An IERI – International Educational Research Institute Journal
- Open access
- Published: 06 June 2020
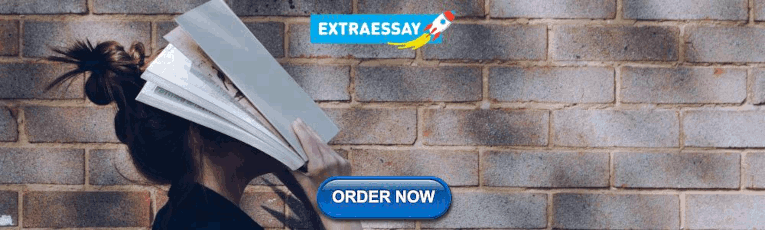
The measure of socio-economic status in PISA: a review and some suggested improvements
- Francesco Avvisati ORCID: orcid.org/0000-0001-9354-5234 1
Large-scale Assessments in Education volume 8 , Article number: 8 ( 2020 ) Cite this article
39k Accesses
96 Citations
7 Altmetric
Metrics details
This article reviews the history of the measure of socio-economic status in PISA and identifies theoretical underpinnings of the index of economic, social and cultural status (ESCS). It then highlights multiple changes in the instruments and scaling methods used by PISA over time, and suggests ways of resolving the tensions behind some of these changes and thereby stabilise the measure of ESCS. A stable definition and operational procedure to derive the ESCS index appears essential to compare the ESCS-achievement relationship over time. Some of the suggestions included in this article were already implemented in the 2018 cycle.
Introduction
The index of economic, cultural and social status (ESCS) is probably, just after student achievement scores, the most used variable in reports and in secondary analysis of data from the Programme for International Student Assessment (PISA). Based on student reports to the context questionnaire, it helps address relevant questions about educational opportunity and inequalities in learning outcomes. While well-established and sometimes used as a reference (if not a standard) for the measurement of socio-economic status (SES) in national assessments of school-aged children (INVALSI 2017 , p. 70; Cowan et al. 2012 ), the ESCS index has also been criticised.
Some scholars, in particular, have challenged the validity, reliability or comparability of the current measurement of socio-economic status or of its components in PISA, calling for revisions and extensions of the index (Rutkowski and Rutkowski 2013 ; Pokropek et al. 2017 ; Willms and Tramonte 2015 ).
This article reviews the history and use of ESCS, and formulates recommendations to strengthen its measurement, based on a set of reporting priorities. The significant advances in statistical methodologies since the early 2000s, and more importantly, concerns about the validity of the current measure of socio-economic status in PISA and of the inferences that are made based on it, justify an effort to revisit this variable. The review takes the PISA 2015 database as a starting point: some of the recommendations in this review were shared, in a preliminary version, with the PISA 2018 consortium and were already implemented in the PISA 2018 database.
What is ESCS, and why is it useful?
The PISA index of economic, social and cultural status is closely related to other measures of socio-economic status (SES) that are commonly used in the education literature, in particular within the North American tradition. A report by the “American Psychological Association Task Force on Socioeconomic Status” (APA 2007 ) described three alternative approaches to define and analyse socio-economic differences in education and in other domains. A first approach (the “materialist” view of SES) summarises the relationship between outcomes (e.g. education) and socio-economic status through the relationship with quantifiable characteristics such as income and wealth. Researchers within this tradition often focus on essential resources and define cut-offs (poverty line, “free-school meal status”) on continuous measures. A second approach (“gradient approaches”) emphasises relative status, and conceives socio-economic status as a unidimensional ranking of individuals in society; this ranking can be informed by multiple dimensions, including, sometimes, subjective ones [such as subjective perceptions of one’s status, measured, for example, through the MacArthur scale (Goodman et al. 2001 )]. A third approach focuses on hierarchies of power and privilege and their reproduction (“class models”); researchers following this approach, which is more closely linked to theories of social stratification [e.g. (Weber 1922 )], focus on categorical measures, which can be ordered or unordered [for example, “the intellectual elite” vs “the business elite”; (Piketty 2018 )].
PISA questionnaire frameworks do not provide an explicit theoretical foundation and definition of economic, social and cultural status (ESCS). The index is operationally defined in the technical reports. For example, the first ESCS variable (constructed for PISA 2000) was simply described as a convenient way to facilitate reporting: “To facilitate the analysis, this chapter combines into a single index the different economic, social and cultural aspects of family background that were examined separately in Chapter 6” (OECD 2001 , p. 185).
The ideas and definition that underpin the construction of ESCS must therefore be found in the broader literature, including secondary literature about PISA. According to Doug Willms, who worked on the construction of the first ESCS variable in PISA, ESCS is closely related to the “gradient approach”. Indeed, in reviewing the measure of socio-economic status used in PISA (Willms and Tramonte 2015 ; Willms 2006 ), he referred to the definition of socio-economic status by Mueller and Parcel ( 1981 , p. 14):
Socio - economic status is the relative position of a family or individual in a social system in which individuals are ranked according to their access to or control over wealth, power and status.
This definition highlights the relative nature of socio-economic scores as reflecting the hierarchical ranking that characterises modern human societies, while “wealth, power and status” refer to the three components of social stratification highlighted in Weber ( 1922 ). In reviewing the broader literature about socio-economic status and achievement, Sirin ( 2005 , p. 418) refers to this same definition as one that can be applied to most studies.
The gradient approach alone however cannot explain PISA’s desire to anchor the measure of socio-economic status on a common scale for all countries and for all years, so as to enable comparisons of “status” across individuals belonging to distinct national societies. The need for such a common scale appears driven by a different definition, which conceptualises socio-economic status not only as one’s position, but as a direct measure of the amount of valued resources and capital that individuals can access and control. This view of “socio-economic status” corresponds to the definition provided by the panel of experts convened at the request of the National Assessment Governing Board to provide recommendations concerning socio-economic status (Cowan et al. 2012 ):
SES can be defined broadly as one’s access to financial, social, cultural and human capital resources. […]
In sum, the current measure of ESCS and its use in PISA analysis appear inspired by both the materialist view and by gradient approaches. A possible definition of ESCS in PISA therefore is:
ESCS is a measure of students’ access to family resources (financial capital, social capital, cultural capital and human capital) which determine the social position of the student’s family/household.
While commonly used in education research, inferences that rest on a composite measure of socio-economic status—whether conceived as a relative measure of position, or as a unidimensional proxy for different kinds of resources—have also been criticised by prominent scholars (Deaton 2002 ; O’Connell 2019 ). Angus Deaton, for example, formulated this critique as follows:
“we have a correlation between socioeconomic status and health and evidence that the correlation is causal, at least in part. [What this implies for policy, however,] depends on what we mean by ‘socioeconomic status’, a term that is convenient as a shorthand for a wide range of possibilities, including income, education, rank, or social class, but that is useless for thinking about policy in the absence of an instrument that acts on them all” (Deaton 2002 ).
A similar critique has also been formulated, within the context of analyses based on PISA data, by Keskpaik and Rocher ( 2011 ), who suggest that the individual components of ESCS provide a more useful description of equity in school systems than the unidimensional analysis based on ESCS: a critique that emphasises the multi-dimensional nature of ESCS and is also closely related to “class models”, more typical of the European tradition.
From a measurement perspective, socio-economic status is often conceptualised as a formative latent variable, rather than a reflective latent variable; and the short discussion in this section reflects different views as to whether it should be seen as a causal formative construct (a latent variable that is caused by the observed variables through which it is measured) or simply as a composite formative construct (a convenient, and entirely artificial summary of somewhat unrelated measures) (Bollen and Bauldry 2011 ).
Our view is that a composite measure of socio-economic status has mainly a practical utility in analysis, avoiding problems that would otherwise arise due to the correlated nature of individual components or due to their interactive effects. In particular, an index such as PISA’s ESCS can be used to build synthetic, high-level descriptive indicators of inequality of opportunity and of outcomes in education, or as a “control” variable to account for the possible confounding effect of pre-existing individual differences on the outcome of interest. While the measure of PISA is inspired by both the gradient and the materialist approach, I suggest to consider ESCS mostly as an artificial composite. In doing so, I make both the individual components and the algebraic operations through which the components are combined part of the definition of ESCS, and redirect questions about the validity, reliability and comparability of the index to its individual components, where such questions are more tractable.
What are the components of socio-economic status?
The definition of ESCS as a composite inspired by the North American tradition of SES measurement suggests constructing ESCS by combining into a single score distinct measures of the financial, social, cultural and human capital resources available to students (and perhaps other resources which are relevant to the family’s position in a particular social hierarchy); this composite score can be thought of as an approximation of individuals’ ranking in a national and global society.
In modern, industrialised societies, it has been common (at least since the first half of the twentieth century) to use formal education credentials, occupation titles converted to a status or prestige scale, and income as the individual components through which socio-economic status is measured. Cowan et al. ( 2012 ) note:
Traditionally, a student’s SES has included, as components, parental educational attainment, parental occupational status, and household or family income, with appropriate adjustment for household or family composition. […]
Education, occupation and income are sometimes referred to as the “big three” (Willms and Tramonte 2019 ); Sirin ( 2005 , p. 418) notes that there is substantial agreement among researchers on the “tripartite nature of SES that incorporates parental income, parental education, and parental occupation as the three main indicators of SES”. Ensminger and Fothergill ( 2003 ) note that “education, income and occupation” are the “three most common measures of SES”, but does not recommend combining them into one scale.
The PISA measure of socio-economic status (ESCS) has traditionally been built as a weighted average of three indices: parental educational attainment (in years), parental occupational status on the “International Socio-Economic Index” (ISEI) scale (Ganzeboom 2010 ; Ganzeboom et al. 1992 ), and a measure of “household possessions”. Two of the three components that inform the composite score of ESCS—parental years of education and parental occupational status—coincide with those used “traditionally”, according to Cowan et al. ( 2012 ). The third component—an index of household possessions, based on the possession or consumption of durable goods—can be thought of as a measure of the household’s income, or more precisely, of its “permanent” component (Friedman 1957 ).
What reporting goals should inform the measure of socio-economic status in PISA?
Having established the artificial nature of ESCS as a “convenient summary”, its construction should be guided by the validity of the inferences and conclusions that are based on it. A review of existing reports leads to identify the following desirable features for the measure of socio-economic status in PISA: Footnote 1
The PISA data set should include a measure of socio-economic status that supports an analysis of the relationship between student socio-economic status and achievement, through a limited number of key indicators;
The PISA data set should include a measure of socio-economic status that enables valid comparisons of the relationship between student socio-economic status and achievement across countries and, within countries, over time;
The PISA data set should include a measure of socio-economic status that enables to classify some students as “vulnerable” or “disadvantaged”, in order to analyse the concentration of such students in particular schools and compare their prevalence, and distribution, over time.
The PISA data set should include one or more measures of socio-economic status to account for individual differences in endowments and prior achievement, in particular when analysing the relationship between achievement and schooling variables (school tracks, school type, teaching practices, learning practices, opportunity-to-learn variables, …).
The PISA data set should include a school-level measure of student advantage/disadvantage, which enables to classify some schools as “advantaged” or “disadvantaged” and which can be used in regression analysis (including for the analysis of individual outcomes);
The development of indicators which capture the essential aspects of the relationship between socio-economic status and achievement, and which enable countries to monitor changes in this relationship over time and to compare themselves to other systems, are valued PISA outputs. Indeed, the relationship between students’ socio-economic profile and their performance is an important indicator of the fairness of education systems, i.e. the extent to which good or adverse circumstances (factors that are outside of students’ own control) influence their opportunities to access quality education and reach good learning outcomes; and distributive values such as equality, adequacy and benefitting the less advantaged are at the heart of many education policy decisions (Brighouse et al. 2015 ).
These needs create a strong rationale for summarising the information about the different components of socio-economic status into a single variable. While it is possible to measure the strength of the relationship between socio-economic status and outcomes in terms of the variation explained by multi-dimensional measures of socio-economic status, this would preclude simple visualisations of this relationship. Multi-dimensional measures also pose challenges for analysing gaps in the average performance of students at different “levels” of socio-economic status (e.g. in different quarters or quintiles), as this would either result in a multiplicity of measures (one per dimension) or in the need to specify distinct discrete profiles or classes to define the levels; in contrast, a single continuous measure allows to simply visualise this relationship and to use simple indicators, such as the gap between the top and bottom quarter of ESCS or the average gap along the continuum (or “slope of the socio-economic gradient”).
Neither of the first two needs mentioned above provides a strong rationale, however, for preferring a particular scale or for assuming a particular distribution for the composite measure of socio-economic status. For example, to avoid choosing a particular scale, a rank- or percentile-measure (which would result in a uniform distribution of socio-economic status) could be used in every country. Non-linear transformations of the composite score, of course, do not result in the same conclusions about the shape of the relationship, and would implicitly re-define the meaning of the “slope”, i.e. of the average gap along the continuum (while a robust, non-parametric definition of the “strength” would be unaffected by such transformations).
Additional reporting needs must be invoked to justify reporting the composite measure of socio-economic status on an interval scale with the same origin in all countries and all cycles. In particular, a common scale presents the advantage of enabling further analyses, such as decomposing the changes in slopes (by distinguishing compositional changes, driven by differences in the underlying distribution of socio-economic status, from shifts in the relationship of socio-economic status and performance). It can also support the development of additional indicators such as the “level” of the socio-economic gradient, i.e. the average outcomes of students at particular points in the distribution of socio-economic status—although due to the artificial nature (and scale) of ESCS, interpretability remains an issue.
The definition of a category of “vulnerable” students, based on some combination of resource indicators, can be a natural by-product of the construction of ESCS or proceed independently. In particular, the definition of levels of vulnerability could explicitly take into account multiple resources and dimensions, without the need to combine them into one measure. At the same time, if the emphasis is on relative disadvantage, a measure that assigns weights to the different dimensions in order to provide a single ranking of students is necessary. In order to enable meaningful comparisons over time or between countries, a composite measure of socio-economic status—or one of its components—should then be reported on an interval scale with the same measurement unit and origin in all countries and over time.
Finally, ESCS is often used as a control variable in regression analyses based on the PISA dataset. The cross-sectional nature of PISA data poses important challenges for the interpretation of analyses that relate resources and processes to outcomes. Measures of family background and socio-economic status can account for possible confounding factors that may create spurious relationships between the outcomes of schooling and the type of school- and out-of-school experiences students have. In such analyses, the direct relationship between outcomes and socio-economic status is of little interest. In some cases, the rationale for including measures of socio-economic status is not to “account” for confounding factors, but simply to increase the precision of estimates; for example, gender can often be expected to be unrelated to family socio-economic status, yet the inclusion of socio-economic status in a regression analysis increases the precision with which gender gaps are estimated.
It is in general preferable to introduce the different components of socio-economic status independently in regression analyses, in order to optimally reduce the variation to be explained and to interpret coefficients on a natural scale, rather than the artificial scale created by the ESCS aggregate. While the different components are related, they are conceptually distinct, and the empirical correlation among different components is unlikely to cause multi-collinearity issues in the large samples that are typical of PISA analysis. However, if interest lies in examining interactive effects (e.g. whether the gender gap is higher among advantaged than among disadvantaged students), focusing on a single measure of socio-economic advantage (which could be a composite measure) greatly facilitates interpretation.
Many policy questions in education are at the school level: how should resources be allocated between schools? When should an additional class be created, or an existing class be closed (and merged with other classes)? Who should decide on the recruitment of new teachers? In analyses related to these questions, it is often interesting to have a school-level measure of advantage and disadvantage. In fact, in many countries, some administrative measures of school advantage exist, because of their use in the funding formula or in staff allocation decisions; for example, “Title I” status in the United States, or the inclusion of the school in a “priority education zone” (ZEP) or “priority education network” (REP) in France. School-level measures that are aggregations of student-level variables, such as the percentage of students eligible for the “National School Lunch Program” (NLSP) in the United States, the percentage of students eligible for “Free School Meals” (FSM) in England, or the percentage of students with low-educated parents, with an immigrant background, or with limited proficiency in the national language, are also often used.
In multi-national studies like PISA, administrative measures are typically not available and not comparable across countries. Instead, one may construct school-level measures of socio-economic (dis)advantage either based on the student sample, or based on specific questions about the entire student body asked to students, teachers, or principals/school administrators. Because of the small within-school samples of students used in PISA, proportions based on the characteristics of sampled students can be affected by significant measurement error, and it may be preferable to use means or medians of continuous measures, rather than proportions of categorical measures. Including more than one measure at the school level can also be problematic, particularly in school-level analyses, due to the small number of schools per country and the high correlation among such measures that can be expected.
In small schools, measures of school advantage that are based on a single grade or cohort of students may give a noisy vision of the school socio-economic profile. The error in the mean estimate is inversely related to the number of students in the sample. In addition, because PISA samples are age-based (as opposed to grade-based), in some cases the PISA sample can give a biased vision of the school profile; such is the case, for example, when the students eligible for PISA are atypical in terms of their grade attainment. Footnote 2
To overcome this problem, school principals could be asked to provide a measure of socio-economic status that refers to the entire student body or to all students in a particular target grade—for example, the proportion of students that lack the basic necessities or advantages of life, such as adequate housing, nutrition or medical care. Indeed, a similar question was introduced in the PISA 2015 School Questionnaire (SC048) and is used in the “Teaching and Learning International Survey” (TALIS).
Another solution for secondary users of PISA data is to restrict the sample for analyses involving school-level aggregates to schools where “average socio-economic status” is better measured, or to conduct sensitivity analyses. The “Effective Teacher Policies” report, for example, restricted its analysis of school advantage/disadvantage to schools that include the modal level of schooling for 15-year-old students (OECD 2018a , p. 87). The “Equity in Education report” addressed the issue of measurement error (and, to some extent, bias) by restricting the analysis of the relationship between student performance and school socio-economic profile (mean and standard error) to schools in which 10 or more students had a valid ESCS index; sensitivity analyses were conducted (OECD 2018b , p. 137).
How can the current measure of ESCS be improved?
The PISA 2015 Technical Report (OECD 2017 , pp. 339–340) describes ESCS as “a composite score derived via principal component analysis (PCA) from the indicators parental education (PARED), highest parental occupation (HISEI), and household possessions (HOMEPOS) including books in the home”:
The next sections will review the instruments and procedures used to derive the composite ESCS index in greater detail. While these instruments have remained similar to when it was first developed, some changes were introduced over time; some of these changes do not appear to reflect methodological advances or new reporting needs. In reviewing each step in the construction of ESCS, the aim is to formulate recommendations for future PISA questionnaires and databases.
For each component, changes in the questionnaire items, and changes in the rules used to derive variables from questionnaire responses will be examined. For the composite measure, changes in the imputation procedure and changes in the weighting scheme will be examined.
Improving the measurement of parents’ or care-takers’ education attainment
The measurement of education attainment in 2015.
In 2015, the “parental education” component of ESCS was measured based on questions about father’s and mother’s level of schooling and father’s and mother’s post-secondary educational qualifications. Footnote 3 These questions were used to identify the highest level of education completed by each parent on a 7-point scale based on the International Standard Classification of Education (ISCED) 1997 (None; ISCED Level 1; ISCED Level 2; ISCED Level 3B or 3C; ISCED Level 3A or 4; ISCED Level 5B; ISCED Level 5A or 6). The highest of the two values was then selected and converted to a years-of-education equivalent (PARED), using conversion tables based on national ISCED mappings, provided by national project managers and documented in an appendix to the technical report.
The following issues affecting the validity, reliability and comparability across countries and over time of the current measurement of “parental education” can be identified.
Increase in immigrant populations
The substantial increase, in many countries, in the proportion of students with an immigrant background, defies the assumptions behind using national and time-invariant mappings to put educational qualifications (which immigrants may have earned in their home countries) on an SES scale.
Over-reporting of post-secondary qualifications compared to national statistics
At the country level, the proportion of students who report their mothers (fathers) to have tertiary education credentials correlates highly with the proportion of 35–54 year-old women (men) who have such credentials, at least for countries covered by the OECD Education at a Glance database. The linear correlation coefficient is 0.86 for mothers/women, and 0.78 for fathers/men. In general, the rate of tertiary qualifications reported for fathers is higher than the corresponding rate among 35–44-year-old men (and similarly for mothers), suggesting that students over-report tertiary degrees. The extent to which this occurs may vary across countries: Poland and Greece, for example, have similar rates of tertiary attainment among men (below 30%); but only about 20% of students report tertiary degrees for their fathers in Poland, Footnote 4 compared to over 40% in Greece. On the other hand, in Russia, more than 80% of students report their fathers to have a tertiary qualification, while labour-force surveys indicate that only about 45% of men to have such qualifications (Fig. 1 ).
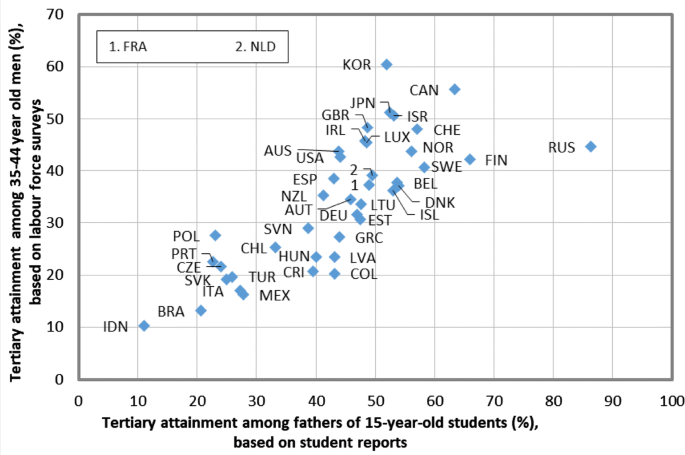
(Source: OECD, PISA 2015 Database; Education at a Glance Database ( https://stats.oecd.org/OECDStat_Metadata/ShowMetadata.ashx?Dataset=EAG_NEAC ))
Tertiary attainment of fathers (PISA) and of 35–44 year-old men (EAG). Pearson’s linear correlation coefficient between the two series is 0.78 (N = 41)
Inconsistency between “level of schooling” and “post-secondary qualifications”
The PISA 2015 measure was based on distinct answer formats for qualifications up to upper-secondary level (ISCED Level 3) and for post-secondary qualifications. By virtue of the hierarchical nature of the ISCED classification, in order to have an ISCED Level 4 degree or higher it is necessary, in theory, to have earned an ISCED Level 3 qualification. Footnote 5 However, a significant number of students report a level lower than ISCED Level 3 as their parents’ highest level of schooling, and at the same time report their parents as having post-secondary qualifications.
Missing answers
The percentage of students with missing reports on both their father’s and mother’s education—and thus for whom no “parental education” component could be computed—is relatively low, but varies considerably across countries and years. The median missing rate across countries for PARED was 1.9% in 2015 (inter-decile range: 0.6–4.5%). The highest missing rate was observed in Germany (17.5%), Footnote 6 the lowest missing rate in Romania (0.04%). The percentage of missing answers fluctuated over time with no clear trend, and no particular change related, for example, to the introduction of computer-based questionnaires in 2015 (Fig. 2 ).
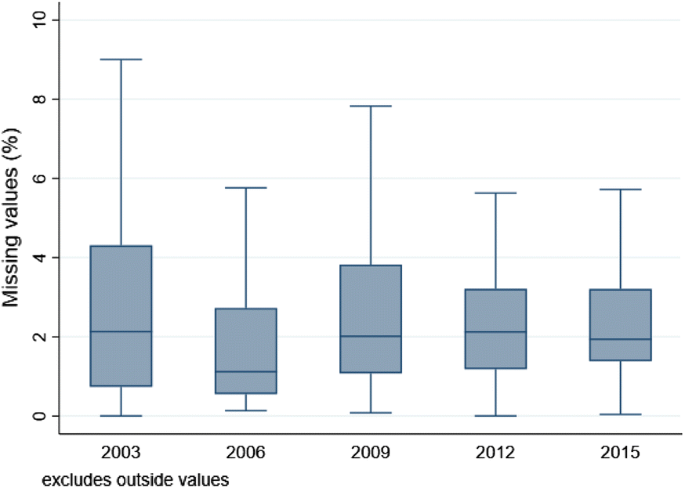
(Source: PISA 2003–2015 database)
Percentage of respondents with missing parental education component. The figure represents the median, interquartile range (shaded area) and adjacent values for country-level missing rates in PARED. Adjacent values are defined as the most extreme missing rates within 1.5 times the interquartile range from the nearest quartile. The following countries have missing rates that exceed the upper adjacent value: 2003: Canada (10.9), Germany (11.0), Luxembourg (13.1), New Zealand (11.9); 2006: Germany (6.2), United Kingdom (8.7), Israel (7.2), Luxembourg (6.8), New Zealand (7.9); 2009: Germany (13.0), United Kingdom (8.0), New Zealand (9.3), Panama (16.7); 2012: Germany (21.5), United Kingdom (7.6), Luxembourg (6.8), New Zealand (8.9); 2015: Brazil (6.6), Germany (17.5), United Kingdom (9.3), New Zealand (8.9)
Misreporting
Researchers have questioned whether students provide valid responses regarding their parents’ education. Studies that were able to compare reports by multiple raters have found that students’ reports of parental education have relatively low correlations with parents’ self reports, lower than, for example, correlations among reports of parents’ occupations; in addition, there appears to be considerable variability across countries in inter-rater agreement (Willms and Tramonte 2019 ; Lien et al. 2001 ; Looker 1989 ; Jerrim and Micklewright 2014 ; Schulz 2005 ).
Changes over time in questionnaire instruments
The international versions of the parental education questions used different wording and response formats in PISA 2000, 2003, 2006 and 2009, and have since not changed until 2018 (Box 1 ).
However, more subtle changes have been introduced all along in national questionnaires, in harmonisation rules to map back national adaptations to international response options, and in rules to derive the year-of-education equivalence:
The mapping of ISCED levels to years of schooling changed a first time in 2006, and was subsequently revised in every cycle in consultation with countries.
Changes in national adaptations of the international questions—e.g. to distinguish a greater number of tertiary qualifications (bachelor, masters, …)—and/or to the mapping of national response options into international variables (“harmonisation”) are poorly documented, but may affect the comparability of data over time. Footnote 7
Alternative measures
Some issues related to the measurement of parental education are difficult to solve: for example, the misreporting of parental education by students. Other issues may be addressed more simply in revised procedures or instruments, such as:
Considering students’ answers about post-secondary qualifications only for those students who reported their parents’ highest level of schooling to be at least (lower) secondary education. This has the potential to reduce mis-reporting and the over-reporting of tertiary qualifications, in particular in developing countries where universal primary education was not yet the rule in the parents’ generation. In future questionnaires, this filter rule could be automatically applied at the time of data collection.
Using a single, international conversion to convert major ISCED levels into their (approximate) years-of-education equivalent. In order to minimise the differences with established patterns, this international conversion could be initially determined by using the modal years of education across countries for each ISCED level. This would eliminate a source of mistakes in the calculation of ESCS and limit arbitrary differences between countries with similar educational structures. The comparability of the parental education component of socio-economic status across countries and over time would therefore rely directly on the comparability of the education levels, before their conversion on an SES scale.
Table 4 ( Appendix ) uses PISA 2015 data to explore the impact of these suggested changes on the measure of parental years of education in PISA, and on the relationship with performance.
Results included in Table 4 ( Appendix ) suggest that the introduction of a filter on secondary education and of a common conversion from ISCED levels to years of education would only minimally affect within-country analyses, and has the potential to improve the cross-country comparability of PARED and its use a component of an international measure of socio-economic status. The alternative that incorporates both changes (PARED2) in particular has marginally higher concurrent and criterion validity, as indicated by correlations with country-level measures based on labour-force surveys, with other ESCS components, and with science performance. The correlation with country-level measures based on labour-force surveys measures increases only minimally, across all countries, but a larger increase is observed for lower-income countries (where over-reporting may be more of an issue). The correlation with the “household possessions” component of ESCS (variable HOMEPOS) was investigated in particular on the share of students with values in the top and bottom international quintile of these measures. Indeed, one use of ESCS as an internationally comparable scale is to generate international categories (typically “deciles” or “quintiles”) of resources; and the analysis typically focuses on the extremes (bottom decile, top decile, etc.). Because parental education (PARED) is the most discrete component of ESCS, the actual values used to convert the top and bottom education category onto the ESCS scale loom large on the percentage of students who are classified in the top and bottom quintiles of ESCS. In particular, in 2015, using the national conversion, countries such as Australia, Israel, France and New Zealand (which have relatively small values, for the PARED conversion of tertiary education) ended up having fewer students than one would expect in the top quintile. An international conversion of education levels to the SES scale would result in international rankings of students on the scale that no longer depend on the values chosen to convert tertiary education into years of education, but directly on the qualifications level distinguished in questionnaires. For the purpose of trend analyses, both suggested changes—using a filter on post-secondary education qualifications and a conversion from ISCED levels to years of education that is common to all countries—could be applied retrospectively to past datasets. Trend comparability of the “education” component of socio-economic status would rely directly on the comparability (over time) of major levels of education distinguished in questionnaires; where this comparability can no longer be guaranteed for all education levels distinguished in questionnaires, because of changes in education structures, it may be necessary to merge questionnaire levels into broader categories that remain comparable.
Parents’ occupational status
The measurement of occupational status in 2015.
The “parental occupation” component of ESCS is measured based on open-ended questions about father’s and mother’s job title/occupation (questions ST014Q01TA and ST014Q02TA in PISA 2015). Countries are then required to use the information provided by students to assign a code based on the International Standard Classification of Occupation (ISCO) to each student. This international classification developed by the International Labour Organisation (ILO) distinguishes over 400 occupations (4-digit codes, e.g. “carpenters”, “stonemasons”, “roofers”, “plasterers”), grouped into 28 groups (defined by the first two digits of their codes, e.g. “Building and related trades workers, excluding electricians”) and 10 major groups (first digit, e.g. “craft and related trades workers”). PISA has expanded the list of ISCO codes with special codes for unemployed and inactive parents (9701 “Doing housework, bringing up children”, 9702 “Learning, studying”, 9703 “Retired, pensioner, on unemployment benefits”). The ISCO codes provided by countries (which form a nominal scale) are then converted into an ordinal or interval scale using prestige rankings or income rankings based on international studies; PISA refers to the “International Socio-Economic Index of occupational status” (ISEI) developed by Ganzeboom ( 2010 ). The highest value for either parent is used in the ESCS composite (HISEI).
Similar to what is proposed above for the education component of socio-economic status, the occupation component already relies on a common mapping, across all countries, of detailed occupational categories on an SES scale. The comparability of the occupation component of socio-economic status across countries and over time therefore relies on the comparability of the occupational codes.
Accuracy of coding
There are no standards about the quality of coding procedures. Some countries do not code occupations to the four-digit level: until 2009, Japan coded occupations only at the 2-digit level; in 2015, the United Kingdom coded occupations only at the 3-digit level.
Validity of the ISEI conversion across time and countries
The prestige, skill level and income of certain occupations can vary significantly across countries and levels of development (to take just one example, “cattle farmer” can correspond to very different income and skill levels, depending on the national context); but Ganzeboom’s work (Ganzeboom et al. 1992 ; Ganzeboom 2010 ) is based on a more restricted set of countries than the set of countries participating in PISA (the most recent conversion of occupational codes to ISEI codes is based on 42 countries or subnational entities that participated in the International Social Survey Programme between 2002 and 2007). Furthermore, the relative prestige and income of certain occupations and the educational requirements to access certain occupations is also subject to change over time (e.g. “teacher”), creating tensions for the conversion of ISCO codes to an ordinal or interval scale.
Missing data
The percentage of students with missing reports on father’s and mother’s occupation is relatively high, and varies significantly across countries. The median missing rate across countries for mother’s occupation (OCOD1) is 12.8% in 2015 (inter-decile range: 6.8–23.8%). The highest missing rate is observed in Algeria (74.8%), the lowest missing rate in Viet Nam (2.0%). The median missing rate across countries for father’s occupation (OCOD2) is 16.6% in 2015 (inter-decile range: 24.5–8.7%). The highest missing rate is observed in Thailand (30.3%), the lowest missing rate in Viet Nam (5.7%). While single-parent households may explain the high levels of missingness to some extent, some scholars attribute high rates of missing data to the open-ended nature of the question (Willms and Tramonte 2015 ). Figure 3 shows that the median rate of missingness has increased over time, and in particular after 2009. The high level of missingness in low-achieving countries suggests that the response burden for this question may be too high for children with low literacy levels.
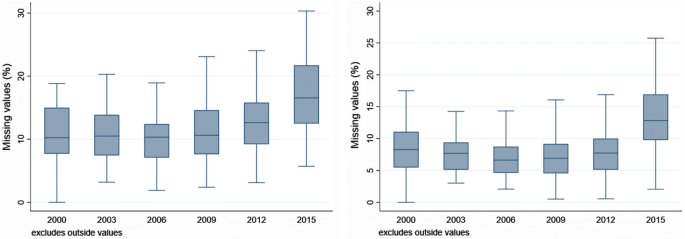
(Source: PISA 2000–2015 database)
Percentage of respondents with missing ISCO codes for parental occupation. Share of missing values for fathers’ (left) and mothers’ (right) occupation codes. The figure represents the median, interquartile range (shaded area) and adjacent values for country-level missing rates in OCOD2 (Father ISCO code) and OCOD1 (Mother ISCO code). Adjacent values are defined as the most extreme missing rates within 1.5 times the interquartile range from the nearest quartile. The following countries have missing rates that exceed the upper adjacent value: Fathers: 2000: Israel (31.1), Japan (65.0), United States (28.3); 2003: Japan (25.4); 2006: Israel (21.7), Japan (22.0), Qatar (44.8); 2009: Georgia (30.3), Panama (25.3), Himachal Pradesh (India) (32.8), Tamil Nadu (India) (26.3); 2012: Albania (31.7), Austria (100), Japan (26.8); 2015: none. Mothers: 2000: Brasil (26.7), Israel (25.5), Japan (66.5), United States (23.1); 2003: Canada (16.4), Germany (16.7), Japan (21.3), Netherlands (15.8), New Zealand (17.8); 2006: Israel (16.5), Japan (16.3), Qatar (31.4); 2009: Canada (16.8), Germany (16.7), Japan (16.2), QTN (24.8); 2012: Albania (27.4), Austria (100), Germany (22.3); 2015: Algeria (74.8), United Kingdom (28.3), Chinese Taipei (37.0), Thailand (29.1), Tunisia (66.2)
In addition, until 2015, no score on the ISEI scale was assigned for the three codes that PISA added to the list of ISCO codes (“Doing housework, bringing up children”, “Learning, studying”, “Retired, pensioner, on unemployment benefits”). As a result, even after combining father’s and mother’s reports in a HISEI value (and thus limiting the impact of single-parent households), missing rates for this component of ESCS remain high. The median missing rate across countries for HISEI is 9.3% in 2015 (inter-decile range: 4.7–17.7%). The highest missing rate is observed in Algeria (24.0%), the lowest missing rate in Viet Nam (4.7%) (Fig. 4 ).
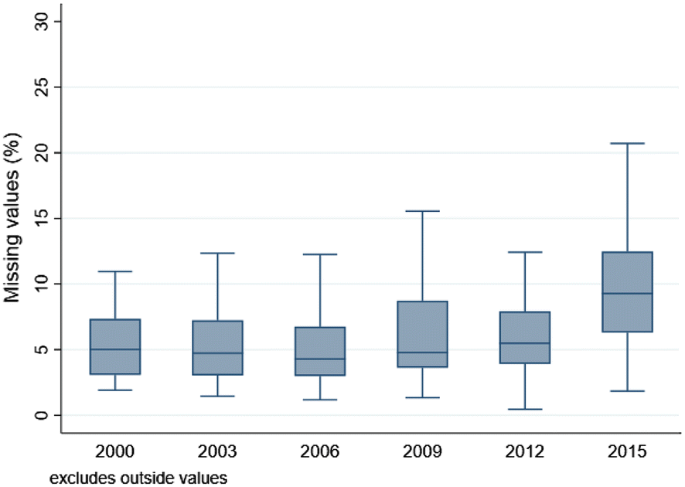
Percentage of respondents with missing parental occupation component of ESCS. The figure represents the median, interquartile range (shaded area) and adjacent values for HISEI. Adjacent values are defined as the most extreme missing rates within 1.5 times the interquartile range from the nearest quartile. The following countries have missing rates that exceed the upper adjacent value: 2000: Israel (26.0), Japan (62.5), United States (14.6); 2003: New Zealand (14.1); 2006: Azerbaidjan (17.0), Israel (16.1), Jordan (20.7), Qatar (41.2); 2009: Georgia (22.4), Himachal Pradesh (India) (32.6), Panama (17.0), Qatar (19.4), Tamil Nadu (India) (23.4); 2012: Germany (19.5), Jordan (21.8), Montenegro (16.2), Qatar (18.3), United Arab Emirates (15.0). 2015: Algeria (23.4), Thailand (22.8)
Coding open-ended questions is costly and requires relatively specialised knowledge that national PISA centres do not necessarily have in house.
Two possible changes to PISA procedures and instruments can address the main issues identified about the parental occupation component of ESCS.
Assign an (approximate) ISEI code to “non-occupations” identified by the pseudo-ISCO codes 9701, 9702 and 9703 (“Doing housework, bringing up children”, “Learning, studying”, “Retired, pensioner, on unemployment benefits”). A possibility is to use the lowest ISEI code for these occupations (11); this would limit the impact of this change for households where only one of the parents has such a code. Following a similar logic, but to avoid extreme values, I suggest using 17 as the ISEI value for these occupations, as this corresponds to the ISEI value used in PISA for occupations generically classified as “elementary occupations” (ISCO08 equal to “9000”). The extension of the ISEI scale to account for the special ISCO codes used in PISA can eliminate one source of cross-country differences in missing rates and thereby improve cross-country comparability.
Reduce the coding scheme to 1-digit or 2-digit codes only or use a closed response format to collect information about occupations. The impact of using only 1-digit or 2-digit coding can be explored by recoding existing data to this level of precision only. In contrast, the impact on the validity and comparability of the data collected from closed response questions about occupations can only be investigated based on field-trial data in which both question formats are administered. While the close response format may significantly reduce missingness and costs, it may also have a higher reading load (since all response categories need to be read) and induce socially desirable answers (e.g. due to order effects) to a greater extent than a text-entry field. Reducing the coding scheme to one or two digits would significantly reduce the cost of ISCO coding. It may also contribute to greater cross-country comparability of occupational codes as a result of higher, and more consistent coder reliability. In the absence of information about the current level of coder reliability, however, these gains remain speculative.
Table 5 (in Appendix ) uses PISA 2015 data to explore the impact of some of the suggested changes on the parental occupation component of socio-economic status, and on its relationship with performance.
Results indicate that the inclusion of pseudo-ISEI values for the additional occupation codes created by PISA (housewife, etc.) not only reduces missingness for this component, but also results in a higher correlation with science achievement ( Appendix , Table 5 ). By reducing the share of students for whom this component is missing, the inclusion of such pseudo-ISEI values can change the country-level average HISEI (and as a consequence, average ESCS) significantly in some cases, as shown by Jordan, whose mean value decreases by about one fourth of a standard deviation.
Results also suggests that analyses based on the parental occupation component of socio-economic status are relatively robust to changes in the coding scheme that would significantly reduce the data-collection costs for countries. In particular, the use of a more limited number of codes—either 34 two-digit codes or 10 one-digit codes—would have only a limited impact on country rankings and on the estimated correlations with achievement ( Appendix , Table 5 ).
- Household possessions
The measurement of household possessions in 2015
In 2015, the “household possessions” component of socio-economic status was based on 25 items in questions ST011 (16 dichotomous items, including 3 chosen by each country), ST012 (8 polytomous items, with a four-point scale) and ST013 (one polytomous item, with a 6-point scale) (see Table 1 ).
A one-dimensional generalised partial credit model was fitted to the data, with some items receiving country-specific item parameters. This was the case for the three national items in ST011, but also for the two indicators about the possession of “classic literature” and “books of poetry”, for which the meaning and the national examples included in the item stem may vary significantly across countries. Items that showed strong evidence of misfit for particular countries were also assigned national items; this was the case for the indicator on “educational software” in Japan.
The following issues affecting the validity, reliability and comparability across countries and over time of the current measurement of household possessions can be identified.
Validity of household possessions in cross-country comparisons
A measure of income or consumption for cross-country comparison should be expected to correlate highly with other measures of national income or household income. The country means of the PISA 2015 measure of household possession have a moderate positive correlation (0.65) with per-capita gross national income measures. The correlation is even higher if small countries with large natural resource revenues or financial sectors are excluded, or if income is expressed in a logarithmic scale (Fig. 5 ).
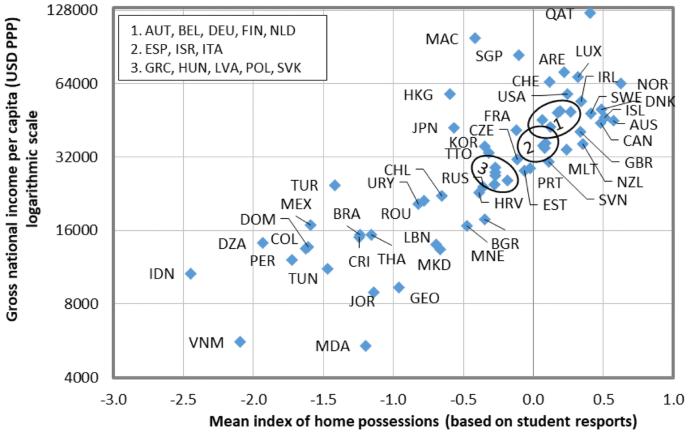
(Source: PISA 2015 Database and https://data.worldbank.org )
Correlation of average HOMEPOS (PISA) and GNI per capita (World Bank). Pearson’s linear correlation coefficient between the two series is equal to 0.65 (0.80 when GNI per capita is measured on a logarithmic scale)
Similarly, the percentage of 15-year-old students with a low level on the international household possession scale (values of HOMEPOS below − 1.77) correlates strongly (r = 0.85) with the percentage of the general population living below the World Bank upper middle-income International Poverty Line, set at USD 5.50 (PPP) (Fig. 6 ).
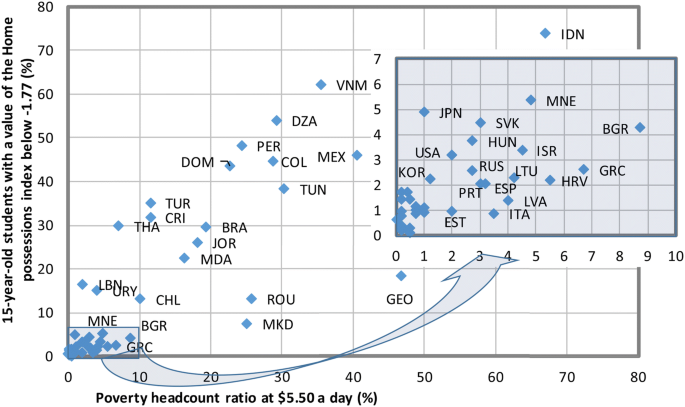
(Source: PISA 2015 database and https://data.worldbank.org )
Students with low household possessions and international poverty rates. The smaller, shaded chart shows an enlargement of the shaded area in the larger chart. Pearson’s linear correlation coefficient between the two series is 0.85. Values for Australia, Austria, Belgium, Canada, Cyprus, the Czech Republic, Germany, Denmark, Finland, France, Ireland, Iceland, Luxembourg, Malta, the Netherlands, Norway, Slovenia, Sweden, Switzerland and the United Kingdom, all countries with a poverty headcount ratio at or below 1%, are also shown but not labelled in the charts
The percentage of students with missing reports about their household possessions is relatively low, compared to other components of ESCS. The use of a proxy of family income instead of direct questions about this therefore seems successful at overcoming problems associated with missing answers. Nevertheless, there has been an increase in the rate of missing answers in 2012 and 2015, compared to previous surveys, and driven in particular by higher missing rates in Germany (Fig. 7 ). Footnote 8
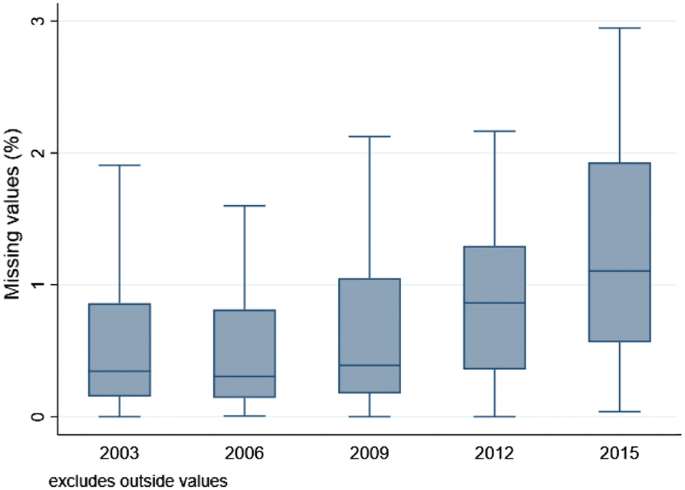
Percentage of respondents with missing household possessions component. The figure represents the median, interquartile range (shaded area) and adjacent values for country-level missing rates in the household possession index (HOMEPOS). Adjacent values are defined as the most extreme missing rates within 1.5 times the interquartile range from the nearest quartile. The following countries have missing rates that exceed the upper adjacent value: 2003: Brasil (2.11), Canada (9.78), Czech Republic (4.14), Germany (5.67), United Kingdom (3.72), Netherlands (3.16); 2006: Bulgaria (2.06), Canada (3.53), Chile (2.05), Germany (3.15), Israel (4.03), Norway (1.98), Qatar (4.47); 2009: Brasil (5.68), Germany (7.04), Panama (6.43); 2012: Albania (6.90), Germany (15.13), Iceland (2.73), Israel (2.86), Qatar (3.24); 2015: Brazil (5.22), Germany (12.64), Russia (4.07), Tunisia (4.17)
Changes in measurement instruments
There have been numerous changes over the years in the instruments used to measure household possessions.
The most visible change is the change in the set of international items in the household possession scale and in the order of items within each question (Table 1 ). The position of the questions within the questionnaire (at the beginning or at the end) has also changed over cycles.
A second type of change is the change in the answer categories for “Books at home”, which were changed in PISA 2003 to better match those used in other educational studies like TIMSS and PIRLS (Table 2 ).
Less visible changes have also happened over the years. In particular:
In 2003, the second set of questions about household possessions [Question 18, items (a) to (e)], asking students to count the “number of… at home”, could not be included in the computation of ESCS since they were deleted from the student data file (OECD 2005a , p. 246). The suppression of student responses was necessary because preliminary analyses revealed that students were confused by the data-entry codes that were printed next to the answer boxes, and which contradicted the answer categories for students provided above the boxes. It must be noted that these small numbers were re-introduced in 2015 for paper-based instruments (in use in a small minority of countries), but no similar action was taken (Fig. 8 ). In 2018, two-digit data-entry codes (“01”, “02”, “03”, “04”) were used in countries that continued to administer paper-based instruments.
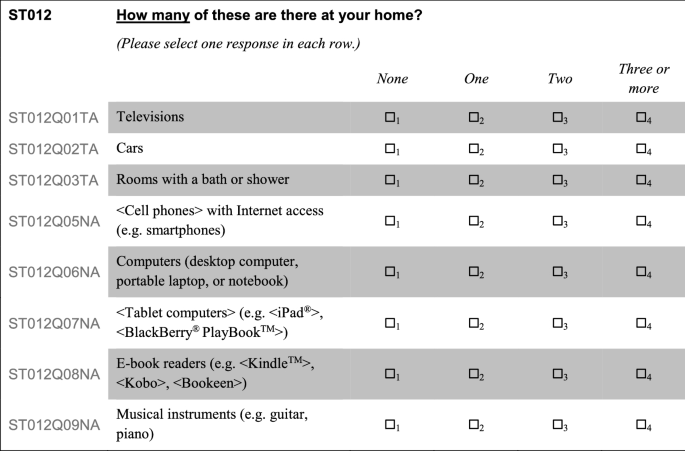
The problem with PISA 2015 paper-based instruments. The data-entry subscripts next to answer boxes are intended to help coders during data entry; they contradict however the labels provided above for respondents, leading to possible confusion. In PISA 2000, 2006, 2009 and 2012, only the last answer box (“three or more”) had a data-entry subscript
In 2006, the item “A room with a bath or shower” was suppressed from the database and the computation of the household possessions scale; the reason for this suppression is not documented in the technical documentation.
Most national items (dichotomous, country-specific items in ST011) have been modified over time.
Change in measurement models
The scaling model and the scaling procedures for the household possession index have changed in almost every cycle of PISA.
For the first PISA database and report (OECD 2001 ), three distinct indices (family wealth, cultural possessions and home educational resources) were derived, and no “overall” household possession index was created to summarise the items in the three indices and the books at home question. The household possession index was first created in PISA 2003, by combining items used in the family wealth, cultural possessions and home educational resources indices with the “books in the home” indicator (OECD 2005b ).
In 2006 and 2009, a country-specific measurement model was assumed for household possessions; scores were put on the same scale through an equating procedure (mean equating on a set of item difficulties in 2006; a linear transformation based on country means in a concurrent scaling in 2009) (OECD 2009 , 2012 ).
In 2009 and 2012, the calibration samples for HOMEPOS were drawn from multiple cycles (in 2012, a higher number of observations from the most recent cycle than from previous cycles was included) (OECD 2009 , 2012 ). In all other cycles, only observations from the most recent cycle contributed to these steps.
In 2012, the scaling of HOMEPOS was performed in two steps: national questionnaire items were not included in the first scaling run, but only in a second run (which was performed separately for each country) where all parameters for the international items were constrained to the values obtained in the first step (OECD 2014 ).
Until 2012, a partial credit model was used in the scaling of HOMEPOS (OECD 2014 ); in 2015, in line with other background questionnaire indices, a generalised partial credit model (including a “slope” or discrimination parameter) was used (OECD 2017 ). The evidence from 2015 shows significant variation in the discrimination parameter, even among items with the same (dichotomous or polytomous) response format: discrimination is only 0.59 for “books to help with your school work”, but 2.45 for “a link to the Internet”.
In 2015, for the first time, all countries’ data were used to scale HOMEPOS; until then, the scaling model for HOMEPOS was calibrated using observations from OECD countries only (OECD 2017 ).
Measurement equivalence across countries and over time
Several scholars have highlighted the weakness of the evidence in favour of a common measure of household possessions in PISA that is valid for all countries (Rutkowski and Rutkowski 2013 ; Pokropek et al. 2017 ).
In 2015, for the first time the PISA consortium introduced an analysis of measurement invariance for the items included in IRT indices, through the inspection of root-mean-square deviation (RMSD) and mean-deviaton (MD) statistics for each item × group interaction. For the purpose of the index of household possessions (HOMEPOS), analyses on the invariance of item parameters across countries, languages and cycles were conducted and unique parameters were assigned if necessary (OECD, PISA 2015 Technical Report, 2017 , p. 342). These analyses led to the use of more country-specific item parameters in the scaling of HOMEPOS. More recently, Lee and von Davier ( 2020 ) analysed the invariance of item parameters both across countries and over time, using concurrent, multiple-group calibration with partial invariance constraints, and concluded that four items in the scale, all related to technology, functioned differently across the PISA cycles, and (among those used in 2015) four other items (i.e. bathroom, classic literature, poetry books, and TV) functioned differently across the majority of participating countries when used to measure family wealth. Several other items used in 2015 exhibited high levels of misfit for a minority of country/language groups; the most notable are the questions about the number of “cars” (40% of country/language groups requiring unique parameters and the number of “computers” (34%).
Even without turning to fit indices and model-based evaluations of equivalence, theoretical considerations and simple comparisons of the mean levels of different household possessions items indicate possible problems of non-invariance across cycles and time. In particular, the extent to which the possession of certain technological goods at home indicates high social class is likely to change, as their price declines and their novelty fades; while for others, and particularly traditional cultural possessions (including books), new substitutes (e.g. e-books) become available. There are clear indications (Fig. 9 ) for example that the availability of “a link to the Internet” at home has moved from being an item indicating high income to an item indicating the availability of basic resources: the upward trend in the availability of “a link to the Internet” is particularly steep in lower-income countries. The top charts in the same figure also show how the relative order of “Cars”, compared to other durable goods, changes between lower-income and higher-income countries; while the bottom charts show similar variation, across countries, for items related to “classic literature”, “poetry books” and “works of art”. At the same time, Fig. 9 indicates also a certain stability and relatively consistent orderings for most items, particularly those indicating durable goods.

Trends in the possession of distinct household possession items, by national income. Lower income countries include countries with a gross national income below USD 28,500 (PPP). This threshold was chosen in order to have an about equal number of countries in the lower- and higher-income group. Only countries/economies that participated in every PISA cycle are included in the analysis. The lower values (compared to a linear trend line) observed in 2003 for many items may be related to the different response format used in that year, which did not distinguish item-non-response from a “no” answer (see notes under Table 1 )
Misreporting, local dependencies and inconsistencies
The items included in the household possession scale are, to some extent, dependent on each other. For example, it is impossible to have “a computer you can use for school work” (question ST011Q04TA in PISA 2015) in the home if there are “no computers” at all (ST012Q06NA) in the home. Similarly, there are multiple questions about books, which are expected to correlate more highly among them than with any other question.
Alternative instruments and scaling procedures
As indicated previously, there is a long history of changes in the measurement and scaling of the household possession components of ESCS. In the initial years, several changes were made to the instruments (or errors in the instruments corrected). Over time PISA has also oscillated between treating the household possession scale as a national scale and treating it as an international scale.
Of the three components of ESCS, the “household possessions” component has been the subject of most scrutiny by researchers; this criticism has also resulted in constructive advice to improve the measurement of this component.
In particular, Lee and von Davier ( 2020 ) show that the tension between the ideal of strong international comparability of a household-possessions component of socio-economic status and the reality of national specificities in consumption preferences and cost schedules, for example with respect to car ownership, can be successfully navigated by relying on multiple-group concurrent calibration with partial invariance constraints. In other words: the tension between a common measurement model for all country/language groups and the reality of model misfit and differential item functioning can be handled (and at the same time, shown explicitly) in a model in which common item parameters are imposed for the majority (but not the totality) of items and groups. In Table 6 ( Appendix ), I estimate such a partial-invariance model and replicate Lee and von Davier’s ( 2020 ) finding that the resulting scale correlates more strongly, on average, with test performance (an indicator of greater within-country accuracy and of criterion validity), while preserving the overall correlation (at country level) with measures of national income or poverty (an indicator of cross-country comparability and of concurrent validity). Lee and von Davier ( 2020 ) further show that the concurrent calibration approach with partial-invariance constraints can be successfully extended to the time dimension, resulting in a scale that finds the best possible balance between comparability (over time and across countries) and accuracy of scores.
Improvements to the instruments may also be considered in parallel. The periodic phasing out and replacement of certain items could be informed by the results of scaling: those items for which invariance constraints cannot be maintained may be replaced by new ones, thus ensuring that the home-possessions scale remains relevant in the presence of changing consumption patterns. In addition, PISA might consider introducing greater coordination, at regional level, in the selection of “national items” (Rutkowski and Rutkowski 2013 ). One possibility would be to introduce an international set of “optional” items from which countries can choose in order to replace one or more of the national items. These optional items would undergo the same translation and verification procedures as international items, and would be treated in scaling as international items (unless there is evidence of misfit for some or all countries), which are missing by design in countries that chose not to administer them. They would strengthen the comparability of the household possessions scale across countries sharing similar levels of economic development, geography, or cultural background.
Improving the composite score
Handling missing data.
In PISA 2015 and PISA 2018, a stochastic regression-based imputation of the missing component was implemented prior to computing a composite measure of socio-economic status. The imputation is applied for those cases where students have values on two out of three components and assumes a multivariate normal distribution for the three variables as well as that data were missing at random (OECD 2017 , 2020 ). While other imputation procedures can be considered (such as the use of multiple-imputation routines, and the inclusion of auxiliary background information in imputation), these changes are unlikely to be consequential for most analyses in which ESCS is used. It would be useful, however, to include in the dataset the imputed and standardised values that are used in the construction of ESCS, not only to document this step in the construction of ESCS, but also to facilitate the equating of measures from other surveys. This would indeed make the scale transformations required when moving from the distinct components to a composite measure visible and replicable.
Weighting scheme
In all past cycles of PISA, empirical weights based on an international PCA were used to combine the three (or five, in PISA 2000) components of socio-economic status into a single score.
The application of principal component weights implies that different components are weighted equally across countries, but differently over time. It can also be criticised as a somewhat unnecessary complication, which makes the definition of ESCS sample-dependent. For example, the optimal component weights for particular national samples can sometimes be very different from those used in the construction of ESCS, which are based on the international sample.
Alternatively, ESCS could be constructed using arbitrary weights; after all, ESCS is just a convenient summary of a multidimensional construct and the definition of these weights can be included in the operational definition of ESCS. Arbitrary weights would also make the ESCS measure more robust to changes in the sample and its construction easier to replicate based on public use files or on data from other surveys.
But how should the weights be determined? The simplest solution would be to use equal weights to combine the three standardised components. In fact, when looking in detail at the variation of component weights across survey cycles, it is striking that they have remained very similar across all cycles and very close to “equal weights” for all three (standardised) components (Table 3 ). In general, the weight for the HOMEPOS component has always been slightly below the weight for PARED and HISEI, but the difference has never been more than 10%, and has tended to reduce over time (perhaps as a consequence of expanding membership in the OECD and the use of all countries in calibration in 2015).
Given the available evidence, the use of arbitrary equal weights for producing the ESCS composite presents several advantages and would create very limited disruptions for trend analyses. Table 7 (in Appendix ) demonstrates this point by comparing the means and correlations based on the original ESCS composite (using principal-component weights) with those based on an alternative composite, using equal weights: differences are hardly noticeable, not only in the aggregate, but also within countries.
This article provides a rationale and theoretical underpinning for the construction of a composite measure of socio-economic status in PISA and in other large-scale international surveys, and suggests practical ways in which the ESCS variable can be improved to strengthen the arguments supporting its validity and comparability, reduce measurement error and missing values, and facilitate the construction of linked measures based on multiple survey years or based on other datasets.
The review highlights the hybrid nature of ESCS as both a measure of resources and a measure of relative social status; and underlines the utility of such a hybrid, composite measure for the construction of indicators of inequality of opportunity in education. It also identifies situations in which it may be preferable to use the individual components of socio-economic status (rather than the composite measure) in analyses based on PISA (or other similar) data.
While ESCS is conceived of as little more than a “convenient summary” of distinct resources that bear a relationship with an individual’s position in society, in order to allow for meaningful comparisons of the indicators based on ESCS over time and between countries, it is important to ensure that the scaling and measurement quality of ESCS remain comparable across different contexts. Yet, in the past, the operational definition of ESCS has changed in almost every cycle; and the measurement quality of the underlying components has received little attention. This review suggests that the validity and cross-country comparability of the components that are summarised in ESCS is, in fact, relatively high, based on concurrent evidence at the country level, and indicates ways in which it can be improved, while also addressing other aspects of measurement quality (such as missingness and reliability). It also suggests simplifying, and stabilising, the way in which the different components are combined, by abandoning the use of empirical weights (based on principal component analysis) in favour of arbitrary weights.
Availability of data and materials
The datasets and questionnaires analysed in this article are available from http://www.oecd.org/pisa .
A similar review was conducted by Willms and Tramonte ( 2015 ).
In France, Italy and Portugal, for example, PISA-eligible students in lower secondary schools are all behind track, below the expected grade for 15-year olds; they tend to be low-achieving and more often come from immigrant or disadvantaged backgrounds than their (younger) schoolmates, which are not eligible to be sampled in the PISA age-based sample. In other countries, such as Australia or Russia, 15-year-old students in upper secondary schools are all ahead of track. They may be more advantaged than the typical (older) students in the schools they attend.
The corresponding variables in the PISA 2015 dataset are ST005Q01TA, ST006Q01TA, ST007Q01TA and ST008Q01TA.
The low value for Poland may also be related to a mistake in the database; indeed, it seems that ST008, indicating fathers’ post-secondary qualifications in the PISA 2015 dataset for Poland, is identical to ST007, indicating mothers’ education.
In practice, there may be exceptions, and multiple ISCED levels may be combined in a single programme; for example, in the Netherlands the typical upper-secondary vocational track leads to an ISCED Level 4 qualification, with no formal degree to sanction the completion of ISCED Level 3.
The high level of missing answers about parental background in Germany is, in part, due to the presence of a filter in German questionnaires, which implies that these questions are not administered to students from the Land of Berlin whose parents have not given explicit consent to the collection of information about the out-of-school environment.
For example, in PISA 2015, students in Denmark were asked about vocational degrees [“En erhvervsfaglig uddannelse (fx elektriker, butiksassistent, smed, SOSU)”] as part of questions on post-secondary qualifications (ST006/ST008), and Danish questionnaires only included ISCED3A qualifications in ST005/ST007; while in PISA 2018, vocational qualifications were included as an additional option in question ST005/ST007.
This increase coincides with the introduction, in February 2011, of a more stringent data protection law in the Land of Berlin, which requires explicit consent for all data collections, including by public actors.
Abbreviations
Economic, social and cultural status
Gross national income
International Labour Organisation
International Standard Classification of Education
International Standard Classification of Occupations
International Socio-Economic Index
Free School Meal
Index of household possessions (variable in the PISA Database)
Mean deviation
National School Lunch Programme
Organisation for Economic Co-Operation and Development
Parental education (variable in the PISA database)
Principal component analysis
Progress in International Reading Literacy Study
Programme for International Student Assessment
Réseau d’éducation prioritaire (Priority education network)
Root mean square deviation
- Socio-economic status
Teaching and Learning International Survey
Trends in mathematics and science study
Zone d’éducation prioritiaire (Priority education zone)
APA. (2007). Report of the APA task force on socioeconomic status. Retrieved January 18, 2019 from https://www.apa.org/pi/ses/resources/publications/task-force-2006.pdf .
Bollen, K., & Bauldry, S. (2011). Three Cs in measurement models: Causal indicators, composite indicators, and covariates. Psychological Methods, 16 (3), 265–284. https://doi.org/10.1037/a0024448 .
Article Google Scholar
Brighouse, H., Ladd, H., Loeb, S., & Swift, A. (2015). Educational goods and values: A framework for decision makers. Theory and Research in Education, 14 (1), 3–25. https://doi.org/10.1177/1477878515620887 .
Cowan, C. D., Hauser, R. M., Levin, H. M., Beale Spencer, M., & Chapman, C. (2012). Improving the measurement of socioeconomic status for the National Assessment of Educational Progress: A theoretical foundation. Retrieved January 18, 2019 from https://nces.ed.gov/nationsreportcard/pdf/researchcenter/Socioeconomic_Factors.pdf .
Deaton, A. (2002). Policy implications of the gradient of health and wealth. Health Affairs, 21 (2), 13–30. https://doi.org/10.1377/hlthaff.21.2.13 .
Ensminger, M., & Fothergill, K. (2003). A decade of measuring SES: What it tells us and where to go from here. In M. Bornstein & R. Bradley (Eds.), Socioeconomic status, parenting, and child development (pp. 13–27). Mahwah: Lawrence Erlbaum.
Google Scholar
Friedman, M. (1957). The permanent income hypothesis. In A theory of the consumption function (pp. 20–37). Princeton: Princeton University Press. Retrieved January 18, 2019 from https://www.nber.org/chapters/c4405.pdf .
Ganzeboom, H. (2010). How was new ISEI - 08 constructed. Retrieved February 20, 2019 from http://www.harryganzeboom.nl/isco08/qa-isei-08.htm .
Ganzeboom, H., De Graaf, P., & Treiman, D. (1992). A standard international socio-economic index of occupational status. Social Science Research, 21 (1), 1–56. https://doi.org/10.1016/0049-089X(92)90017-B .
Goodman, E., Adler, N., Kawachi, I., Frazier, A., Huang, B., & Colditz, G. (2001). Adolescents’ perceptions of social status: Development and evaluation of a new indicator. Pediatrics, 108 (2), e31–e31. https://doi.org/10.1542/peds.108.2.e31 .
INVALSI. (2017). Rilevazioni nazionali degli apprendimenti 2016–17. Retrieved January 18, 2019 from https://invalsi-areaprove.cineca.it/docs/file/Rapporto_Prove_INVALSI_2017.pdf .
Jerrim, J., & Micklewright, J. (2014). Socio-economic gradients in children’s cognitive skills: Are cross-country comparisons robust to who reports family background? European Sociological Review, 30 (6), 766–781. https://doi.org/10.1093/esr/jcu072 .
Keskpaik, S., & Rocher, T. (2011). La mesure de l’équité dans PISA: pour une décomposition des indices statistiques. Revue Éducation et formations, 80 , 69–78. Retrieved January 29, 2019 from http://cache.media.education.gouv.fr/file/revue_80/30/4/Depp-EetF-2011-80-mesure-equite-pisa-indices-statistiques_203304.pdf .
Lee, S., & von Davier, M. (2020). Improving measurement properties of the PISA home possessions scale through partial invariance modeling. Psychological Test and Assessment Modeling, 62 (1), 55–83. Retrieved from https://www.psychologie-aktuell.com/fileadmin/Redaktion/Journale/ptam-2020-1/04_Lee.pdf
Lien, N., Friestad, C., & Klepp, K.-I. (2001). Adolescents’ proxy reports of parents’ socioeconomic status: How valid are they? Journal of Epidemiology and Community Health, 55 (10), 731–737. https://doi.org/10.1136/jech.55.10.731 .
Looker, E. (1989). Accuracy of proxy reports of parental status characteristics. Sociology of Education, 62 (4), 257. https://doi.org/10.2307/2112830 .
Mueller, C., & Parcel, T. (1981). Measures of socioeconomic status: Alternatives and recommendations. Child Development, 52 (1), 13–30.
O’Connell, M. (2019). Is the impact of SES on educational performance overestimated? Evidence from the PISA survey. Intelligence, 75, 41–47. https://doi.org/10.1016/j.intell.2019.04.005 .
OECD. (2001). Knowledge and skills for life: First results from PISA 2000. In PISA. Paris: OECD Publishing. https://doi.org/10.1787/9789264195905-en .
OECD. (2005a). PISA 2003 data analysis manual: SPSS. In PISA. Paris: OECD Publishing. https://doi.org/10.1787/9789264010666-en .
OECD. (2005b). PISA 2003 technical report. In PISA. Paris: OECD Publishing. https://doi.org/10.1787/9789264010543-en .
OECD. (2009). PISA 2006 technical report. In PISA. Paris: OECD Publishing. https://doi.org/10.1787/9789264048096-en .
OECD. (2012). PISA 2009 technical report. In PISA. Paris: OECD Publishing. https://doi.org/10.1787/9789264167872-en.
OECD. (2014). PISA 2012 technical report. Paris: OECD Publishing. Retrieved December 23, 2019 from https://www.oecd.org/pisa/pisaproducts/PISA-2012-technical-report-final.pdf .
OECD. (2017). PISA 2015 technical report. Retrieved July 31, 2017 from http://www.oecd.org/pisa/data/2015-technical-report/ .
OECD. (2018a). Effective teacher policies: Insights from PISA. In PISA. Paris: OECD Publishing. https://doi.org/10.1787/9789264301603-en .
OECD. (2018b). Equity in education: Breaking down barriers to social mobility. In PISA. Paris: OECD Publishing. https://doi.org/10.1787/9789264073234-en .
OECD. (2020). PISA 2018 technical report . Retrieved from https://www.oecd.org/pisa/data/pisa2018technicalreport/ .
Piketty, T. (2018). Brahmin left vs merchant right: Rising inequality and the changing structure of political conflict. WID. World Working Paper.
Pokropek, A., Borgonovi, F., & McCormick, C. (2017). On the cross-country comparability of indicators of socioeconomic resources in PISA. Applied Measurement in Education, 30 (4), 243–258. https://doi.org/10.1080/08957347.2017.1353985 .
Robitzsch, A., Kiefer, T., & Wu, M. (2019). TAM: test analysis modules. Retrieved from https://CRAN.R-project.org/package=TAM .
Rutkowski, D., & Rutkowski, L. (2013). Measuring socioeconomic background in PISA: One size might not fit all. Research in Comparative and International Education, 8 (3), 259–278. https://doi.org/10.2304/rcie.2013.8.3.259 .
Schulz, W. (2005). Measuring the socio - economic background of students and its effect on achievement in PISA 2000 and PISA 2003. Retrieved May 15, 2019 from https://files.eric.ed.gov/fulltext/ED493510.pdf .
Sirin, S. (2005). Socioeconomic status and academic achievement: A meta-analytic review of research. Review of Educational Research, 75 (3), 417–453. https://doi.org/10.3102/00346543075003417 .
Weber, M. (1922). Wirtschaft und Gesellschaft . Tübingen: Mohr.
Willms, J. (2006). Learning divides: Ten policy questions about the performance and equity of schools and schooling systems. UNESCO Institute for Statistics, Montreal. Retrieved February 20, 2019 from https://unesdoc.unesco.org/ark:/48223/pf0000147066 .
Willms, J., & Tramonte, L. (2015). Towards the development of contextual questionnaires for the PISA for development study. In OECD education working papers (Vol. 2015). Paris: OECD Publishing. https://dx.doi.org/10.1787/5js1kv8crsjf-en .
Willms, J. D., & Tramonte, L. (2019). The measurement and use of socioeconomic status in educational research. In L. E. Suter, B. Denman, & E. Smith (Eds.), The SAGE handbook of comparative studies in education . London: Sage.
Download references
Acknowledgements
Camille Marec provided excellent research assistance for all empirical analyses included in this article. This article benefitted from the suggestions and comments of other members of the PISA Analysis and Reporting team at the OECD, of the PISA 2018 Consortium, and of the PISA Technical Advisory Group—the author wishes to acknowledge, in particular, Jonas Bertling, Janine Buchholz, Alfonso Echazarra, Pauline Givord, Rachel Renbarger, Keith Rust, Leslie Rutkowski and Matthias von Davier. The author is solely responsible for all remaining errors and imprecisions.
The author has not received funding.
Author information
Authors and affiliations.
OECD, Directorate for Education and Skills, 2 Rue André Pascal, 75775, Paris Cedex 16, France
Francesco Avvisati
You can also search for this author in PubMed Google Scholar
Contributions
The author read and approved the final manuscript.
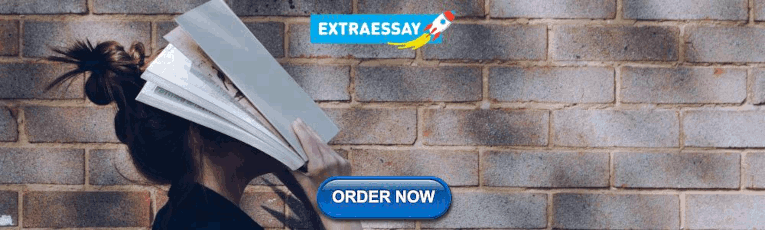
Corresponding author
Correspondence to Francesco Avvisati .
Ethics declarations
Competing interests.
FA was an analyst in the OECD PISA team when he prepared this article. The opinions expressed and arguments employed in this article are those of the author and do not necessarily represent the official view of the OECD or of its member countries.
Additional information
Publisher's note.
Springer Nature remains neutral with regard to jurisdictional claims in published maps and institutional affiliations.
See Tables 4 , 5 , 6 and 7 .
Rights and permissions
Open Access This article is licensed under a Creative Commons Attribution 4.0 International License, which permits use, sharing, adaptation, distribution and reproduction in any medium or format, as long as you give appropriate credit to the original author(s) and the source, provide a link to the Creative Commons licence, and indicate if changes were made. The images or other third party material in this article are included in the article's Creative Commons licence, unless indicated otherwise in a credit line to the material. If material is not included in the article's Creative Commons licence and your intended use is not permitted by statutory regulation or exceeds the permitted use, you will need to obtain permission directly from the copyright holder. To view a copy of this licence, visit http://creativecommons.org/licenses/by/4.0/ .
Reprints and permissions
About this article
Cite this article.
Avvisati, F. The measure of socio-economic status in PISA: a review and some suggested improvements. Large-scale Assess Educ 8 , 8 (2020). https://doi.org/10.1186/s40536-020-00086-x
Download citation
Received : 29 December 2019
Accepted : 27 May 2020
Published : 06 June 2020
DOI : https://doi.org/10.1186/s40536-020-00086-x
Share this article
Anyone you share the following link with will be able to read this content:
Sorry, a shareable link is not currently available for this article.
Provided by the Springer Nature SharedIt content-sharing initiative
- Background questionnaire
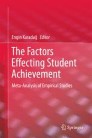
The Factors Effecting Student Achievement pp 171–181 Cite as
The Effect of Socioeconomic Status on Students’ Achievement
- Ş. Koza Çiftçi 2 &
- Firdevs Melis Cin 3
- First Online: 16 May 2017
2968 Accesses
8 Citations
2 Altmetric
The effect of socioeconomic status on student achievement was examined in this meta-analysis study. A total of 187 research studies were collected during the literature review, out of which 66 were included in the meta-analysis. The 66 research studies were compiled to obtain a sample size of 266,817 subjects. The results of the random effect model showed that socioeconomic status has a high level of effect on student achievement . The moderators identified for the study were publication year , publication type , the country in which the study was conducted, the course and class level, out of which publication year, publication type and the class year (grade ) in which the research was conducted were found to be moderator variables .
- Socioeconomic Status
- Research Study
- Student Achievement
- Average Effect
- Equal Opportunity
These keywords were added by machine and not by the authors. This process is experimental and the keywords may be updated as the learning algorithm improves.
This is a preview of subscription content, log in via an institution .
Buying options
- Available as PDF
- Read on any device
- Instant download
- Own it forever
- Available as EPUB and PDF
- Compact, lightweight edition
- Dispatched in 3 to 5 business days
- Free shipping worldwide - see info
- Durable hardcover edition
Tax calculation will be finalised at checkout
Purchases are for personal use only
Note . “*” References marked with an asterisk indicate studies included in the meta-analysis. The in-text citations to studies selected for meta-analysis are not followed by asterisks.
Google Scholar
Alldred, C. C. (2013). A study of eighth grade students ‘self-efficacy as it relates to achievement, gender, and socioeconomic status. Unpublished doctoral dissertation, Liberty University.*
Alordiah, C. O., Akpadaka, G., & Oviogbodu, C. O. (2015). The influence of gender, school location and socio-economic status on students’ academic achievement in mathematics. Journal of Education and Practice , 6 (17), 130–136.*
Barrett, J. A. (2013). Elementary school computer access, socioeconomic status, ethnicity, and grade 5 student achievement . Unpublished dissertation, Sam Houston State University.*
Barry, J. (2006). The effect of socio-economic status on academic achievement. Unpublished doctoral dissertation, Wichita State University.*
Bentzel, E. J. (2012). The combined effects of low socioeconomic status and high mobility on elementary achievement scores in Pennsylvania. Unpublished doctoral dissertation, Capella University.*
Berger, N., & Archer, J. (2016). School socio-economic status and student socio-academic achievement goals in upper secondary contexts. Social Psychology of Education , 19 (1), 175–194.*
Borenstein, M., Hedges, L. V., Higgins, J. P. T., & Rothstein, H. R. (2009). Introduction to meta-analysis . UK: Wiley.
Book Google Scholar
Bornstein, M. C., & Bradley, R. H. (Eds.). (2003). Socioeconmic status, parenting, and child development . Mahwah, NJ: Lawrence Erlbaum.
Coleman, J. S. (1988). Social capital in the creation of human capital. American Journal of Sociology, 94, 95–120.
Article Google Scholar
Coleman, J. S., Campbell, E. Q., Hobson, C. J., McPartland, J., Mood, A. M., Weinfeld, F. D., et al. (1966). Equality of educational opportunity . Washington, DC: Government Printing Office.
Cueto, S., Guerrero, G., Leon, J., Zapata, M., & Freire, S. (2014). The relationship between socioeconomic status at age one, opportunities to learn and achievement in mathematics in fourth grade in Peru. Oxford Review of Education , 40 (1), 50–72.*
Dawson, G. L. (2014). Impact of preschool education on the academic achievement of low socio-economic status elementary students. Unpublished doctoral dissertation, Duquesne University.*
Dotson, L. (2014). Middle grade academic achievement and socioeconomic status on North Carolina State . Unpublished dissertation, North Carolina State University.*
Eni-Olorunda, T., & Adediran, O. A. (2013). Socio-economic status difference in English language comprehension achievement of pupils with intellectual disability. Ife Psychologia , 21 (2), 242.*
Fergusson, D. M., Horwood, L. J., & Boden, J. M. (2008). The transmission of social inequality: Examination of the linkages between family socioeconomic status in childhood and educational achievement in young adulthood. Research in Social Stratification and Mobility , 26 (3), 277–295.*
Feyerherm, J. L. (2008). The relationship between socioeconomic status and reading achievement in English language learners: Dual language vs. English only programs . Unpublished doctoral dissertation, University of Nebraska.*
Hedges, L. V., & Olkin, I. (1985). Statistical method for meta-analysis . United Kingdom: Academic Press.
Hsieh, K. J. (2002). Differences among high school students’ mathematics achievement based on school location, school socioeconomic status, and student ethnicity . Unpublished doctoral dissertation, University of Houston.*
Huang, H. (2015). Can students themselves narrow the socioeconomic-status-based achievement gap through their own persistence and learning time? Education Policy Analysis Archives , 23 (108), n108.*
Jimenez, G. M. (2001). The relationship between socioeconomic status and student achievement of middle school children . Unpublished dissertation, California State University.*
Kurian, N. (2012). Impact of resilience and role model influence on academic achievement of low socio-economic status adolescent students. Indian Journal of Positive Psychology , 3 (3), 250–254.*
Lamdin, D. J. (1996). Evidence of student attendance as an independent variable in education production functions. Journal of Educational Research, 89 (3), 155–162.
Littell, J. H., Corcoran, J., & Pillai, V. (2008). Systematic reviews and meta-analysis . New York: Oxford University.
Ma, X. (2008). A global perspective on socioeconomic differences in learning outcomes . UNESCO: Education for All Global Monitoring Report.
Maxwell, A. F. (2007). A comparison of the academic achievements of intermediate students based on socioeconomic status and participation in an after-school program . Unpublished doctoral dissertation, East Tennessee State University.*
McConney, A., & Perry, L. B. (2010). Socioeconomic status, self-efficacy, and mathematics achievement in Australia: A secondary analysis. Educational Research for Policy and Practice , 9 (2), 77–91.*
McCorvey-Watson, C. (2012). Socioeconomic status and academic achievement of elementary students in Mississippi . Unpublished doctoral dissertation, Walded University.*
McCullough, M. T. (2011). Impact of national board certification, advanced degree, and socio-economic status on the literacy achievement rate of 11th grade students in Arkansas . Unpublished doctoral dissertation, Arkansas State University.*
Mosley, M. L. (2006). The relationship of gender, socioeconomic status, and attendance on mathematics achievement of seventh grade students . Unpublished doctoral dissertation, University of Alabama.*
Norris, T. A. (2000). A longitudinal study of predictors of student achievement related to socioeconomic status, race/ethnicity, attendance, and student mobility . Unpublished doctoral dissertation, University of Nebraska.*
O’Rourke, P. W. (2006). Effects of rural high school size and socioeconomic status on achievement of tenth graders . Unpublished doctoral dissertation, Indiana State University.*
Okoye, N. S., & Okecha, R. E. (2008). The interaction of logical reasoning ability and socio-economic status on achievement in genetics among secondary school students in Nigeria. College Student Journal , 42 (2), 617–624.*
Özdemir, N., Ayral, M., Fındık, L. Y., Ünlü, A., Özarslan, H., & Bozkurt, E. (2014). The relationship between students’ socioeconomic status and their Turkish achievements. Procedia-Social and Behavioral Sciences , 143 , 726–731.*
Pettigrew, E. J. (2009). A study of the impact of socioeconomic status on student achievement in a rural east Tennessee school system . Unpublished doctoral dissertation, East Tennessee State University.*
Reynolds, A. J., & Walberg, H. J. (1992). A process model of mathematics achievement and attitude. Journal for Research in Mathematics Education , 23 (4), 306–328.
Ripple, C. H., & Luthar, S. S. (2000). Academic risk among inner-city adolescents: The role of personal attributes. Journal of School Psychology, 38 (3), 277–298.
Seyfried, S. F. (1998). Academic achievement of African American preadolescents: The influence of teacher perceptions. American Journal of Community Psychology, 26 (3), 381–402.
Sirin, S. R. (2005). Socioeconomic status and academic achievement: A meta-analytic review of research. Review of Educational Research, 75 (3), 417–453.
Smeding, A., Darnon, C., Souchal, C., Toczek-Capelle, M. C., & Butera, F. (2013). Reducing the socio-economic status achievement gap at university by promoting mastery-oriented assessment. PLoS ONE, 8 (8), e71678.
Soden-Hensler, B. (2012). An examination of gene x socioeconomic status interactions for reading achievement . Unpublished doctoral dissertation, Florida State Unsiversity.*
Sutton, A., & Soderstrom, I. (1999). Predicting elementary and secondary school achievement with school-related and demographic factors. Journal of Educational Research, 92 (6), 330–338.
Tok, B. R., & Dupak, S. (2015). A study on academic achievement in relation with socio economic status of secondary school students with reference to east Siang district of Arunachala Pradesh. International Journal of Multidisciplinary Approach and Studies , 2 (5), 37–44.*
Trent, S. E. (2007). A descriptive study of the effect of traditional and year-round calendars, socio-economic status, and teacher tenure status on student achievement in two rural school systems in Tennessee. Unpublished doctoral dissertation, Liberty University.*
Tsai, M., & Liu, F. (2013). Multigroup structural equation approach: Examing the relationship among family socioeconomic status, parent-child interaction, and academic achievement using TASA samples. International Journal of Intelligent Technologies & Applied Statistics , 6 (4), 353–373. doi: 10.6148/IJITAS.2013.0604.03 .
Utne, J. S. (2001). A relationship between student socioeconomic status, perceptions of school environment, academic achievement, and school attendance . Unpublished doctoral dissertation, University of Missouri-Columbia.*
Weers, A. J. (2012). The impact of socioeconomic status on achievement of high school students participating in a one-to-one laptop computer program . Unpublished doctoral dissertation, University of Nebraska.*
White, K. R. (1982). The relation between socioeconomic status and academic achievement. Psychological Bulletin, 91 (3), 461–481.
Yang, Y. (2003). Dimensions of socio-economic status and their relationship to mathematics and science achievement at individual and collective levels. Scandinavian Journal of Educational Research, 47 (1), 21–41.
Download references
Author information
Authors and affiliations.
Akdeniz University, Antalya, Turkey
Ş. Koza Çiftçi
Istanbul Commerce University, Istanbul, Turkey
Firdevs Melis Cin
You can also search for this author in PubMed Google Scholar
Corresponding author
Correspondence to Ş. Koza Çiftçi .
Editor information
Editors and affiliations.
Faculty of Education, Eskisehir Osmangazi University, Eskişehir, Turkey
Engin Karadag
Rights and permissions
Reprints and permissions
Copyright information
© 2017 Springer International Publishing AG
About this chapter
Cite this chapter.
Koza Çiftçi, Ş., Melis Cin, F. (2017). The Effect of Socioeconomic Status on Students’ Achievement. In: Karadag, E. (eds) The Factors Effecting Student Achievement. Springer, Cham. https://doi.org/10.1007/978-3-319-56083-0_10
Download citation
DOI : https://doi.org/10.1007/978-3-319-56083-0_10
Published : 16 May 2017
Publisher Name : Springer, Cham
Print ISBN : 978-3-319-56082-3
Online ISBN : 978-3-319-56083-0
eBook Packages : Education Education (R0)
Share this chapter
Anyone you share the following link with will be able to read this content:
Sorry, a shareable link is not currently available for this article.
Provided by the Springer Nature SharedIt content-sharing initiative
- Publish with us
Policies and ethics
- Find a journal
- Track your research
Academia.edu no longer supports Internet Explorer.
To browse Academia.edu and the wider internet faster and more securely, please take a few seconds to upgrade your browser .
Enter the email address you signed up with and we'll email you a reset link.
- We're Hiring!
- Help Center
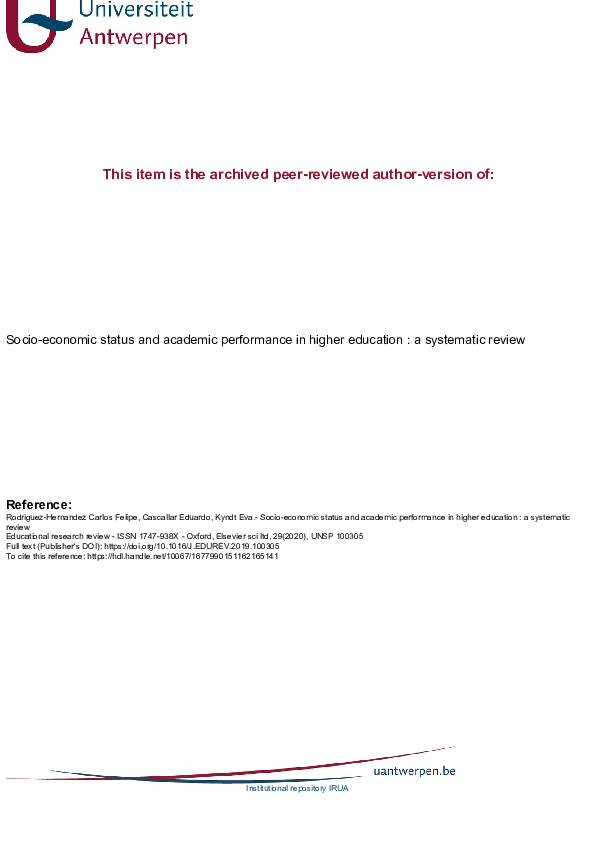
Socio-economic status and academic performance in higher education: A systematic review

2020, Educational Research Review
Related Papers
Evaluation & Research in Education
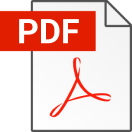
SOCIO-ECONOMIC STATUS CAN BE USED TO EXPLAIN DIFFERENCES IN ACADEMIC PERFORMANCE AMONG PUPILS EXPLAIN
joseph mwelwa
Differential academic performances among pupils in schools is a common phenomenon existing worldwide. Many factors such as political, cultural, environmental, personal and socio-economic status among others explains the differences in academic performance. This piece of writing will only focus on socio-economic status by explaining and discussing how it clearly explains differential academic performance among pupils
gerald hammond
Abstract Education in our country is one of the major topics for discussion. The conversation around education focuses on the failures of our teachers and students in our schools as compared to foreign countries institutions of higher learning. In a nation of divisive politics, education is an area that both major parties can agree to criticize. Critics claims that our schools are failing to prepare our nations youth for the 21st century world. While many of our nations schools, when examined, are indeed falling well short on standards established by local, State, and Federal officials. What is the truth? Are the schools failing our children? In order to understand the reason why too many students still fail to pass academic measurements, it is necessary to understand and define the major causes. Poverty and its effects on our student’s academic performance have long been identified as one of the leading causes of our academic ineptitude. But is it that simple? What is driving this factor? Surely no one is choosing to be poor, there must be a larger factor-taking place in our culture. This study seeks to show the link between poverty and low academic achievement, but it also strives to show that poverty is not a result of ignorance or inferiority that affects one group over another. Poverty is a part of learned culture, for those raised in this culture of despair and hopelessness there is little chance of escape
Dr Yashpal D Netragaonkar
" The present market based global village puts up a barrier in front of those who " cannot read or write or count, and cannot follow written instructions " (Sen, 1998). Education is the basic requirement and the 'Fundamental Right' of the citizens of a nation. While Higher Education is important; the Elementary Education system serves as the base over which the Superstructure of the whole education system is built up. Student's education is closely linked to their life chances, income, and well being. Therefore, it is important to have a clear understanding of what benefits or hinders his/her educational attainment. There are several relevant areas that are most commonly linked to academic performance while the most influencing factor is SocioEconomic Status of the family. Socioeconomic status (SES) is often measured as a combination of education, income, and occupation. Low SES and its correlates, such as lower education, poverty, and poor health, ultimately affect our society as a whole. The main aim of the present paper is to produce a comprehensive literature review of reliable research evidence on the relationship between students' educational attainment and parents' socio economic status.
Greg Thomson
zayreen mae
Review of educational research
Chike Aghadiuno
Not a complete research paper, just wanted to share this as a little help to those in a little need.
gideon adedayo
The purpose of this research work was to investigate the effects of parental socioeconomic status on the academic achievement of primary school pupils. All the pupils studying at primary school level in Ife East local government area, Ile – Ife, Osun state (Nigeria) constituted the population of the study. The study was delimited to only three government primary schools and three private primary schools in Ife East local government area. The study was further delimited to the pupils of final year class (i.e. primary five or six). In order to have adequate representation of the population, 150 primary school pupils were selected through simple random sampling technique. The study was survey type and therefore a self-developed structured questionnaire was used for the collection of data. Data was collected through personal visits. The data was organized, tabulated and analyzed statistically using T-test. Therefore, the researcher concluded that parental socio-economic status; parent’s educational level, mother’s occupational level; and parental income level affect the academic achievement of primary school pupils. Based on the findings, it was suggested that unemployment should be controlled. Poor pupils should be provided scholarships, free books and other stationary. In addition, it was also recommended that government should take steps to raise socioeconomic status of people.
International Journal of Education, Psychology and Counseling
Zeinab Zaremohzzabieh
All students, regardless of socioeconomic status, deserve equitable access to universities. However, many high-achieving students are excluded from this privilege, most of whom come disproportionately from families on the lower rungs of the socioeconomic ladder. This study aims to identify the barriers that influence high achievers from disadvantaged socioeconomic backgrounds to pursue higher education. A total of ten relevant articles from prominent publication databases were chosen for this review. The results show that the majority of researchers believe that parents' negative attitudes, parents' low expectations, first-in-family, vogue career identity, financial factor, and thinking style factor are the primary impediments to high achievers from disadvantaged socioeconomic backgrounds attending university. The results also enable university career counsellors to assist high achievers from disadvantaged socioeconomic backgrounds to overcome any obstacles to effectively jo...
RELATED PAPERS
I2 Innovación e Investigación en Arquitectura y Territorio
Gaizka Altuna Charterina
Revue De Stomatologie Et De Chirurgie Maxillo-faciale - REV STOMATOL CHIR MAXILLO-FAC
Mireille Nadège koffi
Ana Hanisch
British Journal of Haematology
Graham Russell
Rosana Guber
2020 8th International Conference on Information Technology and Multimedia (ICIMU)
Salman Yussof
Rem: Revista Escola de Minas
DEBORA VASCONCELOS DE OLIVEIRA
B98. CONTROL OF VENTILATION: WAKEFULNESS TO SLEEP, ANIMALS TO HUMANS
Jean-paul Marie
Open Journal of Emergency Medicine
Hubertus H Kohle
Magnetic Resonance in Medicine
Thanh Diem Nguyen
Cuadernos de Herpetología
Juan Carlos Troiano
European Journal of Cancer
Nestor Cardoso
Sandeep Raha
The New England journal of medicine
E. Punnoose
EFSA Journal
Maria Rosaria Milana
Optics Letters
István Jánossy
Mihaela Mrazović
federica ferrigno
Anais da V Jornada Acadêmica Internacional da Bioquímica
Danielle Furquim de Almeida
Social Sciences and Missions
Michelle Renshaw
Donna Ginther
Journal of Biological Chemistry
Carolyn Mountford
bioRxiv (Cold Spring Harbor Laboratory)
Tirza Doniger
Asian Academy of Management Journal
See More Documents Like This
RELATED TOPICS
- We're Hiring!
- Help Center
- Find new research papers in:
- Health Sciences
- Earth Sciences
- Cognitive Science
- Mathematics
- Computer Science
- Academia ©2024

An official website of the United States government
The .gov means it’s official. Federal government websites often end in .gov or .mil. Before sharing sensitive information, make sure you’re on a federal government site.
The site is secure. The https:// ensures that you are connecting to the official website and that any information you provide is encrypted and transmitted securely.
- Publications
- Account settings
Preview improvements coming to the PMC website in October 2024. Learn More or Try it out now .
- Advanced Search
- Journal List
- Springer Nature - PMC COVID-19 Collection

Socio-economic factors affecting the academic performance of private university students in Bangladesh: a cross-sectional bivariate and multivariate analysis
Sumaia rahman.
1 Department of Computer Science and Engineering (CSE), Varendra University, Rajshahi, Bangladesh
Al Muktadir Munam
Ahammad hossain, a. s. m. delwar hossain, rejvi ahmed bhuiya.
2 Department of Crop Science and Technology, University of Rajshahi, Rajshahi, Bangladesh
Associated Data
The corresponding author will provide the datasets which were collected from the respondent and analyzed as part of this study upon reasonable request.
Education is considered the most significant factor in producing human resource developments in terms of social, cultural, technological, economic, and overall national perspectives. Higher education is influenced by several factors that affect the quality of students’ education and outcomes. Most students in Bangladesh study at private universities from various socioeconomic conditions. Some of the factors have restricted their ability to attain higher education and as a result, impacted their academic performance. The goal of this study was to explore those socioeconomic factors affecting the academic performance of private university students in Bangladesh. The primary dataset was collected through an online survey from three private universities in Bangladesh named Varendra University (VU), Daffodil International University (DIU), and City University (CU). The Chi-square tests indicate that the age, gender, studying on a subject of their own choice, getting the right direction to make studying comfortable, consulting with the teacher about learning, family status, etc. variables significantly impacted their cumulative grade point average (CGPA). Relationships with parents and the opportunity to share opinions with parents were also significantly associated with their results. According to the multinomial logistic regression model age, gender, employment status, choice of own study field, getting the right direction, previous academic result, consultation with teachers, father's annual income, family status, and relationship with parents are found to be statistically significant determinants of academic performance. Considering the reality and outcomes of this study, to improve academic performance, parental involvement needs to be increased, and they must provide financial and material support to their offspring for their academic success. Moreover, for achieving a satisfactory CGPA, the students need to build friendly relationships with their parents and have regular consultations with their teachers as well.
Introduction
Bangladesh was a country of poverty, famine, drought, low income, and poorly educated people. Despite so many problems, the people of Bangladesh have begun to make progress in various fields, including education, medicine, and economics. At present, as the poverty rate is decreasing in Bangladesh, on the other hand, the literacy rate is increasing (World Bank 2018 ). Bangladesh's higher education sector is one of the fastest-growing private sectors (Tasnima 2008 ). In addition to government educational institutions, many private educational institutions, such as schools, colleges, and universities, have been established. Private universities have proved to be innovative and effective institutions in Bangladesh, just like readymade garments industries and microfinance institutions (Shamsul Haque 2014 ). To consolidate the growth already achieved, everybody involved needs to work together as this subsector has an important role to play in developing a long-term manpower plan for the critical skills required for the economy in Bangladesh (Alam et al. 2007 ). At present various factors affected the academic performance of students and these include students' behavior, socioeconomic conditions, institutional characteristics, family background, and other demographic factors (Amin et al. 2010 ; El Ansari and Stock 2010 ; Imran 2017 ).
The term socio-economic condition/factors generally encompasses two variables: social and economic conditions. The word “Socioeconomic Status” (SES) refers to a person’s relative place in society in terms of family money, political power, educational background, and professional prestige (Parson et al. 2001 ; Hossain et al. 2022 ). It is a consolidated proportion of an individual or family’s monetary and social position compared with others in light of pay, instruction, and occupation (Kessler et al. 2005 ; Saifi et al. 2011 ). Social class, economic status, unemployment, ethnicity, homelessness, detainees, and refugees were also covered by this list (Hossain et al. 2017a , b ; Wales et al. 2018 ; Turner & Flemming 2019 ).
In 2001, research was attempted to determine the association between socioeconomic status and students’ academic performance, and discovered that it has a very significant impact on their achievement (Mirza et al. 2001 ). A parent's money or the social position has a positive impact on a student's exam result (Considine & Zappalà 2002 ). The measurement of students' previous educational outcomes is the most important indicator of students’ future achievement, this means that the student’s academic success in future endeavors will be better if they have a better previous appearance (Durden and Ellis 1995 ). The background of a student’s family and socioeconomic level is important both within and outside of the classroom (Mirza et al. 2001 ). The socioeconomic status of a family is determined by family income, parental education, parental occupation, and social status in the community, which includes community contacts, group affiliation, and the community’s perception of the family (Chen et al. 2018 ).
In different studies, self-efficacy plays a significant role in academic achievement by increasing motivation to succeed as well (Schunk 1991 , 1994 ; Bandura 1997 ; Pietsch et al. 2003 ). It is often regarded as one of the most important non-intellectual determinants of accomplishment in academic contexts. The relationship between self-efficacy and academic performance has regularly been found to be positive in meta-analyses (Multon et al. 1991 ; Honicke and Broadbent 2016 ). Motivation has been well documented in the literature as one of the key aspects of students’ academic performance (Pintrich 2000 ; Pintrich and Schunk 1996 ; Garcia 1995 ; Bandura 1986 ). Researchers have focused on individual student characteristics including motivational orientations that affect students’ chances of persisting (Girelli et al. 2018 ; Dresel and Grassinger 2013 ; Suhre et al. 2013 ). It is challenging and rewarding to develop and implement programs that move students from probation to good standing and finally to graduation (Heisserer and Parette 2002 ). Lacking motivation in a particular class, students frequently miss class and their grades plummet (Brewer and Burgess 2005 ). Students may suffer from poor academic performance as a result of improper course selection or wrong advice (Babad 2001 ). There may be a chance of making a mistake regarding course selection if personal motivations, interests, and objectives do not match those of friends, family, or faculty (Ahmed et al. 2015 ).
Due to family problems, a student may lose focus on studies and have limited motivation and determination to succeed (Simon and Tovar 2004 ). In addition to simple family problems, severe family problems like illness, death, or a break-up between family members can contribute to a more significant adverse effect. A student’s family problems will likely hinder their ability to concentrate and study, resulting in poor academic performance and eventual academic probation (Ahmed et al. 2015 ). However, mental health problems may affect a student’s ability to concentrate and motivate them to study (Olson 1990 ; Lucas 1991 ; Trombley 2000 ). Three types of interventions can have a positive effect on students’ GPAs and these are academic skill instruction, advising and counseling programs, and comprehensive support programs (Pascarella 2006 ).
In addition, effective communication with the teacher in the classroom and the office is crucial to a student’s understanding of confusing topics and grey areas. Students’ poor communication skills appear to affect their ability to perform well in class (Chowdhury 2001 ; Farooqui 2007 ). Generally, a student’s performance in the past, or his or her educational background, tends to influence his or her performance in the future (Ahmed et al. 2015 ). This paper aims to find out the socio-economic factors that affect the academic performance of private university students in Bangladesh. For that reason, in this paper, bivariate and multivariate analyses are carried out to determine the significant factors that affect academic performances. The association between current university CGPA and the socio-economic factors like students’ age, gender, extroversion/introversion status, social media activation status, fathers’ occupation, mothers’ occupation, parents’ income, relationships with parents’, opinions shared with parents, family status and so on are checked using Chi-square test and multinomial logistic regression analysis.
Objectives of the research
This study aims to investigate the socio-economic factors which are hindering the academic performance of private university students in Bangladesh.
Research Questions: This study is conducted to answer the following questions:
- Which socioeconomic factors affect the academic performance of private university students?
- Which socioeconomic factors are significantly and non-significantly associated with academic performance?
Research methodology
Study design.
The current study was a cross-sectional design to investigate the socioeconomic factors that affect the academic performance of private university students. As a community–based countrywide sample survey was not feasible during the COVID–19 pandemic’s post–wave, data were collected online between January and July 2021. The information was widely shared through social media. The questionnaire included several items, including the context of the study, the purpose, the procedures, the confidentiality agreement, and consent. The survey took approximately 6–7 min to complete. To confirm the validity and reliability of the questionnaire, 50 samples were used in a pilot test before data collection, and these data were not included in the final study.
The Cochran technique was used to discover an ideal sample size given the probability of picking a choice, desired confidence level, and the allowed percentage of error in the population. It is particularly effective in circumstances involving large populations and so the following equation was used to compute the sample size (Kamruzzaman et al. 2021 ; Borg et al. 2022 ; Hossain and Munam 2022 ).
Here, n = number of samples, z = 1.96 (95% confidence level), p = the probability of picking a choice = 50% or 0.5 (for highly dispersed populations), q = (1 − p), d = allowed error percentage = 3.272% or 0.03272.
According to the calculation, a sample size of 897 was determined. An online questionnaire survey was conducted with approximately 2300 students from three private universities in Bangladesh. After removing incomplete, biased, and ineligible data from the responses submitted, 897 were reviewed. They were studying in different departments and in different semesters. The respondents were 622 male students (69.3%) and 275 female students (30.7%).
A total of 51 questions were asked in three categories throughout the entire questionnaire. The three categories are—personal information (17 questions/33.33%), family information (14 questions/27.45%), and academic information of the students (20 questions/39.21%). Data were collected from the three private university students in Bangladesh, e.g., Varendra University (VU), Daffodil International University (DIU), and City University (CU). Of 897 participants of the online survey, 637 (71.0%) were from VU, which is located in the Rajshahi division, 184 (20.5%) were from DIU and 76 (8.5%) were from CU, both located in Dhaka, the capital of Bangladesh.
Data analyses
A cross-sectional bivariate and multivariate analysis were employed to check the significant factors affecting the academic performance of private university students in Bangladesh. At 1, 5, 10, and 15% levels of significance, the Chi-square test was employed to evaluate the bivariate relationship (Kamruzzaman et al. 2021 ; Sarwar et al. 2019 ; Hossain and Munam 2022 ). The linear regression analysis has recently been examined in a variety of sectors, including public health, economics, finance, business, environmental science, social sciences, and others (Hossain et al. 2012 , 2015a , b , c , 2016 ; Rahman et al. 2016 ; Hossain et al. 2017a , b ; Tasnim et al. 2019 ). Multinomial logistic regression (MLR) methods were used in this study due to the categorical character of the dependent variables (Hossain et al. 2019 ; Kamruzzaman et al. 2021 ; Hossain and Munam 2022 ). For MLR analysis, current university CGPA was considered as the dependent variable, and other variables like social, academic, demographic, and economic variables were considered as the factors. MLR models were estimated to compute odds ratios (OR) with 95% confidence intervals to determine the influencing factors associated with academic achievement among students. A two-sided level was used to determine statistical significance, with p < 0.01, p < 0.05, and p < 0.10 being judged significant at the 1, 5, and 10% levels, respectively Table Table1 1 .
Category level of letter grade (Current university CGPA)
IBM SPSS version 25 was used to perform different types of statistical analyses, including frequency distributions, bivariate analyses, Chi-square tests, and multivariate analyses. Chi-Square tests were used to analyze the bivariate association between current university CGPA and socioeconomic factors. Current university CGPA and socioeconomic factors were also analyzed using multinomial logistic regression. In multivariate logistic regression, current university CGPA is considered as the dependent variable and socioeconomic factors are considered as the explanatory variables. To analyze the data, the following category level of dependent variable was used:
Bivariate association between current CGPA and its associated attributes
Table Table2 2 provides the students’ personal information related attributes: age, gender, self-employment, extrovert or introvert, everyday routine following, studying on the subject of their own choice, getting the right direction to make study comfortable, spending hours on social media every day, content reading on social media, and their association with the current CGPA. Usually, students between the ages of greater than and equal to 23 years (88.8%) had a satisfactory performance than those who were 19 to 22 years old (84.7%). Among the male students, 84.4% and among the female students, 87.6% had satisfactory academic performance. Academically 83% of self-employed students and 86% of non-self-employed students performed satisfactorily. Among the extrovert students, 85.4% and among the introverted students, again 85.4% did better academically. Students who were following the everyday routine always or frequently among them, 86.7% of students did satisfactory results than those students (84.1%) who were following the everyday routine sometimes or never. Among the students who were studying the subject of their own choice, 13.7% did not achieve satisfactory results. Besides, students who were not studying the subject of their own choice, among them 19.3% students did not achieve satisfactory performance. Among students who were always/sometimes getting the right direction to make their studies comfortable with them, 86.7% of students did satisfactory results, and those who were getting fewer/very fewer or never got the right direction to make their studies comfortable among them 80% of the students outperformed their peers. Of students who had low addiction to social media, 86% of the students did a satisfactory academic performance to those who had medium addiction among them, 83.5% of students did satisfactory results to those who were highly addicted; among them, 85.2% of students did satisfactory results. Students who read entertainment content performed well 85.2%, students who read news content did well 84.4%, and students who read study content, technical content, and other content did well 86%.
The cross table of students’ personal information related attributes with χ2 test statistics and p -value
The bivariate analysis shows that students’ age was significantly associated with their academic performance ( p = 0.053). Students’ gender was significantly associated with their academic performance ( p = 0.005). Studying the subject of their own choice is associated with the academic performance of the students of private universities at a level of 12% significance. Getting the right direction to make the study comfortable was associated with the academic performance ( p = 0.020) of the students at a private university. It was found that academic performance was not significantly associated with the participants’ self-employment status, their introverted or extroverted character, everyday routine following, spending hours on social media, and content reading on social media.
Table Table3 3 provides the students’ family related attributes: father’s occupation, mother’s occupation, father’s annual income, mother’s annual income, family status, relationship with parents, sharing opinions with parents, getting proper educational support from family, and their association with the current CGPA. Students (87.7%) whose fathers did other jobs, like farmers, drivers, actors, and so on, did satisfactorily performance compared to those students whose fathers were businessmen, government employees, and private employees. Of students whose mothers did other jobs like small cottage businesses and so on, 88.9% showed satisfactory academic performance compared to those students whose mothers were government employees, housewives, and private employees. Of students whose father’s annual income was 60,000 BDT to 2,00,000 BDT among them, 86.9% of students did the satisfactory academic performance, whose father’s annual income was 2,00,001 BDT to 5,00,000 BDT among them, 86.3% of students did satisfactorily; and those whose father’s annual income was greater than equal to 5,00,001 BDT, among them, 77.6% of students the did satisfactory academic performance. Of students whose mother’s annual income was 10,000 BDT to 1,00,000 BDT, among them, 87% of students had the satisfactory academic performance; for those whose mother’s annual income was 1,00,001 BDT to 2,00,000 BDT, among them, 83.3% of students had the satisfactory academic performance; and those whose mother’s annual income was greater than equal to 2,00,001 BDT, among them, 85.6% of students had a satisfactory academic performance. Of students who lived in a joint family among them, 88.7% students did satisfactory results, and those who lived in a single-family or nuclear family among them, 84.1% of students did satisfactory results. Of students who had a very friendly relationship with their parents among them 86.7% students did satisfactory results, for those who had a friendly relationship with their parents among them 85.8% students did satisfactorily, and who had a less friendly relationship with their parents among them 79.8% students did a satisfactory academic performance. Of students who always or frequently shared their opinions with their parents among them 86.1% students did satisfactory results and the students who never or sometimes shared their opinions with their parents among them 83.7% did a satisfactory academic performance. Students who were always or frequently getting proper educational support from family among them 85.3% students did satisfactory results and who were sometimes/never getting proper educational support among them 86% students did well. The bivariate analysis shows that students’ family status was associated with their academic performance at the significant level of 12%. Students’ relationship with their parents was significantly associated with their academic performance ( p = 0.027). Besides, sharing opinions with their parents was significantly associated with their academic performance ( p = 0.099). It was found that participants’ academic performance was not significantly associated with their father’s occupation, mother’s occupation, father’s annual income, mother’s annual income, and getting proper educational support from the family.
The cross table of students’ family related attributes with χ2 test statistics and p -value
Table Table4 4 provides the students’ academic information related attributes: SSC result, HSC result, counseling needed before university admission, a consultation with the teacher about learning, attending class regularly at school/college, enjoying the class or not, and their association with current CGPA. The study found that the students who did well (achieved A + , A, and A − grades) in the SSC exam, among whom 85.7% did a satisfactory academic performance at university, and among those who did not do well (achieved B and C grades) in the SSC exam, among whom 78.8% did a satisfactory academic performance at university. With that, the participants who did well (achieved A + , A, and A − grades) in the HSC exam, among whom 86.6% had a satisfactory academic performance at university, and the participants who did not do well (achieved B and C grades) in the HSC exam, among whom 82.8% had a satisfactory academic performance at university. The participants who thought that counseling is needed before university admission, among whom 84.4% of students, did satisfactorily than those who thought that counseling is not needed before university admission, among whom 86.6% of students did satisfactory results academically. Of the participants who consulted with the teacher about their learning, 91.3% of participants achieved satisfactory results compared to those who sometimes or never consulted with the teacher about their learning. Furthermore, students, who always or frequently attended class at school or college, among them 85.5% students did satisfactory results compared to those who occasionally or never attended class at school or college. Among students who enjoyed their class found that 85.7% of students did satisfactory results, and those who did not enjoy the class among them found 83.7% of students did a satisfactory academic performance at university. The bivariate analysis shows that students’ academic performance was significantly associated with their HSC results ( p < 0.01). Students’ academic performance was associated with the consultation with their teacher about learning at a significance level of 14%. Besides, students’ academic performance was associated with their regular class attendance at school or college at a significant level of 15%. Students’ academic performance was associated with their class enjoyment at a significance level of 15%. It was found that participants’ academic performance was not associated with their SSC results and counseling was needed before their university admission.
The cross table of students’ academic information related attributes with χ2 test statistics and p-value
Multivariate association between current CGPA and socio-economic factors
Table Table5 5 presents a summary of the multinomial logistic regression of the university’s current CGPA and its associated factors. Private university students who studied a subject of their choice performed better academically than students who did not study a subject of their choice. Students who always or sometimes got the right direction to make their studies more comfortable were more likely to have better academic performance than those students who got fewer or very few right directions to make their studies comfortable. Besides, students who consulted with their teacher about learning were more likely to have better academic performance than those who consulted with their teacher occasionally. Students who always or frequently attended classes regularly while in school or college had a greater likelihood of having better academic performance than those who sometimes or never attended classes at school or college. Participants who enjoyed their classes had better academic results than those who did not. Students whose father’s annual income was 60,000 BDT to 2,00,000 BDT and 2,00,001 BDT to 5,00,000 BDT, their children’s academic performance was more likely better than those students whose father’s annual income was greater than equal to 5,00,001 BDT. Students who had a friendly or very friendly relationship with their parents had better academic performance than those who had a less friendly relationship with their parents. As well, participants who always or frequently shared their opinions with their parents had better academic results than those who sometimes or never shared their opinions with their parents. Age, gender, self-employment status, studying on a subject of their choice, getting the right direction to make study comfortable, HSC or equivalent result, counseling with the teacher about learning, father’s annual income, family status, and relationship with parents are all statistically significant determinants of academic performance. Whereas regular class attendance at school or college, enjoying the class and discussing ideas with parents are not significant predictors of academic performance, details are given in Table Table5 5 .
Multinomial logistic regression analysis of university current CGPA and its associated factors
OR***, OR**, and OR* indicate the significant level at 1, 5, and 10% respectively
From Table Table6, 6 , some common significant factors from the bivariate and multinomial analysis that affect the academic grades of private university students at the 1, 5, 10, and 15% level of significance were found. The common factors include age, gender, subject of own choice, getting the right direction, and relationship with parents. Some non-significant factors were identified through bivariate and multinomial analysis, contrary to initial expectations. From bivariate analysis, self-employment status, extrovert-introvert characteristics, the habit of following a daily routine, spending time on social media, reading study content on social media, parents’ occupation, getting proper educational support, SSC result, and counseling before getting admitted into university were found to be non-significant. Moreover, attending regular classes at school and colleges, enjoyment of the class, and sharing opinions with parents were found to be non-significant in terms of excellent satisfactory results from multinomial regression analysis.
Significant factors from bivariate and multinomial regression analysis
The primary objective of this study was to investigate and find the socio-economic factors which are responsible for the academic performance of private university students in Bangladesh. We have investigated and found some statistically significant factors and some non-significant factors as well which were found significant in previous studies.
According to a study in 2016, senior students (30–39 years) did better academic results than the middle (23–25 years) and young age (19–21 years) students (Christopher et al. 2016 ). In our study, students between the ages of greater than and equal to 23 years had better academic performance ( OR = 0.607, p = 0.094) than those who were 19–22 years old. But yet this information contradicts certain other research that shows that young students perform better than older students (Lane and Porch 2002 ; Diaz 2003 ). Khan & Golder also found that age was not significantly associated with academic performance (Khan and Golder 2021 ).
Female students have been proven to outperform male students whereas Christopher found that the relationship between gender and the students’ performance was not statistically significant (Cullen et al 2004 ; Garkaz et al 2011 ; Christopher et al. 2016 ). In this study, the academic performance of female students ( OR = 0.501, p = 0.009) was comparatively better than that of male students.
Family structure (i.e., nuclear or joint family) and the main source of income or father’s annual income did not significantly predict the academic performance of a student (Considine et al. 2002 ). But according to our study, fathers’ annual income ( p < 0.05) and family status/structure ( p < 0.10) were significantly associated with academic performance. According to some studies, students thought that consultancy with their teacher is necessary and necessary to do better academic performance (Chau et al. 2020 ; Le Thi Quynh and Nguyen Huu 2020 ). Consulting with teachers was found to be essential for demonstrating better academic performance ( p < 0.05).
Students’ topic choice/self-proposal status had little impact on their academic performance (Murphy et al. 2013 ). According to our research, students who were studying the subject of their own choice ( p < 0.05) performed well academically. Parental involvement in a child’s early education has been proven to be consistently connected with a child’s academic performance, as well as socializing their children (Stevenson and Baker 1987 ; Hara and Burke 1998 ; Marcon 1999 ; Hill and Craft 2003 . The involvement of parents was also found to be significantly related to students’ academic performance ( p < 0.01). McKenzie and Schweitzer showed that the most significant predictor of university performance was found to be students’ previous educational results (McKenzie and Schweitzer 2001 ). According to our study, the previous educational result was significantly associated with the student’s academic performance ( p < 0.01).
According to a previous study, regular class attendance has a positive strong relationship with academic performance (Credé et al. 2010 ). But this statement contradicts the results of our research; because according to our research students’ attended classes regularly and also enjoyed the classes but did not perform well academically.
Our results support some previous work, e.g., in Bangladesh, powerless instructive foundation, off-base course determination, and family and individual issues have a positive impact on a student’s falling into scholarly probation which impacts their scholastic execution unfavorably (Ahmed et al. 2015 ). Different studies endeavor to clarify scholarly disappointment starting with the three components that intercede in instruction: guardians/family causal variables, instructors/scholarly causal components, and students/individual causal components (Díaz 2003 ). From this study, it is shown that academic performance was not significantly associated with spending hours on social media. But Chen found that there was a statistical significance in the differences in scholastic grades ( p < 0.001) and educational gratification ( p < 0.001) between excessive and non-excessive internet users (Chen 2012 ). In comparison with excessive users, non-excessive users had better grades and greater learning satisfaction. Additionally, internet users were found to be gloomy ( p < 0.001), physically unwell ( p < 0.001), and lonely ( p < 0.001). In some ways, our research work is unique. Bayer ( 2012 ) only worked with student-related attributes, semester-related attributes, and social-behavioral data to predict students’ dropouts (Bayer et al. 2012 ; Mafiz et al. 2013 ). And he also found that student performance appears to be correlated with social habits, mainly with the frequency of communication.
But in this study, personal information, family related attributes, and academic information of students were analyzed. In the meantime, some of the studies investigated only the first-year or second-year students, or both. A dropout rate between the first and second years has been identified as one of the most significant indicators of university career quality (Perchinunno et al. 2021 ). But this study examined students from various academic sessions/years and socio-economic backgrounds. However, our study has some limitations. The research was based on data collected from three private universities in Bangladesh, with a limited sample size. It is recommended that future investigations be conducted with a larger population.
The results of this study suggest that a variety of factors, both significant and non-significant, influence the academic performance of private university students in Bangladesh. Socioeconomic variables appear to be particularly important in determining academic success. Additionally, the ability to choose one’s own subjects and having a supportive home environment, including a positive relationship with parents and access to academic support from university staff, were found to be beneficial to student performance. Conversely, regular attendance at prior academic institutions and a lack of guidance or direction were identified as hindrances to academic achievement. University teachers must provide complete academic support to students in areas such as proper course selection, guidance, motivating students to increase their academic participation, and so on. These findings have implications for the improvement of academic performance among private university students in Bangladesh and provide a foundation for future research in the field.
Acknowledgements
It is our sincere pleasure to express our gratitude to the study participants who provided their invaluable opinions.
Not applicable.
Data availability
Declarations.
It is declared that the authors have no conflict of interest.
This study has been approved by Varendra University’s Ethical Review Committee (VU-ERC). During the study, the participants were informed of the objectives and their right to stay in the study or to opt-out of it if they felt uncomfortable. There was a consent process before the study began.
Contributor Information
Sumaia Rahman, Email: moc.liamg@arotnoannono .
Al Muktadir Munam, Email: [email protected] .
Ahammad Hossain, Email: moc.liamg@urtatsdammaha .
A. S. M. Delwar Hossain, Email: [email protected] .
Rejvi Ahmed Bhuiya, Email: db.ca.ur@ivjerza .
- Ahmed JU, Chowdhury MHK, Rahman S, Talukder AMH. Academic probation: an empirical study of private university students. SSRN Electron J. 2015 doi: 10.2139/ssrn.2601259. [ CrossRef ] [ Google Scholar ]
- Alam M, Haque MS, Siddique SF (2007). Private higher education in Bangladesh. IIEP-UNESCO.
- Amin R, Shah NM, Becker S. Socioeconomic factors differentiating maternal and child health-seeking behavior in rural Bangladesh: a cross-sectional analysis. Int J Equity Health. 2010; 9 (1):9. doi: 10.1186/1475-9276-9-9. [ PMC free article ] [ PubMed ] [ CrossRef ] [ Google Scholar ]
- Babad E. Reviewed students course selection: differential considerations for first and last course. Res High Educ. 2001; 42 :469–492. doi: 10.1023/A:1011058926613. [ CrossRef ] [ Google Scholar ]
- Bandura A. Social foundations of thought and action: A social cognitive theory. London: Pearson; 1986. [ Google Scholar ]
- Bandura A. Self-efficacy: the exercise of control. New York: W. W. H. freeman & Company; 1997. [ Google Scholar ]
- Bayer J, Bydžovská H, Géryk J, Obšívač T, Popelínský L (2012). Predicting drop-out from social behaviour of students. In Proceedings of the 5th International Conference on Educational Data Mining.
- Borg DN, Bach AJE, O’Brien JL, Sainani KL. Calculating sample size for reliability studies. PM R. 2022 doi: 10.1002/pmrj.12850. [ PubMed ] [ CrossRef ] [ Google Scholar ]
- Brewer WE, Burgess ND. Professors’ role in motivating students. J Ind Teach Educ. 2005; 42 (3):3. [ Google Scholar ]
- Chau NH. Needs for consultancy of vietnam high school students. Archives Bus Res. 2020 doi: 10.14738/abr.83.7874. [ CrossRef ] [ Google Scholar ]
- Chen R. Institutional characteristics and college student dropout risks: A multilevel event history analysis. Res High Educ. 2012; 53 (5):487–505. doi: 10.1007/s11162-011-9241-4. [ CrossRef ] [ Google Scholar ]
- Chen Q, Kong Y, Gao W, Mo L. Effects of socioeconomic status, parent-child relationship, and learning motivation on reading ability. Front Psychol. 2018; 9 :1297. doi: 10.3389/fpsyg.2018.01297. [ PMC free article ] [ PubMed ] [ CrossRef ] [ Google Scholar ]
- Chowdhury MR. To west or not to west? the question of culture in adopting/adapting CLT in Bangladesh. J Asiat Soc Bangladesh. 2001; 46 :183–194. [ Google Scholar ]
- Christopher MS, Redempta KM. Influence of demographic factors on academic performance among primary teacher trainees-a case study of Machakos teachers college. Int J Educ Stud. 2016; 3 (1):07–11. [ Google Scholar ]
- Considine G, Zappalà G. The influence of social and economic disadvantage in the academic performance of school students in Australia. J Sociol. 2002; 38 (2):129–148. doi: 10.1177/144078302128756543. [ CrossRef ] [ Google Scholar ]
- Credé M, Roch SG, Kieszczynka UM. Class attendance in college: a meta-analytic review of the relationship of class attendance with grades and student characteristics. Rev Educ Res. 2010; 80 (2):272–295. doi: 10.3102/0034654310362998. [ CrossRef ] [ Google Scholar ]
- Cullen MJ, Hardison CM, Sackett PR. Using SAT-grade and ability-job performance relationships to test predictions derived from stereotype threat theory. J Appl Psychol. 2004; 89 (2):220. doi: 10.1037/0021-9010.89.2.220. [ PubMed ] [ CrossRef ] [ Google Scholar ]
- Díaz, A. (2003). Personal, family, and academic factors affecting low achievement in secondary school. Electronic Journal of Research in Educational Psychology and Psycho Pedagogy, 1.
- Dresel M, Grassinger R. Changes in achievement motivation among university freshmen. J Educ Train Stud. 2013 doi: 10.11114/jets.v1i2.147. [ CrossRef ] [ Google Scholar ]
- Durden GC, Ellis LV. The effect of attendance on student learning in principles of economics. Am Econ Rev. 1995; 85 :343–346. [ Google Scholar ]
- El Ansari W, Stock C. Is the health and wellbeing of university students associated with their academic performance? cross sectional findings from the United Kingdom. Int J Environ Res Pub Health. 2010; 7 (2):509–527. doi: 10.3390/ijerph7020509. [ PMC free article ] [ PubMed ] [ CrossRef ] [ Google Scholar ]
- Farooqui S. Developing speaking skills of adult learners in private universities in Bangladesh: problems and solutions. Australian J Adult Learn. 2007; 47 :94–110. [ Google Scholar ]
- Garcia T. The role of motivational strategies in self-regulated learning. New Dir Teach Learn. 1995; 1995 (63):29–42. doi: 10.1002/tl.37219956306. [ CrossRef ] [ Google Scholar ]
- Garkaz M, Banimahd B, Esmaeili H. Factors affecting accounting students’ performance: the case of Students at the slamic azad university. Procedia Soc Behav Sci. 2011; 29 :122–128. doi: 10.1016/j.sbspro.2011.11.216. [ CrossRef ] [ Google Scholar ]
- Girelli L, Alivernini F, Salvatore S, Cozzolino M, Sibilio M, Lucidi F. Affrontare i primi esami: motivazione, supporto all’autonomia e percezione di controllo predicono il rendimento degli studenti universitari del primo anno. ECPS - Educ Cultural Psychol Stud. 2018 doi: 10.7358/ecps-2018-018-gire. [ CrossRef ] [ Google Scholar ]
- Hara SR, Burke DJ. Parent involvement: the key to improved student achievement. School Commun J. 1998; 8 :9–19. [ Google Scholar ]
- Heisserer DL, Parette P. Advising at-risk students in college and university settings. Coll Stud J. 2002; 36 :69–83. [ Google Scholar ]
- Hill NE, Craft SA. Parent-school involvement and school performance: Mediated pathways among socioeconomically comparable African American and Euro-American families. J Educ Psychol. 2003; 95 (1):74–83. doi: 10.1037//0022-0663.95.1.74. [ CrossRef ] [ Google Scholar ]
- Honicke T, Broadbent J. The influence of academic self-efficacy on academic performance: a systematic review. Educ Res Rev. 2016; 17 :63–84. doi: 10.1016/j.edurev.2015.11.002. [ CrossRef ] [ Google Scholar ]
- Hossain A, Karim MS. Prevalence of Depression, Anxiety and Stress: A Case Study among Varendra University Students. Cambridge: Scholars' Press; 2018. [ Google Scholar ]
- Hossain A, Munam AM. Factors influencing facebook addiction among Varendra University students in the lockdown during the COVID-19 outbreak. Comput Hum Behav Reports. 2022 doi: 10.1016/j.chbr.2022.100181. [ CrossRef ] [ Google Scholar ]
- Hossain A, Ali MA, Kamruzzaman M. Volatility analysis and forecasting volume Data of DSE. Cambridge: Academic Publishing; 2015. [ Google Scholar ]
- Hossain A, Kamruzzaman M, Ali MA. ARIMA with GARCH family modeling and projection on share volume of DSE. Econ World. 2015 doi: 10.17265/2328-7144/2015b.0708.003. [ CrossRef ] [ Google Scholar ]
- Hossain A, Kamruzzaman M, Ali MA. Vector autoregressive (VAR) Modeling and Projection of DSE. Chinese Bus Rev. 2015; 14 (6):273–289. [ Google Scholar ]
- Hossain A, Kamruzzaman M, Ali MA. Econometric investigation of DSE’s portfolios through selective micro and macro economic indicators. J Stat Sci Appl. 2016 doi: 10.17265/2328-224x/2016.0506.003. [ CrossRef ] [ Google Scholar ]
- Hossain A, Islam MA, Kamruzzaman M, Khalek MA, Ali MA. Forecasting carbon dioxide emissions in Bangladesh using Box-Jenkins ARIMA models. Int J Stat Sci. 2017; 16 :33–48. [ Google Scholar ]
- Hossain M, Islam M, Biswas B, Hossain M. The impact of students “Socio-economic condition on academic performance in public and national university of Bangladesh” Asian Res J Math. 2017; 7 (3):1–16. doi: 10.9734/arjom/2017/37650. [ CrossRef ] [ Google Scholar ]
- Hossain A, Bhuiya RA, Zulficar Ali M. The association between obesity and depression, anxiety, and stress disorders among university students at Rajshahi city in Bangladesh. J Psychiatry Psychiatric Disorders. 2022 doi: 10.26502/jppd.2572-519x0172. [ CrossRef ] [ Google Scholar ]
- Hossain, A., Ali, M. A., & Ali, A. B. M. S. (2012). Time Varying Volatility Analysis and Forecasting Using ARIMA with Asymmetric GARCH models on Volume Data in Dhaka Stock Exchange. International Conference on Statistical Data Mining for Bioinformatics Health Agriculture and Environment 471–476
- Hossain, A., Mahamud, R., Bishwas, M. R., & Kamruzzaman, M. (2019). Evaluation of Facebook Addiction and its Associated Factors among Varendra University Students: A Cross Sectional Survey Study. 7th Int. Conf. on Data Science & SDG, 677–684
- Imran M, Afshari M, Ghavifekr S, Razak AZA. Demography factors and students academic performance in secondary schools. Mojem Malaysian Online J Educ Manag. 2017; 1 (2):1–9. [ Google Scholar ]
- Kamruzzaman M, Hossain A, Kabir E. Smoker’s characteristics, general health and their perception of smoking in the social environment: a study of smokers in Rajshahi city Bangladesh. J Pub Health. 2021; 30 (6):1–12. doi: 10.1007/s10389-020-01413-w. [ PMC free article ] [ PubMed ] [ CrossRef ] [ Google Scholar ]
- Kessler D, Peters TJ, Lee L, Parr S. Social class and access to specialist palliative care services. Palliat Med. 2005; 19 (2):105–110. doi: 10.1191/0269216305pm980oa. [ PubMed ] [ CrossRef ] [ Google Scholar ]
- Khan AA, Golder P. Factors affecting the academic performance of students: a reality of higher education level in Bangladesh. Int J Trade Commerce-IIARTC. 2021 doi: 10.46333/ijtc/9/2/2. [ CrossRef ] [ Google Scholar ]
- Lane A, Porch M. The impact of background factors on the performance of nonspecialist undergraduate students on accounting modules-a longitudinal study: a research note. Acc Educ. 2002; 11 (1):109–118. doi: 10.1080/09639280210153308. [ CrossRef ] [ Google Scholar ]
- Le Thi Quynh N, Nguyen Huu C. Needs for consultancy of vietnam high school students. Archives Bus Res. 2020; 8 (3):15–22. doi: 10.14738/abr.83.7874. [ CrossRef ] [ Google Scholar ]
- Lucas JS. Evaluation of new probation intervention program at Harper College. Palatine: William Rainey Harper College; 1991. [ Google Scholar ]
- Mafiz AI, Ismail I, Bhuyan MAH. Effects of Socio-economic, demographic and internet exposure factors on school performance among selected students of Nilkhet high school. Bangladesh J Nutr. 2013; 24 :107–120. doi: 10.3329/bjnut.v24i0.14041. [ CrossRef ] [ Google Scholar ]
- Marcon RA. Positive relationships between parent school involvement and public school inner-city preschoolers’ development and academic performance. Sch Psychol Rev. 1999; 28 (3):395–412. doi: 10.1080/02796015.1999.12085973. [ CrossRef ] [ Google Scholar ]
- McKenzie K, Schweitzer R. Who Succeeds at University? Factors predicting academic performance in first year Australian university students. High Educ Res Dev. 2001; 20 (1):21–33. doi: 10.1080/07924360120043621. [ CrossRef ] [ Google Scholar ]
- Mirza M. Relationship of socio-economic stats with achievement. J Element Educ. 2001; 1 (4):18–24. [ Google Scholar ]
- Mozaffor M, Raheem E, Islam MS, Nurunnabi ASM, Hossain MS, Biswas C, Islam S, Arafat SMY. Suicidal behaviors among undergraduate medical students in Bangladesh. Res Develop Med Educ. 2022 doi: 10.34172/rdme.2022.014. [ CrossRef ] [ Google Scholar ]
- Multon KD, Brown SD, Lent RW. Relation of self-efficacy beliefs to academic outcomes: a meta-analytic investigation. J Couns Psychol. 1991; 38 (1):30–38. doi: 10.1037/0022-0167.38.1.30. [ CrossRef ] [ Google Scholar ]
- Murphy MJ, Seneviratne RD, Cochrane L, Davis MH, Mires GJ. Impact of student choice on academic performance: cross-sectional and longitudinal observations of a student cohort. BMC Med Educ. 2013; 13 (1):26. doi: 10.1186/1472-6920-13-26. [ PMC free article ] [ PubMed ] [ CrossRef ] [ Google Scholar ]
- Olson MA. Characteristics of students on academic probation. Commun Junior College. 1990; 14 (4):331–336. doi: 10.1080/0361697900140406. [ CrossRef ] [ Google Scholar ]
- Parson RD, Lewis H, Deborah S. Educational psychology: A practitioner- researcher model of teaching. Boston: Thomson Learning Inc.; 2001. [ Google Scholar ]
- Pascarella ET. How college affects students: Ten directions for future research. J Coll Stud Dev. 2006; 47 (5):508–520. doi: 10.1353/csd.2006.0060. [ CrossRef ] [ Google Scholar ]
- Perchinunno P, Bilancia M, Vitale D. A statistical analysis of factors affecting higher education dropouts. Soc Indic Res. 2021; 156 (2–3):341–362. doi: 10.1007/s11205-019-02249-y. [ CrossRef ] [ Google Scholar ]
- Pietsch J, Walker R, Chapman E. The relationship among self-concept, self-efficacy, and performance in mathematics during secondary school. J Educ Psychol. 2003; 95 (3):589–603. doi: 10.1037/0022-0663.95.3.589. [ CrossRef ] [ Google Scholar ]
- Pintrich RR. Multiple goals, multiple pathways: The role of goal orientations in learning and achievement. J Educ Psychol. 2000; 92 :544–555. doi: 10.1037/0022-0663.92.3.544. [ CrossRef ] [ Google Scholar ]
- Pintrich RR, Schunk DH. Motivation in Education: Theory, Research, and Practice. New Jersey: Prentice Hall; 1996. [ Google Scholar ]
- Rahman ATMS, Kamruzzaman M, Jahan CS, Mazumder QH, Hossain A. Evaluation of spatio-temporal dynamics of water table in NW Bangladesh: an integrated approach of GIS and Statistics. Sustain Water Res Manag. 2016; 2 (3):297–312. doi: 10.1007/s40899-016-0057-4. [ CrossRef ] [ Google Scholar ]
- Saifi S, Mehmood T. Effects of socio-economic status on students achievement. Int J Soc Sci Educ. 2011; 1 (2):119–128. [ Google Scholar ]
- Sarwar, M. N., Hossain, A., & Ali, M. (2019). Impact of Depression, Anxiety and Stress on daily average Sleeping duration: A case study among Varendra University Students. 7th Int. Conf. on Data Science & SDG, Department of Statistics, University of Rajshahi. 285–293
- Schunk D. Self-efficacy and academic motivation. Educ Psychol. 1991; 26 (3):207–231. doi: 10.1207/s15326985ep2603&4_2. [ CrossRef ] [ Google Scholar ]
- Schunk DH. Self-regulation of learning and Performance: Issues and Educational Application. Hillsdale: Lawrence Erlbaum Associates; 1994. [ Google Scholar ]
- Shamsul Haque, M. (2014, May 29). Private universities in Bangladesh: An effective innovation in higher education . The Daily Star. https://www.thedailystar.net/private-universities-in-bangladesh-an-effective-innovation-in-higher-education-26139
- Simon MA, Tovar E. Confirmatory factor analysis of the career factors inventory on a community college sample. J Career Assess. 2004; 12 (3):255–269. doi: 10.1177/1069072703261538. [ CrossRef ] [ Google Scholar ]
- Stevenson DL, Baker DP. The family-school relation and the child’s school performance. Child Dev. 1987; 58 (5):1348–1357. doi: 10.1111/j.1467-8624.1987.tb01463.x. [ PubMed ] [ CrossRef ] [ Google Scholar ]
- Suhre CJM, Jansen EPWA, Torenbeek M. Determinants of timely completion: the impact of bachelor’s degree programme characteristics and student motivation on study progress. High Educ Res Dev. 2013; 32 (3):479–492. doi: 10.1080/07294360.2012.684374. [ CrossRef ] [ Google Scholar ]
- Tasnim, S., Sarkar, P., Hossain, A., & Ali, M. A. (2019). A Classification Approach to Predict Severity of Crime on Boston City Crime Data. 7th Int. Conf. on Data Science & SDG, Department of Statistics, University of Rajshahi. 405–412
- Tasnima T. Determinants of quality in higher education?: Bangladesh perspective through Private Universities. The Hague: Institute of Social Studies; 2008. [ Google Scholar ]
- Trombley CM. Evaluating students on probation and determining intervention strategies: a comparison of probation and good standing students. J College Stud Retent: Res Theory Pract. 2000; 2 (3):239–251. doi: 10.2190/4my9-lb3t-d5y0-apuq. [ CrossRef ] [ Google Scholar ]
- Turner V, Flemming K. Socioeconomic factors affecting access to preferred place of death: a qualitative evidence synthesis. Palliat Med. 2019; 33 (6):607–617. doi: 10.1177/0269216319835146. [ PubMed ] [ CrossRef ] [ Google Scholar ]
- Wales J, Kurahashi AM, Husain A. The interaction of socioeconomic status with place of death: a qualitative analysis of physician experiences. BMC Palliat Care. 2018 doi: 10.1186/s12904-018-0341-1. [ PMC free article ] [ PubMed ] [ CrossRef ] [ Google Scholar ]
- World Bank. (2018). Bangladesh: Reducing poverty and sharing prosperity. Worldbank.Org; World Bank. https://www.worldbank.org/en/results/2018/11/15/bangladesh-reducing-poverty-and-sharing-prosperity
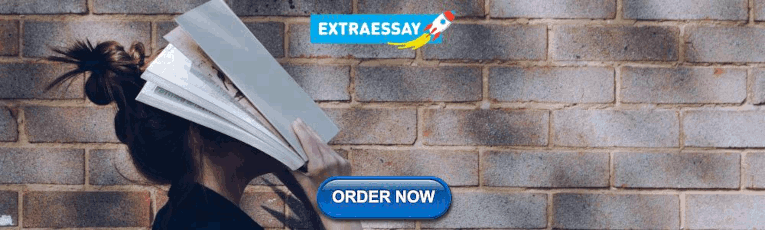
COMMENTS
Chapter 2. A Review of the Literature. on Socioeconomic Status and Educational. Achievement. Abstract The foundations of socioeconomic inequities and the educational. outcomes of efforts to reduce ...
THE EFFECT OF SOCIOECONOMIC STATUS ON ACADEMIC ACHIEVEMENT I have examined the final copy of this thesis for form and content, and recommend that it be accepted in partial fulfillment of the requirement for the degree of Master of Arts with a major in Sociology. _____ Dr. David Wright, Committee Chair We have read this thesis and recommend its ...
Abstract. The foundations of socioeconomic inequities and the educational outcomes of efforts to reduce gaps in socioeconomic status are of great interest to researchers around the world, and narrowing the achievement gap is a common goal for most education systems. This review of the literature focuses on socioeconomic status (SES) and its ...
socioeconomic status - as defined by the combination of the parent/guardian's education, income, and occupation - in their decision to pursue higher education; What perceptions do traditional first generation college students at The University of Alabama have about the role of
The foundations of socioeconomic inequities and the educational outcomes of efforts to reduce gaps in socioeconomic status are of great interest to researchers around the world, and narrowing the achievement gap is a common goal for most education systems. This review of the literature focuses on socioeconomic status (SES) and its related constructs, the association between SES and educational ...
This article reviews the history of the measure of socio-economic status in PISA and identifies theoretical underpinnings of the index of economic, social and cultural status (ESCS). It then highlights multiple changes in the instruments and scaling methods used by PISA over time, and suggests ways of resolving the tensions behind some of these changes and thereby stabilise the measure of ESCS.
Globally, socioeconomic status (SES) is an important determinant of health outcomes. 1-4 Although the relationship between SES and health has been studied extensively, the mechanism by which economic and social status act to influence health is not well understood. In addition to differences in environmental exposures and access
Overall, workers in high-socioeconomic-status categories are much less likely to work in jobs that require proximity to other people compared to their counterparts in low-socioeconomic-status groups. Thus, these data provide further evidence in support of our contention that work context has been the primary cause of differentiated risks by ...
Socioeconomic Status and the Achievement Gap. Gordan and Cui (2016) studied the effects of race on academic achievement in low-. income areas and hypothesized that community poverty has a negative correlation to academic. achievement, black students have a lower rate of academic achievement and the racial gap is.
Table 10.3 presents the meta-analysis results regarding the relationship between socio-economic status and student achievement . The findings support hypothesis H 1 which argues that socio-economic status affects student achievement, and the average standardized effect is at a high level (d = −0.90). This finding shows that students coming from families which have a higher socio-economic ...
In 2010 the World Health. Organization published a report with data supporting the rates of obesity for women of. lower socioeconomic status as higher than men of the same socioeconomic status, with. 48.3% of women being estimated as obese, while 44.2% of men were estimated as obese. (Desilver, 2010).
contribution of socioeconormc status and student perceptions of school effectiveness to academic ach-ievement in engineering students. The variables representing the general factor of socioeconomic status were 1) father's occupation, 2) father's schooling, 3) mother's schooling, 4) family -income, and 5) fam-ily's community population. The ...
similar meaning, such as socioeconomic status and social class. For the research reviewed within this thesis, classism, social class, and socioeconomic status will be non-interchangeable terms as defined by the Classism Attitudinal Profile (CAP) (Colbow, Cannella, Vispoel, Morris, Cederberg, Conrad, Rice, & Liu, 2016), as follows.
The Institutional Repository of Kennesaw State University
This study aims to provide statistical evidence of statistical correlations among these factors and regulations. To achieve this, an online questionnaire with 9 close-ended statements based on 5-Likert scale was distributed to high-school students and school leavers in Thailand. A total of 206 responses were received.
(Unpublished master's thesis), The University of Newcastle, Callaghan, Australia. doi: 10.13140/2.1.2044.9288 ... Purpose This comparative study aims to examine the role of Socio-Economic Status ...
of socio-economic status on academic achievement. To investigate potential mediators and moderators of the socio-economic status-academic achievement relationship. Literature Review An individual's income, education, occupation, and social standing are only some of the factors that make up their Socio-economic status (SES).
Conceptualizing and Measuring Socio-Economic Status (SES) The understanding of students' socio-economic conditions became a major concern for educational researchers when low academic performance at school was observed in students whose parents had low income, low educational level, and were employed at low-status jobs 4 (Cowan et al., 2012).
The term socio-economic condition/factors generally encompasses two variables: social and economic conditions. The word "Socioeconomic Status" (SES) refers to a person's relative place in society in terms of family money, political power, educational background, and professional prestige (Parson et al. 2001; Hossain et al. 2022).