- Open supplemental data
- Reference Manager
- Simple TEXT file
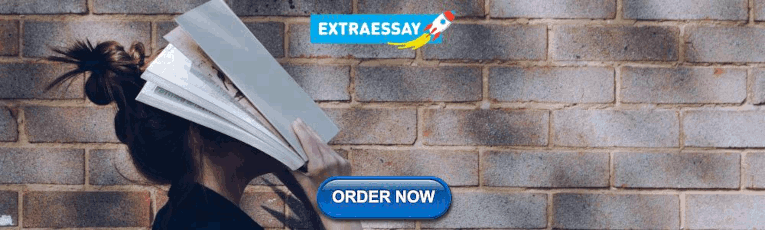
People also looked at
Original research article, when and why did human brains decrease in size a new change-point analysis and insights from brain evolution in ants.
- 1 Department of Anthropology, Dartmouth College, Hanover, NH, United States
- 2 Ecology, Evolution, Environment, and Society, Dartmouth College, Hanover, NH, United States
- 3 Department of Biology, Boston University, Boston, MA, United States
- 4 Graduate Program in Neuroscience, Boston University, Boston, MA, United States
- 5 Department of Anthropology, Boston University, Boston, MA, United States
- 6 Department of Evolution, Ecology, and Organismal Biology, The Ohio State University, Columbus, OH, United States
- 7 Department of Anatomy and Cell Biology, Oklahoma State University Center for Health Sciences, Tulsa, OK, United States
Human brain size nearly quadrupled in the six million years since Homo last shared a common ancestor with chimpanzees, but human brains are thought to have decreased in volume since the end of the last Ice Age. The timing and reason for this decrease is enigmatic. Here we use change-point analysis to estimate the timing of changes in the rate of hominin brain evolution. We find that hominin brains experienced positive rate changes at 2.1 and 1.5 million years ago, coincident with the early evolution of Homo and technological innovations evident in the archeological record. But we also find that human brain size reduction was surprisingly recent, occurring in the last 3,000 years. Our dating does not support hypotheses concerning brain size reduction as a by-product of body size reduction, a result of a shift to an agricultural diet, or a consequence of self-domestication. We suggest our analysis supports the hypothesis that the recent decrease in brain size may instead result from the externalization of knowledge and advantages of group-level decision-making due in part to the advent of social systems of distributed cognition and the storage and sharing of information. Humans live in social groups in which multiple brains contribute to the emergence of collective intelligence. Although difficult to study in the deep history of Homo , the impacts of group size, social organization, collective intelligence and other potential selective forces on brain evolution can be elucidated using ants as models. The remarkable ecological diversity of ants and their species richness encompasses forms convergent in aspects of human sociality, including large group size, agrarian life histories, division of labor, and collective cognition. Ants provide a wide range of social systems to generate and test hypotheses concerning brain size enlargement or reduction and aid in interpreting patterns of brain evolution identified in humans. Although humans and ants represent very different routes in social and cognitive evolution, the insights ants offer can broadly inform us of the selective forces that influence brain size.
We live in a community of knowledge. Everything we do depends on knowledge that is both inside our head as well as out in the world and in other people’s heads.
Steven Slomen 1 , author of Knowledge Illusion: Why We Never Think Alone
The key to the origin of the human condition is not to be found in our species exclusively, because the story did not start and end with humanity.
E. O. Wilson, The Social Conquest of Earth
Only humans and social insects can build and manage large-scale societies according to complex economic decision rules.
Boomsma and Franks, 2006
Introduction
Understanding the causes and consequences of brain evolution in humans—particularly the role of social life—is significant to understanding the nature of humanity. Across diverse clades, sociality is hypothesized to drive brain size and structure. In primates, greater cognitive challenges associated with forming bonded social groups in large societies, among other influences ( DeCasien et al., 2017 ; González-Forero and Gardner, 2018 ; DeCasien and Higham, 2019 ), appear to have selected for increased brain size ( Dunbar, 1998 ; Dunbar and Shultz, 2007 , 2017 ; Meguerditchian et al., 2021 ). A broad phylogenetic perspective can be of significant value in exploring the evolution of nervous systems ( Striedter et al., 2014 ; Keifer and Summers, 2016 ; Shigeno, 2017 ). Although significantly different in sociality, computation and decision-making in humans and social insects are accomplished by physical neuroarchitectures (“solid brains”) as well as “liquid brains” formed by interactions of group members that create collective intelligence ( Couzin, 2009 ; Pagán, 2019 ; Piñero and Solé, 2019 ; Reséndiz-Benhumea et al., 2021 ). Across diverse species that vary in social organization, cognitive demands on individuals may be lower in societies in which group decision-making is more efficacious than individual decision-making ( Surowiecki, 2004 ; Sumpter, 2006 ; Krause et al., 2010 ; Woolley et al., 2010 ; Sasaki and Pratt, 2018 ; Bak-Coleman et al., 2021 ). Superorganismic decentralized “brain” networks characterize humans and ants, the premier social insect. Collective intelligence may reduce brain size in both clades ( Bailey and Geary, 2009 ; Feinerman and Traniello, 2016 ). Therefore, the size of groups and society-level intelligence may affect behavioral performance and cognitive loads and increase or reduce brain size, depending on context.
Over the course of hominin evolution, encephalization has been dynamic (e.g., Miller et al., 2019 ). Australopithecus cranial capacities were on average 20% larger than those of the late Miocene hominins Sahelanthropus and Ardipithecus or modern chimpanzees, despite having chimpanzee-sized bodies ( Wolpoff, 1999 ; Cartmill and Smith, 2009 ; DeSilva, 2011 ). These ∼450 cc brains remained roughly unchanged in size from 3.5 to 2.0 million years ago even though late australopiths (e.g., Paranthropus ) underwent extensive diversification. With the evolution of Homo , brains began to expand but gross neural organization may have remained primitive ( Ponce de León et al., 2021 ). Additionally, brain expansion was not universal in fossil Homo as evidenced by the small-brained Middle and Late Pleistocene hominins Homo naledi ( Berger et al., 2015 ; Montgomery, 2018 ) and Homo floresiensis ( Brown et al., 2004 ; Figure 1 ). Although an almost fourfold increase in brain volume during the last 2 million years is a hallmark in human evolution, it remains unappreciated—but well-documented—that both absolute and relative brain size have decreased since the end of the Pleistocene ( Schwidetzky, 1976 ; Wiercinski, 1979 ; Beals et al., 1984 ; Henneberg, 1988 ; Henneberg and Steyn, 1993 ; Ruff et al., 1997 ; Bailey and Geary, 2009 ; Hawks, 2011 ; Bednarik, 2014 ; Liu et al., 2014 ; Bruner and Gleeson, 2019 ). The precise timing of this decrease in brain size, however, is unclear. Some have placed its origin in the late Pleistocene ∼35 kyr ( Ruff et al., 1997 ) and others in the more recent Holocene ∼10 kyr ( Henneberg, 1988 ; Hawks, 2011 ).
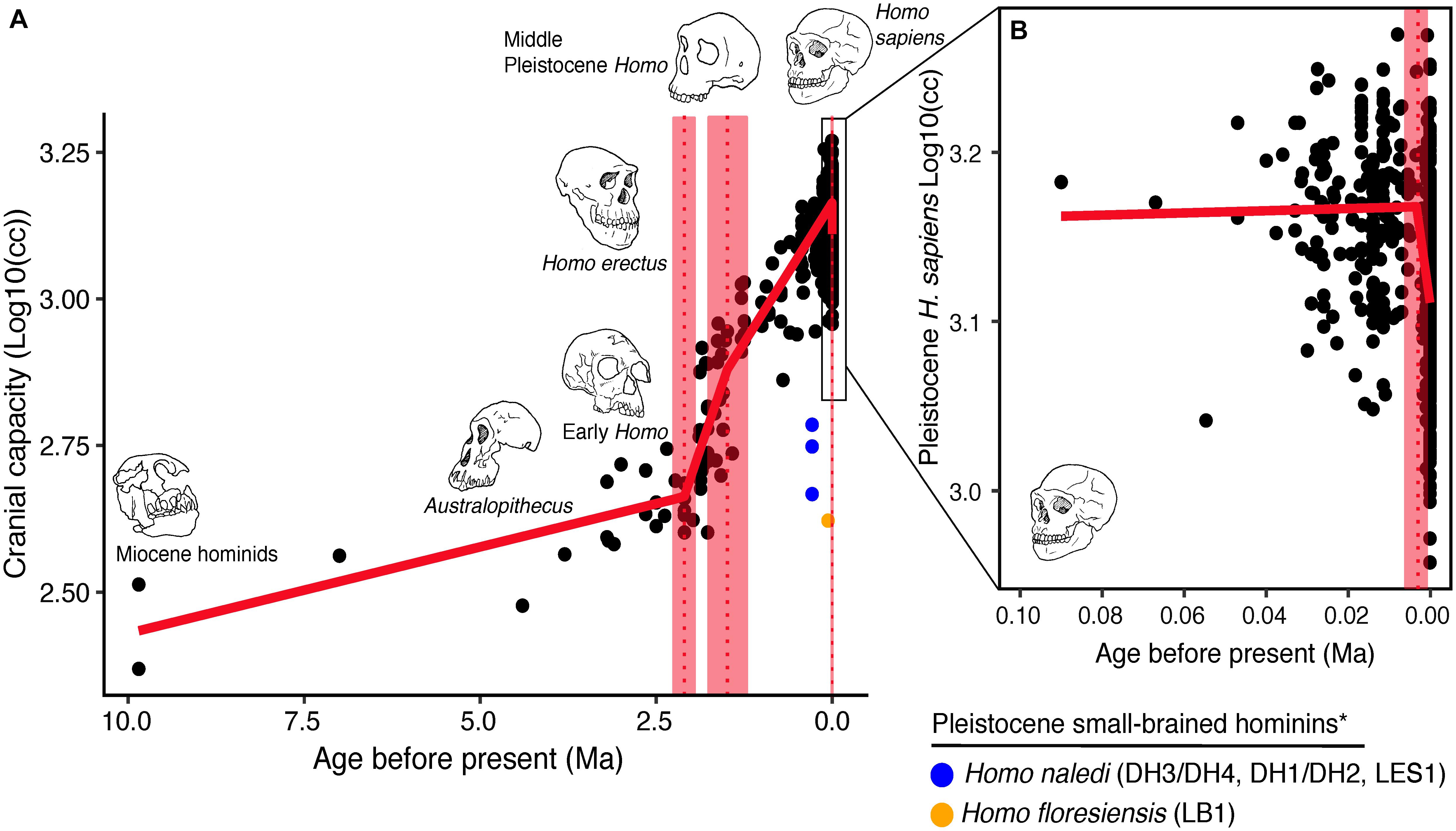
Figure 1. Trends in hominin brain evolution. (A) Cranial capacity in fossil apes (Miocene hominids) and hominins over the last 10 million years. Brain size remained relatively steady throughout the late Miocene and Pliocene, increasing only slightly in Australopithecus compared with earlier hominins. However, by 2 million years ago, there was a dramatic increase in the rate of growth, coinciding with fossil evidence for the earliest members of genus Homo . This change point is illustrated by a red vertical dotted line (95% CI shown as thick pink vertical line). A second change-point is detected at ∼1.5 million years ago and the rate of brain size increase remains steady through the Pleistocene and the evolution of Homo sapiens . If the small-brained Middle and Late Pleistocene hominins H. naledi (blue dots) and H. floresiensis (orange dot) are included, these two change points merge into a single, overlapping encephalization event between 1.97 and 2.21 million years ago. (B) During the last 100,000 years, brain size has remained steady in H. sapiens until a rapid and dramatic change point only 3,000 years ago decreased Holocene human brain size at a rate fifty times greater than the previous increases in Pleistocene brain volume. Each black dot represents an individual fossil skull or osteological specimen.
Here we investigate historical patterns of human brain evolution to date major inflection points of changes in size to attempt to identify selective factors in the environment that may have prevailed during times of significant change in brain size. Because the deep history of neural tissue and its organization is difficult to explore, we use ants to model the broad impacts of social selection on brain size evident in nature to gain insights into the possible selective forces that influenced patterns of human brain evolution. Although phylogenetically remote from humans, ants provide examples of brain evolution that may help identify selective factors and offer neuroarchitectural details to complement the metric of brain size. These advantages may compensate for some of the limitations of behavioral and neurobiological research on extinct hominin forms.
Ants are eusocial insects characterized by reproductive division of labor, cooperative brood care, and overlap of generations. They are exemplars of social life that encompass extant species basal in social structure and highly complex species, enabling comparative studies. Ants are eusocial, and humans have been characterized as eusocial ( Foster and Ratnieks, 2005 ; Boomsma and Franks, 2006 ; Betzig, 2014 ; D’Ettorre, 2017b ), both forming large, complex, kin-oriented societies, including those with agricultural practices and full-time division of labor. In humans, however, division of labor is not associated with a loss of reproductive potential. Ultrasocial species ( Campbell, 1983 ; Gowdy and Krall, 2013 , 2016 ) produce their own food crops and include some ants (and termites) and humans. Sociobiological parallels can be leveraged to understand the general role of advanced social life in brain evolution. While divergent in key aspects of social organization, humans and ants exhibit important convergences, for example, in the ability to act collectively. And although their brains are structurally and functionally different, our understanding of brain size scaling and structural allometries in ants provide opportunities to address general questions of brain evolution—including size reduction — in humans.
Materials and Methods
To understand temporal patterns of human brain evolution, we applied a change-point analysis to identify the timing of inflection points in hominin brain evolution using our brain size dataset of 985 dated log 10 transformed estimates of hominin cranial capacities (cc) compiled from the literature ( Supplementary File 1 ). The dataset represents brain evolution over the last 10 million years of hominid and hominin evolution and includes Rudapithecus ( N = 2), Sahelanthropus ( N = 1), Ardipithecus ( N = 1), Australopithecus (including Paranthropus ) ( N = 29), Early Pleistocene Homo ( N = 37), Middle Pleistocene Homo ( N = 60), Late Pleistocene Homo ( N = 156), and Holocene H. sapiens ( N = 699). We only used published cranial volumes (cc or mL) and not cadaver-derived weights (g) since cranial volume and brain weight are not equivalent (e.g., Tobias, 1970 ). Analysis was limited to individuals that are estimated to have been at least 10 years old and thus had exceeded the age at which modern H. sapiens achieves adult brain volume ( Coqueugniot and Hublin, 2012 ).
Changepoints were determined using the packages changepoint ( Killick and Eckley, 2014 ) and segmented ( Muggeo, 2008 ) in R Studio (Version 1.2. 5019). Changepoint was first used to provide approximate prior estimates for changes in the mean log 1 0 (cc) across the time series using the “BinSeg” method. These prior estimates were then used to fit a piecewise generalized linear model to the data with the segmented package ( Muggeo, 2008 ), which provided estimates of 1) the locations of changepoints (or breakpoints) in the slope of the time series; and 2) the slopes of the lines around each changepoint, which we interpreted as approximate rates of evolutionary change. Because the phylogenetic relationship of the different hominin species remains contentious and because there is genetic evidence for interbreeding between many Late Pleistocene hominin populations (e.g., Gokcumen, 2020 ), we included all hominin specimens in the change point analysis. However, we ran two separate models: one including the small-brained Middle and Late Pleistocene fossils from H. naledi and H. floresiensis and one excluding them. We mainly describe the results for when these small brained species were excluded ( N = 981), but the timing of the decrease in hominin brain size in the Holocene was negligible between these two models.
The best piecewise GLM model fit explained approximately 79% of the variance (adj r 2 = 0.79) in log 1 0 (cc) values and identified three changepoints ( Table 1 ). The first was detected at 2.10 ± 0.07 Ma, coincident with fossil evidence near the first known occurrence of Homo erectus ( Herries et al., 2020 ). At 2.10 Ma, the rate of evolution increased sharply from 0.03 ± 0.01 log 1 0 (cc)/Ma to 0.35 ± 0.05 log 1 0 (cc)/Ma. A second changepoint occurred at 1.49 ± 0.14 Ma when the rate of evolution slowed to 0.19 ± 0.01 log 10 (cc)/Ma. A steady increase in brain size—independent of body size—followed and lasted through the Pleistocene ( Ruff et al., 1997 ; Lee and Wolpoff, 2003 ; Rightmire, 2004 ; Hawks, 2011 ). We identified a third changepoint at 0.003 ± 0.001 Ma at a rate of −17.16 ± 6.69 log 1 0 (cc)/Ma. This rate is 50 times greater than the renowned increase in human brain size. Our data suggest that this reduction may have been more recent—3,000 years ago—than previously suggested. We interpret our result conservatively, and caution that any findings about brain size changes throughout human evolution are contingent on the resolution of the available dataset (e.g., VanSickle et al., 2020 ). Indeed, the inclusion of smaller-brained Pleistocene hominins ( H. naledi and H. floresiensis ) reduced model fit slightly (adj r 2 = 0.74) and had the effect of widening the 95% CIs of the first two changepoints such that they overlapped between the wider time interval of 2.2–1.7 Ma ( Table 2 ). Yet the timing of the third Holocene changepoint in this model was unaffected by the inclusion of these specimens, although its 95%CI also widened slightly ( Table 2 ).

Table 1. Estimates of changepoint dates—excluding small-brained Pleistocene Homo ( N = 981).

Table 2. Estimates of changepoint dates—including small-brained Pleistocene Homo ( N = 985).
Brain Size Increase and Reduction in Humans
The changepoints we identified document trends in brain size but do not reveal underlying causes and mechanisms of encephalization. The expensive tissue hypothesis ( Aiello and Wheeler, 1995 ) posits that a trade-off in allocated resources from one expensive tissue (the brain) to another (the gut) was made possible by shifts to a higher quality diet in Homo , enabled in part by enhanced technological skills ( Lepre et al., 2011 ), exploitation of diverse resources ( Braun et al., 2010 ), and the invention of cooked foods through controlled fire ( Wrangham, 2009 ; Herculano-Houzel, 2016 ). In addition to evidence that energetic constraints on encephalization may have been released by dietary shifts in early Homo , others have posited that brain expansion in early Homo may have been driven by the need for enhanced social intelligence ( Dunbar, 1998 ; Dunbar and Shultz, 2017 ). With growing complexity in social life, perhaps associated with increased group size, brain expansion occurred. Resource sharing within groups and allocare may have provided the energy surplus needed to support the increased energetic cost of a larger brain ( Isler and van Schaik, 2012 ). These hypotheses are not mutually exclusive: a combination of social challenges and ecological pressures and increased dietary quality and breadth drove Pleistocene brain expansion in our ancestors, who were living in increasingly larger groups and likely benefitted from enhanced group-level cognitive abilities and greater cultural intelligence ( van Schaik et al., 2012 ), which could in turn accelerate brain expansion via a feedback loop ( Markov and Markov, 2020 ).
The cause of brain reduction in the Holocene is also unclear. One possibility is that it is associated with a corresponding decrease in body mass ( Henneberg, 1988 ; Ruff et al., 1997 ). The early expansion of the brain in Homo has been explained as an increase in absolute body size ( Ruff et al., 1997 ; Wood and Collard, 1999 ; McHenry and Coffing, 2000 ; Lee and Wolpoff, 2003 ) though Grabowski (2016) later employed quantitative genetics to suggest that selection had favored brain enlargement and body size increase was a by-product. Hawks (2011) contends that the absolute change in brain size from the Pleistocene to the Holocene is greater than expected based on changes in body mass in the same time period, emphasizing that given the correlation between human brain and body size ( Holloway, 1980 ), the observed 5 kg decrease in body size in the Holocene would account for only a 22 mL decrease in brain volume ( Hawks, 2011 ). However, the actual reduction is more than 5x greater, suggesting that body size alone cannot entirely explain the decrease in brain volume. We find here that body size reduction may have preceded brain size reduction by several millennia. Ruff et al. (1997) notes that the decrease in body size in late Pleistocene humans began around 50 kyr, whereas brain reduction appears to been a Holocene phenomenon.
Yet there may be other non-allometric explanations for the reduction in human brain size at the Pleistocene-Holocene transition due to energetic, nutritional ( Wiercinski, 1979 ), and/or developmental constraints ( Hawks, 2011 ). The brain size reduction in Holocene humans parallels that of domesticated animals, suggesting that humans have self-domesticated by deliberately removing highly aggressive individuals from breeding populations, leading to a reduction in intra-population (but not inter-population) aggression ( Leach, 2003 ; Hare and Tomasello, 2005 ; Wrangham, 2018 , 2019 ; Bruner and Gleeson, 2019 ; Hare and Woods, 2020 ). Brain reduction in this case would be a by-product (or cause) of docility, a phenomenon documented recently in domesticated cattle ( Balcarcel et al., 2021 ). Groves (1999) suggests that domesticated dogs have sufficiently co-evolved with humans to have symbiotically become our external senses, thereby decreasing our reliance on brain centers that process sensory information. However, our finding here—that brain size reduction occurred in just within last 3,000 years—is temporally inconsistent with these prior explanations. Human self-domestication is argued to have occurred at the onset of species ∼300,000 years ago ( Wrangham, 2019 ) or coincident with the evolution of what some have called “behavioral modernity” ∼80,000 years ago ( Hare and Woods, 2020 ). Recent fossil and genetic evidence indicate that dogs were domesticated > 20,000 years ago ( Perri et al., 2021 ).
Human and Ant Sociobiology and Evolutionary Neurobiology: Insights Into Brain Elaboration and Reduction
Human social life has long been analyzed in reference to the social organization of ants. Imms (1946) review of Haskins (1939) Of Ants and Men described ants as “predominant” among the “very few living creatures whose social development at all parallels our own.” He emphasized the value of having “a nearly complete series of evolutionary forms among living ants, numerous ‘missing links’ and ‘living fossils”’ and a history “much more complete and much better preserved than that of man.” Comparisons made to identify commonalities in patterns of sociality between these diverse clades continue to be made today ( Boomsma and Franks, 2006 ; Wilson, 2012 ; Crespi, 2014 ; D’Ettorre, 2017a , b ; Friedman and Søvik, 2021 ; Gowdy, 2021 ). The benefits of ant models for the study of human social evolution noted by Imms are made more compelling by an additional 80 + years of new species discovery (roughly 5,000–6,000 circa 1,940 to > 14,000 today), an expanded phylogeny (6 to ∼20 subfamilies), and an eruption of integrative sociobiological and neurobiological research. Ants exhibit striking variation in colony size and demography, and individual and colony-level cognition. Diversity in social phenotypes creates the potential to provide general insights into human social structure and brain evolution.
Acknowledging the limitations of analogies, human and ant comparisons may reveal patterns in nature that broadly suggest social and ecological selective forces relevant to brain evolution. We recognize that the uniqueness of human cognition ( Laland and Seed, 2021 ) cannot be overemphasized, and neurobiological parallels with ants are as constrained as sociobiological comparisons, if not more limited. Although ant and human brains have the common function of processing environmental information to adaptively respond to social signals and cues, they are structured very differently. The size, neuron number, and synaptic connectivity of an ant brain is a minute fraction of that of a human brain ( Herculano-Houzel, 2016 ; Godfrey et al., 2021 ). However, the computational power of an ant brain is remarkable for its size and miniaturization does not appear to constrain behavioral performance and/or higher-order processing ( Muscedere et al., 2014 ), social learning, or consciousness ( Avarguès-Weber and Giurfa, 2013 ; Barron and Klein, 2016 ; Perry et al., 2017 ; Lihoreau et al., 2019 ; Perry and Chittka, 2019 ; Elek et al., 2020 ; D’Ettorre et al., 2021 ). Social organization in ants may “require relatively simple and computationally inexpensive forms of cognition” ( Lihoreau et al., 2012 ), some extrinsic to brain operations, that could mitigate the need for advanced processing capability. Behavior in ants typically involves task routines and kinesthetic performance rather than sophisticated cognition. Unlike the human brain ( Noonan et al., 2018 ), ant brains appear to lack executive function and the ability to mentalize, among other circuitry specialized for human social performance.
Relative needs for social information processing are strikingly different in ants and humans. Olfaction is dominant in ants and involves a relatively small number of chemicals to guide individual actions and organize colony-level behavior. Vision appears to have very limited social functions and mainly functions in navigation during foraging ( Hölldobler and Wilson, 1990 ). The anatomy of ant brains thus largely reflects investment in brain centers such as the antennal lobes and optic lobes responsible for processing olfactory and visual stimuli, respectively. These inputs are mainly integrated in the mushroom bodies, brain compartments specialized in higher-order processing, learning, and memory, and to a lesser extent in the central complex. The mushroom bodies, deeply homologous to the vertebrate cortex ( Tomer et al., 2010 ), have long been consider to be the neuroanatomical seat of intelligence in ants and other insects ( Strausfeld et al., 1998 ), but the nature of ant intelligence is only very loosely comparable to that of humans. Mushroom body elaboration also preceded the evolution of eusociality ( Farris and Schulmeister, 2011 ).
Ants have nevertheless emerged as important models for understanding the role of sociality ( Ilies et al., 2015 ; Kamhi et al., 2016 , 2019 ; Godfrey and Gronenberg, 2019 ) and behavioral performance and cognition ( Muratore and Traniello, 2020 ; Muratore et al., 2021 ) in brain evolution. Analyses of sociality and brain size scaling (e.g., Godfrey and Gronenberg, 2019 ; Muratore and Traniello, 2020 ) and information processing through social interaction (e.g., Davidson et al., 2016 ) can contribute to broadly understanding how social biology may have influenced general aspects of human brain evolution. The allometric scaling of functionally specialized brain centers in ants allows the adaptiveness of brain mosaics to be explored (e.g., Muratore et al., 2021 ) and the metabolic costs of neural tissues that are highly significant to brain evolution ( Aiello and Wheeler, 1995 ) can be recorded in individual ant brains ( Coto and Traniello, 2021 ). Studies of the influences of diet, which is considered significant in primate (and human) brain evolution ( Wrangham, 2009 ; DeCasien et al., 2017 ), are facilitated by the diverse feeding ecologies of ants. Ant nutritional socioecology spans highly specialized as well as generalist predators that vary in colony size and degree of complexity of social organization, allowing the relative roles of diet and social complexity to be separated because specialist and generalist species that each form small and large colonies (Azorsa et al., in preparation). Ants also have culture in the form of tool use ( Zhou et al., 2020 and references therein), and although they do not process food by cooking, they may preserve prey ( Maschwitz et al., 1979 ).
The application of theories of vertebrate brain evolution to social insects has been debated ( Lihoreau et al., 2012 ; Farris, 2016 ) and studies describe variable relationships between sociality, brain and brain compartment size, body size, and group size in eusocial insects ( Farris and Schulmeister, 2011 ; Seid et al., 2011 ; Riveros et al., 2012 ). Workers of ant species characterized by larger colony size have larger brains, suggesting greater social interaction selects for increased brain size ( Wehner et al., 2007 ). Similarly, comparisons of socially basic and social complex ant species suggest larger colony size and collective actions have selected for larger brains and mushroom bodies ( Kamhi et al., 2016 ). In contrast, in monomorphic fungus-growing ants, which have agrarian habits, larger colony size is associated with decreased brain size ( Riveros et al., 2012 ). This latter comparison among agricultural ant species provides insight into how increased group size and sociality in human populations may have reduced brain size ( Bailey and Geary, 2009 ) due to a high level of emergent complexity.
Worker behavior undergoes age-related development in ants. Like human brains, ant worker brains exhibit age-related synaptic remodeling, suggesting synaptic pruning in association with an increasingly diverse, flexible, and efficient behavioral repertoire ( Seid et al., 2005 ; Seid and Traniello, 2006 ; Muscedere et al., 2009 ). Age and behavioral development can be associated with increased brain volume ( Muscedere and Traniello, 2012 ) and declines in the density of mushroom body microglomeruli ( Gordon and Traniello, 2018 )—“microprocessor”-like synaptic structures that underlie plasticity in sensory processing capability and behavior ( Groh and Rössler, 2011 ; Groh et al., 2014 ). Elements of human brain development are thus mirrored in ant brain ontogeny and social behavior. In mole rats, eusocial mammals convergent in social structure with eusocial insects, there is no clear association between social system and relative brain size: eusocial species do not have smaller or larger brains than social or solitary species ( Kverková et al., 2018 ). Although there is an apparent trend between social system and forebrain neuron number, this is likely an artifact of the extreme metabolic adaptations to subterranean life found in H. glaber ( Kverková et al., 2018 ; Browe et al., 2020 ). Ants appear to provide more useful models than eusocial mammals.
Division of Labor and Brain Investment Patterns
Division of labor is a core social trait in humans and ants that potentially influences brain size evolution by unequally distributing behavioral performance needs and cognitive loads across group members. Greater social complexity in ants is correlated with large group size and division of labor among polyphenic workers ( Anderson and McShea, 2001 ; Kappeler, 2019 ). Division of labor by morphologically differentiated and behaviorally specialized workers (physical castes) is an attribute of a relatively small number of ant species. Analyses of brain and behavior in these ants, especially those featuring extraordinary polymorphisms and high degrees of behavioral specialization, can provide important information on how distributed cognition impacts the size and scaling of brain size and structure in relation to body size. For example, mushroom bodies are disproportionally large in the brains of media (mid-size) workers of the fungus-growing ant Atta cephalotes . The diverse and behaviorally challenging task repertoire of these workers encompasses leaf-harvesting, and their social role and neuroanatomy differs from that of smaller and larger workers that perform other tasks ( Muratore et al., 2021 ). Using this ant as a model to understand how agriculture has influenced brain evolution may help identify social conditions, life histories, and ecological factors favoring either an increase or decrease in brain size. It can also help identify how brain centers responsible for higher-order processing have evolved in response to either narrowly circumscribed or pluripotent and flexible task repertoires. In humans, such changes may have occurred during the transition from hunter-gatherer to agrarian habits. Ants provide a window through which such evolutionary processes may have occurred, and neuroanatomical outcomes.
Other strongly polymorphic ants offer additional insights into the role of division of labor in the evolution of brain size and compartmental scaling ( Muscedere and Traniello, 2012 ; Ilies et al., 2015 ; Gordon et al., 2017 , 2019 ). Ecologically different species may have distinct neural phenotypes that vary in the size of sensory input compartments and the mushroom bodies, among other brain centers ( Muscedere and Traniello, 2012 ). Mushroom body size correlates positively with task plasticity, supporting the notion that greater demands on behavioral performance are reflected in greater investment in higher-order processing tissues. Task specialization may also affect brain size and compartmental scaling: larger colonies may show a higher level of task specialization among workers, reflected in relatively larger mushroom bodies ( Amador-Vargas et al., 2015 ). The larger picture is that brain differentiation can occur in association with division of labor in the absence of striking changes in body size, illustrating how selection may have operated in humans as social groups increased in size.
Collective Intelligence and Neuroenergetic Costs
Ponce de León et al. (2008) and Hawks (2011) suggested that reduction of human brain size—without any evidence for intellectual diminishment—implies selection for brain efficiency. In ants, there is evidence of selection for metabolic efficiency in the brain. Kamhi et al. (2016) used cytochrome oxidase (COX) activity, a proxy for neuron metabolism, to contrast brain evolution and social evolution in the weaver ant Oecophylla smaragdina and the garden ant Formica subsericea , two sister clades whose workers are equivalent in body size but differ strongly in social organization and collective intelligence. Increased social complexity in weaver ants—reflected in division of labor by worker physical castes, large colony size, and remarkable decentralized group action including cooperative nest building—was associated with larger mushroom bodies, implying greater needs for higher-order information processing. Weaver ant worker mushroom bodies have reduced COX activity. Therefore, increased brain size in socially complex species may be associated with reduced brain operation costs, contrasting with the assumption that increased brain size increases metabolic costs ( Aiello and Wheeler, 1995 ; Isler and van Schaik, 2009 ).
Social Selection and Brain Evolution
Reduction in brain size may not compromise cognitive performance if intelligence is an attribute of the society rather than the individual. Galton (1907) first described that the accuracy of decision making by human groups could exceed that of any individual group member. This concept of collective intelligence has since been elaborated in studies ranging from insects to humans ( Woolley et al., 2010 ; Ward et al., 2011 ; Sasaki and Pratt, 2018 ; Almaatouq et al., 2020 ). If brain production, maintenance, and operation costs are metabolically significant ( Aiello and Wheeler, 1995 ; Leonard et al., 2003 ; Isler and van Shaik, 2006 ; Chittka and Niven, 2009 ; Navarrete et al., 2011 ; Kuzawa et al., 2014 ; Pontzer et al., 2016 ; Herculano-Houzel, 2017 ), then collective intelligence may reduce demands for neural tissue to support individual cognitive capabilities. Using a multidisciplinary modeling approach, Reséndiz-Benhumea et al. (2021) indeed demonstrated that agents with relatively small brains can through social interaction achieve a level of behavioral performance comparable to those of larger-brained but solitary agents. Further testing of this idea, however, will require a better understanding of whether Holocene human brains reduced isometrically or if specific regions reduced in size.
We suggest that group cognition lowered the demands for neural architechtures required to support some aspects of individual intelligence and decision making ( Bailey and Geary, 2009 ). This effect may have become even more pronounced with the advent of writing ca. 5000 years ago ( Schmandt-Besserat, 2010 ), which falls within the estimated 95% CI for the pronounced reduction in Holocene human brain size ( Figure 1 ). During human history, social groups became larger, social interactions more frequent, social networks more complex, and tracking relationships more demanding ( Bailey and Geary, 2009 ; Foley and Gamble, 2009 ). A rise in sociocultural complexity was not due to particular individuals becoming more intelligent and culturally skilled, but because of the emergence of collective intelligence resulting from a growing population of interconnected humans and interacting human groups. As group size increases, interactions with a dynamic and exceedingly complex social landscapes result in increased demands on the brain ( Bickart et al., 2011 ; Kanai et al., 2012 ). However, because of the metabolic demands of the brain ( Pontzer et al., 2016 ), there may be limits to feedback loops between social network size and brain structure. If group decision-making generated adaptive group responses exceeding the cognitive accuracy and speed of individual decisions and had a fitness consequence, then human brain size may have decreased as a consequence of metabolic cost savings.
Population size expanded dramatically with the advent of agriculture, beginning ∼10 kyr and grew exponentially from an estimated five million to over 100 million by 3000 years ago ( Goldewijk et al., 2011 ). This increase in population coincided with deterioration in individual health ( Armelagos et al., 1991 ; Milner, 2019 ) and increases in infection rate ( Eshed et al., 2010 ), pathogenic load ( Page et al., 2016 ), and virulence ( Menneret et al., 2010 ). It remains possible, then, that the high energetic cost of a heightened immune response ( Wells and Stock, 2020 ), might have been a factor in Holocene brain reduction. In fact, Crabtree ( 2013a ; 2013b ) proposed this immunity-for-intelligence trade-off in his controversial “Idiocracy Hypothesis,” though this idea has been criticized on the basis of flawed assumptions ( Kalinka et al., 2013 ; Mitchell, 2013 ).
Gowdy and Krall (2013) draw parallels between the ultrasocial human superorganism, complete with division of labor and “economic organization around surplus” that arose in the Holocene and the sociobiology of agricultural eusocial insects, including some ants and termites. Brain size reduction occurred in traditional hunter-gatherer human populations ( Wiercinski, 1979 ) that never adopted sedentary agricultural practices, but have complex social networks ( Apicella et al., 2012 ). Foster and Ratnieks (2005) suggest that the presence of post-reproductive female helpers (grandmothers) universally present in human societies is sufficient to characterize humans as a “new eusocial vertebrate,” offering additional support for the value of broad comparisons across unrelated taxa.
We suggest that patterns of human brain evolution were influenced by collective intelligence, a convergent characteristic of diverse group-living animals ( Surowiecki, 2004 ; Sumpter, 2006 ; Woolley et al., 2010 ; Morand-Ferron and Quinn, 2011 ; Reid et al., 2015 ; Biro et al., 2016 ; Bates and Gupta, 2017 ; Sasaki and Biro, 2017 ). The precise role of societal information flow, distribution, and transfer as emergent group properties that may affect brain evolution and neural functioning is not well understood (e.g., Weaverdyck and Parkinson, 2018 ). Large brains may not be required to generate complex behavior, and brain mosaicism and circuitry—rather than overall size—may be important ( Healy and Rowe, 2007 , 2013 ; Chittka and Niven, 2009 ; Avarguès-Weber et al., 2018 ; Logan et al., 2018 ; Godfrey and Gronenberg, 2019 ). Computational models (e.g., Feinerman and Traniello, 2016 ; Reséndiz-Benhumea et al., 2021 ) and patterns in some ant clades ( Muscedere and Traniello, 2012 ; Riveros et al., 2012 ; Muratore et al., 2021 ) suggest that group-level cognition may select for reduced brain size and/or adaptive brain size variation. Moreover, complex systems theory predicts that greater social complexity derives from individual simplicity ( Delgado and Solé, 1997 ), although the neurobiological and behavioral meaning of “simplicity” is unclear. Complexity in eusocial insect colony organization may involve selection for either smaller, neurally differentiated worker brains ( Lihoreau et al., 2012 ; Riveros et al., 2012 ; O’Donnell et al., 2015 ; Feinerman and Traniello, 2016 ) or larger brains ( Wehner et al., 2007 ) able to metabolically offset increased production and operation costs ( Kamhi et al., 2016 ). It seems unlikely that a “theory of theories” will appear to universally and meaningfully explain the multiple roles sociality can play across taxa as evolutionarily as divergent as humans and ants. We advocate for an ecumenical and open-minded approach that integrates theories of social, sociocultural, ecological, and mosaic and metabolic brain evolution to create an awareness of the breadth of the natural landscape of possibilities that can encompass both brain size increase and reduction. Human/ant comparisons have heuristic value and can offer a conceptual compass to guide future research.
Data Availability Statement
The original contributions presented in the study are included in the article/ Supplementary Material , further inquiries can be directed to the corresponding author/s.
Author Contributions
JD and JT conceived the study and wrote the manuscript. JD accumulated the hominin brain dataset. LF ran the change-point analysis and produced Figure 1 . JD and JT edited the manuscript, with contributions from AC and LF. All authors edited the manuscript.
This work was supported by the National Science Foundation grants IOS 1354291 and IOS 1953393 to JT. LF also acknowledges support from a National Science Foundation Graduate Research Fellowship.
Conflict of Interest
The authors declare that the research was conducted in the absence of any commercial or financial relationships that could be construed as a potential conflict of interest.
Publisher’s Note
All claims expressed in this article are solely those of the authors and do not necessarily represent those of their affiliated organizations, or those of the publisher, the editors and the reviewers. Any product that may be evaluated in this article, or claim that may be made by its manufacturer, is not guaranteed or endorsed by the publisher.
Acknowledgments
We gratefully acknowledge the comments of reviewers in revising the manuscript.
Supplementary Material
The Supplementary Material for this article can be found online at: https://www.frontiersin.org/articles/10.3389/fevo.2021.742639/full#supplementary-material
- ^ From Big Picture Science podcast, Collective “Knowledge.” May 17, 2021.
Aiello, L. C., and Wheeler, P. (1995). The expensive-tissue hypothesis: the brain and the digestive system in human and primate evolution. Curr. Anthro. 36, 199–221. doi: 10.1086/204350
CrossRef Full Text | Google Scholar
Almaatouq, A., Noriega-Campero, A., Alotaibi, A., Krafft, P. M., Moussaid, M., and Pentland, A. (2020). Adaptive social networks promote the wisdom of crowds. Proc. Natl. Acad. Sci. U S A. 117, 11379–11386. doi: 10.1073/pnas.1917687117
PubMed Abstract | CrossRef Full Text | Google Scholar
Amador-Vargas, S., Gronenberg, W., Wcislo, W. T., and Mueller, U. (2015). Specialization and group size: brain and behavioural correlates of colony size in ants lacking morphological castes. Proc. R. Soc. B 282:20142502. doi: 10.1098/rspb.2014.2502
Anderson, C., and McShea, D. W. (2001). Individual versus social complexity, with particular reference to ant colonies. Biol. Rev. 76, 211–237. doi: 10.1017/s1464793101005656
Apicella, C. L., Marlowe, F. W., Fowler, J. H., and Christakis, N. A. (2012). Social networks and cooperation in hunter-gatherers. Nature 481, 497–501. doi: 10.1038/nature10736
Armelagos, G. J., Goodman, A. H., and Jacobs, K. H. (1991). The origins of agriculture: population growth during a period of declining health. Popul. Environ. 13, 9–22. doi: 10.1007/bf01256568
Avarguès-Weber, A., d’Amaro, D., Metzler, M., Finke, V., Baracchi, D., and Dyer, A. G. (2018). Does holistic processing require a large brain? insights from honeybees and wasps in fine visual recognition tasks. Front. Psychol. 9:1313. doi: 10.3389/fpsyg.2018.01313
Avarguès-Weber, A., and Giurfa, M. (2013). Conceptual learning by miniature brains. Proc. R. Soc. B: Biol. Sci. 280:20131907. doi: 10.1098/rspb.2013.1907
Bailey, D. H., and Geary, D. C. (2009). Hominid brain evolution. testing climatic, ecological, and social competition models. Hum. Nat. 20, 67–79. doi: 10.1007/s12110-008-9054-0
Bak-Coleman, J. B., Alfano, M., Barfuss, W., Bergstrom, C. T., Centeno, M. A., Couzin, I. D., et al. (2021). Stewardship of global collective behavior. Proc. Natl. Acad. Sci. U.S.A. 118:e2025764118. doi: 10.1073/pnas.2025764118
Balcarcel, A. M., Veitschegger, K., Clauss, M., and Sánchez-Villagra, M. R. (2021). Intensive human contact correlates with smaller brains: differential brain size reduction in cattle types. Proc. R. Soc. Lond. B 288:20210813. doi: 10.1098/rspb.2021.0813
Barron, A. B., and Klein, C. (2016). What insects can tell us about the origins of consciousness. Proc. Natl. Acad. Sci. U.S.A. 113, 4900–4908. doi: 10.1073/pnas.1520084113
Bates, T. C., and Gupta, S. (2017). Smart groups of smart people: evidence for IQ as the origin of collective intelligence in the performance of human groups. Intelligence 60, 46–56. doi: 10.1016/j.intell.2016.11.004
Beals, K. L., Smith, C. L., and Dodd, S. M. (1984). Brain size, cranial morphology, climate and time machines. Curr. Anthropol. 25, 301–330. doi: 10.1086/203138
Bednarik, R. G. (2014). Doing with less: hominin brain atrophy. Homo J. Comp. Hum. Biol. 65, 433–449. doi: 10.1016/j.jchb.2014.06.001
Berger, L. R., Hawks, J., de Ruiter, D. J., Churchill, S. E., Schmid, P., Delezene, L., et al. (2015). Homo naledi , a new species of the genus Homo from the Dinaledi chamber. South Africa. eLife 4:e09560.
Google Scholar
Betzig, L. (2014). Eusociality: from the first foragers to the first states. introduction to the special issue. Hum. Nat. 25, 1–5. doi: 10.1007/s12110-013-9187-7
Bickart, K. C., Wright, C. I., Dautoff, R. J., Dickerson, B. C., and Barrett, L. F. (2011). Amygdala volume and social network size in humans. Nat. Neurosci. 14, 163–164. doi: 10.1038/nn.2724
Biro, D., Sasaki, T., and Portugal, S. J. (2016). Bringing a time-depth perspective to collective animal behaviour. Trends Ecol. Evol. 31, 550–562. doi: 10.1016/j.tree.2016.03.018
Boomsma, J. J., and Franks, N. R. (2006). Social insects: from selfish genes to self organisation and beyond. Trends Ecol. Evol. 21, 303–308. doi: 10.1016/j.tree.2006.04.001
Braun, D. R., Harris, J. W., Levin, N. E., McCoy, J. T., Herries, A. I., Bamford, M. K., et al. (2010). Early hominin diet included diverse terrestrial and aquatic animals 1.95 Ma in East Turkana Kenya. Proc. Natl. Acad. Sci. U.S.A. 107, 10002–10007. doi: 10.1073/pnas.1002181107
Browe, B. M., Vice, E. N., and Park, T. J. (2020). Naked mole-rats: blind, naked, and feeling no pain. Anat. Rec. 303, 77–88. doi: 10.1002/ar.23996
Brown, P., Sutikna, T., Morwood, M. J., Soejono, R. P., Saptomo, E. W., and Due, R. A. (2004). A new small-bodied hominin from the Late Pleistocene of Flores, Indonesia. Nature 431, 1055–1061. doi: 10.1038/nature02999
Bruner, E., and Gleeson, B. T. (2019). Body cognition and self-domestication in human evolution. Front. Psychol. 10:1111. doi: 10.3389/fpsyg.2019.01111
Campbell, D. T. (1983). “The two distinct routes beyond kin selection to ultrasociality: implications for the humanities and social sciences,” in The Nature of Prosocial Development: Theories and Strategies , ed. D. L. Bridgeman (Academic press), 11–41.
Cartmill, M., and Smith, F. H. (2009). The Human Lineage. Hoboken, NJ: John Wiley & Sons.
Chittka, L., and Niven, J. (2009). Are bigger brains better? Curr. Biol. 19, R995–R1008. doi: 10.1016/j.cub.2009.08.023
Coqueugniot, H., and Hublin, J. J. (2012). Age-related changes of digital endocranial volume during human ontogeny: results from an osteological reference collection. Am. J. Phys. Anthropol. 147, 312–318. doi: 10.1002/ajpa.21655
Coto, Z. N., and Traniello, J. F. A. (2021). Brain size, metabolism, and social evolution. Front. Physiol. 12:612865. doi: 10.3389/fphys.2021.612865
Couzin, I. D. (2009). Collective cognition in animal groups. Trends Cogn. Sci. 13, 36–43. doi: 10.1016/j.tics.2008.10.002
Crabtree, G. R. (2013a). Our fragile intellect. Part I. Trends Genet. 29, 1–3. doi: 10.1016/j.tig.2012.10.002
Crabtree, G. R. (2013b). Our fragile intellect. Part II. Trends Genet. 29, 3–5. doi: 10.1016/j.tig.2012.10.003
Crespi, B. (2014). The insectan apes. Hum. Nat. 25, 6–27. doi: 10.1007/s12110-013-9185-9
Davidson, J. D., Arauco-Aliaga, R. P., Crow, S., Gordon, D. M., and Goldman, M. S. (2016). Effect of interactions between harvester ants on forager decisions. Front. Ecol. Evol. 4:115. doi: 10.3389/fevo.2016.00115
DeCasien, A. R., and Higham, J. P. (2019). Primate mosaic brain evolution reflects selection on sensory and cognitive specialization. Nat. Ecol. Evol. 3, 1483–1493. doi: 10.1038/s41559-019-0969-0
DeCasien, A. R., Williams, S. A., and Higham, J. P. (2017). Primate brain size is predicted by diet but not sociality. Nat. Ecol. Evol. 1:0122.
Delgado, J., and Solé, R. V. (1997). Collective-induced computation. Phys. Rev. E 55:2338. doi: 10.1103/physreve.55.2338
DeSilva, J. M. (2011). A shift toward birthing relatively large infants early in human evolution. Proc. Natl. Acad. Sci. U.S.A. 108, 1022–1027. doi: 10.1073/pnas.1003865108
D’Ettorre, P. (2017b). “Invasive eusocieties: commonalities between ants and humans,” in Human Dispersal and Species Movement: from Prehistory to the Present , eds N. Boivin, R. Crassard, and M. Petraglia (Cambridge: Cambridge University Press).
D’Ettorre, P. (2017a). “Distributed agency in ants,” in Distributed Agency , eds N. J. Enfield and P. Kockelman (Oxford, NY: Oxford University Press).
D’Ettorre, P., Meunier, P., Simonelli, P., and Call, J. (2021). Quantitative cognition in carpenter ants. Behav. Ecol. Sociobiol. 75:86.
Dunbar, R. I. M. (1998). The social brain hypothesis. Evol. Anthropol. 6, 178–190.
Dunbar, R. I. M., and Shultz, S. (2007). Evolution in the social brain. Science 317, 1344–1347.
Dunbar, R. I. M., and Shultz, S. (2017). Why are there so many explanations for primate brain evolution? Proc. R. Soc. Lond. B 372:20160244. doi: 10.1098/rstb.2016.0244
Elek, M. I., Roelandt, G., and D’Ettore, P. (2020). A small number of workers with specific personality traits perform tool use in ants. eLife 9:e61298. doi: 10.7554/eLife.61298
Eshed, V., Gopher, A., Pinhasi, R., and Hershkovitz, I. (2010). Paleopathology and the origin of agriculture in the Levant. Am. J. Phys. Anthropol. 143, 121–133. doi: 10.1002/ajpa.21301
Farris, S. M. (2016). Insect societies and the social brain. Curr. Opin. Insect. Sci. 15, 1–8. doi: 10.1016/j.cois.2016.01.010
Farris, S. M., and Schulmeister, S. (2011). Parasitoidism, not sociality, is associated with the evolution of elaborate mushroom bodies in the brains of hymenopteran insects. Proc. R. Soc. Lond. B 278, 940–951. doi: 10.1098/rspb.2010.2161
Feinerman, O., and Traniello, J. F. A. (2016). Social complexity, diet, and brain evolution: modeling the effects of colony size, worker size, brain size, and foraging behavior on colony fitness in ants. Behav. Ecol. Sociobiol. 69, 1–12.
Foley, R., and Gamble, C. (2009). The ecology of social transitions in human evolution. Philos. Trans. R. Soc. B Biol. Sci. 364, 3267–3279. doi: 10.1098/rstb.2009.0136
Foster, K. R., and Ratnieks, F. L. (2005). A new eusocial vertebrate? Trends Ecol. Evol. 20, 363–364. doi: 10.1016/j.tree.2005.05.005
Friedman, D. A., and Søvik, E. (2021). The ant colony as a test for scientific theories of consciousness. Synthese 198, 1457–1480. doi: 10.1007/s11229-019-02130-y
Galton, F. (1907). Vox populi. Nature 75, 450–451.
Godfrey, R. K., and Gronenberg, W. (2019). Brain evolution in social insects: advocating for the comparative approach. J. Comp. Physiol. A 205, 13–32. doi: 10.1007/s00359-019-01315-7
Godfrey, R. K., Swartzlander, M., and Gronenberg, W. (2021). Allometric analysis of brain cell number in Hymenoptera suggests ant brains diverge from general trends. Proc. R. Soc. B 288:20210199. doi: 10.1098/rspb.2021.0199
Gokcumen, O. (2020). Archaic hominin introgression into modern human genomes. Am. J. Phys. Anthropol. 171, 60–73. doi: 10.1002/ajpa.23951
Goldewijk, K. K., Beusen, A., Van Drecht, G., and De Vos, M. (2011). The HYDE 3.1 spatially explicit database of human-induced global land-use change over the past 12,000 years. Global Ecol. Biogeography 20, 73–86. doi: 10.1111/j.1466-8238.2010.00587.x
González-Forero, M., and Gardner, A. (2018). Inference of ecological and social drivers of human brain-size evolution. Nature 557, 554–557. doi: 10.1038/s41586-018-0127-x
Gordon, D. G., Ilieş, I., and Traniello, J. F. A. (2017). Behavior, brain, and morphology in a complex insect society: trait integration and social evolution in the exceptionally polymorphic ant Pheidole rhea. Behav. Ecol. Sociobiol. 71, 1–13. doi: 10.1007/s00265-017-2396-z
Gordon, D. G., and Traniello, J. F. A. (2018). Synaptic organization and division of labor in the exceptionally polymorphic ant Pheidole rhea . Neurosci. Lett. 676, 46–50. doi: 10.1371/journal.pone.0213618
Gordon, D. G., Zelaya, A., Arganda-Carreras, I., Arganda, S., and Traniello, J. F. A. (2019). Division of labor and brain evolution in insect societies: neurobiology of extreme specialization in the turtle ant Cephalotes varians . PLoS One 14:e0213618.
Gowdy, J. (2021). Ultrasocial. Cambridge: Cambridge University Press.
Gowdy, J., and Krall, L. (2013). The ultrasocial origin of the Anthropocene. Ecol. Econ. 95, 137–147. doi: 10.1016/j.ecolecon.2013.08.006
Gowdy, J., and Krall, L. (2016). The economic origins of ultrasociality. Behav. Brain Sci. 36, 563–595. doi: 10.1017/S0140525X1500059X
Grabowski, M. (2016). Bigger brains led to bigger bodies? the correlated evolution of human brain and body size. Curr. Anthro. 57, 174–185. doi: 10.1086/685655
Groh, C., Kelber, C., Grübel, K., and Rössler, W. (2014). Density of mushroom body synaptic complexes limits intraspecies brain miniaturization in highly polymorphic leaf-cutting ant workers. Proc. R. Soc. B 281:20140432. doi: 10.1098/rspb.2014.0432
Groh, C., and Rössler, W. (2011). Comparison of microglomerular structures in the mushroom body calyx of neopteran insects. Arthr. Struc.Devel. 40, 358–367. doi: 10.1016/j.asd.2010.12.002
Groves, C. P. (1999). The advantages and disadvantages of being domesticated. Perspect. Hum. Biol. 4, 1–12.
Hare, B., and Tomasello, M. (2005). The emotional reactivity hypothesis and cognitive evolution. Trends Cogn. Sci. 9, 464–465. doi: 10.1016/j.tics.2005.08.010
Hare, B., and Woods, V. (2020). Survival of the Friendliest: Understanding our Origins and Rediscovering our Common Humanity. New York, NY: Random House.
Haskins, C. P. (1939). Of Ants and Men. Hoboken, NJ: Prentice Hall.
Hawks, J. (2011). Selection for smaller brains in Holocene human evolution. arXiv [preprint]. Available online at: https://arxiv.org/abs/1102.5604#:~:text=Background%3A%20Human%20populations%20during%20the,linear%20measurements%20of%20the%20cranium (accessed 2020).
Healy, S. D., and Rowe, C. (2007). A critique of comparative studies of brain size. Proc. R. Soc. B. 274, 453–464. doi: 10.1098/rspb.2006.3748
Healy, S. D., and Rowe, C. (2013). Costs and benefits of evolving a larger brain: doubts over the evidence that large brains lead to better cognition. Anim. Behav. 86, e1–e3. doi: 10.1016/j.anbehav.2013.05.017
Henneberg, M. (1988). Decrease of human skull size in the Holocene. Hum. Biol. 60, 395–405.
Henneberg, M., and Steyn, M. (1993). Trends in cranial capacity and cranial index in subsaharan Africa during the Holocene. Am. J. Human Biol. 5, 473–479. doi: 10.1002/ajhb.1310050411
Herculano-Houzel, S. (2016). The Human Advantage: How Our Brains Became Remarkable. Cambridge, MA: MIT Press.
Herculano-Houzel, S. (2017). Numbers of neurons as biological correlates of cognitive capability. Curr. Opin. Behav. Sci. 16, 1–7. doi: 10.1016/j.cobeha.2017.02.004
Herries, A. I., Martin, J. M., Leece, A. B., Adams, J. W., Boschian, G., Joannes-Boyau, R., et al. (2020). Contemporaneity of Australopithecus, Paranthropus , and early Homo erectus in South Africa. Science 368:eaaw7293. doi: 10.1126/science.aaw7293
Hölldobler, B., and Wilson, E. O. (1990). The Ants. Cambridge, MA: Harvard University Press.
Holloway, R. L. (1980). Within-species brain-body weight variability: a reexamination of the Danish data and other primate species. Am. J. Phys. Anthropol. 53, 109–121. doi: 10.1002/ajpa.1330530115
Ilies, I., Muscedere, M. L., and Traniello, J. F. A. (2015). Neuroanatomical and morphological trait clusters in the ant genus Pheidole: evidence for modularity and integration in brain structure. Brain Behav. Evol. 85, 63–76. doi: 10.1159/000370100
Imms, A. (1946). Of ants and men. Nature 157, 855–856. doi: 10.1038/157855a0
Isler, K., and van Schaik, C. P. (2009). The expensive brain: a framework for explaining evolutionary changes in brain size. J. Hum. Evol. 57, 392–400. doi: 10.1016/j.jhevol.2009.04.009
Isler, K., and van Schaik, C. P. (2012). Allomaternal care, life history and brain size evolution in mammals. J. Hum. Evol. 63, 52–63. doi: 10.1016/j.jhevol.2012.03.009
Isler, K., and van Shaik, C. P. (2006). Metabolic costs of brain size evolution. Biol. Lett. 2, 557–560. doi: 10.1098/rsbl.2006.0538
Kalinka, A. T., Kelava, I., and Lewitus, E. (2013). Our robust intellect. Trends Genet. 29, 125–127. doi: 10.1016/j.tig.2013.01.008
Kamhi, J. F., Gronenberg, W. G., Robson, S. K. A., and Traniello, J. F. A. (2016). Social complexity influences brain production and operation costs in ants. Proc. R. Soc. B 283:20161949. doi: 10.1098/rspb.2016.1949
Kamhi, J. F., Ilieş, I., and Traniello, J. F. A. (2019). Social complexity and brain evolution: comparative analysis of modularity and integration in formicine ant brain organization. Brain, Behav. Evol. 93, 4–18. doi: 10.1159/000497267
Kanai, R., Bahrami, B., Roylance, R., and Rees, G. (2012). Online social network size is reflected in human brain structure. Proc. R. Soc. B: Biol. Sci. 279, 1327–1334. doi: 10.1098/rspb.2011.1959
Kappeler, P. (2019). A framework for studying social complexity. Behav. Ecol. Sociobiol. 73:13.
Keifer, J., and Summers, C. H. (2016). Putting the “Biology” back into “Neurobiology”: the strength of diversity in animal model systems for neuroscience research. Front. Syst. Neurosci. 10:69. doi: 10.3389/fnsys.2016.00069
Killick, R., and Eckley, I. A. (2014). changepoint: an R package for changepoint analysis. J. Statistical Software 58, 1–19.
Krause, J., Ruxton, G. D., and Krause, S. (2010). Swarm intelligence in animals and humans. Trends Ecol. Evol. 25, 28–34. doi: 10.1016/j.tree.2009.06.016
Kuzawa, C. W., Chugani, H. T., Grossman, L. I., et al. (2014). Metabolic costs and evolutionary implications of human brain development. Proc. Natl. Acad. Sci. U.S.A. 111, 13010–13015. doi: 10.1073/pnas.1323099111
Kverková, K., Olkowicz, S., Pavelkova, Z., et al. (2018). Sociality does not drive the evolution of large brains in eusocial African mole rats. Sci. Rep. 8:9203. doi: 10.1038/s41598-018-26062-8
Laland, K., and Seed, A. (2021). Understanding human cognitive uniqueness. Ann. Rev. Psychol. 72, 689–716. doi: 10.1146/annurev-psych-062220-051256
Leach, H. (2003). Human domestication reconsidered. Curr. Anthropol. 44, 349–368. doi: 10.1086/368119
Lee, S. H., and Wolpoff, M. H. (2003). The pattern of evolution in Pleistocene human brain size. Paleobiology 29, 186–196. doi: 10.1017/s0094837300018054
Leonard, W. R., Robertson, M. L., Snodgrass, J. J., and Kuzawa, C. W. (2003). Metabolic correlates of hominid brain evolution. Comp. Biochem. Physiol. Part A: Mol. Int. Physiol. 136, 5–15. doi: 10.1016/s1095-6433(03)00132-6
Lepre, C. J., Roche, H., Kent, D. V., Harmand, S., Quinn, R. L., Brugal, J. P., et al. (2011). An earlier origin for the Acheulian. Nature 477, 82–85. doi: 10.1038/nature10372
Lihoreau, M., Dubois, T., Gomez-Moracho, T., and Kraus, S. (2019). Putting the ecology back into insect cognition research. Adv. In Insect Phys. 57, 1–25. doi: 10.1016/bs.aiip.2019.08.002
Lihoreau, M., Latty, T., and Chittka, L. (2012). An exploration of the social brain hypothesis in insects. Front. Physiol. 3:442. doi: 10.3389/fphys.2012.00442
Liu, C., Tang, Y., Ge, H., Wang, F., Sun, H., Meng, H., et al. (2014). Increasing breadth of the frontal lobe but decreasing height of the human brain between two Chinese samples from a Neolithic site and from living humans. Am. J. Phys. Anthropol. 154, 94–103. doi: 10.1002/ajpa.22476
Logan, C. J., Avin, S., Boogert, N., Buskell, A., Cross, F. R., Currie, A., et al. (2018). Beyond brain size: uncovering the neural correlates of behavioral and cognitive specialization. Comp. Cog. Behav. Rev. 13, 55–89. doi: 10.3819/CCBR.2018.130008
Markov, A. V., and Markov, M. A. (2020). Runaway brain-culture coevolution as a reason for larger brains: exploring the “cultural drive” hypothesis by computer modeling. Ecol. Evol. 10, 6059–6077. doi: 10.1002/ece3.6350
Maschwitz, U., Hahn, M., and Schönegge, P. (1979). Paralysis of prey in ponerine ants. Naturwiss 66, 213–214. doi: 10.1007/bf00366035
McHenry, H. M., and Coffing, K. (2000). Australopithecus to Homo : transformations in body and mind. Ann. Rev. Anthropol. 29, 125–146.
Meguerditchian, A., Marie, D., Margiotoudi, K., Roth, M., Nazarian, B., Anton, J. L., et al. (2021). Baboons ( Papio anubis ) living in larger social groups have bigger brains. Evol. Hum. Behav. 42, 30–34. doi: 10.1016/j.evolhumbehav.2020.06.010
Menneret, A., Nilsen, F., Ebert, D., and Skorping, A. (2010). Intensive farming: evolutionary implications for parasites and pathogens. Evol. Biol. 37, 59–67. doi: 10.1007/s11692-010-9089-0
Miller, I. F., Barton, R. F., and Nunn, C. L. (2019). Quantitative uniqueness of human brain evolution revealed through phylogenetic comparative analysis. eLife 8:e41250.
Milner, G. R. (2019). Early agriculture’s toll on human health. Proc. Natl. Acad. Sci. U.S.A. 28, 13721–13723. doi: 10.1073/pnas.1908960116
Mitchell, K. J. (2013). Genetic entropy and the human intellect. Trends Genet. 29, 59–60. doi: 10.1016/j.tig.2012.11.010
Montgomery, S. H. (2018). Hominin brain evolution: the only way is up? Curr. Biol. 28, R788–R790.
Morand-Ferron, J., and Quinn, J. L. (2011). Larger groups of passerines are more efficient problem solvers in the wild. Proc. Natl. Acad. Sci. U.S.A. 108, 15898–15903. doi: 10.1073/pnas.1111560108
Muggeo, V. M. R. (2008). segmented: an R package to fit regression models with broken-line relationships. R News 8, 20–25.
Muratore, I. B., Fandozzi, E. M., and Traniello, J. F. A. (2021). Behavioral performance requirements for division of labor influence adaptive brain mosaicism in a socially complex ant. BioRxiv [preprint] doi: 10.1101/2021.07.03.450997
Muratore, I. B., and Traniello, J. F. A. (2020). Fungus-growing ants as models for the integrative analysis of cognition and brain evolution. Front. Behav. Neurosci. 14:599234. doi: 10.3389/fnbeh.2020.599234
Muscedere, M. L., Gronenberg, W., Moreau, C. M., and Traniello, J. F. A. (2014). Investment in higher-order central processing regions is not constrained by brain size in social insects. Proc. R. Soc. B. 281:20140217. doi: 10.1098/rspb.2014.0217
Muscedere, M. L., and Traniello, J. F. A. (2012). Division of labor in the hyperdiverse ant genus Pheidole is associated with distinct patterns of worker brain organization. PLoS One 7:e31618. doi: 10.1371/journal.pone.0031618
Muscedere, M. L., Willey, T. A., and Traniello, J. F. A. (2009). Age and task efficiency in the ant Pheidole dentata : young minor workers are not specialist nurses. Anim. Behav. 77, 911–918. doi: 10.1016/j.anbehav.2008.12.018
Navarrete, A., Van Schaik, C. P., and Isler, K. (2011). Energetics and the evolution of human brain size. Nature 480, 91–93. doi: 10.1038/nature10629
Noonan, M. P., Mars, R. B., Sallet, J., Dunbar, R. I. M., and Fellows, L. K. (2018). The structural and functional brain networks that support human social networks. Behav. Brain Res. 355, 12–23. doi: 10.1016/j.bbr.2018.02.019
O’Donnell, S., Bulova, S. J., DeLeon, S., Khodak, P., Miller, S., and Sulger, E. (2015). Distributed cognition and social brains: reductions in mushroom body investment accompanied the origins of sociality in wasps (Hymenoptera: Vespidae). Proc. R. Soc. B. 282:20150791. doi: 10.1098/rspb.2015.0791
Pagán, O. R. (2019). The brain: a concept in flux. Philos. Trans. R. Soc. B 374:20180383. doi: 10.1098/rstb.2018.0383
Page, A. E., Viguier, S., Dyble, M., Smith, D., Chaudhary, N., Salali, G. D., et al. (2016). Reproductive trade-offs in extant hunter-gatherers suggest adaptive mechanism for the Neolithic expansion. Proc. Natl. Acad. Sci. U.S.A. 113, 4694–4699. doi: 10.1073/pnas.1524031113
Perri, A. R., Feuerborn, T. R., Frantz, L. A., Larson, G., Malhi, R. S., Meltzer, D. J., et al. (2021). Dog domestication and the dual dispersal of people and dogs into the Americas. Proc. Natl. Acad. Sci. U.S.A. 118:e2010083118. doi: 10.1073/pnas.2010083118
Perry, C. J., Barron, A. B., and Chittka, L. (2017). The frontiers of insect cognition. Curr. Opin. Behav. Sci. 16, 111–118. doi: 10.1016/j.cobeha.2017.05.011
Perry, C. J., and Chittka, L. (2019). How foresight might support the behavioral flexibility of arthropods. Curr. Opin. Neurobiol. 54, 171–177. doi: 10.1016/j.conb.2018.10.014
Piñero, J., and Solé, R. (2019). Statistical physics of liquid brains. Philos. Trans. R. Soc. B 374:20180376. doi: 10.1098/rstb.2018.0376
Ponce de León, M. S., Bienvenu, T., Marom, A., Engel, S., Tafforeau, P., et al. (2021). The primitive brain of early Homo. Science 372, 165–171. doi: 10.1126/science.aaz0032
Ponce de León, M. S., Golovanova, L., Doronichev, V., Romanova, G., Akazawa, T., et al. (2008). Neanderthal brain size at birth provides insights into the evolution of human life history. Proc. Natl. Acad. Sci. U.S.A. 105, 13764–13768. doi: 10.1073/pnas.0803917105
Pontzer, H., Brown, M. H., Raichlen, D. A., Dunsworth, H., et al. (2016). Metabolic acceleration and the evolution of human brain size and life history. Nature 533, 390–392. doi: 10.1038/nature17654
Reid, C. R., Lutz, M. J., Powell, S., Kao, A. B., Couzin, I. D., and Garnier, S. (2015). Army ants dynamically adjust living bridges in response to a cost–benefit trade-off. Proc. Natl. Acad. Sci. U.S.A. 112, 15113–15118. doi: 10.1073/pnas.1512241112
Reséndiz-Benhumea, G. M., Sangati, E., Sangati, F., Keshmiri, S., and Froese, T. (2021). Shrunken social brains? a minimal model of the role of social interaction in neural complexity. Front. Neurorobot. 15:634085. doi: 10.3389/fnbot.2021.634085
Rightmire, G. P. (2004). Brain size and encephalization in early to mid-pleistocene homo. Am. J. Phys. Anthropol. 124, 109–123. doi: 10.1002/ajpa.10346
Riveros, A. J., Seid, M. A., and Wcislo, W. T. (2012). Evolution of brain size in class-based societies of fungus-growing ants (Attini). Anim. Behav. 83, 1043–1049. doi: 10.1016/j.anbehav.2012.01.032
Ruff, C. B., Trinkaus, E., and Holliday, T. W. (1997). Body mass and encephalization in Pleistocene Homo . Nature 387, 173–176. doi: 10.1038/387173a0
Sasaki, T., and Biro, D. (2017). Cumulative culture can emerge from collective intelligence in animal groups. Nat. Comm. 8:15049.
Sasaki, T., and Pratt, S. C. (2018). The psychology of superorganisms: collective decision making by insect societies. Ann. Rev. Entomol. 63, 259–275. doi: 10.1146/annurev-ento-020117-043249
Schmandt-Besserat, D. (2010). How Writing Came About. Austin, TX: University of Texas Press.
Schwidetzky, I. (1976). Postpleistocene evolution of the brain? Am. J. Phys. Anthropol. 45, 605–611. doi: 10.1002/ajpa.1330450327
Seid, M. A., Castillo, A., and Wcislo, W. (2011). The allometry of brain miniaturization in ants. Brain Behav. Evol. 77, 5–13. doi: 10.1159/000322530
Seid, M. A., Harris, K. M., and Traniello, J. F. A. (2005). Age-related changes in the number and structure of synapses in the lip region of the mushroom bodies in the ant Pheidole dentata. J. Comp. Neurol. 488, 269–277. doi: 10.1002/cne.20545
Seid, M. A., and Traniello, J. F. A. (2006). Age-related repertoire expansion and division of labor in Pheidole dentata (Hymenoptera: Formicidae): a new perspective on temporal polyethism and behavioral plasticity in ants. Behav. Ecol. Sociobiol. 60, 631–644. doi: 10.1007/s00265-006-0207-z
Shigeno, S. (2017). “Brain evolution by design: from neural origin to cognitive architecture,” in Diversity and Commonality in Animals , eds S. Shigeno, Y. Murakami, and T. Nomura (Tokyo: Springer), doi: 10.1007/978-4-431-56469-0_19
Strausfeld, N. J., Hansen, L., Li, Y., Gomez, R. S., and Ito, K. (1998). Evolution, discovery, and interpretations of arthropod mushroom bodies. Learn. Memory 5, 11–37.
Striedter, G. F., Belgard, T. G., Chen, C., et al. (2014). NSF workshop report: discovering general principles of nervous system organization by comparing brain maps across species. J. Comp. Neurol. 522, 1445–1453. doi: 10.1002/cne.23568
Sumpter, D. J. T. (2006). The principles of collective animal behavior. Proc. R. Soc. B. 361, 5–22.
Surowiecki, J. (2004). The Wisdom of Crowds. London: Little, Brown.
Tobias, P. V. (1970). Brain-size, grey matter and race—fact or fiction? Am. J. Phys. Anthropol. 32, 3–25. doi: 10.1002/ajpa.1330320103
Tomer, R., Denes, A. S., Tessmar-Raible, K., and Arendt, D. (2010). Profiling by image registration reveals common origin of annelid mushroom bodies and vertebrate pallium. Cell 142, 800–809. doi: 10.1016/j.cell.2010.07.043
van Schaik, C. P., Isler, K., and Burkart, J. M. (2012). Explaining brain size variation: from social to cultural brain. Trends Cogn. Sci. 16, 277–284. doi: 10.1016/j.tics.2012.04.004
VanSickle, C., Cofran, Z., and Hunt, D. (2020). Did Neandertals have large brains? factors affecting endocranial volume comparisons. Am. J. Phys. Anthropol. 173, 768–775. doi: 10.1002/ajpa.24124
Ward, A. J., Herbert-Read, J. E., Sumpter, D. J., and Krause, J. (2011). Fast and accurate decisions through collective vigilance in fish shoals. Proc. Natl. Acad. Sci. U.S.A. 108, 2312–2315. doi: 10.1073/pnas.1007102108
Weaverdyck, M. E., and Parkinson, C. (2018). The neural representation of social networks. Curr. Opin. Psych. 24, 58–66.
Wehner, R., Fukushi, T., and Isler, K. (2007). On being small: brain allometry in ants. Brain Behav. Evol. 69, 220–228. doi: 10.1159/000097057
Wells, J. C. K., and Stock, J. T. (2020). Life history transitions at the origins of agriculture: a model for understanding how niche construction impacts human growth, demography and health. Front. Endocrinol. 11:325. doi: 10.3389/fendo.2020.00325
Wiercinski, A. (1979). Has the brain size decreased since the upper paleolithic period? Bull. Mem. Soc. Anthropol. Paris 6, 419–427. doi: 10.3406/bmsap.1979.1979
Wilson, E. O. (2012). The Social Conquest of Earth. New York, NY: WW Norton & Company.
Wolpoff, M. H. (1999). Paleoanthropology. New York, NY: McGraw-Hill Humanities, Social Sciences & World Languages.
Wood, B., and Collard, M. (1999). The human genus. Science 284, 65–71. doi: 10.1126/science.284.5411.65
Woolley, A. W., Chabris, C. F., Pentland, A., Hashmi, N., and Malone, T. W. (2010). Evidence for a collective intelligence factor in the performance of human groups. Science 330, 686–688. doi: 10.1126/science.1193147
Wrangham, R. (2009). Catching Fire: How Cooking Made us Human. New York, NY: Basic books.
Wrangham, R. (2019). The Goodness Paradox: The Strange Relationship between Virtue and Violence in Human Evolution. New York, NY: Vintage.
Wrangham, R. W. (2018). Two types of aggression in human evolution. Proc. Natl. Acad. Sci. U.S.A. 115, 245–253. doi: 10.1073/pnas.1713611115
Zhou, A., Du, Y., and Chen, J. (2020). Ants adjust their tool use strategy in response to foraging risk. Funct. Ecol. 34, 2524–2535. doi: 10.1111/1365-2435.13671
Keywords : brain, evolution, ant, hominin, Holocene
Citation: DeSilva JM, Traniello JFA, Claxton AG and Fannin LD (2021) When and Why Did Human Brains Decrease in Size? A New Change-Point Analysis and Insights From Brain Evolution in Ants. Front. Ecol. Evol. 9:742639. doi: 10.3389/fevo.2021.742639
Received: 16 July 2021; Accepted: 23 September 2021; Published: 22 October 2021.
Reviewed by:
Copyright © 2021 DeSilva, Traniello, Claxton and Fannin. This is an open-access article distributed under the terms of the Creative Commons Attribution License (CC BY) . The use, distribution or reproduction in other forums is permitted, provided the original author(s) and the copyright owner(s) are credited and that the original publication in this journal is cited, in accordance with accepted academic practice. No use, distribution or reproduction is permitted which does not comply with these terms.
*Correspondence: Jeremy M. DeSilva, [email protected]
† These authors have contributed equally to this work
This article is part of the Research Topic
Neuroethology of the Colonial Mind: Ecological and Evolutionary Context of Social Brains
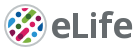
- Research Article
- Evolutionary Biology
- Neuroscience
Quantitative uniqueness of human brain evolution revealed through phylogenetic comparative analysis

- Robert A Barton
- Charles L Nunn
- Princeton University, United States ;
- Duke University, United States ;
- University of Durham, United Kingdom ;
- Open access
- Copyright information
Share this article
Cite this article.
- Ian F Miller
- Copy to clipboard
- Download BibTeX
- Download .RIS
eLife digest
Introduction, materials and methods, data availability, article and author information.
While the human brain is clearly large relative to body size, less is known about the timing of brain and brain component expansion within primates and the relative magnitude of volumetric increases. Using Bayesian phylogenetic comparative methods and data for both extant and fossil species, we identified that a distinct shift in brain-body scaling occurred as hominins diverged from other primates, and again as humans and Neanderthals diverged from other hominins. Within hominins, we detected a pattern of directional and accelerating evolution towards larger brains, consistent with a positive feedback process in the evolution of the human brain. Contrary to widespread assumptions, we found that the human neocortex is not exceptionally large relative to other brain structures. Instead, our analyses revealed a single increase in relative neocortex volume at the origin of haplorrhines, and an increase in relative cerebellar volume in apes.
Humans have much larger brains than other primates, but it is not clear exactly when and how this difference emerged during evolution. Some scientists believe that the expansion of a part of the brain called the neocortex – which handles sight, hearing, conscious decision-making and language – drove the increase in the size of the human brain. Newer studies have challenged that idea.
One way to learn more about how humans evolved bigger brains is to compare the size of the brain, and specific parts of the brain, between humans and our closest relatives: non-human primates. To make accurate comparisons, scientists must account for many factors. Closely related primates may have more similar traits because they more recently shared a common ancestor. This means the evolutionary relationships between species need to be considered. Larger animals also tend to have larger brains so it is important to consider body size, too.
Now, Miller at al. show that the human brain is much larger than expected even after accounting for these factors, and that increases in brain size accelerated over the course of early human evolution. In the analyses, the brain and skull sizes of different living primate species, like chimpanzees and gorillas, and fossils of extinct primates, including Neanderthals, were compared using mathematical models.
These findings suggest that larger brains provided fitness advantages that led to large brain sizes in modern humans and Neanderthals. These increases in brain size were not driven by disproportionate growth in the neocortex alone, but rather by increases in the size of many parts of the brain. Increases in the relative size of the cerebellum, which is essential for balance and movement, were also important.
Primates vary almost a thousand-fold in endocranial volume – a measure which closely approximates brain size – ranging from 1.63 mL in mouse lemurs ( Isler et al., 2008 ) to 1478 mL in humans ( Robson and Wood, 2008 ). Body size is perhaps the most important statistical predictor of brain size across primates, with larger bodied species having larger brains, but substantial variation remains after accounting for the effects of body size ( Isler et al., 2008 ). While numerous comparative studies have sought to identify ecological, behavioral, and cognitive correlates of this variability ( Barton, 1999 ; MacLean et al., 2014 ; DeCasien et al., 2017 ; Powell et al., 2017 ; Noonan et al., 2018 ), much less is known about the evolutionary patterns and processes that generated extant variation in brain size within the primate clade, how these differ for different components of the brain, or the degree to which the brain phenotypes of particular species, such as humans, are the result of exceptional patterns of evolutionary change.
A common approach to investigating human uniqueness is to test whether humans fall ‘significantly’ far from a regression line, for example by regressing brain size on body mass ( Azevedo et al., 2009 ; de Sousa et al., 2010 ; Herculano-Houzel and Kaas, 2011 ). One surprising recent result reported from such an analysis is that the mass of the human brain is only 10% greater than expected for a primate of human body mass ( Azevedo et al., 2009 ). However, such non-phylogenetic methods may give misleading results because they fail to incorporate trait co-variation among species that results from shared evolutionary history. Valid analysis requires methods that account for phylogeny both when estimating scaling parameters and when evaluating deviations from scaling patterns exhibited by individual species ( Garland and Ives, 2000 ; Ross et al., 2004 ; Organ et al., 2011 ). An additional source of error arises if the species being investigated is included in the regression model (e.g. Azevedo et al., 2009 ), particularly when, as for humans, the phenotypic trait lies at the extreme of the distribution for the other species in the analysis. This procedure would reduce the magnitude of deviations from expected trait values for lineages that have undergone exceptional change, and in the case of humans, would bias the results toward failing to detect uniqueness.
Comparative methods make it possible to incorporate phylogeny into analyses and to model phenotypic evolution in ways that uncover hitherto hidden patterns. Such methods are now being applied to a wide variety of traits (e.g. Vining and Nunn, 2016 ; Pagel, 1999 ; Orme, 2013 ), including brain size. Pagel (2002) estimated phylogenetic scaling parameters to characterize the evolutionary trajectory of endocranial volume (ECV) in fossil hominins. His analyses revealed that ECV evolution accelerated towards the present. As this analysis did not account for body size, it is not clear to what extent this pattern reflects changes in brain size independent of body size. Montgomery et al. (2010) used ancestral state reconstruction with fossil data to demonstrate a directional trend in primate brain size evolution and to identify branches in the primate phylogeny along which exceptional evolutionary change occurred. They found that while the absolute change in the mass of the human brain was exceptional, the rate of change relative to body size was not. Phylogenetic methods have also been used to examine how specific brain components evolved and the extent to which the branch leading to humans exhibited unusual amounts or rates of change in the size of these components ( Barton and Venditti, 2013 ; Barton and Venditti, 2014 ). Recently, Lewitus (2018) suggested that comparative analyses of neuroanatomical data can be improved by incorporating and comparing results from different evolutionary models.
Here, we use phylogenetic methods to model the evolution of brain size and to identify exceptional evolutionary change along phylogenetic branches. We employ three methods: The first method models trait evolution both as a multi-optima Ornstein-Uhlenbeck (OU) process (which incorporates stabilizing selection and drift) and as a Brownian motion process ( Felsenstein, 1985 ), and then compares the fit of the two models. In cases where the OU model is favored, exceptional patterns of trait evolution are indicated by recent shifts in adaptive optima in humans’ (or other species’) evolutionary lineage. In cases where the Brownian model is favored, we apply our second method, which is a phylogenetic outlier test that uses phylogenetic generalized least squares (PGLS) to predict a phenotype for a species and then compares observed and predicted values. With this method, we can assess whether humans are a phylogenetic ‘outlier’ relative to expectations based on their phylogenetic position and trait covariation in other primate species. Our last method tests for directional and accelerating evolution by fitting phylogenetic scaling parameters to data on deviation from trait expectations and evolutionary time, building on previous efforts with these approaches ( Pagel, 2002 ).
Using the first two methods, we investigate the evolution of absolute brain size and brain size relative to body mass within primates. Absolute brain volume has been shown to predict cognitive ability in primates better than other metrics that account for body mass ( MacLean et al., 2014 ; Deaner et al., 2007 ). However, brain size is highly correlated with body size ( Isler et al., 2008 ), and as such it is difficult to interpret the significance of brain size alone. Additionally, accounting for body mass gives more insights into the significance of brain size in life history processes, as relative brain size better approximates relative investment in cognitive ability. Accounting for body mass is also important as the relationship between this trait and brain size is associated with scaling effects that reflect conservation of neural function, such as preservation of somatosensory acuity across large surface areas ( St Wecker and Farel, 1994 ) and compensation for increased neural conduction distances in larger animals through (i) larger neuron and axon sizes, increased myelination, and increased white matter volume, all of which result in reduced neuron density ( Barton, 2012 ; Wang et al., 2008 ; Collins et al., 2013 ) and (ii) increased neural resources devoted to prediction-based sensorimotor control that result from escalating neural conduction delays as body size increases ( More et al., 2010 ). Other measures of relative brain size such as encephalization quotients, ratios, and residuals have been used in the past, but all make theoretical assumptions about the underlying relationship between brain and body size evolution that may not hold. Using relative measures can bias parameter estimates and is not recommended as a good statistical practice ( Freckleton, 2002 ). Instead, an empirical approach is preferred in which the covariation of brain size with body size is accounted for within a statistical model that also accounts for phylogenetic history (such as PGLS).
We also apply the first two phylogenetic comparative methods to investigate the evolution of major brain structures involving the neocortex, cerebellum, and medulla. It is widely assumed that the neocortex expanded disproportionately relative to other brain structures during the evolution of anthropoid primates and most particularly in human evolution ( Kriegstein et al., 2006 ; Geschwind and Rakic, 2013 ; Florio and Huttner, 2014 ). Surprisingly however, direct tests of this hypothesis are lacking, despite the focus of much evolutionary and developmental neuroscience on the neocortex as the site of interest for understanding human uniqueness and its developmental mechanisms ( Mitchell and Silver, 2017 ). Recent evidence suggests that the cerebellum may have contributed more to human brain evolution than previously appreciated: it underwent rapid evolutionary expansion in the great ape clade including hominins ( Barton and Venditti, 2014 ; Smaers et al., 2018 ) and has been implicated in shape changes of the brain in hominin fossil endocasts ( Kochiyama et al., 2018 ; Neubauer et al., 2018 ). Molecular evidence now corroborates the proposal that selection on cerebellar function was an important feature of hominoid and hominin brain evolution ( Sousa et al., 2017 ), with changes in protein-coding genes implicated in cerebellar development more likely to have evolved adaptively in apes than those implicated in neocortical development ( Harrison and Montgomery, 2017 ). It therefore appears that the neocortex and cerebellum have had different evolutionary trajectories in primate evolutionary history. More research is needed to document and understand these patterns.
We examined volumetric change in the neocortex and cerebellum relative to both body mass and the volume of the rest of the brain. As a check to establish whether changes in evolutionary patterns for relative neocortex and cerebellum size are primarily attributable to changes in those structures or to changes in the rest of the brain, we investigated the evolution of the rest of the brain relative to body mass. We also conducted analyses of the volume of the medulla relative to body mass and the volume of the rest of the brain. The relative volume of the medulla does not vary significantly across clades ( Barton, 2000 ) and as such it has not been attributed a major role in brain expansion. For the analyses of fossil species, brain component volumes are not available; thus, analyses of these lineages are restricted to overall brain size (ECV).
Although our main focus is on broad patterns across primate phylogeny and on the extent to which human brain evolution fits or departs from these patterns, we also examined brain evolution in other species that are considered to be unusually large-brained, such as the aye-aye ( Daubentonia ) and capuchins ( Cebinae ) ( Isler et al., 2008 ; Pagel and Harvey, 1989 ). Our analyses also help to identify other primate species that have experienced exceptional expansion or reduction of the brain or its components, generating new questions for future research on exceptional brain evolution in primates.
We used our third method to characterize patterns of brain evolution in humans and extinct hominins. Pagel (2002) conducted similar analyses of raw ECV. Our analyses advance his findings in two ways. First, we incorporate body mass as a predictor. Second, we focus on the deviation from brain size expectations, based on the PGLS methods used to assess outlier status. Our findings therefore provide insights to the evolutionary trajectory of exceptional hominin ECV relative to primate-wide brain-body mass scaling relationships.
Comparative data
We compiled ECV and female body mass data on non-human primates ( Isler et al., 2008 ) as well as humans and fossil hominins ( Robson and Wood, 2008 , Tables 1 and 2 ). Given that sex specific body mass estimates are available for ancient humans and extinct hominins ( Robson and Wood, 2008 ), we used female values for body mass because female values are more tightly linked to ecological and life-history factors ( Gordon, 2006 ) and sexual selection can drive increases in male body mass unlinked to ecology, obscuring brain-body scaling relationships ( Fitzpatrick et al., 2012 ). We also compiled data on neocortex, cerebellum, and medulla volume ( Barton and Venditti, 2014 ; Stephan et al., 1981 ; Bush and Allman, 2004 ). Values used to compute predictor variables (described below) for analyses of brain sub-structures were taken from Isler et al. (2008) . We used several phylogenies in our analyses. For analyses of hominin ECV, we constructed a ‘hominin phylogeny’ by combining the hominin consensus tree from Organ et al. (2011) and the non-human primate consensus tree from 10kTrees version 3 ( Arnold et al., 2010 ). To ensure that our results in this set of analyses were not dependent upon the topology of the hominin phylogeny, we repeated them using an ‘alternate hominin phylogeny,’ constructed in a similar manner using another hominin tree from Organ et al. (2011) . Details of the tree construction process are given in Appendix 1. In all other analyses we used either the consensus primate phylogeny or a block of 100 primate phylogenies from 10kTrees, version 3.
Hominin ECV and body mass data details.
All values are from Robson and Wood (2008) .
Human brain data.
To determine whether patterns of exceptional evolution represent absolute or relative changes in scaling, we included several predictor variables in our analyses. To investigate whether the volumes of structures changed relative to body size, we used body mass as a predictor variable, while we used a ‘rest-of-brain’ metric as a predictor variable to investigate whether the volumes of structures changed relative to other brain structures. For the analyses of all structures other than the medulla, the ‘rest-of-brain’ was computed as whole brain volume – (neocortex volume +cerebellum vol). In analyses of the medulla, we calculated ‘rest-of-brain’ volume as brain volume - medulla volume. We also analyzed the volume of the ‘rest-of-brain’ [whole brain volume – (neocortex volume +cerebellum vol)] relative to body mass. The data sets used in all analyses, along with more detailed descriptions, are given in Appendix 1.
Characterizing patterns of phenotypic evolution
We compared the fit of multi-optima Ornstein-Uhlenbeck (OU) models of evolution and Brownian models of evolution using a developmental version of the R package bayou ( Uyeda and Harmon, 2014 ; Uyeda, 2017 ). OU models of evolution incorporate stabilizing selection and drift, while Brownian models only include drift. Bayou fits multi-optima OU models to a phylogeny using a Markov-Chain Monte Carlo (MCMC) approach. A shift in selection regime refers to a change in the parameters that determine the optimum trait value (towards which species evolve) at a specific location on a phylogeny. Thus, inferred changes in selective regime provide insights to how lineages differ. Shifts in selection regime along terminal branches of a tree would provide particularly strong evidence for a species’ uniqueness.
Grabowski et al. (2016) proposed the following OU model to describe the evolution of a trait, y , as a function of a predictor variable, x :
Equation 1 :
Equation 2 :
In these equations, dy is the change in the trait value, α is the magnitude of the selective 'pull' towards the optimum trait value, y 0 , and σ 2 is the variance of the white noise process dB . The variables θ and β can be interpreted as the intercept and slope of the optimum regression line specified in Equation 2 . The optimum regression line represents the state that a species is evolving towards rather than the actual evolutionary trajectory.
This model has limited utility when data for x are only available for the tips of the phylogeny because the values of x must be known along the branches of the phylogeny to infer the expected value of y for a lineage. We utilize two similar models implemented in the developmental version of bayou – the unweighted predictor model and the weighted predictor model (corresponding to ‘immediate’ and ‘alphaweighted’ options for ‘slopechange’ in bayou) – as these circumvent the issue of unknown phenotypes in ancestral lineages while incorporating a predictor variable into the OU model. The weighted predictor model considers the evolutionary history of the predictor variable while fitting models, and the unweighted predictor model only considers the values of the predictor variables at the tips of the phylogeny while fitting models. The details of these two models are provided in Appendix 2.
Bayou uses a MCMC to parameterize the models to fit the data by inferring the location and magnitude of concurrent shifts θ and β on a phylogeny and by inferring the values of α and σ 2 , which remain constant across the phylogeny. The parameters α and σ 2 are used in the calculation of the variance-covariance matrices used in evaluating model fit to the phylogeny. The phylogenetic half-life, the time needed for a trait to evolve halfway to the optimum, is computed as ln(2) / α. We present phylogenetic half-life in units of tree height. A phylogenetic half-life less than tree height indicates that the evolutionary processes can 'pull' parameter values to the optimum within the timescale in question, while a phylogenetic half-life that exceeds tree height or constitutes a large percentage of tree height indicates that evolutionary processes have a weak 'pull' and trait values are not expected to closely approach the optimum during the timescale in question. The expected variance in trait values evolving to the same optima at equilibrium (stationary variance) can be computed as σ 2 2 α .
For each analysis, we ran the weighted and unweighted predictor models. We also ran a Brownian motion model in which the strength of stabilizing selection (α) was fixed at 10 −6 (resulting in a phylogenetic half-life ~9500 times greater than tree height; bayou cannot compute model likelihoods when α is 0), and no shifts away from the root regime were allowed. The predictor variable is still incorporated in the Brownian motion model, but no changes in its coefficient occur on the phylogeny. We used the hominin tree for the analysis of ECV and the consensus tree of extant primates for all other analyses. All MCMCs were run for 5,005,000 time steps, sampling every 10 time steps. The priors used are given in Table 3 . For each analysis, two chains were run and checked for convergence in terms of likelihood, α, and σ 2 (see Appendix 3 for discussion of chain non-convergence issues in analyses of ECV). We also checked for correlation in branch-wise posterior shift probability between chains. Diagnostic plots pertaining to chain convergence are given in Source data 1 . The two chains were combined, with the first 30% of samples being discarded as burn in. We then obtained the likelihood of each model and calculated Bayes factors for each model pairing ( Kass and Raftery, 1995 ; Jeffreys, 1998 ) using the steppingstone algorithm in bayou, which implements the method of Fan et al. (2011) . We imposed a posterior probability cutoff of 0.3 for shift detection.
Priors for bayou MCMC analyses.
* Calculated using ‘cdpois’ option in bayou.
When the multi-optima OU model was selected over the Brownian motion model, we used the location and magnitude of shifts in adaptive optima to assess changes in patterns of evolution. The inference of a shift on a terminal branch would indicate an exceptional pattern of evolution for a given species.
Ho and Ané (2013) identified several potential problems with OU models, including un-identifiability of parameters and over-fitting, but acknowledged that such models may be necessary, and recommended that Bayesian models, specifically bayou, be used to overcome these problems. Several other phylogenetic OU models have been developed (most notably Hansen, 1997 ), but none utilized Bayesian parameter estimation. Cooper et al. (2016) echoed the concerns of Ho and Ané (2013) and again recommended using Bayesian approaches. Additionally, they recommended weighing the fit of an OU model of evolution against that of a Brownian model, which do through our model selection process.
Outlier detection using PGLS
When bayou indicated that the Brownian model of trait evolution was favored over the multi-optima OU model, we conducted a phylogenetic outlier test. This was accomplished using BayesModelS, an R script that generates distributions of predicted trait values for a species or several species based on phylogenetically controlled analyses of trait covariation with predictor variables ( Nunn and Zhu, 2014 ). BayesModelS uses a Markov-Chain Monte Carlo (MCMC) to fit parameters of a PGLS model and assumes a Brownian motion model of evolutionary change. The PGLS models are used to generate trait value predictions for the species of interest. Uncertainty in phylogenetic structure can be accounted for by sampling from a set of trees ( Pagel, 2002 ).
BayesModelS accounts for phylogenetic non-independence of residual trait values by incorporating branch scaling factors when fitting PGLS models. The MCMC samples between two branch length scaling factors, λ and κ, to improve the fit of the models. The parameter λ scales the internal branches of the phylogeny and measures phylogenetic signal ( Nunn, 2011 ). Values for λ were constrained to be in the interval [0, 1]. In the κ model phylogenetic tree branch lengths are raised to the power κ. The value of κ has previously been used to assess support for a ‘speciational’ mode of evolution (see Pagel, 2002 ).
When predicting the value of a trait for a species (or a group of species), its data were excluded from the BayesModelS analysis to avoid biasing the predictions. BayesModelS was then used to generate a posterior probability distribution of predicted values for that species, based on the predictor variable, estimated phylogenetic signal, and estimated trait co-variation with the other species in the analysis. Species were identified as outliers when their trait value was more extreme than 97.5% of the predicted trait values (i.e. when trait values fell outside 95% credible interval). A species was identified as a positive outlier when its true value fell above the majority of predictions, and a negative outlier when the opposite was true.
The analyses conducted using BayesModelS proceeded as follows. First, we investigated whether hominins follow primate brain size to body mass scaling rules by using BayesModelS to predict ECV based on body mass and phylogeny. We tested each hominin species for outlier status while excluding data on all hominins when generating predictions. When computing mean estimates for hominin ECV, we corrected for back transformation bias using the quasi-maximum likelihood estimator method described in Smith (1993) . We used the hominin phylogeny or the alternate hominin phylogeny in these analysis, and the data spanned 225 extant primate species (including humans) and 10 extinct hominin species.
Next, we identified individual primate species that are evolutionary outliers for ECV and other brain structures (neocortex, cerebellum, medulla, rest-of-brain). In these analyses, we accounted for phylogenetic uncertainty by using the block of 100 trees, which included H. sapiens and H. neanderthalensis but no other hominins. We iteratively tested each species in the data set for outlier status. Our analysis for ECV included data from 145 species, and our analyses for other brain structures structures included data from between 39 and 53 species.
MCMC chains were run for 1,000,000 time steps, and the first 200,000 time steps were discarded as burn in. Flat priors were used for all variables being predicted. To assess whether the post-burn in results were drawn from a stable distribution, we used the ‘heidel.diag’ function in the R package coda ( Plummer et al., 2006 ). When post-burn-in results were not drawn from a stable distribution, we discarded an additional portion of the chain (as indicated by ‘heidel-diag’) so that only results drawn from a stable distribution remained. We ensured that the effective sample sizes for the PGLS model parameters (slope, intercept, most frequently selected phylogenetic scaling parameter) were greater than 1000 using the ‘effectiveSize’ function in coda ( Plummer et al., 2006 ). Details of the MCMC diagnostics are given in supplementary materials S6, along with detailed results concerning the posterior predicted distribution and phylogenetic scaling parameters for each species in each analysis.
Characterizing the tempo of ECV evolution in hominins
We investigated the evolutionary trajectory of brain-body scaling in hominins relative to other primates. We calculated the difference between observed ECV and the mean BayesModelS prediction for brain size (generated in the first described BayesModelS analysis in which data for all hominin species was excluded while generating predictions) for each of the hominin species. This difference, which we call ‘brain size deviation’ represents the magnitude and direction of the deviation in brain size from what would be expected under primate brain-body scaling rules. We fit four PGLS model to hominin brain size deviation to examine how brain size deviation covaried with the phylogenetic distance from the hominin- Pan split: First, we fit a ‘Brownian’ model of brain size deviation with no predictor. We fixed λ at one in this and all subsequent models. Next, we fit a ‘directional’ model of brain size deviation predicted by phylogenetic distance from the hominin- Pan split, expecting to find a positive relationship between these variables if brain volume relative to body size has increased since the split of hominins and Pan . To determine whether evolutionary rates in brain size deviation have accelerated over time, we fit an ‘acceleration’ model that included the phylogenetic scaling parameter δ ( Pagel, 2002 ; Pagel, 1999 ). Values of δ greater than one are consistent with accelerating evolution, but not necessarily directional evolution. Finally, we fit a ‘directional acceleration’ model in which we fit the parameter δ and used phylogenetic distance from the hominin- Pan split as a predictor of brain size deviation. In this model, a positive relationship between brain size deviation and phylogenetic distance, along with a value of δ greater than 1, would indicate that brain volume relative to body size has increased at an accelerating rate since the divergence of hominins from Pan. We compared these models using AICc. Analyses were conducted in the R package caper ( Orme, 2013 ).
Endocranial volume (ECV)
In the bayou analysis of ECV predicted by body mass using the hominin phylogeny, the Brownian model was favored over the weighted and unweighted predictor OU models with Bayes factors greater than 22. When we repeated this analysis using the alternate hominin phylogeny, we found that the un-weighted predictor OU model was favored over the weighted predictor OU model and the Brownian model with Bayes factors greater than 42, despite displaying poor convergence in terms of α and σ 2 . However, both chains inferred a similar set of shifts, indicating that this is likely an issue related to parameter identifiability rather than to shift identifiability. In this model, progressive shifts towards larger ECV relative to body mass were detected within the hominin clade along the human lineage ( Figure 1A,B ). Shifts towards larger relative brain size were also detected on the terminal branch leading to D. madagascariensis and the internal branches leading to the Lemuridae and Cebinae, clades, and shifts towards smaller relative brain size were detected on the branch leading to the Alouatta clade, the branch leading to the clade containing the Aotidae and Callitrichidae families, and the branch leading to the Colobinae sub-family ( Figure 1—figure supplement 1 ). The rejected weighted predictor OU model, as well as both OU models that were rejected in the bayou analysis using the hominin phylogeny, detected a very similar set of shifts that included shifts towards progressively larger ECV relative to body mass along the human lineage ( Source data 1 ). Because the Brownian model was favored in the bayou analysis using the hominin phylogeny, we proceeded with BayesModels analyses using both the hominin and alternate hominin phylogenies.
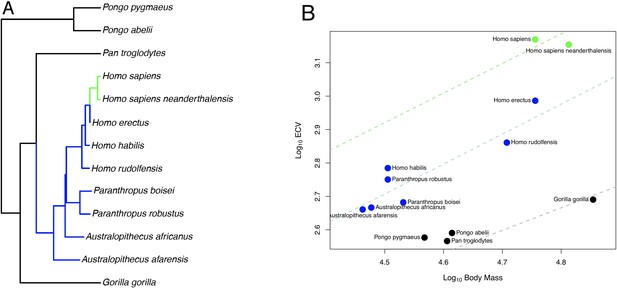
OU Model of ECV Evolution in Primates Panel.
A shows the location of the selection regimes identified in an OU model of ECV predicted by body mass. Panel B shows the corresponding optimum regression lines representing the various selection regimes, along with body mass and ECV data. Data are colored by their corresponding selection regimes. All results are from the un-weighted predictor OU model in the bayou analysis using the alternate hominin phylogeny. Only the great ape clade is shown; selection regimes across the entire primate phylogeny are show in Figure 1—figure supplement 1 .
In the BayesModelS analysis predicting ECV based on body mass while excluding all hominin data, the observed values for H. sapiens and H. neanderthalensis exceeded the mean values predicted by BayesModelS by 7.63 and 6.96 standard deviations respectively ( Figure 2C ). All hominin species were strongly supported positive outliers, with more than 99.9% of predictions falling below the observed values for ECV. The mean ECV prediction for a primate with the body mass of H. sapiens was 438 mL. Remarkably, the observed value for humans is 1478 mL, which is 238% greater than the mean of the predicted posterior distribution. A similar result was found for H. neanderthalensis; the observed ECV for this species exceeded the mean predicted value for a primate of their body mass by 952 mL, or 201%. Humans exceeded their predicted ECV by the greatest percentage, but all hominins exceeded predictions by at least 51% ( Figure 2C , Table 4 ). We obtained similar results using the alternate hominin phylogeny ( Figure 2—figure supplement 1 , Table 5 ).
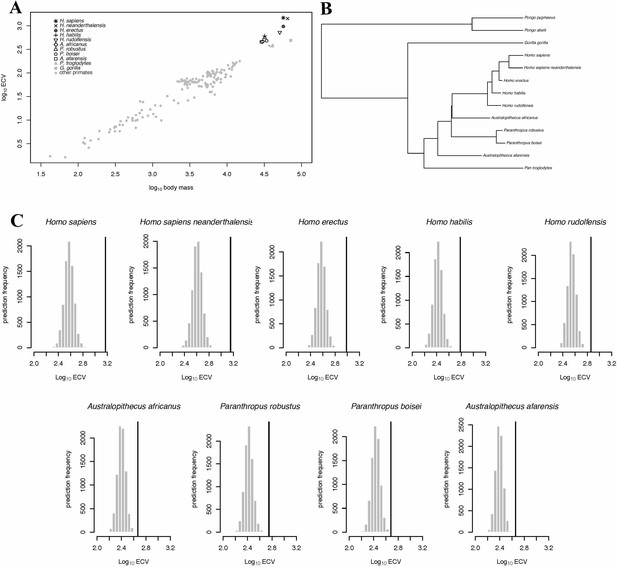
BayesModelS predictions of ECV in hominins.
Panel ( A ) shows a scatter plot of primate ECV and body mass data. Panel ( B ) shows the topology of the great ape portion of the hominin phylogeny used in the BayesModelS analyses of hominin ECV. Panel ( C ) shows the posterior distributions of predicted ECV values generated by BayesModelS for hominin species with body mass used as the predictor variable. Vertical lines indicated observed values.
Predicted Hominin ECV values from BayesModelS analysis using the hominin phylogeny.
Predicted hominin ecv values from bayesmodels analysis using the alternate hominin phylogeny..
When we iteratively predicted ECV based on body mass and phylogeny for each species in the data set (no hominins besides H. sapiens and H. neanderthalensis were included in this analysis) and while using all data to generate predictions. We again found that humans were strongly supported positive outliers (Figure 4A). H. neanderthalensis was not identified as an outlier, perhaps because these analyses included all species except for the one being predicted, and thus inclusion of H. sapiens resulted in a wide posterior distribution when predicting ECV in H. neanderthalensis . Indeed, when we excluded H. sapiens in this analysis we found that H. neanderthalensis was identified as a strongly supported positive outlier ( Source data 1 ). We also identified several other primate species as outliers (see Table 6 and Source data 1 ).
Summary of evidence for exceptional brain evolution among non-human primates.
* The dataset for this analysis did not contain any other gorilla species.
In the bayou analysis of ECV with no predictor variable using the hominin phylogeny, the Brownian model was selected over the un-weighted predictor OU models (in which the influence of the predictor was set to 0) with a Bayes factor >10. No weighted predictor model was run, as it would have been equivalent to the unweighted model given that no predictor variable was incorporated. An equivalent result was found when we repeated the analysis using the alternate hominin phylogeny. We then proceeded with the BayesModelS analysis, iteratively testing the outlier status of each species in the data set. We used the tree block for this analysis, and as such H. sapiens and neanderthalensis were the only hominins included. We found that neither humans nor Neanderthals were detected as an outlier ( figure 4—figure supplement 1 ; Source data 1 ), indicating that without correcting for body mass, the variance in ECV across primates is great enough to prevent humans’ brains from being detected as exceptionally large.
Evolutionary trajectory of ECV in hominins
We conducted PGLS analyses of brain size deviation conducted to characterize the evolution of exceptional brain size in hominins (data shown in Figure 3 ). The analyses revealed evidence for both accelerated evolution of brain size deviation and directional evolution towards larger brain size deviations, as indicated by the directional acceleration model (AICc = −23.38) being favored over the acceleration (AICc = −21.93), directional (AICc = −17.56), and Brownian (AICc = −14.58) evolution models. In this best model, there was evidence of directional evolution towards larger brain size relative to body size (slope = 0.04) over time, and of accelerating evolution (δ = 8.36). These results suggest that the exceptionality of the human brain evolved recently. We found similar results when we repeated this analysis using the alternate hominin phylogeny ( Figure 3—figure supplement 1 ). These analyses therefore support a model of accelerating evolution towards larger brain volume relative to body mass in Homo sapiens .
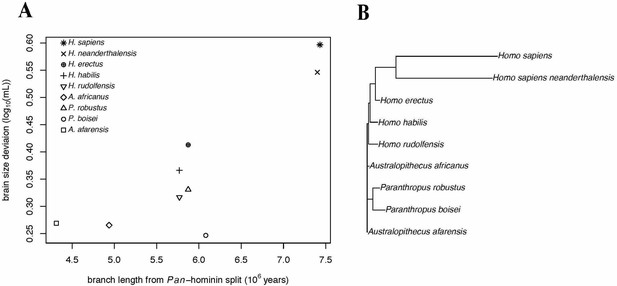
Accelerating Evolution of Brain Size Deviation in Hominins.
( A ) Brain size deviation was calculated as the difference between the mean BayesModelS prediction (made while excluding all hominin data from analysis and using the hominin phylogeny) and the observed value. Phylogenetic distance was measured as time since the shared ancestor of hominins and Pan at 7.43 mya. ( B ) Hominin clade in the hominin phylogeny after δ transformation, with δ = 8.36 following the directional acceleration model.
In the bayou analysis of neocortex volume as predicted by body mass, the Brownian motion model was strongly favored over the weighted and unweighted predictor OU models, with Bayes factors > 18. Humans were detected as strongly supported positive outliers for neocortex volume by BayesModelS when body mass was used as the predictor variable ( Figure 4B ).
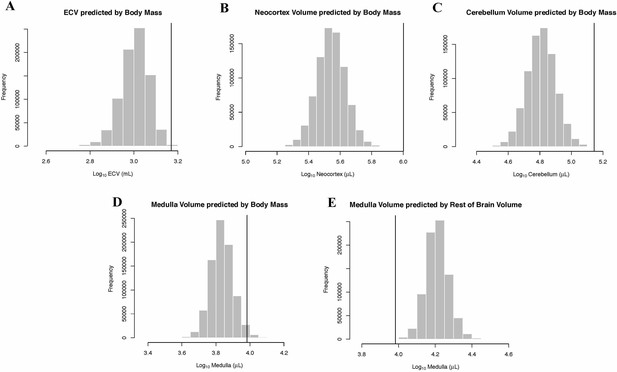
Human Outlier Status for Brain Traits Predicted distributions of trait values generated by BayesModelS are show as histograms.
Vertical bars represent the observed values.
In the bayou analysis of neocortex volume with ‘rest-of-brain’ as the predictor variable, the weighted predictor model was selected over the unweighted predictor and Brownian motion models with Bayes Factors > 9.2. In the weighted predictor model, different scaling patterns were detected for strepsirrhines and haplorhines, with the optimum regression line for haplorhines falling above that of strepsirrhines. The only other detected transition in scaling occurred on the terminal branch leading to Nasalis larvatus, indicating a shift towards lower relative neocortex size ( Figure 5A,B ).
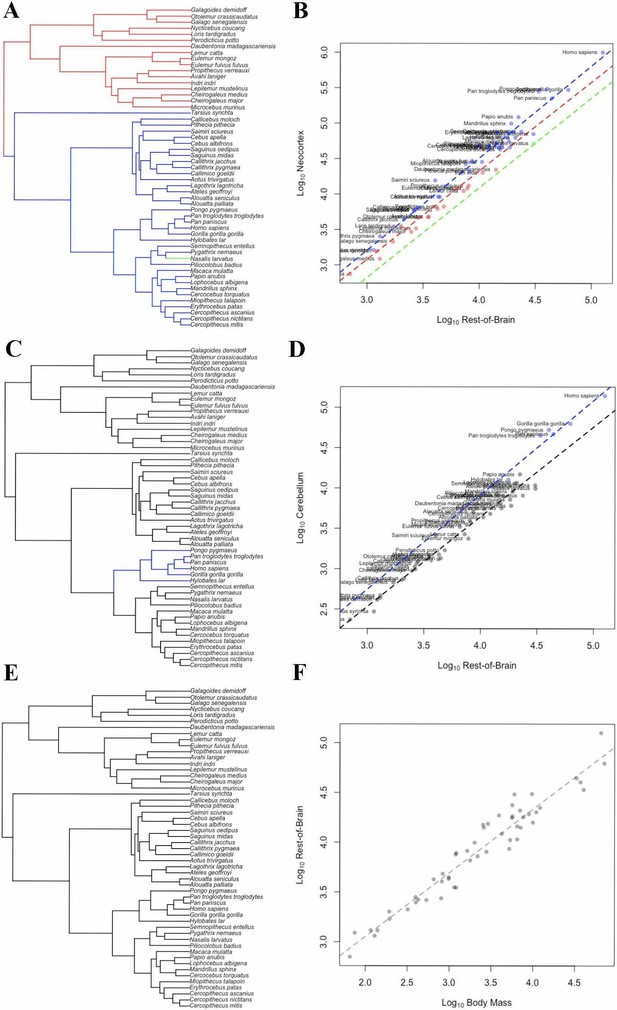
OU Models of Brain Structure Evolution in Primates.
( A and B ) correspond to the OU weighted predictor model of neocortex volume predicted by the rest-of-brain. ( C and D ) correspond to the OU unweighted predictor model of cerebellum volume predicted by the rest-of-brain. ( E and F ) correspond to the OU weighted predictor model of the rest-of-brain volume predicted by body mass. ( A, C ) and ( E ) show the location of selection regimes on the primate phylogeny. ( B, D ) and ( F ) show the optimum regression lines associated with the selection regimes. Points show primate trait and predictor data; colors correspond to the selection regimes. Colors in ( A, C ) and ( E ) match those in ( B, D ) and ( F ).
In the bayou analysis of cerebellar volume predicted by body mass, the Brownian motion model was favored over the weighted predictor and unweighted predictor OU models, with Bayes factors of 11.96 and 22.79, respectively. BayesModelS identified humans as strongly supported positive outliers for cerebellum volume when body mass was used as the predictor variable ( Figure 4C ).
In the bayou analysis of cerebellum volume relative to the rest-of-brain, the comparison between the unweighted predictor model and the Brownian motion model gave a Bayes factor of 10.65, while the comparison between the unweighted and weighted predictor models gave a Bayes factor of 0.20. This indicates that the OU models clearly outperform the Brownian model, but that neither OU model performs significantly better than the other. Both OU models detected a shift on the branch leading to apes associated with an increase in optimum cerebellar volume relative to the ‘rest-of-brain’ volume ( Figure 5C,D ).
In the bayou analysis of medulla volume predicted by body mass, the Brownian motion model was selected over the two OU models with Bayes factors > 7.4. BayesModelS identified humans as strongly supported positive outliers for medulla volume ( Figure 4D ). No other species were identified as exceptional in this analysis. When medulla was predicted by the ‘rest-of-brain’ volume, the Brownian motion model was again selected over the OU models, with Bayes factors > 3.8. Humans were identified as strongly supported negative outliers ( Figure 4E ).
Rest-of-brain
In the bayou analyses of the rest-of-brain relative to body mass, the OU models were selected over the Brownian motion model, with Bayes factors > 13. However, the comparison between the two OU models gave a Bayes factor of 0.20, indicating that neither model is supported relative to the other. No shifts were detected in either model ( Figure 5E,F ).
Our phylogenetic analyses revealed that the human brain is 238% larger than the size expected for a primate of similar body mass and phylogenetic position. The exceptional size of the human brain was achieved through progressive scaling shifts towards larger size over several million years of hominin evolution, and the evolution towards increased brain size relative to expectations based on primate scaling patterns accelerated over time. These findings add an important dimension to previous observations of gradual phyletic increases in hominin brain size. Du et al. (2018) fit six evolutionary models to within- and between-lineage change in hominin brain sizes (random walk, gradualism, stasis, punctuated equilibrium, stasis-random walk and stasis-gradualism), obtaining the best fit for a gradualism model. However, their non-phylogenetic analysis did not test explicitly for accelerating directional increase. Our findings extend the results obtained by Pagel (2002) on absolute cranial volume, as the pattern of accelerating evolution is found even after accounting for body size. The pattern of accelerating brain size increase documented here is consistent with hypotheses that postulate a co-evolutionary positive feedback process driving human brain evolution, such as feedback between brain size and culture or language ( Wills, 1993 ; Deacon, 1998 ) or between the brain sizes of conspecifics engaged in a socio-cognitive evolutionary arms race ( Dunbar, 1998 ; Miller, 2011 ).
While humans clearly have the largest relative brain size among extant primates, anatomically modern humans were closely matched by H. neanderthalensis . However, even when accounting for the close phylogenetic relationship between humans and H. neanderthalensis and the exceptionally large brain of the latter, the human brain is still much larger than expected: humans were identified as strongly supported outliers when their ECV (relative to body mass) was predicted by phenotypic data from all primates, including H. neanderthalensis . This pattern was not reciprocal, however; H. neanderthalensis was not significantly different from other primates when H. sapiens was included in the model.
Significant variation exists between estimates of ECV and body mass made from different fossil specimens of the same hominin species ( Robson and Wood, 2008 ). Thus, using single specimens to represent a species would not be a good statistical practice. We used a dataset in which almost all mean species values were calculated from multiple fossil specimens ( Table 1 ). Unfortunately, we could not explicitly account for intraspecific variation in our analyses, as the multi-optima OU model fitting approach and the outlier test are unable to account for variation in both a trait and predictor variable. It would therefore be worthwhile to revisit our analyses as new phylogenetic comparative methods that can account for intraspecific variation become available. Additionally, data quality will likely improve over time. More hominin fossils will be discovered, increasing sample sizes for estimated ECV and body mass.
The hominin phylogeny will also likely become better resolved and more complete. We accounted for some phylogenetic uncertainty by repeating our analyses with an alternate phylogeny. The use of different phylogenies influenced outcomes of some statistical tests, as the Brownian model favored when we used the hominin phylogeny and OU model was favored when we used the alternate hominin phylogeny. However, we found that all of the OU models we fit inferred the same pattern of evolution towards larger ECV along the human lineage. The results of our outlier tests and PGLS model fitting – which assume a Brownian mode of evolution – also detected this pattern on different phylogenies. Collectively, these results indicate that our findings are likely to be robust to variations in assumed evolutionary relationships, and potentially to assumptions about the mode of evolution.
It is widely assumed that primate brain size evolution in general, and the large size of the human brain in particular, reflects expansion of the neocortex relative to other brain structures ( Kriegstein et al., 2006 ; Rakic, 2009 ). Our results contradict this assumption: human neocortical volume was exceptionally large relative to body mass, but not exceptional relative the volume of the rest of the brain. We documented only one shift in neocortex size relative to the rest of the brain during primate evolution: an increase at the origin of all haplorrhines. This shift may be related to the visual specializations of haplorrhines for high-acuity photic vision, mediated by extensive cortical visual areas that make up over 50% of the cortex in these species ( Drury et al., 1996 ; Barton, 1998 ; Barton, 2007 ). On branches postdating the split between haplorrhines and strepsirrhines, neocortex size is largely predictable from its scaling relationship to the rest of the brain, in line with the proposed importance of cortical-subcortical connectivity in primate brain evolution ( Whiting and Barton, 2003 ).
In contrast, we found that the cerebellum increased in size relative to the rest of the brain on the branch leading to apes. This finding is consistent with the results of recent studies implicating the cerebellum, and especially the lateral cerebellum, in brain expansion in apes and some other mammalian lineages ( Barton and Venditti, 2014 ; Smaers et al., 2018 ; MacLeod et al., 2003 ). Our findings also reinforce the argument that subcortical structures should be given greater consideration in studies of mammalian brain evolution and cognition ( Barton, 2012 ; Miller and Clark, 2018 ). Cerebellar specialization in apes may have been initiated by the demands on motor control and route-planning imposed by arboreal below-branch locomotion and/or by complex extractive foraging ( Barton and Venditti, 2014 ; Barton, 2012 ). The fact that shifts in the relative size of neocortex and cerebellum occurred on different parts of the tree supports the theory of mosaic brain evolution ( Barton and Harvey, 2000 ) and suggests that no single adaptive hypothesis is likely to be capable of accounting for primate brain evolution; rather, different selection pressures, on different information-processing capacities, likely operated at different times on different lineages.
Consistent with previous studies, we found that the medulla expanded in humans (positive outlier status for medulla volume relative to body mass), but to a lesser degree than other structures (negative outlier status for medulla volume relative to the rest of the brain). Relative to body mass, medulla volume has been shown to be much less variable across taxa than other brain structures, particularly compared to the neocortex and cerebellum. For example, unlike neocortex and cerebellum, medulla volume does not differ significantly between insectivores, strepsirrhines and haplorrhines ( Barton, 2000 ). Accordingly, we found that after controlling for either body mass or brain size, the evolution of the medulla was not modulated by selection towards a stationary optimum in the primate clade. These results further support mosaic brain evolution ( Barton and Harvey, 2000 ), and also suggest that scaling constraints related to connectivity with other brain regions ( Montgomery et al., 2016 ) was less critical for the medulla than for the neocortex and cerebellum.
Several non-human primate species exhibited exceptional brain evolution in one trait or another, but only humans showed exceptional brain evolution for multiple brain components. As predicted, we detected shifts towards larger brain size on the terminal branches leading to D. madagascariensis, and on the branch leading to the Cebinae clade. Large brain size in Daubentonia and Cebinae has been attributed to extractive foraging and tool use ( Kaufman, 2005 ; Melin et al., 2014 ; Parker, 2015 ). Although not one of our a priori expectations, we also documented shifts towards smaller brain size on branches leading to several clades, including Alouatta. We also found that two Gorilla species exhibit a smaller brain or neocortex size relative to body mass than expected. Given the extremely large body mass of Gorilla species, these unique traits may be the byproduct of a body mass increase rather than a reduction in brain size. Also unexpectedly, two Pan troglodytes sub-species were found to have exceptionally large and small ECV relative to body mass respectively. However, because more closely related species are weighed more heavily when BayesModelS generates distributions of predicted trait values, sister taxa deviating from expectations in opposite directions could result in both taxa being identified as outliers, even if they both conform to patterns of brain-body scaling for other primates. If the trait distributions for each species overlap significantly, then accounting for intraspecific variation in future analyses could remedy this problem.
The unexpected patterns that we observed amongst non-human primates raise several questions for further research. Given the well-established positive correlation between overall brain size and extended life history ( Isler and van Schaik, 2009 ; Sol, 2009 ; González-Lagos et al., 2010 ), what are the life history implications of mosaic shifts in the sizes of different structures, and do these support any specific interpretations of the correlation between brain size and life histories? One hypothesis, the developmental costs hypothesis, is that large brains simply take longer to grow and mature, leading to extended periods of maternal investment and slower maturation, with other life history correlates of brain size being byproducts of developmental prolongation. Support for this hypothesis is provided by the finding that, amongst mammals, the durations of gestation and lactation have independent effects on pre- and postnatal brain growth, and once these effects are accounted for, other life history correlates are non-significant ( Barton and Capellini, 2011 ). Despite their generally correlated evolution ( MacLeod et al., 2003 ), we found shifts in the relative size of neocortex and cerebellum on different parts of the phylogenetic tree. Because these two structures have different developmental trajectories, the developmental costs hypothesis predicts different life history correlates; this prediction has now received support ( Powell et al., 2019 ). Further work is needed to establish exactly what developmental changes allowed for the neocortex and cerebellum to rest-of-brain scaling rules to change at the origin of haplorrhines and hominoids, respectively.
Another area of interest concerns the cases we found of brain or brain component size reduction. Montgomery et al. (2010) found that brain size reductions were rare during primate evolution, and that there was a general trend for brain size to increase across multiple branches of the phylogeny. This raises questions for future work concerning the causes, developmental mechanisms and functional implications of specific types of size reduction, such as those that we uncovered in brain size relative to body size in Alouatta and other clades, and in neocortex size relative to the rest of the brain in N. larvatus .
Finally, a key question that has attracted considerable attention concerns the ecological and social drivers of brain size and structure across large-scale evolutionary radiations. It has become increasingly apparent that correlations between overall brain size and behavioral ecology needed to be treated with caution ( Powell et al., 2017 ; Healy and Rowe, 2007 ; Wartel et al., 2018 ). However, as suggested by the hypothesis of mosaic brain evolution, correlations between ecology and individual, less functionally heterogenous brain components may be more reliable and robust ( Barton and Venditti, 2014 ; Barton, 2012 ; Barton, 2007 ; Whiting and Barton, 2003 ; Montgomery et al., 2016 ; Barton et al., 1995 ). Our analyses focused on gross subdivisions within the brain, and we suggest that further insights could be obtained by applying the phylogenetic methods used in this paper to more fine-grained neuro-anatomical data, using this approach to tease apart the contributions of correlated and mosaic change among brain components ( Melin et al., 2014 ) and by incorporating ecological, behavioral, and developmental predictor variables that may account for additional variation in the traits of interest.
In conclusion, we provided robust evidence for directional and accelerating selection towards larger brain size over the course of human evolution, resulting in the human brain being exceptionally large for a primate of similar body mass. We also found that the sizes of human brain components – including the neocortex, cerebellum, and the rest of the brain – are not larger or smaller than expected relative to the size of the rest of the brain, but all are larger than expected for a primate of similar body mass. These results suggest that relative neocortical expansion is not a hallmark of our species. The diversity of evolutionary patterns for various brain components that we observed within primates suggests that no single factor fully explains primate brain evolution; instead, comparative research should investigate how different selection pressures influenced the evolution of different neuroanatomical components at different times on different parts of the phylogenetic tree. Additionally, future work should seek to analyze the evolution of other brain traits, including neuronal composition, using similar phylogenetic comparative methods that account for the non-independence of data from related species.
Data Compilation
All data and trees used in our analyses are included in the Source data 2 file. We compiled three data sets for our analyses. The first was used for the analyses of endocranial volume (ECV), the second was used for the analyses of the neocortex and cerebellum, and the third was used for the analyses of the medulla.
In the first data set (‘data set 1.csv’), we compiled ECV and female body mass values for non-human primates from Isler et al. (2008) , who compiled their data set in part from Araújo et al. (2000) , Gordon, 2006 , Smith and Jungers (1997) , and Thalmann and Geissmann (2000) . This dataset was supplemented with fossil data for ancient humans and extinct hominins from Robson and Wood (2008) . These authors provided two taxonomies: one that recognized more species of hominins (the ‘splitting taxonomy’), and another that lumped hominin lineages into fewer taxonomic categories (the ‘lumping taxonomy’). We extracted values from the splitting taxonomy, except those for Australopithecus africanus , which were only available from the lumping taxonomy. We also chose to use values for Homo erectus (sensu lato) from the lumping taxonomy, as these values was calculated from fossils attributed to both H. erectus and H. ergaster , two species that are not differentiated in our phylogeny. We did not include H. heidelbergensis in our analyses because its phylogenetic position is unresolved ( Mounier and Caparros, 2015 ). Sample sizes are given in Table 1 . Museum numbers for the specimens used in calculating species mean values are given in Appendix I of Robson and Wood (2008) .
In the second data set (‘data set 2.csv’), body mass, neocortical volume, and cerebellar volume for humans and extant non-human primates were compiled from the data set of Barton and Venditti ( Barton and Venditti, 2014 ). We also complied brain volumes to use in the calculation of the ‘rest-of-brain’ predictor trait in the analyses of these brain structures. These values were calculated as an average of the values given in Stephan et al. (1981) , MacLeod et al. (2003) , Bush and Allman (2003) , Rilling and Insel (1998) , and Rilling and Insel (1999) . The second data set was limited to extant primates and included values for 55 species, including humans.
The third data set (‘data set 3.csv’) included body mass, brain volumes, and medulla volumes from Stephan et al. (1981) . This data set spanned 41 species.
A summary of all human ECV and body mass estimates and the analyses in which they were used is given in Tables 1 and 2 .
All trait and predictor values were log 10 transformed prior to analyses. When differences between component volumes were used in analyses, we calculated the logarithms after subtraction.
We used several different phylogenetic trees and tree blocks in our analyses. We constructed a ‘hominin phylogeny’ (‘hominin.phylogeny.txt’) that included humans, extinct hominins, and extant primates for use in the analyses of hominin ECV (including the analyses of directional and accelerating evolution); this phylogeny was produced by grafting the ‘combined dataset consensus time tree’ of hominin evolution from Organ et al. (2011) onto the time-scaled consensus tree of extant primates from version 3 of 10kTrees ( Arnold et al., 2010 ). We grafted the clade (including the root branch) containing Pan and all fossil hominins onto the node at which Gorilla diverged from the Pan lineage, and then re-scaled this pasted clade so that the human tip lined up with those of extant primates. We also constructed an ‘alternative hominin phylogeny’ (‘alt.hominin.phylogney.txt’ using the ‘morphology and molecular graft time tree’ from Organ et al. (2011) . To construct this tree, we again grafted the clade (including the root branch) containing Pan and all fossil hominins onto the node at which Gorilla diverged from the Pan lineage, and then shortened the root branch so that the human tip lined up with those of extant primates. We were not able to use this method for constructing the hominin phylogeny because it would have resulted in the branch leading to the clade containing Pan and hominins having a negative length. Both hominin phylogenies we constructed include humans, 300 other extant primates, and 13 extinct hominin species. In other analyses, we used a consensus tree (‘consensus.tree.txt’) of extant primates (for OU model fitting) or a block of 100 primate trees (‘tree.block.txt’) downloaded from version 3 of 10kTrees ( Arnold et al., 2010 for phylogenetic prediction).
Details of Bayou models
The un-weighted predictor model is described by the following equation:
E[y] is the expected value of a species trait. W and θ M represent the evolutionary weight matrix and θ matrix described in Butler and King (2004) . W is a 1 x n matrix whose entries are the weights given to each of the n selection regimes through which the species of interest evolved. The weight of each regime is dependent upon the phylogeny and the value of α. More recent regimes have greater weights, especially when α is high. θ M is an n x 1 matrix of the θ values of the regimes through which the species of interest evolved. The product of W and θ M gives the effective θ value for a species that evolved towards the various optimum θ values specified in θ M . β n is the β value of the parameter regime at the tip of the phylogeny. Therefore, in this model, the expected phenotype for a species is a function of the evolutionarily weighted effective θ value, the coefficient of the predictor variable of the current selection regime, and the value of the predictor at the tip of the phylogeny.
The weighted predictor model is described by a similar equation:
β M is an n x 1 matrix of the optimum β values θ M . of the n regimes through which the species evolved, and is analogous to θ M . Thus, in this model the expected trait value of each species is a function of the species evolutionarily weighted effective θ and β values, and the value of the predictor variable x at the tip of the phylogeny.
Problems with MCMC convergence in bayou
Bayou returned several MCMC chains during the analyses of ECV that did not converge in terms of likelihood, α, and σ 2 . To address this issue, we generated up to six MCMC chains in each analysis for both for the un-weighted predictor, weighted predictor, and Brownian models. Several chains with exceptionally high mean likelihood had σ 2 values approaching zero and very high α values that appeared to be bounded by a maximum value. We infer from these patterns that the chains were settling on an unrealistic pattern of evolution with the stationary variance approaching zero. These chains also inferred shifts erratically; they predicted shifts with posterior probability greater than 0.1 on many branches, but no shifts had a posterior probability greater than 0.3. We discarded these chains, and then selected the two chains with the highest mean likelihood for each analysis for subsequent use.
All data used in our analyses are provided as supplementary material.
- Albuquerque F
- Nascimento M
- Google Scholar
- Matthews LJ
- Carvalho LR
- Grinberg LT
- Ferretti RE
- Jacob Filho W
- Herculano-Houzel S
- Capellini I
- Freckleton RP
- de Sousa AA
- Sherwood CC
- Schleicher A
- van Schaik C
- DeCasien AR
- Williams SA
- Van Essen DC
- Anderson CH
- Felsenstein J
- Fitzpatrick JL
- Gonzalez-Voyer A
- Pennington C
- Geschwind DH
- González-Lagos C
- Grabowski M
- Harrison PW
- Montgomery SH
- Christopher Kirk E
- Albrecht GA
- van Schaik CP
- Kochiyama T
- Ponce de León MS
- Zollikofer CPE
- Kriegstein A
- Martínez-Cerdeño V
- Anderson RC
- DiVincenti LJ
- Hiramatsu C
- Leimgruber KL
- Slocombe KE
- Visalberghi E
- Mosdossy KN
- Hutchinson JR
- Wrangham RW
- Henneberg M
- Gómez-Robles A
- Raghanti MA
- Tebbenkamp ATN
- Kawasawa YI
- Stephenson AR
- Elsworth JD
- Kleinman JE
- Weinberger DR
- Marques-Bonet T
- Gerstein MB
- St Wecker PG
- Geissmann T
- Harrison KH
- Lindenfors P
Author details
- Ecology and Evolutionary Biology, Princeton University, Princeton, United States
- Department of Evolutionary Anthropology, Duke University, Durham, United States
Contribution
For correspondence, competing interests.

- Duke Global Health Institute, Duke University, Durham, United States
National Science Foundation (BCS-1355902)
The funders had no role in study design, data collection and interpretation, or the decision to submit the work for publication.
Acknowledgements
We are grateful to Josef Uyeda for advice on using the developmental version of bayou. We would also like to thank Tom Milledge and Duke Research Computing for assistance with the Duke computing cluster.
Version history
- Received: August 22, 2018
- Accepted: January 29, 2019
- Accepted Manuscript published: January 31, 2019 (version 1)
- Version of Record published: February 18, 2019 (version 2)
© 2019, Miller et al.
This article is distributed under the terms of the Creative Commons Attribution License , which permits unrestricted use and redistribution provided that the original author and source are credited.
- 7,510 Page views
- 846 Downloads
- 37 Citations
Article citation count generated by polling the highest count across the following sources: Crossref , Scopus , PubMed Central .
Download links
Downloads (link to download the article as pdf).
- Article PDF
- Figures PDF
Open citations (links to open the citations from this article in various online reference manager services)
Cite this article (links to download the citations from this article in formats compatible with various reference manager tools), categories and tags.
- brain evolution
- phylogenetic comparative methods
- human evolution
Research organism
Further reading, emergence of catalytic function in prebiotic information-coding polymers.
Life as we know it relies on the interplay between catalytic activity and information processing carried out by biological polymers. Here we present a plausible pathway by which a pool of prebiotic information-coding oligomers could acquire an early catalytic function, namely sequence-specific cleavage activity. Starting with a system capable of non-enzymatic templated replication, we demonstrate that even non-catalyzed spontaneous cleavage would promote proliferation by generating short fragments that act as primers. Furthermore, we show that catalytic cleavage function can naturally emerge and proliferate in this system. Specifically, a cooperative catalytic network with four subpopulations of oligomers is selected by the evolution in competition with chains lacking catalytic activity. The cooperative system emerges through the functional differentiation of oligomers into catalysts and their substrates. The model is inspired by the structure of the hammerhead RNA enzyme as well as other DNA- and RNA-based enzymes with cleavage activity that readily emerge through natural or artificial selection. We identify the conditions necessary for the emergence of the cooperative catalytic network. In particular, we show that it requires the catalytic rate enhancement over the spontaneous cleavage rate to be at least 10 2 –10 3 , a factor consistent with the existing experiments. The evolutionary pressure leads to a further increase in catalytic efficiency. The presented mechanism provides an escape route from a relatively simple pairwise replication of oligomers toward a more complex behavior involving catalytic function. This provides a bridge between the information-first origin of life scenarios and the paradigm of autocatalytic sets and hypercycles, albeit based on cleavage rather than synthesis of reactants.
- Cell Biology
The Hippo kinase cascade regulates a contractile cell behavior and cell density in a close unicellular relative of animals
The genomes of close unicellular relatives of animals encode orthologs of many genes that regulate animal development. However, little is known about the function of such genes in unicellular organisms or the evolutionary process by which these genes came to function in multicellular development. The Hippo pathway, which regulates cell proliferation and tissue size in animals, is present in some of the closest unicellular relatives of animals, including the amoeboid organism Capsaspora owczarzaki . We previously showed that the Capsaspora ortholog of the Hippo pathway nuclear effector Yorkie/YAP/TAZ (coYki) regulates actin dynamics and the three-dimensional morphology of Capsaspora cell aggregates, but is dispensable for cell proliferation control (Phillips et al., 2022). However, the function of upstream Hippo pathway components, and whether and how they regulate coYki in Capsaspora , remained unknown. Here, we analyze the function of the upstream Hippo pathway kinases coHpo and coWts in Capsaspora by generating mutant lines for each gene. Loss of either kinase results in increased nuclear localization of coYki, indicating an ancient, premetazoan origin of this Hippo pathway regulatory mechanism. Strikingly, we find that loss of either kinase causes a contractile cell behavior and increased density of cell packing within Capsaspora aggregates. We further show that this increased cell density is not due to differences in proliferation, but rather actomyosin-dependent changes in the multicellular architecture of aggregates. Given its well-established role in cell density-regulated proliferation in animals, the increased density of cell packing in coHpo and coWts mutants suggests a shared and possibly ancient and conserved function of the Hippo pathway in cell density control. Together, these results implicate cytoskeletal regulation but not proliferation as an ancestral function of the Hippo pathway kinase cascade and uncover a novel role for Hippo signaling in regulating cell density in a proliferation-independent manner.
- Immunology and Inflammation
Caspase-1 activates gasdermin A in non-mammals
Gasdermins oligomerize to form pores in the cell membrane, causing regulated lytic cell death called pyroptosis. Mammals encode five gasdermins that can trigger pyroptosis: GSDMA, B, C, D, and E. Caspase and granzyme proteases cleave the linker regions of and activate GSDMB, C, D, and E, but no endogenous activation pathways are yet known for GSDMA. Here, we perform a comprehensive evolutionary analysis of the gasdermin family. A gene duplication of GSDMA in the common ancestor of caecilian amphibians, reptiles, and birds gave rise to GSDMA–D in mammals. Uniquely in our tree, amphibian, reptile, and bird GSDMA group in a separate clade than mammal GSDMA. Remarkably, GSDMA in numerous bird species contain caspase-1 cleavage sites like YVAD or FASD in the linker. We show that GSDMA from birds, amphibians, and reptiles are all cleaved by caspase-1. Thus, GSDMA was originally cleaved by the host-encoded protease caspase-1. In mammals the caspase-1 cleavage site in GSDMA is disrupted; instead, a new protein, GSDMD, is the target of caspase-1. Mammal caspase-1 uses exosite interactions with the GSDMD C-terminal domain to confer the specificity of this interaction, whereas we show that bird caspase-1 uses a stereotypical tetrapeptide sequence to confer specificity for bird GSDMA. Our results reveal an evolutionarily stable association between caspase-1 and the gasdermin family, albeit a shifting one. Caspase-1 repeatedly changes its target gasdermin over evolutionary time at speciation junctures, initially cleaving GSDME in fish, then GSDMA in amphibians/reptiles/birds, and finally GSDMD in mammals.
Be the first to read new articles from eLife
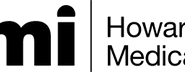
Brain Evolution
Articles (30 in this collection), comparative mri analysis of the forebrain of three sauropsida models, authors (first, second and last of 12).
- I Santos-Álvarez
- Nerea Moreno
- Content type: Original Article
- Open Access
- Published: 28 March 2024
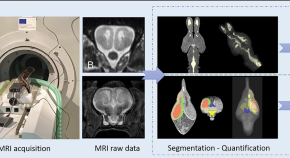
Unveiling the neuroanatomy of Josephoartigasia monesi and the evolution of encephalization in caviomorph rodents
Authors (first, second and last of 7).
- José Darival Ferreira
- Andrés Rinderknecht
- Leonardo Kerber
- Published: 19 March 2024
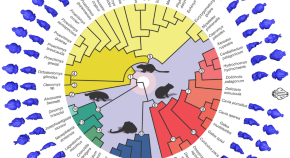
Development of the prosencephalic structures, ganglionic eminence, basal ganglia and thalamus at 11 + 3 to 13 + 6 gestational weeks on 3D transvaginal ultrasound including normative data
- T. Rechberger
- Published: 15 September 2023
- Pages: 2089 - 2101
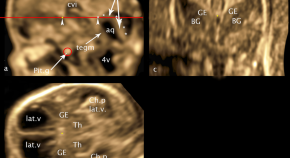
Development of associational fiber tracts in fetal human brain: a cadaveric laboratory investigation
- Davide Tiziano Di Carlo
- Maria Elena Filice
- Paolo Perrini
- Content type: Brief Report
- Published: 02 September 2023
- Pages: 2007 - 2015
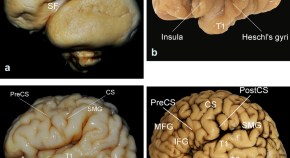
Episodic memory deficit in HIV infection: common phenotype with Parkinson’s disease, different neural substrates
Authors (first, second and last of 11).
- Rosemary Fama
- Eva M. Müller-Oehring
- Tilman Schulte
- Published: 18 April 2023
- Pages: 845 - 858
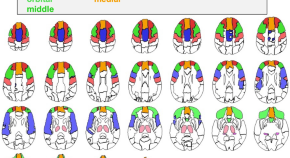
Expression of SATB1 and SATB2 in the brain of bony fishes: what fish reveal about evolution
- Daniel Lozano
- Jesús M. López
- Published: 01 April 2023
- Pages: 921 - 945
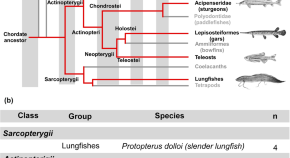
Anatomical organization of forebrain circuits in the primate
- Franco Giarrocco
- Bruno B. Averbeck
- Content type: Review
- Published: 21 October 2022
- Pages: 393 - 411
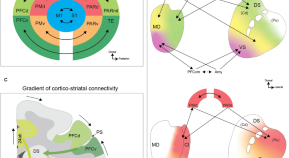
Planum temporale grey matter volume asymmetries in newborn monkeys ( Papio anubis )
Authors (first, second and last of 6).
- Yannick Becker
- Romane Phelipon
- Adrien Meguerditchian
- Content type: Short Communication
- Published: 03 May 2021
- Pages: 463 - 468
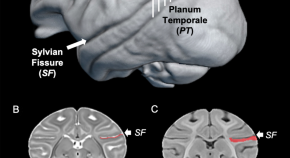
Red nucleus structure and function: from anatomy to clinical neurosciences
Authors (first, second and last of 9).
- Gianpaolo Antonio Basile
- Marina Quartu
- Alberto Cacciola
- Published: 12 November 2020
- Pages: 69 - 91
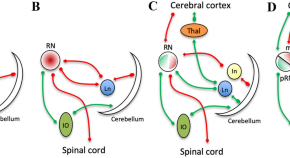
A three-dimensional digital atlas of the Nile crocodile ( Crocodylus niloticus ) forebrain
- Brendon K. Billings
- Mehdi Behroozi
- Felix Ströckens
- Published: 03 February 2020
- Pages: 683 - 703
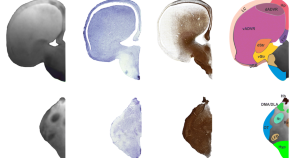
A network approach to brain form, cortical topology and human evolution
- Emiliano Bruner
- Borja Esteve-Altava
- Diego Rasskin-Gutman
- Published: 12 June 2019
- Pages: 2231 - 2245
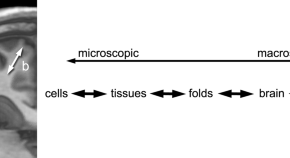
The Structural Model: a theory linking connections, plasticity, pathology, development and evolution of the cerebral cortex
- Miguel Ángel García-Cabezas
- Basilis Zikopoulos
- Helen Barbas
- Published: 09 February 2019
- Pages: 985 - 1008
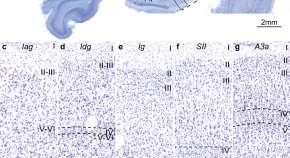
The dark matter of the brain
- Saak V. Ovsepian
- Published: 18 January 2019
- Pages: 973 - 983
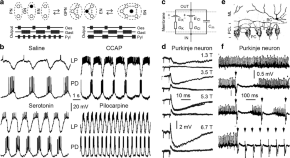
Study of pallial neurogenesis in shark embryos and the evolutionary origin of the subventricular zone
- A. Docampo-Seara
- Published: 06 July 2018
- Pages: 3593 - 3612
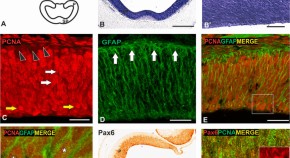
Diffusion tractography reveals pervasive asymmetry of cerebral white matter tracts in the bottlenose dolphin ( Tursiops truncatus )
Authors (first, second and last of 4).
- Alexandra K. Wright
- Rebecca J. Theilmann
- Miriam Scadeng
- Published: 30 November 2017
- Pages: 1697 - 1711
The birth of the synapse
- Published: 13 June 2017
- Pages: 3369 - 3374
Lobular homology in cerebellar hemispheres of humans, non-human primates and rodents: a structural, axonal tracing and molecular expression analysis
- Yuanjun Luo
- Hirofumi Fujita
- Izumi Sugihara
- Published: 15 May 2017
- Pages: 2449 - 2472
The interlaminar glia: from serendipity to hypothesis
- Jorge A. Colombo
- Published: 18 November 2016
- Pages: 1109 - 1129
Evidence for expansion of the precuneus in human evolution
- Todd M. Preuss
- James K. Rilling
- Published: 02 January 2016
- Pages: 1053 - 1060
The extreme capsule fiber complex in humans and macaque monkeys: a comparative diffusion MRI tractography study
Authors (first, second and last of 14).
- Rogier B. Mars
- Sean Foxley
- Matthew F. S. Rushworth
- Published: 01 December 2015
- Pages: 4059 - 4071
From genes to folds: a review of cortical gyrification theory
- Paul C. Fletcher
- Published: 16 December 2014
- Pages: 2475 - 2483
A neuronal aging pattern unique to humans and common chimpanzees
- Emmanuel P. Gilissen
- Karelle Leroy
- Jean-Pierre Brion
- Published: 09 November 2014
- Pages: 647 - 664
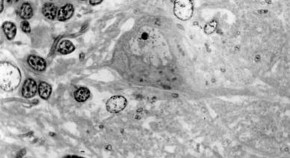
The neocortex of cetartiodactyls: I. A comparative Golgi analysis of neuronal morphology in the bottlenose dolphin ( Tursiops truncatus ), the minke whale ( Balaenoptera acutorostrata ), and the humpback whale ( Megaptera novaeangliae )
- Camilla Butti
- Caroline M. Janeway
- Published: 07 August 2014
- Pages: 3339 - 3368
Acquisition of Paleolithic toolmaking abilities involves structural remodeling to inferior frontoparietal regions
- E. E. Hecht
- D. A. Gutman
- Published: 25 May 2014
- Pages: 2315 - 2331
Diffusion tensor imaging reveals evolution of primate brain architectures
- Degang Zhang
- Tianming Liu
- Published: 08 November 2012
- Pages: 1429 - 1450
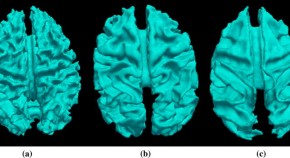
Hemispheric asymmetry in the fusiform gyrus distinguishes Homo sapiens from chimpanzees
- Steven A. Chance
- Eva K. Sawyer
- Timothy J. Crow
- Published: 30 October 2012
- Pages: 1391 - 1405
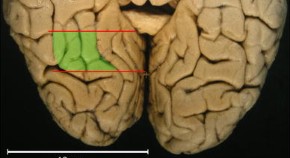
Faster scaling of visual neurons in cortical areas relative to subcortical structures in non-human primate brains
Authors (first, second and last of 5).
- C. E. Collins
- D. B. Leitch
- Suzana Herculano-Houzel
- Published: 09 June 2012
- Pages: 805 - 816
The sea lamprey tryptophan hydroxylase: new insight into the evolution of the serotonergic system of vertebrates
- María Eugenia Cornide-Petronio
- Ramón Anadón
- Antón Barreiro-Iglesias
- Published: 17 April 2012
- Pages: 587 - 593
The insular cortex: a comparative perspective
- Patrick R. Hof
- Content type: Original article
- Published: 29 May 2010
- Pages: 477 - 493
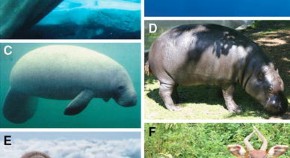
Neocortical neuron types in Xenarthra and Afrotheria: implications for brain evolution in mammals
- Chet C. Sherwood
- Cheryl D. Stimpson
- Published: 15 November 2008
- Pages: 301 - 328
Participating journals
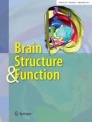
Brain Structure and Function
- Find a journal
- Publish with us
- Track your research
UMass Amherst Biologists Lead Broadest-Ever Study of Primate Brains to See How Gene Expression Influences Brain Evolution
An interdisciplinary team of researchers led by biologists at the University of Massachusetts Amherst recently published the results of a first-of-its-kind study investigating the links between gene expression and brain evolution across 18 primate species. The team collected samples of brain tissue sourced from zoos whose animals had died naturally, as well as from people who had donated their bodies to science, and then sequenced the RNA transcripts from each sample to generate a map of every one of the 17,000 genes expressed in each primate’s brain. The team then compared each species’ fully-sequenced RNA transcriptomes to better understand the links between genomics and evolution and possibly provide insight into the nuances of brain activity as well as neurodegenerative disease.

“We study primate brain evolution,” says Katie Rickelton, lead author of the paper, published in eLife , and doctoral candidate in molecular and cellular biology at UMass Amherst. “Primates, and especially humans, are defined by having very large brains compared to their body size—and yet, humans, chimpanzees and lemurs are all very different, despite having similar DNA sequences. We think that difference can be partly explained by which genes are expressed at higher or lower levels.”
Other researchers have sequenced the RNA in primate brains, but in a much more limited scope. “If we are going to figure out what makes humans unique among primates,” says senior author Courtney Babbitt , associate professor of biology at UMass Amherst, “we’re going to have to study a wider selection of primates, and no one has looked at such a large sample before.”
To conduct their research, Rickelton, Babbitt and their colleagues worked with the National Institute for Child Health and Human Development Brain and Tissue Bank for Developmental Disorders at the University of Maryland, the National Chimpanzee Brain Resource, and a nearly a dozen other institutions that are widely respected for their ethical sourcing of brain tissue. The team obtained samples from four different brain regions—including the prefrontal cortex, primary visual cortex, hippocampus and cerebellum—in each of the 18 species under investigation, and used the genomics core at UMass Amherst’s Institute for Applied Life Sciences to conduct the RNA sequencing.
RNA is the intermediate step between DNA—each species’ master-plan—and the proteins that actually build an individual body. The number and kinds of proteins that can be produced are determined by the amount of RNA, which is mapped with the transcriptomes that Rickelton, Babbitt and their colleagues have generated. And it is an immense task.
“We sequenced every single one of the 17,000 genes expressed in each of the four regions across the 18 species,” says Babbitt. “And we were able to sample them at very high resolution,” adds Rickelton. “This is the best series of transcriptomes that we have for these 18 species’ brains.”
The team was looking for differences related to a host of brain functions related to both cognition and metabolism, because the big and complex brains that we humans share with our primate kin demand a lot of energy. They found a remarkable degree of variation across the species range, from human to pygmy slow loris.
For instance, humans and chimpanzees exhibit a remarkable level of variation compared to the other 16 species, even though humans and chimps branched out from the rest of the great apes relatively recently, leaving little time for natural selection to act. And though there are differences in the four regions of the brain the team sampled, the majority of the variation seems to be primarily explainable by species evolution. The exception, Rickelton points out, is the cerebellum. “It’s evolutionarily the oldest part of the brain,” says Rickelton, “and so has had the most time to evolve in different ways for each of the species.”
Finally, the team’s findings identify particular genes for further study that may help explain the evolution of particular primate’s brains. These genes may help to better understand the nuances of brain activity across each of the four regions, as well as provide insight into various human neurodegenerative disorders, such as Alzheimer’s Disease.
“It’s one of the great evolutionary paradoxes: humans and chimps have pretty much the same genes, and yet we’re so different,” says Babbitt. “To figure out what makes us human, we’re going to have to look at the genetic expression of a wide range of our evolutionary cousins, and that’s exactly what we’ve begun to do with this study.”
This research was supported by the U.S. National Science Foundation, the Wenner Gren Foundation, the James S. McDonnell Foundation, and the National Institutes of Health.
Related: Researchers at UMass Amherst Show How a Small Strand of RNA is Key to Fighting Cancer
A team of UMass Amherst researchers has shown that a single, small strand of microRNA, or miRNA, known as let-7, governs the ability of T-cells to recognize and remember tumor cells.
share this!
March 28, 2024
This article has been reviewed according to Science X's editorial process and policies . Editors have highlighted the following attributes while ensuring the content's credibility:
fact-checked
peer-reviewed publication
trusted source
Comprehensive study explores influence of gene expression on primate brain evolution
by Courtney Babbitt, University of Massachusetts Amherst
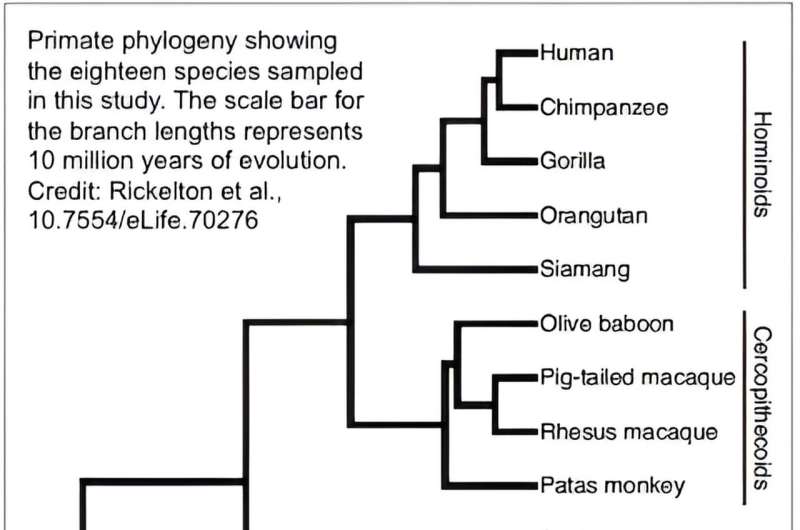
An interdisciplinary team of researchers led by biologists at the University of Massachusetts Amherst recently published the results of a first-of-its-kind study investigating the links between gene expression and brain evolution across 18 primate species.
The team collected samples of brain tissue sourced from zoos whose animals had died naturally, as well as from people who had donated their bodies to science, and then sequenced the RNA transcripts from each sample to generate a map of every one of the 17,000 genes expressed in each primate's brain.
The team then compared each species' fully-sequenced RNA transcriptomes to better understand the links between genomics and evolution and possibly provide insight into the nuances of brain activity as well as neurodegenerative disease.
"We study primate brain evolution ," says Katie Rickelton, lead author of the paper published in eLife , and doctoral candidate in molecular and cellular biology at UMass Amherst.
"Primates, and especially humans, are defined by having very large brains compared to their body size—and yet, humans, chimpanzees, and lemurs are all very different despite having similar DNA sequences. We think that difference can be partly explained by which genes are expressed at higher or lower levels."
Other researchers have sequenced the RNA in primate brains but in a much more limited scope. "If we are going to figure out what makes humans unique among primates," says senior author Courtney Babbitt, associate professor of biology at UMass Amherst, "we're going to have to study a wider selection of primates, and no one has looked at such a large sample before."
To conduct their research, Rickelton, Babbitt, and their colleagues worked with the National Institute for Child Health and Human Development Brain and Tissue Bank for Developmental Disorders at the University of Maryland, the National Chimpanzee Brain Resource, and nearly a dozen other institutions that are widely respected for their ethical sourcing of brain tissue.
The team obtained samples from four different brain regions—including the prefrontal cortex , primary visual cortex , hippocampus, and cerebellum—in each of the 18 species under investigation and used the genomics core at UMass Amherst's Institute for Applied Life Sciences to conduct the RNA sequencing.
RNA is the intermediate step between DNA—each species' master plan—and the proteins that actually build an individual body.
The number and kinds of proteins that can be produced are determined by the amount of RNA, which is mapped with the transcriptomes that Rickelton, Babbitt, and their colleagues have generated. And it is an immense task.
"We sequenced every single one of the 17,000 genes expressed in each of the four regions across the 18 species," says Babbitt. "And we were able to sample them at very high resolution," adds Rickelton. "This is the best series of transcriptomes that we have for these 18 species' brains."
The team was looking for differences related to a host of brain functions related to both cognition and metabolism, because the big and complex brains that we humans share with our primate kin demand a lot of energy. They found a remarkable degree of variation across the species range, from human to pygmy slow loris.
For instance, humans and chimpanzees exhibit a remarkable level of variation compared to the other 16 species, even though humans and chimps branched out from the rest of the great apes relatively recently, leaving little time for natural selection to act. And though there are differences in the four regions of the brain the team sampled, the majority of the variation seems to be primarily explainable by species evolution.
The exception, Rickelton points out, is the cerebellum. "It's evolutionarily the oldest part of the brain," says Rickelton, "and so has had the most time to evolve in different ways for each of the species."
Finally, the team's findings identify particular genes for further study that may help explain the evolution of particular primate's brains. These genes may help to better understand the nuances of brain activity across each of the four regions, as well as provide insight into various human neurodegenerative disorders, such as Alzheimer's Disease.
"It's one of the great evolutionary paradoxes: humans and chimps have pretty much the same genes, and yet we're so different," says Babbitt. "To figure out what makes us human, we're going to have to look at the genetic expression of a wide range of our evolutionary cousins, and that's exactly what we've begun to do with this study."
Journal information: eLife
Provided by University of Massachusetts Amherst
Explore further
Feedback to editors

AI improves monsoon rainfall predictions

82% of EU farm subsidies bolster high emissions foods: Study
2 hours ago

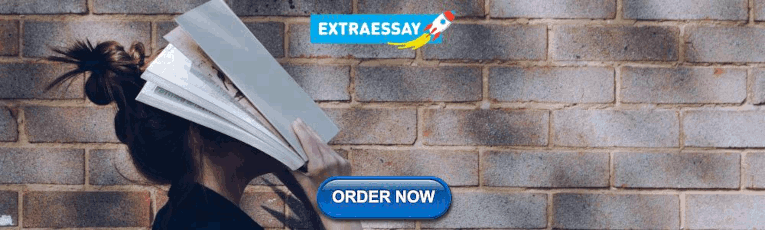
Leaves of three, let it be? Wide variability among poison ivy plants makes identification more challenging

Golfers' risk from pesticides used on turf grass is likely low, studies find

'Frankenstein design' enables 3D printed neutron collimator

New antibiotic class effective against multidrug-resistant bacteria discovered
3 hours ago

Computational tools fuel reconstruction of new and improved bird family tree

New method reveals hidden activity of life below ground

A frozen chunk of genome rewrites our understanding of bird evolution

Engineers 'symphonize' cleaner ammonia production
Relevant physicsforums posts, what do large moles on the body indicate.
Mar 30, 2024
Avian flu - A new study led by a team from the University of Maryland
Mar 27, 2024
Are all biological catabolic reactions exergonic?
Mar 20, 2024
A First of Its Kind: A Calcium-based signal in the Human Brain
Mar 18, 2024
Biological culture and cultural biology
Mar 17, 2024
Potentially fatal dog parasite found in the Colorado River
Mar 15, 2024
More from Biology and Medical
Related Stories

Study reveals genes that set humans apart from other primates in cognitive ability
Dec 6, 2023

Brains of newborns aren't underdeveloped compared to other primates, finds new study
Dec 4, 2023

Organoids research identifies factor involved in brain expansion in humans

Small but distinct differences among species mark evolution of human brain
Nov 23, 2017

Study shows differences between brains of primates—humans, apes and monkeys—are small but significant
Nov 8, 2022

The human brain: not just large but finely shaped
Jul 9, 2020
Recommended for you

Ancient Adélie penguin DNA reveals that small repeats persist for hundreds of millions of years
7 hours ago

Chemists discover a key protein in how lysosomes work
8 hours ago
Let us know if there is a problem with our content
Use this form if you have come across a typo, inaccuracy or would like to send an edit request for the content on this page. For general inquiries, please use our contact form . For general feedback, use the public comments section below (please adhere to guidelines ).
Please select the most appropriate category to facilitate processing of your request
Thank you for taking time to provide your feedback to the editors.
Your feedback is important to us. However, we do not guarantee individual replies due to the high volume of messages.
E-mail the story
Your email address is used only to let the recipient know who sent the email. Neither your address nor the recipient's address will be used for any other purpose. The information you enter will appear in your e-mail message and is not retained by Phys.org in any form.
Newsletter sign up
Get weekly and/or daily updates delivered to your inbox. You can unsubscribe at any time and we'll never share your details to third parties.
More information Privacy policy
Donate and enjoy an ad-free experience
We keep our content available to everyone. Consider supporting Science X's mission by getting a premium account.
E-mail newsletter
Unraveling the Mysteries of Primate Brain Evolution Insights from Gene Expression Studies
T he human brain, with its unparalleled complexity and cognitive abilities, stands as a testament to millions of years of evolution. Yet, the mechanisms underlying the evolution of the primate brain, including our own, remain shrouded in mystery. In a pioneering study, researchers from the University of Massachusetts Amherst have embarked on a quest to decode the genetic blueprint of primate brain evolution. By examining the intricate interplay between gene expression and brain function across a diverse array of primate species, their findings promise to illuminate the evolutionary forces driving the development of the primate mind.
1. Unveiling the Genetic Landscape of Primate Brain Evolution
The study, led by Katie Rickelton and Courtney Babbitt, represents a groundbreaking effort to unravel the genetic underpinnings of primate brain evolution. Leveraging advanced sequencing technologies, the research team embarked on a comprehensive analysis of RNA transcripts extracted from brain tissue samples sourced from 18 primate species. By mapping the expression patterns of over 17,000 genes across multiple brain regions, the researchers sought to elucidate the genetic signatures associated with primate brain evolution.
2. Exploring the Evolutionary Dynamics of Gene Expression
One of the key insights gleaned from the study is the remarkable degree of variation in gene expression observed across primate species. Despite sharing a high degree of genetic similarity, humans and chimpanzees exhibit distinct patterns of gene expression, underscoring the role of evolutionary divergence in shaping the primate brain. Moreover, the researchers identified notable differences in gene expression profiles across different brain regions, shedding light on the functional specialization of distinct brain regions in primates.
3. Evolutionary Paradoxes and Insights
The findings of the study shed light on a longstanding evolutionary paradox: despite sharing nearly identical genetic sequences, humans and chimpanzees display striking differences in brain structure and function. By examining the regulatory mechanisms governing gene expression, the researchers uncovered potential genetic determinants underlying these differences. Moreover, the study highlights the evolutionary significance of the cerebellum, the oldest part of the brain, in shaping primate brain evolution over millions of years.
4. Implications for Understanding Brain Function and Disease
Beyond unraveling the mysteries of primate brain evolution, the study holds profound implications for understanding brain function and disease. By pinpointing specific genes associated with cognitive functions and metabolic processes, the researchers have laid the groundwork for future investigations into the neural basis of primate behavior. Furthermore, insights gained from studying primate gene expression patterns may provide valuable clues for understanding and treating neurodegenerative disorders such as Alzheimer’s disease.
5. Future Directions and Concluding Remarks
As the quest to decipher the genetic basis of primate brain evolution continues, the study underscores the importance of interdisciplinary collaboration and advanced genomic technologies in unraveling the complexities of the primate mind. By elucidating the genetic mechanisms driving brain evolution across diverse primate species, researchers are poised to unlock new insights into the origins of human cognition and behavior. As we journey further into the realm of primate brain evolution, the path ahead promises to be filled with exciting discoveries and transformative insights into the nature of our own humanity.

An official website of the United States government
The .gov means it’s official. Federal government websites often end in .gov or .mil. Before sharing sensitive information, make sure you’re on a federal government site.
The site is secure. The https:// ensures that you are connecting to the official website and that any information you provide is encrypted and transmitted securely.
- Publications
- Account settings
Preview improvements coming to the PMC website in October 2024. Learn More or Try it out now .
- Advanced Search
- Journal List
- Front Neurosci
Evolution of the Human Brain Can Help Determine Pathophysiology of Neurodevelopmental Disorders
Koichiro irie.
1 Department of Neuroscience and Cell Biology, Graduate School of Medicine, Osaka University, Suita, Japan
2 Center for Medical Research and Education, Graduate School of Medicine, Osaka University, Suita, Japan
Noriyoshi Usui
3 United Graduate School of Child Development, Osaka University, Suita, Japan
4 Global Center for Medical Engineering and Informatics, Osaka University, Suita, Japan
5 Addiction Research Unit, Osaka Psychiatric Research Center, Osaka Psychiatric Medical Center, Osaka, Japan
Shoichi Shimada
The evolution of humans brought about a co-occurring evolution of the human brain, which is far larger and more complex than that of many other organisms. The brain has evolved characteristically in humans in many respects, including macro-and micro-anatomical changes in the brain structure, changes in gene expression, and cell populations and ratios. These characteristics are essential for the execution of higher functions, such as sociality, language, and cognition, which express humanity, and are thought to have been acquired over evolutionary time. However, with the acquisition of higher functions also comes the risk of the disease in which they fail. This review focuses on human brain evolution and neurodevelopmental disorders (NDDs) and discusses brain development, molecular evolution, and human brain evolution. Discussing the potential for the development and pathophysiology of NDDs acquired by human brain evolution will provide insights into the acquisition and breakdown of higher functions from a new perspective.
Introduction
The human brain is relatively large, compared with those of other organisms, and it is more complex, harboring a greater capacity for higher functions such as cognition or sociality. A better understanding of the evolution of the human brain and of the pathophysiology of diseases that disrupt cognition might also improve our understanding of the mechanism of acquisition of these higher order functions. Cognitive disorders, such as neurodevelopmental disorders (NDDs) or psychiatric disorders, are thought to be human-specific disorders due to the disruption of higher cognitive functions of the brain, which most other animals lack.
The brains of humans and other primates or rodents differ in a number of neuroanatomical patterns such as wrinkles, size, morphology, and connectivity; cell biological differences such as cell ratios and population distribution; and molecular differences such as gene expression ( Hill and Walsh, 2005 ; Sherwood et al., 2008 ; Defelipe, 2011 ; Preuss, 2011 , 2017 ; Gabi et al., 2016 ; Smaers et al., 2017 ; Li et al., 2018 ; Rilling and van den Heuvel, 2018 ). Structurally, the enlarged human brain is unique in the layered structure of the human cerebral cortex. Unlike other primates and rodents, the structure of the ventricular zone (VZ) and subventricular zone (SVZ) is expanded by characteristic neural progenitor cells ( Hill and Walsh, 2005 ; Lui et al., 2011 ; Cadwell et al., 2019 ). In addition, many unique characteristics of molecular evolution have been reported, such as isoforms and splicing, non-coding RNA contained in untranslated regions, RNA binding proteins (RNAbps), and amino acid substitutions ( Mattick, 2004 ; Rajan et al., 2009 ; Geschwind and Konopka, 2012 ; Gerstberger et al., 2014 ; Zhu et al., 2018 ). The evolution of these molecules has in turn drove the evolution of a mechanism by which one gene has more than one function, thus increasing the number of cognitive functions but without significantly increasing the number of genes.
Despite the advantages of higher cognitive functions, such evolutionary brain structure and molecular complexity foster increases in various risks, such as expression and replication, in decoding genomic information and executing enormous commands. For NDDs such as autism spectrum disorder (ASD, Autism Spectrum Disorders Working Group of The Psychiatric Genomics Consortium, 2017 ) or attention-deficit hyperactivity disorder (ADHD), many large-scale, genome-wide association studies (GWASs) have been carried out using next-generation sequencing (NGS). The causative and related genes of these disorders have been identified, suggesting that mutations in these genes may be directly linked to the cause of NDDs ( Iossifov et al., 2014 ; Satterstrom et al., 2020 ; Wang et al., 2020 ). Most of these causative and related genes are known to play roles in brain development and synaptogenesis ( Tebbenkamp et al., 2014 ; Wang et al., 2014 ; de la Torre-Ubieta et al., 2016 ).
In this review, we look at the latest findings on the relationship between human brain evolution and NDDs, and explore the pathophysiology of NDDs from studies of human brain development, molecular evolution, and comparative genomics. Recently, we reported some results suggesting that the evolution of oligodendrocytes in humans accelerated the evolution of the human brain, therefore, this review also discusses the role of oligodendrocytes in brain function and NDDs.
Neurodevelopmental Disorders
NDDs are complex disorders in which multiple genetic and/or environmental factors are involved in disease onset. Most patients with NDDs begin to show symptoms during childhood. Children with NDDs might experience difficulties with motor skills, learning and/or memory, language and/or non-verbal communication, and/or other neuropsychiatric problems. In the DSM-5, NDDs include disorders such as ASD, ADHD, intellectual disability (ID), communication disorder (CD), specific learning disorders (SLD), and motor disorders (MD).
ASD is a complex NDD characterized by impaired social communication coupled with restricted and repetitive patterns of behaviors or interests. The prevalence of ASD is approximately 1 in every 54 patients (1.85%) in the United States ( Maenner et al., 2020 ). Genetic and environmental factors are both involved in the onset of ASD, and approximately 1,000 genes are thought to be involved ( Toma et al., 2014 ; Griswold et al., 2015 ; Wilkinson et al., 2015 ; Yao et al., 2015 ; Satterstrom et al., 2020 ; Wang et al., 2020 ). ADHD is another NDD that is characterized by attention deficit, hyperactivity, and impulsivity as its main symptoms, and like ASD, it develops in childhood. ADHD affects approximately 8–12% of children worldwide ( Faraone et al., 2003 ; Polanczyk et al., 2015 ). IDs are characterized by the onset of deficits in intellectual and adaptive function during the developmental period. CDs include language disorders, speech or sound disorders, and childhood-onset fluency disorders. SLDs are disorders in which children find it is difficult to read, write, and speak. Finally, MDs are characterized abnormal and involuntary movements, including developmental coordination disorders, stereotypic movement disorders, and tic disorders such as Tourette syndrome.
The commonality among all NDDs is the presence of some neurodevelopmental problem. As the brain develops, interference by genetic or environmental factors that disrupt normal development can lead to higher risk of developing NDDs. Therefore, understanding human brain evolution might contribute to a better understanding of the pathophysiology of some NDDs.
Human Brain Evolution and Development
There are many differences between the human brain and those of other living organisms. For example, the size of synapses and number of spines in the human brain has increased over evolutionary time ( Elston et al., 2001 ; Duan et al., 2003 ; Defelipe, 2011 ).
In human brain development, between gestational weeks (GW) four to five, neuroepithelial cells divide symmetrically at the VZ. Around GW5, neural progenitor cells, called radial glial cells, (RGs) begin to switch from symmetric to asymmetric cell division. During asymmetric division, one daughter cell remains in the VZ as a RG, while the other differentiates into either a post-mitotic neuron or an intermediate progenitor cell (IPCs) ( Pontious et al., 2008 ). In primates, RGs and IPCs are divided into two subpopulations, apical and basal. Apical RGs and IPCs extend bipolar radial fibers between apical and basal surfaces, but basal/outer RGs (bRGs/oRGs) and basal IPCs form basal fibers. A few bRGs are present in the SVZ in rodents, whereas in gyrencephalic species such as humans, most bRGs are located in the outer SVZ (OSVZ), which is a characteristic structure of gyrencephalic species. Asymmetrically dividing bRGs in the OSVZ contribute to cortical growth and folding ( Lui et al., 2011 ).
Around GW7, post-mitotic committed neurons migrate along radial glial fibers from the VZ and SVZ to the apical brain surface and begin to form cortical plates. The cortical plate is initially formed by two layers: the marginal zone and subplate layer. The marginal zone ultimately becomes layer I, which is composed of Cajal-Retzius cells (CR-cells) and plays an important role in lamina formation via reelin secretion ( Goffinet, 1979 ; Causeret et al., 2021 ). Human CR cells specifically express HAR1F , a non-coding RNA gene in human accelerated regions, which are primarily involved in human-specific cortical evolution and acquisition of higher functions ( Pollard et al., 2006 ). The subplate layer functions for thalamocortical axon pathfinding, synapse maturation, and patterning of primary sensory areas in the cortex ( Kanold and Luhmann, 2010 ). Recently, subplate neurons have been shown to control neuronal migration via the multipolar-to-bipolar transition of migrating neurons ( Ohtaka-Maruyama et al., 2018 ). The subplate layer in primates is thicker than in rodents during embryonic brain development, suggesting that the subplate layer may play a distinguishing role in human-specific brain structure and neural circuits. Between GW9 and GW12, neurons generated in the VZ migrate toward the pial surface. Later born neurons pass these newly born neurons in an inside-out manner leading to the development of a mammalian-specific six-layered laminar structure. In human brain development, the formation of such a characteristic layered structure is thought to be involved in the expansion of the brain size and acquisition of higher functions ( Figure 1 ).

Characteristics of human brain development. The human brain has the OSVZ and ISVZ as anatomical features, while the mouse brain has only the SVZ as a thin layer. The OSVZ contains a large number of oRGs that have replication and differentiation potential, and this layer is important for the evolution and expansion of the human brain. Furthermore, the expansion of white matter by oligodendrocytes is thought to have contributed to the evolution of the human brain and the acquisition of higher brain functions. NESC, neuroepithelial stem cell; RG, radial glia cell; oRG, outer radial glia cell; IPC, intermediate progenitor cell, MZ, marginal zone; CP, cortical plate; SP, subplate; IZ, intermediate zone; SVZ, subventricular zone; VZ, ventricular zone; OSVZ, outer subventricular zone; ISVZ, inner subventricular zone; GW, gestational week.
After embryonic neurogenesis, RGs generate glial cells such as astrocytes and oligodendrocytes. Astrocytes play roles in the blood-brain barrier (BBB), regulation of synaptogenesis and synaptic activity, and interactions with oligodendrocytes and microglia ( Khakh and Deneen, 2019 ; Santello et al., 2019 ). Oligodendrocytes form myelin sheaths, which increase the conduction velocity of action potentials by saltatory conduction, and play a role in the metabolism of neurons and astrocytes. When developing, oligodendrocyte progenitor cells (OPCs) differentiate into immature, mature, or myelinated oligodendrocytes ( Fields, 2015 ). Each of these glial cells expressed different cell markers during differentiation. In recent years, single-cell transcriptome studies have revealed the vast diversity of glial cells that play roles in the development of the brain ( Darmanis et al., 2015 ; Polioudakis et al., 2019 ; Fan et al., 2020 ; Huang et al., 2020 ). However, the mechanisms underlying oligodendrogenesis and white matter expansion in human remained largely unknown until recently. Remarkably, Huang et al. (2020) have reported that in human, oRGs produce EGFR-expressing pre-OPC in SVZ, then pre-OPC proliferates itself to increase the numbers of mitotic OPC in SVZ. They also reported that pre-OPC also proliferates and differentiates into early- and late-OPC in SVZ and SP, respectively ( Huang et al., 2020 ). These findings provide the novel insight into the mechanism of oligodendrogenesis and white matter expansion in human brain development.
Molecular Evolution for Human Brain
Functional changes in genes are a critical factor in the evolution of the human brain. Here, we discuss the relationship between the molecular evolution of the gene and human brain evolution and development, mainly focusing on ASD-associated genes.
FOXP2, forkhead box P2 is one of the most well-known genes associated with human brain evolution and ASD. FOXP2 belongs to the forkhead gene family of transcription factors. FOXP2 was first identified in the KE family with speech and language phenotypes ( Lai et al., 2001 ). Mutations of FOXP2 in patients with speech and language have been linked to complete and complex orofacial sequential movements required for normal speech ( Lai et al., 2001 ; Marcus and Fisher, 2003 ; Vargha-Khadem et al., 2005 ; Bacon and Rappold, 2012 ; Usui et al., 2017 ). Additionally, FOXP2 mutations have been identified in patients with ASD ( Gong et al., 2004 ; Li et al., 2005 ; Guo et al., 2019 ; Satterstrom et al., 2020 ; Wang et al., 2020 ). FOXP2 is highly conserved among mammals, but two novel amino acids, T303N and N325S, arose in the protein sequence when the common ancestor of humans and chimpanzees diverged ( Enard et al., 2002 ; Usui et al., 2014 ). These human-specific amino acid changes in FOXP2 have also been confirmed in Neanderthals ( Krause et al., 2007 ; Green et al., 2010 ) and Denisovans ( Reich et al., 2010 ), which further suggests that these two alterations may contribute to an acceleration in the molecular evolution of FOXP2 functions ( Enard et al., 2009 ; Konopka et al., 2009 ; Somel et al., 2013 ; Usui et al., 2014 ). Studies of FOXP2 can provide novel insights into the molecular mechanisms underlying human brain evolution and communication.
CLOCK is a transcriptional factor that acts as a master regulator in controlling circadian rhythms ( Takahashi, 2017 ). A 4q12 copy number variation that includes CLOCK has been linked to ASD ( Sarachana et al., 2010 ; Griswold et al., 2012 ). In addition, a comparative genomics study of human and other primate brains revealed an increased expression of CLOCK only in the human prefrontal cortex (PFC), but not in chimpanzees and macaques ( Konopka et al., 2012 ). Weighted gene co-expression network analysis (WGCNA) have identified CLOCK as a hub gene involved in cognitive disorder genes rather than in known circadian genes ( Konopka et al., 2012 ). We further demonstrated in a previous study that CLOCK regulates human cortical neuronal migration as well as gene networks implicated in cognitive disorders, such as ASD and ID ( Fontenot et al., 2017 ).
Mutations in the ELAVL2 gene have also been identified in patients with ASD ( Iossifov et al., 2014 ). ELAVL2 is an RNAbp-regulating transcriptional and splicing network ( Lenzken et al., 2014 ; Berto et al., 2016 ). RNA splicing plays a key role in brain development and ASD pathology ( Grabowski, 2011 ; Voineagu et al., 2011 ; Irimia et al., 2014 ; Norris et al., 2014 ; Raj et al., 2014 ; Raj and Blencowe, 2015 ; Xiong et al., 2015 ; Bryant and Yazdani, 2016 ; Iijima et al., 2016 ). ELAVL2 is strongly co-expressed with several RNAbps, such as FMRP and RBFOX1, in human brain development ( Berto et al., 2016 ). Additionally, ELAVL2 targets are involved in neuronal development, synaptic function, and neurodegenerative disorders, such as ASD ( Berto et al., 2016 ). Therefore, RNAbps such as ELAVL2 may affect human brain evolution and development via RNA splicing, and mutations or abnormalities in this gene could be a predictive factor of NDDs.
Previous studies on molecular evolution have shown that changes in the nature and function of genes, like those mentioned above, in the evolution of the human brain are likely related to the evolution of higher cognitive functions and the associated risk of brain diseases such as NDDs.
Roles of Oligodendrocyte in Human Brain Function
Human brain evolution was previously characterized by an increase in the number of neurons present over evolutionary time ( Gabi et al., 2016 ; Preuss, 2017 ); however, the number of glia in the human brain, not the number of neurons explicitly, has increased dramatically, and the ratio of neurons to glia is higher in the human brain than in that of rodents and other primates ( Sherwood et al., 2006 ). The human brain undergoes a volumetric expansion of white matter ( Donahue et al., 2018 ; Rilling and van den Heuvel, 2018 ), and approximately 75% of non-neurons in the human cortex are oligodendrocytes ( Pelvig et al., 2008 ; Herculano-Houzel, 2014 ). Oligodendrocytes wrap around neuronal axons and form a sheath of insulating myelin to accelerate the conduction of action potentials ( Fields, 2015 ; de Faria et al., 2021 ). In recent years, it has been reported that oligodendrocytes may also play a role in the metabolism of lactic acid in the brain, and in the supplying of nutrients to nerve cells and astrocytes ( Lee et al., 2012 ), all of which support the development of higher cognitive function. In fact, previous studies have specifically singled out oligodendrocyte dysfunction in both schizophrenia and major depressive disorder (MDD) ( Miyata et al., 2015 ). Other studies have further demonstrated the involvement of oligodendrocytes in human cognitive function ( Voineskos et al., 2013 ; Fields et al., 2014 ), suggesting that oligodendrocytes likely has essential functions in human brain evolution.
To test this idea, we previously carried out a comparative genomic study using postmortem brains of human and other primates, and the results suggested that oligodendrocytes may contribute to human brain evolution ( Berto et al., 2019 ), which supports the findings of other previous studies. A human-specific down-regulated oligodendrocyte module is enriched for genes in a module dysregulated in ASD, schizophrenia, and bipolar disorder (BD), and is linked with transcription and methylation. In contrast, a human-specific upregulated oligodendrocyte module is enriched for genes in a module dysregulated in schizophrenia and linked with splicing, such as RNA metabolism and RNA processing. Furthermore, we demonstrated that human-specific genes are enriched for cognitive disease risk variants, such as ADHD, schizophrenia, BD, and MDD ( Berto et al., 2019 ).
Recent studies have focused on the relationship between oligodendrocytes and these disorders. For example, age-related differences in white matter diffusion have been reported in the uncinate fasciculus, corticospinal tract, inferior longitudinal fasciculus, inferior fronto-occipital fasciculus, anterior thalamic radiation, superior longitudinal fasciculus, and forceps major of ASD ( Thompson et al., 2020 ). Further studies on the severity of ASD and white matter development in early childhood have reported that development with ASD results in lower white matter development than in neurotypical development ( Andrews et al., 2021 ). Furthermore, lipids are the main constituents of myelin; however, dyslipidemia has been reported in children with ASD ( Usui et al., 2020 ). In a recent study using a mouse model, we reported a reduction in the myelinated area in the neocortex of the ASD-associated gene Zbtb16 knockout mice ( Usui et al., 2021 ). In addition, previous studies have reported similar abnormalities in the white matter of patients with ADHD ( Wu et al., 2017 ; Bessette and Stevens, 2019 ). Large white matter tracts that form myelinated fibers are considerably smaller in the brains of patients with ADHD ( Liston et al., 2011 ; van Ewijk et al., 2012 ). NOS1, encoding nitric oxide synthase 1, has been identified as a candidate gene for ADHD ( Hawi et al., 2015 ). NOS1 promotes the growth and arborization of oligodendrocytes ( Garthwaite et al., 2015 ), suggesting that myelination defects might contribute to the presentation of ADHD symptoms. Moreover, it has been reported that the alterations in white matter and oligodendrocyte-related genes regulation are associated with other disorders such as schizophrenia, BD, and MDD ( Barley et al., 2009 ; Haroutunian et al., 2014 ; Srivastava et al., 2016 ; Tonnesen et al., 2018 ).
Together, the findings of these studies suggest that evolutionary trajectories in oligodendrocytes are at risk of association with cognitive diseases as well as NDDs; regardless, oligodendrocytes play a role in human brain evolution and the acquisition of higher functions in the brain ( Figure 2 ).

Roles of oligodendrocyte in the brain functions. Oligodendrocyte plays essential roles for regulation and maintenance of brain functions. It is well known that oligodendrocyte regulates neuronal activities by myelin formation and its remodeling. It also regulates neurotransmission, neuroinflammation, axon protection, and metabolic supports. Oligodendrocytes are one of the major cell types that make up white matter, and in the human brain, the expansion of white matter is thought to have contributed to higher brain functions. Disruption of those oligodendrocyte’s functions is also thought to cause NDDs, psychiatric disorders, and cognitive dysfunction.
In this review, we have taken a multifaceted approach to evaluating the relationship between human brain evolution and NDDs, focusing on several factors such as development, molecular mechanisms, and comparative genomes. It has been suggested that molecular evolution at the gene and protein levels in humans as well as RNA splicing control mechanisms play a role in human brain evolution. Moreover, it is necessary to clarify in future research that oligodendrocytes accelerate brain evolution. Since oligodendrocytes play a human-specific role in metabolism and RNA splicing in the brain, further studies should evaluate the regulation of larger-scale energy metabolism in the evolved human brain and the diversity of various gene regulation mechanisms. In addition, most of the studies in this review are only focused on genetic and molecular mechanisms of evolution, but with little to discuss about the complex interplay of environmental factors that can also affect neurodevelopment. Therefore, future studies could try to integrate environmental factors along with genetic factors to better contextualize the evolution of the human brain and the pathophysiology of NDDs. In conclusion, this review synthesizes recent findings that suggest a role of evolutionary mechanisms in brain development and in the increased risk of NDDs.
Author Contributions
KI: writing - original draft. MD: writing - original draft, and visualization. NU: conceptualization, writing - original draft, writing - review and editing, project administration, and funding acquisition. SS: writing - review and editing, supervision. All authors contributed to the article and approved the submitted version.
Conflict of Interest
The authors declare that the research was conducted in the absence of any commercial or financial relationships that could be construed as a potential conflict of interest.
Publisher’s Note
All claims expressed in this article are solely those of the authors and do not necessarily represent those of their affiliated organizations, or those of the publisher, the editors and the reviewers. Any product that may be evaluated in this article, or claim that may be made by its manufacturer, is not guaranteed or endorsed by the publisher.
This work was supported by the Japan Society for the Promotion of Science (JSPS) Grant-in-Aid for Scientific Research (C) (20K06872) to NU; JSPS Grant-in-Aid for Early-Career Scientists (18K14814) to NU, Uehara Memorial Foundation to NU, Takeda Science Foundation to NU, SENSHIN Medical Research Foundation to NU, Osaka Medical Research Foundation for Intractable Diseases to NU, Public Health Science Foundation to NU, Eli Lilly Japan Research Grant to NU.
- Andrews D. S., Lee J. K., Harvey D. J., Waizbard-Bartov E., Solomon M., Rogers S. J., et al. (2021). A longitudinal study of white matter development in relation to changes in autism severity across early childhood. Biol. Psychiatry 89 424–432. 10.1016/j.biopsych.2020.10.013 [ PMC free article ] [ PubMed ] [ CrossRef ] [ Google Scholar ]
- Autism Spectrum Disorders Working Group of The Psychiatric Genomics Consortium (2017). Meta-analysis of GWAS of over 16,000 individuals with autism spectrum disorder highlights a novel locus at 10q24.32 and a significant overlap with schizophrenia. Mol. Autism 8 : 21 . 10.1186/s13229-017-0137-9 [ PMC free article ] [ PubMed ] [ CrossRef ] [ Google Scholar ]
- Bacon C., Rappold G. A. (2012). The distinct and overlapping phenotypic spectra of FOXP1 and FOXP2 in cognitive disorders. Hum. Genet . 131 1687–1698. 10.1007/s00439-012-1193-z [ PMC free article ] [ PubMed ] [ CrossRef ] [ Google Scholar ]
- Barley K., Dracheva S., Byne W. (2009). Subcortical oligodendrocyte- and astrocyte-associated gene expression in subjects with schizophrenia, major depression and bipolar disorder. Schizophr. Res . 112 54–64. 10.1016/j.schres.2009.04.019 [ PubMed ] [ CrossRef ] [ Google Scholar ]
- Berto S., Mendizabal I., Usui N., Toriumi K., Chatterjee P., Douglas C., et al. (2019). Accelerated evolution of oligodendrocytes in the human brain. Proc. Natl. Acad. Sci. U.S.A . 116 24334–24342. 10.1073/pnas.1907982116 [ PMC free article ] [ PubMed ] [ CrossRef ] [ Google Scholar ]
- Berto S., Usui N., Konopka G., Fogel B. L. (2016). ELAVL2-regulated transcriptional and splicing networks in human neurons link neurodevelopment and autism. Hum. Mol. Genet . 25 2451–2464. 10.1093/hmg/ddw110 [ PMC free article ] [ PubMed ] [ CrossRef ] [ Google Scholar ]
- Bessette K. L., Stevens M. C. (2019). Neurocognitive pathways in attention-deficit/hyperactivity disorder and white matter microstructure. Biol. Psychiatry Cogn. Neurosci. Neuroimaging 4 233–242. 10.1016/j.bpsc.2018.09.007 [ PMC free article ] [ PubMed ] [ CrossRef ] [ Google Scholar ]
- Bryant C. D., Yazdani N. (2016). RNA-binding proteins, neural development and the addictions. Genes Brain Behav . 15 169–186. 10.1111/gbb.12273 [ PMC free article ] [ PubMed ] [ CrossRef ] [ Google Scholar ]
- Cadwell C. R., Bhaduri A., Mostajo-Radji M. A., Keefe M. G., Nowakowski T. J. (2019). Development and arealization of the cerebral cortex. Neuron 103 980–1004. 10.1016/j.neuron.2019.07.009 [ PMC free article ] [ PubMed ] [ CrossRef ] [ Google Scholar ]
- Causeret F., Moreau M. X., Pierani A., Blanquie O. (2021). The multiple facets of Cajal-Retzius neurons. Development 148 : dev199409 . 10.1242/dev.199409 [ PubMed ] [ CrossRef ] [ Google Scholar ]
- Darmanis S., Sloan S. A., Zhang Y., Enge M., Caneda C., Shuer L. M., et al. (2015). A survey of human brain transcriptome diversity at the single cell level. Proc. Natl. Acad. Sci. U.S.A . 112 7285–7290. 10.1073/pnas.1507125112 [ PMC free article ] [ PubMed ] [ CrossRef ] [ Google Scholar ]
- de Faria O., Jr., Pivonkova H., Varga B., Timmler S., Evans K. A., Káradóttir R. T. (2021). Periods of synchronized myelin changes shape brain function and plasticity. Nat. Neurosci . 24 1508–1521. 10.1038/s41593-021-00917-2 [ PubMed ] [ CrossRef ] [ Google Scholar ]
- de la Torre-Ubieta L., Won H., Stein J. L., Geschwind D. H. (2016). Advancing the understanding of autism disease mechanisms through genetics. Nat. Med. 22 345–361. 10.1038/nm.4071 [ PMC free article ] [ PubMed ] [ CrossRef ] [ Google Scholar ]
- Defelipe J. (2011). The evolution of the brain, the human nature of cortical circuits, and intellectual creativity. Front. Neuroanat . 5 : 29 . 10.3389/fnana.2011.00029 [ PMC free article ] [ PubMed ] [ CrossRef ] [ Google Scholar ]
- Donahue C. J., Glasser M. F., Preuss T. M., Rilling J. K., Van Essen D. C. (2018). Quantitative assessment of prefrontal cortex in humans relative to nonhuman primates. Proc. Natl. Acad. Sci. U.S.A . 115 E5183–E5192. 10.1073/pnas.1721653115 [ PMC free article ] [ PubMed ] [ CrossRef ] [ Google Scholar ]
- Duan H., Wearne S. L., Rocher A. B., Macedo A., Morrison J. H., Hof P. R. (2003). Age-related dendritic and spine changes in corticocortically projecting neurons in macaque monkeys. Cereb. Cortex 13 950–961. 10.1093/cercor/13.9.950 [ PubMed ] [ CrossRef ] [ Google Scholar ]
- Elston G. N., Benavides-Piccione R., DeFelipe J. (2001). The pyramidal cell in cognition: a comparative study in human and monkey. J. Neurosci . 21 : Rc163 . 10.1523/JNEUROSCI.21-17-j0002.2001 [ PMC free article ] [ PubMed ] [ CrossRef ] [ Google Scholar ]
- Enard W., Gehre S., Hammerschmidt K., Holter S. M., Blass T., Somel M., et al. (2009). A humanized version of Foxp2 affects cortico-basal ganglia circuits in mice. Cell 137 961–971. 10.1016/j.cell.2009.03.041 [ PubMed ] [ CrossRef ] [ Google Scholar ]
- Enard W., Przeworski M., Fisher S. E., Lai C. S., Wiebe V., Kitano T., et al. (2002). Molecular evolution of FOXP2, a gene involved in speech and language. Nature 418 869–872. 10.1038/nature01025 [ PubMed ] [ CrossRef ] [ Google Scholar ]
- Fan X., Fu Y., Zhou X., Sun L., Yang M., Wang M., et al. (2020). Single-cell transcriptome analysis reveals cell lineage specification in temporal-spatial patterns in human cortical development. Sci. Adv . 6 : eaaz2978 . 10.1126/sciadv.aaz2978 [ PMC free article ] [ PubMed ] [ CrossRef ] [ Google Scholar ]
- Faraone S. V., Sergeant J., Gillberg C., Biederman J. (2003). The worldwide prevalence of ADHD: is it an American condition? World Psychiatry 2 104–113. [ PMC free article ] [ PubMed ] [ Google Scholar ]
- Fields R. D. (2015). A new mechanism of nervous system plasticity: activity-dependent myelination. Nat. Rev. Neurosci . 16 756–767. 10.1038/nrn4023 [ PMC free article ] [ PubMed ] [ CrossRef ] [ Google Scholar ]
- Fields R. D., Araque A., Johansen-Berg H., Lim S. S., Lynch G., Nave K. A., et al. (2014). Glial biology in learning and cognition. Neuroscientist 20 426–431. 10.1177/1073858413504465 [ PMC free article ] [ PubMed ] [ CrossRef ] [ Google Scholar ]
- Fontenot M. R., Berto S., Liu Y., Werthmann G., Douglas C., Usui N., et al. (2017). Novel transcriptional networks regulated by CLOCK in human neurons. Genes Dev . 31 2121–2135. 10.1101/gad.305813.117 [ PMC free article ] [ PubMed ] [ CrossRef ] [ Google Scholar ]
- Gabi M., Neves K., Masseron C., Ribeiro P. F., Ventura-Antunes L., Torres L., et al. (2016). No relative expansion of the number of prefrontal neurons in primate and human evolution. Proc. Natl. Acad. Sci. U.S.A . 113 9617–9622. 10.1073/pnas.1610178113 [ PMC free article ] [ PubMed ] [ CrossRef ] [ Google Scholar ]
- Garthwaite G., Hampden-Smith K., Wilson G. W., Goodwin D. A., Garthwaite J. (2015). Nitric oxide targets oligodendrocytes and promotes their morphological differentiation. Glia 63 383–399. 10.1002/glia.22759 [ PMC free article ] [ PubMed ] [ CrossRef ] [ Google Scholar ]
- Gerstberger S., Hafner M., Tuschl T. (2014). A census of human RNA-binding proteins. Nat. Rev. Genet . 15 829–845. 10.1038/nrg3813 [ PubMed ] [ CrossRef ] [ Google Scholar ]
- Geschwind D. H., Konopka G. (2012). Neuroscience: genes and human brain evolution. Nature 486 481–482. 10.1038/nature11380 [ PMC free article ] [ PubMed ] [ CrossRef ] [ Google Scholar ]
- Goffinet A. M. (1979). An early development defect in the cerebral cortex of the reeler mouse. A morphological study leading to a hypothesis concerning the action of the mutant gene. Anat. Embryol . 157 205–216. 10.1007/bf00305160 [ PubMed ] [ CrossRef ] [ Google Scholar ]
- Gong X., Jia M., Ruan Y., Shuang M., Liu J., Wu S., et al. (2004). Association between the FOXP2 gene and autistic disorder in Chinese population. Am. J. Med. Genet. B Neuropsychiatr. Genet . 127b 113–116. 10.1002/ajmg.b.20162 [ PubMed ] [ CrossRef ] [ Google Scholar ]
- Grabowski P. (2011). Alternative splicing takes shape during neuronal development. Curr. Opin. Genet. Dev . 21 388–394. 10.1016/j.gde.2011.03.005 [ PubMed ] [ CrossRef ] [ Google Scholar ]
- Green R. E., Krause J., Briggs A. W., Maricic T., Stenzel U., Kircher M., et al. (2010). A draft sequence of the Neandertal genome. Science 328 710–722. 10.1126/science.1188021 [ PMC free article ] [ PubMed ] [ CrossRef ] [ Google Scholar ]
- Griswold A. J., Dueker N. D., Van Booven D., Rantus J. A., Jaworski J. M., Slifer S. H., et al. (2015). Targeted massively parallel sequencing of autism spectrum disorder-associated genes in a case control cohort reveals rare loss-of-function risk variants. Mol. Autism 6 : 43 . 10.1186/s13229-015-0034-z [ PMC free article ] [ PubMed ] [ CrossRef ] [ Google Scholar ]
- Griswold A. J., Ma D., Cukier H. N., Nations L. D., Schmidt M. A., Chung R. H., et al. (2012). Evaluation of copy number variations reveals novel candidate genes in autism spectrum disorder-associated pathways. Hum. Mol. Genet . 21 3513–3523. 10.1093/hmg/dds164 [ PMC free article ] [ PubMed ] [ CrossRef ] [ Google Scholar ]
- Guo H., Duyzend M. H., Coe B. P., Baker C., Hoekzema K., Gerdts J., et al. (2019). Genome sequencing identifies multiple deleterious variants in autism patients with more severe phenotypes. Genet. Med . 21 1611–1620. 10.1038/s41436-018-0380-2 [ PMC free article ] [ PubMed ] [ CrossRef ] [ Google Scholar ]
- Haroutunian V., Katsel P., Roussos P., Davis K. L., Altshuler L. L., Bartzokis G. (2014). Myelination, oligodendrocytes, and serious mental illness. Glia 62 1856–1877. 10.1002/glia.22716 [ PubMed ] [ CrossRef ] [ Google Scholar ]
- Hawi Z., Cummins T. D., Tong J., Johnson B., Lau R., Samarrai W., et al. (2015). The molecular genetic architecture of attention deficit hyperactivity disorder. Mol. Psychiatry 20 289–297. 10.1038/mp.2014.183 [ PubMed ] [ CrossRef ] [ Google Scholar ]
- Herculano-Houzel S. (2014). The glia/neuron ratio: how it varies uniformly across brain structures and species and what that means for brain physiology and evolution. Glia 62 1377–1391. 10.1002/glia.22683 [ PubMed ] [ CrossRef ] [ Google Scholar ]
- Hill R. S., Walsh C. A. (2005). Molecular insights into human brain evolution. Nature 437 64–67. 10.1038/nature04103 [ PubMed ] [ CrossRef ] [ Google Scholar ]
- Huang W., Bhaduri A., Velmeshev D., Wang S., Wang L., Rottkamp C. A., et al. (2020). Origins and proliferative states of human oligodendrocyte precursor cells . Cell 182 594–608.e11. 10.1016/j.cell.2020.06.027 [ PMC free article ] [ PubMed ] [ CrossRef ] [ Google Scholar ]
- Iijima T., Hidaka C., Iijima Y.-H. (2016). Spatio-temporal regulations and functions of neuronal alternative RNA splicing in developing and adult brains. Neurosci. Res. 109 1–8. 10.1016/j.neures.2016.01.010 [ PubMed ] [ CrossRef ] [ Google Scholar ]
- Iossifov I., O’Roak B. J., Sanders S. J., Ronemus M., Krumm N., Levy D., et al. (2014). The contribution of de novo coding mutations to autism spectrum disorder. Nature 515 216–221. 10.1038/nature13908 [ PMC free article ] [ PubMed ] [ CrossRef ] [ Google Scholar ]
- Irimia M., Weatheritt R. J., Ellis J. D., Parikshak N. N., Gonatopoulos-Pournatzis T., Babor M., et al. (2014). A highly conserved program of neuronal microexons is misregulated in autistic brains. Cell 159 1511–1523. 10.1016/j.cell.2014.11.035 [ PMC free article ] [ PubMed ] [ CrossRef ] [ Google Scholar ]
- Kanold P. O., Luhmann H. J. (2010). The subplate and early cortical circuits. Annu. Rev. Neurosci . 33 23–48. 10.1146/annurev-neuro-060909-153244 [ PubMed ] [ CrossRef ] [ Google Scholar ]
- Khakh B. S., Deneen B. (2019). The emerging nature of astrocyte diversity. Annu. Rev. Neurosci . 42 187–207. 10.1146/annurev-neuro-070918-050443 [ PubMed ] [ CrossRef ] [ Google Scholar ]
- Konopka G., Bomar J. M., Winden K., Coppola G., Jonsson Z. O., Gao F., et al. (2009). Human-specific transcriptional regulation of CNS development genes by FOXP2. Nature 462 213–217. 10.1038/nature08549 [ PMC free article ] [ PubMed ] [ CrossRef ] [ Google Scholar ]
- Konopka G., Friedrich T., Davis-Turak J., Winden K., Oldham M. C., Gao F., et al. (2012). Human-specific transcriptional networks in the brain. Neuron 75 601–617. 10.1016/j.neuron.2012.05.034 [ PMC free article ] [ PubMed ] [ CrossRef ] [ Google Scholar ]
- Krause J., Lalueza-Fox C., Orlando L., Enard W., Green R. E., Burbano H. A., et al. (2007). The derived FOXP2 variant of modern humans was shared with Neandertals. Curr. Biol . 17 1908–1912. 10.1016/j.cub.2007.10.008 [ PubMed ] [ CrossRef ] [ Google Scholar ]
- Lai C. S., Fisher S. E., Hurst J. A., Vargha-Khadem F., Monaco A. P. (2001). A forkhead-domain gene is mutated in a severe speech and language disorder. Nature 413 519–523. 10.1038/35097076 [ PubMed ] [ CrossRef ] [ Google Scholar ]
- Lee Y., Morrison B. M., Li Y., Lengacher S., Farah M. H., Hoffman P. N., et al. (2012). Oligodendroglia metabolically support axons and contribute to neurodegeneration. Nature 487 443–448. 10.1038/nature11314 [ PMC free article ] [ PubMed ] [ CrossRef ] [ Google Scholar ]
- Lenzken S. C., Achsel T., Carrì M. T., Barabino S. M. (2014). Neuronal RNA-binding proteins in health and disease. Wiley Interdiscip. Rev. RNA 5 565–576. 10.1002/wrna.1231 [ PubMed ] [ CrossRef ] [ Google Scholar ]
- Li H., Yamagata T., Mori M., Momoi M. Y. (2005). Absence of causative mutations and presence of autism-related allele in FOXP2 in Japanese autistic patients. Brain Dev . 27 207–210. 10.1016/j.braindev.2004.06.002 [ PubMed ] [ CrossRef ] [ Google Scholar ]
- Li M., Santpere G., Imamura Kawasawa Y., Evgrafov O. V., Gulden F. O., Pochareddy S., et al. (2018). Integrative functional genomic analysis of human brain development and neuropsychiatric risks. Science 362 : eaat7615 . 10.1126/science.aat7615 [ PMC free article ] [ PubMed ] [ CrossRef ] [ Google Scholar ]
- Liston C., Malter Cohen M., Teslovich T., Levenson D., Casey B. J. (2011). Atypical prefrontal connectivity in attention-deficit/hyperactivity disorder: pathway to disease or pathological end point? Biol. Psychiatry 69 1168–1177. 10.1016/j.biopsych.2011.03.022 [ PubMed ] [ CrossRef ] [ Google Scholar ]
- Lui J. H., Hansen D. V., Kriegstein A. R. (2011). Development and evolution of the human neocortex. Cell 146 18–36. 10.1016/j.cell.2011.06.030 [ PMC free article ] [ PubMed ] [ CrossRef ] [ Google Scholar ]
- Maenner M. J., Shaw K. A., Baio J., Washington A., Patrick M., DiRienzo M., et al. (2020). Prevalence of autism spectrum disorder among children aged 8 years - autism and developmental disabilities monitoring network, 11 sites, United States, 2016. MMWR Surveill. Summ . 69 1–12. 10.15585/mmwr.ss6904a1 [ PMC free article ] [ PubMed ] [ CrossRef ] [ Google Scholar ]
- Marcus G. F., Fisher S. E. (2003). FOXP2 in focus: what can genes tell us about speech and language? Trends Cogn. Sci . 7 257–262. 10.1016/s1364-6613(03)00104-9 [ PubMed ] [ CrossRef ] [ Google Scholar ]
- Mattick J. S. (2004). RNA regulation: a new genetics? Nat. Rev. Genet . 5 316–323. 10.1038/nrg1321 [ PubMed ] [ CrossRef ] [ Google Scholar ]
- Miyata S., Hattori T., Shimizu S., Ito A., Tohyama M. (2015). Disturbance of oligodendrocyte function plays a key role in the pathogenesis of schizophrenia and major depressive disorder. Biomed Res. Int . 2015 : 492367 . 10.1155/2015/492367 [ PMC free article ] [ PubMed ] [ CrossRef ] [ Google Scholar ]
- Norris A. D., Gao S., Norris M. L., Ray D., Ramani A. K., Fraser A. G., et al. (2014). A pair of RNA-binding proteins controls networks of splicing events contributing to specialization of neural cell types. Mol. Cell 54 946–959. 10.1016/j.molcel.2014.05.004 [ PMC free article ] [ PubMed ] [ CrossRef ] [ Google Scholar ]
- Ohtaka-Maruyama C., Okamoto M., Endo K., Oshima M., Kaneko N., Yura K., et al. (2018). Synaptic transmission from subplate neurons controls radial migration of neocortical neurons. Science 360 313–317. 10.1126/science.aar2866 [ PubMed ] [ CrossRef ] [ Google Scholar ]
- Pelvig D. P., Pakkenberg H., Stark A. K., Pakkenberg B. (2008). Neocortical glial cell numbers in human brains. Neurobiol. Aging 29 1754–1762. 10.1016/j.neurobiolaging.2007.04.013 [ PubMed ] [ CrossRef ] [ Google Scholar ]
- Polanczyk G. V., Salum G. A., Sugaya L. S., Caye A., Rohde L. A. (2015). Annual research review: a meta-analysis of the worldwide prevalence of mental disorders in children and adolescents. J. Child Psychol. Psychiatry 56 345–365. 10.1111/jcpp.12381 [ PubMed ] [ CrossRef ] [ Google Scholar ]
- Polioudakis D., de la Torre-Ubieta L., Langerman J., Elkins A. G., Shi X., Stein J. L., et al. (2019). A single-cell transcriptomic atlas of human neocortical development during mid-gestation. Neuron 103 785–801.e8. 10.1016/j.neuron.2019.06.011 [ PMC free article ] [ PubMed ] [ CrossRef ] [ Google Scholar ]
- Pollard K. S., Salama S. R., Lambert N., Lambot M. A., Coppens S., Pedersen J. S., et al. (2006). An RNA gene expressed during cortical development evolved rapidly in humans. Nature 443 167–172. 10.1038/nature05113 [ PubMed ] [ CrossRef ] [ Google Scholar ]
- Pontious A., Kowalczyk T., Englund C., Hevner R. F. (2008). Role of intermediate progenitor cells in cerebral cortex development. Dev. Neurosci . 30 24–32. 10.1159/000109848 [ PubMed ] [ CrossRef ] [ Google Scholar ]
- Preuss T. M. (2011). The human brain: rewired and running hot. Ann. N. Y. Acad. Sci . 1225 E182–E191. 10.1111/j.1749-6632.2011.06001.x [ PMC free article ] [ PubMed ] [ CrossRef ] [ Google Scholar ]
- Preuss T. M. (2017). “ Chapter 8 - the human brain: evolution and distinctive features ,” in On Human Nature , eds Tibayrenc M., Ayala F. J. (San Diego, CA: Academic Press; ), 125–149. [ Google Scholar ]
- Raj B., Blencowe B. J. (2015). Alternative splicing in the mammalian nervous system: recent insights into mechanisms and functional roles. Neuron 87 14–27. 10.1016/j.neuron.2015.05.004 [ PubMed ] [ CrossRef ] [ Google Scholar ]
- Raj B., Irimia M., Braunschweig U., Sterne-Weiler T., O’Hanlon D., Lin Z. Y., et al. (2014). A global regulatory mechanism for activating an exon network required for neurogenesis. Mol. Cell 56 90–103. 10.1016/j.molcel.2014.08.011 [ PMC free article ] [ PubMed ] [ CrossRef ] [ Google Scholar ]
- Rajan P., Elliott D. J., Robson C. N., Leung H. Y. (2009). Alternative splicing and biological heterogeneity in prostate cancer. Nat. Rev. Urol . 6 454–460. 10.1038/nrurol.2009.125 [ PubMed ] [ CrossRef ] [ Google Scholar ]
- Reich D., Green R. E., Kircher M., Krause J., Patterson N., Durand E. Y., et al. (2010). Genetic history of an archaic hominin group from Denisova Cave in Siberia. Nature 468 1053–1060. 10.1038/nature09710 [ PMC free article ] [ PubMed ] [ CrossRef ] [ Google Scholar ]
- Rilling J. K., van den Heuvel M. P. (2018). Comparative primate connectomics. Brain Behav. Evol . 91 170–179. 10.1159/000488886 [ PubMed ] [ CrossRef ] [ Google Scholar ]
- Santello M., Toni N., Volterra A. (2019). Astrocyte function from information processing to cognition and cognitive impairment. Nat. Neurosci . 22 154–166. 10.1038/s41593-018-0325-8 [ PubMed ] [ CrossRef ] [ Google Scholar ]
- Sarachana T., Zhou R., Chen G., Manji H. K., Hu V. W. (2010). Investigation of post-transcriptional gene regulatory networks associated with autism spectrum disorders by microRNA expression profiling of lymphoblastoid cell lines. Genome Med . 2 : 23 . 10.1186/gm144 [ PMC free article ] [ PubMed ] [ CrossRef ] [ Google Scholar ]
- Satterstrom F. K., Kosmicki J. A., Wang J., Breen M. S., De Rubeis S., An J. Y., et al. (2020). Large-scale exome sequencing study implicates both developmental and functional changes in the neurobiology of autism. Cell 180 568–584.e23. 10.1016/j.cell.2019.12.036 [ PMC free article ] [ PubMed ] [ CrossRef ] [ Google Scholar ]
- Sherwood C. C., Stimpson C. D., Raghanti M. A., Wildman D. E., Uddin M., Grossman L. I., et al. (2006). Evolution of increased glia-neuron ratios in the human frontal cortex. Proc. Natl. Acad. Sci. U.S.A . 103 13606–13611. 10.1073/pnas.0605843103 [ PMC free article ] [ PubMed ] [ CrossRef ] [ Google Scholar ]
- Sherwood C. C., Subiaul F., Zawidzki T. W. (2008). A natural history of the human mind: tracing evolutionary changes in brain and cognition. J. Anat . 212 426–454. 10.1111/j.1469-7580.2008.00868.x [ PMC free article ] [ PubMed ] [ CrossRef ] [ Google Scholar ]
- Smaers J. B., Gómez-Robles A., Parks A. N., Sherwood C. C. (2017). Exceptional evolutionary expansion of prefrontal cortex in great apes and humans. Curr. Biol . 27 714–720. 10.1016/j.cub.2017.01.020 [ PubMed ] [ CrossRef ] [ Google Scholar ]
- Somel M., Liu X., Khaitovich P. (2013). Human brain evolution: transcripts, metabolites and their regulators. Nat. Rev. Neurosci . 14 112–127. 10.1038/nrn3372 [ PubMed ] [ CrossRef ] [ Google Scholar ]
- Srivastava S., Bhatia M. S., Bhargava S. K., Kumari R., Chandra S. (2016). A diffusion tensor imaging study using a voxel-based analysis, region-of-interest method to analyze white matter abnormalities in first-episode, treatment-naive major depressive disorder. J. Neuropsychiatry Clin. Neurosci . 28 131–137. 10.1176/appi.neuropsych.15050120 [ PubMed ] [ CrossRef ] [ Google Scholar ]
- Takahashi J. S. (2017). Transcriptional architecture of the mammalian circadian clock. Nat. Rev. Genet . 18 164–179. 10.1038/nrg.2016.150 [ PMC free article ] [ PubMed ] [ CrossRef ] [ Google Scholar ]
- Tebbenkamp A. T., Willsey A. J., State M. W., Sestan N. (2014). The developmental transcriptome of the human brain: implications for neurodevelopmental disorders. Curr. Opin. Neurol . 27 149–156. 10.1097/wco.0000000000000069 [ PMC free article ] [ PubMed ] [ CrossRef ] [ Google Scholar ]
- Thompson A., Shahidiani A., Fritz A., O’Muircheartaigh J., Walker L., D’Almeida V., et al. (2020). Age-related differences in white matter diffusion measures in autism spectrum condition. Mol. Autism 11 : 36 . 10.1186/s13229-020-00325-6 [ PMC free article ] [ PubMed ] [ CrossRef ] [ Google Scholar ]
- Toma C., Torrico B., Hervás A., Valdés-Mas R., Tristán-Noguero A., Padillo V., et al. (2014). Exome sequencing in multiplex autism families suggests a major role for heterozygous truncating mutations. Mol. Psychiatry 19 784–790. 10.1038/mp.2013.106 [ PubMed ] [ CrossRef ] [ Google Scholar ]
- Tonnesen S., Kaufmann T., Doan N. T., Alnaes D., Cordova-Palomera A., Meer D. V., et al. (2018). White matter aberrations and age-related trajectories in patients with schizophrenia and bipolar disorder revealed by diffusion tensor imaging. Sci. Rep . 8 : 14129 . 10.1038/s41598-018-32355-9 [ PMC free article ] [ PubMed ] [ CrossRef ] [ Google Scholar ]
- Usui N., Berto S., Konishi A., Kondo M., Konopka G., Matsuzaki H., et al. (2021). Zbtb16 regulates social cognitive behaviors and neocortical development. Transl. Psychiatry 11 : 242 . 10.1038/s41398-021-01358-y [ PMC free article ] [ PubMed ] [ CrossRef ] [ Google Scholar ]
- Usui N., Co M., Harper M., Rieger M. A., Dougherty J. D., Konopka G. (2017). Sumoylation of FOXP2 regulates motor function and vocal communication through purkinje cell development. Biol. Psychiatry 81 220–230. 10.1016/j.biopsych.2016.02.008 [ PMC free article ] [ PubMed ] [ CrossRef ] [ Google Scholar ]
- Usui N., Co M., Konopka G. (2014). Decoding the molecular evolution of human cognition using comparative genomics. Brain Behav. Evol . 84 103–116. 10.1159/000365182 [ PMC free article ] [ PubMed ] [ CrossRef ] [ Google Scholar ]
- Usui N., Iwata K., Miyachi T., Takagai S., Wakusawa K., Nara T., et al. (2020). VLDL-specific increases of fatty acids in autism spectrum disorder correlate with social interaction. EBioMedicine 58 : 102917 . 10.1016/j.ebiom.2020.102917 [ PMC free article ] [ PubMed ] [ CrossRef ] [ Google Scholar ]
- van Ewijk H., Heslenfeld D. J., Zwiers M. P., Buitelaar J. K., Oosterlaan J. (2012). Diffusion tensor imaging in attention deficit/hyperactivity disorder: a systematic review and meta-analysis. Neurosci. Biobehav. Rev . 36 1093–1106. 10.1016/j.neubiorev.2012.01.003 [ PubMed ] [ CrossRef ] [ Google Scholar ]
- Vargha-Khadem F., Gadian D. G., Copp A., Mishkin M. (2005). FOXP2 and the neuroanatomy of speech and language. Nat. Rev. Neurosci . 6 131–138. 10.1038/nrn1605 [ PubMed ] [ CrossRef ] [ Google Scholar ]
- Voineagu I., Wang X., Johnston P., Lowe J. K., Tian Y., Horvath S., et al. (2011). Transcriptomic analysis of autistic brain reveals convergent molecular pathology. Nature 474 380–384. 10.1038/nature10110 [ PMC free article ] [ PubMed ] [ CrossRef ] [ Google Scholar ]
- Voineskos A. N., Felsky D., Kovacevic N., Tiwari A. K., Zai C., Chakravarty M. M., et al. (2013). Oligodendrocyte genes, white matter tract integrity, and cognition in schizophrenia. Cereb. Cortex 23 2044–2057. 10.1093/cercor/bhs188 [ PMC free article ] [ PubMed ] [ CrossRef ] [ Google Scholar ]
- Wang S. S., Kloth A. D., Badura A. (2014). The cerebellum, sensitive periods, and autism. Neuron 83 518–532. 10.1016/j.neuron.2014.07.016 [ PMC free article ] [ PubMed ] [ CrossRef ] [ Google Scholar ]
- Wang T., Hoekzema K., Vecchio D., Wu H., Sulovari A., Coe B. P., et al. (2020). Large-scale targeted sequencing identifies risk genes for neurodevelopmental disorders. Nat. Commun . 11 : 4932 . 10.1038/s41467-020-18723-y [ PMC free article ] [ PubMed ] [ CrossRef ] [ Google Scholar ]
- Wilkinson B., Grepo N., Thompson B. L., Kim J., Wang K., Evgrafov O. V., et al. (2015). The autism-associated gene chromodomain helicase DNA-binding protein 8 (CHD8) regulates noncoding RNAs and autism-related genes. Transl. Psychiatry 5 : e568 . 10.1038/tp.2015.62 [ PMC free article ] [ PubMed ] [ CrossRef ] [ Google Scholar ]
- Wu Z. M., Bralten J., Cao Q. J., Hoogman M., Zwiers M. P., An L., et al. (2017). White matter microstructural alterations in children with ADHD: categorical and dimensional perspectives. Neuropsychopharmacology 42 572–580. 10.1038/npp.2016.223 [ PMC free article ] [ PubMed ] [ CrossRef ] [ Google Scholar ]
- Xiong H. Y., Alipanahi B., Lee L. J., Bretschneider H., Merico D., Yuen R. K., et al. (2015). RNA splicing. The human splicing code reveals new insights into the genetic determinants of disease. Science 347 : 1254806 . 10.1126/science.1254806 [ PMC free article ] [ PubMed ] [ CrossRef ] [ Google Scholar ]
- Yao P., Lin P., Gokoolparsadh A., Assareh A., Thang M. W., Voineagu I. (2015). Coexpression networks identify brain region-specific enhancer RNAs in the human brain. Nat. Neurosci . 18 1168–1174. 10.1038/nn.4063 [ PubMed ] [ CrossRef ] [ Google Scholar ]
- Zhu Y., Sousa A. M. M., Gao T., Skarica M., Li M., Santpere G., et al. (2018). Spatiotemporal transcriptomic divergence across human and macaque brain development. Science 362 : eaat8077 . 10.1126/science.aat8077 [ PMC free article ] [ PubMed ] [ CrossRef ] [ Google Scholar ]

Not unique to humans but uniquely human: researchers identify factor involved in brain expansion in humans
What makes us human? According to neurobiologists it is our neocortex. This outer layer of the brain is rich in neurons and lets us do abstract thinking, create art, and speak complex languages. An international team led by Dr. Mareike Albert at the Center for Regenerative Therapies Dresden (CRTD) of TUD Dresden University of Technology has identified a new factor that might have contributed to neocortex expansion in humans. The results were published in the EMBO Journal .
The neocortex is the characteristic folded outer layer of the brain that resembles a walnut. It is responsible for higher cognitive functions such as abstract thinking, art, and language. "The neocortex is the most recently evolved part of the brain," says Dr. Mareike Albert, research group leader at the CRTD. "All mammals have a neocortex, but it varies in size and complexity. Human and primate neocortices have folds while, for example, mice have a completely smooth neocortex, without any creases."
The folds characteristic of the human brain increase the surface area of the neocortex. The human neocortex has a greater number of neurons that support complex cognitive functions.
The molecular mechanisms driving neocortex evolution are still largely unknown. "Which genes are responsible for inter-species differences in neocortex size? What factors have contributed to brain expansion in humans? Answering these questions is crucial to understanding human brain development and potentially addressing mental health disorders," explains Dr. Albert.
The Power of Brain Organoids
To search for factors influencing brain expansion, the Albert group compared developing brains of mice and humans. "Stem cells in mice don't divide as much and don't produce as many neurons compared to primates. Humans, on the other hand, have a large number of stem cells in the developing brain. This highly expanded pool of stem cells underlies the increase in number of neurons and brain size," explains Dr. Albert.
The team found a factor that is present in humans but not in mice. Using 3D cell culture technology, the group tested if the newly identified factor could influence the expansion of the neocortex. "Thanks to the research awarded with the Nobel prize in 2012, it is possible to turn any cell into a stem cell. Such a stem cell can then be transformed into a three-dimensional tissue that resembles an organ, e.g., a brain. Human stem cells make it possible to study development and diseases directly in human tissues," explains Dr. Albert.
These 3D brain cultures, or brain organoids, may not resemble brains to an untrained eye, but they mimic the cellular complexity of developing brains. "Most of the cell types of the developing brain are present. They interact, signal, and are similarly arranged as in an actual human brain," says Dr. Albert.
Using 3D brain organoids, the group was able to show that a growth factor, known as epiregulin, indeed promotes the division and expansion of stem cells in the developing brain.
All About the Amount
"Knowing that epiregulin drives expansion of human neocortical stem cells, we looked back at the gene that codes for epiregulin and tried to trace it through the evolutionary tree," says study lead author Paula Cubillos, a doctoral candidate at the CRTD. The gene is not unique to humans, but also present in other primates and even in mice. "Epiregulin is not produced in the developing mouse brain, however, because the gene is permanently shut off and not being used. We were intrigued to understand whether there are any differences in how epiregulin works in humans and other primates," explains Paula Cubillos.
The researchers turned again to the 3D culture technology. Using gorilla stem cells, the researchers generated gorilla brain organoids. "Gorillas are endangered species. We know very little about their brain development. Organoids made from stem cells offer a way to study their brain development without interacting with the species at all," says Dr. Albert.
Comparing the effect of epiregulin in human and gorilla brain organoids, the team found that adding epiregulin to gorilla brain organoids can further promote the expansion of stem cells. However, adding even more epiregulin to human brain organoids did not have the same effect. This might be because the human neocortex has already expanded to a very large extent.
"Unlike previously identified factors, epiregulin as such seems not to be unique to humans. Instead, the amount of the growth factor seems to be the crucial regulator for the inter-species differences," concludes Dr. Albert.
This study not only advances our understanding of human uniqueness but also highlights the importance of new technologies that offer ethical and non-invasive complements to animal research.
The study was performed in collaboration with King's College London, the Medical Faculty Carl Gustav Carus of TU Dresden, the Max Planck Institute of Molecular Cell Biology and Genetics, and Hannover Medical School.
- Brain-Computer Interfaces
- Neuroscience
- Brain Injury
- Intelligence
- Language Acquisition
- Disorders and Syndromes
- Learning Disorders
- Neocortex (brain)
- Memory-prediction framework
- Multiple sclerosis
- Mirror neuron
- Neurobiology
Story Source:
Materials provided by Technische Universität Dresden . Note: Content may be edited for style and length.
Journal Reference :
- Paula Cubillos, Nora Ditzer, Annika Kolodziejczyk, Gustav Schwenk, Janine Hoffmann, Theresa M Schütze, Razvan P Derihaci, Cahit Birdir, Johannes EM Köllner, Andreas Petzold, Mihail Sarov, Ulrich Martin, Katherine R Long, Pauline Wimberger, Mareike Albert. The growth factor EPIREGULIN promotes basal progenitor cell proliferation in the developing neocortex . The EMBO Journal , 2024; DOI: 10.1038/s44318-024-00068-7
Cite This Page :
Explore More
- Simple Brain-Computer Link: Gaming With Thoughts
- Clinical Reasoning: Chatbot Vs Physicians
- Understanding People Who Can't Visualize
- Illuminating Oxygen's Journey in the Brain
- DNA Study IDs Descendants of George Washington
- Heart Disease Risk: More Than One Drink a Day
- Unlocking Supernova Stardust Secrets
- Why Do Some Memories Become Longterm?
- Cell Division Quality Control 'Stopwatch'
- What Controls Sun's Differential Rotation?
Trending Topics
Strange & offbeat.
CRISPR gene drives and the future of evolution
via The Scientist
March 15, 2024
- #bioengineering
- #synthetic biology
- #microbiology
- Kevin Esvelt Associate Professor of Media Arts and Sciences; NEC Career Development Professor of Computer and Communications
- Mice Against Ticks - Preventing tick-borne disease by permanently immunizing mice
- Studying the evolution of gene drive systems
- Understanding Molecular Evolution
- Daisy Drives
- Evolving Decoy ACE2 Receptor Mimics As COVID-19 Therapeutics
- Media Lab Research Theme: Future Worlds
- Media Lab Research Theme: Connected Mind + Body
Share this article
By Hannah Thomasy, PhD
Today, Massachusetts Institute of Technology biologist Kevin Esvelt is well known for his work on guided evolution technologies—creating systems for evolving biomolecules in the lab and developing techniques to shape the evolutionary trajectories of species in the wild—as well as forging new pathways to safeguard these technologies from misuse.1,2
Esvelt’s entanglement with evolution began early. As a child, he visited the Galápagos, and was captivated by the islands’ stunning array of unique wildlife. “That sparked an interest in the evolution of creatures in the natural world,” said Esvelt. “It got me reading Darwin. And I started wondering—could we make things of comparable magnificence?”
So, when he joined David Liu’s research group at Harvard University for his graduate studies in 2004, he jumped into exploring how to put evolutionary processes to work in the lab. “I love solving problems that I am not actually smart enough to solve. And to do that, you need access to something that is effectively smarter than you, or at least can execute search strategies that you can’t,” said Esvelt. “One of the reasons I love playing with different disciplines is they all give you different lenses on the world. And my favorite lens, evolution, is really about search strategies through a hyper astronomically vast space.”
Part 1: Gene Drive; Part 2: Gene Drive and Local Drive
iBiology features two introductory classes from CRISPR expert Kevin Esvelt, head of the Media Lab's Sculpting Evolution group.
Cracking the Genetic Code with Kevin Esvelt
Kevin Esvelt leads the Sculpting Evolution Group at MIT. Their work explores “evolutionary and ecological engineering and responsive science
The public doesn’t trust GMOs. Will it trust CRISPR?
There’s a huge opportunity to improve agriculture with gene editing. But we need to give CRISPR a chance.
Gene Editing: Last Week Tonight with John Oliver
Scientists are developing new ways to alter the genetic code of living organisms. John Oliver explores the risks and rewards.
March 13, 2024
COVID-19 Leaves Its Mark on the Brain. Significant Drops in IQ Scores Are Noted
Research shows that even mild COVID-19 can lead to the equivalent of seven years of brain aging
By Ziyad Al-Aly & The Conversation US
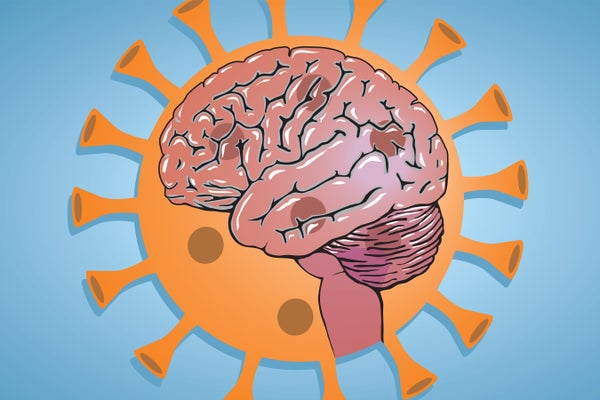
Eva Almqvist/Alamy Stock Vector
From the very early days of the pandemic, brain fog emerged as a significant health condition that many experience after COVID-19.
Brain fog is a colloquial term that describes a state of mental sluggishness or lack of clarity and haziness that makes it difficult to concentrate, remember things and think clearly.
On supporting science journalism
If you're enjoying this article, consider supporting our award-winning journalism by subscribing . By purchasing a subscription you are helping to ensure the future of impactful stories about the discoveries and ideas shaping our world today.
Fast-forward four years and there is now abundant evidence that being infected with SARS-CoV-2 – the virus that causes COVID-19 – can affect brain health in many ways .
In addition to brain fog, COVID-19 can lead to an array of problems , including headaches, seizure disorders, strokes, sleep problems, and tingling and paralysis of the nerves, as well as several mental health disorders .
A large and growing body of evidence amassed throughout the pandemic details the many ways that COVID-19 leaves an indelible mark on the brain. But the specific pathways by which the virus does so are still being elucidated, and curative treatments are nonexistent.
Now, two new studies published in the New England Journal of Medicine shed further light on the profound toll of COVID-19 on cognitive health .
I am a physician scientist , and I have been devoted to studying long COVID since early patient reports about this condition – even before the term “long COVID” was coined. I have testified before the U.S. Senate as an expert witness on long COVID and have published extensively on this topic.
Here are some of the most important studies to date documenting how COVID-19 affects brain health:
Large epidemiological analyses showed that people who had COVID-19 were at an increased risk of cognitive deficits , such as memory problems.
Imaging studies done in people before and after their COVID-19 infections show shrinkage of brain volume and altered brain structure after infection .
A study of people with mild to moderate COVID-19 showed significant prolonged inflammation of the brain and changes that are commensurate with seven years of brain aging .
Severe COVID-19 that requires hospitalization or intensive care may result in cognitive deficits and other brain damage that are equivalent to 20 years of aging .
Laboratory experiments in human and mouse brain organoids designed to emulate changes in the human brain showed that SARS-CoV-2 infection triggers the fusion of brain cells . This effectively short-circuits brain electrical activity and compromises function.
Autopsy studies of people who had severe COVID-19 but died months later from other causes showed that the virus was still present in brain tissue . This provides evidence that contrary to its name, SARS-CoV-2 is not only a respiratory virus, but it can also enter the brain in some individuals. But whether the persistence of the virus in brain tissue is driving some of the brain problems seen in people who have had COVID-19 is not yet clear.
Studies show that even when the virus is mild and exclusively confined to the lungs, it can still provoke inflammation in the brain and impair brain cells’ ability to regenerate .
COVID-19 can also disrupt the blood brain barrier , the shield that protects the nervous system – which is the control and command center of our bodies – making it “leaky.” Studies using imaging to assess the brains of people hospitalized with COVID-19 showed disrupted or leaky blood brain barriers in those who experienced brain fog.
A large preliminary analysis pooling together data from 11 studies encompassing almost one million people with COVID-19 and more than 6 million uninfected individuals showed that COVID-19 increased the risk of development of new-onset dementia in people older than 60 years of age.
Autopsies have revealed devastating damage in the brains of people who died with COVID-19.
Most recently, a new study published in the New England Journal of Medicine assessed cognitive abilities such as memory, planning and spatial reasoning in nearly 113,000 people who had previously had COVID-19. The researchers found that those who had been infected had significant deficits in memory and executive task performance.
This decline was evident among those infected in the early phase of the pandemic and those infected when the delta and omicron variants were dominant. These findings show that the risk of cognitive decline did not abate as the pandemic virus evolved from the ancestral strain to omicron.
In the same study, those who had mild and resolved COVID-19 showed cognitive decline equivalent to a three-point loss of IQ. In comparison, those with unresolved persistent symptoms, such as people with persistent shortness of breath or fatigue, had a six-point loss in IQ. Those who had been admitted to the intensive care unit for COVID-19 had a nine-point loss in IQ. Reinfection with the virus contributed an additional two-point loss in IQ, as compared with no reinfection.
Generally the average IQ is about 100. An IQ above 130 indicates a highly gifted individual, while an IQ below 70 generally indicates a level of intellectual disability that may require significant societal support.
To put the finding of the New England Journal of Medicine study into perspective, I estimate that a three-point downward shift in IQ would increase the number of U.S. adults with an IQ less than 70 from 4.7 million to 7.5 million – an increase of 2.8 million adults with a level of cognitive impairment that requires significant societal support.
Another study in the same issue of the New England Journal of Medicine involved more than 100,000 Norwegians between March 2020 and April 2023. It documented worse memory function at several time points up to 36 months following a positive SARS-CoV-2 test.
Taken together, these studies show that COVID-19 poses a serious risk to brain health, even in mild cases, and the effects are now being revealed at the population level.
A recent analysis of the U.S. Current Population Survey showed that after the start of the COVID-19 pandemic, an additional one million working-age Americans reported having “serious difficulty” remembering, concentrating or making decisions than at any time in the preceding 15 years. Most disconcertingly, this was mostly driven by younger adults between the ages of 18 to 44.
Data from the European Union shows a similar trend – in 2022, 15 percent of people in the EU reported memory and concentration issues .
Looking ahead, it will be critical to identify who is most at risk. A better understanding is also needed of how these trends might affect the educational attainment of children and young adults and the economic productivity of working-age adults. And the extent to which these shifts will influence the epidemiology of dementia and Alzheimer’s disease is also not clear.
The growing body of research now confirms that COVID-19 should be considered a virus with a significant impact on the brain. The implications are far-reaching, from individuals experiencing cognitive struggles to the potential impact on populations and the economy.
Lifting the fog on the true causes behind these cognitive impairments, including brain fog, will require years if not decades of concerted efforts by researchers across the globe. And unfortunately, nearly everyone is a test case in this unprecedented global undertaking.
Thank you for visiting nature.com. You are using a browser version with limited support for CSS. To obtain the best experience, we recommend you use a more up to date browser (or turn off compatibility mode in Internet Explorer). In the meantime, to ensure continued support, we are displaying the site without styles and JavaScript.
- View all journals
- Explore content
- About the journal
- Publish with us
- Sign up for alerts
Collection 10 June 2022
Brain evolution
Eighty-six billion interconnected neurons comprise the human brain, the product of billions of years of evolution. However, the mechanisms supporting the evolution of this remarkable structure over time remain poorly explored.
To develop knowledge in this area, Communications Biology welcomes submissions pertaining to brain evolution in humans and beyond. Accordingly, we are interested in studies exploring neuroimaging in non-human species, palaeoneurology, comparative neuroscience (i.e. across species), neuro-genetics studies for inference of evolutionary mechanisms, neuro-archaeological investigations, and computational models. We welcome any novel lines of enquiry that progress our understanding of brain evolution. Reviews, Perspectives, and Comments covering these topics will also be considered for inclusion in the Collection. All submissions will be subject to the same review process and editorial standards as regular Communications Biology Articles.
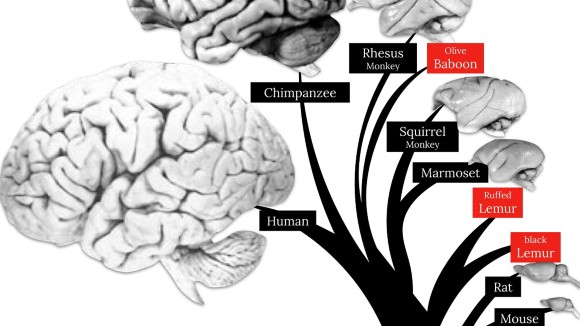
- Michel Thiebaut de Schotten
CNRS, France
- Collection content
- How to submit
- About the Guest Editor
- About this Collection
Reviews and Commentary
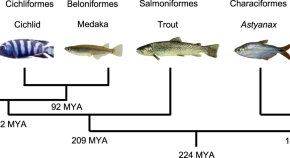
Different ways of evolving tool-using brains in teleosts and amniotes
A neuroanatomical study of the brains of tool-using teleost fishes highlights the pallium and inferior lobe as the putative structures responsible for this behavior, and suggests that there are multiple ways of evolving tool-using brains.
- Pierre Estienne
- Matthieu Simion
- Kei Yamamoto
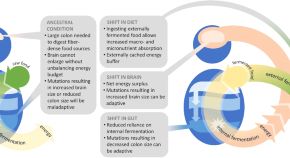
Fermentation technology as a driver of human brain expansion
As brain tissue is metabolically expensive, the evolutionary expansion of human brains required shifts in energy intake and metabolism. The External Fermentation Hypothesis proposes that a shift to consumption of externally fermented foods in human ancestors permitted a reduction in gut tissue required for internal fermentation, facilitating brain expansion.
- Katherine L. Bryant
- Christi Hansen
- Erin E. Hecht
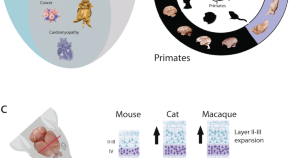
Going beyond established model systems of Alzheimer’s disease: companion animals provide novel insights into the neurobiology of aging
In this Perspective, the authors illustrate how the use of companion animal models will provide insight into of the neurobiology of aging.
- Alexandra A. de Sousa
- Brier A. Rigby Dames
- Christine J. Charvet
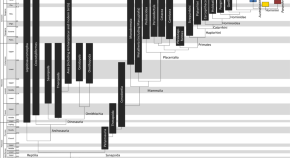
From fossils to mind
Recent advances in paleoneurology are collated together in this comprehensive review, linking neuroanatomy to genes and behavior. We provide guidance to the next generation of researchers to move the field forward.
- Amélie Beaudet
- Yongbin Wei
Comparative neuroscience
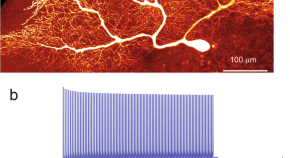
Human Purkinje cells outperform mouse Purkinje cells in dendritic complexity and computational capacity
High-resolution morphological reconstructions and computational models of cerebellar Purkinje cells (PCs) from humans and mice suggest that, while PCs in both species maintain similar spike discharge properties, human PCs developed more complex dendrites.
- Stefano Masoli
- Diana Sanchez-Ponce
- Egidio D’Angelo
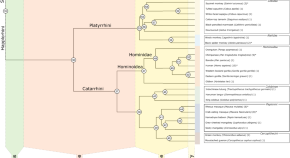
Phylogenetic comparative analysis of the cerebello-cerebral system in 34 species highlights primate-general expansion of cerebellar crura I-II
An evolutionary study in 34 primates provides evidence for a primate-general expansion of cerebellar crura I-II. Specifically, common accelerated scaling may directly explain previously reported high volumetric fractions of the human crura I-II.
- Neville Magielse
- Roberto Toro
- Sofie L. Valk
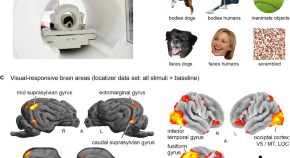
Functionally analogous body- and animacy-responsive areas are present in the dog ( Canis familiaris ) and human occipito-temporal lobe
Comparative fMRI in dogs and humans reveals functionally analogous body- and animacy responsive areas in the occipito-temporal lobe of both species and divergent neural representations of faces and conspecific bodies in dog olfactory regions.
- Magdalena Boch
- Isabella C. Wagner
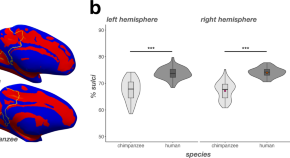
Sulcal morphology of posteromedial cortex substantially differs between humans and chimpanzees
Human and chimpanzee brain MRI scans reveal that the surface anatomy of the posteromedial cortex substantially differs between the species, which improves our understanding of the evolution of neuroanatomical-functional and neuroanatomical-behavioural relationships.
- Ethan H. Willbrand
- Samira A. Maboudian
- Kevin S. Weiner
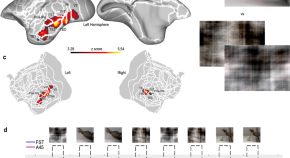
Ultra-high field fMRI identifies an action-observation network in the common marmoset
fMRI analysis of marmosets performing goal- or non-goal-directed grasping tasks suggests they share an action observation network similar to those observed in macaques and humans.
- Alessandro Zanini
- Audrey Dureux
- Stefan Everling
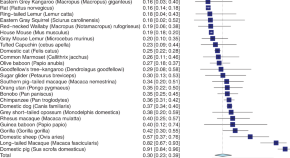
Hemispheric asymmetries and brain size in mammals
Cross-species analysis of brain mass and neuron number suggest that larger-brained species tend to shift towards more right-lateralized individuals, which should be considered in the context of the evolution of hemispheric asymmetries moving forward.
- Sebastian Ocklenburg
- Yasmin El Basbasse
- Anett Müller-Alcazar
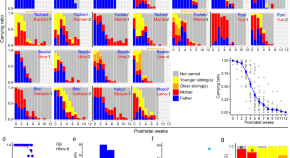
A calcitonin receptor-expressing subregion of the medial preoptic area is involved in alloparental tolerance in common marmosets
Like humans, marmoset caregivers exhibit individual parenting styles. Among these, infant tolerance is in part regulated by a subregion of the medial preoptic area enriched with calcitonin receptor-expressing neurons.
- Kazutaka Shinozuka
- Saori Yano-Nashimoto
- Kumi O. Kuroda
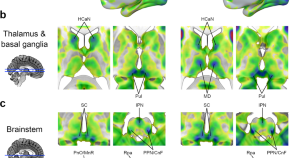
The subcortical and neurochemical organization of the ventral and dorsal attention networks
Integration of functional, structural, and neurochemical data proposes a framework to comprehensively understand the neural basis of attention across different species and neurological diseases.
- Pedro Nascimento Alves
- Stephanie J. Forkel
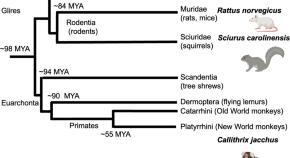
Frontoparietal connectivity as a product of convergent evolution in rodents and primates: functional connectivity topologies in grey squirrels, rats, and marmosets
Comparisons of frontoparietal connectivity between marmosets, rats and grey squirrels suggest the formation of a common frontoparietal network architecture among arboreal species (grey squirrels and marmosets) that might reflect convergent evolution.
- David J. Schaeffer
- Kyle M. Gilbert
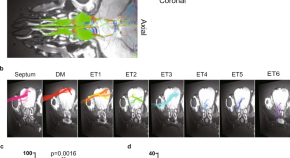
MRI tractography reveals the human olfactory nerve map connecting the olfactory epithelium and olfactory bulb
Combined high-field MRI and DTI analyses in post-mortem mouse, marmoset, and human samples provide insight into the neural connections between nasal cavities and olfactory bulbs.
- Sho Kurihara
- Masayoshi Tei
- Hirotaka James Okano
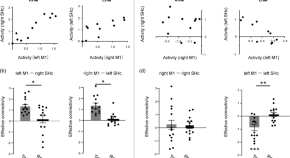
A computational model based on corticospinal functional MRI revealed asymmetrically organized motor corticospinal networks in humans
MRI and network modelling reveal correlation between the degree of involvement of the two corticospinal networks and the lateralization of handedness in humans.
- Eiji Takasawa
- Mitsunari Abe
- Takashi Hanakawa
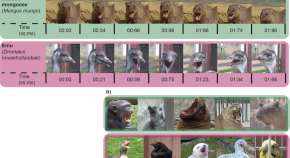
Brain size and neuron numbers drive differences in yawn duration across mammals and birds
Massen, Hartlieb, Martin et al. study the duration of yawns across mammals and birds to test the brain cooling hypothesis. Consistent with this hypothesis, their findings indicate that brain mass and neuron numbers influence yawn duration, and that mammals yawn longer than birds with similar brain and body masses.
- Jorg J. M. Massen
- Margarita Hartlieb
- Andrew C. Gallup
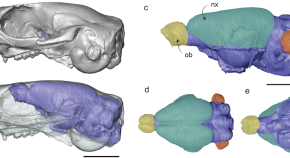
The impact of locomotion on the brain evolution of squirrels and close relatives
Bertrand and colleagues use virtual endocasts from a variety of living and extinct rodent species to assess the effect of locomotion, body mass and phylogeny on the size of the brain and three of its components. Their findings indicate that arboreal and fossorial locomotor types correlate with differential expansion of the neocortex, petrosal lobules, and relative brain size.
- Ornella C. Bertrand
- Hans P. Püschel
- Stephen L. Brusatte
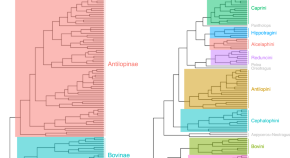
Evolution of the bovid cranium: morphological diversification under allometric constraint
Bibi and Tyler analyse the morphometric variation in the skulls of 96 species across Bovidae, using 3D geometric morphometrics and ecological variables. They show that ecological variables alone appear to have little connection to shape disparity in bovid skulls, instead discovering a deeply conserved association between size and shape that is linked to dietary selection.
- Faysal Bibi
- Joshua Tyler
Neurogenetics
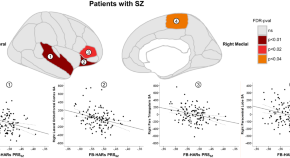
Human-specific evolutionary markers linked to foetal neurodevelopment modulate brain surface area in schizophrenia
The analysis of the schizophrenia polygenic load along the genomic Human Accelerated Regions (HARs) provides insight into the influence of early brain development regulation on the neuroanatomical changes associated with schizophrenia.
- Maria Guardiola-Ripoll
- Carmen Almodóvar-Payá
- Mar Fatjó-Vilas
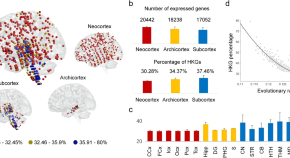
Functional orderly topography of brain networks associated with gene expression heterogeneity
Comparative analysis of human, macaque and mouse function and genetic heterogeneity in the brain reveals links between gene expression and orderly topography of functional networks.
- Ling-Li Zeng
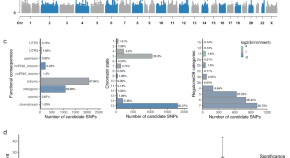
Genome-wide association study of cerebellar volume provides insights into heritable mechanisms underlying brain development and mental health
A genome-wide association study on MRI cerebellar volume in the UK Biobank cohort identifies 30 loci with genome-wide significance that might be relevant to brain structure and cognitive function.
- Elleke Tissink
- Siemon C. de Lange
- Danielle Posthuma
Neuro-archaeology and -palaeology
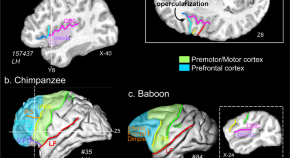
The relevance of the unique anatomy of the human prefrontal operculum to the emergence of speech
Comparisons of fMRI data from humans and chimpanzees suggests that the Prefrontal extent of the Frontal Operculum region recently evolved in humans possibly in relation to the emergence of modern speech.
- Céline Amiez
- Charles Verstraete
- William D. Hopkins
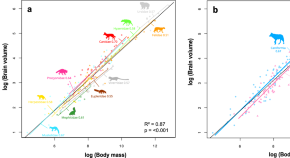
The impact of environmental factors on the evolution of brain size in carnivorans
The brain size of carnivores has evolved to balance a trade-off between increased cognitive function and increased metabolic cost.
- S. L. D. Toussaint
- E. Gilissen
Quick links
- Explore articles by subject
- Guide to authors
- Editorial policies

- International edition
- Australia edition
- Europe edition
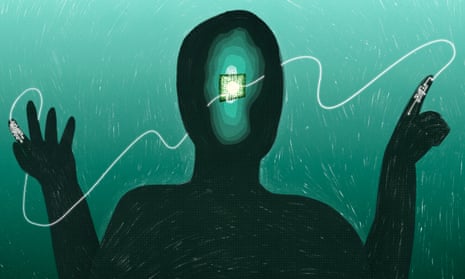
Brain chips: the Sydney researchers ‘miles ahead’ of Elon Musk’s Neuralink
Multiple Australian projects are on the cutting edge of neurotech breakthroughs and man-machine interfaces – raising questions of security and privacy for human minds
- Follow our Australia news live blog for latest updates
- Get our morning and afternoon news emails , free app or daily news podcast
B rain-computer interface technology is at the core of movies such as Ready Player One, The Matrix and Avatar. But outside the realm of science fiction, BCI is being used on Earth to help paralysed people communicate, to study dreams and to control robots.
Billionaire entrepreneur Elon Musk announced in January – to much fanfare – that his neurotechnology company Neuralink had implanted a computer chip into a human for the first time. In February, he announced that the patient was able to control a computer mouse with their thoughts.
Neuralink’s aim is noble: to help people who otherwise can’t communicate and interact with the environment. But details are scant . The project immediately sounded alarm bells about brain privacy, the risk of hacking and other things that could go wrong.
Dr Steve Kassem, a senior research fellow at Neuroscience Research Australia, says “tonnes of grains of salt” should be taken with the Neuralink news. It is not the first company to do a neural implant, he says. In fact, Australia is a “hotspot” for related neurological research.
Do patients dream of electric sheep?
A University of Technology Sydney project that has received millions in funding from the defence department is currently in the third phase of demonstrating how soldiers can use their brain signals to control a robot dog.
“We were successful [demonstrating] that a solder can use their brain to issue a command to assign the dog to reach a destination totally hands-free … so they can use their hands for other purposes,” Prof Lin, the director of the UTS Computational Intelligence and BCI Centre, says.
The soldier uses assisted reality glasses with a special graphene interface to issue brain signal commands to send the robotic dog to different places. Lin says they are working on making the technology multi-user, faster and able to control other vehicles such as drones.
Meanwhile, Sydney company Neurode has created a headset to help people with ADHD by monitoring their brain and delivering electronic pulses to address changes. Another UTS team is working on the DreamMachine , which aims to reconstruct dreams from brain signals. It uses artificial intelligence and electroencephalogram data to generate images from the subconscious.
And then there are the implants.
Good signal
Synchron started at the University of Melbourne and is now also based in New York. It uses a mesh inserted into the brain’s blood vessels that allows patients to use the internet, sending a signal that operates a bit like Bluetooth. People can shop online, email and communicate using the technology to control a computer.
Synchron has developed a Brain-Computer Interface that uses pre-existing technologies such as the stent and catheter to allow insertion into the brain without the need for open brain surgery. pic.twitter.com/Cooh77oukU — CNET (@CNET) September 26, 2023
Synchron has implanted the mesh in a number of patients and is monitoring them, including one in Australia. Patient P4, who has motor neurone disease, has the mesh implanted a few years ago.
“I believe he’s had over 200 sessions,” Gil Rind, Sychron’s senior director of advanced technology, says. “He is still going strong with the implants and has been working very closely with us.
“He’s been able to use his computer through the system … As the disease has progressed it’s really challenging to use physical buttons.
“This has provided him with an alternative method of being able to interact with his computer – for online banking, communication with his carer, [with] loved ones.”
Dr Christina Maher at Sydney University’s Brain and Mind Centre says Synchron’s technology is “miles ahead” of Elon Musk’s and is more sophisticated and safer because it does not require open brain surgery. The researchers have also published more than 25 articles, she says.
“With Neuralink, we don’t know much about it.
“My understanding is that a big priority for them is to test the efficacy and safety of their surgical robots … so they’re a lot more about the robotic side of things, which makes sense from a commercial perspective.”
The need for regulation
Amid the hype and promise of neurotechnology, though, are concerns about who will be able to access the helpful technologies and how they will be protected.
Maher says it is a matter of balancing the need for innovation with proper regulation, while allowing access for those people who really need it. She says the “disparity between the haves and have-nots” is being discussed in Australia and globally.
after newsletter promotion
“When brain-computer interfaces become more common, it’s going to really segregate people into those who can afford it and those who can’t,” she says.
Rind says Synchron is focused on those who have the most to gain, such as people with quadriplegia. “We would like to expand that out as far as we can – we hope we can reach larger markets and help more people in need,” he says.
A personal, pivotal moment for him was seeing the faces of the clinicians, team and family of the first patient to successfully receive the implant, he says.
On Neuralink, Kassem warns there will always be dangers when technology is developed by a company that exists to make profit. “A mobile phone plan for your brain is not what we want,” he says.
“And what about if this is hacked? There is always a risk if it’s not a closed system.”
More likely than that, though, is that Neuralink will use people’s data.
“Just like every single app on your phone and on your computer, Neuralink will monitor as much as it can. Everything it possibly could,” Kassem says.
“It will be stored somewhere.”
Protecting brain data
Maher says hacking will remain a risk if devices are linked to the internet, and agrees that data is a big problem. She says much of our social media, biometric and other data is already out there, but that brain data is different.
“While [BCI companies] are subject to the same data privacy laws … the difference is in a lot of people’s minds is that brain data is quite private, it’s your private thoughts.
“The big picture here is that once we start recording a lot of brain data, there’ll be an absolute megaton of data out there,” she says.
Kassem says despite concerns over privacy, interacting with the brain holds exciting possibilities.
“We need to remember how powerful and significant the brain is … everything you are now, everything you have been, and everything you will be is just your brain, nothing else,” he says.
There are trillions of neural connections in the brain, leading to “boundless opportunities”, he says, quoting the US physicist Emerson Pugh. “If the human brain were so simple that we could understand it, we would be so simple that we couldn’t.”
- Neuroscience
- Medical research
Comments (…)
Most viewed.
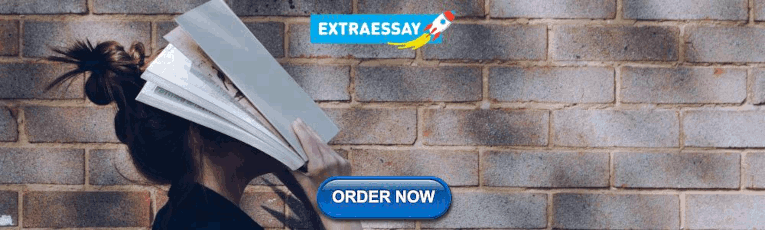
IMAGES
VIDEO
COMMENTS
Our data show that, 300,000 years ago, brain size in early H. sapiens already fell within the range of present-day humans. Brain shape, however, evolved gradually within the H. sapiens lineage, reaching present-day human variation between about 100,000 and 35,000 years ago. This process started only after other key features of craniofacial ...
Introduction. The human brain contains about 100 billion neurons, more than 100,000 km of interconnections, and has an estimated storage capacity of 1.25 × 10 12 bytes (Cherniak, 1990; Hofman, 2012 ). These impressive numbers have led to the idea that our cognitive capabilities are virtually without limit.
Lancaster, M. A. et al. Cerebral organoids model human brain development and microcephaly. Nature 501, 373-379 (2013). This study pioneers a 3D cerebral organoid model derived from hPS cells to ...
A challenge of this research paradigm is how numerous the brain and behavioral modifications have been throughout evolution. The aim of this article is to introduce the concept of a "breakthrough" and use this concept to offer a first approximation explanation of a multitude of both brain and behavioral modifications that occurred during ...
Evolutionary changes can arise from proportional 13 and/or gene regulatory 2,5,10,11,12,18 changes of cell types. Compared to that of non-human primates, the human brain has prolonged myelination ...
Reconstructing the chronology and mode of hominin brain evolution requires a good knowledge of subtle changes in brain areas. In this respect, the inferior part of the frontal lobe, where Broca's cap is located, has been the focus of thorough investigations and intense discussions ().In addition to its use as a critical landmark for brain reorganization, Broca's cap plays a fundamental role in ...
As individuals grow, an increase in brain size allows for an increase in skill, and an increase in body size makes it easier to convert that skill into energy. The skill boost also aids successful ...
pp. 506 - 507. DOI: 10.1126/science.abd1840. References and Notes. eLetters (0) Since early hominids emerged 5 million years ago, humans have evolved sizable brains to support higher cognitive functions. In particular, the human cerebral cortex is greatly expanded, allowing accommodation of the evolutionary increases in the number of cortical ...
Most comparative neuroanatomical research has focused on a tiny fraction of this variety, concentrating on a few primate model species, including common marmosets (Callithrix jacchus), capuchin monkeys ... Summary of the neuroanatomical changes in human brain evolution, including macro- and micro-structural changes (see text for more detail).
Here, we discuss the relationship between the molecular evolution of the gene and human brain evolution and development, mainly focusing on ASD-associated genes. FOXP2, forkhead box P2 is one of the most well-known genes associated with human brain evolution and ASD. FOXP2 belongs to the forkhead gene family of transcription factors.
Human brain evolution is characterized by increase in expression of genes involved in energy metabolism, synaptic function, and plasticity. ... Most comparative neuroanatomical research has focused on a tiny fraction of this variety, concentrating on a few primate model species, including common marmosets (Callithrix jacchus), capuchin monkeys ...
Abstract. Since the last common ancestor shared by modern humans, chimpanzees and bonobos, the lineage leading to Homo sapiens has undergone a substantial change in brain size and organization. As a result, modern humans display striking differences from the living apes in the realm of cognition and linguistic expression.
Human brain size nearly quadrupled in the six million years since Homo last shared a common ancestor with chimpanzees, but human brains are thought to have decreased in volume since the end of the last Ice Age. The timing and reason for this decrease is enigmatic. Here we use change-point analysis to estimate the timing of changes in the rate of hominin brain evolution. We find that hominin ...
Primates vary almost a thousand-fold in endocranial volume - a measure which closely approximates brain size - ranging from 1.63 mL in mouse lemurs (Isler et al., 2008) to 1478 mL in humans (Robson and Wood, 2008).Body size is perhaps the most important statistical predictor of brain size across primates, with larger bodied species having larger brains, but substantial variation remains ...
Credit: Neuroscience News. The neocortex is the characteristic folded outer layer of the brain that resembles a walnut. It is responsible for higher cognitive functions such as abstract thinking, art, and language. "The neocortex is the most recently evolved part of the brain," says Dr. Mareike Albert, research group leader at the CRTD.
From here the species embarked on a slow upward march, reaching more than 1,000 ml by 500,000 years ago. Early Homo sapiens had brains within the range of people today, averaging 1,200 ml or more ...
Rosetta Stone (s) to decode brain evolution. The researchers think not one but multiple mechanisms of evolution helped form the modern human brain. Such mechanisms include: Gene addition, duplication or deletion. Alteration in the protein-coding sequence of genes to create new or modified biochemical functions.
Submission deadline. Brain structure assessments have enriched the current understanding of neuroanatomical function through dissection and histology, tracing, neuroimaging, and modern molecular methods. Another beneficial tactic is to look at the same questions through the lens of evolution. The startlingly —.
Katie Rickelton preparing to sequence primate RNA at the UMass IALS Genomics Core. "We study primate brain evolution," says Katie Rickelton, lead author of the paper, published in eLife, and doctoral candidate in molecular and cellular biology at UMass Amherst."Primates, and especially humans, are defined by having very large brains compared to their body size—and yet, humans ...
Research Highlight; Published: 19 October 2021; BRAIN EVOLUTION. Regulators of human brain evolution. Katherine Whalley 1 Nature Reviews Neuroscience volume 22, pages 720-721 (2021)Cite this article
The power of brain organoids. To search for factors influencing brain expansion, the Albert group compared developing brains of mice and humans. "Stem cells in mice don't divide as much and don't ...
The mammalian ancestral grade has a mean brain size of 1.92 and a mean body size of 6.92 (log scale). The stem cercopithecoid/colobine grade has a mean brain size of 4.40 and a mean body size of 9.04. The difference in mean brain size from the mammalian ancestral grade to the colobine grade is +2.48; that of body size is +2.12.
Comprehensive study explores influence of gene expression on primate brain evolution. Credit: eLife (2024). DOI: 10.7554/eLife.70276. An interdisciplinary team of researchers led by biologists at ...
1. Unveiling the Genetic Landscape of Primate Brain Evolution. The study, led by Katie Rickelton and Courtney Babbitt, represents a groundbreaking effort to unravel the genetic underpinnings of ...
Moreover, it is necessary to clarify in future research that oligodendrocytes accelerate brain evolution. Since oligodendrocytes play a human-specific role in metabolism and RNA splicing in the brain, further studies should evaluate the regulation of larger-scale energy metabolism in the evolved human brain and the diversity of various gene ...
"The neocortex is the most recently evolved part of the brain," says Dr. Mareike Albert, research group leader at the CRTD. ... 2023 — The evolution of higher cognitive functions in humans has ...
By Hannah Thomasy, PhD. Today, Massachusetts Institute of Technology biologist Kevin Esvelt is well known for his work on guided evolution technologies—creating systems for evolving biomolecules in the lab and developing techniques to shape the evolutionary trajectories of species in the wild—as well as forging new pathways to safeguard ...
The following essay is reprinted with permission from The Conversation, an online publication covering the latest research.. From the very early days of the pandemic, brain fog emerged as a ...
Brain evolution. Submission status. Closed. Submission deadline. 30 June 2023. Eighty-six billion interconnected neurons comprise the human brain, the product of billions of years of evolution ...
Dr Christina Maher at Sydney University's Brain and Mind Centre says Synchron's technology is "miles ahead" of Elon Musk's and is more sophisticated and safer because it does not require ...