Academia.edu no longer supports Internet Explorer.
To browse Academia.edu and the wider internet faster and more securely, please take a few seconds to upgrade your browser .
Enter the email address you signed up with and we'll email you a reset link.
- We're Hiring!
- Help Center
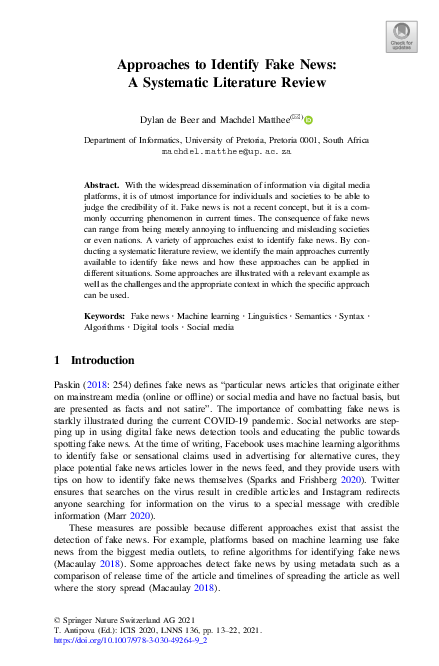
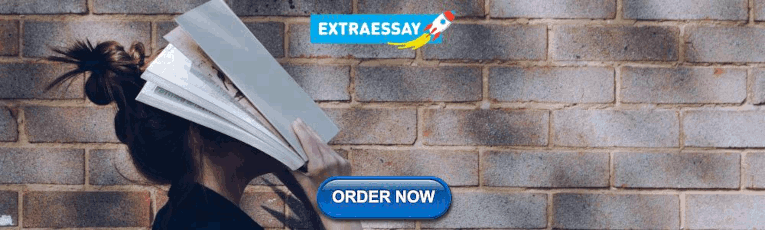
Approaches to Identify Fake News: A Systematic Literature Review

2020, Integrated Science in Digital Age 2020
Related Papers
Mehdi Abbasi
The proliferation of fake news on social media has opened up new directions of research for timely identification and containment of fake news, and mitigation of its widespread impact on public opinion. While much of the earlier research was focused on identification of fake news based on its contents or by exploiting users' engagements with the news on social media, there has been a rising interest in proactive intervention strategies to counter the spread of misinformation and its impact on society. In this survey, we describe the modern-day problem of fake news and, in particular, highlight the technical challenges associated with it. We discuss existing methods and techniques applicable to both identification and mitigation, with a focus on the significant advances in each method and their advantages and limitations. In addition, research has often been limited by the quality of existing datasets and their specific application contexts. To alleviate this problem, we comprehensively compile and summarize characteristic features of available datasets. Furthermore, we outline new directions of research to facilitate future development of effective and interdisciplinary solutions.
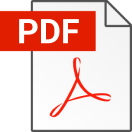
IRJET Journal
False news is the dissemination of false information to grab the audience's attention, which, at first glance, may seem credible. False news is frequently produced by people with personal, political, or economic objectives in mind. It is frequently disseminated online or through conventional media. Fake news can reach dozens and even millions of people via social media, even if it's labeled as fake. In order to lessen the impact of spreading misinformation as well as to eliminate the sources of this false information, all efforts should be made to reduce its impact on citizens. This paper examines and evaluates the many methods for identifying false news.
Álvaro Figueira, PhD
Funding information Nuno Guimaraes thanks the Fundação para a Ciência e Tecnologia (FCT), Portugal for the Ph.D. Grant (SFRH/BD/129708/2017); The work of L. Torgo was undertaken, in part, thanks to funding from the Canada Research Chairs program. Fake news in social media has quickly become one of the most discussed topics in today’s society. With false information proliferating and causing a significant impact in the political, economical, and social domains, research efforts to analyze and automatically identify this type of content have being conducted in the past few years. In this paper, we attempt to summarize the principal findings on the topic of fake news in social media, highlighting the main research path taken and giving a particular focus on the detection of fake news and bot accounts.
Information
Timothy Adeliyi
Sakshini Hangloo
International Journal of Scientific Research in Computer Science, Engineering and Information Technology
International Journal of Scientific Research in Computer Science, Engineering and Information Technology IJSRCSEIT
With increasing popularity in the use of social media for news consumption, the substantial widespread dissemination of fake news has the potential to adversely affect individuals as well as the society as a whole. Even in the midst of the current covid-19 pandemic, false information shared on websites such as WhatsApp, Twitter, and Facebook have the potential to cause panic and shock a large number of people in various parts of the world. These misconceptions obscure healthier habits and encourage incorrect procedures, which aid in the transmission of the virus and, as a result, result in poor physical and psychological health results for individuals. Therefore, it is a research challenge to validate the source, content and publisher of a news article for classifying it as genuine or fake. The existing systems and techniques are not efficient enough to accurately classify a given news based on its statistical rating. Machine learning plays an imperative part in categorizing news data and information, despite some limitations. Our project not only aims on fake news detection but also on generation of real news once the fake news is detected. We propose a user-friendly webpage on which the user enters the news article statement. It is then tested by our machine learning algorithm which then classifies it as genuine or fake, after which the important words are extracted from the statement which helps to get the corresponding genuine news by scraping it from trusted sources and show it to the user. We have compared two machine learning algorithms in this which are- Passive Aggressive Classifier and Naïve Bayes algorithm. We got an accuracy of about 93.5% from Passive Aggressive Classifier and about 83.5% from Naïve Bayes algorithm.
Rhodes University Information Systems Department
Edward de la Rey , Jack Tilbury
Disinformation, misinformation, and fake news have been and are becoming an increasing problem online. The quality of online information is being questioned and the need for validation of data is clear. To overcome this obstacle, where and how information spreads online is examined. Furthermore, detection and mitigation methods that can be employed are discussed. Through this Systematic Literature Review, articles were put through stages of screening to ensure relativity and lastly, they were quality assessed. Once included, data was extracted and results recorded. Three main themes that emerged were the platforms in which we obtain fake news, how fake news spreads online, and the detection and mitigation methods in place. Social networks and search engines are aware of the existence of such content and are reacting accordingly to reduce it. On the other hand, people need to assess information more critically before passing it on. Results indicate that social networks and online information is becoming more and more questioned but detection and mitigation strategies are being adhered to.
IEEE Access
Pitshou Bokoro
Dr Rubaid Ashfaq
In the times of unprecedented epidemic, a lot has been discussed and dictated by a number of experts on varied channels. And unlike any other issue, trend of fake news in this covid19 pandemic is also adding fuel to the fire. Meanwhile, in the hour of crisis, It’s too hard to believe which news sources are authentic and can be believed. However, the researchers of this paper puts in best possible efforts to review fake news problems; its endorsement, mechanism and other distribution vectors. Even though, some of the most scientific solutions are being reviewed and found relevant in advance, leading towards counter-measures positively. Over the years; fake news has evolved out due to multiple factors, such as social media buzz on trending issues, breaking news, and topics in real-time creating a sudden black hole for discussion. We are trying to trace out the measures using reverse engineering of data science to counter machine learning and even deep understanding. We often face mult...
International Journal of Scientific Research in Science, Engineering and Technology
International Journal of Scientific Research in Science, Engineering and Technology IJSRSET
With the growing world in terms of technology and population, the growth of technological use by the population has also increased. The technology has become a part of every human being’s life. It is not just a part of his professional life but also a part of his personal life. There are so many things happening in the world that keeps the world changing. To grow along with this growing world, we need to keep ourselves updated. Media plays an important role in keeping the population updated. The world is kept updated irrespective of the location of the population reading the news and the location of the incident occurring. Fake news is the biggest drawback in this process. We believe what we see and what we read as it the only way to keep ourselves updated. So Fake news hampers the population and may result in unexpected incidents. So it is the need of the hour to understand the difference between real and fake news. This project is for fake news analysis and detection. A dataset of news is considered, pre processing is done and then the fake news and real news are predicted using random forest and xgboost algorithms.
RELATED PAPERS
ANOMOUS RACUN
Geochimica et Cosmochimica Acta
Bruno Kieffer
Hydrology and Earth System Sciences Discussions
Elkamil Tola
MARIA EUGENIA ARRIBAS MOCOROA
Newcode Consultant
Gleison Hidalgo Martins
Nature genetics
Radovan Dvorsky
Magdalena Sobieska
Renato Aguilar
The Journal of neuroscience : the official journal of the Society for Neuroscience
Alfonso Reimúndez Dubra
Journal of Animal and Veterinary Advances
Emese Andrásofszky
Przegląd Prawa Rolnego
Paweł Wojciechowski
Ciência Rural
Paulo Vitor
Damien Féron
Shanthi Rani
Kansas Agricultural Experiment Station Research Reports
Joel Derouchey
Journal of Ethnopharmacology
Tatiana Schoenfelder
IJORER : International Journal of Recent Educational Research
Saidun Hutasuhut
Hundred years of Indian Cinema
Medha Dhapre
Bent Stohlmann
Applied Thermal Engineering
seyfi sevik
MIDAS - Museus e estudos interdisciplinares, 2023
Helena Barranha
Abdul Hapid
Gyankosh: The Journal of Library and Information Management
Jayachristrayar S
See More Documents Like This
RELATED TOPICS
- We're Hiring!
- Help Center
- Find new research papers in:
- Health Sciences
- Earth Sciences
- Cognitive Science
- Mathematics
- Computer Science
- Academia ©2024

An official website of the United States government
The .gov means it’s official. Federal government websites often end in .gov or .mil. Before sharing sensitive information, make sure you’re on a federal government site.
The site is secure. The https:// ensures that you are connecting to the official website and that any information you provide is encrypted and transmitted securely.
- Publications
- Account settings
Preview improvements coming to the PMC website in October 2024. Learn More or Try it out now .
- Advanced Search
- Journal List
- Springer Nature - PMC COVID-19 Collection

A systematic literature review and existing challenges toward fake news detection models
Minal nirav shah.
1 Research Scholar, Department of Computer Science, CSPIT, CHARUSAT, Charotar University of Science and Technology, Changa, Gujarat 388421 India
Amit Ganatra
2 Provost, Parul University, P.O.Limda, Ta.Waghodia, Vadodara, Gujarat 391760 India
Emerging of social media creates inconsistencies in online news, which causes confusion and uncertainty for consumers while making decisions regarding purchases. On the other hand, in existing studies, there is a lack of empirical and systematic examination observed in terms of inconsistency regarding reviews. The spreading of fake news and disinformation on social media platforms has adverse effects on stability and social harmony. Fake news is often emerging and spreading on social media day by day. It results in influencing or annoying and also misleading nations or societies. Several studies aim to recognize fake news from real news on online social media platforms. Accurate and timely detection of fake news prevents the propagation of fake news. This paper aims to conduct a review on fake news detection models that is contributed by a variety of machine learning and deep learning algorithms. The fundamental and well-performing approaches that existed in the past years are reviewed and categorized and described in different datasets. Further, the dataset utilized, simulation platforms, and recorded performance metrics are evaluated as an extended review model. Finally, the survey expedites the research findings and challenges that could have significant implications for the upcoming researchers and professionals to improve the trust worthiness of automated fake news detection models.
Introduction
Fake news identification is one of the eminent research topics, which has been studied in recent years (Sengupta et al. 2021 ). Fake news is often spread by yellow journalism before digital technology with the intention of glorious news like hilarious news, accidents, rumors, and crime news (Islam et al. 2020 ). In the digital era, it is simpler for spreading fake news while a user may distribute fake news to neighbors, their friends, and so on due to the unique characteristics of social media (Habib et al. 2019 ). Thus, fake news can be propagated in a cycle format because of the vast usage of social media by every individual (Singh and Sharma 2021 ). Moreover, comments on fake news can be varied every time that reducing the reliability of real news, the fake news has directly spread in a faster way while comparing to real news (Yang et al. 2021 ). Fake news can have several ranges of impacts by misleading or influencing governments or whole populations (Kim and Ko November 2021 ). Some of the techniques in detecting fake news utilize several approaches like machine learning techniques, language techniques, and knowledge-based techniques (Vereshchaka et al. 2020 ). Some of the strong social media platforms are Twitter, YouTube, Instagram, Facebook, and WhatsApp have offered news and entertainment with the rising employment of mobile devices and simpler WiFi connections (Ribeiro Bezerra 2021 ). Social media and emerging technologies have several profiles for propagating fake news (Sharma 2021 ). Every technology has its extremities and limitations due to the positive effects technology on society and social media (Mridha et al. 2021 ). Moreover, the recent literature analyzes the several advantages of fake new detection.
Nowadays, online fake news has become the main aspect of the growing interest in online media, social-networking sites, and online news portals (Bondielli and Marcelloni 2019 ). However, most people are generally incompetent for spending adequate time cross-checking the references and for ensuring the credibility of news (Zhou and Zafarani 2020 ; D’Ulizia 2021 ). Thus, more attention to fake news detection inspires the research community. In recent days, more research works regarding fake news detection have been implemented (Rama Krishna et al. 2021 ), though several studies only concentrated on news of specific categories like political or e-commerce reviews. Consequently, they have designed and developed certain features with some standard datasets with their topic of interest. These studies face poor performance in detecting news of another topic and also dataset bias (Beer and Matthee 2020 ). Therefore, it is necessary for studying whether these models are suitable for diverse classes of news propagated in social media through the evaluation of diverse datasets on different models and investigating their efficiency or performances (Ahmad et al. 2020 ). On the other hand, conventional studies on fake news detection techniques are focused on either a limited number of models or a particular category of the dataset (Dabbous et al. 2020a ). Thus, there is a need of reviewing a fake news detection model.
The study on fake news detection needs a huge number of evaluations through machine learning techniques on a broad range of datasets (Kansal 2021 ). New methods should obtain deep knowledge regarding the nature of fake news and the way of spreading it over the world. On the other hand, the recent work contributes in this way to implementing a model through novel approaches, which verify the significance of deep learning approaches for detecting fake news (Meneses Silva et al. 2021 ). Among them, the “Convolutional Neural Network (CNN)” model has been utilized and shown a higher competitive performance while comparing with other existing machine learning models. In addition, “long short-term memory (LSTM)” has utilized for analyzing linguistic features and has shown noteworthy performance (Simko et al. 2021 ). More particularly, different variants of CNN can be suggested for detecting fake news. Although deep learning algorithms offer superior efficiency in getting classification results, they suffer from certain challenges like lack of interpretability, the necessity of large training datasets, and complexity in discovering the optimal hyperparameters for every dataset and problem (Silva et al. 2021 ). Thus, recent advancements in bio-inspired approaches permit the optimization of deep learning constraints, and the necessity of advanced intelligent techniques also increases for solving the problems persisted in existing works (Chauhan and Palivela 2021 ). Consequently, there is a need of studying the recent research works in the field of fake news identification models to assist social media users in getting real news.
The major focus of the study on different fake news detection models is given here.
- To prepare an in-depth survey on fake news detection models by collecting noteworthy information from recent studies along with diverse algorithms utilized for achieving it.
- To present a complete study about a chronological review, their related works and contribution to fake news detection models, research designs, and general findings on fake news detection models.
- To analyze the performance metrics, applications focused, datasets used, simulation platforms utilized, and necessary research gap with the challenges present in existing fake news detection models.
The remaining sections of the paper are given here. Section 2 discusses the literature survey, research designs, and general findings on fake news detection with the chronological review. Section 3 specifies the algorithmic classification, feature extraction techniques, and dataset used in the existing fake news detection models. Section 4 gives the simulation platforms and applications focused on the existing fake news detection models. Section 5 describes the architectural view of general fake news detection models and performance measures used in state-of-the-art fake news detection models. Section 6 shows the consequences of fake news and research challenges and the future scope of fake news detection models. Section 7 concludes the survey.
Literature survey, research designs, and general findings on fake news detection with a chronological review
Related works, existing fake news detection model approaches.
In 2019, Ko et al . ( 2019 ) have used a reverse-tracking approach for defining the possibility of fake news in the articles that were taken from the cognitive system, where the designed model has been termed a Fake News Detection System (FNDS). This model has been tested in two case studies, where the first one was posted on February 9, 2017. This article was given about the "blacklisting of extreme right movie studios and Kim Jong Dae, a member of the National Assembly". The second case study was posted in September 2017. This article was about "North Korea, nuclear test, earthquake, Kim Jeong Eun". This model was faster than the conventional models. In 2019, Barbado et al . ( 2019 ) have implemented a feature framework to detect fake reviews in the consumer electronics area, which consisted of four stages of building a dataset to classify fake reviews in four cities in the consumer electronics area through a scraping approach. Secondly, a feature scheme for proposing fake review detection was suggested with the exploitation of the social perspective. It has also focused on selecting fake reviews for organizing and characterizing the features. The results have shown that the AdaBoost classifier has performed better than other existing classifiers. In 2019, Shu et al . ( 2019 ) have implemented a new FakeNewsTracker for gathering the social context and new pieces to create valuable datasets, and then, useful features were extracted for detecting the fake news through Social Article Fusion model, and then, different machine learning models were built for detecting the fake news. In 2020, Henrique and Ferreira ( 2020 ) have implemented a new fake news detection model for detecting fake news from social media texts in Germanic, Latin, and Slavic languages. The detection was carried out through “support vector machines and random forest”. In 2020, Talwar et al. ( 2020 ) have adopted a mixed method for exploring the sharing behavior of fake news. This model has identified six behavioral manifestations correlated with fake news sharing from qualitative data. The control variables were taken as gender and age. This model had created a positive effect on fake news sharing owing to religiosity and lack of time. This model has suggested that active corrective action was engaged by social media users for sharing fake news. In 2020, Xu et al . ( 2020 ) have characterized that numerous real and fake news was shared by comments, reactions, and shares on Facebook in two ways like content understanding and domain reputation. It has revealed that the web sites of news publishers have exhibited various domain popularity, domain ranking, registration timing, and registration behaviors. Additionally, for a certain amount of time, fake news has disappeared. Further, news has been fed to “latent Dirichlet allocation (LDA) topic modeling” and TF-IDF for fake news detection when discovering the document similarity with the word and term vectors. In 2020, Oliveira et al . ( 2020 ) have implemented a “computational-stylistic analysis based on NLP”. This model has used one-class SVM to detect fake news and applied it to data for reducing the dimensionality reduction approaches like data compaction and latent semantic analysis (LSA). In 2020, Li et al. ( 2020 ) have introduced the MCNN for getting the global semantics and local convolutional features for getting the semantic information from the texts for classifying fake news. The weight of sensitive words (TFW) method was used for computing the robust significance of true or fake labels. Thus, MCNN-TFW has focused on extracting the weight of sensitive words and article representation for each news. This model has achieved higher accuracy than other existing approaches to datasets. In 2020, Vereshchaka et al . ( 2020 ) have solved the issue of predicting fake news by getting the socio-cultural and textual characteristics of fake news features and by analyzing and detecting fake news features. Further, data analytics was investigated for constructing a concordance of phase and word frequency. They have formed binary classifiers for extracting the features through deep learning algorithms like GRU, RNN, and LSTM. In 2020, Kaur et al . ( 2020 ) have implemented a new fake news identification model through a multi-level voting ensemble model including 12 classifiers, where the features were extracted using Hashing-Vectorizer (HV), “Count-Vectorizer (CV), and Term Frequency–Inverse Document Frequency (TF–IDF)” through three datasets. It has predicted the textual or fake content from online social media websites. It has verified the performance in terms of less training time, better efficiency, and the trade-off between accuracy and efficiency. Thus, from the analysis, the best classifier was selected for both higher accuracy and efficiency. In 2021, Shahbazi and Byun ( 2021 ) have implemented an integrated model for different criteria of natural language processing and block chain for applying machine learning approaches for detecting fake news and offered a better prediction on posts and accounts on fake users. They used reinforcement learning approach that was used for this process. This scheme utilized the decentralized block chain framework for offering security, which has offered the method of outlining the digital contents. Lastly, the learning rate of the model was predicted for detection to explore the correlation among contents. In 2021, Mehta et al . ( 2021 ) have focused on the fake news classification model through “Bidirectional Encoder Representations from Transformers termed as BERT”. It has required a nominal pre-processing technique and has utilized two diverse versions of BERT, which has shown considerable improvement in terms of the fake news classification model regarding binary classification measures. The designed model has shown higher reliability in terms of multi-label classification. In 2021, Shishah ( 2021 ) has implemented a new fake news detection model through BERT with a joint learning scheme by integrating the Named Entity Recognition (NER) and Relational Features Classification (RFC). In 2021, Jiang et al . ( 2021 ) have investigated the efficiency of three deep learning models and five machine learning models. The superior performance of the designed model was verified while estimating with other existing approaches. In 2021, Kumari and Ekbal ( 2021 ) have implemented a novel multimodal fake news detection scheme with a suitable fusion of multimodal features, which leveraged the information from images and text and tried for maximizing the correlation among them for efficient multimodal distributed depiction. The performance of the designed model was improved by combining the text with images. The experiments have been conducted for validating the efficiency of the designed model that has attained superior performance to others.
Fake news detection model with existing diverse classifiers
In 2018, Jang et al . ( 2018 ) have studied the problem present in the US presidential election in the year 2016. It has gathered 307,738 tweets with 30 real and 30 fake news stories. Thus, there was a need of examining the evolution patterns, producers of the source, and root content. They have utilized the evolution tree modeling method for examining misinformation, the transmission of news, and management. Finally, based on the diverse evolution patterns, fake and real news have been identified. In 2019, Altunbey and Alatas ( 2020 ) have designed a two-step method to identify the “fake news on social media”, which has several steps like pre-processing, vector conversion, and classification. The pre-processing was carried out for converting the unstructured datasets. Initially, the texts in the dataset including the news were depicted by vectors through the attained Document-term matrix and Term- Frequency (TF) weighting method. Secondly, 23 supervised algorithms, like kernel logistic regression (KLR), IBk, decision tree, bagging, sequential minimal optimization (SMO), J48, attribute selected classifier (ASC), simple cart, ordinal learning model (OLM), Ridor, multilayer perceptron (MLP), weighted instances handler wrapper (WIHW), classification via clustering (CvC), locally weighted learning (LWL), logistic model tree (LMT), randomizable filtered classifier (RFC), CV parameter selection (CVPS), stochastic gradient descent (SGD), ZeroR, decision stump, OneR, JRip, and BayesNet, have been experimented in the dataset for transforming the structured format with the text mining algorithms. In 2019, Jadhav and Thepade ( 2019 ) have implemented a new framework for detecting and classifying fake news messages through Deep Structure Semantic Model (DSSM) and improved RNN classifiers. Initially, the twitter data was pre-processed using tokenization, and then, TF-IDF and CV were used for extracting the features. Further, semantic features and multi-layer projection were performed in DSSM, and then, the data were forwarded to the improved RNN for classifying the fake news. In 2020, Kumar et al . ( 2020 ) have developed a new fake news detection model with the help of deep CNN (FNDNet), which has learned the discriminatory features automatically to classify the fake news by several hidden layers. Further, various features were extracted at every layer for maximizing the accuracy of detection. In 2020, Singh et al . ( 2020 ) have explored Bernoulli’s Naive Bayes Classifier with the help of "Multinomial Naive Bayes with predictors as Boolean variables" for detecting fake news. This model has classified the data into two classes 1 or 0, where 1 stand for unique news articles and fake new is represented by 1. In 2020, Hiriyannaiah et al. ( 2020 ) have offered the adverse effects of fake news in society particularly advancements in the vast usage of social media. Generative adversarial networks (GANs) have offered more efficient results in getting fake news detection in terms of validation accuracy than other existing classifiers. It has learned the complex functions for getting a higher accuracy rate. This model has solved the problem of gradient descent in GANs. It has used SeqGAN, where the REINFORCE algorithm was used in the generator for updating their weights by taking the identification of the discriminator network. In 2020, Umer et al . ( 2020 ) have suggested a novel hybrid neural network architecture by LSTM and CNN for classifying the news articles with stance labels, which has also gathered data from the news articles. Initially, the features were gathered from articles using word2vectors, where the dimensionality reduction was carried out for getting the minimized feature set. Finally, the hybrid CNN-LSTM was utilized for detecting fake news for showing its effectiveness. In 2020, Agarwal et al . ( 2020 ) have suggested a new deep learning system for predicting the nature of an article while using an input. They have pre-processed the texts using “word embedding (GloVe)” for constructing a vector space of words and established a lingual relationship. They have combined CNN and RNN for getting the benchmark outcomes while predicting fake news. Moreover, this model has minimized the overfitting problem by the dropout layer and generated higher accurate values. It was shown that the designed model has attained superior outcomes while evaluated with other algorithms. In 2020, Shrivastava et al. ( 2020 ) have investigated the propagation of fake news and described the dissemination of misinformation between groups with the influence of several misinformations refuting metrics. This model has considered the fake news prediction model from online social networks during COVID-19. They have also completely analyzed the equilibrium and stability, which has also prevented the spreading of fake news. It has also been verified via examination of users in online social networks. Several conditions have been evaluated to demonstrate the performance of social network stability and verified theoretical outcomes by experimental outcomes. In 2020, Mahabub ( 2020 ) has suggested an Ensemble Voting Classifier for designing a new fake news detection model among fake and real tasks. The detection was performed by considering several classifiers. Then, better three machine learning algorithms in “Ensemble Voting Classifier” were used after cross-validation. This model has attained superior results while comparing with existing classifiers while detecting fake messages, fake profiles, etc.; finally, the results have verified the superior sufficiency scores of individual classifiers. In 2020, Choudhary and Arora ( 2020 ) have proposed a solution for detecting and classifying fake news through a linguistic model for getting the properties of content for generating the language-driven features. It has also extracted the readability, sentimental, grammatical, and syntactic features of specific news. This model solves the problems of handcrafted features and time-consumption. Thus, for getting superior results in detecting fake news, a neural-based sequential learning model was applied in terms of accuracy and time. In 2021, Ying et al . ( 2021a ) have designed a new "end-to-end Multi-level Multi-modal Cross-attention Network (MMCN)". The high-quality representations have been generated for image regions and text words, respectively, by pre-trained ResNet and BERT models. They have further fused the feature embeddings of the image regions and text words, respectively, for getting diverse as well as duplicate modalities. A multi-level encoding network was used for getting the higher multi-level semantics for enhancing the depictions regarding posts owing to the diverse layers of transformer architecture. In 2021, Li et al . ( 2021a ) have suggested a new automatic model for returning and adding accurate outcomes for assisting the neural network in getting positive sample cases for enhancing the accuracy of the neural network. Initially, this model gathered data, and then, supervised and unsupervised tasks were trained simultaneously through a semi-supervised deep learning network. In 2021, Sivasankari and Vadivu ( 2021 ) have studied a new detection and identification model for learning discriminative features from Facebook posts, tweets content through social network graphs. It has often confirmed the minimization of their propagation. In 2021, Braşoveanu and Andonie ( 2021 ) have improved fake news detection through semantic features. They have suggested a new semantic fake news detection model with relational features such as facts, entities, or sentiment extracted from text. They have mostly considered short texts with several degrees of truth and shown that utilizing semantic features focused on enhancing the accuracy. In 2021, Setiawan et al. ( 2021 ) have implemented the Hybrid “Support Vector Machine (SVM)” for detecting fake news, where the data were gathered from the standard dataset that has been subjected to a feature extraction phase through TF-IDF. Then, the classification was performed by hybrid SVM. In 2021, Raj and Meel ( 2021 ) have implemented coupled ConvNet architecture with image-CNN and text-CNN modules for detecting fake news. Initially, the input data were pre-processed in both modules and then given to CNN. In addition, coupled ConvNet architecture was suggested by extending the usage of CNN. This model was also suitable for larger datasets. In 2021, Kaliyar et al . ( 2021a ) have designed a BERT-based deep learning technique by integrating several parallel blocks of the single layer of deep CNN including filters and several kernel sizes. Thus, it can handle ambiguity and outperform the performance in terms of accuracy while being evaluated with other existing models. It was also carried out with the powerful capability of capturing long-distance and semantic dependencies in sentences. In 2021, Altunbey and Alatas ( 2021 ) have suggested a new model for detecting fake news through “Adaptive Salp swarm optimization with oscillating strategy inertia weight (ASSO-OSIW) using an oscillating inertia weight and nonlinear decreasing coefficient and Grey Wolf Optimizer (GWO)” techniques for finding superior optimal solutions while evaluating online social media contents. It has been performed through flexible fitness functions for getting a superior performance. In 2021, Qureshi et al . ( 2021 ) have introduced a source-based approach focused on the news propagation community consisting of re-tweeters and posters for detecting fake contents from a twitter-based real-world COVID-19 dataset. It has included several features, where the complex network metrics were explored for identifying the news labels and examined the user profile features. Finally, the results have shown superior performance through CATBoost and RNN in detecting fake news. In 2021, Song et al . ( 2021 ) have implemented a new fake news detection model through a temporal propagation framework along with a graph neural network that has fused the temporal information, content semantics, and topological structures. Finally, this model has attained superior performance while evaluating other existing algorithms. In 2021, Saleh et al . ( 2021 ) have adopted an optimized CNN model for detecting fake news, where the feature extraction from input data was performed by N-gram and TF-IDF. They have used several layers for extracting low-level and high-level features. The parameters in every layer were optimized through grid search and hyperopt optimization algorithms. Finally, the high level of accuracy detected fake news efficiently while evaluating with other approaches. In 2021, Ali et al . ( 2021 ) have investigated the reliability of four diverse deep learning architectures like hybrid CNN-RNN, RNN, CNN, and multilayer perceptron (MLP). In addition, the detector complexity was explored, where the robustness of the learned model can be solved with the training loss and input sequence length. This model has also focused on solving the vulnerabilities of recent fake-news detectors. In 2021, Ni et al . ( 2021 ) have suggested a fake news detection model with multi-view attention networks (MVANs) for examining online social media. This model has included propagation structure attention and text semantic attention that has ensured the superior capturing of information. This model has ensured performance in terms of accuracy. It has also some interpretability in both ways of propagation and text structure. In 2021, Li et al . ( 2021b ) have suggested a new fake news detection model with the help of an autoencoder, which has improved the performance. Further, the internal relationship among features and hidden information was obtained by adding the self-attention layer and bidirectional GRU layers into the autoencoder, and further, they reconstructed the remaining for detecting fake news. The experimentation was conducted on two real-world datasets and showed superior and positive results while estimated with other approaches. In 2021, Verma et al . ( 2021 ) have a two-phase benchmark model for solving the authentication of news on social media. They have used word embedding over linguistic features, where initially data pre-processing was performed and validated the veracity of news content through linguistic features. Secondly, the linguistic features with word embedding were merged and applied to voting classification. Finally, the performance of the designed model was evaluated with other existing approaches that have specified superior efficiency in detecting fake news. In 2021, Ying et al . ( 2021b ) have implemented a new end-to-end multi-modal topic memory network (MTMN) that incorporated the topic memory phase for an explicit characterization of final representation. For multimodal fusion, a new blended attention phase was implemented with the ability to exploit the intra-modal correlation within image regions or sentence words, which has also learned the image regions and inter-modal interrelation among sentence words for enhancing and complementing every feature for multimodal and high-quality representations. Lastly, the designed model has depicted better efficiency than others. In 2021, Han et al . ( 2021 ) have implemented a two-stream network for detecting fake videos on the Face-Forensics + + dataset, which can handle low-quality data. Further, the designed model has divided the input videos. Then, spatial-rich model filters were used for leveraging the extracted noise features in the second stream. In addition, considerable improvement was observed by a suggested model with both stream fusion and segmental fusion. It has obtained more state-of-the-art performance than others. In 2020, Dong et al. ( 2021b ) have designed two-path deep semi-supervised learning with CNN for detecting fake news, in which one path was used for unsupervised learning, whereas another path is supervised learning. Here, the unsupervised learning path can learn a large range of unlabeled data, while the supervised learning path focused on learning the limited number of labeled data. These two paths were fed to CNN that were optimized for whole semi-supervised learning. Further, a shared CNN was constructed for getting the low-level features on both unlabeled and labeled data for feeding them into these two paths. The experimental results have verified the higher efficiency while recognizing the fake news with less labeled data. In 2021, Do et al. ( 2021 ) have implemented a generic model that considered both social context and news content for identifying fake news. Particularly, several aspects of the news content were explored through deep and shallow representations. The deep representations were created through transformer-based systems, while the shallow representations were generated with doc2vec and word2vec models. These representations can separately or jointly address the four significant tasks toxicity detection, sentiment analysis, clickbait detection, and bias detection. Additionally, graph CNN and mean-field layers were exploited for specifying the structural information of news articles. Finally, the correlation among the articles was explored by leveraging the social context information. The efficiency of the designed model has been more verified than others. In 2021, Caravanti et al. ( 2021 ) have implemented a network-based technique through label propagation with positive and unlabeled learning, where the classification is performed by transductive and one-class semi-supervised learning techniques. They have considered languages like Portuguese and English and class balancing for specifying the superior balance among datasets. The performance of the designed model was superior to other algorithms like positive and unlabeled learning, and one-class learning. Thus, superior performance was observed even evaluating with unbalanced datasets. In 2021, Kaliyar et al . ( 2021b ) have modeled a new deep neural network architecture for analyzing the social context and news content for getting superior results in terms of detecting fake news. Further, for getting a latent representation of news articles, a joint matrix-tensor factorization has been utilized, where the comparative analysis has been conducted on three techniques like social context-based, news content-based, and a combination of both. Thus, superior results in terms of higher accuracy were observed than existing approaches. In 2021, Kaliyar et al . ( 2021c ) have suggested a new fake new identification model by considering the existence of echo chambers and the content of the new article in the social network. They have designed an efficient deep learning algorithm with tensor factorization. This model was implemented with several counts of filters across every dropout layer with a dense layer. Deep neural network (DNN) was implemented with optimal hyperparameters for classifying the social content and news content-based information individually. The superior efficiency of the designed model was better than the conventional models while detecting fake news. In 2021, Saad et al . ( 2021 ) have implemented an approach through three diverse models trained and created an ensemble of entire models through an aggregation approach to generate final predictions. It has extracted rich information from text reviews through parallel CNNs and bag-of-n-grams. Here, the non-textual and textual linguistic features were used for detecting fake news. In 2021, Choudhary et al . ( 2021 ) have implemented a deep learning model called BerConvoNet with the help of BERT and CNN, where news text was classified into real or fake news with the lowest error. This model has consisted of two major building blocks: multi-scale feature block and a news embedding block. Finally, the experiments with batch size, kernel size, and article embedding have been performed for ensuring the prediction quality. The experimental analysis of the designed model over existing models has presented a superior performance in terms of several performance measures. In 2021, Samadi et al. ( 2021 ) have designed three diverse classifiers like CNN, MLP, and single-layer perceptron (SLP) along with pre-trained models like RoBERTa, GPT2, BERT, and funnel transformer for getting features from deep contextualized representation. The performance analysis was conducted on three datasets that have shown the efficiency of the designed model while estimating with conventional approaches regarding classification accuracy. In 2021, Meel et al . ( 2021 ) have implemented an intelligent CNN-based semi-supervised scheme through self-ensemble theory for considering the stylometric information and leveraging the “linguistic information of annotated news articles”, where the hidden patterns in unlabeled data were explored. This model has achieved the highest classification accuracy in terms of fake news recognition. It has also aimed to save cost, labor, and time and also solve inconsistencies derived during the data annotation procedure. In 2021, Esther et al . ( 2021 ) have implemented the “Attention-based Convolutional Bidirectional Long Short-Term Memory (AC-BiLSTM)” approach to detect fake news and classify them into six classes. The input data were gathered from the standard dataset, which was further given to the AC-BiLSTM for classifying the fake news through several layers. This model has tackled the fake news detection challenges in a multi-class environment that has improved the accuracy of fake news detection. In 2021, Scott et al. ( 2021 ) have presented a “Cross-stitch based Semi-supervised End-to-end neural Attention Network (Cross-SEAN)” model for leveraging the huge range of unlabeled data, which has generalized the fake news in COVID-19 that has learned from suitable external knowledge.
Fake news detection model based on Non-English existing approaches
In 2020, Silva et al . ( 2020 ) have offered a novel fake news detection model for real and fake news in Portuguese with a detailed analysis of machine learning approaches. It has manually constructed reference corpus with fake and true news. Several approaches have been used for evaluating the performance of diverse classes of features like distributed, distributive and linguistic-based features with text representations. The ensemble learning model with SVM, RF, Bagging, and AdaBoost has been utilized for evaluating the performance. In 2021, Zervopoulos et al. ( 2019 ) have detected fake news from tweets that have also focused on predicting patterns in both structures of tweets and linguistic content. A custom filtering process was used through a custom filtering procedure through hash tags co-occurrences. Through the performance analysis, the designed model has improved the performance of conventional deep learning techniques from Hong Kong protests. In 2021, Gokhan et al . ( 2021 ) have used natural language processing approaches for detecting fake news from Turkish-language posts on particular topics on Twitter. Moreover, word embeddings were used for pre-training Turkish language structures, where word2vec and Term Frequency-Inverse Document Frequency (TF-IDF) have given superior performance on fake news detection. The social network analysis has been applied for identifying fake news from Twitter API. In 2021, Mitra et al . ( 2021 ) have presented a new neural network-based approach for detecting fake videos through CNN with a classifier network including Resnet50 and Inception V3. To classify video, the features extracted from convolutional neural network (CNN) classifier were fed to the subsequent classifier. This model has attained lower computational requirements and higher accuracy compared to conventional research works. In 2021, Meesad ( 2021 ) has suggested a new framework for reliable detection of fake news in the Thai language, which has consisted of three major modules. It has also composed of two stages like data collection stage and the building phase of the machine learning model. The web-crawler information retrieval was used for obtaining the data from Thai online news websites in the data collection phase. Then, the data were analyzed for getting suitable features from web data through natural language processing approaches. The detection was performed by LSTM, which was compared to other conventional techniques for detecting fake news.
Fake news detection model with existing diverse analytics approaches
In 2019, Zhang et al . ( 2019 ) have offered a new FakE News Detection (FEND) system. The designed mode was a two-layered method that consisted of identifying the fake events and fake topics. It has grouped the legitimate news into several clusters based on topic, where every cluster can have shared some common topics. Then, the events were extracted from the gathered articles through an event-extraction scheme. Further, they have proposed and implemented a credibility metric to evaluate the authenticity of news by estimating the news authenticity. Then, FEND was focused on detecting fake news by leveraging a huge database with legitimate news. In 2020, Kauffmann et al . ( 2020 ) have designed a methodology for analyzing the reviews automatically that has transformed the positive and negative user opinions into a quantitative score. This model has analyzed the online reviews on Amazon by using sentiment analysis, where the fake reviews have been removed and detected by the designed model from high-tech industries. Based on the consumer sentiments, the rating of brands was performed, which results in getting detailed and appropriate decision-making based on the scores attained.
Fake news detection model with existing diverse ensemble learning approaches
In 2020, Huang and Chen ( 2020 ) have suggested a new fake news detection system by deep learning algorithms. Initially, the pre-processing of news articles has been performed with diverse training models like LIWC, text analysis, grammar analysis, and tokenization words for getting bi-grams and uni-grams. Further, four diverse models like “N-gram CNN, LIWC CNN, depth LSTM, and LSTM” were combined to form an ensemble learning model for detecting fake news. Here, the “Self-Adaptive Harmony Search (SAHS) algorithm was used for optimizing the weights of the ensemble learning” model to get a higher accuracy rate. It has also solved the cross-domain intractability issue by experimenting with it on different domain-oriented datasets. In 2020, Reddy et al . ( 2020 ) have discussed several techniques for detecting fake news by utilizing the features attained from the text of the news without their metadata. They have employed an ensemble learning approach with the integration of text-based vector representations and “stylometric features” for the accurate prediction of fake news. The ensemble learning was designed by considering the classifiers like voting, boosting, and bagging. They have also used the media content in the news articles or no information concerning the users, which was the advantage of the designed model.
Fake news detection model with existing optimization algorithms
In 2021, Sheikhi ( 2021 ) has implemented a new fake news detection model through content-based features and optimized the “Extreme Gradient Boosting Tree (xgbTree) algorithm by the Whale Optimization Algorithm (WOA)”. Initially, the data were collected from the ISOT Fake News dataset and extracted the content-based features for choosing significant features. Further, the extracted features were fed to the WOA-Xgbtree algorithm for classifying the fake news from real news. Finally, the classification outcomes have revealed that the designed model has attained superior performance while evaluated with existing algorithms.
Detecting fake news during the COVID-19 pandemic based on existing approaches
In 2020, Wang et al. ( 2022 ) have revealed the components to determine the acceptance of fake news rebuttals on Sina Weibo. Here, the ELM has been used to analyze the central route, rebuttal acceptance, and peripheral route. The results have recommended the negative and positive effects of the given components. In 2020, Zheng et al. ( 2022 ) have analyzed the Internet users' responses to health-related online fake news (HOFN) for the duration of the coronavirus (COVID-19) pandemic (Nistor and Zadobrischi 2022 ) with the help of the protective action decision model (PADM). The data were investigated using a multi-level linear model. In 2020, Gupta et al. ( 2022 ) have investigated the diverse news across the world. The datasets have been acquired from Twitter based on keywords provided by the Web crawler. Then, the investigation regarding the re-creation of the datasets has been demonstrated by the word clouds through the period of the COVID-19 pandemic (Ncube and Mare 2022 ).
Chronological review
The chronological review generally shows that the information concerned with the count of contributions till now carried out in the field of detecting fake news using deep learning and machine learning-based algorithms. The chronological review of the fake news detection model is depicted in Fig. 1 by considering the total contribution over the years. From the graphical representation, 1.5% of the contribution is taken from the year 2018, 9.2% of the research works are taken from 2019, 30.7% of the papers are reviewed from the year 2020 and finally, 58.4% of the works are taken from 2021, respectively. It inspires other researchers for increasing innovative techniques in the next subsequent years.

A chronological review of the existing fake news detection models
Research designs and general findings
There is no common definition for describing fake news. Fake news can be spread over the world, which can be propagated in any field like COVID-19, politics, e-commerce, marketing, and so on. So, there is a need of analyzing fake news to understand the real news in any particular field. However, some of the writers, publishers, and vendors, posting non-authentic online comments or any third-party monitoring online comments will act as real customers and spreads fake news on online social media for increasing product sales. Similarly, a vast number of users on social media can broadcast fake news based on their opinions. In the case of the tourism field, tweets on social media may propagate fake news based on their imagination without spending at a destination (Das et al. 2021 ). It may lead to the loss of genuine consumers due to fake news on online platforms. In general, fake news is considered one of the huge threats to freedom of expression, journalism, and democracy. It also influences the political impacts, from which the fake news generation can be derived due to the comments, reactions, and shares posted on Facebook, WhatsApp, Instagram, and common websites (Brenes Peralta et al. 2021 ; Chang 2021 ). More specifically, fake news detection can be divided into four perspectives like source-based approaches, propagation-based approaches, style-based approaches, and knowledge-based approaches. Finally, recent advancements in “deep learning have been utilized for detecting” fake news from online social media platforms. Deep learning has several features over machine learning approaches, which are superior accuracy, the capability of extracting high-dimensional features, and “lightly dependent on data pre-processing”. Moreover, the recent broader “availability of data and programming schemes has increased the robustness and utilization of deep learning-based algorithms”. Thus, in the past years, various research articles on fake news detection models have been implemented based on deep learning techniques.
Algorithmic classification, feature extraction techniques, and dataset used in the existing fake news detection models
In this section, the datasets utilized in existing studies for evaluating the performance of their model are listed in Table Table1. 1 . They have utilized benchmark datasets for both training and testing. The major problem in detecting fake news is the lack of a massive dataset and a labeled benchmark dataset with ground-truth labels. For example, some of the datasets are constructed only with political statements like PolitiFact, LIAR, Weibo, etc. The Twitter dataset consists of social media posts, whereas the FNC-1 dataset is built based on news articles. Moreover, datasets can be varied through size, labels, and modalities. Similarly, most of the studies use self-collected data from either news articles or any social media platforms.
The description of publically available datasets used in conventional fake news detection models
NLP techniques used in fake news detection
Natural language processing is an innovative field in machine learning that has the ability of a computer for learning, analyzing, manipulating, and possibly generate human languages. This process has included several tasks like pre-processing, word embedding, and feature extraction techniques. Several fake news detection models utilize data pre-processing as the initial step, which is used for representing obscure attributes, managing lost words, binarization of attributes, and complicated structures with attributes. In the data pre-processing process, various visualization processes are useful. Data pre-processing helps save space and computational time, which solves the noisy data. Secondly, word vectorizing is involved in the mapping of text or words to a list of vectors. Further, a bag of words and TF-IDF are often utilized in several machine learning frameworks to detect fake news. In recent times, fake news identification models have employed pre-trained word-embedding models like word2vec and GloVe due to their ability to train larger datasets. Some of the NLP approaches and word vector models employed in deep learning-based fake news detection models are reviewed in Table Table2 2 .
Benefits and limitations of the word vector models
While analyzing the huge number of variables, a high range of memory and computational power is necessary. Furthermore, classification techniques induce poor samples and overfitting samples. Stylometric features (Reddy et al. 2020 ) have also been utilized for analyzing social media content. Feature extraction is a procedure of constructing combinations of variables for overcoming the above-mentioned complications when describing the data with accurate precision. Few fake news detection models use social context features (Shu et al. 2019 ) for getting suitable features from the news content. N-gram (Vereshchaka et al. 2020 ; Agarwal et al. 2020 ; Saleh et al. 2021 ; Kaliyar et al. 2021b ) generates words and characters from contents with several n-gram orders. Finally, the N-gram vectors are grouped for getting one feature vector for each information. Linguistic feature extraction (Verma et al. Aug. 2021 ) is used for analyzing the performance of fake news, which has several feature classes like quantity features, user credibility, stylistic features, psycho-linguistic features, and readability index. Word embedding (Kaliyar et al. 2021a ; Choudhary et al. 2021 ; Trueman et al. 2021 ; Kumari and Ekbal December 2021 ) generates the word vectors for the downstream tasks. Though, it is complex for constructing the word vectors from scratch with several words on a large-scale dataset. Thus, this review has depicted several tasks with their features and challenges for helping future research works. The contribution of NLP tasks is depicted in Fig. 2 .

Natural language processing tasks utilized in existing fake news detection models
Algorithmic classification
Machine learning is efficiently used for reviewing the fake news detection model, which is divided into two categories, namely supervised and unsupervised learning. Here, unsupervised learning gets useful feature information from unlabeled data which makes it much easy for getting the training data. Conversely, the detection efficiency of unsupervised learning approaches is often inferior to supervised learning approaches. Supervised learning is dependent on the significant information in labeled data, where classification is the most general process, though labeling of data is often “time consuming and expensive”. Similarly, the lack of sufficient labeled data creates a major challenge to supervised learning. Deep learning is a recent research paradigm often utilized for several identification models because of “recent achievements of these techniques in complex natural language processing tasks”. Similarly, fake news detection can be performed by deep learning algorithms. The common algorithms used for fake news identification models are categorized in Fig. 3 .

Algorithmic classification of the existing fake news detection model
The shallow models are also known as traditional machine learning models that have included several algorithms like supervised learning and unsupervised learning, where unsupervised learning includes k-means (Zhang et al. 2019 ) and supervised learning consists of techniques like evolution tree analysis (Jang et al. July 2018 ), SVM (Faustini and Covões November 2020 ; Kauffmann et al. October 2020 ; Oliveira et al. 2020 ), Hybrid SVM (Setiawan et al. 2021 ), Bernoulli’s naive Bayes ( Singh et al. 2020 ), LDA (Reddy et al. 2020 ), and voting (Verma et al. 2021 ) classifier.
Deep learning model has several deep networks, in which unsupervised learning algorithms are GAN (Srinidhi Hiriyannaiah et al. 2020 ), Autoencoder (Li et al. 2021b ), whereas supervised learning (Li et al. 2021a ; Souza et al. 2021 ) algorithms are AdaBoost (Barbado et al. July 2019 ), WOA-Xgbtree (Sheikhi 2021 ), RNN (Shu et al. 2019 ; Agarwal et al. 2020 ), LSTM (Umer et al. 2020 ; Braşoveanu and Andonie 2021 ; Meesad 2021 ), GRU (Vereshchaka et al. 2020 ), DSSM-RNN (Jadhav and Thepade 2019 ), AC-BiLSTM (Trueman et al. 2021 ), Ensemble (Ozbay and Alatas February 2020 ; Huang and Chen November 2020 ; Silva et al. May 2020 ; Reddy et al. 2020 ; Jiang et al. 2021 ; Javed et al. 2021 ; Kumari and Ekbal December 2021 ), ensemble voting (Mahabub 2020 ; Qureshi et al. 2021 ), multi-level voting ensemble (Kaur et al. 2020 ), CNN (Kaliyar et al. June 2020 ; Umer et al. 2020 ; Agarwal et al. 2020 ; Kaliyar et al. 2021a ; Saleh et al. 2021 ; Huu Do et al. 2021 ; Mitra et al. 2021 ; Samadi et al. 2021 ; Meel and Vishwakarma September 2021 ; Dong et al. Dec. 2020 ), MCNN (Li et al. 2020 ), C-LSTM (Zervopoulos et al. 2019 ), Coupled ConvNet (Raj and Meel 2021 ), BerConvoNet (Choudhary et al. 2021 ), MMCN (Ying et al. 2021a ), MVAN (Ni et al. 2021 ), NN (Choudhary and Arora 2020 ; Jain et al. 2021 ), Graph neural network (Song et al. 2021 ), MTMN (Ying et al. 2021b ), DNN (Ali et al. 2021 ; Kaliyar et al. 2021c ), Lyapunov function (Shrivastava et al. Oct. 2020 ), social network graph (Sivasankari and Vadivu 2021 ; Taskin et al. 2021 ), Xception (Han et al. July 2021 ), XGBoost (Kaliyar et al. 2021b ), Cross-SEAN (Paka et al. 2021 ), while reinforcement learning (Shahbazi and Byun 2021 ) includes BERT (Mehta et al. 2021 ; Shishah 2021 ; Kaliyar et al. 2021a ). These algorithms are focused on directly learning the features from the original data like texts and images without the need for manual feature engineering. It can be executed in an end-to-end manner. While compared with the shallow models, deep learning methods have considerable features for large datasets in terms of interpretability, learning capacity, feature representation, number of parameters, and running time. Similarly, some miscellaneous techniques are used for detecting fake news, which is the reverse-tracking approach (Ko et al. June 2019 ), honeycomb framework (Talwar et al. 2020 ), and ASSO-OSIW and GWO (Ozbay and Alatas 2021 ). The most used techniques with some advantages and challenges are listed in Table Table3 3 .
Benefits and limitations of some machine learning-based fake news detection models
Architectural view of fake news detection models and performance measures used in traditional fake news detection models
Architectural view of the fake news detection model.
The overall procedure of the fake news detection model is given in Fig. 4 .

The architecture of the general fake news detection model with deep learning-based models
The major goal of the fake news detection model is to identify fake news to ensure the authenticity of the news by differentiating the fake news from real news. Thus, initially, the standard data can be gathered from any social-networking sites like Hike, WhatsApp, Instagram, Twitter, Facebook, and online news articles. As the gathered data requires special attention, pre-processing is required for adopting machine learning or deep learning algorithms. Various methods have been suggested in recent years to pre-process the text and make it ready for further processing. Secondly, NLP tasks like word embedding and feature extraction will be conducted for getting the most suitable information from the data, which reduces the time and computational complexities. Finally, the features are fed to the classification model, where the classified outcomes in terms of fake vs real news are generated with the help of neural network-based algorithms. At last, the predicted outcomes demonstrate the real news that guarantees higher accuracy in detection.
Performance measures
To evaluate the efficiency of the existing fake news identification models, several performance metrics have been suggested, which are depicted in Table Table4. 4 . The most commonly used techniques are discussed here.
Performance measures considered for evaluating the efficiency of existing fake news identification models
F1 score: “harmonic mean between precision and recall. It is used as a statistical measure to rate performance”.
Precision: It is “the ratio of positive observations that are predicted exactly to the total number of observations that are positively predicted”.
Accuracy: It is a “ratio of the observation of exactly predicted to the whole observations”.
The recall is referred to as “the number of true positive results”
Here, “ T p , T n , F p , F n refer to the true positives, true negatives, false positives, and false negatives”, respectively.
A confusion matrix is “a summary of prediction results on a classification problem. The number of correct and incorrect predictions are summarized with count values and broken down by each class”.
Applications considered in identifying the fake news
The fake news can be propagated in any kind of field like health, education, democracy, politics, COVID-19, etc., that could negatively affect individuals and society. Hence, the applications focused on the recent fake news detection model are diagrammatically represented in Fig. 5 . From the analysis, most of the articles consider politics as their major focus. The remaining studies focus on news articles or social media comments regarding tourism, culture, COVID- 19, marketing, and e-commerce topics. This helps future researchers to focus on the new field to gather information regarding the new field that gives the innovative way of research in the fake news detection model.

Applications focused on the existing fake news detection model
Consequences of fake news and Research challenges, future scope on fake news detection models
Consequences of fake news.
Since the start of human civilization, fake news has often emerged. Though, the propagation of fake news can emerge through the utilization of the global media landscape and modern technologies. Fake news affects several fields including economic, political, and social environments (Aslam et al. 2021 ). On the other hand, fake news and fake information have several faces. Fake news poses tremendous impacts as information molds the view of humans around the world, though critical decisions can be made through fake information, which also leads to wrong decision-making. Similarly, good decisions cannot be made by this fabricated, distorted, false, or fake information on the Internet. The major impacts of fake news affect health, innocent people, democratic impacts, and financial impacts.
Democratic Impact : Fake news has been discussed in media due to its major role in the election. It is also considered an essential democratic problem. Hence, there is a need of predicting fake news and stop spreading fake news.
Financial Impact : Fake news is recently a complicated issue in the business world and industries. For increasing individuals' profits, dishonest businessmen may propagate fake reviews or news. Consequently, fake information can ruin the fame of a business.
Impact on Health : On the internet, health-related news is more vastly searched. People’s lives can be affected due to the emergence of fake news in health. Thus, this is one of the noteworthy problems in recent times. Consequently, social media environments have created some policy changes for banning or limiting the issuing the misinformation on health-related articles as they affect health advocates, lawmakers, and doctors.
Impact on Innocent People : Some specific people can be affected by rumors. Social media harasses these kinds of people. People may also face threats and insults that result in real-life consequences.
Research gaps and future works
This review helps society from the propagation of fake news, which creates awareness of people and their impacts on social media nowadays (Alsaeedi and Al-Sarem 2020 ). The major aim of detecting fake news is to increase the betterment of society. The existing models use several deep learning approaches like LSTM and NNs. This NN-based training model has improved the identification of misleading news (Savyan and Bhanu 2020 ). The identification of fake news has posed several limitations in the existing studies. For eradicating a huge range of fake news from social media platforms, the recognition of fake news among real news can be performed by detecting the fake news subjects and creators (Kapusta and Obonya 2020 ). However, there is a complexity in addressing the fake news detection problems.
The major issue of fake news is inherently a multimodal and multilingual one, which consists of information in an array of languages, auditory, visual or textual forms, and generally involved in communication in a language that may be unfamiliar to users (Hakak et al. 2021 ). Although deep learning-based approaches offer a superior accuracy rate while analyzing with other algorithms, thus, there is a new future perspective in making it more acceptable (Gravanis et al. 2019 ). Fake news detection can also be affected by selecting suitable feature extraction and classifier algorithms. The research studies must consider which classification technique is more applicable for specific features (Al-Ahmad et al. 2021 ). Moreover, the utilization of sequence models requires processing the long textual features. Hence, there is a need for more concentration in choosing the features and classifiers for enhancing performance. Though there are fewer probabilities of inaccurate outcomes through deep learning models, and thus, there is a need of adopting intelligent approaches to detecting (Ambati 2021 ) fake news.
The future direction lies to outstretch and improvise the conventional research studies to implement the conventional works toward designing an automated system for e-commerce websites, where identification of fake news has become considerably significant (Faustini and Covões 2020 ). Future research works concerning fake news detection are only using supervised models, where the texts are not enough for all the cases. This problem can be solved by adding additional information like information regarding authors. The most eminent technique will be designing a knowledge-based automatic fake news detection model (Jwa et al. 2019 ). The results of the designed model will be extracting the information for the text and checking the information related to the dataset, which will alert the clients that the news will be considered fake. Based on this framework, the consumers can obtain awareness to solve the untrusted information (Mouratidis et al. 2021 ). The major problem of the existing technique is to identify the misinformation and health-related fake news, and thus, there is a new future scope for getting fake news regarding health-related fake news.
This paper has offered a detailed review of the fake news detection model with the help of a set of contributions from last year. This survey has presented information concerned with different machine learning and deep learning techniques. Additionally, this model has also given the details regarding datasets, simulation environments, algorithms, and their features and challenges. Furthermore, it has offered performance metrics used for evaluating the performance, and the research gaps and challenges for developing a new fake news detection model. Therefore, this survey can motivate future researchers to focus on novel fake news identification models with intelligent approaches. This will help the researchers in detecting fake news for gaining a concise, better perspective of conventional problems, solutions, and future directions.
Author contributions
Minal Shah and Amit Ganatra designed the model, computational framework and carried out the implementation. Minal Shah performed the calculations and wrote the manuscript with all the inputs. Minal Shah, Amit Ganatra discussed the results and contributed to the final manuscript.
Declarations
The authors declare no competing interests.
Publisher's Note
Springer Nature remains neutral with regard to jurisdictional claims in published maps and institutional affiliations.
Contributor Information
Minal Nirav Shah, Email: moc.liamg@68011lanim .
Amit Ganatra, Email: ni.ca.ytisrevinulurap@tsovorp .
- Agarwal A, Mittal M, Pathak A, Goyal LM. Fake news detection using a blend of neural networks: an application of deep Learning. SN Comput Sci. 2020 doi: 10.1007/s42979-020-00165-4. [ CrossRef ] [ Google Scholar ]
- Ahmad I, Yousaf M, Yousaf S, Ahmad MO (2020) Fake news detection using machine learning ensemble methods, Complexity
- Al-Ahmad B, Al-Zoubi AM, Khurma RA, Aljarah I. An evolutionary fake news detection method for COVID-19 pandemic information. Symmetry. 2021; 13 (6):1091. [ Google Scholar ]
- Ali H, Khan MS, AlGhadhban A, Alazmi M, Alzamil A, Al-Utaibi K, Qadir J. All your fake detector are belong to us: evaluating adversarial robustness of fake-news detectors under black-box settings. IEEE Access. 2021; 9 :81678–81692. [ Google Scholar ]
- Alsaeedi A, Al-Sarem M. Detecting rumors on social media based on a CNN deep learning technique. Arab J Sci Eng. 2020; 45 (12):1–32. [ Google Scholar ]
- Ambati LS, El-Gayar O (2021) Human activity recognition: a comparison of machine learning approaches, J Midwest Assoc Inf Syst, 1
- Aslam N, Khan IU, Alotaibi FS, Aldaej LA, Aldubaikil AK. Fake detect: a deep learning ensemble model for fake news detection. Complexity. 2021; 2021 :1–8. [ Google Scholar ]
- Barbado R, Araque O, Iglesias CA. A framework for fake review detection in online consumer electronics retailers. Inf Process Manage. 2019; 56 (4):1234–1244. [ Google Scholar ]
- Beer DD, Matthee M. Approaches to identify fake news: a systematic literature review. Integr Sci Digit Age. 2020; 136 :13–22. [ Google Scholar ]
- Bondielli A, Marcelloni F. A survey on fake news and rumour detection techniques. Inf Sci. 2019; 497 :38–55. [ Google Scholar ]
- Braşoveanu AMP, Andonie R. Integrating machine learning techniques in semantic fake news detection. Neural Process Lett. 2021; 53 :3055–3072. [ Google Scholar ]
- Brenes Peralta CM, Sánchez RP, González IS (2021) Individual evaluation vs fact-checking in the recognition and willingness to share fake news about Covid-19 via whatsapp, Journal Stud
- Chang C (2021) "Fake news: audience perceptions and concerted coping strategies," Digital Journal, 9(5)
- Chauhan T, Palivela H. Optimization and improvement of fake news detection using deep learning approaches for societal benefit. Int J Inf Manag Data Insights. 2021 doi: 10.1016/j.jjimei.2021.100051. [ CrossRef ] [ Google Scholar ]
- Choudhary A, Arora A. Linguistic feature based learning model for fake news detection and classification. Expert Syst Appl. 2020 doi: 10.1016/j.eswa.2020.114171. [ CrossRef ] [ Google Scholar ]
- Choudhary M, Chouhan SS, Pilli ES, Vipparthi SK (2021) BerConvoNet: a deep learning framework for fake news classification, Appl Soft Comput, 110
- D’Ulizia A, Caschera MC, Ferri F, Grifoni P (2021b) Fake news detection: a survey of evaluation datasets, Peer J Comput Sci, 7 [ PMC free article ] [ PubMed ]
- Dabbous A, Aoun Barakat K, de Quero Navarro B (2020a) Fake news detection and social media trust: a cross-cultural perspective, Behav Inf Technol
- Das SD, Basak A, Dutta S (2021) "A heuristic-driven uncertainty based ensemble framework for fake news detection in tweets and news articles, Neurocomputing
- de Oliveira NR, Medeiros DSV, Mattos DMF. A sensitive stylistic approach to identify fake news on social networking. IEEE Signal Process Lett. 2020; 27 :1250–1254. [ Google Scholar ]
- de Souza MC, Nogueira BM, Rossi RG, Marcacini RM, Dos Santos BN, Rezende SO (2021) A network-based positive and unlabeled learning approach for fake news detection, Mach Learn [ PMC free article ] [ PubMed ]
- Dong X, Victor U, Qian L. Two-path deep semisupervised learning for timely fake news detection. IEEE Trans Comput Soc Syst. 2020; 7 (6):1386–1398. [ Google Scholar ]
- Faustini PHA, Covões TF. Fake news detection in multiple platforms and languages. Expert Syst Appl. 2020 doi: 10.1016/j.eswa.2020.113503. [ CrossRef ] [ Google Scholar ]
- Faustini PH, Covões TF. Fake news detection in multiple platforms and languages. Expert Syst Appl. 2020; 158 :15. [ Google Scholar ]
- Gravanis G, Vakali A, Diamantaras K, Karadais P. Behind the cues: a benchmarking study for fake news detection. Expert Syst Appl. 2019; 128 :201–213. [ Google Scholar ]
- Gupta A, Bansal A, Mamgain K, Gupta A. An exploratory analysis on the unfold of fake news during COVID-19 pandemic. Smart Syst: Innov Comput. 2022; 235 :259–272. [ Google Scholar ]
- Habib A, Asghar MZ, Khan A, Habib A, Khan A (2019) False information detection in online content and its role in decision making: a systematic literature review, Soc Netw Anal Mining, 9(50)
- Hakak S, Alazab M, Khan S, Gadekallu TR, Maddikunta PKR, Khan WZ. An ensemble machine learning approach through effective feature extraction to classify fake news. Futur Gener Comput Syst. 2021; 117 :47–58. [ Google Scholar ]
- Han B, Han X, Zhang H, Li J, Cao X. Fighting fake news: two stream network for deepfake detection via learnable SRM. IEEE Trans Biom Behav Identity Sci. 2021; 3 (3):320–331. [ Google Scholar ]
- Huang YF, Chen PH. Fake news detection using an ensemble learning model based on Self-Adaptive Harmony Search algorithms. Expert Syst Appl. 2020; 159 :30. [ Google Scholar ]
- Huu Do T, Berneman M, Patro J, Bekoulis G, Deligiannis N. Context-aware deep markov random fields for fake news detection. IEEE Access. 2021; 9 :130042–130054. [ Google Scholar ]
- Islam MR, Liu S, Wang X, Xu G (2020) Deep learning for misinformation detection on online social networks: a survey and new perspectives, Soc Netw Anal Mining, 10(82) [ PMC free article ] [ PubMed ]
- Jadhav SS, Thepade SD. Fake news identification and classification using dssm and improved recurrent neural network classifier. Appl Artif Intell Int J. 2019 doi: 10.1080/08839514.2019.1661579. [ CrossRef ] [ Google Scholar ]
- Jain V, Kaliyar RK, Goswami A, Narang P, Sharma Y (2021) "AENeT: an attention-enabled neural architecture for fake news detection using contextual features, Neural Comput Appl [ PMC free article ] [ PubMed ]
- Jang SM, Geng T, Li JY, Xia R, Huang CT, Kim H, Tang J. A computational approach for examining the roots and spreading patterns of fake news: evolution tree analysis. Comput Hum Behav. 2018; 84 :103–113. [ Google Scholar ]
- Javed MS, Majeed H, Mujtaba H, Beg MO. Fake reviews classification using deep learning ensemble of shallow convolutions. J Comput Soc Sci. 2021; 4 :883–902. [ Google Scholar ]
- Jiang T, Li JP, Haq AU, Saboor A, Ali A. A novel stacking approach for accurate detection of fake news. IEEE Access. 2021; 9 :22626–22639. [ Google Scholar ]
- Jwa H, Oh D, Park K, Kang J, Lim H. ExBAKE: automatic fake news detection model based on bidirectional encoder representations from transformers (BERT) Appl Sci. 2019; 9 (19):4062. [ Google Scholar ]
- Kaliyar RK, Goswami A, Narang P, Sinha S. FNDNet—a deep convolutional neural network for fake news detection. Cogn Syst Res. 2020; 61 :32–44. [ Google Scholar ]
- Kaliyar RK, Goswami A, Narang P. FakeBERT: Fake news detection in social media with a BERT-based deep learning approach. Multimed Tools Appl. 2021; 80 :11765–11788. [ PMC free article ] [ PubMed ] [ Google Scholar ]
- Kaliyar RK, Goswami A, Narang P. DeepFakE: improving fake news detection using tensor decomposition-based deep neural network. J Supercomput. 2021; 77 :1015–1037. [ Google Scholar ]
- Kaliyar RK, Goswami A, Narang P. EchoFakeD: improving fake news detection in social media with an efficient deep neural network. Neural Comput Appl. 2021; 33 :8597–8613. [ PMC free article ] [ PubMed ] [ Google Scholar ]
- Kansal A (2021) Fake news detection using pos tagging and machine learning, J Appl Secur Res
- Kapusta J, Obonya J. Improvement of misleading and fake news classification for effective languages by morphological group analysis. Informatics. 2020 doi: 10.3390/informatics7010004. [ CrossRef ] [ Google Scholar ]
- Kauffmann E, Peral J, Gil D, Ferrández A, Sellers R, Mora H. A framework for big data analytics in commercial social networks: a case study on sentiment analysis and fake review detection for marketing decision-making. Ind Mark Manage. 2020; 90 :523–537. [ Google Scholar ]
- Kaur S, Kumar P, Kumaraguru P. Automating fake news detection system using multi-level voting model. Soft Comput. 2020; 24 :9049–9069. [ Google Scholar ]
- Kim G, Ko Y. Effective fake news detection using graph and summarization techniques. Pattern Recogn Lett. 2021; 151 :135–139. [ Google Scholar ]
- Ko H, Hong JY, Kim S, Mesicek L, Na IS. Human-machine interaction: a case study on fake news detection using a backtracking based on a cognitive system. Cogn Syst Res. 2019; 55 :77–81. [ Google Scholar ]
- Kumari R, Ekbal A. AMFB: attention based multimodal factorized bilinear pooling for multimodal fake news detection. Expert Syst Appl. 2021; 184 :1. [ Google Scholar ]
- Li Q, Hu Q, Lu Y, Yang Y, Cheng J. Multi-level word features based on CNN for fake news detection in cultural communication. Pers Ubiquit Comput. 2020; 24 :259–272. [ Google Scholar ]
- Li D, Guo H, Wang Z, Zheng Z. Unsupervised fake news detection based on autoencoder. IEEE Access. 2021; 9 :29356–29365. [ Google Scholar ]
- Li X, Lu P, Hu L, Wang X, Lu L (2021a) A novel self-learning semi-supervised deep learning network to detect fake news on social media, Multimedia Tools Appl [ PMC free article ] [ PubMed ]
- Mahabub A. A robust technique of fake news detection using Ensemble Voting Classifier and comparison with other classifiers. SN Appl Sci. 2020 doi: 10.1007/s42452-020-2326-y. [ CrossRef ] [ Google Scholar ]
- Meel P, Vishwakarma DK. A temporal ensembling based semi-supervised ConvNet for the detection of fake news article. Expert Syst Appl. 2021; 177 :1. [ Google Scholar ]
- Meesad P (2021) Thai fake news detection based on information retrieval, natural language processing and machine learning, SN Comput Sci, 2(425) [ PMC free article ] [ PubMed ]
- Mehta D, Dwivedi A, Patra A, Anand Kumar M (2021) A transformer-based architecture for fake news classification, Social Netw Anal Mining, 11(39)
- Meneses Silva CV, Silva Fontes R, Colaço Júnior M (2021) Intelligent fake news detection: a systematic mapping, J Appl Secur Res, 16(2)
- Mitra A, Mohanty SP, Corcoran P, Kougianos E (2021) A machine learning based approach for deepfake detection in social media through key video frame extraction, SN Comput Sci, 2(98)
- Mouratidis D, Nikiforos MN, Kermanidis KL. Deep learning for fake news detection in a pairwise textual input schema. Computation. 2021 doi: 10.3390/computation9020020. [ CrossRef ] [ Google Scholar ]
- Mridha MF, Keya AJ, Hamid MA, Monowar MM, Rahman MS. A comprehensive review on fake news detection with deep learning. IEEE Access. 2021; 9 :156151–156170. [ Google Scholar ]
- Ncube L, Mare A. Fake news” and multiple regimes of “truth” during the COVID-19 pandemic in Zimbabwe. Afr Journal Stud. 2022; 43 (2):71–89. [ Google Scholar ]
- Ni S, Li J, Kao H-Y. MVAN: multi-view attention networks for fake news detection on social media. IEEE Access. 2021; 9 :106907–106917. [ Google Scholar ]
- Nistor A, Zadobrischi E. The influence of fake news on social media: analysis and verification of web content during the COVID-19 pandemic by advanced machine learning methods and natural language processing. Sustainability. 2022; 14 (17):10466. [ Google Scholar ]
- Ozbay FA, Alatas B. Fake news detection within online social media using supervised artificial intelligence algorithms. Physica A. 2020; 540 :15. [ Google Scholar ]
- Ozbay FA, Alatas B. Adaptive Salp swarm optimization algorithms with inertia weights for novel fake news detection model in online social media. Multimed Tools Appl. 2021; 80 :34333–34357. [ Google Scholar ]
- Paka WS, Bansal R, Kaushik A, Sengupta S, Chakraborty T (2021) Cross-SEAN: a cross-stitch semi-supervised neural attention model for COVID-19 fake news detection, Appl Soft Comput [ PMC free article ] [ PubMed ]
- Qureshi KA, Malick RAS, Sabih M, Cherifi H. Complex network and source inspired COVID-19 fake news classification on twitter. IEEE Access. 2021; 9 :139636–139656. [ Google Scholar ]
- Raj C, Meel P. ConvNet frameworks for multi-modal fake news detection. Appl Intell. 2021; 51 :8132–8148. [ Google Scholar ]
- S. Rama Krishna, S. V. Vasantha, K. Mani Deep (2021) Survey on fake news detection using machine learning algorithms, ICACT, 09(08)
- Reddy H, Raj N, Gala M, Basava A. Text-mining-based fake news detection using ensemble methods. Int J Autom Comput. 2020; 17 :210–221. [ Google Scholar ]
- Ribeiro Bezerra JF. Content-based fake news classification through modified voting ensemble. J Inf Telecommun. 2021 doi: 10.1080/24751839.2021.1963912. [ CrossRef ] [ Google Scholar ]
- Saleh H, Alharbi A, Alsamhi SH. OPCNN-FAKE: optimized convolutional neural network for fake news detection. IEEE Access. 2021; 9 :129471–129489. [ Google Scholar ]
- Samadi M, Mousavian M, Momtazi S (2021) Deep contextualized text representation and learning for fake news detection, Inf Process Manag, 58(6)
- Savyan P, Bhanu SMS. UbCadet: Detection of compromised accounts in Twitter based on user behavioural profiling. Multimed Tools Appl. 2020; 79 :1–37. [ Google Scholar ]
- Sengupta E, Nagpal R, Mehrotra D, Srivastava G. ProBlock: a novel approach for fake news detection. Clust Comput. 2021; 24 :3779–3795. [ PMC free article ] [ PubMed ] [ Google Scholar ]
- Setiawan R, Ponnam VS, Sengan S, Anam M, Subbiah C, Phasinam K, Vairaven M, Ponnusamy S. Certain investigation of fake news detection from facebook and twitter using artificial intelligence approach. Wirel Pers Commun. 2021 doi: 10.1007/s11277-021-08720-9. [ CrossRef ] [ Google Scholar ]
- Shahbazi Z, Byun Y-C. Fake media detection based on natural language processing and blockchain approaches. IEEE Access. 2021; 9 :128442–128453. [ Google Scholar ]
- Sharma DK, Garg S (2021) IFND: a benchmark dataset for fake news detection, Compl Intell Syst [ PMC free article ] [ PubMed ]
- Sheikhi S (2021) An effective fake news detection method using WOA-xgbTree algorithm and content-based features, Appl Soft Comput, 109
- Shishah W. Fake news detection using BERT model with joint learning. Arab J Sci Eng. 2021; 46 :9115–9127. [ Google Scholar ]
- Shrivastava G, Kumar P, Ojha RP, Srivastava PK, Mohan S, Srivastava G. Defensive modeling of fake news through online social networks. IEEE Trans Comput Soc Syst. 2020; 7 (5):1159–1167. [ Google Scholar ]
- Shu K, Mahudeswaran D, Liu H. FakeNewsTracker: a tool for fake news collection, detection, and visualization. Comput Math Organ Theory. 2019; 25 :60–71. [ Google Scholar ]
- Silva RM, Santos RL, Almeida TA, Pardo TA. Towards automatically filtering fake news in Portuguese. Expert Syst Appl. 2020; 146 :15. [ Google Scholar ]
- Silva A, Han Y, Luo L, Karunasekera S, Leckie C. Propagation2Vec: embedding partial propagation networks for explainable fake news early detection. Inf Process Manag. 2021 doi: 10.1016/j.ipm.2021.102618. [ CrossRef ] [ Google Scholar ]
- Simko J, Racsko P, Tomlein M, Hanakova M, Moro R, Bielikova M (2021) A study of fake news reading and annotating in social media context, New Rev Hypermedia Multimed
- Singh M, Bhatt MW, Bedi HS, Mishra U. Performance of bernoulli’s naive bayes classifier in the detection of fake news. Mater Today Proc. 2020 doi: 10.1016/j.matpr.2020.10.896. [ CrossRef ] [ Google Scholar ]
- Singh B, Sharma DK (2021) Predicting image credibility in fake news over social media using multi-modal approach, Neural Comput Appl [ PMC free article ] [ PubMed ]
- Sivasankari S, Vadivu G (2021) Tracing the fake news propagation path using social network analysis, Soft Comput
- Song C, Shu K, Wu B (2021) Temporally evolving graph neural network for fake news detection, Inf Process Manag, 58(6)
- Hiriyannaiah S, Srinivas AM, Shetty GK, Siddesh GM, Srinivasa KG (2020) A computationally intelligent agent for detecting fake news using generative adversarial networks. Hybrid computational intelligence for pattern analysis and understanding, pp. 69–96
- Talwar S, Dhir A, Singh D, Virk GS, Salo J. Sharing of fake news on social media: application of the honeycomb framework and the third-person effect hypothesis. J Retail Consum Serv. 2020 doi: 10.1016/j.jretconser.2020.102197. [ CrossRef ] [ Google Scholar ]
- Taskin SG, Kucuksille EU, Topal K (2021) Detection of Turkish Fake News in Twitter with Machine Learning Algorithms, Arab J Sci Eng [ PMC free article ] [ PubMed ]
- Trueman TE, Kumar A, Narayanasamy P, Vidya J (2021) Attention-based C-BiLSTM for fake news detection, Appl Soft Comput, 110
- Umer M, Imtiaz Z, Ullah S, Mehmood A, Choi GS, On B-W. Fake news stance detection using deep learning architecture (CNN-LSTM) IEEE Access. 2020; 8 :156695–156706. [ Google Scholar ]
- Vereshchaka A, Cosimini S, Dong W. Analyzing and distinguishing fake and real news to mitigate the problem of disinformation. Comput Math Organ Theory. 2020; 26 :350–364. [ Google Scholar ]
- Verma PK, Agrawal P, Amorim I, Prodan R. WELFake: word embedding over linguistic features for fake news detection. IEEE Trans Comput Soc Syst. 2021; 8 (4):881–893. [ Google Scholar ]
- Wang X, Chao F, Yu G, Zhang K. Factors influencing fake news rebuttal acceptance during the COVID-19 pandemic and the moderating effect of cognitive ability. Comput Hum Behav. 2022; 130 :107174. [ PMC free article ] [ PubMed ] [ Google Scholar ]
- Xu K, Wang F, Wang H, Yang B. Detecting fake news over online social media via domain reputations and content understanding. Tsinghua Sci Technol. 2020; 25 (1):20–27. [ Google Scholar ]
- Yang C, Zhou X, Zafarani R (2021) CHECKED: Chinese COVID-19 fake news dataset, Soc Netw Anal Mining, 11(58) [ PMC free article ] [ PubMed ]
- Ying L, Yu H, Wang J, Ji Y, Qian S. Multi-level multi-modal cross-attention network for fake news detection. IEEE Access. 2021; 9 :132363–132373. [ Google Scholar ]
- Ying L, Yu H, Wang J, Ji Y, Qian S. Fake news detection via multi-modal topic memory network. IEEE Access. 2021; 9 :132818–132829. [ Google Scholar ]
- Zervopoulos A, Alvanou AG, Bezas K, Papamichail A, Maragoudakis M, Kermanidis K. "Deep learning for fake news detection on Twitter regarding the 2019 Hong Kong protests. Neur Comput Appl. 2021 doi: 10.1007/s00521-021-06230-0. [ CrossRef ] [ Google Scholar ]
- Zhang C, Gupta A, Kauten C, Deokar AV, Qin X. Detecting fake news for reducing misinformation risks using analytics approaches. Eur J Oper Res. 2019; 279 (3):1036–1052. [ Google Scholar ]
- Zheng L, Elhai JD, Miao M, Wang Y, Wang Y, Gan Y. Health-related fake news during the COVID-19 pandemic: perceived trust and information search. Internet Res. 2022; 32 :768–789. [ Google Scholar ]
- Zhou X, Zafarani R. A survey of fake news: fundamental theories, detection methods, and opportunities. ACM Comput Surv. 2020; 53 (5):1–40. [ Google Scholar ]
Europe PMC requires Javascript to function effectively.
Either your web browser doesn't support Javascript or it is currently turned off. In the latter case, please turn on Javascript support in your web browser and reload this page.
Search life-sciences literature (43,861,538 articles, preprints and more)
- Free full text
Approaches to Identify Fake News: A Systematic Literature Review
Author information, affiliations.
- de Beer D 1
- Matthee M 1
Integrated Science in Digital Age 2020 , 05 May 2020 , 136: 13-22 PMCID: PMC7250114
Abstract
Free full text .

Dylan de Beer
Department of Informatics, University of Pretoria, Pretoria, 0001 South Africa
Machdel Matthee
With the widespread dissemination of information via digital media platforms, it is of utmost importance for individuals and societies to be able to judge the credibility of it. Fake news is not a recent concept, but it is a commonly occurring phenomenon in current times. The consequence of fake news can range from being merely annoying to influencing and misleading societies or even nations. A variety of approaches exist to identify fake news. By conducting a systematic literature review, we identify the main approaches currently available to identify fake news and how these approaches can be applied in different situations. Some approaches are illustrated with a relevant example as well as the challenges and the appropriate context in which the specific approach can be used.
- Introduction
Paskin ( 2018 : 254) defines fake news as “particular news articles that originate either on mainstream media (online or offline) or social media and have no factual basis, but are presented as facts and not satire”. The importance of combatting fake news is starkly illustrated during the current COVID-19 pandemic. Social networks are stepping up in using digital fake news detection tools and educating the public towards spotting fake news. At the time of writing, Facebook uses machine learning algorithms to identify false or sensational claims used in advertising for alternative cures, they place potential fake news articles lower in the news feed, and they provide users with tips on how to identify fake news themselves (Sparks and Frishberg 2020 ). Twitter ensures that searches on the virus result in credible articles and Instagram redirects anyone searching for information on the virus to a special message with credible information (Marr 2020 ).
These measures are possible because different approaches exist that assist the detection of fake news. For example, platforms based on machine learning use fake news from the biggest media outlets, to refine algorithms for identifying fake news (Macaulay 2018 ). Some approaches detect fake news by using metadata such as a comparison of release time of the article and timelines of spreading the article as well where the story spread (Macaulay 2018 ).
The purpose of this research paper is to, through a systematic literature review, categorize current approaches to contest the wide-ranging endemic of fake news.
- The Evolution of Fake News and Fake News Detection
Fake news is not a new concept. Before the era of digital technology, it was spread through mainly yellow journalism with focus on sensational news such as crime, gossip, disasters and satirical news (Stein-Smith 2017 ). The prevalence of fake news relates to the availability of mass media digital tools (Schade 2019 ). Since anyone can publish articles via digital media platforms, online news articles include well researched pieces but also opinion-based arguments or simply false information (Burkhardt 2017 ). There is no custodian of credibility standards for information on these platforms making the spread of fake news possible. To make things worse, it is by no means straightforward telling the difference between real news and semi-true or false news (Pérez-Rosas et al. 2018 ).
The nature of social media makes it easy to spread fake news, as a user potentially sends fake news articles to friends, who then send it again to their friends and so on. Comments on fake news sometimes fuel its ‘credibility’ which can lead to rapid sharing resulting in further fake news (Albright 2017 ).
Social bots are also responsible for the spreading of fake news. Bots are sometimes used to target super-users by adding replies and mentions to posts. Humans are manipulated through these actions to share the fake news articles (Shao et al. 2018 ).
Clickbait is another tool encouraging the spread of fake news. Clickbait is an advertising tool used to get the attention of users. Sensational headlines or news are often used as clickbait that navigate the user to advertisements. More clicks on the advert means more money (Chen et al. 2015 a).
Fortunately, tools have been developed for detecting fake news. For example, a tool has been developed to identify fake news that spreads through social media through examining lexical choices that appear in headlines and other intense language structures (Chen et al. 2015 b). Another tool, developed to identify fake news on Twitter, has a component called the Twitter Crawler which collects and stores tweets in a database (Atodiresei et al. 2018 ). When a Twitter user wants to check the accuracy of the news found they can copy a link into this application after which the link will be processed for fake news detection. This process is built on an algorithm called the NER (Named Entity Recognition) (Atodiresei et al. 2018 ).
There are many available approaches to help the public to identify fake news and this paper aims to enhance understanding of these by categorizing these approaches as found in existing literature.
- Research Method
Research Objective
The purpose of this paper is to categorize approaches used to identify fake news. In order to do this, a systematic literature review was done. This section presents the search terms that were used, the selection criteria and the source selection.
Search Terms
Specific search terms were used to enable the finding of relevant journal articles such as the following:
(“what is fake news” OR “not genuine information” OR “counter fit news” OR “inaccurate report*” OR “forged (NEAR/2) news” OR “mislead* information” OR “false store*” OR “untrustworthy information” OR “hokes” OR “doubtful information” OR “incorrect detail*” OR “false news” OR “fake news” OR “false accusation*”)
AND (“digital tool*” OR “digital approach” OR “automated tool*” OR “approach*” OR “programmed tool*” OR “digital gadget*” OR “digital device*” OR “digital machan*” OR “digital appliance*” OR “digital gizmo” OR “IS gadget*” OR “IS tool*” OR “IS machine*” OR “digital gear*” OR “information device*”)
AND (“fake news detection” OR “approaches to identify fake news” OR “methods to identify fake news” OR “finding fake news” OR “ways to detect fake news”).
Selection Criteria
Inclusion criteria..
Studies that adhere to the following criteria: (1) studies published between 2008 and 2019; (2) studies found in English; (3) with main focus fake news on digital platforms; (4) articles that are published in IT journals or any technology related journal articles (e.g. computers in human behavior) as well as conference proceedings; (5) journal articles that are sited more than 10 times.
Exclusion Criteria.
Studies that adhered to the following criteria: (1) studies not presented in journal articles (e.g. in the form of a slide show or overhead presentation); (2) studies published, not relating to technology or IT; (3) articles on fake news but not the identification of it.
The search terms were used to find relevant articles on ProQuest, ScienceDirect, EBSCOhost and Google Scholar (seen here as ‘other sources’).
Flowchart of Search Process
Figure 1 below gives a flowchart of the search process: the identification of articles, the screening, the selection process and the number of the included articles.

A flowchart of the selection process
In this section of the article we list the categories of approaches that are used to identify fake news. We also discuss how the different approaches interlink with each other and how they can be used together to get a better result.
The following categories of approaches for fake news detection are proposed: (1) language approach, (2) topic-agnostic approach, (3) machine learning approach, (4) knowledge-based approach, (5) hybrid approach.
The five categories mentioned above are depicted in Fig. 2 below. Figure 2 shows the relationship between the different approaches. The sizes of the ellipses are proportional to the number of articles found (given as the percentage of total included articles) in the systematic literature review that refer to that approach.

Categories of fake news detection approaches resulting from the systematic literature review
The approaches are discussed in depth below with some examples for illustration purposes.
Language Approach
This approach focuses on the use of linguistics by a human or software program to detect fake news. Most of the people responsible for the spread of fake news have control over what their story is about, but they can often be exposed through the style of their language (Yang et al. 2018 ). The approach considers all the words in a sentence and letters in a word, how they are structured and how it fits together in a paragraph (Burkhardt 2017 ). The focus is therefore on grammar and syntax (Burkhardt 2017 ). There are currently three main methods that contribute to the language approach:
Bag of Words (BOW):
In this approach, each word in a paragraph is considered of equal importance and as independent entities (Burkhardt 2017 ). Individual words frequencies are analysed to find signs of misinformation. These representations are also called n-grams (Thota et al. 2018 ). This will ultimately help to identify patterns of word use and by investigating these patterns, misleading information can be identified. The bag of words model is not as practical because context is not considered when text is converted into numerical representations and the position of a word is not always taken into consideration (Potthast et al. 2017 ).
Semantic Analysis:
Chen et al. 2017 b explain that truthfulness can be determined by comparing personal experience (e.g. restaurant review) with a profile on the topic derived from similar articles. An honest writer will be more likely to make similar remarks about a topic than other truthful writers. Different compatibly scores are used in this approach.
Deep Syntax:
The deep syntax method is carried out through Probability Context Free Grammars (Stahl 2018 ). The Probability Context Free Grammars executes deep syntax tasks through parse trees that make Context Free Grammar analysis possible. Probabilistic Context Free Grammar is an extension of Context Free Grammars (Zhou and Zafarani 2018 ). Sentences are converted into a set of rewritten rules and these rules are used to analyse various syntax structures. The syntax can be compared to known structures or patterns of lies and can ultimately lead to telling the difference between fake news and real news (Burkhardt 2017 ).
Topic-Agnostic Approach
This category of approaches detect fake news by not considering the content of articles bur rather topic-agnostic features. The approach uses linguistic features and web mark-up capabilities to identify fake news (Castelo et al. 2019 ). Some examples of topic-agnostic features are 1) a large number of advertisements, 2) longer headlines with eye-catching phrases, 3) different text patterns from mainstream news to induce emotive responses 4) presence of an author name (Castelo et al. 2019 ; Horne and Adali 2017 ).
Machine Learning Approach
Machine learning algorithms can be used to identify fake news. This is achieved through using different types of training datasets to refine the algorithms. Datasets enables computer scientists to develop new machine learning approaches and techniques. Datasets are used to train the algorithms to identify fake news. How are these datasets created? One way is through crowdsourcing. Perez-Rosas et al. ( 2018 ) created a fake news data set by first collecting legitimate information on six different categories such as sports, business, entertainment, politics, technology and education (Pérez-Rosas et al. 2018 ). Crowdsourcing was then used and a task was set up which asked the workers to generate a false version of the news stories (Pérez-Rosas et al. 2018 ). Over 240 stories were collected and added to the fake news dataset.
A machine learning approach called the rumor identification framework has been developed that legitimizes signals of ambiguous posts so that a person can easily identify fake news (Sivasangari et al. 2018 ). The framework will alert people of posts that might be fake (Sivasangari et al. 2018 ). The framework is built to combat fake tweets on Twitter and focuses on four main areas; the metadata of tweets, the source of the tweet; the date and area of the tweet, where and when the tweet was developed (Sivasangari et al. 2018 ). By studying these four parts of the tweet the framework can be implemented to check the accuracy of the information and to separate the real from the fake (Sivasangari et al. 2018 ). Supporting this framework, the spread of gossip is collected to create datasets with the use of a Twitter Streaming API (Sivasangari et al. 2018 ).
Twitter has developed a possible solution to identify and prevent the spread of misleading information through fake accounts, likes and comments (Atodiresei et al. 2018 ) - the Twitter crawler, a machine learning approach works by collecting tweets and adding them to a database, making comparison between different tweets possible.
Knowledge Based Approach
Recent studies argue for the integration of machine learning and knowledge engineering to detect fake news. The challenging problem with some of these fact checking methods is the speed at which fake news spreads on social media. Microblogging platforms such as Twitter causes small pieces of false information to spread very quickly to a large number of people (Qazvinian et al. 2011 ). The knowledge-based approach aims at using sources that are external to verify if the news is fake or real and to identify the news before the spread thereof becomes quicker. There are three main categories; (1) Expert Oriented Fact Checking, (2) Computational Oriented Fact Checking, (3) Crowd Sourcing Oriented Fact Checking (Ahmed et al. 2019 ).
Expert Oriented Fact Checking.
With expert oriented fact checking it is necessary to analyze and examine data and documents carefully (Ahmed et al. 2019 ). Expert-oriented fact-checking requires professionals to evaluate the accuracy of the news manually through research and other studies on the specific claim. Fact checking is the process of assigning certainty to a specific element by comparing the accuracy of the text to another which has previously been fact checked (Vlachos and Riedel 2014 ).
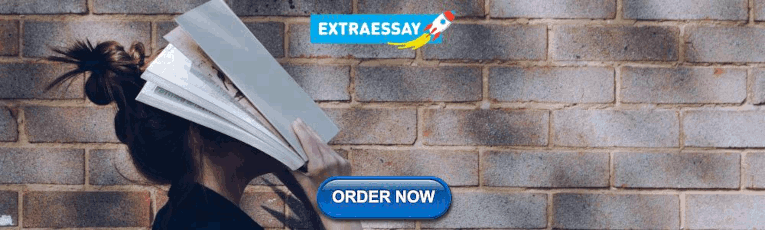
Computational Oriented Fact Checking.
The purpose of computational oriented fact checking is to administer users with an automated fact-checking process that is able to identify if a specific piece of news is true or false (Ahmed et al. 2019 ). An example of computational oriented fact checking is knowledge graphs and open web sources that are based on practical referencing to help distinguish between real and fake news (Ahmed et al. 2019 ). A recent tool called the ClaimBuster has been developed and is an example of how fact checking can automatically identify fake news (Hassan et al. 2017 ). This tool makes use of machine learning techniques combined with natural language processing and a variety of database queries. It analyses context on social media, interviews and speeches in real time to determine ‘facts’ and compares it with a repository that contains verified facts and delivers it to the reader (Hassan et al. 2017 ).
Crowd Sourcing Oriented.
Crowdsourcing gives the opportunity for a group of people to make a collective decision through examining the accuracy of news (Pennycook and Rand 2019 ). The accuracy of the news is completely based on the wisdom of the crowd (Ahmed et al. 2019 ). Kiskkit is an example of a platform that can be used for crowdsourcing where the platform allows a group of people to evaluate pieces of a news article (Hassan et al. 2017 ). After one piece has been evaluated the crowd moves to the next piece for evaluation until the entire news article has been evaluated and the accuracy thereof has been determined by the wisdom of the crowd (Hassan et al. 2017 ).
Hybrid Approach
There are three generally agreed upon elements of fake news articles, the first element is the text of an article, second element is the response that the articles received and lastly the source used that motivate the news article (Ruchansky et al. 2017 ). A recent study has been conducted that proposes a hybrid model which helps to identify fake news on social media through using a combination of human and machine learning to help identify fake news (Okoro et al. 2018 ). Humans only have a 4% chance of identifying fake news if they take a guess and can only identify fake news 54% of the time (Okoro et al. 2018 ). The hybrid model as proven to increase this percentage (Okoro et al. 2018 ). To make the hybrid model effective it combines social media news with machine learning and a network approach (Okoro et al. 2018 ). The purpose of this model is to identify the probability that the news could be fake (Okoro et al. 2018 ). Another hybrid model called CSI (capture, score, integrate) has been developed and functions on the main elements; (1) capture - the process of extracting representations of articles by using a Recurrent Neutral Network (RNN), (2) Score – to create a score and representation vector, (3) Integrate – to integrate the outputs of the capture and score resulting in a vector which is used for classification (Ruchansky et al. 2017 ).
In this paper we discussed the prevalence of fake news and how technology has changed over the last years enabling us to develop tools that can be used in the fight against fake news. We also explored the importance of identifying fake news, the influence that misinformation can have on the public’s decision making and which approaches exist to combat fake news. The current battle against fake news on COVID-19 and the uncertainty surrounding it, shows that a hybrid approach towards fake news detection is needed. Human wisdom as well as digital tools need to be harnessed in this process. Hopefully some of these measures will stay in place and that digital media platform owners and public will take responsibility and work together in detecting and combatting fake news.
- Ahmed, S., Hinkelmann, K., Corradini, F.: Combining machine learning with knowledge engineering to detect fake news in social networks - a survey. In: Proceedings of the AAAI 2019 Spring Symposium, vol. 12 (2019)
- Albright J. Welcome to the era of fake news. Media Commun. 2017; 5 (2):87. 10.17645/mac.v5i2.977. [ CrossRef ] [ Google Scholar ]
- Atodiresei C-S, Tănăselea A, Iftene A. Identifying fake news and fake users on twitter. Procedia Comput. Sci. 2018; 126 :451–461. 10.1016/j.procs.2018.07.279. [ CrossRef ] [ Google Scholar ]
- Burkhardt JM. History of fake news. Libr. Technol. Rep. 2017; 53 (8):37. [ Google Scholar ]
- Castelo, S., Almeida, T., Elghafari, A., Santos, A., Pham, K., Nakamura, E., Freire, J.: A topic-agnostic approach for identifying fake news pages. In: Companion Proceedings of the 2019 World Wide Web Conference on - WWW 2019, pp. 975–980 (2019). 10.1145/3308560.3316739
- Chen, Y., Conroy, N.J., Rubin, V.L.: Misleading online content: recognizing clickbait as false news? In: Proceedings of the 2015 ACM on Workshop on Multimodal Deception Detection - WMDD 2015, Seattle, Washington, USA, pp. 15–19. ACM Press (2015a). 10.1145/2823465.2823467
- Chen Yimin, Conroy Nadia K., Rubin Victoria L. News in an online world: The need for an “automatic crap detector” Proceedings of the Association for Information Science and Technology. 2015; 52 (1):1–4. [ Google Scholar ]
- Hassan, N., Arslan, F., Li, C., Tremayne, M.: Toward automated fact-checking: detecting check-worthy factual claims by claimbuster. In: Proceedings of the 23rd ACM SIGKDD International Conference on Knowledge Discovery and Data Mining - KDD 2017, Halifax, NS, Canada, pp. 1803–1812. ACM Press (2017). 10.1145/3097983.3098131
- Horne, B.D., Adali, S.: This just in: fake news packs a lot in title, uses simpler, repetitive content in text body, more similar to satire than real news. In: International AAAI Conference on Web and Social Media, vol. 8 (2017)
- Macaulay, T.: Can technology solve the fake news problem it helped create? (2018). https://www.techworld.com/startups/can-technology-solve-fake-news-problem-it-helped-create-3672139/
- Marr, B.: Coronavirus fake news: how Facebook, Twitter, and Instagram are tackling the problem. Forbes (2020). https://www.forbes.com/sites/bernardmarr/2020/03/27/finding-the-truth-about-covid-19-how-facebook-twitter-and-instagram-are-tackling-fake-news/
- Okoro EM, Abara BA, Umagba AO, Ajonye AA, Isa ZS. A hybrid approach to fake news detection on social media. Niger. J. Technol. 2018; 37 (2):454. 10.4314/njt.v37i2.22. [ CrossRef ] [ Google Scholar ]
- Paskin D. Real or fake news: who knows? J. Soc. Media Soc. 2018; 7 (2):252–273. [ Google Scholar ]
- Pennycook G, Rand DG. Fighting misinformation on social media using crowdsourced judgments of news source quality. Proc. Natl. Acad. Sci. 2019; 116 (7):2521–2526. 10.1073/pnas.1806781116. [ Europe PMC free article ] [ Abstract ] [ CrossRef ] [ Google Scholar ]
- Pérez-Rosas, V., Kleinberg, B., Lefevre, A., Mihalcea, R.: Automatic detection of fake news. In: Proceedings of the 27th International Conference on Computational Linguistics, Santa Fe, New Mexico, USA, pp. 3391–3401. Association for Computational Linguistics (2018). https://www.aclweb.org/anthology/C18-1287
- Potthast, M., Kiesel, J., Reinartz, K., Bevendorff, J., Stein, B.: A stylometric inquiry into hyperpartisan and fake news (2017). arXiv Preprint arXiv:1702.05638
- Qazvinian, V., Rosengren, E., Radev, D.R., Mei, Q.: Rumor has it: identifying misinformation in microblogs. In: Proceedings of the 2011 Conference on Empirical Methods in Natural Language Processing, EMNLP 2011, pp. 1589–1599 (2011)
- Ruchansky, N., Seo, S., Liu, Y.: CSI: a hybrid deep model for fake news detection. In: Proceedings of the 2017 ACM on Conference on Information and Knowledge Management, CIKM 2017, pp. 797–806 (2017). 10.1145/3132847.3132877
- Schade, U.: Software that can automatically detect fake news. Comput. Sci. Eng. 3 (2019)
- Shao C, Ciampaglia GL, Varol O, Yang K, Flammini A, Menczer F. The spread of low-credibility content by social bots. Nat. Commun. 2018; 9 (1):4787. 10.1038/s41467-018-06930-7. [ Europe PMC free article ] [ Abstract ] [ CrossRef ] [ Google Scholar ]
- Sivasangari V, Anand PV, Santhya R. A modern approach to identify the fake news using machine learning. Int. J. Pure Appl. Math. 2018; 118 (20):10. [ Google Scholar ]
- Sparks, H., Frishberg, H.: Facebook gives step-by-step instructions on how to spot fake news (2020). https://nypost.com/2020/03/26/facebook-gives-step-by-step-instructions-on-how-to-spot-fake-news/
- Stahl, K.: Fake news detection in social media. California State University Stanislaus, 6 (2018)
- Stein-Smith, K.: Librarians, information literacy, and fake news. Strat. Libr. 37 (2017)
- Thota, A., Tilak, P., Ahluwalia, S., Lohia, N.: Fake news detection: a deep learning approach. SMU Data Sci. Rev. 1 (3), 21 (2018)
- Vlachos, A., Riedel, S.: Fact checking: task definition and dataset construction. In: Proceedings of the ACL 2014 Workshop on Language Technologies and Computational Social Science, Baltimore, MD, USA, pp. 18–22. Association for Computational Linguistics (2014). 10.3115/v1/W14-2508
- Yang, Y., Zheng, L., Zhang, J., Cui, Q., Li, Z., Yu, P.S.: TI-CNN: convolutional neural networks for fake news detection (2018). arXiv preprint arXiv:1806.00749
- Zhou, X., Zafarani, R.: Fake news: a survey of research, detection methods, and opportunities (2018). arXiv preprint arXiv:1812.00315
Data behind the article
This data has been text mined from the article, or deposited into data resources.
BioStudies: supplemental material and supporting data
- http://www.ebi.ac.uk/biostudies/studies/S-EPMC7250114?xr=true
Europe PMC is part of the ELIXIR infrastructure
Fake news in business and management literature: a systematic review of definitions, theories, methods and implications
Aslib Journal of Information Management
ISSN : 2050-3806
Article publication date: 16 November 2023
This paper aims to conduct an interdisciplinary systematic literature review (SLR) of fake news research and to advance the socio-technical understanding of digital information practices and platforms in business and management studies.
Design/methodology/approach
The paper applies a focused, SLR method to analyze articles on fake news in business and management journals from 2010 to 2020.
The paper analyzes the definition, theoretical frameworks, methods and research gaps of fake news in the business and management domains. It also identifies some promising research opportunities for future scholars.
Practical implications
The paper offers practical implications for various stakeholders who are affected by or involved in fake news dissemination, such as brands, consumers and policymakers. It provides recommendations to cope with the challenges and risks of fake news.
Social implications
The paper discusses the social consequences and future threats of fake news, especially in relation to social networking and social media. It calls for more awareness and responsibility from online communities to prevent and combat fake news.
Originality/value
The paper contributes to the literature on information management by showing the importance and consequences of fake news sharing for societies. It is among the frontier systematic reviews in the field that covers studies from different disciplines and focuses on business and management studies.
- Disinformation
- Misinformation
- Social media
- Systematic literature review (SLR)
Farhoudinia, B. , Ozturkcan, S. and Kasap, N. (2023), "Fake news in business and management literature: a systematic review of definitions, theories, methods and implications", Aslib Journal of Information Management , Vol. ahead-of-print No. ahead-of-print. https://doi.org/10.1108/AJIM-09-2022-0418
Emerald Publishing Limited
Copyright © 2023, Bahareh Farhoudinia, Selcen Ozturkcan and Nihat Kasap
Published by Emerald Publishing Limited. This article is published under the Creative Commons Attribution (CC BY 4.0) licence. Anyone may reproduce, distribute, translate and create derivative works of this article (for both commercial and non-commercial purposes), subject to full attribution to the original publication and authors. The full terms of this licence may be seen at http://creativecommons.org/licences/by/4.0/legalcode
1. Introduction
Social media is widely regarded as a primary news source for many people. It is accessible, often free and easily promoted, making it easy to spread information, including fake news. The COVID-19 pandemic has only heightened concerns about fake news, as the spread of false information about the pandemic, such as linking 5G cell towers to human immune system issues, has led to serious consequences ( Mourad et al. , 2020 ). In recent years, there has been a growing body of research on fake news, with a particular focus during the pandemic ( Elías and Catalan-Matamoros, 2020 , Hartley and Vu, 2020 , Islam et al ., 2020a , Laato et al. , 2020 , Marin, 2021 , Naeem and Bhatti, 2020 , Pennycook et al ., 2020b ). Twitter was claimed by Thelwall and Thelwall (2020) to have positively impacted information sharing during the COVID-19 pandemic, but it was also widely used to spread fake news. Among many similar electoral events, the 2016 USA presidential election marked a noteworthy milestone in which Facebook-based fake news sources were believed to have significantly impacted the election result ( Meel and Vishwakarma, 2020 ). Barfar (2019) studied the spread of fake political news and observed strong reactions to fake liberal news, including anger. Fake stories spread faster than true ones on Twitter ( Vosoughi et al. , 2018 ), making companies and organizations vulnerable to the consequences of fake news. Fake news about a company could easily influence its stock price, leading to severe financial losses.
The proliferation of health-related fake news on social media is a significant concern with far-reaching consequences. Several studies have reviewed the literature on this issue and identified various types of misinformation, such as false claims about treatments, disease origins and conspiracy theories. The impact of this misinformation on public health has also been discussed, including its promotion of vaccine hesitancy, encouragement of risky health behaviors and erosion of trust in public health authorities.
Several approaches have been suggested to combat the spread of fake news, including fact-checking, social media platform policies, public health campaigns and the use of artificial intelligence. However, these approaches have limitations and require further research and development to improve their accuracy and efficiency.
In this context, we contribute to this emerging field by providing a comprehensive review of the literature related to fake news from a business and management perspective. Our review includes different types of fake news, definitions, theoretical and psychological background and fake news detection approaches through a systematic review. We also highlight the possible consequences of fake news on business and emphasize the importance of this topic from a managerial point of view.
Our review addresses research gaps and provides future research directions for multiple disciplines such as computer science, social science, psychology and policymaking, which cross paths with business and management researchers. We have sourced our collection of research papers from academic journals published within the business and management field by enclosing manuscripts published only in the Academic Journal Guide (AJG)-listed journals [1] . We believe that our review provides interested researchers from various disciplines with valuable insights to advance their academic research on fake news.
The paper is structured as follows: Sections 2 and 3 provide a literature review and methodology, respectively. Section 4 presents the findings. Section 5 discusses limitations, implications and future research opportunities. Finally, Section 6 summarizes the conclusions.
2. Literature review
Many review papers have focused on the topic of fake news detection methods. For instance, Nirav Shah and Ganatra (2022) reviewed 68 articles published between 2015 and 2021 to introduce various challenges in developing effective fake news detection models, such as the absence of standard datasets, various types of fake news and the need for detection models in diverse languages and cultural contexts. Similarly, Thompson et al. (2022) reviewed 62 articles published between 2016 and 2020 to provide an overview of online fake news detection approaches, including content-based, social network-based and hybrid approaches. The authors also discussed the most widely used datasets, evaluation metrics and machine learning algorithms.
In another study, Mridha et al. (2021) conducted a comprehensive review of deep learning techniques for detecting fake news. The paper covers 77 articles published between 2016 and 2021 and highlights the need for more realistic datasets and detection models in diverse languages and cultural contexts. Similarly, Shahzad et al. (2022) provided an overview of the relationship between big data analytics and context-based fake news detection. They reviewed 41 articles published between 2016 and 2021, addressing the methods used for fake news detection, including traditional methods such as fact-checking and new methods based on big data analytics. The authors revealed several research gaps, such as the lack of research on the effectiveness of detection methods, the ethical and legal implications of using big data analytics for fake news detection and the impact of fake news on public opinion and decision-making.
Furthermore, Saquete et al. (2020) reviewed natural language processing methods for fake news detection, including text classification, sentiment analysis and fact-checking. The authors addressed challenges in the field of natural language processing (NLP), such as the problem of biased training data and the need to develop more effective approaches to combat the spread of fake news on social media. Islam et al . (2020b) focused on using deep learning to detect misinformation on social media. They reviewed 70 articles published between 2015 and 2021, covering deep learning methods such as neural networks, convolutional neural networks, recurrent neural networks and attention mechanisms. The authors suggested that future research should focus on developing more advanced deep learning techniques to effectively detect and combat the spread of misinformation on online social networks.
Furthermore, Kaddoura et al. (2022) provided an overview of spam detection and classification. They reviewed 122 papers published between 2011 and 2019, examining the techniques used to detect spam, including rule-based approaches, content-based approaches and machine learning algorithms. The authors highlighted the importance of feature selection in spam detection and the need for large and diverse datasets. They emphasized the need for more research in this area, as spam and fake news pose a significant threat to individuals and organizations.
In a more interdisciplinary approach, Zhou and Zafarani (2020) provided a review of fake news detection methods and challenges and an overview of fundamental theories across various disciplines to encourage interdisciplinary research on fake news. The authors discussed various types of fake news, such as fabricated news, clickbait, satire and propaganda. They addressed the impact of fake news on society and noted that there is no one-size-fits-all solution for detecting fake news. They also highlighted the need for more research in this field.
2.1 Fake news about health
Fake news related to health is a pernicious phenomenon that can lead to serious crises, and as such, it has attracted significant attention from scholars across various disciplines. Wang et al. (2019) conducted a thorough review of the literature on health-related misinformation on social media, examining 40 research papers published between 2010 and 2020. They identified different types of health-related misinformation, including false claims about treatment efficacy, disease origins and conspiracy theories. Moreover, they discussed the deleterious impact of such misinformation on public health, including vaccine hesitancy, risky health behaviors and erosion of trust in public health authorities.
Building upon Wang et al .'s seminal work, Melchior and Oliveira (2022) conducted a comprehensive review of the literature on health-related fake news on social media. They identified key factors contributing to the spread of such fake news on social media platforms and critically reviewed the approaches aimed at combating its proliferation, including fact-checking, social media platform policies and public health campaigns.
Similarly, Balakrishnan et al. (2022) examined the infodemic and fake news related to COVID-19, drawing on 74 research papers published between 2020 and 2021. Their review revealed false claims regarding the virus's origins, conspiracy theories and inaccurate information about the effectiveness of vaccines and treatments. The authors highlighted research gaps, such as a lack of empirical studies on the impact of the infodemic on public health and healthcare systems and a limited understanding of the effectiveness of interventions designed to combat the spread of fake news.
Ahmad et al. (2022) provided an insightful overview of the role of fake news in the COVID-19 pandemic and the use of artificial intelligence to combat its spread. They reviewed 56 research papers published in January and December 2020 and noted that existing approaches have limitations, such as the need for more accurate and efficient natural language processing techniques, better training data and the potential for biases and errors in artificial intelligence (AI)-based systems. They recommended future research that employs interdisciplinary approaches from computer science, communication studies and the social sciences.
Aïmeur et al. (2023) provided a general overview of the literature on fake news, disinformation and misinformation issues. They covered research papers published between 2016 and 2021, exploring the definitions of these concepts and the impact of fake news on society, politics and the economy. The authors highlighted the potential consequences of fake news, including the erosion of trust in institutions and the media, political polarization and the spread of conspiracy theories. They also discussed various approaches and strategies to address fake news, such as fact-checking, media literacy and regulatory interventions, emphasizing the need for more effective methods to detect and combat fake news.
Kim et al. (2021) reviewed fake news from the perspective of news creation and consumption, drawing on 91 research studies published between 2014 and 2020. They provided various approaches to fake news, such as content analysis, social network analysis and machine learning. The authors revealed research gaps, including a limited understanding of psychological and social factors that influence the consumption of fake news. They also highlighted the need for more research on the ethical and legal implications of fake news.
In their recent study, Vasist and Krishnan (2023) conducted a comprehensive literature review, analyzing the relationship between fake news and sustainability-focused innovations from various perspectives. Their review incorporated 31 research papers published between 2016 and 2021, suggesting that fake news can act as a barrier to adopting sustainability-focused innovations by spreading misleading information about their benefits. To combat this issue, the authors recommended that future research focus on developing strategies to counteract the spread of fake news associated with sustainability-focused innovations.
Similarly, Damstra et al. (2021) also provided a literature review, exploring different forms of intentional deception, including fake news, disinformation, propaganda and conspiracy theories. Their analysis highlighted the significant impact that intentional deception can have on both society and individuals, such as the erosion of trust in institutions and the potential for political and social polarization.
Furthermore, Alkhamees et al. (2021) conducted a thorough review of the literature on user trustworthiness in social media, examining 69 papers published between 2004 and 2018. Their paper described various dimensions of user trustworthiness, including reliability, integrity and competence and emphasized the importance of developing effective strategies to enhance user trustworthiness. These strategies included providing users with feedback and incentives and promoting transparency and accountability.
To advance the emerging field of fake news research, we present a comprehensive literature review that explores the topic from the perspective of articles published within the business and management disciplines. Our systematic review offers an overview of different types of fake news, their definitions, theoretical and psychological background and fake news detection approaches. Importantly, we also provide insights into the potential consequences of fake news on businesses and address the significance of this topic from a managerial standpoint, distinguishing our work from previous systematic review papers.
To ensure the rigor of our review, we retrieved a collection of research papers exclusively from journals listed in the AJG, which is a trusted guide of academic journals within the business and management field as endorsed by the Chartered Association of Business Schools (ABS). By addressing research gaps and providing future research directions, our review serves as a valuable resource for multi-disciplinary and interdisciplinary researchers in computer science, social science, psychology, policymaking and management. Our review aims to assist researchers from diverse disciplines in identifying fruitful avenues for further research in the realm of fake news.
The extraordinary growth of fake news and its dangers to societies by threatening democracy, justice, freedom of expression and public trust further intensify the need for emerging research on this topic. This manuscript aims to contribute to the growing information management literature by providing a systematic review of the published business and management literature in the AJG-listed journals between the years 2010 and 2020 to shed light on several research questions: First, we unpack the definition of “fake news” in the business and management literature during the period of our chosen focus. Next, we seek out the most common theoretical frameworks that scholars in business and management use to study fake news. Then, we identify the most commonly employed research methods used in these studies. We explore the major research gaps in past business and management studies included in our dataset. Finally, we uncover some promising research opportunities to point future researchers in the right direction.
3. Methodology
This paper aims to be an unbiased and reproducible study ( Nolan and Garavan, 2016 , Nguyen et al. , 2018 ). We adhere to the systematic review's five-step process that Denyer and Tranfield (2009) outlined. These steps are, namely, (1) formulate the research questions; (2) find studies; (3) select and evaluate studies; (4) analyze the findings; and (5) report the results. The research questions were established first. Then, a search strategy was developed to cover all relevant past research in the field using keywords. The databases were searched accordingly and the results were collected. Filtering criteria were established to refine the results.
The initial search for the term “fake news” and its synonyms, such as “hoax news” and “disinformation,” was conducted in the Scopus, Web of Science, Ebsco, ProQuest, Jstor and Emerald databases. This research focuses on fake news on social media. This review is restricted to English-language articles published in the AJG-listed journals, which are endorsed by the ABS. The time frame of 2010–2020 was selected as this was when fake news on social media became a significant challenge for societies and businesses, while not many studies were published before 2010. Figure 1 illustrates the search steps and the number of papers excluded in every phase, where the funneling led to eighty-one articles being included in this systematic review.
4. Analysis of findings
The analysis indicated that the frequency of articles on the topic showed a dramatic increase in 2019. Figure 2 illustrates the frequency of all articles published with respect to their publication years, ranging from 2010 to 2020. The emerging high frequency of the articles only in recent years could be due to the novelty of the research topic and the 2016 USA presidential election ( Wang et al. , 2019 ; Carlson, 2020 ), marking the relevancy and timeliness of the field.
The articles included in the dataset came from various disciplines that have been published in the business and management literature. Figure 3 indicates the number of articles in each field, including journalism, health, psychology, political science, information science, computer science, management and marketing. Determining a single specific discipline for some articles proved elusive; therefore, they were classified into multiple domains where relevant. Five review articles were found in the literature: Lozano et al. (2020) studied and reviewed computerized veracity assessment methods; Meel and Vishwakarma (2020) focused on theories from different disciplines to solve the problem of truthfulness and credibility analyses of web content; Wang et al. (2019) examined health-related misinformation explicitly by reviewing relevant literature to understand the procedures and mechanisms of misinformation spread; Baccarella et al. (2018) investigated the dark side of social media such as “cyberbullying, addictive use, trolling, online witch hunts, fake news, or privacy abuse.”
4.1 The definition of “fake news”
Fake news takes various forms, including misinformation and disinformation. Misinformation refers to false, inaccurate, or incomplete information (e.g. Berthon and Pitt, 2018 ; Acker and Donovan, 2019 ; Carrieri et al. , 2019 ; Brashier and Schacter, 2020 , Islam et al ., 2020a ). Disinformation refers to incorrect information shared intentionally. Fake news can encompass both definitions, but it is commonly defined as intentionally false, fabricated news articles that can deceive the reader (e.g. Allcott and Gentzkow, 2017 ; Chen and Cheng, 2019 ; Colliander, 2019 ; Flostrand et al. , 2019 ; Kim and Dennis, 2019 ; Kim et al. , 2019 ; Lee et al. , 2019 ; Borges‐Tiago et al. , 2020 ; Di Domenico and Visentin, 2020 ; Kwanda and Lin, 2020 ). According to Ozbay and Alatas (2020) , any low-quality or incomplete news can be fake. Fake news is also referred to as “post-truth,” meaning that emotions and personal beliefs play a greater role in shaping public opinion than objective facts ( Koro-Ljungberg et al. , 2019 ).
Fake news can be in the form of satire, rumors, pictures, or videos. Rumors are unverified information that may be true or false, whereas fake news is false information spread through news outlets ( Wu et al. , 2020 ). User-generated content is any digital content created and posted as videos, pictures, blogs and tweets on social media ( Rajamma et al. , 2019 ). Advances in computer graphics, computer vision and machine learning have made it possible to synthesize fake images or videos ( Agarwal et al. , 2020 ). The term “deepfake” refers to convincing digital content, especially videos. Deepfakes can pose a threat to politicians, celebrities, companies and brands. Career-related fake information on social media is another form of fake information on these platforms ( Sampson et al. , 2018 ). Clickbait is a form of fake news shared on social media. It is a sensational headline that encourages clicks to a separate webpage by providing some information ( Chua et al. , 2021 ).
4.2 Theoretical review
The collective theoretical framework of the papers aims to answer questions such as why people believe and spread fake news (e.g. Al-Rawi et al. , 2019 ; Apuke and Omar 2020 ; Talwar et al ., 2020 ) and identify the characteristics of those who share or contribute to the spread of fake news (e.g. Ben-Gal et al. , 2019 ; Chen and Cheng, 2019 ; Sela et al. , 2020 ; Brashier and Schacter, 2020 ; Duffy et al. , 2020 ). Researchers have stated that fake news sharing can be influenced by confirmation bias, which occurs when individuals prefer to share news that aligns with their existing beliefs ( Kim et al. , 2019 ). Kahneman (2011) proposed that humans are not always rational in decision-making and have two types of cognition: System 1 and System 2. System 1 is fast, intuitive and influenced by confirmation bias, while System 2 is deliberate, slow and requires more effort. Moreover, Kim and Dennis (2019 ) argue that social media users rely on System 1 cognition, leading to the rapid spread of fake news on these platforms. Social media can form echo chambers where beliefs are reinforced without exposure to opposing views ( Meel and Vishwakarma, 2020 ), as the platforms tend to display content that aligns with a user's interests more frequently. As a result, users are less likely to encounter opposing ideas or news, making fake news more credible. Many papers have suggested that the echo chamber effect contributes to the belief and spread of false information on social media (e.g. Allcott and Gentzkow, 2017 ; Berthon and Pitt, 2018 ; Chua and Banerjee, 2018 ; Long et al. , 2019 ; Peterson, 2019 ; Di Domenico and Visentin, 2020 ).
4.3 Methodology review
The papers reviewed in this study had different methodologies depending on their objectives. Papers focusing on the psychology of fake news behavior used surveys or experiments to test theoretical concepts. Papers aimed at detecting and analyzing fake news applied machine learning or deep learning to identify its features. Another group of papers studied the spread of false information using network analysis, resulting in the classification of the papers into three categories.
4.3.1 Experiments and surveys
Psychological research in the reviewed studies aimed to investigate user behavior and motivation toward fake news. The research employed surveys and experiments to test their hypotheses, focusing on the sharing behaviors of social media users. The papers examined the impact of personality traits on an individual's ability to detect, believe and spread fake news ( Lutzke et al. , 2019 ; Thompson et al. , 2020 ; Wolverton and Stevens, 2019 ). For instance, Talwar et al. (2019) proposed that online trust, self-disclosure, fear of missing out (FoMO) and social media fatigue are positively related to sharing behavior. The researchers designed a survey and used Indian WhatsApp users as their sample population. Meanwhile, Laato et al. (2020) suggested that trust in online information is a strong predictor of sharing unverified information. This finding was established through an online survey of 1,000 students. The articles, which included experiments and surveys, were typically published in psychology or marketing journals. Online questionnaire-based survey research from the USA and India confirms that conservatives and collectivists prefer to believe false news more. This study reveals traits that make people more likely to believe bogus news. This study also examines control factors including age, sex and Internet use. Findings indicate conceptual implications and actionable insights ( Gupta et al. , 2023 ). Kim and Dennis (2019) run two online tests to determine the impact of a source assessment icon (a positive or negative summary of the evaluation). They find that positive and negative symbols and details have different effects. Negative symbols lower article credibility, while positive symbols do not affect credibility. They also discover that people are more inclined to check the assessment details when the article content matched their pre-existing attitudes, regardless of icon valence. Mirhoseini et al. (2023) through a laboratory experiment and online survey examines why individuals believe fake news and offers a solution. Behavioral and neurophysiological data suggest closed-mindedness promotes fake news. Performance feedback decreases overconfidence and improves analytical thinking and improves detecting fake news by 14%. This study supports classical thinking and suggests a strategy to improve fake news identification.
4.3.2 Machine learning approach
Machine learning is a field of study that allows machines to perform tasks without explicit programming. It has been widely used in prediction tasks due to its ability to analyze large amounts of data. Machine learning methods have proven to be useful in fake news studies, as they enable researchers to effectively process large datasets ( Ongsulee, 2017 ). In the reviewed literature, several articles utilized machine learning or deep learning methods to detect fake news or identify relevant features. The detection of fake news has garnered attention from researchers across various disciplines, particularly computer scientists. Social media platforms provide ample amounts of big data for research purposes, making machine learning techniques an attractive solution for detecting fake news.
Fake news detection can be accomplished based on the linguistic features of the texts. Faustini and Covões (2020) proposed detecting fake news using only text features. They used K-Nearest Neighbors (KNN), Random Forest, Gaussian Naïve Bayes and Support Vector Machine (SVM) algorithms to detect fake news on social media. Ozbay and Alatas (2020) suggested a two-step method to identify fake news on social media. The first step is preprocessing the data with a term frequency-inverse document frequency (TF-IDF) weighting method. They applied 23 supervised machine learning algorithms combined with text mining features to a dataset containing news stories. Bot detection is another field that has attracted researchers. Since fake news creators do not prefer their identities to be recognized, they often use bots to spread misleading information ( Groshek et al ., 2019 ; Jones, 2019 ; Ross et al. , 2019 ).
Different types of features have been proposed for fake news detection models, including text/content-specific, image-specific, user/account, propagation, temporal, structural and linguistic features. Machine learning methods such as Naive Bayes, SVM, decision tree, random forest and logistic regression have been utilized in this field. However, one disadvantage of these machine learning methods is that they typically rely on manually crafted features, which require human effort and can introduce bias. Recent research has employed deep learning-based models to overcome this limitation.
4.3.3 Deep learning approach
Deep learning models can automatically extract hidden information from text, image, sentiment, or structure and are useful in analyzing tweets to detect fake information online ( Meel and Vishwakarma, 2020 ). Deep learning models excel in text classification due to their ability to learn hierarchical representations of data, revealing hidden patterns. Deep learning models may automatically learn hierarchical data representations due to their many layers and can learn from raw data without human involvement, eliminating the need for manual feature creation ( Goodfellow et al. , 2016 ). Deep learning models can handle enormous data and represent nonlinear connections between characteristics and projected classes. Deep learning has shown effective in computer vision, speech recognition and NLP. Zhang et al. (2023) use behavioral and textual data to identify fake reviewers. Their study consists of two primary parts: A behavior-sensitive feature extractor learns reviewing patterns and a context-aware attention mechanism extracts important traits from online reviews. Two http://Yelp.com datasets are used to assess the modules and architecture against industry standards. They utilized several machine learning and deep-learning models such as SVM, Logistic Regression, Random Forest and Convolutional neural networks. Xia et al. (2023) use a CNN–BiLSTM–AM model to improve the identification of fake news and emphasizes how crucial it is to scientifically disprove erroneous information in order to facilitate public-government dialog and the creation of a trustworthy news release system. The study proposes future approaches for more comprehensive media analysis and enhanced model generalization, while also acknowledging granularity and possible overfitting constraints.
Deep learning algorithms are used in fake news detection research. Rodrigues et al. (2022) evaluate tweets for spam. The research incorporates sentiment analysis. Convolutional neural network (CNN) and long short-term memory (LSTM) networks identify tweet sentiment. They utilize real-time spam identification and sentiment analysis for Twitter fake news detection. Amer et al. (2022) conducted three experiments: one using machine learning classifiers, one using deep learning models and one utilizing transformers. Word embedding is used to extract contextual characteristics from articles in each test. The trials reveal that deep learning models outperform machine learning classifiers and transformers in accuracy. However, while machine learning and deep learning-based models have proven to be useful, research has shown that algorithmic detection methods are not as effective as human intervention ( Marsden et al. , 2020 ).
4.3.4 Transformer-based approaches
Transformer-based approaches are a class of deep learning models. Transformer-based fake news detection methods are becoming more popular due to their natural language comprehension capability. Bidirectional Encoder Representations from Transformers (BERT), Robustly Optimized BERT Approach (RoBERTa), Generative Pre-trained Transformer (GPT) and other transformer models have been pretrained on enormous Internet text corpora. These models have learned extensive word contextual representations and may be fine-tuned for specific tasks ( Devlin et al. , 2018 ). In order to identify COVID-19 fake news, Alghamdi et al. (2023) focus on machine learning techniques and improved transformer-based models like BERT and COVID-Twitter-BERT. On top of these models, they test multiple neural network topologies (CNN and Bidirectional Gate recurrent unit (BiGRU)) with varying parameter settings. The tests conducted using actual COVID-19 fake data demonstrate that the addition of BiGRU to Clinical Trial Information Extraction with BERT (CT-BERT) produces remarkable outcomes, reaching a state-of-the-art F1 score of 98%. In a benchmark research, Khan et al. (2021) compared three different datasets worth of machine learning techniques in order to detect fake news. Even with little data, they discovered that pretrained models like BERT performed better than others. When it comes to languages with little training data, these models are suggested. The study also looked at word count relationships, article subjects and model performance.
4.3.5 Propagation-based approaches
The fake news dissemination process is like the spread of infectious disease and can be understood with network epidemic models. Vosoughi et al. (2018) analyzed a dataset of rumor cascades consisting of tweets and retweets and found that false information spreads faster on social media than accurate information. Lord Ferguson et al. (2019) developed a framework to explain the spread of fake news in the health industry. Giglietto et al . (2019) emphasized that while many researchers have focused on the creation of misleading information and the intention of the creator, it is crucial to focus on the propagation of information to understand how both true and false information spreads. Other researchers have analyzed the role of spreading groups in the propagation of fake news using network analysis on Twitter. They studied the differences between users involved in highly repeated and lowly repeated cascades and found that the distribution of retweets was significantly different (e.g. Sela et al. , 2020 ). They discovered that the messages of a few anonymous Twitter accounts spread more widely than those of well-known accounts. Pantumsinchai (2018) explained how a claim could be perceived as fact or fiction through networks of interactions during major events. Papanastasiou (2020) suggested that individuals are more likely to share the news if their peers have already disseminated it. This indicates that social influence plays a crucial role in the spreading of information on social media. Furthermore, the study highlights the importance of understanding the role of social influence in the spread of information, both true and false.
Table 1 summarizes articles in the primary discipline of computer science with the objective, dataset, and method used in the respective manuscripts classifies articles in the computer sciences into three classes with similar goals and details of the corresponding methods.
4.4 Managerial review
The spread of fake news on social media can have serious consequences for businesses and their brands. Inaccurate information can quickly spread, leading to a negative perception of the company and financial losses. Therefore, it is important for brand managers to have a strategy in place to mitigate the risks posed by fake news. This may include monitoring social media for false information, developing crisis management plans and proactively communicating with customers to dispel rumors and restore trust in the brand. In August 2017, a widely disseminated tweet claimed that Starbucks provided discounts to undocumented immigrants ( Tschiatschek et al. , 2018 ). Starbucks denied the claim by directly responding to users who shared it as a response to the rumor. PepsiCo faced a boycott and a 4% decrease in stock price after false news about the company circulated on social media. The news claimed that the PepsiCo's chief executive officer (CEO) had told Trump supporters to “take their business elsewhere” ( Berthon and Pitt, 2018 ). Kentucky Fried Chicken (KFC) was accused of selling rats instead of chicken ( Pal et al. , 2017 ). These examples demonstrate the impact fake news can have on a company's reputation and financial stability. In the age of social media, false information can spread rapidly, and it can be challenging for companies to effectively combat it. Brand managers must be proactive and have a strategy in place to address fake news and protect their brand's image.
Studies have illustrated the consequences of fake news on brands ( Ryan et al. , 2020 ) and suggest response strategies for managers ( Mills and Robson, 2019 ; Vafeiadis et al. , 2020 ). Marketing and psychology researchers have studied consumer characteristics and the potential factors that influence customers' sharing behaviors ( Beuk et al. , 2019 ; Chen and Cheng, 2019 ; Talwar et al. , 2019 ). These studies help managers understand how fake news affects the public's perception of their brands and the ways in which they can respond to and mitigate the impact of fake news. Table 2 summarizes papers addressing fake news' managerial and marketing impacts with useful findings for brands and managers.
5. Discussion
This paper provides a comprehensive overview of the research on fake news. It highlights that fake news is characterized as false information that spreads through various platforms, including social media. The intention behind creating fake news, whether it is to harm an individual, group or entity, is also an important aspect. Fake news has become a popular research topic since the 2016 USA presidential election and its consequences, with interdisciplinary researchers from fields such as information technology (IT) and psychology contributing to the field. The growth in popularity of social media has also led to an increase in research on fake news spread through these platforms, with the COVID-19 pandemic being a recent event that has contributed to the spread of fake news.
The rise of fake news has led to the emergence of fact-checking services, but their effectiveness in reducing misperceptions and increasing trust among users is still unclear. According to ( Marres, 2018 ), fact-checking may not be successful in reducing misperceptions, especially among people who are prone to believing them. Brandtzaeg and Følstad (2017) found that users with opposing opinions are more concerned about the trustworthiness of fact-checking websites than those with positive perceptions. These findings suggest that the effectiveness of fact-checking services in reducing fake news and increasing trust among users may be limited.
Pennycook et al . (2020a) conduct a study that showed that warnings about fake news titles could actually increase misperception among users. The study found that even if the news is false, it appears more accurate when fact-checking sites label parts of it. This highlights the need for a more human-focused approach to information dissemination, as the sheer amount of information flowing on social media and the fast pace of trending topics can make it difficult for organizations to keep up. As a result, social media platforms, traditional media and institutions must encourage users to spend more time and cognitive effort evaluating news on social media before accepting it as accurate ( Di Domenico and Visentin, 2020 ).
5.1 Managerial implications
Fake news might severely affect marketing brands, organizations and consumer behavior. There are real examples that prove the relevance of the topic for managers. The two businesses that fake news specifically targeted were PepsiCo and Starbucks ( Berthon and Pitt, 2018 , Tschiatschek et al. , 2018 ). The consumers' sharing behaviors differ based on their loyalty to the company. When consumers identify more closely with the brand, the threat to the brand is not very serious, and a trustworthy denial strategy is the best choice ( Mills and Robson, 2019 ). Defining the best response strategy is crucial for companies in the era of fake news. Mustak et al. (2023) reveal that the greatest hazards to organizations are harm to their image, reputation and trustworthiness and the quick obsolescence of technology. To safeguard against market deceptions caused by deepfakes, companies should spend in creating resources and capabilities. This involves investing in technologies to improve a company's deepfake detection and avoidance capabilities. Additionally, they should invest in human resources to mitigate the possible negative consequences of deep-fake technologies. Additionally, managers must address possible customer harm and take preventive efforts to protect them. Cheng et al. (2023) predicts abnormal stock trading behavior using social media data (posts, likes and responses) and decision tree induction. They find that rumor propagation predicts abnormal trading behavior better than management shocks and other factors. Sharif et al. (2022) suggest that businesses must understand how fake news affects behavioral intentions and plan accordingly. Favorable brand experience, trust and credibility create favorable brand behavioral intentions, according to this study businesses that prioritize pleasant brand experience have higher brand trust and credibility and are less influenced by fake news. Rahadian and Nurfitriani (2022) explores the impact of COVID-19-related news on stock market returns, using quantile regression analysis technique they find that fake news and panic can affect stock market returns. Sharif et al. (2022) explore how fake news affects consumer behavior. Positive brand experience, trust and credibility create favorable brand behavioral intentions. They find that businesses that prioritize pleasant brand experiences have higher brand trust and credibility and are less influenced by fake news. Companies must consider fake news as a possible threat and create suitable contingency plans to avoid spreading untrue information about them. Therefore, it is crucial for companies to know and recognize fake news' characteristics and how they propagate in order to detect them. While scholars have conducted valuable research on how fake news can affect businesses and companies, there is still room for further research. Fake news is an essential threat to brands, and future researchers must pay more attention to this field of study.
5.2 Limitations and research gaps
The detection of fake news has been a hot topic in the last few years, making it not a new challenge. Before 2010, fake news was a well-known topic that mainly included traditional media like TV or newspapers. Limitations of this study's findings could be inherited from its design, which purposefully narrowed its sampling to literature published only after 2010 and published in journals included in the AJG-listed journals. Future research could benefit from including a wider range of sources, such as conference proceedings (e.g. Kaliyar, 2018 ; Rana et al. , 2018 ; Zhang et al. , 2018 ) and gray literature, to gain a more comprehensive understanding of the current state of fake news detection. Moreover, the field is rapidly evolving, and new techniques and approaches are emerging all the time, so it is essential to continuously update the knowledge in this area. Thus, the dataset does not include conference papers, while several manuscripts were published as conference proceedings in engineering and computer science, which might contribute to the existing methods and algorithms.
The analysis points to several gaps identified in the existing literature. First, gathering human-labeled datasets has the potential to significantly contribute to the existing literature since only a limited number of datasets have been used. Second, as per methodology, unsupervised machine learning, deep learning and transfer learning can be utilized for better fake news detection. These methods have the potential to enable scholars to utilize social media-based big data. To the best of our knowledge, previous studies rarely applied qualitative research methods simultaneously with social media analysis ( Ozturkcan et al. , 2017 ). The results of these two methods can complement each other to further inform the literature. The emotions associated with fake news and their impacts on engagement are also under-researched areas that require further attention. These gaps in the literature suggest that there is a need for more research in the field of fake news detection and its impact on businesses and consumers.
5.3 Future research
Despite the significant attention given to fake news research in recent years, there is still a need for more research in this area. Social media platforms such as Twitter, Facebook and Instagram offer a vast amount of data for researchers from various disciplines to study fake news. The millions of unlabeled data points available on the web can be used to detect fake news. Future research directions could include the application of unsupervised machine learning or deep learning methods for fake news detection.
Early fake news detection is another important area that requires further research. Early detection can help prevent the spread of fake news, and researchers in computer science should consider developing real-time fake news detection systems that can make a valuable contribution to the field. Using multiple datasets for analysis can improve the quality of research, yet only a small number of studies have used multiple datasets.
Incorporating sentiment analysis into fake news detection could also be a valuable direction for future research. Sentiment analysis methods can be used to analyze the emotions involved in fake news and provide deeper insights into its linguistic and emotional characteristics ( Farhoudinia et al. , 2022 ). Collaboration between data analysts and psychologists could provide a comprehensive understanding of fake news, including how it is formed and spread and the effects it has.
Psychologists have studied the theoretical background of why people believe and share fake news and the impact of personality characteristics on sharing behavior. However, there is still a need for more research in this area, particularly through the use of big data and experiments. The impact of crises such as the COVID-19 pandemic on fake news-sharing behavior also requires further investigation.
Managers need to be equipped with the knowledge of how to respond to fake news spread about their companies, brands, or organizations. Further research is needed to provide guidelines on the most effective response strategies to recover the brand's reputation. There are still many questions that need to be answered, such as the role of social media platforms in dealing with fake news, the limits of freedom of speech and the adequacy of existing policy frameworks.
Researchers in policymaking and law, in collaboration with researchers in library and information science, should ensure that the rules and legislation against fake news do not result in censorship. The balance between protecting against fake news and preserving freedom of speech is an important issue that requires further attention ( Agarwal and Alsaeedi, 2021 ).
6. Conclusion
This systematic review analyzed past research on fake news dissemination on social networks and social media over the last decade. The paper provided an interdisciplinary review of articles from various fields, such as computer and information science, psychology, marketing and journalism, by reviewing the theoretical frameworks, methods and objectives of articles published in AJG-listed journals. The analysis included a definition of fake news, a review of the development of fake news research over the past ten years, an identification of the most used theories in fake news research and an examination of the research methods used. The paper concluded with a discussion of the limitations and gaps in the field and provided suggestions for future research directions.
List of abbreviations
• 5G: the fifth-generation technology standard for broadband cellular networks in telecommunication
• ABS: Chartered Association of Business Schools
• AJG-listed journals: peer-reviewed journals that are included in the AJG listings released by the Chartered Association of Business Schools
• CEO: Chief Executive Officer
• COVID-19: the global pandemic that started in the year 2019.
• KNN: K-Nearest Neighbors Algorithm
• SARS-CoV-2: the infectious coronavirus that led to the beginning of a global pandemic in the year 2019
• SVM: Support Vector Machine Algorithm
• tf–idf: frequency–inverse document frequency
Search steps and review process
Frequency histogram of published articles per year (2010 – 2020)
Number of articles per discipline represented
Articles in computer sciences
Source(s): Created by authors
https://charteredabs.org/academic-journal-guide-2021/
Funding : This research has not received any funding.
Competing interests: The authors declare that there are no competing interests. The authors do not have any competing interests to disclose, whether financial or non-financial, that are directly or indirectly related to the work submitted for publication.
Authors' contributions: All authors have equally contributed to the manuscript.
Acker , A. and Donovan , J. ( 2019 ), “ Data craft: a theory/methods package for critical internet studies ”, Information, Communication and Society , Vol. 22 No. 11 , pp. 1590 - 1609 .
Agarwal , N.K. and Alsaeedi , F. ( 2021 ), “ Creation, dissemination and mitigation: toward a disinformation behavior framework and model ”, Aslib Journal of Information Management , Vol. 73 No. 5 , pp. 639 - 658 , doi: 10.1108/AJIM-01-2021-0034 .
Agarwal , S. , Farid , H. , El-Gaaly , T. and Lim , S.N. ( 2020 , December ), “ Detecting deep-fake videos from appearance and behavior ”, In 2020 IEEE international workshop on information forensics and security (WIFS) , IEEE , pp. 1 - 6 .
Ahmad , T. , Aliaga Lazarte , E.A. and Mirjalili , S. ( 2022 ), “ A systematic literature review on fake news in the COVID-19 pandemic: can AI propose a solution? ”, Applied Sciences , Vol. 12 No. 24 , p. 12727 .
Aïmeur , E. , Sabrine , A. and Gilles , B. ( 2023 ), “ Fake news, disinformation and misinformation in social media: a review ”, Social Network Analysis and Mining , Vol. 13 No. 1 , p. 30 .
Alghamdi , J. , Yuqing , L. and Suhuai , L. ( 2023 ), “ Towards COVID-19 fake news detection using transformer-based models ”, Knowledge-Based Systems , Vol. 274 , 110642 .
Alkhamees , M. , Alsaleem , S. , Al-Qurishi , M. , Al-Rubaian , M. and Hussain , A. ( 2021 ), “ User trustworthiness in online social networks: a systematic review ”, Applied Soft Computing , Vol. 103 , 107159 .
Allcott , H. and Gentzkow , M. ( 2017 ), “ Social media and fake news in the 2016 election ”, Journal of Economic Perspectives , Vol. 31 No. 2 , pp. 211 - 236 .
Al-Rawi , A. , Groshek , J. and Zhang , L. ( 2019 ), “ What the fake? Assessing the extent of networked political spamming and bots in the propagation of# fakenews on Twitter ”, Online Information Review , Vol. 43 No. 1 , pp. 53 - 71 , doi: 10.1108/OIR-02-2018-0065 .
Amer , E. , Kyung-Sup , K. and Shaker , E.-S. ( 2022 ), “ Context-based fake news detection model relying on deep learning models ”, Electronics , Vol. 11 No. 8 , p. 1255 .
Apuke , O.D. and Omar , B. ( 2020 ), “ User motivation in fake news sharing during the COVID-19 pandemic: an application of the uses and gratification theory ”, Online Information Review , Vol. 45 No. 1 , pp. 220 - 239 .
Baccarella , C.V. , Wagner , T , F. , Kietzmann , J.H. and McCarthy , I.P. ( 2018 ), “ Social media? It’s serious! Understanding the dark side of social media ”, European Management Journal , Vol. 36 No. 4 , pp. 431 - 438 .
Balakrishnan , V. , Zhen , N.W. , Chong , S.M. , Han , G.J. and Lee , T.J. ( 2022 ), “ Infodemic and fake news–A comprehensive overview of its global magnitude during the COVID-19 pandemic in 2021: a scoping review ”, International Journal of Disaster Risk Reduction , Vol. 78 , 103144 .
Barfar , A. ( 2019 ), “ Cognitive and affective responses to political disinformation in Facebook ”, Computers in Human Behavior , Vol. 101 , pp. 173 - 179 .
Ben-Gal , I. , Sela , A. , Milo , O. and Kagan , E. ( 2019 ), “ Improving information spread by spreading groups ”, Online Information Review , Vol. 44 No. 1 , pp. 24 - 42 , doi: 10.1108/OIR-08-2018-0245 .
Berthon , P.R. and Pitt , L.F. ( 2018 ), “ Brands, truthiness and post-fact: managing brands in a post-rational world ”, Journal of Macromarketing , Vol. 38 No. 2 , pp. 218 - 227 .
Beuk , F. , Weidner , K. and Bal , A. ( 2019 ), “ Fake news and the willingness to share: a schemer schema and confirmatory bias perspective ”, Journal of Product and Brand Management , Vol. 29 No. 2 , pp. 180 - 187 , doi: 10.1108/JPBM-12-2018-2155 .
Borges‐Tiago , T. , Tiago , F. , Silva , O. , Guaita Martínez , J.M. and Botella‐Carrubi , D. ( 2020 ), “ Online users’ attitudes toward fake news: implications for brand management ”, Psychology and Marketing , Vol. 37 No. 9 , pp. 1171 - 1184 .
Brandtzaeg , P.B. and Følstad , A. ( 2017 ), “ Trust and distrust in online fact-checking services ”, Communications of the ACM , Vol. 60 No. 9 , pp. 65 - 71 .
Brashier , N.M. and Schacter , D.L. ( 2020 ), “ Aging in an era of fake news ”, Current Directions in Psychological Science , Vol. 29 No. 3 , pp. 316 - 323 .
Brigida , M. and Pratt , W.R. ( 2017 ), “ Fake news ”, The North American Journal of Economics and Finance , Vol. 42 , pp. 564 - 573 .
Carlson , M. ( 2020 ), “ Fake news as an informational moral panic: the symbolic deviancy of social media during the 2016 US presidential election ”, Information, Communication and Society , Vol. 23 No. 3 , pp. 374 - 388 .
Carrieri , V. , Madio , L. and Principe , F. ( 2019 ), “ Vaccine hesitancy and (fake) news: Quasi‐experimental evidence from Italy ”, Health Economics , Vol. 28 No. 11 , pp. 1377 - 1382 , available at: https://www.ncbi.nlm.nih.gov/pmc/articles/PMC6851894/pdf/HEC-28-1377.pdf
Chen , Z.F. and Cheng , Y. ( 2019 ), “ Consumer response to fake news about brands on social media: the effects of self-efficacy, media trust, and persuasion knowledge on brand trust ”, Journal of Product and Brand Management , Vol. 29 No. 2 , pp. 188 - 198 .
Chen , Q. , Srivastava , G. , Parizi , R. M. , Aloqaily , M. and Al Ridhawi , I. ( 2020 ), “ An incentive-aware blockchain-based solution for internet of fake media things ”, Information Processing and Management , Vol. 57 No. 6 , 102370 .
Cheng , L.-C. , Lu , W.-T. and Yeo , B. ( 2023 ), “ Predicting abnormal trading behavior from internet rumor propagation: a machine learning approach ”, Financial Innovation , Vol. 9 No. 1 , p. 3 .
Chua , A.Y. and Banerjee , S. ( 2018 ), “ Intentions to trust and share online health rumors: an experiment with medical professionals ”, Computers in Human Behavior , Vol. 87 , pp. 1 - 9 .
Chua , A.Y.K. , Pal , A. and Banerjee , S. ( 2021 ), “ ‘This will blow your mind’: examining the urge to click clickbaits ”, Aslib Journal of Information Management , Vol. 73 No. 2 , pp. 288 - 303 , doi: 10.1108/AJIM-07-2020-0214 .
Colliander , J. ( 2019 ), “ ‘This is fake news’: investigating the role of conformity to other users' views when commenting on and spreading disinformation in social media ”, Computers in Human Behavior , Vol. 97 , pp. 202 - 215 .
Damstra , A. , Boomgaarden , H.G. , Broda , E. , Lindgren , E. , Strömbäck , J. , Tsfati , Y. and Vliegenthart , R. ( 2021 ), “ What does fake look like? A review of the literature on intentional deception in the news and on social media ”, Journalism Studies , Vol. 22 No. 14 , pp. 1947 - 1963 .
Denyer , D. and Tranfield , D. ( 2009 ), “ Creating a Systematic Review ”, In D. A. Buchanan and A. Bryman (Eds.) , The Sage Handbook of Organizational Research Methods , Sage Publications , pp. 671 – 689 , available at: https://www.cebma.org/wpcontent/uploads/Denyer-Tranfield-Producing-a-Systematic-Review.pdf
Devlin , J. , Chang , M.-W. , Lee , K. and Toutanova , K. ( 2018 ), “ Bert: pre-training of deep bidirectional transformers for language understanding ”, arXiv preprint arXiv:1810.04805 .
Di Domenico , G. and Visentin , M. ( 2020 ), “ Fake news or true lies? Reflections about problematic contents in marketing ”, International Journal of Market Research , Vol. 62 No. 4 , pp. 409 - 417 .
Diddi , P. , Xiao , A. , Vafeiadis , M. , Bortree , D.S. and Buckley , C. ( 2019 ), “ Refuting fake news on social media: nonprofits, crisis response strategies and issue involvement ”, Journal of Product and Brand Management , Vol. 29 No. 2 , pp. 209 - 222 .
Duffy , A. , Tandoc , E. and Ling , R. ( 2020 ), “ Too good to be true, too good not to share: the social utility of fake news ”, Information, Communication and Society , Vol. 23 No. 13 , pp. 1965 - 1979 .
Elías , C. and Catalan-Matamoros , D. ( 2020 ), “ Coronavirus in Spain: fear of ‘Official’fake news boosts WhatsApp and alternative sources ”, Media and Communication , Vol. 8 No. 2 , pp. 462 - 466 .
Farhoudinia , B. , Ozturkcan , S. and Kasap , N. ( 2022 ), “ Lexicon-based sentiment analysis of fake news on social media ”, AIRSI2022 Conference: Technologies 4.0 in Tourism , Services and Marketing .
Faustini , P.H.A. and Covões , T.F. ( 2020 ), “ Fake news detection in multiple platforms and languages ”, Expert Systems with Applications , Vol. 158 , 113503 .
Flostrand , A. , Pitt , L. and Kietzmann , J. ( 2019 ), “ Fake news and brand management: a Delphi study of impact, vulnerability and mitigation ”, Journal of Product and Brand Management , Vol. 29 No. 2 , pp. 246 - 254 , doi: 10.1108/JPBM-12-2018-2156 .
Giglietto , F. , et al. ( 2019 ), “ ‘Fake news’ is the invention of a liar: how false information circulates within the hybrid news system ”, Current Sociology , Vol. 67 No. 4 , pp. 625 - 642 .
Goodfellow , I. , Bengio , I. , Courville , A. and Bengio , Y. ( 2016 ), Deep Learning , MIT Press , Cambridge , Vol. 1 .
Groshek , J. , et al. ( 2019 ), “ What the fake? Assessing the extent of networked political spamming and bots in the propagation of #fakenews on Twitter ”, Online Information Review , Vol. 43 No. 1 , pp. 53 - 71 .
Gupta , M. , Dennehy , D. , Parra , C.M. , Mäntymäki , M. and Dwivedi , Y.K. ( 2023 ), “ Fake news believability: the effects of political beliefs and espoused cultural values ”, Information and Management , Vol. 60 No. 2 , 103745 .
Hardalov , M. , Koychev , I. and Nakov , P. ( 2016 ), In Search of Credible News. International Conference on Artificial Intelligence: Methodology, Systems, and Applications , Springer .
Hartley , K. and Vu , M.K. ( 2020 ), “ Fighting fake news in the COVID-19 era: policy insights from an equilibrium model ”, Policy Sciences , Vol. 53 No. 4 , pp. 735 - 758 .
Horne , B. D. , Adali , S. and Sikdar , S. ( 2017 ), “ Identifying the social signals that drive online discussions: a case study of reddit communities ”, 2017 26th International Conference on Computer Communication and Networks (ICCCN) , IEEE .
Islam , A.N. , Laato , S. , Talukder , S. and Sutinen , E. ( 2020a ), “ Misinformation sharing and social media fatigue during COVID-19: an affordance and cognitive load perspective ”, Technological Forecasting and Social Change , Vol. 159 , 120201 .
Islam , M.R. , Liu , S. , Wang , X. and Xu , G. ( 2020b ), “ Deep learning for misinformation detection on online social networks: a survey and new perspectives ”, Social Network Analysis and Mining , Vol. 10 , pp. 1 - 20 .
Jang , S.M. , Geng , T. , Li , J.-Y. Q. , Xia , R. , Huang , C.-T. , Kim , H. and Tang , J. ( 2018 ), “ A computational approach for examining the roots and spreading patterns of fake news: evolution tree analysis ”, Computers in Human Behavior , Vol. 84 , pp. 103 - 113 .
Jones , M.O. ( 2019 ), “ The gulf information war| propaganda, fake news, and fake trends: the weaponization of twitter bots in the gulf crisis ”, International Journal of Communication , Vol. 13 , p. 27 .
Kaddoura , S. , Chandrasekaran , G. , Popescu , D.E. and Duraisamy , J.H. ( 2022 ), “ A systematic literature review on spam content detection and classification ”, PeerJ Computer Science , Vol. 8 , e830 .
Kahneman , D. ( 2011 ), Thinking, Fast and Slow , Macmillan , New York .
Kaliyar , R.K. ( 2018 ), “ Fake news detection using a deep neural network ”, 2018 4th International Conference on Computing Communication and Automation (ICCCA) , IEEE .
Kim , A. and Dennis , A.R. ( 2019 ), “ Says who? The effects of presentation format and source rating on fake news in social media ”, Mis Quarterly , Vol. 43 No. 3 , pp. 1025 - 1039 .
Khan , J.Y. , Khondaker , M.T.I. , Afroz , S. , Uddin , G. and Iqbal , A. ( 2021 ), “ A benchmark study of machine learning models for online fake news detection ”, Machine Learning with Applications , Vol. 4 , 100032 .
Kim , A. , Moravec , P.L. and Dennis , A.R. ( 2019 ), “ Combating fake news on social media with source ratings: the effects of user and expert reputation ratings ”, Journal of Management Information Systems , Vol. 36 No. 3 , pp. 931 - 968 .
Kim , B. , Xiong , A. , Lee , D. and Han , K. ( 2021 ), “ A systematic review on fake news research through the lens of news creation and consumption: research efforts, challenges, and future directions ”, PloS One , Vol. 16 No. 12 , e0260080 .
Koro-Ljungberg , M. , Carlson , D.L. and Montana , A. ( 2019 ), “ Productive forces of post-truth (s)? ”, Qualitative Inquiry , Vol. 25 No. 6 , pp. 583 - 590 .
Kwanda , F.A. and Lin , T.T. ( 2020 ), “ Fake news practices in Indonesian newsrooms during and after the Palu earthquake: a hierarchy-of-influences approach ”, Information, Communication and Society , Vol. 23 No. 6 , pp. 849 - 866 .
Laato , S. , Islam , A.N. , Islam , M.N. and Whelan , E. ( 2020 ), “ What drives unverified information sharing and cyberchondria during the COVID-19 pandemic? ”, European Journal of Information Systems , Vol. 29 No. 3 , pp. 288 - 305 .
Lee , L.W. , Hannah , D. and McCarthy , I.P. ( 2019 ), “ Do your employees think your slogan is ‘fake news?’ A framework for understanding the impact of fake company slogans on employees ”, Journal of Product and Brand Management , Vol. 29 No. 2 , pp. 199 - 208 .
Long , N.V. , Richardson , M. and Stähler , F. ( 2019 ), “ Media, fake news, and debunking ”, Economic Record , Vol. 95 No. 310 , pp. 312 - 324 .
Lord Ferguson , S. , Montecchi , M. and de Regt , A. ( 2019 ), “ A false image of health: how fake news and pseudo-facts spread in the health and beauty industry ”, Journal of Product and Brand Management , Vol. 29 No. 2 , pp. 168 - 179 .
Lozano , M.G. , Brynielsson , J. , Franke , U. , Rosell , M. , Tjörnhammar , E. , Varga , S. and Vlassov , V. ( 2020 ), “ Veracity assessment of online data ”, Decision Support Systems , Vol. 129 , 113132 .
Lutzke , L. , Drummond , C. , Slovic , P. and Árvai , J. ( 2019 ), “ Priming critical thinking: simple interventions limit the influence of fake news about climate change on Facebook ”, Global Environmental Change , Vol. 58 , 101964 .
Marin , L. ( 2021 ), “ Three contextual dimensions of information on social media: lessons learned from the COVID-19 infodemic ”, Ethics and Information Technology , Vol. 23 Suppl 1 , pp. 79 - 86 .
Marres , N. ( 2018 ), “ Why we can't have our facts back ”, Engaging Science, Technology, and Society , Vol. 4 , pp. 423 - 443 .
Marsden , C. , Meyer , T. and Brown , I. ( 2020 ), “ Platform values and democratic elections: how can the law regulate digital disinformation? ”, Computer Law and Security Review , Vol. 36 , 105373 .
Meel , P. and Vishwakarma , D.K. ( 2020 ), “ Fake news, rumor, information pollution in social media and web: a contemporary survey of state-of-the-arts, challenges and opportunities ”, Expert Systems with Applications , Vol. 153 , 112986 .
Melchior , C. and Oliveira , M. ( 2022 ), “ Health-related fake news on social media platforms: a systematic literature review ”, New Media and Society , Vol. 24 No. 6 , pp. 1500 - 1522 .
Mills , A.J. and Robson , K. ( 2019 ), “ Brand management in the era of fake news: narrative response as a strategy to insulate brand value ”, Journal of Product and Brand Management , Vol. 29 No. 2 , pp. 159 - 167 .
Mirhoseini , M. , Early , S. , El Shamy , N. and Hassanein , K. ( 2023 ), “ Actively open-minded thinking is key to combating fake news: a multimethod study ”, Information and Management , Vol. 60 No. 3 , 103761 .
Mourad , A. , Srour , A. , Harmanai , H. , Jenainati , C. and Arafeh , M. ( 2020 ), “ Critical impact of social networks infodemic on defeating coronavirus COVID-19 pandemic: twitter-based study and research directions ”, IEEE Transactions on Network and Service Management , Vol. 17 No. 4 , pp. 2145 - 2155 .
Mridha , M.F. , Keya , A.J. , Hamid , M.A. , Monowar , M.M. and Rahman , M.S. ( 2021 ), “ A comprehensive review on fake news detection with deep learning ”, IEEE Access , Vol. 9 , pp. 156151 - 156170 .
Mustak , M. , Salminen , J. , Mäntymäki , M. , Rahman , A. and Dwivedi , Y.K. ( 2023 ), “ Deepfakes: deceptions, mitigations, and opportunities ”, Journal of Business Research , Vol. 154 , 113368 .
Naeem , S.B. and Bhatti , R. ( 2020 ), “ The COVID‐19 ‘infodemic’: a new front for information professionals ”, Health Information and Libraries Journal , Vol. 37 No. 3 , pp. 233 - 239 .
Nguyen , D.H. , de Leeuw , S. and Dullaert , W.E. ( 2018 ), “ Consumer behaviour and order fulfilment in online retailing: a systematic review ”, International Journal of Management Reviews , Vol. 20 No. 2 , pp. 255 - 276 .
Nirav Shah , M. and Ganatra , A. ( 2022 ), “ A systematic literature review and existing challenges toward fake news detection models ”, Social Network Analysis and Mining , Vol. 12 No. 1 , p. 168 .
Nolan , C.T. and Garavan , T.N. ( 2016 ), “ Human resource development in SMEs: a systematic review of the literature ”, International Journal of Management Reviews , Vol. 18 No. 1 , pp. 85 - 107 .
Nyilasy , G. ( 2019 ), “ Fake news: when the dark side of persuasion takes over ”, International Journal of Advertising , Vol. 38 No. 2 , pp. 336 - 342 .
Ongsulee , P. ( 2017 ), “ Artificial intelligence, machine learning and deep learning ”, 2017 15th International Conference on ICT and Knowledge Engineering (ICT&KE) , IEEE .
Ozbay , F.A. and Alatas , B. ( 2020 ), “ Fake news detection within online social media using supervised artificial intelligence algorithms ”, Physica A: Statistical Mechanics and Its Applications , Vol. 540 , 123174 .
Ozturkcan , S. , Kasap , N. , Cevik , M. and Zaman , T. ( 2017 ), “ An analysis of the Gezi Park social movement tweets ”, Aslib Journal of Information Management , Vol. 69 No. 4 , pp. 426 - 440 .
Pal , A. , Chua , A.Y.K. and Goh , D.H.-L. ( 2017 ), “ Does KFC sell rat? Analysis of tweets in the wake of a rumor outbreak ”, Aslib Journal of Information Management , Vol. 69 No. 6 , pp. 660 - 673 , doi: 10.1108/AJIM-01-2017-0026 .
Pantumsinchai , P. ( 2018 ), “ Armchair detectives and the social construction of falsehoods: an actor–network approach ”, Information, Communication and Society , Vol. 21 No. 5 , pp. 761 - 778 .
Papanastasiou , Y. ( 2020 ), “ Fake news propagation and detection: a sequential model ”, Management Science , Vol. 66 No. 5 , pp. 1826 - 1846 .
Paschen , J. ( 2019 ), “ Investigating the emotional appeal of fake news using artificial intelligence and human contributions ”, Journal of Product and Brand Management , Vol. 29 No. 2 , pp. 223 - 233 .
Pennycook , G. , Bear , A. , Collins , E.T. and Rand , D.G. ( 2020a ), “ The implied truth effect: attaching warnings to a subset of fake news headlines increases perceived accuracy of headlines without warnings ”, Management Science , Vol. 66 No. 11 , pp. 4944 - 4957 .
Pennycook , G. , McPhetres , J. , Zhang , Y. , Lu , J.G. and Rand , D.G. ( 2020b ), “ Fighting COVID-19 misinformation on social media: experimental evidence for a scalable accuracy-nudge intervention ”, Psychological Science , Vol. 31 No. 7 , pp. 770 - 780 .
Peterson , M. ( 2019 ), “ A high-speed world with fake news: brand managers take warning ”, Journal of Product and Brand Management , Vol. 29 No. 2 , pp. 234 - 245 .
Rahadian , D. and Nurfitriani , W. ( 2022 ), “ Impact of news related to Covid-19 on stock market returns in five major ASEAN countries ”, Economics and Business Quarterly Reviews , Vol. 5 No. 2 , pp. 39 - 50 .
Rajamma , R.K. , Paswan , A. and Spears , N. ( 2019 ), “ User-generated content (UGC) misclassification and its effects ”, Journal of Consumer Marketing , Vol. 37 No. 2 , pp. 125 - 138 .
Rana , D.P. , Agarwal , I. and More , A. ( 2018 ), “ A review of techniques to combat the peril of fake news ”, 2018 4th International Conference on Computing Communication and Automation (ICCCA), IEEE .
Robertson , J. , Kirsten , M. and Ferreira , C.C. ( 2019 ), “ The truth (as I see it): philosophical considerations influencing a typology of fake news ”, Journal of Product and Brand Management , Vol. 29 No. 2 , pp. 150 - 158 .
Rodrigues , A.P. , Fernandes , R. , Shetty , A. , Lakshmanna , K. and Shafi , R.M. ( 2022 ), “ Real-time twitter spam detection and sentiment analysis using machine learning and deep learning techniques ”, Computational Intelligence and Neuroscience , pp. 1 - 14 , doi: 10.1155/2022/5211949 .
Ross , B. , Pilz , L. , Cabrera , B. , Brachten , F. , Neubaum , G. and Stieglitz , S. ( 2019 ), “ Are social bots a real threat? An agent-based model of the spiral of silence to analyse the impact of manipulative actors in social networks ”, European Journal of Information Systems , Vol. 28 No. 4 , pp. 394 - 412 .
Ryan , C.D. , Schaul , A.J. , Butner , R. and Swarthout , J.T. ( 2020 ), “ Monetizing disinformation in the attention economy: the case of genetically modified organisms (GMOs) ”, European Management Journal , Vol. 38 No. 1 , pp. 7 - 18 .
Sampson , J.P. , Osborn , D.S. , Kettunen , J. , Hou , P.C. , Miller , A.K. and Makela , J.P. ( 2018 ), “ The validity of social media–based career information ”, The Career Development Quarterly , Vol. 66 No. 2 , pp. 121 - 134 .
Saquete , E. , Tomás , D. , Moreda , P. , Martínez-Barco , P. and Palomar , M. ( 2020 ), “ Fighting post-truth using natural language processing: a review and open challenges ”, Expert Systems with Applications , Vol. 141 , 112943 .
Sela , A. , Milo , O. , Kagan , E. and Ben-Gal , I. ( 2020 ), “ Improving information spread by spreading groups ”, Online Information Review , Vol. 44 No. 1 , pp. 24 - 42 , doi: 10.1108/OIR-08-2018-0245 .
Shahzad , K. , Khan , S.A. , Ahmad , S. and Iqbal , A. ( 2022 ), “ A scoping review of the relationship of big data analytics with context-based fake news detection on digital media in data age ”, Sustainability , Vol. 14 No. 21 , 14365 .
Sharif , A. , Awan , T.M. and Paracha , O.S. ( 2022 ), “ The fake news effect: what does it mean for consumer behavioral intentions towards brands? ”, Journal of Information, Communication and Ethics in Society , Vol. 20 No. 2 , pp. 291 - 307 .
Shin , J. , Jian , L. , Driscoll , K. and Bar , F. ( 2018 ), “ The diffusion of misinformation on social media: temporal pattern, message, and source ”, Computers in Human Behavior , Vol. 83 , pp. 278 - 287 .
Silverman , C. ( 2016 ), “ Viral fake election news outperformed real news on facebook in final months of the us election ”, BuzzFeed News , Vol. 16 .
Song , R. , Kim , H. , Lee , G.M. and Jang , S. ( 2019 ), “ Does deceptive marketing pay? The evolution of consumer sentiment surrounding a pseudo-product-harm crisis ”, Journal of Business Ethics , Vol. 158 No. 3 , pp. 743 - 761 .
Talwar , S. , et al. ( 2020 ), “ Sharing of fake news on social media: application of the honeycomb framework and the third-person effect hypothesis ”, Journal of Retailing and Consumer Services , Vol. 57 , 102197 .
Talwar , S. , Dhir , A. , Kaur , P. , Zafar , N. and Alrasheedy , M. ( 2019 ), “ Why do people share fake news? Associations between the dark side of social media use and fake news sharing behavior ”, Journal of Retailing and Consumer Services , Vol. 51 , pp. 72 - 82 .
Thelwall , M. and Thelwall , S. ( 2020 ), “ A thematic analysis of highly retweeted early COVID-19 tweets: consensus, information, dissent and lockdown life ”, Aslib Journal of Information Management , Vol. 72 No. 6 , pp. 945 - 962 , doi: 10.1108/AJIM-05-2020-0134 .
Thompson , R.C. , Joseph , S. and Adeliyi , T.T. ( 2022 ), “ A systematic literature review and meta-analysis of studies on online fake news detection ”, Information , Vol. 13 No. 11 , p. 527 .
Thompson , N. , Wang , X. and Daya , P. ( 2020 ), “ Determinants of news sharing behavior on social media ”, Journal of Computer Information Systems , Vol. 60 No. 6 , pp. 593 - 601 , doi: 10.1080/08874417.2019.1566803 .
Tschiatschek , S. , Singla , A. , Gomez Rodriguez , M. , Merchant , A. and Krause , A. ( 2018 ), “ Fake news detection in social networks via crowd signals ”, Companion Proceedings of the The Web Conference 2018 .
Vafeiadis , M. , Bortree , D.S. , Buckley , C. , Diddi , P. and Xiao , A. ( 2020 ), “ Refuting fake news on social media: nonprofits, crisis response strategies and issue involvement ”, Journal of Product and Brand Management , Vol. 29 No. 2 , pp. 209 - 222 , doi: 10.1108/JPBM-12-2018-2146 .
Vasist , P.N. and Krishnan , S. ( 2023 ), “ Fake news and sustainability-focused innovations: a review of the literature and an agenda for future research ”, Journal of Cleaner Production , 135933 .
Vosoughi , S. , Roy , D. and Aral , S. ( 2018 ), “ The spread of true and false news online ”, Science , Vol. 359 No. 6380 , pp. 1146 - 1151 .
Wang , Y. , McKee , M. , Torbica , A. and Stuckler , D. ( 2019 ), “ Systematic literature review on the spread of health-related misinformation on social media ”, Social Science and Medicine , Vol. 240 , 112552 .
Wiesenberg , M. and R. Tench ( 2020 ). " Deep strategic mediatization: Organizational leaders’ knowledge and usage of social bots in an era of disinformation ." International Journal of Information Management, Vol. 51 , 102042 .
Wolverton , C. and Stevens , D. ( 2019 ), “ The impact of personality in recognizing disinformation ”, Online Information Review , Vol. 44 No. 1 , pp. 181 - 191 , doi: 10.1108/OIR-04-2019-0115 .
Wu , Z. , Pi , D. , Chen , J. , Xie , M. and Cao , J. ( 2020 ), “ Rumor detection based on propagation graph neural network with attention mechanism ”, Expert Systems with Applications , Vol. 158 , 113595 .
Xia , H. , Wang , Y. , Zhang , J.Z. , Zheng , L.J. , Kamal , M.M. and Arya , V. ( 2023 ), “ COVID-19 fake news detection: a hybrid CNN-BiLSTM-AM model ”, Technological Forecasting and Social Change , Vol. 195 , 122746 .
Zhang , D. , Li , W. , Niu , B. and Wu , C. ( 2018 ), “ Research on text classification for identifying fake news ”, 2018 International Conference on Security, Pattern Analysis, and Cybernetics (SPAC) , IEEE .
Zhang , D. , Wang , Y. and Tan , C. ( 2023 ), “ A deep learning approach for detecting fake reviewers: exploiting reviewing behavior and textual information ”, Decision Support Systems , Vol. 166 , 113911 .
Zhou , X. and Zafarani , R. ( 2020 ), “ A survey of fake news: fundamental theories, detection methods, and opportunities ”, ACM Computing Surveys (CSUR) , Vol. 53 No. 5 , pp. 1 - 40 .
Corresponding author
About the authors.
Bahareh Farhoudinia completed her PhD at the Sabanci Business School.
Selcen Ozturkcan continues her academic career with a permanent faculty position at the School of Business and Economics of Linnaeus University (Sweden) and is affiliated with Sabanci Business School (Turkey). Her research on digital experiences, which appeared as journal articles, books, book chapters and case studies, is accessible at http://www.selcenozturkcan.com .
Nihat Kasap works as Professor of Management Information Systems at the Sabanci Business School, where he also serves as the Dean. His research focuses on social media analytics and data mining, mobile technologies and M-government applications, pricing and quality of service in telecommunication networks, generation expansion planning and investments in the energy sector, mathematical programming and heuristic design and optimization. His publications are accessible at https://sbs.sabanciuniv.edu/en/faculty_members/detail/820
Related articles
We’re listening — tell us what you think, something didn’t work….
Report bugs here
All feedback is valuable
Please share your general feedback
Join us on our journey
Platform update page.
Visit emeraldpublishing.com/platformupdate to discover the latest news and updates
Questions & More Information
Answers to the most commonly asked questions here
How to Identify Fake News on Social Media: A Systematic Literature Review
- Conference paper
- First Online: 17 May 2022
- Cite this conference paper
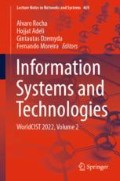
- Russel Jowore 13 &
- Marita Turpin ORCID: orcid.org/0000-0002-4425-2010 13
Part of the book series: Lecture Notes in Networks and Systems ((LNNS,volume 469))
Included in the following conference series:
- World Conference on Information Systems and Technologies
1330 Accesses
2 Citations
Fake news in the form of disinformation on social networks has become a real concern, with serious potential consequences as a result of the massive viral spreading of false information and fake news. To this end, a systematic literature review was performed to investigate how the validity of news on social media can be determined. An initial literature search resulted in identifying 1105 potentially relevant articles, which were taken through a systematic sifting process resulting in 25 articles that were analysed in detail. During a thematic analysis, four themes emerged, related to fake news, social media, the influence of bias and information literacy. This paper discusses the findings related to the four themes. The findings were distilled into a set of five guidelines that may be used to determine the validity of news on social media. The techniques are: consider the source; beware of bots; be aware of bias and make use of multiple sources; beware of mimics; and ways to recognise good sources of information. This paper contributes to the current discourse on how to distinguish between valid news and fake news on social media, and provides guidance to assist with this ever growing and increasingly important information management challenge.
- Social media
- Online media
- Systematic review
This is a preview of subscription content, log in via an institution to check access.
Access this chapter
- Available as PDF
- Read on any device
- Instant download
- Own it forever
- Available as EPUB and PDF
- Compact, lightweight edition
- Dispatched in 3 to 5 business days
- Free shipping worldwide - see info
Tax calculation will be finalised at checkout
Purchases are for personal use only
Institutional subscriptions
Hunt, K., Agarwal, P., Zhuang, J.: Tracking storms of misinformation spread amid disasters. ISE Mag. 51 , 28–32 (2019)
Google Scholar
Musgrove, A.T., Powers, J.R., Rebar, L.C., Musgrove, G.J.: Real or fake? Resources for teaching college students how to identify fake news. Coll. Undergrad. Lib. 25 , 243–260 (2018)
Article Google Scholar
Auberry, K.: Increasing students’ ability to identify fake news through information literacy education and content management systems. Ref. Libr. 59 , 179–187 (2018)
Cooke, N.A.: Fake News and Alternative Facts: Information Literacy in a Post-Truth Era. American Library Association, Chicago (2018)
Bettany-Saltikov, J.: EBOOK: How to do a Systematic Literature Review in Nursing: A step-by-step guide (2016)
de Oliveira, N.R., Medeiros, D.S., Mattos, D.M.: A sensitive stylistic approach to identify fake news on social networking. IEEE Signal Process. Lett. 27 , 1250–1254 (2020)
Calvillo, D.P., Garcia, R.J., Bertrand, K., Mayers, T.A.: Personality factors and self-reported political news consumption predict susceptibility to political fake news. Personality Individ. Differ. 174 , 110666 (2021)
Bondielli, A., Marcelloni, F.: A survey on fake news and rumour detection techniques. Inf. Sci. 497 , 38–55 (2019)
Leeder, C.: How college students evaluate and share “fake news” stories. Libr. Inf. Sci. Res. 41 , 100967 (2019)
De Paor, S., Heravi, B.: Information literacy and fake news: how the field of librarianship can help combat the epidemic of fake news. J. Acad. Librariansh. 46 , 102218 (2020)
Fernández-Torres, M.J., Almansa-Martínez, A., Chamizo-Sánchez, R.: Infodemic and fake news in Spain during the COVID-19 pandemic. Int. J. Environ. Res. Public Health 18 , 1781 (2021)
Nyilasy, G.: Fake news: when the dark side of persuasion takes over. Int. J. Advert. 38 , 336–342 (2019)
Zhang, X., Ghorbani, A.A.: An overview of online fake news: characterization, detection, and discussion. Inf. Process. Manage. 57 , 102025 (2020)
Naeem, S.B., Bhatti, R., Khan, A.: An exploration of how fake news is taking over social media and putting public health at risk. Health Inf. Libr. J. (2020)
Di Domenico, G., Sit, J., Ishizaka, A., Nunan, D.: Fake news, social media and marketing: a systematic review. J. Bus. Res. 124 , 329–341 (2021)
Jang, S.M., Kim, J.K.: Third person effects of fake news: fake news regulation and media literacy interventions. Comput. Hum. Behav. 80 , 295–302 (2018)
Article MathSciNet Google Scholar
Jahng, M.R., Lee, H., Rochadiat, A.: Public relations practitioners’ management of fake news: exploring key elements and acts of information authentication. Public Relat. Rev. 46 , 101907 (2020)
Download references
Acknowledgements
This work is based on research supported by the National Research Foundation.
Author information
Authors and affiliations.
Department of Informatics, University of Pretoria, Pretoria, South Africa
Russel Jowore & Marita Turpin
You can also search for this author in PubMed Google Scholar
Corresponding author
Correspondence to Marita Turpin .
Editor information
Editors and affiliations.
ISEG, Universidade de Lisboa, Lisboa, Portugal
Alvaro Rocha
College of Engineering, The Ohio State University, Columbus, OH, USA
Hojjat Adeli
Institute of Data Science and Digital Te, Vilnius University, Vilnius, Lithuania
Gintautas Dzemyda
DCT, Universidade Portucalense, PORTO, Portugal
Fernando Moreira
Rights and permissions
Reprints and permissions
Copyright information
© 2022 The Author(s), under exclusive license to Springer Nature Switzerland AG
About this paper
Cite this paper.
Jowore, R., Turpin, M. (2022). How to Identify Fake News on Social Media: A Systematic Literature Review. In: Rocha, A., Adeli, H., Dzemyda, G., Moreira, F. (eds) Information Systems and Technologies. WorldCIST 2022. Lecture Notes in Networks and Systems, vol 469. Springer, Cham. https://doi.org/10.1007/978-3-031-04819-7_11
Download citation
DOI : https://doi.org/10.1007/978-3-031-04819-7_11
Published : 17 May 2022
Publisher Name : Springer, Cham
Print ISBN : 978-3-031-04818-0
Online ISBN : 978-3-031-04819-7
eBook Packages : Intelligent Technologies and Robotics Intelligent Technologies and Robotics (R0)
Share this paper
Anyone you share the following link with will be able to read this content:
Sorry, a shareable link is not currently available for this article.
Provided by the Springer Nature SharedIt content-sharing initiative
- Publish with us
Policies and ethics
- Find a journal
- Track your research
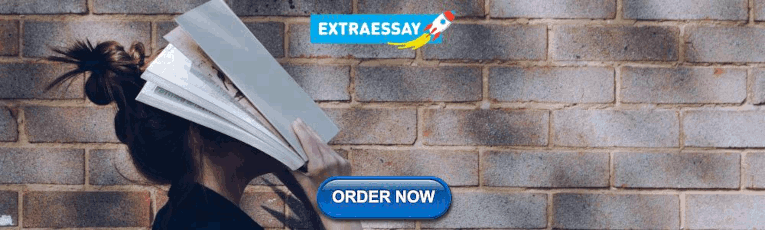
IMAGES
COMMENTS
Some approaches detect fake news by using metadata such as a comparison of release time of the article and timelines of spreading the article as well where the story spread (Macaulay 2018). The purpose of this research paper is to, through a systematic literature review, categorize current approaches to contest the wide-ranging endemic of fake ...
Fake news detection models can be divided based on the following approaches: Knowledge-based approaches, Features-based approaches, and Modality-based approaches [19, 25, 26], as shown in Fig. 2 ...
or even nations. A variety of approaches exist to identify fake news. By con-ducting a systematic literature review, we identify the main approaches currently available to identify fake news and how these approaches can be applied in different situations. Some approaches are illustrated with a relevant example as
By conducting a systematic literature review, this work identifies the main approaches currently available to identify fake news and how these approaches can be applied in different situations. With the widespread dissemination of information via digital media platforms, it is of utmost importance for individuals and societies to be able to judge the credibility of it. Fake news is not a ...
2.1 Related works 2.1.1 Existing fake news detection model approaches. In 2019, Ko et al. have used a reverse-tracking approach for defining the possibility of fake news in the articles that were taken from the cognitive system, where the designed model has been termed a Fake News Detection System (FNDS)This model has been tested in two case studies, where the first one was posted on February ...
Approaches to Identify Fake News: A Systematic Literature Review ... Approaches to Identify Fake News: A Systematic Literature Review. Approaches to Identify Fake News: A Systematic Literature Review. Dylan De Beer. 2020, Integrated Science in Digital Age 2020.
3 3 Research Method 3.1 Research Objective The purpose of this paper is to categorize approaches used to identify fake news. In order to do this, a systematic literature review was done.
By conducting a systematic literature review, we identify the main approaches currently available to identify fake news and how these approaches can be applied in different situations. Some approaches are illustrated with a relevant example as well as the challenges and the appropriate context in which the specific approach can be used.
This article intended to carry out a systematic review of the literature to identify the library practices against fake news. After the data extraction, a final set of 27 documents were analyzed, resulting in a new categorization, grounded in the literature and able to create new insights on the subject.
Fake news detection: a systematic literature review of machine learning algorithms and datasets Villela et al. 20 23 Figure 1. Different approaches proposed for detecting fake news found
The recognition of fake news has become a noticeable area of research, as emphasized in [].Previously, yellow journalism was a standard means of spreading fake news, often aiming to sensationalize stories such as amusing news, accidents, rumors, and crime reports, as mentioned in [].However, in the digital era, the propagation of fake news has become even more straightforward, as users can ...
Existing fake news detection model approaches . In 2019, Ko et al. have used a reverse-tracking approach for defining the possibility of fake news in the articles that were taken from the cognitive system, where the designed model has been termed a Fake News Detection System (FNDS)This model has been tested in two case studies, where the first one was posted on February 9, 2017.
Second, based on the systematic literature review, to identify key research gaps and research problems, and thereby give an outlook in terms of avenues for future research. ... B. B. (2021). Multiple features based approach for automatic fake news detection on social networks using deep learning. Applied Soft Computing, 100, Article 106983 ...
3 Research Method. In this systematic literature review, a qualitative methodology was followed. A thematic approach was implemented to determine the factors and sub-factors that contribute to the sharing and spreading of fake news. The study employed the following search terms: ("Fake News" (NEAR/2) "Social Media") AND (defin* OR ...
The ubiquitous access and exponential growth of information available on social media networks have facilitated the spread of fake news, complicating the task of distinguishing between this and real news. Fake news is a significant social barrier that has a profoundly negative impact on society. Despite the large number of studies on fake news detection, they have not yet been combined to ...
This website requires cookies, and the limited processing of your personal data in order to function. By using the site you are agreeing to this as outlined in our privacy notice and cookie policy.
This paper aims to conduct an interdisciplinary systematic literature review (SLR) of fake news research and to advance the socio-technical understanding of digital information practices and platforms in business and management studies.,The paper applies a focused, SLR method to analyze articles on fake news in business and management journals ...
an organization. These fake news can also harm a society or a political party. The report shows that it is easy to change people opinions by spreading fake news (Levin, 2017). Therefore, there is a need for detecting these fake news from spreading so that the reputation of a person, political party or an organization can be saved. RQ 1
A systematic literature review (SLR) has been performed to identify previous studies on evaluating the credibility of news, and in particular to see what has been done in terms of the use of critical thinking to evaluate online news. During the SLR's sifting process, 22 relevant studies were identified.
Abstract. Fake news in the form of disinformation on social networks has become a real concern, with serious potential consequences as a result of the massive viral spreading of false information and fake news. To this end, a systematic literature review was performed to investigate how the validity of news on social media can be determined.