The Three Most Common Types of Hypotheses
In this post, I discuss three of the most common hypotheses in psychology research, and what statistics are often used to test them.
- Post author By sean
- Post date September 28, 2013
- 37 Comments on The Three Most Common Types of Hypotheses

Simple main effects (i.e., X leads to Y) are usually not going to get you published. Main effects can be exciting in the early stages of research to show the existence of a new effect, but as a field matures the types of questions that scientists are trying to answer tend to become more nuanced and specific. In this post, I’ll briefly describe the three most common kinds of hypotheses that expand upon simple main effects – at least, the most common ones I’ve seen in my research career in psychology – as well as providing some resources to help you learn about how to test these hypotheses using statistics.
Incremental Validity
“Can X predict Y over and above other important predictors?”

This is probably the simplest of the three hypotheses I propose. Basically, you attempt to rule out potential confounding variables by controlling for them in your analysis. We do this because (in many cases) our predictor variables are correlated with each other. This is undesirable from a statistical perspective, but is common with real data. The idea is that we want to see if X can predict unique variance in Y over and above the other variables you include.
In terms of analysis, you are probably going to use some variation of multiple regression or partial correlations. For example, in my own work I’ve shown in the past that friendship intimacy as coded from autobiographical narratives can predict concern for the next generation over and above numerous other variables, such as optimism, depression, and relationship status ( Mackinnon et al., 2011 ).
“Under what conditions does X lead to Y?”
Of the three techniques I describe, moderation is probably the most tricky to understand. Essentially, it proposes that the size of a relationship between two variables changes depending upon the value of a third variable, known as a “moderator.” For example, in the diagram below you might find a simple main effect that is moderated by sex. That is, the relationship is stronger for women than for men:
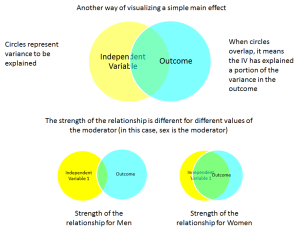
With moderation, it is important to note that the moderating variable can be a category (e.g., sex) or it can be a continuous variable (e.g., scores on a personality questionnaire). When a moderator is continuous, usually you’re making statements like: “As the value of the moderator increases, the relationship between X and Y also increases.”
“Does X predict M, which in turn predicts Y?”
We might know that X leads to Y, but a mediation hypothesis proposes a mediating, or intervening variable. That is, X leads to M, which in turn leads to Y. In the diagram below I use a different way of visually representing things consistent with how people typically report things when using path analysis.

I use mediation a lot in my own research. For example, I’ve published data suggesting the relationship between perfectionism and depression is mediated by relationship conflict ( Mackinnon et al., 2012 ). That is, perfectionism leads to increased conflict, which in turn leads to heightened depression. Another way of saying this is that perfectionism has an indirect effect on depression through conflict.
Helpful links to get you started testing these hypotheses
Depending on the nature of your data, there are multiple ways to address each of these hypotheses using statistics. They can also be combined together (e.g., mediated moderation). Nonetheless, a core understanding of these three hypotheses and how to analyze them using statistics is essential for any researcher in the social or health sciences. Below are a few links that might help you get started:
Are you a little rusty with multiple regression? The basics of this technique are required for most common tests of these hypotheses. You might check out this guide as a helpful resource:
https://statistics.laerd.com/spss-tutorials/multiple-regression-using-spss-statistics.php
David Kenny’s Mediation Website provides an excellent overview of mediation and moderation for the beginner.
http://davidakenny.net/cm/mediate.htm
http://davidakenny.net/cm/moderation.htm
Preacher and Haye’s INDIRECT Macro is a great, easy way to implement mediation in SPSS software, and their MODPROBE macro is a useful tool for testing moderation.
http://afhayes.com/spss-sas-and-mplus-macros-and-code.html
If you want to graph the results of your moderation analyses, the excel calculators provided on Jeremy Dawson’s webpage are fantastic, easy-to-use tools:
http://www.jeremydawson.co.uk/slopes.htm
- Tags mediation , moderation , regression , tutorial
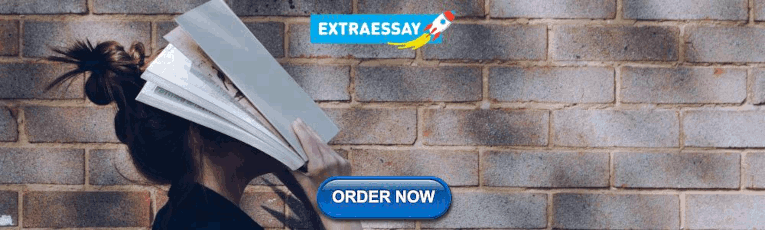
37 replies on “The Three Most Common Types of Hypotheses”
I want to see clearly the three types of hypothesis
Thanks for your information. I really like this
Thank you so much, writing up my masters project now and wasn’t sure whether one of my variables was mediating or moderating….Much clearer now.
Thank you for simplified presentation. It is clearer to me now than ever before.
Thank you. Concise and clear
hello there
I would like to ask about mediation relationship: If I have three variables( X-M-Y)how many hypotheses should I write down? Should I have 2 or 3? In other words, should I have hypotheses for the mediating relationship? What about questions and objectives? Should be 3? Thank you.
Hi Osama. It’s really a stylistic thing. You could write it out as 3 separate hypotheses (X -> Y; X -> M; M -> Y) or you could just write out one mediation hypotheses “X will have an indirect effect on Y through M.” Usually, I’d write just the 1 because it conserves space, but either would be appropriate.
Hi Sean, according to the three steps model (Dudley, Benuzillo and Carrico, 2004; Pardo and Román, 2013)., we can test hypothesis of mediator variable in three steps: (X -> Y; X -> M; X and M -> Y). Then, we must use the Sobel test to make sure that the effect is significant after using the mediator variable.
Yes, but this is older advice. Best practice now is to calculate an indirect effect and use bootstrapping, rather than the causal steps approach and the more out-dated Sobel test. I’d recommend reading Hayes (2018) book for more info:
Hayes, A. F. (2018). Introduction to mediation, moderation, and conditional process analysis: A regression-based approach (2nd ed). Guilford Publications.
Hi! It’s been really helpful but I still don’t know how to formulate the hypothesis with my mediating variable.
I have one dependent variable DV which is formed by DV1 and DV2, then I have MV (mediating variable), and then 2 independent variables IV1, and IV2.
How many hypothesis should I write? I hope you can help me 🙂
Thank you so much!!
If I’m understanding you correctly, I guess 2 mediation hypotheses:
IV1 –> Med –> DV1&2 IV2 –> Med –> DV1&2
Thank you so much for your quick answer! ^^
Could you help me formulate my research question? English is not my mother language and I have trouble choosing the right words. My x = psychopathy y = aggression m = deficis in emotion recognition
thank you in advance
I have mediator and moderator how should I make my hypothesis
Can you have a negative partial effect? IV – M – DV. That is my M will have negative effect on the DV – e.g Social media usage (M) will partial negative mediate the relationship between father status (IV) and social connectedness (DV)?
Thanks in advance
Hi Ashley. Yes, this is possible, but often it means you have a condition known as “inconsistent mediation” which isn’t usually desirable. See this entry on David Kenny’s page:
Or look up “inconsistent mediation” in this reference:
MacKinnon, D. P., Fairchild, A. J., & Fritz, M. S. (2007). Mediation analysis. Annual Review of Psychology, 58, 593-614.
This is very interesting presentation. i love it.
This is very interesting and educative. I love it.
Hello, you mentioned that for the moderator, it changes the relationship between iv and dv depending on its strength. How would one describe a situation where if the iv is high iv and dv relationship is opposite from when iv is low. And then a 3rd variable maybe the moderator increases dv when iv is low and decreases dv when iv is high.
This isn’t problematic for moderation. Moderation just proposes that the magnitude of the relationship changes as levels of the moderator changes. If the sign flips, probably the original relationship was small. Sometimes people call this a “cross-over” effect, but really, it’s nothing special and can happen in any moderation analysis.
i want to use an independent variable as moderator after this i will have 3 independent variable and 1 dependent variable…. my confusion is do i need to have some past evidence of the X variable moderate the relationship of Y independent variable and Z dependent variable.
Dear Sean It is really helpful as my research model will use mediation. Because I still face difficulty in developing hyphothesis, can you give examples ? Thank you
Hi! is it possible to have all three pathways negative? My regression analysis showed significant negative relationships between x to y, x to m and m to y.
Hi, I have 1 independent variable, 1 dependent variable and 4 mediating variable May I know how many hypothesis should I develop?
Hello I have 4 IV , 1 mediating Variable and 1 DV
My model says that 4 IVs when mediated by 1MV leads to 1 Dv
Pls tell me how to set the hypothesis for mediation
Hi I have 4 IVs ,2 Mediating Variables , 1DV and 3 Outcomes (criterion variables).
Pls can u tell me how many hypotheses to set.
Thankyou in advance
I am in fact happy to read this webpage posts which carries tons of useful information, thanks for providing such data.
I see you don’t monetize savvystatistics.com, don’t waste your traffic, you can earn additional bucks every month with new monetization method. This is the best adsense alternative for any type of website (they approve all websites), for more info simply search in gooogle: murgrabia’s tools
what if the hypothesis and moderator significant in regrestion and insgificant in moderation?
Thank you so much!! Your slide on the mediator variable let me understand!
Very informative material. The author has used very clear language and I would recommend this for any student of research/
Hi Sean, thanks for the nice material. I have a question: for the second type of hypothesis, you state “That is, the relationship is stronger for men than for women”. Based on the illustration, wouldn’t the opposite be true?
Yes, your right! I updated the post to fix the typo, thank you!
I have 3 independent variable one mediator and 2 dependant variable how many hypothesis I have 2 write?
Sounds like 6 mediation hypotheses total:
X1 -> M -> Y1 X2 -> M -> Y1 X3 -> M -> Y1 X1 -> M -> Y2 X2 -> M -> Y2 X3 -> M -> Y2
Clear explanation! Thanks!
Leave a Reply Cancel reply
Your email address will not be published. Required fields are marked *
Save my name, email, and website in this browser for the next time I comment.
Bayesian hypothesis testing of mediation: Methods and the impact of prior odds specifications
- Published: 17 May 2022
- Volume 55 , pages 1108–1120, ( 2023 )
Cite this article
- Xiao Liu ORCID: orcid.org/0000-0002-4526-3221 1 ,
- Zhiyong Zhang 1 &
- Lijuan Wang 1
1188 Accesses
1 Altmetric
Explore all metrics
Mediation analysis is widely used to study whether the effect of an independent variable on an outcome is transmitted through a mediator. Bayesian methods have become increasingly popular for mediation analysis. However, limited research has been done on formal Bayesian hypothesis testing of mediation. Although hypothesis testing using Bayes factor for a single path is readily available, how to integrate the Bayes factors of two paths (from input to mediator and from mediator to outcome) while incorporating prior beliefs on the two paths and/or mediation is under-studied. In the current study, we propose a general approach to Bayesian hypothesis testing of mediation. The proposed approach allows researchers to specify prior odds based on the substantive research context and can be used in mediation modeling with latent variables. The impact of prior odds specifications on Bayesian hypothesis test of mediation is demonstrated via both real and hypothetical data examples. Both R functions and a user-friendly R web app are provided for the implementation of the proposed approach. Our study can add to researchers’ toolbox of mediation analysis and raise researchers’ awareness of the importance of prior odds specifications in Bayesian hypothesis testing of mediation.
Similar content being viewed by others
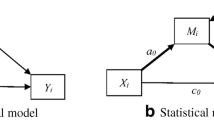
Two-level moderated mediation models with single-level data and new measures of effect sizes
Hongyun Liu, Ke-Hai Yuan & Zhonglin Wen
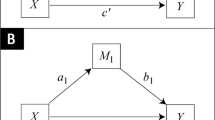
Questions of value, questions of magnitude: An exploration and application of methods for comparing indirect effects in multiple mediator models
Jacob J. Coutts & Andrew F. Hayes
A Multivariate Generalized Linear Model Approach to Mediation Analysis and Application of Confidence Ellipses
Brandie D. Wagner, Miranda Kroehl, … Gary O. Zerbe
Avoid common mistakes on your manuscript.
Introduction
Mediation studies are common in psychology and other social and behavioral science disciplines. Mediation analysis is a useful approach for studying the mechanisms that underlie the effect of an independent variable X on an outcome variable Y . When performing mediation analyses, hypothesis testing is often conducted to test whether the effect of X on Y is transmitted by a mediator M (Baron & Kenny 1986 ; MacKinnon 2008 ).
For mediation analysis, various frequentist methods have bee proposed and evaluated (e.g., MacKinnon, Fritz, Williams, & Lockwood 2007 ; MacKinnon, Lockwood, Hoffman, West, & Sheets 2002 ; Tofighi & Kelley 2020 ). In recent years, Bayesian methods have been developed and becoming increasingly popular for mediation analysis (e.g., Che, Jin, & Zhang 2021 ; Miočević, Gonzalez, Valente, & MacKinnon 2018 ; Wang & Preacher 2015 ; Yuan & MacKinnon 2009 ). For example, Yuan & MacKinnon ( 2009 ) proposed Bayesian approaches for estimating mediation effects for both single-level and multilevel mediation models; Wang & Preacher ( 2015 ) applied Bayesian methods to moderated mediation analysis; and Che, Jin, & Zhang ( 2021 ) utilized Bayesian estimation in mediation analysis with social network data. Compared to the frequentist methods, Bayesian methods allow researchers to incorporate prior knowledge into mediation analysis. For estimation, incorporating prior information has been shown to help reduce uncertainty in posterior distributions of mediation effects and improve precision of mediation effect estimates (e.g., Yuan & MacKinnon 2009 ).
A majority of the developed Bayesian methods for mediation analysis focused on estimation rather than hypothesis testing. Credible interval estimates of mediation effects have been used in Bayesian mediation analysis. While Bayesian parameter estimation is useful, Bayesian hypothesis testing can facilitate theory prediction by (1) quantifying the strength of evidence provided by the data for two hypotheses (null vs. alternative hypotheses) using Bayes factor and (2) making probabilistic statements about the hypotheses using posterior odds given both prior beliefs and data. Formal Bayesian hypothesis testing, however, is still not widely conducted in Bayesian mediation analysis. Yuan & MacKinnon ( 2009 , p. 319) noted that, “One important topic we have not covered in this article is hypothesis testing. An approximation is that we may test the null hypothesis of no mediation effect on the basis of whether the 95% credible interval contains zero. However, this approach has more of the flavor of conventional frequentist hypothesis testing. Strict Bayesian hypothesis testing is based on Bayes factor ...” To reduce the research gap, we study how to conduct Bayesian hypothesis testing for mediation in this paper. Next, we first briefly introduce Bayesian hypothesis testing, and then review the only existing study, to the best of our knowledge, on Bayesian hypothesis testing of mediation.
- Bayesian hypothesis testing
Formal Bayesian hypothesis testing (BHT) is formulated as comparing two or more competing models. Using null hypothesis testing as an example, there are two models: the model under the null hypothesis ( \(H_{0}\) ) and the model under the alternative hypothesis ( \(H_{1}\) ). The two models are both probable to be true. Before collecting data, a researcher evaluates the prior probabilities of the two hypotheses against each other (prior odds; \(PriorOdds=p[H_{1}]/p[H_{0}]\) , with \(p[H_{1}]\) and \(p[H_{0}]\) denoting the prior probabilities of the alternative and null hypotheses respectively) based on prior knowledge. After collecting data ( D ), odds between the two hypotheses are updated to form the posterior odds ( \(PosteriorOdds=p[H_{1}|D]/p[H_{0}|D]\) ) using information in the collected data, by means of the Bayes’ rule. The posterior odds quantifies the relative probabilities of alternative vs. null hypotheses by integrating both prior beliefs and the data information. The Bayes’ rule states that the posterior odds equals the product of the prior odds and the Bayes factor ( BF ):
The Bayes factor (Jeffreys 1961 )
is the relative likelihood of observing the data ( D ) under \(H_{1}\) versus under \(H_{0}\) . Footnote 1 BF can be interpreted as a measure of the relative strength of data evidence in favor of the alternative hypothesis compared to the null hypothesis and is a popular quantity reported in Bayesian hypothesis testing.
During the debate on frequentist null hypothesis significance testing (NHST) over the past few decades (e.g., Berger & Sellke, 1987 ; Harlow et al., 1997 ; Iverson et al., 2009 ; Nickerson, 2000 ), BHT has been promoted as a promising alternative to NHST and becoming increasingly popular in psychology (e.g., Dienes 2019 ; Hoijtink, Mulder, van Lissa, & Gu 2019 ; Ly, Verhagen, & Wagenmakers 2016 ; Mulder & Wagenmakers 2016 ; Wagenmakers et al. 2018 ). In particular, with BHT, researchers can obtain the posterior probability that the null or the alternative hypothesis is true given the observed data to quantify the evidence supporting \(H_{0}\) or \(H_{1}\) (i.e., \(p[H_{0}|D]\) or \(p[H_{1}|D]\) ). In comparison, NHST evaluates the evidence against but not in favor of \(H_{0}\) . The p value yielded by NHST, which is the probability of observing data as extreme or more extreme than the observed one assuming \(H_{0}\) is true, has been found to be liable to misinterpretation in practice (e.g., Anderson 2020 ; Berger & Sellke 1987 ; Ioannidis 2019 ). Furthermore, unlike NHST, BHT allows researchers to incorporate prior information (e.g., from researchers’ substantive knowledge, previous studies, or historical data) into evaluating evidence for \(H_{1}\) vs. \(H_{0}\) . This feature makes BHT a useful approach for cumulative research.and the posterior credible interval approach
In BHT, the null hypothesis can be a point (or “nill”) null hypothesis stating that the parameter of interest is precisely equal to a specific value (e.g., zero), or an interval null hypothesis stating that the value of the parameter belongs to a certain interval (e.g., an interval around zero). There is a large body of literature on the use of BHT for testing point null hypotheses (e.g., Jeffreys 1961 ; Kass & Raftery 1995 ; Wagenmakers et al. 2018 ; Wetzels & Wagenmakers 2012 ). Recent years have also seen great development of BHT approaches for testing interval null hypotheses (e.g., Hoijtink et al., 2008 ; Liao et al., 2020 ; Morey & Rouder, 2011 ). In the current study, we consider point null hypotheses for testing mediation effects. This is because when conducting mediation analysis, researchers are often interested in testing whether the hypothesized mediation effect is present or not.
Bayesian hypothesis testing of mediation
Despite the popularity of BHT, limited research has been done on testing mediation with BHT. Different from the association or direct effect between two variables (e.g., a correlation or regression coefficient), a mediation effect involves at least two relations among three variables: the relation between an independent variable and a mediator (path \(\alpha\) ) and the relation between the mediator and an outcome (path \(\beta\) ) (e.g., MacKinnon 2008 ; Valeri & VanderWeele 2013 ). The mediation effect (i.e., the indirect effect transmitted by the mediator) is present when both paths \(\alpha\) and \(\beta\) are present.
To the best of our knowledge, the only existing article studying BHT for mediation is Nuijten et al. ( 2014 ). In Nuijten et al. ( 2014 ), a three-step BHT procedure was proposed for testing mediation with the simple mediation model. Specifically, in the first two steps, the Bayes factors of paths \(\alpha\) and \(\beta\) were separately computed and transformed to the posterior probabilities that the paths exist, assuming each path has a prior odds of 1. In the third step, the posterior probability that mediation exists was calculated as the product of the two path posterior probabilities assuming the two paths are independent, and then converted to the Bayes factor of mediation, assuming the mediation effect also has a prior odds of 1.
Nuijten et al. ( 2014 ) contributed a useful addition to researchers’ toolbox of mediation analysis. Despite the merits, the approach proposed in Nuijten et al. ( 2014 ) has a few potential limitations. First, the prior odds of the presence of path \(\alpha\) , path \(\beta\) , and mediation are all assumed to equal 1. In order for this assumption to hold, the two paths have to be either both present or both absent, and thus the scenarios where one path is present while the other is absent are not allowed to occur a priori. Footnote 2 Although this specific prior odds specification might be appropriate for some substantive studies, it could occur that researchers may have other prior beliefs about the two paths and/or mediation. In such scenarios, other methods are needed to facilitate the integration of data evidence and researchers’ prior beliefs into BHT of mediation. How the prior odds specifications impact the Bayes factor and posterior odds of mediation also awaits further investigation.
Second, the approach assumes that paths \(\alpha\) and \(\beta\) are independent. However, this assumption is actually violated when the assumed prior odds in Nuijten et al. ( 2014 ) are applied, as we will show later. In addition, when latent variables are used in mediation analysis, the two paths can be dependent. In this case, the three-step procedure is not applicable and BHT methods for testing mediation need further development.
The current study
In this article, we expand the use of BHT for testing mediation with two specific goals. First, we aim to develop a method for BHT of mediation that can be applied to different research contexts. We will propose a general approach to calculating the Bayes factor and posterior odds between the null and alternative mediation hypotheses. The proposed approach is applicable to (1) different substantive scenarios where researchers might have different prior beliefs regarding the two paths and/or mediation effect and (2) different mediation models including the simple mediation and latent variable mediation models.
Second, we aim to examine the impact of prior odds specifications on BHT of mediation. To fulfill this goal, we will assess how the mediation Bayes factor and posterior odds would change with different prior odds specifications, and demonstrate the impact with both real and hypothetical data examples. We hope the examination helps raise researchers’ awareness of the importance of prior odds specifications in BHT of mediation.
In the rest of the article, we first propose a general approach to BHT of mediation. Then we implement the proposed approach in the simple mediation model, provide an illustrative example, and investigate the impact of the prior odds specification. Next, we apply the proposed approach to the latent mediation model, and use a simulated-data example for illustration. We end the article with discussing the implications, limitations of the current study, and future research directions.
The Proposed Approach to Bayesian Hypothesis Testing of Mediation
In this section, we propose a general approach to computing the Bayes factor and posterior odds for hypothesis testing of a mediation effect. Denote the effect of an independent variable on a mediator by \(\alpha\) , and the effect of the mediator on an outcome conditioning on the independent variable by \(\beta\) . Using the product of coefficient method (Baron & Kenny 1986 ; MacKinnon 2008 ), the mediation effect is quantified by \(\alpha \beta\) . Footnote 3 The BHT of mediation is formulated as comparing the null hypothesis (or model) \(H_{0}:\alpha \beta =0\) vs. the alternative \(H_{1}:\alpha \beta \ne 0\) . Other key notations used in the article and their descriptions are summarized in Table 1 .
Following Eq. 2 , the Bayes factor for testing mediation, denoted by \(BF^{med}\) , is the ratio between the likelihoods of observing the data under the alternative vs. null hypotheses. We have
Specifically, under the alternative hypothesis that the mediation effect exists, paths \(\alpha\) and \(\beta\) are both non-zero. Thus, the numerator in Eq. 3 provides the likelihood of observing the data under the alternative hypothesis. Under the null hypothesis of no mediation, there are three possible scenarios: paths \(\alpha\) and \(\beta\) are both zero; path \(\alpha\) is zero and path \(\beta\) is non-zero; or path \(\beta\) is zero and path \(\alpha\) is non-zero. The conditional prior probabilities of the three scenarios under the null hypothesis, \(q_{00|0}\) , \(q_{01|0}\) , and \(q_{10|0}\) , Footnote 4 are used to obtain the weighted average (or marginal) likelihood of observing the data under the null hypothesis (the denominator of Eq. 3 ). The three conditional probabilities sum up to 1 and are determined based on researchers’ prior knowledge about how likely each of the three scenarios occurs if the null is true. For example, \(q_{00|0}\) , \(q_{01|0}\) , and \(q_{10|0}\) are set to be 1/3 when researchers believe the three scenarios under the null are equally likely to occur before collecting data. In the next section, we will discuss how the conditional prior probabilities are related to the prior odds of \(\alpha\) , \(\beta\) , and mediation when \(\alpha\) and \(\beta\) are independent.
The mediation posterior odds \(PosteriorOdds^{med}\) , the relative posterior probabilities of the presence vs. absence of mediation, can be obtained following the Bayes’ rule (Eq. 1 ):
with \(PriorOdds^{med}\) being the prior odds of mediation specified to reflect researchers’ prior belief about the relative probabilities of the presence vs. absence of mediation before collecting data.
Equations 3 and 4 provide a means to conduct BHT of mediation that accommodates different research contexts. First, both equations do not require that paths \(\alpha\) and \(\beta\) are independent. This makes the proposed approach applicable to not only the simple mediation model where paths \(\alpha\) and \(\beta\) are independent but also latent variable mediation models in which the two paths may be dependent. Furthermore, as we will demonstrate, with Eqs. 3 and 4 , prior knowledge can be incorporated into computing the mediation Bayes factor and posterior odds using different ways. This allows the application of the proposed approach across different substantive scenarios in which researchers’ prior beliefs about the mediation process may vary.
Next, we first examine the special case where paths \(\alpha\) and \(\beta\) are independent and then consider the general case where the paths can be dependent. Both R functions ( fun_BHTmed.R ) and an R web app ( BayesianHypothesisTestingMediation ) are developed for implementing the proposed approach, and can be downloaded from https://github.com/xliu12/BHT.med . More details about the developed tools will be provided in the illustrative examples.
Scenario 1: Independent Paths \(\alpha\) and \(\beta\)
In this section, we examine the proposed BHT approach when paths \(\alpha\) and \(\beta\) are independent. We use the simple mediation model where the two paths are independent (Nuijten et al., 2014 ) as an example for illustration. We first present the technical procedure and illustrate it through a real-data example. Then, we investigate the impact of prior odds specification on the BHT results using hypothetical data sets. Technical details (e.g., derivations of Eqs. 7 - 10 ) are given in the supplemental materials ( https://github.com/xliu12/BHT.med/blob/main/bfmed_supplement_technical.pdf ).
Consider the following simple mediation model
where X , M , and Y are centered for the ease of discussion, and the residuals \(\epsilon _{M}\) and \(\epsilon _{Y}\) are assumed to have mean zero and be independent.
Under the assumption that paths α and β are independent, the mediation Bayes factor can be obtained using the Bayes factors of paths \(\alpha\) and \(\beta\) (denoted by \(BF^{\alpha }\) and \(BF^{\beta }\) , respectively; see Table 1 for the mathematical definitions):
Following Eq. 4 , the mediation posterior odds can be obtained as the product of the mediation Bayes factor and mediation prior odds. To compute the individual Bayes factors for paths \(\alpha\) and \(\beta\) , various software programs or packages (e.g., Hoijtink et al., 2019 ; JASP Team, 2020 ; Morey et al., 2018 ; Nuijten et al., 2014 ; Wetzels & Wagenmakers, 2012 ) have been developed and can be used.
When paths \(\alpha\) and \(\beta\) are independent, the prior odds specifications for path \(\alpha\) , path \(\beta\) , and the mediation effect must satisfy the following constraint
where \(PriorOdds^{\alpha }\) and \(PriorOdds^{\beta }\) denote the prior odds of paths \(\alpha\) and \(\beta\) , respectively. Footnote 5
With the equality constraint under the scenario that paths \(\alpha\) and \(\beta\) are independent, the mediation Bayes factor and posterior odds can be obtained by using the Bayes factors and prior odds of paths \(\alpha\) and \(\beta\) :
Here, we recommend researchers to specify prior odds for BHT of mediation using one of two following ways. Which way to use depends on the prior beliefs researchers hold about path \(\alpha\) , path \(\beta\) , and the mediation effect.
The first way is to specify the prior odds for paths \(\alpha\) and \(\beta\) separately. For example, suppose a researcher, Researcher A, specifies the prior odds of paths \(\alpha\) and \(\beta\) as both 1, indicating the prior probability of the presence (or absence) of each path is 0.5 based on prior knowledge. With this specification, according to the constraint in Eq. 8 , the mediation prior odds is 1/3 (not 1 as specified in Nuijten et al., 2014 ), indicating the prior probability is 0.25 for the presence of mediation and 0.75 for the absence of mediation.
The second way is to specify prior odds for the mediation effect and one individual path, for example, path \(\alpha\) . Footnote 6 With the equality constraint, the prior odds for path \(\beta\) is readily solvable. For example, suppose another researcher, Researcher B, specifies the mediation prior odds as 1 to reflect that Researcher B is neutral on the presence and absence of mediation, and the prior odds of paths \(\alpha\) and \(\beta\) to be the same. With the equality constraint, the prior odds of the two individual paths is \(\frac{\sqrt{0.5}}{1-\sqrt{0.5}}\approx 2.41\) . For each individual path ( \(\alpha\) or \(\beta\) ), it is more likely to be present than to be absent in the prior beliefs.
Using either way, Eqs. 9 and 10 can then be used to obtain the Bayes factor and posterior odds of mediation, respectively. Note that with the independence assumption for paths \(\alpha\) and \(\beta\) , the three conditional prior probabilities under the null (i.e., \(q_{00|0}\) , \(q_{01|0}\) , and \(q_{10|0}\) ) are determined with the specified prior odds. They are all 1/3 using Researcher A’s prior odds specification, Footnote 7 suggesting that the three scenarios of no mediation are equally likely to occur. Using Researcher B’s prior odds specifications, under the null of no mediation, the scenarios where one path is present and the other is absent are more likely ( \(q_{10|0}\) and \(q_{01|0}\) being 0.41) than the scenario where the two paths are both absent ( \(q_{00|0}\) being 0.17). Footnote 8 Therefore, different prior odds specifications for \(\alpha\) and \(\beta\) have different implications of the conditional prior probabilities of the three scenarios under the null.
An illustrative example: The PHLAME firefighter study
We use the example presented in Yuan & MacKinnon ( 2009 ) and Nuijten et al. ( 2014 ), the PHLAME (Promoting Healthy Lifestyles: Alternative Models’ Effects) firefighter study (Elliot et al., 2007 ), to illustrate our BHT procedure for the independent paths scenario. We use the prior odds specifications of Researchers A and B to gain insights on the sensitivity of BHT results to prior odds specifications. In the firefighter example, the hypothesized mediation mechanism ( \(H_{1}\) ) is that randomized exposure to an intervention ( X ) affects knowledge of the benefits of eating fruits and vegetables ( M ), which in turn affects reported eating of fruits and vegetables ( Y ). The Bayes factors for paths \(\alpha\) (the exposure-mediator relation) and \(\beta\) (the mediator-outcome relation) were reported in Nuijten et al. ( 2014 ) as \(BF^{\alpha }=10.06\) and \(BF^{\beta }=2.68\) , respectively, indicating that the data are in favor of the presence of each path.
Researcher A’s BHT results can be obtained by running our developed R function pathb.a() as follows:
In the above R code, arguments “PriorOdds.a” and “PriorOdds.b” read in the prior odds Researcher A specified for paths \(\alpha\) and \(\beta\) , respectively. Arguments “BF.a” and “BF.b” read in the Bayes factors of paths \(\alpha\) and \(\beta\) , respectively.
Researcher B’s BHT results can be obtained by running R function med.a() :
In the above R code, arguments “PriorOdds.med” and “PriorOdds.a” read in the prior odds Researcher B specified for the mediation effect and path \(\alpha\) , respectively.
The R functions and R code used in this example can be downloaded from https://github.com/xliu12/BHT.med . The results can also be obtained using our developed R Shiny (RStudio, Inc 2021 ) web app ( https://xiaoliu.shinyapps.io/BayesianHypothesisTestingMediation/ ), and are displayed in Table 2 .
Comparing Tables 2 a and 2 b, one can see that with different prior odds specifications, BHT of mediation yields different results. First, although the Bayes factors of each individual path are the same for both researchers, the mediation Bayes factors are different. For Researcher A, the mediation Bayes factor is 5.887; whereas for Researcher B, the mediation Bayes factor is 4.948.
Second, although Researcher A has a larger mediation Bayes factor than Researcher B, Researcher A’s posterior odds of mediation is nevertheless smaller than Researcher B’s. Specifically, for Researcher A who believes the odds in favor of mediation is 1/3 initially, combining the data evidence with the prior belief leads to a mediation posterior odds of 1.962, with the posterior probability of the presence of mediation being 0.662. In comparison, for Researcher B who specifies a mediation prior odds of 1, the mediation posterior odds is about 4.948, indicating the posterior probability that mediation exists given the data is 0.832. In Nuijten et al. ( 2014 ), their default prior odds specification is that the prior odds of path \(\alpha\) , path \(\beta\) , and the mediation effect all equal 1. Using their method, the Bayes factor and posterior odds of the mediation effect were both computed as 1.94 for the firefighter example. As discussed earlier, the default prior odds specification assumed in Nuijten et al. ( 2014 ) is inconsistent with the independent path assumption required by their method. This inconsistency makes their BHT results difficult to interpret.
As shown in this example, although the data are the same, the mediation Bayes factors and posterior odds change with different prior odds specifications.
Impact of prior odds specifications: Graphical demonstration with hypothetical data examples
In this subsection, we assess the impact of prior odds specification on the BHT of mediation via hypothetical data examples using a variety of prior odds specifications. Each hypothetical data set is characterized by a combination of paths Bayes factors \(BF^{\alpha }\) (1/3, 1, or 3) and \(BF^{\beta }\) (1/3, 1, or 3). In total, there are \(3\times 3=9\) hypothetical data sets. The levels of the Bayes factors are chosen to represent varied strengths of data evidence: a Bayes factor of 1/3 indicates the data support the absence of the path, 1 means the data have no preference for the absence or presence of the path, and 3 suggests the data favor the presence of the path. For each hypothetical set, we apply the proposed BHT approach with varied prior odds specifications. The mediation Bayes factor ( \(BF^{med}\) ) and posterior odds ( \(PosteriorOdds^{med}\) ) are the results of interest.
First, to facilitate the comparison of the BHT for mediation vs. the BHT for a single relation, Fig. 1 demonstrates how the BHT results of path \(\alpha\) , a single regression coefficient, change with varied prior odds specifications for path \(\alpha\) . Specifically, in the left column, the Bayes factor of path \(\alpha\) does not change with the prior odds of path \(\alpha\) Footnote 9 ; and in the right column, the posterior odds of path \(\alpha\) increases monotonically as the prior odds of path \(\alpha\) increases. The results in Fig. 1 are for a single coefficient, which are presented to serve as reference results.
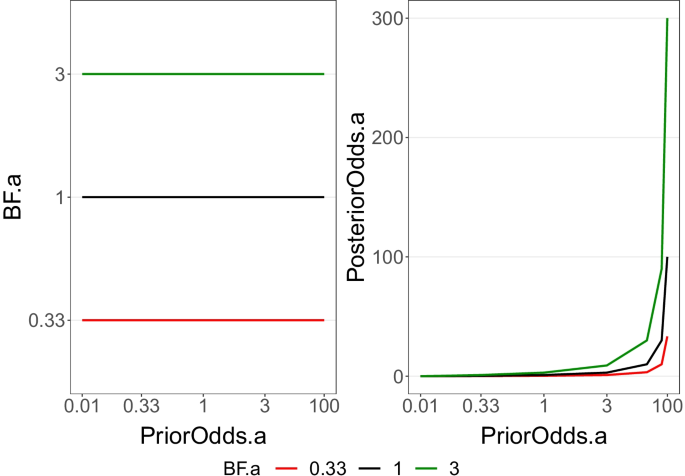
Results from the Bayesian hypothesis testing (BHT) of a single relation, the regression coefficient for path \(\alpha\) . The prior odds of path \(\alpha\) were varied as \(PriorOdds^{\alpha }=1/100,1/30,1/10,1/3,1,3,10,30,\) and 100. Given that the \(PriorOdds^{\alpha }\) values are not equally spaced, we used the prior probabilities of path \(\alpha\) being non-zero (i.e., \(p[\alpha \ne 0]=PriorOdds^{\alpha }/[1+PriorOdds^{\alpha }]\) ) as the breaks of the x-axes. The labels for the breaks in the x-axes are the corresponding prior odds values. The transformation from prior odds to prior probability is monotone and does not influence the increasing/decreasing patterns of the lines. The same plotting strategy is used in Fig. 2 as well
Next, we assessed how the results from the BHT of mediation would change with different prior odds specifications of an individual path or the mediation effect. To do so, we (1) specified the prior odds for mediation as \(PriorOdds^{med}=1\) , and varied that for path \(\alpha\) as \(PriorOdds^{\alpha }=1,3,10,30,\) and 100; or (2) fixed the prior odds of path \(\alpha\) as \(PriorOdds^{\alpha }=1\) and varied the mediation prior odds as \(PriorOdds^{med}=1/100,1/30,1/10,1/3,\) and 1. Footnote 10
The results are demonstrated in Fig. 2 . The mediation Bayes factor does not change with different prior odds specifications of an individual path or the mediation effect when the Bayes factors for individual paths \(\alpha\) and \(\beta\) are both 1.
Under the scenario of fixing the mediation prior odds to be 1 (Fig. 2a ), a higher prior odds for path \(\alpha\) can lead to higher or lower values of the mediation Bayes factor and posterior odds when the individual path Bayes factors are not both 1.
Under the scenario of fixing the prior odds of path \(\alpha\) to be 1 (Fig. 2b ), the posterior odds for the mediation effect increases as the mediation prior odds increases. This is similar to the change pattern of the posterior odds for a single relation (the right column of Fig. 1 ). However, when the individual path Bayes factors are not both 1, the mediation Bayes factor can be higher or lower as the mediation prior odds increases, which is different from the change pattern of the Bayes factor for a single relation (the left column of Fig. 1 ).
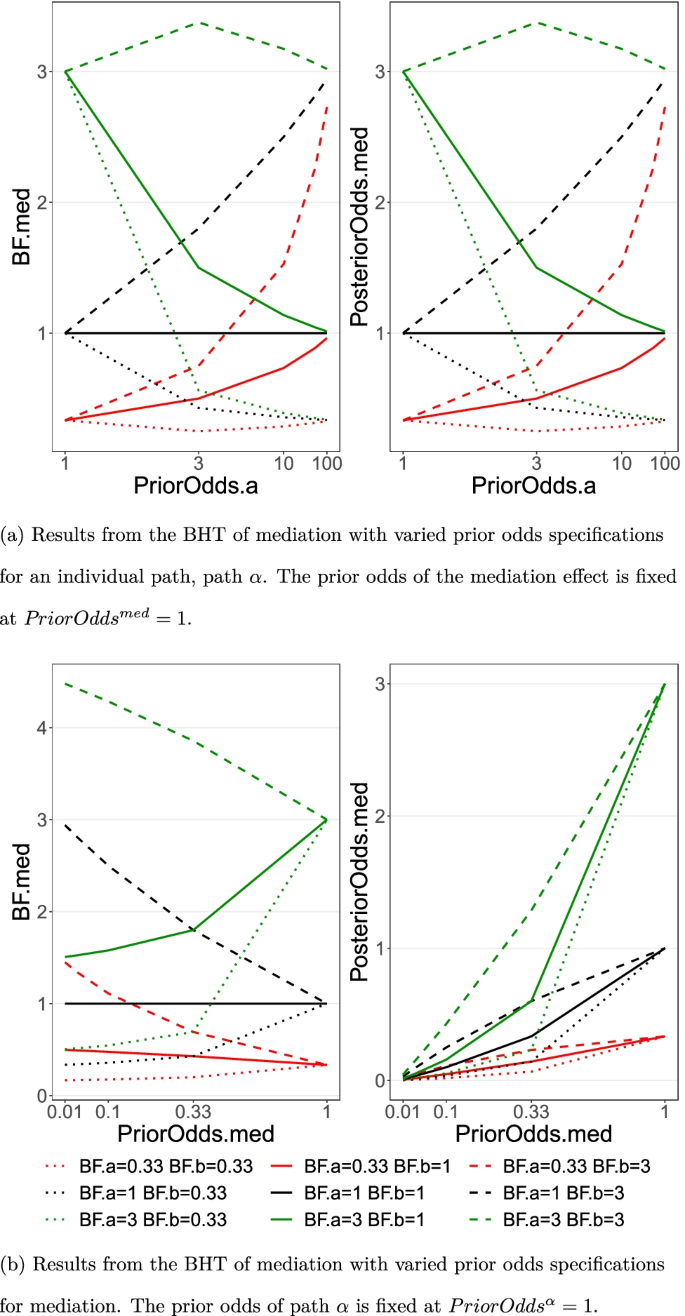
The impact of prior odds specifications on the results from the Bayesian hypothesis testing (BHT) of mediation. Each line in a subplot is plotted for a hypothetical data example with a specific combination of the Bayes factors for paths \(\alpha\) and \(\beta\) (the “BF.a” and “BF.b” in the legend)
In summary, through the hypothetical data examples, we showed that in the BHT of mediation, both the prior odds of an individual path \(\alpha\) (or \(\beta\) ) and the prior odds of the mediation effect can impact the results of BHT. Unlike the BHT for a single relation (e.g., a regression coefficient), the prior odds of the mediation effect can influence not only the mediation posterior odds, but also the mediation Bayes factor. Based on the findings, we emphasize the importance of prior odds specifications in the BHT of mediation. We recommend that researchers should evaluate their prior belief about the mediation process carefully when specifying the prior odds, and provide the prior odds specifications of the mediation effect and of paths \(\alpha\) and \(\beta\) when reporting the results.
Scenario 2: Dependent Paths \(\alpha\) and \(\beta\)
In this section, we apply the proposed BHT approach to the general case where paths \(\alpha\) and \(\beta\) can be dependent. We use a latent mediation model for illustration, as it has been shown that paths \(\alpha\) and \(\beta\) in mediation models involving latent variables can be dependent (e.g., MacKinnon 2008 ; Valente, Gonzalez, Miočević, & MacKinnon 2016 ). We first provide the key BHT idea and then illustrate the implementation through a simulated-data example. Technical details are available in the supplemental material ( https://github.com/xliu12/BHT.med/blob/main/bfmed_supplement_technical.pdf ).
Consider the following latent mediation model:
where \(\xi _{x}\) , \(\eta _{m}\) and \(\eta _{y}\) are the latent independent variable, mediator, and outcome variable respectively, and \(\mathrm {x}, \mathrm {m}\) , and \(\mathrm {y}\) are the corresponding indicators. In Eqs. 11 - 13 , the measurement errors \(\delta _{x}\) , \(\epsilon _{m}\) , and \(\epsilon _{y}\) are assumed to have mean zero, be independent of one another and of the latent variables. In Eq. 14 , the path coefficient \(\alpha\) denotes the effect of \(\xi _{x}\) on \(\eta _{m}\) , the path coefficient \(\beta\) represents the effect of \(\eta _{m}\) on \(\eta _{y}\) conditioning on \(\xi _{x}\) ; and the structural residuals \(\zeta _{y}\) and \(\zeta _{m}\) are assumed to have mean zero, be independent of one another and of \(\xi _{x}\) and the measurement errors. Figure 3 shows the structural relationships among \(\xi _{x}\) , \(\eta _{m}\) , and \(\eta _{y}\) in the latent mediation model.
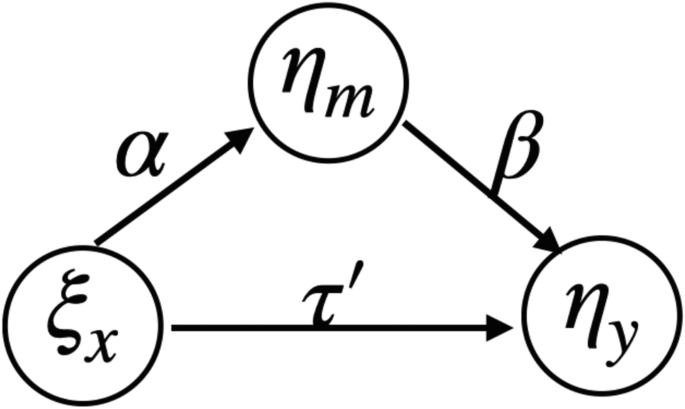
The structural relationships among the latent independent variable \(\xi _{x}\) , mediator \(\eta _{m}\) and outcome variable \(\eta _{y}\) in the latent mediation model (Eqs. 11 to 14 )
For this model, we use the Bayesian information criterion (BIC) approximation approach to obtain the mediation Bayes factor. For complex models such as the latent mediation model, the likelihoods in the Bayes factor formula (Eq. 3 ) are challenging to compute (e.g., Tendeiro & Kiers 2019 ; Bollen, Harden, Ray, & Zavisca 2014 ). For example, to compute the likelihood in the numerator, one needs to integrate the likelihood function over the entire space of the parameters under the alternative hypothesis. For the latent mediation model, the parameters include both those in the measurement model (Eqs. 11 - 13 ) and those in the structural model (Eq. 14 ). The complexity of such integration in latent variable models makes it challenging to obtain exact values of the likelihoods in Eq. 3 . In the BHT literature, a frequently used approach to approximating integrated likelihoods is the BIC approximation (Kass & Raftery 1995 ; Raftery 1995 ), which has been shown to be useful for obtaining Bayes factors for models with latent variables (e.g., Bollen, Harden, Ray, & Zavisca 2014 ; Merkle & Wang 2016 ). Approximating the Bayes factor in Eq. 3 using BIC also makes it easy for researchers to conduct complex BHT of mediation. Let \(\mathrm {BIC}\{\mathbf {M}\}\) denote the BIC value of model \(\mathbf {M}\) . The mediation Bayes factor can be approximated as
where \(\mathbf {M}_{11}\) , \(\mathbf {M}_{01}\) , \(\mathbf {M}_{10}\) , and \(\mathbf {M}_{00}\) denote respectively the unconstrained mediation model, the model where path \(\alpha\) is constrained at zero, the model where path \(\beta\) is constrained at zero, and the model where both paths are constrained at zero. The BIC values can be obtained from, often in the output of, commonly used structural equation modeling software, such as Mplus (Muthén & Muthén 2017 ) and the lavaan R package (Rosseel et al., 2020 ). The mediation posterior odds can then be obtained following the Bayes’ rule (Eq. 1 ).
With Eq. 15 , researchers can incorporate their prior belief into the BHT of mediation in two ways. First, researchers can specify the conditional prior probabilities \(q_{00|0},q_{01|0}\) , and \(q_{10|0}\) for the three scenarios of no mediation under the constraint that they sum up to 1 to obtain the mediation Bayes factor, and specify a prior odds for the mediation effect to obtain the mediation posterior odds following the Bayes’ rule. For example, suppose a researcher, Researcher C, specifies the three conditional prior probabilities under the null hypothesis to all equal 1/3 to reflect the prior belief that the three scenarios of no mediation are equally likely to occur, and specifies the mediation prior odds as 1 to give the presence and absence of mediation equal prior probabilities. With this specification, the prior odds of paths \(\alpha\) and \(\beta\) both equal 2, implying the prior belief that for each of the two paths, its presence is more likely than its absence. Footnote 11 Furthermore, the two paths are not independent: the joint prior probability of paths \(\alpha\) and \(\beta\) being both present under this specification is 1/2, higher than that under the independent paths assumption, which is 4/9. Footnote 12
Second, researchers can specify prior odds for the two paths and the mediation effect under the inequality constraint that the mediation prior odds must not exceed the prior odds of any path. Then, the conditional prior probabilities \(q_{00|0},q_{01|0}\) , and \(q_{10|0}\) for the three scenarios of no mediation are readily obtainable. Footnote 13 Equation 15 and the Bayes’ rule (Eq. 1 ) can then be used to obtain the mediation Bayes factor and posterior odds, respectively. In particular, for the default prior odds specification assumed in Nuijten et al. ( 2014 ) (i.e., the prior odds of path \(\alpha\) , path \(\beta\) , and the mediation effect all equal 1), \(q_{01|0}=q_{10|0}=0\) and \(q_{00|0}=1\) . That is, the scenarios where one path is present and the other is absent are not allowed to occur a priori; and the only possible scenario under \(H_{0}\) is that the two paths are both absent. While this specification does not meet the independent paths constraint, it can occur in the dependent paths scenario.
To illustrate the BHT of mediation in the dependent paths scenario, we simulated a sample of size \(n=100\) from the latent mediation model (Eqs. 11 - 14 ) with the path coefficients \(\alpha =0\) , \(\beta =.39\) and \(\tau ^{\prime }=0\) . That is, the null hypothesis of no mediation is true in the data generation. The latent input variable, mediator, and outcome are each measured by three indicators with loadings 0.9, 0.8, and 0.7; and the variances of the indicators and latent variables are set as 1.
For the simulated sample, we obtained the BIC values of the unconstrained mediation model ( \(\mathrm {BIC}\{\mathbf {M}_{11}\}\approx 2157\) ), the model where path \(\alpha\) is constrained at zero ( \(\mathrm {BIC}\{\mathbf {M}_{01}\}\approx 2153\) ), the model where path \(\beta\) is constrained at zero ( \(\mathrm {BIC}\{\mathbf {M}_{10}\}\approx 2160\) ), and the model where both paths are constrained at zero ( \(\mathrm {BIC}\{\mathbf {M}_{00}\}\approx 2155\) ) using the lavaan package (Rosseel et al., 2020 ) in R.
The BHT results of the simulated data using Researcher C’s prior specifications can be obtained by running our developed R function med.qs() as follows:
In the above R code, argument “PriorOdds.med” reads in the prior odds specified for the mediation effect. Arguments “H0.q10”, “H0.q01”, and “H0.q00” read in the specified values of \(q_{10|0}\) , \(q_{01|0}\) , and \(q_{00|0}\) (the conditional prior probabilities of the three scenarios of no mediation under the null hypothesis), respectively. Note that the three specified values need to sum up to 1. Arguments “BIC11”, “BIC01”, “BIC10”, and “BIC00” read in the BIC values of the unconstrained mediation model, the model where path \(\alpha\) is constrained at zero, the model where path \(\beta\) is constrained at zero, and the model where both paths are constrained at zero, respectively.
The BHT results of specifying the prior odds of the two paths and the mediation effect to all equal 1 can be obtained by running our developed R function med.ab() :
In the above R code, arguments “PriorOdds.med”, “PriorOdds.a”, and “PriorOdds.b” read in the specified prior odds for the mediation effect, path \(\alpha\) , and path \(\beta\) , respectively. Note that these prior odds are all 1, which are the default prior odds in Nuijten et al. ( 2014 ) and can be used when paths \(\alpha\) and \(\beta\) are dependent.
The results are displayed in Table 3 . The R functions and R code used in this example can be downloaded from https://github.com/xliu12/BHT.med . The results can also be obtained using our developed R Shiny (RStudio, Inc, 2021) web app ( https://xiaoliu.shinyapps.io/BayesianHypothesisTestingMediation/ ). Footnote 14
In Table 3 a, the mediation Bayes Factor is 0.216, indicating that the likelihood of observing the data is approximately 5 times as large if mediation is absent than if mediation is present. Given the evidence in the data, the odds between the presence and absence of mediation is lowered from 1 to about 0.216, with the posterior probability of the presence of mediation being 0.178.
In Table 3 b, the Bayes Factor and posterior odds for the mediation effect are 0.282 and 0.220, respectively, which are the same as those for either individual path ( \(\alpha\) or \(\beta\) ). This makes sense given the specified prior odds. Specifically, by specifying the same prior odds for the two paths and mediation, the BHT for either individual path and the BHT for the mediation effect are comparing the same two models: the model where the two paths are both present vs. the model where they are both absent, because the models where one path is present and the other path is absent are impossible to be true given the prior odds specifications.
Mediation analysis plays an important role in theory development and testing in many research fields. To test mediation, many frequentist methods have been proposed and evaluated. Despite the growing popularity of applying Bayesian methods to estimating mediation effects, Bayesian approaches to testing mediation effects are under-developed and under-evaluated. In this article, we studied the use of BHT for mediation testing. Our study can add to the literature in the following ways.
First, we proposed a general approach to performing BHT of mediation (i.e., obtaining mediation Bayes factor and posterior odds). Our approach is applicable to different research contexts and can facilitate the integration of data evidence and researchers’ prior beliefs. Specifically, the proposed approach can be applied to testing mediation effects in both the independent and dependent paths scenarios. For the independent paths scenario, we provided two ways to specify prior odds (for the two paths \(\alpha\) and \(\beta\) ; or for one path and the mediation effect) for implementing the proposed approach, and demonstrated the implementation with the simple mediation model. For the dependent paths scenario, we considered the latent mediation model and implemented the proposed approach with two ways of prior odds/probability specification (specify the mediation prior odds and the conditional prior probabilities under \(H_{0}\) ; specify the mediation prior odds and two path prior odds). Both R functions ( fun_BHTmed.R ) and an R web app ( BayesianHypothesisTestingMediation ) were developed for implementing the techniques presented in the two scenarios, and can be downloaded from https://github.com/xliu12/BHT.med .
Second, we evaluated the sensitivity of BHT of mediation to the prior odds specification. Specifically, through nine hypothetical data examples in the independent paths scenario, we demonstrated that the prior odds of an individual path and the prior odds of the mediation effect can both change the mediation Bayes factor and posterior odds. In particular, we found that the mediation Bayes factor can be influenced by the mediation prior odds, which is unlike the Bayes factor for a single relation (e.g., a regression coefficient). We hope our demonstration can provide insights on the prior odds specification in BHT of mediation.
Recommendations
Based on the findings, we recommend researchers to pay attention to specifying the prior odds/probabilities for BHT of mediation. The specification should be based on their prior knowledge about the mediation process. When there is no prior knowledge available, in the scenario of independent paths a researcher may consider specifying a prior odds of 1 for each path (i.e., \(PriorOdds^{\alpha }=PriorOdds^{\beta }=1\) ) to reflect that the researcher is “in a state of genuine uncertainty” (Freedman 1987 , p. 141) regarding the presence or absence of each path. Note that with this specification, the prior odds in favor of mediation is 1/3. That is, the researcher is conservative about mediation being present.
When the two paths are dependent, a researcher might consider specifying the prior odds of mediation as 1 (i.e., \(PriorOdds^{med}=1\) ), so as to be neutral about the null and alternative mediation hypotheses to be tested. If a researcher has no prior knowledge about which of the three scenarios under the null hypothesis are more/less likely to occur, the researcher may consider specifying the three conditional prior probabilities to be all equal 1/3 (i.e., \(q_{00|0}=q_{01|0}=q_{10|0}=1/3\) ). Note that with this specification, the prior odds of each path is 2 (i.e., \(PriorOdds^{\alpha }=PriorOdds^{\beta }=2\) ). In other words, the researcher believes that each path is more likely to be present than be absent a priori.
Furthermore, we recommend researchers to conduct sensitivity analysis to examine how the mediation Bayes factor and posterior odds would change with a list of reasonable prior odds/probabilities specifications. If the results are relatively stable, the researcher may corroborate his/her posterior belief about the presence vs. absence of mediation. Nonetheless, we suggest that the researcher should report the results from sensitivity analysis and provide a rationale for the chosen prior odds/probability specification.
Last but not least, we echo previous literature (e.g., Tendeiro & Kiers 2019 ) and recommend that researchers should report both the Bayes factor and posterior odds of mediation, and should distinguish between their interpretations. Specifically, the mediation Bayes factor quantifies the relative likelihood of observing the data if mediation is present vs. if mediation is absent; whereas the mediation posterior odds measures the relative posterior probability of the presence vs. absence of mediation given the observed data. We also recommend researchers to provide the Bayes factors of the two paths when the paths are independent, or the BIC values of the four models (i.e., models \(\mathbf {M}_{11}\) , \(\mathbf {M}_{01}\) , \(\mathbf {M}_{10}\) , and \(\mathbf {M}_{00}\) ) when the paths can be dependent. With the information, readers can evaluate the Bayes factor and posterior odds of mediation based on their own prior beliefs.
Limitations and future directions
The current study can be extended in the future. First, although the ability to provide a continuous measure of the relative data evidence and posterior probabilities is argued to be an advantage of the proposed BHT approach, in practice, researchers may be interested in making a binary decision on the presence of mediation. In the NHST framework, a binary decision of rejecting or not rejecting the null is often made by comparing the p -value to a nominal Type I error rate (e.g., 0.05). While formal BHT has no concept of Type I errors, such a binary decision can be made by comparing Bayes factors with a certain cutoff value. For testing a single relation (e.g., a regression coefficient), commonly used Bayes factor cutoffs include the evidence categories proposed by Jeffreys ( 1961 ) and Kass & Raftery ( 1995 ), and the cutoff value 3 for Bayes factors is often used as the counterpart of the cutoff 0.05 for p -values (Hoijtink et al., 2019 ; Kelter, 2020 ). However, the Bayes factor cutoffs for testing a single relation may not be suitable for mediation testing, especially considering the impact of prior odds specifications on the mediation Bayes factor. It is our important future direction to examine what cutoffs for the mediation Bayes factor can be used to maintain certain nominal Type I error rates (e.g., 0.05) given a set of prior odds specifications.
Furthermore, with the determined cutoff values, it is of interest to examine the performance of the proposed BHT in terms of statistical power, and compare the BHT approach with other mediation testing methods such as the frequentist tests (e.g., MacKinnon, Lockwood, Hoffman, West, & Sheets 2002 ) and the posterior credible interval approach (e.g., Yuan & MacKinnon 2009 ). In addition, previous studies have found the power stagnation issue associated with frequentist tests of mediation (e.g., Fritz, Taylor, & MacKinnon 2012 ). Footnote 15 We are interested in investigating whether the BHT approach also has the power stagnation issue.
Second, in complex mediation analyses, there could be multiple purported mediators and researchers may be interested in testing the total mediation effects (e.g., Selig & Preacher 2009 ), or in testing contrasts between two or more mediation effects (e.g., MacKinnon 2000 ). How to extend the proposed BHT approach to testing more complex mediation hypotheses in multiple mediator models is a worthwhile future direction.
Third, in addition to testing a point null hypothesis, BHT methods for testing interval null hypotheses (e.g., Morey & Rouder 2011 ) and for testing informative hypotheses (e.g., Hoijtink, Klugkist, & Boelen 2008 ) have been proposed. It is of interest to explore the feasibility and adaptation of these methods for mediation analysis.
Fourth, in the literature of BHT, it has been shown that the Bayes factor can be sensitive to the within-model prior distributions (e.g., Du, Edwards, & Zhang 2019 ; Liu & Aitkin 2008 ; Tendeiro & Kiers 2019 ). It is an important future direction to examine how the mediation Bayes factor and posterior odds would change with the within-model prior distributions, given a prior odds/probability specification.
Fifth, BHT has been shown to be useful for sequential data analysis (e.g., Hoijtink, Mulder, van Lissa, & Gu 2019 ; Rouder 2014 ). We are interested in utilizing the proposed BHT to develop sequential data analysis procedures for mediation studies.
We hope the current study can contribute a useful BHT approach to researchers’ toolbox of mediation analysis and provide insights on the impact of prior odds specifications in the BHT of mediation.
Availability of data and material
The data in this study are based on simulation. None of the data or materials are related to any experiments.
To simplify notations, throughout the article, the numerators of PriorOdds , PosteriorOdds and BF are for \(H_{1}\) or evaluated under \(H_{1}\) ; and the denominators are for \(H_{0}\) or evaluated under \(H_{0}\) .
If the prior odds of the presence of path \(\alpha\) , path \(\beta\) , and mediation all equal 1, we have \(p\left( \alpha \ne 0\right) =p\left( \beta \ne 0\right) =p(\alpha \ne 0,\beta \ne 0)=0.5\) . It is always true that \(p\left( \alpha \ne 0\right) =p(\alpha \ne 0,\beta \ne 0)+p(\alpha \ne 0,\beta =0)\) and \(p\left( \beta \ne 0\right) =p(\alpha \ne 0,\beta \ne 0)+p(\alpha =0,\beta \ne 0).\) In order for these prior probabilities to hold, both \(p(\alpha \ne 0,\beta =0)=0\) and \(p(\alpha =0,\beta \ne 0)=0\) need to be true.
Besides the product of coefficient method, mediation effects can also be defined using the counterfactual approach (e.g., Holland 1986 ; Pearl 2001 ; Valeri & VanderWeele 2013 ), especially when causal interpretation is of interest. In this article, we use the product of coefficient method because it is widely used in the practice of mediation analysis in psychology and social science research and is relatively easy for substantive researchers to interpret.
That is, \(q_{00|0}=p(\alpha =0,\beta =0|H_{0}:\alpha \beta =0)=\frac{p(\alpha =0,\beta =0)}{p(\alpha \beta =0)}\) , \(q_{01|0}=p(\alpha =0,\beta \ne 0|H_{0}:\alpha \beta =0)=\frac{p(\alpha =0,\beta \ne 0)}{p(\alpha \beta =0)}\) , and \(q_{10|0}=p(\alpha \ne 0,\beta =0|H_{0}:\alpha \beta =0)=\frac{p(\alpha \ne 0,\beta =0)}{p(\alpha \beta =0)}\) .
Nuijten et al. ( 2014 ) assumed paths \(\alpha\) and \(\beta\) are independent and specified the prior odds of path \(\alpha\) , path \(\beta\) , and the mediation effect all as 1 (i.e., \(PriorOdds^{\alpha }=PriorOdds^{\beta }=PriorOdds^{med}=1\) ). Their prior odds specification, however, does not satisfy the equality constraint (Eq. 8 ).
Under the equality constraint, the prior odds specification for mediation should not exceed that for an individual path.
With the independence assumption for paths \(\alpha\) and \(\beta\) , \(q_{00|0}=p(\alpha =0,\beta =0|\alpha \beta =0)=p(\alpha =0)p(\beta =0)/p(\alpha \beta =0)=(0.5*0.5)/0.75=1/3\) ; and similarly, \(q_{01|0}=p(\alpha =0)p(\beta \ne 0)/p(\alpha \beta =0)=(0.5*0.5)/0.75=1/3\) and \(q_{10|0}=p(\alpha \ne 0)p(\beta =0)/p(\alpha \beta =0)=(0.5*0.5)/0.75=1/3\) .
With the independence assumption for paths \(\alpha\) and \(\beta\) , \(q_{10|0}=p(\alpha \ne 0)p(\beta =0)/p(\alpha \beta =0)=\frac{\sqrt{0.5}(1-\sqrt{0.5})}{0.5}\approx 0.41\) , \(q_{01|0}=p(\alpha =0)p(\beta \ne 0)/p(\alpha \beta =0)=\frac{(1-\sqrt{0.5})\sqrt{0.5}}{0.5}\approx 0.41\) , and \(q_{00|0}=p(\alpha =0,\beta =0|\alpha \beta =0)=p(\alpha =0)p(\beta =0)/p(\alpha \beta =0)=\frac{(1-\sqrt{0.5})^{2}}{0.5}\approx 0.17\) .
Although the varied prior odds values range from 0 to 100 in the left column of Fig. 1 , this pattern holds when the prior odds of path \(\alpha\) is larger than 100.
Under the independent paths constraint (Eq. 8 ), the prior odds of path \(\alpha\) must be larger than or equal to the prior odds of mediation.
This can be seen by computing the prior probabilities of the models \(\mathbf {M}_{11}\) , \(\mathbf {M}_{01}\) , \(\mathbf {M}_{10}\) , and \(\mathbf {M}_{00}\) , which are \(p_{11}=p(\alpha \ne 0,\beta \ne 0)=PriorOdds^{med}/(1+PriorOdds^{med})=1/2\) , \(p_{01}=(1-p_{11})q_{01|0}=1/6\) , \(p_{10}=(1-p_{11})q_{10|0}=1/6\) and \(p_{00}=(1-p_{11})q_{00|0}=1/6\) , respectively. Thus, the prior probabilities of paths \(\alpha\) and \(\beta\) are \(p(\alpha \ne 0)=p_{11}+p_{10}=2/3,p(\beta \ne 0)=p_{11}+p_{01}=2/3\) , respectively. The prior odds of the two paths are thus \(PriorOdds^{\alpha }=PriorOdds^{\beta }=\frac{2/3}{1-2/3}=2\) .
See the supplemental material for technical details.
In Table 3 a, the prior odds, Bayes factors and posterior odds of the paths \(\alpha\) and \(\beta\) are computed by first computing the prior probabilities of the models \(\mathbf {M}_{11}\) , \(\mathbf {M}_{01}\) , \(\mathbf {M}_{10}\) , and \(\mathbf {M}_{00}\) as \(p_{11}=PriorOdds^{med}/(1+PriorOdds^{med})\) , \(p_{01}=(1-p_{11})q_{01|0}\) , \(p_{10}=(1-p_{11})q_{10|0}\) and \(p_{00}=(1-p_{11})q_{00|0}\) , respectively. Then the prior odds, Bayes factor, and posterior odds for a path, for example, path \(\alpha\) are computed as \(PriorOdds^{\alpha }=\frac{p_{11}+p_{10}}{p_{01}+p_{00}}\) , \(BF^{\alpha }\overset{}{\approx }\frac{\frac{p_{11}}{p_{11}+p_{10}}e^{-\mathrm {BIC}\{\mathbf {M}_{11}:\alpha \ne 0,\beta \ne 0\}/2}+\frac{p_{10}}{p_{11}+p_{10}}e^{-\mathrm {BIC}\{\mathbf {M}_{10}:\alpha \ne 0,\beta =0\}/2}}{\frac{p_{01}}{p_{01}+p_{00}}e^{-\mathrm {BIC}\{\mathbf {M}_{01}:\alpha =0,\beta \ne 0\}/2}+\frac{p_{00}}{p_{01}+p_{00}}e^{-\mathrm {BIC}\{\mathbf {M}_{00}:\alpha =0,\beta =0\}/2}}\) and \(PosteriorOdds^{\alpha }=PriorOdds^{\alpha }BF^{\alpha }\approx \frac{p_{11}e^{-\mathrm {BIC}\{\mathbf {M}_{11}:\alpha \ne 0,\beta \ne 0\}/2}+p_{10}e^{-\mathrm {BIC}\{\mathbf {M}_{10}:\alpha \ne 0,\beta =0\}/2}}{p_{01}e^{-\mathrm {BIC}\{\mathbf {M}_{01}:\alpha =0,\beta \ne 0\}/2}+p_{00}e^{-\mathrm {BIC}\{\mathbf {M}_{00}:\alpha =0,\beta =0\}/2}}\) , respectively.
That is, as the magnitude of path \(\alpha\) increases, there can be a stagnation or reduction in power of testing mediation.
Anderson, S. F. (2020). Misinterpreting p: The discrepancy between p values and the probability the null hypothesis is true, the influence of multiple testing, and implications for the replication crisis. Psychological Methods, 25 (5), 596–609. https://doi.org/10.1037/met0000248 .
Article PubMed Google Scholar
Baron, R. M., & Kenny, D. A. (1986). The moderator-mediator variable distinction in social psychological research: Conceptual, strategic, and statistical considerations. Journal of Personality and Social Psychology, 51 (6), 1173–1882. https://doi.org/10.1037/0022-3514.51.6.1173 .
Berger, J. O., & Sellke, T. (1987). Testing a point null hypothesis: The irreconcilability of p values and evidence. Journal of the American Statistical Association, 82 (397), 112–122. http://www.jstor.org/stable/2289131
Bollen, K. A., Harden, J. J., Ray, S., & Zavisca, J. (2014). BIC and alternative bayesian information criteria in the selection of structural equation models. Structural Equation Modeling: A Multidisciplinary Journal, 21 (1), 1–19. https://doi.org/10.1080/10705511.2014.856691 .
Che, C., Jin, I. H., & Zhang, Z. (2021). Network mediation analysis using model-based eigenvalue decomposition. Structural Equation Modeling: A Multidisciplinary Journal, 28 (1), 148–161. https://doi.org/10.1080/10705511.2020.1721292 .
Article Google Scholar
Dienes, Z. (2019). How do I know what my theory predicts? Advances in Methods and Practices in Psychological Science, 2 (4), 364–377. https://doi.org/10.1177/2515245919876960 .
Du, H., Edwards, M. C., & Zhang, Z. (2019). Bayes factor in one-sample tests of means with a sensitivity analysis: A discussion of separate prior distributions. Behavior Research Methods, 51 (5), 1998–2021. https://doi.org/10.3758/s13428-019-01262-w .
Elliot, D. L., Goldberg, L., Kuehl, K. S., Moe, E. L., Breger, R. K. R., & Pickering, M. A. (2007). The PHLAME (Promoting Healthy Lifestyles: Alternative Models’ Effects) firefighter study: Outcomes of two models of behavior change. Journal of Occupational and Environmental Medicine, 49 (2), 204–213. https://doi.org/10.1097/jom.0b013e3180329a8d .
Freedman, B. (1987). Equipoise and the ethics of clinical research. New England Journal of Medicine, 317 (3), 141–145. https://doi.org/10.1056/nejm198707163170304 .
Fritz, M. S., Taylor, A. B., & MacKinnon, D. P. (2012). Explanation of two anomalous results in statistical mediation analysis. Multivariate Behavioral Research, 47 (1), 61–87. https://doi.org/10.1080/00273171.2012.640596 .
Article PubMed PubMed Central Google Scholar
Harlow, L. L., Mulaik, S. A., & Steiger, J. H. (1997). What if there were no significance tests? Mahwah, NJ: Lawrence Erlbaum Associates Publishers.
Google Scholar
Hoijtink, H., Klugkist, I., & Boelen, P. A. (Eds.). (2008). Bayesian evaluation of informative hypotheses . New York: Springer.
Hoijtink, H., Mulder, J., van Lissa, C., & Gu, X. (2019). A tutorial on testing hypotheses using the Bayes factor. Psychological Methods, 24 (5), 539–556. https://doi.org/10.1037/met0000201 .
Holland, P. W. (1986). Statistics and causal inference. Journal of the American statistical Association, 81 (396), 945–960. https://doi.org/10.1080/01621459.1986.10478354 .
Ioannidis, J. P. A. (2019). What have we (not) learnt from millions of scientific papers with p values? The American Statistician, 73 (sup1), 20–25. https://doi.org/10.1080/00031305.2018.1447512 .
Iverson, G. J., Lee, M. D., Zhang, S., & Wagenmakers, E.-J. (2009). prep: An agony in five fits. Journal of Mathematical Psychology, 53 (4), 195–202. https://doi.org/10.1016/j.jmp.2008.09.004 .
JASP Team (2020). JASP (Version 0.14.1)[Computer software]. https://jasp-stats.org/
Jeffreys, H. (1961) Theory of probability. Oxford, UK: Oxford University Press, 3rd ed.
Kass, R. E., & Raftery, A. E. (1995). Bayes factors. Journal of the American Statistical Association, 90 (430), 773–795. http://www.jstor.org/stable/2291091
Kelter, R. (2022). Power analysis and type I and type II error rates of Bayesian nonparametric two-sample tests for location-shifts based on the Bayes factor under Cauchy priors. Computational Statistics & Data Analysis, 165, 1–20. https://doi.org/10.1016/j.csda.2021.107326 .
Liao, J. G., Midya, V., & Berg, A. (2020). Connecting and contrasting the Bayes factor and a modified ROPE procedure for testing interval null hypotheses. The American Statistician, 75 (3), 1–19. https://doi.org/10.1080/00031305.2019.1701550 .
Liu, C. C., & Aitkin, M. (2008). Bayes factors: Prior sensitivity and model generalizability. Journal of Mathematical Psychology, 52 (6), 362–375. https://doi.org/10.1016/j.jmp.2008.03.002 .
Ly, A., Verhagen, J., & Wagenmakers, E.-J. (2016). Harold Jeffreys’s default Bayes factor hypothesis tests: Explanation, extension, and application in psychology. Journal of Mathematical Psychology, 72, 19–32. https://doi.org/10.1016/j.jmp.2015.06.004 .
MacKinnon, D. P. (2000). Contrasts in multiple mediator models. In J. S. Rose, L. Chassin, C. C. Presson, & S. J. Sherman (Eds.), Multivariate applications in substance use research: New methods for new questions (pp. 141–160). Lawrence Erlbaum Associates Publishers.
MacKinnon, D. P. (2008). Introduction to statistical mediation analysis . New York, NY: Taylor & Francis Group/Lawrence Erlbaum Associates.
MacKinnon, D. P., Fritz, M. S., Williams, J., & Lockwood, C. M. (2007). Distribution of the product confidence limits for the indirect effect: Program prodclin. Behavior Research Methods, 39 (3), 384–389. https://doi.org/10.3758/BF03193007 .
MacKinnon, D. P., Lockwood, C. M., Hoffman, J. M., West, S. G., & Sheets, V. (2002). A comparison of methods to test mediation and other intervening variable effects. Psychological Methods, 7 (1), 83. https://doi.org/10.1037/1082-989X.7.1.83 .
Merkle, E. C., & Wang, T. (2016). Bayesian latent variable models for the analysis of experimental psychology data. Psychonomic Bulletin & Review, 25 (1), 256–270. https://doi.org/10.3758/s13423-016-1016-7 .
Miočević, M., Gonzalez, O., Valente, M. J., & MacKinnon, D. P. (2018). A tutorial in Bayesian potential outcomes mediation analysis. Structural Equation Modeling: A Multidisciplinary Journal, 25 (1), 121–136. https://doi.org/10.1080/10705511.2017.1342541 .
Morey, RD, Rouder, JN, Jamil, T. (2018) BayesFactor: Computation of Bayes Factors for common designs. R package version 0.9. 12-4.2. [Computer software]. Retrieved from https://CRAN.R-project.org/package=BayesFactor
Morey, R. D., & Rouder, J. N. (2011). Bayes factor approaches for testing interval null hypotheses. Psychological Methods, 16 (4), 406–419. https://doi.org/10.1037/a0024377 .
Mulder, J., & Wagenmakers, E.-J. (2016). Editors’ introduction to the special issue “Bayes factors for testing hypotheses in psychological research: Practical relevance and new developments’’. Journal of Mathematical Psychology, 72, 1–5. https://doi.org/10.1016/j.jmp.2016.01.002 .
Muthén, B., Muthén, L. (2017) Mplus. Chapman and Hall/CRC
Nickerson, R. S. (2000). Null hypothesis significance testing: A review of an old and continuing controversy. Psychological Methods, 5 (2), 241–301. https://doi.org/10.1037/1082-989x.5.2.241 .
Nuijten, M. B., Wetzels, R., Matzke, D., Dolan, C. V., & Wagenmakers, E.-J. (2014). A default Bayesian hypothesis test for mediation. Behavior Research Methods, 47 (1), 85–97. https://doi.org/10.3758/s13428-014-0470-2 .
Pearl, J. (2001) Direct and indirect effects. In: Proceedings of the seventeenth conference on uncertainty in artificial intelligence, UAI’01 (pp. 411–420). San Francisco, CA, USA: Morgan Kaufmann Publishers Inc. http://dl.acm.org/citation.cfm?id=2074022.2074073
Raftery, A. E. (1995). Bayesian model selection in social research. Sociological Methodology, 25, 111–163. http://www.jstor.org/stable/271063
Rosseel, Y., Jorgensen, T.D., Rockwood, N., Oberski, D., Byrnes, J., Vanbrabant, L., Savalei, V., ... et al. (2020). Package ‘lavaan’.
Rouder, J. N. (2014). Optional stopping: No problem for bayesians. Psychonomic Bulletin & Review, 21 (2), 301–308. https://doi.org/10.3758/s13423-014-0595-4 .
RStudio, Inc (2021) Web application framework for R. [Computer software manual]. https://shiny.rstudio.com/ .
Selig, J. P., & Preacher, K. J. (2009). Mediation models for longitudinal data in developmental research. Research in Human Development, 6 (2–3), 144–164. https://doi.org/10.1080/15427600902911247 .
Tendeiro, J. N., & Kiers, H. A. L. (2019). A review of issues about null hypothesis Bayesian testing. Psychological Methods, 24 (6), 774–795. https://doi.org/10.1037/met0000221 .
Tofighi, D., & Kelley, K. (2020). Improved inference in mediation analysis: Introducing the model-based constrained optimization procedure. Psychological Methods, 25 (4), 496–515. https://doi.org/10.1037/met0000259 .
Valente, M. J., Gonzalez, O., Miočević, M., & MacKinnon, D. P. (2016). A note on testing mediated effects in structural equation models: Reconciling past and current research on the performance of the test of joint significance. Educational and Psychological Measurement, 76 (6), 889–911. https://doi.org/10.1177/0013164415618992 .
Valeri, L., & VanderWeele, T. J. (2013). Mediation analysis allowing for exposure-mediator interactions and causal interpretation: Theoretical assumptions and implementation with SAS and SPSS macros. Psychological Methods, 18 (2), 137. https://doi.org/10.1037/a0031034 .
Wagenmakers, E. J., Marsman, M., Jamil, T., Ly, A., Verhagen, J., Love, J., et al. (2018). Bayesian inference for psychology. part I: Theoretical advantages and practical ramifications. Psychonomic Bulletin & Review, 25 (1), 35–57. https://doi.org/10.3758/s13423-017-1343-3 .
Wang, L., & Preacher, K. J. (2015). Moderated mediation analysis using Bayesian methods. Structural Equation Modeling: A Multidisciplinary Journal, 22 (2), 249–263. https://doi.org/10.1080/10705511.2014.935256 .
Wetzels, R., & Wagenmakers, E.-J. (2012). A default Bayesian hypothesis test for correlations and partial correlations. Psychonomic Bulletin & Review, 19 (6), 1057–1064. https://doi.org/10.3758/s13423-012-0295-x .
Yuan, Y., & MacKinnon, D. P. (2009). Bayesian mediation analysis. Psychological Methods, 14 (4), 301. https://doi.org/10.1037/a0016972 .
Download references
Acknowledgements
Lijuan Wang and Zhiyong Zhang are grateful for the financial support from IES grant R305D210023 during the study. Lijuan Wang is also grateful for the financial support from NIH grants R01MD014737, R01HD091235, R01HD088482, and R01HD087319 during the study.
Author information
Authors and affiliations.
Department of Psychology, University of Notre Dame, 390 Corbett Hall, Notre Dame, IN, 46556, USA
Xiao Liu, Zhiyong Zhang & Lijuan Wang
You can also search for this author in PubMed Google Scholar
Corresponding author
Correspondence to Xiao Liu .
Additional information
Publisher’s note.
Springer Nature remains neutral with regard to jurisdictional claims in published maps and institutional affiliations.
Rights and permissions
Reprints and permissions
About this article
Liu, X., Zhang, Z. & Wang, L. Bayesian hypothesis testing of mediation: Methods and the impact of prior odds specifications. Behav Res 55 , 1108–1120 (2023). https://doi.org/10.3758/s13428-022-01860-1
Download citation
Accepted : 11 April 2022
Published : 17 May 2022
Issue Date : April 2023
DOI : https://doi.org/10.3758/s13428-022-01860-1
Share this article
Anyone you share the following link with will be able to read this content:
Sorry, a shareable link is not currently available for this article.
Provided by the Springer Nature SharedIt content-sharing initiative
- Bayes factor
- Mediation analysis
- Find a journal
- Publish with us
- Track your research
Addressing Moderated Mediation Hypotheses: Theory, Methods, and Prescriptions
Affiliations.
- 1 a University of Kansas.
- 2 b Northwestern University.
- 3 c The Ohio State University.
- PMID: 26821081
- DOI: 10.1080/00273170701341316
This article provides researchers with a guide to properly construe and conduct analyses of conditional indirect effects, commonly known as moderated mediation effects. We disentangle conflicting definitions of moderated mediation and describe approaches for estimating and testing a variety of hypotheses involving conditional indirect effects. We introduce standard errors for hypothesis testing and construction of confidence intervals in large samples but advocate that researchers use bootstrapping whenever possible. We also describe methods for probing significant conditional indirect effects by employing direct extensions of the simple slopes method and Johnson-Neyman technique for probing significant interactions. Finally, we provide an SPSS macro to facilitate the implementation of the recommended asymptotic and bootstrapping methods. We illustrate the application of these methods with an example drawn from the Michigan Study of Adolescent Life Transitions, showing that the indirect effect of intrinsic student interest on mathematics performance through teacher perceptions of talent is moderated by student math self-concept.
HYPOTHESIS AND THEORY article
Physical activity and school adaptation among chinese junior high school students: chain mediation of resilience and coping styles.
- Hunan Normal University, Changsha, China
The final, formatted version of the article will be published soon.
Select one of your emails
You have multiple emails registered with Frontiers:
Notify me on publication
Please enter your email address:
If you already have an account, please login
You don't have a Frontiers account ? You can register here
Background:As a crucial juncture in students' educational journey, junior high school presents challenges that profoundly influence well-being and academic performance. Physical activity emerges as a pivotal factor shaping the holistic development of junior high school students. Beyond its recognized impact on physical and mental health, engaging in regular physical activity proves effective in augmenting students' adaptability to school life. Despite its importance, the mechanisms through which physical activity influences school adaptation in junior high school students remain understudied in academic research. Objective: In exploring the potential mechanisms, this study aims to validate the mediating roles of resilience and coping styles by examining the association between physical activity and school adaptation among junior high school students. Methods: This study employed cross-sectional survey approach among junior high school students in China. Through the convenience sampling, 1,488 participants aged from 12 to 16 years old(Average age = 13.59, SD = 1.017) from two Junior high schools in Changsha City, Hunan Province were recruited to complete the Physical Activity Scale, School Adaptation Questionnaire for Junior High School Students, Resilience Scale for Adolescents, and Simple Coping Styles Questionnaire. For data analysis, the SPSS 26.0 and Amos 26.0 were used for statistical processing. Results: The results showed that physical activity exhibited a significant correlation with school adaptation (r = 0.656, p < 0.001). Resilience, positive coping style and negative coping style played partial mediating roles between physical activity and school adaptation, with the effect size were 0.229, 0.170, 0.171. The chain mediation effect size of resilience and positive coping style was 0.042, while the chain mediation effect size of resilience and negative coping style was 0.050. Conclusion: Physical activity positively predicts Chinese junior high school students’ school adaptation through resilience and coping styles, suggesting that junior high school students should engage in regular physical activity, so as to improve their resilience and positive coping styles, mitigating negative coping styles, thus promoting their school adaptation.
Keywords: junior high school students, physical activity, School adaptation, resilience, Coping styles
Received: 25 Jan 2024; Accepted: 12 Apr 2024.
Copyright: © 2024 Wu, Liang, Dong and LU. This is an open-access article distributed under the terms of the Creative Commons Attribution License (CC BY) . The use, distribution or reproduction in other forums is permitted, provided the original author(s) or licensor are credited and that the original publication in this journal is cited, in accordance with accepted academic practice. No use, distribution or reproduction is permitted which does not comply with these terms.
* Correspondence: Junwen Liang, Hunan Normal University, Changsha, China Weixin Dong, Hunan Normal University, Changsha, China Chunxia LU, Hunan Normal University, Changsha, China
Disclaimer: All claims expressed in this article are solely those of the authors and do not necessarily represent those of their affiliated organizations, or those of the publisher, the editors and the reviewers. Any product that may be evaluated in this article or claim that may be made by its manufacturer is not guaranteed or endorsed by the publisher.
Click through the PLOS taxonomy to find articles in your field.
For more information about PLOS Subject Areas, click here .
Loading metrics
Open Access
Peer-reviewed
Research Article
Anxiety, Affect, Self-Esteem, and Stress: Mediation and Moderation Effects on Depression
Affiliations Department of Psychology, University of Gothenburg, Gothenburg, Sweden, Network for Empowerment and Well-Being, University of Gothenburg, Gothenburg, Sweden
Affiliation Network for Empowerment and Well-Being, University of Gothenburg, Gothenburg, Sweden
Affiliations Department of Psychology, University of Gothenburg, Gothenburg, Sweden, Network for Empowerment and Well-Being, University of Gothenburg, Gothenburg, Sweden, Department of Psychology, Education and Sport Science, Linneaus University, Kalmar, Sweden
* E-mail: [email protected]
Affiliations Network for Empowerment and Well-Being, University of Gothenburg, Gothenburg, Sweden, Center for Ethics, Law, and Mental Health (CELAM), University of Gothenburg, Gothenburg, Sweden, Institute of Neuroscience and Physiology, The Sahlgrenska Academy, University of Gothenburg, Gothenburg, Sweden
- Ali Al Nima,
- Patricia Rosenberg,
- Trevor Archer,
- Danilo Garcia
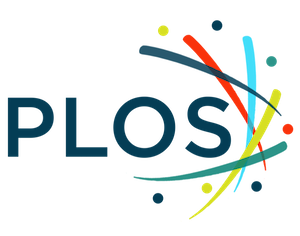
- Published: September 9, 2013
- https://doi.org/10.1371/journal.pone.0073265
- Reader Comments
23 Sep 2013: Nima AA, Rosenberg P, Archer T, Garcia D (2013) Correction: Anxiety, Affect, Self-Esteem, and Stress: Mediation and Moderation Effects on Depression. PLOS ONE 8(9): 10.1371/annotation/49e2c5c8-e8a8-4011-80fc-02c6724b2acc. https://doi.org/10.1371/annotation/49e2c5c8-e8a8-4011-80fc-02c6724b2acc View correction
Mediation analysis investigates whether a variable (i.e., mediator) changes in regard to an independent variable, in turn, affecting a dependent variable. Moderation analysis, on the other hand, investigates whether the statistical interaction between independent variables predict a dependent variable. Although this difference between these two types of analysis is explicit in current literature, there is still confusion with regard to the mediating and moderating effects of different variables on depression. The purpose of this study was to assess the mediating and moderating effects of anxiety, stress, positive affect, and negative affect on depression.
Two hundred and two university students (males = 93, females = 113) completed questionnaires assessing anxiety, stress, self-esteem, positive and negative affect, and depression. Mediation and moderation analyses were conducted using techniques based on standard multiple regression and hierarchical regression analyses.
Main Findings
The results indicated that (i) anxiety partially mediated the effects of both stress and self-esteem upon depression, (ii) that stress partially mediated the effects of anxiety and positive affect upon depression, (iii) that stress completely mediated the effects of self-esteem on depression, and (iv) that there was a significant interaction between stress and negative affect, and between positive affect and negative affect upon depression.
The study highlights different research questions that can be investigated depending on whether researchers decide to use the same variables as mediators and/or moderators.
Citation: Nima AA, Rosenberg P, Archer T, Garcia D (2013) Anxiety, Affect, Self-Esteem, and Stress: Mediation and Moderation Effects on Depression. PLoS ONE 8(9): e73265. https://doi.org/10.1371/journal.pone.0073265
Editor: Ben J. Harrison, The University of Melbourne, Australia
Received: February 21, 2013; Accepted: July 22, 2013; Published: September 9, 2013
Copyright: © 2013 Nima et al. This is an open-access article distributed under the terms of the Creative Commons Attribution License, which permits unrestricted use, distribution, and reproduction in any medium, provided the original author and source are credited.
Funding: The authors have no support or funding to report.
Competing interests: The authors have declared that no competing interests exist.
Introduction
Mediation refers to the covariance relationships among three variables: an independent variable (1), an assumed mediating variable (2), and a dependent variable (3). Mediation analysis investigates whether the mediating variable accounts for a significant amount of the shared variance between the independent and the dependent variables–the mediator changes in regard to the independent variable, in turn, affecting the dependent one [1] , [2] . On the other hand, moderation refers to the examination of the statistical interaction between independent variables in predicting a dependent variable [1] , [3] . In contrast to the mediator, the moderator is not expected to be correlated with both the independent and the dependent variable–Baron and Kenny [1] actually recommend that it is best if the moderator is not correlated with the independent variable and if the moderator is relatively stable, like a demographic variable (e.g., gender, socio-economic status) or a personality trait (e.g., affectivity).
Although both types of analysis lead to different conclusions [3] and the distinction between statistical procedures is part of the current literature [2] , there is still confusion about the use of moderation and mediation analyses using data pertaining to the prediction of depression. There are, for example, contradictions among studies that investigate mediating and moderating effects of anxiety, stress, self-esteem, and affect on depression. Depression, anxiety and stress are suggested to influence individuals' social relations and activities, work, and studies, as well as compromising decision-making and coping strategies [4] , [5] , [6] . Successfully coping with anxiety, depressiveness, and stressful situations may contribute to high levels of self-esteem and self-confidence, in addition increasing well-being, and psychological and physical health [6] . Thus, it is important to disentangle how these variables are related to each other. However, while some researchers perform mediation analysis with some of the variables mentioned here, other researchers conduct moderation analysis with the same variables. Seldom are both moderation and mediation performed on the same dataset. Before disentangling mediation and moderation effects on depression in the current literature, we briefly present the methodology behind the analysis performed in this study.
Mediation and moderation
Baron and Kenny [1] postulated several criteria for the analysis of a mediating effect: a significant correlation between the independent and the dependent variable, the independent variable must be significantly associated with the mediator, the mediator predicts the dependent variable even when the independent variable is controlled for, and the correlation between the independent and the dependent variable must be eliminated or reduced when the mediator is controlled for. All the criteria is then tested using the Sobel test which shows whether indirect effects are significant or not [1] , [7] . A complete mediating effect occurs when the correlation between the independent and the dependent variable are eliminated when the mediator is controlled for [8] . Analyses of mediation can, for example, help researchers to move beyond answering if high levels of stress lead to high levels of depression. With mediation analysis researchers might instead answer how stress is related to depression.
In contrast to mediation, moderation investigates the unique conditions under which two variables are related [3] . The third variable here, the moderator, is not an intermediate variable in the causal sequence from the independent to the dependent variable. For the analysis of moderation effects, the relation between the independent and dependent variable must be different at different levels of the moderator [3] . Moderators are included in the statistical analysis as an interaction term [1] . When analyzing moderating effects the variables should first be centered (i.e., calculating the mean to become 0 and the standard deviation to become 1) in order to avoid problems with multi-colinearity [8] . Moderating effects can be calculated using multiple hierarchical linear regressions whereby main effects are presented in the first step and interactions in the second step [1] . Analysis of moderation, for example, helps researchers to answer when or under which conditions stress is related to depression.
Mediation and moderation effects on depression
Cognitive vulnerability models suggest that maladaptive self-schema mirroring helplessness and low self-esteem explain the development and maintenance of depression (for a review see [9] ). These cognitive vulnerability factors become activated by negative life events or negative moods [10] and are suggested to interact with environmental stressors to increase risk for depression and other emotional disorders [11] , [10] . In this line of thinking, the experience of stress, low self-esteem, and negative emotions can cause depression, but also be used to explain how (i.e., mediation) and under which conditions (i.e., moderation) specific variables influence depression.
Using mediational analyses to investigate how cognitive therapy intervations reduced depression, researchers have showed that the intervention reduced anxiety, which in turn was responsible for 91% of the reduction in depression [12] . In the same study, reductions in depression, by the intervention, accounted only for 6% of the reduction in anxiety. Thus, anxiety seems to affect depression more than depression affects anxiety and, together with stress, is both a cause of and a powerful mediator influencing depression (See also [13] ). Indeed, there are positive relationships between depression, anxiety and stress in different cultures [14] . Moreover, while some studies show that stress (independent variable) increases anxiety (mediator), which in turn increased depression (dependent variable) [14] , other studies show that stress (moderator) interacts with maladaptive self-schemata (dependent variable) to increase depression (independent variable) [15] , [16] .
The present study
In order to illustrate how mediation and moderation can be used to address different research questions we first focus our attention to anxiety and stress as mediators of different variables that earlier have been shown to be related to depression. Secondly, we use all variables to find which of these variables moderate the effects on depression.
The specific aims of the present study were:
- To investigate if anxiety mediated the effect of stress, self-esteem, and affect on depression.
- To investigate if stress mediated the effects of anxiety, self-esteem, and affect on depression.
- To examine moderation effects between anxiety, stress, self-esteem, and affect on depression.
Ethics statement
This research protocol was approved by the Ethics Committee of the University of Gothenburg and written informed consent was obtained from all the study participants.
Participants
The present study was based upon a sample of 206 participants (males = 93, females = 113). All the participants were first year students in different disciplines at two universities in South Sweden. The mean age for the male students was 25.93 years ( SD = 6.66), and 25.30 years ( SD = 5.83) for the female students.
In total, 206 questionnaires were distributed to the students. Together 202 questionnaires were responded to leaving a total dropout of 1.94%. This dropout concerned three sections that the participants chose not to respond to at all, and one section that was completed incorrectly. None of these four questionnaires was included in the analyses.
Instruments
Hospital anxiety and depression scale [17] ..
The Swedish translation of this instrument [18] was used to measure anxiety and depression. The instrument consists of 14 statements (7 of which measure depression and 7 measure anxiety) to which participants are asked to respond grade of agreement on a Likert scale (0 to 3). The utility, reliability and validity of the instrument has been shown in multiple studies (e.g., [19] ).
Perceived Stress Scale [20] .
The Swedish version [21] of this instrument was used to measures individuals' experience of stress. The instrument consist of 14 statements to which participants rate on a Likert scale (0 = never , 4 = very often ). High values indicate that the individual expresses a high degree of stress.
Rosenberg's Self-Esteem Scale [22] .
The Rosenberg's Self-Esteem Scale (Swedish version by Lindwall [23] ) consists of 10 statements focusing on general feelings toward the self. Participants are asked to report grade of agreement in a four-point Likert scale (1 = agree not at all, 4 = agree completely ). This is the most widely used instrument for estimation of self-esteem with high levels of reliability and validity (e.g., [24] , [25] ).
Positive Affect and Negative Affect Schedule [26] .
This is a widely applied instrument for measuring individuals' self-reported mood and feelings. The Swedish version has been used among participants of different ages and occupations (e.g., [27] , [28] , [29] ). The instrument consists of 20 adjectives, 10 positive affect (e.g., proud, strong) and 10 negative affect (e.g., afraid, irritable). The adjectives are rated on a five-point Likert scale (1 = not at all , 5 = very much ). The instrument is a reliable, valid, and effective self-report instrument for estimating these two important and independent aspects of mood [26] .
Questionnaires were distributed to the participants on several different locations within the university, including the library and lecture halls. Participants were asked to complete the questionnaire after being informed about the purpose and duration (10–15 minutes) of the study. Participants were also ensured complete anonymity and informed that they could end their participation whenever they liked.
Correlational analysis
Depression showed positive, significant relationships with anxiety, stress and negative affect. Table 1 presents the correlation coefficients, mean values and standard deviations ( sd ), as well as Cronbach ' s α for all the variables in the study.
- PPT PowerPoint slide
- PNG larger image
- TIFF original image
https://doi.org/10.1371/journal.pone.0073265.t001
Mediation analysis
Regression analyses were performed in order to investigate if anxiety mediated the effect of stress, self-esteem, and affect on depression (aim 1). The first regression showed that stress ( B = .03, 95% CI [.02,.05], β = .36, t = 4.32, p <.001), self-esteem ( B = −.03, 95% CI [−.05, −.01], β = −.24, t = −3.20, p <.001), and positive affect ( B = −.02, 95% CI [−.05, −.01], β = −.19, t = −2.93, p = .004) had each an unique effect on depression. Surprisingly, negative affect did not predict depression ( p = 0.77) and was therefore removed from the mediation model, thus not included in further analysis.
The second regression tested whether stress, self-esteem and positive affect uniquely predicted the mediator (i.e., anxiety). Stress was found to be positively associated ( B = .21, 95% CI [.15,.27], β = .47, t = 7.35, p <.001), whereas self-esteem was negatively associated ( B = −.29, 95% CI [−.38, −.21], β = −.42, t = −6.48, p <.001) to anxiety. Positive affect, however, was not associated to anxiety ( p = .50) and was therefore removed from further analysis.
A hierarchical regression analysis using depression as the outcome variable was performed using stress and self-esteem as predictors in the first step, and anxiety as predictor in the second step. This analysis allows the examination of whether stress and self-esteem predict depression and if this relation is weaken in the presence of anxiety as the mediator. The result indicated that, in the first step, both stress ( B = .04, 95% CI [.03,.05], β = .45, t = 6.43, p <.001) and self-esteem ( B = .04, 95% CI [.03,.05], β = .45, t = 6.43, p <.001) predicted depression. When anxiety (i.e., the mediator) was controlled for predictability was reduced somewhat but was still significant for stress ( B = .03, 95% CI [.02,.04], β = .33, t = 4.29, p <.001) and for self-esteem ( B = −.03, 95% CI [−.05, −.01], β = −.20, t = −2.62, p = .009). Anxiety, as a mediator, predicted depression even when both stress and self-esteem were controlled for ( B = .05, 95% CI [.02,.08], β = .26, t = 3.17, p = .002). Anxiety improved the prediction of depression over-and-above the independent variables (i.e., stress and self-esteem) (Δ R 2 = .03, F (1, 198) = 10.06, p = .002). See Table 2 for the details.
https://doi.org/10.1371/journal.pone.0073265.t002
A Sobel test was conducted to test the mediating criteria and to assess whether indirect effects were significant or not. The result showed that the complete pathway from stress (independent variable) to anxiety (mediator) to depression (dependent variable) was significant ( z = 2.89, p = .003). The complete pathway from self-esteem (independent variable) to anxiety (mediator) to depression (dependent variable) was also significant ( z = 2.82, p = .004). Thus, indicating that anxiety partially mediates the effects of both stress and self-esteem on depression. This result may indicate also that both stress and self-esteem contribute directly to explain the variation in depression and indirectly via experienced level of anxiety (see Figure 1 ).
Changes in Beta weights when the mediator is present are highlighted in red.
https://doi.org/10.1371/journal.pone.0073265.g001
For the second aim, regression analyses were performed in order to test if stress mediated the effect of anxiety, self-esteem, and affect on depression. The first regression showed that anxiety ( B = .07, 95% CI [.04,.10], β = .37, t = 4.57, p <.001), self-esteem ( B = −.02, 95% CI [−.05, −.01], β = −.18, t = −2.23, p = .03), and positive affect ( B = −.03, 95% CI [−.04, −.02], β = −.27, t = −4.35, p <.001) predicted depression independently of each other. Negative affect did not predict depression ( p = 0.74) and was therefore removed from further analysis.
The second regression investigated if anxiety, self-esteem and positive affect uniquely predicted the mediator (i.e., stress). Stress was positively associated to anxiety ( B = 1.01, 95% CI [.75, 1.30], β = .46, t = 7.35, p <.001), negatively associated to self-esteem ( B = −.30, 95% CI [−.50, −.01], β = −.19, t = −2.90, p = .004), and a negatively associated to positive affect ( B = −.33, 95% CI [−.46, −.20], β = −.27, t = −5.02, p <.001).
A hierarchical regression analysis using depression as the outcome and anxiety, self-esteem, and positive affect as the predictors in the first step, and stress as the predictor in the second step, allowed the examination of whether anxiety, self-esteem and positive affect predicted depression and if this association would weaken when stress (i.e., the mediator) was present. In the first step of the regression anxiety ( B = .07, 95% CI [.05,.10], β = .38, t = 5.31, p = .02), self-esteem ( B = −.03, 95% CI [−.05, −.01], β = −.18, t = −2.41, p = .02), and positive affect ( B = −.03, 95% CI [−.04, −.02], β = −.27, t = −4.36, p <.001) significantly explained depression. When stress (i.e., the mediator) was controlled for, predictability was reduced somewhat but was still significant for anxiety ( B = .05, 95% CI [.02,.08], β = .05, t = 4.29, p <.001) and for positive affect ( B = −.02, 95% CI [−.04, −.01], β = −.20, t = −3.16, p = .002), whereas self-esteem did not reach significance ( p < = .08). In the second step, the mediator (i.e., stress) predicted depression even when anxiety, self-esteem, and positive affect were controlled for ( B = .02, 95% CI [.08,.04], β = .25, t = 3.07, p = .002). Stress improved the prediction of depression over-and-above the independent variables (i.e., anxiety, self-esteem and positive affect) (Δ R 2 = .02, F (1, 197) = 9.40, p = .002). See Table 3 for the details.
https://doi.org/10.1371/journal.pone.0073265.t003
Furthermore, the Sobel test indicated that the complete pathways from the independent variables (anxiety: z = 2.81, p = .004; self-esteem: z = 2.05, p = .04; positive affect: z = 2.58, p <.01) to the mediator (i.e., stress), to the outcome (i.e., depression) were significant. These specific results might be explained on the basis that stress partially mediated the effects of both anxiety and positive affect on depression while stress completely mediated the effects of self-esteem on depression. In other words, anxiety and positive affect contributed directly to explain the variation in depression and indirectly via the experienced level of stress. Self-esteem contributed only indirectly via the experienced level of stress to explain the variation in depression. In other words, stress effects on depression originate from “its own power” and explained more of the variation in depression than self-esteem (see Figure 2 ).
https://doi.org/10.1371/journal.pone.0073265.g002
Moderation analysis
Multiple linear regression analyses were used in order to examine moderation effects between anxiety, stress, self-esteem and affect on depression. The analysis indicated that about 52% of the variation in the dependent variable (i.e., depression) could be explained by the main effects and the interaction effects ( R 2 = .55, adjusted R 2 = .51, F (55, 186) = 14.87, p <.001). When the variables (dependent and independent) were standardized, both the standardized regression coefficients beta (β) and the unstandardized regression coefficients beta (B) became the same value with regard to the main effects. Three of the main effects were significant and contributed uniquely to high levels of depression: anxiety ( B = .26, t = 3.12, p = .002), stress ( B = .25, t = 2.86, p = .005), and self-esteem ( B = −.17, t = −2.17, p = .03). The main effect of positive affect was also significant and contributed to low levels of depression ( B = −.16, t = −2.027, p = .02) (see Figure 3 ). Furthermore, the results indicated that two moderator effects were significant. These were the interaction between stress and negative affect ( B = −.28, β = −.39, t = −2.36, p = .02) (see Figure 4 ) and the interaction between positive affect and negative affect ( B = −.21, β = −.29, t = −2.30, p = .02) ( Figure 5 ).
https://doi.org/10.1371/journal.pone.0073265.g003
Low stress and low negative affect leads to lower levels of depression compared to high stress and high negative affect.
https://doi.org/10.1371/journal.pone.0073265.g004
High positive affect and low negative affect lead to lower levels of depression compared to low positive affect and high negative affect.
https://doi.org/10.1371/journal.pone.0073265.g005
The results in the present study show that (i) anxiety partially mediated the effects of both stress and self-esteem on depression, (ii) that stress partially mediated the effects of anxiety and positive affect on depression, (iii) that stress completely mediated the effects of self-esteem on depression, and (iv) that there was a significant interaction between stress and negative affect, and positive affect and negative affect on depression.
Mediating effects
The study suggests that anxiety contributes directly to explaining the variance in depression while stress and self-esteem might contribute directly to explaining the variance in depression and indirectly by increasing feelings of anxiety. Indeed, individuals who experience stress over a long period of time are susceptible to increased anxiety and depression [30] , [31] and previous research shows that high self-esteem seems to buffer against anxiety and depression [32] , [33] . The study also showed that stress partially mediated the effects of both anxiety and positive affect on depression and that stress completely mediated the effects of self-esteem on depression. Anxiety and positive affect contributed directly to explain the variation in depression and indirectly to the experienced level of stress. Self-esteem contributed only indirectly via the experienced level of stress to explain the variation in depression, i.e. stress affects depression on the basis of ‘its own power’ and explains much more of the variation in depressive experiences than self-esteem. In general, individuals who experience low anxiety and frequently experience positive affect seem to experience low stress, which might reduce their levels of depression. Academic stress, for instance, may increase the risk for experiencing depression among students [34] . Although self-esteem did not emerged as an important variable here, under circumstances in which difficulties in life become chronic, some researchers suggest that low self-esteem facilitates the experience of stress [35] .
Moderator effects/interaction effects
The present study showed that the interaction between stress and negative affect and between positive and negative affect influenced self-reported depression symptoms. Moderation effects between stress and negative affect imply that the students experiencing low levels of stress and low negative affect reported lower levels of depression than those who experience high levels of stress and high negative affect. This result confirms earlier findings that underline the strong positive association between negative affect and both stress and depression [36] , [37] . Nevertheless, negative affect by itself did not predicted depression. In this regard, it is important to point out that the absence of positive emotions is a better predictor of morbidity than the presence of negative emotions [38] , [39] . A modification to this statement, as illustrated by the results discussed next, could be that the presence of negative emotions in conjunction with the absence of positive emotions increases morbidity.
The moderating effects between positive and negative affect on the experience of depression imply that the students experiencing high levels of positive affect and low levels of negative affect reported lower levels of depression than those who experience low levels of positive affect and high levels of negative affect. This result fits previous observations indicating that different combinations of these affect dimensions are related to different measures of physical and mental health and well-being, such as, blood pressure, depression, quality of sleep, anxiety, life satisfaction, psychological well-being, and self-regulation [40] – [51] .
Limitations
The result indicated a relatively low mean value for depression ( M = 3.69), perhaps because the studied population was university students. These might limit the generalization power of the results and might also explain why negative affect, commonly associated to depression, was not related to depression in the present study. Moreover, there is a potential influence of single source/single method variance on the findings, especially given the high correlation between all the variables under examination.
Conclusions
The present study highlights different results that could be arrived depending on whether researchers decide to use variables as mediators or moderators. For example, when using meditational analyses, anxiety and stress seem to be important factors that explain how the different variables used here influence depression–increases in anxiety and stress by any other factor seem to lead to increases in depression. In contrast, when moderation analyses were used, the interaction of stress and affect predicted depression and the interaction of both affectivity dimensions (i.e., positive and negative affect) also predicted depression–stress might increase depression under the condition that the individual is high in negative affectivity, in turn, negative affectivity might increase depression under the condition that the individual experiences low positive affectivity.
Acknowledgments
The authors would like to thank the reviewers for their openness and suggestions, which significantly improved the article.
Author Contributions
Conceived and designed the experiments: AAN TA. Performed the experiments: AAN. Analyzed the data: AAN DG. Contributed reagents/materials/analysis tools: AAN TA DG. Wrote the paper: AAN PR TA DG.
- View Article
- Google Scholar
- 3. MacKinnon DP, Luecken LJ (2008) How and for Whom? Mediation and Moderation in Health Psychology. Health Psychol 27 (2 Suppl.): s99–s102.
- 4. Aaroe R (2006) Vinn över din depression [Defeat depression]. Stockholm: Liber.
- 5. Agerberg M (1998) Ut ur mörkret [Out from the Darkness]. Stockholm: Nordstedt.
- 6. Gilbert P (2005) Hantera din depression [Cope with your Depression]. Stockholm: Bokförlaget Prisma.
- 8. Tabachnick BG, Fidell LS (2007) Using Multivariate Statistics, Fifth Edition. Boston: Pearson Education, Inc.
- 10. Beck AT (1967) Depression: Causes and treatment. Philadelphia: University of Pennsylvania Press.
- 21. Eskin M, Parr D (1996) Introducing a Swedish version of an instrument measuring mental stress. Stockholm: Psykologiska institutionen Stockholms Universitet.
- 22. Rosenberg M (1965) Society and the Adolescent Self-Image. Princeton, NJ: Princeton University Press.
- 23. Lindwall M (2011) Självkänsla – Bortom populärpsykologi & enkla sanningar [Self-Esteem – Beyond Popular Psychology and Simple Truths]. Lund:Studentlitteratur.
- 25. Blascovich J, Tomaka J (1991) Measures of self-esteem. In: Robinson JP, Shaver PR, Wrightsman LS (Red.) Measures of personality and social psychological attitudes San Diego: Academic Press. 161–194.
- 30. Eysenck M (Ed.) (2000) Psychology: an integrated approach. New York: Oxford University Press.
- 31. Lazarus RS, Folkman S (1984) Stress, Appraisal, and Coping. New York: Springer.
- 32. Johnson M (2003) Självkänsla och anpassning [Self-esteem and Adaptation]. Lund: Studentlitteratur.
- 33. Cullberg Weston M (2005) Ditt inre centrum – Om självkänsla, självbild och konturen av ditt själv [Your Inner Centre – About Self-esteem, Self-image and the Contours of Yourself]. Stockholm: Natur och Kultur.
- 34. Lindén M (1997) Studentens livssituation. Frihet, sårbarhet, kris och utveckling [Students' Life Situation. Freedom, Vulnerability, Crisis and Development]. Uppsala: Studenthälsan.
- 35. Williams S (1995) Press utan stress ger maximal prestation [Pressure without Stress gives Maximal Performance]. Malmö: Richters förlag.
- 37. Garcia D, Kerekes N, Andersson-Arntén A–C, Archer T (2012) Temperament, Character, and Adolescents' Depressive Symptoms: Focusing on Affect. Depress Res Treat. DOI:10.1155/2012/925372.
- 40. Garcia D, Ghiabi B, Moradi S, Siddiqui A, Archer T (2013) The Happy Personality: A Tale of Two Philosophies. In Morris EF, Jackson M-A editors. Psychology of Personality. New York: Nova Science Publishers. 41–59.
- 41. Schütz E, Nima AA, Sailer U, Andersson-Arntén A–C, Archer T, Garcia D (2013) The affective profiles in the USA: Happiness, depression, life satisfaction, and happiness-increasing strategies. In press.
- 43. Garcia D, Nima AA, Archer T (2013) Temperament and Character's Relationship to Subjective Well- Being in Salvadorian Adolescents and Young Adults. In press.
- 44. Garcia D (2013) La vie en Rose: High Levels of Well-Being and Events Inside and Outside Autobiographical Memory. J Happiness Stud. DOI: 10.1007/s10902-013-9443-x.
- 48. Adrianson L, Djumaludin A, Neila R, Archer T (2013) Cultural influences upon health, affect, self-esteem and impulsiveness: An Indonesian-Swedish comparison. Int J Res Stud Psychol. DOI: 10.5861/ijrsp.2013.228.
- Research article
- Open access
- Published: 15 April 2024
Association and biological pathways between lung function and incident depression: a prospective cohort study of 280,032 participants
- Bao-Peng Liu 1 &
- Cun-Xian Jia 1
BMC Medicine volume 22 , Article number: 160 ( 2024 ) Cite this article
Metrics details
Lung health is increasingly recognized as an essential factor in mental health. However, prospective evidence on lung function with incident depression remains to be determined. The study aimed to examine the prospective association between impaired lung function and incident depression and the underlying biological mechanisms.
This prospective cohort study comprised 280,032 non-depressed individuals with valid lung function measurements from the UK Biobank. Lung function was assessed through the forced vital capacity (FVC) or forced expiratory volume in 1 s (FEV 1 ). Cox proportional hazard models were applied to estimate the associations between lung function and incident depression. Mediation analyses were fitted to investigate the potential mediating role of biomarkers and metabolites in the association.
A total of 9514 participants (3.4%) developed depression during a median follow-up of 13.91 years. Individuals in the highest quartile had a lower risk of depression (FVC % predicted: HR = 0.880, 95% CI = 0.830–0.933; FEV 1 % predicted: HR = 0.854, 95% CI = 0.805–0.905) compared with those in the lowest quartile of the lung function indices. Additionally, the restricted cubic splines suggested lung function indices had reversed J-shaped associations with incident depression (nonlinear P < 0.05 for FVC % predicted and FEV 1 % predicted). Impaired lung function yielded similar risk estimates (HR = 1.124, 95% CI = 1.074–1.176). Biomarkers involving systemic inflammation, erythrocytes, and liver and renal function may be potential mediators in the lung function-depression association.
Conclusions
This study revealed that the higher risk of developing depression was associated with impaired lung function. Also, the association might be partially mediated by biomarkers including systemic inflammation, erythrocytes, and liver and renal function, though these mediation findings should be interpreted with caution due to potential temporal ambiguity.
Peer Review reports
Depression, a mental health condition that impacts the well-being of over 300 million individuals globally [ 1 ], has emerged as the leading contributor to disability-adjusted life years among mental disorders [ 2 ]. Accumulating evidence suggests that depression is associated with a heightened risk of multiple detrimental health outcomes, including all-cause and cancer mortality, as well as cardiovascular disease morbidity and mortality [ 3 , 4 ]. However, traditional therapies for depression (e.g., medication) are costly and of limited efficacy [ 5 ]. Hence, identifying cost-effective strategies to prevent depression is paramount.
Physical health has garnered growing attention as a modifiable protective factor for depression. Whilst research has reported that low grip strength is a significant risk factor for new-onset depression [ 1 ], the role of lung function, which is recognized as an inexpensive, non-invasive, and modifiable indicator for assessing physical fitness, in preventing depression remains poorly understood. A review involving 1,161,632 subjects has shed light on the advantages of maintaining moderate or high levels of pulmonary fitness in the prevention of depression [ 6 ]. However, it remains unclear whether poor lung function, as measured by forced vital capacity (FVC) and forced expiratory volume in 1 s (FEV 1 ), is a direct risk factor for depression. Previous evidence on this topic has primarily focused on either the impact of lung diseases on depression or examined associations in the opposite direction [ 7 , 8 ]. Whilst prior studies have indicated a lung function-depression association, limitations were exhibited in terms of single sex, small samples, cross-sectional designs, and susceptible populations [ 9 , 10 , 11 , 12 ].
Existing epidemiological studies have established links between abnormal lung function and various biomarkers, including liver [ 13 ], kidney [ 14 ], erythrocytes [ 15 ], inflammation [ 16 ], and circulating metabolites [ 17 ]. These biomarkers are also closely associated with the development of depression [ 18 ]. For example, impaired lung function may cause cerebral hypoxia and metabolic disturbances [ 16 ], which in turn trigger a pro-inflammatory state with the release of large amounts of pro-inflammatory factors that elevate the level of the systemic inflammatory response, resulting in the occurrence of depressive symptoms [ 8 ]. Nonetheless, to date, no population-based cohort studies have investigated whether and to what extent these biomarkers may mediate the association between abnormal lung function and depression risks.
To address research gaps, samples from the UK Biobank (UKB) were analyzed to prospectively investigate the association between lung function and incident depression and to delve deeper into the underlying biological mechanisms of this association.
Study population
The UKB, one of the largest prospective cohorts targeting the determinants of a range of complex diseases in middle-aged and older Europeans, recruited over 500,000 participants (aged 37–73) who completed touchscreen questionnaires, physical examination, and biological information at 22 evaluation centers in England, Scotland, and Wales between 2006 and 2010. A comprehensive description of this cohort can be found elsewhere [ 19 ]. In short, the cohort aims to provide a detailed investigation of the socio-demographic, lifestyle, environmental, and genetic determinants of a range of complex diseases. Moreover, the cohort ensures continuous tracking of participant morbidity and mortality by establishing links with electronic records from hospitalization and death registries [ 19 ].
Among participants with complete and acceptable lung function data ( N = 353,243), those with any of the following conditions were also excluded: (1) withdrawal or lost follow-up ( N = 725); (2) suffering from depression ( N = 28,537) or other mental symptoms-related disorders (including dementia, anxiety, schizophrenia, bipolar disorder, Parkinson’s disease, Alzheimer’s disease, or substance abuse; N = 26,854) at baseline; (3) missing values for covariates ( N = 17,005). A total of 280,032 participants were included in formal analyses. Also, our study focused on two subpopulations with complete biomarker ( N = 231,193) or metabolite ( N = 62,488) information for mediating mechanism exploration. The detailed participant selection process is displayed in Fig. 1 .
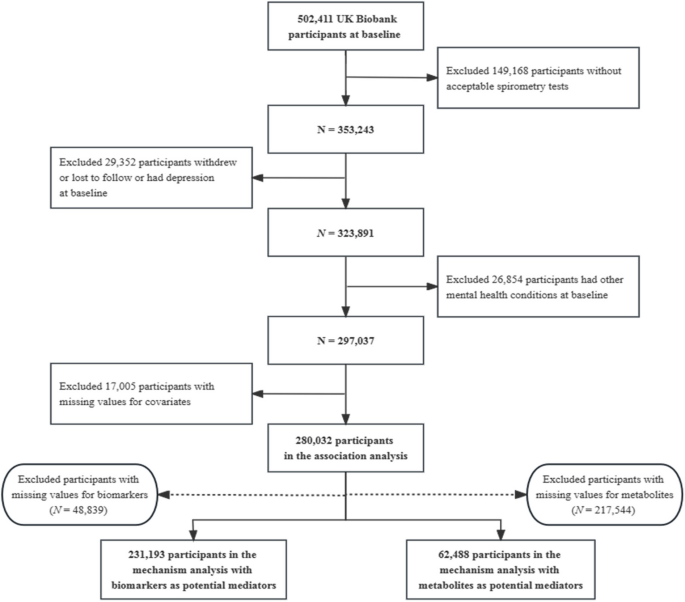
The flow chart of the selection of the study population
The authors assert that all procedures contributing to this work comply with the ethical standards of the relevant national and institutional committees on human experimentation and with the Helsinki Declaration of 1975, as revised in 2008.
- Lung function
Trained healthcare professionals utilized a standard spirometer to measure the respiratory function of participants. Participants were instructed to deliver two to three forceful blows within 6 min, with each blow lasting at least 6 s. If the difference between the first two blows was less than 5%, a third blow was not required. We utilized the Global Lung Function Initiative (GLI) 2012 equations based on age, height, sex, and ethnicity to convert the highest values of FVC and FEV 1 with valid blows into % predicted values for the primary analyses [ 20 ]. Participants with FEV 1 ≥ 80% predicted value and FEV 1 /FVC ≥ 0.70 were considered to possess normal lung function, while the rest had impaired lung function [ 21 ].
Assessment of depression
Depression cases were defined by the International Classification of Diseases, Tenth Revision codes F32 and F33 among the “First occurrence fields”, and were obtained through self-reported medical conditions, primary care, hospital admission, and death registrations [ 22 ]. The same methodology was performed to assess the status of depression or other mental disorders at baseline [ 22 ]. The date of death for participants from England and Wales was obtained from National Health Service Digital, whereas those for participants from Scotland were sourced from the National Health Service Central Register. The follow-up time was computed as the time interval from the recruitment date to the diagnosis date of depression cases, date of death, or the censoring data, whichever occurred first.
Assessment of potential mediators
Based on the evidence of potential pathways [ 13 , 14 , 15 , 16 , 17 , 18 ], 25 blood biomarkers including inflammation, erythrocytes, liver and renal function, and 168 plasma metabolites were selected as potential mediators. In the UKB, blood tests were performed on participants with informed consent at baseline recruitment. Blood samples of about 4 ml were collected, separated by composition, stored in a refrigerator at−80 °C, and analyzed within 24 h using a Beckman Coulter LH750 instrument ( https://biobank.ndph.ox.ac.uk/ukb/ukb/docs/haematology.pdf ). The blood biomarkers with rigorous quality checks have undergone external validation ( https://biobank.ndph.ox.ac.uk/showcase/showcase/docs/serum_biochemistry.pdf ). Plasma samples from about one-fifth of participants in the baseline recruitment were tested for metabolites using Nightingale Health’s NMR-based high-throughput metabolic biomarker analysis platform [ 23 ]. According to the online documentation provided by the UKB ( https://biobank.ndph.ox.ac.uk/ukb/ukb/docs/nmrm_companion_doc.pdf ), a total of 168 metabolites were quantified in molar concentration units, and the metabolite data could be utilized directly for epidemiological analyses without requiring any preprocessing.
Inflammation-related biomarkers included leukocyte count, neutrophil count and percentage, monocyte count and percentage, lymphocyte count and percentage, platelet count, and C reactive protein (CRP). Erythrocyte-related biomarkers suggestive of hypoxia or anemia included erythrocyte count, high light scatter reticulocyte count, reticulocyte count, red blood cell distribution width (RBC), hematocrit percentage, and hemoglobin concentration (HbA1c). Liver function-related biomarkers included alanine aminotransferase (ALT), alkaline phosphatase (ALP), aspartate aminotransferase (AST), gamma-glutamyltransferase (GGT), total bilirubin (TBIL), total protein (TP), and albumin (ALB). Renal function-related biomarkers included cystatin C, urate, and urea. The mean concentration for blood biomarkers and plasma metabolites can be seen in Additional file 1 : Tables S1-S2.
A range of factors that may be related to lung function or depression were regarded as covariates [ 1 , 16 ], including sociodemographic characteristics [(age, sex, height, education level, employment, assessment center, and Townsend deprivation index (TDI)], lifestyles, and medical histories. The TDI covered a wide range of information on social class, employment, and housing and reflected the participant’s area-based socioeconomic status (SES), with higher scores indicating greater deprivation [ 16 ]. Smoking status (never, previous or current), drinking status (never, previous or current), physical activity (active vs inactive), body mass index (BMI, continuous), and sleep duration [short (< 7 h), normal (7–8 h) or long (> 8 h)] were adjusted as lifestyle factors. Physical activity was assessed using the International Physical Activity Questionnaire short questionnaire (IPAQ-SQ). Participants were queried regarding the number of days they engaged in more than 10 min of walking, moderate and vigorous physical activity in a week, and the amount of time they engaged in each activity during the day, with 150 min or more of moderate-intensity activity per week defined as physically active [ 24 ]. Medical histories (yes vs no) were ascertained through self-reported information and medical records, encompassing hypertension, diabetes, heart failure (HF), stroke, coronary heart disease (CHD), ischemic heart disease (IHD), and lung-related diseases (asthma, chronic obstructive pulmonary disease, emphysema/chronic bronchitis, and other respiratory problems). A summary of missing covariates is provided in Additional file 1 : Table S3.
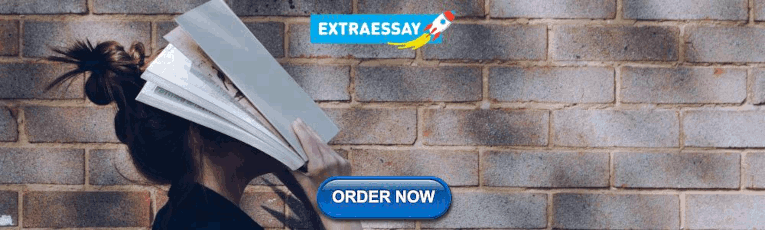
Statistical analysis
The Wilcoxon tests for continuous variables [mean (SD)] or chi-square tests for categorical variables [frequency (%)] were applied to compare baseline characteristics categorized by incident depression or lung function status. Cox proportional hazard models were utilized to estimate the risk of new-onset depression ascribed to low lung function, with results presented as hazard ratios (HRs) and 95% confidence intervals (CIs). The Cox regression model based on the proportional hazards assumption was suitable for analyzing time-to-event data even if the incidence of the outcome event (depression) was relatively low (less than 5%) in this study, as long as the assumption was met. The proportional hazards assumption was assessed by the Schoenfeld residuals test to check for potential time-variant biases, and we found no correlation between residuals and time ( P > 0.05) in this study. Despite the low incidence rate, the Cox models make efficient use of all available data by considering both the time until the event and the occurrence of the event, maximizing the information obtained from the cohort. Furthermore, the Cox models allow adjustment for various confounders, which are essential for accurate estimation of risk factor associations.
To explore the association between different levels of lung function and incident depression, we analyzed FVC and FEV 1 (“rspiro” package in R software) as categorical variables (quartiles), with the lowest quartile (Q1) as the reference group. Dichotomous lung function was employed to examine the longitudinal associations of depression cases with impaired lung function. The restricted cubic spline (“rcssci” package in R software) with four knots was fitted to explore the dose-response relationship between lung function and incident depression. We fitted three Cox models with incremental adjustments for confounders: Model 1 adjusted for sociodemographic covariates; Model 2 additionally accounted for lifestyle factors; Model 3 (priority model) further adjusted for medical histories.
To assess the robustness of results, we conducted several additional analyses. First, we incorporated the cumulative cigarette consumption along with passive smoking duration in Model 3. Cumulative cigarette consumption was quantified as at least 20 cigarettes per day during the year for former or current smokers [ 16 ]. Passive smoking duration was calculated as the number of hours per week in the past year of exposure to tobacco smoke from other people in or outside the home [ 16 ]. Second, considering the susceptibility of lung function to environmental factors, we further controlled for PM 2.5 (particulate matter with a diameter of 2.5 µm or less) and NO 2 (nitrogen dioxide) in Model 3. Third, we excluded individuals with pre-existing lung-related diseases to ascertain the independent impact of lung function. Fourth, to mitigate the potential impact of reverse causality, we eliminated the depression cases occurring within the first 2 years of follow-up. Fifth, covariates with missing values were imputed using the multiple imputations by chained equations method (“mice” package in R software) [ 25 ]. Sixth, we further excluded prevalent depression measured by the Patient Health Questionnaire-2 (PHQ-2) at baseline to re-analyze the associations [ 26 ]. Stratification analyses were conducted by sex, age, education, employment, TDI, smoking status, drinking status, BMI, and sleep duration to assess potential effect modification. By adding product terms to the Cox models, we used the likelihood ratio tests to examine the interactions of lung function and stratification factors on depression risk.
Selected biomarkers and metabolites could be considered as potential mediators by the following analyses. First, multiple linear regression models were applied to evaluate the association of lung function with biomarkers or metabolites. Second, Cox regression models adjusting for covariates in Model 3 were implemented to examine the relationship between lung function, biomarkers, and incident depression. Biomarkers or metabolites that simultaneously exhibited significance in the aforementioned steps would be considered as potential mediators for subsequent mediation analyses [ 16 , 17 , 25 ]. The proportion mediated (PM) was estimated via the “mediation” package, and the non-parametric bootstrap method (1000 draws) was used to calculate 95% CIs of the PM. Raw data for biomarkers and metabolites were standardized ( z -score) before entering formal analysis.
All analyses were conducted by SAS 9.4 (SAS Institute, Cary, NC, USA) and R software (4.0.5). In analyses concerning biomarkers or metabolites, a false discovery rate (FDR) adjusted P < 0.05 was regarded as statistically significant [ 27 ]. In the prospective association analysis, a two-tailed P < 0.05 was regarded as statistically significant.
Of the sample of 280,032 in the association analysis, 147,923 (52.8%) were female, with a mean age (SD) of 56.5 (8.0) years. During more than 3,759,362 person-years of follow-up (median follow-up: 13.91 years), 3.4% of participants without depression or other psychiatric disorders at baseline developed depression. In comparison to the healthy controls, depressed patients were more likely to be female, be less educated, be unemployed, be in a poorer SES situation, smoke but do not regularly drink alcohol, be physically inactive, have a higher BMI, have an abnormal sleep duration, and have a lower FEV 1 and FVC ( P < 0.001) (Table 1 ). When grouped by lung function status, we observed similar baseline characteristics (Additional file 1 : Table S4).
We observed a non-linear dose-response relationship between lung function and risk of depression (nonlinear P < 0.05 for FVC and FEV 1 ) (Fig. 2 ). The prospective lung function-depression association is displayed in Table 2 . We observed a significant protective role of lung function indices for depression risks in the fully adjusted models (model 3). For FVC (% predicted), the HRs of depression were 0.874 (95% CI = 0.827–0.924; P < 0.001), 0.888 (95% CI = 0.839–0.940; P < 0.001), and 0.880 (95% CI = 0.830–0.933; P < 0.001) in quartiles 2 to 4 compared with the lowest quartile. For FEV 1 (% predicted), the HRs of depression were 0.903 (95% CI = 0.855–0.954; P < 0.001), 0.860 (95% CI = 0.813–0.911; P < 0.001), and 0.854 (95% CI = 0.805–0.905; P < 0.001) in quartiles 2 to 4 compared with the lowest quartile. Additionally, individuals with impaired lung function had a 12.4% (HR = 1.124, 95% CI = 1.074–1.176) increased risk of developing depression compared to those with normal lung function. Several sensitivity analyses did not affect the robustness of the findings (Additional file 1 : Tables S5-S8). Stratification analysis discovered that detrimental effects of impaired lung function were stronger among individuals who were older, less educated, non-employed, living in areas with high TDI, and current or former smokers ( P for interaction < 0.05) (Additional file 1 : Fig. S1).
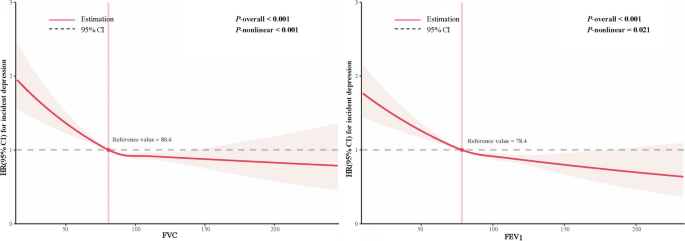
Restricted cubic spline analyses for the association of FVC (% predicted) and FEV 1 (% predicted) with incident depression
Although impaired lung function was substantially associated with all selected biomarkers except monocyte percentage, hematocrit percentage, urea, and ALT, only some of them were significant predictors of depression risk, such as leukocyte count, neutrophil count and percentage, lymphocyte count and percentage, CRP, platelet count, erythrocyte count, RBC distribution width, hemoglobin concentration, cystatin C, urate, ALP, AST, GGT, TBIL, TP, and ALB ( FDR < 0.05) (Additional file 1 : Table S9). Hence, these biomarkers may serve as potential mediators from impaired lung function to incident depression. Additionally, lung function was significantly associated with most metabolites, but none of these metabolites were statistically associated with depression risk (Additional file 1 : Table S10). Therefore, no metabolites are available as potential mediators of the association between impaired lung function and incident depression.
Figure 3 exhibits the PM of these potential mediators that were simultaneously associated with both lung function and incident depression. We observed significant mediating effects of inflammation, suggesting that impaired lung function might increase the risk of depression through the induction of inflammatory dysregulation ( FDR < 0.05). Neutrophil count and CRP might explain 5.5% (95% CI = 3.2–9.8%) and 2.9% (1.0–5.8%) of the association, respectively. Markers related to erythrocytes and renal function might partially account for the increased risk of depression caused by impaired lung function. The overall effect of low lung function leading to an increased risk of depression might be partially explained by biomarkers related to liver function, with PM (95% CI) ranging from 0.4 (0.1–0.8%) to 5.9% (3.5–9.7%).
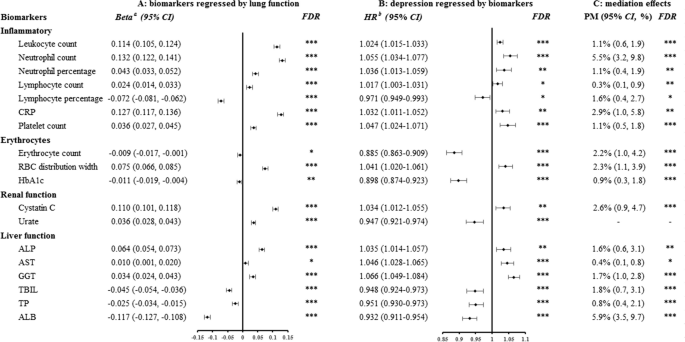
Association of lung function with incident depression mediated by biomarkers
Based on a large prospective cohort with a median follow-up of exceeding 13 years, our findings indicated that participants with impaired lung function had a higher risk of depression, independent of confounders including sociodemographic, lifestyle, environment, and comorbidities. The nonlinear dose-response curves revealed that the risk of new-onset depression was steeper at low lung function. Four biomarker pathways, including inflammation, erythrocytes, liver, and renal function, but not metabolite pathways, might partially mediate the association between impaired lung function and incident depression.
Supporting our findings, prior studies have documented a negative association between lung function and depression, but mostly using small samples, cross-sectional designs, or implemented in susceptible populations. A cross-sectional study from the National Health and Nutrition Examination Survey (NHANES) revealed a significant negative FVC-depression association [ 11 ]. Another cross-sectional survey based on the NHANES also reported an association between impaired lung function and self-reported poor mental health (including depression) [ 12 ]. Moreover, a study including 121 silicosis patients suggested that low FEV 1 and FVC were cross-sectional associated with depressive symptoms [ 10 ]. Notably, Mutz et al., taking advantage of a cross-sectional design, found that lung function in male patients with depression was poorer compared to that of healthy controls, aligning with our findings [ 28 ]. However, the opposite was observed in females [ 28 ], which might be attributed to the protective effect of estrogen on the lungs [ 29 ], shielding them from the detrimental impact of depression. To our awareness, only two studies employed a longitudinal design to investigate the relationship between lung function and incident depression, but they focused on lung disease or were conducted in single-sex populations exclusively. For instance, a longitudinal study enrolling 10,508 Chinese adults with an average follow-up period of 3 years reported that participants with chronic lung disease (CLDs) at baseline had a higher risk of depressive symptoms [ 7 ]. Another prospective study of 1205 middle-aged men revealed that low respiratory function was associated with an increased risk of subsequent depressive symptoms [ 9 ]. Remarkably, the aforementioned studies predominantly relied on self-report measures for the identification of depression rather than clinical diagnosis. Additionally, a previous UK Biobank study using Mendelian randomization (MR) provided evidence of a causal effect of depression on outcomes of impaired lung function, such as asthma [ 30 ]. Combined with our findings, we speculated that there may be a reciprocal causal relationship between lung function and depression. However, to our knowledge, no extant studies have provided evidence of a causal effect of lung function on depression. Based on a large population with long-term follow-up, the study elucidated for the first time the negative associations of lung function with incident depression through adequate adjustment for confounders. Although still observational, our findings may provide insights into causal effects. Future studies are warranted to validate the causality of our findings.
Prior investigations examining the relationship between lung function and adverse outcomes have demonstrated a significant nonlinear association [ 31 , 32 ]. Our study substantiated this and furnished original evidence for the nonlinear association between lung function indicators and depression. Stratified analyses suggested that the lung function-depression association was modified by several sociodemographic and lifestyle factors. First, cigarette consumption exacerbated the negative impact of impaired lung function on depression, which was similar to the trend reported in previous research [ 7 ]. Specifically, although no significant interactions were found, the trend found by Ren et al. that the adverse effect of CLDs at baseline on incident depression was stronger in participants who were current smokers was similar to our findings [ 7 ]. One possible explanation is that the noxious gases and particulate matter generated during tobacco combustion may cause airflow obstruction, which is a primary cause of depression owing to impaired lung function [ 33 ]. Furthermore, the deleterious effects of poor lung function on subsequent depressive symptoms were pronounced in populations with low SES, which was congruent with a previous survey that concluded that individuals with low SES experience a reduction in the beneficial impact of improved lung function on overall health [ 34 ].
Our first exploratory analyses examining potential mediating mechanisms contributed to a deeper understanding of the heightened risk of depression associated with impaired lung function. First, we observed that several inflammatory factors, such as leukocyte count, neutrophil count and percentage, lymphocyte count and percentage, CRP, and platelet, might partially explain the detrimental effects of impaired lung function, which was in accordance with previous observational studies [ 35 , 36 , 37 ]. Impaired lung function can lead to excessive release of pro-inflammatory cytokines [ 35 , 36 ], resulting in elevated plasma levels of glucocorticoids and subsequently contributing to the onset of depression [ 37 ]. Second, erythrocyte-associated markers hinting at hypoxia and anemia (including RBC and HbA1c) may mediate, to some extent, the lung function-depression association, supporting the previously hypothesized underlying pathological mechanisms of hypoxemia and hypercapnia [ 11 ]. Specifically, low lung function that disrupts the exchange of oxygen and carbon dioxide could engender both conditions [ 38 ], causing metabolic dysregulation in brain cells and ultimately triggering depressive symptoms [ 39 ]. Moreover, the increased risk of depression ascribed to impaired lung function might be partially explained by the deterioration in the liver and renal functions, in line with prior studies [ 13 , 14 , 40 , 41 ]. For instance, a cohort study involving more than 370,000 participants revealed a significant association between liver function markers, such as ALT, TBIL, ALB, TP, GGT, and ALP, and an elevated risk of lung disease [ 13 ]. Also, a MR study supported a causal relationship between lung diseases and renal function [ 14 ]. Remarkably, the relationship between liver and renal dysfunction and depression has been well established [ 40 , 41 ].
The main strength of this study was the comprehensive examination of the prospective lung function-depression association and the preliminary exploration of the underlying biological mechanisms of the association. Other strengths comprised large sample sizes, long follow-up, sufficient adjustment for confounders, reliable methods for assessing lung function, and identification of depression from multiple sources including clinical diagnosis.
Several limitations should be considered. First, the participants in the cohort were overwhelmingly white, limiting the extrapolation of our findings. Second, most of the confounders are self-reported, which can introduce recall bias. Third, we did not find any statistical association between metabolites and incident depression cases, which may be due to the low rate of incident depression. Fourth, the methods we used to identify cases of depression may have insufficient power in identifying mild cases, which could lead to misclassification bias and thus obscure the associations found by the study. Nevertheless, sensitivity analyses after excluding possible cases of mild depression additionally identified by PHQ-2 at baseline did not substantially change the robustness of the associations. Fifth, although the UK Biobank cohort has a “healthy volunteer” bias that may affect the representativeness of our findings [ 42 ], the associations of risk factors in the cohort have been demonstrated to be similar to those found in the representative cohort [ 42 ]. Sixth, although we excluded the participants with a history of depression at baseline, the age of onset of depression is usually earlier than the lower age limit (37 years) of the UK Biobank sample, so caution is necessary in applying our findings to a younger population. Seventh, despite our efforts to control for a range of confounders, residual confounding may still exist. Eighth, the inherent nature of observational studies precludes us from drawing causal inferences. Further MR or clinical trials are warranted to confirm causality. Additionally, the mediation analysis method used in this study could only assess one mediator at a time to explore the single pathway of mediating mechanisms for associations between lung function and incident depression. Multiple pathways adopting serial mediation or structural equation model are needed in further studies. Finally, longitudinal mediation analyses necessitate a clear temporal relationship between variables, but the exposure and mediators in the present study were measured at the same time point due to data availability constraints. Nonetheless, the previously proposed lung-brain-axis hypothesis, which suggests that impaired lung function can affect brain structure via the blood pathway, may theoretically support the temporal relationship between the exposures and mediators in our study [ 43 ]. Caution is warranted in interpreting the mediation results, and future studies with well-defined temporal relationships are required to validate the mediating role of these markers.
Based on a large-scale prospective cohort, the study revealed that impaired lung function was associated with an increased risk of developing depression. Regular screening of lung function in routine practice has the potential to facilitate the identification of at-risk populations and the development of personalized interventions, yielding profound clinical and public health implications. Additionally, our findings underscored that the biomarkers involving systemic inflammation, erythrocytes, and liver and renal function may partially mediate this association, but these mediation findings should be interpreted with caution due to potential temporal ambiguity. Further studies with repeatedly measured data are warranted to replicate our mediation findings.
Availability of data and materials
Data supporting the results of this study are available on request from the UK Biobank team ( http://www.ukbiobank.ac.uk/ ).
Abbreviations
Alkaline phosphatase
Alanine aminotransferase
Aspartate aminotransferase
Confidence intervals
Chronic lung disease
C reactive protein
Forced expiratory volume in 1 s
Forced vital capacity
Gamma-glutamyltransferase
Global Lung Function Initiative
Hemoglobin concentration
Hazard ratios
Nitrogen dioxide
Proportion mediated
Particulate matter with a diameter of 2.5 µm or less
Red blood cell
Socioeconomic status
Total bilirubin
Townsend deprivation index
Total protein
Cabanas-Sánchez V, Esteban-Cornejo I, Parra-Soto S, Petermann-Rocha F, Gray SR, Rodríguez-Artalejo F, Ho FK, Pell JP, Martínez-Gómez D, Celis-Morales C. Muscle strength and incidence of depression and anxiety: findings from the UK Biobank prospective cohort study. J Cachexia Sarcopenia Muscle. 2022;13(4):1983–94.
Article PubMed PubMed Central Google Scholar
Liu BP, Jia CX. Shift work schedule and sleep patterns in relation to incident depression: evidence from a prospective cohort study. Psychiatry Res. 2023;321:115076.
Article PubMed Google Scholar
Hare DL, Toukhsati SR, Johansson P, Jaarsma T. Depression and cardiovascular disease: a clinical review. Eur Heart J. 2014;35(21):1365–72.
Liu BP, Jia CX. The associations of physical activity and lifetime depression with all-cause and cause-specific mortality: evidence from a prospective cohort study. Psychiatry Res. 2023;324:115206.
Sinyor M, Schaffer A, Levitt A. The sequenced treatment alternatives to relieve depression (STAR*D) trial: a review. Can J Psychiatry. 2010;55(3):126–35.
Kandola A, Ashdown-Franks G, Stubbs B, Osborn DPJ, Hayes JF. The association between cardiorespiratory fitness and the incidence of common mental health disorders: a systematic review and meta-analysis. J Affect Disord. 2019;257:748–57.
Article CAS PubMed PubMed Central Google Scholar
Ren X, Wang S, He Y, Lian J, Lu Q, Gao Y, Wang Y. Chronic lung diseases and the risk of depressive symptoms based on the China health and retirement longitudinal study: a prospective cohort study. Front Psychol. 2021;12:585597.
Han X, Li C, Zhu Y, Ma Y, Hua R, Xie W, Xie L. Relationships of persistent depressive symptoms with subsequent lung function trajectory and respiratory disease mortality. J Affect Disord. 2022;309:404–10.
Giltay EJ, Nissinen A, Giampaoli S, Zitman FG, Kromhout D. Low respiratory function increases the risk of depressive symptoms in later life in men. Psychosom Med. 2010;72(1):53–60.
Wang C, Yang LS, Shi XH, Yang YF, Liu K, Liu RY. Depressive symptoms in aged Chinese patients with silicosis. Aging Ment Health. 2008;12(3):343–8.
Article CAS PubMed Google Scholar
Peng G, Xin Y, Cao X, Chen Y, Yang Y, Zhang X, Zhou X. Association between depressive symptoms and lung function in the United States adults without pulmonary diseases: a cross-sectional study from NHANES. J Affect Disord. 2023;325:787–93.
Goodwin RD, Chuang S, Simuro N, Davies M, Pine DS. Association between lung function and mental health problems among adults in the United States: findings from the First National Health and Nutrition Examination Survey. Am J Epidemiol. 2007;165(4):383–8.
Du W, Guan H, Wan X, Zhu Z, Yu H, Luo P, Chen L, Su J, Lu Y, Hang D, et al. Circulating liver function markers and the risk of COPD in the UK Biobank. Front Endocrinol (Lausanne). 2023;14:1121900.
Park S, Lee S, Kim Y, Cho S, Kim K, Kim YC, Han SS, Lee H, Lee JP, Joo KW, et al. Kidney function and obstructive lung disease: a bidirectional Mendelian randomisation study. Eur Respir J. 2021;58(6):2100848.
Pan J, Zaigham S, Persson M, Borné Y, Wollmer P, Engström G. The associations between red cell distribution width and lung function measures in a general population. Respir Med. 2021;185:106467.
Ma YH, Shen LX, Li YZ, Leng Y, Yang L, Chen SD, He XY, Zhang YR, Chen RJ, Feng JF, et al. Lung function and risk of incident dementia: a prospective cohort study of 431,834 individuals. Brain Behav Immun. 2023;109:321–30.
Li G, Jankowich MD, Lu Y, Wu L, Shao L, Ke C. Preserved ratio impaired spirometry, metabolomics and the risk of type 2 diabetes. J Clin Endocrinol Metab. 2023;108(9):e769–78.
Nedic Erjavec G, Sagud M, Nikolac Perkovic M, Svob Strac D, Konjevod M, Tudor L, Uzun S, Pivac N. Depression: biological markers and treatment. Prog Neuropsychopharmacol Biol Psychiatry. 2021;105:110139.
Sudlow C, Gallacher J, Allen N, Beral V, Burton P, Danesh J, Downey P, Elliott P, Green J, Landray M, et al. UK biobank: an open access resource for identifying the causes of a wide range of complex diseases of middle and old age. PLoS Med. 2015;12(3):e1001779.
Quanjer PH, Stanojevic S, Cole TJ, Baur X, Hall GL, Culver BH, Enright PL, Hankinson JL, Ip MS, Zheng J, et al. Multi-ethnic reference values for spirometry for the 3–95-yr age range: the global lung function 2012 equations. Eur Respir J. 2012;40(6):1324–43.
Higbee DH, Granell R, Davey Smith G, Dodd JW. Prevalence, risk factors, and clinical implications of preserved ratio impaired spirometry: a UK Biobank cohort analysis. Lancet Respir Med. 2022;10(2):149–57.
Yang T, Wang J, Huang J, Kelly FJ, Li G. Long-term exposure to multiple ambient air pollutants and association with incident depression and anxiety. JAMA Psychiat. 2023;80(4):305–13.
Article Google Scholar
Soininen P, Kangas AJ, Würtz P, Suna T, Ala-Korpela M. Quantitative serum nuclear magnetic resonance metabolomics in cardiovascular epidemiology and genetics. Circ Cardiovasc Genet. 2015;8(1):192–206.
Cao Z, Xu C, Li S, Wang Y, Yang H. Residential greenspace and risk of cancer: a prospective cohort study from the UK Biobank. Sci Total Environ. 2023;871:162145.
Geng T, Zhu K, Lu Q, Wan Z, Chen X, Liu L, Pan A, Liu G. Healthy lifestyle behaviors, mediating biomarkers, and risk of microvascular complications among individuals with type 2 diabetes: a cohort study. PLoS Med. 2023;20(1):e1004135.
Harshfield EL, Pennells L, Schwartz JE, Willeit P, Kaptoge S, Bell S, Shaffer JA, Bolton T, Spackman S, Wassertheil-Smoller S, et al. Association between depressive symptoms and incident cardiovascular diseases. JAMA. 2020;324(23):2396–405.
Benjamini Y, Hochberg Y. Controlling the false discovery rate: a practical and powerful approach to multiple testing. J R Stat Soc Series B Stat Methodol. 1995;57(1):289–300.
Google Scholar
Mutz J, Lewis CM. Lifetime depression and age-related changes in body composition, cardiovascular function, grip strength and lung function: sex-specific analyses in the UK Biobank. Aging (Albany NY). 2021;13(13):17038–79.
Draijer C, Hylkema MN, Boorsma CE, Klok PA, Robbe P, Timens W, Postma DS, Greene CM, Melgert BN. Sexual maturation protects against development of lung inflammation through estrogen. Am J Physiol Lung Cell Mol Physiol. 2016;310(2):L166-174.
Mulugeta A, Zhou A, King C, Hyppönen E. Association between major depressive disorder and multiple disease outcomes: a phenome-wide Mendelian randomisation study in the UK Biobank. Mol Psychiatry. 2020;25(7):1469–76.
Zhou L, Yang H, Zhang Y, Li H, Zhang S, Li D, Ma Y, Hou Y, Lu W, Wang Y. Association of impaired lung function with dementia, and brain magnetic resonance imaging indices: a large population-based longitudinal study. Age Ageing. 2022;51(11):afac269.
Li G, Lu Y, Qiao Y, Hu D, Ke C. Role of pulmonary function in predicting new-onset cardiometabolic diseases and cardiometabolic multimorbidity. Chest. 2022;162(2):421–32.
Zarghami M, Taghizadeh F, Sharifpour A, Alipour AJA. health: Efficacy of smoking cessation on stress, anxiety, and depression in smokers with chronic obstructive pulmonary disease: a randomized controlled clinical trial. Addict Health. 2018;10(3):137.
PubMed PubMed Central Google Scholar
Wan S, Rojas-Rueda D, Pretty J, Roscoe C, James P, Ji JS. Greenspace and mortality in the U.K. Biobank: longitudinal cohort analysis of socio-economic, environmental, and biomarker pathways. SSM Popul Health. 2022;19:101194.
Hancox RJ, Gray AR, Sears MR, Poulton R. Systemic inflammation and lung function: a longitudinal analysis. Respir Med. 2016;111:54–9.
Jiang R, Burke GL, Enright PL, Newman AB, Margolis HG, Cushman M, Tracy RP, Wang Y, Kronmal RA, Barr RG. Inflammatory markers and longitudinal lung function decline in the elderly. Am J Epidemiol. 2008;168(6):602–10.
Lu Y, Feng L, Feng L, Nyunt MS, Yap KB, Ng TP. Systemic inflammation, depression and obstructive pulmonary function: a population-based study. Respir Res. 2013;14(1):53.
Coryell W, Pine D, Fyer A, Klein D. Anxiety responses to CO2 inhalation in subjects at high-risk for panic disorder. J Affect Disord. 2006;92(1):63–70.
Maes M, Yirmyia R, Noraberg J, Brene S, Hibbeln J, Perini G, Kubera M, Bob P, Lerer B, Maj M. The inflammatory & neurodegenerative (I&ND) hypothesis of depression: leads for future research and new drug developments in depression. Metab Brain Dis. 2009;24(1):27–53.
Savage K, Porter C, Bunnett E, Hana M, Keegan A, Ogden E, Stough C, Pipingas A. Liver and inflammatory biomarker relationships to depression symptoms in healthy older adults. Exp Gerontol. 2023;177:112186.
Tanifuji T, Okazaki S, Otsuka I, Mouri K, Horai T, Shindo R, Shirai T, Hishimoto A. Epigenetic clock analysis reveals increased plasma cystatin C levels based on DNA methylation in major depressive disorder. Psychiatry Res. 2023;322:115103.
Batty GD, Gale CR, Kivimäki M, Deary IJ, Bell S. Comparison of risk factor associations in UK Biobank against representative, general population based studies with conventional response rates: prospective cohort study and individual participant meta-analysis. BMJ. 2020;368:m131.
Bajinka O, Simbilyabo L, Tan Y, Jabang J, Saleem SA. Lung-brain axis. Crit Rev Microbiol. 2022;48(3):257–69.
Download references
Acknowledgements
The authors gratefully thank all the participants and professionals contributing to the UK Biobank. The study was conducted under the UK Biobank project application number 91536.
This work was supported by the Shandong Provincial Natural Science Foundation [No: ZR2021QH310].
Author information
Authors and affiliations.
Department of Epidemiology, School of Public Health, Cheeloo College of Medicine, Shandong University, Jinan, China
Wei Hu, Bao-Peng Liu & Cun-Xian Jia
You can also search for this author in PubMed Google Scholar
Contributions
WH wrote the first draft of the paper and analyzed the data. BPL interpreted the data and critically revised the manuscript. CXJ conceptualized this study, interpreted the data, and critically revised the manuscript. All authors approved the final submitted manuscript.
Corresponding authors
Correspondence to Bao-Peng Liu or Cun-Xian Jia .
Ethics declarations
Ethics approval and consent to participate.
The UK Biobank project received ethical approval from the Northwest Multi-center Research Ethics Committee (MREC reference: 21/NW/0157). Informed consent was obtained from all participants before recruitment.
Consent for publication
Not applicable.
Competing interests
The authors declare that they have no competing interests.
Additional information
Publisher’s note.
Springer Nature remains neutral with regard to jurisdictional claims in published maps and institutional affiliations.
Supplementary Information
Additional file 1: table s1..
Mean concentration of biomarkers ( n = 231,193). Table S2. Mean concentration of metabolites ( n = 62,488). Table S3. Summary of missing data of covariates. Table S4. Baseline characteristics by lung function ( n = 280,032). Table S5. Sensitivity analyses for association between incident depression and lung function with further adjustment for covariates. Table S6. Sensitivity analyses for association between incident depression and lung function with different exclusion criteria. Table S7. Sensitivity analyses for association between lung function and risk of incident depression with multiple imputation for missing covariates ( n = 297,037). Table S8. Sensitivity analyses for association between lung function and risk of incident depression with further excluding prevalent depression as measured by the PHQ-2 scale at baseline ( n = 271,122). Table S9. Selection of biomarkers as potential mediators between lung function and incident depression ( n = 231,193). Table S10. Selection of metabolites as potential mediators between lung function and incident depression ( n = 62,488). Fig. S1. Subgroup analysis of the association of lung function on depression by potential risk factors.
Rights and permissions
Open Access This article is licensed under a Creative Commons Attribution 4.0 International License, which permits use, sharing, adaptation, distribution and reproduction in any medium or format, as long as you give appropriate credit to the original author(s) and the source, provide a link to the Creative Commons licence, and indicate if changes were made. The images or other third party material in this article are included in the article's Creative Commons licence, unless indicated otherwise in a credit line to the material. If material is not included in the article's Creative Commons licence and your intended use is not permitted by statutory regulation or exceeds the permitted use, you will need to obtain permission directly from the copyright holder. To view a copy of this licence, visit http://creativecommons.org/licenses/by/4.0/ . The Creative Commons Public Domain Dedication waiver ( http://creativecommons.org/publicdomain/zero/1.0/ ) applies to the data made available in this article, unless otherwise stated in a credit line to the data.
Reprints and permissions
About this article
Cite this article.
Hu, W., Liu, BP. & Jia, CX. Association and biological pathways between lung function and incident depression: a prospective cohort study of 280,032 participants. BMC Med 22 , 160 (2024). https://doi.org/10.1186/s12916-024-03382-3
Download citation
Received : 03 October 2023
Accepted : 08 April 2024
Published : 15 April 2024
DOI : https://doi.org/10.1186/s12916-024-03382-3
Share this article
Anyone you share the following link with will be able to read this content:
Sorry, a shareable link is not currently available for this article.
Provided by the Springer Nature SharedIt content-sharing initiative
- Incident depression
- Mediating mechanisms
BMC Medicine
ISSN: 1741-7015
- Submission enquiries: [email protected]
- General enquiries: [email protected]
- Open access
- Published: 13 April 2024
The effect of commuting time on burnout: the mediation effect of musculoskeletal pain
- Yong-Hsin Chen 1 , 2 , 3 ,
- Jia June Lin 4 , 5 ,
- Ching-wen Yang 3 ,
- Hsiu-Mei Tang 3 ,
- Gwo-Ping Jong 6 , 7 &
- Tsung-Yuan Yang 6 , 7
BMC Health Services Research volume 24 , Article number: 468 ( 2024 ) Cite this article
Metrics details
This study explores the relationship among commuting, musculoskeletal (MS) pain, and burnout.
An observational and cross-sectional study was conducted at a medical university-affiliated hospital in Taichung, Taiwan in 2021. The two questionnaire was used and they included the Copenhagen Burnout Inventory (CBI) and the Nordic Musculoskeletal Questionnaire (NMQ). All participants were invited to complete the cross-sectional survey. A multiple linear regression was assessed correlations between commuting, MS pain, and burnout.
After excluding those with missing data, 1,615 healthcare workers were deemed valid as research participants. In multiple linear regression, commuting time longer than 50 min was associated with personal burnout (PB) in the presence of adjusted confounders; however, long commuting time was not associated with work-related burnout (WB). Furthermore, the choice of commuting method did not affect PB or WB. Notably, both neck and shoulder pain (NBSP) and ankle pain (BAP) increase the risk of PB and WB. The mediation analysis demonstrated that NBSP is a mediating factor, increasing the level of PB and WB for commuting times longer than 50 min.
Conclusions
Healthcare workers who commute for more than 50 min should be considered part of a high-risk group for burnout and musculoskeletal pain. They should also be provided with resources and programs focused on burnout prevention and MS pain relief.
Peer Review reports
Introduction
Burnout is described as physical, emotional, and mental exhaustion resulting from long-term involvement in work situations that are emotionally demanding [ 1 ]. Notably, developing burnout is a multistage process [ 2 ]. In the beginning, people experience at work. Next is the stagnation period, when work pressures cause decreasing enthusiasm. This is followed by the frustration period, when chronic stress gradually causes people to lose enthusiasm and energy for work. Finally, individuals need to seek help and intervention due to poor physical and emotional problems. Symptoms of clinical burnout include emotional exhaustion, physical fatigue, cognitive impairment, disturbed sleep, and functional impairment [ 3 , 4 ]. As for the factors that impact burnout, previous studies have determined that overtime [ 5 ], rotating shift work [ 6 ], lack of sleep [ 6 , 7 ], and chronic diseases [ 8 ] are associated with an increased risk of burnout. In contrast, work experience [ 9 ], regular exercise habits [ 7 ], being married, and being a parent [ 10 ] may help decrease burnout levels.
Commuting is a common problem for people living in cities. In Taiwan, the mean commuting time is 23.15 min, with the proportion of commuters spending over 50 min in commuting being 8% [ 11 ]. It has been reported that increased commuting time was associated with poor physical and mental health. For instance, individuals who experience long commuting times commonly have poor subjective health and visit the general practitioner[ 12 ] more frequently. Moreover, commuting time in compact cities was significantly related to lower satisfaction with life [ 13 ]. Conversely, shorter commute times can improve subjective well-being [ 14 ].
The proportion of commuters that use public transportation and private vehicles or motorcycles in Taichung city in 2010 were 7.4% and 82.3% [ 11 ], respectively. Notably, the method of commuting could be associated with level of satisfaction with life and well-being, and individuals who commute by walking have better mental health [ 15 ] and higher life satisfaction [ 16 ] than those who drive.
Research has demonstrated that stress caused by commuting was positively related to burnout but had no direct association with job satisfaction [ 17 ]. However, the stress of commuting could result from the time spent commuting; for instance, healthcare professionals whose commuting time was more than 30 min seemed to experience higher burnout [ 18 ]. However, another study of commuting in Dublin illustrated six modes of transport, including traveling to work by bus, train, car, tram, cycling and walking, which were not associated with burnout [ 19 ].
Frequently changing positions from sitting to standing or walking could reduce musculoskeletal pain risk [ 20 ]. This result led us to consider whether the long commuting time effect on musculoskeletal (MS) pain resulted from holding the same position for a long time. Some research provided positive evidence to support our opinion, such as, an early study in French nurses found commuting time over one hour was associated with dorsal and lumbar pain [ 21 ]. The individuals who report long commuting time easily suffer from MS pain [ 22 ]. The risk was 7.29 times higher compared to those who reported short-distance commuting [ 23 ]. Coincidentally, not only adults, but also increased commuting times can increase the risk of lower limb dysfunction and low back pain in children [ 24 ].
Past studies confirmed that neck or shoulder pain could be associated with mental health. For instance, individuals with low mood/stress [ 25 ] and burnout [ 26 ] were more likely to develop subsequent neck or shoulder pain. Based on the above, we propose two questions: (1) Does the commuting effect on burnout result from the commuting time, not the commuting method? (2) Is the time spent commuting the main cause of increased burnout resulting from MS pain? To further explore the effect of commuting time on burnout, the present study proposed four hypotheses:
Hypothesis 1
The choice of commuting method is not related to an increased risk of burnout.
Hypothesis 2
The effect of commuting time on burnout is significant.
Hypothesis 3
Commuting time is significantly associated with MS pain.
Hypothesis 4
MS pain is a mediating factor between a long commuting time and the increased risk of burnout.
We believe clarifying the problem could help us understand the cause of the commuting effect on burnout and suggest a full strategy to mitigate burnout in healthcare workers.
Study design
This observational and cross-sectional study was conducted at a medical-university-affiliated hospital in Taichung, Taiwan, between March and April 2021. All 2,531 healthcare workers who have served for one year in the hospital received a QR code by email linking to Google Forms questionnaires. Among them, 1633 (64.52%) individuals filled out full questionnaires. 1,615 (63.81%) of which were deemed valid after excluding those with missing data. The survey included the validated questionnaires of the Copenhagen Burnout Inventory (CBI), the Nordic Musculoskeletal Questionnaire (NMQ), and demographic variables, including family, living habits, work, and physical health. This study was approved by the institutional review board of Chung Shan Medical University in 2021 (No: CS1-21108).
Measuring burnout
The CBI, developed by researchers from Denmark[ 27 ], has very high internal reliability and was formulated to be understandable by and accessible to all people [ 27 ]. It has been used to develop three scales, the personal burnout (PB) scale, work-related burnout (WB) scale, and client burnout scale, that can be applied separately to measure burnout in different occupational fields. According to the definition of CBI for PB and WB [ 27 ], PB is the degree of physical and psychological fatigue and exhaustion that a person experiences; WB is the degree of physical and psychological fatigue and exhaustion that a person perceives as related to his/her work. To reflect the national conditions, we used the Chinese version of the CBI [ 28 ]. Moreover, for the purposes of suitability for all participants, we adopted PB and WB scales to measure burnout for healthcare workers. The scales are listed in Supplementary Information Table S1 . The response options of CBI were “always,” “often,” “sometimes,” “seldom,” and ”never/almost never,” which were scored as 100, 75, 50, 25, and 0, respectively. The 13th item in the scales was an inversely scored item (i.e., the responses were scored by minimum “always” = 0 and maximum “never/almost never” = 100, sequentially). The mean of the PB and WB scores (the sum of scores for items 1–6 and items 7–13) represented the level of PB and WB for participants, respectively.
Measuring musculoskeletal pain
The present study adopted the NMQ, modified and translated by the Taiwan Institute of Occupational Safety and Health. The NMQ measures the presence of pain attributable to work-related factors in the preceding year and is a repeatable, sensitive, and reliable measurement measured of pain [ 29 , 30 , 31 ]; when validity tested against clinical history, the result is less than 20% disagreement [ 32 ]. The response options of NMQ for the presence of pain sites were the neck, left or right shoulder, upper back, waist or lower back, left or right elbow, left or right wrist, left hip/thigh/buttock, right hip/thigh/buttock, left or right knee, and left or right ankle. The options on frequency of each pain site were every day, once a week, once a month, once every half a year, and at least once every half a year (relative scored as 100, 80, 60, 40, and 20 points).
The demographic questionnaires
The demographic questionnaires assessed the participants’ age, education degree (response options: “less than high school,” “Bachelor’s degree,” “Master’s degree,” or “PhD.”), marital status (“married” or “other.”), raising children (“without child,” “one child,” “two children,” “three children,” and “over three children.”), weekly exercise habits (at least once per day, at least once weekly, at least once per month, less than once per month, or never), monthly alcohol use habits (every day, occasionally, or never), sleeping time (“<5 h,” “5–6 h,” “6–7 h,” “7–8 h,” or “>8 h.”), overtime work per month (“seldom,” “less than 45 h per month,” “45–80 h per month,” and “more than 80 h per month.”), shift schedules (“day shift work,” “night shift work,” “irregular shift work,” and “regular shift work.”), professional fields (“physicians,” “nurses,” “professional and technical personnel,” and “administrative staff”), and the presence of one or more chronic diseases (“yes” and “no”). Moreover, the participants were asked if they engaged in leisure activities with family or friends during vacation time. The response options, “always,” “often,” “sometimes,” “seldom,” and”never,” were scored as 100, 75, 50, 25, and 0 points, respectively.
Finally, we surveyed participants’ commuting time and most-used commuting methods. The response options for commuting time were “5–10 minutes,” “10–20 minutes,” “20–30 minutes,” “30–40 minutes,” “40–50 minutes,” and “over 50 minutes.” The options of most-used commuting methods (multiple choice) were “train,” “bus,” “Mass Rapid Transit (MRT),” “walking,” bicycle,” “vehicle,” and “motorcycle.”
Data analysis
The present study adopted four steps to test the four hypotheses presented in the Introduction, as follows.
Step 1: NMQ included complex information for pain sites and occurrence frequency, which is adverse for further statistical analysis. Consequently, we used factor analysis [ 33 ] to determine new underlying variables to effectively explain the questionnaire. Factor analysis uses varimax rotation to obtain the standardized scoring coefficients, which constitute new factor loadings. We retained new factors that featured vector values exceeding 1 according to the principle proposed by Hair et al. [ 33 ] and redefined new variables according to their corresponding significance. For similar reasons, the questionnaire for the most-used commuter transportation also adopted factor analysis to redefined new variables.
Step 2: We adopted simple linear regression to determine if there was an existing significant difference in the level of burnout among variables.
Step 3: The confounders of PB/WB found in step 2 were adjusting variables and were added to multiple linear regression models of PB/WB. These processes could determine if commuting time, commuting method, and MS pain effect on PB/WB.
Step 4: The present study adopts mediation analysis to determine if the MS pain is a mediating factor for the effect of commuting time on increased burnout. We adopted the strategy proposed by Baron and Kenny, [ 34 ] in which (1) the first-stage effect of the independent variable significantly affects the mediating factor; (2) the independent variable significantly affects the dependent variable in the absence of the mediating factor; (3) the second-stage effect of the mediating factor exerts a significant unique effect on the dependent variable; and (4) the effect of the independent variable on the dependent variable weakens upon the addition of a mediating factor to the model. Of them, item (2) is only recommended but not required [ 35 ]. The formulas are as follows:
where a is the linear regression coefficient of the independent variable against the mediating factor, b is the linear regression coefficient of the mediating factor against the dependent variable, c is the linear regression coefficient of the independent variable against the dependent variable in the absence of mediating factors, and c’ is the linear regression coefficient of the independent variable against the dependent variable in the presence of a mediating factor. The standard errors of a and b are represented by s a and s b , respectively. The Z exceeding|1.96|,|2.57|, and|3.90| (for a two-tailed test) are significant at α = 0.05, 0.01, and 0.0001, respectively.
Analyses were performed using SAS Enterprise Guide 7.1 software (SAS Institute Inc., Cary, NC, USA), and significance was set at P < 0.05.
Table 1 presents the demographic variables of all 1,615 participants. This is a female-dominated healthcare worker team (women: 81.36%; 1,314 individuals) and 18.39% (297 individuals) of individuals a master’s degree or higher. The average age of all participants was 38.22 ± 10.20. The proportion of participants who are parents (raising at least one child) was 43.49% (703 individuals) of all individuals. The individuals who reported engaging in regular exercise weekly were 57.78% (933 individuals, including at least once per day and at least once weekly). Regarding alcohol use, individuals who report consuming alcohol in a month were 37.71% (609 individuals: including every day and occasionally). Moreover, individuals who report sleeping fewer than 6 h per day were 38.76% (626 individuals: including < 5 h and 5–6 h per day) of all participants. The participants who worked overtime (less than 45 h per month, or 45–80 h per month, or more than 80 h per month) in the past month are 561 individuals, reaching 34.73% of all individuals. Regarding shift work, most shifts were day shift, night shift, irregular shift, and regular shift work, corresponding to 65.70%, 10.28%, 11.89%, and 12.14% of all individuals, respectively. For professional fields, physicians, nurses, professional and technical personnel, and administrative staff were 8.54% (138 individuals), 37.96% (613 individuals), 17.52% (283 individuals), and 35.98% (581 individuals) of all participants. The individuals who suffered from one or more chronic diseases were 39.50% (638 individuals) of all samples. Regarding the frequency of engaging in leisure activities with family or friends, the proportion of responses for always, often, sometimes, seldom, and never were 6.32%, 30.84%, 47.37%, 14.74%, and 0.74% of all individuals, respectively. The highest proportion of commuters who had a commuting time of 10–20 min was 33.50%, followed by 21.42% for 5–10 min. Other commuting times, from high to low, were 10.46% for 30–40 min, 8.67% for > 50 min, and 6.19% for 40–50 min. The most-used transportation methods from high to low were motorcycle (79.07%), private vehicle (32.63%), walking (24.27%), bus or MRT (11.02%), train (8.92%), and bicycle (4.09%).
Table 2 shows that both shoulders (43.09%), neck (36.22%), waist or lower back (27.93%), and upper back (16.90%) were the common MS pain sites of participants. According to the principle proposed by Hair and Anderson (1995) [ 33 ], the eigenvalues of Factors 1 and 2, 4.93 and 1.55, respectively, were retained because both exceeded 1. The factor loadings were converted into standardized scoring coefficients through varimax rotation, where the relatively large factor loading values for Factors 1 and 2 corresponded to the MS pain sites of the neck, shoulders, and ankles, respectively. Based on this, we defined Factors 1 and 2 as Neck and Both Shoulders Pain (NBSP) and Both Ankles Pain (BAP).
Table 3 transformed the six most commonly used commuter transportation methods by factor analysis as two new underlying variables to effectively explain the transportation used by individuals: private vehicles or motorcycles (Factor 1) and public transportation system (Factor 2).
Table 4 illustrates the statistical association between survey variable and PB/WB by the simple linear regression, where age (B = − 0.25, P < 0.0001), weekly exercise habit (B = − 6.30, P < 0.0001), monthly alcohol use (B = 3.81, P < 0.0001), sleep duration < 6 h per day (B = 8.13, P < 0.0001), overtime per month (B = 9.67, P < 0.0001), irregular and regular shift work (B = 8.47, P < 0.0001; B = 5.20, P = 0.0002), physicians (B = 10.60, P < 0.0001), nurses (B = 9.07, P < 0.0001), PTs (B = 2.50, P = 0.049), the presence of chronic diseases (B = 4.69, P < 0.0001), engaging in leisure activities with family or friends (LAFF) (B = − 0.12, P < 0.0001), NBSP (B = 8.25, P < 0.0001), and commuting time of 10–20 min (B = − 2.74, P = 0.027) were significantly associated with PB.
Moreover, the risk or protective factors of WB were age (B = − 0.31, P < 0.0001), holding a Master’s degree or above (B = − 2.21, P = 0.034), being married (B = − 4.30, P < 0.0001), parenthood (B = − 4.86, P < 0.0001), weekly exercise habit (B = − 5.60, P < 0.0001), monthly alcohol use (B = 3.50, P < 0.0001), sleep duration < 6 h (B = 6.27, P < 0.0001), experience overtime (B = 8.88, P < 0.0001), irregular or regular shift work (B = 8.87; 6.23, both P < 0.0001), night shift work (B = 3.24, P = 0.015), physicians (B = 9.89, P < 0.0001), nurses (B = 8.44, P < 0.0001), the presence of chronic diseases (B = 3.47, P < 0.0001), engaging in LAFF (B = − 0.14, P < 0.0001), NBSP (B = 6.32, P < 0.0001), BAP (B = 1.42, P = 0.003), commuting time of 30–40 min (B = − 3.88, P = 0.011), commuting time of 20–30 min (B = − 3.44, P = 0.006), commuting time of 10–20 min (B = − 2.52, P = 0.024), and private vehicle or motorcycle (B = 1.06, P = 0.045).
Table 5 mainly show if different commuting times or commuter transportation methods impact PB and WB in the presence of adjusted confounders. According to M 1 , M 2 , and M 3 models in Table 5 , commuting times longer than 50 min were significantly associated with increased PB (B = 4.21, P = 0.015; B = 3.39, P = 0.035; B = 4.24, P = 0.015). Moreover, we also found the NBSP effect could explain 19.48% ( \( =\frac{3.39-4.21}{4.21}\) ) of the residual effects of commuting time < 50 on PB according to the M 2 model and itself keep significant in statistic (B = 7.09, P < 0.0001). Nevertheless, the BAP effect on PB is only slightly affected by commuting times longer than 50 min (B value for commuting time < 50 changed from 4.21 to 4.24) according to the M 3 model. Overall, NBSP may play an important role between commuting times longer than 50 min and increased PB. In addition, regarding commuter transportation effect on PB, M 1 , M 2 , and M 3 models in Table 5 , it was determined that commuter transportation was not associated with increased levels of PB ( P < 0.05) in the presence of adjusted variables.
Table 5 shows that regardless of the model (M 1 , M 2 , or M 3 ), commuting time and the commuter transportation method used were not associated with increased levels of WB ( P > 0.05). In contrast with the effect on PB, commuting time is not associated with increased WB.
Based on the above results, we confirmed Hypothesis 1 that the choice of commuter transportation is unrelated to increased burnout. The present study also confirmed Hypothesis 2 that the effect of commuting time on burnout is significant in the presence of adjusting confounders. Nevertheless, we emphasize that only commuting times longer than 50 min are associated with increased burnout; furthermore, the feeling of burnout comes from personal reasons, not work-related reasons.
We found that commuting times longer than 50 min were associated with increased risk of NBSP (Table 6 : B = 0.21, P < 0.01) but not BAP (B = − 0.02, P = 0.802; B = 0.02, P = 0.414). The statistical results confirm Hypothesis 3 that commuting time is significantly associated with MS pain. Additionally, Table 7 also demonstrated the commuting in private vehicles or motorcycles were associated with reduced risk of NBSP (Table 8 : B = − 0.10, P = 0.001). A more valuable finding was that commuting times longer than 50 min increased the risk of MS pain limited to the neck and shoulders.
Based on strategies proposed by Baron and Kenny [ 34 ], only NBSP confirms the first-stage effect (Table 6 , B = 0.21, P = 0.010) and the second-stage effect (Table 8 , b = 8.22, P < 0.001), but BAP does not. Therefore, NBSP will be included in a shortlist of mediating factors. Tables 7 and 8 determined NBSP was a mediating factor (Z = 2.57, P < 0.01; Z = 2.56, P < 0.01) of commuting times longer than 50 min increasing the risk of PB and WB. Notably, NBSP also was a suppression factor between private vehicles or motorcycles and WB (Table 7 , Z = − 3.26, P < 0.01). Namely, commuting in private vehicle or motorcycle reduced neck and both shoulders pain relative to other commuter methods that mitigated WB increases.
BAP did not satisfy the first-stage effect (Table 6 , B = − 0.02, P = 0.802) strategies proposed by Baron and Kenny [ 34 ], thus, it is excluded from the shortlist of mediating factors.
According to the above results, we confirmed Hypothesis 4 that MS pain is a mediating factor between long commuting times and increased risk of burnout. However, for the mediation effect, the sites of the MS pain effect on burnout are specifically associated with neck and both shoulders pain. Another important finding is that commuting times over 50 min indicate that long commuting times led to MS pain, further affecting the risk of increased PB and WB.
Past research had identified many factors that contribute to burnout. For instance, individuals who experience overtime [ 5 ], rotating shift work [ 6 ], lack of sleep [ 6 , 7 ], and suffer from chronic diseases [ 8 ] suffer a higher risk of burnout than others. Similarly, the present study found that individuals who experience overtime had irregular or regular shift work, slept fewer than 6 h, and had chronic diseases had significantly higher levels of PB and WB than others. Notably, some research also identified that factors associated with reduced burnout include work experience [ 9 ], regular exercise habits [ 7 ], being married, having children [ 10 ], etc. These similar protective factors also were found in our study, for instance, individuals who had a weekly exercise habit had lower levels of PB and WB than those who reported no weekly exercise habit. Moreover, our study also confirmed that married individuals or parents reported lower WB levels than others.
Moreover, evidence demonstrated burnout was strongly associated with alcohol use among healthcare workers such as physicians, nurses, and residents [ 36 , 37 ]. Our study found a relationship between burnout and alcohol use: individuals who have ever used alcohol in a month reported higher levels of PB and WB than others. Notably, practicing physicians have a greater prevalence of burnout than individuals in other fields [ 38 , 39 ]. We observed a similar result among participants: physicians experience a markedly personal and work-related burnout compared with other profession fields.
For stressed individuals, engaging in leisure activities can relieve stress, improve emotional health, and maintain physical and mental health [ 40 , 41 , 42 ]. Our study also confirmed this result. Table 4 shows that positively engaging in leisure activities with family or friends was significantly associated with reduced PB and WB.
Musculoskeletal (MS) pain could be associated with burnout. Some studies have suggested that neck or shoulder pain is associated with low mood/stress [ 25 ] and burnout more likely leads to neck or shoulder pain [ 26 ]. Coincidentally, our study also presents the same findings; after adjusting for confounders, neck/shoulder pain and ankle pain were found to be associated with increased risk of personal burnout (PB) and work-related burnout (WB). Moreover, burnout increases the risk of MS pain because of the activation of the autonomic nervous system and the hypothalamic–pituitary–adrenal axis [ 43 ].
Regarding the relationship between the choice of commuter transportation and burnout, a study in Hong Kong on commuting and well-being in 2015 demonstrated that the commuting method was not an independent risk factor for well-being [ 13 ]. Although private vehicle or motorcycle use was associated with a high risk of WB (Table 4 ), the association was not maintained after adjusting for confounders (Table 5 ).
In a Taiwanese study, 8% of individuals had commuting times > 50 min [ 11 ], compared with our study in which 8.67% had commuting times > 50 min, which is slightly higher than the country’s average. Commuting time is associated with subjective health [ 12 ], well-being [ 14 ], and satisfaction with life [ 13 ]. Regarding the influence of family life, studies have demonstrated that individuals have less energy after commuting, which can affect their quality of life [ 44 ] because longer commuting times tend to disrupt work and family life [ 45 ]. These factors lead to a higher sense of family responsibilities [ 46 ], reduced time for leisure activities [ 47 ], and work–family conflict [ 48 ]. Based on these results, does the effect of commuting time on individuals and their families directly or indirectly affect burnout? Using multiple linear regression M1, M2, and M3 models, individuals who reported having commuting times > 50 min sustained a higher risk of PB than others. However, commuting times did not affect WB development despite adjustments for confounders. Long commuting times (such as > 50 min) directly affected PB but not WB.
Although commuting time influenced burnout development (hypothesis 2 ), whether it was a beneficial or detrimental factor was dependent on whether commuting was a source of stress or relaxation. Specifically, commuting is not always a source of stress because it might be a form of mental relaxation and a protective screen between work and family [ 49 ]. A similar effect was observed in this study. For instance, Table 4 demonstrates that all commuting times < 40 min are associated with reduced WB risk, demonstrating that individuals who reported commuting times < 40 min sustained less risk of WB than those who reported commuting times between 5 and 10 min. In addition, individuals who reported commuting times between 10 and 20 min sustained a low risk of burnout. Thus, if commuting relaxes an individual mentally or emotionally, commuting times can be important. Overall, we found that commuting times > 50 min will not mitigate burnout but will worsen it. Therefore, whether commuting is a source of stress or a form of mental relaxation will depend on the commuting time.
This study also confirmed the relationship between commuting time and MS pain (hypothesis 3 ); moreover, evidence showed that a longer commuting time was associated with an increased risk of MS pain. For instance, a study of railway workers in 2015 demonstrated that individuals who experienced commuting time > 60 min had a higher number of complaints of MS pain than those who experienced commuting time < 60 min [ 22 ]. A study of full-time bank employees in Dhaka City between December 2018 and May 2019 demonstrated that the proportions of individuals who experienced MS pain and reported commuting times > 60 min or 31–60 min were 7.29 and 6.35 times higher, respectively, than those who reported commuting time < 15 min [ 23 ]. Compared with previous studies, 50 min was the cut-off point for MS pain, which links this relationship with burnout, MS pain, and commuting time. Table 6 shows that commuting times > 50 min were associated with increased NBSP, which is consistent with the results of previous studies. A study in adults illustrated that increased commuting distance was associated with a higher risk of physical inactivity [ 50 ]. Moreover, sedentary workers have an increased risk of MS pain [ 51 ]. Notably, a study of commuting methods for children found that decreased walking duration and increased sitting duration associated with vehicle commuting induced low back pain [ 24 ]. Therefore, sedentary behavior may be associated with long commuting times and increased risk of MS pain [ 20 ].
We tested hypotheses 1–3 and found that MS pain was associated with commuting time and burnout. Nevertheless, the above relationships lead to a new question about whether MS pain plays a key role in the relationship between long commuting times and increased risk of burnout. We adopted mediation analysis to answer this question. Tables 7 and 8 demonstrate that NBSP is a mediating factor and that commuting time > 50 min increases the risk of PB and WB. Accordingly, healthcare workers who commute for > 50 min are more likely to experience neck and shoulder pain, which may further intensify burnout. Therefore, sedentary behaviors should be avoided during commutes. In addition, hospitals should include individuals with long commutes in the high-risk group for burnout and provide resources and training programs to prevent or mitigate MS pain. To the best of our knowledge, this is the first study to report these findings.
Our study has some limitations. MS pain can be the result of workload, work styles, or posture. Unfortunately, our study did not collect such data in the regression models. Notably, we were unable to determine whether high work stress or emotional exhaustion due to the pandemic affected the findings; thus, a similar study during the nonpandemic period should be replicated and the results compared with the pandemic period. In addition, past studies have shown that working long hours or working overtime does pose a high risk of burnout [ 5 ]. Table 4 found that overtime was a risk factor for PB. In addition, commuting time of more than 50 min remained a risk factor for PB in the presence of adjusted variables including overtime (Table 5 , M 2 , B = 3.39, P = 0.035; M 3 = 4.24, P = 0.015). Therefore, excluding the effect of long working hours on burnout, long commute times still affect burnout. Whether overtime or long working hours are mediating factors needs to be determined in future studies.
Because the mediation model of an observational study could be biased[ 52 ], as causal relationships show a higher risk of judgment. Therefore, we do not conclude a “causal relationship” in our conclusion to avoid misleading readers.
The present study suggests the commuting method chosen is not associated with increased PB and WB. Specifically, commuting times over 50 min will obviously increase the risk of personal burnout, but work-related burnout is not affected. In addition, healthcare workers who commute for > 50 min are at a higher risk than others for neck and shoulder pain, which were associated with increased PB and WB levels. Therefore, healthcare workers with long commuting times should be considered a high-risk group for burnout and MS pain. They should also be provided with resources and programs focused on burnout prevention and MS pain relief.
Data availability
Datasets used and/or analyzed during the current study are available from the corresponding author on reasonable request.
Schaufeli WB, Greenglass ER. Introduction to special issue on burnout and health. Psychol Health. 2001;16(5):501–10.
Article CAS PubMed Google Scholar
De Hert S. Burnout in Healthcare Workers: prevalence, impact and preventative strategies. Local Reg Anesth. 2020;13:171–83.
Article PubMed PubMed Central Google Scholar
Grossi G, Perski A, Osika W, Savic I. Stress-related exhaustion disorder– clinical manifestation of burnout? A review of assessment methods, sleep impairments, cognitive disturbances, and neuro-biological and physiological changes in clinical burnout. Scand J Psychol. 2015;56(6):626–36.
Article PubMed Google Scholar
Schaufeli WB, Bakker AB, Hoogduin K, Schaap C, Kladler A. On the clinical validity of the maslach burnout inventory and the burnout measure. Psychol Health. 2001;16(5):565–82.
Witkoski Stimpfel A, Sloane DM, Aiken LH. The longer the shifts for hospital nurses, the higher the levels of Burnout and Patient Dissatisfaction. Health Aff. 2012;31(11):2501–9.
Article Google Scholar
Gander P, O’Keeffe K, Santos-Fernandez E, Huntington A, Walker L, Willis J. Fatigue and nurses’ work patterns: an online questionnaire survey. Int J Nurs Stud. 2019;98:67–74.
Cai S, Lin H, Hu X, Cai Y-X, Chen K, Cai W-Z. High fatigue and its associations with health and work related factors among female medical personnel at 54 hospitals in Zhuhai, China. Psychol Health Med. 2018;23(3):304–16.
Salvagioni DAJ, Melanda FN, Mesas AE, González AD, Gabani FL. Andrade SMd: physical, psychological and occupational consequences of job burnout: a systematic review of prospective studies. PLoS ONE. 2017;12(10):e0185781.
Bogiatzaki V, Frengidou E, Savakis E, Trigoni M, Galanis P, Anagnostopoulos F. Empathy and Burnout of Healthcare Professionals in Public hospitals of Greece. Int J Caring Sci. 2019;12(2):611–26.
Google Scholar
Maslach C, Jackson SE. The role of sex and family variables in burnout. Sex Roles. 1985;12(7):837–51.
Summary analysis of the survey on the daily use of transportation by the public in 2020. 2020:69.
Künn-Nelen A. Does Commuting Affect Health? Health Economics. 2016;25(8):984–1004.
Sha F, Li B, Law YW, Yip PSF. Associations between commuting and well-being in the context of a compact city with a well-developed public transport system. Journal of Transport & Health. 2019;13:103–114.
Clark B, Chatterjee K, Martin A, Davis A. How commuting affects subjective wellbeing. Transportation. 2020;47(6):2777–2805.
Martin A, Goryakin Y, Suhrcke M. Does active commuting improve psychological wellbeing? Longitudinal evidence from eighteen waves of the British Household Panel Survey. Preventive Medicine. 2014;69:296–303.
Chng S, White M, Abraham C, Skippon S. Commuting and wellbeing in London. The roles of commute mode and local public transport connectivity. Preventive Medicine. 2016;88:182–188.
Amponsah-Tawiah K, Annor F, Arthur BG. Linking commuting stress to job satisfaction and turnover intention: The mediating role of burnout. Journal of Workplace Behavioral Health. 2016;31(2):104–123.
La Torre G, Sestili C, Imeshtari V, Masciullo C, Rizzo F, Guida G, Pagano L, Mannocci A. Association of health status, sociodemographic factors and burnout in healthcare professionals: results from a multicentre observational study in Italy. Public Health. 2021;195:15–17.
Murray R. Commuting in Dublin: an analysis of factors as predictors of occupational burnout. In.
Putsa B, Jalayondeja W, Mekhora K, Bhuanantanondh P, Jalayondeja C. Factors associated with reduced risk of musculoskeletal disorders among office workers: a cross-sectional study 2017 to 2020. BMC Public Health. 2022;22(1):1503.
Niedhammer I, Lert F, Marne MJ. Back pain and associated factors in French nurses. International Archives of Occupational and Environmental Health. 1994;66(5):349–357.
Urhonen T, Lie A, Aamodt G. Associations between long commutes and subjective health complaints among railway workers in Norway. Preventive Medicine Reports. 2016;4:490–495.
Ali M, Uddin Z, Ahsan GU, Hossain A. Association between daily commute and subjective health complaints among the office workers in an urban community. Heliyon. 2021;7(8):e07841.
Kimura M, Kamada H, Tsukagoshi Y, Tomaru Y, Nakagawa S, Tanaka K, Mataki Y, Takeuchi R, Yamazaki M. Influence of commuting methods on low back pain and musculoskeletal function of the lower limbs in elementary school children: A cross-sectional study. Journal of Orthopaedic Science. 2022;27(5):1120–1125.
Smedley J, Inskip H, Trevelyan F, Buckle P, Cooper C, Coggon D. Risk factors for incident neck and shoulder pain in hospital nurses. Occupational and Environmental Medicine. 2003;60(11):864.
Melamed S. Burnout and risk of regional musculoskeletal pain—a prospective study of apparently healthy employed adults. Stress and Health. 2009;25(4):313–321.
Kristensen TS, Borritz M, Villadsen E, Christensen KB. The Copenhagen Burnout Inventory: A new tool for the assessment of burnout. Work Stress. 2005;19(3):192–207.
Yeh W, Cheng Y, Chen M, Chiu AWH. Development and validation of an occupational burnout inventory. Taiwan J Public Health. 2008;27(5):349–364.
Aulia C. Validity and reliability test of the nordic musculoskeletal questionnaire with formal and informal sector workers. In: 7th International Conference on Public Health 2020. Indonesia; 2020. p. 100–106.
Palmer K, Smith G, Kellingray S, Cooper C. Repeatability and validity of an upper limb and neck discomfort questionnaire: the utility of the standardized Nordic questionnaire. Occupational Medicine. 1999;49(3):171–175.
Dahl AG, Havang S, Hagen K. Reliability of a self-administrated musculoskeletal questionnaire: The fourth Trøndelag health study. Musculoskeletal Science and Practice. 2022;57:102496.
Kuorinka I, Jonsson B, Kilbom A, Vinterberg H, Biering-Sørensen F, Andersson G, Jørgensen K. Standardised Nordic questionnaires for the analysis of musculoskeletal symptoms. Applied Ergonomics. 1987;18(3):233–237.
Hair JF, Anderson RE, Tatham RL, Black WC. Multivariate data analysis: with readings, 4 th ed edn: Prentice-Hall, Inc.; 1995.
Baron RM, Kenny DA. The moderator-mediator variable distinction in social psychological research: conceptual, strategic, and statistical considerations. J Pers Soc Psychol. 1986;51(6):1173–1182.
Shrout P, Bolger N. Mediation in Experimental and Nonexperimental Studies: New Procedures and Recommendations. Psychological methods. 2002;7:422–445.
Oreskovich MR, Kaups KL, Balch CM, Hanks JB, Satele D, Sloan J, Meredith C, Buhl A, Dyrbye LN, Shanafelt TD. Prevalence of Alcohol Use Disorders Among American Surgeons. Archives of Surgery. 2012;147(2):168–174.
Alexandrova-Karamanova A, Todorova I, Montgomery A, Panagopoulou E, Costa P, Baban A, Davas A, Milosevic M, Mijakoski D. Burnout and health behaviors in health professionals from seven European countries. International Archives of Occupational and Environmental Health. 2016;89(7):1059–1075.
Dyrbye LN, West CP, Satele D, Boone S, Tan L, Sloan J, Shanafelt TD. Burnout Among U.S. Medical Students, Residents, and Early Career Physicians Relative to the General U.S. Population. Academic Medicine. 2014;89(3):443–451.
Shanafelt TD, Boone S, Tan L, Dyrbye LN, Sotile W, Satele D, West CP, Sloan J, Oreskovich MR. Burnout and Satisfaction With Work-Life Balance Among US Physicians Relative to the General US Population. Archives of Internal Medicine. 2012;172(18):1377–1385.
Lowe R, Bennett P. Exploring coping reactions to work-stress: Application of an appraisal theory. Journal of Occupational and Organizational Psychology. 2003;76(3):393–400.
Yoshi Iwasaki RCM. Hierarchical Dimensions of Leisure Stress Coping. Leisure Sciences. 2000;22(3):163–181.
Cheng T-M, Chang S-Y, Chan Y-Y. I know you are suffering from burnout: The moderated mediation effects of “leisure benefits” and “leisure coping” on the burnout model of flight attendants. Journal of Air Transport Management. 2018;71:119–129.
Mák K, Kapus K, Tóth G, Hesszenberger D, Pohl M, Pusch G, Fejes É, Fehér G, Tibold A. Neuropathic Low Back Pain and Burnout among Hungarian Workers. In: International Journal of Environmental Research and Public Health. 2021;18.
De Geus B, Van Hoof E, Aerts I, Meeusen R. Cycling to work: influence on indexes of health in untrained men and women in Flanders. Coronary heart disease and quality of life. Scandinavian Journal of Medicine & Science in Sports. 2008;18(4):498–510.
Bai B, Gopalan N, Beutell N, Ren F. Impact of Absolute and Relative Commute Time on Work–Family Conflict: Work Schedule Control, Child Care Hours, and Life Satisfaction. Journal of Family and Economic Issues. 2021;42(4):586–600.
Roberts J, Hodgson R, Dolan P. “It’s driving her mad”: Gender differences in the effects of commuting on psychological health. Journal of Health Economics. 2011;30(5):1064–1076.
Hilbrecht M, Shaw SM, Johnson LC, Andrey J. Remixing work, family and leisure: teleworkers’ experiences of everyday life. New Technology, Work and Employment. 2013;28(2):130–144.
Christian TJ. Automobile commuting duration and the quantity of time spent with spouse, children, and friends. Preventive Medicine. 2012;55(3):215–218.
Wheatley D. Good to be home? Time-use and satisfaction levels among home-based teleworkers. New Technology, Work and Employment. 2012;27(3):224–241.
Raza A, Pulakka A, Magnusson Hanson LL, Westerlund H, Halonen JI. Commuting distance and behavior-related health: A longitudinal study. Preventive Medicine. 2021;150:106665.
Hanna F, Daas RN, El-Shareif TJ, Al-Marridi HH, Al-Rojoub ZM, Adegboye OA. The relationship between sedentary behavior, back pain, and psychosocial correlates among university employees. Frontiers in public health. 2019;7:80.
Cheng W, Rolls ET, Ruan H, Feng J. Functional Connectivities in the Brain That Mediate the Association Between Depressive Problems and Sleep Quality. JAMA Psychiatry. 2018;75(10):1052–1061.
Download references
This research received no external funding.
Author information
Authors and affiliations.
The Department of Health Policy and Management, Chung Shan Medical University, Taichung, 402, Taiwan
Yong-Hsin Chen
Department of Public Health, Chung Shan Medical University, Taichung, 402, Taiwan
Department of Occupational Safety and Health, Chung Shan Medical University Hospital, Taichung, 402, Taiwan
Yong-Hsin Chen, Ching-wen Yang & Hsiu-Mei Tang
Department of Endocrinology and Metabolism, Chung Shan Medical University Hospital, Taichung, 402, Taiwan
Jia June Lin
Nursing Department, Chung Shan Medical University Hospital, Taichung, 402, Taiwan
Department of Internal Medicine, Chung Shan Medical University Hospital, Taichung, 402, Taiwan
Gwo-Ping Jong & Tsung-Yuan Yang
Institute of Medicine, Chung Shan Medical University, Taichung, 402, Taiwan
You can also search for this author in PubMed Google Scholar
Contributions
Conceptualization: YHC, JJL, CwY, HMT, GPJ, and TYY; Data curation: YHC and JJL; Investigation: YHC, HMT, and CwY; Methodology: YHC, JJL, CML, and TYY; Supervision: TYY and GPJ; Writing—original draft: YHC; Writing—review and editing, TYY. All authors have read and agreed to the published version of the manuscript.
Corresponding author
Correspondence to Tsung-Yuan Yang .
Ethics declarations
Ethics approval and consent to partecipate.
All procedures performed in this study were approved by the ethical committee of Chung Shan Medical University Hospital. Informed consent was obtained from all subjects involved in the study.
Consent for publication
All authors approved the manuscript and agreed to its submission and publication.
Competing interests
The authors declare no competing interests.
Additional information
Publisher’s note.
Springer Nature remains neutral with regard to jurisdictional claims in published maps and institutional affiliations.
Electronic supplementary material
Below is the link to the electronic supplementary material.
Supplementary Material 1
Rights and permissions.
Open Access This article is licensed under a Creative Commons Attribution 4.0 International License, which permits use, sharing, adaptation, distribution and reproduction in any medium or format, as long as you give appropriate credit to the original author(s) and the source, provide a link to the Creative Commons licence, and indicate if changes were made. The images or other third party material in this article are included in the article’s Creative Commons licence, unless indicated otherwise in a credit line to the material. If material is not included in the article’s Creative Commons licence and your intended use is not permitted by statutory regulation or exceeds the permitted use, you will need to obtain permission directly from the copyright holder. To view a copy of this licence, visit http://creativecommons.org/licenses/by/4.0/ . The Creative Commons Public Domain Dedication waiver ( http://creativecommons.org/publicdomain/zero/1.0/ ) applies to the data made available in this article, unless otherwise stated in a credit line to the data.
Reprints and permissions
About this article
Cite this article.
Chen, YH., Lin, J.J., Yang, Cw. et al. The effect of commuting time on burnout: the mediation effect of musculoskeletal pain. BMC Health Serv Res 24 , 468 (2024). https://doi.org/10.1186/s12913-024-10908-1
Download citation
Received : 03 January 2024
Accepted : 26 March 2024
Published : 13 April 2024
DOI : https://doi.org/10.1186/s12913-024-10908-1
Share this article
Anyone you share the following link with will be able to read this content:
Sorry, a shareable link is not currently available for this article.
Provided by the Springer Nature SharedIt content-sharing initiative
- Commuting time
- Mediation effect
- Musculoskeletal pain
BMC Health Services Research
ISSN: 1472-6963
- General enquiries: [email protected]

An official website of the United States government
The .gov means it’s official. Federal government websites often end in .gov or .mil. Before sharing sensitive information, make sure you’re on a federal government site.
The site is secure. The https:// ensures that you are connecting to the official website and that any information you provide is encrypted and transmitted securely.
- Publications
- Account settings
Preview improvements coming to the PMC website in October 2024. Learn More or Try it out now .
- Advanced Search
- Journal List
- HHS Author Manuscripts

A General Model for Testing Mediation and Moderation Effects
Amanda j. fairchild.
Department of Psychology, University of South Carolina, Barnwell College, 1512 Pendleton St., Columbia, SC 29208, USA
David P. MacKinnon
Research in Prevention Lab, Department of Psychology, Arizona State University, P.O. Box 871104, Tempe, AZ 85287-1104, USA
This paper describes methods for testing mediation and moderation effects in a dataset, both together and separately. Investigations of this kind are especially valuable in prevention research to obtain information on the process by which a program achieves its effects and whether the program is effective for subgroups of individuals. A general model that simultaneously estimates mediation and moderation effects is presented, and the utility of combining the effects into a single model is described. Possible effects of interest in the model are explained, as are statistical methods to assess these effects. The methods are further illustrated in a hypothetical prevention program example.
Relations between variables are often more complex than simple bivariate relations between a predictor and a criterion. Rather these relations may be modified by, or informed by, the addition of a third variable in the research design. Examples of third variables include suppressors, confounders, covariates, mediators, and moderators ( MacKinnon et al. 2000 ). Many of these third variable effects have been investigated in the research literature, and more recent research has examined the influences of more than one third variable effect in an analysis. The importance of investigating mediation and moderation effects together has been recognized for some time in prevention science, but statistical methods to conduct these analyses are only now being developed. Investigations of this kind are especially valuable in prevention research where data may present several mediation and moderation relations.
Previous research has described the differences between mediation and moderation and has provided methods to analyze them separately (e.g., Dearing and Hamilton 2006 ; Frazier et al. 2004 ; Gogineni et al. 1995 ; Rose et al. 2004 ). More recent research has presented models to simultaneously estimate mediation and moderation to investigate how the effects work together (e.g., Edwards and Lambert 2007 ; MacKinnon 2008 ; Muller et al. 2005 ; Preacher et al. 2007 ). A review of the substantive literature illustrates that few applied research examples have used these models, however. Although analyzing mediation and moderation separately for the same data may be useful, as described later in this paper, simultaneous examination of the effects is often relevant and allows for the investigation of more varied, complex research hypotheses.
What Type of Research Questions Can Be Addressed with the Simultaneous Analysis of Mediation and Moderation Effects?
Is the process by which a program has an effect the same across different types of participants.
In prevention and intervention research, the mediation model has been used to understand the mechanism(s) by which program effects occur. To determine the generalizability of these mechanisms or to explain an unexpectedly small mediated effect it may be of interest to investigate whether the mediation relation, or the indirect effect, holds across different subgroups (e.g., men vs. women or low-risk vs. high-risk). To investigate these hypotheses, a researcher asks whether the indirect effect is moderated, or whether the mediated effect depends on levels of another variable. For example, suppose that a business implements a worksite-wellness program (the independent variable, X) to reduce obesity-related health risks in its employees. Program developers hypothesize that by increasing employee knowledge about the benefits of eating fruits and vegetables (the mediator variable, M), employee consumption of fruits and vegetables will increase (the dependent variable, Y), thus reducing health risk. An estimate of the indirect effect of the program on employee fruit and vegetable consumption through employee knowledge of the benefits of eating fruits and vegetables is unexpectedly low. Through talks with employees, it becomes apparent that participants were more or less motivated to gain and use knowledge from the program to improve their diet based on whether they had a family history of obesity-related illness such as diabetes or cardiovascular disease. Program developers hypothesize that participants’ family history of obesity-related illness may moderate the mediation relation in the data, affecting the influence of the program on employee knowledge of fruits and vegetables and its subsequent impact on fruit and vegetable consumption (See Fig. 1 ).

Conceptual diagram for the moderation of an indirect effect example
Can a Mediation Relation Explain an Interaction Effect in My Data?
Suppose a similar worksite-wellness program was implemented in a larger sister company and program effects had been dependent on whether the participant was a full or part-time employee at the company. To investigate the underlying reasons for this unexpected interaction, or moderation relation, program analysts could investigate a mediation hypothesis where the interaction effect predicts a mediator variable which predicts the outcome, defined here as the mediation of a moderator effect . For example, perhaps in addition to increasing employee knowledge of fruit and vegetable benefits with the wellness curriculum, the program (X) also introduced a work culture, or a social norm (M), of healthy eating which contributed to employee fruit and vegetable consumption (Y; See Fig. 2 ). Program developers hypothesize that the more hours an employee worked in a week determined how much they were subjected to the social norm which ultimately influenced their fruit and vegetable consumption.

Conceptual diagram for the mediation of a moderator effect example
Current Research
The purpose of this article is to provide a straightforward, methodological resource on models to simultaneously test mediation and moderation effects for the substantive researcher. To that end, we organize methods for simultaneously testing mediation and moderation into a single framework that allows for point estimation and construction of confidence intervals. Interpretation and effect computation are provided, and the model is applied to a substantive dataset to illustrate the methods. To ensure common ground for this discussion, basic mediation and moderation effects from which the model is formed are first reviewed.
Review of the Mediation Model
The mediation model offers an explanation for how, or why, two variables are related, where an intervening or mediating variable, M, is hypothesized to be intermediate in the relation between an independent variable, X, and an outcome, Y (See Fig. 3 ). Early presentations of mediation in prevention research (e.g., Baron and Kenny 1986 ; Judd and Kenny 1981a ; 1981b ) illustrated causal step methods to test for mediation, but more recent research has supported tests for statistical mediation based on coefficients from two or more of the following regression equations ( MacKinnon and Dwyer 1993 ):

Path diagram for the single-mediator model. Note. X= the independent variable, Y= the dependent variable, and M= the mediating variable. The mediation model decomposes the total effect of X on Y ( c ), into two parts: the indirect effect of X on Y, quantified by ab (the product of a and b ), and the direct effect of X on Y with the effect of the mediator removed, quantified by c ′. c = ab + c ′
Where c is the overall effect of the independent variable on Y; c ′ is the effect of the independent variable on Y controlling for M; b is the effect of the mediating variable on Y; a is the effect of the independent variable on the mediator; i 1 , i 2 , and i 3 are the intercepts for each equation; and e 1 , e 2 , and e 3 are the corresponding residuals in each equation (see Fig. 3 ).
Although there are alternative ways to estimate mediation, the product of coefficients is most easily applied to complex models and is used in this paper. The product of coefficients test computes the mediated effect as the product of the â and b ̂ coefficients from Eq. 2 and 3 . Sobel (1982 , 1986) derived the variance of â b ̂ product based on the multivariate delta method. This formula has been widely used to estimate the normal theory standard error of â b ̂ :
Where s a ^ 2 is the variance of the â coefficient and s b ^ 2 is the variance of the b ̂ coefficient.
MacKinnon et al. (1998) and MacKinnon and Lockwood (2001) showed that tests for the mediated effect based on normal theory can yield inaccurate confidence limits and significance tests, however, as the product of two normally distributed variables is not itself normally distributed. Alternative tests based on the asymmetric distribution of the product of two normally distributed variables are available and have been shown to outperform traditional methods ( MacKinnon et al. 2002 ; MacKinnon et al. 2004 ). A new program called “PRODCLIN” ( MacKinnon et al. 2007 ) has automated computation of the distribution of the product test for mediation so that it is widely accessible. The researcher need only specify values of â , b ̂ , the standard error of â , the standard error of b ̂ , and the statistical significance level desired.
Assumptions of the mediation model include the usual OLS estimation assumptions (e.g., correct specification of the model’s functional form, no omitted variables, no measurement error; Cohen et al. 2003 ). Mediation analysis also assumes correct causal ordering of the variables, no reverse causality effects, and no XM interaction.
Review of the Moderation Model
The moderation model tests whether the prediction of a dependent variable, Y, from an independent variable, X, differs across levels of a third variable, Z (See Fig. 4 ). Moderator variables affect the strength and/or direction of the relation between a predictor and an outcome: enhancing, reducing, or changing the influence of the predictor. Moderation effects are typically discussed as an interaction between factors or variables, where the effects of one variable depend on levels of the other variable in analysis. Detailed descriptions of moderator effects and a framework for their estimation and interpretation were presented in Aiken and West (1991) .

Alternate path diagram representations of the moderation model. Note. X= the independent variable, Y= the dependent variable, Z= the moderator variable, XZ= the product of X and the moderator variable, β 1 = the effect of X on Y, β 2 = the effect of Z on Y, and β 3 = the effect of XZ on Y
Moderation effects are tested with multiple regression analysis, where all predictor variables and their interaction term are centered prior to model estimation to improve interpretation of regression coefficients. A single regression equation forms the basic moderation model:
Where β 1 is the coefficient relating the independent variable, X, to the outcome, Y, when Z = 0, β 2 is the coefficient relating the moderator variable, Z, to the outcome when X = 0, i 5 the intercept in the equation, and e 5 is the residual in the equation.
The regression coefficient for the interaction term, β 3 , provides an estimate of the moderation effect. If β 3 is statistically different from zero, there is significant moderation of the X-Y relation in the data. Plotting interaction effects aids in the interpretation of moderation to show how the slope of Y on X is dependent on the value of the moderator variable. Regression slopes that correspond to the prediction of Y from X at a single value of Z are termed simple slopes.
Assumptions of the moderation model include OLS regression assumptions, as described earlier, and homogeneity of error variance. The latter assumption requires that the residual variance in the outcome that remains after predicting Y from X is equivalent across values of the moderator variable.
Combining Mediation and Moderation Analyses
Analyzing the models separately.
Much of the work combining mediation and moderation analyses has been presented in the context of prevention program design and development, where examining mediation and moderation effects together aims to improve program implementation by combining theory-driven ideas and empirical evidence. For example, Donaldson (2001) indicates that multivariate relations between variables in a treatment program tend to be one of three types: (a) direct effects, (b) mediated effects, and (c) moderated effects. By combining the examination of these effects in a single analysis, the researcher may not only identify mediating processes through which the program achieves its effects but may also identify effective program components and/or particular characteristics of the participants or the environment that moderate the effectiveness of the program. If the theoretical underpinnings of a treatment or prevention program serve as a starting point for its curriculum, separate analyses of mediation and moderation may be used to iteratively refine program theory. These analyses may be used to collect empirical feedback and to conduct pilot work of the program before large-scale implementation of the curriculum (See Fig. 5 ). Specifically, by examining mediation one is able to investigate how effective a program curriculum was in changing target behaviors, and whether the program aimed to alter appropriate mediators of desired outcomes. Analyzing moderation effects in this context allows the researcher to identify variables that may improve or reduce the program’s ability to alter mediating variables, as well as to examine the external validity, or generalizability, of the model across different groups or settings ( Hoyle and Robinson 2003 ). Hypothesized moderator variables may be more or less amenable to program tailoring, however. Although program subgroups may be formed on moderators such as age or gender with little difficulty, forming program subgroups based on other moderator variables such as ethnicity or family risk may be impractical and/or unethical. Nonetheless, the identification of subgroups for which a program is most effective is useful, and the examination of moderation and mediation effects in this context increases the scientific understanding of behaviors and improves program efficacy. West and Aiken (1997) have argued that these analyses are especially useful after the successful implementation and evaluation of a treatment program. This allows for the continual development and improvement of a program, but after an effective first evaluation.

Refining program theory: an empirical-theoretical exchange. Note. Figure is based on Donaldson (2001) diagram that occurs on p. 472 of that text
Analyzing the Models Simultaneously
By simultaneously investigating mediation and moderation, the effects may not only be disentangled and analyzed separately but can also be evaluated together. There have been two primary effects analyzed in the literature: (a) the mediation of a moderator effect, and (b) the moderation of an indirect effect. The mediation of a moderator effect involves exploring mediating mechanisms to explain an overall interaction of XZ in predicting Y, whereas the moderation of an indirect effect involves investigating whether a mediated relation holds across levels of a fourth, moderating variable. These effects have previously been referred to as mediated-moderation and moderated-mediation in the literature, respectively. These alternative descriptions may enhance the distinction between the two.
Previous models to simultaneously test mediation and moderation effects have been presented with varying notation (e.g., Edwards and Lambert 2007 ; James and Brett 1984 ; Muller et al. 2005 ; Preacher et al. 2007 ) or without testable equations (e.g., Baron and Kenny 1986 ; Wegener and Fabrigar 2000 ), making it difficult to understand similarities and differences among the methods. Moreover the criteria for testing the effects have varied across sources, making it hard to extrapolate recommendations for use. It is possible to create a general model to test these effects, however, that subsumes several previous frameworks by including all possible interactions between variables in the mediation and moderation models ( MacKinnon 2008 ). Such a model unifies the methods into a single presentation where different models are represented as special cases of the larger framework. Three regression equations form the model:
where all predictors in the model are centered at zero to improve interpretation of the lower order coefficients. In Eq. 6 , c 1 is the effect of the independent variable on the outcome when Z = 0 (also the average effect of X on Y because the mean of Z = 0), c 2 is the effect of the moderator variable on the outcome when X = 0 (also the average effect of Z on Y because the mean of X = 0), c 3 is the effect of the interaction between the independent variable and the moderator on the outcome, and i 6 and e 6 are the intercept and the residual in the equation, respectively. In Eq. 7 , a 1 is the effect of the independent variable on the mediator when Z = 0 (also the average effect of X on M because the mean of Z = 0), a 2 is the effect of the moderator variable on the mediator (also the average effect of Z on M because the mean of X = 0), a 3 is the effect of the interaction between the independent and moderator variables on the mediator, and i 7 and e 7 are the intercept and the residual in the equation, respectively. In Eq. 8 , c ′ 1 is the effect of the independent variable on the outcome when M = 0 and Z = 0 (the average effect of X on Y), c ′ 2 is the effect of the moderator on the outcome when X = 0 and M = 0 (the average effect of Z on Y), c ′ 3 is the effect of the interaction between the independent and moderator variables on the outcome when M = 0 (the average effect of XZ on Y), b 1 is the effect of the mediator on the outcome when X = 0 and Z = 0 (the average effect of M on Y), b 2 is the effect of the interaction between the moderator and mediator variables on the outcome when X = 0 (the average effect of MZ on Y), h is the effect of the interaction between the independent and mediator variables on the outcome when Z = 0 (the average effect of XM on Y), and j is the effect of the three-way interaction of the mediating, moderating, and independent variables on the outcome. The intercept and residual in Eq. 8 are coded i 8 and e 8 , respectively. A path diagram for the model is presented in Fig. 6 .

MacKinnon (2008) General Joint Analysis Mode. Note. X= the independent variable, Y= the dependent variable, Z= the moderator variable, M= the mediating variable, XZ= the interaction of X and Z, MZ=the interaction of M and Z, XM= the interaction of X and M, and XMZ= the three-way interaction between X, M, and Z
Assumptions of the general model include assumptions of the mediation and moderation models as described earlier. Issues of causal inference in non-additive models may also require additional stipulations for estimation. Note that the presence of any significant two-way interactions in the model implies that the main effects of X and M do not provide a complete interpretation of effects. The presence of a significant three-way interaction in the model also implies that lower order two-way interactions do not provide a complete interpretation of effects. If there are significant interactions, point estimates can be probed with plots and tests of simple effects to probe the interaction effects. Edwards and Lambert (2007) , Preacher et al. (2007) , and Tein et al. (2004) provide methods to perform these analyses.
Testing effects: Criteria for the moderation of an indirect effect
To examine whether an indirect effect is moderated, it is of interest to investigate whether the mediated effect ( ab ) differs across levels of a fourth, moderating variable. Previous sources have argued that this effect can be defined by either a moderated a path, a moderated b path, or both moderated a and b paths in the mediation model ( James and Brett 1984 ; Muller et al. 2005 ; Preacher et al. 2007 ; Wegener and Fabrigar 2000 ), such that if there is moderation in either path of the indirect effect then the mediated relation depends on the level of a moderator variable. There are circumstances, however, in which a heterogeneous a or b path does not imply a heterogeneous ab product term.
Although significant heterogeneity in either the a or b path may imply significant heterogeneity in the ab product term in some cases, examining moderation of the product term or moderation of both paths versus examining moderation of single paths in the mediation model are not conceptually identical. Consider the following example where a moderated a path in the mediation model means something different from both moderated a and b paths in the model. Presume that X is calcium intake, M is bone density, Y is the number of broken bones, and Z is gender. Calcium intake is known to have an effect on the bone density of women, and the relation between calcium intake and bone density is stronger in women than it is in men (i.e., heterogeneity in the a path in the model). Specifically, men have greater bone density in general and thus yield fewer gains from supplemental calcium intake. However, bone density affects the fragility of bones in a constant way across males and females, such that low bone density leads to more broken bones (i.e., no heterogeneity of the b path in the model). Previous models would deem this scenario as moderation of the indirect effect, arguing that moderation of the a path suffices as a test for the effect. There are two problems with this argument. First, testing the heterogeneity of only the a or b path in the mediation model is not a test of mediation because only a single link in the mediated effect is tested in each case. Second, a heterogeneous a path in this model suggests something different from both heterogeneous a and b paths or a heterogeneous ab product. Heterogeneity in both paths of the mediated effect would suggest that gender not only moderates the effect of calcium intake on bone density, but that gender also moderates the effect of bone density on broken bones. Heterogeneity of the product estimate of the mediated effect would suggest that gender moderates the mechanism by which calcium intake affects bone loss; this may or may not be true based on the research literature. Although the moderation of a single path may imply moderation of the product term in some cases, it is critical to differentiate the scenarios as they correspond to different research hypotheses.
There are also numerical examples that show instances when heterogeneity in individual paths of the mediation model does not imply heterogeneity of the product term. Consider the following mediated effect scenarios in two moderator-based subgroups:
In both scenarios the a and b paths are heterogeneous across groups thus satisfying criteria for the moderation of a mediated effect as defined by Edward and Lambert (2007) , James and Brett (1984) , Morgan-Lopez and MacKinnon (2006) , Preacher et al. (2007) , and Wegener and Fabrigar (2000) . However the ab product is identical across groups, indicating that there is no moderation of the indirect effect. Although tests for the moderation of a mediated effect based on the heterogeneity of individual path coefficients in the mediation model will be more powerful than a test based on the heterogeneity of the product term (given the usual low power to detect interactions), these tests may also have elevated Type 1 error rates.
Despite potential problems for making inferences on moderation of the ab product from information on the moderation of individual paths, initial simulation work suggests that extending a test of joint significance (where the test for mediation is based on the significance of component paths in the model such that if both â and b ̂ are significant then the mediated effect â b ̂ is deemed significant) to models for mediation and moderation may be acceptable. Specifically, if conclusions about the moderation of â b ̂ are based on whether both â and b ̂ are significantly affected by the moderator variable, Z, Type 1 error rates never exceed .0550 ( Fairchild 2008 ). Effects of the moderator variable on component paths of the mediation model are examined using Eq. 7 and 8 , where â 3 quantifies the effect of Z on the a path and b ̂ 2 quantifies the effect of Z on the b path, respectively (See Fig. 6 ). If both coefficients are significant, it may be claimed that there is significant moderation of the indirect effect. To obtain either a point estimate or confidence limits for the effect, a product of coefficients test can be used.
To estimate a product of coefficients test for moderation of the indirect effect in the case of a dichotomous moderator variable, separate mediation models can be estimated for each group and equivalence of the â b ̂ point estimates can be compared across moderator-based subgroups. An example of a dichotomous moderator variable might be gender or clinical diagnosis. The null hypothesis associated with the test is that the difference between the two mediated effect point estimates in each group is zero:
If the point estimates in each group are statistically different from one another, there is significant moderation of the indirect effect (i.e., heterogeneity in the ab product), such that the mediated effect is moderated by group membership. To test the estimate in Eq. 9 for statistical significance, the difference is divided by a standard error for the estimate to form a z statistic. If the groups are independent, the standard error of the difference between the two coefficients is:
Where s a ^ b ^ group 1 2 is the variance of the mediated effect in group 1 and s a ^ b ^ group 2 2 is the variance of the mediated effect in group 2.To test heterogeneity of the indirect effect in the case of a continuous moderator variable, variance in the estimates of the ab product across levels of the moderator variable is examined. An example of a continuous moderator variable might be individual motivation to improve. Because a z test as shown above can only accommodate moderator variables with two levels (or a small number of levels with contrasts between two variables) and because levels of continuous moderators often do not represent distinct groups, tests with continuous moderators are more complicated. The question becomes how to assess differences in the ab product across a large number of levels of the moderator variable, and the answer to that question is incomplete at this time. Random coefficient models assess variance in regression coefficients across multiple levels such as multiple levels of a moderator. If the moderator is thought of as a higher order variable across which lower order effects (such as ab ) may vary, the random coefficient modeling framework may be suitable to assess variance in the indirect effect across levels of a continuous moderator. Kenny, Korchmaros, and Bolger (2003) describe an estimate of the variance of ab when a and b are correlated for the case of random effects based on Aroian (1947) :
Bauer, Preacher, and Gil (2006) make an important distinction between the variance of ab and the variance of the average value of ab in the multilevel model. It is the variance of ab that is relevant to the question of whether an indirect effect is moderated as we would like to know if there is substantial variability in ab across levels of the moderator variable.
It is also possible to test whether individual paths in the mediation model differ across levels of a moderator variable. These tests can investigate moderation of the direct effect of X on Y ( c ′) or evaluate the generalizability of action and conceptual theory for a program. The null hypothesis to test homogeneity of a program’s direct effect is:
This hypothesis is tested by examining the significance of c ^ 3 ′ in Eq. 8 ; if the regression coefficient is significant, there is significant moderation of the program’s direct effect.
Recall that conceptual theory for a program corresponds to the b path of the mediation model, which defines the theory that links variables or psychological constructs (e.g., M) to behavioral outcomes. The relationships examined in this piece of the model are driven by previous models or theories presented in the literature that explain motivations for behavior. The null hypothesis to test homogeneity of a program’s conceptual theory across levels of the moderator variable is:
This hypothesis is tested by examining the significance of b ̂ 2 in Eq. 8 ; if the regression coefficient is significant there is significant moderation of the program conceptual theory.
Action theory for a program corresponds to the a path of the mediation model, which defines what components of the program are designed to manipulate the mechanisms of change. This piece of the model illustrates how the program intervenes to modify hypothesized mediators. The null hypothesis to test homogeneity of a program’s action theory across levels of the moderator variable is:
This hypothesis is tested by examining the significance of â 3 in Eq. 7 ; if the regression coefficient is significant there is significant moderation of the program action theory. See Chen (1990) for more details on program action and conceptual theory.
Testing effects: Criteria for the mediation of a moderator effect
A test for the mediation of a moderator effect examines whether the magnitude of an overall interaction effect of the independent variable (X) and the moderator variable (Z) on the dependent variable (Y) is reduced once the mediator is accounted for in the model ( Muller et al. 2005 ). Thus, the examination of the mediation of a moderator effect considers the mediation model as a means to explain why a treatment effect of X on Y is moderated by a third variable, Z. In this way, the mediation of a moderator effect hypothesis probes mediation as a possible process that accounts for the interaction of the treatment and the outcome. There is no need to differentiate methods for categorical and continuous moderator variables here.
One way to test whether an XZ interaction in the data is accounted for, at least in part, by a mediating relation is to examine whether the magnitude of the regression coefficient corresponding to the overall interaction effect, c 3 , is reduced once the mediator is added to the model. Using coefficients from Equations 6 and 8 , where ĉ ′ 3 represents the direct effect of the interaction effect on Y once the mediator is included in the model, a point estimate and standard error of this difference can be computed:
Dividing an estimate of the difference in Eq. 15 by its standard error in Eq. 16 provides a test of significance for the estimate. If there is a significant difference in the coefficients then the overall moderation effect of XZ on Y is significantly explained, at least in part, by a mediated relation. The null hypothesis corresponding to this effect is that there is no reduction in the overall interaction once accounting for the mediator:
A test of the mediation of a moderator effect with a product of coefficients estimator circumvents the need for testing the overall interaction of XZ on Y as shown above. Morgan-Lopez and MacKinnon (2006) presented a point estimator and standard error for the product of coefficients method:
The product of coefficients estimator in Eq. 18 illustrates that the effect of the interaction of the independent and moderator variables on the outcome (represented by â 3 ) is transmitted through a mediating variable (represented by b ̂ 1 ). Dividing the estimate of the product by an estimate of its standard error in Eq. 19 provides a test of significance for the estimate based on normal theory. However, as described for the product of coefficients estimator in the single mediator model, asymmetric confidence intervals for the distribution of the product are more accurate than tests based on the normal distribution and should be implemented (note also that a test of joint significance of the coefficients could be conducted). If the product is significantly different from zero, then the moderator effect is explained, at least in part, by a mediating mechanism. The null hypothesis corresponding to this test is that the product of the coefficients is equal to zero:
Numerical Examples
Returning now to the research scenarios presented at the beginning of this paper, numerical examples can be provided to show how these analyses may appear in practice. Two simulated datasets were created to explore the questions. Scenario #1 asked “ Is the process by which a program has an effect the same across different types of participants? ” The researcher investigates whether the indirect effect is moderated to answer this question. Note that although it is necessary to estimate the XM interaction in Equation 8 to avoid bias in the XZ term ( Yzerbyt, Muller, and Judd 2004 ), the XMZ interaction need not be estimated if there is no supporting hypothesis for its existence. Likewise, Equation 6 need not be estimated if overall effects are not part of the research question. Recall that for this example the independent variable, X, was a worksite-wellness program to reduce obesity-related health risk. The mediating variable, M, was knowledge of the benefits of eating fruits and vegetables, and the outcome, Y, was employee consumption of fruits and vegetables. The moderator variable in analysis, Z, was family history of obesity-related illness with 107 employees having no history of disease (i.e., n group1 = 107 ) and 93 employees having a history of disease (i.e., n group2 = 93 ). The null hypothesis for the research question in Scenario #1 is that there is no difference in the indirect effect of the program on employee fruit and vegetable consumption through knowledge of fruit and vegetable benefits across employees who have a family history of disease versus those who have no family history of disease. To test this hypothesis, an estimate of the mediated effect can be computed in each group and compared for differences. Estimating Equations 2 and 3 , the mediated effect, â b ̂ ( s â b ̂ ), for employees with no family history of obesity-related disease was computed as .0004(.0186), with an asymmetric confidence interval of −.0400, .0413, where â ( s â ) = .0041(.2018)and b ̂ ( s b ̂ ) = .0923(.0969) were used as inputs into the PRODCLIN program ( MacKinnon et al. 2007 ). The mediated effect for employees with a family history of obesity-related disease was .2158(.0821), with an asymmetric confidence interval of .0693, .3909, where â ( s â ) = .5479(.1854) and b ̂ ( s b ̂ ) = .3938(.0687) were used as inputs into the PRODCLIN program ( MacKinnon et al. 2007 ). Initial inspection of the indirect effects shows that the mediation relation in the data is significant for individuals with a family history of obesity-related illness (the simple mediated effect for group 1) and nonsignificant for individuals with no family history of disease (the simple mediated effect for group 2). Statistical inspection of the difference between the two indirect effects shows a difference of .2154. Using Eq. 11 , the pooled standard error for this difference was computed as .0421, yielding a z -statistic of 5.1143. Because the obtained z -value is greater than the critical value (1.96) associated with α = .05, there is significant moderation in the indirect effect such that ab is heterogeneous across moderator-based subgroups. Alternatively estimating Equations 7 and 8 and examining a test of joint significance of the â 3 ( s â 3 ) and b ̂ 2 ( s b ̂ 2 ) coefficients to examine whether the indirect effect is moderated yields the same conclusion. Both parameter estimates are significant: â 3 ( s â 3 ) = .2760(.1386) and b ̂ 2 ( s b ̂ 2 ) = .1919(.0697), respectively. Because the moderator variable in this example only had two levels, there is no need to follow up the finding with simple effects; the difference in the mediated effects occurs between the simple mediated effect in group 1 and the simple mediated effect in group 2. Had there been three or more levels of the moderator variable in the example, the researcher could conduct follow up analyses to investigate simple mediated effects at different levels of the moderator variable to see where specific differences occur. Had there also been a significant XM interaction in the data, the researcher would need to estimate simple mediated effects across levels of X as well.
Scenario #2 asked “ Can a mediation relation explain an interaction effect in my data? ” To answer this question, the researcher investigates whether the interaction effect can predict a mediating variable which in turn predicts the outcome. Recall that X was the worksite-wellness program described above, M was a social norm for eating fruits and vegetables, Z was part-time or full-time work status (191 part-time employees, 209 full-time employees), and Y was fruit and vegetable consumption. The null hypothesis for the research question is that a mediating variable cannot explain any part of the interaction. To test this hypothesis, the â 3 b ̂ 1 point estimate is computed for the data and tested for significance. As indicated in the numerical example for Research Scenario #1, although it is necessary to estimate the XM interaction to avoid bias in the XZ term ( Yzerbyt et al. 2004 ), there is no need to model the XMZ interaction if there is no hypothesis to support its estimation. Estimating Eq. 7 and 8 , â 3 b ̂ 1 was computed as .1781 (.0814), with an asymmetric confidence interval of .0192, .3383, where â 3 ( s â 3 ) = .1550(.0706) and b ̂ 1 ( s b ̂ 1 ) = 1.1495(.0405) were used as inputs into the PRODCLIN program ( MacKinnon et al. 2007 ). Failure to include zero in the confidence interval indicates significance of the point estimate, such that the interaction of the program effect and part-time versus full-time work status is explained at least in part by the mediating mechanism of social norms for eating fruits and vegetables. Example SAS datasets, program code and output, and complete worked examples for the general model to simultaneously test mediation and moderation effects are available from the first author.
Issues of Power in Models to Test Mediation and Moderation Effects
Power to detect interaction effects is often low because of the small effect sizes observed in social science ( Aiken and West 1991 ). Models that simultaneously examine mediation and moderation effects are at an even greater disadvantage as they involve several interaction terms as well as estimation of indirect effects. Fairchild (2008) investigated power for the general model using previous arguments made in the literature that interaction effects in real data typically range from explaining 1% to 3% of the variance in the dependent variable ( Champoux and Peters 1987 ; Evans 1985 ; McClelland and Judd 1993 ). Results showed that when the effect size of the â 3 and b ̂ 2 parameters both explained 1% of the variance, a test of joint significance for moderation of the indirect effect required N ≥ 1000 for .8 power. If the effect size of the parameters both explained 3% of the variance, the sample size requirement for .8 power reduced to N ≥ 500. Morgan-Lopez and MacKinnon (2006) showed similar results for tests to examine the mediation of an interaction effect.
The large sample size requirement of these tests makes investigation of mediation and moderation effects in small samples challenging. Effect size analysis usually offers a guide to the practical utility of effects that is independent of significance and sample size, but effect size measures for models that simultaneously analyze mediation and moderation effects are not yet fully researched. Although increasing sample size has historically been most utilized to increase the power of a test, there are several alternatives that may increase power when accessing additional data is not possible ( Beck 1994 ).
Statistical significance of a test is based on four parameters: sample size, effect size, power, and the alpha-level set for the experiment. Thus to achieve .8 power when sample size is fixed either the effect size of the treatment and/or the alpha level of the experiment may be manipulated to increase sensitivity of the research design. For example, rather than implementing the nominal .05 criterion that allows for errors in null-hypothesis-significance-testing 5 times out of every 100, the researcher may choose to evaluate results against a more liberal .10 criterion that allows for errors 10% of the time. Increasing the Type 1 error rate for the study decreases the possibility of making a Type 2 error, or the failure to find a significant effect when one truly exists in the data. This option may be appropriate when an effect of interest is especially important and avoiding Type 2 errors is critical. A second option for increasing power when sample size is limited in a study is to increase the effect size of the manipulation. This option involves increasing the between-group variance on the independent variable in the design and decreasing the within-group variance, or error variance, of the variables ( Beck 1994 ). To increase the between-group variance on the independent variable the researcher may impose an extreme-groups design (see Borich and Godbout 1974 ) or deliver a stronger manipulation so that differences between the treatment and control groups are exaggerated. When the strength of the intervention is not easily increased or formation of extreme groups is not feasible, researchers may opt to focus on decreasing within-group variance, or error variance, in the design. Statistical methods to reduce error variance include improving reliability of the measurement tools used in analysis and implementing analysis of covariance models that partition covariates out of treatment effects. Research design tools that are able to reduce error variance in a model include blocking and matching designs that attempt to equate treatment and control groups on non-intervention dimensions in which they may differ ( Cook and Campbell 1979 ).
Recommendations
To best implement the simultaneous analysis of mediation and moderator effects, the researcher should first identify which third variable model characterizes his or her primary research question. This a priori model identification acts as the starting point of interpretation and can be guided by theory or previous research. If the primary research question is determined to be mediation, the researcher may examine all possible interaction effects in the model or a subset of theoretically-relevant interactions and discuss which moderated effects exist. Methods for examining the moderation of the indirect effect have been discussed in this manuscript, as well as methods for examining moderation in individual links of the mediation model such as the direct effect. Exploring the moderation of either the total or direct effect in the mediation model is simply a means of examining the significance of the individual regression coefficients corresponding to those effects (i.e., c 3 and c ′ 3 , respectively), and any significant interaction effect in the model will be interpreted with reference to mediation. Interactions may be further probed with simple slopes analyses to examine variable relations across levels of the moderator variable ( Edwards and Lambert 2007 ; Preacher et al. 2007 ; Tein et al. 2004 ).
If the statistical model appropriate to answer the primary research question is determined to be moderation, mediation may be investigated as a means to explain an overall moderated treatment effect. To that end, the interpretation of interaction effects in the model focus on whether a mediating mechanism is responsible, at least in part, for overall moderation in the data. As presented in the manuscript, this assessment may be made with either a difference in coefficients ( c ^ 3 − c ^ 3 ′ ) or product of coefficients ( â 3 b ̂ 1 ) estimator. Both tests examine whether there is a decrease in the overall XZ interaction once a mediating variable is modeled. However, given the availability of more accurate significance testing for the product of coefficients estimator, it is recommended that researchers analyze â 3 b ̂ 1 . Preferably, the exploration of possible interactions in either model are well-thought-out and delineated by theory to avoid confusion and unwieldy models, as well as to facilitate interpretation of effects in the model. Regardless of which third variable model is identified with the primary research question, it is necessary to model the XM interaction to avoid bias in the XZ product term ( Yzerbyt, Muller, and Judd 2004 ).
Limitations and Future Directions
The cost of the generalizability of the general model to test mediation and moderation effects is possible inflation of Type I error, lack of power, and difficulty with interpretation of model parameters if several effects are present. The model may be simplified, however, to represent more specialized cases of mediation and moderation joint effects such as baseline by treatment interactions by constraining paths in the model to be zero.
An additional limitation of models with moderation and mediation is the extensive assumptions required for accurate assessment of relations among variables ( Holland 1988 ). The sensitivity of conclusions to violations of assumptions is not yet known and correct conclusions will likely require repeated applications in any substantive research area. In particular, often the X variable is the only variable that represents random assignment, making interpretation of causal relations between other variables in the model susceptible to omitted variable bias. In many applications, the model results may represent descriptive information about how variables are related rather than elucidating true causal relations among variables. Information on true causal relations will require programs of research to replicate and extend results as well as information from other sources such as qualitative information and replication studies in different substantive research areas.
Estimation of the methods and their performance can be investigated for categorical and longitudinal data. Previous work has explored the needs of categorical data in the single mediator model ( MacKinnon and Dwyer 1993 ) and this research may serve as a resource for extending the general model to test mediation and moderation effects into that domain. With regard to longitudinal data, the current model can be applied to two-wave data by using difference scores or residualized change scores, but data with three or more waves cannot yet be accommodated. The addition of cross-lagged effects in longitudinal data frameworks such as autoregressive models or latent growth models increases the possible number of interactions among variables and whether the investigation of all possible cross-lagged effects is valuable or overly cumbersome will need to be determined.
In summary, many questions in prevention science involve how and for whom a program achieves its effects. Mediation and moderation models are ideal for examining these questions. By investigating both mediation and moderation effects in data from prevention programs information about the mechanisms underlying program effects as well as the generalizability of program effects and curricula can be evaluated. Models that simultaneously estimate mediation and moderation effects not only allow for the examination of these questions, but also permit the evaluation of more complex research hypotheses such as whether a moderator effect in the data can be explained by a mediating mechanism, or whether a mediating mechanism depends on the level of another variable. A broad integration of these models into the substantive research literature will enhance the information drawn from prevention work and will inform our knowledge on prevention models.
Contributor Information
Amanda J. Fairchild, Department of Psychology, University of South Carolina, Barnwell College, 1512 Pendleton St., Columbia, SC 29208, USA.
David P. MacKinnon, Research in Prevention Lab, Department of Psychology, Arizona State University, P.O. Box 871104, Tempe, AZ 85287-1104, USA.
- Aiken LS, West SG. Multiple regression: Testing and interpreting interactions. Newbury Park, CA: Sage; 1991. [ Google Scholar ]
- Aroian LA. The probability function of the product of two normally distributed variables. Annals of Mathematical Statistics. 1947; 18 :265–271. doi: 10.1214/aoms/1177730442. [ CrossRef ] [ Google Scholar ]
- Baron RM, Kenny DA. The moderator-mediator variable distinction in social psychological research: Conceptual, strategic, and statistical considerations. Journal of Personality and Social Psychology. 1986; 51 :1173–1182. doi: 10.1037/0022-3514.51.6.1173. [ PubMed ] [ CrossRef ] [ Google Scholar ]
- Bauer DJ, Preacher KJ, Gil KM. Conceptualizing and testing random indirect effects and moderated mediation in multilevel models: New procedures and recommendations. Psychological Methods. 2006; 11 :142–163. [ PubMed ] [ Google Scholar ]
- Beck CT. Achieving statistical power through research design sensitivity. Journal of Advanced Nursing. 1994; 20 :912–916. [ PubMed ] [ Google Scholar ]
- Borich GD, Godbout RC. Extreme groups designs and the evaluation of statistical power. Educational and Psychological Measurement. 1974; 34 :663–675. [ Google Scholar ]
- Champoux JE, Peters WS. Form, effect size, and power in moderated regression analysis. Journal of Occupational Psychology. 1987; 60 :243–255. [ Google Scholar ]
- Chen HT. Theory driven evaluations. Newbury Park, CA: Sage; 1990. [ Google Scholar ]
- Cohen J, Cohen P, West SG, Aiken LA. Applied multiple regression/correlation analysis for the behavioral sciences. 3. Hillsdale, NJ: Lawrence Erlbaum Associates; 2003. [ Google Scholar ]
- Cook TD, Campbell DT. Quasi-experimentation: Design and analysis issues for field settings. Chicago: Rand McNally; 1979. [ Google Scholar ]
- Dearing E, Hamilton LC. Contemporary advances and classic advice for analyzing mediating and moderating variables. Monographs of the Society for Research in Child Development. 2006 December; 71 :88–104. [ Google Scholar ]
- Donaldson SI. Mediator and moderator analysis in program development. In: Sussman S, editor. Handbook of program development for health and behavior research and practice. Thousand Oaks, CA: Sage; 2001. pp. 470–496. [ Google Scholar ]
- Edwards JR, Lambert LS. Methods for integrating moderation and mediation: A general analytical framework using moderated path analysis. Psychological Methods. 2007; 12 :1–22. doi: 10.1037/1082-989X.12.1.1. [ PubMed ] [ CrossRef ] [ Google Scholar ]
- Evans MG. A Monte Carlo study of the effects of correlated method variance in moderated multiple regression analysis. Organizational Behavior and Human Decisions Processes. 1985; 36 :305–323. [ Google Scholar ]
- Fairchild AJ. A comparison of frameworks for the joint analysis of mediation and moderation effects. Arizona State University; Tempe: 2008. Unpublished doctoral dissertation. [ Google Scholar ]
- Frazier PA, Tix AP, Barron KE. Testing moderator and mediator effects in counseling psychology research. Journal of Counseling Psychology. 2004; 51 :115–134. doi: 10.1037/0022-0167.51.1.115. [ CrossRef ] [ Google Scholar ]
- Gogineni A, Alsup R, Gillespie D. Mediation and moderation in social work research. Social Work Research. 1995; 19 :57–63. [ PubMed ] [ Google Scholar ]
- Holland PW. Causal inference, path analysis, and recursive structural equations models. Sociological Methodology. 1988; 18 :449–484. doi: 10.2307/271055. [ CrossRef ] [ Google Scholar ]
- Hoyle RH, Robinson JC. Mediated and moderated effects in social psychological research: Measurement, design, and analysis issues. In: Sansone C, Morf C, Panter AT, editors. Handbook of methods in social psychology. Thousand Oaks, CA: Sage; 2003. pp. 213–233. [ Google Scholar ]
- James LR, Brett JM. Mediators, moderators, and tests for mediation. The Journal of Applied Psychology. 1984; 69 :307–321. doi: 10.1037/0021-9010.69.2.307. [ CrossRef ] [ Google Scholar ]
- Judd CM, Kenny DA. Process analysis: Estimating mediation in treatment evaluations. Evaluation Review. 1981a; 5 :602–619. doi: 10.1177/0193841X8100500502. [ CrossRef ] [ Google Scholar ]
- Judd CM, Kenny DA. Estimating the effects of social intervention. Cambridge, England: Cambridge University Press; 1981b. [ Google Scholar ]
- Kenny DA, Korchmaros JD, Bolger N. Lower level mediation in multilevel models. Psychological Methods. 2003; 8 :115–128. [ PubMed ] [ Google Scholar ]
- MacKinnon DP. Introduction to statistical mediation analysis. Mahwah, NJ: Earlbaum; 2008. [ Google Scholar ]
- MacKinnon DP, Dwyer JH. Estimating mediated effects in prevention studies. Evaluation Review. 1993; 17 :144–158. doi: 10.1177/0193841X9301700202. [ CrossRef ] [ Google Scholar ]
- MacKinnon DP, Fritz MS, Williams J, Lockwood CM. Distribution of the product confidence limits for the indirect effect: Program PRODCLIN. Behavior Research Methods. 2007; 39 :384–389. [ PMC free article ] [ PubMed ] [ Google Scholar ]
- MacKinnon DP, Krull JL, Lockwood CM. Equivalence of the mediation, confounding, and suppression effect. Prevention Science. 2000; 1 :173–181. doi: 10.1023/A:1026595011371. [ PMC free article ] [ PubMed ] [ CrossRef ] [ Google Scholar ]
- MacKinnon DP, Lockwood CM. Distribution of product tests for the mediated effect: Power and Type I error rates. 2001 Unpublished manuscript. [ Google Scholar ]
- MacKinnon DP, Lockwood CM, Hoffman JM. A new method to test for mediation. Paper presented at the annual meeting of the Society for Prevention Research; Park City, UT. 1998. Jun, [ Google Scholar ]
- MacKinnon DP, Lockwood CM, Hoffman JM, West SG, Sheets V. A comparison of methods to test the significance of mediation and other intervening variable effects. Psychological Methods. 2002; 7 :83–104. doi: 10.1037/1082-989X.7.1.83. [ PMC free article ] [ PubMed ] [ CrossRef ] [ Google Scholar ]
- MacKinnon DP, Lockwood CM, Williams J. Confidence limits for the indirect effect: Distribution of the product and resampling methods. Multivariate Behavioral Research. 2004; 39 :99–128. doi: 10.1207/s15327906mbr3901_4. [ PMC free article ] [ PubMed ] [ CrossRef ] [ Google Scholar ]
- McClelland GH, Judd CM. Statistical difficulties of detecting interactions and moderator effects. Psychological Bulletin. 1993; 114 :376–390. [ PubMed ] [ Google Scholar ]
- Morgan-Lopez AA, MacKinnon DP. Demonstration and evaluation of a method for assessing mediated moderation. Behavior Research Methods. 2006; 38 :77–87. [ PubMed ] [ Google Scholar ]
- Muller D, Judd CM, Yzerbyt VY. When moderation is mediated and mediation is moderated. Journal of Personality and Social Psychology. 2005; 89 :852–863. doi: 10.1037/0022-3514.89.6.852. [ PubMed ] [ CrossRef ] [ Google Scholar ]
- Preacher KJ, Rucker DD, Hayes RF. Addressing moderated mediation hypotheses: Theory, methods, and prescriptions. Multivariate Behavioral Research. 2007; 42 :185–227. [ PubMed ] [ Google Scholar ]
- Rose BM, Holmbeck GN, Coakley RM, Franks EA. Mediator and moderator effects in developmental and behavioral pediatric research. Journal of Developmental and Behavioral Pediatrics. 2004; 25 :58–67. doi: 10.1097/00004703-200402000-00013. [ PubMed ] [ CrossRef ] [ Google Scholar ]
- Sobel ME. Asymptotic confidence intervals for indirect effects in structural equation models. Sociological Methodology. 1982; 13 :290–312. doi: 10.2307/270723. [ CrossRef ] [ Google Scholar ]
- Sobel ME. Some new results on indirect effects and their standard errors in covariance structure models. Sociological Methodology. 1986; 16 :159–186. doi: 10.2307/270922. [ CrossRef ] [ Google Scholar ]
- Tein J, Sandler IN, MacKinnon DP, Wolchik SA. How did it work? Who did it work for? Mediation in the context of a moderated prevention effect for children of divorce. Journal of Consulting and Clinical Psychology. 2004; 72 :617–624. doi: 10.1037/0022-006X.72.4.617. [ PMC free article ] [ PubMed ] [ CrossRef ] [ Google Scholar ]
- Wegener DT, Fabrigar LR. Analysis and design for nonexperimental data addressing causal and noncausal hypotheses. In: Reis HT, Judd CM, editors. Handbook of research methods in social and personality psychology. New York: Cambridge University Press; 2000. pp. 412–450. [ Google Scholar ]
- West SG, Aiken LS. Toward understanding individual effects in multiple component prevention programs: Design and analysis strategies. In: Bryant K, Windle M, West S, editors. The science of prevention: Methodological advances from alcohol and substance abuse research. Washington, DC: American Psychological Association; 1997. pp. 167–209. [ Google Scholar ]
- Yzerbyt VY, Muller D, Judd CM. Adjusting researchers’ approach to adjustment: On the use of covariates when testing interactions. Journal of Experimental Social Psychology. 2004; 40 :424–431. doi: 10.1016/j.jesp.2003.10.001. [ CrossRef ] [ Google Scholar ]
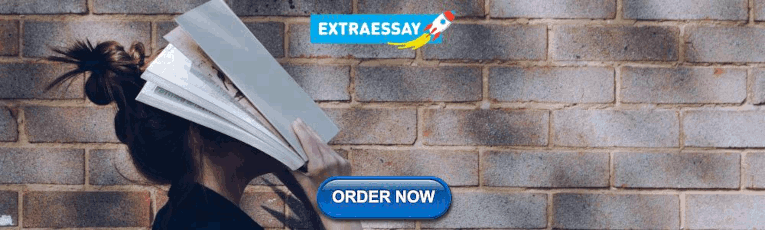
IMAGES
VIDEO
COMMENTS
When a moderator is continuous, usually you're making statements like: "As the value of the moderator increases, the relationship between X and Y also increases.". Mediation. "Does X predict M, which in turn predicts Y?". We might know that X leads to Y, but a mediation hypothesis proposes a mediating, or intervening variable. That is ...
For mediation, researchers generally work with a theory-derived mediation hypothesis and collect data that allows them to test the null hypothesis of no mediation. It is a search for a well-defined form of information, and further the search is considered complete when that information is obtained.
Mediation analysis was developed to assess this "black box," and psychologists and social scientists have utilized this framework particularly frequently. ... it is strongly recommended to construct a directed acyclic graph depicting the central hypothesis before conducting a causal mediation analysis. Open in a separate window.
For mediation, researchers generally work with a theory-derived mediation hypothesis and collect data that allows them to test the null hypothesis of no mediation. It is a search for a well-defined form of information, and further the search is considered complete when that information is obtained.
Calvete and Cardenoso (2005) demonstrated that the effect of gender on depressive symptoms is mediated by need for acceptance, positive thinking, self-focused negative cognitions, and negative problem orientation. Hundreds of new mediation hypotheses are proposed and tested in the literature every year.
The primary hypothesis of interest in a mediation analysis is to see whether the effect of the independent variable (intervention) on the outcome can be mediated by a change in the mediating variable. In a full mediation process, the effect is 100% mediated by the mediator, that is, in the presence of the mediator, the pathway connecting the ...
a mediation hypothesis, specific effects vs. a global model, directness vs. indirectness of causation, effect size vs. null hypothesis testing, and hypothesized vs. alternative explanations.
We discuss five such pairs of perspectives on mediation analysis, their associated advantages and disadvantages, and their implications: with vs. without a mediation hypothesis, specific effects vs. a global model, directness vs. indirectness of causation, effect size vs. null hypothesis testing, and hypothesized vs. alternative explanations.
4.1 Introduction. Mediation analysis is a powerful statistical technique used to understand the relationship between two variables and how one variable influences the other through a mediator variable. To illustrate the importance of mediation analysis, consider the following caselet. Suppose a researcher is interested in examining the ...
In Table 3, Step 4 shows that authoritarian leadership was significantly related to façades of conformity (β = 0.74, p < 0.01), thus supporting Hypothesis 2. Based on the mediation analysis proposed by Baron and Kenny , the results also reported that authoritarian leadership was positively associated with façades of conformity (β = 0.16, p ...
Step 1 refers to hypothesis development for the mediation effect. In this step, we need to develop a mediation hypothesis by supporting theory and literature using the Transmittal Approach or the Segmentation Approach. For instance, suppose a unified theory, a sequential theory such as the value-attitude-behaviour theory, is available to ...
Limitations of the Indirect effect hypothesis. An inherent weakness of Indirect effect hypothesis, and in particular the mediator-based explanation, is that it does not consider the phenomenal experience of the individual who claims that they both experience sadness, and that the sadness itself, for them, forms at least part of the pleasure [e.g., 6].
Basing mediation analysis on hypotheses derived from sound social science theory can help to address concerns about the proposed causal sequence. Current methodological developments will contribute to making mediation analysis an even more versatile tool when it comes to investigating mechanisms in dynamic and interactive contexts. Biographies
Statistical mediation analysis is commonly used in examining a mediational hypothesis, but it is not the default paradigm. The measurement-of-mediation design is limited because it does not allow causal inference between mediators and dependent variables, so designs for experimental mediation analysis are gaining increased attention.
Mediation analysis is widely used to study whether the effect of an independent variable on an outcome is transmitted through a mediator. Bayesian methods have become increasingly popular for mediation analysis. However, limited research has been done on formal Bayesian hypothesis testing of mediation. Although hypothesis testing using Bayes factor for a single path is readily available, how ...
The multilevel moderated mediation analysis was conducted to explore the processes by which knowledge manipulation and reputational concern shaped intergroup cooperation. Contrary to the reputation management hypothesis, we found that common knowledge manipulation increased both ingroup and outgroup cooperation via increased reputational concern.
The second category of mediation methods examines either one or a few mediators that are within a genomic testing unit (e.g., a gene) one at a time. However, different from standard testing approaches, these new methods explicitly account for the composite nature of the null mediation effect hypothesis to obtain calibrated test statistics.
Although this type of experiment does much to reduce alternative explanations of the mediation hypothesis, it may be difficult to implement double randomization in other research contexts. Generally, the most difficult aspect of the design is the ability to randomly assign participants to the levels of the mediator so that the M → Y relation ...
Abstract. This article provides researchers with a guide to properly construe and conduct analyses of conditional indirect effects, commonly known as moderated mediation effects. We disentangle conflicting definitions of moderated mediation and describe approaches for estimating and testing a variety of hypotheses involving conditional indirect ...
HYPOTHESIS AND THEORY article. Front. Psychol. Sec. Sport Psychology Volume 15 ... 0.170, 0.171. The chain mediation effect size of resilience and positive coping style was 0.042, while the chain mediation effect size of resilience and negative coping style was 0.050. Conclusion: Physical activity positively predicts Chinese junior high school ...
Background Mediation analysis investigates whether a variable (i.e., mediator) changes in regard to an independent variable, in turn, affecting a dependent variable. Moderation analysis, on the other hand, investigates whether the statistical interaction between independent variables predict a dependent variable. Although this difference between these two types of analysis is explicit in ...
Mediation is a third-variable effect that explains how or why 2 variables relate. By construction, mediation analysis implies a causal process that connects ≥2 variables. ... As described earlier, although this requirement may appear intuitive, the logic is fallible given the nature of null hypothesis significance testing and other ...
1. Testing Mediation with Regression Analysis. Mediation is a hypothesized causal chain in which one variable affects a second variable that, in turn, affects a third variable. The intervening variable, M, is the mediator. It "mediates" the relationship. between a predictor, X, and an outcome. Graphically, mediation can be depicted in the ...
Mediation Analysis. Statistical Analysis. A mediational hypothesis is a kind of statistical test that assumes that the relationship between an independent and dependent variable is mediated by (or accounted for by) a third variable. This is what is known as a mediating variable. The goal of the mediating variable is to completely mediate the ...
Mediation analyses were fitted to investigate the potential mediating role of biomarkers and metabolites in the association. A total of 9514 participants (3.4%) developed depression during a median follow-up of 13.91 years. ... Nonetheless, the previously proposed lung-brain-axis hypothesis, which suggests that impaired lung function can affect ...
Baron & Kenny's Procedures for Mediational Hypotheses. Mediational hypotheses are the kind of hypotheses in which it is assumed that the affect of an independent variable on a dependent variable is mediated by the process of a mediating variable and the independent variable may still affect the independent variable. In other words, in mediational hypothesis, the mediator variable is the ...
According to the above results, we confirmed Hypothesis 4 that MS pain is a mediating factor between long commuting times and increased risk of burnout. However, for the mediation effect, the sites of the MS pain effect on burnout are specifically associated with neck and both shoulders pain.
1. Introduction. Mediation analysis is of rising interest in clinical trials and epidemiology. The advance of high-throughput technologies has made it possible to interrogate molecular intermediate phenotypes such as gene expression and DNA methylation in a genome-wide fashion, some of which may mediate effects of treatments, external exposures and life-style risk factors on disease risk.
The test results indicated a positive and partial mediation effect of the mediating effect on the research hypothesis. The results of the questionnaire survey revealed the preferred order of consumer decision making based on their psychological needs, including social equity, and perceived value.
Thus, the examination of the mediation of a moderator effect considers the mediation model as a means to explain why a treatment effect of X on Y is moderated by a third variable, Z. In this way, the mediation of a moderator effect hypothesis probes mediation as a possible process that accounts for the interaction of the treatment and the outcome.