- Search Menu
- Advance articles
- Editor's Choice
- Virtual Issue archive
- The Pitelka Award
- Author Guidelines
- Submission Site
- Open Access
- About Behavioral Ecology
- About the International Society for Behavioral Ecology
- Editorial Board
- Advertising and Corporate Services
- Journals Career Network
- Self-Archiving Policy
- Journals on Oxford Academic
- Books on Oxford Academic
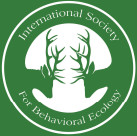
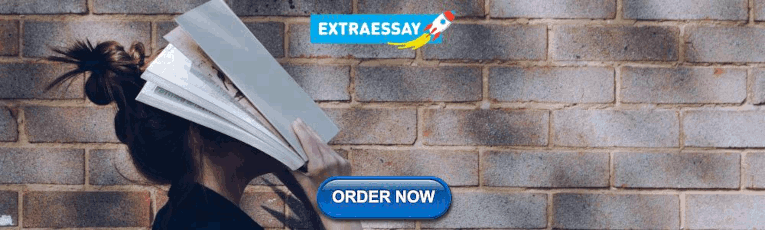
Article Contents
Introduction, supplementary material.
- < Previous
Innovative problem solving in nonhuman animals: the effects of group size revisited
Handling editor: Alexei Maklakov
- Article contents
- Figures & tables
- Supplementary Data
Andrea S. Griffin, David Guez, Innovative problem solving in nonhuman animals: the effects of group size revisited, Behavioral Ecology , Volume 26, Issue 3, May-June 2015, Pages 722–734, https://doi.org/10.1093/beheco/aru238
- Permissions Icon Permissions
Sociality is associated with a variety of costs and benefits, one of which can be to increase the likelihood of individuals solving novel problems. Several hypotheses explaining why groups show higher innovative problem-solving efficiencies than individuals alone have been proposed including the sharing of antipredator vigilance and the pool-of-competence effect, whereby larger groups containing a more diverse range of individuals are more likely to contain individuals with the skills necessary to solve the particular problem at hand. Interference between group members may cause groups to have lower problem solving abilities, however. Using a simulation approach, we model the shape of the relationship between group-level problem-solving probability and group size across a range of facilitation and inhibition scenarios, various population distributions of problem solving, and a task requiring 1 action or 2 actions to be solved. Simulations showed that both sharing of antipredator vigilance and the addition of competent individuals to an existing group lead to positive relationships between group-level problem solving and group size that reach 100% solving probability, whereas interference effects generate group-solving probabilities that rise to a maximum and decrease again, generating a group size for which problem solving is maximized. In contrast, both inhibition and facilitation scenarios generate identical patterns of individual efficiencies. Our results have important implications for our ability to understand the mechanisms that underpin group-size effects on problem solving in nonhumans.
Social systems range from simple aggregations of multiple individuals to complex societies where individuals recognize and negotiate a myriad of transient and lifelong social relationships ( Lott 1991 ; Krause and Ruxton 2002 ; Zuluaga 2013 ). Quantifying the costs and benefits of each of these increasingly more complex social arrangements is central to our understanding of the factors that drive the evolution of sociality ( Giraldeau and Caraco 2000 ; Silk 2007 ). Although the benefits of group living are most typically associated with reduced predation risk ( Elgar 1989 ; Roberts 1996 ; Janson 1998 ; Beauchamp 2010 ), sociality may also benefit individuals by allowing them access to the knowledge and skills of others ( Götmark et al. 1986 ; Galef and Giraldeau 2001 ; Griffin 2004 ). For example, animals that live in groups may use opportunistically the information produced by other, more knowledgeable individuals to detect and/or learn about novel resources ( Galef 1992 ; Reader and Laland 2000 ; Allen and Clarke 2005 ; Aplin et al. 2013 ). More coordinated social interactions may allow individuals to access larger or faster-moving prey, which are difficult to capture alone ( Creel 1995 ; Boesch 2002 ; Hayward and Kerley 2005 ; Lührs et al. 2012 ).
Accessing the skills and knowledge of others may also be beneficial when animals are faced with a novel problem ( Melis et al. 2006 ; Couzin 2009 ; Krause et al. 2010 ; Péron et al. 2011 ; Bräuer et al. 2013 ). For example, sociality may improve problem solving if individuals differ in their personalities, skills, and/or past experiences, such that they vary in their ability to solve particular problems ( Krause et al. 2011 ). Groups containing a more diverse range of individuals should be more likely to contain a problem solver with the knowledge and skills necessary to solve the problem at hand ( Hong and Page 2004 ; Burns and Dyer 2008 ). Once a knowledgeable individual has solved, its behavior becomes available to other individuals to copy. Group-size effects on problem-solving efficiency have long been known in humans ( Hastie 1986 ; Laughlin et al. 2006 ). For example, groups of 3 individuals outperform an equivalent number of single individuals attempting to solve a problem alone or in pairs ( Laughlin et al. 2006 ). Moreover, groups containing individuals with divergent skills have been found to outperform groups of high-performing individuals, suggesting that divergent humans interact synergistically to develop solutions more readily ( Hong and Page 2004 ; Laughlin et al. 2006 ).
Although research investigating the relationship between problem-solving efficiency and group size has yielded mixed results ( Liker and Bókony 2009 ; Overington et al. 2009 ; Morand-Ferron and Quinn 2011 ; Griffin et al. 2013 ), 2 studies in recent years have revealed that nonhuman animals may also show an increase in problem-solving efficiency with increased group size ( Liker and Bókony 2009 ; Morand-Ferron and Quinn 2011 ). In nonhuman animals, solving of novel problems, referred to as innovative problem solving, is most often operationalized by measuring an individual’s success in solving, or latency to solve, an extractive foraging task consisting of some kind of container that must be opened to access food ( Cole et al. 2011 ; Benson-Amram and Holekamp 2012 ; Thornton and Samson 2012 ; Griffin et al. 2013 , 2014 ; for a review, see Griffin and Guez 2014 ). Liker and Bókony (2009) measured the ability of wild-caught, captive-held house sparrows ( Passer domesticus ) to access 3.5-cm diameter wells containing food. Each well was covered with a plastic lid that needed to be removed to access the food. The proportion of individual birds that were successful in accessing a well was significantly larger in groups of 6 birds than in groups of 2. In addition, the per capita number of wells opened was significantly higher in the larger groups. In the second study, Morand-Ferron and Quinn (2011) measured the ability of free-ranging mixed species flocks of Passerines containing blue tits ( Cyanistes caeruleus ) and great tits ( Parus major ) to solve arrays of 6 lever-pulling devices. Two levers needed to be pulled sequentially, in any order, for the food to be released. Results showed that the proportion of devices solved by the group increased with increasing group size. Both studies concluded that increased group diversity offered the most likely explanation for the positive relationship between problem-solving efficiency and group size ( Liker and Bókony 2009 ; Morand-Ferron and Quinn 2011 ). Larger groups contained a more diverse range of individuals making them “more likely to contain individuals with specific skills, individual tendencies, or past experience, making them competent at solving the current problem,” a group-size effect on problem solving coined the “pool-of-competence” effect ( Morand-Ferron and Quinn 2011 ).
However, a prerequisite to establishing a pool-of-competence effect is to eliminate other potential alternative explanations for why problem-solving efficiency may increase in larger groups. First, if the presence of other individuals allows for antipredator vigilance to be shared, as has been found in the context of foraging behavior ( Elgar 1989 ; Beauchamp and Livoreil 1997; Beauchamp 1998; Lima and Bednekoff 1999; Beauchamp and Ruxton 2003; Bednekoff and Lima 2004 ), then problem-solving efficiency should increase in the presence of other individuals. Second, if the presence of other individuals socially facilitates approach and exploration, either via reduced neophobia or increased scramble competition, then individuals should also have higher solving probabilities in the presence of others (Coleman and Mellgren 1994; Visalberghi et al. 1998; Visalberghi and Addessi 2000).
Liker and Bókony (2009) ruled out that increased per capita problem-solving success in house sparrows was attributable to shared vigilance by showing that individual scan rates did not differ significantly among small and large groups. The authors also ruled out a mediating role of neophobia, exploration, and scramble competition by showing that neither individual latency to approach the problem-solving task nor the per capita attempt rates differed between 2-bird and 6-bird groups. Consequently, the possibility that larger groups were more likely to contain a problem solver and that the presence of a problem solver increased the solving rates of other members of the group remains a possible but untested explanation for the positive relationship between problem-solving efficiency and group size in this study ( Liker and Bókony 2009 ).
Morand-Ferron and Quinn (2011) proposed an alterative approach to disentangling a pool-of-competence effect from other facilitation effects. The crux of their argument was that antipredator benefits should diminish as group size becomes larger, whereas the pool-of-competence effect should lead to a linear increase of problem-solving efficiency with group size. This argument hinges on the finding that the antipredator benefits of group size in birds have been found to level off at intermediate group sizes ( Fernández-Juricic et al. 2007 ; Cresswell and Quinn 2011 ). Consequently, if antipredator benefits underpin the positive relationship between problem-solving efficiency and group size, then the relationship should also level off. In contrast, under the assumption that larger pools of individuals become increasingly more likely to contain problem solvers, a pool-of-competence effect should lead to problem-solving efficiency increasing linearly with group size.
Increases in group size may not always be associated with benefits. If problem solving is vulnerable to interference competition, from kleptoparasitism for example, then the frequency of problem solving should decrease in the presence of other individuals when compared with solitary conditions as has been found for food processing behaviors ( Overington et al. 2009 ). If problem solving is vulnerable to a “negotiation over risk,” then problem solving may also be reduced in larger groups ( Stöwe et al. 2006 ; Griffin et al. 2013 ).
Although there has been a substantial effort to model the effects of group size on foraging behavior in nonhuman animals ( Shaw et al. 1995 ; Bednekoff and Lima 1998a , 1998b ; Giraldeau and Caraco 2000 ; Bednekoff and Lima 2004 ), to our knowledge, there have been no previous attempts to model the effects of group size on innovative problem solving. Here, we use a theoretical modeling approach to simulate how the likelihood of a group solving a hypothetical 1-action extractive foraging task varies with group size under different facilitation and inhibitory scenarios. We simulated the effects of group size attributable to adding additional competent individuals to an existing group by assuming that the individual problem-solving probability remained stable as group size increased. Group-size benefits attributable to shared vigilance were modeled by increasing individual problem-solving probabilities each time an additional individual was added to the group, whereas inhibitory effects of group size on group problem solving were modeled by decreasing individual problem-solving probabilities each time an individual was added to the group. Our aim was to evaluate to what extent the relationship between problem-solving efficiency and group size varied in shape across a range of facilitation and inhibition scenarios, allowing for different processes to be identified. Current analyses of problem-solving ability have revealed both within-individual stability in problem-solving propensity and contextual variability ( Laland and Reader 1999 ; Reader 2003 ; Cole et al. 2011 ; Morand-Ferron et al. 2011 ; Griffin et al. 2013 ). Consequently, we modeled the case in which problem solving is assumed to be a stable individual trait, and populations contain both problem solvers and nonproblem solvers. We also modeled the case in which all individuals within a population have a low but equal probability of solving. Finally, we modeled the case in which individuals express specialized problem-solving abilities, and the solving skill of one individual is complementary to the solving skill of another individual.
We considered theoretical populations of animals with varying distributions of problem-solving propensity. We then simulated drawing random samples of individuals without repetition from these populations and calculated a solving probability for each sample. We varied the group size of each sample from 1 to 100 individuals, and for each group size, we calculated an average solving probability by averaging the solving probabilities of 50 independent sampling events.
Consistent with most recent studies on problem solving in nonhuman animals, which operationalize problem solving by measuring their success in opening a container to access food ( Benson-Amram and Holekamp 2012 ; Cole and Quinn 2012 ; Sol et al. 2012 ; Thornton and Samson 2012 ; Bókony et al. 2014 ), we varied the individual probability of solving a hypothetical extractive foraging task. We elected to model separately the effects of solving a 1-action task or a 2-action task. In a 1-action task, animals only need to perform 1 motor action to solve the task, whereas in the 2-action task, animals need to perform 2 motor actions. The 2-action task allowed us to model a scenario in which individuals specialized in 1 kind of motor action interact with another individual specialized a different motor action to solve a given problem. Two-action tasks have recently been proposed to provide a useful assay for measuring innovative problem-solving abilities in nonhumans ( Auersperg et al. 2012 ), so we considered it important for future research to model group-size effects on this type of task.
Theoretical populations
We considered 3 theoretical populations of 1000 individuals each. Each population had a distinct distribution of problem-solving propensity. The first theoretical population had a binomial distribution of problem-solving propensity. The solving probability of problem solvers was fixed at 0.1, whereas that of nonproblem solvers was fixed at 0.01. The frequency of problem solvers within the population was fixed at 10%. The second theoretical population had a bell-like distribution of problem-solving propensity. The population was generated by assuming a beta continuous probability distribution between 0 and 1. The parameters used to generate the population were α = 5 and β = 5. The final population is represented in Figure 1 , panel A. In our third theoretical population, we created a skewed distribution of problem-solving propensity. Once again, the population was generated by assuming a beta continuous probability distribution between 0 and 1, but this time, we assumed that problem solvers were much more rare within the population than nonproblem solvers. The parameters used to generate the population were α = 1 and β = 5. The final population is represented in Figure 1 , panel B. To explore to what extent our results were sensitive to variation in these particular population parameters, we created populations with other parameter sets and repeated our simulations (see below). The distributions of problem-solving propensity obtained using alternative parameter sets are presented in Supplementary Figures S1 and S5 .

Histogram of the distribution of problem-solving propensity within a range of different populations for a 1-action task (A and B) and a 2-action task (C and D). (A) Bell-like probability distribution of problem-solving ability for a 1-action task (beta distribution with α = 5 and β = 5). (B) Skewed distribution of problem-solving ability for a 1-action task (beta distribution with α = 1 and β = 5). (C) Bell-like probability distribution of problem-solving ability for a 2-action task (beta distribution with α = 30 and β = 30). (D) Skewed distribution of problem-solving ability for a 2-action task (beta distribution with α = 0.5 and β = 5).
Simulations
In a first series of simulations, we considered that our random sample of individuals was presented with a 1-action task that required only 1 motor action to be solved. This simulation was done under 3 different theoretical conditions. The first and simplest assumed that group size did not affect individual solving probability. In the second, we assumed that individual problem-solving probability increased with group size uniformly by a small amount. Under natural conditions, this effect would arise if individuals in groups shared antipredator vigilance and could allocate more attention to solving the task at hand. The third theoretical condition assumed that individual problem-solving abilities decreased uniformly by a small amount with group size. An example of a natural correlate of decreasing individual problem-solving probability would be that associated with an increased risk of interference competition (e.g., kleptoparasitism; intragroup aggression) with increasing group size. Increased density of conspecifics is known to be associated with reduced expression of behaviors prone to kleptoparasitism (i.e., food dunking; Morand-Ferron et al. 2004 ). Hence, we considered it reasonable to assume that individual solving probabilities may decrease with increasing group size because individuals would similarly withhold from problem solving.
In a second series of simulations, we considered that our random sample of individuals was presented with a 2-action task that could only be solved using 2 independent motor actions in any order ( Auersperg et al. 2012 ). In these simulations, we considered that each individual within our theoretical population had a different probability of performing each action. Because 2-action tasks involve 2 different actions, and not a repeat of the same action, and that empirical work has demonstrated that solving rates can vary across different kinds of tasks (e.g., Bókony et al. 2014 ), we considered it more realistic to assume that the probabilities of solving would be different for different actions. The probability distribution of the first action conformed to that described for the 1-action task described above. The probability distribution of the second motor action among the theoretical population with a bell-like distribution of problem-solving propensity is depicted in Figure 1 , panel C (beta distribution parameter α = 30 and β = 30), whereas the probability distribution of the second motor action within the theoretical population with skewed distribution of problem-solving propensity is depicted in Figure 1 , panel D (beta distribution parameter α = 0.5 and β = 5). In the case of the theoretical population with a binomial distribution of problem-solving propensity, the frequency of problem solvers within the population was still 10%, but problem solvers had a solving probability of 0.05, whereas nonproblem solvers had a solving probability of 0.005. Under these conditions, a given individual could have a high or low probability of solving via the first motor action, whereas having a high or low probability of solving via the second motor action. In other words, there was no link between the probability of using one action and the probability of using the other action. In the case of the 1-action task, the probability of the group solving was calculated by summing individual solving probabilities because the task could be solved by one group member or another. In the case of a 2-action task, the probability of the group solving was calculated as the product of the sum of the individual probabilities of solving each task. This is because solving the task required that both actions be performed in any order; however, either individual could perform either action. So, if Pa1 and Pa2 are the probabilities of solving using action A of individuals 1 and 2, respectively, and Pb1 and Pb2 are the probabilities of solving using action B for individuals 1 and 2, respectively, then the probability of solving the 2-action task can be calculated as P = (Pa1 + Pa2) × (Pb1 + Pb2).
In addition to the 3 theoretical conditions described above (stable, increasing, and decreasing individual problem-solving probabilities), we modeled group-level problem-solving probability under scenarios where the benefits (or costs) gained by (or imposed on) each additional individual changed exponentially (rather than being a uniform increase or a uniform decrease). First, we assumed that the problem-solving benefit decreased exponentially with each additional individual. Under natural conditions, this would arise if individuals in groups share vigilance, but these individual-level benefits level off beyond certain group sizes ( Elgar 1989 ; Beauchamp and Livoreil 1997; Beauchamp 1998; Lima and Bednekoff 1999; Beauchamp and Ruxton 2003; Bednekoff and Lima 2004 ). Second, we assumed that the problem-solving cost increased exponentially with each individual added to the group, as would arise if the probability of interference competition, such as kleptoparasitism, intragroup aggression, or vigilance toward competitors, increased with group size. Increasing penalties could arise because the probability of a thief being present becomes higher or because the number of individuals available to steal from becomes higher. For the sake of completeness, we also modeled an exponentially increasing benefit and an exponentially decreasing cost, even though we do not think that these conditions have any biological equivalent. As these simulations did not change our general conclusions, we provide the outcomes of these simulations in the supplementary materials (for exponentially decreasing benefits and costs, see Supplementary Figures S9–S11 ; for exponentially increasing benefits and costs, see Supplementary Figures S12–S14 ).
Finally, past empirical work has quantified group-size effects on problem solving either by measuring performance of increasingly large groups (e.g., proportion of devices solved by the group; Morand-Ferron and Quinn 2011 ) or by calculating a per capita solving performance by dividing each group’s performance by the number of group members (e.g., Liker and Bókony 2009 ; Griffin et al. 2013 ). Per capita solving measures (e.g., number of tasks solved per individual or number of tasks solved per individual per unit time) allow for the solving performances of groups of different sizes to be compared and are therefore taken to provide a measure of group efficiency ( Morand-Ferron and Quinn 2011 ). Given that they are calculated at the individual level, however, we refer to them here as “individual efficiencies.” In order to ensure that the outcomes of our models could be compared with empirical data sets, we modeled the effects of increasing group size not only on group-level solving probability but also on individual efficiency for a subset of our theoretical populations and simulation scenarios. Per capita solving performances were calculated for simulations involving binomial, bell-like, and skewed populations and uniformly increasing and decreasing individual solving probabilities, exponentially decreasing costs and benefits, and exponentially increasing costs and benefits for both a 1-action task and a 2-action task. Consistent with the literature where group efficiencies are calculated by dividing a group-level performance measure by the number of individuals in the group, per capita solving performances were calculated by dividing group-level solving probabilities by the number of individuals within the group at each stepwise increase in group size.
All simulations were run using Scilab 5.4.0 software for numerical computation available at www.scilab.org .
One-action task
In this series of simulations, we evaluated the solving probability of an extractive foraging task assuming 3 possible theoretical populations differing in the distribution of their problem-solving propensities (binomial; bell-like, Figure 1A ; skewed, Figure 1B ). We simulated the effect of group size on the probability of solving assuming 1) no variation of individual solving probabilities as a function of group size, 2) a uniformly increased probability of individual solving propensity with group size, and 3) a uniformly decreased probability of individual solving propensity with group size.
Assuming no change in individual solving probability as a function of group size, the probability of solving a 1-action task increased steadily with group size and reached 1 regardless of the distribution of problem-solving propensity within the population ( Figure 2 , left column). This pattern of results did not change when we assumed that the probability of individual problem solving increased with increasing group size, as would be the case if individuals shared antipredator vigilance. Specifically, in a scenario where individuals became slightly more likely to solve each time group size increased, the probability of solving a 1-action task increased steadily with group size and reached 1 regardless of the distribution of problem-solving propensity within the population ( Figure 2 , ✭ symbols). The larger the individual gain associated by increased group size (0.01, 0.005, or 0.0005 for the binomial distribution; 0.01, 0.005, or 0.015 for the bell-like or skewed distributions), the faster the positive relationship between group solving probability and group size increased and reached 1 ( Figure 2 ).

Average solving probability of a hypothetical 1-action problem-solving task as a function of group size in scenarios where individual solving probabilities remained constant (▼ symbols in left column), increased (✭; e.g., via shared antipredator vigilance), or decreased (○ symbols; e.g., via interference competition) with increasing group size. Solving probabilities were increased or decreased by a factor of 0.01, 0.005, and 0.0005 (binomial) or 0.01, 0.005, and 0.015 (bell-like and skewed). For each group size, averages were calculated across 50 independent samples drawn with no repetition from populations with a binomial Figure 1 panel (A), bell “like” Figure 1 panel (B), or skewed Figure 1 panel (C) distributions of problem-solving propensities.
Calculating individual efficiencies at each stepwise increase in group size assuming that individual problem-solving propensity remained stable (i.e., pool-of-competence effect) or increased (e.g., shared vigilance) with increased group size revealed that per capita solving performance consistently increased to a maximum and decreased again as group size increased, and this regardless of the distribution of problem solving within the original population ( Figure 3 , different rows), but also regardless of whether individual solving probability remained stable ( Figure 3 , left column) or increased steadily with group size by any given amount ( Figure 3 , ✭ symbols, different columns).

Individual solving efficiency of a hypothetical 1-action problem-solving task as a function of group size in scenarios where individual solving probabilities remained constant (▼ symbols in left column, e.g., via a pool-of-competence effect), increased (✭ symbols; e.g., via shared antipredator vigilance), or decreased (○ symbols; e.g., via interference competition) with increasing group size. Solving probabilities were increased or decreased by a factor of 0.01, 0.005, and 0.0005 (binomial) or 0.01, 0.005, and 0.015 (bell-like and skewed). For each group size, averages were calculated across 50 independent samples drawn with no repetition from populations with a binomial Figure 1 panel (A), bell-like Figure 1 panel (B), or skewed Figure 1 panel (C) distributions of problem-solving propensities in.
The shape of the relationship between group-solving probability and group size completely changed when we assumed that individual problem-solving propensity decreased with increased group size, as would be the case if the probability of interference competition increased with increasing group size. Under this assumption, group-solving probability increased and then decreased again either side of a group size whereby group-solving probability was maximized ( Figure 2 , ○ symbols). Considered with regard to problem solving, this “optimal” group size varied from 3 to around 40 individuals, depending on the distribution of individual problem-solving probabilities within the population and the amplitude of the reduction in individual problem-solving probability with increasing group size ( Figure 2 , ○ symbols).
In a case where individual problem-solving probability followed a bell-like distribution, group-solving probability reached 100% rapidly with increased group size ( Figure 2 , middle row, ○ symbols) before decreasing sharply when group size increased further. In contrast, in cases where the distribution of individual problem-solving propensity followed a binomial or skewed distribution within the population, maximum group-solving probability was clearly maximized for an optimal group size, but never reached 100%. Assuming a binomial distribution of individual solving probability within the population, and a 0.01 penalty for each additional individual in the group, the group size for which problem solving was maximized was 3–4 and the maximum solving probability reached approximately 5% ( Figure 2 , first row, second column). With a smaller penalty of 0.005 for each additional individual in the group, optimal group size increased to 4 and maximum solving probability to approximately 6% ( Figure 2 , first row, third column). Finally, with an even smaller penalty of 0.0005 for each additional individual in the group, optimal group size increased to between 35 and 40 and maximum solving probability to approximately 40% ( Figure 2 , first row, fourth column).
Assuming that the distribution of problem-solving propensity was skewed within the population, and a 0.005 penalty for each additional individual within the group, the optimal group size was around 18 individuals, and the maximum solving probability fell just short of 100% ( Figure 2 , third row, third column). With a larger penalty of 0.01 per individual added to the group ( Figure 2 , first row, second column), optimal group size decreased to around 12, and the maximum solving probability decreased to approximately 85%. With an even larger penalty of 0.015 ( Figure 2 , third row, fourth column), optimal group size decreased even further to approximately 9, and the maximum solving probability decreased to around 75%.
In sum, within the range of group sizes explored here, the simulations with decreasing individual problem-solving probabilities with increasing group sizes showed that both optimum group size and maximum solving probability changed when the penalty on individual problem solving changed, whether the distribution of problem-solving propensity was binomial ( Figure 2 , first row) or skewed ( Figure 2 , third row) within the population. In contrast, when the distribution of problem-solving propensity within the population followed a bell-like distribution ( Figure 2 , second row), only the optimal group size decreased with increasing penalties for adding additional individuals to the group.
Calculating individual efficiencies at each stepwise increase in group size in scenarios where we assumed that individual problem-solving propensity decreased with increased group size (i.e., interference competition) revealed that per capita solving performance consistently increased to a maximum and decreased again, and this regardless of the distribution of problem solving within the original population ( Figure 3 , different rows) and regardless of the amplitude of the individual penalty associated with increased group size ( Figure 3 , ○ symbols, different columns).
The patterns of group solving probability and individual efficiencies described above remained unchanged when we used alternative parameter sets to describe the distribution of problem solving within the initial populations (see Supplementary Figures S2 and S3 and S6 and S7 ). Our conclusions also remained unchanged when we repeated the simulations assuming that the benefit (or cost) associated with each additional individual decreased (or increased) exponentially as group size increased, as would be the case if individual antipredator vigilance benefits leveled off or if the probability of interference competition (e.g., kleptoparasitism; intraspecific aggression) became gradually higher, with increasing group sizes. These simulations are presented in Supplementary Figures S9–S14 .
Two-action task
The simulations considering that individuals were presented with a 2-action task revealed patterns of problem-solving probabilities that were strikingly similar to those revealed when considering that individuals were presented with a 1-action task. Regardless of the distribution of problem-solving propensity within the population, the probability of group-solving increased with increasing group size, and reached one, whether we assumed that individual problem-solving probability remained constant as additional individuals were added to the group ( Figure 4 , first column) or that individual problem-solving probability increased as additional individuals were added to the group ( Figure 4 , ✭ blue symbols). The only effect of increasing individual problem-solving probabilities as group size increased rather than maintaining them stable was to make the already positive relationship steeper. This effect was most visible when the distribution of problem-solving propensity within the population followed a binomial distribution ( Figure 4 , first row).

Average solving probability of a hypothetical 2-action problem-solving task as a function of group size in scenarios where individual solving probabilities remained constant (black ○ symbols in left column; e.g., via a pool-of-competence effect), increased (✭ blue symbols; e.g., via shared antipredator vigilance), or decreased (○ magenta symbols; e.g., via interference competition) with increasing group size. Solving probabilities were increased or decreased by a factor of 0.01, 0.005, and 0.0005 (binomial) or 0.01, 0.005, and 0.015 (bell-like and skewed). For each group size, averages were calculated as indicated in the legend of Figure 3 .
As for the 1-action task, when we assumed that individual problem-solving propensity decreased with additional individuals added to the group, the positive relationship between group problem solving and group size changed from a positive relationship to one with an optimal group size, for which group problem solving was maximized ( Figure 4 , ○ magenta symbols). The effects of decreasing individual solving probabilities with increasing group size were particularly dramatic if individual problem-solving propensity within the population for a 2-action task followed a binomial distribution. In this case, group-solving probability only rose substantially above 0 if the penalty of adding additional individuals was extremely low. For example, assuming a penalty of 0.0005, the maximal group solving probability plateaued at 0.025, with an optimal group size of around 23 individuals ( Figure 4 , fourth column).
In contrast, assuming that the distribution of individual problem-solving ability within the population followed a bell-like distribution, the requirement of a second motor action to solving generated relationships between solving probabilities and group size that are very similar to those generated using a 1-action task ( Figure 4 , second row, columns 2–4 vs. Figure 2 , second row).
Finally, in the case where the distribution of individual problem-solving ability followed a skewed distribution, introducing the requirement of a second motor action to solve the extractive foraging task decreased not only the optimum group size but also the maximum group-solving probabilities for each group size ( Figure 4 , third row, columns 2–4 vs. Figure 2 , third row).
Calculating individual efficiencies at each stepwise increase in group size when we assumed that individual problem-solving propensity for a 2-action task remained stable, increased uniformly, or decreased uniformly showed the same pattern as for a 1-action task. Per capita solving performance consistently rose to a peak and then decreased again, and this regardless of the distribution of problem solving within the original population ( Supplementary Figure S15 , different rows) and regardless of the amplitude of the individual penalty ( Supplementary Figure S15 , magenta symbols) or gain ( Supplementary Figure S15 , blue symbols) associated with increased group size ( Supplementary Figure S15 , different columns). Assuming that penalties/gains associated with increased group size increased/decreased exponentially, rather than uniformly, as a function of group size for populations with a skewed distribution of problem solving for a 1-action task did not change the shape of the relationship between individual efficiency and group size ( Supplementary Figure S16 ).
Using alternative parameter sets to describe the distribution of problem solving for 2-action tasks within the initial populations did not change the overall pattern of our results ( Supplementary Figures S4 and S7 ), nor did modeling group problem solving probability assuming exponentially increasing and decreasing costs and benefits ( Supplementary Figures S9–S14 ).
It has been suggested that increasing problem-solving efficiencies with increasing group sizes in nonhuman animals is mediated by a pool-of-competence effect. This is the idea that as group size increases, and with it, the diversity of individuals within the group, the presence of a problem solver with the skills suited to solving the particular task at hand becomes more likely, such that larger groups have a higher probability of problem solving than smaller groups ( Liker and Bókony 2009 ; Morand-Ferron and Quinn 2011 ). An alternative reason why performance may increase with increasing numbers of individuals is that each member is able to allocate less time to antipredator vigilance and hence more time to solving the task. In order to determine whether these mechanisms could be disentangled, we modeled the pool-of-competence effect by drawing individuals from a pool of competent and noncompetent individuals with varying distributions of problem-solving probability and adding them to a group without changing their individual problem-solving probabilities. We modeled antipredator benefits by drawing individuals from a pool of competent and noncompetent individuals once again, but this time increasing their problem-solving probabilities as they were added to the group. We found that regardless of the theoretical distribution of problem-solving propensity within the population, and regardless of whether individual problem-solving ability was maintained constant or increased as groups became larger, the relationship between group size and group problem–solving probability was consistently positive and rose to 1, with the only difference that increasing individual problem-solving probabilities at each stepwise increase in group size caused a steeper positive relationship. In addition, calculating per capita solving performance based on group solving probabilities revealed that regardless of the distribution of problem-solving ability within the population and regardless of whether individual problem-solving ability was maintained constant or increased as groups became larger, the relationship between group size and individual efficiency rose to a maximum and then dropped off again as group performance reached 1. These findings did not change when we considered an extractive foraging task that required 2 motor actions to be solved and in which individuals with different sets of skills could cooperate to solve the problem ( Péron et al. 2011 ). To examine what form the relationship between group size and solving probability would take in a case where group members interfered with each other as the group became larger, we simulated scenarios in which adding more individuals to a group decreased the probability of each individual solving. In this case, the likelihood of solving by the group increased to an optimal group size and then decreased again. These findings indicate that the shape of the relationship between group size and group problem solving can be used to distinguish competitive interference from group size–associated benefits. However, within the latter, a pool-of-competence effect cannot be disambiguated from shared antipredator vigilance benefit.
One might argue that individuals could benefit at first from being with others, but that those benefits may plateau as group size continues to increase, as has been found in the foraging context ( Fernández-Juricic et al. 2007 ; Cresswell and Quinn 2011 ). However, explicitly modeling this particular scenario by decreasing the antipredator benefit exponentially each time an individual was added to the group did not change the general pattern of our results. The relationship between group size and group problem–solving probability remained positive, gradually increasing to 1 ( Supplementary Figures S9–S14 ). Intuitively, this consistent increase occurs because even though individual benefits become gradually smaller, adding additional, potentially competent, individuals to the group continues to increase the likelihood of the group solving. In an alternative scenario, increased group size may be beneficial at first, but then become detrimental. For example, in humans, problem-solving performance increases up to groups with 3 members to above those levels exhibited by an equivalent number of individuals alone, and then stabilizes for groups of 4 and 5, an effect attributed to interference between group members ( Laughlin et al. 2006 ). The present results lead to the prediction that switching from benefits to costs at a specific group size would yield a group solving probability that increases at first and then decreases again. It is also important to note that an assumption of all our simulations was that all individuals in a group, independent of its size, had access to the problem to be solved. Failure to meet this assumption would be equivalent to drawing individuals solely from the pool of nonsolvers once the problem became inaccessible. This is the only scenario that produces a leveling off of group problem solving probability as group size increases beyond a certain upper limit.
Past empirical work measuring problem-solving performance of groups has quantified group-size effects using 2 distinct approaches ( Figure 5 ). The first quantifies performance of groups with increasing numbers of members (e.g., proportion of devices solved by the group; Morand-Ferron and Quinn 2011 ), whereas the second quantifies group performance and then calculates a per capita solving performance (e.g., Liker and Bókony 2009 ; Griffin et al. 2013 ). Per capita solving rates, which might be considered a measure of “group efficiency,” are expressed either as a number (or percentage) of problems solved per individual (e.g., Liker and Bókony 2009 ; Griffin et al. 2013 ) or a number (or percentage) of problems solved per individual per unit time ( Laughlin et al. 2006 ) and allow for the performance of groups of different sizes to be compared. Systematically varying individual solving probabilities to model a pool of competency effect, shared antipredator vigilance and interference competition, simulating group-level solving performance, and then back calculating per capita solving performances at each stepwise increase in group size revealed that per capita solving performances rose to a peak and then decreased again regardless of the theoretical distribution of problem-solving propensity within the population and regardless of whether individual problem-solving ability remained constant or changed exponentially as groups became larger. These results indicate that per capita solving performances calculated from measured group-level performance data do not allow for mechanisms underpinning group-size effects to be disentangled ( Figure 5 ). This contrasts with analyses of group level performance relative to group size, which, as discussed above, can be used to distinguish group size–associated costs (e.g., competitive interference) from group size–associated benefits, but within the later, cannot disambiguate pool-of-competence from a shared antipredator vigilance effects ( Figure 5 ).

Conceptual relations between empirical and simulated approaches to studying group-size effects on problem-solving performance. Empirical group performance measures and simulated group solving probabilities are equivalent. In contrast, per capita solving performances (at times used loosely to refer to the efficiency of a group in the literature) and individual solving probabilities are only equivalent if individual solving efficiencies are measured (not shown; e.g., Overington et al. 2009 ) rather than calculated on the basis of group level performance (shown; e.g., Morand-Ferron and Quinn 2011 ). Our simulations show that calculated individual efficiencies cannot be used to disentangle any type of group size–mediating mechanism, whereas group level measures allow for group size–associated costs (i.e., interference competition) to be distinguished from group size–associated benefits, but without identifying a benefit mechanism (i.e., pool of competence vs. shared antipredator vigilance).
The findings from this theoretical analysis are at odds with the prediction that group-size benefits attributable to an increasing number of problem solvers with a diverse range of skills can be distinguished from group-size benefits attributable to shared vigilance by examining the shape of the relationship between problem-solving efficiency and group size ( Morand-Ferron and Quinn 2011 ). It has been suggested that a pool-of-competence effect on problem solving should yield a linear increase in problem-solving efficiency as group size increases, whereas a shared antipredator vigilance should result in a relationship that increases at first and then levels off because the importance of antipredator benefits decrease as group size increases ( Morand-Ferron and Quinn 2011 ). Whereas the empirical approach involves measuring group performance and then calculating group efficiencies to disentangle mechanisms underpinning group-size effects (e.g., shared vigilance), here, we used a modeling approach in which we fixed individual solving probabilities assuming specific underpinning mechanisms and modeled their effect on group performance and individual efficiencies ( Figure 5 ). Modeled at the group level, simulations of both the pool-of-competence effect and shared antipredator vigilance both produced a positive relationship between group problem solving and group size, which reached a solving probability of 100%. Considered at the individual level, a pool-of-competence effect, that is, the increasing likelihood that a group will contain a competent individual as group size increases ( Morand-Ferron and Quinn 2011 ), will be reflected by individual solving rates that remain stable as group size increases. If competent individuals facilitate problem solving in other members of the group, then the individuals that learn will show an improved solving probability, leading, at the group level, to an even steeper increase of solving probability as group size increases compared with the case where no learning occurred. In contrast, at the individual level, a shared antipredator vigilance benefit will be reflected by increasing individual solving rates as groups become larger. If this benefit is maximum for a given group size, individual solving probability will plateau at this group size. However, our simulation shows that none of these distinct patterns of individual-level effects can be detected by calculating individual efficiencies based on group-level performance. This is because both the pool-of-competence effect and shared antipredator vigilance produce calculated individual efficiencies that rise to a peak and then decrease again. In order to demonstrate an antipredator vigilance effect, excluding a pool-of-competence effect, one would need to show that individual solving performances increase in the presence of increased numbers of conspecifics that cannot interact with the problem-solving task ( Overington et al. 2009 ). That is to say that individual solving performance variables need to be measured rather than calculated on the basis of group-level performance data ( Figure 5 ).
Overall, the outcomes of the simulations presented here are consistent with the suggestion that group diversity may underpin a positive relationship between group size and group performance in nonhumans but highlight the need for detailed measurement of individual solving performance in the presence of other individuals that cannot interact with the task ( Overington et al. 2009 ) rather than calculation of per capita solving performances based on group performance to establish with certainty the role of this mechanism. Individual specializations in foraging behavior are well documented across a broad range of vertebrates and can emerge as a consequence of the formation of search images, chance events, and individual learning biases. Skill pool effects have been predicted to maintain individual specializations and therefore diversity in foraging behaviors within species ( Giraldeau 1984 ). The mere addition of individuals with different foraging strategies to a group increases the availability of demonstrators within a group and opportunities for individuals to copy the behavior of others and thereby increase their own foraging efficiencies ( Galef and Giraldeau 2001 ). Liker and Bókony (2009) found that larger groups of sparrows had neither higher proportions of birds trying to solve nor higher attempt rates per capita. However, larger groups contained significantly larger proportions of individuals that succeeded among birds that were trying, indicating a higher conversion rate from trying to succeeding. Hence, with larger numbers of competent individuals available in the group, the pool-of-competence effect, individuals motivated to try presumably copied some aspects of the solving behavior of successful individuals. Social learning of this kind has been demonstrated in pigeons ( Columba livia ) ( Palameta and Lefebvre 1985 ) and in various species of tits ( Parus sp.) and thrushes ( Turdus sp.) ( Sasvari 1985 ; Aplin et al. 2013 ).
Our simulations were based on populations with either a binomial, bell-like, or skewed distribution of problem-solving propensity. Recent analyses indicate that innovative problem-solving ability is stable across time ( Cole et al. 2011 ), tasks ( Griffin and Diquelou 2015 ), and some ( Griffin et al. 2013 ), but not other ( Cole et al. 2011 ; Sol et al. 2012 ), contexts, suggesting that innovative problem solving should be regarded as a personality trait and hence underpinned by a specific genetic makeup. On the other hand, innovative problem solving is influenced by state-dependent variables ( Laland and Reader 1999 ) and motivational factors ( Benson-Amram and Holekamp 2012 ; Thornton and Samson 2012 ; Griffin et al. 2014 ), which most likely interact with personality-dependent expression biases to determine the final probability of problem solving. Although there are currently no descriptions of the distributions of innovation propensity within natural populations, these considerations together with the general view that inventing a solution to a new problem is a rare event within wild populations ( Reader and Laland 2003 ) suggest that either a skewed or a discrete binomial distribution of the type presented here is most likely to be representative of innovative problem-solving propensity within natural populations. For example, if problem-solving propensity was determined by a single gene with 2 alleles, one of which is common and associated with low innovation propensity, the other of which is rare and associated with high innovation tendency, then one would expect innovation propensity to follow a skewed binomial distribution. If, instead, we assume that this single gene has multiple alleles each associated with a discrete problem-solving propensity, with the rare variants being associated with higher innovation propensities, one would expect that innovation propensity would be distributed following a multinomial distribution skewed toward 0. Another possibility is to consider that innovation propensity is the result of the interaction of multiple genes. In this case, that innovation propensity would be best described by a continuous skewed distribution, similar to our skewed distribution of problem solving, with each individual problem-solving propensity the result of the interaction of these genes. Regardless of which of these distributions is appropriate in a given species, the fact that inventing a solution to a new problem appears to be a rare event, which is best described at the population level by a skewed distribution of innovation propensity, suggests that there is a fitness cost to high innovation propensity. These costs may be a consequence of exposure to the risks inherent to innovating ( Greenberg 2003 ). Alternatively, the costs may be of pleiotropic origin where one or more of the genes inducing improved innovation have a detrimental effect on seemingly unrelated phenotypic traits. Detailed studies combining behavioral and population genetic approaches will be useful for future work on mechanisms of innovative problem solving and the causes of group-size effects on this behavior.
Supplementary material can be found at Supplementary Data
Allen T Clarke J . 2005 . Social learning of food preferences by white-tailed ptarmigan chicks . Anim Behav [Internet] . 70 : 305 – 310 .
Google Scholar
Aplin LM Sheldon BC Morand-Ferron J . 2013 . Milk bottles revisited: social learning and individual variation in the blue tit, Cyanistes caeruleus . Anim Behav . 85 : 1225 – 1232 .
Auersperg AM Gajdon GK von Bayern AM . 2012 . A new approach to comparing problem solving, flexibility and innovation . Commun Integr Biol . 5 : 140 – 145 .
Beauchamp G . 2010 . Relaxed predation risk reduces but does not eliminate sociality in birds . Biol Lett . 6 : 472 – 474 .
Beauchamp GUY. 1998. The effect of group size on mean food intake rate in birds. Biol Rev . 73:449–472.
Beauchamp G, Livoreil B. 1997. The effect of group size on vigilance and feeding rate in spice finches ( Lonchura punctulata ). Can J Zool . 75:1526–1531.
Beauchamp G, Ruxton GD. 2003. Changes in vigilance with group size under scamble competition. Am Nat. 161:672–675.
Bednekoff PA Lima SL . 1998a . Re-examining safety in numbers: interactions between risk dilution and collective detection depend upon predator targeting behaviour . Proc R Soc B . 265 : 2021 – 2026 .
Bednekoff PA Lima SL . 1998b . Randomness, chaos and confusion in the study of antipredator vigilance . Trends Ecol Evol . 13 : 284 – 287 .
Bednekoff PA Lima SL . 2004 . Risk allocation and competition in foraging groups: reversed effects of competition if group size varies under risk of predation . Proc R Soc B . 271 : 1491 – 1496 .
Benson-Amram S Holekamp KE . 2012 . Innovative problem solving by wild spotted hyenas . Proc R Soc B . 279 : 4087 – 4095 .
Boesch C . 2002 . Cooperative hunting roles among Taï chimpanzees . Human Nature . 13 : 27 – 46 .
Google Preview
Bókony V Lendvai AZ Vágási CI Pătraş L Pap PL Németh J Vincze E Papp S Preiszner B Seress G et al. 2014 . Necessity or capacity? Physiological state predicts problem-solving performance in house sparrows . Behav Ecol . 25 : 124 – 135 .
Bräuer J Bös M Call J Tomasello M . 2013 . Domestic dogs ( Canis familiaris ) coordinate their actions in a problem-solving task . Anim Cogn . 16 : 273 – 285 .
Burns JG Dyer AG . 2008 . Diversity of speed-accuracy strategies benefits social insects . Curr Biol . 18 : R953 – R954 .
Cole EF Cram DL Quinn JL . 2011 . Individual variation in spontaneous problem-solving performance among wild great tits . Anim Behav . 81 : 491 – 498 .
Cole EF Quinn JL . 2012 . Personality and problem-solving performance explain competitive ability in the wild . Proc R Soc B . 279 : 1168 – 1175 .
Coleman SL, Mellgren RL. 1994. Neophobia when feeding alone or in flocks in zebra finches, Taeniopygia guttata . Anim Behav . 48:903–907. Available from: http://www.scopus.com/inward/record.url?eid=2-s2.0-0028162985&partnerID=40&md5=302a94ad741dd67e4ecc92755423f6fe
Couzin ID . 2009 . Collective cognition in animal groups . Trends Cogn Sci . 13 : 36 – 43 .
Creel S . 1995 . Communal hunting and pack size in African wild dogs, Lycaon pictus . Anim Behav . 50 : 1325 – 1339 .
Cresswell W Quinn JL . 2011 . Predicting the optimal prey group size from predator hunting behaviour . J Anim Ecol . 80 : 310 – 319 .
Elgar MA . 1989 . Predator vigilance and group size in mammals and birds: a critical review of the empirical evidence . Biol Rev . 64 : 13 – 33 .
Fernández-Juricic E Beauchamp G Bastain B . 2007 . Group-size and distance-to-neighbour effects on feeding and vigilance in brown-headed cowbirds . Anim Behav . 73 : 771 – 778 .
Galef BG . 1992 . The question of animal culture . Human nature . 3 : 157 – 178 .
Galef BG Jr Giraldeau LA . 2001 . Social influences on foraging in vertebrates: causal mechanisms and adaptive functions . Anim Behav . 61 : 3 – 15 .
Giraldeau L-A . 1984 . Group foraging: the skill pool effect and frequency-dependent learning . Am Nat . 124 : 72 – 79 .
Giraldeau L-A Caraco T . 2000 . Social foraging theory . Princeton (NJ) : Princeton University Press .
Götmark F Winkler DW Andersson M . 1986 . Flock-feeding on fish schools increases individual success in gulls . Nature . 319 : 589 – 591 .
Greenberg RS . 2003 . The role of neophobia and neophilia in the development of innovative behaviour of birds . In: Reader SM Laland KN , editors. Animal innovation . New York, NY : Oxford University Press . p. 175 – 196 .
Griffin AS . 2004 . Social learning about predators: a review and prospectus . Learn Behav . 32 : 131 – 140 .
Griffin AS Diquelou M . 2015 Innovative problem solving in birds: a cross-species comparison of two highly successful Passerines . Anim Behav 100 : 84 – 94 .
Griffin AS Diquelou M Perea M . 2014 . Innovative problem solving in birds: a key role of motor diversity . Anim Behav . 92 : 221 – 227 .
Griffin AS Guez D . 2014 . Innovation and problem solving: a review of common mechanisms . Behav Process . doi: http://dx.doi.org/10.1016/j.beproc.2014.08.027 .
Griffin AS Lermite F Perea M Guez D . 2013 . To innovate or not: contrasting effects of social groupings on safe and risky foraging in Indian mynahs . Anim Behav . 86 : 1291 – 1300 .
Hastie R . 1986 . Review essay: experimental evidence on group accuracy . In: Owen G Grofman B , editors. Information pooling and group accuracy . Westport (CT) : JAI Press . p. 129 – 157 .
Hayward MW Kerley GIH . 2005 . Prey preferences of the lion ( Panthera leo ) . J Zool . 267 : 309 .
Hong L Page SE . 2004 . Groups of diverse problem solvers can outperform groups of high-ability problem solvers . Proc Natl Acad Sci USA . 101 : 16385 – 16389 .
Janson CH . 1998 . Testing the predation hypothesis for vertebrate sociality: prospects and pitfalls . Behaviour . 135 : 389 – 410 .
Krause J Ruxton GD Krause S . 2010 . Swarm intelligence in animals and humans . Trends Ecol Evol . 25 : 28 – 34 .
Krause L Ruxton GD . 2002 . Living in groups . Oxford : Oxford University Press .
Krause S James R Faria JJ Ruxton GD Krause J . 2011 . Swarm intelligence in humans: diversity can trump ability . Anim Behav . 81 : 941 – 948 .
Laland KN Reader SM . 1999 . Foraging innovation in the guppy . Anim Behav . 57 : 331 – 340 .
Laughlin PR Hatch EC Silver JS Boh L . 2006 . Groups perform better than the best individuals on letters-to-numbers problems: effects of group size . J Pers Soc Psychol . 90 : 644 – 651 .
Liker A Bókony V . 2009 . Larger groups are more successful in innovative problem solving in house sparrows . Proc Natl Acad Sci USA . 106 : 7893 – 7898 .
Lima SL, Bednekoff PA. 1999. Back to the basics of antipredatory vigilance: can nonvigilant animals detect attack? Anim Behav. 58:537–543.
Lott DF . 1991 . Intraspecific variation in the social system of wild vertebrates . Cambridge (UK) : Cambridge University Press .
Lührs M-L Dammhahn M Kappeler P . 2012 . Strength in numbers: males in a carnivore grow bigger when they associate and hunt cooperatively . Behav Ecol . 24 : 21 – 28 .
Melis AP Hare B Tomasello M . 2006 . Chimpanzees recruit the best collaborators . Science . 311 : 1297 – 1300 .
Morand-Ferron J Cole EF Rawles JEC Quinn JL . 2011 . Who are the innovators? A field experiment with 2 passerine species . Behav Ecol . 22 : 1241 – 1248 .
Morand-Ferron J Lefebvre L Reader SM Sol D Elvin S . 2004 . Dunking behaviour in Carib grackles . Anim Behav . 68 : 1267 – 1274 .
Morand-Ferron J Quinn JL . 2011 . Larger groups of passerines are more efficient problem solvers in the wild . Proc Natl Acad Sci USA . 108 : 15898 – 15903 .
Overington SE Cauchard L Morand-Ferron J Lefebvre L . 2009 . Innovation in groups: does the proximity of others facilitate or inhibit performance? Behaviour . 146 : 1543 – 1564 .
Palameta B Lefebvre L . 1985 . The social transmission of a food-finding technique in pigeons: what is learned? Anim Behav . 33 : 892 – 896 .
Péron F Rat-Fischer L Lalot M Nagle L Bovet D . 2011 . Cooperative problem solving in African grey parrots ( Psittacus erithacus ) . Anim Cogn . 14 : 545 – 553 .
Reader SM . 2003 . Innovation and social learning: individual variation and brain evolution . Anim Biol . 53 : 147 – 158 .
Reader SM Laland KN . 2000 . Diffusion of foraging innovations in the guppy . Anim Behav . 60 : 175 – 180 .
Reader SM Laland KN . 2003 . Animal innovation: an introduction . In: Reader SM Laland KN , editors. Animal innovation . Oxford : Oxford University Press . p. 3 – 35 .
Roberts G . 1996 . Why individual vigilance declines as group size increases . Anim Behav . 51 : 1077 – 1086 .
Sasvari L . 1985 . Different observational learning capacity in juvenile and adult individuals of congeneric bird species . Z Tierpsychol . 69 : 293 – 304 .
Shaw JJ Tregenza T Parker GA Harvey IF . 1995 . Evolutionarily stable foraging speeds in feeding scrambles: a model and an experimental test . Proc R Soc B . 260 : 273 – 277 .
Silk JB . 2007 . The adaptive value of sociality in mammalian groups . Philos Trans R Soc Lond B Biol Sci . 362 : 539 – 559 .
Sol D Griffin AS Barthomeus I . 2012 . Consumer and motor innovation in the common myna: the role of motivation and emotional responses . Anim Behav . 83 : 179 – 188 .
Stöwe M Bugnyar T Heinrich B Kotrschal K . 2006 . Effects of group size on approach to novel objects in ravens ( Corvus corax ) . Ethology . 112 : 1079 – 1088 .
Thornton A Samson J . 2012 . Innovative problem solving in wild meerkats . Anim Behav . 83 : 1459 – 1468 .
Visalberghi E, Addessi, E. 2000. Seeing group members eating a familiar food enhances the acceptance of novel foods in capuchin monkeys. Anim Behav . 60:69–76.
Visalberghi E, Valente M, Fragaszy D. 1998. Social context and consumption of unfamiliar foods by capuchin monkeys ( Cebus apella ) over repeated encounters. Am J Primatol. 45:367–380.
Zuluaga GJC . 2013 . Why animals come together, with the special case of mixed-species bird flocks . Rev EIA . 10 : 49 – 66 .
Author notes
Supplementary data, email alerts, citing articles via.
- Recommend to your Library
Affiliations
- Online ISSN 1465-7279
- Copyright © 2024 International Society of Behavioral Ecology
- About Oxford Academic
- Publish journals with us
- University press partners
- What we publish
- New features
- Open access
- Institutional account management
- Rights and permissions
- Get help with access
- Accessibility
- Advertising
- Media enquiries
- Oxford University Press
- Oxford Languages
- University of Oxford
Oxford University Press is a department of the University of Oxford. It furthers the University's objective of excellence in research, scholarship, and education by publishing worldwide
- Copyright © 2024 Oxford University Press
- Cookie settings
- Cookie policy
- Privacy policy
- Legal notice
This Feature Is Available To Subscribers Only
Sign In or Create an Account
This PDF is available to Subscribers Only
For full access to this pdf, sign in to an existing account, or purchase an annual subscription.

An official website of the United States government
The .gov means it’s official. Federal government websites often end in .gov or .mil. Before sharing sensitive information, make sure you’re on a federal government site.
The site is secure. The https:// ensures that you are connecting to the official website and that any information you provide is encrypted and transmitted securely.
- Publications
- Account settings
Preview improvements coming to the PMC website in October 2024. Learn More or Try it out now .
- Advanced Search
- Journal List

Chimpanzees’ ( Pan troglodytes ) problem-solving skills are influenced by housing facility and captive care duration
Sofia forss.
1 Department of Early Prehistory and Quaternary Ecology, Eberhard-Karls-Universität Tübingen, Tübingen, Germany
Alba Motes-Rodrigo
Christine hrubesch.
2 Department of Anthropology, University of Zürich, Leintalzoo, Schwaigern, Germany
Claudio Tennie
Associated data.
The following information was supplied regarding data availability:
All data and code employed in these analyses are available at OSF: Forss, Sofia. 2020. “Fork of Physical Cognition Chimpanzees.” OSF. October 16. doi: 10.17605/OSF.IO/QEYDU .
Although a large body of primate cognition research is done in captive institutions, little is known about how much individuals from different facilities vary in their experiences and cognitive skills. Here we present the results of an experimental study investigating how physical cognitive skills vary between chimpanzees in relation to captive settings and their time in captivity. We tested 59 chimpanzees housed at two different captive facilities (a rehabilitation center (sanctuary) and a zoo) in three problem-solving tasks. Our results showed that chimpanzees at the two housing facilities significantly differed in overall task performance. On average, the sanctuary chimpanzees outperformed the chimpanzees housed at the zoo in the detour reaching task and the honey trap task. However, the zoo chimpanzees performed slightly better on average in the learning task. We propose that, for this particular sample, the documented differences result from a combination of factors, such as prior experience with cognitive testing, motivation levels and varying degrees of human exposure. Within the sanctuary sample, we found that chimpanzees who arrived at an earlier age at the sanctuary and had therefore spent a larger percentage of their lives in a captive environment, were better problem-solvers than those that arrived at a later age to the sanctuary. Thus, rehabilitation and time in captivity contributed to improved physical cognitive skills in sanctuary chimpanzees. Our results highlight the importance of studying intraspecific variation and the effect that previous experience and living conditions might have on physical cognitive skills in non-human apes. Accordingly, we should be cautious when extrapolating findings of cognitive studies from one population to the species as a whole.
Introduction
In order to understand the evolution of cognitive abilities we need to disentangle environmental and genetic influences from cognitive phenotypes in non-human animals. To this end, it is important to consider intraspecific variation and to identify what factors correlate with between-individual differences ( Boesch, 2020 ). Previous research has shown that early life experience can influence cognition in several species: enriched captive conditions during early life increase spatial learning abilities in fish ( Salmo salar ) ( Salvanes et al., 2013 ); the early incubation environment of lizard eggs ( Pogona vitticeps ) influences the adult lizards’ socio-cognitive skills assessed through social learning tasks ( Siviter et al., 2017 ); early maternal separation reduces learning ability in male mice ( Mus musculus ) ( Wang, Jiao & Dulawa, 2011 ) and hand-raised parakeets ( Melopsittacus undulatus ) perform better at object permanence than parent-raised ones ( Funk, 1996 ).
In humans ( Homo sapiens ), part of the observed intraspecific variation in cognitive abilities is explained by genetic inheritance ( Deary, Johnson & Houlihan, 2009 ; Nisbett et al., 2012 ; Bates, Lewis & Weiss, 2013 ). Thus, to identify the variation in cognitive abilities explained by environmental factors and early-life experiences in our species, large research efforts have been deployed into studies of identical twins and adopted children. Such study design has provided insight into how, besides genes, the nurturing environment where a child is raised contributes to outcomes such as educational attainment and income later in life ( Sacerdote, 2011 ). Compared to children from high socio-economic-status (SES) families, children as young as six months old from low SES families already show lowered attentiveness influencing a cascade of cognitive skills ( Clearfield & Niman, 2012 ). Furthermore, studies on adopted institutionalized children have shown that children who move to foster families at an earlier stage of development have better chances at cognitive recovery from early social deprivation ( Nelson et al., 2007 ).
Similar to humans, studies on chimpanzees suggest that great ape (henceforth apes) cognition is influenced both by genetics ( Hopkins, Russell & Schaeffer, 2014 ) and the socio-cultural environment where the individuals develop ( Russon, Bard & Parker, 1998 ; Reader & Laland, 2002 ; Russell et al., 2011 ; van Schaik & Burkart, 2011 ). Ideally, we would assess the influence of experience and early life environments on cognitive abilities in the species’ ecological context. However, it is often challenging to conduct controlled, cognitive experiments in the natural environment of apes. Due to this limitation, most cognitive studies are performed in captive, settings where the learning opportunities during experiments can be controlled for.
Captive apes show large individual variation in cognitive performance ( Herrmann & Call, 2012 ) and even within the same facility, chimpanzees have been shown to differ in their tendencies to use social information during problem-solving tasks ( Watson et al., 2018 ). Thus, when measuring cognitive skills in captive apes, we need to take into consideration this variation as well as its potential underlying factors. Sources of intraspecific variation can be different motivational levels among individuals to participate in an experiment; familiarity with the test apparatuses and methodological procedures and differences in housing conditions or routines between facilities. In addition, the apes’ contact with peers, their degree of human contact and their exposure to human artefacts can also influence the apes’ performance in cognitive tasks ( Tomasello, Savage-Rumbaugh & Kruger, 1993 ; Call & Tomasello, 1996 ; Tomasello & Call, 2004 ; Bering, 2004 ; Furlong, Boose & Boysen, 2008 ; Damerius et al., 2017a ; Damerius et al., 2017b ). Lastly, the rearing background where an individual develops is also likely to influence the individual’s cognitive development. For instance, enculturated apes (defined as apes raised by humans in an anthropomorphic environment and attended to as intentional agents exposed to a wide variety of human cultural experiences; Tomasello, Savage-Rumbaugh & Kruger, 1993 ) show enhanced physical cognitive skills compared to conspecifics reared by their own mother or nursery-reared with peers ( Russell et al., 2011 ).
Therefore, research on ape cognition would benefit from a better understanding of the extent of the variation between captive populations, namely whether chimpanzees at different facilities vary in their physical cognitive skills. In addition, studies exploring the role that different factors play in ape performance within a housing facility can further improve our knowledge regarding sources of intraspecific variation. If, for instance, housing conditions or the duration of human care influence apes’ performance in cognitive tasks, research performed at a single location should not be automatically extrapolated to other populations of the same species or to the species as a whole ( Boesch, 2020 ).
In the present study we systematically compared the problem-solving skills of chimpanzees housed at two different facilities (a zoo and a sanctuary) in several cognitive tasks in order to assess possible between-facility differences. As we were also interested in the individual variation within a single facility, we used the sanctuary sample to evaluate if problem-solving skills differed depending on an individual’s age at arrival at the sanctuary and on the cumulative time they had spent in captive care.
Ethical statement
All problem-solving tests were non-invasive and solely behavioral observations were made. All tests complied with the ethical principles set by the UWA, Ugandan Wildlife Authority (UWA/COD/95/06) and the National Council for Science and Technology (UNCST) (reference number NS27ES). The study was also supported by the BIAZA Animal Care Committee (British and Irish association for Zoos and Aquariums) and approved by the Swiss veterinary institution (2960/ 29815). Barbara Gessmann (Leintal zoo, Schweigern, Germany) provided permission to test the chimpanzees at Leintal zoo.
Subjects and facilities
We tested chimpanzees’ problem-solving skills with three tasks targeting different aspects of physical cognition that yielded six different cognitive measurements ( Table 1 ). The total sample size varied for each task from N = 49 to N = 59, as subjects participated on a voluntarily basis in the tasks ( Table 1 ). We collected part of the data ( N = 40) at Ngamba Island chimpanzee sanctuary in Uganda during September and October 2017. For data collection at Ngamba Island, a field permit was granted from the Chimpanzee Sanctuary and Wildlife Conservation Trust. At the time of data collection, with the exception of two chimpanzees born at the institution (not part of our study), the sanctuary housed a large group of orphan and confiscated chimpanzees, most of them rescued from the illegal pet and bushmeat trade. These chimpanzees have gone through rehabilitation due to traumatic experiences including maternal loss, malnutrition, and periods of restricted movement in small cages. They are currently housed and taken care of by the chimpanzee sanctuary and conservation trust in a semi-natural environment. At Ngamba Island the chimpanzees have access to 95 acres of tropical forest but can return to the facilities voluntarily for feeding multiple times each day as well as for overnighting. The chimpanzees at Ngamba are familiar with being individually separated occasionally for health checkups or research purposes. The sanctuary provided an informative record on the background histories of some of the chimpanzees, including their age at arrival at the sanctuary, health condition upon arrival at the sanctuary and what kind of environment they had been in prior to rescue (i.e., found in cage, malnourished, human held as pet/ “entertainment hostage” or brought straight to sanctuary, described in Table S1 ). This set of information enabled us to address (within the Ngamba Island sanctuary) how the age at arrival at the sanctuary and the percentage of lifetime spent at the sanctuary influenced task performance.
Our second sample was comprised by the chimpanzees housed in Leintal zoo, Germany, during September and October 2017. With a total group size of 33 individuals, this group of chimpanzees represents the largest zoo housed chimpanzee community in Europe. Except for a few training experiments (observational data, Hrubesch 2007–2008), this chimpanzee community had not been targeted for research projects on cognition before the data was collected for this study. The majority of individuals at Leintal zoo were zoo born and mother-reared, with the exception of six individuals, one with an unknown background and five that had lived with humans up to the age of roughly one year. The rearing information of those chimpanzees that participated in the cognitive tasks is included in Table S1 . The chimpanzees at Leintal zoo live in a large outdoor area comprised of multiple enclosures connected to indoor quarters with attached sleeping rooms. Given that the chimpanzees at Leintal zoo were not used to being separated from their group (except for health check-ups) and because they could participate in our cognitive tasks on a voluntarily basis, our zoo sample varies in size between 14 and 19 individuals depending on the task. Due to the large peer group constellation and scarce experience with cognitive tests (including materials and apparatuses), the enculturation of these chimpanzees may be described as minimal for captivity (with the exception of the five hand-reared individuals).
Cognitive tasks and experimental set up
We tested the chimpanzees in three novel (to them) problem-solving tasks (detour reaching task, visible honey trap task and reversal learning task) generating six different measurements on different aspects of physical cognition ( Table 1 ). The tasks were performed in the above listed order, to ensure that all subjects participating in the study had a similar experience through the testing phase. These standardized problem-solving tasks have been previously used to investigate physical cognition in orangutans ( Forss et al., 2016 ; Damerius et al., 2017a ; Damerius et al., 2017b ). Prior to testing the chimpanzees in the problem-solving tasks, we also assessed the level of human orientation of each of the chimpanzees through a previously established Human Orientation Index, HOI ( Damerius et al., 2017a ). In each task, every subject was tested alone and thus for a shorter period separated from its social group. Throughout the study, all chimpanzees were fed according to their normal daily routines and none of the subjects were food deprived during the experiments.
At Ngamba Island, the chimpanzees were tested in their sleeping quarters after the morning feeding before they went into the forest habitat. At Leintal zoo testing took place in the smaller sleeping rooms mostly before mid-day. All tests were recorded with SONY HD handy cameras.
Each subject was tested once on each task (meaning that each problem-solving task was presented only one time to each subject) with the exception of the reversal learning task, which measures working memory and therefore was presented to each subject on four consecutive days. For each task, the subjects’ response towards the test apparatus was coded from video recordings. There were no demonstrations or pre-training trials.
Detour reaching task
The detour reaching task tests for inhibitory control ( Fig. 1A ). In the task, the subject is allowed access to a large, completely transparent, Plexiglas box (100 cm × 30 cm × 30 cm). The front side of the box has one small round hole within a 50 cm distance of a larger rectangular hole where the subjects’ arm can pass through. Inside the box, a food reward (whole banana) is placed behind the small hole. Each subject is given a maximum of five minutes to solve the task. The variables measured in this task that were later used as dependent variables in the statistical models were (a) whether the chimpanzee solved the task and reached for the banana through the big hole (Yes/No) and (b) if the chimpanzee managed to solve the task without any physical trial and error exploration of the box (Yes/No). Explorative actions were defined as touching the Plexiglas box, poking with a finger through the small or big holes, hitting, lifting, or kicking the box.

(A) Detour reaching task, (B) visible honey trap and (C) reversal learning task.
Visible honey trap task
The visible honey trap task (henceforth honey trap task, Fig. 1B ) measures the understanding of tool properties of two types of tools: a rigid stick and a bendable rope. The upper part of the apparatus contains a straight channel that fits a 40 cm long stick. This channel was baited with a stick dipped in a little bit of honey. At the lower part of the trap there is a curved channel filled with much more honey to motivate the subjects to attempt this more difficult problem requiring the innovation of a novel tool (in this case a rope fragment laid out on the floor) to dip for honey. As the stick does not fit in the curved channel, the subjects need to dip a bendable piece of rope into the curved channel to extract the honey. The front side of the apparatus is transparent so that the subjects can see the different shapes of the channels as well as the honey. Each subject is provided with two extra sticks and three pieces of rope on the floor in front of the apparatus. Each trial lasts ten minutes during which the individuals have time to solve both tool tasks. As dependent variables for the statistical analyses we measured (a) if a subject solved the stick task by either re-using the inserted stick or inserting one of the provided sticks into the straight channel (Yes/No) and (b) if a subject solved the rope task by using the rope to obtain honey from the curved channel (Yes/No). We defined a subject as successful when he/she inserted 1/3 of the length of the stick in the straight channel or 1/3 of the rope in the bent channel. Given that this test apparatus was presented to the chimpanzees within their enclosure and they could interact with it for a longer time, we also assessed their motivation to interact with the apparatus, measured as time the chimpanzees spent exploring the apparatus before they found the rope solution or until end of task.
Reversal learning task
The reversal learning task measures working memory, inhibition, and flexibility ( Fig. 1C ). In this task the subjects need to first learn the association between the color of six lids that hide a food reward (black or white) and distinguish those lids from the other color that covers empty holes. A subject is considered to have learned the right color association when, out of the first six lids that he/she touches, five are of the correct/baited color. After learning the color-food association, the subjects need to pass a control trial fulfilling the same criterion. Once this control trial was conducted, we switched the color that hides the food rewards and thereafter the subject needed to learn the reverse pattern. Each individual was tested in this task for four continuous days and subjects were tested in three trials every day for four continuous days. However, if a subject learned the color association in the third trial of a day, we ran the additional control trial that day to confirm that the subject had learned the correct color-food association. In this task we measured (a) if the subjects learnt the correct color-food association (dependent variable) and (b) if the subjects learnt the reverse-food color association (dependent variable).
Human Orientation Index
To quantify variation in human habituation we performed an additional test that measured each ape’s Human Orientation Index (HOI) previously established by Damerius et al. (2017a) . The HOI differs from the standard Human Intruder Test (HIT) ( Gottlieb & Capitanio, 2013 ) because it does not incorporate any threatening positions or movements, instead it aims at testing reactions towards a stranger with and without food. We calculated the HOI of each chimpanzee by testing them individually in three subsequent experimental conditions. In the first condition a male human (to be consistent between facilities) who is unfamiliar to the apes, calmly approaches the enclosure and positions himself 1–1.5 m in front of the enclosure and stands still for 30 s with the side of his body towards the enclosure. In the second condition, the man turns around so that his body frontally faces the subject and tries to establish eye contact with the subject for one minute. Because we additionally wanted to quantify the orientation to humans when food is involved, and when it is not, we conducted one additional condition to those implemented by Damerius et al. (2017a) including an unfamiliar human offering food out of reach of the chimpanzee. In the third condition, the man remains frontally facing the ape but instead of keeping eye contact with the subject, he directs his gaze down at the ground and takes out a preferred food item (peanuts) from his pocket and holds the food beside his body in his hand, out of reach of the subject for 30 s. After condition three is completed, the food reward is placed in front of the enclosure and the test subject can collect it. For each condition we coded the proximity of the ape to the man as follows: 0 = the ape positioned itself as far away as possible from the human; 1 = the ape was more than one meter away from the human; 2 = the ape was within one meter from the human; 3 = the ape placed itself as close to the human as possible. We also scored the subject’s initial behavioral reaction in each condition as follows: 0 = a negative reaction, including retreat, stress vocalization, pilo-erection, nervous swinging or turning away from the human; 1 = a neutral reaction, including resting, moving calmly or play behavior; 2 = a positive reaction, if the ape approached the human; 3 = an active positive reaction including begging gestures (either by using lips or hands) or any other proactive behavior aimed at establishing contact with the human as well as attempts to trade objects from the enclosure for food. In addition, for each condition we scored whether or not the ape engaged in any active positive reactions (begging, trying to get the man’s attention) beyond the initial coded behaviors. The sum of the proximity and reaction scores across conditions constitutes the Human Orientation Index (HOI), which can range from 0 to 21.
Statistical analysis
We conducted the statistical analysis in R (version 3.3.3, R Core Team, 2017) and RStudio (version 1.1.383). We z-transformed covariates (age, age at arrival at the sanctuary and percentage of life at sanctuary) to a mean of 0 and standard deviation of 1 before including them in the models to facilitate the interpretation of the coefficient estimates ( Schielzeth, 2010 ). Factors (facility and sex) were manually dummy coded before being introduced in the models. All data and code employed in these analyses can be found in the link https://osf.io/qeydu/ .
Comparisons between facilities in task performance
To investigate if there were differences in the cognitive measurements between facilities, we fitted a Generalized Linear Mixed Model (glmm) with binomial error structure and logit link function ( Lee & Nelder, 1998 ). The response in Model 1 was a binary variable composed of 1s and 0s with each row indicating the success or failure of an individual in each task. We fitted a single model with a response variable combining the outcomes of all tasks tested in order to reduce the number of models fitted and consequently, the risk of false positives (type I error). As fixed effects, Model 1 included facility, sex and age of the individuals at the time of testing (the last two as control predictors). To prevent pseudoreplication due to the participation of the individuals in several (or all) tasks, we also included the random intercept of individual ID. We adopted a maximal random slope structure ( Barr et al., 2013 ) by including the random slopes of sex, facility (both dummy coded) and age within task. As we were interested in the overall differences in task performance between facilities, the variable of interest in Model 1 was the random slope of facility within task. Task was a factor with six levels (each cognitive measurement). We chose to investigate the differences between facilities in performance across tasks as a random slope rather than an interaction in order to make the model independent of the specific tasks employed and generalizable. Model 1 was fitted with the function glmer from the package lme4 ( Bates et al., 2015 ) using the optimizer bobyqa. The fitted model was checked for collinearity using variance inflation factors (VIF function from package car; ( Fox & Weisberg, 2019 ; Fox & Weisberg, 2011 ), overdispersion and overall stability (see Fig. S1 ) without finding any issues. Inference was drawn by comparing the full model with maximal random slope structure with a reduced (null) model lacking the slope of interest (facility within task, Forstmeier & Schielzeth, 2011 ) but containing all other predictors using a likelihood ratio test (test “Chisq” in the R function anova). Following Bolker et al. (2009) , the p value of the full-null model comparison was divided by 2. R squared was calculated with the function r.squaredGLMM.
Due to the experimental set up and duration of the task, the honey trap allowed us to further compare the motivation of the chimpanzees from the two facilities to interact with the test apparatus (measured as the time spent interacting with the honey trap before innovating the rope solution). We fitted a linear model with the function lm (package lme4) in which the response variable was the time the chimpanzees spent interacting with the honey trap and the test predictor was facility (Model 2). We further controlled for the effects of sex and age. As before, inference was drawn comparing the full model including both control and test predictors with a null model lacking the control predictor (facility) using a likelihood ratio test. When potential influential cases were investigated in Model 2 using the function dffits, it was found that the maximum absolute value was 2, suggesting that influential cases were not an issue. R squared was calculated with the function r2 from the package “performance” ( Lüdecke et al., 2020 ).
Potential differences in the degree of human orientation (HOI) between individuals housed at the two facilities were investigated by fitting a model with the function polr (package MASS; Venables & Ripley, 2002 ) with HOI as response variable and facility as test predictor (Model 3). As before, age and sex were included as control predictors. Inference was drawn as described for Model 2. Given the results of Model 3, two post-hoc analyses were conducted in order to investigate if the HOI differences between facilities were due to the scores in non-food related conditions (conditions 1 and 2, Model 3.1) or in the food related condition (condition 3, Model 3.2). These models were identical to Model 3 except for the response variable, which was the combined score obtained in conditions 1 and 2 (Model 3.1) or the score obtained in condition 3 (Model 3.2). R squared was calculated with the function r2 from the package “performance” ( Lüdecke et al., 2020 ).
Analysis of within facility variation: effects of arrival age and lifetime spent in the sanctuary
The honey trap was the task with the largest sample size that generated the most variability in performance amongst the chimpanzees. Therefore, we used the outcomes of this task (binary response with one row per individual and task) within the sanctuary sample to further investigate intraspecific variation on cognitive performance, while controlling for housing differences (as only one facility was tested). We assessed how the probability of success in the honey trap tasks varied based on how old the chimpanzees were when they first arrived at the sanctuary (Model 4) and the percentage of their lives they had spent in the sanctuary (Model 5). Given that these two variables (percentage of life in sanctuary and age at arrival) were highly correlated (Spearman correlation, rho=-0.91, p < 0.001), they had to be included in different models. This decision was taken based on the fact that the inclusion of correlated variables in the same model increases the uncertainty of the estimates derived from the model, increase the probability of type II errors and makes the assessment of the relative importance of individual predictors in the model unreliable ( Freckleton, 2011 ). Models 4 and 5 were glmms with binomial error structure and logit link function ( Lee & Nelder, 1998 ). The response was binary (composed of 0s and 1s) with one row per individual and task variant (solving the task with a rope or a stick). We included as control predictor in both models the age of the individuals when tests were conducted (z-transformed). The test predictor of interest in these models was the interaction between task (factor with two levels) and the age of the individuals when they arrived at the sanctuary (Model 4) or the percentage of life spent in the sanctuary (Model 5). In Models 4 and 5, task only included two levels corresponding to the two variants of the honey trap task (solved with stick or rope). The random intercept of individual ID was included to prevent pseudoreplication, as individuals were tested in both tasks. To avoid convergence problems, these two models were fitted using the function glmmTBM from the package of the same name ( Brooks et al., 2017 ). Collinearity in Models 4 and 5 was checked with the function vif from the package car without finding any issues. Model stability was visually checked creating stability plots ( Figs. S2 and S3 ) with the packages broom.mixed and dotwhisker ( Bolker, 2016 ). Inference was drawn by comparing the full model containing all control and test predictors with a reduced model lacking the interaction of interest by means of a likelihood ratio test (test set to “Chisq” in function anova). R squared was calculated with the function r2 from the package “performance” ( Lüdecke et al., 2020 ).
Furthermore, we checked inter-rater reliability by having a research assistant (MP) independently code the success variable (Yes/No if a subject solved or not solved the task). The second coder re-coded 15% of the trials, which were randomly selected from all video recorded tasks. The two coders reached a Cohen’s Kappa of 0.81 (N events = 41, p = 0.001), which represents a substantial to almost perfect level of agreement ( Landis & Koch, 1977 ).
Although we tried to additionally account for the rearing background of the individuals included in Model 1 (whether they were hand reared by humans or reared by their mothers), this variable was strongly dependent on the facility and very unbalanced. In Ngamba Island Sanctuary, more than 90% of the individuals had been human reared. On the other hand, in Leintal zoo more than 70% of the individuals had been raised by their mothers. Consequently, the inclusion of this variable in the models created strong model instability and it was decided to exclude it from the main analysis. However, we conducted an additional explorative model (Supplementary Model 1) where we investigated the effect that rearing background had on chimpanzee cognitive performance ( Fig. S4 ). This model is identical to Model 1 except for the substitution of facility by rearing background. Model estimates and BLUPS can be found in Tables S2 and S3 respectively. As in the previous models, the stability ( Fig. S5 ), collinearity and overdispersion of the Supplementary Model 1 were investigated without finding any issues.
Differences between facilities in task performance
We found that facility had a significant effect on the success probability of the chimpanzees in the different cognitive tasks (Model 1; likelihood ratio test between full and reduced model: X 2 = 21.37, df = 1, p = 0.001; R 2 full model=0.57). Table 2 includes the estimates of the fixed effects and Table 3 includes the best linear unbiased prediction (BLUPs) for each of the levels of the random slope of facility within task. The BLUPs (which reveal the variation from the mean slope estimate of facility caused by the different tasks in which the chimpanzees participated) show that in all cognitive measurements from the detour and honey trap tasks, proportionally more chimpanzees at Ngamba Island were successful compared to conspecifics at Leintal zoo (see positive BLUP values in Table 3 and Fig. 2 ).

The area of the dots represents sample size. (A) Detour reaching task. (B) Detour reaching task without exploration. (C) Honey trap task with stick. (D) Honey trap task with rope. (E) color association learning. (F) Reversal learning.
The difference between facilities was larger in the more demanding tasks, such as solving the detour reaching without physical exploration (25/40 successful individuals in Ngamba Island versus 0/19 at Leintal zoo) and solving the honey dipping problem with a rope (15/40 successful individuals at Ngamba Island versus 0/14 at Leintal zoo) ( Table 1 ).
In the reversal learning task, the chimpanzees housed at Leintal zoo had an average success probability higher than the Ngamba Island chimpanzees (negative BLUP values in Table 3 ). This difference was probably driven by the fact that all chimpanzees that participated at Leintal zoo ( N = 14) learned the first color association (first part of task), but only 27/35 of the chimpanzees at Ngamba Island did so ( Table 1 , Table 3 and Fig. 2 ). In the learn-the-reverse part of the task, both facilities had lower proportions of successful chimpanzees compared to the first part of the task (Leinthal zoo: 6/14, Ngamba Island: 10/35, Table 1 ).
Regarding differences in motivation levels (measured as time exploring the honey trap task prior to finding the rope solution) between individuals from the two facilities, we found that Ngamba chimpanzees were significantly more motivated to interact with the test apparatus than chimpanzees housed at Leintal Zoo (Model 2; likelihood ratio test between full and reduced model: X 2 = 120.15, df = 1, p < 0.001; R 2 = 0.26, Fig. 3 , Table 4 ).

N represents the sample sizes in each of the groups. The top and bottom of the boxes represent the 75th percentile and the 25th percentile respectively. The whisker length was calculated as the percentiles plus or minus 1.5 times the interquartile range.
When the degree of human orientation of the chimpanzees at both facilities was compared (HOI), it was found that there were significant differences between the chimpanzees at both facilities, with the chimpanzees housed at Ngamba Island Sanctuary being more human oriented than those housed at Leintal Zoo (Model 3; likelihood ratio test between full and reduced model: X 2 = 5.47, df = 1, p = 0.019; R 2 = 0.14, Fig. 4 , Table 5 ). When these differences were explored further in two post-hoc analyses to determine in which conditions did the chimpanzees from the two facilities differ, no effect of facility was found on the combined score of condition 1 and 2 (no food visible) (Model 3.1: likelihood ratio test between full and reduced model: X 2 = 0.36, df = 1, p = 0.55; R 2 = 0.05, Table 6 ) and a tendency was found on condition 3 (food visible) (Model 3.2: likelihood ratio test between full and reduced model: X 2 = 3.51, df = 1, p = 0.061; R 2 = 0.11, Table 7 ).

Effects of arrival age and lifetime spent in the sanctuary
By examining the performance in the honey trap task in the facility with the largest sample size (Ngamba Island sanctuary), we found a significant interaction between the age of arrival at the Ngamba Sanctuary and the performance in the honey trap tasks. Performance in the honey trap varied between sub-tasks (solved with a stick or a rope) depending on the age at which the chimpanzees had arrived at Ngamba Island Sanctuary (Model 4; likelihood ratio test between full and reduced model: X 2 = 64.76, df = 3, p = 0.001; R 2 = 0.15, Table 8 ; Fig. 5 ).

The size of the points represents sample size (from left to right: N = 16, 11, 2, 6, 1, 2).
Similarly, we found that there was a significant interaction between the proportion of lifetime spent in the sanctuary and the performance in the honey trap task. Problem-solving skills varied between the two sub-tasks (solved with a stick or a rope) depending on the percentage of the life a chimpanzee had lived at Ngamba Island Sanctuary (Model 5; likelihood ratio test between full and reduced model: X 2 = 65.21, df = 3, p = 0.001; R 2 = 0.13, Table 9 ; Fig. 6 ).

The size of the points represents sample size (from left to right: N = 3, 0, 5, 4, 13, 13).
We found overall differences in cognitive performance between two populations of captive chimpanzees housed at a sanctuary and a zoo, with the sanctuary chimpanzees showing an average probability of success across tasks significantly higher than the zoo housed chimpanzees. We observed that in four out of the six cognitive measurements, there was intraspecific variation in problem-solving skills. However, in the reversal learning (in one of which), we did not find observable differences between facilities, even if the zoo chimpanzees had a higher average probability of success than the sanctuary chimpanzees at the first stage of the task (associative learning). In addition, we also found that within the same facility, chimpanzees differed in tool use skills, with those individuals that had arrived to the sanctuary at an earlier age and thereby spent a longer proportion of their lives in captivity being better at flexibly using tools than those individuals who arrived at a later developmental stage and thereby spent a shorter proportion of their lifetime in captivity.
Problem-solving differences between captive facilities
We found that chimpanzees from the Ngamba Island sanctuary had on average a higher probability of success than their conspecifics housed in a Leintal zoo in the detour reaching and honey trap task ( Table 1 , Fig. 2 ). Similarly, prior research applying the Primate Cognitive Test Battery (PCTB) to sanctuary and zoo chimpanzees as well as bonobos ( Pan paniscus ), found that sanctuary apes showed similar, or improved cognitive skills compared to zoo housed conspecifics ( Wobber & Hare, 2011 ). Previous findings on tool use skills of captive apes suggest that the intensity of human contact can impact tool use understanding in chimpanzees ( Furlong, Boose & Boysen, 2008 ) and increase curiosity in orangutans, which in turn can lead them to improve their tool-use skills ( Damerius et al., 2017a ). Most chimpanzees at Ngamba Island sanctuary are orphans due to poaching, habitat destruction, or maternal death and went through years of rehabilitation. Consequently, these chimpanzees were raised and cared for by humans with extensive and close human exposure. One possible factor contributing to the observed variation in task performance between the two facilities could be the different degree of exposure to humans experienced by the chimpanzees at the zoo and the sanctuary. At Ngamba Island volunteers and tourists, who are visiting and supporting the wildlife sanctuary, can participate in chimpanzee feeding routines under the supervision of staff members. Therefore, chimpanzees at Ngamba sanctuary are often exposed to different unfamiliar humans in relatively close contact. Chimpanzees at Leintal zoo on the other hand, are only fed by their familiar caretakers and thus experience less diversity and frequency of humans closely interacting with them during feeding times. These differences in exposure to unfamiliar humans during feeding times might explain why we found differences between facilities in the degree of human orientation in the third condition of the HOI (where an unfamiliar man offered the subjects food, Model 3.2).
Between-facility differences in task performance could also be influenced by the different rearing backgrounds of the majority of chimpanzees housed at the sanctuary and the zoo. Most of the chimpanzees housed at Leintal zoo had been raised by their own mothers, in a large group of conspecifics, representing a developmental socio-environment closer to that of natural chimpanzee communities than that present in Ngamba sanctuary. Such mother-reared individuals may be less influenced by human exposure (being less human oriented, Fig. 4 ) despite living in a captive setting than individuals that have lost their mother and thereby have been mainly hand-reared by humans. However, it is important to note that in the zoo sample of our study, the four chimpanzees that were hand-raised by humans did not solve the more demanding tasks of detour reaching without trial and error exploration and did not use the rope tool to extract honey. Such cases suggest that, in our sample, housing facility and prior exposure to cognitive testing might have a stronger effect on task performance than rearing background. Unfortunately, given the structure of our data, it was not possible to disentangle the individual contributions of rearing background and facility to task performance. Therefore, we encourage future research to investigate the potential role of rearing background during the first years of life on multiple measures of cognitive performance. Likewise, it would be important to disentangle how various factors, such as group compositions, contact with caretakers and diet routines, at different housing facilities affects cognitive development and other behavioral outcomes ( Novak et al., 2014 ). Other factors that could potentially contribute to the observed variation in task performance between facilities are the different levels of familiarity of the chimpanzees with the presented materials and their overall past experience in cognitive tests. The Ngamba Island chimpanzees were more used to being separated from their social group than the chimpanzees at Leintal zoo, which could explain why these chimpanzees showed higher interaction times with the honey trap test apparatus compared to the Leintal chimpanzees ( Fig. 3 ). This higher motivation to manipulate and explore the apparatus could explain why they were more successful at innovating the use of the rope as a tool in this task. Recent findings from avian cognition have shown that Goffin’s cockatoos ( Cacatua goffiniana ) that were temporarily held captive were less motivated to interact with a problem-solving task than those birds that had been hand-raised and lived longer in captivity ( Rössler et al., 2020 ). Together with our study, such results suggest that captivity and human exposure may influence the motivational level rather than the animals’ cognitive capacity per se.
The apparatus used in the detour reaching task was entirely made of Plexiglas ( Fig. 1A ) and previous studies have shown that orangutans with different rearing experiences vary in their problem-solving skills when presented with human-made materials like Plexiglas ( Damerius et al., 2017b ). The Ngamba Island chimpanzee sanctuary has hosted multiple cognitive research projects, some of which have focused on cognitive performance by presenting the chimpanzees with different types of test apparatuses including transparent materials (e.g., Horner & Whiten, 2005 ; Horner & Whiten, 2007 ; Marshall-Pescini & Whiten, 2008 ; Tennie, Call & Tomasello, 2010 ; Bullinger, Melis & Tomasello, 2014 ). Therefore, the Ngamba chimpanzees had previous experience with Plexiglass-like objects at the time of this study. The Leintal zoo chimpanzees on the other hand, have no previous experience with test apparatuses made from Plexiglas (and hardly any experience at all with other testing materials). Since previous experience with certain human-made materials can influence an individual’s reaction and perception of the test apparatus, the chimpanzees less familiar with these materials may need to explore the box more thoroughly before finding a solution. Accordingly, in our experiments, the differences between chimpanzees at Ngamba Island sanctuary and Leintal zoo were stronger when we examined subjects that solved the detour-reaching task without any prior exploration compared to when they solved the task through physical exploration ( Fig. 2 ).
In the reversal learning task, the chimpanzees at Leintal zoo had higher average success probabilities than the sanctuary chimpanzees. This difference was likely driven by the fact that the zoo chimpanzees did better in the first part of the task (learning color association). The success in the second part of the task (reversal learning) was similar in both facilities. Since reversal learning directly depends on how fast an individual learns the first part of the task, one would have expected the zoo chimpanzees to perform better than the sanctuary chimpanzees, as their likelihood of success in the first step of the task was higher than that of sanctuary chimpanzees. However, overall success in the task was low and only 33% of all tested chimpanzees learned the reverse pattern ( Table 1 ). Even though primates’ success in reversal learning tasks has been associated with increased brain size ( Deaner et al., 2007 ), a comparative study across taxa reported that cleaner fish ( Labroides dimidiatus ) outperformed three primate species (Capuchin monkeys, Cebus apella ; orangutans, Pongo spp ; chimpanzees, Pan troglodytes ) in reversal learning tasks ( Salwiczek et al., 2012 ). The superior reversal learning skills of this fish species compared to the primates are arguably the consequence of the species-specific foraging ecology involving unpredictable social interactions with reef fish. In the same study, capuchins outperformed apes in reversal learning tasks ( Salwiczek et al., 2012 ). Reversal learning tasks require a high inhibitory control and as opening the different lids in our study did not impose any direct cost for the chimpanzees, they may not have been motivated enough to inhibit incorrect responses, which could explain why the tested chimpanzees performed particularly poorly in this task.
Tool use differences within the sanctuary
Due to the large sample size in Ngamba, we were able to examine variation in tool use skills while keeping facility constant. Comparing chimpanzees within the Ngamba Island sanctuary revealed that it was harder for the chimpanzees to innovate the rope solution than using the stick in the honey trap task ( Table 1 ). The higher success rate of the stick solution could be due to the generalized use of sticks for varied foraging activities across chimpanzee populations ( Whiten et al., 1999 ; Koops, Furuichi & Hashimoto, 2015 ) –i.e., to a higher likelihood of using sticks than rope as a latent solution in chimpanzees ( Tennie, Call & Tomasello, 2009 ).
Orphans that had arrived at the sanctuary younger than two years old had the highest probability of innovating the rope solution ( Fig. 5 ). We also found that the proportion of lifetime spent at the sanctuary had a significant influence in the success probability in the honey trap task ( Fig. 6 ). Individuals that had spent over 90% of their lives at the sanctuary were more likely to innovate the rope solution. These results suggest that just like in human foster children ( Nelson et al., 2007 ), the arrival age of an infant chimpanzee at the place of care (here the sanctuary) can have an impact on its cognitive skills. Given that the development of chimpanzee brain structure can be influenced by early rearing ( Bogart et al., 2014 ), varying rearing conditions during sensitive developmental periods in the first years of life (see overview by ( Gerhardt, 2014 ) for human infants) could have influenced the chimpanzees’ physical cognition in the tool use task. Since orphans arriving at the sanctuary before reaching their second year (thus deprived from maternal care) performed better than later arriving orphans, physical cognitive skills (at least in this task) may not be negatively influenced by early maternal separation. Previous studies on apes have shown that social interactions with conspecifics and sufficient healthcare provided by humans can compensate for early maternal loss and reverse some of the negative effects that traumatic events can have on social cognition ( Meder, 1989 ; Martin, 2002 ; Bloomsmith et al., 2006 ; Reimers, Schwarzenberger & Preuschoft, 2007 ; Wobber & Hare, 2011 ).
Our study examined within species variation in problem-solving skills by (a) comparing two groups of chimpanzees from different facilities (sanctuary versus zoo) with identical test paradigms and (b) within the same facility by evaluating the influence of time spent in captive rehabilitation. The present study shows intraspecific variation in physical cognition and an effect of facility in the chimpanzees’ motivation to explore test apparatuses, which in turn could influence cognitive performance. Intraspecific differences between facilities in task success probabilities could also be influenced (among others) by previous experience with testing apparatuses and procedures as well as different levels of exposure to humans. Chimpanzees that arrived at an early age to the sanctuary after losing their mothers and that consequently spent large parts of their lives under human care, were better tool innovators than individuals that arrived later and spent less time in the sanctuary. Given the variation between and within housing facilities that we established empirically, researchers should be careful not to automatically extrapolate from one study population towards others without considering the subjects background histories. As such, our findings stress the importance of considering cross-facility differences in primate cognitive performance and the value of multi-institutional studies that allow for further evaluation of experience and developmental effects ( Many Primates et al., 2019 ).
Supplemental Information
Supplemental information 1, acknowledgments.
We would like to thank all the chimpanzee caretakers both at Leintal zoo and at Ngamba Island chimpanzee sanctuary for their enormous help with the logistics of performing the study. A special thanks to research assistance provided by Michael Poglonik during the data collection at Ngamba Island. We are grateful to the owners of Leintal zoo, the Gessmann Family and Lili Ajarova Director of the Chimpanzee trust for the support and collaboration. We thank Matteo Santon and Roger Mundry for statistical advice and Roger Mundry for providing the code used to create Fig. 2 . We also would like to thank our anonymous reviewers and our editor for their useful feedback that helped improved our manuscript.
Funding Statement
Sofia Forss received funding for this work by the Swiss National Science Foundation, Grant Nr: P2ZHP3_171902 and received further financial contribution from Waldemar von Frenckell Foundation in Finland. Alba Motes-Rodrigo and Claudio Tennie received funding from the European Research Council (ERC) under the European Union’s Horizon 2020 research and innovation programme (grant agreement No. 714658). The funders had no role in study design, data collection and analysis, decision to publish, or preparation of the manuscript.
Additional Information and Declarations
The authors declare there are no competing interests.
Sofia Forss conceived and designed the experiments, performed the experiments, prepared figures and/or tables, authored or reviewed drafts of the paper, and approved the final draft.
Alba Motes-Rodrigo analyzed the data, authored or reviewed drafts of the paper, and approved the final draft.
Christine Hrubesch performed the experiments, approved the final draft.
Claudio Tennie authored or reviewed drafts of the paper, and approved the final draft.
The following information was supplied relating to ethical approvals (i.e., approving body and any reference numbers):
Approval for this research was provided by the Ugandan Wildlife Authority, the National Council for Science and Technology (UNCST) (reference number NS27ES), BIAZA Animal Care Committee (British and Irish association for Zoos and Aquariums), Swiss veterinary institution (2960/ 29815).
The following information was supplied relating to field study approvals (i.e., approving body and any reference numbers):
Barbara Gessmann (Leintal Zoo, Schweigern, Germany) provided permission to study the chimpanzees at Leintal Zoo at Leintal Zoo.
Abduction: Can Non-human Animals Make Discoveries?
- Published: 04 August 2017
- Volume 10 , pages 295–313, ( 2017 )
Cite this article
- Mariana Vitti-Rodrigues 1 &
- Claus Emmeche ORCID: orcid.org/0000-0001-6141-7860 1
472 Accesses
8 Citations
1 Altmetric
Explore all metrics
The aim of this paper is to investigate the relationship between information and abductive reasoning in the context of problem-solving , focusing on non-human animals. Two questions guide our investigation: (1) What is the relation between information and abductive reasoning in the context of human and non-human animals? (2) Do non-human animals perform discovery based on inferential processes such as abductive reasoning? In order to answer these questions, we discuss the semiotic concept of information in relation to the concept of abductive reasoning and, more specifically, to the notion of manipulative abduction proposed by Magnani ( 2009 ). Finally, we investigate a case study of corvids’ intelligence, namely, their capacity of causal cognition.
This is a preview of subscription content, log in via an institution to check access.
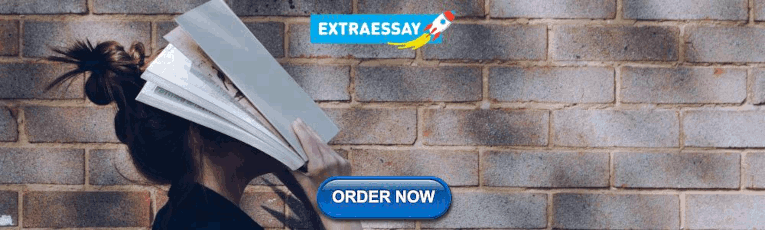
Access this article
Price includes VAT (Russian Federation)
Instant access to the full article PDF.
Rent this article via DeepDyve
Institutional subscriptions
Similar content being viewed by others
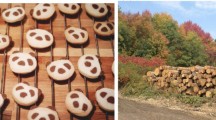
Aleatoric and epistemic uncertainty in machine learning: an introduction to concepts and methods
Eyke Hüllermeier & Willem Waegeman
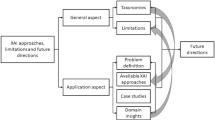
Survey on Explainable AI: From Approaches, Limitations and Applications Aspects
Wenli Yang, Yuchen Wei, … Byeong Kang
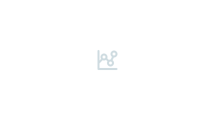
The black box problem revisited. Real and imaginary challenges for automated legal decision making
Bartosz Brożek, Michał Furman, … Bartłomiej Kucharzyk
We acknowledge that reality, according to Peirce, is considered more than the physical reality, encompassing the formal reality of mathematics, the fictional reality of human novels, and the potential reality of future states.
Cf. the long discussion about natural laws as counterfactual conditions, and how this is related to their dispositional aspects: “Would an ordinary match in ordinary conditions light if struck? It seems it would, but only because we presume nature to be regular in certain ways. We think this counterfactual is true because we believe there are laws. Were there no laws, it would not be the case that, if the match were struck, it would light. As a result, it would also not be the case that the match was disposed to ignite, nor the case that striking the match would cause it to light.” (Carroll 2016 )
According to Anderson (1986, p. 148) “Peirce began by viewing abduction as an ‘evidencing process’ and later switched to treating it as the stage of scientific inquiry”. Despite the fact that Peirce has formulated different characterizations of the concept of abductive reasoning, these characterizations are not inconsistent, but reflect a development of Peirce’s ideas (Fann 1970 ). In the present paper, we discuss the concept of abductive reasoning based on the mature work of Peirce, inspired by the interpretations of Fann ( 1970 ), Anderson ( 1987 ), Magnani ( 2009 ), Paavola ( 2004 ), Campos ( 2011 ), Hintikka ( 1998 ), and Minnameier ( 2004 ).
“It must be remembered that abduction, although it is little hampered by logical rules, nevertheless is logical inference, asserting its conclusion only problematically or conjecturally, it is true, but nevertheless having a perfect logical form”. (Peirce, CP 5.188)
This picture is a print screen of the Norman video. It can be found at: < https://www.youtube.com/watch?v=CBJ28V4xGG8 >.
‘Unexpected’ means that the information is “a difference that makes a difference” to the organism, cf. Bateson 1972 . The Batesonian characterization helps us to understand information as a process that involves (in the context of biosemiosis) the relationship between an agent and its environment.
In the second paper, the authors also added a different modification of the solid vs. hollow test, but we do not focus on this modification in the present chapter.
It was a female crow named Kitty (Logan et al. 2014 ).
This is similar to questions about Umwelt , such as: “How could we have access to others’ Umwelt ? In the context of Philosophy of Mind, Nagel notes: “Our own experience provides the basic material for our imagination, whose range is therefore limited. It will not help to try to imagine that one has webbing on one’s arms, which enables one to fly around at dusk and dawn catching insects in one’s mouth; that one has very poor vision, and perceives the surrounding world by a system of reflected high-frequency sound signals; and that one spends the day hanging upside down by one’s feet in an attic. In so far as I can imagine this (which is not very far), it tells me only what it would be like for me to behave as a bat behaves. But that is not the question. I want to know what it is like for a bat to be a bat. Yet if I try to imagine this, I am restricted to the resources of my own mind, and those resources are inadequate to the task. I cannot perform it either by imagining additions to my present experience, or by imagining segments gradually subtracted from it, or by imagining some combination of additions, subtractions, and modifications.” (Nagel 1974 , p. 2–3)
Anderson, D. R. (1987). Creativity and the philosophy of C. S. Peirce . Dordrecht: Martinus Nijhoff.
Book Google Scholar
Bateson, G. (1972). Steps to an ecology of mind: Collected essays in anthropology, psychiatry, evolution, and epistemology . San Francisco: Chandler Publishing Co..
Google Scholar
Blackburn, S. (2016) (ed.). The Oxford Dictionary of Philosophy (2nd rev. ed.) Oxford: Oxford University Press. Current online version.
Campos, D. (2011) On the distinction between Peirce’s abduction and Lipton’s inference to the best explanation. Synthese, v. 180, p. 419–442.
Carroll, J. W. (2016). Laws of nature. In: Zalta E. N. (ed.) The Stanford Encyclopedia of Philosophy (Fall 2016 Edition), URL = http://plato.stanford.edu/archives/fall2016/entries/laws-of-nature/
El-Hani, C. N., Queiroz, J., Emmeche, C. (2009) Genes, Information, and Semiosis. Tartu Semiotics Library 8. Tartu: Tartu University Press.
Fann, K. T. (1970). Peirce's theory of abduction. Martinus Nijhoff, 1970 .
Godfrey-Smith, P., & Sterelny, K. (2016). Biological information. In: Zalta E. N. (ed.) The Stanford Encyclopedia of Philosophy (Summer Edition), URL = http://plato.stanford.edu/archives/sum2016/entries/information-biological/ .
Gonzalez, M.E.Q., Nascimento, T.C.A., Haselager, W.F.G. (2004) Informação e conhecimento: notas para uma taxonomia da informação. In: Ferreira, A., Gonzalez, M.E.Q., Coelho, J.G. (eds.), Encontros com as Ciências Cognitivas. São Paulo: Coleção Estudos Cognitivos, v. 4, p. 195–220.
Hacking, I. (1983). Representing and intervening, introductory topics in the philosophy of natural science . Cambridge: Cambridge University Press.
Hintikka, J. (1998) What is abduction? The fundamental problem of contemporary epistemology. In: Transactions of Charles S. Peirce Society . Summer. 34(3). pp. 503–533.
Hoffmeyer, J. (2008) Biosemiotics: an examination into the Signs of Life and the Life of Signs . Translated by Hoffmeyer J. and Favareau, D. Edited by Favareau, D. University of Scranton Press: Scranton and London.
Holley, A. J. (1993). Do brown hares signal to the foxes? Ethology, 94 , 21–30.
Article Google Scholar
Ibri, I. A. (2006). The heuristic exclusivity of abduction in Peirce's philosophy. In R. F. Leo & S. Marietti (Eds.), Semiotics and Philosophy in C. S. Peirce (pp. 89–111). Cambridge: Cambridge Scholars.
Jelbert, S. A., Taylor, A. H., Cheke, L. G., Clayton, N. S., & Gray, R. D. (2014). Using the Aesop’s fable paradigm to investigate causal understanding of water displacement by new Caledonian crows. PloS One, 9 (3), e92895. doi: 10.1371/journal.pone.0092895 .
Article PubMed PubMed Central Google Scholar
Logan, C. J., Jelbert, S. A., Breen, A. J., Gray, R. D., & Taylor, A. H. (2014). Modifications to the Aesop’s fable paradigm change new Caledonian crow performances. PloS One, 9 (7), e103049. doi: 10.1371/journal.pone.0103049 .
Magnani, L. (2009). Abductive cognition: The epistemological and eco-cognitive dimensions of hypothetical reasoning. In R. Dillmann, Y. Nakamura, S. Schaal, & D. Vernon (Eds.), Cognitive systems monographs volume 3 . Heidelberg: Springer.
Minnameier, G. (2004). Peirce-suit of truth – why inference to the best explanation and abduction ought not to be confused. Erkenntnis, 60 , 75–105.
Nagel, T. (1974). What is it like to be a bat? The Philosophical Review, LXXXIII (4), 435–450.
Paavola, S. (2004). Abduction as a logic and methodology of discovery: The importance of strategies. Foundations of Science, 9 , 267–283.
Peirce, C.S. (1958) The collected papers of Charles Sanders Peirce. Electronic edition. Vols. I-VI, Hartshorne, C., Weiss, P. (eds.), 1931-1935. Vols. VII-VIII, Burks, A.W. (ed.). Charlottesville: Intelex Corporation. Cambridge: Harvard University Press. [Quoted as CP, followed by the volume and paragraph].
Peirce, C.S. (1998) The essential Peirce: Selected philosophical writings . Vol. 2 (1893–1913). Peirce Edition Project (eds.). Bloomington & Indianapolis: Indiana University Press.
Peirce, C.S. (undated) MS 831: Reasoning and instinct. Available at: The Peirce gateway. URL: http://www.iupui.edu/~arisbe/menu/library/bycsp/ms831/ms831.htm . Accessed 15 June 2016.
Shannon, C. (1948). A mathematical theory of communication. The Bell System Technical Journal, 27 (3), 379–423.
Shannon, C. E., & Weaver, W. (1949). The mathematical theory of communication . Urbana: University of Illinois Press (reprinted 1998).
Silveira, L.F.B. (2008) Informação e verdade na filosofia de Peirce. Cognitio , São Paulo, v. 9, n. 2, pp. 281–323.
Stjernfelt, F. (2014). Natural propositions. The actuality of Peirce’s doctrine of Dicisigns . Boston: Docent Press.
Uexküll, J.V. ([1940] 1982). The theory of meaning. Semiotica 42(1): 25–82.
Wilson, M. (2002). Six views of embodied cognition. Psychonomic Bulletin & Review, 9 , 625–636.
Download references
Acknowledgements
This work was supported by a stipend (no. 99999.001457/2015–02) from CAPES, Brazil. We would like to thank two anonymous reviewers, Tommi Vehkavaara (editor of this special issue), Sara Marie Ehrenreich Green, Henrik Kragh Sørensen, and Maria Eunice Quilici Gonzalez for useful comments and criticism. We would also like to thank the members of our research group in History and Philosophy of Science at the Department of Science Education (UCPH), as well the members of GAEC (Academic Group of Cognitive Studies) and the attendees of the 16th Gathering of Biosemiotics, for the inspiring discussions and feedback.
Author information
Authors and affiliations.
Department of Science Education, University of Copenhagen, Copenhagen, Denmark
Mariana Vitti-Rodrigues & Claus Emmeche
You can also search for this author in PubMed Google Scholar
Corresponding author
Correspondence to Mariana Vitti-Rodrigues .
Rights and permissions
Reprints and permissions
About this article
Vitti-Rodrigues, M., Emmeche, C. Abduction: Can Non-human Animals Make Discoveries?. Biosemiotics 10 , 295–313 (2017). https://doi.org/10.1007/s12304-017-9300-0
Download citation
Received : 14 September 2016
Accepted : 14 July 2017
Published : 04 August 2017
Issue Date : July 2017
DOI : https://doi.org/10.1007/s12304-017-9300-0
Share this article
Anyone you share the following link with will be able to read this content:
Sorry, a shareable link is not currently available for this article.
Provided by the Springer Nature SharedIt content-sharing initiative
- Information
- Problem solving
- Animal cognition
- Corvids’ intelligence
- Find a journal
- Publish with us
- Track your research
Thank you for visiting nature.com. You are using a browser version with limited support for CSS. To obtain the best experience, we recommend you use a more up to date browser (or turn off compatibility mode in Internet Explorer). In the meantime, to ensure continued support, we are displaying the site without styles and JavaScript.
- View all journals
- Explore content
- About the journal
- Publish with us
- Sign up for alerts
- Review Article
- Published: 26 May 2016
The neuroscience of working memory capacity and training
- Christos Constantinidis 1 &
- Torkel Klingberg 2
Nature Reviews Neuroscience volume 17 , pages 438–449 ( 2016 ) Cite this article
17k Accesses
284 Citations
85 Altmetric
Metrics details
- Computational neuroscience
- Electrophysiology
- Functional magnetic resonance imaging
- Working memory
The amount of information that can be maintained in working memory (WM) is limited. An individual's WM capacity is predictive of performance in higher cognitive abilities.
Although traditionally viewed as an immutable aptitude, more recently WM has been shown to improve with training. Importantly, improvement in WM can transfer between trained and non-trained tasks.
There are several models for the neural basis of WM. In this Review, we argue that the persistent discharges of prefrontal neurons play the most important part in the maintenance of information in WM.
Effects of WM training include increases in the activity of neurons in the prefrontal cortex, and increases in the strength of connectivity in the prefrontal cortex and between the prefrontal cortex and other areas.
Neural changes after training are found in cortical areas that process spatial information in WM and attention, potentially providing a basis for transfer to other cognitive and behavioural tasks that rely on spatial WM and spatially selective attention.
Working memory — the ability to maintain and manipulate information over a period of seconds — is a core component of higher cognitive functions. The storage capacity of working memory is limited but can be expanded by training, and evidence of the neural mechanisms underlying this effect is accumulating. Human imaging studies and neurophysiological recordings in non-human primates, together with computational modelling studies, reveal that training increases the activity of prefrontal neurons and the strength of connectivity in the prefrontal cortex and between the prefrontal and parietal cortex. Dopaminergic transmission could have a facilitatory role. These changes more generally inform us of the plasticity of higher cognitive functions.
This is a preview of subscription content, access via your institution
Access options
Subscribe to this journal
Receive 12 print issues and online access
176,64 € per year
only 14,72 € per issue
Rent or buy this article
Prices vary by article type
Prices may be subject to local taxes which are calculated during checkout
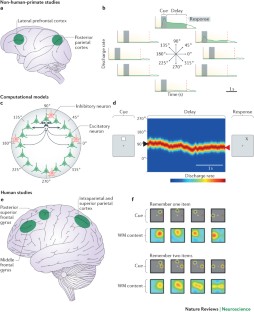
Similar content being viewed by others
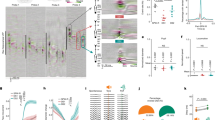
Neural and behavioural state switching during hippocampal dentate spikes
Jordan S. Farrell, Ernie Hwaun, … Ivan Soltesz
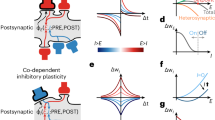
Co-dependent excitatory and inhibitory plasticity accounts for quick, stable and long-lasting memories in biological networks
Everton J. Agnes & Tim P. Vogels
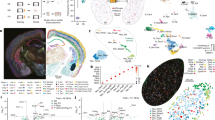
Spatial transcriptomics reveal neuron–astrocyte synergy in long-term memory
Wenfei Sun, Zhihui Liu, … Stephen R. Quake
Miller, G. A., Galanter, E. & Pribram, K. H. Plans and the Structure of Behavior (Rinehart and Winston, 1960).
Book Google Scholar
Baddeley, A. D. & Hitch, G. J. in The Psychology of Learning and Motivation: Advances in Research and Theory (ed. Bower, G. A.) 47–90 (Academic Press, 1974).
Google Scholar
Pribram, K. H., Mishkin, M., Rosvold, H. E. & Kaplan, S. J. Effects on delayed-response performance of lesions of dorsolateral and ventromedial fronal cortex of baboons. J. Comp. Physiol. Psychol. 45 , 565–575 (1952).
Article PubMed CAS Google Scholar
Pribram, K. H., Ahumada, A., Hartog, J. & Ross, L. in The Frontal Granular Cortex and Behavior (eds Warren, J. M. & Akert, K.) 65 (McGraw-Hill, 1964).
Kubota, K. & Niki, H. Prefrontal cortical unit activity and delayed alternation performance in monkey. J. Neurophysiol. 34 , 337–347 (1971).
Fuster, J. M. & Alexander, G. E. Neuron activity related to short-term memory. Science 173 , 652–654 (1971).
Article CAS PubMed Google Scholar
Goldman-Rakic, P. S. in Handbook of Physiology (ed. Mountcastle, V. B.) 373–417 (1987).
Cowan, N. The magical number 4 in short-term memory: a reconsideration of mental storage capacity. Behav. Brain Sci. 24 , 87–185 (2001).
Kane, M. J. et al. For whom the mind wanders, and when: an experience-sampling study of working memory and executive control in daily life. Psychol. Sci. 18 , 614–621 (2007).
Article PubMed Google Scholar
Kyllonen, P. C. & Christal, R. E. Reasoning ability is (little more than) working-memory capacity?! Intelligence 14 , 389–433 (1990).
Article Google Scholar
Gathercole, S. E., Brown, L. H. & Pickering, S. J. Working memory assessments at school entry as longitudinal predictors of National Curriculum attainment levels. Educat. Psychol. 70 , 177–194 (2003).
Klingberg, T., Forssberg, H. & Westerberg, H. Training of working memory in children with ADHD. J. Clin. Exp. Neuropsychol. 24 , 781–791 (2002).
Klingberg, T. et al. Computerized training of working memory in children with ADHD — a randomized, controlled trial. J. Am. Acad. Child Adolesc. Psychiatry 44 , 177–186 (2005).
Jaeggi, S. M., Buschkuehl, M., Jonides, J. & Perrig, W. J. Improving fluid intelligence with training on working memory. Proc. Natl Acad. Sci. USA 105 , 6829–6833 (2008).
Article PubMed PubMed Central CAS Google Scholar
Cortese, S. et al. Cognitive training for attention-deficit/hyperactivity disorder: meta-analysis of clinical and neuropsychological outcomes from randomized controlled trials. J. Am. Acad. Child Adolesc. Psychiatry 54 , 164–174 (2015).
Article PubMed PubMed Central Google Scholar
Schwaighofer, M., Fischer, F. & Buhner, M. Does working memory training transfer? A meta-analysis including training conditions as moderators. Educat. Psychol. 50 , 138–166 (2015).
Peijnenborgh, J. C., Hurks, P. M., Aldenkamp, A. P., Vles, J. S. & Hendriksen, J. G. Efficacy of working memory training in children and adolescents with learning disabilities: a review study and meta-analysis. Neuropsychol. Rehabil. 17 , 1–28 (2015).
Brehmer, Y., Westerberg, H. & Backman, L. Working-memory training in younger and older adults: training gains, transfer, and maintenance. Front. Hum. Neurosci. 6 , 63 (2012).
Green, C. T. et al. Will working memory training generalize to improve off-task behavior in children with attention-deficit/hyperactivity disorder? Neurotherapeutics 9 , 639–648 (2012).
Bigorra, A., Garolera, M., Guijarro, S. & Hervas, A. Long-term far-transfer effects of working memory training in children with ADHD: a randomized controlled trial. Eur. Child Adolesc. Psychiatry http://dx.doi.org/10.1007/s00787-015-0804-3 (2015).
Conklin, H. M. et al. Computerized cognitive training for amelioration of cognitive late effects among childhood cancer survivors: a randomized controlled trial. J. Clin. Oncol. 33 , 3894–3902 (2015).
Funahashi, S., Bruce, C. J. & Goldman-Rakic, P. S. Mnemonic coding of visual space in the monkey's dorsolateral prefrontal cortex. J. Neurophysiol. 61 , 331–349 (1989). This classic paper offers a description of how PFC activity represents spatial information of remembered stimuli, thereby providing a neural code for spatial WM.
Goldman-Rakic, P. S. Cellular basis of working memory. Neuron 14 , 477–485 (1995).
Constantinidis, C. & Wang, X. J. A neural circuit basis for spatial working memory. Neuroscientist 10 , 553–565 (2004).
Freedman, D. J., Riesenhuber, M., Poggio, T. & Miller, E. K. Categorical representation of visual stimuli in the primate prefrontal cortex. Science 291 , 312–316 (2001).
Wallis, J. D., Anderson, K. C. & Miller, E. K. Single neurons in prefrontal cortex encode abstract rules. Nature 411 , 953–956 (2001).
Pasternak, T. & Greenlee, M. W. Working memory in primate sensory systems. Nat. Rev. Neurosci. 6 , 97–107 (2005).
Constantinidis, C. & Procyk, E. The primate working memory networks. Cogn. Affect. Behav. Neurosci. 4 , 444–465 (2004).
Zhou, X. et al. Working memory performance and neural activity in the prefrontal cortex of peri-pubertal monkeys. J. Neurophysiol. 110 , 2648–2660 (2013).
Constantinidis, C., Franowicz, M. N. & Goldman-Rakic, P. S. The sensory nature of mnemonic representation in the primate prefrontal cortex. Nat. Neurosci. 4 , 311–316 (2001).
Wimmer, K., Nykamp, D. Q., Constantinidis, C. & Compte, A. Bump attractor dynamics in prefrontal cortex explains behavioral precision in spatial working memory. Nat. Neurosci. 17 , 431–439 (2014). This study demonstrates that persistent activity in the delay period of a spatial WM task can be modelled as a bump attractor and determines performance in the task.
Watanabe, K. & Funahashi, S. Neural mechanisms of dual-task interference and cognitive capacity limitation in the prefrontal cortex. Nat. Neurosci. 17 , 601–611 (2014).
Wang, M. et al. Neuronal basis of age-related working memory decline. Nature 476 , 210–213 (2011).
Mendoza-Halliday, D., Torres, S. & Martinez-Trujillo, J. C. Sharp emergence of feature-selective sustained activity along the dorsal visual pathway. Nat. Neurosci. 17 , 1255–1262 (2014). This paper illustrates that persistent activity is absent from the visual middle temporal area but emerges for the first time in the medial superior temporal area, and is further transmitted to the PFC.
Buschman, T. J., Siegel, M., Roy, J. E. & Miller, E. K. Neural substrates of cognitive capacity limitations. Proc. Natl Acad. Sci. USA 108 , 11252–11255 (2011).
Heyselaar, E., Johnston, K. & Pare, M. A change detection approach to study visual working memory of the macaque monkey. J. Vis. 11 , 11 (2011).
Lara, A. H. & Wallis, J. D. Capacity and precision in an animal model of visual short-term memory. J. Vis. 12 , 13 (2012).
Stormer, V. S., Alvarez, G. A. & Cavanagh, P. Within-hemifield competition in early visual areas limits the ability to track multiple objects with attention. J. Neurosci. 34 , 11526–11533 (2014).
Wang, X. J. Synaptic reverberation underlying mnemonic persistent activity. Trends Neurosci. 24 , 455–463 (2001).
Compte, A., Brunel, N., Goldman-Rakic, P. S. & Wang, X. J. Synaptic mechanisms and network dynamics underlying spatial working memory in a cortical network model. Cereb. Cortex 10 , 910–923 (2000). This paper documents a biophysically plausible computational model of persistent activity that has been instrumental for the study of spatial WM.
Grill-Spector, K., Henson, R. & Martin, A. Repetition and the brain: neural models of stimulus-specific effects. Trends Cogn. Sci. 10 , 14–23 (2006).
Mongillo, G., Barak, O. & Tsodyks, M. Synaptic theory of working memory. Science 319 , 1543–1546 (2008). The paper provides a computational model that does not depend on persistent activity and can account for some memory phenomena, based on synaptic mechanisms.
Sugase-Miyamoto, Y., Liu, Z., Wiener, M. C., Optican, L. M. & Richmond, B. J. Short-term memory trace in rapidly adapting synapses of inferior temporal cortex. PLoS Comput. Biol. 4 , e1000073 (2008).
Sandberg, A., Tegner, J. & Lansner, A. A working memory model based on fast Hebbian learning. Network 14 , 789–802 (2003).
Stokes, M. G. et al. Dynamic coding for cognitive control in prefrontal cortex. Neuron 78 , 364–375 (2013).
Meyers, E. M., Qi, X. L. & Constantinidis, C. Incorporation of new information into prefrontal cortical activity after learning working memory tasks. Proc. Natl Acad. Sci. USA 109 , 4651–4656 (2012).
Crowe, D. A., Averbeck, B. B. & Chafee, M. V. Neural ensemble decoding reveals a correlate of viewer- to object-centered spatial transformation in monkey parietal cortex. J. Neurosci. 28 , 5218–5228 (2008).
Salazar, R. F., Dotson, N. M., Bressler, S. L. & Gray, C. M. Content-specific fronto-parietal synchronization during visual working memory. Science 338 , 1097–1100 (2012).
Buschman, T. J., Denovellis, E. L., Diogo, C., Bullock, D. & Miller, E. K. Synchronous oscillatory neural ensembles for rules in the prefrontal cortex. Neuron 76 , 838–846 (2012).
Siegel, M., Warden, M. R. & Miller, E. K. Phase-dependent neuronal coding of objects in short-term memory. Proc. Natl Acad. Sci. USA 106 , 21341–21346 (2009).
Lundqvist, M., Herman, P. & Lansner, A. Theta and gamma power increases and alpha/beta power decreases with memory load in an attractor network model. J. Cogn. Neurosci. 23 , 3008–3020 (2011).
Riley, M. R. & Constantinidis, C. Role of prefrontal persistent activity in working memory. Front. Syst. Neurosci. 9 , 181 (2016).
Edin, F. et al. Mechanism for top-down control of working memory capacity. Proc. Natl Acad. Sci. USA 106 , 6802–6807 (2009). This study integrates computational modelling and fMRI to test a model of top-down attention and WM storage.
Macoveanu, J., Klingberg, T. & Tegner, J. Neuronal firing rates account for distractor effects on mnemonic accuracy in a visuo-spatial working memory task. Biol. Cybern. 96 , 407–419 (2007).
Durstewitz, D., Seamans, J. K. & Sejnowski, T. J. Neurocomputational models of working memory. Nat. Neurosci. 3 (Suppl.), 1184–1191 (2000).
Matthey, L., Bays, P. M. & Dayan, P. A probabilistic palimpsest model of visual short-term memory. PLoS Comput. Biol. 11 , e1004003 (2015).
Botvinick, M. & Watanabe, T. From numerosity to ordinal rank: a gain-field model of serial order representation in cortical working memory. J. Neurosci. 27 , 8636–8642 (2007).
Durstewitz, D., Seamans, J. K. & Sejnowski, T. J. Dopamine-mediated stabilization of delay-period activity in a network model of prefrontal cortex. J. Neurophysiol. 83 , 1733–1750 (2000).
Edin, F., Macoveanu, J., Olesen, P., Tegner, J. & Klingberg, T. Stronger synaptic connectivity as a mechanism behind development of working memory-related brain activity during childhood. J. Cogn. Neurosci. 19 , 750–760 (2007).
Rottschy, C. et al. Modelling neural correlates of working memory: a coordinate-based meta-analysis. Neuroimage 60 , 830–846 (2012).
Nee, D. E. et al. A meta-analysis of executive components of working memory. Cereb. Cortex 23 , 264–282 (2013).
Jerde, T. A., Merriam, E. P., Riggall, A. C., Hedges, J. H. & Curtis, C. E. Prioritized maps of space in human frontoparietal cortex. J. Neurosci. 32 , 17382–17390 (2012).
Ikkai, A. & Curtis, C. E. Common neural mechanisms supporting spatial working memory, attention and motor intention. Neuropsychologia 49 , 1428–1434 (2011).
Rowe, J. B., Toni, I., Josephs, O., Frackowiak, R. S. & Passingham, R. E. The prefrontal cortex: response selection or maintenance within working memory? Science 288 , 1656–1660 (2000).
Curtis, C. E., Rao, V. Y. & D'Esposito, M. Maintenance of spatial and motor codes during oculomotor delayed response tasks. J. Neurosci. 24 , 3944–3952 (2004).
Courtney, S. M., Ungerleider, L. G., Keil, K. & Haxby, J. V. Transient and sustained activity in a distributed neural system for human working memory. Nature 386 , 608–611 (1997).
Palva, J. M., Monto, S., Kulashekhar, S. & Palva, S. Neuronal synchrony reveals working memory networks and predicts individual memory capacity. Proc. Natl Acad. Sci. USA 107 , 7580–7585 (2010).
Roux, F. & Uhlhaas, P. J. Working memory and neural oscillations: alpha–gamma versus theta–gamma codes for distinct WM information? Trends Cogn. Sci. 18 , 16–25 (2014).
Ester, E. F., Sprague, T. C. & Serences, J. T. Parietal and frontal cortex encode stimulus-specific mnemonic representations during visual working memory. Neuron 87 , 893–905 (2015). This paper demonstrates that attributes of remembered stimuli can be decoded from fMRI activity of the PFC in humans, reaffirming neurophysiological findings that placed the maintenance of information in the frontal lobe rather than the sensory cortices.
Emrich, S. M., Riggall, A. C., Larocque, J. J. & Postle, B. R. Distributed patterns of activity in sensory cortex reflect the precision of multiple items maintained in visual short-term memory. J. Neurosci. 33 , 6516–6523 (2013).
Goense, J. B. & Logothetis, N. K. Neurophysiology of the BOLD fMRI signal in awake monkeys. Curr. Biol. 18 , 631–640 (2008).
Sereno, M. I., Pitzalis, S. & Martinez, A. Mapping of contralateral space in retinotopic coordinates by a parietal cortical area in humans. Science 294 , 1350–1354 (2001).
Silver, M. A. & Kastner, S. Topographic maps in human frontal and parietal cortex. Trends Cogn. Sci. 13 , 488–495 (2009).
Sprague, T. C., Ester, E. F. & Serences, J. T. Reconstructions of information in visual spatial working memory degrade with memory load. Curr. Biol. 24 , 2174–2180 (2014).
Miller, E. K. & Cohen, J. D. An integrative theory of prefrontal cortex function. Annu. Rev. Neurosci. 24 , 167–202 (2001).
Constantinidis, C., Franowicz, M. N. & Goldman-Rakic, P. S. Coding specificity in cortical microcircuits: a multiple electrode analysis of primate prefrontal cortex. J. Neurosci. 21 , 3646–3655 (2001).
Todd, J. J. & Marois, R. Capacity limit of visual short-term memory in human posterior parietal cortex. Nature 428 , 751–754 (2004).
Xu, Y. & Chun, M. M. Dissociable neural mechanisms supporting visual short-term memory for objects. Nature 440 , 91–95 (2006).
Vogel, E. K. & Machizawa, M. G. Neural activity predicts individual differences in visual working memory capacity. Nature 428 , 748–751 (2004).
Stevens, A. A., Tappon, S. C., Garg, A. & Fair, D. A. Functional brain network modularity captures inter- and intra-individual variation in working memory capacity. PLoS ONE 7 , e30468 (2012).
Holtmaat, A. & Svoboda, K. Experience-dependent structural synaptic plasticity in the mammalian brain. Nat. Rev. Neurosci. 10 , 647–658 (2009).
van Praag, H., Kempermann, G. & Gage, F. H. Neural consequences of environmental enrichment. Nat. Rev. Neurosci. 1 , 191–198 (2000).
Meyer, T., Qi, X. L., Stanford, T. R. & Constantinidis, C. Stimulus selectivity in dorsal and ventral prefrontal cortex after training in working memory tasks. J. Neurosci. 31 , 6266–6276 (2011).
Qi, X. L., Meyer, T., Stanford, T. R. & Constantinidis, C. Changes in prefrontal neuronal activity after learning to perform a spatial working memory task. Cereb. Cortex 21 , 2722–2732 (2011). References 83 and 84 document effects of WM training at the level of single neurons in the PFC of non-human primates.
Qi, X. L., Meyer, T., Stanford, T. R. & Constantinidis, C. Neural correlates of a decision variable before learning to perform a Match/Nonmatch task. J. Neurosci. 32 , 6161–6169 (2012).
Rainer, G. & Miller, E. K. Effects of visual experience on the representation of objects in the prefrontal cortex. Neuron 27 , 179–189 (2000).
Qi, X. L. & Constantinidis, C. Variability of prefrontal neuronal discharges before and after training in a working memory task. PLoS ONE 7 , e41053 (2012).
Qi, X. L. & Constantinidis, C. Correlated discharges in the primate prefrontal cortex before and after working memory training Eur. J. Neurosci. 36 , 3538–3548 (2012).
Garavan, H., Kelley, D., Rosen, A., Rao, S. M. & Stein, E. A. Practice-related functional activation changes in a working memory task. Microsc. Res. Tech. 51 , 54–63 (2000).
Hempel, A. et al. Plasticity of cortical activation related to working memory during training. Am. J. Psychiatry 161 , 745–747 (2004).
Olesen, P. J., Westerberg, H. & Klingberg, T. Increased prefrontal and parietal brain activity after training of working memory. Nat. Neurosci. 7 , 75–79 (2004).
Westerberg, H. et al. Computerized working memory training after stroke — a pilot study. Brain Inj. 21 , 21–29 (2007).
Dahlin, E., Neely, A. S., Larsson, A., Backman, L. & Nyberg, L. Transfer of learning after updating training mediated by the striatum. Science 320 , 1510–1512 (2008).
Jolles, D. D., Grol, M. J., Van Buchem, M. A., Rombouts, S. A. & Crone, E. A. Practice effects in the brain: changes in cerebral activation after working memory practice depend on task demands. Neuroimage 52 , 658–668 (2010).
Mozolic, J. L., Hayasaka, S. & Laurienti, P. J. A cognitive training intervention increases resting cerebral blood flow in healthy older adults. Front. Hum. Neurosci. 4 , 16 (2010).
Schneiders, J. A., Opitz, B., Krick, C. M. & Mecklinger, A. Separating intra-modal and across-modal training effects in visual working memory: an fMRI investigation. Cereb. Cortex 21 , 2555–2564 (2011).
Subramaniam, K. et al. Computerized cognitive training restores neural activity within the reality monitoring network in schizophrenia. Neuron 73 , 842–853 (2012).
Schneiders, J. A. et al. The impact of auditory working memory training on the fronto-parietal working memory network. Front. Hum. Neurosci. 6 , 173 (2012).
Kuhn, S. et al. The dynamics of change in striatal activity following updating training. Hum. Brain Mapp. 34 , 1530–1541 (2013).
Schweizer, S., Grahn, J., Hampshire, A., Mobbs, D. & Dalgleish, T. Training the emotional brain: improving affective control through emotional working memory training. J. Neurosci. 33 , 5301–5311 (2013).
Jolles, D. D., van Buchem, M. A., Crone, E. A. & Rombouts, S. A. Functional brain connectivity at rest changes after working memory training. Hum. Brain Mapp. 34 , 396–406 (2013).
Takeuchi, H. et al. Effects of working memory training on functional connectivity and cerebral blood flow during rest. Cortex 49 , 2106–2125 (2013).
Subramaniam, K. et al. Intensive cognitive training in schizophrenia enhances working memory and associated prefrontal cortical efficiency in a manner that drives long-term functional gains. Neuroimage 99 , 281–292 (2014).
Klingberg, T., Roland, P. E. & Kawashima, R. Activation of multi-modal cortical areas underlies short-term memory. Eur. J. Neurosci. 8 , 1965–1971 (1996).
Thompson, T. W., Waskom, M. L. & Gabrieli, J. D. E. Intensive working memory training produced functional changes in large-scale frontoparietal networks. J. Cogn. Neurosci. 28 , 575–588 (2016).
Kundu, B., Sutterer, D. W., Emrich, S. M. & Postle, B. R. Strengthened effective connectivity underlies transfer of working memory training to tests of short-term memory and attention. J. Neurosci. 33 , 8705–8715 (2013). This study uses transcranial magnetic stimulation and EEG to show increases in connectivity after WM training in children.
Astle, D. E., Barnes, J. J., Baker, K., Colclough, G. L. & Woolrich, M. W. Cognitive training enhances intrinsic brain connectivity in childhood. J. Neurosci. 35 , 6277–6283 (2015). Using resting-state MEG, this study shows how training-induced improvements in WM capacity are associated with increases in functional connectivity.
Gibson, E. M. et al. Neuronal activity promotes oligodendrogenesis and adaptive myelination in the mammalian brain. Science 344 , 1252304 (2014).
Yeung, M. S. et al. Dynamics of oligodendrocyte generation and myelination in the human brain. Cell 159 , 766–774 (2014).
Takeuchi, H. et al. Training of working memory impacts structural connectivity. J. Neurosci. 30 , 3297–3303 (2010).
Zhou, X. et al. Age-dependent changes in prefrontal intrinsic connectivity. Proc. Natl Acad. Sci. USA 111 , 3853–3858 (2014).
McNab, F. et al. Changes in cortical dopamine D1 receptor binding associated with cognitive training. Science 323 , 800–802 (2009). Here, PET is used to map changes in cortical D1R binding associated with WM training.
Sawaguchi, T. & Goldman-Rakic, P. S. D1 dopamine receptors in prefrontal cortex: involvement in working memory. Science 251 , 947–950 (1991).
Wass, C. et al. Dopamine D1 sensitivity in the prefrontal cortex predicts general cognitive abilities and is modulated by working memory training. Learn. Mem. 20 , 617–627 (2013).
Backman, L. et al. Effects of working-memory training on striatal dopamine release. Science 333 , 718 (2011).
Brehmer, Y. et al. Working memory plasticity modulated by dopamine transporter genotype. Neurosci. Lett. 467 , 117–120 (2009).
Soderqvist, S. et al. Dopamine, working memory, and training induced plasticity: implications for developmental research. Dev. Psychol. 48 , 836–843 (2012).
Shang, C. Y. & Gau, S. S. Association between the DAT1 gene and spatial working memory in attention deficit hyperactivity disorder. Int. J. Neuropsychopharmacol. 17 , 9–21 (2014).
Soderqvist, S., Matsson, H., Peyrard-Janvid, M., Kere, J. & Klingberg, T. Polymorphisms in the dopamine receptor 2 gene region influence improvements during working memory training in children and adolescents. J. Cogn. Neurosci. 26 , 54–62 (2014).
Hirvonen, M. et al. C957T polymorphism of the dopamine D2 receptor ( DRD2 ) gene affects striatal DRD2 availability in vivo . Mol. Psychiatry 9 , 1060–1061 (2004).
Nymberg, C. et al. DRD2 / ANKK1 polymorphism modulates the effect of ventral striatal activation on working memory performance. Neuropsychopharmacology 39 , 2357–2365 (2014).
Richmond, L. L., Wolk, D., Chein, J. & Olson, I. R. Transcranial direct current stimulation enhances verbal working memory training performance over time and near transfer outcomes. J. Cogn. Neurosci. 26 , 2443–2454 (2014).
Delatour, B. & Gisquet-Verrier, P. Functional role of rat prelimbic–infralimbic cortices in spatial memory: evidence for their involvement in attention and behavioural flexibility. Behav. Brain Res. 109 , 113–128 (2000).
Erlich, J. C., Bialek, M. & Brody, C. D. A cortical substrate for memory-guided orienting in the rat. Neuron 72 , 330–343 (2011).
Harvey, C. D., Coen, P. & Tank, D. W. Choice-specific sequences in parietal cortex during a virtual-navigation decision task. Nature 484 , 62–68 (2012).
Light, K. R. et al. Working memory training promotes general cognitive abilities in genetically heterogeneous mice. Curr. Biol. 20 , 777–782 (2010).
Cassanelli, P. M. et al. Working memory training triggers delayed chromatin remodeling in the mouse corticostriatothalamic circuit. Prog. Neuropsychopharmacol. Biol. Psychiatry 60 , 93–103 (2015).
Ren, M., Cao, V., Ye, Y., Manji, H. K. & Wang, K. H. Arc regulates experience-dependent persistent firing patterns in frontal cortex. J. Neurosci. 34 , 6583–6595 (2014).
Yuan, Y., Mao, H. & Si, J. Cortical neural responses to previous trial outcome during learning of a directional choice task. J. Neurophysiol. 113 , 1963–1976 (2015).
Logothetis, N. K., Pauls, J., Augath, M., Trinath, T. & Oeltermann, A. Neurophysiological investigation of the basis of the fMRI signal. Nature 412 , 150–157 (2001).
Logothetis, N. K. What we can do and what we cannot do with fMRI. Nature 453 , 869–878 (2008).
Short, S. J. et al. Associations between white matter microstructure and infants' working memory. Neuroimage 64 , 156–166 (2013).
Vestergaard, M. et al. White matter microstructure in superior longitudinal fasciculus associated with spatial working memory performance in children. J. Cogn. Neurosci. 23 , 2135–2146 (2011).
Ullman, H., Almeida, R. & Klingberg, T. Structural maturation and brain activity predict future working memory capacity during childhood development. J. Neurosci. 34 , 1592–1598 (2014).
Ostby, Y., Tamnes, C. K., Fjell, A. M. & Walhovd, K. B. Morphometry and connectivity of the fronto-parietal verbal working memory network in development. Neuropsychologia 49 , 3854–3862 (2011).
Kennedy, K. M. & Raz, N. Aging white matter and cognition: differential effects of regional variations in diffusion properties on memory, executive functions, and speed. Neuropsychologia 47 , 916–927 (2009).
Klingberg, T., Forssberg, H. & Westerberg, H. Increased brain activity in frontal and parietal cortex underlies the development of visuo-spatial working memory capacity during childhood. J. Cogn. Neurosci. 14 , 1–10 (2002).
Crone, E. A., Wendelken, C., Donohue, S., van Leijenhorst, L. & Bunge, S. A. Neurocognitive development of the ability to manipulate information in working memory. Proc. Natl Acad. Sci. USA 103 , 9315–9320 (2006).
Scherf, K. S., Sweeney, J. A. & Luna, B. Brain basis of developmental change in visuospatial working memory. J. Cogn. Neurosci. 18 , 1045–1058 (2006).
Klingberg, T. Childhood cognitive development as a skill. Trends Cogn. Sci. 18 , 573–579 (2014).
Darki, F. & Klingberg, T. The role of fronto-parietal and fronto-striatal networks in the development of working memory: a longitudinal study. Cereb. Cortex 25 , 1587–1595 (2015).
Download references
Acknowledgements
This work was supported by US National Institutes of Health grant R01EY017077 and The Tab Williams Family Endowment (to C.C.).
Author information
Authors and affiliations.
Department of Neurobiology and Anatomy, Wake Forest School of Medicine, Winston-Salem, 27157, North Carolina, USA
- Christos Constantinidis
Deptartment Neuroscience, Karolinska Institutet, Stockholm, 171 77, Sweden
Torkel Klingberg
You can also search for this author in PubMed Google Scholar
Corresponding author
Correspondence to Torkel Klingberg .
Ethics declarations
Competing interests.
The authors declare no competing financial interests.
Supplementary information
Supplementary information s1 (table).
(PDF 790 kb)
PowerPoint slides
Powerpoint slide for fig. 1, powerpoint slide for fig. 2, powerpoint slide for fig. 3.
Probability that the firing rate of one neuron in two identical stimulus conditions is different depending on the subject's choice.
Area of the visual field where a stimulus will elicit the firing of neuron under study.
A phenomenon whereby a stimulus that has been repeated elicits a smaller response than does a stimulus that appears for the first time.
A stable state of activation of a network, with a spatial location maximally activated and adjacent locations activated to a lesser extent, forming a bump.
Mechanism of representing of two variables, whereby the activity of a neuron depends linearly on one continuous variable, multiplied by second variable.
A graph of firing-rate intensity depending on the location (or other dimension) of a stimulus.
The likelihood that activity in one area leads to activity in the other.
Variance of spike counts divided by their mean, per unit of time.
A model or algorithm achieving a classification decision of what a stimulus is, based on the combined activity of multiple neurons or voxels.
Rights and permissions
Reprints and permissions
About this article
Cite this article.
Constantinidis, C., Klingberg, T. The neuroscience of working memory capacity and training. Nat Rev Neurosci 17 , 438–449 (2016). https://doi.org/10.1038/nrn.2016.43
Download citation
Published : 26 May 2016
Issue Date : July 2016
DOI : https://doi.org/10.1038/nrn.2016.43
Share this article
Anyone you share the following link with will be able to read this content:
Sorry, a shareable link is not currently available for this article.
Provided by the Springer Nature SharedIt content-sharing initiative
This article is cited by
Using hypnotic suggestion in the rehabilitation of working memory capacity after acquired brain injury: study protocol for a randomized controlled trial.
- Line Sophie Eide
- Per-Ola Rike
- Marianne Løvstad
Trials (2024)
Nucleus accumbens core dopamine D2 receptors are required for performance of the odor span task in male rats
- Timothy J. Onofrychuk
- Ashton L. Heidt
- John G. Howland
Psychopharmacology (2024)
A cross-sectional study of the association between exposure to violence, intelligence, and executive function in Brazilian youths
- Rhaná Carolina Santos
- Nicole Prigol Dalfovo
- Augusto Buchweitz
Psicologia: Reflexão e Crítica (2023)
Diminished activation of excitatory neurons in the prelimbic cortex leads to impaired working memory capacity in mice
- Li-Xin Jiang
- Geng-Di Huang
BMC Biology (2023)
Neuronal selectivity for stimulus information determines prefrontal LFP gamma power regardless of task execution
- Balbir Singh
- Zhengyang Wang
Communications Biology (2023)
Quick links
- Explore articles by subject
- Guide to authors
- Editorial policies
Sign up for the Nature Briefing newsletter — what matters in science, free to your inbox daily.

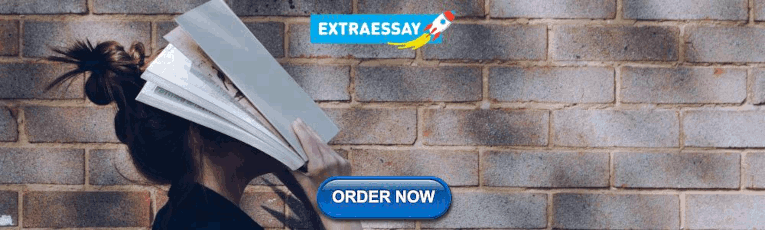
IMAGES
VIDEO
COMMENTS
In addition, calculating per capita solving performance based on group solving probabilities revealed that regardless of the distribution of problem-solving ability within the population and regardless of whether individual problem-solving ability was maintained constant or increased as groups became larger, the relationship between group size ...
Cognitive abilities. As distinct cognitive abilities, we focus on the following traits and properties. Few-shot problem-solving. Few-shot problem-solving is the ability to solve unknown problems ...
Defined as the ability to solve a novel problem or use a novel behavior to solve a familiar problem, innovativeness is among the most commonly measured cognitive abilities in non-human animals 16 ...
Evidence from a number of animal studies clearly demonstrate remarkable reasoning and problem-solving abilities in non-human species (Blaisdell et al., 2006; Taylor et al., 2009; Smirnova et al., 2015), but it is argued that such abilities have reached a higher level in humans (Premack, 1983, 2007; O’Brien and Opie, 2002; Penn et al., 2008).
In order to understand the evolution of cognitive abilities we need to disentangle environmental and genetic influences from cognitive phenotypes in non-human animals. To this end, it is important to consider intraspecific variation and to identify what factors correlate with between-individual differences (Boesch, 2020).
Intelligence is a multidimensional construct that can include a wide range of cognitive abilities. Historically, intelligence in humans and other animals has been measured and defined by flexibility in problem solving, learning, memory, reasoning, abstract thinking, planning, and communication and language. Humans are highly proficient in all ...
However, to date the problem-solving abilities of many human and non-human animals are clearly superior to those of artificial systems. Here, we propose steps to integrate biologically inspired hierarchical mechanisms to enable advanced problem-solving skills in artificial agents. Therefore, we first review the literature
Intelligence in animals can be defined in terms of problem-solving ability, both acquisition to solve problems and the ability to generalize what is learned to novel situations. Intelligence may also be measured in terms of behavioral flexibility, which is comprised of inhibition and innovation components and which concerns adaptability to new ...
The aim of this paper is to investigate the relationship between information and abductive reasoning in the context of problem-solving, focusing on non-human animals. Two questions guide our investigation: (1) What is the relation between information and abductive reasoning in the context of human and non-human animals? (2) Do non-human animals perform discovery based on inferential processes ...
Working memory (WM) — the ability to maintain and manipulate information over a period of seconds — is a key cognitive skill. Constantinidis and Klingberg discuss non-human-primate ...