
- Home
- Research Collections
- Information, School of (SI)
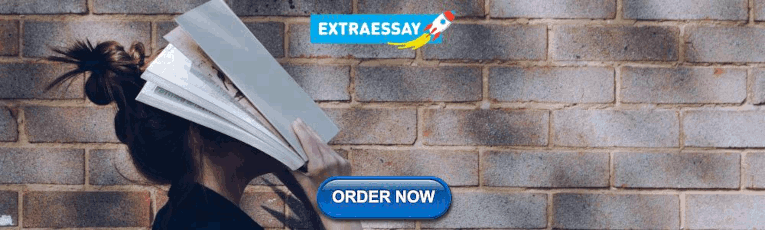
Social Media and Society in India

Deep Blue DOI
Description, collections.
- Interdisciplinary and Peer-Reviewed
The following license files are associated with this item:
- Creative Commons

Remediation of Harmful Language
The University of Michigan Library aims to describe library materials in a way that respects the people and communities who create, use, and are represented in our collections. Report harmful or offensive language in catalog records, finding aids, or elsewhere in our collections anonymously through our metadata feedback form . More information at Remediation of Harmful Language .
Accessibility
If you are unable to use this file in its current format, please select the Contact Us link and we can modify it to make it more accessible to you.
Investigating political polarization in India through the lens of Twitter
- Original Article
- Published: 31 July 2022
- Volume 12 , article number 97 , ( 2022 )
Cite this article
- Anindita Borah 1 &
- Sanasam Ranbir Singh 1
5250 Accesses
3 Citations
10 Altmetric
Explore all metrics
Social media plays a pivotal role in shaping communication among political entities. Substantial research has been carried out for examining the impact of politicians’ social media usage and interactions on political polarization. Analysing political polarization is particularly significant for fragmented political systems like India where collaboration between parties is essential for winning support in parliament. Different topics of discussion between political entities may induce different levels of polarization. This study aims to examine the presence of polarization on Twitter social media platform with respect to different topics of political discussions among Indian politicians. The investigation is based upon two conflicting notions about social media in influencing political polarization. The first notion regards social media as a medium for interaction between different ideological users. The second opinion on the other hand focuses on prevalence of selective exposure in social media leading to polarization. The study will investigate the use of Twitter for forming communication ties in and between parties and the extent of divergence of opinions during political discourse. The investigation performs social network analysis and content analysis of the tweets posted by Indian politicians during some major events in India from 2019 to 2021. For an unbiased topic-specific analysis of polarization, some important topics related to Indian government policies, national security and natural disaster events have been considered. The findings of the study suggest that Twitter not only opens up communication spaces to Indian political users but also makes online political discussions among them polarized. Moreover, the extent of polarization varies with respect to topics of political discussions. Polarization is more for controversial and debatable topics than non-controversial ones.
Similar content being viewed by others
Social Media in Politic: Political Campaign on United States Election 2020 Between Donald Trump and Joe Biden
Blurring Boundaries in Political Journalism? The Digital Authority of Citizens in the Public Debate on Twitter
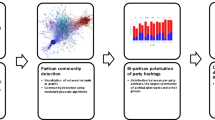
Who polarizes Twitter? Ideological polarization, partisan groups and strategic networked campaigning on Twitter during the 2017 and 2021 German Federal elections 'Bundestagswahlen'
Philipp Darius
Avoid common mistakes on your manuscript.
1 Introduction
Political polarization has become an important subject of deliberation owing to its negative implications on democratic societies. A polarized population is often divided into different groups of same size having opposing polarities or opinions. There has been a constant debate regarding the presence of political polarization on social media due to its growing popularity and usage among the politicians, parties and masses. Politicians use social media platforms for propagating their political views and for supporting or engaging in debates with other politicians. Social media might enable information flow by facilitating direct communication and exchange of ideas between the political entities, but might also induce polarization. Degree of polarization on social media depends on the topics of political discussions to a great extent. Different topics involve different levels of compliance and disagreement that may either unite the political groups or move them far apart. It is fundamental to understand how activities of Indian politicians on social media with respect to different topics influence political polarization.
Among all the social media platforms, Twitter is the most pivotal online places extensively used for political debates. This study focuses on Twitter because of its worldwide popularity, majority of the Indian politicians are having Twitter handles and the data is easily accessible. The primary aim of this study is therefore, to investigate the presence of polarization among Indian politicians on Twitter during political discussions. To investigate the existence of political polarization on social media platforms, this study analyses the usage of Twitter by Indian politicians during some of the major events in India.
1.1 Research questions and hypotheses
Political polarization is a diverse field of research and its investigation in a single dimension may not be sufficient. In order to examine the existence of political polarization on social media, the analysis must be done from different perspectives. This study will therefore, investigate Indian political polarization based on its two broad characteristics: pattern of interaction and opinion divergence. For an unbiased analysis, political polarization will be examined with respect to several topics of political discussions related to some important events in India. The following research questions have been formulated for investing polarization on social media platform Twitter:
What is the pattern of interaction of politicians during discussions on political issues?
Social media on one hand can promote flow of information, ideas and opinions among the political entities and on the other hand, can also induce political polarization. An important question in this regard is whether the political entities use Twitter to communicate with members having different ideologies or their interaction is restricted to only like-minded peers. Therefore, it is crucial to understand how Twitter is affecting the formation of communication ties within and across parties and whether it leads to polarization. The amount of participation and interaction among the politicians varies depending on the topics of political discourse. In this context, the first research question will focus on the way communication ties are formed within and between parties with respect to different topics and whether this leads to polarization. While interacting on Twitter, the politicians make connections with each other, forming complex social network structures. These network structures demonstrate the flow of information and the connections in the networks reflect the sharing of content among politicians.
There are two basic modes of interaction in Twitter: (a) mention , in which one user can mention another user in response to his/her tweets and (b) retweet , in which one user can share or forward another user’s tweets. To identify the pattern of interaction with respect to topics of discussion, this study will analyse the formation of communication ties as well as the degree of polarization among Indian politicians from their mention and retweet networks on different topics.
Existing research on social media polarization demonstrated the presence of cross-ideological exchange in Twitter networks. Mention networks reflect the communication behaviour of political candidates as well as their cross-party interactions. The cross-party connections however, varies based on the layers in which the interaction takes place. In accordance with the first research question and the findings of existing research, the following hypotheses have been formulated:
Mention networks of politicians reflect more cross-party interactions than retweet networks during political discussions
Selective exposure prevails only in retweet networks of politicians and not in mention networks during political discussions
Is there any difference in the opinion of politicians on different political issues?
Social media allow the political entities to articulate and express different opinions which often leads to conflict in their sentiments and views towards an issue . According to social scientists, this divergence in opinion leads to increased polarization among the political entities. In order to reach an agreement regarding the possible solutions about an issue, the political entities must recognize their opponent’s views as valid despite being conflicting. However, refuting their opponents view as invalid or improper might make the political discussions on crucial issues more polarized. The second research question will therefore, try to investigate the extent of divergence of opinions among the political entities with respect to topics of political debate. Selective exposure during political debates has been identified as a major factor influencing the disagreement among politicians. Retweet relations represent agreement among the politicians on their published content. Retweet networks represent the similarity between the interests of the communities. Considering, the retweet relation to be a form of agreement between users and selective exposure a factor influencing opinion divergence, the following hypothesis have been formulated:
Interconnected communities in retweet networks are more similar to each other in terms of opinion
Literature contains ample endeavours intensifying on the phenomenon of political polarization on social media. However, very limited studies can be found concerning the emergence of polarization among political elites on Twitter or other platforms. Study of elite polarization is essential as it eventually gives rise to mass polarization or polarization among the general public. Existing studies investigated polarization either in terms of selective exposure or opinion polarization but not as a whole. Investigating polarization in a single dimension may not be sufficient. Moreover, none of the studies have performed a topic-based analysis on polarization, even though topics of political discourse play a significant role in influencing polarization. The growing polarization in Indian politics has become an important research issue for the political scientists and social media activities of political candidates may prove to be an important indicator for measuring polarization. To the best of our knowledge, this study is the first attempt towards the investigation of Indian political polarization over Twitter with respect to different topics of political discussions.
The remaining paper is organized as follows: Sect. 2 elucidates some existing studies on political polarization. The description of the data used and the methodology adopted for this study is illustrated in Sect. 3 . The findings and observations of the analysis are discussed in greater detail in Sect. 5 . The study is finally summarized and concluded in Sect. 6 .
2 Related work
Several studies can be found in the literature that investigated political polarization over social media. First study towards the same was performed by Adamic and Glance ( 2005 ) to identify the pattern of interaction among the conservative and liberal blogs. They performed network analysis and identified a clustered structure between the hyperlinks of blogs with opposing ideologies. Conover et al. ( 2011 ) did a similar study to identify the extent of polarization among the Twitter users during 2010 U.S. congressional elections. The authors pointed out that users tend to endorse or retweet their politically aligned peers more than users having opposite polarities. Morales et al. ( 2015 ) measured the extent of polarization among the masses based on Twitter conversations about the late Venezuelan president Hugo Chavez. They employed network structures and statistical modelling techniques and identified that the distance between users posting similar contents is comparatively very less. An analysis on user roles during polarized conversations was performed by Recuero et al. ( 2019 ). They pointed out that contents shared in one group are not shared in other groups. Weber et al. ( 2013 ) analysed Egyptian polarization among the Secular and Ismalists users over Twitter. They identified the followers of Egyptian Muslim to be more tightly connected than the Secularists. Olivares et al. ( 2019 ) used opinion distribution as the basis for analysing political polarization during second round of the 2017 Chilean elections. They identified the Twitter conversation to be highly polarized that was continuously increasing till the day of voting.
Garimella et al. carried out several studies on elite polarization in their works in Garimella and Weber ( 2017 ); Garimella et al. ( 2018 ). They performed a temporal analysis of polarization for eight years among the presidential candidates and their parties in Garimella and Weber ( 2017 ). The findings suggest a growth of nearly 10–20% in polarization over the course of 8 years. In Garimella et al. ( 2018 ), through network analysis they pointed out that the retweet networks of polarized discussions have a well-defined structure. Cherepnalkoski and Mozetič ( 2016 ) investigated the community structure of European Parliament members for a period of one year. Their emphasis was primarily on retweet networks. They identified that the retweeting behaviour of the European parliament members is biased towards the members of their own political group. Del Valle and Bravo ( 2018 ) carried out a detailed study on the Twitter networks of Catalan Parliamentarians to analyse the extent of polarization between them. They discovered more cross-ideological interactions in the mention network than the retweet network and the level of polarization is observed to be highest in the relation network. They performed another study in Esteve Del Valle et al. ( 2021 ) about polarization in the Twitter mention networks of the Dutch Member of Parliaments. They identified high degree of cross-party interactions in their mention networks suggesting that the MPs extensively use social media for discussions among different parties. van Vliet et al. ( 2020 ) studied polarization across 26 European Free Trade Association countries by analysing their network of interaction during political discourse. They observed cross-party interactions and cross-national differences in the way of engagement of the political entities.
Political polarization is also analysed using models of opinion dynamics in the literature. Models of opinion dynamics attempt to identify the change in opinion of users in a network with respect to their neighbours. The most popular theoretical model used for analysing the phenomenon of opinion formation is the averaging model. The DeGroot model is a well-known example of averaging models DeGroot ( 1974 ). The model analyses the formation of consensus with the update in individual opinions based on the average of the neighbourhood. User’s opinion is basically updated using the mean of neighbouring opinions. Friedkin and Johnsen ( 1990 ) further extended the model by considering consensus as well as disagreement, thus including both innate and expressed opinion of a user. Several studies employed the existing models of opinion dynamics to study the phenomenon of polarization. Ghezelbash et al. ( 2019 ) utilized the DeGroot model to study polarization in cooperative networks. Alvim et al. ( 2021 ) employed the concepts of DeGroot model to establish the fact that polarization might not vanish in case of weakly connected graphs.
3 Data and methods
This section discusses the data collected for the study and the methodologies used for analysis. To perform a topic-wise analysis of Indian political polarization on social media, politicians tweets based on some major events in India are collected. Important topics related to government policies, natural disaster and national security during the last 2 years have been considered. The data has been collected from the Twitter handles of 823 politicians belonging to the major national and state political parties of India. Twitter handles of most active politicians have been considered. Table 1 elicits the number of members from each political party whose data has been collected.
India has witnessed some important events during 2019 and 2020 in terms of government policy formulation, national security and natural disaster management. For an unbiased study on Indian political polarization, topic-specific Twitter data has been collected related to all the major events. Among government policies, the Citizenship Amendment Act (CAA) during 2019 and the recent Farm Bills have been considered. Indian parliament passed CAA in 2019 for granting the eligibility of citizenship to religious minorities in countries like Afghanistan, Pakistan and Bangladesh. Three acts of Farm Bills were passed by parliament of India in 2020 as per which intra- and inter-state farmer’s produce trade is allowed beyond the physical premises of Agricultural Produce Market Committee (APMC). Topics related to national security like Balakot airstrikes of 2019 and India China Stand-Off in 2020 have been considered for the study. In 2019, Indian warplanes conducted a bombing raid against an alleged terrorist training camp in Balakot, Pakistan. In 2020, Indian and Chinese troops got engaged in skirmishes and face offs along the Sino-Indian border leading to several casualties of soldiers on both sides. Apart from these topics, the recent natural disaster of COVID-19 has also been considered. Initially identified in Wuhan, China, the Coronavirus Disease (COVID-19) has spread exponentially throughout the world affecting millions of lives. India is among the worst affected countries due to COVID-19 in terms of mortality and number of cases. The nation has suffered extensive economic, political and social crisis due to this sudden outbreak.
For collecting topic-specific data, the most popular hashtags capturing a particular topic of interest have been manually identified. For analysing the data, a hybrid approach of social network analysis and content analysis has been adopted. Table 2 elicits the statistics of data collected for this study. The statistics depict the number of tweets collected for a particular topic, the number of politicians participating in the political discussions about the topic and the number of hashtags considered for collecting topic-specific tweets.
The Twitter activities of Indian politicians were monitored from 2019 to 2020. The Twitter search API has been used to select the tweets posted by the politicians on important topics on natural disaster, national security and government policies during that duration. The collected tweets are then used for constructing social networks and identifying communities in those networks. The communication flow among the communities and their opinions on the selected topics is then analysed. The process of identifying the interaction patterns of communities and their opinions on the topics consists of two steps. Initially, the network of politicians mentioning and retweeting each other is constructed and the densely connected communities are identified. Secondly, the content published by the communities is analysed to identify the convergence and divergence in opinions within and across communities. Figure 1 illustrates the proposed methodology and roadmap for this study. The detailed methodology for analysing the pattern of interaction and convergence and divergence of opinions of the communities is discussed below:
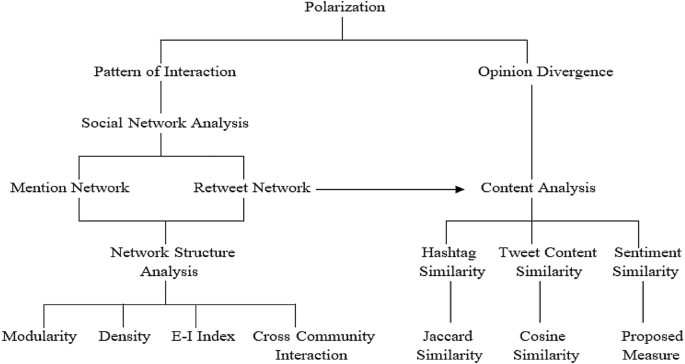
Proposed roadmap for the study
3.1 Pattern of interaction
The first line of research in this study is based on identifying the pattern of interaction and formation of communication ties among the political candidates. Twitter interactions among the politicians using mentions and retweet induce different network structures. The structure of networks also varies with respect to the topics of discussion. These network structures reflect the mechanism underlying the formation of communication ties between the politicians. Employing social network analysis for solving a broad range of political issues has been widely accepted. Concepts of social network analysis can be used to identify the factors influencing the formation of ties in political networks and the nature and meaning of those ties. A social network analysis approach has therefore been, adopted to examine the pattern of interactions in Twitter political networks of Indian politicians. The degree of party polarization in the politicians interaction networks(retweet and mention) is examined using network visualization and network polarization analysis. The interaction patterns of politicians and its effect on polarization can be determined based on the following analysis of Twitter interaction networks:
Do politicians form distinct communities while contributing to a particular topic?
Politicians on social media often tend to interact and share their ideas more with their own party people while discussing any topic of interest. Communities identified in the political networks can reveal the existence of selective exposure and polarization based on their size, content and level of interconnections. Hence, the first step towards investigating the existence of selective exposure and polarization is identification of distinct communities in the Twitter mention and retweet networks.
From the topic-specific Twitter data, networks of politicians participating in discussions are mapped and the connections are created based on their mention and retweet relationships. Open source network exploration software Gephi is used for network visualization for anticipating the retweet and mention network structures Bastian et al. ( 2009 ). Each node in the network represents a politicians Twitter account and an edge between 2 nodes reflects the relationship (mention or retweet) between the politicians. For better visualization, only those politicians or nodes are considered that received a minimum of 5 mentions and retweets.
What is the level of interconnectedness and information flow among the communities during discussions?
To measure the level of interconnectedness and information flow among the identified communities, modularity of the network is computed using Newman’s measurement of modularity Newman ( 2004 ). Measurement of modularity has been used to analyse the strength of divisions of the communities identified in the networks. Modularity values range from 0 to 1. Higher modularity values indicate the communities to be more distinct or separated. Studies reveal that networks having modularity value higher than 0.6 shows little or no increase in the separation of communities. For this study, value of 0.6 and above has been considered as higher modularity, between 0.4 and 0.6 medium modularity and values less than 0.4 as low modularity. A higher value of modularity indicates the presence of selective exposure, where the communities are more exposed to their own content rather than the contents posted by users in other communities.
The degree of cohesiveness among the politicians at the party level is examined by computing the network density of the retweet and mention networks. The value of network density varies from 0 to 1, where 0 indicates absence of any ties while 1 indicates that all ties are connected.
What is the extent of party polarization and cross-community interaction during topical discussions?
Communities reveal significant information regarding polarization. Once the communities are identified in the politicians interaction networks, it is essential to identify the extent to which these communities are polarized and closely associated with each other. Hence, it is crucial to examine the degree of polarization and homophily within and across the communities. This can be done by comparing the number of connections formed within and across those communities or groups. Several measures are identified as indicators of selective exposure and polarization.
The degree of party polarization and homophily has been computed using the measure of external–internal index (E–I index), developed by Krackhardt and Stern ( 1988 ). E–I index is a measure of relative density of internal ties within a group with respect to the number of external ties across the group. The value of the index ranges from –1 representing complete homophily to +1 indicating that all connections are external to the group. Cross-community interactions have been analysed to verify the extent of polarization. The cross-ideological interaction ratio measure developed by Conover et al. ( 2011 ) has been modified to calculate the cross-community interaction ratio. Cross-community interaction has been computed as the ratio between the observed and expected number of connections between nodes belonging to different groups or communities. Let C 1, C 2 and C 3 be 3 communities in a network. If K \(_{C1}\) is the total number of connections arising from community C 1 and U \(_{C1}\) , U \(_{C2}\) and U \(_{C3}\) are the number of users in community C 1, C 2 and C 3, then the expected number of connections from C 1 to C 2 is computed as:
3.2 Interest and opinion on political issues
The second research question of this study focuses on agreement and disagreement among Indian politicians on political issues. Retweet relations signify agreement between users on posted contents. Hence, for analysing the pattern of agreement and disagreement of the politicians on different issues, the retweet networks constructed in the previous phase have been considered. The process of identifying common interest and leaning of the politicians involves 3 steps. Densely connected communities in the retweet networks of politicians are initially identified. Secondly, the content shared by the communities is analysed to identify their common interest. Finally, the sentiment of the communities is obtained to identify their sentiment towards respective topics of political discussion.
Which communities share similar content on different political issues?
To identify common interest and leaning of the politicians on various issues, it is essential to analyse their posted tweet contents and the hashtags used. The similarity between the contents published by the communities and the hashtags used reveal their shared interest. The hashtag similarity between communities is initially computed based on Jaccard similarity. Jaccard Similarity computes the number of common hashtags between two communities \(C_i\) and \(C_j\) with respect to their total hashtags. The Jaccard Similarity between communities \(C_i\) and \(C_j\) is computed as:
where H ( x ) represents the number of hashtags in community x .
The tweet content similarity is calculated using cosine similarity. Term Frequency Inverse Document Frequency (TFIDF) approach has been used to identify the importance of a term in a set of documents and cosine similarity has been used to obtain the similarity between that set of documents. To examine the content similarity of the communities, a standard text mining approach has been adopted:
For each community \(C_i\) , where i \(\epsilon\) \(\{1,...,N\}\) , document D \(_i\) is created containing the content published by all the users of C \(_i\) .
The set of terms used by communities \({C_1,....,C_N}\) is obtained from documents \({D_1,....,D_N}\) and the term frequency of each term t is computed. Term Frequency \(TF_i(t)\) for a term t signifies its number of appearances in a document \(D_i\) .
The document frequency DF ( t ) for each term t is calculated, that represents the number of documents in which t appears.
A Bag of Words (BoW) vector is constructed for each document \({D_1,....,D_N}\) , where each value in the vector is the value of a term t from the set of terms:
The cosine similarity between each document \({D_1,....,D_N}\) , represented by vectors is computed. Each document \(D_i\) represents a community \(C_i\) and the similarity between documents is considered as the similarity between the respective communities. The cosine similarity between documents \(D_i\) and \(D_j\) is computed as:
Which communities have similar sentiments towards political issues?
This analysis for identifying the sentiment similarity is done in 2 steps. Initially, the sentiment polarity of the tweets are identified using TextBlob. The tweets having polarity value between –1 to 0 are considered as positive tweets, value of 0 as neutral tweets and value between 0 and 1 as positive tweets. To identify the communities having similar sentiments, the sentiment similarity among each community is identified based on the common hashtags shared. Instead of considering the sentiment score, the average number of positive, negative and neutral tweets in each community has been considered. The following approach has been proposed to compute the hashtag-based sentiment similarity between communities:
For each topic, identify the set of common hashtags \(C_{HT}\) between communities \(C_i\) and \(C_j\) .
Identify the average number of positive tweets \(PT_\mathrm{avg}(C_i)\) and \(PT_\mathrm{avg}(C_j)\) , for communities \(C_i\) and \(C_j\) based on \(C_{HT}\) .
Identify the average number of negative tweets \(NT_\mathrm{avg}(C_i)\) and \(NT_\mathrm{avg}(C_j)\) , for communities \(C_i\) and \(C_j\) based on \(C_{HT}\) .
Identify the average number of neutral tweets \(NTT_\mathrm{avg}(C_i)\) and \(NTT_\mathrm{avg}(C_j)\) , for communities \(C_i\) and \(C_j\) based on \(C_{HT}\) .
Compute similarity between \(C_i\) and \(C_j\) as:
Twitter data of Indian politicians related to some important topics of discussion have been collected initially. Separate datasets are then generated for each topic based on the presence of selected hashtags in the tweets. Each dataset is then mapped onto a network using network analysis techniques based on the relationships among the politicians. Analysis has been done to explore the two research questions of this study as discussed next. The results have been discussed for each topic-specific dataset separately.
To visually observe the level of polarization of Indian politicians on the considered topics of discussion, the mention and retweet networks are initially generated. Force Atlas algorithm in Gephi visualization tool has been used to analyse and cluster the networks into sub-groups. The nodes are coloured based on their party affiliation. Each identified community represents a political party and is named from C 1 to C 7. The communities identified are represented using different colours as follows: C 1 (BJP) = Violet, C 2 (INC) = Green, C 3 (AITMC) = Light Blue, C 4 (AAP) = Orange, C 5 (SP) = Pink, C 6 (RJD) = Red, C 7 (SS)= Dark Blue. Figures 2 and 3 depict the mention and retweet networks generated for all the topics. The statistics for mention and retweet networks generated for all topics are illustrated in Table 3 .
4.1 Do politicians form distinct communities while contributing to a particular topic?
The mention networks generated for Indian politicians formed distinct communities while engaging in political discussions on all the considered topics. The COVID-19 mention network shown in Fig. 2 a, contains 524 nodes and 1370 edges. Seven distinct communities are identified in the network accounting for nearly 72.3% of the entire network. Remaining 27.7% of the network contains 8 small communities containing 2–18 nodes. Only communities within 72.2% of the networks have been considered for visualization. 3 large communities are identified: one with 178 users linked by 385 connections ( C 1), second with 112 users linked by 291 connections ( C 2) and the third with 72 users with 176 connections ( C 3). These three major communities constituted nearly 69.1% of the total connected users and 62.2% of the total connections in the network. The mention network on Citizenship Amendment Act shown in Fig. 2 b, contains 256 nodes and 517 edges. Seven distinct communities are identified in the network accounting for nearly 78.2% of the entire network. A single large community is identified containing 86 users linked by 165 connections ( C 1). The Farm Bill mention network in Fig. 2 c contains 285 nodes and 515 edges. The 6 communities identified in the mention network constitute nearly 74.6% of the entire network. 2 large communities are identified in the mention network. The largest community ( C 1) comprises of 105 users linked by 232 connections and the second community ( C 2) contains 85 users connected by 115 links. These 2 communities together constituted nearly 71.6% of the total connected users in the network. The mention network shown in Fig. 2 d, contains 164 nodes and 327 edges. Seven distinct communities are identified in the network accounting for nearly 75.6% of the entire network. A single large community is identified containing 86 users linked by 165 connections ( C 1). The mention network shown in Fig. 2 e, contains 114 nodes and 324 edges. Six distinct communities are identified in the network accounting for nearly 78.3% of the entire network. 2 large communities are identified: one with 58 users ( C 1) and second with 42 users ( C 2). These two major communities constituted nearly 79.1% of the total connected users. From Fig. 2 , it can be observed that the mention networks of all the datasets contain more number of cross-ideological and cross-party connections.
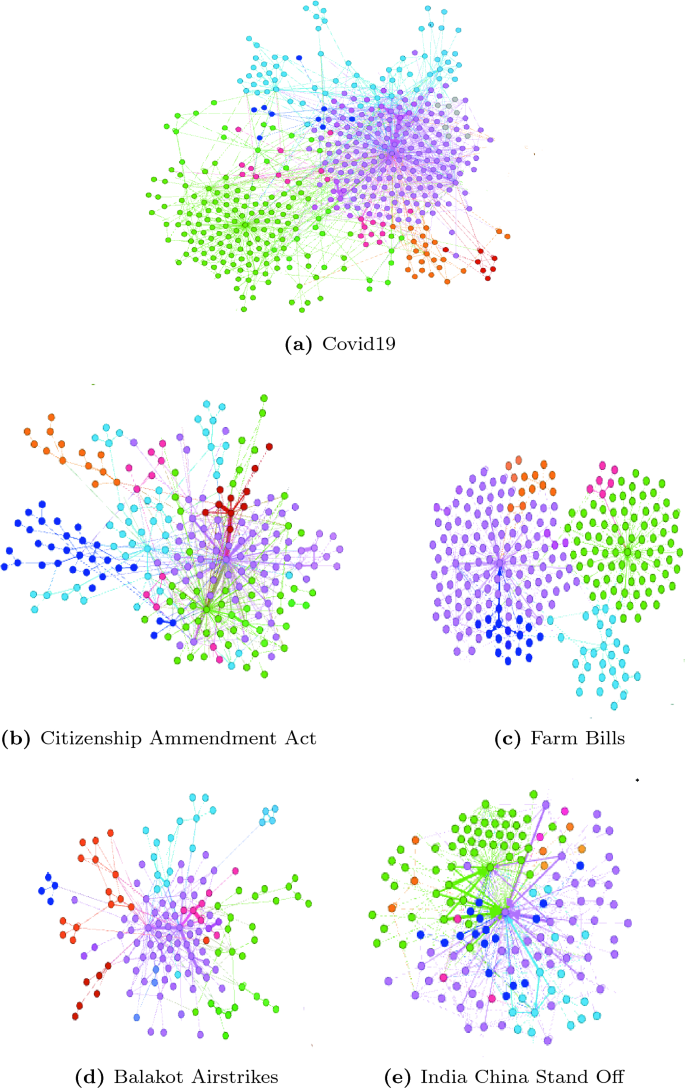
Mention networks on all topics
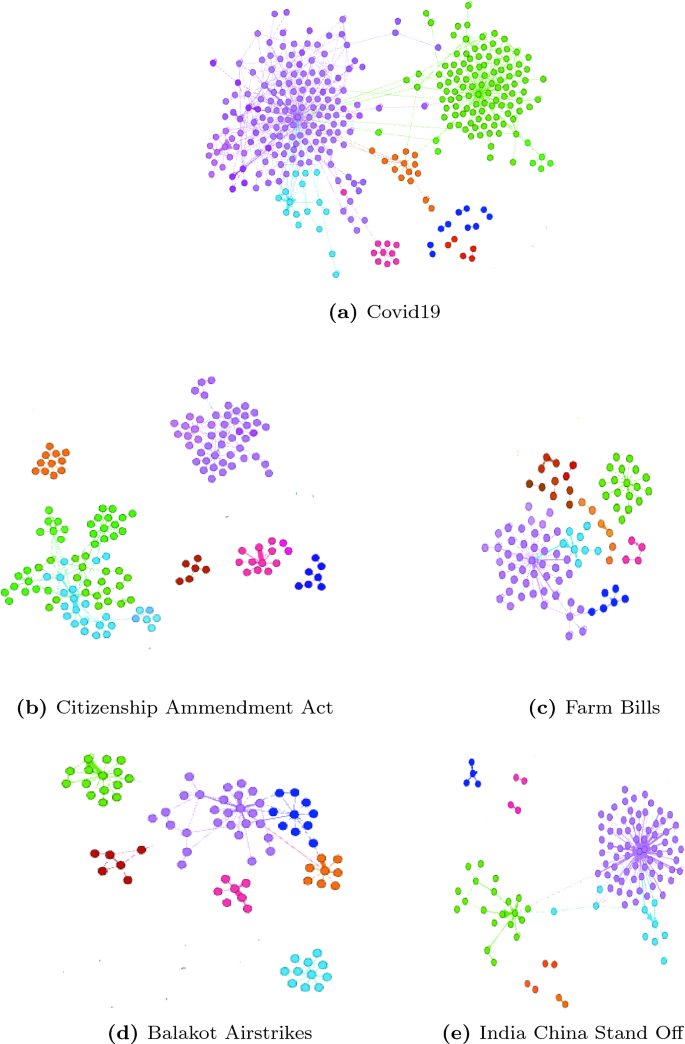
Retweet networks on all datasets
The retweet networks also formed distinct communities similar to mention networks. The COVID-19 retweet network in Fig. 3 a, contains 359 nodes and 576 edges. Similar to the mention network, 7 distinct communities have been identified in the retweet network as well that constitute around 78.4% of the entire network. Two large distinct communities have been identified in the retweet network. The largest community contains 134 users linked by 236 connections ( C 1) while the second network includes 98 users connected by 142 connections ( C 2). The 2 large communities together accounted for nearly 64.6% of the total connected users and 65.6% of the total connections. The Citizenship Amendment Act retweet network in Fig. 3 b, contains 125 nodes and 136 edges. Similar to the mention network, 7 distinct communities have been identified in the retweet network as well that constitute around 75.3% of the entire network. As observed in Fig. 3 c, the retweet network on Farm Bill contains 115 nodes with 156 edges. Seven distinct communities were identified in the network. The communities are completely separated and disjoint from each other with connections only between 3 communities: C 1, C 3 and C 7. Users in communities C 4 and C 6 formed separate connections while retweeting their community users. Communities C 1 and C 2 formed the majority accounting for 62.7% of total users in the network. The Balakot Airstrikes retweet network in Fig. 3 d, contains 75 nodes and 96 edges. Similar to the mention network, 7 distinct communities have been identified in the retweet network that constitute around 74.8% of the entire network. The retweet network in Fig. 3 e, contains 86 nodes and 105 edges. Six distinct communities have been identified in the retweet network that constitute around 71.4% of the entire network. One large community has been identified in the retweet network that contains 46 users with 62 connections ( C 1). The single large community accounted for nearly 54.6% of the total connected users.
4.2 What is the level of interconnectedness and information flow among the communities during discussions?
The level of interconnectedness and information flow among the communities are identified using the measurements of modularity and network density. The modularity value of the mention network of COVID-19 is observed to 0.524, that suggests medium modularity and the network to be medium separated. As discussed previously, modularity values between 0.4 and 0.6 have been considered to be medium modularity for the networks. The retweet network on the other hand, has a high modularity of 0.757. For Citizenship Amendment Act, the modularity value of the mention network is observed to be 0.519, suggesting medium modularity and medium separation. The retweet network on the other hand, has a high modularity of 0.794. The mention network on Farm Bills has a moderate modularity value of 0.483 while the retweet network has a high modularity value of 0.814. The mention network is therefore, highly interconnected having many cross-community interactions. The retweet network on the other hand, is more separated forming distinct clusters and have lesser number of cross-community interactions. The modularity value of Balakot Airstrikes mention network is observed to 0.582, suggesting medium modularity while the retweet network has a high modularity of 0.837. The modularity of the mention network of India China Stand-Off is observed to have a low value of 0.283. The retweet network on the other hand, has a high modularity of 0.732. The medium modularity of mention network on all topics indicates medium level of separation among the communities while the high modularity of retweet network suggests the presence of selective exposure to a great extent.
The mention network of COVID-19 has a density of 0.326 indicating that there is 32% chance of forming all the possible connection in the network. On the other hand, retweet network has a density of 0.085 suggesting a chance of only 8% of forming all possible connections. The mention network for COVID-19 is well-connected while the retweet network is loosely connected. Mention network of Citizenship Amendment Act has a density of 0.253 indicating that there is 25% chance of forming all the possible connections in the network. On the other hand, retweet network has a density of 0.073 suggesting a chance of only 7% of forming all possible connections. Density of mention network of Farm Bills is 0.216 while retweet network has a value of 0.048. This indicates that there are 21% chances of forming all the possible connections in the mention network while the chance is only 4.8% in case of retweet networks. The mention network of Balakot Airstrikes has a density of 0.286 indicating 28% chance of forming all the possible connections in the network. On the other hand, retweet network has a density of 0.063 suggesting a chance of only 6% of forming all possible connections. The mention network on India China Stand-Off has a density of 0.289 indicating that there is 28.9% chance of forming all the possible connection in the network while retweet network has a density of 0.056 suggesting only 5.6% chances of forming all possible connections. The results on network density reveals that mention network is well-connected than retweet network and selective exposure is present more in case of retweet than mention network. The amount of information flow is therefore, more in case of mention network than retweet networks.
4.3 What is the extent of party polarization and cross-community interaction during topical discussions?
To further assess the existence of selective exposure in the interaction networks of Indian politicians, the level of intra- and inter-party connections are identified. To examine party or community polarization during political discourse on the considered topics, the E–I index of both the interaction networks is computed. Table 4 reports the rescaled E–I index values for the mention and retweet networks of all the topics. The E–I index values indicate the interaction networks to be polarized. The polarization values however, varies with the type of interaction. The degree of polarization is very high in case of retweet network while for the mention network, it is almost null.
To investigate the degree of polarization in every community, E–I index is computed for each community separately. The plots in Figs. 4 and 5 report the E–I index of each community for the mention and retweet networks of all topics. E–I index values between 0.2 and 0.5 have been considered as medium cross-party interactions while above 0.5 has been regarded as high cross-party interactions. For the mention network of COVID-19, the results reveal that only community C 4 and C 5 have a negative value (− 0.146 and − 0.275), indicating them to be highly homophilic. Rest of the 5 communities have shown high to moderate level of cross-party interactions and are therefore, less polarized. The degree of polarization is highest for community C 6 while lowest for community C 2. For Citizenship Amendment Act mention network, community C 1 and C 7 have been found to be highly polarized while other communities are less polarized. In the Farm Bill mention network, Community C 2 has the highest number of cross-party interactions. Community C 1 has been identified to be homophilic, mentioning own party politicians more. For the mention network of Balakot Airstrikes, only community C 1 has a negative value and hence high homophily. Rest of the 6 communities have shown high to moderate level of cross-party interactions with less polarization. In the Indian China Stand-Off mention network, all the communities have a positive value except community C 1, indicating C 1 to be homophilic. Community C 2 has the highest number of cross-party interactions.
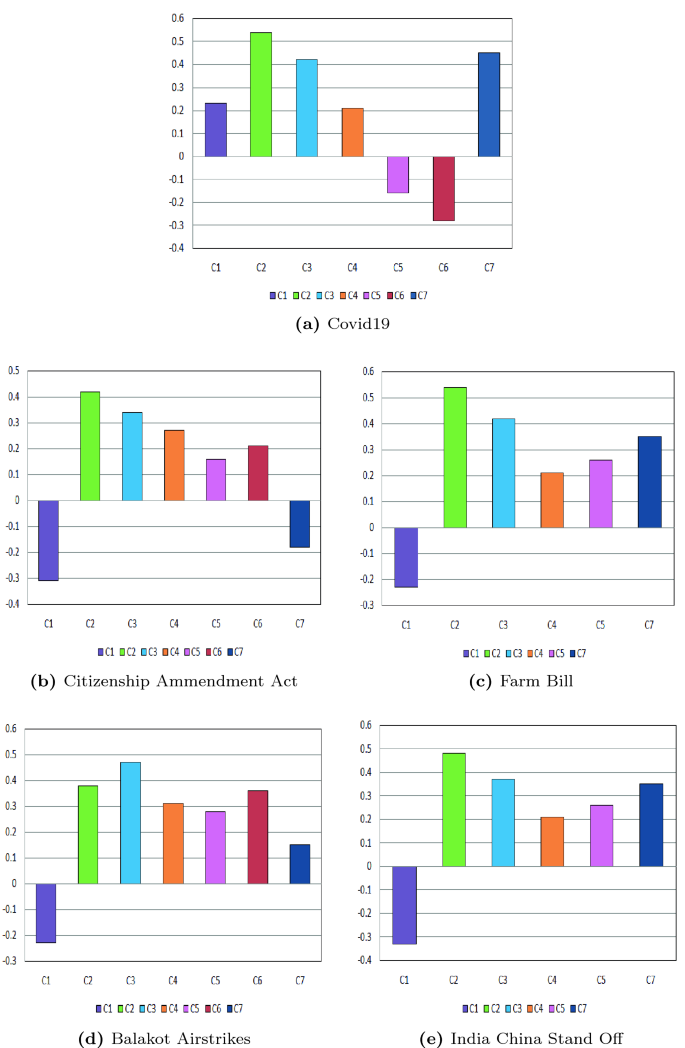
E–I index of communities in the mention networks of all topics
The analysis of retweet networks has revealed a complete different scenario. All the communities from C 1 to C 7 in COVID-19 retweet network have been found to be homophilic and polarized. Communities C 2, C 3 and C 5 have been found to be less homophilic while community C 1 has shown slightly higher homophily. Community C 6 and C 7 have been identified to be completely homophilic indicating that all ties are internal to the communities. Similarly for Citizenship Amendment Act, all the communities from C 1 to C 7 have been found to be homophilic and polarized. Communities C 2 and C 3 have been found to be less homophilic while other communities have been identified to be completely homophilic. All the communities for Farm Bills have high polarization, with community C 2, C 4, C 5 and C 6 being completely polarized. In the retweet network of Balakot Airstrikes and India China Stand-Off, all the communities from C 1 to C 7 have been found to be homophilic and polarized. For Balakot Airstrikes, communities C 2, C 3, C 5 and C 6 while for India China Stand-Off, community C 4, C 5 and C 7 have shown complete homophily.
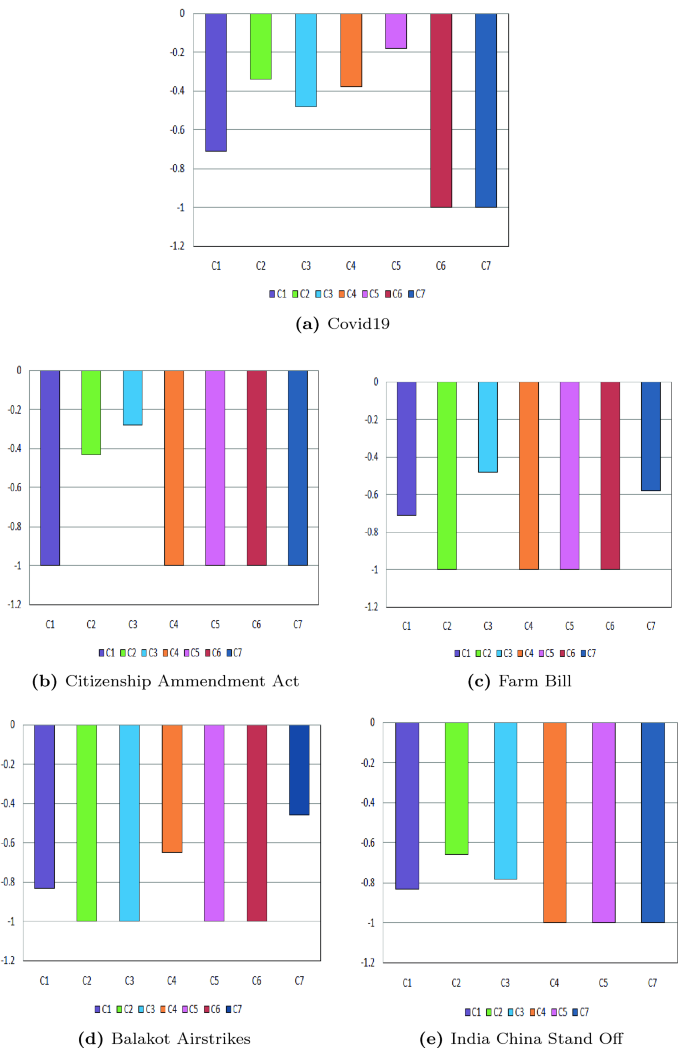
E–I index of communities in the retweet networks of all topics
In order to examine the amount of cross-community interactions, the interaction ratio within and across community is computed. For mention network, the observed and expected number of links between the communities is identified and the cross-community interaction ratio is computed accordingly. Table 5 depicts the ratio between observed and expected number of connections between politicians belonging to different political communities on COVID-19. From the table, it can be observed that the amount of cross-community mentions is more for most of the communities. Community C 6 and C 7 however have more intra-community mentions. The politicians are more likely to interact with the members of their own community during retweets. The amount of interaction in both mention and retweet networks is more within community and less across community. A value of 0 between 2 communities indicates that there are no interactions within those communities. Largest communities C 1 and C 2 have received the highest amount of cross-community mentions and retweets. Interestingly, the number of incoming mentions is more than outgoing mentions for these communities. For the smallest communities C 6 and C 7, the amount of cross-community mentions is very less and there are no cross-community retweets.
Table 6 depicts the cross-community interaction ratio for Citizenship Amendment Act. It can be observed that the amount of cross-community mentions is more for most of the communities. Community C 1 and C 7 however have more intra-community mentions. Community C 1 has received the highest amount of cross-community mentions. Except communities C 2 and C 3, there are no cross-community retweets. The cross-community interaction ratio for Farm Bill is illustrated in Table 7 . Community C 1 has received the highest number of external mentions among all the communities followed by C 2. The mention network on Farm Bills has only 6 communities. Community C 6 has no mention on Farm Bills. Hence, the column representing the mention values for C6 is null. The retweet network has very high level of selective exposure. Community C 2, C 4, C 5 and C 6 has no cross-community retweets.
Table 8 depicts the cross-community ratio for Balakot Airstrikes. The amount of interaction in retweet network is found to be more within community and less across community. Community C 1 has received the highest amount of cross-community mentions and retweets. For communities C 2, C 3, C 5 and C 6, there are no cross-community retweets. Cross-community ratio for India China Stand-Off is shown in Table 9 . From the table, it can be observed that the amount of cross-community mentions is more for all the communities except C 1. Largest community C 1 has received the highest amount of cross-community mentions and retweets. Apart from communities C 1, C 2 and C 3, there are no cross-community retweets among other communities.
To analyse the interest and opinion of the politicians on different issues, a content analysis approach has been adopted. The communities identified in the retweet network have been considered for analysis. The analysis is done in two phases. Initially, the tweet content similarity of all the communities on the considered topics is examined. In the next phase, sentiments of the tweets are identified and sentiment similarity among all the communities is computed.
4.4 Which communities share similar content on different political issues?
The similarity in contents of the communities is computed in two ways. The hashtag similarity between each community is computed based on Jaccard similarity. The tweet content similarity is then calculated based on cosine similarity between documents. The heatmap visualizations of the hashtag and tweet content similarities across communities are shown in Figs. 6 and 7 . Darker shades in the heatmap indicate higher similarity between communities. Since the similarity across own community is highest, the darkest shade can be seen diagonally.
Figure 6 a depicts the hashtag similarity between communities on COVID-19. Communities C 1, C 2 and C 3 have been identified to be the most similar communities in terms of the hashtags shared. For communities C 4 and C 5, the most similar community is C 1 while for C 6 and C 7, it is community C 2. Figure 6 b depicts the hashtag similarity between communities on Citizenship Amendment Act. Community C 1 is closest to C 3 followed by C 5 in terms of hashtags shared. Community C 2 is similar to C 1 and C 4, C 3 is similar to C 1 and C 7, C 4 is similar to C 3 and C 7. The hashtag similarity across each community on Farm Bill is illustrated in Fig. 6 c. In terms of hashtags used, community C 4 has been identified to be most similar to C 1 while C 3 has been identified to be most similar to C 2 and vice versa. Figure 6 d depicts the hashtag similarity between communities on Balakot Airstrikes. Communities C 1, C 3, C 5 and C 7 have been identified to be the most similar communities in terms of hashtags shared. For communities C 2, the most similar communities are C 4 and C 6 while for C 6, it is community C 2. Hashtag similarity on India China Stand-Off is illustrated in Fig. 6 e. Communities C 1 has been found to be most similar to C 2 and C 3 in terms of the hashtags shared. For communities C 2, the most similar community is C 4 and C 7 while for C3, it is community C 5. C 4 is most similar to C 1 and C 2, C 5 is most similar to C 3 and C 7 is most similar to C 2.
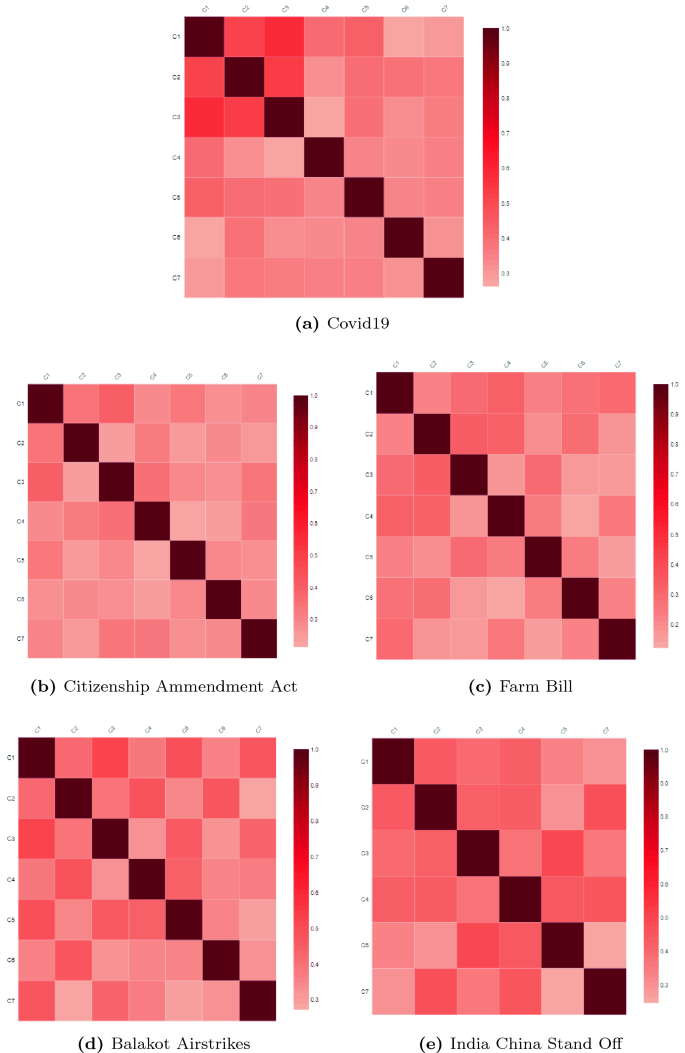
Hashtag similarity of communities for all topics
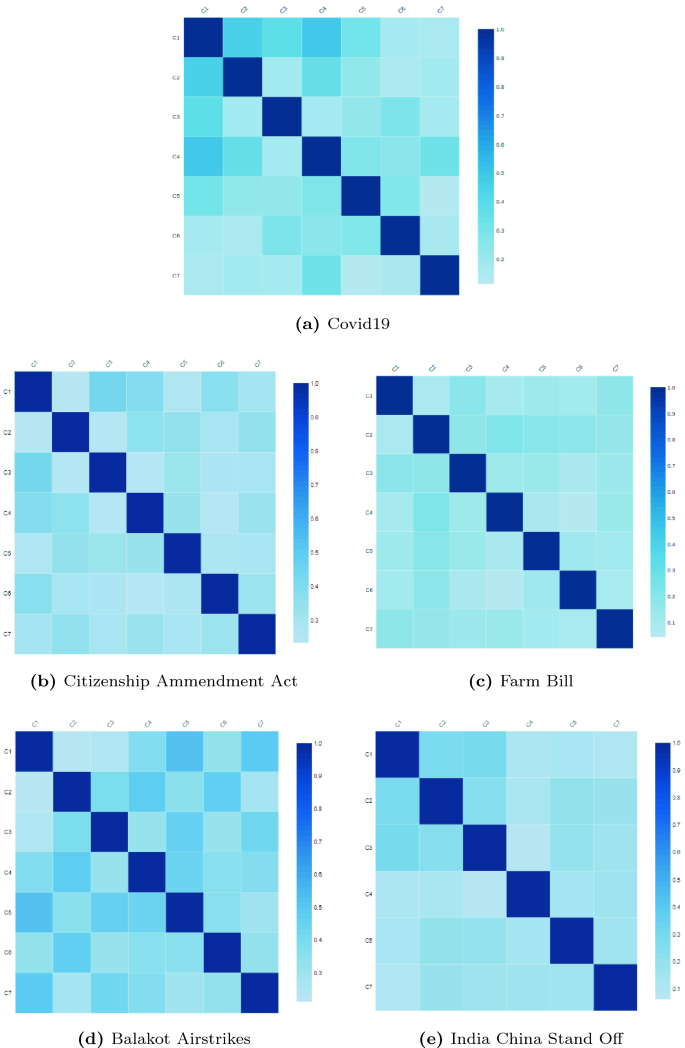
Tweet content similarity of communities for all topics
Figure 7 a represents the tweet content similarity between communities on COVID-19. Community C 1 has been found to be most similar to C 2, C 3, C 4 and C 5. C 3 is the most similar community for C 6 while C 4 is the most similar community for C 7 in terms of the tweet content published. Figure 7 b represents the tweet content similarity between communities. Community C 1 has been found to be most similar to C 3, C 4 and C 6. C 2 is the most similar community for C 4, C 5 and C 7 while C 5 is the most similar community for C 2 in terms of the tweet content published. The hashtag similarity across each community on Farm Bill is illustrated in Fig. 7 c. In terms of tweet content, community C 3 is most similar to C 5, C 2 is most similar to C 6 and C 1 is most similar to C 7. Figure 7 d represents the tweet content similarity between communities on Balakot Airstrikes. Community C 1 has been found to be most similar to C 5 and C 7. C 4 is the most similar community for C 2 while for C 4 it is C 2 and C 5. Tweet content similarity on India China Stand-Off is illustrated in Fig. 7 e. Community C 1 has been found to be most similar to C 2 and C 3 and vice versa. C 7 is the most similar community for C 4 while for C 5 and C 7, the most similar community is C 2 in terms of the tweet content published.
4.5 Which communities have similar sentiments towards political issues?
The sentiments of the tweets on every topic posted by each community are initially evaluated. The average number of positive, negative and neutral tweets are identified. The heatmap visualization of sentiment similarities between communities is shown in Fig. 8 . The similarity is more if difference in value is less. Hence, a lower value indicates higher similarity. The similarity between each community is computed using the methodology discussed in Sect. 3.2 . The lesser the difference in the number of positive, negative and neutral tweets shared by the communities, the more similar the communities are. Therefore, the similarity across the same community has been identified as 0. The values can be observed diagonally in the heatmap. For COVID-19, as shown in Fig. 8 a, both communities C 1 and C 2 are most similar to C 3, the level of similarity however is more between C 1 and C 3. Community C 4 is most similar to C 1, C 5– C 7, C 6– C 4 and C 7– C 5. As shown in Fig. 8 b for Citizenship Amendment Act, community C 3 is close to community C 1, C 4 and C 5 in terms of similarity. For C 2, the most similar community is C 4, for C 6 it is C 1 and for C 7 it is C 4. In case of Farm Bills as shown in Fig. 8 c, for community C 1, the most similar community is C 7 and for C 2 it is C 4 and vice versa. Most similar community for C 3 is C 1 and for C 5 and C 6 it is C 7. The least similar community for C 1, C 3, C 5 and C 7 is C 2, for C 2 it is C 1 and for C 4 and C 6 it is C 3. Figure 8 d illustrates the sentiment similarity for Balakot Airstrikes. For community C 1, most similar communities are C 3, C 4 and C 7, for C 2 it is C 5 and C 6, for C 3 it is C 1, C 5 and C 6, for C 4 it is C 1 and C 7. Community C 5 is most closest to C 2, C 3 and C 7, C 6– C 2 and C 3 and C 7– C 1, C 4 and C 5. For India China Stand-Off as shown in Fig. 8 e, community C 1 and C 3 are most similar to each other, C 2 is most similar to C 5 and C 4 is most similar to C 1. Community C 5 is most similar to C 3 and C 7– C 5.
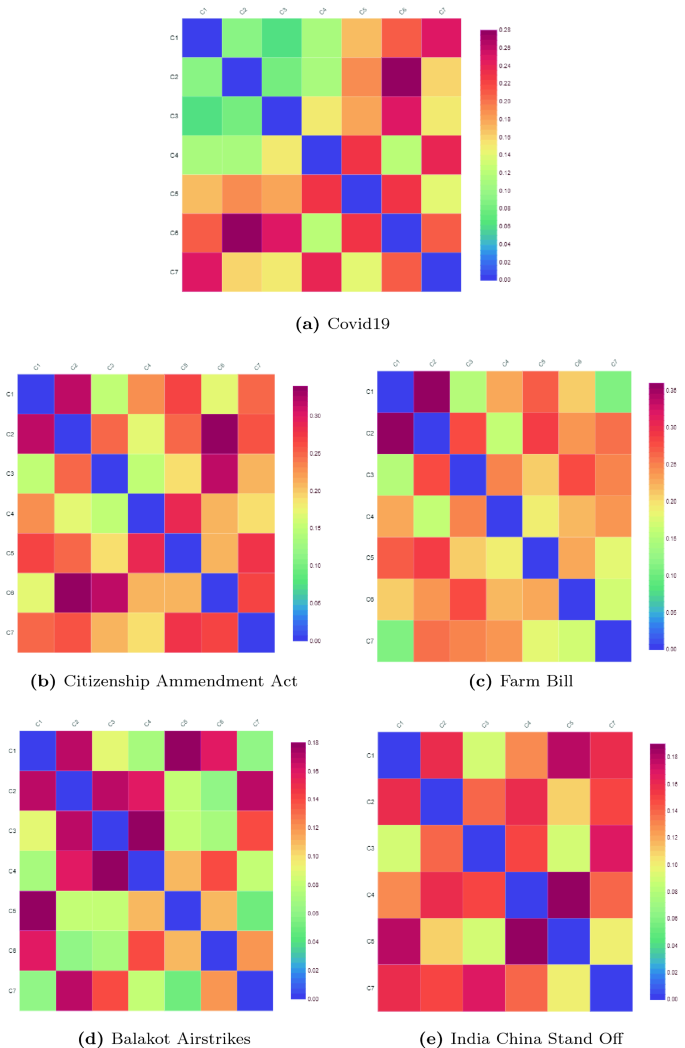
Sentiment similarity of communities for all topics
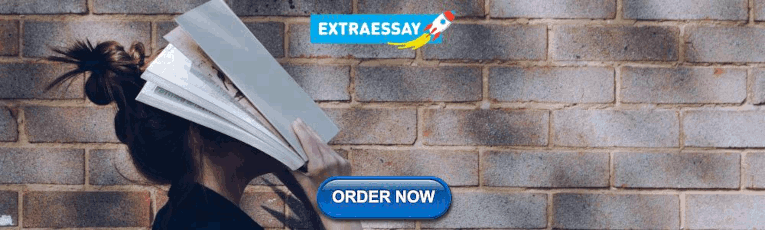
5 Discussion
This study investigates whether the online activities of Indian politicians on Twitter lead to polarization and how topics of political discussions influence the same. The paper performs an in-depth analysis of political polarization with respect to the pattern of interaction and opinions of the politicians on different political issues. This study is the first attempt to examine Indian political polarization on Twitter social media platform. We considered 5 major events in India during 2019 and 2020 and analysed the pattern of interaction of the politicians and their similarities and dissimilarities in opinion regarding those political events. This section provides a comparative analysis of the findings on all the 5 topics with respect to the hypotheses formulated for the study:
The analysis of the mention networks revealed different levels of cross-party interactions with respect to topics of political discussion. At the network level, for all the topics considered, clear evidence of cross-party interactions has been found in the mention networks of politicians. However, the degree of such interactions is different for different topics of political discourse. For COVID-19, the degree of cross-party interactions was identified to be high (E–I index = 0.263). Topics of government policies like Citizenship Amendment Act and Farm Bill revealed highest amount of cross-party interactions (0.316 and 0.378). The level of such cross-party interaction is lowest in case of national security issues Balakot Airstrikes and India China Stand-Off (0.163 and 0.184). The observations support the hypothesis formulated that mention networks reflect more cross-party interactions during political discussions. The findings of the study refute the existence of selective exposure in the mention networks of Indian politicians and also support the fact that social media opens up conversation spaces between politicians and political parties.
The degree of cross-party connections in the mention networks of Indian politicians has been found to be quite high than retweet networks. These findings are in line with some existing studies Conover et al. ( 2011 ); Del Valle and Bravo ( 2018 ); Esteve Del Valle et al. ( 2021 ); Chamberlain et al. ( 2021 ) that suggest the mention networks to be reflective of cross-party interactions. One possible reason for this could be the nature of different interaction networks of Twitter. Retweet networks are basically considered as support and endorsement networks while the mention networks are more indicative of a dialogical or communication network. The politicians use mention networks to engage with fellow politicians with different ideologies. Moreover, the intensity of cross-party interactions in the mention networks can be explained by the fact that politicians engage with one another through mentions either in agreement Del Valle et al. ( 2020 ) or in disapproval Laaksonen et al. ( 2017 ). This view can be supported from the findings of the analysis. The high intensity of cross-party interactions in controversial government policy topics is indicative of the fact that politicians are using mentions mostly as a means of criticism. The level of disagreement and criticism is more in case of controversial topical discussions like government policies and less for comparatively non-controversial topics of natural disaster and national security.
Moreover, interesting differences in the degree of polarization have been observed for different communities or political parties. For instance, in all the topics except COVID-19, mentions among the politicians of the largest community C 1 has been found to be more homophilic than other smaller communities. The largest community C 1 represents the party in power thus indicating that the party forming the government is more homophilic in terms of mentions than other parties. In addition to that, community C 1 has received the highest amount of mentions from other parties suggesting that the party in governance is also likely to receive higher cross-party mentions. This study reveals that the governing party politicians prefers to limit their political conversations within themselves while the opposing politicians tend to engage more with other parties. This is in line with the findings of Tromble ( 2018 ) that suggested the governing parties to be more engaging with their own party politicians and Esteve Del Valle and Borge Bravo ( 2018 ) that revealed the smaller parties to be less homophilic. Out of all the opposing parties, party or community C 2 and C 3 has been identified to have lowest homophily and more cross-party mentions. Interestingly, most of the outgoing mentions of C 2 is towards the governing community C 1, particularly during discussions on Citizenship Amendment Act and Farm Bills. Our analysis also reveals interesting association between the level of participation and number of mentions received. It has been observed that higher participation of politicians in political discussions increases their likelihood of being mentioned. This can be another reason for community C 1 receiving highest mentions as C 1 has shown highest participation from its members.
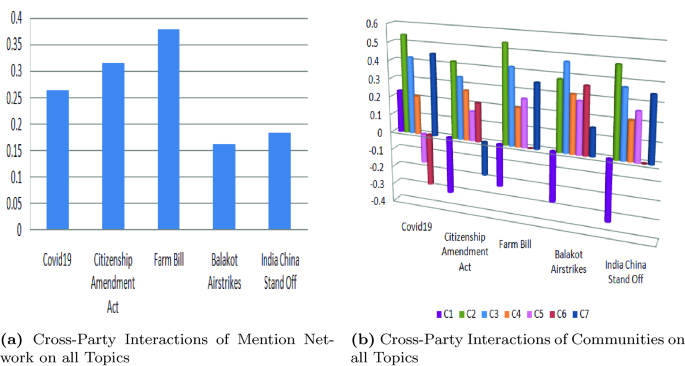
Cross-party interactions on all topics
Figure 9 depicts the cross-party interactions among parties or communities across all topics. From Fig. 9 a, it can be observed that highest cross-party interactions have taken place during the political discussions on Farm Bills and Citizenship Amendment Act, two important policies framed by Indian government. Further analysis of the data revealed that the politicians have used mentions mostly for disapproving and debating about the respective topic of discussion. The intra-party mentions were mostly in support of a political party or politician while the cross-party mentions were mostly used as a form of disagreement. This can be one of the possible reasons for the debate on government policies getting highest cross-party mentions. Discussions on COVID-19 too received considerable amount of cross-party mentions, particularly for lockdown and migration of workers. Balakot airstrikes and India China Stand-Off being comparatively less controversial topics, received lowest number of cross-party mentions. Fig. 9 b elicits the degree of polarization of each community in terms of mentions for all the topics. Community C1 being the governing party has been found to be homophilic in all political discussions except COVID-19. Highest cross-party mentions have been identified for community C2 on all topics followed by community C3. However, for Balakot Airstrikes highest cross-party mention was from C3. Interestingly, some smaller communities like C5, C6 and C7 have been found to be homophilic during discussions on COVID-19 and Citizenship Amendment Act.
Analysis of the retweet networks disclosed patterns of selective exposure, suggesting that the politicians participated in fragmented interactions and formed separate groups during discussions. Retweets are a form of endorsement and the network is a representative of a support network. A politician retweeting another politician or party is an indication of his/her support or agreement towards the political party or politician. The retweet networks of politicians on all topics appeared to be highly divided and segregated. The degree of polarization, however was different for different topics. The rate of polarization is highest for Farm Bills and Citizenship Amendment Act (– 0.521 and – 0.431) followed by Balakot Airstrikes and India China Stand-Off (− 0.326 and − 0.293). Least polarization has been observed in case of COVID-19 (− 0.193). The findings support the formulated hypothesis that selective exposure prevails in the retweet networks of Indian politicians. This further establishes the dual nature of social media that it not only open up conversation spaces to users but can also make the communication polarized.
At the network level, values of modularity also revealed the retweet networks to be highly segregated. The level of segregation is more for controversial topics of government policies like Farm Bills and Citizenship Amendment Act in comparison to other less controversial topics. The intensity of polarization is also different for different communities. All the communities have been identified to be homophilic for all the topics. The extent of homophily is more for government policy topics followed by national security topics and COVID-19. The analysis revealed the presence of ’echo chambers’ in the retweet networks of politicians. Our findings are in line with some of the existing studies Conover et al. ( 2011 ); Del Valle and Bravo ( 2018 ); Himelboim et al. ( 2013 ) that disclosed the ’echo chamber’ view in the retweet networks.
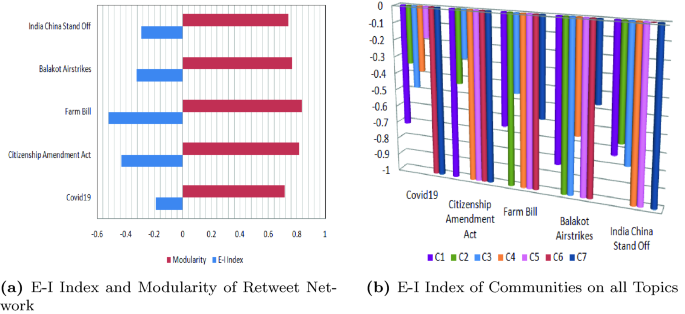
Selective exposure in retweet networks on all topics
Figure 10 elicits the pattern of selective exposure in the retweet networks of all topics. The comparative analysis of the degree of polarization and modularity in the retweet networks of the topics are depicted in Fig. 10 a. The extent of polarization is highest for government policy related topics Farm Bill and Citizenship Amendment Act. A possible reason for this could be the fact that the governing party frames the policies and the opposing parties are in disagreement most of the time. As retweet basically signifies support and endorsement, the politicians usually support their own parties and people forming distinct groups. The extent of polarization further increases in case of controversial and debatable topics. The results on modularity reflect the same observations. The modularity values of retweet network are highest for government policy topics, suggesting the networks to be highly segregated. Figure 10 b shows the E–I index of the retweet networks for all the communities. All the communities have been found to be highly homophilic. Some communities like C 6 and C 7 even found to be completely homophilic in most of the topics.
The communities identified in the retweet networks have been used for similarity computation. The similarity is computed based on three factors: hashtag usage, tweet content posted and average sentiment towards a topic. For calculating the average sentiment, instead of sentiment scores, average number of positive, negative and neutral tweets posted have been considered. In terms of hashtag usage, the similarity identified between the communities does not completely comply with the retweet network structure. Some polarized communities in terms of pattern of interaction have been found to be similar in their usage of hashtags particularly for COVID-19. One important reason for this could be the higher rate of participation of politicians from these communities. Larger communities therefore, have been identified to be more similar than smaller communities. This similarity however, is comparatively less for debatable topics of government policies. The use of similar hashtags basically reflects the amount of participation of the politicians and their interests rather than their opinion towards an issue. The retweet network structure is based on the pattern of interaction of the politicians. The observations indicate that the politicians belonging to different communities or parties are using similar hashtags even though they are not retweeting each other. This refutes the third hypothesis in terms of hashtag usage that only the connected communities in the retweet network are similar to each other.
Findings on tweet content similarity revealed that the content posted by politicians on a certain topic is directly related to their pattern of communication in the retweet networks. The interconnected communities in the retweet network have higher similarity in terms of tweet content published. For instance, content on COVID-19 posted by some of the large interconnected communities like C 1, C 2 and C 3 have been found to be similar. Similarly, for Farm Bill and Citizenship Amendment Act, the interconnected communities have been found to be more similar. Sentiment similarity observations have also revealed a similar trend. The overall sentiment of the interconnected communities on a specific topic has been found to be more similar. Tweet content published and the overall sentiment on that topic reflects the opinion of the politician towards that issue. Since retweet networks are a form of support network, a politician retweeting another politician signifies agreement and thus similar opinions. However, in contradiction to the hypothesis, there are some interesting and exceptional findings on tweet content and sentiment similarity. Some non-connected communities in the retweet networks have also been found similar to each other in terms of tweet content and sentiment. For instance, community C 1 and C 3 have been found to be quiet similar in their opinions on Citizenship Amendment Act despite being highly polarized in their retweet interactions. This may be due to the fact that some communities might not have retweeted each other but the content shared and the overall sentiment of the community for the topic might be same. Similarly, some interconnected communities have been found to be dissimilar in terms of their tweet content and sentiment. A possible explanation of this could be that few politicians of a community might have retweeted another community but the overall tweet content and sentiment of both the communities are different. Few differences identified in the results of polarization based on pattern of interaction and opinion divergence only partially supports the hypothesis that only interconnected communities in the retweet networks are similar.
To better understand the difference in results, a comparative analysis has been done on polarization identified between communities in terms of both pattern of interaction and opinion divergence. Table 10 depicts the polarization between communities in terms of their pattern of interaction in the retweet networks. As already discussed, the amount of selective exposure in the retweet networks is very high and the network is therefore, highly polarized. From the table, it can be observed that most of the communities or parties are homophilic and tend to interact with their own community. This makes them polarized towards each other. Moreover, the extent of polarization is higher for debatable topics like Farm Bills and Citizenship Amendment Act. For these topics, since the overall degree of polarization is highest, almost all the communities are polarized towards each other. Smaller communities C 6 and C 7 have shown higher homophily and polarization for almost all the topics, suggesting that extent of homophily depends on the amount of participation. Politicians with lesser participation, retweets very less and whenever does, retweets only their own party politicians.
Table 11 illustrates the polarization between communities in terms of opinion divergence. The combined results of tweet content and sentiment similarity have been used as it reflects the opinion of a politician towards an issue. From the table, it can be seen that the amount of polarization for each community is highest in case of government policy topics similar to polarization in terms of pattern of interaction. However, there are some differences in the number of polarized communities identified for each community. As discussed earlier, the difference in results might be due to two factors. Some non-connected communities might not have retweeted each other but may have posted similar tweet content and have similar opinion towards an issue. Similarly, among the interconnected communities, some politicians from one community might have retweeted another community, but the overall similarity in the tweet content and sentiment is very less. Thus, based on these findings, it can be concluded that polarization based on pattern of interaction and opinion divergence might be different. For ease of understanding, the main findings of this study are summarized in Table 12 .
6 Conclusion
In this study, we have investigated the existence of Indian political polarization on Twitter social media platform based on two broad characteristics of polarization: pattern of interaction and opinion divergence. Social network analysis and content analysis methods have been used to analyse the tweets posted by Indian politicians during some major events in India from 2019 and 2020. The findings of the study illustrate that political polarization does exist on social media platforms like Twitter and the topic of political discourse plays an important role in the extent of polarization. High polarization exists between politicians and parties during retweets while for mention the polarization is almost null. Controversial and debatable topics are followed by higher level of polarization compared to less controversial topics. With respect to pattern of interaction, it has been identified that Indian politicians behave strategically on Twitter depending on the layer of communication. A clear tendency of homophily was observed in the retweet networks while mention networks basically includes cross-party connections. Same party mention reflects support and agreement while cross-party mention reflects disagreement. In terms of opinion divergence, it has been observed that pattern of interaction in retweet networks does not always reflect the similarity in interest and opinions among the politicians. Hashtag similarity is based on the amount of participation rather than interconnections in retweet network. Furthermore, findings on tweet content similarity and sentiment similarity revealed that interconnected communities in the retweet networks need not necessarily be similar in terms of opinion.
This study is topic specific and hence is limited to network analysis and content analysis of tweets of Indian politicians on selected topics. The results obtained therefore, might not be generalized to all political conversations on Twitter. Since follower information of politicians Twitter handle does not change with respect to topic, follower relations are not used in this study. As a future work, one can perform a more generalized study considering the follower relations along with mention and retweet. Moreover, the study has been done at the political party level, where the degree of polarization is examined across different parties. Another essential work direction could be the investigation of polarization at individual politicians level. Such examination could be helpful in identifying outliers in a political party. The analysis of opinion divergence carried out in the current study is based on retweet networks. Retweets indicate endorsement and support which better characterize the opinion difference among the politicians. Links between politicians in the mention network may not necessarily imply similar opinion. However, utilizing the mention networks as signed networks can be useful in identifying the same. The positive and negative sign of the edges between politicians in the mention network would indicate their exact sentiment or opinion towards each other.
Data availability
The datasets generated and analysed during the current study are not publicly available due to sensitivity of information but are available from the corresponding author on reasonable request.
Adamic LA, Glance N (2005) The political blogosphere and the 2004 us election: divided they blog. In: Proceedings of the 3rd international workshop on Link discovery, pp 36–43
Alvim MS, Amorim B, Knight S, Quintero S, Valencia F (2021) A multi-agent model for polarization under confirmation bias in social networks. In: International conference on formal techniques for distributed objects, components, and systems, Springer, pp 22–41
Bastian M, Heymann S, Jacomy M (2009) Gephi: an open source software for exploring and manipulating networks. In: Proceedings of the international AAAI conference on web and social media, vol 3
Chamberlain JM, Spezzano F, Kettler JJ, Dit B (2021) A network analysis of twitter interactions by members of the us congress. ACM Transact Soc Comput 4(1):1–22
Article Google Scholar
Cherepnalkoski D, Mozetič I (2016) Retweet networks of the European parliament: evaluation of the community structure. Appl Netw Sci 1(1):1–20
Conover M, Ratkiewicz J, Francisco M, Gonçalves B, Menczer F, Flammini A (2011) Political polarization on twitter. In: Proceedings of the international AAAI conference on web and social media, vol 5
DeGroot MH (1974) Reaching a consensus. J Am Stat Assoc 69(345):118–121
Article MATH Google Scholar
Del Valle ME, Bravo RB (2018) Echo chambers in parliamentary twitter networks: the catalan case. Int J Commun 12:21
Google Scholar
Del Valle ME, Sijtsma R, Stegeman H, Borge R (2020) Online deliberation and the public sphere: Developing a coding manual to assess deliberation in twitter political networks. Javnost-The Public 27(3):211–229
Esteve Del Valle M, Borge Bravo R (2018) Leaders or brokers? Potential influencers in online parliamentary networks. Policy Internet 10(1):61–86
Esteve Del Valle M, Broersma M, Ponsioen A (2021) Political interaction beyond party lines: communication ties and party polarization in parliamentary twitter networks. Soc Sci Comput Rev p 0894439320987569
Friedkin NE, Johnsen EC (1990) Social influence and opinions. J Math Sociol 15(3–4):193–206
Garimella K, Morales GDF, Gionis A, Mathioudakis M (2018) Quantifying controversy on social media. ACM Transact Soc Comput 1(1):1–27
Garimella VRK, Weber I (2017) A long-term analysis of polarization on twitter. In: Proceedings of the international AAAI conference on web and social media, vol 11
Ghezelbash E, Yazdanpanah MJ, Asadpour M (2019) Polarization in cooperative networks through optimal placement of informed agents. Phys A Stat Mech Appl 536:120936
Article MathSciNet MATH Google Scholar
Himelboim I, Smith M, Shneiderman B (2013) Tweeting apart: applying network analysis to detect selective exposure clusters in twitter. Commun Methods Meas 7(3–4):195–223
Krackhardt D, Stern RN (1988) Informal networks and organizational crises: an experimental simulation. Soc Psycho Q pp 123–140
Laaksonen SM, Nelimarkka M, Tuokko M, Marttila M, Kekkonen A, Villi M (2017) Working the fields of big data: Using big-data-augmented online ethnography to study candidate-candidate interaction at election time. J Inf Technol Polit 14(2):110–131
Morales AJ, Borondo J, Losada JC, Benito RM (2015) Measuring political polarization Twitter shows the two sides of Venezuela. Chaos Interdiscip J Nonlinear Sci 25(3):033114
Newman ME (2004) Detecting community structure in networks. Eur Phys J B 38(2):321–330
Olivares G, Cárdenas JP, Losada JC, Borondo J (2019) Opinion polarization during a dichotomous electoral process. Complexity
Recuero R, Zago G, Soares F (2019) Using social network analysis and social capital to identify user roles on polarized political conversations on twitter. Soc Med Soc 5(2):2056305119848745
Tromble R (2018) The great leveler? Comparing citizen-politician twitter engagement across three western democracies. Eur Polit Sci 17:223–239
van Vliet L, Törnberg P, Uitermark J (2020) The twitter parliamentarian database: analyzing twitter politics across 26 countries. PloS One 15(9):e0237073
Weber I, Garimella VRK, Batayneh A (2013) Secular vs. islamist polarization in egypt on twitter. In: Proceedings of the 2013 IEEE/ACM international conference on advances in social networks analysis and mining, pp 290–297
Download references
Author information
Authors and affiliations.
Indian Institute of Technology Guwahati, Guwahati, Assam, India
Anindita Borah & Sanasam Ranbir Singh
You can also search for this author in PubMed Google Scholar
Corresponding author
Correspondence to Anindita Borah .
Ethics declarations
Conflict of interest.
The authors declare that there is no conflict of interest.
Additional information
Publisher’s note.
Springer Nature remains neutral with regard to jurisdictional claims in published maps and institutional affiliations.
Rights and permissions
Springer Nature or its licensor holds exclusive rights to this article under a publishing agreement with the author(s) or other rightsholder(s); author self-archiving of the accepted manuscript version of this article is solely governed by the terms of such publishing agreement and applicable law.
Reprints and permissions
About this article
Borah, A., Singh, S.R. Investigating political polarization in India through the lens of Twitter. Soc. Netw. Anal. Min. 12 , 97 (2022). https://doi.org/10.1007/s13278-022-00939-z
Download citation
Received : 16 December 2021
Revised : 14 July 2022
Accepted : 16 July 2022
Published : 31 July 2022
DOI : https://doi.org/10.1007/s13278-022-00939-z
Share this article
Anyone you share the following link with will be able to read this content:
Sorry, a shareable link is not currently available for this article.
Provided by the Springer Nature SharedIt content-sharing initiative
- Polarization
- Social networks
- Social media
- Content analysis
- Find a journal
- Publish with us
- Track your research
ISSN 2581-5369
HeinOnline, MANUPATRA, Google Scholar Indexed
Social Media and It’s Regulations In India
Dornala sai mahitha.
- Show Author Details
LLM Student at NALSAR, Hyderabad, India
- img Download Full Paper
- img Export Citation
Export citation
During the pandemic, social media was the only means through which we were able to interact with one another. This extensive use of these platforms facilitated interactions during this period, but it also revealed a number of disadvantages associated with them. Because social media is a platform where no one individual is referred to as a producer or a consumer, and because everyone has the ability to share information with others all over the world virtually, these characteristics together have contributed to the recent growth in the number of cyber crimes. Efforts by the government to regulate these mediums have stalled, and social media platforms themselves are subjected to a number of unwarranted restrictions placed by the government on their policies, which are interfering with the rights of users and causing turmoil in cyberspace. On the subject of Information Technology Act 2001 and Amendment of 2008, this paper addresses the advances in law as well as lacunae that have been identified. It also includes a discussion of Information Technology (Intermediary Guidelines and Digital Media Ethics Code) Rules 2021 and charges that have been brought against them. This study also examines theories of regulation, as well as how to regulate and not regulate social media platforms, among other topics. It also makes a recommendation for a Uniform Social Media Framework, which would eliminate the obstacles of territorial jurisdiction and differences in national laws that prevent offenders from being convicted.
- Social Media
- The Information Technology Act- 2000
- The IT Rules-2021
- Regulation of Social Media
Research Paper
Information
International Journal of Law Management and Humanities, Volume 4, Issue 6, Page 1560 - 1572
Creative Commons

This is an Open Access article, distributed under the terms of the Creative Commons Attribution -NonCommercial 4.0 International (CC BY-NC 4.0) (https://creativecommons.org/licenses/by-nc/4.0/), which permits remixing, adapting, and building upon the work for non-commercial use, provided the original work is properly cited.
Copyright © IJLMH 2021
Total number of HTML views: 2798
Total number of pdf downloaded: 745, open access.
https://doij.org/10.10000/IJLMH.112426
Recent content
1 need for deep sea diving and exploration regulation in india.
By Aryan Clement and Dr. Khushboo Malik
Volume: 7 Issue : 2 Page: 3074 - 3085
2 Balancing Act: Navigating Artificial Intelligence, Data Privacy, and Legal Challenges in the Digital Age
By Prajeet Sen
Volume: 7 Issue : 2 Page: 3062 - 3073
3 John Rawls Theory of Justice
By Insha Afreen
Volume: 7 Issue : 2 Page: 3051 - 3061
4 Unravelling Criminal Psychopathy in India: A Study of Punishments, Treatment Approaches, and Rehabilitation Strategies
By Dharmashri Pratapaneni
Volume: 7 Issue : 2 Page: 3036 - 3050
5 Introduction to Delegated Legislation and it’s Judicial Control
By Manas Shrivastava
Volume: 7 Issue : 2 Page: 3018 - 3035
International Journal of Law Management & Humanities
Typically replies within 24 hours.
Any questions related to the journal or your submission?
WhatsApp Us
🟢 We will respond within 24 hours, maybe less.
WhatsApp us.
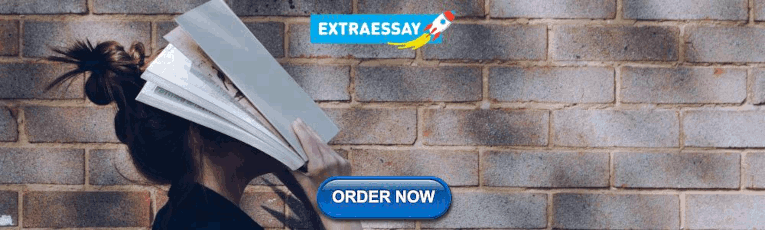
IMAGES
VIDEO
COMMENTS
762 IJRAR- International Journal of Research and Analytical Reviews Research Paper. Social Media users in India: A Futuristic Approac h. Jamal Abdul Nasir* 1 & Aaisha Khatoon & Shubhangi Bharadwaj ...
Broadly speaking, Media can be classified as Broadcast Media, Print Media and the newly added Social Media. While Broadcast Media primarily deals with telecasts on Television and other electronic devices with efficient powers of addressing large number of people at a time, Print Media is basically Newspapers, Journals and Magazines and Social ...
Social media has become a daily habit in most people's lives. People of all ages participate in social media, and the average Indian devotes nearly 2.4 hours per day to this one activity alone.
Social media subscribers in India are growing in a constant rate due to the deep penetration of internet. connectivity in both urban and rural parts of the country (TRAI, 2018). Digital India ...
This collection of papers and talks from the proceedings of the Social Media and Society in India conference held at the University of Michigan, Ann Arbor, in April 2023. The talk summaries cover the conversations with practitioners on various domains from the impact of social media on journalism and activism to mental health, culture, and ...
This paper is intended to provide a snapshot of research on social media in Indian context and has followed rapid literature review method. This can be operationalized in the lines of rapid evidence summaries or assessments followed in the field of health or medicine (Haby et al., 2016).This method is a diluted version of systematic review (Snilstveit, 2012), in terms of shortened timeframe ...
The remainder of the paper is structured as follows. First, we introduce the background of the study, namely, the role of social media and users' engagement. ... and media sharing (Dolan, Seo, & Kemper, 2019; Zeng & Gerritsen, 2014). Although tourism research in social media context is still in the early stages, scholars (e.g., Luo & Zhong ...
Here I discuss the first of the broad questions, namely effects of social media on journalism. Three major themes emerged: (1) social media as a useful tool for journalists, (2) social media adversely affects news industry practices, and (3) social media has changed news dissemination process.
Social media plays a pivotal role in shaping communication among political entities. Substantial research has been carried out for examining the impact of politicians' social media usage and interactions on political polarization. Analysing political polarization is particularly significant for fragmented political systems like India where collaboration between parties is essential for ...
paper. Consequences and context, being the major themes driving this research effort. Why India as a society is a hotspot for misinformation, how social media platforms have failed the general public ... address this situation by reinforcing existing research on regulation codes and social media practices. This paper makes a strong business and ...
This study aimed to examine the impact of content characteristics of social media posts shared by destination marketing organisations (DMOs) on tourist engagement. An analysis of over 12,000 posts and 3.65 million tourist impressions recorded on 16 official Indian DMOs' Facebook and Twitter handles was undertaken.
A descriptive and analytical stance is taken when discussing social media and its effects on young people, both good and bad, in this study, which heavily rely on the thematic analysis tool MAXQDA to draw a conclusion. Social media has become a daily habit in most people's lives. People of all ages participate in social media, and the average Indian devotes nearly 2.4 hours per day to this ...
The extensive use of social media in India has been on the rise among the new generation youths. In today's world, use of social media has become an integral part of everyday life of human being. This paper throws a light on pattern of social media usage and its impact on youth.
India's social media research output came from several organizations and authors, of which the top 10 contributed 30.42% and 17.87% share respectively of its total output during 2004-13.
The usage of social media reduced learning and research capabilities, reduction in real human contact, Reduces command over language usage and creative writing skills, Time wastage, Low grades, and Loss of motivation ... Panigrahi (2019) rightly analysed the impacts of Social Media in his paper Impacts of Social Media upon India youth ...
The aim of this paper is to capture the historical developments of social media over a period of time. Keywords: Social Media, Bulletin Board Systems, UseNet. Suggested Citation: Dhingra, Manish and Mudgal, Rakesh K., Historical Evolution of Social Media: An Overview (March 15, 2019). International Conference on Advances in Engineering Science ...
This paper studies the use of social media in the Indian Lok Sabha and assembly elections. This ... Research Scholar, Maulana Azad National Urdu University, Hyderabad, Telangana, 500032 ... it was through social media that people in India and abroad were getting updates regarding this incident. Social media was used in the 2009
: We collected data from undergraduate medical students (MBBS) in Delhi, India using a self-administered 20-item social media addiction questionnaire (SMAQ) to measure addiction-like behavior, and the Pittsburgh Sleep Quality Index (PSQI) to assess sleep quality. Results: We enrolled 264 (62.3%) male and 160 (37.7%) female participants of mean (standard deviation) age 19.83 (1.6) years. The ...
PDF | On Mar 8, 2018, Kanagavalli G. published Impact of Social Media Marketing in India | Find, read and cite all the research you need on ResearchGate
IJCRT2106583 20International Journal of Creative Research Thoughts (IJCRT) www.ijcrt.org f THE ROLE OF SOCIAL MEDIA MARKETING IN INDIA AFTER CRISES OF COVID-19: AN OVERVIEW Prabhat Bain Masters of Commerce ... articles, research papers, social media sites, journal and internet source also.
A study was conducted to determine a possible relation between social media usage and. various aspects of psychological wellbeing (anxiety, depressive mood, self-control, positive. wellbeing ...
Because social media is a platform where no one individual is referred to as a producer or a consumer, and because everyone has the ability to share information with others all over the world virtually, these characteristics together have contributed to the recent growth in the number of cyber crimes. Efforts by the government to regulate these ...
The present paper is based on secondary data which tries to access both positive and negative impact of social media on society and particular on women through major social media sites such as ...