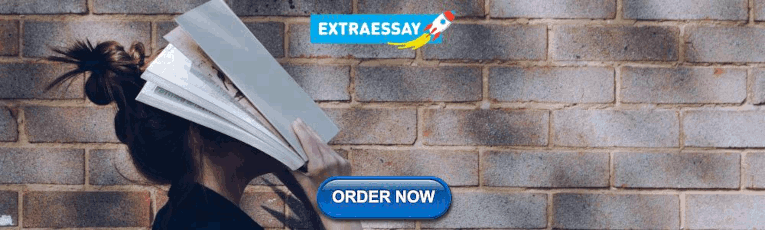
Journal of Population Research
This is a transformative journal , you may have access to funding.
- Santosh Jatrana
Societies and partnerships
Latest articles
Hidden numbers, hidden people: family violence among south asian australians.
- Heshani Samantha De Silva
- Stephane M. Shepherd
- Troy E. McEwan
Accuracy of small area mortality prediction methods: evidence from Poland
- Agnieszka Orwat-Acedańska
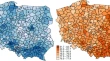
Estimating the civilian noninstitutional population for small areas: a modified cohort component approach using public use data
- Andrew C. Forrester
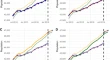
A global and regional assessment of the timing of birth registration using DHS and MICS survey data
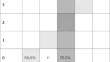
Decomposing the differences in healthy life expectancy between migrants and natives: the ‘healthy migrant effect’ and its age variations in Australia
- Guogui Huang
- Marika Franklin
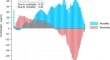
Journal updates
Australian population association.
Published in cooperation with the Australian Population Association
Journal information
- Astrophysics Data System (ADS)
- Australian Business Deans Council (ABDC) Journal Quality List
- CAB Abstracts
- Emerging Sources Citation Index
- Engineering Village – GEOBASE
- Google Scholar
- Norwegian Register for Scientific Journals and Series
- OCLC WorldCat Discovery Service
- TD Net Discovery Service
- UGC-CARE List (India)
Rights and permissions
Springer policies
© Springer Nature B.V.
- Find a journal
- Publish with us
- Track your research
ENCYCLOPEDIC ENTRY
Demography is the statistical study of human populations. Demographers use census data, surveys, and statistical models to analyze the size, movement, and structure of populations.
Old London Demographic Notebook
Early demographic studies were often carried out by insurance agents to determine life insurance rates. Here is a demographic notebook from London, England.
Photograph by whitemay
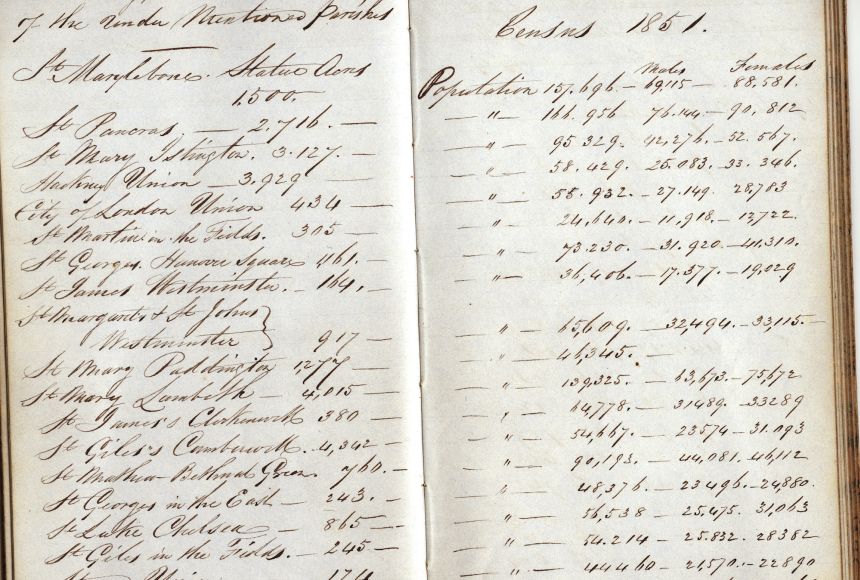
Demography is the statistical study of human populations. Demography examines the size, structure, and movements of populations over space and time. It uses methods from history, economics, anthropology, sociology, and other fields. Demography is useful for governments and private businesses as a means of analyzing and predicting social, cultural, and economic trends related to population. While basic demographic studies, such as censuses , were conducted in the ancient world as far back as 6,000 years ago, demographers as we know them, such as John Graunt from the United Kingdom, came about in the 16th century. The earliest statistical studies were concerned mostly with mortality (how many people died and at what age). Through studying baptism and burial records, Graunt could estimate the number of men of military age, and the number of women of childbearing age. His study represents one of the earliest statistical examinations of the population of a region. Demographic studies were often carried out by early insurance agents to determine life insurance rates. These early demographic studies were mostly concerned with mortality . However, in the 19th century, studies showed that there was a decline in the number of births, and researchers began to study fertility as well as mortality . These studies led to the idea of “ differential fertility .” Differential fertility suggests that different groups within a population have different numbers of children due to factors, such as religion, cultural attitudes, poverty, and employment. Migration of people is the last main factor in demographic studies. It is these three variables ( mortality , fertility , and migration) that contribute to population change. Demographers gather data mainly through government censuses and government registries of births and deaths. However, these sources can be inaccurate depending on the precision of government records. Demographers also gather data indirectly through surveying smaller groups within a population. These samples are then examined using statistical models to draw conclusions about the whole population.
Media Credits
The audio, illustrations, photos, and videos are credited beneath the media asset, except for promotional images, which generally link to another page that contains the media credit. The Rights Holder for media is the person or group credited.
Production Managers
Program specialists, last updated.
October 19, 2023
User Permissions
For information on user permissions, please read our Terms of Service. If you have questions about how to cite anything on our website in your project or classroom presentation, please contact your teacher. They will best know the preferred format. When you reach out to them, you will need the page title, URL, and the date you accessed the resource.
If a media asset is downloadable, a download button appears in the corner of the media viewer. If no button appears, you cannot download or save the media.
Text on this page is printable and can be used according to our Terms of Service .
Interactives
Any interactives on this page can only be played while you are visiting our website. You cannot download interactives.
Related Resources
Demography's Changing Intellectual Landscape: A Bibliometric Analysis of the Leading Anglophone Journals, 1950–2020
Corresponding author: [email protected]

- Standard View
- Article contents
- Figures & tables
- Supplementary Data
- Peer Review
- Open the PDF for in another window
- Permissions
- Cite Icon Cite
- Search Site
M. Giovanna Merli , James Moody , Ashton Verdery , Mark Yacoub; Demography's Changing Intellectual Landscape: A Bibliometric Analysis of the Leading Anglophone Journals, 1950–2020. Demography 1 June 2023; 60 (3): 865–890. doi: https://doi.org/10.1215/00703370-10714127
Download citation file:
- Reference Manager
Much of what we know about the intellectual landscape of anglophone demography comes from two sources: subjective narratives authored by leaders in the field, whose reviews and observations are derived from their research experience and field-specific knowledge; and professional histories covering the field's foundational controversies, which tend to focus on individuals, institutions, and influence. Here we use bibliographic information from all articles published in the three leading journals of anglophone demography— Demography , Population Studies , and Population and Development Review —to survey the changing contours of anglophone demography's key research areas over the past 70 years. We characterize the field of demography by applying a two-pronged, data-grounded approach from the sociology of science. The first uses natural language processing that lets the substance of the field emerge from the contents of publication records and applies social network analyses to identify groups of papers that talk about the same thing. The second uses bibliometric tools to capture the “conversations” of demography with other disciplines. Our goals are to (1) identify the primary topics of demography since the discipline first gained prominence as an organized field; (2) assess changes in the field's intellectual cohesion and the topical areas that have grown or shrunk; and (3) examine how demographers place their work in relationship to other disciplines, the visibility and influence of demographic research in the broader scientific literature, and the cross-disciplinary translational reach of demographic research. Results provide a dynamic view of the field's scientific development in the second half of the twentieth century and the first two decades of the twenty-first century.
- Introduction
Since its origins as an organized scientific field in research and training more than 70 years ago ( Caselli 2002 ; Merchant 2021 ), the field of demography convened around clearly defined topics and methods, focused on accurate measurement and the faithful representation of the relationship between vital rates and changes in aggregate population structures. The boundaries of the field have since shifted. Demography has morphed into a multidisciplinary field concerned with interpreting and explaining the individual- and macro-level causes and consequences of population change and structures and applying theories and analyses that span life stages and multiple generations linked by interconnected events and environments.
The changing boundaries of demography have spurred semiregular assessments. One important source of knowledge about the field comes from historians who have catalogued demography's foundational period and the webs of people, power, and ideas that underpin several of its key controversies, such as its close ties with eugenics research ( Ramsden 2002 , 2003 ), engagement with the birth control movement ( McCann 1999 ), and the development of the notion of a “population bomb” from long-running mercantilist versus Malthusian debates about the consequences of population growth ( Merchant 2021 ). These histories tend to focus on individuals, institutions, and influence. They use archival research, personal correspondence, contemporaneous and retrospective interviews, and the published literature to chart the “whos, whats, and whys” behind key moments in the field's foundation and its early fault lines of debate. As histories, these works tend to focus on the period before 1950, when the field's professional identity coalesced and its intellectual standing became relatively solidified. A partial exception to these efforts are recent computational text analyses ( Merchant 2017 ; Merchant and Alexander 2022 ), which trace both the historical processes underpinning the field's foundation (e.g., funding sources and the creation of population centers, professional associations, and journals) as well as how the concerns of funders were reflected in the types of scholarship published in the 1915–1984 period and beyond.
Assessments of more recently published work come primarily from self-reflection by members of the community of demographers. In these efforts, people and places figure strongly, but much of the focus rests on the evolution of the field's intellectual traditions—and, bucking the constraints of professional historians, many of these assessments consider what they see as the field's future. Earlier concerns focused on demography's narrowing scope: that it might contract to its “accountancy core, with behavioral excursions governed wholly by survey datasets and packages of statistical software” ( McNicoll 1992 :414), and that efforts to establish itself as a stand-alone, “academically recognized independent discipline” ( Demeny 1988 ) might lead to diminishing conversations with other disciplines. These concerns have given way to a preoccupation with what some perceive to be an erosion of the core of demography, motivated by a shift in funding focus for population research ( Lee 2001 ) and loss of disciplinary integrity ( McNicoll 2007 ). This view that demography has abandoned its core was summarized by Lee ( 2001 :1) in a panel presentation on Micro–Macro Issues at the 2001 annual meeting of the Population Association of America: “There is less aggregate level (macro) analysis and more individual level (micro) analysis. There is less emphasis on process and dynamics, and more emphasis on individual decisions about demographic behavior. There is less formal demography, and more data analysis. There is much less funding of aggregate demography and formal demography, and much more funding of micro level empirical studies.” Fears were also voiced that demography's fragmentation into different objects of research, levels and methods of analysis, and explanatory factors may lead to compartmentalization ( Tabutin 2007 ), leaving the discipline without an integrated research program.
We propose an alternative view that considers demography as a successful research program (or progressive, see Lakatos 1978 ) 1 as opposed to a disintegrating or fragmented research program. According to this view, demography is organized around a set of rules, propositions (its mathematical apparatus), and heuristics resulting in the construction of models whereby new evidence highlights regularities as well as anomalies, leading to the integration of new expectations with earlier ones and, ultimately, to the development of more comprehensive theories. If viewed from this vantage point, demography can be considered a program that has succeeded in preserving its core while benefiting from the availability of new empirical evidence distilled from a growing variety of data sources and facilitated by the advantageous encroachment from and conversation with the allied disciplines. This infusion of new data and disciplinary perspectives has enabled new analyses of the processes of individual decision-making and behaviors across time and space, the development of more comprehensive theories that predict new regularities and new facts, and the opening up of new opportunities for scientific advancement. This explanation provides a more dynamic view of our field's scientific development in the second half of the twentieth century and the first two decades of the twenty-first century.
These different views and perceptions motivate questions regarding the development and current intellectual boundaries of the field. Has demography become topically more disintegrated? Has the core of demography truly eroded? Have demography's disciplinary boundaries become more porous? To what extent has demography relied on knowledge from other disciplines, or breached the boundaries of other disciplines? What do these shifts imply for the influence and relevance of demography in the broader scientific community?
Much of what we know about the intellectual evolution of demography in recent decades comes from subjective narratives authored by leaders in the field, whose reviews and observations are grounded in broad knowledge of the field. For instance, explanations of changes in the field point to the collection of increasingly complex, often longitudinal or experimental study designs and new measurement techniques that have increased the depth and breadth of what demographers can speak to with the data available ( McNicoll 2007 ). This growth in the complexity of data sets and analyses was made possible by “changes in the technology available for information processing” ( Chasteland et al. 2004 ; Crimmins 1993 :579). Whereas some emphasize the role of more data and more sophisticated methods, others focus on institutional drivers. Some have noted that, because demography is a small field “lacking security in academic structures, [it has been particularly] sensitive to demand factors including those associated with perceived population problems” ( Preston 1993 :593) and the priorities of funding agencies ( Morgan and Lynch 2001 ; Tabutin 2007 ). Historical work shows that such factors played an outsized role in the field's foundation ( McCann 2016 ; Merchant 2015 , 2021 ) and determined the relative space afforded to certain ideas in its early intellectual traditions ( Merchant 2017 ). Others have noted that the evolution and expansion of the field have occurred in conjunction with developments in other disciplines (sociology, economics, epidemiology), in the sense that demography, as a productive field, has enhanced its core by drawing its theoretical and interpretative baggage from these allied disciplines while applying its unique demographic tool kit to ideas generated in these disciplines ( Goldman 2002 ; Palloni 2002 ). These processes have spurred the emergence of new analytic approaches, levels of analysis, and topics in demographic research.
Earlier quantitative assessments of changes in demography's landscape in terms of topics, number, and gender composition of authors of papers published in demography's key intellectual outlets have relied on content analyses of decades of articles grouped into predefined and well-recognized subfields (e.g., mortality, fertility, family, migration, methods) according to coding schemes that use a modified version of the field's conventional subject headings ( Teachman et al. 1993 ) or assign papers to subject areas based on lists of keywords ( Krapf et al. 2016 ). Other analyses have relied on large demography paper corpuses and computational modes of textual analysis, such as Latent Dirichlet Allocation (LDA)—commonly described as topic modeling—that ascribe to topics common vocabularies shared by papers and track the prevalence of a prespecified number of topics across journals or fields (e.g., Merchant 2017 ; Mills and Rahal 2021 ). These prior studies have focused primarily on bounded historical periods (e.g., the earlier decades of the field's evolution), questions about the field's political and policy engagement, or a single journal. Similarly, studies that have relied on bibliometric approaches to examine the pattern of demographic knowledge dissemination have captured only a short snapshot of time (e.g., 1991–1995, see van Dalen and Henkens 1999 ).
In this article, we complement prior work with a broad overview of the developing intellectual structure of the field over the past 70 years by examining what is published in the field's leading outlets that constitute the intellectual home base of many anglophone demographers, temporal changes in the field's core topics, and the field's engagement with other scientific communities over time. We rely on a large corpus of demography papers and a two-pronged, “bottom-up” approach from the sociology of science. We first use a combination of natural language processing and social network analysis tools that describe the topical contours of the field by letting clusters of papers emerge from the substance and contents of publications records without the need to prespecify the number of topic clusters. We then use bibliometric tools to capture demographers' scientific conversations with other disciplines and the influence of the knowledge produced by demography journals on the scientific community (e.g., Boyack 2004 ; van Dalen and Henkens 1999 ).
Our goal is to focus primarily on papers' content in order to (1) identify the primary topics of anglophone demography after the field first established its key intellectual outlets and gained prominence as an organized field, with special attention to how the field is organized into core and noncore areas; and (2) assess changes in the field's intellectual cohesion, the topical areas that have grown or shrunk, and the expansion of the boundaries of demography. We then consider demographers' conversations with other disciplines by focusing on (3) demographers' reliance on the allied disciplines with an analysis of citations in the reference lists of demography papers; (4) the influence of demography's knowledge on the broad scientific literature with an analysis of citations received by demography papers; and (5) time trends of where demographers working in the core areas place their publications outside of the leading demography outlets as one means to assess demographers' communication with their scientific peers and demography's cross-disciplinary and translational reach.
Our analyses generalize to publications in the three leading journals of demography that together span the last seven decades of anglophone demography. Our main corpus consists of all articles published in Demography , Population Studies , and Population and Development Review ( PDR ) between the year of a journal's first issue through the last issue of 2020, excluding comments, replies, and book reviews. While demography as a field emerged from interdisciplinary efforts in the first half of the twentieth century ( Merchant 2017 , 2021 ), our focus is on the period when the field established its key outlets of intellectual and scholarly communication. Population Studies is the oldest of the three, established in 1947 with funds from the Rockefeller Foundation. The Population Association of America established its flagship journal, Demography , in 1964, with a grant from the Ford Foundation. Population and Development Review was established in 1975 and became an outlet for demographic studies that did not fit the traditional mold. At the time of its founding by the Population Council, Population and Development Review devoted considerably less space to quantitative and formal analyses than did either of the other two journals ( Merchant 2015 :577). These three journals offer the longest, continuous coverage of the last 70 years of anglophone demography publications. They have been recognized as the triad defining the field of population research in terms of citations to and from other demography journals during the 1990s ( van Dalen and Henkens 1999 :247) and, as journals specifically concerned with demography, they have maintained the highest impact factors of all demography journals during the first two decades of the twenty-first century, according to Journal Citation Reports. 2
Most papers in our corpus were downloaded from JSTOR (5,767 publications) and updated for the most recent issues or supplemented with earlier paper records from the Web of Science (WoS) abstract and citation database, yielding a total of 6,252 papers published between 1947 and 2020.
To assess how demographers position their work with respect to other disciplines, we rely on out-citations (i.e., citations from papers published in the three journals considered) drawn from reference lists in the papers in our corpus. These lists provide an indication of the influence of other disciplines on demography. Citations also form the most visible source of recognition in science and can be used to gauge the influence of demography in the scientific community. To assess this influence, we use measures based on the crude citation counts to our corpus drawn from the WoS. Because journals are a chief venue of scholarly communication across disciplines, we also evaluate demographers' communication with their scientific peers by searching for demographers' papers published in outlets outside of the three leading journals. To conduct this search, we use Scopus instead of WoS because it allows for the automatic retrieval of authors' publications through a dedicated application programming interface (API) (rather than having to do it manually in WoS) that matches an author's name and affiliation to a unique author ID, reducing the ambiguity name problem. We limit this search to contemporary demographers who have published at least two papers on core demography topics 3 in our three leading anglophone demography journals over the last three decades. Of the 5,387 authors of papers in our paper corpus, 486 unique authors published at least two papers on core demography topics since 1990 and authored a total of 23,456 unique articles published across a multitude of outlets between January 1990 and November 2021. This period coincides with the exponential increase in digital journals, many of which are open access, a shift that may have contributed not only to growing communications of authors of demography papers with their scientific peers but also to the diffusion, application, and translation of demographic tools and analytic approaches.
Topical Structure of Demography
To identify topics in demography, we use text-network models ( Bail 2016 ; Moody and Light 2006 ) to build and then cluster a network of papers linked by similar terms. The first step is to preprocess the text content (abstract, title, keywords) of each article to identify substantively meaningful terms. This involves removing words that have little substantive meaning based on part of speech and taking advantage of a common English-language stop words list augmented with corpus-specific, noninformative terms. We then use a natural language processing tool that combines derivative terms to a common parent term (i.e., “marriages” → “marriage”) and automatically identifies noun groups (such as “birth order” or “demographic transition”), entities (“National Institutes of Health”), and proper names (“Gabon”). Each parent term is then weighted according to the “term-frequency, inverse-document frequency” scheme ( Spärck Jones 1972 ), which discounts terms that are common in a corpus. Each paper is thus reduced to a vector of weighted counts of terms. The similarity score for each pair of papers is then calculated as the cosine similarity of the two vectors. Two papers that use the same set of terms will have a cosine similarity score of 1, while those with no overlapping terms will have a score of 0.
We create a network from these similarity scores linking papers to each other using a minimum cosine similarity of .25. Because text networks tend to be very dense, it is common to use a “backbone” procedure to highlight the most consequential edges ( Bail 2016 ). We do this by selecting edges (the source and the target of a link between two papers) such that they are within the top-15 most heavily weighted links for one of the papers. The weight of the link between each paper is defined by the sum of the term frequency–inverse document frequency for the overlapping terms. We then cluster the network using the Louvain community detection algorithm ( Blondel et al. 2008 ), applied recursively to large clusters and implemented in the Pajek network visualization system. These clusters form the topics we study.
Labeling clusters is done through our reading of the most common heavily weighted terms (e.g., fertility/family/size/family size/preferences/desires → “Number of Children”; health/mortality/old/age → “Health and Aging”) and of the central papers in each cluster. We visually inspect each cluster for obvious subclusters and, when found, we force a second split. To avoid small idiosyncratic clusters, we discuss only those with at least a dozen papers.
To visualize the results, we construct two-dimensional maps of the topic space by applying a network layout routine ( Fruchterman and Reingold 1991 ) that places nodes (or papers) near each other if they share many neighbors. 4 Because large dense networks are difficult to see as traditional point-and-line diagrams, we overlay a contour map that reflects paper density in the topic space ( Light 2014 ; Moody 2004 ; Moody and Light 2006 , 2020 ). In general, the locations of topics with respect to one another reflect shared content. This map allows us to qualitatively augment the formal analysis by identifying sets of papers that form the topical core of a cluster so that topics that are similar to each other are close to each other in the layout space. However, because any two-dimensional visualization of an n -dimensional space inherently distorts the information, such linkages are generally approximate. Figure S1 in the online supplement walks through an example of this process (all figures and tables designated with an “S” are available in the online supplement).
Demographers' Conversations With Other Disciplines: Citations by and to Our Corpus
where R is the total number of matched references in our corpus, r d is the number of references to discipline d (demography, economics, sociology, all other disciplines, according to the WoS broad subject categories), and the summation is over all disciplines. 5
where i indexes papers in our corpus, r d , i refers to a paper's references to discipline d (economics or sociology), r dem,i refers to references to demography journals, R i refers to all references in a paper, and Year i × Journal i is an interaction term between year and journal ( Demography , Population Studies , or Population and Development Review ) to capture journal-specific time trends of a paper's reliance on economics or sociology references. The logistic transformation of the proportion bounds model predictions to stay within 0,1.
To assess the influence of the knowledge produced by papers in our corpus, we estimate a negative binomial model 6 of the count of WoS citations (in any journals indexed by WoS) to paper i , which can be written as
where Y i is the count of WoS citations to paper i and Y e a r i 2 is a quadratic function, which is needed to capture the typical pattern of a citation count's increase and then decline after a paper's publication. 7
Demographers' Papers in Outlets Outside of Demography
After identifying a list of contemporary demographers who published at least two papers since 1990 on core demography topics that have emerged from the text-network models (i.e., the topics below the diagonal in Figure 1 ), we use the Scopus Author Search API to retrieve each author's unique Scopus ID by matching first name, last name, and author's affiliation. We then use the Scopus Author Retrieval API to obtain the journal publications of each of these authors in the scientific literature universe indexed by Scopus and provide counts of publications by journal and decade. Names in the database were manually harmonized for homonyms, errors in the order of last and first names (frequently found with Chinese transliterated names), and name changes. Publications for ambiguous names were checked using Google Scholar, JSTOR, or an author's online CV to ensure author matches.
The contour map of demography's intellectual landscape as modeled from our text-network analyses is shown in Figure 1 . Underlying this map is a network consisting of 6,252 papers, connected by edges indicating high levels of topic co-term similarity. The map displays 35 identified topic clusters, each with 12 or more papers, that emerged from the data. The topology of this topic network resembles a ring structure with multiple topic-centered “peaks”—indicated by darker shading and generated by the number of associated papers—joined at their periphery to neighboring topics.
Because the network visualization layout we use positions papers that talk about similar things close to each other, physical proximity in this layout space suggests substantive interrelations not only among papers but also among topics. Cardinal or ordinal orientations do not matter (i.e., the map could be rotated 45 degrees, 180 degrees, or any other amount and the meanings would not change), although we use directional descriptions based on the figure's layout to draw attention to specific areas.
Face validity is good, with topics familiar to demographers. The highest (most densely populated) peak consists of work related to the “Demographic Transition.” It is surrounded by the smaller clusters “Child Mortality,” “Political Demography,” and “Population Policy.” To the west of “Population Policy” is “China,” which mostly consists of papers on China's fertility decline, and next to “China” is “Family Planning.” This latter cluster is close to work on the other proximate determinants of fertility and biometric models of fertility (e.g., “Abortion,” “Birth Intervals,” “Fecundability,” and “Birth Spacing”; see Menken 1975 ). In the south of the map, we see “Life Expectancy and Longevity,” which is close to “Mortality Transition” and “Demographic Techniques”; the latter cluster consists of work on the collection and evaluation of demographic data and mortality estimation techniques. “Demographic Techniques” is close to work on the formal, theoretical aspects of the dynamics of population change labeled “Mathematical Demography.”
To the east of “Demographic Techniques” is “Population Growth,” which consists of applications of the demographic tool kit to population growth models and the consequences of population change (economic development and climate change). “Health and Aging”—consisting of work on health differentials, disability, risk factors of health and survival, and early precursors of adult health and disease—is just north of “Population Growth” and east of “Demographic Transition.” Moving to the far east of the map across a valley, we find work on “Migration” (international and internal) next to “Immigration” and “Racial Segregation,” the natural proximity of the latter two clusters owing to work on residential segregation and immigration. In the northeast, we find “Child Well-being” (consisting of work on the effects of parental characteristics, behaviors, and inputs on child outcomes), which links to work on, moving counterclockwise, “Households and Living Arrangements,” “Divorce,” “Marriage and Cohabitation,” “Marriage Patterns,” “Number of Children,” “Income and Poverty,” “Education,” and “Women's Labor Force Participation.” In the far north, linking to “Marriage and Cohabitation,” is the small but distinct topic of “Same-Sex Families.” 8
It is important to note that the relative position of clusters in this map is determined by the entire population of papers in our corpus spanning multiple decades. This broad time frame gives us a good overview of the field over the past seven decades, allowing us to smooth over one-time phenomena such as special issues in journals. Had we disaggregated our text-network analyses by decade, or were we, in the future, to add the network of papers published in later years, we would have/will observe(d) shifts in the relative position of clusters and the emergence or disappearance of clusters because nodes in the graph move as links between papers change.
After inspection of the central papers in each cluster, we manually traced a roughly diagonal line from the northwest to the southeast of the topical landscape portrayed in Figure 1 . Below this diagonal is mostly work on the technical and formal aspects of demography, the collection and evaluation of demographic data, and the determinants and consequences of demographic change. These topics are regarded by insiders as the “core” of demography ( Lee 2001 ; Morgan and Lynch 2001 ; Preston 1993 ) and emphasize macro-level analyses and dynamic models of vital rates and population structures. Above the diagonal are largely topics that can be classified as social and behavioral demography, including work that emphasizes the compositional variables of population (race and ethnicity, occupation, marital status, and living arrangements), the micro-level determinants of demographic behaviors, the relationship between demography and inequality, parental inputs and child outcomes, family studies, and migration; however, work on population-level analyses and dynamic interactions of population processes, such as migration, fertility, and the evolution of household structure, which might be considered “core” demography, is also found in a few clusters above the diagonal (e.g., “Number of Children,” “Households and Living Arrangements”). Topics above the diagonal rely on the perspectives of demography's allied disciplines, primarily sociology and economics. “HIV/AIDS” and “Health and Aging,” found just above the diagonal, are topics that primarily focus on health and illness and often rely on epidemiological perspectives.
Topical coverage is diverse in anglophone demography, with our largest topic—“Demographic Transition”—containing only 9% of papers. The next largest clusters are “Demographic Techniques,” “Population Growth,” “Migration,” “Number of Children,” “Life Expectancy and Longevity,” “Family Planning,” and “Racial Segregation,” each accounting for between 5% and 6% of all publications, while all remaining topics represent less than 5% each. The full distribution of topics is shown in Figure S2 .
The Ebbs and Flows of Topics in Demography
The evolution of demography as seen in the pages of the three leading anglophone journals is characterized by external drivers and demand factors—for example, early U.S. Cold War policy-making needs and the mission of private funders to advance research on family planning, followed by public funding agencies seeking to address emerging social problems through research and intervention ( Demeny 1988 ; Merchant 2017 ; Merchant and Alexander 2022 ; Morgan and Lynch 2001 ; Preston 1993 )—but also by the application of the demographic approach to new topics, as well as by demography's conversations with the allied disciplines offering new opportunities for advancement. These factors have enabled an expansion in the topical breadth of demography, introduced disciplinary heterogeneity in the training of population researchers, and led to a growth in the volume of articles published in the leading anglophone demography journals. The volume of articles published each year in these journals has increased, with more papers published in recent decades, a trend that is dominated by Demography . While the number of articles in Demography in the 2010s is more than double that in the 1980s, the volume of articles in the other two journals has remained more or less stable between 1980 and 2020. As a result, in the 2010s, Demography articles account for 60% of the articles in our corpus, up from 40% in the 1980s. A visualization of the growing volume of articles scattered across the landscape is shown in Figure S3 .
Figure 2 illustrates the percentage of papers published in each decade by topic cluster. 9 If we consider dominant topics those that represent more than 5% of all papers in a given decade, we see that in earlier decades the dominant topics were “Demographic Techniques,” “Demographic Transition,” “Population Growth,” and “Family Planning,” 10 while in the last two decades the dominant topics were “Child Well-being,” “Health and Aging,” “Life Expectancy and Longevity,” “Migration,” and “Racial Segregation.” “Demographic Transition” and “Number of Children” have maintained dominant positions throughout the decades, possibly owing to the persistent prominence of the former topic with recent and current work on late-transitional societies and, for the latter topic, with research concerned with small family size replacing research concerned with large family size.
When we group each journal's publications into demography core topics (clusters below the diagonal line in Figure 1 ) and compare this group with social and behavioral demography topics (clusters above the diagonal line), we find that the trend has shifted in favor of the latter group. In the 1960s, the ratio of papers on core demography topics to papers on social and behavioral demography across all three journals was about 2:1, while in the 2010s it was close to 1:2. This shift is largely driven by an expansion in the count of papers on social and behavioral demography topics rather than by a reduction in the number of papers on core demography topics, suggesting complementarity rather than competition. Although the three journals are trending in the same direction, Demography dominates this trend with a significant expansion in the count of published papers on social and behavioral demography, especially after 2010. (Counts and ratios by decade are shown graphically in Figure S4 .)
Figure 3 depicts the temporal distribution of each topic. We see that the distribution of publications on several core demography topics is relatively even across decades, with the exception of papers on “Fecundability,” “Birth Intervals,” “Abortion,” “Birth Spacing,” “Family Planning,” “Demographic Techniques,” and “Mathematical Demography,” which appear more often in the 1960s, 1970s, and 1980s, and papers on “Life Expectancy and Longevity,” which appear more often in the 2000s and 2010s. Papers on this burgeoning topic rely heavily on the methods and materials of demography. Among the social and behavioral demography topics, most papers on “Same-Sex Families,” “Child Well-being,” “Racial Segregation,” “Education,” “Health and Aging,” and “HIV/AIDS” were published since 2000, while the majority of papers on classic social demography topics (“Occupational Mobility,” “Status of Women”) were published in earlier decades.
In sum, as represented in the three leading journals of anglophone demography, scientific production in demography during the 1960s, 1970s, and 1980s was concentrated on the technical aspects of demography, the demographic transition, the mortality transition, population growth, biometric models of fertility, family planning, and the other proximate determinants of fertility. In the 1990s, the topical structure of demography became more expansive, but this trend is most visible in the 2000s and 2010s. Papers on the core topics of demography that are best addressed by macro-level analyses and utilize demography's tool kit are still getting published, with the core methodology of demography being applied to increasingly prominent topics such as “Life Expectancy and Longevity,” which benefit from its adoption. However, over the 2000s and 2010s, these journals, with Demography in the lead, have increasingly covered topics that integrate demographic approaches with theories, models, and measurement of individual behavior grounded in the allied disciplines and made possible by the collection of increasingly complex, often longitudinal data that integrate biosocial perspectives. During this period, these journals have also featured papers on “Health and Aging” and “HIV/AIDS” that bridge the gap between demography's emphasis on mortality measurement and distribution and epidemiology's emphasis on risk factors and the disease process leading to death.
Citation Patterns in Reference Lists of Papers in Our Corpus
The changes in the topical composition of the paper corpus we examined suggest demography's expanding boundaries and spheres of interest. Next, we analyze the lists of references in our corpus to assess the intellectual influence of the allied disciplines on demography.
Scholars publishing in the leading demography journals have been expansive in their citation practices, with a strong linear trend in the number of papers referenced (from an average of about 20 per paper in the 1950s to nearly 60 now), a trend also observed across other disciplines ( Moody et al. 2022 ; Petersen et al. 2019 ). As might be expected given the overall increase in citations over time, citations have also become more heterogeneous with respect to disciplines, with the heterogeneity score introduced in Eq. (1) increasing linearly from just over .7 in 1950 to about .9 in 2020 (a graphic representation of this trend is shown in Figure S5 ).
According to the subject areas indexed by WoS, the top three disciplinary areas cited by papers in Demography , Population Studies , and Population and Development Review are Demography, Economics, and Sociology, followed by Public/Environmental/Occupational Health or Public Health, 11 Family Studies (includes journals focused on research on families, children, and adolescents), and Medicine 12 (for a full ranking of citations by subject area see Table S1 ). The rank-order of cited disciplinary journals has changed over time. With demography journals always at the top, sociology and economics journals have traded places for the second and third spots. Previously highly cited disciplines have fallen in ranking (e.g., Probability and Statistics), and by 2000, citations to Public Health and Family Studies journals have solidified their positions in fourth and fifth place, respectively (see Figure S6 ).
With an increase in the numbers of papers and citations, overall disciplinary heterogeneity would increase even if individual papers cite exclusively one discipline. To address within-paper disciplinary heterogeneity, Figure 4 plots the distribution of papers pooled across all years according to the proportional balance score introduced in Eq. (2) . On the left are papers where economics cites exceed sociology cites, and on the right are papers where sociology cites exceed economics cites. There is evidence for disciplinary partiality in papers that cite sociology but also in papers that cite economics; that is, the proportion of papers with all or most citations to sociology (i.e., those with a proportional balance score between 1 and .5) or to economics (proportional balance score between ‒1 and ‒.5) is higher than the proportion of papers with balanced citations (those with a proportional balance score of 0). However, the proportion of papers that cite economics but not sociology (proportional balance score of ‒1) is much smaller than the proportion of papers that cite sociology but not economics (proportional balance score of 1). After all, economists who publish in demography journals are entering a field dominated by scholars trained in sociology, because the majority of demography programs rely heavily on sociology coursework or are embedded in sociology departments, and so they forgo their own disciplinary norm of citing exclusively within economics ( Fourcade et al. 2015 ; Moody and Light 2006 ). It could also be that sociologists who engage topics that are of direct relevance to research in applied economics cite both disciplines or are members of multidisciplinary teams. These are hypotheses, however, that we cannot verify because of the absence of information on authors' discipline in our data set.
We expect papers on the core topics of demography to cite more heavily within the discipline, while we expect papers on social and behavioral demography topics to cite across the disciplines. Figure 5 plots the proportion of references to demography journals for each paper averaged over all papers within a topic cluster. Topics lie on a spectrum ranging from .1 (or 10%) to more than .6 (or 60%) of the references in a topic's constituent papers being to demography journals. Topics that rely most heavily on demography references are technical and substantive core topics such as “Mathematical Demography,” “Demographic Techniques,” the biometric aspects of fertility, “Demographic Transition,” and classic topics in social demography such as “Occupational Mobility” and “Status of Women.” Topics that rely the least on demography references are those that intersect other disciplines, such as “Health and Aging,” “HIV/AIDS,” “Racial Segregation,” “Child Well-being,” “Education,” “Income and Poverty,” and “Women's Labor Force.” Other core demography topics—such as “Life Expectancy and Longevity,” “Population Growth,” and “Mortality Transition”—are close to the middle of the range.
The regression models of citation patterns specified in Eq. (3) help disentangle subfield effects from growth in citations over time and journal proclivity. Since these models control for a paper's total number of references and proportionate references to demography papers, they are mainly set to contrast a paper's reliance on economics or on sociology references compared with demography references. Figure 6 illustrates the proportions predicted from these models of a paper's references to economics and sociology by year and journal (accompanying model coefficients are presented in Table S2 ). This figure visualizes differences in the temporal citation trend across the three journals (with other variables at their means/modes). Panel a shows that papers published in Demography have smaller proportions of citations to economics early on, relative to the other two journals, but this proportion and the rate at which it is changing grow over time, surpassing the other two journals. Panel b shows the drop over time of the proportion of references to sociology and, again, this trend is most marked for Demography . Additional results of these models (see Figure S7 ) indicate that papers that rely most heavily on the economics literature are primarily those on “Income and Poverty” and “Women's Labor Force,” followed by “Migration,” “Population Growth,” and “Immigration.” Papers that rely more heavily on the sociology literature are those focused on “China,” “Divorce,” “Marriage and Cohabitation,” “Occupational Mobility,” and “Racial Segregation,” followed by “Demographic Transition,” “Number of Children,” “Households and Living Arrangements,” and “Sex Ratios.”
Citations to Papers in Our Corpus: The Visibility and Influence of Demography in the Scientific Literature
With demography's expanding scope and growing conversations with and reliance on allied disciplines, the question then becomes one of demography's influence and visibility in the broader scientific literature. Which topics are most visible in the scientific literature and are these topics the ones that rely most heavily on allied disciplines? As with the foregoing analyses of reference lists cited by papers in the top three anglophone journals of demography, the volume of citations to papers in these journals depends on date of publication and journal visibility, as well as topical area. The negative binomial regression model of the count of WoS citations in Eq. (4) predicts the number of times a paper in our corpus is cited as a function of topic area, year of publication, and journal and accounts for citation aging. Model coefficients are presented in Table S3 . Figure 7 presents a mosaic plot of the predicted citation counts by topic. Predicted counts range from 20 or fewer citations (dark blue) to 60 or more citations (dark red).
The papers that receive the most citations are those on “Child Well-being,” “Child Mortality,” “Education,” and “Marriage and Cohabitation,” followed by “HIV/AIDS,” “Divorce,” “Racial Segregation,” “Sex Ratio,” “Status of Women,” “Life Expectancy and Longevity,” and “Health and Aging.” Many papers on these topics require the contribution of conceptual frameworks, theories, and models of sociology, economics, and epidemiology. Some (e.g., papers on “Life Expectancy and Longevity” and “Child Mortality”) require the application of unique demographic tools and approaches, highlighting the demographic core's translational focus and the value of applications of demography to population and societal problems that are of converging interest to multiple disciplines. Despite the high volume of papers on “Population Growth” and “Demographic Techniques” appearing in the top three anglophone demography journals, papers on these topics are among the least popular in terms of citations received, as they cover concerns specific to the field of demography.
Where Do Demographers Working in the Core Areas Publish Outside of Demography Journals?
To indicate cross-disciplinary outreach and diffusion of papers on core demography topics, we next consider the outlets outside of the three leading anglophone journals where contemporary core demographers (i.e., authors of at least two publications on core demography topics lying below the diagonal in Figure 1 ) have published their work over the past three decades. Figure 8 shows the top 20 journal outlets. It is clear from the figure that the earlier dominance of Demography , Population Studies , and Population and Development Review has diminished over time, with a shift to public health and epidemiology journals, the open-access Demographic Research (launched in 1999), open-access multidisciplinary science journals, and medical journals. 13 Demographic Research and Social Science and Medicine rank first and third, respectively, in the 2000s. The open-access multidisciplinary journal Public Library of Science ( PLoS ) One , established in 2006, is the top outlet for work by demographers working on core demography topics in the 2010s. At the dawn of the 2020s, open-access multidisciplinary science journals ( PLoS One and PNAS ) and multidisciplinary medical journals (such as BMJ Open and The Lancet ) represent four of the top six outlets in which research by demographers working on core demography topics is found.
The dominance of outlets other than the three leading anglophone demography journals is even clearer for papers published between 1990 and 2021 with “Life Expectancy,” “Longevity,” or “Life Span” in the article title, as well as for papers published in 2020–2021 with “COVID-19” in the title, by our group of contemporary core demographers. These are topics that rely heavily on the application of demographic tools, including demography's best-known analytic tool—the life table—and that appeal to a large scientific audience, highlighting the demographic tool kit's translational reach in the broader scientific literature (for a graphic representation of these findings, see Figure S8 ).
- Discussion and Conclusions
Our results highlight key features of the field of demography as reflected by work published in demography's three leading anglophone journals —Demography , Population Studies , and Population and Development Review . From a field that first coalesced around a relatively narrow scope, anglophone demography has become a broad, diverse field of research, with articles published in its three leading journals touching on an expansive range of topics. Our findings suggest that, over the last 70 years, the intellectual landscape of anglophone demography has broadened and its subjects have increasingly diversified.
Consistent with experts' narratives, demography has expanded from an emphasis on core demographic topics and methods and aggregate-level demographic analyses of the linkages between vital rates and population structures to a broad focus on social, behavioral, and health demography topics that blend demographic thinking with ideas and theories about individual behaviors, health, and disease grounded in allied disciplines.
In contrast to earlier expert narratives highlighting the decline of demography's core, our results suggest a research program characterized by the application of a methodological core to new topics that benefit from its adoption, and a dynamic exchange with allied disciplines that has benefited from the availability of new empirical evidence grounded in a variety of new data sources. Much of the field's growth in publications (with more issues per journal and more papers per issue) has been nurtured by social, behavioral, and health demography topics, though not at the expense of core demography topics. Although all three journals are trending in the same direction, Demography , the flagship journal of the Population Association of America and an institution representing multiple disciplines, dominates this trend, with a significant expansion of published work on social and behavioral demography, especially during the 2010–2020 period. This expansion is healthy, suggesting that the field has grown its purview while maintaining its core.
Regarding demography's conversation with other disciplines, as shown by the reference lists of the papers in our corpus, topics in social and behavioral demography rely more strongly on demography's closely connected disciplines, primarily sociology and economics, and less on demography references. As a proportion of citations, references to economics journals are rising and references to sociology journals are slowly declining, even after controlling for the general growth in citations, with Demography leading this trend.
Topics that engage with ideas central to the allied disciplines also have higher visibility in the broader scientific literature. But it is also clear that core demography topics that require the application of unique demographic tools and models, such as “Life Expectancy and Longevity” and “Child Mortality,” have good visibility, as shown by the count of citations in the broader scientific literature to papers on these topics.
We also examined where demographers working in the core areas place their publications outside of demography journals, showing that open-access multidisciplinary science, public health, and medical journals have risen to become competing venues where work by demographers specializing in demography's core topics is published. Although this shift may be due to a variety of factors—for example, demography journals' editor biases and authors' preferences to publish in high-impact journals with unrestricted circulation, demands on reviewers' speed, and shorter article length—the fact that these high-impact, multidisciplinary journals are among the main outlets where research by scholars who contribute to the stability of core demography topics is published is a sign of the translational reach of the demographic tool kit and approach.
Our study yields a picture of a topical network of papers linked by ideas, not a social network of authors linked by interactions. What our study did not do is analyze authors' collaborations across disciplines, characterize paper authorship by gender, track authors' transitions across topics, or incorporate funding sources in our analyses. Future analyses by the current or other authors will allow a deeper engagement with the questions of demography's integration with other disciplines, the gender composition of authorship, and the role of key authors, key approaches and tools, and funding agencies in driving the structure of the network and the generation of new topics.
New directions for the field may pose more challenges. Whereas the number of articles published in the three leading journals of anglophone demography expanded significantly over the decades of our analysis, leaving room for the growth in new topics without sacrificing the core, it is uncertain whether such expansion will continue in these journals. For example, our analysis of the publication outlets of work by core demographers on current time-sensitive topics—such as the measurement and demographic drivers and impacts of COVID-19 infection and mortality that command urgency for knowledge and intervention—suggests that the leading demography journals are not attracting much of the growth of applications of core demography approaches on these topics. This may be more of an issue of publication timing and demand for reviewers' speed than of openness to new directions, as these journals are slow to produce certified knowledge. With new capacities for digital publication, there may be room for the leading demography journals to create more publication space to accommodate growth in new topics of converging multidisciplinary interest. Digital formats might also allow room for a greater diversity of article types, which could spur more growth in new areas. The field can learn from its past in ways that can prepare it better for its future.
- Acknowledgments
The authors thank Sara Curran, Noreen Goldman, Daniel Goodkind, Mark Hayward, Jennifer Johnson-Hanks, Grant Miller, Angela O'Rand, Samuel Preston, Herbert Smith, and four anonymous reviewers for their constructive comments on early versions of this article. Support for this research was provided by NICHD grant P2CHD065563.
Lakatos’s principles of a progressive research program were applied by Lesthaeghe ( 1997 ) to explain the evolution of demographic theories of fertility, by O’Rand ( 1992 ) to explain the early development and diffusion of game theory in the quantitative social sciences, and by Weintraub ( 1985 ) to highlight the role of general equilibrium analysis in the growth of economic knowledge.
The choice of these three journals notably excludes two anglophone journals specifically concerned with demography. Population Index , established in 1937 as a reference tool, also handled a small number of papers, mainly on demographic methods, but it ceased publication in 1999. Demographic Research is a monthly open-access online journal published by the Max Planck Institute for Demographic Research that started publication only in 1999. The corpus of papers we analyze is also necessarily smaller than the increasingly diverse universe of demography publications, and it represents a declining fraction of all articles in demography journals according to the Web of Science (WoS) subject category. This decline—from 100% in 1950, when Population Studies was the only demography journal indexed by WoS, to about 70% in the mid-1960s, 25% in the 1970–1990 period, and 12% in the 2010s—has coincided with a transformation in the format of journals into digital versions and a growth in the number of titles, many of which are on specialized population topics, and is consistent with overall changes in journal publishing since the late 1960s but especially since the 1990s (Tenopir and King 2014 ).
Core demography topics refer to topics on the technical and formal aspects of demography, the collection and evaluation of demographic data, and the determinants and consequences of changes in population size and structures. These are some of the topics uncovered by our text-network analyses described in the following Methods and Results sections.
Substantively, the Fruchterman–Rheingold approach on a weighted network is very similar to a metric multidimensional scaling.
This excludes citations to books or journals not indexed by WoS.
We have tested zero-inflated versions as well with little substantive difference.
This approach addresses concerns about citations “aging” and the pros and cons of short (e.g., 2–3 years) and long citation windows (Aksnes et al. 2019 ; Wang 2013 ).
Co-word similarity mapping does not come without challenges and limitations. For example, the clusters labeled “Africa” and “HIV/AIDS” result from two splits, where the first split included work on fertility in Africa, HIV and fertility, and the behavioral determinants of HIV transmission, and the second split resulted in two substantively distinct clusters on fertility and its behavioral determinants in Africa (labeled “Africa”) and the behavioral determinants of HIV/AIDS transmission (labeled “HIV/AIDS”). In terms of limitations, the location of the “Sex Ratio” cluster in the same region as the “Africa” cluster is due to terms like “sex” and “sexual behavior” being shared by these clusters, which draws the two clusters close to each other.
The 2010 decade in Figures 2 , 3 , 7 , S3, S4, and S6 includes one extra year, 2010 to 2020 inclusive.
The dominant position of papers on family planning, population growth, and number of children in the 1960s and 1970s reflects the field’s preoccupation with overpopulation and its close ties to U.S. Cold War policy needs of population control (Demeny 1988 ), including a 1968 special issue of Demography devoted to “Progress and Problems of Fertility Control Around the World” (Merchant 2015 ).
Citations include American Journal of Epidemiology , American Journal of Public Health , Social Science and Medicine , BMC Public Health , etc.
Citations include The Lancet , BMJ , JAMA , New England Journal of Medicine , Journal of the American Medical Association , Annals of Internal Medicine , etc.
Demography went open access in 2020.
Supplementary data
Data & figures.

Demography intellectual landscape from leading anglophone journals, 1947–2020. Contour represents 6,252 papers published in Demography , Population and Development Review , and Population Studies between 1947 and 2020. Below the diagonal line lies work mostly on technical and formal aspects of demography, including the collection and evaluation of demographic data, and the determinants and consequences of demographic change; above the diagonal is work mostly on topics that can be classified as social and behavioral demography.

Percentage of papers published in each decade by topic cluster. Data points (circles) are percentages by decade; to visualize trends, lines are fitted to the data points with locally weighted smoothing (LOESS). Papers published before 1960 are excluded because they are very sparse.

Proportion of papers by topic and decade. Counts of papers by topic are listed above each column.

Distribution of papers by the proportional balance score. The absolute value of the score is calculated as (proportion in the majority) / (proportion sociology + proportion economics). The sign is negative if economics is in the majority, and positive if sociology is in the majority.

Mean proportion of references to demography journals by topic cluster. Circle size is proportional to the number of publications in the cluster.

Predicted proportions of paper references to economics and sociology by year and journal, estimated from logit models of citation patterns, 1947–2020. Shading represents 95% confidence intervals. PDR = Population and Development Review .

Predicted counts of citations to papers in Demography , Population and Development Review , and Population Studies by topic, estimated from negative binominal models of citation counts. Bar width is proportional to the number of papers in the topic cluster.

Top 20 journals and journal subject categories in which contemporary core demographers published their work, by decade. In this figure, the 2020 decade includes 2020 and 2021. N for 1990–1999 = 4,040; N for 2000–2009 = 6,792; N for 2010–2019 = 10,471; N for 2020–2021 = 2,153.

- Previous Issue
- Previous Article
- Next Article
Advertisement
Supplements
Citing articles via, email alerts, related articles, related topics, related book chapters, affiliations.
- About Demography
- Editorial Board
- For Authors
- Rights and Permissions Inquiry
- Online ISSN 1533-7790
- Print ISSN 0070-3370
- Copyright © 2024
- Duke University Press
- 905 W. Main St. Ste. 18-B
- Durham, NC 27701
- (888) 651-0122
- International
- +1 (919) 688-5134
- Information For
- Advertisers
- Book Authors
- Booksellers/Media
- Journal Authors/Editors
- Journal Subscribers
- Prospective Journals
- Licensing and Subsidiary Rights
- View Open Positions
- email Join our Mailing List
- catalog Current Catalog
- Accessibility
- Get Adobe Reader
This Feature Is Available To Subscribers Only
Sign In or Create an Account
- Advertisers
- Agents and Vendors
- Book Authors and Editors
- Booksellers / Media / Review Copies
- Librarians and Consortia
- Journal Authors and Editors
- Licensing and Subsidiary Rights
- Mathematics Authors and Editors
- Prospective Journals
- Scholarly Publishing Collective
- Explore Subjects
- Authors and Editors
- Society Members and Officers
- Prospective Societies
- Open Access
- Job Opportunities
- Conferences

Demography is a Subscribe to Open journal, starting with the 2024 volume year. Read more about its funding model.
- For Authors
For information on how to submit an article, visit submission guidelines .
Academic Editor: Sara R. Curran
Since its founding in 1964, the population research journal Demography has mirrored the vitality, diversity, high intellectual standard, and wide impact of the field on which it reports. Demography presents the highest-quality original research of scholars in a broad range of disciplines that includes anthropology, biology, economics, geography, history, psychology, public health, sociology, and statistics. The journal encompasses a wide variety of methodological approaches to population research. Its geographic focus is global, with articles addressing demographic matters from around the planet. Its temporal scope is broad, as represented by research that explores demographic phenomena from past to present and reaching toward the future. Demography is the flagship journal of the Population Association of America. The journal will be a Subscribe to Open journal, starting with the 2024 volume. Subscription fees will support publishing costs, and the current content for that year will be made fully OA if the funding threshold is met. The 2021, 2022, 2023 volumes that were published open access will remain open. Subscribers have perpetual access to the 2024 volume and term access to the journal's entire backlist (1964-2020). Articles with corresponding authors who are affiliated with S2O-subscribing institutions are guaranteed to be open access in perpetuity.

Read Online
Subscribers and institutions with electronic access can read the journal online.
- Buy an Issue
- Related Sites
- Abstractors & Indexers
- Advertising
- Online Access
- Institutional Pricing
- Additional Information

Demography 61:2 (61:2)

Demography 61:1 (61:1)

Demography 60:6 (60:6)

Demography 60:5 (60:5)

Demography 60:4 (60:4)

Demography 60:3 (60:3)

Demography 60:2 (60:2)

Demography 60:1 (60:1)

Demography 59:6 (59:6)

Demography 59:5 (59:5)

Demography 59:4 (59:4)

Demography 59:3 (59:3)

Demography 59:2 (59:2)

Demography 59:1 (59:1)

Demography 58:6 (58:6)

Demography 58:5 (58:5)

Demography 58:4 (58:4)

Demography 58:2 (58:2)

Demography 58:1 (58:1)
More advertising information can be found on our Information for Advertisers page . To reserve an ad or to submit artwork, email [email protected] . No insertion is required. Please specify in which Duke University Press journal your ad should appear.
- Also Viewed
- Also Purchased
Designs for the Pluriverse

Police and the Empire City

TSQ: Transgender Studies Quarterly

Necropolitics

The Affect Theory Reader

On Decoloniality

Intersectionality as Critical Social Theory

Cruel Optimism

The Right to Maim

A peer-reviewed, open-access journal of population sciences
Recent articles.
10 April 2024 | research article
The influence of parental cancer on the mental health of children and young adults: Evidence from Norwegian register data on healthcare consultations
Øystein Kravdal , Jonathan Wörn , Rannveig Hart , Bjørn-Atle Reme
Volume: 50 Article ID: 27 Pages: 763–796
09 April 2024 | research article
The importance of education for understanding variability of dementia onset in the United States
Hyungmin Cha , Mateo Farina , Chi-Tsun Chiu , Mark D. Hayward
Volume: 50 Article ID: 26 Pages: 733–762
05 April 2024 | research article
The importance of correcting for health-related survey non-response when estimating health expectancies: Evidence from The HUNT Study
Fred Schroyen
Volume: 50 Article ID: 25 Pages: 667–732
More Articles
04 April 2024 | formal relationship
How lifespan and life years lost equate to unity
Annette Baudisch , Jose Manuel Aburto
Volume: 50 Article ID: 24 Pages: 643–666
03 April 2024 | descriptive finding
Age-heterogamous partnerships: Prevalence and partner differences by marital status and gender composition
Tony Silva , Christine Percheski
Volume: 50 Article ID: 23 Pages: 625–642
28 March 2024 | research article
Subnational contribution to life expectancy and life span variation changes: Evidence from the United States
Wen Su , Alyson van Raalte , Jose Manuel Aburto , Vladimir Canudas-Romo
Volume: 50 Article ID: 22 Pages: 583–624
27 March 2024 | research article
Religion and contraceptive use in Kazakhstan: A study of mediating mechanisms
Volume: 50 Article ID: 21 Pages: 547–582
26 March 2024 | research article
Differences in mortality before retirement: The role of living arrangements and marital status in Denmark
Serena Vigezzi , Cosmo Strozza
Volume: 50 Article ID: 20 Pages: 515–546
22 March 2024 | descriptive finding
Housework time and task segregation: Revisiting gender inequality among parents in 15 European countries
Joan García Román , Ariane Ophir
Volume: 50 Article ID: 19 Pages: 503–514
19 March 2024 | research article
Mortality inequalities at retirement age between migrants and non-migrants in Denmark and Sweden
Julia Callaway , Cosmo Strozza , Sven Drefahl , Eleonora Mussino , Ilya Kashnitsky
Volume: 50 Article ID: 18 Pages: 473–502
Fewer Articles
Editorial on p-values
23 october 2022.
In the last few years, the American Statistical Association has published position statements on the correct use and interpretation of p-values and on avoiding possible traps in applied statistical work. As much of empirical research in demography relies on statistical methods, in order to meet the challenge, Demographic Research has now also issued editorial guidance on our expectations regarding statistical rigour and replicability in empirical work. Please find the full editorial here: demographic-research.org/…/vol41/32
Recent Response Letters
02 February 2024
Do Ultra-Orthodox Jews Exhibit Natural Fertility?
25 January 2024
Fertility of Ultra-Orthodox Jews in USA
Meet our Authors
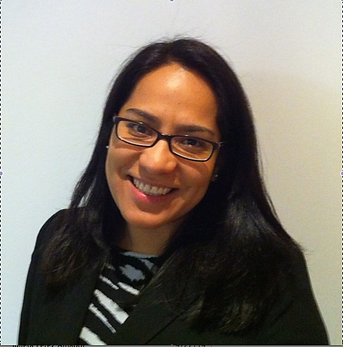
Julieta Pérez Amador : Julieta Perez-Amador specializes in family demography and demography of inequality. She is currently Assistant Professor at the Center for Demographic, Urban and Environmental Studies at El Colegio de Mexico. Previously she was a Demography Trainee in the Center for Demography and Ecology at the University of Wisconsin–Madison, where she earned her PhD in Sociology. She also worked at the National Statistics Office of Mexico (INEGI) and at the Luxembourg Income Study (LIS). Her research interests focus on nuptiality, living arrangements, and the transition to adulthood; she is especially interested in the relationship between sociodemographic family behaviors and inequality and in the intergenerational transmission of demographic behavior. Author Page
Recent Article:
Continuity and change of cohabitation in Mexico By Julieta Pérez Amador
Inside the Cover
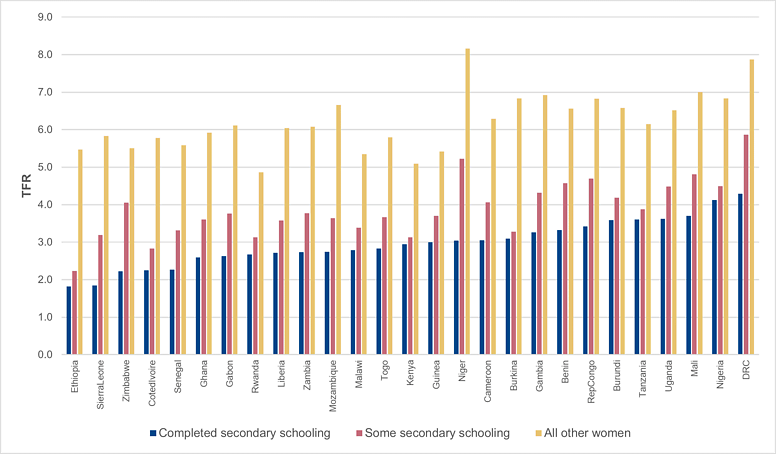
TFR for women with completed secondary education, some secondary education, and the rest of the population (women aged 20–49), by country, 2010–2019, DHS, in 27 countries in West, Central, and East Africa Full View
From Article:
Fertility among better-off women in sub-Saharan Africa: Nearing late transition levels across the region By Jamaica Corker, Clémentine Rossier, Lonkila Moussa Zan
News from the web
Conférence inaugurale AFÉPOP
Population Studies
A modal age at death approach to forecasting adult mortality
Journal of Population Economics
The minimum wage and cross-community crime disparities
Global household trends: converging sizes, divergent structures
Population Research and Policy Review
Between Marketization and Demarketization: Reconfiguration of the Migration Industry in the Agricultural Sector in Israel
European Journal of Population
A Precarious Path to Partnership? The Moderating Effects of Labour Market Regulations on the Relationship Between Unstable Employment and Union Formation in Europe
Max Planck Institute for Demographic Research
Do Food and Drink Preferences Influence Migration Flows?
Population & Environment
Correction to: Spatio-temporal patterns of pre-eclampsia and eclampsia in relation to drinking water salinity at the district level in Bangladesh from 2016 to 2018

An official website of the United States government
The .gov means it’s official. Federal government websites often end in .gov or .mil. Before sharing sensitive information, make sure you’re on a federal government site.
The site is secure. The https:// ensures that you are connecting to the official website and that any information you provide is encrypted and transmitted securely.
- Publications
- Account settings
Preview improvements coming to the PMC website in October 2024. Learn More or Try it out now .
- Advanced Search
- Journal List
- HHS Author Manuscripts
- PMC10235209

An Ethics and Social Justice Approach to Collecting and Using Demographic Data for Psychological Researchers
Christine c. call.
1 Department of Psychiatry, University of Pittsburgh
Kristen L. Eckstrand
Steven w. kasparek.
2 Department of Psychology, Harvard University
Cassandra L. Boness
3 Center on Alcohol, Substance use, And Addictions, University of New Mexico
Lorraine Blatt
4 Department of Psychology, University of Pittsburgh
Nabila Jamal-Orozco
Derek m. novacek.
5 Department of Psychiatry and Biobehavioral Sciences, UCLA and Desert Pacific Mental Illness Research, Education, and Clinical Center, VA Greater Los Angeles Healthcare System
6 Department of Psychological Sciences, Purdue University
The collection and use of demographic data in psychological sciences has the potential to aid in transforming inequities brought about by unjust social conditions towards equity. However, many current methods surrounding demographic data do not achieve this goal. Some methods function to reduce, but not eliminate, inequities, while others may perpetuate harmful stereotypes, invalidate minoritized identities, and exclude key groups from research participation or access to disseminated findings. This paper aims to (1) review key ethical and social justice dilemmas inherent to working with demographic data in psychological research, and (2) introduce a framework positioned in ethics and social justice to help psychologists and researchers in social science fields make thoughtful decisions about the collection and use of demographic data. Although demographic data methods vary across sub-disciplines and research topics, we assert that these core issues – and solutions – are relevant to all research within the psychological sciences, including basic and applied research. Our overarching aim is to support key stakeholders in psychology (e.g., researchers, funding agencies, journal editors, peer reviewers) in making ethical and socially just decisions about the collection, analysis, reporting, interpretation, and dissemination of demographic data.
The study of demography and collection of demographic data are quintessential aspects of human research. Demography refers to the characteristics that encapsulate communities of people such as sex, race, marital status, or socioeconomic status ( Caldwell, 1996 ; Furler et al., 2012 ). Demographic data, on the other hand, describe the quantitative assessment of these characteristics ( Vogt & Johnson, 2011 ). In research, demographic data are almost always used to characterize the sample at hand, which provides critical information for comparing findings across studies. Data are also commonly used to determine whether specific demographic groups are disproportionately associated with, or affected by, phenomena ( Hughes et al., 2016 ). Findings from such research are used to make data-driven economic, political, and social decisions. For example, the United States (U.S.) relies on demographic data from the U.S. Census to directly shape policies and distribute federal funds based on the demographic composition of different areas of the country ( Fernandez et al., 2016 ). Given these downstream societal impacts, the collection and use of demographic data require thoughtful decisions.
Specific to psychological science, demographic data are used in many ways including, but not limited to, understanding differences in psychological phenomena or outcomes among social groups, identifying population trends over time, or examining the relevance and generalizability of statistical findings from a research sample to specific populations ( Figure 1A ). Although psychology tends to focus on the study of individuals, many psychological phenomena have structural causes. Therefore, consideration of demographic characteristics can help to situate the experiences of individuals within broader social and structural contexts, especially when contending with inequities (e.g., C.S. Brown et al., 2019 ; Roberts et al., 2020 ; Trent et al., 2019 ). However, many demographic variables represent fundamental aspects of personhood ( Fernandez et al., 2016 ), may be considered protected (e.g., collection of sexual orientation in healthcare settings; Sanders et al., 2013 ), and are intricately tied to structural forces of inequity (e.g., distribution of services) that may cause harm. The harms that may arise from demographic data disproportionately impact minoritized 1 communities and may, in turn, contribute to structural inequities.

(A) Typical approach to demographic data that seeks to collect and use demographics as standard research conduct which functions to maintain or, at best, reduce inequity; (B) Ethics and social justice framework for demographic data highlighting the psychologist’s role in ethical data use and critical points for giving those who could benefit from the research the capability to choose whether, and how, to engage and apply research towards transforming well-being and restoring justice.
Recent efforts across fields of research (e.g., the QuantCrit framework in education; Castillo & Gillborn, 2022 ) are challenging long held assumptions about data objectivity by characterizing ways in which demographic data may cause harm. While there is obvious benefit to the intentional use of demographic data to identify inequities and disproportionalities, the potential harms from processes of demographic data collection, analysis, interpretation, and dissemination necessitate an ethical approach to demographic data use. Further, if one value of using demographic data is to identify disparities or disproportionalities and reduce inequities, the collection and use of demographics must be situated within contexts that aim to address the forces perpetuating inequities (e.g., social injustice). A framework that addresses the ethical and social justice imperatives of demographic data collection in psychology research is particularly critical at a time when large-scale data collection efforts are increasingly called upon for reproducible science ( Taylor, 2017 ). An ethical, social justice framework for demographic data collection and use could lead to more accurate scientific conclusions, reduce “deficit-driven” research that positions minoritized groups as disadvantaged compared to majoritized groups, and support the development of evidence- and equity-based solutions (e.g., Cogua et al., 2019 ).
Not all researchers who examine psychological processes do so with human participants, which for some may call into question the role of demographic data collection in such studies. Still, this research is often performed with an ultimate goal of providing a lens into human experiences. Thus, it is important for psychological researchers to understand the implications of their research in translation to humans. Experimental and basic research, whether conducted in humans or non-human animals, is often intended to create an empirical basis to test theories. In these cases, research likely prioritizes internal validity without goals of achieving ecological validity, and thus generalizability to all populations may not be a priority ( Mook, 1983 ). However, regulatory bodies do recommend collection of some variables that are relevant to human demographics. For example, the National Institutes of Health (NIH) recommends the inclusion of sex into research design, including in non-human research ( NIH, 2015a ), because the inclusion of sex can support equity in pre-clinical to clinical translation ( Waltz et al., 2021 ). Consistent with these guidelines, preclinical researchers should also be able to discuss what demographic variables, such as sex, are relevant to their research and consider how such variables can support preclinical to clinical translation. It is true that there is not a ‘one-size fits all’ approach to demographic data collection; the appropriate scope and depth of demographic characteristics measured within a study may vary across sub-disciplines and projects depending on the research question ( Figure 1 ). However, as a field, psychological researchers of all kinds should be willing to examine assumptions about what identity information is, or is not, important in order to avoid furthering or creating new inequities in the research translation process ( Snell-Rood et al., 2021 ). Indeed, in order for researchers to build on existing research with eventual goals of generalizability, it is critical that they have access to a suitable demographic characterization of the initial research – even if that research did not have goals of generalizability – to inform their approach. By collecting and reporting on demographic data (or animal data that is related to human demographic data) experimental and basic researchers can facilitate the translation of their findings more efficiently, which is likely to increase the impact of their work and the field of psychology as a whole.
Through an ethics and social justice lens that includes acknowledgment of the inequities within research, this paper (1) provides a review of the ethical and social justice challenges that arise when using demographic data in psychological research and (2) proposes a framework to aid psychologists and allied social science fields in responsibly collecting and using demographic data. The overarching goal of this manuscript is to support key stakeholders in psychology (e.g., researchers, funding agencies, journal editors, peer reviewers) in making ethical and socially just decisions related to demographic data. The discussion largely focuses on U.S.-based research although aspects may be relevant to research globally. We acknowledge that there are likely important considerations for other geographical regions that warrant discussion that are outside the scope of this paper.
Review of Ethical and Social Justice Challenges Related to the Use of Demographic Data
Researchers regularly face dilemmas in navigating the collection, analysis, reporting, and dissemination of demographic data. Additional challenges arise during the peer review process, as reviewers consider demographic data in grant applications or submitted manuscripts. Before deciding how to navigate these challenges, it is first critical that researchers become aware of these dilemmas, which may not be obvious at the outset, particularly if a researcher, lab, or institution is accustomed to handling demographic data in certain ways. Below, we highlight key challenges or dilemmas that arise when working with demographic data at each step of the research process (data collection, analysis, reporting, dissemination, and peer review) and review scholarship related to these issues.
Collection of Demographic Data
Recruitment: the implicit exclusion of minoritized groups from research samples.
Before demographic data can be collected, researchers must recruit participants, a critical step in the research process that impacts the examination of demographic data. Historically, “basic science” methods that prioritize internal validity at the expense of heterogeneous samples have been conferred disproportionate legitimacy compared to “applied science” methods where context is inherent ( Lewis Jr., 2021 ). This is harmful when findings from “basic science” are assumed to generalize to populations and contexts that were not considered in the research, including in the absence of data demonstrating generalizability ( Lewis Jr., 2021 ). Bias in research sampling is an increasingly recognized problem and is sometimes formally referred to as the “WEIRD”, or White, Educated, Industrialized, Rich, and Democratic, problem. Although WEIRD samples are common, including in psychological science, only about 12% of the world’s population are actually WEIRD, suggesting a major gap in generalizability to non-WEIRD communities for whom such research could benefit (see Arnett, 2008 for a discussion). For example, White samples are over represented in therapeutic research proportional to their representation in the population while racially and ethnically minoritized samples are under represented in therapeutic research ( George et al., 2014 ; Miranda et al., 2003 ; Scharff et al., 2010 ; Walsh & Ross, 2003 ). The lack of inclusion of minoritized groups from research samples limits the confidence by which research can be applied to minoritized communities, raising ethical and social justice issues and impacting scientific integrity.
Underrepresentation of minoritized groups in research samples may be due to recruitment challenges as well as consequence of historical maltreatment of minoritized groups in clinical and psychological research (e.g., Auguste et al., 2022 ). Mistrust of psychological research and lack of access to information are commonly reported barriers to research participation by minoritized communities ( George et al., 2014 ; Rowley & Camacho, 2015 ; Scharff et al., 2010 ). These barriers can be exacerbated by recruitment methods that rely on research participants to seek out studies as opposed to methods that build trust with minoritized communities that researchers can then recruit from. The latter approach is necessary to right historical wrongs and conduct research with respect and care for minoritized communities to ensure a positive experience and maximize the benefits of research within these communities.
Underrepresentation in psychological research may also contribute to growing health inequities if findings are selectively validated among homogenous, majoritized groups. White, heterosexual norms are often equated with objectivity and impartiality, an assumption that can harm minoritized communities ( Lewis Jr., 2021 ). For example, neuropsychology relies on normed tests to aid in diagnosis. These norms are influenced by sociocultural factors (e.g., acculturation), for which demographic variables often serve as proxies. When research is conducted in relatively homogenous samples and without adequate assessment of sociocultural factors known to impact test performance, norms fail to account for diverse sociocultural experiences, which in turn has downstream consequences for diagnosis and treatment ( Byrd & Rivera-Mindht, 2022 ).
Assessment: Balancing Respect for Participants with Generalizability
When considering how to assess demographic data, researchers face decisions about using inclusive approaches sensitive to participants' identities versus methods that allow for aggregating data. The former emphasizes respect for participants while the latter can facilitate the comparison across studies and scientific growth. The spectra of demographic collection methods can range from most inclusive and least prescriptive (e.g., open-text responses for all demographic questions; Strunk & Hoover, 2019 ; Hughes et al., 2016 ; Moody et al., 2013 ) to least inclusive and most prescriptive (e.g., forced, single-answer choice to a limited list of demographic categories). Choosing an approach presents ethical and social justice dilemmas.
There are numerous reasons to take a more inclusive approach, which typically means less prescriptive or constrained assessment of identity. Forcing participants to incorrectly select an identity from a list of identities that do not apply to them is an act of oppression ( Strunk & Hoover, 2019 ) and can reinforce the sense that psychological research does not recognize or accept their identity. It can also lead to uncertainty about how to respond or frustration with the research, which may contribute to participants from minoritized groups opting out of research, thus exacerbating existing inequities ( Hughes et al., 2016 ) or potentially causing emotional harm. On the other hand, giving participants more freedom to report their identities can validate their lived experiences, convey respect, and build trust in the research process.
Despite the clear drawbacks to less inclusive approaches, there are certain ethical and social justice reasons for being more prescriptive in the assessment of demographic data. To promote the wellbeing of minoritized groups, it is crucial that we can identify, aggregate, and compare data from these groups. It is clear that minoritized groups are underrepresented in research, limiting the ability to draw inferences from existing studies, create policies, and develop interventions that serve minoritized groups. Less prescriptive approaches can make it challenging to aggregate or compare data about minoritized groups across studies (e.g., for a meta-analysis or review). These challenges also arise if the categories reported on are not actually representative of the participants’ identities, either because the questions were not sufficiently inclusive to adequately capture identity or because data were collapsed into categories that are not representative of participants’ identities. Still, there may be benefits to collecting demographic data in ways that are more confined and therefore more easily and accurately compared across studies.
Researchers have proposed practices that may provide balance between less versus more prescriptive approaches in the interest of furthering science while supporting inclusivity. For example, Moody and colleagues (2013) propose a two-step process involving asking participants for free-text responses to demographic questions, and then applying a standardized coding scheme for those responses. Hughes and colleagues (2016) build on and modify the questionnaire and coding scheme provided by Moody and colleagues (2013) . Strunk and Hoover (2019) propose a similar concept in the field of education research. Still, there is not a one-size-fits all answer to how best to handle this tension.
In secondary data analyses, researchers may be faced with using demographic data that they did not initially collect. In these cases, the challenge becomes how to responsibly analyze and report on the data. This challenge is particularly pronounced when the researcher conducting the secondary analysis believes that demographic data were assessed in a way that compromises ethics or perpetuates injustices in the field. Given the dramatic rise in data sharing and open-science, this dilemma is likely to be of increasing relevance.
Analysis of Demographic Data
Both ethical and social justice dilemmas arise during statistical analysis. Perhaps because there is ambiguity in if, when, and how to examine demographic data, researchers may not pre-specify a plan for analyzing such data in the same way that they would for a primary outcome variable. Ad hoc statistical approaches (e.g., multiple analyses) may increase the risk of false positives, particularly when analyzing associations between demographic characteristics and phenomena ( Simmons et al., 2011 ). False positives related to demographic data have implications for research integrity and reproducibility, as well as equity and social justice in that they may reinforce inaccurate biases or divert attention away from true inequities.
Prior to conducting statistical analyses, aggregating or collapsing subsets of socially-defined communities (e.g., gay, lesbian, bisexual, transgender, queer) into larger, less descriptive categories (e.g., LGBTQ+) for analyses conceals variation between groups that may be important ( Strunk & Hoover, 2019 ). Such practices also falsely imply that the collapsed categories share key similarities when their differences may be clinically important to acknowledge. The practice of collapsing across categories is often done when the number of individuals in a given category is too small to conduct valid inferential statistical analyses. Collapsing within minoritized identities while majoritized groups (e.g., straight or heterosexual participants) are rarely collapsed conveys that psychological science perceives identities to be variables which can be arranged at the discretion of the researchers, or that altering identity data may be acceptable under circumstances deemed “appropriate” by researchers but without permission of those whose identities are being permuted. Keeping categories more descriptive and nuanced rather than collapsing categories may provide a more accurate representation of who was included in the research and, thus, which populations the research can be generalized to ( Hughes et al., 2016 ).
During statistical analyses, attempts to account for confounding variables can be problematic when significant effects related to minoritized communities are obscured through statistical correction or aggregation ( Kauh et al., 2021 ). For example, race, ethnicity, and other demographic variables that are not outcomes of interest but are related to dependent variables are often seen as adjustable ( Kaufman & Cooper, 2001 ). If a demographic variable is not an outcome of interest but is related to outcomes, it is common to statistically control for the demographic variable ( Kaufman & Cooper, 2001 ). However, as is discussed in more detail later, this adjustment is done at the expense of other social determinants (e.g., systemic racism) and often without thoughtful explanation of where demographics and social determinants intersect and why ( Noroña-Zhou & Bush, 2021 ; Ross et al., 2020 ). Finally, when analyzing demographic variables, it is common practice to set the most privileged group as the comparison (e.g., including White vs. “other” racial identities), which can reinforce societal hierarchies of how social groups are compared and erase heterogeneity within reference or “other” categories ( Noroña-Zhou & Bush, 2021 ).
Reporting and Interpreting Demographic Data
After demographic data have been collected and analyzed, researchers are faced with decisions about how to report and interpret these data in publications and elsewhere. It is common for publications in psychology and related fields to omit demographic data during reporting ( Buchanan & Wiklund, 2020 ). For example, in a review of all studies published in the American Journal of Psychiatry between 2019-2020 ( N =125), Pedersen and colleagues (2022) found that data on age were omitted in 10% of studies, gender/sex in 16% of studies, race and ethnicity in 57% of studies, and sexual orientation identity in 99% of studies. Although there have been many calls for psychological researchers to shift from conceptualizing identity as one-dimensional to intersectional, reporting intersectional identities in published psychology articles remains rare ( Cole, 2009 ; McCormick-Huhn et al., 2019 ; Sabik et al., 2021 ).
The presentation of analyses involving demographic data is also important to consider. When research has focused on experiences of minoritized individuals, the conclusions drawn have focused largely on negative consequences and deleterious effects of being a minoritized person (i.e., “deficit” models). This can include, for example, increased symptoms of psychopathology and experiences of stereotype threat, in minoritized communities ( Barnett et al., 2019 ). Both the framing of “negative” demographic-related effects and saturation of research articles reporting “deficit” model understandings of being a minoritized person contribute to perceptions of minoritized groups as inherently flawed or struggling psychologically. This practice risks perpetuating trauma through stigmatization and stereotypes and impacts communities’ trust in research participation.
Reporting of demographic data in publications, when presented without certain context or appropriate elaboration, can facilitate spurious misinterpretations of key findings ( Okazaki & Sue, 1995 ; Helms et al., 2005 ). Misattributions of effects that arise from systemic or contextual influences related to demographics can lead to the furtherance of biases and stereotypes in science and wider society, harming minoritized populations and creating deterministic pathways for populations ( Lett et al., 2022 ). For example, much research in the history of psychological science attempted to elucidate biological predispositions for violence among male youths with minoritized racial and ethnic identities ( Washington, 2006 , Chapter 11). These studies often use overly broad demographic criteria for inclusion in their studies and leave many other collinear variables, such as low socioeconomic status, lack of access to resources, and other systemic variables, unmeasured, facilitating the erroneous conclusion that violence among males is primarily related to minoritized racial and ethnic identities. Presenting associations between violence and minoritized racial and ethnic identities without the context of broader systemic considerations limits the ability to target addressable socio-political and environmental factors that may improve outcomes among these populations. Beyond erroneous conclusions, these studies reify stereotypes about minoritized groups that lead to serious consequences for members of these groups. For example, misperceptions of Black men as larger and more intimidating are informed by racial stereotypes and contribute to justifications for the use of physical force in police alterations ( Wilson et al., 2017 ). Using methodological and statistical approaches that position demographic variables as proxies for social conditions, rather than biological differences, shifts the focus from disparities to inequities, thus allowing for system-level change to occur ( Lett et al., 2022 ).
Misinterpretations are also facilitated when psychological research conflates distinct demographic variables. For example, sex and gender are often used interchangeably, sometimes even within the same publication. The National Academies of Science, Engineering, and Medicine (NASEM) defines sex as a multidimensonal construct of anatomical and physical traits including internal and external reproductive organs, secondary sex characteristics, chromosomes and hormones whereas gender unites gender identity, gender expression, and sociocultural expectations associated with sex traits ( National Academies of Science, Engineering, and Medicine, 2022 ; Rubin et al., 2020 ), where variations exist across cultures, societies, and eras. Research that does not parse sex/gender in meaningful ways limits interpretations of effects and generalizability to populations, perhaps among communities who may benefit from specificity in research ( Lindqvist et al., 2021 ). Omission of gender/sex during research often occurs due to limited consensus on how and when, to assess sex and gender in research. The absence of tools for assessing gender and sex has led to research where gender/sex was collected with binary categorical labels (e.g., “male/female” or “boy/girl”), which precludes gender- and sex-diverse individuals being able to identify themselves within categories that reflect their experiences ( Cameron & Stinson, 2019 ). NASEM specifically recommends that researchers use terminology that is specific to the construct of interest, report which components of sex and/or gender are collected, and collect sex and gender when there is a clear, well-defined goal for collection.
Dissemination of Findings Related to Demographic Data
Research that is inclusive of minoritized groups, or which seeks to examine psychological phenomena related to experiences of minoritized identities, is only beneficial insofar as it is effectively and widely disseminated to communities that participated in the research, the larger scientific community, and society at large. Researchers and institutions rarely create methods for disseminating findings to minoritized communities that have participated in research and those that are supporting these communities, which further exploits minoritized communities ( K.S. Brown et al., 2019 ; Lewis Jr. & Wai, 2021 ). The exclusion of studies on these topics from higher impact journals that reach broader audiences implicitly dismisses the validity of these topics of study. Recent evidence shows that a disproportionate majority of psychological science articles are authored by White individuals, and that most (83%) editors-in-chief of psychology journals are White ( Roberts et al., 2020 ). Having disproportionately White authors and editors results in majoritized communities determining which topics are worth studying, how findings are interpreted, and which findings should be published and disseminated ( Lewis Jr. & Wai, 2021 ). This is consequential because White scientists and editors are less likely to study and publish research centering experiences of racially diverse populations ( Roberts et al., 2020 ). In a study by Roberts and colleagues (2020) examining over 26,000 publications in cognitive, developmental, and social psychology over the last five decades, only 5% of publications highlighted race explicitly. White editors published significantly fewer articles highlighting race (4%) compared with editors who are people of color (11%) and selected significantly fewer editorial board members who are people of color (6%) than editors-in-chief who are people of color (17%). Finally, White participants were more common in papers authored by White scientists whereas participants of color were more common in papers authored by scientists of color.
The Peer Review Process: A Note for Funding Agencies, Journal Editors, and Peer Reviewers
The use of demographic data also presents challenges during peer review. Important data can be dismissed based on reviewers’ critiques of how demographic data were handled; alternatively, research in which demographic data are handled in unethical ways may make its way through the review process. Investigators of trials funded by the NIH are currently required to report on certain demographic characteristics of their samples (e.g., race and ethnicity) using language that is predetermined by the funding agency and mirrors U.S. Census categories ( NIH, 2015b ). This is meant to provide a “common language” that allows for comparison across or aggregation of research from various studies to facilitate scientific growth, to promote generalizability of findings to the broader population, and ensure that certain groups are not excluded from research. While this may increase equity and facilitate science, the execution can introduce new dilemmas. The language of identity is constantly evolving, often at a faster pace than funding agencies or the U.S. Census are updated, creating a mismatch between demographic data and individuals’ identities. For example, before 2000, Americans could only select one racial identity on the U.S. census, leaving those identifying as multiracial without the option of selecting multiple racial identities, a practice that both yielded inaccurate data and undermined multiracial identities ( A. Brown, 2020 ). Further, individuals who identify as Middle Eastern or North African (MENA) are categorized as White in the U.S. Census despite most MENA individuals self-identifying and being perceived by others as MENA rather than White ( Maghbouleh et al.2022 ).
These challenges have led to calls for NIH and other funding agencies to modify demographic reporting requirements in ways that promotes equity, fund research focused on minoritized groups and structural inequities, and fund research conducted by minoritized researchers. Journal editors can similarly help grow the amount of research on minoritized groups and topics related to marginalization (e.g., racism) by establishing which demographic information is required of all published articles, explicitly encouraging submissions on topics related to these issues, and providing guidance for editors and reviewers to check the cited literature for adequate representation of topics and authors ( Galán et al., 2021 ; Schwabish & Feng, 2021 ).
An Ethical and Social Justice Framework for Thinking Critically in Regard to Demographic Data Collection and Use
The discussed challenges and harms with demographic data in psychology, and their consequent impact on individuals and communities who could benefit from psychological research, highlight the ethical and social justice conflicts arising from the current dominant practices of demographic data collection and use in psychological science. Given the importance of demographic data for the recognition of inequities and redistribution of resources, it is imperative that researchers in psychology have a framework through which to consider responsible demographic data collection and use. To build such a framework, we call on three foundational models for ethics and social justice. We describe each model and its application to demographic data in psychological science separately and then integrate the three into a proposed framework.
Applying the APA Code of Ethics to Demographic Data
First, we recognize the American Psychological Association’s (APA) Code of Ethics ( APA, 2016 ) that applies broadly across the profession of psychology, including research. The APA Code of Ethics provides “a common set of principles and standards upon which psychologists build their professional and scientific work,” underscoring the commitment of psychology in “[improving] the condition of individuals, organizations, and society” while also supporting freedom of inquiry. The APA Code of Ethics is comprised of five ethical principles: (1) Beneficence and Nonmaleficence, seeking to do work that has benefit, without harm; (2) Fidelity and Responsibility to professional standards of conduct in psychology; (3) Integrity to the accuracy, honesty, and truthfulness of scientific conduct; (4) Justice in ensuring that all persons can access and benefit from psychological contributions; and (5) Respect for People's Rights and Dignity, including self-determination and respect for cultural, individual, and role differences across individuals. Ethical decisions about data use are inherent to research (e.g., confidentiality, storage), however the application of ethical decision-making in research is context-dependent ( Birnbacher, 1999 ) and may evolve as understanding regarding the challenges of demographic data emerges. Specifically, demographic methods that met a prior ethical standard may not meet the same standard in the future if such methodology, in a new context, violates one or more ethical principles. For example, as language around identity evolves, ethical assessment of demographic characteristics requires researchers to use the most current, bias-free, and affirming language (see the APA’s guide to bias-free language; APA, 2019 ). This may mean changing the word choice on a demographic questionnaire if a term is now considered pejorative or adding additional response options given that the omission of a response option can invalidate and “other” participants’ identities.
Consider a questionnaire that asks for a participant’s “sex” and provides the possible responses of “male” and “female.” Consistent with NASEM recommendations, we would recommend (1) changing “sex” to “sex assigned at birth” or “sex listed on birth certificate” to reduce bias and (2) include a second question on current gender, as this allows participants to have their identity respected during data collection and to be counted in research with the identities they hold in order to support translation of research within their communities 2 . When researchers proactively adapt their demographic questionnaires to use affirming, bias-free language, they exemplify the APA Code of Ethics in the following ways: (1) Beneficence and Nonmaleficence by conducting research that aims to benefit all individuals and groups (whereas using biased, stigmatized, or oppressive language may do harm to participants, consumers of the research, and society as a whole); (2) Fidelity and Responsibility by striving to remain up-to-date on research and guidelines surrounding affirming language for identity; (3) Integrity by ensuring their research accurately captures the identities of participants; (4) Justice by building trust with minoritized communities, thus encouraging research participation by those who are often underrepresented in research; and (5) Respect for People's Rights and Dignity, by affirming individuals’ identity or culture. This is just one example of how the APA Code of Ethics can be applied by researchers when working with demographic data; below, we suggest additional points in the research process that necessitate consideration of the APA Code of Ethics with regard to demographic data.
Applying Sen’s Capability Approach to Demographic Data
Second, and consistent with the commitment of psychology to improving the health condition of individuals, organizations, and society, we recognize Sen’s Capability Approach ( Sen, 1985 ) and its relationship to human health ( Nussbaum, 2011 ; Sen, 1989 ). Briefly, the Capability Approach focuses on the moral importance of individual abilities to realize the life they value. In contrast to objective metrics of a successful or valued life, this approach focuses on subjective well-being and the “capability sets” one has to achieve it. In this context, capability sets are combinations of real “functionings” (e.g., wealth or health) to which one has access to and uses to realize their valued life. Societal deficiencies arise when individuals, or collectives of people, lack necessary capability sets or can only achieve capabilities that are incompatible with human dignity ( Nussbaum, 2011 ). Social, institutional, and environmental conditions can function as conversion factors, supporting an individual in converting resources into capability sets, suggesting that such systems have a moral obligation to reduce capability shortfalls ( Drydyk, 2012 ). In the context of psychology research, notably few in society have the capability to enact and produce research that influences their own well-being. However, as an institution, psychology’s use of demographic data could serve as a conversion factor that supports individuals or collectives to guide research that facilitates the achievement of a valuable life ( Taylor, 2016 , 2017 ).
Researchers can draw on Sen’s Capability Approach to identify the inequities related to their research that arise from social deficiencies and impact capability sets. These inequities might be evident in representation in research (i.e., the exclusion of certain demographic groups from research), in inaccuracies or misrepresentations in characterizing demographic groups in research, or in the outcome the researcher is studying (e.g., health inequities faced by certain demographic groups). Each of these inequities hinders the capability sets needed to achieve a valued life. Once these inequities are identified, researchers can rework their approach to demographic data to serve as a conversion factor, for example by including underrepresented groups in their research, ensuring that those groups are accurately described, and analyzing demographic data in such a way that helps elucidate inequities.
Applying Fraser’s Theory of Social Justice to Demographic Data
Lastly, because the Capability Approach focuses on the means to individual outcomes of value, we recognize Fraser’s Theory of Social Justice to describe an outcome of justice ( Fraser, 2009 ). Fraser’s model includes three dimensions critical for justice: (1) recognition vs. misrecognition, which highlights status inequality between groups of people, leading to unfair biases and attributions; (2) redistribution vs. maldistribution, which acknowledges the unequal distribution of resources that limits equal participation in society; and (3) representation vs. misrepresentation, which considers who is included in a system, thus influencing who has the right to frame discourse and policies within a system. This model considers these dimensions from two perspectives. The affirmative perspective considers these dimensions from within a defined state, wherein addressing injustice does not change the state itself and instead produces reforms meant to ameliorate injustice. From this perspective, injustice may be reduced, but the structures producing the injustice are affirmed, thus maintaining a state in which future injustice may arise. In contrast, the transformative perspective seeks to restructure the boundaries of a defined state, rather than redistribute resources within the state, to address the root causes of injustice to promote multiculturalism and parity. As detailed above, demographic data collection and use has historically limited accurate recognition within research, which consequently impacts on resource distribution and societal representation and affirms existing structures that perpetuate inequities. Researchers can draw from Fraser’s model to work towards a transformative approach to demographic data.
Proposed Ethical and Social Justice Framework for Working with Demographic Data
With these models in mind, we propose an ethical and social justice framework for demographic data collection and use ( Figure 1B ). Table 1 provides questions that researchers can ask themselves and procedures they might use at each stage of the research process as they apply this framework. Our framework acknowledges, per the APA Code of Ethics, that researchers have the ability to maintain freedom of inquiry in their research question and process; however, this framework highlights pivotal points at which ethical and socially just demographic data practices could be applied throughout the research process. After selection of the research question, researchers should seek input on - rather than assume - who may benefit from the research in building a valued life, and how the research should be conducted to enhance that value. The capability set to make such decisions places functional value in the knowledge and perspectives of communities the research is meant to support, both in determining whether the research question is one that is valued by the community and, if so, how to best collect demographic data to ensure accurate representation.
Suggested questions to consider and corresponding examples for navigating demographic data use through an ethics and social justice lens
Note. Our suggestions related to demographic data draw from theories of social justice ( Fraser, 2009 ; Sen, 1985 ) and the American Psychological Association’s General Principles of Ethics ( APA, 2016 ): (1) Beneficence/maleficence: Maximizing benefits and minimizing harms to research participants and the broader community; (2) Fidelity and responsibility: Justifying decisions related to demographic data by remaining up-to-date on empirical and theoretical knowledge; (3) Integrity: Ensuring that demographic data accurately capture identities and clearly communicating the limits of the data; (4) Justice: Attending to who is included and excluded from the research, who is affected by research findings, and how we can utilize research findings to address root causes of inequity and restore wellbeing; (5) Respect for people’s rights and dignity: Partnering with individuals from the community to center their voices in the research process in order to affirm identities, communicate respect, and promote wellbeing.
Ethical and socially just choices may vary considerably based on the research project and other contextual factors, so we emphasize the importance of justifying and clearly reporting on each choice using our framework and Table 1 as guides. To this end, prior to collecting data, researchers should consider utilizing pre-registration options to share how they plan to analyze certain variables, including how they will define and utilize demographic data and how decisions were made regarding the use of demographic data in their analyses. This step would greatly improve the extent of forethought and consideration given to possible roles and repercussions of demographic data use in psychological research.
Once demographic data are collected, researchers should articulate the ethical use or non-use of demographic data in analyses in the write-up of their findings, with a focus on APA principles of benefit without harm, research integrity and fidelity, justice and respect for persons. Specifically, it is imperative that researchers describe the methods used to gather demographic data from participants and report how said data are operationalized to formulate the demographic variables used in their statistical analyses. Researchers should also develop competency in explaining the limits of their demographic data. Scientific journals should update publication guidelines to include recommendations such as these for the methods and results sections of empirical articles.
In addition, researchers should be attuned to how analyses benefit communities and support justice, while also minimizing inadvertent harms. This is consistent with emerging recommendations for research conduct from psychology organizations, peer reviewed journals, and select funding agencies ( APA Task Force on Race and Ethnicity Guidelines in Psychology, 2019 ; Buchanan et al., 2021 ; Flanagin et al., 2021 ). Following completion of ethical analyses that address the research question, researchers should consider whether sharing the data publicly is an appropriate step. Sharing demographic data openly provides the maximum level of transparency and informs the generalizability of the findings, consistent with APA Ethics Principles of research integrity and fidelity. However, it is also an ethical imperative (e.g., Beneficence and Nonmaleficence) to protect the identities of minoritized groups or groups that have been historically oppressed via research (e.g., Indigenous communities), especially in cases when research findings may easily be traced back to individuals or used to further denigrate minoritized groups (e.g., Lui et al., 2022 ). Thus, the decision to share data openly and the decision to use open data should be considered within our ethical framework.
As yet another step toward an ethical and social justice approach for utilizing demographic data in research, researchers should seek input on the functional value of the results of their research rather than assuming their application. Without such input, researchers run the risk of implicitly supporting defined states (i.e., affirmative functioning) that may not have value to impacted communities or only reduces or redirects the impact of injustice rather than addressing root causes. In contrast, supporting communities in defining the research value using their capabilities may lead to a transformative outcome that leads to a just restructuring, social equity, and parity.
As previously discussed, numerous barriers exist to the seeking of input from, recruiting, and retaining diverse perspectives in research. In this framework, we acknowledge the role of social, institutional, and environmental conversion factors that would support community-driven capabilities in the research process. One simple way to do this would be for researchers and departments to promote the use of evidence-based demographic tools that have already been developed (e.g., PhenX Toolkit; Hamilton et al., 2011 ). Some researchers may have access to Clinical and Translational Science Institutes (CTSIs) that can serve to enhance the capabilities of individuals from diverse backgrounds in research or support researchers in making ethical analytic choices. We also encourage research collaborations that include expertise in community-based participatory methods and for research institutions and departments to consider equitable strategies that allow for stronger community engagement (e.g., funding a research advisory board). Importantly, community engagement needs to be built on equitable, participatory principles that aim to increase trust and engagement without placing additional or unnecessary burdens on communities themselves ( Collins et al., 2018 ; Israel et al., 2005 ; Smith et al., 2015 ). However, given the importance of transformative outcomes in research, ongoing commitments to establishing and enabling social, institutional, and environmental conversion factors is critical to the implementation of this ethical and social justice framework for demographic data.
Researchers in psychological science are regularly faced with critical decision points related to the incorporation of demographic data into their studies. These decisions can either reinforce practices that perpetuate inequities and bias, or can move the field towards greater diversity, inclusivity, and equity. As such, we implore researchers to proceed thoughtfully when collecting, analyzing, reporting, interpreting, and disseminating the results of demographic data, and to regularly review and update their practices given the rapid pace at which society’s understanding of identity and demography shift.
While we have provided a framework to help researchers think critically about decisions related to demographic data and critical opportunities for stakeholder input, additional research in this area is needed to provide guidelines. Qualitative and quantitative research should examine the preferences of individuals with minoritized identities regarding how demographic data are collected, analyzed, and reported. Additionally, community-based participatory research involving individuals with minoritized identities who can advise researchers on their handling of demographic data may be appropriate in many cases. 3
Training in the ethical and socially just use of demographic data is also needed. To decrease inequities in the psychological sciences, recent calls have focused on revamping graduate curricula to ensure that it does not continue to reinforce oppressive systems ( Galán et al., 2021 ). Graduate programs could benefit from substantively incorporating issues regarding demographic data use into various classes. For example, research methods courses could explicitly discuss ethical and socially just methods for engaging underrepresented participants in research, obtaining their input about the value and methods of a research question, accurately assessing demographic data, and disseminating findings related to demographic data. Statistical analysis courses could engage students in dialogue about how to appropriately decide how to utilize demographic data in analyses (e.g., as a covariate, predictor, or not at all). Departments could require that thesis or dissertation proposals include a section that specifically discusses decision-making around demographic data, and committee members could weigh in on this section.
We emphasize the need for continued conversations among researchers, journal editors, grant and peer reviewers, and other key stakeholders regarding the use of demographic data. To facilitate such conversations, we have created an open reader commentary page ( https://osf.io/gmbpf/?view_only=c4f51c3f72fb4f49b6add6d5fd935215 ), where stakeholders can provide feedback on our manuscript and offer ideas for additional recommendations that can be considered in future efforts to create a valuable framework for addressing the issues identified in this publication.
Acknowledgments
C.C.C. and K.L.E. receive funding from the National Institute of Mental Health (T32 MH018269). C.L.B. receives funding from the National Institute on Alcohol Abuse and Alcoholism (K08 AA030301). D.M.N. receives funding from the VA Advanced Fellowship in Mental Illness Research and Treatment. S.W.K. receives funding from The National Science Foundation Graduate Research Fellowship (DGE1745303).
We have no conflicts of interest to disclose.
Positionality Statement: One author identifies as a non-Hispanic white cishet woman from a low-income background; one as a white, cishet woman; one as a Black cis gay man; one as a multiracial (Black and white), queer, gender-non-conforming, first-generation college person from a low-income background; one as a white, queer person; one as a non-Hispanic white cisgender queer woman from a low-income background; one as a non-Hispanic white cishet man; one as a multiethnic middle-eastern Latina, first generation American and college person from a low-income background. Together, the authors represent a group of United States-based early- and mid-career scholars across several sub-disciplines within and outside of psychology who are invested in moving academia towards equity and social justice. We acknowledge that our identities - as well as our position as academics - influences our biases when it comes to decentering dominant or majoritized identities in research and thinking about demographic data.
CRediT Authorship Contribution Statement: Christine C. Call: Conceptualization, Project Administration, Writing – original draft, Writing – review & editing; Kristen L. Eckstrand: Conceptualization, Visualization, Writing – original draft, Writing – review & editing; Steven W. Kasparek: Conceptualization, Writing – original draft, Writing – review & editing; Cassandra L. Boness: Conceptualization, Project Administration, Writing – original draft, Writing – review & editing; Lorraine Blatt: Conceptualization, Project Administration, Writing – original draft, Writing – review & editing; Nabila Jamal-Orozco: Conceptualization, Writing – original draft, Writing – review & editing; Derek M. Novacek: Conceptualization, Writing – original draft, Writing – review & editing; Dan Foti: Conceptualization, Writing – review & editing
1 We use the term “minoritized” throughout to refer to groups, communities, or individuals who experience historic and ongoing oppression due social and structural inequities that create and systematically privilege “majoritized” groups. We acknowledge that other terms, such as “marginalized,” also capture this sentiment and may be preferred by some readers.
2 A recent experience by one of our authors offers another example of a failure to validate an individual’s identity with demographic items. When collecting ethnic identity data, the author unintentionally omitted “Arab” from a prescriptive list of options and in a text entry field, a participant responded: “Arab for the love of god why is there never Araaaaaaaaab”.
3 For a recent review of the many benefits of community-based participatory research and an overview of several studies that have successfully used this approach see Kia-Keating & Juang (2022) .
- American Psychological Association (APA). (2016). Revision of ethical standard 3.04 of the “Ethical Principles of Psychologists and Code of Conduct” (2002, as amended 2010) . American Psychologist , 71 , 900. 10.1037/amp0000102 [ PubMed ] [ CrossRef ] [ Google Scholar ]
- American Psychological Association (APA). (2019, August ). Bias-Free Language . https://apastyle.apa.org/style-grammar-guidelines/bias-free-language
- APA Task Force on Race and Ethnicity Guidelines in Psychology. (2019). Race and Ethnicity Guidelines in Psychology: Promoting Responsiveness and Equity . American Psychological Association . http://www.apa.org/about/policy/race-and-ethnicity-in-psychology.pdf [ Google Scholar ]
- Arnett JJ (2008). The neglected 95%: Why American psychology needs to become less American . American Psychologist , 63 ( 7 ), 602–614. 10.1037/0003-066X.63.7.602 [ PubMed ] [ CrossRef ] [ Google Scholar ]
- Auguste E, Bowdring MA, Kasparek SW, McPhee J, Tabachnick A, Tung I, & Galán C (2022). Psychology's contributions to anti-Blackness in the United States within psychological research, criminal justice, and mental health . 10.31234/osf.io/f5yk6 [ PMC free article ] [ PubMed ] [ CrossRef ] [ Google Scholar ]
- Barnett AP, del Río-González AM, Parchem B, Pinho V, Aguayo-Romero R, Nakamura N, Calabrese SK, Poppen PJ, & Zea MC (2019). Content analysis of psychological research with lesbian, gay, bisexual, and transgender people of color in the United States: 1969 – 2018 . American Psychologist , 74 ( 8 ), 898–911. 10.1037/amp0000562 [ PMC free article ] [ PubMed ] [ CrossRef ] [ Google Scholar ]
- Birnbacher D (1999). Ethics and social science: Which kind of co-operation? . Ethical Theory and Moral Practice , 2 ( 4 ), 319–336. https://www.jstor.org/stable/27504102 [ Google Scholar ]
- Brown A (2020, February 25). The changing categories the US census has used to measure race . Pew Research Center . https://www.pewresearch.org/fact-tank/2020/02/25/the-changing-categories-the-u-s-has-used-to-measure-race/ [ Google Scholar ]
- Brown KS, Kijakazi K, Runes C, & Turner MA (2019, February 19). Confronting structural racism in research and policy analysis . Urban Institute. https://www.urban.org/research/publication/confronting-structural-racism-research-and-policy-analysis [ Google Scholar ]
- Brown CS, Mistry RS, & Yip T (2019). Moving from the margins to the mainstream:Equity and justice as key considerations for developmental science . Child Development Perspectives , 13 ( 4 ), 235–240. 10.1111/cdep.12340 [ PMC free article ] [ PubMed ] [ CrossRef ] [ Google Scholar ]
- Buchanan NT, Perez M, Prinstein MJ, & Thurston IB (2021). Upending racism in psychological science: Strategies to change how science is conducted, reported, reviewed, and disseminated . American Psychologist , 76 ( 7 ), 1097–1112. 10.1037/amp0000905 [ PubMed ] [ CrossRef ] [ Google Scholar ]
- Buchanan NT, & Wiklund LO (2020). Why clinical science must change or die: Integrating intersectionality and social justice . Women & Therapy , 43 ( 3-4 ), 309–329. 10.1080/02703149.2020.1729470 [ CrossRef ] [ Google Scholar ]
- Byrd DA, & Rivera-Mindt MG (2022). Neuropsychology’s race problem does not begin or end with demographically adjusted norms . Nature Reviews Neurology . 10.1038/s41582-021-00607-4 [ PubMed ] [ CrossRef ] [ Google Scholar ]
- Caldwell JC (1996). Demography and social science . Population Studies , 50 ( 3 ), 305–333. 10.1080/0032472031000149516 [ PubMed ] [ CrossRef ] [ Google Scholar ]
- Cameron JJ, & Stinson DA (2019). Gender (mis)measurement: Guidelines for respecting gender diversity in psychological research . Social and Personality Psychology Compass , 13 ( 11 ), e12506. 10.1111/spc3.12506 [ CrossRef ] [ Google Scholar ]
- Castillo W & Gillborn D (2022). How to “QuantCrit:” Practices and questions for education data researchers and users .(EdWorkingPaper: 22-546). Retrieved from Annenberg Institute at Brown University: 10.26300/v5kh-dd65 [ CrossRef ] [ Google Scholar ]
- Chandran A, Knapp E, Liu T, & Dean LT (2021). A new era: Improving use of sociodemographic constructs in the analysis of pediatric cohort study data . Pediatric Research , 90 ( 6 ), 1132–1138. 10.1038/s41390-021-01386-w [ PMC free article ] [ PubMed ] [ CrossRef ] [ Google Scholar ]
- Cogua J, Ho KY, & Mason WA (2019). The peril and promise of racial and ethnic subgroup analysis in health disparities research . Journal of Evidence-Based Social Work , 16 ( 3 ), 311–321. 10.1080/26408066.2019.1591317 [ CrossRef ] [ Google Scholar ]
- Cole ER (2009). Intersectionality and research in psychology . American psychologist , 64 ( 3 ), 170–180. 10.1037/a0014564 [ PubMed ] [ CrossRef ] [ Google Scholar ]
- Collins SE, Clifasefi SL, Stanton J, Straits KJ, Gil-Kashiwabara E, Rodriguez Espinosa P, Andrasik MP, Miller KA, Orfaly VE, The LEAP Advisory Board, Gil-Kashiwabara E, Nicasio AV, Hawes SM, Nelson LA, Duran BM & Wallerstein N (2018). Community-based participatory research (CBPR): Towards equitable involvement of community in psychology research . American Psychologist , 73 ( 7 ), 884. 10.1037/amp0000167 [ PMC free article ] [ PubMed ] [ CrossRef ] [ Google Scholar ]
- Drydyk J (2012). A capability approach to justice as a virtue . Ethical Theory and Moral Practice , 15 , 23–38. 10.1007/s10677-011-9327-2 [ CrossRef ] [ Google Scholar ]
- Fernandez T, Godwin A, Doyle J, Verdin D, Boone H, Kirn A, Benson L, & Potvin G (2016). More comprehensive and inclusive approaches to demographic data collection . School of Engineering Education Graduate Student Series . Paper 60. https://docs.lib.purdue.edu/enegs/60/ [ Google Scholar ]
- Flanagin A, Frey T, Christiansen SL, & AMA Manual of Style Committee. (2021). Updated guidance on the reporting of race and ethnicity in medical and science journals . Journal of the American Medical Association , 326 ( 7 ), 621–627. 10.1001/jama.2021.13304 [ PubMed ] [ CrossRef ] [ Google Scholar ]
- Ford KS, Rosinger KO, Choi J, & Pulido G (2021). Toward gender-inclusive postsecondary data collection . Educational Researcher , 50 ( 2 ), 127–131. 10.3102/0013189X20966589 [ CrossRef ] [ Google Scholar ]
- Furler J, Magin P, Pirotta M, & van Driel M (2012). Participant demographics reported in "Table 1" of randomised controlled trials: a case of" inverse evidence"? . International Journal for Equity in Health , 11 ( 1 ), 1–4. 10.1186/1475-9276-11-14 [ PMC free article ] [ PubMed ] [ CrossRef ] [ Google Scholar ]
- Fraser N (2009). Scales of justice: reimagining political space in a globalizing world . New York: Columbia University Press. [ Google Scholar ]
- Galán CA, Bekele B, Boness CL, Bowdring M, Call CC, Hails K, McPhee J, Mendes SH, Moses J, Northrup J, Rupert P, Savell S, Sequeira S, Tervo-Clemmens B, Tung I, Vanwoerden S, Womack S & Yilmaz B (2021). A call to action for an antiracist clinical science . Journal of Clinical Child & Adolescent Psychology , 50 ( 1 ), 12–57. 10.1080/15374416.2020.1860066 [ PubMed ] [ CrossRef ] [ Google Scholar ]
- George S, Duran N, & Norris K (2014). A Systematic Review of Barriers and Facilitators to Minority Research Participation Among African Americans, Latinos, Asian Americans, and Pacific Islanders . American Journal of Public Health , 104 ( 2 ), e16–e31. 10.2105/AJPH.2013.301706 [ PMC free article ] [ PubMed ] [ CrossRef ] [ Google Scholar ]
- Hamilton CM, Strader LC, Pratt JG, Maiese D, Hendershot T, Kwok RK, Hammond JA, Huggins W, Jackman D, Pan H, Nettles DS, Beaty TH, Farrer LA, Kraft P, Marazita ML, Ordovas JM, Pato CN, Spitz MR, Wagener D, Williams M, Junkins HA, Harlan WR, Ramos EM, & Haines J (2011). The PhenX Toolkit: Get the Most From Your Measures . American Journal of Epidemiology , 174 ( 3 ), 253–260. 10.1093/aje/kwr193 [ PMC free article ] [ PubMed ] [ CrossRef ] [ Google Scholar ]
- Helms JE, Jernigan M, & Mascher J (2005). The meaning of race in psychology and how to change it: a methodological perspective . American Psychologist , 60 ( 1 ), 27–36. 10.1037/0003-066X.60.1.27 [ PubMed ] [ CrossRef ] [ Google Scholar ]
- Hughes JL, Camden AA, & Yangchen T (2016). Rethinking and updating demographic questions: Guidance to improve descriptions of research samples [Editorial] . Psi Chi Journal of Psychological Research , 21 ( 3 ), 138–151. https://www.rwu.edu/sites/default/files/demographic%20recs.pdf [ Google Scholar ]
- Hyde JS, Bigler RS, Joel D, Tate CC, & van Anders SM (2019). The future of sex and gender in psychology: Five challenges to the gender binary . American Psychologist , 74 ( 2 ), 171. 10.1037/amp0000307 [ PubMed ] [ CrossRef ] [ Google Scholar ]
- Ioannidis JP, Powe NR, & Yancy C (2021). Recalibrating the use of race in medical research . Journal of the American Medical Association , 325 ( 7 ), 623–624. 10.1001/jama.2021.0003 [ PubMed ] [ CrossRef ] [ Google Scholar ]
- Israel BA, Eng E, Schulz AJ, & Parker EA (2005). Introduction to methods in community-based participatory research for health. In Israel BA, Eng E, Schulz AJ, & Parker EA (Eds.), Methods in community-based participatory research for health (Vol. 3 , pp. 3–26). Jossey-Bass. [ Google Scholar ]
- Kaufman JS, & Cooper RS (2001). Commentary: Considerations for use of racial/ethnic classification in etiologic research . American Journal of Epidemiology , 154 ( 4 ), 291–298. 10.1093/aje/154.4.291 [ PubMed ] [ CrossRef ] [ Google Scholar ]
- Kauh TJ, Read JNG, & Scheitler AJ (2021). The critical role of racial/ethnic data disaggregation for health equity . Population Research and Policy Review , 40 ( 1 ), 1–7. 10.1007/s11113-020-09631-6 [ PMC free article ] [ PubMed ] [ CrossRef ] [ Google Scholar ]
- Kia-Keating M, & Juang LP (2022). Participatory science as a decolonizing methodology: Leveraging collective knowledge from partnerships with refugee and immigrant communities . Cultural Diversity and Ethnic Minority Psychology . Advance online publication. 10.1037/cdp0000514 [ PubMed ] [ CrossRef ] [ Google Scholar ]
- Ledgerwood A, Pickett C, Navarro D, Remedios JD, & Lewis NA (2022). The unbearable limitations of solo science: Team science as a path for more rigorous and relevant research . The Behavioral and brain sciences , 45 , e81. 10.1017/S0140525X21000844 [ PubMed ] [ CrossRef ] [ Google Scholar ]
- Lett E, Asabor E, Beltrán S, Cannon M, & Arah OA (2022). Conceptualizing, contextualizing, and operationalizing race in quantitative health sciences research . Annals of Family Medicine , 20 . Advanced online publication. 10.1370/afm.2792 [ PMC free article ] [ PubMed ] [ CrossRef ] [ Google Scholar ]
- Lewis NA Jr (2021). What counts as good science? How the battle for methodological legitimacy affects public psychology . American Psychologist , 76 ( 8 ), 1323–1333. 10.1037/amp0000870 [ PubMed ] [ CrossRef ] [ Google Scholar ]
- Lewis NA Jr, & Wai J (2021). Communicating what we know and what isn’t so: Science communication in psychology . Perspectives on Psychological Science , 16 ( 6 ), 1242–1254. 10.1177/1745691620964062 [ PubMed ] [ CrossRef ] [ Google Scholar ]
- Lindqvist A, Sendén MG, & Renström EA (2021). What is gender, anyway: A review of the options for operationalising gender . Psychology & Sexuality , 12 ( 4 ), 332–344. 10.1080/19419899.2020.1729844 [ CrossRef ] [ Google Scholar ]
- Lou S & Yang M (2020). A beginner's guide to data ethics . https://medium.com/big-data-at-berkeley/things-you-need-to-know-before-you-become-a-data-scientist-a-beginners-guide-to-data-ethics-8f9aa21af742 [ Google Scholar ]
- Lui PP, Gobrial S, Pham S, Giadolor W, Adams N, & Rollock D (2022). Open science and multicultural research: Some data, considerations, and recommendations . Cultural Diversity & Ethnic Minority Psychology . Advance online publication. 10.1037/cdp0000541 [ PubMed ] [ CrossRef ] [ Google Scholar ]
- Maghbouleh N, Schachter A, & Flores RD (2022). Middle Eastern and North Africans may not be perceived, nor perceive themselves, to be White . Proceedings of the National Academy of Sciences of the United States of America , 119 ( 7 ), 1–9. 10.1073/pnas.2117940119 [ PMC free article ] [ PubMed ] [ CrossRef ] [ Google Scholar ]
- McCormick-Huhn K, Warner LR, Settles IH, & Shields SA (2019). What if psychology took intersectionality seriously? Changing how psychologists think about participants . Psychology of Women Quarterly , 43 ( 4 ), 445–456. 10.1177/0361684319866430 [ CrossRef ] [ Google Scholar ]
- Miranda J, Nakamura R, & Bernal G (2003). Including ethnic minorities in mental health intervention research: A practical approach to a long-standing problem . Culture, Medicine and Psychiatry , 27 ( 4 ), 467–486. 10.1023/B:MEDI.0000005484.26741.79 [ PubMed ] [ CrossRef ] [ Google Scholar ]
- Moody C, Obear K, Gasser H, Cheah S, & Fechter T (2013, December 5). ACPA standards for demographic questions . https://drive.google.com/file/d/1kRI8S_TxCJMvXghGgd7eKlexEAlYb3jL/view [ Google Scholar ]
- Mook DG (1983). In defense of external invalidity . American psychologist , 38 ( 4 ), 379–387. 10.1037/0003-066X.38.4.379 [ CrossRef ] [ Google Scholar ]
- Msimang P (2020). Lessons in our faults: Fault lines on race and research ethics . South African Journal of Science , 116 ( 9-10 ), 1–3. 10.17159/sais.2020/8449 [ CrossRef ] [ Google Scholar ]
- National Academies of Sciences, Engineering, and Medicine. (2022). Measuring Sex, Gender Identity, and Sexual Orientation . The NationalAcademies Press. 10.17226/26424 [ PubMed ] [ CrossRef ] [ Google Scholar ]
- National Institutes of Health (NIH). (2015a). NOT-OD-15-102: consideration of sex as a biological variable in NIH-funded Research . https://grants.nih.gov/grants/guide/notice-files/.html
- National Institutes of Health (NIH). (2015b). NOT-OD-15-089: racial and ethnic categories and definitions for NIH diversity programs and for other reporting purposes . https://grants.nih.gov/grants/guide/notice-files/not-od-15-089.html
- Noroña-Zhou A, & Bush NR (2021, April 13). Considerations regarding the responsible use of categorical race/ethnicity within health research . 10.31234/osf.io/kfa57 [ CrossRef ] [ Google Scholar ]
- Nussbaum M (2011). Creating capabilities: The human development approach . Harvard University Press. [ Google Scholar ]
- Okazaki S, & Sue S (1995). Methodological issues in assessment research with ethnic minorities . Psychological Assessment , 7 ( 3 ), 367–375. 10.1037/14805-015 [ CrossRef ] [ Google Scholar ]
- Pedersen SL, Lindstrom R, Powe PM, Louie K, & Escobar-Viera C (2022). Lack of representation in psychiatric research: A data-driven example from scientific articles published in 2019 and 2020 in the American Journal of Psychiatry . American Journal of Psychiatry , 179 ( 5 ), 388–392. 10.1176/appi.ajp.21070758 [ PMC free article ] [ PubMed ] [ CrossRef ] [ Google Scholar ]
- Roberts SO, Bareket-Shavit C, Dollins FA, Goldie PD, & Mortenson E (2020). Racial inequality in psychological research: Trends of the past and recommendations for the future . Perspectives on Psychological Science , 15 ( 6 ), 1295–1309. 10.1177/1745691620927709 [ PubMed ] [ CrossRef ] [ Google Scholar ]
- Ross PT, Hart-Johnson T, Santen SA, & Zaidi NLB (2020). Considerations for using race and ethnicity as quantitative variables in medical education research . Perspectives on Medical Education , 9 ( 5 ), 318–323. 10.1007/s40037-020-00602-3 [ PMC free article ] [ PubMed ] [ CrossRef ] [ Google Scholar ]
- Rowley SJ, & Camacho TC (2015). Increasing diversity in cognitive developmental research: Issues and solutions . Journal of Cognition and Development , 16 ( 5 ), 683–692. 10.1080/15248372.2014.976224 [ CrossRef ] [ Google Scholar ]
- Rubin JD, Atwood S, & Olson KR (2020). Studying Gender Diversity . Trends in Cognitive Sciences , 24 ( 3 ), 163–165. 10.1016/j.tics.2019.12.011 [ PubMed ] [ CrossRef ] [ Google Scholar ]
- Sabik NJ, Matsick JL, McCormick-Huhn K, & Cole ER (2021). Bringing an intersectional lens to “open” science: An analysis of representation in the reproducibility project . Psychology of Women Quarterly , 45 ( 4 ), 475–492. 10.1177/03616843211035678 [ CrossRef ] [ Google Scholar ]
- Sablan JR (2019). Can you really measure that? Combining critical race theory and quantitative methods . American Educational Research Journal , 56 ( 1 ), 178–203. 10.3102/0002831218798325 [ CrossRef ] [ Google Scholar ]
- Sanders JQ, Feit MN & Alper J (Eds.). (2013). Collecting sexual orientation and gender identity data in electronic health records: workshop summary . National Academies Press. [ PubMed ] [ Google Scholar ]
- Scharff DP, Mathews KJ, Jackson P, Hoffsuemmer J, Martin E, & Edwards D (2010). More than Tuskegee: Understanding mistrust about research participation . Journal of Health Care for the Poor and Underserved , 21 ( 3 ), 879–897. 10.1353/hpu.0.0323 [ PMC free article ] [ PubMed ] [ CrossRef ] [ Google Scholar ]
- Schwabish J, & Feng A (2021, June 9). Do no harm guide: Applying equity awareness in data visualization . Urban Institute. https://www.urban.org/research/publication/do-no-harm-guide-applying-equity-awareness-data-visualization [ Google Scholar ]
- Sen A (1985). Commodities and capabilities . North-Holland. [ Google Scholar ]
- Sen A (1989). Development as capability expansion . Journal of Development Planning , 19 , 41–58. [ Google Scholar ]
- Simmons JP, Nelson LD, & Simonsohn U (2011). False-positive psychology: Undisclosed flexibility in data collection and analysis allows presenting anything as significant . Psychological Science , 22 ( 11 ), 1359–1366. 10.1177/0956797611417632 [ PubMed ] [ CrossRef ] [ Google Scholar ]
- Simons DJ, Shoda Y, Lindsay DS (2017). Constraints on generality (COG): A proposed addition to all empirical papers . Perspectives on Psychological Science , 12 ( 6 ), 1123–1128. 10.1177/1745691617708630 [ PubMed ] [ CrossRef ] [ Google Scholar ]
- Smith SA, Whitehead MS, Sheats JQ, Ansa BE, Coughlin SS, & Blumenthal DS (2015). Community-based participatory research principles for the African American community . Journal of the Georgia Public Health Association , 5 ( 1 ), 52–56. http://hdl.handle.net/10675.2/618545 [ PMC free article ] [ PubMed ] [ Google Scholar ]
- Snell-Rood C, Jaramillo ET, Hamilton AB, Raskin SE, Nicosia FM, & Willging C (2021). Advancing health equity through a theoretically critical implementation science . Translational Behavioral Medicine , 11 ( 8 ), 1617–1625. 10.1093/tbm/ibab008 [ PMC free article ] [ PubMed ] [ CrossRef ] [ Google Scholar ]
- Strunk KK, & Hoover PD (2019). Quantitative methods for social justice and equity: Theoretical and practical considerations. In Strunk KK & Locke LA (Eds.), Research methods for social justice and equity in education (pp. 191–201). Palgrave Macmillan. 10.1007/978-3-030-05900-2 [ CrossRef ] [ Google Scholar ]
- Taylor L (2017). What is data justice? The case for connecting digital rights and freedoms globally . Big Data & Society , 4 ( 2 ), 1–14. 10.1177/2053951717736335 [ CrossRef ] [ Google Scholar ]
- Taylor L (2016) The ethics of big data as a public good: Which public? Whose good? Philosophical Transactions of the Royal Society A 374 , 1–13. 10.1098/rsta.2016.0126 [ PMC free article ] [ PubMed ] [ CrossRef ] [ Google Scholar ]
- Trent M, Dooley DG, Dougé J, SECTION ON ADOLESCENT HEALTH, COUNCIL ON COMMUNITY PEDIATRICS, COMMITTEE ON ADOLESCENCE, Cavanaugh RM Jr, Lacroix AE, Fanburg J, Rahmandar MH, Hornberger LL, Schneider MB, Yen S, Chilton LA, Green AE, Dilley KJ, Gutierrez JR, Duffee JH, Keane VA, Krugman SD, McKelvey CD, Linton JM, Nelson JL, Mattson G, Breuner CC, Alderman EM, Grubb LK, Lee J, Powers ME, Rahmandar MH, Upadhya KK, & Wallace SB (2019). The Impact of racism on child and adolescent health . Pediatrics , 144 ( 2 ), e20191765. 10.1542/peds.2019-1765 [ PubMed ] [ CrossRef ] [ Google Scholar ]
- Viano S, & Baker DJ (2020). How administrative data collection and analysis can better reflect racial and ethnic identities . Review of Research in Education , 44 ( 1 ), 301–331. [ Google Scholar ]
- Vogt WP & Johnson B (2011). Dictionary of statistics & methodology: A nontechnical guide for the social sciences . Sage. [ Google Scholar ]
- Walsh C & Ross LF (2003). Are minority children under- or overrepresented in pediatric research? Pediatrics , 112 ( 4 ), 890–895. 10.1542/peds.112.4.890 [ PubMed ] [ CrossRef ] [ Google Scholar ]
- Waltz M, Fisher JA, Lyerly AD, & Walker RL (2021). Evaluating the National Institutes of Health's Sex as a Biological Variable Policy: Conflicting Accounts from the Front Lines of Animal Research . Journal of Women's Health , 30 ( 3 ), 348–354. 10.1089/jwh.2020.8674 [ PMC free article ] [ PubMed ] [ CrossRef ] [ Google Scholar ]
- Washington HA (2006). Medical apartheid: The dark history of medical experimentation on Black Americans from colonial times to the present . Doubleday. [ Google Scholar ]
- Wilson JP, Hugenberg K, & Rule NO (2017). Racial bias in judgments of physical size and formidability: From size to threat . Journal of Personality and Social Psychology , 113 ( 1 ), 59–80. 10.1037/pspi0000092 [ PubMed ] [ CrossRef ] [ Google Scholar ]
- Open access
- Published: 10 April 2024
Understanding social needs screening and demographic data collection in primary care practices serving Maryland Medicare patients
- Claire M. Starling 1 ,
- Marjanna Smith 1 ,
- Sadaf Kazi 2 , 3 ,
- Arianna Milicia 3 ,
- Rachel Grisham 4 ,
- Emily Gruber 4 ,
- Joseph Blumenthal 5 &
- Hannah Arem 1 , 6
BMC Health Services Research volume 24 , Article number: 448 ( 2024 ) Cite this article
60 Accesses
Metrics details
Health outcomes are strongly impacted by social determinants of health, including social risk factors and patient demographics, due to structural inequities and discrimination. Primary care is viewed as a potential medical setting to assess and address individual health-related social needs and to collect detailed patient demographics to assess and advance health equity, but limited literature evaluates such processes.
We conducted an analysis of cross-sectional survey data collected from n = 507 Maryland Primary Care Program (MDPCP) practices through Care Transformation Requirements (CTR) reporting in 2022. Descriptive statistics were used to summarize practice responses on social needs screening and demographic data collection. A stepwise regression analysis was conducted to determine factors predicting screening of all vs. a targeted subset of beneficiaries for unmet social needs.
Almost all practices (99%) reported conducting some form of social needs screening and demographic data collection. Practices reported variation in what screening tools or demographic questions were employed, frequency of screening, and how information was used. More than 75% of practices reported prioritizing transportation, food insecurity, housing instability, financial resource strain, and social isolation.
Conclusions
Within the MDPCP program there was widespread implementation of social needs screenings and demographic data collection. However, there was room for additional supports in addressing some challenging social needs and increasing detailed demographics. Further research is needed to understand any adjustments to clinical care in response to identified social needs or application of data for uses such as assessing progress towards health equity and the subsequent impact on clinical care and health outcomes .
Peer Review reports
There is increasing attention on the impact of factors such as economic stability, education, neighborhood, and built environment on healthcare outcomes and, in particular, how primary care settings can assess and address individual level health-related social needs (HRSN) [ 1 , 2 ]. In turn, the American Academy of Pediatrics (AAP) and the American Academy of Family Physicians (AAFP) both recommend that primary care providers screen and address social needs as part of routine primary care visits [ 3 ]. Patients with unmet social needs are at a higher risk of missing appointments, frequent emergency room visits, and hospitalization and rehospitalization [ 4 , 5 ]. Identifying social needs and collecting detailed patient demographics in primary care can be used to tailor care, allocate resources effectively, and advocate for equitable policies, making these workflows a critical step towards advancing health equity [ 1 , 2 , 3 ].
Despite acknowledgement of the importance of integrating social care in clinical settings including a recent mandate by the Centers for Medicare and Medicaid services for screening in inpatient settings, the implementation of social needs screening and demographic data collection is complex and resource intensive [ 6 , 7 ]. Furthermore, patients who screen positive for social needs may decline assistance to address those needs. These occurrences may prove frustrating to those conducting screening if they lack sufficient training on delivering screening or assisting individuals with addressing social needs [ 8 ]. Additionally, while many practices already collect basic demographic data such as age, ethnicity, and race, demographic information is not always collected in a culturally sensitive or inclusive manner. Demographic data collection processes are not standardized, and many demographic fields (e.g., education level, sexual orientation, and disability status) are sometimes not asked at all. As part of a contract to provide technical assistance to Maryland Primary Care Program (MDPCP) practices to support social needs screening and demographic data collection, we explored collected survey data to understand current practices around social needs screening and demographic data collection as well as potential areas for growth in screening delivery.
Study population
MDPCP is a voluntary program for eligible primary care practices that provides funding and support for the delivery of advanced primary care for Medicare beneficiaries throughout Maryland. MDPCP supports the overall health care transformation process and allows primary care providers to play an increased role in disease prevention, management of chronic disease, and prevention of unnecessary hospital utilization [ 9 ]. The primary goal of MDPCP is the sustainable transformation of primary care across Maryland to include all the elements of advanced primary care to support the health needs of state residents [ 9 ]. MDPCP is co-administered by teams at the Maryland Department of Health and the Center for Medicare and Medicaid Innovation (CMMI). At the time of the survey, the MDPCP network included n = 507 participating primary care practices representative of every county in Maryland.
MDPCP offers a combination of financial incentives and other supports tailored to primary care practices. These incentives encompass non-visit-based payments specifically designed for care coordination initiatives, as well as performance-based incentives, rewarding practices for achieving clinical quality, patient experience, and utilization benchmarks. In addition to financial incentives, MDPCP provides a variety of additional supports for care transformation MDPCP practices are paired with a Practice Transformation Coaches, who provide guidance, answer questions, and work directly with practices to improve processes that improve quality of care and decrease costs. In addition to Coaches, practices have access to the MDPCP Learning System encompassing a myriad of learning opportunities including User Groups, All-Practice Calls, and other collaborative forums for practices to learn from subject matter experts and fellow participants. Practices also have access to a handful of Guides including the Advancing Primary Care Guide, which provides information on MDPCP requirements, tactics for advancing the functions of primary care, and achieving care transformation. Additionally, practices have the option to partner with a Care Transformation Organization (CTO), who can assist with care management or other related patient services.
Data collection
Care transformation requirement (CTR) reporting questions ask MDPCP participants about progress on specific MDPCP requirements that span the five comprehensive primary care functions (Appendix 1 ). The five key functions of advanced primary care are care management, access and continuity, comprehensiveness, and coordination across the continuum of care, beneficiary and caregiver experience, and planned care for health outcomes. The questionnaire is developed by CMMI, and MDPCP participants respond in the online Centers for Medicare and Medicaid Services (CMS) program portal twice annually, as a requirement of program participation (Appendix 2 ). The survey used in this analysis was collected in the third quarter of 2022. This analysis was deemed exempt by the Georgetown/MedStar Institutional Review Board (Study 4698).
Statistical analysis
We used descriptive statistics to review social needs screening and demographic data collection responses from MDPCP practices . We conducted additional analysis to investigate responses by practice characteristics including practice size (small 1–2, medium 3–7, large 8 + providers) and hospital affiliation (yes or no). Further, a stepwise regression analysis was used to determine factors predicting the routine screening of beneficiaries for unmet social needs, comparing all beneficiaries to a specific targeted subsection. Variables used in the model were practice size, and hospital affiliation. 487 of the 507 records were used for regression analyses. We excluded practices if they did not report screening beneficiaries ( n = 4), practice size ( n = 1), or hospital affiliation status ( n = 15). SAS 9.4 (Cary, NC) was used in all analyses.
Practice responses on social needs screening and referral processes are presented in Table 1 . Among the MDPCP practices, nearly all reported some form of social needs screening for all (63%) or at least some (36%) beneficiaries. Many practices reported utilizing a social needs screening tool developed by the practice or affiliated health system (32%). Other practices reported screening using standardized screening tools, including, an unspecified standardized tool (21%); EHR-specific tool (19%); Accountable Health Communities (14%); and PRAPARE (5%). There was substantial variation in EHR vendors, with 23% of practices using EPIC, 17% using eClinicalWorks, 14% using Cerner, and 11% using Athenahealth. Approximately half (49.5%) of the practices reported conducting social needs screening annually, while 18% of practices reported conducting screenings at every visit and 15% when indicated based on reason for visit. Just over a quarter (27%) of practices reported linking responses to discrete ICD-10 or Social Determinants of Health (SDOH) Z codes.
Survey responses revealed variability regarding which patients receive social needs screening, screening frequency, EHR integration and use of Z-codes based on practice characteristics (Appendix 3 ). In an exploratory multivariate logistic regression we found that practices with a hospital affiliation were more likely to screen a targeted population than all patients (OR = 1.54, 95% CI = 1.05–2.27) and practices that were small- (1–2 providers) or medium-sized (3–7 providers) were more likely to screen all patients. (OR = 0.46, 95% CI = 0.26–0.80; OR = 0.46, 95% CI = 0.27–0.78, respectively; data shown in text only). Practices had the opportunity to describe which beneficiaries were targeted. Responses included individuals at high risk ( n = 67) or experiencing recent mental or clinical health events ( n = 18), participants in care management or care coordination programs ( n = 82), Health Equity Advancement Resource and Transformation (HEART) patients ( n = 25), and attendees of annual wellness visits ( n = 40).
When practices were asked to select social needs that they prioritize, common responses were transportation (93%), food insecurity (89%), housing instability (86%), financial resource strain (85%), and social isolation (84%) (Table 2 ). The least common needs prioritized were internet access (42%), phone access (46%), employment (48%), and language access (51%). Practices also reported which social needs were most challenging to support. The greatest challenges came with addressing housing instability (31%), internet access (31%), financial resource strain (30%), and medication affordability (30%).
Nearly all practices reported collecting patient demographics in some capacity (99%), with most practices reporting that demographic data are collected by a staff member (70%), collected at every visit (51%), annually (23%), or only at the patient’s initial visit (20%). Race and primary language were collected by nearly all practices (96%), gender identity was collected by 92%, relationship status by 87%, ethnicity by 87%, and employment status by 84% of practices. Other demographic factors were less commonly asked: only 49% of practices reported asking about sexual orientation, 48% asked about disability status, and 38% asked about highest level of education.
In this study we found that primary care practices participating in the MDPCP program overall had a high rate of social risk factor screening, with many using screeners that had been developed to meet individual practice needs. Commonly prioritized domains included transportation, food insecurity, housing instability, financial strain, and social isolation, the last being a commonly cited problem among older adults. Describing patterns of screening and demographics in this sample of practices across the state increase understanding of successes and challenges in real-world practice settings and informs potential future interventions.
Determining which patients should be screened and by whom in a busy primary care setting, as well as who can respond to identified needs, can be challenging. In our study there were differences both in which patients were screened and how often by practice [ 10 , 11 ]. Open ended responses suggested that among some MDPCP practices, screening was performed only for individuals who qualify for extra social assistance through the MDPCP program (i.e., those who qualify due to medical complexity and area deprivation index). Although we did not find other published literature focused specifically on Medicare patients at the state level, we found literature on programs focused on social needs screening among Medicaid populations in several states. Like Maryland practices, standardized measures and consistent approaches to measuring social needs have not been adopted or required in many states [ 12 , 13 , 14 ]. Further, a high percentage of the Maryland practices reported using home grown and standardized screening tools with additional questions to meet the practices’ needs. While the ability to aggregate social needs data across care settings can be challenging with different screeners, there is national movement to harmonize domains across various social risk factor screeners through the Gravity Project and the Office of the National Coordinator [ 12 , 15 ]. Notably, CMS has mandated social needs reporting in the inpatient setting beginning January 2024 for five specific domains, but has not specified a single tool or set of tools given that while there are some validated subsets of questions (e.g., Hunger Vital Signs), there is currently no gold standard tool [ 16 ]. Potential hurdles in requiring specific tools may include limitations on EHR technology, referral processes, and provider or staff level comfort and training in asking specific questions. Furthermore, implementing screening without supports for training the staff on trauma-informed approaches and how to respond to identified needs has the potential to cause more harm than benefit to patients. Thus, toolkits established by various professional societies and public health societies may be useful to determine which tools are most appropriate for a given practice and how to integrate them into care where practices have not yet started screening or encounter challenges [ 17 , 18 , 19 ].
Regarding practices with a hospital affiliation being more likely to screen a targeted population, one possibility is that practices affiliated with hospitals may have access to additional resources and supports that facilitate targeted screening efforts. Hospitals often have established practices including social risk factor screening for targeted subpopulations to address costly hospital readmissions, which may encourage affiliated practices to deliver more targeted screening practices. While it is unclear why small or medium-sized practices were more likely to screen all patients than a sub-population, it may have to do with more autonomy in workflow process, less customization of the EHR to target sub-populations, or differences in staffing and provider to patient ratios. While we cannot explain these differences from the survey alone, findings suggest that the size and affiliation of practices play a role in their screening practices, highlighting the importance of considering practice characteristics when designing specific supportive interventions or policies aimed at increasing screening rates.
It is important to highlight that MDPCP practices have achieved impressive levels of social needs screening and demographic data collection implementation. This success could be attributed largely to the program’s requirements and incentives to screen beneficiaries for social needs and collect demographic information. Additionally, the program provides technical support and resources to meet these requirements and to stand up social needs screening workflows if not already in place. By joining MDPCP, participating practices have demonstrated a commitment to advanced primary care, further indicating MDPCP participation may be associated with higher uptake of these workflows, as opposed to primary care practices who do not participate in similar value-based programs. Other states considering such programs may look to some of these supports when rolling out new requirements or incentives.
While the findings highlight the high level of social needs screening and demographic data collection, challenges in addressing identified needs may also be due to various factors including complexity of workflows and staffing, patients with social needs declining assistance, or limited local resource availability [ 20 ]. Previous research suggests patients may decline social needs assistance in healthcare settings if they do not feel like they need help, are confused about what is offered, are not confident that the assistance would be helpful, have experienced previous negative experiences, or feel fear and mistrust related to disclosing personal information [ 8 ]. In areas that posed the greatest referral challenges, policy efforts may be needed to deliver services and bridge the gaps to access. For example, the challenge of addressing housing needs is not newly identified; previous literature has shown increasing costs and declining supply have contributed to national housing availability and affordability challenges [ 21 , 22 ]. Medication cost continues to be a major problem cited in the literature, especially for older populations with a higher incidence of chronic diseases [ 23 , 24 ]. Financial strain among individuals often poses a challenge as financial needs fluctuate frequently, and changes can be dramatic; further, these changing needs over time are often not resolved by a one-time intervention and require long-term involvements [ 11 ]. Though research on the effects of internet access and health outcomes is still emerging, literature suggests investment in digital infrastructure by federal, state, and local governments is needed for further development of the internet as a means of addressing long-standing inequality in health [ 25 , 26 ]. While food insecurity and transportation were top needs prioritized within MDPCP practices, they did not present the same level of challenge to practices, perhaps due to wider availability of resources, partnerships, and supports such as transportation vouchers.
Although addressing connection to resources continues to be a challenge for practices, there are opportunities to leverage information from social needs screenings and demographic data collection in several other ways to improve care. Aggregate screening and demographic data can be used for quality improvement initiatives within primary care practices by analyzing trends and patterns in social needs data to help practices identify areas of unmet need, track outcomes, and update protocols for screening and referral processes. Additionally, data can be used to advocate for policy changes to address systemic issues affecting patients’ health outcomes. However, challenges in utilizing information from social needs screening and demographic data collection may still exist due to limited resources and capacity and lack of provider awareness and training availability.
Increased collection of detailed demographic data, particularly regarding sexual orientation, education level, and disability status presents an opportunity for improvement in primary care. Furthermore, collecting detailed demographic information can better allow practices to understand the need for targeted educational materials, track quality indicators, and address challenges faced by historically marginalized populations [ 26 , 27 ]. Still, even with good data collection approaches, some practices do not have the infrastructure or resources to analyze data to assess disparities in care or outcomes.
This study's strengths lie in its comprehensive analysis of a diverse range of primary care practices across Maryland. The inclusion of 507 practices with variations in size, location, and demographics enhances the representativeness of the findings and improves the generalizability of the results to a broader population. Consequently, the findings derived from studying a large population can contribute to a stronger evidence base for decision-making in healthcare and support the development of effective interventions and policies. A limitation of the study is the reliance on self-report, which may depend on the participants’ perspectives. Additionally, MDPCP practices meet eligibility criteria and voluntarily select to join the program, so these practices may be better equipped to join a value-based program that includes requirements or incentives to screen for social needs. Despite the limitations, our findings are novel in that few published studies highlight current practices at scale on social risk factor screening and referral in outpatient primary care settings for adults. Future research is warranted to show what strategies effectively increase uptake and drive meaningful change in social-needs responsive healthcare delivery.
MDPCP practices have demonstrated widespread adoption of social risk factor screenings and needs prioritization. While practices have implemented strategies to link patients to resources to address needs, challenges remain with providing social needs resources to beneficiaries from the primary care setting. Additionally, there is room for improvement in collecting certain demographic data fields within primary care practices. As the present analysis was based on cross-sectional data, future studies are needed to understand how to effect change in implementing or scaling social risk factor screening and detailed demographic data collection at the practice level. Additionally, future work is needed to understand how care is adjusted in response to identified social needs and how that impacts outcomes at the patient level.
Availability of data and materials
To access the datasets examined in this study, interested parties must follow the procedure outlined by the CMS. Requests should be submitted through the CMS website (cms.gov), and any queries can be directed to [email protected].
Abbreviations
Maryland Primary Care Program
Care Transformation Requirements
Center for Medicare and Medicaid Innovation
Centers for Medicare and Medicaid Services
Electronic Health Record
Social Determinants of Health
Social Determinants of Health. World Health Organization, 2023, at https://www.who.int/health-topics/social-determinants-of-health#tab=tab_1 . Accessed 9 Apr 2023.
DeVoe JE, Bazemore AW, Cottrell EK, et al. Perspectives in Primary Care: A Conceptual Framework and Path for Integrating Social Determinants of Health into Primary Care Practice. Ann Fam Med. 2016;14(2):104–8. https://doi.org/10.1370/afm.1917 .
Article PubMed PubMed Central Google Scholar
Lax Y, Bathory E, Braganza S. Pediatric primary care and subspecialist providers’ comfort, attitudes and practices screening and referring for social determinants of health. BMC Health Serv Res. 2021;21(1):956. https://doi.org/10.1186/s12913-021-06975-3 . PMID:34511119;PMCID:PMC8436516.
Andermann A; CLEAR Collaboration. Taking action on the social determinants of health in clinical practice: a framework for health professionals. CMAJ. 2016;188(17–18):E474–83. https://doi.org/10.1503/cmaj.160177 .
Article Google Scholar
Berkowitz SA, Seligman HK, Meigs JB, Basu S. Food insecurity, healthcare utilization, and high cost: a longitudinal cohort study. Am J Manag Care. 2018;24(9):399–404. https://pubmed.ncbi.nlm.nih.gov/33989068/ . PMID: 30222918; PMCID: PMC6426124.
PubMed PubMed Central Google Scholar
McQueen A, Li L, Herrick CJ, Verdecias N, Brown DS, Broussard DJ, Smith RE, Kreuter M. Social Needs, Chronic Conditions, and Health Care Utilization among Medicaid Beneficiaries. Popul Health Manag. 2021;24(6):681–90. https://doi.org/10.1089/pop.2021.0065 . PMID: 33989068; PMCID: PMC8713253.
National Academies of Sciences, Engineering, and Medicine. Integrating Social Care into the Delivery of Health Care: Moving Upstream to Improve the Nation’s Health. Washington: The National Academies Press; 2019. https://doi.org/10.17226/25467 .
Book Google Scholar
Pfeiffer EJ, De Paula CL, Flores WO, Lavallee AJ. Barriers to Patients’ Acceptance of Social Care Interventions in Clinic Settings. Am J Prev Med. 2022;63(3 Suppl 2):S116–21. https://doi.org/10.1016/j.amepre.2022.03.035 . Epub 2022 Aug 17 PMID: 35987523.
Article PubMed Google Scholar
Centers for Medicare and Medicaid Services, Centers for Medicare and Medicaid Innovation Sate Innovations Group. https://innovation.cms.gov/files/x/mdtcocm-rfa.pdf . Accessed 9 Apr 2023.
Sandhu S, Xu J, Eisenson H, Prvu Bettger J. Workforce Models to Screen for and Address Patients’ Unmet Social Needs in the Clinic Setting: A Scoping Review. J Prim Care Community Health. 2021;12:21501327211021020. https://doi.org/10.1177/21501327211021021 . PMID: 34053370; PMCID: PMC8772357.
Kreuter MW, Thompson T, McQueen A, Garg R. Addressing Social Needs in Health Care Settings: Evidence, Challenges, and Opportunities for Public Health. Annu Rev Public Health. 2021;42:329–44. https://doi.org/10.1146/annurev-publhealth-090419-102204 . Epub 2021 Dec 16. PMID: 33326298; PMCID: PMC8240195.
Measuring Social Determinants of Health among Medicaid Beneficiaries: Early State Lessons. Center for Health Care Strategies, Inc. 2016. https://www.chcs.org/media/CHCS-SDOH-Measures-Brief_120716_FINAL.pdf . Accessed 21 June 2023.
States Reporting Social Determinants of Health Related Policies Required in Medicaid Managed Care. Kaiser Family Foundation. 2022. https://www.kff.org/other/state-indicator/states-reporting-social-determinant-of-health-related-policies-required-in-medicaid-managed-care-contracts/?currentTimeframe=0&sortModel=%7B%22colId%22:%22Location%22,%22sort%22:%22asc%22%7D . Accessed 21 June 2023.
Social Determinants of Health Measurement Work Group: Final Report. Oregon Health Authority. 2021. https://www.oregon.gov/oha/HPA/ANALYTICS/SDOH%20Page%20Documents/SDOH%20final%20report%202_10_21.pdf . Accessed 21 June 2023.
The Gravity Project: Accelerating National Social Determinants of Health (SDOH) Data Standards. Gravity Project. 2022. https://confluence.hl7.org/download/attachments/46892727/Gravity%20Overview%20One%20Pager%2020220209.pdf?version=1&modificationDate=1644529823670&api=v2 . Accessed 21 June 2023.
Quality ID #487: Screening for Social Drivers of Health. Centers for Medicare & Medicaid Services. 2022. https://qpp.cms.gov/docs/QPP_quality_measure_specifications/CQM-Measures/2023_Measure_487_MIPSCQM.pdf . Accessed 21 June 2023.
Identifying and Addressing Social Needs in Primary Care Settings. Agency for Healthcare Research and Quality. 2021. https://www.ahrq.gov/sites/default/files/wysiwyg/evidencenow/tools-and-materials/social-needs-tool.pdf . Accessed 21 June 2023.
The Health Leads Social Health Data Toolkit. Health Leads. 2021. https://healthleadsusa.org/wp-content/uploads/2021/02/Health-Leads-Social-Health-Data-Toolkit.pdf . Accessed 21 June 2023.
A Guide to Using the Accountable Health Communities Health-Related Social Needs Screening Took: Promising Practices and Key Insights. Centers for Medicare & Medicaid Services. 2023. https://www.cms.gov/priorities/innovation/media/document/ahcm-screeningtool-companion . Accessed 21 June 2023.
Beidler LB, Razon N, Lang H, Fraze TK. “More than just giving them a piece of paper”: Interviews with Primary Care on Social Needs Referrals to Community-Based Organizations. J Gen Intern Med. 2022;37(16):4160–7. https://doi.org/10.1007/s11606-022-07531-3 . Epub 2022 Apr 14. PMID: 35426010; PMCID: PMC9708990.
Child Care and Housing: Big Expenses with Too Little Help Available. Center on Budget and Policy Priortities. 2019. https://www.cbpp.org/research/housing/child-care-and-housing-big-expenses-with-too-little-help-available . Accessed 13 July 2023.
Key facts about housing affordability in the U.S. Pew Research Center. 2022. https://www.pewresearch.org/short-reads/2022/03/23/key-facts-about-housing-affordability-in-the-u-s/ . Accessed 13 July 2023.
Soumerai SB, Pierre-Jacques M, Zhang F, et al. Cost-related medication nonadherence among elderly and disabled medicare beneficiaries: a national survey 1 year before the medicare drug benefit. Arch Intern Med. 2006;166(17):1829–35. https://doi.org/10.1001/archinte.166.17.1829 . PMID: 17000938.
Naci H, Soumerai SB, Ross-Degnan D, Zhang F, Briesacher BA, Gurwitz JH, Madden JM. Medication affordability gains following Medicare Part D are eroding among elderly with multiple chronic conditions. Health Aff (Millwood). 2014;33(8):1435–43. https://doi.org/10.1377/hlthaff.2013.1067 . PMID:25092846;PMCID:PMC4340076.
Studies and Data Analytics on Broadband and Health. Federal Communications Commision. 2022. https://www.fcc.gov/health/sdoh/studies-and-data-analytics . Accessed 13 July 2023.
Yu J, Meng S. Impacts of the Internet on Health Inequality and Healthcare Access: A Cross-Country Study. Front Public Health. 2022;9(10):935608. https://doi.org/10.3389/fpubh.2022.935608 . PMID:35757602;PMCID:PMC9218541.
Grasso C, McDowell MJ, Goldhammer H, Keuroghlian AS. Planning and implementing sexual orientation and gender identity data collection in electronic health records. J Am Med Inform Assoc. 2019;26(1):66–70. https://doi.org/10.1093/jamia/ocy137 . PMID:30445621;PMCID:PMC6657380.
Download references
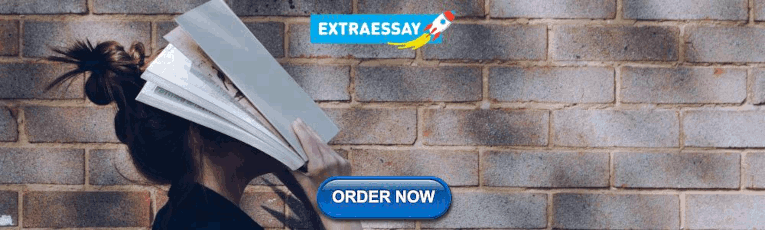
Acknowledgements
Not applicable
This study was supported by the Centers for Disease Control and Prevention’s National Initiative to Address COVID-19 Health Disparities Among Populations at High-Risk and Underserved, Including Racial and Ethnic Minority Populations and Rural Communities grant number OT21-2103 through the Maryland Department of Health.
Author information
Authors and affiliations.
Implementation Science, Healthcare Delivery Research Program, MedStar Health Research Institute, 6525 Belcrest Road, Suite 700, Hyattsville, MD, 20782, USA
Claire M. Starling, Marjanna Smith & Hannah Arem
Department of Emergency Medicine, Georgetown University School of Medicine, 3900 Reservoir Road, Washington, NWDC, 20007, USA
National Center for Human Factors in Healthcare, MedStar Health Research Institute, 3007 Tilden St.Suite 6N, Washington, NWDC, 20008, USA
Sadaf Kazi & Arianna Milicia
Maryland Primary Care Program, Maryland Department of Health, 201 W. Preston Street, Baltimore, MD, 21201, USA
Rachel Grisham & Emily Gruber
MedStar Center for Biostatistics, Informatics and Data Science, MedStar Health Research Institute, 3007 Tilden St.Suite 6N, Washington, NWDC, 20008, USA
Joseph Blumenthal
Department of Oncology, Georgetown University School of Medicine, 3900 Reservoir Road, Washington, NWDC, 20007, USA
Hannah Arem
You can also search for this author in PubMed Google Scholar
Contributions
CMS and HA analyzed and interpreted the data and drafted the manuscript. MS, SK, AM, RG, EG, and JB contributed to revising the manuscript. All authors approved the version to be published and agreed to be accountable for the accuracy and integrity of the data.
Corresponding author
Correspondence to Claire M. Starling .
Ethics declarations
Ethics approval and consent to participate.
No consent was obtained to collect this data originally as it is mandated as part of CMS reporting. Using this data in the aggregate for publication was reviewed by the Georgetown University/Medstar Health IRB and deemed exempt (study 4698, modification approval date: December 15, 2022). CMS and the Maryland Department of Health approved the Georgetown/MedStar IRB decision.
Consent for publication
Not Applicable.
Competing interests
The authors declare no competing interests.
Additional information
Publisher’s note.
Springer Nature remains neutral with regard to jurisdictional claims in published maps and institutional affiliations.
Supplementary Information
Supplementary material 1., supplementary material 2., supplementary material 3., rights and permissions.
Open Access This article is licensed under a Creative Commons Attribution 4.0 International License, which permits use, sharing, adaptation, distribution and reproduction in any medium or format, as long as you give appropriate credit to the original author(s) and the source, provide a link to the Creative Commons licence, and indicate if changes were made. The images or other third party material in this article are included in the article's Creative Commons licence, unless indicated otherwise in a credit line to the material. If material is not included in the article's Creative Commons licence and your intended use is not permitted by statutory regulation or exceeds the permitted use, you will need to obtain permission directly from the copyright holder. To view a copy of this licence, visit http://creativecommons.org/licenses/by/4.0/ . The Creative Commons Public Domain Dedication waiver ( http://creativecommons.org/publicdomain/zero/1.0/ ) applies to the data made available in this article, unless otherwise stated in a credit line to the data.
Reprints and permissions
About this article
Cite this article.
Starling, C.M., Smith, M., Kazi, S. et al. Understanding social needs screening and demographic data collection in primary care practices serving Maryland Medicare patients. BMC Health Serv Res 24 , 448 (2024). https://doi.org/10.1186/s12913-024-10948-7
Download citation
Received : 22 January 2024
Accepted : 03 April 2024
Published : 10 April 2024
DOI : https://doi.org/10.1186/s12913-024-10948-7
Share this article
Anyone you share the following link with will be able to read this content:
Sorry, a shareable link is not currently available for this article.
Provided by the Springer Nature SharedIt content-sharing initiative
- Social needs screening
- Demographic data collection
- Primary care
- Community resources
BMC Health Services Research
ISSN: 1472-6963
- General enquiries: [email protected]

- SUGGESTED TOPICS
- The Magazine
- Newsletters
- Managing Yourself
- Managing Teams
- Work-life Balance
- The Big Idea
- Data & Visuals
- Reading Lists
- Case Selections
- HBR Learning
- Topic Feeds
- Account Settings
- Email Preferences
What Demographics Forms Say About Inclusivity at Your Company
- Devon Proudfoot

Two low-cost, low-risk suggestions that can boost belonging.
For members of minority groups, finding their identity omitted in an organization’s demographics forms can make them question whether their identity is valued and respected by that organization. The seemingly mundane choices companies make when designing demographics forms — such as those used in job applications or employee engagement surveys — can have relatively major implications. Decision makers may not recognize these implications, though. In this article, the authors summarize recent research on identity omission in demographics forms and offer two low-cost, low-risk suggestions for how organizations can boost the inclusivity of the demographics forms they use.
Picture yourself filling out a job application online. You arrive at a question asking you to provide some demographic information, like your gender or race. Although several response options are provided, you notice that your own identity group is not included in the list. At this point in the process, how would you feel about this potential employer? Would you expect to feel a sense of belonging if hired?

- SF Sean Fath is an Assistant Professor of Organizational Behavior at Cornell’s ILR School. His research interests include managerial decision making, bias reduction in social evaluations, and perceptions of social and organizational hierarchy.
- DP Devon Proudfoot is an Assistant Professor of Human Resource Studies at Cornell’s ILR School. Her research focuses on identity, stereotyping and bias, and creativity at work.
Partner Center
Thank you for visiting nature.com. You are using a browser version with limited support for CSS. To obtain the best experience, we recommend you use a more up to date browser (or turn off compatibility mode in Internet Explorer). In the meantime, to ensure continued support, we are displaying the site without styles and JavaScript.
- View all journals
- My Account Login
- Explore content
- About the journal
- Publish with us
- Sign up for alerts
- Open access
- Published: 07 April 2023
Using machine learning to predict student retention from socio-demographic characteristics and app-based engagement metrics
- Sandra C. Matz 1 ,
- Christina S. Bukow 2 ,
- Heinrich Peters 1 ,
- Christine Deacons 3 ,
- Alice Dinu 5 na1 &
- Clemens Stachl 4
Scientific Reports volume 13 , Article number: 5705 ( 2023 ) Cite this article
7793 Accesses
2 Citations
35 Altmetric
Metrics details
- Human behaviour
An Author Correction to this article was published on 21 June 2023
This article has been updated
Student attrition poses a major challenge to academic institutions, funding bodies and students. With the rise of Big Data and predictive analytics, a growing body of work in higher education research has demonstrated the feasibility of predicting student dropout from readily available macro-level (e.g., socio-demographics or early performance metrics) and micro-level data (e.g., logins to learning management systems). Yet, the existing work has largely overlooked a critical meso-level element of student success known to drive retention: students’ experience at university and their social embeddedness within their cohort. In partnership with a mobile application that facilitates communication between students and universities, we collected both (1) institutional macro-level data and (2) behavioral micro and meso-level engagement data (e.g., the quantity and quality of interactions with university services and events as well as with other students) to predict dropout after the first semester. Analyzing the records of 50,095 students from four US universities and community colleges, we demonstrate that the combined macro and meso-level data can predict dropout with high levels of predictive performance (average AUC across linear and non-linear models = 78%; max AUC = 88%). Behavioral engagement variables representing students’ experience at university (e.g., network centrality, app engagement, event ratings) were found to add incremental predictive power beyond institutional variables (e.g., GPA or ethnicity). Finally, we highlight the generalizability of our results by showing that models trained on one university can predict retention at another university with reasonably high levels of predictive performance.
Similar content being viewed by others
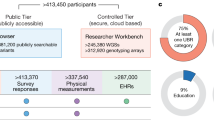
Genomic data in the All of Us Research Program
The All of Us Research Program Genomics Investigators
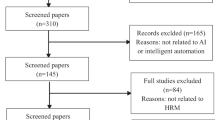
Ethics and discrimination in artificial intelligence-enabled recruitment practices
Zhisheng Chen
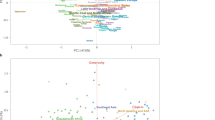
Principal component analysis
Michael Greenacre, Patrick J. F. Groenen, … Elena Tuzhilina
Introduction
In the US, only about 60% of full-time students graduate from their program 1 , 2 with the majority of those who discontinue their studies dropping out during their first year 3 These high attrition rates pose major challenges to students, universities, and funding bodies alike 4 , 5 .
Dropping out of university without a degree negatively impacts students’ finances and mental health. Over 65% of US undergraduate students receive student loans to help pay for college, causing them to incur heavy debts over the course of their studies 6 . According to the U.S. Department of Education, students who take out a loan but never graduate are three times more likely to default on loan repayment than students who graduate 7 . This is hardly surprising, given that students who drop out of university without a degree, earn 66% less than university graduates with a bachelor's degree and are far more likely to be unemployed 2 . In addition to financial losses, the feeling of failure often adversely impacts students’ well-being and mental health 8 .
At the same time, student attrition negatively impacts universities and federal funding bodies. For universities, student attrition results in an average annual revenue reduction of approximately $16.5 billion per year through the loss of tuition fees 9 , 10 . Similarly, student attrition wastes valuable resources provided by states and federal governments. For example, the US Department of Education Integrated Postsecondary Education Data System (IPEDS) shows that between 2003 and 2008, state and federal governments together provided more than $9 billion in grants and subsidies to students who did not return to the institution where they were enrolled for a second year 11 .
Given the high costs of attrition, the ability to predict at-risk students – and to provide them with additional support – is critical 12 , 13 . As most dropouts occur during the first year 14 , such predictions are most valuable if they can identify at-risk students as early as possible 13 , 15 , 16 . The earlier one can identify students who might struggle, the better the chances that interventions aimed at protecting them from gradually falling behind – and eventually discontinuing their studies – will be effective 17 , 18 .
Indicators of student retention
Previous research has identified various predictors of student retention, including previous academic performance, demographic and socio-economic factors, and the social embeddedness of a student in their home institution 19 , 20 , 21 , 22 , 23 .
Prior academic performance (e.g., high school GPA, SAT and ACT scores or college GPA) has been identified as one of the most consistent predictors of student retention: Students who are more successful academically are less likely to drop out 17 , 21 , 24 , 25 , 26 , 27 , 28 , 29 . Similarly, research has highlighted the role of demographic and socio-economic variables, including age, gender, and ethnicity 12 , 19 , 25 , 27 , 30 as well as socio-economic status 31 in predicting a students’ likelihood of persisting. For example, women are more likely to continue their studies than men 12 , 30 , 32 , 33 while White and Asian students are more likely to persist than students from other ethnic groups 19 , 27 , 30 . Moreover, a student’s socio-economic status and immediate financial situation have been shown to impact retention. Students are more likely to discontinue their studies if they are first generation students 34 , 35 , 36 or experience high levels of financial distress, (e.g., due to student loans or working nearly full time to cover college expenses) 37 , 38 . In contrast, students who receive financial support that does not have to be repaid post-graduation are more likely to complete their degree 39 , 40 .
While most of the outlined predictors of student retention are relatively stable intrapersonal characteristics and oftentimes difficult or costly to change, research also points to a more malleable pillar of retention: the students’ experience at university. In particular, the extent to which they are successfully integrated and socialized into the institution 16 , 22 , 41 , 42 . As Bean (2005) notes, “few would deny that the social lives of students in college and their exchanges with others inside and outside the institution are important in retention decisions” (p. 227) 41 . The extent to which a student is socially integrated and embedded into their institution has been studied in a number of ways, relating retention to the development of friendships with fellow students 43 , the student’s position in the social networks 16 , 29 , the experience of social connectedness 44 and a sense of belonging 42 , 45 , 46 . Taken together, these studies suggest that interactions with peers as well as faculty and staff – for example through participation in campus activities, membership of organizations, and the pursuit of extracurricular activities – help students better integrate into university life 44 , 47 . In contrast, a lack of social integration resulting from commuting (i.e., not living on campus with other students) has shown to negatively impact a student’s chances of completing their degree 48 , 49 , 50 , 51 . In short, the stronger a student is embedded and feels integrated into the university community – particularly in their first year – the less likely the student will drop out 42 , 52 .
Predicting retention using machine learning
A large proportion of research on student attrition has focused on understanding and explaining drivers of student retention. However, alongside the rise of computational methods and predictive modeling in the social sciences 53 , 54 , 55 , educational researchers and practitioners have started exploring the feasibility and value of data-driven approaches in supporting institutional decision making and educational effectiveness (for excellent overviews of the growing field see 56 , 57 ). In line with this broader trend, a growing body of work has shown the potential of predicting student dropout with the help of machine learning. In contrast to traditional inferential approaches, machine learning approaches are predominantly concerned with predictive performance (i.e., the ability to accurately forecast behavior that has not yet occurred) 54 . In the context of student retention this means: How accurately can we predict whether a student is going to complete or discontinue their studies (in the future) by analyzing their demographic and socio-economic characteristics, their past and current academic performance, as well as their current embeddedness in the university system and culture?
Echoing the National Academy of Education’s statement (2017) that “in the educational context, big data typically take the form of administrative data and learning process data, with each offering their own promise for educational research” (p.4) 58 , the vast majority of existing studies have focused on the prediction of student retention from demographic and socio-economic characteristics as well as students’ academic history and current performance 13 , 59 , 60 , 61 , 62 , 63 , 64 , 65 , 66 . In a recent study, Aulck and colleagues trained a model on the administrative data of over 66,000 first-year students enrolled in a public US university (e.g., race, gender, highschool GPA, entrance exam scores and early college performance/transcript data) to predict whether they would re-enroll in the second year and eventually graduate 59 . Specifically, they used a range of linear and non-linear machine learning models (e.g., regularized logistic regression, k-nearest neighbor, random forest, support vector machine, and gradient boosted trees) to predict retention out-of-sample using a standard cross-validation procedures. Their model was able to predict dropouts with an accuracy of 88% and graduation with an accuracy of 81% (where 50% is chance).
While the existing body of work provides robust evidence for the potential of predictive models for identifying at-risk students, it is based on similar sets of macro-level data (e.g., institutional data, academic performance) or micro-level data (e.g., click-stream data). Almost entirely absent from this research is data on students’ daily experience and engagement with both other students and the university itself (meso-level). Although there have been a small number of studies trying to capture part of this experience by inferring social networks from smart card transactions that were made by students in the same time and place 16 or engagement metrics with an open online course 67 , none of the existing work has offered a more holistic and comprehensive view on students’ daily experience. One potential explanation for this gap is that information about students’ social interactions with classmates or their day-to-day engagement with university services and events is difficult to track. While universities often have access to demographic or socio-economic variables through their Student Information Systems (SISs), and can easily track their academic performance, most universities do not have an easy way of capturing student’s deeper engagement with the system.
Research overview
In this research, we partner with an educational software company – READY Education – that offers a virtual one-stop interaction platform in the form of a smartphone application to facilitate communication between students, faculty, and staff. Students receive relevant information and announcements, can manage their university activities, and interact with fellow students in various ways. For example, the app offers a social media experience like Facebook, including private messaging, groups, public walls, and friendships. In addition, it captures students’ engagement with the university asking them to check into events (e.g., orientation, campus events, and student services) using QR code functionality and prompting them to rate their experience afterwards (see Methods for more details on the features we extracted from this data). As a result, the READY Education app allows us to observe a comprehensive set of information about students that include both (i) institutional data (i.e., demographic, and socio-economic characteristics as well as academic performance), and (ii) their idiosyncratic experience at university captured by their daily interactions with other students and the university services/events. Combining the two data sources captures a student’s profile more holistically and makes it possible to consider potential interactions between the variable sets. For example, being tightly embedded in a social support network of friends might be more important for retention among first-generation students who might not receive the same level of academic support or learn about implicit academic norms and rules from their parents.
Building on this unique dataset, we use machine learning models to predict student retention (i.e., dropout) from both institutional and behavioral engagement data. Given the desire to identify at-risk students as early as possible, we only use information gathered in the students’ first semester to predict whether the student dropped out at any point in time during their program. To thoroughly validate and scrutinize our analytical approach, generate insights for potential interventions, and probe the generalizability of our predictive models across different universities, we investigate the following three research questions:
How accurately can we predict a student's likelihood of discontinuing their studies using information from the first term of their studies (i.e., institutional data, behavioral engagement data, and a combination of both)?
Which features are the most predictive of student retention?
How well do the predictive models generalize across universities (i.e., how well can we predict student retention of students from one university if we use the model trained on data from another university and vice versa)?
Participants
We analyze de-identified data from four institutions with a total of 50,095 students (min = 476, max = 45,062). All students provided informed consent to the use of the anonymized data by READY Education and research partners. All experimental protocols were approved by the Columbia University Ethics Board, and all methods carried out were in accordance with the Board’s guidelines and regulations. The data stem from two sources: (a) Institutional data and (b) behavioral engagement data. The institutional data collected by the universities contain socio-demographics (e.g., gender, ethnicity), general study information (e.g., term of admission, study program), financial information (e.g., pell eligibility), students’ academic achievement scores (e.g., GPA, ACT) as well as the retention status. The latter indicated whether students continued or dropped out and serves as the outcome variable. As different universities collect different information about their students, the scope of institutional data varied between universities. Table 1 provides a descriptive overview of the most important sociodemographic characteristics for each of the four universities. In addition, it provides a descriptive overview of the app usage, including the average number of logs per student, the total number of sessions and logs, as well as the percentage of students in a cohort using the app (i.e., coverage). The broad coverage of students using the app, ranging between 70 and 98%, results in a largely representative sample of the student populations at the respective universities.
Notably, Universities 1–3 are traditional university campuses, while University 4 is a combination of 16 different community colleges. Given that there is considerable heterogeneity across campuses, the predictive accuracies for University 4 are a-priori expected to be lower than those observed for universities 1–3 (and partly speak to the generalizability of findings already). The decision to include University 4 as a single entity was based on the fact that separating out the 16 colleges would have resulted in an over-representation of community colleges that all share similar characteristics thereby artificially inflating the observed cross-university accuracies. Given these limitations (and the fact that the University itself collapsed the college campuses for many of their internal reports), we decided to analyze it as a single unit, acknowledging that this approach brings its own limitations.
The behavioral engagement data were generated through the app (see Table 1 for the specific data collection windows at each University). Behavioral engagement data were available in the form of time-stamped event-logs (i.e., each row in the raw data represented a registered event such as a tab clicked, a comment posted, a message sent). Each log could be assigned to a particular student via an anonymized, unique identifier. Across all four universities, the engagement data contained 7,477,630 sessions (Mean = 1,869,408, SD = 3,329,852) and 17,032,633 logs (Mean = 4,258,158, SD = 6,963,613) across all universities. For complete overview of all behavioral engagement metrics including a description see Table S1 in the Supplementary Materials.
Pre-processing and feature extraction
As a first step, we cleaned both the institutional and app data. For the institutional data, we excluded students who did not use the app and therefore could not be assigned a unique identifier. In addition, we excluded students without a term of admission to guarantee that we are only observing the students’ first semester. Lastly, we removed duplicate entries resulting from dual enrollment in different programs. For the app usage data, we visually inspected the variables in our data set for outliers that might stem from technical issues. We pre-processed data that reflected clicking through the app, named “clicked_[…]” and “viewed_[…]” (see Table S1 in the Supplementary Materials). A small number of observations showed unrealistically high numbers of clicks on the same tab in a very short period, which is likely a reflection of a student repeatedly clicking on a tab due to long loading time or other technical issues. To avoid oversampling these behaviors, we removed all clicks of the same type which were made by the same person less than one minute apart.
We extracted up to 462 features for each university across two broad categories: (i) institutional features and (ii) engagement features, using evidence from previous research as a reference point (see Table S2 in the Supplementary Materials for a comprehensive overview of all features and their availability for each of the universities). Institutional features contain students’ demographic, socio-economic and academic information. The engagement features represent the students’ behavior during their first term of studies. They can be further divided into app engagement and community engagement. The app engagement features represent the students’ behavior related to app usage, such as whether the students used the app before the start of the semester, how often they clicked on notifications or the community tabs, or whether their app use increased over the course of the semester. The community involvement features reflect social behavior and interaction with others, e.g., the number of messages sent, posts and comments made, events visited or a student’s position in the network as inferred from friendships and direct messages. Importantly, many of the features in our dataset will be inter-correlated. For example, living in college accommodation could signal higher levels of socio-economic status, but also make it more likely that students attend campus events and connect with other students living on campus. While intercorrelations among predictors is a challenge with standard inferential statistical techniques such as regression analyses, the methods we apply in this paper can account for a large number of correlated predictors.
Institutional features were directly derived from the data recorded by the institutions. As noted above, not all features were available for all universities, resulting in slightly different feature sets across universities. The engagement features were extracted from the app usage data. As we focused on an early prediction of drop-out, we restricted the data to event-logs that were recorded in the respective students' first term. Notably, the data captures students’ engagement as a time-stamped series of events, offering fine-grained insights into their daily experience. For reasons of simplicity and interpretability (see research question 2), we collapse the data into a single entry for each student. Specifically, we describe a student’s overall experience during the first semester, by calculating distribution measures for each student such as the arithmetic mean, standard deviation, kurtosis, skewness, and sum values. For example, we calculate how many daily messages a particular student sent or received during their first semester, or how many campus events they attended in total. However, we also account for changes in a student’s behavior over time by calculating more complex features such as entropy (e.g., the extent to which a person has frequent contact with few people or the same degree of contact with many people) and the development of specific behaviors over time measured by the slope of regression analyses, as well as features representing the regularity of behavior (e.g., the deviation of time between sending messages). Overall, the feature set was aimed at describing a student’s overall engagement with campus resources and other students during the first semester as well as changed in engagement over time. Finally, we extracted some of the features separately for weekdays and weekends to account for differences and similarities in students’ activities during the week and the weekend. For example, little social interaction on weekdays might predict retention differently than little social interaction on the weekend.
We further cleaned the data by discarding participants for whom the retention status was missing and those in which 95% or more of the values were zero or missing. Furthermore, features were removed if they showed little or no variance across participants, which makes them essentially meaningless in a prediction task. Specifically, we excluded numerical features which showed the same values for more than 90% of observations and categorical features which showed the same value for all observations.
In addition to these general pre-processing procedures, we integrated additional pre-processing steps into the resampling prior to training the models to avoid an overestimation of model performance 68 . To prevent problems with categorical features that occur when there are fewer levels in the test than in the training data, we first removed categories that did not occur in the training data. Second, we removed constant categorical features containing a single value only (and therefore no variation). Third, we imputed missing values using the following procedures: Categorical features were imputed with the mode. Following commonly used approaches to dealing with missing data, the imputation of numeric features varied between the learners. For the elastic net, we imputed those features with the median. For the random forest, we used twice the maximum to give missing values a distinct meaning that would allow the model to leverage this information. Lastly, we used the "Synthetic Minority Oversampling Technique" (SMOTE) to create artificial examples for the minority class in the training data 69 . The only exception was University 4 which followed a different procedure due to the large sample size and estimated computing power for implementing SMOTE. Instead of oversampling minority cases, we downsampled majority cases such that the positive and negative class were balanced. This was done to address the class imbalance caused by most students continuing their studies rather than dropping out 12 .
Predictive modeling approach
We predicted the retention status (1 = dropped out, 0 = continued) in a binary prediction task, with three sets of features: (1) institutional features (2) engagement features, and (3) a combined set of all features. To ensure the robustness of our predictions and to identify the model which is best suited for the current prediction context 54 , we compared a linear classifier ( elastic net; implemented in glmnet 4.1–4) 70 , 71 and a nonlinear classifier ( random forest ; implemented in randomForest 4.7–1) 72 , 73 . Both models are particularly well suited for our prediction context and are common choices in computational social science. That is, simple linear or logistic regression models are not suitable to work with datasets that have many inter-correlated predictors (in our case, a total of 462 predictors many of which are highly correlated) due to a high risk of overfitting. Both the elastic net and the random forest algorithm can effectively utilize large feature sets while reducing the risk of overfitting. We evaluate the performance of our six models for each school (2 algorithms and 3 feature sets), using out-of-sample benchmark experiments that estimate predictive performance and compare it against a common non-informative baseline model. The baseline represents a null-model that does not include any features, but instead always predicts the majority class, which in our samples means “continued.” 74 Below, we provide more details about the specific algorithms (i.e., elastic net and random forest), the cross-validation procedure, and the performance metrics we used for model evaluation.
Elastic net model
The elastic net is a regularized regression approach that combines advantages of ridge regression 75 with those of the LASSO 76 and is motivated by the need to handle large feature sets. The elastic net shrinks the beta-coefficients of features that add little predictive value (e.g., intercorrelated, little variance). Additionally, the elastic net can effectively remove variables from the model by reducing the respective beta coefficients to zero 70 . Unlike classical regression models, the elastic net does not aim to optimize the sum of least squares, but includes two penalty terms (L1, L2) that incentivize the model to reduce the estimated beta value of features that do not add information to the model. Combining the L1 (the sum of absolute values of the coefficients) and L2 (the sum of the squared values of the coefficients) penalties, elastic net addresses the limitations of alternative linear models such as LASSO regression (not capable of handling multi-collinearity) and Ridge Regression (may not produce sparse-enough solutions) 70 .
Formally, following Hastie & Qian (2016) the model equation of elastic net for binary classification problems can be written as follows 77 . Suppose the response variable takes values in G = {0,1}, y i denoted as I(g i = 1), the model formula is written as
After applying the log-odds transformation, the model formula can be written as
The objective function for logistic regression is the penalized negative binomial log-likelihood
where λ is the regularization parameter that controls the overall strength of the regularization, α is the mixing parameter that controls the balance between L1 and L2 regularization with α values closer to zero to result in sparser models (lasso regression α = 1, ridge regression α = 0). β represents coefficients of the regression model, ||β|| 1 is the is the L1 norm of the coefficients (the sum of absolute values of the coefficients), ||β|| 2 is the L2 norm of the coefficients (the sum of the squared values of the coefficients).
The regularized regression approach is especially relevant for our model because many of the app-based engagement features are highly correlated (e.g., the number of clicks is related to the number of activities registered in the app). In addition, we favored the elastic net algorithm over more complex alternatives, because the regularized beta coefficients can be interpreted as feature importance, allowing insights into which predictors are most informative of college dropout 78 , 79 .
Random forest model
Random forest models are a widely used ensemble learning method that grows many bagged and decorrelated decision trees to come up with a “collective” prediction of the outcome (i.e., the outcome that is chosen by most trees in a classification problem) 72 . Individual decision trees recursively split the feature space (rules to distinguish classes) with the goal to separate the different classes of the criterion (drop out vs. remain in our case). For a detailed description of how individual decision trees operate and translate to a random forest see Pargent, Schoedel & Stachl 80 .
Unlike the elastic net, random forest models can account for nonlinear associations between features and criterion and automatically include multi-dimensional interactions between features. Each decision tree in a random forest considers a random subset of bootstrapped cases and features, thereby increasing the variance of predictions across trees and the robustness of the overall prediction. For the splitting in each node of each tree, a random subset of features (mtry hyperparameter that we optimize in our models) are used by randomly drawing from the total set. For each split, all combinations of split variables and split points are compared, with the model choosing the splits that optimize the separation between classes 72 .
The random forest algorithm can be formally described as follows (verbatim from Hastie et al., 2016, p. 588):
For b = 1 to B:
Draw a bootstrap sample of size N from the training data.
Grow a decision tree to the bootstrapped data, by recursively repeating the following steps for each terminal node of the tree, until the minimum node size is reached.
Select m variables at random from the p variables.
Pick the best variable/split-point among the m according to the loss function (in our case Gini-impurity decrease)
Split the node into two daughter nodes.
Output the ensemble of trees
New predictions can then be made by generating a prediction for each tree and aggregating the results using majority vote.
The aggregation of predictions across trees in random forests improves the prediction performance compared to individual decision trees, as it can benefit from the trees’ variance and greatly reduces it to arrive at a single prediction 72 , 81 .
(Nested) Cross-validation: Out-of-sample model evaluation
We evaluate the performance of our predictive models using an out-of-sample validation approach. The idea behind out-of-sample validation is to increase the likelihood that a model will accurately predict student dropout on new data (e.g. new students) by using different datasets when training and evaluating the model. A commonly used, efficient technique for out-of-sample validation is to repeatedly fit (cf. training) and evaluate (cf. testing) models on non-overlapping parts of the same datasets and to combine the individual estimates across multiple iterations. This procedure – known as cross-validation – can also be used for model optimization (e.g., hyperparameter-tuning, pre-processing, variable selection), by repeatedly evaluating different settings for optimal predictive performance. When both approaches are combined, evaluation and optimization steps need to be performed in a nested fashion to ensure a strict separation of training and test data for a realistic out-of-sample performance estimation. The general idea is to emulate all modeling steps in each fold of the resampling as if it were a single in-sample model. Here, we use nested cross-validation to estimate the predictive performance of our models, to optimize model hyperparameters, and to pre-process data. We illustrate the procedure in Fig. 1 .
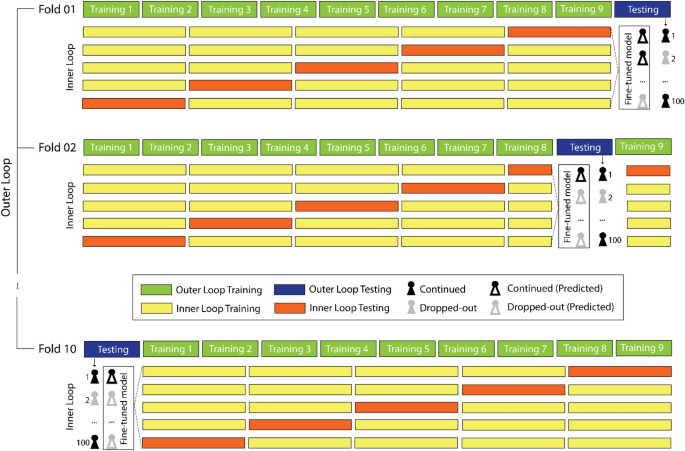
Schematic cross-validation procedure for out-of-sample predictions. The figure shows a tenfold cross-validation in the outer loop which is used to estimate the overall performance of the model by comparing the predicted outcomes for each student in the previously unseen test set with their actual outcomes. Within each of the 10 outer loops, a fivefold cross-validation in the inner loop is used to finetune model hyperparameters by evaluating different model settings.
The cross-validation procedure works as follows: Say we have a dataset with 1,000 students. In a first step, the dataset is split into ten different subsamples, each containing data from 100 students. In the first round, nine of these subsamples are used for training (i.e., fitting the model to estimate parameters, green boxes). That means, the data from the first 900 students will be included in training the model to relate the different features to the retention outcome. Once training is completed, the model’s performance can be evaluated on the data of the remaining 100 students (i.e., test dataset, blue boxes). For each student, the actual outcome (retained or discontinued, grey and black figures) is compared to the predicted outcome (retained or discontinued, grey and black figures). This comparison allows for the calculation of various performance metrics (see “ Performance metrics ” section below for more details). In contrast to the application of traditional inferential statistics, the evaluation process in predictive models separates the data used to train a model from the data used to evaluate these associations. Hence any overfitting that occurs at the training stage (e.g., using researcher degrees of freedom or due to the model learning relationships that are unique to the training data), hurts the predictive performance in the testing stage. To further increase the robustness of findings and leverage the entire dataset, this process is repeated for all 10 subsamples, such that each subsample is used nine times for training and once for testing. Finally, the obtained estimates from those ten iterations are aggregated to arrive at a cross-validated estimate of model performance. This tenfold cross validation procedure is referred to as the “outer loop”.
In addition to the outer loop, our models also contain an “inner loop”. The inner loop consists of an additional cross-validation procedure that is used to identify ideal hyperparameter settings (see “ Hyperparameter tuning ” section below). That is, in each of the ten iterations of the outer loop, the training sample is further divided into a training and test set to identify the best parameter constellations before model evaluation in the outer loop. We used fivefold cross-validation in the inner loop. All analyses scripts for the pre-processing and modeling steps are available on OSF ( https://osf.io/bhaqp/?view_only=629696d6b2854aa9834d5745425cdbbc ).
Performance metrics
We evaluate model performance based on four different metrics. Our main metric for model performance is AUC (area under the received operating characteristics curve). AUC is commonly used to assess the performance of a model over a 50%-chance baseline, and can range anywhere between 0 and 1. The AUC metric captures the area under the receiver operating characteristic (ROC) curve, which plots the true positive rate (TPR or recall; i.e. the percentage of correctly classified dropouts among all students who actually dropped out), against the false positive rate (FPR; i.e. the percentage of students erroneously classified as dropouts among all the students who actually continued). When the AUC is 0.5, the model’s predictive performance is equal to chance or a coin flip. The closer to 1, the higher the model’s predictive performance in distinguishing between students who continued and those who dropped out.
In addition, we report the F1 score, which ranges between 0 and 1 82 . The F1 score is based on the model’s positive predictive value (or precision, i.e., the percentage of correctly classified dropouts among all students predicted to have dropped out) as well as the model's TPR. A high F1 score hence indicates that there are both few false positives and few false negatives.
Given the specific context, we also report the TPR and the true negative rates (TNR, i.e. the percentage of students predicted to continue among all students who actually continued). Depending on their objective, universities might place a stronger emphasis on optimizing the TPR to make sure no student who is at risk of dropping out gets overlooked or on optimizing the TNR to save resources and assure that students do not get overly burdened. Notably, in most cases, universities are likely to strive for a balance between the two, which is reflected in our main AUC measure. All reported performance metrics represent the mean predictive performance across the 10 cross-validation folds of the outer loop 54 .
Hyperparameter tuning
We used a randomized search with 50 iterations and fivefold cross-validation for hyperparameter tuning in the inner loop of our cross-validation. The randomized search algorithm fits models with hyperparameter configurations randomly selected from a previously defined hyperparameter space and then picks the model that shows the best generalized performance averaged over the five cross-validation folds. The best hyperparamter configuration is used for training in the outer resampling loop to evaluate model performance.
For the elastic net classifier, we tuned the regularization parameter lambda, the decision rule used to choose lambda, and the L1-ratio parameter. The search space for lambda encompassed the 100 glmnet default values 71 . The space of decision rules for lambda included lambda.min which chooses the value of lambda that results in the minimum mean cross-validation error, and lambda.1se which chooses the value of lambda that results in the most regularized model such that the cross-validation error remains within one standard error of the minimum. The search space for the L1-ratio parameter included the range of values between 0 (ridge) to 1 (lasso). For the random forest classifier, we tuned the number of features selected for each split within a decision tree (mtry) and the minimum node size (i.e., how many cases are required to be left in the resulting end-nodes of the tree). The search space for the number of input features per decision tree was set to a range of 1 to p, where p represents the dimensionality of the feature space. The search space for minimum node size was set to a range of 1 to 5. Additionally, for both models, we tuned the oversampling rate and the number or neighbors used to generate new samples utilized by the SMOTE algorithm. The oversampling rate was set to a range of 2 to 15 and the number of nearest neighbors was set to a range of 1 to 10.
RQ1: How accurately can we predict a student's likelihood of discontinuing their studies using information from the first term of their studies?
Figure 2 displays AUC scores (Y-axis) across the different universities (rows), separated by the different feature sets (colors) and predictive algorithms (X-axis labels). The figure displays the distribution of AUC accuracies across the 10 cross-validation folds, alongside their mean and standard deviation. Independent t-tests using Holm corrections for multiple comparisons indicate statistical differences in the predictive performance across the different models and feature sets within each university. Table 2 provides the predictive performance across all four metrics.

AUC performance across the four universities for different feature sets and model. Inst. = Institutional data. Engag. = Engagement data. (EN) = Elastic Net. (RF) = Random Forest.
Overall, our models showed high levels of predictive accuracies across universities, models, feature sets and performance metrics, significantly outperforming the baseline in all instances. The main performance metric AUC reached an average of 73% (where 50% is chance), with a maximum of 88% for the random forest model and the full feature set in University 1. Both institutional features and engagement features significantly contributed to predictive performance, highlighting the fact that a student’s likelihood to drop out is both a function of their more stable socio-demographic characteristics as well as their experience of campus life. In most cases, the joint model (i.e., the combination of institutional and engagement features) performed better than each of the individual models alone. Finally, the random forest models produced higher levels of predictive performance than the elastic net in most cases (average AUC elastic net = 70%, AUC random forest = 75%), suggesting that the features are likely to interact with one another in predicting student retention and might not always be linearly related to the outcome.
RQ2: Which features are the most predictive of student retention?
To provide insights into the underlying relationships between student retention and socio-demographic as well as behavioral features, we examined two indicators of feature importance that both offer unique insights. First, we calculated the zero-order correlations between the features and the outcome for each of the four universities. We chose zero-order correlations over elastic net coefficients as they represent the relationships unaltered by the model’s regularization procedure (i.e., the relationship between a feature and the outcome is shown independently of the importance of the other features in the model). To improve the robustness of our findings, we only included the variables that passed the threshold for data inclusion in our models and had less than 50% of the data imputed. The top third of Table 3 displays the 10 most important features (i.e., highest absolute correlation with retention). The sign in brackets indicates the direction of the effects with ( +) indicating a protective factor and (−) indicating a risk factor. Features that showed up in the top 10 for more than 1 university are highlighted in bold.
Second, we calculated permutation variable importance scores for the elastic net and random forest models. For the elastic net model, feature importance is reported as the model coefficient after shrinking the coefficients according to their incremental predictive power. Compared to the zero-order correlation, the elastic net coefficients hence identify the features that have the strongest unique variance. For the random forest models, feature importance is reported as a model-agnostic metric that estimates the importance of a feature by observing the drop in model predictive performance when the actual association between the feature and the outcome is broken by randomly shuffling observations 72 , 83 . A feature is considered important if shuffling its values increases the model error (and therefore decreases the model’s predictive performance). In contrast to the coefficients from the elastic net model, the permutation feature importance scores are undirected and do not provide insights into the specific nature of the relationship between the feature and the outcome. However, they account for the fact that some features might not be predictive themselves but could still prove valuable in the overall model performance because they moderate the impact of other features. For example, minority or first-generation students might benefit more from being embedded in a strong social network than majority students who do not face the same barriers and are likely to have a stronger external support network. The bottom of Table 3 displays the 10 most important features in the elastic net and random forest models (i.e., highest permutation variable importance).
Supporting the findings reported in RQ1, the zero-order correlations confirm that both institutional and behavioral engagement features play an important role in predicting student retention. Aligned with prior work, students’ performance (measured by GPA or ACT) repeatedly appeared as one of the most important predictors across universities and models. In addition, many of the engagement features (e.g., services attended, chat messages network centrality) are related to social activities or network features, supporting the notion that a student’s social connections and support play a critical role in student retention. In addition, the extent to which students are positively engaged with their institutions (e.g., by attending events and rating them highly) appears to play a critical role in preventing dropout.
RQ3: How well do the predictive models generalize across universities?
To test the generalizability of our models across universities, we used the predictive model trained on one university (e.g., University 1) to predict retention of the remaining three universities (e.g., Universities 2–4). Figures 3 A,B display the AUCs across all possible pairs, indicating which university was used for training (X-axis) and which was used for testing (Y-axis, see Figure S1 in the SI for graphs illustrating the findings for F1, TNR and TPR).
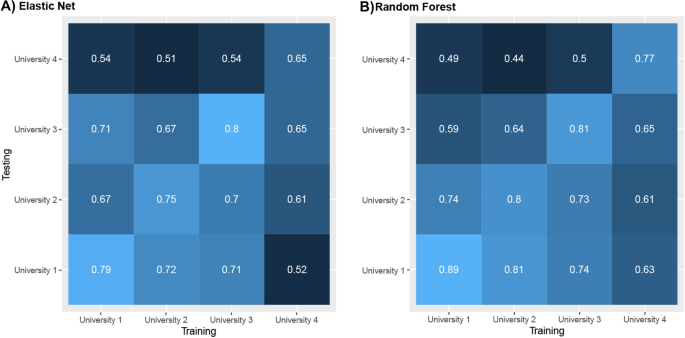
Performance (average AUC) of cross-university predictions.
Overall, we observed reasonably high levels of predictive performance when applying a model trained on one university to the data of another. The average AUC observed was 63% (for both the elastic net and the random forest), with the highest predictive performance reaching 74% (trained on University 1, predicting University 2), just 1%-point short of the predictive performance observed for the prediction from the universities own model (trained on University 2, predicting University 2). Contrary to the findings in RQ1, the random forest models did not perform better than the elastic net when making predictions for other universities. This suggests that the benefits afforded by the random forest models capture complex interaction patterns that are somewhat unique to each university but might not generalize well to new contexts. The main outlier in generalizability was University 4, where none of the other models reached accuracies much better than chance, and whose model produced relatively low levels of accuracies when predicting student retention across universities 1–2. This is likely a result of the fact that University 4 was qualitatively different from the other universities in several ways, including the fact that University 4 was a community college and consisted of 16 different campuses that were merged for the purpose of this analysis (see Methods for more details).
We show that student retention can be predicted from institutional data, behavioral engagement data, and their combination. Using data from over 50,000 students across four Universities, our predictive models achieve out-of-sample accuracies of up to 88% (where 50% is chance). Notably, while both institutional data and behavioral engagement data significantly predict retention, the combination of the two performs best in most instances. This finding is further supported by our feature importance analyses which suggest that both institutional and behavioral engagement features are among the most important predictors of student retention. Specifically, academic performance as measured by GPA and behavioral metrics associated with campus engagement (e.g., event attendances or ratings) or a student’s position in the network (e.g., closeness or centrality) were shown to consistently act as protective factors. Finally, we highlight the generalizability of our models across universities. Models trained on one university were able to predict student retention at another with reasonably high levels of predictive performance. As one might expect, the generalizability across universities heavily depends on the extent to which the universities are similar on important structural dimensions, with prediction accuracies dropping radically in cases where similarity is low (see low cross-generalization for University 4).
Contributions to the scientific literature
Our findings contribute to the existing literature in several ways. First, they respond to recent calls for more predictive research in psychology 54 , 55 as well as the use of Big Data analytics in education research 56 , 57 . Not only do our models consider socio-demographic characteristics that are collected by universities, but they also capture students’ daily experience and university engagement by tracking behaviors via the READY Education app. Our findings suggest, these more psychological predictors of student retention can improve the performance of predictive models above and beyond socio-demographic variables. This is consistent with previous findings suggesting that the inclusion of engagement metrics improves the performance of predictive models 16 , 84 , 85 . Overall, our models showed superior accuracies to models of former studies that were trained only on demographics and transcript records 15 , 25 or less comprehensive behavioral features 16 and provided results comparable to those reported in studies that additionally included a wide range of socio-economic variables 12 . Given that the READY Education app captures only a fraction of the students' actual experience, the high predictive accuracies make an even stronger case for the importance of student engagement in college retention.
Second, our findings provide insights into the features that are most important in predicting whether a student is going to drop out or not. By doing so they complement our predictive approach with layers of understanding that are conducive to not only validating our models but also generating insights into potential protective and risk factors. Most importantly, our findings highlight the relevance of the behavioral engagement metrics for predicting student retention. Most features identified as being important in the prediction were related to app and community engagement. In line with previous research, features indicative of early and deep social integration, such as interactions with peers and faculty or the development of friendships and social networks, were found to be highly predictive 16 , 41 . For example, it is reasonable to assume that a short time between app registration and the first visit of a campus event (one of the features identified as important) has a positive impact on retention, because campus events offer ideal opportunities for students to socialize 86 . Early participation in a campus event implies early integration and networking with others, protecting students from perceived stress 87 and providing better social and emotional support 88 . In contrast, a student who never attends an event or does so very late in the semester may be less connected to the campus life and the student community which in turn increases the likelihood of dropping out. This interpretation is strengthened by the fact that a high proportion of positive event ratings was identified as an important predictor of a student continuing their studies. Students who enjoy an event are likely to feel more comfortable, be embedded in the university life, make more connections, and build stronger connections. This might result in a virtuous cycle in which students continue attending events and over time create a strong social connection to their peers. As in most previous work, a high GPA score was consistently related to a higher likelihood of continuing one’s studies 21 , 24 . Although their importance varied across universities, ethnicity was also found to play a major role for retention, with consistent inequalities replicating in our predictive models 12 , 19 , 47 . For example, Black students were on average more likely to drop-out, suggesting that universities should dedicate additional resources to protect this group. Importantly, all qualitative interpretations are post-hoc. While many of the findings are intuitive and align with previous research on the topic, future studies should validate our results and investigate the causality underlying the effects in experimental or longitudinal within-person designs 54 , 78 .
Finally, our findings are the first to explore the extent to which the relationships between certain socio-demographic and behavioral characteristics might be idiosyncratic and unique to a specific university. By being able to compare the models across four different universities, we were able to show that many of the insights gained from one university can be leveraged to predict student retention at another. However, our findings also point to important boundary conditions: The more dissimilar universities are in their organizational structures and student experience, the more idiosyncratic the patterns between certain socio-demographic and behavioral features with student retention will be and the harder it is to merely translate general insights to the specific university campus.
Practical contributions
Our findings also have important practical implications. In the US, student attrition results in an average annual revenue loss of approximately $16.5 billion per year 9 , 10 and over $9 billion wasted in federal and state grants and subsidies that are awarded to students who do not finish their degree 11 . Hence, it is critical to predict potential dropouts as early and as accurately as possible to be able to offer dedicated support and allocate resources where they are needed the most. Our models rely exclusively on data collected in the first semester at university and are therefore an ideal “early warning” system for universities who want to predict whether their students will likely continue their studies or drop out at some point. Depending on the university’s resources and goals, the predictive models can be optimized for different performance measures. Indeed, a university might decide to focus on the true positive rate to capture as many dropouts as possible. While this would mean erroneously classifying “healthy “ students as potential dropouts, universities might decide that the burden of providing “unnecessary “ support to these healthy students is worth the reduced risk of missing a dropout. Importantly, our models go beyond mere socio-demographic variables and allow for a more nuanced, personal model that considers not just “who someone is” but also what their experience on campus looks like. As such, our models make it possible to acknowledge individuality rather than using over-generalized assessments of entire socio-demographic segments.
Importantly, however, it is critical to subject these models to continuous quality assurance. While predictive models could allow universities to flag at-risk students early, they could also perpetuate biases that get calcified in the predictive models themselves. For example, students who are traditionally less likely to discontinue their studies might have to pass a much higher level of dysfunctional engagement behavior before their file gets flagged as “at-risk”. Similarly, a person from a traditionally underrepresented group might receive an unnecessarily high volume of additional check-ins even though they are generally flourishing in their day-to-day experience. Given that being labeled as “at-risk” can be associated with stigma that could reinforce stigmas around historically marginalized groups, it will be critical to monitor both the performance of the model over time as well as the perception of its helpfulness among administrators, faculty, and students.
Limitations and future research
Our study has several limitations and highlights avenues for future research. First, our sample consisted of four US universities. Thus, our results are not necessarily generalizable to countries with more collectivistic cultures and other education systems such as Asia, where the reasons for dropping out might be different 89 , 90 , or Europe where most students work part-time jobs and live off-campus. Future research should investigate the extent to which our models can generalize to other cultural contexts and identify the features of student retention that are universally valid across contexts.
Second, our predictive models relied on app usage data. Therefore, our predictive approach could only be applied to students who decided to use the app. This selection, in and by itself, is likely to introduce a sampling bias, as students who decide to use the app might be more likely to retain in the first place, restricting the variance in observations, and excluding students for whom app usage data was not available. However, as our findings suggest, the institutional data alone provide predictive performance independent of the app features, making this a viable alternative for students who do not use the app.
Third, our predictive models rely on cross-sectional predictions. That is, we observe a students’ behavior over the course of an entire semester and based on the patterns observed in other students we predict whether that student is likely to drop out or not. Future research could try to improve both the predictive performance of the model and its usefulness for applied contexts by modeling within-person trends dynamically. Given enough data, the model could observe a person’s baseline behavior and identify changes from that baseline as potentially problematic. In fact, more social contact with other students might be considered a protective factor in our cross-sectional model. However, there are substantial individual differences in how much social contact individuals seek out and enjoy 91 . Hence, sending 10 chat messages a week might be considered a lot for one person, but very little for another. Future research should hence investigate whether the behavioral engagement features allow for a more dynamic within-person model that makes it possible to take base rates into account and provide a dynamic, momentary assessment of a student’s likelihood to drop out.
Fourth, although the engagement data was captured as a longitudinal time series with time-stamped events, we collapsed the data into a single set of cross-sectional features for each student. Although some of these features captures variation in behaviors over time (e.g., entropy and linear trends), future research should try to implement more advanced machine learning models to account for this time series data directly. For example, long short-term memory models (LSTMs) 92 – a type of recurrent neural network – are capable of learning patterns in longitudinal, sequential data like ours.
Fifth, even though the current research provides initial insights into the workings of the models by highlighting the importance of certain features, the conclusions that can be drawn from these analyses are limited as the importance metrics are calculated for the overall population. Future research could aim to calculate the importance of certain features at the individual level to test whether their importance varies across certain socio-demographic features. Estimating the importance of a person’s position in the social network on an individual level, for example, would make it possible to see whether the importance is correlated with institutional data such as minority or first-generation status.
Finally, our results lay the foundation for developing interventions that foster retention through shaping students’ experience at university 93 . Interventions which have been shown to have a positive effect on retention, include orientation programs and academic advising 94 , student support services like mentoring and coaching as well as need-based grants 95 . However, to date, the first-year experience programs meant to strengthen social integration of first year students, do not seem to have yielded positive results 96 , 97 . Our findings could support the development of interventions aimed at improving and maintaining student integration on campus. On a high level, the insights into the most important features provide an empirical path for developing relevant interventions that target the most important levers of student retention. For example, the fact that the time between registration and the first event attendance has such a big impact on student retention means that universities should do everything they can to get students to attend events as early as possible. Similarly, they could develop interventions that lead to more cohesive networks among cohorts and make sure that all students connect to their community. On a deeper, more sophisticated level, new approaches to model explainability could allow universities to tailor their intervention to each student 98 , 99 . For example, explainable AI makes it possible to derive decision rules for each student, indicating which features were critical in predicting the students’ outcome. While student A might be predicted to drop out because they are disconnected from the network, student B might be predicted to drop out because they don’t access the right information on the app. Given this information, universities would be able to personalize their offerings to the specific needs of the student. While student A might be encouraged to spend more time socializing with other students, student B might be reminded to check out important course information. Hence, predictive models could not only be used to identify students at risk but also provide an automated path to offering personalized guidance and support.
For every study that is discontinued, an educational dream shatters. And every shattered dream has a negative long-term impact both on the student and the university the student attended. In this study we introduce an approach to accurately predicting student retention after the first term. Our results show that student retention can be predicted with relatively high levels of predictive performance when considering institutional data, behavioral engagement data, or a combination of the two. By combining socio-demographic characteristics with passively observed behavioral traces reflecting a student’s daily activities, our models offer a holistic picture of students' university experiences and its relation to retention. Overall, such predictive models have great potential both for the early identification of at-risk students and for enabling timely, evidence-based interventions.
Data availability
Raw data are not publicly available due to their proprietary nature and the risks associated with de-anonymization, but they are available from the corresponding author on reasonable request. The pre-processed data and all analyses codes are available on OSF ( https://osf.io/bhaqp/ ) to facilitate reproducibility of our work. Data were analyzed using R, version 4.0.0 (R Core Team, 2020; see subsections for specific packages and versions used). The study’s design relies on secondary data and the analyses were not preregistered.
Change history
21 june 2023.
A Correction to this paper has been published: https://doi.org/10.1038/s41598-023-36579-2
Ginder, S. A., Kelly-Reid, J. E. & Mann, F. B. Graduation Rates for Selected Cohorts, 2009–14; Outcome Measures for Cohort Year 2009–10; Student Financial Aid, Academic Year 2016–17; and Admissions in Postsecondary Institutions, Fall 2017. First Look (Provisional Data). NCES 2018–151. National Center for Education Statistics (2018).
Snyder, T. D., de Brey, C. & Dillow, S. A. Digest of Education Statistics 2017 NCES 2018-070. Natl. Cent. Educ. Stat. (2019).
NSC Research Center. Persistence & Retention – 2019. NSC Research Center https://nscresearchcenter.org/snapshotreport35-first-year-persistence-and-retention/ (2019).
Bound, J., Lovenheim, M. F. & Turner, S. Why have college completion rates declined? An analysis of changing student preparation and collegiate resources. Am. Econ. J. Appl. Econ. 2 , 129–157 (2010).
Article PubMed PubMed Central Google Scholar
Bowen, W. G., Chingos, M. M. & McPherson, M. S. Crossing the finish line. in Crossing the Finish Line (Princeton University Press, 2009).
McFarland, J. et al. The Condition of Education 2019. NCES 2019-144. Natl. Cent. Educ. Stat. (2019).
Education, U. S. D. of. Fact sheet: Focusing higher education on student success. [Fact Sheet] (2015).
Freudenberg, N. & Ruglis, J. Peer reviewed: Reframing school dropout as a public health issue. Prev. Chronic Dis. 4 , 4 (2007).
Google Scholar
Raisman, N. The cost of college attrition at four-year colleges & universities-an analysis of 1669 US institutions. Policy Perspect. (2013).
Wellman, J., Johnson, N. & Steele, P. Measuring (and Managing) the Invisible Costs of Postsecondary Attrition. Policy brief. Delta Cost Proj. Am. Instit. Res. (2012).
Schneider, M. Finishing the first lap: The cost of first year student attrition in America’s four year colleges and universities (American Institutes for Research, 2010).
Delen, D. A comparative analysis of machine learning techniques for student retention management. Decis. Support Syst. 49 , 498–506 (2010).
Article Google Scholar
Yu, R., Lee, H. & Kizilcec, R. F. Should College Dropout Prediction Models Include Protected Attributes? in Proceedings of the Eighth ACM Conference on Learning@ Scale 91–100 (2021).
Tinto, V. Reconstructing the first year of college. Plan. High. Educ. 25 , 1–6 (1996).
Ortiz-Lozano, J. M., Rua-Vieites, A., Bilbao-Calabuig, P. & Casadesús-Fa, M. University student retention: Best time and data to identify undergraduate students at risk of dropout. Innov. Educ. Teach. Int. 57 , 74–85 (2020).
Ram, S., Wang, Y., Currim, F. & Currim, S. Using big data for predicting freshmen retention. in 2015 international conference on information systems: Exploring the information frontier, ICIS 2015 (Association for Information Systems, 2015).
Levitz, R. S., Noel, L. & Richter, B. J. Strategic moves for retention success. N. Dir. High. Educ. 1999 , 31–49 (1999).
Veenstra, C. P. A strategy for improving freshman college retention. J. Qual. Particip. 31 , 19–23 (2009).
Astin, A. W. How, “good” is your institution’s retention rate?. Res. High. Educ. 38 , 647–658 (1997).
Coleman, J. S. Social capital in the creation of human capital. Am. J. Sociol. 94 , S95–S120 (1988).
Reason, R. D. Student variables that predict retention: Recent research and new developments. J. Stud. Aff. Res. Pract. 40 , 704–723 (2003).
Tinto, V. Dropout from higher education: A theoretical synthesis of recent research. Rev Educ Res 45 , 89–125 (1975).
Tinto, V. Completing college: Rethinking institutional action (University of Chicago Press, 2012).
Book Google Scholar
Astin, A. Retaining and Satisfying Students. Educ. Rec. 68 , 36–42 (1987).
Aulck, L., Velagapudi, N., Blumenstock, J. & West, J. Predicting student dropout in higher education. arXiv preprint arXiv:1606.06364 (2016).
Bogard, M., Helbig, T., Huff, G. & James, C. A comparison of empirical models for predicting student retention (Western Kentucky University, 2011).
Murtaugh, P. A., Burns, L. D. & Schuster, J. Predicting the retention of university students. Res. High. Educ. 40 , 355–371 (1999).
Porter, K. B. Current trends in student retention: A literature review. Teach. Learn. Nurs. 3 , 3–5 (2008).
Thomas, S. L. Ties that bind: A social network approach to understanding student integration and persistence. J. High. Educ. 71 , 591–615 (2000).
Peltier, G. L., Laden, R. & Matranga, M. Student persistence in college: A review of research. J. Coll. Stud. Ret. 1 , 357–375 (2000).
Nandeshwar, A., Menzies, T. & Nelson, A. Learning patterns of university student retention. Expert Syst. Appl. 38 , 14984–14996 (2011).
Boero, G., Laureti, T. & Naylor, R. An econometric analysis of student withdrawal and progression in post-reform Italian universities. (2005).
Tinto, V. Leaving college: Rethinking the causes and cures of student attrition (ERIC, 1987).
Choy, S. Students whose parents did not go to college: Postsecondary access, persistence, and attainment. Findings from the condition of education, 2001. (2001).
Ishitani, T. T. Studying attrition and degree completion behavior among first-generation college students in the United States. J. High. Educ. 77 , 861–885 (2006).
Thayer, P. B. Retention of students from first generation and low income backgrounds. (2000).
Britt, S. L., Ammerman, D. A., Barrett, S. F. & Jones, S. Student loans, financial stress, and college student retention. J. Stud. Financ. Aid 47 , 3 (2017).
McKinney, L. & Burridge, A. B. Helping or hindering? The effects of loans on community college student persistence. Res. High Educ. 56 , 299–324 (2015).
Hochstein, S. K. & Butler, R. R. The effects of the composition of a financial aids package on student retention. J. Stud. Financ. Aid 13 , 21–26 (1983).
Singell, L. D. Jr. Come and stay a while: Does financial aid effect retention conditioned on enrollment at a large public university?. Econ. Educ. Rev. 23 , 459–471 (2004).
Bean, J. P. Nine themes of college student. Coll. Stud. Retent. Formula Stud. Success 215 , 243 (2005).
Tinto, V. Through the eyes of students. J. Coll. Stud. Ret. 19 , 254–269 (2017).
Cabrera, A. F., Nora, A. & Castaneda, M. B. College persistence: Structural equations modeling test of an integrated model of student retention. J. High. Educ. 64 , 123–139 (1993).
Roberts, J. & Styron, R. Student satisfaction and persistence: Factors vital to student retention. Res. High. Educ. J. 6 , 1 (2010).
Gopalan, M. & Brady, S. T. College students’ sense of belonging: A national perspective. Educ. Res. 49 , 134–137 (2020).
Hoffman, M., Richmond, J., Morrow, J. & Salomone, K. Investigating, “sense of belonging” in first-year college students. J. Coll. Stud. Ret. 4 , 227–256 (2002).
Terenzini, P. T. & Pascarella, E. T. Toward the validation of Tinto’s model of college student attrition: A review of recent studies. Res. High Educ. 12 , 271–282 (1980).
Astin, A. W. The impact of dormitory living on students. Educational record (1973).
Astin, A. W. Student involvement: A developmental theory for higher education. J. Coll. Stud. Pers. 25 , 297–308 (1984).
Terenzini, P. T. & Pascarella, E. T. Studying college students in the 21st century: Meeting new challenges. Rev. High Ed. 21 , 151–165 (1998).
Thompson, J., Samiratedu, V. & Rafter, J. The effects of on-campus residence on first-time college students. NASPA J. 31 , 41–47 (1993).
Tinto, V. Research and practice of student retention: What next?. J. Coll. Stud. Ret. 8 , 1–19 (2006).
Lazer, D. et al. Computational social science. Science 1979 (323), 721–723 (2009).
Yarkoni, T. & Westfall, J. Choosing prediction over explanation in psychology: Lessons from machine learning. Perspect. Psychol. Sci. 12 , 1100–1122 (2017).
Peters, H., Marrero, Z. & Gosling, S. D. The Big Data toolkit for psychologists: Data sources and methodologies. in The psychology of technology: Social science research in the age of Big Data. 87–124 (American Psychological Association, 2022). doi: https://doi.org/10.1037/0000290-004 .
Fischer, C. et al. Mining big data in education: Affordances and challenges. Rev. Res. Educ. 44 , 130–160 (2020).
Hilbert, S. et al. Machine learning for the educational sciences. Rev. Educ. 9 , e3310 (2021).
National Academy of Education. Big data in education: Balancing the benefits of educational research and student privacy . (2017).
Aulck, L., Nambi, D., Velagapudi, N., Blumenstock, J. & West, J. Mining university registrar records to predict first-year undergraduate attrition. Int. Educ. Data Min. Soc. (2019).
Beaulac, C. & Rosenthal, J. S. Predicting university students’ academic success and major using random forests. Res. High Educ. 60 , 1048–1064 (2019).
Berens, J., Schneider, K., Görtz, S., Oster, S. & Burghoff, J. Early detection of students at risk–predicting student dropouts using administrative student data and machine learning methods. Available at SSRN 3275433 (2018).
Dawson, S., Jovanovic, J., Gašević, D. & Pardo, A. From prediction to impact: Evaluation of a learning analytics retention program. in Proceedings of the seventh international learning analytics & knowledge conference 474–478 (2017).
Dekker, G. W., Pechenizkiy, M. & Vleeshouwers, J. M. Predicting students drop Out: A case study. Int. Work. Group Educ. Data Min. (2009).
del Bonifro, F., Gabbrielli, M., Lisanti, G. & Zingaro, S. P. Student dropout prediction. in International Conference on Artificial Intelligence in Education 129–140 (Springer, 2020).
Hutt, S., Gardner, M., Duckworth, A. L. & D’Mello, S. K. Evaluating fairness and generalizability in models predicting on-time graduation from college applications. Int. Educ. Data Min. Soc. (2019).
Jayaprakash, S. M., Moody, E. W., Lauría, E. J. M., Regan, J. R. & Baron, J. D. Early alert of academically at-risk students: An open source analytics initiative. J. Learn. Anal. 1 , 6–47 (2014).
Balakrishnan, G. & Coetzee, D. Predicting student retention in massive open online courses using hidden markov models. Elect. Eng. Comput. Sci. Univ. Calif. Berkeley 53 , 57–58 (2013).
Hastie, T., Tibshirani, R. & Friedman, J. The elements of statistical learning (Springer series in statistics, New York, NY, USA, 2001).
Book MATH Google Scholar
Chawla, N. V., Bowyer, K. W., Hall, L. O. & Kegelmeyer, W. P. SMOTE: Synthetic minority over-sampling technique. J. Artif. Intell. Res. 16 , 321–357 (2002).
Article MATH Google Scholar
Zou, H. & Hastie, T. Regularization and variable selection via the elastic net. J. R. Stat. Soc. Seri. B Stat. Methodol. 67 , 301–320 (2005).
Article MathSciNet MATH Google Scholar
Friedman, J., Hastie, T. & Tibshirani, R. Regularization paths for generalized linear models via coordinate descent. J. Stat. Softw. 33 , 1 (2010).
Breiman, L. Random forests. Mach. Learn. 45 , 5–32 (2001).
Liaw, A. & Wiener, M. Classification and regression by randomForest. R News 2 , 18–22 (2002).
Pargent, F., Schoedel, R. & Stachl, C. An introduction to machine learning for psychologists in R. Psyarxiv (2022).
Hoerl, A. E. & Kennard, R. W. Ridge Regression. in Encyclopedia of Statistical Sciences vol. 8 129–136 (John Wiley & Sons, Inc., 2004).
Tibshirani, R. Regression shrinkage and selection via the Lasso. J. R. Stat. Soc. Ser. B (Methodol.) 58 , 267–288 (1996).
MathSciNet MATH Google Scholar
Hastie, T. & Qian, J. Glmnet vignette. vol. 9 1–42 https://hastie.su.domains/Papers/Glmnet_Vignette.pdf (2016).
Orrù, G., Monaro, M., Conversano, C., Gemignani, A. & Sartori, G. Machine learning in psychometrics and psychological research. Front. Psychol. 10 , 2970 (2020).
Pargent, F. & Albert-von der Gönna, J. Predictive modeling with psychological panel data. Z Psychol (2019).
Pargent, F., Schoedel, R. & Stachl, C. Best practices in supervised machine learning: A tutorial for psychologists. Doi: https://doi.org/10.31234/osf.io/89snd (2023).
Friedman, J., Hastie, T. & Tibshirani, R. The elements of statistical learning Vol. 1 (Springer series in statistics, 2001).
MATH Google Scholar
Rijsbergen, V. & Joost, C. K. Information Retrieval Butterworths London. Google Scholar Google Scholar Digital Library Digital Library (1979).
Molnar, C. Interpretable machine learning . (Lulu. com, 2020).
Aguiar, E., Ambrose, G. A., Chawla, N. v, Goodrich, V. & Brockman, J. Engagement vs Performance: Using Electronic Portfolios to Predict First Semester Engineering Student Persistence . Journal of Learning Analytics vol. 1 (2014).
Chai, K. E. K. & Gibson, D. Predicting the risk of attrition for undergraduate students with time based modelling. Int. Assoc. Dev. Inf. Soc. (2015).
Saenz, T., Marcoulides, G. A., Junn, E. & Young, R. The relationship between college experience and academic performance among minority students. Int. J. Educ. Manag (1999).
Pidgeon, A. M., Coast, G., Coast, G. & Coast, G. Psychosocial moderators of perceived stress, anxiety and depression in university students: An international study. Open J. Soc. Sci. 2 , 23 (2014).
Wilcox, P., Winn, S. & Fyvie-Gauld, M. ‘It was nothing to do with the university, it was just the people’: The role of social support in the first-year experience of higher education. Stud. High. Educ. 30 , 707–722 (2005).
Guiffrida, D. A. Toward a cultural advancement of Tinto’s theory. Rev. High Ed. 29 , 451–472 (2006).
Triandis, H. C., McCusker, C. & Hui, C. H. Multimethod probes of individualism and collectivism. J. Pers. Soc. Psychol. 59 , 1006 (1990).
Watson, D. & Clark, L. A. Extraversion and its positive emotional core. in Handbook of personality psychology 767–793 (Elsevier, 1997).
Greff, K., Srivastava, R. K., Koutník, J., Steunebrink, B. R. & Schmidhuber, J. LSTM: A search space odyssey. IEEE Trans. Neural Netw. Learn. Syst. 28 , 2222–2232 (2017).
Article MathSciNet PubMed Google Scholar
Arnold, K. E. & Pistilli, M. D. Course signals at Purdue: Using learning analytics to increase student success. in Proceedings of the 2nd international conference on learning analytics and knowledge 267–270 (2012).
Braxton, J. M. & McClendon, S. A. The fostering of social integration and retention through institutional practice. J. Coll. Stud. Ret. 3 , 57–71 (2001).
Sneyers, E. & de Witte, K. Interventions in higher education and their effect on student success: A meta-analysis. Educ. Rev. (Birm) 70 , 208–228 (2018).
Jamelske, E. Measuring the impact of a university first-year experience program on student GPA and retention. High Educ. (Dordr) 57 , 373–391 (2009).
Purdie, J. R. & Rosser, V. J. Examining the academic performance and retention of first-year students in living-learning communities and first-year experience courses. Coll. Stud. Aff. J. 29 , 95 (2011).
Lundberg, S. M. et al. From local explanations to global understanding with explainable AI for trees. Nat. Mach. Intell. 2 , 56–67 (2020).
Ramon, Y., Farrokhnia, R. A., Matz, S. C. & Martens, D. Explainable AI for psychological profiling from behavioral data: An application to big five personality predictions from financial transaction records. Information 12 , 518 (2021).
Download references
Author information
Alice Dinu is an Independent Researcher.
Authors and Affiliations
Columbia University, New York, USA
Sandra C. Matz & Heinrich Peters
Ludwig Maximilian University of Munich, Munich, Germany
Christina S. Bukow
Ready Education, Montreal, Canada
Christine Deacons
University of St. Gallen, St. Gallen, Switzerland
Clemens Stachl
Montreal, Canada
You can also search for this author in PubMed Google Scholar
Contributions
S.C.M., C.B, A.D., H.P., and C.S. designed the research. C.D. and A.D. provided the data. S.C.M, C.B. and H.P. analyzed the data. S.C.M and C.B. wrote the manuscript. All authors reviewed the manuscript. Earlier versions of thi research were part of the C.B.’s masters thesis which was supervised by S.C.M. and C.S.
Corresponding author
Correspondence to Sandra C. Matz .
Ethics declarations
Competing interests.
C.D. is a former employee of Ready Education. None of the other authors have conflict of interests related to this submission.
Additional information
Publisher's note.
Springer Nature remains neutral with regard to jurisdictional claims in published maps and institutional affiliations.
The original online version of this Article was revised: Alice Dinu was omitted from the author list in the original version of this Article. The Author Contributions section now reads: “S.C.M., C.B, A.D., H.P., and C.S. designed the research. C.D. and A.D. provided the data. S.C.M, C.B. and H.P. analyzed the data. S.C.M and C.B. wrote the manuscript. All authors reviewed the manuscript. Earlier versions of this research were part of the C.B.’s masters thesis which was supervised by S.C.M. and C.S.” Additionally, the Article contained an error in Data Availability section and the legend of Figure 2 was incomplete.
Supplementary Information
Supplementary information., rights and permissions.
Open Access This article is licensed under a Creative Commons Attribution 4.0 International License, which permits use, sharing, adaptation, distribution and reproduction in any medium or format, as long as you give appropriate credit to the original author(s) and the source, provide a link to the Creative Commons licence, and indicate if changes were made. The images or other third party material in this article are included in the article's Creative Commons licence, unless indicated otherwise in a credit line to the material. If material is not included in the article's Creative Commons licence and your intended use is not permitted by statutory regulation or exceeds the permitted use, you will need to obtain permission directly from the copyright holder. To view a copy of this licence, visit http://creativecommons.org/licenses/by/4.0/ .
Reprints and permissions
About this article
Cite this article.
Matz, S.C., Bukow, C.S., Peters, H. et al. Using machine learning to predict student retention from socio-demographic characteristics and app-based engagement metrics. Sci Rep 13 , 5705 (2023). https://doi.org/10.1038/s41598-023-32484-w
Download citation
Received : 09 August 2022
Accepted : 28 March 2023
Published : 07 April 2023
DOI : https://doi.org/10.1038/s41598-023-32484-w
Share this article
Anyone you share the following link with will be able to read this content:
Sorry, a shareable link is not currently available for this article.
Provided by the Springer Nature SharedIt content-sharing initiative
By submitting a comment you agree to abide by our Terms and Community Guidelines . If you find something abusive or that does not comply with our terms or guidelines please flag it as inappropriate.
Quick links
- Explore articles by subject
- Guide to authors
- Editorial policies
Sign up for the Nature Briefing newsletter — what matters in science, free to your inbox daily.

Read our research on: Gun Policy | International Conflict | Election 2024
Regions & Countries
About half of americans say public k-12 education is going in the wrong direction.
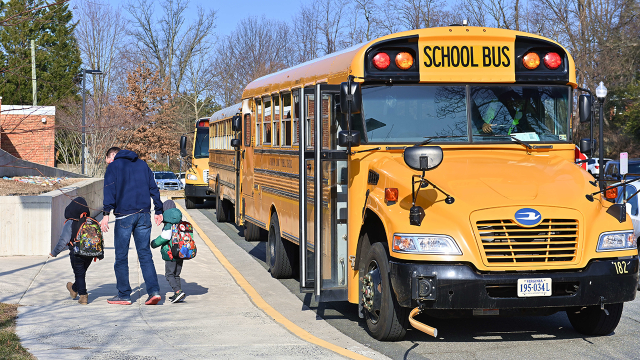
About half of U.S. adults (51%) say the country’s public K-12 education system is generally going in the wrong direction. A far smaller share (16%) say it’s going in the right direction, and about a third (32%) are not sure, according to a Pew Research Center survey conducted in November 2023.
Pew Research Center conducted this analysis to understand how Americans view the K-12 public education system. We surveyed 5,029 U.S. adults from Nov. 9 to Nov. 16, 2023.
The survey was conducted by Ipsos for Pew Research Center on the Ipsos KnowledgePanel Omnibus. The KnowledgePanel is a probability-based web panel recruited primarily through national, random sampling of residential addresses. The survey is weighted by gender, age, race, ethnicity, education, income and other categories.
Here are the questions used for this analysis , along with responses, and the survey methodology .
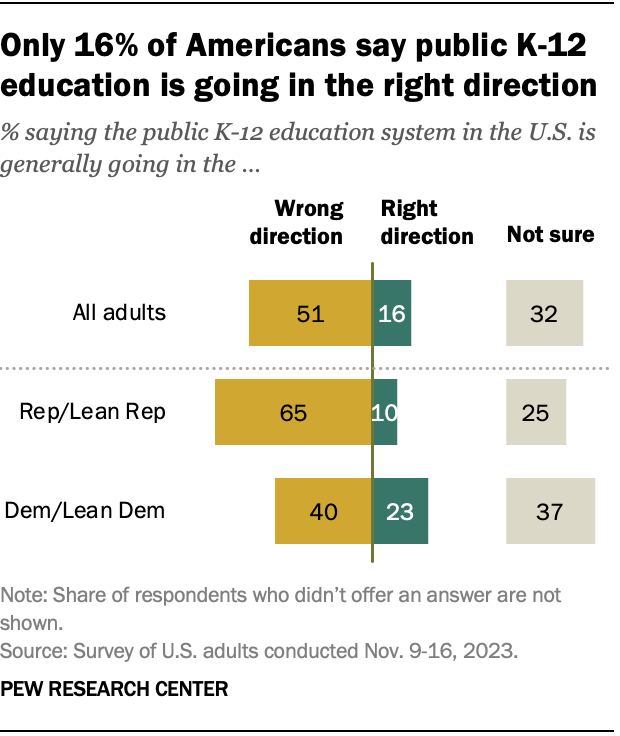
A majority of those who say it’s headed in the wrong direction say a major reason is that schools are not spending enough time on core academic subjects.
These findings come amid debates about what is taught in schools , as well as concerns about school budget cuts and students falling behind academically.
Related: Race and LGBTQ Issues in K-12 Schools
Republicans are more likely than Democrats to say the public K-12 education system is going in the wrong direction. About two-thirds of Republicans and Republican-leaning independents (65%) say this, compared with 40% of Democrats and Democratic leaners. In turn, 23% of Democrats and 10% of Republicans say it’s headed in the right direction.
Among Republicans, conservatives are the most likely to say public education is headed in the wrong direction: 75% say this, compared with 52% of moderate or liberal Republicans. There are no significant differences among Democrats by ideology.
Similar shares of K-12 parents and adults who don’t have a child in K-12 schools say the system is going in the wrong direction.
A separate Center survey of public K-12 teachers found that 82% think the overall state of public K-12 education has gotten worse in the past five years. And many teachers are pessimistic about the future.
Related: What’s It Like To Be A Teacher in America Today?
Why do Americans think public K-12 education is going in the wrong direction?
We asked adults who say the public education system is going in the wrong direction why that might be. About half or more say the following are major reasons:
- Schools not spending enough time on core academic subjects, like reading, math, science and social studies (69%)
- Teachers bringing their personal political and social views into the classroom (54%)
- Schools not having the funding and resources they need (52%)
About a quarter (26%) say a major reason is that parents have too much influence in decisions about what schools are teaching.
How views vary by party
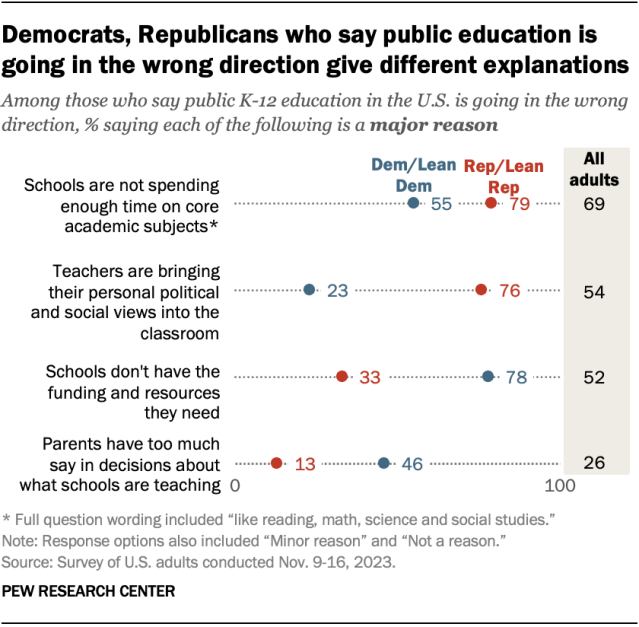
Americans in each party point to different reasons why public education is headed in the wrong direction.
Republicans are more likely than Democrats to say major reasons are:
- A lack of focus on core academic subjects (79% vs. 55%)
- Teachers bringing their personal views into the classroom (76% vs. 23%)
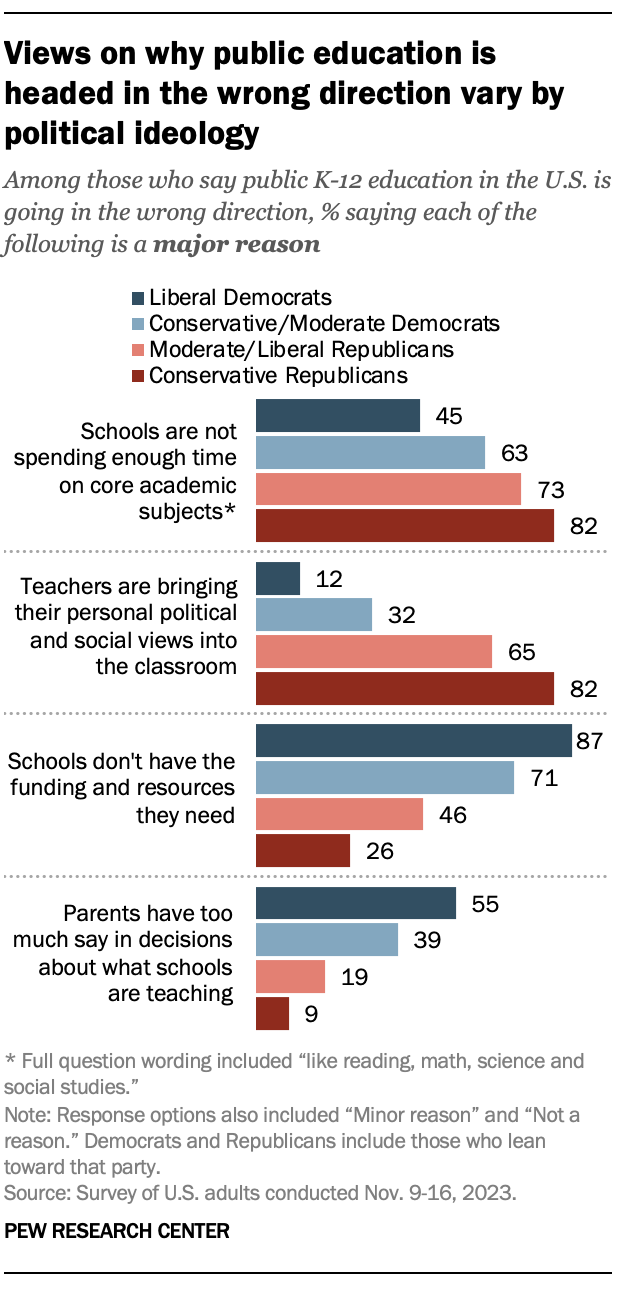
In turn, Democrats are more likely than Republicans to point to:
- Insufficient school funding and resources (78% vs. 33%)
- Parents having too much say in what schools are teaching (46% vs. 13%)
Views also vary within each party by ideology.
Among Republicans, conservatives are particularly likely to cite a lack of focus on core academic subjects and teachers bringing their personal views into the classroom.
Among Democrats, liberals are especially likely to cite schools lacking resources and parents having too much say in the curriculum.
Note: Here are the questions used for this analysis , along with responses, and the survey methodology .
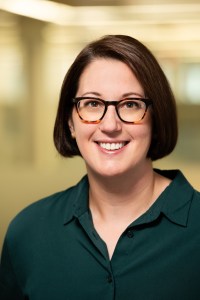
Sign up for our weekly newsletter
Fresh data delivered Saturday mornings
‘Back to school’ means anytime from late July to after Labor Day, depending on where in the U.S. you live
Among many u.s. children, reading for fun has become less common, federal data shows, most european students learn english in school, for u.s. teens today, summer means more schooling and less leisure time than in the past, about one-in-six u.s. teachers work second jobs – and not just in the summer, most popular.
About Pew Research Center Pew Research Center is a nonpartisan fact tank that informs the public about the issues, attitudes and trends shaping the world. It conducts public opinion polling, demographic research, media content analysis and other empirical social science research. Pew Research Center does not take policy positions. It is a subsidiary of The Pew Charitable Trusts .
Advertisement
Supported by
More Voters Shift to Republican Party, Closing Gap With Democrats
The trend toward the Republican Party among white voters without a college degree has continued, and Democrats have lost ground among Hispanic voters, too.
- Share full article

By Ruth Igielnik
In the run-up to the 2020 election, more voters across the country identified as Democrats than Republicans. But four years into Joseph R. Biden Jr.’s presidency, that gap has shrunk, and the United States now sits almost evenly divided between Democrats and Republicans.
Republicans have made significant gains among voters without a college degree, rural voters and white evangelical voters, according to a new report from the Pew Research Center . At the same time, Democrats have held onto key constituencies, such as Black voters and younger voters, and have gained ground with college-educated voters.
The report offers a window into how partisan identification — that is, the party that voters tell pollsters they identify with or lean toward — has shifted over the past three decades. The report groups independents, who tend to behave like partisans even if they eschew the label, with the party they lean toward.
Voters are evenly divided between Democrats and Republicans.
Among all registered voters
“The Democratic and Republican parties have always been very different demographically, but now they are more different than ever,” said Carroll Doherty, the director of political research at Pew.
The implications of the trend, which has also shown up in party registration data among newly registered voters , remains uncertain, as a voter’s party affiliation does not always predict who he or she will select in an election. But partisan affiliation patterns do offer clues to help understand how the shifting coalitions over the last quarter century have shaped recent political outcomes. During the Trump administration, the Democratic Party’s coalition grew, helping to bring about huge victories in the 2018 midterm elections and a victory for President Biden in 2020.
The G.O.P. has long struggled with the fact that there have generally been fewer Americans who identified as Republicans than as Democrats. After Barack Obama was re-elected as president in 2012, the Republican Party produced an autopsy report that concluded that in order to be successful in future elections, the party would need to widen its tent to include Black and Hispanic voters, who were not traditionally aligned with the G.O.P.
Twelve years later, the party has made some small gains with Hispanic voters. But it is growth with the white working class and with rural voters that has propelled Republicans to equity with Democrats.
The catch is that white working-class voters are slowly declining as a share of registered voters, so the Republican strategy of relying heavily on the group may not be sustainable in the long term.
At the same time, a much talked-about broad political realignment among Black and Hispanic voters has yet to materialize, at least by the metric of party identification.
The education gap among white voters has grown dramatically since 2010.
Percent who identify as Republican or lean toward the Republican party
Republicans’ growing strength with white working-class voters represents one of the biggest political schisms in the country over the past 15 years. In the 1990s and early 2000s, Democrats had a slight partisan identification advantage among voters without a college degree, while college-educated voters were more evenly divided between the two parties. Beginning in the early 2010s — and accelerating during the presidency of Donald J. Trump — voters without a college degree, in particular white voters without a degree, increasingly moved toward the Republican Party.
Now, nearly two-thirds of all white noncollege voters identify as Republicans or lean toward the Republican Party.
And Republicans are making gains among white women, as well. In 2018, a year after the Women’s March that attracted millions to protest Mr. Trump’s policies, the group was split about evenly between Democrats and Republicans. But since then, Republicans have slowly been gaining ground. They now hold a 10 percentage point partisanship advantage.
Overall, over most of the last 30 years, white voters have been more likely to identify as Republicans than Democrats, though the gap closed briefly in the mid-2000s.
While Hispanic voters are still far more likely to identify as Democrats, the party’s edge with the group has narrowed in the past few years. Currently, 61 percent of Hispanic voters identify as Democrats or lean toward the Democratic Party, down from nearly 70 percent in 2016. That trend mirrors polling in 2020 and 2024 that has shown the potential for support for Mr. Trump to grow among Hispanic voters.
That change appears most notable among Hispanic voters who do not have a college degree or who identify as Protestant. As recently as 2017, the latter group leaned Democratic; now, it is more likely to identify as Republican, even as Hispanic Catholics are still more likely to identify as Democrats.
These shifts in partisanship fall short of what some predicted to be a political realignment, said Bernard Fraga, an associate professor of political science at Emory University who studies Latino voters.
But Latino voters care deeply about the economy, Mr. Fraga noted, and Latinos who are ideologically conservative are interested in Republicans and their plans for the country.
“It is also important to remember that the Latino population is extremely dynamic,” Mr. Fraga said. “There are a tremendous number of newly eligible voters in every election cycle. And what we perceive as a change or shift for Latinos is going to be disproportionately due to new voters.”
“About one third of Latino voters weren’t even in the electorate before 2016,” he added.
More Hispanic voters now identify as Republicans than in 2016.
Percent who identify as Republican or lean toward the Republican Party
Black voters still overwhelmingly associate with the Democratic Party: Eighty-three percent of Black voters identify as Democrats or lean Democratic. There has been a small decline since 2020, when the share of Black voters who identified as Democrats was about five percentage points higher. Among Black men, 15 percent currently identify as Republicans, the same share as 30 years ago.
Ruth Igielnik is a polling editor for The Times, where she writes and analyzes surveys. She was previously a senior researcher at the Pew Research Center. More about Ruth Igielnik
Our Coverage of the 2024 Election
Presidential Race
The democratic party is unifying around a blunt message on abortion, solely blaming Donald Trump for the country’s shift, ahead of Kamala Harris’s trip to Arizona, where Democrats hope to keep Republicans reeling.
Trump and Mike Johnson, the G.O.P. speaker, at odds over many issues, are making a common cause on “election integrity,” ahead of Johnson’s trip to Mar-a-Lago.
Price pressures aren’t easing fast enough to guarantee the interest-rate cuts President Biden had hoped to see , so his message is evolving as he casts Trump and Republicans as uninterested in the actual policy work of fighting inflation and as barriers to his own proposals.
The political prediction markets — which allow traders to place bets on the outcome of the November election — show that the presidential race is tight, giving Trump an even chance of winning the election . So far, it appears the market doesn't care either way.
Trump’s penchant for bending the truth has been well documented, but a close study of how he does so reveals a kind of technique to his dishonesty .
Primaries in three Mid-Atlantic House districts will test whether the battle cry of “save democracy” will be enough even for Democratic voters who have many other concerns.
In Arizona’s crucial Senate race, Ruben Gallego, who has long embraced his progressive background, is striking a moderate tone .
- Rest of World News
- Japan's elderly population living alone to jump 47% by 2050: Research
Japan's elderly population living alone to jump 47% by 2050: Research
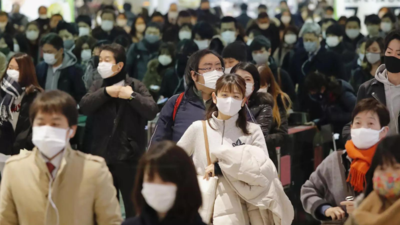
Visual Stories
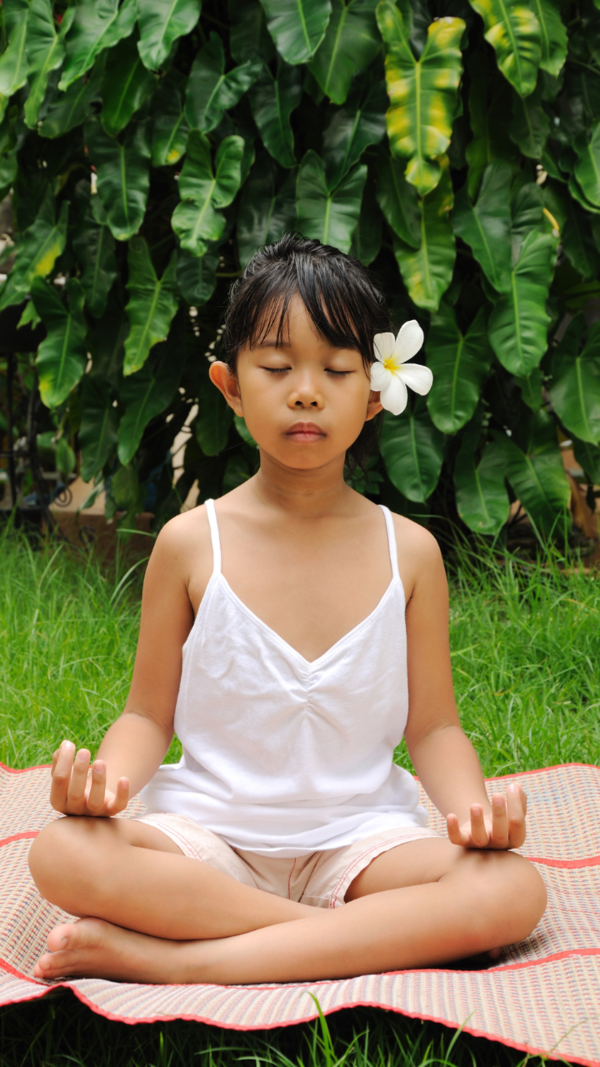
Wall Street Journal poll shows Biden losing support from Black men
Fox News political analyst Gianno Caldwell tells 'The Story' that the Democratic Party has taken the Black community for granted.
America is split in political party affiliation heading into the 2024 presidential election , seeing one of the most evenly split electorates in the past two decades, resurfaced research reveals.
A Pew Research Center analysis examined voter identification across different ages, races, religions and education levels, comparing how voters identified in 1996 to new data from 2023.
Fifty-one percent of Americans said they identified with the Republican Party in 1994, while 47% identified as Democrats. The tables turned over the years, with 5% more of American voters identifying as Democrats over Republicans in 2020. However, the Pew results from 2023 reveal a significant shift in party affiliation this cycle, reporting that 49% of voters identify as Democrat or leaning Democrat, while 48% identify as Republican or leaning Republican.
Additionally, about 33% of respondents said they identify as being conservative or moderate in 2023, while the other side of the aisle only sees 23% identifying as liberal Democrats or leaning liberal.
BLACK GEORGIA VOTERS ABANDONING BIDEN SAY THEY'RE SENDING MESSAGES ON GAZA: ‘DEMOCRATS SHOULD LISTEN’
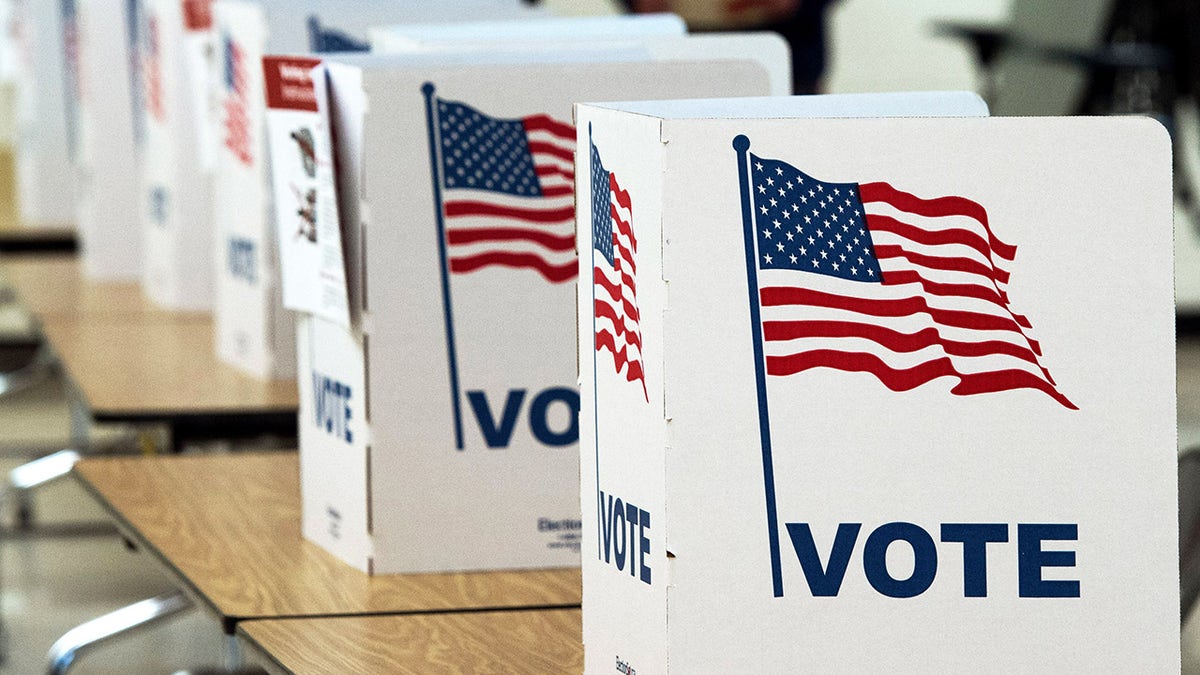
Ranked choice voting comes in multiple forms and is used in a wide variety of states and localities around the U.S. (Paul Richards)
The poll found that while Democrats remain the party of choice for most Hispanic, Black and Asian voters, party support among non-Hispanic White Democratic voters has dropped 21 percentage points since 1996, falling from 77% to 56% in 2023.
Recent polls have found that despite their advantage, Democratic support among minority voters is shrinking. A recent Gallup poll found that 19% of Black adults said they identify as lean Republican or Republican, while 66% identify as Democrat or lean Democrat, the "smallest Gallup has recorded in its polling, dating back to 1999."
UNDECIDED BATTLEGROUND VOTERS UNANIMOUSLY BLAST BIDEN ON ECONOMY: ‘ABSOLUTELY DISASTROUS’
Among different age groups, Democrats maintain their advantage among young voters, while the majority of older individuals are Republican affiliated.
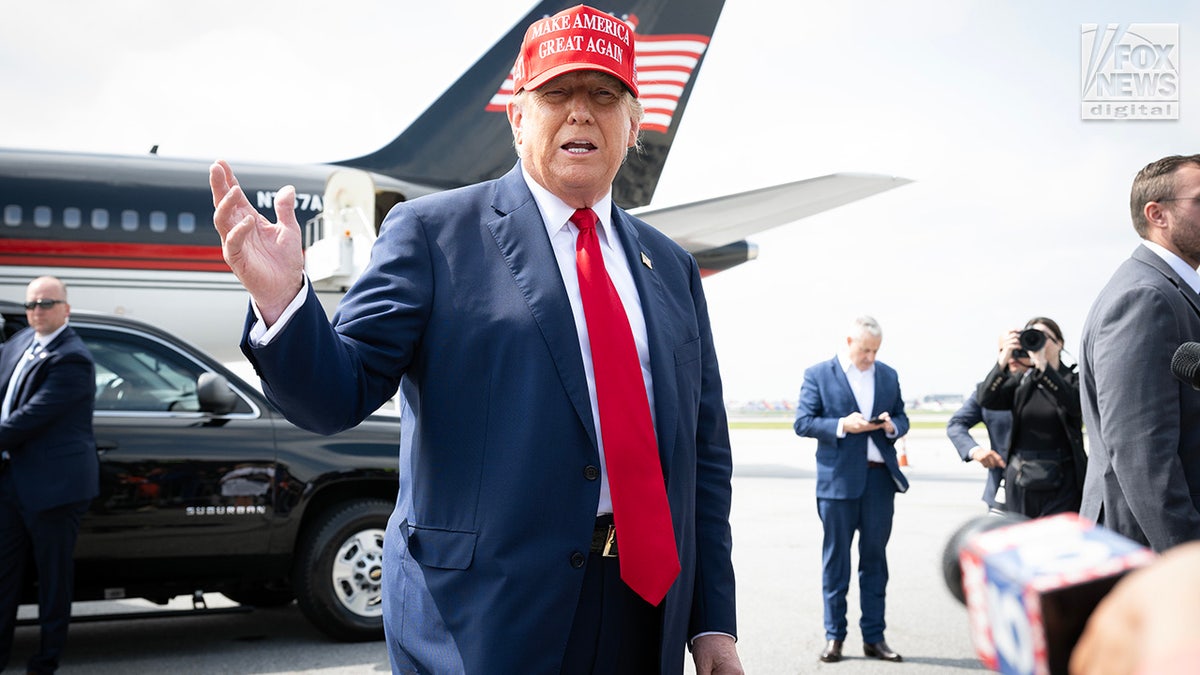
Former President Donald Trump arrives at Atlanta’s Hartsfield-Jackson Airport in Georgia on Wednesday to host a campaign fundraising event. (Robin Rayne for Fox News Digital)
Republicans have gained ground among Hispanic voters in recent years, tripling affiliation with their base in the demographic over the past two decades from 3% to 9%.
Rural voters also appear to be shifting towards the GOP, with the new poll showing the party holds a 25-point advantage over Democrats, 60% to 35%, after the parties were evenly split among voters in 2008.
President Biden and former President Donald Trump are expected to compete in a presidential election rematch in November. While Biden won the 2020 election against Trump, Pew's analysis reveals a potential shift in the political landscape that could be echoed on the ballot this fall.
CLICK HERE TO GET THE FOX NEWS APP
Pew Research Center conducted the surveys via telephone for the results dating 1994 to 2018, and via online surveys from 2019 to 2023 among registered voters.
Aubrie Spady is a Production Assistant for Fox News Digital.
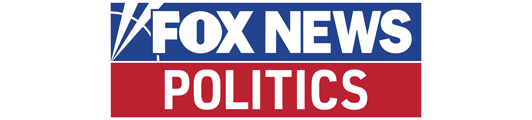
Get the latest updates from the 2024 campaign trail, exclusive interviews and more Fox News politics content.
You've successfully subscribed to this newsletter!
More from Politics
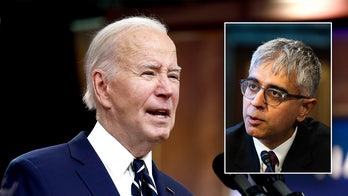
Biden resists pulling controversial judicial nominee Adeel Mangi despite Democrat defectors
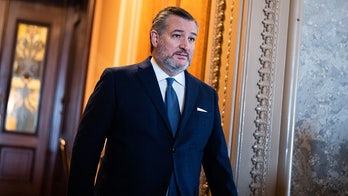
Texas showdown: Sen. Ted Cruz steps up his game as conservative firebrand faces bruising re-election race
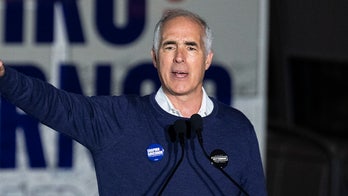
Battleground state Dem distances himself from defund movement, but political record shows different story
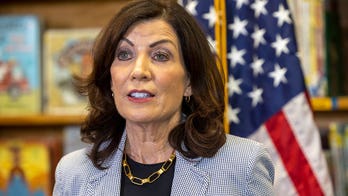
NY ballot initiative could ban parents from approving child's trans surgery, critics warn in fiery campaign
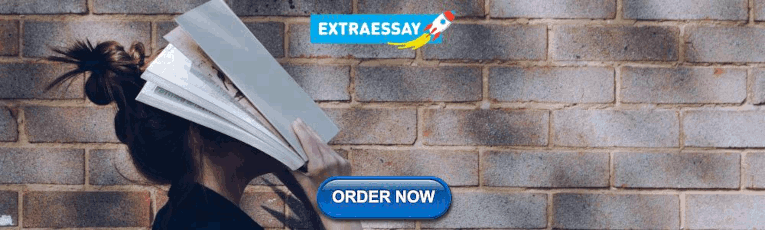
IMAGES
VIDEO
COMMENTS
Introduction. For 75 years, the journal Population Studies has published work advancing our knowledge of demography and population, from substantive topics in the areas of fertility, mortality, migration, and families to innovations in theory, methods, policy, and practice. Demographic topics, theories, and methods have drawn from multiple ...
Demography is the official journal of the Population Association of America. It is an interdisciplinary peer-reviewed periodical that publishes articles of general interest to population scientists. Fields represented in its contents include geography, history, biology, statistics, business, epidemiology, and public health, in addition to social scientific disciplines such as sociology ...
Dr. Abhishek Singh, is a Professor in the Department of Public Health and Mortality Studies at the International Institute for Population Sciences (IIPS), Mumbai India.Dr. Singh has published more than 100 research papers in peer-reviewed national/international journals. Dr. Singh's areas of interest are mortality analysis (including maternal mortality), maternal and child health, gender ...
Demographic research monographs (a series of the Max Planck Institute for Demographic Research). Springer, Dordrecht 2019: 167-183. View in Article Scopus (6) Crossref; Google Scholar; Article info Publication history. Published: 14 August 2021. Identification. DOI: ...
Overview. The Journal of Population Research publishes peer-reviewed papers on demography and population-related issues. International in scope, the journal presents original research papers, perspectives, review articles and shorter technical research notes. The range of coverage extends to substantive empirical analyses, theoretical works ...
Its geographic focus is global, with articles addressing demographic matters from around the planet. Its temporal scope is broad, as represented by research that explores demographic phenomena from past to present and reaching toward the future. Demography is the flagship journal of the Population Association of America.
noun. arrangement or spread of people or organisms living in a given area. statistics. noun. the collection and analysis of sets of numbers. Demography is the statistical study of human populations. Demographers use census data, surveys, and statistical models to analyze the size, movement, and structure of populations.
This article was most recently revised and updated by Michael Ray. Demography, statistical study of human populations, especially with reference to size and density, distribution, and vital statistics (births, marriages, deaths, etc.). Contemporary demographic concerns include the "population explosion," the interplay between population and ...
Demographic Research is a peer-reviewed, open-access journal of population sciences published by the Max Planck Institute for Demographic Research in Rostock, Germany. Contributions are generally published within one month of final acceptance. Journal information. 2023 (Vol. 49)
Abstract. This article reviews some key strands of demographic research on past trends in human longevity and explores possible future trends in life expectancy at birth. Demographic data on age-specific mortality are used to estimate life expectancy, and validated data on exceptional life spans are used to study the maximum length of life.
Demographic Research is a monthly open-access online journal published by the Max Planck Institute for Demographic Research that started publication only in 1999. The corpus of papers we analyze is also necessarily smaller than the increasingly diverse universe of demography publications, and it represents a declining fraction of all articles ...
Its geographic focus is global, with articles addressing demographic matters from around the planet. Its temporal scope is broad, as represented by research that explores demographic phenomena from past to present and reaching toward the future. Demography is the flagship journal of the Population Association of America.
In research, there are 2 kinds of populations: the target population and the accessible population. The accessible population is exactly what it sounds like, the subset of the target population that we can easily get our hands on to conduct our research. While our target population may be Caucasian females with a GFR of 20 or less who are ...
About Pew Research Center Pew Research Center is a nonpartisan fact tank that informs the public about the issues, attitudes and trends shaping the world. It conducts public opinion polling, demographic research, media content analysis and other empirical social science research. Pew Research Center does not take policy positions.
Irene Barbiera is currently research fellow at the Department of Statistical Sciences, University of Padova, Italy, where she works since 2015. She has been research fellow at the Central European University between 2011 and 2013. In 2007-2008 she has been research fellow at the Academy of Sciences of Vienna, in the frame of the Wittgenstein project entitled: Ethnic Identities in Early ...
The main impediment to the study of family demography and related research in India is first the lack of a comprehensive conceptual framework and second lack of reliable data. Understandably, family demography is currently in its nascent stages as a branch of demography and population studies. Fundamental intricacy inherent in demographic ...
The study of demography and collection of demographic data are quintessential aspects of human research. Demography refers to the characteristics that encapsulate communities of people such as sex, race, marital status, or socioeconomic status (Caldwell, 1996; Furler et al., 2012).Demographic data, on the other hand, describe the quantitative assessment of these characteristics (Vogt & Johnson ...
Background Health outcomes are strongly impacted by social determinants of health, including social risk factors and patient demographics, due to structural inequities and discrimination. Primary care is viewed as a potential medical setting to assess and address individual health-related social needs and to collect detailed patient demographics to assess and advance health equity, but limited ...
About Pew Research Center Pew Research Center is a nonpartisan fact tank that informs the public about the issues, attitudes and trends shaping the world. It conducts public opinion polling, demographic research, media content analysis and other empirical social science research. Pew Research Center does not take policy positions.
Decision makers may not recognize these implications, though. In this article, the authors summarize recent research on identity omission in demographics forms and offer two low-cost, low-risk ...
Similarly, research has highlighted the role of demographic and socio-economic variables, including age, gender, and ethnicity 12,19,25,27,30 as well as socio-economic status 31 in predicting a ...
About Pew Research Center Pew Research Center is a nonpartisan fact tank that informs the public about the issues, attitudes and trends shaping the world. It conducts public opinion polling, demographic research, media content analysis and other empirical social science research. Pew Research Center does not take policy positions.
April 9, 2024. In the run-up to the 2020 election, more voters across the country identified as Democrats than Republicans. But four years into Joseph R. Biden Jr.'s presidency, that gap has ...
Aging research is helping us understand the deep biological implications of this advice. Eating a variety of healthy foods in moderation can prevent the health risks of obesity.
Of those one-person households, senior citizens aged 65 or older will likely represent 46.5% in 2050, compared with 34.9% in 2020, the institute's estimates showed. Japan, one of the world's most ...
A Pew Research Center analysis examined voter identification across different ages, races, religions and education levels, comparing how voters identified in 1996 to new data from 2023.