How to conduct a meta-analysis in eight steps: a practical guide
- Open access
- Published: 30 November 2021
- Volume 72 , pages 1–19, ( 2022 )
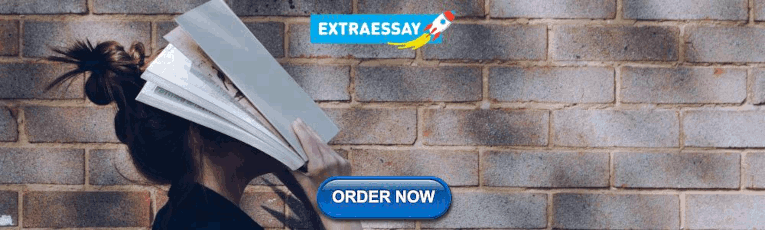
Cite this article
You have full access to this open access article
- Christopher Hansen 1 ,
- Holger Steinmetz 2 &
- Jörn Block 3 , 4 , 5
142k Accesses
44 Citations
157 Altmetric
Explore all metrics
Avoid common mistakes on your manuscript.
1 Introduction
“Scientists have known for centuries that a single study will not resolve a major issue. Indeed, a small sample study will not even resolve a minor issue. Thus, the foundation of science is the cumulation of knowledge from the results of many studies.” (Hunter et al. 1982 , p. 10)
Meta-analysis is a central method for knowledge accumulation in many scientific fields (Aguinis et al. 2011c ; Kepes et al. 2013 ). Similar to a narrative review, it serves as a synopsis of a research question or field. However, going beyond a narrative summary of key findings, a meta-analysis adds value in providing a quantitative assessment of the relationship between two target variables or the effectiveness of an intervention (Gurevitch et al. 2018 ). Also, it can be used to test competing theoretical assumptions against each other or to identify important moderators where the results of different primary studies differ from each other (Aguinis et al. 2011b ; Bergh et al. 2016 ). Rooted in the synthesis of the effectiveness of medical and psychological interventions in the 1970s (Glass 2015 ; Gurevitch et al. 2018 ), meta-analysis is nowadays also an established method in management research and related fields.
The increasing importance of meta-analysis in management research has resulted in the publication of guidelines in recent years that discuss the merits and best practices in various fields, such as general management (Bergh et al. 2016 ; Combs et al. 2019 ; Gonzalez-Mulé and Aguinis 2018 ), international business (Steel et al. 2021 ), economics and finance (Geyer-Klingeberg et al. 2020 ; Havranek et al. 2020 ), marketing (Eisend 2017 ; Grewal et al. 2018 ), and organizational studies (DeSimone et al. 2020 ; Rudolph et al. 2020 ). These articles discuss existing and trending methods and propose solutions for often experienced problems. This editorial briefly summarizes the insights of these papers; provides a workflow of the essential steps in conducting a meta-analysis; suggests state-of-the art methodological procedures; and points to other articles for in-depth investigation. Thus, this article has two goals: (1) based on the findings of previous editorials and methodological articles, it defines methodological recommendations for meta-analyses submitted to Management Review Quarterly (MRQ); and (2) it serves as a practical guide for researchers who have little experience with meta-analysis as a method but plan to conduct one in the future.
2 Eight steps in conducting a meta-analysis
2.1 step 1: defining the research question.
The first step in conducting a meta-analysis, as with any other empirical study, is the definition of the research question. Most importantly, the research question determines the realm of constructs to be considered or the type of interventions whose effects shall be analyzed. When defining the research question, two hurdles might develop. First, when defining an adequate study scope, researchers must consider that the number of publications has grown exponentially in many fields of research in recent decades (Fortunato et al. 2018 ). On the one hand, a larger number of studies increases the potentially relevant literature basis and enables researchers to conduct meta-analyses. Conversely, scanning a large amount of studies that could be potentially relevant for the meta-analysis results in a perhaps unmanageable workload. Thus, Steel et al. ( 2021 ) highlight the importance of balancing manageability and relevance when defining the research question. Second, similar to the number of primary studies also the number of meta-analyses in management research has grown strongly in recent years (Geyer-Klingeberg et al. 2020 ; Rauch 2020 ; Schwab 2015 ). Therefore, it is likely that one or several meta-analyses for many topics of high scholarly interest already exist. However, this should not deter researchers from investigating their research questions. One possibility is to consider moderators or mediators of a relationship that have previously been ignored. For example, a meta-analysis about startup performance could investigate the impact of different ways to measure the performance construct (e.g., growth vs. profitability vs. survival time) or certain characteristics of the founders as moderators. Another possibility is to replicate previous meta-analyses and test whether their findings can be confirmed with an updated sample of primary studies or newly developed methods. Frequent replications and updates of meta-analyses are important contributions to cumulative science and are increasingly called for by the research community (Anderson & Kichkha 2017 ; Steel et al. 2021 ). Consistent with its focus on replication studies (Block and Kuckertz 2018 ), MRQ therefore also invites authors to submit replication meta-analyses.
2.2 Step 2: literature search
2.2.1 search strategies.
Similar to conducting a literature review, the search process of a meta-analysis should be systematic, reproducible, and transparent, resulting in a sample that includes all relevant studies (Fisch and Block 2018 ; Gusenbauer and Haddaway 2020 ). There are several identification strategies for relevant primary studies when compiling meta-analytical datasets (Harari et al. 2020 ). First, previous meta-analyses on the same or a related topic may provide lists of included studies that offer a good starting point to identify and become familiar with the relevant literature. This practice is also applicable to topic-related literature reviews, which often summarize the central findings of the reviewed articles in systematic tables. Both article types likely include the most prominent studies of a research field. The most common and important search strategy, however, is a keyword search in electronic databases (Harari et al. 2020 ). This strategy will probably yield the largest number of relevant studies, particularly so-called ‘grey literature’, which may not be considered by literature reviews. Gusenbauer and Haddaway ( 2020 ) provide a detailed overview of 34 scientific databases, of which 18 are multidisciplinary or have a focus on management sciences, along with their suitability for literature synthesis. To prevent biased results due to the scope or journal coverage of one database, researchers should use at least two different databases (DeSimone et al. 2020 ; Martín-Martín et al. 2021 ; Mongeon & Paul-Hus 2016 ). However, a database search can easily lead to an overload of potentially relevant studies. For example, key term searches in Google Scholar for “entrepreneurial intention” and “firm diversification” resulted in more than 660,000 and 810,000 hits, respectively. Footnote 1 Therefore, a precise research question and precise search terms using Boolean operators are advisable (Gusenbauer and Haddaway 2020 ). Addressing the challenge of identifying relevant articles in the growing number of database publications, (semi)automated approaches using text mining and machine learning (Bosco et al. 2017 ; O’Mara-Eves et al. 2015 ; Ouzzani et al. 2016 ; Thomas et al. 2017 ) can also be promising and time-saving search tools in the future. Also, some electronic databases offer the possibility to track forward citations of influential studies and thereby identify further relevant articles. Finally, collecting unpublished or undetected studies through conferences, personal contact with (leading) scholars, or listservs can be strategies to increase the study sample size (Grewal et al. 2018 ; Harari et al. 2020 ; Pigott and Polanin 2020 ).
2.2.2 Study inclusion criteria and sample composition
Next, researchers must decide which studies to include in the meta-analysis. Some guidelines for literature reviews recommend limiting the sample to studies published in renowned academic journals to ensure the quality of findings (e.g., Kraus et al. 2020 ). For meta-analysis, however, Steel et al. ( 2021 ) advocate for the inclusion of all available studies, including grey literature, to prevent selection biases based on availability, cost, familiarity, and language (Rothstein et al. 2005 ), or the “Matthew effect”, which denotes the phenomenon that highly cited articles are found faster than less cited articles (Merton 1968 ). Harrison et al. ( 2017 ) find that the effects of published studies in management are inflated on average by 30% compared to unpublished studies. This so-called publication bias or “file drawer problem” (Rosenthal 1979 ) results from the preference of academia to publish more statistically significant and less statistically insignificant study results. Owen and Li ( 2020 ) showed that publication bias is particularly severe when variables of interest are used as key variables rather than control variables. To consider the true effect size of a target variable or relationship, the inclusion of all types of research outputs is therefore recommended (Polanin et al. 2016 ). Different test procedures to identify publication bias are discussed subsequently in Step 7.
In addition to the decision of whether to include certain study types (i.e., published vs. unpublished studies), there can be other reasons to exclude studies that are identified in the search process. These reasons can be manifold and are primarily related to the specific research question and methodological peculiarities. For example, studies identified by keyword search might not qualify thematically after all, may use unsuitable variable measurements, or may not report usable effect sizes. Furthermore, there might be multiple studies by the same authors using similar datasets. If they do not differ sufficiently in terms of their sample characteristics or variables used, only one of these studies should be included to prevent bias from duplicates (Wood 2008 ; see this article for a detection heuristic).
In general, the screening process should be conducted stepwise, beginning with a removal of duplicate citations from different databases, followed by abstract screening to exclude clearly unsuitable studies and a final full-text screening of the remaining articles (Pigott and Polanin 2020 ). A graphical tool to systematically document the sample selection process is the PRISMA flow diagram (Moher et al. 2009 ). Page et al. ( 2021 ) recently presented an updated version of the PRISMA statement, including an extended item checklist and flow diagram to report the study process and findings.
2.3 Step 3: choice of the effect size measure
2.3.1 types of effect sizes.
The two most common meta-analytical effect size measures in management studies are (z-transformed) correlation coefficients and standardized mean differences (Aguinis et al. 2011a ; Geyskens et al. 2009 ). However, meta-analyses in management science and related fields may not be limited to those two effect size measures but rather depend on the subfield of investigation (Borenstein 2009 ; Stanley and Doucouliagos 2012 ). In economics and finance, researchers are more interested in the examination of elasticities and marginal effects extracted from regression models than in pure bivariate correlations (Stanley and Doucouliagos 2012 ). Regression coefficients can also be converted to partial correlation coefficients based on their t-statistics to make regression results comparable across studies (Stanley and Doucouliagos 2012 ). Although some meta-analyses in management research have combined bivariate and partial correlations in their study samples, Aloe ( 2015 ) and Combs et al. ( 2019 ) advise researchers not to use this practice. Most importantly, they argue that the effect size strength of partial correlations depends on the other variables included in the regression model and is therefore incomparable to bivariate correlations (Schmidt and Hunter 2015 ), resulting in a possible bias of the meta-analytic results (Roth et al. 2018 ). We endorse this opinion. If at all, we recommend separate analyses for each measure. In addition to these measures, survival rates, risk ratios or odds ratios, which are common measures in medical research (Borenstein 2009 ), can be suitable effect sizes for specific management research questions, such as understanding the determinants of the survival of startup companies. To summarize, the choice of a suitable effect size is often taken away from the researcher because it is typically dependent on the investigated research question as well as the conventions of the specific research field (Cheung and Vijayakumar 2016 ).
2.3.2 Conversion of effect sizes to a common measure
After having defined the primary effect size measure for the meta-analysis, it might become necessary in the later coding process to convert study findings that are reported in effect sizes that are different from the chosen primary effect size. For example, a study might report only descriptive statistics for two study groups but no correlation coefficient, which is used as the primary effect size measure in the meta-analysis. Different effect size measures can be harmonized using conversion formulae, which are provided by standard method books such as Borenstein et al. ( 2009 ) or Lipsey and Wilson ( 2001 ). There also exist online effect size calculators for meta-analysis. Footnote 2
2.4 Step 4: choice of the analytical method used
Choosing which meta-analytical method to use is directly connected to the research question of the meta-analysis. Research questions in meta-analyses can address a relationship between constructs or an effect of an intervention in a general manner, or they can focus on moderating or mediating effects. There are four meta-analytical methods that are primarily used in contemporary management research (Combs et al. 2019 ; Geyer-Klingeberg et al. 2020 ), which allow the investigation of these different types of research questions: traditional univariate meta-analysis, meta-regression, meta-analytic structural equation modeling, and qualitative meta-analysis (Hoon 2013 ). While the first three are quantitative, the latter summarizes qualitative findings. Table 1 summarizes the key characteristics of the three quantitative methods.
2.4.1 Univariate meta-analysis
In its traditional form, a meta-analysis reports a weighted mean effect size for the relationship or intervention of investigation and provides information on the magnitude of variance among primary studies (Aguinis et al. 2011c ; Borenstein et al. 2009 ). Accordingly, it serves as a quantitative synthesis of a research field (Borenstein et al. 2009 ; Geyskens et al. 2009 ). Prominent traditional approaches have been developed, for example, by Hedges and Olkin ( 1985 ) or Hunter and Schmidt ( 1990 , 2004 ). However, going beyond its simple summary function, the traditional approach has limitations in explaining the observed variance among findings (Gonzalez-Mulé and Aguinis 2018 ). To identify moderators (or boundary conditions) of the relationship of interest, meta-analysts can create subgroups and investigate differences between those groups (Borenstein and Higgins 2013 ; Hunter and Schmidt 2004 ). Potential moderators can be study characteristics (e.g., whether a study is published vs. unpublished), sample characteristics (e.g., study country, industry focus, or type of survey/experiment participants), or measurement artifacts (e.g., different types of variable measurements). The univariate approach is thus suitable to identify the overall direction of a relationship and can serve as a good starting point for additional analyses. However, due to its limitations in examining boundary conditions and developing theory, the univariate approach on its own is currently oftentimes viewed as not sufficient (Rauch 2020 ; Shaw and Ertug 2017 ).
2.4.2 Meta-regression analysis
Meta-regression analysis (Hedges and Olkin 1985 ; Lipsey and Wilson 2001 ; Stanley and Jarrell 1989 ) aims to investigate the heterogeneity among observed effect sizes by testing multiple potential moderators simultaneously. In meta-regression, the coded effect size is used as the dependent variable and is regressed on a list of moderator variables. These moderator variables can be categorical variables as described previously in the traditional univariate approach or (semi)continuous variables such as country scores that are merged with the meta-analytical data. Thus, meta-regression analysis overcomes the disadvantages of the traditional approach, which only allows us to investigate moderators singularly using dichotomized subgroups (Combs et al. 2019 ; Gonzalez-Mulé and Aguinis 2018 ). These possibilities allow a more fine-grained analysis of research questions that are related to moderating effects. However, Schmidt ( 2017 ) critically notes that the number of effect sizes in the meta-analytical sample must be sufficiently large to produce reliable results when investigating multiple moderators simultaneously in a meta-regression. For further reading, Tipton et al. ( 2019 ) outline the technical, conceptual, and practical developments of meta-regression over the last decades. Gonzalez-Mulé and Aguinis ( 2018 ) provide an overview of methodological choices and develop evidence-based best practices for future meta-analyses in management using meta-regression.
2.4.3 Meta-analytic structural equation modeling (MASEM)
MASEM is a combination of meta-analysis and structural equation modeling and allows to simultaneously investigate the relationships among several constructs in a path model. Researchers can use MASEM to test several competing theoretical models against each other or to identify mediation mechanisms in a chain of relationships (Bergh et al. 2016 ). This method is typically performed in two steps (Cheung and Chan 2005 ): In Step 1, a pooled correlation matrix is derived, which includes the meta-analytical mean effect sizes for all variable combinations; Step 2 then uses this matrix to fit the path model. While MASEM was based primarily on traditional univariate meta-analysis to derive the pooled correlation matrix in its early years (Viswesvaran and Ones 1995 ), more advanced methods, such as the GLS approach (Becker 1992 , 1995 ) or the TSSEM approach (Cheung and Chan 2005 ), have been subsequently developed. Cheung ( 2015a ) and Jak ( 2015 ) provide an overview of these approaches in their books with exemplary code. For datasets with more complex data structures, Wilson et al. ( 2016 ) also developed a multilevel approach that is related to the TSSEM approach in the second step. Bergh et al. ( 2016 ) discuss nine decision points and develop best practices for MASEM studies.
2.4.4 Qualitative meta-analysis
While the approaches explained above focus on quantitative outcomes of empirical studies, qualitative meta-analysis aims to synthesize qualitative findings from case studies (Hoon 2013 ; Rauch et al. 2014 ). The distinctive feature of qualitative case studies is their potential to provide in-depth information about specific contextual factors or to shed light on reasons for certain phenomena that cannot usually be investigated by quantitative studies (Rauch 2020 ; Rauch et al. 2014 ). In a qualitative meta-analysis, the identified case studies are systematically coded in a meta-synthesis protocol, which is then used to identify influential variables or patterns and to derive a meta-causal network (Hoon 2013 ). Thus, the insights of contextualized and typically nongeneralizable single studies are aggregated to a larger, more generalizable picture (Habersang et al. 2019 ). Although still the exception, this method can thus provide important contributions for academics in terms of theory development (Combs et al., 2019 ; Hoon 2013 ) and for practitioners in terms of evidence-based management or entrepreneurship (Rauch et al. 2014 ). Levitt ( 2018 ) provides a guide and discusses conceptual issues for conducting qualitative meta-analysis in psychology, which is also useful for management researchers.
2.5 Step 5: choice of software
Software solutions to perform meta-analyses range from built-in functions or additional packages of statistical software to software purely focused on meta-analyses and from commercial to open-source solutions. However, in addition to personal preferences, the choice of the most suitable software depends on the complexity of the methods used and the dataset itself (Cheung and Vijayakumar 2016 ). Meta-analysts therefore must carefully check if their preferred software is capable of performing the intended analysis.
Among commercial software providers, Stata (from version 16 on) offers built-in functions to perform various meta-analytical analyses or to produce various plots (Palmer and Sterne 2016 ). For SPSS and SAS, there exist several macros for meta-analyses provided by scholars, such as David B. Wilson or Andy P. Field and Raphael Gillet (Field and Gillett 2010 ). Footnote 3 Footnote 4 For researchers using the open-source software R (R Core Team 2021 ), Polanin et al. ( 2017 ) provide an overview of 63 meta-analysis packages and their functionalities. For new users, they recommend the package metafor (Viechtbauer 2010 ), which includes most necessary functions and for which the author Wolfgang Viechtbauer provides tutorials on his project website. Footnote 5 Footnote 6 In addition to packages and macros for statistical software, templates for Microsoft Excel have also been developed to conduct simple meta-analyses, such as Meta-Essentials by Suurmond et al. ( 2017 ). Footnote 7 Finally, programs purely dedicated to meta-analysis also exist, such as Comprehensive Meta-Analysis (Borenstein et al. 2013 ) or RevMan by The Cochrane Collaboration ( 2020 ).
2.6 Step 6: coding of effect sizes
2.6.1 coding sheet.
The first step in the coding process is the design of the coding sheet. A universal template does not exist because the design of the coding sheet depends on the methods used, the respective software, and the complexity of the research design. For univariate meta-analysis or meta-regression, data are typically coded in wide format. In its simplest form, when investigating a correlational relationship between two variables using the univariate approach, the coding sheet would contain a column for the study name or identifier, the effect size coded from the primary study, and the study sample size. However, such simple relationships are unlikely in management research because the included studies are typically not identical but differ in several respects. With more complex data structures or moderator variables being investigated, additional columns are added to the coding sheet to reflect the data characteristics. These variables can be coded as dummy, factor, or (semi)continuous variables and later used to perform a subgroup analysis or meta regression. For MASEM, the required data input format can deviate depending on the method used (e.g., TSSEM requires a list of correlation matrices as data input). For qualitative meta-analysis, the coding scheme typically summarizes the key qualitative findings and important contextual and conceptual information (see Hoon ( 2013 ) for a coding scheme for qualitative meta-analysis). Figure 1 shows an exemplary coding scheme for a quantitative meta-analysis on the correlational relationship between top-management team diversity and profitability. In addition to effect and sample sizes, information about the study country, firm type, and variable operationalizations are coded. The list could be extended by further study and sample characteristics.

Exemplary coding sheet for a meta-analysis on the relationship (correlation) between top-management team diversity and profitability
2.6.2 Inclusion of moderator or control variables
It is generally important to consider the intended research model and relevant nontarget variables before coding a meta-analytic dataset. For example, study characteristics can be important moderators or function as control variables in a meta-regression model. Similarly, control variables may be relevant in a MASEM approach to reduce confounding bias. Coding additional variables or constructs subsequently can be arduous if the sample of primary studies is large. However, the decision to include respective moderator or control variables, as in any empirical analysis, should always be based on strong (theoretical) rationales about how these variables can impact the investigated effect (Bernerth and Aguinis 2016 ; Bernerth et al. 2018 ; Thompson and Higgins 2002 ). While substantive moderators refer to theoretical constructs that act as buffers or enhancers of a supposed causal process, methodological moderators are features of the respective research designs that denote the methodological context of the observations and are important to control for systematic statistical particularities (Rudolph et al. 2020 ). Havranek et al. ( 2020 ) provide a list of recommended variables to code as potential moderators. While researchers may have clear expectations about the effects for some of these moderators, the concerns for other moderators may be tentative, and moderator analysis may be approached in a rather exploratory fashion. Thus, we argue that researchers should make full use of the meta-analytical design to obtain insights about potential context dependence that a primary study cannot achieve.
2.6.3 Treatment of multiple effect sizes in a study
A long-debated issue in conducting meta-analyses is whether to use only one or all available effect sizes for the same construct within a single primary study. For meta-analyses in management research, this question is fundamental because many empirical studies, particularly those relying on company databases, use multiple variables for the same construct to perform sensitivity analyses, resulting in multiple relevant effect sizes. In this case, researchers can either (randomly) select a single value, calculate a study average, or use the complete set of effect sizes (Bijmolt and Pieters 2001 ; López-López et al. 2018 ). Multiple effect sizes from the same study enrich the meta-analytic dataset and allow us to investigate the heterogeneity of the relationship of interest, such as different variable operationalizations (López-López et al. 2018 ; Moeyaert et al. 2017 ). However, including more than one effect size from the same study violates the independency assumption of observations (Cheung 2019 ; López-López et al. 2018 ), which can lead to biased results and erroneous conclusions (Gooty et al. 2021 ). We follow the recommendation of current best practice guides to take advantage of using all available effect size observations but to carefully consider interdependencies using appropriate methods such as multilevel models, panel regression models, or robust variance estimation (Cheung 2019 ; Geyer-Klingeberg et al. 2020 ; Gooty et al. 2021 ; López-López et al. 2018 ; Moeyaert et al. 2017 ).
2.7 Step 7: analysis
2.7.1 outlier analysis and tests for publication bias.
Before conducting the primary analysis, some preliminary sensitivity analyses might be necessary, which should ensure the robustness of the meta-analytical findings (Rudolph et al. 2020 ). First, influential outlier observations could potentially bias the observed results, particularly if the number of total effect sizes is small. Several statistical methods can be used to identify outliers in meta-analytical datasets (Aguinis et al. 2013 ; Viechtbauer and Cheung 2010 ). However, there is a debate about whether to keep or omit these observations. Anyhow, relevant studies should be closely inspected to infer an explanation about their deviating results. As in any other primary study, outliers can be a valid representation, albeit representing a different population, measure, construct, design or procedure. Thus, inferences about outliers can provide the basis to infer potential moderators (Aguinis et al. 2013 ; Steel et al. 2021 ). On the other hand, outliers can indicate invalid research, for instance, when unrealistically strong correlations are due to construct overlap (i.e., lack of a clear demarcation between independent and dependent variables), invalid measures, or simply typing errors when coding effect sizes. An advisable step is therefore to compare the results both with and without outliers and base the decision on whether to exclude outlier observations with careful consideration (Geyskens et al. 2009 ; Grewal et al. 2018 ; Kepes et al. 2013 ). However, instead of simply focusing on the size of the outlier, its leverage should be considered. Thus, Viechtbauer and Cheung ( 2010 ) propose considering a combination of standardized deviation and a study’s leverage.
Second, as mentioned in the context of a literature search, potential publication bias may be an issue. Publication bias can be examined in multiple ways (Rothstein et al. 2005 ). First, the funnel plot is a simple graphical tool that can provide an overview of the effect size distribution and help to detect publication bias (Stanley and Doucouliagos 2010 ). A funnel plot can also support in identifying potential outliers. As mentioned above, a graphical display of deviation (e.g., studentized residuals) and leverage (Cook’s distance) can help detect the presence of outliers and evaluate their influence (Viechtbauer and Cheung 2010 ). Moreover, several statistical procedures can be used to test for publication bias (Harrison et al. 2017 ; Kepes et al. 2012 ), including subgroup comparisons between published and unpublished studies, Begg and Mazumdar’s ( 1994 ) rank correlation test, cumulative meta-analysis (Borenstein et al. 2009 ), the trim and fill method (Duval and Tweedie 2000a , b ), Egger et al.’s ( 1997 ) regression test, failsafe N (Rosenthal 1979 ), or selection models (Hedges and Vevea 2005 ; Vevea and Woods 2005 ). In examining potential publication bias, Kepes et al. ( 2012 ) and Harrison et al. ( 2017 ) both recommend not relying only on a single test but rather using multiple conceptionally different test procedures (i.e., the so-called “triangulation approach”).
2.7.2 Model choice
After controlling and correcting for the potential presence of impactful outliers or publication bias, the next step in meta-analysis is the primary analysis, where meta-analysts must decide between two different types of models that are based on different assumptions: fixed-effects and random-effects (Borenstein et al. 2010 ). Fixed-effects models assume that all observations share a common mean effect size, which means that differences are only due to sampling error, while random-effects models assume heterogeneity and allow for a variation of the true effect sizes across studies (Borenstein et al. 2010 ; Cheung and Vijayakumar 2016 ; Hunter and Schmidt 2004 ). Both models are explained in detail in standard textbooks (e.g., Borenstein et al. 2009 ; Hunter and Schmidt 2004 ; Lipsey and Wilson 2001 ).
In general, the presence of heterogeneity is likely in management meta-analyses because most studies do not have identical empirical settings, which can yield different effect size strengths or directions for the same investigated phenomenon. For example, the identified studies have been conducted in different countries with different institutional settings, or the type of study participants varies (e.g., students vs. employees, blue-collar vs. white-collar workers, or manufacturing vs. service firms). Thus, the vast majority of meta-analyses in management research and related fields use random-effects models (Aguinis et al. 2011a ). In a meta-regression, the random-effects model turns into a so-called mixed-effects model because moderator variables are added as fixed effects to explain the impact of observed study characteristics on effect size variations (Raudenbush 2009 ).
2.8 Step 8: reporting results
2.8.1 reporting in the article.
The final step in performing a meta-analysis is reporting its results. Most importantly, all steps and methodological decisions should be comprehensible to the reader. DeSimone et al. ( 2020 ) provide an extensive checklist for journal reviewers of meta-analytical studies. This checklist can also be used by authors when performing their analyses and reporting their results to ensure that all important aspects have been addressed. Alternative checklists are provided, for example, by Appelbaum et al. ( 2018 ) or Page et al. ( 2021 ). Similarly, Levitt et al. ( 2018 ) provide a detailed guide for qualitative meta-analysis reporting standards.
For quantitative meta-analyses, tables reporting results should include all important information and test statistics, including mean effect sizes; standard errors and confidence intervals; the number of observations and study samples included; and heterogeneity measures. If the meta-analytic sample is rather small, a forest plot provides a good overview of the different findings and their accuracy. However, this figure will be less feasible for meta-analyses with several hundred effect sizes included. Also, results displayed in the tables and figures must be explained verbally in the results and discussion sections. Most importantly, authors must answer the primary research question, i.e., whether there is a positive, negative, or no relationship between the variables of interest, or whether the examined intervention has a certain effect. These results should be interpreted with regard to their magnitude (or significance), both economically and statistically. However, when discussing meta-analytical results, authors must describe the complexity of the results, including the identified heterogeneity and important moderators, future research directions, and theoretical relevance (DeSimone et al. 2019 ). In particular, the discussion of identified heterogeneity and underlying moderator effects is critical; not including this information can lead to false conclusions among readers, who interpret the reported mean effect size as universal for all included primary studies and ignore the variability of findings when citing the meta-analytic results in their research (Aytug et al. 2012 ; DeSimone et al. 2019 ).
2.8.2 Open-science practices
Another increasingly important topic is the public provision of meta-analytical datasets and statistical codes via open-source repositories. Open-science practices allow for results validation and for the use of coded data in subsequent meta-analyses ( Polanin et al. 2020 ), contributing to the development of cumulative science. Steel et al. ( 2021 ) refer to open science meta-analyses as a step towards “living systematic reviews” (Elliott et al. 2017 ) with continuous updates in real time. MRQ supports this development and encourages authors to make their datasets publicly available. Moreau and Gamble ( 2020 ), for example, provide various templates and video tutorials to conduct open science meta-analyses. There exist several open science repositories, such as the Open Science Foundation (OSF; for a tutorial, see Soderberg 2018 ), to preregister and make documents publicly available. Furthermore, several initiatives in the social sciences have been established to develop dynamic meta-analyses, such as metaBUS (Bosco et al. 2015 , 2017 ), MetaLab (Bergmann et al. 2018 ), or PsychOpen CAMA (Burgard et al. 2021 ).
3 Conclusion
This editorial provides a comprehensive overview of the essential steps in conducting and reporting a meta-analysis with references to more in-depth methodological articles. It also serves as a guide for meta-analyses submitted to MRQ and other management journals. MRQ welcomes all types of meta-analyses from all subfields and disciplines of management research.
Gusenbauer and Haddaway ( 2020 ), however, point out that Google Scholar is not appropriate as a primary search engine due to a lack of reproducibility of search results.
One effect size calculator by David B. Wilson is accessible via: https://www.campbellcollaboration.org/escalc/html/EffectSizeCalculator-Home.php .
The macros of David B. Wilson can be downloaded from: http://mason.gmu.edu/~dwilsonb/ .
The macros of Field and Gillet ( 2010 ) can be downloaded from: https://www.discoveringstatistics.com/repository/fieldgillett/how_to_do_a_meta_analysis.html .
The tutorials can be found via: https://www.metafor-project.org/doku.php .
Metafor does currently not provide functions to conduct MASEM. For MASEM, users can, for instance, use the package metaSEM (Cheung 2015b ).
The workbooks can be downloaded from: https://www.erim.eur.nl/research-support/meta-essentials/ .
Aguinis H, Dalton DR, Bosco FA, Pierce CA, Dalton CM (2011a) Meta-analytic choices and judgment calls: Implications for theory building and testing, obtained effect sizes, and scholarly impact. J Manag 37(1):5–38
Google Scholar
Aguinis H, Gottfredson RK, Joo H (2013) Best-practice recommendations for defining, identifying, and handling outliers. Organ Res Methods 16(2):270–301
Article Google Scholar
Aguinis H, Gottfredson RK, Wright TA (2011b) Best-practice recommendations for estimating interaction effects using meta-analysis. J Organ Behav 32(8):1033–1043
Aguinis H, Pierce CA, Bosco FA, Dalton DR, Dalton CM (2011c) Debunking myths and urban legends about meta-analysis. Organ Res Methods 14(2):306–331
Aloe AM (2015) Inaccuracy of regression results in replacing bivariate correlations. Res Synth Methods 6(1):21–27
Anderson RG, Kichkha A (2017) Replication, meta-analysis, and research synthesis in economics. Am Econ Rev 107(5):56–59
Appelbaum M, Cooper H, Kline RB, Mayo-Wilson E, Nezu AM, Rao SM (2018) Journal article reporting standards for quantitative research in psychology: the APA publications and communications BOARD task force report. Am Psychol 73(1):3–25
Aytug ZG, Rothstein HR, Zhou W, Kern MC (2012) Revealed or concealed? Transparency of procedures, decisions, and judgment calls in meta-analyses. Organ Res Methods 15(1):103–133
Begg CB, Mazumdar M (1994) Operating characteristics of a rank correlation test for publication bias. Biometrics 50(4):1088–1101. https://doi.org/10.2307/2533446
Bergh DD, Aguinis H, Heavey C, Ketchen DJ, Boyd BK, Su P, Lau CLL, Joo H (2016) Using meta-analytic structural equation modeling to advance strategic management research: Guidelines and an empirical illustration via the strategic leadership-performance relationship. Strateg Manag J 37(3):477–497
Becker BJ (1992) Using results from replicated studies to estimate linear models. J Educ Stat 17(4):341–362
Becker BJ (1995) Corrections to “Using results from replicated studies to estimate linear models.” J Edu Behav Stat 20(1):100–102
Bergmann C, Tsuji S, Piccinini PE, Lewis ML, Braginsky M, Frank MC, Cristia A (2018) Promoting replicability in developmental research through meta-analyses: Insights from language acquisition research. Child Dev 89(6):1996–2009
Bernerth JB, Aguinis H (2016) A critical review and best-practice recommendations for control variable usage. Pers Psychol 69(1):229–283
Bernerth JB, Cole MS, Taylor EC, Walker HJ (2018) Control variables in leadership research: A qualitative and quantitative review. J Manag 44(1):131–160
Bijmolt TH, Pieters RG (2001) Meta-analysis in marketing when studies contain multiple measurements. Mark Lett 12(2):157–169
Block J, Kuckertz A (2018) Seven principles of effective replication studies: Strengthening the evidence base of management research. Manag Rev Quart 68:355–359
Borenstein M (2009) Effect sizes for continuous data. In: Cooper H, Hedges LV, Valentine JC (eds) The handbook of research synthesis and meta-analysis. Russell Sage Foundation, pp 221–235
Borenstein M, Hedges LV, Higgins JPT, Rothstein HR (2009) Introduction to meta-analysis. John Wiley, Chichester
Book Google Scholar
Borenstein M, Hedges LV, Higgins JPT, Rothstein HR (2010) A basic introduction to fixed-effect and random-effects models for meta-analysis. Res Synth Methods 1(2):97–111
Borenstein M, Hedges L, Higgins J, Rothstein H (2013) Comprehensive meta-analysis (version 3). Biostat, Englewood, NJ
Borenstein M, Higgins JP (2013) Meta-analysis and subgroups. Prev Sci 14(2):134–143
Bosco FA, Steel P, Oswald FL, Uggerslev K, Field JG (2015) Cloud-based meta-analysis to bridge science and practice: Welcome to metaBUS. Person Assess Decis 1(1):3–17
Bosco FA, Uggerslev KL, Steel P (2017) MetaBUS as a vehicle for facilitating meta-analysis. Hum Resour Manag Rev 27(1):237–254
Burgard T, Bošnjak M, Studtrucker R (2021) Community-augmented meta-analyses (CAMAs) in psychology: potentials and current systems. Zeitschrift Für Psychologie 229(1):15–23
Cheung MWL (2015a) Meta-analysis: A structural equation modeling approach. John Wiley & Sons, Chichester
Cheung MWL (2015b) metaSEM: An R package for meta-analysis using structural equation modeling. Front Psychol 5:1521
Cheung MWL (2019) A guide to conducting a meta-analysis with non-independent effect sizes. Neuropsychol Rev 29(4):387–396
Cheung MWL, Chan W (2005) Meta-analytic structural equation modeling: a two-stage approach. Psychol Methods 10(1):40–64
Cheung MWL, Vijayakumar R (2016) A guide to conducting a meta-analysis. Neuropsychol Rev 26(2):121–128
Combs JG, Crook TR, Rauch A (2019) Meta-analytic research in management: contemporary approaches unresolved controversies and rising standards. J Manag Stud 56(1):1–18. https://doi.org/10.1111/joms.12427
DeSimone JA, Köhler T, Schoen JL (2019) If it were only that easy: the use of meta-analytic research by organizational scholars. Organ Res Methods 22(4):867–891. https://doi.org/10.1177/1094428118756743
DeSimone JA, Brannick MT, O’Boyle EH, Ryu JW (2020) Recommendations for reviewing meta-analyses in organizational research. Organ Res Methods 56:455–463
Duval S, Tweedie R (2000a) Trim and fill: a simple funnel-plot–based method of testing and adjusting for publication bias in meta-analysis. Biometrics 56(2):455–463
Duval S, Tweedie R (2000b) A nonparametric “trim and fill” method of accounting for publication bias in meta-analysis. J Am Stat Assoc 95(449):89–98
Egger M, Smith GD, Schneider M, Minder C (1997) Bias in meta-analysis detected by a simple, graphical test. BMJ 315(7109):629–634
Eisend M (2017) Meta-Analysis in advertising research. J Advert 46(1):21–35
Elliott JH, Synnot A, Turner T, Simmons M, Akl EA, McDonald S, Salanti G, Meerpohl J, MacLehose H, Hilton J, Tovey D, Shemilt I, Thomas J (2017) Living systematic review: 1. Introduction—the why, what, when, and how. J Clin Epidemiol 91:2330. https://doi.org/10.1016/j.jclinepi.2017.08.010
Field AP, Gillett R (2010) How to do a meta-analysis. Br J Math Stat Psychol 63(3):665–694
Fisch C, Block J (2018) Six tips for your (systematic) literature review in business and management research. Manag Rev Quart 68:103–106
Fortunato S, Bergstrom CT, Börner K, Evans JA, Helbing D, Milojević S, Petersen AM, Radicchi F, Sinatra R, Uzzi B, Vespignani A (2018) Science of science. Science 359(6379). https://doi.org/10.1126/science.aao0185
Geyer-Klingeberg J, Hang M, Rathgeber A (2020) Meta-analysis in finance research: Opportunities, challenges, and contemporary applications. Int Rev Finan Anal 71:101524
Geyskens I, Krishnan R, Steenkamp JBE, Cunha PV (2009) A review and evaluation of meta-analysis practices in management research. J Manag 35(2):393–419
Glass GV (2015) Meta-analysis at middle age: a personal history. Res Synth Methods 6(3):221–231
Gonzalez-Mulé E, Aguinis H (2018) Advancing theory by assessing boundary conditions with metaregression: a critical review and best-practice recommendations. J Manag 44(6):2246–2273
Gooty J, Banks GC, Loignon AC, Tonidandel S, Williams CE (2021) Meta-analyses as a multi-level model. Organ Res Methods 24(2):389–411. https://doi.org/10.1177/1094428119857471
Grewal D, Puccinelli N, Monroe KB (2018) Meta-analysis: integrating accumulated knowledge. J Acad Mark Sci 46(1):9–30
Gurevitch J, Koricheva J, Nakagawa S, Stewart G (2018) Meta-analysis and the science of research synthesis. Nature 555(7695):175–182
Gusenbauer M, Haddaway NR (2020) Which academic search systems are suitable for systematic reviews or meta-analyses? Evaluating retrieval qualities of Google Scholar, PubMed, and 26 other resources. Res Synth Methods 11(2):181–217
Habersang S, Küberling-Jost J, Reihlen M, Seckler C (2019) A process perspective on organizational failure: a qualitative meta-analysis. J Manage Stud 56(1):19–56
Harari MB, Parola HR, Hartwell CJ, Riegelman A (2020) Literature searches in systematic reviews and meta-analyses: A review, evaluation, and recommendations. J Vocat Behav 118:103377
Harrison JS, Banks GC, Pollack JM, O’Boyle EH, Short J (2017) Publication bias in strategic management research. J Manag 43(2):400–425
Havránek T, Stanley TD, Doucouliagos H, Bom P, Geyer-Klingeberg J, Iwasaki I, Reed WR, Rost K, Van Aert RCM (2020) Reporting guidelines for meta-analysis in economics. J Econ Surveys 34(3):469–475
Hedges LV, Olkin I (1985) Statistical methods for meta-analysis. Academic Press, Orlando
Hedges LV, Vevea JL (2005) Selection methods approaches. In: Rothstein HR, Sutton A, Borenstein M (eds) Publication bias in meta-analysis: prevention, assessment, and adjustments. Wiley, Chichester, pp 145–174
Hoon C (2013) Meta-synthesis of qualitative case studies: an approach to theory building. Organ Res Methods 16(4):522–556
Hunter JE, Schmidt FL (1990) Methods of meta-analysis: correcting error and bias in research findings. Sage, Newbury Park
Hunter JE, Schmidt FL (2004) Methods of meta-analysis: correcting error and bias in research findings, 2nd edn. Sage, Thousand Oaks
Hunter JE, Schmidt FL, Jackson GB (1982) Meta-analysis: cumulating research findings across studies. Sage Publications, Beverly Hills
Jak S (2015) Meta-analytic structural equation modelling. Springer, New York, NY
Kepes S, Banks GC, McDaniel M, Whetzel DL (2012) Publication bias in the organizational sciences. Organ Res Methods 15(4):624–662
Kepes S, McDaniel MA, Brannick MT, Banks GC (2013) Meta-analytic reviews in the organizational sciences: Two meta-analytic schools on the way to MARS (the Meta-Analytic Reporting Standards). J Bus Psychol 28(2):123–143
Kraus S, Breier M, Dasí-Rodríguez S (2020) The art of crafting a systematic literature review in entrepreneurship research. Int Entrepreneur Manag J 16(3):1023–1042
Levitt HM (2018) How to conduct a qualitative meta-analysis: tailoring methods to enhance methodological integrity. Psychother Res 28(3):367–378
Levitt HM, Bamberg M, Creswell JW, Frost DM, Josselson R, Suárez-Orozco C (2018) Journal article reporting standards for qualitative primary, qualitative meta-analytic, and mixed methods research in psychology: the APA publications and communications board task force report. Am Psychol 73(1):26
Lipsey MW, Wilson DB (2001) Practical meta-analysis. Sage Publications, Inc.
López-López JA, Page MJ, Lipsey MW, Higgins JP (2018) Dealing with effect size multiplicity in systematic reviews and meta-analyses. Res Synth Methods 9(3):336–351
Martín-Martín A, Thelwall M, Orduna-Malea E, López-Cózar ED (2021) Google Scholar, Microsoft Academic, Scopus, Dimensions, Web of Science, and OpenCitations’ COCI: a multidisciplinary comparison of coverage via citations. Scientometrics 126(1):871–906
Merton RK (1968) The Matthew effect in science: the reward and communication systems of science are considered. Science 159(3810):56–63
Moeyaert M, Ugille M, Natasha Beretvas S, Ferron J, Bunuan R, Van den Noortgate W (2017) Methods for dealing with multiple outcomes in meta-analysis: a comparison between averaging effect sizes, robust variance estimation and multilevel meta-analysis. Int J Soc Res Methodol 20(6):559–572
Moher D, Liberati A, Tetzlaff J, Altman DG, Prisma Group (2009) Preferred reporting items for systematic reviews and meta-analyses: the PRISMA statement. PLoS medicine. 6(7):e1000097
Mongeon P, Paul-Hus A (2016) The journal coverage of Web of Science and Scopus: a comparative analysis. Scientometrics 106(1):213–228
Moreau D, Gamble B (2020) Conducting a meta-analysis in the age of open science: Tools, tips, and practical recommendations. Psychol Methods. https://doi.org/10.1037/met0000351
O’Mara-Eves A, Thomas J, McNaught J, Miwa M, Ananiadou S (2015) Using text mining for study identification in systematic reviews: a systematic review of current approaches. Syst Rev 4(1):1–22
Ouzzani M, Hammady H, Fedorowicz Z, Elmagarmid A (2016) Rayyan—a web and mobile app for systematic reviews. Syst Rev 5(1):1–10
Owen E, Li Q (2021) The conditional nature of publication bias: a meta-regression analysis. Polit Sci Res Methods 9(4):867–877
Page MJ, McKenzie JE, Bossuyt PM, Boutron I, Hoffmann TC, Mulrow CD, Shamseer L, Tetzlaff JM, Akl EA, Brennan SE, Chou R, Glanville J, Grimshaw JM, Hróbjartsson A, Lalu MM, Li T, Loder EW, Mayo-Wilson E,McDonald S,McGuinness LA, Stewart LA, Thomas J, Tricco AC, Welch VA, Whiting P, Moher D (2021) The PRISMA 2020 statement: an updated guideline for reporting systematic reviews. BMJ 372. https://doi.org/10.1136/bmj.n71
Palmer TM, Sterne JAC (eds) (2016) Meta-analysis in stata: an updated collection from the stata journal, 2nd edn. Stata Press, College Station, TX
Pigott TD, Polanin JR (2020) Methodological guidance paper: High-quality meta-analysis in a systematic review. Rev Educ Res 90(1):24–46
Polanin JR, Tanner-Smith EE, Hennessy EA (2016) Estimating the difference between published and unpublished effect sizes: a meta-review. Rev Educ Res 86(1):207–236
Polanin JR, Hennessy EA, Tanner-Smith EE (2017) A review of meta-analysis packages in R. J Edu Behav Stat 42(2):206–242
Polanin JR, Hennessy EA, Tsuji S (2020) Transparency and reproducibility of meta-analyses in psychology: a meta-review. Perspect Psychol Sci 15(4):1026–1041. https://doi.org/10.1177/17456916209064
R Core Team (2021). R: A language and environment for statistical computing . R Foundation for Statistical Computing, Vienna, Austria. URL https://www.R-project.org/ .
Rauch A (2020) Opportunities and threats in reviewing entrepreneurship theory and practice. Entrep Theory Pract 44(5):847–860
Rauch A, van Doorn R, Hulsink W (2014) A qualitative approach to evidence–based entrepreneurship: theoretical considerations and an example involving business clusters. Entrep Theory Pract 38(2):333–368
Raudenbush SW (2009) Analyzing effect sizes: Random-effects models. In: Cooper H, Hedges LV, Valentine JC (eds) The handbook of research synthesis and meta-analysis, 2nd edn. Russell Sage Foundation, New York, NY, pp 295–315
Rosenthal R (1979) The file drawer problem and tolerance for null results. Psychol Bull 86(3):638
Rothstein HR, Sutton AJ, Borenstein M (2005) Publication bias in meta-analysis: prevention, assessment and adjustments. Wiley, Chichester
Roth PL, Le H, Oh I-S, Van Iddekinge CH, Bobko P (2018) Using beta coefficients to impute missing correlations in meta-analysis research: Reasons for caution. J Appl Psychol 103(6):644–658. https://doi.org/10.1037/apl0000293
Rudolph CW, Chang CK, Rauvola RS, Zacher H (2020) Meta-analysis in vocational behavior: a systematic review and recommendations for best practices. J Vocat Behav 118:103397
Schmidt FL (2017) Statistical and measurement pitfalls in the use of meta-regression in meta-analysis. Career Dev Int 22(5):469–476
Schmidt FL, Hunter JE (2015) Methods of meta-analysis: correcting error and bias in research findings. Sage, Thousand Oaks
Schwab A (2015) Why all researchers should report effect sizes and their confidence intervals: Paving the way for meta–analysis and evidence–based management practices. Entrepreneurship Theory Pract 39(4):719–725. https://doi.org/10.1111/etap.12158
Shaw JD, Ertug G (2017) The suitability of simulations and meta-analyses for submissions to Academy of Management Journal. Acad Manag J 60(6):2045–2049
Soderberg CK (2018) Using OSF to share data: A step-by-step guide. Adv Methods Pract Psychol Sci 1(1):115–120
Stanley TD, Doucouliagos H (2010) Picture this: a simple graph that reveals much ado about research. J Econ Surveys 24(1):170–191
Stanley TD, Doucouliagos H (2012) Meta-regression analysis in economics and business. Routledge, London
Stanley TD, Jarrell SB (1989) Meta-regression analysis: a quantitative method of literature surveys. J Econ Surveys 3:54–67
Steel P, Beugelsdijk S, Aguinis H (2021) The anatomy of an award-winning meta-analysis: Recommendations for authors, reviewers, and readers of meta-analytic reviews. J Int Bus Stud 52(1):23–44
Suurmond R, van Rhee H, Hak T (2017) Introduction, comparison, and validation of Meta-Essentials: a free and simple tool for meta-analysis. Res Synth Methods 8(4):537–553
The Cochrane Collaboration (2020). Review Manager (RevMan) [Computer program] (Version 5.4).
Thomas J, Noel-Storr A, Marshall I, Wallace B, McDonald S, Mavergames C, Glasziou P, Shemilt I, Synnot A, Turner T, Elliot J (2017) Living systematic reviews: 2. Combining human and machine effort. J Clin Epidemiol 91:31–37
Thompson SG, Higgins JP (2002) How should meta-regression analyses be undertaken and interpreted? Stat Med 21(11):1559–1573
Tipton E, Pustejovsky JE, Ahmadi H (2019) A history of meta-regression: technical, conceptual, and practical developments between 1974 and 2018. Res Synth Methods 10(2):161–179
Vevea JL, Woods CM (2005) Publication bias in research synthesis: Sensitivity analysis using a priori weight functions. Psychol Methods 10(4):428–443
Viechtbauer W (2010) Conducting meta-analyses in R with the metafor package. J Stat Softw 36(3):1–48
Viechtbauer W, Cheung MWL (2010) Outlier and influence diagnostics for meta-analysis. Res Synth Methods 1(2):112–125
Viswesvaran C, Ones DS (1995) Theory testing: combining psychometric meta-analysis and structural equations modeling. Pers Psychol 48(4):865–885
Wilson SJ, Polanin JR, Lipsey MW (2016) Fitting meta-analytic structural equation models with complex datasets. Res Synth Methods 7(2):121–139. https://doi.org/10.1002/jrsm.1199
Wood JA (2008) Methodology for dealing with duplicate study effects in a meta-analysis. Organ Res Methods 11(1):79–95
Download references
Open Access funding enabled and organized by Projekt DEAL. No funding was received to assist with the preparation of this manuscript.
Author information
Authors and affiliations.
University of Luxembourg, Luxembourg, Luxembourg
Christopher Hansen
Leibniz Institute for Psychology (ZPID), Trier, Germany
Holger Steinmetz
Trier University, Trier, Germany
Erasmus University Rotterdam, Rotterdam, The Netherlands
Wittener Institut Für Familienunternehmen, Universität Witten/Herdecke, Witten, Germany
You can also search for this author in PubMed Google Scholar
Corresponding author
Correspondence to Jörn Block .
Ethics declarations
Conflict of interest.
The authors have no relevant financial or non-financial interests to disclose.
Additional information
Publisher's note.
Springer Nature remains neutral with regard to jurisdictional claims in published maps and institutional affiliations.
See Table 1 .
Rights and permissions
Open Access This article is licensed under a Creative Commons Attribution 4.0 International License, which permits use, sharing, adaptation, distribution and reproduction in any medium or format, as long as you give appropriate credit to the original author(s) and the source, provide a link to the Creative Commons licence, and indicate if changes were made. The images or other third party material in this article are included in the article's Creative Commons licence, unless indicated otherwise in a credit line to the material. If material is not included in the article's Creative Commons licence and your intended use is not permitted by statutory regulation or exceeds the permitted use, you will need to obtain permission directly from the copyright holder. To view a copy of this licence, visit http://creativecommons.org/licenses/by/4.0/ .
Reprints and permissions
About this article
Hansen, C., Steinmetz, H. & Block, J. How to conduct a meta-analysis in eight steps: a practical guide. Manag Rev Q 72 , 1–19 (2022). https://doi.org/10.1007/s11301-021-00247-4
Download citation
Published : 30 November 2021
Issue Date : February 2022
DOI : https://doi.org/10.1007/s11301-021-00247-4
Share this article
Anyone you share the following link with will be able to read this content:
Sorry, a shareable link is not currently available for this article.
Provided by the Springer Nature SharedIt content-sharing initiative
- Find a journal
- Publish with us
- Track your research
- Skip to main content
- Skip to ChatBot Assistant
- Academic Writing
- What is a Research Paper?
- Steps in Writing a Research Paper
- Critical Reading and Writing
- Punctuation
- Writing Exercises
- ELL/ESL Resources
Analysis in Research Papers
To analyze means to break a topic or concept down into its parts in order to inspect and understand it, and to restructure those parts in a way that makes sense to you. In an analytical research paper, you do research to become an expert on a topic so that you can restructure and present the parts of the topic from your own perspective.
For example, you could analyze the role of the mother in the ancient Egyptian family. You could break down that topic into its parts--the mother's duties in the family, social status, and expected role in the larger society--and research those parts in order to present your general perspective and conclusion about the mother's role.
Need Assistance?
If you would like assistance with any type of writing assignment, learning coaches are available to assist you. Please contact Academic Support by emailing [email protected].
Questions or feedback about SUNY Empire's Writing Support?
Contact us at [email protected] .
Smart Cookies
They're not just in our classes – they help power our website. Cookies and similar tools allow us to better understand the experience of our visitors. By continuing to use this website, you consent to SUNY Empire State University's usage of cookies and similar technologies in accordance with the university's Privacy Notice and Cookies Policy .
- Skip to main content
- Skip to primary sidebar
- Skip to footer
- QuestionPro

- Solutions Industries Gaming Automotive Sports and events Education Government Travel & Hospitality Financial Services Healthcare Cannabis Technology Use Case NPS+ Communities Audience Contactless surveys Mobile LivePolls Member Experience GDPR Positive People Science 360 Feedback Surveys
- Resources Blog eBooks Survey Templates Case Studies Training Help center

Home Market Research
Data Analysis in Research: Types & Methods
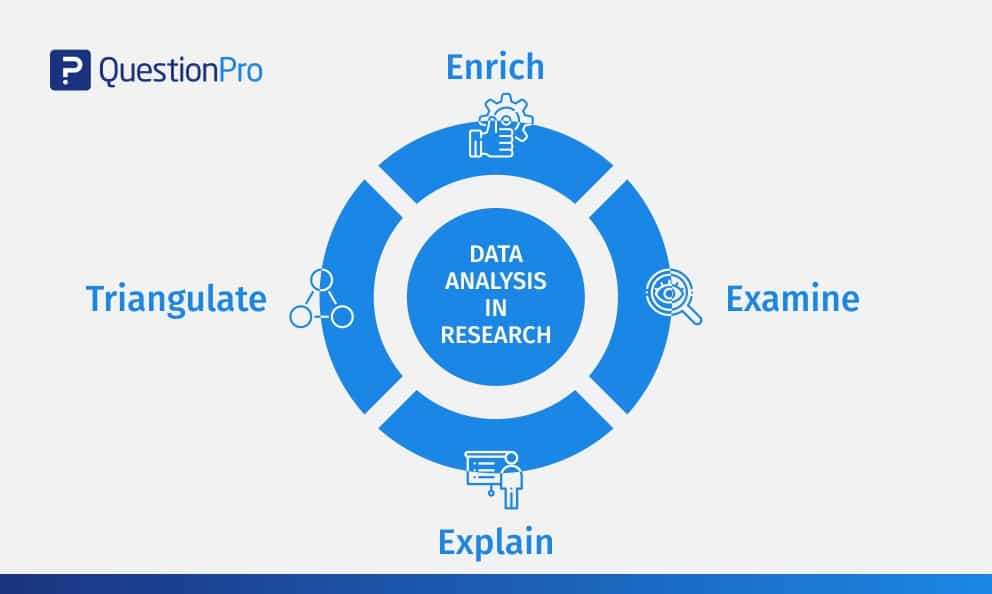
Content Index
Why analyze data in research?
Types of data in research, finding patterns in the qualitative data, methods used for data analysis in qualitative research, preparing data for analysis, methods used for data analysis in quantitative research, considerations in research data analysis, what is data analysis in research.
Definition of research in data analysis: According to LeCompte and Schensul, research data analysis is a process used by researchers to reduce data to a story and interpret it to derive insights. The data analysis process helps reduce a large chunk of data into smaller fragments, which makes sense.
Three essential things occur during the data analysis process — the first is data organization . Summarization and categorization together contribute to becoming the second known method used for data reduction. It helps find patterns and themes in the data for easy identification and linking. The third and last way is data analysis – researchers do it in both top-down and bottom-up fashion.
LEARN ABOUT: Research Process Steps
On the other hand, Marshall and Rossman describe data analysis as a messy, ambiguous, and time-consuming but creative and fascinating process through which a mass of collected data is brought to order, structure and meaning.
We can say that “the data analysis and data interpretation is a process representing the application of deductive and inductive logic to the research and data analysis.”
Researchers rely heavily on data as they have a story to tell or research problems to solve. It starts with a question, and data is nothing but an answer to that question. But, what if there is no question to ask? Well! It is possible to explore data even without a problem – we call it ‘Data Mining’, which often reveals some interesting patterns within the data that are worth exploring.
Irrelevant to the type of data researchers explore, their mission and audiences’ vision guide them to find the patterns to shape the story they want to tell. One of the essential things expected from researchers while analyzing data is to stay open and remain unbiased toward unexpected patterns, expressions, and results. Remember, sometimes, data analysis tells the most unforeseen yet exciting stories that were not expected when initiating data analysis. Therefore, rely on the data you have at hand and enjoy the journey of exploratory research.
Create a Free Account
Every kind of data has a rare quality of describing things after assigning a specific value to it. For analysis, you need to organize these values, processed and presented in a given context, to make it useful. Data can be in different forms; here are the primary data types.
- Qualitative data: When the data presented has words and descriptions, then we call it qualitative data . Although you can observe this data, it is subjective and harder to analyze data in research, especially for comparison. Example: Quality data represents everything describing taste, experience, texture, or an opinion that is considered quality data. This type of data is usually collected through focus groups, personal qualitative interviews , qualitative observation or using open-ended questions in surveys.
- Quantitative data: Any data expressed in numbers of numerical figures are called quantitative data . This type of data can be distinguished into categories, grouped, measured, calculated, or ranked. Example: questions such as age, rank, cost, length, weight, scores, etc. everything comes under this type of data. You can present such data in graphical format, charts, or apply statistical analysis methods to this data. The (Outcomes Measurement Systems) OMS questionnaires in surveys are a significant source of collecting numeric data.
- Categorical data: It is data presented in groups. However, an item included in the categorical data cannot belong to more than one group. Example: A person responding to a survey by telling his living style, marital status, smoking habit, or drinking habit comes under the categorical data. A chi-square test is a standard method used to analyze this data.
Learn More : Examples of Qualitative Data in Education
Data analysis in qualitative research
Data analysis and qualitative data research work a little differently from the numerical data as the quality data is made up of words, descriptions, images, objects, and sometimes symbols. Getting insight from such complicated information is a complicated process. Hence it is typically used for exploratory research and data analysis .
Although there are several ways to find patterns in the textual information, a word-based method is the most relied and widely used global technique for research and data analysis. Notably, the data analysis process in qualitative research is manual. Here the researchers usually read the available data and find repetitive or commonly used words.
For example, while studying data collected from African countries to understand the most pressing issues people face, researchers might find “food” and “hunger” are the most commonly used words and will highlight them for further analysis.
LEARN ABOUT: Level of Analysis
The keyword context is another widely used word-based technique. In this method, the researcher tries to understand the concept by analyzing the context in which the participants use a particular keyword.
For example , researchers conducting research and data analysis for studying the concept of ‘diabetes’ amongst respondents might analyze the context of when and how the respondent has used or referred to the word ‘diabetes.’
The scrutiny-based technique is also one of the highly recommended text analysis methods used to identify a quality data pattern. Compare and contrast is the widely used method under this technique to differentiate how a specific text is similar or different from each other.
For example: To find out the “importance of resident doctor in a company,” the collected data is divided into people who think it is necessary to hire a resident doctor and those who think it is unnecessary. Compare and contrast is the best method that can be used to analyze the polls having single-answer questions types .
Metaphors can be used to reduce the data pile and find patterns in it so that it becomes easier to connect data with theory.
Variable Partitioning is another technique used to split variables so that researchers can find more coherent descriptions and explanations from the enormous data.
LEARN ABOUT: Qualitative Research Questions and Questionnaires
There are several techniques to analyze the data in qualitative research, but here are some commonly used methods,
- Content Analysis: It is widely accepted and the most frequently employed technique for data analysis in research methodology. It can be used to analyze the documented information from text, images, and sometimes from the physical items. It depends on the research questions to predict when and where to use this method.
- Narrative Analysis: This method is used to analyze content gathered from various sources such as personal interviews, field observation, and surveys . The majority of times, stories, or opinions shared by people are focused on finding answers to the research questions.
- Discourse Analysis: Similar to narrative analysis, discourse analysis is used to analyze the interactions with people. Nevertheless, this particular method considers the social context under which or within which the communication between the researcher and respondent takes place. In addition to that, discourse analysis also focuses on the lifestyle and day-to-day environment while deriving any conclusion.
- Grounded Theory: When you want to explain why a particular phenomenon happened, then using grounded theory for analyzing quality data is the best resort. Grounded theory is applied to study data about the host of similar cases occurring in different settings. When researchers are using this method, they might alter explanations or produce new ones until they arrive at some conclusion.
LEARN ABOUT: 12 Best Tools for Researchers
Data analysis in quantitative research
The first stage in research and data analysis is to make it for the analysis so that the nominal data can be converted into something meaningful. Data preparation consists of the below phases.
Phase I: Data Validation
Data validation is done to understand if the collected data sample is per the pre-set standards, or it is a biased data sample again divided into four different stages
- Fraud: To ensure an actual human being records each response to the survey or the questionnaire
- Screening: To make sure each participant or respondent is selected or chosen in compliance with the research criteria
- Procedure: To ensure ethical standards were maintained while collecting the data sample
- Completeness: To ensure that the respondent has answered all the questions in an online survey. Else, the interviewer had asked all the questions devised in the questionnaire.
Phase II: Data Editing
More often, an extensive research data sample comes loaded with errors. Respondents sometimes fill in some fields incorrectly or sometimes skip them accidentally. Data editing is a process wherein the researchers have to confirm that the provided data is free of such errors. They need to conduct necessary checks and outlier checks to edit the raw edit and make it ready for analysis.
Phase III: Data Coding
Out of all three, this is the most critical phase of data preparation associated with grouping and assigning values to the survey responses . If a survey is completed with a 1000 sample size, the researcher will create an age bracket to distinguish the respondents based on their age. Thus, it becomes easier to analyze small data buckets rather than deal with the massive data pile.
LEARN ABOUT: Steps in Qualitative Research
After the data is prepared for analysis, researchers are open to using different research and data analysis methods to derive meaningful insights. For sure, statistical analysis plans are the most favored to analyze numerical data. In statistical analysis, distinguishing between categorical data and numerical data is essential, as categorical data involves distinct categories or labels, while numerical data consists of measurable quantities. The method is again classified into two groups. First, ‘Descriptive Statistics’ used to describe data. Second, ‘Inferential statistics’ that helps in comparing the data .
Descriptive statistics
This method is used to describe the basic features of versatile types of data in research. It presents the data in such a meaningful way that pattern in the data starts making sense. Nevertheless, the descriptive analysis does not go beyond making conclusions. The conclusions are again based on the hypothesis researchers have formulated so far. Here are a few major types of descriptive analysis methods.
Measures of Frequency
- Count, Percent, Frequency
- It is used to denote home often a particular event occurs.
- Researchers use it when they want to showcase how often a response is given.
Measures of Central Tendency
- Mean, Median, Mode
- The method is widely used to demonstrate distribution by various points.
- Researchers use this method when they want to showcase the most commonly or averagely indicated response.
Measures of Dispersion or Variation
- Range, Variance, Standard deviation
- Here the field equals high/low points.
- Variance standard deviation = difference between the observed score and mean
- It is used to identify the spread of scores by stating intervals.
- Researchers use this method to showcase data spread out. It helps them identify the depth until which the data is spread out that it directly affects the mean.
Measures of Position
- Percentile ranks, Quartile ranks
- It relies on standardized scores helping researchers to identify the relationship between different scores.
- It is often used when researchers want to compare scores with the average count.
For quantitative research use of descriptive analysis often give absolute numbers, but the in-depth analysis is never sufficient to demonstrate the rationale behind those numbers. Nevertheless, it is necessary to think of the best method for research and data analysis suiting your survey questionnaire and what story researchers want to tell. For example, the mean is the best way to demonstrate the students’ average scores in schools. It is better to rely on the descriptive statistics when the researchers intend to keep the research or outcome limited to the provided sample without generalizing it. For example, when you want to compare average voting done in two different cities, differential statistics are enough.
Descriptive analysis is also called a ‘univariate analysis’ since it is commonly used to analyze a single variable.
Inferential statistics
Inferential statistics are used to make predictions about a larger population after research and data analysis of the representing population’s collected sample. For example, you can ask some odd 100 audiences at a movie theater if they like the movie they are watching. Researchers then use inferential statistics on the collected sample to reason that about 80-90% of people like the movie.
Here are two significant areas of inferential statistics.
- Estimating parameters: It takes statistics from the sample research data and demonstrates something about the population parameter.
- Hypothesis test: I t’s about sampling research data to answer the survey research questions. For example, researchers might be interested to understand if the new shade of lipstick recently launched is good or not, or if the multivitamin capsules help children to perform better at games.
These are sophisticated analysis methods used to showcase the relationship between different variables instead of describing a single variable. It is often used when researchers want something beyond absolute numbers to understand the relationship between variables.
Here are some of the commonly used methods for data analysis in research.
- Correlation: When researchers are not conducting experimental research or quasi-experimental research wherein the researchers are interested to understand the relationship between two or more variables, they opt for correlational research methods.
- Cross-tabulation: Also called contingency tables, cross-tabulation is used to analyze the relationship between multiple variables. Suppose provided data has age and gender categories presented in rows and columns. A two-dimensional cross-tabulation helps for seamless data analysis and research by showing the number of males and females in each age category.
- Regression analysis: For understanding the strong relationship between two variables, researchers do not look beyond the primary and commonly used regression analysis method, which is also a type of predictive analysis used. In this method, you have an essential factor called the dependent variable. You also have multiple independent variables in regression analysis. You undertake efforts to find out the impact of independent variables on the dependent variable. The values of both independent and dependent variables are assumed as being ascertained in an error-free random manner.
- Frequency tables: The statistical procedure is used for testing the degree to which two or more vary or differ in an experiment. A considerable degree of variation means research findings were significant. In many contexts, ANOVA testing and variance analysis are similar.
- Analysis of variance: The statistical procedure is used for testing the degree to which two or more vary or differ in an experiment. A considerable degree of variation means research findings were significant. In many contexts, ANOVA testing and variance analysis are similar.
- Researchers must have the necessary research skills to analyze and manipulation the data , Getting trained to demonstrate a high standard of research practice. Ideally, researchers must possess more than a basic understanding of the rationale of selecting one statistical method over the other to obtain better data insights.
- Usually, research and data analytics projects differ by scientific discipline; therefore, getting statistical advice at the beginning of analysis helps design a survey questionnaire, select data collection methods , and choose samples.
LEARN ABOUT: Best Data Collection Tools
- The primary aim of data research and analysis is to derive ultimate insights that are unbiased. Any mistake in or keeping a biased mind to collect data, selecting an analysis method, or choosing audience sample il to draw a biased inference.
- Irrelevant to the sophistication used in research data and analysis is enough to rectify the poorly defined objective outcome measurements. It does not matter if the design is at fault or intentions are not clear, but lack of clarity might mislead readers, so avoid the practice.
- The motive behind data analysis in research is to present accurate and reliable data. As far as possible, avoid statistical errors, and find a way to deal with everyday challenges like outliers, missing data, data altering, data mining , or developing graphical representation.
LEARN MORE: Descriptive Research vs Correlational Research The sheer amount of data generated daily is frightening. Especially when data analysis has taken center stage. in 2018. In last year, the total data supply amounted to 2.8 trillion gigabytes. Hence, it is clear that the enterprises willing to survive in the hypercompetitive world must possess an excellent capability to analyze complex research data, derive actionable insights, and adapt to the new market needs.
LEARN ABOUT: Average Order Value
QuestionPro is an online survey platform that empowers organizations in data analysis and research and provides them a medium to collect data by creating appealing surveys.
MORE LIKE THIS
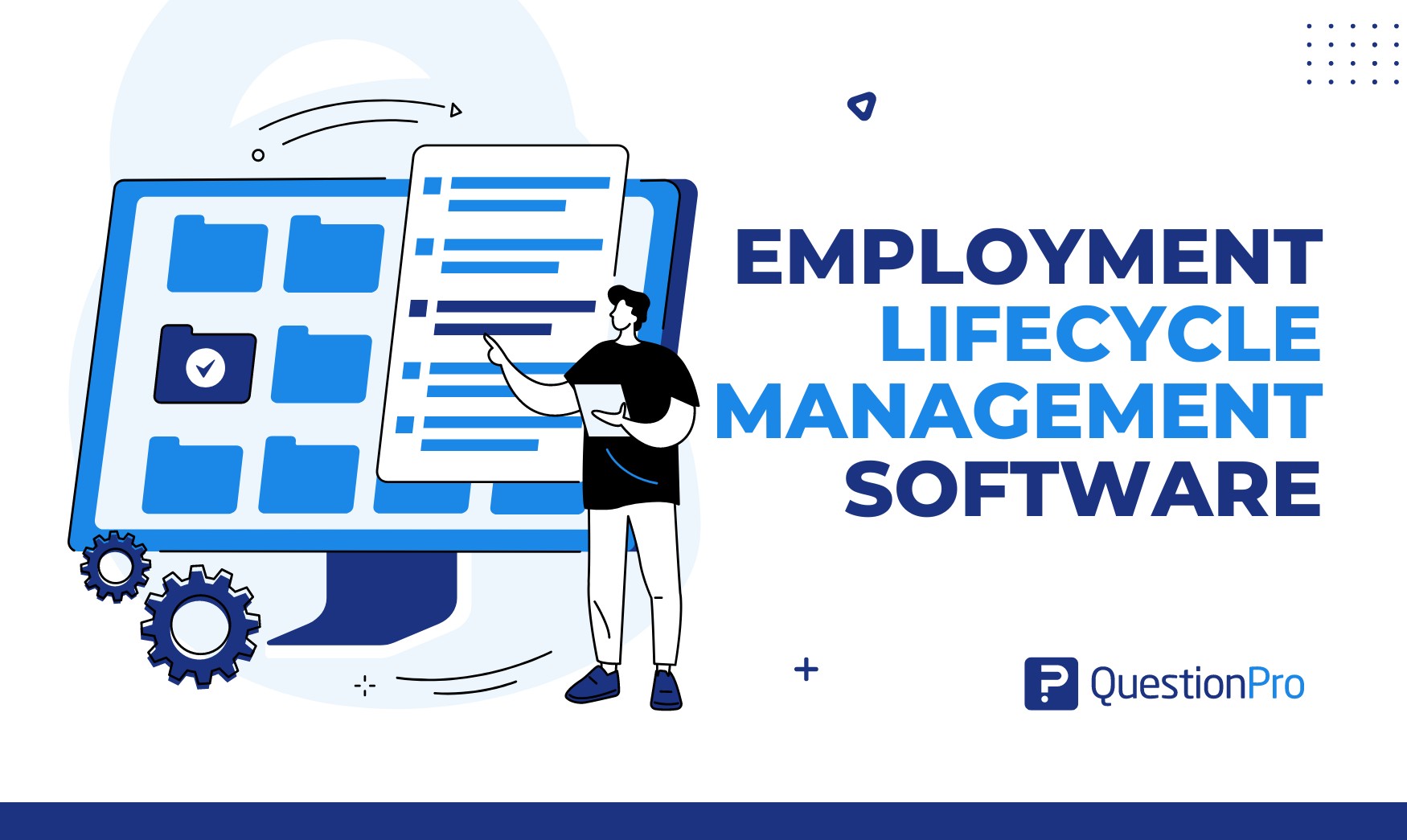
Employee Lifecycle Management Software: Top of 2024
Apr 15, 2024
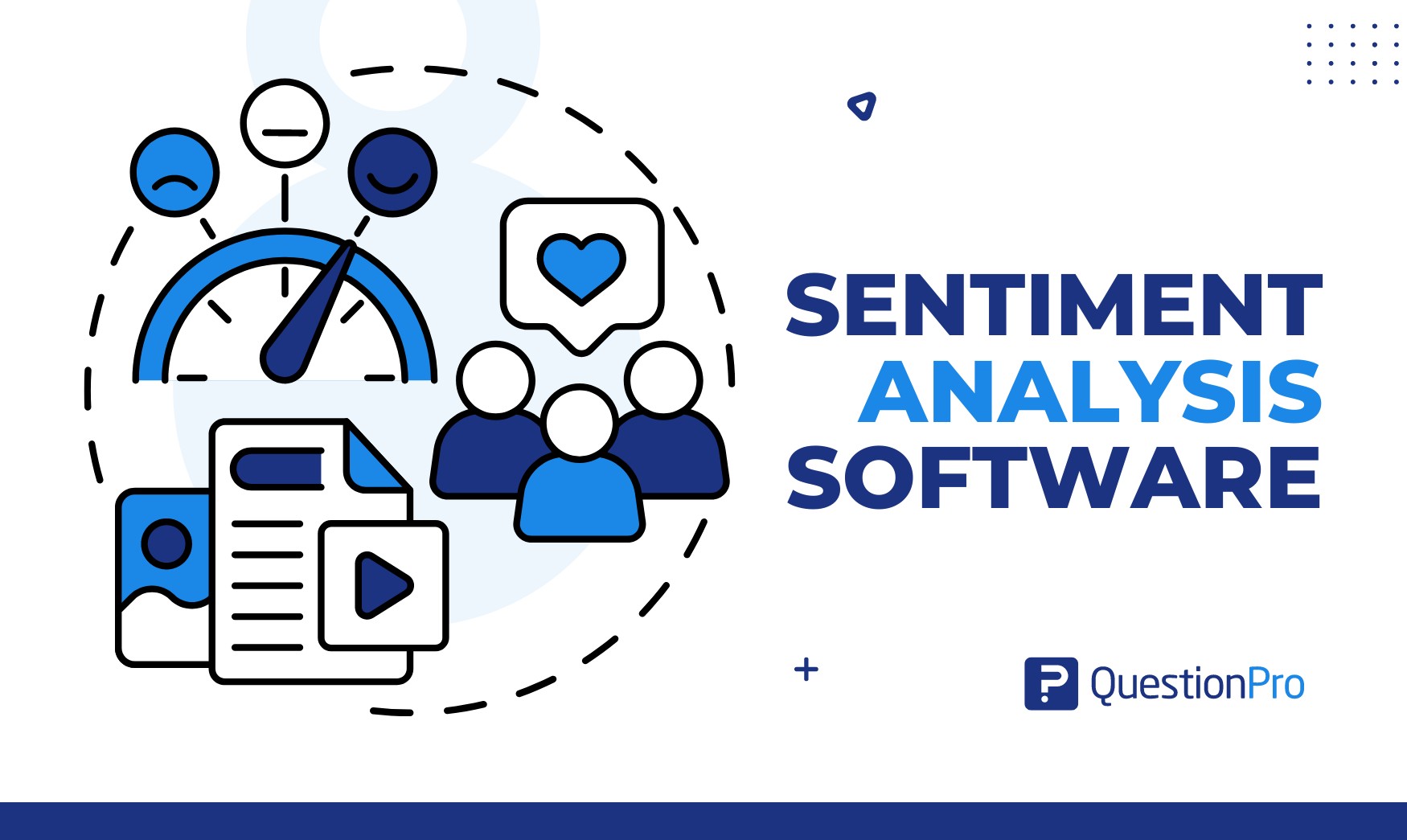
Top 15 Sentiment Analysis Software That Should Be on Your List
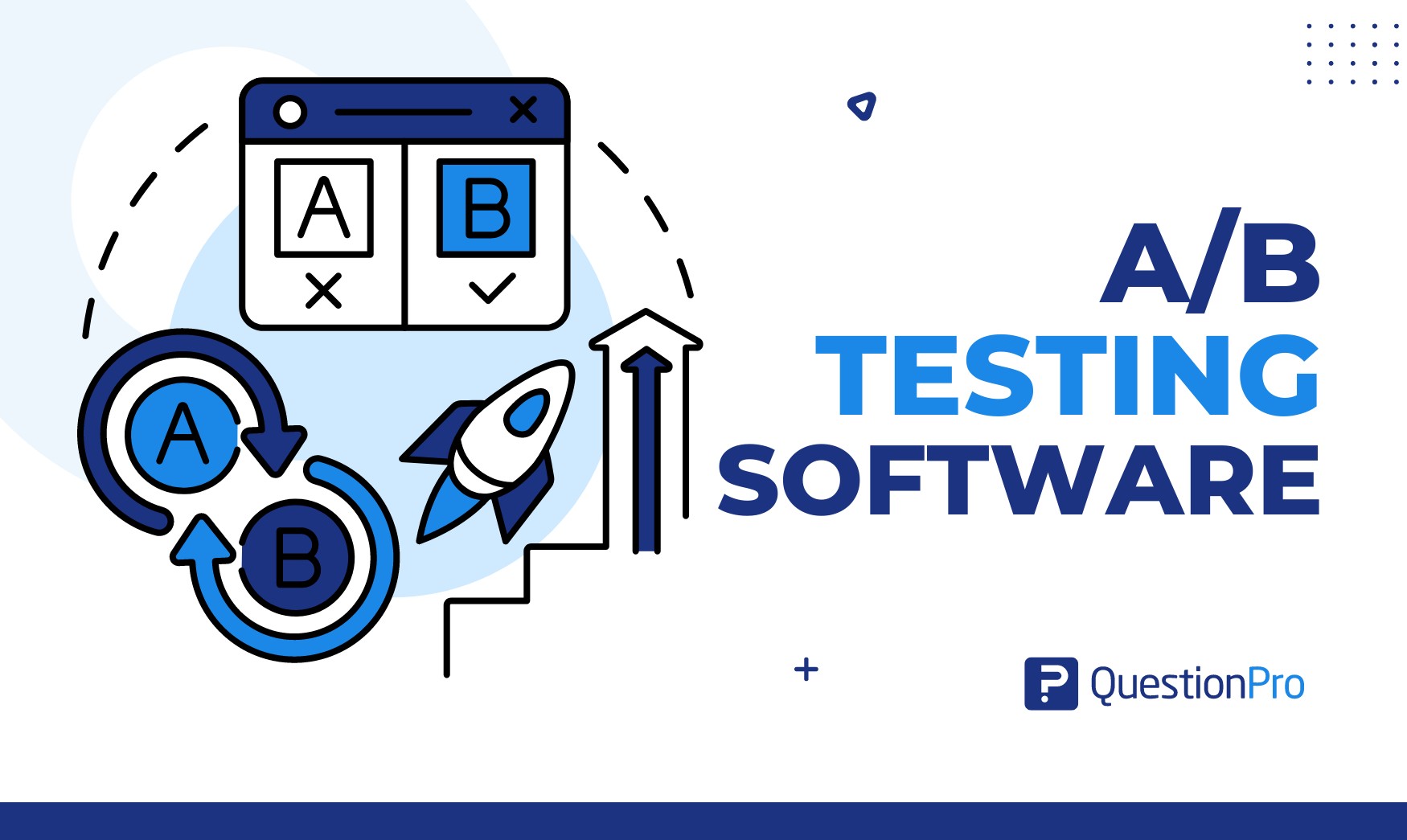
Top 13 A/B Testing Software for Optimizing Your Website
Apr 12, 2024
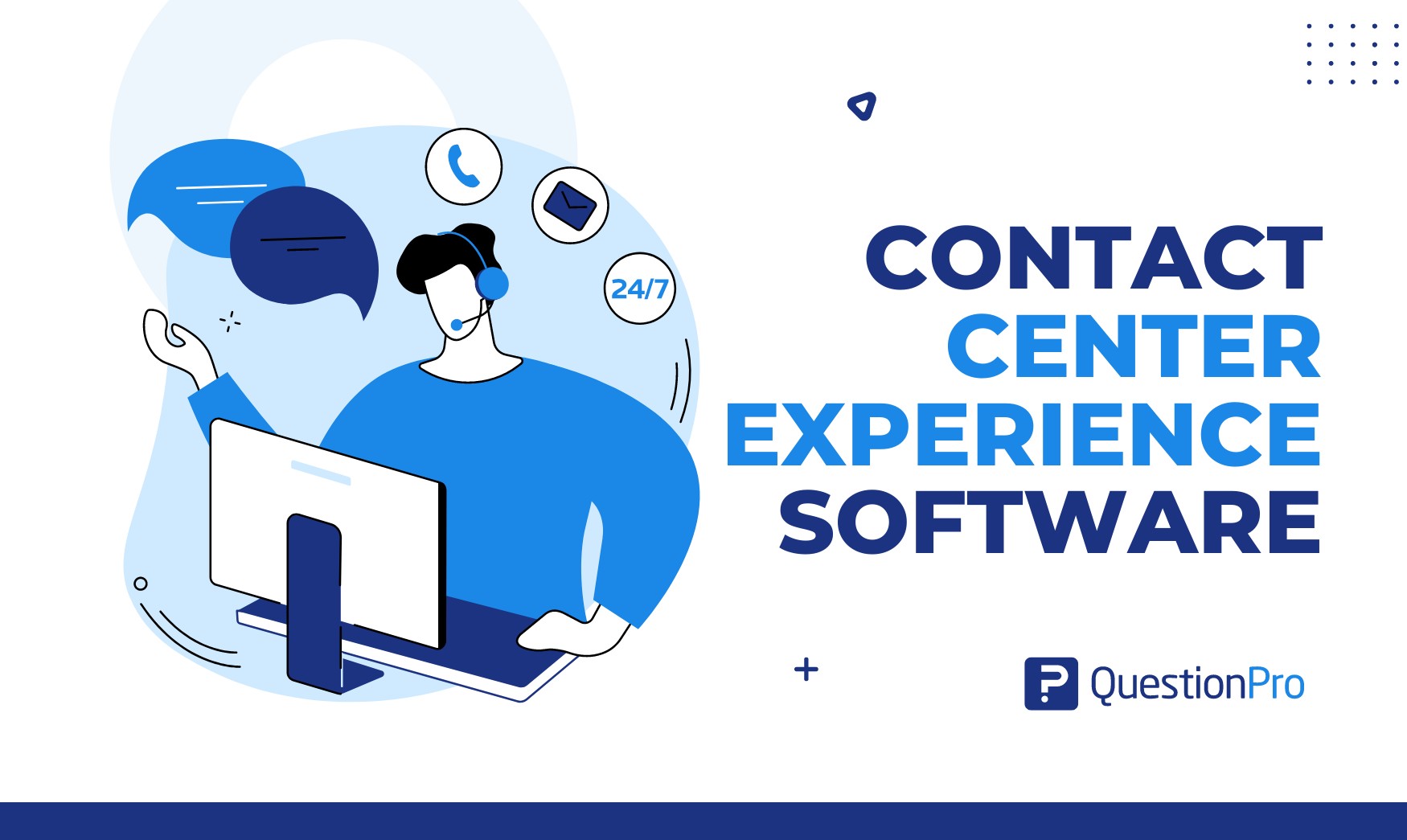
21 Best Contact Center Experience Software in 2024
Other categories.
- Academic Research
- Artificial Intelligence
- Assessments
- Brand Awareness
- Case Studies
- Communities
- Consumer Insights
- Customer effort score
- Customer Engagement
- Customer Experience
- Customer Loyalty
- Customer Research
- Customer Satisfaction
- Employee Benefits
- Employee Engagement
- Employee Retention
- Friday Five
- General Data Protection Regulation
- Insights Hub
- Life@QuestionPro
- Market Research
- Mobile diaries
- Mobile Surveys
- New Features
- Online Communities
- Question Types
- Questionnaire
- QuestionPro Products
- Release Notes
- Research Tools and Apps
- Revenue at Risk
- Survey Templates
- Training Tips
- Uncategorized
- Video Learning Series
- What’s Coming Up
- Workforce Intelligence
Research Paper Analysis: How to Analyze a Research Article + Example
Why might you need to analyze research? First of all, when you analyze a research article, you begin to understand your assigned reading better. It is also the first step toward learning how to write your own research articles and literature reviews. However, if you have never written a research paper before, it may be difficult for you to analyze one. After all, you may not know what criteria to use to evaluate it. But don’t panic! We will help you figure it out!
In this article, our team has explained how to analyze research papers quickly and effectively. At the end, you will also find a research analysis paper example to see how everything works in practice.
- 🔤 Research Analysis Definition
📊 How to Analyze a Research Article
✍️ how to write a research analysis.
- 📝 Analysis Example
- 🔎 More Examples
🔗 References
🔤 research paper analysis: what is it.
A research paper analysis is an academic writing assignment in which you analyze a scholarly article’s methodology, data, and findings. In essence, “to analyze” means to break something down into components and assess each of them individually and in relation to each other. The goal of an analysis is to gain a deeper understanding of a subject. So, when you analyze a research article, you dissect it into elements like data sources , research methods, and results and evaluate how they contribute to the study’s strengths and weaknesses.
📋 Research Analysis Format
A research analysis paper has a pretty straightforward structure. Check it out below!
Research articles usually include the following sections: introduction, methods, results, and discussion. In the following paragraphs, we will discuss how to analyze a scientific article with a focus on each of its parts.
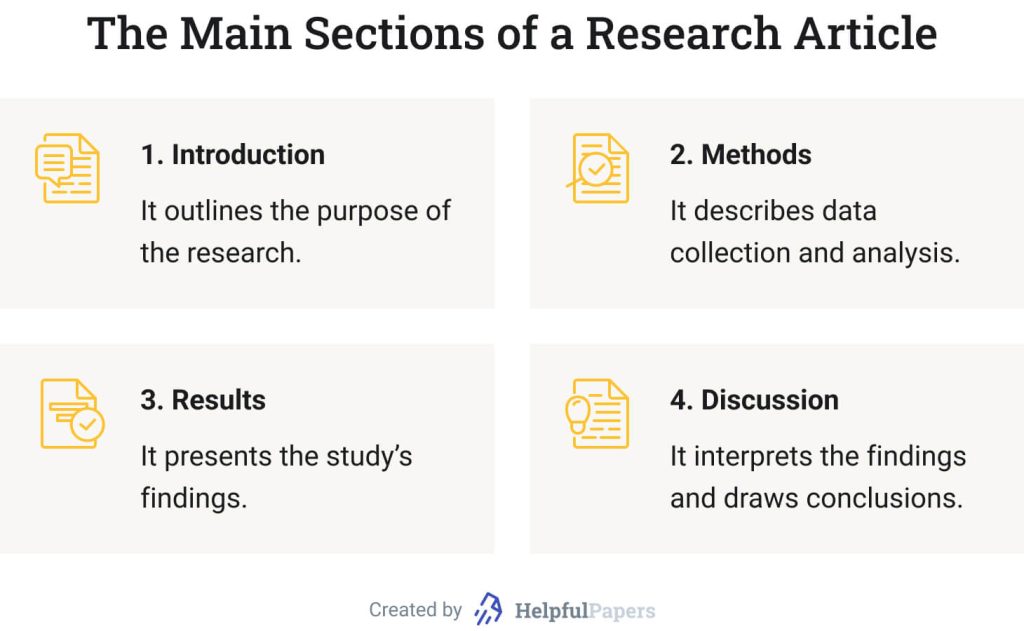
How to Analyze a Research Paper: Purpose
The purpose of the study is usually outlined in the introductory section of the article. Analyzing the research paper’s objectives is critical to establish the context for the rest of your analysis.
When analyzing the research aim, you should evaluate whether it was justified for the researchers to conduct the study. In other words, you should assess whether their research question was significant and whether it arose from existing literature on the topic.
Here are some questions that may help you analyze a research paper’s purpose:
- Why was the research carried out?
- What gaps does it try to fill, or what controversies to settle?
- How does the study contribute to its field?
- Do you agree with the author’s justification for approaching this particular question in this way?
How to Analyze a Paper: Methods
When analyzing the methodology section , you should indicate the study’s research design (qualitative, quantitative, or mixed) and methods used (for example, experiment, case study, correlational research, survey, etc.). After that, you should assess whether these methods suit the research purpose. In other words, do the chosen methods allow scholars to answer their research questions within the scope of their study?
For example, if scholars wanted to study US students’ average satisfaction with their higher education experience, they could conduct a quantitative survey . However, if they wanted to gain an in-depth understanding of the factors influencing US students’ satisfaction with higher education, qualitative interviews would be more appropriate.
When analyzing methods, you should also look at the research sample . Did the scholars use randomization to select study participants? Was the sample big enough for the results to be generalizable to a larger population?
You can also answer the following questions in your methodology analysis:
- Is the methodology valid? In other words, did the researchers use methods that accurately measure the variables of interest?
- Is the research methodology reliable? A research method is reliable if it can produce stable and consistent results under the same circumstances.
- Is the study biased in any way?
- What are the limitations of the chosen methodology?
How to Analyze Research Articles’ Results
You should start the analysis of the article results by carefully reading the tables, figures, and text. Check whether the findings correspond to the initial research purpose. See whether the results answered the author’s research questions or supported the hypotheses stated in the introduction.
To analyze the results section effectively, answer the following questions:
- What are the major findings of the study?
- Did the author present the results clearly and unambiguously?
- Are the findings statistically significant ?
- Does the author provide sufficient information on the validity and reliability of the results?
- Have you noticed any trends or patterns in the data that the author did not mention?
How to Analyze Research: Discussion
Finally, you should analyze the authors’ interpretation of results and its connection with research objectives. Examine what conclusions the authors drew from their study and whether these conclusions answer the original question.
You should also pay attention to how the authors used findings to support their conclusions. For example, you can reflect on why their findings support that particular inference and not another one. Moreover, more than one conclusion can sometimes be made based on the same set of results. If that’s the case with your article, you should analyze whether the authors addressed other interpretations of their findings .
Here are some useful questions you can use to analyze the discussion section:
- What findings did the authors use to support their conclusions?
- How do the researchers’ conclusions compare to other studies’ findings?
- How does this study contribute to its field?
- What future research directions do the authors suggest?
- What additional insights can you share regarding this article? For example, do you agree with the results? What other questions could the researchers have answered?
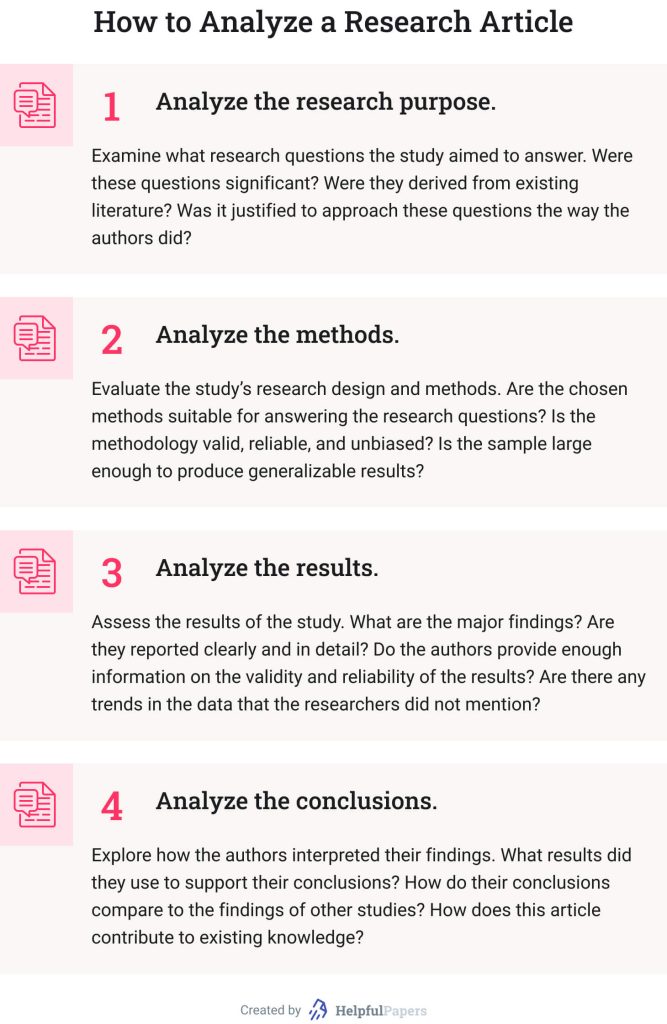
Now, you know how to analyze an article that presents research findings. However, it’s just a part of the work you have to do to complete your paper. So, it’s time to learn how to write research analysis! Check out the steps below!
1. Introduce the Article
As with most academic assignments, you should start your research article analysis with an introduction. Here’s what it should include:
- The article’s publication details . Specify the title of the scholarly work you are analyzing, its authors, and publication date. Remember to enclose the article’s title in quotation marks and write it in title case .
- The article’s main point . State what the paper is about. What did the authors study, and what was their major finding?
- Your thesis statement . End your introduction with a strong claim summarizing your evaluation of the article. Consider briefly outlining the research paper’s strengths, weaknesses, and significance in your thesis.
Keep your introduction brief. Save the word count for the “meat” of your paper — that is, for the analysis.
2. Summarize the Article
Now, you should write a brief and focused summary of the scientific article. It should be shorter than your analysis section and contain all the relevant details about the research paper.
Here’s what you should include in your summary:
- The research purpose . Briefly explain why the research was done. Identify the authors’ purpose and research questions or hypotheses .
- Methods and results . Summarize what happened in the study. State only facts, without the authors’ interpretations of them. Avoid using too many numbers and details; instead, include only the information that will help readers understand what happened.
- The authors’ conclusions . Outline what conclusions the researchers made from their study. In other words, describe how the authors explained the meaning of their findings.
If you need help summarizing an article, you can use our free summary generator .
3. Write Your Research Analysis
The analysis of the study is the most crucial part of this assignment type. Its key goal is to evaluate the article critically and demonstrate your understanding of it.
We’ve already covered how to analyze a research article in the section above. Here’s a quick recap:
- Analyze whether the study’s purpose is significant and relevant.
- Examine whether the chosen methodology allows for answering the research questions.
- Evaluate how the authors presented the results.
- Assess whether the authors’ conclusions are grounded in findings and answer the original research questions.
Although you should analyze the article critically, it doesn’t mean you only should criticize it. If the authors did a good job designing and conducting their study, be sure to explain why you think their work is well done. Also, it is a great idea to provide examples from the article to support your analysis.
4. Conclude Your Analysis of Research Paper
A conclusion is your chance to reflect on the study’s relevance and importance. Explain how the analyzed paper can contribute to the existing knowledge or lead to future research. Also, you need to summarize your thoughts on the article as a whole. Avoid making value judgments — saying that the paper is “good” or “bad.” Instead, use more descriptive words and phrases such as “This paper effectively showed…”
Need help writing a compelling conclusion? Try our free essay conclusion generator !
5. Revise and Proofread
Last but not least, you should carefully proofread your paper to find any punctuation, grammar, and spelling mistakes. Start by reading your work out loud to ensure that your sentences fit together and sound cohesive. Also, it can be helpful to ask your professor or peer to read your work and highlight possible weaknesses or typos.
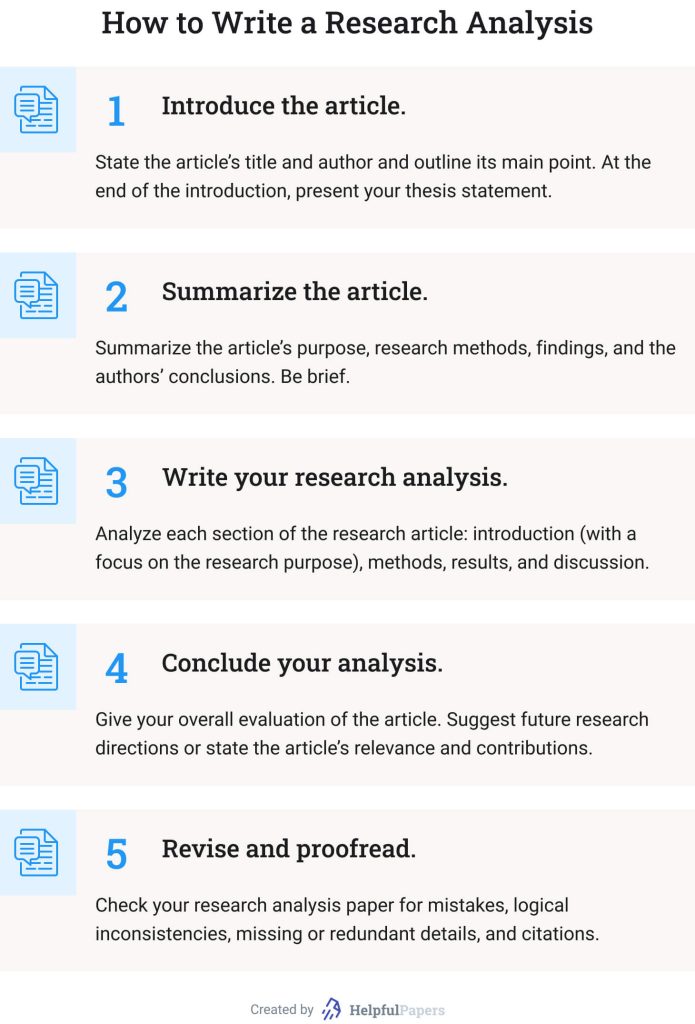
📝 Research Paper Analysis Example
We have prepared an analysis of a research paper example to show how everything works in practice.
No Homework Policy: Research Article Analysis Example
This paper aims to analyze the research article entitled “No Assignment: A Boon or a Bane?” by Cordova, Pagtulon-an, and Tan (2019). This study examined the effects of having and not having assignments on weekends on high school students’ performance and transmuted mean scores. This article effectively shows the value of homework for students, but larger studies are needed to support its findings.
Cordova et al. (2019) conducted a descriptive quantitative study using a sample of 115 Grade 11 students of the Central Mindanao University Laboratory High School in the Philippines. The sample was divided into two groups: the first received homework on weekends, while the second didn’t. The researchers compared students’ performance records made by teachers and found that students who received assignments performed better than their counterparts without homework.
The purpose of this study is highly relevant and justified as this research was conducted in response to the debates about the “No Homework Policy” in the Philippines. Although the descriptive research design used by the authors allows to answer the research question, the study could benefit from an experimental design. This way, the authors would have firm control over variables. Additionally, the study’s sample size was not large enough for the findings to be generalized to a larger population.
The study results are presented clearly, logically, and comprehensively and correspond to the research objectives. The researchers found that students’ mean grades decreased in the group without homework and increased in the group with homework. Based on these findings, the authors concluded that homework positively affected students’ performance. This conclusion is logical and grounded in data.
This research effectively showed the importance of homework for students’ performance. Yet, since the sample size was relatively small, larger studies are needed to ensure the authors’ conclusions can be generalized to a larger population.
🔎 More Research Analysis Paper Examples
Do you want another research analysis example? Check out the best analysis research paper samples below:
- Gracious Leadership Principles for Nurses: Article Analysis
- Effective Mental Health Interventions: Analysis of an Article
- Nursing Turnover: Article Analysis
- Nursing Practice Issue: Qualitative Research Article Analysis
- Quantitative Article Critique in Nursing
- LIVE Program: Quantitative Article Critique
- Evidence-Based Practice Beliefs and Implementation: Article Critique
- “Differential Effectiveness of Placebo Treatments”: Research Paper Analysis
- “Family-Based Childhood Obesity Prevention Interventions”: Analysis Research Paper Example
- “Childhood Obesity Risk in Overweight Mothers”: Article Analysis
- “Fostering Early Breast Cancer Detection” Article Analysis
- Lesson Planning for Diversity: Analysis of an Article
- Journal Article Review: Correlates of Physical Violence at School
- Space and the Atom: Article Analysis
- “Democracy and Collective Identity in the EU and the USA”: Article Analysis
- China’s Hegemonic Prospects: Article Review
- Article Analysis: Fear of Missing Out
- Article Analysis: “Perceptions of ADHD Among Diagnosed Children and Their Parents”
- Codependence, Narcissism, and Childhood Trauma: Analysis of the Article
- Relationship Between Work Intensity, Workaholism, Burnout, and MSC: Article Review
We hope that our article on research paper analysis has been helpful. If you liked it, please share this article with your friends!
- Analyzing Research Articles: A Guide for Readers and Writers | Sam Mathews
- Summary and Analysis of Scientific Research Articles | San José State University Writing Center
- Analyzing Scholarly Articles | Texas A&M University
- Article Analysis Assignment | University of Wisconsin-Madison
- How to Summarize a Research Article | University of Connecticut
- Critique/Review of Research Articles | University of Calgary
- Art of Reading a Journal Article: Methodically and Effectively | PubMed Central
- Write a Critical Review of a Scientific Journal Article | McLaughlin Library
- How to Read and Understand a Scientific Paper: A Guide for Non-scientists | LSE
- How to Analyze Journal Articles | Classroom
How to Write an Animal Testing Essay: Tips for Argumentative & Persuasive Papers
Descriptive essay topics: examples, outline, & more.
Purdue Online Writing Lab Purdue OWL® College of Liberal Arts
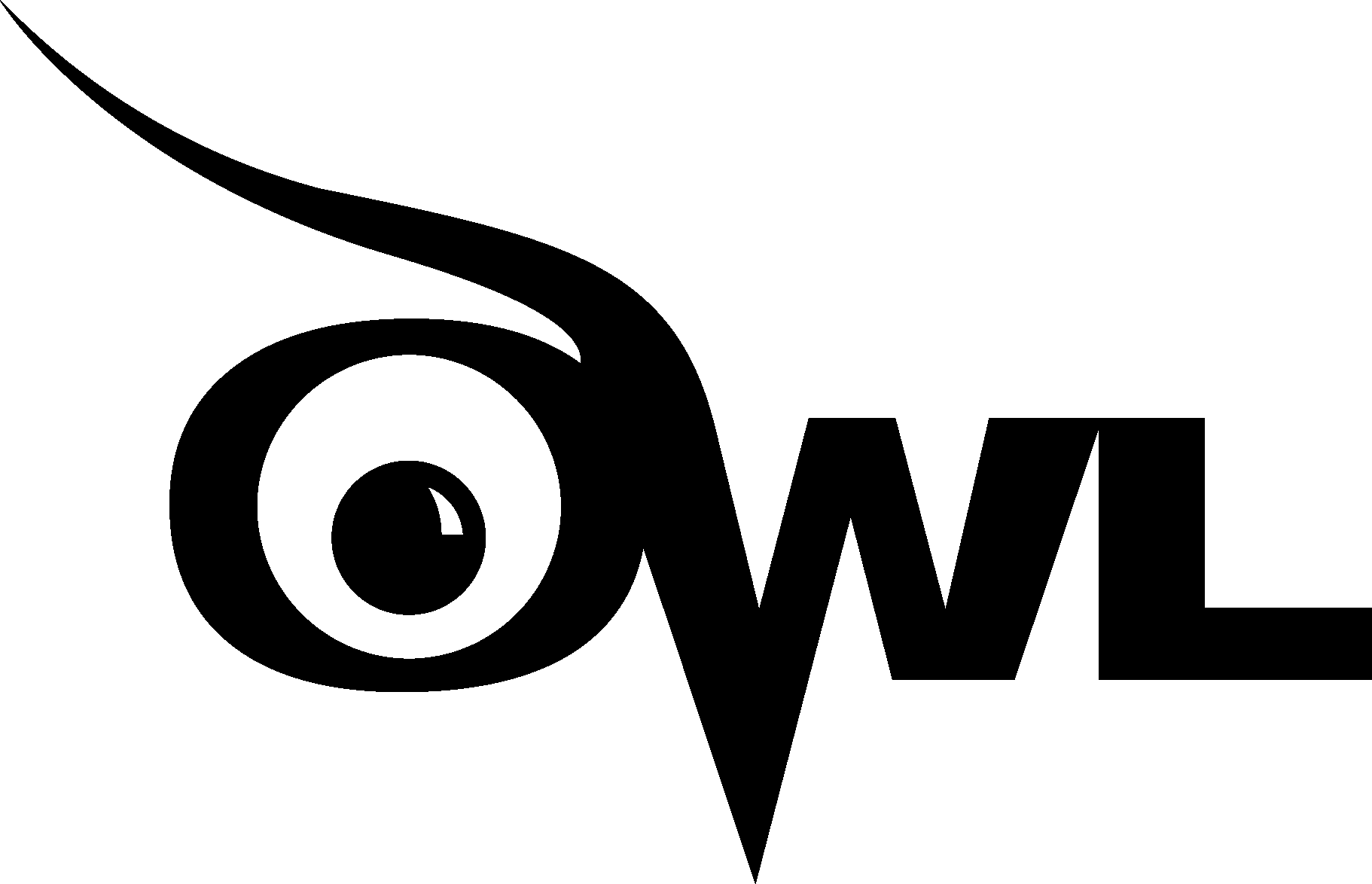
Welcome to the Purdue OWL
This page is brought to you by the OWL at Purdue University. When printing this page, you must include the entire legal notice.
Copyright ©1995-2018 by The Writing Lab & The OWL at Purdue and Purdue University. All rights reserved. This material may not be published, reproduced, broadcast, rewritten, or redistributed without permission. Use of this site constitutes acceptance of our terms and conditions of fair use.
Primary research involves collecting data about a given subject directly from the real world. This section includes information on what primary research is, how to get started, ethics involved with primary research and different types of research you can do. It includes details about interviews, surveys, observations, and analysis.
Analysis is a type of primary research that involves finding and interpreting patterns in data, classifying those patterns, and generalizing the results. It is useful when looking at actions, events, or occurrences in different texts, media, or publications. Analysis can usually be done without considering most of the ethical issues discussed in the overview, as you are not working with people but rather publicly accessible documents. Analysis can be done on new documents or performed on raw data that you yourself have collected.
Here are several examples of analysis:
- Recording commercials on three major television networks and analyzing race and gender within the commercials to discover some conclusion.
- Analyzing the historical trends in public laws by looking at the records at a local courthouse.
- Analyzing topics of discussion in chat rooms for patterns based on gender and age.
Analysis research involves several steps:
- Finding and collecting documents.
- Specifying criteria or patterns that you are looking for.
- Analyzing documents for patterns, noting number of occurrences or other factors.

An official website of the United States government
The .gov means it’s official. Federal government websites often end in .gov or .mil. Before sharing sensitive information, make sure you’re on a federal government site.
The site is secure. The https:// ensures that you are connecting to the official website and that any information you provide is encrypted and transmitted securely.
- Publications
- Account settings
Preview improvements coming to the PMC website in October 2024. Learn More or Try it out now .
- Advanced Search
- Journal List
- Indian J Anaesth
- v.60(9); 2016 Sep
Basic statistical tools in research and data analysis
Zulfiqar ali.
Department of Anaesthesiology, Division of Neuroanaesthesiology, Sheri Kashmir Institute of Medical Sciences, Soura, Srinagar, Jammu and Kashmir, India
S Bala Bhaskar
1 Department of Anaesthesiology and Critical Care, Vijayanagar Institute of Medical Sciences, Bellary, Karnataka, India
Statistical methods involved in carrying out a study include planning, designing, collecting data, analysing, drawing meaningful interpretation and reporting of the research findings. The statistical analysis gives meaning to the meaningless numbers, thereby breathing life into a lifeless data. The results and inferences are precise only if proper statistical tests are used. This article will try to acquaint the reader with the basic research tools that are utilised while conducting various studies. The article covers a brief outline of the variables, an understanding of quantitative and qualitative variables and the measures of central tendency. An idea of the sample size estimation, power analysis and the statistical errors is given. Finally, there is a summary of parametric and non-parametric tests used for data analysis.
INTRODUCTION
Statistics is a branch of science that deals with the collection, organisation, analysis of data and drawing of inferences from the samples to the whole population.[ 1 ] This requires a proper design of the study, an appropriate selection of the study sample and choice of a suitable statistical test. An adequate knowledge of statistics is necessary for proper designing of an epidemiological study or a clinical trial. Improper statistical methods may result in erroneous conclusions which may lead to unethical practice.[ 2 ]
Variable is a characteristic that varies from one individual member of population to another individual.[ 3 ] Variables such as height and weight are measured by some type of scale, convey quantitative information and are called as quantitative variables. Sex and eye colour give qualitative information and are called as qualitative variables[ 3 ] [ Figure 1 ].

Classification of variables
Quantitative variables
Quantitative or numerical data are subdivided into discrete and continuous measurements. Discrete numerical data are recorded as a whole number such as 0, 1, 2, 3,… (integer), whereas continuous data can assume any value. Observations that can be counted constitute the discrete data and observations that can be measured constitute the continuous data. Examples of discrete data are number of episodes of respiratory arrests or the number of re-intubations in an intensive care unit. Similarly, examples of continuous data are the serial serum glucose levels, partial pressure of oxygen in arterial blood and the oesophageal temperature.
A hierarchical scale of increasing precision can be used for observing and recording the data which is based on categorical, ordinal, interval and ratio scales [ Figure 1 ].
Categorical or nominal variables are unordered. The data are merely classified into categories and cannot be arranged in any particular order. If only two categories exist (as in gender male and female), it is called as a dichotomous (or binary) data. The various causes of re-intubation in an intensive care unit due to upper airway obstruction, impaired clearance of secretions, hypoxemia, hypercapnia, pulmonary oedema and neurological impairment are examples of categorical variables.
Ordinal variables have a clear ordering between the variables. However, the ordered data may not have equal intervals. Examples are the American Society of Anesthesiologists status or Richmond agitation-sedation scale.
Interval variables are similar to an ordinal variable, except that the intervals between the values of the interval variable are equally spaced. A good example of an interval scale is the Fahrenheit degree scale used to measure temperature. With the Fahrenheit scale, the difference between 70° and 75° is equal to the difference between 80° and 85°: The units of measurement are equal throughout the full range of the scale.
Ratio scales are similar to interval scales, in that equal differences between scale values have equal quantitative meaning. However, ratio scales also have a true zero point, which gives them an additional property. For example, the system of centimetres is an example of a ratio scale. There is a true zero point and the value of 0 cm means a complete absence of length. The thyromental distance of 6 cm in an adult may be twice that of a child in whom it may be 3 cm.
STATISTICS: DESCRIPTIVE AND INFERENTIAL STATISTICS
Descriptive statistics[ 4 ] try to describe the relationship between variables in a sample or population. Descriptive statistics provide a summary of data in the form of mean, median and mode. Inferential statistics[ 4 ] use a random sample of data taken from a population to describe and make inferences about the whole population. It is valuable when it is not possible to examine each member of an entire population. The examples if descriptive and inferential statistics are illustrated in Table 1 .
Example of descriptive and inferential statistics

Descriptive statistics
The extent to which the observations cluster around a central location is described by the central tendency and the spread towards the extremes is described by the degree of dispersion.
Measures of central tendency
The measures of central tendency are mean, median and mode.[ 6 ] Mean (or the arithmetic average) is the sum of all the scores divided by the number of scores. Mean may be influenced profoundly by the extreme variables. For example, the average stay of organophosphorus poisoning patients in ICU may be influenced by a single patient who stays in ICU for around 5 months because of septicaemia. The extreme values are called outliers. The formula for the mean is

where x = each observation and n = number of observations. Median[ 6 ] is defined as the middle of a distribution in a ranked data (with half of the variables in the sample above and half below the median value) while mode is the most frequently occurring variable in a distribution. Range defines the spread, or variability, of a sample.[ 7 ] It is described by the minimum and maximum values of the variables. If we rank the data and after ranking, group the observations into percentiles, we can get better information of the pattern of spread of the variables. In percentiles, we rank the observations into 100 equal parts. We can then describe 25%, 50%, 75% or any other percentile amount. The median is the 50 th percentile. The interquartile range will be the observations in the middle 50% of the observations about the median (25 th -75 th percentile). Variance[ 7 ] is a measure of how spread out is the distribution. It gives an indication of how close an individual observation clusters about the mean value. The variance of a population is defined by the following formula:

where σ 2 is the population variance, X is the population mean, X i is the i th element from the population and N is the number of elements in the population. The variance of a sample is defined by slightly different formula:

where s 2 is the sample variance, x is the sample mean, x i is the i th element from the sample and n is the number of elements in the sample. The formula for the variance of a population has the value ‘ n ’ as the denominator. The expression ‘ n −1’ is known as the degrees of freedom and is one less than the number of parameters. Each observation is free to vary, except the last one which must be a defined value. The variance is measured in squared units. To make the interpretation of the data simple and to retain the basic unit of observation, the square root of variance is used. The square root of the variance is the standard deviation (SD).[ 8 ] The SD of a population is defined by the following formula:

where σ is the population SD, X is the population mean, X i is the i th element from the population and N is the number of elements in the population. The SD of a sample is defined by slightly different formula:

where s is the sample SD, x is the sample mean, x i is the i th element from the sample and n is the number of elements in the sample. An example for calculation of variation and SD is illustrated in Table 2 .
Example of mean, variance, standard deviation

Normal distribution or Gaussian distribution
Most of the biological variables usually cluster around a central value, with symmetrical positive and negative deviations about this point.[ 1 ] The standard normal distribution curve is a symmetrical bell-shaped. In a normal distribution curve, about 68% of the scores are within 1 SD of the mean. Around 95% of the scores are within 2 SDs of the mean and 99% within 3 SDs of the mean [ Figure 2 ].

Normal distribution curve
Skewed distribution
It is a distribution with an asymmetry of the variables about its mean. In a negatively skewed distribution [ Figure 3 ], the mass of the distribution is concentrated on the right of Figure 1 . In a positively skewed distribution [ Figure 3 ], the mass of the distribution is concentrated on the left of the figure leading to a longer right tail.

Curves showing negatively skewed and positively skewed distribution
Inferential statistics
In inferential statistics, data are analysed from a sample to make inferences in the larger collection of the population. The purpose is to answer or test the hypotheses. A hypothesis (plural hypotheses) is a proposed explanation for a phenomenon. Hypothesis tests are thus procedures for making rational decisions about the reality of observed effects.
Probability is the measure of the likelihood that an event will occur. Probability is quantified as a number between 0 and 1 (where 0 indicates impossibility and 1 indicates certainty).
In inferential statistics, the term ‘null hypothesis’ ( H 0 ‘ H-naught ,’ ‘ H-null ’) denotes that there is no relationship (difference) between the population variables in question.[ 9 ]
Alternative hypothesis ( H 1 and H a ) denotes that a statement between the variables is expected to be true.[ 9 ]
The P value (or the calculated probability) is the probability of the event occurring by chance if the null hypothesis is true. The P value is a numerical between 0 and 1 and is interpreted by researchers in deciding whether to reject or retain the null hypothesis [ Table 3 ].
P values with interpretation

If P value is less than the arbitrarily chosen value (known as α or the significance level), the null hypothesis (H0) is rejected [ Table 4 ]. However, if null hypotheses (H0) is incorrectly rejected, this is known as a Type I error.[ 11 ] Further details regarding alpha error, beta error and sample size calculation and factors influencing them are dealt with in another section of this issue by Das S et al .[ 12 ]
Illustration for null hypothesis

PARAMETRIC AND NON-PARAMETRIC TESTS
Numerical data (quantitative variables) that are normally distributed are analysed with parametric tests.[ 13 ]
Two most basic prerequisites for parametric statistical analysis are:
- The assumption of normality which specifies that the means of the sample group are normally distributed
- The assumption of equal variance which specifies that the variances of the samples and of their corresponding population are equal.
However, if the distribution of the sample is skewed towards one side or the distribution is unknown due to the small sample size, non-parametric[ 14 ] statistical techniques are used. Non-parametric tests are used to analyse ordinal and categorical data.
Parametric tests
The parametric tests assume that the data are on a quantitative (numerical) scale, with a normal distribution of the underlying population. The samples have the same variance (homogeneity of variances). The samples are randomly drawn from the population, and the observations within a group are independent of each other. The commonly used parametric tests are the Student's t -test, analysis of variance (ANOVA) and repeated measures ANOVA.
Student's t -test
Student's t -test is used to test the null hypothesis that there is no difference between the means of the two groups. It is used in three circumstances:

where X = sample mean, u = population mean and SE = standard error of mean

where X 1 − X 2 is the difference between the means of the two groups and SE denotes the standard error of the difference.
- To test if the population means estimated by two dependent samples differ significantly (the paired t -test). A usual setting for paired t -test is when measurements are made on the same subjects before and after a treatment.
The formula for paired t -test is:

where d is the mean difference and SE denotes the standard error of this difference.
The group variances can be compared using the F -test. The F -test is the ratio of variances (var l/var 2). If F differs significantly from 1.0, then it is concluded that the group variances differ significantly.
Analysis of variance
The Student's t -test cannot be used for comparison of three or more groups. The purpose of ANOVA is to test if there is any significant difference between the means of two or more groups.
In ANOVA, we study two variances – (a) between-group variability and (b) within-group variability. The within-group variability (error variance) is the variation that cannot be accounted for in the study design. It is based on random differences present in our samples.
However, the between-group (or effect variance) is the result of our treatment. These two estimates of variances are compared using the F-test.
A simplified formula for the F statistic is:

where MS b is the mean squares between the groups and MS w is the mean squares within groups.
Repeated measures analysis of variance
As with ANOVA, repeated measures ANOVA analyses the equality of means of three or more groups. However, a repeated measure ANOVA is used when all variables of a sample are measured under different conditions or at different points in time.
As the variables are measured from a sample at different points of time, the measurement of the dependent variable is repeated. Using a standard ANOVA in this case is not appropriate because it fails to model the correlation between the repeated measures: The data violate the ANOVA assumption of independence. Hence, in the measurement of repeated dependent variables, repeated measures ANOVA should be used.
Non-parametric tests
When the assumptions of normality are not met, and the sample means are not normally, distributed parametric tests can lead to erroneous results. Non-parametric tests (distribution-free test) are used in such situation as they do not require the normality assumption.[ 15 ] Non-parametric tests may fail to detect a significant difference when compared with a parametric test. That is, they usually have less power.
As is done for the parametric tests, the test statistic is compared with known values for the sampling distribution of that statistic and the null hypothesis is accepted or rejected. The types of non-parametric analysis techniques and the corresponding parametric analysis techniques are delineated in Table 5 .
Analogue of parametric and non-parametric tests

Median test for one sample: The sign test and Wilcoxon's signed rank test
The sign test and Wilcoxon's signed rank test are used for median tests of one sample. These tests examine whether one instance of sample data is greater or smaller than the median reference value.
This test examines the hypothesis about the median θ0 of a population. It tests the null hypothesis H0 = θ0. When the observed value (Xi) is greater than the reference value (θ0), it is marked as+. If the observed value is smaller than the reference value, it is marked as − sign. If the observed value is equal to the reference value (θ0), it is eliminated from the sample.
If the null hypothesis is true, there will be an equal number of + signs and − signs.
The sign test ignores the actual values of the data and only uses + or − signs. Therefore, it is useful when it is difficult to measure the values.
Wilcoxon's signed rank test
There is a major limitation of sign test as we lose the quantitative information of the given data and merely use the + or – signs. Wilcoxon's signed rank test not only examines the observed values in comparison with θ0 but also takes into consideration the relative sizes, adding more statistical power to the test. As in the sign test, if there is an observed value that is equal to the reference value θ0, this observed value is eliminated from the sample.
Wilcoxon's rank sum test ranks all data points in order, calculates the rank sum of each sample and compares the difference in the rank sums.
Mann-Whitney test
It is used to test the null hypothesis that two samples have the same median or, alternatively, whether observations in one sample tend to be larger than observations in the other.
Mann–Whitney test compares all data (xi) belonging to the X group and all data (yi) belonging to the Y group and calculates the probability of xi being greater than yi: P (xi > yi). The null hypothesis states that P (xi > yi) = P (xi < yi) =1/2 while the alternative hypothesis states that P (xi > yi) ≠1/2.
Kolmogorov-Smirnov test
The two-sample Kolmogorov-Smirnov (KS) test was designed as a generic method to test whether two random samples are drawn from the same distribution. The null hypothesis of the KS test is that both distributions are identical. The statistic of the KS test is a distance between the two empirical distributions, computed as the maximum absolute difference between their cumulative curves.
Kruskal-Wallis test
The Kruskal–Wallis test is a non-parametric test to analyse the variance.[ 14 ] It analyses if there is any difference in the median values of three or more independent samples. The data values are ranked in an increasing order, and the rank sums calculated followed by calculation of the test statistic.
Jonckheere test
In contrast to Kruskal–Wallis test, in Jonckheere test, there is an a priori ordering that gives it a more statistical power than the Kruskal–Wallis test.[ 14 ]
Friedman test
The Friedman test is a non-parametric test for testing the difference between several related samples. The Friedman test is an alternative for repeated measures ANOVAs which is used when the same parameter has been measured under different conditions on the same subjects.[ 13 ]
Tests to analyse the categorical data
Chi-square test, Fischer's exact test and McNemar's test are used to analyse the categorical or nominal variables. The Chi-square test compares the frequencies and tests whether the observed data differ significantly from that of the expected data if there were no differences between groups (i.e., the null hypothesis). It is calculated by the sum of the squared difference between observed ( O ) and the expected ( E ) data (or the deviation, d ) divided by the expected data by the following formula:

A Yates correction factor is used when the sample size is small. Fischer's exact test is used to determine if there are non-random associations between two categorical variables. It does not assume random sampling, and instead of referring a calculated statistic to a sampling distribution, it calculates an exact probability. McNemar's test is used for paired nominal data. It is applied to 2 × 2 table with paired-dependent samples. It is used to determine whether the row and column frequencies are equal (that is, whether there is ‘marginal homogeneity’). The null hypothesis is that the paired proportions are equal. The Mantel-Haenszel Chi-square test is a multivariate test as it analyses multiple grouping variables. It stratifies according to the nominated confounding variables and identifies any that affects the primary outcome variable. If the outcome variable is dichotomous, then logistic regression is used.
SOFTWARES AVAILABLE FOR STATISTICS, SAMPLE SIZE CALCULATION AND POWER ANALYSIS
Numerous statistical software systems are available currently. The commonly used software systems are Statistical Package for the Social Sciences (SPSS – manufactured by IBM corporation), Statistical Analysis System ((SAS – developed by SAS Institute North Carolina, United States of America), R (designed by Ross Ihaka and Robert Gentleman from R core team), Minitab (developed by Minitab Inc), Stata (developed by StataCorp) and the MS Excel (developed by Microsoft).
There are a number of web resources which are related to statistical power analyses. A few are:
- StatPages.net – provides links to a number of online power calculators
- G-Power – provides a downloadable power analysis program that runs under DOS
- Power analysis for ANOVA designs an interactive site that calculates power or sample size needed to attain a given power for one effect in a factorial ANOVA design
- SPSS makes a program called SamplePower. It gives an output of a complete report on the computer screen which can be cut and paste into another document.
It is important that a researcher knows the concepts of the basic statistical methods used for conduct of a research study. This will help to conduct an appropriately well-designed study leading to valid and reliable results. Inappropriate use of statistical techniques may lead to faulty conclusions, inducing errors and undermining the significance of the article. Bad statistics may lead to bad research, and bad research may lead to unethical practice. Hence, an adequate knowledge of statistics and the appropriate use of statistical tests are important. An appropriate knowledge about the basic statistical methods will go a long way in improving the research designs and producing quality medical research which can be utilised for formulating the evidence-based guidelines.
Financial support and sponsorship
Conflicts of interest.
There are no conflicts of interest.
AI Index Report
Welcome to the seventh edition of the AI Index report. The 2024 Index is our most comprehensive to date and arrives at an important moment when AI’s influence on society has never been more pronounced. This year, we have broadened our scope to more extensively cover essential trends such as technical advancements in AI, public perceptions of the technology, and the geopolitical dynamics surrounding its development. Featuring more original data than ever before, this edition introduces new estimates on AI training costs, detailed analyses of the responsible AI landscape, and an entirely new chapter dedicated to AI’s impact on science and medicine.
Read the 2024 AI Index Report
The AI Index report tracks, collates, distills, and visualizes data related to artificial intelligence (AI). Our mission is to provide unbiased, rigorously vetted, broadly sourced data in order for policymakers, researchers, executives, journalists, and the general public to develop a more thorough and nuanced understanding of the complex field of AI.
The AI Index is recognized globally as one of the most credible and authoritative sources for data and insights on artificial intelligence. Previous editions have been cited in major newspapers, including the The New York Times, Bloomberg, and The Guardian, have amassed hundreds of academic citations, and been referenced by high-level policymakers in the United States, the United Kingdom, and the European Union, among other places. This year’s edition surpasses all previous ones in size, scale, and scope, reflecting the growing significance that AI is coming to hold in all of our lives.
Steering Committee Co-Directors
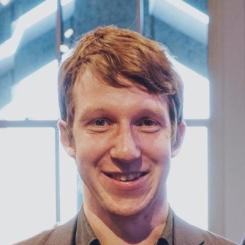
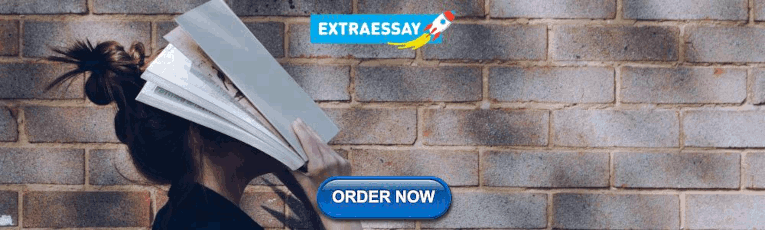
Ray Perrault
Steering committee members.
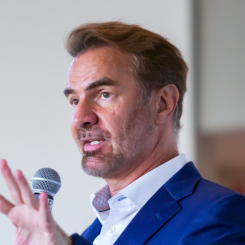
Erik Brynjolfsson
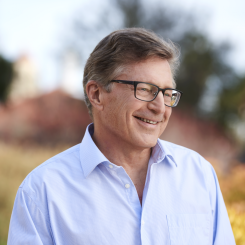
John Etchemendy
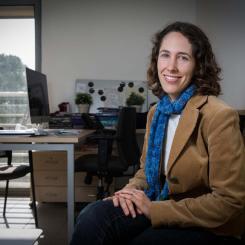
Katrina Ligett
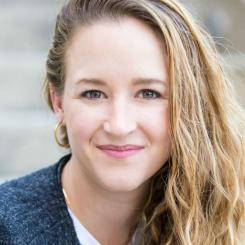
Terah Lyons
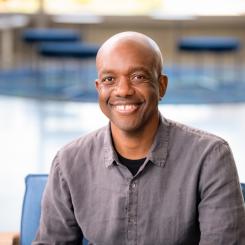
James Manyika
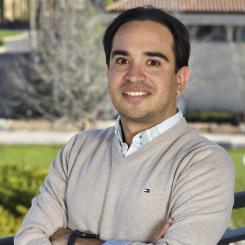
Juan Carlos Niebles
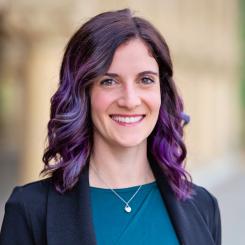
Vanessa Parli
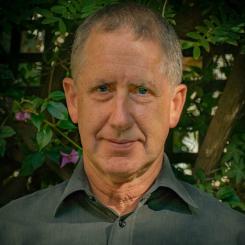
Yoav Shoham
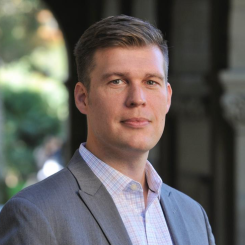
Russell Wald
Staff members.
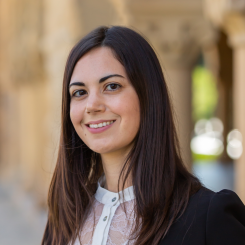
Loredana Fattorini
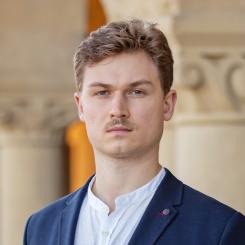
Nestor Maslej
Letter from the co-directors.
A decade ago, the best AI systems in the world were unable to classify objects in images at a human level. AI struggled with language comprehension and could not solve math problems. Today, AI systems routinely exceed human performance on standard benchmarks.
Progress accelerated in 2023. New state-of-the-art systems like GPT-4, Gemini, and Claude 3 are impressively multimodal: They can generate fluent text in dozens of languages, process audio, and even explain memes. As AI has improved, it has increasingly forced its way into our lives. Companies are racing to build AI-based products, and AI is increasingly being used by the general public. But current AI technology still has significant problems. It cannot reliably deal with facts, perform complex reasoning, or explain its conclusions.
AI faces two interrelated futures. First, technology continues to improve and is increasingly used, having major consequences for productivity and employment. It can be put to both good and bad uses. In the second future, the adoption of AI is constrained by the limitations of the technology. Regardless of which future unfolds, governments are increasingly concerned. They are stepping in to encourage the upside, such as funding university R&D and incentivizing private investment. Governments are also aiming to manage the potential downsides, such as impacts on employment, privacy concerns, misinformation, and intellectual property rights.
As AI rapidly evolves, the AI Index aims to help the AI community, policymakers, business leaders, journalists, and the general public navigate this complex landscape. It provides ongoing, objective snapshots tracking several key areas: technical progress in AI capabilities, the community and investments driving AI development and deployment, public opinion on current and potential future impacts, and policy measures taken to stimulate AI innovation while managing its risks and challenges. By comprehensively monitoring the AI ecosystem, the Index serves as an important resource for understanding this transformative technological force.
On the technical front, this year’s AI Index reports that the number of new large language models released worldwide in 2023 doubled over the previous year. Two-thirds were open-source, but the highest-performing models came from industry players with closed systems. Gemini Ultra became the first LLM to reach human-level performance on the Massive Multitask Language Understanding (MMLU) benchmark; performance on the benchmark has improved by 15 percentage points since last year. Additionally, GPT-4 achieved an impressive 0.97 mean win rate score on the comprehensive Holistic Evaluation of Language Models (HELM) benchmark, which includes MMLU among other evaluations.
Although global private investment in AI decreased for the second consecutive year, investment in generative AI skyrocketed. More Fortune 500 earnings calls mentioned AI than ever before, and new studies show that AI tangibly boosts worker productivity. On the policymaking front, global mentions of AI in legislative proceedings have never been higher. U.S. regulators passed more AI-related regulations in 2023 than ever before. Still, many expressed concerns about AI’s ability to generate deepfakes and impact elections. The public became more aware of AI, and studies suggest that they responded with nervousness.
Ray Perrault Co-director, AI Index
Our Supporting Partners
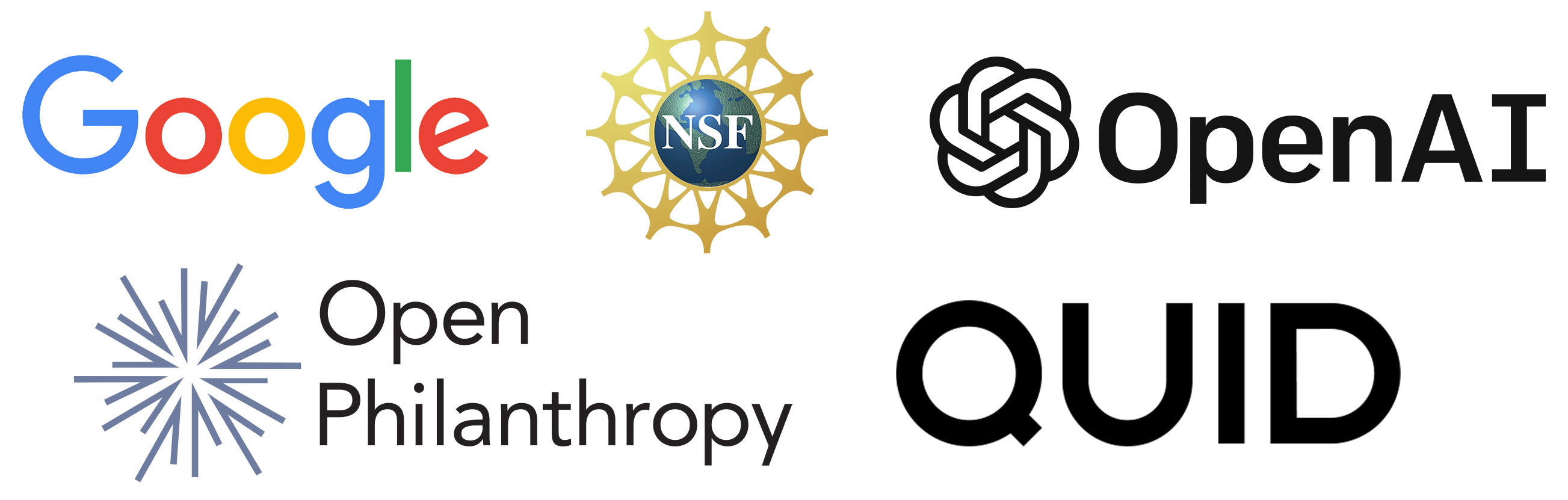
Analytics & Research Partners
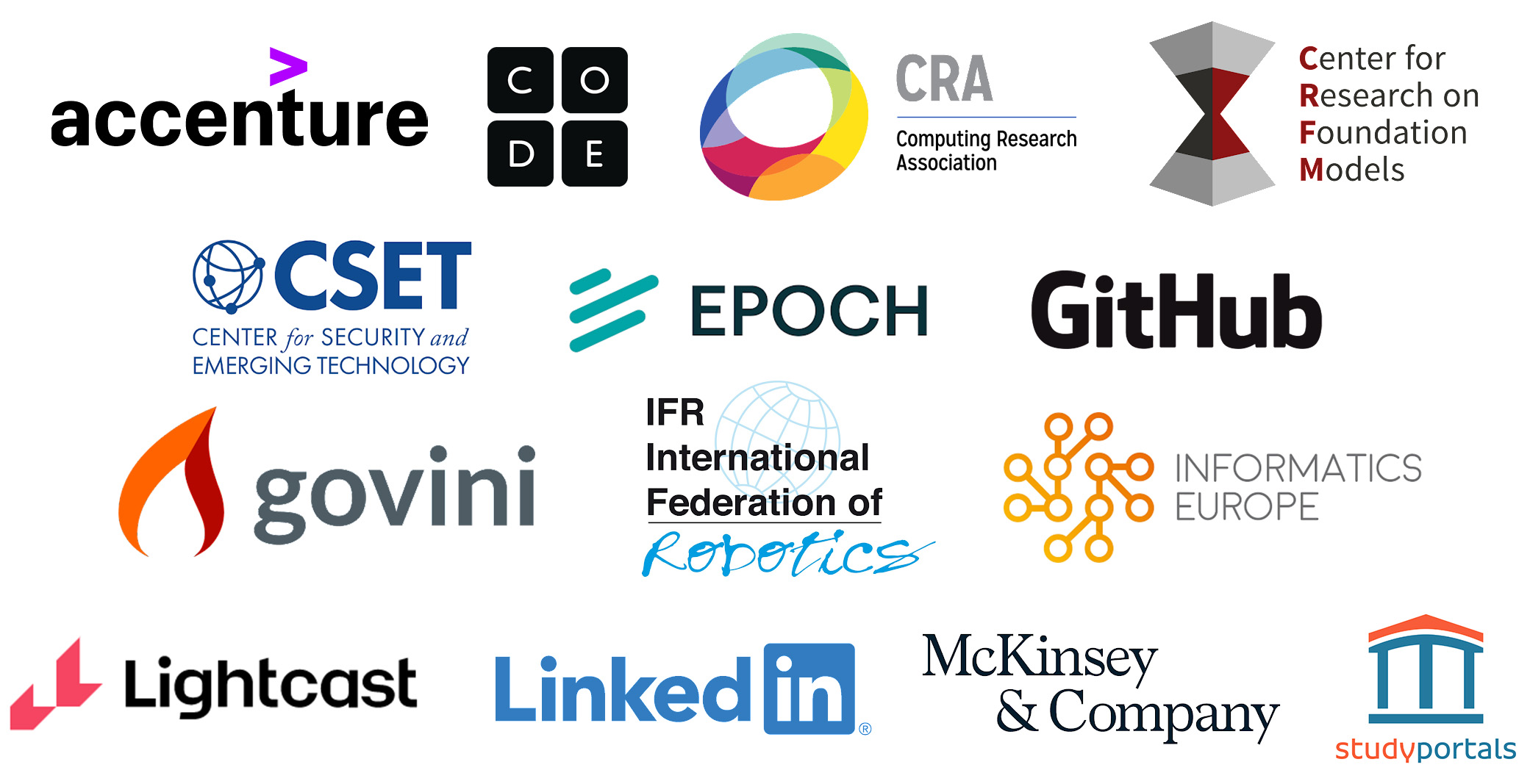
Stay up to date on the AI Index by subscribing to the Stanford HAI newsletter.
Thank you for visiting nature.com. You are using a browser version with limited support for CSS. To obtain the best experience, we recommend you use a more up to date browser (or turn off compatibility mode in Internet Explorer). In the meantime, to ensure continued support, we are displaying the site without styles and JavaScript.
- View all journals
- My Account Login
- Explore content
- About the journal
- Publish with us
- Sign up for alerts
- Open access
- Published: 13 April 2024
Psycholinguistic and emotion analysis of cryptocurrency discourse on X platform
- Moein Shahiki Tash 1 ,
- Olga Kolesnikova nAff1 ,
- Zahra Ahani 1 &
- Grigori Sidorov 1
Scientific Reports volume 14 , Article number: 8585 ( 2024 ) Cite this article
212 Accesses
5 Altmetric
Metrics details
- Computational science
- Computer science
- Human behaviour
- Scientific data
This paper provides an extensive examination of a sizable dataset of English tweets focusing on nine widely recognized cryptocurrencies, specifically Cardano, Binance, Bitcoin, Dogecoin, Ethereum, Fantom, Matic, Shiba, and Ripple. Our goal was to conduct a psycholinguistic and emotional analysis of social media content associated with these cryptocurrencies. Such analysis can enable researchers and experts dealing with cryptocurrencies to make more informed decisions. Our work involved comparing linguistic characteristics across the diverse digital coins, shedding light on the distinctive linguistic patterns emerging in each coin’s community. To achieve this, we utilized advanced text analysis techniques. Additionally, this work unveiled an understanding of the interplay between these digital assets. By examining which coin pairs are mentioned together most frequently in the dataset, we established co-mentions among different cryptocurrencies. To ensure the reliability of our findings, we initially gathered a total of 832,559 tweets from X. These tweets underwent a rigorous preprocessing stage, resulting in a refined dataset of 115,899 tweets that were used for our analysis. Overall, our research offers valuable perception into the linguistic nuances of various digital coins’ online communities and provides a deeper understanding of their interactions in the cryptocurrency space.
Similar content being viewed by others
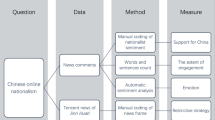
Chinese online nationalism as imaginary engagement: an automated sentiment analysis of Tencent news comments on the 2012 Diaoyu (Senkaku) Islands incident
Qiaoqi Zhang & Cheng-Jun Wang
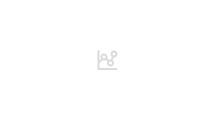
Ideological asymmetries in online hostility, intimidation, obscenity, and prejudice
Vivienne Badaan, Mark Hoffarth, … John T. Jost
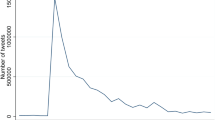
Earthquake conspiracy discussion on Twitter
Dmitry Erokhin & Nadejda Komendantova
Introduction
How people employ words daily can reveal a wealth of information about their beliefs, fears, thought processes, social connections, and personal characteristics 1 . Nowadays, online social media platforms significantly affect human life, and people freely pen their thoughts on social networks 2 . Furthermore, the extensive use of social media platforms has been instrumental in spreading awareness about groundbreaking projects. The proliferation of digital technologies has been facilitated by the process of globalization 3 . Owing to cryptocurrencies’ digital character, wide-ranging conversations occur in online forums and on social media platforms, including X (formerly known as Twitter) and Facebook. These platforms serve as significant determinants of the prevailing sentiment among the general public regarding cryptocurrencies and to a certain extent, influence their market valuations 4 , 5 . Notably, these networks are home to a huge user base, encompassing billions of individuals and a vast, intricate web of interconnected relationships among them 6 .
On January 29, 2021, Elon Musk, the world’s wealthiest individual at that time 7 , took a surprising step by adding the hashtag #bitcoin to his X bio. This unexpected move triggered an outpouring of excitement and prompted a surge in cryptocurrency enthusiasts rushing to buy Bitcoin. Remarkably, this seemingly minor action had a significant impact, quickly driving the price of Bitcoin from around $32,000 to over $38,000, ultimately leading to a remarkable increase of $111 billion in the cryptocurrency’s market capitalization 8 .
The majority of data produced on social networks is unstructured, making it challenging to quantify. As a result, it is typically analyzed using various characteristic features 9 . In the above instance, we observed a significant role that social media plays in shaping the cryptocurrency market.
Prior research in the field of cryptocurrency and blockchain technology has explored a wide range of subjects and methodologies. For instance, scholars have utilized natural language processing (NLP) techniques to analyze various aspects such as miner extractable value (MEV) in social media discussions 10 . Similarly, others developed strategies for maximizing wealth through Initial Coin Offerings (ICOs) in blockchain ventures 11 . Additionally, there have been endeavors to predict cryptocurrency prices and investigate the societal ramifications of these emerging technologies in contemporary business environments 12 . However, very few studies have addressed the psycholinguistics and emotions associated with the discourse on cryptocurrencies in social media. To contribute to these existing avenues of inquiry, our study aims to bridge a significant gap in the literature. Specifically, we intend to conduct psycholinguistic and emotion analyses, alongside with assessing the readability of cryptocurrency comments on social platforms, with NLP methods. By adopting this innovative approach, we seek to get more knowledge of the psychological and emotional dimensions in cryptocurrency discourse, which have thus far received limited attention in scholarly research. Our objective in this endeavor is twofold: to enhance awareness among newcomers in digital marketing to prevent misguided investments, and to offer support to traders who rely on metrics such as the fear and greed index in their trading strategies.
We analyzed nine distinct digital coins using psycholinguistic methods to assist cryptocurrency enthusiasts. The cryptocurrencies examined in this paper encompass Bitcoin 13 , Ethereum 14 , Ripple 15 , Binance 16 , Dogecoin 17 , Shiba 18 , Fantom 19 , Matic 20 , and Cardano 21 . Psycholinguistics is the examination of how linguistic elements and psychological aspects are interconnected. It is important to emphasize that we did not consider user-specific characteristics; our primary focus was solely on textual data. To clarify, we utilized psycholinguistic attributes that often convey the underlying meaning communicated by text. The text analysis we conducted comprises the following categories:
1. LIWC (Linguistic Inquiry and Word Count) 22
2. Sentiment analysis 23
3. Emotion analysis 24
4. Assessment of readability 25 , 26 , 27 , 28
Concerning the features we used for our computerized text analysis, first, we employed subcategories of LIWC 29 . We utilized only a selection of such subcategories, including Analytical Thinking, Clout, Drives, Affect, Money, Hope, Attention, Netspeak, and Filler. This internal dictionary encompasses an extensive compilation of more than 12,000 words, word stems, phrases, and specific emoticons. Each dictionary entry is associated with one or more categories, or subdictionaries, strategically designed to evaluate a wide range of psychosocial constructs 22 .
Investors typically initiate an assessment of public sentiment surrounding a particular cryptocurrency before making investment decisions 30 . Consequently, sentiment and emotion analysis in cryptocurrency markets has gained significant prominence 31 . Research indicates that tweets expressing positive sentiments can exert a substantial influence on cryptocurrency demand, and conversely, negative sentiments can have a similar effect 32 , 33 .
Readability refers to the level of ease with which a piece of writing can be understood or comprehended, primarily influenced by its writing style and presentation 34 . Readability not only relates to how easily a text can be understood with respect to its writing style but also takes into account how well readers comprehend it, read it at an appropriate speed, and find it engaging 35 .
Moreover, we went a step further by investigating the “reasons and significance” aspect. In simpler terms, we sought to determine which characteristics among the aforementioned four hold more importance for novice investors. To accomplish this, we explored the following research questions.
RQ1: Do psycholinguistic characteristics vary among digital coins?
RQ2: What are the dominant feelings expressed by X users regarding the cryptocurrencies under study?
RQ3: Does the readability level of tweets exhibit uniformity across all selected digital currencies?
RQ4: Is there any co-mention among different cryptocurrencies?
To address these research inquiries, we analyzed tweets related to nine distinct cryptocurrencies. We conducted psycholinguistic investigations and emotion analysis to respond to RQ1, RQ2, and RQ3 and extracted the above categories of features from the dataset, including LIWC, Readability, Sentiment, and Emotions analysis. To answer RQ4, we established a co-mention among different cryptocurrency coins, identifying which two coins tend to be mentioned together more frequently.
Related work
A cryptocurrency is a form of digital currency designed for use as a means of exchange. It relies on robust cryptographic techniques to secure financial transactions, regulate the creation of additional units, and validate asset transfers 36 . Because of their substantial market values, cryptocurrencies have gained considerable interest, with some individuals regarding them as legitimate currencies and others as attractive investment prospects 33 .
Sentiment and emotion analysis
Aslam et al. 37 focused on sentiment analysis and emotion detection in cryptocurrency-related tweets collected using specific hashtags such as ’#cryptocurrency’, ’#cryptomarket’, and ’#Bitcoin’, amassing a total of 40,000 tweets. The authors employed traditional feature extraction methods like Bag-of-Words (BoW), TF-IDF, and Word2Vec, along with machine learning models including Random Forest (RF), Decision Tree (DT), k-Nearest Neighbors (KNN), Support Vector Machine (SVM), Gaussian Naive Bayes (GNB), and Logistic Regression (LR). Additionally, they leveraged advanced deep learning techniques, specifically a combination of Convolutional Neural Networks (CNN) and Long Short-Term Memory (LSTM) networks, to classify tweet sentiments as positive, negative, or neutral. Notably, they introduced an ensemble model that merges LSTM and Gated Recurrent Unit (GRU) 38 , 39 , achieving remarkable accuracy scores of 99% for sentiment analysis and 92% for emotion prediction.
Research in Ibrahim et al. 40 centered on predicting early market movements of Bitcoin by harnessing sentiment analysis of X data 41 , 42 . The primary objective of their work was to introduce a Composite Ensemble Prediction Model (CEPM) built upon sentiment analysis. They employed a combination of data mining techniques, machine learning algorithms, and natural language processing to decipher public sentiment and mood states pertaining to cryptocurrencies. The research evaluated various models, such as Logistic Regression, Binary Classified Vector Prediction, Support Vector Machine, Naïve Bayes, and a single XGBoost 43 for sentiment analysis. The remarkable point to be highlighted is the CEPM’s outperformance of other approaches, demonstrating its effectiveness in forecasting early Bitcoin market movements via the analysis of sentiment in X data.
Shahzad et al. 44 presented a framework for performing sentiment analysis on X data with the aim of predicting the future price of Bitcoin. They highlighted the significance of NLP in bridging the gap between human communication and digital data and emphasized the growing importance of sentiment analysis in the field. The authors utilized three artificial intelligence tools, namely, LR, LSTM, and Deep Neural Network (DNN) Regressor to evaluate their performance in predicting Bitcoin prices. The best performance was demonstrated by the LSTM model.
Rahman et al. 45 explored the usage of various natural language processing models for sentiment analysis in the context of cryptocurrency and financial market prediction. They used a dataset of approximately 100,000 news items, including tweets and Reddit posts, gathered from 77 public X timelines and Reddit subreddits over a six-month period from July to December 2021. The study also examined the creation of ensemble models, encompassing all 22 selected models as well as a subset of the top three models labeled as “ensemble (all) “ and “ensemble (top 3) “, which included Aigents+, Aigents, and FinBERT. The “ensemble (top 3)” method exhibited a higher degree of correlation with other models compared to the rest.
Huang et al. 46 collected a substantial dataset comprising 24,000 cryptocurrency-related tweets and 70,000 comments from Sina-Weibo using specific keywords. The study adopted a methodology that utilized a training dataset consisting of posts from the top 100 crypto investor’s accounts on Sina-Weibo over the most recent seven days, while the subsequent day’s posts served as testing data. Remarkably, their sentiment analysis approach based on LSTM surpassed the time series auto-regression (AR) method by 87.0% in precision and 92.5% in recall.
The authors of 47 aimed to detect sentiment and emotion in X posts and utilized this information for recommendations. They used a dataset containing tweets and user data and manually annotated 7,246 tweets and replies. Their approach involved text preprocessing and applying a Naïve Bayes classifier with cross-validation. The findings demonstrated that analyzing the entire text provided superior accuracy compared to focusing on specific words (NAVA). Moreover, as the number of cross-validation folds increased, the accuracy showed improvement. Specifically, in the realm of emotion analysis, the Naïve Bayes classifier achieved an accuracy of 47.34%. Furthermore, in sentiment analysis, Naïve Bayes outperformed other classifiers significantly, attaining an accuracy of 66.86%.
The researchers in 48 utilized the AIT-2018 dataset 49 to construct a model for detecting emotions expressed in tweets. The dataset of tweets was acquired through the X API by extracting tweets containing emotion-related hashtags such as ’#angry’, ’#annoyed’, ’#panic’, ’#happy’, ’#love’, and ’#surprised’. The proposed model integrated lexical-based approaches, employing emotion lexicons like WordNet-Affect and EmoSenticNet, along with supervised classifiers to autonomously classify multi-class emotions from the dataset. The authors conducted experiments employing three machine learning classifiers: Naïve Bayes, DT, and SVM. Their findings demonstrated that when filtering tweets using EmoSenticNet words, the precision in detecting emotions significantly improved. Specifically, the SVM classifier achieved a high precision rate of 89.28% in the Anger class, surpassing previous results obtained using logistic regression.
Psycholinguistic analysis
Psycholinguistics utilizes various methods to comprehend language in the context of psychological processes. These methods encompass observational research, analysis, experimental studies, and the application of neuroimaging techniques 50 . Researchers also make use of text analysis models to interpret findings related to the language system. This section explores the methodologies employed by researchers in this field. Butt et al. 29 presented a comprehensive analysis of the psycholinguistic aspects of rumors on online social media (OSM). Using the PHEME dataset 51 , which encompasses nine breaking news events, the researchers examined source tweets (rumor and non-rumor) and response tweets. They integrated various psycholinguistic features, including LIWC, SenticNet 52 , readability indices, and emotions to uncover user behavior patterns. Rumor source tweets were found to be characterized by language related to the past, prepositions, and motivations associated with reward, risk, and power. In contrast, non-rumor source tweets exhibited affective and cognitive processes, present-oriented language, and motivations linked to affiliation and achievement. Emotional analysis revealed that non-rumor tweets tended towards neutrality, while rumor-source tweets evoked fear and grief, subsequently prompting anger and fear in reactions.
Narman et al. 53 reported an analysis of Reddit comments employing seven readability techniques to discern the education levels of users interested in eight cryptocurrencies. The data collection process involved gathering comments data from subreddits of eight cryptocurrencies by selecting ten to seventy top posts for each coin to collect distinct usernames. For education level information, they used Reddit.com to gather and categorize the collected comments data. The analysis was performed using seven text readability techniques. Interestingly, the results indicate that a majority, approximately 60%, possess an education level equivalent to middle school, with 30% at the high school level, while the remaining 10% span other educational levels.
The researches in 54 aimed to assess the readability of tweets for English language learners. Their task involved collecting a dataset of 14,659 tweets and obtaining readability judgments from participants representing different language groups. For methodology, they analyzed various linguistic and content-related factors in the tweets, including emojis, hashtags, mentions, and links, as well as traditional readability measures like Flesch Reading Ease and Dale-Chall scores. The results revealed that demographic factors, such as language proficiency and education, were stronger predictors of tweet readability than any other single feature.
The proposal in 55 included a framework to analyze linguistic features and cultural distinctions in climate-related tweets from the UK and Nigeria. A dataset of 81,507 English-language tweets was collected, comprising 44,071 from the UK and 37,436 from Nigeria. The study combined transformer networks with linguistic feature analysis, including the application of the (LIWC-22) software 56 ,version 15.0, to address small dataset limitations and identify cultural differences 22 . Findings reveal that Nigerians tend to use more leadership language and informal words in climate change discussions on X, emphasizing the urgency of the issue. In contrast, UK discourse on climate change is characterized by more formality, logic, and longer words per sentence. The study also confirmed the geographical attribution of tweets using DistilBERT 55 , achieving an 83% accuracy rate.
This section provides a detailed overview of the data acquisition processes we employed. We clarify the exact steps undertaken during preprocessing and explore the complexities of conducting co-mention analyses among various cryptocurrency coins.
Data collection
The data collection process commenced with the acquisition of X data pertaining to nine popular cryptocurrencies: Cardano, Bitcoin, Binance, Dogecoin, Ethereum, Fantom, Matic, Shiba, and Ripple 57 . These specific cryptocurrencies were selected for inclusion in the dataset due to their widespread usage across various research studies conducted by different scholars 18 , 19 , 20 , 58 , 59 . This endeavor yielded a substantial dataset comprising 832,559 tweets spanning from September 2021 to March 2023. After undergoing essential preprocessing steps, the dataset available for analysis was refined, resulting in a curated dataset consisting of 115,899 tweets. Table 1 presents dataset statistics both before and after preprocessing. Additionally, it lists the names of the coins and their respective symbols, which we utilized as keywords for extracting tweets from X. This extraction process was conducted separately for each coin, using both the name and the symbol as search criteria.
Data preprocessing
The utilization of the Tweepy 60 API was instrumental in our tweet data collection procedure, as it empowered us to filter tweets according to diverse criteria, including date, location, language, and various tweet attributes, for example, the number of retweets. In the final phase, we focused exclusively on English-language tweets, excluding unnecessary fields such as ’username’, ’id’, ’date’, ’likeCount’, and ’retweetCount’ retaining only the actual tweet content. After obtaining the dataset, we conducted a multi-step data preprocessing procedure to refine and enhance the data. This procedure involved the following key steps:
URL Removal: We applied a regular expression pattern to identify and subsequently remove any URLs. Text Cleaning: This step included the removal of special characters, such as punctuation marks, with the assistance of a designated dictionary of special characters. Additionally, we excluded words that had a length less than or equal to two characters. The result was a cleaned version of the text data.
Data labeling
In the process of data labeling, we examined each tweet systematically, with the primary objective of identifying any references to the selected cryptocurrencies. Notably, the search encompassed both “Bitcoin” and “Btc” in a case-insensitive manner, with any discovery leading to the classification of the tweet as Btc. This procedure was iteratively applied to all cryptocurrencies listed in Table 1 , encompassing both their complete nomenclature and associated abbreviations.
Further, we encountered instances where tweets discussed multiple cryptocurrencies simultaneously which was uncovered as co-mention among these cryptocurrencies. The results of this co-occurrence analysis are considered in Section 3.4. To tackle this challenge effectively, a comprehensive set encompassing the names of all pertinent cryptocurrencies was devised. For instance, to annotate tweets as of Bitcoin, tweets mentioning any other cryptocurrency present in this predefined set were systematically excluded. The set itself comprised a roster of cryptocurrency names, notably including “Cardano”, “Ada”, “Fantom”, “Ftm”, “Matic”, “Shiba”, “Shib”, “Dogecoin”, “Doge”, “Ripple”,“Xrp”, “Ethereum”, “Eth”, “Binance” and, “Bnb“.
Subsequently, the inclusion of both Bitcoin and Btc into this enumerated list facilitated the resolution of similar issues encountered with other cryptocurrencies, with the same process being replicated across each cryptocurrency to ensure comprehensive data labeling. As an example, in the dataset, a tweet was identified as featuring the keyword Matic. The content of the tweet is provided below: APompliano Good day sir, I have 100$ to invest in a coin right, small but what I can afford for now. So, Im thinking It I should rather go for $ada $matic, $doge What do you suggest fam.
This tweet was acquired using the keyword Matic, and the keywords to be examined for the Matic coin included:’Cardano’, ’Ada’, ’Fantom’, ’Ftm’, ’Bitcoin’, ’Btc’, ’Shiba’, ’Shib’, ’Dogecoin’, ’Doge’, ’Ripple’, ’Xrp’, ’Ethereum’, ’Eth’, ’Binance’ and, ’Bnb’. The exclusion criterion described above ensured that if any of these keywords were present in a tweet, except for the keywords related to the specific coin for which we used keywords to extract tweets, that tweet should be removed. In our example, it’s evident that the tweet contains both ’ada’ and ’doge’ keywords, indicating that it should be removed.
Figure 1 illustrates the processing steps for a tweet.
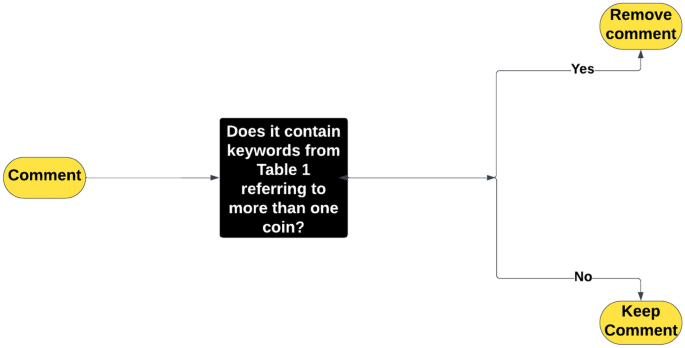
Example tweet processing.
Cryptocurrencies co-mention
During the labeling process, we examined co-mention and co-occurrence among various cryptocurrencies in tweets. Such analysis resulted in an interesting observation: multiple cryptocurrencies often co-occurred in the same tweets, indicating a significant level of co-mention, which led us to reconsider our labeling model, as previously detailed.
In this section, we explore specific, noteworthy co-mentions among cryptocurrency coins. These co-mentions provide valuable information for our investigation, enhancing our understanding of the relationships and trends emerging in the cryptocurrency ecosystem as reflected in social media discourse. The co-mention matrix provided in Table 2 serves as a tool for assessing the relationships between different cryptocurrencies, particularly concerning their trends and market dynamics, as opposed to a sole focus on price movements. An illustrative example lies in the substantial positive co-mention of Bitcoin (Btc) and Ethereum (Eth) in 53.52% of tweets. This significant co-occurrence indicates that when Bitcoin undergoes an upward trend, or garners increased market attention, Ethereum frequently follows suit. This co-occurrence can be attributed to the prominent positions both cryptocurrencies occupy in the market, as well as to their substantial influence on overall market sentiment.
In contrast, co-mention values nearing 0% in Table 2 signify a lack of substantial co-occurrence among cryptocurrencies. This absence of mention underscores the potential for diversification strategies for designing a cryptocurrency portfolio. Cryptocurrencies exhibiting low or negative co-mention can be strategically employed to diversify a portfolio, potentially resulting in reduced overall portfolio risk. Conversely, cryptocurrencies demonstrating high positive co-mention may offer limited diversification benefits as they tend to move in sync with one another.
Inside the domain of cryptocurrency portfolio management and risk mitigation, these co-mention observations underscore the critical importance of accurate asset selection and allocation, especially in light of the observed co-mention among cryptocurrencies. Such strategic decision-making becomes paramount in achieving diversified and risk-optimized cryptocurrency portfolios.
In this research, we examined cryptocurrency data, concentrating on a specific group of cryptocurrencies. Our choice of these particular coins was driven by their significant popularity among users, as well as the limited availability of substantial data for other coins. To interpret the data, we applied four analytical methods explained in section " Introduction ". Here we present the outcomes of our analysis for each of the aforementioned cryptocurrencies. The selection of features was made considering their past influence 29 , 61 . In the analysis conducted, LIWC assessments were applied to nine cryptocurrencies, resulting in an extensive collection of nine distinct analyses. We selected values that were highly informative for extracting linguistic interpretations relevant to cryptocurrencies. Our choice was made to capture key aspects of sentiment, linguistic style, and thematic content pertinent to discussions around cryptocurrencies. By narrowing down our focus to these particular features, we aimed to mine information from the psychological and linguistic dimensions of cryptocurrency discourse, thus aligning analysis with our goals. these categories encompass analytical thinking (metric of logical, formal thinking), clout (language of leadership), drives (related to personal motivations and psychological desires), affect (linguistic expressions associated with emotional and affective states expressed by a given text), money (refers to a set of linguistic cues or indicators related to financial terms, wealth, and economic aspects, Want (a human ability that allows individuals to envision future events with flexibility), attention (crucial subset of the “Perception” category), netspeak (represents a subset of the conversational category) and filler (non-essential sounds, words, or phrases, commonly used in speech to fill in pauses and maintain the flow of conversation without altering its meaning). In the drives and affect categories, additional features will be elaborated upon in the following discussion. Our examination indicated that Fantom attracts a larger number of tweets centered on technical aspects and holds a higher level of trust in comparison to other cryptocurrencies. For Binance, our observations revealed that the tweets predominantly revolve around themes of affiliation, achievements, and the pursuit of power and wealth. This pattern in discussions on Binance suggests a focus on notable accomplishments and financial success, indicative of a unique narrative and sentiment surrounding the coin. For Matic, the tweets primarily center around emotional impact compared to other cryptocurrencies. This emphasis on affective responses suggests that the coin is particularly influenced by emotional novelty. This distinctive characteristic could be considered a contributing factor to the fluctuations in the coin’s price, as emotional sentiment plays a significant role in shaping market dynamics and investor behavior. Our analysis revealed that Dogecoin exhibits a higher prevalence of netspeak, the informal language commonly used on the internet, compared to other cryptocurrencies. Conversely, Ethereum appears to attract more attention relative to other coins. This distinction suggests that Dogecoin is characterized by a more casual and internet-centric communication style, while Ethereum stands out for its ability to capture increased Attention and interest. A deeper understanding of the communication dynamics and community sentiment surrounding different coins may aid investors in making more informed choices, aligning their investment strategies with the unique qualities and trends associated with each cryptocurrency. From an emotional perspective, most cryptocurrencies exhibit a generally moderate and harmonious emotional profile. Notably, there is a distinct focus on the emotional category of Anticipation, with Dogecoin taking the forefront in this aspect. In this context, Anticipation likely signifies the expectation or excitement surrounding the future prospects, developments, or events associated with these cryptocurrencies.The outcomes of our analysis are presented in Table 5 . In terms of readability, the analysis revealed that Dogecoin’s tweets are relatively more challenging to read and comprehend, as indicated by lower scores on the Flesch Reading Ease measure. The Flesch-Kincaid and Dale-Chall Measures suggest an average reading difficulty level akin to content tailored for college graduates. Conversely, Ethereum’s tweets, as per the Gunning Fog Index, demand a higher level of reading proficiency, indicating a more complex and advanced readability suitable for individuals with a college-level education and vocabulary. To explore additional results, refer to Figs. 5 and 6 s, as well as Table 6 .
The LIWC model revolutionized psychological research by making the analysis of language data more robust, accessible, and scientifically rigorous than ever before. LIWC-22 examines over 100 textual dimensions, all of which have undergone validation by esteemed research institutions globally. With over 20,000 scientific publications utilizing LIWC, it has become a widely recognized and trusted tool in the field 62 giving way to novel approaches in analysis 63 , 64 . Although LIWC provides several benefits, it has its limitations. One drawback is its dependence on predefined linguistic categories, which might not encompass nuances and variations present in natural language. Furthermore, LIWC may encounter challenges in accurately deciphering sarcasm, irony, and other subtle forms of language usage, potentially resulting in text misinterpretation.
To effectively convey the outcomes of our analysis, average values among all the tweets were computed for each of LIWC categories. Averages can help identify broadscale sentiment trends over time. By tracking changes in average scores across key linguistic categories, such as sentiment, emotion, or cognitive processes, one can observe shifts in user sentiment and attitudes towards cryptocurrencies, market developments, or external events. Therefore, the average was calculated by summing up the scores of all comments related to each coin for each LIWC feature and then dividing by the total number of comments for that coin. These computed averages provide information along the linguistic and psychological dimensions intertwined with the selected digital currencies. A comprehensive presentation of these average values for each category can be found in Table 3 .
Analytical thinking and clout
Analytical Thinking, when showing high numerical values, signifies a formal, logical, and hierarchical thought process. Conversely, lower numbers suggest a more informal, personal, present-focused, and narrative style of thinking 65 . The values of this category computed for tweets related to cryptocurrency, reach their highest average score of 67.76 in texts mentioning Fantom. This fact indicates that, on average, discussions in this domain exhibit a relatively high level of logical and formal thinking. Conversely, the lowest average score of 52.00 was found for Ripple, which might suggest that discussions concerning this particular cryptocurrency place slightly less emphasis on logical and analytical thinking compared to the cryptocurrency domain’s average.
Clout is one of the four summary variables in LIWC designed to assess the degree of confidence and certainty conveyed in the text 66 , 67 . Our analysis revealed that the cryptocurrency Fantom exhibits a relatively high Clout score, with an average result of 70.91. This suggests that discussions and conversations related to Fantom often convey a strong sense of confidence and certainty. This high Clout score may also indicate a substantial degree of assurance in Fantom stability. In contrast, the cryptocurrency Ripple demonstrates a comparatively lower Clout score with an average result of 43.39. Figure 2 presents a comparative evaluation of Analytical Thinking and Clout scores across different cryptocurrencies. This suggests that discussions related to Ripple may not consistently display the same level of confidence and certainty found in the Fantom discussions. In essence, when Fantom demonstrates higher Clout values, it signifies that the users who composed the tweets are expressing increased confidence. This, in turn, leads us to infer a heightened level of knowledge on their part. In both analyses, we observed that Fantom consistently had the highest scores, indicating a higher level of analytical thinking and confidence in discussions related to it. Conversely, Ripple consistently had the lowest scores in both categories, suggesting a relatively lower emphasis on analytical thinking and a lower degree of expressed confidence in discussions related to it. While these observations suggest a correlation between analytical thinking and confidence in these specific cryptocurrency discussions, it’s important to note that correlation does not imply causation. Other factors, such as market conditions, community sentiment, and news events, can also influence these results. For example, when we examined Binance, we foound that it ranks as the second-highest in terms of Analytical Thinking scores among the various cryptocurrencies. However, when we assess it as the position in the Clout category, Binance ranks fifth. The results of Analytical Thinking and Clout analysis related to digital currencies can be viewed in Table 3 .
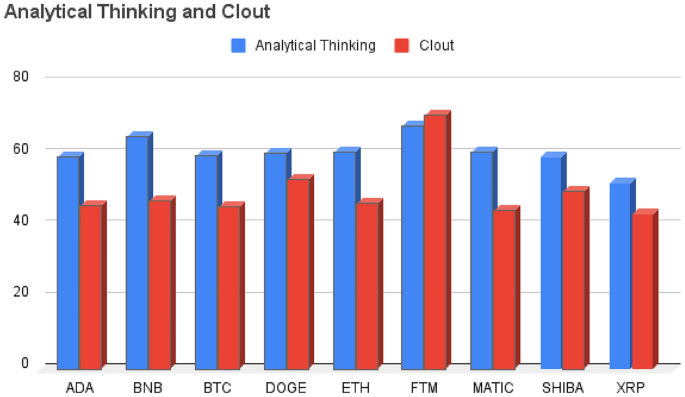
Comparative evaluation of analytical thinking and clout scores across different cryptocurrencies.
Drives and affect
Drives is a comprehensive dimension that encapsulates various needs and motives 65 . In our LIWC analysis, we concentrated on the Drives, particularly examining the aspects of Affiliation, Achievement, and Power. We observed that the presence of Affiliation-related language (such as “us” and “help“) is comparatively lower in discussions related to Cardano, while it appears more frequently in conversations about Dogecoin. Similarly, in terms of Achievement-related language (including “work”, “better”, and “best“), Dogecoin tends to have fewer instances compared to Matic. Furthermore, when examining Power-related language (like “allow” and “power“), we found that Dogecoin exhibits a lower frequency, while Bitcoin discussions tend to feature a greater occurrence of such language. These patterns highlight variations in linguistic expressions across different cryptocurrencies, shedding light on the distinctive characteristics of discussions over different digital coins. Upon closer examination, it became evident that tweets originating from Binance sources tended to include a higher frequency of words associated with Drives, whereas Fantom source tweets had a notably lower occurrence of Drives-related words. Additional details can be found in Fig. 3 .
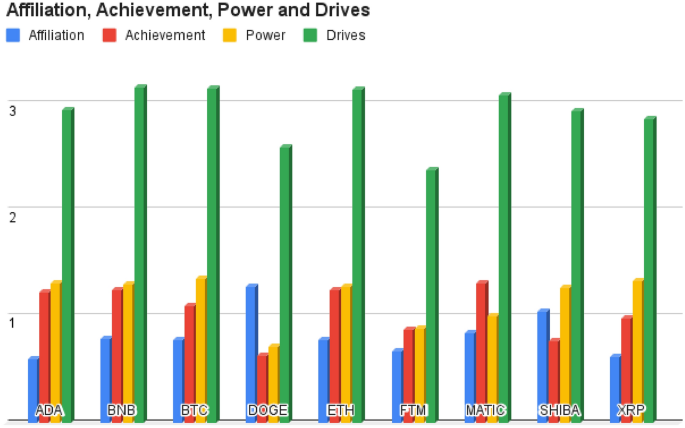
Frequency of language associated with affiliation, achievement, power, and drives across different cryptocurrency discussions.
In the Affect 1 subset, our analysis encompassed various emotional dimensions, including Positive Emotion, Negative Emotion, Anxiety, Anger, Sadness, and Swear Words. In the upcoming Emotion section, we delve deeper into affective analysis. However, in this preliminary report, we provide an overview of the affective processes observed in the LIWC analysis. It can be observed in Table 3 that there is a variation in affective (good, well, new, love) content among different cryptocurrencies. Notably, Matic coin exhibits a higher level of affective language, while Ada appears to have a lower level. This distinction becomes clearer when we explore the affective subcategories including Positive tone (new, love), Negative tone (bad, wrong, too much, hate), Emotion (good, love, happy, hope), and Swear words (shit, fuckin*, fuck, damn), as depicted in Fig. 4 . It becomes evident that Matic coin scores higher in Positive tone and Emotion, while Bitcoin registers a higher Negative tone. Additionally, Ripple stands out with a higher score in Swear words, indicating potential user dissatisfaction. When we further break down the Emotion category into its subsets, which encompass Anxiety (worry, fear, afraid, nervous), Anger (hate, mad, angry, frustr), and Sadness (sad, disappoint, cry), we notice that Dogecoin exhibits a higher score in Anxiety, Ripple in Anger, while most of the nine analyzed coins show similar values for Sadness. These observations contribute to our analysis and highlight the varying affective language usage across different cryptocurrencies, which we explore in greater detail in the subsequent Emotion section.
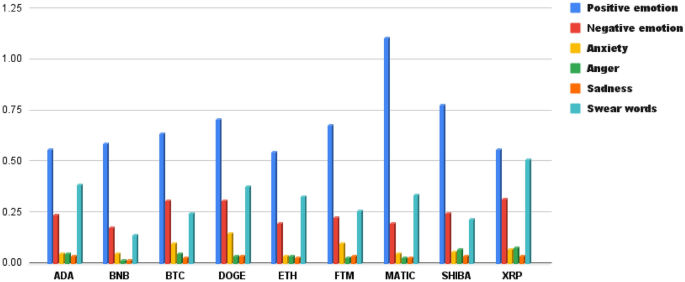
Comparative analysis of affective language dimensions-positive tone, negative tone, emotion, and swear words-across different cryptocurrencies.
Want words signify the author’s desires or preferences. Typically, wants are philosophically differentiated from needs by conceptualizing needs as innate and essential for survival, while wants are learned and generally linked to additional satisfaction beyond basic necessities 68 . What is important for cryptocurrency analysis in this category is the aspect of hope (want, hope, wanted, wish) as Want, or Hope, is a remarkable human ability that allows individuals to envision future events and their potential outcomes with flexibility 69 . Many users have high hopes for the future of cryptocurrency, anticipating greater benefits from their investments. From Table 3 , it becomes evident that Shiba is the cryptocurrency that garners most hope among users. The range of hope scores falls between 0.19 and 0.41, with the lowest level of hope associated with Fantom. This data suggests that Shiba is particularly promising in the eyes of cryptocurrency enthusiasts, while Fantom elicits comparatively less optimism.
Another important LIWC category is Money (business, pay, price, and market) 22 . The range of Money scores, from 2.46 for Shiba to 10.51 for Binance, indicates varying degrees of discussion or emphasis on cryptocurrency financial aspects. Notably, Binance stands out with the highest score, suggesting a significant emphasis on business and financial aspects in discussions related to this coin. Conversely, Shiba has the lowest score, indicating relatively less emphasis on these financial terms in conversations related to it. These findings offer a glimpse into the importance placed on financial and business-related aspects and potentially shed light on the perception and use of the cryptocurrencies in the broader context of market and economy.
At the dawn of experimental psychology, William James wrote that everyone knows what attention is. It is the taking possession by the mind, in a clear and vivid manner 70 . When users include the term Attention in their tweets, it signifies their intention to draw focus to a significant event or topic. Upon reviewing Table 3 , it becomes evident that Ethereum tweets receive more attention than tweets about the other cryptocurrencies, indicating a heightened interest or emphasis on Ethereum-related matters. Conversely, tweets concerning Dogecoin appear to attract less attention when compared to tweets about the other coins, suggesting a relatively lower level of interest or engagement in discussions related to it. For Shiba, our observations indicate a prevalent sense of hope and an increased use of filler words compared to the other cryptocurrencies. This heightened expression of hope suggests a more optimistic sentiment surrounding Shiba when contrasted with the other coins. Additionally, the frequent use of filler words, including expressions like “wow”, “sooo”, and “youknow” signifies a more conversational and engaged discourse. This linguistic pattern may reflect a greater level of enthusiasm and interaction among Shiba enthusiasts.
Netspeak and filler
This analysis includes words commonly used in social media and text messaging, such as “bae”, “lol” and basic punctuation-based emoticons like “:)” and “;)” 65 , 71 . This mode of communication is widely employed by netizens during computer-mediated communication (CMC). In the context of cryptocurrency discussions, which predominantly transpire on online forums, social media platforms, and chat groups, it is customary for participants to incorporate netspeak into their interactions. Through the analysis of netspeak, researchers can understand more the degree of user engagement and interaction. Notably, the adoption of terms such as “HODL” (a deliberate misspelling of “hold”, indicating a long-term investment strategy) or “moon” (indicating an expectation of significant price increases) serves as meaningful pointers to user sentiment and active participation in discussions. In the obtained results, Matic stands out prominently with a notably high netspeak score, signaling the prevalence of internet-specific expressions and informal language related to it. The results can be found in Table 3 . Fillers (wow, sooo, youknow) are non-essential sounds, words, or phrases, such as “well”, “erm” or “hmm” commonly used in speech to occupy pauses and maintain the flow of conversation without altering its meaning 65 , 72 , 73 . The filler analysis results highlight that Shiba and Dogecoin exhibit higher scores in this category compared to the other cryptocurrencies, with scores ranging between 0.02 and 0.04 for the remaining coins, as depicted in Table 3 . In the sentiment analysis, it’s clear that Fantom distinguishes itself with a notably elevated positive score in comparison to the other cryptocurrencies. A consistently positive sentiment can enhance investor confidence, attract new stakeholders, and contribute to a more favorable market perception. Table 3 presents the remaining outcomes for the other cryptocurrencies.
Sentiment and emotions analysis
Table 4 provides a detailed sentiment analysis, encompassing positive, neutral, and negative percentages for various digital coins. In the world of cryptocurrency investments, it’s common for investors to assess public sentiment before making their decisions, as highlighted in prior research 30 . Consequently, sentiment analysis has gained substantial importance on cryptocurrency markets 74 . Studies have shown that tweets expressing positive emotions wield substantial influence over cryptocurrency demand, while negative sentiments can have the opposite effect 32 , 33 .
Analyzing the data in Table 4 , it becomes apparent that Fantom distinguishes itself by displaying a notably higher positive sentiment percentage in comparison to its digital counterparts, which strongly suggests an elevated degree of interest and enthusiasm among investors towards this digital coin.
Examining opinions involves another aspect known as emotion detection. In contrast to sentiment, which can be positive, negative, or neutral, emotions offer richer categorization over personality traits by revealing experiences of joy, anger, and more. Automated methods for emotion detection have been developed to enhance the analysis of individual sentiments. The primary goal of emotion analysis is to identify the specific words or sentences conveying emotions 75 . To achieve such analysis, we employed the NRCLex library to extract and categorize emotions from text 24 . NRCLex is a Python library designed for natural language processing and sentiment analysis. The acronym stands for “Natural Resources Canada Lexicon”, and it is particularly focused on assessing sentiment in text based on word associations. NRCLex is built upon a lexicon that assigns sentiment scores to words, allowing users to analyze the sentiment of individual words, sentences, or entire documents 76 . Table 5 provides the outcomes of our emotion analysis, revealing a narrow range of results for various emotions: Anger (0.02-0.04), Surprise (0.01-0.02), Sadness (0.01-0.03), Disgust (0.01-0.02), and Joy (0.02-0.04). These consistent findings suggest that most of the coins evoke similar emotional responses, highlighting their emotional proximity.
In contrast, when it comes to emotions such as Fear and Trust, there are more noticeable differences between the coins. For instance, when examining the sentiment of Cardano, the fear score is 0.0324, while the trust score is higher at 0.1252. Similarly, for Ripple, the fear score is 0.0416, with a trust score of 0.1172. The scores provide a difference in the emotional tones associated with these cryptocurrencies, indicating the levels of fear and trust expressed in the analyzed content.
Furthermore, the emotion of Anticipation stands out with higher scores in tweets, indicating that many users are keen on anticipating the future of these coins. Notably, Dogecoin (0.3752) and Shiba (0.3467) generate more anticipation among users when compared to the other coins.
Readability
In this section, we pay attention to the readability of data, utilizing metrics such as the Flesch Reading Ease 25 , Flesch-Kincaid Grade Level 26 , Gunning Fog Index 27 , and Dale-Chall Readability Score 28 . Assessing readability helps distinguish between text that is straightforward to grasp and text that is complex and demands a high level of education or intelligence to comprehend. Numerous readability metrics exist for text evaluation, and we have chosen to employ the above four measures as the most widely recognized tests to assess tweets.
Table 6 presents the significant differences in readability scores across tweets related to nine different digital coins.
The Flesch Reading Ease score provides an indication of how easily a text can be understood, with higher scores indicating greater readability. Flesch Reading Ease score can be observed in Fig. 5 . The Flesch-Kincaid Grade Level is a metric that estimates the educational grade level required to understand a piece of text based on factors like sentence length and word complexity. Analyzing the readability scores for the tweets related to each digital coin shows the linguistic complexity employed in discussions surrounding these coins. The presence of significant differences in readability scores suggests variations in the accessibility and comprehension levels required to engage with these tweets. Negative scores in some readability metrics, such as the Flesch Reading Ease and Flesch-Kincaid Grade Level, indicate higher levels of complexity, while positive scores indicate greater ease of comprehension. Refer to Fig. 6 for the necessary details to assess the readability levels of the specified analyses (Flesch-Kincaid Grade Level, Gunning Fog Index, Dale-Chall Readability Score). Table 6 provides evidence on the fact that Dogecoin possesses a notably lower score in Flesch Reading Ease compared to the other cryptocurrencies, which suggests that the communication pertaining to Dogecoin might present hurdles in accessibility and comprehension for the typical reader. Getting rid of such readability obstacles have the potential to amplify the effectiveness of communication, expand audience involvement, and cultivate heightened comprehension and acceptance of cryptocurrencies among varied stakeholders. This observation aligns with Fig. 5 77 , where we notice a pronounced level of complexity in comprehending tweets related to Dogecoin. To gain a better understanding of the varied readability levels, it’s essential to consider both Fig. 5 78 , 79 and Table 6 . When examining the Flesch-Kincaid Grade Level and Dale-Chall Readability in Table 6 , Dogecoin emerges with higher values compared to the other cryptocurrencies, signifying an average grade level and a college reading level, respectively. Furthermore, an examination of the results pertaining to the Gunning Fog Index, as depicted in Table 6 and Fig. 6 , reveals that Ethereum stands out with a higher score. This observation implies that understanding tweets related to Ethereum requires a reading comprehension level equivalent to a college education.
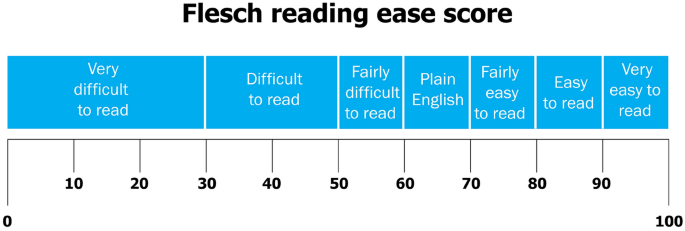
Flesch reading ease score.
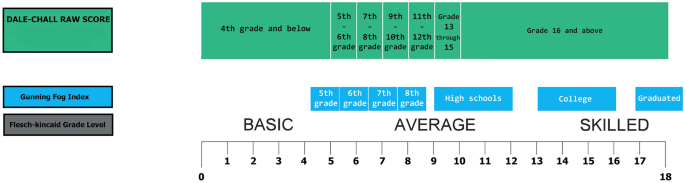
Dale-Chall Readability Score, Gunning Fog Index, Flesch-Kincaid Grade Level.
In the process of labeling our data, we identified a notable co-mention among various cryptocurrencies. We resolved this issue by excluding tweets that mentioned more than one coin or used abbreviations for coins not relevant to our research. Consequently, we focused our analysis on the specific set of cryptocurrencies pertinent to our study.
During the utilization of the LIWC software in our analysis, it became apparent that not all its components contributed substantively to the objectives of our research. In light of this, we exercised judicious discernment in the curation of our selection, focusing exclusively on those specific LIWC analyses that bore direct relevance to the concerns and interests of researchers, investors, and individuals engaged in the realm of digital marketing. Additionally, we imposed constraints on our scrutiny of emotional aspects, as certain LIWC framework components exhibited redundancy with one another.
When comparing the Analytical Thinking and Clout aspects to other LIWC features, we could see that these two scores are higher across all coins. This suggests that the tweets generally lean toward logical and formal thought. Notably, among all the coins, Fantom has the highest scores in these aspects, indicating that discussions regarding it are particularly characterized by logical and formal thinking compared to the other cryptocurrencies. After conducting a LIWC analysis, it became apparent that the highest scores were associated with two features that we considered above with concepts such as money, personal drives, and emotional affect. Furthermore, concerning the category of money and personal drives, Binance displayed notably higher scores compared to the other cryptocurrencies. Notably, Matic exhibited significantly higher levels of emotional affect when compared to the other cryptocurrencies. In contrast, other features such as Hope, Attention, Netspeak, and Filler exhibited remarkably low scores, nearly hovering around one percent, when compared to the features we previously discussed. This suggests that the tweets of users are primarily centered on analytical thinking, clout, personal drives, emotional affect, and financial matters. In the sentiment analysis, Fantom stands out with a higher positive score when compared to the other cryptocurrencies. On the contrary, Ripple registers a significantly elevated negative score. This information suggests that Fantom is generating a higher level of positive sentiment, possibly due to positive news, community sentiment, or price performance, while Ripple is experiencing more negative sentiment, which could be linked to negative news or market sentiment surrounding the coin. Emotionally, the majority of cryptocurrencies displayed a relatively modest and well-balanced emotional profile. Interestingly, there was an emphasis on the emotional category of Anticipation, and in this aspect, Dogecoin took the lead. Anticipation in this context likely refers to the expectation or excitement surrounding the future prospects, developments, or events related to these cryptocurrencies. The reason could be upcoming upgrades, partnerships, or any other factors that create a sense of anticipation among the cryptocurrency community. Regarding readability as assessed by Flesch Reading Ease, Dogecoin’s tweets scored lower on this measure. This implies that the content related to Dogecoin is relatively more difficult to read and comprehend, as its language and sentence structure are complex. Concerning Flesch-Kincaid and Dale-Chall measures, Dogecoin’s tweets received higher scores on these measures, indicating that the reading difficulty is at an average level, similar to what one might expect from a college graduate. While the Flesch-Kincaid measure estimates the U.S. grade level needed to understand the text, the Dale-Chall measure also assesses reading difficulty and is often used as a more accurate indicator for texts aimed at older audiences. Speaking of the Gunning Fog Index, Ethereum’s content, on the other hand, registered higher scores on this measure, implying a need for college-level reading proficiency. This means that content related to Ethereum is more challenging to read and understand, requiring a higher level of education and vocabulary.
Limitations
One significant challenge encountered during the data collection phase revolved around sentences containing references to multiple cryptocurrencies. Deciphering the intended cryptocurrency from such sentences posed a considerable complexity, leading to inaccurate analysis for each coin. Therefore, these data were excluded for a more precise analysis of psycholinguistics and emotions for each coin. Additionally, the sheer volume of data presented logistical hurdles, rendering manual labeling impractical in terms of both time and financial resources.
Moreover, the dynamic nature of the cryptocurrency landscape poses another limitation, as sudden events or developments can influence user comments and sentiments, leading to shifts in behavior and sentiment. For instance, specific events like the collapse of Terra Luna or Celsius in 2022 80 , 81 , led to significant market price decreases. Despite efforts to mitigate these impacts through regular monitoring and updates, the inherent volatility of the cryptocurrency market presents challenges in maintaining the consistency and relevance of the dataset over time.
These limitations underscore the necessity for a cautious interpretation of the study’s findings. Future research endeavors in this domain should strive to address such methodological challenges through enhanced data collection techniques and strategies tailored to the dynamic nature of cryptocurrency discourse.
Conclusions and future work
This paper presents a substantial dataset of English tweets related to cryptocurrencies, which were labeled using cryptocurrency keywords and abbreviations (e.g., ADA for Cardano, Ftm for Fantom, Matic for Matic, Btc for Bitcoin, Shib for Shiba, Dogecoin for Doge, Xrp for Ripple, Eth for Ethereum, and Bnb for Binance). Initially, we collected 832,559 tweets, which were reduced to 115,899 tweets after preprocessing. These tweets span from September 2021 to March 2023 and pertain to nine digital coins, namely Cardano, Bitcoin, Binance, Dogecoin, Ethereum, Fantom, Matic, Shiba, and Ripple.
This study conducted psycholinguistic and sentiment analyses on this dataset, utilizing tools such as LIWC, Emotion, Sentiment, and Readability analysis. To avoid LIWC framework redundancy, constraints were applied to the examination of emotional aspects. Our analysis revealed distinct linguistic characteristics and sentiment patterns associated with various cryptocurrencies.
Our investigation into the psycholinguistic characteristics of digital coins showed notable variations among different cryptocurrencies. Through detailed analysis of tweets related to nine distinct digital currencies, we discerned prevalent sentiments expressed by users, assessed the consistency of readability levels across various coins, and identified co-mention between different cryptocurrencies. Leveraging techniques such as psycholinguistic investigations, emotion analysis, and co-mention studies, we obtained valuable estimation of users’ perceptions and interactions.
In a broader context, our study revealed significant psycholinguistic differences among cryptocurrency data. We observed variations in sentiment and emotion analyses, as well as disparities in the readability levels associated with different cryptocurrencies. In future research, we aim to diversify our analysis techniques to delve deeper into the psychological aspects of cryptocurrency discourse. Specifically, we plan to explore sentiments of hope 69 , 82 and regret 83 in textual data using various Natural Language Processing (NLP) methodologies. Additionally, we intend to leverage Large Language Models (LLMs) to conduct psycholinguistic analyses, with an expectation to a deeper analysis of underlying linguistic patterns and emotional tones present in cryptocurrency discussions. Furthermore, our future work will involve classification algorithms with diverse machine learning approaches to distinguish bullish and bearish sentiments in comments, utilizing manual labeling for training data.
Data availability
The datasets generated and/or analysed during the current study are available in the GitHub repository, https://github.com/moeintash72/cryptocurrency-data- .
Pennebaker, J. W., Boyd, R. L., Jordan, K. & Blackburn, K. The development and psychometric properties of liwc2015 (Tech Rep, 2015).
Balouchzahi, F., Sidorov, G. & Shashirekha, H. L. Fake news spreaders profiling using n-grams of various types and shap-based feature selection. J. Intell. Fuzzy Syst. 42 , 4437–4448 (2022).
Article Google Scholar
Blanco-González-Tejero, C., Cano-Marin, E., Ulrich, K. & Giralt-Escobar, S. Leveraging blockchain for industry funding: A social media analysis. Sustain. Technol. Entrep. 3 , 100071 (2024).
Google Scholar
Barber, S., Boyen, X., Shi, E. & Uzun, E. Bitter to better-how to make bitcoin a better currency. In Financial Cryptography and Data Security: 16th International Conference, FC 2012, Kralendijk, Bonaire, Februray 27–March 2, 2012, Revised Selected Papers 16 , 399–414 (Springer, 2012).
Reid, F. & Harrigan, M. An Analysis of Anonymity in the Bitcoin System (Springer, 2013).
Book Google Scholar
Mulahuwaish, A., Loucks, M., Qolomany, B. & Al-Fuqaha, A. Topic modeling based on two-step flow theory: Application to tweets about bitcoin. IT Prof. 25 , 52–63. https://doi.org/10.1109/MITP.2023.3253103 (2023).
Klebnikov, S. Elon musk is the richest person in the world-again [www document]. Forbes. https://www.forbes.com/sites/sergeiklebnikov/2021/01/14/elon-musk-is-the-richestperson-in-the-world-again/ . Aaccessed 31 Jan 2021 (2021).
Musk, E. Am considering taking tesla private at \$420. funding secured. Retrieved June 1, 2019 (2018).
Cano-Marin, E., Mora-Cantallops, M. & Sánchez-Alonso, S. Twitter as a predictive system: A systematic literature review. J. Bus. Res. 157 , 113561 (2023).
Fu, Y., Zhuang, Z. & Zhang, L. Ai ethics on blockchain: Topic analysis on twitter data for blockchain security. In Science and Information Conference , 82–100 (Springer, 2023).
Choi, Y., Kim, B. & Lee, S. Blockchain ventures and initial coin offerings. Int. J. Technoentrep. 4 , 32–46 (2020).
Park, J. & Seo, Y.-S. Twitter sentiment analysis-based adjustment of cryptocurrency action recommendation model for profit maximization. In IEEE Access (2023).
Nakamoto, S. Bitcoin: A peer-to-peer electronic cash system. Decent. Bus. Rev. 20 , 20 (2008).
Wood, G. et al. Ethereum: A secure decentralised generalised transaction ledger. Ethereum Project Yellow Pap. 151 , 1–32 (2014).
Schwartz, D. et al. The ripple protocol consensus algorithm. Ripple Labs Inc White Pap. 5 , 151 (2014).
Disli, M., Abd Rabbo, F., Leneeuw, T. & Nagayev, R. Cryptocurrency comovements and crypto exchange movement: The relocation of binance. Financ. Res. Lett. 48 , 102989 (2022).
Nani, A. The doge worth 88 billion dollars: A case study of dogecoin. Convergence 28 , 1719–1736 (2022).
Pagariya, P., Shinde, S., Shivpure, R., Patil, S. & Jarali, A. Cryptocurrency analysis and forecasting. In 2022 2nd Asian Conference on Innovation in Technology (ASIANCON) , 1–6. https://doi.org/10.1109/ASIANCON55314.2022.9909168 (2022).
David, H. Investing In Fantom (FTM)—everything you need to know (2024). https://www.securities.io/investing-in-fantom/ .
Cointelegraph. Polygon blockchain explained: A beginner’s guide to MATIC (2024). https://cointelegraph.com/learn/polygon-blockchain-explained-a-beginners-guide-to-matic .
Stilt. What is Cardano? (2024). https://www.stilt.com/blog/2021/10/what-is-cardano/ .
Boyd, R. L., Ashokkumar, A., Seraj, S. & Pennebaker, J. W. The Development and Psychometric Properties of liwc-22 1–47 (University of Texas at Austin, 2022).
TextBlob. TextBlob: Simplified text processing (2024). https://textblob.readthedocs.io/en/dev/ .
Mohammad, S. M. & Turney, P. D. Nrc emotion lexicon. Natl. Res. Council Can. 2 , 234 (2013).
Farr, J. N., Jenkins, J. J. & Paterson, D. G. Simplification of flesch reading ease formula. J. Appl. Psychol. 35 , 333 (1951).
Kincaid, J., Fishburne, R., Rogers, R. & Chissom, B. Derivation of New Readability Formula for Navy Enlisted Personnel (Navy Research Branch, 1975).
Gunning, R. The fog index after twenty years. J. Bus. Commun. 6 , 3–13 (1969).
Flesch, R. A new readability yardstick. J. Appl. Psychol. 32 , 221 (1948).
Article CAS PubMed Google Scholar
Butt, S., Sharma, S., Sharma, R., Sidorov, G. & Gelbukh, A. What goes on inside rumour and non-rumour tweets and their reactions: A psycholinguistic analyses. Comput. Hum. Behav. 135 , 107345 (2022).
Inamdar, A., Bhagtani, A., Bhatt, S. & Shetty, P. M. Predicting cryptocurrency value using sentiment analysis. In 2019 International Conference on Intelligent Computing and Control Systems (ICCS) , 932–934 (IEEE, 2019).
Chuen, D., Guo, L. & Wang, Y. Cryptocurrency: A new investment opportunity?. SSRN Electron. J. https://doi.org/10.2139/ssrn.2994097 (2017).
Wołk, K. Advanced social media sentiment analysis for short-term cryptocurrency price prediction. Expert. Syst. 37 , e12493 (2020).
Lamon, C., Nielsen, E. & Redondo, E. Cryptocurrency price prediction using news and social media sentiment. SMU Data Sci. Rev 1 , 1–22 (2017).
Klare, G. R. The measurement of readability. (No Title) (1963).
Dale, E. & Chall, J. S. The concept of readability. Elem. Engl. 26 , 19–26 (1949).
Mohapatra, S., Ahmed, N. & Alencar, P. Kryptooracle: A real-time cryptocurrency price prediction platform using twitter sentiments. In 2019 IEEE International Conference on Big Data (Big Data) , 5544–5551 (IEEE, 2019).
Aslam, N., Rustam, F., Lee, E., Washington, P. B. & Ashraf, I. Sentiment analysis and emotion detection on cryptocurrency related tweets using ensemble lstm-gru model. IEEE Access 10 , 39313–39324 (2022).
Jamil, R. et al. Detecting sarcasm in multi-domain datasets using convolutional neural networks and long short term memory network model. PeerJ Comput. Sci. 7 , e645 (2021).
Article PubMed PubMed Central Google Scholar
Mujahid, M. et al. Sentiment analysis and topic modeling on tweets about online education during covid-19. Appl. Sci. 11 , 8438 (2021).
Article CAS Google Scholar
Ibrahim, A. Forecasting the early market movement in bitcoin using twitter’s sentiment analysis: An ensemble-based prediction model. In 2021 IEEE International IOT, Electronics and Mechatronics Conference (IEMTRONICS) , 1–5 (IEEE, 2021).
Pano, T. & Kashef, R. A corpus of btc tweets in the era of covid-19. In 2020 IEEE International IOT, Electronics and Mechatronics Conference (IEMTRONICS) , 1–4 (IEEE, 2020).
Pano, T. & Kashef, R. A complete vader-based sentiment analysis of bitcoin (btc) tweets during the era of covid-19. Big Data Cogn. Comput. 4 , 33 (2020).
Wang, L., Wang, X., Chen, A., Jin, X. & Che, H. Prediction of type 2 diabetes risk and its effect evaluation based on the xgboost model. In Healthcare , vol. 8, 247 (MDPI, 2020).
Shahzad, M. K. et al. Bpte: Bitcoin price prediction and trend examination using twitter sentiment analysis. In 2021 International Conference on Information and Communication Technology Convergence (ICTC) , 119–122 (IEEE, 2021).
Raheman, A., Kolonin, A., Fridkins, I., Ansari, I. & Vishwas, M. Social media sentiment analysis for cryptocurrency market prediction. arXiv:2204.10185 (arXiv preprint) (2022).
Huang, X. et al. Lstm based sentiment analysis for cryptocurrency prediction. In Database Systems for Advanced Applications: 26th International Conference, DASFAA 2021, Taipei, Taiwan, April 11–14, 2021, Proceedings, Part III 26 , 617–621 (Springer, 2021).
Sailunaz, K. & Alhajj, R. Emotion and sentiment analysis from twitter text. J. Comput. Sci. 36 , 101003 (2019).
Shah, F. M., Reyadh, A. S., Shaafi, A. I., Ahmed, S. & Sithil, F. T. Emotion detection from tweets using ait-2018 dataset. In 2019 5th International Conference on Advances in Electrical Engineering (ICAEE) , 575–580 (IEEE, 2019).
Mohammad, S., Bravo-Marquez, F., Salameh, M. & Kiritchenko, S. Semeval-2018 task 1: Affect in tweets. In Proceedings of the 12th International Workshop on Semantic Evaluation , 1–17 (2018).
BCcampus. Accessibility Toolkit-2nd Edition-Open Textbook (2024). https://opentextbc.ca/accessibilitytoolkit/ .
Kochkina, E., Liakata, M. & Zubiaga, A. All-in-one: Multi-task learning for rumour verification. arXiv:1806.03713 (arXiv preprint) (2018).
Cambria, E., Gelbukh, A., Poria, S. & Kwok, K. Sentic api: A common-sense based api for concept-level sentiment analysis. In CEUR Workshop Proceedings , vol. 1141, 19–24 (CEUR-WS, 2014).
Narman, H. S., Uulu, A. D. & Liu, J. Profile analysis for cryptocurrency in social media. In 2018 IEEE International Symposium on Signal Processing and Information Technology (ISSPIT) , 229–234. https://doi.org/10.1109/ISSPIT.2018.8642634 (2018).
Jacob, P. & Uitdenbogerd, A. L. Readability of twitter tweets for second language learners. In Proceedings of the The 17th Annual Workshop of the Australasian Language Technology Association , 19–27 (2019).
Wuraola, I., Dethlefs, N. & Marciniak, D. Linguistic pattern analysis in the climate change-related tweets from UK and Nigeria. In Proceedings of the 2023 CLASP Conference on Learning with Small Data (LSD) , 90–97 (2023).
LIWC. LIWC : A linguistic inquiry and word count standard (2024). https://www.liwc.app/download (2024).
James, R. 12 most popular types of cryptocurrency (2024). https://finance.yahoo.com/news/12-most-popular-types-cryptocurrency-221243578.html .
Singh, P. K., Pandey, A. K. & Bose, S. A new grey system approach to forecast closing price of bitcoin, bionic, cardano, dogecoin, ethereum, xrp cryptocurrencies. Qual. Quantity 57 , 2429–2446 (2023).
Vidal-Tomás, D., Briola, A. & Aste, T. Ftx’s downfall and Binance’s consolidation: The fragility of centralised digital finance. Phys. A 625 , 129044 (2023).
Tweepy. API-tweepy 4.14.0 documentation (2024). https://docs.tweepy.org/en/stable/api.html .
Lee, C. J. & Chua, H. N. Using linguistics and psycholinguistics features in machine learning for fake news classification through twitter. In Proceedings of International Conference on Data Science and Applications: ICDSA 2021, Volume 1 , 717–730 (Springer, 2022).
LIWC. Linguistic Inquiry and Word Count (LIWC, 2024). https://www.liwc.app/ .
Lyu, S., Ren, X., Du, Y. & Zhao, N. Detecting depression of Chinese microblog users via text analysis: Combining linguistic inquiry word count (liwc) with culture and suicide related lexicons. Front. Psych. 14 , 1121583 (2023).
Bojić, L. M. The patterns of influence: Liwc analysis of leading news portals’ impact and communication accommodation theory on twitter. Ethnoanthropol. Probl. 18 , 589–612 (2023).
Pennebaker, J. W., Francis, M. E. & Booth, R. J. Linguistic Inquiry and Word Count: Liwc 2001 Vol. 71 (Lawrence Erlbaum Associates, 2001).
Kacewicz, E., Pennebaker, J. W., Davis, M., Jeon, M. & Graesser, A. C. Pronoun use reflects standings in social hierarchies. J. Lang. Soc. Psychol. 33 , 125–143 (2014).
Pennebaker, J. W., Booth, R. J., Boyd, R. L. & Francis, M. E. Linguistic Inquiry and Word Count: LIWC2015 Operator’s Manual (2015).
Oliver, R. L. Satisfaction: A Behavioral Perspective on the Consumer (Routledge, 2014).
Balouchzahi, F., Sidorov, G. & Gelbukh, A. Polyhope: Two-level hope speech detection from tweets. Expert Syst. Appl. 225 , 120078 (2023).
Chun, M. M., Golomb, J. D. & Turk-Browne, N. B. A taxonomy of external and internal attention. Annu. Rev. Psychol. 62 , 73–101 (2011).
Article PubMed Google Scholar
Liu, W. & Liu, W. Analysis on the word-formation of English netspeak neologism. J. Arts Human. 3 , 22–30 (2014).
Baalen, I. V. Male and female language: Growing together. Retrieved on September 25, 2018 (2001).
Santos, N. M. B., Alarcón, M. M. H. & Pablo, I. M. Fillers and the development of oral strategic competence in foreign language learning. Porta Linguarum 191 , 201 (2016).
Chuen, D. L. K., Guo, L. & Wang, Y. Cryptocurrency: A new investment opportunity?. J. Altern. Invest. 20 , 16–40 (2017).
Chen, Y.-L., Chang, C.-L. & Yeh, C.-S. Emotion classification of youtube videos. Decis. Support Syst. 101 , 40–50 (2017).
TutorialsPoint. Emotion classification using NRC Lexicon in Python (2024). https://www.tutorialspoint.com/emotion-classification-using-nrc-lexicon-in-python .
Rock Content. Flesch Reading Ease Score: Is It Still Relevant in 2024? (2024). https://rockcontent.com/blog/flesch-reading-ease-score/ .
Zamanian, M. & Heydari, P. Readability of texts: State of the art. Theory Pract. Lang. Stud. 2 , 43–53 (2012).
Readable. Flesch reading ease and the Flesch Kincaid grade level (2024). https://readable.com/readability/flesch-reading-ease-flesch-kincaid-grade-level/ .
Briola, A., Vidal-Tomás, D., Wang, Y. & Aste, T. Anatomy of a stablecoin’s failure: The terra-luna case. Financ. Res. Lett. 51 , 103358 (2023).
Anthony Clarke. 7 biggest crypto collapses of 2022 the industry would like to forget (2022). https://cointelegraph.com/news/7-biggest-crypto-collapses-of-2022-the-industry-would-like-to-forget (2022).
Sidorov, G., Balouchzahi, F., Butt, S. & Gelbukh, A. Regret and hope on transformers: An analysis of transformers on regret and hope speech detection datasets. Appl. Sci. 13 , 3983 (2023).
Balouchzahi, F., Butt, S., Sidorov, G. & Gelbukh, A. Reddit: Regret detection and domain identification from text. Expert Syst. Appl. 225 , 120099 (2023).
Download references
Acknowledgements
The work was done with partial support from the Mexican Government through the grant A1-S-47854 of CONACYT, Mexico, grants 20241816, 20241819, and 20240951 of the Secretaría de Investigación y Posgrado of the Instituto Politécnico Nacional, Mexico. The authors thank the CONACYT for the computing resources brought to them through the Plataforma de Aprendizaje Profundo para Tecnologas del Lenguaje of the Laboratorio de Supercómputo of the INAOE, Mexico and acknowledge the support of Microsoft through the Microsoft Latin America PhD Award.
Author information
Olga Kolesnikova
Present address: Instituto Politécnico Nacional (IPN), Centro de Investigación en Computación (CIC), Mexico, Mexico
Authors and Affiliations
Instituto Politécnico Nacional (IPN), Centro de Investigación en Computación (CIC), Mexico, Mexico
Moein Shahiki Tash, Zahra Ahani & Grigori Sidorov
You can also search for this author in PubMed Google Scholar
Contributions
M.S.T., O.K., Z.A., and G.S. contributed to this manuscript as follows: M.S.T. conceived and designed the study, collected and analyzed the Twitter data, performed the psycholinguistic and emotion analysis, and drafted the manuscript. O.K. contributed to the data analysis, provided expertise in statistical modeling, and critically revised the manuscript for important intellectual content. Z.A. assisted in data collection, conducted literature review, and contributed to the interpretation of results. G.S. provided guidance on computational methodologies, supervised the research process, and contributed to manuscript revisions.
Corresponding authors
Correspondence to Moein Shahiki Tash or Olga Kolesnikova .
Ethics declarations
Competing interests.
The authors declare no competing interests.
Additional information
Publisher's note.
Springer Nature remains neutral with regard to jurisdictional claims in published maps and institutional affiliations.
Rights and permissions
Open Access This article is licensed under a Creative Commons Attribution 4.0 International License, which permits use, sharing, adaptation, distribution and reproduction in any medium or format, as long as you give appropriate credit to the original author(s) and the source, provide a link to the Creative Commons licence, and indicate if changes were made. The images or other third party material in this article are included in the article’s Creative Commons licence, unless indicated otherwise in a credit line to the material. If material is not included in the article’s Creative Commons licence and your intended use is not permitted by statutory regulation or exceeds the permitted use, you will need to obtain permission directly from the copyright holder. To view a copy of this licence, visit http://creativecommons.org/licenses/by/4.0/ .
Reprints and permissions
About this article
Cite this article.
Tash, M.S., Kolesnikova, O., Ahani, Z. et al. Psycholinguistic and emotion analysis of cryptocurrency discourse on X platform. Sci Rep 14 , 8585 (2024). https://doi.org/10.1038/s41598-024-58929-4
Download citation
Received : 21 November 2023
Accepted : 04 April 2024
Published : 13 April 2024
DOI : https://doi.org/10.1038/s41598-024-58929-4
Share this article
Anyone you share the following link with will be able to read this content:
Sorry, a shareable link is not currently available for this article.
Provided by the Springer Nature SharedIt content-sharing initiative
- Cryptocurrency
- Psycholinguistic
- Digital coins
- Reliability
By submitting a comment you agree to abide by our Terms and Community Guidelines . If you find something abusive or that does not comply with our terms or guidelines please flag it as inappropriate.
Quick links
- Explore articles by subject
- Guide to authors
- Editorial policies
Sign up for the Nature Briefing: AI and Robotics newsletter — what matters in AI and robotics research, free to your inbox weekly.

Countries for Old Men: An Analysis of the Age Pay Gap
This study investigates the growing wage disparity between older and younger workers in high-income countries. We propose a conceptual framework of the labor market in which firms cannot change the contracts of older employees and cannot freely add higher-ranked positions to their organizations. In this model, a larger supply of older workers and declining economic growth restrict younger workers’ access to higher-paying roles and widen the age pay gap in favor of older workers. Drawing on extensive administrative and survey data, we document that the characteristics of these negative spillovers on younger workers’ careers align with the model’s predictions. As older workers enjoy more successful careers, younger workers become less likely to hold higher-ranked jobs and fall toward the bottom of the wage distribution. The pay gap between younger and older workers increases more in slower-growing, older, and larger firms and in firms with higher mean wages, where these negative spillovers on younger workers are larger in magnitude. Moreover, younger employees become less likely to work for higher-paying firms, whose share of older workers disproportionately increases over time. Finally, we show that alternative explanations for these findings receive little empirical support.
We thank Jaime Arellano-Bover, David Autor, Alexander Bartik, Barbara Biasi, Christian Dustmann, Andrew Garin, Luigi Guiso, Ben Jones, Hyejin Ku, Salvatore Lattanzio, Attila Lindner, Niko Matouschek, Sara Moreira, Paolo Naticchioni, Michael Powell, Raffaele Saggio, Elia Sartori, Uta Schoenberg, Liangjie Wu, as well as participants at various seminars and conferences for helpful comments. We thank Thomas Barden, Sean Chen, Alessandra Grimaldi, Chuqiao Nan, and Georgii Zherebilov for outstanding research assistance. The realization of the present article was possible thanks to the sponsorship of the “VisitINPS Scholars” program. This study uses the Cross-sectional model of the Linked-Employer-Employee Data (LIAB) (Version 2, Years 1993-2017) from the IAB. Data access was provided via on-site use at the Research Data Centre (FDZ) of the German Federal Employment Agency (BA) at the Institute for Employment Research (IAB) in Berlin and subsequently via remote data access (project number: fdz1968/1969). The views expressed herein are those of the authors and do not necessarily reflect the views of the National Bureau of Economic Research.
MARC RIS BibTeΧ
Download Citation Data
Working Groups
More from nber.
In addition to working papers , the NBER disseminates affiliates’ latest findings through a range of free periodicals — the NBER Reporter , the NBER Digest , the Bulletin on Retirement and Disability , the Bulletin on Health , and the Bulletin on Entrepreneurship — as well as online conference reports , video lectures , and interviews .
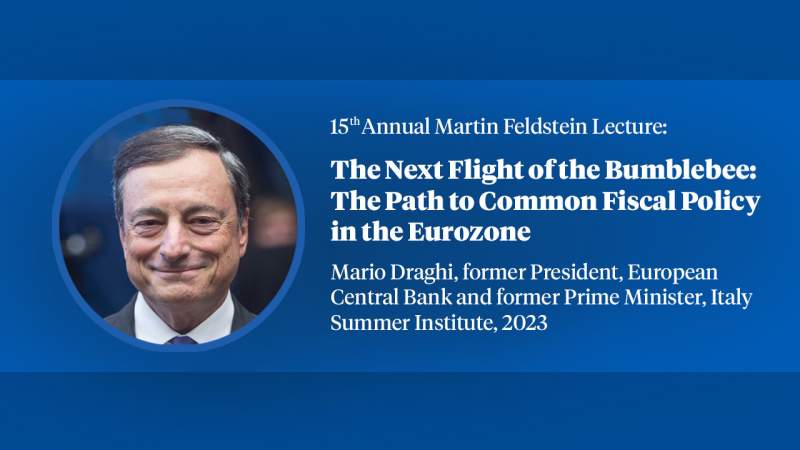
Mobile Menu Overlay
The White House 1600 Pennsylvania Ave NW Washington, DC 20500
The Economics of Administration Action on Student Debt
Higher education financing allows many Americans from lower- and middle-income backgrounds to invest in education. However, over the past 30 years, college tuition prices have increased faster than median incomes, leaving many Americans with large amounts of student debt that they struggle or are unable to, pay off.
Recognizing the burden of this debt, the Biden-Harris Administration has pursued two key strategies for debt reduction and cancellation. The first, student debt relief (SDR), aims to address the ill effects of flaws in the student debt system for borrowers. The second, the SAVE plan, reforms the federal student loan system, improving student loan affordability for future students and providing current graduates with breathing room during the beginning of a new career.
This issue brief examines the factors that precipitated the current student debt landscape, and details how both SDR and SAVE will enhance the economic status of millions of Americans with student debt: enabling them to allocate more funds towards basic necessities, take career risks, start businesses, and purchase homes. This brief highlights credible research, underscoring how the Administration’s student debt relief could boost consumption in the short-term by billions of dollars and could have important impacts on borrower mental health, financial security, and outcomes such as homeownership and entrepreneurship. This brief also details how the SAVE plan makes repaying college costs more affordable for current borrowers and future generations. CEA simulations show that, under SAVE, an average borrower with a bachelor’s degree could save $20,000 in loan payments, while a borrower with an associate degree could see nearly 90 percent savings compared to the standard loan repayment plan. These changes enable more people to pursue education and contribute to the broader economy.
Why do borrowers need relief?
Over the last 20 years especially, the sticker price of college has risen significantly. Despite recent minor declines, sticker prices at public universities (which over 70% of undergraduate students in the United States attend) are 56% higher today than two decades ago. [1] While there are many reasons for this trend, the most rapid increases in tuition often occur during economic downturns as tuitions grow to fill the budgetary holes that are left when states cut their support to public colleges ( Webber, 2017 ; Deming and Walters 2018 ). This is especially problematic given many people choose to return to school during economic downturns ( Betts and MacFarland 1995 ; Hillman and Orians 2013 ). Unfortunately, contracting state appropriations have played a role in shifting the responsibility of financing away from public subsidies and toward students and families ( Turner and Barr, 2013 ; Bound et al., 2019 )–leading many students to take on more debt.
At the same time that college sticker prices have risen, the wage premium (the earnings difference between college goers and high school graduates) has not seen analogous growth. While obtaining a college degree remains a reliable entry point to the middle class, the relative earning gains for degree holders began to stagnate in the early 2000s after increasing for several decades. As shown in Figure 1b, since 2000, the wage premium for both bachelor’s degree holders and those with “some college” education (which includes anyone who enrolled in college but didn’t earn a BA) saw declines around the 2001-02 and 2008-10 recessions and a slow, inconsistent recovery thereafter. The decline is particularly notable for students who didn’t complete a four-year degree, a group that includes two-year college enrollees who have among the highest student loan default rates. [2]
Traditional economic theory tells us that individuals choose to invest in post-secondary education based on the expected costs and wage returns associated with the investment. But rapid and unforeseeable rises in prices and declines in college wage premia have contributed to decades of “unlucky” college-entry cohorts affected by a form of recessionary scarring . For example, a student who entered college in 2006 would have expected a sticker price of roughly $8,800 per year for a four-year college, but actually faced tuition of over $10,000 in their final year of college, a roughly 15% difference. This same student, upon graduation if they worked full time, would have earned about $3,500 less, on average, than what they would have expected upon entering. This example illustrates that many borrowers made sound borrowing decisions with available information, but as a result of these trends ended up with more debt than they could afford to pay off. [3] Consistent with this notion, the default rate for “unlucky” college entry cohorts of the 2000s is much higher than those of other cohorts, with undergraduate default rates doubling between 2000 and 2010: in 2017, 21 percent of undergraduate loan holders and 6 percent of graduate loan holders defaulted within 3 years ( CBO, 2020 ).
It is important to note that sticker prices for public institutions have declined 7 percent since 2021, the same period over which college wage premiums have been rising. Declining tuition, for the first time in decades, coincided with increased investment in higher education through pandemic-era legislation such as the American Rescue Plan, which allocated $40 billion in 2021 to support institutes of higher education and their students. Despite these improvements, as well as significant advances in the return on college investments over the last three years, many current borrowers still need some relief. The Administration has taken significant action to protect future cohorts from similar risks.
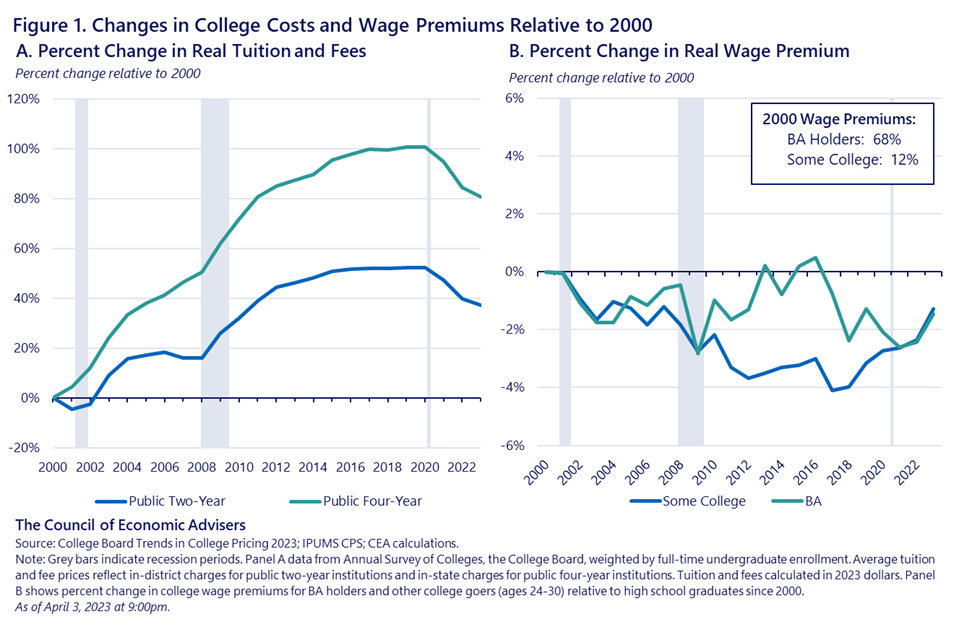
How the Administration is providing relief
Retrospective: Student Debt Relief Helps Existing Borrowers
In a commitment to help those who are overburdened with debt, the Administration has already approved Federal student debt cancellation for nearly 4 million Americans through various actions. Today , the Administration announced details of proposed rules that, if finalized as proposed, would provide relief to over 30 million borrowers when taken together with actions to date.
Importantly, much of this debt forgiveness comes from correcting program administration and improving regulations related to laws that were on the books before this Administration took office. This debt relief has affected borrowers from all walks of life, including nearly 900,000 Americans who have dedicated their lives to public service (such as teachers, social workers, nurses, firefighters, police officers, and others), borrowers who were misled and cheated by their institutions, and borrowers who are facing total or permanent disability, including many veterans. By relieving these borrowers of long-held, and in some cases very large burdens of debt, relief can have significant meaning and impact for borrowers, families, and their communities.
By reducing debtors’ liabilities, debt relief raises net worth (assets, including income less liabilities). Debt relief can also ease the financial burden of making payments—leading to greater disposable income for borrowers and their families, which enhances living standards and could positively influence decisions about employment, home buying, and mobility. While there are few direct estimates of the effect of debt cancelation in the literature, estimates based on the relationship between wealth and consumption suggest that this forgiveness could increase consumption by several billions of dollars each year in the next five to ten years.
Additionally, a recent study suggests that student debt cancellation can lead to increased earnings (due to greater geographic and career mobility), improved credit scores, and lower delinquency rates on other debts ( Di Maggio, Kalda, and Yao, 2019 ). This can facilitate access to capital for starting a business or buying a car or home. As home mortgages often require a certain debt-to-income ratio and depend heavily on credit scores, student debt cancellation could potentially increase home ownership. Indeed, based on the mechanical relationship between housing industry affordability standards and debt-to-income ratios, industry sources have suggested that those without student debt could afford to take out substantially larger mortgages ( Zillow, 2018 ). Other research also indicates a negative correlation between student loan debt and homeownership ( Mezza et al., 2020 ).
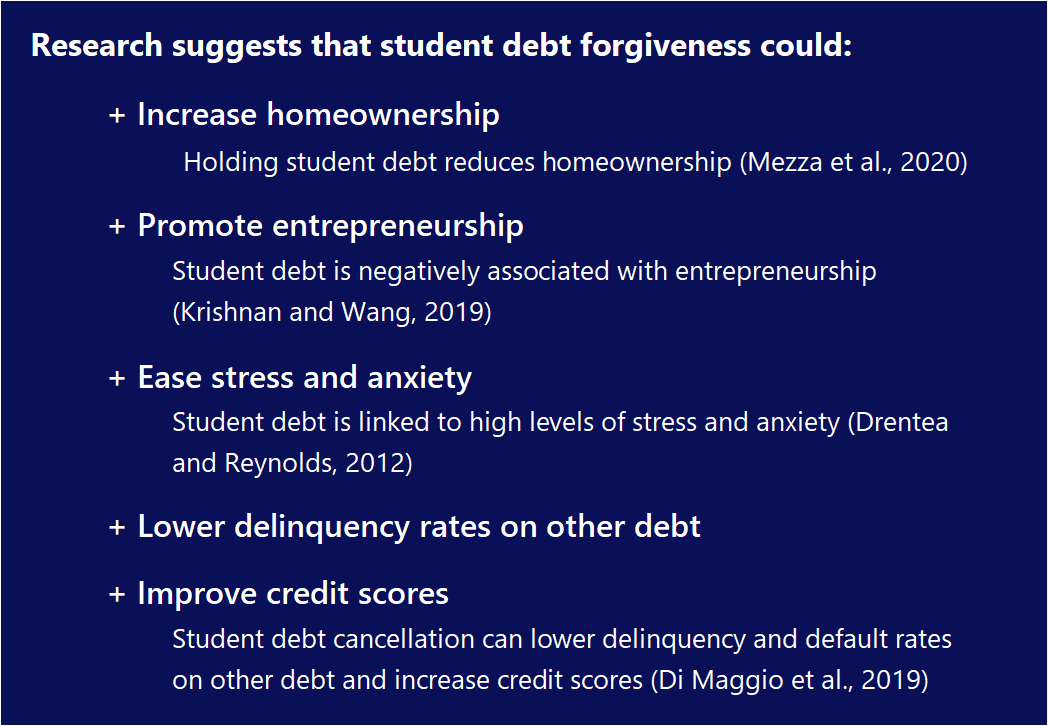
It is important to note that, while these pecuniary benefits are important, the benefits associated with debt relief are not merely financial. Experimental evidence has linked holding debt to heightened levels of stress and anxiety ( Drentea and Reynolds, 2012 ), worse self-reported physical health ( Sweet et al., 2013 ), and reduced cognitive capacity ( Robb et al., 2012 ; Ong et al., 2019 ). Studies also show that holding student debt can be a barrier to positive life cycle outcomes such as entrepreneurship ( Krishnan and Wang, 2019 ), and marriage ( Gicheva, 2016 ; Sieg and Wang, 2018 ). Student debt relief has the potential to improve these key outcomes for millions of borrowers.
Prospective: The SAVE Plan Helps Prevent Future Challenges
To address unaffordable education financing moving forward, the Administration has also introduced the Saving on a Valuable Education (SAVE) loan repayment program. The SAVE plan prospectively helps student borrowers by ensuring that once they graduate, they never have to pay more than they can afford towards their student loan debt. Importantly, the SAVE plan protects borrowers from being “unlucky” by ensuring that high tuition or low earnings do not result in loan payments that borrowers can’t afford. The CEA has detailed the real benefits of SAVE for borrowers in issue briefs and blogs , underscoring that SAVE is the most affordable student loan repayment program in U.S. history. By substantially reducing monthly payment amounts compared to previous income driven repayment (IDR) plans and reducing time to forgiveness to as little as 10 years for people who borrowed smaller amounts, the SAVE plan can mean tens of thousands of dollars in real savings for borrowers over the course of repayment.
Figure 2 gives the example of two representative borrowers. Take the first, a 4-year college graduate who has $31,000 in debt and earns about $40,500 per year. Under a standard repayment plan, this borrower would pay roughly $330 dollars each month for 10 years. Under SAVE, this borrower would pay about $50 per month for the first ten years, and on average about $130 per month for the next 10 years. Over a 20-year period, this borrower would make roughly $17,500 less in payments, not accounting for inflation over that period. This represents a 56 percent reduction in total payments compared to the standard repayment plan and includes considerable loan forgiveness. Similarly, the representative 2-year college graduate has $10,000 in debt and earns about $32,000 per year. Under a standard plan, this borrower would pay $110 dollars each month for 10 years. Under SAVE, this borrower would pay $0 per month for the first two years, and under $20 per month for the next eight years before their debt is forgiven at year 10. Overall, this borrower would be responsible for roughly $11,700 less in lifetime payments, not accounting for inflation. This borrower sees nearly 90 percent savings compared to the standard plan and receives considerable loan forgiveness.
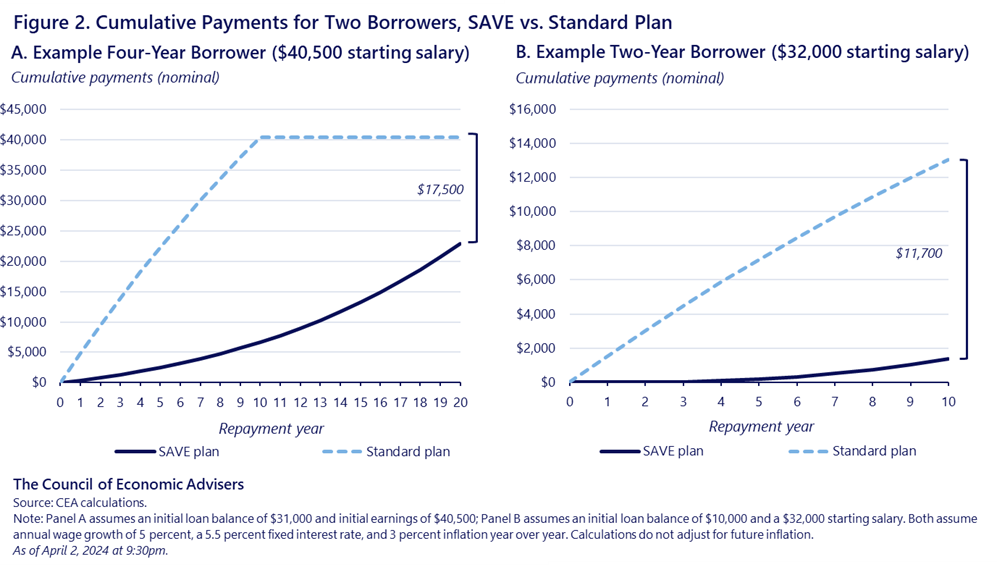
SAVE can also have benefits beyond the individual borrower. More money in borrowers’ pockets due to lower payment obligations under SAVE could boost consumption and give borrowers breathing room to make payments on other debt. This consumption effect is bolstered by a large literature documenting the benefits of easing liquidity constraints (see, for example, Aydin, 2022 ; Parker et al., 2022 ). Additionally, by shortening time to forgiveness for undergraduate borrowers, SAVE can lead to positive debt-relief outcomes (as discussed above) for many more borrowers.
Another key aspect of income-driven repayment plans like SAVE is that they protect borrowers from having to make large payments when incomes are low. Specifically, the required payments are not based on the initial loan balance, but on one’s income and household size so that those cohorts who need to borrow more to pay for college do not make larger payments unless they make more income. SAVE also protects more of a borrower’s income as discretionary and, when the full plan is implemented in Summer 2024, will limit monthly payments on undergraduate loans to 5 percent of discretionary income. In fact, for single borrowers who make less than $33,000 per year, the required monthly payments will be zero dollars. From a finance perspective, the SAVE plan provides a form of insurance against tuition spikes and economic downturns–taking some of the risk out of investing in one’s education while also bringing costs down.
A common concern, and one that could mute these benefits, is that increases in the generosity of education financing may encourage institutions to raise tuition and fees in response, a phenomenon commonly referred to as the Bennett Hypothesis (for an excellent overview of research, see Dynarski et al., 2022 ). Theoretically, in a market when sellers are maximizing profits, any policy that increases demand will also increase prices. However, this is less likely to impact the over 70% of U.S. undergraduates who attend public colleges, which are not profit-driven and often have statutorily set tuition. Consistent with this notion, the evidence in support of the Bennett Hypothesis primarily comes from for-profit colleges, which are highly reliant on students who receive federal financial aid ( Cellini and Goldin, 2014 ; Baird et al, 2022 ). [4] Importantly, although the for-profit sector enrolls some of the country’s most vulnerable students, enrollment in the sector in 2021 accounted for only 5 percent of total undergraduate enrollment, suggesting that aggregate tuition increases in response to changes in education financing may be modest. Furthermore, the Biden-Harris Administration has taken action to crack down on for-profit colleges that take advantage of, or mislead, their students. And, recent regulations, such as the Gainful Employment ( GE ) rule, add safeguards against unaffordable debt regardless of more generous education financing.
Although the SAVE plan stands to benefit borrowers of all backgrounds, the plan has important racial and socioeconomic equity implications because it is particularly beneficial for those borrowers with the lowest incomes. Centuries of inequities have led to Black, Hispanic, and Native households being more likely than their White peers to fall in the low end of the income distribution. This means that, mechanically, the SAVE plan’s benefits could accrue disproportionately to these groups. Indeed, using completion data from recent years, an Urban Institute analysis estimates that 59 percent of credentials earned by Black students and 53 percent of credentials earned by Hispanic students are likely to be eligible for some amount of loan forgiveness under SAVE, compared to 42 percent of credentials earned by White students ( Delisle and Cohn, 2023 ). Finally, the interest subsidy described in an August 2023 CEA blog , prevents ballooning balances when a borrower cannot cover their entire monthly interest payment, a phenomenon that has historically led to many borrowers in general, and Black borrowers in particular, to see loan balances that are higher than their original loan amount, even several years out from graduating with a bachelor’s degree ( NCES, 2023 ).
Broader economic impacts
The benefits associated with SDR and SAVE for millions of Americans are considerable. In the short run, under both SDR and SAVE, those who receive relief may be able to spend more in their communities and contribute to their local economies. Summing the likely consumption effects of the Administration’s student debt relief and SAVE programs results in billions of dollars in additional consumption annually. Despite the modest effect on the macroeconomy as a whole (note that the U.S. economy is roughly $28 trillion with a population of roughly 320 million), these consumption effects represent incredibly meaningful impacts on individual borrowers’ financial security and the economic wellbeing of their communities.
SAVE, because it brings down the cost of taking out loans to go to college, has the potential to lead to longer-term economic growth if it leads to greater educational attainment. This increased attainment can occur both through improved retention and completion of post-secondary education, and also the movement of students into college who would not have otherwise enrolled. There is a long macroeconomics literature linking educational attainment in a nation to GDP growth (see, for example, Lucas, 1988 ; Hanushek and Woesmann, 2008 ). While identifying the causal effect of schooling on GDP is challenging, researchers, using a variety of approaches, find that a one-year increase in average education (for the entire working population) would increase the real GDP level by between 5 and 12 percent ( Barro and Lee, 2013 ; Soto, 2002 ) —a result that is in line with the micro-founded relationship between years of education and earnings ( Lovenheim and Smith, 2022 ).
To put this relationship in perspective and highlight the growth potential of increasing educational attainment, the CEA simulated the hypothetical effect on GDP of increasing the college-going rate by 1, 3, and 5 percentage points, respectively. This range represents the kinds of changes in college going that have been observed over several years: the college enrollment rate for 18- to 24-year-olds declined 4 percentage points between 2011 and 2021 after increasing by 6 percentage points between 2000 and 2011 ( NCES 2023 ). CEA simulations show that by 2055, a policy that increased the college going rate by 1, 3, and 5 percentage points could increase the level of GDP in 2055 (thirty years from now) by 0.2, 0.6, and 1 percent respectively. This represents hundreds of billions of dollars of additional economic activity in the long run.
While increased growth is an exciting possibility, it would only occur insofar as SAVE leads to increased educational attainment, which is uncertain. The academic literature has found that student loans can promote academic performance ( Barr, et. al. 2021 ), and increase educational attainment by increasing transfers from 2-year to 4-year colleges and increasing college completion among enrollees ( Marx and Turner, 2019 ). At the same time, increases in college-going due to SAVE are by no means guaranteed. While, historically, policies that reduce the cost of college through direct means—such as providing students with generous grant aid, or reducing tuition—have succeeded at raising college enrollment levels ( Dynarski, 2003 ; Turner, 2011 ), a pair of recent studies show that prospective students may only respond to cost changes when they are salient, i.e., they are framed and marketed in the right way ( Dynarski et al., 2021 ), and relatively certain ( Burland et al., 2022 ). However, evidence suggests that there is demand for plans like SAVE ( Balakrishnan et al., 2024 ), particularly as SAVE can provide sizable benefits to borrowers in terms of reducing their long-term debt burden and keep monthly payments low (dependent on a borrower’s income) after they finish school.
This highlights the importance of communicating the benefits of the SAVE program to prospective students who otherwise would not enroll in college due to cost concerns, including potential barriers to paying off student loans in the future. Doing so could lead to meaningful increases in college enrollment, and the resulting improvements in productive capacity could increase the size of the U.S. economy for years to come.
Concluding remarks
The Biden-Harris Administration has taken bold action to address a student debt problem that has been decades in the making. This student debt cancellation will provide well-deserved relief for borrowers who have paid their fair share, many of whom had the proverbial rug pulled out from under them with concurrent rapidly rising tuition and declining returns to a college degree. The relief has and will improve economic health and wellbeing of those who have devoted years of their life to public service, those who were defrauded or misled by their institutions, and those who have been doing all they can to make payments, but have still seen their loan balances grow. Looking to future generations, the Administration implemented the SAVE plan to protect borrowers against tuition spikes and poorer than expected labor market outcomes that often plague students graduating into a period of economic downturn ( Rothstein, 2021 ; Schwandt and von Wachter, 2023 ).
Both student debt relief and SAVE will enhance the economic status of millions of Americans with student debt: enable them to allocate more funds towards basic necessities, take career risks, start businesses, and purchase homes with the understanding that they will never have to pay more than they can afford towards their student loans. Moreover, the SAVE plan makes repayment more affordable for future generations, which helps borrowers manage monthly payments, but also enables more people from all walks of life to explore their full potential and pursue higher education, enhancing the potential of the U.S. workforce and the economy more broadly.
[1] In 2021, 51% of total undergraduates attended public 4-year universities and 21% attended public 2-years in 2021.
[2] The BA group excludes those with a graduate degree, or any education beyond a bachelor’s degree.
[3] Recent research shows that, despite a positive return on investment (ROI) for many, including the average student, the distribution of ROI has widened over the last several decades such that the likelihood of negative ROI is higher than it has historically been, particularly so for underrepresented minority students ( Webber 2022 ).
[4] There is also some evidence in support of the Bennett Hypothesis at the graduate level ( Black et al. 2023 ).
Stay Connected
We'll be in touch with the latest information on how President Biden and his administration are working for the American people, as well as ways you can get involved and help our country build back better.
Opt in to send and receive text messages from President Biden.
Have a language expert improve your writing
Run a free plagiarism check in 10 minutes, generate accurate citations for free.
- Knowledge Base
Methodology
Research Methods | Definitions, Types, Examples
Research methods are specific procedures for collecting and analyzing data. Developing your research methods is an integral part of your research design . When planning your methods, there are two key decisions you will make.
First, decide how you will collect data . Your methods depend on what type of data you need to answer your research question :
- Qualitative vs. quantitative : Will your data take the form of words or numbers?
- Primary vs. secondary : Will you collect original data yourself, or will you use data that has already been collected by someone else?
- Descriptive vs. experimental : Will you take measurements of something as it is, or will you perform an experiment?
Second, decide how you will analyze the data .
- For quantitative data, you can use statistical analysis methods to test relationships between variables.
- For qualitative data, you can use methods such as thematic analysis to interpret patterns and meanings in the data.
Table of contents
Methods for collecting data, examples of data collection methods, methods for analyzing data, examples of data analysis methods, other interesting articles, frequently asked questions about research methods.
Data is the information that you collect for the purposes of answering your research question . The type of data you need depends on the aims of your research.
Qualitative vs. quantitative data
Your choice of qualitative or quantitative data collection depends on the type of knowledge you want to develop.
For questions about ideas, experiences and meanings, or to study something that can’t be described numerically, collect qualitative data .
If you want to develop a more mechanistic understanding of a topic, or your research involves hypothesis testing , collect quantitative data .
You can also take a mixed methods approach , where you use both qualitative and quantitative research methods.
Primary vs. secondary research
Primary research is any original data that you collect yourself for the purposes of answering your research question (e.g. through surveys , observations and experiments ). Secondary research is data that has already been collected by other researchers (e.g. in a government census or previous scientific studies).
If you are exploring a novel research question, you’ll probably need to collect primary data . But if you want to synthesize existing knowledge, analyze historical trends, or identify patterns on a large scale, secondary data might be a better choice.
Descriptive vs. experimental data
In descriptive research , you collect data about your study subject without intervening. The validity of your research will depend on your sampling method .
In experimental research , you systematically intervene in a process and measure the outcome. The validity of your research will depend on your experimental design .
To conduct an experiment, you need to be able to vary your independent variable , precisely measure your dependent variable, and control for confounding variables . If it’s practically and ethically possible, this method is the best choice for answering questions about cause and effect.
Receive feedback on language, structure, and formatting
Professional editors proofread and edit your paper by focusing on:
- Academic style
- Vague sentences
- Style consistency
See an example
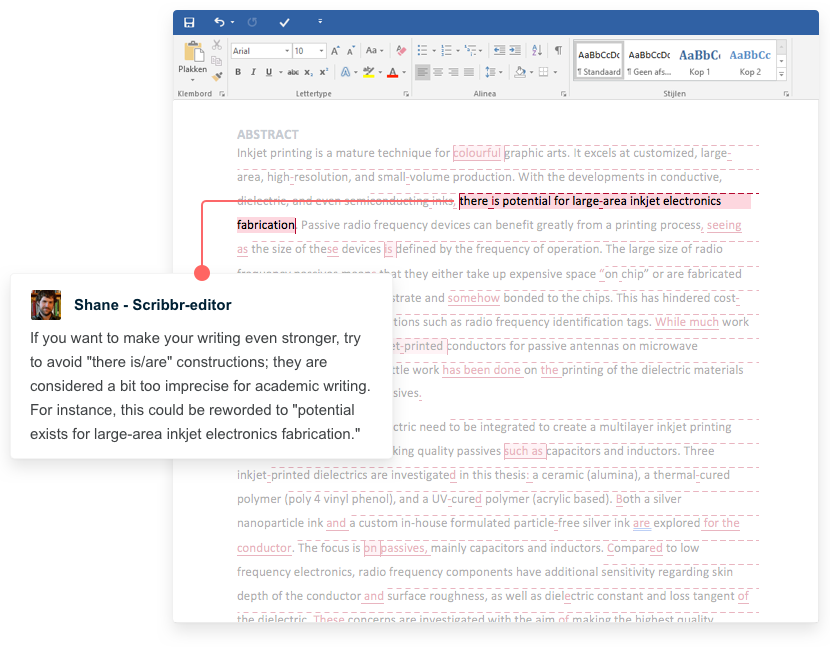
Your data analysis methods will depend on the type of data you collect and how you prepare it for analysis.
Data can often be analyzed both quantitatively and qualitatively. For example, survey responses could be analyzed qualitatively by studying the meanings of responses or quantitatively by studying the frequencies of responses.
Qualitative analysis methods
Qualitative analysis is used to understand words, ideas, and experiences. You can use it to interpret data that was collected:
- From open-ended surveys and interviews , literature reviews , case studies , ethnographies , and other sources that use text rather than numbers.
- Using non-probability sampling methods .
Qualitative analysis tends to be quite flexible and relies on the researcher’s judgement, so you have to reflect carefully on your choices and assumptions and be careful to avoid research bias .
Quantitative analysis methods
Quantitative analysis uses numbers and statistics to understand frequencies, averages and correlations (in descriptive studies) or cause-and-effect relationships (in experiments).
You can use quantitative analysis to interpret data that was collected either:
- During an experiment .
- Using probability sampling methods .
Because the data is collected and analyzed in a statistically valid way, the results of quantitative analysis can be easily standardized and shared among researchers.
If you want to know more about statistics , methodology , or research bias , make sure to check out some of our other articles with explanations and examples.
- Chi square test of independence
- Statistical power
- Descriptive statistics
- Degrees of freedom
- Pearson correlation
- Null hypothesis
- Double-blind study
- Case-control study
- Research ethics
- Data collection
- Hypothesis testing
- Structured interviews
Research bias
- Hawthorne effect
- Unconscious bias
- Recall bias
- Halo effect
- Self-serving bias
- Information bias
Quantitative research deals with numbers and statistics, while qualitative research deals with words and meanings.
Quantitative methods allow you to systematically measure variables and test hypotheses . Qualitative methods allow you to explore concepts and experiences in more detail.
In mixed methods research , you use both qualitative and quantitative data collection and analysis methods to answer your research question .
A sample is a subset of individuals from a larger population . Sampling means selecting the group that you will actually collect data from in your research. For example, if you are researching the opinions of students in your university, you could survey a sample of 100 students.
In statistics, sampling allows you to test a hypothesis about the characteristics of a population.
The research methods you use depend on the type of data you need to answer your research question .
- If you want to measure something or test a hypothesis , use quantitative methods . If you want to explore ideas, thoughts and meanings, use qualitative methods .
- If you want to analyze a large amount of readily-available data, use secondary data. If you want data specific to your purposes with control over how it is generated, collect primary data.
- If you want to establish cause-and-effect relationships between variables , use experimental methods. If you want to understand the characteristics of a research subject, use descriptive methods.
Methodology refers to the overarching strategy and rationale of your research project . It involves studying the methods used in your field and the theories or principles behind them, in order to develop an approach that matches your objectives.
Methods are the specific tools and procedures you use to collect and analyze data (for example, experiments, surveys , and statistical tests ).
In shorter scientific papers, where the aim is to report the findings of a specific study, you might simply describe what you did in a methods section .
In a longer or more complex research project, such as a thesis or dissertation , you will probably include a methodology section , where you explain your approach to answering the research questions and cite relevant sources to support your choice of methods.
Is this article helpful?
Other students also liked, writing strong research questions | criteria & examples.
- What Is a Research Design | Types, Guide & Examples
- Data Collection | Definition, Methods & Examples
More interesting articles
- Between-Subjects Design | Examples, Pros, & Cons
- Cluster Sampling | A Simple Step-by-Step Guide with Examples
- Confounding Variables | Definition, Examples & Controls
- Construct Validity | Definition, Types, & Examples
- Content Analysis | Guide, Methods & Examples
- Control Groups and Treatment Groups | Uses & Examples
- Control Variables | What Are They & Why Do They Matter?
- Correlation vs. Causation | Difference, Designs & Examples
- Correlational Research | When & How to Use
- Critical Discourse Analysis | Definition, Guide & Examples
- Cross-Sectional Study | Definition, Uses & Examples
- Descriptive Research | Definition, Types, Methods & Examples
- Ethical Considerations in Research | Types & Examples
- Explanatory and Response Variables | Definitions & Examples
- Explanatory Research | Definition, Guide, & Examples
- Exploratory Research | Definition, Guide, & Examples
- External Validity | Definition, Types, Threats & Examples
- Extraneous Variables | Examples, Types & Controls
- Guide to Experimental Design | Overview, Steps, & Examples
- How Do You Incorporate an Interview into a Dissertation? | Tips
- How to Do Thematic Analysis | Step-by-Step Guide & Examples
- How to Write a Literature Review | Guide, Examples, & Templates
- How to Write a Strong Hypothesis | Steps & Examples
- Inclusion and Exclusion Criteria | Examples & Definition
- Independent vs. Dependent Variables | Definition & Examples
- Inductive Reasoning | Types, Examples, Explanation
- Inductive vs. Deductive Research Approach | Steps & Examples
- Internal Validity in Research | Definition, Threats, & Examples
- Internal vs. External Validity | Understanding Differences & Threats
- Longitudinal Study | Definition, Approaches & Examples
- Mediator vs. Moderator Variables | Differences & Examples
- Mixed Methods Research | Definition, Guide & Examples
- Multistage Sampling | Introductory Guide & Examples
- Naturalistic Observation | Definition, Guide & Examples
- Operationalization | A Guide with Examples, Pros & Cons
- Population vs. Sample | Definitions, Differences & Examples
- Primary Research | Definition, Types, & Examples
- Qualitative vs. Quantitative Research | Differences, Examples & Methods
- Quasi-Experimental Design | Definition, Types & Examples
- Questionnaire Design | Methods, Question Types & Examples
- Random Assignment in Experiments | Introduction & Examples
- Random vs. Systematic Error | Definition & Examples
- Reliability vs. Validity in Research | Difference, Types and Examples
- Reproducibility vs Replicability | Difference & Examples
- Reproducibility vs. Replicability | Difference & Examples
- Sampling Methods | Types, Techniques & Examples
- Semi-Structured Interview | Definition, Guide & Examples
- Simple Random Sampling | Definition, Steps & Examples
- Single, Double, & Triple Blind Study | Definition & Examples
- Stratified Sampling | Definition, Guide & Examples
- Structured Interview | Definition, Guide & Examples
- Survey Research | Definition, Examples & Methods
- Systematic Review | Definition, Example, & Guide
- Systematic Sampling | A Step-by-Step Guide with Examples
- Textual Analysis | Guide, 3 Approaches & Examples
- The 4 Types of Reliability in Research | Definitions & Examples
- The 4 Types of Validity in Research | Definitions & Examples
- Transcribing an Interview | 5 Steps & Transcription Software
- Triangulation in Research | Guide, Types, Examples
- Types of Interviews in Research | Guide & Examples
- Types of Research Designs Compared | Guide & Examples
- Types of Variables in Research & Statistics | Examples
- Unstructured Interview | Definition, Guide & Examples
- What Is a Case Study? | Definition, Examples & Methods
- What Is a Case-Control Study? | Definition & Examples
- What Is a Cohort Study? | Definition & Examples
- What Is a Conceptual Framework? | Tips & Examples
- What Is a Controlled Experiment? | Definitions & Examples
- What Is a Double-Barreled Question?
- What Is a Focus Group? | Step-by-Step Guide & Examples
- What Is a Likert Scale? | Guide & Examples
- What Is a Prospective Cohort Study? | Definition & Examples
- What Is a Retrospective Cohort Study? | Definition & Examples
- What Is Action Research? | Definition & Examples
- What Is an Observational Study? | Guide & Examples
- What Is Concurrent Validity? | Definition & Examples
- What Is Content Validity? | Definition & Examples
- What Is Convenience Sampling? | Definition & Examples
- What Is Convergent Validity? | Definition & Examples
- What Is Criterion Validity? | Definition & Examples
- What Is Data Cleansing? | Definition, Guide & Examples
- What Is Deductive Reasoning? | Explanation & Examples
- What Is Discriminant Validity? | Definition & Example
- What Is Ecological Validity? | Definition & Examples
- What Is Ethnography? | Definition, Guide & Examples
- What Is Face Validity? | Guide, Definition & Examples
- What Is Non-Probability Sampling? | Types & Examples
- What Is Participant Observation? | Definition & Examples
- What Is Peer Review? | Types & Examples
- What Is Predictive Validity? | Examples & Definition
- What Is Probability Sampling? | Types & Examples
- What Is Purposive Sampling? | Definition & Examples
- What Is Qualitative Observation? | Definition & Examples
- What Is Qualitative Research? | Methods & Examples
- What Is Quantitative Observation? | Definition & Examples
- What Is Quantitative Research? | Definition, Uses & Methods
Unlimited Academic AI-Proofreading
✔ Document error-free in 5minutes ✔ Unlimited document corrections ✔ Specialized in correcting academic texts
- International edition
- Australia edition
- Europe edition
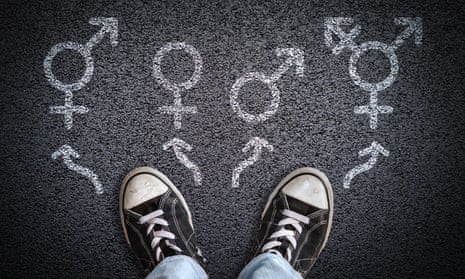
Gender medicine ‘built on shaky foundations’, Cass review finds
Analysis finds most research underpinning clinical guidelines, hormone treatments and puberty blockers to be low quality
Review of gender services has major implications for mental health services
The head of the world’s largest review into children’s care has said that gender medicine is “built on shaky foundations”.
Dr Hilary Cass, the paediatrician commissioned to conduct a review of the services provided by the NHS to children and young people questioning their gender identity, said that while doctors tended to be cautious in implementing new findings in emerging areas of medicine, “quite the reverse happened in the field of gender care for children”.
Cass commissioned the University of York to conduct a series of analyses as part of her review.
Two papers examined the quality and development of current guidelines and recommendations for managing gender dysphoria in children and young people. Most of the 23 clinical guidelines reviewed were not independent or evidence based, the researchers found.
A third paper on puberty blockers found that of 50 studies, only one was of high quality.
Similarly, of 53 studies included in a fourth paper on the use of hormone treatment, only one was of sufficiently high quality, with little or only inconsistent evidence on key outcomes.
Here are the main findings of the reviews:
Clinical guidelines
Increasing numbers of children and young people experiencing gender dysphoria are being referred to specialist gender services. There are various guidelines outlining approaches to the clinical care of these children and adolescents.
In the first two papers, the York researchers examined the quality and development of published guidelines or clinical guidance containing recommendations for managing gender dysphoria in children and young people up to the age of 18.
They studied a total of 23 guidelines published in different countries between 1998 and 2022. All but two were published after 2010.
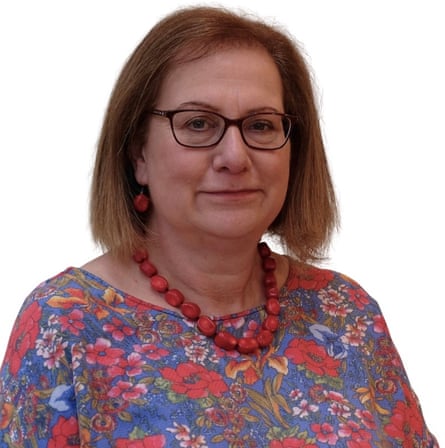
Most of them lacked “an independent and evidence-based approach and information about how recommendations were developed”, the researchers said.
Few guidelines were informed by a systematic review of empirical evidence and they lack transparency about how their recommendations were developed. Only two reported consulting directly with children and young people during their development, the York academics found.
“Healthcare services and professionals should take into account the poor quality and interrelated nature of published guidance to support the management of children and adolescents experiencing gender dysphoria/incongruence,” the researchers wrote.
Writing in the British Medical Journal (BMJ) , Cass said that while medicine was usually based on the pillars of integrating the best available research evidence with clinical expertise, and patient values and preferences, she “found that in gender medicine those pillars are built on shaky foundations”.
She said the World Professional Association of Transgender Healthcare (WPATH) had been “highly influential in directing international practice, although its guidelines were found by the University of York’s appraisal to lack developmental rigour and transparency”.
In the foreword to her report, Cass said while doctors tended to be cautious in implementing new findings “quite the reverse happened in the field of gender care for children”.
In one example, she said a single Dutch medical study, “suggesting puberty blockers may improve psychological wellbeing for a narrowly defined group of children with gender incongruence”, had formed the basis for their use to “spread at pace to other countries”. Subsequently, there was a “greater readiness to start masculinising/feminising hormones in mid-teens”.
She added: “Some practitioners abandoned normal clinical approaches to holistic assessment, which has meant that this group of young people have been exceptionalised compared to other young people with similarly complex presentations. They deserve very much better.”
Both papers repeatedly pointed to a key problem in this area of medicine: a dearth of good data.
She said: “Filling this knowledge gap would be of great help to the young people wanting to make informed choices about their treatment.”
Cass said the NHS should put in place a “full programme of research” looking at the characteristics, interventions and outcomes of every young person presenting to gender services, with consent routinely sought for enrolment in a research study that followed them into adulthood.
Gender medicine was “an area of remarkably weak evidence”, her review found, with study results also “exaggerated or misrepresented by people on all sides of the debate to support their viewpoint”.
Alongside a puberty blocker trial, which could be in place by December, there should be research into psychosocial interventions and the use of the masculinising and feminising hormones testosterone and oestrogen, the review found.
Hormone treatment
Many trans people who seek medical intervention in their transition opt to take hormones to masculinise or feminise their body, an approach that has been used in transgender adults for decades.
“It is a well-established practice that has transformed the lives of many transgender people,” the Cass review notes, adding that while these drugs are not without long-term problems and side-effects, for many they are dramatically outweighed by the benefits.
For birth-registered females, the approach means taking testosterone, which brings about changes including the growth of facial hair and a deepening of the voice, while for birth-registered males, it involves taking hormones including oestrogen to promote changes including the growth of breasts and an increase in body fat. Some of these changes may be irreversible.
However, in recent years a growing proportion of adolescents have begun taking these cross-sex, or gender-affirming, hormones, with the vast majority who are prescribed puberty blockers subsequently moving on to such medication.
This growing take-up among young people has led to questions over the impact of these hormones in areas ranging from mental health to sexual functioning and fertility.
Now researchers at the University of York have carried out a review of the evidence, comprising an analysis of 53 previously published studies, in an attempt to set out what is known – and what is not – about the risks, benefits and possible side-effects of such hormones on young people.
All but one study, which looked at side-effects, were rated of moderate or low quality, with the researchers finding limited evidence for the impact of such hormones on trans adolescents with respect to outcomes, including gender dysphoria and body satisfaction.
The researchers noted inconsistent findings around the impact of such hormones on growth, height, bone health and cardiometabolic effects, such as BMI and cholesterol markers. In addition, they found no study assessed fertility in birth-registered females, and only one looked at fertility in birth-registered males.
“These findings add to other systematic reviews in concluding there is insufficient and/or inconsistent evidence about the risks and benefits of hormone interventions in this population,” the authors write.
However, the review did find some evidence that masculinising or feminising hormones might help with psychological health in young trans people. An analysis of five studies in the area suggested hormone treatment may improve depression, anxiety and other aspects of mental health in adolescents after 12 months of treatment, with three of four studies reporting an improvement around suicidality and/or self-harm (one reported no change).
But unpicking the precise role of such hormones is difficult. “Most studies included adolescents who received puberty suppression, making it difficult to determine the effects of hormones alone,” the authors write, adding that robust research on psychological health with long-term follow-up was needed.
The Cass review has recommended NHS England should review the current policy on masculinising or feminising hormones, advising that while there should be the option to provide such drugs from age 16, extreme caution was recommended, and there should be a clear clinical rationale for not waiting until an individual reached 18.
Puberty blockers
Treatments to suppress puberty in adolescents became available through routine clinical practice in the UK a decade ago.
While the drugs have long been used to treat precocious puberty – when children start puberty at an extremely young age – they have only been used off-label in children with gender dysphoria or incongruence since the late 1990s. The rationale for giving puberty blockers, which originated in the Netherlands, was to buy thinking time for young people and improve their ability to smooth their transition in later life.
Data from gender clinics reported in the Cass review showed the vast majority of people who started puberty suppression went on to have masculinising or feminising hormones, suggesting that puberty blockers did not buy people time to think.
To understand the broader effects of puberty blockers, researchers at the University of York identified 50 papers that reported on the effects of the drugs in adolescents with gender dysphoria or incongruence. According to their systematic review, only one of these studies was high quality, with a further 25 papers regarded as moderate quality. The remaining 24 were deemed too weak to be included in the analysis.
Many of the reports looked at how well puberty was suppressed and the treatment’s side-effects, but fewer looked at whether the drugs had their intended benefits.
Of two studies that investigated gender dysphoria and body satisfaction, neither found a change after receiving puberty blockers. The York team found “very limited” evidence that puberty blockers improved mental health.
Overall, the researchers said “no conclusions” could be drawn about the impact on gender dysphoria, mental and psychosocial health or cognitive development, though there was some evidence bone health and height may be compromised during treatment.
Based on the York work, the Cass review finds that puberty blockers offer no obvious benefit in helping transgender males to help their transition in later life, particularly if the drugs do not lead to an increase in height in adult life. For transgender females, the benefits of stopping irreversible changes such as a deeper voice and facial hair have to be weighed up against the need for penile growth should the person opt for vaginoplasty, the creation of a vagina and vulva.
In March, NHS England announced that children with gender dysphoria would no longer receive puberty blockers as routine practice. Instead, their use will be confined to a trial that the Cass review says should form part of a broader research programme into the effects of masculinising and feminising hormones.
- Transgender
- Young people
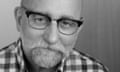
Veteran trans campaigner: ‘Cass review has potential for positive change’
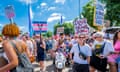
Cass review must be used as ‘watershed moment’ for NHS gender services, says Streeting
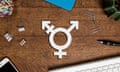
‘This isn’t how good scientific debate happens’: academics on culture of fear in gender medicine research
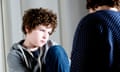
Five thousand children with gender-related distress awaiting NHS care in England
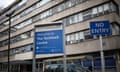
Ban on children’s puberty blockers to be enforced in private sector in England
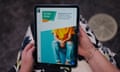
What Cass review says about surge in children seeking gender services
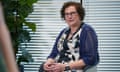
Adult transgender clinics in England face inquiry into patient care
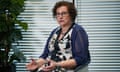
‘Children are being used as a football’: Hilary Cass on her review of gender identity services
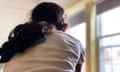
Thousands of children unsure of gender identity ‘let down by NHS’, report finds
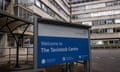
Most viewed
How Quickly Do Prices Respond to Monetary Policy?
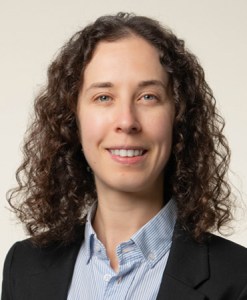
Download PDF (238 KB)
FRBSF Economic Letter 2024-10 | April 8, 2024
With inflation still above the Federal Reserve’s 2% objective, there is renewed interest in understanding how quickly federal funds rate hikes typically affect inflation. Beyond monetary policy’s well-known lagged effect on the economy overall, new analysis highlights that not all prices respond with the same strength or speed. Results suggest that inflation for the most responsive categories of goods and services has come down substantially from recent highs, likely due in part to more restrictive monetary policy. As a result, the contributions of these categories to overall inflation have fallen.
Monetary policy affects inflation with a lag. This means that, although interest rates react quickly when the Federal Reserve raises the federal funds rate, the effects on inflation are slower and indirect. Higher interest rates increase borrowing costs, slowing investment and overall demand, which ultimately eases the pressure on prices. Understanding the timing and strength of this mechanism is key for policymakers.
Many researchers have estimated the speed and strength of the economy’s response to monetary policy, notably Romer and Romer (2004). The focus is typically a broader measure of inflation, such as headline or core, which reflects an average across many goods and services. However, not all prices of the component goods and services react to monetary policy in the same way. For example, food and energy prices, which are excluded from core but included in headline inflation, often move more in response to global market fluctuations, such as changes in international oil prices, rather than to changes in domestic monetary policy.
In this Economic Letter , we estimate how prices of different goods and services respond to changes in the federal funds rate and use those estimates to build a monetary policy-responsive inflation index. We find substantial variation in how prices react to monetary policy, which suggests that understanding the makeup of overall inflation can provide insights into the transmission of monetary policy to inflation. The extent to which categories that are more responsive to the federal funds rate contribute to inflation affects how much slowing in economic activity is needed to reduce overall inflation. Our analysis indicates that recent ups and downs of inflation have been focused in categories that are most sensitive to monetary policy. Inflation rates for the most sensitive categories—and their contributions to headline inflation—rose from the first half of 2020 through mid-2022, reaching a higher peak than headline inflation, and then began to decline. The inflation rate for this most responsive group of goods and services categories is now close to its pre-2020 rate. Our findings suggest that the Fed’s rate hikes that began in March 2022 are exerting downward pressure on prices and will continue to do so in the near term. Our estimated lags are consistent with the view that the full effects of past policy tightening are still working their way through the economy.
Measuring how prices react to monetary policy
To understand which goods and services are most responsive to monetary policy, we need to determine how their prices react to changes in the federal funds rate, the Federal Reserve’s main policy rate. Because the Federal Reserve adjusts the federal funds rate target in response to macroeconomic developments, including inflation, we use a transformation of the federal funds rate in our estimation. This transformed series, developed by Romer and Romer (2004) and updated by Wieland and Yang (2020), captures the differences between Federal Reserve staff forecasts and the chosen target rate, leaving only policy shocks, or movements in the federal funds rate that are not driven by actual or anticipated changes in economic conditions. We use this series as a so-called instrument for the federal funds rate, such that our results can account for how the federal funds rate itself, rather than its transformation, affects inflation.
We use an approach developed by Jordà (2005) that compares two forecasts—with and without rate shocks—to estimate how the federal funds rate affects price movements over time. Specifically, we estimate the relationship between the federal funds rate and the cumulative percent change in prices, controlling for recent trends in the federal funds rate, inflation, and economic activity. Repeating this estimation over multiple horizons produces a forecast comparison, or impulse response function, that gives us an estimate of the expected percent change in prices following a rate increase. For example, applying this method to the headline personal consumption expenditures (PCE) price index indicates that four years after a 1 percentage point increase in the federal funds rate, overall prices are typically about 2.5% below what they would have been without the rate increase.
Creating a policy-responsive inflation index
We estimate impulse response functions separately for the 136 goods and services categories that collectively make up headline PCE inflation. Figure 1 shows examples of the largest cumulative percent price declines over a four-year period in response to a 1 percentage point increase in the federal funds rate. The goods and services categories selected as examples account for large shares of total expenditures in headline PCE inflation. We also include one example of the few categories where prices do not decline, higher education, shown as a small positive value.
Figure 1 Reaction to a policy rate increase: Selected PCE categories
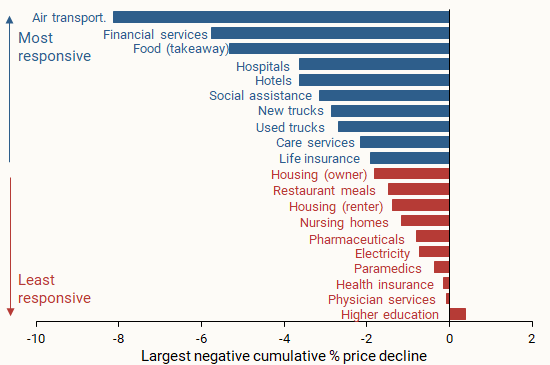
The takeaway from Figure 1 is that headline PCE inflation is made up of categories that differ in their responsiveness to increases in the federal funds rate. Some respond more strongly, such as those with larger typical cumulative price declines, while others respond less strongly, such as those with smaller typical price declines. Focusing on the most responsive categories can shed light on how monetary policy has influenced the path of inflation over the post-pandemic period. We use our results to divide the categories into two groups of goods and services. The most responsive group (blue bars) contains goods and services whose largest cumulative percent price decline over a four-year window is in the top 50% of all such declines. The least responsive group (red bars) contains goods and services in the bottom 50%.
Following the methods in Shapiro (2022), we use these two groups, along with the share of total expenditures for each good or service, to create two new aggregate PCE inflation measures. Figure 2 shows their 12-month percent changes over time. The blue shading marks the period from mid-2019 until early 2020 when the Federal Reserve lowered the federal funds rate. The vertical yellow line marks the start of the most recent tightening cycle in March 2022. Inflation in the most responsive categories (blue line) is more volatile than overall headline PCE inflation (green line) from the Bureau of Economic Analysis (BEA), and inflation in the least responsive categories is less volatile (red line).
Figure 2 Most and least responsive inflation rates
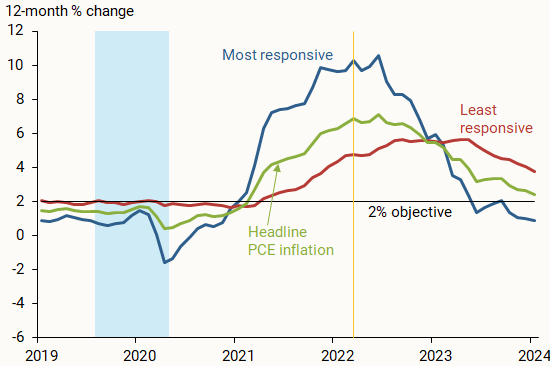
After the start of the 2020 recession, inflation rates for both categories rose but have since come down from their recent peaks. This pattern is particularly pronounced for the most responsive inflation group, for which inflation peaked at 10.5% in mid-2022 and has fallen to 0.9% as of January 2024; this is just under its average of 1% from 2012, when the Federal Reserve officially adopted a numerical inflation objective, to 2019. Inflation in the least responsive group peaked later, in early 2023, and has fallen only slightly to 3.8% as of January 2024; it remains well above its 2012–2019 average of 1.8%.
How does policy-responsive inflation react to rate increases?
The inflation rates of categories in the most and least responsive groups can move for reasons beyond changes in the federal funds rate, such as global or national macroeconomic developments. To assess the specific role of policy rate increases, we use the methodology described earlier to estimate how the most and least responsive inflation groups tend to react to rate hikes.
The results in Figure 3 suggest that an increase in the federal funds rate typically starts exerting downward pressure on the most responsive prices after about 18 months, when the line showing the impulse response function falls below zero. Month-to-month price changes start falling after a little over a year, depicted when the slope drops below zero and stays negative. This is quicker than the response of overall headline prices from the BEA (not shown), which becomes negative after a little over 24 months and shows month-to-month declines after about 18 months.
Figure 3 Reaction of most and least responsive prices to rate hikes
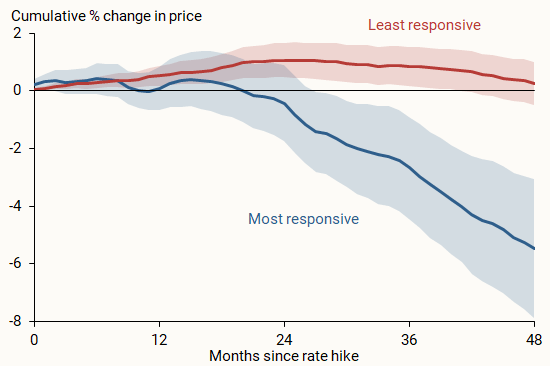
Because we grouped inflation categories based on the size of their response, there is not necessarily a tie-in to the speed of each categories’ change. However, our results suggest that looking at the most responsive goods and services may also be a useful way of assessing how quickly monetary policy affects inflation.
Applying the typical impact timing of the most responsive group of goods and services to the most recent tightening cycle, shown by the federal funds rate line in Figure 4, leads to several conclusions. First, rate cuts from 2019 to early 2020 could have contributed upward price pressures starting in mid- to late 2020 and thus could explain some of the rise in inflation over this period. Second, the tightening cycle that began in March 2022 likely started putting downward pressure on prices in mid-2023 and will continue to do so in the near term. This is consistent with the view that the full effects of monetary policy tightening have yet to be felt. Finally, though inflation for the most responsive categories has been falling since mid-2022, the early part of this decline was likely to have been driven more by changes in prevailing economic conditions than by policy tightening, given estimated policy lags. Some research has considered whether policy lags have shortened (see, for example, Doh and Foerster 2021); however, because inflation began falling mere months after the first rate hike, the drop in inflation may have been too soon to be caused by policy action.
Figure 4 Headline inflation contributions and the federal funds rate
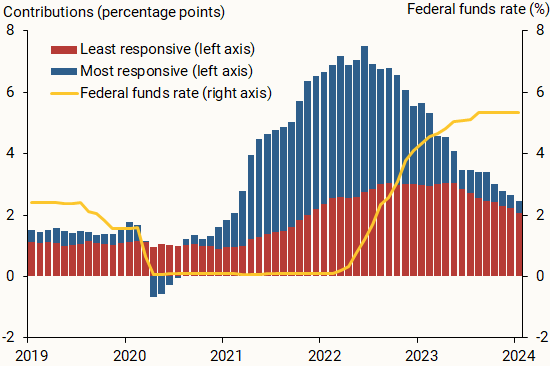
Our findings in this Letter are useful for broadening our understanding of how monetary policy affects inflation. For example, if inflation and the contributions to overall headline inflation are high in a set of categories that are more responsive to monetary policy, as was the case in early 2022, then rate hikes during the most recent tightening cycle are likely to continue to reduce inflation due to policy lags. On the other hand, though inflation in the least responsive categories may come down because of other economic forces, less inflation is currently coming from categories that are most responsive to monetary policy, perhaps limiting policy impacts going forward.
Doh, Taeyoung, and Andrew T. Foerster. 2022. “ Have Lags in Monetary Policy Transmission Shortened? ” FRB Kansas City Economic Bulletin (December 21).
Jordà, Òscar. 2005. “Estimation and Inference of Impulse Responses by Local Projections.” American Economic Review 95(1), pp. 161–182.
Romer, Christina, and David Romer. 2004. “A New Measure of Monetary Shocks: Derivation and Implications.” American Economic Review 94(4), pp. 1,055–1,084.
Shapiro, Adam. 2022. “ A Simple Framework to Monitor Inflation .” FRB San Francisco Working Paper 2020-29.
Wieland, Johannes, and Mu‐Jeung Yang. 2020. “Financial Dampening.” Journal of Money, Credit and Banking 52(1), pp. 79–113.
Opinions expressed in FRBSF Economic Letter do not necessarily reflect the views of the management of the Federal Reserve Bank of San Francisco or of the Board of Governors of the Federal Reserve System. This publication is edited by Anita Todd and Karen Barnes. Permission to reprint portions of articles or whole articles must be obtained in writing. Please send editorial comments and requests for reprint permission to [email protected]
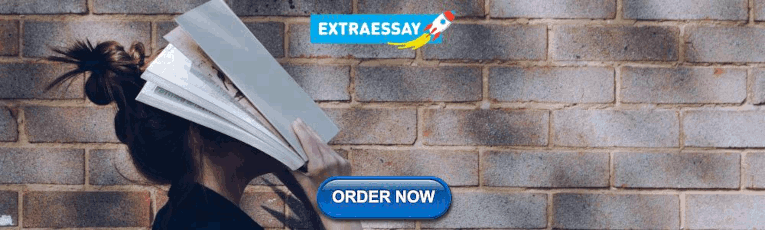
IMAGES
VIDEO
COMMENTS
On the basis of Rocco (2010), Storberg-Walker's (2012) amended list on qualitative data analysis in research papers included the following: (a) the article should provide enough details so that reviewers could follow the same analytical steps; (b) the analysis process selected should be logically connected to the purpose of the study; and (c ...
The analysis shows that you can evaluate the evidence presented in the research and explain why the research could be important. Summary. The summary portion of the paper should be written with enough detail so that a reader would not have to look at the original research to understand all the main points. At the same time, the summary section ...
This article is a practical guide to conducting data analysis in general literature reviews. The general literature review is a synthesis and analysis of published research on a relevant clinical issue, and is a common format for academic theses at the bachelor's and master's levels in nursing, physiotherapy, occupational therapy, public health and other related fields.
Communications Program. 79 John F. Kennedy Street Cambridge, Massachusetts 02138. TIPS FOR WRITING ANALYTIC RESEARCH PAPERS. • Papers require analysis, not just description. When you describe an existing situation (e.g., a policy, organization, or problem), use that description for some analytic purpose: respond to it, evaluate it according ...
Table of contents. Step 1: Write your hypotheses and plan your research design. Step 2: Collect data from a sample. Step 3: Summarize your data with descriptive statistics. Step 4: Test hypotheses or make estimates with inferential statistics.
2.1 Step 1: defining the research question. The first step in conducting a meta-analysis, as with any other empirical study, is the definition of the research question. Most importantly, the research question determines the realm of constructs to be considered or the type of interventions whose effects shall be analyzed.
A research paper is a piece of academic writing that provides analysis, interpretation, and argument based on in-depth independent research. Research papers are similar to academic essays , but they are usually longer and more detailed assignments, designed to assess not only your writing skills but also your skills in scholarly research.
When to use thematic analysis. Thematic analysis is a good approach to research where you're trying to find out something about people's views, opinions, knowledge, experiences or values from a set of qualitative data - for example, interview transcripts, social media profiles, or survey responses. Some types of research questions you might use thematic analysis to answer:
Analysis in Research Papers. To analyze means to break a topic or concept down into its parts in order to inspect and understand it, and to restructure those parts in a way that makes sense to you. In an analytical research paper, you do research to become an expert on a topic so that you can restructure and present the parts of the topic from ...
Definition of research in data analysis: According to LeCompte and Schensul, research data analysis is a process used by researchers to reduce data to a story and interpret it to derive insights. The data analysis process helps reduce a large chunk of data into smaller fragments, which makes sense. Three essential things occur during the data ...
Save the word count for the "meat" of your paper — that is, for the analysis. 2. Summarize the Article. Now, you should write a brief and focused summary of the scientific article. It should be shorter than your analysis section and contain all the relevant details about the research paper.
A research question is what a study aims to answer after data analysis and interpretation. The answer is written in length in the discussion section of the paper. Thus, the research question gives a preview of the different parts and variables of the study meant to address the problem posed in the research question.1 An excellent research ...
Analysis is a type of primary research that involves finding and interpreting patterns in data, classifying those patterns, and generalizing the results. It is useful when looking at actions, events, or occurrences in different texts, media, or publications. Analysis can usually be done without considering most of the ethical issues discussed ...
It is easy to confuse systematic reviews and meta-analyses. A systematic review is an objective, reproducible method to find answers to a certain research question, by collecting all available studies related to that question and reviewing and analyzing their results. A meta-analysis differs from a systematic review in that it uses statistical ...
Definition: Research Paper is a written document that presents the author's original research, analysis, and interpretation of a specific topic or issue. It is typically based on Empirical Evidence, and may involve qualitative or quantitative research methods, or a combination of both. The purpose of a research paper is to contribute new ...
Abstract. Statistical methods involved in carrying out a study include planning, designing, collecting data, analysing, drawing meaningful interpretation and reporting of the research findings. The statistical analysis gives meaning to the meaningless numbers, thereby breathing life into a lifeless data. The results and inferences are precise ...
A subsequent paper by Kaczynski et al. ... R provides a flexible and extensible free environment to conduct research and analysis. Researchers can contribute open-source routines and packages, which promotes reproducibility. R's package ecosystem is one of its major advantages; packages are available for most widely used statistical, data ...
The AI Index report tracks, collates, distills, and visualizes data related to artificial intelligence (AI). Our mission is to provide unbiased, rigorously vetted, broadly sourced data in order for policymakers, researchers, executives, journalists, and the general public to develop a more thorough and nuanced understanding of the complex field ...
Research in Ibrahim et al. 40 centered on predicting early market movements of Bitcoin by harnessing sentiment analysis of X data 41,42. The primary objective of their work was to introduce a ...
The scope of ES&T is broad and comprehensive, covering diverse areas associated with preserving and restoring human health and the environment. This includes air and water quality, remediation technologies, natural processes, and supporting infrastructures. This wide-ranging scope ensures that the most impactful publications involve not only rigorous scientific and technological research but ...
The most logical way to structure quantitative results is to frame them around your research questions or hypotheses. For each question or hypothesis, share: A reminder of the type of analysis you used (e.g., a two-sample t test or simple linear regression). A more detailed description of your analysis should go in your methodology section.
Charlie Mace, associate professor of chemistry, thought of a way to help. He and his team created a paper device called a patterned dried blood spot card, in which the paper is engineered with channels and collection zones that measure out defined volumes of blood and allow it to dry in place for later analysis.
Working Paper 32340. DOI 10.3386/w32340. Issue Date April 2024. This study investigates the growing wage disparity between older and younger workers in high-income countries. We propose a conceptual framework of the labor market in which firms cannot change the contracts of older employees and cannot freely add higher-ranked positions to their ...
1.1. Roles of canids in South American human societies. Ethnographic research has demonstrated the different types of relationships between wild animals and humans that can arise from their interactions, from that of prey to that of pet [15,16].For instance, in Amazonian indigenous communities, young wild canid species are commonly adopted, become part of the family and are treated like humans ...
CEA simulations show that by 2055, a policy that increased the college going rate by 1, 3, and 5 percentage points could increase the level of GDP in 2055 (thirty years from now) by 0.2, 0.6, and ...
Research methods are specific procedures for collecting and analyzing data. Developing your research methods is an integral part of your research design. When planning your methods, there are two key decisions you will make. First, decide how you will collect data. Your methods depend on what type of data you need to answer your research question:
Analysis finds most research underpinning clinical guidelines, hormone treatments and puberty blockers to be low quality. Review of gender services has major implications for mental health services
With inflation still above the Federal Reserve's 2% objective, there is renewed interest in understanding how quickly federal funds rate hikes typically affect inflation. Beyond monetary policy's well-known lagged effect on the economy overall, new analysis highlights that not all prices respond with the same strength or speed. Results suggest that inflation for the most responsive ...