- ACRIS instructions
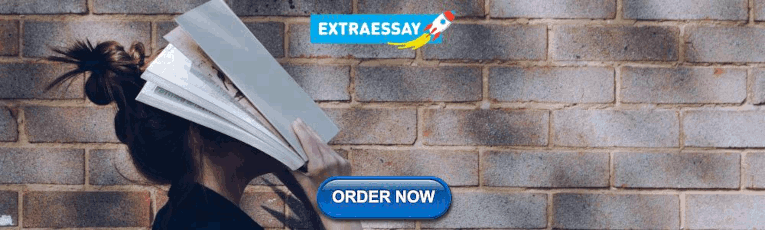
Global citation recommendation using knowledge graphs
- Helsinki Institute for Information Technology (HIIT)
- Department of Computer Science
- Hungarian Academy of Sciences
- Eötvös Loránd University
- University of Lyon
Research output : Contribution to journal › Article › Scientific › peer-review
Scholarly search engines, reference management tools, and academic social networks enable modern researchers to organize their scientific libraries. Moreover, they often provide recommendations for scientific publications that might be of interest to researchers. Because of the exponentially increasing volume of publications, effective citation recommendation is of great importance to researchers, as it reduces the time and effort spent on retrieving, understanding, and selecting research papers. In this context, we address the problem of citation recommendation, i.e., the task of recommending citations for a new paper. Current research investigates this task in different settings, including cases where rich user metadata is available (e.g., user profile, publications, citations). This work focus on a setting where the user provides only the abstract of a new paper as input. Our proposed approach is to expand the semantic features of the given abstract using knowledge graphs - and, combine them with other features (e.g., indegree, recency) to fit a learning to rank model. This model is used to generate the citation recommendations. By evaluating on real data, we show that the expanded semantic features lead to improving the quality of the recommendations measured by nDCG@10.
- Citation recommendations
- knowledge graphs
- recommender systems
Access to Document
- 10.3233/JIFS-169493
OpenUrl availability
Fingerprint.
- User Computer Science 100%
- Models Computer Science 66%
- Semantic Feature Computer Science 66%
- Contexts Computer Science 33%
- Research Paper Computer Science 33%
- Social Networks Computer Science 33%
- References Computer Science 33%
- Metadata Computer Science 33%
Projects per year
Network structure from group response
Xiao, H., Gionis, A. , Garimella, K., Vitale, F., Parotsidis, N., Zhang, G., Rozenshtein, P., Galbrun, E., Tatti, N., Scepanovic, S., Matakos, A. & Muniyappa, S.
01/09/2015 → 31/08/2019
Project : Academy of Finland: Other research funding
- Models 100%
- Algorithms 88%
- Classifier 70%
- Application 70%
- Networks 70%
T1 - Global citation recommendation using knowledge graphs
AU - Ayala-Gomez, Frederick
AU - Daroczy, Balint
AU - Benczur, Andras
AU - Mathioudakis, Michael
AU - Gionis, Aristides
N1 - | openaire: EC/H2020/654024/EU//SoBigData
N2 - Scholarly search engines, reference management tools, and academic social networks enable modern researchers to organize their scientific libraries. Moreover, they often provide recommendations for scientific publications that might be of interest to researchers. Because of the exponentially increasing volume of publications, effective citation recommendation is of great importance to researchers, as it reduces the time and effort spent on retrieving, understanding, and selecting research papers. In this context, we address the problem of citation recommendation, i.e., the task of recommending citations for a new paper. Current research investigates this task in different settings, including cases where rich user metadata is available (e.g., user profile, publications, citations). This work focus on a setting where the user provides only the abstract of a new paper as input. Our proposed approach is to expand the semantic features of the given abstract using knowledge graphs - and, combine them with other features (e.g., indegree, recency) to fit a learning to rank model. This model is used to generate the citation recommendations. By evaluating on real data, we show that the expanded semantic features lead to improving the quality of the recommendations measured by nDCG@10.
AB - Scholarly search engines, reference management tools, and academic social networks enable modern researchers to organize their scientific libraries. Moreover, they often provide recommendations for scientific publications that might be of interest to researchers. Because of the exponentially increasing volume of publications, effective citation recommendation is of great importance to researchers, as it reduces the time and effort spent on retrieving, understanding, and selecting research papers. In this context, we address the problem of citation recommendation, i.e., the task of recommending citations for a new paper. Current research investigates this task in different settings, including cases where rich user metadata is available (e.g., user profile, publications, citations). This work focus on a setting where the user provides only the abstract of a new paper as input. Our proposed approach is to expand the semantic features of the given abstract using knowledge graphs - and, combine them with other features (e.g., indegree, recency) to fit a learning to rank model. This model is used to generate the citation recommendations. By evaluating on real data, we show that the expanded semantic features lead to improving the quality of the recommendations measured by nDCG@10.
KW - Citation recommendations
KW - knowledge graphs
KW - recommender systems
U2 - 10.3233/JIFS-169493
DO - 10.3233/JIFS-169493
M3 - Article
SN - 1064-1246
JO - Journal of Intelligent and Fuzzy Systems
JF - Journal of Intelligent and Fuzzy Systems
Scholarly Paper Recommendation via Related Path Analysis in Knowledge Graph
Ieee account.
- Change Username/Password
- Update Address
Purchase Details
- Payment Options
- Order History
- View Purchased Documents
Profile Information
- Communications Preferences
- Profession and Education
- Technical Interests
- US & Canada: +1 800 678 4333
- Worldwide: +1 732 981 0060
- Contact & Support
- About IEEE Xplore
- Accessibility
- Terms of Use
- Nondiscrimination Policy
- Privacy & Opting Out of Cookies
A not-for-profit organization, IEEE is the world's largest technical professional organization dedicated to advancing technology for the benefit of humanity. © Copyright 2024 IEEE - All rights reserved. Use of this web site signifies your agreement to the terms and conditions.
Help | Advanced Search
Computer Science > Information Retrieval
Title: sequential recommendation with latent relations based on large language model.
Abstract: Sequential recommender systems predict items that may interest users by modeling their preferences based on historical interactions. Traditional sequential recommendation methods rely on capturing implicit collaborative filtering signals among items. Recent relation-aware sequential recommendation models have achieved promising performance by explicitly incorporating item relations into the modeling of user historical sequences, where most relations are extracted from knowledge graphs. However, existing methods rely on manually predefined relations and suffer the sparsity issue, limiting the generalization ability in diverse scenarios with varied item relations. In this paper, we propose a novel relation-aware sequential recommendation framework with Latent Relation Discovery (LRD). Different from previous relation-aware models that rely on predefined rules, we propose to leverage the Large Language Model (LLM) to provide new types of relations and connections between items. The motivation is that LLM contains abundant world knowledge, which can be adopted to mine latent relations of items for recommendation. Specifically, inspired by that humans can describe relations between items using natural language, LRD harnesses the LLM that has demonstrated human-like knowledge to obtain language knowledge representations of items. These representations are fed into a latent relation discovery module based on the discrete state variational autoencoder (DVAE). Then the self-supervised relation discovery tasks and recommendation tasks are jointly optimized. Experimental results on multiple public datasets demonstrate our proposed latent relations discovery method can be incorporated with existing relation-aware sequential recommendation models and significantly improve the performance. Further analysis experiments indicate the effectiveness and reliability of the discovered latent relations.
Submission history
Access paper:.
- HTML (experimental)
- Other Formats
References & Citations
- Google Scholar
- Semantic Scholar
BibTeX formatted citation

Bibliographic and Citation Tools
Code, data and media associated with this article, recommenders and search tools.
- Institution
arXivLabs: experimental projects with community collaborators
arXivLabs is a framework that allows collaborators to develop and share new arXiv features directly on our website.
Both individuals and organizations that work with arXivLabs have embraced and accepted our values of openness, community, excellence, and user data privacy. arXiv is committed to these values and only works with partners that adhere to them.
Have an idea for a project that will add value for arXiv's community? Learn more about arXivLabs .
Knowledge graph enhanced citation recommendation model for patent examiners
- Published: 11 March 2024
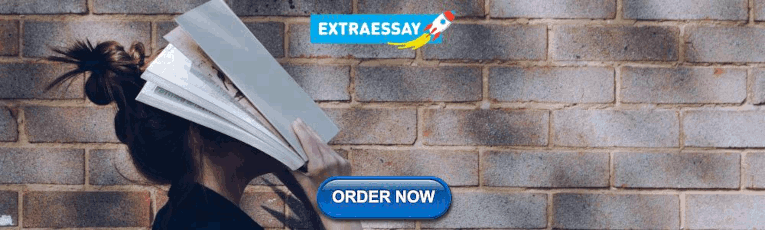
Cite this article
- Yonghe Lu 1 , 2 ,
- Xinyu Tong 1 ,
- Xin Xiong 1 &
- Hou Zhu ORCID: orcid.org/0000-0002-6843-9795 1
67 Accesses
Explore all metrics
In the face of a growing volume of patents, patent examiners grapple with prolonged examination cycles, prompting the need for more effective citation recommendations. To address this, we introduce the patent knowledge graph embedded in Bert (PK-Bert) model. This innovation combines a patent knowledge graph with semantic information in an advanced Transformer framework, outperforming conventional common-sense knowledge graph embedding. PK-Bert exhibits substantial improvements, boosting the recall of accurate citation recommendations by 2.15% over the benchmark model Bert and 1.25% over K-Bert with CnDBpedia. Ablation experiments highlight the significance of knowledge graph elements, with the inventor proving most influential, followed by the IPC number and assignee. At the same time, publication time and title information have a minor impact. Moreover, PK-Bert excels when trained with earlier data and evaluated for patents issued post-November 2023. Our study not only advances patent examiner recommendations but also presents an efficient integration method for knowledge graph-enhanced semantic patent characterization.
This is a preview of subscription content, log in via an institution to check access.
Access this article
Price includes VAT (Russian Federation)
Instant access to the full article PDF.
Rent this article via DeepDyve
Institutional subscriptions
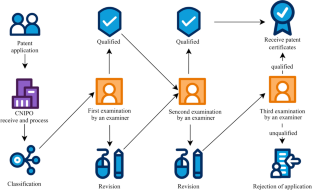
Similar content being viewed by others
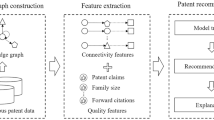
Interpretable patent recommendation with knowledge graph and deep learning
Han Chen & Weiwei Deng
PatentMiner: Patent Vacancy Mining via Context-Enhanced and Knowledge-Guided Graph Attention
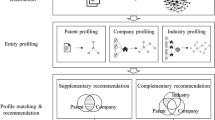
A knowledge graph approach for recommending patents to companies
Weiwei Deng & Jian Ma
https://github.com/google-research/bert .
https://github.com/autoliuweijie/K-BERT .
https://github.com/dbiir/UER-py .
http://kw.fudan.edu.cn/cndbpedia/intro/ .
https://dbpedia.org/page/DBpedia .
Alcácer, J., & Gittelman, M. (2006). Patent citations as a measure of knowledge flows: The influence of examiner citations. The Review of Economics and Statistics, 88 (4), 774–779.
Article Google Scholar
An, X., Li, J., Xu, S., Chen, L., & Sun, W. (2021). An improved patent similarity measurem-ent based on entities and semantic relations. Journal of Informetrics, 15 (2), 101135. https://doi.org/10.1016/j.joi.2021.101135
Arts, S., Cassiman, B., & Gomez, J. C. (2018). Text matching to measure patent similarity. Strategic Management Journal, 39 (1), 62–84. https://doi.org/10.1002/smj.2699
Arts, S., Hou, J., & Gomez, J. C. (2021). Natural language processing to identify the creation and impact of new technologies in patent text: Code, data, and new measures. Research Policy, 50 (2), 104144. https://doi.org/10.1016/j.respol.2020.104144
Brooks, T. A. (1985). Private acts and public objects: An investigation of citer motivations. Journal of the American Society for Information Science, 36 (4), 223–229. https://doi.org/10.1002/asi.4630360402
Chen, H., & Deng, W. (2023). Interpretable patent recommendation with knowledge graph and deep learning. Scientific Reports, 13 (1), 2586. https://doi.org/10.1038/s41598-023-28766-y
Article ADS CAS PubMed PubMed Central Google Scholar
Chen, J., Chen, J., Zhao, S., Zhang, Y., & Tang, J. (2020). Exploiting word embedding for he-erogeneous topic model towards patent recommendation. Scientometrics, 125 (3), 2091–2108. https://doi.org/10.1007/s11192-020-03666-4
Article CAS Google Scholar
Chen, L. (2017). Do patent citations indicate knowledge linkage? The evidence from text simil-arities between patents and their citations. Journal of Informetrics, 11 (1), 63–79. https://doi.org/10.1016/j.joi.2016.04.018
China National Intellectual Property Office (CNIPO). (2010). Guidelines for patent examination 2010 . Intellectual Property Publishing House Co., Ltd.
Google Scholar
China National Intellectual Property Office (CNIPO). (2020). Process of patent application examination and approval. Retrieved December 10, 2023, from https://www.cnipa.gov.cn/art/2020/6/5/art_1517_92471.html
Choi, J., & Yoon, J. (2022). Measuring knowledge exploration distance at the patent level: Application of network embedding and citation analysis. Journal of Informetrics, 16 (2), 101286. https://doi.org/10.1016/j.joi.2022.101286
Choi, J., Lee, J., Yoon, J., Jang, S., Kim, J., & Choi, S. (2022a). A two-stage deep learning- based system for patent citation recommendation. Scientometrics, 127 (11), 6615–6636. https://doi.org/10.1007/s11192-022-04301-0
Choi, S., Lee, H., Park, E., & Choi, S. (2022b). Deep learning for patent landscaping using transformer and graph embedding. Technological Forecasting and Social Change, 175 , 121413. https://doi.org/10.1016/j.techfore.2021.121413
DeGrazia, C. A. W., Pairolero, N. A., & Teodorescu, M. H. M. (2021). Examination incentives, learning, and patent office outcomes: The use of examiner’s amendments at the USPTO. Research Policy, 50 (10), 104360. https://doi.org/10.1016/j.respol.2021.104360
Deng, W., & Ma, J. (2022). A knowledge graph approach for recommending patents to companies. Electronic Commerce Research, 22 (4), 1435–1466. https://doi.org/10.1007/s10660-021-09471-2
Devlin, J., Chang, M.W., Lee, K., & Toutanova, K. (2019). BERT: Pre-training of Deep Bidirectional Transformers for Language Understanding. In J. Burstein, C. Doran, & T. Solorio (Eds.), Proceedings of the 2019 conference of the North American chapter of the association for computational linguistics: human language technologies, volume 1 (long and short papers) (pp. 4171–4186). https://doi.org/10.18653/v1/N19-1423
Dietz, L., Kotov, A., & Meij, E. (2018). Utilizing knowledge graphs for text-centric information retrieval. In: The 41st international ACM SIGIR conference on research & development in information retrieval (pp. 1387–1390). https://doi.org/10.1145/3209978.3210187
European Patent Office (EPO). (2023). Guidelines for examination. Retrieved December 10, 2023, from https://www.epo.org/en/legal/guidelines-epc/2023/index.html
Färber, M., & Jatowt, A. (2020). Citation recommendation: Approaches and datasets. International Journal on Digital Libraries, 21 , 375–405. https://doi.org/10.1007/s00799-020-00288-2
Fu, T.Y., Lei, Z., & Lee, W.C. (2015). Patent Citation Recommendation for Examiners. In Proceedings of the 2015 IEEE international conference on data mining (ICDM) (pp. 751–756). https://doi.org/10.1109/ICDM.2015.151
Hain, D. S., Jurowetzki, R., Buchmann, T., & Wolf, P. (2022). A text-embedding-based approach to measuring patent-to-patent technological similarity. Technological Forecasting and Social Change, 177 , 121559. https://doi.org/10.1016/j.techfore.2022.121559
Harhoff, D., & Wagner, S. (2009). The duration of patent examination at the European patent office. Management Science, 55 (12), 1969–1984. https://doi.org/10.1287/mnsc.1090.1069
Japan Patent Office (JPO). (2015). Examination guidelines for patent and utility model in Japan. Retrieved December 10, 2023, from https://www.jpo.go.jp/e/system/laws/rule/guideline/patent/tukujitu_kijun/index.html
Ji, S., Pan, S., Cambria, E., Marttinen, P., & Yu, P. S. (2022). A survey on knowledge graphs: Representation, acquisition, and applications. IEEE Transactions on Neural Networks and Learning Systems, 33 (2), 494–514. https://doi.org/10.1109/TNNLS.2021.3070843
Article MathSciNet PubMed Google Scholar
Kim, Y. K., & Oh, J. B. (2017). Examination workloads, grant decision bias and examination quality of patent office. Research Policy, 46 (5), 1005–1019. https://doi.org/10.1016/j.respol.2017.03.007
Krestel, R., Chikkamath, R., Hewel, C., & Risch, J. (2021). A survey on deep learning for patent analysis. World Patent Information, 65 , 102035. https://doi.org/10.1016/j.wpi.2021.102035
Kuhn, J. M. (2011). Information overload at the U.S. patent and trademark office: Reframing the duty of disclosure in patent law as a search and filter problem. Journal of Law and Technology, 13 (3), 90–139. https://doi.org/10.1016/j.wpi.2021.102035
Lee, J., & Sohn, S. Y. (2021). Recommendation system for technology convergence opportunit-ies based on self-supervised representation learning. Scientometrics, 126 (1), 1–25. https://doi.org/10.1007/s11192-020-03731-y
Lin, D., Sun, J., Hao, T., & Wang, C. (2016). Research on the applicability of patent citation in patent value evaluation. Journal of Intelligence, 35 (12), 150–154.
Liu, W., Zhou, P., Zhao, Z., Wang, Z., Ju, Q., Deng, H., & Wang, P. (2020). K-BERT: Enabling language representation with knowledge graph. Proceedings of the AAAI Conference on Artificial Intelligence, 34 (03), 2901–2908. https://doi.org/10.1609/aaai.v34i03.5681
Lu, Y., Xiong, X., Zhang, W., Liu, J., & Zhao, R. (2020). Research on classification and simi-larity of patent citation based on deep learning. Scientometrics, 123 , 813–839. https://doi.org/10.1007/s11192-020-03385-w
Meyer, M. (2000). What is special about patent citations? Differences between scientific and patent citations. Scientometrics, 49 (1), 93–123. https://doi.org/10.1023/A:1005613325648
Ou, G., Pang, N., & Wu, J. (2022). Influencing factors of patent examination cycle: Case study of artificial intelligence in China. Data Analysis and Knowledge Discovery, 8 (6), 20–30.
Ridho, R., Edgar, M., & Maarten, D. R. (2020). Knowledge graphs: An information retrieval perspective . Now Foundations and Trends.
Shalaby, W., & Zadrozny, W. (2019). Patent retrieval: A literature review. Knowledge and Information Systems, 61 (2), 631–660. https://doi.org/10.1007/s10115-018-1322-7
Shi, K., Cai, X., Yang, L., & Zhao, J. (2023). Enriched entity representation of knowledge graph for text generation. Complex & Intelligent Systems, 9 (2), 2019–2030. https://doi.org/10.1007/s40747-022-00898-0
Teng, H., Wang, N., Zhao, H., Hu, Y., & Jin, H. (2024). Enhancing semantic text similarity with functional semantic knowledge (FOP) in patents. Journal of Informetrics, 18 (1), 101467. https://doi.org/10.1016/j.joi.2023.101467
Tong, T. W., Zhang, K., He, Z., & Zhang, Y. (2018). What determines the duration of patent examination in China? An outcome-specific duration analysis of invention patent applications at SIPO. Research Policy, 47 (3), 583–591. https://doi.org/10.1016/j.respol.2018.01.002
United States Patent Office (USPTO). (2020). Understanding the patent examination process. Retrieved December 10, 2023, from https://www.uspto.gov/sites/default/files/documents/InventionCon2020_Understanding_the_Patent_Examination_Process.pdf
Vaswani, A., Shazeer, N., Parmar, N., Uszkoreit, J., Jones, L., Gomez, A. N., Polosukhin, I. (2017). Attention is all you need. In Proceedings of the 31st international conference on neural information processing systems (pp. 6000–6010). Curran Associates Inc
Wada, T. (2018). The choice of examiner patent citations for refusals: Evidence from the trilateral offices. Scientometrics, 117 (2), 825–843. https://doi.org/10.1007/s11192-018-2885-5
Wang, X., Ren, H., Chen, Y., Liu, Y., Qiao, Y., & Huang, Y. (2019). Measuring patent similarity with SAO semantic analysis. Scientometrics, 121 (1), 1–23. https://doi.org/10.1007/s11192-019-03191-z
Wang, Z., & Liu, Y. (2022). SEA-PS: Semantic embedding with attention to measuring patent similarity by leveraging various text fields. Journal of Information Science . https://doi.org/10.1177/01655515221106651
Wu, J., Li, B., Ji, Y., Tian, J., & Xiang, Y. (2022). Text-enhanced knowledge graph representation model in hyperbolic space. In J. Jiang & W. Chen (Eds.), Advanced data mining and applications. Springer. https://doi.org/10.1007/978-3-030-95408-6_11
Chapter Google Scholar
Xiao, Y., Li, C., & Thürer, M. (2023). A patent recommendation method based on KG repres-entation learning. Engineering Applications of Artificial Intelligence, 126 , 106722. https://doi.org/10.1016/j.engappai.2023.106722
Xu, B., Xu, Y., Liang, J., Xie, C., Liang, B., Cui, W., Xu, B., & Xiao, Y. (2017). CN-DBpedia: A never-ending Chinese knowledge extraction system. In S. Benferhat, K. Tabia, & M. Ali (Eds.), Advances in artificial intelligence: From theory to practice. Springer.
Yamauchi, I., & Nagaoka, S. (2015). Does the outsourcing of prior art search increase the efficiency of patent examination? Evidence from Japan. Research Policy, 44 (8), 1601–1614. https://doi.org/10.1016/j.respol.2015.05.003
Yin, M. J., Wang, B., & Ling, C. (2024). A fast local citation recommendation algorithm scal-able to multi-topics. Expert Systems with Applications, 238 , 122031. https://doi.org/10.1016/j.eswa.2023.122031
Zhang, Y., Shang, L., Huang, L., Porter, A. L., Zhang, G., Lu, J., & Zhu, D. (2016). A hybrid similarity measure method for patent portfolio analysis. Journal of Informetrics, 10 (4), 1108–1130. https://doi.org/10.1016/j.joi.2016.09.006
Zhao, Z., Chen, H., Zhang, J., Zhao, X., Liu, T., Lu, W., Du, X. (2019). UER: An open-source toolkit for pre-training models. In Proceedings of the 2019 conference on empirical methods in natural language processing and the 9th international joint conference on natural language processing (EMNLP-IJCNLP): System demonstrations (pp. 241–246). https://doi.org/10.18653/v1/D19-3041
Zhao, Y., & Wen, T. (2017). Motivation analysis of patent citation. Information Studies Theory & Application, 40 (7), 28–32.
Download references
Acknowledgements
The authors warmly thank reviewers for their valuable suggestions. This research was partly supported by Key-Area Research and Development Program of Guangdong Province (NO.2021B0101420004), National Natural Science Foundation of China (NO.71801229).
Funding was provided by Key-Area Research and Development Program of Guangdong Province (Grant No. 2021B0101420004), National Natural Science Foundation of China (Grant No. 71801229).
Author information
Authors and affiliations.
School of Information Management, Sun Yat-sen University, Guangzhou, China
Yonghe Lu, Xinyu Tong, Xin Xiong & Hou Zhu
School of Artificial Intelligence, Sun Yat-sen University, Zhuhai, China
You can also search for this author in PubMed Google Scholar
Contributions
YL: Investigation, Writing—review and editing. XT: Methodology, Investigation, Writing—review and editing. XX: Methodology, Software, Writing—original draft. HZ: Supervision, Funding acquisition, Writing—review and editing.
Corresponding author
Correspondence to Hou Zhu .
Ethics declarations
Competing interests.
The authors declare no competing interests.
Additional information
Publisher's note.
Springer Nature remains neutral with regard to jurisdictional claims in published maps and institutional affiliations.
See Table 9 .
Rights and permissions
Springer Nature or its licensor (e.g. a society or other partner) holds exclusive rights to this article under a publishing agreement with the author(s) or other rightsholder(s); author self-archiving of the accepted manuscript version of this article is solely governed by the terms of such publishing agreement and applicable law.
Reprints and permissions
About this article
Lu, Y., Tong, X., Xiong, X. et al. Knowledge graph enhanced citation recommendation model for patent examiners. Scientometrics (2024). https://doi.org/10.1007/s11192-024-04966-9
Download citation
Received : 23 April 2023
Accepted : 08 February 2024
Published : 11 March 2024
DOI : https://doi.org/10.1007/s11192-024-04966-9
Share this article
Anyone you share the following link with will be able to read this content:
Sorry, a shareable link is not currently available for this article.
Provided by the Springer Nature SharedIt content-sharing initiative
- Knowledge graph
- Patent citation recommendation
- Patent examiner citation
- Deep learning
- Find a journal
- Publish with us
- Track your research
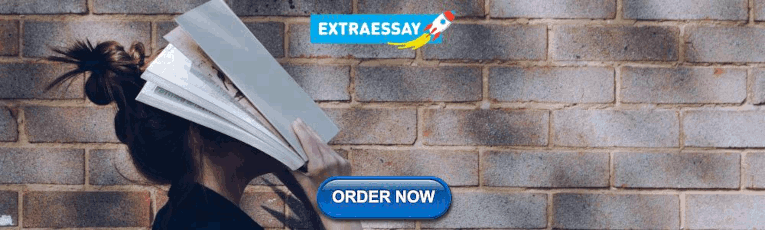
IMAGES
VIDEO
COMMENTS
Download PDF Abstract: Citation recommendation for research papers is a valuable task that can help researchers improve the quality of their work by suggesting relevant related work. Current approaches for this task rely primarily on the text of the papers and the citation network. In this paper, we propose to exploit an additional source of information, namely research knowledge graphs (KG ...
Here, we briefly review research KGs and approaches for citation recommendation. 2.1 Research Knowledge Graphs. Various KGs interlink research papers through metadata (e.g. authors, venues) and citations [13, 22], or through research artefacts (e.g. datasets) [1, 23].Other initiatives organise scientific knowledge in a structured manner with community effort, such as Gene Ontology [], WikiData ...
Experimental results are presented for the STM-KG (STM: Science, Technology, Medicine), which is an automatically populated knowledge graph based on the scientific concepts extracted from papers ...
In this paper, we propose to exploit an additional source of information, namely research knowledge graphs (KGs) that interlink research papers based on mentioned scientific concepts. Our experimental results demonstrate that the combination of information from research KGs with existing state-of-the-art approaches is beneficial.
The overall knowledge graph and multi-task learning-based model for citation recommendation (KMCR) is shown in Fig. 1. The model is composed of three parts: a citation recommendation task module, a knowledge graph link prediction task module, and a feature sharing module. The left side is the citation recommendation task module.
Citation Recommendation for Research Papers via Knowledge Graphs. 10 Jun 2021 · Arthur Brack , Anett Hoppe , Ralph Ewerth ·. Edit social preview. Citation recommendation for research papers is a valuable task that can help researchers improve the quality of their work by suggesting relevant related work. Current approaches for this task rely ...
The experimental results demonstrate that the combination of information from research KGs with existing state-of-the-art approaches is beneficial and outperforms the state of the art with a mean average precision of 20.6% (+0.8) for the top-50 retrieved results. Citation recommendation for research papers is a valuable task that can help researchers improve the quality of their work by ...
Keywords: Information retrieval · Research knowledge graph · Research paper citation recommendation 1 Introduction Citations are a core part of research articles as they enable the reader to posi-tion the novel contribution in the scientific context. Moreover, relating own contributions with relevant research via references can also improve ...
Citation Recommendation for Research Papers via Knowledge Graphs. September 2021. DOI: 10.1007/978-3-030-86324-1_20. In book: Linking Theory and Practice of Digital Libraries (pp.165-174) Authors ...
Citation Recommendation for Research Papers via Knowledge Graphs Arthur Brack 1[0000 00021428 5348], Anett Hoppe 1452 9509], and Ralph Ewerth1;2[0000 0003 0918 6297] 1 TIB - Leibniz Information ...
In this context, we address the problem of citation recommendation, i.e., the task of recommending citations for a new paper. Current research investigates this task in different settings, including cases where rich user metadata is available (e.g., user profile, publications, citations).
This work focus on a setting where the user provides only the abstract of a new paper as input. Our proposed approach is to expand the semantic features of the given abstract using knowledge graphs - and, combine them with other features (e.g., indegree, recency) to fit a learning to rank model. This model is used to generate the citation ...
Therefore, this paper proposes a Content-based and knowledge Graph-based Paper Recommendation method (CGPRec), which uses a two-layer self-attention block to obtain global features of texts for more complete explicit user preferences, and proposes an improved graph convolutional network for modeling high-order associations on the knowledge ...
This work focuses on a setting where the user provides only the abstract of a new paper as input, and proposes a model to expand the semantic features of the given abstract using knowledge graphs and combine them with other features to fit a learning to rank model. Scholarly search engines, reference management tools, and academic social networks enable modern researchers to organize their ...
Citation recommendation for research papers is a valuable task that can help researchers improve the quality of their work by suggesting relevant related work. Current approaches for this task rely primarily on the text of the papers and the citation network. In this paper, we propose to exploit an additional source of information, namely research knowledge graphs (KG) that interlink research ...
Recommending helpful and interesting scholarly papers for researchers from a large number of scholarly papers is the main way to improve research efficiency. Traditional collaborative filtering or content-based recommendation methods do not have a better-fused knowledge graph and have method bottlenecks such as cold start and poor interpretation. Based on the knowledge-aware path recurrent ...
In the academic field, Ayala-Gomez et al. proposed to use knowledge graph to extend the semantic features of a given abstract and combine them with other features such as indegree and recency to ...
A Context-Aware Citation Recommendation Model with BERT and Graph Convolutional Networks. TeamLab/bert-gcn-for-paper-citation • • 15 Mar 2019. Many researchers have utilized the text data called the context sentence, which surrounds the citation tag, and the metadata of the target paper to find the appropriate cited research. 1.
To better use the abundant attributes and interaction information, the knowledge graph is introduced to recommendation system recently. We construct a multi-task learning-based model for citation ...
Automatic recommendation of citations has been a focal point of research in scholarly digital libraries. Many graph-based citation recommendation algorithms have been proposed; however, most of them utilize local citation behavior from the citation network that results in recommending papers in the same proximity as the query article. In this paper, we propose to capture the global citation ...
View a PDF of the paper titled Sequential Recommendation with Latent Relations based on Large Language Model, by Shenghao Yang and 6 other authors ... where most relations are extracted from knowledge graphs. However, existing methods rely on manually predefined relations and suffer the sparsity issue, limiting the generalization ability in ...
Graph Convolutional Neural Networks (GCNs) possess strong capabilities for processing graph data in non-grid domains. They can capture the topological logical structure and node features in graphs and integrate them into nodes' final representations. GCNs have been extensively studied in various fields, such as recommendation systems, social networks, and protein molecular structures. With the ...
In the face of a growing volume of patents, patent examiners grapple with prolonged examination cycles, prompting the need for more effective citation recommendations. To address this, we introduce the patent knowledge graph embedded in Bert (PK-Bert) model. This innovation combines a patent knowledge graph with semantic information in an advanced Transformer framework, outperforming ...