- Product Management
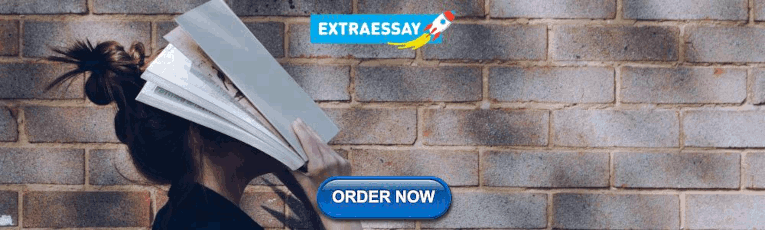
How to Generate and Validate Product Hypotheses
What is a product hypothesis.
A hypothesis is a testable statement that predicts the relationship between two or more variables. In product development, we generate hypotheses to validate assumptions about customer behavior, market needs, or the potential impact of product changes. These experimental efforts help us refine the user experience and get closer to finding a product-market fit.
Product hypotheses are a key element of data-driven product development and decision-making. Testing them enables us to solve problems more efficiently and remove our own biases from the solutions we put forward.
Here’s an example: ‘If we improve the page load speed on our website (variable 1), then we will increase the number of signups by 15% (variable 2).’ So if we improve the page load speed, and the number of signups increases, then our hypothesis has been proven. If the number did not increase significantly (or not at all), then our hypothesis has been disproven.
In general, product managers are constantly creating and testing hypotheses. But in the context of new product development , hypothesis generation/testing occurs during the validation stage, right after idea screening .
Now before we go any further, let’s get one thing straight: What’s the difference between an idea and a hypothesis?
Idea vs hypothesis
Innovation expert Michael Schrage makes this distinction between hypotheses and ideas – unlike an idea, a hypothesis comes with built-in accountability. “But what’s the accountability for a good idea?” Schrage asks. “The fact that a lot of people think it’s a good idea? That’s a popularity contest.” So, not only should a hypothesis be tested, but by its very nature, it can be tested.
At Railsware, we’ve built our product development services on the careful selection, prioritization, and validation of ideas. Here’s how we distinguish between ideas and hypotheses:
Idea: A creative suggestion about how we might exploit a gap in the market, add value to an existing product, or bring attention to our product. Crucially, an idea is just a thought. It can form the basis of a hypothesis but it is not necessarily expected to be proven or disproven.
- We should get an interview with the CEO of our company published on TechCrunch.
- Why don’t we redesign our website?
- The Coupler.io team should create video tutorials on how to export data from different apps, and publish them on YouTube.
- Why not add a new ‘email templates’ feature to our Mailtrap product?
Hypothesis: A way of framing an idea or assumption so that it is testable, specific, and aligns with our wider product/team/organizational goals.
Examples:
- If we add a new ‘email templates’ feature to Mailtrap, we’ll see an increase in active usage of our email-sending API.
- Creating relevant video tutorials and uploading them to YouTube will lead to an increase in Coupler.io signups.
- If we publish an interview with our CEO on TechCrunch, 500 people will visit our website and 10 of them will install our product.
Now, it’s worth mentioning that not all hypotheses require testing . Sometimes, the process of creating hypotheses is just an exercise in critical thinking. And the simple act of analyzing your statement tells whether you should run an experiment or not. Remember: testing isn’t mandatory, but your hypotheses should always be inherently testable.
Let’s consider the TechCrunch article example again. In that hypothesis, we expect 500 readers to visit our product website, and a 2% conversion rate of those unique visitors to product users i.e. 10 people. But is that marginal increase worth all the effort? Conducting an interview with our CEO, creating the content, and collaborating with the TechCrunch content team – all of these tasks take time (and money) to execute. And by formulating that hypothesis, we can clearly see that in this case, the drawbacks (efforts) outweigh the benefits. So, no need to test it.
In a similar vein, a hypothesis statement can be a tool to prioritize your activities based on impact. We typically use the following criteria:
- The quality of impact
- The size of the impact
- The probability of impact
This lets us organize our efforts according to their potential outcomes – not the coolness of the idea, its popularity among the team, etc.
Now that we’ve established what a product hypothesis is, let’s discuss how to create one.
Start with a problem statement
Before you jump into product hypothesis generation, we highly recommend formulating a problem statement. This is a short, concise description of the issue you are trying to solve. It helps teams stay on track as they formalize the hypothesis and design the product experiments. It can also be shared with stakeholders to ensure that everyone is on the same page.
The statement can be worded however you like, as long as it’s actionable, specific, and based on data-driven insights or research. It should clearly outline the problem or opportunity you want to address.
Here’s an example: Our bounce rate is high (more than 90%) and we are struggling to convert website visitors into actual users. How might we improve site performance to boost our conversion rate?
How to generate product hypotheses
Now let’s explore some common, everyday scenarios that lead to product hypothesis generation. For our teams here at Railsware, it’s when:
- There’s a problem with an unclear root cause e.g. a sudden drop in one part of the onboarding funnel. We identify these issues by checking our product metrics or reviewing customer complaints.
- We are running ideation sessions on how to reach our goals (increase MRR, increase the number of users invited to an account, etc.)
- We are exploring growth opportunities e.g. changing a pricing plan, making product improvements , breaking into a new market.
- We receive customer feedback. For example, some users have complained about difficulties setting up a workspace within the product. So, we build a hypothesis on how to help them with the setup.
BRIDGES framework for ideation
When we are tackling a complex problem or looking for ways to grow the product, our teams use BRIDGeS – a robust decision-making and ideation framework. BRIDGeS makes our product discovery sessions more efficient. It lets us dive deep into the context of our problem so that we can develop targeted solutions worthy of testing.
Between 2-8 stakeholders take part in a BRIDGeS session. The ideation sessions are usually led by a product manager and can include other subject matter experts such as developers, designers, data analysts, or marketing specialists. You can use a virtual whiteboard such as Figjam or Miro (see our Figma template ) to record each colored note.
In the first half of a BRIDGeS session, participants examine the Benefits, Risks, Issues, and Goals of their subject in the ‘Problem Space.’ A subject is anything that is being described or dealt with; for instance, Coupler.io’s growth opportunities. Benefits are the value that a future solution can bring, Risks are potential issues they might face, Issues are their existing problems, and Goals are what the subject hopes to gain from the future solution. Each descriptor should have a designated color.
After we have broken down the problem using each of these descriptors, we move into the Solution Space. This is where we develop solution variations based on all of the benefits/risks/issues identified in the Problem Space (see the Uber case study for an in-depth example).
In the Solution Space, we start prioritizing those solutions and deciding which ones are worthy of further exploration outside of the framework – via product hypothesis formulation and testing, for example. At the very least, after the session, we will have a list of epics and nested tasks ready to add to our product roadmap.
How to write a product hypothesis statement
Across organizations, product hypothesis statements might vary in their subject, tone, and precise wording. But some elements never change. As we mentioned earlier, a hypothesis statement must always have two or more variables and a connecting factor.
1. Identify variables
Since these components form the bulk of a hypothesis statement, let’s start with a brief definition.
First of all, variables in a hypothesis statement can be split into two camps: dependent and independent. Without getting too theoretical, we can describe the independent variable as the cause, and the dependent variable as the effect . So in the Mailtrap example we mentioned earlier, the ‘add email templates feature’ is the cause i.e. the element we want to manipulate. Meanwhile, ‘increased usage of email sending API’ is the effect i.e the element we will observe.
Independent variables can be any change you plan to make to your product. For example, tweaking some landing page copy, adding a chatbot to the homepage, or enhancing the search bar filter functionality.
Dependent variables are usually metrics. Here are a few that we often test in product development:
- Number of sign-ups
- Number of purchases
- Activation rate (activation signals differ from product to product)
- Number of specific plans purchased
- Feature usage (API activation, for example)
- Number of active users
Bear in mind that your concept or desired change can be measured with different metrics. Make sure that your variables are well-defined, and be deliberate in how you measure your concepts so that there’s no room for misinterpretation or ambiguity.
For example, in the hypothesis ‘Users drop off because they find it hard to set up a project’ variables are poorly defined. Phrases like ‘drop off’ and ‘hard to set up’ are too vague. A much better way of saying it would be: If project automation rules are pre-defined (email sequence to responsible, scheduled tickets creation), we’ll see a decrease in churn. In this example, it’s clear which dependent variable has been chosen and why.
And remember, when product managers focus on delighting users and building something of value, it’s easier to market and monetize it. That’s why at Railsware, our product hypotheses often focus on how to increase the usage of a feature or product. If users love our product(s) and know how to leverage its benefits, we can spend less time worrying about how to improve conversion rates or actively grow our revenue, and more time enhancing the user experience and nurturing our audience.
2. Make the connection
The relationship between variables should be clear and logical. If it’s not, then it doesn’t matter how well-chosen your variables are – your test results won’t be reliable.
To demonstrate this point, let’s explore a previous example again: page load speed and signups.
Through prior research, you might already know that conversion rates are 3x higher for sites that load in 1 second compared to sites that take 5 seconds to load. Since there appears to be a strong connection between load speed and signups in general, you might want to see if this is also true for your product.
Here are some common pitfalls to avoid when defining the relationship between two or more variables:
Relationship is weak. Let’s say you hypothesize that an increase in website traffic will lead to an increase in sign-ups. This is a weak connection since website visitors aren’t necessarily motivated to use your product; there are more steps involved. A better example is ‘If we change the CTA on the pricing page, then the number of signups will increase.’ This connection is much stronger and more direct.
Relationship is far-fetched. This often happens when one of the variables is founded on a vanity metric. For example, increasing the number of social media subscribers will lead to an increase in sign-ups. However, there’s no particular reason why a social media follower would be interested in using your product. Oftentimes, it’s simply your social media content that appeals to them (and your audience isn’t interested in a product).
Variables are co-dependent. Variables should always be isolated from one another. Let’s say we removed the option “Register with Google” from our app. In this case, we can expect fewer users with Google workspace accounts to register. Obviously, it’s because there’s a direct dependency between variables (no registration with Google→no users with Google workspace accounts).
3. Set validation criteria
First, build some confirmation criteria into your statement . Think in terms of percentages (e.g. increase/decrease by 5%) and choose a relevant product metric to track e.g. activation rate if your hypothesis relates to onboarding. Consider that you don’t always have to hit the bullseye for your hypothesis to be considered valid. Perhaps a 3% increase is just as acceptable as a 5% one. And it still proves that a connection between your variables exists.
Secondly, you should also make sure that your hypothesis statement is realistic . Let’s say you have a hypothesis that ‘If we show users a banner with our new feature, then feature usage will increase by 10%.’ A few questions to ask yourself are: Is 10% a reasonable increase, based on your current feature usage data? Do you have the resources to create the tests (experimenting with multiple variations, distributing on different channels: in-app, emails, blog posts)?
Null hypothesis and alternative hypothesis
In statistical research, there are two ways of stating a hypothesis: null or alternative. But this scientific method has its place in hypothesis-driven development too…
Alternative hypothesis: A statement that you intend to prove as being true by running an experiment and analyzing the results. Hint: it’s the same as the other hypothesis examples we’ve described so far.
Example: If we change the landing page copy, then the number of signups will increase.
Null hypothesis: A statement you want to disprove by running an experiment and analyzing the results. It predicts that your new feature or change to the user experience will not have the desired effect.
Example: The number of signups will not increase if we make a change to the landing page copy.
What’s the point? Well, let’s consider the phrase ‘innocent until proven guilty’ as a version of a null hypothesis. We don’t assume that there is any relationship between the ‘defendant’ and the ‘crime’ until we have proof. So, we run a test, gather data, and analyze our findings — which gives us enough proof to reject the null hypothesis and validate the alternative. All of this helps us to have more confidence in our results.
Now that you have generated your hypotheses, and created statements, it’s time to prepare your list for testing.
Prioritizing hypotheses for testing
Not all hypotheses are created equal. Some will be essential to your immediate goal of growing the product e.g. adding a new data destination for Coupler.io. Others will be based on nice-to-haves or small fixes e.g. updating graphics on the website homepage.
Prioritization helps us focus on the most impactful solutions as we are building a product roadmap or narrowing down the backlog . To determine which hypotheses are the most critical, we use the MoSCoW framework. It allows us to assign a level of urgency and importance to each product hypothesis so we can filter the best 3-5 for testing.
MoSCoW is an acronym for Must-have, Should-have, Could-have, and Won’t-have. Here’s a breakdown:
- Must-have – hypotheses that must be tested, because they are strongly linked to our immediate project goals.
- Should-have – hypotheses that are closely related to our immediate project goals, but aren’t the top priority.
- Could-have – hypotheses of nice-to-haves that can wait until later for testing.
- Won’t-have – low-priority hypotheses that we may or may not test later on when we have more time.
How to test product hypotheses
Once you have selected a hypothesis, it’s time to test it. This will involve running one or more product experiments in order to check the validity of your claim.
The tricky part is deciding what type of experiment to run, and how many. Ultimately, this all depends on the subject of your hypothesis – whether it’s a simple copy change or a whole new feature. For instance, it’s not necessary to create a clickable prototype for a landing page redesign. In that case, a user-wide update would do.
On that note, here are some of the approaches we take to hypothesis testing at Railsware:
A/B testing
A/B or split testing involves creating two or more different versions of a webpage/feature/functionality and collecting information about how users respond to them.
Let’s say you wanted to validate a hypothesis about the placement of a search bar on your application homepage. You could design an A/B test that shows two different versions of that search bar’s placement to your users (who have been split equally into two camps: a control group and a variant group). Then, you would choose the best option based on user data. A/B tests are suitable for testing responses to user experience changes, especially if you have more than one solution to test.
Prototyping
When it comes to testing a new product design, prototyping is the method of choice for many Lean startups and organizations. It’s a cost-effective way of collecting feedback from users, fast, and it’s possible to create prototypes of individual features too. You may take this approach to hypothesis testing if you are working on rolling out a significant new change e.g adding a brand-new feature, redesigning some aspect of the user flow, etc. To control costs at this point in the new product development process , choose the right tools — think Figma for clickable walkthroughs or no-code platforms like Bubble.
Deliveroo feature prototype example
Let’s look at how feature prototyping worked for the food delivery app, Deliveroo, when their product team wanted to ‘explore personalized recommendations, better filtering and improved search’ in 2018. To begin, they created a prototype of the customer discovery feature using web design application, Framer.
One of the most important aspects of this feature prototype was that it contained live data — real restaurants, real locations. For test users, this made the hypothetical feature feel more authentic. They were seeing listings and recommendations for real restaurants in their area, which helped immerse them in the user experience, and generate more honest and specific feedback. Deliveroo was then able to implement this feedback in subsequent iterations.
Asking your users
Interviewing customers is an excellent way to validate product hypotheses. It’s a form of qualitative testing that, in our experience, produces better insights than user surveys or general user research. Sessions are typically run by product managers and involve asking in-depth interview questions to one customer at a time. They can be conducted in person or online (through a virtual call center , for instance) and last anywhere between 30 minutes to 1 hour.
Although CustDev interviews may require more effort to execute than other tests (the process of finding participants, devising questions, organizing interviews, and honing interview skills can be time-consuming), it’s still a highly rewarding approach. You can quickly validate assumptions by asking customers about their pain points, concerns, habits, processes they follow, and analyzing how your solution fits into all of that.
Wizard of Oz
The Wizard of Oz approach is suitable for gauging user interest in new features or functionalities. It’s done by creating a prototype of a fake or future feature and monitoring how your customers or test users interact with it.
For example, you might have a hypothesis that your number of active users will increase by 15% if you introduce a new feature. So, you design a new bare-bones page or simple button that invites users to access it. But when they click on the button, a pop-up appears with a message such as ‘coming soon.’
By measuring the frequency of those clicks, you could learn a lot about the demand for this new feature/functionality. However, while these tests can deliver fast results, they carry the risk of backfiring. Some customers may find fake features misleading, making them less likely to engage with your product in the future.
User-wide updates
One of the speediest ways to test your hypothesis is by rolling out an update for all users. It can take less time and effort to set up than other tests (depending on how big of an update it is). But due to the risk involved, you should stick to only performing these kinds of tests on small-scale hypotheses. Our teams only take this approach when we are almost certain that our hypothesis is valid.
For example, we once had an assumption that the name of one of Mailtrap ’s entities was the root cause of a low activation rate. Being an active Mailtrap customer meant that you were regularly sending test emails to a place called ‘Demo Inbox.’ We hypothesized that the name was confusing (the word ‘demo’ implied it was not the main inbox) and this was preventing new users from engaging with their accounts. So, we updated the page, changed the name to ‘My Inbox’ and added some ‘to-do’ steps for new users. We saw an increase in our activation rate almost immediately, validating our hypothesis.
Feature flags
Creating feature flags involves only releasing a new feature to a particular subset or small percentage of users. These features come with a built-in kill switch; a piece of code that can be executed or skipped, depending on who’s interacting with your product.
Since you are only showing this new feature to a selected group, feature flags are an especially low-risk method of testing your product hypothesis (compared to Wizard of Oz, for example, where you have much less control). However, they are also a little bit more complex to execute than the others — you will need to have an actual coded product for starters, as well as some technical knowledge, in order to add the modifiers ( only when… ) to your new coded feature.
Let’s revisit the landing page copy example again, this time in the context of testing.
So, for the hypothesis ‘If we change the landing page copy, then the number of signups will increase,’ there are several options for experimentation. We could share the copy with a small sample of our users, or even release a user-wide update. But A/B testing is probably the best fit for this task. Depending on our budget and goal, we could test several different pieces of copy, such as:
- The current landing page copy
- Copy that we paid a marketing agency 10 grand for
- Generic copy we wrote ourselves, or removing most of the original copy – just to see how making even a small change might affect our numbers.
Remember, every hypothesis test must have a reasonable endpoint. The exact length of the test will depend on the type of feature/functionality you are testing, the size of your user base, and how much data you need to gather. Just make sure that the experiment running time matches the hypothesis scope. For instance, there is no need to spend 8 weeks experimenting with a piece of landing page copy. That timeline is more appropriate for say, a Wizard of Oz feature.
Recording hypotheses statements and test results
Finally, it’s time to talk about where you will write down and keep track of your hypotheses. Creating a single source of truth will enable you to track all aspects of hypothesis generation and testing with ease.
At Railsware, our product managers create a document for each individual hypothesis, using tools such as Coda or Google Sheets. In that document, we record the hypothesis statement, as well as our plans, process, results, screenshots, product metrics, and assumptions.
We share this document with our team and stakeholders, to ensure transparency and invite feedback. It’s also a resource we can refer back to when we are discussing a new hypothesis — a place where we can quickly access information relating to a previous test.
Understanding test results and taking action
The other half of validating product hypotheses involves evaluating data and drawing reasonable conclusions based on what you find. We do so by analyzing our chosen product metric(s) and deciding whether there is enough data available to make a solid decision. If not, we may extend the test’s duration or run another one. Otherwise, we move forward. An experimental feature becomes a real feature, a chatbot gets implemented on the customer support page, and so on.
Something to keep in mind: the integrity of your data is tied to how well the test was executed, so here are a few points to consider when you are testing and analyzing results:
Gather and analyze data carefully. Ensure that your data is clean and up-to-date when running quantitative tests and tracking responses via analytics dashboards. If you are doing customer interviews, make sure to record the meetings (with consent) so that your notes will be as accurate as possible.
Conduct the right amount of product experiments. It can take more than one test to determine whether your hypothesis is valid or invalid. However, don’t waste too much time experimenting in the hopes of getting the result you want. Know when to accept the evidence and move on.
Choose the right audience segment. Don’t cast your net too wide. Be specific about who you want to collect data from prior to running the test. Otherwise, your test results will be misleading and you won’t learn anything new.
Watch out for bias. Avoid confirmation bias at all costs. Don’t make the mistake of including irrelevant data just because it bolsters your results. For example, if you are gathering data about how users are interacting with your product Monday-Friday, don’t include weekend data just because doing so would alter the data and ‘validate’ your hypothesis.
- Not all failed hypotheses should be treated as losses. Even if you didn’t get the outcome you were hoping for, you may still have improved your product. Let’s say you implemented SSO authentication for premium users, but unfortunately, your free users didn’t end up switching to premium plans. In this case, you still added value to the product by streamlining the login process for paying users.
- Yes, taking a hypothesis-driven approach to product development is important. But remember, you don’t have to test everything . Use common sense first. For example, if your website copy is confusing and doesn’t portray the value of the product, then you should still strive to replace it with better copy – regardless of how this affects your numbers in the short term.
Wrapping Up
The process of generating and validating product hypotheses is actually pretty straightforward once you’ve got the hang of it. All you need is a valid question or problem, a testable statement, and a method of validation. Sure, hypothesis-driven development requires more of a time commitment than just ‘giving it a go.’ But ultimately, it will help you tune the product to the wants and needs of your customers.
If you share our data-driven approach to product development and engineering, check out our services page to learn more about how we work with our clients!
How to Generate and Validate Product Hypotheses

Every product owner knows that it takes effort to build something that'll cater to user needs. You'll have to make many tough calls if you wish to grow the company and evolve the product so it delivers more value. But how do you decide what to change in the product, your marketing strategy, or the overall direction to succeed? And how do you make a product that truly resonates with your target audience?
There are many unknowns in business, so many fundamental decisions start from a simple "what if?". But they can't be based on guesses, as you need some proof to fill in the blanks reasonably.
Because there's no universal recipe for successfully building a product, teams collect data, do research, study the dynamics, and generate hypotheses according to the given facts. They then take corresponding actions to find out whether they were right or wrong, make conclusions, and most likely restart the process again.
On this page, we thoroughly inspect product hypotheses. We'll go over what they are, how to create hypothesis statements and validate them, and what goes after this step.
What Is a Hypothesis in Product Management?
A hypothesis in product development and product management is a statement or assumption about the product, planned feature, market, or customer (e.g., their needs, behavior, or expectations) that you can put to the test, evaluate, and base your further decisions on . This may, for instance, regard the upcoming product changes as well as the impact they can result in.
A hypothesis implies that there is limited knowledge. Hence, the teams need to undergo testing activities to validate their ideas and confirm whether they are true or false.
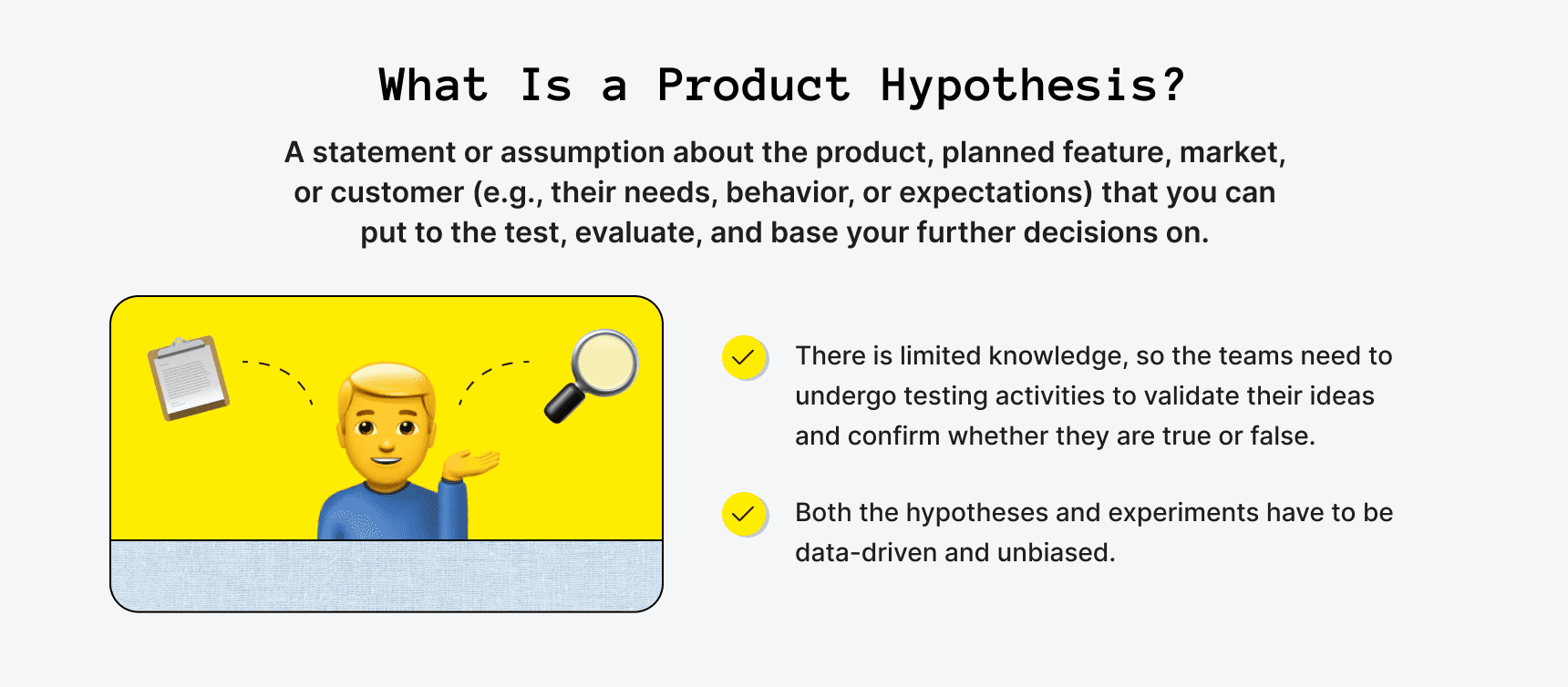
Hypotheses guide the product development process and may point at important findings to help build a better product that'll serve user needs. In essence, teams create hypothesis statements in an attempt to improve the offering, boost engagement, increase revenue, find product-market fit quicker, or for other business-related reasons.
It's sort of like an experiment with trial and error, yet, it is data-driven and should be unbiased . This means that teams don't make assumptions out of the blue. Instead, they turn to the collected data, conducted market research , and factual information, which helps avoid completely missing the mark. The obtained results are then carefully analyzed and may influence decision-making.
Such experiments backed by data and analysis are an integral aspect of successful product development and allow startups or businesses to dodge costly startup mistakes .
When do teams create hypothesis statements and validate them? To some extent, hypothesis testing is an ongoing process to work on constantly. It may occur during various product development life cycle stages, from early phases like initiation to late ones like scaling.
In any event, the key here is learning how to generate hypothesis statements and validate them effectively. We'll go over this in more detail later on.
Idea vs. Hypothesis Compared
You might be wondering whether ideas and hypotheses are the same thing. Well, there are a few distinctions.
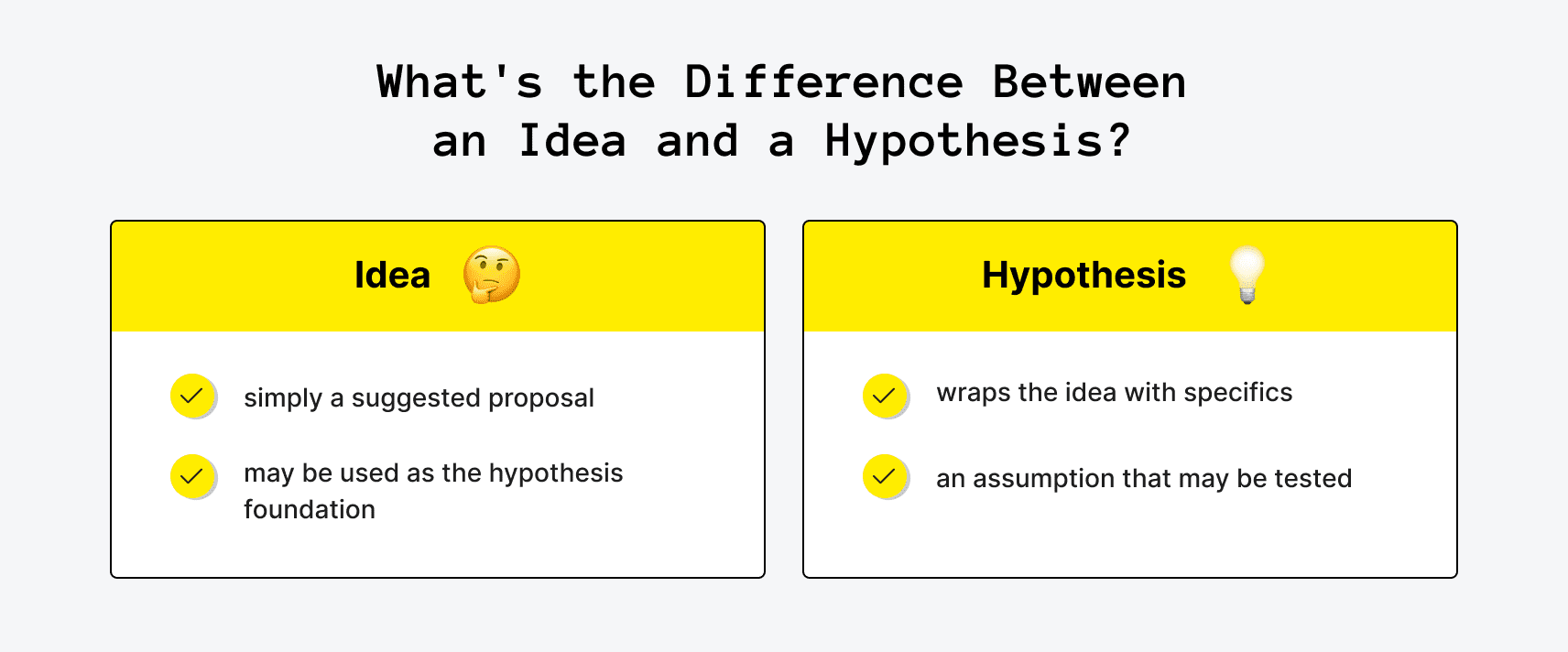
An idea is simply a suggested proposal. Say, a teammate comes up with something you can bring to life during a brainstorming session or pitches in a suggestion like "How about we shorten the checkout process?". You can jot down such ideas and then consider working on them if they'll truly make a difference and improve the product, strategy, or result in other business benefits. Ideas may thus be used as the hypothesis foundation when you decide to prove a concept.
A hypothesis is the next step, when an idea gets wrapped with specifics to become an assumption that may be tested. As such, you can refine the idea by adding details to it. The previously mentioned idea can be worded into a product hypothesis statement like: "The cart abandonment rate is high, and many users flee at checkout. But if we shorten the checkout process by cutting down the number of steps to only two and get rid of four excessive fields, we'll simplify the user journey, boost satisfaction, and may get up to 15% more completed orders".
A hypothesis is something you can test in an attempt to reach a certain goal. Testing isn't obligatory in this scenario, of course, but the idea may be tested if you weigh the pros and cons and decide that the required effort is worth a try. We'll explain how to create hypothesis statements next.
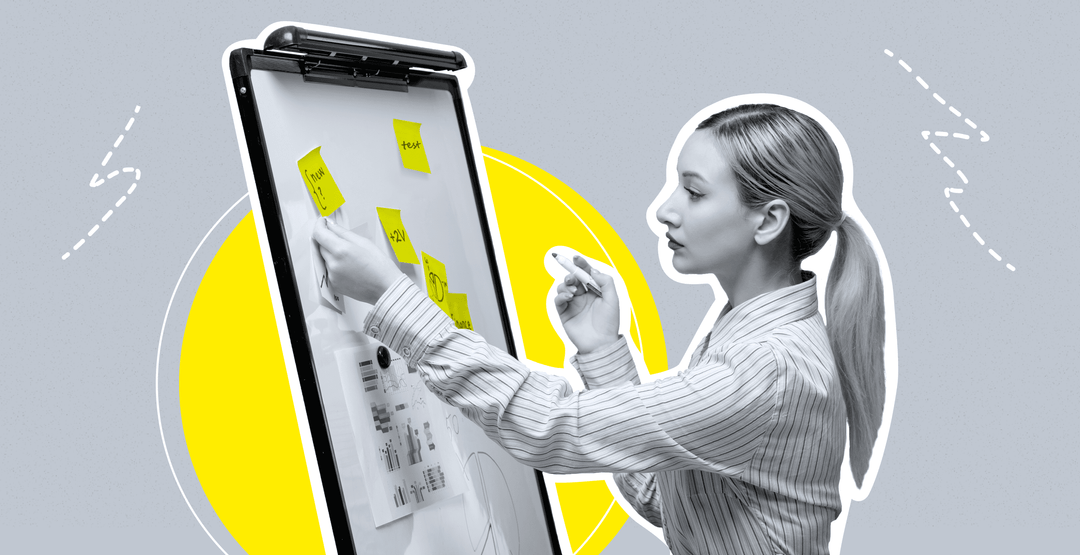
How to Generate a Hypothesis for a Product
The last thing those developing a product want is to invest time and effort into something that won't bring any visible results, fall short of customer expectations, or won't live up to their needs. Therefore, to increase the chances of achieving a successful outcome and product-led growth , teams may need to revisit their product development approach by optimizing one of the starting points of the process: learning to make reasonable product hypotheses.
If the entire procedure is structured, this may assist you during such stages as the discovery phase and raise the odds of reaching your product goals and setting your business up for success. Yet, what's the entire process like?
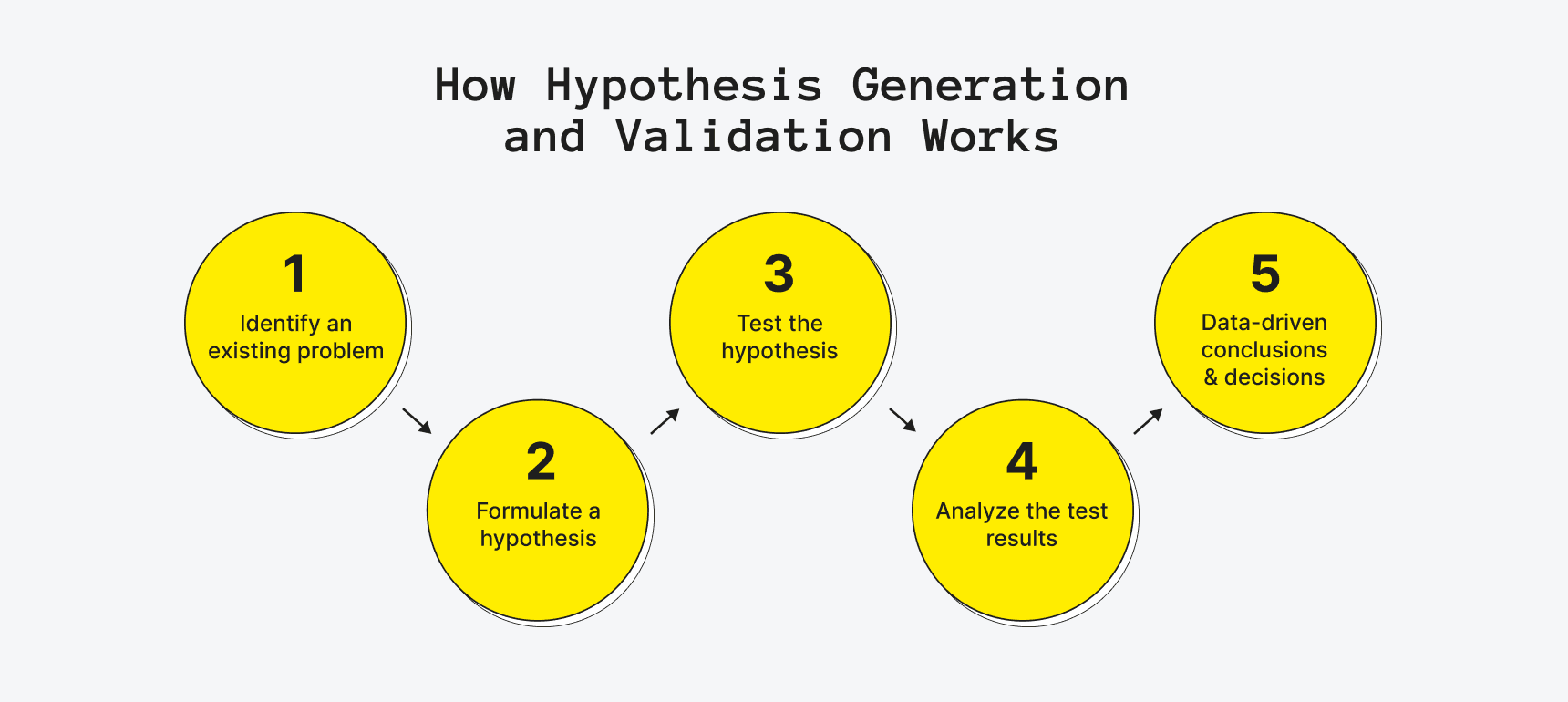
- It all starts with identifying an existing problem . Is there a product area that's experiencing a downfall, a visible trend, or a market gap? Are users often complaining about something in their feedback? Or is there something you're willing to change (say, if you aim to get more profit, increase engagement, optimize a process, expand to a new market, or reach your OKRs and KPIs faster)?
- Teams then need to work on formulating a hypothesis . They put the statement into concise and short wording that describes what is expected to achieve. Importantly, it has to be relevant, actionable, backed by data, and without generalizations.
- Next, they have to test the hypothesis by running experiments to validate it (for instance, via A/B or multivariate testing, prototyping, feedback collection, or other ways).
- Then, the obtained results of the test must be analyzed . Did one element or page version outperform the other? Depending on what you're testing, you can look into various merits or product performance metrics (such as the click rate, bounce rate, or the number of sign-ups) to assess whether your prediction was correct.
- Finally, the teams can make conclusions that could lead to data-driven decisions. For example, they can make corresponding changes or roll back a step.
How Else Can You Generate Product Hypotheses?
Such processes imply sharing ideas when a problem is spotted by digging deep into facts and studying the possible risks, goals, benefits, and outcomes. You may apply various MVP tools like (FigJam, Notion, or Miro) that were designed to simplify brainstorming sessions, systemize pitched suggestions, and keep everyone organized without losing any ideas.
Predictive product analysis can also be integrated into this process, leveraging data and insights to anticipate market trends and consumer preferences, thus enhancing decision-making and product development strategies. This approach fosters a more proactive and informed approach to innovation, ensuring products are not only relevant but also resonate with the target audience, ultimately increasing their chances of success in the market.
Besides, you can settle on one of the many frameworks that facilitate decision-making processes , ideation phases, or feature prioritization . Such frameworks are best applicable if you need to test your assumptions and structure the validation process. These are a few common ones if you're looking toward a systematic approach:
- Business Model Canvas (used to establish the foundation of the business model and helps find answers to vitals like your value proposition, finding the right customer segment, or the ways to make revenue);
- Lean Startup framework (the lean startup framework uses a diagram-like format for capturing major processes and can be handy for testing various hypotheses like how much value a product brings or assumptions on personas, the problem, growth, etc.);
- Design Thinking Process (is all about interactive learning and involves getting an in-depth understanding of the customer needs and pain points, which can be formulated into hypotheses followed by simple prototypes and tests).
Need a hand with product development?
Upsilon's team of pros is ready to share our expertise in building tech products.
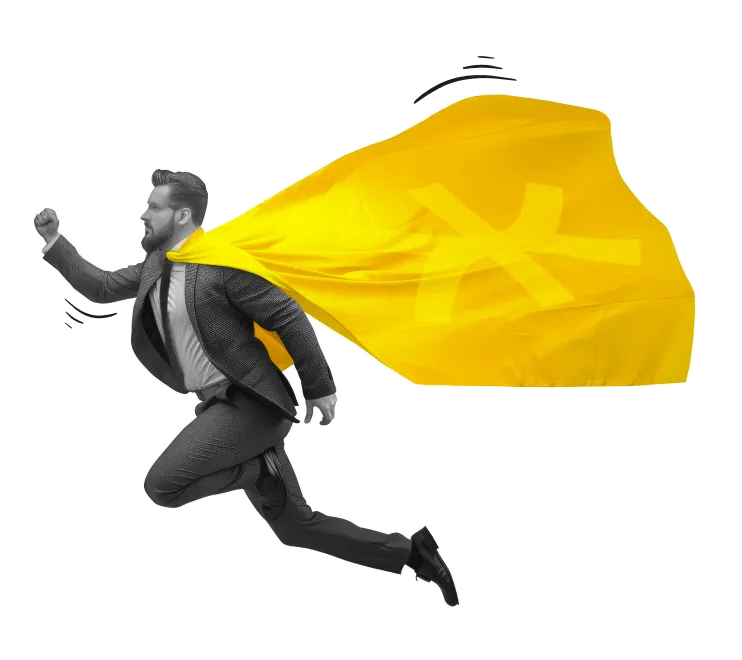
How to Make a Hypothesis Statement for a Product
Once you've indicated the addressable problem or opportunity and broken down the issue in focus, you need to work on formulating the hypotheses and associated tasks. By the way, it works the same way if you want to prove that something will be false (a.k.a null hypothesis).
If you're unsure how to write a hypothesis statement, let's explore the essential steps that'll set you on the right track.
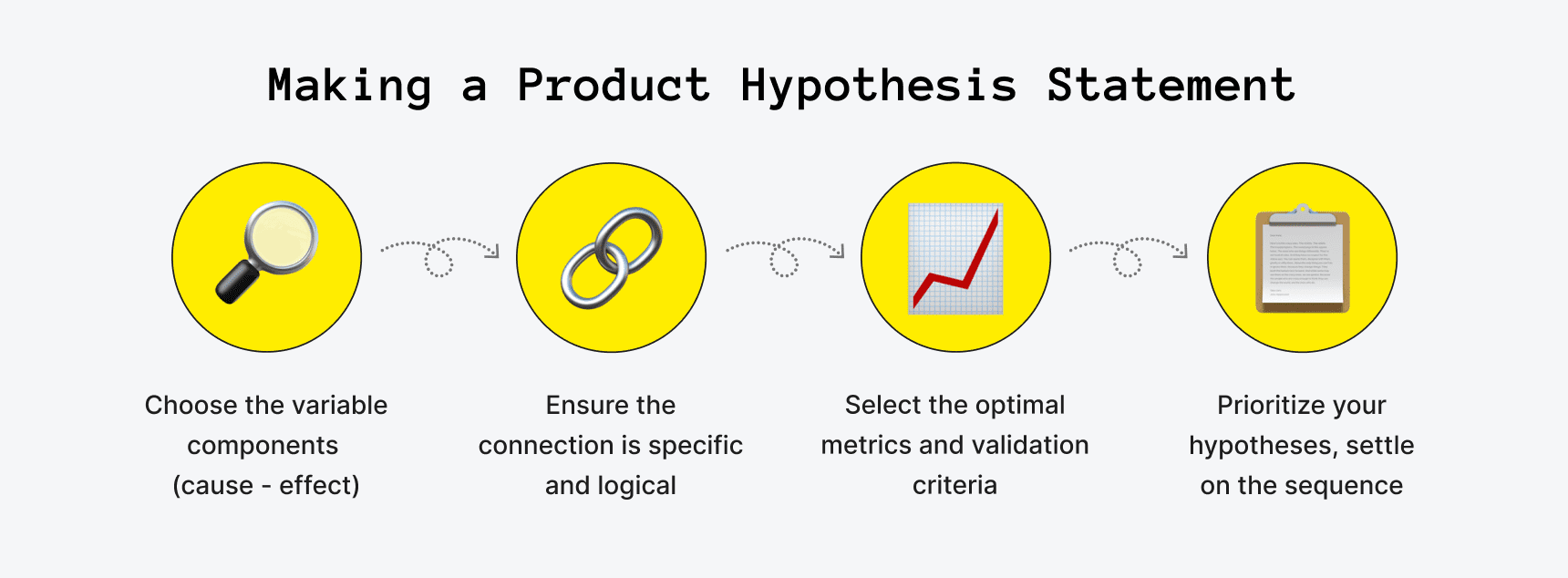
Step 1: Allocate the Variable Components
Product hypotheses are generally different for each case, so begin by pinpointing the major variables, i.e., the cause and effect . You'll need to outline what you think is supposed to happen if a change or action gets implemented.
Put simply, the "cause" is what you're planning to change, and the "effect" is what will indicate whether the change is bringing in the expected results. Falling back on the example we brought up earlier, the ineffective checkout process can be the cause, while the increased percentage of completed orders is the metric that'll show the effect.
Make sure to also note such vital points as:
- what the problem and solution are;
- what are the benefits or the expected impact/successful outcome;
- which user group is affected;
- what are the risks;
- what kind of experiments can help test the hypothesis;
- what can measure whether you were right or wrong.
Step 2: Ensure the Connection Is Specific and Logical
Mind that generic connections that lack specifics will get you nowhere. So if you're thinking about how to word a hypothesis statement, make sure that the cause and effect include clear reasons and a logical dependency .
Think about what can be the precise and link showing why A affects B. In our checkout example, it could be: fewer steps in the checkout and the removed excessive fields will speed up the process, help avoid confusion, irritate users less, and lead to more completed orders. That's much more explicit than just stating the fact that the checkout needs to be changed to get more completed orders.
Step 3: Decide on the Data You'll Collect
Certainly, multiple things can be used to measure the effect. Therefore, you need to choose the optimal metrics and validation criteria that'll best envision if you're moving in the right direction.
If you need a tip on how to create hypothesis statements that won't result in a waste of time, try to avoid vagueness and be as specific as you can when selecting what can best measure and assess the results of your hypothesis test. The criteria must be measurable and tied to the hypotheses . This can be a realistic percentage or number (say, you expect a 15% increase in completed orders or 2x fewer cart abandonment cases during the checkout phase).
Once again, if you're not realistic, then you might end up misinterpreting the results. Remember that sometimes an increase that's even as little as 2% can make a huge difference, so why make 50% the merit if it's not achievable in the first place?
Step 4: Settle on the Sequence
It's quite common that you'll end up with multiple product hypotheses. Some are more important than others, of course, and some will require more effort and input.
Therefore, just as with the features on your product development roadmap , prioritize your hypotheses according to their impact and importance. Then, group and order them, especially if the results of some hypotheses influence others on your list.
Product Hypothesis Examples
To demonstrate how to formulate your assumptions clearly, here are several more apart from the example of a hypothesis statement given above:
- Adding a wishlist feature to the cart with the possibility to send a gift hint to friends via email will increase the likelihood of making a sale and bring in additional sign-ups.
- Placing a limited-time promo code banner stripe on the home page will increase the number of sales in March.
- Moving up the call to action element on the landing page and changing the button text will increase the click-through rate twice.
- By highlighting a new way to use the product, we'll target a niche customer segment (i.e., single parents under 30) and acquire 5% more leads.
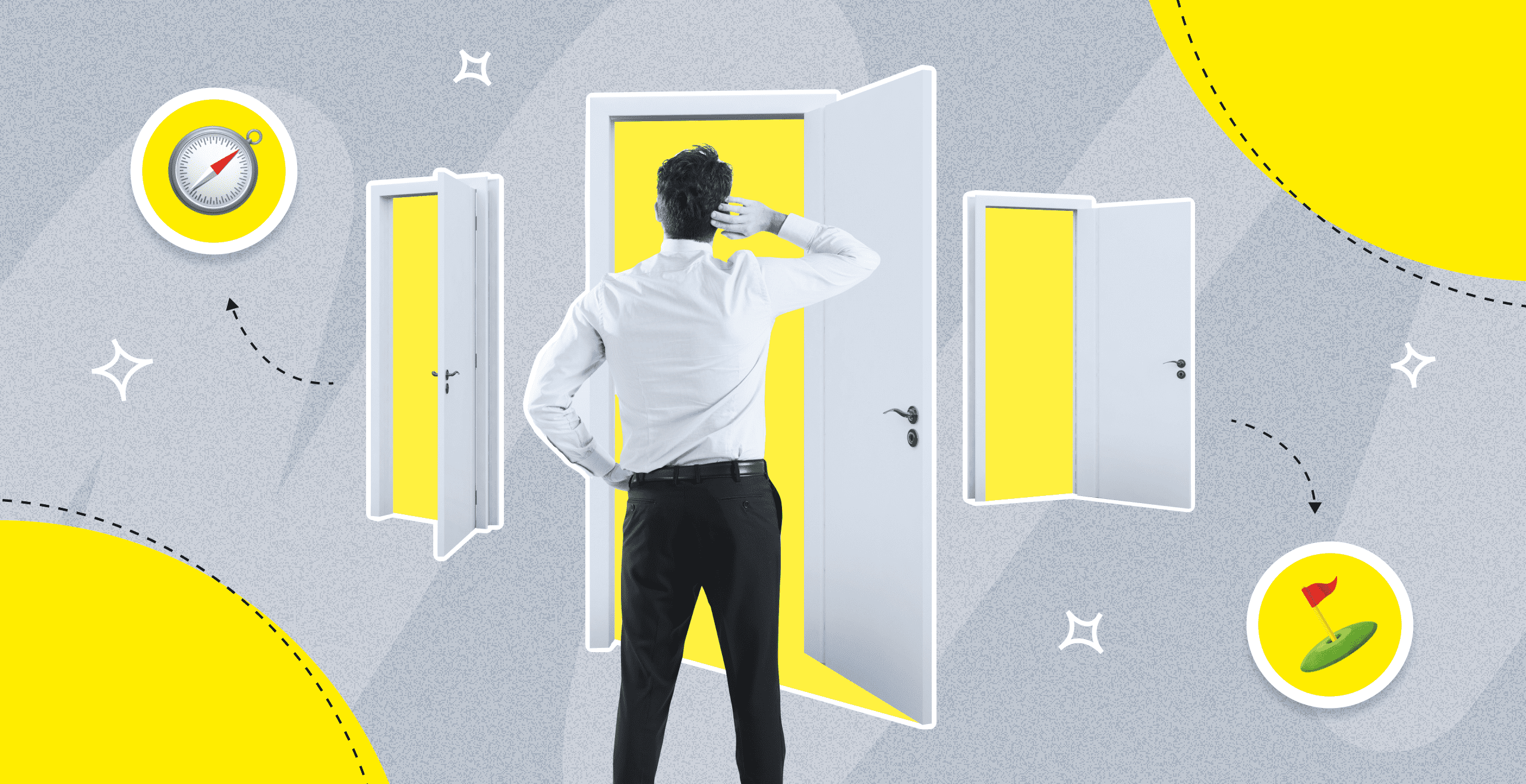
How to Validate Hypothesis Statements: The Process Explained
There are multiple options when it comes to validating hypothesis statements. To get appropriate results, you have to come up with the right experiment that'll help you test the hypothesis. You'll need a control group or people who represent your target audience segments or groups to participate (otherwise, your results might not be accurate).
What can serve as the experiment you may run? Experiments may take tons of different forms, and you'll need to choose the one that clicks best with your hypothesis goals (and your available resources, of course). The same goes for how long you'll have to carry out the test (say, a time period of two months or as little as two weeks). Here are several to get you started.
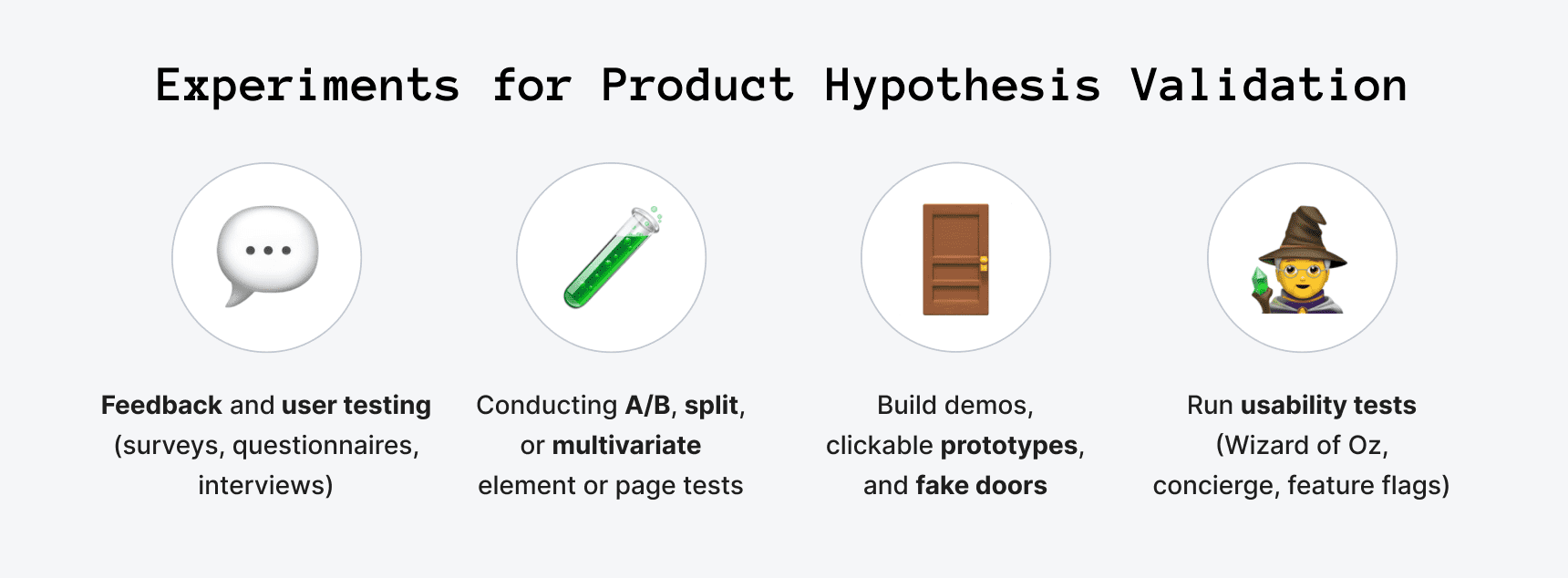
Feedback and User Testing
Talking to users, potential customers, or members of your own online startup community can be another way to test your hypotheses. You may use surveys, questionnaires, or opt for more extensive interviews to validate hypothesis statements and find out what people think. This assumption validation approach involves your existing or potential users and might require some additional time, but can bring you many insights.
Conduct A/B or Multivariate Tests
One of the experiments you may develop involves making more than one version of an element or page to see which option resonates with the users more. As such, you can have a call to action block with different wording or play around with the colors, imagery, visuals, and other things.
To run such split experiments, you can apply tools like VWO that allows to easily construct alternative designs and split what your users see (e.g., one half of the users will see version one, while the other half will see version two). You can track various metrics and apply heatmaps, click maps, and screen recordings to learn more about user response and behavior. Mind, though, that the key to such tests is to get as many users as you can give the tests time. Don't jump to conclusions too soon or if very few people participated in your experiment.
Build Prototypes and Fake Doors
Demos and clickable prototypes can be a great way to save time and money on costly feature or product development. A prototype also allows you to refine the design. However, they can also serve as experiments for validating hypotheses, collecting data, and getting feedback.
For instance, if you have a new feature in mind and want to ensure there is interest, you can utilize such MVP types as fake doors . Make a short demo recording of the feature and place it on your landing page to track interest or test how many people sign up.
Usability Testing
Similarly, you can run experiments to observe how users interact with the feature, page, product, etc. Usually, such experiments are held on prototype testing platforms with a focus group representing your target visitors. By showing a prototype or early version of the design to users, you can view how people use the solution, where they face problems, or what they don't understand. This may be very helpful if you have hypotheses regarding redesigns and user experience improvements before you move on from prototype to MVP development.
You can even take it a few steps further and build a barebone feature version that people can really interact with, yet you'll be the one behind the curtain to make it happen. There were many MVP examples when companies applied Wizard of Oz or concierge MVPs to validate their hypotheses.
Or you can actually develop some functionality but release it for only a limited number of people to see. This is referred to as a feature flag , which can show really specific results but is effort-intensive.
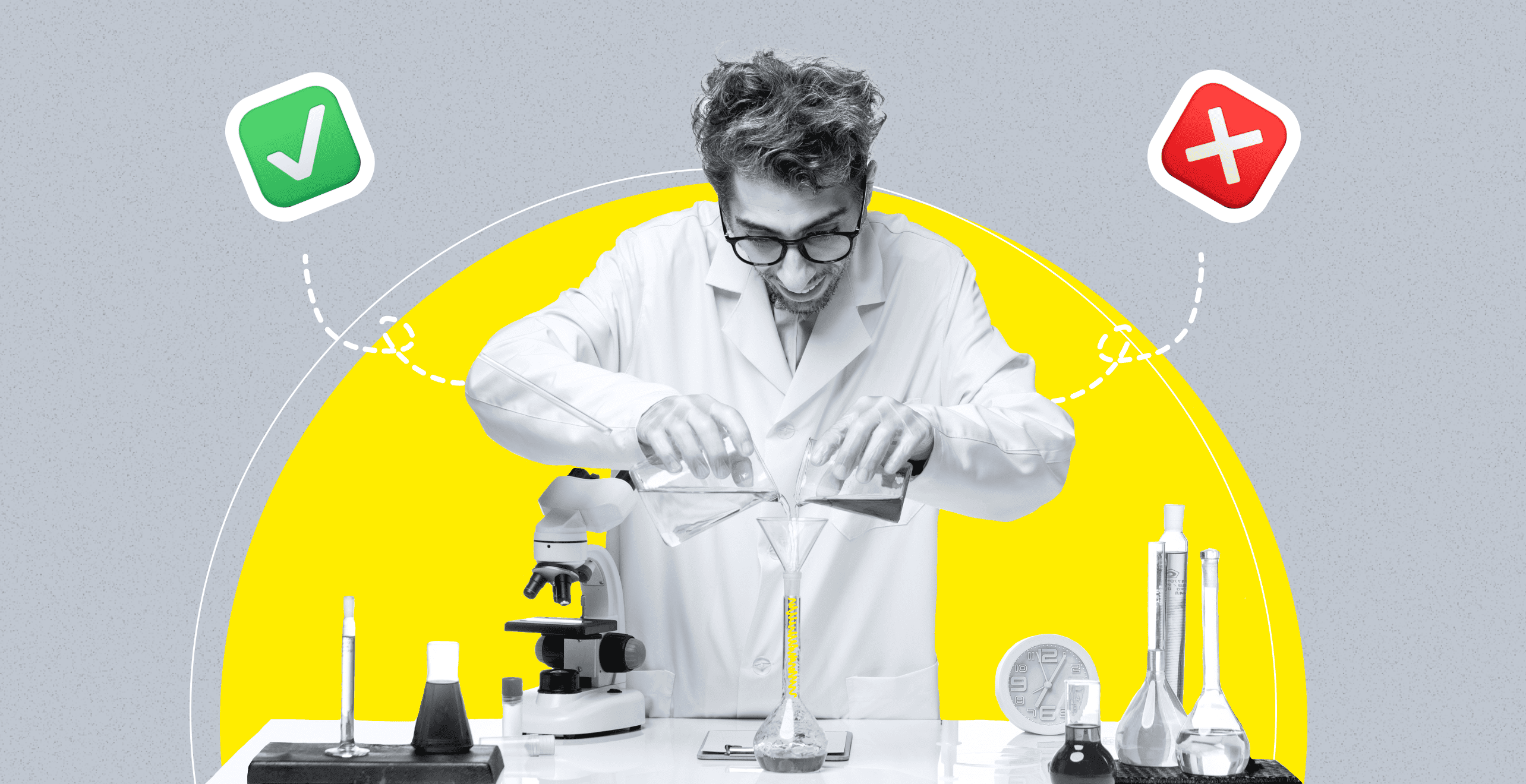
What Comes After Hypothesis Validation?
Analysis is what you move on to once you've run the experiment. This is the time to review the collected data, metrics, and feedback to validate (or invalidate) the hypothesis.
You have to evaluate the experiment's results to determine whether your product hypotheses were valid or not. For example, if you were testing two versions of an element design, color scheme, or copy, look into which one performed best.
It is crucial to be certain that you have enough data to draw conclusions, though, and that it's accurate and unbiased . Because if you don't, this may be a sign that your experiment needs to be run for some additional time, be altered, or held once again. You won't want to make a solid decision based on uncertain or misleading results, right?
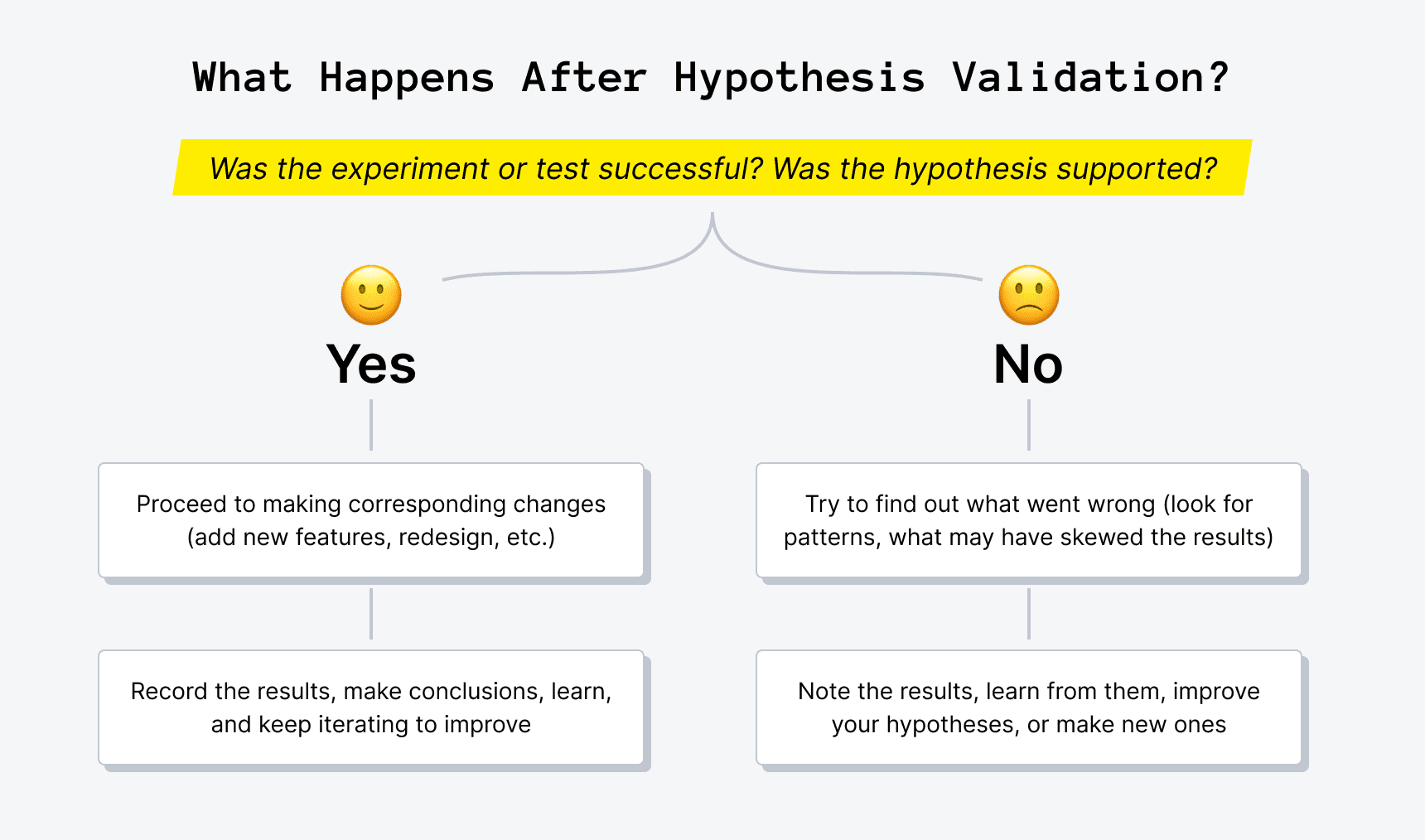
- If the hypothesis was supported , proceed to making corresponding changes (such as implementing a new feature, changing the design, rephrasing your copy, etc.). Remember that your aim was to learn and iterate to improve.
- If your hypothesis was proven false , think of it as a valuable learning experience. The main goal is to learn from the results and be able to adjust your processes accordingly. Dig deep to find out what went wrong, look for patterns and things that may have skewed the results. But if all signs show that you were wrong with your hypothesis, accept this outcome as a fact, and move on. This can help you make conclusions on how to better formulate your product hypotheses next time. Don't be too judgemental, though, as a failed experiment might only mean that you need to improve the current hypothesis, revise it, or create a new one based on the results of this experiment, and run the process once more.
On another note, make sure to record your hypotheses and experiment results . Some companies use CRMs to jot down the key findings, while others use something as simple as Google Docs. Either way, this can be your single source of truth that can help you avoid running the same experiments or allow you to compare results over time.
Have doubts about how to bring your product to life?
Upsilon's team of pros can help you build a product most optimally.
Final Thoughts on Product Hypotheses
The hypothesis-driven approach in product development is a great way to avoid uncalled-for risks and pricey mistakes. You can back up your assumptions with facts, observe your target audience's reactions, and be more certain that this move will deliver value.
However, this only makes sense if the validation of hypothesis statements is backed by relevant data that'll allow you to determine whether the hypothesis is valid or not. By doing so, you can be certain that you're developing and testing hypotheses to accelerate your product management and avoiding decisions based on guesswork.
Certainly, a failed experiment may bring you just as much knowledge and findings as one that succeeds. Teams have to learn from their mistakes, boost their hypothesis generation and testing knowledge, and make improvements according to the results of their experiments. This is an ongoing process, of course, as no product can grow if it isn't iterated and improved.
If you're only planning to or are currently building a product, Upsilon can lend you a helping hand. Our team has years of experience providing product development services for growth-stage startups and building MVPs for early-stage businesses , so you can use our expertise and knowledge to dodge many mistakes. Don't be shy to contact us to discuss your needs!
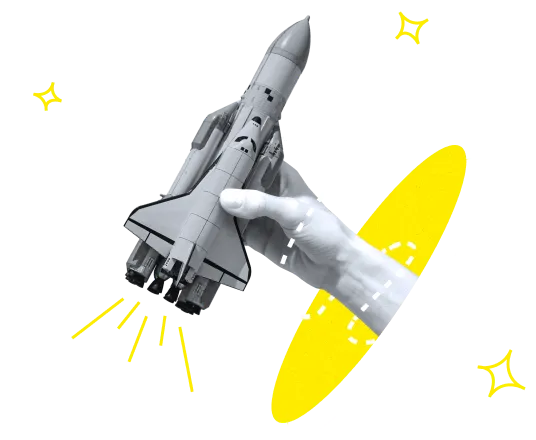
Information Architecture Design: A Product Discovery Step
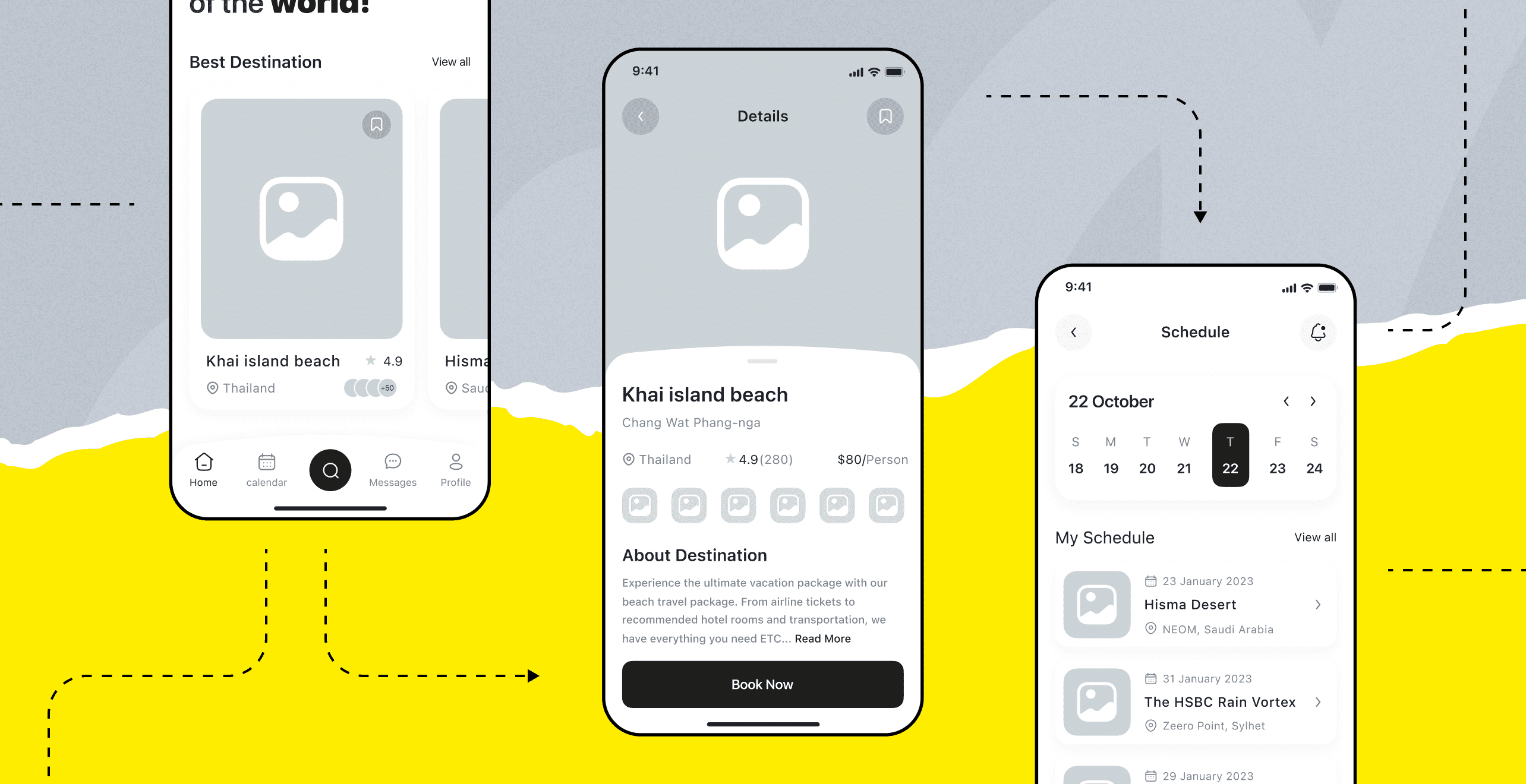
How to Prototype a Product: Steps, Tips, and Best Practices
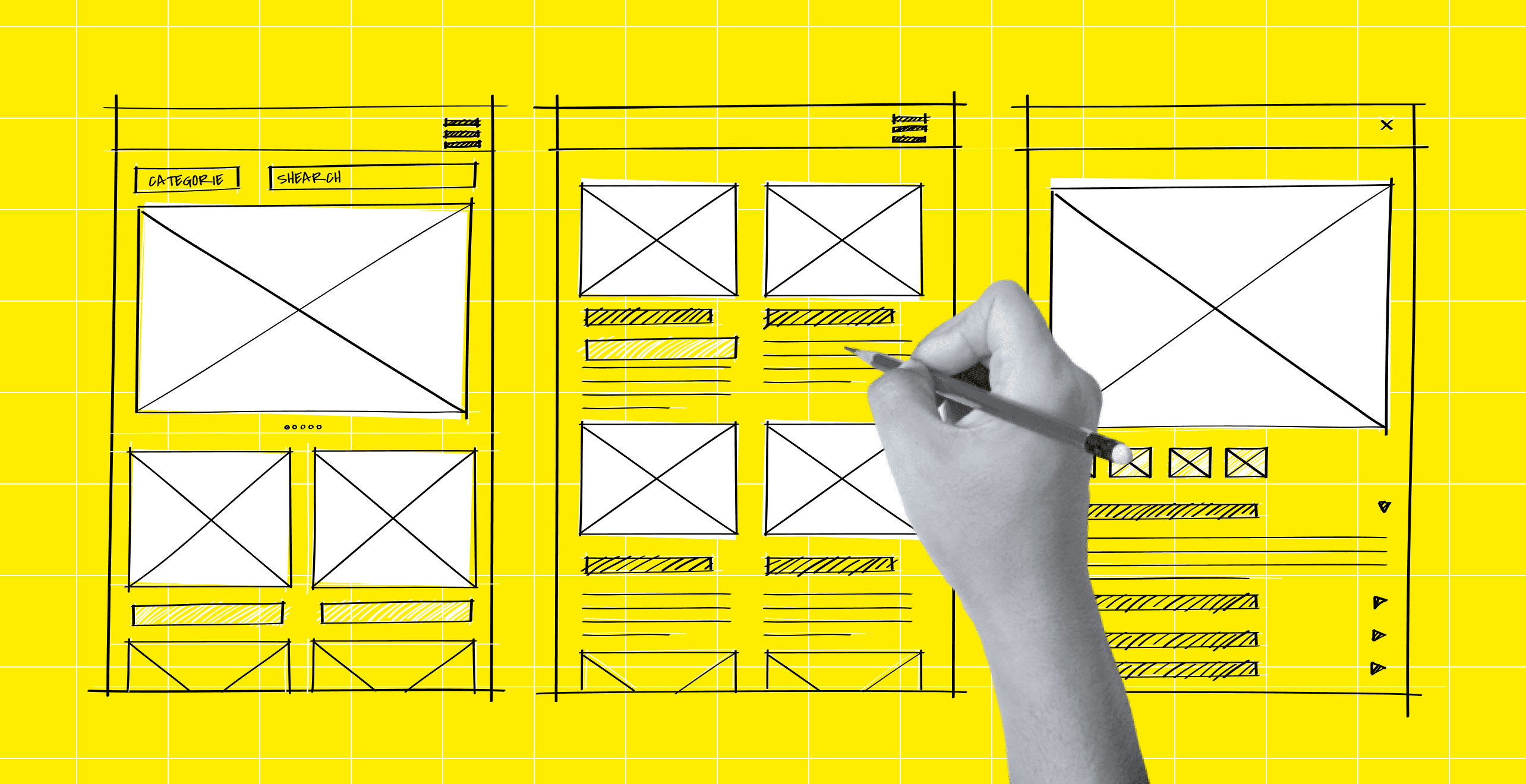
What Is Wireframing and Its Role in Product Development
Never miss an update.

SHARE THIS POST
Product best practices
Product hypothesis - a guide to create meaningful hypotheses.
13 December, 2023
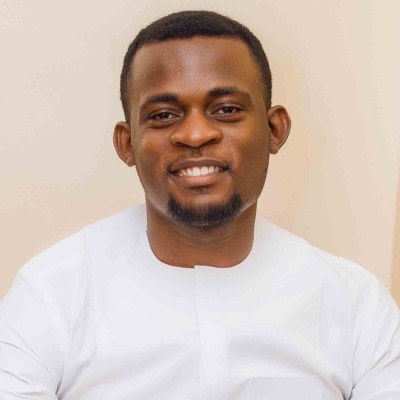
Growth Manager
Data-driven development is no different than a scientific experiment. You repeatedly form hypotheses, test them, and either implement (or reject) them based on the results. It’s a proven system that leads to better apps and happier users.
Let’s get started.
What is a product hypothesis?
A product hypothesis is an educated guess about how a change to a product will impact important metrics like revenue or user engagement. It's a testable statement that needs to be validated to determine its accuracy.
The most common format for product hypotheses is “If… than…”:
“If we increase the font size on our homepage, then more customers will convert.”
“If we reduce form fields from 5 to 3, then more users will complete the signup process.”
At UXCam, we believe in a data-driven approach to developing product features. Hypotheses provide an effective way to structure development and measure results so you can make informed decisions about how your product evolves over time.
Take PlaceMakers , for example.
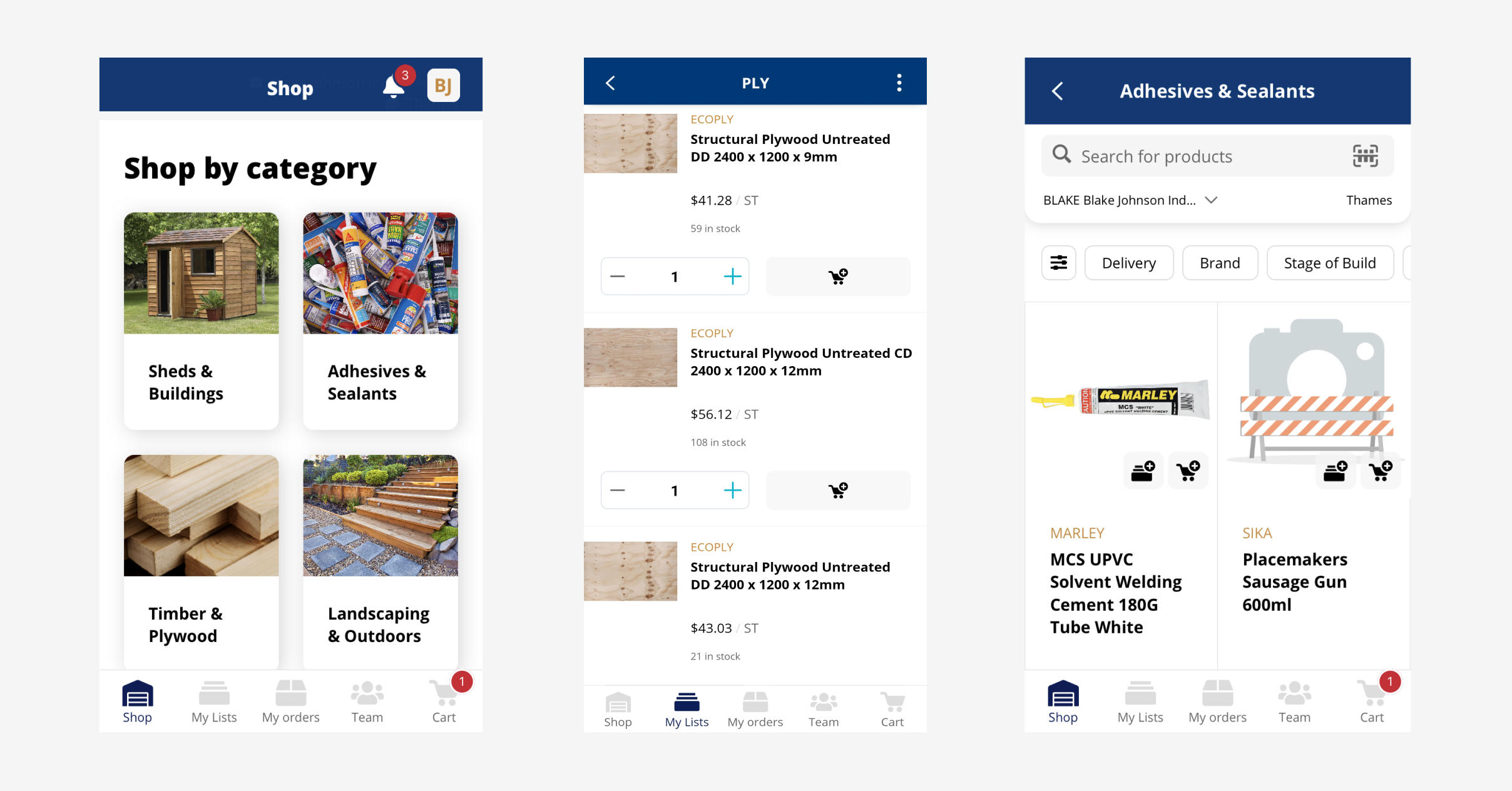
PlaceMakers faced challenges with their app during the COVID-19 pandemic. Due to supply chain shortages, stock levels were not being updated in real-time, causing customers to add unavailable products to their baskets. The team added a “Constrained Product” label, but this caused sales to plummet.
The team then turned to UXCam’s session replays and heatmaps to investigate, and hypothesized that their messaging for constrained products was too strong. The team redesigned the messaging with a more positive approach, and sales didn’t just recover—they doubled.
Types of product hypothesis
1. counter-hypothesis.
A counter-hypothesis is an alternative proposition that challenges the initial hypothesis. It’s used to test the robustness of the original hypothesis and make sure that the product development process considers all possible scenarios.
For instance, if the original hypothesis is “Reducing the sign-up steps from 3 to 1 will increase sign-ups by 25% for new visitors after 1,000 visits to the sign-up page,” a counter-hypothesis could be “Reducing the sign-up steps will not significantly affect the sign-up rate.
2. Alternative hypothesis
An alternative hypothesis predicts an effect in the population. It’s the opposite of the null hypothesis, which states there’s no effect.
For example, if the null hypothesis is “improving the page load speed on our mobile app will not affect the number of sign-ups,” the alternative hypothesis could be “improving the page load speed on our mobile app will increase the number of sign-ups by 15%.”
3. Second-order hypothesis
Second-order hypotheses are derived from the initial hypothesis and provide more specific predictions.
For instance, “if the initial hypothesis is Improving the page load speed on our mobile app will increase the number of sign-ups,” a second-order hypothesis could be “Improving the page load speed on our mobile app will increase the number of sign-ups.”
Why is a product hypothesis important?
Guided product development.
A product hypothesis serves as a guiding light in the product development process. In the case of PlaceMakers, the product owner’s hypothesis that users would benefit from knowing the availability of items upfront before adding them to the basket helped their team focus on the most critical aspects of the product. It ensured that their efforts were directed towards features and improvements that have the potential to deliver the most value.
Improved efficiency
Product hypotheses enable teams to solve problems more efficiently and remove biases from the solutions they put forward. By testing the hypothesis, PlaceMakers aimed to improve efficiency by addressing the issue of stock levels not being updated in real-time and customers adding unavailable products to their baskets.
Risk mitigation
By validating assumptions before building the product, teams can significantly reduce the risk of failure. This is particularly important in today’s fast-paced, highly competitive business environment, where the cost of failure can be high.
Validating assumptions through the hypothesis helped mitigate the risk of failure for PlaceMakers, as they were able to identify and solve the issue within a three-day period.
Data-driven decision-making
Product hypotheses are a key element of data-driven product development and decision-making. They provide a solid foundation for making informed, data-driven decisions, which can lead to more effective and successful product development strategies.
The use of UXCam's Session Replay and Heatmaps features provided valuable data for data-driven decision-making, allowing PlaceMakers to quickly identify the problem and revise their messaging approach, leading to a doubling of sales.
How to create a great product hypothesis
Map important user flows
Identify any bottlenecks
Look for interesting behavior patterns
Turn patterns into hypotheses
Step 1 - Map important user flows
A good product hypothesis starts with an understanding of how users more around your product—what paths they take, what features they use, how often they return, etc. Before you can begin hypothesizing, it’s important to map out key user flows and journey maps that will help inform your hypothesis.
To do that, you’ll need to use a monitoring tool like UXCam .
UXCam integrates with your app through a lightweight SDK and automatically tracks every user interaction using tagless autocapture. That leads to tons of data on user behavior that you can use to form hypotheses.
At this stage, there are two specific visualizations that are especially helpful:
Funnels : Funnels are great for identifying drop off points and understanding which steps in a process, transition or journey lead to success.
In other words, you’re using these two tools to define key in-app flows and to measure the effectiveness of these flows (in that order).
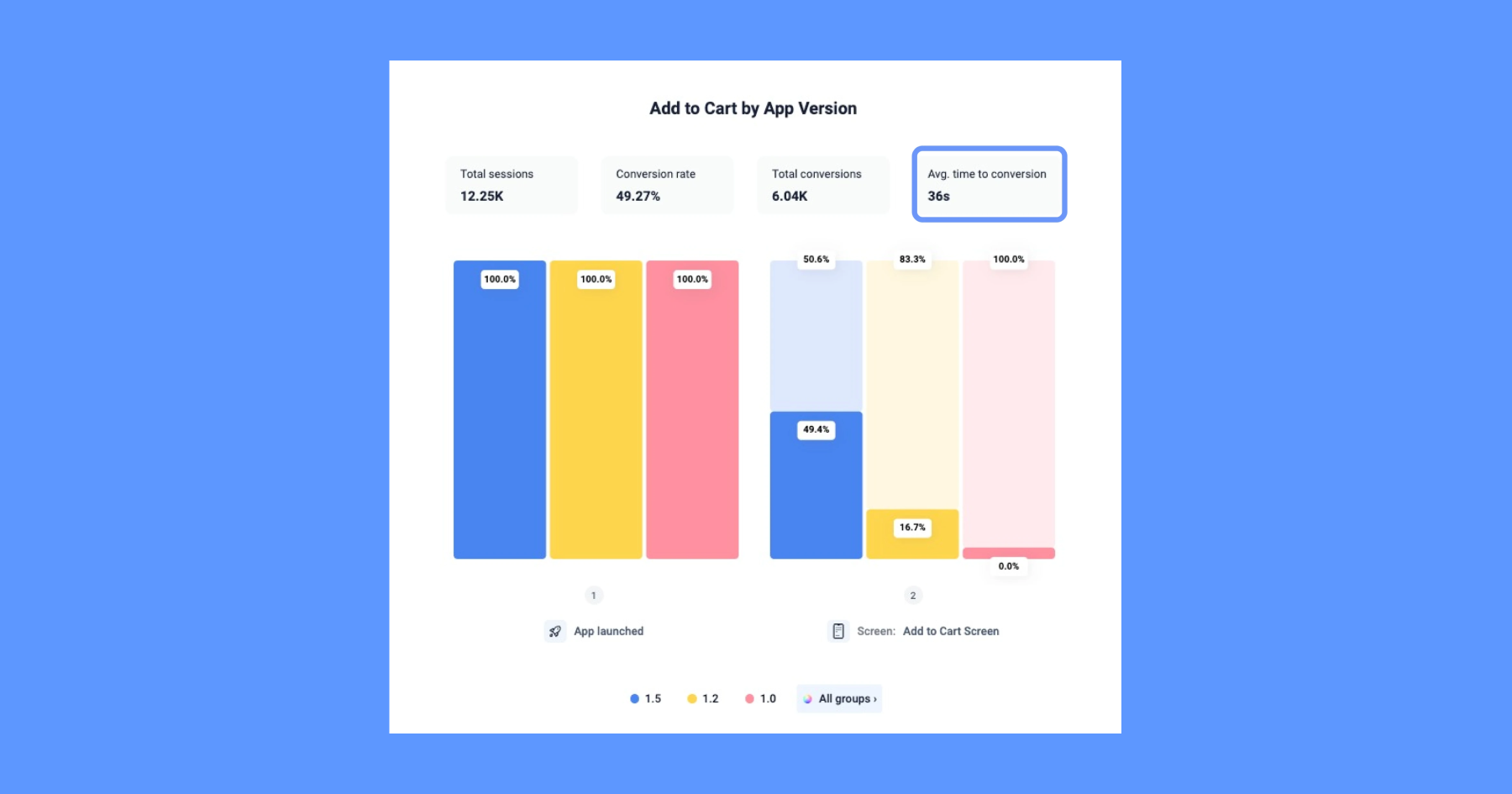
Average time to conversion in highlights bar.
Step 2 - Identify any bottlenecks
Once you’ve set up monitoring and have started collecting data, you’ll start looking for bottlenecks—points along a key app flow that are tripping users up. At every stage in a funnel, there’s going to be dropoffs, but too many dropoffs can be a sign of a problem.
UXCam makes it easy to spot dropoffs by displaying them visually in every funnel. While there’s no benchmark for when you should be concerned, anything above a 10% dropoff could mean that further investigation is needed.
How do you investigate? By zooming in.
Step 3 - Look for interesting behavior patterns
At this stage, you’ve noticed a concerning trend and are zooming in on individual user experiences to humanize the trend and add important context.
The best way to do this is with session replay tools and event analytics. With a tool like UXCam, you can segment app data to isolate sessions that fit the trend. You can then investigate real user sessions by watching videos of their experience or by looking into their event logs. This helps you see exactly what caused the behavior you’re investigating.
For example, let’s say you notice that 20% of users who add an item to their cart leave the app about 5 minutes later. You can use session replay to look for the behavioral patterns that lead up to users leaving—such as how long they linger on a certain page or if they get stuck in the checkout process.
Step 4 - Turn patterns into hypotheses
Once you’ve checked out a number of user sessions, you can start to craft a product hypothesis.
This usually takes the form of an “If… then…” statement, like:
“If we optimize the checkout process for mobile users, then more customers will complete their purchase.”
These hypotheses can be tested using A/B testing and other user research tools to help you understand if your changes are having an impact on user behavior.
Product hypothesis emphasizes the importance of formulating clear and testable hypotheses when developing a product. It highlights that a well-defined hypothesis can guide the product development process, align stakeholders, and minimize uncertainty.
UXCam arms product teams with all the tools they need to form meaningful hypotheses that drive development in a positive direction. Put your app’s data to work and start optimizing today— sign up for a free account .
You might also be interested in these;
Product experimentation framework for mobile product teams
7 Best AB testing tools for mobile apps
A practical guide to product experimentation
5 Best product experimentation tools & software
How to use data to challenge the HiPPO
Ardent technophile exploring the world of mobile app product management at UXCam.
Get the latest from UXCam
Stay up-to-date with UXCam's latest features, insights, and industry news for an exceptional user experience.
Related articles
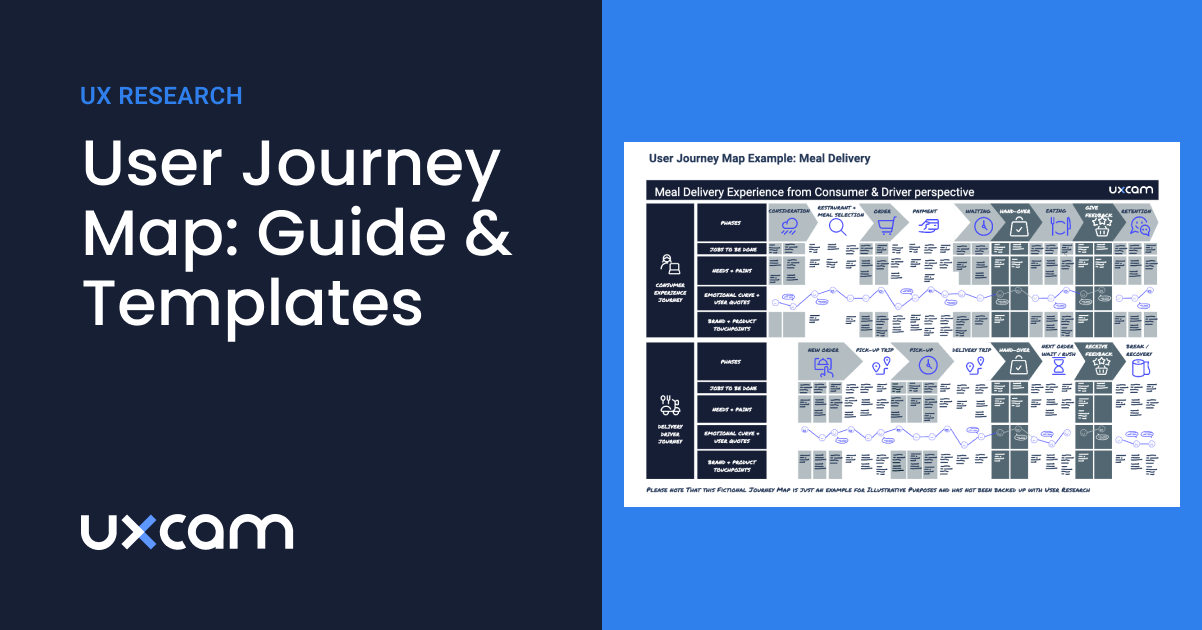
User Journey Map Guide with Examples & FREE Templates
Learn experience mapping basics and benefits using templates and examples with mixed-methods UX researcher Alice...
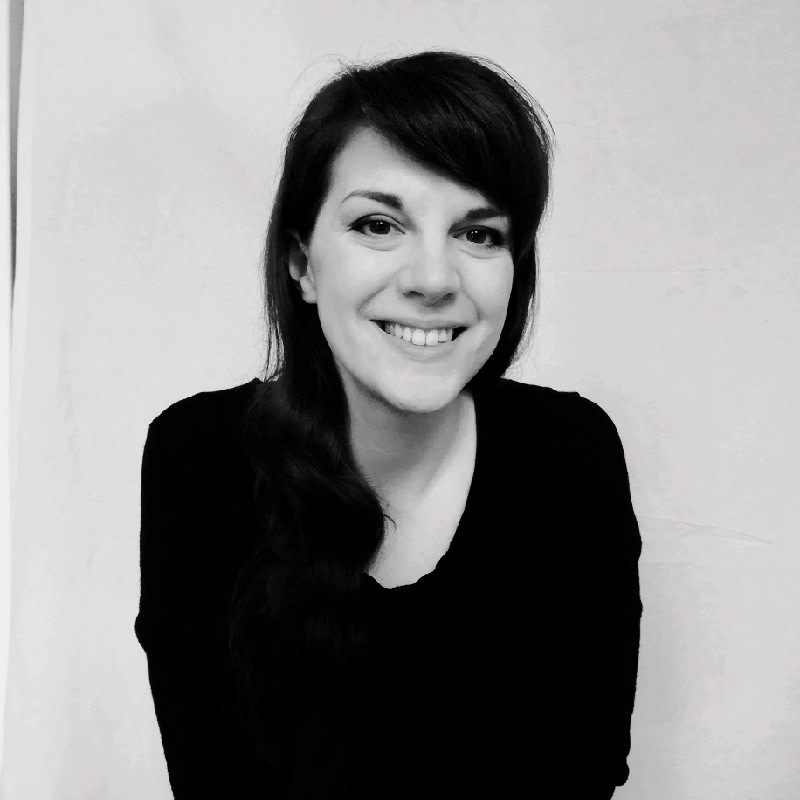
Alice Ruddigkeit
Senior UX Researcher
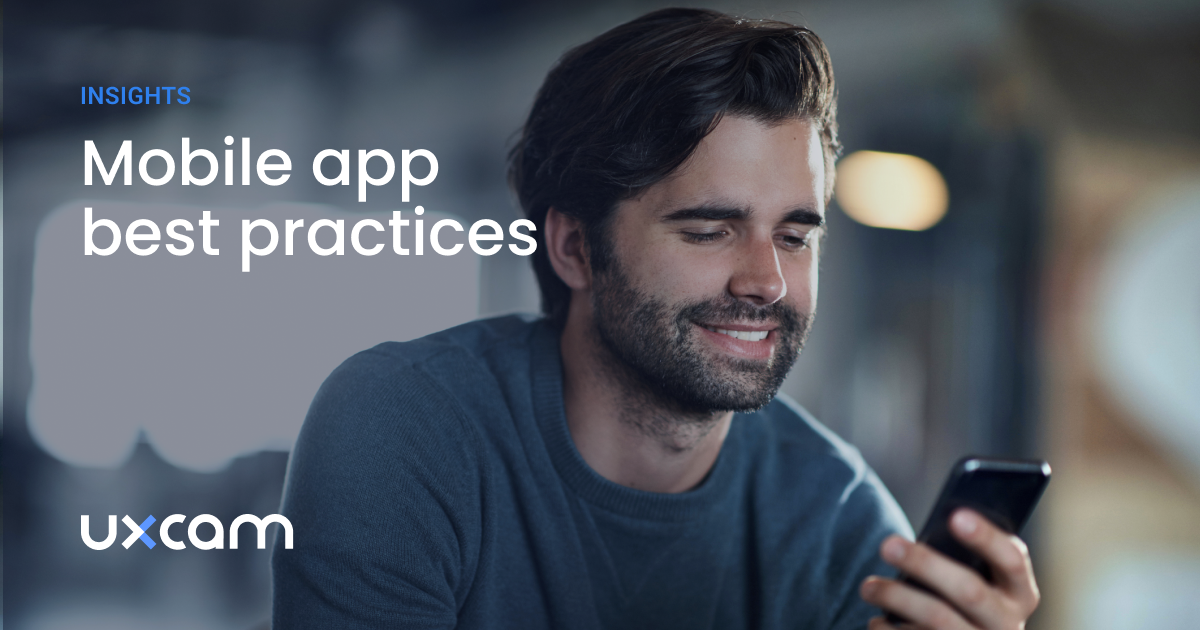
45 Mobile App Best Practices: The Ultimate List 2024
Proven best practices to improve user experience and performance of your mobile...
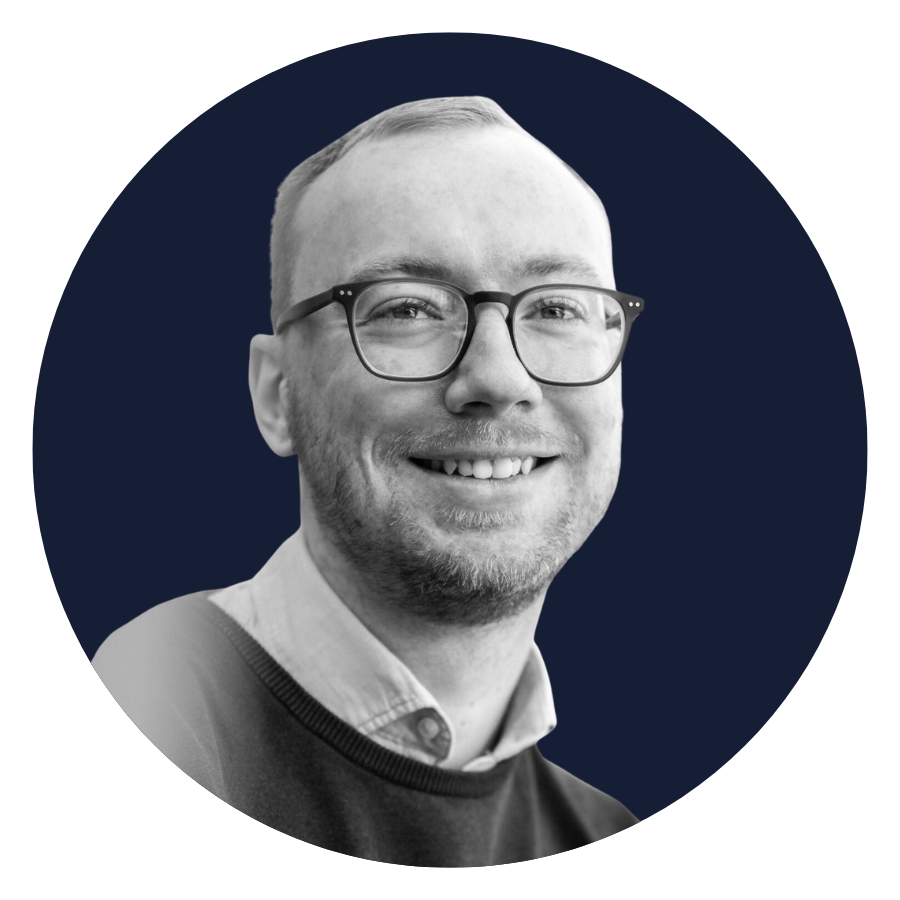
Jonas Kurzweg
Growth Lead
North Star Metric Examples from Tech Giants
Discover 9 North Star Metric examples to guide your business growth strategy, from user engagement to revenue, and align your team's...
Shipping Your Product in Iterations: A Guide to Hypothesis Testing
Glancing at the App Store on any phone will reveal that most installed apps have had updates released within the last week. Software products today are shipped in iterations to validate assumptions and hypotheses about what makes the product experience better for users.
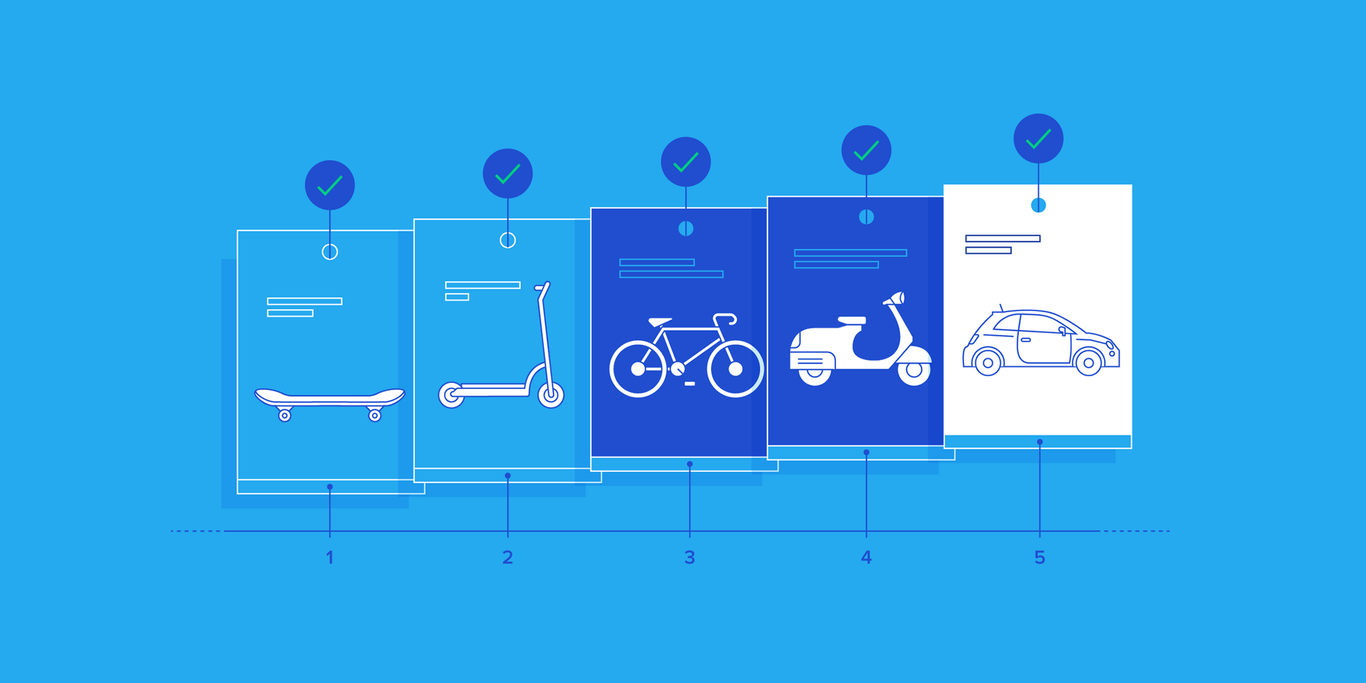
By Kumara Raghavendra
Kumara has successfully delivered high-impact products in various industries ranging from eCommerce, healthcare, travel, and ride-hailing.
PREVIOUSLY AT
A look at the App Store on any phone will reveal that most installed apps have had updates released within the last week. A website visit after a few weeks might show some changes in the layout, user experience, or copy.
Today, software is shipped in iterations to validate assumptions and the product hypothesis about what makes a better user experience. At any given time, companies like booking.com (where I worked before) run hundreds of A/B tests on their sites for this very purpose.
For applications delivered over the internet, there is no need to decide on the look of a product 12-18 months in advance, and then build and eventually ship it. Instead, it is perfectly practical to release small changes that deliver value to users as they are being implemented, removing the need to make assumptions about user preferences and ideal solutions—for every assumption and hypothesis can be validated by designing a test to isolate the effect of each change.
In addition to delivering continuous value through improvements, this approach allows a product team to gather continuous feedback from users and then course-correct as needed. Creating and testing hypotheses every couple of weeks is a cheaper and easier way to build a course-correcting and iterative approach to creating product value .
What Is Hypothesis Testing in Product Management?
While shipping a feature to users, it is imperative to validate assumptions about design and features in order to understand their impact in the real world.
This validation is traditionally done through product hypothesis testing , during which the experimenter outlines a hypothesis for a change and then defines success. For instance, if a data product manager at Amazon has a hypothesis that showing bigger product images will raise conversion rates, then success is defined by higher conversion rates.
One of the key aspects of hypothesis testing is the isolation of different variables in the product experience in order to be able to attribute success (or failure) to the changes made. So, if our Amazon product manager had a further hypothesis that showing customer reviews right next to product images would improve conversion, it would not be possible to test both hypotheses at the same time. Doing so would result in failure to properly attribute causes and effects; therefore, the two changes must be isolated and tested individually.
Thus, product decisions on features should be backed by hypothesis testing to validate the performance of features.
Different Types of Hypothesis Testing
A/b testing.
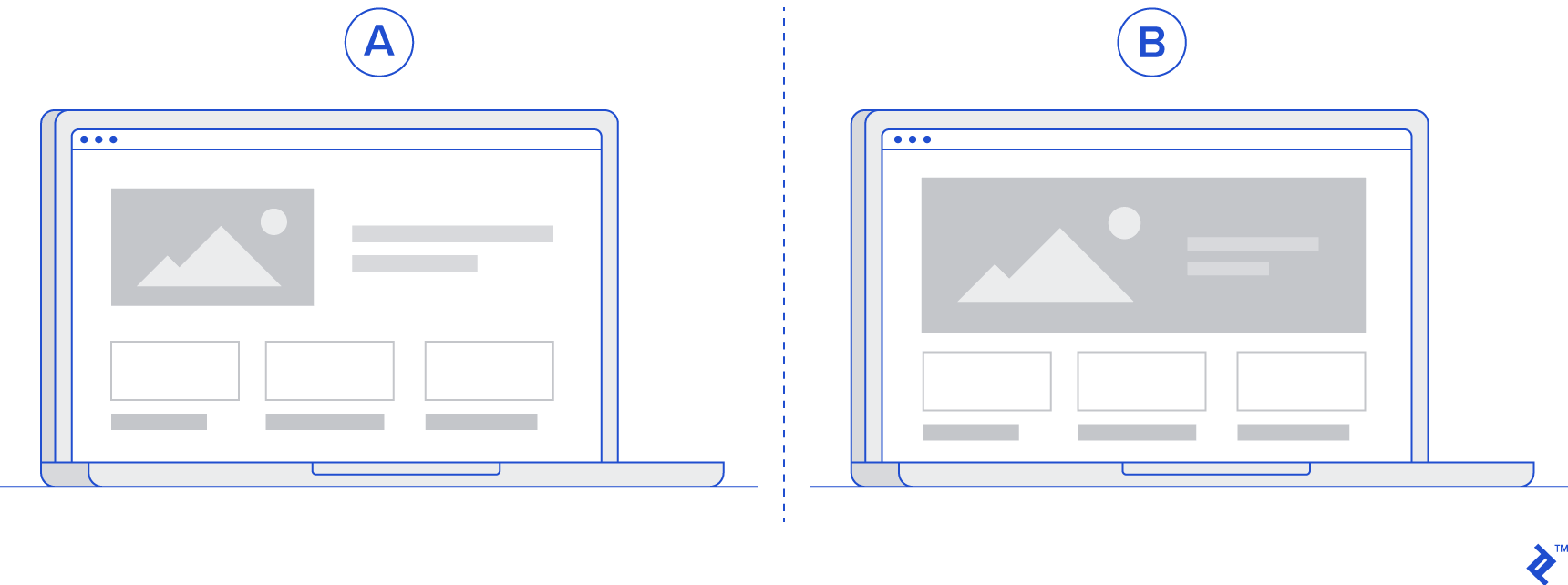
One of the most common use cases to achieve hypothesis validation is randomized A/B testing, in which a change or feature is released at random to one-half of users (A) and withheld from the other half (B). Returning to the hypothesis of bigger product images improving conversion on Amazon, one-half of users will be shown the change, while the other half will see the website as it was before. The conversion will then be measured for each group (A and B) and compared. In case of a significant uplift in conversion for the group shown bigger product images, the conclusion would be that the original hypothesis was correct, and the change can be rolled out to all users.
Multivariate Testing
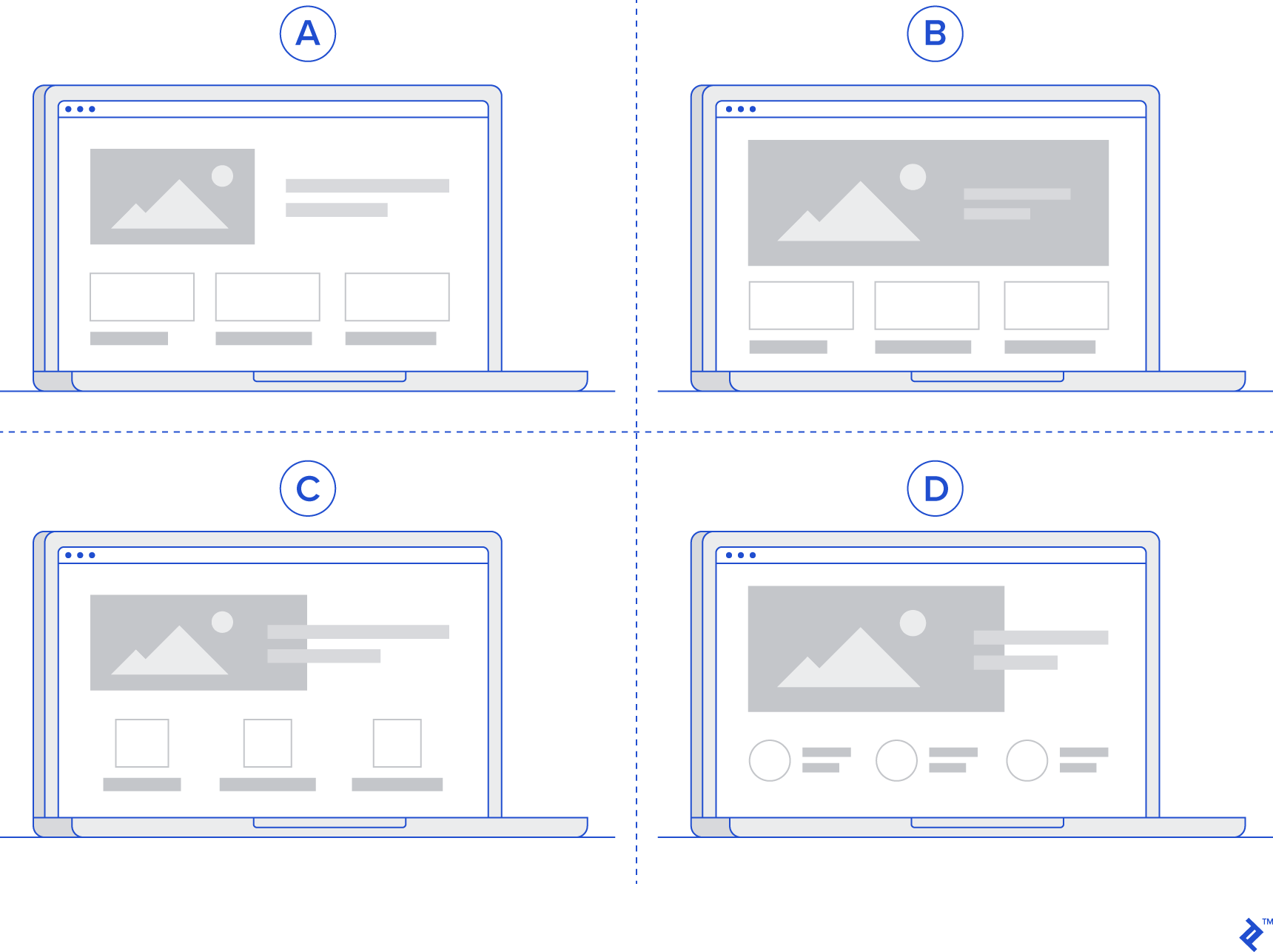
Ideally, each variable should be isolated and tested separately so as to conclusively attribute changes. However, such a sequential approach to testing can be very slow, especially when there are several versions to test. To continue with the example, in the hypothesis that bigger product images lead to higher conversion rates on Amazon, “bigger” is subjective, and several versions of “bigger” (e.g., 1.1x, 1.3x, and 1.5x) might need to be tested.
Instead of testing such cases sequentially, a multivariate test can be adopted, in which users are not split in half but into multiple variants. For instance, four groups (A, B, C, D) are made up of 25% of users each, where A-group users will not see any change, whereas those in variants B, C, and D will see images bigger by 1.1x, 1.3x, and 1.5x, respectively. In this test, multiple variants are simultaneously tested against the current version of the product in order to identify the best variant.
Before/After Testing
Sometimes, it is not possible to split the users in half (or into multiple variants) as there might be network effects in place. For example, if the test involves determining whether one logic for formulating surge prices on Uber is better than another, the drivers cannot be divided into different variants, as the logic takes into account the demand and supply mismatch of the entire city. In such cases, a test will have to compare the effects before the change and after the change in order to arrive at a conclusion.

However, the constraint here is the inability to isolate the effects of seasonality and externality that can differently affect the test and control periods. Suppose a change to the logic that determines surge pricing on Uber is made at time t , such that logic A is used before and logic B is used after. While the effects before and after time t can be compared, there is no guarantee that the effects are solely due to the change in logic. There could have been a difference in demand or other factors between the two time periods that resulted in a difference between the two.
Time-based On/Off Testing

The downsides of before/after testing can be overcome to a large extent by deploying time-based on/off testing, in which the change is introduced to all users for a certain period of time, turned off for an equal period of time, and then repeated for a longer duration.
For example, in the Uber use case, the change can be shown to drivers on Monday, withdrawn on Tuesday, shown again on Wednesday, and so on.
While this method doesn’t fully remove the effects of seasonality and externality, it does reduce them significantly, making such tests more robust.
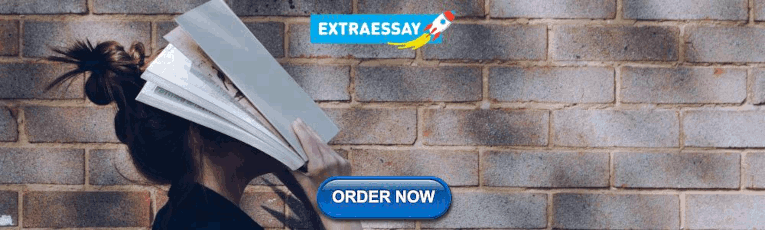
Test Design
Choosing the right test for the use case at hand is an essential step in validating a hypothesis in the quickest and most robust way. Once the choice is made, the details of the test design can be outlined.
The test design is simply a coherent outline of:
- The hypothesis to be tested: Showing users bigger product images will lead them to purchase more products.
- Success metrics for the test: Customer conversion
- Decision-making criteria for the test: The test validates the hypothesis that users in the variant show a higher conversion rate than those in the control group.
- Metrics that need to be instrumented to learn from the test: Customer conversion, clicks on product images
In the case of the product hypothesis example that bigger product images will lead to improved conversion on Amazon, the success metric is conversion and the decision criteria is an improvement in conversion.
After the right test is chosen and designed, and the success criteria and metrics are identified, the results must be analyzed. To do that, some statistical concepts are necessary.
When running tests, it is important to ensure that the two variants picked for the test (A and B) do not have a bias with respect to the success metric. For instance, if the variant that sees the bigger images already has a higher conversion than the variant that doesn’t see the change, then the test is biased and can lead to wrong conclusions.
In order to ensure no bias in sampling, one can observe the mean and variance for the success metric before the change is introduced.
Significance and Power
Once a difference between the two variants is observed, it is important to conclude that the change observed is an actual effect and not a random one. This can be done by computing the significance of the change in the success metric.
In layman’s terms, significance measures the frequency with which the test shows that bigger images lead to higher conversion when they actually don’t. Power measures the frequency with which the test tells us that bigger images lead to higher conversion when they actually do.
So, tests need to have a high value of power and a low value of significance for more accurate results.
While an in-depth exploration of the statistical concepts involved in product management hypothesis testing is out of scope here, the following actions are recommended to enhance knowledge on this front:
- Data analysts and data engineers are usually adept at identifying the right test designs and can guide product managers, so make sure to utilize their expertise early in the process.
- There are numerous online courses on hypothesis testing, A/B testing, and related statistical concepts, such as Udemy , Udacity , and Coursera .
- Using tools such as Google’s Firebase and Optimizely can make the process easier thanks to a large amount of out-of-the-box capabilities for running the right tests.
Using Hypothesis Testing for Successful Product Management
In order to continuously deliver value to users, it is imperative to test various hypotheses, for the purpose of which several types of product hypothesis testing can be employed. Each hypothesis needs to have an accompanying test design, as described above, in order to conclusively validate or invalidate it.
This approach helps to quantify the value delivered by new changes and features, bring focus to the most valuable features, and deliver incremental iterations.
- How to Conduct Remote User Interviews [Infographic]
- A/B Testing UX for Component-based Frameworks
- Building an AI Product? Maximize Value With an Implementation Framework
Further Reading on the Toptal Blog:
- Evolving UX: Experimental Product Design with a CXO
- How to Conduct Usability Testing in Six Steps
- 3 Product-led Growth Frameworks to Build Your Business
- A Product Designer’s Guide to Competitive Analysis
Understanding the basics
What is a product hypothesis.
A product hypothesis is an assumption that some improvement in the product will bring an increase in important metrics like revenue or product usage statistics.
What are the three required parts of a hypothesis?
The three required parts of a hypothesis are the assumption, the condition, and the prediction.
Why do we do A/B testing?
We do A/B testing to make sure that any improvement in the product increases our tracked metrics.
What is A/B testing used for?
A/B testing is used to check if our product improvements create the desired change in metrics.
What is A/B testing and multivariate testing?
A/B testing and multivariate testing are types of hypothesis testing. A/B testing checks how important metrics change with and without a single change in the product. Multivariate testing can track multiple variations of the same product improvement.
Kumara Raghavendra
Dubai, United Arab Emirates
Member since August 6, 2019
About the author
World-class articles, delivered weekly.
By entering your email, you are agreeing to our privacy policy .
Toptal Product Managers
- Artificial Intelligence Product Managers
- Blockchain Product Managers
- Business Systems Analysts
- Cloud Product Managers
- Data Science Product Managers
- Digital Marketing Product Managers
- Digital Product Managers
- Directors of Product
- eCommerce Product Managers
- Enterprise Product Managers
- Enterprise Resource Planning Product Managers
- Freelance Product Managers
- Interim CPOs
- Jira Product Managers
- Kanban Product Managers
- Lean Product Managers
- Mobile Product Managers
- Product Consultants
- Product Development Managers
- Product Owners
- Product Portfolio Managers
- Product Strategy Consultants
- Product Tour Consultants
- Robotic Process Automation Product Managers
- Robotics Product Managers
- SaaS Product Managers
- Salesforce Product Managers
- Scrum Product Owner Contractors
- Web Product Managers
- View More Freelance Product Managers
Join the Toptal ® community.
Advisory boards aren’t only for executives. Join the LogRocket Content Advisory Board today →
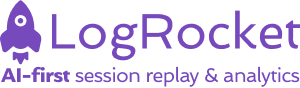
- Product Management
- Solve User-Reported Issues
- Find Issues Faster
- Optimize Conversion and Adoption
How to write an effective hypothesis
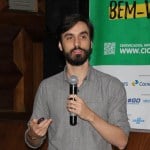
Hypothesis validation is the bread and butter of product discovery. Understanding what should be prioritized and why is the most important task of a product manager. It doesn’t matter how well you validate your findings if you’re trying to answer the wrong question.
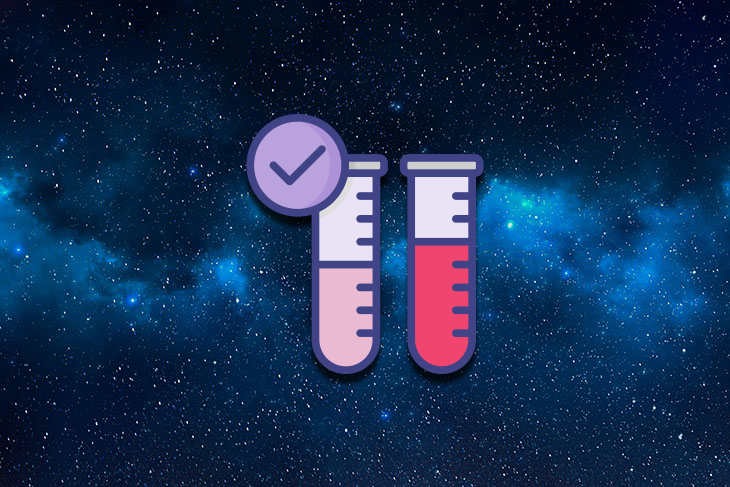
A question is as good as the answer it can provide. If your hypothesis is well written, but you can’t read its conclusion, it’s a bad hypothesis. Alternatively, if your hypothesis has embedded bias and answers itself, it’s also not going to help you.
There are several different tools available to build hypotheses, and it would be exhaustive to list them all. Apart from being superficial, focusing on the frameworks alone shifts the attention away from the hypothesis itself.
In this article, you will learn what a hypothesis is, the fundamental aspects of a good hypothesis, and what you should expect to get out of one.
The 4 product risks
Mitigating the four product risks is the reason why product managers exist in the first place and it’s where good hypothesis crafting starts.
The four product risks are assessments of everything that could go wrong with your delivery. Our natural thought process is to focus on the happy path at the expense of unknown traps. The risks are a constant reminder that knowing why something won’t work is probably more important than knowing why something might work.
These are the fundamental questions that should fuel your hypothesis creation:
Is it viable for the business?
Is it relevant for the user, can we build it, is it ethical to deliver.
Is this hypothesis the best one to validate now? Is this the most cost-effective initiative we can take? Will this answer help us achieve our goals? How much money can we make from it?
Has the user manifested interest in this solution? Will they be able to use it? Does it solve our users’ challenges? Is it aesthetically pleasing? Is it vital for the user, or just a luxury?
Do we have the resources and know-how to deliver it? Can we scale this solution? How much will it cost? Will it depreciate fast? Is it the best cost-effective solution? Will it deliver on what the user needs?
Is this solution safe both for the user and for the business? Is it inclusive enough? Is there a risk of public opinion whiplash? Is our solution enabling wrongdoers? Are we jeopardizing some to privilege others?
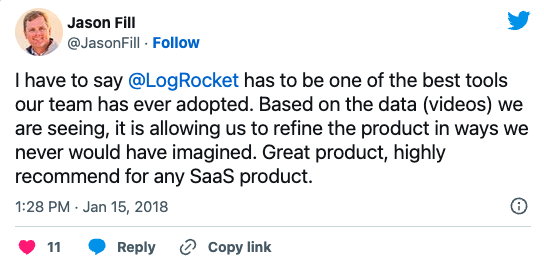
Over 200k developers and product managers use LogRocket to create better digital experiences

There is an infinite amount of questions that can surface from these risks, and most of those will be context dependent. Your industry, company, marketplace, team composition, and even the type of product you handle will impose different questions, but the risks remain the same.
How to decide whether your hypothesis is worthy of validation
Assuming you came up with a hefty batch of risks to validate, you must now address them. To address a risk, you could do one of three things: collect concrete evidence that you can mitigate that risk, infer possible ways you can mitigate a risk and, finally, deep dive into that risk because you’re not sure about its repercussions.
This three way road can be illustrated by a CSD matrix :
Certainties
Suppositions.
Everything you’re sure can help you to mitigate whatever risk. An example would be, on the risk “how to build it,” assessing if your engineering team is capable of integrating with a certain API. If your team has made it a thousand times in the past, it’s not something worth validating. You can assume it is true and mark this particular risk as solved.
To put it simply, a supposition is something that you think you know, but you’re not sure. This is the most fertile ground to explore hypotheses, since this is the precise type of answer that needs validation. The most common usage of supposition is addressing the “is it relevant for the user” risk. You presume that clients will enjoy a new feature, but before you talk to them, you can’t say you are sure.
Doubts are different from suppositions because they have no answer whatsoever. A doubt is an open question about a risk which you have no clue on how to solve. A product manager that tries to mitigate the “is it ethical to deliver” risk from an industry that they have absolute no familiarity with is poised to generate a lot of doubts, but no suppositions or certainties. Doubts are not good hypothesis sources, since you have no idea on how to validate it.
A hypothesis worth validating comes from a place of uncertainty, not confidence or doubt. If you are sure about a risk mitigation, coming up with a hypothesis to validate it is just a waste of time and resources. Alternatively, trying to come up with a risk assessment for a problem you are clueless about will probably generate hypotheses disconnected with the problem itself.
That said, it’s important to make it clear that suppositions are different from hypotheses. A supposition is merely a mental exercise, creativity executed. A hypothesis is a measurable, cartesian instrument to transform suppositions into certainties, therefore making sure you can mitigate a risk.
How to craft a hypothesis
A good hypothesis comes from a supposed solution to a specific product risk. That alone is good enough to build half of a good hypothesis, but you also need to have measurable confidence.
More great articles from LogRocket:
- How to implement issue management to improve your product
- 8 ways to reduce cycle time and build a better product
- What is a PERT chart and how to make one
- Discover how to use behavioral analytics to create a great product experience
- Explore six tried and true product management frameworks you should know
- Advisory boards aren’t just for executives. Join LogRocket’s Content Advisory Board. You’ll help inform the type of content we create and get access to exclusive meetups, social accreditation, and swag.
You’ll rarely transform a supposition into a certainty without an objective. Returning to the API example we gave when talking about certainties, you know the “can we build it” risk doesn’t need validation because your team has made tens of API integrations before. The “tens” is the quantifiable, measurable indication that gives you the confidence to be sure about mitigating a risk.
What you need from your hypothesis is exactly this quantifiable evidence, the number or hard fact able to give you enough confidence to treat your supposition as a certainty. To achieve that goal, you must come up with a target when creating the hypothesis. A hypothesis without a target can’t be validated, and therefore it’s useless.
Imagine you’re the product manager for an ecommerce app. Your users are predominantly mobile users, and your objective is to increase sales conversions. After some research, you came across the one click check-out experience, made famous by Amazon, but broadly used by ecommerces everywhere.
You know you can build it, but it’s a huge endeavor for your team. You best make sure your bet on one click check-out will work out, otherwise you’ll waste a lot of time and resources on something that won’t be able to influence the sales conversion KPI.
You identify your first risk then: is it valuable to the business?
Literature is abundant on the topic, so you are almost sure that it will bear results, but you’re not sure enough. You only can suppose that implementing the one click functionality will increase sales conversion.
During case study and data exploration, you have reasons to believe that a 30 percent increase of sales conversion is a reasonable target to be achieved. To make sure one click check-out is valuable to the business then, you would have a hypothesis such as this:
We believe that if we implement a one-click checkout on our ecommerce, we can grow our sales conversion by 30 percent
This hypothesis can be played with in all sorts of ways. If you’re trying to improve user-experience, for example, you could make it look something like this:
We believe that if we implement a one-click checkout on our ecommerce, we can reduce the time to conversion by 10 percent
You can also validate different solutions having the same criteria, building an opportunity tree to explore a multitude of hypothesis to find the better one:
We believe that if we implement a user review section on the listing page, we can grow our sales conversion by 30 percent
Sometimes you’re clueless about impact, or maybe any win is a good enough win. In that case, your criteria of validation can be a fact rather than a metric:
We believe that if we implement a one-click checkout on our ecommerce, we can reduce the time to conversion
As long as you are sure of the risk you’re mitigating, the supposition you want to transform into a certainty, and the criteria you’ll use to make that decision, you don’t need to worry so much about “right” or “wrong” when it comes to hypothesis formatting.
That’s why I avoided following up frameworks on this article. You can apply a neat hypothesis design to your product thinking, but if you’re not sure why you’re doing it, you’ll extract nothing out of it.
What comes after a good hypothesis?
The final piece of this puzzle comes after the hypothesis crafting. A hypothesis is only as good as the validation it provides, and that means you have to test it.
If we were to test the first hypothesis we crafted, “we believe that if we implement a one-click checkout on our ecommerce, we can grow our sales conversion by 30 percent,” you could come up with a testing roadmap to build up evidence that would eventually confirm or deny your hypothesis. Some examples of tests are:
A/B testing — Launch a quick and dirty one-click checkout MVP for a controlled group of users and compare their sales conversion rates against a control group. This will provide direct evidence on the effect of the feature on sales conversions
Customer support feedback — Track any inquiries or complaints related to the checkout process. You can use organic user complaints as an indirect measure of latent demand for one-click checkout feature
User survey — Ask why carts were abandoned for a cohort of shoppers that left the checkout step close to completion. Their reasons might indicate the possible success of your hypothesis
Effective hypothesis crafting is at the center of product management. It’s the link between dealing with risks and coming up with solutions that are both viable and valuable. However, it’s important to recognize that the formulation of a hypothesis is just the first step.
The real value of a hypothesis is made possible by rigorous testing. It’s through systematic validation that product managers can transform suppositions into certainties, ensuring the right product decisions are made. Without validation, even the most well-thought-out hypothesis remains unverified.
Featured image source: IconScout
LogRocket generates product insights that lead to meaningful action
Get your teams on the same page — try LogRocket today.
Share this:
- Click to share on Twitter (Opens in new window)
- Click to share on Reddit (Opens in new window)
- Click to share on LinkedIn (Opens in new window)
- Click to share on Facebook (Opens in new window)
- #product strategy
- #project management
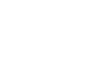
Stop guessing about your digital experience with LogRocket
Recent posts:.
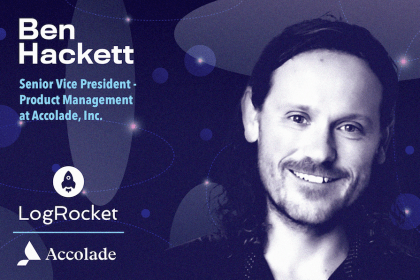
Leader Spotlight: How first principles tie into ruthless prioritization, with Ben Hackett
Ben Hackett discusses the balance of revenue and core cultural values and how ruthless prioritization comes from first-principles thinking.
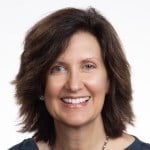
An overview of project baselines
A project baseline acts as a reference point so you can compare project progress against your intended plan.
Leader Spotlight: The benefits of being a full-stack PM, with Kunal Thadani
Kunal Thadani shares necessary qualities of a full-stack PM, including being resourceful, process-driven, and willing to wear many hats.
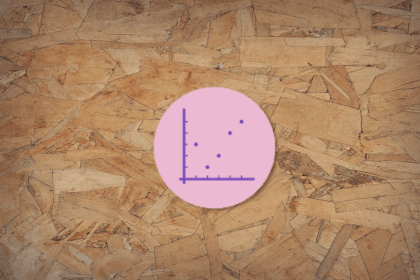
A guide to data product managers
A data product manager (DPM) is a type of PM who specializes in managing products that are heavily reliant on data.
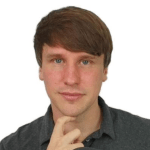
Leave a Reply Cancel reply
Product Talk
Make better product decisions.
The 5 Components of a Good Hypothesis
November 12, 2014 by Teresa Torres
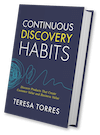
Update: I’ve since revised this hypothesis format. You can find the most current version in this article:
- How to Improve Your Experiment Design (And Build Trust in Your Product Experiments)
“My hypothesis is …”
These words are becoming more common everyday. Product teams are starting to talk like scientists. Are you?
The internet industry is going through a mindset shift. Instead of assuming we have all the right answers, we are starting to acknowledge that building products is hard. We are accepting the reality that our ideas are going to fail more often than they are going to succeed.
Rather than waiting to find out which ideas are which after engineers build them, smart product teams are starting to integrate experimentation into their product discovery process. They are asking themselves, how can we test this idea before we invest in it?
This process starts with formulating a good hypothesis.
These Are Not the Hypotheses You Are Looking For
When we are new to hypothesis testing, we tend to start with hypotheses like these:
- Fixing the hard-to-use comment form will increase user engagement.
- A redesign will improve site usability.
- Reducing prices will make customers happy.
There’s only one problem. These aren’t testable hypotheses. They aren’t specific enough.
A good hypothesis can be clearly refuted or supported by an experiment. – Tweet This
To make sure that your hypotheses can be supported or refuted by an experiment, you will want to include each of these elements:
- the change that you are testing
- what impact we expect the change to have
- who you expect it to impact
- by how much
- after how long
The Change: This is the change that you are introducing to your product. You are testing a new design, you are adding new copy to a landing page, or you are rolling out a new feature.
Be sure to get specific. Fixing a hard-to-use comment form is not specific enough. How will you fix it? Some solutions might work. Others might not. Each is a hypothesis in its own right.
Design changes can be particularly challenging. Your hypothesis should cover a specific design not the idea of a redesign.
In other words, use this:
- This specific design will increase conversions.
- Redesigning the landing page will increase conversions.
The former can be supported or refuted by an experiment. The latter can encompass dozens of design solutions, where some might work and others might not.
The Expected Impact: The expected impact should clearly define what you expect to see as a result of making the change.
How will you know if your change is successful? Will it reduce response times, increase conversions, or grow your audience?
The expected impact needs to be specific and measurable. – Tweet This
You might hypothesize that your new design will increase usability. This isn’t specific enough.
You need to define how you will measure an increase in usability. Will it reduce the time to complete some action? Will it increase customer satisfaction? Will it reduce bounce rates?
There are dozens of ways that you might measure an increase in usability. In order for this to be a testable hypothesis, you need to define which metric you expect to be affected by this change.
Who Will Be Impacted: The third component of a good hypothesis is who will be impacted by this change. Too often, we assume everyone. But this is rarely the case.
I was recently working with a product manager who was testing a sign up form popup upon exiting a page.
I’m sure you’ve seen these before. You are reading a blog post and just as you are about to navigate away, you get a popup that asks, “Would you like to subscribe to our newsletter?”
She A/B tested this change by showing it to half of her population, leaving the rest as her control group. But there was a problem.
Some of her visitors were already subscribers. They don’t need to subscribe again. For this population, the answer to this popup will always be no.
Rather than testing with her whole population, she should be testing with just the people who are not currently subscribers.
This isn’t easy to do. And it might not sound like it’s worth the effort, but it’s the only way to get good results.
Suppose she has 100 visitors. Fifty see the popup and fifty don’t. If 45 of the people who see the popup are already subscribers and as a result they all say no, and of the five remaining visitors only 1 says yes, it’s going to look like her conversion rate is 1 out of 50, or 2%. However, if she limits her test to just the people who haven’t subscribed, her conversion rate is 1 out of 5, or 20%. This is a huge difference.
Who you test with is often the most important factor for getting clean results. – Tweet This
By how much: The fourth component builds on the expected impact. You need to define how much of an impact you expect your change to have.
For example, if you are hypothesizing that your change will increase conversion rates, then you need to estimate by how much, as in the change will increase conversion rate from x% to y%, where x is your current conversion rate and y is your expected conversion rate after making the change.
This can be hard to do and is often a guess. However, you still want to do it. It serves two purposes.
First, it helps you draw a line in the sand. This number should determine in black and white terms whether or not your hypothesis passes or fails and should dictate how you act on the results.
Suppose you hypothesize that the change will improve conversion rates by 10%, then if your change results in a 9% increase, your hypothesis fails.
This might seem extreme, but it’s a critical step in making sure that you don’t succumb to your own biases down the road.
It’s very easy after the fact to determine that 9% is good enough. Or that 2% is good enough. Or that -2% is okay, because you like the change. Without a line in the sand, you are setting yourself up to ignore your data.
The second reason why you need to define by how much is so that you can calculate for how long to run your test.
After how long: Too many teams run their tests for an arbitrary amount of time or stop the results when one version is winning.
This is a problem. It opens you up to false positives and releasing changes that don’t actually have an impact.
If you hypothesize the expected impact ahead of time than you can use a duration calculator to determine for how long to run the test.
Finally, you want to add the duration of the test to your hypothesis. This will help to ensure that everyone knows that your results aren’t valid until the duration has passed.
If your traffic is sporadic, “how long” doesn’t have to be defined in time. It can also be defined in page views or sign ups or after a specific number of any event.
Putting It All Together
Use the following examples as templates for your own hypotheses:
- Design x [the change] will increase conversions [the impact] for search campaign traffic [the who] by 10% [the how much] after 7 days [the how long].
- Reducing the sign up steps from 3 to 1 will increase signs up by 25% for new visitors after 1,000 visits to the sign up page.
- This subject line will increase open rates for daily digest subscribers by 15% after 3 days.
After you write a hypothesis, break it down into its five components to make sure that you haven’t forgotten anything.
- Change: this subject line
- Impact: will increase open rates
- Who: for daily digest subscribers
- By how much: by 15%
- After how long: After 3 days
And then ask yourself:
- Is your expected impact specific and measurable?
- Can you clearly explain why the change will drive the expected impact?
- Are you testing with the right population?
- Did you estimate your how much based on a baseline and / or comparable changes? (more on this in a future post)
- Did you calculate the duration using a duration calculator?
It’s easy to give lip service to experimentation and hypothesis testing. But if you want to get the most out of your efforts, make sure you are starting with a good hypothesis.
Did you learn something new reading this article? Keep learning. Subscribe to the Product Talk mailing list to get the next article in this series delivered to your inbox.
Get the latest from Product Talk right in your inbox.
Never miss an article.
May 21, 2017 at 2:11 am
Interesting article, I am thinking about making forming a hypothesis around my product, if certain customers will find a proposed value useful. Can you kindly let me know if I’m on the right track.
“Certain customer segment (AAA) will find value in feature (XXX), to tackle their pain point ”
Change: using a feature (XXX)/ product Impact: will reduce monetary costs/ help solve a problem Who: for certain customers segment (AAA) By how much: by 5% After how long: 10 days
April 4, 2020 at 12:33 pm
Hi! Could you throw a little light on this: “Suppose you hypothesize that the change will improve conversion rates by 10%, then if your change results in a 9% increase, your hypothesis fails.”
I understood the rationale behind having a number x (10% in this case) associated with “by how much”, but could you explain with an example of how to ballpark a figure like this?
Popular Resources
- Product Discovery Basics: Everything You Need to Know
- Visualize Your Thinking with Opportunity Solution Trees
- Customer Interviews: How to Recruit, What to Ask, and How to Synthesize What You Learn
- Assumption Testing: Everything You Need to Know to Get Started
Recent Posts
- Join 3 Free Product Discovery Webinars in May 2024
- Product in Practice: Shifting from a Feature Factory to Continuous Discovery at Doodle
- Story-Based Customer Interviews Uncover Much-Needed Context
Agile Learning Labs
Scrum training, coaching and consulting.

Measuring Value With Product Hypotheses
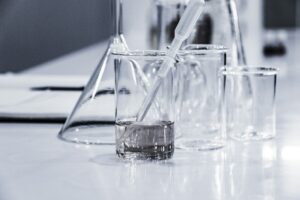
Photo by Hans Reniers
Sadly, most teams never measure the value of what they create, and thus they continue to invest in building those aspects that aren’t delivering value. Measuring value allows the product owner to direct the team’s focus toward the 20 percent of features that hold most of the value.
The first step in measuring the value that we are producing is moving from assumptions to hypotheses. An assumption is something that we believe based on limited evidence. A scrum team might capture their assumptions like this:
As a [type of stakeholder], I want [what we believe the stakeholder wants] So that [some value is created].
When developing a hypothesis, we want to ask ourselves, “How will we know our stakeholder got that value?” We want to identify measurable criteria that will answer that question. We can add this criteria as a fourth line to our user story:
As a [type of stakeholder], I want [what we believe the stakeholder wants] So that [some value is created]. This will be confirmed when [criteria that will confirm the value].
This last line is not an acceptance criteria though there are similarities. Acceptance criteria let us know when the team has built what was intended, that they created the expected output. The fourth line above tests whether that output has created the desired outcome, the actual value.
Example Product Hypothesis
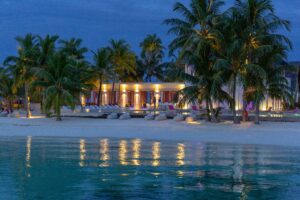
Photo by Saad Khan
As a businessperson, I want a self-serve business center with printing, copying, and faxing capabilities, so that I can get a little work done without leaving the resort.
We can turn this into a testable hypothesis by adding one more line.
As a businessperson, I want a self-serve business center with printing, copying, and faxing capabilities, so that I can get a little work done without leaving the resort. This will be confirmed when at least ten guests do printing, copying, or faxing each week during the first month that the self-serve business center is open.
This additional line gives us a way to measure the value of what the team has built. The hypothesis statement should include:
- The data we will collect
- How long the experiment will last
- The expected result from the experiment
The data we gather will inform decisions about what to build in the future. If we get the predicted result:
At least ten guests do printing, copying, or faxing each week during the first month that the business center is open
then we can feel justified in continuing to invest in this area of our product.
But what if we build a self-serve business center and nobody uses it? Should we still move forward with upgrading it to full service? Probably not! The data doesn’t support our original belief that our customers want to work while on vacation.
Techniques To Measure Value
Here are some approaches to measuring the value of what the scrum team has built. Any of these can be used as the means to test a product hypothesis.
A/B Testing
A/B testing involves directing some users to the new version of what the team has built and the rest of the users to the pre-existing version. We then measure how the two groups behave with regard to whatever value our product hypothesis is testing. For example, we might be looking for 20% more users to make a purchase when they get the new page vs. the pre-existing page.
Learn More About A/B Testing
Multivariate Testing
Multivariate testing is A/B testing made really complicated. Instead of testing one change, we are testing a combination of changes, trying to find the best combination. In a website, we might be experimenting with page layout, images, and ‘Buy Now!’ button design all at the same time.
Learn More About Multivariate Testing
- Multivariate Testing: What, Why, How
- Wikipedia Entry on multivariate testing
- Video: A/B & Multivariate Testing
Usage Tracking
When we implement a new functionality in our product, we add the ability to track how often it gets used. For example, if we added an AI-enhanced grammar checker to a word processor, we could track how often users activated the feature and how often they accepted the suggestions from the grammar checker.
Learn More About Usage Tracking
- Software Usage Analytics Guide for Product Managers
- What Is Product Usage Analytics And How To Implement It
Web Analytics
Web analytics is a specific type of usage tracking for websites. It enables us to see what parts of the site are most visited, how people navigate around the site, where they enter our site, and where they leave it.
Learn More About Web Analytics
- Web Analytics Playbook
- Wikipedia Entry on web analytics
Usability Testing
Usability testing involves watching users as they try to accomplish a task using our product. It enables us to understand how they interact with the product, what they expect, and where they get stuck.
Learn More About Usability Testing
- How to Do Usability Testing
- Wikipedia Entry on usability testing
User Interviews
A simple way to know what parts of your product are valued by users is to ask them. After deploying a new feature, you can ask users if they have used it, how they have used it, what they like/dislike about it, and so on.
Learn More About User Interviews
- How To Do a Customer Interview
- How to Conduct User Interviews
Further Learning
- Pirate Metrics
Leave a Reply Cancel reply
Your email address will not be published. Required fields are marked *
Save my name, email, and website in this browser for the next time I comment.
Upcoming Events
Online certified scrummaster workshop in four 4-hour sessions, certified scrum product owner workshop in four 4-hour sessions, online experience scrum workshop in four 2-hour sessions, online office hour, advanced certified scrum product owner workshop, inclusion is the 4th wave of agile, online certified scrummaster workshop, copy short link.
- Product Management Tutorial
- What is Product Management
- Product Life Cycle
- Product Management Process
- General Availability
- Product Manager
- PM Interview Questions
- Courses & Certifications
- Project Management Tutorial
- Agile Methodology
- Software Engineering Tutorial
- Software Development Tutorial
- Software Testing Tutorial
How do you define and measure your product hypothesis?
- What Is Product Hunt and How Do You Use It?
- How to define a Target Market for a Product?
- How to measure product-market fit
- How to Ensure Your Product Meets User Needs
- Product-Market Fit : Definition, Importance and Example
- What is Product Discovery? | Definition and Overview
- What is Product Marketing? Strategy, Importance and Evolution
- Challenges in Product Management and How to Overcome them.
- What is a Product Portfolio Strategy and How to Develop It?
- How to Become an AI Product Manager?
- Product Segmentation: Definition, Importance and Examples
- How to Become a Product Manager Without Experience ?
- Product Research: Definition, Importance, and Stages
- What does a Product Manager do?
- Difference Between Product Design and Product Development
- What is "Lean Product Development"? Principles and Benefits
- How to Find a P-Value from a t-Score in Python?
- Product Design | Definition, Tools and Challenges
- Measuring Product Success in Product Management: Tips and Tricks
- What is a Product in Product Management?
- What are Product Pillars? Definition and Real-life Examples
- How to balance short-term and long-term goals in product management?
- Learn Product Management | Beginner to Advanced Tutorial
- Chief Product Officer | Definition, Role, and Responsibility
- Gross Domestic Product- History, Types, Formula and Estimation
- How to Write a Research Hypothesis- Step-By-Step Guide With Examples
- Measurement of Area, Volume and Density
- Means and Function of Production
- Difference between Program and Product
Hypothesis in product management is like making an educated guess or assumption about something related to a product, such as what users need or how a new feature might work. It’s a statement that you can test to see if it’s true or not, usually by trying out different ideas and seeing what happens. By testing hypotheses, product managers can figure out what works best for the product and its users, helping to make better decisions about how to improve and develop the product further.
Table of Content
What Is a Hypothesis in Product Management?
How does the product management hypothesis work, how to generate a hypothesis for a product, how to make a hypothesis statement for a product, how to validate hypothesis statements:, the process explained what comes after hypothesis validation, final thoughts on product hypotheses, product management hypothesis example, conclusion: product hypothesis, faqs: product hypothesis.
In product management, a hypothesis is a proposed explanation or assumption about a product, feature, or aspect of the product’s development or performance. It serves as a statement that can be tested, validated, or invalidated through experimentation and data analysis. Hypotheses play a crucial role in guiding product managers’ decision-making processes, informing product development strategies , and prioritizing initiatives. In summary, hypotheses in product management serve as educated guesses or assertions about the relationship between product changes and their impact on user behaviour or business outcomes.
Product management hypotheses work by guiding product managers through a structured process of identifying problems, proposing solutions, and testing assumptions to drive product development and improvement. Here’s how the process typically works:
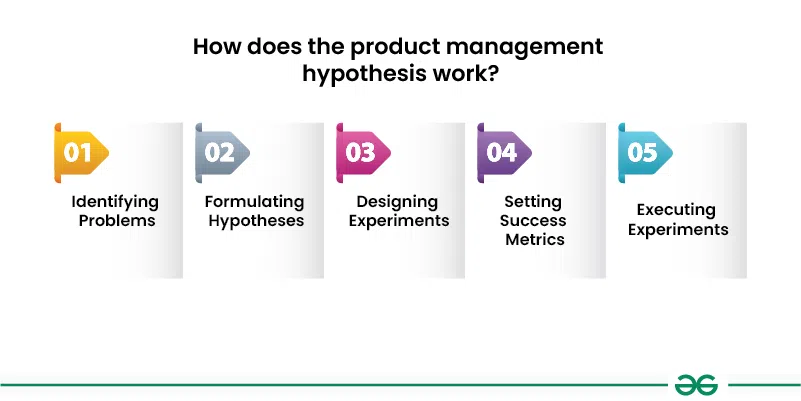
How does the product management hypothesis work
- Identifying Problems : Product managers start by identifying potential problems or opportunities for improvement within their product. This could involve gathering feedback from users, analyzing data, conducting market research, or observing user behaviour.
- Formulating Hypotheses : Based on the identified problems or opportunities, product managers formulate hypotheses that articulate their assumptions about the causes of these issues and potential solutions. Hypotheses are typically written as clear, testable statements that specify what the expected outcomes will be if the hypothesis is true.
- Designing Experiments : Product managers design experiments or tests to validate or invalidate their hypotheses. This could involve implementing changes to the product, such as introducing new features, modifying existing functionalities, or adjusting user experiences. Experiments may also involve collecting data through surveys, interviews, user testing, or analytics tools.
- Setting Success Metrics : Product managers define success metrics or key performance indicators (KPIs) that will be used to measure the effectiveness of the experiments. These metrics should be aligned with the goals of the hypothesis and provide quantifiable insights into whether the proposed solution is achieving the desired outcomes.
- Executing Experiments : Product managers implement the planned changes or interventions in the product and monitor their impact on the defined success metrics. This could involve conducting A/B tests, where different versions of the product are presented to different groups of users, or running pilot programs to gather feedback from a subset of users.
Generating a hypothesis for a product involves systematically identifying potential problems, proposing solutions, and formulating testable assumptions about how changes to the product could address user needs or improve performance. Here’s a step-by-step process for generating hypotheses:
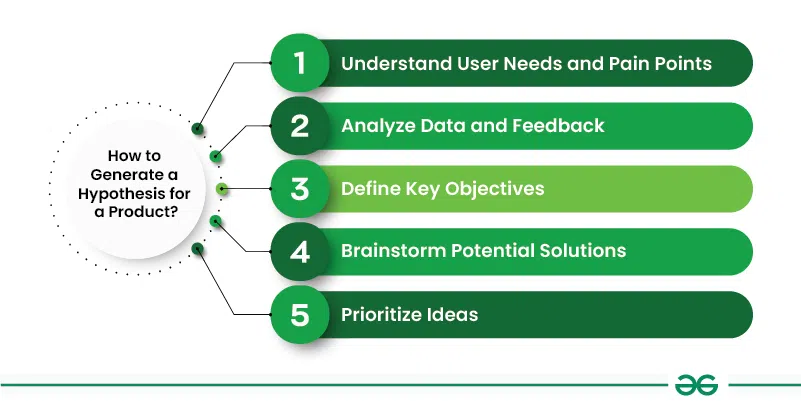
How to Generate a Hypothesis for a Product
- Start by gaining a deep understanding of your target users and their needs, preferences, and pain points. Conduct user research, including surveys, interviews, usability tests, and behavioral analysis, to gather insights into user behavior and challenges they face when using your product.
- Review qualitative and quantitative data collected from user interactions, analytics tools, customer support inquiries, and feedback channels. Look for patterns, trends, and recurring issues that indicate areas where the product may be falling short or where improvements could be made.
- Clarify the goals and objectives you want to achieve with your product. This could include increasing user engagement, improving retention rates, boosting conversion rates, or enhancing overall user satisfaction. Align your hypotheses with these objectives to ensure they are focused and actionable.
- Brainstorm potential solutions or interventions that could address the identified user needs or pain points. Encourage creativity and divergent thinking within your product team to generate a wide range of ideas. Consider both incremental improvements and more radical changes to the product.
- Evaluate and prioritize the potential solutions based on factors such as feasibility, impact on user experience, alignment with strategic goals, and resource constraints. Focus on solutions that are likely to have the greatest impact on addressing user needs and achieving your objectives.
To make a hypothesis statement for a product, follow these steps:
- Identify the Problem : Begin by identifying a specific problem or opportunity for improvement within your product. This could be based on user feedback, data analysis, market research, or observations of user behavior.
- Define the Proposed Solution : Determine what change or intervention you believe could address the identified problem or opportunity. This could involve introducing a new feature, improving an existing functionality, changing the user experience, or addressing a specific user need.
- Formulate the Hypothesis : Write a clear, specific, and testable statement that articulates your assumption about the relationship between the proposed solution and its expected impact on user behavior or business outcomes. Your hypothesis should follow the structure: If [proposed solution], then [expected outcome].
- Specify Success Metrics : Define the key metrics or performance indicators that will be used to measure the success of your hypothesis. These metrics should be aligned with your objectives and provide quantifiable insights into whether the proposed solution is achieving the desired outcomes.
- Consider Constraints and Assumptions : Take into account any constraints or assumptions that may affect the validity of your hypothesis. This could include technical limitations, resource constraints, dependencies on external factors, or assumptions about user behavior.
Validating hypothesis statements in product management involves testing the proposed solutions or interventions to determine whether they achieve the desired outcomes. Here’s a step-by-step guide on how to validate hypothesis statements:
- Design Experiments or Tests : Based on your hypothesis statement, design experiments or tests to evaluate the proposed solution’s effectiveness. Determine the experimental setup, including the control group (no changes) and the experimental group (where the proposed solution is implemented).
- Define Success Metrics : Specify the key metrics or performance indicators that will be used to measure the success of your hypothesis. These metrics should be aligned with your objectives and provide quantifiable insights into whether the proposed solution is achieving the desired outcomes.
- Collect Baseline Data : Before implementing the proposed solution, collect baseline data on the identified metrics from both the control group and the experimental group. This will serve as a reference point for comparison once the experiment is conducted.
- Implement the Proposed Solution : Implement the proposed solution or intervention in the experimental group while keeping the control group unchanged. Ensure that the implementation is consistent with the hypothesis statement and that any necessary changes are properly documented.
- Monitor and Collect Data : Monitor the performance of both the control group and the experimental group during the experiment. Collect data on the defined success metrics, track user behavior, and gather feedback from users to assess the impact of the proposed solution.
After hypothesis validation in product management , the process typically involves several key steps to leverage the findings and insights gained from the validation process. Here’s what comes after hypothesis validation:
- Data Analysis and Interpretation : Once the hypothesis has been validated (or invalidated), product managers analyze the data collected during the experiment to gain deeper insights into user behavior, product performance, and the impact of the proposed solution. This involves interpreting the results in the context of the hypothesis statement and the defined success metrics.
- Documentation of Findings : Document the findings of the hypothesis validation process, including the outcomes of the experiment, key insights gained, and any lessons learned. This documentation serves as a valuable reference for future decision-making and helps ensure that knowledge is shared across the product team and organization.
- Knowledge Sharing and Communication : Communicate the results of the hypothesis validation process to relevant stakeholders, including product team members, leadership, and other key decision-makers. Share insights, lessons learned, and recommendations for future action to ensure alignment and transparency within the organization.
- Iterative Learning and Adaptation : Use the insights gained from hypothesis validation to inform future iterations of the product development process . Apply learnings from the experiment to refine the product strategy, adjust feature priorities, and make data-driven decisions about product improvements.
- Further Experimentation and Testing : Based on the validated hypothesis and the insights gained, identify new areas for experimentation and testing. Continuously test new ideas, features, and hypotheses to drive ongoing product innovation and improvement. This iterative process of experimentation and learning helps product managers stay responsive to user needs and market dynamics.
product hypotheses serve as a cornerstone of the product management process, guiding decision-making, fostering innovation, and driving continuous improvement. Here are some final thoughts on product hypotheses:
- Foundation for Experimentation : Hypotheses provide a structured framework for formulating, testing, and validating assumptions about product changes and their impact on user behavior and business outcomes. By systematically testing hypotheses, product managers can gather valuable insights, mitigate risks, and make data-driven decisions.
- Focus on User-Centricity : Effective hypotheses are rooted in a deep understanding of user needs, preferences, and pain points. By prioritizing user-centric hypotheses, product managers can ensure that product development efforts are aligned with user expectations and deliver meaningful value to users.
- Iterative and Adaptive : The process of hypothesis formulation and validation is iterative and adaptive, allowing product managers to learn from experimentation, refine their assumptions, and iterate on their product strategies over time. This iterative approach enables continuous innovation and improvement in the product.
- Data-Driven Decision Making : Hypothesis validation relies on empirical evidence and data analysis to assess the impact of proposed changes. By leveraging data to validate hypotheses, product managers can make informed decisions, mitigate biases, and prioritize initiatives based on their expected impact on key metrics.
- Collaborative and Transparent : Formulating and validating hypotheses is a collaborative effort that involves input from cross-functional teams, stakeholders, and users. By fostering collaboration and transparency, product managers can leverage diverse perspectives, align stakeholders, and build consensus around product priorities.
Here’s an example of a hypothesis statement in the context of product management:
- Problem: Users are abandoning the onboarding process due to confusion about how to set up their accounts.
- Proposed Solution: Implement a guided onboarding tutorial that walks users through the account setup process step-by-step.
- Hypothesis Statement: If we implement a guided onboarding tutorial that walks users through the account setup process step-by-step, then we will see a decrease in the dropout rate during the onboarding process and an increase in the percentage of users completing account setup.
- Percentage of users who complete the onboarding process
- Time spent on the onboarding tutorial
- Feedback ratings on the effectiveness of the tutorial
Experiment Design:
- Control Group: Users who go through the existing onboarding process without the guided tutorial.
- Experimental Group: Users who go through the onboarding process with the guided tutorial.
- Duration: Run the experiment for two weeks to gather sufficient data.
- Data Collection: Track the number of users who complete the onboarding process, the time spent on the tutorial, and collect feedback ratings from users.
Expected Outcome: We anticipate that users who go through the guided onboarding tutorial will have a higher completion rate and spend more time on the tutorial compared to users who go through the existing onboarding process without guidance.
By testing this hypothesis through an experiment and analyzing the results, product managers can validate whether implementing a guided onboarding tutorial effectively addresses the identified problem and improves the user experience.
In conclusion, hypothesis statements are invaluable tools in the product management process, providing a structured approach to identifying problems, proposing solutions, and validating assumptions. By formulating clear, testable hypotheses, product managers can drive innovation, mitigate risks, and make data-driven decisions that ultimately lead to the development of successful products.
Q. What is the lean product hypothesis?
Lean hypothesis testing is a strategy within agile product development aimed at reducing risk, accelerating the development process, and refining product-market fit through the creation and iterative enhancement of a minimal viable product (MVP).
Q. What is the product value hypothesis?
The value hypothesis centers on the worth of your product to customers and is foundational to achieving product-market fit. This hypothesis is applicable to both individual products and entire companies, serving as a crucial element in determining alignment with market needs.
Q. What is the hypothesis for a minimum viable product?
Hypotheses for minimum viable products are testable assumptions supported by evidence. For instance, one hypothesis to validate could be whether people will be interested in the product at a certain price point; if not, adjusting the price downwards may be necessary.
Please Login to comment...
Similar reads.
- Dev Scripter 2024
- Dev Scripter
- Product Management

Improve your Coding Skills with Practice
What kind of Experience do you want to share?
Product Hypothesis Canvas
We are increasingly shifting from projects centered on the demands of customers or users to projects focused on product hypotheses. There are several reasons for this.
On the one hand, we are expected to implement new functions within increasingly shorter deadlines. That’s because the competition is getting fiercer, and the world, thanks to modern technology, keeps speeding up and getting more complex.
On the other hand, having more diverse groups of users means facing more diverse needs. We are moving at full speed towards an entirely customizable world. And this creates an even greater demand for instantaneous product adjustments.
If “demands” require implementation, then “hypotheses,” above all, need to be tested. But before that, the hypotheses must be coherently articulated. Which is not always as easy as we would have wanted.
We believe that the more coherent the hypothesis, the more fruitful testing it will be. Testing in this sense covers not just the verification of the actual hypothesis, but also any possible insights that may be gathered in the process. To structure and simplify the process of articulating your hypothesis, we have singled out the following questions, which you can answer sequentially.
The Product Hypothesis Canvas helps you create more effective hypotheses. Keep in mind that the canvas does not do the work for you; it simply helps you focus on the task at hand and reach a more effective solution.
The steps for filling out the Product Hypothesis Canvas
We believe that…
Here, we describe what we plan to develop.
2. For (whom)…
In this block, we define our target audience and, if required, evaluate its role in our project.
This step is very important, as it will later help us rank our hypotheses by their relevance to our project. Sometimes, project team members become utterly seduced by an interesting idea, and end up forgetting that it is only applicable to a few isolated cases.
In fact, if the author is unable to coherently explain whom their hypothesis is going to benefit, it’s very likely that they are just indulging in random guesswork. That is like when a pool player breaks with a powerful strike, hoping to pocket a ball at random. In the same way, product managers and designers generate hypotheses with no connection to the users, hoping that at least someone is going to be interested. Be really careful with such hypotheses; it may be worth the time to think about them in greater detail.
3. To achieve…
It is also important to determine what kind of result we are expecting from our experiment. Moreover, the result should preferably be measurable in specific terms. Don’t write, “We must do better!” It’s better to express your expectations like, “We must improve [product] by 5%.”
Depending on the hypothesis, we may have different expectations for short-term and long-term results. Many people prefer to focus on short-term results and avoid working with hypotheses that have more far-reaching goals. But when we create our hypothesis, we must be aware of how long it will take to test it: a day, a week, a month, or maybe even longer. With that in mind, we will later be able to plan a backlog of our experiment.
4. How do we measure this?
The ability to measure the results is the key parameter for testing product hypotheses. While we already mentioned what we are going to measure in the previous step, here we describe the kinds of tools we are going to use for this. What signals will indicate that the opportunity we have created is impactful? Which key performance indicators (qualitative or quantitative) are we going to measure to prove our experiment was successful?
5. Impact, positive or negative
We have introduced this block in case we want to approach our hypothesis as something beyond a single objective. Filling it in is not mandatory.
In some cases, the introduction of a certain function has a negative impact on other parameters within the system. For example, we add an extensive, informative presentation
to our home page, hoping to increase user engagement. However, the presentation impacts the page’s loading speed, which, contrary to our intent, increases the bounce rate, potentially reducing engagement. In this specific case, the higher bounce rate is probably not caused by the functionality itself but by its bulkiness and poor implementation.
- Product Management
- Research & Design
- Meetings & Workshops
- Brainstorming
Submit your template →
Do you have a great board to share with the world? We' ll help you turn it into a template to share with the community.
Similar templates
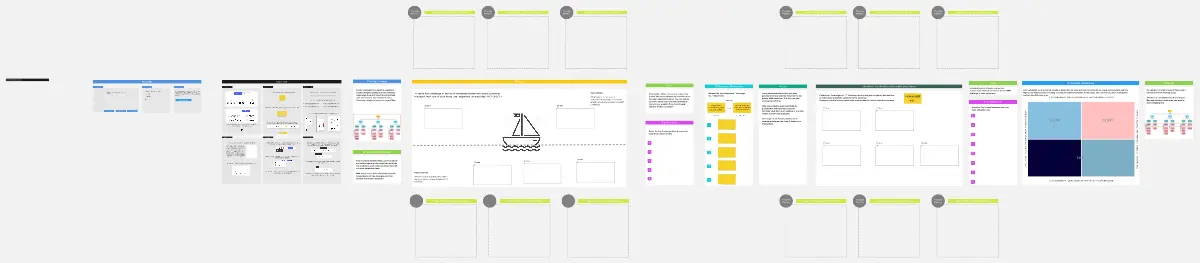
Product Discovery Ideation Session
Value-Effort Matrix (with CSV export)
Idea Canvas
- Product management
- Product strategy
- What is product-market fit?
Product-market fit: Essential steps to measure and achieve it
Product-market fit describes how well your product meets the demand of a viable market. (That is a textbook definition, anyway.) Here is another definition that we like better: Product-market fit is when your product is something that people really want to buy and you have enough customers to support your growth over time.
Finding product-market fit is vital to getting product development right. Maybe this seems obvious — who would build a product that no one wants to buy? No one. At least, not intentionally. But it happens more often than you might think.
Deliver more value with Aha! Roadmaps. Sign up for a free trial .
You can find countless cautionary tales. Many well-meaning founders and product builders have put passion above product-market fit. Those that do so often confuse their own excitement for a solution to a problem that may not exist in the market. If a product does not fit, it tends to fail.
Product-market fit is essential to lasting product success. It is like a special ingredient with no substitutions. But the process of finding it (and recognizing when you have) is not always straightforward. Many product experts have shared their take on what product-market fit really looks like. As a product builder, it is wise to seek out varied perspectives to inform your understanding of the topic. But this guide will walk you through the fundamentals as a start.
Skip ahead to any section:
Why is product-market fit important?
Components of product-market fit
How to find product-market fit
Who is responsible for product-market fit, how to measure product-market fit.
Product-market fit examples
You can have a brilliant idea. A compelling story. A talented product team. And even a fully functional product that manifests all of these things. But if no one wants to buy what you are offering, then you will not get anywhere. This is why product-market fit matters.
While you may think product-market fit is primarily a startup issue, it is just as important for established companies with successful product portfolios . New and existing products require the same level of attention to product-market fit.
Some startups fall into the trap of conflating early enthusiasm from a few people with a real market opportunity. For established businesses, the challenge is looking beyond positive feedback and requests from a few to an actual need by many. No matter how innovative you believe your idea is or how badly someone wishes your product had a certain feature — this does not mean you should build it.
- What is product development?
- How to structure your product team meeting
Product-led vs. engineering-led organizations
In both scenarios, product-market fit is a counterbalance to egos and excitement. If you cannot confirm that enough demand exists to sustain growth and eventually turn a profit, it will be hard to win. Maybe even impossible. Finding product-market fit requires you to question what you think you know and prove how you will succeed. This is challenging, ongoing work — it is core to ensuring your long-term success and continuously delivering product value .
Of course, those other elements still contribute. A solid strategy , bold leadership, and a high-achieving product development team — getting all of these strong building blocks in place bodes well for the future of any business.
Certain frameworks can also help you to validate your likelihood of product-market fit success. For example, completing a proof of concept upfront is one way to examine the feasibility of your product idea from multiple angles. Aha! software includes this template:
Start using this template now
What are the components of product-market fit?
Product-market fit has two main components: your product and the market. Self-explanatory? Yes. But these components are usually expressed in terms of value and growth. Both can be written as a hypothesis explaining what you believe will drive success.
Product-market fit can be elusive. Some product builders will describe it as the stuff of legends but it is certainly obtainable. You can assume that any highly successful company has found product-market fit at least once.
There is no rulebook but many successful companies follow similar pathways to find product-market fit. Above all, the most important component is market research . You need extensive, up-to-date knowledge of potential markets to unearth viable opportunities.
This comes with a few caveats. Sometimes a market will not yet exist for your product. It is difficult to establish a new market but it has been done — though it requires much greater innovation, customer research, and confidence to do so. In other cases, external factors like world events or faster competitors may affect your success, even in a good market.
With that in mind, here are five steps to finding product-market fit that apply to most scenarios:
Identify the growth opportunity. First, conduct market research to find a good market. The definition of a "good market" can vary, but usually means there is a sufficient number of existing users, potential for growth, and ease of customer acquisition. Writing a market requirements document can help you articulate the market opportunity. Summarize the results in your growth hypothesis.
Understand customer needs. Dig into your target market and research potential customers . Seek to understand their deepest pain points and underserved needs. Then, develop customer personas based on what you learn.
Define product value. Think hard about what value means to your business — then capture how you plan to deliver it and solve the problems you have identified in the market by forming your value hypothesis.
Build value first. Develop an initial version of your product to start validating your hypotheses. (You may know this as a Minimum Viable Product — or a Minimum Lovable Product as we call it.)
Iterate and adapt as necessary. Despite your best research, you may not achieve product-market fit on the first try. Even if you do — it takes diligence to maintain. Keep working to improve your understanding of market opportunities and enhancing your product to meet them.
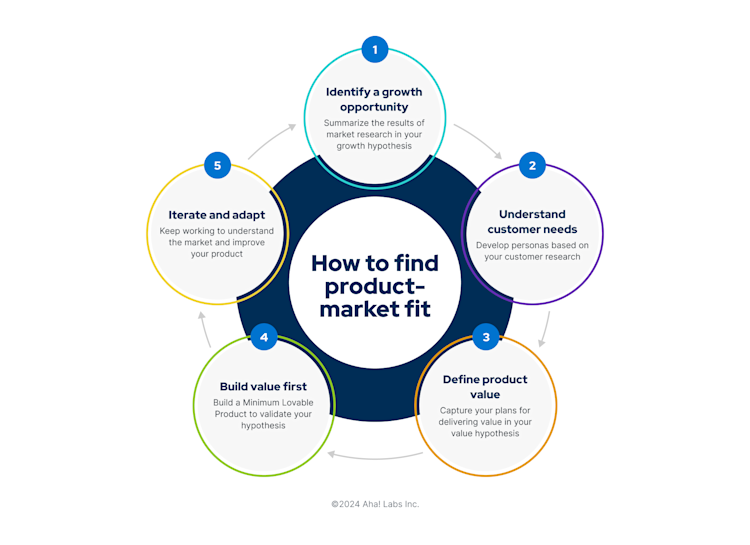
This varies by company stage. In startups, finding product-market fit is what founders do. Much of it falls under executive leadership in established companies too as part of setting the overall business strategy. Product management also plays a considerable role — conducting customer and market research, validating ideas and opportunities, and delivering the product itself. Typically all of these inputs will be used to do a formal analysis of the product's strengths, weaknesses, opportunities, and threats (SWOT).
You can conduct a SWOT analysis of your own using the whiteboard template below. (A similar template is also built into Aha! Roadmaps .)
Start using this template now
That said, product-market fit should be the concern of the entire product development team . It affects everyone's ability to deliver value to customers and meet business goals. For example, engineering should consider how the product's technical foundation supports user needs — while product marketing must consider how product positioning will resonate with the target market.
It can be difficult to pinpoint successful product-market fit. Some say to measure it with benchmarks while others describe it as a feeling . This ambiguity is tricky — it can even lead some companies to believe they have found product-market fit when they have not. But the most obvious sign of product-market fit is a steady stream of customers excitedly buying from you.
Keep in mind that product-market fit does not suddenly happen one day. Reaching it is gradual — it relies on you consistently prioritizing, delivering, and measuring product value in your decision-making. Practicing value-based product development is integral to how successful companies encourage this deeper level of thinking.
In addition, some other metrics and methods can be indicators of product-market fit:
Growing demand for new product features
Major increases in product usage
High Net Promoter Scores (NPS)
Responses to customer survey questions (e.g., "Would you be disappointed if you could no longer use this product?")
Long-term customer retention and/or low churn
Examples of product-market fit
We can learn from plenty of good product-market fit examples (Uber, Netflix, and Slack) and bad product-market fit examples (Quibi, Segway, and MoviePass). You can also read about examples of very similar products that failed at first but gained traction later on with better product-market fit (Vine and TikTok).
Many of these examples are of failed startups, but as we mentioned before, product-market fit is just as pertinent for established companies. Let's look at two examples from Google. Google has experienced both sides of the product-market fit problem. The examples below help to show how product-market fit is usually a greater success factor than ambitious technology, well-known branding, vast resources, and even highly-skilled development teams.
Finding product-market fit and building a product that fulfills customer needs is complex work. If your team is still using disparate tools like spreadsheets and documents to store information, it can be challenging to connect the dots between your research, strategy, and daily product work.
That is why most modern product development teams rely on purpose-built software that brings all of these elements together in one place — so you can create real value for customers and the business.
Aha! is the world's #1 product development software. Start a free 30-day trial today .
- What is a business model?
- What is customer experience?
- What is the Complete Product Experience (CPE)?
- What is a customer journey map?
- What is product-led growth?
- What are the types of business transformation?
- What is enterprise transformation?
- What is digital transformation?
- What is the role of product management in enterprise transformation?
- What is a Minimum Viable Product (MVP)?
- What is a Minimum Lovable Product (MLP)?
- What is product vision?
- How to set product strategy
- What is product differentiation?
- How to position your product
- How to price your product
- What are product goals and initiatives?
- How to set product goals
- How to set product initiatives
- What is product value?
- What is value-based product development?
- Introduction to marketing strategy
- Introduction to marketing templates
- What is a marketing strategy?
- How to set marketing goals
- Marketing vs. advertising
- What is a creative brief?
- How to define buyer personas
- Understanding the buyer's journey
- What is competitive differentiation?
- 10Ps marketing matrix
2x2 prioritization matrix
- Business model
Customer journey map
- Decision log
- Decision tree
- Fit gap analysis
- Gap analysis
- Lean canvas
- Marketing strategy
- MoSCoW model
- Opportunity canvas
- Porter's 5 forces
- Pricing and packaging research
- Pricing plan chart
- Pricing strategies (Kotler)
Product positioning
- Product vision
- Segment profile
- SMART goals
- Strategic roadmap
- Strategy mountain
- SWOT analysis
- Value proposition
- VMOST analysis
- Working backwards
- Collections: Business model
- Collections: SWOT
- Collections: Objectives and key results (OKR)
- Collections: Product positioning
- Collections: Market positioning
- Collections: Marketing strategy
- Collections: Marketing messaging
- What is product discovery?
- How to do market research
- How to define customer personas
- How to research competitors
- How to gather customer feedback
- Asking the right questions to drive innovation
- Approaches table
- Competitive analysis
- Customer empathy map
- Customer interview
- Customer research plan
- PESTLE analysis
- Problem framing
- Product comparison chart
- Pros and cons
- Target audience
- Collections: Customer research
- Collections: Competitor analysis
- Collections: Marketing competitor analysis
- How to brainstorm product ideas
- Brainstorming techniques for product builders
- Why product teams need an internal knowledge hub
- Why product teams need virtual whiteboarding software
- What is idea management?
- 4 steps for product ideation
- How to estimate the value of new product ideas
- How to prioritize product ideas
- What is idea management software?
- Introduction to marketing idea management
- How to gather marketing feedback from teammates
- Brainstorming new marketing ideas
- How to estimate the value of new marketing ideas
- Brainstorming meeting
- Brainstorming session
- Concept map
- Data flow diagram
- Fishbone diagram
- Ideas portal guide
- Jobs to be done
- Process flow diagram
- Proof of concept
- Sticky note pack
- User story map
- Workflow diagram
- Roadmapping: Your starter guide
- Agile roadmap
- Business roadmap
- Features roadmap
- Innovation roadmap
- Marketing roadmap
- Product roadmap
- Product portfolio roadmap
- Project roadmap
- Strategy roadmap
- Technology roadmap
- How to choose a product roadmap tool
- How to build a brilliant roadmap
- What to include on your product roadmap
- How to visualize data on your product roadmap
- What milestones should be included on a roadmap?
- How often should roadmap planning happen?
- How to build a roadmap for a new product
- How to build an annual product roadmap
- How to customize the right roadmap for your audience
- Product roadmap examples
- How to report on progress against your roadmap
- How to communicate your product roadmap to customers
- What is a content marketing roadmap?
- What is a digital marketing roadmap?
- What is an integrated marketing roadmap?
- What is a go-to-market roadmap?
- What is a portfolio marketing roadmap?
- How to choose a marketing roadmap tool
- Epics roadmap
- Now, Next, Later roadmap
- Portfolio roadmap
- Release roadmap
- Collections: Product roadmap
- Collections: Product roadmap presentation
- Collections: Marketing roadmap
- What is product planning?
- How to diagram product use cases
- How product managers use Gantt charts
- How to use a digital whiteboard for product planning
- Introduction to release management
- How to plan product releases across teams
- What is a product backlog?
- Product backlog vs. release backlog vs. sprint backlog
- How to refine the product backlog
- Capacity planning for product managers
- What is requirements management?
- What is a market requirements document (MRD)?
- How to manage your product requirements document (PRD)
- What is a product feature?
- What is user story mapping?
- How to prioritize product features
- Common product prioritization frameworks
- JTBD prioritization framework
- Introduction to marketing plans
- What is a marketing plan?
- How to create a marketing plan
- What is a digital marketing plan?
- What is a content marketing plan?
- Why is content marketing important?
- What is a social media plan?
- How to create a marketing budget
- 2023 monthly calendar
- 2024 monthly calendar
- Feature requirement
- Kanban board
- Market requirements document
- Problem statement
- Product requirements document
- SAFe® Program board
- Stakeholder analysis
- Stakeholder map
- Timeline diagram
- Collections: Product development process
- Collections: MRD
- Collections: PRD
- Collections: Gantt chart
- Collections: User story
- Collections: User story mapping
- Collections: Feature definition checklist
- Collections: Feature prioritization templates
- Collections: Marketing plan templates
- Collections: Marketing calendar templates
- Product design basics
- What is user experience design?
- What is the role of a UX designer?
- What is the role of a UX manager?
- How to use a wireframe in product management
- Wireframe vs. mockup vs. prototype
- 18 expert tips for wireframing
- Analytics dashboard wireframe
- Product homepage wireframe
- Signup wireframe
- Collections: Creative brief
- Common product development methodologies
- Common agile development methodologies
- What is agile product management?
- What is agile software development?
- What is agile project management?
- What is the role of a software engineer?
- What is waterfall product management?
- What is agile transformation?
- Agile vs. lean
- Agile vs. waterfall
- What is an agile roadmap?
- What is an agile retrospective?
- Best practices of agile development teams
- What is a burndown chart?
- What is issue tracking?
- What is unit testing?
- Introduction to agile metrics
- Agile glossary
- What is kanban?
- How development teams implement kanban
- How is kanban used by product managers?
- How to set up a kanban board
- Kanban vs. scrum
- What is scrum?
- What are scrum roles?
- What is a scrum master?
- What is the role of a product manager in scrum?
- What is a sprint?
- What is a sprint planning meeting?
- What is a daily standup?
- What is a sprint review?
- Product release vs. sprint in scrum
- Themes, epics, stories, and tasks
- How to implement scrum
- How to choose a scrum certification
- What is the Scaled Agile Framework®?
- What is the role of a product manager in SAFe®?
- SAFe® PI planning
- SAFe® PI retrospective
- SAFe® Sprint planning
- Sprint planning
- Sprint retrospective
- Sprint retrospective meeting
- UML class diagram
- Collections: Sprint retrospective
- How to test your product before launch
- What is a go-to-market strategy?
- How to write excellent release notes
- How to plan a marketing launch
- Knowledge base article
- Product launch plan
- Product updates
- Release notes
- Collections: Product launch checklist
- Collections: Marketing launch checklist
- How to make data-driven product decisions
- How to measure product value
- What is product analytics?
- What are product metrics?
- What is a product?
- What is a product portfolio?
- What is product management?
- What is the role of a product manager?
- What is portfolio product management?
- What is program management?
- What is product operations?
- What are the stages of product development?
- What is the product lifecycle?
- What is a product management maturity model?
- What is product development software?
- How to create internal product documentation
- What to include in an internal product documentation hub
- Internal vs. external product documentation
- How to build a product knowledge base
- Introduction to marketing methods
- What is agile marketing?
- What is digital marketing?
- What is product marketing?
- What is social media marketing?
- What is B2B marketing?
- Collections: Product management
- 15 tips for running effective product team meetings
- Daily standup meeting
- Meeting agenda
- Meeting notes
- Product backlog refinement meeting
- Product feature kickoff meeting
- Product operations meeting
- Product strategy meeting
- Sprint planning meeting
- What are the types of product managers?
- 10 skills to succeed as a product manager
- Common product management job titles
- What does a product manager do each day?
- What is the role of a product operations manager?
- What is the role of a program manager?
- Templates for program and portfolio management
- How to become a product manager
- How to prepare for a product manager interview
- Interview questions for product managers
- Typical salary for product managers
- Tips for new product managers
- How to choose a product management certification
- Introduction to marketing
- What are some marketing job titles?
- What is the role of a marketing manager?
- What is the role of a product marketing manager?
- How are marketing teams organized?
- Which tools do marketers use?
- Interview questions for marketing managers
- Typical salary for marketing managers
- How to make a career switch into marketing
- Job interview
- Negotiating an offer
- Product manager resume
- Collections: Product manager resume
- How to structure your product development team
- Best practices for managing a product development team
- Which tools do product managers use?
- How to streamline your product management tools
- Tips for effective collaboration between product managers and engineers
- How do product managers work with other teams?
- How product managers achieve stakeholder alignment
- Aha! record map
- Creative brief
- Marketing calendar
- Organizational chart
- Presentation slides
- Process improvement
- Collections: Product management meeting
- Collections: Diagrams, flowcharts for product teams
- Collections: Whiteboarding
- Collections: Templates to run product meetings
- Product development definitions
- Marketing definitions
Popular templates
Evaluate options to make better tradeoff decisions.
Articulate the key benefits of your product and what you solve.
Sketch the complete user journey to understand customer touch points more deeply.
- Privacy policy
- Terms of service
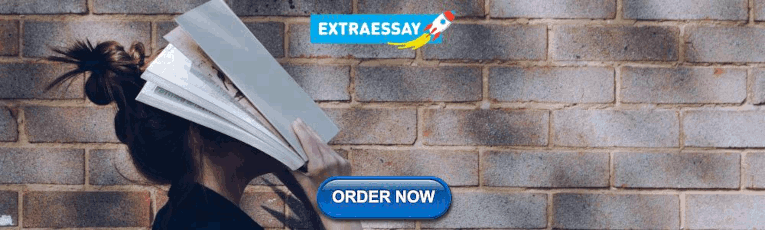
IMAGES
VIDEO
COMMENTS
Product Hypothesis statements can come in many different forms so pick what's most comfortable for the team and business to understand. However they should always include the following key details:
What is a product hypothesis? A hypothesis is a testable statement that predicts the relationship between two or more variables. In product development, we generate hypotheses to validate assumptions about customer behavior, market needs, or the potential impact of product changes.
Product Hypothesis Examples. To demonstrate how to formulate your assumptions clearly, here are several more apart from the example of a hypothesis statement given above: Adding a wishlist feature to the cart with the possibility to send a gift hint to friends via email will increase the likelihood of making a sale and bring in additional sign-ups.
A product hypothesis serves as a guiding light in the product development process. In the case of PlaceMakers, the product owner's hypothesis that users would benefit from knowing the availability of items upfront before adding them to the basket helped their team focus on the most critical aspects of the product.
Product management teams in every organization must make it a point to highlight the importance of conducting hypothesis-driven experiments. The product-design hypothesis is an iterative measure that defines and explores assumptions, followed by conducting suitable experiments and validating the outcome based on user feedback.
Which brings us to the next step, writing hypotheses. Take all your ideas and turn them into testable hypotheses. Do this by rewriting each idea as a prediction that claims the causes proposed in Step 2 will be overcome, and furthermore that a change will occur to the metrics you outlined in Step 1 (your outcome).
A/B Testing. One of the most common use cases to achieve hypothesis validation is randomized A/B testing, in which a change or feature is released at random to one-half of users (A) and withheld from the other half (B). Returning to the hypothesis of bigger product images improving conversion on Amazon, one-half of users will be shown the ...
May 17, 2019. --. Key Takeaways: You need a hypothesis because it clearly defines a change you want to make and the impact you expect to have on your product. A good hypothesis can be proven false ...
How to write an effective hypothesis. Hypothesis validation is the bread and butter of product discovery. Understanding what should be prioritized and why is the most important task of a product manager. It doesn't matter how well you validate your findings if you're trying to answer the wrong question. A question is as good as the answer ...
A product hypothesis is a statement that expresses your belief about how your product will solve a specific problem or meet a specific need for your target audience. It is based on your research ...
Learn how to test your product idea with a null and alternative hypothesis, and how to validate it with data and metrics. Find out the basics of hypothesis testing, how to figure out the right lever to pull, and how to use your numbers or qualitative methods to generate the hypothesis.
A product hypothesis is a statement that proposes a connection between two or more variables and is crucially testable. When creating a product, we generate hypotheses to validate assumptions about customer behavior, market needs, or the potential impact of product changes.
A product hypothesis is a testable statement that expresses your belief about how your product will solve a specific problem or deliver a certain value for your target audience. In this article ...
As a product manager, you need to get comfortable saying "NO"! As previously described in Part 1 of this series, Product Hypothesis Testing: Generating The Hypothesis, t he first step in hypothesis testing involves setting up two competing hypotheses, the null hypothesis and the alternative hypothesis. Null hypothesis: states the "status quo".
Hypothesis Testing: The 5 Components of a Good Hypothesis. To make sure that your hypotheses can be supported or refuted by an experiment, you will want to include each of these elements: the change that you are testing. what impact we expect the change to have. who you expect it to impact.
Example Product Hypothesis. Photo by Saad Khan. Imagine a team whose product is a tropical resort. The team running the resort believes that some guests really want to work while they are at the resort. They have populated their product backlog with items (stories) including: self-serve business center, full-service business center, and meeting ...
What Is a Hypothesis in Product Management? In product management, a hypothesis is a proposed explanation or assumption about a product, feature, or aspect of the product's development or performance. It serves as a statement that can be tested, validated, or invalidated through experimentation and data analysis.
Product Hypothesis. Once we clearly understand our target market, customer profile, and the problem we aim to solve, it's time to do just that by constructing and deploying an MVP. The main goal ...
The Product Hypothesis Canvas helps you create more effective hypotheses. Keep in mind that the canvas does not do the work for you; it simply helps you focus on the task at hand and reach a more effective solution. The steps for filling out the Product Hypothesis Canvas. We believe that… Here, we describe what we plan to develop. 2. For ...
Product-market fit component. Description. Value hypothesis or proposition. A value hypothesis or proposition outlines what makes your product attractive to customers. It is a short statement that describes the problems you want to solve and how you will do it differently than anyone else.
Product Life Cycle: The product life cycle describes the period of time over which an item is developed, brought to market and eventually removed from the market. The cycle is broken into four ...
Developing a hypothesis (with example) Step 1. Ask a question. Writing a hypothesis begins with a research question that you want to answer. The question should be focused, specific, and researchable within the constraints of your project. Example: Research question.
Study with Quizlet and memorize flashcards containing terms like One can expect their sales volume to be _____ when they are in product/market fit. [Elements of the Product/Market Fit Hypothesis], Product/market fit is making a product or _____ that many people want. [Elements of the Product/Market Fit Hypothesis], A business must provide customers with quality products to maintain customer