
An official website of the United States government
The .gov means it’s official. Federal government websites often end in .gov or .mil. Before sharing sensitive information, make sure you’re on a federal government site.
The site is secure. The https:// ensures that you are connecting to the official website and that any information you provide is encrypted and transmitted securely.
- Publications
- Account settings
Preview improvements coming to the PMC website in October 2024. Learn More or Try it out now .
- Advanced Search
- Journal List
- J Korean Med Sci
- v.37(16); 2022 Apr 25

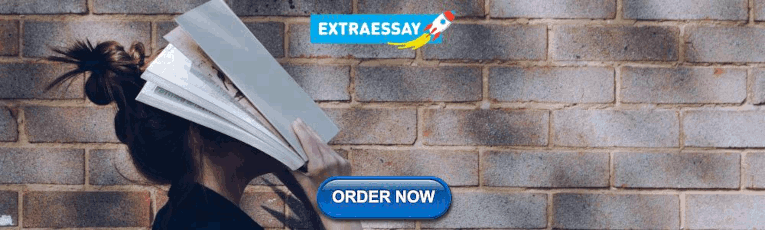
A Practical Guide to Writing Quantitative and Qualitative Research Questions and Hypotheses in Scholarly Articles
Edward barroga.
1 Department of General Education, Graduate School of Nursing Science, St. Luke’s International University, Tokyo, Japan.
Glafera Janet Matanguihan
2 Department of Biological Sciences, Messiah University, Mechanicsburg, PA, USA.
The development of research questions and the subsequent hypotheses are prerequisites to defining the main research purpose and specific objectives of a study. Consequently, these objectives determine the study design and research outcome. The development of research questions is a process based on knowledge of current trends, cutting-edge studies, and technological advances in the research field. Excellent research questions are focused and require a comprehensive literature search and in-depth understanding of the problem being investigated. Initially, research questions may be written as descriptive questions which could be developed into inferential questions. These questions must be specific and concise to provide a clear foundation for developing hypotheses. Hypotheses are more formal predictions about the research outcomes. These specify the possible results that may or may not be expected regarding the relationship between groups. Thus, research questions and hypotheses clarify the main purpose and specific objectives of the study, which in turn dictate the design of the study, its direction, and outcome. Studies developed from good research questions and hypotheses will have trustworthy outcomes with wide-ranging social and health implications.
INTRODUCTION
Scientific research is usually initiated by posing evidenced-based research questions which are then explicitly restated as hypotheses. 1 , 2 The hypotheses provide directions to guide the study, solutions, explanations, and expected results. 3 , 4 Both research questions and hypotheses are essentially formulated based on conventional theories and real-world processes, which allow the inception of novel studies and the ethical testing of ideas. 5 , 6
It is crucial to have knowledge of both quantitative and qualitative research 2 as both types of research involve writing research questions and hypotheses. 7 However, these crucial elements of research are sometimes overlooked; if not overlooked, then framed without the forethought and meticulous attention it needs. Planning and careful consideration are needed when developing quantitative or qualitative research, particularly when conceptualizing research questions and hypotheses. 4
There is a continuing need to support researchers in the creation of innovative research questions and hypotheses, as well as for journal articles that carefully review these elements. 1 When research questions and hypotheses are not carefully thought of, unethical studies and poor outcomes usually ensue. Carefully formulated research questions and hypotheses define well-founded objectives, which in turn determine the appropriate design, course, and outcome of the study. This article then aims to discuss in detail the various aspects of crafting research questions and hypotheses, with the goal of guiding researchers as they develop their own. Examples from the authors and peer-reviewed scientific articles in the healthcare field are provided to illustrate key points.
DEFINITIONS AND RELATIONSHIP OF RESEARCH QUESTIONS AND HYPOTHESES
A research question is what a study aims to answer after data analysis and interpretation. The answer is written in length in the discussion section of the paper. Thus, the research question gives a preview of the different parts and variables of the study meant to address the problem posed in the research question. 1 An excellent research question clarifies the research writing while facilitating understanding of the research topic, objective, scope, and limitations of the study. 5
On the other hand, a research hypothesis is an educated statement of an expected outcome. This statement is based on background research and current knowledge. 8 , 9 The research hypothesis makes a specific prediction about a new phenomenon 10 or a formal statement on the expected relationship between an independent variable and a dependent variable. 3 , 11 It provides a tentative answer to the research question to be tested or explored. 4
Hypotheses employ reasoning to predict a theory-based outcome. 10 These can also be developed from theories by focusing on components of theories that have not yet been observed. 10 The validity of hypotheses is often based on the testability of the prediction made in a reproducible experiment. 8
Conversely, hypotheses can also be rephrased as research questions. Several hypotheses based on existing theories and knowledge may be needed to answer a research question. Developing ethical research questions and hypotheses creates a research design that has logical relationships among variables. These relationships serve as a solid foundation for the conduct of the study. 4 , 11 Haphazardly constructed research questions can result in poorly formulated hypotheses and improper study designs, leading to unreliable results. Thus, the formulations of relevant research questions and verifiable hypotheses are crucial when beginning research. 12
CHARACTERISTICS OF GOOD RESEARCH QUESTIONS AND HYPOTHESES
Excellent research questions are specific and focused. These integrate collective data and observations to confirm or refute the subsequent hypotheses. Well-constructed hypotheses are based on previous reports and verify the research context. These are realistic, in-depth, sufficiently complex, and reproducible. More importantly, these hypotheses can be addressed and tested. 13
There are several characteristics of well-developed hypotheses. Good hypotheses are 1) empirically testable 7 , 10 , 11 , 13 ; 2) backed by preliminary evidence 9 ; 3) testable by ethical research 7 , 9 ; 4) based on original ideas 9 ; 5) have evidenced-based logical reasoning 10 ; and 6) can be predicted. 11 Good hypotheses can infer ethical and positive implications, indicating the presence of a relationship or effect relevant to the research theme. 7 , 11 These are initially developed from a general theory and branch into specific hypotheses by deductive reasoning. In the absence of a theory to base the hypotheses, inductive reasoning based on specific observations or findings form more general hypotheses. 10
TYPES OF RESEARCH QUESTIONS AND HYPOTHESES
Research questions and hypotheses are developed according to the type of research, which can be broadly classified into quantitative and qualitative research. We provide a summary of the types of research questions and hypotheses under quantitative and qualitative research categories in Table 1 .
Research questions in quantitative research
In quantitative research, research questions inquire about the relationships among variables being investigated and are usually framed at the start of the study. These are precise and typically linked to the subject population, dependent and independent variables, and research design. 1 Research questions may also attempt to describe the behavior of a population in relation to one or more variables, or describe the characteristics of variables to be measured ( descriptive research questions ). 1 , 5 , 14 These questions may also aim to discover differences between groups within the context of an outcome variable ( comparative research questions ), 1 , 5 , 14 or elucidate trends and interactions among variables ( relationship research questions ). 1 , 5 We provide examples of descriptive, comparative, and relationship research questions in quantitative research in Table 2 .
Hypotheses in quantitative research
In quantitative research, hypotheses predict the expected relationships among variables. 15 Relationships among variables that can be predicted include 1) between a single dependent variable and a single independent variable ( simple hypothesis ) or 2) between two or more independent and dependent variables ( complex hypothesis ). 4 , 11 Hypotheses may also specify the expected direction to be followed and imply an intellectual commitment to a particular outcome ( directional hypothesis ) 4 . On the other hand, hypotheses may not predict the exact direction and are used in the absence of a theory, or when findings contradict previous studies ( non-directional hypothesis ). 4 In addition, hypotheses can 1) define interdependency between variables ( associative hypothesis ), 4 2) propose an effect on the dependent variable from manipulation of the independent variable ( causal hypothesis ), 4 3) state a negative relationship between two variables ( null hypothesis ), 4 , 11 , 15 4) replace the working hypothesis if rejected ( alternative hypothesis ), 15 explain the relationship of phenomena to possibly generate a theory ( working hypothesis ), 11 5) involve quantifiable variables that can be tested statistically ( statistical hypothesis ), 11 6) or express a relationship whose interlinks can be verified logically ( logical hypothesis ). 11 We provide examples of simple, complex, directional, non-directional, associative, causal, null, alternative, working, statistical, and logical hypotheses in quantitative research, as well as the definition of quantitative hypothesis-testing research in Table 3 .
Research questions in qualitative research
Unlike research questions in quantitative research, research questions in qualitative research are usually continuously reviewed and reformulated. The central question and associated subquestions are stated more than the hypotheses. 15 The central question broadly explores a complex set of factors surrounding the central phenomenon, aiming to present the varied perspectives of participants. 15
There are varied goals for which qualitative research questions are developed. These questions can function in several ways, such as to 1) identify and describe existing conditions ( contextual research question s); 2) describe a phenomenon ( descriptive research questions ); 3) assess the effectiveness of existing methods, protocols, theories, or procedures ( evaluation research questions ); 4) examine a phenomenon or analyze the reasons or relationships between subjects or phenomena ( explanatory research questions ); or 5) focus on unknown aspects of a particular topic ( exploratory research questions ). 5 In addition, some qualitative research questions provide new ideas for the development of theories and actions ( generative research questions ) or advance specific ideologies of a position ( ideological research questions ). 1 Other qualitative research questions may build on a body of existing literature and become working guidelines ( ethnographic research questions ). Research questions may also be broadly stated without specific reference to the existing literature or a typology of questions ( phenomenological research questions ), may be directed towards generating a theory of some process ( grounded theory questions ), or may address a description of the case and the emerging themes ( qualitative case study questions ). 15 We provide examples of contextual, descriptive, evaluation, explanatory, exploratory, generative, ideological, ethnographic, phenomenological, grounded theory, and qualitative case study research questions in qualitative research in Table 4 , and the definition of qualitative hypothesis-generating research in Table 5 .
Qualitative studies usually pose at least one central research question and several subquestions starting with How or What . These research questions use exploratory verbs such as explore or describe . These also focus on one central phenomenon of interest, and may mention the participants and research site. 15
Hypotheses in qualitative research
Hypotheses in qualitative research are stated in the form of a clear statement concerning the problem to be investigated. Unlike in quantitative research where hypotheses are usually developed to be tested, qualitative research can lead to both hypothesis-testing and hypothesis-generating outcomes. 2 When studies require both quantitative and qualitative research questions, this suggests an integrative process between both research methods wherein a single mixed-methods research question can be developed. 1
FRAMEWORKS FOR DEVELOPING RESEARCH QUESTIONS AND HYPOTHESES
Research questions followed by hypotheses should be developed before the start of the study. 1 , 12 , 14 It is crucial to develop feasible research questions on a topic that is interesting to both the researcher and the scientific community. This can be achieved by a meticulous review of previous and current studies to establish a novel topic. Specific areas are subsequently focused on to generate ethical research questions. The relevance of the research questions is evaluated in terms of clarity of the resulting data, specificity of the methodology, objectivity of the outcome, depth of the research, and impact of the study. 1 , 5 These aspects constitute the FINER criteria (i.e., Feasible, Interesting, Novel, Ethical, and Relevant). 1 Clarity and effectiveness are achieved if research questions meet the FINER criteria. In addition to the FINER criteria, Ratan et al. described focus, complexity, novelty, feasibility, and measurability for evaluating the effectiveness of research questions. 14
The PICOT and PEO frameworks are also used when developing research questions. 1 The following elements are addressed in these frameworks, PICOT: P-population/patients/problem, I-intervention or indicator being studied, C-comparison group, O-outcome of interest, and T-timeframe of the study; PEO: P-population being studied, E-exposure to preexisting conditions, and O-outcome of interest. 1 Research questions are also considered good if these meet the “FINERMAPS” framework: Feasible, Interesting, Novel, Ethical, Relevant, Manageable, Appropriate, Potential value/publishable, and Systematic. 14
As we indicated earlier, research questions and hypotheses that are not carefully formulated result in unethical studies or poor outcomes. To illustrate this, we provide some examples of ambiguous research question and hypotheses that result in unclear and weak research objectives in quantitative research ( Table 6 ) 16 and qualitative research ( Table 7 ) 17 , and how to transform these ambiguous research question(s) and hypothesis(es) into clear and good statements.
a These statements were composed for comparison and illustrative purposes only.
b These statements are direct quotes from Higashihara and Horiuchi. 16
a This statement is a direct quote from Shimoda et al. 17
The other statements were composed for comparison and illustrative purposes only.
CONSTRUCTING RESEARCH QUESTIONS AND HYPOTHESES
To construct effective research questions and hypotheses, it is very important to 1) clarify the background and 2) identify the research problem at the outset of the research, within a specific timeframe. 9 Then, 3) review or conduct preliminary research to collect all available knowledge about the possible research questions by studying theories and previous studies. 18 Afterwards, 4) construct research questions to investigate the research problem. Identify variables to be accessed from the research questions 4 and make operational definitions of constructs from the research problem and questions. Thereafter, 5) construct specific deductive or inductive predictions in the form of hypotheses. 4 Finally, 6) state the study aims . This general flow for constructing effective research questions and hypotheses prior to conducting research is shown in Fig. 1 .

Research questions are used more frequently in qualitative research than objectives or hypotheses. 3 These questions seek to discover, understand, explore or describe experiences by asking “What” or “How.” The questions are open-ended to elicit a description rather than to relate variables or compare groups. The questions are continually reviewed, reformulated, and changed during the qualitative study. 3 Research questions are also used more frequently in survey projects than hypotheses in experiments in quantitative research to compare variables and their relationships.
Hypotheses are constructed based on the variables identified and as an if-then statement, following the template, ‘If a specific action is taken, then a certain outcome is expected.’ At this stage, some ideas regarding expectations from the research to be conducted must be drawn. 18 Then, the variables to be manipulated (independent) and influenced (dependent) are defined. 4 Thereafter, the hypothesis is stated and refined, and reproducible data tailored to the hypothesis are identified, collected, and analyzed. 4 The hypotheses must be testable and specific, 18 and should describe the variables and their relationships, the specific group being studied, and the predicted research outcome. 18 Hypotheses construction involves a testable proposition to be deduced from theory, and independent and dependent variables to be separated and measured separately. 3 Therefore, good hypotheses must be based on good research questions constructed at the start of a study or trial. 12
In summary, research questions are constructed after establishing the background of the study. Hypotheses are then developed based on the research questions. Thus, it is crucial to have excellent research questions to generate superior hypotheses. In turn, these would determine the research objectives and the design of the study, and ultimately, the outcome of the research. 12 Algorithms for building research questions and hypotheses are shown in Fig. 2 for quantitative research and in Fig. 3 for qualitative research.

EXAMPLES OF RESEARCH QUESTIONS FROM PUBLISHED ARTICLES
- EXAMPLE 1. Descriptive research question (quantitative research)
- - Presents research variables to be assessed (distinct phenotypes and subphenotypes)
- “BACKGROUND: Since COVID-19 was identified, its clinical and biological heterogeneity has been recognized. Identifying COVID-19 phenotypes might help guide basic, clinical, and translational research efforts.
- RESEARCH QUESTION: Does the clinical spectrum of patients with COVID-19 contain distinct phenotypes and subphenotypes? ” 19
- EXAMPLE 2. Relationship research question (quantitative research)
- - Shows interactions between dependent variable (static postural control) and independent variable (peripheral visual field loss)
- “Background: Integration of visual, vestibular, and proprioceptive sensations contributes to postural control. People with peripheral visual field loss have serious postural instability. However, the directional specificity of postural stability and sensory reweighting caused by gradual peripheral visual field loss remain unclear.
- Research question: What are the effects of peripheral visual field loss on static postural control ?” 20
- EXAMPLE 3. Comparative research question (quantitative research)
- - Clarifies the difference among groups with an outcome variable (patients enrolled in COMPERA with moderate PH or severe PH in COPD) and another group without the outcome variable (patients with idiopathic pulmonary arterial hypertension (IPAH))
- “BACKGROUND: Pulmonary hypertension (PH) in COPD is a poorly investigated clinical condition.
- RESEARCH QUESTION: Which factors determine the outcome of PH in COPD?
- STUDY DESIGN AND METHODS: We analyzed the characteristics and outcome of patients enrolled in the Comparative, Prospective Registry of Newly Initiated Therapies for Pulmonary Hypertension (COMPERA) with moderate or severe PH in COPD as defined during the 6th PH World Symposium who received medical therapy for PH and compared them with patients with idiopathic pulmonary arterial hypertension (IPAH) .” 21
- EXAMPLE 4. Exploratory research question (qualitative research)
- - Explores areas that have not been fully investigated (perspectives of families and children who receive care in clinic-based child obesity treatment) to have a deeper understanding of the research problem
- “Problem: Interventions for children with obesity lead to only modest improvements in BMI and long-term outcomes, and data are limited on the perspectives of families of children with obesity in clinic-based treatment. This scoping review seeks to answer the question: What is known about the perspectives of families and children who receive care in clinic-based child obesity treatment? This review aims to explore the scope of perspectives reported by families of children with obesity who have received individualized outpatient clinic-based obesity treatment.” 22
- EXAMPLE 5. Relationship research question (quantitative research)
- - Defines interactions between dependent variable (use of ankle strategies) and independent variable (changes in muscle tone)
- “Background: To maintain an upright standing posture against external disturbances, the human body mainly employs two types of postural control strategies: “ankle strategy” and “hip strategy.” While it has been reported that the magnitude of the disturbance alters the use of postural control strategies, it has not been elucidated how the level of muscle tone, one of the crucial parameters of bodily function, determines the use of each strategy. We have previously confirmed using forward dynamics simulations of human musculoskeletal models that an increased muscle tone promotes the use of ankle strategies. The objective of the present study was to experimentally evaluate a hypothesis: an increased muscle tone promotes the use of ankle strategies. Research question: Do changes in the muscle tone affect the use of ankle strategies ?” 23
EXAMPLES OF HYPOTHESES IN PUBLISHED ARTICLES
- EXAMPLE 1. Working hypothesis (quantitative research)
- - A hypothesis that is initially accepted for further research to produce a feasible theory
- “As fever may have benefit in shortening the duration of viral illness, it is plausible to hypothesize that the antipyretic efficacy of ibuprofen may be hindering the benefits of a fever response when taken during the early stages of COVID-19 illness .” 24
- “In conclusion, it is plausible to hypothesize that the antipyretic efficacy of ibuprofen may be hindering the benefits of a fever response . The difference in perceived safety of these agents in COVID-19 illness could be related to the more potent efficacy to reduce fever with ibuprofen compared to acetaminophen. Compelling data on the benefit of fever warrant further research and review to determine when to treat or withhold ibuprofen for early stage fever for COVID-19 and other related viral illnesses .” 24
- EXAMPLE 2. Exploratory hypothesis (qualitative research)
- - Explores particular areas deeper to clarify subjective experience and develop a formal hypothesis potentially testable in a future quantitative approach
- “We hypothesized that when thinking about a past experience of help-seeking, a self distancing prompt would cause increased help-seeking intentions and more favorable help-seeking outcome expectations .” 25
- “Conclusion
- Although a priori hypotheses were not supported, further research is warranted as results indicate the potential for using self-distancing approaches to increasing help-seeking among some people with depressive symptomatology.” 25
- EXAMPLE 3. Hypothesis-generating research to establish a framework for hypothesis testing (qualitative research)
- “We hypothesize that compassionate care is beneficial for patients (better outcomes), healthcare systems and payers (lower costs), and healthcare providers (lower burnout). ” 26
- Compassionomics is the branch of knowledge and scientific study of the effects of compassionate healthcare. Our main hypotheses are that compassionate healthcare is beneficial for (1) patients, by improving clinical outcomes, (2) healthcare systems and payers, by supporting financial sustainability, and (3) HCPs, by lowering burnout and promoting resilience and well-being. The purpose of this paper is to establish a scientific framework for testing the hypotheses above . If these hypotheses are confirmed through rigorous research, compassionomics will belong in the science of evidence-based medicine, with major implications for all healthcare domains.” 26
- EXAMPLE 4. Statistical hypothesis (quantitative research)
- - An assumption is made about the relationship among several population characteristics ( gender differences in sociodemographic and clinical characteristics of adults with ADHD ). Validity is tested by statistical experiment or analysis ( chi-square test, Students t-test, and logistic regression analysis)
- “Our research investigated gender differences in sociodemographic and clinical characteristics of adults with ADHD in a Japanese clinical sample. Due to unique Japanese cultural ideals and expectations of women's behavior that are in opposition to ADHD symptoms, we hypothesized that women with ADHD experience more difficulties and present more dysfunctions than men . We tested the following hypotheses: first, women with ADHD have more comorbidities than men with ADHD; second, women with ADHD experience more social hardships than men, such as having less full-time employment and being more likely to be divorced.” 27
- “Statistical Analysis
- ( text omitted ) Between-gender comparisons were made using the chi-squared test for categorical variables and Students t-test for continuous variables…( text omitted ). A logistic regression analysis was performed for employment status, marital status, and comorbidity to evaluate the independent effects of gender on these dependent variables.” 27
EXAMPLES OF HYPOTHESIS AS WRITTEN IN PUBLISHED ARTICLES IN RELATION TO OTHER PARTS
- EXAMPLE 1. Background, hypotheses, and aims are provided
- “Pregnant women need skilled care during pregnancy and childbirth, but that skilled care is often delayed in some countries …( text omitted ). The focused antenatal care (FANC) model of WHO recommends that nurses provide information or counseling to all pregnant women …( text omitted ). Job aids are visual support materials that provide the right kind of information using graphics and words in a simple and yet effective manner. When nurses are not highly trained or have many work details to attend to, these job aids can serve as a content reminder for the nurses and can be used for educating their patients (Jennings, Yebadokpo, Affo, & Agbogbe, 2010) ( text omitted ). Importantly, additional evidence is needed to confirm how job aids can further improve the quality of ANC counseling by health workers in maternal care …( text omitted )” 28
- “ This has led us to hypothesize that the quality of ANC counseling would be better if supported by job aids. Consequently, a better quality of ANC counseling is expected to produce higher levels of awareness concerning the danger signs of pregnancy and a more favorable impression of the caring behavior of nurses .” 28
- “This study aimed to examine the differences in the responses of pregnant women to a job aid-supported intervention during ANC visit in terms of 1) their understanding of the danger signs of pregnancy and 2) their impression of the caring behaviors of nurses to pregnant women in rural Tanzania.” 28
- EXAMPLE 2. Background, hypotheses, and aims are provided
- “We conducted a two-arm randomized controlled trial (RCT) to evaluate and compare changes in salivary cortisol and oxytocin levels of first-time pregnant women between experimental and control groups. The women in the experimental group touched and held an infant for 30 min (experimental intervention protocol), whereas those in the control group watched a DVD movie of an infant (control intervention protocol). The primary outcome was salivary cortisol level and the secondary outcome was salivary oxytocin level.” 29
- “ We hypothesize that at 30 min after touching and holding an infant, the salivary cortisol level will significantly decrease and the salivary oxytocin level will increase in the experimental group compared with the control group .” 29
- EXAMPLE 3. Background, aim, and hypothesis are provided
- “In countries where the maternal mortality ratio remains high, antenatal education to increase Birth Preparedness and Complication Readiness (BPCR) is considered one of the top priorities [1]. BPCR includes birth plans during the antenatal period, such as the birthplace, birth attendant, transportation, health facility for complications, expenses, and birth materials, as well as family coordination to achieve such birth plans. In Tanzania, although increasing, only about half of all pregnant women attend an antenatal clinic more than four times [4]. Moreover, the information provided during antenatal care (ANC) is insufficient. In the resource-poor settings, antenatal group education is a potential approach because of the limited time for individual counseling at antenatal clinics.” 30
- “This study aimed to evaluate an antenatal group education program among pregnant women and their families with respect to birth-preparedness and maternal and infant outcomes in rural villages of Tanzania.” 30
- “ The study hypothesis was if Tanzanian pregnant women and their families received a family-oriented antenatal group education, they would (1) have a higher level of BPCR, (2) attend antenatal clinic four or more times, (3) give birth in a health facility, (4) have less complications of women at birth, and (5) have less complications and deaths of infants than those who did not receive the education .” 30
Research questions and hypotheses are crucial components to any type of research, whether quantitative or qualitative. These questions should be developed at the very beginning of the study. Excellent research questions lead to superior hypotheses, which, like a compass, set the direction of research, and can often determine the successful conduct of the study. Many research studies have floundered because the development of research questions and subsequent hypotheses was not given the thought and meticulous attention needed. The development of research questions and hypotheses is an iterative process based on extensive knowledge of the literature and insightful grasp of the knowledge gap. Focused, concise, and specific research questions provide a strong foundation for constructing hypotheses which serve as formal predictions about the research outcomes. Research questions and hypotheses are crucial elements of research that should not be overlooked. They should be carefully thought of and constructed when planning research. This avoids unethical studies and poor outcomes by defining well-founded objectives that determine the design, course, and outcome of the study.
Disclosure: The authors have no potential conflicts of interest to disclose.
Author Contributions:
- Conceptualization: Barroga E, Matanguihan GJ.
- Methodology: Barroga E, Matanguihan GJ.
- Writing - original draft: Barroga E, Matanguihan GJ.
- Writing - review & editing: Barroga E, Matanguihan GJ.
Click through the PLOS taxonomy to find articles in your field.
For more information about PLOS Subject Areas, click here .
Loading metrics
Open Access
Peer-reviewed
Research Article
Recent quantitative research on determinants of health in high income countries: A scoping review
Roles Conceptualization, Data curation, Formal analysis, Investigation, Methodology, Project administration, Software, Visualization, Writing – original draft, Writing – review & editing
* E-mail: [email protected]
Affiliation Centre for Health Economics Research and Modelling Infectious Diseases, Vaccine and Infectious Disease Institute, University of Antwerp, Antwerp, Belgium

Roles Conceptualization, Data curation, Funding acquisition, Project administration, Resources, Supervision, Validation, Visualization, Writing – review & editing
- Vladimira Varbanova,
- Philippe Beutels
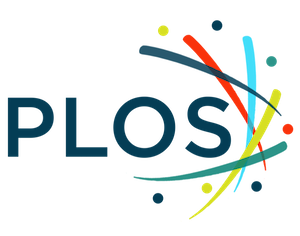
- Published: September 17, 2020
- https://doi.org/10.1371/journal.pone.0239031
- Peer Review
- Reader Comments
Identifying determinants of health and understanding their role in health production constitutes an important research theme. We aimed to document the state of recent multi-country research on this theme in the literature.
We followed the PRISMA-ScR guidelines to systematically identify, triage and review literature (January 2013—July 2019). We searched for studies that performed cross-national statistical analyses aiming to evaluate the impact of one or more aggregate level determinants on one or more general population health outcomes in high-income countries. To assess in which combinations and to what extent individual (or thematically linked) determinants had been studied together, we performed multidimensional scaling and cluster analysis.
Sixty studies were selected, out of an original yield of 3686. Life-expectancy and overall mortality were the most widely used population health indicators, while determinants came from the areas of healthcare, culture, politics, socio-economics, environment, labor, fertility, demographics, life-style, and psychology. The family of regression models was the predominant statistical approach. Results from our multidimensional scaling showed that a relatively tight core of determinants have received much attention, as main covariates of interest or controls, whereas the majority of other determinants were studied in very limited contexts. We consider findings from these studies regarding the importance of any given health determinant inconclusive at present. Across a multitude of model specifications, different country samples, and varying time periods, effects fluctuated between statistically significant and not significant, and between beneficial and detrimental to health.
Conclusions
We conclude that efforts to understand the underlying mechanisms of population health are far from settled, and the present state of research on the topic leaves much to be desired. It is essential that future research considers multiple factors simultaneously and takes advantage of more sophisticated methodology with regards to quantifying health as well as analyzing determinants’ influence.
Citation: Varbanova V, Beutels P (2020) Recent quantitative research on determinants of health in high income countries: A scoping review. PLoS ONE 15(9): e0239031. https://doi.org/10.1371/journal.pone.0239031
Editor: Amir Radfar, University of Central Florida, UNITED STATES
Received: November 14, 2019; Accepted: August 28, 2020; Published: September 17, 2020
Copyright: © 2020 Varbanova, Beutels. This is an open access article distributed under the terms of the Creative Commons Attribution License , which permits unrestricted use, distribution, and reproduction in any medium, provided the original author and source are credited.
Data Availability: All relevant data are within the manuscript and its Supporting Information files.
Funding: This study (and VV) is funded by the Research Foundation Flanders ( https://www.fwo.be/en/ ), FWO project number G0D5917N, award obtained by PB. The funder had no role in study design, data collection and analysis, decision to publish, or preparation of the manuscript.
Competing interests: The authors have declared that no competing interests exist.
Introduction
Identifying the key drivers of population health is a core subject in public health and health economics research. Between-country comparative research on the topic is challenging. In order to be relevant for policy, it requires disentangling different interrelated drivers of “good health”, each having different degrees of importance in different contexts.
“Good health”–physical and psychological, subjective and objective–can be defined and measured using a variety of approaches, depending on which aspect of health is the focus. A major distinction can be made between health measurements at the individual level or some aggregate level, such as a neighborhood, a region or a country. In view of this, a great diversity of specific research topics exists on the drivers of what constitutes individual or aggregate “good health”, including those focusing on health inequalities, the gender gap in longevity, and regional mortality and longevity differences.
The current scoping review focuses on determinants of population health. Stated as such, this topic is quite broad. Indeed, we are interested in the very general question of what methods have been used to make the most of increasingly available region or country-specific databases to understand the drivers of population health through inter-country comparisons. Existing reviews indicate that researchers thus far tend to adopt a narrower focus. Usually, attention is given to only one health outcome at a time, with further geographical and/or population [ 1 , 2 ] restrictions. In some cases, the impact of one or more interventions is at the core of the review [ 3 – 7 ], while in others it is the relationship between health and just one particular predictor, e.g., income inequality, access to healthcare, government mechanisms [ 8 – 13 ]. Some relatively recent reviews on the subject of social determinants of health [ 4 – 6 , 14 – 17 ] have considered a number of indicators potentially influencing health as opposed to a single one. One review defines “social determinants” as “the social, economic, and political conditions that influence the health of individuals and populations” [ 17 ] while another refers even more broadly to “the factors apart from medical care” [ 15 ].
In the present work, we aimed to be more inclusive, setting no limitations on the nature of possible health correlates, as well as making use of a multitude of commonly accepted measures of general population health. The goal of this scoping review was to document the state of the art in the recent published literature on determinants of population health, with a particular focus on the types of determinants selected and the methodology used. In doing so, we also report the main characteristics of the results these studies found. The materials collected in this review are intended to inform our (and potentially other researchers’) future analyses on this topic. Since the production of health is subject to the law of diminishing marginal returns, we focused our review on those studies that included countries where a high standard of wealth has been achieved for some time, i.e., high-income countries belonging to the Organisation for Economic Co-operation and Development (OECD) or Europe. Adding similar reviews for other country income groups is of limited interest to the research we plan to do in this area.
In view of its focus on data and methods, rather than results, a formal protocol was not registered prior to undertaking this review, but the procedure followed the guidelines of the PRISMA statement for scoping reviews [ 18 ].
We focused on multi-country studies investigating the potential associations between any aggregate level (region/city/country) determinant and general measures of population health (e.g., life expectancy, mortality rate).
Within the query itself, we listed well-established population health indicators as well as the six world regions, as defined by the World Health Organization (WHO). We searched only in the publications’ titles in order to keep the number of hits manageable, and the ratio of broadly relevant abstracts over all abstracts in the order of magnitude of 10% (based on a series of time-focused trial runs). The search strategy was developed iteratively between the two authors and is presented in S1 Appendix . The search was performed by VV in PubMed and Web of Science on the 16 th of July, 2019, without any language restrictions, and with a start date set to the 1 st of January, 2013, as we were interested in the latest developments in this area of research.
Eligibility criteria
Records obtained via the search methods described above were screened independently by the two authors. Consistency between inclusion/exclusion decisions was approximately 90% and the 43 instances where uncertainty existed were judged through discussion. Articles were included subject to meeting the following requirements: (a) the paper was a full published report of an original empirical study investigating the impact of at least one aggregate level (city/region/country) factor on at least one health indicator (or self-reported health) of the general population (the only admissible “sub-populations” were those based on gender and/or age); (b) the study employed statistical techniques (calculating correlations, at the very least) and was not purely descriptive or theoretical in nature; (c) the analysis involved at least two countries or at least two regions or cities (or another aggregate level) in at least two different countries; (d) the health outcome was not differentiated according to some socio-economic factor and thus studied in terms of inequality (with the exception of gender and age differentiations); (e) mortality, in case it was one of the health indicators under investigation, was strictly “total” or “all-cause” (no cause-specific or determinant-attributable mortality).
Data extraction
The following pieces of information were extracted in an Excel table from the full text of each eligible study (primarily by VV, consulting with PB in case of doubt): health outcome(s), determinants, statistical methodology, level of analysis, results, type of data, data sources, time period, countries. The evidence is synthesized according to these extracted data (often directly reflected in the section headings), using a narrative form accompanied by a “summary-of-findings” table and a graph.
Search and selection
The initial yield contained 4583 records, reduced to 3686 after removal of duplicates ( Fig 1 ). Based on title and abstract screening, 3271 records were excluded because they focused on specific medical condition(s) or specific populations (based on morbidity or some other factor), dealt with intervention effectiveness, with theoretical or non-health related issues, or with animals or plants. Of the remaining 415 papers, roughly half were disqualified upon full-text consideration, mostly due to using an outcome not of interest to us (e.g., health inequality), measuring and analyzing determinants and outcomes exclusively at the individual level, performing analyses one country at a time, employing indices that are a mixture of both health indicators and health determinants, or not utilizing potential health determinants at all. After this second stage of the screening process, 202 papers were deemed eligible for inclusion. This group was further dichotomized according to level of economic development of the countries or regions under study, using membership of the OECD or Europe as a reference “cut-off” point. Sixty papers were judged to include high-income countries, and the remaining 142 included either low- or middle-income countries or a mix of both these levels of development. The rest of this report outlines findings in relation to high-income countries only, reflecting our own primary research interests. Nonetheless, we chose to report our search yield for the other income groups for two reasons. First, to gauge the relative interest in applied published research for these different income levels; and second, to enable other researchers with a focus on determinants of health in other countries to use the extraction we made here.
- PPT PowerPoint slide
- PNG larger image
- TIFF original image
https://doi.org/10.1371/journal.pone.0239031.g001
Health outcomes
The most frequent population health indicator, life expectancy (LE), was present in 24 of the 60 studies. Apart from “life expectancy at birth” (representing the average life-span a newborn is expected to have if current mortality rates remain constant), also called “period LE” by some [ 19 , 20 ], we encountered as well LE at 40 years of age [ 21 ], at 60 [ 22 ], and at 65 [ 21 , 23 , 24 ]. In two papers, the age-specificity of life expectancy (be it at birth or another age) was not stated [ 25 , 26 ].
Some studies considered male and female LE separately [ 21 , 24 , 25 , 27 – 33 ]. This consideration was also often observed with the second most commonly used health index [ 28 – 30 , 34 – 38 ]–termed “total”, or “overall”, or “all-cause”, mortality rate (MR)–included in 22 of the 60 studies. In addition to gender, this index was also sometimes broken down according to age group [ 30 , 39 , 40 ], as well as gender-age group [ 38 ].
While the majority of studies under review here focused on a single health indicator, 23 out of the 60 studies made use of multiple outcomes, although these outcomes were always considered one at a time, and sometimes not all of them fell within the scope of our review. An easily discernable group of indices that typically went together [ 25 , 37 , 41 ] was that of neonatal (deaths occurring within 28 days postpartum), perinatal (fetal or early neonatal / first-7-days deaths), and post-neonatal (deaths between the 29 th day and completion of one year of life) mortality. More often than not, these indices were also accompanied by “stand-alone” indicators, such as infant mortality (deaths within the first year of life; our third most common index found in 16 of the 60 studies), maternal mortality (deaths during pregnancy or within 42 days of termination of pregnancy), and child mortality rates. Child mortality has conventionally been defined as mortality within the first 5 years of life, thus often also called “under-5 mortality”. Nonetheless, Pritchard & Wallace used the term “child mortality” to denote deaths of children younger than 14 years [ 42 ].
As previously stated, inclusion criteria did allow for self-reported health status to be used as a general measure of population health. Within our final selection of studies, seven utilized some form of subjective health as an outcome variable [ 25 , 43 – 48 ]. Additionally, the Health Human Development Index [ 49 ], healthy life expectancy [ 50 ], old-age survival [ 51 ], potential years of life lost [ 52 ], and disability-adjusted life expectancy [ 25 ] were also used.
We note that while in most cases the indicators mentioned above (and/or the covariates considered, see below) were taken in their absolute or logarithmic form, as a—typically annual—number, sometimes they were used in the form of differences, change rates, averages over a given time period, or even z-scores of rankings [ 19 , 22 , 40 , 42 , 44 , 53 – 57 ].
Regions, countries, and populations
Despite our decision to confine this review to high-income countries, some variation in the countries and regions studied was still present. Selection seemed to be most often conditioned on the European Union, or the European continent more generally, and the Organisation of Economic Co-operation and Development (OECD), though, typically, not all member nations–based on the instances where these were also explicitly listed—were included in a given study. Some of the stated reasons for omitting certain nations included data unavailability [ 30 , 45 , 54 ] or inconsistency [ 20 , 58 ], Gross Domestic Product (GDP) too low [ 40 ], differences in economic development and political stability with the rest of the sampled countries [ 59 ], and national population too small [ 24 , 40 ]. On the other hand, the rationales for selecting a group of countries included having similar above-average infant mortality [ 60 ], similar healthcare systems [ 23 ], and being randomly drawn from a social spending category [ 61 ]. Some researchers were interested explicitly in a specific geographical region, such as Eastern Europe [ 50 ], Central and Eastern Europe [ 48 , 60 ], the Visegrad (V4) group [ 62 ], or the Asia/Pacific area [ 32 ]. In certain instances, national regions or cities, rather than countries, constituted the units of investigation instead [ 31 , 51 , 56 , 62 – 66 ]. In two particular cases, a mix of countries and cities was used [ 35 , 57 ]. In another two [ 28 , 29 ], due to the long time periods under study, some of the included countries no longer exist. Finally, besides “European” and “OECD”, the terms “developed”, “Western”, and “industrialized” were also used to describe the group of selected nations [ 30 , 42 , 52 , 53 , 67 ].
As stated above, it was the health status of the general population that we were interested in, and during screening we made a concerted effort to exclude research using data based on a more narrowly defined group of individuals. All studies included in this review adhere to this general rule, albeit with two caveats. First, as cities (even neighborhoods) were the unit of analysis in three of the studies that made the selection [ 56 , 64 , 65 ], the populations under investigation there can be more accurately described as general urban , instead of just general. Second, oftentimes health indicators were stratified based on gender and/or age, therefore we also admitted one study that, due to its specific research question, focused on men and women of early retirement age [ 35 ] and another that considered adult males only [ 68 ].
Data types and sources
A great diversity of sources was utilized for data collection purposes. The accessible reference databases of the OECD ( https://www.oecd.org/ ), WHO ( https://www.who.int/ ), World Bank ( https://www.worldbank.org/ ), United Nations ( https://www.un.org/en/ ), and Eurostat ( https://ec.europa.eu/eurostat ) were among the top choices. The other international databases included Human Mortality [ 30 , 39 , 50 ], Transparency International [ 40 , 48 , 50 ], Quality of Government [ 28 , 69 ], World Income Inequality [ 30 ], International Labor Organization [ 41 ], International Monetary Fund [ 70 ]. A number of national databases were referred to as well, for example the US Bureau of Statistics [ 42 , 53 ], Korean Statistical Information Services [ 67 ], Statistics Canada [ 67 ], Australian Bureau of Statistics [ 67 ], and Health New Zealand Tobacco control and Health New Zealand Food and Nutrition [ 19 ]. Well-known surveys, such as the World Values Survey [ 25 , 55 ], the European Social Survey [ 25 , 39 , 44 ], the Eurobarometer [ 46 , 56 ], the European Value Survey [ 25 ], and the European Statistics of Income and Living Condition Survey [ 43 , 47 , 70 ] were used as data sources, too. Finally, in some cases [ 25 , 28 , 29 , 35 , 36 , 41 , 69 ], built-for-purpose datasets from previous studies were re-used.
In most of the studies, the level of the data (and analysis) was national. The exceptions were six papers that dealt with Nomenclature of Territorial Units of Statistics (NUTS2) regions [ 31 , 62 , 63 , 66 ], otherwise defined areas [ 51 ] or cities [ 56 ], and seven others that were multilevel designs and utilized both country- and region-level data [ 57 ], individual- and city- or country-level [ 35 ], individual- and country-level [ 44 , 45 , 48 ], individual- and neighborhood-level [ 64 ], and city-region- (NUTS3) and country-level data [ 65 ]. Parallel to that, the data type was predominantly longitudinal, with only a few studies using purely cross-sectional data [ 25 , 33 , 43 , 45 – 48 , 50 , 62 , 67 , 68 , 71 , 72 ], albeit in four of those [ 43 , 48 , 68 , 72 ] two separate points in time were taken (thus resulting in a kind of “double cross-section”), while in another the averages across survey waves were used [ 56 ].
In studies using longitudinal data, the length of the covered time periods varied greatly. Although this was almost always less than 40 years, in one study it covered the entire 20 th century [ 29 ]. Longitudinal data, typically in the form of annual records, was sometimes transformed before usage. For example, some researchers considered data points at 5- [ 34 , 36 , 49 ] or 10-year [ 27 , 29 , 35 ] intervals instead of the traditional 1, or took averages over 3-year periods [ 42 , 53 , 73 ]. In one study concerned with the effect of the Great Recession all data were in a “recession minus expansion change in trends”-form [ 57 ]. Furthermore, there were a few instances where two different time periods were compared to each other [ 42 , 53 ] or when data was divided into 2 to 4 (possibly overlapping) periods which were then analyzed separately [ 24 , 26 , 28 , 29 , 31 , 65 ]. Lastly, owing to data availability issues, discrepancies between the time points or periods of data on the different variables were occasionally observed [ 22 , 35 , 42 , 53 – 55 , 63 ].
Health determinants
Together with other essential details, Table 1 lists the health correlates considered in the selected studies. Several general categories for these correlates can be discerned, including health care, political stability, socio-economics, demographics, psychology, environment, fertility, life-style, culture, labor. All of these, directly or implicitly, have been recognized as holding importance for population health by existing theoretical models of (social) determinants of health [ 74 – 77 ].
https://doi.org/10.1371/journal.pone.0239031.t001
It is worth noting that in a few studies there was just a single aggregate-level covariate investigated in relation to a health outcome of interest to us. In one instance, this was life satisfaction [ 44 ], in another–welfare system typology [ 45 ], but also gender inequality [ 33 ], austerity level [ 70 , 78 ], and deprivation [ 51 ]. Most often though, attention went exclusively to GDP [ 27 , 29 , 46 , 57 , 65 , 71 ]. It was often the case that research had a more particular focus. Among others, minimum wages [ 79 ], hospital payment schemes [ 23 ], cigarette prices [ 63 ], social expenditure [ 20 ], residents’ dissatisfaction [ 56 ], income inequality [ 30 , 69 ], and work leave [ 41 , 58 ] took center stage. Whenever variables outside of these specific areas were also included, they were usually identified as confounders or controls, moderators or mediators.
We visualized the combinations in which the different determinants have been studied in Fig 2 , which was obtained via multidimensional scaling and a subsequent cluster analysis (details outlined in S2 Appendix ). It depicts the spatial positioning of each determinant relative to all others, based on the number of times the effects of each pair of determinants have been studied simultaneously. When interpreting Fig 2 , one should keep in mind that determinants marked with an asterisk represent, in fact, collectives of variables.
Groups of determinants are marked by asterisks (see S1 Table in S1 Appendix ). Diminishing color intensity reflects a decrease in the total number of “connections” for a given determinant. Noteworthy pairwise “connections” are emphasized via lines (solid-dashed-dotted indicates decreasing frequency). Grey contour lines encircle groups of variables that were identified via cluster analysis. Abbreviations: age = population age distribution, associations = membership in associations, AT-index = atherogenic-thrombogenic index, BR = birth rate, CAPB = Cyclically Adjusted Primary Balance, civilian-labor = civilian labor force, C-section = Cesarean delivery rate, credit-info = depth of credit information, dissatisf = residents’ dissatisfaction, distrib.orient = distributional orientation, EDU = education, eHealth = eHealth index at GP-level, exch.rate = exchange rate, fat = fat consumption, GDP = gross domestic product, GFCF = Gross Fixed Capital Formation/Creation, GH-gas = greenhouse gas, GII = gender inequality index, gov = governance index, gov.revenue = government revenues, HC-coverage = healthcare coverage, HE = health(care) expenditure, HHconsump = household consumption, hosp.beds = hospital beds, hosp.payment = hospital payment scheme, hosp.stay = length of hospital stay, IDI = ICT development index, inc.ineq = income inequality, industry-labor = industrial labor force, infant-sex = infant sex ratio, labor-product = labor production, LBW = low birth weight, leave = work leave, life-satisf = life satisfaction, M-age = maternal age, marginal-tax = marginal tax rate, MDs = physicians, mult.preg = multiple pregnancy, NHS = Nation Health System, NO = nitrous oxide emissions, PM10 = particulate matter (PM10) emissions, pop = population size, pop.density = population density, pre-term = pre-term birth rate, prison = prison population, researchE = research&development expenditure, school.ref = compulsory schooling reform, smoke-free = smoke-free places, SO = sulfur oxide emissions, soc.E = social expenditure, soc.workers = social workers, sugar = sugar consumption, terror = terrorism, union = union density, UR = unemployment rate, urban = urbanization, veg-fr = vegetable-and-fruit consumption, welfare = welfare regime, Wwater = wastewater treatment.
https://doi.org/10.1371/journal.pone.0239031.g002
Distances between determinants in Fig 2 are indicative of determinants’ “connectedness” with each other. While the statistical procedure called for higher dimensionality of the model, for demonstration purposes we show here a two-dimensional solution. This simplification unfortunately comes with a caveat. To use the factor smoking as an example, it would appear it stands at a much greater distance from GDP than it does from alcohol. In reality however, smoking was considered together with alcohol consumption [ 21 , 25 , 26 , 52 , 68 ] in just as many studies as it was with GDP [ 21 , 25 , 26 , 52 , 59 ], five. To aid with respect to this apparent shortcoming, we have emphasized the strongest pairwise links. Solid lines connect GDP with health expenditure (HE), unemployment rate (UR), and education (EDU), indicating that the effect of GDP on health, taking into account the effects of the other three determinants as well, was evaluated in between 12 to 16 studies of the 60 included in this review. Tracing the dashed lines, we can also tell that GDP appeared jointly with income inequality, and HE together with either EDU or UR, in anywhere between 8 to 10 of our selected studies. Finally, some weaker but still worth-mentioning “connections” between variables are displayed as well via the dotted lines.
The fact that all notable pairwise “connections” are concentrated within a relatively small region of the plot may be interpreted as low overall “connectedness” among the health indicators studied. GDP is the most widely investigated determinant in relation to general population health. Its total number of “connections” is disproportionately high (159) compared to its runner-up–HE (with 113 “connections”), and then subsequently EDU (with 90) and UR (with 86). In fact, all of these determinants could be thought of as outliers, given that none of the remaining factors have a total count of pairings above 52. This decrease in individual determinants’ overall “connectedness” can be tracked on the graph via the change of color intensity as we move outwards from the symbolic center of GDP and its closest “co-determinants”, to finally reach the other extreme of the ten indicators (welfare regime, household consumption, compulsory school reform, life satisfaction, government revenues, literacy, research expenditure, multiple pregnancy, Cyclically Adjusted Primary Balance, and residents’ dissatisfaction; in white) the effects on health of which were only studied in isolation.
Lastly, we point to the few small but stable clusters of covariates encircled by the grey bubbles on Fig 2 . These groups of determinants were identified as “close” by both statistical procedures used for the production of the graph (see details in S2 Appendix ).
Statistical methodology
There was great variation in the level of statistical detail reported. Some authors provided too vague a description of their analytical approach, necessitating some inference in this section.
The issue of missing data is a challenging reality in this field of research, but few of the studies under review (12/60) explain how they dealt with it. Among the ones that do, three general approaches to handling missingness can be identified, listed in increasing level of sophistication: case-wise deletion, i.e., removal of countries from the sample [ 20 , 45 , 48 , 58 , 59 ], (linear) interpolation [ 28 , 30 , 34 , 58 , 59 , 63 ], and multiple imputation [ 26 , 41 , 52 ].
Correlations, Pearson, Spearman, or unspecified, were the only technique applied with respect to the health outcomes of interest in eight analyses [ 33 , 42 – 44 , 46 , 53 , 57 , 61 ]. Among the more advanced statistical methods, the family of regression models proved to be, by and large, predominant. Before examining this closer, we note the techniques that were, in a way, “unique” within this selection of studies: meta-analyses were performed (random and fixed effects, respectively) on the reduced form and 2-sample two stage least squares (2SLS) estimations done within countries [ 39 ]; difference-in-difference (DiD) analysis was applied in one case [ 23 ]; dynamic time-series methods, among which co-integration, impulse-response function (IRF), and panel vector autoregressive (VAR) modeling, were utilized in one study [ 80 ]; longitudinal generalized estimating equation (GEE) models were developed on two occasions [ 70 , 78 ]; hierarchical Bayesian spatial models [ 51 ] and special autoregressive regression [ 62 ] were also implemented.
Purely cross-sectional data analyses were performed in eight studies [ 25 , 45 , 47 , 50 , 55 , 56 , 67 , 71 ]. These consisted of linear regression (assumed ordinary least squares (OLS)), generalized least squares (GLS) regression, and multilevel analyses. However, six other studies that used longitudinal data in fact had a cross-sectional design, through which they applied regression at multiple time-points separately [ 27 , 29 , 36 , 48 , 68 , 72 ].
Apart from these “multi-point cross-sectional studies”, some other simplistic approaches to longitudinal data analysis were found, involving calculating and regressing 3-year averages of both the response and the predictor variables [ 54 ], taking the average of a few data-points (i.e., survey waves) [ 56 ] or using difference scores over 10-year [ 19 , 29 ] or unspecified time intervals [ 40 , 55 ].
Moving further in the direction of more sensible longitudinal data usage, we turn to the methods widely known among (health) economists as “panel data analysis” or “panel regression”. Most often seen were models with fixed effects for country/region and sometimes also time-point (occasionally including a country-specific trend as well), with robust standard errors for the parameter estimates to take into account correlations among clustered observations [ 20 , 21 , 24 , 28 , 30 , 32 , 34 , 37 , 38 , 41 , 52 , 59 , 60 , 63 , 66 , 69 , 73 , 79 , 81 , 82 ]. The Hausman test [ 83 ] was sometimes mentioned as the tool used to decide between fixed and random effects [ 26 , 49 , 63 , 66 , 73 , 82 ]. A few studies considered the latter more appropriate for their particular analyses, with some further specifying that (feasible) GLS estimation was employed [ 26 , 34 , 49 , 58 , 60 , 73 ]. Apart from these two types of models, the first differences method was encountered once as well [ 31 ]. Across all, the error terms were sometimes assumed to come from a first-order autoregressive process (AR(1)), i.e., they were allowed to be serially correlated [ 20 , 30 , 38 , 58 – 60 , 73 ], and lags of (typically) predictor variables were included in the model specification, too [ 20 , 21 , 37 , 38 , 48 , 69 , 81 ]. Lastly, a somewhat different approach to longitudinal data analysis was undertaken in four studies [ 22 , 35 , 48 , 65 ] in which multilevel–linear or Poisson–models were developed.
Regardless of the exact techniques used, most studies included in this review presented multiple model applications within their main analysis. None attempted to formally compare models in order to identify the “best”, even if goodness-of-fit statistics were occasionally reported. As indicated above, many studies investigated women’s and men’s health separately [ 19 , 21 , 22 , 27 – 29 , 31 , 33 , 35 , 36 , 38 , 39 , 45 , 50 , 51 , 64 , 65 , 69 , 82 ], and covariates were often tested one at a time, including other covariates only incrementally [ 20 , 25 , 28 , 36 , 40 , 50 , 55 , 67 , 73 ]. Furthermore, there were a few instances where analyses within countries were performed as well [ 32 , 39 , 51 ] or where the full time period of interest was divided into a few sub-periods [ 24 , 26 , 28 , 31 ]. There were also cases where different statistical techniques were applied in parallel [ 29 , 55 , 60 , 66 , 69 , 73 , 82 ], sometimes as a form of sensitivity analysis [ 24 , 26 , 30 , 58 , 73 ]. However, the most common approach to sensitivity analysis was to re-run models with somewhat different samples [ 39 , 50 , 59 , 67 , 69 , 80 , 82 ]. Other strategies included different categorization of variables or adding (more/other) controls [ 21 , 23 , 25 , 28 , 37 , 50 , 63 , 69 ], using an alternative main covariate measure [ 59 , 82 ], including lags for predictors or outcomes [ 28 , 30 , 58 , 63 , 65 , 79 ], using weights [ 24 , 67 ] or alternative data sources [ 37 , 69 ], or using non-imputed data [ 41 ].
As the methods and not the findings are the main focus of the current review, and because generic checklists cannot discern the underlying quality in this application field (see also below), we opted to pool all reported findings together, regardless of individual study characteristics or particular outcome(s) used, and speak generally of positive and negative effects on health. For this summary we have adopted the 0.05-significance level and only considered results from multivariate analyses. Strictly birth-related factors are omitted since these potentially only relate to the group of infant mortality indicators and not to any of the other general population health measures.
Starting with the determinants most often studied, higher GDP levels [ 21 , 26 , 27 , 29 , 30 , 32 , 43 , 48 , 52 , 58 , 60 , 66 , 67 , 73 , 79 , 81 , 82 ], higher health [ 21 , 37 , 47 , 49 , 52 , 58 , 59 , 68 , 72 , 82 ] and social [ 20 , 21 , 26 , 38 , 79 ] expenditures, higher education [ 26 , 39 , 52 , 62 , 72 , 73 ], lower unemployment [ 60 , 61 , 66 ], and lower income inequality [ 30 , 42 , 53 , 55 , 73 ] were found to be significantly associated with better population health on a number of occasions. In addition to that, there was also some evidence that democracy [ 36 ] and freedom [ 50 ], higher work compensation [ 43 , 79 ], distributional orientation [ 54 ], cigarette prices [ 63 ], gross national income [ 22 , 72 ], labor productivity [ 26 ], exchange rates [ 32 ], marginal tax rates [ 79 ], vaccination rates [ 52 ], total fertility [ 59 , 66 ], fruit and vegetable [ 68 ], fat [ 52 ] and sugar consumption [ 52 ], as well as bigger depth of credit information [ 22 ] and percentage of civilian labor force [ 79 ], longer work leaves [ 41 , 58 ], more physicians [ 37 , 52 , 72 ], nurses [ 72 ], and hospital beds [ 79 , 82 ], and also membership in associations, perceived corruption and societal trust [ 48 ] were beneficial to health. Higher nitrous oxide (NO) levels [ 52 ], longer average hospital stay [ 48 ], deprivation [ 51 ], dissatisfaction with healthcare and the social environment [ 56 ], corruption [ 40 , 50 ], smoking [ 19 , 26 , 52 , 68 ], alcohol consumption [ 26 , 52 , 68 ] and illegal drug use [ 68 ], poverty [ 64 ], higher percentage of industrial workers [ 26 ], Gross Fixed Capital creation [ 66 ] and older population [ 38 , 66 , 79 ], gender inequality [ 22 ], and fertility [ 26 , 66 ] were detrimental.
It is important to point out that the above-mentioned effects could not be considered stable either across or within studies. Very often, statistical significance of a given covariate fluctuated between the different model specifications tried out within the same study [ 20 , 49 , 59 , 66 , 68 , 69 , 73 , 80 , 82 ], testifying to the importance of control variables and multivariate research (i.e., analyzing multiple independent variables simultaneously) in general. Furthermore, conflicting results were observed even with regards to the “core” determinants given special attention, so to speak, throughout this text. Thus, some studies reported negative effects of health expenditure [ 32 , 82 ], social expenditure [ 58 ], GDP [ 49 , 66 ], and education [ 82 ], and positive effects of income inequality [ 82 ] and unemployment [ 24 , 31 , 32 , 52 , 66 , 68 ]. Interestingly, one study [ 34 ] differentiated between temporary and long-term effects of GDP and unemployment, alluding to possibly much greater complexity of the association with health. It is also worth noting that some gender differences were found, with determinants being more influential for males than for females, or only having statistically significant effects for male health [ 19 , 21 , 28 , 34 , 36 , 37 , 39 , 64 , 65 , 69 ].
The purpose of this scoping review was to examine recent quantitative work on the topic of multi-country analyses of determinants of population health in high-income countries.
Measuring population health via relatively simple mortality-based indicators still seems to be the state of the art. What is more, these indicators are routinely considered one at a time, instead of, for example, employing existing statistical procedures to devise a more general, composite, index of population health, or using some of the established indices, such as disability-adjusted life expectancy (DALE) or quality-adjusted life expectancy (QALE). Although strong arguments for their wider use were already voiced decades ago [ 84 ], such summary measures surface only rarely in this research field.
On a related note, the greater data availability and accessibility that we enjoy today does not automatically equate to data quality. Nonetheless, this is routinely assumed in aggregate level studies. We almost never encountered a discussion on the topic. The non-mundane issue of data missingness, too, goes largely underappreciated. With all recent methodological advancements in this area [ 85 – 88 ], there is no excuse for ignorance; and still, too few of the reviewed studies tackled the matter in any adequate fashion.
Much optimism can be gained considering the abundance of different determinants that have attracted researchers’ attention in relation to population health. We took on a visual approach with regards to these determinants and presented a graph that links spatial distances between determinants with frequencies of being studies together. To facilitate interpretation, we grouped some variables, which resulted in some loss of finer detail. Nevertheless, the graph is helpful in exemplifying how many effects continue to be studied in a very limited context, if any. Since in reality no factor acts in isolation, this oversimplification practice threatens to render the whole exercise meaningless from the outset. The importance of multivariate analysis cannot be stressed enough. While there is no “best method” to be recommended and appropriate techniques vary according to the specifics of the research question and the characteristics of the data at hand [ 89 – 93 ], in the future, in addition to abandoning simplistic univariate approaches, we hope to see a shift from the currently dominating fixed effects to the more flexible random/mixed effects models [ 94 ], as well as wider application of more sophisticated methods, such as principle component regression, partial least squares, covariance structure models (e.g., structural equations), canonical correlations, time-series, and generalized estimating equations.
Finally, there are some limitations of the current scoping review. We searched the two main databases for published research in medical and non-medical sciences (PubMed and Web of Science) since 2013, thus potentially excluding publications and reports that are not indexed in these databases, as well as older indexed publications. These choices were guided by our interest in the most recent (i.e., the current state-of-the-art) and arguably the highest-quality research (i.e., peer-reviewed articles, primarily in indexed non-predatory journals). Furthermore, despite holding a critical stance with regards to some aspects of how determinants-of-health research is currently conducted, we opted out of formally assessing the quality of the individual studies included. The reason for that is two-fold. On the one hand, we are unaware of the existence of a formal and standard tool for quality assessment of ecological designs. And on the other, we consider trying to score the quality of these diverse studies (in terms of regional setting, specific topic, outcome indices, and methodology) undesirable and misleading, particularly since we would sometimes have been rating the quality of only a (small) part of the original studies—the part that was relevant to our review’s goal.
Our aim was to investigate the current state of research on the very broad and general topic of population health, specifically, the way it has been examined in a multi-country context. We learned that data treatment and analytical approach were, in the majority of these recent studies, ill-equipped or insufficiently transparent to provide clarity regarding the underlying mechanisms of population health in high-income countries. Whether due to methodological shortcomings or the inherent complexity of the topic, research so far fails to provide any definitive answers. It is our sincere belief that with the application of more advanced analytical techniques this continuous quest could come to fruition sooner.
Supporting information
S1 checklist. preferred reporting items for systematic reviews and meta-analyses extension for scoping reviews (prisma-scr) checklist..
https://doi.org/10.1371/journal.pone.0239031.s001
S1 Appendix.
https://doi.org/10.1371/journal.pone.0239031.s002
S2 Appendix.
https://doi.org/10.1371/journal.pone.0239031.s003
- View Article
- Google Scholar
- PubMed/NCBI
- 75. Dahlgren G, Whitehead M. Policies and Strategies to Promote Equity in Health. Stockholm, Sweden: Institute for Future Studies; 1991.
- 76. Brunner E, Marmot M. Social Organization, Stress, and Health. In: Marmot M, Wilkinson RG, editors. Social Determinants of Health. Oxford, England: Oxford University Press; 1999.
- 77. Najman JM. A General Model of the Social Origins of Health and Well-being. In: Eckersley R, Dixon J, Douglas B, editors. The Social Origins of Health and Well-being. Cambridge, England: Cambridge University Press; 2001.
- 85. Carpenter JR, Kenward MG. Multiple Imputation and its Application. New York: John Wiley & Sons; 2013.
- 86. Molenberghs G, Fitzmaurice G, Kenward MG, Verbeke G, Tsiatis AA. Handbook of Missing Data Methodology. Boca Raton: Chapman & Hall/CRC; 2014.
- 87. van Buuren S. Flexible Imputation of Missing Data. 2nd ed. Boca Raton: Chapman & Hall/CRC; 2018.
- 88. Enders CK. Applied Missing Data Analysis. New York: Guilford; 2010.
- 89. Shayle R. Searle GC, Charles E. McCulloch. Variance Components: John Wiley & Sons, Inc.; 1992.
- 90. Agresti A. Foundations of Linear and Generalized Linear Models. Hoboken, New Jersey: John Wiley & Sons Inc.; 2015.
- 91. Leyland A. H. (Editor) HGE. Multilevel Modelling of Health Statistics: John Wiley & Sons Inc; 2001.
- 92. Garrett Fitzmaurice MD, Geert Verbeke, Geert Molenberghs. Longitudinal Data Analysis. New York: Chapman and Hall/CRC; 2008.
- 93. Wolfgang Karl Härdle LS. Applied Multivariate Statistical Analysis. Berlin, Heidelberg: Springer; 2015.
Advertisement
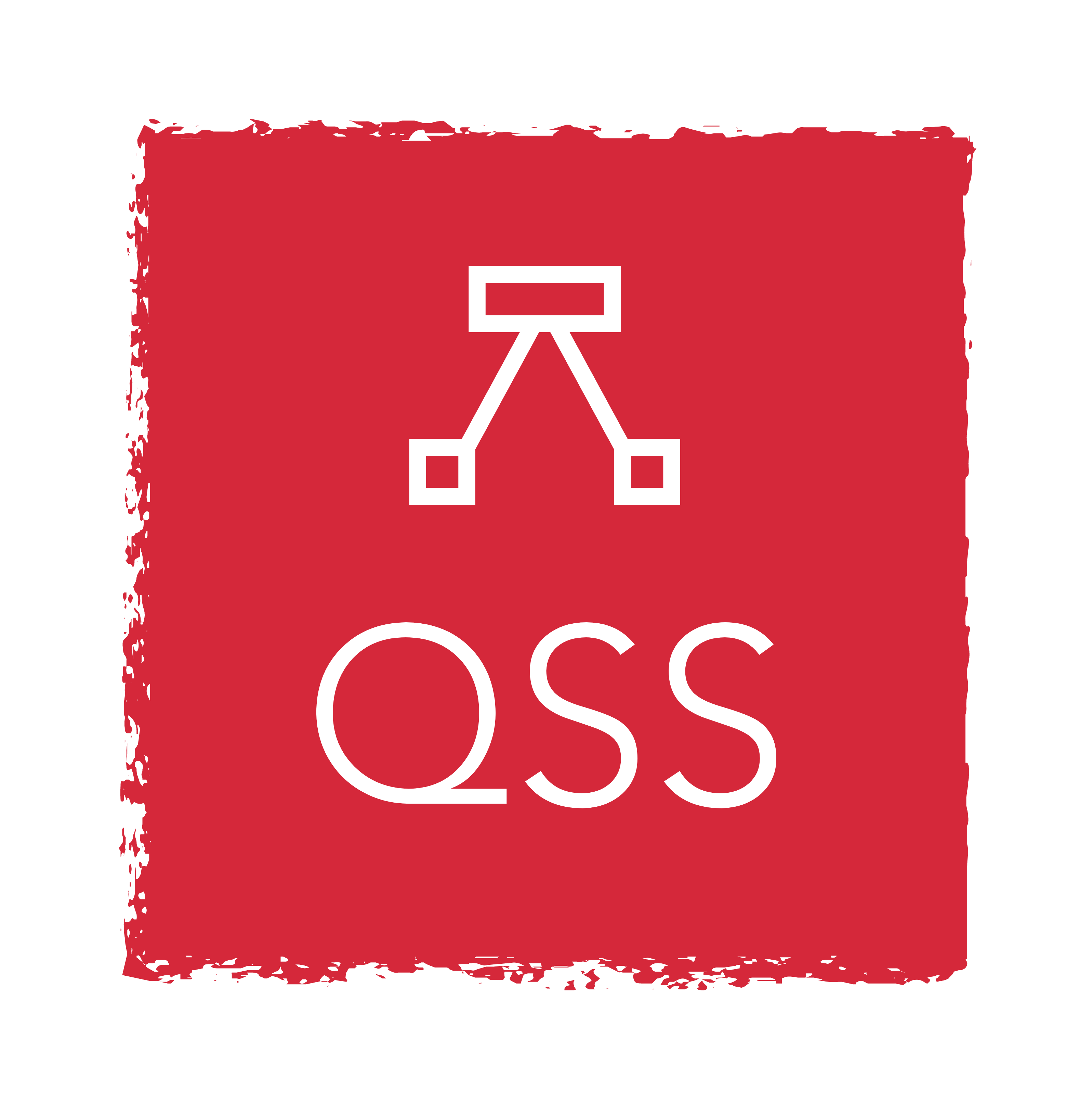
- Previous Issue
Special Issue: Editorial
Special issue: articles, research articles, bridging the divide between qualitative and quantitative science studies, numbers or no numbers in science studies, against method: exploding the boundary between qualitative and quantitative studies of science, is “the time ripe” for quantitative research on misconduct in science, the impact of j. d. bernal’s thoughts in the science of science upon china: implications for today’s quantitative studies of science, powerful numbers: exemplary quantitative studies of science that had policy impact, quantitative science studies should be framed with middle-range theories and concepts from the social sciences, whose text, whose mining, and to whose benefit, gender, science, and academic rank: key issues and approaches, geography of scientific knowledge: a proximity approach, beyond networks: aligning qualitative and computational science studies, past as prologue: approaches to the study of confirmation in science, from indicators to indicating interdisciplinarity: a participatory mapping methodology for research communities in-the-making, pandemic publishing: medical journals strongly speed up their publication process for covid-19, covid-19 publications: database coverage, citations, readers, tweets, news, facebook walls, reddit posts, mapping scholarly publications related to the sustainable development goals: do independent bibliometric approaches get the same results, a longitudinal analysis of university rankings, proposal success in horizon 2020: a study of the influence of consortium characteristics, concentration of danish research funding on individual researchers and research topics: patterns and potential drivers, longitudinal variation in national research publication portfolios: steps required to index balance and evenness, a typology of scientific breakthroughs, frequently cocited publications: features and kinetics, are disruption index indicators convergently valid the comparison of several indicator variants with assessments by peers, a gender equality paradox in academic publishing: countries with a higher proportion of female first-authored journal articles have larger first-author gender disparities between fields, greater female first author citation advantages do not associate with reduced or reducing gender disparities in academia, recency predicts bursts in the evolution of author citations, noncumulative measures of researcher citation impact, informed peer review for publication assessments: are improved impact measures worth the hassle, all downhill from the phd the typical impact trajectory of u.s. academic careers, product(s) added to cart, email alerts, affiliations.
- Online ISSN 2641-3337
A product of The MIT Press
Mit press direct.
- About MIT Press Direct
Information
- Accessibility
- For Authors
- For Customers
- For Librarians
- Direct to Open
- Open Access
- Media Inquiries
- Rights and Permissions
- For Advertisers
- About the MIT Press
- The MIT Press Reader
- MIT Press Blog
- Seasonal Catalogs
- MIT Press Home
- Give to the MIT Press
- Direct Service Desk
- Terms of Use
- Privacy Statement
- Crossref Member
- COUNTER Member
- The MIT Press colophon is registered in the U.S. Patent and Trademark Office
This Feature Is Available To Subscribers Only
Sign In or Create an Account
Your browser is not supported
Sorry but it looks as if your browser is out of date. To get the best experience using our site we recommend that you upgrade or switch browsers.
Find a solution
- Skip to main content
- Skip to navigation
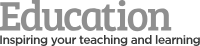
- Back to parent navigation item
- Primary teacher
- Secondary/FE teacher
- Early career or student teacher
- Higher education
- Curriculum support
- Literacy in science teaching
- Periodic table
- Interactive periodic table
- Climate change and sustainability
- Resources shop
- Collections
- Post-lockdown teaching support
- Remote teaching support
- Starters for ten
- Screen experiments
- Assessment for learning
- Microscale chemistry
- Faces of chemistry
- Classic chemistry experiments
- Nuffield practical collection
- Anecdotes for chemistry teachers
- On this day in chemistry
- Global experiments
- PhET interactive simulations
- Chemistry vignettes
- Context and problem based learning
- Journal of the month
- Chemistry and art
- Art analysis
- Pigments and colours
- Ancient art: today's technology
- Psychology and art theory
- Art and archaeology
- Artists as chemists
- The physics of restoration and conservation
- Ancient Egyptian art
- Ancient Greek art
- Ancient Roman art
- Classic chemistry demonstrations
- In search of solutions
- In search of more solutions
- Creative problem-solving in chemistry
- Solar spark
- Chemistry for non-specialists
- Health and safety in higher education
- Analytical chemistry introductions
- Exhibition chemistry
- Introductory maths for higher education
- Commercial skills for chemists
- Kitchen chemistry
- Journals how to guides
- Chemistry in health
- Chemistry in sport
- Chemistry in your cupboard
- Chocolate chemistry
- Adnoddau addysgu cemeg Cymraeg
- The chemistry of fireworks
- Festive chemistry
- Education in Chemistry
- Teach Chemistry
- On-demand online
- Live online
- Selected PD articles
- PD for primary teachers
- PD for secondary teachers
- What we offer
- Chartered Science Teacher (CSciTeach)
- Teacher mentoring
- UK Chemistry Olympiad
- Who can enter?
- How does it work?
- Resources and past papers
- Top of the Bench
- Schools' Analyst
- Regional support
- Education coordinators
- RSC Yusuf Hamied Inspirational Science Programme
- RSC Education News
- Supporting teacher training
- Interest groups
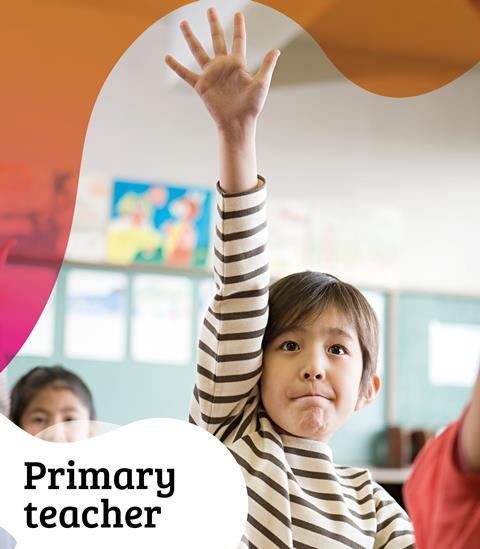
- More from navigation items
All Quantitative research articles
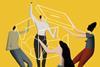
Harness self-regulation to nurture independent study skills
2020-10-29T10:15:00Z
Follow these tips to engage students with learning processes
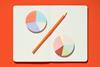
Why declining science scores are no reason to panic
2020-02-05T10:31:00Z
PISA provides an interesting background to teaching, but is it only for policymakers?
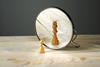
Dunning-Kruger: the gap between prediction and performance
2018-03-19T14:15:00Z
Improve expectations to improve learning
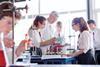
Encouraging inquiry-based approaches
2016-09-28T00:00:00Z
Manage the load for students
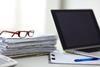
Transforming education research
2016-09-14T00:00:00Z
New project to investigate the opportunities and challenges for teachers and researchers
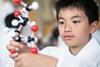
The value of modelling molecules
2016-08-10T00:00:00Z
Challenge of visual-spatial representations
![article of quantitative research Education research shutterstock 139305425 300tb[1]](https://d1ymz67w5raq8g.cloudfront.net/Pictures/100x67/5/6/4/113564_educationresearch_shutterstock_139305425_300tb1.jpg)
Why don't teachers use education research in teaching?
2016-08-09T07:57:00Z
Paul MacLellan digs into the problem with research from Durham, a secondary school teacher and a journal editor
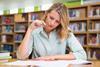
What influences future science study?
2016-07-27T00:00:00Z
Study beyond GCSE linked to confidence and perceptions
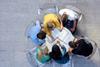
It’s good to talk
2016-06-08T00:00:00Z
Facilitating peer group learning
![article of quantitative research Micer shutterstock 348717923 300tb[1]](https://d1ymz67w5raq8g.cloudfront.net/Pictures/100x67/5/9/9/113599_micer_shutterstock_348717923_300tb1.jpg)
The community of chemistry education research
2016-03-03T15:11:00Z
Michael Seery talks about being part of the chemistry education research community in the UK and Ireland
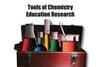
Tools of chemistry education research
2015-11-09T00:00:00Z
Methods and strategies
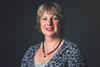
Understanding education
2015-11-06T00:00:00Z
Raising awareness of teaching and learning opportunities all around us
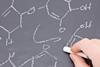
Organic confusion
Rote memorising v deep understanding
![article of quantitative research Img 0013 300tb[1]](https://d1ymz67w5raq8g.cloudfront.net/Pictures/100x67/6/3/3/113633_img_0013_300tb1.jpg)
Variety in Chemistry Education 2015
2015-08-24T16:14:00Z
Michael Seery reports from the conference for chemistry teaching and learning in higher education
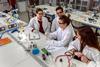
The case against inquiry-based learning
2015-05-26T10:44:00Z
Michael Seery takes a critical look at inquiry-based learning
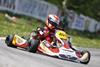
Rationalising reasoning
2015-05-11T00:00:00Z
Is contextualisation the best solution?
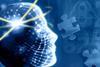
Analysing analogies
Teacher CPD could support analogical thinking
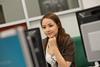
Flipped chemistry revisited
2015-03-05T00:00:00Z
Successful organic chemistry teaching
![article of quantitative research Sl india 300tb[1]](https://d1ymz67w5raq8g.cloudfront.net/Pictures/100x67/6/8/1/113681_slindia_300tb1.jpg)
International Conference on Education in Chemistry, 2014
2015-01-20T13:20:00Z
Simon Lancaster reports on his visit to ICEC-2014 in Mumbai
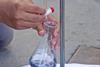
Moles and titrations
2015-01-06T00:00:00Z
Dorothy Warren describes some of the difficulties with teaching this topic and shows how you can help your students to master aspects of quantitative chemistry
- Previous Page
- Contributors
- Email alerts
Site powered by Webvision Cloud
- Open access
- Published: 01 April 2024
Strategies to implement evidence-informed decision making at the organizational level: a rapid systematic review
- Emily C. Clark 1 ,
- Trish Burnett 1 ,
- Rebecca Blair 1 ,
- Robyn L. Traynor 1 ,
- Leah Hagerman 1 &
- Maureen Dobbins 1 , 2
BMC Health Services Research volume 24 , Article number: 405 ( 2024 ) Cite this article
Metrics details
Achievement of evidence-informed decision making (EIDM) requires the integration of evidence into all practice decisions by identifying and synthesizing evidence, then developing and executing plans to implement and evaluate changes to practice. This rapid systematic review synthesizes evidence for strategies for the implementation of EIDM across organizations, mapping facilitators and barriers to the COM-B (capability, opportunity, motivation, behaviour) model for behaviour change. The review was conducted to support leadership at organizations delivering public health services (health promotion, communicable disease prevention) to drive change toward evidence-informed public health.
A systematic search was conducted in multiple databases and by reviewing publications of key authors. Articles that describe interventions to drive EIDM within teams, departments, or organizations were eligible for inclusion. For each included article, quality was assessed, and details of the intervention, setting, outcomes, facilitators and barriers were extracted. A convergent integrated approach was undertaken to analyze both quantitative and qualitative findings.
Thirty-seven articles are included. Studies were conducted in primary care, public health, social services, and occupational health settings. Strategies to implement EIDM included the establishment of Knowledge Broker-type roles, building the EIDM capacity of staff, and research or academic partnerships. Facilitators and barriers align with the COM-B model for behaviour change. Facilitators for capability include the development of staff knowledge and skill, establishing specialized roles, and knowledge sharing across the organization, though staff turnover and subsequent knowledge loss was a barrier to capability. For opportunity, facilitators include the development of processes or mechanisms to support new practices, forums for learning and skill development, and protected time, and barriers include competing priorities. Facilitators identified for motivation include supportive organizational culture, expectations for new practices to occur, recognition and positive reinforcement, and strong leadership support. Barriers include negative attitudes toward new practices, and lack of understanding and support from management.
This review provides a comprehensive analysis of facilitators and barriers for the implementation of EIDM in organizations for public health, mapped to the COM-B model for behaviour change. The existing literature for strategies to support EIDM in public health illustrates several facilitators and barriers linked to realizing EIDM. Knowledge of these factors will help senior leadership develop and implement EIDM strategies tailored to their organization, leading to increased likelihood of implementation success.
Review registration
PROSPERO CRD42022318994.
Peer Review reports
There exist expectations that decisions and programs that affect public and population health are informed by the best available evidence from research, local context, and political will [ 1 , 2 , 3 ]. To achieve evidence-informed public health, it is important that public health organizations engage in and support evidence-informed decision making (EIDM). For this review, “public health organizations” refers to organizations that implement public health programs, including health promotion, injury and disease prevention, population health monitoring, emergency preparedness and response, and other critical functions [ 4 ]. EIDM, at an organizational level, involves the integration of evidence into all practice decisions by identifying and synthesizing evidence, then developing and executing plans to implement and evaluate changes to practice [ 2 , 5 , 6 ]. EIDM considers research evidence along with other factors such as context, resources, experience, and patient/community input to influence decision making and program implementation [ 2 , 3 , 7 , 8 ]. When implemented, EIDM results in efficient use of scarce resources, encourages stakeholder involvement resulting in more effective programs and decisions, improves transparency and accountability of organizations, improves health outcomes, and reduces harm [ 3 , 7 , 8 ]. Therefore, it is important that EIDM is integrated into organizations serving public health.
Driving organizational change for EIDM is challenging due to the need for multifaceted interventions [ 9 ].While there are systematic reviews of the implementation of specific evidence-informed initiatives, reviews of implementation of organization-wide EIDM are lacking. For example, Mathieson et al. and Li et al. examined the barriers and facilitators to the implementation of evidence-informed interventions in community nursing and Paci et al. examined barriers in physiotherapy [ 10 , 11 , 12 ]. Li et al. found that implementation of evidence-informed practices is associated with an organizational culture for EIDM where staff at all levels value and contribute to EIDM [ 12 ]. Similarly, Mathieson et al. and Paci et al. found that organizational context plays an important role in evidence-informed practice implementation along with organizational support and resources [ 10 , 11 ]. While these reviews identify organizational context, culture and support as crucial for the implementation of a particular evidence-informed practice, they do not identify and describe sufficiently what and how an organization evolves to consistently be evidence-informed for all decisions and programs and services it delivers.
Primary studies have explored how building capacity for staff to find, interpret and synthesize evidence to develop practice and program recommendations may contribute to EIDM [ 13 , 14 , 15 , 16 ]. In 2019, Saunders et al. completed an overview of systematic reviews on primary health care professionals’ EIDM competencies and found that implementation of EIDM across studies was low [ 9 ]. Participants reported insufficient knowledge and skills to implement EIDM in daily practice despite positive EIDM beliefs and attitudes [ 9 ]. In 2014, Sadeghi-Bazargani et al. and in 2018, Barzkar et al. also explored the implementation of EIDM and found similar results, listing inadequate skills and lack of knowledge amongst the most common barriers to EIDM [ 17 , 18 ].
An underlying current in research for organizational EIDM is a focus on organizational change [ 13 , 14 , 19 , 20 ]. To achieve EIDM across an organization, significant organizational change is usually necessary, resulting in substantial impact on the entire organization, as well as for individuals working there. However, while there are reviews of individual capacity for EIDM, there is minimal synthesized evidence describing EIDM capacity at the organizational level. This review seeks to address this research gap by identifying, appraising, and synthesizing research evidence from studies seeking to understand the process of embedding EIDM across an organization, with a focus on public health organizations.
The COM-B model for behaviour change was used as a guide for contextualizing the findings across studies. By integrating causal components of behaviour change, the COM-B model supports the development of interventions that can sustain behaviour change in the long-term. While there are numerous models available to support implementation and organizational change, the COM-B model was chosen, in part, for its simple visual representation of concepts, as well as its contributions to the sustainability of behaviours [ 21 ]. This model is designed to guide organizational change initiatives and distill complex systems that influence behaviour into simpler, visual representations. Specifically, this model looks at capability (C), opportunity (O) and motivation (M) as three key influencers of behaviour (B). The capability section of the COM-B model reflects whether the intended audience possess the knowledge and skills for a new behaviour. Opportunity reflects whether there is opportunity for new behaviour to occur, while motivation reflects whether there is sufficient motivation for a new behaviour to occur. All three components interact to create behaviour and behaviours can, in turn, alter capability, motivation and opportunity [ 21 ]. Selection of the COM-B model was also driven by authors’ extensive experience supporting public health organizations in implementing EIDM, which observed enablers for EIDM that align well with the COM-B model, such as team-wide capacity-building for EIDM, integration of EIDM into processes, and support from senior leadership [ 20 , 22 , 23 ]. The COM-B model has been used to map findings from systematic reviews examining the barriers and facilitators of various health interventions including nicotine replacement, chlamydia testing and lifestyle management of polycystic ovary syndrome [ 24 , 25 , 26 ]. This review has a broader focus and maps barriers and facilitators for organization-wide EIDM to the COM-B model.
Overall, EIDM is expected to be a foundation at public health organizations to achieve optimal health of populations. However, the capacity of public health organizations to realize EIDM varies considerably from organization to organization [ 14 , 22 , 27 , 28 , 29 ]. This rapid review aims to examine the implementation of EIDM at the organizational level to inform change efforts at Canadian public health organizations. The findings of this review can be applied more broadly and will support public health organizations beyond Canada to implement change efforts to practice in an evidence-informed way.
Study design
The review protocol was registered with the International Prospective Register of Systematic Reviews (PROSPERO; Registration CRD42022318994). The review was conducted and reported following the Preferred Reporting Items for Systematic Reviews and Meta-Analyses (PRISMA) statement for reporting systematic reviews and meta-analyses [ 30 ]. A rapid review approach was used, since the review was requested to be completed by the National Collaborating Centre for Methods and Tools’ Rapid Evidence Service within a specific timeline, in order to inform an organizational change initiative at a provincial public health organization in Canada [ 31 ]. Given the nature of the research question, a mixed methods rapid systematic review approach was taken, with guidance from the Joanna Briggs Institute (JBI) Manual for Evidence Synthesis [ 32 ].
Information sources and search strategy
The search was conducted on March 18, 2022. The following databases were searched from 2012 onward: Medline, Embase, Emcare, Global Health Database, PsycINFO, Web of Science. Each database was searched using combinations and variations of the terms “implement*”, “knowledge broker*”, “transform*”, “organizational culture”, “change management”, “evidence-based”, “knowledge translation”, and “knowledge mobilization”. Additionally, publications by key contributors to the field were reviewed. The full search strategy is included in Appendix 1 .
Studies were screened using DistillerSR software. Titles and abstracts of retrieved studies were screened by a single reviewer. Full texts of included studies were screened by a second reviewer and reviewed by a third. Screening was not completed in duplicate, consistent with a rapid review protocol [ 31 ]. To minimize the risk of bias, a subset of 100 retrieved articles were screened in duplicate at the title and abstract stage to ensure consistency across reviewers. Of this subset, there were four articles with conflicting decisions, which were discussed amongst screeners to clarify inclusion criteria.
Eligibility criteria
English-language, published primary studies with experimental or observational designs were eligible for inclusion. Review papers, such as literature and systematic reviews, were excluded to ensure that details regarding implementation of initiatives were captured without re-interpretation or generalization by review authors. Grey literature was not included. Eligibility criteria are outlined below in terms of a PICO (Population, Intervention, Comparison, Outcome) structure [ 33 ].
Studies conducted with public sector health-related service-delivery organizations were eligible for inclusion. This included public health departments and authorities, health care settings and social services. Studies focused on departments or teams within an organization, or on entire organizations, were also eligible for inclusion. Studies conducted in private sectors or academic institutions were excluded to narrow the focus of the review.
Intervention
Interventions designed and implemented to shift teams, departments, or organizations to EIDM in all decisions were eligible for inclusion. These can include initiatives where organizations establish roles or teams to drive organizational change for EIDM, or efforts to build and apply the knowledge and skill of staff for EIDM. These are distinct from implementation strategies for evidence-informed interventions. Eligible interventions were applied to a team, department, or organization to drive change toward evidence use in decision making at all levels of the organizations.
Studies that included any comparator or no comparator were included, recognizing that literature was likely to include case reports.
Outcomes measured either quantitatively or qualitatively were considered. These included behaviour change, confidence and skills, patient-level data such as quality indicators, evidence of EIDM embedded in organizational and decision-making processes, changes in organizational culture, and changes to budget allocation. Studies that reported primarily on implementation fidelity were excluded, since studies of implementation fidelity focus on whether an intervention is delivered as intended, rather than drivers for organizational change.
Studies conducted in the 38 member countries of the Organization for Economic Co-operation and Development (OECD) were included in this review to best align with the Canadian context and to inform organizational change efforts in public health within Canada [ 34 ].
Quality assessment
The methodological rigour of included studies was evaluated using the JBI suite of critical appraisal tools [ 35 ]. Ratings of low, moderate, or high quality were assigned based on the critical appraisal results. Quality assessment was completed by one reviewer and verified by a second. Conflicts were resolved through discussion or by consulting a third reviewer.
Data extraction
Data extraction was completed by a single reviewer and reviewed by a second. Data on the study design, setting, sector (e.g., public health, primary care, etc.), participants, intervention (e.g., description of learning initiatives, implementation strategies, etc.), outcome measures, and findings were extracted. To minimize the risk of bias, a subset of three included articles underwent data extraction in duplicate to ensure consistency across reviewers. There was good agreement between duplicate extraction, with variations in the format of extracted data but consistency in content.
Data analysis
Quantitative and qualitative data were synthesized simultaneously, using a convergent integrated approach [ 32 ]. Quantitative data underwent narrative synthesis, where findings that caused benefit were compared with those that caused harm or no effect [ 36 ]. Vote counting based on the direction of effect was used to determine whether most studies found a positive or negative effect [ 36 ]. For qualitative findings, studies were grouped according to common strategies. Within these common strategies, findings were reviewed for trends in reported facilitators and barriers. These trends were deductively mapped to the COM-B model for behaviour change [ 37 ].
Due to the heterogeneity in study outcomes, the Grading of Recommendations, Assessment, Development and Evaluations (GRADE) [ 38 ] approach was not used for this review. Overall certainty of evidence was determined based on the risk of bias of included study designs and study quality.
Database searching retrieved 7067 records. After removing duplicates, 4174 records were screened by title and abstract, resulting in 1370 reports for full text review. Of those 1370 records, 35 articles were included. Scanning the publication lists of key authors retrieved 187 records, of which eight were retrieved for full text review and two were included, for a total of 37 articles included in this review. See Fig. 1 for a PRISMA flow chart illustrating the article search and selection process.
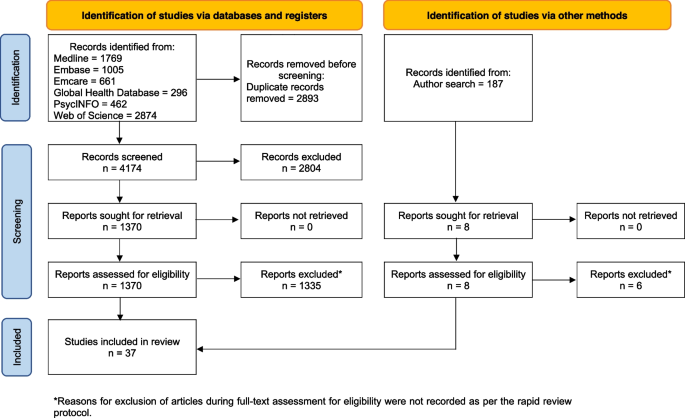
PRISMA 2020 flow chart
Study characteristics
The overall characteristics of included studies are summarized in Table 1 . Of 37 included studies, most were conducted in primary care settings ( n = 16) and public health settings ( n = 16), with some in social services ( n = 3), child and youth mental health ( n = 1), and occupational health ( n = 1). Most studies were conducted in the USA ( n = 17), followed by Canada ( n = 12), Australia ( n = 5), and Europe ( n = 3).
Study designs included case reports ( n = 18), single group pre-/post-test studies ( n = 10), qualitative studies ( n = 7), and randomized controlled trials (RCTs) ( n = 2). Both RCTs evaluated the implementation of organizational EIDM.
Studies reported quantitative ( n = 11), qualitative ( n = 20), or both quantitative and qualitative results ( n = 6). For the studies that reported quantitative results, measures included EIDM implementation, EIDM-related beliefs and behaviours, organizational priorities for EIDM, and patient care quality indicators. Quantitative measures were heterogenous and did not allow meta-analysis. Qualitative findings were generated through formal qualitative analysis ( n = 19) or descriptive case reports ( n = 7). Most qualitative results included facilitators and barriers to implementation ( n = 16).
Study quality
The critical appraisal checklist used to assess each study is indicated in Table 1 . Single group, pre-/post-test studies were evaluated according to the JBI Checklist for Quasi-experimental Studies [ 35 ].
A lack of control groups contributed to the risk of bias. Most included studies were rated Moderate or High quality according to their respective quality assessment tools. Full quality assessments for each article are included in Appendix 2 . Therefore, the overall methodological quality for this body of literature was rated as Moderate.
Strategies for implementing organization-wide EIDM
Due to the heterogeneity of study designs, interventions, and outcomes, it was not possible to determine which EIDM implementation strategies are more effective compared to others. Implementation strategies included the establishment of Knowledge Broker-type roles, building the EIDM capacity of staff, and research or academic partnerships. These strategies are listed in Table 2 .
Evaluation of strategies implemented by studies in this review was often qualitative and described facilitators and barriers, rather than quantitatively measuring effectiveness. However, it is possible to explore EIDM implementation strategies and factors that appear to contribute to or inhibit success. The most common strategy implemented in included studies was the establishment of Knowledge Broker-type roles [ 20 , 41 , 44 , 47 , 48 , 51 , 52 , 54 , 55 , 56 , 57 , 59 , 60 , 62 , 63 , 64 , 65 , 66 , 67 , 69 , 71 , 72 ]. Studies described roles differently (e.g., “Evidence-based Practice Facilitator”, “Evidence Facilitator”, “EIDM Mentor”). These roles all served to support EIDM across organizations through knowledge sharing, evidence synthesis, implementation, and other EIDM-related activities. In some studies, new staff were hired to Knowledge Broker roles, or developed among existing staff, while in others, Knowledge Brokers were contracted from external organizations. Knowledge Broker strategies were mostly implemented in parallel with other EIDM implementation strategies, such as capacity building for staff, integrating EIDM into decision-making processes and development of leadership to support EIDM. When these strategies were evaluated quantitatively for organizational capacity, culture and implementation of EIDM, most studies found positive results, such as increased scores for organizational climates supporting EIDM, improved attitudes toward EIDM, or the integration of EIDM into processes [ 44 , 52 , 54 , 62 , 66 , 67 , 71 , 72 ], although some studies found no change [ 55 , 60 ] following implementation of Knowledge Broker roles. Qualitatively, most studies described facilitators and barriers to EIDM, either through formal qualitative analysis or case report [ 14 , 20 , 39 , 40 , 41 , 42 , 43 , 45 , 47 , 48 , 52 , 55 , 57 , 59 , 60 , 61 , 64 , 65 , 68 ]. Facilitators included organizational culture with supportive leadership and staff buy-in, expectations to use evidence to inform decisions, accessible knowledge, and integration of EIDM into processes and templates. Barriers included limited time and competing priorities, staff turnover, and lack of understanding and support from management.
Ten included studies focused primarily on building EIDM capacity of existing staff at the organization, often at multiple levels (e.g., front-line service providers, managers, and leadership) [ 13 , 14 , 39 , 40 , 42 , 43 , 46 , 49 , 50 , 58 , 61 ]. Capacity building was typically done through EIDM-focused workshops, often with ongoing follow up support from workshop facilitators. While studies often measured changes in individual knowledge and skill for EIDM for workshop participants, organizational change for EIDM was reported qualitatively, either through formal qualitative analysis or through a case report. Facilitators for EIDM in these ten studies included organizational culture with supportive leadership and staff buy-in, dedicated staff roles to support EIDM, opportunities to meet and discuss EIDM (e.g., communities of practice, journal clubs), knowledge sharing across the organization, expectations to use evidence to inform decisions, accessible knowledge, and integration of EIDM into processes and templates. Barriers included limited time and competing priorities, staff turnover, and negative attitudes toward EIDM.
Research or academic partnerships and networks were the main strategy described in three case reports [ 45 , 53 , 68 ]. These involved establishing collaborations, either through universities or non-governmental health organizations, that provided direct EIDM support. These strategies were not evaluated quantitatively but described facilitators and barriers to effective cross-sector collaborations. Facilitators for EIDM included supportive leadership and management, dedicated staff roles to support EIDM, EIDM knowledge and skill development for staff, and regular communication between partners. Barriers included limited time and competing priorities, preference for experiential over research evidence, and negative attitudes toward EIDM.
Overall, studies described successes in implementing EIDM across organizations, citing several common key facilitators and barriers. To instigate behaviour change, strategies must address capability for change, which may be achieved by building staff capacity, establishing dedicated support roles, improving access to evidence, and sharing knowledge across the organization. Strategies must also enable opportunities for change, which may be supported through forums for EIDM learning and practice, protecting time for EIDM, integrating EIDM into new or existing roles, and adding EIDM to processes and templates. Behaviour change also requires motivation, which may be built through a supportive organizational culture, expectations to use EIDM, recognition and positive reinforcement, and strong support from leadership.
Key considerations for implementing EIDM
Many of the facilitators and barriers to EIDM are common across strategies explored by the studies included in this review. To conceptualize these factors, they were mapped to the COM-B model for behaviour change [ 21 ] in Fig. 2 .
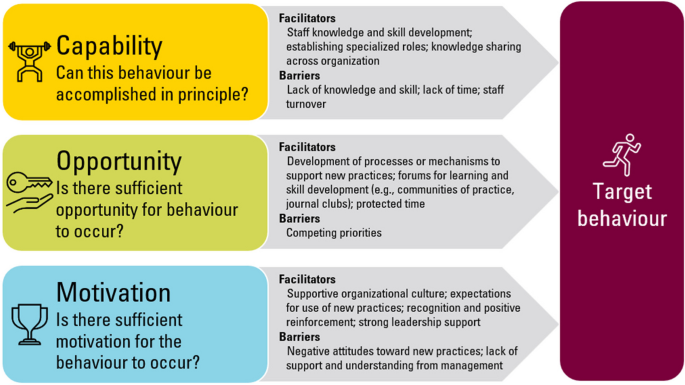
COM-B Model for behaviour change with facilitators and barriers for implementation of organization-wide EIDM
Within the capability component of the COM-B model, staff knowledge and skill development were included as a facilitator. Studies included in this review demonstrated that knowledge and skill for EIDM supported the use of evidence in decision making [ 13 , 14 , 39 , 40 , 42 , 43 , 46 , 49 , 50 , 58 , 61 ]. The establishment of specialized or dedicated roles for EIDM, such as Knowledge Broker roles, was included in the capability component of the COM-B model, since Knowledge Broker roles support the capacity of organizations and their staff to use evidence-informed approaches [ 20 , 41 , 44 , 47 , 48 , 51 , 52 , 54 , 55 , 56 , 57 , 59 , 60 , 62 , 63 , 64 , 65 , 66 , 67 , 69 , 71 , 72 ]. Finally, knowledge sharing across organizations was described as a facilitator for EIDM by several of the studies that built staff capacity for EIDM or established Knowledge Broker roles [ 13 , 48 , 49 , 51 , 52 , 54 , 56 , 59 , 61 , 65 ]. Barriers to the capability for EIDM behaviours include staff turnover and subsequent knowledge loss [ 14 , 20 , 56 ]. Staff turnover is especially challenging for interventions that involve staff in dedicated Knowledge Broker roles and interventions that build the knowledge and skill for staff to engage in evidence use [ 14 , 20 , 56 ]. In some cases, individuals who are trained in the Knowledge Broker role are then promoted to new roles or management and have fewer opportunities to apply their Knowledge Broker skills [ 20 ].
The opportunity portion of the COM-B model reflects whether there is opportunity for new behaviour to occur. The development of processes and mechanisms that support new practices can act as a reminder for staff, and may include re-design of planning or decision-making templates to capture supporting evidence, or adding EIDM-related items to agendas for regular meetings [ 41 , 47 , 53 , 60 ]. Forums for learning and skill development provide staff with opportunities to gain knowledge and practice newly acquired skills in group settings, such as communities of practice or journal clubs [ 48 , 56 , 61 , 65 ]. Finally, protected time to apply EIDM was found to be a facilitator for opportunity in the COM-B model [ 20 , 47 , 57 , 59 , 65 ], while competing priorities were found to be a barrier [ 20 , 39 , 40 , 52 , 55 , 57 , 60 , 64 , 65 ].
The final influencer in the COM-B model, motivation, reflects whether there is sufficient motivation for a new behaviour to occur. Facilitators include supportive organizational culture [ 14 , 20 , 43 , 47 , 57 , 59 ], expectations for new practices to occur [ 20 , 40 ], recognition and positive reinforcement [ 52 , 59 , 60 , 65 ], and strong leadership support [ 14 , 20 , 39 , 40 , 43 , 47 , 56 , 59 , 65 , 68 ]. Barriers to motivation included a lack of understanding or support from management [ 20 ], and negative attitudes toward change [ 20 , 52 , 59 , 68 ].
Strategies to implement EIDM across organizations include establishing specialized roles, providing staff education and training, developing processes or mechanisms to support new practices, and demonstrating leadership support. Facilitators and barriers for these strategies align with the COM-B model for behaviour change, which outlines capability, opportunity, and motivation as influencers of behaviour (Fig. 2 ). The COM-B model provides a comprehensive framework for the factors that influence behaviour change and has provided a valuable structure for examining barriers and facilitators to behaviour change in public health and related fields [ 73 , 74 , 75 , 76 ].
The capability section of the COM-B model reflects whether the intended audience possess the knowledge and skill for a new behaviour. Findings from this review establish facilitators for EIDM implementation capability, including the development of staff knowledge and skill, establishing specialized roles, and knowledge sharing across the organization. The development of staff knowledge and skill for EIDM are a necessary component to ensure EIDM in practice, however, literature has found that the organization-wide impact of conducting only individual-level knowledge and skill development is limited [ 77 , 78 , 79 ]. While knowledge and skill development are a necessary component to EIDM practice, they must be supported by other components to have an impact beyond the individual. Other strategies that support the use of newly gained knowledge and skills include the establishment of specialized roles for EIDM. Another strategy to support the use of EIDM is the establishment of dedicated staff roles, such as Knowledge Brokers. Knowledge Broker roles have been used across diverse contexts and show promise in supporting organization-wide EIDM implementation [ 20 , 22 , 23 , 67 , 80 , 81 , 82 , 83 ]. One facilitator for Knowledge Broker roles was knowledge sharing across the organization. Factors that influence the success of staff in Knowledge Broker roles align with those mapped to opportunity and motivation in the COM-B model, including the integration of EIDM into processes, knowledge sharing, and supportive organizational culture [ 20 , 22 , 47 , 67 , 84 , 85 ]. Knowledge Brokers can also help facilitate knowledge sharing across the organization, which was another facilitator mapped to the capability level of the model [ 20 , 47 , 84 , 85 ]. Knowledge sharing refers to the shared learning, knowledge products and resources for EIDM. At large public health organizations, it can be challenging to facilitate knowledge sharing between teams and departments [ 86 , 87 ]. Integrating technology can help; there have been some advances driven by the COVID-19 pandemic, such as the development of knowledge sharing platforms [ 88 , 89 , 90 , 91 ]. Public health organizations seeking to implement EIDM should invest in their knowledge sharing infrastructure.
At the capability level of the COM-B model, staff turnover was a barrier to EIDM implementation. Organizations that implement these strategies should be cognizant of the potential for knowledge loss due to staff turnover when selecting staff for Knowledge Broker roles or capacity building opportunities.
Facilitators for organizational EIDM opportunity include the development of processes or mechanisms to support new practices, forums for learning and skill development, and protected time. The use of reminders for organizational behaviour change and implementation of clinical practice guidelines has been shown to be an effective strategy across many contexts [ 92 , 93 , 94 , 95 ]. Organizations seeking to implement EIDM should consider revising current templates and processes to support their initiatives. Another facilitator included forums for shared learning and skill development. Other literature shows that these forums can be effective in developing knowledge and skill and should foster an environment of learning without fear of reprisal [ 96 , 97 ]. Finally, protected time for EIDM was a facilitator and competing priorities were a barrier. In public health practice, staff are often challenged with high workloads, so that EIDM may be viewed as an additional burden rather than a means to improve practice [ 98 , 99 ]. For an EIDM approach to be practiced, staff must be provided with sufficient time to apply and practice skills. Organizations should consider involving middle management who oversee staff time allocations, rather than only senior leadership, to help ensure that staff are provided with the time they need and that expectations are adjusted accordingly [ 20 , 23 ].
At the motivation level of the COM-B model, supportive organizational culture was mapped as a facilitator. The influence of organizational culture on evidence-informed practice at health organizations has been explored in a previous systematic review by Li et al. [ 100 ]. This systematic review of organizational contextual factors that influence evidence-based practice included 37 studies conducted in healthcare-related settings. Findings align with facilitators identified above, especially leadership support, which was found to impact evidence-based practice as well as all other factors that influence evidence-based practice [ 100 ]. The review also found that monitoring and feedback contributed to implementation of evidence-based practice, which aligns with recognition and positive reinforcement in the COM-B model above [ 100 ]. Notably, another factor that was mapped to the COM-B model was the expectation for new practices to occur, which was not explicitly identified as an influence on practice [ 100 ]. While Li et al. acknowledge that leadership that neglects to hold staff accountable are detrimental to implementation of EIDM, this accountability and clear expectations for change practice were a stronger finding in this current rapid systematic review.
The need for leadership support aligns with opportunity, since it is often management that determines the allocation of staff time for EIDM [ 20 , 23 ]. Attitudes and the belief that EIDM is associated with positive outcomes is a key factor in overall competence for EIDM [ 101 ]. Efforts to address negative attitudes within staff, especially at the leadership level, may improve implementation of EIDM.
While this review provides a comprehensive overview of interventions to support EIDM in public health and related organizations, it does have some limitations. Given the heterogeneity of included studies, it was not possible to discern which implementation strategies for EIDM are more effective compared to others. Knowledge Broker roles, building capacity for EIDM, and research-academic partnerships were all shown to contribute to EIDM, but study findings do not support one strategy as superior to others. Given the highly contextual nature of these interventions, it is likely that the relative effectiveness of different interventions depends on the organization’s unique set of characteristics. Evaluation is also critical to determine if change efforts are successful or need to be adjusted. It is possible that a combination of strategies would maximize the likelihood that diverse needs of staff are met. Rigorous studies to evaluate this hypothesis are needed.
Most studies included in this review are non-randomized studies of interventions. Given the importance of context in organizational change, randomized controlled trial designs may not be well-suited to evaluate studies of EIDM implementation [ 102 ]. High-quality single-group studies, such as prospective cohort analytic studies evaluated with validated measures or qualitative descriptive analyses of case studies with thorough descriptions of interventions and context, may be more appropriate designs for designing future initiatives in this field. However, arguments have been made for the use of randomized trial designs in implementation research [ 103 ]. Foy et al. advocate for overcoming contextual barriers by using innovative trial designs, such as the multiphase optimization strategy approach, where a series of trials identify the most promising single or combined intervention components, or the sequential multiple assignment randomized trial approach, where early results inform tailoring of adaptive interventions [ 103 ]. These designs may be a promising approach to conducting trials within highly contextual settings. Another viewpoint is that perhaps it may not be essential to determine if one strategy is superior to another, but rather that strategies build a larger, multi-strategy approach to implementation [ 104 ]. There may be greater benefit to determining the conditions under which various strategies are effective [ 104 ].
A limitation in this review’s methodology is that the review was completed following a rapid review protocol to ensure timely completion. Modifications of a systematic review approach included the use of a single reviewer for screening and using an unblinded reviewer to check quality assessment and data extraction. This may have contributed to some bias within the review, due to the reviewers’ interpretations of studies. To minimize this bias, there were efforts to calibrate screening, quality assessment and data extraction using a subset of studies.
This review provides a synthesis of strategies for the organization-wide implementation of EIDM, and an in-depth analysis of their facilitators and barriers in public health organizations. Facilitators and barriers mapped to the COM-B model for behaviour change can be used by organizational leadership to drive organizational change toward EIDM.
This rapid systematic review explored the implementation of EIDM at the organizational level of public health and related organizations. Despite the similarity of these implementation challenges, studies used distinct strategies for implementation, including the establishment of dedicated roles to support EIDM, building staff capacities, research or academic partnerships, and integrating evidence into processes or mechanisms. Facilitators and barriers mapped to the COM-B model provide key guidance for driving organizational change to evidence-informed approaches for all decisions.
Availability of data and materials
All data generated or analysed during this study are included in this published article and its supplementary information files.
Abbreviations
Evidence-informed Decision Making
Evidence-based Practice
Evidence-informed Practice
Grading of Recommendations, Assessment, Development and Evaluations
Joanna Briggs Institute
Knowledge Translation
Randomized Controlled Trial
Public Health Agency of Canada. Core Competencies for Public Health in Canada. 1st ed. 2008.
Google Scholar
National Collaborating Centre for Methods and Tools. Evidence-Informed Decision Making in Public Health 2022. Available from: https://www.nccmt.ca/tools/eiph .
World Health Organization. WHO guide for evidence-informed decision-making. Evidence, policy, impact. 2021.
Canadian Public Health Association. Public health: a conceptual framework. Ottawa: Canadian Public Health Association; 2017.
Brownson RC, Gurney JG, Land GH. Evidence-based decision making in public health. J Public Health Manag Pract. 1999;5(5):86–97.
Article CAS PubMed Google Scholar
Kohatsu ND, Robinson JG, Torner JC. Evidence-based public health: an evolving concept. Am J Prev Med. 2004;27(5):417–21.
PubMed Google Scholar
Titler MG. The evidence for evidence-based practice implementation. In: Hughes RG, editor. Patient safety and quality: an evidence-based handbook for nurses. Advances in Patient Safety. Rockville (MD); 2008.
Pan American Health Organization. A guide for evidence-informed decision-making, including in health emergencies. 2022.
Saunders H, Gallagher-Ford L, Kvist T, Vehvilainen-Julkunen K. Practicing Healthcare professionals’ evidence-based practice competencies: an overview of systematic reviews. Worldviews Evid Based Nurs. 2019;16(3):176–85.
Article PubMed Google Scholar
Paci M, Faedda G, Ugolini A, Pellicciari L. Barriers to evidence-based practice implementation in physiotherapy: a systematic review and meta-analysis. Int J Qual Health Care. 2021;33(2):mzab093.
Mathieson A, Grande G, Luker K. Strategies, facilitators and barriers to implementation of evidence-based practice in community nursing: a systematic mixed-studies review and qualitative synthesis. Prim Health Care Res Dev. 2019;20:e6.
Li S, Cao M, Zhu X. Evidence-based practice: knowledge, attitudes, implementation, facilitators, and barriers among community nurses-systematic review. Med (Baltim). 2019;98(39):e17209.
Article Google Scholar
Ward M, Mowat D. Creating an organizational culture for evidence-informed decision making. Healthc Manage Forum. 2012;25(3):146–50.
Peirson L, Ciliska D, Dobbins M, Mowat D. Building capacity for evidence informed decision making in public health: a case study of organizational change. BMC Public Health. 2012;12:137.
Article PubMed PubMed Central Google Scholar
Allen P, Parks RG, Kang SJ, Dekker D, Jacob RR, Mazzucca-Ragan S, et al. Practices among Local Public Health Agencies to support evidence-based decision making: a qualitative study. J Public Health Manag Pract. 2023;29(2):213–25.
Ellen ME, Leon G, Bouchard G, Ouimet M, Grimshaw JM, Lavis JN. Barriers, facilitators and views about next steps to implementing supports for evidence-informed decision-making in health systems: a qualitative study. Implement Sci. 2014;9:179.
Sadeghi-Bazargani H, Tabrizi JS, Azami-Aghdash S. Barriers to evidence-based medicine: a systematic review. J Eval Clin Pract. 2014;20(6):793–802.
Barzkar F, Baradaran HR, Koohpayehzadeh J. Knowledge, attitudes and practice of physicians toward evidence-based medicine: a systematic review. J Evid Based Med. 2018;11(4):246–51.
Clark E, Dobbins M, Hagerman L, Neumann S, Akaraci S. What is known about strategies to implement evidence-informed practice at an organizational level? Prospero; 2022.
Clark EC, Dhaliwal B, Ciliska D, Neil-Sztramko SE, Steinberg M, Dobbins M. A pragmatic evaluation of a public health knowledge broker mentoring education program: a convergent mixed methods study. Implement Sci Commun. 2022;3(1):18.
Michie S, van Stralen MM, West R. The behaviour change wheel: a new method for characterising and designing behaviour change interventions. Implement Sci. 2011;6:42.
Dobbins M, Hanna SE, Ciliska D, Manske S, Cameron R, Mercer SL, et al. A randomized controlled trial evaluating the impact of knowledge translation and exchange strategies. Implement Sci. 2009;4:61.
Dobbins M, Traynor RL, Workentine S, Yousefi-Nooraie R, Yost J. Impact of an organization-wide knowledge translation strategy to support evidence-informed public health decision making. BMC Public Health. 2018;18(1):1412.
McDonagh LK, Saunders JM, Cassell J, Curtis T, Bastaki H, Hartney T, et al. Application of the COM-B model to barriers and facilitators to chlamydia testing in general practice for young people and primary care practitioners: a systematic review. Implement Sci. 2018;13(1):130.
Mersha AG, Gould GS, Bovill M, Eftekhari P. Barriers and facilitators of adherence to nicotine replacement therapy: a systematic review and analysis using the capability, opportunity, motivation, and Behaviour (COM-B) Model. Int J Environ Res Public Health. 2020;17(23):8895.
Article CAS PubMed PubMed Central Google Scholar
Pirotta S, Joham AJ, Moran LJ, Skouteris H, Lim SS. Implementation of evidence-based PCOS lifestyle management guidelines: perceived barriers and facilitators by consumers using the theoretical domains Framework and COM-B Model. Patient Educ Couns. 2021;104(8):2080–8.
Dubois A, Lévesque M. Canada’s National Collaborating centres: facilitating evidence-informed decision-making in public health. Can Commun Dis Rep. 2020;46(2–3):31–5.
Martin W, Wharf Higgins J, Pauly BB, MacDonald M. Layers of translation - evidence literacy in public health practice: a qualitative secondary analysis. BMC Public Health. 2017;17(1):803.
van der Graaf P, Forrest LF, Adams J, Shucksmith J, White M. How do public health professionals view and engage with research? A qualitative interview study and stakeholder workshop engaging public health professionals and researchers. BMC Public Health. 2017;17(1):892.
Page MJ, McKenzie JE, Bossuyt PM, Boutron I, Hoffmann TC, Mulrow CD, et al. The PRISMA 2020 statement: an updated guideline for reporting systematic reviews. BMJ. 2021;372:n71.
Neil-Sztramko SE, Belita E, Traynor RL, Clark E, Hagerman L, Dobbins M. Methods to support evidence-informed decision-making in the midst of COVID-19: creation and evolution of a rapid review service from the National Collaborating Centre for Methods and Tools. BMC Med Res Methodol. 2021;21(1):231.
Lizarondo L, Stern C, Carrier J, Godfrey C, Rieger K, Salmond S, Apostolo J, Kirkpatrick P, Loveday H. Chapter 8: mixed methods systematic reviews. Aromataris EMZ. 2020.
Thomas J, Kneale D, McKenzie JE, Brennan SE, Bhaumik S. In: Chandler J, Cumpston M, Li T, Page MJ, Welch VA, editors. Chapter 2: determining the scope of the review and the questions it will address. editor: Cochrane: Higgins JPT TJ; 2023.
Organisation for Economic Co-operation and Development. List of OECD Member countries - Ratification of the Convention on the OECD; 2021. Available from: https://www.oecd.org/about/document/ratification-oecd-convention.htm .
Joanna Briggs Institute. Available from: https://jbi.global/critical-appraisal-tools .
McKenzie JE, Brennan SE. Chapter 12. Synthesizing and presenting findings using other methods. 2021.
Brogly C, Bauer MA, Lizotte DJ, Press ML, MacDougall A, Speechley M, et al. An app-based Surveillance System for undergraduate students’ Mental Health during the COVID-19 pandemic: protocol for a prospective cohort study. JMIR Res Protoc. 2021;10(9):e30504.
Guyatt G, Oxman AD, Akl EA, Kunz R, Vist G, Brozek J, et al. GRADE guidelines: 1. Introduction-GRADE evidence profiles and summary of findings tables. J Clin Epidemiol. 2011;64(4):383–94.
Allen P, O’Connor JC, Best LA, Lakshman M, Jacob RR, Brownson RC. Management practices to build evidence-based decision-making capacity for Chronic Disease Prevention in Georgia: a Case Study. Prev Chronic Dis. 2018;15:E92.
Allen P, Jacob RR, Lakshman M, Best LA, Bass K, Brownson RC. Lessons learned in promoting evidence-based Public Health: perspectives from Managers in State Public Health Departments. J Community Health. 2018;43(5):856–63.
Augustino LR, Braun L, Heyne RE, Shinn A, Lovett-Floom L, King H, et al. Implementing evidence-based practice facilitators: a Case Series. Mil Med. 2020;185(Suppl 2):7–14.
Awan S, Samokhvalov AV, Aleem N, Hendershot CS, Irving JA, Kalvik A, et al. Development and implementation of an Ambulatory Integrated Care Pathway for Major Depressive Disorder and Alcohol Dependence. Psychiatr Serv. 2015;66(12):1265–7.
Bennett S, Whitehead M, Eames S, Fleming J, Low S, Caldwell E. Building capacity for knowledge translation in occupational therapy: learning through participatory action research. BMC Med Educ. 2016;16(1):257.
Breckenridge-Sproat ST, Throop MD, Raju D, Murphy DA, Loan LA, Patrician PA. Building a unit-level Mentored Program to sustain a culture of Inquiry for evidence-based practice. Clin Nurse Spec. 2015;29(6):329–37.
Brodowski ML, Counts JM, Gillam RJ, Baker L, Collins VS, Winkle E, Skala J, Stokes K, Gomez R, Redmon J. Translating evidence-based policy to practice: a Multilevel Partnership using the interactive systems Framework. J Contemp Social Serv. 2018;94(3):141–9.
Brownson RC, Allen P, Jacob RR, deRuyter A, Lakshman M, Reis RS, et al. Controlling Chronic diseases through evidence-based decision making: a Group-Randomized Trial. Prev Chronic Dis. 2017;14:E121.
Dobbins M, Greco L, Yost J, Traynor R, Decorby-Watson K, Yousefi-Nooraie R. A description of a tailored knowledge translation intervention delivered by knowledge brokers within public health departments in Canada. Health Res Policy Syst. 2019;17(1):63.
Elliott MJ, Allu S, Beaucage M, McKenzie S, Kappel J, Harvey R, et al. Defining the scope of knowledge translation within a National, Patient-Oriented Kidney Research Network. Can J Kidney Health Dis. 2021;8:20543581211004803.
Fernandez ME, Melvin CL, Leeman J, Ribisl KM, Allen JD, Kegler MC, et al. The cancer prevention and control research network: an interactive systems approach to advancing cancer control implementation research and practice. Cancer Epidemiol Biomarkers Prev. 2014;23(11):2512–21.
Flaherty HB, Bornheimer LA, Hamovitch E, Garay E, Mini de Zitella ML, Acri MC, et al. Examining organizational factors supporting the adoption and use of evidence-based interventions. Community Ment Health J. 2021;57(6):1187–94.
Gallagher-Ford L. Implementing and sustaining EBP in real world healthcare settings: transformational evidence-based leadership: redesigning traditional roles to promote and sustain a culture of EBP. Worldviews Evid Based Nurs. 2014;11(2):140–2.
Gifford W, Lefebre N, Davies B. An organizational intervention to influence evidence-informed decision making in home health nursing. J Nurs Adm. 2014;44(7/8):395–402.
Haynes A, Rowbotham S, Grunseit A, Bohn-Goldbaum E, Slaytor E, Wilson A, et al. Knowledge mobilisation in practice: an evaluation of the Australian Prevention Partnership Centre. Health Res Policy Syst. 2020;18(1):13.
Hitch D, Lhuede K, Vernon L, Pepin G, Stagnitti K. Longitudinal evaluation of a knowledge translation role in occupational therapy. BMC Health Serv Res. 2019;19(1):154.
Hooge N, Allen DH, McKenzie R, Pandian V. Engaging advanced practice nurses in evidence-based practice: an e-mentoring program. Worldviews Evid Based Nurs. 2022;19(3):235–44.
Humphries S, Hampe T, Larsen D, Bowen S. Building organizational capacity for evidence use: the experience of two Canadian healthcare organizations. Healthc Manage Forum. 2013;26(1):26–32.
Irwin MM, Bergman RM, Richards R. The experience of implementing evidence-based practice change: a qualitative analysis. Clin J Oncol Nurs. 2013;17(5):544–9.
Kaplan L, Zeller E, Damitio D, Culbert S, Bayley KB. Improving the culture of evidence-based practice at a Magnet(R) hospital. J Nurses Prof Dev. 2014;30(6):274–80. quiz E1-2.
Kimber M, Barwick M, Fearing G. Becoming an evidence-based service provider: staff perceptions and experiences of organizational change. J Behav Health Serv Res. 2012;39(3):314–32.
Mackay HJ, Campbell KL, van der Meij BS, Wilkinson SA. Establishing an evidenced-based dietetic model of care in haemodialysis using implementation science. Nutr Diet. 2019;76(2):150–7.
Martin-Fernandez J, Aromatario O, Prigent O, Porcherie M, Ridde V, Cambon L. Evaluation of a knowledge translation strategy to improve policymaking and practices in health promotion and disease prevention setting in French regions: TC-REG, a realist study. BMJ Open. 2021;11(9):e045936.
Melnyk BM, Fineout-Overholt E, Giggleman M, Choy K. A test of the ARCC(c) model improves implementation of evidence-based practice, Healthcare Culture, and patient outcomes. Worldviews Evid Based Nurs. 2017;14(1):5–9.
Miro A, Perrotta K, Evans H, Kishchuk NA, Gram C, Stanwick RS, et al. Building the capacity of health authorities to influence land use and transportation planning: lessons learned from the healthy Canada by Design CLASP Project in British Columbia. Can J Public Health. 2014;106(1 Suppl 1):eS40–52.
Parke B, Stevenson L, Rowe M. Scholar-in-Residence: an Organizational Capacity-Building Model to move evidence to action. Nurs Leadersh (Tor Ont). 2015;28(2):10–22.
Plath D. Organizational processes supporting evidence-based practice. Adm Social work. 2013;37(2):171–88.
Roberts M, Reagan DR, Behringer B. A Public Health Performance Excellence Improvement Strategy: Diffusion and Adoption of the Baldrige Framework within Tennessee Department of Health. J Public Health Manag Pract. 2020;26(1):39–45.
Traynor R, DeCorby K, Dobbins M. Knowledge brokering in public health: a tale of two studies. Public Health. 2014;128(6):533–44.
van der Zwet RJM, Beneken genaamd Kolmer DM, Schalk R, Van Regenmortel T. Implementing evidence-based practice in a Dutch Social Work Organisation: A Shared responsibility. Br J Social Work. 2020;50(7):2212–32.
Waterman H, Boaden R, Burey L, Howells B, Harvey G, Humphreys J, et al. Facilitating large-scale implementation of evidence based health care: insider accounts from a co-operative inquiry. BMC Health Serv Res. 2015;15:60.
Williams NJ, Wolk CB, Becker-Haimes EM, Beidas RS. Testing a theory of strategic implementation leadership, implementation climate, and clinicians’ use of evidence-based practice: a 5-year panel analysis. Implement Sci. 2020;15(1):10.
Williams C, van der Meij BS, Nisbet J, McGill J, Wilkinson SA. Nutrition process improvements for adult inpatients with inborn errors of metabolism using the i-PARIHS framework. Nutr Diet. 2019;76(2):141–9.
Williams NJ, Glisson C, Hemmelgarn A, Green P. Mechanisms of change in the ARC Organizational Strategy: increasing Mental Health clinicians’ EBP adoption through improved Organizational Culture and Capacity. Adm Policy Ment Health. 2017;44(2):269–83.
Alexander KE, Brijnath B, Mazza D. Barriers and enablers to delivery of the healthy kids check: an analysis informed by the theoretical domains Framework and COM-B model. Implement Sci. 2014;9:60.
McArthur C, Bai Y, Hewston P, Giangregorio L, Straus S, Papaioannou A. Barriers and facilitators to implementing evidence-based guidelines in long-term care: a qualitative evidence synthesis. Implement Sci. 2021;16(1):70.
Moffat A, Cook EJ, Chater AM. Examining the influences on the use of behavioural science within UK local authority public health: qualitative thematic analysis and deductive mapping to the COM-B model and theoretical domains Framework. Front Public Health. 2022;10:1016076.
De Leo A, Bayes S, Bloxsome D, Butt J. Exploring the usability of the COM-B model and theoretical domains Framework (TDF) to define the helpers of and hindrances to evidence-based practice in midwifery. Implement Sci Commun. 2021;2(1):7.
Morshed AB, Ballew P, Elliott MB, Haire-Joshu D, Kreuter MW, Brownson RC. Evaluation of an online training for improving self-reported evidence-based decision-making skills in cancer control among public health professionals. Public Health. 2017;152:28–35.
Jones K, Armstrong R, Pettman T, Waters E. Knowledge translation for researchers: developing training to support public health researchers KTE efforts. J Public Health (Oxf). 2015;37(2):364–6.
Dreisinger M, Leet TL, Baker EA, Gillespie KN, Haas B, Brownson RC. Improving the public health workforce: evaluation of a training course to enhance evidence-based decision making. J Public Health Manag Pract. 2008;14(2):138–43.
Mendell J, Richardson L. Integrated knowledge translation to strengthen public policy research: a case study from experimental research on income assistance receipt among people who use drugs. BMC Public Health. 2021;21(1):153.
Russell DJ, Rivard LM, Walter SD, Rosenbaum PL, Roxborough L, Cameron D, et al. Using knowledge brokers to facilitate the uptake of pediatric measurement tools into clinical practice: a before-after intervention study. Implement Sci. 2010;5:92.
Brown KM, Elliott SJ, Robertson-Wilson J, Vine MM, Leatherdale ST. Can knowledge exchange support the implementation of a health-promoting schools approach? Perceived outcomes of knowledge exchange in the COMPASS study. BMC Public Health. 2018;18(1):351.
Langeveld K, Stronks K, Harting J. Use of a knowledge broker to establish healthy public policies in a city district: a developmental evaluation. BMC Public Health. 2016;16:271.
Bornbaum CC, Kornas K, Peirson L, Rosella LC. Exploring the function and effectiveness of knowledge brokers as facilitators of knowledge translation in health-related settings: a systematic review and thematic analysis. Implement Sci. 2015;10:162.
Sarkies MN, Robins LM, Jepson M, Williams CM, Taylor NF, O’Brien L, et al. Effectiveness of knowledge brokering and recommendation dissemination for influencing healthcare resource allocation decisions: a cluster randomised controlled implementation trial. PLoS Med. 2021;18(10):e1003833.
Jansen MW, De Leeuw E, Hoeijmakers M, De Vries NK. Working at the nexus between public health policy, practice and research. Dynamics of knowledge sharing in the Netherlands. Health Res Policy Syst. 2012;10:33.
Sibbald SL, Kothari A. Creating, synthesizing, and sharing: the management of knowledge in Public Health. Public Health Nurs. 2015;32(4):339–48.
Barnes SJ. Information management research and practice in the post-COVID-19 world. Int J Inf Manage. 2020;55:102175.
Dwivedi YH, Coombs DL, Constantiniou C, Duan I, Edwards Y, Gupta JS, Lal B, Misra B, Prashant S, Raman P, Rana R, Sharma NP, Upadhyay SK. Impact of COVID-19 pandemic on information management research and practice: transforming education, work and life. Int J Inf Manag. 2020;55:102211.
Krausz M, Westenberg JN, Vigo D, Spence RT, Ramsey D. Emergency response to COVID-19 in Canada: platform development and implementation for eHealth in Crisis Management. JMIR Public Health Surveill. 2020;6(2):e18995.
Smith RW, Jarvis T, Sandhu HS, Pinto AD, O’Neill M, Di Ruggiero E, et al. Centralization and integration of public health systems: perspectives of public health leaders on factors facilitating and impeding COVID-19 responses in three Canadian provinces. Health Policy. 2023;127:19–28.
Pereira VC, Silva SN, Carvalho VKS, Zanghelini F, Barreto JOM. Strategies for the implementation of clinical practice guidelines in public health: an overview of systematic reviews. Health Res Policy Syst. 2022;20(1):13.
Tomsic I, Heinze NR, Chaberny IF, Krauth C, Schock B, von Lengerke T. Implementation interventions in preventing surgical site infections in abdominal surgery: a systematic review. BMC Health Serv Res. 2020;20(1):236.
Harrison R, Fischer S, Walpola RL, Chauhan A, Babalola T, Mears S, et al. Where do models for Change Management, improvement and implementation meet? A systematic review of the applications of Change Management models in Healthcare. J Healthc Leadersh. 2021;13:85–108.
Correa VC, Lugo-Agudelo LH, Aguirre-Acevedo DC, Contreras JAP, Borrero AMP, Patino-Lugo DF, et al. Individual, health system, and contextual barriers and facilitators for the implementation of clinical practice guidelines: a systematic metareview. Health Res Policy Syst. 2020;18(1):74.
Valizadeh L, Zamanzadeh V, Alizadeh S, Namadi Vosoughi M. Promoting evidence-based nursing through journal clubs: an integrative review. J Res Nurs. 2022;27(7):606–20.
Portela Dos Santos O, Melly P, Hilfiker R, Giacomino K, Perruchoud E, Verloo H, et al. Effectiveness of educational interventions to increase skills in evidence-based practice among nurses: the EDITcare. Syst Rev Healthc (Basel). 2022;10(11):2204.
Shelton RC, Lee M. Sustaining evidence-based interventions and policies: recent innovations and future directions in implementation science. Am J Public Health. 2019;109(S2):S132–4.
Brownson RC, Fielding JE, Green LW. Building Capacity for evidence-based Public Health: reconciling the pulls of Practice and the push of Research. Annu Rev Public Health. 2018;39:27–53.
Li SA, Jeffs L, Barwick M, Stevens B. Organizational contextual features that influence the implementation of evidence-based practices across healthcare settings: a systematic integrative review. Syst Rev. 2018;7(1):72.
Belita E, Yost J, Squires JE, Ganann R, Dobbins M. Development and content validation of a measure to assess evidence-informed decision-making competence in public health nursing. PLoS One. 2021;16(3):e0248330.
Dobbins M, Robeson P, Ciliska D, Hanna S, Cameron R, O’Mara L, et al. A description of a knowledge broker role implemented as part of a randomized controlled trial evaluating three knowledge translation strategies. Implement Sci. 2009;4:23.
Foy R, Ivers NM, Grimshaw JM, Wilson PM. What is the role of randomised trials in implementation science? Trials. 2023;24(1):537.
Pawson R. Pragmatic trials and implementation science: grounds for divorce? BMC Med Res Methodol. 2019;19(1):176.
Download references
Acknowledgements
The authors would like to acknowledge the NCCMT’s Rapid Evidence Service, particularly Alyssa Kostopoulos, Sophie Neumann and Selin Akaraci, for their contributions to this review.
The National Collaborating Centre for Methods and Tools is hosted by McMaster University and funded by the Public Health Agency of Canada. The views expressed herein do not necessarily represent the views of the Public Health Agency of Canada. The funder had no role in the design of the study, collection, analysis, or interpretation of data or in writing the manuscript.
Author information
Authors and affiliations.
National Collaborating Centre for Methods and Tools, McMaster University, McMaster Innovation Park, 175 Longwood Rd S, Suite 210a, Hamilton, ON, L8P 0A1, Canada
Emily C. Clark, Trish Burnett, Rebecca Blair, Robyn L. Traynor, Leah Hagerman & Maureen Dobbins
School of Nursing, McMaster University, Health Sciences Centre, 2J20, 1280 Main St W, Hamilton, ON, L8S 4K1, Canada
Maureen Dobbins
You can also search for this author in PubMed Google Scholar
Contributions
E.C.C. and M.D. designed the study. E.C.C., L.H., R.B., R.L.T., and T.B. completed screening, quality assessment and data extraction. E.C. and M.D. analyzed study results. E.C.C. and T.B. wrote the manuscript in consultation with M.D. All authors read and approved the final manuscript.
Corresponding author
Correspondence to Maureen Dobbins .
Ethics declarations
Ethics approval and consent to participate.
Not applicable.
Consent for publication
Competing interests.
The authors declare no competing interests.
Additional information
Publisher’s note.
Springer Nature remains neutral with regard to jurisdictional claims in published maps and institutional affiliations.
Supplementary Information
Supplementary material 1., supplementary material 2., rights and permissions.
Open Access This article is licensed under a Creative Commons Attribution 4.0 International License, which permits use, sharing, adaptation, distribution and reproduction in any medium or format, as long as you give appropriate credit to the original author(s) and the source, provide a link to the Creative Commons licence, and indicate if changes were made. The images or other third party material in this article are included in the article's Creative Commons licence, unless indicated otherwise in a credit line to the material. If material is not included in the article's Creative Commons licence and your intended use is not permitted by statutory regulation or exceeds the permitted use, you will need to obtain permission directly from the copyright holder. To view a copy of this licence, visit http://creativecommons.org/licenses/by/4.0/ . The Creative Commons Public Domain Dedication waiver ( http://creativecommons.org/publicdomain/zero/1.0/ ) applies to the data made available in this article, unless otherwise stated in a credit line to the data.
Reprints and permissions
About this article
Cite this article.
Clark, E.C., Burnett, T., Blair, R. et al. Strategies to implement evidence-informed decision making at the organizational level: a rapid systematic review. BMC Health Serv Res 24 , 405 (2024). https://doi.org/10.1186/s12913-024-10841-3
Download citation
Received : 23 October 2023
Accepted : 07 March 2024
Published : 01 April 2024
DOI : https://doi.org/10.1186/s12913-024-10841-3
Share this article
Anyone you share the following link with will be able to read this content:
Sorry, a shareable link is not currently available for this article.
Provided by the Springer Nature SharedIt content-sharing initiative
- Evidence-informed decision making
- Evidence-based practice
- Knowledge translation
- Knowledge mobilization
- Implementation
- Organizational change
BMC Health Services Research
ISSN: 1472-6963
- General enquiries: [email protected]
Thank you for visiting nature.com. You are using a browser version with limited support for CSS. To obtain the best experience, we recommend you use a more up to date browser (or turn off compatibility mode in Internet Explorer). In the meantime, to ensure continued support, we are displaying the site without styles and JavaScript.
- View all journals
- My Account Login
- Explore content
- About the journal
- Publish with us
- Sign up for alerts
- Open access
- Published: 26 March 2024
Predicting and improving complex beer flavor through machine learning
- Michiel Schreurs ORCID: orcid.org/0000-0002-9449-5619 1 , 2 , 3 na1 ,
- Supinya Piampongsant 1 , 2 , 3 na1 ,
- Miguel Roncoroni ORCID: orcid.org/0000-0001-7461-1427 1 , 2 , 3 na1 ,
- Lloyd Cool ORCID: orcid.org/0000-0001-9936-3124 1 , 2 , 3 , 4 ,
- Beatriz Herrera-Malaver ORCID: orcid.org/0000-0002-5096-9974 1 , 2 , 3 ,
- Christophe Vanderaa ORCID: orcid.org/0000-0001-7443-5427 4 ,
- Florian A. Theßeling 1 , 2 , 3 ,
- Łukasz Kreft ORCID: orcid.org/0000-0001-7620-4657 5 ,
- Alexander Botzki ORCID: orcid.org/0000-0001-6691-4233 5 ,
- Philippe Malcorps 6 ,
- Luk Daenen 6 ,
- Tom Wenseleers ORCID: orcid.org/0000-0002-1434-861X 4 &
- Kevin J. Verstrepen ORCID: orcid.org/0000-0002-3077-6219 1 , 2 , 3
Nature Communications volume 15 , Article number: 2368 ( 2024 ) Cite this article
39k Accesses
749 Altmetric
Metrics details
- Chemical engineering
- Gas chromatography
- Machine learning
- Metabolomics
- Taste receptors
The perception and appreciation of food flavor depends on many interacting chemical compounds and external factors, and therefore proves challenging to understand and predict. Here, we combine extensive chemical and sensory analyses of 250 different beers to train machine learning models that allow predicting flavor and consumer appreciation. For each beer, we measure over 200 chemical properties, perform quantitative descriptive sensory analysis with a trained tasting panel and map data from over 180,000 consumer reviews to train 10 different machine learning models. The best-performing algorithm, Gradient Boosting, yields models that significantly outperform predictions based on conventional statistics and accurately predict complex food features and consumer appreciation from chemical profiles. Model dissection allows identifying specific and unexpected compounds as drivers of beer flavor and appreciation. Adding these compounds results in variants of commercial alcoholic and non-alcoholic beers with improved consumer appreciation. Together, our study reveals how big data and machine learning uncover complex links between food chemistry, flavor and consumer perception, and lays the foundation to develop novel, tailored foods with superior flavors.
Similar content being viewed by others
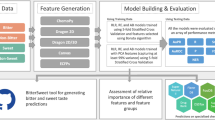
BitterSweet: Building machine learning models for predicting the bitter and sweet taste of small molecules
Rudraksh Tuwani, Somin Wadhwa & Ganesh Bagler
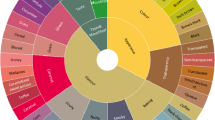
Sensory lexicon and aroma volatiles analysis of brewing malt
Xiaoxia Su, Miao Yu, … Tianyi Du
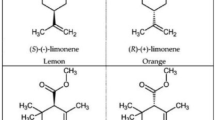
Predicting odor from molecular structure: a multi-label classification approach
Kushagra Saini & Venkatnarayan Ramanathan
Introduction
Predicting and understanding food perception and appreciation is one of the major challenges in food science. Accurate modeling of food flavor and appreciation could yield important opportunities for both producers and consumers, including quality control, product fingerprinting, counterfeit detection, spoilage detection, and the development of new products and product combinations (food pairing) 1 , 2 , 3 , 4 , 5 , 6 . Accurate models for flavor and consumer appreciation would contribute greatly to our scientific understanding of how humans perceive and appreciate flavor. Moreover, accurate predictive models would also facilitate and standardize existing food assessment methods and could supplement or replace assessments by trained and consumer tasting panels, which are variable, expensive and time-consuming 7 , 8 , 9 . Lastly, apart from providing objective, quantitative, accurate and contextual information that can help producers, models can also guide consumers in understanding their personal preferences 10 .
Despite the myriad of applications, predicting food flavor and appreciation from its chemical properties remains a largely elusive goal in sensory science, especially for complex food and beverages 11 , 12 . A key obstacle is the immense number of flavor-active chemicals underlying food flavor. Flavor compounds can vary widely in chemical structure and concentration, making them technically challenging and labor-intensive to quantify, even in the face of innovations in metabolomics, such as non-targeted metabolic fingerprinting 13 , 14 . Moreover, sensory analysis is perhaps even more complicated. Flavor perception is highly complex, resulting from hundreds of different molecules interacting at the physiochemical and sensorial level. Sensory perception is often non-linear, characterized by complex and concentration-dependent synergistic and antagonistic effects 15 , 16 , 17 , 18 , 19 , 20 , 21 that are further convoluted by the genetics, environment, culture and psychology of consumers 22 , 23 , 24 . Perceived flavor is therefore difficult to measure, with problems of sensitivity, accuracy, and reproducibility that can only be resolved by gathering sufficiently large datasets 25 . Trained tasting panels are considered the prime source of quality sensory data, but require meticulous training, are low throughput and high cost. Public databases containing consumer reviews of food products could provide a valuable alternative, especially for studying appreciation scores, which do not require formal training 25 . Public databases offer the advantage of amassing large amounts of data, increasing the statistical power to identify potential drivers of appreciation. However, public datasets suffer from biases, including a bias in the volunteers that contribute to the database, as well as confounding factors such as price, cult status and psychological conformity towards previous ratings of the product.
Classical multivariate statistics and machine learning methods have been used to predict flavor of specific compounds by, for example, linking structural properties of a compound to its potential biological activities or linking concentrations of specific compounds to sensory profiles 1 , 26 . Importantly, most previous studies focused on predicting organoleptic properties of single compounds (often based on their chemical structure) 27 , 28 , 29 , 30 , 31 , 32 , 33 , thus ignoring the fact that these compounds are present in a complex matrix in food or beverages and excluding complex interactions between compounds. Moreover, the classical statistics commonly used in sensory science 34 , 35 , 36 , 37 , 38 , 39 require a large sample size and sufficient variance amongst predictors to create accurate models. They are not fit for studying an extensive set of hundreds of interacting flavor compounds, since they are sensitive to outliers, have a high tendency to overfit and are less suited for non-linear and discontinuous relationships 40 .
In this study, we combine extensive chemical analyses and sensory data of a set of different commercial beers with machine learning approaches to develop models that predict taste, smell, mouthfeel and appreciation from compound concentrations. Beer is particularly suited to model the relationship between chemistry, flavor and appreciation. First, beer is a complex product, consisting of thousands of flavor compounds that partake in complex sensory interactions 41 , 42 , 43 . This chemical diversity arises from the raw materials (malt, yeast, hops, water and spices) and biochemical conversions during the brewing process (kilning, mashing, boiling, fermentation, maturation and aging) 44 , 45 . Second, the advent of the internet saw beer consumers embrace online review platforms, such as RateBeer (ZX Ventures, Anheuser-Busch InBev SA/NV) and BeerAdvocate (Next Glass, inc.). In this way, the beer community provides massive data sets of beer flavor and appreciation scores, creating extraordinarily large sensory databases to complement the analyses of our professional sensory panel. Specifically, we characterize over 200 chemical properties of 250 commercial beers, spread across 22 beer styles, and link these to the descriptive sensory profiling data of a 16-person in-house trained tasting panel and data acquired from over 180,000 public consumer reviews. These unique and extensive datasets enable us to train a suite of machine learning models to predict flavor and appreciation from a beer’s chemical profile. Dissection of the best-performing models allows us to pinpoint specific compounds as potential drivers of beer flavor and appreciation. Follow-up experiments confirm the importance of these compounds and ultimately allow us to significantly improve the flavor and appreciation of selected commercial beers. Together, our study represents a significant step towards understanding complex flavors and reinforces the value of machine learning to develop and refine complex foods. In this way, it represents a stepping stone for further computer-aided food engineering applications 46 .
To generate a comprehensive dataset on beer flavor, we selected 250 commercial Belgian beers across 22 different beer styles (Supplementary Fig. S1 ). Beers with ≤ 4.2% alcohol by volume (ABV) were classified as non-alcoholic and low-alcoholic. Blonds and Tripels constitute a significant portion of the dataset (12.4% and 11.2%, respectively) reflecting their presence on the Belgian beer market and the heterogeneity of beers within these styles. By contrast, lager beers are less diverse and dominated by a handful of brands. Rare styles such as Brut or Faro make up only a small fraction of the dataset (2% and 1%, respectively) because fewer of these beers are produced and because they are dominated by distinct characteristics in terms of flavor and chemical composition.
Extensive analysis identifies relationships between chemical compounds in beer
For each beer, we measured 226 different chemical properties, including common brewing parameters such as alcohol content, iso-alpha acids, pH, sugar concentration 47 , and over 200 flavor compounds (Methods, Supplementary Table S1 ). A large portion (37.2%) are terpenoids arising from hopping, responsible for herbal and fruity flavors 16 , 48 . A second major category are yeast metabolites, such as esters and alcohols, that result in fruity and solvent notes 48 , 49 , 50 . Other measured compounds are primarily derived from malt, or other microbes such as non- Saccharomyces yeasts and bacteria (‘wild flora’). Compounds that arise from spices or staling are labeled under ‘Others’. Five attributes (caloric value, total acids and total ester, hop aroma and sulfur compounds) are calculated from multiple individually measured compounds.
As a first step in identifying relationships between chemical properties, we determined correlations between the concentrations of the compounds (Fig. 1 , upper panel, Supplementary Data 1 and 2 , and Supplementary Fig. S2 . For the sake of clarity, only a subset of the measured compounds is shown in Fig. 1 ). Compounds of the same origin typically show a positive correlation, while absence of correlation hints at parameters varying independently. For example, the hop aroma compounds citronellol, and alpha-terpineol show moderate correlations with each other (Spearman’s rho=0.39 and 0.57), but not with the bittering hop component iso-alpha acids (Spearman’s rho=0.16 and −0.07). This illustrates how brewers can independently modify hop aroma and bitterness by selecting hop varieties and dosage time. If hops are added early in the boiling phase, chemical conversions increase bitterness while aromas evaporate, conversely, late addition of hops preserves aroma but limits bitterness 51 . Similarly, hop-derived iso-alpha acids show a strong anti-correlation with lactic acid and acetic acid, likely reflecting growth inhibition of lactic acid and acetic acid bacteria, or the consequent use of fewer hops in sour beer styles, such as West Flanders ales and Fruit beers, that rely on these bacteria for their distinct flavors 52 . Finally, yeast-derived esters (ethyl acetate, ethyl decanoate, ethyl hexanoate, ethyl octanoate) and alcohols (ethanol, isoamyl alcohol, isobutanol, and glycerol), correlate with Spearman coefficients above 0.5, suggesting that these secondary metabolites are correlated with the yeast genetic background and/or fermentation parameters and may be difficult to influence individually, although the choice of yeast strain may offer some control 53 .
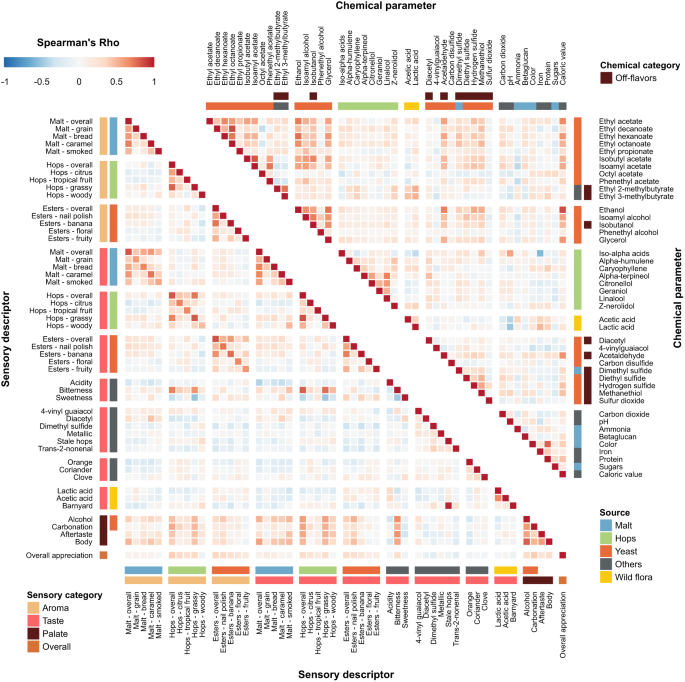
Spearman rank correlations are shown. Descriptors are grouped according to their origin (malt (blue), hops (green), yeast (red), wild flora (yellow), Others (black)), and sensory aspect (aroma, taste, palate, and overall appreciation). Please note that for the chemical compounds, for the sake of clarity, only a subset of the total number of measured compounds is shown, with an emphasis on the key compounds for each source. For more details, see the main text and Methods section. Chemical data can be found in Supplementary Data 1 , correlations between all chemical compounds are depicted in Supplementary Fig. S2 and correlation values can be found in Supplementary Data 2 . See Supplementary Data 4 for sensory panel assessments and Supplementary Data 5 for correlation values between all sensory descriptors.
Interestingly, different beer styles show distinct patterns for some flavor compounds (Supplementary Fig. S3 ). These observations agree with expectations for key beer styles, and serve as a control for our measurements. For instance, Stouts generally show high values for color (darker), while hoppy beers contain elevated levels of iso-alpha acids, compounds associated with bitter hop taste. Acetic and lactic acid are not prevalent in most beers, with notable exceptions such as Kriek, Lambic, Faro, West Flanders ales and Flanders Old Brown, which use acid-producing bacteria ( Lactobacillus and Pediococcus ) or unconventional yeast ( Brettanomyces ) 54 , 55 . Glycerol, ethanol and esters show similar distributions across all beer styles, reflecting their common origin as products of yeast metabolism during fermentation 45 , 53 . Finally, low/no-alcohol beers contain low concentrations of glycerol and esters. This is in line with the production process for most of the low/no-alcohol beers in our dataset, which are produced through limiting fermentation or by stripping away alcohol via evaporation or dialysis, with both methods having the unintended side-effect of reducing the amount of flavor compounds in the final beer 56 , 57 .
Besides expected associations, our data also reveals less trivial associations between beer styles and specific parameters. For example, geraniol and citronellol, two monoterpenoids responsible for citrus, floral and rose flavors and characteristic of Citra hops, are found in relatively high amounts in Christmas, Saison, and Brett/co-fermented beers, where they may originate from terpenoid-rich spices such as coriander seeds instead of hops 58 .
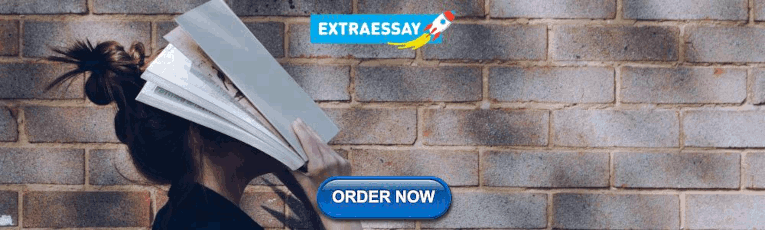
Tasting panel assessments reveal sensorial relationships in beer
To assess the sensory profile of each beer, a trained tasting panel evaluated each of the 250 beers for 50 sensory attributes, including different hop, malt and yeast flavors, off-flavors and spices. Panelists used a tasting sheet (Supplementary Data 3 ) to score the different attributes. Panel consistency was evaluated by repeating 12 samples across different sessions and performing ANOVA. In 95% of cases no significant difference was found across sessions ( p > 0.05), indicating good panel consistency (Supplementary Table S2 ).
Aroma and taste perception reported by the trained panel are often linked (Fig. 1 , bottom left panel and Supplementary Data 4 and 5 ), with high correlations between hops aroma and taste (Spearman’s rho=0.83). Bitter taste was found to correlate with hop aroma and taste in general (Spearman’s rho=0.80 and 0.69), and particularly with “grassy” noble hops (Spearman’s rho=0.75). Barnyard flavor, most often associated with sour beers, is identified together with stale hops (Spearman’s rho=0.97) that are used in these beers. Lactic and acetic acid, which often co-occur, are correlated (Spearman’s rho=0.66). Interestingly, sweetness and bitterness are anti-correlated (Spearman’s rho = −0.48), confirming the hypothesis that they mask each other 59 , 60 . Beer body is highly correlated with alcohol (Spearman’s rho = 0.79), and overall appreciation is found to correlate with multiple aspects that describe beer mouthfeel (alcohol, carbonation; Spearman’s rho= 0.32, 0.39), as well as with hop and ester aroma intensity (Spearman’s rho=0.39 and 0.35).
Similar to the chemical analyses, sensorial analyses confirmed typical features of specific beer styles (Supplementary Fig. S4 ). For example, sour beers (Faro, Flanders Old Brown, Fruit beer, Kriek, Lambic, West Flanders ale) were rated acidic, with flavors of both acetic and lactic acid. Hoppy beers were found to be bitter and showed hop-associated aromas like citrus and tropical fruit. Malt taste is most detected among scotch, stout/porters, and strong ales, while low/no-alcohol beers, which often have a reputation for being ‘worty’ (reminiscent of unfermented, sweet malt extract) appear in the middle. Unsurprisingly, hop aromas are most strongly detected among hoppy beers. Like its chemical counterpart (Supplementary Fig. S3 ), acidity shows a right-skewed distribution, with the most acidic beers being Krieks, Lambics, and West Flanders ales.
Tasting panel assessments of specific flavors correlate with chemical composition
We find that the concentrations of several chemical compounds strongly correlate with specific aroma or taste, as evaluated by the tasting panel (Fig. 2 , Supplementary Fig. S5 , Supplementary Data 6 ). In some cases, these correlations confirm expectations and serve as a useful control for data quality. For example, iso-alpha acids, the bittering compounds in hops, strongly correlate with bitterness (Spearman’s rho=0.68), while ethanol and glycerol correlate with tasters’ perceptions of alcohol and body, the mouthfeel sensation of fullness (Spearman’s rho=0.82/0.62 and 0.72/0.57 respectively) and darker color from roasted malts is a good indication of malt perception (Spearman’s rho=0.54).
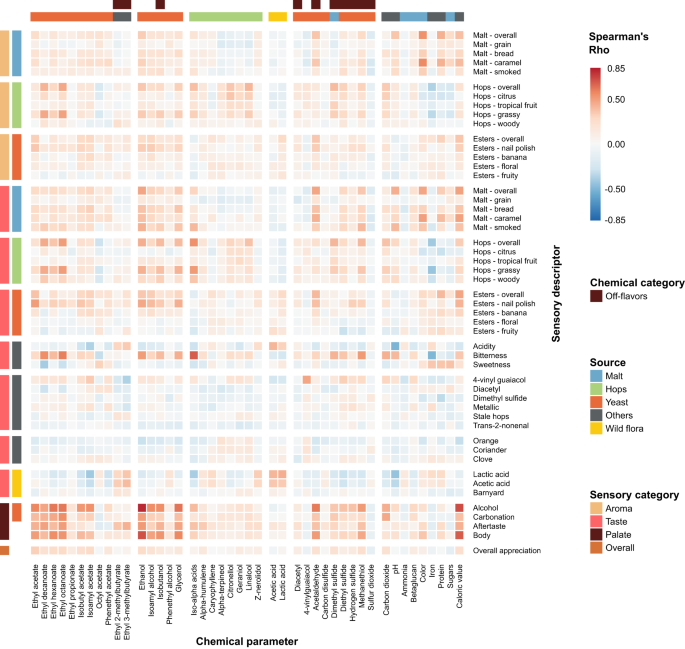
Heatmap colors indicate Spearman’s Rho. Axes are organized according to sensory categories (aroma, taste, mouthfeel, overall), chemical categories and chemical sources in beer (malt (blue), hops (green), yeast (red), wild flora (yellow), Others (black)). See Supplementary Data 6 for all correlation values.
Interestingly, for some relationships between chemical compounds and perceived flavor, correlations are weaker than expected. For example, the rose-smelling phenethyl acetate only weakly correlates with floral aroma. This hints at more complex relationships and interactions between compounds and suggests a need for a more complex model than simple correlations. Lastly, we uncovered unexpected correlations. For instance, the esters ethyl decanoate and ethyl octanoate appear to correlate slightly with hop perception and bitterness, possibly due to their fruity flavor. Iron is anti-correlated with hop aromas and bitterness, most likely because it is also anti-correlated with iso-alpha acids. This could be a sign of metal chelation of hop acids 61 , given that our analyses measure unbound hop acids and total iron content, or could result from the higher iron content in dark and Fruit beers, which typically have less hoppy and bitter flavors 62 .
Public consumer reviews complement expert panel data
To complement and expand the sensory data of our trained tasting panel, we collected 180,000 reviews of our 250 beers from the online consumer review platform RateBeer. This provided numerical scores for beer appearance, aroma, taste, palate, overall quality as well as the average overall score.
Public datasets are known to suffer from biases, such as price, cult status and psychological conformity towards previous ratings of a product. For example, prices correlate with appreciation scores for these online consumer reviews (rho=0.49, Supplementary Fig. S6 ), but not for our trained tasting panel (rho=0.19). This suggests that prices affect consumer appreciation, which has been reported in wine 63 , while blind tastings are unaffected. Moreover, we observe that some beer styles, like lagers and non-alcoholic beers, generally receive lower scores, reflecting that online reviewers are mostly beer aficionados with a preference for specialty beers over lager beers. In general, we find a modest correlation between our trained panel’s overall appreciation score and the online consumer appreciation scores (Fig. 3 , rho=0.29). Apart from the aforementioned biases in the online datasets, serving temperature, sample freshness and surroundings, which are all tightly controlled during the tasting panel sessions, can vary tremendously across online consumers and can further contribute to (among others, appreciation) differences between the two categories of tasters. Importantly, in contrast to the overall appreciation scores, for many sensory aspects the results from the professional panel correlated well with results obtained from RateBeer reviews. Correlations were highest for features that are relatively easy to recognize even for untrained tasters, like bitterness, sweetness, alcohol and malt aroma (Fig. 3 and below).
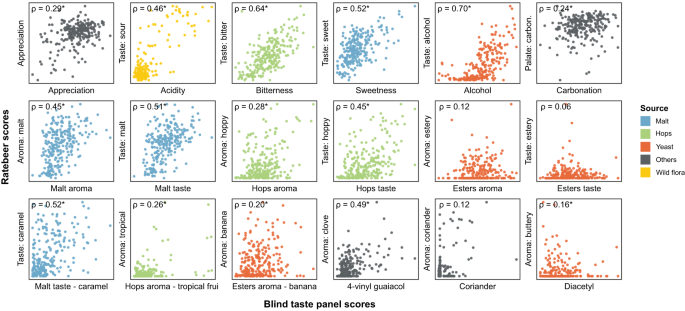
RateBeer text mining results can be found in Supplementary Data 7 . Rho values shown are Spearman correlation values, with asterisks indicating significant correlations ( p < 0.05, two-sided). All p values were smaller than 0.001, except for Esters aroma (0.0553), Esters taste (0.3275), Esters aroma—banana (0.0019), Coriander (0.0508) and Diacetyl (0.0134).
Besides collecting consumer appreciation from these online reviews, we developed automated text analysis tools to gather additional data from review texts (Supplementary Data 7 ). Processing review texts on the RateBeer database yielded comparable results to the scores given by the trained panel for many common sensory aspects, including acidity, bitterness, sweetness, alcohol, malt, and hop tastes (Fig. 3 ). This is in line with what would be expected, since these attributes require less training for accurate assessment and are less influenced by environmental factors such as temperature, serving glass and odors in the environment. Consumer reviews also correlate well with our trained panel for 4-vinyl guaiacol, a compound associated with a very characteristic aroma. By contrast, correlations for more specific aromas like ester, coriander or diacetyl are underrepresented in the online reviews, underscoring the importance of using a trained tasting panel and standardized tasting sheets with explicit factors to be scored for evaluating specific aspects of a beer. Taken together, our results suggest that public reviews are trustworthy for some, but not all, flavor features and can complement or substitute taste panel data for these sensory aspects.
Models can predict beer sensory profiles from chemical data
The rich datasets of chemical analyses, tasting panel assessments and public reviews gathered in the first part of this study provided us with a unique opportunity to develop predictive models that link chemical data to sensorial features. Given the complexity of beer flavor, basic statistical tools such as correlations or linear regression may not always be the most suitable for making accurate predictions. Instead, we applied different machine learning models that can model both simple linear and complex interactive relationships. Specifically, we constructed a set of regression models to predict (a) trained panel scores for beer flavor and quality and (b) public reviews’ appreciation scores from beer chemical profiles. We trained and tested 10 different models (Methods), 3 linear regression-based models (simple linear regression with first-order interactions (LR), lasso regression with first-order interactions (Lasso), partial least squares regressor (PLSR)), 5 decision tree models (AdaBoost regressor (ABR), extra trees (ET), gradient boosting regressor (GBR), random forest (RF) and XGBoost regressor (XGBR)), 1 support vector regression (SVR), and 1 artificial neural network (ANN) model.
To compare the performance of our machine learning models, the dataset was randomly split into a training and test set, stratified by beer style. After a model was trained on data in the training set, its performance was evaluated on its ability to predict the test dataset obtained from multi-output models (based on the coefficient of determination, see Methods). Additionally, individual-attribute models were ranked per descriptor and the average rank was calculated, as proposed by Korneva et al. 64 . Importantly, both ways of evaluating the models’ performance agreed in general. Performance of the different models varied (Table 1 ). It should be noted that all models perform better at predicting RateBeer results than results from our trained tasting panel. One reason could be that sensory data is inherently variable, and this variability is averaged out with the large number of public reviews from RateBeer. Additionally, all tree-based models perform better at predicting taste than aroma. Linear models (LR) performed particularly poorly, with negative R 2 values, due to severe overfitting (training set R 2 = 1). Overfitting is a common issue in linear models with many parameters and limited samples, especially with interaction terms further amplifying the number of parameters. L1 regularization (Lasso) successfully overcomes this overfitting, out-competing multiple tree-based models on the RateBeer dataset. Similarly, the dimensionality reduction of PLSR avoids overfitting and improves performance, to some extent. Still, tree-based models (ABR, ET, GBR, RF and XGBR) show the best performance, out-competing the linear models (LR, Lasso, PLSR) commonly used in sensory science 65 .
GBR models showed the best overall performance in predicting sensory responses from chemical information, with R 2 values up to 0.75 depending on the predicted sensory feature (Supplementary Table S4 ). The GBR models predict consumer appreciation (RateBeer) better than our trained panel’s appreciation (R 2 value of 0.67 compared to R 2 value of 0.09) (Supplementary Table S3 and Supplementary Table S4 ). ANN models showed intermediate performance, likely because neural networks typically perform best with larger datasets 66 . The SVR shows intermediate performance, mostly due to the weak predictions of specific attributes that lower the overall performance (Supplementary Table S4 ).
Model dissection identifies specific, unexpected compounds as drivers of consumer appreciation
Next, we leveraged our models to infer important contributors to sensory perception and consumer appreciation. Consumer preference is a crucial sensory aspects, because a product that shows low consumer appreciation scores often does not succeed commercially 25 . Additionally, the requirement for a large number of representative evaluators makes consumer trials one of the more costly and time-consuming aspects of product development. Hence, a model for predicting chemical drivers of overall appreciation would be a welcome addition to the available toolbox for food development and optimization.
Since GBR models on our RateBeer dataset showed the best overall performance, we focused on these models. Specifically, we used two approaches to identify important contributors. First, rankings of the most important predictors for each sensorial trait in the GBR models were obtained based on impurity-based feature importance (mean decrease in impurity). High-ranked parameters were hypothesized to be either the true causal chemical properties underlying the trait, to correlate with the actual causal properties, or to take part in sensory interactions affecting the trait 67 (Fig. 4A ). In a second approach, we used SHAP 68 to determine which parameters contributed most to the model for making predictions of consumer appreciation (Fig. 4B ). SHAP calculates parameter contributions to model predictions on a per-sample basis, which can be aggregated into an importance score.
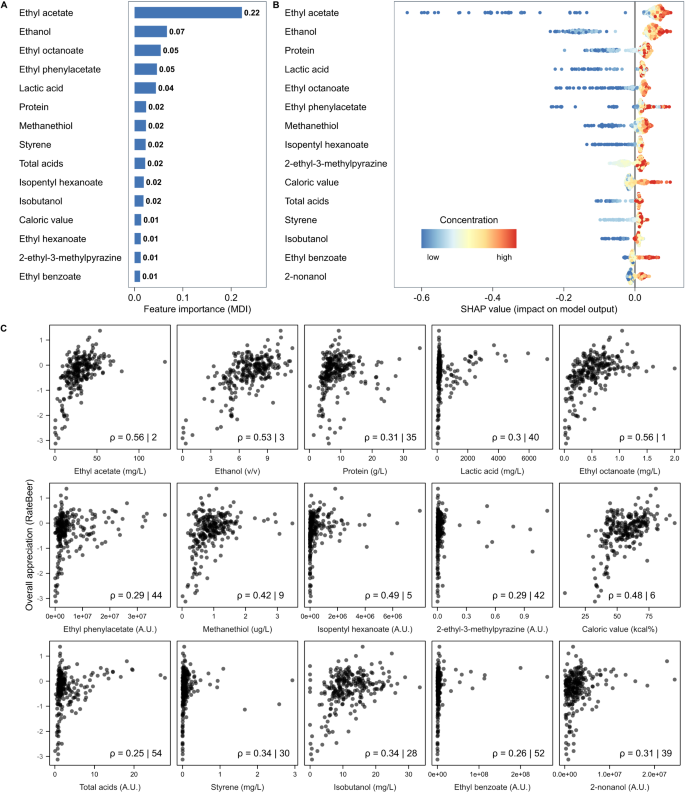
A The impurity-based feature importance (mean deviance in impurity, MDI) calculated from the Gradient Boosting Regression (GBR) model predicting RateBeer appreciation scores. The top 15 highest ranked chemical properties are shown. B SHAP summary plot for the top 15 parameters contributing to our GBR model. Each point on the graph represents a sample from our dataset. The color represents the concentration of that parameter, with bluer colors representing low values and redder colors representing higher values. Greater absolute values on the horizontal axis indicate a higher impact of the parameter on the prediction of the model. C Spearman correlations between the 15 most important chemical properties and consumer overall appreciation. Numbers indicate the Spearman Rho correlation coefficient, and the rank of this correlation compared to all other correlations. The top 15 important compounds were determined using SHAP (panel B).
Both approaches identified ethyl acetate as the most predictive parameter for beer appreciation (Fig. 4 ). Ethyl acetate is the most abundant ester in beer with a typical ‘fruity’, ‘solvent’ and ‘alcoholic’ flavor, but is often considered less important than other esters like isoamyl acetate. The second most important parameter identified by SHAP is ethanol, the most abundant beer compound after water. Apart from directly contributing to beer flavor and mouthfeel, ethanol drastically influences the physical properties of beer, dictating how easily volatile compounds escape the beer matrix to contribute to beer aroma 69 . Importantly, it should also be noted that the importance of ethanol for appreciation is likely inflated by the very low appreciation scores of non-alcoholic beers (Supplementary Fig. S4 ). Despite not often being considered a driver of beer appreciation, protein level also ranks highly in both approaches, possibly due to its effect on mouthfeel and body 70 . Lactic acid, which contributes to the tart taste of sour beers, is the fourth most important parameter identified by SHAP, possibly due to the generally high appreciation of sour beers in our dataset.
Interestingly, some of the most important predictive parameters for our model are not well-established as beer flavors or are even commonly regarded as being negative for beer quality. For example, our models identify methanethiol and ethyl phenyl acetate, an ester commonly linked to beer staling 71 , as a key factor contributing to beer appreciation. Although there is no doubt that high concentrations of these compounds are considered unpleasant, the positive effects of modest concentrations are not yet known 72 , 73 .
To compare our approach to conventional statistics, we evaluated how well the 15 most important SHAP-derived parameters correlate with consumer appreciation (Fig. 4C ). Interestingly, only 6 of the properties derived by SHAP rank amongst the top 15 most correlated parameters. For some chemical compounds, the correlations are so low that they would have likely been considered unimportant. For example, lactic acid, the fourth most important parameter, shows a bimodal distribution for appreciation, with sour beers forming a separate cluster, that is missed entirely by the Spearman correlation. Additionally, the correlation plots reveal outliers, emphasizing the need for robust analysis tools. Together, this highlights the need for alternative models, like the Gradient Boosting model, that better grasp the complexity of (beer) flavor.
Finally, to observe the relationships between these chemical properties and their predicted targets, partial dependence plots were constructed for the six most important predictors of consumer appreciation 74 , 75 , 76 (Supplementary Fig. S7 ). One-way partial dependence plots show how a change in concentration affects the predicted appreciation. These plots reveal an important limitation of our models: appreciation predictions remain constant at ever-increasing concentrations. This implies that once a threshold concentration is reached, further increasing the concentration does not affect appreciation. This is false, as it is well-documented that certain compounds become unpleasant at high concentrations, including ethyl acetate (‘nail polish’) 77 and methanethiol (‘sulfury’ and ‘rotten cabbage’) 78 . The inability of our models to grasp that flavor compounds have optimal levels, above which they become negative, is a consequence of working with commercial beer brands where (off-)flavors are rarely too high to negatively impact the product. The two-way partial dependence plots show how changing the concentration of two compounds influences predicted appreciation, visualizing their interactions (Supplementary Fig. S7 ). In our case, the top 5 parameters are dominated by additive or synergistic interactions, with high concentrations for both compounds resulting in the highest predicted appreciation.
To assess the robustness of our best-performing models and model predictions, we performed 100 iterations of the GBR, RF and ET models. In general, all iterations of the models yielded similar performance (Supplementary Fig. S8 ). Moreover, the main predictors (including the top predictors ethanol and ethyl acetate) remained virtually the same, especially for GBR and RF. For the iterations of the ET model, we did observe more variation in the top predictors, which is likely a consequence of the model’s inherent random architecture in combination with co-correlations between certain predictors. However, even in this case, several of the top predictors (ethanol and ethyl acetate) remain unchanged, although their rank in importance changes (Supplementary Fig. S8 ).
Next, we investigated if a combination of RateBeer and trained panel data into one consolidated dataset would lead to stronger models, under the hypothesis that such a model would suffer less from bias in the datasets. A GBR model was trained to predict appreciation on the combined dataset. This model underperformed compared to the RateBeer model, both in the native case and when including a dataset identifier (R 2 = 0.67, 0.26 and 0.42 respectively). For the latter, the dataset identifier is the most important feature (Supplementary Fig. S9 ), while most of the feature importance remains unchanged, with ethyl acetate and ethanol ranking highest, like in the original model trained only on RateBeer data. It seems that the large variation in the panel dataset introduces noise, weakening the models’ performances and reliability. In addition, it seems reasonable to assume that both datasets are fundamentally different, with the panel dataset obtained by blind tastings by a trained professional panel.
Lastly, we evaluated whether beer style identifiers would further enhance the model’s performance. A GBR model was trained with parameters that explicitly encoded the styles of the samples. This did not improve model performance (R2 = 0.66 with style information vs R2 = 0.67). The most important chemical features are consistent with the model trained without style information (eg. ethanol and ethyl acetate), and with the exception of the most preferred (strong ale) and least preferred (low/no-alcohol) styles, none of the styles were among the most important features (Supplementary Fig. S9 , Supplementary Table S5 and S6 ). This is likely due to a combination of style-specific chemical signatures, such as iso-alpha acids and lactic acid, that implicitly convey style information to the original models, as well as the low number of samples belonging to some styles, making it difficult for the model to learn style-specific patterns. Moreover, beer styles are not rigorously defined, with some styles overlapping in features and some beers being misattributed to a specific style, all of which leads to more noise in models that use style parameters.
Model validation
To test if our predictive models give insight into beer appreciation, we set up experiments aimed at improving existing commercial beers. We specifically selected overall appreciation as the trait to be examined because of its complexity and commercial relevance. Beer flavor comprises a complex bouquet rather than single aromas and tastes 53 . Hence, adding a single compound to the extent that a difference is noticeable may lead to an unbalanced, artificial flavor. Therefore, we evaluated the effect of combinations of compounds. Because Blond beers represent the most extensive style in our dataset, we selected a beer from this style as the starting material for these experiments (Beer 64 in Supplementary Data 1 ).
In the first set of experiments, we adjusted the concentrations of compounds that made up the most important predictors of overall appreciation (ethyl acetate, ethanol, lactic acid, ethyl phenyl acetate) together with correlated compounds (ethyl hexanoate, isoamyl acetate, glycerol), bringing them up to 95 th percentile ethanol-normalized concentrations (Methods) within the Blond group (‘Spiked’ concentration in Fig. 5A ). Compared to controls, the spiked beers were found to have significantly improved overall appreciation among trained panelists, with panelist noting increased intensity of ester flavors, sweetness, alcohol, and body fullness (Fig. 5B ). To disentangle the contribution of ethanol to these results, a second experiment was performed without the addition of ethanol. This resulted in a similar outcome, including increased perception of alcohol and overall appreciation.
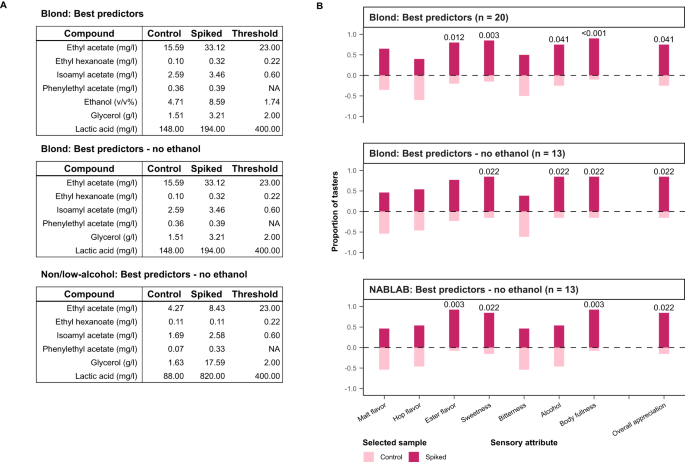
Adding the top chemical compounds, identified as best predictors of appreciation by our model, into poorly appreciated beers results in increased appreciation from our trained panel. Results of sensory tests between base beers and those spiked with compounds identified as the best predictors by the model. A Blond and Non/Low-alcohol (0.0% ABV) base beers were brought up to 95th-percentile ethanol-normalized concentrations within each style. B For each sensory attribute, tasters indicated the more intense sample and selected the sample they preferred. The numbers above the bars correspond to the p values that indicate significant changes in perceived flavor (two-sided binomial test: alpha 0.05, n = 20 or 13).
In a last experiment, we tested whether using the model’s predictions can boost the appreciation of a non-alcoholic beer (beer 223 in Supplementary Data 1 ). Again, the addition of a mixture of predicted compounds (omitting ethanol, in this case) resulted in a significant increase in appreciation, body, ester flavor and sweetness.
Predicting flavor and consumer appreciation from chemical composition is one of the ultimate goals of sensory science. A reliable, systematic and unbiased way to link chemical profiles to flavor and food appreciation would be a significant asset to the food and beverage industry. Such tools would substantially aid in quality control and recipe development, offer an efficient and cost-effective alternative to pilot studies and consumer trials and would ultimately allow food manufacturers to produce superior, tailor-made products that better meet the demands of specific consumer groups more efficiently.
A limited set of studies have previously tried, to varying degrees of success, to predict beer flavor and beer popularity based on (a limited set of) chemical compounds and flavors 79 , 80 . Current sensitive, high-throughput technologies allow measuring an unprecedented number of chemical compounds and properties in a large set of samples, yielding a dataset that can train models that help close the gaps between chemistry and flavor, even for a complex natural product like beer. To our knowledge, no previous research gathered data at this scale (250 samples, 226 chemical parameters, 50 sensory attributes and 5 consumer scores) to disentangle and validate the chemical aspects driving beer preference using various machine-learning techniques. We find that modern machine learning models outperform conventional statistical tools, such as correlations and linear models, and can successfully predict flavor appreciation from chemical composition. This could be attributed to the natural incorporation of interactions and non-linear or discontinuous effects in machine learning models, which are not easily grasped by the linear model architecture. While linear models and partial least squares regression represent the most widespread statistical approaches in sensory science, in part because they allow interpretation 65 , 81 , 82 , modern machine learning methods allow for building better predictive models while preserving the possibility to dissect and exploit the underlying patterns. Of the 10 different models we trained, tree-based models, such as our best performing GBR, showed the best overall performance in predicting sensory responses from chemical information, outcompeting artificial neural networks. This agrees with previous reports for models trained on tabular data 83 . Our results are in line with the findings of Colantonio et al. who also identified the gradient boosting architecture as performing best at predicting appreciation and flavor (of tomatoes and blueberries, in their specific study) 26 . Importantly, besides our larger experimental scale, we were able to directly confirm our models’ predictions in vivo.
Our study confirms that flavor compound concentration does not always correlate with perception, suggesting complex interactions that are often missed by more conventional statistics and simple models. Specifically, we find that tree-based algorithms may perform best in developing models that link complex food chemistry with aroma. Furthermore, we show that massive datasets of untrained consumer reviews provide a valuable source of data, that can complement or even replace trained tasting panels, especially for appreciation and basic flavors, such as sweetness and bitterness. This holds despite biases that are known to occur in such datasets, such as price or conformity bias. Moreover, GBR models predict taste better than aroma. This is likely because taste (e.g. bitterness) often directly relates to the corresponding chemical measurements (e.g., iso-alpha acids), whereas such a link is less clear for aromas, which often result from the interplay between multiple volatile compounds. We also find that our models are best at predicting acidity and alcohol, likely because there is a direct relation between the measured chemical compounds (acids and ethanol) and the corresponding perceived sensorial attribute (acidity and alcohol), and because even untrained consumers are generally able to recognize these flavors and aromas.
The predictions of our final models, trained on review data, hold even for blind tastings with small groups of trained tasters, as demonstrated by our ability to validate specific compounds as drivers of beer flavor and appreciation. Since adding a single compound to the extent of a noticeable difference may result in an unbalanced flavor profile, we specifically tested our identified key drivers as a combination of compounds. While this approach does not allow us to validate if a particular single compound would affect flavor and/or appreciation, our experiments do show that this combination of compounds increases consumer appreciation.
It is important to stress that, while it represents an important step forward, our approach still has several major limitations. A key weakness of the GBR model architecture is that amongst co-correlating variables, the largest main effect is consistently preferred for model building. As a result, co-correlating variables often have artificially low importance scores, both for impurity and SHAP-based methods, like we observed in the comparison to the more randomized Extra Trees models. This implies that chemicals identified as key drivers of a specific sensory feature by GBR might not be the true causative compounds, but rather co-correlate with the actual causative chemical. For example, the high importance of ethyl acetate could be (partially) attributed to the total ester content, ethanol or ethyl hexanoate (rho=0.77, rho=0.72 and rho=0.68), while ethyl phenylacetate could hide the importance of prenyl isobutyrate and ethyl benzoate (rho=0.77 and rho=0.76). Expanding our GBR model to include beer style as a parameter did not yield additional power or insight. This is likely due to style-specific chemical signatures, such as iso-alpha acids and lactic acid, that implicitly convey style information to the original model, as well as the smaller sample size per style, limiting the power to uncover style-specific patterns. This can be partly attributed to the curse of dimensionality, where the high number of parameters results in the models mainly incorporating single parameter effects, rather than complex interactions such as style-dependent effects 67 . A larger number of samples may overcome some of these limitations and offer more insight into style-specific effects. On the other hand, beer style is not a rigid scientific classification, and beers within one style often differ a lot, which further complicates the analysis of style as a model factor.
Our study is limited to beers from Belgian breweries. Although these beers cover a large portion of the beer styles available globally, some beer styles and consumer patterns may be missing, while other features might be overrepresented. For example, many Belgian ales exhibit yeast-driven flavor profiles, which is reflected in the chemical drivers of appreciation discovered by this study. In future work, expanding the scope to include diverse markets and beer styles could lead to the identification of even more drivers of appreciation and better models for special niche products that were not present in our beer set.
In addition to inherent limitations of GBR models, there are also some limitations associated with studying food aroma. Even if our chemical analyses measured most of the known aroma compounds, the total number of flavor compounds in complex foods like beer is still larger than the subset we were able to measure in this study. For example, hop-derived thiols, that influence flavor at very low concentrations, are notoriously difficult to measure in a high-throughput experiment. Moreover, consumer perception remains subjective and prone to biases that are difficult to avoid. It is also important to stress that the models are still immature and that more extensive datasets will be crucial for developing more complete models in the future. Besides more samples and parameters, our dataset does not include any demographic information about the tasters. Including such data could lead to better models that grasp external factors like age and culture. Another limitation is that our set of beers consists of high-quality end-products and lacks beers that are unfit for sale, which limits the current model in accurately predicting products that are appreciated very badly. Finally, while models could be readily applied in quality control, their use in sensory science and product development is restrained by their inability to discern causal relationships. Given that the models cannot distinguish compounds that genuinely drive consumer perception from those that merely correlate, validation experiments are essential to identify true causative compounds.
Despite the inherent limitations, dissection of our models enabled us to pinpoint specific molecules as potential drivers of beer aroma and consumer appreciation, including compounds that were unexpected and would not have been identified using standard approaches. Important drivers of beer appreciation uncovered by our models include protein levels, ethyl acetate, ethyl phenyl acetate and lactic acid. Currently, many brewers already use lactic acid to acidify their brewing water and ensure optimal pH for enzymatic activity during the mashing process. Our results suggest that adding lactic acid can also improve beer appreciation, although its individual effect remains to be tested. Interestingly, ethanol appears to be unnecessary to improve beer appreciation, both for blond beer and alcohol-free beer. Given the growing consumer interest in alcohol-free beer, with a predicted annual market growth of >7% 84 , it is relevant for brewers to know what compounds can further increase consumer appreciation of these beers. Hence, our model may readily provide avenues to further improve the flavor and consumer appreciation of both alcoholic and non-alcoholic beers, which is generally considered one of the key challenges for future beer production.
Whereas we see a direct implementation of our results for the development of superior alcohol-free beverages and other food products, our study can also serve as a stepping stone for the development of novel alcohol-containing beverages. We want to echo the growing body of scientific evidence for the negative effects of alcohol consumption, both on the individual level by the mutagenic, teratogenic and carcinogenic effects of ethanol 85 , 86 , as well as the burden on society caused by alcohol abuse and addiction. We encourage the use of our results for the production of healthier, tastier products, including novel and improved beverages with lower alcohol contents. Furthermore, we strongly discourage the use of these technologies to improve the appreciation or addictive properties of harmful substances.
The present work demonstrates that despite some important remaining hurdles, combining the latest developments in chemical analyses, sensory analysis and modern machine learning methods offers exciting avenues for food chemistry and engineering. Soon, these tools may provide solutions in quality control and recipe development, as well as new approaches to sensory science and flavor research.
Beer selection
250 commercial Belgian beers were selected to cover the broad diversity of beer styles and corresponding diversity in chemical composition and aroma. See Supplementary Fig. S1 .
Chemical dataset
Sample preparation.
Beers within their expiration date were purchased from commercial retailers. Samples were prepared in biological duplicates at room temperature, unless explicitly stated otherwise. Bottle pressure was measured with a manual pressure device (Steinfurth Mess-Systeme GmbH) and used to calculate CO 2 concentration. The beer was poured through two filter papers (Macherey-Nagel, 500713032 MN 713 ¼) to remove carbon dioxide and prevent spontaneous foaming. Samples were then prepared for measurements by targeted Headspace-Gas Chromatography-Flame Ionization Detector/Flame Photometric Detector (HS-GC-FID/FPD), Headspace-Solid Phase Microextraction-Gas Chromatography-Mass Spectrometry (HS-SPME-GC-MS), colorimetric analysis, enzymatic analysis, Near-Infrared (NIR) analysis, as described in the sections below. The mean values of biological duplicates are reported for each compound.
HS-GC-FID/FPD
HS-GC-FID/FPD (Shimadzu GC 2010 Plus) was used to measure higher alcohols, acetaldehyde, esters, 4-vinyl guaicol, and sulfur compounds. Each measurement comprised 5 ml of sample pipetted into a 20 ml glass vial containing 1.75 g NaCl (VWR, 27810.295). 100 µl of 2-heptanol (Sigma-Aldrich, H3003) (internal standard) solution in ethanol (Fisher Chemical, E/0650DF/C17) was added for a final concentration of 2.44 mg/L. Samples were flushed with nitrogen for 10 s, sealed with a silicone septum, stored at −80 °C and analyzed in batches of 20.
The GC was equipped with a DB-WAXetr column (length, 30 m; internal diameter, 0.32 mm; layer thickness, 0.50 µm; Agilent Technologies, Santa Clara, CA, USA) to the FID and an HP-5 column (length, 30 m; internal diameter, 0.25 mm; layer thickness, 0.25 µm; Agilent Technologies, Santa Clara, CA, USA) to the FPD. N 2 was used as the carrier gas. Samples were incubated for 20 min at 70 °C in the headspace autosampler (Flow rate, 35 cm/s; Injection volume, 1000 µL; Injection mode, split; Combi PAL autosampler, CTC analytics, Switzerland). The injector, FID and FPD temperatures were kept at 250 °C. The GC oven temperature was first held at 50 °C for 5 min and then allowed to rise to 80 °C at a rate of 5 °C/min, followed by a second ramp of 4 °C/min until 200 °C kept for 3 min and a final ramp of (4 °C/min) until 230 °C for 1 min. Results were analyzed with the GCSolution software version 2.4 (Shimadzu, Kyoto, Japan). The GC was calibrated with a 5% EtOH solution (VWR International) containing the volatiles under study (Supplementary Table S7 ).
HS-SPME-GC-MS
HS-SPME-GC-MS (Shimadzu GCMS-QP-2010 Ultra) was used to measure additional volatile compounds, mainly comprising terpenoids and esters. Samples were analyzed by HS-SPME using a triphase DVB/Carboxen/PDMS 50/30 μm SPME fiber (Supelco Co., Bellefonte, PA, USA) followed by gas chromatography (Thermo Fisher Scientific Trace 1300 series, USA) coupled to a mass spectrometer (Thermo Fisher Scientific ISQ series MS) equipped with a TriPlus RSH autosampler. 5 ml of degassed beer sample was placed in 20 ml vials containing 1.75 g NaCl (VWR, 27810.295). 5 µl internal standard mix was added, containing 2-heptanol (1 g/L) (Sigma-Aldrich, H3003), 4-fluorobenzaldehyde (1 g/L) (Sigma-Aldrich, 128376), 2,3-hexanedione (1 g/L) (Sigma-Aldrich, 144169) and guaiacol (1 g/L) (Sigma-Aldrich, W253200) in ethanol (Fisher Chemical, E/0650DF/C17). Each sample was incubated at 60 °C in the autosampler oven with constant agitation. After 5 min equilibration, the SPME fiber was exposed to the sample headspace for 30 min. The compounds trapped on the fiber were thermally desorbed in the injection port of the chromatograph by heating the fiber for 15 min at 270 °C.
The GC-MS was equipped with a low polarity RXi-5Sil MS column (length, 20 m; internal diameter, 0.18 mm; layer thickness, 0.18 µm; Restek, Bellefonte, PA, USA). Injection was performed in splitless mode at 320 °C, a split flow of 9 ml/min, a purge flow of 5 ml/min and an open valve time of 3 min. To obtain a pulsed injection, a programmed gas flow was used whereby the helium gas flow was set at 2.7 mL/min for 0.1 min, followed by a decrease in flow of 20 ml/min to the normal 0.9 mL/min. The temperature was first held at 30 °C for 3 min and then allowed to rise to 80 °C at a rate of 7 °C/min, followed by a second ramp of 2 °C/min till 125 °C and a final ramp of 8 °C/min with a final temperature of 270 °C.
Mass acquisition range was 33 to 550 amu at a scan rate of 5 scans/s. Electron impact ionization energy was 70 eV. The interface and ion source were kept at 275 °C and 250 °C, respectively. A mix of linear n-alkanes (from C7 to C40, Supelco Co.) was injected into the GC-MS under identical conditions to serve as external retention index markers. Identification and quantification of the compounds were performed using an in-house developed R script as described in Goelen et al. and Reher et al. 87 , 88 (for package information, see Supplementary Table S8 ). Briefly, chromatograms were analyzed using AMDIS (v2.71) 89 to separate overlapping peaks and obtain pure compound spectra. The NIST MS Search software (v2.0 g) in combination with the NIST2017, FFNSC3 and Adams4 libraries were used to manually identify the empirical spectra, taking into account the expected retention time. After background subtraction and correcting for retention time shifts between samples run on different days based on alkane ladders, compound elution profiles were extracted and integrated using a file with 284 target compounds of interest, which were either recovered in our identified AMDIS list of spectra or were known to occur in beer. Compound elution profiles were estimated for every peak in every chromatogram over a time-restricted window using weighted non-negative least square analysis after which peak areas were integrated 87 , 88 . Batch effect correction was performed by normalizing against the most stable internal standard compound, 4-fluorobenzaldehyde. Out of all 284 target compounds that were analyzed, 167 were visually judged to have reliable elution profiles and were used for final analysis.
Discrete photometric and enzymatic analysis
Discrete photometric and enzymatic analysis (Thermo Scientific TM Gallery TM Plus Beermaster Discrete Analyzer) was used to measure acetic acid, ammonia, beta-glucan, iso-alpha acids, color, sugars, glycerol, iron, pH, protein, and sulfite. 2 ml of sample volume was used for the analyses. Information regarding the reagents and standard solutions used for analyses and calibrations is included in Supplementary Table S7 and Supplementary Table S9 .
NIR analyses
NIR analysis (Anton Paar Alcolyzer Beer ME System) was used to measure ethanol. Measurements comprised 50 ml of sample, and a 10% EtOH solution was used for calibration.
Correlation calculations
Pairwise Spearman Rank correlations were calculated between all chemical properties.
Sensory dataset
Trained panel.
Our trained tasting panel consisted of volunteers who gave prior verbal informed consent. All compounds used for the validation experiment were of food-grade quality. The tasting sessions were approved by the Social and Societal Ethics Committee of the KU Leuven (G-2022-5677-R2(MAR)). All online reviewers agreed to the Terms and Conditions of the RateBeer website.
Sensory analysis was performed according to the American Society of Brewing Chemists (ASBC) Sensory Analysis Methods 90 . 30 volunteers were screened through a series of triangle tests. The sixteen most sensitive and consistent tasters were retained as taste panel members. The resulting panel was diverse in age [22–42, mean: 29], sex [56% male] and nationality [7 different countries]. The panel developed a consensus vocabulary to describe beer aroma, taste and mouthfeel. Panelists were trained to identify and score 50 different attributes, using a 7-point scale to rate attributes’ intensity. The scoring sheet is included as Supplementary Data 3 . Sensory assessments took place between 10–12 a.m. The beers were served in black-colored glasses. Per session, between 5 and 12 beers of the same style were tasted at 12 °C to 16 °C. Two reference beers were added to each set and indicated as ‘Reference 1 & 2’, allowing panel members to calibrate their ratings. Not all panelists were present at every tasting. Scores were scaled by standard deviation and mean-centered per taster. Values are represented as z-scores and clustered by Euclidean distance. Pairwise Spearman correlations were calculated between taste and aroma sensory attributes. Panel consistency was evaluated by repeating samples on different sessions and performing ANOVA to identify differences, using the ‘stats’ package (v4.2.2) in R (for package information, see Supplementary Table S8 ).
Online reviews from a public database
The ‘scrapy’ package in Python (v3.6) (for package information, see Supplementary Table S8 ). was used to collect 232,288 online reviews (mean=922, min=6, max=5343) from RateBeer, an online beer review database. Each review entry comprised 5 numerical scores (appearance, aroma, taste, palate and overall quality) and an optional review text. The total number of reviews per reviewer was collected separately. Numerical scores were scaled and centered per rater, and mean scores were calculated per beer.
For the review texts, the language was estimated using the packages ‘langdetect’ and ‘langid’ in Python. Reviews that were classified as English by both packages were kept. Reviewers with fewer than 100 entries overall were discarded. 181,025 reviews from >6000 reviewers from >40 countries remained. Text processing was done using the ‘nltk’ package in Python. Texts were corrected for slang and misspellings; proper nouns and rare words that are relevant to the beer context were specified and kept as-is (‘Chimay’,’Lambic’, etc.). A dictionary of semantically similar sensorial terms, for example ‘floral’ and ‘flower’, was created and collapsed together into one term. Words were stemmed and lemmatized to avoid identifying words such as ‘acid’ and ‘acidity’ as separate terms. Numbers and punctuation were removed.
Sentences from up to 50 randomly chosen reviews per beer were manually categorized according to the aspect of beer they describe (appearance, aroma, taste, palate, overall quality—not to be confused with the 5 numerical scores described above) or flagged as irrelevant if they contained no useful information. If a beer contained fewer than 50 reviews, all reviews were manually classified. This labeled data set was used to train a model that classified the rest of the sentences for all beers 91 . Sentences describing taste and aroma were extracted, and term frequency–inverse document frequency (TFIDF) was implemented to calculate enrichment scores for sensorial words per beer.
The sex of the tasting subject was not considered when building our sensory database. Instead, results from different panelists were averaged, both for our trained panel (56% male, 44% female) and the RateBeer reviews (70% male, 30% female for RateBeer as a whole).
Beer price collection and processing
Beer prices were collected from the following stores: Colruyt, Delhaize, Total Wine, BeerHawk, The Belgian Beer Shop, The Belgian Shop, and Beer of Belgium. Where applicable, prices were converted to Euros and normalized per liter. Spearman correlations were calculated between these prices and mean overall appreciation scores from RateBeer and the taste panel, respectively.
Pairwise Spearman Rank correlations were calculated between all sensory properties.
Machine learning models
Predictive modeling of sensory profiles from chemical data.
Regression models were constructed to predict (a) trained panel scores for beer flavors and quality from beer chemical profiles and (b) public reviews’ appreciation scores from beer chemical profiles. Z-scores were used to represent sensory attributes in both data sets. Chemical properties with log-normal distributions (Shapiro-Wilk test, p < 0.05 ) were log-transformed. Missing chemical measurements (0.1% of all data) were replaced with mean values per attribute. Observations from 250 beers were randomly separated into a training set (70%, 175 beers) and a test set (30%, 75 beers), stratified per beer style. Chemical measurements (p = 231) were normalized based on the training set average and standard deviation. In total, three linear regression-based models: linear regression with first-order interaction terms (LR), lasso regression with first-order interaction terms (Lasso) and partial least squares regression (PLSR); five decision tree models, Adaboost regressor (ABR), Extra Trees (ET), Gradient Boosting regressor (GBR), Random Forest (RF) and XGBoost regressor (XGBR); one support vector machine model (SVR) and one artificial neural network model (ANN) were trained. The models were implemented using the ‘scikit-learn’ package (v1.2.2) and ‘xgboost’ package (v1.7.3) in Python (v3.9.16). Models were trained, and hyperparameters optimized, using five-fold cross-validated grid search with the coefficient of determination (R 2 ) as the evaluation metric. The ANN (scikit-learn’s MLPRegressor) was optimized using Bayesian Tree-Structured Parzen Estimator optimization with the ‘Optuna’ Python package (v3.2.0). Individual models were trained per attribute, and a multi-output model was trained on all attributes simultaneously.
Model dissection
GBR was found to outperform other methods, resulting in models with the highest average R 2 values in both trained panel and public review data sets. Impurity-based rankings of the most important predictors for each predicted sensorial trait were obtained using the ‘scikit-learn’ package. To observe the relationships between these chemical properties and their predicted targets, partial dependence plots (PDP) were constructed for the six most important predictors of consumer appreciation 74 , 75 .
The ‘SHAP’ package in Python (v0.41.0) was implemented to provide an alternative ranking of predictor importance and to visualize the predictors’ effects as a function of their concentration 68 .
Validation of causal chemical properties
To validate the effects of the most important model features on predicted sensory attributes, beers were spiked with the chemical compounds identified by the models and descriptive sensory analyses were carried out according to the American Society of Brewing Chemists (ASBC) protocol 90 .
Compound spiking was done 30 min before tasting. Compounds were spiked into fresh beer bottles, that were immediately resealed and inverted three times. Fresh bottles of beer were opened for the same duration, resealed, and inverted thrice, to serve as controls. Pairs of spiked samples and controls were served simultaneously, chilled and in dark glasses as outlined in the Trained panel section above. Tasters were instructed to select the glass with the higher flavor intensity for each attribute (directional difference test 92 ) and to select the glass they prefer.
The final concentration after spiking was equal to the within-style average, after normalizing by ethanol concentration. This was done to ensure balanced flavor profiles in the final spiked beer. The same methods were applied to improve a non-alcoholic beer. Compounds were the following: ethyl acetate (Merck KGaA, W241415), ethyl hexanoate (Merck KGaA, W243906), isoamyl acetate (Merck KGaA, W205508), phenethyl acetate (Merck KGaA, W285706), ethanol (96%, Colruyt), glycerol (Merck KGaA, W252506), lactic acid (Merck KGaA, 261106).
Significant differences in preference or perceived intensity were determined by performing the two-sided binomial test on each attribute.
Reporting summary
Further information on research design is available in the Nature Portfolio Reporting Summary linked to this article.
Data availability
The data that support the findings of this work are available in the Supplementary Data files and have been deposited to Zenodo under accession code 10653704 93 . The RateBeer scores data are under restricted access, they are not publicly available as they are property of RateBeer (ZX Ventures, USA). Access can be obtained from the authors upon reasonable request and with permission of RateBeer (ZX Ventures, USA). Source data are provided with this paper.
Code availability
The code for training the machine learning models, analyzing the models, and generating the figures has been deposited to Zenodo under accession code 10653704 93 .
Tieman, D. et al. A chemical genetic roadmap to improved tomato flavor. Science 355 , 391–394 (2017).
Article ADS CAS PubMed Google Scholar
Plutowska, B. & Wardencki, W. Application of gas chromatography–olfactometry (GC–O) in analysis and quality assessment of alcoholic beverages – A review. Food Chem. 107 , 449–463 (2008).
Article CAS Google Scholar
Legin, A., Rudnitskaya, A., Seleznev, B. & Vlasov, Y. Electronic tongue for quality assessment of ethanol, vodka and eau-de-vie. Anal. Chim. Acta 534 , 129–135 (2005).
Loutfi, A., Coradeschi, S., Mani, G. K., Shankar, P. & Rayappan, J. B. B. Electronic noses for food quality: A review. J. Food Eng. 144 , 103–111 (2015).
Ahn, Y.-Y., Ahnert, S. E., Bagrow, J. P. & Barabási, A.-L. Flavor network and the principles of food pairing. Sci. Rep. 1 , 196 (2011).
Article CAS PubMed PubMed Central Google Scholar
Bartoshuk, L. M. & Klee, H. J. Better fruits and vegetables through sensory analysis. Curr. Biol. 23 , R374–R378 (2013).
Article CAS PubMed Google Scholar
Piggott, J. R. Design questions in sensory and consumer science. Food Qual. Prefer. 3293 , 217–220 (1995).
Article Google Scholar
Kermit, M. & Lengard, V. Assessing the performance of a sensory panel-panellist monitoring and tracking. J. Chemom. 19 , 154–161 (2005).
Cook, D. J., Hollowood, T. A., Linforth, R. S. T. & Taylor, A. J. Correlating instrumental measurements of texture and flavour release with human perception. Int. J. Food Sci. Technol. 40 , 631–641 (2005).
Chinchanachokchai, S., Thontirawong, P. & Chinchanachokchai, P. A tale of two recommender systems: The moderating role of consumer expertise on artificial intelligence based product recommendations. J. Retail. Consum. Serv. 61 , 1–12 (2021).
Ross, C. F. Sensory science at the human-machine interface. Trends Food Sci. Technol. 20 , 63–72 (2009).
Chambers, E. IV & Koppel, K. Associations of volatile compounds with sensory aroma and flavor: The complex nature of flavor. Molecules 18 , 4887–4905 (2013).
Pinu, F. R. Metabolomics—The new frontier in food safety and quality research. Food Res. Int. 72 , 80–81 (2015).
Danezis, G. P., Tsagkaris, A. S., Brusic, V. & Georgiou, C. A. Food authentication: state of the art and prospects. Curr. Opin. Food Sci. 10 , 22–31 (2016).
Shepherd, G. M. Smell images and the flavour system in the human brain. Nature 444 , 316–321 (2006).
Meilgaard, M. C. Prediction of flavor differences between beers from their chemical composition. J. Agric. Food Chem. 30 , 1009–1017 (1982).
Xu, L. et al. Widespread receptor-driven modulation in peripheral olfactory coding. Science 368 , eaaz5390 (2020).
Kupferschmidt, K. Following the flavor. Science 340 , 808–809 (2013).
Billesbølle, C. B. et al. Structural basis of odorant recognition by a human odorant receptor. Nature 615 , 742–749 (2023).
Article ADS PubMed PubMed Central Google Scholar
Smith, B. Perspective: Complexities of flavour. Nature 486 , S6–S6 (2012).
Pfister, P. et al. Odorant receptor inhibition is fundamental to odor encoding. Curr. Biol. 30 , 2574–2587 (2020).
Moskowitz, H. W., Kumaraiah, V., Sharma, K. N., Jacobs, H. L. & Sharma, S. D. Cross-cultural differences in simple taste preferences. Science 190 , 1217–1218 (1975).
Eriksson, N. et al. A genetic variant near olfactory receptor genes influences cilantro preference. Flavour 1 , 22 (2012).
Ferdenzi, C. et al. Variability of affective responses to odors: Culture, gender, and olfactory knowledge. Chem. Senses 38 , 175–186 (2013).
Article PubMed Google Scholar
Lawless, H. T. & Heymann, H. Sensory evaluation of food: Principles and practices. (Springer, New York, NY). https://doi.org/10.1007/978-1-4419-6488-5 (2010).
Colantonio, V. et al. Metabolomic selection for enhanced fruit flavor. Proc. Natl. Acad. Sci. 119 , e2115865119 (2022).
Fritz, F., Preissner, R. & Banerjee, P. VirtualTaste: a web server for the prediction of organoleptic properties of chemical compounds. Nucleic Acids Res 49 , W679–W684 (2021).
Tuwani, R., Wadhwa, S. & Bagler, G. BitterSweet: Building machine learning models for predicting the bitter and sweet taste of small molecules. Sci. Rep. 9 , 1–13 (2019).
Dagan-Wiener, A. et al. Bitter or not? BitterPredict, a tool for predicting taste from chemical structure. Sci. Rep. 7 , 1–13 (2017).
Pallante, L. et al. Toward a general and interpretable umami taste predictor using a multi-objective machine learning approach. Sci. Rep. 12 , 1–11 (2022).
Malavolta, M. et al. A survey on computational taste predictors. Eur. Food Res. Technol. 248 , 2215–2235 (2022).
Lee, B. K. et al. A principal odor map unifies diverse tasks in olfactory perception. Science 381 , 999–1006 (2023).
Mayhew, E. J. et al. Transport features predict if a molecule is odorous. Proc. Natl. Acad. Sci. 119 , e2116576119 (2022).
Niu, Y. et al. Sensory evaluation of the synergism among ester odorants in light aroma-type liquor by odor threshold, aroma intensity and flash GC electronic nose. Food Res. Int. 113 , 102–114 (2018).
Yu, P., Low, M. Y. & Zhou, W. Design of experiments and regression modelling in food flavour and sensory analysis: A review. Trends Food Sci. Technol. 71 , 202–215 (2018).
Oladokun, O. et al. The impact of hop bitter acid and polyphenol profiles on the perceived bitterness of beer. Food Chem. 205 , 212–220 (2016).
Linforth, R., Cabannes, M., Hewson, L., Yang, N. & Taylor, A. Effect of fat content on flavor delivery during consumption: An in vivo model. J. Agric. Food Chem. 58 , 6905–6911 (2010).
Guo, S., Na Jom, K. & Ge, Y. Influence of roasting condition on flavor profile of sunflower seeds: A flavoromics approach. Sci. Rep. 9 , 11295 (2019).
Ren, Q. et al. The changes of microbial community and flavor compound in the fermentation process of Chinese rice wine using Fagopyrum tataricum grain as feedstock. Sci. Rep. 9 , 3365 (2019).
Hastie, T., Friedman, J. & Tibshirani, R. The Elements of Statistical Learning. (Springer, New York, NY). https://doi.org/10.1007/978-0-387-21606-5 (2001).
Dietz, C., Cook, D., Huismann, M., Wilson, C. & Ford, R. The multisensory perception of hop essential oil: a review. J. Inst. Brew. 126 , 320–342 (2020).
CAS Google Scholar
Roncoroni, Miguel & Verstrepen, Kevin Joan. Belgian Beer: Tested and Tasted. (Lannoo, 2018).
Meilgaard, M. Flavor chemistry of beer: Part II: Flavor and threshold of 239 aroma volatiles. in (1975).
Bokulich, N. A. & Bamforth, C. W. The microbiology of malting and brewing. Microbiol. Mol. Biol. Rev. MMBR 77 , 157–172 (2013).
Dzialo, M. C., Park, R., Steensels, J., Lievens, B. & Verstrepen, K. J. Physiology, ecology and industrial applications of aroma formation in yeast. FEMS Microbiol. Rev. 41 , S95–S128 (2017).
Article PubMed PubMed Central Google Scholar
Datta, A. et al. Computer-aided food engineering. Nat. Food 3 , 894–904 (2022).
American Society of Brewing Chemists. Beer Methods. (American Society of Brewing Chemists, St. Paul, MN, U.S.A.).
Olaniran, A. O., Hiralal, L., Mokoena, M. P. & Pillay, B. Flavour-active volatile compounds in beer: production, regulation and control. J. Inst. Brew. 123 , 13–23 (2017).
Verstrepen, K. J. et al. Flavor-active esters: Adding fruitiness to beer. J. Biosci. Bioeng. 96 , 110–118 (2003).
Meilgaard, M. C. Flavour chemistry of beer. part I: flavour interaction between principal volatiles. Master Brew. Assoc. Am. Tech. Q 12 , 107–117 (1975).
Briggs, D. E., Boulton, C. A., Brookes, P. A. & Stevens, R. Brewing 227–254. (Woodhead Publishing). https://doi.org/10.1533/9781855739062.227 (2004).
Bossaert, S., Crauwels, S., De Rouck, G. & Lievens, B. The power of sour - A review: Old traditions, new opportunities. BrewingScience 72 , 78–88 (2019).
Google Scholar
Verstrepen, K. J. et al. Flavor active esters: Adding fruitiness to beer. J. Biosci. Bioeng. 96 , 110–118 (2003).
Snauwaert, I. et al. Microbial diversity and metabolite composition of Belgian red-brown acidic ales. Int. J. Food Microbiol. 221 , 1–11 (2016).
Spitaels, F. et al. The microbial diversity of traditional spontaneously fermented lambic beer. PLoS ONE 9 , e95384 (2014).
Blanco, C. A., Andrés-Iglesias, C. & Montero, O. Low-alcohol Beers: Flavor Compounds, Defects, and Improvement Strategies. Crit. Rev. Food Sci. Nutr. 56 , 1379–1388 (2016).
Jackowski, M. & Trusek, A. Non-Alcohol. beer Prod. – Overv. 20 , 32–38 (2018).
Takoi, K. et al. The contribution of geraniol metabolism to the citrus flavour of beer: Synergy of geraniol and β-citronellol under coexistence with excess linalool. J. Inst. Brew. 116 , 251–260 (2010).
Kroeze, J. H. & Bartoshuk, L. M. Bitterness suppression as revealed by split-tongue taste stimulation in humans. Physiol. Behav. 35 , 779–783 (1985).
Mennella, J. A. et al. A spoonful of sugar helps the medicine go down”: Bitter masking bysucrose among children and adults. Chem. Senses 40 , 17–25 (2015).
Wietstock, P., Kunz, T., Perreira, F. & Methner, F.-J. Metal chelation behavior of hop acids in buffered model systems. BrewingScience 69 , 56–63 (2016).
Sancho, D., Blanco, C. A., Caballero, I. & Pascual, A. Free iron in pale, dark and alcohol-free commercial lager beers. J. Sci. Food Agric. 91 , 1142–1147 (2011).
Rodrigues, H. & Parr, W. V. Contribution of cross-cultural studies to understanding wine appreciation: A review. Food Res. Int. 115 , 251–258 (2019).
Korneva, E. & Blockeel, H. Towards better evaluation of multi-target regression models. in ECML PKDD 2020 Workshops (eds. Koprinska, I. et al.) 353–362 (Springer International Publishing, Cham, 2020). https://doi.org/10.1007/978-3-030-65965-3_23 .
Gastón Ares. Mathematical and Statistical Methods in Food Science and Technology. (Wiley, 2013).
Grinsztajn, L., Oyallon, E. & Varoquaux, G. Why do tree-based models still outperform deep learning on tabular data? Preprint at http://arxiv.org/abs/2207.08815 (2022).
Gries, S. T. Statistics for Linguistics with R: A Practical Introduction. in Statistics for Linguistics with R (De Gruyter Mouton, 2021). https://doi.org/10.1515/9783110718256 .
Lundberg, S. M. et al. From local explanations to global understanding with explainable AI for trees. Nat. Mach. Intell. 2 , 56–67 (2020).
Ickes, C. M. & Cadwallader, K. R. Effects of ethanol on flavor perception in alcoholic beverages. Chemosens. Percept. 10 , 119–134 (2017).
Kato, M. et al. Influence of high molecular weight polypeptides on the mouthfeel of commercial beer. J. Inst. Brew. 127 , 27–40 (2021).
Wauters, R. et al. Novel Saccharomyces cerevisiae variants slow down the accumulation of staling aldehydes and improve beer shelf-life. Food Chem. 398 , 1–11 (2023).
Li, H., Jia, S. & Zhang, W. Rapid determination of low-level sulfur compounds in beer by headspace gas chromatography with a pulsed flame photometric detector. J. Am. Soc. Brew. Chem. 66 , 188–191 (2008).
Dercksen, A., Laurens, J., Torline, P., Axcell, B. C. & Rohwer, E. Quantitative analysis of volatile sulfur compounds in beer using a membrane extraction interface. J. Am. Soc. Brew. Chem. 54 , 228–233 (1996).
Molnar, C. Interpretable Machine Learning: A Guide for Making Black-Box Models Interpretable. (2020).
Zhao, Q. & Hastie, T. Causal interpretations of black-box models. J. Bus. Econ. Stat. Publ. Am. Stat. Assoc. 39 , 272–281 (2019).
Article MathSciNet Google Scholar
Hastie, T., Tibshirani, R. & Friedman, J. The Elements of Statistical Learning. (Springer, 2019).
Labrado, D. et al. Identification by NMR of key compounds present in beer distillates and residual phases after dealcoholization by vacuum distillation. J. Sci. Food Agric. 100 , 3971–3978 (2020).
Lusk, L. T., Kay, S. B., Porubcan, A. & Ryder, D. S. Key olfactory cues for beer oxidation. J. Am. Soc. Brew. Chem. 70 , 257–261 (2012).
Gonzalez Viejo, C., Torrico, D. D., Dunshea, F. R. & Fuentes, S. Development of artificial neural network models to assess beer acceptability based on sensory properties using a robotic pourer: A comparative model approach to achieve an artificial intelligence system. Beverages 5 , 33 (2019).
Gonzalez Viejo, C., Fuentes, S., Torrico, D. D., Godbole, A. & Dunshea, F. R. Chemical characterization of aromas in beer and their effect on consumers liking. Food Chem. 293 , 479–485 (2019).
Gilbert, J. L. et al. Identifying breeding priorities for blueberry flavor using biochemical, sensory, and genotype by environment analyses. PLOS ONE 10 , 1–21 (2015).
Goulet, C. et al. Role of an esterase in flavor volatile variation within the tomato clade. Proc. Natl. Acad. Sci. 109 , 19009–19014 (2012).
Article ADS CAS PubMed PubMed Central Google Scholar
Borisov, V. et al. Deep Neural Networks and Tabular Data: A Survey. IEEE Trans. Neural Netw. Learn. Syst. 1–21 https://doi.org/10.1109/TNNLS.2022.3229161 (2022).
Statista. Statista Consumer Market Outlook: Beer - Worldwide.
Seitz, H. K. & Stickel, F. Molecular mechanisms of alcoholmediated carcinogenesis. Nat. Rev. Cancer 7 , 599–612 (2007).
Voordeckers, K. et al. Ethanol exposure increases mutation rate through error-prone polymerases. Nat. Commun. 11 , 3664 (2020).
Goelen, T. et al. Bacterial phylogeny predicts volatile organic compound composition and olfactory response of an aphid parasitoid. Oikos 129 , 1415–1428 (2020).
Article ADS Google Scholar
Reher, T. et al. Evaluation of hop (Humulus lupulus) as a repellent for the management of Drosophila suzukii. Crop Prot. 124 , 104839 (2019).
Stein, S. E. An integrated method for spectrum extraction and compound identification from gas chromatography/mass spectrometry data. J. Am. Soc. Mass Spectrom. 10 , 770–781 (1999).
American Society of Brewing Chemists. Sensory Analysis Methods. (American Society of Brewing Chemists, St. Paul, MN, U.S.A., 1992).
McAuley, J., Leskovec, J. & Jurafsky, D. Learning Attitudes and Attributes from Multi-Aspect Reviews. Preprint at https://doi.org/10.48550/arXiv.1210.3926 (2012).
Meilgaard, M. C., Carr, B. T. & Carr, B. T. Sensory Evaluation Techniques. (CRC Press, Boca Raton). https://doi.org/10.1201/b16452 (2014).
Schreurs, M. et al. Data from: Predicting and improving complex beer flavor through machine learning. Zenodo https://doi.org/10.5281/zenodo.10653704 (2024).
Download references
Acknowledgements
We thank all lab members for their discussions and thank all tasting panel members for their contributions. Special thanks go out to Dr. Karin Voordeckers for her tremendous help in proofreading and improving the manuscript. M.S. was supported by a Baillet-Latour fellowship, L.C. acknowledges financial support from KU Leuven (C16/17/006), F.A.T. was supported by a PhD fellowship from FWO (1S08821N). Research in the lab of K.J.V. is supported by KU Leuven, FWO, VIB, VLAIO and the Brewing Science Serves Health Fund. Research in the lab of T.W. is supported by FWO (G.0A51.15) and KU Leuven (C16/17/006).
Author information
These authors contributed equally: Michiel Schreurs, Supinya Piampongsant, Miguel Roncoroni.
Authors and Affiliations
VIB—KU Leuven Center for Microbiology, Gaston Geenslaan 1, B-3001, Leuven, Belgium
Michiel Schreurs, Supinya Piampongsant, Miguel Roncoroni, Lloyd Cool, Beatriz Herrera-Malaver, Florian A. Theßeling & Kevin J. Verstrepen
CMPG Laboratory of Genetics and Genomics, KU Leuven, Gaston Geenslaan 1, B-3001, Leuven, Belgium
Leuven Institute for Beer Research (LIBR), Gaston Geenslaan 1, B-3001, Leuven, Belgium
Laboratory of Socioecology and Social Evolution, KU Leuven, Naamsestraat 59, B-3000, Leuven, Belgium
Lloyd Cool, Christophe Vanderaa & Tom Wenseleers
VIB Bioinformatics Core, VIB, Rijvisschestraat 120, B-9052, Ghent, Belgium
Łukasz Kreft & Alexander Botzki
AB InBev SA/NV, Brouwerijplein 1, B-3000, Leuven, Belgium
Philippe Malcorps & Luk Daenen
You can also search for this author in PubMed Google Scholar
Contributions
S.P., M.S. and K.J.V. conceived the experiments. S.P., M.S. and K.J.V. designed the experiments. S.P., M.S., M.R., B.H. and F.A.T. performed the experiments. S.P., M.S., L.C., C.V., L.K., A.B., P.M., L.D., T.W. and K.J.V. contributed analysis ideas. S.P., M.S., L.C., C.V., T.W. and K.J.V. analyzed the data. All authors contributed to writing the manuscript.
Corresponding author
Correspondence to Kevin J. Verstrepen .
Ethics declarations
Competing interests.
K.J.V. is affiliated with bar.on. The other authors declare no competing interests.
Peer review
Peer review information.
Nature Communications thanks Florian Bauer, Andrew John Macintosh and the other, anonymous, reviewer(s) for their contribution to the peer review of this work. A peer review file is available.
Additional information
Publisher’s note Springer Nature remains neutral with regard to jurisdictional claims in published maps and institutional affiliations.
Supplementary information
Supplementary information, peer review file, description of additional supplementary files, supplementary data 1, supplementary data 2, supplementary data 3, supplementary data 4, supplementary data 5, supplementary data 6, supplementary data 7, reporting summary, source data, source data, rights and permissions.
Open Access This article is licensed under a Creative Commons Attribution 4.0 International License, which permits use, sharing, adaptation, distribution and reproduction in any medium or format, as long as you give appropriate credit to the original author(s) and the source, provide a link to the Creative Commons licence, and indicate if changes were made. The images or other third party material in this article are included in the article’s Creative Commons licence, unless indicated otherwise in a credit line to the material. If material is not included in the article’s Creative Commons licence and your intended use is not permitted by statutory regulation or exceeds the permitted use, you will need to obtain permission directly from the copyright holder. To view a copy of this licence, visit http://creativecommons.org/licenses/by/4.0/ .
Reprints and permissions
About this article
Cite this article.
Schreurs, M., Piampongsant, S., Roncoroni, M. et al. Predicting and improving complex beer flavor through machine learning. Nat Commun 15 , 2368 (2024). https://doi.org/10.1038/s41467-024-46346-0
Download citation
Received : 30 October 2023
Accepted : 21 February 2024
Published : 26 March 2024
DOI : https://doi.org/10.1038/s41467-024-46346-0
Share this article
Anyone you share the following link with will be able to read this content:
Sorry, a shareable link is not currently available for this article.
Provided by the Springer Nature SharedIt content-sharing initiative
By submitting a comment you agree to abide by our Terms and Community Guidelines . If you find something abusive or that does not comply with our terms or guidelines please flag it as inappropriate.
Quick links
- Explore articles by subject
- Guide to authors
- Editorial policies
Sign up for the Nature Briefing: Translational Research newsletter — top stories in biotechnology, drug discovery and pharma.

- USC Libraries
- Research Guides
Organizing Your Social Sciences Research Paper
- Quantitative Methods
- Purpose of Guide
- Design Flaws to Avoid
- Independent and Dependent Variables
- Glossary of Research Terms
- Reading Research Effectively
- Narrowing a Topic Idea
- Broadening a Topic Idea
- Extending the Timeliness of a Topic Idea
- Academic Writing Style
- Choosing a Title
- Making an Outline
- Paragraph Development
- Research Process Video Series
- Executive Summary
- The C.A.R.S. Model
- Background Information
- The Research Problem/Question
- Theoretical Framework
- Citation Tracking
- Content Alert Services
- Evaluating Sources
- Primary Sources
- Secondary Sources
- Tiertiary Sources
- Scholarly vs. Popular Publications
- Qualitative Methods
- Insiderness
- Using Non-Textual Elements
- Limitations of the Study
- Common Grammar Mistakes
- Writing Concisely
- Avoiding Plagiarism
- Footnotes or Endnotes?
- Further Readings
- Generative AI and Writing
- USC Libraries Tutorials and Other Guides
- Bibliography
Quantitative methods emphasize objective measurements and the statistical, mathematical, or numerical analysis of data collected through polls, questionnaires, and surveys, or by manipulating pre-existing statistical data using computational techniques . Quantitative research focuses on gathering numerical data and generalizing it across groups of people or to explain a particular phenomenon.
Babbie, Earl R. The Practice of Social Research . 12th ed. Belmont, CA: Wadsworth Cengage, 2010; Muijs, Daniel. Doing Quantitative Research in Education with SPSS . 2nd edition. London: SAGE Publications, 2010.
Need Help Locating Statistics?
Resources for locating data and statistics can be found here:
Statistics & Data Research Guide
Characteristics of Quantitative Research
Your goal in conducting quantitative research study is to determine the relationship between one thing [an independent variable] and another [a dependent or outcome variable] within a population. Quantitative research designs are either descriptive [subjects usually measured once] or experimental [subjects measured before and after a treatment]. A descriptive study establishes only associations between variables; an experimental study establishes causality.
Quantitative research deals in numbers, logic, and an objective stance. Quantitative research focuses on numeric and unchanging data and detailed, convergent reasoning rather than divergent reasoning [i.e., the generation of a variety of ideas about a research problem in a spontaneous, free-flowing manner].
Its main characteristics are :
- The data is usually gathered using structured research instruments.
- The results are based on larger sample sizes that are representative of the population.
- The research study can usually be replicated or repeated, given its high reliability.
- Researcher has a clearly defined research question to which objective answers are sought.
- All aspects of the study are carefully designed before data is collected.
- Data are in the form of numbers and statistics, often arranged in tables, charts, figures, or other non-textual forms.
- Project can be used to generalize concepts more widely, predict future results, or investigate causal relationships.
- Researcher uses tools, such as questionnaires or computer software, to collect numerical data.
The overarching aim of a quantitative research study is to classify features, count them, and construct statistical models in an attempt to explain what is observed.
Things to keep in mind when reporting the results of a study using quantitative methods :
- Explain the data collected and their statistical treatment as well as all relevant results in relation to the research problem you are investigating. Interpretation of results is not appropriate in this section.
- Report unanticipated events that occurred during your data collection. Explain how the actual analysis differs from the planned analysis. Explain your handling of missing data and why any missing data does not undermine the validity of your analysis.
- Explain the techniques you used to "clean" your data set.
- Choose a minimally sufficient statistical procedure ; provide a rationale for its use and a reference for it. Specify any computer programs used.
- Describe the assumptions for each procedure and the steps you took to ensure that they were not violated.
- When using inferential statistics , provide the descriptive statistics, confidence intervals, and sample sizes for each variable as well as the value of the test statistic, its direction, the degrees of freedom, and the significance level [report the actual p value].
- Avoid inferring causality , particularly in nonrandomized designs or without further experimentation.
- Use tables to provide exact values ; use figures to convey global effects. Keep figures small in size; include graphic representations of confidence intervals whenever possible.
- Always tell the reader what to look for in tables and figures .
NOTE: When using pre-existing statistical data gathered and made available by anyone other than yourself [e.g., government agency], you still must report on the methods that were used to gather the data and describe any missing data that exists and, if there is any, provide a clear explanation why the missing data does not undermine the validity of your final analysis.
Babbie, Earl R. The Practice of Social Research . 12th ed. Belmont, CA: Wadsworth Cengage, 2010; Brians, Craig Leonard et al. Empirical Political Analysis: Quantitative and Qualitative Research Methods . 8th ed. Boston, MA: Longman, 2011; McNabb, David E. Research Methods in Public Administration and Nonprofit Management: Quantitative and Qualitative Approaches . 2nd ed. Armonk, NY: M.E. Sharpe, 2008; Quantitative Research Methods. Writing@CSU. Colorado State University; Singh, Kultar. Quantitative Social Research Methods . Los Angeles, CA: Sage, 2007.
Basic Research Design for Quantitative Studies
Before designing a quantitative research study, you must decide whether it will be descriptive or experimental because this will dictate how you gather, analyze, and interpret the results. A descriptive study is governed by the following rules: subjects are generally measured once; the intention is to only establish associations between variables; and, the study may include a sample population of hundreds or thousands of subjects to ensure that a valid estimate of a generalized relationship between variables has been obtained. An experimental design includes subjects measured before and after a particular treatment, the sample population may be very small and purposefully chosen, and it is intended to establish causality between variables. Introduction The introduction to a quantitative study is usually written in the present tense and from the third person point of view. It covers the following information:
- Identifies the research problem -- as with any academic study, you must state clearly and concisely the research problem being investigated.
- Reviews the literature -- review scholarship on the topic, synthesizing key themes and, if necessary, noting studies that have used similar methods of inquiry and analysis. Note where key gaps exist and how your study helps to fill these gaps or clarifies existing knowledge.
- Describes the theoretical framework -- provide an outline of the theory or hypothesis underpinning your study. If necessary, define unfamiliar or complex terms, concepts, or ideas and provide the appropriate background information to place the research problem in proper context [e.g., historical, cultural, economic, etc.].
Methodology The methods section of a quantitative study should describe how each objective of your study will be achieved. Be sure to provide enough detail to enable the reader can make an informed assessment of the methods being used to obtain results associated with the research problem. The methods section should be presented in the past tense.
- Study population and sampling -- where did the data come from; how robust is it; note where gaps exist or what was excluded. Note the procedures used for their selection;
- Data collection – describe the tools and methods used to collect information and identify the variables being measured; describe the methods used to obtain the data; and, note if the data was pre-existing [i.e., government data] or you gathered it yourself. If you gathered it yourself, describe what type of instrument you used and why. Note that no data set is perfect--describe any limitations in methods of gathering data.
- Data analysis -- describe the procedures for processing and analyzing the data. If appropriate, describe the specific instruments of analysis used to study each research objective, including mathematical techniques and the type of computer software used to manipulate the data.
Results The finding of your study should be written objectively and in a succinct and precise format. In quantitative studies, it is common to use graphs, tables, charts, and other non-textual elements to help the reader understand the data. Make sure that non-textual elements do not stand in isolation from the text but are being used to supplement the overall description of the results and to help clarify key points being made. Further information about how to effectively present data using charts and graphs can be found here .
- Statistical analysis -- how did you analyze the data? What were the key findings from the data? The findings should be present in a logical, sequential order. Describe but do not interpret these trends or negative results; save that for the discussion section. The results should be presented in the past tense.
Discussion Discussions should be analytic, logical, and comprehensive. The discussion should meld together your findings in relation to those identified in the literature review, and placed within the context of the theoretical framework underpinning the study. The discussion should be presented in the present tense.
- Interpretation of results -- reiterate the research problem being investigated and compare and contrast the findings with the research questions underlying the study. Did they affirm predicted outcomes or did the data refute it?
- Description of trends, comparison of groups, or relationships among variables -- describe any trends that emerged from your analysis and explain all unanticipated and statistical insignificant findings.
- Discussion of implications – what is the meaning of your results? Highlight key findings based on the overall results and note findings that you believe are important. How have the results helped fill gaps in understanding the research problem?
- Limitations -- describe any limitations or unavoidable bias in your study and, if necessary, note why these limitations did not inhibit effective interpretation of the results.
Conclusion End your study by to summarizing the topic and provide a final comment and assessment of the study.
- Summary of findings – synthesize the answers to your research questions. Do not report any statistical data here; just provide a narrative summary of the key findings and describe what was learned that you did not know before conducting the study.
- Recommendations – if appropriate to the aim of the assignment, tie key findings with policy recommendations or actions to be taken in practice.
- Future research – note the need for future research linked to your study’s limitations or to any remaining gaps in the literature that were not addressed in your study.
Black, Thomas R. Doing Quantitative Research in the Social Sciences: An Integrated Approach to Research Design, Measurement and Statistics . London: Sage, 1999; Gay,L. R. and Peter Airasain. Educational Research: Competencies for Analysis and Applications . 7th edition. Upper Saddle River, NJ: Merril Prentice Hall, 2003; Hector, Anestine. An Overview of Quantitative Research in Composition and TESOL . Department of English, Indiana University of Pennsylvania; Hopkins, Will G. “Quantitative Research Design.” Sportscience 4, 1 (2000); "A Strategy for Writing Up Research Results. The Structure, Format, Content, and Style of a Journal-Style Scientific Paper." Department of Biology. Bates College; Nenty, H. Johnson. "Writing a Quantitative Research Thesis." International Journal of Educational Science 1 (2009): 19-32; Ouyang, Ronghua (John). Basic Inquiry of Quantitative Research . Kennesaw State University.
Strengths of Using Quantitative Methods
Quantitative researchers try to recognize and isolate specific variables contained within the study framework, seek correlation, relationships and causality, and attempt to control the environment in which the data is collected to avoid the risk of variables, other than the one being studied, accounting for the relationships identified.
Among the specific strengths of using quantitative methods to study social science research problems:
- Allows for a broader study, involving a greater number of subjects, and enhancing the generalization of the results;
- Allows for greater objectivity and accuracy of results. Generally, quantitative methods are designed to provide summaries of data that support generalizations about the phenomenon under study. In order to accomplish this, quantitative research usually involves few variables and many cases, and employs prescribed procedures to ensure validity and reliability;
- Applying well established standards means that the research can be replicated, and then analyzed and compared with similar studies;
- You can summarize vast sources of information and make comparisons across categories and over time; and,
- Personal bias can be avoided by keeping a 'distance' from participating subjects and using accepted computational techniques .
Babbie, Earl R. The Practice of Social Research . 12th ed. Belmont, CA: Wadsworth Cengage, 2010; Brians, Craig Leonard et al. Empirical Political Analysis: Quantitative and Qualitative Research Methods . 8th ed. Boston, MA: Longman, 2011; McNabb, David E. Research Methods in Public Administration and Nonprofit Management: Quantitative and Qualitative Approaches . 2nd ed. Armonk, NY: M.E. Sharpe, 2008; Singh, Kultar. Quantitative Social Research Methods . Los Angeles, CA: Sage, 2007.
Limitations of Using Quantitative Methods
Quantitative methods presume to have an objective approach to studying research problems, where data is controlled and measured, to address the accumulation of facts, and to determine the causes of behavior. As a consequence, the results of quantitative research may be statistically significant but are often humanly insignificant.
Some specific limitations associated with using quantitative methods to study research problems in the social sciences include:
- Quantitative data is more efficient and able to test hypotheses, but may miss contextual detail;
- Uses a static and rigid approach and so employs an inflexible process of discovery;
- The development of standard questions by researchers can lead to "structural bias" and false representation, where the data actually reflects the view of the researcher instead of the participating subject;
- Results provide less detail on behavior, attitudes, and motivation;
- Researcher may collect a much narrower and sometimes superficial dataset;
- Results are limited as they provide numerical descriptions rather than detailed narrative and generally provide less elaborate accounts of human perception;
- The research is often carried out in an unnatural, artificial environment so that a level of control can be applied to the exercise. This level of control might not normally be in place in the real world thus yielding "laboratory results" as opposed to "real world results"; and,
- Preset answers will not necessarily reflect how people really feel about a subject and, in some cases, might just be the closest match to the preconceived hypothesis.
Research Tip
Finding Examples of How to Apply Different Types of Research Methods
SAGE publications is a major publisher of studies about how to design and conduct research in the social and behavioral sciences. Their SAGE Research Methods Online and Cases database includes contents from books, articles, encyclopedias, handbooks, and videos covering social science research design and methods including the complete Little Green Book Series of Quantitative Applications in the Social Sciences and the Little Blue Book Series of Qualitative Research techniques. The database also includes case studies outlining the research methods used in real research projects. This is an excellent source for finding definitions of key terms and descriptions of research design and practice, techniques of data gathering, analysis, and reporting, and information about theories of research [e.g., grounded theory]. The database covers both qualitative and quantitative research methods as well as mixed methods approaches to conducting research.
SAGE Research Methods Online and Cases
- << Previous: Qualitative Methods
- Next: Insiderness >>
- Last Updated: Mar 26, 2024 10:40 AM
- URL: https://libguides.usc.edu/writingguide
Identification and validation of tryptophan metabolism-related lncRNAs in lung adenocarcinoma prognosis and immune response
- Open access
- Published: 01 April 2024
- Volume 150 , article number 171 , ( 2024 )
Cite this article
You have full access to this open access article
- Mingjun Gao 1 na1 ,
- Mengmeng Wang 1 na1 ,
- Yong Chen 1 ,
- Siding Zhou 2 ,
- Wenbo He 2 ,
- Yusheng Shu 3 &
- Xiaolin Wang 3
Tryptophan (Trp) is an essential amino acid. Increasing evidence suggests that tryptophan metabolism plays a complex role in immune escape from Lung adenocarcinoma (LUAD). However, the role of long non-coding RNAs (lncRNAs) in tryptophan metabolism remains to be investigated.
This study uses The Cancer Genome Atlas (TCGA)-LUAD dataset as the training cohort, and several datasets from the Gene Expression Omnibus (GEO) database are merged into the validation cohort. Genes related to tryptophan metabolism were identified from the Molecular Signatures Database (MSigDB) database and further screened for lncRNAs with Trp-related expression. Subsequently, a prognostic signature of lncRNAs related to tryptophan metabolism was constructed using Cox regression analysis, (Least absolute shrinkage and selection operator regression) and LASSO analysis. The predictive performance of this risk score was validated by Kaplan–Meier (KM) survival analysis, (receiver operating characteristic) ROC curves, and nomograms. We also explored the differences in immune cell infiltration, immune cell function, tumor mutational load (TMB), tumor immune dysfunction and exclusion (TIDE), and anticancer drug sensitivity between high- and low-risk groups. Finally, we used real-time fluorescence quantitative PCR, CCK-8, colony formation, wound healing, transwell, flow cytometry, and nude mouse xenotransplantation models to elucidate the role of ZNF8-ERVK3-1 in LUAD.
We constructed 16 tryptophan metabolism-associated lncRNA prognostic models in LUAD patients. The risk score could be used as an independent prognostic indicator for the prognosis of LUAD patients. Kaplan–Meier survival analysis, ROC curves, and risk maps validated the prognostic value of the risk score. The high-risk and low-risk groups showed significant differences in phenotypes, such as the percentage of immune cell infiltration, immune cell function, gene mutation frequency, and anticancer drug sensitivity. In addition, patients with high-risk scores had higher TMB and TIDE scores compared to patients with low-risk scores. Finally, we found that ZNF8-ERVK3-1 was highly expressed in LUAD tissues and cell lines. A series of in vitro experiments showed that knockdown of ZNF8-ERVK3-1 inhibited cell proliferation, migration, and invasion, leading to cell cycle arrest in the G0/G1 phase and increased apoptosis. In vivo experiments with xenografts have shown that knocking down ZNF8-ERVK3-1 can significantly inhibit tumor size and tumor proliferation.
We constructed a new prognostic model for tryptophan metabolism-related lncRNA. The risk score was closely associated with common clinical features such as immune cell infiltration, immune-related function, TMB, and anticancer drug sensitivity. Knockdown of ZNF8-ERVK3-1 inhibited LUAD cell proliferation, migration, invasion, and G0/G1 phase blockade and promoted apoptosis.
Avoid common mistakes on your manuscript.
Lung cancer (LC) is one of the most common malignancies worldwide, and lung adenocarcinoma is the most common pathological type, accounting for nearly 40% of all lung cancer subtypes (Sung et al. 2021 ; Lortet-Tieulent et al. 2014 ). In recent decades, the development of molecularly targeted therapies and immune checkpoint inhibitors (ICIs) has improved the survival of LUAD patients. However, LUAD survival remains poor, with a 5-year survival rate of less than 20% (Hirsch et al. 2017 ). Therefore, developing an effective prognostic model to predict LUAD prognosis is crucial.
Tryptophan is an essential amino acid. Increasing evidence suggests that tryptophan metabolism is involved in tumor development through various mechanisms. Trp metabolism has a complex and multifaceted role in immune escape from LC cells and cancer-associated cells (Godin-Ethier et al. 2011 ). Trp is degraded via the kynurenine (KYN) pathway and metabolized to form serotonin or other metabolites (Schwarcz and Stone 2017 ). Indoleamine 2,3-dioxygenase 1 (IDO1) and tryptophan 2,3-dioxygenase (TDO) catalyze the first and rate-limiting steps of tryptophan metabolism (Cervenka et al. 2017 ). IDO1 inhibitors have been used in clinical trials for cancer immunotherapy and have emerged as a critical target for tumor immunotherapy. IDO1 blockers are used with ICIs to inhibit tumor growth (Yentz and Smith 2018 ). The IDO1 inhibitor, Epacadostat, shows potent anti-IDO1 activity by promoting T-cell activation and inhibiting regulatory T-cell function (Komiya and Huang 2018 ; Jochems et al. 2016 ). Recent studies have shown that IDO1-associated Try metabolites are strongly associated with the development of lung cancer (Yoshida et al. 1981 ). It has been demonstrated that increased IDO1 activity was detected in lung cancer patients with recurrent metastases after receiving immunotherapy (Agulló-Ortuño et al. 2020 ). LC cells with high expression of IDO1 have enhanced invasive ability in vitro and distant metastasis to the brain, liver, and bone in vivo, whereas IDO1 inhibition attenuates their invasion and distant metastasis (Tang et al. 2017 ). Similarly, IDO1 inhibition suppresses lung metastasis in breast cancer (Smith et al. 2012 ; Levina et al. 2012 ). Tryptophan metabolism is a key target for tumor immunotherapy. Therefore, a comprehensive analysis of the Trp pathway may improve survival and provide a potential strategy for precise treatment of LUAD patients.
lncRNAs are a class of RNA molecules with transcripts longer than 200 nt (Volders et al. 2019 ). In many cancers, lncRNAs are aberrantly expressed and regulate biological properties such as cell proliferation, cell invasion, and cell cycle (Chen et al. 2017 ). Various studies have shown that lncRNAs are implicated in tumorigenesis, disease progression, and drug resistance in LUAD (Feng et al. 2021 ; Chen et al. 2021 ; Yu et al. 2020 ). Down-regulation of the lncRNA LHFPL3-AS2 reduces its specific interaction with SFPQ, leading to more SFPQ binding to the TXNIP promoter, causing transcriptional repression of TXNIP, which ultimately promotes metastasis in non-small cell lung cancer (Cheng et al. 2022 ). In addition, M2 macrophage-derived exosomal lncRNA AGAP2-AS1 enhances immunity to radiotherapy in lung cancer by decreasing miR-296 and elevating NOTCH2 (Zhang et al. 2021 ).
Currently, studies on the role of Trp metabolism-associated lncRNA genes and LUAD patients still need to be made available. Therefore, potential Trp metabolism-associated lncRNAs were mined based on the TCGA-LUAD cohort to construct a risk model for the prognosis of LUAD patients. The applicability of this risk profile has been validated in the GEO cohort. The mechanism of Trp metabolism-associated lncRNAs in lung adenocarcinoma and TME heterogeneity were explored by functional enrichment and immune infiltration analyses. The risk model also helped to differentiate the response of LUAD patients to targeted therapeutic and chemotherapeutic agents. Finally, we also preliminarily verified the mechanism by which ZNF8-ERVK3-1 promotes lung adenocarcinoma development by in vitro cellular experiments. In this study, we attempted to elucidate the clinical value of Trp metabolism-associated lncRNAs in LUAD patients using bioinformatics analysis to provide theoretical support for individualized treatment of LUAD patients.
Data acquisition
RNA sequencing data and corresponding clinical information were extracted from the TCGA database for 516 patients with LUAD. 9 patients were excluded due to lack of available survival information, and 507 patients with LUAD were included for further analysis. Ensembl IDs were then converted to official gene symbols, and the data were log2 processed. Mutation data for the included LUAD patients were also obtained from TCGA. These patients were randomly divided into a training group ( n = 253) and a test group ( n = 254) in a 1:1 ratio using the "caret" R package (Kuhn 2008 ). No statistically significant difference was found in clinical features between the training group and the test group ( p > 0.05).
lncRNAs and mRNAs were isolated by sorting the downloaded transcriptome data using Perl software. Ethics committee approval was not required as the clinical information of the patients participating in this study was obtained from the TCGA database, and the TCGA publication guidelines were strictly adhered to. We included four external datasets with lncRNA expression profiles and prognostic information to validate the models: the GSE30219 (85 LUAD samples), the GSE37745 (106 LUAD samples), the GSE50081 (127 LUAD samples), and the GSE31210 (226 LUAD samples). In the GEO database, all files were received from the platform with the GPL570 standard, and all data were log2 transformed. The software package "SVA" was used to run the algorithm cbind function to integrate the four datasets and the ComBat function to remove batch effects and normalize. A final cohort of 544 LUAD patients will be included in the GEO validation cohort.
Identification of tryptophan metabolism-related lncRNAs
We extracted the genes involved in the tryptophan metabolic pathway "KEGG tryptophan metabolism" from the MSigDB database ( https://www.gsea-msigdb.org/gsea/msigdb ) of Kyoto Encyclopedia of Genes and Genomes (KEGG) (Liberzon et al. 2015 ), and a total of 59 genes were extracted (Table S1 ). The association coefficients between lncRNAs and Trp-related genes were calculated using the R software "limma" package. The screening criteria were |cor|> 0.4 and P-value < 0.001. Sankey diagrams were obtained using the R package "galluvial".
Model construction
To identify lncRNA profiles associated with tryptophan metabolism, we first used univariate Cox analysis to screen for lncRNAs associated with LUAD overall survival. Then LASSO analysis was performed for further screening (Tibshirani 1997 ). Finally, multivariate Cox regression analysis was finalized, identifying of 16 lncRNAs associated with tryptophan metabolism for a more robust prognostic risk model. Patients in the training cohort were categorized into low-risk and high-risk groups based on the median risk score. The risk score was calculated using the following formula:
where exp lncRNAi is the relative expression of tryptophan metabolism-related lncRNAs and βi is the regression coefficient.
Risk assessment models
The test cohort and the whole cohort were used for model validation, and the risk score algorithm was used to calculate each person's risk score. Subjects were assigned to a high-risk group (risk score above threshold) or a low-risk group (risk score below threshold) based on a threshold (median risk score). Overall survival (OS) and progression-free survival (PFS) were analyzed using the Kaplan–Meier method. The PFS dataset was obtained from UCSC Xena ( http://xena.ucsc.edu/ ). Univariate and multivariate Cox regression analyses were used to investigate whether the risk model was an independent risk factor excluding other clinical characteristics (age, sex, and stage). Heatmaps of patient survival status and expression of lncRNAs based on risk scores were plotted using the pheatmap software package. The "timeROC" R software package was used to perform ROC analyses and area under the curve (AUC) calculations, and the c-index curves were plotted using the R software "survival", "rms", and "pec" packages to evaluate the predictive ability of prognostic characteristics. Principal component analysis (PCA) was used to assess the ability to group genome-wide, tryptophan metabolism genes, all tryptophan lncRNAs, and tryptophan lncRNA features of the risk model. Patients were categorized into stages I–II and III–IV to determine the suitability of risk profiles for patients with different stages of LUAD.
Construction of forecast line charts
Clinicopathological factors were combined with our constructed risk scores to construct column charts for predicting 1-, 3-, and 5-year OS rates in LUAD patients. The "rms" R package was used to construct predictive column charts and corresponding calibration curves. The closer the calibration curve is to the diagonal, the better the prognostic predictive performance of the column chart.
Validation of risk models
The risk score for each case in the GEO cohort was calculated using the formula used in the TCGA cohort. Using the median risk score from the TCGA cohort as a criterion for risk setting in the GEO cohort, we assigned 272 cases to the low-risk group. The remaining 272 cases were included in the high-risk group. The risk model was analyzed using a similar approach in the TCGA-LUAD training set to determine whether it was an independent prognostic factor in the validation cohort. The validity of predicting prognosis was validated using KM curves to assess survival outcomes in the risk group in the GEO validation dataset. The predictive power of prognostic features was calculated by ROC analysis. Univariate and multivariate Cox regression analyses were performed to investigate whether the risk model was an independent risk factor excluding other clinical characteristics (age, sex, and stage).
Functional enrichment analysis
Differentially expressed genes (DEGs) were analyzed between high- and low-risk groups using the "limma "R package, |log2FC|> 1 and False Discovery Rate (FDR) < 0.05.Tryptophan metabolism-associated lncRNAs were analyzed using the clusterProfiler package for Gene Ontology (GO) and KEGG enrichment (Kanehisa and Goto 2000 ). lncRNAs were analyzed for GO and KEGG enrichment.
Tumor mutational burden (TMB) analysis and immune-related functional analysis
We downloaded TMB-related data from the TCGA samples and analyzed the number of mutations in both subgroups of LUAD patients using the R package (Maftools package). We used the survival package to determine the difference between the survival of patients with high and low TMB. P -values < 0.05 were considered statistically significant. Immunological scores of LUAD patients were calculated by the ESTIMATE algorithm (Yoshihara et al. 2013 ). Score scores for 22 immune cell subtypes in each tumor sample were identified by CIBERSORT (cell type identification by estimating the relative subtypes of RNA transcripts) (Newman et al. 2015 ). The relative abundance of different immune cell types in the low and high-risk groups was quantified and assessed to compare and predict immune cell infiltration between the two groups. Correlation analysis of immune function was based on ssGSEA (Subramanian et al. 2005 ).
Predicting response to immunotherapy and chemotherapy
NSCLC immune dysfunction and rejection were obtained by TIDE ( http://tide.dfci.harvard.edu/ ). TIDE scores were analyzed using the "limma" and "ggpubr" R packages in the high- and low-risk groups. TIDE scores accurately predicted the efficacy of immunotherapeutic drugs received by patients (Jiang et al. 2018 ), with higher TIDE scores predicting poor response to immunotherapy. Higher TIDE scores predict poor response to immunotherapy.
The Genomics of Drug Sensitivity in Cancer (GDSC) is a public dataset containing information about drug sensitivity and molecular markers of drug response in cancer cells (Iorio et al. 2016 ). The "oncoPredict" package was used to predict the drug sensitivity of LUAD samples to various antineoplastic drugs (Maeser et al. 2021 ).
Tissue sample collection and lung adenocarcinoma cell line culture
All tissue samples were collected from the Department of Thoracic Surgery of the People's Hospital of Northern Jiangsu Province and approved by the hospital's Medical Ethics Committee. We obtained informed consent from each relevant patient before collection. Sixteen pairs of samples, including tumor tissue (T) and paired normal tissue (N), were obtained from patients with lung adenocarcinoma who underwent tumor resection between January 2019 and January 2022, and the pathological type of all LUAD cases was lung adenocarcinoma. All samples were stored at -80 °C. HBE, A549, H1975, H1299, and PC9 cell lines were obtained from the China Cell Resource Centre (Shanghai, China). Cells were cultured in RPMI 1640 (Solarbio) medium supplemented with 10% fetal bovine serum (Procell). Cells were incubated in a humidified incubator (Thermo Scientific, China) with 5% CO2, 37 °C. The cells were incubated in a humidified incubator (Thermo Scientific, China) with 5% CO2.
RNA extraction and quantitative real-time polymerase chain reaction (qRT-PCR)
RNA was extracted from tissues and cells using TRIzol reagent (Vazyme). We measured RNA concentration using a spectrophotometer and stored the samples at – 80 °C cDNA was synthesized using the Hifair®III 1st Strand cDNA Synthesis SuperMix for qPCR (gDNA digester plus) (Yeasen Biotechnology, Shanghai, China). Quantitative real-time PCR was performed using Hieff®qPCR SYBR Green Master Mix (High Rox Plus) (Yeasen Biotechnology, Shanghai, China) in StepOne Plus real-time PCR System (Applied Biosystems). The relative expression of ZNF8-ERVK3-1 was normalized to the endogenous control GAPDH using the 2-ΔΔCt method, respectively. The primer sequences were:ZNF8-ERVK3-1:F:′-CAAGCATCACGCAAGGAAGAGG-3′, R:5′- TGGTGGGATAAGGAGCATCTGTC-3′;GAPDH:F:5′-TCATTTCCTGGGACACGA-3′,R:5′-GTCTTACTCCTTGGAGGCC-3′.
Cell transfection
The siRNA and siRNA negative control (siNC) were purchased from GenePharma (Shanghai, China). siRNA sequences were as follows: ZNF8-ERVK3-1 siNC sense:5′-UUCUCCGAACGUGUCACGUTT -3′, antisense:5′- acgugacacguucagaat -3′; siRNA1 sense:5′-GAAGGUCUGUCCUCGUGUUTT -3′, antisense:5′-AACACGAGGACAGACCUUCTT-3′; siRNA2 sense:5′- GCGAGACUGUGGGAGAACUTT -3′, antisense:5′-AGUUCUCCCACAGUCUCGCTT -3ʹ; siRNA3 sense:5′- GUGACCUGGAACAACAAUATT -3′, antisense:5′- UAUUGUUGUUUCCAGGUCACTT -3′. Cells were incubated in 6-well plates, and transfection was started when cell density reached 60%. Transfection was performed using gp -transfection-mate (GenePharma). Transfection efficiency was detected using qRT-PCR.
Cell proliferation assay
The cell proliferation assay was performed on a panel of 96 wells, and 1000 cells were added to each well after counting. After 24, 48, 72, and 96 h, 10 μL of CCK-8 solution (Yeasen) was added to each well and incubated for 1 h. Absorbance (OD) at 450 nm was detected in each well by an enzyme labeling instrument (Skanlt RE 7.0).
Cell migration and invasion assay
We used 8 µm pore size Transwell chambers (Corning, USA) in 24-well plates; 200ul of cell suspension containing 10,000 FBS-free cells was added to the upper chambers with matrix gel (BD Biocoat) or without matrix gel (Corning), and 1640 500 ul containing 10% FBS was added to the lower chambers. The cells were cultured in a cell incubator for 48 h. Cells suspended in the chambers were rinsed with phosphate buffer saline (PBS), cells were fixed with 4% paraformaldehyde for 15 min, stained with 0.1% crystal violet solution for 5 min, washed with PBS three times, and cells on the upper surface of the bottom chamber were gently wiped with a cotton swab. Cells on the lower surface of the bottom of the drying chamber, images were taken with an inverted microscope (OLYMPUS-CKX53). Cell counting was performed using Image J.
Wound healing test
Wound healing assay was performed to assess the migratory capacity of the cells. Transfected cells (15 × 10 4 /well) were inoculated in six-well plates with a monolayer of cells evenly distributed on the bottom of the plate. The cell layer was scraped with a 200 μl pipette tip, washed with PBS, and FBS-free medium was added to each well. Images were then taken under an inverted microscope at 0 and 48 h (OLYMPUS-CKX53, China). The images were analyzed using Image J software.
Colony formation assay
siNC and siRNA3 targeting the ZNF8-ERVK3-1 gene were used to transfect cells. 1000 cells were inoculated into each well of a 6-well culture plate and incubated for 2 weeks. 4% paraformaldehyde fixed the cells for 15 min, 0.1% crystal violet solution stained for 10 min, air-dried and photographed, and colonies were counted using Image J software.
Flow cytometry
Cell cycle: PBS washed 3 times, trypsin digested for 3 min, fixed in 70% ethanol at 4℃ for 30 min, and then incubated in 500 μl of propidium iodide staining solution (PI) (Beyotime) at 37 ℃ for 30 min. Apoptosis: PBS washed 3 times, trypsin digested for 3 min, and then annexin-FITC reagent (Beyotime) was added in order, and incubated for 20 min, protected from light. Cell cycle and apoptosis were detected by flow cytometry (BD Biosciences, USA), and the results were analyzed using flowJo software.
Immunohistochemical staining (IHC)
Sections of 3 μm thick paraffin-embedded tissues were dewaxed and hydrated, and antigenic repair was performed in pH 6.0 sodium citrate antigen repair solution in a microwave oven for 2 min on high and 15 min on bottom. Peroxidase activity was blocked by incubation in endogenous peroxide blocking solution (Beyotime, code:P0100A) for 25 min. Non-specific staining was blocked by confinement in immunostaining confinement solution (Beyotime, code:P0260) for 15 min. Sections were incubated overnight with anti-PCNA (Cell Signaling, code:13,110, 1:500 dilution) and anti-ki67 (proteintech, code:27,309–1-AP, 1: 2000 dilution). Placed at room temperature, the sections were washed 3 times with PBS, followed by incubation with secondary anti-IgG antibody (Servicebio, product number:G1215-200T) at room temperature for 1 h. DAB was used as a chromogen. The nucleus was stained with hematoxylin solution. The sections were scanned with KScanner software.
Xenograft model
The Laboratory Animal Ethics Committee of Yangzhou University approved animal experiments. A mouse xenograft model was established to explore the functional role of ZNF8-ERVK3-1 in vivo. H1975 cells were plated in six-well plates and transfected with siNC and siRNA3. Ten BALB/c nude mice from Nanjing Ji Biotechnology Co., Ltd. were randomly divided into two groups, and the above-treated cells (8 × 10 5 ) were injected into the axillary pit of nude mice. Tumor volume was monitored every seven days, and the formula was as follows: V = (Length × Width 2 ) × 0.5, nude mice were sacrificed after four weeks, and tumor size was recorded after anesthesia with isoflurane, nude mice suffered from cervical dislocation to death. Animal experiments were performed by animal care guidelines and approved by the Ethics Committee.
Identification of tryptophan-related lncRNAs in LUAD
A total of 16,876 lncRNAs were identified in the TCGA-LUAD database. 59 tryptophan metabolism-related genes were obtained from the GSEA database (Table S1 ), and a network of co-expression of tryptophan metabolism-related lncRNAs was constructed to identify the lncRNAs related to tryptophan metabolism.2578 final screened tryptophan metabolism-related lncRNAs (|cor|> 0.4, P -value < 0.001) (Fig. 1 A). Cox regression analysis was used to initially screen 137 target lncRNAs associated with the prognosis of LUAD patients (Table S2 ), and a forest plot was drawn (Fig. S1 ).
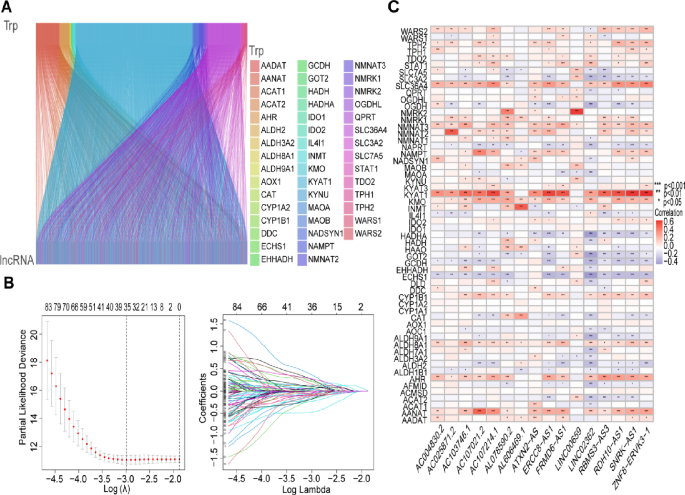
Identification of tryptophan metabolism gene-related lncRNAs and construction of prognostic models. A Sankey diagram showing co-expression of tryptophan metabolism-related genes and tryptophan metabolism-related lncRNAs. B The selection process of the optimal cross-validation parameter λ in the LASSO model and the trajectory plot of each variable. C Correlation heatmap showing the relationship between tryptophan metabolism-related lncRNAs and tryptophan metabolism-related genes. Red color indicates positive correlation and blue color indicates negative correlation
Prognosis-based risk modeling
Further screening and analyses were then performed by LASSO regression to reduce the overfitting of the data. Thirty-five tryptophan metabolism-associated lncRNAs with high prognostic values were identified, and lasso regression coefficient profiles were plotted (Fig. 1 B). Finally, multivariate Cox regression analysis was used to identify 16 tryptophan metabolism-related lncRNAs with prognostic value in LUAD patients, and the LUAD prognostic model was constructed Riskscore = (– 0.786293899438286*RBMS3-AS3) + (0.729525271199102*AC107214.1) + (– 0.594981659042687*AL078590.2) + (– 0.488362224373613*ATXN2-AS) + (– 1.26851214948258*ERCC8-AS1) + (0.691321177315073*AC004830.2) + (0.396799898336932*AC107021.2) + (– 0.366051764081276*RDH10-AS1) + (– 0.869907297975532*SNRK-AS1) + (0.448278641184027*LINC00659) + (1.04921620930125*ZNF8-ERVK3-1) + (0.743359529448505*AL606469.1) + (0.550402175045129*FRMD6-AS1) + (– 0.839764551688578*AL606469.1) + (– 0.352579344066772*LINC02362) + (– 0.61899293624872*AC025871.2). The associated heatmap also shows the relationship between tryptophan metabolism -related genes and lncRNAs (Fig. 1 C).
Evaluation and validation of LUAD lncRNA signature associated with tryptophan metabolism
Based on the median risk score, lung adenocarcinoma patients were categorized into high-risk and low-risk groups, and OS and PFS were compared. OS and PFS were lower in the high-risk group than in the low-risk group in the training set, validation set, and overall set (Fig. 2 A–D). According to the risk score and survival status display, we found that mortality increased with a higher score (Fig. 2 E–G). Heatmap showed the expression of 16 tryptophan metabolism lncRNAs in high and low-risk groups.LINC00659, ZNF8-ERVK3-1, and FRMD6-AS1 were high-risk lncRNAs, while ERCC8-AS1,ATXN2-AS,RDH10-AS1,and LINC02362 were low-risk lncRNAs (Fig. 2 E–G).
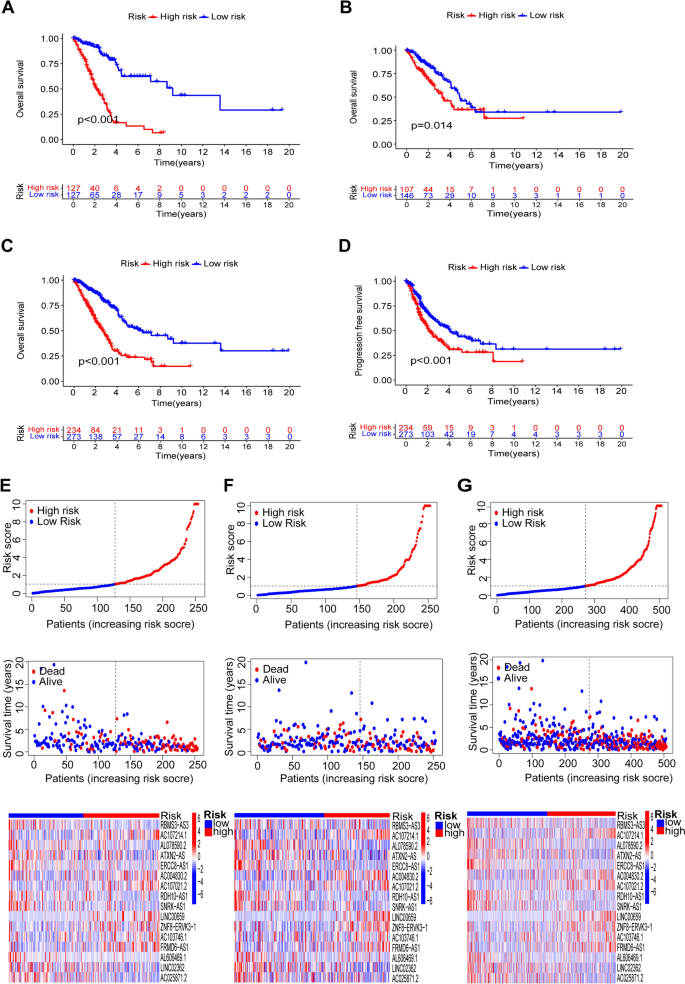
Kaplan–Meier survival analysis of patients in the high- and low-risk groups and 16 lncRNA risk score maps, survival status maps, and heat maps. A Training set OS. B Test set OS. C Overall set OS. D Overall set PFS. E Training set. F Test set. G Overall set
Independent analysis of prognostic factors
The results of univariate and multivariate COX regression analyses showed that the risk score could be used as an independent prognostic indicator for the prognosis of patients with LUAD (all P < 0.001) (Fig. 3 A, B ). In addition, we used ROC curves to assess the predictive accuracy of risk scores. The AUC values for the 1-, 3-, and 5-year risk scores for the training set were 0.742, 0.830, and 0.875, respectively, and the AUC values for the 1-, 3-, and 5-year values for the test set were 0.703, 0.604, and 0.568, respectively (Fig. 3 C–E). Next, we performed a series of analyses based on clinical characteristics, and ROC analyses showed that risk scores had more substantial prognostic power than other clinical characteristics (Fig. 3 F).
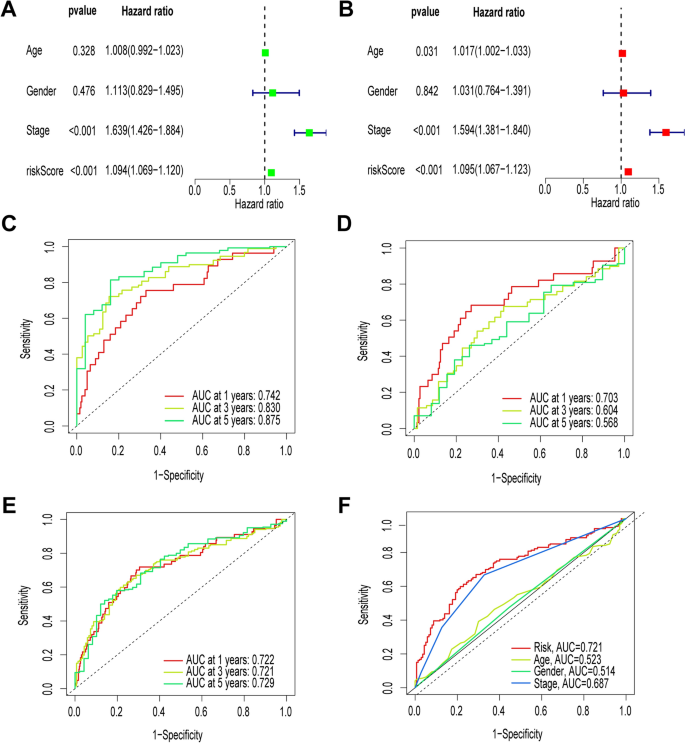
Independent predictive value of risk models. A , B Univariate Cox and multivariate Cox analyses to assess model independence from other clinical parameters. C – E ROC curves for risk scores at 1, 3, and 5 years for the training set and test set, and overall set. F Showing that risk scores accurately predict survival better than common clinical parameters
Construction and validation of column-line diagrams
Column line graphs were constructed for LUAD patients based on gender, age, risk score, and clinical stage (Fig. 4 A). The calibration curves showed that the predicted OS of the 1-, 3-, and 5-year column-line diagrams were generally consistent with the corresponding observed OS of LUAD patients (Fig. 4 B). We also found that the C-index value of the risk score was higher than other clinical characteristics (Fig. 4 C). We further analyzed the significant difference ( P < 0.05) in OS between patients in the high-risk and low-risk groups at different stages (Stages I–II and III–IV) (Fig. 4 D, E), which suggests that the model has high predictive accuracy and can be used to compare the survival of patients at different stages. Finally, we performed PCA to observe the distribution of all genes, tryptophan metabolism-related genes, tryptophan metabolism-related lncRNAs, and risk lncRNAs in LUAD patients, and the results showed a clear distribution, suggesting that these lncRNAs can be reliably used to construct the model (Fig. S2 ).
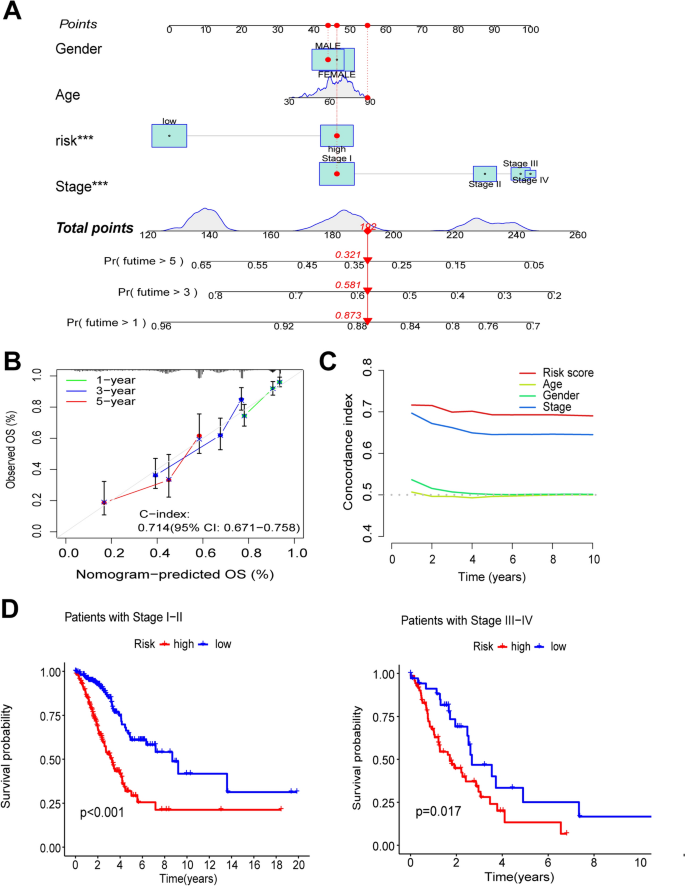
Creation of a column-line graph for predicting prognosis in patients with LUAD. A Nomogram survival prediction for LUAD patients with risk scores. B nomogram correction plot. C C-index curve of the risk model. D Risk score applied to LUAD patients with stages I–II and III–IV
Validation of risk profiles in GEO cohorts
Kaplan–Meier survival analysis showed that patients in the high-risk group had poorer OS than those in the low-risk group (Fig. 5 A). Risk scores and survival outcomes are shown in Fig. 5 B. We also included risk models and clinical characteristics in univariate and multivariate Cox regression analyses (Fig. 5 C). Univariate Cox regression analysis showed that risk score was an independent prognostic factor (HR: 1.034, 95% CI 1.010–1.058, P < 0.05). After adjusting for potential confounders in the multivariate Cox regression analysis, the correlation between risk score and OS remained significant (HR : 1.039; 95% CI 1.013–1.065, P < 0.05). ROC curves revealed reliable predictive efficacy of our model in the GEO cohort (Fig. 5 D). The AUC for 1 year, 3 years, and 5 years were 0.698, 0.608, and 0.599, respectively. Next, our ROC analysis based on clinical characteristics showed that the risk score also had good prognostic power (Fig. 5 E).
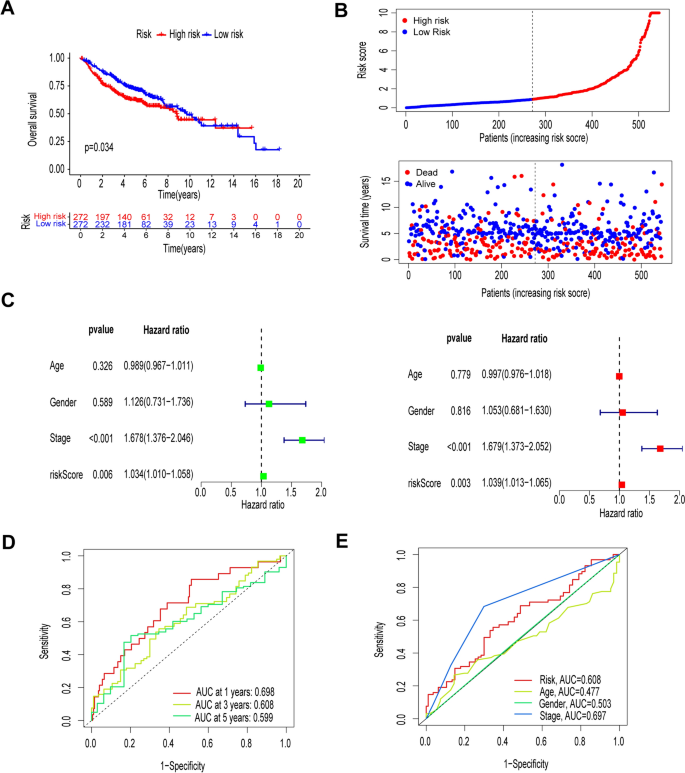
Validation of risk profiles in GEO cohorts. A Kaplan–Meier survival analysis of overall survival (OS) in the GEO cohorts. B Risk score maps, survival status maps. C Univariate and multivariate Cox analyses assessed the independence of the model from other clinical parameters. D ROC curves for risk scores at 1, 3, and 5 years. E ROC curves for clinical characteristics and risk scores. CI, confidence interval; HR: risk ratio; OS, overall survival; ROC, subject operating characteristic curve
Gene set enrichment analysis in LUAD patients
551 DEGs were identified between the high- and low-risk groups (Table S3 ). To reveal the biological pathways of lncRNAs associated with tryptophan metabolism, GO functional enrichment and KEGG pathway enrichment were analyzed based on the DEGs between the high- and low-risk groups. The GO analysis showed that tryptophan metabolism-associated lncRNAs in biological processes (BP) were mainly concentrated in epidermis development, and cellular components (CC) were mainly concentrated in collagen-containing, extracellular matrix, and cytoplasmic region categories. Categories, etc. Molecular functions (MF) mainly focused on endopeptidase inhibitor activity and peptidase inhibitor activity categories (Fig. 6 A). The KEGG pathway indicated that tryptophan metabolism-related lncRNAs were involved in tryptophan metabolism. The KEGG pathway indicated that lncRNAs related to tryptophan metabolism were involved in Complement and coagulation cascades, Hematopoietic cell lineage, and Amoebiasis (Fig. 6 B).
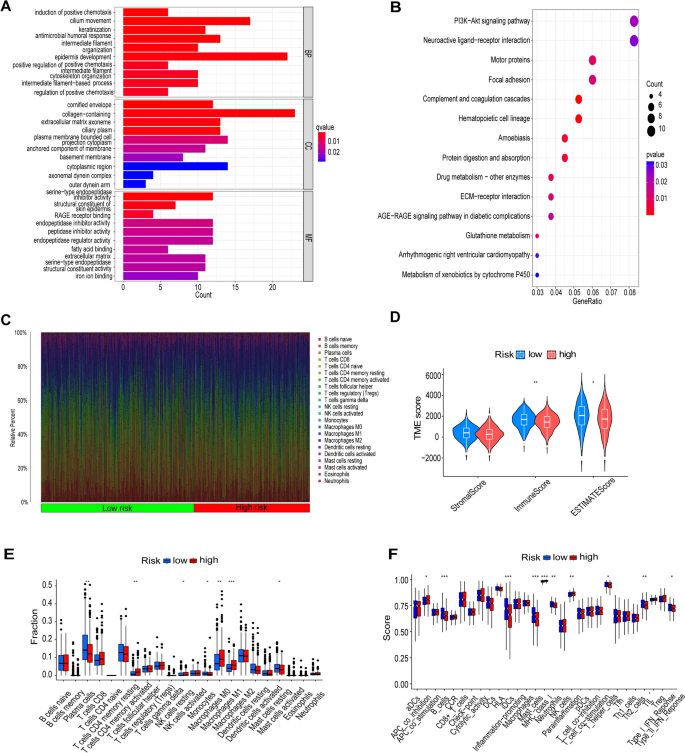
GO and KEGG analyses and TME characteristics of high and low-risk groups. A Barplot of the first 10 GO-enriched conditions. B Bubble plot of the first 30 KEGG-enriched terms. C , E Proportion of immune cells between the two groups. D Stromal scores, immune scores, and estimated scores between the two groups. F Differences in immune-related functions between the two groups. Asterisks indicate statistical significance, *, P < 0.05; **, P < 0.01; ***, P < 0.001
Tumor immunoscape based on risk modeling
To further explore the relationship between tryptophan metabolism-associated lncRNAs and the tumor microenvironment in LUAD patients, we determined the landscape of immune cell infiltration in all LUAD patients from the TCGA database using the CIBERSORT algorithm. The proportions of each type of immune cell (Fig. 6 C) are shown. To determine the difference in infiltrating immune cells between the high- and low-risk groups, we assessed this by the immunity score (immune cell infiltration in tumor tissue) and the estimated score (sum of stromal and immunity scores for individual cases), which were both significantly higher ( P < 0.01) in the low-risk group (Fig. 6 D). In addition, we compared the proportion of each immune cell between the high-risk and low-risk groups and found significant differences in Plasma cells, T cells CD4 memory activated, M1 and M0 macrophages, Monocytes, Mast cells resting, and NK cells resting between the two groups (Fig. 6 E). We further explored the relationship between risk scores and immune-related features, and ssGSEA analysis showed that higher risk scores were significantly associated with reduced levels of most immune-related features (Fig. 6 F), including immune cell infiltration (e.g., B cells, iDCs, Mast_cells, Neutrophils, T_helper_cells, and TIL).
Tumor mutation load analysis and prediction of response to drug therapy
We used the maftools algorithm to observe mutations in the high-risk and low-risk groups, and the high-risk group showed a wider range of somatic mutations than the low-risk group (TP53: low risk, 40%; high risk 52%, TTN: low risk, 36%; high risk, 52%, and CSMD3: low risk, 33%; high risk, 44%) (Fig. 7 A). There was a difference in TMB difference between high- and low-risk groups ( P < 0.05) (Fig. 7 B). Kaplan–Meier survival curves showed that patients with higher TMB had better OS than those with lower TMB. The combination of risk scores and TMB showed greater prognostic value for patients with LUAD (Fig. 7 C).
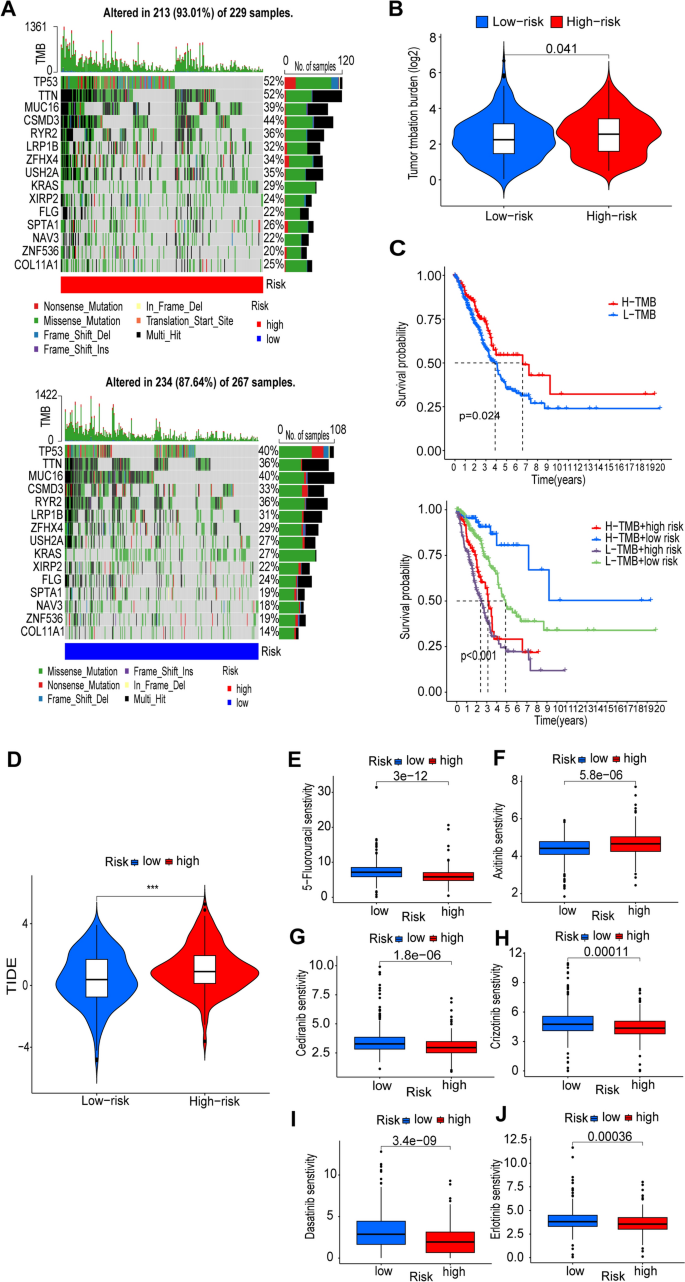
Mutation analysis of tumor somatic cells and prediction of response to drug therapy. A Waterfall plots showing the top 15 mutated genes in LUAD in the high-risk group (213 samples) and low-risk group (234 samples). B Differences in TMB between the two groups. C KM curves of OS in the high and low TMB groups. KM curves of OS in patients stratified by risk score and TMB subgroups. TMB tumor mutational load, H high, L low, LUAD lung adenocarcinoma, OS overall survival. D Correlation between risk scores and response to immunotherapy. E – J Drug sensitivity of 5-fluorouracil, axitinib, cediranib, crizotinib, dasatinib, and erlotinib was observed. * indicates statistical significance, *, P < 0.05; **, P < 0.01; ***, P < 0.001
The risk of tumor immune escape was calculated using the TIDE algorithm. The results showed that the TIDE score was higher in the high-risk group than in the low-risk group, indicating a higher probability of immune escape ( P < 0.001) (Fig. 7 D). We further explored the potential effective therapeutic agents for LUAD patients using the "oncoPredict" R package. Among the drugs commonly used in the treatment of LUAD, 5-fluorouracil, cediranib, crizotinib, dasatinib, and erlotinib were found to have lower IC50 values (50% inhibition of cell growth) in the high-risk group of patients, suggesting that these drugs may be more effective in high-risk patients. However, low-risk patients may benefit more from axitinib (Fig. 7 E–J).
ZNF8-ERVK3-1 expression was significantly elevated in LUAD tissues and cells
Differential expression of ZNF8-ERVK3-1 in LUAD cell line and normal human bronchial epithelioid cell line (HBE) was verified by qRT-PCR assay, and it was found that the expression of LUAD cell line was higher than that of HBE (Fig. 8 A). Further analysis of the RNA levels of ZNF8-ERVK3-1 in lung adenocarcinoma tissues and paired normal tissues showed that the expression of ZNF8-ERVK3-1 was significantly higher in LUAD tissues (Fig. 8 B).
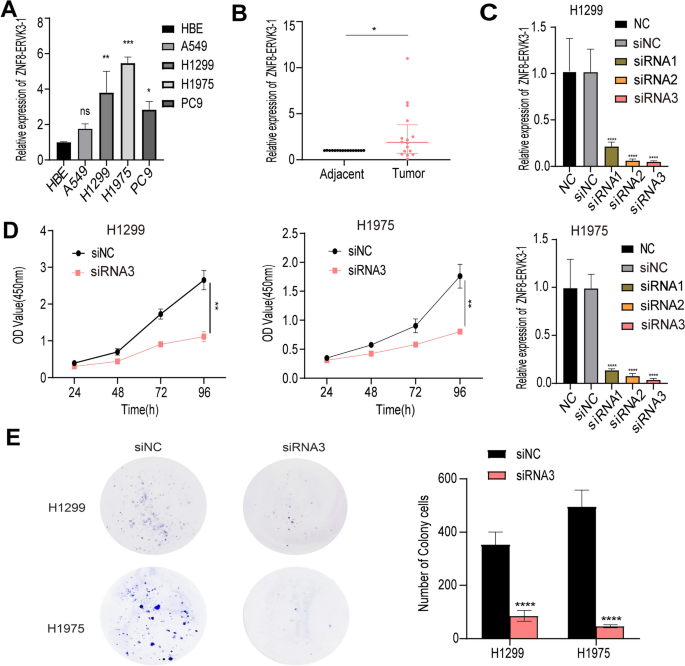
ZNF8-ERVK3-1 expression was upregulated in LUAD tissues and cells. ZNF8-ERVK3-1 knockdown inhibited tumor cell proliferation, migration, and invasion. A The expression of ZNF8-ERVK3-1 in LUAD cells (A549, H1299, H1975, PC9) and HBE was determined by qRT-PCR. *, P < 0.05; **, P < 0.01; ***, P < 0.001. B ZNF8-ERVK3-1 expression was detected in 16 pairs of LUAD tissues and adjacent non-tumor tissues by qRT-PCR. C The efficiency of ZNF8-ERVK3-1 knockdown in H1299 and H1975 cells transfected with siRNA1 / 2 / 3 was determined by both qRT-PCR. D , E The viability and proliferation ability of H1299 and H1975 cells transfected with siRNA3 were determined by CCK-8 and clone formation assay
ZNF8-ERVK3-1 knockdown inhibits tumor cell proliferation, migration, invasion, and G1 phase inhibits and promotes apoptosis
H1299 and H1975 cells had high expression of ZNF8-ERVK3-1, so H1299 and H1975 cells were transfected using si-ZNF8-ERVK3-1, and the knockdown efficiency was verified by qPCR (Fig. 8 C). siRNA3 was selected to perform a series of functional experiments. CCK-8, clone formation experiments showed that knockdown of ZNF8-ERVK3-1 gene resulted in growth retardation of H1299 and H1975 cells (Fig. 8 D, E). The complete original image of clone formation experiments is supplemented in Fig. S3 .
Wound healing and transwell assays showed that ZNF8-ERVK3-1 knockdown resulted in a significant decrease in cell migration (Fig. 9 A) and a dramatic decrease in their invasive ability (Fig. 9 B). In addition, we applied flow cytometry to explore whether ZNF8-ERVK3-1 knockdown leads to LUAD cell cycle arrest and increased apoptosis. In H1975 cells, the proportion of cells in the G0/G1 phase was significantly increased in both the si-ZNF8-ERVK3-1 group compared with the si-NC group, while the proportion of cells in S and G2/M phases was decreased (Fig. 9 C). We further found that the apoptosis rate was significantly increased after ZNF8-ERVK3-1 knockdown (Fig. 9 D).
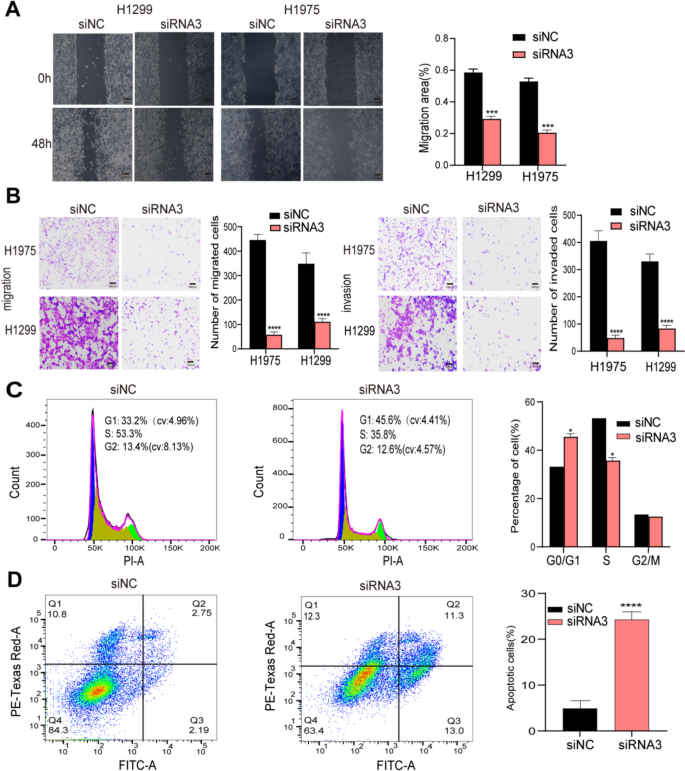
ZNF8-ERVK3-1 knockdown inhibited tumor cell migration, invasion and G0/G1 cell cycle arrest and apoptosis were increased. A Wound healing assay examining the mobility of H1299 and H1975 cells transfected with siNC and siRNA3. B The number of H1299 and H1975 cells transfected with siNC and siRNA3 migrated and invaded were assessed by transwell assay. C The effect of ZNF8-ERVK3-1 knockdown on H1975 cells cycle was detected using Cell Cycle and Apoptosis Analysis Kit. D The effect of ZNF8-ERVK3-1 knockdown on apoptosis of H1975 cells was detected using Annexin V Apoptosis Detection Kit. * P < 0.05, ** P < 0.01, **** P < 0.0001
ZNF8-ERVK3-1 promotes tumorigenesis in vivo
A xenograft nude mouse model was established to elucidate the role of ZNF8-ERVK3-1 in LUAD in vivo. Tumor changes were closely monitored after injection of H1975 cells from transfected siNC and siRNA3. The results showed that ZNF8-ERVK3-1 knockdown inhibited tumor growth, i.e., a significant reduction in tumor size and weight (Fig. 10 A–D). Immunohistochemical analysis showed that the expression level of ki67 and PCNA, which are closely related to tumor proliferation, were significantly lower in the siRNA3 group than in siNC (Fig. 10 E).
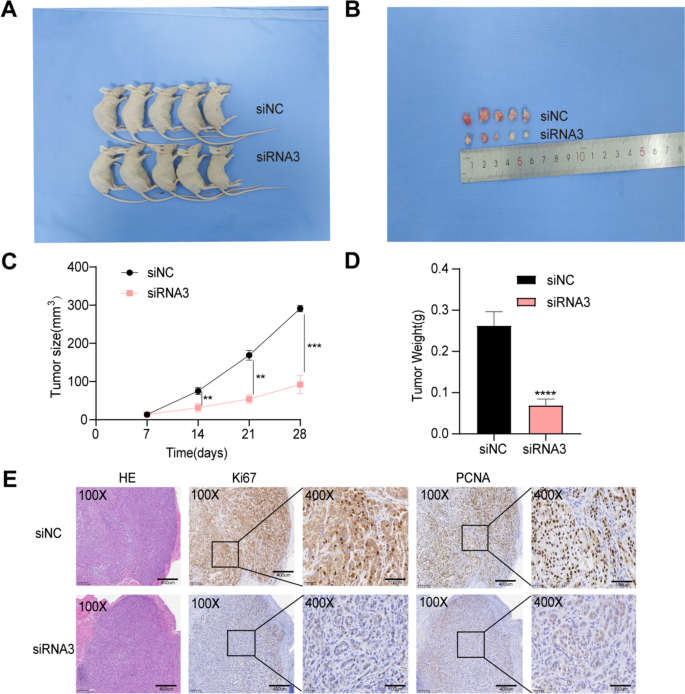
ZNF8-ERVK3-1 promotes in vivo tumorigenesis. A , B Xenograft tumors in nude mouse models. C Tumor size. D Tumor weight. E The expression of ki67 and PCNA in siNC and siRNA3 subcutaneous xenografts was analyzed by immunohistochemistry. * P < 0.05, ** P < 0.01
Lung adenocarcinoma is one of the most common pathological types of lung cancer. Despite advances in the fields of surgery, radiotherapy, chemotherapy, and immunotherapy, the overall survival of LUAD remains poor (Allemani et al. 2015 ). Therefore, we want to continue to explore novel molecular biomarkers for individualized prediction of LUAD prognosis and provide new targets for future LUAD treatment. An increasing number of lncRNAs have been shown to play critical roles in the onset and progression of LUAD (Qu et al. 2021 ; Loewen et al. 2014 ). For example, lncRNA UPLA1 can be a prognostic marker to promote lung adenocarcinoma progression through Wnt/β-linker protein signaling (Han et al. 2020 ). Jiang et al. found that lncRNA HCP5 acts as a novel regulator in the TGFβ/SMAD signaling pathway to promote LUAD tumor growth and metastasis (Jiang et al. 2019 ). Recently, tryptophan metabolism was found to be closely related to regulating immunity and tumorigenesis (Kwiatkowska et al. 2021 ). Meanwhile, the tryptophan metabolic pathway and its metabolites have multiple functions in lung cancer pathogenesis, including regulating the tumor microenvironment and promoting immunosuppression and drug resistance (Li and Zhao 2021 ). In this study, we successfully established a prognostic risk profile based on tryptophan metabolism-associated lncRNAs for predicting overall survival in LUAD patients. In addition, we preliminarily validated the oncogenic role of ZNF8-ERVK3-1 in LUAD. We found that inhibition of ZNF8-ERVK3-1 may inhibit the proliferation, migration, and invasion of LUAD cells and can also lead to cellular G0/G1 phase cycle blockage and increased apoptosis. In vivo experiments showed that ZNF8-ERVK3-1 promoted LUAD tumorigenesis.
Pearson correlation analysis showed that 2578 lncRNAs were significantly associated with 60 tryptophan metabolism-related genes ( P < 0.001). By univariate Cox regression analysis, 137 lncRNAs were identified as independent prognostic factors for LUAD ( P < 0.05). Finally, 16 lncRNAs were identified by multivariate Cox regression analysis to establish prognostic models, which were accurate for predicting OS and PFS in LUAD. Overexpression of RBMS3-AS3 inhibited cell proliferation, migration, invasion, and angiogenesis as well as tumorigenicity of prostate cancer, and RBMS3-AS3 acted as a miR-4534 sponge to inhibit cell proliferation, migration, invasion, and angiogenesis. Inhibits prostate cancer development by up-regulating VASH1 (Jiang et al. 2020 ). Li et al. found that ATXN2-AS may be associated with spinal cerebellar ataxia type 2 (SCA2) and amyotrophic lateral sclerosis (ALS) (Li et al. 2016 ). ERCC8-AS1 and RDH10-AS1 were markedly upregulated in osteosarcoma tissues, which may serve as biomarkers for osteosarcoma and potential therapeutic targets (Rothzerg et al. 2021 ). LINC00659 as an oncogene, Sheng et al. found that LINC00659 could promote gastric carcinogenesis by promoting SUZ12 expression (Sheng et al. 2020 ). Another group found that cancer-associated fibroblast (CAF)-derived exosome LINC00659 promotes colorectal cancer cell proliferation, invasion, and migration through the miR-342-3p / ANXA2 axis (Zhou et al. 2021 ). Wu et al. found that FRMD6-AS1 as necrotic apoptosis-associated lncRNA was significantly elevated in lung adenocarcinoma cells and tissues (Wu et al. 2022 ). We then used ROC analysis to assess the predictive performance of the risk model we constructed. Our model's AUC of the ROC curves for 1-, 3- and 5-year OS were 0.742, 0.83, and 0.87, respectively. In addition, the AUC of the ROC curves of our model were more significant than the conventional clinical characteristics of the patients. All LUAD samples were randomly divided into a training set (50%) and a test set (50%) to validate the confidence of our risk model. The training and test sets were divided into high-risk and low-risk groups based on the median risk score. For the training set, the overall survival of LUAD patients in the high-risk group was significantly shorter than that in the low-risk group ( P < 0.001). Moreover, the results of the training set were similar to those of the test set ( P = 0.014). Univariate and multivariate COX regression analyses showed that risk score and clinical stage were independent indicators affecting the prognosis of LUAD patients ( P < 0.05). We constructed a column-line graph combining risk scores and clinical characteristics to reliably predict the prognosis of patients with LUAD. The predictive model showed the same predictive ability in the GEO-LUAD validation cohort.
We performed an immune cell infiltration analysis using CIBERSORT and examined the correlation between immune cell infiltration and risk scores.M1-type macrophages have pro-inflammatory, immunogenic, and anti-tumor properties (Ginhoux and Guilliams 2016 ). In our study, we found that patients with higher risk scores had higher M1 macrophage infiltration scores, suggesting that tumors in high-risk patients may have higher M1 macrophage infiltration.
TMB, defined as the number of somatic mutations per megabase, is often used as a predictive biomarker for immune checkpoint blockade in lung cancer (Fusco et al. 2021 ). We analyzed the TMB status of lung adenocarcinoma patients in the high-risk and low-risk groups. The high-risk group exhibited higher TMB than the low-risk group. some mutations were strongly associated with risk scores. For example, mutations in TP53, TTN, and CSMD3 were the top three mutations in the high-risk group.TP53 is the most commonly mutated gene in patients with NSCLC. The tumor-suppressor function of the p53 protein is reversed in TP53-mutated individuals, who exhibit pro-cancer effects and have a poorer prognosis (Bykov et al. 2018 ). TTN is associated with increased TMB in a variety of solid tumors and is closely related to the objective response to ICB (Jia et al. 2019 ). Liu et al. found that CSMD3, a common mutated gene in lung cancer, and CSMD3 deletion resulted in increased proliferation of airway epithelial cells (Liu et al. 2012 ). In addition, the combination of TMB and risk modeling has brought more accurate survival analysis to patients.
TIDE is a computational method used to predict ICB response (Jiang et al. 2018 ). According to the TIDE prediction results, patients in the high-risk group had higher TIDE values. This finding suggests high-risk patients have a higher potential for tumor immune escape. We used the "oncoPredict" R package to investigate the potential effective therapeutic agents for LUAD patients. Drug sensitivity analyses showed that patients with high-risk scores might be more sensitive to 5-fluorouracil, cediranib, crizotinib, dasatinib, and erlotinib, a tyrosine kinase inhibitor with a broad spectrum of anti-tumor activity in non-small cell lung cancer (Nikolinakos and Heymach 2008 ). Studies have shown that crizotinib prolongs the survival of patients with ALK mutation-positive non-small cell lung cancer (Solomon et al. 2018 ). Dasatinib, a multi-targeted protein tyrosine kinase inhibitor targeting the BCR-ABL and SRC family of kinases, has been successfully used in the treatment of chronic myeloid leukemia (CML), and several studies have shown that dasatinib inhibits the lung cancer cell proliferation in vitro and tumor growth in vitro (Zhang et al. 2020 , 2023 ; Redin et al. 2021 ).
Finally, we analyzed the role of ZNF8-ERVK3-1, a lncRNA associated with tryptophan metabolism, in LUAD. We verified that ZNF8-ERVK3-1 expression was significantly elevated in LUAD tissues and cells. We also explored the proliferation, migration, and invasion of ZNF8-ERVK3-1 using CCK-8, clone formation, wound healing, and Transwell assays. We found that the knockdown of ZNF8-ERVK3-1 inhibited the proliferation, migration, and invasion of LUAD cells. We also found that the knockdown of ZNF8-ERVK3-1 resulted in G0/G1 phase cycle block and increased apoptosis in LUAD cells by flow cytometry analysis. In vivo experiments further confirmed that ZNF8-ERVK3-1 promoted LUAD tumorigenesis.
Although our findings have been validated in an independent cohort, there are some limitations. First, our study is a retrospective study based on the publicly available TCGA database, and prognostic models need to be validated in prospective studies for clinical use. Second, the underlying mechanisms of how these lncRNAs affect tryptophan metabolism remain unknown. Further studies are necessary to investigate the relationship between these lncRNAs and tryptophan metabolism. Finally, other cohorts have not validated the correlation between our drug sensitivity prediction and immunotherapy response.
Conclusions
In summary, we constructed a robust prognostic model of 16 tryptophan metabolism-associated lncRNAs in lung adenocarcinoma, providing new insights for predicting the prognosis of lung adenocarcinoma patients. The prognostic risk score was strongly correlated with common clinical characteristics such as immune cell infiltration, immune-related function, TMB, and anticancer drug sensitivity, which may improve the benefit rate of patients. In conclusion, we preliminarily verified by in vitro experiments that ZNF8-ERVK3-1 promotes lung adenocarcinoma proliferation, migration, and invasion and that knockdown of ZNF8-ERVK3-1 leads to G0/G1 phase cycle blockage and increased apoptosis. In vivo experiments confirmed that ZNF8-ERVK3-1 promoted LUAD tumorigenesis. It provides a theoretical basis for individualized treatment of lung adenocarcinoma.
Availability of data and materials
Data supporting the findings of this study may be obtained from the respective authors upon reasonable request.
Abbreviations
The Cancer Genome Atlas
Gene Expression Omnibus
- Lung adenocarcinoma
Tumor mutational load
Tumor immune dysfunction and exclusion
Indoleamine 2,3-dioxygenase 1
Lung cancer
Immune checkpoint inhibitors
Long non-coding RNAs
Molecular Signatures Database
Least absolute shrinkage and selection operator regression
Overall survival
Progression-free survival
Receiver operating characteristic
Area under the curve
Principal component analysis
Differentially expressed genes
False discovery rate
Gene Ontology
Kyoto Encyclopedia of Genes and Genomes
Genomics of Drug Sensitivity in Cancer
Quantitative real-time polymerase chain reaction
Phosphate buffer saline
Propidium iodide
Agulló-Ortuño MT, Gómez-Martín Ó, Ponce S, Iglesias L, Ojeda L, Ferrer I et al (2020) Blood predictive biomarkers for patients with non-small-cell lung cancer associated with clinical response to nivolumab. Clin Lung Cancer 21(1):75–85. https://doi.org/10.1016/j.cllc.2019.08.006
Article CAS PubMed Google Scholar
Allemani C, Weir HK, Carreira H, Harewood R, Spika D, Wang XS et al (2015) Global surveillance of cancer survival 1995–2009: analysis of individual data for 25,676,887 patients from 279 population-based registries in 67 countries (CONCORD-2). Lancet (london, England). 385(9972):977–1010. https://doi.org/10.1016/s0140-6736(14)62038-9
Article PubMed Google Scholar
Bykov VJN, Eriksson SE, Bianchi J, Wiman KG (2018) Targeting mutant p53 for efficient cancer therapy. Nat Rev Cancer 18(2):89–102. https://doi.org/10.1038/nrc.2017.109
Cervenka I, Agudelo LZ, Ruas JL (2017) Kynurenines: Tryptophan’s metabolites in exercise, inflammation, and mental health. Science (new York, NY). https://doi.org/10.1126/science.aaf9794
Article Google Scholar
Chen X, Yan CC, Zhang X, You ZH (2017) Long non-coding RNAs and complex diseases: from experimental results to computational models. Briefings Bioinform 18(4):558–576. https://doi.org/10.1093/bib/bbw060
Article CAS Google Scholar
Chen J, Zhang K, Zhi Y, Wu Y, Chen B, Bai J et al (2021) Tumor-derived exosomal miR-19b-3p facilitates M2 macrophage polarization and exosomal LINC00273 secretion to promote lung adenocarcinoma metastasis via Hippo pathway. Clin Transl Med 11(9):e478. https://doi.org/10.1002/ctm2.478
Article CAS PubMed PubMed Central Google Scholar
Cheng Z, Lu C, Wang H, Wang N, Cui S, Yu C et al (2022) Long noncoding RNA LHFPL3-AS2 suppresses metastasis of non-small cell lung cancer by interacting with SFPQ to regulate TXNIP expression. Cancer Lett 531:1–13. https://doi.org/10.1016/j.canlet.2022.01.031
Feng J, Li J, Qie P, Li Z, Xu Y, Tian Z (2021) Long non-coding RNA (lncRNA) PGM5P4-AS1 inhibits lung cancer progression by up-regulating leucine zipper tumor suppressor (LZTS3) through sponging microRNA miR-1275. Bioengineered 12(1):196–207. https://doi.org/10.1080/21655979.2020.1860492
Fusco MJ, West HJ, Walko CM (2021) Tumor mutation burden and cancer treatment. JAMA Oncol 7(2):316. https://doi.org/10.1001/jamaoncol.2020.6371
Ginhoux F, Guilliams M (2016) Tissue-resident macrophage ontogeny and homeostasis. Immunity 44(3):439–449. https://doi.org/10.1016/j.immuni.2016.02.024
Godin-Ethier J, Hanafi LA, Piccirillo CA, Lapointe R (2011) Indoleamine 2,3-dioxygenase expression in human cancers: clinical and immunologic perspectives. Clin Cancer Res 17(22):6985–6991. https://doi.org/10.1158/1078-0432.ccr-11-1331
Han X, Jiang H, Qi J, Li J, Yang J, Tian Y et al (2020) Novel lncRNA UPLA1 mediates tumorigenesis and prognosis in lung adenocarcinoma. Cell Death Dis 11(11):999. https://doi.org/10.1038/s41419-020-03198-y
Hirsch FR, Scagliotti GV, Mulshine JL, Kwon R, Curran WJ Jr, Wu YL et al (2017) Lung cancer: current therapies and new targeted treatments. Lancet (london, England). 389(10066):299–311. https://doi.org/10.1016/s0140-6736(16)30958-8
Iorio F, Knijnenburg TA, Vis DJ, Bignell GR, Menden MP, Schubert M et al (2016) A landscape of pharmacogenomic interactions in cancer. Cell 166(3):740–754. https://doi.org/10.1016/j.cell.2016.06.017
Jia Q, Wang J, He N, He J, Zhu B (2019) Titin mutation associated with responsiveness to checkpoint blockades in solid tumors. JCI Insight. https://doi.org/10.1172/jci.insight.127901
Article PubMed PubMed Central Google Scholar
Jiang P, Gu S, Pan D, Fu J, Sahu A, Hu X et al (2018) Signatures of T cell dysfunction and exclusion predict cancer immunotherapy response. Nat Med 24(10):1550–1558. https://doi.org/10.1038/s41591-018-0136-1
Jiang L, Wang R, Fang L, Ge X, Chen L, Zhou M et al (2019) HCP5 is a SMAD3-responsive long non-coding RNA that promotes lung adenocarcinoma metastasis via miR-203/SNAI axis. Theranostics. 9(9):2460–2474. https://doi.org/10.7150/thno.31097
Jiang Z, Zhang Y, Chen X, Wu P, Chen D (2020) Long noncoding RNA RBMS3-AS3 acts as a microRNA-4534 sponge to inhibit the progression of prostate cancer by upregulating VASH1. Gene Ther 27(3–4):143–156. https://doi.org/10.1038/s41434-019-0108-1
Jochems C, Fantini M, Fernando RI, Kwilas AR, Donahue RN, Lepone LM et al (2016) The IDO1 selective inhibitor epacadostat enhances dendritic cell immunogenicity and lytic ability of tumor antigen-specific T cells. Oncotarget 7(25):37762–37772. https://doi.org/10.18632/oncotarget.9326
Kanehisa M, Goto S (2000) KEGG: kyoto encyclopedia of genes and genomes. Nucl Acids Res 28(1):27–30. https://doi.org/10.1093/nar/28.1.27
Komiya T, Huang CH (2018) Updates in the Clinical Development of Epacadostat and Other Indoleamine 2,3-Dioxygenase 1 Inhibitors (IDO1) for Human Cancers. Front Oncol 8:423. https://doi.org/10.3389/fonc.2018.00423
Kuhn M (2008) Building predictive models in R Using the caret Package. J Stat Softw 28(5):1–26. https://doi.org/10.18637/jss.v028.i05
Kwiatkowska I, Hermanowicz JM, Przybyszewska-Podstawka A, Pawlak D (2021) Not only immune escape-the confusing role of the TRP metabolic pathway in carcinogenesis. Cancers. https://doi.org/10.3390/cancers13112667
Levina V, Su Y, Gorelik E (2012) Immunological and nonimmunological effects of indoleamine 2,3-dioxygenase on breast tumor growth and spontaneous metastasis formation. Clin Dev l Immunol 2012:173029. https://doi.org/10.1155/2012/173029
Li C (2021) Tryptophan and its metabolites in lung cancer: basic functions and clinical significance. Front Oncol 11:707277. https://doi.org/10.3389/fonc.2021.707277
Li PP, Sun X, Xia G, Arbez N, Paul S, Zhu S et al (2016) ATXN2-AS, a gene antisense to ATXN2, is associated with spinocerebellar ataxia type 2 and amyotrophic lateral sclerosis. Ann Neurol 80(4):600–615. https://doi.org/10.1002/ana.24761
Liberzon A, Birger C, Thorvaldsdóttir H, Ghandi M, Mesirov JP, Tamayo P (2015) The Molecular Signatures Database (MSigDB) hallmark gene set collection. Cell Syst 1(6):417–425. https://doi.org/10.1016/j.cels.2015.12.004
Liu P, Morrison C, Wang L, Xiong D, Vedell P, Cui P et al (2012) Identification of somatic mutations in non-small cell lung carcinomas using whole-exome sequencing. Carcinogenesis 33(7):1270–1276. https://doi.org/10.1093/carcin/bgs148
Loewen G, Jayawickramarajah J, Zhuo Y, Shan B (2014) Functions of lncRNA HOTAIR in lung cancer. J Hematol & Oncol 7:90. https://doi.org/10.1186/s13045-014-0090-4
Lortet-Tieulent J, Soerjomataram I, Ferlay J, Rutherford M, Weiderpass E, Bray F (2014) International trends in lung cancer incidence by histological subtype: adenocarcinoma stabilizing in men but still increasing in women. Lung Cancer (amsterdam, Netherlands). 84(1):13–22. https://doi.org/10.1016/j.lungcan.2014.01.009
Maeser D, Gruener RF, Huang RS (2021) oncoPredict: an R package for predicting in vivo or cancer patient drug response and biomarkers from cell line screening data. Briefings Bioinform. https://doi.org/10.1093/bib/bbab260
Newman AM, Liu CL, Green MR, Gentles AJ, Feng W, Xu Y et al (2015) Robust enumeration of cell subsets from tissue expression profiles. Nat Methods 12(5):453–457. https://doi.org/10.1038/nmeth.3337
Nikolinakos P, Heymach JV (2008) The tyrosine kinase inhibitor cediranib for non-small cell lung cancer and other thoracic malignancies. J Thorac Oncol 3(6 Suppl 2):S131–S134. https://doi.org/10.1097/JTO.0b013e318174e910
Qu S, Jiao Z, Lu G, Yao B, Wang T, Rong W et al (2021) PD-L1 lncRNA splice isoform promotes lung adenocarcinoma progression via enhancing c-Myc activity. Genome Biol 22(1):104. https://doi.org/10.1186/s13059-021-02331-0
Redin E, Garmendia I, Lozano T, Serrano D, Senent Y, Redrado M et al (2021) SRC family kinase (SFK) inhibitor dasatinib improves the antitumor activity of anti-PD-1 in NSCLC models by inhibiting Treg cell conversion and proliferation. J Immunother Cancer. https://doi.org/10.1136/jitc-2020-001496
Rothzerg E, Ho XD, Xu J, Wood D, Märtson A, Kõks S (2021) Upregulation of 15 antisense long non-coding RNAs in osteosarcoma. Genes. https://doi.org/10.3390/genes12081132
Schwarcz R, Stone TW (2017) The kynurenine pathway and the brain: Challenges, controversies and promises. Neuropharmacology 112(Pt B):237–247. https://doi.org/10.1016/j.neuropharm.2016.08.003
Sheng Y, Han C, Yang Y, Wang J, Gu Y, Li W et al (2020) Correlation between LncRNA-LINC00659 and clinical prognosis in gastric cancer and study on its biological mechanism. J Cell Mol Med 24(24):14467–14480. https://doi.org/10.1111/jcmm.16069
Smith C, Chang MY, Parker KH, Beury DW, Du Hadaway JB, Flick HE et al (2012) IDO is a nodal pathogenic driver of lung cancer and metastasis development. Cancer Discov 2(8):722–735. https://doi.org/10.1158/2159-8290.cd-12-0014
Solomon BJ, Kim DW, Wu YL, Nakagawa K, Mekhail T, Felip E et al (2018) Final overall survival analysis from a study comparing first-line crizotinib versus chemotherapy in ALK-mutation-positive non-small-cell lung cancer. J Clin Oncol 36(22):2251–2258. https://doi.org/10.1200/jco.2017.77.4794
Subramanian A, Tamayo P, Mootha VK, Mukherjee S, Ebert BL, Gillette MA et al (2005) Gene set enrichment analysis: a knowledge-based approach for interpreting genome-wide expression profiles. Proc Natl Acad Sci USA 102(43):15545–15550. https://doi.org/10.1073/pnas.0506580102
Sung H, Ferlay J, Siegel RL, Laversanne M, Soerjomataram I, Jemal A et al (2021) Global Cancer Statistics 2020: GLOBOCAN estimates of incidence and mortality worldwide for 36 cancers in 185 countries. CA A Cancer J Clin 71(3):209–249. https://doi.org/10.3322/caac.21660
Tang D, Yue L, Yao R, Zhou L, Yang Y, Lu L et al (2017) P53 prevent tumor invasion and metastasis by down-regulating IDO in lung cancer. Oncotarget 8(33):54548–54557. https://doi.org/10.18632/oncotarget.17408
Tibshirani R (1997) The lasso method for variable selection in the Cox model. Stat Med 16(4):385–395. https://doi.org/10.1002/(sici)1097-0258(19970228)16:4%3c385::aid-sim380%3e3.0.co;2-3
Volders PJ, Anckaert J, Verheggen K, Nuytens J, Martens L, Mestdagh P et al (2019) LNCipedia 5: towards a reference set of human long non-coding RNAs. Nucleic Acids Res 47(D1):D135–D139. https://doi.org/10.1093/nar/gky1031
Wu J, Song D, Zhao G, Chen S, Ren H, Zhang B (2022) Cross-talk between necroptosis-related lncRNAs to construct a novel signature and predict the immune landscape of lung adenocarcinoma patients. Front Genet 13:966896. https://doi.org/10.3389/fgene.2022.966896
Yentz S, Smith D (2018) Indoleamine 2,3-dioxygenase (IDO) inhibition as a strategy to augment cancer immunotherapy. BioDrugs Clin Immunotherap BiopharmGene Therapy. 32(4):311–317. https://doi.org/10.1007/s40259-018-0291-4
Yoshida R, Imanishi J, Oku T, Kishida T, Hayaishi O (1981) Induction of pulmonary indoleamine 2,3-dioxygenase by interferon. Proc Natl Acad Sci USA 78(1):129–132. https://doi.org/10.1073/pnas.78.1.129
Yoshihara K, Shahmoradgoli M, Martínez E, Vegesna R, Kim H, Torres-Garcia W et al (2013) Inferring tumour purity and stromal and immune cell admixture from expression data. Nat Commun 4:2612. https://doi.org/10.1038/ncomms3612
Yu T, Bai W, Su Y, Wang Y, Wang M, Ling C (2020) Enhanced expression of lncRNA ZXF1 promotes cisplatin resistance in lung cancer cell via MAPK axis. Exp Mol Pathol 116:104484. https://doi.org/10.1016/j.yexmp.2020.104484
Zhang M, Tian J, Wang R, Song M, Zhao R, Chen H et al (2020) Dasatinib inhibits lung cancer cell growth and patient derived tumor growth in mice by targeting LIMK1. Front Cell Dev Biol 8:556532. https://doi.org/10.3389/fcell.2020.556532
Zhang F, Sang Y, Chen D, Wu X, Wang X, Yang W et al (2021) M2 macrophage-derived exosomal long non-coding RNA AGAP2-AS1 enhances radiotherapy immunity in lung cancer by reducing microRNA-296 and elevating NOTCH2. Cell Death Dis 12(5):467. https://doi.org/10.1038/s41419-021-03700-0
Zhang C, Zhao X, Wang Z, Gong T, Zhao H, Zhang D et al (2023) Dasatinib in combination with BMS-754807 induce synergistic cytotoxicity in lung cancer cells through inhibiting lung cancer cell growth, and inducing autophagy as well as cell cycle arrest at the G1 phase. Invest New Drugs 41(3):438–452. https://doi.org/10.1007/s10637-023-01360-9
Zhou L, Li J, Tang Y, Yang M (2021) Exosomal LncRNA LINC00659 transferred from cancer-associated fibroblasts promotes colorectal cancer cell progression via miR-342–3p/ANXA2 axis. J Transl Med 19(1):8. https://doi.org/10.1186/s12967-020-02648-7
Download references
This work was supported by Yangzhou City Science and Technology Bureau social development-clinical frontier technology project [No.YZ2021078] and Jiangsu Provincial Health Commission Elderly Health Research Project (No. LKZ2022019).
Author information
Mingjun Gao, Mengmeng Wang have contributed equally to this work.
Authors and Affiliations
Dalian Medical University, Dalian, 116000, China
Mingjun Gao, Mengmeng Wang & Yong Chen
Clinical Medical College, Yangzhou University, Yangzhou, 225000, China
Jun Wu, Siding Zhou & Wenbo He
Department of Thoracic Surgery, Northern Jiangsu People’s Hospital, No. 98 Nantong West Road, Yangzhou, 225000, Jiangsu, China
Yusheng Shu & Xiaolin Wang
You can also search for this author in PubMed Google Scholar
Contributions
MJG: Conceptualization, Supervision, Formal analysis, Writing-original draft, Writing—review and editing. MMW: Formal analysis, Writing—review and editing. YC,JW,SDZ,WBH: contributed to discussions and suggestions. YSS, XLW: Reviewed and approved the final version of the manuscript.
Corresponding authors
Correspondence to Yusheng Shu or Xiaolin Wang .
Ethics declarations
Conflict of interest.
The authors declare no competing interests.
Ethics approval and consent to participate
The study was approved by the ethics committee under the Northern Jiangsu People’s Hospital (2021ky012-1). Obtain informed written consent from each patient prior to enrollment. The utilization and program of animals were approved by the Experimental Animal Ethics Committee of Yangzhou University (Ethics number: yzu-lcyxy-s036). All methods are carried out in accordance with relevant guidelines and regulations. The study was conducted in accordance with ARRIVE guidelines.
Consent for publication
Not applicable.
Additional information
Publisher's note.
Springer Nature remains neutral with regard to jurisdictional claims in published maps and institutional affiliations.
Supplementary Information
Below is the link to the electronic supplementary material.
Supplementary file1 (TIF 6680 KB)
Supplementary file2 (tif 1872 kb), supplementary file3 (tif 17354 kb), supplementary file4 (xlsx 10 kb), supplementary file5 (xlsx 20 kb), supplementary file6 (xlsx 60 kb), rights and permissions.
Open Access This article is licensed under a Creative Commons Attribution 4.0 International License, which permits use, sharing, adaptation, distribution and reproduction in any medium or format, as long as you give appropriate credit to the original author(s) and the source, provide a link to the Creative Commons licence, and indicate if changes were made. The images or other third party material in this article are included in the article's Creative Commons licence, unless indicated otherwise in a credit line to the material. If material is not included in the article's Creative Commons licence and your intended use is not permitted by statutory regulation or exceeds the permitted use, you will need to obtain permission directly from the copyright holder. To view a copy of this licence, visit http://creativecommons.org/licenses/by/4.0/ .
Reprints and permissions
About this article
Gao, M., Wang, M., Chen, Y. et al. Identification and validation of tryptophan metabolism-related lncRNAs in lung adenocarcinoma prognosis and immune response. J Cancer Res Clin Oncol 150 , 171 (2024). https://doi.org/10.1007/s00432-024-05665-x
Download citation
Received : 07 December 2023
Accepted : 23 February 2024
Published : 01 April 2024
DOI : https://doi.org/10.1007/s00432-024-05665-x
Share this article
Anyone you share the following link with will be able to read this content:
Sorry, a shareable link is not currently available for this article.
Provided by the Springer Nature SharedIt content-sharing initiative
- Tryptophan metabolism
- Find a journal
- Publish with us
- Track your research
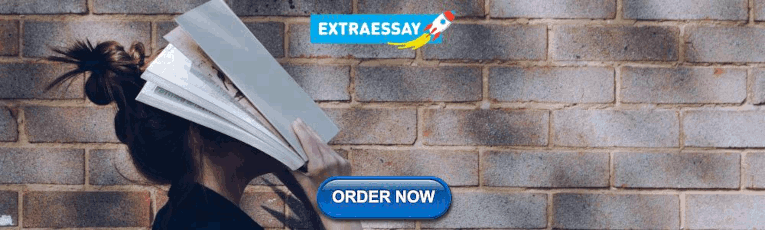
IMAGES
VIDEO
COMMENTS
INTRODUCTION. Scientific research is usually initiated by posing evidenced-based research questions which are then explicitly restated as hypotheses.1,2 The hypotheses provide directions to guide the study, solutions, explanations, and expected results.3,4 Both research questions and hypotheses are essentially formulated based on conventional theories and real-world processes, which allow the ...
Background Identifying determinants of health and understanding their role in health production constitutes an important research theme. We aimed to document the state of recent multi-country research on this theme in the literature. Methods We followed the PRISMA-ScR guidelines to systematically identify, triage and review literature (January 2013—July 2019). We searched for studies that ...
Quantitative Research for the Qualitative Researcher. 2014. SAGE Knowledge. Book chapter . Issues in Validity and Reliability. Show details Hide details. Daniel J. Boudah. Conducting Educational Research: Guide to Completing a Major Project. 2011. SAGE Knowledge. Entry . Quantitative Research.
The current Editorial presents an overview of the special issue, and describes the underlying, translational issues embedded within the issue. We highlight three main themes that emerged, and describe how this work will help to fuel the future directions of quantitative-based research within the Psychological Sciences.
Quantitative research in the social sciences is undergoing a change. After years of scholarship on the oppressive history of quantitative methods, quantitative scholars are grappling with the ways that our preferred methodology reinforces social injustices (Zuberi, 2001).Among others, the emerging fields of CritQuant (critical quantitative studies) and QuantCrit (quantitative critical race ...
By design: Planning research on higher education. By design: Planning research on higher education. (Harvard University Press, 1990). Ioannidis, J. P. A. Why most published research findings are ...
We share how critical quantitative approaches are definite shifts within the quantitative research paradigm, highlight relevant assumptions, and share strategies and future directions for applied practice in this emergent field. Get full access to this article. View all access and purchase options for this article.
A gender equality paradox in academic publishing: Countries with a higher proportion of female first-authored journal articles have larger first-author gender disparities between fields. Mike Thelwall, Amalia Mas-Bleda. Quantitative Science Studies (2020) 1 (3): 1260-1282. Abstract.
Definition. Quantitative method is the collection and analysis of numerical data to answer scientific research questions. Quantitative method is used to summarize, average, find patterns, make predictions, and test causal associations as well as generalizing results to wider populations.
Quantitative research methods are concerned with the planning, design, and implementation of strategies to collect and analyze data. Descartes, the seventeenth-century philosopher, suggested that how the results are achieved is often more important than the results themselves, as the journey taken along the research path is a journey of discovery. . High-quality quantitative research is ...
Revised on June 22, 2023. Quantitative research is the process of collecting and analyzing numerical data. It can be used to find patterns and averages, make predictions, test causal relationships, and generalize results to wider populations. Quantitative research is the opposite of qualitative research, which involves collecting and analyzing ...
The research should pertain to in-service school teachers. 3. The research must be situated in the context of school education. 4. The research should encompass both the operationalization and measurement of teacher digital competences. 5. The research should adopt a quantitative or mixed-methods approach. Exclusion criteria: 1.
Citations are the number of other articles citing this article, calculated by Crossref and updated daily. Find more information about Crossref citation counts. The Altmetric Attention Score is a quantitative measure of the attention that a research article has received online.
Moles and titrations. 5 January 2015. Dorothy Warren describes some of the difficulties with teaching this topic and shows how you can help your students to master aspects of quantitative chemistry. Previous. 1. 2. Next. All Quantitative research articles in RSC Education.
Quantitative research is relatively uncommon in socio-legal studies, which tend, on the whole, to make use of qualitative methodology or take a mixed methodological approach to empirical research. One exception to this was a large-scale randomised telephone survey carried out in the late 1990s in the United Kingdom. This produced some ...
There exist expectations that decisions and programs that affect public and population health are informed by the best available evidence from research, local context, and political will [1,2,3].To achieve evidence-informed public health, it is important that public health organizations engage in and support evidence-informed decision making (EIDM).
For each beer, we measure over 200 chemical properties, perform quantitative descriptive sensory analysis with a trained tasting panel and map data from over 180,000 consumer reviews to train 10 ...
Quantitative research is a research strategy that focuses on quantifying the collection and analysis of data. It is formed from a deductive approach where emphasis is placed on the testing of theory, shaped by empiricist and positivist philosophies.. Associated with the natural, applied, formal, and social sciences this research strategy promotes the objective empirical investigation of ...
5.1 Quantitative Research Methods. Quantitative research uses methods that seek to explain phenomena by collecting numerical data, which are then analysed mathematically, typically by statistics. With quantitative approaches, the data produced are always numerical; if there are no numbers, then the methods are not quantitative.
Revised on 10 October 2022. Quantitative research is the process of collecting and analysing numerical data. It can be used to find patterns and averages, make predictions, test causal relationships, and generalise results to wider populations. Quantitative research is the opposite of qualitative research, which involves collecting and ...
Quantitative research is the process of collecting and analyzing numerical data to describe, predict, or control variables of interest. This type of research helps in testing the causal relationships between variables, making predictions, and generalizing results to wider populations. The purpose of quantitative research is to test a predefined ...
Deductive theory evaluation and testing are typically associated with quantitative research methods (Bitektine, 2008). However, DQA is a qualitative methodology that allows for systematic empirical investigation of existing theory, thus expanding the utility of qualitative research. As with any theory, the results of DQA studies are provisional ...
Quantitative methods emphasize objective measurements and the statistical, mathematical, or numerical analysis of data collected through polls, questionnaires, and surveys, or by manipulating pre-existing statistical data using computational techniques.Quantitative research focuses on gathering numerical data and generalizing it across groups of people or to explain a particular phenomenon.
When collecting and analyzing data, quantitative research deals with numbers and statistics, while qualitative research deals with words and meanings. Both are important for gaining different kinds of knowledge. Quantitative research. Quantitative research is expressed in numbers and graphs. It is used to test or confirm theories and assumptions.
Edible bird's nests have a variety of biological activities, the main components of which are sialic acids. Sialic acids are a group of nine-carbon N-acetylated derivatives of neuraminic acid containing a keto group at position C2 and play important roles in many biological processes. To verify whether the oral administration of edible bird's nests would change the content and distribution ...
Differences in methodological strengths and weaknesses between quantitative and qualitative research are discussed, followed by a data mining exercise on 1,089 journal articles published in Adult Education Quarterly, Studies in Continuing Education, and International Journal of Lifelong Learning. A categorization of quantitative adult education ...
Background Tryptophan (Trp) is an essential amino acid. Increasing evidence suggests that tryptophan metabolism plays a complex role in immune escape from Lung adenocarcinoma (LUAD). However, the role of long non-coding RNAs (lncRNAs) in tryptophan metabolism remains to be investigated. Methods This study uses The Cancer Genome Atlas (TCGA)-LUAD dataset as the training cohort, and several ...