Child Obesity Grew During the Pandemic. How Schools Can Help Reverse the Trend

- Share article
Anxiety, depression, and stalled social development are a few of the troubling developments that research has linked to the pandemic. Add obesity to that list.
Recent research has found a significant rise in obesity rates among children and teens over the course of the pandemic, accelerating an already troubling upward trend.
Schools, a key source of proper nutrition and physical education, are positioned to help head off this trend. But efforts to tackle the problem face stiff competition for educators’ attention alongside the ongoing pandemic, staff shortages, and the need to make up for lost academic ground.
“It is concerning to me and all school nurses because the disease of obesity has some big impacts on the health and learning of children,” said Kate King, the president elect of the National Association of School Nurses and a school nurse at World Language Middle School in Columbus, Ohio.
While school building closures, excessive amounts of screen time, and much less exercise likely fueled the rise in obesity among children during the first year or so of the pandemic, simply returning kids to in-person learning won’t solve the problem, said King.
“It’s not that easy to take that weight off again,” she said. “I think it’s going to be a longer haul—I don’t think we’re just going to even out again in a year.”
King understands that the pandemic continues to drain resources from schools. The time school nurses would normally have spent conducting health screenings or creating programing to promote healthy eating and exercise among students has been gobbled up by pandemic-related tasks such as contact tracing and COVID-19 testing.
But educators must be purposeful about addressing the rise in obesity among school-aged children, she said.
Studies and surveys have found that children spent more time in front of a screen, ate more processed and fast foods, and spent less time exercising when school buildings were closed. All of that likely contributed to weight gain among children, suggests the U.S. Centers for Disease Control and Prevention in a study published last fall. The study analyzed body mass index, or BMI, data on more than 432,000 children and teens ages 2 to 19 between August 2019 and August 2020.
The percentage of children and teens who were obese rose from 19 to 22 percent in just one year, an increase described by one of the study’s authors as “substantial and alarming,” according to the Associated Press. Children in preschool and elementary school, and kids who already had moderate or severe obesity before the pandemic, saw the biggest weight gains.
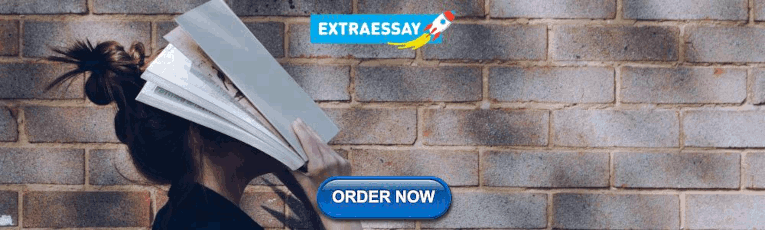
Obesity hurts kids’ learning and mental health
Weight gain linked to the pandemic is not limited to the United States or just children and adolescents. Childhood obesity rates also increased substantially in the United Kingdom during the pandemic, and 42 percent of U.S. adults told the American Psychological Associatio n and The Harris Poll in a February 2021 survey they had gained more weight than they intended since the pandemic started. The median weight gain among those adults was 15 pounds.
Obesity can lead to several other negative health conditions in children —both physical and mental, according to the CDC—including high blood pressure and cholesterol, breathing problems, type 2 diabetes, anxiety, and depression.
When you have a kid who has less than 10 minutes to eat a lunch, it is heartbreaking to see them try and shovel a salad into their mouths as they’re walking to the trash.
King emphasized that physical movement, even just small breaks during class time to move around, is connected to learning and good mental health.
“We know that exercise releases endorphins, brain chemicals, that make us think and feel better,” she said. “We know that taking those mental breaks, which can be physical as well, helps our brain to process what we are learning.”
Obesity is an equity issue, too, said King, citing research that shows that Black and brown children are more likely to have obesity.
In addition, low-income families can find it particularly hard to get a good diet and enough exercise, said King. Kids with parents working multiple jobs might eat more cheap, processed foods, she said.
To make matters worse, King pointed out that children and teens who live in areas with high crime rates or near busy roads likely spend less time exercising; many extracurricular activities such as sports take time and money that low-income families simply don’t have; and supply chain disruptions and rising food costs are only going to make these disparities worse.
What schools can do to combat obesity
Childhood obesity may look like too big of a problem for schools to tackle. Or maybe it’s simply secondary to other more pressing crises caused by the pandemic. Even so, school health experts say there are small steps that educators can take to help students get more physical activity and better nutrition during the school day.
School leaders should get rid of vending machines filled with candy, chips, and soft drinks, and avoid using food as a reward, said King. They should also never take away recess from students to punish them for misbehavior.
Lunch should also follow recess in school schedules, not the other way around, said Jessica Shelly, the director of dining services for the Cincinnati public schools. Kids are more likely to eat their food if they have had a chance to run around and play before mealtime.
While the types of food schools serve are largely dictated by federal rules around providing nutritious meals to students ( and whatever they can procure with all the disruptions to the supply chain ), there are other adjustments to the meal schedule schools can make to ensure kids are eating their food. One relatively easy adjustment is to extend mealtimes, said Shelly.
To address social distancing measures during the pandemic, the city’s largest high school added one extra lunch bell and increased all other lunch periods to 40 minutes. As a result, participation in the school lunch program rose dramatically. “Participation doubled. Doubled! Because they had time to come down, come through the line, get a meal, eat the meal,” said Shelly.

Healthier foods also take more time to eat, Shelly added, comparing chewing an apple to slurping down apple sauce.
“When you have a kid who has less than 10 minutes to eat a lunch, it is heartbreaking to see them try and shovel a salad into their mouths as they’re walking to the trash,” she said.
For teachers, there are many ways they can incorporate more physical activity into their classrooms, said Terri Drain, a longtime physical education teacher and the president of SHAPE America, a national organization of physical education instructors.
“Kids learn better after they get up and move,” said Drain. “Insert some physical activity and power breaks, but also provide kids the opportunity to learn with movement.”
That might mean using basketball to teach about inertia, force, and momentum; or a game of bowling to teach fractions by asking students to convert the number of pins they knocked down into a fraction.
Drain also recommends assigning discussion questions for students to tackle as they walk around the schoolyard in pairs instead of sitting at their desks, and giving homework that requires students to go outside instead of spending more time on a screen.
Those small movement breaks add up and can have a big impact, said King, the school nurse. She believes schools should make it a policy to set aside five minutes at the beginning of every class period to move around and stretch.
“All of this does make a difference,” said King. “Just like teachers and staff and all of us modeling healthy behaviors, creating this culture of health in schools will stick with children for the rest of their lives.”
Sign Up for The Savvy Principal
Edweek top school jobs.
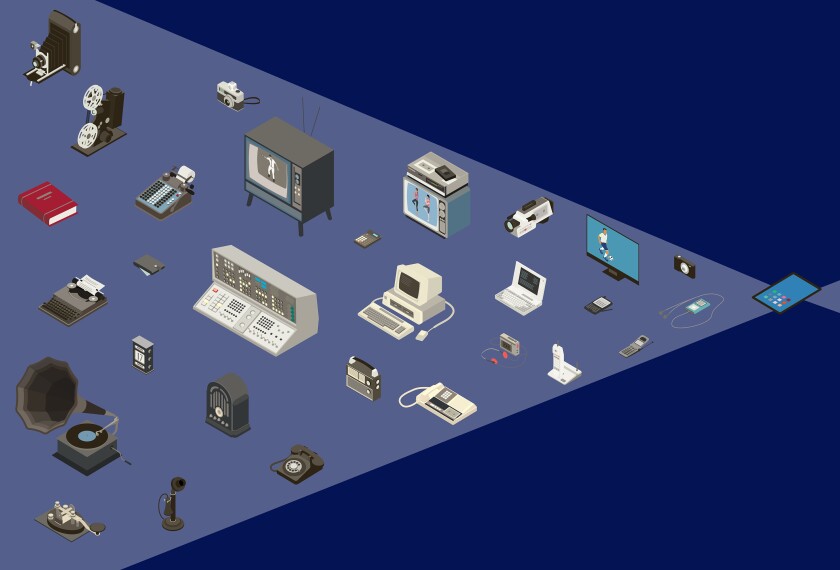
Sign Up & Sign In


Essay on Obesity Among Students
Students are often asked to write an essay on Obesity Among Students in their schools and colleges. And if you’re also looking for the same, we have created 100-word, 250-word, and 500-word essays on the topic.
Let’s take a look…
100 Words Essay on Obesity Among Students
What is obesity.
Obesity means having too much body fat. It is not the same as being overweight, which means weighing too much. For students, it can happen for many reasons, like eating too much and not exercising enough.
Why Are Students Getting Obese?
Many students are getting obese because they eat unhealthy food like chips and soda and don’t move around much. They sit a lot, watching TV or playing video games, and sometimes they feel too busy with schoolwork to play outside.
Problems Caused by Obesity
Being obese can lead to sickness. Students may develop diabetes, have trouble breathing, or get heart disease. It also makes them feel tired and can make it hard to keep up with friends.
Preventing Obesity
To prevent obesity, students should eat fruits and vegetables and be active. Playing sports, riding bikes, or just running around can help. Also, drinking water instead of sugary drinks is good for staying at a healthy weight.
250 Words Essay on Obesity Among Students
Understanding obesity in students.
Obesity is when a person has too much body fat. It’s a big health problem that many students face today. This can happen from eating too much food, especially the kind that’s not healthy, and not moving around or playing enough to burn off the energy.
Why Are More Students Becoming Obese?
Many students prefer snacks like chips and soda over fruits and vegetables. Also, with lots of homework and video games, they might not go outside to play as much. Schools sometimes offer food that’s tasty but not always good for you, and that can add to the problem.
The Health Risks
Being very overweight can lead to health troubles like diabetes, which is when your blood sugar is too high, and heart disease, which can make it hard for your heart to work right. It can also make students feel tired and hurt their feelings if others are unkind about their weight.
What Can Be Done?
Schools can help by giving healthier meals and making sure students have time for sports or play. At home, families can choose better food and spend time being active together. It’s important to remember that everyone’s body is different, and being healthy is what really matters.
Obesity among students is a concern, but we can all work together to make things better. By eating right and staying active, students can live healthier and happier lives. It’s not about being the skinniest; it’s about being healthy enough to enjoy life and do all the things you love.
500 Words Essay on Obesity Among Students
Obesity is when someone has too much body fat. It is not just about looking a certain way; it is a serious health issue. When doctors want to find out if someone is obese, they look at something called the Body Mass Index, or BMI. This tells them if a person’s weight is too much for how tall they are. If the BMI is very high, the person is said to be obese.
Why Are Some Students Obese?
There are many reasons why a student might become obese. One big reason is eating too much food that is not good for the body, like chips, candy, and fast food. These foods are high in calories, which means they give the body more energy than it needs, and this extra energy turns into fat.
Another reason is not being active. Many kids love to watch TV, play video games, or use computers for hours. This means they are sitting down a lot and not moving their bodies enough to burn off the energy from the food they eat.
Sometimes, a student might become obese because their family has a history of obesity. This means that their parents or other family members might also be obese, and this can sometimes make it more likely for the student to be obese too.
Being obese can cause a lot of problems for students. They might get sick more often and have health issues like diabetes, which is when your blood sugar is too high, or high blood pressure, which can hurt your heart. It can also make it hard for them to breathe well or sleep well.
Obesity can also make students feel bad about themselves. They might get teased by other kids, which can make them sad or angry. It can also make it hard for them to join in sports or play with friends, which is not fun at all.
How to Help Students Stay Healthy
To help students not become obese, schools and families can do many things. One important thing is to teach kids about eating healthy foods like fruits, vegetables, and whole grains. These foods are better for the body and do not have too many calories.
Schools can also make sure that students have time to play and be active. This can be during gym class, recess, or after-school sports. Being active helps burn off the extra energy from food.
Families can help by making sure there are healthy food options at home and by being active together. Going for walks, riding bikes, or playing sports as a family can make being healthy fun.
Obesity among students is a big problem, but we can all help to fix it. By eating the right foods and staying active, students can stay at a healthy weight and avoid the problems that come with obesity. Schools and families have a big part to play in this, and by working together, they can make sure that students are happy, healthy, and ready to learn.
That’s it! I hope the essay helped you.
If you’re looking for more, here are essays on other interesting topics:
- Essay on Nutrition And Exercise
- Essay on Nursing As A Career
- Essay on Nurse
Apart from these, you can look at all the essays by clicking here .
Happy studying!
Leave a Reply Cancel reply
Your email address will not be published. Required fields are marked *
Save my name, email, and website in this browser for the next time I comment.
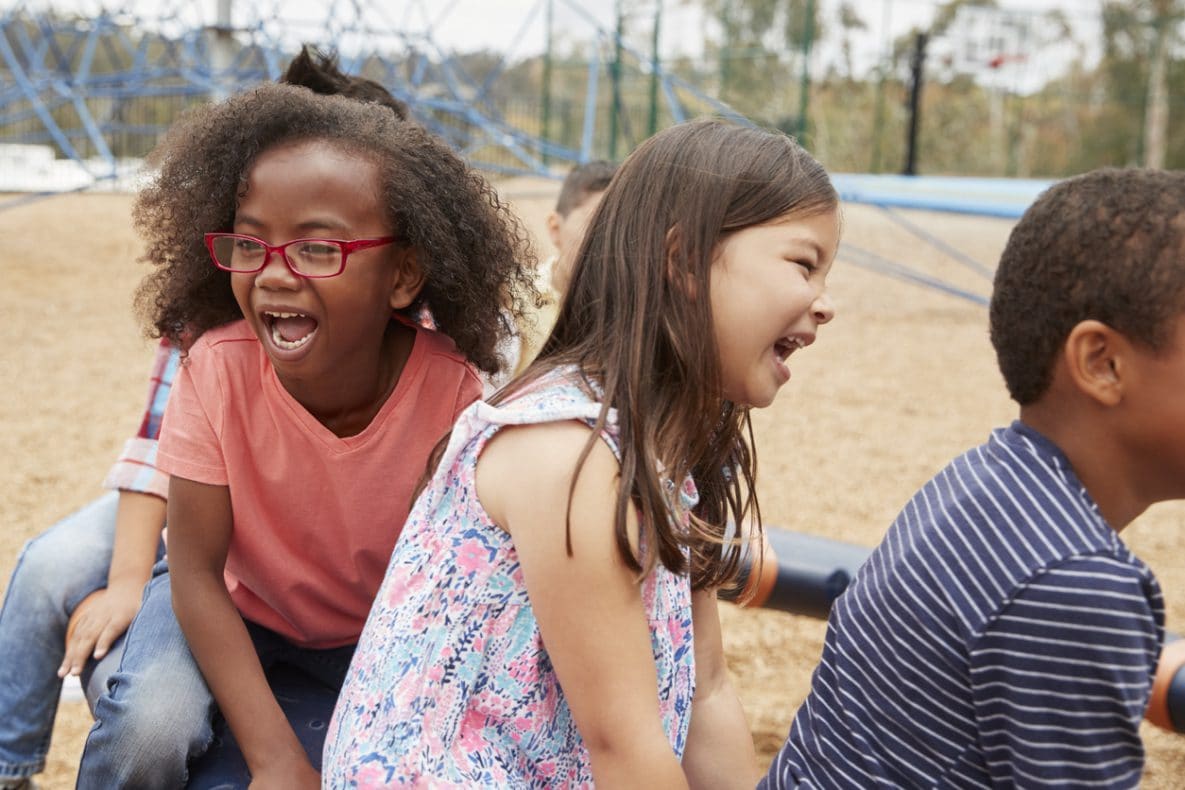
In the United States, the percentage of children and adolescents affected by obesity has more than tripled since the 1970s. 1 In 2017–2018, about 1 in 5 school-aged children were affected by obesity (20.3% of all 6-11 year olds, 21.2% of all 12-19 year olds) 1 . The prevalence of obesity among 2-19 year old children was 25.6% for Hispanic children, 24.2% for Black children, 16.1% for White children, and 8.7% for Asian children. 1 Many factors contribute to childhood obesity, including: 2-6
- Metabolism—how your body changes food and oxygen into energy it can use.
- Eating and physical activity behaviors.
- Community and neighborhood design and safety.
- Short sleep duration.
- Negative childhood events.
Genetic factors cannot be changed. However, people and places can play a role in helping children achieve and maintain a healthy weight. Changes in the environments where young people spend their time—like homes, schools, and community settings—can make it easier for youths to access nutritious foods and be physically active. Schools can adopt policies and practices that help young people eat more fruits and vegetables, eat fewer foods and beverages that are high in added sugars or solid fats, and increase daily minutes of physical activity. 3, 7-9 These kinds of school-based and after-school programs and policies can be cost-effective and even cost-saving. 10,11
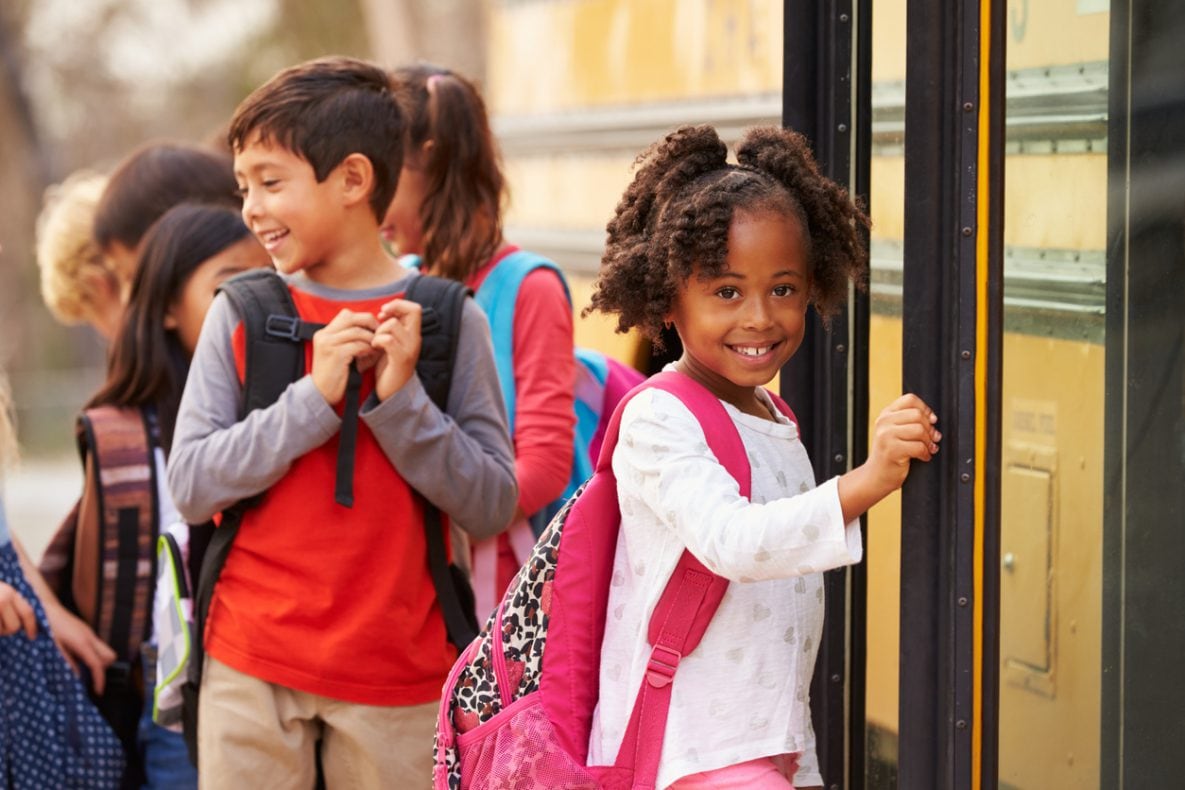
The COVID-19 pandemic has disrupted students’ routines and access to the school environment. Children gained weight at a faster rate during the pandemic (June 1-November 2020) compared to pre-pandemic, with younger school-aged children (6-11) experiencing a rate of BMI change that was 2.5 higher than before the pandemic. 15
Schools are a priority setting for obesity prevention efforts because they reach the vast majority of school-aged youth, provide regularly scheduled opportunities for physical activity, and offer nutritious foods through school meal programs. 3
With a widespread return to in person learning, children can have consistent access to physical activity opportunities, healthy meals, and services provided by school nurses and counselors.
A comprehensive approach is effective at addressing childhood obesity in schools, especially for elementary and middle school students. 13-15 Scientists know less about what school-based obesity prevention approaches are effective for teenagers. 13-15 A comprehensive approach means directing attention to nutrition and physical activity in schools and even involving school nurses, parents, caregivers, and other community members (e.g., pediatricians, after-school program providers) in the process. This approach aims to support the health and well-being of all students. It does not single out students according to their weight status or body size. Overweight and obesity are sensitive issues for students and families and must be addressed with compassion, understanding, and care. 16 School-based programs to promote physical activity and improved diet quality do not appear to contribute to increases in depression, anxiety, or body-dissatisfaction; however, many studies do not collect information about these unintended effects. 15 To avoid embarrassing or shaming students, schools should not emphasize physical appearances or reinforce negative stereotypes about obesity. 17
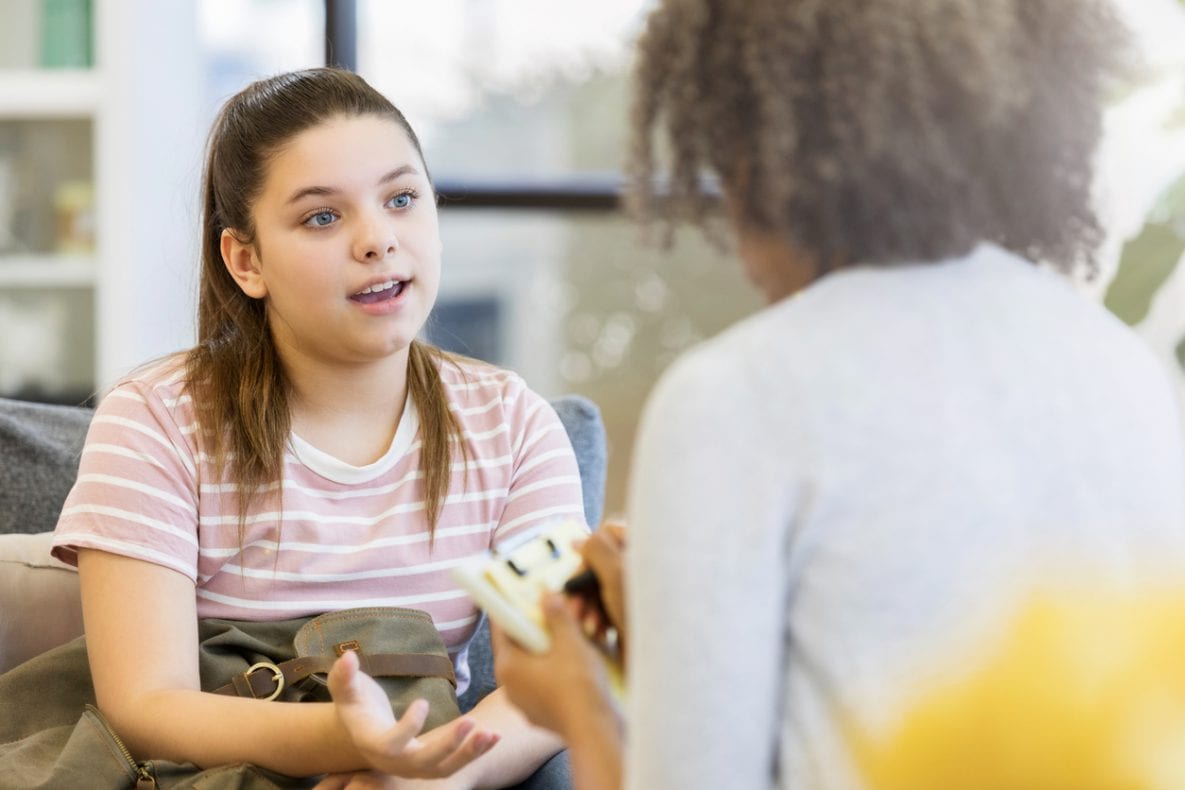
School nurses play an important role to prevent and reduce student overweight and obesity prevalence. School nurses can address the complex physical, social, and health education needs of children and adolescents who are overweight or who have obesity. 15, 16 School nurses have the knowledge and skills to do the following:
- Create a culture of health and wellness in school.
- Promote and implement school-based policies and strategies for healthy eating and physical activity.
- Coordinate care with families and health care professionals.
- Lead the school community to influence policy changes that reinforce healthy eating, physical education, and physical activity before, during, and after school.
For more information about childhood obesity, visit Child & Teen Healthy Weight and Obesity .
- CDC MMWR : BMI Among Children and Adolescents During the COVID-19 Pandemic
- School Health Guidelines
- School Nutrition
- Physical Education and Physical Activity
- Parents for Healthy Schools
- Local School Wellness Policy
- Tips for Parents
- Fryar CD, Carroll MD, Afful J. Prevalence of overweight, obesity, and severe obesity among children and adolescents aged 2–19 years: United States, 1963–1965 through 2017–2018. NCHS Health E-Stats. 2020. https://www.cdc.gov/nchs/data/hestat/obesity-child-17-18/overweight-obesity-child-H.pdf
- Swinburn BA, Sacks G, Hall KD, et al. The global obesity pandemic: shaped by global drivers and local environments. Lancet . 2011;378(9793):804–814.
- Institute of Medicine. Accelerating Progress in Obesity Prevention: Solving the Weight of the Nation . Washington, DC: National Academies Press; 2012.
- Eisenburg LK, can Wijk KJE, Liefbroer AC, Smidt N. Accumulation of adverse childhood events and overweight in children: a systematic review and meta-analysis. Obesity . 2017;25(5):820–832.
- Danese A, Tan M. Childhood maltreatment and obesity: systematic review and meta-analysis. Mol Psychiatry. 2014;19:544–54.
- Fatima Y, Doi SAR, Mamun AA. Longitudinal impact of sleep on overweight and obesity in children and adolescents: a systematic review and bias-adjusted meta-analysis . Obes Rev . 2015;15(2):137–149.
- US Department of Health and Human Services. The Surgeon General’s Vision for a Healthy and Fit Nation . Rockville, MD: US Dept of Health and Human Services; 2010.
- Micha R, Karageorgou D, Bakogianni I, et al. Effectiveness of school food environment policies on children’s dietary behaviors: A systematic review and meta-analysis. PLoS ONE 2018;13(3):e0194555.
- The Community Guide. Interventions to Increase Healthy Eating and Physical Activity in Schools. 2019. Retrieved from https://www.thecommunityguide.org/content/interventions-increase-healthy-eating-and-physical-activity-schoolsexternal icon .
- Gortmarker SL, Want CY, Long MW et al. Three interventions that reduce childhood obesity are projected to save more than they cost to implement. Health Affairs. 2015;11(34):1932–1939.
- Cradock AL, Barrett JL, Kenney EL. Using cost-effectiveness analysis to prioritize policy and programmatic approaches to physical activity promotion and obesity prevention in childhood. Prev Med. 2017;95(S):S17–27.
- Lange SJ, Kompaniyets L, Freedman DS, et al. Longitudinal Trends in Body Mass Index Before and During the COVID-19 Pandemic Among Persons Aged 2–19 Years — United States, 2018–2020. MMWR Morb Mortal Wkly Rep 2021;70:1278–1283. DOI: http://dx.doi.org/10.15585/mmwr.mm7037a3external icon
- Wang Y, Cai L, Wu Y, Wilson RF, Weston C, Fawole O, Bleich SN, Cheskin LJ, Showell NN, Lau BD, Chiu DT. What childhood obesity prevention programmes work? A systematic review and meta‐ Obes Rev. 2015;16(7):547-65.
- Sobol‐Goldberg S, Rabinowitz J, Gross R. School‐based obesity prevention programs: a meta‐analysis of randomized controlled trials. 2013;21(12):2422–2428.
- Brown T, Moore THM, Hooper L, Gao Y, Zayegh A, Ijaz S, Elwenspoek M, Foxen SC, Magee L, O’Malley C, Waters E, Summerbell CD. Interventions for preventing obesity in children. Cochrane Database of Systematic Reviews 2019. DOI: 10.1002/14651858.CD001871.pub4.
- National Association of School Nurses. Overweight and obesity in children and adolescents in schools -The role of the school nurse (Position Statement). Silver Spring, MD: Author. 2018. Retrieved from https://www.nasn.org/advocacy/professional-practice-documents/position-statements/ps-overweightexternal icon . Accessed November 9, 2021.
- Brownell KD, Schwartz MB, Puhl RM, Henderson KE, Harris JL. The need for bold action to prevent adolescent obesity. J Adolesc Health . 2009;45(suppl 3):S8–S17.
- Schroeder K, Travers J, Smaldone A. Are school nurses an overlooked resource in reducing childhood obesity? A systematic review and meta-analysis. J Sch Health . 2016;86(5):309–321. doi: 10.1111/josh.12386
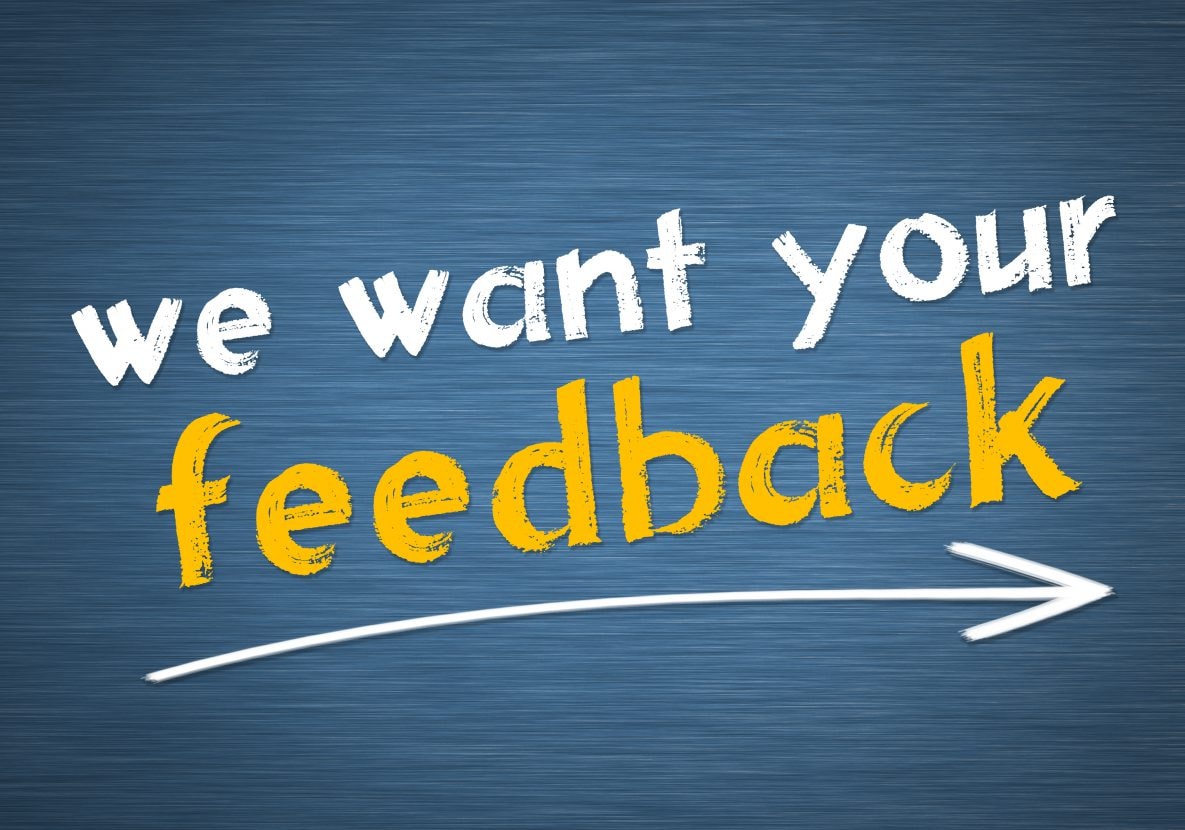
Healthy Youth
To receive email updates about this page, enter your email address:
Exit Notification / Disclaimer Policy
- The Centers for Disease Control and Prevention (CDC) cannot attest to the accuracy of a non-federal website.
- Linking to a non-federal website does not constitute an endorsement by CDC or any of its employees of the sponsors or the information and products presented on the website.
- You will be subject to the destination website's privacy policy when you follow the link.
- CDC is not responsible for Section 508 compliance (accessibility) on other federal or private website.
- Open access
- Published: 21 March 2024
Prevalence and risk factors of obesity among undergraduate student population in Ghana: an evaluation study of body composition indices
- Christian Obirikorang 1 , 2 ,
- Evans Asamoah Adu 1 , 2 ,
- Enoch Odame Anto 3 , 4 ,
- Anthony Afum-Adjei Awuah 1 , 2 ,
- Angela Nana Bosowah Fynn 2 ,
- George Osei-Somuah 3 ,
- Patience Nyarkoa Ansong 5 ,
- Alexander Owusu Boakye 1 , 2 ,
- Ivy Ofori-Boadu 3 ,
- Yaa Obirikorang 5 ,
- Austin Gideon Adobasom-Anane 2 ,
- Eric NY Nyarko 6 &
- Lois Balmer 4
BMC Public Health volume 24 , Article number: 877 ( 2024 ) Cite this article
510 Accesses
Metrics details
Obesity is a classified risk factor for several of the world’s leading causes of death. In this study, we combined information contained in body mass index (BMI), total percentage body fat (TPBF) and relative fat mass (RFM) to estimate obesity prevalence and examine the risk factors associated with obesity.
The study recruited 1027 undergraduate students aged between 16 and 25 years using a cross-sectional study design and two-stage stratified random sampling between January and April 2019 from the Kwame Nkrumah University of Science and Technology, Kumasi, Ghana. Demographic, lifestyle, and family history of chronic disease data, were collected using a structured questionnaire. Bioelectrical impedance, along with height, weight, age, and gender, were used to estimate BMI and TPBF. The RFM was calculated using a published equation. The TPBF and RFM ranges were evaluated based on standard BMI thresholds and an informative combined obesity prevalence estimated in a Bayesian framework. Multiple logistic regression analysis was used to evaluate potential risk factors of overweight/obesity.
Concordance between BMI, TPBF and RFM for obesity classification was 84% among female and 82.9% among male students. The Bayesian analysis revealed a combined prevalence means of obesity of 9.4% (95%CI: 6.9-12.2%) among female students and 6.7% (95%CI:4.3-9.5%) among male students. The odds of obesity were increased between 1.8 and 2.5 for females depending on the classification index. A significant increasing trend of obesity was observed with university-level. A family history of obesity was associated with a high estimate of general, central, and high TPBF.
Using multiple adiposity indicators conjointly in a Bayesian framework offers a greater power to examine obesity prevalence. We have applied this and reported high obesity prevalence, especially among female students. University level and family history of obesity were key determinants for obesity among the student population.
Peer Review reports
Introduction
Obesity is a classified risk factor for several of the world’s leading causes of death including cardiovascular diseases, diabetes, and various types of cancers [ 1 ]. It represents the 5th and 6th major level two public health problem among women and men, respectively, leading to the toll of death and disability worldwide [ 2 ]. Obesity stands out among the top leading causes of attributable disability-adjusted life years (DALYs) this is due to the rate of exposure increasing by more than 0.5% per year [ 3 ]. The prevalence of obesity has increased in pandemic dimensions over the past 50 years [ 4 ] with 650 million adults, 340 million adolescents and 39 million children classified as obese [ 5 ]. As the obesity pandemic continues, estimates indicate that approximately 167 million adults and children will become less healthy due to being overweight or obese by 2025 [ 4 ]. Especially in developing countries, the possible implications of obesity on current and future population health and healthcare spending are likely to be enormous [ 6 ].
According to existing nationwide data, the prevalence of being overweight and obese is estimated at 25.4% and 17.1%, respectively [ 7 ]. Among the adult Ghanaian population, obesity is higher in women than men and mimics the level of urbanization [ 7 ]. A meta-analysis involving 29,160 Ghanaian children (≤ 19 years) across sixteen studies reported 8.6% obesity and 10.7% overweight [ 8 ]. There exists a significant number of studies that quantify the burden of obesity in Ghana with a special focus on the general adult population [ 9 , 10 , 11 , 12 , 13 , 14 ], women [ 15 , 16 , 17 , 18 , 19 , 20 , 21 ], school-aged children [ 22 , 23 , 24 ], adolescents [ 25 , 26 , 27 ], healthcare workers [ 28 , 29 , 30 , 31 , 32 ], civil servants [ 33 , 34 , 35 , 36 , 37 , 38 ] and commercial workers [ 39 ]. However, knowledge and data about the experiences of being overweight and obese among young Ghanaian adults are inadequate. Among the few existing studies in Ghana [ 20 , 40 , 41 , 42 ], there is an inconclusive estimate of those who are overweight/obese (4.2-39.3%) among the young adult population. This is due to population non-representativeness, that is, varying lifestyle habits and health-related behaviours of these age groups.
Among undergraduate students, which mainly represent the young adult population group, poor lifestyle habits, including decreased quality of diet and physical activity, sedentary lifestyle, alcohol use and smoking, as well as decreased quality sleep, are associated with obesity [ 42 , 43 , 44 ]. Also, the concurrence of altered eating behaviours (emotional eating, uncontrolled eating, and restrained eating), depression and poor sleep are estimated to be high among undergraduate students, mainly females [ 45 ]. These are fundamental factors driving the obesity epidemic [ 1 ]. Thus, exploring obesity experiences using representative sampling among undergraduate students will allow for the acquisition of information related to young Ghanaian adults. This knowledge will go a long way in informing strategies to combat the obesity epidemic and hopefully, related medical conditions among university students and the general young adult population.
We have mainly relied on the routine use of the body mass index (BMI) as an obesity measure. However, BMI has a limitation in differentiating between body composition and body fat distribution [ 46 , 47 , 48 , 49 ]. Alternative measures, including the bioelectrical impedance analysis (BIA) and BIA-derived body fat indices [ 50 ], like the body adiposity index [ 51 ] and relative fat mass (RFM) [ 52 ], have been proposed. These measures claim to adjust the limitations of BMI and alternatively represent cost-effective indices to appropriately identify individuals with accuracy close to that of underwater weighing [ 53 ] and dual-energy X-ray absorptiometry [ 54 ]. In particular, RFM and total percentage body fat (TPBF) have been validated as being a more accurate measure compared to BMI to estimate whole-body fat percentage, in addition to improving body fat-defined obesity misclassification among different population groups [ 55 ].
In this study, we have combined information contained in body mass index (BMI), TPBF and relative fat mass (RFM) to estimate an informative obesity prevalence. Because there is no single universally accepted measure of adiposity and each index has its drawbacks, we performed an evaluation analysis of waist-to-height derived RFM, corresponding to central fatness and TPBF corresponding to overall adiposity based on the routinely used weight-to-height derived BMI thresholds. By using data from the evaluation analysis assessing concordance and the estimate of measurement properties of TPBF and RFM with BMI, we combined this classification in a Bayesian framework. Thus, we reported an informative obesity prevalence corresponding to central and general adiposity, with much power. Our governing hypothesis was that a combined estimate of obesity in a Bayesian framework does not offer a more representative estimate than commonly used BMI, RFM and TPBF in isolation.
Because it is common in population surveys to have one or multiple measures investigating the same condition, the Bayesian framework has been useful in drawing inferences on disease prevalence and measurement properties while adjusting for the possibility of conditional dependence between several disease measures [ 56 , 57 , 58 ]. In practice, two aspects exist, that can be used to estimate uncertainty and improve the accuracy of population estimate of prevalence. The first is to use the prior information from existing studies, while the second requires the integration of multiple population-based measures into one estimate [ 57 , 58 ]. In our case, we employed the second approach for this study.
Methodology
This was a cross-sectional study undertaken at the Kwame Nkrumah University of Science and Technology (KNUST), Kumasi, between August 2018 and July 2019. All students provided written informed consent for their participation in the study. Ethical approval with reference ID (CHRPE/AP/030/19) was obtained from the Committee on Human Research, Publications and Ethics (CHRPE), School of Medicine and Dentistry, Kwame Nkrumah University of Science & Technology.
Sample design
A two-stage stratified random sampling was used to select 1027 first to fourth-year undergraduate students aged 16–25 years. These students were selected to cover the six Colleges in KNUST including the College of Science (CoS), Art and Built Environment (CABE), Humanities and Social Sciences (CoHSS), Health Sciences (CoHS), Engineering (CoE), and Agric and Natural Resources (CANR). Students who were feverish, bodybuilders or highly trained athletes, and students with osteoporosis or oedema (swelling in the body) were excluded.
The targeted population consisted of undergraduate students’ population from 1st to 4th year of their academic level, across the six colleges of KNUST. The operational definition of a student’s year directly depended on the recruitment dates between January and April 2019. This period represents the second semester where first-year students have spent at least one complete semester in the college. We used a two-stage stratified cluster survey design. The study population and sampling consisted of the entire student population at KNUST (43,757) during the 2018/2019 academic year. Considering the low probability of sampling 5th and 6th -year students, available only for health sciences, were excluded from the sample. The first stage of clustering involved censoring all colleges with probabilities relative to the number of departments. From each selected college, a fixed number of departments was sampled. Eight students (two students, male and female, from levels 1st, 2nd, 3 rd, and 4th year) are sampled at random at the department level.
Sample size
The required sample size to assess overweight/obesity prevalence among students was calculated assuming p = 0.18 [ 41 ], level of acceptable precision d = 0.05 (or ± 5%) at 95% CI corresponds to 227 which relate to 28 departments of 8 students in each. Using analysis of data from a previous study [ 41 ] within the same KNUST student population, the design effect was estimated at 1.9 for overweight/obesity. This was based on 6 clusters with an average of 50 students per cluster (n = 300) and an intra-cluster coefficient of 0.1. Considering these figures and while assuming a college response rate of 90% and individual students response rate at 85%, the actual sample size was estimated to be 83 departments of 8 students each (n = 664) with male and female students having equal proportion of being sampled. In the end, we recruited 1027 participants to increase the power of our estimate. Table 1 illustrates the minimum possible sampling expectations.
The equation for sample size calculation:
\(n=(z^2 \times p(1-p\left)\right(DEEF\left)\right)/\left(\right(j\left)\right(k\left)\right(l\left)\right(d^2\left)\right)\) [ 59 ].
Z was taken at 1.96, j = is the expected response rate as a proportion (0.85 × 0.9); k is the average department size (n = 8). The proportion of the student population accounted for by the targeted of interest (l) was set at 0.8. DEFF is the design effect.
Data collection and anthropometric measurement
A structured questionnaire was used to collect data on socio-demographic characteristics, lifestyle risk factors and family history of obesity, diabetes and hypertension (Table 2 ). socio-demographic data included age, sex, year of study, and college. Lifestyle data included alcohol intake, smoking and exercise history. Height was measured with a portable height rod Stadiometer with students in a straight posture, feet placed together and flat on the ground. Waist circumference (WC) was measured using a tape measure at the point of the umbilicus and maximum gluteal protrusion. Each participant was asked to stand straight on the main unit of the OMRON BF511 Clinically Validated Full Body Composition Monitor with 8 high-precision sensors for hand-to-foot measurement (OMRON HEALTHCARE Co., Ltd.), looking straight, barefooted and with arms horizontally raised holding a display unit, extended at a 90° angle for weight, body fat mass (BFM) and TPBF estimation. The machine conforms to EN60601-1-2:2015 Electro Magnetic Compatibility (EMC) standard and uses the bioelectrical impedance, along with height, weight, age and gender information to generate results based on the OMRON’s data of body composition [ 60 ]. The Omron Full Body Sensor Body Composition Monitor and Scale estimates the TPBF by the Bioelectrical Impedance Method. The instrument sends an extremely weak electrical current of 50 kHz and less than 500 µA through the participant’s body to determine the amount of water in each tissue. The instrument takes measurements from both hands and feet to reduce the influence of water movement on body composition results. The output of the OMRON BF511 monitor included TPBF, relative visceral fat content, body mass index (BMI), and skeletal muscle. We included the TPBF and BMI output together with RFM for the analysis. RFM was calculated from WC and height:
Obesity was defined based on BMI thresholds for overweight (≥ 25 Kg/m 2 ), and obesity (≥ 30 Kg/m 2 ) according to the World Health Organization’s criteria [ 1 ].
Statistical analyses
Patient characteristics were stratified by primary clusters (colleges). Counts and corresponding percentages were used to describe categorical variables and compared using the Chi-square test. Mean and standard deviations were used to describe continuous variables if they followed the Gaussian normal distribution. Median and interquartile ranges were used if otherwise distributed. Either the One-way analysis of variance or Kruskal-Willi’s test was used to compare continuous variables among primary clusters. Multiple comparison analysis with Bonferroni correction was performed when the probability value was < 0.05. Sex-stratified prevalence estimates for obesity were determined according to BMI thresholds and the corresponding TPBF and RFM thresholds for age. The Passing and Bablok regression analysis was used to evaluate the measurement agreement and possible systematic bias for TPBF and RFM against BMI [ 61 ]. The diagnostic accuracy of TPBF and RFM was estimated based on the optimal cut-off, sensitivity, and specificity analysis, considering the area under the curve (AUC) estimated with the receiver-operating characteristic curves (ROC) analysis. We integrated the results from TPBF and RFM for obesity definition based on BMI threshold in a Bayesian framework, to report a combined obesity prevalence. Twenty chains were used to sample 50,000 samples per chain (25,000 warmups and 25,000 post warmups). Posterior densities were estimated using the Hamiltonian Monte Carlo (HMC) method. Summaries of posterior distributions including the mean and 95% credible interval were used to interpret the results. Multiple logistic regression analysis was used to evaluate potential risk factors of overweight/obesity. A two-sided p -value of 0.05 was considered statistically significant. Statistical analyses were performed using R version 4.3.0 (2023-04-21 ucrt) and MedCalc software Bvba, version 18.9.1.
Table 2 displays the characteristics of the study participants by sampling strata. There was a significant over-representation of female students at CoHSS and underrepresentation at CABE and CoE (p-value < 0.001). The proportion of students that consume alcohol was comparatively low in CoHS (p-value = 0.039). Compared with CANR and CoHSS, a significant proportion of students from CoHS (31.2%), CoE and CoS (18.4% each) never engaged in regular exercise (p-value < 0.001). We observed significant variance in TPBF% measurements compared across the colleges (p-value < 0.001).
Prevalence of being overweight and Obese based on standard BMI thresholds
Using BMI ≥ 25.0 Kg/m 2 , approximately 31% and 15% of female and male students were classified as overweight/obese. However, only 2.4% of male students and 8.0% of female students were classified as obese using BMI ≥ 30.0 Kg/m 2 (Table 3 ). We observed a trend towards increased overweight/obesity with age. Among students < 20 years, 26.3% and 13.6% female and male, respectively, were classified as being overweight and/or obese. Among ≥ 20 years female and male students, 34.5% and 15.5% were classified as overweight and/or obese.
Evaluation of RFM and BAI-derived TPBF based on BMI
Passing and Bablok regression analysis are shown in Table S1 . Here we emphasised the interpretation based on the observed random difference. The null assumption was that the observed random differences within ± 1.96 residual standard deviation (RSD) > 10%. Linearity between the variables was evaluated based on the custom test for linearity probability value (Table S1 , Figure S1 ). Concordance was observed between TPBF and BMI (RSD = 1.60, ± 1.96 = -3.14 to 3.14) compared with RFM (RSD = 2.64, ± 1.96 = -5.17 to 5.17) among male students. Similarly, among female students, TPBF demonstrated good agreement with BMI (RSD = 2.23, ± 1.96 = -4.37 to 4.37) compared to RFM (RSD = 2.98, ± 1.96 = -5.83 to 5.83). The linearity test revealed a significant deviation from linearity between TPBF and BMI (p-value < 0.01) and between BMI and RFM (p-value > 0.05). The ROC curve analysis (Fig. 1 ) identified TPBF threshold values of > 20.3% and > 20.8% and was associated with high information values for defining being overweight (BMI > 29.9 Kg/m 2 ) among male students: 15–19 years (AUC = 0.941, sensitivity = 100.0%, specificity = 87.8%) and 20–25 years (AUC = 0.942, sensitivity = 94.4%, specificity = 89.6%), respectively. Also, TPBF threshold values for defining obesity (BMI ≥ 30.0 Kg/m 2 ) among 15–19 years and 20–25 years female students were > 35.4% and > 35.2%, respectively (Fig. 1 ).
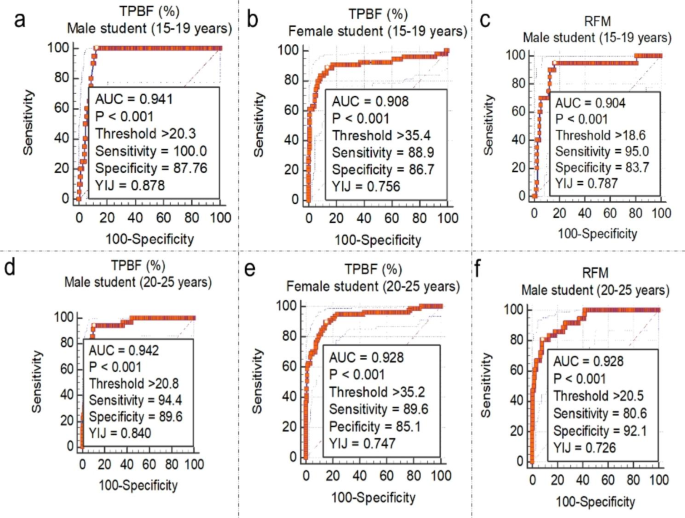
Threshold of TPBF and RFM estimates corresponding to WHO-defined BMI thresholds for overweight among male and female students
An optimal threshold value for TPBF > 26.2% (AUC = 0.981, sensitivity = 100.0%, specificity = 95.2%) for males (15–19 years) and > 24.4% (AUC = 0.938, sensitivity = 80.0%, specificity = 92.0%) had exceptional diagnostic accuracy for obesity (BMI ≥ 29.9 Kg/m 2 ) among male students (Fig. 2 ). The TPBF threshold of > 35.4% and > 35.2 was optimal for defining overweight female students 15–19 years and 20–25 years, respectively. TPBF values > 41.8% and 44.3% were optimal for defining obesity among female students 15–19 years and 20–25 years.
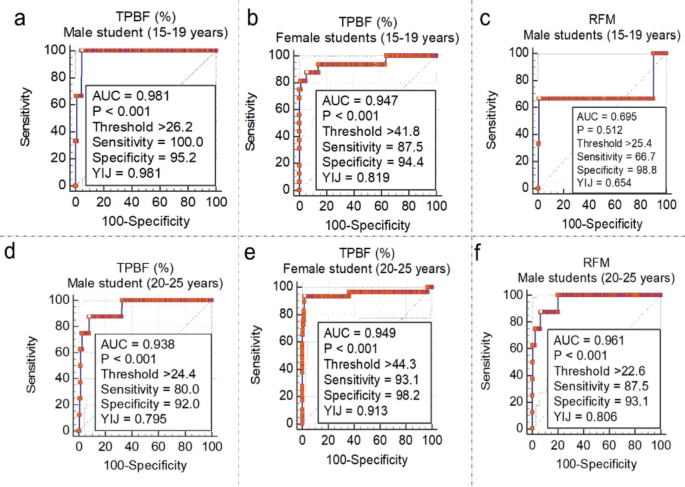
Threshold of TPBF and RFM estimates corresponding to WHO-defined BMI thresholds for obesity among male and female students
The ROC curve analysis of RFM for defining overweightness revealed an optimal cut-off of > 18.6 and > 20.5 among 15–19 years and 20–25 years male students, respectively (Fig. 1 c and f). RFM thresholds for defining obesity were > 25.4 and > 22.6 among male students 15–19 years and 20–25 years respectively (Fig. 2 a and f). Among female students (Fig. 3 a and b), RFM thresholds of > 20.7 and > 20.9 were associated with high information values for defining overweight among 15–19 years and 20 − 15 years, respectively. Moreover, obesity definition thresholds were > 24.5 and > 25.2, respectively among female students 15–19 years and 20 − 15 years (Fig. 3 c and d).
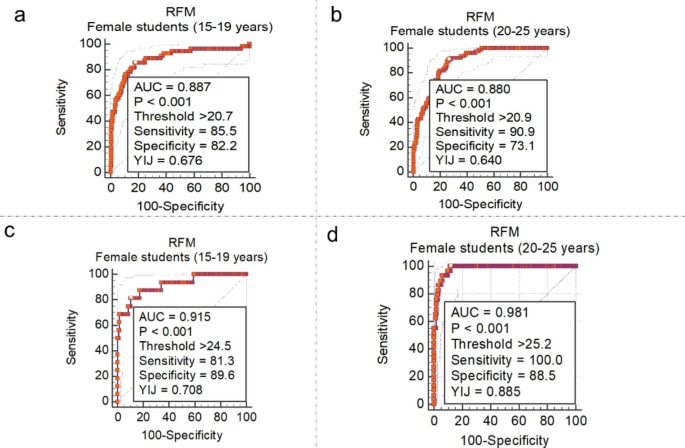
Threshold of RFM estimates corresponding to WHO-defined BMI thresholds for overweight and obesity among female students
The concordance between BMI, TPBF and RFM for obesity classification was 84% (95% lower limit = 82.0%) among female students and 82.9% (95% lower limit = 80.5%) among male students. The findings of the posterior predictive checks using the simulated data are presented in Figure S3 . The results of the Bayesian analysis suggest that the combined prevalence mean of overweight/obesity for TPBF and RFM were 33.8% (95%CI: 29.2-38.6%) among female students and 17.0% (95%CI: 13.1-21.3%) among male students. The combined prevalence mean of obesity for TPBF and RFM was 9.4% (95%CI: 6.9-12.2%) among female students and 6.7% (95%CI: 4.3-9.5%) among female students (Fig. 4 ).
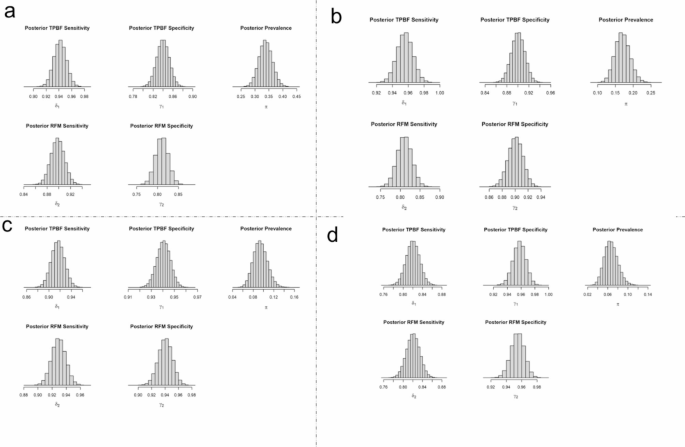
Marginal posterior density for the prevalence of obesity using combined data from TBPF and RFM. Note: π represents posterior prevalence using both TPBF and RFM data, δ1 represents sensitivity for TPBF data, γ1 represents specificity for TPBF data, δ2 represents sensitivity for RFM data, and γ2 represents specificity for RFM data
Factors associated with overweight/obesity among students
From the Multiple Logistic Regression analysis sex and family history of education were found to be consistent factors associated with general and central adiposity and percentage body fat distribution. The odds of being overweight were increased between 1.8 and 2.5, for women, depending on the classification criteria (Table 4 ). Family history of obesity was associated with increased odds of general obesity (OR = 3.48, 95%CI: 2.04–5.91), central obesity (OR = 1.98, 95%CI: 1.18–3.30) and high percentage body fat distribution (OR = 2.36, 95%CI: 1.42–3.94). Compared with first years students, the odds of central obesity and high percentage body fat were increased among third year students: OR = 2.77(1.59–4.82) and OR = 1.79(1.05–3.08), and fourth year students: OR = 3.26(1.76–6.04) and OR = 2.34(1.29–4.23), respectively.
This study sought to investigate the prevalence and risk factors of obesity among undergraduate students using multiple adiposity indices in a Byersian framework. In general the prevalence of being overweight/obese in this age group of young adults was high: 33.8% among female students and 17.0% among male students. Significant association were found between being overweight/obese and potential factors including sex, family history of obesity and university level.
Using the combined informative estimate, we observed an obesity prevalence of 6.7% among male students and 9.4% among female students. More generally, 17.0% of male students and 33.8% of female students were classified as having weight status corresponding to abnormal central and general adiposity as well as high body fat accumulation. These estimates are within the obesity prevalence range of 1.7–19.0% as estimated by a previous study in the same population group using different anthropometric indices [ 41 ]. Among the university student population in Botswana [ 43 ] and Ghana [ 44 ], similar estimates of overweight and/or obesity prevalence have been reported. In a larger study representing university students from 22 countries [ 62 ], 14.1% and 5.2% of female students and 18.9% and 5.8% of male students were reported to be overweight and obese, respectively. These data highlight the significant burden of obesity among undergraduate university students, which has a potential future health impact. In line with the current 16.2%, tertiary enrolment rate in Ghana [ 63 ], the current estimate of obesity reflects a significant national obesity problem among the young adult population with significant future health consequences.
We believe our estimate may be a true reflection of the obesity burden among student populations. First, TPBF and RFM contain high information values for obesity and fat distribution classification. Second, RFM is less accurate than BMI in lean individuals [ 64 ] whiles BAI-derived TPBF is less accurate than BMI in obese individuals [ 65 ]. Thus, combining these measures in a population estimation of obesity would provide a value informed by a broader distribution of obesity and fat distribution among the population group. Third, we relied on prior information from the concordance between both RFM and TPBF with standard BMI thresholds and a previous study from the same population [ 41 ]. Finally, the method adapted for the estimation of the combined prevalence of obesity has been successfully applied elsewhere [ 66 ]. Because this approach could be more flexible and adaptable, there is a need to test its performance in other settings with other related adiposity estimates.
An important observation of concern was the proportion of overweight/obese female students, which was higher compared with male students. This observation is consistent in several other studies [ 40 , 41 , 43 , 62 ], suggesting an increased risk for weight gain in young women and the critical need for interventions to prevent obesity and the host of associated adverse health outcomes. The evidence has been confirmed in several nationally representative surveys, where greater increases in weight are observed in young women aged 18 to 35 years compared with those seen in older women [ 67 ]. In a study among young Ghanain women aged between 15 and 24 years [ 20 ], overweight/obesity increased by 49% between 1993 and 2014 and projected a future prevalence of 35% by the year 2040. Fat deposition in women usually begins with the onset of puberty and continues unless consciously controlled [ 68 ]. Some studies has reported that female transition from adolescence to adulthood is associated with certain obesogenic dietary and physical activity behaviours to satisfy a historic valorization of large body size as a function of beauty, sexual attraction, prosperity, health and prestige [ 20 , 69 , 70 ].
In a prospective analysis of mother-daughter dyads and father-son dyads, the study reported a large and concerning increase in obesity rates over two generations of young adults, especially females [ 71 ]. These findings indicate that young adulthood represents periods of crucial importance regarding the establishment of life-long lifestyle habits and skills to control obesity. Studies have attributed this to a lack of knowledge and skills around food and nutrition, depression, anxiety, stress, satiety, neural responses, and possibly sleep patterns and premenstrual cravings [ 42 , 43 , 44 , 67 , 72 ]. Thus, there is the need to study these factors and their relationship with obesity among undergraduate students in Ghana, which can benefit future interventions.
We observed a trend in increasing obesity prevalence with academic level such that third- and fourth-year students had significantly increased obesity prevalence than first- and second-year students. Similar findings have been observed in other related studies [ 41 , 73 , 74 ] but not all [ 75 ]. This relationship may suggest the role of other factors of obesity associated with progressive academic level, which was not the focus of this study. We recommend future research to focus on changing lifestyle and eating habits of students related to progressive academic level. We replicated the association between obesity and family history and increased risk of obesity prevalence. This finding contributes to the evidence that genetics play an important role in the onset of obesity and the severity of obesity [ 76 , 77 ]. In several studies, sendentary life has been strsongly associated with being overweight/obese [ 11 , 17 , 27 , 28 ]. However, we did not find a significant association between being overweight/obese and students engagement in regular physical activity.
We would like to acknowledge some limitations of this study. First, Bayesian modelling is reliance on prior information, in our case we used the prior prevalence, concordance and diagnostic estimates obtained from the linked data and previous studies within the same population. As such, our analyses are limited by the accuracy of standard BMI thresholds for classifying obesity. Second, female students were over-represented in the dataset, which could bias the estimation of obesity prevalence. Although, we considered this in the analysis by reporting sex-specific prevalence estimates. Also, the generalizability of our estimate may be limited as data were obtained from only one tertiary institution in Ghana. This approach is significant to fill a gap in the current lack of consensus on the appropriate adiposity index and serves as the opportunity to unique data linkage and novel analytical techniques to improve obesity surveillance.
As different adiposity indices become increasingly available, multiple indicators used in combination may offer a greater power to examine obesity prevalence. We have demonstrated this by integrating central adiposity and percentage body fat criteria relative to standard BMI thresholds in a Bayesian framework and reported high obesity prevalence, especially among female students. We also demonstrated that obesity prevalence increases with university level and among students with a family history of obesity. The study suggests that the prevalence of being overweight or obese is expected to increase in the coming years, leading to several health issues. It emphasizes the requirement for public health efforts and interventions at a national level to control the problem and its associated costs and co-morbidities. Furthermore, interventions against obesity should be customized to target the socio-demographic disparities highlighted in the study.
Data Availability
The datasets and codes used and/or analysed during the current study are within the manuscript, and available at the GitHub repository ( https://github.com/EvansKCCR/obesity_among_students ).
Abbreviations
Relative fat mass
Total percentage of body fat
Body mass index
Bioelectrical impedance analysis
Kwame Nkrumah University of Science and Technology
Design effect
Receiver operative characteristics curve
Area under the curve
Hamiltonian Monte Carlo
Residual standard deviation
Obesity. and overweight. In: Department of Sustainable Development and Healthy Environments World Health Organization; 2021.
Murray CJL, Aravkin AY, Zheng P, Abbafati C, Abbas KM, Abbasi-Kangevari M, Abd-Allah F, Abdelalim A, Abdollahi M, Abdollahpour I et al. Global burden of 87 risk factors in 204 countries and territories, 1990–2019:a systematic analysis for the global burden of Disease Study 2019. The Lancet. 2020;396(10258):1223–49.
Murray CJL, Abbafati C, Abbas KM, Abbasi M, Abbasi-Kangevari M, Abd-Allah F, Abdollahi M, Abedi P, Abedi A, Abolhassani H, et al. Five insights from the global burden of Disease Study 2019. The Lancet. 2020;396(10258):1135–59.
Article Google Scholar
Blüher M. Obesity: global epidemiology and pathogenesis. Nat Reviews Endocrinol. 2019;15(5):288–98.
World Obesity Day. 2022 – Accelerating action to stop obesity. In.: World Health Organization; 2022.
Nugent R. Chronic Diseases in developing countries: health and economic burdens. Ann N Y Acad Sci. 2008;1136(1):70–9.
Article PubMed Google Scholar
Ofori-Asenso R, Agyeman AA, Laar A, Boateng D. Overweight and obesity epidemic in Ghana—a systematic review and meta-analysis. BMC Public Health. 2016;16(1):1–18.
Akowuah PK, Kobia-Acquah EJJoN. Metabolism: Childhood obesity and overweight in Ghana: a systematic review and meta-analysis. Journal of Nutrition Metabolism 2020, 2020.
Amegah A, Lumor S, Vidogo FJAJF. Agriculture, Nutrition, Development: prevalence and determinants of overweight and obesity in adult residents of Cape Coast, Ghana: a hospital-based study. Afr J Food Agric Nutr Dev 2011, 11(3).
Anderson AK. Prevalence of anemia, overweight/obesity, and undiagnosed Hypertension and Diabetes among residents of selected communities in Ghana. Int J Chronic Dis 2017, 2017.
Boateng GO, Adams EA, Odei Boateng M, Luginaah IN, Taabazuing M-M. Obesity and the burden of health risks among the elderly in Ghana: a population study. PLoS ONE. 2017;12(11):e0186947.
Article PubMed PubMed Central Google Scholar
Nyakotey DA, Ananga AS, Apprey C. Assessing physical activity, nutrient intake and obesity in middle-aged adults in Akuse, Lower Manya Krobo, Ghana. J Health Res. 2022;36(2):199–208.
Obirikorang C, Osakunor DNM, Anto EO, Amponsah SO, Adarkwa OK. Obesity and cardio-metabolic risk factors in an urban and rural population in the Ashanti Region-Ghana: a comparative cross-sectional study. PLoS ONE. 2015;10(6):e0129494.
William K. Obesity and cardiovascular risk factors in a Pentecostal population in Kumasi-Ghana. J Med Sci. 2008;8(8):682–90.
Agbeko MP, Akwasi K-K, Andrews DA, Gifty OB. Predictors of overweight and obesity among women in Ghana. Open Obes J 2013, 5(1).
Asosega KA, Adebanji AO, Abdul IW. Spatial analysis of the prevalence of obesity and overweight among women in Ghana. BMJ open. 2021;11(1):e041659.
Asosega KA, Aidoo EN, Adebanji AO, Owusu-Dabo E. Examining the risk factors for overweight and obesity among women in Ghana: A multilevel perspective. Heliyon 2023.
Marquis GS, Colecraft EK, Bannerman B, Thompson-Colón T. Overweight and obesity among women in a predominantly rural district of Ghana. The FASEB Journal 2016, 30:667.669-667.669.
Tuoyire DA. Television exposure and overweight/obesity among women in Ghana. BMC Obes. 2018;5(1):1–10.
Tuoyire DA. Overweight/obesity among 15-to 24-year-old women in Ghana: 21-year trend, future projections and socio-demographic correlates. J Biosoc Sci. 2021;53(6):839–55.
Tuoyire DA, Kumi-Kyereme A, Doku DT. Socio-demographic trends in overweight and obesity among parous and nulliparous women in Ghana. BMC Obes. 2016;3(1):1–14.
Adom T, De Villiers A, Puoane T, Kengne AP. Prevalence and correlates of overweight and obesity among school children in an urban district in Ghana. BMC Obes. 2019;6:1–11.
Aryeetey R, Lartey A, Marquis GS, Nti H, Colecraft E, Brown P. Prevalence and predictors of overweight and obesity among school-aged children in urban Ghana. BMC Obes. 2017;4:1–8.
Mogre V, Gaa PK, Abukari RNS. Overweight, obesity and thinness and associated factors among school-aged children (5–14 years) in Tamale, Northern Ghana. Eur Sci J 2013, 9(20).
Akoto S, Tandoh MA, Nsiah K, Asamoah-Boakye O, Annaful VT. Lifestyle habits, macronutrient intake, and obesity prevalence among adolescents in rural-periurban community senior high schools in the Ho municipality of Ghana. Front Nutr 2022, 9.
Peltzer K, Pengpid S. Overweight and obesity and associated factors among school-aged adolescents in Ghana and Uganda. Int J Environ Res Public Health. 2011;8(10):3859–70.
Amoh I, Appiah-Brempong E. Prevalence and risk factors of obesity among senior high school students in the Adansi North district of Ghana. Int J Community Med Public Health. 2017;4(10):3762–9.
Aryee P, Helegbe G, Baah B, Sarfo-Asante R, Quist-Therson R. Prevalence and risk factors for overweight and obesity among nurses in the tamale metropolis of Ghana. J Med Biomedical Sci. 2013;2(4):13–23.
Duodu C, Awuni TK, Attito P, Zotor FB. Assessment of overweight and obesity prevalence among practicing nurses and midwives in the Hohoe Municipality of the Volta Region, Ghana. Sci J Public Health. 2015;3(6):842–51.
Obirikorang Y, Obirikorang C, Enoch O, Acheampong E, Tuboseiyefah P, Miriam A, Emmanuella N. Prevalence and risk factors of obesity among practicing nurses at three selected hospitals in Kumasi Metropolis, Ghana. J Med Biomedical Sci. 2016;5(3):45–55.
Amo-Mensah P, Vanotoo L, Davies-Teye B. Prevalence of overweight and Obesity among Health Personnel in an urban Ghana: a descriptive cross-sectional study, 2017. Value in Health. 2017;20(9):A544.
Abubakar MB, Uthman YA, Ibrahim KG. Prevalence of overweight and obesity among health-care workers in Ghana: a systematic review. Nigerian J Experimental Clin Biosci. 2021;9(1):47.
Addo J, Smeeth L, Leon D. Obesity in urban civil servants in Ghana: association with pre-adult wealth and adult socio-economic status. Public Health. 2009;123(5):365–70.
Article CAS PubMed Google Scholar
Addo P. Prevalence of obesity and overweight and Associated factors among Financial Institution workers in Accra Metropolis, Ghana: a Case Control Study. Value in Health. 2016;19(7):A579.
Addo PN, Nyarko KM, Sackey SO, Akweongo P, Sarfo B. Prevalence of obesity and overweight and associated factors among financial institution workers in Accra Metropolis, Ghana: a cross sectional study. BMC Res Notes. 2015;8(1):1–8.
Atuahene M, Ganle JK, Adjuik M, Atuahene NF, Kampitib GB. Overweight and obesity prevalence among public servants in Nadowli district, Ghana, and associated risk factors: a cross-sectional study. BMC Obes. 2017;4(1):1–8.
Kumah DB. Prevalence of overweight and obesity among staff of a Tertiary Institution in Kumasi, Ghana. EC Nutr. 2016;5:1277–81.
Google Scholar
Nuertey BD, Alhassan AI, Nuertey AD, Mensah IA, Adongo V, Kabutey C, Addai J, Biritwum RB. Prevalence of obesity and overweight and its associated factors among registered pensioners in Ghana; a cross sectional studies. BMC Obes. 2017;4(1):1–12.
Anto EO, Owiredu W, Adua E, Obirikorang C, Fondjo LA, Annani-Akollor ME, Acheampong E, Asamoah EA, Roberts P, Wang W. Prevalence and lifestyle-related risk factors of obesity and unrecognized Hypertension among bus drivers in Ghana. Heliyon. 2020;6(1):e03147.
Mogre V, Nyaba R, Aleyira S. Lifestyle risk factors of general and abdominal obesity in students of the school of medicine and health science of the University of Development Studies, Tamale, Ghana. International Scholarly Research Notices 2014, 2014.
Obirikorang C, Anto EO, Addai P, Obirikorang Y, Acheampong E. Prevalence and risks factors of overweight/obesity among undergraduate students: an institutional based cross-sectional study, Ghana. J Med Biomedical Sci. 2017;6(1):24–34.
Agyei A, Yorke E, Boima V. Prevalence of overweight and obesity and its relation to Diet and physical activity among medical students in Accra, Ghana. Afr J Health Sci. 2022;35(2):99–113.
Tapera R, Merapelo MT, Tumoyagae T, Maswabi TM, Erick P, Letsholo B, Mbongwe B. The prevalence and factors associated with overweight and obesity among University of Botswana students. Cogent Med. 2017;4(1):1357249.
Mogre V, Nyaba R, Aleyira S. Lifestyle risk factors of general and abdominal obesity in students of the school of medicine and health science of the University of Development Studies, Tamale, Ghana. ISRN obesity 2014, 2014.
Amoako M, Amoah-Agyei F, Du C, Fenton JI, Tucker RM. Emotional Eating among Ghanaian University Students: Associations with Physical and Mental Health Measures. 2023, 15(6):1526.
Dolan CM, Kraemer H, Browner W, Ensrud K, Kelsey JL. Associations between body composition, anthropometry, and mortality in women aged 65 years and older. Am J Public Health. 2007;97(5):913–8.
Kontogianni MD, Panagiotakos DB, Skopouli FN. Does body mass index reflect adequately the body fat content in perimenopausal women? Maturitas. 2005;51(3):307–13.
Obirikorang C, Obirikorang Y, Acheampong E, Anto EO, Toboh E, Asamoah EA, Amakwaa B, Batu EN, Brenya P. Association of wrist circumference and waist-to-height ratio with cardiometabolic risk factors among type II diabetics in a Ghanaian population. Journal of diabetes research 2018, 2018.
Frankenfield DC, Rowe WA, Cooney RN, Smith JS, Becker D. Limits of body mass index to detect obesity and predict body composition. Nutrition. 2001;17(1):26–30.
Sun SS, Chumlea WC, Heymsfield SB, Lukaski HC, Schoeller D, Friedl K, Kuczmarski RJ, Flegal KM, Johnson CL, Hubbard VS. Development of bioelectrical impedance analysis prediction equations for body composition with the use of a multicomponent model for use in epidemiologic surveys. Am J Clin Nutr. 2003;77(2):331–40.
Freedman DS, Thornton JC, Pi-Sunyer FX, Heymsfield SB, Wang J, Pierson RN Jr, Blanck HM, Gallagher D. The body adiposity index (hip circumference ÷ height1. 5) is not a more accurate measure of adiposity than is BMI, waist circumference, or hip circumference. Obesity. 2012;20(12):2438–44.
Senkus KE, Crowe-White KM, Locher JL, Ard JD. Relative fat mass assessment estimates changes in adiposity among female older adults with obesity after a 12-month exercise and diet intervention. Ann Med. 2022;54(1):1160–6.
Article CAS PubMed PubMed Central Google Scholar
Brodowicz GR, Mansfield RA, McClung MR, Althoff SA. Measurement of body composition in the elderly: dual energy x-ray absorptiometry, underwater weighing, bioelectrical impedance analysis, and anthropometry. Gerontology. 1994;40(6):332–9.
Bi Y, Wang R. Application of DEXA to body composition measurement. Sports Sci Res. 2008;29(1):17.
Woolcott OO, Bergman RN. Relative fat mass (RFM) as a new estimator of whole-body fat percentage a cross-sectional study in American adult individuals. Sci Rep. 2018;8(1):10980.
Joseph L, Gyorkos TW, Coupal L. Bayesian estimation of Disease Prevalence and the parameters of diagnostic tests in the absence of a Gold Standard. Am J Epidemiol. 1995;141(3):263–72.
Erkanli A, Soyer R, Stangl D. Hierarchical bayesian analysis for Prevalence Estimation. Case studies in bayesian statistics: 1997// 1997; New York. NY: Springer New York; 1997. pp. 325–46.
Chapter Google Scholar
Speybroeck N, Devleesschauwer B, Joseph L, Berkvens DJI. Misclassification errors in prevalence estimation: bayesian handling with care. 2013, 58:791–5.
Arya R, Antonisamy B, Kumar SJTIJP. Sample size estimation in prevalence studies. 2012, 79:1482–8.
Manual OI. Full body Sensor Body Composition Monitor and Scale Model HBF–510. China: Omron Healthcare 2008.
Passing H, Bablok. A new biometrical procedure for testing the equality of measurements from two different analytical methods. Application of linear regression procedures for method comparison studies in clinical chemistry, part I. J Clin Chem Clin Biochem Z fur Klinische Chemie Und Klinische Biochemie. 1983;21(11):709–20.
CAS Google Scholar
Peltzer K, Pengpid S, Samuels TA, Özcan NK, Mantilla C, Rahamefy OH, Wong ML, Gasparishvili A. Prevalence of overweight/obesity and its associated factors among university students from 22 countries. Int J Environ Res Public Health. 2014;11(7):7425–41.
Ghana - Gross. enrolment ratio in tertiary education [ https://knoema.com/atlas/Ghana/topics/Education/Tertiary-Education/Gross-enrolment-ratio-in-tertiary-education ].
Woolcott OO, Bergman RN. Relative Fat Mass as an estimator of whole-body fat percentage among children and adolescents: a cross-sectional study using NHANES. Sci Rep. 2019;9(1):15279.
Li YC, Li CI, Lin WY, Liu CS, Hsu HS, Lee CC, Chen FN, Li TC, Lin CC. Percentage of body fat assessment using bioelectrical impedance analysis and dual-energy X-ray absorptiometry in a weight loss program for obese or overweight Chinese adults. PLoS ONE. 2013;8(4):e58272.
Edwards J, Pananos AD, Thind A, Stranges S, Chiu M, Anderson KK. A bayesian approach to estimating the population prevalence of mood and anxiety disorders using multiple measures. Epidemiol Psychiatric Sci. 2021;30:e4.
Pegington M, French DP, Harvie MN. Why young women gain weight: a narrative review of influencing factors and possible solutions. 2020, 21(5):e13002.
Ersoy C, Imamoglu S. Comparison of the obesity risk and related factors in employed and unemployed (housewife) premenopausal urban women. Diabetes Res Clin Pract. 2006;72(2):190–6.
Cohen E, Boetsch G, Palstra FP, Pasquet P. Social valorisation of stoutness as a determinant of obesity in the context of nutritional transition in Cameroon: the Bamiléké case. Social science & medicine ( 1982 ) 2013, 96:24–32.
Jokela M, Elovainio M, Kivimäki M. Lower fertility associated with obesity and underweight: the US National Longitudinal Survey of Youth. Am J Clin Nutr. 2008;88(4):886–93.
Alati R, Betts KS, Williams GM, Najman JM, Zalbahar N, Mamun A. Generational increase in obesity among young women: a prospective analysis of mother–daughter dyads. Int J Obes. 2016;40(1):176–80.
Article CAS Google Scholar
Eating Patterns of Young Women (18–25 y) with Overweight and Obesity: A Preliminary Investigation. 2023, 15(7):1652.
Elham AA, Anas A, Ikram K, Jayakumary M. Obesity among university students: a cross-sectional study in Ajman, UAE. 2015.
Ghazawy ER, Mahfouz EM, Rahman A-E, Ahmed T. Emam SAJMJoMR: Obesity/Overweight among University students. Minia Egypt. 2022;33(3):30–6.
Boukrim M, Obtel M, Lahlou L, Razine R. University students’ perceptions and factors contributing to obesity and overweigh in Southern of Morocco. Afr Health Sci. 2021;21(2):942–50.
Corica D, Aversa T, Valenzise M, Messina MF, Alibrandi A, De Luca F. Wasniewska MJFie: Does family history of obesity, cardiovascular, and metabolic diseases influence onset and severity of childhood obesity? 2018, 9:187.
van der Sande MA, Walraven GE, Milligan PJ, Banya WA, Ceesay SM, Nyan OA. McAdam KPJBotWho: family history: an opportunity for early interventions and improved control of Hypertension, obesity and Diabetes. 2001, 79(4):321–8.
Download references
Acknowledgements
We would like to thank the students of KNUST who dedicated their time and participation to this study. We also express our gratitude to the KNUST Counselling unit and the University Hospital for their support during the data collection.
This research did not receive any specific grant from funding agencies in the public, commercial, or not-for-profit sectors.
Author information
Authors and affiliations.
Department of Molecular Medicine, School of Medical Science, Kwame Nkrumah University of Science and Technology (KNUST), Kumasi, Ghana
Christian Obirikorang, Evans Asamoah Adu, Anthony Afum-Adjei Awuah & Alexander Owusu Boakye
Kumasi Centre for Collaborative Research, Kumasi, Ghana
Christian Obirikorang, Evans Asamoah Adu, Anthony Afum-Adjei Awuah, Angela Nana Bosowah Fynn, Alexander Owusu Boakye & Austin Gideon Adobasom-Anane
Department of Medical Diagnostics, Faculty of Allied Health Sciences, Kwame Nkrumah University of Science and Technology, Kumasi, Ghana
Enoch Odame Anto, George Osei-Somuah & Ivy Ofori-Boadu
Centre for Precision Health, School of Medical and Health Sciences, Edith Cowan University, Western Australia, Australia
Enoch Odame Anto & Lois Balmer
Department of Nursing, Faculty of Health Sciences, Garden City University College, Kumasi, Ghana
Patience Nyarkoa Ansong & Yaa Obirikorang
Department of Chemical Pathology, University of Ghana Medical School, University of Ghana, Accra, Ghana
Eric NY Nyarko
You can also search for this author in PubMed Google Scholar
Contributions
CO, LB, and AAA conceptualized, designed the study, and edited the manuscript. EAA, EOA and AOB analyzed, interpreted the data, and drafted the paper. ANB, GOS and PNA assisted in data acquisition and measurement. YO, IOB, ENYN and AGA contributed to writing and editing the manuscript. All authors have read and approved the final version before submission.
Corresponding author
Correspondence to Christian Obirikorang .
Ethics declarations
Ethical approval and consent to participate.
Ethical approval with reference ID (CHRPE/AP/030/19) was obtained from the Committee on Human Research, Publications and Ethics (CHRPE), School of Medicine and Dentistry, Kwame Nkrumah University of Science & Technology. Written informed consent was obtained from participants 18 years and above and assent from minors (< 18 years) who opted to participate in the study. Additionally, informed consent to participate was taken from the legal guardians of minor participants. All methods were performed following the guidelines and regulations of the Helsinki Declaration.
Consent for publication
Not applicable.
Competing interests
The authors declare no competing interests.
Additional information
Publisher’s note.
Springer Nature remains neutral with regard to jurisdictional claims in published maps and institutional affiliations.
Electronic supplementary material
Below is the link to the electronic supplementary material.
Supplementary Material 1
Rights and permissions.
Open Access This article is licensed under a Creative Commons Attribution 4.0 International License, which permits use, sharing, adaptation, distribution and reproduction in any medium or format, as long as you give appropriate credit to the original author(s) and the source, provide a link to the Creative Commons licence, and indicate if changes were made. The images or other third party material in this article are included in the article’s Creative Commons licence, unless indicated otherwise in a credit line to the material. If material is not included in the article’s Creative Commons licence and your intended use is not permitted by statutory regulation or exceeds the permitted use, you will need to obtain permission directly from the copyright holder. To view a copy of this licence, visit http://creativecommons.org/licenses/by/4.0/ . The Creative Commons Public Domain Dedication waiver ( http://creativecommons.org/publicdomain/zero/1.0/ ) applies to the data made available in this article, unless otherwise stated in a credit line to the data.
Reprints and permissions
About this article
Cite this article.
Obirikorang, C., Adu, E.A., Anto, E.O. et al. Prevalence and risk factors of obesity among undergraduate student population in Ghana: an evaluation study of body composition indices. BMC Public Health 24 , 877 (2024). https://doi.org/10.1186/s12889-023-17175-5
Download citation
Received : 26 June 2023
Accepted : 07 November 2023
Published : 21 March 2024
DOI : https://doi.org/10.1186/s12889-023-17175-5
Share this article
Anyone you share the following link with will be able to read this content:
Sorry, a shareable link is not currently available for this article.
Provided by the Springer Nature SharedIt content-sharing initiative
- Body composition
- Undergraduate students
- Risk factors
BMC Public Health
ISSN: 1471-2458
- Submission enquiries: [email protected]
- General enquiries: [email protected]
- Skip to main content
- Keyboard shortcuts for audio player

- Your Health
- Treatments & Tests
- Health Inc.
- Public Health
Shots - Health News
Arkansas led the nation sending letters home from school about obesity. did it help.
Kavitha Cardoza

Since Arkansas started sending the obesity letters to parents, the state's childhood obesity rates rose to nearly 24% from 21%. During the pandemic, the state obesity rate hit a high of more than 26%. M. Spencer Green/AP hide caption
Since Arkansas started sending the obesity letters to parents, the state's childhood obesity rates rose to nearly 24% from 21%. During the pandemic, the state obesity rate hit a high of more than 26%.
LITTLE ROCK, Ark. — Sixth-grade boys were lining up to be measured in the Mann Arts and Science Magnet Middle School library. As they took off their shoes and emptied their pockets, they joked about being the tallest.
"It's an advantage," said one. "You can play basketball," said another. "A taller dude can get more girls!" a third student offered.
Everyone laughed. What they didn't joke about was their weight.
Anndrea Veasley, the school's registered nurse, had them stand one by one. One boy, Christopher, slumped as she measured his height. "Chin up slightly," she said. Then Veasley asked him to stand backward on a scale so he didn't see the numbers. She silently noted his height as just shy of 4 feet, 7 inches, and his weight as 115.6 pounds.

Lifesaving or stigmatizing? Parents wrestle with obesity treatment options for kids
His parents later would be among thousands to receive a letter beginning, "Many children in Arkansas have health problems caused by their weight." The letter includes each student's measurements as well as their calculated body mass index. The BMI number categorizes each child as "underweight," "normal," "overweight," or "obese." Christopher's BMI of 25.1 put him in the obese range.
In 2003, Arkansas became the first state to send home BMI reports about all students as part of a broader anti-obesity initiative. But in the 20 years since, the state's childhood obesity rates have risen to nearly 24% from 21%, reflecting a similar, albeit higher, trajectory than national rates. During the pandemic, the state obesity rate hit a high of more than 26%.
Still, at least 23 states followed Arkansas' lead and required height and weight assessments of students. Some have since scaled back their efforts after parents raised concerns.
One school district in Wyoming used to include a child's BMI score in report cards , a practice it has since stopped. Ohio allows districts to opt in, and last year just two of 611 school districts reported BMI information to the state. And Massachusetts stopped sending letters home . Even Arkansas changed its rules to allow parents to opt out.
Multiple studies have shown that these reports, or "fat letters" as they're sometimes mockingly called, have had no effect on weight loss. And some nutritionists, psychologists, and parents have criticized the letters, saying they can lead to weight stigma and eating disorders.
BMI as a tool has come under scrutiny, too, because it does not consider differences across racial and ethnic groups, sex, gender, and age. In 2023, the American Medical Association called the BMI "imperfect" and suggested it be used alongside other tools such as visceral fat measurements, body composition, and genetic factors.
All that highlights a question: What purpose do BMI school measurements and letters serve? Nearly 20% of American children were classified as obese just before the pandemic — up from only 5% some 50 years ago — and lockdowns made the problem worse. It's unclear what sorts of interventions might reverse the trend.
Joe Thompson, a pediatrician who helped create Arkansas' program and now leads the Arkansas Center for Health Improvement , said BMI letters are meant to be a screening tool, not a diagnostic test, to make parents aware if their child is at risk of developing serious health issues, such as heart disease, diabetes, and respiratory problems.
Sharing this information with them is critical, he said, given that many don't see it as a problem because obesity is so prevalent. Arkansas is also a rural state, so many families don't have easy access to pediatricians, he said.

Don't Focus On Kids' Weight Gain. Focus On Healthy Habits Instead
Thompson said he's heard from many parents who have acted on the letters. "To this day, they are still our strongest advocates," he said.
The program also led to new efforts to reduce obesity. Some school districts in Arkansas have instituted " movement breaks ," while others have added vegetable gardens , cooking classes , and walking trails . One district sought funding for bicycles . The state does not study whether these efforts are working.
Researchers say the BMI data also serves an important purpose in illuminating population-level trends , even if it isn't helpful to individuals.
Parents are generally supportive of weighing children in school, and the letters have helped increase their awareness of obesity, research shows. At the same time, few parents followed up with a health care provider or made changes to their child's diet or physical activity after getting a BMI letter, several other studies have found.
In what is considered the gold-standard study of BMI letters , published in 2020, researchers in California found that the letters home had no effect on students' weight. Hannah Thompson , a University of California-Berkeley assistant professor who co-authored the study, said most parents didn't even remember getting the letters. "It's such a tiny-touch behavioral intervention," she said.
Arkansas now measures all public school students in even grades annually — except for 12th graders because by that stage, the pediatrician Joe Thompson said, the students are "beyond the opportunities for schools to have an impact." The change also came after many boys in one school wore leg weights under their jeans as a prank, he said.
Kimberly Collins, 50, remembers being confused by the BMI letters sent to her from the Little Rock School District stating that all her children were overweight, and that one daughter was considered obese.
"It offended me as a mama," she said. "It made me feel like I wasn't doing my job."
She didn't think her children looked overweight and the family pediatrician had never brought it up as a concern.
Hannah Thompson, the researcher from California, said that's the biggest problem with BMI letters: Parents don't know what to do with the information. Without support to help change behavior, she said, the letters don't do much.
"You find out your child is asthmatic, and you can get an inhaler, right?" she said. "You find out that your child is overweight and where do you even go from there? What do you do?"
Kevin Gee , a professor at the University of California-Davis, who has studied BMI letters , said the mailings miss cultural nuances. In some communities, for example, people prefer their children to be heavier, associating it with comfort and happiness. Or some eat foods that they know aren't very nutritious but are an important way of expressing love and traditions.
"There's a lot of rich contextual pieces that we know influences rates of obesity," Gee said. "And so how do we balance that information?"
Collins' daughter, now 15, said that as she's grown older she increasingly feels uncomfortable about her weight. People stare at her and sometimes make comments. (Collins' mother asked that her daughter's name not be published because of her age and the sensitive nature of the subject.)
"On my birthday, I went to get my allergy shots and one of the nurses told me, 'You are getting chubbier,'" she said. "That didn't make me feel the best."

How Doctors Can Stop Stigmatizing — And Start Helping — Kids With Obesity
Collins said it pains her to see her soft-spoken daughter cover herself with her arms as if she's trying to hide. The teenager has also begun sneaking food and avoids the mirror by refusing to turn on the bathroom light, Collins said. The girl signed up for tennis but stopped after other children made fun of her, her mother said.
Looking back, Collins said, while she wishes she had paid more attention to the BMI letters, she also would have liked practical suggestions on what to do. Collins said she had already been following the short list of recommended healthy practices, including feeding her children fruits and vegetables and limiting screen time. She isn't sure what else she could have done.
Now everyone has an opinion on her daughter's weight, Collins said. One person told her to put a lock on the fridge. Another told her to buy vegan snacks. Her mother bought them a scale.
"It's a total uphill climb," Collins said with a sigh.
This article was produced as a part of a project for the Spencer Education Journalism Fellowship .
KFF Health News is a national newsroom that produces in-depth journalism about health issues and is one of the core operating programs at KFF — the independent source for health policy research, polling, and journalism.
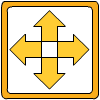
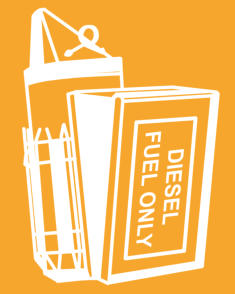
An official website of the United States government
The .gov means it’s official. Federal government websites often end in .gov or .mil. Before sharing sensitive information, make sure you’re on a federal government site.
The site is secure. The https:// ensures that you are connecting to the official website and that any information you provide is encrypted and transmitted securely.
- Publications
- Account settings
Preview improvements coming to the PMC website in October 2024. Learn More or Try it out now .
- Advanced Search
- Journal List
- HHS Author Manuscripts

Junk Food in Schools and Childhood Obesity
Ashlesha datar.
RAND Corporation, 1776 Main Street, P.O. Box 2138, Santa Monica, CA 90407, USA, gro.dnar@ratad , Phone: 1-310-393-0411 x7367, Fax: 1-310-260-8161
Nancy Nicosia
RAND Corporation, 20 Park Plaza, 7th Floor, Suite 720, Boston, MA 02116, USA, gro.dnar@aisocin , Phone: 1-617-338-2059 x4227
Despite limited empirical evidence, there is growing concern that junk food availability in schools has contributed to the childhood obesity epidemic. In this paper, we estimate the effects of junk food availability on BMI, obesity, and related outcomes among a national sample of fifth-graders. Unlike previous studies, we address the endogeneity of the school food environment by controlling for children’s BMI at school entry and estimating instrumental variables regressions that leverage variation in the school’s grade span. Our main finding is that junk food availability does not significantly increase BMI or obesity among this fifth grade cohort despite the increased likelihood of in-school junk food purchases. The results are robust to alternate measures of junk food availability including school administrator reports of sales during school hours, school administrator reports of competitive food outlets, and children’s reports of junk food availability. Moreover, the absence of any effects on overall food consumption and physical activity further support the null findings for BMI and obesity.
1. Introduction
The prevalence of childhood obesity in the US is at an all-time high with nearly one-third of all children and adolescents now considered overweight or obese ( Ogden et al 2008 ). Considerable attention has been focused on schools in an attempt to identify policy levers that will help reverse the obesity epidemic. In particular, the availability of “competitive foods”, defined as foods and beverages available or sold in schools outside of the school lunch and breakfast programs, has been a much debated issue. On the one hand, opponents question the nutritional value of competitive foods and consider them the primary source of “junk foods” in schools. Indeed, the available evidence suggests that these foods are higher in fat compared with foods sold as part of the school meal programs ( Gordon et al 2007b , Harnack et al 2000 , Wechsler et al 2000, Story, Hayes & Kalina 1996 ). On the other hand, supporters argue that revenues from these food sales provide much-needed funding for schools, especially in times of budgetary pressures ( Gordon et al 2007a ).
The debate draws from largely cross-sectional research that rarely addresses the potential endogeneity of the school food environment. Our paper advances the literature by attempting to isolate the causal effect of junk food availability on children’s food consumption and BMI. We use longitudinal data on BMI for a national sample of fifth graders from the Early Childhood Longitudinal Study – Kindergarten Class (ECLS-K) and an instrumental variables (IV) approach that leverages the well-documented fact that junk foods are significantly more prevalent in middle and high schools relative to elementary schools ( Finkelstein, Hill and Whitaker 2008 ). Plausibly exogenous variation in junk food availability across a cohort of fifth graders is identified using the grade structure in their schools. We argue that a fifth grader attending a combined (e.g. K-8, K-12) or middle school (e.g. 5–8) is more likely to be exposed to junk foods compared to a fifth grader in an elementary school (e.g. K-5, K-6), but that the school’s grade span has no direct effect on a child’s weight. First-stage regressions confirm that combined school attendance is a strong predictor of junk food availability. Further tests for instrument validity including an examination of sorting and peer effects support our use of the instrument.
We find that junk food availability has small positive associations with BMI and obesity in basic OLS models that only control for a limited set of covariates, but those associations become insignificant when controls for BMI at school entry and state fixed effects are added. Our IV models, which address potential bias in the OLS models, generate somewhat larger, albeit less precise, point estimates that are also not statistically significant. Even if the IV point estimates were statistically significant, they would still represent only minor increases in BMI and obesity, generally one-third of one percent. Moreover, reduced form estimates, which are more precisely estimated than IV estimates, provide further support because combined school attendance has no significant effects on 5 th graders’ BMI and obesity. These results are robust to alternative measures of junk food availability and sample restrictions. The models also produce the expected findings on various falsification tests.
While we acknowledge their limitations, ancillary analyses of children’s in-school junk food purchases, total consumption of healthy and unhealthy foods, and physical activity are consistent with our null findings for BMI and obesity. Our estimates suggest that the caloric contributions of in-school junk food purchases are likely to be small. Moreover, we find evidence consistent with substitution between in- and out-of-school consumption. Specifically, the total amount of soda and fast food consumed in- and out-of-school, is not significantly higher among those children with greater exposure to junk food in school (i.e. attending a combined school). And, finally, we find little support for the notion that children substitute calories from healthy foods or increase their physical activity to compensate for increased junk food intake.
The remainder of this paper is organized as follows. We first discuss junk food availability in schools and the findings from the existing literature in Section 2. Section 3 describes our data and relevant analysis variables. In Section 4, we describe our empirical strategy, which leverages longitudinal information on BMI and implements an instrumental variables approach to identify the causal impact of junk food availability. In Section 5, we first discuss our main results for children’s BMI and obesity and then support these findings with robustness checks and falsification tests. We also present supporting evidence from models of in-school purchases of junk food, total consumption of various healthy and unhealthy items, and physical activity. Finally, Section 6 concludes with the policy implications of our findings.
2. Background and Literature
Competitive foods are sold through a la carte lines, vending machines, school canteens/stores, and fundraisers and, in contrast to the federally-reimbursable school meal programs, are not subject to federal nutritional standards. As a result, competitive foods account for much of the variation in the food environment across schools. Competitive foods are available in a large share of schools, although the availability of these foods varies significantly across elementary, middle, and high schools. For example, as many as 97% of high schools and 82% of middle schools have vending machines compared to only 17% of elementary schools ( Gordon et al 2007a ). However, a la carte lines, which are the predominant source of competitive food sales, operate not only in most high (93%) and middle (92%) schools, but also in a large proportion of elementary schools (71%) ( Gordon et al 2007b ).
Sales of competitive foods have the potential to generate significant revenues for schools. During 2005–2006, middle and high schools earned an average of $10,850 and $15,233, respectively, from a la carte sales alone ( Gordon et al 2007a ). In addition, nearly a third of high schools and middle schools earned between $1,000–$9,999 during that same year from vending machines, another ten percent earned between $10,000–$50,000, and a small number earned in excess of $50,000 per year. These revenues may in turn be supplemented by on-site school stores and pouring contracts with beverage companies. While availability and revenues were less common in elementary schools, nearly half of elementary schools had pouring rights contracts, and competitive food sales from fundraising activities were also common.
The U.S. Department of Agriculture’s regulations on competitive foods in schools had been comprehensive, but in 1983, a successful lawsuit by the National Soft Drink Association limited the scope of these regulations to food service areas during meal hours ( Institute of Medicine 2007 ). In recent years, several states, districts, and schools have enacted competitive food policies that are more restrictive than federal regulations. And, between 2003 and 2005, approximately 200 pieces of legislation were introduced in US state legislatures to establish nutritional standards in schools or to address the availability or quality of competitive foods ( Boehmer et al 2007 ). At the federal level, legislation was passed in 2004 requiring local education agencies to develop a “wellness policy” by 2006 that included nutrition guidelines for all of the foods available in schools. More recently, there has been debate in the US Congress over enacting an amendment to the farm bill that would further restrict the sale of unhealthy foods and beverages in schools ( Black 2007 ). At the local level, two of the largest school districts in the nation, New York City Public School District and Los Angeles Unified School District, imposed a ban on soda vending in schools in 2003 and 2004, respectively.
Despite the growing support for competitive food regulation, it is hard to deny opponents’ claims that the evidence against competitive foods is limited. Existing research does show that competitive food availability is associated with a decline in nutritional quality of meals consumed at school ( Cullen et al 2000 , Cullen & Zakeri 2004 ; Templeton, Marlette & Panemangalore 2005 ). 1 However, less is known about the effects on overall diet quality (consumed both in and out of school) and children’s weight. The literature does provide some evidence of substitution of caloric intake across meals and locations among adults ( Anderson and Matsa 2011 ), but the evidence is less clear regarding children for whom parental oversight can also play a role. Only Kubik and colleagues have examined 24 hour dietary recall (2003) and BMI (2005) among children, however these studies are based on small cross-sectional samples and do not address the potential endogeneity of the school food environment. 2 , 3
The only effort to address endogeneity is in Anderson and Butcher (2006) , who use national data on adolescents aged 14–20 years to examine whether various school food policies influence BMI (based on self-reported height and weight data). In the absence of a single data source containing information on school food policies and BMI among adolescents, the authors use a two-sample IV approach that employs county, state, and regional characteristics as instruments to capture budgetary pressures on schools. They find that a 10 percentage point increase in the proportion of schools in the county that offer junk foods leads to a 1 percent increase in BMI. But this effect is primarily driven by adolescents with an overweight parent, which the authors interpret as a measure of family susceptibility. 4 Their IV approach constitutes an innovation over the literature, but the authors acknowledge that their results may be undermined by a weak first stage.
Our paper adds to the existing literature in its sample, methodology and scope. First, to our knowledge, ours is the only study that addresses the endogeneity of the school food environment among younger children. The focus on fifth graders is useful because junk food regulations are increasingly targeting elementary and middle schools. 5 And our national sample of children provides a larger and more representative sample with significant variation in school environments. Second, our data contain actual measurements of children’s height and weight, unlike the self-reports from other national datasets that have been used to examine this question previously. Third, our approach improves on the common cross-sectional designs by controlling for children’s BMI at school entry and state fixed-effects, and leveraging variation in schools’ grade spans to estimate IV models. Finally, unlike previous studies, we also provide evidence on the underlying mechanisms by examining effects on food consumption and physical activity.
The ECLS-K is a panel dataset on a nationally representative cohort of kindergarteners in the U.S. who entered school in fall 1998. In the fall and spring of kindergarten and the spring of the first, third, and fifth grades, the study collected information from the children and their parents, teachers, and schools on children′s cognitive, social, emotional, physical development (including BMI), and their home, classroom, and school environments. One limitation is that the information on the school food environment and children’s food consumption was collected only in the fifth grade. Our analysis sample includes the approximately 9,380 children attending the fifth grade in public and private schools in the 2003–04 school year. 6 In this section, we describe the key variables for our analyses.
3.1. Dependent Variables Measuring BMI, Food Consumption and Physical Activity
Body mass index (bmi).
A distinct advantage of the ECLS-K is that it collected height and weight measurements from children at kindergarten (school) entry and in the spring of kindergarten and first, third, and fifth grades. Measurements are superior to self- or parent-reported height and weight data that may introduce non-random measurement error. These measurements are used to compute BMI, defined as weight in kilograms divided by height in meters squared. The average BMI in our sample during the fifth grade is 20.4 ( Table 1 ). Approximately 20% of the ECLS-K sample is categorized as obese – this is nearly identical to prevalence rates among 6–11 years olds from the 2007–8 National Health and Nutrition Examination Survey ( Ogden et al 2010 ). 7
Descriptive Statistics in the Fifth Grade
Notes: N=9,380. Means are unweighted. Standard deviation in parentheses.
Junk Food Purchase in School
The food consumption questionnaire collected information on in-school junk food purchase during the fifth grade. These questions asked children about their purchases of sweets, salty snack foods, and sweetened beverages (hereafter, referred to as “soda”) during the previous week. 8 A substantial majority of the children did not purchase junk food in school during the reference week: 77% for sweets, 84% for salty snacks, and 88% for soda (see Appendix Table A1 ). But a large share of these children did not have junk food available in their schools (see Section 3.2). Conditional on availability, about half the sample purchased any of these unhealthy foods at least once a week in school. Among those who did purchase, the modal response was 1 to 2 purchases per week: 68 percent for sweets, 72 percent for salty snacks, and 70 percent for soda. 9
In-School and Total Food Consumption in Fifth Grade
Notes: N=9,380. Percentages are unweighted. Figures in the top panel are not conditional on availability in school.
Total Consumption of Selected Foods and Beverages
The child food consumption questionnaire asked about the frequency of overall consumption of specific food items during the past week. Children were asked to include foods they ate at home, at school, at restaurants, or anywhere else. We examine the consumption of two unhealthy items - soda and fast food, and six healthy food items – milk, green salad, potatoes 10 , carrots, other vegetables, and fruits. The percentage of children not consuming any soda or fast food during the previous week was 16 and 29 percent, respectively, with modal responses at 1 to 3 times per week (see Appendix Table A1 ). Among the healthy foods, green salad, carrots and potatoes were consumed most infrequently with nearly half of children reporting no consumption during the past week. The modal responses for the other healthy foods were 1 to 3 times during the past week.
3.2. Junk Food Availability
Detailed information on junk food availability in schools was collected from the school administrators and from children in the fifth grade. School administrators were asked whether students could purchase 17 individual food and beverage items, either from vending machines, school store, canteen, snack bar or a la carte items from the cafeteria during school hours. From these responses, we constructed an indicator variable of junk food availability in school that equals 1 if the administrator reports that students can purchase food and beverage items containing high sodium and/or sugar, including candy, chocolate, baked foods (e.g. cookies), salty snacks (e.g. potato chips), ice cream or frozen yogurt, or sweetened beverages during school hours, and zero otherwise. 11 Based on these school administrator reports, approximately 61 percent of the children had junk food availability in school. For robustness checks, we also considered two alternative measures of availability. The first is based on whether the modal child at each school reports that foods containing sugar, salty snacks, or sweetened beverages can be purchased at school. Based on this measure, about 75 percent of the children had junk foods available. And the second is based on whether the administrator reports any of the following competitive food outlets operate in the school: vending machines, school stores, canteens, snack bars, and a la carte lines. About 60 percent of the sample had at least one competitive food outlet. 12
4. Empirical Approach
4.1. econometric model.
The relationship between junk food availability and children’s BMI in fifth grade can be estimated cross-sectionally using the following linear regression model.
where, BMI iks , denotes fifth grade BMI for child i attending school k located in state s , JF k captures junk food availability in the child’s school, X i and S k are the vectors of individual/family (gender, age, age interacted with gender, race/ethnicity, mother’s education, household income) and school characteristics (private/public, percent minority, enrollment, urbanicity, state/region), respectively, and ε iks is the error term. The child’s baseline BMI (BBMI i ) is included to address potential heterogeneity that can bias OLS estimates such as student demand for junk foods, genetic susceptibility, and sorting. Because junk food availability is collected only in fifth grade, we do not know the length of exposure during prior school years. Therefore, BMI at school entry is the preferred baseline because it is measured prior to any exposure to the school food environment. Finally, since states differ markedly in terms of obesity prevalence in their populations as well as the policy environment geared towards combating obesity, we include state fixed effects (θ s ) to control for state-specific time-invariant unobserved heterogeneity that may be correlated with school food environments and children’s weight.
The parameter of interest in Equation (1) is β 1 . Obtaining an unbiased estimate of β 1 is challenging because the school food environment is not exogenous to the outcomes of interest. Schools that serve high-fat, energy-dense junk foods may differ on many observable and unobservable factors that are correlated with children’s weight and dietary behavior. In particular, the decision to offer junk foods in schools may be influenced by a variety of factors including budgetary pressures, demands of the student population, parental involvement, and state/district policies. These factors could independently influence children’s weight as well. For example, budgetary pressures may induce schools or districts to scale back or eliminate physical education programs, which might increase children’s weight. As a result, coefficient estimates from the ordinary least squares (OLS) estimation of Equation 1 would be biased.
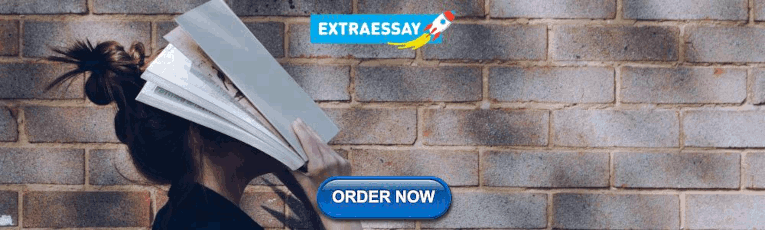
4.2. Addressing Endogeneity of Junk Food Availability in Schools
We address the potential endogeneity of junk food availability using instrumental variables. Specifically, we estimate the model in Equations (2.1) and (2.2) using Two-Stage Least Squares.
Equation 2.1 represents the first-stage regression where junk food availability (JF k ) is regressed on the combined school attendance instrument (CS k ), individual (X i ) and school (S k ) characteristics, baseline BMI (BBMI i ), and state fixed effects (θ s ). Equation 2.2 represents the second stage where children’s BMI (or obesity) is regressed on the predicted availability of junk foods from the first stage (ĴF k ) in addition to the common covariates.
We also report results from the reduced form, which regresses BMI or obesity directly on the instrument ( Equation 3 ). These results have the advantage of being unbiased and providing evidence of whether a causal relationship exists in the regression of interest. 13
4.2.1. Instrument
Our sample consists of a single cohort of 5 th graders attending schools with a variety of grade spans. Given that junk food availability is significantly higher in middle and high schools compared to elementary schools, a potentially useful instrument for junk food availability is whether the 5 th grader attends a combined school (defined as the highest grade is seventh or higher) or whether the 5 th grader is in an elementary school (defined as highest grade is 5 th or 6 th ). Our instrument considers only this dichotomy of school type: elementary versus combined. Over 70 percent of our sample attends elementary schools while the remainder attends combined schools usually with grade spans of K-8, K-12 and 5–8 (see Appendix Table A2 ).
Variation in Grade Span in Fifth Grade
Notes: N=9380. “Combined” schools are defined as schools with highest grade equal to 7 or higher.
For combined school attendance to be a valid instrument, it must be the case that the school’s grade span has no direct effect on children’s weight except through the junk food environment. One potential concern is that there may be unobserved factors that are correlated with both the likelihood of combined school attendance as well as BMI. For example, it is well known that states differ markedly in the prevalence of childhood obesity. But, states are also likely to differ in terms of factors that contribute to school grade span such as: (1) the size of the school-age population, (2) its distribution within the state, (3) differences in the educational systems and policies, as well as (4) education budgets. Similarly, school grade span can vary across urban versus rural areas (even within states), with the latter more likely to have combined schools largely because of a smaller school-age population. The inclusion of state and urbanicity dummies in our regressions controls for unobserved differences across states and across rural/urban areas that may be correlated with combined school attendance (or grade span, more generally) and BMI.
Another potential concern with this identification strategy is that variation in grade span exposes children to older peers who may influence obesogenic behaviors. Peers, defined broadly, have been shown to influence a wide range of adolescent behaviors and outcomes. 14 However, of particular relevance to our identification strategy is the literature examining a specific type of peer effect, namely, the effect of exposure to older peers due to school grade span.
Several studies have examined peer effects on academic, social-behavioral and substance use outcomes by leveraging variation in school grade span ( Clark and Folk 2007 ; Clark and Loheac 2007 ; Eisenberg 2004 ; Bedard and Do 2005 ; Cook et al 2008 ). Most studies compare students in the same grade who attend middle versus combined schools or middle versus elementary schools . 15 These studies generally find that 6 th or 7 th graders who attend middle school fare poorly compared to those who attend elementary or combined schools. 16 However, we are not aware of any studies that compare children in the same grade level who attend elementary versus combined schools . The exception is Rickles (2005) , whose findings suggest inconsistent effects of elementary versus combined schools attendance on achievement.
Furthermore, there is very limited evidence on the influence of older peers on food choices. Cullen and Zakeri (2004) compared changes in food consumption of 4 th graders who transitioned to middle school in 5 th grade and gained access to school snack bars to changes in food consumption of 5 th graders who were already in middle school. Fourth graders who transitioned to middle school consumed fewer healthy foods compared with the previous school year, but it is not clear whether this was due to the presence of older peers or the change in school food environment.
Overall, the literature suggests that the presence of older peers may adversely affect academic and social behavioral outcomes, but there is less evidence to support effects on their eating behaviors. Nevertheless, if such an effect exists, the potential bias in our IV estimates due to peer effects is likely to be upward. That is, 5 th graders might emulate older peers who are more likely to consume junk foods in school and would therefore tend to be overweight, independent of the school food environment. In that case, an insignificant finding is unlikely to be undermined.
4.2.2. Checks for Instrument Validity
Identification in our IV models relies on the assumption that, conditional on state and urbanicity dummies, the school’s grade span does not influence BMI except through differences in the availability of junk foods. Districts typically determine the grade span at the time of the schools’ opening based on a number of factors including transportation costs, length of bus ride, desired number of transitions, population size, site availability, preferred school size, and likelihood of parental involvement ( Paglin and Fager 1997 ) rather than children’s health outcomes. Changes in grade span over time are possible, but infrequent and similarly-motivated. For example, in our ECLS-K sample, less than 4 percent of the children who remained in the same school between kindergarten and fifth grade experienced a grade-span change from combined to elementary school or vice-versa. While unlikely, it is nevertheless possible that schools may change grade span in response to children’s physical size. Therefore, below we report results from several tests that support the validity of our instruments. These analyses are based on our preferred specification, which controls for the full set of covariates, including state and urbanicity dummies and baseline BMI.
First, we report first-stage estimates of the effect of our instrument – combined school attendance – on junk food availability in school. The first-stage estimates show that combined school attendance significantly increases the likelihood of junk food availability with an F-statistic on the instrument that exceeds 22 ( Table 2 ).
First Stage Regression Estimates of Junk Food Availability in Fifth Grade
Notes: Figures in brackets are robust standard errors clustered at the school level. Other covariates in the model include male, age (months), male*age, race/ethnicity, kindergarten BMI, mother’s education, income, private school dummy, categories for percent minority in school and school enrollment, and state and urbanicity dummies.
Second, since our instrument leverages across school variation we might be concerned that selection into different schools (or communities) might undermine the validity of our instrument. To test for differential selection into combined versus elementary schools, we regress BMI, obesity, test scores, social-behavioral outcomes, and parental involvement measured in kindergarten on combined school attendance in 5 th grade ( Table 3 ). 17 Because these outcomes are determined prior to exposure to school, these comparisons allow us to test for selection. The results suggest that, conditional on observed characteristics, combined school attendance is uncorrelated with pre-exposure BMI, obesity, test scores, social-behavioral outcomes and parental involvement.
Effect of Attending a Combined School on Kindergarten Outcomes
Notes: Each estimate represents a separate regression. Other covariates in the models include age, male, age*male, race/ethnicity, kindergarten BMI (not in model in Columns 1 and 2), mother’s education, income, private school dummy, categories for percent minority in school and school enrollment, and state and urbanicity dummies. Robust standard errors clustered at school level are shown in brackets. For reading, math, self control, and interpersonal skills, higher skills indicate better outcomes. For externalizing and internalizing behavior problems, higher scores indicate worse outcomes. Parent involvement is measured as the sum of the number of times/week that the parent engages in 9 activities with the child (e.g. reading books, talk about nature, do science projects, tell stories).
Third, another concern is that combined school attendance might generate peer effects on BMI, obesity, food consumption and physical activity, independent of junk food availability. We test for the presence of peer effects by regressing these outcomes on combined school attendance using only the sample of schools that do not offer junk foods ( Table 4 ). The results do not provide any support for peer effects on BMI, obesity, food consumption or physical activity. 18
Effect of Combined School Attendance on BMI, Obesity and Related Behaviors Without Junk Food Availability in Fifth Grade
Notes: Each estimate represents a separate regression. All models control for the full set of covariates. Robust standard errors clustered at school level are shown in brackets.
Overall, the instrument appears to be strongly predictive of junk food availability and there is no evidence that selection or peer effects threaten its validity.
We now turn to our main results, which examine the effects of junk food availability on BMI and other outcomes. We first estimate basic OLS models of BMI and obesity, then augment with state fixed effects and baseline BMI to address omitted variable bias and selection, and finally estimate the IV and reduced form specifications (Section 5.1). In Section 5.2, we examine the sensitivity of our results to alternate measures of junk food availability and various sample restrictions. We also report findings from falsification tests. And finally, in Section 5.3, we describe results from ancillary regressions that explore the potential mechanisms underlying our BMI findings. In particular, we examine in-school and total consumption of selected foods and beverages and the availability of and participation in physical activity.
5.1. BMI and Obesity
Our main results focus on whether the availability of junk foods increases BMI and obesity among 5 th graders ( Table 5 ). Columns 1 and 4 in Panel A show the results of basic OLS regressions of log BMI and obesity, respectively, on junk food availability controlling for child, household, and school characteristics. 19 These regressions yield a statistically significant increase in both BMI and obesity when junk food is available, although the point estimates are small. The inclusion of state fixed effects and urbanicity dummies (Panel A, columns 2 and 5) and then baseline BMI measured in kindergarten (Panel A, columns 3 and 6) eliminates the significant coefficients. The fully-specified OLS models have very small, precisely estimated, and statistically insignificant point estimates.
Effects of Junk Food Availability on BMI and Obesity in Fifth Grade
Notes: N=9,380. Robust standard errors clustered at school level are shown in brackets. Other covariates in the model include male, age (months), male*age, race/ethnicity, kindergarten BMI, mother’s education, income, private school dummy, categories for percent minority in school and school enrollment, and state and urbanicity dummies. First stage results are shown in Table 2 .
However, the coefficients from these OLS models may be biased if junk food availability is related to unobserved determinants of children’s BMI. For example, districts with a large population of students at risk for obesity may adopt more stringent nutritional policies that reduce the availability of junk foods in school. In such situations, OLS regressions may show no significant relationship or even a negative relationship between junk food availability and BMI. OLS estimates might also suffer from attenuation bias due to the presence of measurement error in the junk food availability measures.
To address these issues, we estimate instrumental variables (IV) and reduced form regressions using grade span as the instrument: whether the 5 th grader attends a combined school with older peers. 20 The IV point estimates are relatively larger than the OLS estimates, but less precisely estimated rendering them statistically insignificant ( Table 5 , Panel B). 21 , 22 IV estimates from models that do not control for state and urbanicity dummies and baseline BMI (columns 1 and 4) are much larger than those in our preferred specification (Columns 3 and 6), although they are not statistically significantly different from each other. Even if the IV point estimates in our preferred specification (columns 3 and 6) were significant, they would represent only small increases in BMI and obesity of less than one-third of one percent. Hausman tests that check for the endogeneity of junk food availability by comparing estimates from the fully-specified OLS regression with the IV cannot reject the null hypothesis that both estimates are consistent. Therefore, we also report the reduced form estimates of BMI and obesity regressed directly on our instrument ( Table 5 , Panel C). The coefficients on the instrument are close to zero and very precisely estimated, which further confirm the null findings. Given concerns about unobserved heterogeneity in the OLS specifications and the larger standard errors in the IV specifications, the reduced form estimates are preferred.
5.2. Sensitivity and Falsification Checks
We conducted a number of sensitivity analyses to test the robustness of our findings. In this section, we report results from a few key analyses and then turn to falsification tests. 23 These analyses control for the full set of covariates, including state and urbanicity dummies and baseline BMI.
For the sensitivity analyses, we first re-estimate our BMI and obesity regressions with the two alternate measures of junk food availability ( Table 6 ). Both the child-reported measure of junk food availability and the school-administrator reported measure of competitive food outlet show no effect of junk food availability on BMI or obesity. Next, we re-estimate the models with the exclusion of three particular groups that might confound our instrument ( Table 7 ). First, because combined schools are much more likely to be private, our instruments may simply capture variation across public versus private schools students, even though the regressions control for private school attendance. We re-estimate the models on a sample that excludes children who attend private schools ( Table 7 , Panel A) and find no effects on BMI and obesity. 24 Second, even though Section 4.2.2 suggests there are no peer effects on BMI and related behaviors, we test the sensitivity of our results to exclusion of the oldest peers (e.g., grade 9 or higher), but still find no evidence of an effect on BMI and obesity ( Table 7 , Panel B). Finally, children who switch schools for unobservable reasons potentially related to junk food availability may bias our estimates, but estimates from models that exclude children who changed schools between kindergarten and fifth grade confirm no effects ( Table 7 , Panel C). The point estimates from the OLS, IV and reduced form regressions for these sensitivity checks are essentially zero, though less precisely estimated in the IV models. 25
Effects of Alternate Measures of Junk Food Availability on BMI and Obesity in Fifth Grade
Notes: N=9,380
Effects of Junk Food Availability on BMI and Obesity in Fifth Grade with Alternate Sample Restrictions
Notes: All models include the full set of covariates. Robust standard errors clustered at school level are shown in brackets. Hausman tests for consistency of OLS estimates could not be rejected in any case. The tests are not reported in the table.
As falsification tests, we examined whether junk food availability in the fifth grade influenced children’s height in the fifth grade and their pre-exposure BMI. Height should clearly be unrelated. And indeed, the coefficients are essentially zero and insignificant ( Table 8 ). Because BMI and obesity in kindergarten is measured prior to exposure to junk foods in school, any effects would suggest unobserved heterogeneity. The OLS, IV and reduced form point estimates are close to zero (though the IV estimates are less precise) and the reduced form specifications also show no relationship ( Table 9 , Panel A). Results for BMI and obesity measured in first and third grade likewise confirm insignificant effects of junk food availability during fifth grade ( Table 9 , Panels B and C). However, because our data do not contain information on junk food availability prior to 5 th grade, these results are also consistent with the absence of junk foods in earlier grades.
Effect of Junk Food Availability in School on Height in Fifth Grade
Note: N=9,380. Robust standard errors clustered at school level are shown in brackets.
Effects of Junk Food Availability on BMI and Obesity in Kindergarten, First, and Third Grade
Notes: Each estimate represents a separate regression. All models include the full set of covariates. Robust standard errors clustered at school level are shown in brackets.
5.3. Effects of Junk Food Availability on Food Consumption and Physical Activity
The consistent lack of significant findings for BMI and obesity raises questions regarding how the energy balance equation is affected by junk food availability. While we cannot measure children’s energy intake and expenditure explicitly with these data, we can examine whether junk food availability influences general food consumption patterns and physical activity. Unlike BMI and obesity, the consumption and physical activity measures are based on parents’ and children’s reports . As a result, they are subject to measurement error and consequently produce noisier estimates particularly for the IV models. Nevertheless, they represent our best opportunity for understanding important mechanisms underlying our null finding. Therefore, for the in-school junk food purchases, total consumption, and physical activity analyses, we focus mainly on the reduced form results (though we provide OLS results for comparison). 26
5.3.1 In-School Purchases and Overall Consumption
One potential explanation for our null findings for BMI and obesity may be that availability does not impact overall food consumption. This may happen for several different reasons. First, young children may not purchase significant amounts of junk food in school either due to limited access to such foods or fewer discretionary resources to purchase them. Second, children may not change their total consumption of junk food because junk food purchased in school simply substitutes for junk food brought from home. Or third, children may not change their overall consumption during the day, but simply substitute between junk food consumed in-school and out-of-school.
Unfortunately, we cannot completely separate out these possible explanations because the ECLS-K does not provide us with full information about the daily dietary intake of each child. However, we do have information about in-school purchases of foods with sugar, salty snacks, and sweetened beverages for those children with in-school availability. We also have total (in-school plus out-of-school) consumption of soda, fast food, and a variety of healthy foods for all children in the sample. While not definitive, we can use this information to gain some insight into underlying eating behaviors and lend support for our BMI and obesity findings.
Not surprisingly, our analysis of in-school consumption of junk foods does confirm that children purchase junk food when it is available. 27 The OLS estimates show a significant relationship for purchases of all types of junk food when junk foods are available in schools ( Table 10 , Panel A). And the reduced form estimates show that children in combined schools are between 5 and 9 percentage points more likely to purchase junk foods compared to those in elementary schools Table 10 , Panel B).
Effect of Junk Food Availability on In-School Junk Food Purchases in Fifth Grade
Notes: N=9380. Each estimate represents a separate regression. Dependent variables in columns (1)–(3) are dichotomous and capture whether any purchase of that item was made in school during the last week. All regressions include the full set of covariates. Robust standard errors clustered at school level are shown in brackets.
To provide a sense of the caloric contribution of these purchases, we multiplied the increase in the probability of purchase from attending a combined school by the median number of times that food was purchased among children who purchased at least once, by the number of the calories per unit. 28 Summing across the three junk food groups yields 50 calories per week (7 calories per day) from in-school junk food purchases. The caloric contribution of in-school purchases is much higher (435 calories per week or 62 calories per day for the median child) among children who purchase these foods (as opposed to merely having them available). But even the 62 calories per day represents less than a quarter (23 percent) of the daily discretionary calorie allowance (267 calories) for a moderately active fifth grader. 29
It is possible that children substitute in-school purchases for snacks brought from home or eaten at home either due to satiation or parental monitoring. With our simple dietary recall measures, we cannot explicitly test the nature of potential substitution. We can, however, examine the total intake of soda and fast food consumed in and out of school. Soda is of particular interest because it is the only item for which children were asked about both their in-school and total consumption separately. Fast food, on the other hand, does not correspond exactly to the in-school snack food consumption categories. We find that junk food availability is not associated with significant increases in children’s total consumption of soda or fast foods ( Table 11 , Columns 1 and 2). 30 The OLS regressions show negative, though generally insignificant, estimates. 31 More importantly, the reduced form estimates confirm that there is no relationship between combined school attendance and total consumption of soda and fast food. The fact that children who consume soda and other junk food in schools show no evidence of an increase in total consumption provides support for the substitution hypothesis. This finding is also consistent with the literature, which indicates that only 27 percent of soda and sweetened drinks consumed in elementary schools are bought at school compared to 67 percent brought from home ( Briefel et al 2009 ).
Effect of Junk Food Availability on Total Consumption of Selected Unhealthy and Healthy Foods in Fifth Grade
Notes: N=9380. Each estimate represents a separate regression. Dependent variable captures the number of times the food or beverage item was consumed during the last 7 days. All models include the full set of covariates. Robust standard errors clustered at school level are shown in brackets.
While BMI is a widely-used outcome measure, it does not capture nutritional changes. Just because children are not gaining weight does not mean that their diets are not adversely affected by junk food availability. If children are consuming junk food in lieu of healthy foods, there may still be concerns about their nutrition. Columns 3 through 8 of Table 11 examine whether children with in-school availability of junk foods consume less milk, green salad, carrots, potatoes, other vegetables, and fruit. The OLS results show no significant associations with junk food availability. Moreover, reduced form regressions also show that combined school attendance does not significantly impact total consumption of the healthy foods. 32
Physical Activity
The absence of any effects of junk food availability on BMI despite the in-school purchases of junk food also raises questions regarding potential compensatory changes in the availability of and participation in physical activity. For example, revenues from junk food sales may be used to fund playgrounds or pay for physical education instructors. Or it may be that combined schools simply offer more opportunities for physical activity due to their scale and organization relative to elementary schools. Another possibility is that parents or children may increase children’s physical activity to balance junk food intake. If physical activity is greater, then we may find no change in BMI or obesity despite an increase in caloric intake.
OLS and reduced form estimates for school- and parent-reported physical activity measures are reported in Table 12 . OLS estimates show no relationship between junk food availability and minutes per week of physical education at school, minutes per week of recess at school, and parent-reported participation in physical activity (measured as the number of days per week that the child engaged in exercise that causes rapid heart beat for 20 continuous minutes or more). The reduced form regressions show no significant effects of combined school attendance on minutes per week of physical education instruction. Children attending combined school have fewer minutes of recess ( Table 12 , Column 2), but slightly higher days of parent-reported physical activity ( Table 12 , Column 3) though neither finding is statistically significant at.conventional levels. Overall, the regressions do not provide consistent evidence that increased energy expenditure explains the null finding for BMI and obesity.
Effects of Junk Food Availability on Physical Education, Recess and Physical Activity in Fifth Grade
Notes: Each estimate represents a separate regression. All models include the full set of covariates as well as the baseline (kindergarten) measure of the dependent variable. Robust standard errors clustered at school level are shown in brackets.
6. Conclusion
Junk food availability is a prominent issue for middle and high schools in the U.S. However, there is also widespread legislation and regulation targeting junk foods even in elementary school ( Trust for American’s Health 2009 ). Young children’s access to junk foods in school is an important concern due to the strong correlation between childhood overweight and obesity in adolescence and adulthood ( Institute of Medicine 2005 ). In this paper, we examined whether junk food availability increased BMI and obesity among a national sample of 5th graders. Those 5th graders who attend a combined school are much more likely to have junk food availability relative to those in elementary school. While estimates from naïve models that only control for a limited set of covariates suggest a positive association between junk foods in school and BMI and obesity, fully-specified OLS models that control for BMI at school entry and state fixed-effects demonstrate no statistically or economically significant relationships among these young children. Likewise, the IV and reduced form models, which are not subject to the potential bias undermining OLS models, confirm the null findings for BMI and obesity. These results are not sensitive to various robustness checks including alternate measures of junk food availability and sample restrictions.
Finally, we provide further support for the null findings by examining in-school and overall food consumption patterns as well as physical activity. The null effects on BMI and obesity cannot be explained entirely by limited access or limited discretionary resources among young children because 5 th graders do purchase junk food when it is available in schools. However, our results suggest that the caloric contribution of in-school purchases is likely to be small. Moreover, we find no evidence of significant changes in the overall frequency of consumption of soda and fast food, which is consistent with children substituting in-school purchases of junk food for that taken from or eaten at home. Alternative explanations such as compensatory changes children’s consumption of healthy foods and in their opportunities for and participation in physical activity do not appear to play a significant role in explaining our null findings for BMI and obesity.
Our findings may have implications in the current economic environment. Half of the states are projecting budget shortfalls that threaten staffing, compensation, extracurricular activities, and policy initiatives such as mandated limits on class size. 33 Many schools subsidize their funding with revenue from the sale of junk foods. In total, elementary schools earn approximately $442 million annually from junk food sales ( Institute of Medicine 2007 ). In light of our findings, certain policy measures, such as outright bans on junk food sales (at least among elementary school children), might appear premature given that they remove a key source of discretionary funds.
While our results are robust, we caution that we could not consider the full range of consequences of junk food availability. Not only are the dietary intake measures in the ECLS-K limited, but we are also not able to examine whether related health outcomes such as diet quality or dental caries are influenced by junk food availability. Also, we are unable to examine the generalizability of our findings to older children who may have greater junk food access and intake both in and outside school. And finally, we could not consider whether exclusive contracts between schools and beverage/snack companies influence students’ food choices in the longer run through product or brand recognition. Additional research is necessary to fully understand the potential consequences before costly legislation is implemented. Such research might also consider the consequences of junk food regulations on school finances and the extent to which these financial consequences could be mitigated by the sale of more nutritious alternatives or through alternative financing mechanisms.
Means by Attendance in Elementary Versus Combined School and by Private/Public
Notes: N=9,380.
Effect of Grade-Span on Academic and Social-Behavioral Outcomes Among Schools Without Junk Food Availability in Fifth Grade
Acknowledgments
This research was funded by grants from the Robert Wood Johnson Foundation’s Healthy Eating Research Program, NIH R01 HD057193, the Bing Center for Health Economics at RAND, and the RAND Labor and Population Program. All opinions are those of the authors and do not represent opinions of the funding agencies.
1 Other studies have examined the effects of price reductions, increases in availability, and promotion of low-fat foods in secondary schools on sales and purchases of these foods ( French et al 2004 , 2001 , 1997a , 1997b , Jeffery et al 1994 ) as well as their consumption ( Perry et al 2004 ) within experimental settings and found positive effects.
2 Kubik et al (2003) find that a la carte availability in school is negatively associated with overall intake of fruits and vegetables and positively associated with total and saturated fat intake among 7 th graders attending 16 Minneapolis-St Paul schools. Using the same data, Kubik et al (2005) show that using competitive foods as rewards and incentives is positively associated with BMI.
3 Also, using the ECLS-K, Fernandes (2008) found small positive associations between soda availability in schools and both in-school and overall soda consumption of fifth graders.
4 Their results for the other school policies, pouring rights contracts, and food and beverage advertisements are smaller and less precise.
5 For example, California’s first nutrition policy (SB 677) implemented beverage standards for elementary and middle schools, not high schools.
6 All sample sizes have been rounded to the nearest 10 per the ECLS-K’s restricted-use data agreement.
7 Obesity is defined as BMI greater than the 95 th percentile for age and gender on the Center for Disease Control growth charts.
8 Sweets include candy, ice cream, cookies, brownies or other sweets; salty snack foods include potato chips, corn chips, Cheetos, pretzels, popcorn, crackers or other salty snacks, and sweetened beverages include soda pop, sports drinks or fruit drinks that are not 100 percent juice.
9 To validate the ECLS-K estimates, we examined the Third School Nutrition and Dietary Assessment Study (SNDA-III), which collected 24-hour dietary recall from 2,300 children attending a nationally representative sample of public schools in 2005. Similar to the ECLS-K, eighty percent of elementary school children reported no competitive food purchases. Among children who made a purchase, the median daily caloric intake from these foods was 185 calories. The SNDA estimate is higher than our ECLS-K estimates (62 calories reported in Section 5) because it includes healthy foods purchased from competitive food venues: for example, milk was by far the most popular item purchased from competitive food venues and yogurt also ranked highly.
10 The “potatoes” category excluded French fries, fried potatoes, and potato chips.
11 The questionnaire separately asked about availability of high- and low-fat options for baked foods, salty snacks, and ice cream/frozen yogurt/sherbert. We include both the low- and high-fat options in our measure, however, in sensitivity analyses, we used only the high-fat versions to construct our school-administrator based measure of junk food availability and found results to be similar.
12 We rely mainly on the first measure of junk food availability because it is the most specific with respect to the quality of foods and because school-level policies regarding junk food availability are frequently set by school principals and staff ( Gordon et al 2007a ). We prefer this measure over the simple dichotomy of having any (unregulated) competitive food outlets because the outlet-based measure does not differentiate the type of foods sold (e.g. milk vs. soda). We also prefer it over the child-report because children who do not consume junk foods are less likely to accurately report availability and because children reported only the availability of any sweets, salty snacks, or sweetened beverages, but did not differentiate specific items (e.g. low-fat vs. high-fat).
13 The value of reduced form regressions has been highlighted by Angrist and Krueger (2001) and, more recently, Chernozhukov and Hansen (2008) formally show that the test for instrument irrelevance in the reduced form regression can be viewed as a weak-instrument-robust test of the hypothesis that the coefficient on the endogenous variable in the structural equation is zero.
14 This literature examines peer effects on a wide range of outcomes including substance use ( Lundborg 2006 ; Eisenberg 2004 ; Case and Katz 1991 ; Gaviria and Raphael 2001 ), crime ( Case and Katz 1991 ; Glaeser, Sacerdote, and Scheinkman 1996 ; Regnerus 2002 ), teenage pregnancy ( Crane 1991 ; Evans, Oates and Schwab 1992 ), discipline ( Cook et al 2008 ), academic achievement ( Hanushek et al 2003 ; Cook et al 2008 ), adolescent food choices ( Perry, Kelder, Komro 1993 ; Cullen et al 2001 ; French et al 2004 ) and weight ( Trogdon, Nonnemaker and Pais 2008 ).
15 However, Clark and Loheac (2007) estimate how substance use behavior of students within the same school who are one year older influences adolescent substance use and find a positive relationship.
16 One exception is Eisenberg (2004) who finds that 7 th and 8 th graders who attend schools with older peers are no more likely to use substances relative to those who attend schools with younger peers.
17 We also examined unadjusted differences in children’s individual, family and school characteristics during the 5 th grade (see Appendix Table A3 ). There were slight differences for some of the covariates. However, there was no overall pattern in the socioeconomic factors that would threaten the validity of the IV approach: that is, some differences imply better BMI outcomes for one group and others worse. For example, in our sample, elementary school students are more likely to be Hispanic and Asian while combined school students are more likely to be white. There are no differences in the share that are Black. Similarly, there is no consistent pattern in maternal education. Elementary school students are more likely to have poorly and highly educated mothers (less than high school, more than Bachelors).
18 To check whether these null findings are merely due to lack of power instead of absence of peer effects, we estimated the same models using social-behavioral outcomes and test scores as dependent variables because the literature finds evidence of peer effects on these outcomes. We were able to identify statistically significant peer effects on social-behavioral outcomes (but not test scores), which suggests that lack of power is an unlikely explanation for the finding of null peer effects on BMI and related outcomes.
19 In all models, we estimate robust standard errors clustered at the school level.
20 In alternate analyses, we used continuous measures of the highest and lowest grades in the school as instruments. In these over-identified models, both instruments had a strong positive association with junk food availability (i.e. increases in the highest and lowest grades available at the school were strongly predictive of junk food availability). This approach yielded qualitatively similar results as the exactly-identified models (available upon request).
21 The IV regressions were also estimated without baseline BMI. The point estimates, first-stage F-statistics, and Hausman tests yield similar results (available upon request).
22 A concern with our IV specification estimated via two-stage least-squares is that our first stage models do not account for the dichotomous nature of the treatment variable ( Maddala 1983 ). Estimates from binary treatment effect IV models confirm that the effects of junk food availability on BMI are neither substantive nor significant (available upon request).
23 We also conducted additional sensitivity analyses not reported here. First, given that we do not know the exposure to junk food in previous grades and given concerns that genetic susceptibility may not have a constant proportional effect on BMI at every point in the life cycle, we controlled for 1 st or 3 rd grade BMI instead of BMI in Kindergarten and obtained similar results. Second, inclusion of controls for school meal participation did not change our findings. Third, we used BMI z-scores as the dependent variable to accurately control for age and gender influences on BMI and obtained qualitatively similar results. Fourth, we estimated quantile regressions to test whether the effects of junk food availability varied across the BMI distribution, but found no evidence for heterogeneous effects. Finally, we also re-estimated our BMI and obesity models separately for each gender. The results for junk food availability mirrored those for the full sample. The OLS, IV, and RF models show no significant effects of junk food availability for either boys or girls. Still we may be concerned about differential peer effects, for example, if girls are influenced by older peers’ concerns about body image, which would bias our IV estimates downward. Restricting the sample to those boys and girls attending schools without junk food availability, the coefficients from the reduced form were nearly identical to those based on the full sample of boys and girls, which suggests that peer effects are not an issue even when regressions are gender-specific.
24 Estimates based only on the sample of private schools yield small and statistically insignificant effects of competitive food availability on BMI in both OLS and IV specifications, although the F-statistics for the instrument in the first stage were smaller (Results available upon request).
25 Hausman tests cannot reject the consistency of fully-specified OLS estimates in any of our sensitivity checks.
26 Although not shown, the IV (Wald) estimates are easily calculated by dividing the reduced form estimates in Table 10 – Table 12 by 0.2 (first stage estimate from Table 2 ). The IV coefficients are never significant in part due to the larger standard errors in the regressions of reported eating behaviors and physical activity.
27 We dichotomize the in-school purchase variables and estimate linear probability models since much of the variation in junk food purchases at school occurs on the extensive margin.
28 The median number of times an item is purchased in school among children who purchase at least once is 1.5 times (1–2 times per week). We assume that salty snacks add 140 calories (typical calories from a bag of potato chips), sweets add 200 calories (typically calories from a candy bar), and soda adds 150 calories. Given the limitations of the consumption data in the ECLS-K, we caution the reader to treat these caloric intake calculations as approximations.
29 Discretionary calories are the difference between an individual’s total energy requirement and the energy necessary to meet nutrient requirements. According to Dietary Guidelines for Americans, the discretionary allowance for a 2000 calorie diet is 267 calories. See: http://www.health.gov/dietaryguidelines/dga2005/document/html/chapter2.htm#table3 accessed August 22, 2008.
30 The total consumption variables are not dichotomized because there is sufficient variation on the intensive margin.
31 Negative binomial models with a binary treatment variable to account for the count-data distribution of the total consumption variable and the binary nature of junk food availability produced qualitatively similar results. (Results available upon request).
32 Given the limitations of the ECLS-K’s consumption variables, we again examined the SNDA-III data and found no evidence that combined school attendance increases total caloric intake.
33 “Schools expect budget cuts as economy sours: State problems, decline in property values eat away at district funds”. Available at: http://www.msnbc.msn.com/id/23116409/ (Accessed February 10, 2009).
Contributor Information
Ashlesha Datar, RAND Corporation, 1776 Main Street, P.O. Box 2138, Santa Monica, CA 90407, USA, gro.dnar@ratad , Phone: 1-310-393-0411 x7367, Fax: 1-310-260-8161.
Nancy Nicosia, RAND Corporation, 20 Park Plaza, 7th Floor, Suite 720, Boston, MA 02116, USA, gro.dnar@aisocin , Phone: 1-617-338-2059 x4227.
- Anderson PM, Butcher KM. Reading, Writing, and Refreshments: Are School Finances Contributing to Children’s Obesity? Journal of Human Resources. 2006; 41 (3):467–494. [ Google Scholar ]
- Anderson ML, Matsa DA. Are Restaurants Really Supersizing America? American Economic Journal: Applied Economics. 2011; 3 (1):152–188. [ Google Scholar ]
- Angrist J, Krueger A. Instrumental Variables and the Search for Identification: From Supply and Demand to Natural Experiments. Journal of Economic Perspectives. 2001; 15 (4):69–85. [ Google Scholar ]
- Bedard K, Do C. Are Middle Schools More Effective? The Impact of School Structure on Student Outcomes. Journal of Human Resources. 2005; 40 (3):660–682. [ Google Scholar ]
- Black J. Senate drops measure to greatly reduce sugar and fat in food at schools. Washington Post: 2007. Dec 15, p. A02. [ Google Scholar ]
- Boehmer TK, Brownson RC, Haire–Joshu D, Dreisinger ML. Patterns of childhood obesity prevention legislation in the United States. Prev Chronic Disease. 2007; 4 (3):A56. [ PMC free article ] [ PubMed ] [ Google Scholar ]
- Briefel RR, Crepinsek MK, Cabili C, Wilson A, Gleason PM. School food environments and practices affect dietary behaviors of US public school children. Journal of the American Dietetic Association. 2009; 109 (2 Suppl):S91–S107. [ PubMed ] [ Google Scholar ]
- Case AC, Katz L. The Company You Keep: The Effects of Family and Neighborhood on Disadvantaged Youths. National Bureau of Economic Research Working paper. 1991 # 3705. [ Google Scholar ]
- Chernozhukov V, Hansen C. An IV Model of Quantile Treatment Effects. Econometrica. 2005; 73 (1):245–261. [ Google Scholar ]
- Chernozhukov V, Hansen C. The Reduced Form: A Simple Approach to Inference with Weak Instruments. Economics Letters. 2008; 100 :68–71. [ Google Scholar ]
- Clark C, Folk J. Mimeo. Milledgeville, GA: Georgia College and State University; 2007. Do Peers Matter? Evidence from the Sixth Grade Experiment. [ Google Scholar ]
- Clark AE, Loheac Y. “It wasn’t me, it was them!” Social influence in risky behavior by adolescents. Journal of Health Economics. 2007; 26 :763–784. [ PubMed ] [ Google Scholar ]
- Cook P, MacCoun R, Muschkin C, Vigdor JL. The Negative Impacts of Starting Middle School in Sixth Grade. Journal of Policy Analysis and Management. 2008; 27 (1):104–121. [ Google Scholar ]
- Crane J. The Epidemic Theory of Ghettos and Neighborhood Effects on Dropping Out and Teenage Childbearing. American Journal of Sociology. 1991; 96 (5):1226–1260. [ Google Scholar ]
- Cullen KW, Eagan J, Baranowski T, et al. Effect of à la carte and snack bar foods at school on children’s lunchtime intake of fruits and vegetables. Journal of American Dietetic Association. 2000; 100 :1482–1486. 2000. [ PubMed ] [ Google Scholar ]
- Cullen KW, Zakeri I. Fruits, vegetables, milk, and sweetened beverages consumption and access to a la carte/snack bar meals at school. Am J Public Health. 2004 Mar; 94 (3):463–467. [ PMC free article ] [ PubMed ] [ Google Scholar ]
- Cullen KW, Baranowski T, Rittenberry L, et al. Child-reported family and peer influences on fruit, juice and vegetable consumption: reliability and validity of measures. Health Education Research. 2001 Apr; 16 (2):187–200. [ PubMed ] [ Google Scholar ]
- Eisenberg D. Mimeo. Ann Arbor: University of Michigan; 2004. Peer Effects for Adolescent Substance Use: Do They Really Exist? [ Google Scholar ]
- Evans WM, Oates WE, Schwab RM. Measuring Peer Group Effects: A Study of Teenage Behavior. Journal of Political Economy. 1992; 100 (5):966–991. [ Google Scholar ]
- Fernandes M. The Effect of Soft Drink Availability in Elementary Schools on Consumption. Journal of the American Dietetic Association. 2008; 108 (9):1445–1452. [ PubMed ] [ Google Scholar ]
- Finkelstein DM, Hill EL, Whitaker RC. School food environments and policies in US public schools. Pediatrics. 2008; 122 (1):e251–e259. [ PubMed ] [ Google Scholar ]
- French S, Story M, Fulkerson JA, Hannan P. An environmental intervention to promote lower fat food choices in secondary schools: Outcomes from the TACOS study. American Journal of Public Health. 2004; 94 :1507–1512. [ PMC free article ] [ PubMed ] [ Google Scholar ]
- French SA, Jeffery RW, Story M, et al. Pricing and promotion effects on low-fat vending snack purchases: the CHIPS Study. Am J Public Health. 2001; 91 (1):112–117. [ PMC free article ] [ PubMed ] [ Google Scholar ]
- French SA, Jeffery RW, Story M, Hannan P, Snyder MP. A pricing strategy to promote low-fat snack choices through vending machines. Am J Public Health. 1997; 87 (5):849–851. [ PMC free article ] [ PubMed ] [ Google Scholar ]
- French SA, Story M, Jeffery RW, et al. Pricing strategy to promote fruit and vegetable purchase in high school cafeterias. J Am Diet Assoc. 1997; 97 :1008–1010. [ PubMed ] [ Google Scholar ]
- Froelich M, Melly B. [Retrieved 2009-02-10]; Estimation of quantile treatment effects with STATA: mimeo. 2008 from http://www.alexandria.unisg.ch/publications/46580 .
- Gaviria A, Raphael S. School Based Peer Effects and Juvenile Behavior. Review of Economics and Statistics. 2001; 83 (2):257–268. [ Google Scholar ]
- Glaeser EL, Sacerdote B, Scheinkman JA. Crime and Social Interactions. Quarterly Journal of Economics. 1996; 111 (2):507–548. [ Google Scholar ]
- Gordon A, Crepinsek MK, Nogales R, Elizabeth Condon. School Nutrition Dietary Assessment Study-III: Volume I: School Foodservice, School Food Environment, and Meals Offered and Served. 2007a Report No. CN-07-SNDA-III. [ Google Scholar ]
- Gordon A, Crepinsek MK, Nogales R, Elizabeth Condon. School Nutrition Dietary Assessment Study-III: Volume II: Student Participation and Dietary Intakes. 2007b Report No. CN-07-SNDA-III, November. [ Google Scholar ]
- Gresham F, Elliott S. Social skills rating system. Circle Pines, MN: American Guidance Service; 1990. [ Google Scholar ]
- Hanushek EA, Kain JF, Markman JM, Rivkin SG. Does peer ability affect student achievement? Journal of Applied Econometrics. 2003; 18 (5):527–544. [ Google Scholar ]
- Harnack L, Snyder P, Story M, et al. Availability of a la carte food items in junior and senior high schools: a needs assessment. J Am Diet Assoc. 2000; 100 :701–703. [ PubMed ] [ Google Scholar ]
- Institute of Medicine. Committee on Prevention of Obesity in Children and Youth, Preventing Childhood Obesity:Health in the Balance. Washington, D.C: National Academies Press; 2005. [ PubMed ] [ Google Scholar ]
- Institute of Medicine. Nutrition Standards For Foods in Schools: Leading the Way Towards Healthier Youth. Washington, D.C.: The National Academies Press; 2007. [ Google Scholar ]
- Jeffery RW, French SA, Raether C, Baxter JE. An environmental intervention to increase fruit and salad purchases in a cafeteria. Prev Med. 1994; 23 :788–792. [ PubMed ] [ Google Scholar ]
- Kubik MY, Lytle LA, Hannan PJ, et al. The association of the school food environment with dietary behaviors of young adolescents. Am J Public Health. 2003; 93 :1168–1173. [ PMC free article ] [ PubMed ] [ Google Scholar ]
- Lundborg P. Having the wrong friends? Peer effects in adolescent substance use. Journal of Health Economics. 2006; 25 (2):214–233. [ PubMed ] [ Google Scholar ]
- Maddala GS. Limited Dependent and Qualitative Variables in Econometrics. Cambridge University Press; 1983. [ Google Scholar ]
- Ogden CL, Carroll MD, et al. Prevalence of high body mass index in US children and adolescents, 2007–2008. JAMA. 2010; 303 (3):242–249. [ PubMed ] [ Google Scholar ]
- Paglin C, Fager J. Grade configuration: Who goes where? By request series. Portland, OR: Northwest Regional Educational Laboratory; 1997. [ Google Scholar ]
- Perry CL, Bishop DB, Taylor GL, et al. A Randomized School Trial of Environmental Strategies to Encourage Fruit and Vegetable Consumption among Children. Health Educ Behav. 2004; 31 :65. [ PubMed ] [ Google Scholar ]
- Perry CL, Kelder SH, Komro KA. In: The social world of adolescents: families, peers, school and the community. SG Millstein, AC Petersen, EO Nightingale., editors. New York, NY: Oxford University Press; 1993. pp. 73–96. Promoting the Health of Adolescents: New Directions for the Twenty-First Century. [ Google Scholar ]
- Rickles JH. Achievement Gains for Students in Schools with Alternative Grade Spans. Planning, Assessment and Research Division Publication No. 251. Los Angeles Unified School District, Los Angeles, CA: Program Evaluation and Research Branch; 2005. [ Google Scholar ]
- Regnerus MD. Friends’ influence on adolescent theft and minor delinquency: A developmental test of peer-reported effects. Social Science Research. 2002; 31 :681–705. [ Google Scholar ]
- Story M, Hayes M, Kalina B. Availability of foods in high schools: is there cause for concern? J Am Diet Assoc. 1996; 96 :123–126. [ PubMed ] [ Google Scholar ]
- Templeton SB, Marlette MA, Panemangalore M. Competitive foods increase the intake of energy and decrease the intake of certain nutrients by adolescents consuming school lunch. J Am Diet Assoc. 2005 Feb; 105 (2):215–220. [ PubMed ] [ Google Scholar ]
- Tourangeau K, Nord C, Lê T, et al. U.S. Department of Education. Washington, DC: National Center for Education Statistics; 2006. Early childhood longitudinal study, kindergarten class of 1998–99 (ECLS-K), Combined User’s Manual for the ECLS-K Fifth-Grade Data Files and Electronic Codebooks (NCES 2006–032) [ Google Scholar ]
- Trogdon JG, Nonnemaker J, Pais J. Peer effects in adolescent overweight. Journal of Health Economics. 2008; 27 (5):1388–1399. [ PubMed ] [ Google Scholar ]
- Trust for American’s Health. F as in Fat. How Obesity Policies are Failing in America. Tech. rep. 2009 Trust for American’s Health. [ Google Scholar ]
- Wechsler H, Brener NC, Kuester S, Miller C. Food service and foods and beverages available at school: results from the School Health Policies and Programs Study 2000. J Sch Health. 2001; 71 :313–324. [ PubMed ] [ Google Scholar ]
- Wolraich ML, Wilson DB, White JW. The effect of sugar on behavior or cognition in children: a meta-analysis. JAMA. 1995; 274 :1617–1621. [ PubMed ] [ Google Scholar ]

Turn Your Curiosity Into Discovery
Latest facts.
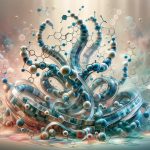
Follistatin344 Peptide Considerations
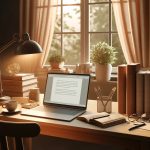
Approach for Using 5 Tips To Help You Write Your Dissertation
40 facts about elektrostal.
Written by Lanette Mayes
Modified & Updated: 02 Mar 2024

Reviewed by Jessica Corbett
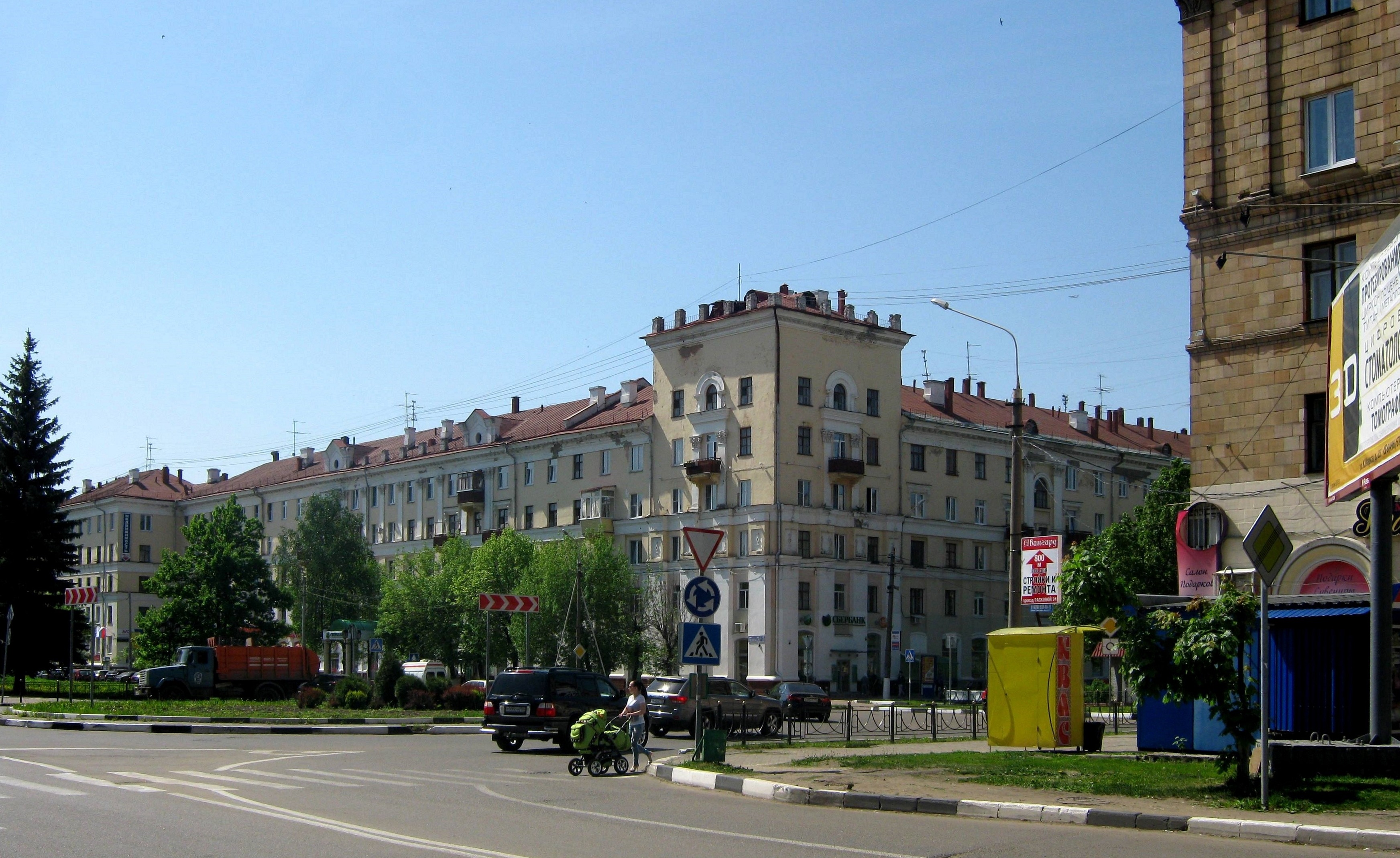
Elektrostal is a vibrant city located in the Moscow Oblast region of Russia. With a rich history, stunning architecture, and a thriving community, Elektrostal is a city that has much to offer. Whether you are a history buff, nature enthusiast, or simply curious about different cultures, Elektrostal is sure to captivate you.
This article will provide you with 40 fascinating facts about Elektrostal, giving you a better understanding of why this city is worth exploring. From its origins as an industrial hub to its modern-day charm, we will delve into the various aspects that make Elektrostal a unique and must-visit destination.
So, join us as we uncover the hidden treasures of Elektrostal and discover what makes this city a true gem in the heart of Russia.
Key Takeaways:
- Elektrostal, known as the “Motor City of Russia,” is a vibrant and growing city with a rich industrial history, offering diverse cultural experiences and a strong commitment to environmental sustainability.
- With its convenient location near Moscow, Elektrostal provides a picturesque landscape, vibrant nightlife, and a range of recreational activities, making it an ideal destination for residents and visitors alike.
Known as the “Motor City of Russia.”
Elektrostal, a city located in the Moscow Oblast region of Russia, earned the nickname “Motor City” due to its significant involvement in the automotive industry.
Home to the Elektrostal Metallurgical Plant.
Elektrostal is renowned for its metallurgical plant, which has been producing high-quality steel and alloys since its establishment in 1916.
Boasts a rich industrial heritage.
Elektrostal has a long history of industrial development, contributing to the growth and progress of the region.
Founded in 1916.
The city of Elektrostal was founded in 1916 as a result of the construction of the Elektrostal Metallurgical Plant.
Located approximately 50 kilometers east of Moscow.
Elektrostal is situated in close proximity to the Russian capital, making it easily accessible for both residents and visitors.
Known for its vibrant cultural scene.
Elektrostal is home to several cultural institutions, including museums, theaters, and art galleries that showcase the city’s rich artistic heritage.
A popular destination for nature lovers.
Surrounded by picturesque landscapes and forests, Elektrostal offers ample opportunities for outdoor activities such as hiking, camping, and birdwatching.
Hosts the annual Elektrostal City Day celebrations.
Every year, Elektrostal organizes festive events and activities to celebrate its founding, bringing together residents and visitors in a spirit of unity and joy.
Has a population of approximately 160,000 people.
Elektrostal is home to a diverse and vibrant community of around 160,000 residents, contributing to its dynamic atmosphere.
Boasts excellent education facilities.
The city is known for its well-established educational institutions, providing quality education to students of all ages.
A center for scientific research and innovation.
Elektrostal serves as an important hub for scientific research, particularly in the fields of metallurgy, materials science, and engineering.
Surrounded by picturesque lakes.
The city is blessed with numerous beautiful lakes, offering scenic views and recreational opportunities for locals and visitors alike.
Well-connected transportation system.
Elektrostal benefits from an efficient transportation network, including highways, railways, and public transportation options, ensuring convenient travel within and beyond the city.
Famous for its traditional Russian cuisine.
Food enthusiasts can indulge in authentic Russian dishes at numerous restaurants and cafes scattered throughout Elektrostal.
Home to notable architectural landmarks.
Elektrostal boasts impressive architecture, including the Church of the Transfiguration of the Lord and the Elektrostal Palace of Culture.
Offers a wide range of recreational facilities.
Residents and visitors can enjoy various recreational activities, such as sports complexes, swimming pools, and fitness centers, enhancing the overall quality of life.
Provides a high standard of healthcare.
Elektrostal is equipped with modern medical facilities, ensuring residents have access to quality healthcare services.
Home to the Elektrostal History Museum.
The Elektrostal History Museum showcases the city’s fascinating past through exhibitions and displays.
A hub for sports enthusiasts.
Elektrostal is passionate about sports, with numerous stadiums, arenas, and sports clubs offering opportunities for athletes and spectators.
Celebrates diverse cultural festivals.
Throughout the year, Elektrostal hosts a variety of cultural festivals, celebrating different ethnicities, traditions, and art forms.
Electric power played a significant role in its early development.
Elektrostal owes its name and initial growth to the establishment of electric power stations and the utilization of electricity in the industrial sector.
Boasts a thriving economy.
The city’s strong industrial base, coupled with its strategic location near Moscow, has contributed to Elektrostal’s prosperous economic status.
Houses the Elektrostal Drama Theater.
The Elektrostal Drama Theater is a cultural centerpiece, attracting theater enthusiasts from far and wide.
Popular destination for winter sports.
Elektrostal’s proximity to ski resorts and winter sport facilities makes it a favorite destination for skiing, snowboarding, and other winter activities.
Promotes environmental sustainability.
Elektrostal prioritizes environmental protection and sustainability, implementing initiatives to reduce pollution and preserve natural resources.
Home to renowned educational institutions.
Elektrostal is known for its prestigious schools and universities, offering a wide range of academic programs to students.
Committed to cultural preservation.
The city values its cultural heritage and takes active steps to preserve and promote traditional customs, crafts, and arts.
Hosts an annual International Film Festival.
The Elektrostal International Film Festival attracts filmmakers and cinema enthusiasts from around the world, showcasing a diverse range of films.
Encourages entrepreneurship and innovation.
Elektrostal supports aspiring entrepreneurs and fosters a culture of innovation, providing opportunities for startups and business development.
Offers a range of housing options.
Elektrostal provides diverse housing options, including apartments, houses, and residential complexes, catering to different lifestyles and budgets.
Home to notable sports teams.
Elektrostal is proud of its sports legacy, with several successful sports teams competing at regional and national levels.
Boasts a vibrant nightlife scene.
Residents and visitors can enjoy a lively nightlife in Elektrostal, with numerous bars, clubs, and entertainment venues.
Promotes cultural exchange and international relations.
Elektrostal actively engages in international partnerships, cultural exchanges, and diplomatic collaborations to foster global connections.
Surrounded by beautiful nature reserves.
Nearby nature reserves, such as the Barybino Forest and Luchinskoye Lake, offer opportunities for nature enthusiasts to explore and appreciate the region’s biodiversity.
Commemorates historical events.
The city pays tribute to significant historical events through memorials, monuments, and exhibitions, ensuring the preservation of collective memory.
Promotes sports and youth development.
Elektrostal invests in sports infrastructure and programs to encourage youth participation, health, and physical fitness.
Hosts annual cultural and artistic festivals.
Throughout the year, Elektrostal celebrates its cultural diversity through festivals dedicated to music, dance, art, and theater.
Provides a picturesque landscape for photography enthusiasts.
The city’s scenic beauty, architectural landmarks, and natural surroundings make it a paradise for photographers.
Connects to Moscow via a direct train line.
The convenient train connection between Elektrostal and Moscow makes commuting between the two cities effortless.
A city with a bright future.
Elektrostal continues to grow and develop, aiming to become a model city in terms of infrastructure, sustainability, and quality of life for its residents.
In conclusion, Elektrostal is a fascinating city with a rich history and a vibrant present. From its origins as a center of steel production to its modern-day status as a hub for education and industry, Elektrostal has plenty to offer both residents and visitors. With its beautiful parks, cultural attractions, and proximity to Moscow, there is no shortage of things to see and do in this dynamic city. Whether you’re interested in exploring its historical landmarks, enjoying outdoor activities, or immersing yourself in the local culture, Elektrostal has something for everyone. So, next time you find yourself in the Moscow region, don’t miss the opportunity to discover the hidden gems of Elektrostal.
Q: What is the population of Elektrostal?
A: As of the latest data, the population of Elektrostal is approximately XXXX.
Q: How far is Elektrostal from Moscow?
A: Elektrostal is located approximately XX kilometers away from Moscow.
Q: Are there any famous landmarks in Elektrostal?
A: Yes, Elektrostal is home to several notable landmarks, including XXXX and XXXX.
Q: What industries are prominent in Elektrostal?
A: Elektrostal is known for its steel production industry and is also a center for engineering and manufacturing.
Q: Are there any universities or educational institutions in Elektrostal?
A: Yes, Elektrostal is home to XXXX University and several other educational institutions.
Q: What are some popular outdoor activities in Elektrostal?
A: Elektrostal offers several outdoor activities, such as hiking, cycling, and picnicking in its beautiful parks.
Q: Is Elektrostal well-connected in terms of transportation?
A: Yes, Elektrostal has good transportation links, including trains and buses, making it easily accessible from nearby cities.
Q: Are there any annual events or festivals in Elektrostal?
A: Yes, Elektrostal hosts various events and festivals throughout the year, including XXXX and XXXX.
Was this page helpful?
Our commitment to delivering trustworthy and engaging content is at the heart of what we do. Each fact on our site is contributed by real users like you, bringing a wealth of diverse insights and information. To ensure the highest standards of accuracy and reliability, our dedicated editors meticulously review each submission. This process guarantees that the facts we share are not only fascinating but also credible. Trust in our commitment to quality and authenticity as you explore and learn with us.
Share this Fact:
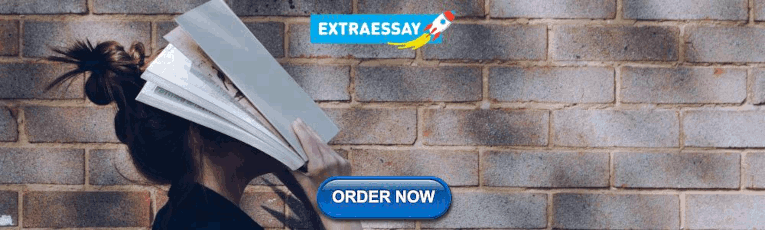
IMAGES
COMMENTS
This updated synthesis of obesity prevention interventions for children aged 6-18 years, found a small beneficial impact on child BMI for school-based obesity prevention interventions. A more comprehensive assessment of interventions is required to identify mechanisms of effective interventions to inform future obesity prevention public health policy, which may be particularly salient in for ...
Introduction. Childhood and adolescent obesity have reached epidemic levels in the United States, affecting the lives of millions of people. In the past 3 decades, the prevalence of childhood obesity has more than doubled in children and tripled in adolescents. 1 The latest data from the National Health and Nutrition Examination Survey show that the prevalence of obesity among US children and ...
Obesity Essay: Obesity is a condition that occurs when a person puts on excess body fat. It is a sudden and unusual increase in body fat. It can lead to heart-related diseases, blood pressure, hypertension, cholesterol, and various other health issues. The main cause of obesity is over-eating. Consuming junk food and staying away for physical ...
The time school nurses would normally have spent conducting health screenings or creating programing to promote healthy eating and exercise among students has been gobbled up by pandemic-related ...
A school-based obesity prevalence study carried out in Bengaluru by Sunil Kumar et al. documented that prevalence of overweight and obesity among school children was 7.09% and 4.08%. A study conducted by Ramesh among high school students of Trivandrum city, Kerala, reported that the prevalence of overweight was 12% and obesity was 6.3% among ...
During elementary. school, the prevalence of overweight rises from 26.1% in kindergarten to 40.5% in fifth grade, and over the same period the prevalence of obesity roughly doubles from 11.8% to 22.4%. Appendix Table 1 describes the PE requirements for elementary schools that prevailed in.
This Review describes current knowledge on the epidemiology and causes of child and adolescent obesity, considerations for assessment, and current management approaches. Before the COVID-19 pandemic, obesity prevalence in children and adolescents had plateaued in many high-income countries despite levels of severe obesity having increased. However, in low-income and middle-income countries ...
Findings about PAFs help demonstrate the importance of promoting physical activity, healthy diet, and other healthy lifestyles in reducing obesity among high school students in the United States. Top. Introduction. The prevalence of obesity among children and adolescents in the United States increased from 10.6% to 13.9% during 1999 through ...
Identify the physical activity score in the. 21 schools. 5. Make recommendations to strengthen the school environment for the prevention of overweight and obesity among children. . 1. Identify the most effective actions for preventing overweight and obesity in schools, based on a systematic literature review.
The introduction of school lunch and meal subsidy brought about more promising dietary benefits as compared to when students packed lunches themselves [73, 74].Studies suggested that a low-energy-dense diet will attenuate BMI and obesity risk among school-goers. Although findings about the direct interaction between diet pattern approach and ...
Abstract. Obesity is a complex condition that interweaves biological, developmental, environmental, behavioral, and genetic factors; it is a significant public health problem. The most common cause of obesity throughout childhood and adolescence is an inequity in energy balance; that is, excess caloric intake without appropriate caloric ...
The School Health Policies and Practices Study 2014 indicates that among U.S. high schools: Health Education. 88% required students to receive instruction on health topics as part of a specific course. 86% required students to receive instruction on nutrition and dietary behavior. 80% required students to receive instruction on physical activity.
In adolescent students, 72.4% and 34% have used at least one type of fast foods in recent month and in recent week. The obesity prevalence based on BMI and WHR was 21.3 % (18.2% in females vs 26.3% in males) and 33.2% (40.1% in females vs 21.9% in males), respectively. Fast food consumption was associated with WHR, while was not related to BMI.
Risk Factors of Overweight and Obesity Among School Children Aged 6 to 18 Years: A Scoping Review. Nur Zakiah Mohd Saat 1 Centre for Community Health ... Tsegaye M, Alemu D. Risk factors of overweight and obesity among high school students in Bahir Dar city, north west Ethiopia: school based cross-sectional study. Adv Prev Med. 2015;2015:1-9 ...
Students are often asked to write an essay on Obesity Among Students in their schools and colleges. And if you're also looking for the same, we have created 100-word, 250-word, and 500-word essays on the topic. ... This can be during gym class, recess, or after-school sports. Being active helps burn off the extra energy from food. Families ...
In the United States, the percentage of children and adolescents affected by obesity has more than tripled since the 1970s. 1 In 2017-2018, about 1 in 5 school-aged children were affected by obesity (20.3% of all 6-11 year olds, 21.2% of all 12-19 year olds) 1 . The prevalence of obesity among 2-19 year old children was 25.6% for Hispanic children, 24.2% for Black children, 16.1% for White ...
Obesity is a classified risk factor for several of the world's leading causes of death. In this study, we combined information contained in body mass index (BMI), total percentage body fat (TPBF) and relative fat mass (RFM) to estimate obesity prevalence and examine the risk factors associated with obesity. The study recruited 1027 undergraduate students aged between 16 and 25 years using a ...
Since Arkansas started sending the obesity letters to parents, the state's childhood obesity rates rose to nearly 24% from 21%. During the pandemic, the state obesity rate hit a high of more than 26%.
Furthermore, Ahrens et al 128 conducted a population-based cohort study on a sample of 16,228 school students aged 2-9 years and found that bone stiffness, genetic susceptibility, and specific metabolic biomarkers were significant risk factors for overweight and obesity among European school children. In a study by Blair et al, it was found ...
Welcome to the 628DirtRooster website where you can find video links to Randy McCaffrey's (AKA DirtRooster) YouTube videos, community support and other resources for the Hobby Beekeepers and the official 628DirtRooster online store where you can find 628DirtRooster hats and shirts, local Mississippi honey and whole lot more!
Abstract. Despite limited empirical evidence, there is growing concern that junk food availability in schools has contributed to the childhood obesity epidemic. In this paper, we estimate the effects of junk food availability on BMI, obesity, and related outcomes among a national sample of fifth-graders. Unlike previous studies, we address the ...
English Report Writing for Students - 9+ Examples, Format, Pdf 9+ English Report Writing Examples for Students - PDF School reports are a big part of a student's academic life. In fact, students are asked to write reports so often that they are almost as common as lunch breaks.... Report Writing Format for Class 10th to 12th.
Elektrostal is a city in Moscow Oblast, Russia, located 58 kilometers east of Moscow. Elektrostal has about 158,000 residents. Mapcarta, the open map.
40 Facts About Elektrostal. Elektrostal is a vibrant city located in the Moscow Oblast region of Russia. With a rich history, stunning architecture, and a thriving community, Elektrostal is a city that has much to offer. Whether you are a history buff, nature enthusiast, or simply curious about different cultures, Elektrostal is sure to ...