
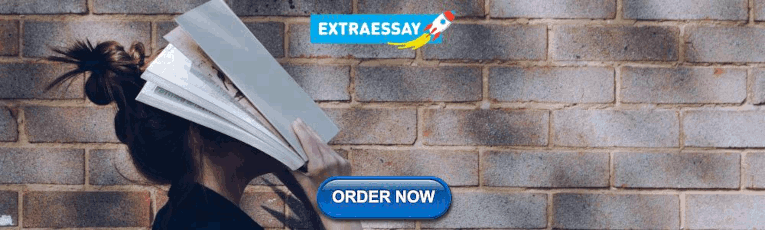
Gateway Drug Hypothesis
Page last updated July 22, 2023 by Doug McVay, Editor.
Gateway Drug Use
- Living reference work entry
- First Online: 01 January 2016
- Cite this living reference work entry
- Wayne D. Hall 2 &
- Rosa Alati 2
193 Accesses
Research on adolescent drug use over the past three decades in the USA and other developed countries has found a common pattern of drug initiation and progression. Alcohol and tobacco are used first, followed by cannabis, which, in turn, is followed by the amphetamines, heroin, and cocaine. Some of the drugs in this sequence have been called “gateway drugs”: that is, drugs whose use in some unspecified sense is a cause of the use of later drugs in the sequence. Traditionally, cannabis has been the drug of most concern as a possible gateway to the use of cocaine and heroin (Kandel 2002 ). More recently, attention has focused on the role of tobacco as a gateway drug to cannabis use (Patton et al. 2005 ) and more recently still on the possible role of electronic cigarettes as a gateway to conventional combustible cigarette smoking (Kandel and Kandel 2015 ; Phillips 2015 ).
The status of the “gateway hypothesis” has been controversial in part because it has not always been clear what...
This is a preview of subscription content, log in via an institution to check access.
Access this chapter
Institutional subscriptions
Badiani, A., Boden, J., De Pirro, S., et al. (2015). Tobacco smoking and cannabis use in a longitudinal birth cohort: Evidence of reciprocal causal relationships. Drug and Alcohol Dependence, 150 , 69–76. doi:10.1016/j.drugalcdep.2015.02.015.
Article PubMed Google Scholar
Botvin, G., Scheier, L., & Griffin, K. (2002). Preventing the onset and developmental progression of adolescent drug use: Implications for the gateway hypothesis. In D. Kandel (Ed.), Stages and pathways of drug involvement: Examining the gateway hypothesis (pp. 115–138). New York: Cambridge University Press.
Chapter Google Scholar
Cleveland, H., & Wiebe, R. (2008). Understanding the association between adolescent marijuana use and later serious drug use: Gateway effect or developmental trajectory? Development and Psychopathology, 20 (2), 615–632. doi:10.1017/S0954579408000308.
Dutra, L., & Glantz, S. (2014). Electronic cigarettes and conventional cigarette use among U.S. adolescents: A cross-sectional study. JAMA Pediatrics, 168 (7), 610–617. doi:10.1001/jamapediatrics.2013.5488.
Article PubMed PubMed Central Google Scholar
Fergusson, D., & Horwood, L. (2000). Does cannabis use encourage other forms of illicit drug use? Addiction, 95 (4), 505–520. doi:10.1046/j.1360-0443.2000.9545053.x.
Fergusson, D., Horword, L., & Swain-Campbell, N. (2002). Cannabis use and psychosocial adjustment in adolescence and young adulthood. Addiction, 97 , 1123–1135. doi:10.1046/j.1360-0443.2002.00103.x.
Fergusson, D., Boden, J., & Horwood, L. (2006). Cannabis use and other illicit drug use: Testing the cannabis gateway hypothesis. Addiction, 101 (4), 556–569. doi:10.1111/j.1360-0443.2005.01322.x.
Grana, R., Benowitz, N., & Glantz, S. (2013). Background paper on e-cigarettes (electronic nicotine delivery systems). WHO Tobacco Control Papers . San Francisco: Center for Tobacco Control Research and Education, UC San Francisco.
Google Scholar
Hall, W. D., & Lynskey, M. (2005). Is cannabis a gateway drug? Testing hypotheses about the relationship between cannabis use and the use of other illicit drugs. Drug and Alcohol Review, 24 (1), 39–48. doi:10.1080/09595230500126698.
Kandel, D. (2002). Stages and pathways of drug involvement: Examining the gateway hypothesis . New York: Cambridge University Press.
Book Google Scholar
Kandel, E., & Kandel, D. (2014). Shattuck lecture. A molecular basis for nicotine as a gateway drug. New England Journal of Medicine, 371 (10), 932–943. doi:10.1056/NEJMsa1405092.
Kandel, D., & Kandel, E. (2015). The gateway hypothesis of substance abuse: Developmental, biological and societal perspectives. Acta Paediatrica, 104 (2), 130–137. doi:10.1111/apa.12851.
Lee, S., Grana, R., & Glantz, S. (2014). Electronic cigarette use among Korean adolescents: A cross-sectional study of market penetration, dual use, and relationship to quit attempts and former smoking. Journal of Adolescent Health, 54 (6), 684–690. doi:10.1016/j.jadohealth.2013.11.003.
Lessem, J., Hopfer, C., Haberstick, B., et al. (2006). Relationship between adolescent marijuana use and young adult illicit drug use. Behavior Genetics, 36 (4), 498–506. doi:10.1007/s10519-006-9064-9.
Lin, H. C., Jester, J. M., & Buu, A. (2016). The relationships of cigarette and alcohol use with the initiation, reinitiation, and persistence of cannabis use. Journal of Studies on Alcohol and Drugs, 77 (1), 113–120. doi:10.15288/jsad.2016.77.113.
Lynskey, M., Heath, A., Bucholz, K., et al. (2003). Escalation of drug use in early-onset cannabis users vs co-twin controls. JAMA: Journal of the American Medical Association, 289 (4), 427–433. doi:10.1001/jama.289.4.427.
McKee, M., Chapman, S., Daube, M., et al. (2014). The debate on electronic cigarettes. The Lancet, 384 (9960), 2107. doi:10.1016/S0140-6736(14)62366-7.
Article Google Scholar
Morral, A., McCaffrey, D., & Paddock, S. (2002). Reassessing the marijuana gateway effect. Addiction, 97 (12), 1493–1504. doi:10.1046/j.1360-0443.2002.00280.x.
Patton, G., Coffey, C., Carlin, J., et al. (2005). Reverse gateways? Frequent cannabis use as a predictor of tobacco initiation and nicotine dependence. Addiction, 100 (10), 1518–1525. doi:10.1111/j.1360-0443.2005.01220.x.
Phillips, C. (2015). Gateway effects: Why the cited evidence does not support their existence for low-risk tobacco products (and what evidence would). International Journal of Environmental Research and Public Health, 12 (5), 5439–5464. doi:10.3390/ijerph120505439.
Rebellon, C., & Van Gundy, K. (2006). Can social psychological delinquency theory explain the link between marijuana and other illicit drug use? A longitudinal analysis of the gateway hypothesis. Journal of Drug Issues, 36 (3), 515–539. doi:10.1177/002204260603600302.
Timberlake, D., Haberstick, B., Hopfer, C., et al. (2007). Progression from marijuana use to daily smoking and nicotine dependence in a national sample of U.S. adolescents. Drug and Alcohol Dependence, 88 (2–3), 272–281. doi:10.1016/j.drugalcdep.2006.11.005.
Wagner, F., & Anthony, J. (2002). Into the world of illegal drug use: Exposure opportunity and other mechanisms linking the use of alcohol, tobacco, marijuana, and cocaine. American Journal of Epidemiology, 155 (10), 918–925. doi:10.1093/aje/155.10.918.
Download references
Author information
Authors and affiliations.
School of Population Health and Centre for Youth Substance Abuse Research, University of Queensland, 4006, Herston, QLD, Australia
Wayne D. Hall & Rosa Alati
You can also search for this author in PubMed Google Scholar
Corresponding author
Correspondence to Wayne D. Hall .
Editor information
Editors and affiliations.
Indiana University, Bloomington, Indiana, USA
Roger J.R. Levesque
Rights and permissions
Reprints and permissions
Copyright information
© 2016 Springer International Publishing Switzerland
About this entry
Cite this entry.
Hall, W.D., Alati, R. (2016). Gateway Drug Use. In: Levesque, R. (eds) Encyclopedia of Adolescence. Springer, Cham. https://doi.org/10.1007/978-3-319-32132-5_79-2
Download citation
DOI : https://doi.org/10.1007/978-3-319-32132-5_79-2
Received : 03 May 2016
Accepted : 31 May 2016
Published : 05 July 2016
Publisher Name : Springer, Cham
Online ISBN : 978-3-319-32132-5
eBook Packages : Springer Reference Behavioral Science and Psychology Reference Module Humanities and Social Sciences Reference Module Business, Economics and Social Sciences
- Publish with us
Policies and ethics
- Find a journal
- Track your research
The Gateway Hypothesis, Common Liability to Addictions or the Route of Administration Model A Modelling Process Linking the Three Theories
Affiliation.
- 1 Centre d'x00C9;pidx00E9;miologie et de Santx00E9; Publique des Armx00E9;es, INSERM - IRD - Universitx00E9; Aix-Marseille, Marseille, France.
- PMID: 26431216
- DOI: 10.1159/000439564
Background: The aim of this study was to describe the transitions between tobacco (T), cannabis (C) and other illicit drugs (OIDs) initiations, to simultaneously explore several substance use theories: gateway theory (GT), common liability model (CLM) and route of administration model (RAM).
Methods: Data from 2 French nationwide surveys conducted in 2005 and 2010 were used (16,421 subjects aged 18-34). Using reported ages at initiations, we reconstituted a retrospective cohort describing all initiation sequences between T, C and OID. Transition probabilities between the substances were computed using a Markov multi-state model that also tested the effect of 2 latent variables (item response theory scores reflecting propensity for early onset and further substance use) on all transitions.
Results: T initiation was associated with increased likelihood of subsequent C initiation, but the reverse relationship was also observed. While the most likely initiation sequence among subjects who initiated the 3 groups of substances was the 'gateway' sequence T x2192; C x2192; OID, this pattern was not associated with substance use propensity more than alternative sequences. Early use propensity was associated with the 'gateway' sequence but also with some alternative ones beginning with T, C or OID.
Conclusion: If the gateway sequence appears as the most likely pattern, in line with GT, the effects of early onset and substance use propensities were also observed for some alternative sequences, which is more in line with CLM. RAM could explain reciprocal interactions observed between T and C. This suggests shared influences of individual (personality traits) and environmental (substance availability, peer influence) characteristics.
© 2015 S. Karger AG, Basel.
- Behavior, Addictive / etiology*
- Marijuana Abuse / etiology*
- Models, Psychological*
- Retrospective Studies
- Substance-Related Disorders / etiology*
- Tobacco Use Disorder / etiology*
- Young Adult
- Newsletters
Site search
- Israel-Hamas war
- Home Planet
- 2024 election
- Supreme Court
- TikTok’s fate
- All explainers
- Future Perfect
Filed under:
Is marijuana a gateway drug? Here's what the research says.
Share this story.
- Share this on Facebook
- Share this on Twitter
- Share this on Reddit
- Share All sharing options
Share All sharing options for: Is marijuana a gateway drug? Here's what the research says.
Is marijuana a gateway drug? Much of the conventional wisdom and middle school drug education say yes. But the research tells a different story.
The gateway hypothesis says marijuana inspires users to try other drugs: Once they get a taste of how fun pot is, they're more likely to want to see how fun other drugs are. So if marijuana is more easily available through legalization, believers of the hypothesis say, it could push people to harder drugs.
But this is an empirical claim — one that can be verified or refuted by research. So far, there's no solid evidence to support the gateway hypothesis. And there's some evidence that legalizing marijuana may actually reduce the use of other drugs.
There's no good evidence for the gateway hypothesis
:no_upscale()/cdn.vox-cdn.com/uploads/chorus_asset/file/6407061/73245308.jpg)
Supporters of the gateway theory point to the correlation between marijuana use and the use of other drugs. Robert DuPont, president of the Institute for Behavior and Health, argued in the New York Times :
Marijuana use is positively correlated with alcohol use and cigarette use, as well as illegal drugs like cocaine and methamphetamine. This does not mean that everyone who uses marijuana will transition to using heroin or other drugs, but it does mean that people who use marijuana also consume more, not less, legal and illegal drugs than do people who do not use marijuana. People who are addicted to marijuana are three times more likely to be addicted to heroin.
It's true that marijuana use correlates with harder drug use. But so does alcohol and tobacco use. There doesn't have to be a causal link between marijuana or alcohol and harder drugs to explain this; it could just be that the things that drive someone to marijuana or alcohol — boredom, depression, social circles — can just as easily drive them to other drugs. Perhaps the correlation is actually exposing those underlying factors, not some gateway effect.
If that's the case, why do harder drug users tend to start with alcohol and marijuana before they end up at harder drugs? There's an easy explanation: Alcohol and marijuana are much more accessible, because they're generally cheaper than harder drugs and part of much bigger markets.
A 2002 report by RAND's Drug Policy Research Center said that this explanation is at least as plausible as the gateway hypothesis:
The new DPRC research thus demonstrates that the phenomena supporting claims that marijuana is a gateway drug also support the alternative explanation: that it is not marijuana use but individuals' opportunities and unique propensities to use drugs that determine their risk of initiating hard drugs. The research does not disprove the gateway theory; it merely shows that another explanation is plausible.
Back in 1999, the highly prestigious Institute of Medicine looked at the evidence and reached a similar conclusion:
Patterns in progression of drug use from adolescence to adulthood are strikingly regular. Because it is the most widely used illicit drug, marijuana is predictably the first illicit drug most people encounter. Not surprisingly, most users of other illicit drugs have used marijuana first. In fact, most drug users begin with alcohol and nicotine before marijuana — usually before they are of legal age. In the sense that marijuana use typically precedes rather than follows initiation of other illicit drug use, it is indeed a "gateway" drug. But because underage smoking and alcohol use typically precede marijuana use, marijuana is not the most common, and is rarely the first, "gateway" to illicit drug use. There is no conclusive evidence that the drug effects of marijuana are causally linked to the subsequent abuse of other illicit drugs.
Since then, other studies have prodded at the question, similarly failing to find hard evidence of marijuana leading people to harder drugs.
So there is no good causal evidence for the gateway hypothesis. There is some weak correlational evidence, but it can be easily support with an entirely different idea — drug users tend to start with more accessible drugs — over the gateway hypothesis.
That doesn't rule out the gateway effect entirely. But it should give proponents of it pause.
Marijuana legalization could lead to an anti-gateway effect
To the extent there is any gateway effect, it might be due to marijuana's illegality.
As drug policy experts Jon Caulkins, Beau Kilmer, and Mark Kleiman explained in their newly released book, Marijuana Legalization: What Everyone Needs to Know , it's possible that people may be exposed to harder drugs when they, for example, interact with their pot dealer. Once that dealer knows a marijuana user is a reliable customer, he may push his customer to trying harder drugs, such as heroin or cocaine.
There's some weak evidence behind this idea. After the Dutch liberalized their marijuana policies, a 2010 report by RAND researcher Robert MacCoun found "some evidence" for a "weakened gateway." This is by no means conclusive, but it suggests that marijuana legalization could actually prevent people from going to harder drugs by separating the cocaine and heroin dealers from the marijuana sellers.
Other research suggests that marijuana can act as a sort of anti-gateway. As the nation deals with an opioid epidemic , some opioid painkiller users are moving on to other opioids — heroin and fentanyl — to satisfy their addiction. But several studies have found that medical marijuana legalization can actually reduce opioid deaths, perhaps because patients can use pot to treat their chronic pain — without the risk of overdose and less of a risk for addiction — instead of highly addictive, deadly opioids.
Similarly, marijuana legalization also may lead people to substitute their alcohol use with marijuana use. This could be hugely beneficial to public health and safety, since alcohol is a fairly dangerous drug linked to violent crimes, poisonings, and fatal accidents, while legal pot isn't linked to violent crimes or poisonings and less likely to cause accidents .
The evidence on alcohol substitution is mixed: A 2015 study by UCLA researcher Rosanna Smart linked liberalized marijuana policies to fewer alcohol and opioid deaths among older adults, but more alcohol deaths among adolescents and young adults. But the research in this area is still fairly early, so it's hard to draw definitive conclusions from it.
Either way, the evidence suggests that marijuana's illegality — not the drug itself — may cause a gateway effect, and loosening marijuana policy may push some people away from much more dangerous drugs.
The verdict: Marijuana, by itself, probably won't lead people to use harder drugs
:no_upscale()/cdn.vox-cdn.com/uploads/chorus_asset/file/4314219/459780027.0.jpg)
Putting all of this together, there's no good evidence that marijuana is a "gateway drug" or that any gateway effect would be worsened by legalization. The evidence suggests, in fact, that pot could stop some people from using harder, more dangerous drugs if it was legalized.
None of this means marijuana is harmless. Its potential risks include dependence and overuse , accidents , non-deadly overdoses that lead to mental anguish and anxiety, and marijuana use potentially causing psychotic episodes .
But the gateway hypothesis isn't supported by the evidence. Until that changes, the debates about marijuana should focus on real issues that are evidence-based.
Will you support Vox today?
We believe that everyone deserves to understand the world that they live in. That kind of knowledge helps create better citizens, neighbors, friends, parents, and stewards of this planet. Producing deeply researched, explanatory journalism takes resources. You can support this mission by making a financial gift to Vox today. Will you join us?
We accept credit card, Apple Pay, and Google Pay. You can also contribute via
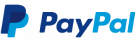
Next Up In Politics
Sign up for the newsletter today, explained.
Understand the world with a daily explainer plus the most compelling stories of the day.
Thanks for signing up!
Check your inbox for a welcome email.
Oops. Something went wrong. Please enter a valid email and try again.

Bird flu in milk is alarming — but not for the reason you think

What the backlash to student protests over Gaza is really about
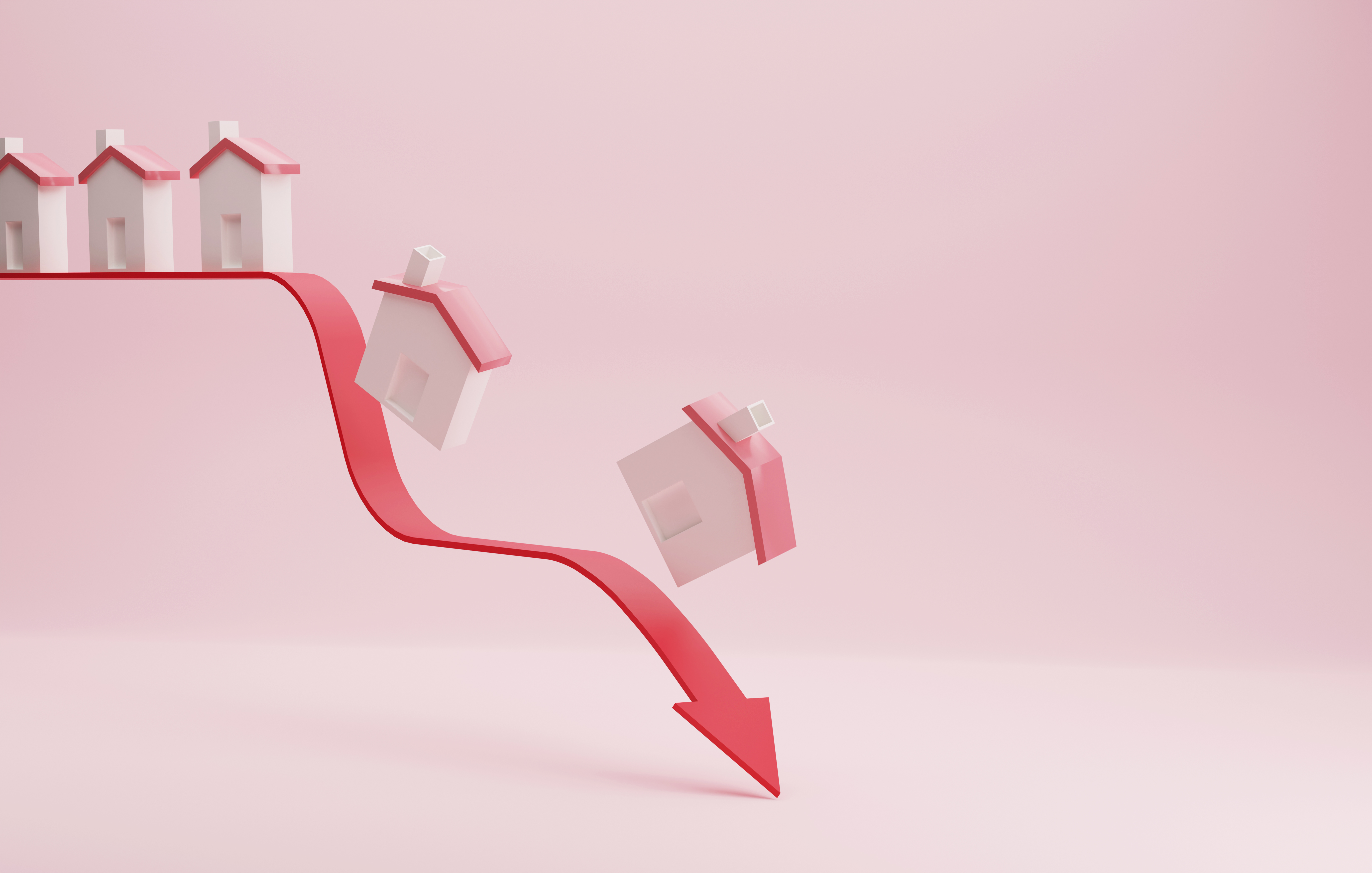
Could a major lawsuit against realtors mean lower home prices?

Lawmakers are overreacting to crime

How the Supreme Court weaponizes its own calendar

Imagining an internet without TikTok
Cannabis (Marijuana) Research Report Is marijuana a gateway drug?
Some research suggests that marijuana use is likely to precede use of other licit and illicit substances 45 and the development of addiction to other substances. For instance, a study using longitudinal data from the National Epidemiological Study of Alcohol Use and Related Disorders found that adults who reported marijuana use during the first wave of the survey were more likely than adults who did not use marijuana to develop an alcohol use disorder within 3 years; people who used marijuana and already had an alcohol use disorder at the outset were at greater risk of their alcohol use disorder worsening. 46 Marijuana use is also linked to other substance use disorders including nicotine addiction.
Early exposure to cannabinoids in adolescent rodents decreases the reactivity of brain dopamine reward centers later in adulthood. 47 To the extent that these findings generalize to humans, this could help explain the increased vulnerability for addiction to other substances of misuse later in life that most epidemiological studies have reported for people who begin marijuana use early in life. 48 It is also consistent with animal experiments showing THC’s ability to "prime" the brain for enhanced responses to other drugs. 49 For example, rats previously administered THC show heightened behavioral response not only when further exposed to THC but also when exposed to other drugs such as morphine—a phenomenon called cross-sensitization . 50
These findings are consistent with the idea of marijuana as a "gateway drug." However, the majority of people who use marijuana do not go on to use other, "harder" substances. Also, cross-sensitization is not unique to marijuana. Alcohol and nicotine also prime the brain for a heightened response to other drugs 51 and are, like marijuana, also typically used before a person progresses to other, more harmful substances.
It is important to note that other factors besides biological mechanisms, such as a person’s social environment, are also critical in a person’s risk for drug use. An alternative to the gateway-drug hypothesis is that people who are more vulnerable to drug-taking are simply more likely to start with readily available substances such as marijuana, tobacco, or alcohol, and their subsequent social interactions with others who use drugs increases their chances of trying other drugs. Further research is needed to explore this question.
- NORML FAcebook
- NORML Twitter
- NORML Insta

Working to Reform Marijuana Laws Since 1970
Marijuana and the ‘gateway theory’.

Experts agree that the use of marijuana is not causally linked to the use of other illicit substances
Is recreational marijuana use a gateway to harder drug use and crime national bureau of economic research, 2021, is marijuana really a gateway drug a nationally representative test of the marijuana gateway hypothesis using a propensity score matching design, nature communications, 2021, the benjamin center for public policy initiatives at suny new paltz university, the marijuana gateway fallacy, 2017, ready for retirement: the gateway drug hypothesis, substance use & misuse, 2015, reassessing the marijuana gateway effect, addiction, 2002, report of the special senate committee on illegal drugs, cannabis: our position for a canadian public policy, 2002, national academy of sciences, marijuana and medicine: assessing the science base, 1999, sequentially, most consumers of illicit substances first experimented with either alcohol or tobacco, first use of cannabis compared to first use of alcohol and tobacco: associations with single and poly-substance use behavior, drug and alcohol dependence, 2023, prioritizing alcohol prevention: establishing alcohol as the gateway drug and linking age of first drink with illicit drug use, the journal of school health, 2016.
- RESOURCES: Relationship between substance initiation sequence and further substance use: A French nationwide prospective study , Addictive Behaviors, 2016
- Birth cohorts analysis of adolescent cigarette smoking and subsequent marijuana and cocaine use , American Journal of Public Health, 2016
Statistically, the overwhelming majority of people to try marijuana do not go on to use other illicit drugs, and most typically cease their use of marijuana by middle age
Us national institute on drug abuse, “is marijuana a gateway drug” factsheet, predictors of marijuana use among married couples: the influence of one’s spouse, drug and alcohol dependence, 2007, in jurisdictions where marijuana is legally accessible, adults typically report decreasing their use of other controlled substances. in this sense, marijuana appears to act more as a potential ‘exit drug’ rather than as an alleged ‘gateway’, trends in alcohol, cigarette, e-cigarette, and nonprescribed pain reliever use among young adults in washington state after legalization of nonmedical cannabis, journal of adolescent health, 2022, rationale for cannabis-based interventions in the opioid overdose crisis, harm reduction journal, 2017, substitution of medical cannabis for pharmaceutical agents for pain, anxiety, and sleep, journal of psychopharmacology, 2017, medical cannabis access, use, and substitution for prescription opioids and other substances: a survey of authorized medical cannabis patients, international journal of drug policy, 2017, cannabis as a substitute for prescription drugs – a cross-sectional study, journal of pain research, 2017, substituting cannabis for prescription drugs, alcohol and other substances among medical cannabis patients: the impact of contextual factors, drug and alcohol review, 2015, in clinical settings, marijuana use is associated with reduced cravings for cocaine and opiates, intentional cannabis use to reduce crack cocaine in a canadian setting: a longitudinal analysis, addictive behaviors, 2017, the effects of dronabinol during detoxification and the initiation of treatment with extended release naltrexone, drug and alcohol dependence, 2015, does cannabis use predict poor outcome for heroin-dependent patients on maintenance treatment a review of past findings, and more evidence against, addiction, 2003, therapeutic use of cannabis by crack addicts in brazil, journal of psychoactive drugs, 1999, share this:, contact to listing owner.
Home › Blog › Science
A Brief Overview of the Gateway Hypothesis
December 15, 2021
Dane O'Leary
Most experts agree on the general facts surrounding the issue, but when it comes to the gateway hypothesis, there's no consensus on whether there is a causal link between the use of soft drugs and the later use of hard drugs.
The gateway hypothesis attempts to explain why it appears that people who use “soft” drugs seem to have an increased probability of using other, “harder” drugs. The hypothesis proposes that there is something about using less addictive drugs that leads to the use of more addictive drugs. The idea has existed in some form for almost a century. But while there are studies that support the gateway hypothesis, there are conflicting studies as well.
While most experts agree on the general facts surrounding the issue (e.g., if someone uses hard drugs, they probably used soft drugs at some point before that), there is no consensus on whether there is a causal link between the use of soft drugs and the later use of hard drugs.
A Brief History of the Gateway Hypothesis
The gateway hypothesis was initially advanced by Dr. Denise Kandel in 1975 although it didn’t really enter the public consciousness until the ’80s when American leadership really began to wage what we still refer to as the War on Drugs. However, similar ideas were around much earlier.
In fact, the gateway hypothesis has its origins in the 1930s. At that time, the stepping-stone theory held that “consumption of a ‘soft’ drug such as marijuana inexorably sets an individual on a trajectory to addiction to hard drugs.”
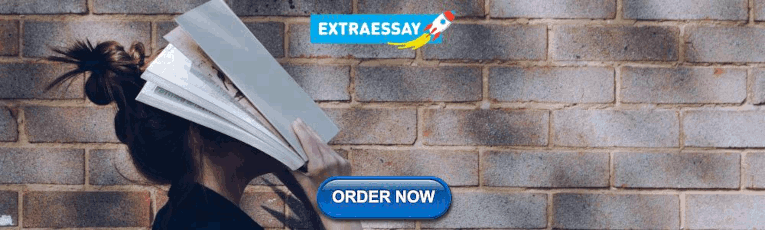
What is a Gateway Drug?
Theoretically, a gateway drug is known by its effects. The effect of using gateway drugs is an increased risk of physical and psychological dependence on drugs in the future. It is a soft drug that increases the probability that a person will later move on to hard drug use. But what is a soft drug?
Soft drugs are drugs that are thought to be less likely to lead to dependence. That’s not to say that they are harmless. There are risks involved with using soft drugs, but the medical consensus is that these drugs are less likely to lead to physical or psychological addiction Hallucinogens like cannabis, mescaline, psilocybin, LSD, ayahuasca, iboga, and DMT are all considered soft drugs.
Examples of Gateway Drugs
There are many soft drugs that can lead to dependence on other drugs in the future. However, the National Institute on Drug Abuse (NIDA) released the Marijuana Research Report in 2020, which identified marijuana as a gateway drug.
Like marijuana, “alcohol and nicotine also prime the brain for a heightened response to other drugs and are, like marijuana, also typically used before a person progresses to other, more harmful substances,” according to the same report.
Gateway Drugs & the Stages of Addiction
Dr. Nora Volkow , director of NIDA, has conducted research on how people become addicted, breaks the addiction process down into three phases: (1) binge and intoxication, (2) withdrawal and negative effects, and (3) preoccupation and anticipation.
In the first stage, a person takes a drug and their brain releases dopamine, a “feel-good” transmitter . After that, the person experiences withdrawal symptoms in stage two. This can lead them to seek more of the drug. At some point, people who become addicted begin to be preoccupied with the drug and anticipate their next dose in the final stage.
It stands to reason that, as a person’s tolerance for a drug increases and their brain “adjusts by producing fewer neurotransmitters in the reward circuit, or by reducing the number of receptors that can receive signals” they may begin to search for harder drugs in order to achieve the high they experienced before. But there the scientific community has not reached a consensus on the question.
Mixed Evidence for Gateway Hypothesis
While the Marijuana Research Report found that marijuana leads to dependence on other substances, it also found that “the majority of people who use marijuana do not go on to use other, harder substances.” The study’s support for the claim that marijuana is a gateway drug was based on the researchers finding that marijuana users were more likely to develop a dependence on alcohol and nicotine.
The report notes that it is also possible that “people who are more vulnerable to drug-taking are simply more likely to start with readily available substances such as marijuana, tobacco, or alcohol, and their subsequent social interactions with others who use drugs increase their chances of trying other drugs.” And, even if there is a causal link between soft and hard drug use, there is still research to be done in order to determine exactly what the mechanism is that explains the link.
Do Soft Drugs Lead to Dependence on Harder Drugs?
Though the gateway hypothesis and similar variations of the concept have been around for almost a century, there is still more research to be done in order to determine whether there is a causal link between soft drug use and a later dependence on drugs. But whether the gateway hypothesis is correct or not, addiction is certainly real.
If you or someone you know is harming themselves with drug abuse, contact Never Alone Recovery to learn about the treatment options available for gateway drug abuse and addiction.
gateway drugs , gateway hypothesis
We're On Medium!
We're sharing the recovery stories of our community members. Be sure to check out Never Alone Recovery on Medium to read those featured stories.
YOU MAY ALSO LIKE...
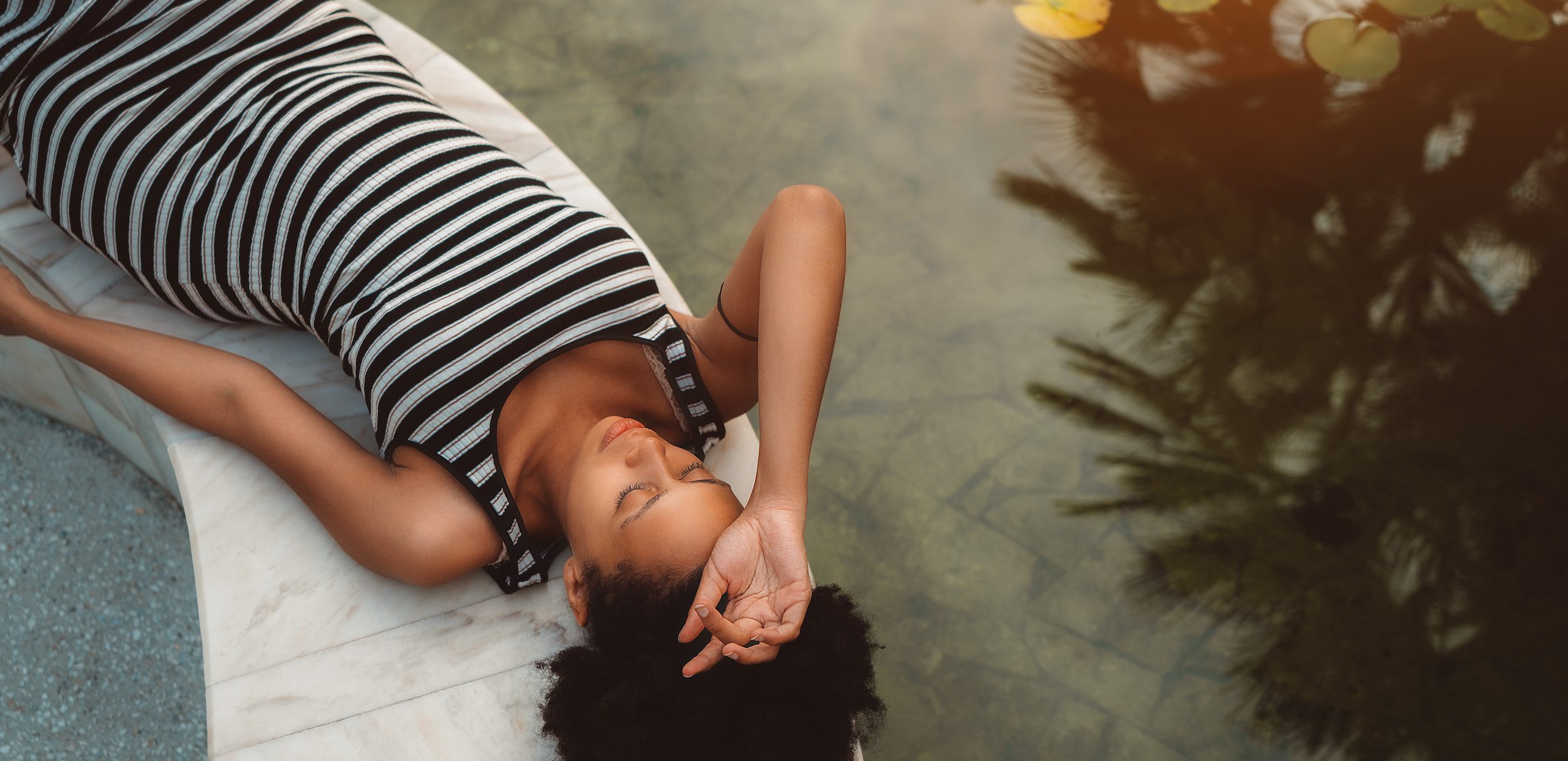
January 11, 2024
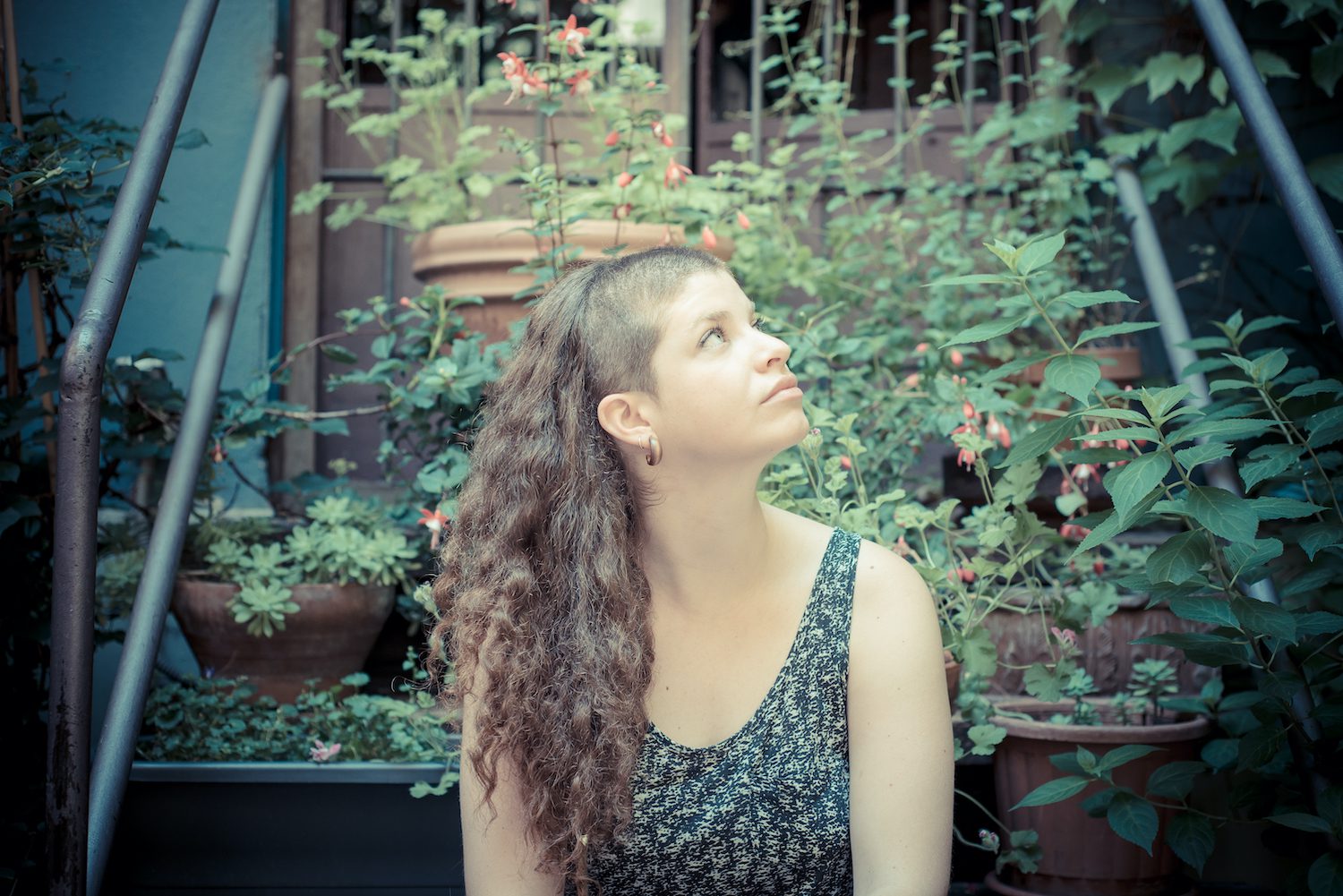
December 26, 2023
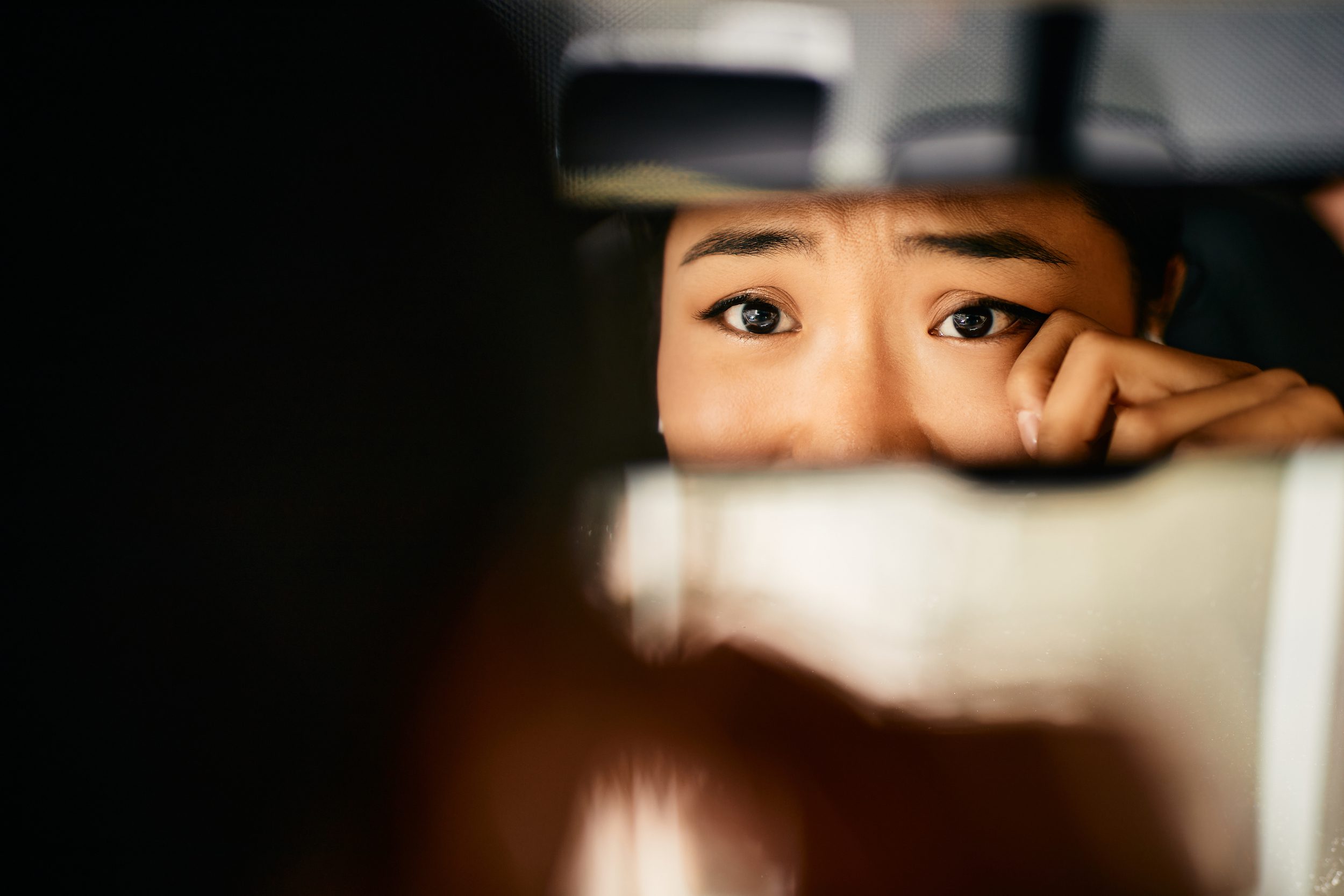
February 17, 2023
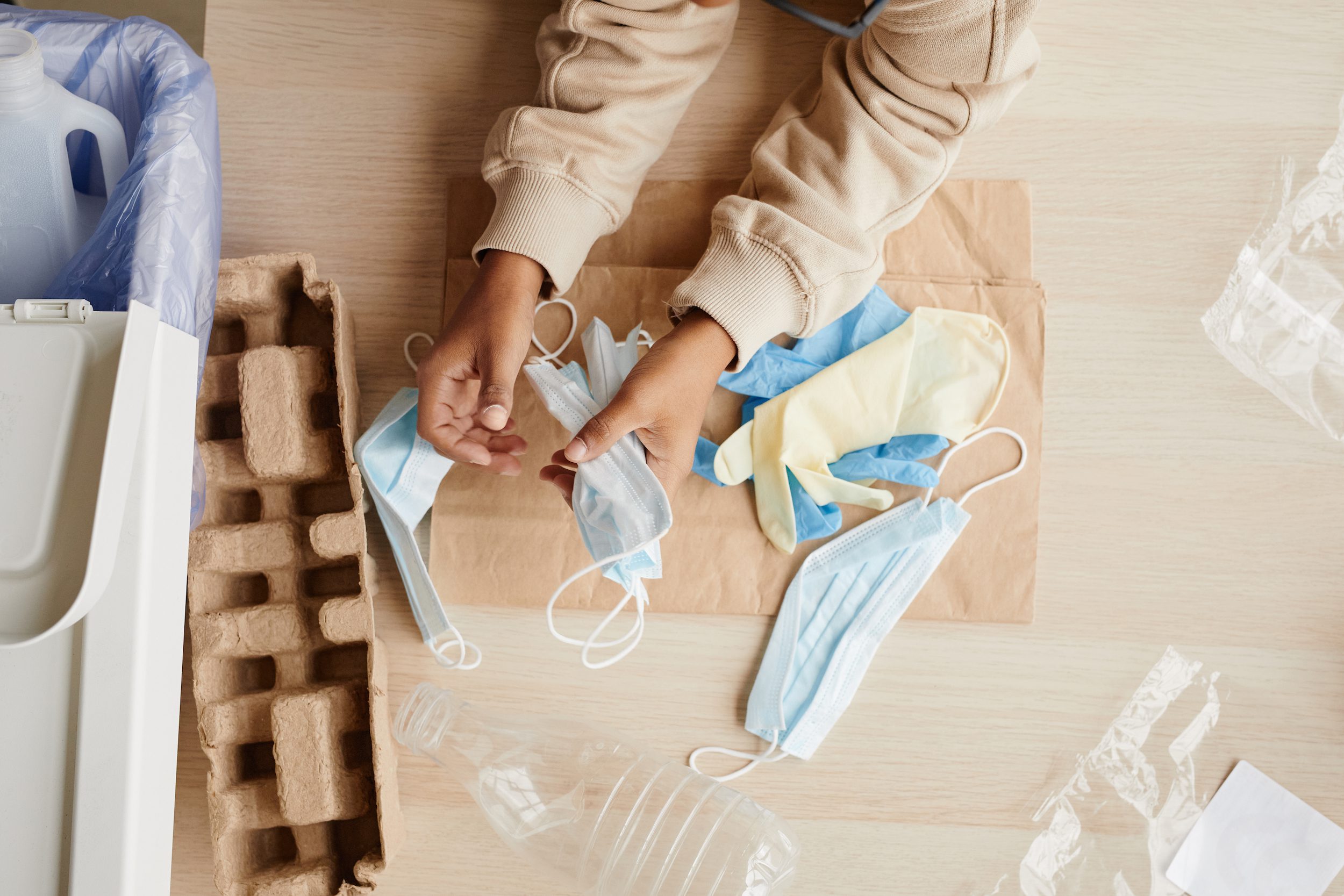
January 21, 2023
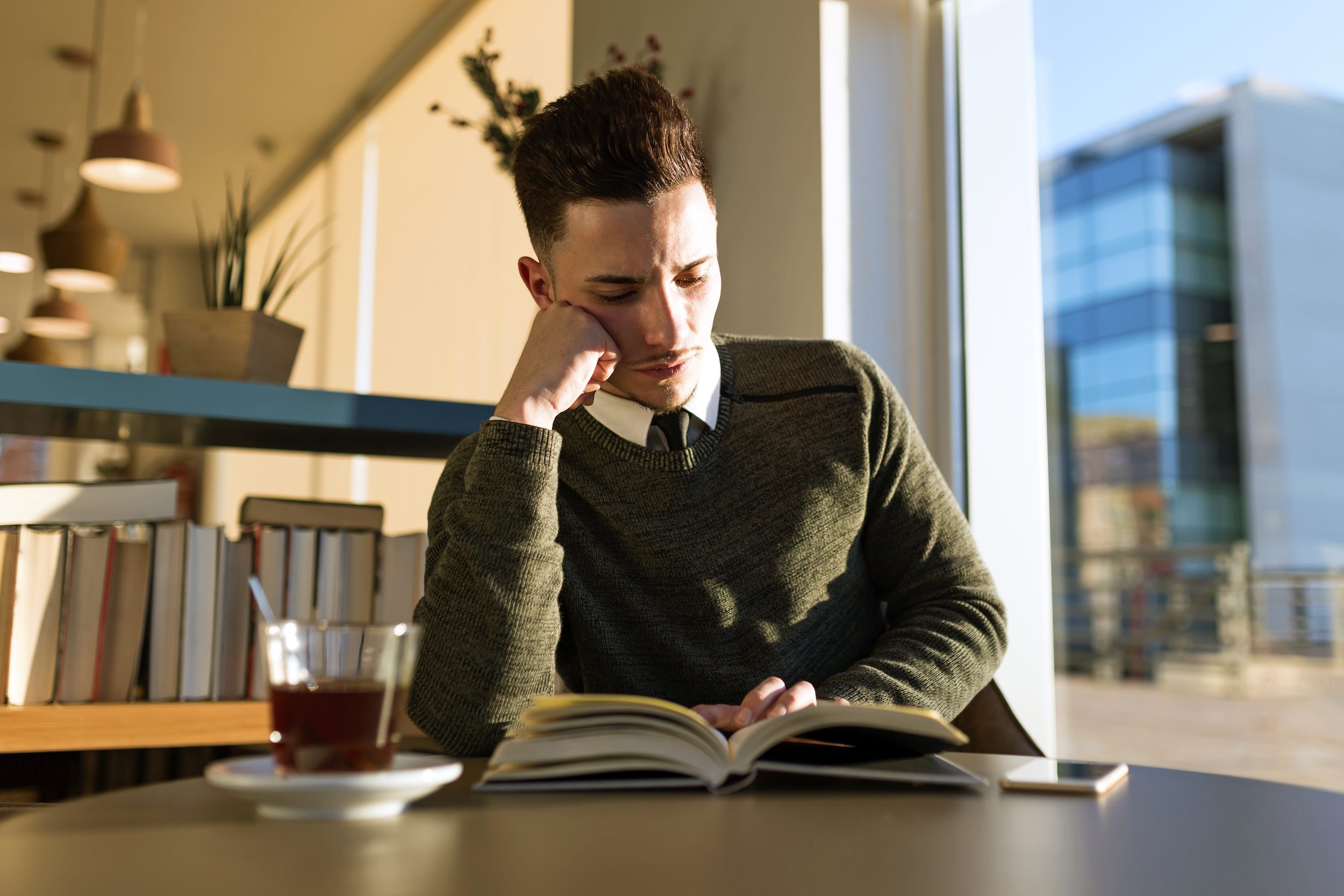
April 27, 2023
Get Your Free Consultation Now
No matter where you are or what you're going through, send us a secure message and someone from our team will get back to you.
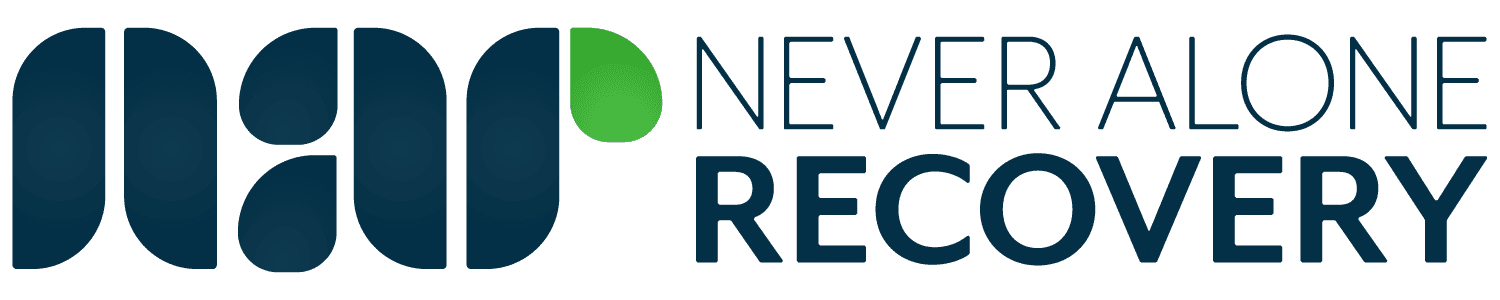
Check Out Our eBook
Surviving your loved one's addiction, $19.95 value.
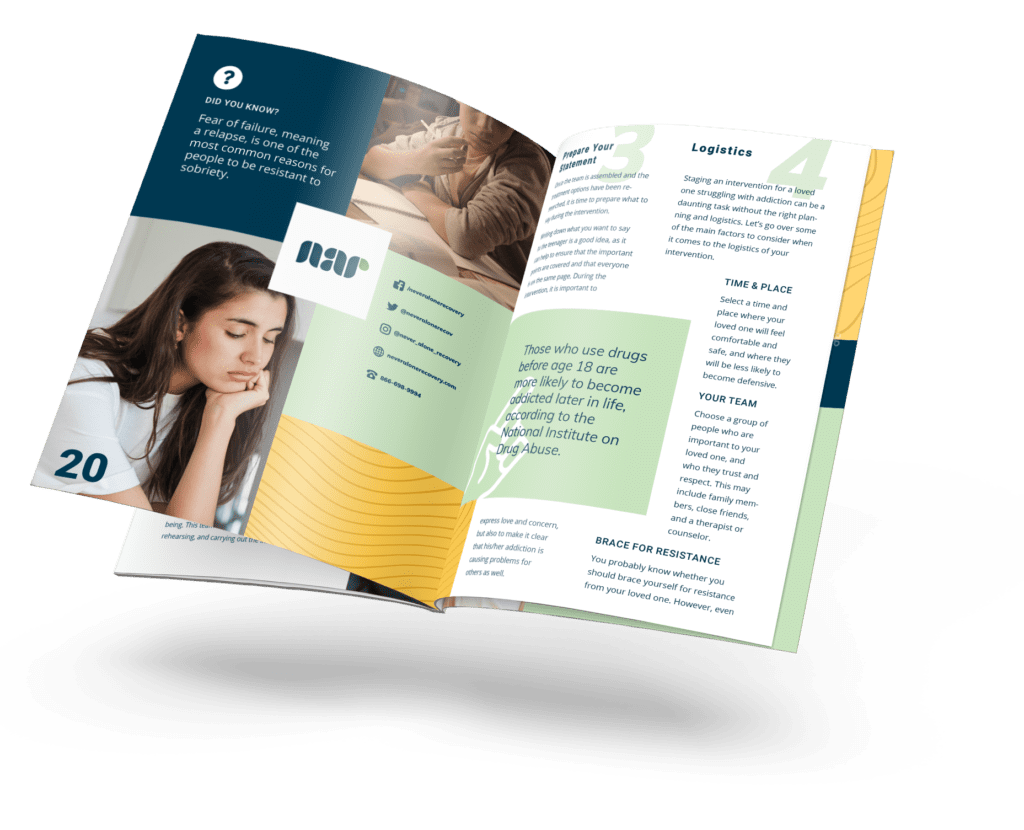
Smithsonian Voices
From the Smithsonian Museums
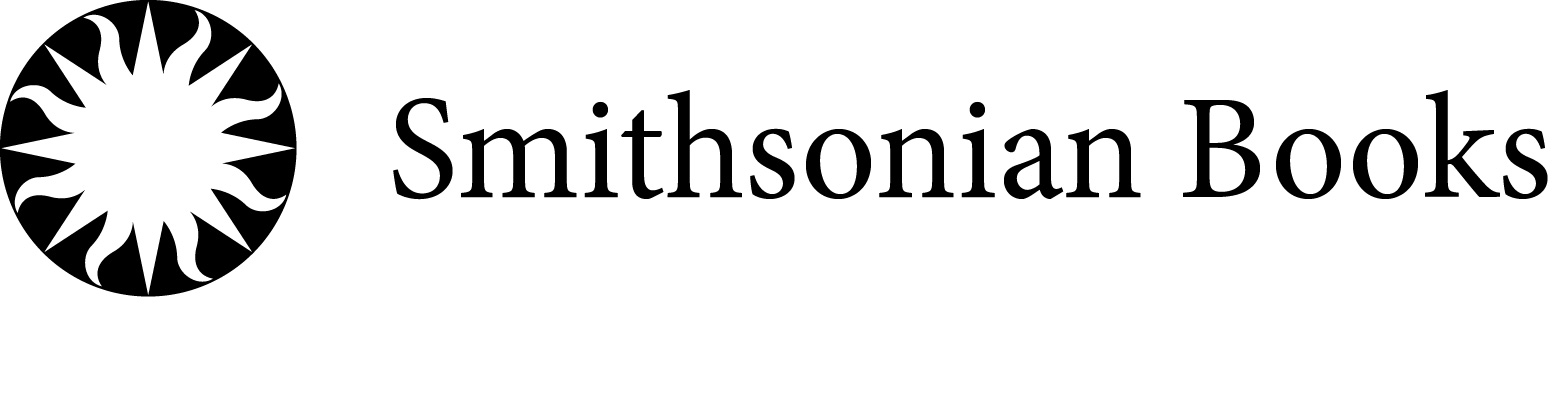
SMITHSONIAN BOOKS
Where Is Everybody in Our Universe?
Two physicists contemplate life on other planets in this excerpt from “Exoplanets”
Michael Summers and James Trefil
:focal(812x502:813x503)/https://tf-cmsv2-smithsonianmag-media.s3.amazonaws.com/filer_public/07/79/077988b2-736a-422d-b78a-1b0fcad1f55b/exoplanet.jpg)
The story is that it all started one day in 1950, when a group of prominent physicists— all veterans of the Manhattan Project—were walking to lunch at the Fuller Lodge in Los Alamos. They were discussing the spate of recent UFO sightings that had been claimed in the area, and the conversation turned to the topic of extraterrestrial civilizations. Out of the blue, Enrico Fermi (1901–54), a man well known for his ability to see to the heart of a problem, asked a simple question: Where is everybody? In the years since then, scientists have come to realize that Fermi’s offhand question is, in fact, the deepest question we can ask about life in our galaxy. The fact that there is no evidence for the existence of extraterrestrials in spite of the calculations suggesting that they should exist is known as the Fermi paradox.
So why has his offhand question played such an important role in the debate about extraterrestrials? To understand this, we can go back to our old device of compressing the lifetime of the universe into a single year. In this scheme, the Sun and our solar system formed in the late summer (Labor Day is a convenient approximation), modern humans showed up a few minutes before midnight on New Year’s Eve, and all of recorded history took place while the ball is descending in Times Square, with modern science appearing in the last second of that descent.
The point is this: if there really are other technological civilizations out there, it is extremely unlikely that they developed science after we did—after all, they had the whole year to discover the laws of nature. To understand what follows from this statement, let’s look at a possible future for the human race.
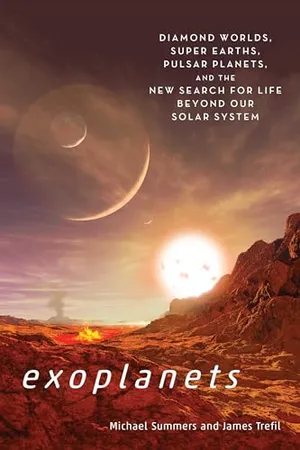
Exoplanets: Diamond Worlds, Super Earths, Pulsar Planets, and the New Search for Life beyond Our Solar System
In Exoplanets, astronomer Michael Summers and physicist James Trefil explore remarkable recent discoveries: planets revolving around pulsars, planets made of diamond, planets that are mostly water, and numerous rogue planets wandering through the emptiness of space.
We’ll start at Princeton University in the 1970s, where physicist Gerard O’Neill (1927–92) was teaching a seminar centered around an interesting question: is the surface of a planet really the best place for a technological civilization? The answer the class came up with was “no,” and from their deliberations came the design for a structure now called an O’Neill colony.
Imagine a hollow doughnut, a mile or more across, rotating slowly in space. In O’Neill’s vision, people live inside the doughnut, and the centrifugal force associated with its rotation substitutes for gravity. Using solar or nuclear power, possibly with ancillary doughnuts for raising crops, such a system could be self-sustaining, a true move of humanity away from our home planet. It is almost within our technological capabilities to build such a structure right now, if not within our budgets. In any case, we should expect that any extraterrestrial race that has come to our level of technical sophistication should also be able to build something like an O’Neill colony.
Let’s imagine how something like O’Neill colonies might play out in our future. Eventually, we can expect that people in colonies like this would leave the space around Earth and move to the truly prime real estate in the solar system, the asteroid belt, where ample material and solar power are available.
It’s the next step that has enormous implications for the Fermi paradox. After a few generations have spent their lives in something like an O’Neill colony, will it really matter to them if their colony is on the way to another star system rather than in the asteroid belt? As the best locations in our own system fill up, it is reasonable to suppose that future space colonists will follow the example of their forebears and “light out for the territories,” except that, in this case, that would mean moving to other solar systems. In essence, we suggest that they would turn their colonies into interstellar starships. How hard would that be?
Let’s make two extraordinarily conservative assumptions. Let’s assume that (1) there is no way to get around the speed-of-light barrier—no “warp drive”—and (2) no major technological advances will be made in the next couple of centuries. The immense distance between stars would require travel times of a century or more, which would mean that the starship would be multigenerational—you get on, your grandchildren get off. Several propulsion systems for such a trip have been proposed—for example, one in which the ship scoops up rarefied interstellar hydrogen to run its power and propulsion systems. The idea of such a multigenerational starship is already a staple of science fiction.
The point of this exercise in futurology is that once a civilization reaches our level of sophistication, it is only a matter of a few centuries before it can start colonizing other star systems. If we can imagine ourselves doing it, then there’s no reason extraterrestrials couldn’t do it as well. The important point for our discussion is that we are talking about a time span of only a few hundred years. In terms of our galactic year analogy, this amounts to only one second. Basically, as soon as the ball touches down in Times Square, Earth could be the center of an expanding wave of human colonization. No one would even have time to say, “Happy New Year.”
How long would it take that wave to engulf the entire galaxy? Most calculations give times on the order of 30 million years or so. And while this is an extremely long time on a human scale, it is only one day in our galactic year. So if extraterrestrial civilizations have been popping up throughout the galactic year, and if at least some of those civilizations are as scientifically adept as we are, there should have been multiple waves of colonization sweeping over the solar system.
So . . . where is everybody?
That, in essence, is a modern look at the question Fermi asked over a half century ago, one we still haven’t been able to answer. His point can be stated this way: we shouldn’t be looking for extraterrestrials out there, as we do in SETI (the search for extraterrestrial intelligence)—we should be looking for them right here. And if we ignore the silliness of UFOs and ancient astronauts, we can say that there is no evidence whatsoever for extraterrestrials being here now or in the past.
Where is everybody? Why the Great Silence?
/https://tf-cmsv2-smithsonianmag-media.s3.amazonaws.com/filer_public/fe/79/fe7925dc-075e-4aa6-8e13-05d0593294e2/exoplanets.jpg)
William of Ockham was an English scholar who is famous for one throwaway line in an otherwise turgid theological treatise. Called Occam’s razor, it says, “Plurality must never be posited without necessity.” In essence, it tells us that when we have a question to answer, the simplest solution is the one we should choose. The concept shaves away complexity; hence the word razor .
There is no doubt that the simplest answer to the questions “Why the Great Silence? Why don’t we hear any SETI signals?” is that we don’t hear signals because no one is sending them. There are a number of other explanations that have been put forward, and we can look at them briefly before taking William of Ockham seriously. Basically, the explanations can be divided into three categories:
1. They really are out there, but they’re not interested in us.
2. They really are out there, but they’re protecting us.
3. They really are out there, and we’re going to get it unless we mend our ways.
An example of the first category would be a race of extraterrestrials living in a Dyson sphere, happy as clams with their star’s energy and supremely uninterested in anyone else. Another possibility would be extraterrestrials on a rogue planet who can’t imagine a planet near a star being inhabitable. An example of the second item in the list is seen in the Star Trek series, where spacefarers obey the Prime Directive, which forbids them from interfering with the development of other life forms. The last category is portrayed in the classic 1950s film The Day the Earth Stood Still , in which an extraterrestrial visitor warns that Earth will be destroyed unless we control our use of atomic weapons:
Klaatu barrada nikto!
All these schemes have two things in common. First, there is no evidence to support any of them, and, second, they are all somewhat improbable in a galaxy with thousands of different advanced civilizations. Some might indeed retreat to Dyson spheres or refuse to go near stars, but to suppose that all of them would is something of a stretch. Similar arguments can be made for the other explanations.
One way to approach the question posed by the Great Silence is to think of each term in the Drake equation as a gateway or valve on the way to an advanced technological civilization. If even one of those terms has a numerical value much less than we have assumed, the effect would be to greatly reduce our estimate of the number of extraterrestrials out there. In essence, that term would act as a kind of filter, blocking the orderly progression implied in the equation. To use a term introduced by economist Robin Hanson, our colleague at George Mason, somewhere in the chain of events in the Drake equation there might be a “Great Filter” that effectively blocks the development of civilizations that might be trying to communicate with us.
Some scientists have argued that the existence of periodic ice ages played an important role in producing the kind of social interactions needed to take humans beyond the hunter-gatherer stage. In one scenario, for example, the need to protect the nutritionally rich shellfish beds along the African coast—a dependable source of food—during an ice age is what led to both the kind of cooperativeness and the kind of aggressiveness that have characterized our species ever since. Again, if you accept this sort of argument, you are saying that the Great Filter is located at the point where intelligence progresses into advanced society. If this is true, there will be lots of planets with the equivalent of dinosaurs out there, but none (or very few) with radio telescopes.
Arguments that say, in effect, that there is something special about Earth that is unlikely to be duplicated elsewhere in the galaxy—go under the name of the Rare Earth Hypothesis. They are put forward most completely in a book titled Rare Earth , by geologist Peter Ward and astronomer Donald Brownlee. Ward and Brownlee’s central thrust is that we have been blindly accepting the Copernican principle—the idea that Earth is not special—and ignoring the fact that there are many unusual things about our home planet. In essence, they look at all the things that are unique about Earth and argue that if they are all necessary for an advanced civilization to develop, then we could well be the only such civilization in the galaxy. For example, if, besides an Earth-sized planet in the CHZ of its star, you need a star located a certain distance from the galactic center, a Jupiter farther out, plate tectonics, the right planetary tilt to produce ice ages, and a large moon to stabilize the planet’s axis of rotation and produce tidal pools (Darwin’s warm little pond), Earth might well be the only planet like that in the galaxy. The Rare Earth answer to the Fermi paradox is thus quite simple: there’s nobody here because there’s nobody there. We are indeed alone.
Those who don’t accept the Rare Earth Hypothesis assert that any specific event you want to talk about is extremely unlikely, and that simply reciting that fact proves nothing. Think, for example, of the chain of unlikely events that led to your reading these words. Your parents had to meet, you had to attend a certain school, learn to read, acquire an interest in science, and so on. There’s no point in harping on this improbability, though, because if you weren’t reading this book, you’d be doing something else equally improbable. In the same way, other types of improbable intelligences could have developed in the galaxy following their own improbable chain of events, and there could be an infinite number of those improbable paths. For these critics, all the Rare Earth Hypothesis proves is that there is at least one improbable path to an advanced civilization (our own); it says absolutely nothing about the possible existence of other paths.
The scenarios we have considered all have one thing in common: they all assume that the Great Filter is behind us, that by some combination of luck or providence, Homo sapiens has made it through all the filters and bottlenecks that stood in our way. But there is another, much more frightening possibility. What if none of these events in our past constitutes the Great Filter? What if the Great Filter is still in front of us?
To understand the importance of this question, let’s think for a moment about the nature of the evolutionary process. Natural selection is driven by one criterion and one criterion only: the need to get an organism’s genes into the next generation. Winners in the evolutionary game, in other words, are not determined by moral or ethical considerations. Consider the history of our own species as an example of this statement. The appearance of Homo sapiens in any region once we left Africa was accompanied by the disappearance of competing hominids (think Neanderthals and Denisovans) and just about every large animal (think woolly mammoths and giant tree sloths). We became the dominant life form on the planet by wiping out our competitors, either directly or indirectly. Given this history, we think it’s fair to say that Homo sapiens is not the sort of species you’d want to meet in a dark alley, and the same will be true of any other winner of the evolutionary game who became the dominant species on their planet.
The “Great Filter is in front of us” argument goes like this: despite the Rare Earth Hypothesis, there really doesn’t seem to be anything all that special about the way that life developed on Earth, and given the abundance of planets out there, there is no reason that complex life shouldn’t be quite common. On the other hand, from what we know about the process of evolution, we can expect the winners of the evolutionary game on other planets to be no more benevolent than Homo sapiens . In this case, the coming Great Filter is easy to see. Once an aggressive, warlike species discovers science, they are likely to turn their discoveries against one another and, in essence, wipe themselves out.
The picture of galactic history that comes from this argument is a disturbing one. From the very beginning, intelligent, technologically advanced societies have appeared only to disappear in a short time as they succumb to their own dark inner nature—a nature produced by the laws of natural selection. No one is out there, in other words, because they’ve all wiped themselves out long ago, before we started listening.
Exoplanets is available from Smithsonian Books. Visit Smithsonian Books’ website to learn more about its publications and a full list of titles.
Excerpt condensed for print from Exoplanets: Diamond Worlds, Super Earths, Pulsar Planets, and the New Search for Life beyond Our Solar System © 2017 by Michaels Summers and James Trefil
A Note to our Readers Smithsonian magazine participates in affiliate link advertising programs. If you purchase an item through these links, we receive a commission.

An official website of the United States government
The .gov means it’s official. Federal government websites often end in .gov or .mil. Before sharing sensitive information, make sure you’re on a federal government site.
The site is secure. The https:// ensures that you are connecting to the official website and that any information you provide is encrypted and transmitted securely.
- Publications
- Account settings
Preview improvements coming to the PMC website in October 2024. Learn More or Try it out now .
- Advanced Search
- Journal List
- Int J Environ Res Public Health

Gateway Effects: Why the Cited Evidence Does Not Support Their Existence for Low-Risk Tobacco Products (and What Evidence Would)
It is often claimed that low-risk drugs still create harm because of “gateway effects”, in which they cause the use of a high-risk alternative. Such claims are popular among opponents of tobacco harm reduction, claiming that low-risk tobacco products (e.g., e-cigarettes, smokeless tobacco) cause people to start smoking, sometimes backed by empirical studies that ostensibly support the claim. However, these studies consistently ignore the obvious alternative causal pathways, particularly that observed associations might represent causation in the opposite direction (smoking causes people to seek low-risk alternatives) or confounding (the same individual characteristics increase the chance of using any tobacco product). Due to these complications, any useful analysis must deal with simultaneity and confounding by common cause. In practice, existing analyses seem almost as if they were designed to provide teaching examples about drawing simplistic and unsupported causal conclusions from observed associations. The present analysis examines what evidence and research strategies would be needed to empirically detect such a gateway effect, if there were one, explaining key methodological concepts including causation and confounding, examining the logic of the claim, identifying potentially useful data, and debunking common fallacies on both sides of the argument, as well as presenting an extended example of proper empirical testing. The analysis demonstrates that none of the empirical studies to date that are purported to show a gateway effect from tobacco harm reduction products actually does so. The observations and approaches can be generalized to other cases where observed association of individual characteristics in cross-sectional data could result from any of several causal relationships.
1. Introduction
An often-claimed downside of harm reduction strategies is that the lower-risk alternative to the high-risk behavior is a “gateway” to the high-risk behavior. That is, the availability or promotion of the low-risk option causes some people who would not otherwise have done so to adopt the high-risk behavior. Various studies have purported to show that such effects exist but, at least for the case of tobacco, none of the evidence actually supports the claim. Rather, they demonstrate the faulty understanding of the authors about what evidence would support the claim.
Most of the analysis presented here applies to any gateway claim, but the presentation focuses on tobacco harm reduction (THR) products, low-risk alternatives to smoking, including smokeless tobacco and e-cigarettes. The analysis also generalizes to any situation where cross-sectional data is used to test a particular causal claim when competing causal pathways appear to be much stronger causes of the association. In the THR case, there is clear confounding due to a common cause, which can be thought of as the generic unspecified common cause, typically designated “U” in the methods literature, or specifically in terms of a propensity toward liking the effects of tobacco/nicotine and being willing to resist the social pressures that demand abstinence. There is also clear causation in the opposite direction of the gateway: low-risk tobacco products, especially e-cigarettes, are almost exclusively used as a substitute for smoking, causing an association between their use and the use of cigarettes. Any methodology attempting to identify the effects of one causal pathway among those three—especially if, as with the THR gateway claim, the pathway of interest undoubtedly contributes less to the association than the others—requires a great deal of thought about how to use data.
Gateway claims became common starting in the 1950s, as a U.S. Drug War tactic aimed at demonizing cannabis use. (The term itself appears to have been coined in the 1970s as part of that effort and of the still ongoing studies by one researcher who does not appear to have seriously considered the complications presented in the present paper [ 1 , 2 ].) The biological and social effects of typical patterns of cannabis use are inadequate to justify a prohibitionist war, and there was even less evidence suggesting it was harmful at that time. So the claim was adopted that it is seriously harmful because it causes some users to “move on” to “hard drugs”. A similar tactic has been adopted by opponents of THR strategies, in which they claim that their use causes substantial harm because it causes some people to start smoking (e.g., [ 3 , 4 , 5 , 6 , 7 ]). It has even been claimed by the coiner of the term “gateway” that e-cigarettes are a gateway to cocaine [ 2 ], though that claim is easily debunked [ 8 ].
Gateway claims can serve as a refuge for activists who profess concern about health or welfare, but really oppose a particular behavior for some other reason and cannot find any health- or welfare-based objection. The claims serve as a rationalization that is sufficiently ominous to influence the thinking of an uncritical target audience due to the common tendency to fixate on the consequences of a possible negative outcome, ignoring its probability and thus net expected value. The consistent lack of quantification in the claims ( i.e. , how many gateway cases have occurred or are predicted) tends to suggest that their only goal is to trigger this cognitive bias.
Opponents of THR invoke the gateway claim in discussions about eliminating the products entirely (which would stop any gateway effect), but also about regulating where the products can be used (which would have no apparent effect on any gateway). They invoke the same claims when the discussion is about whether to encourage the use of low-risk alternatives among current smokers (which could not possibly create a gateway). This suggests the gateway is, to them, merely a rhetorical tactic, not a genuine concern. Indeed, a leading anti-THR strategy is to claim that the low-risk products are much higher risk than they are, which, if believed, tends to increase , rather than decrease, any gateway effect because it tells anyone using those products that they might as well smoke [ 9 ]. It has recently been argued that propaganda campaigns against e-cigarettes have caused many vapers (users of e-cigarettes) to switch back to smoking [ 10 , 11 ]. These observations suggest that the stated worry about gateway effects is disingenuous.
But even if the gateway claim is primarily used as a rationalization for other goals, there is a legitimate scientific question about the extent to which it occurs. There are certainly policy-makers and other observers who have been persuaded to believe there are substantial effects that should motivate policy. Thus, there is value in analyzing what empirical evidence is potentially useful in assessing whether such effects exist. Even if the conclusion is that there is little chance of demonstrating an effect, there is value in identifying what research could (and thus what research could not) detect it, because it serves to point out which claims are groundless.
The following analysis explains why most of the evidence that is typically cited in support of gateway claims is invalid, and is perfectly consistent with there being no gateway effect or even with extreme alternative hypotheses. The empirically-based claims that are made only support the hypothesis that people who use one tobacco product are more likely than the average person to use another. They do not support a gateway claim over two other candidate causes of such an association. However, the present analysis also points out that some of the arguments most frequently made against gateway claims are also faulty. It concludes with an assessment of what empirical evidence could be used to support or refute gateway claims and an example of applying such reasoning.
While some of the particulars of this analysis are rather unusual, there are many general lessons about analyzing cross-sectional data. The most obvious is about not confusing causation in one direction with confounding or causation in the other direction, a straightforward point but one that can always benefit from additional teaching examples. More subtle potentially generalizable lessons can be found in the proposed strategies for dealing with that challenge, in particular how to devise severe hypothesis tests using data that is not as rich as we might prefer.
2. Analysis
2.1. the logic of the gateway claim.
Describing the mechanism by which a cause might lead to an effect is frequently useful. Cause-effect relationships are often established without knowledge of a mechanism when studying the complexities of human biology. But in a relatively straightforward process of preferences, decisions, and volition, as in the present case, it is possible to characterize the necessary mechanism. Doing so offers some clues about useful empirical research while also emphasizing that this is an extraordinary claim that calls for extraordinary evidence.
Gateways certainly can exist. For example, medical opiates appear to sometimes be a gateway to heroin, with some people who never would have considered using heroin acquiring a strong preference for consuming opiates due to medical usage (which is often labeled addiction or dependence, though such characterizations are immaterial for present purposes), and then discovering that the net benefits of heroin are even greater, considering both the effectiveness and the relative cost of obtaining it. But for tobacco products there is no analogous story. The barriers to starting smoking are quite low, in contrast with heroin use, so there are not formidable obstacles that require a barrier-lowering experience or “ramping up” effect. Moreover, while some THR alternatives are a bit more difficult to acquire than cigarettes in some places due to bans or anti-THR taxes, they rarely become unavailable to a user who became accustomed to them as can happen with medical opiates. Thus, the most obvious real gateway problem that currently exists has little resemblance to use of tobacco products.
Consider the scenario that is needed to create a gateway case of smoking: Someone chooses abstinence over smoking, though the latter is an easy option. Assume that this individual is among the approximately half of the population that benefits from using tobacco (and thus is even a candidate to start using the products). The choice of abstinence shows she is among the approximately half of that subpopulation who decide that the costs of smoking outweigh the benefits (which is why she does not smoke in spite of those benefits). She is then exposed to a low-risk alternative that she prefers to abstinence. Why, exactly, would she then switch from this product to what had been her least-preferred option, smoking? The logic of this is difficult to conceive and is never explained by those who claim there is such a gateway effect.
It is not impossible, of course. At the moment, smoking delivers nicotine more effectively than existing low-risk alternatives, and includes other psychoactive agents that are not present in extracted nicotine products such as e-cigarettes. It is thus possible that someone who never even tried smoking because of concerns about its health effects could discover how much she would like it by discovering she likes the low-risk alternative. This could conceivably lead her to conclude that smoking has benefits that are greater than its costs. It is also possible that she had tried smoking and was not enthralled, but acquires a growing taste for nicotine via the use of a low-risk alternative which then makes the better delivery more attractive than it was initially. However, in both cases, the new net marginal appeal of smoking would have to be positive. That is, the advantages of smoking over and above those already provided by the low-risk product would have to be greater than the health costs of smoking (net of the trivial health costs of the alternative product), and enough to get her to overcome the inertia to switch products. Even if the change in total appeal promoted smoking over abstinence, a much greater change would be required to elevate it over the low-risk product. It is difficult to imagine this scenario occurring.
It is significant that proponents of the gateway claim never even attempt to present a scenario for why someone would ever make the gateway switch. This is presumably because presenting this scenario would illustrate that our prior beliefs should be that the effect is improbable and rare.
It is possible to engineer a gateway from low-risk tobacco products to smoking via public policy, similar to the opiates story. A spike in the punitive smokeless tobacco taxes in Canada caused some nonsmoking smokeless tobacco users to switch to cigarettes, at least occasionally, because they became much cheaper than the smokeless product they preferred [ 12 ]. A ban (either literal or de facto ) on e-cigarettes, which is still possible in many jurisdictions, could drive some non-smokers to smoke, though almost all would be former smokers who resume smoking, so it is not clear this should be called a gateway. Thus an incentive can be created to switch from a low-risk tobacco product to smoking, but little such incentive exists naturally.
2.2. Causation
As is common when terms from political rhetoric find their way into scientific analysis, the word “gateway” is seldom defined by those who use it. For the gateway claim to match the policy discussion that surrounds it, it must be interpreted as the low-risk product causing the use of the high-risk product. This is sometimes stated explicitly and can be inferred in other cases. (The claim that the high-risk behavior is caused to persist as a result of the low-risk alternative, but not caused to initiate, has similar implications to the gateway claim. But it is a fundamentally different phenomenon, requiring different research, and so is not included here and does not seem to be properly included in the “gateway” label.)
An alternative potential meaning, “C (the ostensible cause) merely precedes E (the ostensible effect)” is clearly not what is meant, though there is sometimes a bait-and-switch use of such a definition. “C is a convenient step on the way to E, which is the ultimate goal” is the literal interpretation of the metaphor (you pass through a portal because you are trying to arrive at something on the other side of it, so the destination causes the use of the gateway, not the other way around), but clearly this is not the intended interpretation. Similarly, sometimes THR proponents attempt to hijack the term, saying “yes, there is a gateway, but only away from smoking.” While it is easy to understand the temptation, this is not a legitimate argument; the word has an accepted meaning and trying to redefine it does not constitute an argument that the phenomenon does not exist.
Though the philosophical nuances of the concept of causation are extensively debated, for practical purposes the statement “C causes E” is defined by the counterfactual statement, “if C is true then E will occur and if C is not true then E will not occur, holding constant everything else that is not caused by C.” Granted the last condition, as phrased, is a bit circular (technically it is an infinite recursion), but it gets the point across for practical purposes. Counterfactual refers to the fact that if one of those if-then scenarios is observed then the other cannot be, and so is necessarily counter to the facts. This is why causation can never be observed or proven, only inferred.
The previous paragraph describes causation at the level of a single event or individual. At the population level, a cause is a factor that for some individuals has the properties of C in the definition. When causation is inferred from data about a population (as it almost always is in social science), it will not be known which particular cases of E were caused by C and which were not (unless C is a necessary cause—see below). That C is causing some cases of E can be inferred from particular patterns in data. However, as explained below, the simplest observation about the data—a correlation between the variables that are candidates for C and E—is not in itself sufficient information, and further analysis is required before the claim is established.
Every worldly outcome has multiple causes. Someone becoming a smoker on a particular day might be caused by a friend handing her a first cigarette, but it is also caused by her living long enough to become a smoker and by the historical fact that the proto-tobacco plant evolved and was discovered and cultivated by humans. Practical discussion tend to only address causes like the first, the one that might not be a cause of her ever becoming a smoker. If the world were changed such that tobacco did not exist, she would never become a smoker. However, if the world were changed so she was home sick instead of experiencing the particular friend event, she might not have become a smoker that day, but some other event in her life could have caused her to become a smoker.
The friend event is the only one on that list that is not a necessary cause of her ever becoming a smoker. A necessary cause is anything whose absence would ensure that the outcome would not occur no matter what else was true. Put another way, if you listed every set of causes that would lead to the outcome if all in the set were true, a necessary cause would appear in every such list. In social sciences, necessary causes are seldom very interesting, though the failure to understand the concept sometimes creates confusion.
A sufficient cause is a cause that produces the effect no matter what else is true. So if we created the list of each combination of causes that result in an outcome, one of the entries on the list is the sufficient cause by itself. Single sufficient causes do not exist in the real world, except when they are tautological, definitional, or a constructed conjunction. Smoking your 100th cigarette causes you to become an ever-smoker if “ever-smoker” is defined as having smoked at least 100 cigarettes. It is always possible to take any list of causes that together are sufficient and combine them into one long conjunction and call it a cause. Indeed, in epidemiology that conjunction is often described as a sufficient cause, following the terminology of Rothman’s “sufficient-component cause model”. But familiarity with this convention can distract from the fact that what we think of as a single event (not a conjunction) is never a sufficient cause, and failure to understand the difference between sufficient cause and cause creates confusion. For example, it is sometimes suggested that since most smokers who have tried e-cigarettes are still smokers, it must be that e-cigarettes must not cause smoking cessation. This is wrong because it merely shows that trying an e-cigarette is not sufficient for smoking cessation; it is still a cause (for some individuals, and thus at the population level).
A critical observation is that many effects are overdetermined , meaning that all the causes of two or more of the sets in the list are met. This means that if you changed the world so that one set of causes were no longer all true, the outcome would still be caused by another set if it remained unchanged. In the above example, if the subject, who we know has an inclination to smoke, was not handed her first cigarette by a particular friend on a particular day, there is still a good chance that she would become a smoker because some other set would be completed (e.g., a month later she asked for a puff from a different friend). In common language, each item on each redundant set of potential causes for an overdetermined effect is often called a cause, though the counterfactual definition says that none of them (except those that appear in every list like “tobacco exists”) are causes for the outcome ever occurring because it still would have occurred in their absence.
The reader may have noticed the seemingly tortured phrasing distinguishing causes of “being a smoker” or other conditions ever coming into being—sometime and somehow—from causes of specific event occurring at a specific time. This is a critical distinction. Even if e-cigarette use caused the particular chain of events that led to someone taking up smoking, it might not have caused him to be a smoker . If he would have started smoking in the absence of e-cigarettes due to some other prompt, then the outcome “being a smoker” was overdetermined and so e-cigarettes did not cause that outcome, and thus were not a gateway. Note the relationship to a common misunderstanding about epidemiologic statements like “smoking causes millions of deaths”: Some observers balk at such claims, protesting that those individuals would have died whether or not they smoked. But the unstated caveat in the epidemiologic claim is “caused deaths to occur at a particular time that would not have otherwise occurred at that time .” The confusion is created by using phrasing that in common language could mean either that or “caused deaths that would have never occurred otherwise”.
This is not a philosophical or semantic technicality; it is crucial to understanding the gateway claim and how to test it. A meaningful gateway claim is a “would have never occurred otherwise” claim, not an “at that time” claim. If that person would have become a smoker in a world without e-cigarettes, then e-cigarette use cannot be blamed for the ultimate outcome even if it was the trigger for the particular path he followed. Of course, it may be useful to study the very different question “what are people’s proximate triggers for taking up smoking?”, apart from whether they are actually the causes of them becoming a smoker. But since the practical gateway claims are about an exposure creating smokers from would-be nonsmokers, the technical logical definition clarifies what is really being claimed.
Confounding and Reverse Causation
Confounding, because of the way it is presented in the context of statistical analyses, is sometimes not understood to be a statement about causation. The association between C and E in a population is confounded if there is a difference in the rate of E between the subpopulation who have C and subpopulation who do not have C that is not caused by C . That is, if you could change the world such that no one had C, but all else was the same, the rate of E would still be different between the two subpopulations. A simplistic comparison of the groups, then, would attribute this difference to C even though it was not caused by C.
Notice that the definition of confounding does not make reference to the concept of confounders , which refers to either other variables that might be the causes of that difference or, more typically, to other variables that could be put into a statistical analysis that might allow you to sort out the effect of C from the confounding. These are quite different concepts, and the latter—available variables that help control for confounding—are better labeled deconfounders [ 13 ]. A true confounder would include some characteristic U that is a cause of E and is also a cause of C. There are other more complicated causal roles true confounders also play, but this phenomenon—a common cause —creates the greatest challenge when exploring gateway claims. Liking the effects of nicotine, for example, causes the use of cigarettes and the use of smoke-free alternatives.
It might be possible to measure U and thus use the measurement as a deconfounder variable, or to measure proxies for U and use them as deconfounders. In practice in public health research, “controlling for confounding” consists of throwing in whatever variables the researcher has that might be (though often probably are not) proxies for real confounders. While methods have been developed in epidemiology to improve the choices of deconfounder variables (e.g., causal flowcharts), they are almost never employed. Many covariates included in epidemiology models seem about as likely to “adjust” the measure of effect further from the true value as to adjust it closer. For example, the habit of controlling for race, because the variable happens to be in the dataset, when there is no reason to believe that race confounds or modifies the ostensible causes of interest, is functionally equivalent to controlling for a column of random numbers. Even worse, controlling for intermediate factors on a causal pathway (e.g., for blood pressure when estimating the effects of smoking on cardiovascular disease) will tend to mask the real effect.
But even when researchers are skilled at identifying optimal deconfounders, it is often the case that either no available variables offer good proxies for the postulated confounders or that they are not measured accurately enough for statistical adjustment to occur. For example, a researcher trying to assess whether an exposure causes smoking may realize that he needs to control for propensity for depression and willingness to defy social pressure, but the available covariates (age, race, level of education, income, etc. ) offer little deconfounding for these. Controlling for the heterogeneous preferences for nicotine seems nearly impossible. Seldom do researchers even discuss what hypothesized confounder variables they are attempting to proxy for, let alone offer any argument that the control variables they threw into their model achieve the goal of deconfounding.
Another causal pathway that has similar implications to confounding is reverse causation . That is, E is actually causing C. Association of two variables always works in both directions: if E is associated with C, then C is associated with E. Thus, some additional analysis, beyond observing a correlation in the data, is needed to draw conclusions about which direction the causation flows, even in the absence of confounding. For many areas of research, the reverse pathway is so implausible that it may be valid to completely ignore it, but for some questions—particularly including analysis of constellations of behavioral choices—this is not so. ( Association is most easily interpreted as “knowing the value of one variable for an individual affects your probability of the other”. Correlation refers to the same phenomenon, but is a mathematical property of population data rather than a reference to worldly phenomena that is meaningful at the individual level. Note also that associations or correlations can be positive (C true increases the likelihood of E true) or negative (decreases the likelihood), but when used without clarification the words generally refer to the positive.)
The logic of the gateway claim shows it to be an extraordinary claim, while alternative explanations inevitably create an association. Still, co-occurrence of all three of those causal pathways is possible, and thus there could also be a gateway effect, but further evidence is needed to affirmatively support such a claim.
2.3. Use of Similar Drugs is Inevitably Associated
Give the above observations, it is impossible to imagine that use of low-risk tobacco products would not be associated with smoking. Still, there is value in further exposition to clarify the thinking of serious researchers and to push back against those who implicitly deny the obvious.
Someone who smokes a particular pack of Marlboros will almost certainly smoke another pack of Marlboros, though obviously the fact that the particular first pack was shipped to his local store and he bought it did not cause him to smoke the other. He is also far more likely than the average person to smoke or have smoked a pack of another brand of cigarettes. The probability drops somewhat when the other brand is menthol; the closer the similarity of the products, the stronger is the association of their use. The association is smaller, but still far from null for non-cigarette tobacco products. We would also predict a greater probability of smoking cannabis or using cocaine than would be average for someone of the particular age, cohort, and demographics, since he has demonstrated a greater desire and willingness to use drugs compared to the average person. It should be obvious that none of those other higher probabilities were caused by the fact that the particular pack of Marlboros in question found its way to that consumer’s corner store.
This is a cartoon example, and yet, blaming that particular pack for the other behaviors is exactly the logic of most empirically-based gateway claims. Common gateway claims are based on the simple observation that a larger proportion of those who smoke have used a low-risk alternative as compared to those who do not smoke.
For example, Haddock et al. [ 14 ] claimed to demonstrate a gateway effect by observing a mere association between smokeless tobacco use and smoking. They also reported a strong association with other risk-taking or socially disparaged behaviors (e.g., motorcycle riding) that were obviously not caused by smokeless tobacco use, but were apparently unaware that this illustrated the fallacy of their conclusion. More recently, Glantz et al. have claimed that e-cigarettes are causing teenagers to smoke, when their data [ 15 , 16 ] actually provided no support for that claim and instead better supported the claim that those who already smoked were seeking a substitute [ 17 ].
2.4. Precedence Has Epistemic Value, but It Is Generally Overstated
A variation on the claim that mere association indicates a gateway is to suggest that the association must be causal if use of the low-risk product preceded the use of the high-risk product. Some gateway claims are based on merely showing that some individuals used the low-risk product before becoming smokers, and then discussing the result as if it supported the much stronger claim of a gateway. This is clearly invalid, not just because of the change in definition, but because there is no reason to care about non-causal precedence. The classic example is that almost every heroin user consumed milk before ever touching heroin. No one who is not trying to mislead uses the term gateway to refer to mere precedence.
A slightly more informative approach is to combine association and precedence, observing that nonsmokers who use or have tried a low-risk alternative are more likely to later become smokers than similar nonsmokers who have never used tobacco products. But while this substantially reduces (but does not eliminate) the problem of reverse causation, it offers minimal reduction in confounding and so remains inadequate evidence for a gateway.
However, contrary to a large portion of criticisms of gateway claims, the lack of the “right” temporal ordering does not disprove the gateway claim. That is, just because someone tried a cigarette before ever using the low-risk alternative does not mean that the low-risk alternative is not the cause of later smoking. Trialing smoking in the past, or even a period of regular smoking followed by cessation, does not immunize someone against the possibility of another product causing later smoking. Causation of someone trying his first cigarette could be ruled out by the simplest measures of temporality, but that is not the outcome of interest. Temporality data is not useless; it can be used to identify individuals who are much more likely to be candidates for the gateway, as is done below. But simply declaring that someone who used a cigarette first cannot be a gateway case is incorrect.
2.5. Testimony is Critical, though Overdetermination Complicates Inference
A good method for assessing causation is to ask subjects what they perceive to be a cause. This is not definitive, for obvious reasons, but is potentially among the most useful data when it is an option ( i.e ., the objects of study are people and the apparent link between cause and effect is easily observable). It is a neglected research option in epidemiology, which seems largely to result from researchers’ failure to acknowledge that they are engaged in social science rather than clinical research [ 18 ], although obvious political motivations are often the explanation for ignoring the available testimonial evidence (e.g., [ 19 ]). An individual’s testimony about what caused his cancer is worthless because there is no way anyone can know that. But his testimony about why he changed his behavior is useful scientific evidence.
However, even if someone (accurately) communicates a proximate cause of smoking such as “my friends and I were using e-cigarettes all the time, and I wanted to try the real thing”, it does not mean that e-cigarettes were a cause because of overdetermination. It could be that the individual would have also started smoking in a world that lacked e-cigarettes, triggered by some other event. People’s testimony about the proximate trigger for smoking initiation can be reliable, but their assessment about whether the outcome was overdetermined will be much less reliable.
By contrast, someone’s testimony about conscious adoption of THR is quite reliable. Anyone who testifies that her history of product usage includes both smoking and a low-risk alternative, and that she intentionally switched from the former to the latter, is almost certainly correct. One simple survey question is sufficient to rule out a gateway effect in favor of reverse causation in such cases, and the failure to ask that question indicates a lack of serious interest in whether there really is a gateway.
Overdetermination is not a problem here. The overdetermination challenge does reemerge if we try to extend the causal statement to the common stronger claim “…and I would still be smoking were it not for THR” (though other details can help support this claim, and thus the testimonial evidence can still be compelling). But even if the smoker would have quit via other means, the testimony about the switching pattern is sufficient to rule out the gateway.
Testimonial evidence is seldom collected in a manner that allows estimation of population-level statistics. But testimonials can rule out gateway cases, as noted, and can also identify potential true gateway cases, though overdetermination would prevent these from being sufficient evidence. It is noteworthy that those who claim that e-cigarettes are a gateway to smoking have failed, to my knowledge, to produce even a single testimonial in which someone reports being such a potential case.
2.6. Use of Population Prevalence Data is Not Promising
If use of low-risk tobacco products becomes popular in a population, then a theoretically testable prediction of the gateway claim is there will be more smoking. In the only population (Sweden) where a low-risk tobacco product (snus) has been sufficiently prevalent that such an effect could have be seen, Rodu et al. argued that there was no apparent gateway effect [ 20 ]. It is not possible to test this prediction during a transition period, where many people are switching from smoking to the alternative (either literally or via cohort replacement); under those circumstances, there could be a gateway effect, but it is masked by the stronger reverse causation trend. However, in Sweden the rate of male snus use stabilized at a high level while smoking rates remained low, which is contrary to the implications of a substantial gateway effect.
By contrast, recent similar claims that e-cigarettes cannot be causing a gateway because smoking continues to decline as e-cigarette use becomes more popular are fallacious. E-cigarette usage prevalence is low compared to smoking (e-cigarette trialing incidence rates among younger people appear to be similar to smoking in populations where e-cigarettes have become popular, but an actual usage practice appears to be remaining much lower). E-cigarette use is dominated by former smokers who intentionally used e-cigarettes to quit smoking, and thus are unlikely candidates for a gateway effect, and indeed are arguably not at risk of it (depending on the exact definition). Thus, when we consider the very few people who adopted e-cigarette use other than for THR, even if half of them were caused to start smoking (presumably far more than even gateway proponents suggest could happen), it would not be detectable. Any signal would be completely swamped by the trends in smoking prevalence and the noise in those estimates, to say nothing of the countervailing THR effect.
The gateway hypothesis could be amended to say that a low-risk alternative causes uptake of cigarettes in a population where cigarettes are currently a lot more popular than the alternative . This caveat might be seen as cynical expedience, but it is not unreasonable. The claim would be that when people start using any tobacco product, it causes some to drift among multiple tobacco products, and social forces will cause more to drift toward and settle on the most popular product. Indeed, this is the obvious explanation for observations that a larger portion of people who use smokeless tobacco in the USA equilibrate as smokers rather than the other way around, while the converse occurs in Sweden. With the addition of this nuance, no population prevalence data could ever effectively test the hypothesis: Once the alternative was sufficiently popular to extract the signal from the noise, smoking would be sufficiently unpopular that it nullified the claim.
Thus, while the Swedish data provides evidence against a simplistic gateway claim, no such data will be available for other products or other populations for the foreseeable future, and even then it would not rule out that there is still a gateway while smoking is far more popular.
2.7. Experimental Studies
Though not a realistic option, it is useful to consider the implications of a gateway experiment, where abstainers are assigned to use low-risk tobacco products to see if that causes smoking. The advantage of experiments (a.k.a. randomized controlled trials) is that they can eliminate systematic confounding and reverse causation as explanations, and are not affected by population trends. However, the differences between any operationalizable experiment and the real exposure of interest, inevitably introduced by an artificial controlled setting when studying complicated human behaviors, tend to be worse than the problems that are solved. It is often claimed that the reason that randomized trials of tobacco use behavior are not promising is that they would be unethical and impractical; while that is largely true, it is not actually the fundamental problem.
The conceptually ideal experiment, always useful to consider when contemplating research design, would be to run two histories of the world, one where the low-risk alternative existed and one where it did not. This would allow observations about how many nonsmokers in the latter world are smokers in the former (with the added bonus of observing how many smokers quit only because of THR). Monitoring corresponding individuals in the parallel worlds would solve the problem of merely analyzing aggregate counts, which shows only the net change from gateway and THR effects. Many would argue that from a practical standpoint only the net effect matters (though there are valid ethical objections to such netting), but it does not answer the specific question about a gateway.
The conceivable real-world experiment, which always falls far short of the theoretical ideal in social science, consists of selecting a random collection of nonusers of tobacco products, from the population (age group, etc. ) of interest, and conscripting a random sample of them into using a low-risk alternative. If they became smokers more often than those who were not conscripted or were conscripted to a null-treatment arm, it would suggest there is a gateway effect. But conscripted use would obviously be quite different from real-world adoption so this experiment would tell us little.
Observational studies in epidemiology are typically misinterpreted as if they were an experiment, but the fundamental differences are obvious in this case. People who voluntarily try the low-risk products are not a random sample from all nonusers ( i.e ., there is important confounding), those who transition from trialing to using are not a random sample of them (further confounding), and many of them may be trying to substitute for their inclination to smoke, even those who are not currently smokers (reverse causation). Using the only available evidence of real-world behavior—which is observational—is much more difficult than interpreting results from an ideal experiment.
2.8. Magnitude Matters
Before considering what available evidence would be useful to support or refute the hypothesis, it is important to quantify the hypothesis. If it is merely “there is one person who has been caused to start smoking due to use of the low-risk alternative” then there are no realistic statistics that could address the claim. A testimonial by an individual could support that claim, though the overdetermination problem would call the “I would have otherwise never started smoking” aspect of the testimony into serious doubt unless the individual is an older never-smoker. If the hypothesis is “half of would-be never-smokers who use the alternative product will become smokers,” available statistics, used properly, would address the claim. It would be easy to observe that more than half (to account for those destined to become smokers anyway) of never-smoker users of the alternative become smokers.
Presumably the hypothesis falls somewhere in between the former inconsequential claim and the latter implausible claim. “Presumably”, because proponents of gateway effect never quantify the claim they are making. This is a serious problem, both for the policy discourse and the epistemology; without quantification, the claim is disingenuous and research is meaningless.
To make the hypothesis of interest both plausible and large enough to matter, it probably makes sense to test a claim in the order of 5% of would-be never-smokers who take up the alternative product are caused to smoke.
2.9. How Can Researchers Assess Whether There is a Gateway?
It is not entirely clear that any systematic study can detect gateway behavior of that magnitude, given how overwhelming the confounding is. If there is any hope, it needs to be based on thoughtful analysis about what constitutes evidence.
2.9.1. The Need for Testimonial Evidence
Testimony-based research would be useful for directing quantitative analysis. Given that the proposed gateway behavior defies the welfare-maximizing logic described above, it is difficult to know what data to collect without some research about the nature, motives, and dynamics of apparent gateway cases. Naively hunting for evidence of a gateway effect in quantitative data is likely to be a dead-end, doing nothing to resolve competing hypotheses. But if the specifics of apparent gateway cases were documented, it might be possible to refine the statistical analysis.
For example, it might be that most purported examples of gateway cases would include a story like, “I tried a few packs of cigarettes a while ago, but did not really like them; but then I used e-cigarettes every day for six months and found myself thinking that a real cigarette sounded good after all.” If that were the case, the systematic research should focus on those who had previously consumed some quantity of cigarettes but had never become a smoker, and then later used e-cigarettes for a substantial period. It is also possible that such testimonials would reveal potential mediation analyses (statistical analyses based on intermediate variables that are a step along a causal pathway), with testable implications that would not be lost in the noise as the aggregate values are. If it were found that self-identified gateway cases mostly followed a particular pattern of smoking adoption that was generally not common among those starting smoking via other causal pathways, that pattern could be investigated in population statistics. This is a generalizable strategy when attempting to distinguish a weak causal relationship from stronger confounding effects.
Currently such hypotheticals are moot because it appears that no gateway proponent has ever offered the testimony-based prima facie evidence that there are any instances of gateway behavior. Statistics alone, absent case studies that suggest plausibility, cannot justify such an economically extraordinary claim. Without evidence of even a single case, the claim is not even a defensible hypothesis and is more like a religious myth or philosopher’s demon theory, something made up from whole cloth for rhetorical purposes.
2.9.2. Bayesian Analysis Shows How to Avoid Garbage-In-Garbage-Out
Obvious Bayesian reasoning shows the lack of useful information in the recent studies of e-cigarettes. The observed statistics reduce one’s posterior probabilities that there is no association (which should have a very low prior in the first place), but do not change the relative probabilities of the competing explanations for the association. This means that gateway claims based on associations are examples of “faith-based Bayesianism”.
To take an example I presented previously [ 17 ], consider someone whose prior beliefs are that there is a 50% chance that e-cigarettes cause smoking, a 1% chance that e-cigarettes are used for THR, a 1% chance that there is a strong association caused by confounding, with the remaining probability that there is no association. Further assume that there is an 80% probability a study will show an association under the first three hypotheses and a 5% chance under the last. (This characterization is not really proper since magnitude matters for updating the priors, not just this dichotomous characterization that is typical in epidemiology, but set that aside). The posterior probabilities after observing an association, applying the Bayes Theorem, would be 0.91, 0.02, 0.02, and 0.05, which appears to be strong support for the first hypothesis. But, of course, the observation increased the probabilities of each of the first three hypotheses by the same ratio, at the expense of the fourth, and so the overwhelming support for the first compared to the second and third is just an echo of the prior beliefs. This is not valid empirical reasoning, and represents the common critique of simplistic Bayesian analysis: garbage in, garbage out.
2.9.3. Hypothetico-Deductive Reasoning
A good hypothetico-deductive test is based on asking, “what would we expect to observe if this hypothesis were true which we would not expect to observe it were false ?” Such reasoning has recently been discussed in epidemiology under the rubric “negative controls for confounding.” An example of this is observing that the association of better health and getting an influenza vaccination in an elderly population is not stronger during flu season, which is not what we would expect if the association were caused by the vaccine working rather than by confounding [ 21 ]. Similarly, if an ostensible cause is strongly associated with an outcome variable that it cannot conceivably cause or be caused by, and this persists after “controlling for confounding”, it is apparent that there is uncontrolled residual confounding that may explain the main relationship of interest (as with the example of motorcycling being associated with smokeless tobacco use).
It is often possible to devise hypothetico-deductive tests that can be performed on a particular dataset. Unfortunately there is no easy recipe for identifying them, and they need to come from ad hoc scientific reasoning. An example of this appears below.
Another possible application of hypothetico-deductive reasoning is to take advantage of natural experiments. Where there is spatial heterogeneity of low-risk tobacco product use within a similar population, the gateway hypothesis predicts corresponding heterogeneity of smoking adoption rates. The popularity of e-cigarettes among teenagers appears to have involved substantial spatial heterogeneity across schools, due to contagion effects [ 22 ] ( i.e ., it is a social process that is either adopted in a social group or not). For years there were reports of some American high schools repeatedly confiscating e-cigarettes from students, while contemporaries at other schools reported never having seen one. Though overall smoking trends are uninformative for the reasons cited above, the gateway hypothesis makes both positive and negative prediction regarding this heterogeneity, which could provide a good test. Something similar might be possible among Norwegian users of smokeless tobacco, where there has been explosive adoption, unlike the relatively steady state in Sweden. Anyone genuinely interested in detecting a gateway effect should take advantage of this situation before the heterogeneity disappears. Indeed, it may already be too late to collect such data, but existing recent data might be available and new examples might be found as e-cigarettes become popular in countries where they are not yet.
2.9.4. Propensity Scores: Mimicking the Ideal Experiment
The idealized experiment shows that we want to identify people who would not have been a smoker in a world that lacked the alternative product. The real world does not allow such definitive identification, but tools like propensity scoring or multi-stage regression statistically predict the probability someone would have become a smoker from available variables. With a sufficiently good predictor, we could notice if the ostensible gateway product was substantially changing the likelihood of someone smoking. Apparently only one study ever attempted this for THR products, and found no evidence of a gateway effect [ 23 ].
This approach is common in some social sciences, but is largely absent from substance-use epidemiology even though the concept of propensities is a natural fit there. Epidemiology’s use of arbitrary and largely untested covariates as “control” variables is an inadequate substitute for propensity scoring or other hypothesis-based statistical models, though it can still be sufficient to show that confounding may explain an uncontrolled result [ 24 ].
A proper propensity score is a reasonable predictor of the effect (smoking) using variables that are mostly independent of the ostensible cause (low-risk product use). The “controlling for confounding” approach could use the same demographic and personality covariates (neighborhood, career track, propensity for risk taking, etc. ), but even for the best possible application of that approach, measurement error, simultaneity, and imperfect proxying for the true common causes will leave residual confounding that will inevitably create an association. By contrast, an effective propensity score eliminates simultaneity and the need for careful consideration of causal pathways, and though imperfect prediction means that the effect size estimate will be attenuated, any apparent effect will be better evidence the phenomenon exists.
Ideally the variables used to create the propensity score are completely independent of the causal variable of interest. This is unlikely to be possible in the present context since the main problem is that of common causes. (As an aside, note that this means that the similar strategy, use of instrumental variables to deal with simultaneous causation, is not promising. This is largely moot, however, since the instrumental variables are particularly valuable when analyzing time series data. When examining cross-sectional data, there is little reason to use this method rather than a full-on two stage method.) However, this is not a fatal problem. So long as the variables used to measure propensity cannot plausibly be caused by low-risk product use and are not intermediate steps on a pathway by which low-risk product use causes smoking, the approach is still valid. The addition of temporal data can help determine the direction of causation.
In the case of e-cigarettes, fairly recent data from before e-cigarettes were available could be used to create the score, which eliminates the possibility that e-cigarette use affected any of the variables. A recurring theme here is that researchers interested in honest attempts to detect a gateway effect need to take advantage of a temporary window, before almost-random heterogeneity disappears and propensity data from before the ostensible gateway product became popular is too old. Once this window closes across the populations of interest, it is not clear there is much hope of sorting out the effects of competing causes until such a time that Swedish-style stability is reached.
2.10. A Checklist for Evaluating Empirical Research about Gateway Effects
To summarize the above, the following considerations should be applied to the design and interpretation of any empirical study of the gateway effect. Many of these principles generalize to other attempts to distinguish among the three possible causes of an association in cross-sectional data.
1. Is the research based on (a) a clear statement of what “gateway” means and (b) a hypothesized quantification of what is being tested or claimed? Failing either of those, the effort is only propaganda, not science. Is there any presentation of (c) a theory of why there would ever be gateway behavior or (d) testimonial evidence suggesting there is any such case and how it came about? Failing both of those, it is unlikely that the statistical analysis will be anything other than a misguided fishing expedition.
2. Does the result or potential result make any effort to discriminate between a gateway effect and causation in the other direction (THR) or confounding? If not, it is completely uninformative about whether a gateway, rather than just one of those established sources of association, is occurring.
3. Is the attempt to deal with the inevitable confounding based on some theory of the nature of the confounding ( i.e ., addressing the counterfactual or hypothetical experiment concept) and is it empirically tested (e.g., by checking the sensitivity to combinations of variables), or does it just consist of throwing in whatever covariates are conveniently available? If the latter, it is probably little better than no attempt to control for confounding, as is likely to be demonstrated by the persistence of negative control associations. If the former, the stated confidence in its success should still be epistemically modest.
4. Do the study results support any prediction that would be true under the hypothesis but unlikely if the hypothesis is false? If the data is highly consistent with the hypothesis being false, it obviously does not provide much support for the hypothesis.
5. Is there any attempt to detect temporal ordering of behaviors? As noted, this is neither necessary nor sufficient for a gateway, but the right ordering tends to better support the claim for obvious reasons. In particular, if the data shows that most of the association results from people who are already smokers trying the other product (as is likely for e-cigarettes and other THR products), and if it appears that the association is causal, then the causation is apparently flowing in the other direction.
6. Did the authors report enough different cuts at the data to make clear that any conclusion the data supports a particular hypothesis is apparently not an artifact of the specific models and results that are reported? Researchers can easily check how their results change based on innocuous-seeming details in their models (such as choosing among multiple candidate definitions of smoking, which covariates to control for, which cohorts from a dataset to use, how to categorize continuous variables, etc. ) and choose the result they like best, leaving the readers (including journal reviewers) unable to detect this was done. This is an overarching problem with epidemiology, not specific to the gateway question [ 25 ]. But it is especially critical for highly politicized hunts for a tiny signal amidst a lot of noise. It is trivial to cherry-pick models and results that tend to support the author’s preferred claim. Adoption of methods that mitigate this is clearly beneficial (e.g., [ 26 ]).
2.11. An Application of These Principles
It is possible to apply these principles to a recent article by Dutra and Glantz (2014) [ 15 ] that was widely claimed to show there is a gateway effect. This included claims by the authors in various concurrent and subsequent communications [e.g., 27 , 28 , 29 , 30 ], which suggest this claim was their main interest, though they avoided the term “gateway” in the paper itself. (Details of their claims can be found in my previous analysis [ 17 ].) Doing so shows:
- (a) The results completely failed to support what the authors and other commentators claimed, and
- (b) the variables available in their dataset were really inadequate to answer the gateway question, however
- (c) further analysis of the dataset shows that the data can be used more effectively, and that it shows the authors’ claim is even less well supported than the originally reported statistics implied.
The paper used the 2011 and 2012 U.S. National Youth Tobacco Survey to look at the association, among teenage students, between smoking and what they refer to as e-cigarette “use”. (This is a misleading term, since the survey only measures trying e-cigarettes and does not distinguish what we would think of as use.) The analysis was limited to subjects who reported having ever smoked, at least once, and the endpoints consisted of the available measures of smoking, specifically smoking a total of 100 cigarettes and having smoked (at all) in the last 30 days. Note that the original authors made odd use of the available data (e.g., omitting anyone who had never tried a cigarette; using at least one idiosyncratic definition) and only reported the results from a single model without explaining their choice. But the present analysis is not an attempt to critique their entire approach. In order to focus on the above-cited methodological points, what follows is based on replicating their approach—methods, models, and definitions—as closely as possible. This should not be seen as an endorsement of that approach.
The authors observed strong associations between measures of smoking and measures of e-cigarette trying (their Table 2 and Table 3 ), which serves as the entire basis for the gateway claims. A partial replication of their main results appears in Table 1 (note: all tables are in the Appendix ). They claimed emphatically that their results were evidence that e-cigarettes were a gateway to smoking.
Point (a) was presented in detail in my previous analysis. In particular, the original paper involved no attempt to discriminate between a gateway effect and either confounding (which the authors did not even seem to recognize exists; they never even used the word) or reverse causation. If the exposure and endpoint variables are reversed, the association remains, as will always be the case (this is illustrated in Table 2 for concreteness). If having tried an e-cigarette predicts being a smoker (the gateway phrasing), then being a smoker predicts having tried an e-cigarette (the THR phrasing). The results support no prediction that would be true under the gateway hypothesis that would not also be true if the hypothesis were false (unless the only alternative hypothesis considered is the implausible alternative that there is no association between using one tobacco product and using another).
Moreover, the authors and others who made gateway claims about this data offered no theory of why or under what circumstance a gateway might occur, nor did they give any indication of what magnitude of gateway effect they were testing for or claim to have found. This makes clear that they were not making a serious attempt to empirically validate a scientific hypothesis.
Given the lack of acknowledgment of confounding in the original paper, it is no surprise that there was no serious attempt to control for confounding. Moreover (point (b)), the NYTS dataset is simply not rich enough to do so. The original authors controlled for age (which is obviously critical when studying a “have you ever…” phenomena), gender (also important for drug-use behavior), and race (which is rather less useful, though might be a very rough proxy for some cultural phenomena). They control for nothing else (other than which year of data collection a subject was in), and indeed, there is very little else they could have done with this data to assess propensity to use tobacco products. It should be obvious that those few variables do not come close to serving as good propensity estimators or proxies for underlying common causes. Thus there is simply no way this dataset can be used to discriminate a gateway effect from the inevitable confounding.
However, the data can be used more effectively than the original authors attempted. The original authors ignored data that would help predict, albeit roughly, which subjects were smokers before they ever tried e-cigarettes. The dataset is very limited, asking temporal questions only about cigarettes, but since e-cigarettes were a very new phenomenon at the time of the survey, it is safe to infer that almost everyone who tried e-cigarettes had first done so recently.
Reiterating the point that temporality is not decisive (one way or the other) but is useful (since precedence is undoubtedly associated with causation), the data shows that of those defined as “current smokers” who had tried an e-cigarette, about three quarters (from the 2011 survey) or half (from the 2012 survey) tried their first cigarette before 2009 ( Table 3 ). (This is based on their age at the time of the survey and their reported age of trying their first cigarette, as detailed in the Appendix ; there is obviously imperfect correspondence between this and calendar year, but it is described in calendar terms for clarity.) This almost certainly means they tried a cigarette before trying an e-cigarette. Of course, many of those who first tried a cigarette later than 2009 also did so before trying an e-cigarette. While this cannot rule out gateway cases for this subpopulation ( trying a cigarette does not immunize you from a later event causing you to become a smoker ), it seems to provide better support for the reverse-causation THR claim than the gateway claim. A clear majority of the smokers who tried an e-cigarette tried a cigarette first, and we can surmise that most of them were smokers before trying an e-cigarette.
We can improve on this simplistic cut at the temporality data with a more formal hypothetico-deductive test. It makes use of the novelty of e-cigarettes as described above— i.e. , gateway cases would have to have started smoking fairly recently. (We could do much better if we had temporal data on when subjects first tried an e-cigarette.)
If the association of smoking and e-cigarette trialing or use is substantially explained by the gateway effect, then the association should increase as we narrow the population of smokers toward those who are more likely to be gateway cases. Those who more recently tried their first cigarette are more likely to be gateway cases because they are more likely to have become smokers recently. If many of them were caused to smoke by their (necessarily) recent e-cigarette use, the association will become stronger when we remove the dilution that results from including those who were more likely to have started smoking before they ever saw an e-cigarette. If there is a stronger association among the group that tried a cigarette earlier (in calendar time), and thus almost certainly has more established smokers, it would suggest that THR or some other reverse-causation is more likely because established smokers are the ones who are more often adopting e-cigarettes. If there is little difference, it will tend to support (though obviously not definitively) the hypothesis that confounding dominates both of those explanations. (It is worth noting the epistemically important fact that this test was devised and this paragraph was drafted before the data was analyzed.)
The simple counts of when subjects first tried a cigarette show earlier smoking among those who had tried e-cigarettes as compared to those who never tried e-cigarettes, which by itself is enough to favor the THR interpretation over the gateway interpretation. Repeating the original methodology for subpopulations divided by when they first tried a cigarette confirms this (details in the Appendix ). For parsimony the two waves of the survey are combined, with a covariate for survey wave. I recognize that odds ratios (ORs), which were used to follow the original analysis, are a terribly misleading statistic for comparing proportions. However, they are adequate for showing whether associations are stronger or weaker between different subpopulations, which is the present goal.
For an endpoint of having ever smoked 100 cigarettes, the OR for ever trying an e-cigarette for those who tried their first cigarette before 2009 is 7.7, versus 5.2 for those who tried their first cigarette more recently ( Table 4 ). These numbers should not be over-interpreted: The results are similar, though the contrast is weaker, for “current smokers”. We do not see a trend when we step the cutoff through 2007, 2009, and 2010 ( Table 5 and Table 6 ), and the result does not always show up when we look at the “last 30 days” e-cigarettes exposure variable ( Table 7 , Table 8 and Table 9 ). Still, this does show that the available data could be used to test a prediction of the hypothesis in question, and when that is done, the results provide somewhat better support for the THR explanation than they do the gateway hypothesis. The association between smoking and trying an e-cigarette is stronger when we select for long-term smokers and weaker as we select for those who might actually be gateway cases.
This analysis was easy to perform, and recognizing its potential value in answering the scientific question took only a bit of serious contemplation. Its absence from the original authors’ analysis, along with the more blatant omissions already noted, suggest that the authors were seeking to support the conclusion rather than test the hypothesis. Moreover, journal editors and reviewers still signed off on publishing the paper, demonstrating a lack of critical analysis on their parts.
In addition, this analysis illustrates the ease of cherrypicking results that support a particular claim when authors report only one of many potential results. The results, all considered, still support the conclusion that THR better explains the association than does the gateway claim. But had I followed the common practice in epidemiology and public health, reporting only the “best” numerical results (the left side of Table 4 or Table 5 or Table 7 ), it would have misled the reader into believing the support for the conclusion is stronger than it really is. Instead, this truth-seeking method of reporting of the results, showing the results for the other cuts at the data that occurred to me to check, shows that the support for that conclusion is rather weaker than the cherrypicked strongest numbers would have shown. A typical health journal article would not have even mentioned that the other calculations had been done, implying that the “best” model was the only one ever considered by the authors.
It seems likely that such hints of support are the best we are likely to get on this topic, pointing out the need for careful methods, including sufficient reporting to show that authors are not cherrypicking much “clearer” results than the data really supports.
3. Conclusions
Searching for some signal of a gateway effect amidst overwhelming confounding and reverse causation requires more rigorous methods than are typical in public health epidemiology. This generalizes to any attempt to use cross-sectional data to sort out causation in a particular direction from confounding or reverse causation. When seeking epidemiologic associations where confounding is minimal or relatively simple in its causes, the typical methods used in the field are still far from optimal, but the empirical results might still be basically useful. That is not the case in this context. While it might never be possible to convincingly demonstrate a gateway effect given the challenges, and statistical analyses have no hope of detecting a tiny effect, there are clearly better and worse ways to pursue the question.
Acknowledgments
Catherine Nissen, CASAA Research Associate, provided research assistance. Thanks to members of Clive Bates’s Nicotine Policy Google Group and two anonymous reviewers for helpful suggestions. Thanks also to the journal editors who, at the suggestion of the author, attempted to recruit as peer reviewers proponents of gateway claims, particularly the authors of [ 15 ], in the interest of ensuring that this paper did not contain material that could not withstand critical scrutiny; unfortunately, they all refused to participate in the peer-review process. The author’s work on this paper was supported by CASAA. CASAA is a public health NGO that represents the interests of consumers; it is dedicated to protecting consumers’ access to THR products and to promoting THR. Some of the research incorporated here, though not the creation of this paper itself, took place in 2014 when the author was the recipient of an unrestricted grant from British American Tobacco for support of his research. The author is the recipient of grant (unrestricted except for the general subject matter) from Imperial Tobacco Group for research on peer review in the health sciences; that topic is tangential to the main analysis, but some observations address it.
See the main text for context and how these results were used. The first table replicates the 2011 survey wave results from Table 3 of Dutra and Glantz (2014) [ 15 ]. The numbers are not quite identical because, as is typical for public health research, the methodology was not reported sufficiently to permit exact replication, but they are close enough to show that the present methodology replicates that from the original paper. As noted in the text, the original authors made various dubious modeling choices, but this is not an attempt to broadly critique their paper. Replicating, to the extent possible, their methodology is sufficient to make the points at hand.
The original authors defined “current smoking” to mean ever smoked 100 cigarettes and smoked (at all) in the last 30 days. The two e-cigarette variables are based on ever trying or using an e-cigarette (at all) and trying or using an e-cigarette (at all) in the last 30 days.
Many authors, including Dutra and Glantz, refer to these as “use” and “current use.” This is controversial, despite its parallel with the traditional characterization of smoking status, and has been used in political rhetoric to intentionally exaggerate e-cigarette use among teens. Any use in the last 30 days is a decent proxy for a current practice of smoking in an adult, since smoking tends to either be an established habit or entirely absent. But this is not so accurate for teenagers and especially for e-cigarettes, which are a novelty that teenagers might try in social settings (and since someone is unlikely to give away their e-cigarette, in contrast with the norm of giving a whole cigarette, so it could easily just be a single puff), or that smokers might use as an occasional convenient substitute. The “use” statistics are presented without such caveats, and the typical reader is likely to interpret “uses” to mean something like “many times per day, every day” even though an affirmative survey answer might refer only to taking a single puff ever, which happened to have been within 30 days.
Following the original, the estimated associations are presented as odds ratios (ORs), which are not the proper statistics to use when the endpoint is a proportion, but adequate for all present purposes.
Partial replication of main Dutra-Glantz result: Association of smoking and e-cigarette trialing.
The next table shows the obvious result that the association remains if you reverse the ostensible cause and the ostensible effect ( i.e ., the dependent variable and the independent variable of interest).
Association from Table 1 , with dependent and main independent variables reversed.
The following counts identify the portion of the “current smokers” study population that tried their first cigarette more and less recently. Note that the description of this is simplified to “before 2009” vs. not, because calendar time is important for the analysis, based on the emerging availability of e-cigarettes. The survey, however, did not ask about calendar time of first trying, but age at first trying. This along with current age of the subject allows a rough calculation of calendar time, but obviously the step functions do not line up perfectly. The definition of “before 2009” is {if [current age] − [age at first trying] >3}, for data collected in 2012, using >2 for data collected in 2011. Someone who is 18 on a given day in 2012 and tried a first cigarette at 14 (defined as “before 2009”) probably did so in 2008 (assuming every distribution in sight is close to flat), but it might have been 2007 or 2009.
Time of trying first cigarette for current smokers, by e-cigarette trialing status.
The following table follows the original methodology, as described above, with the exception of pooling the two survey waves and adding a covariate for which wave included the record. The population is divided into those who first tried a cigarette before 2009 versus more recently, as well as can be calculated per the above. The purpose of this analysis is explained in the main text.
Association of smoking and e-cigarette ever trying, by time of first trying a cigarette: before 2009 vs. later (ORs and 95% CIs).
The following two tables repeat the previous table’s approach, substituting “before 2007” and “before 2010” for “before 2009” (the former two being defined analogously to the latter).
Association of smoking and e-cigarette ever trying, by time of first trying a cigarette: before 2007 vs. later (ORs and 95% CIs).
Association of smoking and e-cigarette ever trying, by time of first trying a cigarette: before 2010 vs. later (ORs and 95% CIs).
The following three tables repeat the above three tables, for recently trying or using an e-cigarette rather than ever trying.
Association of smoking and e-cigarette recent trying, by time of first trying a cigarette: before 2009 vs. later (ORs and 95% CIs).
Association of smoking and e-cigarette recent trying, by time of first trying a cigarette: before 2007 vs. later (ORs and 95% CIs).
Association of smoking and e-cigarette recent trying, by time of first trying a cigarette: before 2010 vs. later (ORs and 95% CIs).
Conflicts of Interest
The author is an advocate of THR and much of his work involves debunking junk science claims in the space, interests that motivated the creation of this work. See also the Acknowledgements.
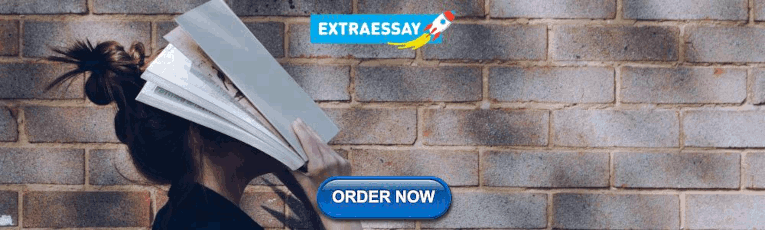
IMAGES
VIDEO
COMMENTS
Testing the Gateway Hypothesis. The gateway drug hypothesis refers to the pattern of substance use during adolescence whereby legal substances, such as nicotine and alcohol, precede the progressive use of illicit substances like cocaine and heroin. This concept of a gateway progression related to addiction vulnerability has had important ...
1. Introduction. The concept of "gateway hypothesis" has been studied since the 1970s (Kandel, 1975, Kandel and Faust, 1975) as the theory suggests that an adolescent's early experimentation with alcohol or tobacco or cannabis escalates to more addictive illicit drugs later in adulthood (Lynskey et al., 2003).Most commonly used illicit substances include heroin/opioids, cocaine and or ...
The Gateway Hypothesis describes how tobacco or alcohol use precedes marijuana and other illicit drug use. We review the epidemiological data, explore the underlying molecular mechanisms in mice and discuss the societal implications of the hypothesis, including the use of e-cigarettes by young people. Conclusion: Our mouse model identifies ...
"The gateway hypothesis holds that abusable drugs occupy distinct ranks in a hierarchy as well as definite positions in a temporal sequence. Accordingly, substance use is theorized to progress through a sequence of stages, beginning with legal, socially acceptable compounds that are low in the hierarchy, followed by use of illegal 'soft' and ...
Neuropsychopharmacology 42 , 985-986 ( 2017) Cite this article. The gateway drug hypothesis refers to the pattern of substance use during adolescence whereby legal substances, such as nicotine ...
The gateway drug effect (alternatively, stepping-stone theory, escalation hypothesis, or progression hypothesis) is a comprehensive catchphrase for the often observed effect that the use of a psychoactive substance is coupled to an increased probability of the use of further substances. Possible reasons for the connection include environmental influence, impulsive people seeking both soft and ...
Testing the Gateway Hypothesis. Michael L Miller1 and Yasmin L Hurd*,1. 1Departments of Psychiatry and Neuroscience, Friedman Brain Institute, Icahn School of Medicine at Mount Sinai, New York, NY ...
The "gateway hypothesis" (GH) connects initiation of use of various drugs by a sequence stated to represent a developmentally staged process. The alternative model of common (general) liability to addiction (CLA) posits non-specific liability to all drug addictions, regardless of the order of use initiation. The two perspectives have ...
Revisiting the Association Between "Gateway Hypothesis" of Early Drug Use and Drug Use Progression: A Cohort Analysis of Peer Influences on Drug Use Progression Among a Population Cohort Subst Use Misuse. 2020;55(6):998-1007. doi: 10.1080/10826084.2020.1720245. ...
In the early 1980s, the term Gateway drug began to be used to refer to alcohol and cigarettes, the drugs that are used prior to the use of illicit drugs. Soon, the usage was extended to include the use of marijuana as a precursor to the use of other illicit drugs, such as cocaine or heroin, and more rarely even to cocaine as a precursor to heroin.
The status of the "gateway hypothesis" has been controversial in part because it has not always been clear what its advocates mean. Very different policies have been based on assumptions about what factors explain the gateway sequence. In the USA, for example, the gateway pattern has been used to justify prevention programs that attempt to ...
Background: The aim of this study was to describe the transitions between tobacco (T), cannabis (C) and other illicit drugs (OIDs) initiations, to simultaneously explore several substance use theories: gateway theory (GT), common liability model (CLM) and route of administration model (RAM). Methods: Data from 2 French nationwide surveys conducted in 2005 and 2010 were used (16,421 subjects ...
The gateway hypothesis proposes a specific relationship between the use of one drug and later use of other drugs, whereas the common liability model suggests that individuals who develop an abuse issue to any drug are at a greater risk to develop any number of mental health disorders, including an increased risk for further abuse of other drugs
The gateway hypothesis says marijuana inspires users to try other drugs: Once they get a taste of how fun pot is, they're more likely to want to see how fun other drugs are. So if marijuana is ...
An alternative to the gateway-drug hypothesis is that people who are more vulnerable to drug-taking are simply more likely to start with readily available substances such as marijuana, tobacco, or alcohol, and their subsequent social interactions with others who use drugs increases their chances of trying other drugs. Further research is needed ...
The gateway hypothesis, in its simplest form, is the theory that initial use of legal 'gateway' drugs, including tobacco and alcohol, may lead to illicit drug use such as cannabis, cocaine and opioids [3, 4, 5].
National Bureau of Economic Research, 2021. "Marijuana use has been proposed to serve as a 'gateway' that increases the likelihood that users will engage in subsequent use of harder and more harmful substances, known as the marijuana gateway hypothesis (MGH). The current study refines and extends the literature on the MGH by testing the ...
The gateway hypothesis was initially advanced by Dr. Denise Kandel in 1975 although it didn't really enter the public consciousness until the '80s when American leadership really began to wage what we still refer to as the War on Drugs. However, similar ideas were around much earlier. In fact, the gateway hypothesis has its origins in the ...
Testing of Hypothesis 2: The Intensity of Passenger Flow in the Entrance Groups of the Station Has an Impact on the Satisfaction of the Passengers Since the model described above confirmed the impact of passenger flow on the satisfaction of visitors to the stations, it was necessary to identify problems that arise in passengers due to the ...
2. They really are out there, but they're protecting us. 3. They really are out there, and we're going to get it unless we mend our ways. An example of the first category would be a race of ...
The presented model allows us to conclude that the first hypothesis is proved, and that satisfaction with the work of the station depends on passenger traffic. However, such factors as the organization of recreation areas, meals, areas for work, the proper functioning of the ticket-selling terminal also have a significant impact on satisfaction.
The gateway hypothesis could be amended to say that a low-risk alternative causes uptake of cigarettes in a population where cigarettes are currently a lot more popular than the alternative. This caveat might be seen as cynical expedience, but it is not unreasonable. The claim would be that when people start using any tobacco product, it causes ...
for this hypothesis is provided by the surprising fact that Moscow has the lowest divorce rate of all regions in which ethnic Russians pr edominate, much lower , in particular, than the boomto wns ...
the first hypothesis was tested that the intensity of flow at the station a ... Gateway (person / min.) Number of Passengers per Minute. 2019 ^ y cp ^ y max. Y aroslavsky 249-332 46 79.