- Skip to main content
- Skip to primary sidebar
- Skip to footer
- QuestionPro

- Solutions Industries Gaming Automotive Sports and events Education Government Travel & Hospitality Financial Services Healthcare Cannabis Technology Use Case NPS+ Communities Audience Contactless surveys Mobile LivePolls Member Experience GDPR Positive People Science 360 Feedback Surveys
- Resources Blog eBooks Survey Templates Case Studies Training Help center

Home Market Research
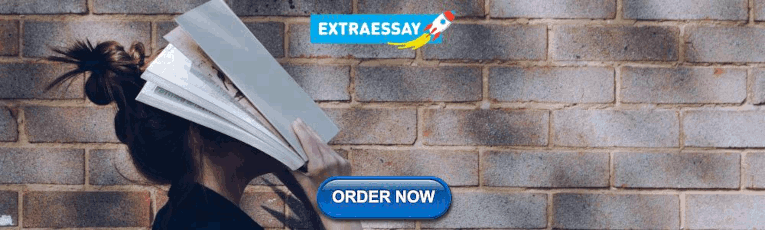
Data Analysis in Research: Types & Methods
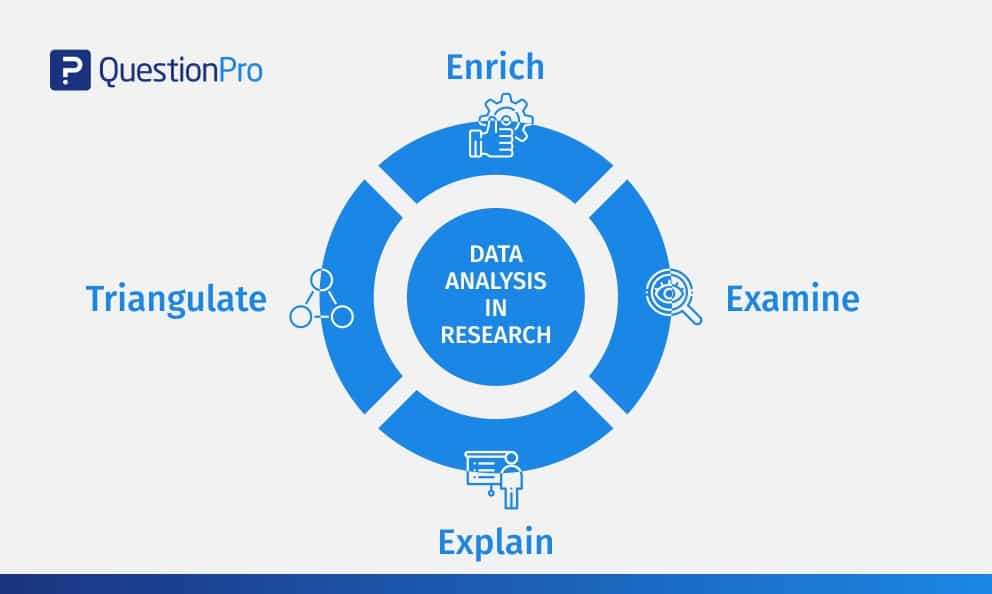
Content Index
Why analyze data in research?
Types of data in research, finding patterns in the qualitative data, methods used for data analysis in qualitative research, preparing data for analysis, methods used for data analysis in quantitative research, considerations in research data analysis, what is data analysis in research.
Definition of research in data analysis: According to LeCompte and Schensul, research data analysis is a process used by researchers to reduce data to a story and interpret it to derive insights. The data analysis process helps reduce a large chunk of data into smaller fragments, which makes sense.
Three essential things occur during the data analysis process — the first is data organization . Summarization and categorization together contribute to becoming the second known method used for data reduction. It helps find patterns and themes in the data for easy identification and linking. The third and last way is data analysis – researchers do it in both top-down and bottom-up fashion.
LEARN ABOUT: Research Process Steps
On the other hand, Marshall and Rossman describe data analysis as a messy, ambiguous, and time-consuming but creative and fascinating process through which a mass of collected data is brought to order, structure and meaning.
We can say that “the data analysis and data interpretation is a process representing the application of deductive and inductive logic to the research and data analysis.”
Researchers rely heavily on data as they have a story to tell or research problems to solve. It starts with a question, and data is nothing but an answer to that question. But, what if there is no question to ask? Well! It is possible to explore data even without a problem – we call it ‘Data Mining’, which often reveals some interesting patterns within the data that are worth exploring.
Irrelevant to the type of data researchers explore, their mission and audiences’ vision guide them to find the patterns to shape the story they want to tell. One of the essential things expected from researchers while analyzing data is to stay open and remain unbiased toward unexpected patterns, expressions, and results. Remember, sometimes, data analysis tells the most unforeseen yet exciting stories that were not expected when initiating data analysis. Therefore, rely on the data you have at hand and enjoy the journey of exploratory research.
Create a Free Account
Every kind of data has a rare quality of describing things after assigning a specific value to it. For analysis, you need to organize these values, processed and presented in a given context, to make it useful. Data can be in different forms; here are the primary data types.
- Qualitative data: When the data presented has words and descriptions, then we call it qualitative data . Although you can observe this data, it is subjective and harder to analyze data in research, especially for comparison. Example: Quality data represents everything describing taste, experience, texture, or an opinion that is considered quality data. This type of data is usually collected through focus groups, personal qualitative interviews , qualitative observation or using open-ended questions in surveys.
- Quantitative data: Any data expressed in numbers of numerical figures are called quantitative data . This type of data can be distinguished into categories, grouped, measured, calculated, or ranked. Example: questions such as age, rank, cost, length, weight, scores, etc. everything comes under this type of data. You can present such data in graphical format, charts, or apply statistical analysis methods to this data. The (Outcomes Measurement Systems) OMS questionnaires in surveys are a significant source of collecting numeric data.
- Categorical data: It is data presented in groups. However, an item included in the categorical data cannot belong to more than one group. Example: A person responding to a survey by telling his living style, marital status, smoking habit, or drinking habit comes under the categorical data. A chi-square test is a standard method used to analyze this data.
Learn More : Examples of Qualitative Data in Education
Data analysis in qualitative research
Data analysis and qualitative data research work a little differently from the numerical data as the quality data is made up of words, descriptions, images, objects, and sometimes symbols. Getting insight from such complicated information is a complicated process. Hence it is typically used for exploratory research and data analysis .
Although there are several ways to find patterns in the textual information, a word-based method is the most relied and widely used global technique for research and data analysis. Notably, the data analysis process in qualitative research is manual. Here the researchers usually read the available data and find repetitive or commonly used words.
For example, while studying data collected from African countries to understand the most pressing issues people face, researchers might find “food” and “hunger” are the most commonly used words and will highlight them for further analysis.
LEARN ABOUT: Level of Analysis
The keyword context is another widely used word-based technique. In this method, the researcher tries to understand the concept by analyzing the context in which the participants use a particular keyword.
For example , researchers conducting research and data analysis for studying the concept of ‘diabetes’ amongst respondents might analyze the context of when and how the respondent has used or referred to the word ‘diabetes.’
The scrutiny-based technique is also one of the highly recommended text analysis methods used to identify a quality data pattern. Compare and contrast is the widely used method under this technique to differentiate how a specific text is similar or different from each other.
For example: To find out the “importance of resident doctor in a company,” the collected data is divided into people who think it is necessary to hire a resident doctor and those who think it is unnecessary. Compare and contrast is the best method that can be used to analyze the polls having single-answer questions types .
Metaphors can be used to reduce the data pile and find patterns in it so that it becomes easier to connect data with theory.
Variable Partitioning is another technique used to split variables so that researchers can find more coherent descriptions and explanations from the enormous data.
LEARN ABOUT: Qualitative Research Questions and Questionnaires
There are several techniques to analyze the data in qualitative research, but here are some commonly used methods,
- Content Analysis: It is widely accepted and the most frequently employed technique for data analysis in research methodology. It can be used to analyze the documented information from text, images, and sometimes from the physical items. It depends on the research questions to predict when and where to use this method.
- Narrative Analysis: This method is used to analyze content gathered from various sources such as personal interviews, field observation, and surveys . The majority of times, stories, or opinions shared by people are focused on finding answers to the research questions.
- Discourse Analysis: Similar to narrative analysis, discourse analysis is used to analyze the interactions with people. Nevertheless, this particular method considers the social context under which or within which the communication between the researcher and respondent takes place. In addition to that, discourse analysis also focuses on the lifestyle and day-to-day environment while deriving any conclusion.
- Grounded Theory: When you want to explain why a particular phenomenon happened, then using grounded theory for analyzing quality data is the best resort. Grounded theory is applied to study data about the host of similar cases occurring in different settings. When researchers are using this method, they might alter explanations or produce new ones until they arrive at some conclusion.
LEARN ABOUT: 12 Best Tools for Researchers
Data analysis in quantitative research
The first stage in research and data analysis is to make it for the analysis so that the nominal data can be converted into something meaningful. Data preparation consists of the below phases.
Phase I: Data Validation
Data validation is done to understand if the collected data sample is per the pre-set standards, or it is a biased data sample again divided into four different stages
- Fraud: To ensure an actual human being records each response to the survey or the questionnaire
- Screening: To make sure each participant or respondent is selected or chosen in compliance with the research criteria
- Procedure: To ensure ethical standards were maintained while collecting the data sample
- Completeness: To ensure that the respondent has answered all the questions in an online survey. Else, the interviewer had asked all the questions devised in the questionnaire.
Phase II: Data Editing
More often, an extensive research data sample comes loaded with errors. Respondents sometimes fill in some fields incorrectly or sometimes skip them accidentally. Data editing is a process wherein the researchers have to confirm that the provided data is free of such errors. They need to conduct necessary checks and outlier checks to edit the raw edit and make it ready for analysis.
Phase III: Data Coding
Out of all three, this is the most critical phase of data preparation associated with grouping and assigning values to the survey responses . If a survey is completed with a 1000 sample size, the researcher will create an age bracket to distinguish the respondents based on their age. Thus, it becomes easier to analyze small data buckets rather than deal with the massive data pile.
LEARN ABOUT: Steps in Qualitative Research
After the data is prepared for analysis, researchers are open to using different research and data analysis methods to derive meaningful insights. For sure, statistical analysis plans are the most favored to analyze numerical data. In statistical analysis, distinguishing between categorical data and numerical data is essential, as categorical data involves distinct categories or labels, while numerical data consists of measurable quantities. The method is again classified into two groups. First, ‘Descriptive Statistics’ used to describe data. Second, ‘Inferential statistics’ that helps in comparing the data .
Descriptive statistics
This method is used to describe the basic features of versatile types of data in research. It presents the data in such a meaningful way that pattern in the data starts making sense. Nevertheless, the descriptive analysis does not go beyond making conclusions. The conclusions are again based on the hypothesis researchers have formulated so far. Here are a few major types of descriptive analysis methods.
Measures of Frequency
- Count, Percent, Frequency
- It is used to denote home often a particular event occurs.
- Researchers use it when they want to showcase how often a response is given.
Measures of Central Tendency
- Mean, Median, Mode
- The method is widely used to demonstrate distribution by various points.
- Researchers use this method when they want to showcase the most commonly or averagely indicated response.
Measures of Dispersion or Variation
- Range, Variance, Standard deviation
- Here the field equals high/low points.
- Variance standard deviation = difference between the observed score and mean
- It is used to identify the spread of scores by stating intervals.
- Researchers use this method to showcase data spread out. It helps them identify the depth until which the data is spread out that it directly affects the mean.
Measures of Position
- Percentile ranks, Quartile ranks
- It relies on standardized scores helping researchers to identify the relationship between different scores.
- It is often used when researchers want to compare scores with the average count.
For quantitative research use of descriptive analysis often give absolute numbers, but the in-depth analysis is never sufficient to demonstrate the rationale behind those numbers. Nevertheless, it is necessary to think of the best method for research and data analysis suiting your survey questionnaire and what story researchers want to tell. For example, the mean is the best way to demonstrate the students’ average scores in schools. It is better to rely on the descriptive statistics when the researchers intend to keep the research or outcome limited to the provided sample without generalizing it. For example, when you want to compare average voting done in two different cities, differential statistics are enough.
Descriptive analysis is also called a ‘univariate analysis’ since it is commonly used to analyze a single variable.
Inferential statistics
Inferential statistics are used to make predictions about a larger population after research and data analysis of the representing population’s collected sample. For example, you can ask some odd 100 audiences at a movie theater if they like the movie they are watching. Researchers then use inferential statistics on the collected sample to reason that about 80-90% of people like the movie.
Here are two significant areas of inferential statistics.
- Estimating parameters: It takes statistics from the sample research data and demonstrates something about the population parameter.
- Hypothesis test: I t’s about sampling research data to answer the survey research questions. For example, researchers might be interested to understand if the new shade of lipstick recently launched is good or not, or if the multivitamin capsules help children to perform better at games.
These are sophisticated analysis methods used to showcase the relationship between different variables instead of describing a single variable. It is often used when researchers want something beyond absolute numbers to understand the relationship between variables.
Here are some of the commonly used methods for data analysis in research.
- Correlation: When researchers are not conducting experimental research or quasi-experimental research wherein the researchers are interested to understand the relationship between two or more variables, they opt for correlational research methods.
- Cross-tabulation: Also called contingency tables, cross-tabulation is used to analyze the relationship between multiple variables. Suppose provided data has age and gender categories presented in rows and columns. A two-dimensional cross-tabulation helps for seamless data analysis and research by showing the number of males and females in each age category.
- Regression analysis: For understanding the strong relationship between two variables, researchers do not look beyond the primary and commonly used regression analysis method, which is also a type of predictive analysis used. In this method, you have an essential factor called the dependent variable. You also have multiple independent variables in regression analysis. You undertake efforts to find out the impact of independent variables on the dependent variable. The values of both independent and dependent variables are assumed as being ascertained in an error-free random manner.
- Frequency tables: The statistical procedure is used for testing the degree to which two or more vary or differ in an experiment. A considerable degree of variation means research findings were significant. In many contexts, ANOVA testing and variance analysis are similar.
- Analysis of variance: The statistical procedure is used for testing the degree to which two or more vary or differ in an experiment. A considerable degree of variation means research findings were significant. In many contexts, ANOVA testing and variance analysis are similar.
- Researchers must have the necessary research skills to analyze and manipulation the data , Getting trained to demonstrate a high standard of research practice. Ideally, researchers must possess more than a basic understanding of the rationale of selecting one statistical method over the other to obtain better data insights.
- Usually, research and data analytics projects differ by scientific discipline; therefore, getting statistical advice at the beginning of analysis helps design a survey questionnaire, select data collection methods, and choose samples.
LEARN ABOUT: Best Data Collection Tools
- The primary aim of data research and analysis is to derive ultimate insights that are unbiased. Any mistake in or keeping a biased mind to collect data, selecting an analysis method, or choosing audience sample il to draw a biased inference.
- Irrelevant to the sophistication used in research data and analysis is enough to rectify the poorly defined objective outcome measurements. It does not matter if the design is at fault or intentions are not clear, but lack of clarity might mislead readers, so avoid the practice.
- The motive behind data analysis in research is to present accurate and reliable data. As far as possible, avoid statistical errors, and find a way to deal with everyday challenges like outliers, missing data, data altering, data mining , or developing graphical representation.
LEARN MORE: Descriptive Research vs Correlational Research The sheer amount of data generated daily is frightening. Especially when data analysis has taken center stage. in 2018. In last year, the total data supply amounted to 2.8 trillion gigabytes. Hence, it is clear that the enterprises willing to survive in the hypercompetitive world must possess an excellent capability to analyze complex research data, derive actionable insights, and adapt to the new market needs.
LEARN ABOUT: Average Order Value
QuestionPro is an online survey platform that empowers organizations in data analysis and research and provides them a medium to collect data by creating appealing surveys.
MORE LIKE THIS
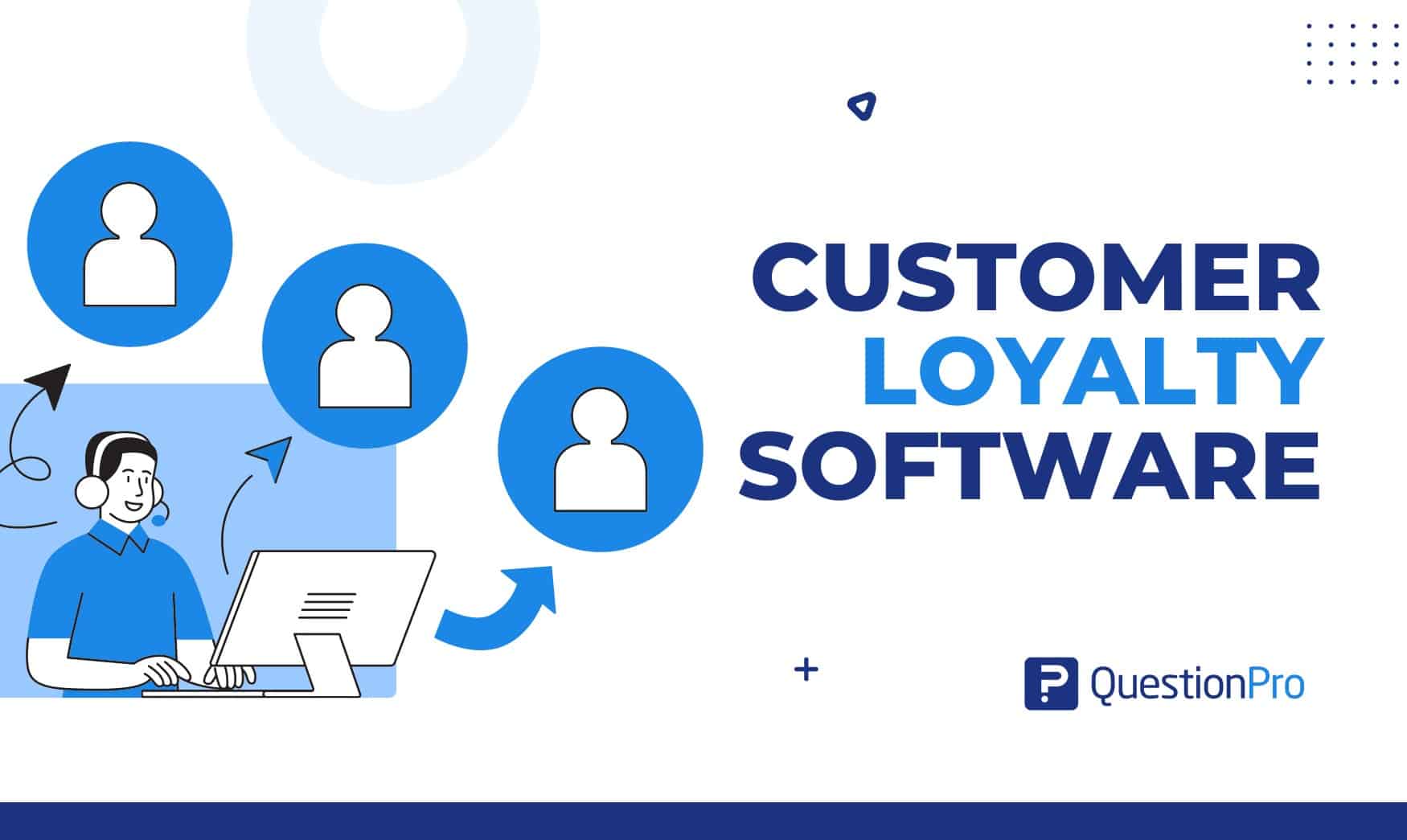
10 Top Customer Loyalty Software to Boost Your Business
Mar 25, 2024
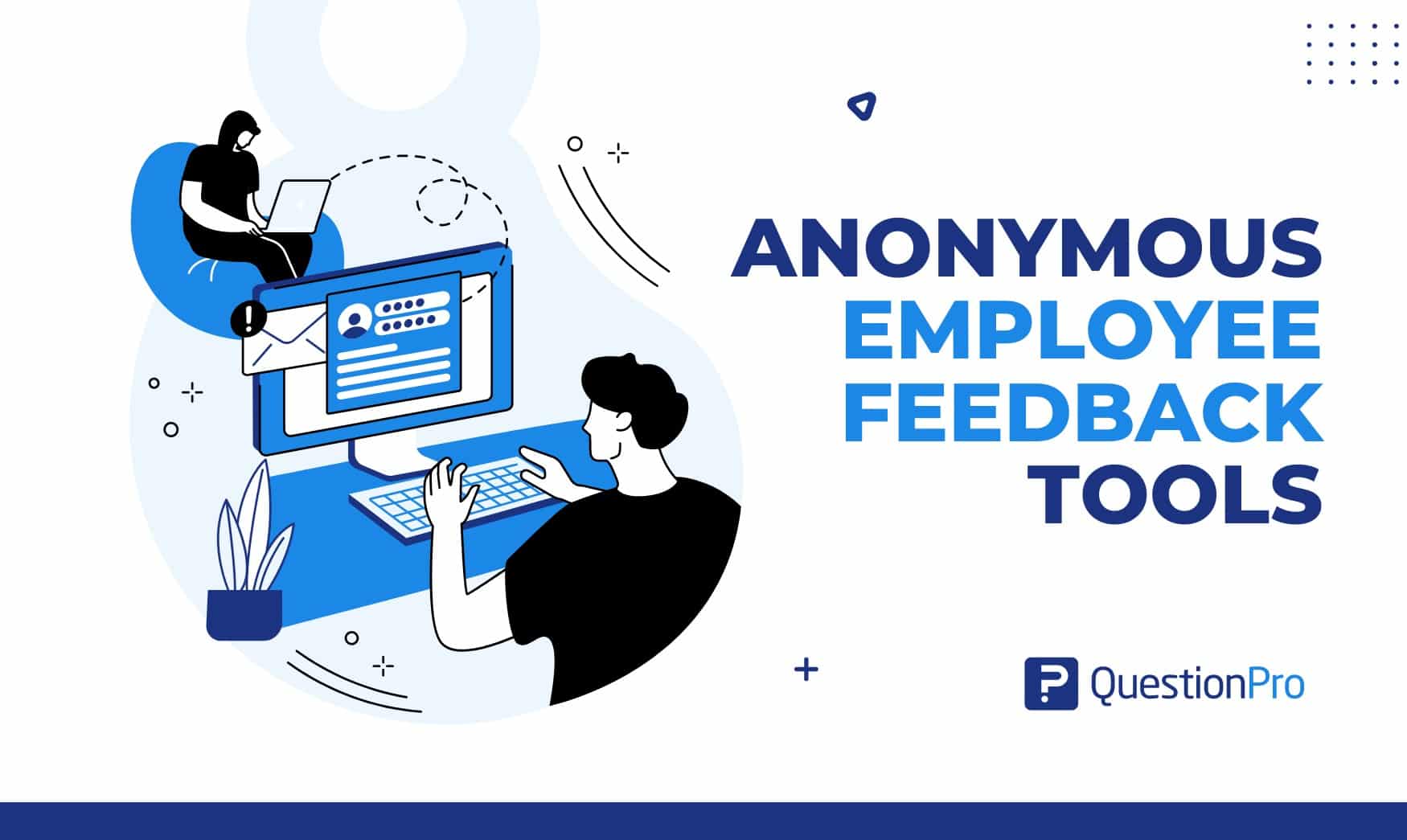
Top 13 Anonymous Employee Feedback Tools for 2024
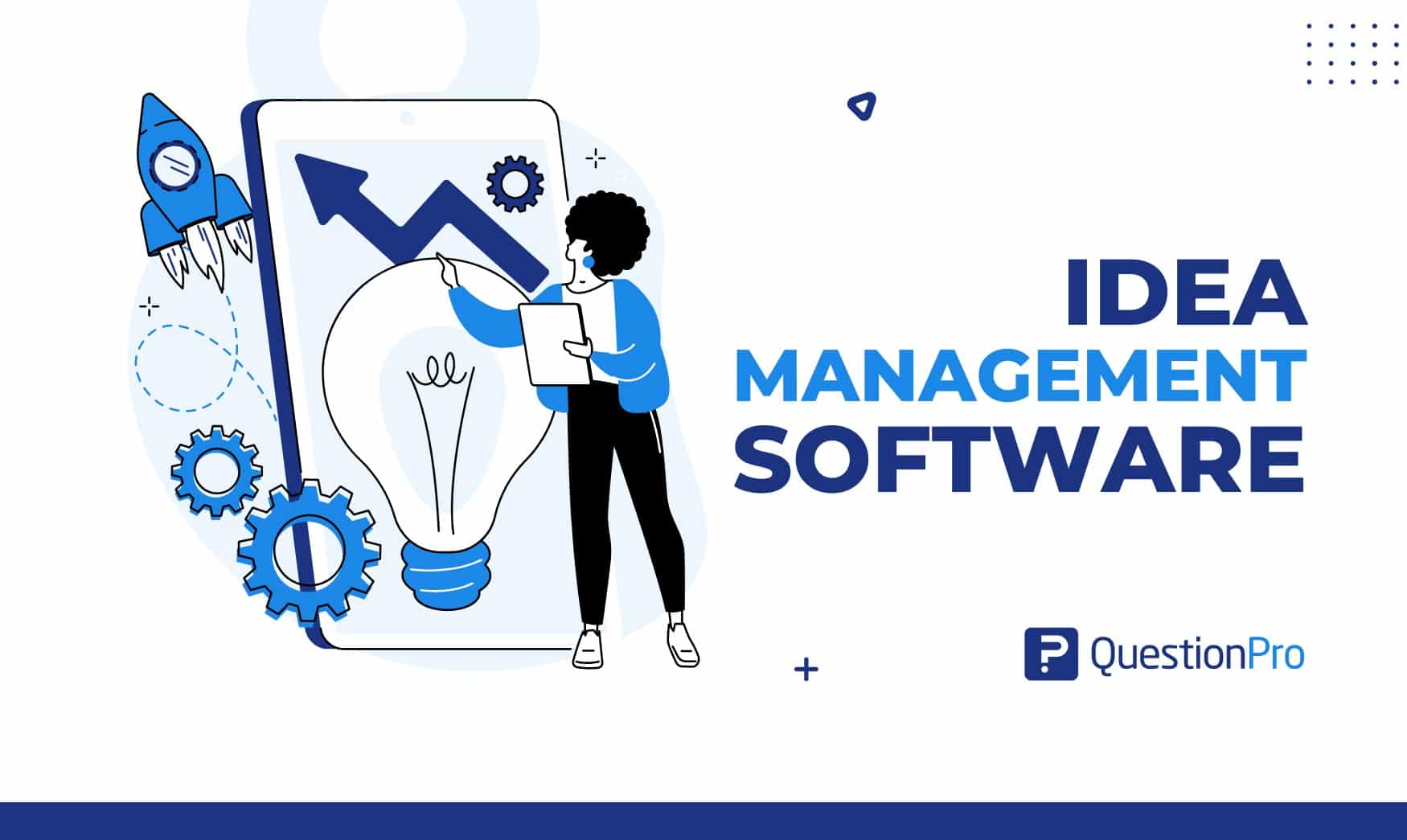
Unlocking Creativity With 10 Top Idea Management Software
Mar 23, 2024
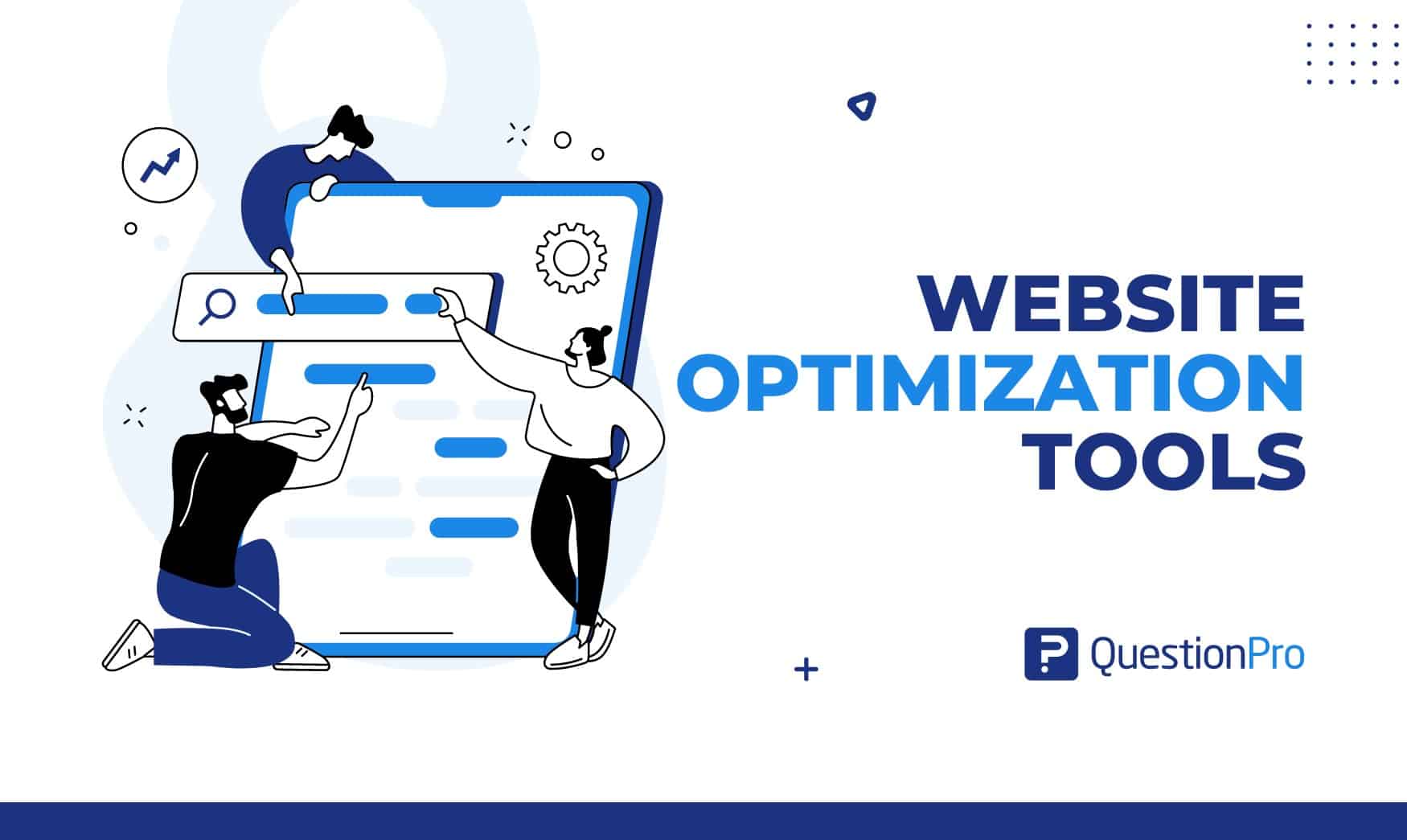
20 Best Website Optimization Tools to Improve Your Website
Mar 22, 2024
Other categories
- Academic Research
- Artificial Intelligence
- Assessments
- Brand Awareness
- Case Studies
- Communities
- Consumer Insights
- Customer effort score
- Customer Engagement
- Customer Experience
- Customer Loyalty
- Customer Research
- Customer Satisfaction
- Employee Benefits
- Employee Engagement
- Employee Retention
- Friday Five
- General Data Protection Regulation
- Insights Hub
- Life@QuestionPro
- Market Research
- Mobile diaries
- Mobile Surveys
- New Features
- Online Communities
- Question Types
- Questionnaire
- QuestionPro Products
- Release Notes
- Research Tools and Apps
- Revenue at Risk
- Survey Templates
- Training Tips
- Uncategorized
- Video Learning Series
- What’s Coming Up
- Workforce Intelligence
Data Analysis
- Introduction to Data Analysis
- Quantitative Analysis Tools
- Qualitative Analysis Tools
- Mixed Methods Analysis
- Geospatial Analysis
- Further Reading
What is Data Analysis?
According to the federal government, data analysis is "the process of systematically applying statistical and/or logical techniques to describe and illustrate, condense and recap, and evaluate data" ( Responsible Conduct in Data Management ). Important components of data analysis include searching for patterns, remaining unbiased in drawing inference from data, practicing responsible data management , and maintaining "honest and accurate analysis" ( Responsible Conduct in Data Management ).
In order to understand data analysis further, it can be helpful to take a step back and understand the question "What is data?". Many of us associate data with spreadsheets of numbers and values, however, data can encompass much more than that. According to the federal government, data is "The recorded factual material commonly accepted in the scientific community as necessary to validate research findings" ( OMB Circular 110 ). This broad definition can include information in many formats.
Some examples of types of data are as follows:
- Photographs
- Hand-written notes from field observation
- Machine learning training data sets
- Ethnographic interview transcripts
- Sheet music
- Scripts for plays and musicals
- Observations from laboratory experiments ( CMU Data 101 )
Thus, data analysis includes the processing and manipulation of these data sources in order to gain additional insight from data, answer a research question, or confirm a research hypothesis.
Data analysis falls within the larger research data lifecycle, as seen below.
( University of Virginia )
Why Analyze Data?
Through data analysis, a researcher can gain additional insight from data and draw conclusions to address the research question or hypothesis. Use of data analysis tools helps researchers understand and interpret data.
What are the Types of Data Analysis?
Data analysis can be quantitative, qualitative, or mixed methods.
Quantitative research typically involves numbers and "close-ended questions and responses" ( Creswell & Creswell, 2018 , p. 3). Quantitative research tests variables against objective theories, usually measured and collected on instruments and analyzed using statistical procedures ( Creswell & Creswell, 2018 , p. 4). Quantitative analysis usually uses deductive reasoning.
Qualitative research typically involves words and "open-ended questions and responses" ( Creswell & Creswell, 2018 , p. 3). According to Creswell & Creswell, "qualitative research is an approach for exploring and understanding the meaning individuals or groups ascribe to a social or human problem" ( 2018 , p. 4). Thus, qualitative analysis usually invokes inductive reasoning.
Mixed methods research uses methods from both quantitative and qualitative research approaches. Mixed methods research works under the "core assumption... that the integration of qualitative and quantitative data yields additional insight beyond the information provided by either the quantitative or qualitative data alone" ( Creswell & Creswell, 2018 , p. 4).
- Next: Planning >>
- Last Updated: Mar 18, 2024 2:56 PM
- URL: https://guides.library.georgetown.edu/data-analysis

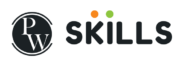
Data Analysis Techniques in Research – Methods, Tools & Examples
Data analysis techniques in research are essential because they allow researchers to derive meaningful insights from data sets to support their hypotheses or research objectives.
Data Analysis Techniques in Research : While various groups, institutions, and professionals may have diverse approaches to data analysis, a universal definition captures its essence. Data analysis involves refining, transforming, and interpreting raw data to derive actionable insights that guide informed decision-making for businesses.
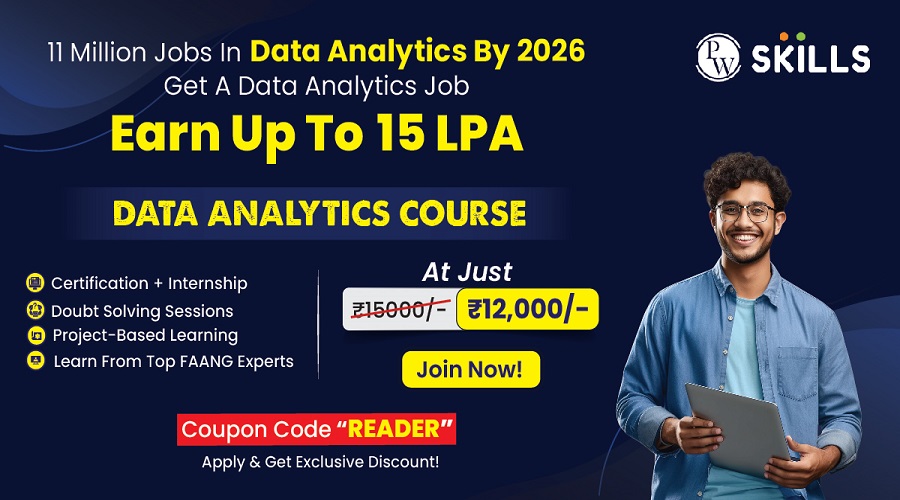
A straightforward illustration of data analysis emerges when we make everyday decisions, basing our choices on past experiences or predictions of potential outcomes.
If you want to learn more about this topic and acquire valuable skills that will set you apart in today’s data-driven world, we highly recommend enrolling in the Data Analytics Course by Physics Wallah . And as a special offer for our readers, use the coupon code “READER” to get a discount on this course.
Table of Contents
What is Data Analysis?
Data analysis is the systematic process of inspecting, cleaning, transforming, and interpreting data with the objective of discovering valuable insights and drawing meaningful conclusions. This process involves several steps:
- Inspecting : Initial examination of data to understand its structure, quality, and completeness.
- Cleaning : Removing errors, inconsistencies, or irrelevant information to ensure accurate analysis.
- Transforming : Converting data into a format suitable for analysis, such as normalization or aggregation.
- Interpreting : Analyzing the transformed data to identify patterns, trends, and relationships.
Types of Data Analysis Techniques in Research
Data analysis techniques in research are categorized into qualitative and quantitative methods, each with its specific approaches and tools. These techniques are instrumental in extracting meaningful insights, patterns, and relationships from data to support informed decision-making, validate hypotheses, and derive actionable recommendations. Below is an in-depth exploration of the various types of data analysis techniques commonly employed in research:
1) Qualitative Analysis:
Definition: Qualitative analysis focuses on understanding non-numerical data, such as opinions, concepts, or experiences, to derive insights into human behavior, attitudes, and perceptions.
- Content Analysis: Examines textual data, such as interview transcripts, articles, or open-ended survey responses, to identify themes, patterns, or trends.
- Narrative Analysis: Analyzes personal stories or narratives to understand individuals’ experiences, emotions, or perspectives.
- Ethnographic Studies: Involves observing and analyzing cultural practices, behaviors, and norms within specific communities or settings.
2) Quantitative Analysis:
Quantitative analysis emphasizes numerical data and employs statistical methods to explore relationships, patterns, and trends. It encompasses several approaches:
Descriptive Analysis:
- Frequency Distribution: Represents the number of occurrences of distinct values within a dataset.
- Central Tendency: Measures such as mean, median, and mode provide insights into the central values of a dataset.
- Dispersion: Techniques like variance and standard deviation indicate the spread or variability of data.
Diagnostic Analysis:
- Regression Analysis: Assesses the relationship between dependent and independent variables, enabling prediction or understanding causality.
- ANOVA (Analysis of Variance): Examines differences between groups to identify significant variations or effects.
Predictive Analysis:
- Time Series Forecasting: Uses historical data points to predict future trends or outcomes.
- Machine Learning Algorithms: Techniques like decision trees, random forests, and neural networks predict outcomes based on patterns in data.
Prescriptive Analysis:
- Optimization Models: Utilizes linear programming, integer programming, or other optimization techniques to identify the best solutions or strategies.
- Simulation: Mimics real-world scenarios to evaluate various strategies or decisions and determine optimal outcomes.
Specific Techniques:
- Monte Carlo Simulation: Models probabilistic outcomes to assess risk and uncertainty.
- Factor Analysis: Reduces the dimensionality of data by identifying underlying factors or components.
- Cohort Analysis: Studies specific groups or cohorts over time to understand trends, behaviors, or patterns within these groups.
- Cluster Analysis: Classifies objects or individuals into homogeneous groups or clusters based on similarities or attributes.
- Sentiment Analysis: Uses natural language processing and machine learning techniques to determine sentiment, emotions, or opinions from textual data.
Also Read: AI and Predictive Analytics: Examples, Tools, Uses, Ai Vs Predictive Analytics
Data Analysis Techniques in Research Examples
To provide a clearer understanding of how data analysis techniques are applied in research, let’s consider a hypothetical research study focused on evaluating the impact of online learning platforms on students’ academic performance.
Research Objective:
Determine if students using online learning platforms achieve higher academic performance compared to those relying solely on traditional classroom instruction.
Data Collection:
- Quantitative Data: Academic scores (grades) of students using online platforms and those using traditional classroom methods.
- Qualitative Data: Feedback from students regarding their learning experiences, challenges faced, and preferences.
Data Analysis Techniques Applied:
1) Descriptive Analysis:
- Calculate the mean, median, and mode of academic scores for both groups.
- Create frequency distributions to represent the distribution of grades in each group.
2) Diagnostic Analysis:
- Conduct an Analysis of Variance (ANOVA) to determine if there’s a statistically significant difference in academic scores between the two groups.
- Perform Regression Analysis to assess the relationship between the time spent on online platforms and academic performance.
3) Predictive Analysis:
- Utilize Time Series Forecasting to predict future academic performance trends based on historical data.
- Implement Machine Learning algorithms to develop a predictive model that identifies factors contributing to academic success on online platforms.
4) Prescriptive Analysis:
- Apply Optimization Models to identify the optimal combination of online learning resources (e.g., video lectures, interactive quizzes) that maximize academic performance.
- Use Simulation Techniques to evaluate different scenarios, such as varying student engagement levels with online resources, to determine the most effective strategies for improving learning outcomes.
5) Specific Techniques:
- Conduct Factor Analysis on qualitative feedback to identify common themes or factors influencing students’ perceptions and experiences with online learning.
- Perform Cluster Analysis to segment students based on their engagement levels, preferences, or academic outcomes, enabling targeted interventions or personalized learning strategies.
- Apply Sentiment Analysis on textual feedback to categorize students’ sentiments as positive, negative, or neutral regarding online learning experiences.
By applying a combination of qualitative and quantitative data analysis techniques, this research example aims to provide comprehensive insights into the effectiveness of online learning platforms.
Also Read: Learning Path to Become a Data Analyst in 2024
Data Analysis Techniques in Quantitative Research
Quantitative research involves collecting numerical data to examine relationships, test hypotheses, and make predictions. Various data analysis techniques are employed to interpret and draw conclusions from quantitative data. Here are some key data analysis techniques commonly used in quantitative research:
1) Descriptive Statistics:
- Description: Descriptive statistics are used to summarize and describe the main aspects of a dataset, such as central tendency (mean, median, mode), variability (range, variance, standard deviation), and distribution (skewness, kurtosis).
- Applications: Summarizing data, identifying patterns, and providing initial insights into the dataset.
2) Inferential Statistics:
- Description: Inferential statistics involve making predictions or inferences about a population based on a sample of data. This technique includes hypothesis testing, confidence intervals, t-tests, chi-square tests, analysis of variance (ANOVA), regression analysis, and correlation analysis.
- Applications: Testing hypotheses, making predictions, and generalizing findings from a sample to a larger population.
3) Regression Analysis:
- Description: Regression analysis is a statistical technique used to model and examine the relationship between a dependent variable and one or more independent variables. Linear regression, multiple regression, logistic regression, and nonlinear regression are common types of regression analysis .
- Applications: Predicting outcomes, identifying relationships between variables, and understanding the impact of independent variables on the dependent variable.
4) Correlation Analysis:
- Description: Correlation analysis is used to measure and assess the strength and direction of the relationship between two or more variables. The Pearson correlation coefficient, Spearman rank correlation coefficient, and Kendall’s tau are commonly used measures of correlation.
- Applications: Identifying associations between variables and assessing the degree and nature of the relationship.
5) Factor Analysis:
- Description: Factor analysis is a multivariate statistical technique used to identify and analyze underlying relationships or factors among a set of observed variables. It helps in reducing the dimensionality of data and identifying latent variables or constructs.
- Applications: Identifying underlying factors or constructs, simplifying data structures, and understanding the underlying relationships among variables.
6) Time Series Analysis:
- Description: Time series analysis involves analyzing data collected or recorded over a specific period at regular intervals to identify patterns, trends, and seasonality. Techniques such as moving averages, exponential smoothing, autoregressive integrated moving average (ARIMA), and Fourier analysis are used.
- Applications: Forecasting future trends, analyzing seasonal patterns, and understanding time-dependent relationships in data.
7) ANOVA (Analysis of Variance):
- Description: Analysis of variance (ANOVA) is a statistical technique used to analyze and compare the means of two or more groups or treatments to determine if they are statistically different from each other. One-way ANOVA, two-way ANOVA, and MANOVA (Multivariate Analysis of Variance) are common types of ANOVA.
- Applications: Comparing group means, testing hypotheses, and determining the effects of categorical independent variables on a continuous dependent variable.
8) Chi-Square Tests:
- Description: Chi-square tests are non-parametric statistical tests used to assess the association between categorical variables in a contingency table. The Chi-square test of independence, goodness-of-fit test, and test of homogeneity are common chi-square tests.
- Applications: Testing relationships between categorical variables, assessing goodness-of-fit, and evaluating independence.
These quantitative data analysis techniques provide researchers with valuable tools and methods to analyze, interpret, and derive meaningful insights from numerical data. The selection of a specific technique often depends on the research objectives, the nature of the data, and the underlying assumptions of the statistical methods being used.
Also Read: Analysis vs. Analytics: How Are They Different?
Data Analysis Methods
Data analysis methods refer to the techniques and procedures used to analyze, interpret, and draw conclusions from data. These methods are essential for transforming raw data into meaningful insights, facilitating decision-making processes, and driving strategies across various fields. Here are some common data analysis methods:
- Description: Descriptive statistics summarize and organize data to provide a clear and concise overview of the dataset. Measures such as mean, median, mode, range, variance, and standard deviation are commonly used.
- Description: Inferential statistics involve making predictions or inferences about a population based on a sample of data. Techniques such as hypothesis testing, confidence intervals, and regression analysis are used.
3) Exploratory Data Analysis (EDA):
- Description: EDA techniques involve visually exploring and analyzing data to discover patterns, relationships, anomalies, and insights. Methods such as scatter plots, histograms, box plots, and correlation matrices are utilized.
- Applications: Identifying trends, patterns, outliers, and relationships within the dataset.
4) Predictive Analytics:
- Description: Predictive analytics use statistical algorithms and machine learning techniques to analyze historical data and make predictions about future events or outcomes. Techniques such as regression analysis, time series forecasting, and machine learning algorithms (e.g., decision trees, random forests, neural networks) are employed.
- Applications: Forecasting future trends, predicting outcomes, and identifying potential risks or opportunities.
5) Prescriptive Analytics:
- Description: Prescriptive analytics involve analyzing data to recommend actions or strategies that optimize specific objectives or outcomes. Optimization techniques, simulation models, and decision-making algorithms are utilized.
- Applications: Recommending optimal strategies, decision-making support, and resource allocation.
6) Qualitative Data Analysis:
- Description: Qualitative data analysis involves analyzing non-numerical data, such as text, images, videos, or audio, to identify themes, patterns, and insights. Methods such as content analysis, thematic analysis, and narrative analysis are used.
- Applications: Understanding human behavior, attitudes, perceptions, and experiences.
7) Big Data Analytics:
- Description: Big data analytics methods are designed to analyze large volumes of structured and unstructured data to extract valuable insights. Technologies such as Hadoop, Spark, and NoSQL databases are used to process and analyze big data.
- Applications: Analyzing large datasets, identifying trends, patterns, and insights from big data sources.
8) Text Analytics:
- Description: Text analytics methods involve analyzing textual data, such as customer reviews, social media posts, emails, and documents, to extract meaningful information and insights. Techniques such as sentiment analysis, text mining, and natural language processing (NLP) are used.
- Applications: Analyzing customer feedback, monitoring brand reputation, and extracting insights from textual data sources.
These data analysis methods are instrumental in transforming data into actionable insights, informing decision-making processes, and driving organizational success across various sectors, including business, healthcare, finance, marketing, and research. The selection of a specific method often depends on the nature of the data, the research objectives, and the analytical requirements of the project or organization.
Also Read: Quantitative Data Analysis: Types, Analysis & Examples
Data Analysis Tools
Data analysis tools are essential instruments that facilitate the process of examining, cleaning, transforming, and modeling data to uncover useful information, make informed decisions, and drive strategies. Here are some prominent data analysis tools widely used across various industries:
1) Microsoft Excel:
- Description: A spreadsheet software that offers basic to advanced data analysis features, including pivot tables, data visualization tools, and statistical functions.
- Applications: Data cleaning, basic statistical analysis, visualization, and reporting.
2) R Programming Language:
- Description: An open-source programming language specifically designed for statistical computing and data visualization.
- Applications: Advanced statistical analysis, data manipulation, visualization, and machine learning.
3) Python (with Libraries like Pandas, NumPy, Matplotlib, and Seaborn):
- Description: A versatile programming language with libraries that support data manipulation, analysis, and visualization.
- Applications: Data cleaning, statistical analysis, machine learning, and data visualization.
4) SPSS (Statistical Package for the Social Sciences):
- Description: A comprehensive statistical software suite used for data analysis, data mining, and predictive analytics.
- Applications: Descriptive statistics, hypothesis testing, regression analysis, and advanced analytics.
5) SAS (Statistical Analysis System):
- Description: A software suite used for advanced analytics, multivariate analysis, and predictive modeling.
- Applications: Data management, statistical analysis, predictive modeling, and business intelligence.
6) Tableau:
- Description: A data visualization tool that allows users to create interactive and shareable dashboards and reports.
- Applications: Data visualization , business intelligence , and interactive dashboard creation.
7) Power BI:
- Description: A business analytics tool developed by Microsoft that provides interactive visualizations and business intelligence capabilities.
- Applications: Data visualization, business intelligence, reporting, and dashboard creation.
8) SQL (Structured Query Language) Databases (e.g., MySQL, PostgreSQL, Microsoft SQL Server):
- Description: Database management systems that support data storage, retrieval, and manipulation using SQL queries.
- Applications: Data retrieval, data cleaning, data transformation, and database management.
9) Apache Spark:
- Description: A fast and general-purpose distributed computing system designed for big data processing and analytics.
- Applications: Big data processing, machine learning, data streaming, and real-time analytics.
10) IBM SPSS Modeler:
- Description: A data mining software application used for building predictive models and conducting advanced analytics.
- Applications: Predictive modeling, data mining, statistical analysis, and decision optimization.
These tools serve various purposes and cater to different data analysis needs, from basic statistical analysis and data visualization to advanced analytics, machine learning, and big data processing. The choice of a specific tool often depends on the nature of the data, the complexity of the analysis, and the specific requirements of the project or organization.
Also Read: How to Analyze Survey Data: Methods & Examples
Importance of Data Analysis in Research
The importance of data analysis in research cannot be overstated; it serves as the backbone of any scientific investigation or study. Here are several key reasons why data analysis is crucial in the research process:
- Data analysis helps ensure that the results obtained are valid and reliable. By systematically examining the data, researchers can identify any inconsistencies or anomalies that may affect the credibility of the findings.
- Effective data analysis provides researchers with the necessary information to make informed decisions. By interpreting the collected data, researchers can draw conclusions, make predictions, or formulate recommendations based on evidence rather than intuition or guesswork.
- Data analysis allows researchers to identify patterns, trends, and relationships within the data. This can lead to a deeper understanding of the research topic, enabling researchers to uncover insights that may not be immediately apparent.
- In empirical research, data analysis plays a critical role in testing hypotheses. Researchers collect data to either support or refute their hypotheses, and data analysis provides the tools and techniques to evaluate these hypotheses rigorously.
- Transparent and well-executed data analysis enhances the credibility of research findings. By clearly documenting the data analysis methods and procedures, researchers allow others to replicate the study, thereby contributing to the reproducibility of research findings.
- In fields such as business or healthcare, data analysis helps organizations allocate resources more efficiently. By analyzing data on consumer behavior, market trends, or patient outcomes, organizations can make strategic decisions about resource allocation, budgeting, and planning.
- In public policy and social sciences, data analysis is instrumental in developing and evaluating policies and interventions. By analyzing data on social, economic, or environmental factors, policymakers can assess the effectiveness of existing policies and inform the development of new ones.
- Data analysis allows for continuous improvement in research methods and practices. By analyzing past research projects, identifying areas for improvement, and implementing changes based on data-driven insights, researchers can refine their approaches and enhance the quality of future research endeavors.
However, it is important to remember that mastering these techniques requires practice and continuous learning. That’s why we highly recommend the Data Analytics Course by Physics Wallah . Not only does it cover all the fundamentals of data analysis, but it also provides hands-on experience with various tools such as Excel, Python, and Tableau. Plus, if you use the “ READER ” coupon code at checkout, you can get a special discount on the course.
For Latest Tech Related Information, Join Our Official Free Telegram Group : PW Skills Telegram Group
Data Analysis Techniques in Research FAQs
What are the 5 techniques for data analysis.
The five techniques for data analysis include: Descriptive Analysis Diagnostic Analysis Predictive Analysis Prescriptive Analysis Qualitative Analysis
What are techniques of data analysis in research?
Techniques of data analysis in research encompass both qualitative and quantitative methods. These techniques involve processes like summarizing raw data, investigating causes of events, forecasting future outcomes, offering recommendations based on predictions, and examining non-numerical data to understand concepts or experiences.
What are the 3 methods of data analysis?
The three primary methods of data analysis are: Qualitative Analysis Quantitative Analysis Mixed-Methods Analysis
What are the four types of data analysis techniques?
The four types of data analysis techniques are: Descriptive Analysis Diagnostic Analysis Predictive Analysis Prescriptive Analysis
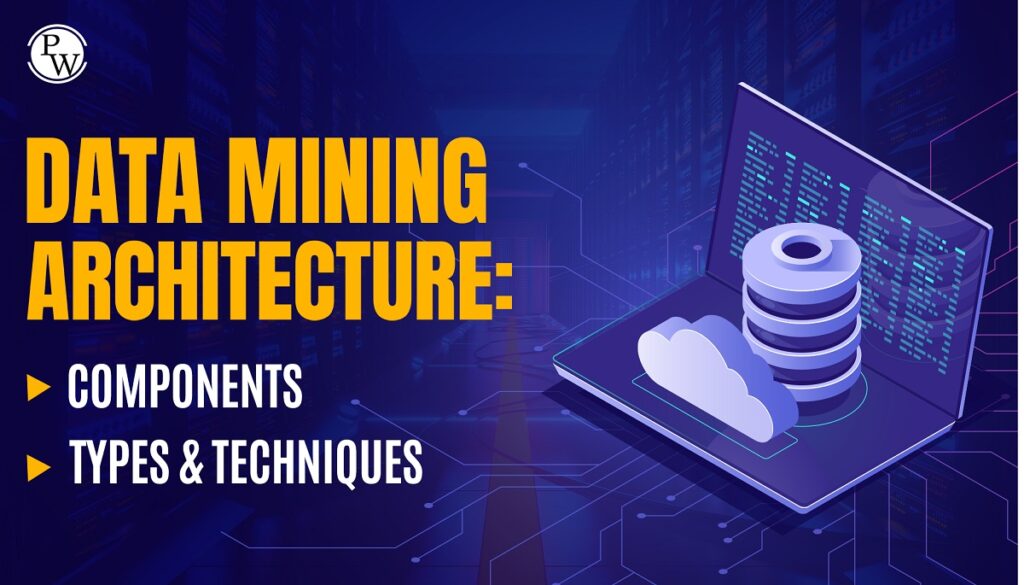
Data Mining Architecture: Components, Types & Techniques
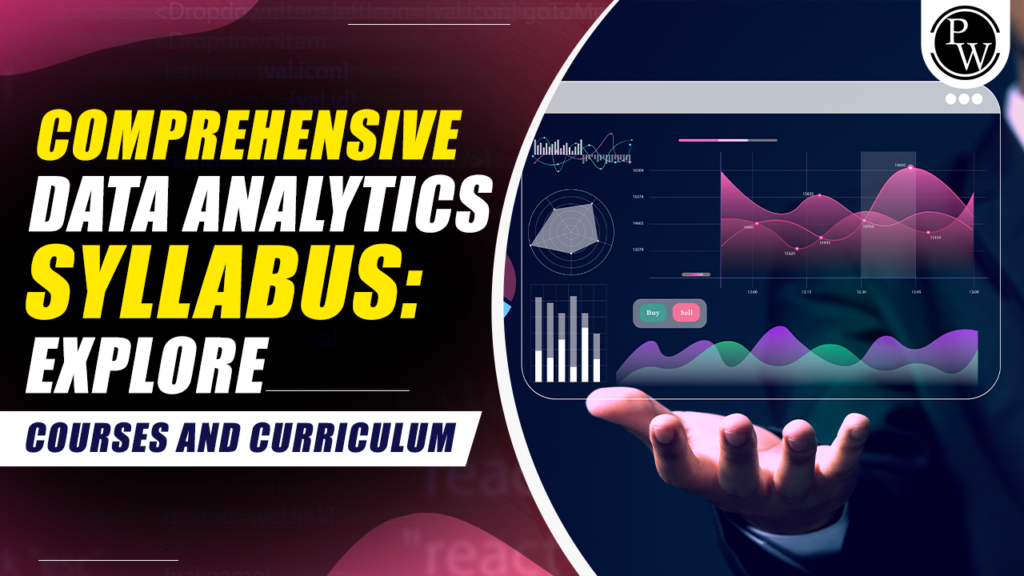
Comprehensive Data Analytics Syllabus: Courses and Curriculum
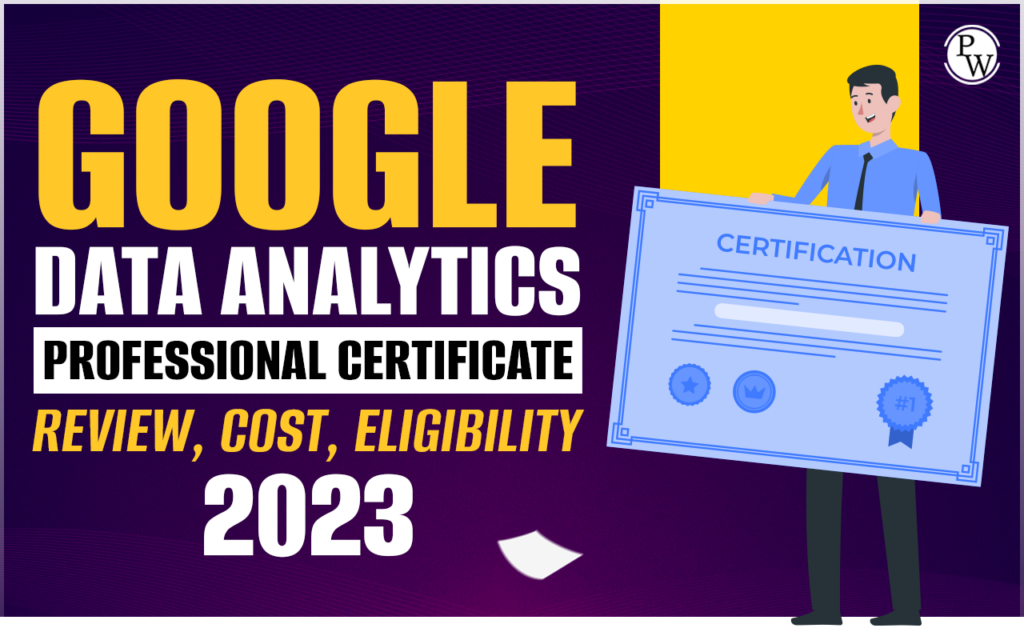
Google Data Analytics Professional Certificate Review, Cost, Eligibility 2023
Have a language expert improve your writing
Run a free plagiarism check in 10 minutes, generate accurate citations for free.
- Knowledge Base
Methodology
Research Methods | Definitions, Types, Examples
Research methods are specific procedures for collecting and analyzing data. Developing your research methods is an integral part of your research design . When planning your methods, there are two key decisions you will make.
First, decide how you will collect data . Your methods depend on what type of data you need to answer your research question :
- Qualitative vs. quantitative : Will your data take the form of words or numbers?
- Primary vs. secondary : Will you collect original data yourself, or will you use data that has already been collected by someone else?
- Descriptive vs. experimental : Will you take measurements of something as it is, or will you perform an experiment?
Second, decide how you will analyze the data .
- For quantitative data, you can use statistical analysis methods to test relationships between variables.
- For qualitative data, you can use methods such as thematic analysis to interpret patterns and meanings in the data.
Table of contents
Methods for collecting data, examples of data collection methods, methods for analyzing data, examples of data analysis methods, other interesting articles, frequently asked questions about research methods.
Data is the information that you collect for the purposes of answering your research question . The type of data you need depends on the aims of your research.
Qualitative vs. quantitative data
Your choice of qualitative or quantitative data collection depends on the type of knowledge you want to develop.
For questions about ideas, experiences and meanings, or to study something that can’t be described numerically, collect qualitative data .
If you want to develop a more mechanistic understanding of a topic, or your research involves hypothesis testing , collect quantitative data .
You can also take a mixed methods approach , where you use both qualitative and quantitative research methods.
Primary vs. secondary research
Primary research is any original data that you collect yourself for the purposes of answering your research question (e.g. through surveys , observations and experiments ). Secondary research is data that has already been collected by other researchers (e.g. in a government census or previous scientific studies).
If you are exploring a novel research question, you’ll probably need to collect primary data . But if you want to synthesize existing knowledge, analyze historical trends, or identify patterns on a large scale, secondary data might be a better choice.
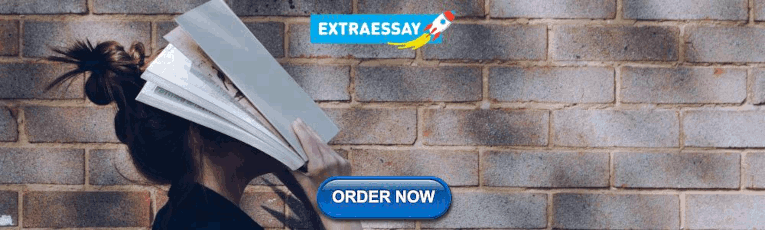
Descriptive vs. experimental data
In descriptive research , you collect data about your study subject without intervening. The validity of your research will depend on your sampling method .
In experimental research , you systematically intervene in a process and measure the outcome. The validity of your research will depend on your experimental design .
To conduct an experiment, you need to be able to vary your independent variable , precisely measure your dependent variable, and control for confounding variables . If it’s practically and ethically possible, this method is the best choice for answering questions about cause and effect.
Receive feedback on language, structure, and formatting
Professional editors proofread and edit your paper by focusing on:
- Academic style
- Vague sentences
- Style consistency
See an example
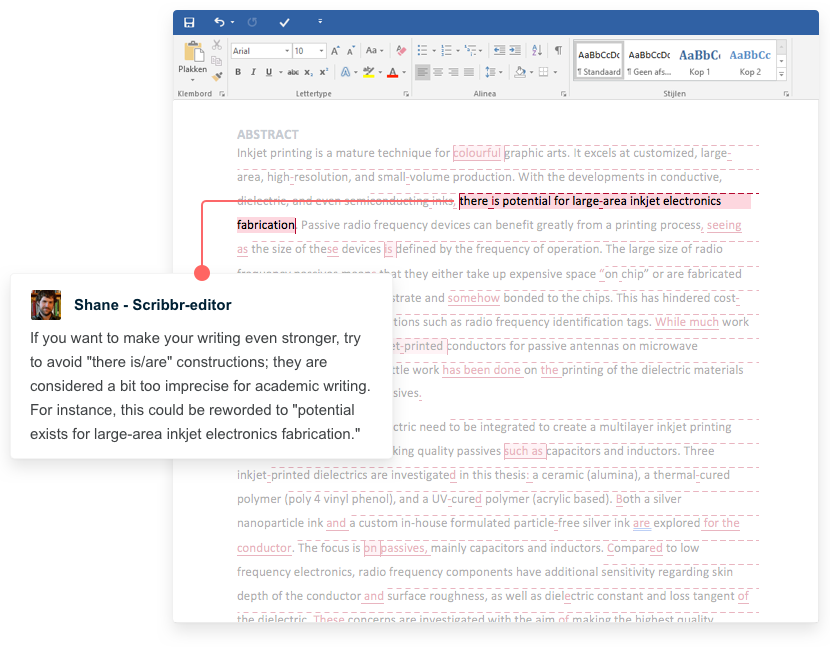
Your data analysis methods will depend on the type of data you collect and how you prepare it for analysis.
Data can often be analyzed both quantitatively and qualitatively. For example, survey responses could be analyzed qualitatively by studying the meanings of responses or quantitatively by studying the frequencies of responses.
Qualitative analysis methods
Qualitative analysis is used to understand words, ideas, and experiences. You can use it to interpret data that was collected:
- From open-ended surveys and interviews , literature reviews , case studies , ethnographies , and other sources that use text rather than numbers.
- Using non-probability sampling methods .
Qualitative analysis tends to be quite flexible and relies on the researcher’s judgement, so you have to reflect carefully on your choices and assumptions and be careful to avoid research bias .
Quantitative analysis methods
Quantitative analysis uses numbers and statistics to understand frequencies, averages and correlations (in descriptive studies) or cause-and-effect relationships (in experiments).
You can use quantitative analysis to interpret data that was collected either:
- During an experiment .
- Using probability sampling methods .
Because the data is collected and analyzed in a statistically valid way, the results of quantitative analysis can be easily standardized and shared among researchers.
If you want to know more about statistics , methodology , or research bias , make sure to check out some of our other articles with explanations and examples.
- Chi square test of independence
- Statistical power
- Descriptive statistics
- Degrees of freedom
- Pearson correlation
- Null hypothesis
- Double-blind study
- Case-control study
- Research ethics
- Data collection
- Hypothesis testing
- Structured interviews
Research bias
- Hawthorne effect
- Unconscious bias
- Recall bias
- Halo effect
- Self-serving bias
- Information bias
Quantitative research deals with numbers and statistics, while qualitative research deals with words and meanings.
Quantitative methods allow you to systematically measure variables and test hypotheses . Qualitative methods allow you to explore concepts and experiences in more detail.
In mixed methods research , you use both qualitative and quantitative data collection and analysis methods to answer your research question .
A sample is a subset of individuals from a larger population . Sampling means selecting the group that you will actually collect data from in your research. For example, if you are researching the opinions of students in your university, you could survey a sample of 100 students.
In statistics, sampling allows you to test a hypothesis about the characteristics of a population.
The research methods you use depend on the type of data you need to answer your research question .
- If you want to measure something or test a hypothesis , use quantitative methods . If you want to explore ideas, thoughts and meanings, use qualitative methods .
- If you want to analyze a large amount of readily-available data, use secondary data. If you want data specific to your purposes with control over how it is generated, collect primary data.
- If you want to establish cause-and-effect relationships between variables , use experimental methods. If you want to understand the characteristics of a research subject, use descriptive methods.
Methodology refers to the overarching strategy and rationale of your research project . It involves studying the methods used in your field and the theories or principles behind them, in order to develop an approach that matches your objectives.
Methods are the specific tools and procedures you use to collect and analyze data (for example, experiments, surveys , and statistical tests ).
In shorter scientific papers, where the aim is to report the findings of a specific study, you might simply describe what you did in a methods section .
In a longer or more complex research project, such as a thesis or dissertation , you will probably include a methodology section , where you explain your approach to answering the research questions and cite relevant sources to support your choice of methods.
Is this article helpful?
Other students also liked, writing strong research questions | criteria & examples.
- What Is a Research Design | Types, Guide & Examples
- Data Collection | Definition, Methods & Examples
More interesting articles
- Between-Subjects Design | Examples, Pros, & Cons
- Cluster Sampling | A Simple Step-by-Step Guide with Examples
- Confounding Variables | Definition, Examples & Controls
- Construct Validity | Definition, Types, & Examples
- Content Analysis | Guide, Methods & Examples
- Control Groups and Treatment Groups | Uses & Examples
- Control Variables | What Are They & Why Do They Matter?
- Correlation vs. Causation | Difference, Designs & Examples
- Correlational Research | When & How to Use
- Critical Discourse Analysis | Definition, Guide & Examples
- Cross-Sectional Study | Definition, Uses & Examples
- Descriptive Research | Definition, Types, Methods & Examples
- Ethical Considerations in Research | Types & Examples
- Explanatory and Response Variables | Definitions & Examples
- Explanatory Research | Definition, Guide, & Examples
- Exploratory Research | Definition, Guide, & Examples
- External Validity | Definition, Types, Threats & Examples
- Extraneous Variables | Examples, Types & Controls
- Guide to Experimental Design | Overview, Steps, & Examples
- How Do You Incorporate an Interview into a Dissertation? | Tips
- How to Do Thematic Analysis | Step-by-Step Guide & Examples
- How to Write a Literature Review | Guide, Examples, & Templates
- How to Write a Strong Hypothesis | Steps & Examples
- Inclusion and Exclusion Criteria | Examples & Definition
- Independent vs. Dependent Variables | Definition & Examples
- Inductive Reasoning | Types, Examples, Explanation
- Inductive vs. Deductive Research Approach | Steps & Examples
- Internal Validity in Research | Definition, Threats, & Examples
- Internal vs. External Validity | Understanding Differences & Threats
- Longitudinal Study | Definition, Approaches & Examples
- Mediator vs. Moderator Variables | Differences & Examples
- Mixed Methods Research | Definition, Guide & Examples
- Multistage Sampling | Introductory Guide & Examples
- Naturalistic Observation | Definition, Guide & Examples
- Operationalization | A Guide with Examples, Pros & Cons
- Population vs. Sample | Definitions, Differences & Examples
- Primary Research | Definition, Types, & Examples
- Qualitative vs. Quantitative Research | Differences, Examples & Methods
- Quasi-Experimental Design | Definition, Types & Examples
- Questionnaire Design | Methods, Question Types & Examples
- Random Assignment in Experiments | Introduction & Examples
- Random vs. Systematic Error | Definition & Examples
- Reliability vs. Validity in Research | Difference, Types and Examples
- Reproducibility vs Replicability | Difference & Examples
- Reproducibility vs. Replicability | Difference & Examples
- Sampling Methods | Types, Techniques & Examples
- Semi-Structured Interview | Definition, Guide & Examples
- Simple Random Sampling | Definition, Steps & Examples
- Single, Double, & Triple Blind Study | Definition & Examples
- Stratified Sampling | Definition, Guide & Examples
- Structured Interview | Definition, Guide & Examples
- Survey Research | Definition, Examples & Methods
- Systematic Review | Definition, Example, & Guide
- Systematic Sampling | A Step-by-Step Guide with Examples
- Textual Analysis | Guide, 3 Approaches & Examples
- The 4 Types of Reliability in Research | Definitions & Examples
- The 4 Types of Validity in Research | Definitions & Examples
- Transcribing an Interview | 5 Steps & Transcription Software
- Triangulation in Research | Guide, Types, Examples
- Types of Interviews in Research | Guide & Examples
- Types of Research Designs Compared | Guide & Examples
- Types of Variables in Research & Statistics | Examples
- Unstructured Interview | Definition, Guide & Examples
- What Is a Case Study? | Definition, Examples & Methods
- What Is a Case-Control Study? | Definition & Examples
- What Is a Cohort Study? | Definition & Examples
- What Is a Conceptual Framework? | Tips & Examples
- What Is a Controlled Experiment? | Definitions & Examples
- What Is a Double-Barreled Question?
- What Is a Focus Group? | Step-by-Step Guide & Examples
- What Is a Likert Scale? | Guide & Examples
- What Is a Prospective Cohort Study? | Definition & Examples
- What Is a Retrospective Cohort Study? | Definition & Examples
- What Is Action Research? | Definition & Examples
- What Is an Observational Study? | Guide & Examples
- What Is Concurrent Validity? | Definition & Examples
- What Is Content Validity? | Definition & Examples
- What Is Convenience Sampling? | Definition & Examples
- What Is Convergent Validity? | Definition & Examples
- What Is Criterion Validity? | Definition & Examples
- What Is Data Cleansing? | Definition, Guide & Examples
- What Is Deductive Reasoning? | Explanation & Examples
- What Is Discriminant Validity? | Definition & Example
- What Is Ecological Validity? | Definition & Examples
- What Is Ethnography? | Definition, Guide & Examples
- What Is Face Validity? | Guide, Definition & Examples
- What Is Non-Probability Sampling? | Types & Examples
- What Is Participant Observation? | Definition & Examples
- What Is Peer Review? | Types & Examples
- What Is Predictive Validity? | Examples & Definition
- What Is Probability Sampling? | Types & Examples
- What Is Purposive Sampling? | Definition & Examples
- What Is Qualitative Observation? | Definition & Examples
- What Is Qualitative Research? | Methods & Examples
- What Is Quantitative Observation? | Definition & Examples
- What Is Quantitative Research? | Definition, Uses & Methods
"I thought AI Proofreading was useless but.."
I've been using Scribbr for years now and I know it's a service that won't disappoint. It does a good job spotting mistakes”
Child Care and Early Education Research Connections
Data analysis.
Different statistics and methods used to describe the characteristics of the members of a sample or population, explore the relationships between variables, to test research hypotheses, and to visually represent data are described. Terms relating to the topics covered are defined in the Research Glossary .
Descriptive Statistics
Tests of Significance
Graphical/Pictorial Methods
Analytical techniques.
Descriptive statistics can be useful for two purposes:
To provide basic information about the characteristics of a sample or population. These characteristics are represented by variables in a research study dataset.
To highlight potential relationships between these characteristics, or the relationships among the variables in the dataset.
The four most common descriptive statistics are:
Proportions, Percentages and Ratios
Measures of central tendency, measures of dispersion, measures of association.
One of the most basic ways of describing the characteristics of a sample or population is to classify its individual members into mutually exclusive categories and counting the number of cases in each of the categories. In research, variables with discrete, qualitative categories are called nominal or categorical variables. The categories can be given numerical codes, but they cannot be ranked, added, or multiplied. Examples of nominal variables include gender (male, female), preschool program attendance (yes, no), and race/ethnicity (White, African American, Hispanic, Asian, American Indian). Researchers calculate proportions, percentages and ratios in order to summarize the data from nominal or categorical variables and to allow for comparisons to be made between groups.
Proportion —The number of cases in a category divided by the total number of cases across all categories of a variable.
Percentage —The proportion multiplied by 100 (or the number of cases in a category divided by the total number of cases across all categories of a value times 100).
Ratio —The number of cases in one category to the number of cases in a second category.
A researcher selects a sample of 100 students from a Head Start program. The sample includes 20 White children, 30 African American children, 40 Hispanic children and 10 children of mixed-race/ethnicity.
Proportion of Hispanic children in the program = 40 / (20+30+40+10) = .40.
Percentage of Hispanic children in the program = .40 x 100 = 40%.
Ratio of Hispanic children to White children in the program = 40/20 = 2.0, or the ratio of Hispanic to White children enrolled in the Head Start program is 2 to 1.
Proportions, percentages and ratios are used to summarize the characteristics of a sample or population that fall into discrete categories. Measures of central tendency are the most basic and, often, the most informative description of a population's characteristics, when those characteristics are measured using an interval scale. The values of an interval variable are ordered where the distance between any two adjacent values is the same but the zero point is arbitrary. Values on an interval scale can be added and subtracted. Examples of interval scales or interval variables include household income, years of schooling, hours a child spends in child care and the cost of child care.
Measures of central tendency describe the "average" member of the sample or population of interest. There are three measures of central tendency:
Mean —The arithmetic average of the values of a variable. To calculate the mean, all the values of a variable are summed and divided by the total number of cases.
Median —The value within a set of values that divides the values in half (i.e. 50% of the variable's values lie above the median, and 50% lie below the median).
Mode —The value of a variable that occurs most often.
The annual incomes of five randomly selected people in the United States are $10,000, $10,000, $45,000, $60,000, and $1,000,000.
Mean Income = (10,000 + 10,000 + 45,000 + 60,000 + 1,000,000) / 5 = $225,000.
Median Income = $45,000.
Modal Income = $10,000.
The mean is the most commonly used measure of central tendency. Medians are generally used when a few values are extremely different from the rest of the values (this is called a skewed distribution). For example, the median income is often the best measure of the average income because, while most individuals earn between $0 and $200,000 annually, a handful of individuals earn millions.
Measures of dispersion provide information about the spread of a variable's values. There are three key measures of dispersion:
Range is simply the difference between the smallest and largest values in the data. Researchers often report simply the values of the range (e.g., 75 – 100).
Variance is a commonly used measure of dispersion, or how spread out a set of values are around the mean. It is calculated by taking the average of the squared differences between each value and the mean. The variance is the standard deviation squared.
Standard deviation , like variance, is a measure of the spread of a set of values around the mean of the values. The wider the spread, the greater the standard deviation and the greater the range of the values from their mean. A small standard deviation indicates that most of the values are close to the mean. A large standard deviation on the other hand indicates that the values are more spread out. The standard deviation is the square root of the variance.
Five randomly selected children were administered a standardized reading assessment. Their scores on the assessment were 50, 50, 60,75 and 90 with a mean score of 65.
Range = 90 - 50 = 40.
Variance = [(50 - 65)2 + (50 - 65)2 + (60 - 65)2 + (75 - 65)2 + (90 - 65)2] / 5 = 300.
Standard Deviation = Square Root (150,540,000,000) = 17.32.
Skewness and Kurtosis
The range, variance and standard deviation are measures of dispersion and provide information about the spread of the values of a variable. Two additional measures provide information about the shape of the distribution of values.
Skew is a measure of whether some values of a variable are extremely different from the majority of the values. Skewness refers to the tendency of the values of a variable to depart from symmetry. A distribution is symmetric if one half of the distribution is exactly equal to the other half. For example, the distribution of annual income in the U.S. is skewed because most people make between $0 and $200,000 a year, but a handful of people earn millions. A variable is positively skewed (skewed to the right) if the extreme values are higher than the majority of values. A variable is negatively skewed (skewed to the left) if the extreme values are lower than the majority of values. In the example of students' standardized test scores, the distribution is slightly positively skewed.
Kurtosis measures how outlier-prone a distribution is. Outliers are values of a variable that are much smaller or larger than most of the values found in a dataset. The kurtosis of a normal distribution is 0. If the kurtosis is different from 0, then the distribution produces outliers that are either more extreme (positive kurtosis) or less extreme (negative kurtosis) than are produced by the normal distribution.
Measures of association indicate whether two variables are related. Two measures are commonly used:
Chi-square test of independence
Correlation
Chi-Square test of independence is used to evaluate whether there is an association between two variables. (The chi-square test can also be used as a measure of goodness of fit, to test if data from a sample come from a population with a specific distribution, as an alternative to Anderson-Darling and Kolmogorov-Smirnov goodness-of-fit tests.)
It is most often used with nominal data (i.e., data that are put into discrete categories: e.g., gender [male, female] and type of job [unskilled, semi-skilled, skilled]) to determine whether they are associated. However, it can also be used with ordinal data.
Assumes that the samples being compared (e.g., males, females) are independent.
Tests the null hypothesis of no difference between the two variables (i.e., type of job is not related to gender).
To test for associations, a chi-square is calculated in the following way: Suppose a researcher wants to know whether there is a relationship between gender and two types of jobs, construction worker and administrative assistant. To perform a chi-square test, the researcher counts the number of female administrative assistants, the number of female construction workers, the number of male administrative assistants, and the number of male construction workers in the data. These counts are compared with the number that would be expected in each category if there were no association between job type and gender (this expected count is based on statistical calculations). The association between the two variables is determined to be significant (the null hypothesis is rejected), if the value of the chi-square test is greater than or equal to the critical value for a given significance level (typically .05) and the degrees of freedom associated with the test found in a chi-square table. The degrees of freedom for the chi-square are calculated using the following formula: df = (r-1)(c-1) where r is the number of rows and c is the number of columns in a contingency or cross-tabulation table. For example, the critical value for a 2 x 2 table with 1 degree of freedom ([2-1][2-1]=1) is 3.841.
Correlation coefficient is used to measure the strength and direction of the relationship between numeric variables (e.g., weight and height).
The most common correlation coefficient is the Pearson's product-moment correlation coefficient (or simply Pearson's r ), which can range from -1 to +1.
Values closer to 1 (either positive or negative) indicate that a stronger association exists between the two variables.
A positive coefficient (values between 0 and 1) suggests that larger values of one of the variables are accompanied by larger values of the other variable. For example, height and weight are usually positively correlated because taller people tend to weigh more.
A negative association (values between 0 and -1) suggests that larger values of one of the variables are accompanied by smaller values of the other variable. For example, age and hours slept per night are often negatively correlated because older people usually sleep fewer hours per night than younger people.
The findings reported by researchers are typically based on data collected from a single sample that was drawn from the population of interest (e.g., a sample of children selected from the population of children enrolled in Head Start or Early Head Start). If additional random samples of the same size were drawn from this population, the estimated percentages and means calculated using the data from each of these other samples might differ by chance somewhat from the estimates produced from one sample. Researchers use one of several tests to evaluate whether their findings are statistically significant.
Statistical significance refers to the probability or likelihood that the difference between groups or the relationship between variables observed in statistical analyses is not due to random chance (e.g., that differences between the average scores on a measure of language development between 3- and 4-year-olds are likely to be “real” rather than just observed in this sample by chance). If there is a very small probability that an observed difference or relationship is due to chance, the results are said to reach statistical significance. This means that the researcher concludes that there is a real difference between two groups or a real relationship between the observed variables.
Significance tests and the associated p- value only tell us how likely it is that a statistical result (e.g., a difference between the means of two or more groups, or a correlation between two variables) is due to chance. The p-value is the probability that the results of a statistical test are due to chance. In the social and behavioral sciences, a p-value less than or equal to .05 is usually interpreted to mean that the results are statistically significant (that the statistical results would occur by chance 5 times or fewer out of 100), although sometimes researchers use a p-value of .10 to indicate whether a result is statistically significant. The lower the p-value, the less likely a statistical result is due to chance. Lower p-values are therefore a more rigorous criteria for concluding significance.
Researchers use a variety of approaches to test whether their findings are statistically significant or not. The choice depends on several factors, including the number of groups being compared, whether the groups are independent from one another, and the type of variables used in the analysis. Three widely used tests are the t-test, F-test, and Chi-square test.
Three of the more widely used tests of statistical significance are described briefly below.
Chi-Square test is used when testing for associations between categorical variables (e.g., differences in whether a child has been diagnosed as having a cognitive disability by gender or race/ethnicity). It is also used as a goodness-of-fit test to determine whether data from a sample come from a population with a specific distribution.
t-test is used to compare the means of two independent samples (independent t-test), the means of one sample at different times (paired sample t-test) or the mean of one sample against a known mean (one sample t-test). For example, when comparing the mean assessment scores of boys and girls or the mean scores of 3- and 4-year-old children, an independent t-test would be used. When comparing the mean assessment scores of girls only at two time points (e.g., fall and spring of the program year) a paired t-test would be used. A one sample t-test would be used when comparing the mean scores of a sample of children to the mean score of a population of children. The t- test is appropriate for small sample sizes (less than 30) although it is often used when testing group differences for larger samples. It is also used to test whether correlation and regression coefficients are significantly different from zero.
F-test is an extension of the t-test and is used to compare the means of three or more independent samples (groups). The F-test is used in Analysis of Variance (ANOVA) to examine the ratio of the between groups to within groups variance. It is also used to test the significance of the total variance explained by a regression model with multiple independent variables.
Significance tests alone do not tell us anything about the size of the difference between groups or the strength of the association between variables. Because significance test results are sensitive to sample size, studies with different sample sizes with the same means and standard deviations would have different t statistics and p values. It is therefore important that researchers provide additional information about the size of the difference between groups or the association and whether the difference/association is substantively meaningful.
See the following for additional information about descriptive statistics and tests of significance:
Descriptive analysis in education: A guide for researchers (PDF)
Basic Statistics
Effect Sizes and Statistical Significance
Summarizing and Presenting Data
There are several graphical and pictorial methods that enhance understanding of individual variables and the relationships between variables. Graphical and pictorial methods provide a visual representation of the data. Some of these methods include:
Line graphs
Scatter plots.
Geographical Information Systems (GIS)
Bar charts visually represent the frequencies or percentages with which different categories of a variable occur.
Bar charts are most often used when describing the percentages of different groups with a specific characteristic. For example, the percentages of boys and girls who participate in team sports. However, they may also be used when describing averages such as the average boys and girls spend per week participating in team sports.
Each category of a variable (e.g., gender [boys and girls], children's age [3, 4, and 5]) is displayed along the bottom (or horizontal or X axis) of a bar chart.
The vertical axis (or Y axis) includes the values of the statistic on that the groups are being compared (e.g., percentage participating in team sports).
A bar is drawn for each of the categories along the horizontal axis and the height of the bar corresponds to the frequency or percentage with which that value occurs.
A pie chart (or a circle chart) is one of the most commonly used methods for graphically presenting statistical data.
As its name suggests, it is a circular graphic, which is divided into slices to illustrate the proportion or percentage of a sample or population that belong to each of the categories of a variable.
The size of each slice represents the proportion or percentage of the total sample or population with a specific characteristic (found in a specific category). For example, the percentage of children enrolled in Early Head Start who are members of different racial/ethnic groups would be represented by different slices with the size of each slice proportionate to the group's representation in the total population of children enrolled in the Early Head Start program.
A line graph is a type of chart which displays information as a series of data points connected by a straight line.
Line graphs are often used to show changes in a characteristic over time.
It has an X-axis (horizontal axis) and a Y axis (vertical axis). The time segments of interest are displayed on the X-axis (e.g., years, months). The range of values that the characteristic of interest can take are displayed along the Y-axis (e.g., annual household income, mean years of schooling, average cost of child care). A data point is plotted coinciding with the value of the Y variable plotted for each of the values of the X variable, and a line is drawn connecting the points.
Scatter plots display the relationship between two quantitative or numeric variables by plotting one variable against the value of another variable
The values of one of the two variables are displayed on the horizontal axis (x axis) and the values of the other variable are displayed on the vertical axis (y axis)
Each person or subject in a study would receive one data point on the scatter plot that corresponds to his or her values on the two variables. For example, a scatter plot could be used to show the relationship between income and children's scores on a math assessment. A data point for each child in the study showing his or her math score and family income would be shown on the scatter plot. Thus, the number of data points would equal the total number of children in the study.
Geographic Information Systems (GIS)
A Geographic Information System is computer software capable of capturing, storing, analyzing, and displaying geographically referenced information; that is, data identified according to location.
Using a GIS program, a researcher can create a map to represent data relationships visually. For example, the National Center for Education Statistics creates maps showing the characteristics of school districts across the United States such as the percentage of children living in married couple households, median family incomes and percentage of population that speaks a language other than English. The data that are linked to school district location come from the American Community Survey.
Display networks of relationships among variables, enabling researchers to identify the nature of relationships that would otherwise be too complex to conceptualize.
See the following for additional information about different graphic methods:
Graphical Analytic Techniques
Geographic Information Systems
Researchers use different analytical techniques to examine complex relationships between variables. There are three basic types of analytical techniques:
Regression Analysis
Grouping methods, multiple equation models.
Regression analysis assumes that the dependent, or outcome, variable is directly affected by one or more independent variables. There are four important types of regression analyses:
Ordinary least squares (OLS) regression
OLS regression (also known as linear regression) is used to determine the relationship between a dependent variable and one or more independent variables.
OLS regression is used when the dependent variable is continuous. Continuous variables, in theory, can take on any value with a range. For example, family child care expenses, measured in dollars, is a continuous variable.
Independent variables may be nominal, ordinal or continuous. Nominal variables, which are also referred to as categorical variables, have two or more non-numeric or qualitative categories. Examples of nominal variables are children's gender (male, female), their parents' marital status (single, married, separated, divorced), and the type of child care children receive (center-based, home-based care). Ordinal variables are similar to nominal variables except it is possible to order the categories and the order has meaning. For example, children's families’ socioeconomic status may be grouped as low, middle and high.
When used to estimate the associations between two or more independent variables and a single dependent variable, it is called multiple linear regression.
In multiple regression, the coefficient (i.e., standardized or unstandardized regression coefficient for each independent variable) tells you how much the dependent variable is expected to change when that independent variable increases by one, holding all the other independent variables constant.
Logistic regression
Logistic regression (or logit regression) is a special form of regression analysis that is used to examine the associations between a set of independent or predictor variables and a dichotomous outcome variable. A dichotomous variable is a variable with only two possible values, e.g. child receives child care before or after the Head Start program day (yes, no).
Like linear regression, the independent variables may be either interval, ordinal, or nominal. A researcher might use logistic regression to study the relationships between parental education, household income, and parental employment and whether children receive child care from someone other than their parents (receives nonparent care/does not receive nonparent care).
Hierarchical linear modeling (HLM)
Used when data are nested. Nested data occur when several individuals belong to the same group under study. For example, in child care research, children enrolled in a center-based child care program are grouped into classrooms with several classrooms in a center. Thus, the children are nested within classrooms and classrooms are nested within centers.
Allows researchers to determine the effects of characteristics for each level of nested data, classrooms and centers, on the outcome variables. HLM is also used to study growth (e.g., growth in children’s reading and math knowledge and skills over time).
Duration models
Used to estimate the length of time before a given event occurs or the length of time spent in a state. For example, in child care policy research, duration models have been used to estimate the length of time that families receive child care subsidies.
Sometimes referred to as survival analysis or event history analysis.
Grouping methods are techniques for classifying observations into meaningful categories. Two of the most common grouping methods are discriminant analysis and cluster analysis.
Discriminant analysis
Identifies characteristics that distinguish between groups. For example, a researcher could use discriminant analysis to determine which characteristics identify families that seek child care subsidies and which identify families that do not.
It is used when the dependent variable is a categorical variable (e.g., family receives child care subsidies [yes, no], child enrolled in family care [yes, no], type of child care child receives [relative care, non-relative care, center-based care]). The independent variables are interval variables (e.g., years of schooling, family income).
Cluster analysis
Used to classify similar individuals together. It uses a set of measured variables to classify a sample of individuals (or organizations) into a number of groups such that individuals with similar values on the variables are placed in the same group. For example, cluster analysis would be used to group together parents who hold similar views of child care or children who are suspended from school.
Its goal is to sort individuals into groups in such a way that individuals in the same group (cluster) are more similar to each other than to individuals in other groups.
The variables used in cluster analysis may be nominal, ordinal or interval.
Multiple equation modeling, which is an extension of regression, is used to examine the causal pathways from independent variables to the dependent variable. For example, what are the variables that link (or explain) the relationship between maternal education (independent variable) and children's early reading skills (dependent variable)? These variables might include the nature and quality of mother-child interactions or the frequency and quality of shared book reading.
There are two main types of multiple equation models:
Path analysis
Structural equation modeling
Path analysis is an extension of multiple regression that allows researchers to examine multiple direct and indirect effects of a set of variables on a dependent, or outcome, variable. In path analysis, a direct effect measures the extent to which the dependent variable is influenced by an independent variable. An indirect effect measures the extent to which an independent variable's influence on the dependent variable is due to another variable.
A path diagram is created that identifies the relationships (paths) between all the variables and the direction of the influence between them.
The paths can run directly from an independent variable to a dependent variable (e.g., X→Y), or they can run indirectly from an independent variable, through an intermediary, or mediating, variable, to the dependent variable (e.g. X1→X2→Y).
The paths in the model are tested to determine the relative importance of each.
Because the relationships between variables in a path model can become complex, researchers often avoid labeling the variables in the model as independent and dependent variables. Instead, two types of variables are found in these models:
Exogenous variables are not affected by other variables in the model. They have straight arrows emerging from them and not pointing to them.
Endogenous variables are influenced by at least one other variable in the model. They have at least one straight arrow pointing to them.
Structural equation modeling (SEM)
Structural equation modeling expands path analysis by allowing for multiple indicators of unobserved (or latent) variables in the model. Latent variables are variables that are not directly observed (measured), but instead are inferred from other variables that are observed or directly measured. For example, children's school readiness is a latent variable with multiple indicators of children's development across multiple domains (e.g., children's scores on standardized assessments of early math and literacy, language, scores based on teacher reports of children's social skills and problem behaviors).
There are two parts to a SEM analysis. First, the measurement model is tested. This involves examining the relationships between the latent variables and their measures (indicators). Second, the structural model is tested in order to examine how the latent variables are related to one another. For example, a researcher might use SEM to investigate the relationships between different types of executive functions and word reading and reading comprehension for elementary school children. In this example, the latent variables word reading and reading comprehension might be inferred from a set of standardized reading assessments and the latent variables cognitive flexibility and inhibitory control from a set of executive function tasks. The measurement model of SEM allows the researcher to evaluate how well children's scores on the standardized reading assessments combine to identify children's word reading and reading comprehension. Assuming that the results of these analyses are acceptable, the researcher would move on to an evaluation of the structural model, examining the predicted relationships between two types of executive functions and two dimensions of reading.
SEM has several advantages over traditional path analysis:
Use of multiple indicators for key variables reduces measurement error.
Can test whether the effects of variables in the model and the relationships depicted in the entire model are the same for different groups (e.g., are the direct and indirect effects of parent investments on children's school readiness the same for White, Hispanic and African American children).
Can test models with multiple dependent variables (e.g., models predicting several domains of child development).
See the following for additional information about multiple equation models:
Finding Our Way: An Introduction to Path Analysis (Streiner)
An Introduction to Structural Equation Modeling (Hox & Bechger) (PDF)
- Share full article
Advertisement
Supported by
What the Data Says About Pandemic School Closures, Four Years Later
The more time students spent in remote instruction, the further they fell behind. And, experts say, extended closures did little to stop the spread of Covid.

By Sarah Mervosh , Claire Cain Miller and Francesca Paris
Four years ago this month, schools nationwide began to shut down, igniting one of the most polarizing and partisan debates of the pandemic.
Some schools, often in Republican-led states and rural areas, reopened by fall 2020. Others, typically in large cities and states led by Democrats, would not fully reopen for another year.
A variety of data — about children’s academic outcomes and about the spread of Covid-19 — has accumulated in the time since. Today, there is broad acknowledgment among many public health and education experts that extended school closures did not significantly stop the spread of Covid, while the academic harms for children have been large and long-lasting.
While poverty and other factors also played a role, remote learning was a key driver of academic declines during the pandemic, research shows — a finding that held true across income levels.
Source: Fahle, Kane, Patterson, Reardon, Staiger and Stuart, “ School District and Community Factors Associated With Learning Loss During the COVID-19 Pandemic .” Score changes are measured from 2019 to 2022. In-person means a district offered traditional in-person learning, even if not all students were in-person.
“There’s fairly good consensus that, in general, as a society, we probably kept kids out of school longer than we should have,” said Dr. Sean O’Leary, a pediatric infectious disease specialist who helped write guidance for the American Academy of Pediatrics, which recommended in June 2020 that schools reopen with safety measures in place.
There were no easy decisions at the time. Officials had to weigh the risks of an emerging virus against the academic and mental health consequences of closing schools. And even schools that reopened quickly, by the fall of 2020, have seen lasting effects.
But as experts plan for the next public health emergency, whatever it may be, a growing body of research shows that pandemic school closures came at a steep cost to students.
The longer schools were closed, the more students fell behind.
At the state level, more time spent in remote or hybrid instruction in the 2020-21 school year was associated with larger drops in test scores, according to a New York Times analysis of school closure data and results from the National Assessment of Educational Progress , an authoritative exam administered to a national sample of fourth- and eighth-grade students.
At the school district level, that finding also holds, according to an analysis of test scores from third through eighth grade in thousands of U.S. districts, led by researchers at Stanford and Harvard. In districts where students spent most of the 2020-21 school year learning remotely, they fell more than half a grade behind in math on average, while in districts that spent most of the year in person they lost just over a third of a grade.
( A separate study of nearly 10,000 schools found similar results.)
Such losses can be hard to overcome, without significant interventions. The most recent test scores, from spring 2023, show that students, overall, are not caught up from their pandemic losses , with larger gaps remaining among students that lost the most ground to begin with. Students in districts that were remote or hybrid the longest — at least 90 percent of the 2020-21 school year — still had almost double the ground to make up compared with students in districts that allowed students back for most of the year.
Some time in person was better than no time.
As districts shifted toward in-person learning as the year went on, students that were offered a hybrid schedule (a few hours or days a week in person, with the rest online) did better, on average, than those in places where school was fully remote, but worse than those in places that had school fully in person.
Students in hybrid or remote learning, 2020-21
80% of students
Some schools return online, as Covid-19 cases surge. Vaccinations start for high-priority groups.
Teachers are eligible for the Covid vaccine in more than half of states.
Most districts end the year in-person or hybrid.
Source: Burbio audit of more than 1,200 school districts representing 47 percent of U.S. K-12 enrollment. Note: Learning mode was defined based on the most in-person option available to students.
Income and family background also made a big difference.
A second factor associated with academic declines during the pandemic was a community’s poverty level. Comparing districts with similar remote learning policies, poorer districts had steeper losses.
But in-person learning still mattered: Looking at districts with similar poverty levels, remote learning was associated with greater declines.
A community’s poverty rate and the length of school closures had a “roughly equal” effect on student outcomes, said Sean F. Reardon, a professor of poverty and inequality in education at Stanford, who led a district-level analysis with Thomas J. Kane, an economist at Harvard.
Score changes are measured from 2019 to 2022. Poorest and richest are the top and bottom 20% of districts by percent of students on free/reduced lunch. Mostly in-person and mostly remote are districts that offered traditional in-person learning for more than 90 percent or less than 10 percent of the 2020-21 year.
But the combination — poverty and remote learning — was particularly harmful. For each week spent remote, students in poor districts experienced steeper losses in math than peers in richer districts.
That is notable, because poor districts were also more likely to stay remote for longer .
Some of the country’s largest poor districts are in Democratic-leaning cities that took a more cautious approach to the virus. Poor areas, and Black and Hispanic communities , also suffered higher Covid death rates, making many families and teachers in those districts hesitant to return.
“We wanted to survive,” said Sarah Carpenter, the executive director of Memphis Lift, a parent advocacy group in Memphis, where schools were closed until spring 2021 .
“But I also think, man, looking back, I wish our kids could have gone back to school much quicker,” she added, citing the academic effects.
Other things were also associated with worse student outcomes, including increased anxiety and depression among adults in children’s lives, and the overall restriction of social activity in a community, according to the Stanford and Harvard research .
Even short closures had long-term consequences for children.
While being in school was on average better for academic outcomes, it wasn’t a guarantee. Some districts that opened early, like those in Cherokee County, Ga., a suburb of Atlanta, and Hanover County, Va., lost significant learning and remain behind.
At the same time, many schools are seeing more anxiety and behavioral outbursts among students. And chronic absenteeism from school has surged across demographic groups .
These are signs, experts say, that even short-term closures, and the pandemic more broadly, had lasting effects on the culture of education.
“There was almost, in the Covid era, a sense of, ‘We give up, we’re just trying to keep body and soul together,’ and I think that was corrosive to the higher expectations of schools,” said Margaret Spellings, an education secretary under President George W. Bush who is now chief executive of the Bipartisan Policy Center.
Closing schools did not appear to significantly slow Covid’s spread.
Perhaps the biggest question that hung over school reopenings: Was it safe?
That was largely unknown in the spring of 2020, when schools first shut down. But several experts said that had changed by the fall of 2020, when there were initial signs that children were less likely to become seriously ill, and growing evidence from Europe and parts of the United States that opening schools, with safety measures, did not lead to significantly more transmission.
“Infectious disease leaders have generally agreed that school closures were not an important strategy in stemming the spread of Covid,” said Dr. Jeanne Noble, who directed the Covid response at the U.C.S.F. Parnassus emergency department.
Politically, though, there remains some disagreement about when, exactly, it was safe to reopen school.
Republican governors who pushed to open schools sooner have claimed credit for their approach, while Democrats and teachers’ unions have emphasized their commitment to safety and their investment in helping students recover.
“I do believe it was the right decision,” said Jerry T. Jordan, president of the Philadelphia Federation of Teachers, which resisted returning to school in person over concerns about the availability of vaccines and poor ventilation in school buildings. Philadelphia schools waited to partially reopen until the spring of 2021 , a decision Mr. Jordan believes saved lives.
“It doesn’t matter what is going on in the building and how much people are learning if people are getting the virus and running the potential of dying,” he said.
Pandemic school closures offer lessons for the future.
Though the next health crisis may have different particulars, with different risk calculations, the consequences of closing schools are now well established, experts say.
In the future, infectious disease experts said, they hoped decisions would be guided more by epidemiological data as it emerged, taking into account the trade-offs.
“Could we have used data to better guide our decision making? Yes,” said Dr. Uzma N. Hasan, division chief of pediatric infectious diseases at RWJBarnabas Health in Livingston, N.J. “Fear should not guide our decision making.”
Source: Fahle, Kane, Patterson, Reardon, Staiger and Stuart, “ School District and Community Factors Associated With Learning Loss During the Covid-19 Pandemic. ”
The study used estimates of learning loss from the Stanford Education Data Archive . For closure lengths, the study averaged district-level estimates of time spent in remote and hybrid learning compiled by the Covid-19 School Data Hub (C.S.D.H.) and American Enterprise Institute (A.E.I.) . The A.E.I. data defines remote status by whether there was an in-person or hybrid option, even if some students chose to remain virtual. In the C.S.D.H. data set, districts are defined as remote if “all or most” students were virtual.
An earlier version of this article misstated a job description of Dr. Jeanne Noble. She directed the Covid response at the U.C.S.F. Parnassus emergency department. She did not direct the Covid response for the University of California, San Francisco health system.
How we handle corrections
Sarah Mervosh covers education for The Times, focusing on K-12 schools. More about Sarah Mervosh
Claire Cain Miller writes about gender, families and the future of work for The Upshot. She joined The Times in 2008 and was part of a team that won a Pulitzer Prize in 2018 for public service for reporting on workplace sexual harassment issues. More about Claire Cain Miller
Francesca Paris is a Times reporter working with data and graphics for The Upshot. More about Francesca Paris
Help | Advanced Search
Computer Science > Computer Vision and Pattern Recognition
Title: mm1: methods, analysis & insights from multimodal llm pre-training.
Abstract: In this work, we discuss building performant Multimodal Large Language Models (MLLMs). In particular, we study the importance of various architecture components and data choices. Through careful and comprehensive ablations of the image encoder, the vision language connector, and various pre-training data choices, we identified several crucial design lessons. For example, we demonstrate that for large-scale multimodal pre-training using a careful mix of image-caption, interleaved image-text, and text-only data is crucial for achieving state-of-the-art (SOTA) few-shot results across multiple benchmarks, compared to other published pre-training results. Further, we show that the image encoder together with image resolution and the image token count has substantial impact, while the vision-language connector design is of comparatively negligible importance. By scaling up the presented recipe, we build MM1, a family of multimodal models up to 30B parameters, including both dense models and mixture-of-experts (MoE) variants, that are SOTA in pre-training metrics and achieve competitive performance after supervised fine-tuning on a range of established multimodal benchmarks. Thanks to large-scale pre-training, MM1 enjoys appealing properties such as enhanced in-context learning, and multi-image reasoning, enabling few-shot chain-of-thought prompting.
Submission history
Access paper:.
- Download PDF
- Other Formats
References & Citations
- Google Scholar
- Semantic Scholar
BibTeX formatted citation

Bibliographic and Citation Tools
Code, data and media associated with this article, recommenders and search tools.
- Institution
arXivLabs: experimental projects with community collaborators
arXivLabs is a framework that allows collaborators to develop and share new arXiv features directly on our website.
Both individuals and organizations that work with arXivLabs have embraced and accepted our values of openness, community, excellence, and user data privacy. arXiv is committed to these values and only works with partners that adhere to them.
Have an idea for a project that will add value for arXiv's community? Learn more about arXivLabs .
- Open supplemental data
- Reference Manager
- Simple TEXT file
People also looked at
Original research article, causal relationship between immune cells and prostate cancer: a mendelian randomization study.
- 1 State Key Laboratory of Oncology in South China, Guangdong Provincial Clinical Research Center for Cancer, Sun Yat-Sen University Cancer Center, Guangzhou, China
- 2 Department of Oncology, The First Affiliated Hospital of Jinan University, Guangzhou, China
Introduction: Despite the abundance of research indicating the participation of immune cells in prostate cancer development, establishing a definitive cause-and-effect relationship has proven to be a difficult undertaking.
Methods: This study employs Mendelian randomization (MR), leveraging genetic variables related to immune cells from publicly available genome-wide association studies (GWAS), to investigate this association. The primary analytical method used in this study is inverse variance weighting (IVW) analysis. Comprehensive sensitivity analyses were conducted to assess the heterogeneity and horizontal pleiotropy of the results.
Results: The study identifies four immune cell traits as causally contributing to prostate cancer risk, including CD127- CD8+ T cell %CD8+ T cell (OR = 1.0042, 95%CI:1.0011–1.0073, p = 0.0077), CD45RA on CD39+ resting CD4 regulatory T cell (OR = 1.0029, 95%CI:1.0008–1.0050, p = 0.0065), CD62L− Dendritic Cell Absolute Count (OR = 1.0016; 95%CI:1.0005–1.0026; p = 0.0039), CX3CR1 on CD14+ CD16− monocyte (OR = 1.0024, 95%CI:1.0007–1.0040, p = 0.0060). Additionally, two immune cell traits are identified as causally protective factors: CD4 on monocyte (OR = 0.9975, 95%CI:0.9958–0.9992, p = 0.0047), FSC-A on plasmacytoid Dendritic Cell (OR = 0.9983, 95%CI:0.9970–0.9995, p = 0.0070). Sensitivity analyses indicated no horizontal pleiotropy.
Discussion: Our MR study provide evidence for a causal relationship between immune cells and prostate cancer, holding implications for clinical diagnosis and treatment.
Introduction
Prostate cancer is a prevalent malignant tumor in elderly men, ranking as the most common solid malignancy in men in western countries, with an increasing incidence year by year ( Rebello et al., 2021 ). Current research indicates that the occurrence of prostate cancer is primarily associated with factors such as age, hormones, race, and genetics ( Bergengren et al., 2023 ). However, its etiology and pathogenesis are not fully understood. Treatment for prostate cancer primarily includes surgery, radiation therapy, and androgen deprivation therapy ( Cha et al., 2020 ). Emerging treatment modalities have provided patients with a greater range of options. For example, the use of nanomaterials in conjunction with circRNA enhance the sensitivity of tumor cells to treatment ( Ghorbani et al., 2023 ; Su et al., 2023 ; Wang et al., 2023 ; Xie et al., 2023 ; Zetrini et al., 2023 ; Zhou et al., 2023 ). However, currently the efficacy for recurrent, drug-resistant, and metastatic prostate cancer is limited ( Antonarakis et al., 2010 ; Gao et al., 2023 ; Guo et al., 2023 ; Sooi et al., 2023 ; Su et al., 2023 ). Therefore, it is imperative to investigate the etiology, pathogenesis, and explore new treatment methods for prostate cancer.
Increasing research indicates that immune cells were involved in the development of prostate cancer. Various cell types involved in the regulation of prostate cancer have been identified ( Fridlender et al., 2009 ; Sagnak et al., 2011 ; Shi et al., 2023 ). NK cells and CD8 + T lymphocytes are pivotal forces in anti-tumor immunity, effectively eliminating cancer cells. Conversely, tumor-associated macrophages and other cells exert inhibitory effects on anti-tumor immunity, and their excessive activation may be associated with the occurrence and progression of tumors ( Luo et al., 2021 ; Shi et al., 2023 ). While there is a preliminary understanding of the roles of certain immune cell types in the pathogenesis of prostate cancer, the specific functions of various subtypes of immune cells and whether there is a causal relationship between these cells and tumor development remain unclear. Clarifying the causal relationship between immune cells and the onset of prostate cancer is a critical topic in current prostate cancer research.
However, the majority of research methods currently employed still face significant limitations in establishing a causal relationship between these factors. Mendelian randomization (MR), utilizing genetic variations as instrumental variables, is a valuable tool for establishing causal relationships. MR improves study validity by reducing bias and enabling causal inference in experimental designs. Mendelian randomization analysis offers advantages over randomized controlled trials (RCTs) by utilizing genetic variants as instrumental variables, providing insights into long-term exposures and outcomes, reducing confounding bias inherent in observational studies, and offering cost-effective alternatives in situations where RCTs are impractical or unethical ( Smith and Ebrahim, 2003 ; Larsson and Burgess, 2022 ). In this study, we employ MR to investigate the causal relationship between immune cells and prostate cancer. This approach is advantageous for illustrating the relationship between immune cells and prostate cancer, laying the groundwork for immunotherapeutic interventions in prostate cancer.
Data sources
This study utilized a population-based immune profiling analysis reported in the Nature Genetics journal. The research included a cohort of 3,757 individuals from the Sardinian population. The comprehensive investigation encompassed a wide range of 731 immunophenotypes, comprising absolute cell counts (n = 118), median fluorescence intensities (n = 389), morphological parameters (n = 32), and relative cell counts (n = 192) ( Orru et al., 2020 ).
The prostate cancer data used in this study were sourced from the Integrative Epidemiology Unit Open GWAS database ( https://gwas.mrcieu.ac.uk/ ). The study included 9,132 European male prostate cancer patients as the study group and 173,493 European males without prostate cancer as the control group. A total of 12,097,504 SNPs were screened for their impact on prostate cancer. The diagnostic criteria for prostate cancer are derived from ICD-10 code C61 and ICD-9 code 185 ( Kimberley Burrows, 2021 ). Figure 1 illustrates the study’s specific research approach, while Table 1 provides specific details on data sources and features.
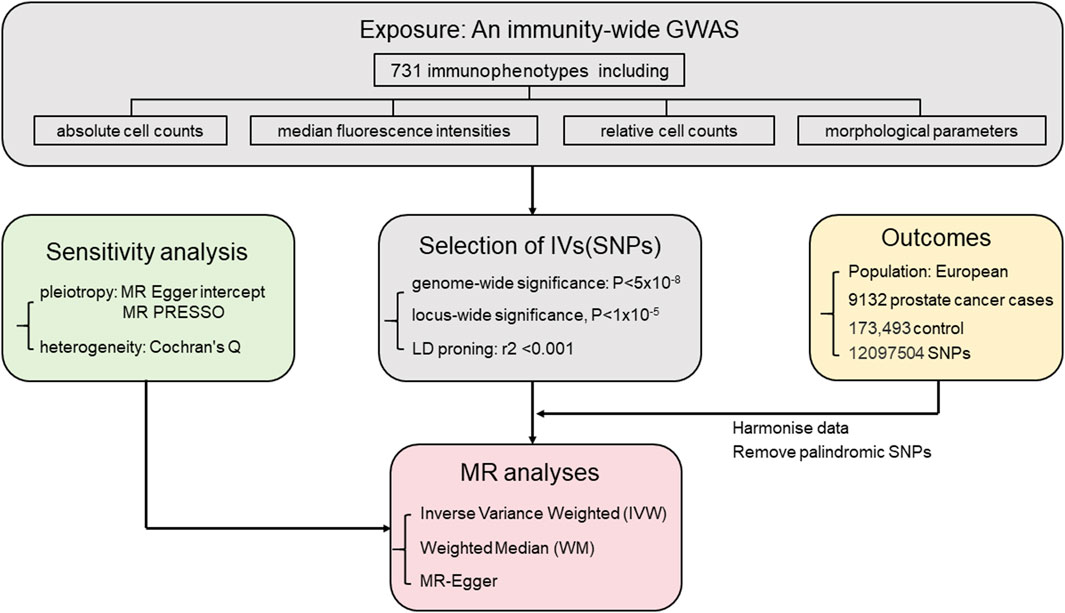
FIGURE 1 . Mendelian randomization study workflow on the association between immune cell types and prostate cancer.

TABLE 1 . Detailed information on the analyzed data.
Instrumental variables (IVs)
MR employs genetic variants as instrumental variables. The selection of instrumental variables needs to satisfy three assumptions: 1) the genetic variants have strong association with the exposure factor; 2) the genetic variants were independent from confounding factors. 3) the genetic variants affect the outcome through the exposure factor ( Smith and Ebrahim, 2004 ; Richmond and Davey Smith, 2022 ). The process of selecting genetic variants as instrumental variables is illustrated in Figure 2 .
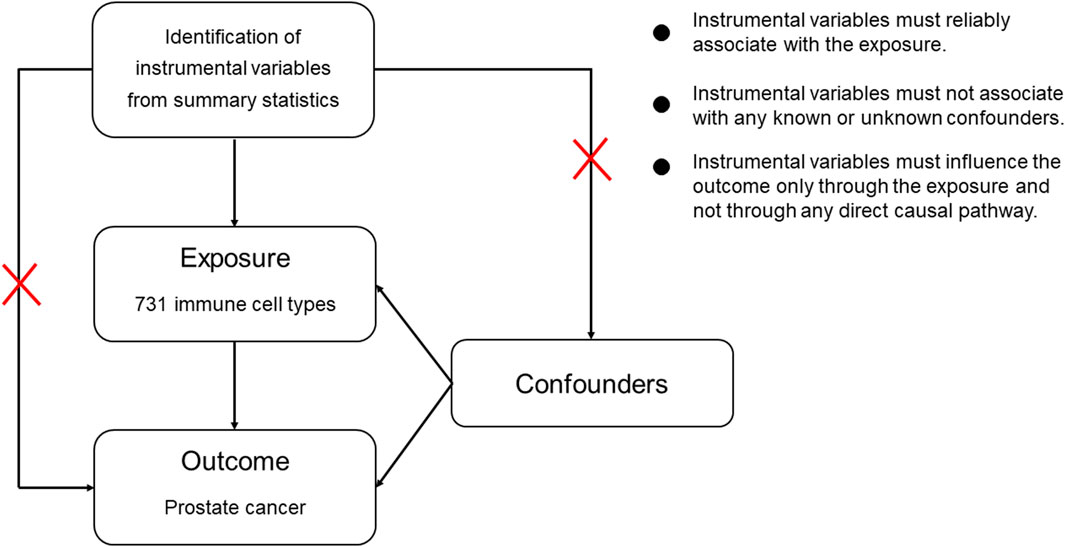
FIGURE 2 . Assumptions in MR studies: a brief overview.
The selection and analysis of IVs for immune traits were conducted meticulously, employing a significance level of 1 × 10 −5 . To ensure the independence of loci, a clumping window of 10,000 kb and a linkage disequilibrium (LD) threshold of r2 < 0.001 were employed. The implementation was carried out using the “TwoSampleMR” package and 1,000 Genomes EUR data. Palindrome SNPs may introduce uncertainty regarding the effect allele in GWAS. To ensure reliability, we excluded palindrome SNPs with effect allele frequencies between 0.3 and 0.7. Additionally, instrument strength was assessed using F-statistics, where a variance ratio (b 2 /se 2 ) exceeding 10 indicates minimal weak instrument bias ( Burgess et al., 2011 ).
Statistical analysis
In this research, various analytical methods including the Inverse Variance Weighted (IVW) method, weighted median method, and MR-Egger regression analysis were employed. The IVW method, widely used in MR studies for its excellent accuracy in effect estimation, was applied in this study with a higher screening threshold ( p -value <0.01) to ensure result accuracy. Random-effects IVW provides an unbiased estimate by considering heterogeneity among studies and appropriately weighting the effects based on their precision. The IVW method was chosen as the primary research method used in this study ( Hemani et al., 2018b ). The MR Egger method accurately evaluates causal relationships, effectively addresses sample selection bias, and enhances statistical power and robustness of data ( Verbanck et al., 2018 ). The weighted median approach provides a reliable estimate by accounting for the distribution of weights assigned to each data point ( Hemani et al., 2018b ). Cochran’s Q test was used to assess heterogeneity ( Bowden et al., 2019 ). MR Egger intercept analysis serves to assess and correct for potential bias caused by horizontal pleiotropy. MR-PRESSO analysis method is designed to detect and correct for horizontal pleiotropy ( Verbanck et al., 2018 ). The application of multiple statistical techniques contributes to the reliability and rigor of the study, facilitating a deeper understanding of the intricate relationship between immune cells and prostate cancer ( Hemani et al., 2018a ). All analyses were conducted with the “TwoSampleMR” package (v.0.5.7) in R (v.4.3.0).
The main results of the analysis of the association between 731 immune cell types and the risk of prostate cancer.
F-statistics were calculated for all 731 immune cell types, ranging from 19.55 to 2381.77. The F-values for all results exceeded 10, surpassing the minimum threshold for weak instrument bias, indicating that they are all strong instrument variables. Detailed information on single nucleotide polymorphisms (SNPs) for each immune cell type is provided in Supplementary Table S1 . The MR results for all features and their associations with prostate cancer are summarized in Supplementary Table S3 , revealing six immune cell types with potential correlations detected using the IVW method, as shown in Figure 1 . The IVs used for immune traits are presented in Supplementary Tables S1, S2 . This MR analysis identified a causal relationship between six immune cell types and the risk of prostate cancer, as illustrated in Figure 3 and detailed in Supplementary Table S4 . The study provides additional evidence to establish potential connections between specific types of immune cells and the risk of prostate cancer.
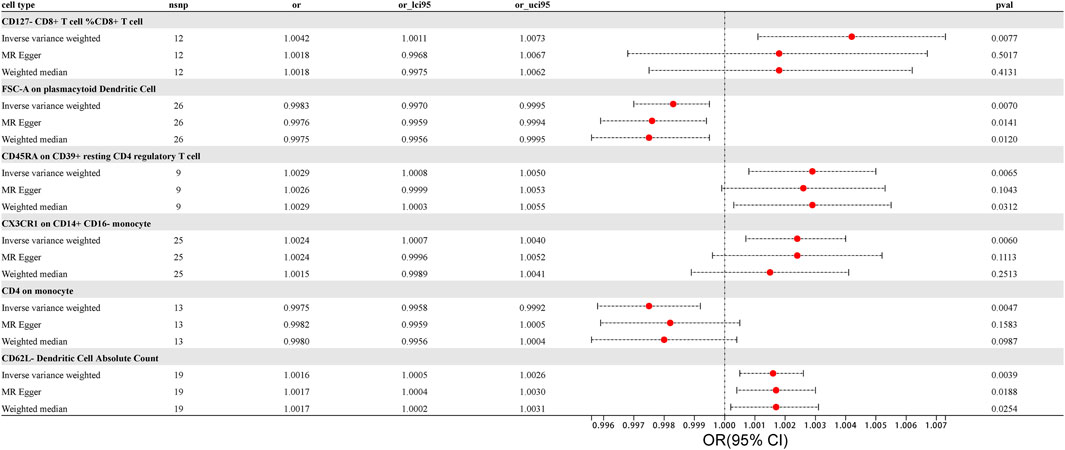
FIGURE 3 . Forest Plot: Associations of Genetically Determined immune traits with prostate cancer risk.
Using the IVW method, we found a clear association between T lymphocytes, monocytes-macrophages, dendritic cells, and the occurrence of prostate cancer. The IVW analysis revealed a positive correlation between CD127 - CD8 + T cell %CD8 + T cell and the risk of prostate cancer (OR = 1.0042, 95%CI:1.0011–1.0073, p = 0.0077). The MR-Egger and weighted median analysis methods did not reveal specific association. Both the MR-Egger intercept assessment ( p = 0.2416) and MR-PRESSO global test ( p = 0.3970) analysis did not indicate horizontal pleiotropy ( Supplementary Tables S5, S6 ).
Similarly, CD45RA on CD39 + resting CD4 regulatory T cell, belonging to T cells, was confirmed to be positively correlated with prostate cancer risk through IVW analysis (OR = 1.0029, 95% CI: 1.0008–1.0050, p = 0.0065), with no significant association in weighted median and MR-Egger. No evidence of horizontal pleiotropy in the MR-Egger intercept assessment ( p = 0.7244) and MR-PRESSO global test analysis ( p = 0.8420) ( Supplementary Tables S5, S6 ).
CX3CR1 on CD14 + CD16 − monocyte was identified through IVW analysis to be positively associated with the risk of prostate cancer (OR = 1.0024, 95%CI:1.0007–1.0040, p = 0.0060). This association was not significant in MR-Egger and weighted median analyses. Both the MR-Egger intercept assessment ( p = 0.9813) and MR-PRESSO global test analysis ( p = 0.8660) did not reveal horizontal pleiotropy ( Supplementary Tables S5, S6 ).
For dendritic cells, CD62L − Dendritic Cell Absolute Count was confirmed to be positively correlated with the risk of prostate cancer through IVW analysis (IVW: OR = 1.0016; 95%CI:1.0005–1.0026; p = 0.0039), with no significant association in MR-Egger and weighted median, and no evidence of horizontal pleiotropy in the MR-Egger intercept assessment ( p = 0.7088) as well as MR-PRESSO global test analysis ( p = 0.4650). The results, analyzed and tested using various methods while excluding outliers and heterogeneity, provided more accurate causal associations and offered new evidence in exploring which immune cells may promote the occurrence of prostate cancer ( Supplementary Tables S5, S6 ).
In contrast to the cells positively correlated with the risk of prostate cancer mentioned above, we also identified immune cells negatively correlated with prostate cancer risk using the IVW method. In monocytes-macrophages, CD4 on monocyte was found to be negatively correlated with prostate cancer risk through IVW testing (OR = 0.9975, 95%CI:0.9958–0.9992, p = 0.0047). This correlation was not significant in MR-Egger and weighted median analyses, and the MR-Egger intercept assessment ( p = 0.3962) as well as MR-PRESSO global test analysis ( p = 0.7580) did not reveal horizontal pleiotropy. Similarly, in dendritic cells, FSC-A on plasmacytoid Dendritic Cell was identified through IVW testing to be negatively correlated with the risk of prostate cancer (OR = 0.9983, 95%CI:0.9970–0.9995, p = 0.0070). However, MR Egger and weighted median analyses did not find a significant association between these immune cells and the risk of prostate cancer, and intercept of MR-Egger analysis ( p = 0.3206) as well as MR-PRESSO global test ( p = 0.4020) analysis did not reveal horizontal pleiotropy in both cases ( Supplementary Tables S5, S6 ).
Scatterplot of genetic association between immune traits and prostate cancer were shown in Figure 4 . We found no significant heterogeneity among immune cells instrumental variables, which further indicates that immune cells play a complex and crucial role in the development of prostate cancer ( Supplementary Table S7 ). Some cells promote the occurrence of prostate cancer, while others have the potential to inhibit the onset of prostate cancer. These findings provide new insights into the pathogenesis and treatment of prostate cancer.
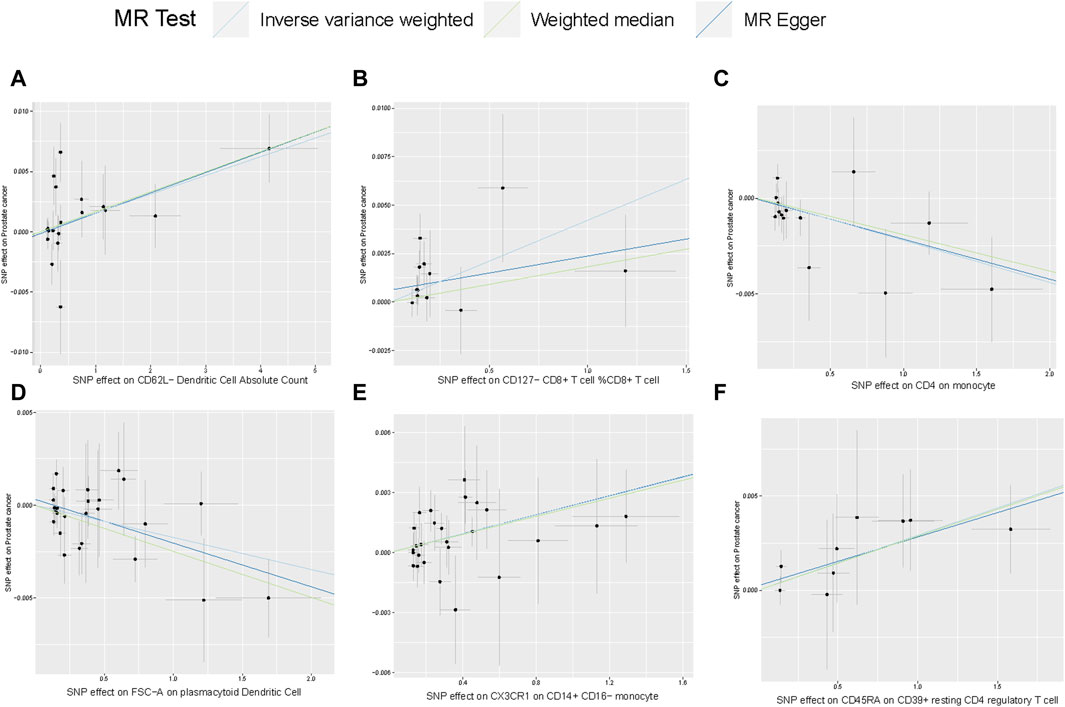
FIGURE 4 . Scatterplot of genetic association between immune traits and prostate cancer. (A) Genetic association of CD62L− Dendritic Cell Absolute Count with prostate cancer Causal effect of Desulfovibrionales on CKD; (B–F) Potential causal effect of five other immune traits on prostate cancer.
In this MR analysis, we identified six types of immune cells related to the risk of prostate cancer, primarily including T cells, monocytes, and dendritic cells. These three categories exhibit different phenotypes and causal relationships with prostate cancer.
The study revealed a positive correlation between CD127 - CD8 + T cell %CD8 + T cell and the development of prostate cancer. CD127, also known as the IL-7 receptor alpha chain, is a component of the IL-7 receptor ( Aloufi et al., 2021 ). IL-7 assumes a vital role in the progression of lymphocyte development ( Lundstrom et al., 2012 ). In normal circumstances, immune cells, especially T cells, receive IL-7 signals through CD127, enhancing their survival and functionality to combat abnormal cells, including cancer cells. CD127 - cells, lacking the ability to receive IL-7 signals, result in immune cells becoming inactive or ineffective in the tumor microenvironment, leading to immune tolerance ( Joshi et al., 2007 ). Therefore, the research findings indicating a positive correlation between CD127 - CD8 + T cell %CD8 + T cell and prostate cancer development are mechanistically reasonable. This provides a favorable basis for further exploring the exact role of CD127 in tumors.
In investigating the interplay between T cells and prostate cancer, we uncovered another intriguing result: CD45RA on CD39 + resting CD4 regulatory T cells is also positively correlated with prostate cancer development. CD45RA is typically expressed on unactivated, resting immune cells, especially unstimulated T cells ( Hermiston et al., 2003 ). CD39, also known as NTPDase1, degrades extracellular ATP. Since ATP has pro-inflammatory effects outside the cells, CD39, by converting ATP to ADP and AMP, indirectly reduces extracellular ATP concentration, thus inhibiting inflammatory reactions ( Timperi and Barnaba, 2021 ). Regulatory T cells (Tregs) suppress the activity of immune cells through various mechanisms.
In the tumor microenvironment, an excessive presence of Tregs may restrict the attack of other immune cells on tumor cells, promoting tumor escape ( Ji et al., 2020 ). Therefore, CD45RA on CD39 + resting CD4 regulatory T cells, by degrading the pro-inflammatory factor ATP and inhibiting the anti-tumor effects of other immune cells, creates a more permissive immune environment for tumor cells. This allows tumor cells to evade immune system surveillance and attacks.
In the analysis of the interaction between immune cells and prostate cancer using MR analysis, a complex relationship was identified, such as a positive correlation between CX3CR1 on CD14 + CD16 − monocytes and the development of prostate cancer, and a negative correlation between CD4 on monocytes and prostate cancer development. CX3CR1 is a chemokine receptor that, by influencing monocyte chemotaxis and tumor angiogenesis ( Pawelec et al., 2020 ), can alter the tumor immune microenvironment, thereby affecting tumor development and immune responses ( Schmall et al., 2015 ). On the other hand, CD14 can interact with receptors such as TLR4, recognizing and binding to molecular patterns of bacteria ( Marchesi et al., 2010 ). However, the role of CD14 in tumor development may be more complex. They can participate in anti-tumor immune responses ( Pallett et al., 2023 ) and produce inhibitory cytokines that promote immune escape by tumors ( Cheah et al., 2015 ). CD16 can bind to the Fc region of antibodies, forming complexes with antibodies. When these complexes bind to antigens on the surface of target cells, they activate natural killer cells, triggering ADCC ( Bhatnagar et al., 2014 ). Based on the above, it is speculated that the possible mechanism by which CX3CR1 on CD14 + CD16 − monocytes promotes prostate cancer development is through the CX3CR1/CX3CL1 signaling pathway, promoting tumor angiogenesis, migration, and infiltration, while inhibiting ADCC, thereby weakening the body’s anti-tumor effects.
The expression of CD4 on monocytes is likely a marker of monocyte activation. Activated monocytes may participate in regulating immune responses, limiting tumor growth. However, as the tumor microenvironment changes ( Zhen et al., 2014 ), the immune regulatory function of monocytes may be inhibited. The exact role of CD4 on monocytes in tumor development depends on the specific tumor type and individual differences among patients. Therefore, further experimental and clinical studies are needed. In summary, the MR analysis revealed complex interactions between monocytes and prostate cancer. This MR analysis is crucial for understanding the role of monocytes in cancer development and exploring new treatment methods.
Dendritic cells, a subset of antigen-presenting cells (APCs), play a crucial role in initiating and activating T cells, enhance the immune regulation of natural killer cells, and exhibit cytotoxic capabilities ( Laginha et al., 2022 ). Presently, there have been encouraging outcomes observed in the use of immunotherapy utilizing dendritic cells for the management of prostate cancer ( Jahnisch et al., 2010 ). This MR analysis provides the first confirmation that CD62L − Dendritic Cell Absolute Count is positively correlated with the development of prostate cancer, while FSC-A on plasmacytoid Dendritic Cell shows a negative correlation. CD62L, also known as L-selectin, is a cell adhesion molecule that participates in leukocyte rolling, adhesion, and migration by binding to ligands on endothelial cells ( Ivetic et al., 2019 ). Decreased expression of CD62L results in reduced chemotactic ability of dendritic cells, leading to a weakened anti-tumor inflammatory response. FSC-A is a crucial parameter used in flow cytometry to measure forward scatter signals and estimate cell size. Plasmacytoid dendritic cells are among the most potent regulators of antiviral immune responses in the body, producing large amounts of type I interferons ( Mitchell et al., 2018 ), such as IFN-α. However, there is currently no experimental data supporting the negative correlation between FSC-A on plasmacytoid Dendritic Cell and prostate cancer. This finding provides new experimental avenues for exploring the relationship between dendritic cells and prostate cancer.
The intricate complexity lies in the interplay between prostate cancer and immune cells. These findings provide important insights into the roles of T cells, monocytes, and dendritic cells in the risk of prostate cancer, contributing to the advancement of immunotherapy for prostate cancer. However, there are certain limitations to consider. Firstly, the causal relationship between the identified six immune cells and prostate cancer was not strong. The causality links’ low power may result from the heterogeneity of the outcomes such as prostate cancer’s stage, severity, and duration. However, at this stage, there is still a lack of data on prostate cancer GWAS sequencing with specific clinical characteristics. Secondly, the population included in the Genome-Wide Association Study (GWAS) mainly comprises individuals of European ancestry. Genetic differences between populations may result in variations in the relationship between immune cells and prostate cancer, introducing a potential ethnic bias to the MR study results. Thirdly, the use of a low threshold value ( p < 1.0 × 10 −5 ) during the tool variable selection may lead to false positives or overlook important genetic variations related to immune cell features. Fourthly, the lack of independent cohort studies to validate the research findings. Fifthly, our research has only demonstrated partial correlation between immune cells and the development of prostate cancer, lacking experimental evidence to further explore and uncover the underlying mechanisms. In the future, we will conduct biological experiments to delve deeper into our findings and investigate potential mechanisms.
Data availability statement
The original contributions presented in the study are included in the article/ Supplementary Material , further inquiries can be directed to the corresponding authors.
Author contributions
ZY: Conceptualization, Data curation, Formal Analysis, Writing–original draft, Writing–review and editing. XD: Data curation, Writing–original draft, Writing–review and editing. JinZ: Data curation, Writing–original draft. RS: Formal Analysis, Writing–original draft. CS: Formal Analysis, Writing–original draft. JiaZ: Conceptualization, Project administration, Writing–review and editing, Writing–original draft. HT: Conceptualization, Project administration, Writing–review and editing, Writing–original draft.
The author(s) declare that no financial support was received for the research, authorship, and/or publication of this article.
Conflict of interest
The authors declare that the research was conducted in the absence of any commercial or financial relationships that could be construed as a potential conflict of interest.
Publisher’s note
All claims expressed in this article are solely those of the authors and do not necessarily represent those of their affiliated organizations, or those of the publisher, the editors and the reviewers. Any product that may be evaluated in this article, or claim that may be made by its manufacturer, is not guaranteed or endorsed by the publisher.
Supplementary material
The Supplementary Material for this article can be found online at: https://www.frontiersin.org/articles/10.3389/fcell.2024.1381920/full#supplementary-material
Aloufi, N. A., Ali, A. K., Burke Schinkel, S. C., Molyer, B., Barros, P. O., Mcbane, J. E., et al. (2021). Soluble CD127 potentiates IL-7 activity in vivo in healthy mice. Immun. Inflamm. Dis. 9, 1798–1808. doi:10.1002/iid3.530
PubMed Abstract | CrossRef Full Text | Google Scholar
Antonarakis, E. S., Carducci, M. A., and Eisenberger, M. A. (2010). Novel targeted therapeutics for metastatic castration-resistant prostate cancer. Cancer Lett. 291, 1–13. doi:10.1016/j.canlet.2009.08.012
Bergengren, O., Pekala, K. R., Matsoukas, K., Fainberg, J., Mungovan, S. F., Bratt, O., et al. (2023). 2022 update on prostate cancer epidemiology and risk factors-A systematic review. Eur. Urol. 84, 191–206. doi:10.1016/j.eururo.2023.04.021
Bhatnagar, N., Ahmad, F., Hong, H. S., Eberhard, J., Lu, I. N., Ballmaier, M., et al. (2014). FcγRIII (CD16)-mediated ADCC by NK cells is regulated by monocytes and FcγRII (CD32). Eur. J. Immunol. 44, 3368–3379. doi:10.1002/eji.201444515
Bowden, J., Del Greco, M. F., Minelli, C., Zhao, Q., Lawlor, D. A., Sheehan, N. A., et al. (2019). Improving the accuracy of two-sample summary-data Mendelian randomization: moving beyond the NOME assumption. Int. J. Epidemiol. 48, 728–742. doi:10.1093/ije/dyy258
Burgess, S., Thompson, S. G., and Collaboration, C. C. G. (2011). Avoiding bias from weak instruments in Mendelian randomization studies. Int. J. Epidemiol. 40, 755–764. doi:10.1093/ije/dyr036
Cha, H. R., Lee, J. H., and Ponnazhagan, S. (2020). Revisiting immunotherapy: a focus on prostate cancer. Cancer Res. 80, 1615–1623. doi:10.1158/0008-5472.CAN-19-2948
Cheah, M. T., Chen, J. Y., Sahoo, D., Contreras-Trujillo, H., Volkmer, A. K., Scheeren, F. A., et al. (2015). CD14-expressing cancer cells establish the inflammatory and proliferative tumor microenvironment in bladder cancer. Proc. Natl. Acad. Sci. U. S. A. 112, 4725–4730. doi:10.1073/pnas.1424795112
Fridlender, Z. G., Sun, J., Kim, S., Kapoor, V., Cheng, G., Ling, L., et al. (2009). Polarization of tumor-associated neutrophil phenotype by TGF-beta: "N1" versus "N2" TAN. Cancer Cell 16, 183–194. doi:10.1016/j.ccr.2009.06.017
Gao, K., Li, X., Ni, J., Wu, B., Guo, J., Zhang, R., et al. (2023). Non-coding RNAs in enzalutamide resistance of castration-resistant prostate cancer. Cancer Lett. 566, 216247. doi:10.1016/j.canlet.2023.216247
Ghorbani, R., Gharbavi, M., Sharafi, A., Rismani, E., Rezaeejam, H., Mortazavi, Y., et al. (2023). Targeted anti-tumor synergistic effects of Myc decoy oligodeoxynucleotides-loaded selenium nanostructure combined with chemoradiotherapy on LNCaP prostate cancer cells. Oncol. Res. 32, 101–125. doi:10.32604/or.2023.044741
Guo, X., Gao, C., Yang, D. H., and Li, S. (2023). Exosomal circular RNAs: a chief culprit in cancer chemotherapy resistance. Drug Resist Updat 67, 100937. doi:10.1016/j.drup.2023.100937
Hemani, G., Bowden, J., and Davey Smith, G. (2018a). Evaluating the potential role of pleiotropy in Mendelian randomization studies. Hum. Mol. Genet. 27, R195–R208. doi:10.1093/hmg/ddy163
Hemani, G., Zheng, J., Elsworth, B., Wade, K. H., Haberland, V., Baird, D., et al. (2018b). The MR-Base platform supports systematic causal inference across the human phenome. Elife 7, e34408. doi:10.7554/eLife.34408
Hermiston, M. L., Xu, Z., and Weiss, A. (2003). CD45: a critical regulator of signaling thresholds in immune cells. Annu. Rev. Immunol. 21, 107–137. doi:10.1146/annurev.immunol.21.120601.140946
Ivetic, A., Hoskins Green, H. L., and Hart, S. J. (2019). L-Selectin: a major regulator of leukocyte adhesion, migration and signaling. Front. Immunol. 10, 1068. doi:10.3389/fimmu.2019.01068
Jahnisch, H., Fussel, S., Kiessling, A., Wehner, R., Zastrow, S., Bachmann, M., et al. (2010). Dendritic cell-based immunotherapy for prostate cancer. Clin. Dev. Immunol. 2010, 517493. doi:10.1155/2010/517493
Ji, D., Song, C., Li, Y., Xia, J., Wu, Y., Jia, J., et al. (2020). Combination of radiotherapy and suppression of Tregs enhances abscopal antitumor effect and inhibits metastasis in rectal cancer. J. Immunother. Cancer 8, e000826. doi:10.1136/jitc-2020-000826
Joshi, N. S., Cui, W., Chandele, A., Lee, H. K., Urso, D. R., Hagman, J., et al. (2007). Inflammation directs memory precursor and short-lived effector CD8(+) T cell fates via the graded expression of T-bet transcription factor. Immunity 27, 281–295. doi:10.1016/j.immuni.2007.07.010
Kimberley Burrows, P. H. (2021). Genome-wide association study of cancer risk in UK biobank. Available: http://www.nealelab.is/uk-biobank/ .
Google Scholar
Laginha, P. A., Arcoverde, F. V. L., Riccio, L. G. C., Andres, M. P., and Abrao, M. S. (2022). The role of dendritic cells in endometriosis: a systematic review. J. Reprod. Immunol. 149, 103462. doi:10.1016/j.jri.2021.103462
Larsson, S. C., and Burgess, S. (2022). Appraising the causal role of smoking in multiple diseases: a systematic review and meta-analysis of Mendelian randomization studies. EBioMedicine 82, 104154. doi:10.1016/j.ebiom.2022.104154
Lundstrom, W., Fewkes, N. M., and Mackall, C. L. (2012). IL-7 in human health and disease. Semin. Immunol. 24, 218–224. doi:10.1016/j.smim.2012.02.005
Luo, Z. W., Xia, K., Liu, Y. W., Liu, J. H., Rao, S. S., Hu, X. K., et al. (2021). Extracellular vesicles from akkermansia muciniphila elicit antitumor immunity against prostate cancer via modulation of CD8(+) T cells and macrophages. Int. J. Nanomedicine 16, 2949–2963. doi:10.2147/IJN.S304515
Marchesi, F., Locatelli, M., Solinas, G., Erreni, M., Allavena, P., and Mantovani, A. (2010). Role of CX3CR1/CX3CL1 axis in primary and secondary involvement of the nervous system by cancer. J. Neuroimmunol. 224, 39–44. doi:10.1016/j.jneuroim.2010.05.007
Mitchell, D., Chintala, S., and Dey, M. (2018). Plasmacytoid dendritic cell in immunity and cancer. J. Neuroimmunol. 322, 63–73. doi:10.1016/j.jneuroim.2018.06.012
Orru, V., Steri, M., Sidore, C., Marongiu, M., Serra, V., Olla, S., et al. (2020). Complex genetic signatures in immune cells underlie autoimmunity and inform therapy. Nat. Genet. 52, 1036–1045. doi:10.1038/s41588-020-0684-4
Pallett, L. J., Swadling, L., Diniz, M., Maini, A. A., Schwabenland, M., Gasull, A. D., et al. (2023). Tissue CD14(+)CD8(+) T cells reprogrammed by myeloid cells and modulated by LPS. Nature 614, 334–342. doi:10.1038/s41586-022-05645-6
Pawelec, P., Ziemka-Nalecz, M., Sypecka, J., and Zalewska, T. (2020). The impact of the cx3cl1/cx3cr1 Axis in neurological disorders. Cells 9, 2277. doi:10.3390/cells9102277
Rebello, R. J., Oing, C., Knudsen, K. E., Loeb, S., Johnson, D. C., Reiter, R. E., et al. (2021). Prostate cancer. Nat. Rev. Dis. Prim. 7, 9. doi:10.1038/s41572-020-00243-0
Richmond, R. C., and Davey Smith, G. (2022). Mendelian randomization: concepts and scope. Cold Spring Harb. Perspect. Med. 12, a040501. doi:10.1101/cshperspect.a040501
Sagnak, L., Topaloglu, H., Ozok, U., and Ersoy, H. (2011). Prognostic significance of neuroendocrine differentiation in prostate adenocarcinoma. Clin. Genitourin. Cancer 9, 73–80. doi:10.1016/j.clgc.2011.07.003
Schmall, A., Al-Tamari, H. M., Herold, S., Kampschulte, M., Weigert, A., Wietelmann, A., et al. (2015). Macrophage and cancer cell cross-talk via CCR2 and CX3CR1 is a fundamental mechanism driving lung cancer. Am. J. Respir. Crit. Care Med. 191, 437–447. doi:10.1164/rccm.201406-1137OC
Shi, W., Wang, Y., Zhao, Y., Kim, J. J., Li, H., Meng, C., et al. (2023). Immune checkpoint B7-H3 is a therapeutic vulnerability in prostate cancer harboring PTEN and TP53 deficiencies. Sci. Transl. Med. 15, eadf6724. doi:10.1126/scitranslmed.adf6724
Smith, G. D., and Ebrahim, S. (2003). Mendelian randomization': can genetic epidemiology contribute to understanding environmental determinants of disease? Int. J. Epidemiol. 32, 1–22. doi:10.1093/ije/dyg070
Smith, G. D., and Ebrahim, S. (2004). Mendelian randomization: prospects, potentials, and limitations. Int. J. Epidemiol. 33, 30–42. doi:10.1093/ije/dyh132
Sooi, K., Walsh, R., Kumarakulasinghe, N., Wong, A., and Ngoi, N. (2023). A review of strategies to overcome immune resistance in the treatment of advanced prostate cancer. Cancer Drug Resist 6, 656–673. doi:10.20517/cdr.2023.48
Su, Y., Jin, G., Zhou, H., Yang, Z., Wang, L., Mei, Z., et al. (2023). Development of stimuli responsive polymeric nanomedicines modulating tumor microenvironment for improved cancer therapy. Med. Rev. 3, 4–30. doi:10.1515/mr-2022-0048
CrossRef Full Text | Google Scholar
Timperi, E., and Barnaba, V. (2021). CD39 regulation and functions in T cells. Int. J. Mol. Sci. 22, 8068. doi:10.3390/ijms22158068
Verbanck, M., Chen, C. Y., Neale, B., and Do, R. (2018). Detection of widespread horizontal pleiotropy in causal relationships inferred from Mendelian randomization between complex traits and diseases. Nat. Genet. 50, 693–698. doi:10.1038/s41588-018-0099-7
Wang, H., Liu, J., Zhu, X., Yang, B., He, Z., and Yao, X. (2023). AZGP1P2/UBA1/RBM15 cascade mediates the fate determinations of prostate cancer stem cells and promotes therapeutic effect of docetaxel in castration-resistant prostate cancer via TPM1 m6A modification. Res. (Wash D C) 6, 0252. doi:10.34133/research.0252
Xie, J., Ye, F., Deng, X., Tang, Y., Liang, J. Y., Huang, X., et al. (2023). Circular RNA: a promising new star of vaccine. J. Transl. Int. Med. 11, 372–381. doi:10.2478/jtim-2023-0122
Zetrini, A. E., Lip, H., Abbasi, A. Z., Alradwan, I., Ahmed, T., He, C., et al. (2023). Remodeling tumor immune microenvironment by using polymer-lipid-manganese dioxide nanoparticles with radiation therapy to boost immune response of castration-resistant prostate cancer. Res. (Wash D C) 6, 0247. doi:10.34133/research.0247
Zhen, A., Krutzik, S. R., Levin, B. R., Kasparian, S., Zack, J. A., and Kitchen, S. G. (2014). CD4 ligation on human blood monocytes triggers macrophage differentiation and enhances HIV infection. J. Virol. 88, 9934–9946. doi:10.1128/JVI.00616-14
Zhou, Z., Qin, J., Song, C., Wu, T., Quan, Q., Zhang, Y., et al. (2023). circROBO1 promotes prostate cancer growth and enzalutamide resistance via accelerating glycolysis. J. Cancer 14, 2574–2584. doi:10.7150/jca.86940
Keywords: prostate cancer, immune cells, Mendelian randomization, single nucleotide polymorphism, genome-wide association studies
Citation: Ye Z, Deng X, Zhang J, Shao R, Song C, Zhao J and Tang H (2024) Causal relationship between immune cells and prostate cancer: a Mendelian randomization study. Front. Cell Dev. Biol. 12:1381920. doi: 10.3389/fcell.2024.1381920
Received: 04 February 2024; Accepted: 08 March 2024; Published: 19 March 2024.
Reviewed by:
Copyright © 2024 Ye, Deng, Zhang, Shao, Song, Zhao and Tang. This is an open-access article distributed under the terms of the Creative Commons Attribution License (CC BY). The use, distribution or reproduction in other forums is permitted, provided the original author(s) and the copyright owner(s) are credited and that the original publication in this journal is cited, in accordance with accepted academic practice. No use, distribution or reproduction is permitted which does not comply with these terms.
*Correspondence: Jianfu Zhao, [email protected] ; Hailin Tang, [email protected]
† These authors have contributed equally to this work
This article is part of the Research Topic
Perspectives on Omics Analysis in Solid Tumors: Advancing Cancer Research

An official website of the United States government
The .gov means it's official. Federal government websites often end in .gov or .mil. Before sharing sensitive information, make sure you're on a federal government site.
The site is secure. The https:// ensures that you are connecting to the official website and that any information you provide is encrypted and transmitted securely.
- Publications
- Account settings
- Browse Titles
NCBI Bookshelf. A service of the National Library of Medicine, National Institutes of Health.
StatPearls [Internet]. Treasure Island (FL): StatPearls Publishing; 2024 Jan-.

StatPearls [Internet].
Qualitative study.
Steven Tenny ; Janelle M. Brannan ; Grace D. Brannan .
Affiliations
Last Update: September 18, 2022 .
- Introduction
Qualitative research is a type of research that explores and provides deeper insights into real-world problems. [1] Instead of collecting numerical data points or intervene or introduce treatments just like in quantitative research, qualitative research helps generate hypotheses as well as further investigate and understand quantitative data. Qualitative research gathers participants' experiences, perceptions, and behavior. It answers the hows and whys instead of how many or how much. It could be structured as a stand-alone study, purely relying on qualitative data or it could be part of mixed-methods research that combines qualitative and quantitative data. This review introduces the readers to some basic concepts, definitions, terminology, and application of qualitative research.
Qualitative research at its core, ask open-ended questions whose answers are not easily put into numbers such as ‘how’ and ‘why’. [2] Due to the open-ended nature of the research questions at hand, qualitative research design is often not linear in the same way quantitative design is. [2] One of the strengths of qualitative research is its ability to explain processes and patterns of human behavior that can be difficult to quantify. [3] Phenomena such as experiences, attitudes, and behaviors can be difficult to accurately capture quantitatively, whereas a qualitative approach allows participants themselves to explain how, why, or what they were thinking, feeling, and experiencing at a certain time or during an event of interest. Quantifying qualitative data certainly is possible, but at its core, qualitative data is looking for themes and patterns that can be difficult to quantify and it is important to ensure that the context and narrative of qualitative work are not lost by trying to quantify something that is not meant to be quantified.
However, while qualitative research is sometimes placed in opposition to quantitative research, where they are necessarily opposites and therefore ‘compete’ against each other and the philosophical paradigms associated with each, qualitative and quantitative work are not necessarily opposites nor are they incompatible. [4] While qualitative and quantitative approaches are different, they are not necessarily opposites, and they are certainly not mutually exclusive. For instance, qualitative research can help expand and deepen understanding of data or results obtained from quantitative analysis. For example, say a quantitative analysis has determined that there is a correlation between length of stay and level of patient satisfaction, but why does this correlation exist? This dual-focus scenario shows one way in which qualitative and quantitative research could be integrated together.
Examples of Qualitative Research Approaches
Ethnography
Ethnography as a research design has its origins in social and cultural anthropology, and involves the researcher being directly immersed in the participant’s environment. [2] Through this immersion, the ethnographer can use a variety of data collection techniques with the aim of being able to produce a comprehensive account of the social phenomena that occurred during the research period. [2] That is to say, the researcher’s aim with ethnography is to immerse themselves into the research population and come out of it with accounts of actions, behaviors, events, etc. through the eyes of someone involved in the population. Direct involvement of the researcher with the target population is one benefit of ethnographic research because it can then be possible to find data that is otherwise very difficult to extract and record.
Grounded Theory
Grounded Theory is the “generation of a theoretical model through the experience of observing a study population and developing a comparative analysis of their speech and behavior.” [5] As opposed to quantitative research which is deductive and tests or verifies an existing theory, grounded theory research is inductive and therefore lends itself to research that is aiming to study social interactions or experiences. [3] [2] In essence, Grounded Theory’s goal is to explain for example how and why an event occurs or how and why people might behave a certain way. Through observing the population, a researcher using the Grounded Theory approach can then develop a theory to explain the phenomena of interest.
Phenomenology
Phenomenology is defined as the “study of the meaning of phenomena or the study of the particular”. [5] At first glance, it might seem that Grounded Theory and Phenomenology are quite similar, but upon careful examination, the differences can be seen. At its core, phenomenology looks to investigate experiences from the perspective of the individual. [2] Phenomenology is essentially looking into the ‘lived experiences’ of the participants and aims to examine how and why participants behaved a certain way, from their perspective . Herein lies one of the main differences between Grounded Theory and Phenomenology. Grounded Theory aims to develop a theory for social phenomena through an examination of various data sources whereas Phenomenology focuses on describing and explaining an event or phenomena from the perspective of those who have experienced it.
Narrative Research
One of qualitative research’s strengths lies in its ability to tell a story, often from the perspective of those directly involved in it. Reporting on qualitative research involves including details and descriptions of the setting involved and quotes from participants. This detail is called ‘thick’ or ‘rich’ description and is a strength of qualitative research. Narrative research is rife with the possibilities of ‘thick’ description as this approach weaves together a sequence of events, usually from just one or two individuals, in the hopes of creating a cohesive story, or narrative. [2] While it might seem like a waste of time to focus on such a specific, individual level, understanding one or two people’s narratives for an event or phenomenon can help to inform researchers about the influences that helped shape that narrative. The tension or conflict of differing narratives can be “opportunities for innovation”. [2]
Research Paradigm
Research paradigms are the assumptions, norms, and standards that underpin different approaches to research. Essentially, research paradigms are the ‘worldview’ that inform research. [4] It is valuable for researchers, both qualitative and quantitative, to understand what paradigm they are working within because understanding the theoretical basis of research paradigms allows researchers to understand the strengths and weaknesses of the approach being used and adjust accordingly. Different paradigms have different ontology and epistemologies . Ontology is defined as the "assumptions about the nature of reality” whereas epistemology is defined as the “assumptions about the nature of knowledge” that inform the work researchers do. [2] It is important to understand the ontological and epistemological foundations of the research paradigm researchers are working within to allow for a full understanding of the approach being used and the assumptions that underpin the approach as a whole. Further, it is crucial that researchers understand their own ontological and epistemological assumptions about the world in general because their assumptions about the world will necessarily impact how they interact with research. A discussion of the research paradigm is not complete without describing positivist, postpositivist, and constructivist philosophies.
Positivist vs Postpositivist
To further understand qualitative research, we need to discuss positivist and postpositivist frameworks. Positivism is a philosophy that the scientific method can and should be applied to social as well as natural sciences. [4] Essentially, positivist thinking insists that the social sciences should use natural science methods in its research which stems from positivist ontology that there is an objective reality that exists that is fully independent of our perception of the world as individuals. Quantitative research is rooted in positivist philosophy, which can be seen in the value it places on concepts such as causality, generalizability, and replicability.
Conversely, postpositivists argue that social reality can never be one hundred percent explained but it could be approximated. [4] Indeed, qualitative researchers have been insisting that there are “fundamental limits to the extent to which the methods and procedures of the natural sciences could be applied to the social world” and therefore postpositivist philosophy is often associated with qualitative research. [4] An example of positivist versus postpositivist values in research might be that positivist philosophies value hypothesis-testing, whereas postpositivist philosophies value the ability to formulate a substantive theory.
Constructivist
Constructivism is a subcategory of postpositivism. Most researchers invested in postpositivist research are constructivist as well, meaning they think there is no objective external reality that exists but rather that reality is constructed. Constructivism is a theoretical lens that emphasizes the dynamic nature of our world. “Constructivism contends that individuals’ views are directly influenced by their experiences, and it is these individual experiences and views that shape their perspective of reality”. [6] Essentially, Constructivist thought focuses on how ‘reality’ is not a fixed certainty and experiences, interactions, and backgrounds give people a unique view of the world. Constructivism contends, unlike in positivist views, that there is not necessarily an ‘objective’ reality we all experience. This is the ‘relativist’ ontological view that reality and the world we live in are dynamic and socially constructed. Therefore, qualitative scientific knowledge can be inductive as well as deductive.” [4]
So why is it important to understand the differences in assumptions that different philosophies and approaches to research have? Fundamentally, the assumptions underpinning the research tools a researcher selects provide an overall base for the assumptions the rest of the research will have and can even change the role of the researcher themselves. [2] For example, is the researcher an ‘objective’ observer such as in positivist quantitative work? Or is the researcher an active participant in the research itself, as in postpositivist qualitative work? Understanding the philosophical base of the research undertaken allows researchers to fully understand the implications of their work and their role within the research, as well as reflect on their own positionality and bias as it pertains to the research they are conducting.
Data Sampling
The better the sample represents the intended study population, the more likely the researcher is to encompass the varying factors at play. The following are examples of participant sampling and selection: [7]
- Purposive sampling- selection based on the researcher’s rationale in terms of being the most informative.
- Criterion sampling-selection based on pre-identified factors.
- Convenience sampling- selection based on availability.
- Snowball sampling- the selection is by referral from other participants or people who know potential participants.
- Extreme case sampling- targeted selection of rare cases.
- Typical case sampling-selection based on regular or average participants.
Data Collection and Analysis
Qualitative research uses several techniques including interviews, focus groups, and observation. [1] [2] [3] Interviews may be unstructured, with open-ended questions on a topic and the interviewer adapts to the responses. Structured interviews have a predetermined number of questions that every participant is asked. It is usually one on one and is appropriate for sensitive topics or topics needing an in-depth exploration. Focus groups are often held with 8-12 target participants and are used when group dynamics and collective views on a topic are desired. Researchers can be a participant-observer to share the experiences of the subject or a non-participant or detached observer.
While quantitative research design prescribes a controlled environment for data collection, qualitative data collection may be in a central location or in the environment of the participants, depending on the study goals and design. Qualitative research could amount to a large amount of data. Data is transcribed which may then be coded manually or with the use of Computer Assisted Qualitative Data Analysis Software or CAQDAS such as ATLAS.ti or NVivo. [8] [9] [10]
After the coding process, qualitative research results could be in various formats. It could be a synthesis and interpretation presented with excerpts from the data. [11] Results also could be in the form of themes and theory or model development.
Dissemination
To standardize and facilitate the dissemination of qualitative research outcomes, the healthcare team can use two reporting standards. The Consolidated Criteria for Reporting Qualitative Research or COREQ is a 32-item checklist for interviews and focus groups. [12] The Standards for Reporting Qualitative Research (SRQR) is a checklist covering a wider range of qualitative research. [13]
Examples of Application
Many times a research question will start with qualitative research. The qualitative research will help generate the research hypothesis which can be tested with quantitative methods. After the data is collected and analyzed with quantitative methods, a set of qualitative methods can be used to dive deeper into the data for a better understanding of what the numbers truly mean and their implications. The qualitative methods can then help clarify the quantitative data and also help refine the hypothesis for future research. Furthermore, with qualitative research researchers can explore subjects that are poorly studied with quantitative methods. These include opinions, individual's actions, and social science research.
A good qualitative study design starts with a goal or objective. This should be clearly defined or stated. The target population needs to be specified. A method for obtaining information from the study population must be carefully detailed to ensure there are no omissions of part of the target population. A proper collection method should be selected which will help obtain the desired information without overly limiting the collected data because many times, the information sought is not well compartmentalized or obtained. Finally, the design should ensure adequate methods for analyzing the data. An example may help better clarify some of the various aspects of qualitative research.
A researcher wants to decrease the number of teenagers who smoke in their community. The researcher could begin by asking current teen smokers why they started smoking through structured or unstructured interviews (qualitative research). The researcher can also get together a group of current teenage smokers and conduct a focus group to help brainstorm factors that may have prevented them from starting to smoke (qualitative research).
In this example, the researcher has used qualitative research methods (interviews and focus groups) to generate a list of ideas of both why teens start to smoke as well as factors that may have prevented them from starting to smoke. Next, the researcher compiles this data. The research found that, hypothetically, peer pressure, health issues, cost, being considered “cool,” and rebellious behavior all might increase or decrease the likelihood of teens starting to smoke.
The researcher creates a survey asking teen participants to rank how important each of the above factors is in either starting smoking (for current smokers) or not smoking (for current non-smokers). This survey provides specific numbers (ranked importance of each factor) and is thus a quantitative research tool.
The researcher can use the results of the survey to focus efforts on the one or two highest-ranked factors. Let us say the researcher found that health was the major factor that keeps teens from starting to smoke, and peer pressure was the major factor that contributed to teens to start smoking. The researcher can go back to qualitative research methods to dive deeper into each of these for more information. The researcher wants to focus on how to keep teens from starting to smoke, so they focus on the peer pressure aspect.
The researcher can conduct interviews and/or focus groups (qualitative research) about what types and forms of peer pressure are commonly encountered, where the peer pressure comes from, and where smoking first starts. The researcher hypothetically finds that peer pressure often occurs after school at the local teen hangouts, mostly the local park. The researcher also hypothetically finds that peer pressure comes from older, current smokers who provide the cigarettes.
The researcher could further explore this observation made at the local teen hangouts (qualitative research) and take notes regarding who is smoking, who is not, and what observable factors are at play for peer pressure of smoking. The researcher finds a local park where many local teenagers hang out and see that a shady, overgrown area of the park is where the smokers tend to hang out. The researcher notes the smoking teenagers buy their cigarettes from a local convenience store adjacent to the park where the clerk does not check identification before selling cigarettes. These observations fall under qualitative research.
If the researcher returns to the park and counts how many individuals smoke in each region of the park, this numerical data would be quantitative research. Based on the researcher's efforts thus far, they conclude that local teen smoking and teenagers who start to smoke may decrease if there are fewer overgrown areas of the park and the local convenience store does not sell cigarettes to underage individuals.
The researcher could try to have the parks department reassess the shady areas to make them less conducive to the smokers or identify how to limit the sales of cigarettes to underage individuals by the convenience store. The researcher would then cycle back to qualitative methods of asking at-risk population their perceptions of the changes, what factors are still at play, as well as quantitative research that includes teen smoking rates in the community, the incidence of new teen smokers, among others. [14] [15]
Qualitative research functions as a standalone research design or in combination with quantitative research to enhance our understanding of the world. Qualitative research uses techniques including structured and unstructured interviews, focus groups, and participant observation to not only help generate hypotheses which can be more rigorously tested with quantitative research but also to help researchers delve deeper into the quantitative research numbers, understand what they mean, and understand what the implications are. Qualitative research provides researchers with a way to understand what is going on, especially when things are not easily categorized. [16]
- Issues of Concern
As discussed in the sections above, quantitative and qualitative work differ in many different ways, including the criteria for evaluating them. There are four well-established criteria for evaluating quantitative data: internal validity, external validity, reliability, and objectivity. The correlating concepts in qualitative research are credibility, transferability, dependability, and confirmability. [4] [11] The corresponding quantitative and qualitative concepts can be seen below, with the quantitative concept is on the left, and the qualitative concept is on the right:
- Internal validity--- Credibility
- External validity---Transferability
- Reliability---Dependability
- Objectivity---Confirmability
In conducting qualitative research, ensuring these concepts are satisfied and well thought out can mitigate potential issues from arising. For example, just as a researcher will ensure that their quantitative study is internally valid so should qualitative researchers ensure that their work has credibility.
Indicators such as triangulation and peer examination can help evaluate the credibility of qualitative work.
- Triangulation: Triangulation involves using multiple methods of data collection to increase the likelihood of getting a reliable and accurate result. In our above magic example, the result would be more reliable by also interviewing the magician, back-stage hand, and the person who "vanished." In qualitative research, triangulation can include using telephone surveys, in-person surveys, focus groups, and interviews as well as surveying an adequate cross-section of the target demographic.
- Peer examination: Results can be reviewed by a peer to ensure the data is consistent with the findings.
‘Thick’ or ‘rich’ description can be used to evaluate the transferability of qualitative research whereas using an indicator such as an audit trail might help with evaluating the dependability and confirmability.
- Thick or rich description is a detailed and thorough description of details, the setting, and quotes from participants in the research. [5] Thick descriptions will include a detailed explanation of how the study was carried out. Thick descriptions are detailed enough to allow readers to draw conclusions and interpret the data themselves, which can help with transferability and replicability.
- Audit trail: An audit trail provides a documented set of steps of how the participants were selected and the data was collected. The original records of information should also be kept (e.g., surveys, notes, recordings).
One issue of concern that qualitative researchers should take into consideration is observation bias. Here are a few examples:
- Hawthorne effect: The Hawthorne effect is the change in participant behavior when they know they are being observed. If a researcher was wanting to identify factors that contribute to employee theft and tells the employees they are going to watch them to see what factors affect employee theft, one would suspect employee behavior would change when they know they are being watched.
- Observer-expectancy effect: Some participants change their behavior or responses to satisfy the researcher's desired effect. This happens in an unconscious manner for the participant so it is important to eliminate or limit transmitting the researcher's views.
- Artificial scenario effect: Some qualitative research occurs in artificial scenarios and/or with preset goals. In such situations, the information may not be accurate because of the artificial nature of the scenario. The preset goals may limit the qualitative information obtained.
- Clinical Significance
Qualitative research by itself or combined with quantitative research helps healthcare providers understand patients and the impact and challenges of the care they deliver. Qualitative research provides an opportunity to generate and refine hypotheses and delve deeper into the data generated by quantitative research. Qualitative research does not exist as an island apart from quantitative research, but as an integral part of research methods to be used for the understanding of the world around us. [17]
- Enhancing Healthcare Team Outcomes
Qualitative research is important for all members of the health care team as all are affected by qualitative research. Qualitative research may help develop a theory or a model for health research that can be further explored by quantitative research. Much of the qualitative research data acquisition is completed by numerous team members including social works, scientists, nurses, etc. Within each area of the medical field, there is copious ongoing qualitative research including physician-patient interactions, nursing-patient interactions, patient-environment interactions, health care team function, patient information delivery, etc.
- Review Questions
- Access free multiple choice questions on this topic.
- Comment on this article.
Disclosure: Steven Tenny declares no relevant financial relationships with ineligible companies.
Disclosure: Janelle Brannan declares no relevant financial relationships with ineligible companies.
Disclosure: Grace Brannan declares no relevant financial relationships with ineligible companies.
This book is distributed under the terms of the Creative Commons Attribution-NonCommercial-NoDerivatives 4.0 International (CC BY-NC-ND 4.0) ( http://creativecommons.org/licenses/by-nc-nd/4.0/ ), which permits others to distribute the work, provided that the article is not altered or used commercially. You are not required to obtain permission to distribute this article, provided that you credit the author and journal.
- Cite this Page Tenny S, Brannan JM, Brannan GD. Qualitative Study. [Updated 2022 Sep 18]. In: StatPearls [Internet]. Treasure Island (FL): StatPearls Publishing; 2024 Jan-.
In this Page
Bulk download.
- Bulk download StatPearls data from FTP
Related information
- PMC PubMed Central citations
- PubMed Links to PubMed
Similar articles in PubMed
- Suicidal Ideation. [StatPearls. 2024] Suicidal Ideation. Harmer B, Lee S, Duong TVH, Saadabadi A. StatPearls. 2024 Jan
- Folic acid supplementation and malaria susceptibility and severity among people taking antifolate antimalarial drugs in endemic areas. [Cochrane Database Syst Rev. 2022] Folic acid supplementation and malaria susceptibility and severity among people taking antifolate antimalarial drugs in endemic areas. Crider K, Williams J, Qi YP, Gutman J, Yeung L, Mai C, Finkelstain J, Mehta S, Pons-Duran C, Menéndez C, et al. Cochrane Database Syst Rev. 2022 Feb 1; 2(2022). Epub 2022 Feb 1.
- Macromolecular crowding: chemistry and physics meet biology (Ascona, Switzerland, 10-14 June 2012). [Phys Biol. 2013] Macromolecular crowding: chemistry and physics meet biology (Ascona, Switzerland, 10-14 June 2012). Foffi G, Pastore A, Piazza F, Temussi PA. Phys Biol. 2013 Aug; 10(4):040301. Epub 2013 Aug 2.
- Review Evidence Brief: The Effectiveness Of Mandatory Computer-Based Trainings On Government Ethics, Workplace Harassment, Or Privacy And Information Security-Related Topics [ 2014] Review Evidence Brief: The Effectiveness Of Mandatory Computer-Based Trainings On Government Ethics, Workplace Harassment, Or Privacy And Information Security-Related Topics Peterson K, McCleery E. 2014 May
- Review Public sector reforms and their impact on the level of corruption: A systematic review. [Campbell Syst Rev. 2021] Review Public sector reforms and their impact on the level of corruption: A systematic review. Mugellini G, Della Bella S, Colagrossi M, Isenring GL, Killias M. Campbell Syst Rev. 2021 Jun; 17(2):e1173. Epub 2021 May 24.
Recent Activity
- Qualitative Study - StatPearls Qualitative Study - StatPearls
Your browsing activity is empty.
Activity recording is turned off.
Turn recording back on
Connect with NLM
National Library of Medicine 8600 Rockville Pike Bethesda, MD 20894
Web Policies FOIA HHS Vulnerability Disclosure
Help Accessibility Careers
- Open access
- Published: 27 March 2024
Threshold-modifying effect of the systemic inflammatory response index on kidney function decline in hypertensive patients
- Xing Wei 1 , 2 ,
- Jing Wei 1 , 2 ,
- Jun Feng 1 ,
- Chao Li 1 ,
- Zhipeng Zhang 1 , 2 ,
- Ben Hu 1 , 2 ,
- Nv Long 1 , 2 &
- Chunmiao Luo 1 , 2
European Journal of Medical Research volume 29 , Article number: 202 ( 2024 ) Cite this article
Metrics details
Chronic kidney disease (decreased kidney function) is common in hypertensive patients. The SIRI is a novel immune biomarker. We investigated the correlation between the SIRI and kidney function in hypertensive patients.
The present study analyzed data from participants who suffered from hypertension in the NHANES from 2009 to 2018. Multivariate regression analysis and subgroup analysis were used to clarify whether the SIRI was an independent risk factor for decreased kidney function. RCSs were utilized to evaluate the correlation between the SIRI and the eGFR and between the SIRI and the ACR. In addition, we modeled the mediating effect of the SIRI on the eGFR and the ACR using blood pressure as a mediating variable.
The highest SIRI was an independent risk factor for a decreased eGFR [odds ratio (OR) = 1.46, 95% CI (1.15, 1.86)] and an increased ACR [OR = 2.26, 95% CI (1.82, 2.82)] when the lowest quartile was used as the reference. The RCS results indicated an inverted U-shaped relationship between the SIRI and the eGFR and between the SIRI and the ACR (the inflection points were 1.86 and 3.09, respectively). The mediation effect analysis revealed that the SIRI was the main factor influencing kidney function, and diastolic blood pressure was a mediating variable. In particular, there was a fully mediating effect between the SIRI and UCr, with a mediating effect value of -0.61 (-0.90, -0.36).
Conclusions
The association between the SIRI and renal function in hypertensive patients was significant and was particularly dominated by the association between the SIRI and the ACR. This difference may be due to the mediating effect of diastolic blood pressure.
Introduction
Hypertension is a significant determinant of kidney disease progression and is exacerbated by kidney failure [ 1 ]. In addition, both hypertension and chronic kidney disease (CKD) are independent risk factors for cardiovascular disease (CVD), and the morbidity and mortality of CVD significantly increase when both coexist [ 2 , 3 ]. Multiple mechanisms contribute to the development of hypertensive nephropathy; as the estimated glomerular filtration rate (eGFR) decreases, the renin–angiotensin–aldosterone system is upregulated, promoting sodium retention and increasing blood pressure, exacerbating hypertensive kidney damage [ 2 , 4 ]. In addition, several factors, including oxidative stress and the resulting relative renal hypoxia, may further exacerbate the development of hypertension and CKD [ 5 ]. CKD is characterized by reduced renal function, defined as an eGFR of less than 60 ml/min/1.73 m 2 and/or a urinary albumin (UAlb)-to-creatinine ratio (ACR) of more than 30 mg/g for more than three months [ 6 , 7 ]. A decreased eGFR reflects the extent of kidney damage [ 8 ], and increased UAlb excretion is an independent predictor of the progression of kidney damage [ 9 ]. Proteinuria quantification can also stratify the risk of kidney damage and can be utilized as a marker of response to treatment [ 10 ]. Several studies have shown that the ACR is superior to 24-h UAlb excretion for predicting CKD [ 11 ]. CKD affects many adults worldwide, and a persistent chronic low-grade inflammatory state is a significant contributor to its development and progression [ 12 ]. The immune-inflammatory mechanism is now recognized as a component of the multifactorial etiology of hypertension and related organ damage [ 13 ]. Chronic low-grade inflammation leads to elevated blood pressure in various experimental models of hypertension, resulting in target organ damage [ 14 ]. The systemic inflammatory response index (SIRI) has been proposed as a novel inflammatory biomarker involving single inflammatory markers such as neutrophils, monocytes, and lymphocytes that can more accurately predict poor prognosis in patients with GI tumors [ 15 , 16 ]. Previous studies have shown that induction of neutrophil apoptosis by CD39 on regulatory T cells during RAAS activation attenuates hypertension, suggesting that neutrophils may be one of the factors contributing to elevated blood pressure [ 17 ]. Furthermore, monocytes can release pro-inflammatory cytokines, such as IL-6, IL-1β, and TNF-α [ 18 ]. All of the above inflammatory response mechanisms can play a role in promoting the development of hypertension. Chronic low-grade inflammation has been associated with a variety of health outcomes, and recent investigations have shown that SIRI is related to the development of all-cause mortality and cardiovascular mortality in adults [ 19 ]. However, there are no studies on the association between the SIRI and renal impairment in hypertensive patients, and this study aimed to investigate the association between the SIRI and kidney function in adult hypertensive patients in the U.S. using data from the National Health and Nutrition Examination Survey (NHANES).
Materials and methods
Research population.
We investigated a total of 49,693 participants in five cycles of the NHANES from 2009 through 2018. Among these participants, those who met the following criteria were excluded from the analysis: individuals with missing creatinine (Cr) and ACR data; pregnant individuals and female individuals who could not specify whether they were pregnant or not pregnant; adolescent individuals aged less than 20 years old; nonhypertensive individuals; and individuals with missing data on race, marital status, education level, BMI, and personal history. The final sample consisted of 5446 individuals (Fig. 1 ).
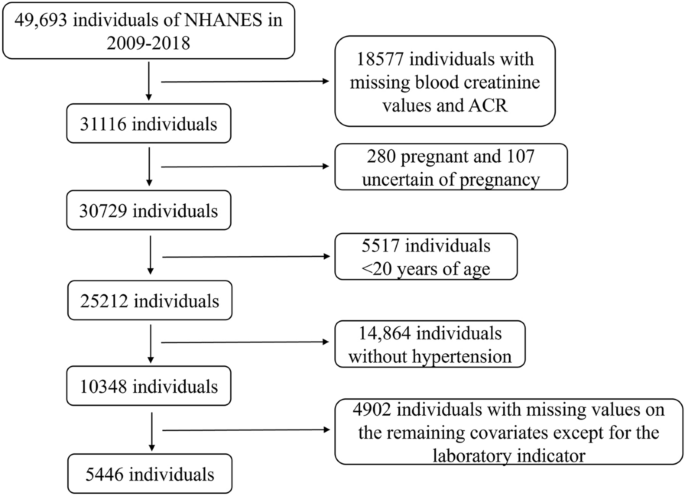
Research flow chart
NHANES is a nationally representative survey of the general population conducted by the National Center for Health Statistics (NCHS). Detailed data is obtainable at the https://www.cdc.gov/nchs/nhanes/ . All study protocols of NHANES were approved by the Research Ethics Review Board of the NCHS. The study was exempt from ethical review because the NHANES database is open to the public. All participants provided written informed consent. The study adheres to the Declaration of Helsinki.
Exposure variables and outcome variables
Routine blood samples from the study participants were analyzed via automated hematology analysis equipment. The exposure variable (the SIRI) was calculated as (neutrophil count) × (monocyte count)/(lymphocyte count) [ 15 ].
The primary outcome of this study was an eGFR ≤ 60 mL/minute/1.73 m 2 [The glomerular filtration rate was estimated using the following formula [ 20 ]: 175 × Scr −1.154 × age −0.203 × 1.212 (if black) × 0.742 (if female)]. The secondary outcome was defined as an ACR ≥ 30 mg/g. Serum Cr concentrations were analyzed by the Jaffe rate method with a Beckman UniCel DxC800 Synchron (Beckman, Fullerton, CA, USA). UAlb was measured from a spot sample using a solid-phase fluorescent immunoassay. Urinary creatinine (UCr) was measured using Roche/Hitachi Modular P Chemistry and Roche/Hitachi Cobas 6000 chemistry Analyzer using an enzymatic (creatinase) method immunoassay.
Covariates and definitions
Baseline demographic information about the participants in this study was obtained from demographic data. History of alcohol consumption, smoking, diabetes, and hypertension were obtained from the participants’ questionnaires. Regarding laboratory indices that may be potential confounders, platelet (PLT), hemoglobin (Hb), alanine aminotransferase (ALT), aspartate aminotransferase (AST), high-density lipoprotein cholesterol (HDL-C), total cholesterol (TC), triglycerides (TG), albumin (ALB), glucose, and glycosylated hemoglobin (HbA1c) were collected in this study.
Hypertension was defined as 1. having been explicitly told that they had hypertension previously, 2. being on antihypertensive medication, 3. we calculated the mean of systolic and diastolic blood pressure (DBP) using the patient’s three resting state measurements for participants who did not have a history of this condition or who were taking medication for this condition, and we chose to use the mean of the participant’s blood pressure from the first two resting state measurements for participants who had a third missing measurement, and were defined as having high blood pressure when the participants were defined as hypertensive when the mean systolic blood pressure (SBP) was ≥ 140 mmHg or the mean DBP was ≥ 90 mmHg. Definition of diabetes mellitus: 1. fasting blood glucose ≥ 11.1 mmol/L, 2. HbA1c ≥ 6.5%, 3. previous notification of diabetes mellitus, and 4. ongoing insulin use. Smoking history was defined as “Never” for participants who had not smoked more than 100 cigarettes in their lifetime, "Smoking former" for those who had smoked more than 100 cigarettes in their lifetime but were no longer current smokers, and “Smoking now” for those who were current smokers. Drinking history was defined as “Rarely drinker” for participants who had not consumed 12 drinks in their lifetime, “Light drinker” for participants who had consumed more than 12 drinks in their lifetime but not more than 12 drinks in a year, and “Excessive drinker” for participants who had consumed more than 12 drinks in a year.
This investigation was divided into two groups based on the primary outcome event (eGFR ≤ 60 mL/minute/1.73 m 2 and eGFR > 60 mL/minute/1.73 m 2 ). It was divided into two groups based on secondary outcome (ACR < 30 mg/g and ACR ≥ 30 mg/g). Participants were categorized into four grades based on SIRI level quartiles: Q1 ( n = 1361, SIRI ≤ 0.73), Q2 ( n = 1362, 0.73 < SIRI ≤ 1.11), Q3 ( n = 1361, 1.11 < SIRI ≤ 1.66), and Q4 ( n = 1362, SIRI > 1.66).
Statistical analysis
In this research, all continuous numerical variables are expressed as medians (quartiles) after the normality test and were analyzed using the nonparametric rank-sum test. Categorical variables were compared using the Chi-square test. Binary logistic regression analyses were performed with group Q1 as the reference group. In the regression model with eGFR ≤ 60 mL/minute/1.73 m 2 as the endpoint event, the minimum-adjusted model was adjusted for age, sex, smoking status, drinking status, history of diabetes, race, education, and marital status. Fully adjusted models were adjusted for age, sex, smoking status, drinking status, history of diabetes mellitus, race, education, marriage, BMI, HDL-C, ALT, AST, TG, TC, ALB, PLT, Hb, glucose, and HbA1c. In regression models with ACR ≥ 30 mg/g as the outcome, the minimally adjusted model was adjusted for age, sex, smoking history, drinking history, history of diabetes mellitus, race, education level, and marital status, and the fully adjusted model was adjusted for age, sex, smoking history, drinking history, history of diabetes mellitus, race, education level, marital status, BMI, ALT, HDL-C, TG, TC, ALB, PLT, Hb, glucose, and HbA1c. The median of the four sets of SIRI was taken for a dummy variable setting and then tested for trend. We utilized the restricted cubic spline curves (RCS) to fit smoothed curves and perform threshold effects analysis (the adjustment strategy was the same as the fully adjusted regression model). This was followed by interaction tests in subgroup analyses. To further investigate the relationship between SIRI, blood pressure, and renal function, we developed a mediated effect model using SBP and DBP as mediating variables. In addition, we performed sensitivity analyses for two populations (1. Excluding those with recent-onset hypertension. 2. Excluding those with current blood pressure below 140/90 mmHg). This study discarded laboratory indicators with missing values > 20%, and for laboratory indicators with missing values < 20%, this study used multiple sampling interpolation to interpolate the variables. In this study, we used unweighted estimation. Because this study limited the inclusion members to the hypertensive population, after excluding participants who did not meet the inclusion criteria, the inclusion members were unevenly distributed from cycle to cycle, resulting in large variations in the weights of the combined samples. Unweighted estimation is recommended when sample weights vary significantly or when covariates used to calculate weights (such as age, gender, and race) are already included in the regression model [ 21 ]. Statistical analyses were performed using R Studio (version R4.2.3) and EmpowerStats (version 4.1). All tests were two-tailed, and P < 0.05 was considered to indicate statistical significance.
Comparison of participants' baseline information
This study enrolled 5446 participants, including 2721 males (49.96%, mean age 58.22 ± 15.31 years) and 2725 females (50.04%, mean age 60.43 ± 14.39 years). eGFR levels increased by race, education level, and marital status, and were lower in older, female, diabetic, and non-smoking drinkers. ACR levels increased by race, education level, and marital status. ACR levels increased significantly with advancing age, and albuminuria was more common in diabetes, nonsmoking participants, and participants who consumed alcohol. In addition, The SIRI was substantially greater in patients with a low eGFR and albuminuria (Table 1 ). Compared with those in the low-level group, patients in the high-SIRI group were generally older; had a greater proportion of males; had a greater prevalence of obesity, diabetes, smoking and drinking; were non-Hispanic white; were more likely to have elevated platelet counts and hemoglobin, triglyceride and fasting glucose levels; were more likely to have a greater ACR; and were more likely to have lower high-density lipoprotein cholesterol, total cholesterol and ALB levels as well as a lower eGFR (Additional file 1 : Table S1).
Correlation between the SIRI and kidney function
Among the two unadjusted univariate regression models with an eGFR ≤ 60 mL/minute/1.73 m 2 and an ACR ≥ 30 mg/g as the outcome event, the SIRI was associated with a decrease in the eGFR (≤ 60 mL/minute/1.73 m 2 ) (Q1 vs. Q2: HR, 0.99 [95% CI 0.80, 1.23]; Q3: HR, 1.18 [95% CI 0.96, 1.45]; Q4: HR, 1.70 [95% CI 1.40, 2.07]; P for trend < 0.001), elevated ACR (≥ 30 mg/g) (Q1 vs. Q2: HR, 1.22 [95% CI 1.00, 1.50]; Q3: HR, 1.44 [95% CI 1.18, 1.76]; Q4: HR, 2.29 [95% CI 1.89, 2.76]; P for trend < 0.001) were associated. The results of the regression models adjusted for all confounders were consistent, with a high SIRI associated with a decreased eGFR (≤ 60 mL/minute/1.73 m2) (Q1 vs. Q4: HR, 1.46 [95% CI 1.15, 1.86]) and an elevated ACR (≥ 30 mg/g) (Q1 vs. Q4: HR, 2.26 [95% CI 1.82, 2.82]) (Table 2 ).
The RCS results showed that the SIRI was associated with an inverted U-shaped nonlinear increase in the risk of both a decreased eGFR ( P = 0.014) and an elevated ACR ( P < 0.001) (the adjustment strategy was the same as that for the fully adjusted logistic regression model) (Fig. 2 ). We further performed a threshold effect analysis, which revealed that in the standard linear regression model, SIRI was associated with a decrease in the eGFR ( P = 0.0451) and an increase in the ACR ( P < 0.001), respectively; after adjusting for confounders, we built a two-stage linear regression model, which detected an inverted “U” shape with inflection points of 1.86 and 3.09 for the eGFR and the ACR, respectively (Table 3 ).
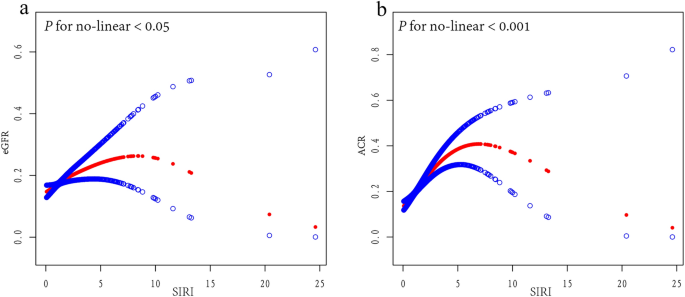
Restricted cubic spline curve. The adjustment strategy is the same as the fully adjusted regression model. a Fitting a smoothed curve with SIRI as the independent variable and eGFR as the dependent variable results in an inverted U-shaped curve (inflection point 1.86). b Fitting a smoothed curve with SIRI as the independent variable and ACR as the dependent variable results in an inverted U-shaped curve (inflection point 3.09)
Subgroup analysis
In subgroup analyses, the SIRI was significantly associated with a decreased eGFR in patients with advanced age, female sex, high BMI, diabetes mellitus status, nonsmoking status, and consumption of alcohol, with interactions showing that the results were robust (Additional file 1 : Table S2). In addition, the SIRI was significantly associated with an elevated ACR in individuals who were older, male, had a high BMI, were married, had diabetes mellitus, and consumed alcohol. According to the analyses of the other subgroups excluding alcohol consumption, the interaction results remained robust (Additional file 1 : Table S3).
Mediation effect analysis with blood pressure as a mediating variable
Correlation analysis revealed that the SIRI, SBP, and DBP were weakly correlated with UAlb, UCr, the ACR, and the eGFR (All correlation coefficients r < 0.2) (Fig. 3 a). Among those correlations, SBP had weak positive correlations with the ACR ( r = 0.15, P < 0.001), Cr ( r = 0.04, P < 0.01), and UAlb ( r = 0.14, P < 0.001) and negative correlations with the eGFR ( r = − 0.05, P < 0.001) and UCr (r = -0.08, P < 0.001); DBP was negatively correlated with the eGFR ( r = 0.17, P < 0.001) and UCr ( r = 0.09, P < 0.001) and had weak positive correlations with the SIRI ( r = − 0.09, P < 0.001) and Cr ( r = − 0.07, P < 0.001) (Fig. 3 b).
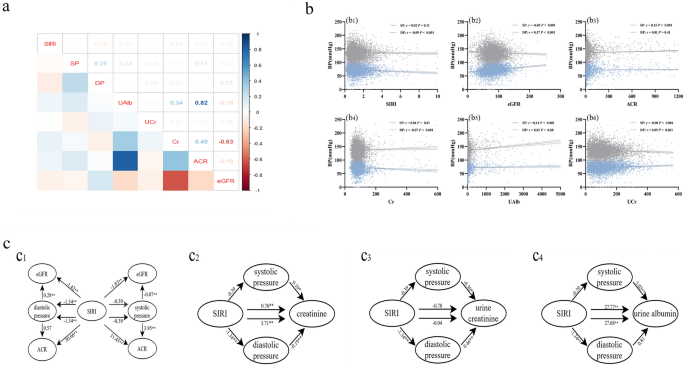
Analysis of intermediation effects . r: correlation coefficient, *: P < 0.05, **: P < 0.01, ***: P < 0.001。 SIRI Systemic Inflammation Response Index, SBP systolic blood pressure, DBP diastolic blood pressure, UAlb urine albumin, UCr urinary creatinine, Cr creatinine, ACR Urine Albumin Creatinine Ratio, eGFR estimated glomerular filtration rate. a Correlation analysis between SIRI, SBP, DBP and kidney function indicators; b Scatter plot of correlation between SBP and DBP as dependent variables and SIRI, eGFR, ACR, Cr, UAlb, UCr; c All mediated effects models had SIRI as the independent variable and systolic and diastolic blood pressure as the mediating variables. Model c1 had eGFR and ACR as dependent variables, model c2 had Cr as dependent variable, model c3 had UCr as dependent variable, and model c4 had UAlb as dependent variable
Based on the results, we conducted a mediation effect analysis with the SIRI as the independent variable; SBP and DBP as the mediating variables; and the eGFR, the ACR, Cr, UCr, and UAlb as the dependent variables in this study. The results indicated that a direct effect was observed between the SIRI and the eGFR ( β = − 1.83, P < 0.01) and between the SIRI and the ACR ( β = 31.45, P < 0.01) in the model with SBP as the mediating variable. In the model with DBP as the mediating variable, there was a partial mediating effect between the SIRI and the eGFR, with a mediating effect value of − 0.38 (− 0.53, − 0.25), which accounted for 0.21 of the total impact; however, there was a direct effect model between the SIRI and the ACR ( β = 30.66, P < 0.01). We further tested the mediating effects with Cr, UCr, and UAlb selected as the dependent variables. The results indicated that DBP partially mediated the effect between the SIRI and Cr, and its mediating effect was 0.25 (0.11, 0.43), accounting for 0.06 of the total effect ratio; in addition, DBP and fully mediated the effect between the SIRI and UCr, with a mediating effect of − 0.61 (− 0.90, − 0.36). The rest of the models showed all direct effects and no mediating effects (Fig. 3 c).
Sensitivity analysis
Sensitivity analyses were performed in this investigation.1. We excluded people with new-onset hypertension. The results showed that the risk of a decreased eGFR and the risk of an increased ACR associated with the SIRI were greater in the fully adjusted model (eGFR: Q1vs. Q4 (OR, 1.37 [95% CI 1.06, 1.75])), (ACR: Q1vs. Q4 (OR, 1.37 [95% CI 1.06, 1.75])) (Additional file 1 : Table S4). 2. We performed regression analyses again for those with mean blood pressure higher than 140/90 mmHg in this research. The results showed that the SIRI was more strongly correlated with a decrease in the eGFR according to the fully adjusted regression model (Q1vs. Q4 (OR, 1.81 [95% CI 1.25, 2.62]) (Additional file 1 : Table S5). 3. We performed propensity score matching based on confounders between the SIRI and the outcomes. As shown in Additional file 1 : Tables S6 and S7, the results remain solid, and the difference in SIRI between the two groups is statistically significant ( P < 0.001).
In the present study, we demonstrated that a high SIRI was strongly associated with kidney insufficiency in hypertensive patients. The correlation coefficient remained significant after adjusting for confounding factors such as baseline population information and laboratory data. We further fitted the smoothed curves and found that the SIRI had inverted U-shaped relationships with a decreased eGFR and an increased ACR in hypertensive patients (inflection points of 1.86 and 3.09, respectively). In the subgroup analyses, we applied an identical adjustment strategy, and the results were robust. In addition, to further analyze the association between the SIRI, blood pressure, and kidney function, we performed a mediation effect analysis. The results revealed that in the model with DBP as the mediating variable, DBP had a partial mediating effect on the relationship between the SIRI and the eGFR. In addition, DBP had a partial mediating effect on the relationship between the SIRI and Cr. Moreover, DBP fully mediated the relationship between the SIRI and UCr. This finding implies that the SIRI may modulate kidney function through DBP in hypertensive patients. However, we did not observe the same effect on SBP. This deserves further study. The pathophysiology of hypertensive kidney injury is complex and results from a variety of factors. Chronic low-grade inflammation, reduced number of kidney units, expansion of extracellular fluid volume due to increased water and sodium retention, overactivity of the sympathetic and RASS systems, and endothelial dysfunction have been suggested as potential mechanisms [ 12 , 22 , 23 ]. Meanwhile, kidney injury, loss of functional kidney units, and increased RASS activity greatly increase the salt sensitivity of blood pressure, which can contribute to further elevation of blood pressure, thus creating a vicious cycle. These changes within the microcirculation eventually result in chronic ischemia and fibrosis and are characterized by increased proteinuria and blood creatinine [ 24 ].
Many diseases, such as metabolic syndrome, cardiovascular disease, and diabetes mellitus, are associated with chronic low-grade inflammation [ 25 ]. Distinct inflammatory markers each play different roles in the kidney. TNF-α activates the endothelial inflammatory response, leading to capillary leakage and allowing entry of immune cells. Simultaneously, immune cells (monocytes and macrophages) are attracted to MCP-1. During this time, neutrophils are chemotactically attracted to IL-8, and IL-23 upregulates the proliferation of Th17 cells, which triggers a more proinflammatory response [ 26 ]. The SIRI integrates neutrophils, monocytes, and lymphocytes, reflecting the interaction between immunity and inflammation [ 15 , 19 ]. In a retrospective cohort study from China on the SIRI and long-term outcomes of patients with type B aortic dissection, when the researchers adjusted for confounders, they identified a significant correlation between the SIRI and poor prognosis [ 27 ]. One study demonstrated that the SIRI can be used as a predictor of stroke risk in elderly hypertensive patients [ 28 ]. In addition, in a recent retrospective cohort study, researchers used the MIMIC-III database to analyze the predictive value of SIRI in the prognosis of patients with stroke, and the study showed that, after adjusting for several covariates, SIRI was correlated with all-cause mortality of patients with stroke, and as the SIRI rises, the mortality rate also increases [ 29 ]. This finding demonstrated that the SIRI is related not only to the occurrence of stroke but also to the prognosis of stroke. The above studies suggest that the SIRI is closely related to cardiovascular and cerebrovascular diseases. Two recent retrospective analyses of diabetic patients suggest that the SIRI is an independent risk factor for deteriorating kidney function [ 30 , 31 ]. In earlier studies, increased neutrophil and monocyte counts as well as decreased lymphocyte counts were associated with decreased kidney function. This may clarify the relationship between the SIRI and kidney function [ 32 , 33 , 34 ]. In addition, in a prospective clinical study exploring postoperative metastasis of kidney cell carcinoma, researchers found that the SIRI had high predictive value for metastasis (AUC of 0.737) [ 35 ]. However, there are no studies on the correlation between the SIRI and renal insufficiency in hypertensive patients.
Under pathological conditions, increased production of reactive oxygen species (ROS) triggers oxidative stress, which plays a role in vascular changes associated with hypertension, including endothelial dysfunction, vascular reactivity, and arterial remodeling [ 36 ]. Furthermore, oxidative stress is associated with inflammation, as ROS production activates inflammatory cells and enhances the production of inflammatory mediators. Relatively, inflammation increases ROS release, creating a vicious cycle [ 37 ]. ROS has an undertaking relationship between blood pressure and inflammation. And there is an intrinsic correlation between blood pressure and kidney function. Above-target SBP or no nocturnal drop is associated with a higher risk of cardiovascular and kidney disease progression in patients with CKD, regardless of ambulatory blood pressure, a multicenter cohort study suggests [ 38 ]. The high prevalence of hypertension at all stages of CKD and the dual benefit of effective antihypertensive therapy in reducing renal and cardiovascular risk amply demonstrate the bidirectional relationship between hypertension and renal function 1 .
An intrinsic link exists between inflammation, blood pressure, and kidney function. We employed mediation effect analysis to clarify the relationship between the SIRI, blood pressure, and renal function. In the mediation effect analysis, it was observed that DBP mediated the relationship between the SIRI and kidney function, playing a fully mediating role in the association between the SIRI and UCr. These findings indicate that changes in DBP play a more critical role in the decline in renal function in hypertensive patients. In the present study, we noticed a more significant correlation between the SIRI and the ACR than between the SIRI and the eGFR. An elevated ACR is a marker of early kidney disease [ 10 ]. The above analysis revealed that early in the disease course, special attention should be given to the SIRI in hypertensive patients.
This research has several limitations. First, we used only the mean of three blood pressure measurements for each of the participants as the study variable in the mediation effect analysis, but to accurately assess the mediating effect of participants’ blood pressure, ambulatory blood pressure values should have been used as the study variable. Second, the participants included in the analysis of this study were all hypertensive patients, and some of them may have taken relevant medications for antihypertensive purposes, which is a confounding factor, but we had no way of knowing the application of appropriate medications by the participants. Third, the blood cell-based assay was performed only once, and the concentration of these blood cells may be affected by other factors and changes. We believe that further relevant cohort studies are necessary to explore the associations of the SIRI with blood pressure and kidney function in hypertensive patients.
The present investigation confirmed a correlation between the SIRI and kidney function in hypertensive patients. This difference may be mediated by increases in diastolic blood pressure leading to decreased kidney function. These findings indicate that immune inflammation should be treated in hypertensive patients.
Data availability statement
This study analyzed datasets from the publicly available database NHANES from 2009 to 2018. These data can be found here: https://www.cdc.gov/nchs/nhanes/ .
Abbreviations
Systemic inflammatory response index
National Health and Nutrition Examination Survey
National Center for Health Statistics
Estimated glomerular filtration rate
Albumin creatinine ratio
Urinary albumin
Urinary creatinine
Chronic kidney disease
Cardiovascular disease
Alanine aminotransferase
Aspartate aminotransferase
High-density lipoprotein cholesterol
Total cholesterol
Triglycerides
Glycosylated hemoglobin
Diastolic blood pressure
Systolic blood pressure
Restricted cubic spline curves
Confidence interval
Burnier M, Damianaki A. Hypertension as cardiovascular risk factor in chronic kidney disease. Circ Res. 2023;132(8):1050–63.
Article CAS PubMed Google Scholar
Pugh D, Gallacher PJ, Dhaun N. Management of hypertension in chronic kidney disease. Drugs. 2019;79(4):365–79.
Article PubMed PubMed Central Google Scholar
Gansevoort RT, Correa-Rotter R, Hemmelgarn BR, Jafar TH, Heerspink HJ, Mann JF, Matsushita K, Wen CP. Chronic kidney disease and cardiovascular risk: epidemiology, mechanisms, and prevention. Lancet (London, England). 2013;382(9889):339–52.
Article PubMed Google Scholar
Maaliki D, Itani MM, Itani HA. Pathophysiology and genetics of salt-sensitive hypertension. Front Physiol. 2022;13:1001434.
Fine LG, Norman JT. Chronic hypoxia as a mechanism of progression of chronic kidney diseases: from hypothesis to novel therapeutics. Kidney Int. 2008;74(7):867–72.
Stevens PE, Levin A. Evaluation and management of chronic kidney disease: synopsis of the kidney disease: improving global outcomes 2012 clinical practice guideline. Ann Intern Med. 2013;158(11):825–30.
Murton M, Goff-Leggett D, Bobrowska A, Garcia Sanchez JJ, James G, Wittbrodt E, Nolan S, Sörstadius E, Pecoits-Filho R, Tuttle K. Burden of chronic kidney disease by kdigo categories of glomerular filtration rate and albuminuria: a systematic review. Adv Ther. 2021;38(1):180–200.
Kibria GMA, Crispen R. Prevalence and trends of chronic kidney disease and its risk factors among US adults: an analysis of NHANES 2003–18. Prev Med Rep. 2020;20: 101193.
Matsushita K, van der Velde M, Astor BC, Woodward M, Levey AS, de Jong PE, Coresh J, Gansevoort RT. Association of estimated glomerular filtration rate and albuminuria with all-cause and cardiovascular mortality in general population cohorts: a collaborative meta-analysis. Lancet. 2010;375(9731):2073–81.
Qin Z, Li H, Wang L, Geng J, Yang Q, Su B, Liao R. Systemic immune-inflammation index is associated with increased urinary albumin excretion: a population-based study. Front Immunol. 2022;13: 863640.
Article CAS PubMed PubMed Central Google Scholar
Lambers Heerspink HJ, Gansevoort RT, Brenner BM, Cooper ME, Parving HH, Shahinfar S, de Zeeuw D. Comparison of different measures of urinary protein excretion for prediction of renal events. J Am Soc Nephrol. 2010;21(8):1355–60.
Kadatane SP, Satariano M, Massey M, Mongan K, Raina R. The role of inflammation in CKD. Cells. 2023;12(12):1581.
Deussen A, Kopaliani I. Targeting inflammation in hypertension. Curr Opin Nephrol Hypertens. 2023;32(2):111–7.
Lu X, Crowley SD. Inflammation in salt-sensitive hypertension and renal damage. Curr Hypertens Rep. 2018;20(12):103.
Geng Y, Zhu D, Wu C, Wu J, Wang Q, Li R, Jiang J, Wu C. A novel systemic inflammation response index (SIRI) for predicting postoperative survival of patients with esophageal squamous cell carcinoma. Int Immunopharmacol. 2018;65:503–10.
Xie QK, Chen P, Hu WM, Sun P, He WZ, Jiang C, Kong PF, Liu SS, Chen HT, Yang YZ, Wang D, Yang L, Xia LP. The systemic immune-inflammation index is an independent predictor of survival for metastatic colorectal cancer and its association with the lymphocytic response to the tumor. J Transl Med. 2018;16(1):273.
Fabbiano S, Menacho-Márquez M, Robles-Valero J, Pericacho M, Matesanz-Marín A, García-Macías C, Sevilla MA, Montero MJ, Alarcón B, López-Novoa JM, Martín P, Bustelo XR. Immunosuppression-independent role of regulatory T cells against hypertension-driven renal dysfunctions. Mol Cell Biol. 2015;35(20):3528–46.
Loperena R, Van Beusecum JP, Itani HA, Engel N, Laroumanie F, Xiao L, Elijovich F, Laffer CL, Gnecco JS, Noonan J, Maffia P, Jasiewicz-Honkisz B, Czesnikiewicz-Guzik M, Mikolajczyk T, Sliwa T, Dikalov S, Weyand CM, Guzik TJ, Harrison DG. Hypertension and increased endothelial mechanical stretch promote monocyte differentiation and activation: roles of STAT3, interleukin 6 and hydrogen peroxide. Cardiovasc Res. 2018;114(11):1547–63.
Xia Y, Xia C, Wu L, Li Z, Li H, Zhang J. Systemic immune inflammation index (SII), system inflammation response index (siri) and risk of all-cause mortality and cardiovascular mortality: a 20-year follow-up cohort study of 42,875 US adults. J Clin Med. 2023;12(3):1128.
Levey AS, Coresh J, Greene T, Stevens LA, Zhang YL, Hendriksen S, Kusek JW, Van Lente F. Using standardized serum creatinine values in the modification of diet in renal disease study equation for estimating glomerular filtration rate. Ann Intern Med. 2006;145(4):247–54.
Kim S, Kim S, Won S, Choi K. Considering common sources of exposure in association studies—urinary benzophenone-3 and DEHP metabolites are associated with altered thyroid hormone balance in the NHANES 2007–2008. Environ Int. 2017;107:25–32.
Hall JE, de Carmo JM, da Silva AA, Wang Z, Hall ME. Obesity, kidney dysfunction and hypertension: mechanistic links. Nature reviews. Nephrology. 2019;15(6):367–85.
PubMed Google Scholar
Scurt FG, Ganz MJ, Herzog C, Bose K, Mertens PR, Chatzikyrkou C. Association of metabolic syndrome and chronic kidney disease. Obesity Rev. 2023;25:e13649.
Article Google Scholar
Cortinovis M, Perico N, Ruggenenti P, Remuzzi A, Remuzzi G. Glomerular hyperfiltration. Nat Rev Nephrol. 2022;18(7):435–51.
Akchurin OM, Kaskel F. Update on inflammation in chronic kidney disease. Blood Purif. 2015;39(1–3):84–92.
Sheu JN, Chen MC, Cheng SL, Lee IC, Chen SM, Tsay GJ. Urine interleukin-1beta in children with acute pyelonephritis and renal scarring. Nephrology. 2007;12(5):487–93.
Zhao Y, Hong X, Xie X, Guo D, Chen B, Fu W, Wang L. Preoperative systemic inflammatory response index predicts long-term outcomes in type B aortic dissection after endovascular repair. Front Immunol. 2022;13: 992463.
Cai X, Song S, Hu J, Wang L, Shen D, Zhu Q, Yang W, Luo Q, Hong J, Li N. Systemic inflammation response index as a predictor of stroke risk in elderly patients with hypertension: a cohort study. J Inflamm Res. 2023;16:4821–32.
Zhang Y, Xing Z, Zhou K, Jiang S. The predictive role of systemic inflammation response index (SIRI) in the prognosis of stroke patients. Clin Interv Aging. 2021;16:1997–2007.
Liu W, Zheng S, Du X. Association of systemic immune-inflammation index and systemic inflammation response index with diabetic kidney disease in patients with type 2 diabetes mellitus. Diabetes Metabol Syndr Obes Targets Ther. 2024;17:517–31.
CAS Google Scholar
Li X, Wang L, Liu M, Zhou H, Xu H. Association between neutrophil-to-lymphocyte ratio and diabetic kidney disease in type 2 diabetes mellitus patients: a cross-sectional study. Front Endocrinol. 2023;14:1285509.
Bronze-da-Rocha E, Santos-Silva A. Neutrophil elastase inhibitors and chronic kidney disease. Int J Biol Sci. 2018;14(10):1343–60.
Heine GH, Ortiz A, Massy ZA, Lindholm B, Wiecek A, Martínez-Castelao A, Covic A, Goldsmith D, Süleymanlar G, London GM, Parati G, Sicari R, Zoccali C, Fliser D. Monocyte subpopulations and cardiovascular risk in chronic kidney disease. Nat Rev Nephrol. 2012;8(6):362–9.
Sharma R, Kinsey GR. Regulatory T cells in acute and chronic kidney diseases. Am J Physiol Renal Physiol. 2018;314(5):F679-f698.
Arı E, Köseoğlu H, Eroğlu T. Predictive value of SIRI and SII for metastases in RCC: a prospective clinical study. BMC Urol. 2024;24(1):14.
Camargo LL, Wang Y, Rios FJ, McBride M, Montezano AC, Touyz RM. Oxidative stress and endoplasmic reticular stress interplay in the vasculopathy of hypertension. Can J Cardiol. 2023. https://doi.org/10.1016/j.cjca.2023.10.012 .
Saucedo R, Ortega-Camarillo C, Ferreira-Hermosillo A, Díaz-Velázquez MF, Meixueiro-Calderón C, Valencia-Ortega J. Role of oxidative stress and inflammation in gestational diabetes mellitus. Antioxidants. 2023;12(10):1812.
Borrelli S, Garofalo C, Gabbai FB, Chiodini P, Signoriello S, Paoletti E, Ravera M, Bussalino E, Bellizzi V, Liberti ME, De Nicola L, Minutolo R. Dipping status, ambulatory blood pressure control, cardiovascular disease, and kidney disease progression: a multicenter cohort study of CKD. Am J Kidney Dis. 2023;81(1):15-24.e1.
Download references
Acknowledgements
For their platforms and for uploading their valuable datasets, we acknowledge the NHANES database.
There were no external funding sources for this study.
Author information
Authors and affiliations.
Department of Cardiology, The Second People’s Hospital of Hefei, Hefei Hospital Affiliated to Anhui Medical University, Hefei, 230011, Anhui, China
Xing Wei, Jing Wei, Jun Feng, Chao Li, Zhipeng Zhang, Ben Hu, Nv Long & Chunmiao Luo
The Fifth Clinical School of Medicine, Anhui Medical University, Hefei, 230032, Anhui, China
Xing Wei, Jing Wei, Zhipeng Zhang, Ben Hu, Nv Long & Chunmiao Luo
You can also search for this author in PubMed Google Scholar
Contributions
CML, XW, JW, JF and CL designed the study. XW, BH, ZPZ and JW engaged in data analysis. XW authored the first draft. XW and NL revised the initial draft. CML and JF examined and revised the paper. All authors read and approved the final manuscript.
Corresponding author
Correspondence to Chunmiao Luo .
Ethics declarations
Ethics approval and consent to participate.
All study protocols of NHANES were approved by the Research Ethics Review Board of the National Center for Health Statistics (NCHS). All participants provided written informed consent ( https://www.cdc.gov/nchs/nhanes/index.htm).All the methods were performed in accordance with the relevant guidelines and regulations.
Consent for publication
All authors agree to publish this work.
Competing interests
The authors have no competing interests.
Additional information
Publisher's note.
Springer Nature remains neutral with regard to jurisdictional claims in published maps and institutional affiliations.
Supplementary Information
Additional file 1: table s1..
Baseline information table based on SIRI quartiles. Table S2. Subgroup analysis of outcome events with eGFR ≤60 mL/minute/1.73 m 2 . Table S3. Subgroup analysis of outcome events with ACR≥30 mg/g. Table S4. Regression analysis of exclusion of people with new-onset hypertension. Table S5. Regression analysis with exclusion of people with blood pressure less than 140/90 mmHg. Table S6. Propensity score matching for outcome events with eGFR ≤60 mL/minute/1.73 m 2 . Table S7. Propensity score matching for outcome events with ACR≥30 mg/g.
Rights and permissions
Open Access This article is licensed under a Creative Commons Attribution 4.0 International License, which permits use, sharing, adaptation, distribution and reproduction in any medium or format, as long as you give appropriate credit to the original author(s) and the source, provide a link to the Creative Commons licence, and indicate if changes were made. The images or other third party material in this article are included in the article's Creative Commons licence, unless indicated otherwise in a credit line to the material. If material is not included in the article's Creative Commons licence and your intended use is not permitted by statutory regulation or exceeds the permitted use, you will need to obtain permission directly from the copyright holder. To view a copy of this licence, visit http://creativecommons.org/licenses/by/4.0/ . The Creative Commons Public Domain Dedication waiver ( http://creativecommons.org/publicdomain/zero/1.0/ ) applies to the data made available in this article, unless otherwise stated in a credit line to the data.
Reprints and permissions
About this article
Cite this article.
Wei, X., Wei, J., Feng, J. et al. Threshold-modifying effect of the systemic inflammatory response index on kidney function decline in hypertensive patients. Eur J Med Res 29 , 202 (2024). https://doi.org/10.1186/s40001-024-01804-9
Download citation
Received : 19 December 2023
Accepted : 20 March 2024
Published : 27 March 2024
DOI : https://doi.org/10.1186/s40001-024-01804-9
Share this article
Anyone you share the following link with will be able to read this content:
Sorry, a shareable link is not currently available for this article.
Provided by the Springer Nature SharedIt content-sharing initiative
- Hypertension
- Systemic inflammation response index
European Journal of Medical Research
ISSN: 2047-783X
- Submission enquiries: [email protected]
- General enquiries: [email protected]
Read our research on: Abortion | Podcasts | Election 2024
Regions & Countries
What the data says about abortion in the u.s..
Pew Research Center has conducted many surveys about abortion over the years, providing a lens into Americans’ views on whether the procedure should be legal, among a host of other questions.
In a Center survey conducted nearly a year after the Supreme Court’s June 2022 decision that ended the constitutional right to abortion , 62% of U.S. adults said the practice should be legal in all or most cases, while 36% said it should be illegal in all or most cases. Another survey conducted a few months before the decision showed that relatively few Americans take an absolutist view on the issue .
Find answers to common questions about abortion in America, based on data from the Centers for Disease Control and Prevention (CDC) and the Guttmacher Institute, which have tracked these patterns for several decades:
How many abortions are there in the U.S. each year?
How has the number of abortions in the u.s. changed over time, what is the abortion rate among women in the u.s. how has it changed over time, what are the most common types of abortion, how many abortion providers are there in the u.s., and how has that number changed, what percentage of abortions are for women who live in a different state from the abortion provider, what are the demographics of women who have had abortions, when during pregnancy do most abortions occur, how often are there medical complications from abortion.
This compilation of data on abortion in the United States draws mainly from two sources: the Centers for Disease Control and Prevention (CDC) and the Guttmacher Institute, both of which have regularly compiled national abortion data for approximately half a century, and which collect their data in different ways.
The CDC data that is highlighted in this post comes from the agency’s “abortion surveillance” reports, which have been published annually since 1974 (and which have included data from 1969). Its figures from 1973 through 1996 include data from all 50 states, the District of Columbia and New York City – 52 “reporting areas” in all. Since 1997, the CDC’s totals have lacked data from some states (most notably California) for the years that those states did not report data to the agency. The four reporting areas that did not submit data to the CDC in 2021 – California, Maryland, New Hampshire and New Jersey – accounted for approximately 25% of all legal induced abortions in the U.S. in 2020, according to Guttmacher’s data. Most states, though, do have data in the reports, and the figures for the vast majority of them came from each state’s central health agency, while for some states, the figures came from hospitals and other medical facilities.
Discussion of CDC abortion data involving women’s state of residence, marital status, race, ethnicity, age, abortion history and the number of previous live births excludes the low share of abortions where that information was not supplied. Read the methodology for the CDC’s latest abortion surveillance report , which includes data from 2021, for more details. Previous reports can be found at stacks.cdc.gov by entering “abortion surveillance” into the search box.
For the numbers of deaths caused by induced abortions in 1963 and 1965, this analysis looks at reports by the then-U.S. Department of Health, Education and Welfare, a precursor to the Department of Health and Human Services. In computing those figures, we excluded abortions listed in the report under the categories “spontaneous or unspecified” or as “other.” (“Spontaneous abortion” is another way of referring to miscarriages.)
Guttmacher data in this post comes from national surveys of abortion providers that Guttmacher has conducted 19 times since 1973. Guttmacher compiles its figures after contacting every known provider of abortions – clinics, hospitals and physicians’ offices – in the country. It uses questionnaires and health department data, and it provides estimates for abortion providers that don’t respond to its inquiries. (In 2020, the last year for which it has released data on the number of abortions in the U.S., it used estimates for 12% of abortions.) For most of the 2000s, Guttmacher has conducted these national surveys every three years, each time getting abortion data for the prior two years. For each interim year, Guttmacher has calculated estimates based on trends from its own figures and from other data.
The latest full summary of Guttmacher data came in the institute’s report titled “Abortion Incidence and Service Availability in the United States, 2020.” It includes figures for 2020 and 2019 and estimates for 2018. The report includes a methods section.
In addition, this post uses data from StatPearls, an online health care resource, on complications from abortion.
An exact answer is hard to come by. The CDC and the Guttmacher Institute have each tried to measure this for around half a century, but they use different methods and publish different figures.
The last year for which the CDC reported a yearly national total for abortions is 2021. It found there were 625,978 abortions in the District of Columbia and the 46 states with available data that year, up from 597,355 in those states and D.C. in 2020. The corresponding figure for 2019 was 607,720.
The last year for which Guttmacher reported a yearly national total was 2020. It said there were 930,160 abortions that year in all 50 states and the District of Columbia, compared with 916,460 in 2019.
- How the CDC gets its data: It compiles figures that are voluntarily reported by states’ central health agencies, including separate figures for New York City and the District of Columbia. Its latest totals do not include figures from California, Maryland, New Hampshire or New Jersey, which did not report data to the CDC. ( Read the methodology from the latest CDC report .)
- How Guttmacher gets its data: It compiles its figures after contacting every known abortion provider – clinics, hospitals and physicians’ offices – in the country. It uses questionnaires and health department data, then provides estimates for abortion providers that don’t respond. Guttmacher’s figures are higher than the CDC’s in part because they include data (and in some instances, estimates) from all 50 states. ( Read the institute’s latest full report and methodology .)
While the Guttmacher Institute supports abortion rights, its empirical data on abortions in the U.S. has been widely cited by groups and publications across the political spectrum, including by a number of those that disagree with its positions .
These estimates from Guttmacher and the CDC are results of multiyear efforts to collect data on abortion across the U.S. Last year, Guttmacher also began publishing less precise estimates every few months , based on a much smaller sample of providers.
The figures reported by these organizations include only legal induced abortions conducted by clinics, hospitals or physicians’ offices, or those that make use of abortion pills dispensed from certified facilities such as clinics or physicians’ offices. They do not account for the use of abortion pills that were obtained outside of clinical settings .
(Back to top)
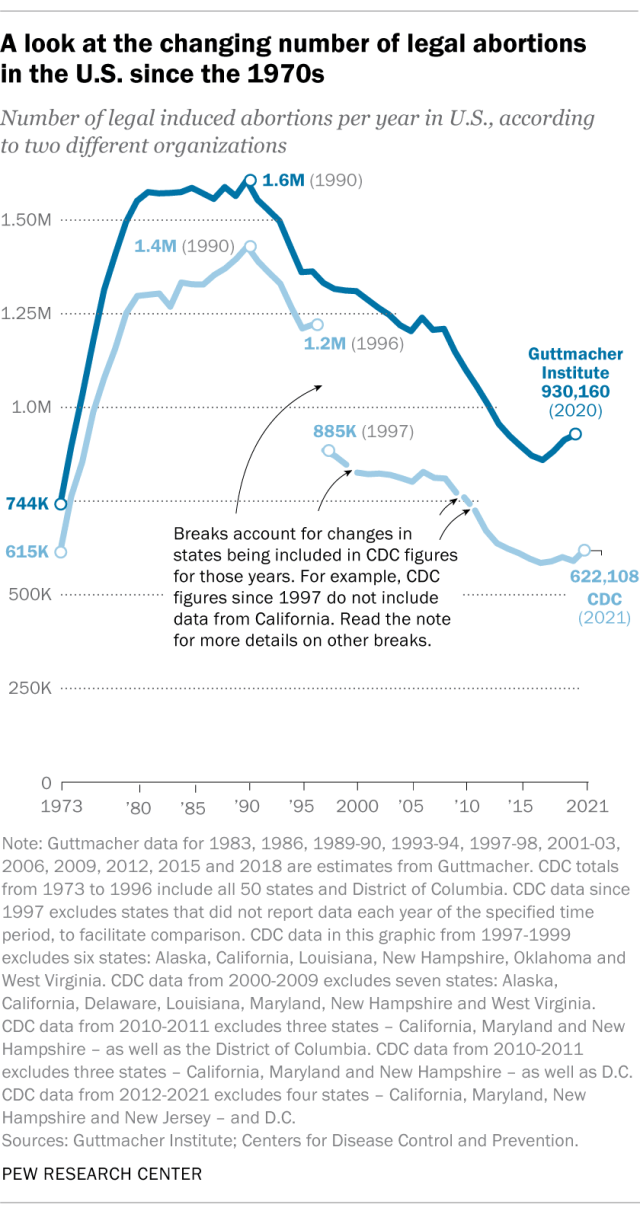
The annual number of U.S. abortions rose for years after Roe v. Wade legalized the procedure in 1973, reaching its highest levels around the late 1980s and early 1990s, according to both the CDC and Guttmacher. Since then, abortions have generally decreased at what a CDC analysis called “a slow yet steady pace.”
Guttmacher says the number of abortions occurring in the U.S. in 2020 was 40% lower than it was in 1991. According to the CDC, the number was 36% lower in 2021 than in 1991, looking just at the District of Columbia and the 46 states that reported both of those years.
(The corresponding line graph shows the long-term trend in the number of legal abortions reported by both organizations. To allow for consistent comparisons over time, the CDC figures in the chart have been adjusted to ensure that the same states are counted from one year to the next. Using that approach, the CDC figure for 2021 is 622,108 legal abortions.)
There have been occasional breaks in this long-term pattern of decline – during the middle of the first decade of the 2000s, and then again in the late 2010s. The CDC reported modest 1% and 2% increases in abortions in 2018 and 2019, and then, after a 2% decrease in 2020, a 5% increase in 2021. Guttmacher reported an 8% increase over the three-year period from 2017 to 2020.
As noted above, these figures do not include abortions that use pills obtained outside of clinical settings.
Guttmacher says that in 2020 there were 14.4 abortions in the U.S. per 1,000 women ages 15 to 44. Its data shows that the rate of abortions among women has generally been declining in the U.S. since 1981, when it reported there were 29.3 abortions per 1,000 women in that age range.
The CDC says that in 2021, there were 11.6 abortions in the U.S. per 1,000 women ages 15 to 44. (That figure excludes data from California, the District of Columbia, Maryland, New Hampshire and New Jersey.) Like Guttmacher’s data, the CDC’s figures also suggest a general decline in the abortion rate over time. In 1980, when the CDC reported on all 50 states and D.C., it said there were 25 abortions per 1,000 women ages 15 to 44.
That said, both Guttmacher and the CDC say there were slight increases in the rate of abortions during the late 2010s and early 2020s. Guttmacher says the abortion rate per 1,000 women ages 15 to 44 rose from 13.5 in 2017 to 14.4 in 2020. The CDC says it rose from 11.2 per 1,000 in 2017 to 11.4 in 2019, before falling back to 11.1 in 2020 and then rising again to 11.6 in 2021. (The CDC’s figures for those years exclude data from California, D.C., Maryland, New Hampshire and New Jersey.)
The CDC broadly divides abortions into two categories: surgical abortions and medication abortions, which involve pills. Since the Food and Drug Administration first approved abortion pills in 2000, their use has increased over time as a share of abortions nationally, according to both the CDC and Guttmacher.
The majority of abortions in the U.S. now involve pills, according to both the CDC and Guttmacher. The CDC says 56% of U.S. abortions in 2021 involved pills, up from 53% in 2020 and 44% in 2019. Its figures for 2021 include the District of Columbia and 44 states that provided this data; its figures for 2020 include D.C. and 44 states (though not all of the same states as in 2021), and its figures for 2019 include D.C. and 45 states.
Guttmacher, which measures this every three years, says 53% of U.S. abortions involved pills in 2020, up from 39% in 2017.
Two pills commonly used together for medication abortions are mifepristone, which, taken first, blocks hormones that support a pregnancy, and misoprostol, which then causes the uterus to empty. According to the FDA, medication abortions are safe until 10 weeks into pregnancy.
Surgical abortions conducted during the first trimester of pregnancy typically use a suction process, while the relatively few surgical abortions that occur during the second trimester of a pregnancy typically use a process called dilation and evacuation, according to the UCLA School of Medicine.
In 2020, there were 1,603 facilities in the U.S. that provided abortions, according to Guttmacher . This included 807 clinics, 530 hospitals and 266 physicians’ offices.
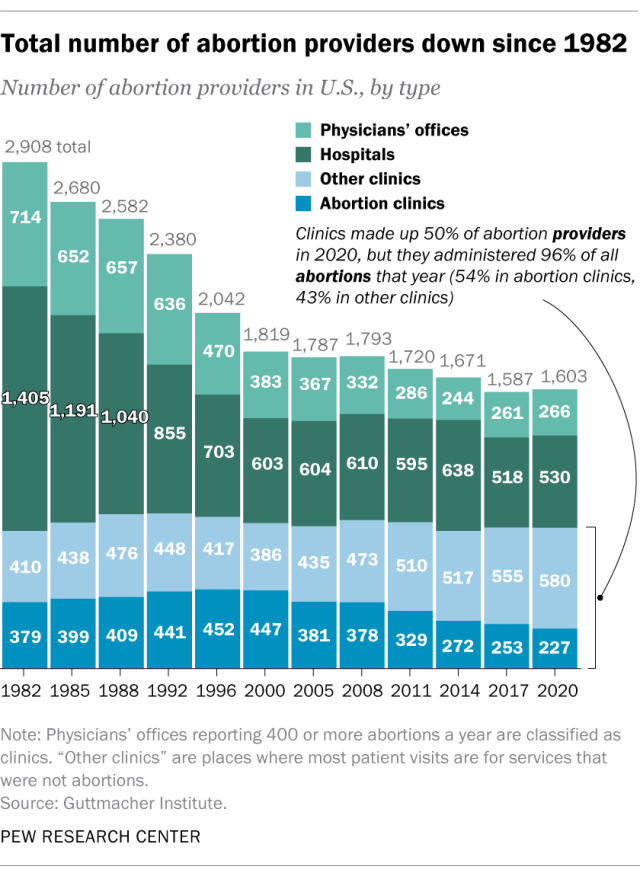
While clinics make up half of the facilities that provide abortions, they are the sites where the vast majority (96%) of abortions are administered, either through procedures or the distribution of pills, according to Guttmacher’s 2020 data. (This includes 54% of abortions that are administered at specialized abortion clinics and 43% at nonspecialized clinics.) Hospitals made up 33% of the facilities that provided abortions in 2020 but accounted for only 3% of abortions that year, while just 1% of abortions were conducted by physicians’ offices.
Looking just at clinics – that is, the total number of specialized abortion clinics and nonspecialized clinics in the U.S. – Guttmacher found the total virtually unchanged between 2017 (808 clinics) and 2020 (807 clinics). However, there were regional differences. In the Midwest, the number of clinics that provide abortions increased by 11% during those years, and in the West by 6%. The number of clinics decreased during those years by 9% in the Northeast and 3% in the South.
The total number of abortion providers has declined dramatically since the 1980s. In 1982, according to Guttmacher, there were 2,908 facilities providing abortions in the U.S., including 789 clinics, 1,405 hospitals and 714 physicians’ offices.
The CDC does not track the number of abortion providers.
In the District of Columbia and the 46 states that provided abortion and residency information to the CDC in 2021, 10.9% of all abortions were performed on women known to live outside the state where the abortion occurred – slightly higher than the percentage in 2020 (9.7%). That year, D.C. and 46 states (though not the same ones as in 2021) reported abortion and residency data. (The total number of abortions used in these calculations included figures for women with both known and unknown residential status.)
The share of reported abortions performed on women outside their state of residence was much higher before the 1973 Roe decision that stopped states from banning abortion. In 1972, 41% of all abortions in D.C. and the 20 states that provided this information to the CDC that year were performed on women outside their state of residence. In 1973, the corresponding figure was 21% in the District of Columbia and the 41 states that provided this information, and in 1974 it was 11% in D.C. and the 43 states that provided data.
In the District of Columbia and the 46 states that reported age data to the CDC in 2021, the majority of women who had abortions (57%) were in their 20s, while about three-in-ten (31%) were in their 30s. Teens ages 13 to 19 accounted for 8% of those who had abortions, while women ages 40 to 44 accounted for about 4%.
The vast majority of women who had abortions in 2021 were unmarried (87%), while married women accounted for 13%, according to the CDC , which had data on this from 37 states.
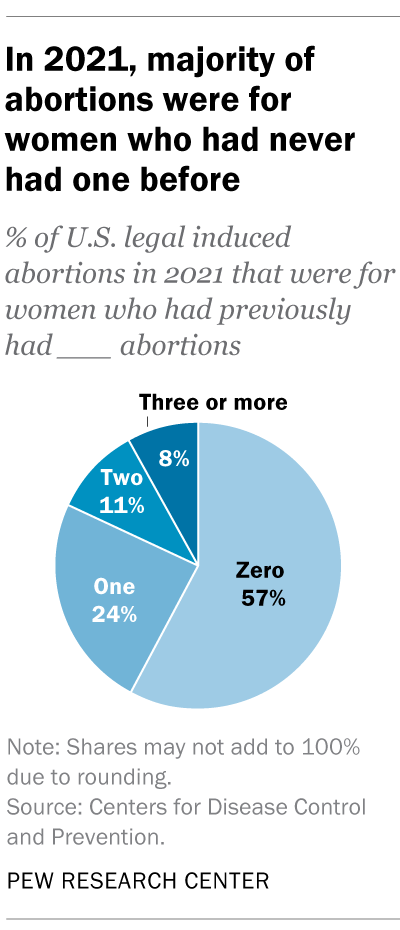
In the District of Columbia, New York City (but not the rest of New York) and the 31 states that reported racial and ethnic data on abortion to the CDC , 42% of all women who had abortions in 2021 were non-Hispanic Black, while 30% were non-Hispanic White, 22% were Hispanic and 6% were of other races.
Looking at abortion rates among those ages 15 to 44, there were 28.6 abortions per 1,000 non-Hispanic Black women in 2021; 12.3 abortions per 1,000 Hispanic women; 6.4 abortions per 1,000 non-Hispanic White women; and 9.2 abortions per 1,000 women of other races, the CDC reported from those same 31 states, D.C. and New York City.
For 57% of U.S. women who had induced abortions in 2021, it was the first time they had ever had one, according to the CDC. For nearly a quarter (24%), it was their second abortion. For 11% of women who had an abortion that year, it was their third, and for 8% it was their fourth or more. These CDC figures include data from 41 states and New York City, but not the rest of New York.
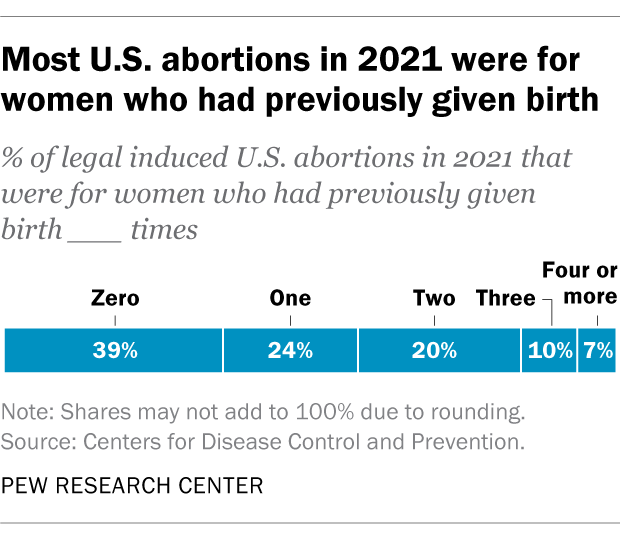
Nearly four-in-ten women who had abortions in 2021 (39%) had no previous live births at the time they had an abortion, according to the CDC . Almost a quarter (24%) of women who had abortions in 2021 had one previous live birth, 20% had two previous live births, 10% had three, and 7% had four or more previous live births. These CDC figures include data from 41 states and New York City, but not the rest of New York.
The vast majority of abortions occur during the first trimester of a pregnancy. In 2021, 93% of abortions occurred during the first trimester – that is, at or before 13 weeks of gestation, according to the CDC . An additional 6% occurred between 14 and 20 weeks of pregnancy, and about 1% were performed at 21 weeks or more of gestation. These CDC figures include data from 40 states and New York City, but not the rest of New York.
About 2% of all abortions in the U.S. involve some type of complication for the woman , according to an article in StatPearls, an online health care resource. “Most complications are considered minor such as pain, bleeding, infection and post-anesthesia complications,” according to the article.
The CDC calculates case-fatality rates for women from induced abortions – that is, how many women die from abortion-related complications, for every 100,000 legal abortions that occur in the U.S . The rate was lowest during the most recent period examined by the agency (2013 to 2020), when there were 0.45 deaths to women per 100,000 legal induced abortions. The case-fatality rate reported by the CDC was highest during the first period examined by the agency (1973 to 1977), when it was 2.09 deaths to women per 100,000 legal induced abortions. During the five-year periods in between, the figure ranged from 0.52 (from 1993 to 1997) to 0.78 (from 1978 to 1982).
The CDC calculates death rates by five-year and seven-year periods because of year-to-year fluctuation in the numbers and due to the relatively low number of women who die from legal induced abortions.
In 2020, the last year for which the CDC has information , six women in the U.S. died due to complications from induced abortions. Four women died in this way in 2019, two in 2018, and three in 2017. (These deaths all followed legal abortions.) Since 1990, the annual number of deaths among women due to legal induced abortion has ranged from two to 12.
The annual number of reported deaths from induced abortions (legal and illegal) tended to be higher in the 1980s, when it ranged from nine to 16, and from 1972 to 1979, when it ranged from 13 to 63. One driver of the decline was the drop in deaths from illegal abortions. There were 39 deaths from illegal abortions in 1972, the last full year before Roe v. Wade. The total fell to 19 in 1973 and to single digits or zero every year after that. (The number of deaths from legal abortions has also declined since then, though with some slight variation over time.)
The number of deaths from induced abortions was considerably higher in the 1960s than afterward. For instance, there were 119 deaths from induced abortions in 1963 and 99 in 1965 , according to reports by the then-U.S. Department of Health, Education and Welfare, a precursor to the Department of Health and Human Services. The CDC is a division of Health and Human Services.
Note: This is an update of a post originally published May 27, 2022, and first updated June 24, 2022.
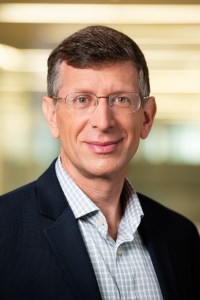
Sign up for our weekly newsletter
Fresh data delivered Saturday mornings
Key facts about the abortion debate in America
Public opinion on abortion, three-in-ten or more democrats and republicans don’t agree with their party on abortion, partisanship a bigger factor than geography in views of abortion access locally, do state laws on abortion reflect public opinion, most popular.
About Pew Research Center Pew Research Center is a nonpartisan fact tank that informs the public about the issues, attitudes and trends shaping the world. It conducts public opinion polling, demographic research, media content analysis and other empirical social science research. Pew Research Center does not take policy positions. It is a subsidiary of The Pew Charitable Trusts .
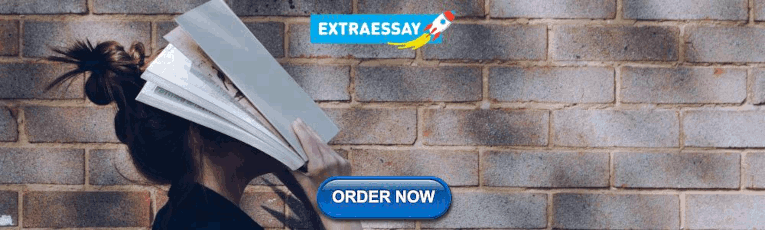
IMAGES
VIDEO
COMMENTS
Definition of research in data analysis: According to LeCompte and Schensul, research data analysis is a process used by researchers to reduce data to a story and interpret it to derive insights. The data analysis process helps reduce a large chunk of data into smaller fragments, which makes sense. Three essential things occur during the data ...
For many researchers unfamiliar with qualitative research, determining how to conduct qualitative analyses is often quite challenging. Part of this challenge is due to the seemingly limitless approaches that a qualitative researcher might leverage, as well as simply learning to think like a qualitative researcher when analyzing data. From framework analysis (Ritchie & Spencer, 1994) to content ...
This article is a practical guide to conducting data analysis in general literature reviews. The general literature review is a synthesis and analysis of published research on a relevant clinical issue, and is a common format for academic theses at the bachelor's and master's levels in nursing, physiotherapy, occupational therapy, public health and other related fields.
Data analysis can be quantitative, qualitative, or mixed methods. Quantitative research typically involves numbers and "close-ended questions and responses" (Creswell & Creswell, 2018, p. 3).Quantitative research tests variables against objective theories, usually measured and collected on instruments and analyzed using statistical procedures (Creswell & Creswell, 2018, p. 4).
Importance of Data Analysis in Research. The importance of data analysis in research cannot be overstated; it serves as the backbone of any scientific investigation or study. Here are several key reasons why data analysis is crucial in the research process: Data analysis helps ensure that the results obtained are valid and reliable.
Discover data analysis techniques, methods, and approaches, and study examples of data analysis in research papers. Updated: 11/21/2023 Table of Contents
Step 1: Consider your aims and approach. Step 2: Choose a type of research design. Step 3: Identify your population and sampling method. Step 4: Choose your data collection methods. Step 5: Plan your data collection procedures. Step 6: Decide on your data analysis strategies. Other interesting articles.
data analysis, the process of systematically collecting, cleaning, transforming, describing, modeling, and interpreting data, generally employing statistical techniques. Data analysis is an important part of both scientific research and business, where demand has grown in recent years for data-driven decision making.Data analysis techniques are used to gain useful insights from datasets, which ...
Step 1: Write your hypotheses and plan your research design. To collect valid data for statistical analysis, you first need to specify your hypotheses and plan out your research design. Writing statistical hypotheses. The goal of research is often to investigate a relationship between variables within a population. You start with a prediction ...
Written by Coursera Staff • Updated on Nov 20, 2023. Data analysis is the practice of working with data to glean useful information, which can then be used to make informed decisions. "It is a capital mistake to theorize before one has data. Insensibly one begins to twist facts to suit theories, instead of theories to suit facts," Sherlock ...
According to Belotto (2018), data analysis refers to the process of data management, description, evaluation, and interpretation. Thematic analysis is a method of data analysis in qualitative ...
Methodologies like experimental research and case study research, which aim to verify or validate preexisting hypotheses, frequently take this approach. Naeem and Ozuem (2022a) used TORT and PMT to do a deductive thematic analysis of their data. Methodologies that want to both discover new phenomena and validate or develop current theories may ...
For those interested in conducting qualitative research, previous articles in this Research Primer series have provided information on the design and analysis of such studies. 2, 3 Information in the current article is divided into 3 main sections: an overview of terms and concepts used in data analysis, a review of common methods used to ...
To analyze data collected in a statistically valid manner (e.g. from experiments, surveys, and observations). Meta-analysis. Quantitative. To statistically analyze the results of a large collection of studies. Can only be applied to studies that collected data in a statistically valid manner.
Data Analysis. Different statistics and methods used to describe the characteristics of the members of a sample or population, explore the relationships between variables, to test research hypotheses, and to visually represent data are described. Terms relating to the topics covered are defined in the Research Glossary. Descriptive Statistics.
A systematic review collects all possible studies related to a given topic and design, and reviews and analyzes their results [ 1 ]. During the systematic review process, the quality of studies is evaluated, and a statistical meta-analysis of the study results is conducted on the basis of their quality. A meta-analysis is a valid, objective ...
Data analysis in qualitative research is defined as the process of systematically searching and arranging the interview transcripts, ... To work with NVivo, first and foremost, the researcher has to create a Project to hold the data or study information. Once a project is created, the Project pad appears (Figure 2).
Qualitative research and quantitative research both have data analysis procedures. Qualitative research is the process that focuses on gaining insight and understanding of an individual or ...
Analyzing research data is a crucial skill for any researcher, whether you are conducting a survey, an experiment, a case study, or any other type of research. Data analysis helps you answer your ...
For closure lengths, the study averaged district-level estimates of time spent in remote and hybrid learning compiled by the Covid-19 School Data Hub (C.S.D.H.) and American Enterprise Institute ...
The study included data for NHANES participants who were at least 20 years old at enrollment, between 2003-2018, and had completed two 24-hour dietary recall questionnaires within the first year of enrollment. ... were not included in the analysis. Future research may examine the biological mechanisms that underly the associations between a ...
Abstract. This paper aims to provide an overview of the use and assessment of qualitative research methods in the health sciences. Qualitative research can be defined as the study of the nature of phenomena and is especially appropriate for answering questions of why something is (not) observed, assessing complex multi-component interventions ...
In this work, we discuss building performant Multimodal Large Language Models (MLLMs). In particular, we study the importance of various architecture components and data choices. Through careful and comprehensive ablations of the image encoder, the vision language connector, and various pre-training data choices, we identified several crucial design lessons. For example, we demonstrate that ...
New Guttmacher Institute research from the Monthly Abortion Provision Study shows that there were approximately 642,700 medication abortions in the United States in 2023, accounting for 63% of all abortions in the formal health care system. This is an increase from 2020, when medication abortions accounted for 53% of all abortions.
Introduction:Despite the abundance of research indicating the participation of immune cells in prostate cancer development, establishing a definitive cause-and-effect relationship has proven to be a difficult undertaking.Methods:This study employs Mendelian randomization (MR), leveraging genetic variables related to immune cells from publicly available genome-wide association studies (GWAS ...
A case study is one of the most commonly used methodologies of social research. This article attempts to look into the various dimensions of a case study research strategy, the different epistemological strands which determine the particular case study type and approach adopted in the field, discusses the factors which can enhance the effectiveness of a case study research, and the debate ...
Employee Engagement Create a culture that ensures employees are involved, enthusiastic and highly productive in their work and workplace.; Employee Experience Analyze and improve the experiences across your employee life cycle, so your people and organization can thrive.; Leadership Identify and enable future-ready leaders who can inspire exceptional performance.
Qualitative research is a type of research that explores and provides deeper insights into real-world problems.[1] Instead of collecting numerical data points or intervene or introduce treatments just like in quantitative research, qualitative research helps generate hypotheses as well as further investigate and understand quantitative data. Qualitative research gathers participants ...
Background Chronic kidney disease (decreased kidney function) is common in hypertensive patients. The SIRI is a novel immune biomarker. We investigated the correlation between the SIRI and kidney function in hypertensive patients. Methods The present study analyzed data from participants who suffered from hypertension in the NHANES from 2009 to 2018. Multivariate regression analysis and ...
The CDC data that is highlighted in this post comes from the agency's "abortion surveillance" reports, which have been published annually since 1974 (and which have included data from 1969). Its figures from 1973 through 1996 include data from all 50 states, the District of Columbia and New York City - 52 "reporting areas" in all.