- Business Essentials
- Leadership & Management
- Credential of Leadership, Impact, and Management in Business (CLIMB)
- Entrepreneurship & Innovation
- Digital Transformation
- Finance & Accounting
- Business in Society
- For Organizations
- Support Portal
- Media Coverage
- Founding Donors
- Leadership Team
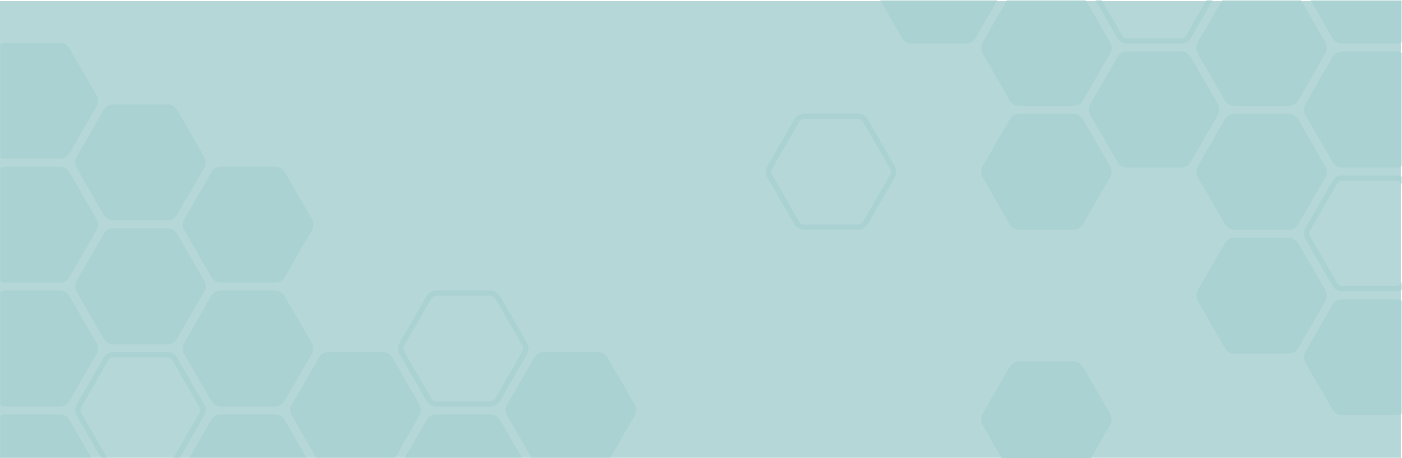
- Harvard Business School →
- HBS Online →
- Business Insights →
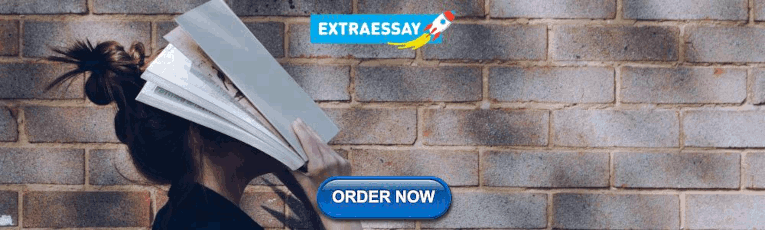
Business Insights
Harvard Business School Online's Business Insights Blog provides the career insights you need to achieve your goals and gain confidence in your business skills.
- Career Development
- Communication
- Decision-Making
- Earning Your MBA
- Negotiation
- News & Events
- Productivity
- Staff Spotlight
- Student Profiles
- Work-Life Balance
- AI Essentials for Business
- Alternative Investments
- Business Analytics
- Business Strategy
- Business and Climate Change
- Design Thinking and Innovation
- Digital Marketing Strategy
- Disruptive Strategy
- Economics for Managers
- Entrepreneurship Essentials
- Financial Accounting
- Global Business
- Launching Tech Ventures
- Leadership Principles
- Leadership, Ethics, and Corporate Accountability
- Leading with Finance
- Management Essentials
- Negotiation Mastery
- Organizational Leadership
- Power and Influence for Positive Impact
- Strategy Execution
- Sustainable Business Strategy
- Sustainable Investing
- Winning with Digital Platforms
The History of the Case Study at Harvard Business School
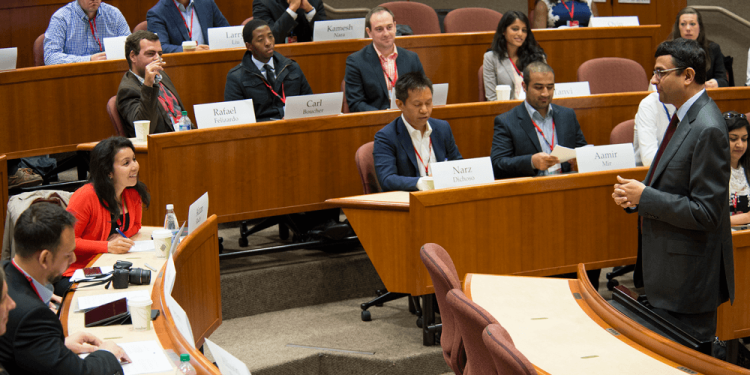
- 28 Feb 2017
Many first-time HBS Online participants are surprised to learn that, often, the professor is not at the center of their learning experience. Instead of long faculty lectures, the HBS Online learning model centers on smaller, more digestible pieces of content that require participants to interact with each other, test concepts, and learn from real-world examples.
Often, the professor fades into the background and lets the focus shift to interviews with executives, industry leaders, and small business owners. Some students might be left thinking, "Wait, where did that professor go? Why am I learning about a grocery store in Harvard Square?"
In the words of The Hitchhiker's Guide to the Galaxy , “Don’t panic.” These interviews, or cases, feature leaders at companies of all sizes and provide valuable examples of business concepts in action. This case study method forms the backbone of the Harvard Business School curriculum.
Back in the 1920s, HBS professors decided to develop and experiment with innovative and unique business instruction methods. As the first school in the world to design a signature, distinctive program in business, later to be called the MBA, there was a need for a teaching method that would benefit this novel approach.
HBS professors selected and took a few pages to summarize recent events, momentous challenges, strategic planning, and important decisions undertaken by major companies and organizations. The idea was, and remains to this day, that through direct contact with a real-world case, students will think independently about those facts, discuss and compare their perspectives and findings with their peers, and eventually discover a new concept on their own.
Central to the case method is the idea that students are not provided the "answer" or resolution to the problem at hand. Instead, just like a board member, CEO, or manager, the student is forced to analyze a situation and find solutions without full knowledge of all methods and facts. Without excluding more traditional aspects, such as interaction with professors and textbooks, the case method provides the student with the opportunity to think and act like managers.
Since 1924, the case method has been the most widely applied and successful teaching instrument to come out of HBS, and it is used today in almost all MBA and Executive Education courses there, as well as in hundreds of other top business schools around the world. The application of the case method is so extensive that HBS students will often choose to rely on cases, instead of textbooks or other material, for their research. Large corporations use the case method as well to approach their own challenges, while competing universities create their own versions for their students.
This is what the case method does—it puts students straight into the game, and ensures they acquire not just skills and abstract knowledge, but also a solid understanding of the outside world.

About the Author
What is the Case Study Method?
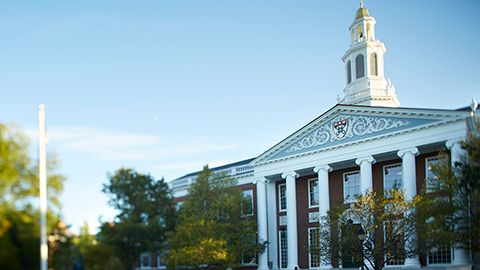
Overview Dropdown up
Overview dropdown down, celebrating 100 years of the case method at hbs.
The 2021-2022 academic year marks the 100-year anniversary of the introduction of the case method at Harvard Business School. Today, the HBS case method is employed in the HBS MBA program, in Executive Education programs, and in dozens of other business schools around the world. As Dean Srikant Datar's says, the case method has withstood the test of time.
Case Discussion Preparation Details Expand All Collapse All
In self-reflection in self-reflection dropdown down, in a small group setting in a small group setting dropdown down, in the classroom in the classroom dropdown down, beyond the classroom beyond the classroom dropdown down, how the case method creates value dropdown up, how the case method creates value dropdown down, in self-reflection, in a small group setting, in the classroom, beyond the classroom.
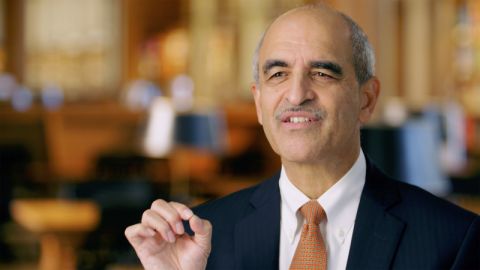
How Cases Unfold In the Classroom
How cases unfold in the classroom dropdown up, how cases unfold in the classroom dropdown down, preparation guidelines expand all collapse all, read the professor's assignment or discussion questions read the professor's assignment or discussion questions dropdown down, read the first few paragraphs and then skim the case read the first few paragraphs and then skim the case dropdown down, reread the case, underline text, and make margin notes reread the case, underline text, and make margin notes dropdown down, note the key problems on a pad of paper and go through the case again note the key problems on a pad of paper and go through the case again dropdown down, how to prepare for case discussions dropdown up, how to prepare for case discussions dropdown down, read the professor's assignment or discussion questions, read the first few paragraphs and then skim the case, reread the case, underline text, and make margin notes, note the key problems on a pad of paper and go through the case again, case study best practices expand all collapse all, prepare prepare dropdown down, discuss discuss dropdown down, participate participate dropdown down, relate relate dropdown down, apply apply dropdown down, note note dropdown down, understand understand dropdown down, case study best practices dropdown up, case study best practices dropdown down, participate, what can i expect on the first day dropdown down.
Most programs begin with registration, followed by an opening session and a dinner. If your travel plans necessitate late arrival, please be sure to notify us so that alternate registration arrangements can be made for you. Please note the following about registration:
HBS campus programs – Registration takes place in the Chao Center.
India programs – Registration takes place outside the classroom.
Other off-campus programs – Registration takes place in the designated facility.
What happens in class if nobody talks? Dropdown down
Professors are here to push everyone to learn, but not to embarrass anyone. If the class is quiet, they'll often ask a participant with experience in the industry in which the case is set to speak first. This is done well in advance so that person can come to class prepared to share. Trust the process. The more open you are, the more willing you’ll be to engage, and the more alive the classroom will become.
Does everyone take part in "role-playing"? Dropdown down
Professors often encourage participants to take opposing sides and then debate the issues, often taking the perspective of the case protagonists or key decision makers in the case.
View Frequently Asked Questions
Subscribe to Our Emails
Case Study Research Method in Psychology
Saul Mcleod, PhD
Editor-in-Chief for Simply Psychology
BSc (Hons) Psychology, MRes, PhD, University of Manchester
Saul Mcleod, PhD., is a qualified psychology teacher with over 18 years of experience in further and higher education. He has been published in peer-reviewed journals, including the Journal of Clinical Psychology.
Learn about our Editorial Process
Olivia Guy-Evans, MSc
Associate Editor for Simply Psychology
BSc (Hons) Psychology, MSc Psychology of Education
Olivia Guy-Evans is a writer and associate editor for Simply Psychology. She has previously worked in healthcare and educational sectors.
On This Page:
Case studies are in-depth investigations of a person, group, event, or community. Typically, data is gathered from various sources using several methods (e.g., observations & interviews).
The case study research method originated in clinical medicine (the case history, i.e., the patient’s personal history). In psychology, case studies are often confined to the study of a particular individual.
The information is mainly biographical and relates to events in the individual’s past (i.e., retrospective), as well as to significant events that are currently occurring in his or her everyday life.
The case study is not a research method, but researchers select methods of data collection and analysis that will generate material suitable for case studies.
Freud (1909a, 1909b) conducted very detailed investigations into the private lives of his patients in an attempt to both understand and help them overcome their illnesses.
This makes it clear that the case study is a method that should only be used by a psychologist, therapist, or psychiatrist, i.e., someone with a professional qualification.
There is an ethical issue of competence. Only someone qualified to diagnose and treat a person can conduct a formal case study relating to atypical (i.e., abnormal) behavior or atypical development.
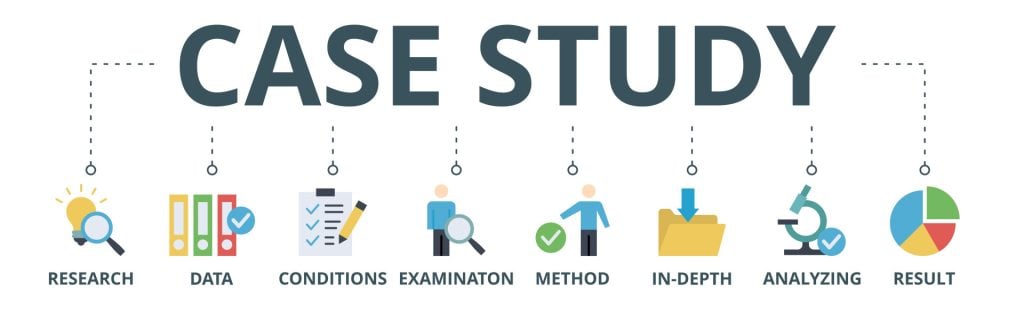
Famous Case Studies
- Anna O – One of the most famous case studies, documenting psychoanalyst Josef Breuer’s treatment of “Anna O” (real name Bertha Pappenheim) for hysteria in the late 1800s using early psychoanalytic theory.
- Little Hans – A child psychoanalysis case study published by Sigmund Freud in 1909 analyzing his five-year-old patient Herbert Graf’s house phobia as related to the Oedipus complex.
- Bruce/Brenda – Gender identity case of the boy (Bruce) whose botched circumcision led psychologist John Money to advise gender reassignment and raise him as a girl (Brenda) in the 1960s.
- Genie Wiley – Linguistics/psychological development case of the victim of extreme isolation abuse who was studied in 1970s California for effects of early language deprivation on acquiring speech later in life.
- Phineas Gage – One of the most famous neuropsychology case studies analyzes personality changes in railroad worker Phineas Gage after an 1848 brain injury involving a tamping iron piercing his skull.
Clinical Case Studies
- Studying the effectiveness of psychotherapy approaches with an individual patient
- Assessing and treating mental illnesses like depression, anxiety disorders, PTSD
- Neuropsychological cases investigating brain injuries or disorders
Child Psychology Case Studies
- Studying psychological development from birth through adolescence
- Cases of learning disabilities, autism spectrum disorders, ADHD
- Effects of trauma, abuse, deprivation on development
Types of Case Studies
- Explanatory case studies : Used to explore causation in order to find underlying principles. Helpful for doing qualitative analysis to explain presumed causal links.
- Exploratory case studies : Used to explore situations where an intervention being evaluated has no clear set of outcomes. It helps define questions and hypotheses for future research.
- Descriptive case studies : Describe an intervention or phenomenon and the real-life context in which it occurred. It is helpful for illustrating certain topics within an evaluation.
- Multiple-case studies : Used to explore differences between cases and replicate findings across cases. Helpful for comparing and contrasting specific cases.
- Intrinsic : Used to gain a better understanding of a particular case. Helpful for capturing the complexity of a single case.
- Collective : Used to explore a general phenomenon using multiple case studies. Helpful for jointly studying a group of cases in order to inquire into the phenomenon.
Where Do You Find Data for a Case Study?
There are several places to find data for a case study. The key is to gather data from multiple sources to get a complete picture of the case and corroborate facts or findings through triangulation of evidence. Most of this information is likely qualitative (i.e., verbal description rather than measurement), but the psychologist might also collect numerical data.
1. Primary sources
- Interviews – Interviewing key people related to the case to get their perspectives and insights. The interview is an extremely effective procedure for obtaining information about an individual, and it may be used to collect comments from the person’s friends, parents, employer, workmates, and others who have a good knowledge of the person, as well as to obtain facts from the person him or herself.
- Observations – Observing behaviors, interactions, processes, etc., related to the case as they unfold in real-time.
- Documents & Records – Reviewing private documents, diaries, public records, correspondence, meeting minutes, etc., relevant to the case.
2. Secondary sources
- News/Media – News coverage of events related to the case study.
- Academic articles – Journal articles, dissertations etc. that discuss the case.
- Government reports – Official data and records related to the case context.
- Books/films – Books, documentaries or films discussing the case.
3. Archival records
Searching historical archives, museum collections and databases to find relevant documents, visual/audio records related to the case history and context.
Public archives like newspapers, organizational records, photographic collections could all include potentially relevant pieces of information to shed light on attitudes, cultural perspectives, common practices and historical contexts related to psychology.
4. Organizational records
Organizational records offer the advantage of often having large datasets collected over time that can reveal or confirm psychological insights.
Of course, privacy and ethical concerns regarding confidential data must be navigated carefully.
However, with proper protocols, organizational records can provide invaluable context and empirical depth to qualitative case studies exploring the intersection of psychology and organizations.
- Organizational/industrial psychology research : Organizational records like employee surveys, turnover/retention data, policies, incident reports etc. may provide insight into topics like job satisfaction, workplace culture and dynamics, leadership issues, employee behaviors etc.
- Clinical psychology : Therapists/hospitals may grant access to anonymized medical records to study aspects like assessments, diagnoses, treatment plans etc. This could shed light on clinical practices.
- School psychology : Studies could utilize anonymized student records like test scores, grades, disciplinary issues, and counseling referrals to study child development, learning barriers, effectiveness of support programs, and more.
How do I Write a Case Study in Psychology?
Follow specified case study guidelines provided by a journal or your psychology tutor. General components of clinical case studies include: background, symptoms, assessments, diagnosis, treatment, and outcomes. Interpreting the information means the researcher decides what to include or leave out. A good case study should always clarify which information is the factual description and which is an inference or the researcher’s opinion.
1. Introduction
- Provide background on the case context and why it is of interest, presenting background information like demographics, relevant history, and presenting problem.
- Compare briefly to similar published cases if applicable. Clearly state the focus/importance of the case.
2. Case Presentation
- Describe the presenting problem in detail, including symptoms, duration,and impact on daily life.
- Include client demographics like age and gender, information about social relationships, and mental health history.
- Describe all physical, emotional, and/or sensory symptoms reported by the client.
- Use patient quotes to describe the initial complaint verbatim. Follow with full-sentence summaries of relevant history details gathered, including key components that led to a working diagnosis.
- Summarize clinical exam results, namely orthopedic/neurological tests, imaging, lab tests, etc. Note actual results rather than subjective conclusions. Provide images if clearly reproducible/anonymized.
- Clearly state the working diagnosis or clinical impression before transitioning to management.
3. Management and Outcome
- Indicate the total duration of care and number of treatments given over what timeframe. Use specific names/descriptions for any therapies/interventions applied.
- Present the results of the intervention,including any quantitative or qualitative data collected.
- For outcomes, utilize visual analog scales for pain, medication usage logs, etc., if possible. Include patient self-reports of improvement/worsening of symptoms. Note the reason for discharge/end of care.
4. Discussion
- Analyze the case, exploring contributing factors, limitations of the study, and connections to existing research.
- Analyze the effectiveness of the intervention,considering factors like participant adherence, limitations of the study, and potential alternative explanations for the results.
- Identify any questions raised in the case analysis and relate insights to established theories and current research if applicable. Avoid definitive claims about physiological explanations.
- Offer clinical implications, and suggest future research directions.
5. Additional Items
- Thank specific assistants for writing support only. No patient acknowledgments.
- References should directly support any key claims or quotes included.
- Use tables/figures/images only if substantially informative. Include permissions and legends/explanatory notes.
- Provides detailed (rich qualitative) information.
- Provides insight for further research.
- Permitting investigation of otherwise impractical (or unethical) situations.
Case studies allow a researcher to investigate a topic in far more detail than might be possible if they were trying to deal with a large number of research participants (nomothetic approach) with the aim of ‘averaging’.
Because of their in-depth, multi-sided approach, case studies often shed light on aspects of human thinking and behavior that would be unethical or impractical to study in other ways.
Research that only looks into the measurable aspects of human behavior is not likely to give us insights into the subjective dimension of experience, which is important to psychoanalytic and humanistic psychologists.
Case studies are often used in exploratory research. They can help us generate new ideas (that might be tested by other methods). They are an important way of illustrating theories and can help show how different aspects of a person’s life are related to each other.
The method is, therefore, important for psychologists who adopt a holistic point of view (i.e., humanistic psychologists ).
Limitations
- Lacking scientific rigor and providing little basis for generalization of results to the wider population.
- Researchers’ own subjective feelings may influence the case study (researcher bias).
- Difficult to replicate.
- Time-consuming and expensive.
- The volume of data, together with the time restrictions in place, impacted the depth of analysis that was possible within the available resources.
Because a case study deals with only one person/event/group, we can never be sure if the case study investigated is representative of the wider body of “similar” instances. This means the conclusions drawn from a particular case may not be transferable to other settings.
Because case studies are based on the analysis of qualitative (i.e., descriptive) data , a lot depends on the psychologist’s interpretation of the information she has acquired.
This means that there is a lot of scope for Anna O , and it could be that the subjective opinions of the psychologist intrude in the assessment of what the data means.
For example, Freud has been criticized for producing case studies in which the information was sometimes distorted to fit particular behavioral theories (e.g., Little Hans ).
This is also true of Money’s interpretation of the Bruce/Brenda case study (Diamond, 1997) when he ignored evidence that went against his theory.
Breuer, J., & Freud, S. (1895). Studies on hysteria . Standard Edition 2: London.
Curtiss, S. (1981). Genie: The case of a modern wild child .
Diamond, M., & Sigmundson, K. (1997). Sex Reassignment at Birth: Long-term Review and Clinical Implications. Archives of Pediatrics & Adolescent Medicine , 151(3), 298-304
Freud, S. (1909a). Analysis of a phobia of a five year old boy. In The Pelican Freud Library (1977), Vol 8, Case Histories 1, pages 169-306
Freud, S. (1909b). Bemerkungen über einen Fall von Zwangsneurose (Der “Rattenmann”). Jb. psychoanal. psychopathol. Forsch ., I, p. 357-421; GW, VII, p. 379-463; Notes upon a case of obsessional neurosis, SE , 10: 151-318.
Harlow J. M. (1848). Passage of an iron rod through the head. Boston Medical and Surgical Journal, 39 , 389–393.
Harlow, J. M. (1868). Recovery from the Passage of an Iron Bar through the Head . Publications of the Massachusetts Medical Society. 2 (3), 327-347.
Money, J., & Ehrhardt, A. A. (1972). Man & Woman, Boy & Girl : The Differentiation and Dimorphism of Gender Identity from Conception to Maturity. Baltimore, Maryland: Johns Hopkins University Press.
Money, J., & Tucker, P. (1975). Sexual signatures: On being a man or a woman.
Further Information
- Case Study Approach
- Case Study Method
- Enhancing the Quality of Case Studies in Health Services Research
- “We do things together” A case study of “couplehood” in dementia
- Using mixed methods for evaluating an integrative approach to cancer care: a case study

- > Master in Management (MiM)
- > Master in Research in Management (MRM)
- > PhD in Management
- > Executive MBA
- > Global Executive MBA
- > Individuals
- > Organizations
- > Founders (School of Founders)
- > Certificate in AI & Digital Transformation
- > Sustainability & ESG
- > Foundations of Scaling
- CHOOSE YOUR MBA
- IESE PORTFOLIO
- PROGRAM FINDER
- > Faculty Directory
- > Academic Departments
- > Initiatives
- > Competitive Projects
- > Academic Events
- > Behavioral Lab
- > Limitless Learning
- > Learning Methodologies
- > The Case Method
- > IESE Insight: Research-Based Ideas
- > IESE Business School Insight Magazine
- > StandOut: Career Inspiration
- > Professors' Blogs
- > Alumni Learning Program
- > IESE Publishing
- SEARCH PUBLICATIONS
- IESE NEWSLETTERS
- > Our Mission, Vision and Values
- > Our History
- > Our Governance
- > Our Alliances
- > Our Impact
- > Diversity at IESE
- > Sustainability at IESE
- > Accreditations
- > Annual Report
- > Roadmap for 2023-25
- > Frequently Asked Questions (FAQs)
- > Barcelona
- > São Paulo
- > Security & Campus Access
- > Loans and Scholarships
- > Chaplaincy
- > IESE Shop
- > Jobs @IESE
- > Compliance Channel
- > Contact Us
- GIVING TO IESE
- WORK WITH US

The Case Study method: 100 years young
On its 100th anniversary, the discussion-based learning method keeps evolving
Case study discussions can also take place in a variety of different contexts, whether studied in person, in hybrid formats or through our virtual classroom.
May 3, 2021

This year commemorates the 100 th anniversary of the first written case study, produced by Harvard Business School back in April 1921. IESE joins in the celebration because it’s one of the academic institutions that has helped spearhead the use of the case method outside the U.S. , educating more than 60,000 professionals with this methodology and producing around 6,200 original cases through more than 60 years of history.
But what is the case study method exactly, and why is it still relevant now?
The main reason the case method is still relevant today is that it works. It’s a dynamic, practical way to study, which puts students in the shoes of senior executives, allowing them to practice solving real-life business problems and making strategic decisions.
It is also an example of a learning methodology based on the exchange of ideas and debate. At IESE, we recognize that the best way to learn is through interacting with others, and having your perspectives and assumptions challenged and stretched . The case study is one example of how we do this, alongside a variety of other discussion-based learning methodologies , such as business simulations, coaching or experiential learning.
For the case method, this means that passively sitting in a lecture room doesn’t cut it. Instead, all participants are required to discuss and reflect on the issues at hand. Here, the professor acts more as a facilitator, guiding the conversation and teasing out the various ethical and business implications of each case. The discussions also draw upon the diverse industry experiences, cultural backgrounds and mindsets of each individual in class, further enriching the learning process.
New content and formats, same impact
Throughout the last 100 years, the case method has been able to adapt with the times by constantly evolving . Indeed, in the last three years, 200 new cases have been written by IESE professors on the most pressing issues happening now. An example of this is the case of how Barcelona-based Vall d’Hebron University Hospital managed the first wave of the COVID-19 outbreak.
Our cases also have a distinct international perspective, reflecting the diversity of the school, faculty and students. While many assume most case studies just focus on big North American companies, at IESE our cases cover not only big name companies like YouTube and Spotify but also companies in emerging countries and young startups. We also have one of the largest collections in the world of Spanish-language cases.
Having a diverse set of cases to study – and a diverse student body to discuss them with – is the key to encouraging those lively and enriching debates that are so crucial to broadening participants’ perspectives.
In addition to content, nowadays cases are available in a variety of formats , such as audio cases that allow participants to listen to a case as they would a podcast, and simulations, among others.
Case study discussions can also take place in a variety of different contexts, whether studied in person, in hybrid formats or through our virtual classroom. This reflects the fact that today’s managers require educational solutions that allow for flexibility , but that also guarantee interaction with others and personalized follow-up.
IESE has one of the largest collections of Spanish-language cases in the world. The full catalog is available in the IESE Publishing online store, which also distributes the cases of 15 leading universities. Here, we present some of the more recent challenges from cases that we have produced .
“At IESE, our focus is on delivering the best learning experience possible, regardless of the format or context. As technologies advance, the case method also continues to evolve. Yet the essence of how executives learn best – and the value that comes from sharing knowledge and exchanging viewpoints with a diverse set of peers – remains the same,” said Prof. Julia Prats .
- case method
Related stories
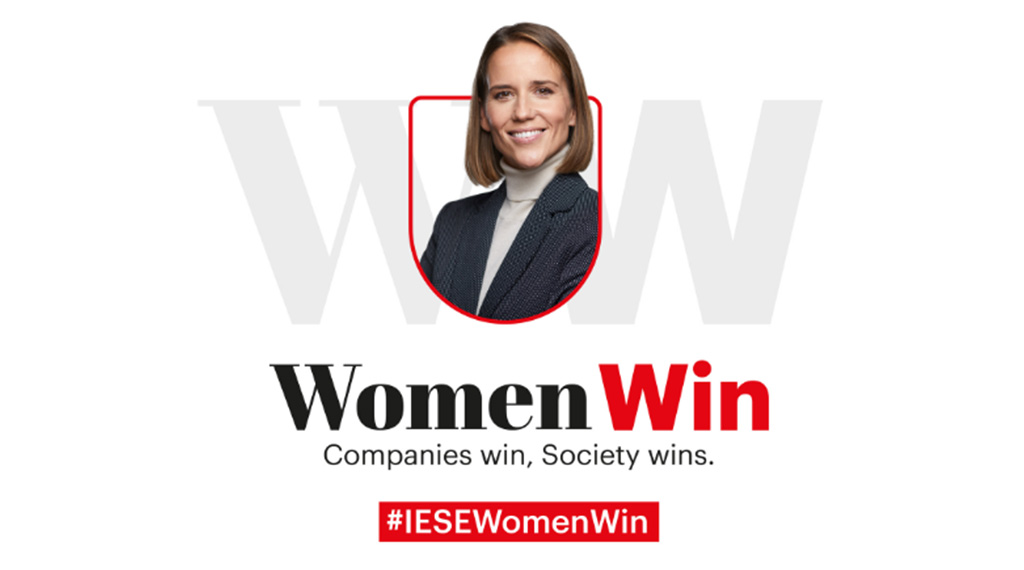
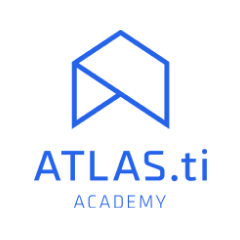
The Ultimate Guide to Qualitative Research - Part 1: The Basics
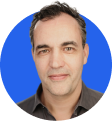
- Introduction and overview
- What is qualitative research?
- What is qualitative data?
- Examples of qualitative data
- Qualitative vs. quantitative research
- Mixed methods
- Qualitative research preparation
- Theoretical perspective
- Theoretical framework
- Literature reviews
Research question
- Conceptual framework
- Conceptual vs. theoretical framework
Data collection
- Qualitative research methods
- Focus groups
- Observational research
What is a case study?
Applications for case study research, what is a good case study, process of case study design, benefits and limitations of case studies.
- Ethnographical research
- Ethical considerations
- Confidentiality and privacy
- Power dynamics
- Reflexivity
Case studies
Case studies are essential to qualitative research , offering a lens through which researchers can investigate complex phenomena within their real-life contexts. This chapter explores the concept, purpose, applications, examples, and types of case studies and provides guidance on how to conduct case study research effectively.
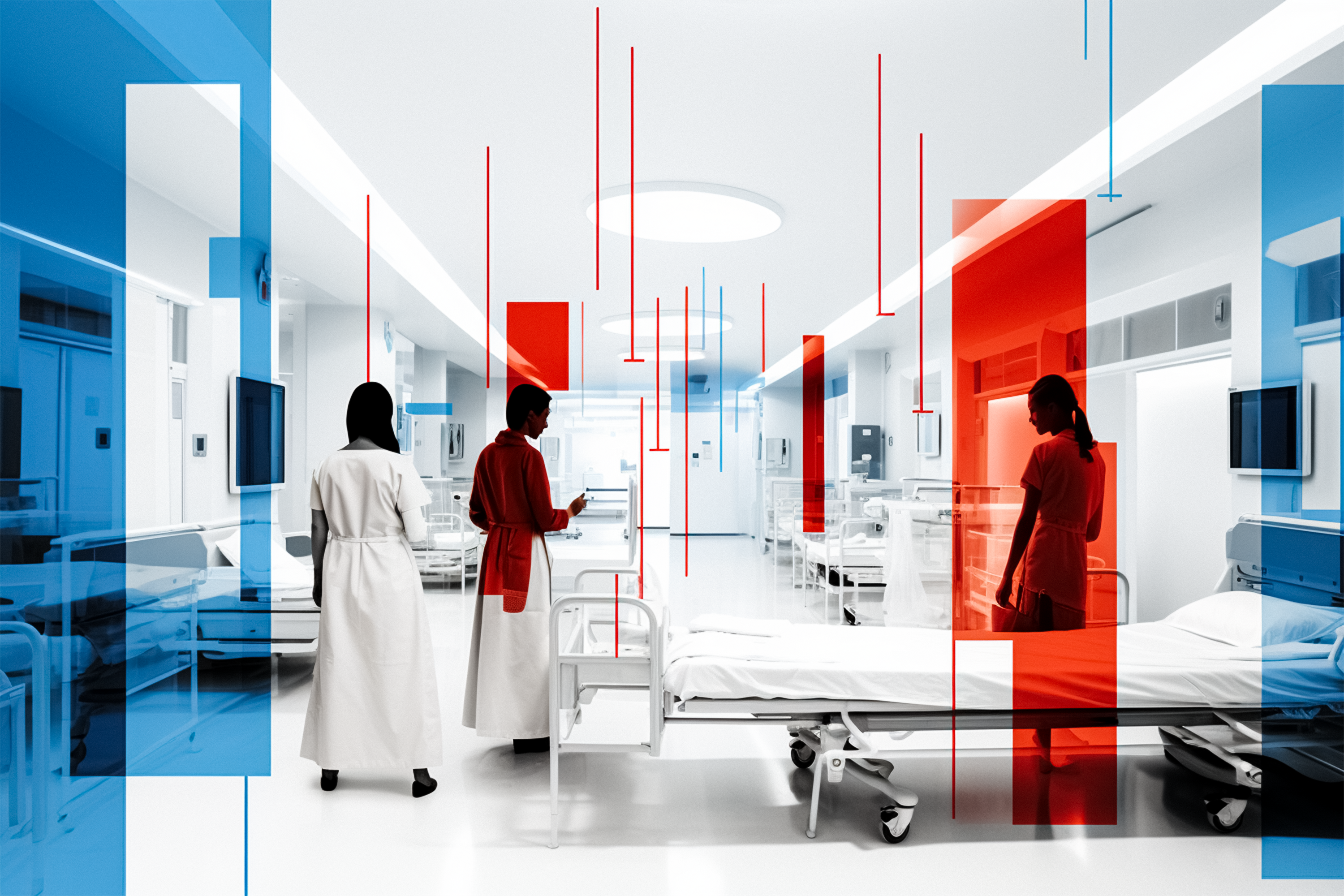
Whereas quantitative methods look at phenomena at scale, case study research looks at a concept or phenomenon in considerable detail. While analyzing a single case can help understand one perspective regarding the object of research inquiry, analyzing multiple cases can help obtain a more holistic sense of the topic or issue. Let's provide a basic definition of a case study, then explore its characteristics and role in the qualitative research process.
Definition of a case study
A case study in qualitative research is a strategy of inquiry that involves an in-depth investigation of a phenomenon within its real-world context. It provides researchers with the opportunity to acquire an in-depth understanding of intricate details that might not be as apparent or accessible through other methods of research. The specific case or cases being studied can be a single person, group, or organization – demarcating what constitutes a relevant case worth studying depends on the researcher and their research question .
Among qualitative research methods , a case study relies on multiple sources of evidence, such as documents, artifacts, interviews , or observations , to present a complete and nuanced understanding of the phenomenon under investigation. The objective is to illuminate the readers' understanding of the phenomenon beyond its abstract statistical or theoretical explanations.
Characteristics of case studies
Case studies typically possess a number of distinct characteristics that set them apart from other research methods. These characteristics include a focus on holistic description and explanation, flexibility in the design and data collection methods, reliance on multiple sources of evidence, and emphasis on the context in which the phenomenon occurs.
Furthermore, case studies can often involve a longitudinal examination of the case, meaning they study the case over a period of time. These characteristics allow case studies to yield comprehensive, in-depth, and richly contextualized insights about the phenomenon of interest.
The role of case studies in research
Case studies hold a unique position in the broader landscape of research methods aimed at theory development. They are instrumental when the primary research interest is to gain an intensive, detailed understanding of a phenomenon in its real-life context.
In addition, case studies can serve different purposes within research - they can be used for exploratory, descriptive, or explanatory purposes, depending on the research question and objectives. This flexibility and depth make case studies a valuable tool in the toolkit of qualitative researchers.
Remember, a well-conducted case study can offer a rich, insightful contribution to both academic and practical knowledge through theory development or theory verification, thus enhancing our understanding of complex phenomena in their real-world contexts.
What is the purpose of a case study?
Case study research aims for a more comprehensive understanding of phenomena, requiring various research methods to gather information for qualitative analysis . Ultimately, a case study can allow the researcher to gain insight into a particular object of inquiry and develop a theoretical framework relevant to the research inquiry.
Why use case studies in qualitative research?
Using case studies as a research strategy depends mainly on the nature of the research question and the researcher's access to the data.
Conducting case study research provides a level of detail and contextual richness that other research methods might not offer. They are beneficial when there's a need to understand complex social phenomena within their natural contexts.
The explanatory, exploratory, and descriptive roles of case studies
Case studies can take on various roles depending on the research objectives. They can be exploratory when the research aims to discover new phenomena or define new research questions; they are descriptive when the objective is to depict a phenomenon within its context in a detailed manner; and they can be explanatory if the goal is to understand specific relationships within the studied context. Thus, the versatility of case studies allows researchers to approach their topic from different angles, offering multiple ways to uncover and interpret the data .
The impact of case studies on knowledge development
Case studies play a significant role in knowledge development across various disciplines. Analysis of cases provides an avenue for researchers to explore phenomena within their context based on the collected data.
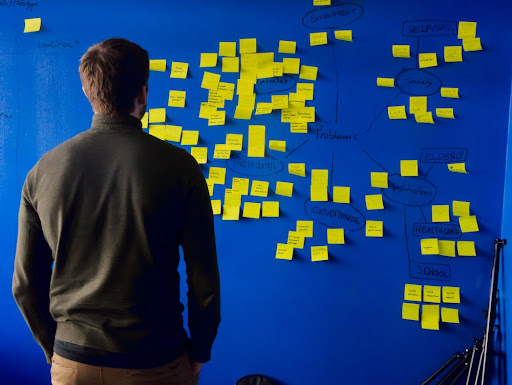
This can result in the production of rich, practical insights that can be instrumental in both theory-building and practice. Case studies allow researchers to delve into the intricacies and complexities of real-life situations, uncovering insights that might otherwise remain hidden.
Types of case studies
In qualitative research , a case study is not a one-size-fits-all approach. Depending on the nature of the research question and the specific objectives of the study, researchers might choose to use different types of case studies. These types differ in their focus, methodology, and the level of detail they provide about the phenomenon under investigation.
Understanding these types is crucial for selecting the most appropriate approach for your research project and effectively achieving your research goals. Let's briefly look at the main types of case studies.
Exploratory case studies
Exploratory case studies are typically conducted to develop a theory or framework around an understudied phenomenon. They can also serve as a precursor to a larger-scale research project. Exploratory case studies are useful when a researcher wants to identify the key issues or questions which can spur more extensive study or be used to develop propositions for further research. These case studies are characterized by flexibility, allowing researchers to explore various aspects of a phenomenon as they emerge, which can also form the foundation for subsequent studies.
Descriptive case studies
Descriptive case studies aim to provide a complete and accurate representation of a phenomenon or event within its context. These case studies are often based on an established theoretical framework, which guides how data is collected and analyzed. The researcher is concerned with describing the phenomenon in detail, as it occurs naturally, without trying to influence or manipulate it.
Explanatory case studies
Explanatory case studies are focused on explanation - they seek to clarify how or why certain phenomena occur. Often used in complex, real-life situations, they can be particularly valuable in clarifying causal relationships among concepts and understanding the interplay between different factors within a specific context.
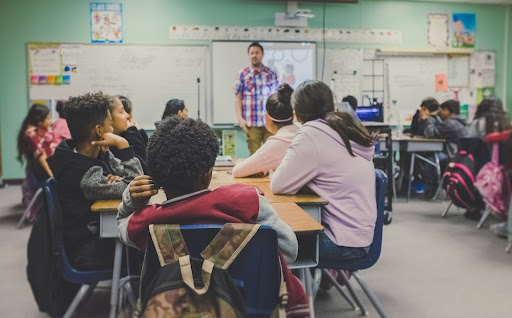
Intrinsic, instrumental, and collective case studies
These three categories of case studies focus on the nature and purpose of the study. An intrinsic case study is conducted when a researcher has an inherent interest in the case itself. Instrumental case studies are employed when the case is used to provide insight into a particular issue or phenomenon. A collective case study, on the other hand, involves studying multiple cases simultaneously to investigate some general phenomena.
Each type of case study serves a different purpose and has its own strengths and challenges. The selection of the type should be guided by the research question and objectives, as well as the context and constraints of the research.
The flexibility, depth, and contextual richness offered by case studies make this approach an excellent research method for various fields of study. They enable researchers to investigate real-world phenomena within their specific contexts, capturing nuances that other research methods might miss. Across numerous fields, case studies provide valuable insights into complex issues.
Critical information systems research
Case studies provide a detailed understanding of the role and impact of information systems in different contexts. They offer a platform to explore how information systems are designed, implemented, and used and how they interact with various social, economic, and political factors. Case studies in this field often focus on examining the intricate relationship between technology, organizational processes, and user behavior, helping to uncover insights that can inform better system design and implementation.
Health research
Health research is another field where case studies are highly valuable. They offer a way to explore patient experiences, healthcare delivery processes, and the impact of various interventions in a real-world context.
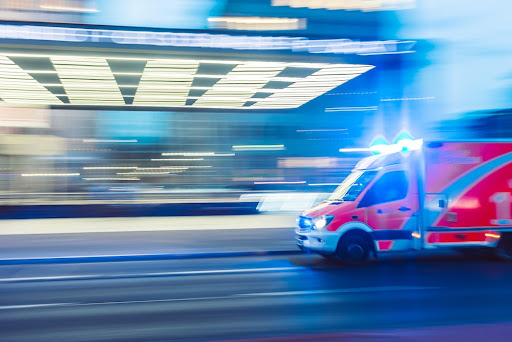
Case studies can provide a deep understanding of a patient's journey, giving insights into the intricacies of disease progression, treatment effects, and the psychosocial aspects of health and illness.
Asthma research studies
Specifically within medical research, studies on asthma often employ case studies to explore the individual and environmental factors that influence asthma development, management, and outcomes. A case study can provide rich, detailed data about individual patients' experiences, from the triggers and symptoms they experience to the effectiveness of various management strategies. This can be crucial for developing patient-centered asthma care approaches.
Other fields
Apart from the fields mentioned, case studies are also extensively used in business and management research, education research, and political sciences, among many others. They provide an opportunity to delve into the intricacies of real-world situations, allowing for a comprehensive understanding of various phenomena.
Case studies, with their depth and contextual focus, offer unique insights across these varied fields. They allow researchers to illuminate the complexities of real-life situations, contributing to both theory and practice.
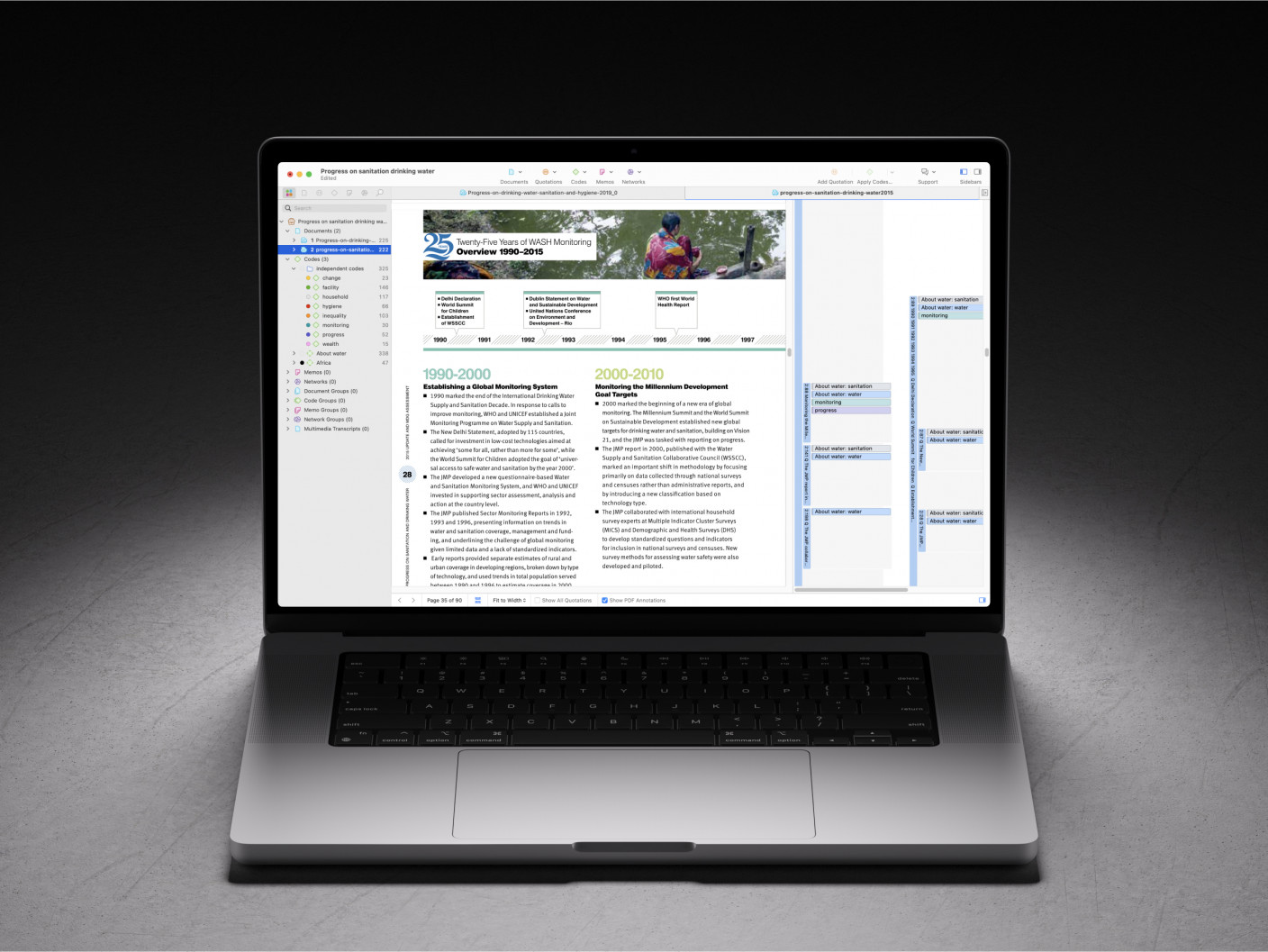
Whatever field you're in, ATLAS.ti puts your data to work for you
Download a free trial of ATLAS.ti to turn your data into insights.
Understanding the key elements of case study design is crucial for conducting rigorous and impactful case study research. A well-structured design guides the researcher through the process, ensuring that the study is methodologically sound and its findings are reliable and valid. The main elements of case study design include the research question , propositions, units of analysis, and the logic linking the data to the propositions.
The research question is the foundation of any research study. A good research question guides the direction of the study and informs the selection of the case, the methods of collecting data, and the analysis techniques. A well-formulated research question in case study research is typically clear, focused, and complex enough to merit further detailed examination of the relevant case(s).
Propositions
Propositions, though not necessary in every case study, provide a direction by stating what we might expect to find in the data collected. They guide how data is collected and analyzed by helping researchers focus on specific aspects of the case. They are particularly important in explanatory case studies, which seek to understand the relationships among concepts within the studied phenomenon.
Units of analysis
The unit of analysis refers to the case, or the main entity or entities that are being analyzed in the study. In case study research, the unit of analysis can be an individual, a group, an organization, a decision, an event, or even a time period. It's crucial to clearly define the unit of analysis, as it shapes the qualitative data analysis process by allowing the researcher to analyze a particular case and synthesize analysis across multiple case studies to draw conclusions.
Argumentation
This refers to the inferential model that allows researchers to draw conclusions from the data. The researcher needs to ensure that there is a clear link between the data, the propositions (if any), and the conclusions drawn. This argumentation is what enables the researcher to make valid and credible inferences about the phenomenon under study.
Understanding and carefully considering these elements in the design phase of a case study can significantly enhance the quality of the research. It can help ensure that the study is methodologically sound and its findings contribute meaningful insights about the case.
Ready to jumpstart your research with ATLAS.ti?
Conceptualize your research project with our intuitive data analysis interface. Download a free trial today.
Conducting a case study involves several steps, from defining the research question and selecting the case to collecting and analyzing data . This section outlines these key stages, providing a practical guide on how to conduct case study research.
Defining the research question
The first step in case study research is defining a clear, focused research question. This question should guide the entire research process, from case selection to analysis. It's crucial to ensure that the research question is suitable for a case study approach. Typically, such questions are exploratory or descriptive in nature and focus on understanding a phenomenon within its real-life context.
Selecting and defining the case
The selection of the case should be based on the research question and the objectives of the study. It involves choosing a unique example or a set of examples that provide rich, in-depth data about the phenomenon under investigation. After selecting the case, it's crucial to define it clearly, setting the boundaries of the case, including the time period and the specific context.
Previous research can help guide the case study design. When considering a case study, an example of a case could be taken from previous case study research and used to define cases in a new research inquiry. Considering recently published examples can help understand how to select and define cases effectively.
Developing a detailed case study protocol
A case study protocol outlines the procedures and general rules to be followed during the case study. This includes the data collection methods to be used, the sources of data, and the procedures for analysis. Having a detailed case study protocol ensures consistency and reliability in the study.
The protocol should also consider how to work with the people involved in the research context to grant the research team access to collecting data. As mentioned in previous sections of this guide, establishing rapport is an essential component of qualitative research as it shapes the overall potential for collecting and analyzing data.
Collecting data
Gathering data in case study research often involves multiple sources of evidence, including documents, archival records, interviews, observations, and physical artifacts. This allows for a comprehensive understanding of the case. The process for gathering data should be systematic and carefully documented to ensure the reliability and validity of the study.
Analyzing and interpreting data
The next step is analyzing the data. This involves organizing the data , categorizing it into themes or patterns , and interpreting these patterns to answer the research question. The analysis might also involve comparing the findings with prior research or theoretical propositions.
Writing the case study report
The final step is writing the case study report . This should provide a detailed description of the case, the data, the analysis process, and the findings. The report should be clear, organized, and carefully written to ensure that the reader can understand the case and the conclusions drawn from it.
Each of these steps is crucial in ensuring that the case study research is rigorous, reliable, and provides valuable insights about the case.
The type, depth, and quality of data in your study can significantly influence the validity and utility of the study. In case study research, data is usually collected from multiple sources to provide a comprehensive and nuanced understanding of the case. This section will outline the various methods of collecting data used in case study research and discuss considerations for ensuring the quality of the data.
Interviews are a common method of gathering data in case study research. They can provide rich, in-depth data about the perspectives, experiences, and interpretations of the individuals involved in the case. Interviews can be structured , semi-structured , or unstructured , depending on the research question and the degree of flexibility needed.
Observations
Observations involve the researcher observing the case in its natural setting, providing first-hand information about the case and its context. Observations can provide data that might not be revealed in interviews or documents, such as non-verbal cues or contextual information.
Documents and artifacts
Documents and archival records provide a valuable source of data in case study research. They can include reports, letters, memos, meeting minutes, email correspondence, and various public and private documents related to the case.
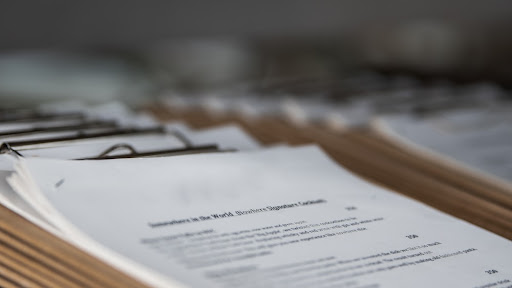
These records can provide historical context, corroborate evidence from other sources, and offer insights into the case that might not be apparent from interviews or observations.
Physical artifacts refer to any physical evidence related to the case, such as tools, products, or physical environments. These artifacts can provide tangible insights into the case, complementing the data gathered from other sources.
Ensuring the quality of data collection
Determining the quality of data in case study research requires careful planning and execution. It's crucial to ensure that the data is reliable, accurate, and relevant to the research question. This involves selecting appropriate methods of collecting data, properly training interviewers or observers, and systematically recording and storing the data. It also includes considering ethical issues related to collecting and handling data, such as obtaining informed consent and ensuring the privacy and confidentiality of the participants.
Data analysis
Analyzing case study research involves making sense of the rich, detailed data to answer the research question. This process can be challenging due to the volume and complexity of case study data. However, a systematic and rigorous approach to analysis can ensure that the findings are credible and meaningful. This section outlines the main steps and considerations in analyzing data in case study research.
Organizing the data
The first step in the analysis is organizing the data. This involves sorting the data into manageable sections, often according to the data source or the theme. This step can also involve transcribing interviews, digitizing physical artifacts, or organizing observational data.
Categorizing and coding the data
Once the data is organized, the next step is to categorize or code the data. This involves identifying common themes, patterns, or concepts in the data and assigning codes to relevant data segments. Coding can be done manually or with the help of software tools, and in either case, qualitative analysis software can greatly facilitate the entire coding process. Coding helps to reduce the data to a set of themes or categories that can be more easily analyzed.
Identifying patterns and themes
After coding the data, the researcher looks for patterns or themes in the coded data. This involves comparing and contrasting the codes and looking for relationships or patterns among them. The identified patterns and themes should help answer the research question.
Interpreting the data
Once patterns and themes have been identified, the next step is to interpret these findings. This involves explaining what the patterns or themes mean in the context of the research question and the case. This interpretation should be grounded in the data, but it can also involve drawing on theoretical concepts or prior research.
Verification of the data
The last step in the analysis is verification. This involves checking the accuracy and consistency of the analysis process and confirming that the findings are supported by the data. This can involve re-checking the original data, checking the consistency of codes, or seeking feedback from research participants or peers.
Like any research method , case study research has its strengths and limitations. Researchers must be aware of these, as they can influence the design, conduct, and interpretation of the study.
Understanding the strengths and limitations of case study research can also guide researchers in deciding whether this approach is suitable for their research question . This section outlines some of the key strengths and limitations of case study research.
Benefits include the following:
- Rich, detailed data: One of the main strengths of case study research is that it can generate rich, detailed data about the case. This can provide a deep understanding of the case and its context, which can be valuable in exploring complex phenomena.
- Flexibility: Case study research is flexible in terms of design , data collection , and analysis . A sufficient degree of flexibility allows the researcher to adapt the study according to the case and the emerging findings.
- Real-world context: Case study research involves studying the case in its real-world context, which can provide valuable insights into the interplay between the case and its context.
- Multiple sources of evidence: Case study research often involves collecting data from multiple sources , which can enhance the robustness and validity of the findings.
On the other hand, researchers should consider the following limitations:
- Generalizability: A common criticism of case study research is that its findings might not be generalizable to other cases due to the specificity and uniqueness of each case.
- Time and resource intensive: Case study research can be time and resource intensive due to the depth of the investigation and the amount of collected data.
- Complexity of analysis: The rich, detailed data generated in case study research can make analyzing the data challenging.
- Subjectivity: Given the nature of case study research, there may be a higher degree of subjectivity in interpreting the data , so researchers need to reflect on this and transparently convey to audiences how the research was conducted.
Being aware of these strengths and limitations can help researchers design and conduct case study research effectively and interpret and report the findings appropriately.
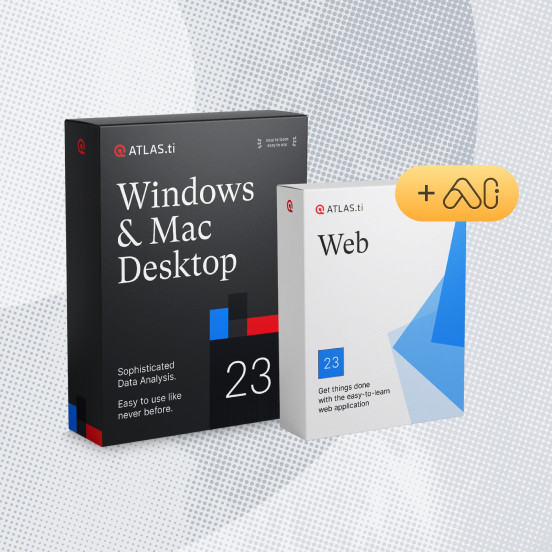
Ready to analyze your data with ATLAS.ti?
See how our intuitive software can draw key insights from your data with a free trial today.
- Privacy Policy

Home » Case Study – Methods, Examples and Guide
Case Study – Methods, Examples and Guide
Table of Contents

A case study is a research method that involves an in-depth examination and analysis of a particular phenomenon or case, such as an individual, organization, community, event, or situation.
It is a qualitative research approach that aims to provide a detailed and comprehensive understanding of the case being studied. Case studies typically involve multiple sources of data, including interviews, observations, documents, and artifacts, which are analyzed using various techniques, such as content analysis, thematic analysis, and grounded theory. The findings of a case study are often used to develop theories, inform policy or practice, or generate new research questions.
Types of Case Study
Types and Methods of Case Study are as follows:
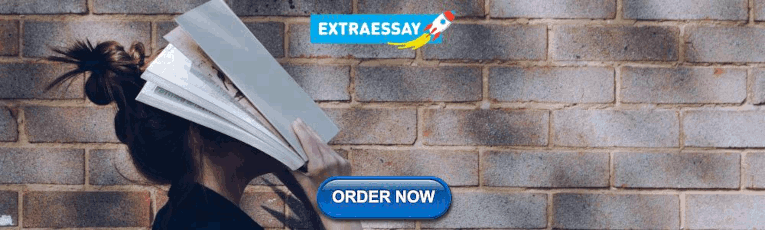
Single-Case Study
A single-case study is an in-depth analysis of a single case. This type of case study is useful when the researcher wants to understand a specific phenomenon in detail.
For Example , A researcher might conduct a single-case study on a particular individual to understand their experiences with a particular health condition or a specific organization to explore their management practices. The researcher collects data from multiple sources, such as interviews, observations, and documents, and uses various techniques to analyze the data, such as content analysis or thematic analysis. The findings of a single-case study are often used to generate new research questions, develop theories, or inform policy or practice.
Multiple-Case Study
A multiple-case study involves the analysis of several cases that are similar in nature. This type of case study is useful when the researcher wants to identify similarities and differences between the cases.
For Example, a researcher might conduct a multiple-case study on several companies to explore the factors that contribute to their success or failure. The researcher collects data from each case, compares and contrasts the findings, and uses various techniques to analyze the data, such as comparative analysis or pattern-matching. The findings of a multiple-case study can be used to develop theories, inform policy or practice, or generate new research questions.
Exploratory Case Study
An exploratory case study is used to explore a new or understudied phenomenon. This type of case study is useful when the researcher wants to generate hypotheses or theories about the phenomenon.
For Example, a researcher might conduct an exploratory case study on a new technology to understand its potential impact on society. The researcher collects data from multiple sources, such as interviews, observations, and documents, and uses various techniques to analyze the data, such as grounded theory or content analysis. The findings of an exploratory case study can be used to generate new research questions, develop theories, or inform policy or practice.
Descriptive Case Study
A descriptive case study is used to describe a particular phenomenon in detail. This type of case study is useful when the researcher wants to provide a comprehensive account of the phenomenon.
For Example, a researcher might conduct a descriptive case study on a particular community to understand its social and economic characteristics. The researcher collects data from multiple sources, such as interviews, observations, and documents, and uses various techniques to analyze the data, such as content analysis or thematic analysis. The findings of a descriptive case study can be used to inform policy or practice or generate new research questions.
Instrumental Case Study
An instrumental case study is used to understand a particular phenomenon that is instrumental in achieving a particular goal. This type of case study is useful when the researcher wants to understand the role of the phenomenon in achieving the goal.
For Example, a researcher might conduct an instrumental case study on a particular policy to understand its impact on achieving a particular goal, such as reducing poverty. The researcher collects data from multiple sources, such as interviews, observations, and documents, and uses various techniques to analyze the data, such as content analysis or thematic analysis. The findings of an instrumental case study can be used to inform policy or practice or generate new research questions.
Case Study Data Collection Methods
Here are some common data collection methods for case studies:
Interviews involve asking questions to individuals who have knowledge or experience relevant to the case study. Interviews can be structured (where the same questions are asked to all participants) or unstructured (where the interviewer follows up on the responses with further questions). Interviews can be conducted in person, over the phone, or through video conferencing.
Observations
Observations involve watching and recording the behavior and activities of individuals or groups relevant to the case study. Observations can be participant (where the researcher actively participates in the activities) or non-participant (where the researcher observes from a distance). Observations can be recorded using notes, audio or video recordings, or photographs.
Documents can be used as a source of information for case studies. Documents can include reports, memos, emails, letters, and other written materials related to the case study. Documents can be collected from the case study participants or from public sources.
Surveys involve asking a set of questions to a sample of individuals relevant to the case study. Surveys can be administered in person, over the phone, through mail or email, or online. Surveys can be used to gather information on attitudes, opinions, or behaviors related to the case study.
Artifacts are physical objects relevant to the case study. Artifacts can include tools, equipment, products, or other objects that provide insights into the case study phenomenon.
How to conduct Case Study Research
Conducting a case study research involves several steps that need to be followed to ensure the quality and rigor of the study. Here are the steps to conduct case study research:
- Define the research questions: The first step in conducting a case study research is to define the research questions. The research questions should be specific, measurable, and relevant to the case study phenomenon under investigation.
- Select the case: The next step is to select the case or cases to be studied. The case should be relevant to the research questions and should provide rich and diverse data that can be used to answer the research questions.
- Collect data: Data can be collected using various methods, such as interviews, observations, documents, surveys, and artifacts. The data collection method should be selected based on the research questions and the nature of the case study phenomenon.
- Analyze the data: The data collected from the case study should be analyzed using various techniques, such as content analysis, thematic analysis, or grounded theory. The analysis should be guided by the research questions and should aim to provide insights and conclusions relevant to the research questions.
- Draw conclusions: The conclusions drawn from the case study should be based on the data analysis and should be relevant to the research questions. The conclusions should be supported by evidence and should be clearly stated.
- Validate the findings: The findings of the case study should be validated by reviewing the data and the analysis with participants or other experts in the field. This helps to ensure the validity and reliability of the findings.
- Write the report: The final step is to write the report of the case study research. The report should provide a clear description of the case study phenomenon, the research questions, the data collection methods, the data analysis, the findings, and the conclusions. The report should be written in a clear and concise manner and should follow the guidelines for academic writing.
Examples of Case Study
Here are some examples of case study research:
- The Hawthorne Studies : Conducted between 1924 and 1932, the Hawthorne Studies were a series of case studies conducted by Elton Mayo and his colleagues to examine the impact of work environment on employee productivity. The studies were conducted at the Hawthorne Works plant of the Western Electric Company in Chicago and included interviews, observations, and experiments.
- The Stanford Prison Experiment: Conducted in 1971, the Stanford Prison Experiment was a case study conducted by Philip Zimbardo to examine the psychological effects of power and authority. The study involved simulating a prison environment and assigning participants to the role of guards or prisoners. The study was controversial due to the ethical issues it raised.
- The Challenger Disaster: The Challenger Disaster was a case study conducted to examine the causes of the Space Shuttle Challenger explosion in 1986. The study included interviews, observations, and analysis of data to identify the technical, organizational, and cultural factors that contributed to the disaster.
- The Enron Scandal: The Enron Scandal was a case study conducted to examine the causes of the Enron Corporation’s bankruptcy in 2001. The study included interviews, analysis of financial data, and review of documents to identify the accounting practices, corporate culture, and ethical issues that led to the company’s downfall.
- The Fukushima Nuclear Disaster : The Fukushima Nuclear Disaster was a case study conducted to examine the causes of the nuclear accident that occurred at the Fukushima Daiichi Nuclear Power Plant in Japan in 2011. The study included interviews, analysis of data, and review of documents to identify the technical, organizational, and cultural factors that contributed to the disaster.
Application of Case Study
Case studies have a wide range of applications across various fields and industries. Here are some examples:
Business and Management
Case studies are widely used in business and management to examine real-life situations and develop problem-solving skills. Case studies can help students and professionals to develop a deep understanding of business concepts, theories, and best practices.
Case studies are used in healthcare to examine patient care, treatment options, and outcomes. Case studies can help healthcare professionals to develop critical thinking skills, diagnose complex medical conditions, and develop effective treatment plans.
Case studies are used in education to examine teaching and learning practices. Case studies can help educators to develop effective teaching strategies, evaluate student progress, and identify areas for improvement.
Social Sciences
Case studies are widely used in social sciences to examine human behavior, social phenomena, and cultural practices. Case studies can help researchers to develop theories, test hypotheses, and gain insights into complex social issues.
Law and Ethics
Case studies are used in law and ethics to examine legal and ethical dilemmas. Case studies can help lawyers, policymakers, and ethical professionals to develop critical thinking skills, analyze complex cases, and make informed decisions.
Purpose of Case Study
The purpose of a case study is to provide a detailed analysis of a specific phenomenon, issue, or problem in its real-life context. A case study is a qualitative research method that involves the in-depth exploration and analysis of a particular case, which can be an individual, group, organization, event, or community.
The primary purpose of a case study is to generate a comprehensive and nuanced understanding of the case, including its history, context, and dynamics. Case studies can help researchers to identify and examine the underlying factors, processes, and mechanisms that contribute to the case and its outcomes. This can help to develop a more accurate and detailed understanding of the case, which can inform future research, practice, or policy.
Case studies can also serve other purposes, including:
- Illustrating a theory or concept: Case studies can be used to illustrate and explain theoretical concepts and frameworks, providing concrete examples of how they can be applied in real-life situations.
- Developing hypotheses: Case studies can help to generate hypotheses about the causal relationships between different factors and outcomes, which can be tested through further research.
- Providing insight into complex issues: Case studies can provide insights into complex and multifaceted issues, which may be difficult to understand through other research methods.
- Informing practice or policy: Case studies can be used to inform practice or policy by identifying best practices, lessons learned, or areas for improvement.
Advantages of Case Study Research
There are several advantages of case study research, including:
- In-depth exploration: Case study research allows for a detailed exploration and analysis of a specific phenomenon, issue, or problem in its real-life context. This can provide a comprehensive understanding of the case and its dynamics, which may not be possible through other research methods.
- Rich data: Case study research can generate rich and detailed data, including qualitative data such as interviews, observations, and documents. This can provide a nuanced understanding of the case and its complexity.
- Holistic perspective: Case study research allows for a holistic perspective of the case, taking into account the various factors, processes, and mechanisms that contribute to the case and its outcomes. This can help to develop a more accurate and comprehensive understanding of the case.
- Theory development: Case study research can help to develop and refine theories and concepts by providing empirical evidence and concrete examples of how they can be applied in real-life situations.
- Practical application: Case study research can inform practice or policy by identifying best practices, lessons learned, or areas for improvement.
- Contextualization: Case study research takes into account the specific context in which the case is situated, which can help to understand how the case is influenced by the social, cultural, and historical factors of its environment.
Limitations of Case Study Research
There are several limitations of case study research, including:
- Limited generalizability : Case studies are typically focused on a single case or a small number of cases, which limits the generalizability of the findings. The unique characteristics of the case may not be applicable to other contexts or populations, which may limit the external validity of the research.
- Biased sampling: Case studies may rely on purposive or convenience sampling, which can introduce bias into the sample selection process. This may limit the representativeness of the sample and the generalizability of the findings.
- Subjectivity: Case studies rely on the interpretation of the researcher, which can introduce subjectivity into the analysis. The researcher’s own biases, assumptions, and perspectives may influence the findings, which may limit the objectivity of the research.
- Limited control: Case studies are typically conducted in naturalistic settings, which limits the control that the researcher has over the environment and the variables being studied. This may limit the ability to establish causal relationships between variables.
- Time-consuming: Case studies can be time-consuming to conduct, as they typically involve a detailed exploration and analysis of a specific case. This may limit the feasibility of conducting multiple case studies or conducting case studies in a timely manner.
- Resource-intensive: Case studies may require significant resources, including time, funding, and expertise. This may limit the ability of researchers to conduct case studies in resource-constrained settings.
About the author
Muhammad Hassan
Researcher, Academic Writer, Web developer
You may also like

Questionnaire – Definition, Types, and Examples

Observational Research – Methods and Guide

Quantitative Research – Methods, Types and...

Qualitative Research Methods

Explanatory Research – Types, Methods, Guide

Survey Research – Types, Methods, Examples
The Case Study as Research Method: A Practical Handbook
Qualitative Research in Accounting & Management
ISSN : 1176-6093
Article publication date: 21 June 2011
Scapens, R.W. (2011), "The Case Study as Research Method: A Practical Handbook", Qualitative Research in Accounting & Management , Vol. 8 No. 2, pp. 201-204. https://doi.org/10.1108/11766091111137582
Emerald Group Publishing Limited
Copyright © 2011, Emerald Group Publishing Limited
This book aims to provide case‐study researchers with a step‐by‐step practical guide to “help them conduct the study with the required degree of rigour” (p. xi).
It seeks to “demonstrate that the case study is indeed a scientific method” (p. 104) and to show “the usefulness of the case method as one tool in the researcher's methodological arsenal” (p. 105). The individual chapters cover the various stages in conducting case‐study research, and each chapter sets out a number of practical steps which have to be taken by the researcher. The following are the eight stages/chapters and, in brackets, the number of steps in each stages:
Assessing appropriateness and usefulness (4).
Ensuring accuracy of results (21).
Preparation (6).
Selecting cases (4).
Collecting data (7).
Analyzing data (4).
Interpreting data (3).
Reporting results (4).
It is particularly noticeable that ensuring accuracy of results has by far the largest number of number of steps – 21 steps compared to seven or fewer steps in the other stages. This reflects Gagnon's concern to demonstrate the scientific rigour of case‐study research. In the forward, he explains that the book draws on his experience in conducting his own PhD research, which was closely supervised by three professors, one of whom was inclined towards quantitative research. Consequently, his research was underpinned by the principles and philosophy of quantitative research. This is clearly reflected in the approach taken in this book, which seeks to show that case‐study research is just as rigorous and scientific as quantitative research, and it can produce an objective and accurate representation of the observed reality.
There is no discussion of the methodological issues relating to the use of case‐study research methods. This is acknowledged in the forward, although Gagnon refers to them as philosophical or epistemological issues (p. xii), as he tends to use the terms methodology and method interchangeably – as is common in quantitative research. Although he starts (step 1.1) by trying to distance case and other qualitative research from the work of positivists, arguing that society is socially constructed, he nevertheless sees social reality as objective and independent of the researcher. So for Gagnon, the aim of case research is to accurately reflect that reality. At various points in the book the notion of interpretation is used – evidence is interpreted and the (objective) case findings have to be interpreted.
So although there is a distancing from positivist research (p. 1), the approach taken in this book retains an objective view of the social reality which is being researched; a view which is rather different to the subjective view of reality taken by many interpretive case researchers. This distinction between an objective and a subjective view of the social reality being researched – and especially its use in contrasting positivist and interpretive research – has its origins the taxonomy of Burrell and Morgan (1979) . Although there have been various developments in the so‐called “objective‐subjective debate”, and recently some discussion in relation to management accounting research ( Kakkuri‐Knuuttila et al. , 2008 ; Ahrens, 2008 ), this debate is not mentioned in the book. Nevertheless, it is clear that Gagnon is firmly in the objective camp. In a recent paper, Johnson et al. (2006, p. 138) provide a more contemporary classification of the different types of qualitative research. In their terms, the approach taken in this book could be described as neo‐empiricist – an approach which they characterise as “qualitative positivists”.
The approach taken in this handbook leaves case studies open to the criticisms that they are a small sample, and consequently difficult to generalise, and to arguments that case studies are most appropriate for exploratory research which can subsequently be generalised though quantitative research. Gagnon explains that this was the approach he used after completing his thesis (p. xi). The handbook only seems to recognise two types of case studies, namely exploratory and raw empirical case studies – the latter being used where “the researcher is interested in a subject without having formed any preconceived ideas about it” (p. 15) – which has echoes of Glaser and Strauss (1967) . However, limiting case studies to these two types ignores other potential types; in particular, explanatory case studies which are where interpretive case‐study research can make important contributions ( Ryan et al. , 2002 ).
This limited approach to case studies comes through in the practical steps which are recommended in the handbook, and especially in the discussion of reliability and validity. The suggested steps seem to be designed to keep very close to the notions of reliability and validity used in quantitative research. There is no mention of the recent discussion of “validity” in interpretive accounting research, which emphasises the importance of authenticity and credibility and their implications for writing up qualitative and case‐study research ( Lukka and Modell, 2010 ). Although the final stage of Gagnon's handbook makes some very general comments about reporting the results, it does not mention, for example, Baxter and Chua's (2008) paper in QRAM which discusses the importance of demonstrating authenticity, credibility and transferability in writing qualitative research.
Despite Gagnon's emphasis on traditional notions of reliability and validity the handbook provides some useful practical advice for all case‐study researchers. For example, case‐study research needs a very good research design; case‐study researchers must work hard to gain access to and acceptance in the research settings; a clear strategy is needed for data collection; the case researcher should create field notes (in a field notebook, or otherwise) to record all the thoughts, ideas, observations, etc. that would not otherwise be collected; and the vast amount of data that case‐study research can generate needs to be carefully managed. Furthermore, because of what Gagnon calls the “risk of mortality” (p. 54) (i.e. the risk that access to a research site may be lost – for instance, if the organisation goes bankrupt) it is crucial for some additional site(s) to be selected at the outset to ensure that the planned research can be completed. This is what I call “insurance cases” when talking to my own PhD students. Interestingly, Gagnon recognises the ethical issues involved in doing case studies – something which is not always mentioned by the more objectivist type of case‐study researchers. He emphasises that it is crucial to honour confidentiality agreements, to ensure data are stored securely and that commitments are met and promises kept.
There is an interesting discussion of the advantages and disadvantages of using computer methods in analysing data (in stage 6). However, the discussion of coding appears to be heavily influenced by grounded theory, and is clearly concerned with producing an accurate reflection of an objective reality. In addition, Gagnon's depiction of case analysis is overly focussed on content analysis – possibly because it is a quantitative type of technique. There is no reference to the other approaches available to qualitative researchers. For example, there is no mention of the various visualisation techniques set out in Miles and Huberman (1994) .
To summarise, Gagnon's book is particularly useful for case‐study researchers who see the reality they are researching as objective and researcher independent. However, this is a sub‐set of case‐study researchers. Although some of the practical guidance offered is relevant for other types of case‐study researchers, those who see multiple realities in the social actors and/or recognise the subjectivity of the research process might have difficulty with some of the steps in this handbook. Gagnon's aim to show that the case study is a scientific method, gives the handbook a focus on traditional (quantitatively inspired) notions rigour and validity, and a tendency to ignore (or at least marginalise) other types of case study research. For example, the focus on exploratory cases, which need to be supplemented by broad based quantitative research, overlooks the real potential of case study research which lies in explanatory cases. Furthermore, Gagnon is rather worried about participant research, as the researcher may play a role which is “not consistent with scientific method” (p. 42), and which may introduce researcher bias and thereby damage “the impartiality of the study” (p. 53). Leaving aside the philosophical question about whether any social science research, including quantitative research, can be impartial, this stance could severely limit the potential of case‐study research and it would rule out both the early work on the sociology of mass production and the recent calls for interventionist research. Clearly, there could be a problem where a researcher is trying to sell consulting services, but there is a long tradition of social researchers working within organisations that they are studying. Furthermore, if interpretive research is to be relevant for practice, researchers may have to work with organisations to introduce new ideas and new ways of analysing problems. Gagnon would seem to want to avoid all such research – as it would not be “impartial”.
Consequently, although there is some good practical advice for case study researchers in this handbook, some of the recommendations have to be treated cautiously, as it is a book which sees case‐study research in a very specific way. As mentioned earlier, in the Forward Gagnon explicitly recognises that the book does not take a position on the methodological debates surrounding the use of case studies as a research method, and he says that “The reader should therefore use and judge this handbook with these considerations in mind” (p. xii). This is very good advice – caveat emptor .
Ahrens , T. ( 2008 ), “ A comment on Marja‐Liisa Kakkuri‐Knuuttila ”, Accounting, Organizations and Society , Vol. 33 Nos 2/3 , pp. 291 ‐ 7 , Kari Lukka and Jaakko Kuorikoski.
Baxter , J. and Chua , W.F. ( 2008 ), “ The field researcher as author‐writer ”, Qualitative Research in Accounting & Management , Vol. 5 No. 2 , pp. 101 ‐ 21 .
Burrell , G. and Morgan , G. ( 1979 ), Sociological Paradigms and Organizational Analysis , Heinneman , London .
Glaser , B.G. and Strauss , A.L. ( 1967 ), The Discovery of Grounded Theory: Strategies for Qualitative Research , Aldine , New York, NY .
Johnson , P. , Buehring , A. , Cassell , C. and Symon , G. ( 2006 ), “ Evaluating qualitative management research: towards a contingent critieriology ”, International Journal of Management Reviews , Vol. 8 No. 3 , pp. 131 ‐ 56 .
Kakkuri‐Knuuttila , M.‐L. , Lukka , K. and Kuorikoski , J. ( 2008 ), “ Straddling between paradigms: a naturalistic philosophical case study on interpretive research in management accounting ”, Accounting, Organizations and Society , Vol. 33 Nos 2/3 , pp. 267 ‐ 91 .
Lukka , K. and Modell , S. ( 2010 ), “ Validation in interpretive management accounting research ”, Accounting, Organizations and Society , Vol. 35 , pp. 462 ‐ 77 .
Miles , M.B. and Huberman , A.M. ( 1994 ), Qualitative Data Analysis: A Source Book of New Methods , 2nd ed. , Sage , London .
Ryan , R.J. , Scapens , R.W. and Theobald , M. ( 2002 ), Research Methods and Methodology in Finance and Accounting , 2nd ed. , Thomson Learning , London .
Related articles
We’re listening — tell us what you think, something didn’t work….
Report bugs here
All feedback is valuable
Please share your general feedback
Join us on our journey
Platform update page.
Visit emeraldpublishing.com/platformupdate to discover the latest news and updates
Questions & More Information
Answers to the most commonly asked questions here
From David E. Gray \(2014\). Doing Research in the Real World \(3rd ed.\) London, UK: Sage.
- Open access
- Published: 27 June 2011
The case study approach
- Sarah Crowe 1 ,
- Kathrin Cresswell 2 ,
- Ann Robertson 2 ,
- Guro Huby 3 ,
- Anthony Avery 1 &
- Aziz Sheikh 2
BMC Medical Research Methodology volume 11 , Article number: 100 ( 2011 ) Cite this article
774k Accesses
1037 Citations
37 Altmetric
Metrics details
The case study approach allows in-depth, multi-faceted explorations of complex issues in their real-life settings. The value of the case study approach is well recognised in the fields of business, law and policy, but somewhat less so in health services research. Based on our experiences of conducting several health-related case studies, we reflect on the different types of case study design, the specific research questions this approach can help answer, the data sources that tend to be used, and the particular advantages and disadvantages of employing this methodological approach. The paper concludes with key pointers to aid those designing and appraising proposals for conducting case study research, and a checklist to help readers assess the quality of case study reports.
Peer Review reports
Introduction
The case study approach is particularly useful to employ when there is a need to obtain an in-depth appreciation of an issue, event or phenomenon of interest, in its natural real-life context. Our aim in writing this piece is to provide insights into when to consider employing this approach and an overview of key methodological considerations in relation to the design, planning, analysis, interpretation and reporting of case studies.
The illustrative 'grand round', 'case report' and 'case series' have a long tradition in clinical practice and research. Presenting detailed critiques, typically of one or more patients, aims to provide insights into aspects of the clinical case and, in doing so, illustrate broader lessons that may be learnt. In research, the conceptually-related case study approach can be used, for example, to describe in detail a patient's episode of care, explore professional attitudes to and experiences of a new policy initiative or service development or more generally to 'investigate contemporary phenomena within its real-life context' [ 1 ]. Based on our experiences of conducting a range of case studies, we reflect on when to consider using this approach, discuss the key steps involved and illustrate, with examples, some of the practical challenges of attaining an in-depth understanding of a 'case' as an integrated whole. In keeping with previously published work, we acknowledge the importance of theory to underpin the design, selection, conduct and interpretation of case studies[ 2 ]. In so doing, we make passing reference to the different epistemological approaches used in case study research by key theoreticians and methodologists in this field of enquiry.
This paper is structured around the following main questions: What is a case study? What are case studies used for? How are case studies conducted? What are the potential pitfalls and how can these be avoided? We draw in particular on four of our own recently published examples of case studies (see Tables 1 , 2 , 3 and 4 ) and those of others to illustrate our discussion[ 3 – 7 ].
What is a case study?
A case study is a research approach that is used to generate an in-depth, multi-faceted understanding of a complex issue in its real-life context. It is an established research design that is used extensively in a wide variety of disciplines, particularly in the social sciences. A case study can be defined in a variety of ways (Table 5 ), the central tenet being the need to explore an event or phenomenon in depth and in its natural context. It is for this reason sometimes referred to as a "naturalistic" design; this is in contrast to an "experimental" design (such as a randomised controlled trial) in which the investigator seeks to exert control over and manipulate the variable(s) of interest.
Stake's work has been particularly influential in defining the case study approach to scientific enquiry. He has helpfully characterised three main types of case study: intrinsic , instrumental and collective [ 8 ]. An intrinsic case study is typically undertaken to learn about a unique phenomenon. The researcher should define the uniqueness of the phenomenon, which distinguishes it from all others. In contrast, the instrumental case study uses a particular case (some of which may be better than others) to gain a broader appreciation of an issue or phenomenon. The collective case study involves studying multiple cases simultaneously or sequentially in an attempt to generate a still broader appreciation of a particular issue.
These are however not necessarily mutually exclusive categories. In the first of our examples (Table 1 ), we undertook an intrinsic case study to investigate the issue of recruitment of minority ethnic people into the specific context of asthma research studies, but it developed into a instrumental case study through seeking to understand the issue of recruitment of these marginalised populations more generally, generating a number of the findings that are potentially transferable to other disease contexts[ 3 ]. In contrast, the other three examples (see Tables 2 , 3 and 4 ) employed collective case study designs to study the introduction of workforce reconfiguration in primary care, the implementation of electronic health records into hospitals, and to understand the ways in which healthcare students learn about patient safety considerations[ 4 – 6 ]. Although our study focusing on the introduction of General Practitioners with Specialist Interests (Table 2 ) was explicitly collective in design (four contrasting primary care organisations were studied), is was also instrumental in that this particular professional group was studied as an exemplar of the more general phenomenon of workforce redesign[ 4 ].
What are case studies used for?
According to Yin, case studies can be used to explain, describe or explore events or phenomena in the everyday contexts in which they occur[ 1 ]. These can, for example, help to understand and explain causal links and pathways resulting from a new policy initiative or service development (see Tables 2 and 3 , for example)[ 1 ]. In contrast to experimental designs, which seek to test a specific hypothesis through deliberately manipulating the environment (like, for example, in a randomised controlled trial giving a new drug to randomly selected individuals and then comparing outcomes with controls),[ 9 ] the case study approach lends itself well to capturing information on more explanatory ' how ', 'what' and ' why ' questions, such as ' how is the intervention being implemented and received on the ground?'. The case study approach can offer additional insights into what gaps exist in its delivery or why one implementation strategy might be chosen over another. This in turn can help develop or refine theory, as shown in our study of the teaching of patient safety in undergraduate curricula (Table 4 )[ 6 , 10 ]. Key questions to consider when selecting the most appropriate study design are whether it is desirable or indeed possible to undertake a formal experimental investigation in which individuals and/or organisations are allocated to an intervention or control arm? Or whether the wish is to obtain a more naturalistic understanding of an issue? The former is ideally studied using a controlled experimental design, whereas the latter is more appropriately studied using a case study design.
Case studies may be approached in different ways depending on the epistemological standpoint of the researcher, that is, whether they take a critical (questioning one's own and others' assumptions), interpretivist (trying to understand individual and shared social meanings) or positivist approach (orientating towards the criteria of natural sciences, such as focusing on generalisability considerations) (Table 6 ). Whilst such a schema can be conceptually helpful, it may be appropriate to draw on more than one approach in any case study, particularly in the context of conducting health services research. Doolin has, for example, noted that in the context of undertaking interpretative case studies, researchers can usefully draw on a critical, reflective perspective which seeks to take into account the wider social and political environment that has shaped the case[ 11 ].
How are case studies conducted?
Here, we focus on the main stages of research activity when planning and undertaking a case study; the crucial stages are: defining the case; selecting the case(s); collecting and analysing the data; interpreting data; and reporting the findings.
Defining the case
Carefully formulated research question(s), informed by the existing literature and a prior appreciation of the theoretical issues and setting(s), are all important in appropriately and succinctly defining the case[ 8 , 12 ]. Crucially, each case should have a pre-defined boundary which clarifies the nature and time period covered by the case study (i.e. its scope, beginning and end), the relevant social group, organisation or geographical area of interest to the investigator, the types of evidence to be collected, and the priorities for data collection and analysis (see Table 7 )[ 1 ]. A theory driven approach to defining the case may help generate knowledge that is potentially transferable to a range of clinical contexts and behaviours; using theory is also likely to result in a more informed appreciation of, for example, how and why interventions have succeeded or failed[ 13 ].
For example, in our evaluation of the introduction of electronic health records in English hospitals (Table 3 ), we defined our cases as the NHS Trusts that were receiving the new technology[ 5 ]. Our focus was on how the technology was being implemented. However, if the primary research interest had been on the social and organisational dimensions of implementation, we might have defined our case differently as a grouping of healthcare professionals (e.g. doctors and/or nurses). The precise beginning and end of the case may however prove difficult to define. Pursuing this same example, when does the process of implementation and adoption of an electronic health record system really begin or end? Such judgements will inevitably be influenced by a range of factors, including the research question, theory of interest, the scope and richness of the gathered data and the resources available to the research team.
Selecting the case(s)
The decision on how to select the case(s) to study is a very important one that merits some reflection. In an intrinsic case study, the case is selected on its own merits[ 8 ]. The case is selected not because it is representative of other cases, but because of its uniqueness, which is of genuine interest to the researchers. This was, for example, the case in our study of the recruitment of minority ethnic participants into asthma research (Table 1 ) as our earlier work had demonstrated the marginalisation of minority ethnic people with asthma, despite evidence of disproportionate asthma morbidity[ 14 , 15 ]. In another example of an intrinsic case study, Hellstrom et al.[ 16 ] studied an elderly married couple living with dementia to explore how dementia had impacted on their understanding of home, their everyday life and their relationships.
For an instrumental case study, selecting a "typical" case can work well[ 8 ]. In contrast to the intrinsic case study, the particular case which is chosen is of less importance than selecting a case that allows the researcher to investigate an issue or phenomenon. For example, in order to gain an understanding of doctors' responses to health policy initiatives, Som undertook an instrumental case study interviewing clinicians who had a range of responsibilities for clinical governance in one NHS acute hospital trust[ 17 ]. Sampling a "deviant" or "atypical" case may however prove even more informative, potentially enabling the researcher to identify causal processes, generate hypotheses and develop theory.
In collective or multiple case studies, a number of cases are carefully selected. This offers the advantage of allowing comparisons to be made across several cases and/or replication. Choosing a "typical" case may enable the findings to be generalised to theory (i.e. analytical generalisation) or to test theory by replicating the findings in a second or even a third case (i.e. replication logic)[ 1 ]. Yin suggests two or three literal replications (i.e. predicting similar results) if the theory is straightforward and five or more if the theory is more subtle. However, critics might argue that selecting 'cases' in this way is insufficiently reflexive and ill-suited to the complexities of contemporary healthcare organisations.
The selected case study site(s) should allow the research team access to the group of individuals, the organisation, the processes or whatever else constitutes the chosen unit of analysis for the study. Access is therefore a central consideration; the researcher needs to come to know the case study site(s) well and to work cooperatively with them. Selected cases need to be not only interesting but also hospitable to the inquiry [ 8 ] if they are to be informative and answer the research question(s). Case study sites may also be pre-selected for the researcher, with decisions being influenced by key stakeholders. For example, our selection of case study sites in the evaluation of the implementation and adoption of electronic health record systems (see Table 3 ) was heavily influenced by NHS Connecting for Health, the government agency that was responsible for overseeing the National Programme for Information Technology (NPfIT)[ 5 ]. This prominent stakeholder had already selected the NHS sites (through a competitive bidding process) to be early adopters of the electronic health record systems and had negotiated contracts that detailed the deployment timelines.
It is also important to consider in advance the likely burden and risks associated with participation for those who (or the site(s) which) comprise the case study. Of particular importance is the obligation for the researcher to think through the ethical implications of the study (e.g. the risk of inadvertently breaching anonymity or confidentiality) and to ensure that potential participants/participating sites are provided with sufficient information to make an informed choice about joining the study. The outcome of providing this information might be that the emotive burden associated with participation, or the organisational disruption associated with supporting the fieldwork, is considered so high that the individuals or sites decide against participation.
In our example of evaluating implementations of electronic health record systems, given the restricted number of early adopter sites available to us, we sought purposively to select a diverse range of implementation cases among those that were available[ 5 ]. We chose a mixture of teaching, non-teaching and Foundation Trust hospitals, and examples of each of the three electronic health record systems procured centrally by the NPfIT. At one recruited site, it quickly became apparent that access was problematic because of competing demands on that organisation. Recognising the importance of full access and co-operative working for generating rich data, the research team decided not to pursue work at that site and instead to focus on other recruited sites.
Collecting the data
In order to develop a thorough understanding of the case, the case study approach usually involves the collection of multiple sources of evidence, using a range of quantitative (e.g. questionnaires, audits and analysis of routinely collected healthcare data) and more commonly qualitative techniques (e.g. interviews, focus groups and observations). The use of multiple sources of data (data triangulation) has been advocated as a way of increasing the internal validity of a study (i.e. the extent to which the method is appropriate to answer the research question)[ 8 , 18 – 21 ]. An underlying assumption is that data collected in different ways should lead to similar conclusions, and approaching the same issue from different angles can help develop a holistic picture of the phenomenon (Table 2 )[ 4 ].
Brazier and colleagues used a mixed-methods case study approach to investigate the impact of a cancer care programme[ 22 ]. Here, quantitative measures were collected with questionnaires before, and five months after, the start of the intervention which did not yield any statistically significant results. Qualitative interviews with patients however helped provide an insight into potentially beneficial process-related aspects of the programme, such as greater, perceived patient involvement in care. The authors reported how this case study approach provided a number of contextual factors likely to influence the effectiveness of the intervention and which were not likely to have been obtained from quantitative methods alone.
In collective or multiple case studies, data collection needs to be flexible enough to allow a detailed description of each individual case to be developed (e.g. the nature of different cancer care programmes), before considering the emerging similarities and differences in cross-case comparisons (e.g. to explore why one programme is more effective than another). It is important that data sources from different cases are, where possible, broadly comparable for this purpose even though they may vary in nature and depth.
Analysing, interpreting and reporting case studies
Making sense and offering a coherent interpretation of the typically disparate sources of data (whether qualitative alone or together with quantitative) is far from straightforward. Repeated reviewing and sorting of the voluminous and detail-rich data are integral to the process of analysis. In collective case studies, it is helpful to analyse data relating to the individual component cases first, before making comparisons across cases. Attention needs to be paid to variations within each case and, where relevant, the relationship between different causes, effects and outcomes[ 23 ]. Data will need to be organised and coded to allow the key issues, both derived from the literature and emerging from the dataset, to be easily retrieved at a later stage. An initial coding frame can help capture these issues and can be applied systematically to the whole dataset with the aid of a qualitative data analysis software package.
The Framework approach is a practical approach, comprising of five stages (familiarisation; identifying a thematic framework; indexing; charting; mapping and interpretation) , to managing and analysing large datasets particularly if time is limited, as was the case in our study of recruitment of South Asians into asthma research (Table 1 )[ 3 , 24 ]. Theoretical frameworks may also play an important role in integrating different sources of data and examining emerging themes. For example, we drew on a socio-technical framework to help explain the connections between different elements - technology; people; and the organisational settings within which they worked - in our study of the introduction of electronic health record systems (Table 3 )[ 5 ]. Our study of patient safety in undergraduate curricula drew on an evaluation-based approach to design and analysis, which emphasised the importance of the academic, organisational and practice contexts through which students learn (Table 4 )[ 6 ].
Case study findings can have implications both for theory development and theory testing. They may establish, strengthen or weaken historical explanations of a case and, in certain circumstances, allow theoretical (as opposed to statistical) generalisation beyond the particular cases studied[ 12 ]. These theoretical lenses should not, however, constitute a strait-jacket and the cases should not be "forced to fit" the particular theoretical framework that is being employed.
When reporting findings, it is important to provide the reader with enough contextual information to understand the processes that were followed and how the conclusions were reached. In a collective case study, researchers may choose to present the findings from individual cases separately before amalgamating across cases. Care must be taken to ensure the anonymity of both case sites and individual participants (if agreed in advance) by allocating appropriate codes or withholding descriptors. In the example given in Table 3 , we decided against providing detailed information on the NHS sites and individual participants in order to avoid the risk of inadvertent disclosure of identities[ 5 , 25 ].
What are the potential pitfalls and how can these be avoided?
The case study approach is, as with all research, not without its limitations. When investigating the formal and informal ways undergraduate students learn about patient safety (Table 4 ), for example, we rapidly accumulated a large quantity of data. The volume of data, together with the time restrictions in place, impacted on the depth of analysis that was possible within the available resources. This highlights a more general point of the importance of avoiding the temptation to collect as much data as possible; adequate time also needs to be set aside for data analysis and interpretation of what are often highly complex datasets.
Case study research has sometimes been criticised for lacking scientific rigour and providing little basis for generalisation (i.e. producing findings that may be transferable to other settings)[ 1 ]. There are several ways to address these concerns, including: the use of theoretical sampling (i.e. drawing on a particular conceptual framework); respondent validation (i.e. participants checking emerging findings and the researcher's interpretation, and providing an opinion as to whether they feel these are accurate); and transparency throughout the research process (see Table 8 )[ 8 , 18 – 21 , 23 , 26 ]. Transparency can be achieved by describing in detail the steps involved in case selection, data collection, the reasons for the particular methods chosen, and the researcher's background and level of involvement (i.e. being explicit about how the researcher has influenced data collection and interpretation). Seeking potential, alternative explanations, and being explicit about how interpretations and conclusions were reached, help readers to judge the trustworthiness of the case study report. Stake provides a critique checklist for a case study report (Table 9 )[ 8 ].
Conclusions
The case study approach allows, amongst other things, critical events, interventions, policy developments and programme-based service reforms to be studied in detail in a real-life context. It should therefore be considered when an experimental design is either inappropriate to answer the research questions posed or impossible to undertake. Considering the frequency with which implementations of innovations are now taking place in healthcare settings and how well the case study approach lends itself to in-depth, complex health service research, we believe this approach should be more widely considered by researchers. Though inherently challenging, the research case study can, if carefully conceptualised and thoughtfully undertaken and reported, yield powerful insights into many important aspects of health and healthcare delivery.
Yin RK: Case study research, design and method. 2009, London: Sage Publications Ltd., 4
Google Scholar
Keen J, Packwood T: Qualitative research; case study evaluation. BMJ. 1995, 311: 444-446.
Article CAS PubMed PubMed Central Google Scholar
Sheikh A, Halani L, Bhopal R, Netuveli G, Partridge M, Car J, et al: Facilitating the Recruitment of Minority Ethnic People into Research: Qualitative Case Study of South Asians and Asthma. PLoS Med. 2009, 6 (10): 1-11.
Article Google Scholar
Pinnock H, Huby G, Powell A, Kielmann T, Price D, Williams S, et al: The process of planning, development and implementation of a General Practitioner with a Special Interest service in Primary Care Organisations in England and Wales: a comparative prospective case study. Report for the National Co-ordinating Centre for NHS Service Delivery and Organisation R&D (NCCSDO). 2008, [ http://www.sdo.nihr.ac.uk/files/project/99-final-report.pdf ]
Robertson A, Cresswell K, Takian A, Petrakaki D, Crowe S, Cornford T, et al: Prospective evaluation of the implementation and adoption of NHS Connecting for Health's national electronic health record in secondary care in England: interim findings. BMJ. 2010, 41: c4564-
Pearson P, Steven A, Howe A, Sheikh A, Ashcroft D, Smith P, the Patient Safety Education Study Group: Learning about patient safety: organisational context and culture in the education of healthcare professionals. J Health Serv Res Policy. 2010, 15: 4-10. 10.1258/jhsrp.2009.009052.
Article PubMed Google Scholar
van Harten WH, Casparie TF, Fisscher OA: The evaluation of the introduction of a quality management system: a process-oriented case study in a large rehabilitation hospital. Health Policy. 2002, 60 (1): 17-37. 10.1016/S0168-8510(01)00187-7.
Stake RE: The art of case study research. 1995, London: Sage Publications Ltd.
Sheikh A, Smeeth L, Ashcroft R: Randomised controlled trials in primary care: scope and application. Br J Gen Pract. 2002, 52 (482): 746-51.
PubMed PubMed Central Google Scholar
King G, Keohane R, Verba S: Designing Social Inquiry. 1996, Princeton: Princeton University Press
Doolin B: Information technology as disciplinary technology: being critical in interpretative research on information systems. Journal of Information Technology. 1998, 13: 301-311. 10.1057/jit.1998.8.
George AL, Bennett A: Case studies and theory development in the social sciences. 2005, Cambridge, MA: MIT Press
Eccles M, the Improved Clinical Effectiveness through Behavioural Research Group (ICEBeRG): Designing theoretically-informed implementation interventions. Implementation Science. 2006, 1: 1-8. 10.1186/1748-5908-1-1.
Article PubMed Central Google Scholar
Netuveli G, Hurwitz B, Levy M, Fletcher M, Barnes G, Durham SR, Sheikh A: Ethnic variations in UK asthma frequency, morbidity, and health-service use: a systematic review and meta-analysis. Lancet. 2005, 365 (9456): 312-7.
Sheikh A, Panesar SS, Lasserson T, Netuveli G: Recruitment of ethnic minorities to asthma studies. Thorax. 2004, 59 (7): 634-
CAS PubMed PubMed Central Google Scholar
Hellström I, Nolan M, Lundh U: 'We do things together': A case study of 'couplehood' in dementia. Dementia. 2005, 4: 7-22. 10.1177/1471301205049188.
Som CV: Nothing seems to have changed, nothing seems to be changing and perhaps nothing will change in the NHS: doctors' response to clinical governance. International Journal of Public Sector Management. 2005, 18: 463-477. 10.1108/09513550510608903.
Lincoln Y, Guba E: Naturalistic inquiry. 1985, Newbury Park: Sage Publications
Barbour RS: Checklists for improving rigour in qualitative research: a case of the tail wagging the dog?. BMJ. 2001, 322: 1115-1117. 10.1136/bmj.322.7294.1115.
Mays N, Pope C: Qualitative research in health care: Assessing quality in qualitative research. BMJ. 2000, 320: 50-52. 10.1136/bmj.320.7226.50.
Mason J: Qualitative researching. 2002, London: Sage
Brazier A, Cooke K, Moravan V: Using Mixed Methods for Evaluating an Integrative Approach to Cancer Care: A Case Study. Integr Cancer Ther. 2008, 7: 5-17. 10.1177/1534735407313395.
Miles MB, Huberman M: Qualitative data analysis: an expanded sourcebook. 1994, CA: Sage Publications Inc., 2
Pope C, Ziebland S, Mays N: Analysing qualitative data. Qualitative research in health care. BMJ. 2000, 320: 114-116. 10.1136/bmj.320.7227.114.
Cresswell KM, Worth A, Sheikh A: Actor-Network Theory and its role in understanding the implementation of information technology developments in healthcare. BMC Med Inform Decis Mak. 2010, 10 (1): 67-10.1186/1472-6947-10-67.
Article PubMed PubMed Central Google Scholar
Malterud K: Qualitative research: standards, challenges, and guidelines. Lancet. 2001, 358: 483-488. 10.1016/S0140-6736(01)05627-6.
Article CAS PubMed Google Scholar
Yin R: Case study research: design and methods. 1994, Thousand Oaks, CA: Sage Publishing, 2
Yin R: Enhancing the quality of case studies in health services research. Health Serv Res. 1999, 34: 1209-1224.
Green J, Thorogood N: Qualitative methods for health research. 2009, Los Angeles: Sage, 2
Howcroft D, Trauth E: Handbook of Critical Information Systems Research, Theory and Application. 2005, Cheltenham, UK: Northampton, MA, USA: Edward Elgar
Book Google Scholar
Blakie N: Approaches to Social Enquiry. 1993, Cambridge: Polity Press
Doolin B: Power and resistance in the implementation of a medical management information system. Info Systems J. 2004, 14: 343-362. 10.1111/j.1365-2575.2004.00176.x.
Bloomfield BP, Best A: Management consultants: systems development, power and the translation of problems. Sociological Review. 1992, 40: 533-560.
Shanks G, Parr A: Positivist, single case study research in information systems: A critical analysis. Proceedings of the European Conference on Information Systems. 2003, Naples
Pre-publication history
The pre-publication history for this paper can be accessed here: http://www.biomedcentral.com/1471-2288/11/100/prepub
Download references
Acknowledgements
We are grateful to the participants and colleagues who contributed to the individual case studies that we have drawn on. This work received no direct funding, but it has been informed by projects funded by Asthma UK, the NHS Service Delivery Organisation, NHS Connecting for Health Evaluation Programme, and Patient Safety Research Portfolio. We would also like to thank the expert reviewers for their insightful and constructive feedback. Our thanks are also due to Dr. Allison Worth who commented on an earlier draft of this manuscript.
Author information
Authors and affiliations.
Division of Primary Care, The University of Nottingham, Nottingham, UK
Sarah Crowe & Anthony Avery
Centre for Population Health Sciences, The University of Edinburgh, Edinburgh, UK
Kathrin Cresswell, Ann Robertson & Aziz Sheikh
School of Health in Social Science, The University of Edinburgh, Edinburgh, UK
You can also search for this author in PubMed Google Scholar
Corresponding author
Correspondence to Sarah Crowe .
Additional information
Competing interests.
The authors declare that they have no competing interests.
Authors' contributions
AS conceived this article. SC, KC and AR wrote this paper with GH, AA and AS all commenting on various drafts. SC and AS are guarantors.
Rights and permissions
This article is published under license to BioMed Central Ltd. This is an Open Access article distributed under the terms of the Creative Commons Attribution License ( http://creativecommons.org/licenses/by/2.0 ), which permits unrestricted use, distribution, and reproduction in any medium, provided the original work is properly cited.
Reprints and permissions
About this article
Cite this article.
Crowe, S., Cresswell, K., Robertson, A. et al. The case study approach. BMC Med Res Methodol 11 , 100 (2011). https://doi.org/10.1186/1471-2288-11-100
Download citation
Received : 29 November 2010
Accepted : 27 June 2011
Published : 27 June 2011
DOI : https://doi.org/10.1186/1471-2288-11-100
Share this article
Anyone you share the following link with will be able to read this content:
Sorry, a shareable link is not currently available for this article.
Provided by the Springer Nature SharedIt content-sharing initiative
- Case Study Approach
- Electronic Health Record System
- Case Study Design
- Case Study Site
- Case Study Report
BMC Medical Research Methodology
ISSN: 1471-2288
- General enquiries: [email protected]
Deep learning for prediction of energy consumption: an applied use case in an office building
- Open access
- Published: 30 April 2024
Cite this article
You have full access to this open access article
- Roberto Morcillo-Jimenez ORCID: orcid.org/0000-0002-8771-5636 1 ,
- Jesús Mesa 1 ,
- Juan Gómez-Romero 1 ,
- M. Amparo Vila 1 &
- Maria J. Martin-Bautista 1
104 Accesses
Explore all metrics
Non-residential buildings are responsible for more than a third of global energy consumption. Estimating building energy consumption is the first step towards identifying inefficiencies and optimizing energy management policies. This paper presents a study of Deep Learning techniques for time series analysis applied to building energy prediction with real environments. We collected multisource sensor data from an actual office building under normal operating conditions, pre-processed them, and performed a comprehensive evaluation of the accuracy of feed-forward and recurrent neural networks to predict energy consumption. The results show that memory-based architectures (LSTMs) perform better than stateless ones (MLPs) even without data aggregation (CNNs), although the lack of ample usable data in this type of problem avoids making the most of recent techniques such as sequence-to-sequence (Seq2Seq).
Graphical abstract
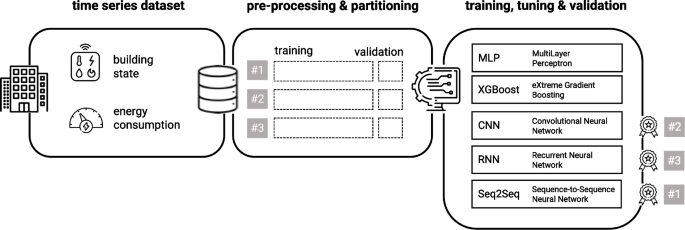
Similar content being viewed by others
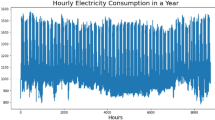
Deep learning-based forecasting of electricity consumption
Interpretable Deep Attention Model for Multivariate Time Series Prediction in Building Energy Systems
DeepEnergy: Prediction of Appliances Energy with Long-Short Term Memory Recurrent Neural Network
Avoid common mistakes on your manuscript.
1 Introduction
The current unstable situation in Europe has led to a decrease in the amount of energy supplied to the European continent, causing an exorbitant increase in prices across the continent. It is therefore necessary to reduce energy consumption in general, to prevent inflation from increasing further and the energy crisis from continuing to grow. Our work focuses on increasing energy efficiency in office buildings, as this is one of the most energy intensive areas due to the number of hours they are in operation. Reducing energy consumption by controlling consumption through algorithmic artificial intelligence techniques is one of the goals of our society in the coming years and our case study.
According to [ 26 ], residential, office, and industrial buildings account for 20-40% of global energy consumption. In 2010 in the USA, buildings energy consumption accounted for 41% of their total energy consumption, with 75% of this energy coming from fossil fuels [ 17 ]. Meanwhile, in 2012 in the European Union, buildings consumed approximately 40% of the total energy used [ 17 ]. More than two-thirds of the energy consumed by buildings goes to heating systems (37%), water heating (12%), air conditioning (10%) and lighting (9%). Several case studies have shown that the operational phase is the most energy-consuming stage of the buildings life cycle, accounting for 90% in conventional buildings and 50% in low-energy buildings [ 29 ].
In the current context in Europe, it is important to reduce energy consumption due to the high prices being achieved in the various energy markets. It is not surprising that interest in improving the energy efficiency of buildings has increased. According to [ 6 ] and [ 4 ], this interest is driven by three factors: rising energy prices, increasingly restrictive environmental regulations and increased environmental awareness among citizens. All over the world, public policies are being developed to increase this efficiency, as reflected in the Unsustainable Development Goals and the European Green Deal.
One of the first steps towards improving building energy efficiency is studying how consumption happens and knowing the factors that significantly impact it. In particular, predicting energy consumption from historical data allows building operators and managers to anticipate peaks in energy demand, modify building uses to shift this demand and plan equipment operation appropriately. Likewise, accurate consumption prediction allows assessing the improvement in building performance when making improvements or implementing new energy policies by comparing the actual consumption with the estimated consumption (or baseline). Data Science has emerged as an effective tool to address these objectives [ 21 ].
In the literature, there can be found numerous proposals for estimating energy consumption in buildings throughout time series prediction. Traditionally, these approaches were based on numerical regression or moving average models, which have limitations regarding multivariate series and series with changing trends. In contrast, modern machine learning methods based on neural networks have shown more effective in those scenarios [ 34 ]. However, the application of these techniques is often hampered by the noisy and incomplete nature of building energy data in real environments [ 9 ], which results in a gap between theoretical and practical works.
The rationale behind this work is to perform a comprehensive evaluation of neural network methods in a real-world scenario and to draw conclusions for practical application in similar contexts. More specifically, this paper studies the accuracy of several methods for heating consumption prediction, namely XGBoost, MLPs, RNNs, CNNs and Seq2Seq. The need and impact of preprocessing, which is applied to remove noisy and missing values and for data reduction, is also discussed.
The dataset was collected in the ICPE office building located in Bucharest, one of the pilot buildings considered in the Energy IN TIME Footnote 1 . Our starting hypothesis is that modern neural network techniques improve the performance of other approaches, and within them, memory-based architectures (RNNs, Seq2Seq) are superior. The results show that the hypothesis holds, despite the risk of overfitting these techniques when applied to not very large datasets.
The remainder of this paper is structured as follows. We first provide a review of related works on prediction of building energy consumption (Section 2 ). Next, we describe the data used in the study (Section 3 ), the methodology (Section 4 ), and the experimentation (Section 5 ). At the end of the paper conclusions and directions for future research work (Section 6 ) will be exposed.
2 Related work
Energy consumption forecasting models can be differentiated into categories based on their respective energy end-uses, such as cooling, heating, space heating, primary, natural gas, electricity, and steam load consumption [ 35 ]. Regarding the application of the models, [ 33 ] identified two primary categories: (1) model-based control, demand response, and optimization of energy consumption in buildings; and (2) design and modernization of building parameters, including energy planning and assessing the impact of buildings on climate change.
Also in [ 33 ], numerous factors impacting energy consumption were also identified, mainly the number and type of buildings under consideration. The temporal horizon of the prediction and the resolution of the sensor data are also relevant. Remarkably, natural time-based groupings (i.e., hours, days, months, and years) were proved superior in [ 35 ] to the more generic short-, medium-, and long-term ranking schemes proposed in [ 1 ].
Many data-driven techniques have proved effective for estimating building energy consumption, ranging from classical statistical regression to modern deep learning architectures. Regarding the former, [ 15 ] evaluated four models incorporating exogenous inputs, specifically autoregressive moving average models with exogenous inputs (ARMAX). [ 10 ] proposed a system utilizing a seasonal autoregressive integrated moving average model (SARIMA) and a least squares support vector regression model based on firefly metaheuristic algorithms (MetaFA-LSSVR). The prediction system yielded highly accurate and reliable day-ahead predictions of building energy consumption, with an overall error rate of 1.18%.
Another interesting study is [ 19 ], which investigated two stochastic models for short-term time series prediction of energy consumption, namely the conditional constrained Boltzmann machine (CRBM) and the factored conditional constrained Boltzmann machine (FCRBM). In the comparison, the results showed that the FCRBM outperformed the artificial neural network, support vector machine, recurrent neural networks and CRBM. The work was extended to include a deep belief network with automated feature extraction for the short-term building energy modeling process [ 20 ]. Other relevant work applying classical learning techniques is [ 24 ], which used a decision tree method (C4.5). In addition to obtaining accurate results, this algorithm was able to identify the factors contributing to building energy use. A sophisticated regression tree algorithm (Chi-Square Automatic Interaction Automatic Detector) was used in [ 11 ] to predict short-term heating and cooling load.
Raza and Khosravi [ 28 ] provided a comprehensive review of artificial intelligence-based load demand forecasting techniques for smart grids and buildings. The authors explored various machine learning algorithms used in load forecasting, including artificial neural networks, fuzzy logic, genetic algorithms, and support vector regression. [ 3 ] also reviewed the applications of artificial neural networks (ANNs) and support vector machines (SVMs) for building electrical energy consumption forecasting. The authors compared the performance of ANNs and SVMs with traditional statistical methods used in energy forecasting. [ 30 ] explored the different ML algorithms used in the field, including artificial neural networks, support vector regression, decision trees, and clustering methods. They also discussed the challenges associated with accurate data acquisition and modelling and the limitations of different ML algorithms. More recently, [ 14 ] emphasized that applying machine learning and statistical analysis techniques can lead to significant energy savings and cost reductions. [ 37 ] examined the advantages and limitations of different ML algorithms, including artificial neural networks, decision trees, and support vector machines, and explore their application in different building load prediction scenarios. [ 27 ] proposed using deep recurrent neural networks (DRNNs) for predicting heating, ventilation, and air conditioning (HVAC) loads in commercial buildings. The authors describde the architecture and training process of the DRNN model, which included a combination of convolutional neural networks (CNNs) and long short-term memory (LSTM) layers.
Finally, other works showed the importance of preprocessing in building energy forecasting, e.g., data cleaning and feature selection For instance, [ 2 ] reviewed the current development of machine learning (ML) techniques for predicting building energy consumption and discussed the challenges associated with data acquisition, feature selection, and model validation.
Table 1 summarises the related works mentioned in this section.
Our data was collected from the ICPE building (Institute of Technologies for Sustainable Development) in Bucarest (Romania). This is a three-building, each one divided into areas. For the experiments, we defined a pilot zone through a transversely cut of the building covering three areas (D1, D2, D5/2) of the three floors (see Figs. 1 , 2 ).
The building is equipped with sensors to measure room temperatures and meters measuring electricity, heating, and water consumption in distinct zones of the building. We focused on predicting heating meter values, given the higher contribution of this subsystem to the total energy consumption. Due to the low temperatures in these countries, buildings are powered by a system called district heating, which is used to prevent pipes from freezing outside, and then energy is consumed inside the building to raise the temperature of the water before it is distributed to the radiators inside.
We considered as prediction targets the three heating meters that respectively cover the area D1, D2 and D5/2 from buildings. However, we found a problem with area D2 data was not available because of errors in sensors, we only selected zone D1 and zone D5/2 of our building. The variables that we select for the D1 zone of our building are H-F123-D1, where all the heating consumption of the D1 zone in the floor 1, 2, and 3 are grouped, and for the D5/2 zone are H-F123-D5/2W and H-F123-D5/2E, where the heating consumption are grouped as the previous one, both for the West zone and for the East zone. We have other external variables that are related to our building, these variables are the outdoor temperature and the building occupancy, which are crucial for our experiments.
We collected data in 2017 before the implementation of the new control system. We focused on heating, and only the cold season (January, February and March) was considered in the experimentation. We re-sampled all variables at 15-minute intervals and calculated the cumulative consumption values. As a result, we obtained a data set with 8640 samples. The dataset is not publicly available but an excerpt can be obtained on demand.
4 Methodology
The methodological approach of the experiments followed the workflow of the Data Science process applied to energy data presented in [ 21 ]. As shown in Fig. 3 , we retrieved the data through an API REST and carried out data preprocessing, including outliers removal, missing value imputation, normalization and feature selection. Afterwards, we continued splitting these data by months to apply the learning algorithms and get the best prediction models for each period. Next, we describe how data is partitioned, as well as the parameters used to train the models and the evaluation metrics.
4.1 Definition of the prediction problem and data partition
The problem we aim to solve is to predict the energy usage of a building for the next 12 hours. We need to partition the original dataset into training and validation samples in chronological order to train our model. Given the different energy consumption patterns at the beginning and the end of the data collection period, we decided to split the full dataset into smaller chunks (namely, subproblems) and then make the training and validation partitions within each one. This implies that we have a different model trained specifically for each subproblem, which must be conveniently selected during the test phase to calculate the predictions depending on the date of the observations.
To perform the splits, we applied time series decomposition to analyze the evolution of the energy consumption variables and identify potential cut points, focusing on trend change. This study, which is explained below, showed that we could safely split the data in months since the time series have consistent behaviour in each of these periods. Furthermore, a preliminary analysis of the stability of the training, in terms of errors obtained with slightly different splits, showed that the differences were not significant. While this is a rough approximation of a more fine-grained splitting, it has the advantage of facilitating the selection of the model to be applied for prediction. It remains for future work to apply a more sophisticated time series splitting algorithm [ 18 ] and a more comprehensive analysis of the impact of the splitting.
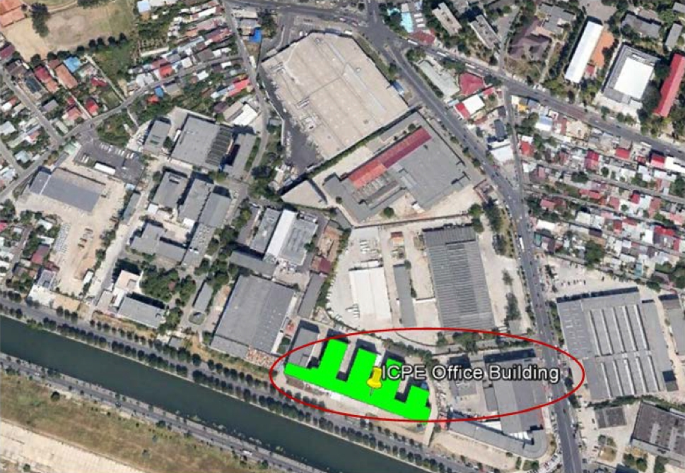
Aerial view
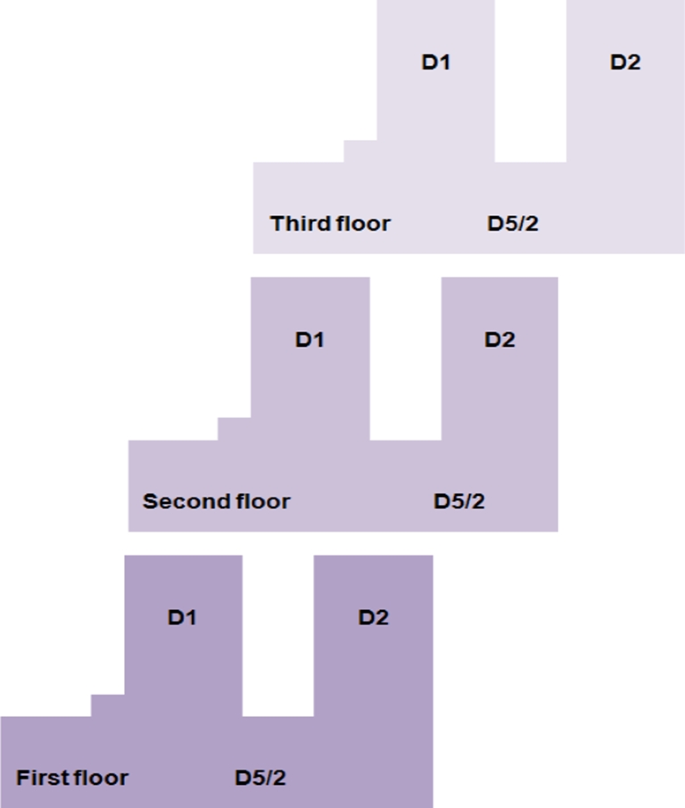
The analysis of the trend change to assess the monthly splitting was based on the decomposition of the time series with STL technique (Seasonal-Trend decomposition using LOESS), which has been previously used for energy demand time series [ 25 ]. Figure 4 depicts the decomposition of the heating consumption variables into three components: trend, i.e., long-term evolution of values; seasonality, i.e., repetitions in fixed periods; and irregularity, i.e., random patterns that remain after removing the other two components. It can be seen that there is a clear pattern of increasingly high consumption in January, followed by a steady decline in consumption in February and a plateau in March. The irregularity component throughout the whole series remains quite stable, meaning that the trend and season components capture quite well the changes in the series. Therefore, we proceeded to split the dataset in two parts, training and validation. Specifically, the selected training samples were 70% of each month’s observations (e.g., from 2016-01-05 00:15:00 until 2016-01-21 23:45:00 in January), while the remaining values were used for validation.
4.2 Methods
The methods used to build the predictions models are XGBoost [ 8 ] and a selection of neural networks including CNNs [ 7 ], RNNs [ 31 ], MLPs [ 32 ] and Seq2Seq [ 12 ]. The results with Seq2Seq suggested that other techniques, such as transformers, would not be very useful given the relatively small size of each partition, in line with the recent literature [ 36 ].
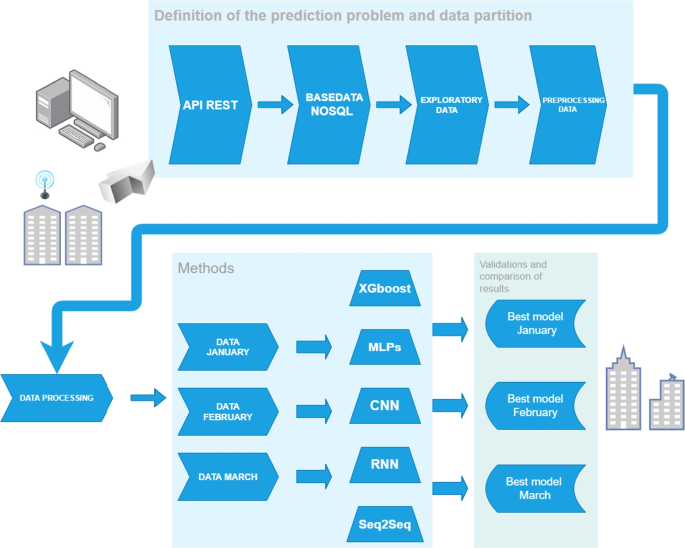
Methodology
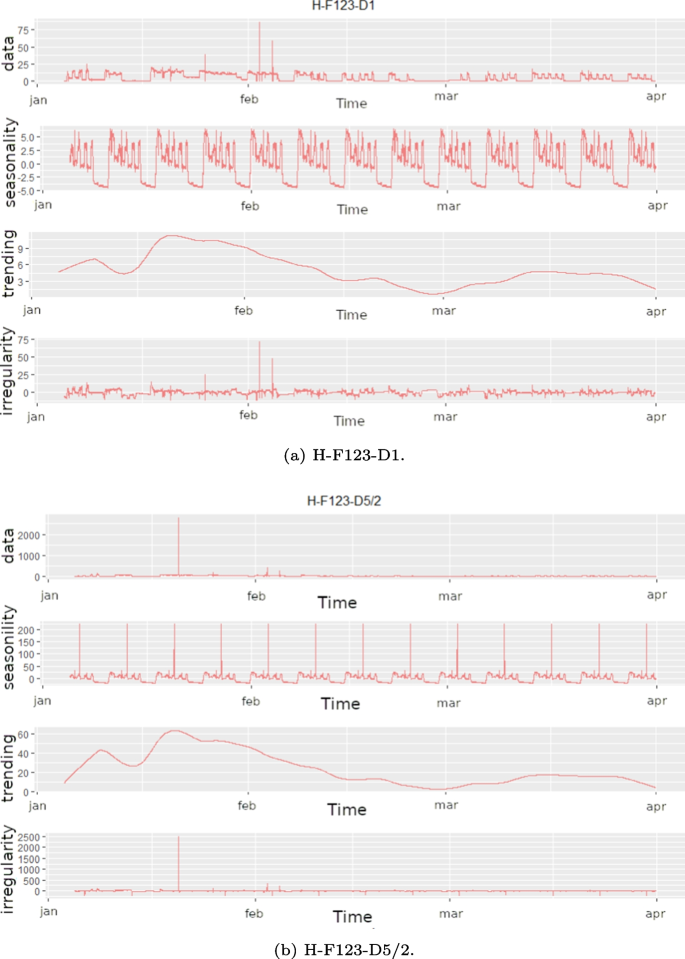
Decomposition of the heating consumption variables of plants 1, 2, and 3 in the pilot zone of the ICPE building
Table 2 summarises the configuration of the models and the hyperparameters probed with each technique. For XGBoost, we reflect the number of trees, maximum depth, and learning rate. For CNN, we describe the number of convolutional layers, max pooling 1D, and the number of filters. For MLP, RNN and Seq2Seq, the table lists the number of hidden layers, dropout layers, and layer size. The best configurations obtained with the training data are highlighted in section 5.2 .
4.3 Metrics
To validate and compare the different results of our experiments, we must define the error metrics to be minimized. Since we have a regression problem, we use MAE (Mean Absolute Error), which aggregates at m the absolute difference between the predictions and the actual values of delay data points:
We also use the normalized MAE, namely NMAE, which averages the error for a batch of size N .
5 Experiments and results
This section shows the results of the experiments after data preprocessing and model training and validation with the algorithms mentioned above: decision trees, XGBoost, and neural networks (MLPs, CNNs, RNNs and Seq2Seq). The implementation of the preprocessing and the prediction algorithms was developed with TSxtend [ 23 ], our open source library for batch analysis of sensor data.
5.1 Preprocessing and data preparation
In our experiment, we used processing data techniques such as aggregation, data modification and removal, data transformation, outliers detection, missing values detection, normalization and feature selection. We discarded heating consumption with null values to discard rows with no heating consumption. Then, we eliminated the variables with extreme values and finally joined the variables measuring the same consumption type on the same floor.
The variables in the collected dataset store aggregated values, e.g., summing up new values at each instant. The transformation of these variables was done with the differences between each instant of time, thus obtaining the actual consumption of each variable at each instant of time. These variables were used as inputs for the execution of our prediction algorithms.
In the subsequent step, we employed techniques to detect and remove outliers for each consumption variable. We calculated percentiles for each variable and searched for values outside this range. Such values were substituted with more conventional values (mean, median, etc...). This approach, however, leads to a loss of information. Fortunately, the number of outliers in our case is limited.
We had in our dataset missing values in February when the system did not gather data, for which we applied missing values imputation algorithms. Particularly, we used Interp to fill gaps by interpolation of known values. Interpolation techniques are utilized to fill gaps by interpolating known values, as mentioned in work by [ 22 ]. Specifically, this approach involves the utilization of Interp, a commonly employed software for performing interpolation. This methodology aims to accurately estimate values within a given range based on the available data. The interpolation process involves estimating each data point’s value by considering its neighbouring data points. Applying this technique makes the resulting dataset complete and can be analyzed more effectively for the intended purpose. Figure 5 depicts data after imputation for three variables.
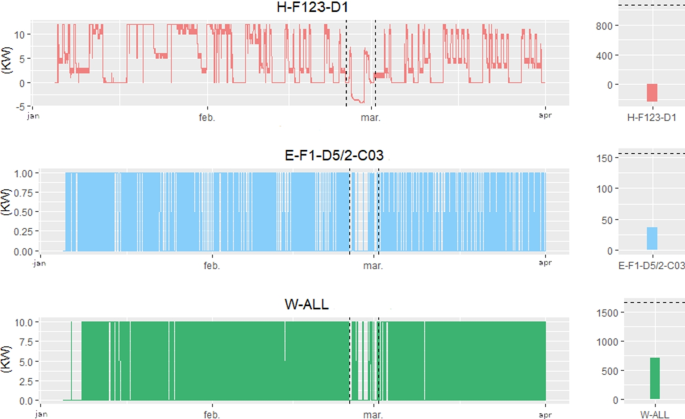
Imputation of missing values ((end February) with Interpolate for variables Energy Zone D1, E-F1-D5/2-C03 and W-ALL
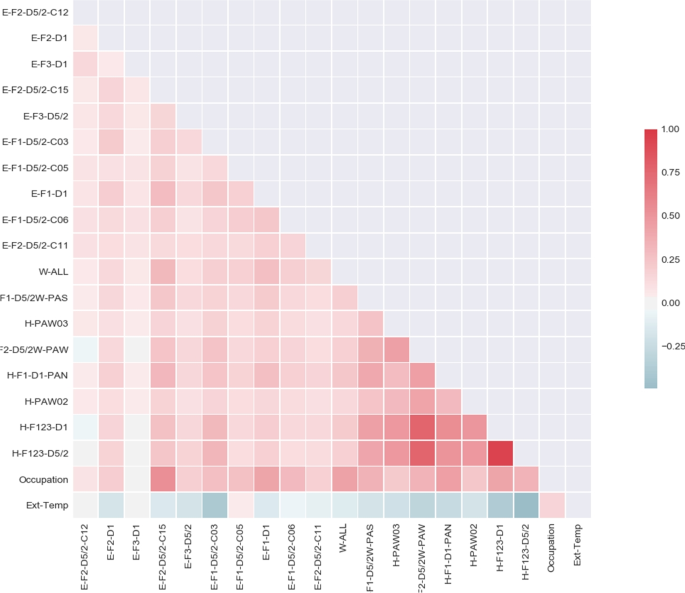
Heatmap showing correlations between variables
Then, we selected the most relevant variables for predicting energy consumption as Energy Zone D1 and Energy Zone D5/2. We calculated the cross-correlation between pairs of variables and grouped them by levels according to their correlation to the prediction target. Figure 6 indicates few dependencies between the electrical sensors. There is a high correlation between the heating consumption variables, while water consumption does not correlate. In the case of the occupancy variables, there are more correlations, but it is not high because the values are estimated. We can also observe energy consumption when the building is empty.
Then we used XGBoost algorithm for the assessment of variable importance. In Fig. 7 , we can see that the most relevant variables for energy consumption are occupancy, outside temperature and heating consumption (Energy Zone D1 and Energy Zone D5/2). With this information, we performed the selection of variables, being the most important ones (not surprisingly) Outside Temperature, Occupation, Energy Zone D5/2 and Energy Zone D1.
The last step in order to preprocess data is to normalize the dataset. To do this, we calculated the mean and standard deviation of each variable, and then transformed the values to the range [0, 1].
5.2 Results and discussion
We experimented with the January, February and March data using the selected algorithms. We applied grid search to obtain the best configurations and hyperparameter, yielding the values depicted in Table 3 . We can observe that the best configurations are similar for each subproblem.
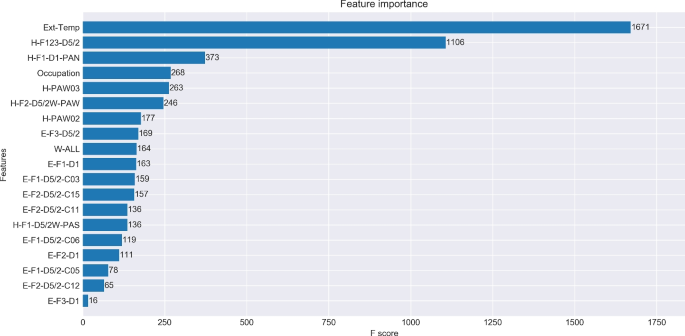
Ranking of variables by importance with XGBoost
The validation errors of the best models are shown in Tables 4 and 5 , while Figs. 8 , 9 , 10 , 11 , 12 , and 13 depict the predicted vs the real values. In January, the algorithm that offered the best results was Seq2Seq, which achieved a validation NMAE of 0.21 for the prediction of consumption in zone D1 and 0.20 for zone D5/2, significantly improving the performance of the other models. The best model for February in both zones was CNNs. Overfitting was lower than that with other algorithms, at the cost of having worse validation metrics —the model tends to ignore or lessen the consumption peaks, as it can be seen in the chart. A second reason to explain these results is that we had many more missing values to impute in this period. The best method for March was RNNs, with an NMAE of 0.29 in both zones, almost twice better than MLP and XGBoost. Overall, we confirmed the ability of memory-based models (Seq2Seq and RNN) to extract characteristics from the input time series and learn the predictions in more diverse scenarios, while CNNs were slightly better when there was a uniform trend and fewer data. In all cases, the best models’ MAE was around 5 kW, which is small enough for this type of applications.
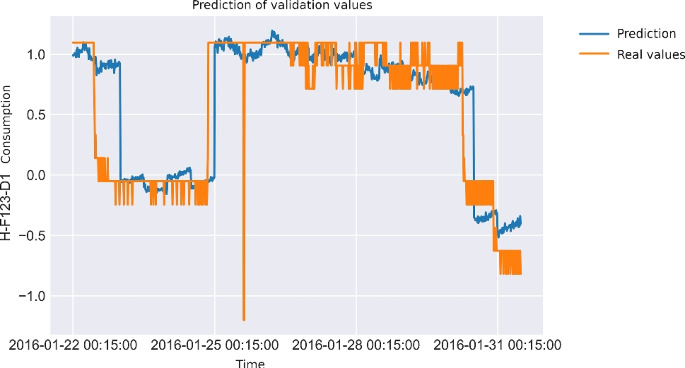
Prediction of the Validation Values in January with Seq2Seq Energy Zone D1
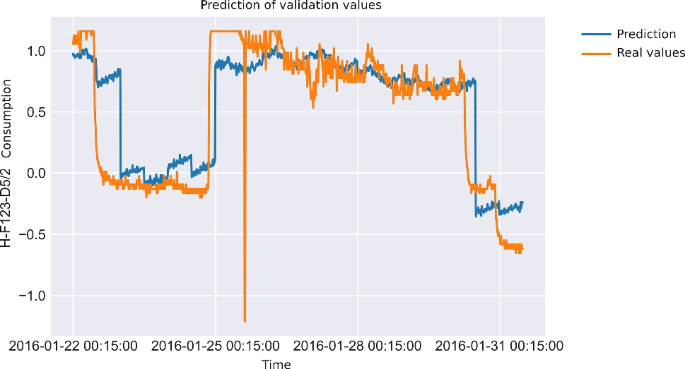
Prediction of the Validation Values in January with Seq2Seq Energy Zone D5/2
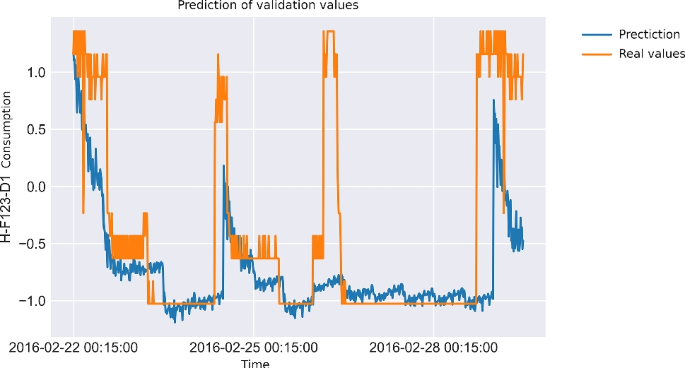
Prediction of the Validation Values in February with CNNs Energy Zone D1
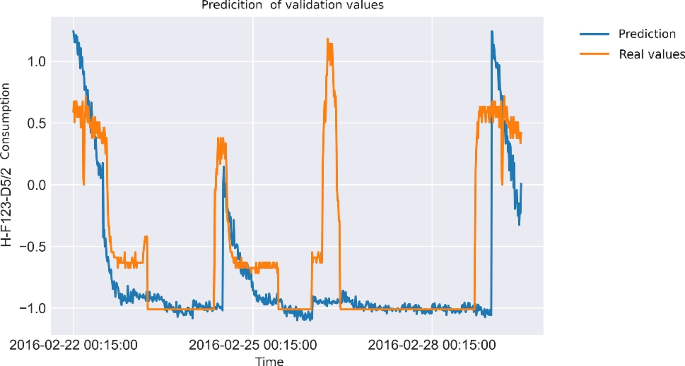
Prediction of the Validation Values in February with CNNs Energy Zone D5/2
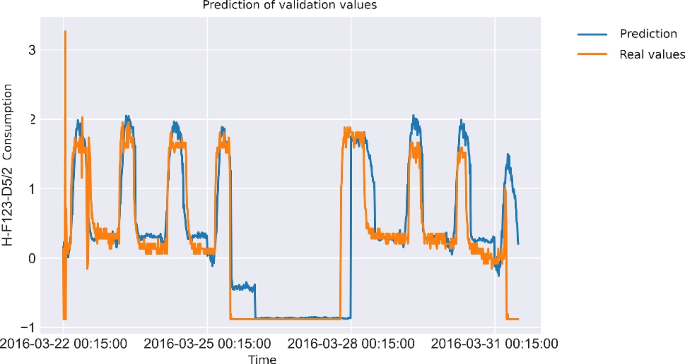
Prediction of the Validation Values in March with RNNs Energy Zone D1
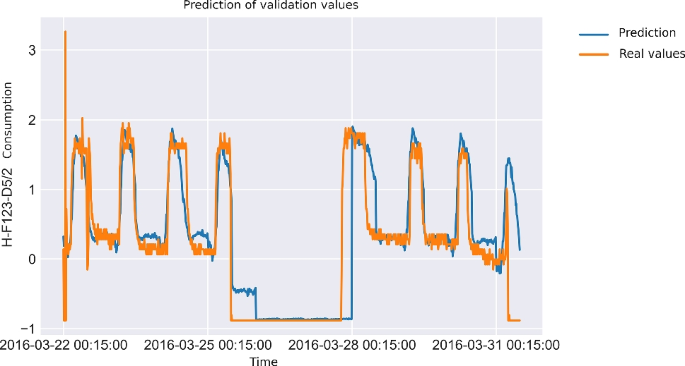
Prediction of the Validation Values in March with RNNs Energy Zone D5/2
Regarding the drawback of having limited data for training and validation, it resulted in that the more complex models did not learn the prediction of heating consumption as well as it could be expected. Hence, we suggest that more sophisticated techniques (Seq2Seq, but also transformer-based architectures) might not be necessary in this kind of problems or under similar circumstances. Instead, RNNs or CNNs with proper data pre-processing could be precise enough and less prone to overfitting.
6 Conclusions and future work
Concluding our discussion, we can see how the XGBoost model shows worse results, as XGBoost does not extract the dependencies between the variables it receives as input to perform the energy consumption prediction. With MLP models we have shown that they do not provide better results than other models because they do not allow to follow a chronological order in our dataset. With respect to the use of RNNs on our dataset, we have been able to reduce the NMAE error function by half, as these algorithms remember the information by processing the data in chronological order. Furthermore, we have applied Seq2Seq, which has allowed us to observe that, like RNNs, they obtain good results in general but do not perform very well on datasets with too many missing values (i.e., smaller in size). Finally, convolution networks (CNNs) have been found to perform better than RNNs and Seq2Seq algorithms on sections of the dataset with a large number of missing values and steady trends.
One of the problems we have in this study has been the limited amount and quality of data, since we have only worked with the ICPE building sensors for three months including many missing values. In the future we can take the ICPE building sensors from other years to have a more extensive training and validation. Another option may be to generate artificial values with building simulation models. We can also use recurrent neural network models using attention mechanisms to improve synthetic data generation and missing values imputation [ 5 ]. Additionally, peak changes could be addressed with noise reduction techniques to smooth abrupt oscillations, e.g., as the filter proposed in [ 16 ].
Energy IN TIME was an European project running in 2013-2017. The aim of the project was to implement a model-predictive control system to improve the energy efficiency of non-residential buildings [ 13 ].
Ahmad T, Chen H (2018) Short and medium-term forecasting of cooling and heating load demand in building environment with data-mining based approaches. Energy Build 166:460–476. https://doi.org/10.1016/J.ENBUILD.2018.01.066
Article Google Scholar
Ahmad T, Chen H, Guo Y et al (2018) A comprehensive overview on the data driven and large scale based approaches for forecasting of building energy demand: A review. Energy Build 165:301–320. https://doi.org/10.1016/j.enbuild.2018.01.017
Arpanahi G, Javadi M (2018) A review on applications of artificial neural networks and support vector machines for building electrical energy consumption forecasting. Renew Sustain Energy Rev 82:1814–1832. https://doi.org/10.1016/j.rser.2014.01.069
Benedetti M (2015) A proposal for energy services classification including a product service systems perspective. Procedia CIRP 30:251–256. https://doi.org/10.1016/j.procir.2015.02.121
Bülte C, Kleinebrahm M, Yilmaz HÜ et al (2023) Multivariate time series imputation for energy data using neural networks. Energy and AI 13:100,239. https://doi.org/10.1016/j.egyai.2023.100239 , https://www.sciencedirect.com/science/article/pii/S2666546823000113
Chau C, Leung T, Ng W (2015) A review on life cycle assessment, life cycle energy assessment and life cycle carbon emissions assessment on buildings. Appl Energy 143:395–413. https://doi.org/10.1016/j.apenergy.2015.01.023
Chauhan R, Ghanshala KK, Joshi RC (2018) Convolutional Neural Network (CNN) for Image Detection and Recognition. ICSCCC 2018 - 1st International Conference on Secure Cyber Computing and Communications pp 278–282. https://doi.org/10.1109/ICSCCC.2018.8703316
Chen T, Guestrin C (2016) Xgboost: A scalable tree boosting system pp 785–794. https://doi.org/10.1145/2939672.2939785
Chen W, Zhou K, Yang S et al (2017) Data quality of electricity consumption data in a smart grid environment. Renew Sustain Energy Rev 75:98–105. https://doi.org/10.1016/j.rser.2016.10.054
Chou JS, Ngo NT (2016) Time series analytics using sliding window metaheuristic optimization-based machine learning system for identifying building energy consumption patterns. Appl Energy 177:751–770. https://doi.org/10.1016/J.APENERGY.2016.05.074
Ezan MA, Uçan ON, Kalfa M (2017) Predicting short-term building heating and cooling load using regression tree algorithm. J Build Perform Simul 10(5):487–502. https://doi.org/10.1080/19401493.2016.1202888
Gong G (2019) Research on short-term load prediction based on Seq2Seq model. Energies 12:3199. https://doi.org/10.3390/en12163199
Gómez-Romero J, Fernández-Basso CJ, Cambronero MV et al (2019) A probabilistic algorithm for predictive control with full-complexity models in non-residential buildings. IEEE Access 7:38,748–38,765. https://doi.org/10.1109/ACCESS.2019.2906311
Khalil M, McGough AS, Pourmirza Z et al (2022) Machine learning, deep learning and statistical analysis for forecasting building energy consumption - a systematic review. Eng Appl Artif Intell 115(105):287. https://doi.org/10.1016/j.engappai.2022.105287
Lago J, Marcjasz G, De Schutter B et al (2021) Forecasting day-ahead electricity prices: A review of state-of-the-art algorithms, best practices and an open-access benchmark. Appl Energy 293(116):983. https://doi.org/10.1016/j.apenergy.2021.116983
Ma W, Wang W, Wu X et al (2019) Control strategy of a hybrid energy storage system to smooth photovoltaic power fluctuations considering photovoltaic output power curtailment. Sustain 11(5). https://doi.org/10.3390/su11051324 , https://www.mdpi.com/2071-1050/11/5/1324
Marino DL, Amarasinghe K, Manic M (2016) Building energy load forecasting using deep neural networks pp 7046–7051. https://doi.org/10.1109/IECON.2016.7793413
Micheletti A, Aletti G, Ferrandi G et al (2020) A weighted \(\chi ^2\) test to detect the presence of a major change point in non-stationary markov chains. Stat Methods Appl 29(4):899–912. https://doi.org/10.1007/s10260-020-00510-0 , https://doi.org/10.1007/s10260-020-00510-0
Mocanu E, Nguyen PH, Gibescu M et al (2016) Deep learning for estimating building energy consumption. Sustain Energy, Grids Netw 6:91–99. https://doi.org/10.1016/j.segan.2016.02.005
Mocanu E, Nguyen PH, Kling WL et al (2016) Unsupervised energy prediction in a smart grid context using reinforcement cross-building transfer learning. Energy and Buildings 116:646–655. https://doi.org/10.1016/J.ENBUILD.2016.01.030
Molina-Solana M, Ros M, Ruiz MD et al (2017) Data science for building energy management: A review. Renew Sustain Energy Rev 70:598–609. https://doi.org/10.1016/j.rser.2016.11.132
Montero-Manso P, Hyndman RJ (2021) Principles and algorithms for forecasting groups of time series: Locality and globality. Int J Forecast 37(4):1632–1653. https://doi.org/10.1016/j.ijforecast.2021.03.004
Morcillo-Jimenez R, Gutiérrez-Batista K, Gómez-Romero J (2023) Tsxtend: A tool for batch analysis of temporal sensor data. Energies 16(4). https://doi.org/10.3390/en16041581 , https://www.mdpi.com/1996-1073/16/4/1581
Pachauri N, Ahn CW (2022) Regression tree ensemble learning-based prediction of the heating and cooling loads of residential buildings. Build Simul 15(11):2003–2017. https://doi.org/10.1007/s12273-022-0908-x
Phinikarides A, Makrides G, Zinsser B et al (2015) Analysis of photovoltaic system performance time series: Seasonality and performance loss. Renew Energy 77:51–63. https://doi.org/10.1016/j.renene.2014.11.091 , https://www.sciencedirect.com/science/article/pii/S0960148114008222
Pérez-Lombard L, Ortiz J, Pout C (2008) A review on buildings energy consumption information. Energy Build 40(3):394–398. https://doi.org/10.1016/j.enbuild.2007.03.007
Rahman A, Srikumar V, Smith AD (2018) Predicting electricity consumption for commercial and residential buildings using deep recurrent neural networks. Appl Energy 212:372–385. https://doi.org/10.1016/j.apenergy.2017.12.051 , https://www.sciencedirect.com/science/article/pii/S0306261917317658
Raza MQ, Khosravi A (2015) A review on artificial intelligence based load demand forecasting techniques for smart grid and buildings. Renew Sustain Energy Rev 50:1352–1372. https://doi.org/10.1016/j.rser.2015.04.065
Schmidt M, Åhlund C (2018) Smart buildings as cyber-physical systems: Data-driven predictive control strategies for energy efficiency. Renew Sustain Energy Rev 90:742–756. https://doi.org/10.1016/j.rser.2018.04.013
Seyedzadeh S, Rahimian FP, Glesk I et al (2018) Machine learning for estimation of building energy consumption and performance: a review. Vis Eng 6:1–20. https://doi.org/10.1186/s40327-018-0064-7
Sherstinsky A (2020) Fundamentals of recurrent neural network (RNN) and long short-term memory (LSTM) network. Physica D: Nonlinear Phenom 404(132):306. https://doi.org/10.1016/j.physd.2019.132306
Taud H, Mas J (2018) Multilayer perceptron (MLP) pp 451–455. https://doi.org/10.1007/978-3-319-60801-3_27
Tien PW, Wei S, Darkwa J et al (2022) Machine learning and deep learning methods for enhancing building energy efficiency and indoor environmental quality - a review. Energy AI 10:100,198. https://doi.org/10.1016/j.egyai.2022.100198 , https://www.sciencedirect.com/science/article/pii/S2666546822000441
Torres JF (2021) Deep learning for time series forecasting: A survey. Big Data 9:3–21. https://doi.org/10.1089/big.2020.0159
Wang Z, Srinivasan RS (2016) A review of artificial intelligence based building energy prediction with a focus on ensemble prediction models. pp 3438–3448. https://doi.org/10.1109/WSC.2015.7408504
Zeng A, Chen M, Zhang L et al (2023) Are transformers effective for time series forecasting?
Zhang L, Wen J, Li Y et al (2021) A review of machine learning in building load prediction. Appl Energy. https://doi.org/10.1016/j.apenergy.2021.116452
Download references
Acknowledgements
This work was funded by the European Union NextGenerationEU/PRTR through the IA4TES project (MIA.2021.M04.0008); by MICIU/AEI/10.13039/501100011033 through the SINERGY project (PID2021.125537NA.I00); by ERDF/Junta de Andalucía through the D3S project (P21.00247); by the FEDER programme 2014-2020 (B-TIC-145-UGR18 and P18-RT-1765); and by the European Union (Energy IN TIME EeB.NMP.2013-4, No. 608981).
Funding for open access publishing: Universidad de Granada/CBUA.
Author information
Authors and affiliations.
Department of Computer Science and Artificial Intelligence, Escuela Técnica Superior de Ingenierías Informática y de Telecomunicación, Universidad de Granada, Granada, 18071, Spain
Roberto Morcillo-Jimenez, Jesús Mesa, Juan Gómez-Romero, M. Amparo Vila & Maria J. Martin-Bautista
You can also search for this author in PubMed Google Scholar
Corresponding author
Correspondence to Roberto Morcillo-Jimenez .
Additional information
Publisher's note.
Springer Nature remains neutral with regard to jurisdictional claims in published maps and institutional affiliations.
Rights and permissions
Open Access This article is licensed under a Creative Commons Attribution 4.0 International License, which permits use, sharing, adaptation, distribution and reproduction in any medium or format, as long as you give appropriate credit to the original author(s) and the source, provide a link to the Creative Commons licence, and indicate if changes were made. The images or other third party material in this article are included in the article’s Creative Commons licence, unless indicated otherwise in a credit line to the material. If material is not included in the article’s Creative Commons licence and your intended use is not permitted by statutory regulation or exceeds the permitted use, you will need to obtain permission directly from the copyright holder. To view a copy of this licence, visit http://creativecommons.org/licenses/by/4.0/ .
Reprints and permissions
About this article
Morcillo-Jimenez, R., Mesa, J., Gómez-Romero, J. et al. Deep learning for prediction of energy consumption: an applied use case in an office building. Appl Intell (2024). https://doi.org/10.1007/s10489-024-05451-9
Download citation
Accepted : 07 April 2024
Published : 30 April 2024
DOI : https://doi.org/10.1007/s10489-024-05451-9
Share this article
Anyone you share the following link with will be able to read this content:
Sorry, a shareable link is not currently available for this article.
Provided by the Springer Nature SharedIt content-sharing initiative
- Energy consumption forecasting
- Time series
- Deep learning
- Multilayer perceptron
- Recurrent neural networks
- Convolutional neural networks
- Sequence to sequence networks
- Find a journal
- Publish with us
- Track your research
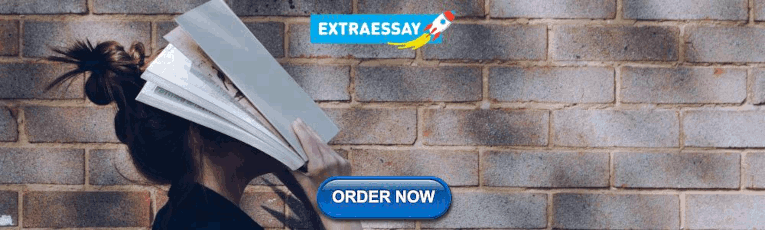
IMAGES
VIDEO
COMMENTS
The purpose of case study research is twofold: (1) to provide descriptive information and (2) to suggest theoretical relevance. Rich description enables an in-depth or sharpened understanding of the case. It is unique given one characteristic: case studies draw from more than one data source. Case studies are inherently multimodal or mixed ...
"The case study approach allows researchers to focus in-depth on a case and to retain holistic and real-world perspectives" (Yin, 2018, p. 5). In addition, the case study method was especially ...
This case study method forms the backbone of the Harvard Business School curriculum. Back in the 1920s, HBS professors decided to develop and experiment with innovative and unique business instruction methods. As the first school in the world to design a signature, distinctive program in business, later to be called the MBA, there was a need ...
Overview. Simply put, the case method is a discussion of real-life situations that business executives have faced. On average, you'll attend three to four different classes a day, for a total of about six hours of class time (schedules vary). To prepare, you'll work through problems with your peers. Read More.
A case study is one of the most commonly used methodologies of social research. This article attempts to look into the various dimensions of a case study research strategy, the different epistemological strands which determine the particular case study type and approach adopted in the field, discusses the factors which can enhance the effectiveness of a case study research, and the debate ...
Case studies are in-depth investigations of a person, group, event, or community. Typically, data is gathered from various sources using several methods (e.g., observations & interviews). The case study research method originated in clinical medicine (the case history, i.e., the patient's personal history). In psychology, case studies are ...
A case study is a detailed study of a specific subject, such as a person, group, place, event, organization, or phenomenon. Case studies are commonly used in social, educational, clinical, and business research. A case study research design usually involves qualitative methods, but quantitative methods are sometimes also used.
The term "case study" refers to both a specific research design or methodology, and a method of analysis for examining a problem. Mills et al. ( 2010) note that case study, both as a methodology and as a method—unlike many qualitative methodologies—is frequently used to generalize across populations.
This year commemorates the 100 th anniversary of the first written case study, produced by Harvard Business School back in April 1921. IESE joins in the celebration because it's one of the academic institutions that has helped spearhead the use of the case method outside the U.S., educating more than 60,000 professionals with this methodology and producing around 6,200 original cases through ...
The definitions of case study evolved over a period of time. Case study is defined as "a systematic inquiry into an event or a set of related events which aims to describe and explain the phenomenon of interest" (Bromley, 1990).Stoecker defined a case study as an "intensive research in which interpretations are given based on observable concrete interconnections between actual properties ...
A case study protocol outlines the procedures and general rules to be followed during the case study. This includes the data collection methods to be used, the sources of data, and the procedures for analysis. Having a detailed case study protocol ensures consistency and reliability in the study.
Defnition: A case study is a research method that involves an in-depth examination and analysis of a particular phenomenon or case, such as an individual, organization, community, event, or situation. It is a qualitative research approach that aims to provide a detailed and comprehensive understanding of the case being studied.
First is to provide a step-by-step guideline to research students for conducting case study. Second, an analysis of authors' multiple case studies is presented in order to provide an application of step-by-step guideline. This article has been divided into two sections. First section discusses a checklist with four phases that are vital for ...
A case study is a research approach that is used to generate an in-depth, multi-faceted understanding of a complex issue in its real-life context. It is an established research design that is used extensively in a wide variety of disciplines, particularly in the social sciences. A case study can be defined in a variety of ways (Table.
Explicitly attending to the design of your case study serves as the first important way of using more systematic procedures when doing case study research. The ... CHAPTER 1. A (VERY) BRIEF REFRESHER ON THE CASE STUDY METHOD 7 study. At the same time, case studies also can have nested units within the main unit (see "embedded subcases" in ...
This book aims to provide case‐study researchers with a step‐by‐step practical guide to "help them conduct the study with the required degree of rigour" (p. xi). It seeks to "demonstrate that the case study is indeed a scientific method" (p. 104) and to show "the usefulness of the case method as one tool in the researcher's ...
The final case study in this chapter brings together many of the principles of case study design that we have discussed. These include the role of theoretical propositions, the design of clear research methodologies and data gathering tools and the use of multiple sources of evidence. CASE STUDY 11.4.
cipline-specific applications of case study methods and describe the appropriate research questions addressed by case studies. I follow this description with methods considerations, including case study design, research questions, sample size, data collection, and data analysis. Note that there are many approaches and styles to case study research.
In this special issue we have asked the contributors to make a case for the case study. The guest editors, Jeffrey Longhofer, Jerry Floersch and Eric Hartmann, intergrate ideas from across the disciplines to explore the complexties of case study methods and theory. In education, Gary Thomas explores the importance of ethnographic case studies in understanding the relationships among schools ...
TRM studies have increased over time, ... The first type of case study develops a common structure for a specific type of technology roadmap based on one case (e.g., Kim et al., ... the strengths and limitations of the case study methods applied in TRM literature; and 2) future research directions identified through the themes. ...
The case studies describe implementation research in various disease areas in LMICs around the world. This commentary highlights the value of case study methods commonly used in law and business schools as a source of "thick" (i.e., context-rich) description and a teaching tool for global implementation researchers.
A case study is a research approach that is used to generate an in-depth, multi-faceted understanding of a complex issue in its real-life context. It is an established research design that is used extensively in a wide variety of disciplines, particularly in the social sciences. A case study can be defined in a variety of ways (Table 5 ), the ...
Ground subsidence often occurs over a large area. Although traditional monitoring methods have high accuracy, they cannot perform wide-area ground deformation monitoring. Synthetic Aperture Radar (SAR) interferometry (InSAR) technology utilizes phase information in SAR images to extract surface deformation information in a low-cost, large-scale, high-precision, and high-efficiency manner.
Background Economic evaluation of one-time therapies during reimbursement decision-making is challenging due to uncertain long-term outcomes. The availability of 5-year outcome data from the ELIANA trial and real-world evidence of tisagenlecleucel, the first chimeric antigen receptor T-cell (CAR-T) therapy, presents an opportunity to re-evaluate the predictions of prior cost-effectiveness ...
To achieve the failure warning of marine systems and their equipment (MSAE), the threshold is one of the most prominent issues that should be solved first. In this study, a fusion model based on sparse Bayes and probabilistic statistical methods is applied to determine a new and more accurate adaptive alarm threshold. A multistep relevance vector machine (RVM) model is established to realize ...
Abstract Non-residential buildings are responsible for more than a third of global energy consumption. Estimating building energy consumption is the first step towards identifying inefficiencies and optimizing energy management policies. This paper presents a study of Deep Learning techniques for time series analysis applied to building energy prediction with real environments. We collected ...