Click through the PLOS taxonomy to find articles in your field.
For more information about PLOS Subject Areas, click here .
Loading metrics
Open Access
Peer-reviewed
Research Article
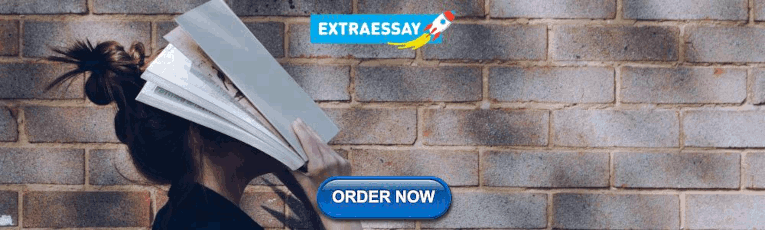
Breast cancer survival and its prognostic factors in the United Arab Emirates: A retrospective study
Roles Conceptualization, Data curation, Formal analysis, Validation, Writing – original draft, Writing – review & editing
Affiliation Department of Medical and Health Sciences, Khawarizmi International College, Abu Dhabi, United Arab Emirates

Roles Conceptualization, Investigation, Validation
Affiliations Department of Medical and Health Sciences, Khawarizmi International College, Abu Dhabi, United Arab Emirates, Cancer Registry, Tawam Hospital, SEHA, Al Ain, United Arab Emirates
Roles Conceptualization, Methodology, Resources, Supervision
Affiliation Institute of Public Health, College of Medicine and Health Sciences, United Arab Emirates University, Al Ain, United Arab Emirates
Roles Data curation, Formal analysis, Investigation, Methodology, Validation
Roles Conceptualization, Methodology, Supervision, Writing – review & editing
Affiliation Department of Pharmacology and Therapeutics, College of Medicine and Health Sciences, United Arab Emirates University, Al-Ain, United Arab Emirates
Roles Investigation, Methodology, Supervision, Validation, Writing – review & editing
Affiliation Department of Surgery, College of Medicine and Health Sciences, United Arab Emirates University, Al Ain, United Arab Emirates
Roles Formal analysis, Methodology, Supervision, Validation, Writing – review & editing
Roles Conceptualization, Data curation, Formal analysis, Investigation, Methodology, Software, Supervision, Validation, Writing – original draft, Writing – review & editing
* E-mail: [email protected]
Affiliations Institute of Public Health, College of Medicine and Health Sciences, United Arab Emirates University, Al Ain, United Arab Emirates, Zayed Center for Health Sciences, United Arab Emirates University, Al Ain, United Arab Emirates
- Yusra Elobaid,
- Maria Aamir,
- Michal Grivna,
- Abubaker Suliman,
- Samir Attoub,
- Hussam Mousa,
- Luai A. Ahmed,
- Abderrahim Oulhaj
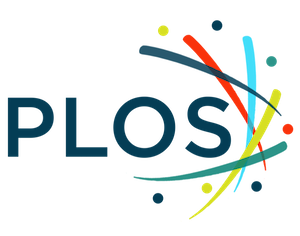
- Published: May 5, 2021
- https://doi.org/10.1371/journal.pone.0251118
- Reader Comments
Data on breast cancer survival and its prognostic factors are lacking in the United Arab Emirates (UAE). Sociodemographic and pathologic factors have been studied widely in western populations but are very limited in this region. This study is the first to report breast cancer survival and investigate prognostic factors associated with its survival in the UAE.
This is a retrospective cohort study involving 988 patients who were diagnosed and histologically confirmed with breast cancer between January 2008 and December 2012 at Tawam hospital, Al Ain, UAE. Patient were followed from the date of initial diagnosis until the date of death from any cause, lost-to-follow up or the end of December 2018. The primary outcome is overall survival (OS). The Kaplan-Meier method was used to estimate the survival curve along with the 2- and 5-year survivals. Different group of patients categorized according to prognostic factors were compared using the log-rank test. Multiple Cox proportional hazards models was used to examine the impact of several prognostic factors on the overall survival.
The median study follow-up was 35 months. Of the 988 patients, 62 had died during their follow-up, 56 were lost to follow-up and 870 were still alive at the end of the study. The average age of patients was 48 years. The majority of patients presented to the hospital with grade II or III, 24% with at least stage 3 and 9.2% had metastasis. The 2-year and 5-year survivals were estimated to 97% and 89% respectively. Results of the multiple Cox proportional hazard model show that tumor grade, and stage of cancer at presentation are jointly significantly associated with survival.
The 2- and 5-year survival are within the norms compared to other countries. Significant clinical and pathological prognostic factors associated with survival were tumor grade, and the stage of cancer at presentation.
Citation: Elobaid Y, Aamir M, Grivna M, Suliman A, Attoub S, Mousa H, et al. (2021) Breast cancer survival and its prognostic factors in the United Arab Emirates: A retrospective study. PLoS ONE 16(5): e0251118. https://doi.org/10.1371/journal.pone.0251118
Editor: Elda Tagliabue, Fondazione IRCCS Istituto Nazionale dei Tumori, ITALY
Received: November 12, 2020; Accepted: April 20, 2021; Published: May 5, 2021
Copyright: © 2021 Elobaid et al. This is an open access article distributed under the terms of the Creative Commons Attribution License , which permits unrestricted use, distribution, and reproduction in any medium, provided the original author and source are credited.
Data Availability: The data are not publicly available owing to privacy or ethical restrictions, as they contain sensitive information. The data are held by the Tawam Hospital Cancer Registry. Requests to access the data can be sent to Dr. Khalid Balaraj, Chairman of Oncology Department, Tawam Hospital, Al Ain, UAE ( [email protected] ). The authors also confirm that the authors did not receive any special privileges to access the data set that other researchers would not receive.
Funding: The authors received no specific funding for this work.
Competing interests: The authors have declared that no competing interests exist.
Introduction
Breast cancer is a significant global public health concern, since it is the most common cancer among women [ 1 ]. Survival rates differ across the globe with higher survival rates in developed compared to less developed countries. For instance, the 5-year survival rate in developed countries such as USA and UK was in the range of 85–90% between the years 2017–2019 [ 2 , 3 ]. In developing countries this rate ranged between 40–60% [ 1 , 4 , 5 ]. The poorer survival in developing countries could be related to low awareness of screening needs, lack of early detection programs and lack of diagnosis and treatment facilities [ 6 ].
Many improvements have been made in the last 20 years in the management of breast cancer due to the identification of prognostic factors capable of providing information on the progression of the disease. These prognostic markers are usually indicators of growth, invasion, and metastatic potential [ 7 ]. These include tumor size, lymph nodes, histologic grade, stage, estrogen and progesterone receptors and HER2/neu (erB-2) oncogene alteration [ 8 ].
In the United Arab Emirates (UAE), breast cancer constitutes approximately 20% of all cases of cancer and is considered to be the second leading cause of death among women after cardiovascular diseases [ 9 ]. Although, the burden of breast cancer is not systematically investigated in the country, the available information indicates that the cumulative probability of developing breast cancer in the UAE increased over the past three decades, being 2% in 1980, 2.4% in 1990, 3.9% in 2000 and 5.2% in 2010 [ 10 ]. Although this rise in cumulative probability could be explained by the improvement in screening program, this rising incidence is not the only cause of concern in the UAE, but also the advanced stage at presentation [ 11 ]. Metastasis is also common among women in the UAE where around 15% of cases are metastatic [ 9 ].
Despite few studies have been published in the UAE on breast cancer research, there is still a gap of knowledge in this field. There is very limited data in the UAE and the region on the survival rates and prognostic factors for breast cancer. To the best of our knowledge, no study has been yet carried out in the UAE to estimate the survival rates of breast cancer. The aim of this study is to estimate the survival curve along with the 2-year and 5-year survival in breast cancer patients in the UAE, and also to evaluate the impact of multiple prognostic factors on the breast cancer survival. Knowledge of these prognostic factors in the UAE population will be the foundation for planning treatment and predicting the outcome for patients with breast cancer.
Population and study design
This is a retrospective cohort study involving all patients (n = 988) who were diagnosed (and histologically confirmed) with breast cancer between January 2008 and December 2012 at the department of oncology at Tawam hospital, a tertiary care and teaching hospital located in Al Ain (Eastern region of the Emirate of Abu Dhabi). Each patient was followed up from the date of initial diagnosis until the date of death from any cause or until 31 st December, 2018. Data collected at baseline (date of diagnosis) included demographic variables (such as age and sex), anatomical site and quadrant (primary site), tumor size, pathological nodal status, clinical stage, histopathological type and grade and treatment modalities. The lymph node and tumor size status were pathologically evaluated. Outcome data (at follow-up) included date of death if occurred or date of last contact with the patient. This information was collected using a pre-formed questionnaire. Ethical approval to conduct the study was obtained from the Al Ain District Human Ethics Committee before the commencement of the study.
Determination of histological type, grade and stage using TNM classification.
Determination of histological type and grade was established by Modified Bloom-Richardson score system which scores for tubular formation, nuclear pleomorphic and mitotic rate within tumor cells. TNM was used to assign the clinical stage of the disease to each patient [ 12 ]. This is a staging system which is expression of anatomical extent of disease based on extent of primary tumor (T), absence or presence of and extent of regional lymph node metastasis (N) and absence or presence of distant metastasis (M).
Statistical analysis
Baseline characteristics were summarized using descriptive statistics including mean, median, and standard deviation for continuous measures, and frequencies tables for categorical variables. Categorical variables were compared using the chi square or Fisher’s exact test and continuous variables using the unpaired t-test. The primary outcome is overall survival (OS). The survival time was defined as the duration of time from the date of initial diagnosis until the date of death from any cause, date lost-to-follow up or the end of December 2018. Survival curves were estimated and plotted using the Kaplan-Meier method. Survival curves of different groups were compared using the log-rank test. Cox proportional hazards models were applied to examine the impact of prognostic factors on overall survival. These included the age at diagnosis, treatment delay (duration of time from the date of initial diagnosis to the date of starting the treatment), tumor grade, stage of cancer, metastasis, primary site and laterality. Pairwise associations between all these prognostic factors was first carried out, using the Goodman and Kruskal’s tau [ 13 ], in order to identify factors providing redundant or similar information on overall survival. This is done to avoid issues of multi-collinearity when fitting Cox proportional hazards models. Lasso procedure was used as a selection method to identify the set of risk factors that are jointly significantly associated with overall survival. The 2-year and 5-year chance of survival were derived from the fitted model. The results of Cox proportional hazard model are presented as hazard ratios along with their 95% confidence intervals. All statistical tests were two-sided, and p-values < .05 were considered statistically significant. All the analysis was conducted using R software version 3.6 [ 14 ].
Baseline characteristics of the patients
The final data set includes 988 breast cancer female patients having complete information on their demographic, prognosis factors and follow-up duration. Of these 988 patients, 62 had died during the follow-up, 56 were lost to follow-up and 870 were still alive at their last follow-up. The maximum follow-up time was 125 months and the median follow-up was of 35 months.
The average age at diagnosis was 48 years. The distribution of demographics, clinical and pathological characteristics at baseline, overall and according to the age group of diagnosis (< 50 years versus > 50 years) are presented in Table 1 . The majority of patients are non-Emirati (81%). The average duration of time between the date of initial diagnosis and the date of first treatment was 18 days.
- PPT PowerPoint slide
- PNG larger image
- TIFF original image
https://doi.org/10.1371/journal.pone.0251118.t001
The majority of patients (88%) were presented with grade II or III and approximately 54% had left laterality. Regarding the stage of breast cancer, 80% of the patients were diagnosed with stage 2 at least. There was no statistically significant association between the tumor grade and the age at diagnosis. The distribution of tumor grades was similar in younger (< 50 years) compared to older woman (> 50 years) (P-value = 0.204). The same conclusions apply to other prognostic factors such as metastasis and stage of cancer except for laterality where older women were found to be more likely to have breast cancer in the left side compared to younger women.
Survival curves.
The unadjusted survival curve of patients, estimated using Kaplan-Meier method, is illustrated in Fig 1 . Unadjusted survival curve. The 2-years survival was 97% (95% CI; 96%-99%) meaning that 97% of breast cancer women survived beyond 2 the first two years after their initial diagnosis and the 5-years survival was estimated to 89% (95% CI; 86%-92%).
https://doi.org/10.1371/journal.pone.0251118.g001
Fig 2 shows the estimated survival functions according to the stage of cancer, tumor grade and metastasis. The log-rank test shows significant differences in survival curves between groups of each prognosis factor (all p-values were < 0.001). Patients with tumor grade I were observed to have higher 5-years survival of 99% (95% CI; 96%-100%) compared to those with tumor grade III who had a low 5-years survival rate of 85% (95% CI; 80%-90%). Similarly, patients with M0 metastasis had higher 5-years survival of 93% (95% CI; 90%-95%) compared to patients with M1 metastasis who had a 5-years survival of 48% (95% CI; 33%-70%). Regarding the stage of breast cancer, it is clear that those diagnosed with advanced stage have worse survival diagnosis 48% (95% CI; 33%-70%) in comparison to those with stage 1 or less 97% (95% CI; 94%-100%).
https://doi.org/10.1371/journal.pone.0251118.g002
Cox proportional hazard model.
The hazard ratios obtained from separate univariate cox proportional hazard models fitted for each potential prognosis factor show no evidence for the survival curve to be affected by the age at diagnosis or the relative delay in treatment (in months). However, among clinical and pathological factors investigated, only the tumor grade and stage of cancer were statistically significantly associated with the survival ( Table 2 ).
https://doi.org/10.1371/journal.pone.0251118.t002
The final multiple Cox proportional hazard model, derived from the stepwise backward and the lasso variable selection procedures, retained two prognosis factors that are jointly significantly associated with survival. These include tumor grade and the stage of cancer. The results of the fit of the final Cox proportional hazard model, along with the estimated hazard ratio and their corresponding 95% confidence intervals are provided in Table 3 .
https://doi.org/10.1371/journal.pone.0251118.t003
The hazard ratio of death in patients with tumor grade III was 10.24 compared to those with Grade I. Patients presented at earlier stage were more likely to survive compared to those presented at late stage. More specifically, the hazard ratio was 4.85 for patients with stage 3 and 25.76 in patients with stage 4 compared to those with stage I.
Clinical and histological information of a large number of breast cancer patients recruited from a tertiary hospital-based cancer registry in the UAE were analyzed. The aim of the study is to estimate the overall survival curve and the 2- and 5-year survival in breast cancer patients, and also to identify the set of important prognostic factors affecting survival in these patients. The average age of diagnosis was 48 years with the majority of patients presenting to the hospital with grade II or grade III, 24% with at least stage 3, and 9.2% had metastasis. We showed that among the clinical and pathological risk factors investigated, the tumor grade and the stage of cancer at presentation and metastasis were statistically significantly associated with the overall survival when analyzed separately. However, when added together into the Cox proportional hazard model, only tumor grade and stage of cancer were found to be jointly significantly associated with overall survival. The 5-year risk of death from breast cancer was estimated to 11% and the 2-year risk to 3%.
Based on the CONCORD 3 study [ 15 ], in the gulf region, breast cancer survival rates increased between 1995–1999 and 2010–2014. In Saudi Arabia, between 1995–1999 and 2005–2009 the overall 5- year’s survival increased from 70.9% to 78.4%. In Bahrain, between 2000 and 2004 the survival was reported to be 63%. In Kuwait, between 2000–2004 and 2010–2014, the survival increased from 68.3% to 75.2% and in Qatar, it has increased from 59.2% to 71.9%. In the UAE, there is no previously reported disease survival rate, this is the first study to report it. Based on our findings, the 5 years survival of breast cancer in the UAE was 89%, this is similar to western countries such as Australia 89.5% and Canada 88.2% [ 15 ]. The 5 year survival rate is also good compared to other countries in the same region such as Qatar (71.95%) and Kuwait (75.2%). This may reflect improved treatment and intensified efforts to tackle the burden of breast cancer in the country through the introduction of the national screening program. Previous studies have established numerous prognostic factors of breast cancer survival including age at diagnosis, tumor size, axillary lymph node involvement, tumor grade, stage of cancer, and metastasis. In our study, the average age at diagnosis of women was 48 years supporting the findings of other studies from the region claiming that Arab women are more likely to develop breast cancer at an earlier age [ 16 , 17 ]. In fact, the median age at diagnosis of breast cancer in the UAE is 10 to 15 years younger than in North America and Europe [ 18 ]. Women at younger ages generally suffer from a more aggressive type of breast cancer, an advanced stage at presentation, and worse outcome [ 19 ]. A study conducted in southern Iran showed a relatively low 5-year survival (58%) of breast cancer [ 20 ]. However, in our data set, the hazard ratios obtained from separate univariate cox proportional hazard models fitted for each variable show no evidence for the survival function to be affected by age at diagnosis. In this study, the median patient delay in seeking treatment was 18 days, this is relatively similar to other studies in Europe [ 21 , 22 ]. The percentage of stage 1 and II breast cancer in Australia, Canada, Denmark, Norway, Sweden, and the United Kingdom was 61–62% between 2000–2007 [ 23 ]. In our study, around 66% of patients were diagnosed at stage I or II versus 34% in stage III or IV. This was comparable to data from developed countries.
In this study, tumor stage was related to decreased survival, similar to other researches [ 24 – 26 ]. Saadatmand et al (2015) reported that in univariable and multivariable analyses, both stage and lymph node status had major influence on overall survival. Stage at diagnosis still influence overall survival considerably [ 27 ].
In our study, the hazard ratio for death in patients with grade III compared to those with Grade I was 10.59 (95% CI 1.45–77.44). This finding is in agreement with the literature, suggesting that more advanced grading has worse prognoses for breast cancer [ 28 , 29 ].
This is the first study to report estimates of survival rates and prognostics factors of breast cancer based on cancer registry data in the UAE. The study was based on robust cancer registry data. The follow-up duration was sufficient to capture and adequately estimate the 2-year and 5-year risk of death due to the fact that 50% of subjects were followed up for more than 35 months and 25% of patients followed up for more than 56 months. However, as this was hospital-based registry, generalizability of the study findings should be interpreted with caution. The sample does not represent the whole UAE, but it captures a good proportion of breast cancer patients referred to Tawam Hospital as it is used to be the main referral center for oncology cases in the UAE and has the only breast health center in the country. Moreover, as the analysis was based on secondary data, other potential confounding factors such as presenting symptoms and signs, family history, and lifestyle factors including diet and physical activity were unavailable and could not be included in the study analysis.
The 2-year survival was estimated to 97% and the 5-year survival to 89%. Tumor grade and the stage of cancer were found to be jointly significantly associated with survival. The findings of this research enable the estimate of the long-term breast cancer survival, as well as reemphasizes the importance of early diagnosis and screening through mammography which can prevent local systemic metastasis and therefore advanced stage diagnosis.
Acknowledgments
We would like to acknowledge Tawam Hospital Cancer registry staff for their cooperation.
- View Article
- Google Scholar
- 5. GLOBOCAN, 784-united-arab-emirates-fact-sheets.pdf. (2018). Retrieved on March 15, 2020 from http://gco.iarc.fr/today/data/factsheets/populations/784-united-arab-emirates-fact-sheets.pdf
- 7. Foukakis, T., & Bergh, j. Prognostic and predictive factors in early, non-metastatic breast cancer. UptoDate 2013. Retreived on 15/12/2013 from http://www.uptodate.com/contents/prognostic-and-predictive-factors-in-early-non-metastatic-breast-cancer
- 8. Rubin E., & Reisner H. Essentials of Rubin’s Pathology (5th ed.). Lippincott Williams & Wilkins, 2009, Philadelphia, USA.
- 9. Cancer Incidence in United Arab Emirates Annual Report of the UAE National Cancer Registry- 2017 www.mohap.gov.ae Statistics and Research Center https://www.mohap.gov.ae/Files/MOH_OpenData/1585/CANCER%20INCIDENCE%20IN%20UNITED%20ARAB%20EMIRATES%20ANNUAL%20REPORT%20OF%20THE%20UAE%20-%20NATIONAL%20CANCER%20REGISTRY%20-%202017.pdf
- PubMed/NCBI
- 11. Elobaid, Y. (2014). Breast Cancer Presentation Delay among Women in the United Arab Emirates. Thesis. United Arab Emirates University. Available at: https://scholarworks.uaeu.ac.ae/all_dissertations/31/ (Accessed: 18 October 2020).
- 12. American Joint Committee: AJCC Cancer Staging Manual. 5th edition. Philadelphia, New York. Lippincott-Raven; 1997.
- 14. R Development Core Team. (2013). a language and environment for statistical computing: reference index . R Foundation for Statistical Computing. http://www.polsci.wvu.edu/duval/PS603/Notes/R/fullrefman.pdf
- 17. World Health Statistics by World Health Organization. Electronic version http://www.who.int.whosis/whostat/EN_WHSo8_Full.pdf (2008). Google Scholar
- 18. National Cancer Registry (UAE) . (2015). Retrieved April 11, 2020, from https://smartapps.moh.gov.ae/ords/f?p=105:512:10387122530459::NO:RP,RP,RIR,512:IR_YEAR_OF_DIAGNOSIS:2015&cs=1glSOv-Qci-yb_CJ3qHk9Q0O_YJc
- Cancer Survival Research Paper
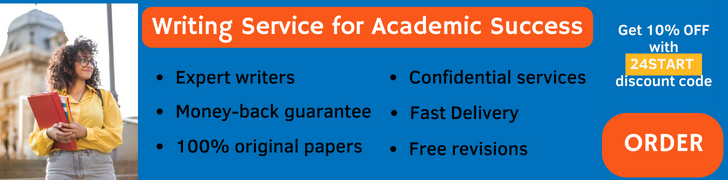
View sample cancer research paper on cancer survival. Browse other research paper examples for more inspiration. If you need a thorough research paper written according to all the academic standards, you can always turn to our experienced writers for help. This is how your paper can get an A! Feel free to contact our writing service for professional assistance. We offer high-quality assignments for reasonable rates.
Introduction
It is of considerable interest to know the outcome of treatment for all cancer patients in a population. Clinical trials or studies of hospital records cannot provide this information. Trials include patients who are selected on the basis of strict inclusion and exclusion criteria such as a restricted age range or a lack of serious comorbidity. Trials focus on a pragmatic comparison of efficacy between treatment regimens: They are generally quite small (a few hundred or occasionally a few thousand patients), and in general fewer than 10% of all adult cancer patients are included in trials, even in developed countries. Hospital statistics can only indicate the fate of patients who have been referred to that hospital. The criteria for referral to a given hospital are not usually explicit, and the statistics on outcome are most unlikely to be representative of any population.
Academic Writing, Editing, Proofreading, And Problem Solving Services
Get 10% off with 24start discount code.
In contrast, the public health interest in cancer survival is in the outcome for all cancer patients. Population-based cancer survival estimates are used to monitor trends in survival over time, as one measure of overall progress in cancer control. They can display breakthroughs in cancer treatment, as happened for childhood cancers in the 1970s and 1980s. They provide a basis for resource estimation for cancer care and a baseline for planning of the clinical trials. They are used to assess inequality in the outcome of cancer between population subgroups defined by age, sex, social class or degree of affluence, and race or ethnic group, or to compare survival between regions within a country or with other countries. Survival estimates provide a crucial measure of the overall efficacy of the entire cancer patient pathway, from the initial health-seeking behavior of a person with symptoms that may be due to cancer, through primary care, referral to secondary care for investigation and diagnosis, and the efficacy and accessibility of available treatment resources. All these uses of cancer survival figures require that the estimates be statistically robust and adequately comparable.
Population-based survival estimates should include the outcome for all cancer patients in a given population. For this reason, the data are usually derived from a population-based cancer registry that collects information on all persons diagnosed with cancer who are normally resident in the region or country covered by that registry. Further reference here to cancer survival will be to population-based estimates, unless otherwise stated.
It is worth considering whether the follow-up of cancer patients, to ascertain their vital status, is in fact required in order to estimate cancer survival, since cancer mortality rates should also reflect survival, and they are routinely available in many countries. Mortality rates cannot be used for this purpose, however. First, mortality reflects both incidence and survival; in other words, the number of deaths from a given cancer will reflect the number of people who are diagnosed with it, as well as their chances of surviving it. In a steady state, therefore, mortality from a very common cancer with good survival could be higher than mortality from an uncommon cancer with poor survival. Second, the deaths from cancer in a given year will arise not just in patients who were diagnosed in that year, but also those diagnosed up to ten or more years earlier, and this backscatter of diagnoses will vary with the lethality of the cancer and with any recent advances in treatment for that cancer. For this reason, mortality trends can rarely offer more than a delayed and blurred reflection of the public health impact of advances in treatment. Third, the accuracy of cancer mortality statistics depends on the accuracy of death certification, and of the coding of the underlying cause of death; both these components of mortality statistics vary with the type of cancer, as well as between countries and over time. For these reasons, mortality statistics are not an adequate surrogate for cancer survival in the context of public health.
Public interest in international comparisons of population-based cancer survival has increased in recent years (Berrino et al., 2003). Such comparisons can be useful for understanding national survival data and for the interpretation of incidence rates. They serve as broad indicators of the quality of patient care. For example, the EUROCARE studies have clearly demonstrated lower survival among cancer patients in several Eastern European countries than in Western European countries, differences that are directly attributable to inferior diagnosis and treatment.
Observed Patient Survival
Survival and the duration of life after diagnosis have been considered to be among the most important indicators of success in the management of cancer patients. In what follows, the analysis of survival is demonstrated in detail. The method is also applicable to the analysis of other clinical outcomes, e.g., relapses or side effects of treatment.
A characteristic problem with follow-up studies is that the patients have different potential follow-up times. A patient diagnosed a long time ago can, potentially, be followed up for much longer than a patient who was diagnosed only recently. It is much more likely that the death of a patient with a long potential follow-up time will be observed than the death of a patient with a short potential follow-up time. Because the times of death are not usually available for all patients, it is not advisable to calculate a mean of the observed survival times, whether of all patients or only of those who have died. Again, because of these differences in potential follow-up time, it is also inappropriate to calculate direct proportions of survivors after certain fixed durations of follow-up time. Those proportions can be obtained by special methods, such as the life table method or the product-limit (Kaplan-Meier) method.
Let us take the colon cancer patients diagnosed in Finland during 1992–2002 as an example. The follow-up time of the patients starts on the date of diagnosis and ends with the date of death, or emigration or 31 December 2003, whichever comes first. Thus, the potential follow-up time for patients diagnosed during 1999–2002 was less than 5 years, whereas it was more than 10 years for patients diagnosed during 1992–93. The actual follow-up was shorter than the potential follow-up for any patient who died or emigrated before 31 December 2003.
Nonlocalized colon cancer was diagnosed in 1230 males aged 60–74 (Table 1). During the first month of follow-up, 53 died from colon cancer and nine from other causes of death. During the second month, 90 died, 79 from colon cancer and 11 from other causes.

The life table has a row for each interval (month) of follow-up i, including the number of patients alive and under follow-up at the beginning of the interval (l i ), the number of deaths during the interval (d i ), and the number of persons who were alive when withdrawn or lost to follow-up at some point during the interval (w i ). For months 13–24, the number of withdrawals includes patients diagnosed in 2002 who were still alive on 31 December 2003, when follow-up in the study ceased. Those patients had only been followed up for 1–2 years; their follow-up is said to have been censored (i.e., cut short) after the last possible date on which their death could have been observed.
Loss to follow-up is a good reason for censoring a patient’s follow-up time in the analysis, since the patient is no longer under observation, and a death could not be observed. In Finland, it is rare for cancer patients to move abroad, and because of highly efficient population and death registration systems, it is also rare to lose patients from follow-up. In many other countries, however, withdrawals will also include both patients known to have emigrated and patients who have been lost to follow-up.
The interval-specific observed survival proportion in Table 1, pi, is an estimate of the probability that a patient who is alive at the beginning of interval i will survive until the end of it. This proportion is often incorrectly called a rate (Elandt-Johnson, 1975). The observed survival proportion can be calculated from the formula:
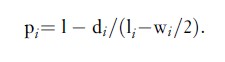
This is simply the complement of the proportion of deaths among those who started the interval, with a correction for censoring, in which we assume that the w i patients who did not die but who were not followed up throughout the entire interval were, on average, followed up until the mid-point of the interval.
The cumulative observed survival proportion up to a given time interval since diagnosis, which is an estimate of the corresponding probability of survival, is obtained by calculation of the product:
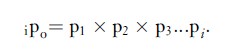
This quantity gives an estimate of the probability of surviving i intervals (months) from the start of follow-up. For the 24th month, for example, the observed survival proportion is p 24 = 0.98394, while the 24-month (2-year) cumulative observed survival proportion is 24 p o = 0.40147 (Table 1).
This formula for the survival proportion is based on an assumption that the probability of surviving for those who could not be followed after interval i is the same as the probability of survival for those who were successfully followed up during subsequent intervals. This is called the assumption of independence between dying and censoring.
The assumption may well be valid, but even if follow-up information is complete, problems can arise when the data set includes patients who were diagnosed a long time ago. Obviously, patients diagnosed a long time ago have longer potential follow-up times than patients diagnosed recently, for whom the observed follow-up will thus end more frequently with the patient being censored alive (death has not been observed). Patients whose follow-up times are censored are assumed to have had the same pattern of survival after censoring as patients whom it was possible to follow up for longer, i.e., generally those patients who were diagnosed a longer time ago. Since survival generally improves with time, however, this assumption is not necessarily valid. Under those circumstances, therefore, the cumulative observed survival proportion is likely to be somewhat too low.
The consecutive estimates of the cumulative observed survival proportion can be joined up to produce observed survival curves (Figure 1). The older the patients, the lower is the cumulative observed survival. The use of straight lines to connect the survival estimates illustrates the idea that deaths within each time interval are evenly spaced in time.
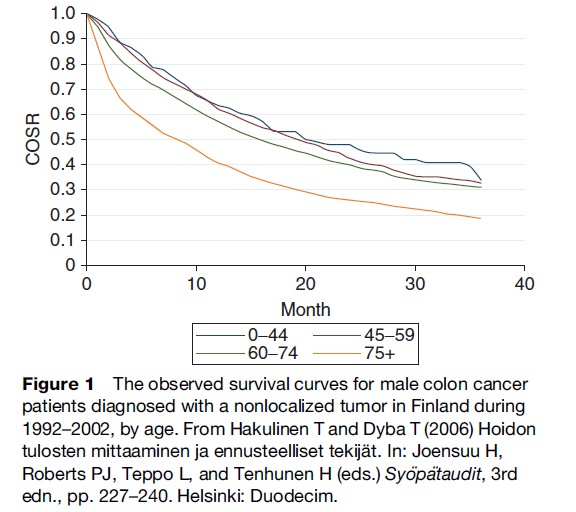
With the product-limit (Kaplan-Meier) method, it is assumed that the times of different events are exactly known. The survival times are then tabulated individually by observed lengths, not by fixed subintervals as in Table 1. In order to apply the product-limit method to the material in Table 1, a decision must be made on how to treat apparently simultaneous deaths and censorings, i.e., those that occur during the same interval. Most computer programs are set to assume that withdrawals (events in the w i column) would happen slightly later than deaths, although there is no particular logical basis for this choice. The survival proportion pi will then be calculated from the formula:
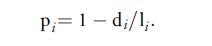
The number of withdrawals is used only to obtain a new value l i+1 = l i – d i – w i . Otherwise, the calculations are the same as with the usual life-table method outlined above.
Observed survival curves obtained with the productlimit method are step functions, that is, they run horizontally (constant survival proportion) in the intervals between deaths, then drop vertically when deaths occur. In practice, the life-table method and the product-limit method give nearly the same results when the time intervals are short, on the order of 1 month.
The 2-year cumulative observed survival proportion can also be estimated by annual intervals; results are virtually identical. The choice of subintervals for the life-table method depends on the cancer and on the desired degree of detail. The intervals may be unequal, e.g., short intervals at the start of follow-up, when deaths are more frequent, and longer intervals later on, when deaths are less frequent. Intervals should not be longer than 1 year. The assumption of exact event times with the product-limit method requires intervals no longer than 1 month. The life-table method with longer, say, yearly intervals is efficient in summarizing survival information when the data set is large, as is typically the case with population-based cancer registries.
Cause-Specific Survival
Figure 1 The observed survival curves for male colon cancer patients diagnosed with a nonlocalized tumor in Finland during 1992–2002, by age. From Hakulinen T and Dyba T (2006) Hoidon tulosten mittaaminen ja ennusteelliset tekija¨ t. In: Joensuu H, Roberts PJ, Teppo L, and Tenhunen H (eds.) Syo¨pa¨taudit, 3rd edn., pp. 227–240. Helsinki: Duodecim.
We cannot conclude from Figure 1 that colon cancer is more fatal in older patients. The probability of survival for older people is lower in part because of mortality due to other causes of death. If the cause of death of each patient is known, it is possible to calculate cause-specific survival proportions to estimate the probability of survival in the hypothetical situation where colon cancer is the only cause of death. The cause-specific survival proportion in each interval (Table 1) can be obtained as:
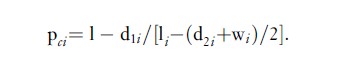
Here, deaths from the cancer of interest in the ith interval are counted as events, d1i, while deaths from other causes (d2i) are considered as censorings (compare this formula with that for observed survival in the previous section). Thus the follow-up time has been cut short (censored) by the death of the patient, but the death is not considered as an event of interest for this particular analysis, if it is not a death from the cancer being studied and is therefore not included in the numerator of the estimate of the proportion who have died. In this context, therefore, as with other reasons for censoring the follow-up, deaths from other causes than the cancer of interest are assumed to occur, on average, at the mid-point of the interval.
The cumulative, cause-specific survival proportion is simply the product of the interval-specific proportions, as before:

At the end of the 2nd year of follow-up, the cumulative cause-specific survival proportion 24pco ¼ 0.43116 (Table 2) is slightly higher than the observed survival proportion 0.40147 (Table 2).
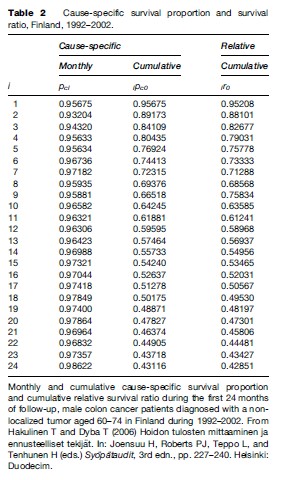
With the product-limit approach to estimating causespecific survival, the assumption of independence is more difficult to accept than for observed survival, particularly if the patient group is very heterogeneous with respect to age. This is because the risk of death from the cancer and the risk of death from other causes are usually both higher in older patients. This introduces dependence between the risk of death (from the specific cancer) and the risk of censoring (death from another cause). The usual approach to eliminating most of this dependence is to analyze survival by age group. If this is not done, the resulting survival estimate will be too high, because the follow-up of older patients will more often be censored due to death from other causes, and censored patients are assumed to have the same survival experience as those who were not censored, who are on average younger, and in fact have better survival. In other words, censoring for other causes removes from the analysis a group of patients (the elderly) whose actual survival is lower than average, leading to an inflated estimate of overall survival.
The cumulative cause-specific survival proportions may be used to construct cumulative cause-specific survival curves. While the range of the observed 3-year cumulative survival proportions is from 19% (patients aged 75 years or more at diagnosis) to 34% (those aged 0–44 years), the range of the cause-specific survival proportions is 24–34%. Colon cancer is thus more fatal for older patients.
Relative Survival
If the cause of death of the cancer patients is not known, or unreliable, the cause-specific survival can be estimated by using the relative survival ratio, i.e., the ratio of the survival actually observed in the cancer patients and the survival that would be expected in the absence of cancer. Relative survival is thus a ratio of two survival proportions.
The expected survival of the cancer patients is estimated from mortality in the general population, using life tables by sex, age, calendar period, and possibly also other factors such as region, social class or degree of deprivation, and race. This can be done in Finland for the patients in Table 1 by using the general population life tables by sex, age, and time. Thus, if the patients in Table 1 were a sample of the general population in Finland, their expected survival proportion would be p i * = 0.9974 during each month within the 1st year of follow-up and p i * = 0.9972 during each of the following 12 months. The 2-year expected survival proportion would then be 24 p 0 * = 0.93725.
Relative survival in the 1st month of follow-up for the patients in Table 1 is thus r 1 = p 1 /p 1 * = 0.94959/0.9974 = 0.95208. The ratio in the 2nd month is r 2 = p 2 /p 2 * = 0.92295/0.9974 = 0.92535. The 2-year cumulative relative survival is 24 p 0 / 24 p 0 * = 0.40147/0.93725 = 0.42851 (Table 2).
Relative survival can be interpreted as the cause-specific survival, i.e., an estimate of the probability of survival if the patient’s cancer were the only cause of death. Because of the method of calculation, this interpretation is not always strictly possible. Sometimes, particularly when the life table intervals are short and the mortality in the cancer patients is low, the relative survival in a particular time interval may exceed the value of one. This leads to slight upward turns in a survival curve, such as the curve for the youngest age group in Figure 2.
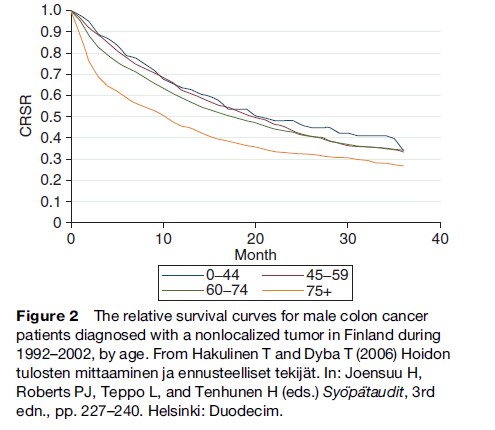
Five-year relative survival for colon cancer is highest in females when the tumor is localized and lowest in males with nonlocalized tumors (Figure 3). Relative survival is lowest for the oldest patients. The variation in relative survival by age is much smaller than for the corresponding observed survival estimates, particularly when the tumor is localized at diagnosis.

The 5-year relative survival ratios and the cause- specific survival proportions for colon cancer patients are generally similar (Table 3). The differences increase with age and with follow-up time since diagnosis. It is difficult to conclude which estimates of survival are more correct when the differences are large. Knowledge that the patient has cancer may influence the physician’s assessment and certification of the cause of death. On the other hand, cause-specific 15-year survival of those aged 75 years or more at diagnosis may not be of such great interest in practice.
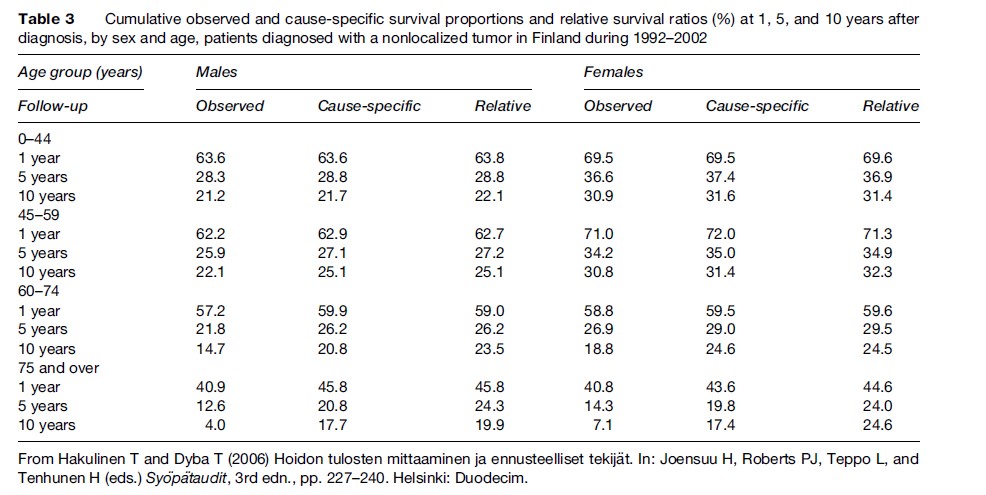
It is important to know when cancer patients are cured. This can be estimated from either the relative survival ratio or the cause-specific survival proportion within successive intervals of time since diagnosis. The estimates should be close to 100% for cured patients. For cause-specific survival, cure implies that patients surviving up to that point would no longer die from their disease. For relative survival, cure would imply that patients surviving up to that point have approximately the same mortality as a comparable group of the general population. On the basis of these quantities, the point of cure for Finnish colon cancer patients occurs around 9 years after diagnosis (Figure 4).
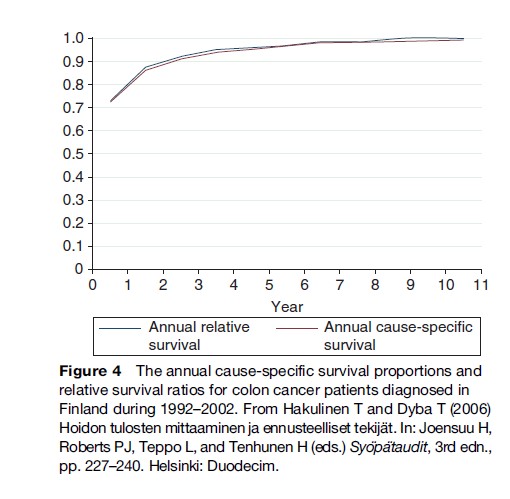
Confidence Intervals And Tests
The survival proportion is an estimate of the probability of survival. A confidence interval expresses the range of values in which the true survival probability is most probably located. In a hypothetical repetition of 100 identical studies, the estimates from 95 studies would on average produce a 95% confidence interval covering the true value of the probability (provided that the statistical model used is appropriate). The confidence interval of the survival proportion (Table 4) is often based on a standard error obtained by Greenwood’s formula and an approximation based on the normal distribution.

For cause-specific survival, the numbers of deaths due to other causes are regarded as fixed. For relative survival, the expected survival is assumed to lack random error because it is usually based on the mortality experience of the (large) general population.
Differences between groups of patients in observed, cause-specific, and relative survival can be tested for statistical significance. The logrank test is most commonly used for observed and cause-specific survival, but it lacks power when the data are heavily grouped, e.g., with annual follow-up intervals. Alternatives with better power are available, which can also be used to test for differences in relative survival (Hakulinen et al., 1987).
Simple testing of the difference between survival proportions or ratios in observational studies is problematic, because the groups being compared were not formed by random allocation. The groups will usually differ with respect to many factors that should be controlled for in testing. This control in testing, including the logrank method and its extensions, is best done by proper statistical modeling (Dickman et al., 2004), which will be introduced in the section for regression analysis. Modeling both facilitates proper statistical testing and produces estimates that are adjusted for important prognostic factors.
The Maximally Updated Survival Estimates
The colon cancer patients in the example used here were diagnosed in Finland during 1992–2002 and followed up to the end of 2003. The problem with the survival estimates is that they tend to be outdated. For example, one can think that the 5-year survival analysis consists of a combination of five analyses, each specific to a follow-up year. The 1st-year analysis is based on follow-up that occurred in 1992–93 for those diagnosed in 1992, in 1993–94 for those diagnosed in 1993, and so on until in 2002–2003 for those diagnosed in 2002. A similar check reveals that also the 2nd-year, 3rd-year, etc., analyses are based on follow-up that occurred a longer time ago.
In demography, the current expectation of life is based on age-specific mortality rates of the most recent year. This principle can also be followed successfully in making an estimate for the current survival, based on the most recent 1st-year, 2nd-year, etc., follow-up year-specific figures. It is usual to use at least two recent calendar years, to avoid statistical uncertainty in the estimates arising from small numbers of events.
Many cancer registries use this period analysis (Brenner et al., 2004b) to produce maximally updated estimates of patient survival. For example in Finland, when all five follow-up year-specific analyses are based on follow-up that took place in 2001–2003, the 5-year relative survival for female colon cancer patients is 62%, whereas it is 59% based on the patients diagnosed during 1992–2002.
The period analysis result is based on patients who were followed up in 2001–2003. Patients diagnosed during 2000–2002 had their 1st year of follow-up within that time window 2001–2003 (no patients diagnosed in 2003 were included); patients diagnosed during 1999–2002 had their 2nd year of follow-up within that window, those diagnosed during 1998–2001 their 3rd year, etc., and those diagnosed during 1996–99 had their 5th year of follow-up within that time window.
In period analysis, both the period of diagnosis and that of the follow-up should be reported. Here, patients diagnosed during the period 1996–2002 and followed up during the period 2001–2003 were the basis of the analysis. Of these two periods, the period of follow-up, here 2001–2003, gives the name period analysis for 2001–2003 to the method.
Prognostic Factors
Prognostic factors can be divided into four groups: those related to the patient, the tumor, the diagnosis, and the treatment. Prognostic factors related to the patient include age, sex, symptoms, weight, genetic background, and immunological status. Tumor-related factors include tumor size, stage, histologic type, degree of malignancy, presence and location of metastases, hormone receptor status, and biochemical, molecular, and genetic markers. Diagnosis-related factors include the various diagnostic methods that may have been deployed and for solid tumors, the numbers of lymph nodes examined. Treatment-related factors also include the treatment techniques and their quality, e.g., dose intensity of cytostatic treatment and the total treatment time in radiation therapy.
All these factors may have independent effects on cancer survival, and they may also modify each other’s’ effects. The effects of certain factors may also be confounded by other factors. It may also depend on the context whether a certain effect should be regarded as confounding or not.
For example, one geographic region may have lower relative survival than another. Patients diagnosed in this region may have a less favorable distribution of disease stage. If diagnosing the tumors early has not been of high priority in that region and a comparison is made between the health system performances, it may be improper to adjust for stage, because stage is then a part of the outcome being studied, and thus an intervening variable on the causal pathway between the exposure under consideration (the performance of the health-care system) and the effect of that exposure (cancer-specific survival). On the other hand, it may only be of interest to compare cancer treatment services between the areas, and then adjustment for stage may be important. Thus, when survival comparisons are made between population groups, great care should be taken whether or not to adjust for the disease stage.
Population-based cancer registries normally collect information on a limited set of these variables, to enable monitoring of outcome over time and between population subgroups. In so-called high-resolution studies (Sant et al., 2003), this basic set of variables is extended, for representative samples of registered patients, by collection of additional data items from clinical and laboratory sources, e.g., diagnostic determinants or data on state-of-the-art treatment. This enables the explanatory impact of such variables on relative survival to be modeled.
Cause-Specific And Excess Mortality
With a large number of prognostic factors, estimating survival within each of the strata defined by the levels of those factors becomes difficult, because the required number of strata increases rapidly. The solution is statistical regression modeling of survival times, which can be based on individual data or on strata formed by individuals.
The most commonly used regression model is the Cox proportional hazards model, included in many software packages. It is possible and important to check and, when necessary, to give up the proportionality assumption in this method. This model can be used to study observed and cause-specific mortality. Observed (overall) mortality has two components, the first related to the cancer, the second due to other causes of death. It is unlikely that the second component would have the same prognostic factor background as the first.
In cause-specific analysis, only the first component of the overall risk of death is modeled. That is viable when the patients’ causes of death are available and can be assessed in a reliable and similar way in all the population subgroups to be compared and over time if the purpose of the study includes monitoring over time. Frequently, this ideal situation is not available. Death certification has changed over time and certification and coding practices have varied between countries and population subgroups to be compared. The solution is to model relative survival.
The Cox proportional hazards model has been generalized to relative survival. This allows relaxation of the assumption of proportionality, which does not usually hold in population-based data. In relative survival regression, the two components of mortality are explicitly recognized. The second component, the expected mortality, is estimated from life tables, and the difference between the total mortality and the expected mortality is then modeled. This difference, the excess mortality in the cancer patients, can generally be considered as attributable to the cancer. It may not always be true, for example if certain social classes or geographic areas are under or overrepresented among the cancer patients and this has not been taken into account in the selection of the general population comparison group, i.e., in the choice of life tables. For analyses comparing survival between social groups and geographic areas, it is particularly important to use the appropriate data on general mortality. Other factors such as smoking may make it difficult to identify a truly comparable general population group. For example, lung cancer patients tend to have a higher mortality due to many other smoking-related causes of death than just the lung cancer itself.
Finally, one might consider it important to exclude from the general population (or background) mortality that component of overall mortality that is due to the cancer being studied. This can be done, but the effect is generally small, because even the most common cancer typically accounts for only a small proportion of the total mortality in any one age group. For all cancers combined, however, it may be important if relative survival is to be used as an overall indicator of cancer survival when other causes of death have been eliminated. Such an analysis may be justified if relative survival is to be used as an index of the overall excess mortality of cancer patients.
Statistical modeling is based on the concept of hazard, the details of which will not be covered here. A mortality rate is an estimate of the hazard and for simplicity here it can be used synonymously. It is simply defined as the ratio between the number of deaths and the amount of persontime at risk.
Let us take the patients in Table 1 as an example. In the first interval, 62 deaths occurred. On the assumption that the deaths occurred, on average, in the middle of the interval, the patient group had n 1 = 1230 – 62/2 = 1199 person-months at risk in that interval; there were no withdrawals (w i is zero). In general terms, the approximate formula for person-months at risk in interval i is n i = l i – (d i + w i )/2. The Finnish Cancer Registry data do not allow greater accuracy because the date of diagnosis does not include the day.
The observed mortality in the 1st month is thus 62/1199 person-months = 5.2/100 person-months or 62/100 person-years. Cause-specific mortality rates are 53/100 person-years for colon cancer and 9/100 person-years for other causes of death. In the section titled ‘Relative survival,’ it was noted that expected survival in each month of the 1st year was p 1 * = 0.9974, based on the corresponding general population mortality. This corresponds to an expected mortality of –ln[p 1 *] = 2.6/ 1000 person-months or 3.1/100 person-years (Dickman et al., 2004). In the 1st month, therefore, observed mortality among the cancer patients even due to causes of death other than cancer exceeds the expected mortality by almost threefold. Only nine such deaths were observed in that month, however, and after the 3rd month of followup, the numbers of deaths due to other causes are rather close to the expected number (Table 1). For example, in the 4th month of follow-up, the number of person-months at risk is n 4 =1009 – 47/2 = 985.5, the expected mortality is 2.6/1000 person-months, as above, and so the expected number of deaths from other causes is 0.9855×2.6 = 2.56, close to the observed number of three deaths from other causes.
The excess mortality of the cancer patients is the difference between the total mortality rate and the expected mortality rate. In the first month in Table 1, it is 62/100 – 3.1/100 = 58.9/100 person-years. In the 4th month, the total mortality is 47/985.5 person-months or 57.2/100 person-years and the excess mortality thus 57.2/ 100 – 3.1/100 = 54.1/100 person-years, slightly lower than in the 1st month.
Regression Analyses
Regression analysis with the Cox proportional hazards model and its extension for relative survival (Dickman et al., 2004) can be conducted with statistical software packages such as SAS, STATA, R and S+. Let us now look at modeling the cause-specific and relative survival during the first 5 years of follow-up for the patients in Table 3.
The results (Table 5) show the ratios between the cause-specific mortality rates (cause-specific hazard ratios) in different age groups and time intervals since diagnosis, and, similarly, ratios between the excess mortality rates (excess hazard ratios). For example, the cause-specific hazard ratio for women is 0.95 that of men (reference) and the excess mortality hazard ratio is 0.93, i.e. women have a 5–7% advantage over the males. According to this model, the advantage holds true for the 5 years of follow-up. The hazard ratios are significantly lower by 15–17% for patients diagnosed during 1998–2002 than for those diagnosed during 1992–97.
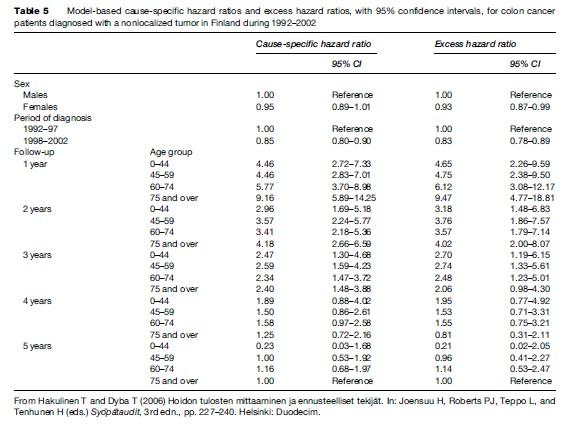
The models for cause-specific and relative survival both introduce an interaction between age and time since diagnosis, meaning that the cause-specific and excess hazards do not retain their proportionality between age groups throughout the duration of follow-up. The differences in the hazard between the age groups are the largest in the first interval and nearly disappear by the third interval. Methods exist to smooth these effects and to provide a continuous representation for the interaction (Remontet et al., 2007).
It is important that the analysis does not stop after the coefficients of Table 5 have been obtained. When the model adequately describes the data, model-based estimates with standard errors should be calculated for the cause-specific survival proportions and the relative survival ratios. The model-based estimates have smaller standard errors than the empirical estimates, because the entire data set has been used in the model, rather than a particular subset (Table 6). The modeling has thus removed unnecessary randomness from the estimates.
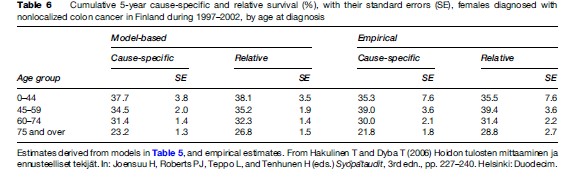
In principle, the modeled results are also free from problems caused by differences in potential follow-up times by age group. The validity of the independence assumption between the causes of death can be improved by a finer stratification by age.
Proportion Cured, Parametric Methods
It was roughly estimated from Figure 4 that the point of cure for Finnish colon cancer patients diagnosed in 1992–2002 was achieved after approximately 9 years of follow-up. Thus the proportion cured is practically the same as the 10-year cumulative relative survival by sex and age (Table 3).
It is possible to study cure systematically by fitting parametric excess mortality models that explicitly include the possibility of cure for a proportion of patients (De Angelis et al., 1999), and that the proportion cured may depend on prognostic factors. The cured patients are considered as subject to general population mortality only, i.e., having no excess mortality. The proportion of patients who are not cured (fatal cases), or those bound to die from their cancer in the absence of competing risks of death, may be characterized by the mean or median duration of survival.
The proportion cured and the mean duration of survival for fatal cases have both increased for Finnish colon cancer patients. For males aged 65–74 years at diagnosis, some 20% of those diagnosed during 1953–59 were cured, whereas the proportion rose to 44% for those diagnosed during 1980–92. At the same time, the mean survival time for fatal cases increased from 0.59 to 1.51 years. Earlier diagnosis and more effective treatment may both be involved in these favorable trends.
Summarizing Results, Age Standardization
The results in Tables 5 and 6 can conveniently be used to summarize survival trends over time and differences between populations. However, there is still a need for conventional methods as for direct standardization, particularly if model-based results are not available. Model-based results may also be standardized for age, particularly when the empirical stratum-specific results have large standard errors and are thus very inaccurately estimated.
The standardization of survival proportions and particularly of relative survival ratios is much more complicated than that for incidence rates, because of the follow-up time dimension. The choice of method depends on the desired interpretation of the standardized ratio.
The traditional interpretation of a directly age standardized survival proportion is the survival that would be observed in the index population of cancer patients under study if its age structure were the same as that in a chosen standard population. The solution is to stratify the analysis by age and to take a weighted average of the age-specific survival curves as the age-standardized survival curve.
For example, due to demographic changes the number of old patients can increase by calendar time, whereas that of young patients does not. As a consequence, the old patients have, on average, shorter potential follow-up times than the young patients, and the more favorable survival of the young patients that can be followed longer will be predicted for the old patients after the time of censoring. The result is that the nonstandardized survival proportions will be higher than the age-standardized proportions even when the age distribution of the patients themselves is used as a standard. This is not a problem for colon cancer patients diagnosed in Finland in 1992–2002 (Table 7).
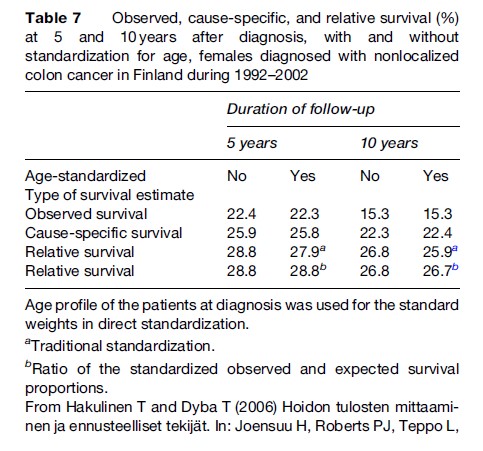
Two new methods of age standardization have been proposed by Brenner and Hakulinen (2003) and Brenner et al. (2004a). They both use time-varying weights and the newer of them does not presuppose any age stratification, which is very advantageous with smaller data sets. They are both consistent with the interpretation of relative survival as a ratio and not an estimate of cancer-specific survival probability.
Traditional standardization is required at least in longer-term follow-up for probability interpretation of the relative survival ratio even when there is no aim at comparisons between populations. Standardization controls both the effect of informative censoring and that of dependence between the causes of death. Standardization may also be needed for comparisons between patient populations, even when the age structures of the populations do not differ when there is informative censoring by age.
With the exception of one standardization method for the ratio interpretation of the relative survival ratio (Brenner et al., 2004a), all the age standardization methods presented depend on survival analyses stratified by age.
There are three different methods for calculation of the expected survival, and hence for the relative survival ratio, two proposed by Ederer et al. (1959, 1961) and one proposed by Hakulinen (1982). With stratified analyses, such as age standardization with the direct method, and nonstratified analyses up to 10 years of follow-up, it does not in practice matter which one of the methods is used. In longer-term follow-up for nonstratified and nonstandardized analyses, as well as in the standardization method of Brenner et al. (2004a), the method proposed by Hakulinen should be used because it gives the best control against informative censoring.
Concluding Practical Remarks
The relative survival ratio was proposed in 1950 by Berkson and Gage (Berkson and Gage, 1950). Ever since then, it has been used to monitor progress in population-based cancer survival, in the United States (the SEER project), in Europe (the EUROCARE project), in the world (the CONCORD project), and in many countries and cancer registration areas.
Socioeconomic differences generally determine the differences observed. Thus, for example, differences in relative survival could directly be related to the degree of deprivation of the population in England and Wales (Coleman et al., 1999), whereas differences within the Nordic countries by region and in Finland by social class were small (Dickman et al., 1997). Diagnostic determinants, e.g., numbers of lymph nodes examined, explain differences observed in relative survival between European countries and between Europe and the United States. Comparability of disease definition is still an issue in attempts to further elucidate the advantage that American patients tend to have in relative survival compared to European patients. To aid in the interpretation of relative survival, it is useful to examine the corresponding incidence and mortality rates (Dickman and Adami, 2006).
Estimates of relative survival do not depend on the certified cause of death, or on temporal trends or socio-geographic differences in death certification practices. This property of relative survival is extremely valuable for examining trends or differences in survival, in direct contrast with cause-specific survival proportions. In Finland, where death certification is accurate and where the cancer registry uses causes of death that, when necessary, have been corrected by the registry, relative and cause-specific survival analyses quite often give results that agree with each other. This provides further empirical support to the validity of relative survival as an estimate of cause-specific survival.
Nevertheless, interpretation of relative survival requires care. The general population group must be comparable with the patient group, particularly for analyses specific to region, social class, or degree of deprivation (Dickman et al., 1998).
Tumors are increasingly diagnosed early. As a result, survival times become longer even when there is no true improvement in the sense of postponement of death. Screening or particularly intense diagnostic activity, especially with prostatic cancer, may also lead to the diagnoses of cases that would not otherwise have been detected during the person’s lifetime. These cases, naturally, have favorable survival.
The methods of tumor diagnosis have changed over time. Consider the spectrum of disease progression in just two categories, localized and nonlocalized. In the past, when diagnostic facilities were less effective at detecting metastatic spread than they are now, cases described as localized would have included some that would now be classified, more correctly, as nonlocalized. As a result, newly diagnosed cases described as localized are now, on average, even less advanced than in the past. Similarly, nonlocalized cases now include some that are less advanced, and on average, nonlocalized cases are also less advanced than in the past. This was reflected in trends of survival from cancer of the oral cavity in females in Finland over the period 1953–74: Relative survival increased with time both for localized and for nonlocalized cases, whereas for all cases combined, there was no change (Hakulinen, 1983). This problem, called stage migration, does not just apply over time; it also affects stage-specific comparisons between populations.
Newly diagnosed patients included in cancer registries normally include a small proportion of cases for whom the diagnosis is based solely on a death certificate or autopsy. These cases are often excluded from survival analyses because the diagnosis is based on persons who are already dead, and for whom the date of diagnosis (and thus the duration of survival) is unknown. Some cancer registries, more than others, try to trace back the individuals of whom the first notification came through a death certificate. Sometimes this is successful. On average, these so-called death-certificate-initiated cases have poorer survival than the other patients. Thus, attempts to trace back (or follow-back) individuals may confer the cancer registry area a somewhat lower patient survival compared with another area where follow-back is not done.
On the other hand, a large proportion of cases in the cancer registry’s database with diagnosis based solely on a death certificate or autopsy is likely to be an indication that the registry may miss the recording of many nonfatal cases. This leads to survival that is unduly low. This has been one of the possible explanations behind the observation that the relative survival in England and Wales tends to be lower than in the other countries of Western Europe.
A high proportion of cases in which there is microscopic confirmation of the diagnosis is also important for unbiased survival analysis. If survival is high for patients with a cancer that is usually fatal, this may indicate poor quality of diagnostic information.
High survival figures are also observed when there are problems in the follow-up of patients. Relative survival is again a very good indicator of data quality: If relative survival ratios within successive intervals show that patients survive more favorably in the long term than the general population, there is reason to suspect incompleteness of the cancer registry’s follow-up procedures to ascertain the deaths of registered patients.
Population-based relative survival is a challenging concept to use. It can be used for many important purposes within cancer control, and its use has certainly led to changes in public health policy to improve the diagnosis, treatment, and care of cancer patients. But the use of relative survival requires considerable care and a sound understanding of the basic data, as well as of the underlying principles and methods.
Bibliography:
- Berkson J and Gage RP (1950) Calculation of survival rates for cancer. Proceedings of Staff Meetings of the Mayo Clinic 25: 270–286.
- Berrino F, Capocaccia R Coleman MP, et al. (eds.) (2003) Survival of Cancer Patients in Europe: the EUROCARE-3 study. Annals of Oncology 14(supplement 5): 1–155.
- Brenner H and Hakulinen T (2003) On crude and age-adjusted relative survival rates. Journal of Clinical Epidemiology 56: 1185–1191.
- Brenner H, Arndt V, Gefeller O, and Hakulinen T (2004a) An alternative approach to age adjustment of cancer survival rates. European Journal of Cancer 40: 2317–2322.
- Brenner H, Gefeller O, and Hakulinen T (2004b) Period analysis for ‘‘up-to-date’’ cancer survival data: Theory, empirical evaluation, computational realisation and applications. European Journal Cancer 40: 326–335.
- Coleman MP, Babb P, Damiecki P, et al. (1999) Cancer Survival Trends in England and Wales 1971–1995: Deprivation and NHS Region. London: The Stationery Office.
- DeAngelis R, Capocaccia R, Hakulinen T, So¨ derman B, and Verdecchia A (1999) Mixture models for cancer survival analysis: Application to population-based data with covariates. Statistics in Medicine 18: 441–454.
- Dickman P and Adami H-O (2006) Interpreting trends in cancer patient survival. Journal of Internal Medicine 260: 103–117.
- Dickman PW, Gibberd RW, and Hakulinen T (1997) Estimating potential savings in cancer deaths by eliminating regional and social class variation in cancer survival in the Nordic countries. Journal of Epidemiology and Community Health 51: 289–298.
- Dickman PW, Auvinen A, Voutilainen ET, and Hakulinen T (1998) Measuring social class differences in cancer patient survival: Is it necessary to control for social class differences in general population mortality? A Finnish population-based study. Journal of Epidemiology and Community Health 52: 1–8.
- Dickman PW, Sloggett A, Hills M, and Hakulinen T (2004) Regression models for relative survival. Statistics in Medicine 23: 51–64.
- Ederer F and Heise H (1959) Instructions to IBM 650 Programmes in Processing Survival Computations. Methodological Note No. 10. Bethesda, MD: End Results Evaluation Section. National Cancer Institute.
- Ederer F, Axtell LM, and Cutter SJ (1961) The Relative Survival Rate: A Statistical Methodology. UICC Technical Report Series. pp. 101–121. Bethesda, MD: National Cancer Institute
- Elandt-Johnson RC (1975) Definition of rates: Some remarks on their use and misuse. American Journal of Epidemiology 102: 267–271.
- Hakulinen T (1982) Cancer survival corrected for heterogeneity in patient withdrawal. Biometrics 38: 933–942.
- Hakulinen T (1983) A comparison of nationwide cancer survival statistics in Finland and Norway. World Health Statistics Quarterly 36: 35–46.
- Hakulinen T and Dyba T (2006) Hoidon tulosten mittaaminen ja ennusteelliset tekija¨ t. In: Joensuu H, Roberts PJ, Teppo L, and Tenhunen H (eds.) Syo¨pa¨taudit, 3rd edn., pp. 227–240. Helsinki Finland: Duodecim.
- Hakulinen T, Tenkanen L, Abeywickrama K, and Pa¨ iva¨ rinta L (1987) Testing equality of relative survival patterns based on aggregated data. Biometrics 43: 313–325.
- Remontet L, Bossard N, Belot A, Este` ve J and the French Network of Cancer Registries FRANCIM (2007) An overall strategy based on regression models to estimate relative survival and model the effects of prognostic factors in cancer survival studies. Statistics in Medicine 26: 2214–2228.
- Sant M, Allemani C, Capocaccia R, et al. (2003) Stage at diagnosis is a key explanation of differences in breast cancer survival across Europe. International Journal of Cancer 106: 416–422; 107: 1058 (erratum).
- Brenner H and Hakulinen T (2006) Up-to-date and precise estimates of cancer patient survival: Model-based period analysis. American Journal of Epidemiology 164: 689–696.
- Hakama M and Hakulinen T (1977) Estimating the expectation of life in cancer survival studies with incomplete follow-up information. Journal of Chronic Diseases 30: 585–597.
- Hakulinen T (1977) On long-term relative survival rates. Journal of Chronic Diseases 30: 431–443.
- Heina¨ vaara S, Teppo L, and Hakulinen T (2002) Cancer-specific survival of patients with multiple cancers: An application to patients with multiple breast cancers. Statistics in Medicine 21: 3183–3195.
- Parkin DM and Hakulinen T (1991) Analysis of survival. In: Jensen OM, Parkin DM, MacLennan R, Muir CS, and Skeet RG (eds.) Cancer Registration: Principles and Methods. IARC Scientific Publications No 95. pp. 159–176. Lyon, France: International Agency for Research on Cancer.
- Verdecchia A, DeAngelis G, and Capocaccia R (2002) Estimation and projections of cancer prevalence from cancer registry data. Statistics in Medicine 21: 3511–3526.
- http://www.eurocare.it/ – EUROCARE, Survival of cancer patients in Europe.
- http://unimed.mf.uni-lj.si/relsurv/index.pl – European Information Network-Ethics in Medicine and Biotechnology, Calculate your individual relative survival.
- https://seer.cancer.gov/ – National Cancer Institute, Surveillance, Epidemiology and End Results.
More Cancer Research Paper Examples:
- Bladder Cancer Research Paper
- Brain Tumours Research Paper
- Breast Cancer Research Paper
- Cancer and Senescence Research Paper
- Cancer Care Research Paper
- Cancer Economics Research Paper
- Cancer Epidemiology Research Paper
- Cancer Mortality Research Paper
- Cancer Screening Research Paper
- Colorectal Cancer Research Paper
- Diet and Cancer Research Paper
- Environmental Carcinogens Research Paper
- Esophageal Cancer Research Paper
- Gastric Cancer Research Paper
- Leukemia Research Paper
- Liver Cancer Research Paper
- Lung Cancer Research Paper
- Lymphoma Research Paper
- Meat Consumption and Cancer Research Paper
- Oral Cancer Research Paper
- Ovarian Cancer Research Paper
- Pancreatic Cancer Research Paper
- Pediatric Cancers Research Paper
- Prostate Cancer Research Paper
- Radiation Therapy Research Paper
- Testicular Cancer Research Paper
ORDER HIGH QUALITY CUSTOM PAPER

- Alzheimer's disease & dementia
- Arthritis & Rheumatism
- Attention deficit disorders
- Autism spectrum disorders
- Biomedical technology
- Diseases, Conditions, Syndromes
- Endocrinology & Metabolism
- Gastroenterology
- Gerontology & Geriatrics
- Health informatics
- Inflammatory disorders
- Medical economics
- Medical research
- Medications
- Neuroscience
- Obstetrics & gynaecology
- Oncology & Cancer
- Ophthalmology
- Overweight & Obesity
- Parkinson's & Movement disorders
- Psychology & Psychiatry
- Radiology & Imaging
- Sleep disorders
- Sports medicine & Kinesiology
- Vaccination
- Breast cancer
- Cardiovascular disease
- Chronic obstructive pulmonary disease
- Colon cancer
- Coronary artery disease
- Heart attack
- Heart disease
- High blood pressure
- Kidney disease
- Lung cancer
- Multiple sclerosis
- Myocardial infarction
- Ovarian cancer
- Post traumatic stress disorder
- Rheumatoid arthritis
- Schizophrenia
- Skin cancer
- Type 2 diabetes
- Full List »
share this!
April 24, 2024
This article has been reviewed according to Science X's editorial process and policies . Editors have highlighted the following attributes while ensuring the content's credibility:
fact-checked
peer-reviewed publication
trusted source
New potential avenues for cancer therapies through RNA-binding proteins
by King's College London
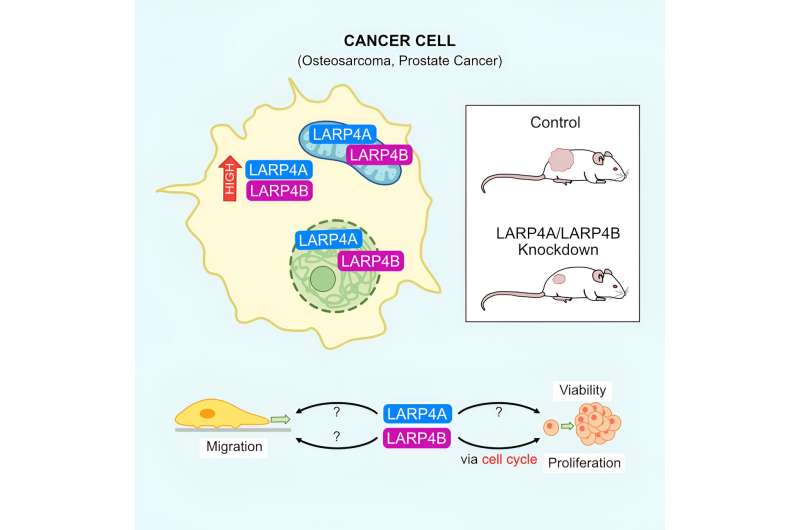
A new paper describes the role of two RNA-binding proteins in the development of sarcoma and carcinoma cancers, highlighting the important and emerging role of RNA-binding proteins in cancer research and offering a new avenue for therapeutic targets.
This research was a key component of the Ph.D. thesis of Jen Coleman, an MRC-DTP student who has since won a prize for best Ph.D. thesis. The findings are published in the journal iScience .
The traditional understanding of cancer is of a genetic disease driven largely by mutations to oncogenes, a loss of tumor suppressor genes, or a combination of both. Research in the last decade has also begun to look at RNA-binding proteins, which control what genes are expressed in health and disease.
Two RNA-binding proteins, called LARP4A and LARP4B, which have unclear roles in the development of cancer, was the focus of the new publication from the Conte and Grigoriadis labs at King's.
The authors look at their role in the growth of sarcoma and carcinoma cancers. Blocking LARP4A and LARP4B was found to prevent the formation of cancers tumors, showing that they promote cancer growth. Their research also found that they play different, distinct roles within cancer cells .
Their results highlight for the first time the differences between LARP4A and LARP4B, and their role in cancer development. The authors hope that this can provide new avenues for drugs to therapeutically target LARP4A and LARP4B, and more broadly to realize the importance of RNA-binding proteins in cancer research .
"RNA-binding proteins play crucial roles in almost all aspects of cellular biology and therefore it is not surprising that their dysregulation is linked to cancer. However, it has been difficult to put RNA binding proteins at the forefront of cancer biology. Our results show that two such proteins, LARP4A and LARP4B, promote cancer growth and spread that, if targeted, might contribute to cancer therapy ," said Professor Conte.
The next steps for Conte and Grigoriadis labs will be to focus on further understanding the precise mechanisms that LARP4A and LARP4B use to promote cancer growth, which can be used identify targets for therapies.
Explore further
Feedback to editors

Living at higher altitudes in India linked to increased risk of childhood stunting
3 hours ago

Study confirms effectiveness of bivalent COVID-19 vaccine
4 hours ago

How does aging start? Scientists explain how IgG antibodies are a driving factor

Study reveals emotional turmoil experienced after dog-theft is like that of a caregiver losing a child
5 hours ago

Targeting specific protein regions offers a new treatment approach in medulloblastoma

Study recommends exposing deaf children to sign language before and after cochlear implantation

Study reveals racial disparities in COVID-19 testing delays among health care workers
6 hours ago

Study reports new compound that halts replication of COVID by targeting 'Mac-1' protein in cell models

Shoulder surgeons should rethink a common practice, new study suggests

Study finds vitamin D alters mouse gut bacteria to give better cancer immunity
7 hours ago
Related Stories

New tools reveal how genes work and cells organize
Apr 3, 2024

New insights into the growth and spread of cancer cells
Mar 7, 2024

Scientists get sly, use deception to fight cancer
Apr 15, 2019

Tiny proteins found across the animal kingdom may play a key role in cancer spread
May 15, 2023

One protein is key to the spread of lung cancer: New study finds a way to stop it
Nov 27, 2023

How common genetic alterations cause kidney cancer
Aug 9, 2022
Recommended for you

Blocking gene may halt growth of breast cancer cells
8 hours ago

Researchers create an AI-powered digital imaging system to speed up cancer biopsy results
11 hours ago

Study reveals tai chi benefits for sleep quality in advanced lung cancer patients
12 hours ago
Let us know if there is a problem with our content
Use this form if you have come across a typo, inaccuracy or would like to send an edit request for the content on this page. For general inquiries, please use our contact form . For general feedback, use the public comments section below (please adhere to guidelines ).
Please select the most appropriate category to facilitate processing of your request
Thank you for taking time to provide your feedback to the editors.
Your feedback is important to us. However, we do not guarantee individual replies due to the high volume of messages.
E-mail the story
Your email address is used only to let the recipient know who sent the email. Neither your address nor the recipient's address will be used for any other purpose. The information you enter will appear in your e-mail message and is not retained by Medical Xpress in any form.
Newsletter sign up
Get weekly and/or daily updates delivered to your inbox. You can unsubscribe at any time and we'll never share your details to third parties.
More information Privacy policy
Donate and enjoy an ad-free experience
We keep our content available to everyone. Consider supporting Science X's mission by getting a premium account.
E-mail newsletter
- Seeking Patient Care?
Home / News / Research and Innovation / Cancer
Death Rates from Breast Cancer Linked to Neighborhood

- Breast cancer patients from disadvantaged neighborhoods have a higher mortality rate from the disease than patients from advantaged neighborhoods, according to a study led by Sylvester researchers.
- The disparity was observed after controlling for multiple known risk factors.
- Unknown neighborhood-linked factors contribute to differences in breast cancer outcomes, concluded the researchers.
Breast cancer patients from disadvantaged neighborhoods face a higher risk of dying than patients from advantaged, wealthier neighborhoods, according to a new study .
The data from the nationwide study, released April 18, dovetails with similar results from breast cancer patients in the Miami area, published last year .
“Even after controlling for multiple other variables, women living in disadvantaged neighborhoods still have disparate survival outcomes,“ said Neha Goel, M.D., M.P.H., a surgeon-scientist and social epidemiologist at Sylvester Comprehensive Cancer Center at the University of Miami Miller School of Medicine, who led both studies.
Dr. Goel, also an assistant professor in the Miller School’s DeWitt Daughtry Family Department of Surgery, and her colleagues are now conducting research to find out what accounts for the differences in breast cancer survival.
Deep Data on Breast Cancer Survival Rates
The new study on survival rates examined de-identified data from 350,824 breast cancer patients, collected by the National Cancer Institute (NCI) from 2016 to 2018. The data captured information such as tumor treatment regimens and access to health insurance, as well as socioeconomic measurements from patient neighborhoods, at the census-tract level.
Neighborhood measurements included factors such as:
• Median household income
• House value
• Median rent
• Education index
• Percentage of residents who were working class or unemployed
The dataset was pulled from 21 geographic areas, representing 36.7% of the U.S. population.
Dr. Goel and her team found that neighborhood disparities remained even after accounting for race and other factors known to affect outcomes, including access to health insurance, stage of cancer at diagnosis and type of treatment.

After crunching the numbers and controlling for known factors, they report that patients from the most disadvantaged neighborhoods had about a 40% higher risk of dying from the disease than patients from the most advantaged neighborhoods.
The new study was published in the Journal of the American Medical Association (JAMA) Network Open . While other studies have shown that neighborhood disadvantage can affect cancer outcomes, this study covered a larger geographic area and assessed a broader patient population, said Dr. Goel.
The new study also highlights the power of giant datasets like the NCI’s.
“They allow us to look closer at findings we first identify locally and validate them nationally,” said Dr. Goel.
That’s just what Dr. Goel did, tapping into the NCI database after first studying populations in the Miami area and reporting neighborhood-related differences in breast cancer mortality. That earlier study was also published in JAMA Network Open .
Unknown Factors Impacting Cancer Outcomes
The new findings have limitations, caution the researchers. It’s possible that the NCI dataset didn’t capture all characteristics that can affect outcomes, they note. Other individual, tumor and treatment measures might still come into play, such as trust in health care practitioners.
Dr. Goel thinks there may also be something about where people live that affects the outcome.
“Even after controlling for all these factors that we know are associated with disparities in breast cancer survival, there’s a residual disparity,” said Dr. Goel. “That disparity is associated with neighborhood-level disadvantage.”
Last year’s study on Miami-area neighborhoods ruled out a major contribution from air pollution. But there may be other environmental factors, such as exposure to toxins from contaminated sites that could play a role, she speculated.
Dr. Goel is investigating another potential factor: higher levels of stress in people living in disadvantaged neighborhoods. After all, poverty is associated with elevated stress levels, and some studies have hinted at a link between stress and breast cancer biology.
In their new research, Dr. Goel and her colleagues are examining genome-level changes in tumor biology that correlate with stress and other factors and linking them with neighborhood data.
“My lab is taking a ‘zip code to genomic code’ approach to understand how where one lives can impact tumor biology and ultimately breast cancer outcomes,” said Dr. Goel.
Tags: breast cancer , Dr. Neha Goel , Health Equity , Sylvester Comprehensive Cancer Center
Sylvester Team Leads Talks on Cancer Disparities for People of African Descent
Sylvester Comprehensive Cancer Center researchers presented cancer findings on disparities and outcomes among populations of African descent.
Fostering Collaboration and Innovation in Medical Education
The University of Miami Miller School of Medicine played a significant role at Lead Serve Lead 2023: The AAMC Annual Meeting.
Dr. Nima Sharifi Pens JAMA Commentary on Prostate Cancer Variant His Team Identified
Nima Sharifi, M.D., coauthored a JAMA Open Network commentary discussing the implications of the HSD3B1 gene variant.
- Clinical Care
- Community Outreach
- Grants and Awards
- Medical Education
- Research and Innovation
- Infectious Diseases
- Neurosciences
- Ophthalmology
- Orthopaedics
- Otolaryngology
- Philanthropy
- Cardiovascular Medicine
- Digestive Health and Liver Diseases
Share this Story
This article was printed from The Miller School of Medicine Medical News at the following URL: https://news.med.miami.edu/jama-open-commentary-on-prostate-cancer-gene/
Copyright © 2024 University of Miami Health System
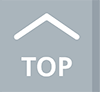
Thank you for visiting nature.com. You are using a browser version with limited support for CSS. To obtain the best experience, we recommend you use a more up to date browser (or turn off compatibility mode in Internet Explorer). In the meantime, to ensure continued support, we are displaying the site without styles and JavaScript.
- View all journals
- Explore content
- About the journal
- Publish with us
- Sign up for alerts
- Review Article
- Published: 21 July 2023
The global burden of lung cancer: current status and future trends
- Amanda Leiter ORCID: orcid.org/0000-0001-9072-5512 1 ,
- Rajwanth R. Veluswamy 2 , 4 &
- Juan P. Wisnivesky 3
Nature Reviews Clinical Oncology volume 20 , pages 624–639 ( 2023 ) Cite this article
9560 Accesses
67 Citations
16 Altmetric
Metrics details
- Cancer epidemiology
- Health policy
- Non-small-cell lung cancer
- Public health
Lung cancer is the leading cause of cancer-related death worldwide. However, lung cancer incidence and mortality rates differ substantially across the world, reflecting varying patterns of tobacco smoking, exposure to environmental risk factors and genetics. Tobacco smoking is the leading risk factor for lung cancer. Lung cancer incidence largely reflects trends in smoking patterns, which generally vary by sex and economic development. For this reason, tobacco control campaigns are a central part of global strategies designed to reduce lung cancer mortality. Environmental and occupational lung cancer risk factors, such as unprocessed biomass fuels, asbestos, arsenic and radon, can also contribute to lung cancer incidence in certain parts of the world. Over the past decade, large-cohort clinical studies have established that low-dose CT screening reduces lung cancer mortality, largely owing to increased diagnosis and treatment at earlier disease stages. These data have led to recommendations that individuals with a high risk of lung cancer undergo screening in several economically developed countries and increased implementation of screening worldwide. In this Review, we provide an overview of the global epidemiology of lung cancer. Lung cancer risk factors and global risk reduction efforts are also discussed. Finally, we summarize lung cancer screening policies and their implementation worldwide.
Lung cancer is the leading cause of cancer death globally, with incidence and mortality trends varying greatly by country and largely reflecting differences in tobacco smoking trends.
Cigarette smoking is the most prevalent lung cancer risk factor, although environmental exposures, such as biomass fuels, asbestos, arsenic and radon, are all important lung factor risk factors with levels of exposure that vary widely across the globe.
Lung cancer incidence and mortality rates are highest in economically developed countries in which tobacco smoking peaked several decades ago, although these rates have mostly now peaked and are declining.
Reductions in lung cancer mortality in economically developed countries reflect decreased incidence (mirroring declines in tobacco smoking) and improvements in treatment of patients with advanced-stage disease, including immunotherapies and targeted therapies.
In low-income and middle-income countries at the later stages of the tobacco epidemic, both lung cancer incidence and mortality are increasing, thus highlighting the importance of tobacco mitigation policies for reducing the global burden of lung cancer.
Low-dose CT-based lung cancer screening reduces lung cancer mortality, although adoption of lung cancer screening programmes has been slow, with limited uptake compared with other cancer screening programmes.
This is a preview of subscription content, access via your institution
Access options
Access Nature and 54 other Nature Portfolio journals
Get Nature+, our best-value online-access subscription
24,99 € / 30 days
cancel any time
Subscribe to this journal
Receive 12 print issues and online access
195,33 € per year
only 16,28 € per issue
Buy this article
- Purchase on Springer Link
- Instant access to full article PDF
Prices may be subject to local taxes which are calculated during checkout
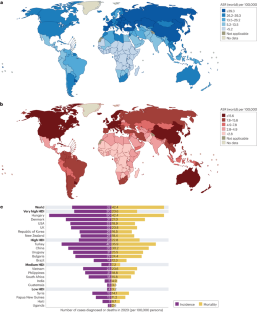
Similar content being viewed by others
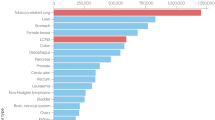
Lung cancer in patients who have never smoked — an emerging disease
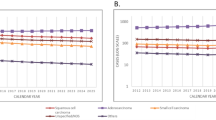
Trends in lung cancer incidence by age, sex and histology from 2012 to 2025 in Catalonia (Spain)
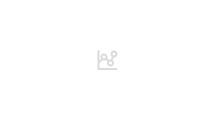
The fraction of lung cancer attributable to smoking in the Norwegian Women and Cancer (NOWAC) Study
Sung, H. et al. Global cancer statistics 2020: GLOBOCAN estimates of incidence and mortality worldwide for 36 cancers in 185 countries. CA Cancer J. Clin. 71 , 209–249 (2021).
Article PubMed Google Scholar
Travis, W. D., Brambilla, E., Burke, A. P., Marx, A. & Nicholson, A. G. WHO Classification of Tumours of the Lung, Pleura, Thymus and Heart (IARC, 2015).
Schabath, M. B. & Cote, M. L. Cancer progress and priorities: lung cancer. Cancer Epidemiol. Biomark. Prev. 28 , 1563–1579 (2019).
Article Google Scholar
Lortet-Tieulent, J. et al. International trends in lung cancer incidence by histological subtype: adenocarcinoma stabilizing in men but still increasing in women. Lung Cancer 84 , 13–22 (2014).
Article CAS PubMed Google Scholar
Wakelee, H. A. et al. Lung cancer incidence in never smokers. J. Clin. Oncol. 25 , 472–478 (2007).
United Nations Development Programme. Human development report 2021-22. UNDP http://report.hdr.undp.org (2022).
Jemal, A., Ma, J., Rosenberg, P. S., Siegel, R. & Anderson, W. F. Increasing lung cancer death rates among young women in southern and midwestern states. J. Clin. Oncol. 30 , 2739–2744 (2012).
Article PubMed PubMed Central Google Scholar
Jemal, A. et al. Higher lung cancer incidence in young women than young men in the united states. N. Engl. J. Med. 378 , 1999–2009 (2018).
Islami, F. et al. Proportion and number of cancer cases and deaths attributable to potentially modifiable risk factors in the United states. CA Cancer J. Clin. 68 , 31–54 (2018).
Siegel, D. A., Fedewa, S. A., Henley, S. J., Pollack, L. A. & Jemal, A. Proportion of never smokers among men and women with lung cancer in 7 US states. JAMA Oncol. 7 , 302–304 (2021).
Sakoda, L. C. et al. Trends in smoking-specific lung cancer incidence rates within a US integrated health system, 2007-2018. Chest https://doi.org/10.1016/j.chest.2023.03.016 (2023).
Pelosof, L. et al. Proportion of never-smoker non-small cell lung cancer patients at three diverse institutions. J. Natl Cancer Inst. https://doi.org/10.1093/jnci/djw295 (2017).
Meza, R., Meernik, C., Jeon, J. & Cote, M. L. Lung cancer incidence trends by gender, race and histology in the United States, 1973-2010. PLoS ONE 10 , e0121323 (2015).
Haiman, C. A. et al. Ethnic and racial differences in the smoking-related risk of lung cancer. N. Engl. J. Med. 354 , 333–342 (2006).
Murphy, S. E. Biochemistry of nicotine metabolism and its relevance to lung cancer. J. Biol. Chem. 296 , 100722 (2021).
Article CAS PubMed PubMed Central Google Scholar
Siegel, R. L., Miller, K. D., Fuchs, H. E. & Jemal, A. Cancer statistics, 2021. CA Cancer J. Clin. 71 , 7–33 (2021).
Siegel, R. L., Miller, K. D., Fuchs, H. E. & Jemal, A. Cancer statistics, 2022. CA Cancer J. Clin. 72 , 7–33 (2022).
Howlader, N. et al. The effect of advances in lung-cancer treatment on population mortality. N. Engl. J. Med. 383 , 640–649 (2020).
Singh, G. K. & Jemal, A. Socioeconomic and racial/ethnic disparities in cancer mortality, incidence, and survival in the United States, 1950-2014: over six decades of changing patterns and widening inequalities. J. Environ. Public Health 2017 , 2819372 (2017).
Blom, E. F., Ten Haaf, K., Arenberg, D. A. & de Koning, H. J. Disparities in receiving guideline-concordant treatment for lung cancer in the United States. Ann. Am. Thorac. Soc. 17 , 186–194 (2020).
Sineshaw, H. M. et al. County-level variations in receipt of surgery for early-stage non-small cell lung cancer in the United States. Chest 157 , 212–222 (2020).
GBD 2019 Tobacco Collaborators. Spatial, temporal, and demographic patterns in prevalence of smoking tobacco use and attributable disease burden in 204 countries and territories, 1990-2019: a systematic analysis from the Global Burden of Disease Study 2019. Lancet 397 , 2337–2360 (2021).
Allemani, C. et al. Global surveillance of trends in cancer survival 2000-14 (CONCORD-3): analysis of individual records for 37 513 025 patients diagnosed with one of 18 cancers from 322 population-based registries in 71 countries. Lancet 391 , 1023–1075 (2018).
Jani, C. et al. Lung cancer mortality in Europe and the USA between 2000 and 2017: an observational analysis. ERJ Open. Res. https://doi.org/10.1183/23120541.00311-2021 (2021).
Malvezzi, M. et al. European cancer mortality predictions for the year 2023 with focus on lung cancer. Ann. Oncol. 34 , 410–419 (2023).
Carioli, G. et al. European cancer mortality predictions for the year 2020 with a focus on prostate cancer. Ann. Oncol. 31 , 650–658 (2020).
Alves, L., Bastos, J. & Lunet, N. Trends in lung cancer mortality in Portugal (1955-2005). Rev. Port. Pneumol. 15 , 575–587 (2009).
Martínez, C., Guydish, J., Robinson, G., Martínez-Sánchez, J. M. & Fernández, E. Assessment of the smoke-free outdoor regulation in the WHO European region. Prev. Med. 64 , 37–40 (2014).
Forsea, A. M. Cancer registries in Europe – going forward is the only option. Ecancermedicalscience 10 , 641 (2016).
Cho, B. C. et al. Genomic landscape of non-small cell lung cancer (NSCLC) in East Asia using circulating tumor DNA (ctDNA) in clinical practice. Curr. Oncol. 29 , 2154–2164 (2022).
Mathias, C. et al. Lung cancer in Brazil. J. Thorac. Oncol. 15 , 170–175 (2020).
Souza, M. C., Vasconcelos, A. G. & Cruz, O. G. Trends in lung cancer mortality in Brazil from the 1980s into the early 21st century: age-period-cohort analysis. Cad. Saude Publica 28 , 21–30 (2012).
Jiang, D. et al. Trends in cancer mortality in China from 2004 to 2018: a nationwide longitudinal study. Cancer Commun. 41 , 1024–1036 (2021).
Parascandola, M. & Xiao, L. Tobacco and the lung cancer epidemic in China. Transl. Lung Cancer Res. 8 , S21–S30 (2019).
Hosgood, H. D. 3rd et al. In-home coal and wood use and lung cancer risk: a pooled analysis of the International Lung Cancer Consortium. Env. Health Perspect. 118 , 1743–1747 (2010).
Article CAS Google Scholar
Kurmi, O. P., Arya, P. H., Lam, K. B., Sorahan, T. & Ayres, J. G. Lung cancer risk and solid fuel smoke exposure: a systematic review and meta-analysis. Eur. Respir. J. 40 , 1228–1237 (2012).
Qiu, A. Y., Leng, S., McCormack, M., Peden, D. B. & Sood, A. Lung effects of household air pollution. J. Allergy Clin. Immunol. Pract. 10 , 2807–2819 (2022).
Zhang, M. et al. Trends in smoking prevalence in urban and rural China, 2007 to 2018: findings from 5 consecutive nationally representative cross-sectional surveys. PLoS Med. 19 , e1004064 (2022).
Pineros, M., Znaor, A., Mery, L. & Bray, F. A global cancer surveillance framework within noncommunicable disease surveillance: making the case for population-based cancer registries. Epidemiol. Rev. 39 , 161–169 (2017).
Wei, W. et al. Cancer registration in China and its role in cancer prevention and control. Lancet Oncol. 21 , e342–e349 (2020).
Mathur, P. et al. Cancer statistics, 2020: report from National Cancer Registry Programme, India. JCO Glob. Oncol. 6 , 1063–1075 (2020).
Nath, A., Sathishkumar, K., Das, P., Sudarshan, K. L. & Mathur, P. A clinicoepidemiological profile of lung cancers in India – results from the National Cancer Registry Programme. Indian J. Med. Res. 155 , 264–272 (2022).
Singh, N. et al. Lung cancer in India. J. Thorac. Oncol. 16 , 1250–1266 (2021).
Kaur, H. et al. Evolving epidemiology of lung cancer in India: reducing non-small cell lung cancer–not otherwise specified and quantifying tobacco smoke exposure are the key. Indian J. Cancer 54 , 285–290 (2017).
Mohan, A. et al. Clinical profile of lung cancer in North India: a 10-year analysis of 1862 patients from a tertiary care center. Lung India 37 , 190–197 (2020).
Shaikh, R., Janssen, F. & Vogt, T. The progression of the tobacco epidemic in India on the national and regional level, 1998-2016. BMC Public Health 22 , 317 (2022).
India State-Level Disease Burden Initiative Cancer Collaborators. The burden of cancers and their variations across the states of India: the Global Burden of Disease Study 1990–2016. Lancet Oncol. 19 , 1289–1306 (2022).
Google Scholar
& Piñeros, M. et al. An updated profile of the cancer burden, patterns and trends in Latin America and the Caribbean. Lancet Reg. Health Am. 13 , 100294 (2022).
PubMed PubMed Central Google Scholar
Raez, L. E. et al. The burden of lung cancer in Latin-America and challenges in the access to genomic profiling, immunotherapy and targeted treatments. Lung Cancer 119 , 7–13 (2018).
Pakzad, R., Mohammadian-Hafshejani, A., Ghoncheh, M., Pakzad, I. & Salehiniya, H. The incidence and mortality of lung cancer and their relationship to development in Asia. Transl. Lung Cancer Res. 4 , 763–774 (2015).
Hamdi, Y. et al. Cancer in Africa: the untold story. Front. Oncol. 11 , 650117 (2021).
Centers for Disease Control and Prevention. What are the risk factors for lung cancer? CDC https://www.cdc.gov/cancer/lung/basic_info/risk_factors.htm (2022).
Peto, R. et al. Smoking, smoking cessation, and lung cancer in the UK since 1950: combination of national statistics with two case-control studies. Br. Med. J. 321 , 323–329 (2000).
Boffetta, P. et al. Cigar and pipe smoking and lung cancer risk: a multicenter study from Europe. J. Natl Cancer Inst. 91 , 697–701 (1999).
Pednekar, M. S., Gupta, P. C., Yeole, B. B. & Hébert, J. R. Association of tobacco habits, including bidi smoking, with overall and site-specific cancer incidence: results from the Mumbai cohort study. Cancer Causes Control. 22 , 859–868 (2011).
Proctor, R. N. The history of the discovery of the cigarette–lung cancer link: evidentiary traditions, corporate denial, global toll. Tob. Control. 21 , 87–91 (2012).
Doll, R. & Hill, A. B. The mortality of doctors in relation to their smoking habits. Br. Med. J. 1 , 1451 (1954).
US Department of Health, Education, and Welfare. Smoking and health: report of the Advisory Committee to the Surgeon General of the Public Health Service (US Public Health Service, 1964).
Brawley, O. W., Glynn, T. J., Khuri, F. R., Wender, R. C. & Seffrin, J. R. The first Surgeon General’s report on smoking and health: the 50th anniversary. CA Cancer J. Clin. 64 , 5–8 (2014).
WHO Framework Convention on Tobacco Control. 2021 global progress report on implementation of the WHO Framework Convention on Tobacco Control (WHO FCTC, 2022).
Oberg, M., Jaakkola, M. S., Woodward, A., Peruga, A. & Prüss-Ustün, A. Worldwide burden of disease from exposure to second-hand smoke: a retrospective analysis of data from 192 countries. Lancet 377 , 139–146 (2011).
Office on Smoking and Health. The Health Consequences of Involuntary Exposure to Tobacco Smoke: a Report of the Surgeon General (Centers for Disease Control and Prevention, 2006).
Yousuf, H. et al. Estimated worldwide mortality attributed to secondhand tobacco smoke exposure, 1990-2016. JAMA Netw. Open 3 , e201177 (2020).
Bracken-Clarke, D. et al. Vaping and lung cancer – a review of current data and recommendations. Lung Cancer 153 , 11–20 (2021).
Centers for Disease Control and Prevention. Trends in tobacco use among youth. CDC https://www.cdc.gov/tobacco/data_statistics/fact_sheets/fast_facts/trends-in-tobacco-use-among-youth.html (2022).
Sindelar, J. L. Regulating vaping – policies, possibilities, and perils. N. Engl. J. Med. 382 , e54 (2020).
Campus, B., Fafard, P., St Pierre, J. & Hoffman, S. J. Comparing the regulation and incentivization of e-cigarettes across 97 countries. Soc. Sci. Med. 291 , 114187 (2021).
Bruce, N. et al. Does household use of biomass fuel cause lung cancer? A systematic review and evaluation of the evidence for the GBD 2010 study. Thorax 70 , 433–441 (2015).
Woolley, K. E. et al. Effectiveness of interventions to reduce household air pollution from solid biomass fuels and improve maternal and child health outcomes in low- and middle-income countries: a systematic review protocol. Syst. Rev. 10 , 33 (2021).
Johnston, F. H. et al. Estimated global mortality attributable to smoke from landscape fires. Environ. Health Perspect. 120 , 695–701 (2012).
Korsiak, J. et al. Long-term exposure to wildfires and cancer incidence in Canada: a population-based observational cohort study. Lancet Planet. Health 6 , e400–e409 (2022).
Rousseau, M.-C., Straif, K. & Siemiatycki, J. IARC carcinogen update. Environ. Health Perspect. 113 , A580–A581 (2005).
Yuan, T., Zhang, H., Chen, B., Zhang, H. & Tao, S. Association between lung cancer risk and inorganic arsenic concentration in drinking water: a dose-response meta-analysis. Toxicol. Res. 7 , 1257–1266 (2018).
Shankar, S., Shanker, U. & Shikha Arsenic contamination of groundwater: a review of sources, prevalence, health risks, and strategies for mitigation. Sci. World J. 2014 , 304524 (2014).
D’Ippoliti, D. et al. Arsenic in drinking water and mortality for cancer and chronic diseases in central Italy, 1990-2010. PLoS ONE 10 , e0138182 (2015).
Ferdosi, H. et al. Arsenic in drinking water and lung cancer mortality in the United States: an analysis based on US counties and 30 years of observation (1950-1979). J. Environ. Public Health 2016 , 1602929 (2016).
Ferreccio, C. et al. Arsenic, tobacco smoke, and occupation: associations of multiple agents with lung and bladder cancer. Epidemiol 24 , 898–905 (2013).
Wu, M. M., Kuo, T. L., Hwang, Y. H. & Chen, C. J. Dose-response relation between arsenic concentration in well water and mortality from cancers and vascular diseases. Am. J. Epidemiol. 130 , 1123–1132 (1989).
Oberoi, S., Barchowsky, A. & Wu, F. The global burden of disease for skin, lung, and bladder cancer caused by arsenic in food. Cancer Epidemiol. Biomark. Prev. 23 , 1187–1194 (2014).
UNICEF. Arsenic Primer: Guidance on the Investigation and Mitigation of Arsenic Contamination (UNICEF, 2018).
Turner, M. C. et al. Radon and lung cancer in the American Cancer Society Cohort. Cancer Epidemiol. Biomark. Prev. 20 , 438–448 (2011).
Ngoc, L. T. N., Park, D. & Lee, Y. C. Human health impacts of residential radon exposure: updated systematic review and meta-analysis of case-control studies. Int. J. Environ. Res. Public Health 20 , 97 (2012).
Shan, X. et al. A global burden assessment of lung cancer attributed to residential radon exposure during 1990-2019. Indoor Air 32 , e13120 (2022).
World Health Organization. WHO Handbook on Indoor Radon: a Public Health Perspective (WHO, 2009).
US Environmental Protection Agency. The national radon action plan – a strategy for saving lives. EPA https://www.epa.gov/radon/national-radon-action-plan-strategy-saving-lives (2023).
IARC Working Group on the Evaluation of Carcinogenic Risks to Humans. A Review of Human Carcinogens. Part F: Chemical Agents and Related Occupations (IARC, 2012).
Pira, E., Donato, F., Maida, L. & Discalzi, G. Exposure to asbestos: past, present and future. J. Thorac. Dis. 10 , S237–S245 (2018).
Villeneuve, P. J., Parent, M., Harris, S. A. & Johnson, K. C. Occupational exposure to asbestos and lung cancer in men: evidence from a population-based case-control study in eight Canadian provinces. BMC Cancer 12 , 595 (2012).
Lash, T. L., Crouch, E. A. & Green, L. C. A meta-analysis of the relation between cumulative exposure to asbestos and relative risk of lung cancer. Occup. Environ. Med. 54 , 254–263 (1997).
Nelson, H. H. & Kelsey, K. T. The molecular epidemiology of asbestos and tobacco in lung cancer. Oncogene 21 , 7284–7288 (2002).
Markowitz, S. B., Levin, S. M., Miller, A. & Morabia, A. Asbestos, asbestosis, smoking, and lung cancer. New findings from the North American Insulator cohort. Am. J. Respir. Crit. Care Med. 188 , 90–96 (2013).
Mossman B. T., Gualtieri A. F. in Occupational Cancers (eds.Anttila S. & Boffetta P.) 239–256 (Springer, 2020).
Thives, L. P., Ghisi, E., Thives Júnior, J. J. & Vieira, A. S. Is asbestos still a problem in the world? A current review. J. Environ. Manag. 319 , 115716 (2022).
Benbrahim-Tallaa, L. et al. Carcinogenicity of diesel-engine and gasoline-engine exhausts and some nitroarenes. Lancet Oncol. 13 , 663–664 (2012).
Ge, C. et al. Diesel engine exhaust exposure, smoking, and lung cancer subtype risks. a pooled exposure-response analysis of 14 case-control studies. Am. J. Respir. Crit. Care Med. 202 , 402–411 (2020).
Garshick, E. et al. Lung cancer and elemental carbon exposure in trucking industry workers. Env. Health Perspect. 120 , 1301–1306 (2012).
Silverman, D. T. et al. The diesel exhaust in miners study: a nested case-control study of lung cancer and diesel exhaust. J. Natl Cancer Inst. 104 , 855–868 (2012).
Vermeulen, R. et al. Exposure-response estimates for diesel engine exhaust and lung cancer mortality based on data from three occupational cohorts. Environ. Health Perspect. 122 , 172–177 (2024).
Young, R. P. et al. COPD prevalence is increased in lung cancer, independent of age, sex and smoking history. Eur. Respir. J. 34 , 380–386 (2009).
de Torres, J. P. et al. Lung cancer in patients with chronic obstructive pulmonary disease-incidence and predicting factors. Am. J. Respir. Crit. Care Med. 184 , 913–919 (2011).
Durham, A. L. & Adcock, I. M. The relationship between COPD and lung cancer. Lung Cancer 90 , 121–127 (2015).
Young, R. P. et al. Individual and cumulative effects of GWAS susceptibility loci in lung cancer: associations after sub-phenotyping for COPD. PLoS ONE 6 , e16476 (2011).
Saber Cherif, L. et al. The nicotinic receptor polymorphism rs16969968 is associated with airway remodeling and inflammatory dysregulation in COPD patients. Cells 11 , 2937 (2022).
Sigel, K., Makinson, A. & Thaler, J. Lung cancer in persons with HIV. Curr. Opin. Hiv. AIDS 12 , 31–38 (2017).
Shiels, M. S., Cole, S. R., Mehta, S. H. & Kirk, G. D. Lung cancer incidence and mortality among HIV-infected and HIV-uninfected injection drug users. J. Acquir. Immune Defic. Syndr. 55 , 510–515 (2010).
Sigel, K. et al. HIV as an independent risk factor for incident lung cancer. AIDS 26 , 1017–1025 (2012).
Engels, E. A. et al. Elevated incidence of lung cancer among HIV-infected individuals. J. Clin. Oncol. 24 , 1383–1388 (2006).
Chaturvedi, A. K. et al. Elevated risk of lung cancer among people with AIDS. AIDS 21 , 207–213 (2007).
Kirk, G. D. et al. HIV infection is associated with an increased risk for lung cancer, independent of smoking. Clin. Infect. Dis. 45 , 103–110 (2007).
Parker, M. S., Leveno, D. M., Campbell, T. J., Worrell, J. A. & Carozza, S. E. AIDS-related bronchogenic carcinoma: fact or fiction? Chest 113 , 154–161 (1998).
Patel, P. et al. Incidence of types of cancer among HIV-infected persons compared with the general population in the United States, 1992-2003. Ann. Intern. Med. 148 , 728–736 (2008).
Winstone, T. A., Man, S. F., Hull, M., Montaner, J. S. & Sin, D. D. Epidemic of lung cancer in patients with HIV infection. Chest 143 , 305–314 (2013).
Hessol, N. A. et al. Lung cancer incidence and survival among HIV-infected and uninfected women and men. AIDS 29 , 1183–1193 (2015).
Bearz, A. et al. Lung cancer in HIV positive patients: the GICAT experience. Eur. Rev. Med. Pharmacol. Sci. 18 , 500–508 (2014).
CAS PubMed Google Scholar
O’Connor, E. A. et al. Vitamin and mineral supplements for the primary prevention of cardiovascular disease and cancer: updated evidence report and systematic review for the US Preventive Services Task Force. J. Am. Med. Assoc. 327 , 2334–2347 (2022).
Wei, X. et al. Diet and risk of incident lung cancer: a large prospective cohort study in UK Biobank. Am. J. Clin. Nutr. 114 , 2043–2051 (2021).
Xue, X. J. et al. Red and processed meat consumption and the risk of lung cancer: a dose-response meta-analysis of 33 published studies. Int. J. Clin. Exp. Med. 7 , 1542–1553 (2014).
Vieira, A. R. et al. Fruits, vegetables and lung cancer risk: a systematic review and meta-analysis. Ann. Oncol. 27 , 81–96 (2016).
Amararathna, M., Johnston, M. R. & Rupasinghe, H. P. V. Plant polyphenols as chemopreventive agents for lung cancer. Int. J. Mol. Sci. 17 , 1352 (2016).
Alsharairi, N. A. The effects of dietary supplements on asthma and lung cancer risk in smokers and non-smokers: a review of the literature. Nutrients 11 , 725 (2016).
The Lung Cancer Cohort Consortium.Circulating folate, vitamin B6, and methionine in relation to lung cancer risk in the Lung Cancer Cohort Consortium (LC3). J. Natl Cancer Inst. 110 , 57–67 (2018).
Slatore, C. G., Littman, A. J., Au, D. H., Satia, J. A. & White, E. Long-term use of supplemental multivitamins, vitamin C, vitamin E, and folate does not reduce the risk of lung cancer. Am. J. Respir. Crit. Care Med. 177 , 524–530 (2008).
Verbeek, J. H. et al. An approach to quantifying the potential importance of residual confounding in systematic reviews of observational studies: a GRADE concept paper. Environ. Int. 157 , 106868 (2021).
Cortés-Jofré, M., Rueda, J. R., Asenjo-Lobos, C., Madrid, E. & Bonfill Cosp, X. Drugs for preventing lung cancer in healthy people. Cochrane Database Syst. Rev. 3 , Cd002141 (2020).
PubMed Google Scholar
The Alpha-Tocopherol Beta Carotene Cancer Prevention Study Group. The effect of vitamin E and beta carotene on the incidence of lung cancer and other cancers in male smokers. N. Engl. J. Med. 330 , 1029–1035 (1994).
Omenn, G. S. et al. Effects of a combination of beta carotene and vitamin A on lung cancer and cardiovascular disease. N. Engl. J. Med. 334 , 1150–1155 (1996).
Pearson-Stuttard, J. et al. Worldwide burden of cancer attributable to diabetes and high body-mass index: a comparative risk assessment. Lancet Diabetes Endocrinol. 6 , e6–e15 (2018).
Lennon, H., Sperrin, M., Badrick, E. & Renehan, A. G. The obesity paradox in cancer: a review. Curr. Oncol. Rep. 18 , 56 (2016).
Duan, P. et al. Body mass index and risk of lung cancer: systematic review and dose-response meta-analysis. Sci. Rep. 5 , 16938 (2015).
Ardesch, F. H. et al. The obesity paradox in lung cancer: associations with body size versus body shape. Front. Oncol. 10 , 591110 (2020).
Yu, D. et al. Overall and central obesity and risk of lung cancer: a pooled analysis. J. Natl Cancer Inst. 110 , 831–842 (2018).
Leiter, A. et al. Assessing the association of diabetes with lung cancer risk. Transl. Lung Cancer Res. 10 , 4200–4208 (2021).
Yi, Z. H. et al. Association between diabetes mellitus and lung cancer: meta-analysis. Eur. J. Clin. Invest. 50 , e13332 (2020).
Carreras-Torres, R. et al. Obesity, metabolic factors and risk of different histological types of lung cancer: a Mendelian randomization study. PLoS ONE 12 , e0177875 (2017).
Dziadziuszko, R., Camidge, D. R. & Hirsch, F. R. The insulin-like growth factor pathway in lung cancer. J. Thorac. Oncol. 3 , 815–818 (2008).
Li, S. et al. Coexistence of EGFR with KRAS, or BRAF, or PIK3CA somatic mutations in lung cancer: a comprehensive mutation profiling from 5125 Chinese cohorts. Br. J. Cancer 110 , 2812–2820 (2014).
Kris, M. G. et al. Using multiplexed assays of oncogenic drivers in lung cancers to select targeted drugs. JAMA 311 , 1998–2006 (2014).
Zhang, Y. L. et al. The prevalence of EGFR mutation in patients with non-small cell lung cancer: a systematic review and meta-analysis. Oncotarget 7 , 78985–78993 (2016).
Swanton, C. & Govindan, R. Clinical implications of genomic discoveries in lung cancer. N. Engl. J. Med. 374 , 1864–1873 (2016).
AACR Project GENIE Consortium et al. AACR Project Genie: Powering precision medicine through an international consortium. Cancer Discov. 7 , 818–831 (2017).
Dogan, S. et al. Molecular epidemiology of EGFR and KRAS mutations in 3,026 lung adenocarcinomas: higher susceptibility of women to smoking-related KRAS-mutant cancers. Clin. Cancer Res. 18 , 6169–6177 (2012).
Etzel, C. J., Amos, C. I. & Spitz, M. R. Risk for smoking-related cancer among relatives of lung cancer patients. Cancer Res. 63 , 8531–8535 (2003).
Matakidou, A., Eisen, T. & Houlston, R. S. Systematic review of the relationship between family history and lung cancer risk. Br. J. Cancer 93 , 825–833 (2005).
Coté, M. L. et al. Increased risk of lung cancer in individuals with a family history of the disease: a pooled analysis from the International Lung Cancer Consortium. Eur. J. Cancer 48 , 1957–1968 (2012).
Mucci, L. A. et al. Familial risk and heritability of cancer among twins in Nordic countries. J. Am. Med. Assoc. 315 , 68–76 (2016).
Caron, O., Frebourg, T., Benusiglio, P. R., Foulon, S. & Brugières, L. Lung adenocarcinoma as part of the Li–Fraumeni syndrome spectrum: preliminary data of the LIFSCREEN randomized clinical trial. JAMA Oncol. 3 , 1736–1737 (2017).
Gazdar, A. et al. Hereditary lung cancer syndrome targets never smokers with germline EGFR gene T790M mutations. J. Thorac. Oncol. 9 , 456–463 (2014).
McKay, J. D. et al. Large-scale association analysis identifies new lung cancer susceptibility loci and heterogeneity in genetic susceptibility across histological subtypes. Nat. Genet. 49 , 1126–1132 (2017).
Klein, R. J. & Gümüş, Z. H. Are polygenic risk scores ready for the cancer clinic? – a perspective. Transl. Lung Cancer Res. 11 , 910–919 (2022).
Hung, R. J. et al. Assessing lung cancer absolute risk trajectory based on a polygenic risk model. Cancer Res. 81 , 1607–1615 (2021).
Dai, J. et al. Identification of risk loci and a polygenic risk score for lung cancer: a large-scale prospective cohort study in Chinese populations. Lancet Respir. Med. 7 , 881–891 (2019).
Surveillance, Epidemiology, and End Results (SEER) Program. SEER*Stat Database: Incidence – SEER Research Data, 8 Registries, Nov 2021 Sub (1975-2020) – Linked To County Attributes – Time Dependent (1990–2020) Income/Rurality, 1969–2020 Counties. https://seer.cancer.gov/statistics-network/explorer (National Cancer Institute, 2023).
Surveillance, Epidemiology, and End Results (SEER) Program. SEER*Stat Database: Mortality – All COD, Aggregated With State, Total U.S. (1969-2020), Katrina/Rita Population Adjustment. https://seer.cancer.gov/statistics-network/explorer (National Cancer Institute, 2022).
Paci, E. et al. Mortality, survival and incidence rates in the ITALUNG randomised lung cancer screening trial. Thorax 72 , 825–831 (2017).
Infante, M. et al. Long-term follow-up results of the DANTE trial, a randomized study of lung cancer screening with spiral computed tomography. Am. J. Respir. Crit. Care Med. 191 , 1166–1175 (2015).
Saghir, Z. et al. CT screening for lung cancer brings forward early disease. The randomised Danish Lung Cancer Screening Trial: status after five annual screening rounds with low-dose CT. Thorax 67 , 296–301 (2012).
Becker, N. et al. Lung cancer mortality reduction by LDCT screening – results from the randomized German LUSI trial. Int. J. Cancer 146 , 1503–1513 (2020).
de Koning, H. J. et al. Reduced lung-cancer mortality with volume CT screening in a randomized trial. N. Engl. J. Med. 382 , 503–513 (2020).
Aberle, D. R. et al. Reduced lung-cancer mortality with low-dose computed tomographic screening. N. Engl. J. Med. 365 , 395–409 (2011).
Krist, A. H. et al. Screening for lung cancer: US Preventive Services Task Force recommendation statement. J. Am. Med. Assoc. 325 , 962–970 (2021).
Canadian Task Force on Preventive Health Care. Recommendations on screening for lung cancer. Can. Med. Assoc. J. 188 , 425–432 (2016).
Oudkerk, M. et al. European position statement on lung cancer screening. Lancet Oncol. 18 , e754–e766 (2017).
UK National Screening Committee. Adult screening programme: lung cancer. GOV.UK https://view-health-screening-recommendations.service.gov.uk/lung-cancer/ (2022).
Bach, P. B. et al. Benefits and harms of CT screening for lung cancer: a systematic review. JAMA 307 , 2418–2429 (2012).
Japan Radiological Society The Japanese imaging guideline 2013. Japan Radiological Society http://www.radiology.jp/content/files/diagnostic_imaging_guidelines_2013_e.pdf (2013).
Zhou, Q. et al. China national lung cancer screening guideline with low-dose computed tomography (2018 version) [Chinese]. Zhongguo Fei Ai Za Zhi 21 , 67–75 (2018).
Jang, S. H. et al. The Korean guideline for lung cancer screening. J. Korean Med. Assoc. 58 , 291–301 (2015).
Triphuridet, N. & Henschke, C. Landscape on CT screening for lung cancer in Asia. Lung Cancer 10 , 107–124 (2019).
Sagawa, M., Nakayama, T., Tanaka, M., Sakuma, T. & Sobue, T. A randomized controlled trial on the efficacy of thoracic CT screening for lung cancer in non-smokers and smokers of <30 pack-years aged 50–64 years (JECS study): research design. Jpn. J. Clin. Oncol. 42 , 1219–1221 (2012).
dos Santos, R. S. et al. Do current lung cancer screening guidelines apply for populations with high prevalence of granulomatous disease? Results from the first Brazilian lung cancer screening trial (BRELT1). Ann. Thorac. Surg. 101 , 481–486 (2016).
Ministéro Saúde. Protocolos clínicos e diretrizes terapêuticas em oncologia. Ministéro Saúde https://www.gov.br/saude/pt-br/assuntos/protocolos-clinicos-e-diretrizes-terapeuticas-pcdt/arquivos/2014/livro-pcdt-oncologia-2014.pdf (2014).
Toumazis, I. et al. Cost-effectiveness evaluation of the 2021 US Preventive Services Task Force recommendation for lung cancer screening. JAMA Oncol. 7 , 1833–1842 (2021).
Criss, S. D., Sheehan, D. F., Palazzo, L. & Kong, C. Y. Population impact of lung cancer screening in the United States: projections from a microsimulation model. PLoS Med. 15 , e1002506 (2018).
Kee, D., Wisnivesky, J. & Kale, M. S. Lung cancer screening uptake: analysis of BRFSS 2018. J. Gen. Intern. Med. 36 , 2897–2899 (2021).
Cao, W. et al. Uptake of lung cancer screening with low-dose computed tomography in China: a multi-centre population-based study. EClinicalMedicine 52 , 101594 (2022).
Quaife, S. L. et al. Lung screen uptake trial (LSUT): randomized controlled clinical trial testing targeted invitation materials. Am. J. Respir. Crit. Care Med. 201 , 965–975 (2020).
National Cancer Institute. Breast, cervical, and colorectal cancers – early detection summary table. NIH https://progressreport.cancer.gov/tables/breast-cervical (2022).
Jonnalagadda, S. et al. Beliefs and attitudes about lung cancer screening among smokers. Lung Cancer 77 , 526–531 (2012).
Carter-Harris, L., Ceppa, D. P., Hanna, N. & Rawl, S. M. Lung cancer screening: what do long-term smokers know and believe? Health Expect. 20 , 59–68 (2017).
Gesthalter, Y. B. et al. Evaluations of implementation at early-adopting lung cancer screening programs: lessons learned. Chest 152 , 70–80 (2017).
Medicare. Lung cancer screenings. Medicare.gov https://www.medicare.gov/coverage/lung-cancer-screenings (2023).
Carter-Harris, L. & Gould, M. K. Multilevel barriers to the successful implementation of lung cancer screening: Why does it have to be so hard? Ann. Am. Thorac. Soc. 14 , 1261–1265 (2017).
Modin, H. E. et al. Pack-year cigarette smoking history for determination of lung cancer screening eligibility. comparison of the electronic medical record versus a shared decision-making conversation. Ann. Am. Thorac. Soc. 14 , 1320–1325 (2017).
American Lung Association. State of lung cancer. American Lung Association https://www.lung.org/research/state-of-lung-cancer (2022).
Jia, Q., Chen, H., Chen, X. & Tang, Q. Barriers to low-dose CT lung cancer screening among middle-aged Chinese. Int. J. Environ. Res. Public Health 2020 17 , 7107 (2020).
Novellis, P. et al. Lung cancer screening: who pays? Who receives? The European perspectives. Transl. Lung Cancer Res. 10 , 2395–2406 (2021).
Crosbie, P. A. et al. Implementing lung cancer screening: baseline results from a community-based ‘Lung Health Check’ pilot in deprived areas of Manchester. Thorax 74 , 405–409 (2019).
Verghese, C., Redko, C. & Fink, B. Screening for lung cancer has limited effectiveness globally and distracts from much needed efforts to reduce the critical worldwide prevalence of smoking and related morbidity and mortality. J. Glob. Oncol. 4 , 1–7 (2018).
Shankar, A. et al. Feasibility of lung cancer screening in developing countries: challenges, opportunities and way forward. Transl. Lung Cancer Res. 8 , S106–S121 (2019).
Fitzgerald, R. C., Antoniou, A. C., Fruk, L. & Rosenfeld, N. The future of early cancer detection. Nat. Med. 28 , 666–677 (2022).
Liu, M. C., Oxnard, G. R., Klein, E. A., Swanton, C. & Seiden, M. V. Sensitive and specific multi-cancer detection and localization using methylation signatures in cell-free DNA. Ann. Oncol. 31 , 745–759 (2020).
Hubbell, E., Clarke, C. A., Aravanis, A. M. & Berg, C. D. Modeled reductions in late-stage cancer with a multi-cancer early detection test. Cancer Epidemiol. Biomark. Prev. 30 , 460–468 (2021).
Hackshaw, A. et al. Estimating the population health impact of a multi-cancer early detection genomic blood test to complement existing screening in the US and UK. Br. J. Cancer 125 , 1432–1442 (2021).
Mouritzen, M. T. et al. Nationwide survival benefit after implementation of first-line immunotherapy for patients with advanced NSCLC – real world efficacy. Cancers 13 , 4846 (2021).
Smeltzer, M. P. et al. The International Association for the Study of Lung Cancer global survey on molecular testing in lung cancer. J. Thorac. Oncol. 15 , 1434–1448 (2020).
Febbraro, M. et al. Barriers to access: global variability in implementing treatment advances in lung cancer. Am. Soc. Clin. Oncol. Educ. Book 42 , 1–7 (2022).
US Environmental Protection Agency. Learn about impacts of diesel exhaust and the Diesel Emissions Reduction Act (DERA). EPA https://www.epa.gov/dera/learn-about-impacts-diesel-exhaust-and-diesel-emissions-reduction-act-dera (2023).
Ervik, M. et al. Global Cancer Observatory: Cancer Over Time ( International Agency for Research on Cancer, accessed 1 May 2022); https://gco.iarc.fr/overtime .
Soda, M. et al. Identification of the transforming EML4–ALK fusion gene in non-small-cell lung cancer. Nature 448 , 561–566 (2007).
Shaw, A. T. et al. Clinical features and outcome of patients with non-small-cell lung cancer who harbor EML4-ALK. J. Clin. Oncol. 27 , 4247–4253 (2009).
Kim, H. R. et al. Distinct clinical features and outcomes in never-smokers with nonsmall cell lung cancer who harbor EGFR or KRAS mutations or ALK rearrangement. Cancer 118 , 729–739 (2012).
Download references
Author information
Authors and affiliations.
Division of Endocrinology, Diabetes, and Bone Diseases, Department of Medicine, Icahn School of Medicine at Mount Sinai, New York, NY, USA
Amanda Leiter
Division of Hematology and Oncology, Department of Medicine, Icahn School of Medicine at Mount Sinai, New York, NY, USA
Rajwanth R. Veluswamy
Division of General Internal Medicine, Department of Medicine, Icahn School of Medicine at Mount Sinai, New York, NY, USA
Juan P. Wisnivesky
Tisch Cancer Institute, Icahn School of Medicine at Mount Sinai, New York, NY, USA
You can also search for this author in PubMed Google Scholar
Contributions
All authors made a substantial contribution to all aspects of the preparation of this manuscript.
Corresponding author
Correspondence to Amanda Leiter .
Ethics declarations
Competing interests.
R.V. has acted as an adviser and/or consultant to AstraZeneca, Beigene, BerGenBio, Bristol-Myers Squibb, Merck, Novartis, Novocure and Regeneron, and has received research grants from AstraZeneca, Boehringer Ingelheim, Bristol-Myers Squibb, and Onconova Therapeutics. J.P.W. has acted as an adviser and/or consultant to Atea, Banook, PPD and Sanofi and has received research grants from Arnold Consultants, Regeneron and Sanofi. A.L. declares no competing interests.
Peer review
Peer review information.
Nature Reviews Clinical Oncology thanks D. Christiani and the other, anonymous reviewers for the peer-review of this work.
Additional information
Publisher’s note Springer Nature remains neutral with regard to jurisdictional claims in published maps and institutional affiliations.
Rights and permissions
Springer Nature or its licensor (e.g. a society or other partner) holds exclusive rights to this article under a publishing agreement with the author(s) or other rightsholder(s); author self-archiving of the accepted manuscript version of this article is solely governed by the terms of such publishing agreement and applicable law.
Reprints and permissions
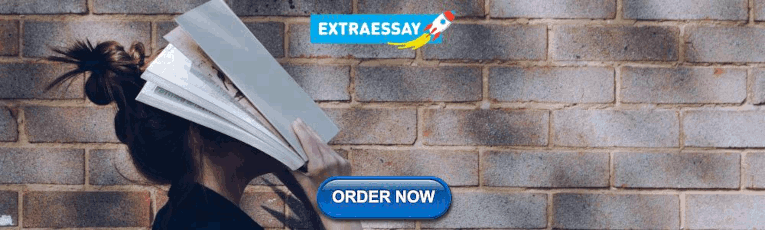
About this article
Cite this article.
Leiter, A., Veluswamy, R.R. & Wisnivesky, J.P. The global burden of lung cancer: current status and future trends. Nat Rev Clin Oncol 20 , 624–639 (2023). https://doi.org/10.1038/s41571-023-00798-3
Download citation
Accepted : 20 June 2023
Published : 21 July 2023
Issue Date : September 2023
DOI : https://doi.org/10.1038/s41571-023-00798-3
Share this article
Anyone you share the following link with will be able to read this content:
Sorry, a shareable link is not currently available for this article.
Provided by the Springer Nature SharedIt content-sharing initiative
This article is cited by
A cuproptosis score model and prognostic score model can evaluate clinical characteristics and immune microenvironment in nsclc.
- Tianyi Wang
Cancer Cell International (2024)
Exercise accelerates recruitment of CD8+ T cell to promotes anti-tumor immunity in lung cancer via epinephrine
- Sai-Nan Miao
- Meng-Qi Chai
BMC Cancer (2024)
Enhanced recovery after surgery program focusing on chest tube management improves surgical recovery after video-assisted thoracoscopic surgery
Journal of Cardiothoracic Surgery (2024)
Can aerosol optical depth unlock the future of air quality monitoring and lung cancer prevention?
- Izni Zahidi
- Dag Øivind Madsen
Environmental Sciences Europe (2024)
Advancing accuracy in breath testing for lung cancer: strategies for improving diagnostic precision in imbalanced data
- Ke-Cheng Chen
- Shuenn-Wen Kuo
- Hsiao-Yu Yang
Respiratory Research (2024)
Quick links
- Explore articles by subject
- Guide to authors
- Editorial policies
Sign up for the Nature Briefing: Cancer newsletter — what matters in cancer research, free to your inbox weekly.

Help | Advanced Search
Computer Science > Human-Computer Interaction
Title: "i wish there were an ai": challenges and ai potential in cancer patient-provider communication.
Abstract: Patient-provider communication has been crucial to cancer patients' survival after their cancer treatments. However, the research community and patients themselves often overlook the communication challenges after cancer treatments as they are overshadowed by the severity of the patient's illness and the variety and rarity of the cancer disease itself. Meanwhile, the recent technical advances in AI, especially in Large Language Models (LLMs) with versatile natural language interpretation and generation ability, demonstrate great potential to support communication in complex real-world medical situations. By interviewing six healthcare providers and eight cancer patients, our goal is to explore the providers' and patients' communication barriers in the post-cancer treatment recovery period, their expectations for future communication technologies, and the potential of AI technologies in this context. Our findings reveal several challenges in current patient-provider communication, including the knowledge and timing gaps between cancer patients and providers, their collaboration obstacles, and resource limitations. Moreover, based on providers' and patients' needs and expectations, we summarize a set of design implications for intelligent communication systems, especially with the power of LLMs. Our work sheds light on the design of future AI-powered systems for patient-provider communication under high-stake and high-uncertainty situations.
Submission history
Access paper:.
- HTML (experimental)
- Other Formats

References & Citations
- Google Scholar
- Semantic Scholar
BibTeX formatted citation

Bibliographic and Citation Tools
Code, data and media associated with this article, recommenders and search tools.
- Institution
arXivLabs: experimental projects with community collaborators
arXivLabs is a framework that allows collaborators to develop and share new arXiv features directly on our website.
Both individuals and organizations that work with arXivLabs have embraced and accepted our values of openness, community, excellence, and user data privacy. arXiv is committed to these values and only works with partners that adhere to them.
Have an idea for a project that will add value for arXiv's community? Learn more about arXivLabs .
This paper is in the following e-collection/theme issue:
Published on 25.4.2024 in Vol 26 (2024)
Digital Therapeutic (Mika) Targeting Distress in Patients With Cancer: Results From a Nationwide Waitlist Randomized Controlled Trial
Authors of this article:

Original Paper
- Franziska Springer 1 * , MSc ;
- Ayline Maier 2 * , PhD ;
- Michael Friedrich 1 , PhD ;
- Jan Simon Raue 2 , PhD ;
- Gandolf Finke 2 , PhD ;
- Florian Lordick 3, 4 , Prof Dr ;
- Guy Montgomery 5 , Prof Dr ;
- Peter Esser 1 , PhD ;
- Hannah Brock 1 , MSc ;
- Anja Mehnert-Theuerkauf 1 , Prof Dr
1 Department of Medical Psychology and Medical Sociology, Comprehensive Cancer Center Central Germany, University Medical Center Leipzig, Leipzig, Germany
2 Fosanis GmbH, Berlin, Germany
3 Department of Medicine II, University Medical Center Leipzig, Leipzig, Germany
4 University Cancer Center Leipzig, Comprehensive Cancer Center Central Germany, Leipzig, Germany
5 Center for Behavioral Oncology, Department of Population Health Science and Policy, Icahn School of Medicine at Mount Sinai, New York, NY, United States
*these authors contributed equally
Corresponding Author:
Anja Mehnert-Theuerkauf, Prof Dr
Department of Medical Psychology and Medical Sociology, Comprehensive Cancer Center Central Germany, University Medical Center Leipzig
Philipp-Rosenthal-Str. 55, Haus W
Leipzig, 04103
Phone: 49 341 97 18800
Email: [email protected]
Background: Distress is highly prevalent among patients with cancer, but supportive care needs often go unmet. Digital therapeutics hold the potential to overcome barriers in cancer care and improve health outcomes.
Objective: This study conducted a randomized controlled trial to investigate the efficacy of Mika, an app-based digital therapeutic designed to reduce distress across the cancer trajectory.
Methods: This nationwide waitlist randomized controlled trial in Germany enrolled patients with cancer across all tumor entities diagnosed within the last 5 years. Participants were randomized into the intervention (Mika plus usual care) and control (usual care alone) groups. The participants completed web-based assessments at baseline and at 2, 6, and 12 weeks. The primary outcome was the change in distress from baseline to week 12, as measured by the National Comprehensive Cancer Network Distress Thermometer. Secondary outcomes included depression, anxiety (Hospital Anxiety and Depression Scale), fatigue (Functional Assessment of Chronic Illness Therapy-Fatigue), and quality of life (Clinical Global Impression-Improvement Scale). Intention-to-treat and per-protocol analyses were performed. Analyses of covariance were used to test for outcome changes over time between the groups, controlling for baseline.
Results: A total of 218 patients (intervention: n=99 and control: n=119) were included in the intention-to-treat analysis. Compared with the control group, the intervention group reported greater reductions in distress ( P =.03; ηp²=0.02), depression ( P< .001; ηp²=0.07), anxiety ( P= .03; ηp²=0.02), and fatigue ( P= .04; ηp²=0.02). Per-protocol analyses revealed more pronounced treatment effects, with the exception of fatigue. No group difference was found for quality of life.
Conclusions: Mika effectively diminished distress in patients with cancer. As a digital therapeutic solution, Mika offers accessible, tailored psychosocial and self-management support to address the unmet needs in cancer care.
Trial Registration: German Clinical Trials Register (DRKS) DRKS00026038; https://drks.de/search/en/trial/DRKS00026038
Introduction
In addition to somatic symptoms such as pain [ 1 ], patients with cancer report elevated levels of distress, anxiety, and depression [ 2 , 3 ]. Epidemiological data show that the prevalence of clinically substantial psychological distress typically ranges from 30% to 60% among patients with cancer [ 2 , 4 ]. Psychological distress can persist long after the end of treatment and is associated with reduced quality of life (QoL), lower cancer treatment adherence, and lower survival rates [ 5 ].
Supportive care interventions to prevent and manage the adverse psychological and physical effects of cancer across the cancer trajectory effectively improve outcomes such as emotional distress, QoL, and fatigue [ 6 ]. Optimal supportive care is holistic and patient centered, that is, based on the needs of each individual patient [ 7 ]. However, access to supportive care is often limited by a lack of specialist staff, organizational deficiencies, and barriers that cause patients to avoid or delay their treatment [ 8 - 10 ]. Thus, emerging or persistent supportive care needs across the cancer trajectory often go unmet, with detrimental psychosocial and emotional impacts on patients with cancer [ 11 ]. Moreover, the number of patients living with cancer has increased rapidly in recent years [ 12 ] due to improved early detection, diagnosis, and oncological treatments, posing a growing challenge to health systems worldwide to ensure adequate and long-term care for all patients with cancer [ 13 ].
The increasing use of digital health has ushered in a new era of patient-centered cancer care due to its potential for cancer care delivery [ 14 ]. Digital health interventions provide multiple benefits: they facilitate easy and low-threshold access to care, can overcome barriers to care (eg, location, time, and health status), may enhance symptom management through real-time symptom assessment, are scalable, and provide cost-effective and efficient information sharing [ 14 ]. Growing literature suggests that digital therapeutics, a subset of digital health interventions providing evidence-based treatments driven by software, play a useful role in addressing the unmet needs of patients with cancer [ 15 ]. For instance, various mobile apps have proven to be effective in catering to specific needs of patients with cancer, such as pain, anxiety, or QoL, by using different types of interventions, such as psychoeducation, physical exercises, or coping skills training (eg, [ 16 - 19 ]). Moreover, large analyses such as systematic reviews and meta-analyses evaluating the efficacy of app-based interventions for patients with cancer show positive effects on patient-relevant outcomes, such as distress, QoL, anxiety, depression, pain, and fatigue [ 20 - 23 ].
Existing app-based supportive care interventions provide various intervention modules, such as symptom monitoring, psychoeducation, mindfulness exercises, physical exercises, and cognitive behavioral therapy (CBT) techniques [ 24 ]. However, most of these apps are limited in their scope, targeting only specific symptoms (eg, fatigue) [ 25 ] and health behaviors (eg, physical activity) [ 26 ], or provide only a single function (eg, mindfulness training or symptom tracking) [ 27 - 29 ]. Furthermore, some of these apps were originally developed for non–oncology patient populations and have only been slightly adapted for patients with cancer [ 30 ]. Only a few apps offer a broader range of intervention modules [ 25 , 31 ], but they target specific subgroups of patients with cancer (eg, patients with 1 tumor entity or with specific symptoms).
Despite the evident need, there is yet no digital therapeutic that comprehensively addresses the problems faced by all patients with cancer and simultaneously offers tailored support for each individual patient. Therefore, we investigated the efficacy of Mika (developed by Fosanis GmbH), an app-based digital therapeutic that addresses all patients with cancer transdiagnostically and provides a holistic supportive care intervention. The app incorporates evidence-based supportive care elements, such as distress and symptom monitoring [ 32 ], CBT-based coping skills training [ 33 ], mindfulness-based stress reduction (MBSR) [ 34 , 35 ], strength and flexibility training [ 36 ], and patient education [ 37 ], thus targeting different aspects of psychological distress. An artificial intelligence algorithm individually tailors the content of the app to patients’ needs, considering cancer type, cancer treatment stage, and use behavior. A previously conducted pilot study of 70 patients with gynecological cancer indicated Mika’s feasibility and potential efficacy [ 38 ]. Considering the significant prevalence and impact of psychological distress among patients with cancer, this condition was selected as the primary end point of our study. This is underscored by the app’s integrated features for distress tracking and management alongside the widespread recommendation for distress screening in routine clinical care. Distress is recognized as a crucial clinical marker for assessing the efficacy of interventions across various tumor types and catering to the immediate and long-term supportive care needs of this patient group.
The primary aim of this waitlist randomized controlled trial (RCT) was to examine the efficacy of the Mika app for general distress in patients with cancer. The secondary aim was to assess the efficacy of the Mika app on anxiety, depression, fatigue, and QoL. We hypothesized that participants receiving access to the Mika app plus usual care (UC) for 12 weeks would report greater reductions in distress, anxiety, depression, and fatigue and greater improvements in QoL compared to participants receiving UC only.
Study Design
This nationwide unblinded 2-arm waitlist RCT evaluated the efficacy of the app-based digital therapeutic Mika in reducing distress in patients with cancer and was conducted fully decentralized in Germany, that is, participant recruitment, delivery of the study intervention, and outcome data collection were conducted without involving in-person contact between the study team and the participants. In this RCT, participants were assigned to either (1) access to the Mika app plus UC (intervention group [IG]), or (2) UC alone (control group [CG]). Participants were assessed at baseline (t0), 2 weeks (t1), 6 weeks (t2), and 12 weeks (t3) using self-report questionnaires. Once the participants in the CG completed the 12-week questionnaire, they also received access to the Mika app.
Ethical Considerations
The trial was approved by the Ethics Committee of the Medical Faculty of Leipzig University (404/21-ek) and was registered at the German Clinical Trials Register (DRKS00026038) in October 2021. All participants provided written informed consent prior to their participation in the study and retained the autonomy to withdraw from the study at any time. All personal data collected and used for this study underwent deidentification to safeguard the anonymity of participants. Monetary compensation was not provided to participants for their involvement in the study.
Participants
Textbox 1 shows the inclusion and exclusion criteria for this study. We only included patients who had been diagnosed with cancer or relapse within the last 5 years as they are likely to feel burdened by the physical and psychological effects of the disease and its treatment and therefore require supportive care. Epidemiological data indicate that supportive care needs typically decline in the years of long-term survivorship (cancer or relapse diagnosis ≥5 years ago) [ 39 ]. Participants were required to confirm their cancer diagnosis during the course of the study by submitting a letter from their treating physician. The study team enrolled patients after they had provided written informed consent, which had to be completed at home and submitted by email or mail.
Inclusion criteria
- Age≥18 years
- Cancer diagnosis or relapse diagnosis within the last 5 years (10th revision of the International Statistical Classification of Diseases and Related Health Problems: C00-C97)
- Access to a smartphone or tablet
- Ability to provide informed consent
Exclusion criteria
- Insufficient German language skills
- Inability to use a smartphone or tablet
- Prior use of the investigated digital therapeutic
Random Assignment
Participants were randomly assigned (1:1) to either the IG or CG using permuted block randomization with blocks of 4 based on an a priori created randomization list. The allocation sequence was concealed from the study investigators until assignment. Due to the nature of the intervention, it was not feasible to blind participants or the study team to the group assignment.
Recruitment and Procedure
Between September and November 2021, patients were recruited via social media advertising campaigns (Facebook and Instagram, Meta Inc) and informational emails to cancer support groups that directed patients to the trial website with a contact form for study registration. In addition, patients were recruited from a participant pool consisting of participants from previous independent studies at the University Medical Center Leipzig. Patients from the participant pool were approached directly by the study team via phone.
All interested patients were screened by phone to determine eligibility. To identify patients who were already users of the digital therapeutic, the study team asked participants about their use of digital support, however, without referring to the publicly available digital therapeutic by name to prevent CG patients from accessing the digital therapeutic before their enrollment in the study. Eligible patients received study information in the form of a video and text via email. Patients were informed that they were required to submit a physician’s letter confirming their cancer diagnosis via a secure cloud data-sharing service (TeamDrive, Crunchbase) during the course of their study participation. After providing informed consent, the participants were randomized into the IG or CG and completed the baseline questionnaires. Participants were informed about their group assignment following a completed baseline assessment. IG participants received a study access code to activate the app after downloading it from the app stores for either Android or iOS smartphones, allowing free use. The questionnaire battery was administered electronically using LimeSurvey (LimeSurvey GmbH). All participants received email invitations and reminders at 2, 6, and 12 weeks to complete the questionnaire. This RCT focused on changes in outcomes from baseline (t0) to week 12 (t3). The 2 assessments in between (t1 and t2) were not part of the analysis; an analysis of the trajectory of the symptoms is planned for the future. Once the CG participants completed the 12-week questionnaire, they also received a study access code that could be used to activate the app. All the participants received information about the app’s content and technical application via a standardized telephone introduction to the app. All participants were contacted for an exploratively structured telephone interview after completing the 12-week questionnaire. During this interview, the use of psychotherapeutic support during study participation was assessed. Data collection ended in March 2022.
Data monitoring was performed via standardized phone calls following questionnaire completion of each participant across all measurement time points to ensure data validity. These phone calls served to ask participants to provide missing questionnaire data, to allow participants to clarify difficulties in understanding single questionnaire items, and to provide assistance with limited app functionality. Missing questionnaire data were entered directly into the database by the study team, with a study team member reading the unanswered questions and associated response options to participants verbatim, prompting them to select their response option.
Self-reported adverse reactions and side effects of the investigated digital therapeutic were assessed at each measurement time point as part of the web-based questionnaire battery.
Intervention
Mika is an app-based digital therapeutic that provides a personalized supportive intervention aiming to reduce distress associated with cancer and its medical treatment, thus improving patients’ QoL. Mika comprises 3 modules: Check-Up , Discover, and Journeys . The Check-Up module allows for the monitoring of distress and symptom monitoring with electronic patient-reported outcomes that can be shared and discussed with the attending physician. The Discover module delivers coaching via articles and videos on cancer types and medical treatments, psychological well-being, physical activity, diet, and social and financial issues, which are based on scientific evidence and presented in a clear and understandable manner for patients. The Journeys module provides users with evidence-based, resource-activating training courses combining psychoeducation and exercises to help patients cope with the mental and physical effects of cancer, for example, coping with stress and fatigue, making decisions, or living with immunotherapy (for more details on the app modules, refer to Table 1 and Figure 1 ). An artificial intelligence algorithm within the app customizes the content for each patient. This includes personalized recommendations based on cancer type, cancer treatment stage, and crucially; the nature and severity of reported symptoms; and ensuring personalized support for each individual. This customization process not only accounts for general patient information but also actively incorporates real-time symptom tracking data and user reading behavior using an attentional factorization machine that predicts a patient’s likelihood of engaging with specific content. This approach focuses on important feature interactions related to content consumption [ 40 ], ensuring that recommendations are dynamically adjusted as patients report changes in symptoms and interact with the content. In addition, the algorithm uses a Dirichlet loss function to estimate the uncertainty in predictions [ 41 ], allowing the content to be ranked and presented based on the estimated read probability. The model undergoes monthly updates using historical data, optimizing through hyperparameter tuning evaluated by 7-fold time series cross-validation.
It is hypothesized that the digital therapeutic empowers patients with cancer by improving their health literacy and self-management along the cancer trajectory using evidence-based methods, such as symptom monitoring, patient education, MBSR, strength and flexibility training, acceptance and commitment therapy, and CBT-based coping skills training.
The Mika app was developed by Fosanis GmbH in collaboration with leading research institutions, such as the Charité University Hospital Berlin, University Hospital Leipzig, and the National Center for Tumor Diseases Heidelberg. All content of the app was carefully reviewed by experts (eg, oncologists, psychotherapists, nutritionists, and physiotherapists) before publication. The feasibility and preliminary efficacy of Mika were investigated in a previously conducted randomized pilot study involving 70 patients with gynecological cancer [ 38 ]. Mika is available for download free of charge in German and the United Kingdom app stores for Android and iOS smartphones.
IG participants could freely choose the modules to work on. While regular app use was recommended, participants were instructed to use the app at least 3 times a week.
a PRO: patient-reported outcome.

UC Condition
UC consisted of all health care that patients in Germany usually receive. There were no restrictions on health care use.
Outcome Assessment
Primary outcome.
The primary outcome was the change in psychological distress from baseline to 12 weeks, measured using the validated German version of the National Comprehensive Cancer Network Distress Thermometer [ 42 ]. Distress Thermometer is a well-established single-item self-report measure that assesses the global level of distress on a 0 (no distress) to 10 (extreme distress)-point Likert scale. It shows excellent psychometric properties across various cancer populations worldwide and is recommended as a clinical tool for routine clinical care [ 43 ]. A score ≥5 indicates clinically significant levels of distress.
Secondary Outcomes
The secondary outcomes included changes in anxiety and depression symptoms, fatigue from baseline to 12 weeks, and QoL at 12 weeks. Anxiety and depression symptoms were measured using the Hospital Anxiety and Depression Scale [ 44 ], a 14-item self-report measure of anxiety and depression, with 7 items measuring each subscale. Scores for each subscale range from 0 to 21, with a higher score indicating higher levels of anxiety or depression and a cutoff score of ≥8 for each subscale. Fatigue was assessed using the Functional Assessment of Chronic Illness Therapy-Fatigue [ 45 ], a 13-item measure that assesses self-reported tiredness, weakness, and difficulty in performing usual activities due to fatigue. The Functional Assessment of Chronic Illness Therapy-Fatigue score ranges from 0 to 52, with higher scores representing less fatigue. Self-reported QoL was measured using an adapted version of the Clinical Global Impression-Improvement Scale [ 46 ], a single-item 7-point measure that assesses the overall improvement of a patient’s disease relative to a baseline state at the beginning of the intervention. In this trial, the Clinical Global Impression-Improvement Scale measured improvement in QoL relative to the beginning of the study, with a value of 4 indicating no change, <4 indicating improvement, and >4 indicating deterioration in QoL.
Intervention Safety
The safety of the digital therapeutic was assessed by the number and type of self-reported adverse reactions and side effects during the trial duration.
Intervention Adherence and Engagement
Adherence to the intervention was assessed by tracking app activities. IG participants were considered active once they activated the app using the study access code and consented to the Mika app’s privacy terms. Subsequently, their pseudonymized in-app activities were automatically recorded as log data. These log data facilitated the evaluation of intervention adherence, defined as the number of days with ≥1 app activity during each of the three 4-week periods (0-4, 5-8, and 9-12 weeks) within the 12-week intervention. Such an approach enabled us to capture the frequency and diversity of app engagement, thus embodying a comprehensive definition of adherence. In addition, engagement across the app’s 3 modules—Check-Up, Discover, and Journeys—was analyzed.
Statistical Analysis
Given an estimated dropout rate of 20% (50/250), a priori sample calculations showed that a sample of 2×125 (N=250) at baseline was needed to detect a change of 1 scale point (SD 2; α=.05; 1−β=.8) in the primary outcome.
Primary analyses were performed using the intention-to-treat (ITT) principle, which included all randomized participants with a confirmed cancer diagnosis by a physician’s letter. Analyses were also performed per-protocol (PP), which was restricted to participants who (1) completed the self-report questionnaire at all measurement time points, (2) did not receive psychotherapeutic support during study participation, (3) did not use the investigated digital therapeutic before receiving access during study participation, and (4) used the investigated digital therapeutic at least 1 time per period up to the 5- to 8-week period of the 12-week intervention period (only IG).
Analysis of covariance was used to examine changes in distress, depression, anxiety, and fatigue outcomes between the trial arms from baseline to 12 weeks, controlling for baseline scores. Exploratory regression analyses were conducted to investigate potential variables influencing the primary outcome. These analyses focused exclusively on sociodemographic and clinical factors that showed differences between the IG and CG in the initial group comparison. Partial eta–squared was reported as the effect size for all analyses of covariance, with effect sizes interpreted as small, medium, and large at ≥0.01, ≥0.06, and ≥0.14 [ 47 ], respectively. Differences in QoL between trial arms at follow-up (12 weeks) were analyzed with a 2-tailed 2-sample t test, using Hedges g ' as a measure of effect size (≥0.2=small effect, ≥0.5=medium effect, and ≥0.8=large effect [ 47 ]).
Missing outcome data at random were imputed using the expectation-maximization algorithm. For dropouts, the last observation carried forward was used. For deceased participants, the worst possible values were assumed. Dropouts were participants who failed to complete the baseline or follow-up questionnaires or failed to provide a physician’s letter confirming their cancer diagnosis. A dropout analysis was performed to compare the variables of age, sex, and baseline distress between study noncompleters (dropouts) and study completers using chi-square and t tests. Furthermore, to model the robustness of the primary efficacy analysis under different assumptions for missing data mechanisms, an explorative sensitivity analysis using reference-based multiple imputation (jump-to-reference) [ 48 ] was performed in the extended ITT population (all randomized participants). For this purpose, monotone missing values were replaced using the jump-to-reference approach, whereas sporadic missing values were replaced under the assumption of missing at random. For jump-to-control and jump-to-reference imputation, 50 data sets were generated to minimize the loss of statistical power. The results were then aggregated across the imputed data sets [ 49 ].
All statistical tests were 2-tailed, with a significance level of 5%. Analyses were performed using R (version 4.1.0; R Foundation for Statistical Computing) [ 50 ].
Study Sample
Over the 3-month recruitment period, 517 persons were screened for eligibility and 321 were determined eligible. Of the 321 participants, 248 (77.3%) gave informed consent and were randomly assigned to the IG and the CG ( Figure 2 ). Of the 248 participants, 37 (14.9%) were considered dropouts because they did not complete baseline or follow-up assessments (n=7), failed to confirm their cancer diagnosis by submission of a physician’s letter (n=7), or both (n=23). Age and sex of study dropouts and study completers did not differ ( P age =.89 and P sex =.23), but participants who dropped out showed higher distress levels at baseline compared to study completers ( P =.02). Participants without a verified cancer diagnosis (30/248, 12.1%) were excluded from the ITT analysis, resulting in an ITT population of 218 participants (n=99, 45.4% IG and n=119, 54.6% CG). Of the 218 participants, 173 (79%) were recruited via social media advertisements and cancer support groups and 45 (21%) were recruited using the participant pool of prior studies.

Baseline characteristics were balanced between the groups ( Table 2 ), but participants in the IG were younger compared with those in the CG ( P =.02). No baseline differences in the primary and secondary outcome parameters were observed between the groups, with P values as follows: P =.99 (distress), P =.25 (depression), P =.47 (anxiety), and P =.21 (fatigue). On average, participants were 56 (SD 11) years old, and 60.6% (132/218) of the participants were female and had been diagnosed with cancer 25 (SD 17) months earlier. The most frequently reported cancer types were breast cancer (74/218, 33.9%) and hematological cancer (61/218, 28%), with 8.7% (19/218) of participants reporting a diagnosis of relapsed cancer. The PP population comprised 124 participants, following the exclusion of 94 participants. The primary reasons for exclusion were psychotherapeutic support during study participation and prior use of the investigated digital therapeutic.
a Intervention=12-week access to digital therapeutic app intervention+usual care.
b Control=usual care.
c NCCN Distress Thermometer: National Comprehensive Cancer Network Distress Thermometer (at baseline, clinically significant level of distress≥5).
d HADS-A: Hospital Anxiety and Depression Scale, anxiety subscale (German version, at baseline, cutoff score ≥8).
e HADS-D: Hospital Anxiety and Depression Scale, depression subscale (German version, at baseline, cutoff score ≥8).
f Multiple reasons are possible within 1 patient, and cases do not add up to the total number.
After 12 weeks, participants in the IG reported a reduced level of distress compared to participants in the CG in the ITT population ( F 1,215 =4.7; P =.03; ηp²=0.02; Table 3 ). The observed treatment effect was more pronounced in the PP population ( F 1,121 =6.9; P =.01; ηp²=0.05). The analysis revealed that higher levels of baseline distress predicted a greater change in distress after 12 weeks in the IG. An exploratory regression analysis yielded no predictive effect of age on the change in distress. The explorative sensitivity analysis among all randomized participants (n=248) showed comparable treatment effects (jump-to-control: F 1,19375.1 =5.3; P =.02; ηp²=0.02 and jump-to-intervention: F 1,15314.8 =5.9; P =.02; ηp²=0.02).
a An analysis of covariance was used to test for differences in change in distress levels between groups from baseline to follow-up (12 weeks), controlling for baseline. The partial eta–squared is the reported standardized effect size for the mean difference. The effect sizes can be interpreted as small, medium, or large at ≥0.01, ≥0.06, and ≥0.14, respectively. The results of the intention-to-treat and per-protocol analysis are reported.
b Intervention=12-week access to digital therapeutic app intervention+usual care.
c Control=usual care.
d N/A: not applicable.
In the ITT population, symptoms of anxiety ( F 1,215 =4.8; P =.03; ηp²=0.02), depression ( F 1,215 =15.5; P <.001; ηp²=0.07), and fatigue ( F 1,215 =4.4; P =.04; ηp²=0.02) improved in participants in the IG from baseline to 12 weeks compared to participants in the CG ( Table 4 ). The observed treatment effects on anxiety and depression were more pronounced in the PP population (anxiety: F 1,121 =7.2; P =.01; ηp²=0.06 and depression: F 1,121 =14.9; P <.001; ηp²=0.11). A trend-to-significant treatment effect was observed for fatigue symptoms in the PP population ( F 1,121 =3.8; P =.05; ηp²=0.03). QoL did not differ significantly between the groups at 12 weeks (ITT: t 216 =0.88; P =.38; g=0.12 and PP: t 122 =1.63; P =.11; g=0.30).
c HADS-A: Hospital Anxiety and Depression Scale, anxiety subscale (German version, at baseline, cutoff score ≥8).
d ITT: intention-to-treat.
e N/A: not applicable.
f Italicized values are significant at P <.05.
g PP: per-protocol.
h HADS-D: Hospital Anxiety and Depression Scale, depression subscale (German version, at baseline, cutoff score ≥8).
i FACIT-F Functional Assessment of Chronic Illness Therapy–Fatigue.
j CGI-I: Clinical Global Impression Improvement.
Safety Outcomes
IG participants reported no adverse reactions or side effects of digital therapeutic during the study.
Of the 99 participants in the IG (ITT), 98 (99%), 78 (79%), and 67 (68%) used the digital therapeutic intervention at 0- to 4-, 5- to 8-, and 9- to 12-week periods of the 12-week intervention, respectively, demonstrating good initial adherence to the intervention, which decreased moderately over time. App use (module use and days spent on the app) decreased over time ( Table 5 ). IG participants accessed content from various categories at different frequencies. The most accessed categories were cancer therapy, symptoms and side effects, and nutrition in cancer, with 80% (79/99), 83% (82/99), and 80% (79/99) of users accessing the content in these categories, respectively. Conversely, partnership and family, relaxation, and recipes were accessed less, with 29% (28/99), 34% (33/99), and 32% (31/99) of users, respectively.
Principal Findings
This nationwide waitlist RCT examined the efficacy of Mika, an app-based digital therapeutic that provides a personalized supportive intervention for patients with cancer. Participants who had access to the Mika app for 12 weeks showed significant improvements in perceived distress (ie, the primary outcome) and symptoms of anxiety, depression, and fatigue (ie, the secondary outcomes) compared to participants who received UC. The observed treatment effects were similar in the ITT and PP populations but more pronounced in the PP population, indicating the overall robustness of the findings. We observed no group difference in the QoL after 12 weeks. Intervention adherence was good, and no adverse reactions or side effects of the investigated digital therapeutic were reported.
Comparison With Prior Work
While a growing body of research shows evidence of the efficacy of app-based interventions for oncological populations on distress, fatigue, anxiety, and depression [ 20 , 25 , 27 , 31 , 51 ], this is the first study to examine the efficacy of a single holistic app-based digital therapeutic based on multiple intervention modules on these patient-relevant outcomes. Although the improvement in the primary outcome was modest, it reflects the nuanced nature of psycho-oncological interventions, where even modest changes can have significant clinical relevance. Furthermore, we conducted comprehensive testing of the effects of the investigated digital therapeutic on patients with cancer across all tumor entities, using a larger sample size compared to most previous studies [ 25 , 27 , 31 , 51 ].
In contrast to the findings of this study, however, other studies found an effect of app-based supportive interventions on QoL [ 23 , 27 , 31 ]. This difference in findings could be due to differences in the operationalization and measurement of QoL. In this study, participants’ global QoL was assessed using a single-item questionnaire after a 12-week intervention period. However, global QoL has been shown to be less affected in patients with cancer compared to specific components of QoL, such as social or cognitive functioning and symptom burden from fatigue or insomnia [ 52 ]. Further research using different QoL assessment tools could provide more insights into the efficacy of the investigated digital therapeutic on specific aspects of QoL.
A significant level of intervention adherence and engagement with the digital therapeutic, with varying degrees of interaction across the different app modules, indicates good acceptability and perceived subjective benefit of the investigated digital therapeutic and allows for reliable conclusions about its efficacy in oncological settings. The broad range of engagement, as illustrated by the IQRs, underscores the personalized nature of app use, catering to diverse participant needs and preferences. The variability in engagement levels across different app modules highlights the importance of personalizing digital therapeutics to increase adherence and maximize therapeutic effects.
As we evaluated the app intervention holistically, future studies should examine the impact of the app’s individual components.
While the dropout rate in the IG was slightly higher than that in the CG, the dropout rate in the IG as well as the overall dropout rate was low compared to other app-based supportive interventions [ 25 , 30 ]. Considering that patients with cancer have been found to have a positive attitude toward digital health [ 53 , 54 ], the findings of this study add to the notion that digital health interventions have the potential to overcome barriers associated with access to supportive care in oncological populations [ 55 ].
We found a positive effect of the investigated digital therapeutic on general psychological distress and a broad range of specific distress-associated parameters. Importantly, improvements in psychological symptoms, that is, depression and anxiety, can also have a positive tertiary preventive effect on cancer progression [ 5 ]. The effect sizes in this study ranged from small (ηp²=0.02) to medium (ηp²=0.07) in the ITT population and were more pronounced in the PP population (ηp²=0.05-0.11). The primary outcome improvement, while subtle, aligns with the expected outcomes in psycho-oncological interventions, highlighting the importance of considering the broad spectrum of therapeutic impacts. The medium to large effects observed in secondary end points, together with the primary outcome, illustrate the broad therapeutic impact and highlight the digital therapeutic’s capacity to significantly improve key aspects of psychological well-being in patients with cancer. Small-to-medium effect sizes are common in in-person supportive care interventions [ 6 ]. Our results also compare well with other app-based supportive care interventions, such as small effect sizes reported for a CBT and psychoeducation self-management apps on fatigue [ 25 ] or small to medium effects of a web-based mindfulness-based intervention on anxiety and depression [ 56 ]. This is further supported by the results of several systematic reviews [ 20 , 21 ]. The fact that such effect sizes can be achieved with minimal cost and personnel effort via a digital approach further supports the significant potential for accessibility, reach, and impact of digital therapeutics.
Clinical Implications
The multifaceted intervention modules of the investigated digital therapeutic aim to support patients holistically. The investigated digital therapeutic hereby translates widely used evidence-based intervention methods within supportive care, such as symptom monitoring; patient education; modules of CBT, MBSR, and acceptance and commitment therapy; and strength and flexibility training, into a digital format. The intervention modules of the app are designed to help patients learn about their disease and prepare for discussions with clinicians in an informed decision-making process. This may reduce anxiety and insecurities across the cancer trajectory, while empowering patients and strengthening their self-efficacy.
While it is acknowledged that digital therapeutic interventions might not fully replicate the “in-person” experience, the scope and utility of these tools in the realm of oncology are substantial. For instance, a study evaluating a mobile app designed for tracking patient-reported daily activities found that when supervised by a physician, the data collected were more accurate than when used without guidance [ 57 ]. Conversely, a music app was equally effective in alleviating pain and anxiety in emergency department patients irrespective of supervision [ 58 ]. This suggests that certain interventions, such as symptom tracking, might be more prone to inaccuracies without proper guidance than passive activities, such as listening to music. In addition, CBT, which is traditionally the most effective in face-to-face settings, has generated interest in the digital domain. A study on the digital adaptation of mindfulness-based cognitive therapy for patients with cancer experiencing distress found the therapeutic connection between therapist and patient to be as potent as in in-person sessions [ 59 ]. This underlines the evolving role of digital therapeutics and its potential to reshape therapeutic avenues in oncology, thus paving the way for enhanced patient care.
Furthermore, considering the increasing number of patients with cancer experiencing psychosocial distress and the limited availability of health care professionals, digital therapeutics could present scalable and cost-effective solutions. These solutions can address symptoms and bolster the quality and accessibility of supportive care [ 55 , 60 , 61 ]. Recognizing patients’ diverse needs, tools such as the Mika app leverage artificial intelligence to deliver real-time, tailored support. This has the potential to benefit a broad spectrum of patients with cancer globally while also reducing the pressure on health care infrastructure and professionals. Therefore, digital therapeutics offer a patient-focused approach that is adaptable to specific clinical and lifestyle challenges such as disease management, emotional support, and health-related determinants. They might also further enhance medication adherence, tolerance to chemotherapy, and overall survival rate in the cancer care continuum [ 15 ]. Incorporating these digital tools into routine oncological supportive care can augment patient-centric care and enrich patient experience, safety, and interactions with clinicians [ 15 , 61 ]. However, while there is a consensus among medical professionals and stakeholders regarding the revolutionary potential of digital health in addressing cancer treatment challenges, the path to universal adoption remains intricate. Future studies should delve into the assimilation of digital therapeutics, such as Mika, into standard care across varied clinical environments and evaluate hurdles such as digital literacy and the acceptance of digital tools by both patients and health care professionals [ 62 - 64 ].
Strengths and Limitations
The main strength of this study was the app itself. It addresses the overreaching problem areas faced by all patients with cancer while providing tailored support for population-specific areas of burden (ie, cancer type, treatment status, and use behavior). Its flexible and easily accessible use allows for seamless integration into patients’ daily lives and continuity of supportive treatment. In addition, the low overall dropout rate and data monitoring led to very little missing data. Similar findings in the ITT, PP, and extended ITT populations suggest overall robustness of the results.
This study has several limitations. First, the web-based recruitment procedure may have led to study registration from patients with cancer who were particularly motivated, digitally literate, and highly functioning in seeking support during their cancer journey, which may limit the generalizability of the study. However, the use of additional recruitment pathways (support groups and participant pool) likely resulted in the recruitment of a more heterogeneous sample, possibly compensating for potential selection bias. Future studies might investigate the impact of various recruitment channels on the efficacy of digital therapeutics, and thus, which population may be particularly responsive to digital interventions. Second, the higher number of dropouts in the IG compared to the CG may reflect treatment dissatisfaction or lost interest in the treatment of some participants, potentially confounding the study’s results. Dropouts, who are more likely to show elevated levels of distress, may have been made aware of the increased need for support through the intervention modules. Patients with clinically significant levels of distress or mental disorders might have accessed support services with more guidance from a health care professional, such as psychotherapy or psycho-oncological counseling. However, no side effects or adverse events were reported in the IG, and the overall robust pattern of results in the ITT, PP, and extended ITT populations suggests a low risk of attrition bias. The fact that participants who dropped out of the study showed higher baseline distress levels may have led to an underestimation of the intervention effect as higher baseline distress levels predicted a greater change in outcome after treatment. Third, due to the nature of the intervention, the group allocation could not be blinded. While experimenter bias was reduced due to a predefined, standardized monitoring procedure and statistical analysis plan, IG participants may have anticipated potential effects. Fourth, the intervention, along with its adherence, was assessed as a whole, which requires the evaluation of specific modules and any potential dose-response relationship in the future. In addition, there was no specific measure to evaluate the subjective usefulness or satisfaction with the digital therapeutic under investigation. Incorporating such a measure could have provided targeted insights into the participants’ perceptions and experiences with the app. However, the observed use behavior, characterized by participants repeatedly accessing the app and actively engaging with its content, may serve as an indirect indicator of the app’s value to the participants. Future studies should aim to validate this interpretation. Finally, the study sample included participants with a wide variety of cancer diagnoses, which did not allow for the examination of diagnosis-specific intervention effects. However, the sample composition is consistent with the target population of the investigated digital therapeutic, which includes patients with cancer of all entities, and strengthens the study’s generalizability and clinical utility. Moreover, a large body of data shows that while variables such as cancer type, treatment status, disease progression, and sex may influence the magnitude of treatment response to supportive therapy, the beneficial effects of supportive therapy are present across various cancer subpopulations [ 65 - 67 ]. In addition, there is a consensus that psychosocial support needs to be integrated into routine cancer care for all cancer types [ 68 , 69 ].
Conclusions
In summary, this RCT demonstrated that Mika, an app-based digital therapeutic that provides a personalized supportive care intervention, can effectively reduce psychological distress and further alleviate symptoms of anxiety, depression, and fatigue in patients with cancer. Digital therapeutics, such as Mika, deliver easily accessible, patient-centered, and effective psychosocial and self-management support for patients with cancer across the course of the disease. Digital therapeutics may present scalable solutions to support patients with cancer worldwide and thus help fill the supportive care gap. Further research is needed to explore the integration of Mika into routine cancer care and its efficacy in diverse clinical settings.
Acknowledgments
The clinical trial was funded by Fosanis GmbH, Berlin.
Data Availability
The data set generated during and analyzed during this study, including individual participant data that underlie the results reported in this article after deidentification (text, tables, and figures), clinical study report, informed consent form, and analytic code, are available from AMT beginning 3 months and ending 5 years following article publication. Access to the data will be granted to investigators whose proposed use of the data has been approved by an independent review committee identified for this purpose, for individual patient data meta-analysis. Proposals for accessing the data may be submitted up to 36 months following article publication.
Authors' Contributions
JSR and GF provided financial support. FS, AM, and HB provided administrative support, with FS also contributing to the collection and assembly of data. MF contributed to data curation. FS, AM, and MF contributed to data analysis and interpretation. FS and AM equally contributed to writing the original draft. All authors contributed to reviewing and editing the draft and provided final approval of the manuscript. AMT, FS, and JSR contributed to the conception and design.
Conflicts of Interest
FS, MF, and HB received research funding for this trial from Fosanis GmbH, which was paid to their institution. AM is an employee at the company Fosanis GmbH. JSR and GF work for the company Fosanis GmbH. They are the managing directors and board members of Fosanis GmbH and own shares of Fosanis GmbH. All other authors declare no other conflicts of interest.
CONSORT-eHEALTH checklist (V 1.6.1).
- Pachman DR, Barton DL, Swetz KM, Loprinzi CL. Troublesome symptoms in cancer survivors: fatigue, insomnia, neuropathy, and pain. J Clin Oncol. Oct 20, 2012;30(30):3687-3696. [ CrossRef ] [ Medline ]
- Mehnert A, Hartung TJ, Friedrich M, Vehling S, Brähler E, Härter M, et al. One in two cancer patients is significantly distressed: prevalence and indicators of distress. Psychooncology. Jan 16, 2018;27(1):75-82. [ CrossRef ] [ Medline ]
- Mitchell AJ, Chan M, Bhatti H, Halton M, Grassi L, Johansen C, et al. Prevalence of depression, anxiety, and adjustment disorder in oncological, haematological, and palliative-care settings: a meta-analysis of 94 interview-based studies. Lancet Oncol. Feb 2011;12(2):160-174. [ CrossRef ] [ Medline ]
- Meggiolaro E, Berardi MA, Andritsch E, Nanni MG, Sirgo A, Samorì E, et al. Cancer patients' emotional distress, coping styles and perception of doctor-patient interaction in European cancer settings. Pall Supp Care. Jul 09, 2015;14(3):204-211. [ CrossRef ] [ Medline ]
- Brown KW, Levy AR, Rosberger Z, Edgar L. Psychological distress and cancer survival: a follow-up 10 years after diagnosis. Psychosom Med. 2003;65(4):636-643. [ CrossRef ] [ Medline ]
- Faller H, Schuler M, Richard M, Heckl U, Weis J, Küffner R. Effects of psycho-oncologic interventions on emotional distress and quality of life in adult patients with cancer: systematic review and meta-analysis. J Clin Oncol. Feb 20, 2013;31(6):782-793. [ CrossRef ] [ Medline ]
- Epstein RM, Street RL. Patient-centered communication in cancer care: promoting healing and reducing suffering. National Cancer Institute. 2007. URL: https://cancercontrol.cancer.gov/sites/default/files/2020-06/pcc_monograph.pdf [accessed 2024-03-05]
- Alcalde Castro M, Chavarri Guerra Y, Ramos-Lopez WA, Covarrubias-Gómez A, Sanchez S, Quiroz P, et al. Patient-reported barriers for accessing supportive care among patients with metastatic cancer treated at a public cancer center in Mexico. J Clin Oncol. Dec 01, 2018;36(34_suppl):124. [ CrossRef ]
- Carrieri D, Peccatori FA, Boniolo G. Supporting supportive care in cancer: the ethical importance of promoting a holistic conception of quality of life. Crit Rev Oncol Hematol. Nov 2018;131:90-95. [ CrossRef ] [ Medline ]
- Kumar P, Casarett D, Corcoran A, Desai K, Li Q, Chen J, et al. Utilization of supportive and palliative care services among oncology outpatients at one academic cancer center: determinants of use and barriers to access. J Palliat Med. Aug 2012;15(8):923-930. [ FREE Full text ] [ CrossRef ] [ Medline ]
- Bellas O, Kemp E, Edney L, Oster C, Roseleur J. The impacts of unmet supportive care needs of cancer survivors in Australia: a qualitative systematic review. Eur J Cancer Care (Engl). Nov 12, 2022;31(6):e13726. [ CrossRef ] [ Medline ]
- Siegel RL, Miller KD, Jemal A. Cancer statistics, 2019. CA Cancer J Clin. Jan 08, 2019;69(1):7-34. [ FREE Full text ] [ CrossRef ] [ Medline ]
- Atun R, Cavalli F. The global fight against cancer: challenges and opportunities. Lancet. Feb 2018;391(10119):412-413. [ CrossRef ]
- Penedo FJ, Oswald LB, Kronenfeld JP, Garcia SF, Cella D, Yanez B. The increasing value of eHealth in the delivery of patient-centred cancer care. Lancet Oncol. May 2020;21(5):e240-e251. [ FREE Full text ] [ CrossRef ] [ Medline ]
- Gussoni G, Ravot E, Zecchina M, Recchia G, Santoro E, Ascione R, et al. Digital therapeutics in oncology: findings, barriers and prospects. A narrative review. Ann Res Oncol. Feb 2022;02(01):55. [ FREE Full text ] [ CrossRef ]
- Yang J, Weng L, Chen Z, Cai H, Lin X, Hu Z, et al. Development and testing of a mobile app for pain management among cancer patients discharged from hospital treatment: randomized controlled trial. JMIR Mhealth Uhealth. May 29, 2019;7(5):e12542. [ FREE Full text ] [ CrossRef ] [ Medline ]
- Ghanbari E, Yektatalab S, Mehrabi M. Effects of psychoeducational interventions using mobile apps and mobile-based online group discussions on anxiety and self-esteem in women with breast cancer: randomized controlled trial. JMIR Mhealth Uhealth. May 18, 2021;9(5):e19262. [ FREE Full text ] [ CrossRef ] [ Medline ]
- Hou IC, Lin HY, Shen SH, Chang KJ, Tai HC, Tsai AJ, et al. Quality of life of women after a first diagnosis of breast cancer using a self-management support mHealth app in Taiwan: randomized controlled trial. JMIR Mhealth Uhealth. Mar 04, 2020;8(3):e17084. [ FREE Full text ] [ CrossRef ] [ Medline ]
- Keum J, Chung MJ, Kim Y, Ko H, Sung MJ, Jo JH, et al. Usefulness of smartphone apps for improving nutritional status of pancreatic cancer patients: randomized controlled trial. JMIR Mhealth Uhealth. Aug 31, 2021;9(8):e21088. [ FREE Full text ] [ CrossRef ] [ Medline ]
- Hernandez Silva E, Lawler S, Langbecker D. The effectiveness of mHealth for self-management in improving pain, psychological distress, fatigue, and sleep in cancer survivors: a systematic review. J Cancer Surviv. Feb 2019;13(1):97-107. [ CrossRef ] [ Medline ]
- Matis J, Svetlak M, Slezackova A, Svoboda M, Šumec R. Mindfulness-based programs for patients with cancer via eHealth and mobile health: systematic review and synthesis of quantitative research. J Med Internet Res. Nov 16, 2020;22(11):e20709. [ FREE Full text ] [ CrossRef ] [ Medline ]
- Adriaans DJ, Dierick-van Daele AT, van Bakel MJ, Nieuwenhuijzen GA, Teijink JA, Heesakkers FF, et al. Digital self-management support tools in the care plan of patients with cancer: review of randomized controlled trials. J Med Internet Res. Jun 29, 2021;23(6):e20861. [ FREE Full text ] [ CrossRef ] [ Medline ]
- Qin M, Chen B, Sun S, Liu X. Effect of mobile phone app-based interventions on quality of life and psychological symptoms among adult cancer survivors: systematic review and meta-analysis of randomized controlled trials. J Med Internet Res. Dec 19, 2022;24(12):e39799. [ FREE Full text ] [ CrossRef ] [ Medline ]
- Springer F, Mehnert-Theuerkauf A. Content features and its implementation in novel app-based psycho-oncological interventions for cancer survivors: a narrative review. Curr Opin Oncol. Jul 01, 2022;34(4):313-319. [ CrossRef ] [ Medline ]
- Spahrkäs SS, Looijmans A, Sanderman R, Hagedoorn M. Beating cancer-related fatigue with the Untire mobile app: results from a waiting-list randomized controlled trial. Psychooncology. Nov 2020;29(11):1823-1834. [ FREE Full text ] [ CrossRef ] [ Medline ]
- Mayer DK, Landucci G, Awoyinka L, Atwood AK, Carmack CL, Demark-Wahnefried W, et al. SurvivorCHESS to increase physical activity in colon cancer survivors: can we get them moving? J Cancer Surviv. Feb 9, 2018;12(1):82-94. [ FREE Full text ] [ CrossRef ] [ Medline ]
- Lengacher CA, Reich RR, Ramesar S, Alinat CB, Moscoso M, Cousin L, et al. Feasibility of the mobile mindfulness-based stress reduction for breast cancer (mMBSR(BC)) program for symptom improvement among breast cancer survivors. Psychooncology. Feb 2018;27(2):524-531. [ FREE Full text ] [ CrossRef ] [ Medline ]
- Mikolasek M, Witt CM, Barth J. Effects and implementation of a mindfulness and relaxation app for patients with cancer: mixed methods feasibility study. JMIR Cancer. Jan 13, 2021;7(1):e16785. [ FREE Full text ] [ CrossRef ] [ Medline ]
- Gustafson DH, DuBenske LL, Atwood AK, Chih MY, Johnson RA, McTavish F, et al. Reducing symptom distress in patients with advanced cancer using an e-alert system for caregivers: pooled analysis of two randomized clinical trials. J Med Internet Res. Nov 14, 2017;19(11):e354. [ FREE Full text ] [ CrossRef ] [ Medline ]
- Chung IY, Jung M, Park YR, Cho D, Chung H, Min YH, et al. Exercise promotion and distress reduction using a mobile app-based community in breast cancer survivors. Front Oncol. 2019;9:1505. [ FREE Full text ] [ CrossRef ] [ Medline ]
- Greer JA, Jacobs J, Pensak N, MacDonald JJ, Fuh C, Perez GK, et al. Randomized trial of a tailored cognitive-behavioral therapy mobile application for anxiety in patients with incurable cancer. Oncologist. Aug 25, 2019;24(8):1111-1120. [ FREE Full text ] [ CrossRef ] [ Medline ]
- Basch E, Deal AM, Kris MG, Scher HI, Hudis CA, Sabbatini P, et al. Symptom monitoring with patient-reported outcomes during routine cancer treatment: a randomized controlled trial. J Clin Oncol. Feb 20, 2016;34(6):557-565. [ FREE Full text ] [ CrossRef ] [ Medline ]
- Greer JA, Park ER, Prigerson HG, Safren SA. Tailoring cognitive-behavioral therapy to treat anxiety comorbid with advanced cancer. J Cogn Psychother. Jan 01, 2010;24(4):294-313. [ FREE Full text ] [ CrossRef ] [ Medline ]
- Carlson LE, Speca M, Faris P, Patel KD. One year pre-post intervention follow-up of psychological, immune, endocrine and blood pressure outcomes of mindfulness-based stress reduction (MBSR) in breast and prostate cancer outpatients. Brain Behav Immun. Nov 2007;21(8):1038-1049. [ CrossRef ] [ Medline ]
- Speca M, Carlson LE, Goodey E, Angen M. A randomized, wait-list controlled clinical trial: the effect of a mindfulness meditation-based stress reduction program on mood and symptoms of stress in cancer outpatients. Psychosom Med. 2000;62(5):613-622. [ CrossRef ] [ Medline ]
- Winters-Stone KM, Dobek J, Nail L, Bennett JA, Leo MC, Naik A, et al. Strength training stops bone loss and builds muscle in postmenopausal breast cancer survivors: a randomized, controlled trial. Breast Cancer Res Treat. Jun 19, 2011;127(2):447-456. [ FREE Full text ] [ CrossRef ] [ Medline ]
- Adam R, Bond C, Murchie P. Educational interventions for cancer pain. A systematic review of systematic reviews with nested narrative review of randomized controlled trials. Patient Educ Couns. Mar 2015;98(3):269-282. [ CrossRef ] [ Medline ]
- Wolff J, Stupin J, Olschewski J, Pirmorady Sehouli A, Maier A, Fofana M, et al. Digital therapeutic to improve cancer-related well-being: a pilot randomized controlled trial. Int J Gynecol Cancer. Jul 03, 2023;33(7):1118-1124. [ CrossRef ] [ Medline ]
- Harrison SE, Watson EK, Ward AM, Khan NF, Turner D, Adams E, et al. Primary health and supportive care needs of long-term cancer survivors: a questionnaire survey. J Clin Oncol. May 20, 2011;29(15):2091-2098. [ CrossRef ] [ Medline ]
- Xiao J, Ye H, He X, Zhang H, Wu F, Chua TS. Attentional factorization machines: learning the weight of feature interactions via attention networks. arXiv. Preprint posted online August 15, 2017. 2017. [ FREE Full text ] [ CrossRef ]
- Sensoy M, Kaplan L, Kandemir M. Evidential deep learning to quantify classification uncertainty. In: Proceedings of the 32nd Conference on Neural Information Processing Systems. 2018. Presented at: NeurIPS '18; December 3-8, 2018; Montreal, QC. URL: https://www.proceedings.com/48413.html
- Mehnert A, Müller D, Lehmann C, Koch U. Die Deutsche version des NCCN distress-thermometers. Z Für Psychiatr Psychol Psychother. Jan 2006;54(3):213-223. [ CrossRef ]
- National Comprehensive Cancer Network. Distress management. Clinical practice guidelines. J Natl Compr Canc Netw. Jul 01, 2003;1(3):344-374. [ CrossRef ] [ Medline ]
- Petermann F. Hospital anxiety and depression scale, Deutsche version (HADS-D). Z Für Psychiatr Psychol Psychother. Jul 2011;59(3):251-253. [ CrossRef ]
- Lai J, Cella D, Chang C, Bode RK, Heinemann AW. Item banking to improve, shorten and computerize self-reported fatigue: an illustration of steps to create a core item bank from the FACIT-Fatigue Scale. Qual Life Res. Aug 2003;12(5):485-501. [ CrossRef ] [ Medline ]
- Guy W. Clinical global impressions scale. Psychiatry. 1976. [ CrossRef ]
- Cohen J. Statistical Power Analysis for the Behavioral Sciences. Oxfordshire, UK. Routledge; 2013.
- Carpenter JR, Roger JH, Kenward MG. Analysis of longitudinal trials with protocol deviation: a framework for relevant, accessible assumptions, and inference via multiple imputation. J Biopharm Stat. Oct 18, 2013;23(6):1352-1371. [ CrossRef ] [ Medline ]
- Enders CK. Applied Missing Data Analysis. New York, NY. The Guilford Press; 2022.
- R Core Team. R: a language and environment for statistical computing. R Foundation for Statistical Computing. 2021. URL: https://www.R-project.org/ [accessed 2024-03-05]
- Ham K, Chin S, Suh YJ, Rhee M, Yu ES, Lee HJ, et al. Preliminary results from a randomized controlled study for an app-based cognitive behavioral therapy program for depression and anxiety in cancer patients. Front Psychol. Jul 25, 2019;10:1592. [ FREE Full text ] [ CrossRef ] [ Medline ]
- Hinz A, Mehnert A, Dégi C, Reissmann DR, Schotte D, Schulte T. The relationship between global and specific components of quality of life, assessed with the EORTC QLQ-C30 in a sample of 2019 cancer patients. Eur J Cancer Care (Engl). Mar 16, 2017;26(2):e12416. [ CrossRef ] [ Medline ]
- Jansen F, van Uden-Kraan CF, van Zwieten V, Witte BI, Verdonck-de Leeuw IM. Cancer survivors' perceived need for supportive care and their attitude towards self-management and eHealth. Support Care Cancer. Jun 26, 2015;23(6):1679-1688. [ CrossRef ] [ Medline ]
- Kessel KA, Vogel MM, Kessel C, Bier H, Biedermann T, Friess H, et al. Mobile health in oncology: a patient survey about app-assisted cancer care. JMIR Mhealth Uhealth. Jun 14, 2017;5(6):e81. [ FREE Full text ] [ CrossRef ] [ Medline ]
- Marthick M, McGregor D, Alison J, Cheema B, Dhillon H, Shaw T. Supportive care interventions for people with cancer assisted by digital technology: systematic review. J Med Internet Res. Oct 29, 2021;23(10):e24722. [ FREE Full text ] [ CrossRef ] [ Medline ]
- Nissen ER, O'Connor M, Kaldo V, Højris I, Borre M, Zachariae R, et al. Internet-delivered mindfulness-based cognitive therapy for anxiety and depression in cancer survivors: a randomized controlled trial. Psychooncology. Jan 18, 2020;29(1):68-75. [ FREE Full text ] [ CrossRef ] [ Medline ]
- Egbring M, Far E, Roos M, Dietrich M, Brauchbar M, Kullak-Ublick GA, et al. A mobile app to stabilize daily functional activity of breast cancer patients in collaboration with the physician: a randomized controlled clinical trial. J Med Internet Res. Sep 06, 2016;18(9):e238. [ FREE Full text ] [ CrossRef ] [ Medline ]
- Chai PR, Schwartz E, Hasdianda MA, Azizoddin DR, Kikut A, Jambaulikar GD, et al. A brief music app to address pain in the emergency department: prospective study. J Med Internet Res. May 20, 2020;22(5):e18537. [ FREE Full text ] [ CrossRef ] [ Medline ]
- Bisseling E, Cillessen L, Spinhoven P, Schellekens M, Compen F, van der Lee M, et al. Development of the therapeutic alliance and its association with internet-based mindfulness-based cognitive therapy for distressed cancer patients: secondary analysis of a multicenter randomized controlled trial. J Med Internet Res. Oct 18, 2019;21(10):e14065. [ FREE Full text ] [ CrossRef ] [ Medline ]
- Parikh RB, Basen-Enquist KM, Bradley C, Estrin D, Levy M, Lichtenfeld JL, et al. Digital health applications in oncology: an opportunity to seize. J Natl Cancer Inst. Oct 06, 2022;114(10):1338-1339. [ FREE Full text ] [ CrossRef ] [ Medline ]
- Aapro M, Bossi P, Dasari A, Fallowfield L, Gascón P, Geller M, et al. Digital health for optimal supportive care in oncology: benefits, limits, and future perspectives. Support Care Cancer. Oct 12, 2020;28(10):4589-4612. [ FREE Full text ] [ CrossRef ] [ Medline ]
- Rankin NM, Butow PN, Thein T, Robinson T, Shaw JM, Price MA, et al. Everybody wants it done but nobody wants to do it: an exploration of the barrier and enablers of critical components towards creating a clinical pathway for anxiety and depression in cancer. BMC Health Serv Res. Jan 22, 2015;15(1):28. [ FREE Full text ] [ CrossRef ] [ Medline ]
- Leader AE, Capparella LM, Waldman LB, Cammy RB, Petok AR, Dean R, et al. Digital literacy at an urban cancer center: implications for technology use and vulnerable patients. JCO Clinical Cancer Informatics. Dec 2021;(5):872-880. [ CrossRef ]
- den Bakker CM, Schaafsma FG, Huirne JA, Consten EC, Stockmann HB, Rodenburg CJ, et al. Cancer survivors' needs during various treatment phases after multimodal treatment for colon cancer - is there a role for eHealth? BMC Cancer. Dec 04, 2018;18(1):1207. [ FREE Full text ] [ CrossRef ] [ Medline ]
- Cillessen L, Johannsen M, Speckens AE, Zachariae R. Mindfulness-based interventions for psychological and physical health outcomes in cancer patients and survivors: a systematic review and meta-analysis of randomized controlled trials. Psychooncology. Dec 11, 2019;28(12):2257-2269. [ FREE Full text ] [ CrossRef ] [ Medline ]
- Tauber NM, O'Toole MS, Dinkel A, Galica J, Humphris G, Lebel S, et al. Effect of psychological intervention on fear of cancer recurrence: a systematic review and meta-analysis. J Clin Oncol. Nov 01, 2019;37(31):2899-2915. [ FREE Full text ] [ CrossRef ] [ Medline ]
- Merluzzi TV, Pustejovsky JE, Philip EJ, Sohl SJ, Berendsen M, Salsman JM. Interventions to enhance self-efficacy in cancer patients: a meta-analysis of randomized controlled trials. Psychooncology. Sep 09, 2019;28(9):1781-1790. [ FREE Full text ] [ CrossRef ] [ Medline ]
- Jacobsen PB, Wagner LI. A new quality standard: the integration of psychosocial care into routine cancer care. J Clin Oncol. Apr 10, 2012;30(11):1154-1159. [ CrossRef ] [ Medline ]
- Fann JR, Ell K, Sharpe M. Integrating psychosocial care into cancer services. J Clin Oncol. Apr 10, 2012;30(11):1178-1186. [ CrossRef ] [ Medline ]
Abbreviations
Edited by YH Lin; submitted 21.08.23; peer-reviewed by F Denis, A Haussmann, N Schaeffeler, P Chow; comments to author 24.01.24; accepted 23.02.24; published 25.04.24.
©Franziska Springer, Ayline Maier, Michael Friedrich, Jan Simon Raue, Gandolf Finke, Florian Lordick, Guy Montgomery, Peter Esser, Hannah Brock, Anja Mehnert-Theuerkauf. Originally published in the Journal of Medical Internet Research (https://www.jmir.org), 25.04.2024.
This is an open-access article distributed under the terms of the Creative Commons Attribution License (https://creativecommons.org/licenses/by/4.0/), which permits unrestricted use, distribution, and reproduction in any medium, provided the original work, first published in the Journal of Medical Internet Research, is properly cited. The complete bibliographic information, a link to the original publication on https://www.jmir.org/, as well as this copyright and license information must be included.

An official website of the United States government
The .gov means it’s official. Federal government websites often end in .gov or .mil. Before sharing sensitive information, make sure you’re on a federal government site.
The site is secure. The https:// ensures that you are connecting to the official website and that any information you provide is encrypted and transmitted securely.
- Publications
- Account settings
Preview improvements coming to the PMC website in October 2024. Learn More or Try it out now .
- Advanced Search
- Journal List
- SAGE Open Med
New approaches and procedures for cancer treatment: Current perspectives
Dejene tolossa debela.
1 Center for Innovative Drug Development and Therapeutic Trials for Africa (CDT-Africa), College of Health Sciences, Addis Ababa University, Addis Ababa, Ethiopia
Seke GY Muzazu
2 Enteric Diseases and Vaccines Research Unit, Centre for Infectious Disease Research in Zambia (CIDRZ), Lusaka, Zambia
Kidist Digamo Heraro
3 Wachemo University, Hossana, Ethiopia
Maureen Tayamika Ndalama
Betelhiem woldemedhin mesele.
4 Kotebe Metropolitan University, Addis Ababa, Ethiopia
Dagimawi Chilot Haile
5 University of Gondar, Gondar, Ethiopia
Sophia Khalayi Kitui
Tsegahun manyazewal.
Cancer is a global health problem responsible for one in six deaths worldwide. Treating cancer has been a highly complex process. Conventional treatment approaches, such as surgery, chemotherapy, and radiotherapy, have been in use, while significant advances are being made in recent times, including stem cell therapy, targeted therapy, ablation therapy, nanoparticles, natural antioxidants, radionics, chemodynamic therapy, sonodynamic therapy, and ferroptosis-based therapy. Current methods in oncology focus on the development of safe and efficient cancer nanomedicines. Stem cell therapy has brought promising efficacy in regenerating and repairing diseased or damaged tissues by targeting both primary and metastatic cancer foci, and nanoparticles brought new diagnostic and therapeutic options. Targeted therapy possessed breakthrough potential inhibiting the growth and spread of specific cancer cells, causing less damage to healthy cells. Ablation therapy has emerged as a minimally invasive procedure that burns or freezes cancers without the need for open surgery. Natural antioxidants demonstrated potential tracking down free radicals and neutralizing their harmful effects thereby treating or preventing cancer. Several new technologies are currently under research in clinical trials, and some of them have already been approved. This review presented an update on recent advances and breakthroughs in cancer therapies.
Introduction
Cancer is a global health problem responsible for one in six deaths worldwide. In 2020, there were an estimated 19.3 million new cancer cases and about 10 million cancer deaths globally. Cancer is a very complicated sequence of disease conditions progressing gradually with a generalized loss of growth control. 1 – 3 There were only a few options of cancer treatment for patients for many decades which include surgery, radiation therapy, and chemotherapy as single treatments or in combination. 4 , 5 But recently, many pathways involved in cancer therapy progression and how they can be targeted has improved dramatically, with combinatorial strategies, involving multiple targeted therapies or “traditional” chemotherapeutics, such as the taxanes and platinum compounds, being found to have a synergistic effect. 6 New approaches, such as drugs, biological molecules, and immune-mediated therapies, are being used for treatment even if the excepted therapy level has not reached that resists the mortality rate and decreases the prolonged survival time for metastatic cancer.
The creation of a new revolution in neoplastic cancer or targeting drugs depends on the pathways and characteristics of different tumor entities. 7 Chemotherapy is considered the most effective and widely used modality in treating cancers as used alone or in combination with radiotherapy. Genotoxicity is how chemotherapy drugs target the tumor cells mainly producing reactive oxygen species that largely destroy tumor cells. 8 Hormonal treatments are also widely used for cancer malignancies and considered as cytostatic because it restricts tumor development by limiting the hormonal growth factors acting through the direction of hypothalamic–pituitary–gonadal axis (HPGA), hormone receptor blockage, and limiting of adrenal steroid synthesis. 9
In this narrative review, a general overview of the most advanced and novel cancer therapies was provided. In addition, also new strategies currently under investigation at the research stage that should overwhelm the drawbacks of standard therapies; different strategies to cancer diagnosis and therapy; and their current status in the clinical context, underlining their impact as innovative anti-cancer approaches.
Cancer treatment modalities
We can see cancer treatment modalities by dividing them into conventional (traditional) and advanced or novel or modern categories. In this era worldwide, over half of all ongoing medical treatment trials are focusing on cancer treatments. 7 Entities, such as the type of cancer, its site, and severity, guide to select treatment options and its progress. The most widely used traditional treatment methods are surgery, chemotherapy, and radiotherapy, while modern modalities include hormone therapy, anti-angiogenic, stem cell therapies, immunotherapy, and dendritic cell-based immunotherapy. 10
Conventional cancer therapies
The most recommended conventional cancer treatment strategies include surgical resection of the tumors followed by radiotherapy with x-rays and/or chemotherapy. 11 Of these modalities, surgery is most effective at an early stage of disease progression. Radiation therapy can damage healthy cells, organs, and tissues. Although chemotherapy has reduced morbidity and mortality, virtually all chemotherapeutic agents damage healthy cells, especially rapidly dividing and growing cells. 12 Drug resistance, a major problem with chemotherapy, is a phenomenon wherein cancer cells that initially were suppressed by an anti-cancer drug develop resistance to the drug. This is caused primarily by reduced drug uptake and increased drug efflux. 13 Limitations of conventional chemotherapeutic modality, such as dosage selection difficulty, lack of specificity, rapid drug metabolism, and mainly harmful side effects. 14
Advanced and innovative cancer therapies
Among the obstacles of cancer, drug resistance and its delivery systems are the most problem in cancer cure and decreasing signs and symptoms; but currently, there are many approved treatment approaches and drugs. The efficiency of conventional cancer is reduced due to tumor pathology and architectural abnormality of tumor tissue blood vessels. 15 The following are the advanced and innovative cancer therapy types with their benefits and challenges.
Stem cells therapy
Stem cells are undifferentiated cells present in the bone marrow (BM) with an ability to differentiate into any type of body cell. Stem cell therapeutic strategy is also one of the treatment options for cancer which are considered to be safe and effective. Application of stem cell is yet in the experimental clinical trial; for example, their use in the regeneration of other damaged tissue is being explored. Mesenchymal stem cells (MSCs) are currently being used in trials that are delivered from the BM, fat tissues, and connective tissues. 16
Pluripotent stem cells
Embryonic stem cells (ESCs) isolated from the uniform inner mass cells of the embryo possess the flexibility to administer rise to any or all kinds of cells except those within the placenta. In 2006, the invention of Yamanaka factors to induce pluripotent stem cells (iPSCs) from physical cells in a culture marked a breakthrough in cell biology. 17 Avoiding ethical issues from embryo destruction, iPSCs and ESCs have the same characteristics. Hematopoietic embryonic stem cells (hESCs) and iPSCs are currently used for the induction of effector T cells and natural killer (NK) cells, 18 and anti-tumor vaccine preparation. 19
Adult stem cells
Adult stem cells (ASCs) groups often used in tumor therapy include hematopoietic stem cells (HSCs), MSCs, and neural stem cells (NSCs). HSCs, located in BM, can form all mature blood cells in the body. Currently, only approved by the Food and Drug Administration (FDA) is the infusion of HSCs derived from cord blood to treat multiple myeloma and leukemia. 20 MSCs are found in many tissues and organs, playing important roles in tissue repair and regeneration into cells, such as osteocytes, adipocytes, and chondrocytes. MSCs have special biological characteristics and are used as complimentary with other approaches in treating tumors. 21 NSCs can self-renew and generate new neurons and glial cells and are used for treating both primary and metastatic breast and other tumors. 22
Cancer stem cells
Cancer stem cells (CSCs) are generated in normal stem cells or precursor/progenitor cells by the epigenetic mutations process. Their role in tumor treatment includes cancer growth, metastasis, and recurrence, so that it could give promise in the treatment of solid tumors. 23 Stem cells have several action mechanisms in treating the tumor. The homing process is one mechanism which is a rapid migration of HSCs into defined stem cell niches in BM after that the transplants undergo the engraftment process before giving rise to specialized blood cells. This mechanism is dependent on the active interaction between stem cell CXCR4 receptors and requires their interaction with endothelial cells through LFA-1, VLA-4/5, CD44, and the secretion of matrix degradable enzyme MMP-2/9. 22 The second mechanism is the tumor-tropic effect in which the migration of MSCs toward tumor microenvironment (TM) after attraction by CXCL16, SDF-1, CCL-25, and IL-6 secreted by tumor cells and differentiation of MSCs within the tumor cells which contributes to tumor stromal development. 24 Stem cells also act by paracrine factor secretion, including extracellular vesicles (EVs) and soluble materials, 25 and their differentiation capacity, such as transplanted HSCs, can give rise to all blood cell types. 26
Generally, cancer treatment using stem cell therapy by various strategies, including transplantation of HSC, 27 MSC infusion, 28 therapeutic carriers, 29 generation of immune effector cells, 30 and vaccine production. 31 The stem cell cancer therapy approach confronted the following side effects: (1) tumorigenesis, (2) adverse events in allogeneic HSC transplantation, (3) drug toxicity and drug resistance, (4) increased immune responses and autoimmunity, and (5) viral infection. 22 Despite several successes, there are challenges, such as therapeutic dose control, low cell targeting, and retention in tumor sites, that should be investigated and overcome in the future. In addition, existing results from stem cell technologies are highly encouraging for tumor treatment but it still needs further efforts to improve the safety and efficacy before they could enter clinical trials. Table 1 summarized the licensed list of stem cell therapies.
Licensed stem cell therapies.
AML: acute myelogenous leukemia; FDA: The US Food and Drug Administration; iPSC: induced pluripotent stem cell; MSC-INβ: mesenchymal stem cells with interferon beta.
Targeted drug therapy
Targeted cancer therapies are drugs or other substances which are sometimes interchangeably used as “molecularly targeted drugs,” “molecularly targeted therapies,” and “precision medicines.” Those drugs’ mechanism of action is by interfering with growth molecules which leads to blocking the growth and spreading of cancer. 34 Tumor initiation and progression are determined by the TM of an atypical tumor which comprises endothelial cells, pericytes, smooth muscle cells, fibroblasts, various inflammatory cells, dendritic cells, and CSCs. There are various signaling mechanisms and pathways that TM-forming cells dynamically interact with the cancerous cells which are suitable for sustaining a reasonably high cellular proliferation. So, it is the area of research interest using TM conditions to mediate effective targeting measures for cancer therapy. 35
Selectively treating cancer cells with conventional chemotherapy is difficult since it is similar to normal cells. So those problems are intervened by cellular mechanisms, such as cell cycle arrest, apoptosis induction, proliferation prevention, and interfering with metabolic reprogramming by targeted drug therapy agents. 36 Modifying TM and targeting TM for drug delivery for effective treatment are two strategies that can be used for the treatment of cancer. 37 Targeted therapy drugs do work in different ways from standard chemotherapy drugs treatment like attacking cancer cells while doing less damage to normal cells which is a programming that sets them apart from normal, healthy cells. 38
Using targeted therapy markedly increased the survival rate for some diseases, for example, from 17% to 24% in patients with advanced pancreatic cancer, the addition of erlotinib to standard chemotherapy. Imatinib has had a dramatic effect on chronic myeloid leukemia, and rituximab, sunitinib, and trastuzumab have revolutionized the treatment of renal cell carcinoma and breast cancer, respectively. 39
We can classify the targeted cell agents based on the mechanism of their work or their target site. Some enzymes serve as signals for cancer cells to grow. Some targeted therapies inhibit enzymes that are signals for cancer cells to grow. These drugs are called enzyme inhibitors. Blocking these cell signals can inhibit cancer from getting bigger and spreading. 40
Some targeted therapies are called apoptosis-inducing drugs because they are aimed right at the parts of the cell that control whether cells live or die. The examples are serine/threonine kinase, protein kinase B (PKB/Akt), which promotes cell survival, and inhibitors of this protein are in the preclinical phase. 41
These agents stop the tumors from making new blood vessels which helps cut off the tumors’ blood supply so that tumors cannot grow. In addition, they arrest tumor growth that involves by curtailing blood supply to the tumor by inhibiting angiogenic factors, such as vascular endothelial growth factor (VEGF) or its receptors. The study showed the survival of patients with advanced colorectal carcinoma extended by months after the use of Avastin (bevacizumab) in combination with 5-fluorouracil-based chemotherapy. 42
Types of target agents
Monoclonal antibodies.
Antibody drugs are man-made versions of immune system proteins administered intravenously to attack certain targets on cancer cells. They contain a more proportion of human components than murine components. 43 Their attack mechanisms of action are recruiting host immune functions to attack the target cell, binding to ligands or receptors thereby interrupting essential cancer cell processes, and carrying a lethal payload, such as radioisotope or toxin, to the target cell. 44 Gemtuzumab is an example of a CD-33-specific monoclonal antibody currently used for AML treatment by conjugating with calicheamicin. 45 In addition, ibritumomab tiuxetan is an anti-CD20, a 90Y metal isotope-based is developed in clinical therapy. 46 Delivery of active therapeutics, prodrug activation enzymes, and chemotherapy toxins are also another use of target agents of monoclonal antibodies. 47
Small molecule inhibitors
These are smaller protein in size (⩽500 Da) than monoclonal antibodies, so that they can simply translocate through plasma membranes and can be taken orally. Their main function is interrupting cellular processes by interfering with the intracellular signaling of tyrosine kinases which leads to the inhibition of tyrosine kinase signaling and initiates a molecular cascade that can lead to the inhibition of cell growth, proliferation, migration, and angiogenesis in malignant tissues. 48 Examples of small molecule inhibitors are gefitinib and erlotinib which inhibit epidermal growth factor receptor (EGFR) kinase and EGFR in non-small cell lung cancer (NSCLC) patients, respectively. There are also lapatinib and sorafenib which act on the inhibition of EGFR/Erb-B2 Receptor Tyrosine Kinase 2 (ERBB2) for ERBB2-positive breast cancer and VEGFR kinase, in renal cancer. 49
Ablation cancer therapy
Ablation is a treatment technique that destroys tumors without removing them mostly indicated for small-size tumors of less than 3 cm and the surgical option is contraindicated. Ablation is also used with embolization for larger tumors. However, this technique might not be indicated for treating tumors near major blood vessels, the diaphragm, or major bile ducts due to destroying some of the normal tissue around the tumor. 50
Thermal ablation
This technique uses extreme hyperthermia or hypothermia to destruct tumor tissue concentrating on a focal zone in and around the tumor. Similar to surgery, thermal ablation removes the tumor and a 5–10 mm thick margin of seemingly normal tissue but the tissue is killed in situ and then absorbed by the body later. The procedure is similar to surgery using an open, laparoscopic, or endoscopic approach but is commonly applied using a percutaneous or non-invasive approach. The type of tumor, site, physician’s choice, and health status determine the approach. 51
Radiofrequency ablation (RFA), microwave ablation, high-intensity focused ultrasound, and cryoablation are currently being used in the clinical setting. Cryoablation uses a hypothermic modality to induce tissue damage by a freeze-thaw process against others. All these treatments operate on the principle of hyperthermia except cryoablation. Of all the ablation techniques, cryoablation demonstrated the highest potential to elicit a post-ablative immunogenic response. 52
Recent studies showed additional to tissue disruption RFA and cryoablation can modulate the immune system that they were applied as therapy on TM and in the systematic circulation. Evidence has shown that ablation procedures affect carcinogenesis due to its local inflammatory response leading to an immunogenic gene signature. 53
The advantage of this procedure over surgery is that it provides a minimal (e.g. percutaneously or laparoscopically) or non-invasive approach to cancer therapy and gets attention as an alternative to standard surgical therapies. 54
Cryoablation
Cryoablation is one of the ablation techniques which ablates the extensive tissue by freezing to lethal temperatures followed by liquid formation, causing extensive tissue. Benign and malignant primary tumors are mostly treated by this therapy. 55 James Arnott reported that the freezing temperatures can impair cancer cell viability after he attempted the usage of cold temperatures by salt and ice solutions for the generation of local numbness before surgical operations in the nineteenth century. He suggested cryoablation as an attractive therapeutic option and increased a patient’s survival. 56
Cryoablation techniques are based on the principle of the Joule–Thomson effect which was studied in the 1930s by many researchers and concluded using liquid CO 2 under high pressure, liquid air, and liquid oxygen to achieve the cooling effect and the subsequent formation of ice crystals so employed to treat lesions, warts, and keratosis. However, after 1950, Allington replaced liquid N 2 for the treatment of various skin lesion disorders. 47
RFA therapy
RFA is a minimally invasive procedure and an image-guided technique using hyperthermic (high-frequency electrical currents) conditions to destroy cancer cells. Imaging techniques, such as ultrasound, computed tomography (CT), or magnetic resonance imaging (MRI), guide needle electrodes into a tumor cell. Generally, RFA is the most effective approach for treating small-size tumors of less than 3 cm in diameter. RFA can be used in combination with other conventional cancer treatment options. 57 After starting the use of deployable devices or multiple-electrode systems, RFA can treat medium tumors (up to 5 cm diameter). 58
Gene therapy
Gene therapy is the insertion of a normal copy of a defective gene in the genome to cure a specific disorder. The first application dates back to 1990 when a retroviral vector was exploited to deliver the adenosine deaminase (ADA) gene to T cells in patients with severe combined immunodeficiency (SCID). Approximately, about 2900 gene therapy clinical trials are currently ongoing, two-third of which are related to cancer. Strategies, such as expression of proapoptotic and chemosensitizing genes, expression of wild-type tumor suppressor genes, expression of genes able to solicit specific anti-tumor immune responses, and targeted silencing of oncogenes, are under evaluation for cancer gene therapy. 47
Thymidine kinase (TK) gene delivery is effective for the administration of prodrug ganciclovir to activate its expression and induce specific cytotoxicity. 59 The p53 tumor suppressor gene which is vectors carrying has been assessed for the clinical purpose very recently. ONYX-015 has been tested in NSCLC patients and gave a high response rate when given alone or combined with chemotherapy. 60 Gendicine, a recombinant adenovirus carrying wild-type p53-induced complete disease regression in head and neck squamous cell cancer had similar success when combined with radiotherapy. 61
Some challenges that have been faced with gene therapy are the selection of the right conditions and the choice of the best delivery mechanism. Identified drawbacks of this therapy are genome integration, limited efficacy in specific subsets of patients, and high chances of being neutralized by the immune system. Basic research and medical translation used RNA interference (RNAi) as an efficient technology that able to produce targeted gene silencing. 62 RNA-induced silencing complex (RISC) mediates the targeted gene silencing process by cleaving the messenger RNA (mRNA) and interference with protein synthesis. 63 A siRNAs can be designed to block desired targets, involving cell proliferation and metastatic invasion; hence, precise molecular mechanisms are a triggering factor for tumor formation. This method relies on siRNA-mediated gene silencing of anti-apoptotic proteins, transcription factors (i.e. c-myc gene), 64 , 65 or cancer mutated genes (i.e. K-RAS). 66
Advantages of siRNA-based drugs are safety, high efficacy, specificity, few side effects, and low costs of production. 67 However, occasionally, they can induce off-target effects or elicit innate immune responses, followed by specific inflammation. 68 Delivery methods currently under study are chemical modification (insertion of a phosphorothioate at 3’ end, introduction of a 2’ O-methyl group, and modification by 2,4-dinitrophenol) and lipid encapsulation, or conjugation with organic molecules (polymers, peptides, lipids, antibodies, small molecules) efficiently target to spontaneously cross cell membranes of naked siRNAs. 69 Interaction of cationic liposomes with negatively charged nucleic acids facilitates easy transfection by simple electrostatic interactions. 70 They can be constituted by 1,2-dioleoyl-3-trimethylammonium propane (DOTAP) and N-[1-(2,3-dioleoyloxy) propyl]-N, Ntrimethylammonium methyl sulfate (DOTMA). 71 Currently, a Phase I clinical trial is recruiting patients for evaluating the safety of Eph receptor A2 (EphA2) targeting 1,2-dioleoyl-sn-glycero-3-phosphocholine (DOPC) encapsulated siRNA (siRNA-EphA2- DOPC) in patients with advanced and recurrent cancer. 72 siRNAs can be concentrated in cationic polymers, such as chitosan, cyclodextrin, and polyethyleneimine (PEI). 73 CALAA-01 is one of the cyclodextrin polymers conjugated with human transferrin is being entered a Phase I clinical trial. PEI has been used as an anti-cancer by forming small cationic nanoparticles and loading with human epidermal growth factor receptor 2 (HER-2 receptor)-specific siRNA. 74 Phase II clinical trial has been started to evaluate Local Drug EluteR (siG12D LODER) directed to mutated Kirsten rat sarcoma (K-RAS) oncogene for the treatment of advanced pancreatic cancer. Conjugating to peptides, antibodies, and aptamers improves stability during circulation and enhances cellular uptake of siRNAs. 75 The introduction of nanocarriers has largely improved siRNAs stability, pharmacokinetics and biodistribution properties, and targeting specificity. Polyallylamine phosphate nanocarriers have been developed to release siRNAs in the cytoplasm after disassembly at low endosomal pH. 76
Dose correction and variabilities between individuals and different stages of disease are challenging issues on clinical translation of the siRNA-based approach. In the future, the needed research is on setting up the best-personalized therapy and toward controlled release to reach only specific targets on treating the tumor. Table 2 summarizes the gene therapy drugs based on their mechanism of action and induction.
Summary of gene therapy approaches.
Natural antioxidants
Day to day, the anatomy undergoes many exogenous insults, such as ultraviolet (UV) rays, pollution, and tobacco smoke, that end in the assembly of reactive species, particularly oxidants and free radicals, liable for the onset of many diseases, together with cancer. These molecules can even be made as a consequence of clinical administration of medication; however, they are additionally naturally created within our cells and tissues by mitochondria and peroxisomes, and from macrophages metabolism, throughout traditional physiological aerobic processes. 47
Oxidative stress and radical oxygen species can significantly change the regulation of transcription factors by damaging the DNA and other bio-macromolecule. 77
Vitamins, polyphenols, and plant-derived bioactive compounds are natural antioxidants used as preventive and therapeutic drugs against these molecules that damage the body due to their anti-inflammatory and antioxidant properties. 78 Studies added to cancer therapy after appreciating their anti-proliferative and proapoptotic properties. Compounds, such as vitamins, alkaloids, flavonoids, carotenoids, curcumin, berberine, quercetin, and others, are examples of natural antioxidants screened in vitro and in vivo. 79
Limited bioavailability and/ or toxicity is one of the challenges of natural drugs while their translation into clinical practice. 47 Curcumin has cytotoxic effects in different kinds of tumors, such as the brain, lung, leukemia, pancreatic, and hepatocellular carcinoma, 80 while sparing normal cells at effective therapeutic doses. The curcumin’s biological properties, treatment duration and efficient therapeutic doses are under study. 80 This day, about 27 clinical trials are done, while 40 are under study on curcumin.
Berberine is an alkaloid compound that has been studied to be effective against different cancers as a chemopreventive agent, modulating many signaling pathways. Different nanotechnological strategies have been developed to facilitate its delivery across cell membranes due to their poorly soluble in water. 81 Six clinical trials are under study and two have been completed.
Quercetin is another natural plant origin agent that is effective alone and also in combination with chemotherapeutic agents in treating many cancers, such as lung, prostate, liver, colon, and breast cancers. 82 Quercetin’s mechanism of action is by binding to cellular receptors and the interference of several signaling pathways. 83 Currently, six clinical trials are under study and seven studies have been completed.
Current clinical trials
In recent years, analysis of cancer medication has taken outstanding steps toward more practical, precise, and fewer invasive cancer treatments in the research of clinical trials ( Figure 1 ).

Current status of clinical trials of innovative and novel strategies of cancer therapy.
Currently, the most frequent entries focusing on cancer therapies in the database of clinical trials ( www.clinicalTrials.gov ) include the terminologies stem cell, targeted therapy, immunotherapy, and gene therapy because they are very promising and effective. Table 3 summarizes the potential advantages and disadvantages of the new treatment approaches.
Comparison of advantageous and disadvantageous of new cancer therapies.
MRI: magnetic resonance imaging.
Table 4 summarizes the approaches to advanced cancer therapies and their respective delivery systems with examples.
Advanced therapy approaches and delivery systems.
Current methods in oncology focus on the development of safe and efficient cancer nanomedicines. Targeted medical care helped rising the biodistribution of recent or already tested chemotherapeutical agents around the specific tissue to be treated; different methods, such as sequence medical care, siRNAs delivery, therapy, and inhibitor molecules, supply new potentialities to cancer patients. Gene therapy acts by direct in situ insertion of exogenous genes into benign tumors. Noticeably, stem cells can be used as regenerative medicine, therapeutic carriers, drug targeting, and generation of immune cells because of having unique biological actions on other cells. 22 On the opposite hand, thermal ablation and magnetic hyperthermia are promising alternatives to the growth surgical process. Finally, radionics and pathomics approaches facilitate the management of huge knowledge sets from cancer patients to enhance prognosis and outcomes. Much progress has been made, but many others are likely to come soon, producing more and more ad hoc personalized therapies. Further development and refinement of drug delivery systems are essential for improving therapeutic outcomes.
Acknowledgments
The authors thank the Center for Innovative Drug Development and Therapeutic Trials for Africa (CDT-Africa), Addis Ababa University, for the support rendered.
Author contributions: D.T.D. is a major contributor in writing the manuscript. All others reviewed and approved the manuscript.
Declaration of conflicting interests: The author(s) declared no potential conflicts of interest with respect to the research, authorship, and/or publication of this article.
Funding: The author(s) received no financial support for the research, authorship, and/or publication of this article.

- Coronavirus Updates
- Education at MUSC
- Adult Patient Care
- Children's Health
- Hollings Cancer Center
- Research at MUSC
- MUSC Catalyst News
- Prolonging T cell survival
Improving cancer immunotherapy by prolonging T-cell survival
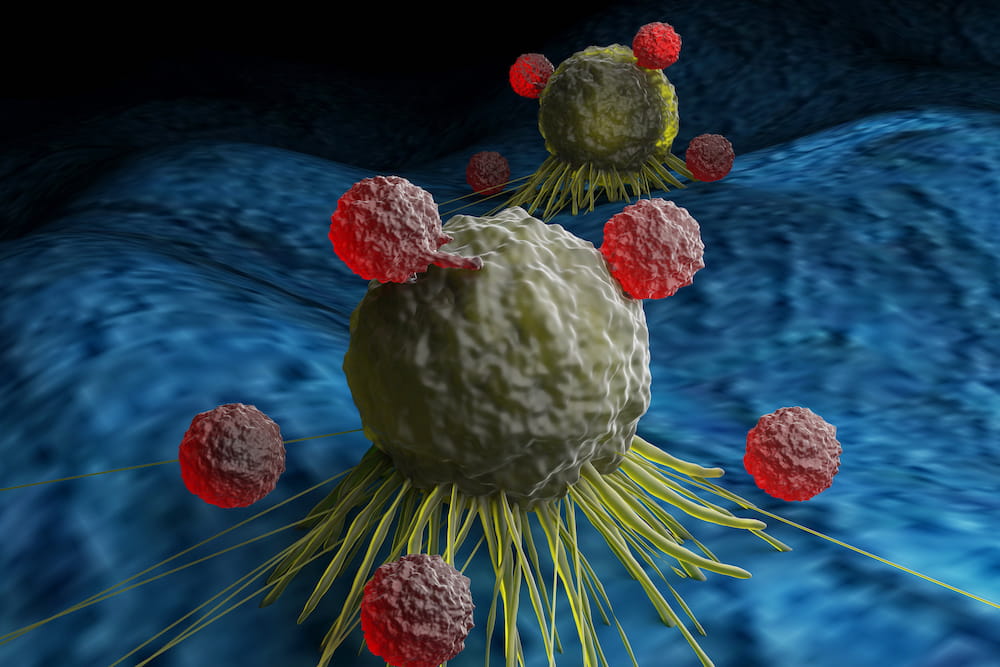
In the past decade, immunotherapy has emerged as the fourth pillar of cancer treatment, joining surgery, radiotherapy and chemotherapy. It is an approved treatment in 15 cancers, including melanoma and some types of lymphomas and leukemias, but it is not effective in all cancers or in all patients.
Immunotherapy uses a patient’s own cancer-fighting cells, or T-cells, and adapts them so that they do a better job at killing cancer cells. T-cells are excellent at identifying and attacking tumor cells but can become exhausted in the tumor microenvironment, where they can become overworked and overstimulated and are starved of glucose and other nutrients by tumor cells. Helping these cells to function better and live longer could improve immunotherapy responses.
“T-cell exhaustion has been a key factor in preventing optimal responses to immunotherapy,” said Shikhar Mehrotra, Ph.D. co-leader of the Cancer Biology & Immunology Research Program and scientific director of the Center for Cellular Therapy at MUSC Hollings Cancer Center.
Research in the Mehrotra Lab suggests that overcoming T-cell exhaustion could improve responses to two types of immunotherapies – anti-PD1 therapy and CAR-T therapy.
In a recent study reported in the Proceedings of the National Academies of Science , Mehrotra and his team, which included Shilpak Chatterjee, Ph.D., showed that T-cell exhaustion limited the efficacy of anti-PD1 therapy. That therapy works by unmasking tumor cells, allowing T-cells to see and target them.
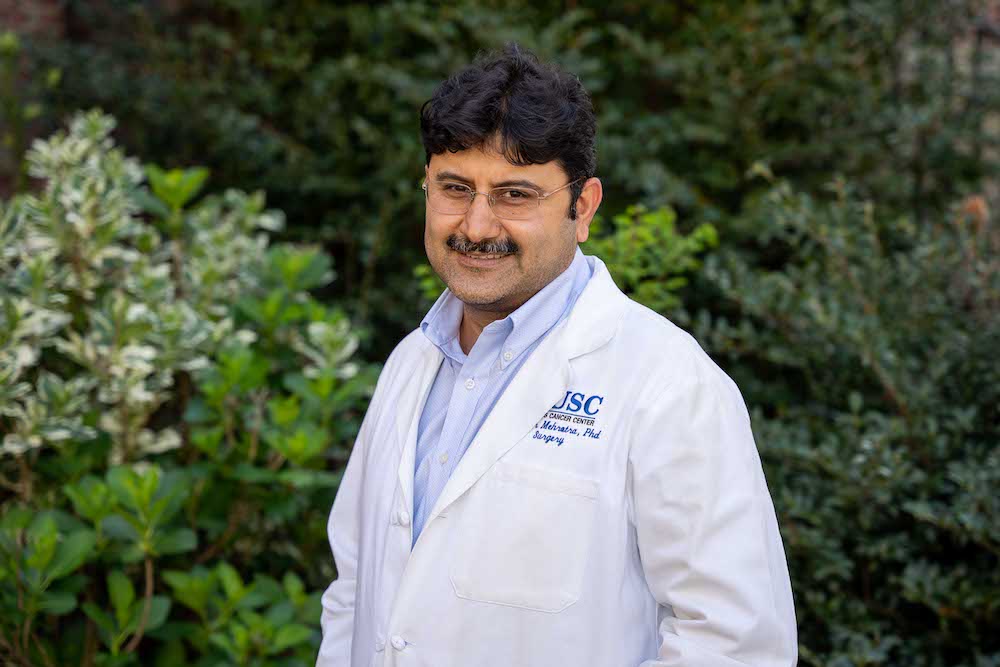
The study showed that T-cells expressing the cell receptor CD38 were more susceptible to exhaustion. Using an anti-CD38 antibody, the research team was able to block CD38 and overcome T-cell exhaustion. They then showed in a mouse model that overcoming T-cell exhaustion by blocking CD38 restored the efficacy of anti-PD1 therapy against cancer. However, the anti-CD38 antibody they used in the mouse model would not be appropriate for use in humans.
To find a way to combat T-cell exhaustion in humans, and specifically for CAR-T therapy, Mehrotra and Chatterjee have developed and patented a cytokine “cocktail” that can be used to reduce CD38 expression on T-cells. In CAR-T therapy, the patient’s own immune cells are harvested, enhanced to detect and fight the cancer cells more effectively and then put back into the patient.
Although the processing of these cells outside the body makes them better able to fight cancer, it also exhausts them, making it difficult for them to survive long in the nutrient-depleted tumor microenvironment. Treating the cells with the cytokine cocktail while they are out of the body not only reduces their expression of CD38, a marker of exhaustion, but it also helps to ensure their function and longevity by giving them the traits of both Th1 (effector) and Th17 (stemness) cells.
"In the clinical setting, we want these T-cells to be ‘marathon runners,’ not sprinters in a 100-meter dash.” -- Dr. Shikhar Mehrotra
Th1 cells can mount an anti-cancer response but are short-lived, while Th17 cells have greater longevity but are unable to mount a response. Combining the two harnesses the strengths of both, producing T-cells that live longer and can mount an effective response. These cells are also less likely to depend on glucose and better able to withstand the nutrient-deprived tumor microenvironment.
Mehrotra believes that the cocktail could be an important key to overcoming exhaustion in T-cells, ensuring their long-term effectiveness and improving responses to immunotherapy.
“In the clinical setting, we want these T-cells to be ‘marathon runners,’ not sprinters in a 100-meter dash,” said Mehrotra.
The cocktail is currently being used in a phase 1 clinical trial of CD19 CAR-T-cells in adult patients with certain relapsed or refractory B-cell cancers (ClinicalTrials.gov Identifier: NCT05702853 ). It allows the cells to continue dividing and remain persistent as they attack the tumor environment.
Thus far, two patients have been enrolled and both have tolerated the treatment well and achieved complete responses without experiencing any of the side effects that are otherwise commonly associated with CAR-T-cell therapy. Based on these results, a second set of patients is being enrolled in this trial, which is now open at MUSC’s Hollings Cancer Center.
Kar A, Ghosh P, Gautam A, Chowdhury Sasak D, Sarkar I, Bhoumik A, Barman S, Chakraborty P, Mukhopadhyay A, Mehrotra S, Ganesan SK, Paul S, Chatterjee S. CD38-RyR2 axis-mediated signaling impedes CD8 + T cell response to anti-PD1 therapy in cancer. Proc Natl Acad Sci U S A. 2024 Mar 12;121(11):e2315989121. doi: 10.1073/pnas.2315989121. Epub 2024 Mar 7. PMID: 38451948; PMCID: PMC10945783.
About the Author
Sarah Allen Sarah Allen is a Master's student at MUSC and a science communications intern in the College of Graduate Studies.
Categories: Cancer , Research
- Skip to main content
- Keyboard shortcuts for audio player

- Your Health
- Treatments & Tests
Health Inc.
- Public Health
Oncologists' meetings with drug reps don't help cancer patients live longer
Sydney Lupkin

Drug companies often do one-on-one outreach to doctors. A new study finds these meetings with drug reps lead to more prescriptions for cancer patients, but not longer survival. Chris Hondros/Getty Images hide caption
Drug companies often do one-on-one outreach to doctors. A new study finds these meetings with drug reps lead to more prescriptions for cancer patients, but not longer survival.
Pharmaceutical company reps have been visiting doctors for decades to tell them about the latest drugs. But how does the practice affect patients? A group of economists tried to answer that question.
When drug company reps visit doctors, it usually includes lunch or dinner and a conversation about a new drug. These direct-to-physician marketing interactions are tracked as payments in a public database, and a new study shows the meetings work. That is, doctors prescribe about five percent more oncology drugs following a visit from a pharmaceutical representative, according to the new study published by the National Bureau of Economic Research this month.
But the researchers also found that the practice doesn't make cancer patients live longer.
"It does not seem that this payment induces physicians to switch to drugs with a mortality benefit relative to the drug the patient would have gotten otherwise," says study author Colleen Carey , an assistant professor of economics and public policy at Cornell University.
For their research, she and her colleagues used Medicare claims data and the Open Payments database , which tracks drug company payments to doctors.
While the patients being prescribed these new cancer drugs didn't live longer, Carey also points out that they didn't live shorter lives either. It was about equal.
The pharmaceutical industry trade group, which is known as PhRMA, has a code of conduct for how sales reps should interact with doctors. The code was most recently updated in 2022, says Jocelyn Ulrich, the group's vice president of policy and research .
"We're ensuring that there is a constant attention from the industry and ensuring that these are very meaningful and important interactions and that they're compliant," she explains.
The code says that if drug reps are buying doctors a meal, it must be modest and can't be part of an entertainment or recreational event. The goal should be education.
Ulrich also points out that cancer deaths in the U.S. have declined by 33 percent since the 1990s , and new medicines are a part of that.
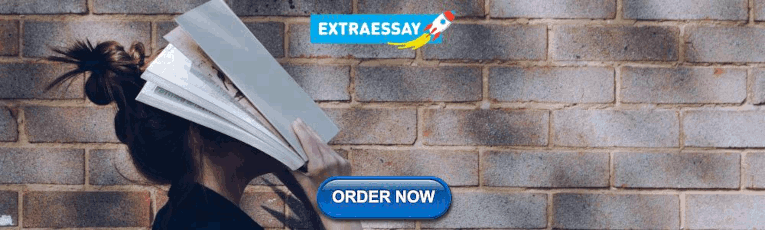
IMAGES
VIDEO
COMMENTS
Cancer therapies have evolved considerably in recent decades, substantially improving the quality of life and survival of patients with cancer. In this issue, we launch our Series on Cancer ...
According to the International Agency for Research on Cancer (IARC), in 2020 there were approximately 19.3 million new cases of cancer, and 10 million deaths by this disease, 6 while 23.8 million cases and 13.0 million deaths are projected to occur by 2030. 73 In this regard, it is clear the increasing role that environmental factors ...
Innovative measures of cancer survival will be included in forthcoming papers and made publicly available via an online tool to facilitate the next generation of benchmarking studies. ... Great advances in cancer research, treatment, and survival have clearly been made—for example, in the UK, cancer mortality is now half that recorded in the ...
Survival statistics are of great interest to clinicians, researchers, patients, and policy makers. Numerous methods and measures of cancer survival for cancer registry data have been developed, but not all are well known or in common use. This paper is an attempt to introduce the main cancer registry survival measures to a broad audience.
The anti-cancer compounds derived from either natural sources or their synthetic chemical analogs can kill cancer cells or stop their development. 52, 53, 54 Notably, various other approaches are emerging to treat the cancer cells, including small molecule inhibitors, 55 a combination of anti-cancer agents and immunotherapy, 56 nanotechnology ...
Abstract. A proactive approach to detecting cancer at an early stage can make treatments more effective, with fewer side effects and improved long-term survival. However, as detection methods ...
An outline for future research in lung cancer survival prediction. ... In this paper, we review and explore studies using machine learning to predict lung cancer survival based on clinical data. It provides an overview of data sources, patient cohort, data pre-processing, feature selection algorithms, selected features, ML algorithms, and ...
Recent papers have used SVMs in the context of cancer survival analysis. Papers [3, 37, 38] ... Kaur, Ishleen et al. [2] is a review that focused on the progression of the research of cancer survival in recent years. This study concluded that Artificial Neural Networks, Decision Trees and ensemble approaches are some of the widely used ...
In this Special Issue entitled, 'Treatment strategies and survival outcomes in breast cancer', a number of original research articles are included covering a diversity of studies, from pre-clinical and translational biomarker studies to clinical trials and population-based studies. ... Fann et al. evaluated the 15-year adjusted cumulative ...
Here we highlight some of the tabled results. For the testing pool of 82 lymph node positive patients, we obtain PPV 0.77 and NPV 0.78 (odds ratio 11.50); for the pool of 61 lymph node negative ...
Background Accurately predicting the survival rate of breast cancer patients is a major issue for cancer researchers. Machine learning (ML) has attracted much attention with the hope that it could provide accurate results, but its modeling methods and prediction performance remain controversial. The aim of this systematic review is to identify and critically appraise current studies regarding ...
The assessment of cancer survival at the population level is essential for monitoring progress in cancer control. We aimed to assess cancer survival and its trends in adults in Spain. Individual records of 601,250 adults with primary cancer diagnosed during 2002-2013 and followed up to 2015 were included from 13 population-based cancer registries. We estimated net survival up to five years ...
Pembrolizumab, an anti-programmed death 1 (PD-1) antibody, was approved in 2021 as adjuvant treatment for patients with renal-cell carcinoma who were at an intermediate-to-high or high risk for ...
Tumor grade and the stage of cancer were found to be jointly significantly associated with survival. The findings of this research enable the estimate of the long-term breast cancer survival, as well as reemphasizes the importance of early diagnosis and screening through mammography which can prevent local systemic metastasis and therefore ...
New Cases. More than 228,000 people will be diagnosed with lung cancer this year, with the rate of new cases varying by state. The report finds that Utah has the nation's best lung cancer rate while Kentucky has the worst at almost 2.5 times the incidence rate of Utah. Over the last five years, the rate of new cases decreased 9% nationally.
Cancer survival is becoming more common which means that there is now a growing population of cancer survivors, in whom pain may be common. However, its prevalence has hardly been addressed systematically. ... Feature papers represent the most advanced research with significant potential for high impact in the field. A Feature Paper should be a ...
Extracellular vesicles for cancer diagnosis and therapy. EVs are classified in two categories based on their biogenesis. Specifically, exosomes are small vesicles of around 30-150 nm originated from endosomes in physiological and pathological conditions and released by a fusion of multivesicular bodies (MVBs) to the cell membrane [55, 56], while shed microvesicles (sMVs), with a typical size ...
Research Paper Cancer survival in patients from a hospital -based cancer ... worst survival rate was observed in pancreatic cancer, with survival rates of 12.50%, 1.39%, 1.39%, and 1.39%, respectively (Table 2). The survival ves curof the top 20 cancer sites are shown in Figure 2.
Cause-specific mortality rates are 53/100 person-years for colon cancer and 9/100 person-years for other causes of death. In the section titled 'Relative survival,' it was noted that expected survival in each month of the 1st year was p 1 * = 0.9974, based on the corresponding general population mortality.
Papers recommending treatments based on the cancer survival outcome. v. Papers selecting or validating features necessary for predicting cancer survival. vi. Papers not dealing with trials for cancer treatment. After removing the duplicates and excluding the articles not dealing with data mining models for cancer survival outcomes, finally, 117 ...
Lung cancer remains the most lethal form of the disease, killing about 135,000 Americans a year - more than breast, prostate and colon cancer combined - which is why many people still think of ...
A new paper describes the role of two RNA-binding proteins in the development of sarcoma and carcinoma cancers, highlighting the important and emerging role of RNA-binding proteins in cancer ...
The new study on survival rates examined de-identified data from 350,824 breast cancer patients, collected by the National Cancer Institute (NCI) from 2016 to 2018. The data captured information such as tumor treatment regimens and access to health insurance, as well as socioeconomic measurements from patient neighborhoods, at the census-tract ...
Environmental exposure to arsenic, a group 1 carcinogen according to the International Agency for Research on Cancer (IARC), is known to increase the risk of lung cancer 72,73,74,75,76,77,78.
Patient-provider communication has been crucial to cancer patients' survival after their cancer treatments. However, the research community and patients themselves often overlook the communication challenges after cancer treatments as they are overshadowed by the severity of the patient's illness and the variety and rarity of the cancer disease itself. Meanwhile, the recent technical advances ...
Background: Distress is highly prevalent among patients with cancer, but supportive care needs often go unmet. Digital therapeutics hold the potential to overcome barriers in cancer care and improve health outcomes. Objective: This study conducted a randomized controlled trial to investigate the efficacy of Mika, an app-based digital therapeutic designed to reduce distress across the cancer ...
Cancer is a global health problem responsible for one in six deaths worldwide. Treating cancer has been a highly complex process. Conventional treatment approaches, such as surgery, chemotherapy, and radiotherapy, have been in use, while significant advances are being made in recent times, including stem cell therapy, targeted therapy, ablation therapy, nanoparticles, natural antioxidants ...
The study showed that T-cells expressing the cell receptor CD38 were more susceptible to exhaustion. Using an anti-CD38 antibody, the research team was able to block CD38 and overcome T-cell exhaustion. They then showed in a mouse model that overcoming T-cell exhaustion by blocking CD38 restored the efficacy of anti-PD1 therapy against cancer.
Drug company reps commonly visit doctors to talk about new medications. A team of economists wanted to know if that helps patients live longer. They found that for cancer patients, the answer is no.
Deep Convolutional Neural Network CNNs is used to identify or label a medical image in some research papers. Diagnosed lung cancer in 2015 with a multiscal two-layer CNN ... Prostate cancer survival is also examined in context, including artificial neural networks, decision trees and logistical regression. In the segment, data on patients ...