are well-understood proxies for risk. As we show there, experts who understand pretrial (public defenders and legal aid society staff) do not recognize the signal about judge decision making that the algorithm has discovered in the mug shot. These considerations as a whole—that measured rearrest is itself biased, the bounding exercise, and the failure of experts to recreate this signal—together lead us to tentatively conclude that it is unlikely that what the algorithm is finding in the face is merely a well-understood proxy for risk, but reflects errors in the judicial decision-making process. Of course, that presumption is not essential for the rest of the article, which asks: what exactly has the algorithm discovered in the face?
IV.C. Is the Algorithm Discovering Something New?
Previous studies already tell us a number of things about what shapes the decisions of judges and other people. For example, we know people stereotype by gender ( Avitzour et al. 2020 ), age ( Neumark, Burn, and Button 2016 ; Dahl and Knepper 2020 ), and race or ethnicity ( Bertrand and Mullainathan 2004 ; Arnold, Dobbie, and Yang 2018 ; Arnold, Dobbie, and Hull 2020 ; Fryer 2020 ; Hoekstra and Sloan 2022 ; Goncalves and Mello 2021 ). Is the algorithm just rediscovering known determinants of people’s decisions, or discovering something new? We address this in two ways. We first ask how much of the algorithm’s predictions can be explained by already-known features ( Table II ). We then ask how much of the algorithm’s predictive power in explaining actual judges’ decisions is diminished when we control for known factors ( Table III ). We carry out both analyses for three sets of known facial features: (i) demographic characteristics, (ii) psychological features, and (iii) incentivized human guesses. 44
Is the Algorithm Rediscovering Known Facial Features?
Notes. The table presents the results of regressing an algorithmic prediction of judge detention decisions against each of the different explanatory variables as listed in the rows, where each column represents a different regression specification (the specific explanatory variables in each regression are indicated by the filled-in coefficients and standard errors in the table). The algorithm was trained using mug shots from the training data set; the regressions reported here are carried out using data from the validation data set. Data on skin tone, attractiveness, competence, dominance, and trustworthiness comes from asking subjects to assign feature ratings to mug shot images from the Mecklenburg County, NC, Sheriff’s Office public website (see the text). The human guess about the judges’ decision comes from showing workers on the Prolific platform pairs of mug shot images and asking them to report which defendant they believe the judge would be more likely to detain. Regressions follow a linear probability model and also include indicators for unknown race and unknown gender. * p < .1; ** p < .05; *** p < .01.
Does the Algorithm Predict Judge Behavior after Controlling for Known Factors?
Notes. This table reports the results of estimating a linear probability specification of judges’ detain decisions against different explanatory variables in the validation set described in Table I . Each row represents a different explanatory variable for the regression, while each column reports the results of a separate regression with different combinations of explanatory variables (as indicated by the filled-in coefficients and standard errors in the table). The algorithmic predictions of the judges’ detain decision come from our convolutional neural network algorithm built using the defendants’ face image as the only feature, using data from the training data set. Measures of defendant demographics and current arrest charge come from government administrative data obtained from a combination of Mecklenburg County, NC, and state agencies. Measures of skin tone, attractiveness, competence, dominance, and trustworthiness come from subject ratings of mug shot images (see the text). Human guess variable comes from showing subjects pairs of mug shot images and asking subjects to identify the defendant they think the judge would be more likely to detain. Regression specifications also include indicators for unknown race and unknown gender. * p < .1; ** p < .05; *** p < .01.
Table II , columns (1)–(3) show the relationship of the algorithm’s predictions to demographics. The predictions vary enormously by gender (men have predicted detention likelihoods 11.9 percentage points higher than women), less so by age, 45 and by different indicators of race or ethnicity. With skin tone scored on a 0−1 continuum, defendants whom independent raters judge to be at the lightest end of the continuum are 4.4 percentage points less likely to be detained than those rated to have the darkest skin tone (column (3)). Conditional on skin tone, Black defendants have a 1.9 percentage point lower predicted likelihood of detention compared with whites. 46
Table II , column (4) shows how the algorithm’s predictions relate to facial features implicated by past psychological studies as shaping people’s judgments of one another. These features also help explain the algorithm’s predictions of judges’ detention decisions: people judged by independent raters to be one standard deviation more attractive, competent, or trustworthy have lower predicted likelihood of detention equal to 0.55, 0.91, and 0.48 percentage points, respectively, or |$2.2\%$| , |$3.6\%$| , and |$1.8\%$| of the base rate. 47 Those whom subjects judge are one standard deviation more dominant-looking have a higher predicted likelihood of detention of 0.37 percentage points (or |$1.5\%)$| .
How do we know we have controlled for everything relevant from past research? The literature on what shapes human judgments in general is vast; perhaps there are things that are relevant for judges’ decisions specifically that we have inadvertently excluded? One way to solve this problem would be to do a comprehensive scan of past studies of human judgment and decision making, and then decide which results from different non–criminal justice contexts might be relevant for criminal justice. But that itself is a form of human-driven hypothesis generation, bringing us right back to where we started.
To get out of this box, we take a different approach. Instead of enumerating individual characteristics, we ask people to embody their beliefs in a guess, which ought to be the compound of all these characteristics. Then we can ask whether the algorithm has rediscovered this human guess (and later whether it has discovered more). We ask independent subjects to look at pairs of mug shots matched by gender, race, and five-year age bins and forecast which defendant is more likely to be detained by a judge. We provide a financial incentive for accurate guesses to increase the chances that subjects take the exercise seriously. 48 We also provide subjects with an opportunity to learn by showing subjects 50 image pairs with feedback after each pair about which defendant the judge detained. We treat the first 10 image pairs from each subject as learning trials and only use data from the last 40 image pairs. This approach is intended to capture anything that influences judges’ decisions that subjects could recognize, from subtle signs of things like socioeconomic status or drug use or mood, to things people can recognize but not articulate.
It turns out subjects are modestly good at this task ( Table II ). Participants guess which mug shot is more likely to be detained at a rate of |$51.4\%$| , which is different to a statistically significant degree from the |$50\%$| random-guessing threshold. When we regress the algorithm’s predicted detention rate against these subject guesses, the coefficient is 3.99 percentage points, equal to |$17.1\%$| of the base rate.
The findings in Table II are somewhat remarkable. The only input the algorithm had access to was the raw pixel values of each mug shot, yet it has rediscovered findings from decades of previous research and human intuition.
Interestingly, these features collectively explain only a fraction of the variation in the algorithm’s predictions: the R 2 is only 0.2228. That by itself does not necessarily mean the algorithm has discovered additional useful signal. It is possible that the remaining variation is prediction error—components of the prediction that do not explain actual judges’ decisions.
In Table III , we test whether the algorithm uncovers any additional signal for actual judge decisions, above and beyond the influence of these known factors. The algorithm by itself produces an R 2 of 0.0331 (column (1)), substantially higher than all previously known features taken together, which produce an R 2 of 0.0162 (column (5)), or the human guesses alone which produce an R 2 of 0.0025 (so we can see the algorithm is much better at predicting detention from faces than people are). Another way to see that the algorithm has detected signal above and beyond these known features is that the coefficient on the algorithm prediction when included alone in the regression, 0.6963 (column (1)), changes only modestly when we condition on everything else, now equal to 0.6171 (column (7)). The algorithm seems to have discovered some novel source of signal that better predicts judge detention decisions. 49
The algorithm has made a discovery: something about the defendant’s face explains judge decisions, above and beyond the facial features implicated by existing research. But what is it about the face that matters? Without an answer, we are left with a discovery of an unsatisfying sort. We have simply replaced one black box hypothesis generation procedure (human creativity) with another (the algorithm). In what follows we demonstrate how existing methods like saliency maps cannot solve this challenge in our application and then discuss our solution to that problem.
V.A. The Challenge of Explanation
The problem of algorithm-human communication stems from the fact that we cannot simply look inside the algorithm’s “black box” and see what it is doing because m ( x ), the algorithmic predictor, is so complicated. A common solution in computer science is to forget about looking inside the algorithmic black box and focus instead on drawing inferences from curated outputs of that box. Many of these methods involve gradients: given a prediction function m ( x ), we can calculate the gradient |$\nabla m(x) = \frac{\mathrm{d}{m}}{\mathrm{d}x}(x)$| . This lets us determine, at any input value, what change in the input vector maximally changes the prediction. 50 The idea of gradients is useful for image classification tasks because it allows us to tell which pixel image values are most important for changing the predicted outcome.
For example, a widely used method known as saliency maps uses gradient information to highlight the specific pixels that are most important for predicting the outcome of interest ( Baehrens et al. 2010 ; Simonyan, Vedaldi, and Zisserman 2014 ). This approach works well for many applications like determining whether a given picture contains a given type of animal, a common task in ecology ( Norouzzadeh et al. 2018 ). What distinguishes a cat from a dog? A saliency map for a cat detector might highlight pixels around, say, the cat’s head: what is most cat-like is not the tail, paws, or torso, but the eyes, ears, and whiskers. But more complicated outcomes of the sort social scientists study may depend on complicated functions of the entire image.
Even if saliency maps were more selective in highlighting pixels in applications like ours, for hypothesis generation they also suffer from a second limitation: they do not convey enough information to enable people to articulate interpretable hypotheses. In the cat detector example, a saliency map can tell us that something about the cat’s (say) whiskers are key for distinguishing cats from dogs. But what about that feature matters? Would a cat look more like a dog if its whiskers were longer? Or shorter? More (or less?) even in length? People need to know not just what features matter but how they must change to change the prediction. For hypothesis generation, the saliency map undercommunicates with humans.
To test the ability of saliency maps to help with our application, we focused on a facial feature that people already understand and can easily recognize from a photo: age. We first build an algorithm that predicts each defendant’s age from their mug shot. For a representative image, as in the top left of Figure III , we can highlight which pixels are most important for predicting age, shown in the top right. 51 A key limitation of saliency maps is easy to see: because age (like many human facial features) is a function of almost every part of a person’s face, the saliency map highlights almost everything.

Candidate Algorithm-Human Communication Vehicles for a Known Facial Feature: Age
Panel A shows a randomly selected point in the GAN latent space for a non-Hispanic white male defendant. Panel B shows a saliency map that highlights the pixels that are most important for an algorithmic model that predicts the defendant’s age from the mug shot image. Panel C shows an image changed or “morphed” in the direction of older age, based on the gradient of the image-based age prediction, using the “naive” morphing procedure that does not constrain the new image to lie on the face manifold (see the text). Panel D shows the image morphed to the maximum age using our actual preferred morphing procedure.
An alternative to simply highlighting high-leverage pixels is to change them in the direction of the gradient of the predicted outcome, to—ideally—create a new face that now has a different predicted outcome, what we call “morphing.” This new image answers the counterfactual question: “How would this person’s face change to increase their predicted outcome?” Our approach builds on the ability of people to comprehend ideas through comparisons, so we can show morphed image pairs to subjects to have them name the differences that they see. Figure IV summarizes our semiautomated hypothesis generation pipeline. (For more details see Online Appendix B .) The benefit of morphed images over actual mug shot images is to isolate the differences across faces that matter for the outcome of interest. By reducing noise, morphing also reduces the risk of spurious discoveries.

Hypothesis Generation Pipeline
The diagram illustrates all the algorithmic components in our procedure by presenting a full pipeline for algorithmic interpretation.
Figure V illustrates how this morphing procedure works in practice and highlights some of the technical challenges that arise. Let the box in the top panel represent the space of all possible images—all possible combinations of pixel values for, say, a 512 × 512 image. Within this space, we can apply our mug shot–based predictor of the known facial feature, age, to identify all images with the same predicted age, as shown by the contour map of the prediction function. Imagine picking some random initial mug shot image. We could follow the gradient to find an image with a higher predicted value of the outcome y .

Morphing Images for Detention Risk On and Off the Face Manifold
The figure shows the difference between an unconstrained (naive) morphing procedure and our preferred new morphing approach. In both panels, the background represents the image space (set of all possible pixel values) and the blue line (color version available online) represents the set of all pixel values that correspond to any face image (the face manifold). The orange lines show all images that have the same predicted outcome (isoquants in predicted outcome). The initial face (point on the outermost contour line) is a randomly selected face in GAN face space. From there we can naively follow the gradients of an algorithm that predicts some outcome of interest from face images. As shown in Panel A, this takes us off the face manifold and yields a nonface image. Alternatively, with a model of the face manifold, we can follow the gradient for the predicted outcome while ensuring that the new image is again a realistic instance as shown in Panel B.
The challenge is that most points in this image space are not actually face images. Simply following the gradient will usually take us off the data distribution of face images, as illustrated abstractly in the top panel of Figure V . What this means in practice is shown in the bottom left panel of Figure III : the result is an image that has a different predicted outcome (in the figure, illustrated for age) but no longer looks like a real instance—that is, no longer looks like a realistic face image. This “naive” morphing procedure will not work without some way to ensure the new point we wind up on in image space corresponds to a realistic face image.
V.B. Building a Model of the Data Distribution
To ensure morphing leads to realistic face images, we need a model of the data distribution p ( x )—in our specific application, the set of images that are faces. We rely on an unsupervised learning approach to this problem. 52 Specifically, we use generative adversarial networks (GANs), originally introduced to generate realistic new images for a variety of tasks (see Goodfellow et al. 2014 ). 53
A GAN is built by training two algorithms that “compete” with each another, the generator G and the classifier C : the generator creates synthetic images and the classifier (or “discriminator”), presented with synthetic or real images, tries to distinguish which is which. A good discriminator pressures the generator to produce images that are harder to distinguish from real; in turn, a good generator pressures the classifier to get better at discriminating real from synthetic images. Data on actual faces are used to train the discriminator, which results in the generator being trained as it seeks to fool the discriminator. With machine learning, the performance of C and G improve with successive iterations of training. A perfect G would output images where the classifier C does no better than random guessing. Such a generator would by definition limit itself to the same input space that defines real images, that is, the data distribution of faces. (Additional discussion of GANs in general and how we construct our GAN specifically are in Online Appendix B .)
To build our GAN and evaluate its expressiveness we use standard training metrics, which turn out to compare favorably to what we see with other widely used GAN models on other data sets (see Online Appendix B.C for details). A more qualitative way to judge our GAN comes from visual inspection; some examples of synthetic face images are in Figure II . Most importantly, the GAN we build (as is true of GANs in general) is not generic. GANs are specific. They do not generate “faces” but instead seek to match the distribution of pixel combinations in the training data. For example, our GAN trained using mug shots would never generate generic Facebook profile photos or celebrity headshots.
Figure V illustrates how having a model such as the GAN lets morphing stay on the data distribution of faces and produce realistic images. We pick a random point in the space of faces (mug shots) and then use the algorithmic predictor of the outcome of interest m ( x ) to identify nearby faces that are similar in all respects except those relevant for the outcome. Notice this procedure requires that faces closer to one another in GAN latent space should look relatively more similar to one another to a human in pixel space. Otherwise we might make a small movement along the gradient and wind up with a face that looks different in all sorts of other ways that are irrelevant to the outcome. That is, we need the GAN not just to model the support of the data but also to provide a meaningful distance metric.
When we produce these morphs, what can possibly change as we morph? In principle there is no limit. The changes need not be local: features such as skin color, which involves many pixels, could change. So could features such as attractiveness, where the pixels that need to change to make a face more attractive vary from face to face: the “same” change may make one face more attractive and another less so. Anything represented in the face could change, as could anything else in the image beyond the face that matters for the outcome (if, for example, localities varied in both detention rates and the type of background they have someone stand in front of for mug shots).
In practice, though, there is a limit. What can change depends on how rich and expressive the estimated GAN is. If the GAN fails to capture a certain kind of face or a dimension of the face, then we are unlikely to be able to morph on that dimension. The morphing procedure is only as complete as the GAN is expressive. Assuming the GAN expresses a feature, then if m ( x ) truly depends on that feature, morphing will likely display it. Nor is there any guarantee that in any given application the classifier m ( x ) will find novel signal for the outcome y , or that the GAN successfully learns the data distribution ( Nalisnick et al. 2018 ), or that subjects can detect and articulate whatever signal the classifier algorithm has discovered. Determining the general conditions under which our procedure will work is something we leave to future research. Whether our procedure can work for the specific application of judge decisions is the question to which we turn next. 54
V.C. Validating the Morphing Procedure
We return to our algorithmic prediction of a known facial feature—age—and see what morphing by age produces as a way to validate or test our procedure. When we follow the gradient of the predicted outcome (age), by constraining ourselves to stay on the GAN’s latent space of faces we wind up with a new age-morphed face that does indeed look like a realistic face image, as shown in the bottom right of Figure III . We seem to have successfully developed a model of the data distribution and a way to move around on that surface to create realistic new instances.
To figure out if algorithm-human communication occurs, we run these age-morphed image pairs through our experimental pipeline ( Figure IV ). Our procedure is only useful if it is replicable—that is, if it does not depend on the idiosyncratic insights of any particular person. For that reason, the people looking at these images and articulating what they see should not be us (the investigators) but a sample of external, independent study subjects. In our application, we use Prolific workers (see Online Appendix Table A.III ). Reliability or replicability is indicated by the agreement in the subject responses: lots of subjects see and articulate the same thing in the morphed images.
We asked subjects to look at 50 age-morphed image pairs selected at random from a population of 100 pairs, and told them the images in each pair differ on some hidden dimension but did not tell them what that was. 55 We asked subjects to guess which image expresses that hidden feature more, gave them feedback about the right answer, treated the first 10 image pairs as learning examples, and calculated accuracy on the remaining 40 images. Subjects correctly selected the older image |$97.8\%$| of the time.
The final step was to ask subjects to name what differs in image pairs. Making sense of these responses requires some way to group them into semantic categories. Each subject comment could include several concepts (e.g., “wrinkles, gray hair, tired”). We standardized these verbal descriptions by removing punctuation, using only lowercase characters, and removing stop words. We gave three research assistants not otherwise involved in the project these responses and asked them to create their own categories that would capture all the responses (see Online Appendix Figure A.XIII ). We also gave them an illustrative subject comment and highlighted the different “types” of categories (descriptive physical features, i.e., “thick eyebrows,” descriptive impression category, i.e., “energetic,” but also an illustration of a category of comment that is too vague to lend itself to useful measurement, i.e., “ears”). In our validation exercise |$81.5\%$| of subject reports fall into the semantic categories of either age or the closely related feature of hair color. 56
V.D. Understanding the Judge Detention Predictor
Having validated our algorithm-human communication procedure for the known facial feature of age, we are ready to apply it to generate a new hypothesis about what drives judge detention decisions. To do this we combine the mug shot algorithm predictor of judges’ detention decisions, m ( x ), with our GAN of the data distribution of mug shot images, then create new synthetic image pairs morphed with respect to the likelihood the judge would detain the defendant (see Figure IV ).
The top panel of Figure VI shows a pair of such images. Underneath we show an “image strip” of intermediate steps, along with each image’s predicted detention rate. With an overall detention rate of |$23.3\%$| in our validation data set, morphing takes us from about one-half the base rate ( |$13\%$| ) up to nearly twice the base rate ( |$41\%$| ). Additional examples of morphed image pairs are shown in Figure VII .

Illustration of Morphed Faces along the Detention Gradient
Panel A shows the result of selecting a random point on the GAN latent face space for a white non-Hispanic male defendant, then using our new morphing procedure to increase the predicted detention risk of the image to 0.41 (left) or reduce the predicted detention risk down to 0.13 (right). The overall average detention rate in the validation data set of actual mug shot images is 0.23 by comparison. Panel B shows the different intermediate images between these two end points, while Panel C shows the predicted detention risk for each of the images in the middle panel.

Examples of Morphing along the Gradients of the Face-Based Detention Predictor
We showed 54 subjects 50 detention-risk-morphed image pairs each, asked them to predict which defendant would be detained, offered them financial incentives for correct answers, 57 and gave them feedback on the right answer. Online Appendix Figure A.XV shows how accurate subjects are as they get more practice across successive morphed image pairs. With the initial image-pair trials, subjects are not much better than random guessing, in the range of what we see when subjects look at pairs of actual mugshots (where accuracy is |$51.4\%$| across the final 40 mug shot pairs people see). But unlike what happens when subjects look at actual images, when looking at morphed image pairs subjects seem to quickly learn what the algorithm is trying to communicate to them. Accuracy increased by over 10 percentage points after 20 morphed image pairs and reached |$67\%$| after 30 image pairs. Compared to looking at actual mugshots, the morphing procedure accomplished its goal of making it easier for subjects to see what in the face matters most for detention risk.
We asked subjects to articulate the key differences they saw across morphed image pairs. The result seems to be a reliable hypothesis—a facial feature that a sizable share of subjects name. In the top panel of Figure VIII , we present a histogram of individual tokens (cleaned words from worker comments) in “word cloud” form, where word size is approximately proportional to frequency. 58 Some of the most common words are “shaved,” “cleaner,” “length,” “shorter,” “moustache,” and “scruffy.” To form semantic categories, we use a procedure similar to what we describe for our validation exercise for the known feature of age. 59 Grouping tokens into semantic categories, we see that nearly |$40\%$| of the subjects see and name a similar feature that they think helps explain judge detention decisions: how well-groomed the defendant is (see the bottom panel of Figure VIII ). 60

Subject Reports of What They See between Detention-Risk-Morphed Image Pairs
Panel A shows a word cloud of subject reports about what they see as the key difference between image pairs where one is a randomly selected point in the GAN latent space and the other is morphed in the direction of a higher predicted detention risk. Words are approximately proportionately sized to the frequency of subject mentions. Panel B shows the frequency of semantic groupings of those open-ended subject reports (see the text for additional details).
Can we confirm that what the subjects think the algorithm is seeing is what the algorithm actually sees? We asked a separate set of 343 independent subjects (MTurk workers) to label the 32,881 mug shots in our combined training and validation data sets for how well-groomed each image was perceived to be on a nine-point scale. 61 For data sets of our size, these labeling costs are fairly modest, but in principle those costs could be much more substantial (or even prohibitive) in some applications.
Table IV suggests algorithm-human communication has successfully occurred: our new hypothesis, call it h 1 ( x ), is correlated with the algorithm’s prediction of the judge, m ( x ). If subjects were mistaken in thinking they saw well-groomed differences across images, there would be no relationship between well-groomed and the detention predictions. Yet what we actually see is the R 2 from regressing the algorithm’s predictions against well-groomed equals 0.0247, or |$11\%$| of the R 2 we get from a model with all the explanatory variables (0.2361). In a bivariate regression the coefficient (−0.0172) implies that a one standard deviation increase in well-groomed (1.0118 points on our 9-point scale) is associated with a decline in predicted detention risk of 1.74 percentage points, or |$7.5\%$| of the base rate. Another way to see the explanatory power of this hypothesis is to note that this coefficient hardly changes when we add all the other explanatory variables to the regression (equal to −0.0153 in the final column) despite the substantial increase in the model’s R 2 .
Correlation between Well-Groomed and the Algorithm’s Prediction
Notes. This table shows the results of estimating a linear probability specification regressing algorithmic predictions of judges’ detain decision against different explanatory variables, using data from the validation set of cases from Mecklenburg County, NC. Each row of the table represents a different explanatory variable for the regression, while each column reports the results of a separate regression with different combinations of explanatory variables (as indicated by the filled-in coefficients and standard errors in the table). Algorithmic predictions of judges’ decisions come from applying an algorithm built with face images in the training data set to validation set observations. Data on well-groomed, skin tone, attractiveness, competence, dominance, and trustworthiness come from subject ratings of mug shot images (see the text). Human guess variable comes from showing subjects pairs of mug shot images and asking subjects to identify the defendant they think the judge would be more likely to detain. Regression specifications also include indicators for unknown race and unknown gender. * p < .1; ** p < .05; *** p < .01.
V.E. Iteration
Our procedure is iterable. The first novel feature we discovered, well-groomed, explains some—but only some—of the variation in the algorithm’s predictions of the judge. We can iterate our procedure to generate hypotheses about the remaining residual variation as well. Note that the order in which features are discovered will depend on how important each feature is in explaining the judge’s detention decision and on how salient each feature is to the subjects who are viewing the morphed image pairs. So explanatory power for the judge’s decisions need not monotonically decline as we iterate and discover new features.
To isolate the algorithm’s signal above and beyond what is explained by well-groomed, we wish to generate a new set of morphed image pairs that differ in predicted detention but hold well-groomed constant. That would help subjects see other novel features that might differ across the detention-risk-morphed images, without subjects getting distracted by differences in well-groomed. 62 But iterating the procedure raises several technical challenges. To see these challenges, consider what would in principle seem to be the most straightforward way to orthogonalize, in the GAN’s latent face space:
use training data to build predictors of detention risk, m ( x ), and the facial features to orthogonalize against, h 1 ( x );
pick a point on the GAN latent space of faces;
collect the gradients with respect to m ( x ) and h 1 ( x );
use the Gram-Schmidt process to move within the latent space toward higher predicted detention risk m ( x ), but orthogonal to h 1 ( x ); and
show new morphed image pairs to subjects, have them name a new feature.
The challenge with implementing this playbook in practice is that we do not have labels for well-groomed for the GAN-generated synthetic faces. Moreover, it would be infeasible to collect this feature for use in this type of orthogonalization procedure. 63 That means we cannot orthogonalize against well-groomed, only against predictions of well-groomed. And orthogonalizing with respect to a prediction is an error-prone process whenever the predictor is imperfect (as it is here). 64 The errors in the process accumulate as we take many morphing steps. Worse, that accumulated error is not expected to be zero on average. Because we are morphing in the direction of predicted detention and we know predicted detention is correlated with well-groomed, the prediction error will itself be correlated with well-groomed.
Instead we use a different approach. We build a new detention-risk predictor with a curated training data set, limited to pairs of images matched on the features to be orthogonalized against. For each detained observation i (such that y i = 1), we find a released observation j (such that y j = 0) where h 1 ( x i ) = h 1 ( x j ). In that training data set y is now orthogonal to h 1 ( x ), so we can use the gradient of the orthogonalized detention risk predictor to move in GAN latent space to create new morphed images with different detention odds but are similar with respect to well-groomed. 65 We call these “orthogonalized morphs,” which we then feed into the experimental pipeline shown in Figure IV . 66 An open question for future work is how many iterations are possible before the dimensionality of the matching problem required for this procedure would create problems.
Examples from this orthogonalized image-morphing procedure are in Figure IX . Changes in facial features across morphed images are notably different from those in the first iteration of morphs as in Figure VI . From these examples, it appears possible that orthogonalization may be slightly imperfect; sometimes they show subtle differences in “well-groomed” and perhaps age. As with the first iteration of the morphing procedure, the second (orthogonalized) iteration of the procedure again generates images that vary substantially in their predicted risk, from 0.07 up to 0.27 (see Online Appendix Figure A.XVIII ).

Examples of Morphing along the Orthogonal Gradients of the Face-Based Detention Predictor
Still, there is a salient new signal: when presented to subjects they name a second facial feature, as shown in Figure X . We showed 52 subjects (Prolific workers) 50 orthogonalized morphed image pairs and asked them to name the differences they see. The word cloud shown in the top panel of Figure X shows that some of the most common terms reported by subjects include “big,” “wider,” “presence,” “rounded,” “body,” “jaw,” and “head.” When we ask independent research assistants to group the subject tokens into semantic groups, we can see as in the bottom of the figure that a sizable share of subject comments (around |$22\%$| ) refer to a similar facial feature, h 2 ( x ): how “heavy-faced” or “full-faced” the defendant is.

Subject Reports of What They See between Detention-Risk-Morphed Image Pairs, Orthogonalized to the First Novel Feature Discovered (Well-Groomed)
Panel A shows a word cloud of subject reports about what they see as the key difference between image pairs, where one is a randomly selected point in the GAN latent space and the other is morphed in the direction of a higher predicted detention risk, where we are moving along the detention gradient orthogonal to well-groomed and skin tone (see the text). Panel B shows the frequency of semantic groupings of these open-ended subject reports (see the text for additional details).
This second facial feature (like the first) is again related to the algorithm’s prediction of the judge. When we ask a separate sample of subjects (343 MTurk workers, see Online Appendix Table A.III ) to independently label our validation images for heavy-facedness, we can see the R 2 from regressing the algorithm’s predictions against heavy-faced yields an R 2 of 0.0384 ( Table V , column (1)). With a coefficient of −0.0182 (0.0009), the results imply that a one standard deviation change in heavy-facedness (1.1946 points on our 9-point scale) is associated with a reduced predicted detention risk of 2.17 percentage points, or |$9.3\%$| of the base rate. Adding in other facial features implicated by past research substantially boosts the adjusted R 2 of the regression but barely changes the coefficient on heavy-facedness.
Correlation between Heavy-Faced and the Algorithm’s Prediction
Notes. This table shows the results of estimating a linear probability specification regressing algorithmic predictions of judges’ detain decision against different explanatory variables, using data from the validation set of cases from Mecklenburg County, NC. Each row of the table represents a different explanatory variable for the regression, while each column reports the results of a separate regression with different combinations of explanatory variables (as indicated by the filled-in coefficients and standard errors in the table). Algorithmic predictions of judges’ decisions come from applying the algorithm built with face images in the training data set to validation set observations. Data on heavy-faced, well-groomed, skin tone, attractiveness, competence, dominance, and trustworthiness come from subject ratings of mug shot images (see the text). Human guess variable comes from showing subjects pairs of mug shot images and asking subjects to identify the defendant they think the judge would be more likely to detain. Regression specifications also include indicators for unknown race and unknown gender. * p < .1; ** p < .05; *** p < .01.
In principle, the procedure could be iterated further. After all, well-groomed, heavy-faced plus previously known facial features all together still only explain |$27\%$| of the variation in the algorithm’s predictions of the judges’ decisions. As long as there is residual variation, the hypothesis generation crank could be turned again and again. Because our goal is not to fully explain judges’ decisions but to illustrate that the procedure works and is iterable, we leave this for future work (ideally done on data from other jurisdictions as well).
Here we consider whether the new hypotheses our procedure has generated meet our final criterion: empirical plausibility. We show that these facial features are new not just to the scientific literature but also apparently to criminal justice practitioners, before turning to whether these correlations might reflect some underlying causal relationship.
VI.A. Do These Hypotheses Predict What Judges Actually Do?
Empirical plausibility need not be implied by the fact that our new facial features are correlated with the algorithm’s predictions of judges’ decisions. The algorithm, after all, is not a perfect predictor. In principle, well-groomed and heavy-faced might be correlated with the part of the algorithm’s prediction that is unrelated to judge behavior, or m ( x ) − y .
In Table VI , we show that our two new hypotheses are indeed empirically plausible. The adjusted R 2 from regressing judges’ decisions against heavy-faced equals 0.0042 (column (1)), while for well-groomed the figure is 0.0021 (column (2)) and for both together the figure equals 0.0061 (column (3)). As a benchmark, the adjusted R 2 from all variables (other than the algorithm’s overall mug shot–based prediction) in explaining judges’ decisions equals 0.0218 (column (6)). So the explanatory power of our two novel hypotheses alone equals about |$28\%$| of what we get from all the variables together.
Do Well-Groomed and Heavy-Faced Correlate with Judge Decisions?
Notes. This table reports the results of estimating a linear probability specification of judges’ detain decisions against different explanatory variables in the validation set described in Table I . The algorithmic predictions of the judges’ detain decision come from our convolutional neural network algorithm built using the defendants’ face image as the only feature, using data from the training data set. Measures of defendant demographics and current arrest charge come from Mecklenburg County, NC, administrative data. Data on heavy-faced, well-groomed, skin tone, attractiveness, competence, dominance, and trustworthiness come from subject ratings of mug shot images (see the text). Human guess variable comes from showing subjects pairs of mug shot images and asking subjects to identify the defendant they think the judge would be more likely to detain. Regression specifications also include indicators for unknown race and unknown gender. * p < .1; ** p < .05; *** p < .01.
For a sense of the magnitude of these correlations, the coefficient on heavy-faced of −0.0234 (0.0036) in column (1) and on well-groomed of −0.0198 (0.0043) in column (2) imply that one standard deviation changes in each variable are associated with reduced detention rates equal to 2.8 and 2.0 percentage points, respectively, or |$12.0\%$| and |$8.9\%$| of the base rate. Interestingly, column (7) shows that heavy-faced remains statistically significant even when we control for the algorithm’s prediction. The discovery procedure led us to a facial feature that, when measured independently, captures signal above and beyond what the algorithm found. 67
VI.B. Do Practitioners Already Know This?
Our procedure has identified two hypotheses that are new to the existing research literature and to our study subjects. Yet the study subjects we have collected data from so far likely have relatively little experience with the criminal justice system. A reader might wonder: do experienced criminal justice practitioners already know that these “new” hypotheses affect judge decisions? The practitioners might have learned the influence of these facial features from day-to-day experience.
To answer this question, we carried out two smaller-scale data collections with a sample of N = 15 staff at a public defender’s office and a legal aid society. We first asked an open-ended question: on what basis do judges decide to detain versus release defendants pretrial? Practitioners talked about judge misunderstandings of the law, people’s prior criminal records, and judge underappreciation for the social contexts in which criminal records arise. Aside from the defendant’s race, nothing about the appearance of defendants was mentioned.
We showed practitioners pairs of actual mug shots and asked them to guess which person is more likely to be detained by a judge (as we had done with MTurk and Prolific workers). This yields a sample of 360 detention forecasts. After seeing these mug shots practitioners were asked an open-ended question about what they think matters about the defendant’s appearance for judge detention decisions. There were a few mentions of well-groomed and one mention of something related to heavy-faced, but these were far from the most frequently mentioned features, as seen in Online Appendix Figure A.XX .
The practitioner forecasts do indeed seem to be more accurate than those of “regular” study subjects. Table VII , column (5) shows that defendants whom the practitioners predict will be detained are 29.2 percentage points more likely to actually be detained, even after controlling for the other known determinants of detention from past research. This is nearly four times the effect of forecasts made by Prolific workers, as shown in the last column of Table VI . The practitioner guesses (unlike the regular study subjects) are even about as accurate as the algorithm; the R 2 from the practitioner guess (0.0165 in column (1)) is similar to the R 2 from the algorithm’s predictions (0.0166 in column (6)).
Results from the Criminal Justice Practitioner Sample
Notes. This table shows the results of estimating judges’ detain decisions using a linear probability specification of different explanatory variables on a subset of the validation set. The criminal justice practitioner’s guess about the judge’s decision comes from showing 15 different public defenders and legal aid society members actual mug shot images of defendants and asking them to report which defendant they believe the judge would be more likely to detain. The pairs are selected to be congruent in gender and race but discordant in detention outcome. The algorithmic predictions of judges’ detain decisions come from applying the algorithm, which is built with face images in the training data set, to validation set observations. Measures of defendant demographics and current arrest charge come from Mecklenburg County, NC, administrative data. Data on heavy-faced, well-groomed, skin tone, attractiveness, competence, dominance, and trustworthiness come from subject ratings of mug shot images (see the text). Regression specifications also include indicators for unknown race and unknown gender. * p < .1; ** p < .05; *** p < .01.
Yet practitioners do not seem to already know what the algorithm has discovered. We can see this in several ways in Table VII . First, the sum of the adjusted R 2 values from the bivariate regressions of judge decisions against practitioner guesses and judge decisions against the algorithm mug shot–based prediction is not so different from the adjusted R 2 from including both variables in the same regression (0.0165 + 0.0166 = 0.0331 from columns (1) plus (6), versus 0.0338 in column (7)). We see something similar for the novel features of well-groomed and heavy-faced specifically as well. 68 The practitioners and the algorithm seem to be tapping into largely unrelated signal.
VI.C. Exploring Causality
Are these novel features actually causally related to judge decisions? Fully answering that question is clearly beyond the scope of the present article. But we can present some additional evidence that is at least suggestive.
For starters we can rule out some obvious potential confounders. With the specific hypotheses in hand, identifying the most important concerns with confounding becomes much easier. In our application, well-groomed and heavy-faced could in principle be related to things like (say) the degree to which the defendant has a substance-abuse problem, is struggling with mental health, or their socioeconomic status. But as shown in a series of Online Appendix tables, we find that when we have study subjects independently label the mug shots in our validation data set for these features and then control for them, our novel hypotheses remain correlated with the algorithmic predictions of the judge and actual judge decisions. 69 We might wonder whether heavy-faced is simply a proxy for something that previous mock-trial-type studies suggest might matter for criminal justice decisions, “baby-faced” ( Berry and Zebrowitz-McArthur 1988 ). 70 But when we have subjects rate mug shots for baby-facedness, our full-faced measure remains strongly predictive of the algorithm’s predictions and actual judge decisions; see Online Appendix Tables A.XII and A.XVI .
In addition, we carried out a laboratory-style experiment with Prolific workers. We randomly morphed synthetic mug shot images in the direction of either higher or lower well-groomed (or full-faced), randomly assigned structured variables (current charge and prior record) to each image, explained to subjects the detention decision judges are asked to make, and then asked them which from each pair of subjects they would be more likely to detain if they were the judge. The framework from Mobius and Rosenblat (2006) helps clarify what this lab experiment gets us: appearance might affect how others treat us because others are reacting to something about our own appearance directly, because our appearance affects our own confidence, or because our appearance affects our effectiveness in oral communication. The experiment’s results shut down these latter two mechanisms and isolate the effects of something about appearance per se, recognizing it remains possible well-groomed and heavy-faced are correlated with some other aspect of appearance. 71
The study subjects recommend for detention those subjects with higher-risk structured variables (like current charge and prior record), which at the very least suggests they are taking the task seriously. Holding these other case characteristics constant, we find that the subjects are more likely to recommend for detention those defendants who are less well-groomed or less heavy-faced (see Online Appendix Table A.XVII ). Qualitatively, these results support the idea that well-groomed and heavy-faced could have a causal effect. It is not clear that the magnitudes in these experiments necessarily have much meaning: the subjects are not actual judges, and the context and structure of choice is very different from real detention decisions. Still, it is worth noting that the magnitudes implied by our results are nontrivial. Changing well-groomed or heavy-faced has the same effect on subject decisions as a movement within the predicted rearrest risk distribution of 4 and 6 percentile points, respectively (see Online Appendix C for details). Of course only an actual field experiment could conclusively determine causality here, but carrying out that type of field experiment might seem more worthwhile to an investigator in light of the lab experiment’s results.
Is this enough empirical support for these hypotheses to justify incurring the costs of causal testing? The empirical basis for these hypotheses would seem to be at least as strong as (or perhaps stronger than) the informal standard currently used to decide whether an idea is promising enough to test, which in our experience comes from some combination of observing the world, brainstorming, and perhaps some exploratory investigator-driven correlational analysis.
What might such causal testing look like? One possibility would follow in the spirit of Goldin and Rouse (2000) and compare detention decisions in settings where the defendant is more versus less visible to the judge to alter the salience of appearance. For example, many jurisdictions have continued to use some version of virtual hearings even after the pandemic. 72 In Chicago the court system has the defendant appear virtually but everyone else is in person, and the court system of its own volition has changed the size of the monitors used to display the defendant to court participants. One could imagine adding some planned variation to screen size or distance or angle to the judge. These video feeds could in principle be randomly selected for AI adjustment to the defendant’s level of well-groomedness or heavy-facedness (this would probably fall into a legal gray area). In the case of well-groomed, one could imagine a field experiment that changed this aspect of the defendant’s actual appearance prior to the court hearing. We are not claiming these are the right designs but intend only to illustrate that with new hypotheses in hand, economists are positioned to deploy the sort of creativity and rigorous testing that have become the hallmark of the field’s efforts at causal inference.
We have presented a new semi-automated procedure for hypothesis generation. We applied this new procedure to a concrete, socially important application: why judges jail some defendants and not others. Our procedure suggests two novel hypotheses: some defendants appear more well-groomed or more heavy-faced than others.
Beyond the specific findings from our illustrative application, our empirical analysis also illustrates a playbook for other applications. Start with a high-dimensional predictor m ( x ) of some behavior of interest. Build an unsupervised model of the data distribution, p ( x ). Then combine the models for m ( x ) and p ( x ) in a morphing procedure to generate new instances that answer the counterfactual question: what would a given instance look like with higher or lower likelihood of the outcome? Show morphed pairs of instances to participants and get them to name what they see as the differences between morphed instances. Get others to independently rate instances for whatever the new hypothesis is; do these labels correlate with both m ( x ) and the behavior of interest, y ? If so, we have a new hypothesis worth causal testing. This playbook is broadly applicable whenever three conditions are met.
The first condition is that we have a behavior we can statistically predict. The application we examine here fits because the behavior is clearly defined and measured for many cases. A study of, say, human creativity would be more challenging because it is not clear that it can be measured ( Said-Metwaly, Van den Noortgate, and Kyndt 2017 ). A study of why U.S. presidents use nuclear weapons during wartime would be challenging because there have been so few cases.
The second condition relates to what input data are available to predict behavior. Our procedure is likely to add only modest value in applications where we only have traditional structured variables, because those structured variables already make sense to people. Moreover the structured variables are usually already hypothesized to affect different behaviors, which is why economists ask about them on surveys. Our procedure will be more helpful with unstructured, high-dimensional data like images, language, and time series. The deeper point is that the collection of such high-dimensional data is often incidental to the scientific enterprise. We have images because the justice system photographs defendants during booking. Schools collect text from students as part of required assignments. Cellphones create location data as part of cell tower “pings.” These high-dimensional data implicitly contain an endless number of “features.”
Such high-dimensional data have already been found to predict outcomes in many economically relevant applications. Student essays predict graduation. Newspaper text predicts political slant of writers and editors. Federal Open Market Committee notes predict asset returns or volatility. X-ray images or EKG results predict doctor diagnoses (or misdiagnoses). Satellite images predict the income or health of a place. Many more relationships like these remain to be explored. From such prediction models, one could readily imagine human inspection of morphs leading to novel features. For example, suppose high-frequency data on volume and stock prices are used to predict future excess returns, for example, to understand when the market over- or undervalues a stock. Morphs of these time series might lead us to discover the kinds of price paths that produce overreaction. After all, some investors have even named such patterns (e.g., “head and shoulders,” “double bottom”) and trade on them.
The final condition is to be able to morph the input data to create new cases that differ in the predicted outcome. This requires some unsupervised learning technique to model the data distribution. The good news is that a number of such techniques are now available that work well with different types of high-dimensional data. We happen to use GANs here because they work well with images. But our procedure can accomodate a variety of unsupervised models. For example for text we can use other methods like Bidirectional Encoder Representations from Transformers ( Devlin et al. 2018 ), or for time series we could use variational auto-encoders ( Kingma and Welling 2013 ).
An open question is the degree to which our experimental pipeline could be changed by new technologies, and in particular by recent innovations in generative modeling. For example, several recent models allow people to create new synthetic images from text descriptions, and so could perhaps (eventually) provide alternative approaches to the creation of counterfactual instances. 73 Similarly, recent generative language models appear to be able to process images (e.g., GPT-4), although they are only recently publicly available. Because there is inevitably some uncertainty in forecasting what those tools will be able to do in the future, they seem unlikely to be able to help with the first stage of our procedure’s pipeline—build a predictive model of some behavior of interest. To see why, notice that methods like GPT-4 are unlikely to have access to data on judge decisions linked to mug shots. But the stage of our pipeline that GPT-4 could potentially be helpful for is to substitute for humans in “naming” the contrasts between the morphed pairs of counterfactual instances. Though speculative, such innovations potentially allow for more of the hypothesis generation procedure to be automated. We leave the exploration of these possibilities to future work.
Finally, it is worth emphasizing that hypothesis generation is not hypothesis testing. Each follows its own logic, and one procedure should not be expected to do both. Each requires different methods and approaches. What is needed to creatively produce new hypotheses is different from what is needed to carefully test a given hypothesis. Testing is about the curation of data, an effort to compare comparable subsets from the universe of all observations. But the carefully controlled experiment’s focus on isolating the role of a single prespecified factor limits the ability to generate new hypotheses. Generation is instead about bringing as much data to bear as possible, since the algorithm can only consider signal within the data available to it. The more diverse the data sources, the more scope for discovery. An algorithm could have discovered judge decisions are influenced by football losses, as in Eren and Mocan (2018) , but only if we thought to merge court records with massive archives of news stories as for example assembled by Leskovec, Backstrom, and Kleinberg (2009) . For generating ideas, creativity in experimental design useful for testing is replaced with creativity in data assembly and merging.
More generally, we hope to raise interest in the curious asymmetry we began with. Idea generation need not remain such an idiosyncratic or nebulous process. Our framework hopefully illustrates that this process can also be modeled. Our results illustrate that such activity could bear actual empirical fruit. At a minimum, these results will hopefully spur more theoretical and empirical work on hypothesis generation rather than leave this as a largely “prescientific” activity.
This is a revised version of Chicago Booth working paper 22-15 “Algorithmic Behavioral Science: Machine Learning as a Tool for Scientific Discovery.” We gratefully acknowledge support from the Alfred P. Sloan Foundation, Emmanuel Roman, and the Center for Applied Artificial Intelligence at the University of Chicago, and we thank Stephen Billings for generously sharing data. For valuable comments we thank Andrei Shleifer, Larry Katz, and five anonymous referees, as well as Marianne Bertrand, Jesse Bruhn, Steven Durlauf, Joel Ferguson, Emma Harrington, Supreet Kaur, Matteo Magnaricotte, Dev Patel, Betsy Levy Paluck, Roberto Rocha, Evan Rose, Suproteem Sarkar, Josh Schwartzstein, Nick Swanson, Nadav Tadelis, Richard Thaler, Alex Todorov, Jenny Wang, and Heather Yang, plus seminar participants at Bocconi, Brown, Columbia, ETH Zurich, Harvard, the London School of Economics, MIT, Stanford, the University of California Berkeley, the University of Chicago, the University of Pennsylvania, the University of Toronto, the 2022 Behavioral Economics Annual Meetings, and the 2022 NBER Summer Institute. For invaluable assistance with the data and analysis we thank Celia Cook, Logan Crowl, Arshia Elyaderani, and especially Jonas Knecht and James Ross. This research was reviewed by the University of Chicago Social and Behavioral Sciences Institutional Review Board (IRB20-0917) and deemed exempt because the project relies on secondary analysis of public data sources. All opinions and any errors are our own.
The question of hypothesis generation has been a vexing one in philosophy, as it appears to follow a process distinct from deduction and has been sometimes called “abduction” (see Schickore 2018 for an overview). A fascinating economic exploration of this topic can be found in Heckman and Singer (2017) , which outlines a strategy for how economists should proceed in the face of surprising empirical results. Finally, there is a small but growing literature that uses machine learning in science. In the next section we discuss how our approach is similar in some ways and different in others.
See Einav and Levin (2014) , Varian (2014) , Athey (2017) , Mullainathan and Spiess (2017) , Gentzkow, Kelly, and Taddy (2019) , and Adukia et al. (2023) on how these changes can affect economics.
In practice, there are a number of additional nuances, as discussed in Section III.A and Online Appendix A.A .
This is calculated for some of the most commonly used measures of predictive accuracy, area under the curve (AUC) and R 2 , recognizing that different measures could yield somewhat different shares of variation explained. We emphasize the word predictable here: past work has shown that judges are “noisy” and decisions are hard to predict ( Kahneman, Sibony, and Sunstein 2022 ). As a consequence, a predictive model of the judge can do better than the judge themselves ( Kleinberg et al. 2018 ).
In Section IV.B , we examine whether the mug shot’s predictive power can be explained by underlying risk differences. There, we tentatively conclude that the predictive power of the face likely reflects judicial error, but that working assumption is not essential to either our results or the ultimate goal of the article: uncovering hypotheses for later careful testing.
For reviews of the interpretability literature, see Doshi-Velez and Kim (2017) and Marcinkevičs and Vogt (2020) .
See Liu et al. (2019) , Narayanaswamy et al. (2020) , Lang et al. (2021) , and Ghandeharioun et al. (2022) .
For example, if every dog photo in a given training data set had been taken outdoors and every cat photo was taken indoors, the algorithm might learn what animal is in the image based in part on features of the background, which would lead the algorithm to perform poorly in a new data set of more representative images.
For example, for canonical computer science applications like image classification (does this photo contain an image of a dog or of a cat?), predictive accuracy (AUC) can be on the order of 0.99. In contrast, our model of judge decisions using the face only achieves an AUC of 0.625.
Of course even if the hypotheses that are generated are the result of idiosyncratic creativity, this can still be useful. For example, Swanson (1986 , 1988) generated two novel medical hypotheses: the possibility that magnesium affects migraines and that fish oil may alleviate Raynaud’s syndrome.
Conversely, given a data set, our procedure has a built-in advantage: one could imagine a huge number of hypotheses that, while possible, are not especially useful because they are not measurable. Our procedure is by construction guaranteed to generate hypotheses that are measurable in a data set.
For additional discussion, see Ludwig and Mullainathan (2023a) .
For example, isolating the causal effects of gender on labor market outcomes is a daunting task, but the clever test in Goldin and Rouse (2000) overcomes the identification challenges by using variation in screening of orchestra applicants.
See the clever paper by Grogger and Ridgeway (2006) that uses this source of variation to examine this question.
This is related to what Autor (2014) called “Polanyi’s paradox,” the idea that people’s understanding of how the world works is beyond our capacity to explicitly describe it. For discussions in psychology about the difficulty for people to access their own cognition, see Wilson (2004) and Pronin (2009) .
Consider a simple example. Suppose x = ( x 1 , …, x k ) is a k -dimensional binary vector, all possible values of x are equally likely, and the true function in nature relating x to y only depends on the first dimension of x so the function h 1 is the only true hypothesis and the only empirically plausible hypothesis. Even with such a simple true hypothesis, people can generate nonplausible hypotheses. Imagine a pair of data points ( x 0 , 0) and ( x 1 , 1). Since the data distribution is uniform, x 0 and x 1 will differ on |$\frac{k}{2}$| dimensions in expectation. A person looking at only one pair of observations would have a high chance of generating an empirically implausible hypothesis. Looking at more data, the probability of discovering an implausible hypothesis declines. But the problem remains.
Some canonical references include Breiman et al. (1984) , Breiman (2001) , Hastie et al. (2009) , and Jordan and Mitchell (2015) . For discussions about how machine learning connects to economics, see Belloni, Chernozhukov, and Hansen (2014) , Varian (2014) , Mullainathan and Spiess (2017) , Athey (2018) , and Athey and Imbens (2019) .
Of course there is not always a predictive signal in any given data application. But that is equally an issue for human hypothesis generation. At least with machine learning, we have formal procedures for determining whether there is any signal that holds out of sample.
The intuition here is quite straightforward. If two predictor variables are highly correlated, the weight that the algorithm puts on one versus the other can change from one draw of the data to the next depending on the idiosyncratic noise in the training data set, but since the variables are highly correlated, the predicted outcome values themselves (hence predictive accuracy) can be quite stable.
See Online Appendix Figure A.I , which shows the top nine eigenfaces for the data set we describe below, which together explain |$62\%$| of the variation.
Examples of applications of this type include Carleo et al. (2019) , He et al. (2019) , Davies et al. (2021) , Jumper et al. (2021) , and Pion-Tonachini et al. (2021) .
As other examples, researchers have found that retinal images alone can unexpectedly predict gender of patient or macular edema ( Narayanaswamy et al. 2020 ; Korot et al. 2021 ).
Sheetal, Feng, and Savani (2020) use machine learning to determine which of the long list of other survey variables collected as part of the World Values Survey best predict people’s support for unethical behavior. This application sits somewhat in between an investigator-generated hypothesis and the development of an entirely new hypothesis, in the sense that the procedure can only choose candidate hypotheses for unethical behavior from the set of variables the World Values Survey investigators thought to include on their questionnaire.
Closest is Miller et al. (2019) , which morphs EKG output but stops at the point of generating realistic morphs and does not carry this through to generating interpretable hypotheses.
Additional details about how the system works are found in Online Appendix A .
For Black non-Hispanics, the figures for Mecklenburg County versus the United States were |$33.3\%$| versus |$13.6\%$| . See https://www.census.gov/programs-surveys/sis/resources/data-tools/quickfacts.html .
Details on how we operationalize these variables are found in Online Appendix A .
The mug shot seems to have originated in Paris in the 1800s ( https://law.marquette.edu/facultyblog/2013/10/a-history-of-the-mug-shot/ ). The etymology of the term is unclear, possibly based on “mug” as slang for either the face or an “incompetent person” or “sucker” since only those who get caught are photographed by police ( https://www.etymonline.com/word/mug-shot ).
See https://mecksheriffweb.mecklenburgcountync.gov/ .
We partition the data by arrestee, not arrest, to ensure people show up in only one of the partitions to avoid inadvertent information “leakage” across data partitions.
As the Online Appendix tables show, while there are some changes to a few of the coefficients that relate the algorithm’s predictions to factors known from past research to shape human decisions, the core findings and conclusions about the importance of the defendant’s appearance and the two specific novel facial features we identify are similar.
Using the data on arrests up to July 17, 2019, we randomly reassign arrestees to three groups of similar size to our training, validation, and lock-box hold-out data sets, convert the data to long format (with one row for each arrest-and-variable) and calculate an F -test statistic for the joint null hypothesis that the difference in baseline characteristics are all zero, clustering standard errors by arrestee. We store that F -test statistic, rerun this procedure 1,000 times, and then report the share of splits with an F -statistic larger than the one observed for the original data partition.
For an example HIT task, see Online Appendix Figure A.II .
For age and skin tone, we calculated the average pairwise correlation between two labels sampled (without replacement) from the 10 possibilities, repeated across different random pairs. The Pearson correlation was 0.765 for skin tone, 0.741 for age, and between age assigned labels versus administrative data, 0.789. The maximum correlation between the average of the first k labels collected and the k + 1 label is not all that much higher for k = 1 than k = 9 (0.733 versus 0.837).
For an example of the consent form and instructions given to labelers, see Online Appendix Figures A.IV and A.V .
We actually collected at least three and at least five, but the averages turned out to be very close to the minimums, equal to 3.17 and 5.07, respectively.
For example, in Oosterhof and Todorov (2008) , Supplemental Materials Table S2, they report Cronbach’s α values of 0.95 for attractiveness, and 0.93 for both trustworthy and dominant.
See Online Appendix Figure A.VIII , which shows that the change in the correlation between the ( k + 1)th label with the mean of the first k labels declines after three labels.
For an example, see Online Appendix Figure A.IX .
We use the validation data set to estimate |$\hat{\beta }$| and then evaluate the accuracy of m p ( x ). Although this could lead to overfitting in principle, since we are only estimating a single parameter, this does not matter much in practice; we get very similar results if we randomly partition the validation data set by arrestee, use a random |$30\%$| of the validation data set to estimate the weights, then measure predictive performance in the other random |$70\%$| of the validation data set.
The mean squared area for a linear probability model’s predictions is related to the Brier score ( Brier 1950 ). For a discussion of how this relates to AUC and calibration, see Murphy (1973) .
Note how this comparison helps mitigate the problem that police arrest decisions could depend on a person’s face. When we regress rearrest against the mug shot, that estimated coefficient may be heavily influenced by how police arrest decisions respond to the defendant’s appearance. In contrast when we regress judge detention decisions against predicted rearrest risk, some of the variation across defendants in rearrest risk might come from the effect of the defendant’s appearance on the probability a police officer makes an arrest, but a great deal of the variation in predicted risk presumably comes from people’s behavior.
The average mug shot–predicted detention risk for the bottom and top quartiles equal 0.127 and 0.332; that difference times 2.880 implies a rearrest risk difference of 59.0 percentage points. By way of comparison, the difference in rearrest risk between those who are arrested for a felony crime rather than a less serious misdemeanor crime is equal to just 7.8 percentage points.
In our main exhibits, we impose a simple linear relationship between the algorithm’s predicted detention risk and known facial features like age or psychological variables, for ease of presentation. We show our results are qualitatively similar with less parametric specifications in Online Appendix Tables A.VI, A.VII, and A.VIII .
With a coefficient value of 0.0006 on age (measured in years), the algorithm tells us that even a full decade’s difference in age has |$5\%$| the impact on detention likelihood compared to the effects of gender (10 × 0.0006 = 0.6 percentage point higher likelihood of detention, versus 11.9 percentage points).
Online Appendix Table A.V shows that Hispanic ethnicity, which we measure from subject ratings from looking at mug shots, is not statistically significantly related to the algorithm’s predictions. Table II , column (2) showed that conditional on gender, Black defendants have slightly higher predicted detention odds than white defendants (0.3 percentage points), but this is not quite significant ( t = 1.3). Online Appendix Table A.V , column (1) shows that conditioning on Hispanic ethnicity and having stereotypically Black facial features—as measured in Eberhardt et al. (2006) —increases the size of the Black-white difference in predicted detention odds (now equal to 0.8 percentage points) as well as the difference’s statistical significance ( t = 2.2).
This comes from multiplying the effect of each 1 unit change in our 9-point scale associated, equal to 0.55, 0.91, and 0.48 percentage points, respectively, with the standard deviation of the average label for each psychological feature for each image, which equal 0.923, 0.911, and 0.844, respectively.
As discussed in Online Appendix Table A.III , we offer subjects a |${\$}$| 3.00 base rate for participation plus an incentive of 5 cents per correct guess. With 50 image pairs shown to each participant, they could increase their earnings by another |${\$}$| 2.50, or up to |$83\%$| above the base compensation.
Table III gives us another way to see how much of previously known features are rediscovered by the algorithm. That the algorithm’s prediction plus all previously known features yields an R 2 of just 0.0380 (column (7)), not much larger than with the algorithm alone, suggests the algorithm has discovered most of the signal in these known features. But not necessarily all: these other known features often do remain statistically significant predictors of judges’ decisions even after controlling for the algorithm’s predictions (last column). One possible reason is that, given finite samples, the algorithm has only imperfectly reconstructed factors such as “age” or “human guess.” Controlling for these factors directly adds additional signal.
Imagine a linear prediction function like |$m(x_1,x_2) = \widehat{\beta }_1 x_1 + \widehat{\beta }_2 x_2$| . If our best estimates suggested |$\widehat{\beta }_2=0$| , the maximum change to the prediction comes from incrementally changing x 1 .
As noted already, to avoid contributing to the stereotyping of minorities in discussions of crime, in our exhibits we show images for non-Hispanic white men, although in our HITs we use images representative of the larger defendant population.
Modeling p ( x ) through a supervised learning task would involve assembling a large set of images, having subjects label each image for whether they contain a realistic face, and then predicting those labels using the image pixels as inputs. But this supervised learning approach is costly because it requires extensive annotation of a large training data set.
Kaji, Manresa, and Pouliot (2020) and Athey et al. (2021 , 2022) are recent uses of GANs in economics.
Some ethical issues are worth considering. One is bias. With human hypothesis generation there is the risk people “see” an association that impugns some group yet has no basis in fact. In contrast our procedure by construction only produces empirically plausible hypotheses. A different concern is the vulnerability of deep learning to adversarial examples: tiny, almost imperceptible changes in an image changing its classification for the outcome y , so that mug shots that look almost identical (that is, are very “similar” in some visual image metric) have dramatically different m ( x ). This is a problem because tiny changes to an image don’t change the nature of the object; see Szegedy et al. (2013) and Goodfellow, Shlens, and Szegedy (2014) . In practice such instances are quite rare in nature, indeed, so rare they usually occur only if intentionally (maliciously) generated.
Online Appendix Figure A.XII gives an example of this task and the instructions given to participating subjects to complete it. Each subject was tested on 50 image pairs selected at random from a population of 100 images. Subjects were told that for every pair, one image was higher in some unknown feature, but not given details as to what the feature might be. As in the exercise for predicting detention, feedback was given immediately after selecting an image, and a 5 cent bonus was paid for every correct answer.
In principle this semantic grouping could be carried out in other ways, for example, with automated procedures involving natural-language processing.
See Online Appendix Table A.III for a high-level description of this human intelligence task, and Online Appendix Figure A.XIV for a sample of the task and the subject instructions.
We drop every token of just one or two characters in length, as well as connector words without real meaning for this purpose, like “had,” “the,” and “and,” as well as words that are relevant to our exercise but generic, like “jailed,” “judge,” and “image.”
We enlisted three research assistants blinded to the findings of this study and asked them to come up with semantic categories that captured all subject comments. Since each assistant mapped each subject comment to |$5\%$| of semantic categories on average, if the assistant mappings were totally uncorrelated, we would expect to see agreement of at least two assistant categorizations about |$5\%$| of the time. What we actually see is if one research assistant made an association, |$60\%$| of the time another assistant would make the same association. We assign a comment to a semantic category when at least two of the assistants agree on the categorization.
Moreover what subjects see does not seem to be particularly sensitive to which images they see. (As a reminder, each subject sees 50 morphed image pairs randomly selected from a larger bank of 100 morphed image pairs). If we start with a subject who says they saw “well-groomed” in the morphed image pairs they saw, for other subjects who saw 21 or fewer images in common (so saw mostly different images) they also report seeing well-groomed |$31\%$| of the time, versus |$35\%$| among the population. We select the threshold of 21 images because this is the smallest threshold in which at least 50 pairs of raters are considered.
See Online Appendix Table A.III and Online Appendix Figure A.XVI . This comes to a total of 192,280 individual labels, an average of 3.2 labels per image in the training set and an average of 10.8 labels per image in the validation set. Sampling labels from different workers on the same image, these ratings have a correlation of 0.14.
It turns out that skin tone is another feature that is correlated with well-groomed, so we orthogonalize on that as well as well-groomed. To simplify the discussion, we use “well-groomed” as a stand-in for both features we orthogonalize against, well-groomed plus skin tone.
To see why, consider the mechanics of the procedure. Since we orthogonalize as we create morphs, we would need labels at each morphing step. This would entail us producing candidate steps (new morphs), collecting data on each of the candidates, picking one that has the same well-groomed value, and then repeating. Moreover, until the labels are collected at a given step, the next step could not be taken. Since producing a final morph requires hundreds of such intermediate morphing steps, the whole process would be so time- and resource-consuming as to be infeasible.
While we can predict demographic features like race and age (above/below median age) nearly perfectly, with AUC values close to 1, for predicting well-groomed, the mean absolute error of our OOS prediction is 0.63, which is plus or minus over half a slider value for this 9-point-scaled variable. One reason it is harder to predict well-groomed is because the labels, which come from human subjects looking at and labeling mug shots, are themselves noisy, which introduces irreducible error.
For additional details see Online Appendix Figure A.XVII and Online Appendix B .
There are a few additional technical steps required, discussed in Online Appendix B . For details on the HIT we use to get subjects to name the new hypothesis from looking at orthogonalized morphs, and the follow-up HIT to generate independent labels for that new hypothesis or facial feature, see Online Appendix Table A.III .
See Online Appendix Figure A.XIX .
The adjusted R 2 of including the practitioner forecasts plus well-groomed and heavy-facedness together (column (3), equal to 0.0246) is not that different from the sum of the R 2 values from including just the practitioner forecasts (0.0165 in column (1)) plus that from including just well-groomed and heavy-faced (equal to 0.0131 in Table VII , column (2)).
In Online Appendix Table A.IX we show that controlling for one obvious indicator of a substance abuse issue—arrest for drugs—does not seem to substantially change the relationship between full-faced or well-groomed and the predicted detention decision. Online Appendix Tables A.X and A.XI show a qualitatively similar pattern of results for the defendant’s mental health and socioeconomic status, which we measure by getting a separate sample of subjects to independently rate validation–data set mug shots. We see qualitatively similar results when the dependent variable is the actual rather than predicted judge decision; see Online Appendix Tables A.XIII, A.XIV, and A.XV .
Characteristics of having a baby face included large eyes, narrow chin, small nose, and high, raised eyebrows. For a discussion of some of the larger literature on how that feature shapes the reactions of other people generally, see Zebrowitz et al. (2009) .
For additional details, see Online Appendix C .
See https://www.nolo.com/covid-19/virtual-criminal-court-appearances-in-the-time-of-the-covid-19.html .
See https://stablediffusionweb.com/ and https://openai.com/product/dall-e-2 .
The data underlying this article are available in the Harvard Dataverse, https://doi.org/10.7910/DVN/ILO46V ( Ludwig and Mullainathan 2023b ).
Adukia Anjali , Eble Alex , Harrison Emileigh , Birali Runesha Hakizumwami , Szasz Teodora , “ What We Teach about Race and Gender: Representation in Images and Text of Children’s Books ,” Quarterly Journal of Economics , 138 ( 2023 ), 2225 – 2285 . https://doi.org/10.1093/qje/qjad028
Google Scholar
Angelova Victoria , Dobbie Will S. , Yang Crystal S. , “ Algorithmic Recommendations and Human Discretion ,” NBER Working Paper no. 31747, 2023 . https://doi.org/10.3386/w31747
Arnold David , Dobbie Will S. , Hull Peter , “ Measuring Racial Discrimination in Bail Decisions ,” NBER Working Paper no. 26999, 2020. https://doi.org/10.3386/w26999
Arnold David , Dobbie Will , Yang Crystal S. , “ Racial Bias in Bail Decisions ,” Quarterly Journal of Economics , 133 ( 2018 ), 1885 – 1932 . https://doi.org/10.1093/qje/qjy012
Athey Susan , “ Beyond Prediction: Using Big Data for Policy Problems ,” Science , 355 ( 2017 ), 483 – 485 . https://doi.org/10.1126/science.aal4321
Athey Susan , “ The Impact of Machine Learning on Economics ,” in The Economics of Artificial Intelligence: An Agenda , Ajay Agrawal, Joshua Gans, and Avi Goldfarb, eds. (Chicago: University of Chicago Press , 2018 ), 507 – 547 .
Athey Susan , Imbens Guido W. , “ Machine Learning Methods That Economists Should Know About ,” Annual Review of Economics , 11 ( 2019 ), 685 – 725 . https://doi.org/10.1146/annurev-economics-080217-053433
Athey Susan , Imbens Guido W. , Metzger Jonas , Munro Evan , “ Using Wasserstein Generative Adversarial Networks for the Design of Monte Carlo Simulations ,” Journal of Econometrics , ( 2021 ), 105076. https://doi.org/10.1016/j.jeconom.2020.09.013
Athey Susan , Karlan Dean , Palikot Emil , Yuan Yuan , “ Smiles in Profiles: Improving Fairness and Efficiency Using Estimates of User Preferences in Online Marketplaces ,” NBER Working Paper no. 30633 , 2022 . https://doi.org/10.3386/w30633
Autor David , “ Polanyi’s Paradox and the Shape of Employment Growth ,” NBER Working Paper no. 20485 , 2014 . https://doi.org/10.3386/w20485
Avitzour Eliana , Choen Adi , Joel Daphna , Lavy Victor , “ On the Origins of Gender-Biased Behavior: The Role of Explicit and Implicit Stereotypes ,” NBER Working Paper no. 27818 , 2020 . https://doi.org/10.3386/w27818
Baehrens David , Schroeter Timon , Harmeling Stefan , Kawanabe Motoaki , Hansen Katja , Müller Klaus-Robert , “ How to Explain Individual Classification Decisions ,” Journal of Machine Learning Research , 11 ( 2010 ), 1803 – 1831 .
Baltrušaitis Tadas , Ahuja Chaitanya , Morency Louis-Philippe , “ Multimodal Machine Learning: A Survey and Taxonomy ,” IEEE Transactions on Pattern Analysis and Machine Intelligence , 41 ( 2019 ), 423 – 443 . https://doi.org/10.1109/TPAMI.2018.2798607
Begall Sabine , Červený Jaroslav , Neef Julia , Vojtěch Oldřich , Burda Hynek , “ Magnetic Alignment in Grazing and Resting Cattle and Deer ,” Proceedings of the National Academy of Sciences , 105 ( 2008 ), 13451 – 13455 . https://doi.org/10.1073/pnas.0803650105
Belloni Alexandre , Chernozhukov Victor , Hansen Christian , “ High-Dimensional Methods and Inference on Structural and Treatment Effects ,” Journal of Economic Perspectives , 28 ( 2014 ), 29 – 50 . https://doi.org/10.1257/jep.28.2.29
Berry Diane S. , Zebrowitz-McArthur Leslie , “ What’s in a Face? Facial Maturity and the Attribution of Legal Responsibility ,” Personality and Social Psychology Bulletin , 14 ( 1988 ), 23 – 33 . https://doi.org/10.1177/0146167288141003
Bertrand Marianne , Mullainathan Sendhil , “ Are Emily and Greg More Employable than Lakisha and Jamal? A Field Experiment on Labor Market Discrimination ,” American Economic Review , 94 ( 2004 ), 991 – 1013 . https://doi.org/10.1257/0002828042002561
Bjornstrom Eileen E. S. , Kaufman Robert L. , Peterson Ruth D. , Slater Michael D. , “ Race and Ethnic Representations of Lawbreakers and Victims in Crime News: A National Study of Television Coverage ,” Social Problems , 57 ( 2010 ), 269 – 293 . https://doi.org/10.1525/sp.2010.57.2.269
Breiman Leo , “ Random Forests ,” Machine Learning , 45 ( 2001 ), 5 – 32 . https://doi.org/10.1023/A:1010933404324
Breiman Leo , Friedman Jerome H. , Olshen Richard A. , Stone Charles J. , Classification and Regression Trees (London: Routledge , 1984 ). https://doi.org/10.1201/9781315139470
Google Preview
Brier Glenn W. , “ Verification of Forecasts Expressed in Terms of Probability ,” Monthly Weather Review , 78 ( 1950 ), 1 – 3 . https://doi.org/10.1175/1520-0493(1950)078<0001:VOFEIT>2.0.CO;2
Carleo Giuseppe , Cirac Ignacio , Cranmer Kyle , Daudet Laurent , Schuld Maria , Tishby Naftali , Vogt-Maranto Leslie , Zdeborová Lenka , “ Machine Learning and the Physical Sciences ,” Reviews of Modern Physics , 91 ( 2019 ), 045002 . https://doi.org/10.1103/RevModPhys.91.045002
Chen Daniel L. , Moskowitz Tobias J. , Shue Kelly , “ Decision Making under the Gambler’s Fallacy: Evidence from Asylum Judges, Loan Officers, and Baseball Umpires ,” Quarterly Journal of Economics , 131 ( 2016 ), 1181 – 1242 . https://doi.org/10.1093/qje/qjw017
Chen Daniel L. , Philippe Arnaud , “ Clash of Norms: Judicial Leniency on Defendant Birthdays ,” Journal of Economic Behavior & Organization , 211 ( 2023 ), 324 – 344 . https://doi.org/10.1016/j.jebo.2023.05.002
Dahl Gordon B. , Knepper Matthew M. , “ Age Discrimination across the Business Cycle ,” NBER Working Paper no. 27581 , 2020 . https://doi.org/10.3386/w27581
Davies Alex , Veličković Petar , Buesing Lars , Blackwell Sam , Zheng Daniel , Tomašev Nenad , Tanburn Richard , Battaglia Peter , Blundell Charles , Juhász András , Lackenby Marc , Williamson Geordie , Hassabis Demis , Kohli Pushmeet , “ Advancing Mathematics by Guiding Human Intuition with AI ,” Nature , 600 ( 2021 ), 70 – 74 . https://doi.org/10.1038/s41586-021-04086-x
Devlin Jacob , Chang Ming-Wei , Lee Kenton , Toutanova Kristina , “ BERT: Pre-Training of Deep Bidirectional Transformers for Language Understanding ,” arXiv preprint arXiv:1810.04805 , 2018 . https://doi.org/10.48550/arXiv.1810.04805
Dobbie Will , Goldin Jacob , Yang Crystal S. , “ The Effects of Pretrial Detention on Conviction, Future Crime, and Employment: Evidence from Randomly Assigned Judges ,” American Economic Review , 108 ( 2018 ), 201 – 240 . https://doi.org/10.1257/aer.20161503
Dobbie Will , Yang Crystal S. , “ The US Pretrial System: Balancing Individual Rights and Public Interests ,” Journal of Economic Perspectives , 35 ( 2021 ), 49 – 70 . https://doi.org/10.1257/jep.35.4.49
Doshi-Velez Finale , Kim Been , “ Towards a Rigorous Science of Interpretable Machine Learning ,” arXiv preprint arXiv:1702.08608 , 2017 . https://doi.org/10.48550/arXiv.1702.08608
Eberhardt Jennifer L. , Davies Paul G. , Purdie-Vaughns Valerie J. , Lynn Johnson Sheri , “ Looking Deathworthy: Perceived Stereotypicality of Black Defendants Predicts Capital-Sentencing Outcomes ,” Psychological Science , 17 ( 2006 ), 383 – 386 . https://doi.org/10.1111/j.1467-9280.2006.01716.x
Einav Liran , Levin Jonathan , “ The Data Revolution and Economic Analysis ,” Innovation Policy and the Economy , 14 ( 2014 ), 1 – 24 . https://doi.org/10.1086/674019
Eren Ozkan , Mocan Naci , “ Emotional Judges and Unlucky Juveniles ,” American Economic Journal: Applied Economics , 10 ( 2018 ), 171 – 205 . https://doi.org/10.1257/app.20160390
Frieze Irene Hanson , Olson Josephine E. , Russell June , “ Attractiveness and Income for Men and Women in Management ,” Journal of Applied Social Psychology , 21 ( 1991 ), 1039 – 1057 . https://doi.org/10.1111/j.1559-1816.1991.tb00458.x
Fryer Roland G., Jr , “ An Empirical Analysis of Racial Differences in Police Use of Force: A Response ,” Journal of Political Economy , 128 ( 2020 ), 4003 – 4008 . https://doi.org/10.1086/710977
Fudenberg Drew , Liang Annie , “ Predicting and Understanding Initial Play ,” American Economic Review , 109 ( 2019 ), 4112 – 4141 . https://doi.org/10.1257/aer.20180654
Gentzkow Matthew , Kelly Bryan , Taddy Matt , “ Text as Data ,” Journal of Economic Literature , 57 ( 2019 ), 535 – 574 . https://doi.org/10.1257/jel.20181020
Ghandeharioun Asma , Kim Been , Li Chun-Liang , Jou Brendan , Eoff Brian , Picard Rosalind W. , “ DISSECT: Disentangled Simultaneous Explanations via Concept Traversals ,” arXiv preprint arXiv:2105.15164 2022 . https://doi.org/10.48550/arXiv.2105.15164
Goldin Claudia , Rouse Cecilia , “ Orchestrating Impartiality: The Impact of ‘Blind’ Auditions on Female Musicians ,” American Economic Review , 90 ( 2000 ), 715 – 741 . https://doi.org/10.1257/aer.90.4.715
Goncalves Felipe , Mello Steven , “ A Few Bad Apples? Racial Bias in Policing ,” American Economic Review , 111 ( 2021 ), 1406 – 1441 . https://doi.org/10.1257/aer.20181607
Goodfellow Ian , Pouget-Abadie Jean , Mirza Mehdi , Xu Bing , Warde-Farley David , Ozair Sherjil , Courville Aaron , Bengio Yoshua , “ Generative Adversarial Nets ,” Advances in Neural Information Processing Systems , 27 ( 2014 ), 2672 – 2680 .
Goodfellow Ian J. , Shlens Jonathon , Szegedy Christian , “ Explaining and Harnessing Adversarial Examples ,” arXiv preprint arXiv:1412.6572 , 2014 . https://doi.org/10.48550/arXiv.1412.6572
Grogger Jeffrey , Ridgeway Greg , “ Testing for Racial Profiling in Traffic Stops from Behind a Veil of Darkness ,” Journal of the American Statistical Association , 101 ( 2006 ), 878 – 887 . https://doi.org/10.1198/016214506000000168
Hastie Trevor , Tibshirani Robert , Friedman Jerome H. , Friedman Jerome H. , The Elements of Statistical Learning: Data Mining, Inference, and Prediction , vol. 2 (Berlin: Springer , 2009 ).
He Siyu , Li Yin , Feng Yu , Ho Shirley , Ravanbakhsh Siamak , Chen Wei , Póczos Barnabás , “ Learning to Predict the Cosmological Structure Formation ,” Proceedings of the National Academy of Sciences , 116 ( 2019 ), 13825 – 13832 . https://doi.org/10.1073/pnas.1821458116
Heckman James J. , Singer Burton , “ Abducting Economics ,” American Economic Review , 107 ( 2017 ), 298 – 302 . https://doi.org/10.1257/aer.p20171118
Heyes Anthony , Saberian Soodeh , “ Temperature and Decisions: Evidence from 207,000 Court Cases ,” American Economic Journal: Applied Economics , 11 ( 2019 ), 238 – 265 . https://doi.org/10.1257/app.20170223
Hoekstra Mark , Sloan CarlyWill , “ Does Race Matter for Police Use of Force? Evidence from 911 Calls ,” American Economic Review , 112 ( 2022 ), 827 – 860 . https://doi.org/10.1257/aer.20201292
Hunter Margaret , “ The Persistent Problem of Colorism: Skin Tone, Status, and Inequality ,” Sociology Compass , 1 ( 2007 ), 237 – 254 . https://doi.org/10.1111/j.1751-9020.2007.00006.x
Jordan Michael I. , Mitchell Tom M. , “ Machine Learning: Trends, Perspectives, and Prospects ,” Science , 349 ( 2015 ), 255 – 260 . https://doi.org/10.1126/science.aaa8415
Jumper John , Evans Richard , Pritzel Alexander , Green Tim , Figurnov Michael , Ronneberger Olaf , Tunyasuvunakool Kathryn , Bates Russ , Žídek Augustin , Potapenko Anna et al. , “ Highly Accurate Protein Structure Prediction with AlphaFold ,” Nature , 596 ( 2021 ), 583 – 589 . https://doi.org/10.1038/s41586-021-03819-2
Jung Jongbin , Concannon Connor , Shroff Ravi , Goel Sharad , Goldstein Daniel G. , “ Simple Rules for Complex Decisions ,” SSRN working paper , 2017 . https://doi.org/10.2139/ssrn.2919024
Kahneman Daniel , Sibony Olivier , Sunstein C. R , Noise (London: HarperCollins , 2022 ).
Kaji Tetsuya , Manresa Elena , Pouliot Guillaume , “ An Adversarial Approach to Structural Estimation ,” University of Chicago, Becker Friedman Institute for Economics Working Paper No. 2020-144 , 2020 . https://doi.org/10.2139/ssrn.3706365
Kingma Diederik P. , Welling Max , “ Auto-Encoding Variational Bayes ,” arXiv preprint arXiv:1312.6114 , 2013 . https://doi.org/10.48550/arXiv.1312.6114
Kleinberg Jon , Lakkaraju Himabindu , Leskovec Jure , Ludwig Jens , Mullainathan Sendhil , “ Human Decisions and Machine Predictions ,” Quarterly Journal of Economics , 133 ( 2018 ), 237 – 293 . https://doi.org/10.1093/qje/qjx032
Korot Edward , Pontikos Nikolas , Liu Xiaoxuan , Wagner Siegfried K. , Faes Livia , Huemer Josef , Balaskas Konstantinos , Denniston Alastair K. , Khawaja Anthony , Keane Pearse A. , “ Predicting Sex from Retinal Fundus Photographs Using Automated Deep Learning ,” Scientific Reports , 11 ( 2021 ), 10286 . https://doi.org/10.1038/s41598-021-89743-x
Lahat Dana , Adali Tülay , Jutten Christian , “ Multimodal Data Fusion: An Overview of Methods, Challenges, and Prospects ,” Proceedings of the IEEE , 103 ( 2015 ), 1449 – 1477 . https://doi.org/10.1109/JPROC.2015.2460697
Lang Oran , Gandelsman Yossi , Yarom Michal , Wald Yoav , Elidan Gal , Hassidim Avinatan , Freeman William T , Isola Phillip , Globerson Amir , Irani Michal , et al. , “ Explaining in Style: Training a GAN to Explain a Classifier in StyleSpace ,” paper presented at the IEEE/CVF International Conference on Computer Vision , 2021. https://doi.org/10.1109/ICCV48922.2021.00073
Leskovec Jure , Backstrom Lars , Kleinberg Jon , “ Meme-Tracking and the Dynamics of the News Cycle ,” paper presented at the 15th ACM SIGKDD international conference on Knowledge discovery and data mining, 2009. https://doi.org/10.1145/1557019.1557077
Little Anthony C. , Jones Benedict C. , DeBruine Lisa M. , “ Facial Attractiveness: Evolutionary Based Research ,” Philosophical Transactions of the Royal Society B: Biological Sciences , 366 ( 2011 ), 1638 – 1659 . https://doi.org/10.1098/rstb.2010.0404
Liu Shusen , Kailkhura Bhavya , Loveland Donald , Han Yong , “ Generative Counterfactual Introspection for Explainable Deep Learning ,” paper presented at the IEEE Global Conference on Signal and Information Processing (GlobalSIP) , 2019. https://doi.org/10.1109/GlobalSIP45357.2019.8969491
Ludwig Jens , Mullainathan Sendhil , “ Machine Learning as a Tool for Hypothesis Generation ,” NBER Working Paper no. 31017 , 2023a . https://doi.org/10.3386/w31017
Ludwig Jens , Mullainathan Sendhil , “ Replication Data for: ‘Machine Learning as a Tool for Hypothesis Generation’ ,” ( 2023b ), Harvard Dataverse. https://doi.org/10.7910/DVN/ILO46V .
Marcinkevičs Ričards , Vogt Julia E. , “ Interpretability and Explainability: A Machine Learning Zoo Mini-Tour ,” arXiv preprint arXiv:2012.01805 , 2020 . https://doi.org/10.48550/arXiv.2012.01805
Miller Andrew , Obermeyer Ziad , Cunningham John , Mullainathan Sendhil , “ Discriminative Regularization for Latent Variable Models with Applications to Electrocardiography ,” paper presented at the International Conference on Machine Learning , 2019.
Mobius Markus M. , Rosenblat Tanya S. , “ Why Beauty Matters ,” American Economic Review , 96 ( 2006 ), 222 – 235 . https://doi.org/10.1257/000282806776157515
Mobley R. Keith , An Introduction to Predictive Maintenance (Amsterdam: Elsevier , 2002 ).
Mullainathan Sendhil , Obermeyer Ziad , “ Diagnosing Physician Error: A Machine Learning Approach to Low-Value Health Care ,” Quarterly Journal of Economics , 137 ( 2022 ), 679 – 727 . https://doi.org/10.1093/qje/qjab046
Mullainathan Sendhil , Spiess Jann , “ Machine Learning: an Applied Econometric Approach ,” Journal of Economic Perspectives , 31 ( 2017 ), 87 – 106 . https://doi.org/10.1257/jep.31.2.87
Murphy Allan H. , “ A New Vector Partition of the Probability Score ,” Journal of Applied Meteorology and Climatology , 12 ( 1973 ), 595 – 600 . https://doi.org/10.1175/1520-0450(1973)012<0595:ANVPOT>2.0.CO;2
Nalisnick Eric , Matsukawa Akihiro , Whye Teh Yee , Gorur Dilan , Lakshminarayanan Balaji , “ Do Deep Generative Models Know What They Don’t Know? ,” arXiv preprint arXiv:1810.09136 , 2018 . https://doi.org/10.48550/arXiv.1810.09136
Narayanaswamy Arunachalam , Venugopalan Subhashini , Webster Dale R. , Peng Lily , Corrado Greg S. , Ruamviboonsuk Paisan , Bavishi Pinal , Brenner Michael , Nelson Philip C. , Varadarajan Avinash V. , “ Scientific Discovery by Generating Counterfactuals Using Image Translation ,” in International Conference on Medical Image Computing and Computer-Assisted Intervention , (Berlin: Springer , 2020), 273 – 283 . https://doi.org/10.1007/978-3-030-59710-8_27
Neumark David , Burn Ian , Button Patrick , “ Experimental Age Discrimination Evidence and the Heckman Critique ,” American Economic Review , 106 ( 2016 ), 303 – 308 . https://doi.org/10.1257/aer.p20161008
Norouzzadeh Mohammad Sadegh , Nguyen Anh , Kosmala Margaret , Swanson Alexandra , S. Palmer Meredith , Packer Craig , Clune Jeff , “ Automatically Identifying, Counting, and Describing Wild Animals in Camera-Trap Images with Deep Learning ,” Proceedings of the National Academy of Sciences , 115 ( 2018 ), E5716 – E5725 . https://doi.org/10.1073/pnas.1719367115
Oosterhof Nikolaas N. , Todorov Alexander , “ The Functional Basis of Face Evaluation ,” Proceedings of the National Academy of Sciences , 105 ( 2008 ), 11087 – 11092 . https://doi.org/10.1073/pnas.0805664105
Peterson Joshua C. , Bourgin David D. , Agrawal Mayank , Reichman Daniel , Griffiths Thomas L. , “ Using Large-Scale Experiments and Machine Learning to Discover Theories of Human Decision-Making ,” Science , 372 ( 2021 ), 1209 – 1214 . https://doi.org/10.1126/science.abe2629
Pierson Emma , Cutler David M. , Leskovec Jure , Mullainathan Sendhil , Obermeyer Ziad , “ An Algorithmic Approach to Reducing Unexplained Pain Disparities in Underserved Populations ,” Nature Medicine , 27 ( 2021 ), 136 – 140 . https://doi.org/10.1038/s41591-020-01192-7
Pion-Tonachini Luca , Bouchard Kristofer , Garcia Martin Hector , Peisert Sean , Bradley Holtz W. , Aswani Anil , Dwivedi Dipankar , Wainwright Haruko , Pilania Ghanshyam , Nachman Benjamin et al. “ Learning from Learning Machines: A New Generation of AI Technology to Meet the Needs of Science ,” arXiv preprint arXiv:2111.13786 , 2021 . https://doi.org/10.48550/arXiv.2111.13786
Popper Karl , The Logic of Scientific Discovery (London: Routledge , 2nd ed. 2002 ). https://doi.org/10.4324/9780203994627
Pronin Emily , “ The Introspection Illusion ,” Advances in Experimental Social Psychology , 41 ( 2009 ), 1 – 67 . https://doi.org/10.1016/S0065-2601(08)00401-2
Ramachandram Dhanesh , Taylor Graham W. , “ Deep Multimodal Learning: A Survey on Recent Advances and Trends ,” IEEE Signal Processing Magazine , 34 ( 2017 ), 96 – 108 . https://doi.org/10.1109/MSP.2017.2738401
Rambachan Ashesh , “ Identifying Prediction Mistakes in Observational Data ,” Harvard University Working Paper, 2021 . www.nber.org/system/files/chapters/c14777/c14777.pdf
Said-Metwaly Sameh , Van den Noortgate Wim , Kyndt Eva , “ Approaches to Measuring Creativity: A Systematic Literature Review ,” Creativity: Theories–Research-Applications , 4 ( 2017 ), 238 – 275 . https://doi.org/10.1515/ctra-2017-0013
Schickore Jutta , “ Scientific Discovery ,” in The Stanford Encyclopedia of Philosophy , Edward N. Zalta, ed. (Stanford, CA: Stanford University , 2018).
Schlag Pierre , “ Law and Phrenology ,” Harvard Law Review , 110 ( 1997 ), 877 – 921 . https://doi.org/10.2307/1342231
Sheetal Abhishek , Feng Zhiyu , Savani Krishna , “ Using Machine Learning to Generate Novel Hypotheses: Increasing Optimism about COVID-19 Makes People Less Willing to Justify Unethical Behaviors ,” Psychological Science , 31 ( 2020 ), 1222 – 1235 . https://doi.org/10.1177/0956797620959594
Simonyan Karen , Vedaldi Andrea , Zisserman Andrew , “ Deep Inside Convolutional Networks: Visualising Image Classification Models and Saliency Maps ,” paper presented at the Workshop at International Conference on Learning Representations , 2014.
Sirovich Lawrence , Kirby Michael , “ Low-Dimensional Procedure for the Characterization of Human Faces ,” Journal of the Optical Society of America A , 4 ( 1987 ), 519 – 524 . https://doi.org/10.1364/JOSAA.4.000519
Sunstein Cass R. , “ Governing by Algorithm? No Noise and (Potentially) Less Bias ,” Duke Law Journal , 71 ( 2021 ), 1175 – 1205 . https://doi.org/10.2139/ssrn.3925240
Swanson Don R. , “ Fish Oil, Raynaud’s Syndrome, and Undiscovered Public Knowledge ,” Perspectives in Biology and Medicine , 30 ( 1986 ), 7 – 18 . https://doi.org/10.1353/pbm.1986.0087
Swanson Don R. , “ Migraine and Magnesium: Eleven Neglected Connections ,” Perspectives in Biology and Medicine , 31 ( 1988 ), 526 – 557 . https://doi.org/10.1353/pbm.1988.0009
Szegedy Christian , Zaremba Wojciech , Sutskever Ilya , Bruna Joan , Erhan Dumitru , Goodfellow Ian , Fergus Rob , “ Intriguing Properties of Neural Networks ,” arXiv preprint arXiv:1312.6199 , 2013 . https://doi.org/10.48550/arXiv.1312.6199
Todorov Alexander , Oh DongWon , “ The Structure and Perceptual Basis of Social Judgments from Faces. in Advances in Experimental Social Psychology , B. Gawronski, ed. (Amsterdam: Elsevier , 2021 ), 189–245.
Todorov Alexander , Olivola Christopher Y. , Dotsch Ron , Mende-Siedlecki Peter , “ Social Attributions from Faces: Determinants, Consequences, Accuracy, and Functional Significance ,” Annual Review of Psychology , 66 ( 2015 ), 519 – 545 . https://doi.org/10.1146/annurev-psych-113011-143831
Varian Hal R. , “ Big Data: New Tricks for Econometrics ,” Journal of Economic Perspectives , 28 ( 2014 ), 3 – 28 . https://doi.org/10.1257/jep.28.2.3
Wilson Timothy D. , Strangers to Ourselves (Cambridge, MA: Harvard University Press , 2004 ).
Yuhas Ben P. , Goldstein Moise H. , Sejnowski Terrence J. , “ Integration of Acoustic and Visual Speech Signals Using Neural Networks ,” IEEE Communications Magazine , 27 ( 1989 ), 65 – 71 . https://doi.org/10.1109/35.41402
Zebrowitz Leslie A. , Luevano Victor X. , Bronstad Philip M. , Aharon Itzhak , “ Neural Activation to Babyfaced Men Matches Activation to Babies ,” Social Neuroscience , 4 ( 2009 ), 1 – 10 . https://doi.org/10.1080/17470910701676236
Supplementary data
Email alerts, citing articles via.
- Recommend to Your Librarian
Affiliations
- Online ISSN 1531-4650
- Print ISSN 0033-5533
- Copyright © 2024 President and Fellows of Harvard College
- About Oxford Academic
- Publish journals with us
- University press partners
- What we publish
- New features
- Open access
- Institutional account management
- Rights and permissions
- Get help with access
- Accessibility
- Advertising
- Media enquiries
- Oxford University Press
- Oxford Languages
- University of Oxford
Oxford University Press is a department of the University of Oxford. It furthers the University's objective of excellence in research, scholarship, and education by publishing worldwide
- Copyright © 2024 Oxford University Press
- Cookie settings
- Cookie policy
- Privacy policy
- Legal notice
This Feature Is Available To Subscribers Only
Sign In or Create an Account
This PDF is available to Subscribers Only
For full access to this pdf, sign in to an existing account, or purchase an annual subscription.
Help | Advanced Search
Computer Science > Artificial Intelligence
Title: hypothesis generation with large language models.
Abstract: Effective generation of novel hypotheses is instrumental to scientific progress. So far, researchers have been the main powerhouse behind hypothesis generation by painstaking data analysis and thinking (also known as the Eureka moment). In this paper, we examine the potential of large language models (LLMs) to generate hypotheses. We focus on hypothesis generation based on data (i.e., labeled examples). To enable LLMs to handle arbitrarily long contexts, we generate initial hypotheses from a small number of examples and then update them iteratively to improve the quality of hypotheses. Inspired by multi-armed bandits, we design a reward function to inform the exploitation-exploration tradeoff in the update process. Our algorithm is able to generate hypotheses that enable much better predictive performance than few-shot prompting in classification tasks, improving accuracy by 31.7% on a synthetic dataset and by 13.9%, 3.3% and, 24.9% on three real-world datasets. We also outperform supervised learning by 12.8% and 11.2% on two challenging real-world datasets. Furthermore, we find that the generated hypotheses not only corroborate human-verified theories but also uncover new insights for the tasks.
Submission history
Access paper:.
- HTML (experimental)
- Other Formats

References & Citations
- Google Scholar
- Semantic Scholar
BibTeX formatted citation

Bibliographic and Citation Tools
Code, data and media associated with this article, recommenders and search tools.
- Institution
arXivLabs: experimental projects with community collaborators
arXivLabs is a framework that allows collaborators to develop and share new arXiv features directly on our website.
Both individuals and organizations that work with arXivLabs have embraced and accepted our values of openness, community, excellence, and user data privacy. arXiv is committed to these values and only works with partners that adhere to them.
Have an idea for a project that will add value for arXiv's community? Learn more about arXivLabs .
New hypothesis emerges on Parkinson's disease's origins and spread
T he nose or the gut? For the past two decades, the scientific community has debated the wellspring of the toxic proteins at the source of Parkinson's disease. In 2003, a German pathologist, Heiko Braak, MD, first proposed that the disease begins outside the brain.
More recently, Per Borghammer, MD, with Aarhus University Hospital in Denmark, and his colleagues argue that the disease is the result of processes that start in either the brain's smell center (brain-first) or the body's intestinal tract (body-first).
A new hypothesis paper appearing in the Journal of Parkinson's Disease on World Parkinson's Day unites the brain- and body-first models with some of the likely causes of the disease–environmental toxicants that are either inhaled or ingested.
The authors of the new study, who include Borghammer, argue that inhalation of certain pesticides, common dry cleaning chemicals, and air pollution predispose to a brain-first model of the disease. Other ingested toxicants, such as tainted food and contaminated drinking water, lead to body-first model of the disease.
"In both the brain-first and body-first scenarios the pathology arises in structures in the body closely connected to the outside world," said Ray Dorsey, MD, a professor of Neurology at the University of Rochester Medical Center and co-author of the piece.
"Here we propose that Parkinson's is a systemic disease and that its initial roots likely begin in the nose and in the gut and are tied to environmental factors increasingly recognized as major contributors, if not causes, of the disease. This further reinforces the idea that Parkinson's, the world's fastest growing brain disease, may be fueled by toxicants and is therefore largely preventable."
Different pathways to the brain, different forms of disease
A misfolded protein called alpha-synuclein has been in scientists' sights for the last 25 years as one of the driving forces behind Parkinson's. Over time, the protein accumulates in the brain in clumps, called Lewy bodies, and causes progressive dysfunction and death of many types of nerve cells, including those in the dopamine-producing regions of the brain that control motor function. When first proposed, Braak thought that an unidentified pathogen, such as a virus, may be responsible for the disease.
The new piece argues that toxins encountered in the environment, specifically the dry cleaning and degreasing chemicals trichloroethylene (TCE) and perchloroethylene (PCE), the weed killer paraquat, and air pollution, could be common causes for the formation of toxic alpha-synuclein.
TCE and PCE contaminates thousands of former industrial, commercial, and military sites, most notably the Marine Corps base Camp Lejeune, and paraquat is one of the most widely used herbicides in the US, despite being banned for safety concerns in more than 30 countries, including the European Union and China. Air pollution was at toxic levels in nineteenth century London when James Parkinson, whose 269th birthday is celebrated today, first described the condition.
The nose and the gut are lined with a soft permeable tissue, and both have well established connections to the brain. In the brain-first model, the chemicals are inhaled and may enter the brain via the nerve responsible for smell. From the brain's smell center, alpha-synuclein spreads to other parts of the brain principally on one side, including regions with concentrations of dopamine-producing neurons.
The death of these cells is a hallmark of Parkinson's disease. The disease may cause asymmetric tremor and slowness in movement and, a slower rate of progression after diagnosis, and only much later, significant cognitive impairment or dementia.
When ingested, the chemicals pass through the lining of the gastrointestinal tract. Initial alpha-synuclein pathology may begin in the gut's own nervous system from where it can spread to both sides of the brain and spinal cord.
This body-first pathway is often associated with Lewy body dementia, a disease in the same family as Parkinson's, which is characterized by early constipation and sleep disturbance, followed by more symmetric slowing in movements and earlier dementia, as the disease spreads through both brain hemispheres.
New models to understand and study brain diseases
"These environmental toxicants are widespread and not everyone has Parkinson's disease," said Dorsey. "The timing, dose, and duration of exposure and interactions with genetic and other environmental factors are probably key to determining who ultimately develops Parkinson's. In most instances, these exposures likely occurred years or decades before symptoms develop."
Pointing to a growing body of research linking environmental exposure to Parkinson's disease, the authors believe the new models may enable the scientific community to connect specific exposures to specific forms of the disease. This effort will be aided by increasing public awareness of the adverse health effects of many chemicals in our environment.
The authors conclude that their hypothesis "may explain many of the mysteries of Parkinson's disease and open the door toward the ultimate goal–prevention."
In addition to Parkinson's, these models of environmental exposure may advance understanding of how toxicants contribute to other brain disorders, including autism in children, ALS in adults, and Alzheimer's in seniors.
Dorsey and his colleagues at the University of Rochester have organized a symposium on the Brain and the Environment in Washington, DC, on May 20 that will examine the role toxicants in our food, water, and air are playing in all these brain diseases. Additional authors of the hypothesis paper include Briana De Miranda, Ph.D., with the University of Alabama at Birmingham, and Jacob Horsager, MD, Ph.D., with Aarhus University Hospital in Denmark.
More information: E. Ray Dorsey et al, The Body, the Brain, the Environment, and Parkinson's Disease, Journal of Parkinson's Disease (2024). DOI: 10.3233/JPD-240019
Provided by University of Rochester Medical Center
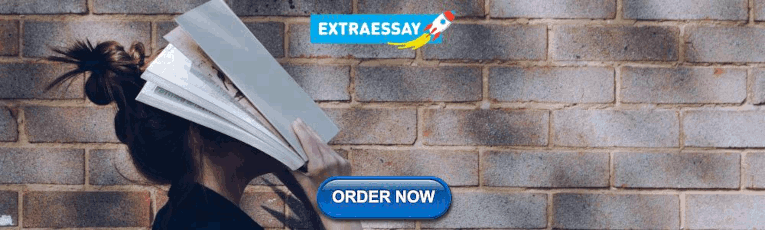
IMAGES
VIDEO
COMMENTS
Scientific Revolution, drastic change in scientific thought that took place during the 16th and 17th centuries.A new view of nature emerged during the Scientific Revolution, replacing the Greek view that had dominated science for almost 2,000 years. Science became an autonomous discipline, distinct from both philosophy and technology, and it came to be regarded as having utilitarian goals.
The Scientific Revolution was a revolution in the sense that old theories and methods were discarded, new technology opened up new fields of inquiry, and the scientific method (where controlled experiments are subjected to peer review) came to be regarded as the best way to improve humanity's knowledge.
The Scientific Revolution was a series of events that marked the emergence of modern science during the early modern period, when ... experiment. The true method of experience first lights the candle [hypothesis], and then by means of the candle shows the way [arranges and delimits the experiment]; commencing as it does with experience duly ...
The scientific method, developed during the Scientific Revolution (1500-1700), changed theoretical philosophy into practical science when experiments to demonstrate observable results were used to confirm, adjust, or deny specific hypotheses. Experimental results were then shared and critically reviewed by peers until universal laws could be made.
3.2.3 Kuhn: Scientific Revolution. Before we move to the idea of "scientific revolution" from a Philosophy-of-Science perspective, it is important to keep in mind that the designation "The Scientific Revolution" is commonly used in reference to a period in modern Western history - the 16th, 17th, and 18th centuries.
The Scientific Revolution. The scientific revolution was the emergence of modern science during the early modern period, when developments in mathematics, physics, astronomy, biology (including human anatomy), and chemistry transformed societal views about nature. The scientific revolution began in Europe toward the end of the Renaissance ...
Examples of scientific revolutions are the Copernican revolution in astronomy, the Einsteinian revolution in physics, and the Darwinian revolution in biology. Each of these revolutions led to a fundamental change in the scientific world‐view - the overthrow of an existing set of ideas by a completely different set.
Arguably, the first time that the term "revolution" was borrowed to describe scientific change was also in the seventeenth century. Sir William Temple (1628-1699), in an essay entitled "Of Health and Long Life," regarded the development in the history of medicine from Hippocrates to William Harvey's work on the circulation of blood as the "great changes or revolutions in the ...
The Scientific Revolution was really mainly a mindset change for scientists, and like the article said, probably didn't directly result in much application immediately. But however, some of the new techniques of reason such as the scientific method, better knowledge of anatomy, and better navigation instruments laid the foundation to increase ...
An important focus of Kuhn's interest in The Structure of Scientific Revolutions was on the nature of perception and how it may be that what a scientist observes can change as a result of scientific revolution. He developed what has become known as the thesis of the theory-dependence of observation, building on the work of N. R. Hanson (1958 ...
The Scientific Revolution (1543 - 1687) describes quite accurately what took place in the scientific community. ... Both these theories did not have a need for fact-gathering, hypothesis writing, and controlled experimentation. Robert Boyle (1627-1691), an Irish-born English scientist, was an early supporter of the scientific method and the ...
A scientific hypothesis is a tentative, testable explanation for a phenomenon in the natural world. It's the initial building block in the scientific method. Many describe it as an "educated guess ...
The scientific revolution was the emergence of modern science during the early modern period, when developments in mathematics, physics, astronomy, biology (including human anatomy), and chemistry transformed societal views about nature. The scientific revolution began in Europe toward the end of the Renaissance period, and continued through ...
The Scientific Revolution was the topic around which the field of history of science itself came to maturity. Kuhn's popularization of the idea that even the mature natural sciences undergo deep conceptual change stimulated much general intellectual interest in the history of science during the 1960s and 1970s.
The Scientific Revolution began in 1543, toward the end of the Renaissance period, ... Robert Boyle's book The Sceptical Chymist presents his hypothesis that every phenomenon was a result of collisions of particles in motion. It has the earliest modern ideas of atoms, molecules, and chemical reactions. ...
Nicolaus Copernicus. In a way, you can say that the scientific revolution started out as the Copernican Revolution. The man who started it all, Nicolaus Copernicus, was a Renaissance mathematician and astronomer who was born and raised in the Polish city of Toruń. He attended the University of Cracow, later continuing his studies in Bologna ...
Before the Scientific Revolution, most educated people who studied the world took guidance from the explanations given by authorities like ancient Greek writers and Catholic Church officials. ... Forming a hypothesis. A hypothesis is a solution that the scientist proposes to solve the problem. A hypothesis differs from a theory in that a ...
The scientific revolution was the emergence of modern science during the early modern period, when developments in mathematics, physics, astronomy, biology (including human anatomy), and chemistry transformed societal views about nature. The scientific revolution began in Europe toward the end of the Renaissance period, and continued through ...
By the end of the following century, the Scientific Revolution had given birth to an Industrial Revolution which dramatically transformed the daily lives of people around the world. Western society has been moving forward on Bacon's model for the past three hundred years. Perhaps though, we are in danger of forgetting the vital role doubt ...
The astronomers of the Scientific Revolution rejected long-held theories of ancient thinkers like Claudius Ptolemy and Aristotle and instead set out to systematically observe the heavens in order to create a model of the universe that fit observable facts rather than preconceived theories.. Astronomers like Nicolaus Copernicus, Tycho Brahe, Galileo Galilei, Johannes Hevelius, Edmond Halley ...
While hypothesis testing is a highly formalized activity, hypothesis generation remains largely informal. ... . 10 Put differently, a single research team's idiosyncratic judgments lack the kind of replicability we desire of a scientific procedure. To overcome this problem, it is key that we use independent (nonresearcher) subjects to inspect ...
Hypothesis Generation with Large Language Models. Effective generation of novel hypotheses is instrumental to scientific progress. So far, researchers have been the main powerhouse behind hypothesis generation by painstaking data analysis and thinking (also known as the Eureka moment). In this paper, we examine the potential of large language ...
During the Scientific Revolution, however, the greatest flaw in the device was the lack of technology to make high-grade lenses. Galileo was streets ahead of everyone else in this area but would not reveal exactly how he ground his fine lenses. Despite the technical problems, the leap forward in massively extending human sight was a marvel that ...
A new hypothesis paper appearing in the Journal of Parkinson's Disease on World Parkinson's Day unites the brain- and body-first models with some of the likely causes of the disease ...